Volume 21 Supplement 9

Selected Articles from the 20th International Conference on Bioinformatics & Computational Biology (BIOCOMP 2019)
- Introduction
- Open access
- Published: 03 December 2020
Current trend and development in bioinformatics research
- Yuanyuan Fu 1 ,
- Zhougui Ling 1 , 2 ,
- Hamid Arabnia 3 &
- Youping Deng 1
BMC Bioinformatics volume 21 , Article number: 538 ( 2020 ) Cite this article
10k Accesses
16 Citations
4 Altmetric
Metrics details
This is an editorial report of the supplements to BMC Bioinformatics that includes 6 papers selected from the BIOCOMP’19—The 2019 International Conference on Bioinformatics and Computational Biology. These articles reflect current trend and development in bioinformatics research.
The supplement to BMC Bioinformatics was proposed to launch during the BIOCOMP’19—The 2019 International Conference on Bioinformatics and Computational Biology held from July 29 to August 01, 2019 in Las Vegas, Nevada. In this congress, a variety of research areas was discussed, including bioinformatics which was one of the major focuses due to the rapid development and requirement of using bioinformatics approaches in biological data analysis, especially for omics large datasets. Here, six manuscripts were selected after strict peer review, providing an overview of the bioinformatics research trend and its application for interdisciplinary collaboration.
Cancer is one of the leading causes of morbidity and mortality worldwide. There exists an urgent need to identify new biomarkers or signatures for early detection and prognosis. Mona et al. identified biomarker genes from functional network based on the 407 differential expressed genes between lung cancer and healthy populations from a public Gene Expression Omnibus dataset. The lower expression of sixteen gene signature is associated with favorable lung cancer survival, DNA repair, and cell regulation [ 1 ]. A new class of biomarkers such as alternative splicing variants (ASV) have been studied in recent years. Various platforms and methods, for example, Affymetrix Exon-Exon Junction Array, RNA-seq, and liquid chromatography tandem mass spectrometry (LC–MS/MS), have been developed to explore the role of ASV in human disease. Zhang et al. have developed a bioinformatics workflow to combine LC–MS/MS with RNA-seq which provide new opportunities in biomarker discovery. In their study, they identified twenty-six alternative splicing biomarker peptides with one single intron event and one exon skipping event; further pathways indicated the 26 peptides may be involved in cancer, signaling, metabolism, regulation, immune system and hemostasis pathways which validated by the RNA-seq analysis [ 2 ].
Proteins serve crucial functions in essentially all biological processes and the function directly depends on their three-dimensional structures. Traditional approaches to elucidation of protein structures by NMR spectroscopy are time consuming and expensive, however, the faster and more cost-effective methods are critical in the development of personalized medicine. Cole et al. improved the REDRAFT software package in the important areas of usability, accessibility, and the core methodology which resulted in the ability to fold proteins [ 3 ].
The human microbiome is the aggregation of microorganisms that reside on or within human bodies. Rebecca et al. discussed the tissue-associated microbial detection in cancer using next generation sequencing (NGS). Various computational frameworks could shed light on the role of microbiota in cancer pathogenesis [ 4 ]. How to analyze the human microbiome data efficiently is a huge challenge. Zhang et al. developed a nonparametric test based on inter-point distance to evaluate statistical significance from a Bayesian point of view. The proposed test is more efficient and sensitive to the compositional difference compared with the traditional mean-based method [ 5 ].
Human disease is also considered as the cause of the interaction between genetic and environmental factors. In the last decades, there was a growing interest in the effect of metal toxicity on human health. Evaluating the toxicity of chemical mixture and their possible mechanism of action is still a challenge for humans and other organisms, as traditional methods are very time consuming, inefficient, and expensive, so a limited number of chemicals can be tested. In order to develop efficient and accurate predictive models, Yu et al. compared the results among a classification algorithm and identified 15 gene biomarkers with 100% accuracy for metal toxicant using a microarray classifier analysis [ 6 ].
Currently, there is a growing need to convert biological data into knowledge through a bioinformatics approach. We hope these articles can provide up-to-date information of research development and trend in bioinformatics field.
Availability of data and materials
Not applicable.
Abbreviations
The 2019 International Conference on Bioinformatics and Computational Biology
Liquid chromatography tandem mass spectrometry
Alternative splicing variants
Nuclear Magnetic Resonance
Residual Dipolar Coupling based Residue Assembly and Filter Tool
Next generation sequencing
Mona Maharjan RBT, Chowdhury K, Duan W, Mondal AM. Computational identification of biomarker genes for lung cancer considering treatment and non-treatment studies. 2020. https://doi.org/10.1186/s12859-020-3524-8 .
Zhang F, Deng CK, Wang M, Deng B, Barber R, Huang G. Identification of novel alternative splicing biomarkers for breast cancer with LC/MS/MS and RNA-Seq. Mol Cell Proteomics. 2020;16:1850–63. https://doi.org/10.1186/s12859-020-03824-8 .
Article Google Scholar
Casey Cole CP, Rachele J, Valafar H. Increased usability, algorithmic improvements and incorporation of data mining for structure calculation of proteins with REDCRAFT software package. 2020. https://doi.org/10.1186/s12859-020-3522-x .
Rebecca M, Rodriguez VSK, Menor M, Hernandez BY, Deng Y. Tissue-associated microbial detection in cancer using human sequencing data. 2020. https://doi.org/10.1186/s12859-020-03831-9 .
Qingyang Zhang TD. A distance based multisample test for high-dimensional compositional data with applications to the human microbiome . 2020. https://doi.org/10.1186/s12859-020-3530-x .
Yu Z, Fu Y, Ai J, Zhang J, Huang G, Deng Y. Development of predicitve models to distinguish metals from non-metal toxicants, and individual metal from one another. 2020. https://doi.org/10.1186/s12859-020-3525-7 .
Download references
Acknowledgements
This supplement will not be possible without the support of the International Society of Intelligent Biological Medicine (ISIBM).
About this supplement
This article has been published as part of BMC Bioinformatics Volume 21 Supplement 9, 2020: Selected Articles from the 20th International Conference on Bioinformatics & Computational Biology (BIOCOMP 2019). The full contents of the supplement are available online at https://bmcbioinformatics.biomedcentral.com/articles/supplements/volume-21-supplement-9 .
Publication of this supplement has been supported by NIH grants R01CA223490 and R01 CA230514 to Youping Deng and 5P30GM114737, P20GM103466, 5U54MD007601 and 5P30CA071789.
Author information
Authors and affiliations.
Department of Quantitative Health Sciences, John A. Burns School of Medicine, University of Hawaii at Manoa, Honolulu, HI, 96813, USA
Yuanyuan Fu, Zhougui Ling & Youping Deng
Department of Pulmonary and Critical Care Medicine, The Fourth Affiliated Hospital of Guangxi Medical University, Liuzhou, 545005, China
Zhougui Ling
Department of Computer Science, University of Georgia, Athens, GA, 30602, USA
Hamid Arabnia
You can also search for this author in PubMed Google Scholar
Contributions
YF drafted the manuscript, ZL, HA, and YD revised the manuscript. All authors read and approved the final manuscript.
Corresponding author
Correspondence to Youping Deng .
Ethics declarations
Ethics approval and consent to participate, consent for publication, competing interests.
The authors declare that they have no competing interests.
Additional information
Publisher's note.
Springer Nature remains neutral with regard to jurisdictional claims in published maps and institutional affiliations.
Rights and permissions
Open Access This article is licensed under a Creative Commons Attribution 4.0 International License, which permits use, sharing, adaptation, distribution and reproduction in any medium or format, as long as you give appropriate credit to the original author(s) and the source, provide a link to the Creative Commons licence, and indicate if changes were made. The images or other third party material in this article are included in the article's Creative Commons licence, unless indicated otherwise in a credit line to the material. If material is not included in the article's Creative Commons licence and your intended use is not permitted by statutory regulation or exceeds the permitted use, you will need to obtain permission directly from the copyright holder. To view a copy of this licence, visit http://creativecommons.org/licenses/by/4.0/ . The Creative Commons Public Domain Dedication waiver ( http://creativecommons.org/publicdomain/zero/1.0/ ) applies to the data made available in this article, unless otherwise stated in a credit line to the data.
Reprints and permissions
About this article
Cite this article.
Fu, Y., Ling, Z., Arabnia, H. et al. Current trend and development in bioinformatics research. BMC Bioinformatics 21 (Suppl 9), 538 (2020). https://doi.org/10.1186/s12859-020-03874-y
Download citation
Published : 03 December 2020
DOI : https://doi.org/10.1186/s12859-020-03874-y
Share this article
Anyone you share the following link with will be able to read this content:
Sorry, a shareable link is not currently available for this article.
Provided by the Springer Nature SharedIt content-sharing initiative
- Bioinformatics
- Human disease
BMC Bioinformatics
ISSN: 1471-2105
- General enquiries: [email protected]
- Director’s Welcome
- Participating Departments
- Frontiers in Computational Biosciences Seminar Series
- Current Ph.D. Students
- Current M.S. Students
- Bioinformatics Department Handbook
- B.I.G. Summer Institute
- The Collaboratory
- Diversity and Inclusiveness
- Helpful Information for Current Students
- Joint UCLA-USC Meeting
- Student Blog and Twitter Feed
- Social Gatherings
- Introduction to the Program
- Admissions Information
- Admissions FAQs
- Student Funding
- Curriculum and Graduate Courses
- Research Rotations
- Qualifying Exams
- Doctoral Dissertation
- Student Publications
- Capstone Project
- Undergraduate Courses
- Undergraduate and Masters Research
- Bioinformatics Minor Course Requirements
- Bioinformatics Minor FAQs
- Bioinformatics Minor End-of-Year Celebration
- For Engineering Students

General Information There are plenty of opportunities for Bioinformatics research projects at UCLA. This program is designed to help interested students find research projects related to Bioinformatics across campus. Typically, these projects are for credit; in exceptional circumstances they may offer funding. Participation in research projects can both significantly improve your chances of admittance into top graduate programs and make you a much more competitive employment candidate. Even better, it gives you something to talk about during an interview. Feel free to contact us even if you do not know exactly whether or not you want to work on a research project or know the field you wish to research in. Please remember that every undergraduate and masters student is welcome to participate in research, regardless of your background or year in the program. Undergraduates are STRONGLY encouraged to participate in research as early as possible in their careers. Ideally, you should start a research project during your sophomore year, but it is never too late or to early to start! Undergraduate students may receive up to 8 units credit toward the minor with enrollment in Computer Science 194/199 or Bioinformatics 194/199.
General Procedure If you are reasonably sure which project you would like to work on, use the contact information listed under the project to contact the person responsible for the project directly to set up a meeting. If you are not sure, but you are even slightly interested in research, feel free to email us or drop in to help chose an appropriate project. Most students take a project for course credit, although funding may be available in some cases. You can contact Eleazar Eskin (eeskin [at] cs [dot] ucla [dot] edu) if you have any questions.
Research Projects Below is a list of research projects that are accepting undergraduate researchers.
Featured News
Researchers awarded $4.7 million to study genomic variation in stem cell production, dr. nandita garud recognized for her research on gut microbiome, ucla study reveals how immune cells can be trained to fight infections, ucla scientists decode the ‘language’ of immune cells, dr. eran halperin elected as fellow of international society for computational biology, upcoming events, ghayda mirzaa seminar, jana lipkova seminar, memorial day holiday, katherine andriole seminar, spring 2024 quarter instruction ends, recent student publications.
RECENT STUDENT PUBLICATIONS LINK-PLEASE CLICK!
Updates Coming Soon!
Thank you for visiting nature.com. You are using a browser version with limited support for CSS. To obtain the best experience, we recommend you use a more up to date browser (or turn off compatibility mode in Internet Explorer). In the meantime, to ensure continued support, we are displaying the site without styles and JavaScript.
- View all journals
- My Account Login
- Explore content
- About the journal
- Publish with us
- Sign up for alerts
- Open access
- Published: 08 April 2022
Challenges in large-scale bioinformatics projects
- Sarah Morrison-Smith ORCID: orcid.org/0000-0002-4959-807X 1 ,
- Christina Boucher 2 ,
- Aleksandra Sarcevic 3 ,
- Noelle Noyes 4 ,
- Catherine O’Brien 1 ,
- Nazaret Cuadros 1 &
- Jaime Ruiz 2
Humanities and Social Sciences Communications volume 9 , Article number: 125 ( 2022 ) Cite this article
4445 Accesses
3 Citations
1 Altmetric
Metrics details
- Science, technology and society
Biological and biomedical research is increasingly conducted in large, interdisciplinary collaborations to address problems with significant societal impact, such as reducing antibiotic resistance, identifying disease sub-types, and identifying genes that control for drought tolerance in plants. Many of these projects are data driven and involve the collection and analysis of biological data at a large-scale. As a result, life-science projects, which are frequently diverse, large and geographically dispersed, have created unique challenges for collaboration and training. We examine the communication and collaboration challenges in multidisciplinary research through an interview study with 20 life-science researchers. Our results show that both the inclusion of multiple disciplines and differences in work culture influence collaboration in life science. Using these results, we discuss opportunities and implications for designing solutions to better support collaborative tasks and workflows of life scientists. In particular, we show that life science research is increasingly conducted in large, multi-institutional collaborations, and these large groups rely on “mutual respect” and collaboration. However, we found that the interdisciplinary nature of these projects cause technical language barriers and differences in methodology affect trust. We use these findings to guide our recommendations for technology to support life science. We also present recommendations for life science research training programs and note the necessity for incorporating training in project management, multiple language, and discipline culture.
Similar content being viewed by others
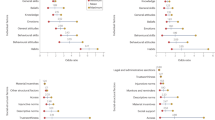
Determinants of behaviour and their efficacy as targets of behavioural change interventions
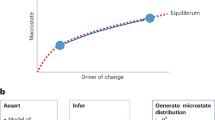
Entropy, irreversibility and inference at the foundations of statistical physics
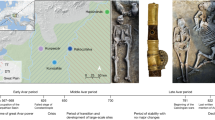
Network of large pedigrees reveals social practices of Avar communities
Introduction.
Life-science research—which encapsulates a wide range of biological and biomedical research—is responsible for many major scientific findings in the past decade. Such findings include the completion of a near perfect error-free human genome (Miga, 2020 ), the rapid sequencing and assembly of the SARS-COVID19 genome (Fernandes, 2020 ), the identification of nearly 70,000 extant vertebrate species (Rhie, 2021 ), and the definition of Parkinson’s disease sub-types for efficient therapeutic development (Kenneth, 2018 )—just to name a few. One of the main drivers of these, and countless other discoveries in life science has been the development of technologies that are capable of identified the DNA, RNA or amino acid sequence corresponding to a biological sample. One of the first technologies—so called first generation sequencing technologies —was capable of sequencing a couple of thousand nucleotides of a DNA sample per day. This technology was fundamental to the sequencing and assembly of the first human genome (International Human Genome Sequencing Consortium, 2001 ; Venter, 2001 ). Since then sequencing technologies have increased their throughput (meaning the amount of DNA they can sequence at a given time), decreased their cost, and became highly accurate (Bansal and Boucher, 2019 ; Lang, 2020 ). Currently, it is now feasible to sequence an entire human genome in less than day and for less than one thousand dollars (Giani et al., 2020 ).
Sequencing technologies take as input a biological sample and produce a sequence of signals that are then interpreted to produce the string of DNA, RNA or amino acid. In the case of next generation sequencing, the signal produced is fluorescent light that can be viewed under a microscope that can be translated into a nucleotide (A, C, G, or T) sequence, and in the case of mass spectrometer there is ion/mass charge that is interpreted to produce an amino acid sequence corresponding to a peptide. All of these technologies have been dramatically advanced over the past couple decades, and other laboratory methods (such as optical mapping (Mukherjee, 2018 )) have been automated (Giani et al., 2020 ). Even though one of the drivers of this advancements has been human genetics, very few other life science areas remain untouched by the discovery and advancement of sequencing technologies. Plant biology (Waese, 2017 ), soil sciences (Bajpai et al., 2021 ), species evolution (Funk et al., 2018 ; i5K Consortium, 2013 ; Luikart et al., 2018 ; Rhie, 2021 ), extinction of animal species (Humble, 2020 ; Shapiro, 2017 ), and creation of synthetic species have all been advanced due to the creation of these technologies.
What is underlying these technologies is the analysis of data, which frequently requires more time than the generation of the data itself. Pollack ( 2011 ) states that “The field of genomics is caught in a data deluge. DNA sequencing is becoming faster and cheaper at a pace far outstripping Moore’s law, which describes the rate at which computing gets faster and cheaper. The result is that the ability to determine DNA sequences is starting to outrun the ability of researchers to store, transmit and especially to analyze the data.” Prior research (Morrison-Smith et al., 2015 ) showed that in order to overcome the challenges of analyzing data, life science researchers collaborate with researchers and trainees in different disciplines, locations, and institutions. Yet earlier research on interdisciplinary science showed that scientific projects that depend on a large number of institutions and disciplines are less successful than those relying on fewer (Cummings and Kiesler, 2005 ; Kiesler and Cummings, 2002 ); here, success was defined to include metrics such as graduate and post-graduate supervision, the number of related projects, the frequency of project meetings, and the likelihood of having created a project-related course. These findings are compounded by prior work showing that the development of communication technology has negatively impacted projects by hindering information sharing (Hinds and Mortensen, 2005 ), delaying outcomes (Espinosa, 2004 ), and causing misunderstandings (Cramton, 2001 ). Because technology has advanced substantially since these prior works, it is natural to ask whether advances in the development of new technology and refinement of existing tools have circumvented these problems of coordination—and if not, what issues remain.
This paper focuses on the challenges of interdisciplinary collaboration in life science. We aim to uncover how researchers from various backgrounds and expertize communicate to perform data analysis from the perspective of life scientists that generate data as a means towards a scientific goal. Follow-up work could reverse this perspective to understand the challenges faced by the researchers in computer science, statistics and data analysis. In light of this focus, we conducted semi-structured interviews with life science researchers from nine research institutions. We performed a bottom-up analysis by constructing an affinity diagram to identify themes related to our goals, which we then correlated to themes from prior work. Our results show that both interdisciplinarity and differences in work culture and practices affect collaboration in life science. We contextualize our result in current research and hence, show that many of the challenges identified in prior work (Olson and Olson, 2000 , 2006 ) persist today in spite of technological advancements. We conclude by offering new perspectives insights for interface and software development, and providing recommendations for training programs to better prepare trainees for collaboration in life science research.
Related work
Here, we examine studies that have identified the factors influencing scientific collaboration and training.
Collaboration has been extensively studied over the past several decades from a variety of perspectives including the sciences (Armenteras, 2021 ; Olson and Olson, 2000 , 2006 ) and the humanities (Balestrini et al., 2021 ; Canfield, 2020 ; Cooke et al., 2017 ). This research has uncovered challenges resulting from lack of adequate support for collaboration across geographical, institutional, and disciplinary boundaries (Jirotka et al., 2006 ). Life-science research is commonly multi-institutional, and therefore may face challenges related to remote work including, but not limited to, the lack of the motivational sense of the presence of others (Olson and Olson, 2006 ), difficulty establishing and maintaining trust (McDonough et al., 2001 ; Olson and Olson, 2006 ; Sarker et al., 2011 ), increased intra-team conflict due to us-vs-them attitudes (Armstrong and Cole, 2002 ; Cramton, 2001 ), and coordination difficulties caused by reduced number of overlapping work hours between collaboration sites (Battin et al., 2001 ; Casey and Richardson, 2004 ; Kiel, 2003 ). In addition, due to the interdisciplinary nature of these collaborations, it is possible that life scientists also face challenges related to group composition due to lack of common ground (Cundill, 2019 ; Maynard and Gilson, 2014 ), socio-cultural distance (Hinds and Bailey, 2003 ; Mortensen and Hinds, 2001 ; Swigger et al., 2004 ), and differences in work culture (Cundill, 2019 ). Each of these challenges has been extensively discussed in recent work exploring the challenges associated with remote work by Morrison-Smith and Ruiz ( 2020 ). However, despite decades of research focusing on collaboration challenges, recent work has revealed that life scientists are still encountering problems when collaborating (Morrison-Smith et al., 2015 ). This indicates that there is a clear need to further investigate the collaboration challenges faced by life science researchers.
A number of prior works have focused on the importance of transdisciplinary and interdisciplinary training in a variety of contexts including, but not limited to social work (Kemp and Nurius, 2015 ), medicine (Nash, 2008 ), and multidisciplinary research in general (Stokols, 2013 ). However, despite what we know about collaboration, there has been a lack of research focusing on collaboration-based training in the life sciences. There is an abundance of research focusing on supplementing life science education by training life scientists to program (Goodman and Dekhtyar, 2014 ; Mangul et al., 2017 ; Mariano et al., 2019 ; Qin, 2009 ), use bioinformatics software (Attwood et al., 2017 ; Miskowski et al., 2007 ; Ranganathan, 2005 ), and conduct data science (Emery et al., 2021 ) in order to carry out their research. However, despite calls for increasing multidisciplinary training in life science (Cech et al., 2000 ; The National Research Council, 2000 ) research exploring training life scientists to work and communicate in interdisciplinary collaborations has been much more limited.
In 2008, Stokols et al. ( 2008 ) identified that models to guide the development of transdisciplinary training curricula remain to be developed and tested. Since then, Misra et al. ( 2011 ) has identified institutional factors that facilitate transdisciplinary training in health research. In the process, they recommended that transdisciplinary training strengthen individuals’ communication skills that build and sustain cooperation among team members, management strategies for resolving interpersonal conflict, and foster the ability to reach a consensus regarding research goals and visions to reduce task-related uncertainty. However, these recommendations appear highly general and lack insight into how they could be integrated into training specific to life science. Additionally, Sturner et al. ( 2017 ) recently implemented a one-hour professional development course aimed at undergraduate students participating in the National Institute for Mathematical and Biological Synthesis Summer Research Experience that focused on developing collaboration skills. They identified that among other considerations, communication, setting concrete goals, and developing a shared mental model were the top three most important skills that students identified they needed to foster in order to facilitate teamwork. However, further research is required to identify methods for training these skills in a manner that prepares students for life science research. Thus, the question of how to prepare trainees for collaboration in life science research remains open.
Furthermore despite what we know about collaboration, there has been a lack of research focusing on collaboration-based training in the life sciences. There is an abundance of research focusing on supplementing life science education by training life scientists to program (Mangul et al., 2017 ; Mariano et al., 2019 ; Qin, 2009 ), use bioinformatics software (Attwood et al., 2017 ; Miskowski et al., 2007 ; Ranganathan, 2005 ), and conduct data science (Emery et al., 2021 ) in order to carry out their research. However, despite calls for increasing multidisciplinary training in life science (Cech et al., 2000 ; The National Research Council, 2000 ) research exploring training life scientists to work and communicate in interdisciplinary collaborations has been much more limited.
In this section, we describe the participant recruitment, their demographics and workflow, the data collection, and the data analysis. All procedures performed in studies involving human participants were in accordance with the ethical standards of the institutional and with the 1964 Helsinki Declaration and its later amendments or comparable ethical standards. The study was approved by the University of Florida Institutional Review Board (IRB201602178).
Participants
A total of 20 life science researchers aged 28 to 64 (mean = 41.26, standard deviation = 9.64, 10 female) from nine universities and research institutions located in the United States, Canada, Australia, and China participated in this study. We used data saturation (Bonde, 2013 ) to establish our sample size, i.e., data collection terminated once further sessions resulted in minimal new information. We recruited participants via science discussions on Reddit (Reddit.com, 2017 ) and email. Each participant was in life science (Table 1 ). Their current scientific projects varied significantly by size, ranging from two collaborators to over 50. We recruited several participants (P1-9) from an existing collaborative project; whereas the remaining were independent of each other.
Our participants communicated that they seek to answer a variety of scientific questions that include the following:
Immunology: prevention, mitigation, and control of infectious diseases affecting humans and animals.
Animal sciences: improving public food safety associated with consumption of meat products, or consumer demand and satisfaction with these products.
Plant biology: understanding and mitigating the physiological, genetic and biological effects of environmental stressors on plants.
This is not an exhaustive list but rather gives some specific examples of the breadth of research that our participants aimed to advance. Although our participants are seeking to answer a diverse set of research questions, we found that their projects followed very similar workflows, which can be broadly defined as collection, extraction, preparation, and sequencing of biological material. The resulting data are then transferred to the participants’ computer or server and analyzed.
During the collection phase, cells or other biological material of interest are acquired naturally or purchased from a scientific vendor. Upon arrival in the lab, an experimental protocol is performed. After the experiment, the biological material (DNA or RNA) is extracted from the organisms using laboratory methods. These samples are first prepared for sequencing and then sent to an off-site sequencing facility, where each sample is provided as input to sequencing machines. The output of the sequencing phase is a specialized text file (e.g., fastq), which is then transferred from the sequencing facility back to the participant’s computer via SFTP, Windows Remote Desktop, or, when data is too large to easily transmit, a portable hard drive. The sequencing facility can be extremely geographically distant (e.g., the Beijing Genome Institute in China) or near (e.g., at the same institution). Data analysis frequently consists of some combination of bioinformatics and statistics, and may result in scientific conclusions or suggestions for follow-up studies. These steps may be done by multiple scientists or institutions, since specific expertize is needed for each step.
Data collection
We collected data through a series of semi-structured interviews focusing on the identified research questions, allowing us to cover additional topics as they occurred, and thus lessening the probability that important issues would be missed (Lazar et al., 2010 ). We interviewed local participants at their primary workspace (office or lab), and interviewed the remaining participants via Skype or over the phone. The interviews were ~30 to 60 min in duration and were recorded in audio format, then later transcribed verbatim. Our protocol was approved by our Institutional Review Board (IRB201602178). Participation in interviews was voluntary and participants did not receive compensation. Data is publicly available at https://anonymous.4open.science/r/collaboration-data-sharing-data-7F0E .
Data analysis
We performed a bottom-up analysis of participants’ responses by constructing an affinity diagram—which is also known as the KJ method (Beyer and Holtzblatt, 1998 ; Subramonyam et al., 2019 )—to expose prevailing themes in the scientists’ research goals and work practices. This approach follows methodology for qualitative analysis via coding as outlined by Auerbach and Silverstein ( 2003 ). Unlike in qualitative coding, however, instead of each researcher independently organizing data followed by calculating the group’s inter-rater reliability, a quantitative measure, the five researchers analyzing the data came to a consensus on all responses. This is appropriate for semi-structured interviews as qualitative coding results in the possibility of applying the same code to different sections of the interview (Jun et al., 2018 ). We then examined themes from prior work, which enhanced our interpretation of the interview data and allowed us to draw comparisons between our findings and prior knowledge, highlighting new discoveries. This insight facilitated the recommendations for design and training.
We found a clear relationship between the size, distribution, and number of varied disciplines included in a team. Our participants expressed that this was primarily due to the need for expertize and resources—it is unlikely that one researcher has all the necessary expertize to answer a research question with a significant societal or scientific impact.
I have limited abilities, there are some things I know how to do and a bunch of things I don’t. A lot of the collaborations have addressed scientific questions that I would have otherwise not have been able to do with my skill set. (P16)
This need for expertize was one of the primary drivers in dictating the size, geography and disciplines needed of a project. Here, we summarize our main findings that largely stem from the interdisciplinary nature of the projects. Table 2 highlights data supporting each key finding.
Communication barriers reduce project efficiency
Team members who have shared experiences (e.g., share a common vocabulary) have fewer difficulties collaborating remotely (Olson and Olson, 2000 ). This phenomenon is supported by our data in that 17 of our participants with different backgrounds, i.e., less common ground, encountered language barriers that inhibited collaboration by requiring extra effort to successfully discuss project goals and tasks. Hence, we found our participants encountered technical language barriers because they lacked the background necessary to understand all portions of the project. These language barriers affected the ability of researchers to communicate about project goals and individual tasks, making it difficult to understand how their research fit into the whole. The predominance of jargon and the tendency for some words to have different meanings in the context of other scientific fields further exacerbated these difficulties To address these challenges, our participants attempted to mimic the language of their collaborator(s) by describing their methodology at a high level, which they thought was easier for their collaborator(s) to understand.
Sometimes it’s taking something that is complicated and explaining so it’s understandable. That’s difficult. You have to speak in terms of their language. (P7)
Aside from language, twelve of our participants indicated that interdisciplinary and multi-institutional teams experienced differences in scientific methodology or standards. Moreover, these disparities had a significant impact on the project by requiring that the participants have additional discussions to come to a consensus regarding protocol and, in some cases, redo aspects of the project using different techniques. In some projects, experiments cannot be redone, in which case the participants felt they must reformat the data or “work with what [they] get" (P7). In a worst-case scenario, improper technique can make the data unusable:
She’s probably spent 50 to 100 thousand dollars on sequencing and has nothing to show for it simply because proper controls weren’t done. (P8)
Lastly, since participants needed to provide a substantial amount of background when discussing almost any aspects of a project, they felt that the processes of initiating and participating in this dialog can be time-consuming and inefficient to achieving the project goals. Thus, the participants often felt that the only solution was to trust that their collaborator(s) knew what they were doing for their part of the project and vice versa.
Mutual respect and trust is necessary for project engagement
The interdisciplinary projects our participants engaged in often also resulted in different methodological approaches to science, which could influence the participants ability to trust their collaborator(s). In turn, this sometimes led to feelings on mistrust in a collaborator’s competence, impeding progress when previous portions of the project are redone or verified. This is expressed by P7 when they question the methodology, leading to perceptions of quality of work, and translating into mistrust regarding the quality of a collaborator’s output or data:
It’s hard because if you’re receiving samples from them, or you’re receiving a protocol, or they’re sharing information, the way they would have done it, the method or technique, is much different from what you did. And there might be some disparities there, or I might have even an issue with how it was done and the quality of how it was done. (P7)
Ultimately, fourteen of our participants felt that the projects where there was a significant amount of “ mutual respect ” (P8,P17) were more successful than those where some team members felt under-appreciated and under-prioritized. Interestingly, we found instances where the work culture shifted from collaborative to competitive—namely when interdisciplinary teams increased expertize in a specific area, this frequently lead to territorial issues. Our participants, such as P3, expressed that the addition of collaborators in a project can pose challenges if the expertize overlaps because there is potential for territorial actions that foster animosity and jeopardize the project’s success (e.g., competition for funding sources):
Because you are working in the same field and you are doing the same stuff, there is more potential for territorial actions versus when you are working with people totally outside, they have their own funding sources. (P3)
The outcome of mistrust or lack of mutual respect within the project was frequently interpersonal conflict leading to detachment or disconnection of the project. This detachment, in turn, can cause researchers to drop out of the project, leading to increased costs in terms of time and funding to complete the project. In some cases, interpersonal conflict could interfere with the publication of a completed project.
People creating conflict. If they were sort of ad-hoc projects where there were not a lot of funding in place, then they would tend to fade away you know, evaporate.... If there was funding on the line and the project was centered here, I end up having to pick up the pieces for what they were doing. And it usually took longer. Sometimes we had to find additional funding because of those failures. Sometimes, and almost inevitably put publication of the research in jeopardy. And some of those projects, well they may have been completed, but they’ve never been published. (P6)
Large, distributed teams lead to reduced engagement
We found that eight of our participants were more likely to feel disconnected when collaborating in large distributed groups than smaller ones. These participants felt that large group meetings are a “ waste of time ” (P16) due to both inefficiency and a tendency for conversation topics to interest only a few collaborators at a time. This lack of interest is partially due to the structure of the projects consisting of multiple phases, each of which is more interesting to some researchers than others.
There were little bits that were sometimes important to me that I had to share but I would usually sit there with my phone on mute, doing something else waiting for my ears to perk up for something that was like what’s going on with this. So it was probably a fair amount of wasted of time. The fraction of the call that was important to the average individual, was quite small. (P16)
In these situations, our participants viewed discussions about a phase that they are not particularly concerned with as going into too much unnecessary detail, and thus lose interest. They described this issue as being especially prevalent in large, interdisciplinary projects where collaborators are perceived to be apathetic or uninterested in the details of the project that are not directly related to their field.
It is pretty easy for some researchers to drop out of the mix in a large group and that can ultimately be, you know, a herald of death for the whole thing, right? (P12)
One participant (P19) observed that enthusiasm varies throughout the life of the project, depending on how much the researcher cares about that stage. They observed that researchers are typically the most excited at the beginning of the project and later are interested in the results, but lose focus during the middle. This fluctuation in interest levels has important implications for design of training programs to ensure the engagement of the trainees.
Perceived priorities are difficult to gauge
We identified differences in priorities—or perceived priorities—of collaborators as a challenge associated with interpersonal dynamics that especially affected six of our life scientist participants. Researchers are frequently involved with multiple scientific projects, and, thus, they prioritize their efforts.
One of the biggest difficulties is having collaborators who are really busy and they have multiple projects going on and your collaborative project may not be their highest priority. (P13)
Our participants found it frequently difficult to tell whether a collaborator is prioritizing the project and thus, when they are not “ pulling their weight ” (P17). This is frequently manifested when lack of informal interactions led to doubts about their collaborators’ prioritization of the project, creating concern that a vital part of the project would not be completed. Hence, this sense of project involvement is particularly important when researchers are concerned that improper prioritization jeopardizes the project’s timeline. In these situations, our participants sometimes used email to gauge their interest—a collaborator who responds quickly to email is more likely to be prioritizing the project than one who takes weeks or even months to respond.
It can be really hard to tell where they put our collaboration project into priority, but you can tell from email comment—sometimes you can tell they’re working and I get email back really soon, but sometimes it’s like after a couple of weeks may be months then I get a response. (P18)
In some cases, collaborators are even encouraged to drop out of a project, sometimes with a recommendation for a new collaborator, if they are unable to complete their portion in a timely manner. Hence, resolving this issue is complicated and frequently requires additional work on the side of the researcher who is waiting. This issue sometimes caused researchers to make extra work for themselves—such as doing everything in their power to make it more convenient for their collaborators to complete their part of the project.
First I do what I can do on my side and then try to make everything easier and convenient for my collaborators (P18)
Over the course of our investigation into the challenges faced by life science researchers, we identified several key issues that specifically affect life science collaborations: “mutual respect” is important; interdisciplinary causes technical language barriers; differences in methodology affect trust; and perception of a collaborator’s priorities can cause unnecessary work. These key findings demonstrate that collaboration challenges are still impacting life science, despite years of collaboration research. In this section, we discuss the implications of our results for the design of technology and for training in life science.
Implications for design of technology
Support documentation and discussion of scientific knowledge.
Our results show that involving various disciplines creates language barriers that delay life science projects. Despite this effect, we also find that multidisciplinary collaborations are likely to continue to be the norm for these types of projects, given their potential to assist in answering broad scientific questions. Therefore, new tools are needed to lower the language barriers and support discussions around scientific knowledge that go beyond current communication tools that simply support communication (e.g., email, Zoom (Zoom Video Communications Inc., 2020 ), and Slack (Salesforce Inc., 2021c )) over geographical distance. We envision systems that enable teams to both document and discuss activities and methods throughout the project life cycle while providing tools and techniques to minimize language barriers. For example, we envision a specialized word processor similar to Microsoft Word Online (Microsoft Inc., 2021b ) or Google Docs (Google Inc., 2021a ) that enable remote collaborators to collaboratively work on a document and easily import output of computational programs. To minimize the language barriers, the system could provide easy lookup of specific terminology, method, and datasets through a context menu (i.e., right clicking or hovering over a specific text). This would enable a researcher unfamiliar with a term or method to look-up more information. The context menu can also enable easy linking to shared datasets. These methods not only help minimize language barriers, which will promote more discussion across all members of the team, but also provide more transparency on the approach individual team members are taking so that their is less concern around differences in research methodologies.
Support creation of collaborations with mutual respect and trust
Participants frequently stated that mutual respect and trust were necessary for project engagement and success. However, the need to find expertize in an specific area often results in the creation of a team where trust has not been previously established. To avoid these issues researchers often constrain teams to their current network of collaborators and when forced to reach out rely on the collaborators of trusted collaborators. For example, one participant stated:
So they had collaborated with my advisor before and so they needed some of that specific skill. So they called us for other grants. It’s usually because the people they’re like, first of all, we know them. We know we can work with them. (P3)
This suggest the need of to capitalize systems that focus on social networking to create a space that enable researchers to precisely specify their expertize and specify their past and current collaborators. This would create a social network where researchers who are starting a new project can find new collaborators by searching for a particular expertize, and by determining how the potential collaborators relate to their prior collaborations. The ability to know how the new collaborator fits within their research network, new teams would have more common collaborators, which would result in higher initial trust and respect. In addition, the network should also enable to see how potential collaborators’ expertize may or may not overlap with the current teams expertize. This is important as participants mentioned that too much overlapping of expertize can result in conflict and unhealthy internal competition.
Greater support for management of scientific projects
Participants from our study often commented on that since their collaborators often worked on several projects, they had a difficultly gauging status of their collaborators’ tasks, which lead to them questioning their collaborators’ priorities. Although software has been developed to assist in creating and running data analysis pipelines (e.g., Galaxy (Afgan et al., 2018 )), there is a need for a project management system explicitly tailored to life science pipelines and life science project workflows. We envision a system developed to support a life science project life cycle similar to those that have been developed to support software engineering (e.g., agile development software). These systems are unique in that they not only would require task management, but also enable effective sharing of datasets, analysis pipelines, and project results. To support task management, these systems should enable to collaborators to create milestones and tasks, assign tasks, and specify the status of tasks. The system should also enable researchers to specify tasks that are dependent on one another. In this case, the system should notify members of a task chain notifying them that the dependent task has been completed and the new tasks may now begin. This would enable the researchers to focus on doing science instead of managing the handoff of tasks. Together, these the proposed features would enable a shared sense of ownership of the project while also providing a better sense of each members progress toward the shared goals. We envision that these systems could also provide support for automation. For example, a data analysis pipeline can be set up such that when new datasets are added to the shared project, the pipeline is automatically run with the new dataset and the results are stored and shared. The system would also allow researchers to view and visualize (when appropriate) all results of a pipeline to enable comparisons between pipeline executions and datasets.
Implications for training in life science
Life science requires multidisciplinary training.
Despite the issues related to interdisciplinarity in life science collaborations, it is unlikely that future collaborations will consist only of collaborators from the same field due to the need for specific expertize and resources to answer broad research questions with significant societal or scientific impact. Furthermore, researchers appreciate the resulting increase in the range of scientific perspectives and potential for gaining new insight, making it more likely that multidisciplinary collaborations will be pursued. Addressing the challenges associated with the inclusion of multiple disciplines in a collaboration will therefore be crucial in life science training programs. Moreover, the participants were more engaged and had higher perception of their colleagues work when they were knowledgeable of that proponent of the project.
Therefore, one—perhaps most obvious—finding of our work is need for life science training programs to be multidisciplinary. Our participants felt that although they may not need to actively participate in all aspects of the project, they would be more engaged if they had knowledge of each proponent of the scientific process. Moreover, our finding suggest that this would lead to an increased appreciation of colleagues’ contributions. Training programs also need to accommodate the discussion and negotiation process as methodologies and data sharing standards evolve, rather than just facilitate a decision of which techniques should be used.
Training should break down language barriers via standardization
Cummings and Kiesler (Cummings and Kiesler, 2005 ) work found that projects incorporating multiple disciplines had as many positive outcomes as projects involving fewer. Our findings, however, indicate that projects with high-field heterogeneity face challenges in the form of language and background barriers, and thus align with the findings of Kiesler and Cummings ( 2002 ) that discipline influences project success. This finding is particularly problematic for life science since the bulk of the researchers’ work is highly technical and requires conveying specific knowledge of terminology and methodology. While prior work has examined the challenges resulting from differences in project-related terminology (Morrison-Smith et al., 2015 ). For example, “test procedure” and “phase completion” are not necessarily analogous to the all scientific fields, and terms are frequently specific to a sub-discipline of life science, e.g, “contig” is used to define “a set of overlapping DNA segments that together represent a consensus region of DNA”. Furthermore, the transfer of this knowledge is unavoidable since it is impossible for a single researcher to have all the expertize required to complete a project with high societal impact. Participating in additional dialogs to overcome these barriers can further slow the progression of the project. Training programs should provide mechanisms for facilitating, simplifying, and documenting these conversations. Documentation should be done in a manner that allows users to search for abstract representations of information, as discussed by Olson et al. ( 2008 ). One way to achieve this is through standardization of terminology, which implies instruction on contextualizing scientific terms.
Life science requires training in project management
The need for explicit management details how collaborations require additional management to overcome the challenges associated with being dispersed geographically or larger in size (Olson and Olson, 2006 ). We found several instances of this additional work, particularly when coordinating meetings (especially across multiple time zones and in different languages) and ensuring that everyone is up-to-date with the project status. While it is well documented that scientists do not like explicit management (Olson and Olson, 2006 )—and our own findings suggest that scientists are not interested in micromanagement and rather prefer ad-hoc collaborations—several participants specifically acknowledged this need for explicit management by describing “ a good PI” as someone who dedicates time to ensure that all portions of the project are “moving forward ”(P19).
Our findings suggest that mechanisms for training in explicit management should be available, as they are necessary for large-scale project and may not encounter as much resistance as previously thought. For example, funding agencies could mandate or strongly suggest a formal management training program for the Co-PIs, so that they are better able to execute the type of explicit management that is required for large collaborative projects. In addition, our results imply that formal and explicit training in project management should also be available for students in life science. We recommend that universities and other training intuitions offer certificate programs in project management. The curriculum for these certificate programs could include formal pedagogy from the team science research literature, as well as experiential activities.
Work culture of disciplines needs to be incorporated into training programs for life science
Our results provide answers to the research question about the influence of work culture on life science research. While Walsh and Maloney ( 2007 ) asserted that remote collaborations do not experience more challenges associated with culture than co-located teams, results from our study demonstrate that differences in work culture, particularly work practices regarding methodology and data sharing, profoundly affect collaboration in life science. Like McDonough et al. ( 2001 ), we found that differences in work practices and culture pose additional challenges in project management and coordination. For life science projects, differences in methodology can call into question the quality of completed work, resulting in delays caused by redoing procedures. Hence, our recommendation is that scientific programs in life science (bioinformatics being inclusive of that definition) take a short, required course in differences in related to discipline culture, which include methodology, data sharing, grant writing procedures, and determination of authorship and co-authorship.
Size of training programs in life sciences needs to be varied
Our study demonstrated that participants felt disconnected to the goals of a project in large groups. Our participants frequently felt this translated into “ mutual respect ” for each other’s contribution, and attributed this to being tied to the size of the research group. In addition, in large groups the participants they sometimes perceived their collaborators as being apathetic or uninterested in the details of the project. Thus, one recommendation that stems from our findings is to have training programs of varied sizes and disciplines; smaller discussion sections or research project groups will allow further engagement and understanding into the scientific progress.
While our study is limited by the sole use of interview data, we were able to elicit many new insights about the collaboration and training faced by life scientists. Future work includes supplementing our results with observations or journaling of work. It is clear that discipline, language, project management, engagement and work culture will vary in future scientific projects and, therefore, the creation of training programs that either overcomes the barriers of all of these factors remains imperative–regardless of their individual impact–or prepares life science researchers with the necessary skills to overcome these barriers.
We presented the results of semi-structured interviews that examined the challenges associated with collaborative life science research. We identified several key issues that specifically affect life science collaborations: “ mutual respect ” is important; interdisciplinarity causes technical language barriers; differences in methodology affect trust; and perception of a collaborator’s priorities can cause unnecessary work. These key findings demonstrate that collaboration and training challenges are still impacting life science, despite years of collaboration research. Our contributions include (1) new insights into the communication and collaboration challenges that hinder life science research, particularly on the effects of discipline, work practices, and culture; and (2) recommendations for designing training programs to better support life science. Lastly, we note that identifying opportunities for the bioinformatics community to engage with life scientists to design tools that support collaboration and communication in this domain warrants future investigation.
Data availability
The datasets generated during and/or analyzed during the current study are available from the corresponding author on reasonable request.
Afgan E, Baker D, Batut B, vandenBeek M, Bouvier D, Čech M, Chilton J, Clements D, Coraor N, Grüning B, Guerler A, Hillman-Jackson J, Hiltemann S, Jalili V, Rasche H, Soranzo N, Goecks J, Taylor J, Nekrutenko A, Blankenberg D (2018) The Galaxy platform for accessible, reproducible and collaborative biomedical analyses: 2018 update. Nucleic Acids Res 46(W1 (may)):W537–W544. ISSN 0305-1048
Article CAS PubMed PubMed Central Google Scholar
Armenteras D (2021) Guidelines for healthy global scientific collaborations. Nat Ecol Evol. 5(9):1193–1194
Armstrong DJ, Cole P (2002) Managing distances and differences in geographically distributed work groups. In: Distributed work. The MIT Press, Cambridge, MA, USA. pp. 167–186
Attwood TK, Blackford S, Brazas MD, Davies A, Schneider MV (2017) A global perspective on evolving bioinformatics and data science training needs. Brief Bioinform 20(2 (August)):398–404. ISSN 1477-4054
PubMed Central Google Scholar
Auerbach C, Silverstein LB (2003) Qualitative data: an introduction to coding and analysis, vol 21. NYU Press
Bajpai R, Meher J, Rashid MM, Lingayat D (2021) Metatranscriptomics: a recent advancement to explore and understand rhizosphere. In: Nath M, Bhatt D, Bhargava P, Choudhary DK (eds.) Microbial metatranscriptomics belowground. Springer
Balestrini M, Kotsev A, Ponti M, Schade S (2021) Collaboration matters: capacity building, up-scaling, spreading, and sustainability in citizen-generated data projects. Humanit Soc Sci Commun 8(1):169
Article Google Scholar
Bansal V, Boucher C (2019) Sequencing technologies and analyses: Where have we been and where are we going? iScience 18:37–41
Article ADS PubMed PubMed Central Google Scholar
Battin RD, Crocker R, Kreidler J, Subramanian K (2001) Leveraging resources in global software development. IEEE Softw 18(2):70–77
Beyer H, Holtzblatt K (1998) Contextual design: defining customer-centered systems. Morgan Kaufmann Publishers Inc., San Francisco, CA, USA
Bonde D (2013) Qualitative interviews: when enough is enough. Research by Design
Canfield KN et al. (2020) Science communication demands a critical approach that centers inclusion, equity, and intersectionality. Frontiers in Communication 5:2
Casey V, Richardson I (2004) Practical experience of virtual team software development. In: Proc of European Software Process Improvement (Euro SPI)
Cech TR, Bond EC, Stevens J (2000) The role of the private sector in training the next generation of biomedical scientists. In: Proc. of a conference sponsored by the American Cancer Society, the Burroughs Wellcome Fund, and the Howard Hughes Medical Institute
Cooke SJ, Gallagher AJ, Sopinka NM, Nguyen VM, Skubel RA, Hammerschlag N, Boon S, Young N, Danylchuk AJ (2017) Considerations for effective science communication. FACETS 2:233–248
Cramton CD (2001) The mutual knowledge problem and its consequences for dispersed collaboration. Organ Sci 12(3):346–371
Cummings JN, Kiesler S (2005) Collaborative research across disciplinary and organizational boundaries. Soc Stud Sci 35(5):703–722
Cundill G et al. (2019) Large-scale transdisciplinary collaboration for adaptation research: Challenges and insights. Glob Challenge 3(4):1700132
Emery N, Crispo E, Supp SR, Kerkhoff AJ, Farrell KJ, Bledsoe EK, O’Donnell KL, McCall AC, Aiello-Lammens M (2021) Training data: how can we best prepare instructors to teach data science in undergraduate biology and environmental science courses? Preprint at bioRxiv https://doi.org/10.1101/2021.01.25.428169
Espinosa JA, Carmel E (2004) The effect of time separation on coordination costs in global software teams: a dyad model. In: Proc. of the 37th Annual Hawaii International Conference on, 10–pp
Fernandes JD et al. (2020) The UCSC SARS-CoV-2 genome browser. Nat Genet 52:991–998
Article PubMed PubMed Central Google Scholar
Funk WC, Zamudio KR, Crawford AJ (2018) Advancing understanding of amphibian evolution, ecology, behavior, and conservation with massively parallel sequencing. In: Hohenlohe PA, Rajora OP (eds.) Population genomics: wildlife. population genomics. Springer
Giani AM, Gallo GR, Gianfranceschi L, Formentic G (2020) Long walk to genomics: history and current approaches to genome sequencing and assembly. Comput Struct Biotechnol 18:9–19
Article CAS Google Scholar
Goodman AL, Dekhtyar A (2014) Teaching bioinformatics in concert. PLoS Comput Biol 10(11):e1003896
Google Inc. (2021a) Google docs. https://docs.google.com
Hinds PJ, Bailey DE (2003) Out of sight, out of sync: understanding conflict in distributed teams. Organ Sci 14(6):615–632. ISSN 1047-7039
Hinds PJ, Mortensen M (2005) Understanding conflict in geographically distributed teams: the moderating effects of shared identity, shared context, and spontaneous communication. Organ Sci 16(3):290–307
Humble E et al. (2020) Chromosomal-level genome assembly of the scimitar-horned oryx: Insights into diversity and demography of a species extinct in the wild. Mol Ecol Resour 20(6):1668–1681
Article PubMed Google Scholar
International Human GenomeSequencing Consortium (2001) Initial sequencing and analysis of the humangenome. Nature 409:860–921
i5K Consortium (2013) The i5Kinitiative: advancing arthropod genomics for knowledge, human health,agriculture, and the environment. J Hered 104(5):595–600
Jirotka M, Procter R, Rodden T, Bowker GC (2006) Special issue: collaboration in e-research. Comput Support Coop Work (CSCW) 15(4):251–255
Jun E, Jo BA, Oliveira N, Reinecke K (2018) Digestif: promoting science communication in online experiments. In: Proc of the ACM on Human-Computer Interaction, vol 2 (CSCW), pp. 1–26
Kemp SP, Nurius PS (2015) Preparing emerging doctoral scholars for transdisciplinary research: a developmental approach. J Teach Soc Work 35(1-2):131–150
Kenneth M et al. (2018) The Parkinsonas progression markers initiative (PPMI)-establishing a PD biomarker cohort. Ann Clin Transl Neurol 5(12):1460–1477
Kiel L (2003) Experiences in distributed development: a case study. In Proc. of International Workshop on Global Software Development at ICSE
Kiesler S, Cummings JN (2002) What do we know about proximity and distance in work groups? A legacy of research. Distrib Work 1:57–80
Google Scholar
Lang D et al. (2020) Comparison of the two up-to-date sequencing technologies for genome assembly: HiFi reads of Pacific Biosciences Sequel II system and ultralong reads of Oxford Nanopore. GigaScience 9(12):giaa123
Lazar J, Feng JH, Hochheiser H (2010) Research methods in human-computer interaction. Wiley Publishing
Luikart G, Kardos M, Hand BK, Rajora OP, Aitken SN, Hohenlohe PA (2018) Population genomics: advancing understanding of nature. In Population genomics, Springer, pp. 3–79
Mangul S, Martin LS, Hoffmann A, Pellegrini M, Eskin E (2017) Addressing the digital divide in contemporary biology: lessons from teaching UNIX. Trend Biotechnol 35(10):901–903
Mariano D, Martins P, HeleneSantos L, de Melo-Minardi RC (2019) Introducing programming skills for life science students. Biochem Mol Biol Educ 47(3):288–295
Maynard MT, Gilson LL (2014) The role of shared mental model development in understanding virtual team effectiveness. Group Organ Manag 39(1):3–32
McDonough EF, Kahnb KB, Barczaka G (2001) An investigation of the use of global, virtual, and colocated new product development teams. J Prod Innov Manag 18(2):110–120
Microsoft Inc. (2021b) Microsoft word online. https://office.live.com
Miga KH et al. (2020) Telomere-to-telomere assembly of a complete human X chromosome. Nature 585:79–84
Article ADS CAS PubMed PubMed Central Google Scholar
Miskowski JA, Howard DR, Abler ML, Grunwald SK (2007) Design and implementation of an interdepartmental bioinformatics program across life science curricula. Biochem Mole Biol Educ 35(1):9–15
Misra S, Stokols D, Hall K, Feng A (2011) Transdisciplinary training in health research: distinctive features and future directions. In: Converging disciplines. Springer, pp. 133–147
Morrison-Smith S, Ruiz J (2020) Challenges and barriers in virtual teams: a literature review. SN Appl Sci 2(6):1096
Morrison-Smith S, Boucher C, Bunt A, Ruiz J (2015) Elucidating the role and use of bioinformatics software in life science research. In: Proceedings of the 2015 British HCI Conference. ACM, pp. 230–238
Mortensen M, Hinds PJ (2001) Conflict and shared identity in geographically distributed teams. Int J Confl Manag 12(3):212–238. ISSN 1044-4068
Mukherjee K et al. (2018) Error correcting optical mapping data. GigaScience 7(6):giy061
Article PubMed Central Google Scholar
Nash JM (2008) Transdisciplinary training: key components and prerequisites for success. Am J Prevent Med 35(2):S133–S140
Olson GM, Olson JS (2000) Distance matters. Hum Comput Interact 15(2):139–178
Olson JS, Olson GM (2006) Bridging distance: empirical studies of distributed teams. Hum Comput Interact Manage Inform Syst2:27–30
Olson GM, Zimmerman A, Bos N (2008) Scientific collaboration on the Internet. The MIT Press
Pollack A (2011) DNA sequencing caught in deluge of data. New York Times
Qin H (2009) Teaching computational thinking through bioinformatics to biology students. In: Proc. of the 40th ACM Technical Symposium on Computer Science Education (SIGCSE). pp. 188–191
Ranganathan S (2005) Bioinformatics education–perspectives and challenges. PLOS Comput Biol 1(6 (nov)):e52
Reddit.com. (2017) AskScience: Got Questions? Get Answers. https://www.reddit.com/r/askscience/
Rhie A et al. (2021) Towards complete and error-free genome assemblies of all vertebrate species. Nature 592(7856):737–746. ISSN 1476-4687
Salesforce Inc. (2021c) Slack. https://slack.com
Sarker S, Ahuja M, Sarker S, Kirkeby S (2011) The role of communication and trust in global virtual teams: a social network perspective. J Manag Inform Syst 28(1):273–310
Shapiro B (2017) Pathways to de-extinction: how close can we get to resurrection of an extinct species? Funct Ecol 31(5):996–1002
Stokols D, Hall KL, Taylor BK, Moser RP (2008) The science of team science: overview of the field and introduction to the supplement. Am J Prevent Med 35(2):S77–S89
Stokols D (2013) Training the next generation of transdisciplinarians. In: O’Rourke M, Crowley S, Eigenbrode SD, Wulfhorst JD (eds.), Enhancing communication & collaboration in interdisciplinary research, ch. 4. Sage Publications
Sturner KK, Bishop P, Lenhart SM (2017) Developing collaboration skills in team undergraduate research experiences. Primus 27(3):370–388
Subramonyam H, Drucker SM, Adar E (2019) Affinity lens: data-assisted affinity diagramming with augmented reality. In Proc. of the 2019 CHI Conference on Human Factors in Computing Systems. pp. 1–13
Swigger K, Alpaslan F, Brazile R, Monticino M (2004) Effects of culture on computer-supported international collaborations. Int J Hum Comput Stud 60(3):365–380
The National Research Council (2000) Addressing the nation’s changing needs for biomedical and behavioral scientists. The National Research Council
Venter JC et al. (2001) The sequence of the human genome. Science 291:1304–1351
Article ADS CAS PubMed Google Scholar
Waese J et al. (2017) ePlant: visualizing and exploring multiple levels of data for hypothesis generation in plant biology. Plant Cell 29(8):1806–1821
Walsh JP, Maloney NG (2007) Collaboration structure, communication media, and problems in scientific work teams. J Comput Mediat Commun 12(2):712–732
Zoom Video Communications Inc. (2020) Zoom for video, conferencing, and phones. https://zoom.us/
Download references
Acknowledgements
This work is partially supported by National Science Foundation Grant Award #IIS-2013998. Any opinions, findings, and conclusions or recommendations expressed in this paper are those of the authors and do not necessarily reflect these agencies’ views. The authors would like to thank Isaac Wang, Courtney Sanchez, Megan Hofmann, Julia Chang, Ariel Goldman, Aditi Patil, Dipashreya Sur, Luiza Leschziner, and Christopher Dean for assisting in the data analysis and related works.
Author information
Authors and affiliations.
Barnard College, New York, NY, USA
Sarah Morrison-Smith, Catherine O’Brien & Nazaret Cuadros
University of Florida, Gainesville, FL, USA
Christina Boucher & Jaime Ruiz
Drexel University, Philadelphia, PA, USA
Aleksandra Sarcevic
University of Minnesota, Minneapolis, MN, USA
Noelle Noyes
You can also search for this author in PubMed Google Scholar
Corresponding author
Correspondence to Sarah Morrison-Smith .
Ethics declarations
Competing interests.
The authors declare no competing interests.
Ethical approval
As stated in section “Methods”, all procedures performed in studies involving human participants were in accordance with the ethical standards of the institutional and with the 1964 Helsinki Declaration and its later amendments or comparable ethical standards. The study was approved by the University of Florida Institutional Review Board (IRB201602178).
Informed consent
As stated in section “Methods”, this study was approved by the University of Florida Institutional Review Board (IRB201602178). As such, all participants provided informed consent before participating.
Additional information
Publisher’s note Springer Nature remains neutral with regard to jurisdictional claims in published maps and institutional affiliations.
Rights and permissions
Open Access This article is licensed under a Creative Commons Attribution 4.0 International License, which permits use, sharing, adaptation, distribution and reproduction in any medium or format, as long as you give appropriate credit to the original author(s) and the source, provide a link to the Creative Commons license, and indicate if changes were made. The images or other third party material in this article are included in the article’s Creative Commons license, unless indicated otherwise in a credit line to the material. If material is not included in the article’s Creative Commons license and your intended use is not permitted by statutory regulation or exceeds the permitted use, you will need to obtain permission directly from the copyright holder. To view a copy of this license, visit http://creativecommons.org/licenses/by/4.0/ .
Reprints and permissions
About this article
Cite this article.
Morrison-Smith, S., Boucher, C., Sarcevic, A. et al. Challenges in large-scale bioinformatics projects. Humanit Soc Sci Commun 9 , 125 (2022). https://doi.org/10.1057/s41599-022-01141-4
Download citation
Received : 28 May 2021
Accepted : 21 March 2022
Published : 08 April 2022
DOI : https://doi.org/10.1057/s41599-022-01141-4
Share this article
Anyone you share the following link with will be able to read this content:
Sorry, a shareable link is not currently available for this article.
Provided by the Springer Nature SharedIt content-sharing initiative
Quick links
- Explore articles by subject
- Guide to authors
- Editorial policies

Current Topics in Bioinformatics Spring 2024 Schedule

The Harvard Bioinformatics Core is excited to announce the Spring 2024 schedule for the Current Topics in Bioinformatics series: Big data? Big computer! The skill set you need to succeed. These free, hands-on workshops are available to all Harvard affiliates and cover a variety of bioinformatics topics & related skills. The first workshop is a pre-requisite for the next three workshops. All workshops are currently held remotely via Zoom and typically meet the 3rd Wednesday of each month. The topics are provided in the table above, along with prerequisites. You can find more information and register here .
News from the School
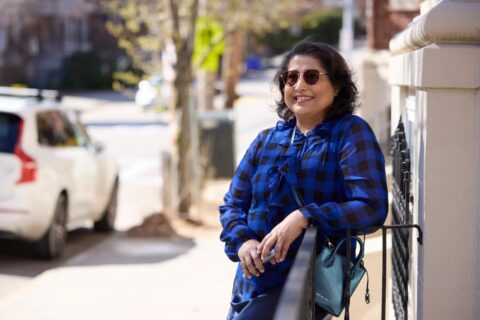
From public servant to public health student
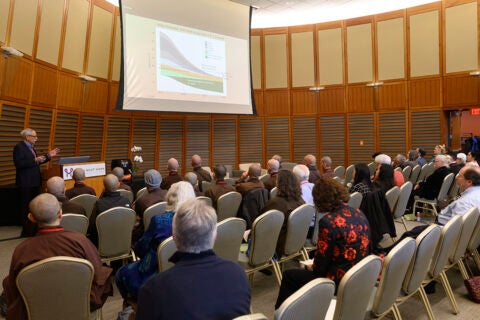
Exploring the intersection of health, mindfulness, and climate change
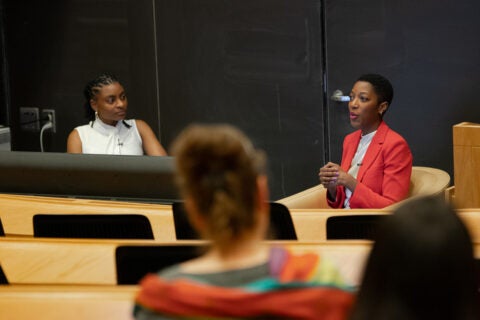
Conference aims to help experts foster health equity
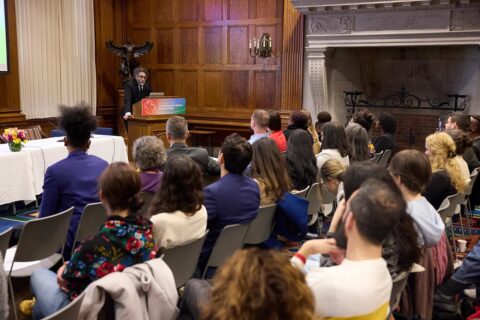
Building solidarity to face global injustice
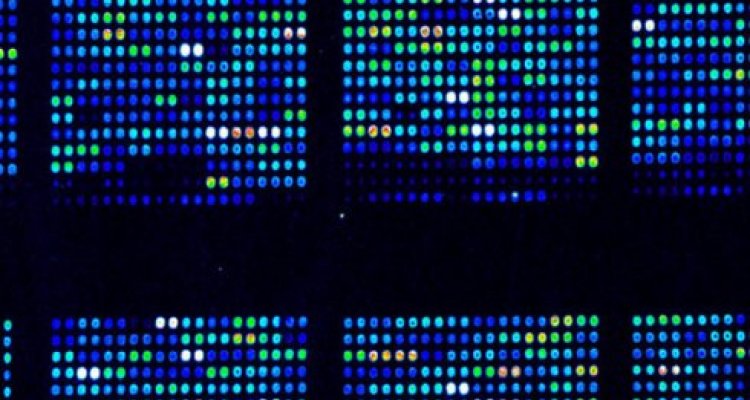

BSc and MSc Thesis Subjects of the Bioinformatics Group
On this page you can find an overview of the BSc and MSc thesis topics that are offered by our group. The procedure to find the right thesis project for you is described below.
MSc thesis: In the Bioinformatics group, we offer a wide range of MSc thesis projects, from applied bioinformatics to computational method development. Here is a list of available MSc thesis projects . Besides the fact that these topics can be pursued for a MSc thesis, they can also be pursued as part of a Research Practice .
BSc thesis: As a BSc student you will work as an apprentice alongside one of the PhD students or postdocs in the group. You will work on your own research project, closely guided by your supervisor. You will be expected to work with several tools and/or databases, be creative and potentially overcome technical challenges. Below you will find short descriptions of the research projects of our PhDs and Postdocs. In addition you can take a look at the list of MSc thesis projects above.
Procedure for WUR students:
- Request an intake meeting with one of our thesis coordinators by filling out the MSc intake form or BSc intake form and sending it to [email protected]
- Contact project supervisors to discuss specific projects that fit your background and interest
- Upon a match, take care of the required thesis administration together with your supervisor(s) and enroll in the thesis BrightSpace site to find more information on a thesis in the Bioinformatics group
Procedure for non-WUR students or students in other non-standard situations: We have limited space for interns from other institutes. If you are interested, please email our thesis coordinators at [email protected]; please attach your CV and indicate what are your main research interests.
BSc thesis topics
Integrative omics for the discovery of biosynthetic pathways in plants, molecular function prediction of natural products, linking the metabolome and genome, linking metagenomics and metatranscriptomics to study the endophytic root microbiome, exploiting variation in lettuce and its wild relatives.
- Frontiers in Computational Neuroscience
- Research Topics
Bioinformatics for Modern Neuroscience
Total Downloads
Total Views and Downloads
About this Research Topic
The current field of neuroscience is increasingly using bioinformatics, which has provided new research avenues, thus enhancing our understanding of brain functions. Some well-known examples include high-throughput analysis of single-cell transcriptomics, comparative genomics, networks & neurophysiology, the ...
Keywords : bioinformatics, computational neuroscience
Important Note : All contributions to this Research Topic must be within the scope of the section and journal to which they are submitted, as defined in their mission statements. Frontiers reserves the right to guide an out-of-scope manuscript to a more suitable section or journal at any stage of peer review.
Topic Editors
Topic coordinators, recent articles, submission deadlines.
Submission closed.
Participating Journals
Total views.
- Demographics
No records found
total views article views downloads topic views
Top countries
Top referring sites, about frontiers research topics.
With their unique mixes of varied contributions from Original Research to Review Articles, Research Topics unify the most influential researchers, the latest key findings and historical advances in a hot research area! Find out more on how to host your own Frontiers Research Topic or contribute to one as an author.
Loading metrics
Open Access
A Quick Guide for Building a Successful Bioinformatics Community
* E-mail: [email protected] (AB); [email protected] (MC); [email protected] (MDB); [email protected] (JCF)
Affiliation Structural and Computational Biology (SCB) Unit, European Molecular Biology Laboratory (EMBL), Heidelberg, Germany
Affiliation The Genome Analysis Centre (TGAC), Norwich Research Park, Norwich, United Kingdom
Affiliation Ontario Institute for Cancer Research, MaRS Centre, West Tower, Toronto, Ontario, Canada
Affiliation Heidelberg Institute for Theoretical Studies (HITS) gGmbH, Heidelberg, Germany
Affiliation The Computational Biology Institute, George Washington University, Innovation Hall, Virginia, United States of America
Affiliation Computational Biology Group, Institute of Infectious Disease and Molecular Medicine (IDM), University of Cape Town Faculty of Health Sciences, Cape Town, South Africa
Affiliation Computational Cancer Biology, Netherlands Cancer Institute, Amsterdam, the Netherlands
Affiliations Ontario Institute for Cancer Research, MaRS Centre, West Tower, Toronto, Ontario, Canada, Department of Cell and Systems Biology, University of Toronto, Toronto, Canada
Affiliation The Software Sustainability Institute, School of Computer Science, University of Manchester, Manchester, United Kingdom
Affiliation ELIXIR Hub, Wellcome Trust Genome Campus, Cambridge, United Kingdom
- Aidan Budd,
- Manuel Corpas,
- Michelle D. Brazas,
- Jonathan C. Fuller,
- Jeremy Goecks,
- Nicola J. Mulder,
- Magali Michaut,
- B. F. Francis Ouellette,
- Aleksandra Pawlik,
- Niklas Blomberg
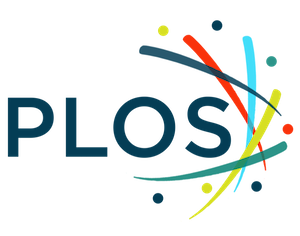
Published: February 5, 2015
- https://doi.org/10.1371/journal.pcbi.1003972
- Reader Comments
“Scientific community” refers to a group of people collaborating together on scientific-research-related activities who also share common goals, interests, and values. Such communities play a key role in many bioinformatics activities. Communities may be linked to a specific location or institute, or involve people working at many different institutions and locations. Education and training is typically an important component of these communities, providing a valuable context in which to develop skills and expertise, while also strengthening links and relationships within the community. Scientific communities facilitate: (i) the exchange and development of ideas and expertise; (ii) career development; (iii) coordinated funding activities; (iv) interactions and engagement with professionals from other fields; and (v) other activities beneficial to individual participants, communities, and the scientific field as a whole. It is thus beneficial at many different levels to understand the general features of successful, high-impact bioinformatics communities; how individual participants can contribute to the success of these communities; and the role of education and training within these communities. We present here a quick guide to building and maintaining a successful, high-impact bioinformatics community, along with an overview of the general benefits of participating in such communities. This article grew out of contributions made by organizers, presenters, panelists, and other participants of the ISMB/ECCB 2013 workshop “The ‘How To Guide’ for Establishing a Successful Bioinformatics Network” at the 21st Annual International Conference on Intelligent Systems for Molecular Biology (ISMB) and the 12th European Conference on Computational Biology (ECCB).
Citation: Budd A, Corpas M, Brazas MD, Fuller JC, Goecks J, Mulder NJ, et al. (2015) A Quick Guide for Building a Successful Bioinformatics Community. PLoS Comput Biol 11(2): e1003972. https://doi.org/10.1371/journal.pcbi.1003972
Editor: Amarda Shehu, George Mason University, UNITED STATES
Copyright: © 2015 Budd et al. This is an open access article distributed under the terms of the Creative Commons Attribution License , which permits unrestricted use, distribution, and reproduction in any medium, provided the original author and source are credited
Funding: The work of AB was supported by the European Molecular Biology Laboratory ( http://www.embl.org/ ). MC is supported by BBSRC's ( http://www.bbsrc.ac.uk ) strategic funding. MDB is supported by the Ontario Institute for Cancer Research (OICR, http://oicr.on.ca/ ) with funding from the Government of Ontario ( https://www.ontario.ca/government/government ). JCF gratefully acknowledges the financial support of the Bundesministerium für Bildung und Forschung (BMBF, http://www.bmbf.de/ ) Virtual Liver Network (grant no. 0315749) and the Klaus Tschira Foundation ( http://www.klaus-tschira-stiftung.de/ ). Galaxy is supported in part by American Recovery and Reinvestment Act (ARRA) funds through grant number HG005542 from the National Human Genome Research Institute, National Institutes of Health, as well as grants HG005133 and HG006620 ( http://www.genome.gov/ ). H3ABioNet is funded by the National Institutes of Health Common Fund under grant number U41HG006941 ( http://commonfund.nih.gov/ ). MM was supported by the Netherlands Cancer Institute ( http://www.nki.nl/ ) and a travel fellowship from the International Society for Computational Biology ( http://www.iscb.org/ ). AP was supported by the UK Engineering and Physical Sciences Research Council (EPSRC, http://www.epsrc.ac.uk/ ) Grant EP/H043160/1 for the UK Software Sustainability Institute. The work of NB is supported by ELIXIR ( http://www.elixir-europe.org/ ). The funders had no role in the preparation of the manuscript.
Competing interests: The authors have declared that no competing interests exist.
Introduction
In many cases, bioinformatics communities play a central role in the success of highly complex scientific projects and consortia. This guide was inspired by a workshop, “The ‘How To Guide’ for Establishing a Successful Bioinformatics Network,” that took place as part of the ISMB/ECCB 2013 conference. During the workshop, organizers, presenters, and panelists shared insights gained from participating in a range of successful bioinformatics communities with an audience of more than 100 additional participants. One session of the workshop was an interactive, small-group discussion in which all participants described their opinions on the benefits and disadvantages of contributing to bioinformatics communities. The opinions and ideas presented in this guide are a synthesis of the experiences and opinions contributed by all participants of the workshop.
Communities, Networks, and Organizations
While the terms “communities,” “networks,” and “organizations” are sometimes used interchangeably, in other contexts they refer to distinct kinds of social structures. This guide concerns, in part, interactions between these different structures. Thus, to avoid confusion, we begin by defining our use of these terms in this article.
Borrowing from anthropology and learning science, as well as management and organizational behaviour studies, we define “network” as a set of “relationships, personal interactions, and connections” [ 1 ] between a group of people and “community” as a group of people possessing a shared identity around a topic or set of challenges of joint interest, linked to a collective intention of working together to build knowledge and solutions around this topic or challenges (see also The Art of Community by Jono Bacon [ 2 ]). We also define “organization” as an organized or cohesive group of people working together to achieve commonly agreed goals and objectives, in which specific roles and responsibilities are openly acknowledged for some or all members of the group. This explicit acknowledgment of specific roles for members of the group is often linked to the establishment of the group as a legal entity [ 3 ].
A successful community or organization is one that effectively and efficiently achieves its goals; such success is easier to assess in the context of explicitly stated goals. Working with the definitions above, explicit goals and missions are typically associated with organizations rather than with communities. Thus, it is typically organizations associated with communities, rather than communities themselves, that are identified as successful.
Benefits of Participating in Bioinformatics Communities: Opportunities for Collaborative Research, Professional Development, Education, and Training
You are almost certainly a member of one or more professional networks or organizations, for example, professional societies such as the International Society for Computational Biology (ISCB) [ 4 ], scientific consortia such as the Encyclopedia of DNA Elements (ENCODE) [ 5 ], social media networks such as LinkedIn or ResearchGate, or the groups and institutions associated with your workplace (the group, the institute, the department you work in). By actively engaging and collaborating with others in these networks and organizations, on topics of mutual interest, you are also a member of one or more professional communities.
Scientists participating in research-driven communities have delivered a wide range of high-impact publications and life science data sets. These have typically been associated with projects (time-boxed, funded activities with agreed outcomes) such as ENCODE [ 5 ], the Human Microbiome Project [ 6 ], Galaxy [ 7 , 8 ], Bioconductor [ 9 ], BioJS [ 10 ], and the Virtual Liver Network [ 11 , 12 ].
Recent analyses highlight the increase in the proportion of science carried out in such communities of collaboration, and also the increase in the size of these collaborations, particularly when considering international collaborations [ 13 – 16 ]. This increase in the importance of collaborative science has even spawned its own field of social science research, the Science of Team Science (SciTS) [ 17 – 21 ].
Beyond these research-project-focused communities, other common bioinformatics-related activities are carried out in communities including professional/career development (especially training and education) or tool and resource development. Examples of such development-focused communities include interactions between groups of fellows and students (e.g., ISCB Student Council and Regional Student Groups [RSGs] [ 22 ] or the Software Sustainability Institute's [SSI] [ 23 ] Fellowship Program http://www.software.ac.uk/fellowship-programme ), local geographically-focused groups of bioinformaticians (e.g., TorBUG http://www.torbug.org or Heidelberg Unseminars in Bioinformatics [HUB] [ 24 ] http://www.hub-hub.de ), bioinformatics trainers and educators (e.g., GOBLET [ 25 ]), heads of nodes and technical coordinators involved in bioinformatics infrastructure development and capacity building (e.g., ELIXIR http://www.elixir-europe.org or H3ABioNet http://www.h3abionet.org ), and cooperations between software/tool developers and their larger user communities (e.g., Bioconductor [ 9 ] or Galaxy [ 7 , 8 , 26 ]).
Communities focused around a common set of knowledge, skills, and tasks (“communities of practice” [ 27 – 29 ]), such as the bioinformatics communities discussed in this guide, have been recognized as crucial for training, development, and education. Bioinformatics communities provide a forum for participants to share information and experiences with each other, to learn from each other, and to develop themselves professionally. More formally, training events and activities delivered by bioinformatics communities are opportunities both to share expertise and knowledge within the community and to promote and strengthen the interactions (between and within trainers and trainees) that are the basis of successful communities.
Different Kinds of Communities
Communities can be classified as “bottom-up” (or “grassroots”) or “top-down.” A “bottom-up” community is established by a group of people who find each other through their desire to collaborate around a topic of shared interest, typically with little or no funding or support from larger communities or organizations and little or no formal structure (although, as described by Jo Freeman in her essay on the inherent structures of groups that claim to be “unstructured,” there will inevitably be some form of structure, with an associated hierarchy, within these organizations [ 30 ]). An example of such a community, Heidelberg Unseminars in Bioinformatics (HUB) [ 24 ], was conceived through informal discussions between people from several Heidelberg organizations who were interested in bioinformatics and exploring alternative meeting formats. HUB participants collaborate together as volunteers, in a relatively informal fashion, via regular local meetings on these topics (unconferences or unseminars [ 31 ]). HUB recently established an organization linked to HUB and is currently in the process of establishing itself as a legal entity. This move towards formalizing the community into an organization was motivated by issues of liability limitation, the benefits of establishing clarity of goals and structure described elsewhere in this guide, and the opportunities it provides for setting up an organizational bank account and being eligible to apply for funding. Other examples of such “bottom-up” organizations described in this guide include the pan-Canadian Bioinformatics User Groups (BUG) of VanBUG, TorBUG, and MonBUG organizations and the ISCB Student Council and Regional Student Groups [ 22 ]. Guidelines for starting new communities of this kind have been described based on the experience of establishing more than 20 different RSGs [ 32 ].
"Top-down" communities linked to organizations are typically created as a result of a strategic decision. The goals of such communities are typically goals shared by the founding stakeholders. A major challenge faced in establishing such organizations is to reach agreement between large numbers of stakeholders on the common goals, costs, and expected return on the investments. A further challenge is to find ways to effectively foster collaborations across geographical and organizational boundaries. ELIXIR ( www.elixir-europe.org ), the European infrastructure for biological information, is an example of such a “top-down” organization, growing out of a community effort. In 2007, a consortium of bioinformaticians across Europe were awarded European Union (EU) funding to plan and prepare the building of a trans-European infrastructure to support the transfer, storage, and analysis of biological information. After more than six years of intense planning and high-level political discussions with science funders and potential member states, ELIXIR was established through an agreement between its member states in December 2013. Thus, ELIXIR represents the evolution from: a shared idea of a group of researchers to a large, funded, project to a transnational organization with a set of clearly defined goals, organizational governance structures, legal framework, and funding processes. Members of the community linked to ELIXIR are bioinformaticians involved in service and infrastructure delivery across Europe, collaborating together to “orchestrate the collection, quality control and archiving of large amounts of biological data produced by life science experiment.”
Communities can also be classified according to their progression through a “community lifecycle” [ 33 ]. Millington [ 34 ] provides specific advice on building a community during different phases of the lifecycle. In particular, he focuses on a shift from an initial phase where most activity, and new members, comes from the activity of the founders of the community and/or a community manager to a more mature phase where community growth and activity is driven by the activities of others. This change is accompanied by a shift in the importance for founders and community managers from more tactical, hands-on, one-to-one activities within the community to more macro-level, strategic activities. This often necessitates a formalization of the community objectives and processes, e.g., through the introduction of formal code reviews and release processes or introduction of subgroups focusing on specific tasks or deliverables.
A Quick Guide to Building a Successful Bioinformatics Community
Analyses of successful, high-impact collaborations, organizations, networks, and communities have identified several common features of such groups including effective interactions, communication, and leadership; a clear, shared vision of the aims of the group; and a passionate commitment by participants to the goals of the group [ 2 , 34 – 38 ]. To gain further insight into features of highly successful bioinformatics communities, we hosted a workshop on bioinformatics communities at the ISMB/ECCB 2013 meeting in Berlin. Based on the discussions and presentations featured in the workshop, we provide a “Quick Guide” of practical actions you can take to build and develop successful bioinformatics communities. Where relevant, we describe examples provided by the participants of the workshop to reinforce these points.
As described above, there is considerable diversity in bioinformatics communities, for example, in terms of size, funding, shared interests and goals, influence, organizational structures, and maturity. However, despite this diversity, the points included in this guide are, we believe, relevant and beneficial to the success of any such community.
1. Ensure membership in a community brings obvious benefits to its members
People choose to be part of a community because they perceive that they will benefit from this participation. This could range from simply enjoying the company of collaborators within the community to providing opportunities to participate in projects with a direct benefit to professional development (e.g., contributing to high-impact publications). Making these benefits clear motivates newcomers to join the community and existing members to continue (and perhaps increase) their levels of participation in the community. An example of this can be seen in the activities of the ISCB Student Council and Regional Student Groups [ 22 ]. They strongly emphasize (in their communications with members and the wider public) that participating in the activities of the organization brings clear benefits in terms of opportunities for training, gaining experience of leadership and organizing events, and building a stronger professional network. The clarity of these benefits to existing members of these organizations is described as essential for their success by creating a core group of highly motivated, competent members who work hard to achieve the mission of the organization.
2. Provide a description of the goals, vision, and mission of the community that are accessible, clear, and concise
A clear, concise set of goals describing the aims of a community is invaluable for communicating the focus and purpose of the mission to members of the community, and to other stakeholders. This can, for example, be very useful for helping potential members decide whether or not their interests align with those of the community. By providing a context to assess the utility and appropriateness of community activities, a description of the goals, vision, and mission of the community can also be a useful aid for setting priorities and making decisions within the community, i.e., when trying to choose between several alternative courses of action, the best decision would be the one that helps the community best achieve its goals.
Note that, as mentioned in the introduction, explicit goals and missions are typically associated with a specific organization, rather than the community linked to the organization. Writing and agreeing on these can be a major challenge, particularly for “top-down” organizations, as this process will involve prioritization and trade-off between funders and other stakeholders.
The mission of the ISCB Student Council [ 22 ], for example, is to “promote the development of the next generation of computational biologists.” It is a clear mission, attainable and of great perceived value to its members. A key factor for the success of the Bioconductor project [ 9 ] was described as being the clear, shared vision for the direction and methods of software development, including emphasis on high-powered statistics and commitment to common interfaces and containers. The importance of a shared, clearly described mission was also described as important for the success of ELIXIR, whose mission is “to build a sustainable European infrastructure for biological information, supporting life science research and its translation to medicine and the environment, the bio-industries and society.”
3. Facilitate communication between members of the group
Communication is an essential component of any collaboration. One cannot collaborate without communication. Therefore, a successful community must be built around effective communication.
Different means of communication are better suited to different purposes. For example, announcing upcoming events to a large, geographically distributed community is well suited to electronic communication and social media such as email, Twitter, LinkedIn, wikis, and other online resources. With minimal effort, these means of communication can easily deliver information to many different people. In contrast, discussions of complex, urgent issues involving input from many participants are typically best carried out in face-to-face meetings. Thus, communication within a community is typically a mixture of face-to-face meetings and distant/remote/electronic communication.
It is important to be aware that people have different preferences for communication. Some people are email enthusiasts, but refuse to use social media tools such as LinkedIn, Twitter, or Facebook, while others may find it intimidating to edit a wiki. Thus, it may be useful to communicate using a range of different media and challenges. At the same time, the more modes of communication you use, the more resources are required. If communication appears to be effective within your group, it may be best to avoid introducing additional communication channels that take more resources to support and use than they bring benefit to the group.
All participants in the workshop emphasized the importance of effective, regular communication for the success of a community. The Galaxy project [ 7 , 8 , 26 ] provides an excellent example of this, using a range of different ways in which community members can communicate with each other, including a substantial wiki, Twitter, mailing lists, a custom Biostar [ 39 ] forum ( https://biostar.usegalaxy.org ), regular face-to-face meetings, and conference calls. Open, wide-reaching communication is also important in the project for acknowledging and publicizing contributions to the project, in particular using social media. The importance of community building and outreach for Galaxy is reflected in the allocation of approximately half of the project’s budget to these activities. In a similar way, the success of the Bioconductor project [ 9 ] is also linked to its lively mailing list, many face-to-face developer meetings, and regular interactions between users and developers at training courses.
4. Establish and communicate a clear, transparent organizational structure
Even groups that claim to be “unstructured” have a structure. This structure might be an equal distribution of power between all members or an unequal distribution of power between different members together with the existence of (unacknowledged) subgroups (cliques) of stronger relationships within the group [ 30 ]. Such unacknowledged power structures are non-transparent, difficult to identify and understand, and tend to promote distrust within the group. Thus, it is strongly recommended that an organization linked to, or representing, a community, has a clearly defined, transparent, leadership structure that is communicated to all members of the community. This helps everyone to understand which responsibilities and decision-making powers are held by which members of the organization. A clear decision-making structure can also help reduce the time needed to make important decisions.
By considering the role and presence of structure in communities, we highlight a key difference between a community and an organization linked to that community. Explicit structures of this kind are typically associated with organizations rather than the communities associated with these organizations (as the word suggests, organizations are more “organized” than communities). Thus, in this section of the article, we focus on the structure of organizations strongly linked to specific bioinformatics communities.
Different organizations have been successful with different kinds of organizational structures [ 40 , 41 ]. For relatively small, low-resource organizations, such as HUB, a very simple structure has been successful, with a board consisting of three members, all other members having the same organizational status. At the other end of the spectrum, ELIXIR, where national states rather than individuals or organizations are the formal members, has a structure with a clear division of tasks, assigned accountabilities, and the level of governance and oversight necessary for transnational collaboration. The ELIXIR operational structure consists of a central, jointly funded Hub linked to nodes in the member states. The governing structure is made up of the ELIXIR Board (with representation from national science funders or ministries) with operational and scientific responsibility provided by the ELIXIR director together with national node directors through the “Heads-of-Nodes” committee.
Several speakers in the workshop that inspired this article highlighted the importance of a clear management structure for the success of communities, including the H3ABioNet project and the ISCB Student Council and Regional Student Groups [ 22 ]. A clear structure, and, importantly, assigned roles and responsibilities, provides a framework for collaboration. Forming subgroups with clearly assigned tasks and responsibilities allows the individual contributors to focus on their activities without feeling pressured to consult widely before implementation.
At a more operational level, clear rules for submitting code to Bioconductor [ 9 ] are described as important for the project’s success [ 42 ]. These rules, written by core developers of the project, facilitate the integration of contributions from diverse developers into the Bioconductor code base, while clear communication of the guidelines to potential code contributors helps manage expectations and reduce the wasted efforts by contributors. This is also an example of the use of authority, in this case of the leadership of the Bioconductor project, to facilitate and coordinate the activities of the community; if the leadership were not prepared to insist on this set of rules, the rate of growth, and utility of the project, would be expected to suffer.
5. Whenever possible, make your communications open and transparent
Open communication is important for building trust within the group. Giving everyone access to the information on which decisions have been made, and for what reasons, goes a long way to avoiding misunderstandings and distrust amongst community members.
The Bioconductor project [ 9 ] is strongly focused on a shared vision of open source and open development, both of which make a major contribution to the transparency and openness of interactions within the project. Openness and transparency in reporting structure was also emphasized as important for the ISCB Student Council and RSGs [ 22 ]. An example of the policy and utility of such openness is HUB [ 24 ], where the minutes and agendas of all planning and other meetings associated with the project are posted for all to read on the HUB wiki.
6. Make it easy and enjoyable to participate in the activities of the community
Participation increases the amount of activity of the community, and at the same time fosters a sense of ownership that is a key motivator for further participation. An essential component of the success of the Bioconductor project [ 9 ] is that everyone is welcome and encouraged to participate in the project by contributing to discussions on mailing lists, code, code documentation, and joining courses and conferences linked to the project. The H3ABioNet project also makes a concerted effort to welcome and make it easy for a wide range of different people to contribute and remain active within the project, thus harnessing the good will and expertise available to the project and giving participants a feeling of ownership of and belonging to the network. The Galaxy project [ 7 , 8 , 26 ] also focuses considerable efforts on enabling and empowering the community to contribute to code, documentation, and discussions associated with the project. To achieve this, Galaxy and its web resources are designed to promote and facilitate sharing analyses, data, tools, and curation.
7. Acknowledge and highlight contributions to the community
If participants see that their work within a community is acknowledged and visible to all members of the community, and perhaps also to people outside the community, this can be a key motivator to further engage with the community. Openly acknowledging all contributions to Bioconductor [ 9 ] and Galaxy [ 7 , 8 , 26 ] code and resources was described as important for the success of the projects. Within the Galaxy project, a conscious effort is made to publicize and praise contributions, in particular using Bitbucket pull requests to integrate attributed software enhancements to the project’s codebase and a special “contributors” section in software release briefs. Small-group discussions during the workshop with all participants (not just organizers, speakers, and panelists) also described the provision of attribution/reward for contributions, particularly for junior researchers, as an important feature of successful communities.
8. Be aware that resources are essential to achieve the goals of a community
All activity expends resources; community activities are no exception. For example, organizing a TorBUG event requires many person-hours for planning the speaker and trainee sessions within an event, access to a web server to host the TorBUG website used for communicating with the community about the event, printed posters to advertise the event, a physical space to hold the meeting, refreshments and other consumables for use during the meeting, and laptops and a projector/beamer for presentations during the meeting. Of all of these, person-hours are of particular importance and value to the success of an event and thus the TorBUG community; without them, there would be no community.
Expending resources, particularly on facilitating and promoting communication, is therefore essential for achieving the goals of a community. Organizations seeking to promote community activity are thus strongly recommended to invest in establishing resources for the community. Part of this involves infrastructure and consumables (audiovisual [AV] equipment, computers, servers, stationary, web platforms), but, perhaps more importantly, it also involves providing funding for salaries for people committed to facilitating communications and other aspects of community growth and activity, i.e., funding a role of “community manager.” The Software Sustainability Institute [ 23 ], for example, uses significant resources to provide salary and other funding for a community leader position.
We have established some general guidelines for building successful bioinformatics communities. Ever-improving access to higher-speed Internet, huge data production, and open source projects empower distributed international projects, which, in turn, feed into the development of new communities. In this article we describe the importance of openness and communication for the establishment and effective functioning of bioinformatics collaborations and communities. Perhaps our most important conclusion is that scientific communities are at the heart of many fulfilling bioinformatics-related careers. This highlights the importance for scientists of finding and participating in communities that align with their interests, goals, and values.
Organizers, Presenters, and Panelists of the ISMB/ECCB 2013 Workshop “The ‘How To Guide’ for Establishing a Successful Bioinformatics Network”
The ideas, comments, and advice presented in this article are based on presentations and discussions from the ISMB/ECCB 2013 workshop “The ‘How To Guide’ for Establishing a Successful Bioinformatics Network.” These were carried out by people with experience in establishing, building, and maintaining a range of different bioinformatics communities and networks. To give context and provenance to these ideas, comments, and advice, we provide below the complete list of all organizers, presenters, and panelists from the workshop, with brief summaries of some of their activities in such networks and communities.
Niklas Blomberg : Director of ELIXIR. Leads ELIXIR, the European infrastructure for biological information. Has been an industry advisor in national eScience initiatives and an active participant in cross-industry research programmes.
Aidan Budd : Senior Project Manager for Bioinformatics at the European Molecular Biology Laboratory (EMBL) in Heidelberg, Germany. Manages an internal network for bioinformatics at EMBL in Heidelberg. Co-founder of the Heidelberg Unseminars in Bioinformatics series of events [ 24 ].
Michelle Brazas : Coordinator of the Canadian Bioinformatics Workshops (bioinformatics.ca) [ 43 ] and Manager of Bioinformatics Education at the Ontario Institute for Cancer Research. Involved in organizing the Toronto Bioinformatics User Group (TorBUG) networking and community-building events.
Manuel Corpas : Project Leader at The Genome Analysis Centre, UK. Technical coordinator of the ELIXIR UK node. Coordinator of the BioJS project [ 10 ]. Inaugural chair of ISCB’s Student Council [ 22 ].
Jonathan Fuller : postdoctoral fellow with Professor Rebecca Wade at the Heidelberg Institute for Theoretical Studies, in Germany. Member of the Virtual Liver Network [ 11 , 12 ]. Co-founding member of Heidelberg Unseminars in Bioinformatics (HUB) [ 24 ].
Jeremy Goecks : Assistant Professor of Computational Biology at George Washington University. A lead investigator for the Galaxy project [ 7 , 8 , 26 ], a Web-based platform for doing accessible, reproducible, and collaborative computational biomedical research.
Wolfgang Huber : group leader and senior scientist at the EMBL in Heidelberg, Germany. Long-term participant in the Bioconductor project [ 9 ]. Writer and maintainer of several software packages. Current member of the Bioconductor Advisory Board.
Magali Michaut : computational biologist in the Computational Cancer Biology (CCB) group of the Netherlands Cancer Institute. Involved in the ISCB Student Council since 2007 [ 22 ], served as elected secretary in 2009. Founded ISCB SC Regional Student Groups (RSGs [ 32 ]) in France and Europe.
Nicola Mulder : Associate Professor at the University of Cape Town heading the Computational Biology Group. Coordinator of H3ABioNet, a Pan African bioinformatics network for H3Africa [ 44 ]. President of the African Society for Bioinformatics and Computational Biology [ 45 ].
Francis Ouellette : Senior Scientist, Associate Director of the Informatics and Biocomputing platform at the Ontario Institute for Cancer Research (OICR); Associate Professor in the department of Cell and Systems Biology at the University of Toronto. Scientific coordinator and instructor with the Canadian Bioinformatics Workshop series (CBW—bioinformatics.ca) [ 43 ]. Involved in establishing Bioinformatics User Groups in Vancouver and Toronto (VanBUG.org and TorBUG.org). Advisor to SGD, Galaxy, GenomeSpace, HMP, H3ABionet, and Genome Canada.
Aleksandra Pawlik : Training Leader at the Software Sustainability Institute [ 23 ] supporting development of research software communities in various disciplines. Co-manages the Institute's Fellows' network.
Reinhard Schneider : Head of Bioinformatics Core Facility at the Luxembourg Centre for Systems Biology. Treasurer of the ISCB[ 4 ]. Actively involved in various international networks and advisory boards.
- 1. Wenger E, Trayner B, de Laat M (2011) Promoting and assessing value creation in communities and networks: a conceptual framework. Ruud de Moor Centrum, Open University of the Netherlands. https://doi.org/10.1080/17437199.2011.587961 pmid:25473706
- 2. Bacon J (2012) The Art of Community: Building the new age of participation (theory in practice). O'Reilly Media. 527 p.
- 3. Allen JM, Sawhney RS (2010) Chapter 1: Defining Management and Organization. editors. Administration and Management in Criminal Justice: A Service Quality Approach. SAGE Publications, Inc. pp. 1–26.
- View Article
- PubMed/NCBI
- Google Scholar
- 15. The Royal Society (2011) Knowledge, networks and nations. Global scientific collaboration in the 21st century. RS Policy Documents 03/11 DES2096
- 16. The National Science Board (2012) Science and engineering indicators http://www.nsf.gov/statistics/seind12/ .
- 18. Spring B, Moller AC, Falk-Krzesinski H (2011) Teamscience.net. Available: http://teamscience.net/ via the Internet.
- 20. Iorns E (2013) Research 2.0.2: how research is conducted. Nature Blogs http://blogs.nature.com/soapboxscience/2013/06/13/research-2-0-2-how-research-is-conducted
- 21. Williams S (2013) Team Science—the science of collaborative research. Nature Blogs http://blogs.nature.com/soapboxscience/2013/03/15/team-science-the-science-of-collaborative-research
- 27. Smith MK (2003) Jean Lave, Etienne Wenger and communities of practice. Encyclopedia of Informal Education Available: http://infed.org/mobi/jean-lave-etienne-wenger-and-communities-of-practice/ via the Internet.
- 28. Wenger E (2009) Communities of Practice: A Brief Introduction. Available: http://wenger-trayner.com/theory/ via the Internet.
- 29. Lave J, Wenger E (1991) Situated Learning: Legitimate Peripheral Participation. Cambridge, UK: CUP. 138 p. pmid:25144101
- 30. Freeman J (1970) The Tyranny of Structurelessness. Available: http://uic.edu/orgs/cwluherstory/jofreeman/joreen/tyranny.htm via the Internet.
- 34. Millington R (2012) Buzzing communities.
- 38. Bennett LM, Gadlin H, Levine-Finley S (2010) Collaboration & team science: a field guide. NIH Publications 10–7660: https://doi.org/10.1111/j.1747-4477.2010.00267.x pmid:22432820
- 40. Robbins SP, Judge TA, Campbell TT (2010) Organizational Behaviour. Harlow, UK: Pearson Education Limited. 589 p. pmid:25506974
- 41. Goold M, Campbell A (2002) Do you have a well-designed organization? Harvard Bus Rev 5–11.
- 42. Bioconductor Package Guidelines. Available: http://www.bioconductor.org/developers/package-guidelines/ via the Internet.
at the Department of Biology: Biology, Molecular biology, Bioinformatics
The browser you are using is not supported by this website. All versions of Internet Explorer are no longer supported, either by us or Microsoft (read more here: https://www.microsoft.com/en-us/microsoft-365/windows/end-of-ie-support ).
Please use a modern browser to fully experience our website, such as the newest versions of Edge, Chrome, Firefox or Safari etc.
Research projects in Bioinformatics
BINP35, BINP37, BINP39
During a research project, you learn how to plan and carry out a project from start to end. These courses are available only for students within the Master’s programme in Bioinformatics at the Department of Biolog. You may choose to do a 7.5, 15 or 30 credits research project. You can carry out the project full time or part time, during the semester or in the summer. You can do a project at a University in Sweden or abroad. You can also do a project at a company.
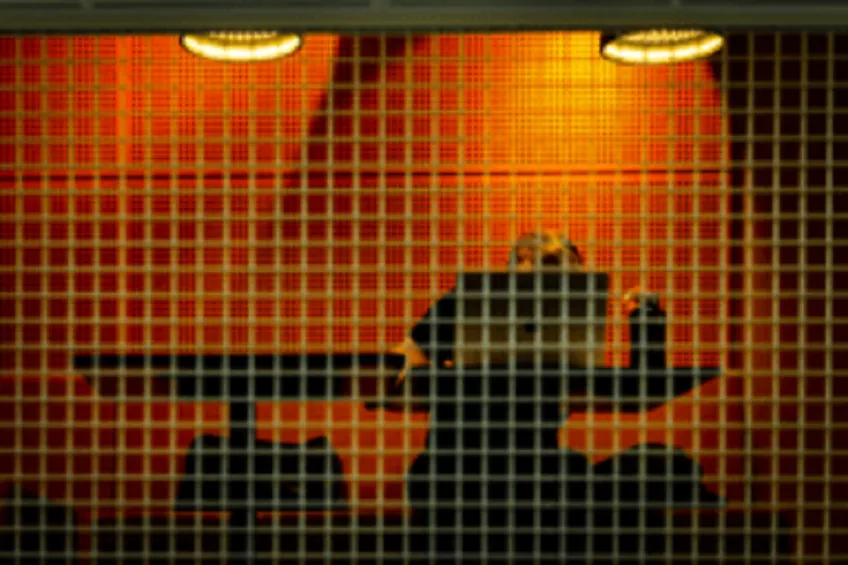
Look for projects on our Blog
Biology education project suggestions
Before the start
Contact a supervisor within the area of your interest and discuss the general outline of the project. Before proceeding, you should show your CV and LADOK excerpt to the supervisor. Note that the project can be conducted outside the university, for example at a company.
Write a project plan together with your supervisor when you have decided about a project. The plan should be brief, but give a clear description of your specific project (2–3 A4 pages). It should contain:
- Project title
- Name and e-mail addresses to you and the supervisor
- Topic, time, and number of credits
- Introduction, with a theoretical background to the project and key references
- The specific aim(s) of your project
- Time plan (rough planning of the project). Remember to include time for writing of the report and preparations for the seminar.
During the project
Carefully document your work. You should document your work systematically in a README file. With the aid of the README file, you or another person should be able to recreate the current results from the original data. The README file may be used in the final examination and grading of your project. You should submit the README file together with the final report. Try to participate in group meetings, seminars, and such that may be arranged in the group or at the department where you are working. It is advisable to start writing the report as soon as possible. Parts of the introduction and materials and methods can be written in parallel with your practical/theoretical work.
The project shall be presented as a scientific report in English. The format of your report should follow the instructions on how to write manuscripts for PLOS One. Author instructions are found on PLOS One's website . Discuss with your supervisor whether your report may deviate from these instructions. Your final report can be in manuscript form or you may choose a layout that is more similar to a printed paper.
Plagiarism. You are not allowed to present someone else’s work, such as text, figures or results, without giving proper reference. You may of course refer to the works of others, but you must write about it in your own words and refer to the source of information in a correct way.
The oral examination
Anna Runemark, Dag Ahrén and Eran Elhaik will act as examiners. The supervisor will take part in the discussion, but not in the decision. If the supervisor is not present, the examiner will contact the supervisor for his or her opinion.
All project work seminars are presented during the allocated examination period, three or four occasions each year. At the examination, you will give a presentation (about 10-15 min). After your presentation, the examiner will ask you questions and discuss your report and project, and thereafter the audience will be invited to ask questions.
Application
Detailed information and application in the l earning platform Canvas for Bioinformatics, Research Project, at Lund University
Research Project BINP35, 7,5 credits, 5 weeks (pdf)
Research Project BINP37, 15 credits, 10 weeks (pdf)
Research Project BINP39, 15 credits, 20 weeks (pdf)
Lotta Persmark, Study advisor, biology and bioinformatics
Telephone : +46 46 222 37 28 Email : Lotta [dot] Persmark [at] biol [dot] lu [dot] se (Lotta[dot]Persmark[at]biol[dot]lu[dot]se)
Anna Runemark - information at Lund University Research Porta l

College of Agricultural Sciences

Project Examples
In this section.
- Bioinformatics
Here are some examples of Bioinformatic analyses we have expertise in conducting.
We have experience working with many diverse data and organism types, so even if your topic is not listed in our project examples, we are likely to be able to assist you.
Deliverables for Basic/Standard Analysis
MAX Turnaround time – 2 months depending on application and sample size
1. Whole Genome Sequencing
Prokaryotes.
- RE-SEQUENCING: Raw Data QC and Report, Alignment Statistics and Report, Variation Calling Report (SNP, InDels), Gene Annotation Table with Variations.
- DENOVO: Raw Data QC and Report, Assembly Statistics and Report, Genome Finishing using Closest homolog, rRNA identification and analysis report, Phage Identification and analysis report, Plasmid Identification, and analysis report, RAST Annotation.
- RE-SEQUENCING/TARGETED/EXOME: Raw Data QC and Report, Alignment Report, Variation calling Report (SNP, InDels), Basic Variation Annotation, and Effect Analysis Report.
- DENOVO: Raw Data QC and Report, Assembly Statistics and Report, Gene Prediction and Annotation Report. Data generation depends on predicted genome size
2. Transcriptome Sequencing
- RE-SEQUENCING: Ribo Depletion (rRNA Depletion) – Raw Data QC and Report, Read Alignment to reference genome and transcript Identification, Comprehensive Transcript Annotation, Functional Classification of Annotated Transcript, Expression Profiling, Quantification & Expression Profiling of transcripts, Differential Analysis among the conditions, Biological Significance Analysis of differentials.
- RE-SEQUENCING: – Raw Data QC and Report, Read Alignment to reference genome and transcript Identification, Quantification & Expression Profiling of transcripts, Differential, Analysis among the conditions, Biological Significance Analysis of differentials (n-1). All pictorial representations of comparisons will be according to n-1
- DENOVO: Raw Data QC and Report, De novo assembly, Assembly Evaluation & Filtering, Sequence homology-based Transcript Annotation using Blast2Go – REFSEQ, Expression Profiling, Differential Analysis among the conditions, Biological Significance Analysis of differentials (n-1). ALL pictorial representation LL of comparisons will be according to n-1.
3. Chip Sequencing
Raw Data QC and Report, Alignment Report, Peak Identification, and Enrichment Report, Peak Annotation Report
4. Metagenome Sequencing
Sample Grouping or individual as per experimental design, Group-wise OTU Clustering and abundance Report, OTU identification and taxonomic annotation Report (Sample Wise – Genius Level) and OTU Fasta file will be provided, Pie chart representation TOP 10 taxonomic classification; phylum to species-level.
5. SmallRNA Sequencing
Sample wise Raw Data QC, Unique tags and abundance Report, Known Small RNA analysis report, Identification and Quantitation of Known miRNAs, Expression Profiling and Differential Expression Analysis of Known miRNAs.
6. Microbiome Sequencing
Pre-processing of reads including Quality Filtering, trimming low-quality reads, De-Replication, Sequence reconstruction and grouping, Gene prediction, Functional Annotation.
Deliverables for Advanced Analysis
MAX TAT – 3 months depending on the project requirement and sample size
- RE-SEQUENCING: Raw Data QC and Report, Alignment Statistics and Report, Variation Calling Report (SNP, InDels), Gene Annotation Table with Variations, Structural Variations (Inversion, Deletion, Insertion, Translocation, Transversion) analysis report, Comparative Genome analysis – Across selected genomes, High SNP and Low SNP Region, Generic and NonGeneic SNPs, SNP Density Analysis, Synonymous and Non-synonymous SNPs, Effect of Frameshift Indels on Gene Prediction, Submitting Data to NCBI -SRA, Support in providing write up on methods for the manuscript purpose (Time Limit: 3-6 month)
- DENOVO: Raw Data QC and Report, Assembly Statistics and Report, Genome Finishing using Closest homolog, rRNA identification and analysis report, Phage Identification and analysis report, Plasmid Identification and analysis report, Phylogeny 16s RNA based, COG Analysis, Interproscan Analysis, AAI and ANI analysis with the selected reference genome, Antibiotic resistance gene analysis with reference to transposable elements, PAN and Core genome analysis, Synteny Analysis, Chromosome Mapping, Plasmid Re-construction from whole-genome, Submitting Data to NCBI- SRA, Support in providing write up on methods for the manuscript purpose (Time Limit: 3-6 month)
- RE-SEQUENCING/TARGETED/EXOME: Raw Data QC and Report, Alignment Report, Variation calling Report (SNP, InDels), Basic Variation Annotation and Effect Analysis Report, All the deliverables from Standard Analysis, Structural Variation Analysis Report, Variation Effect Analysis Report, Pathway and GO analysis of variations, Copy Number Variation Analysis, Data Submission to NCBI, Comparative Exome Analysis, Submitting Data to NCBI- SRA, Support in providing write up on methods for the manuscript purpose.
- DENOVO: Raw Data QC and Report, Assembly Statistics and Report, Gene Prediction and Annotation Report, Prediction of rRNAs, tRNAs, Repeat Analysis, Identification of Transposons, Domain Identification, Analysis of Virulence genes, Analysis of CaZymes, Synteny Analysis, Comparative Exome Analysis, Submitting Data to NCBI- SRA, Support in providing write up on methods for the manuscript purpose.
- RE-SEQUENCING: Ribo Depletion (rRNA Depletion) – Raw Data QC and Report, Read Alignment to reference genome and transcript Identification, Comprehensive Transcript Annotation, Functional Classification of Annotated Transcript, Expression Profiling, Quantification & Expression Profiling of transcripts, Differential Analysis among the conditions, Biological Significance Analysis of differentials, Inter and Intra Gene List Comparisons, Gene and Pathway enrichment analysis, GO and Pathways based Gene Regulatory Network Modelling, Submitting Data to NCBI- SRA, Support in providing write up on methods for the manuscript purpose.
- RE-SEQUENCING: Raw Data QC and Report, Read Alignment to reference genome and transcript Identification, Expression Profiling, Quantification & Expression Profiling of transcripts, Differential Analysis among the conditions, Biological Significance Analysis of differentials, Inter and Intra Gene List Comparisons, Gene and Pathway enrichment analysis, GO and Pathways based Gene Regulatory Network Modeling, Functional classification of expressed transcripts Submitting, Data to NCBI-SRA, Support in providing write up on methods for the manuscript purpose.
- DENOVO: Raw Data QC and Report, De novo assembly, Assembly Evaluation & Filtering, Sequence homology-based Transcript Annotation using Blast2Go – NRDB, Expression Profiling, Differential Analysis among the conditions, Biological Significance Analysis of differentials, Sequence homology-based Transcript Annotation against the customized database, Inter and Intra Gene List Comparisons, Gene and Pathway enrichment analysis, Functional Classification of Annotated Transcript, GO and Pathways based Gene Regulatory Network Modeling, Data to NCBI-SRA, Support in providing write up on methods for the manuscript purpose.
Raw Data QC and Report, Alignment Report, Peak Identification, and Enrichment Report, Peak Annotation Report, Motif Identification, Statistical analysis of Peak Reproducibility (If replicates are provided), Significant GO and Pathway Analysis, Data to NCBI-SRA, Support in providing write up on methods for the manuscript purpose.
Sample Grouping/Individual (either one) as per experimental design, Group-wise OTU Clustering and abundance Report, OTU identification and taxonomic annotation Report (Sample Wise – Genius Level) and OTU Fasta file will be provided, Pie chart representation TOP 10 taxonomic classification (Phylum to Species-level), Differential Metagenome based on sample conditions, Diversity Analysis (Alpha and Beta), Rarefaction Curves, PCoA Plot (required minimum six samples), Krona Plot at the genus level, Heat-Maps for comparisons, Species-level annotation (If V3 & V4 is covered), Data to NCBI-SRA, Support in providing write up on methods for the manuscript purpose.
Raw Data QC and Report, Known Small RNA analysis report, Identification and Quantitation of Known miRNAs, Expression Profiling and Differential Expression Analysis of Known miRNAs, Novel miRNA Identification (In case of reference genome availability) and analysis report, Characterization of other small RNAs like siRNA, piRNA, snoRNA, miRNA Target Prediction / Identification, Significant GO and Pathway Analysis of targets of differentially expressed miRNAs, DData to NCBI-SRA, Support in providing write up on methods for the manuscript purpose.
Pre-processing of reads including Quality Filtering, Trimming low quality reads, De-Replication, Sequence reconstruction and grouping, Gene and regulatory element prediction, Functional Annotation, Differential Microbiome based on sample parameters, Statistical analysis of Microbiome based on OTUs, Diversity Analysis (Alpha and Beta), Rarefaction Curves, Species-level annotation, Seed Subsystem classification, COG, KEGG Analysis, Gene Ontology and Pathway Analysis (Functional Microbiome Analysis), Data to NCBI-SRA, Support in providing write up on methods for the manuscript purpose.
Bioinformatics Project Ideas/Topics Collection For Engineering Students
- Predicting Cellular Localization . Eukaryotic cells contain several sub-compartments, the Cellular Localization problem consists of predicting which compartment a protein is most likely to be found, on the basis of sequence information alone. The project may consist of a review of the literature and/or a novel analysis (I have access to a data-set that has never been used in a predictive context).
- Regulatory-motifs . Review of the literature on algorithms to automatically determine regulatory motifs (short sequence signals) in DNA sequence data. I have a Java library that can be used to implement a prototype application; see suffix tree below.
- SNP (Single Nucleotide Polymorphism) . Review the literature of the methods for detecting SNPs, as well as their application. Single nucleotide polymorphisms (SNPs) are common DNA sequence variations among individuals. They promise to significantly advance our ability to understand and treat human disease. (Excerpt fromsnp.cshl.org). See also Linkage analysis. (S)
- Metabolic Pathways . Proteins interact together to perform specific functions. Such network of interaction is called a molecular pathway. There are two main aspects to this field: how to infer/determine the connections and how to simulate cellular processes. There exist several computational approaches to model molecular pathways, including Petri-net.
- Molecular -arrays . Todays technology (which borrows from inkjet technology) allows to fix tens of thousands of different macromolecules (DNA or protein molecules) onto a small surface. This technology allows to reveal which macromolecule is expressed, at different times, within different tissues, or different cellular states (disease vs non-disease). In the case of DNA chips, they measure the levels of expression of each gene.
- Mass spectrometry (MS) . MS produces a spectrum of all the masses of all the compounds that are present in a sample. When an input protein is cut at specific sites, it will produce a specific spectrum. Such technology can now be used to fingerprint the content of a cell.
- Expression data + motif discovery . DNA--arrays allows to find genes that are simultaneously expressed. Those genes are most likely co-regulated, i.e. they share a common sequence signal in their promoter region. Daniela Cerna implemented a suffix tree library in Java, in the context of her honours project. Here, we would be re-using the library to help finding conserved motifs.
- Expression data + cell localization . Can the use of (predicted and experimental) data on cellular localization help distinguish between true and false positive when expression data is analyzed to find actors and inhibitors?
- Genome comparison . Implementing a MUMMER-like algorithm using Danielas suffix tree (Java) library. This involves writing a hybrid algorithm k-bands dynamic programming algorithm + suffix trees.
- Genome rearrangements . Genomes are evolving at several scales: from point mutations to large rearrangements. It the late 80s, it became evident that several closely related genomes had genes that were extremely similar (say 99 pid), one to another, but the order of genes along the chromosomes was not preserved. Review and present the main algorithms to compare entire genomes. Topics include: sorting by reversals (Sankoff), break point graph, Hannenhalli and Pevzner algorithm.
- Accurate Phylogenetic Reconstruction from Gene-Order Data.
- Ontologies . What is an ontology? What tools and knowledge representation formalisms (languages) are available to support the development of ontologies. Give examples of ontologies. Expose the problems associated with ontologies.An ontology is a controlled vocabulary (e.g. gene ontology). It allows to resolve some of the problems associated with data integration.
- Genome assembly . Because of physical limitations, only relatively short DNA sequences can be read (some 500nt). For processing a complete genome, one approach, called shut-gun, consists of sampling small reads (500 nt) at random location along the chromosomes. The total number of reads is chosen so that the likelihood that each nucleotide is included into more than one read is high (typically each nt is part of 3, 5 or 10 reads). Computers are then used to stitch the reads together. One solution to this problem is related to the shortest super-string problem.
- Grammatical frameworks for RNA structure . RNA secondary structure information can be represented using context-free grammars. As with most biological data, the information is better represented within a statistical framework. A Stochastic Context-Free Grammar (SCFG) has probabilities attached to its production rules. The two main issues with SCFGs are the parsing and the induction of the grammar. Review the literature on SCFGs (this includes COVE, infernal and pfold), and build a prototype parser in Java.
- Predicting Gene-Gene (Protein-Protein) interactions . There exist a vast number of algorithms that allow to predict if two genes will be interacting. This includes: text-mining, co-location along the chromosomes, phylogenetic footprinting, etc.
- Lattice models . Predicting the three-dimensional structure of a protein is a notoriously difficult problem. So much that alternative problems have been devised to circumvent it: secondary structure prediction, inverse folding problem, etc. Some authors have also been studying simpler systems, such as 2D and 3D lattices. Create your own implementation; this includes an algorithm to efficiently search the folding space and a scoring function. Run some simulations.
- Structure comparison methods . Review the literature on 3D structure comparison. Implement at least one algorithm. Input: 2 three-dimensional structures, output: a measure of distance (typically root-mean-square deviation expressed in ), and a list of equivalent residues.
- Methods for detecting trans-membrane helices . There is class of transmenbrane proteins whose secondary structure can be reliably predicted. Those proteins are mainly made of helices, such that if the loop connecting the helices i and i + i is exposed to the inside of the cell, then the next one will be exposed to the outside of the cell. Use a Hidden Markov Model or Neural Network to reproduce this result.
- Secondary Structure Prediction . Implement a secondary structure prediction method and compare its accuracy to known methods. Common choices for your implementation include: Neural Networks, Hidden Markov Models, and possibly decision trees.
- Surface/Interior . Implement a algorithm to predict the solvent accessibility. Common choices for your implementation include: Neural Networks, Hidden Markov Models, and possibly decision trees.
- Applications of suffix trees . Use Daniela Cerneas suffix tree library and implement some of the following algorithms: linear time algorithm for finding the longest common substring of k strings (interestingly, Knuth had predicted that no linear time algorithm would be found for solving this problem), finding all maximal repetitive substrings in linear time, finding all maximum palindromes, k -mismatch algorithm.
- Bio-Ethics . Bioinformatics deals with biological and medical data, according there are numerous related ethical issues: should patenting genes be allowed? how to handle patient data? how to deal with genomic data, imagine that the analysis of a dataset allows to draw conclusions about a population, a religious group, people who live in a specific region, etc. The consequences can be sever: it could be that this group will be more likely to suffer from certain diseases, such information could be used by insurance companies, employers, etc. to screen candidate.
- Genome motifs viewer . Construct a flexible graphical using interface to visualize shared motifs. Suggestions: make it 3D to ease viewing multiple strings. Motifs would be extracted from a suffix tree.
- Teaching tools : interactive linear time construction of a suffix tree, showing the suffix links, interactive tools for software alignments.
- Expectation-Maximization (EM) algorithm and some of its applications in molecular biology . EM is used for training certain Hidden Markov Models, Covariance Models and building phylogenetic trees. What is it? What are the main applications? Prototype implementation. (S)
- Gibbs sampling . This technique forms the basis for several motif detection tools. What is it? What are the main applications? Prototype implementation. (S)
- Bayesian networks . What are bayesian networks? What is interesting about them? What are the bioinformatics applications of bayesian networks? Carry out a small experiment. (S)
- Predicting Phenotype from Patterns of Annotation, -arrays, etc . One of the goals of bioinformatics research is to transform molecular biology into a predictive science. For example, given a certain pattern of gene expression, detected by -arrays for example, what would be the best treatment (personalized medicine)? Survey the literature on the use of bioinformatics techniques to assist medical diagnosis, prognosis and treatments. Where are we heading? When will personalized medicine be true? How much data? Remaining problems to be solved?
- Statistics behind BLAST . Good candidate for a multiple teams work, where one team would focus on the statistics of word matching while the other would focus on hashing. Produce a Java implementation of hashing techniques for speeding up the sequence alignment problem. The part on the statistical analysis of hits requires a statistical background (S) but not the algorithmic part.
- Constructing phylogenetic trees . Read an overview of the construction of phylogenetic trees using a neighbour-joining approach. For this project, you will produce a prototype implementation, in Java, of a modern method such as: quartet method, maximum likelihood or maximum parsimony. (s)
- QSAR . One of the main bioinformatics contributions to drug discovery is the Quantitative Structure Activity Relationship analysis (QSAR); the other is molecular docking. QSAR analyses take as input a set of compounds and their relative activity/efficacy. It then finds the commonalities between those molecules. The commonalities are then used to design new/better drugs.
- Molecular docking consists of predicting how two molecules will interact. This can either be two proteins or one protein and a small compound, such as a new drug. The two main factors that are taken into account are the shape and electrostatics of the two molecules.
- BioJava is a large collection of classes for solving bioinformatics problems. See #-Link-Snipped-#.
- Java3D . A protein viewer was developed two years ago in the context of a CSI 4900 project. Extensions of this project could be considered.
- Tandem repeats . Review the literature on tandem repeats detection and implement a prototype application. Tandem repeats are repeats of the form n , s.t. 2
- Simultaneous alignment and structure prediction for two RNA sequences . Implement a simplified version of dynalign, where the secondary structure prediction is calculated using the Nissinov algorithm; i.e. finds the maximum number of base pairs.
- 3 way genome alignments .
- 1. Testing for absence of secondary structure in combinatorial sets of DNA strands.

Akshay Sanap "Resolving Complexity of Bioinformatic Algorithms using Python".

Hi, I'm umer currently trying to find out a better final project for masters in bioinformatics. Can u plz send me extra details related to " Predicting Gene-Gene (Protein-Protein) interactions . There exist a vast number of algorithms that allow to predict if two genes will be interacting. This includes: text-mining, co-location along the chromosomes, phylogenetic footprinting, etc. " this topic at [email protected]

Hello I am a student of bioinformatics ..I am final year student and I want to select molecular docking as my final year project.. So kindly provide me a dataset of this project and its coding as well ..

Hi, I am a student of bioinformatics, final year. I want to do good programming project using Neural Networks and deep learning. Suggest any idea or dataset to work upon.

can I get help with next-generation sequencing technique topic, to gene related to breast , pancreatic or lung cancer

good day! I am an undergraduate and I need project topics on biomedical informatics
You are reading an archived discussion.
Related Posts
Gis project ideas topics collection for engineering students - 2023, how does buchholz relay work in a transformer, radio frequency transmitter and reciever, how to become a successful engineer, cloud computing questions.
Suggestions or feedback?
MIT News | Massachusetts Institute of Technology
- Machine learning
- Social justice
- Black holes
- Classes and programs
Departments
- Aeronautics and Astronautics
- Brain and Cognitive Sciences
- Architecture
- Political Science
- Mechanical Engineering
Centers, Labs, & Programs
- Abdul Latif Jameel Poverty Action Lab (J-PAL)
- Picower Institute for Learning and Memory
- Lincoln Laboratory
- School of Architecture + Planning
- School of Engineering
- School of Humanities, Arts, and Social Sciences
- Sloan School of Management
- School of Science
- MIT Schwarzman College of Computing
Professor Emeritus David Lanning, nuclear engineer and key contributor to the MIT Reactor, dies at 96
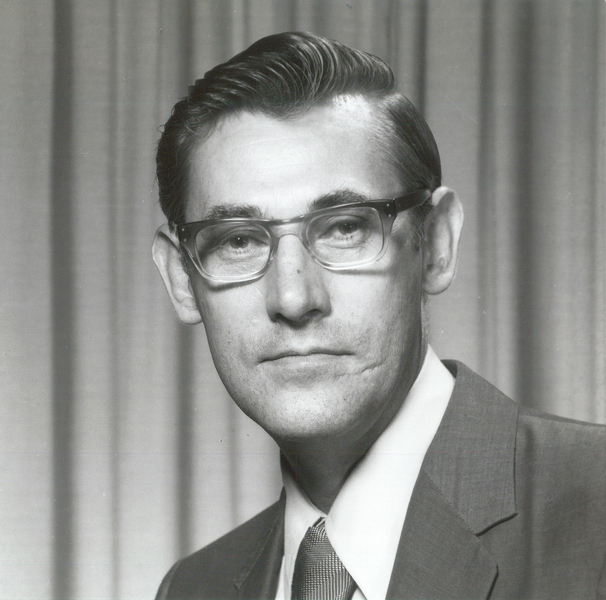
Previous image Next image
David Lanning, MIT professor emeritus of nuclear science and engineering and a key contributor to the MIT Reactor project, passed away on April 26 at the Lahey Clinic in Burlington, Massachusetts, at the age of 96.
Born in Baker, Oregon, on March 30, 1928, Lanning graduated in 1951 from the University of Oregon with a BS in physics. While taking night classes in nuclear engineering, in lieu of an available degree program at the time, he started his career path working for General Electric in Richland, Washington. There he conducted critical-mass studies for handling and designing safe plutonium-bearing systems in separation plants at the Hanford Atomic Products Operation, making him a pioneer in nuclear fuel cycle management.
Lanning was then involved in the design, construction, and startup of the Physical Constants Testing Reactor (PCTR). As one of the few people qualified to operate the experimental reactor, he trained others to safely assess and handle its highly radioactive components.
Lanning supervised experiments at the PCTR to find the critical conditions of various lattices in a safe manner and conduct reactivity measurements to determine relative flux distributions. This primed him to be an indispensable asset to the MIT Reactor (MITR), which was being constructed on the opposite side of the country.
An early authority in nuclear engineering comes to MIT
Lanning came to MIT in 1957 to join what was being called the “MIT Reactor Project” after being recruited by the MITR’s designer and first director, Theos “Tommy” J. Thompson, to serve as one of the MITR’s first operating supervisors. With only a handful of people on the operations team at the time, Lanning also completed the emergency plan and startup procedures for the MITR, which achieved criticality on July 21, 1958.
In addition to becoming a faculty member in the Department of Nuclear Engineering in 1962, Lanning’s roles at the MITR went from reactor operations superintendent in the 1950s and early 1960s, to assistant director in 1962, and then acting director in 1963, when Thompson went on sabbatical.
In his faculty position, Lanning took responsibility for supervising lab subjects and research projects at the MITR, including the Heavy Water Lattice Project. This project supported the thesis work of more than 30 students doing experimental studies of sub-critical uranium fuel rods — including Lanning’s own thesis. He received his PhD in nuclear engineering from MIT in fall 1963.
Lanning decided to leave MIT in July 1965 and return to Hanford as the manager of their Reactor Neutronics Section. Despite not having plans to return to work for MIT, Lanning agreed when Thompson requested that he renew his MITR operator’s license shortly after leaving.
“Because of his thorough familiarity with our facility, it is anticipated that Dr. Lanning may be asked to return to MIT for temporary tours of duty at our reactor. It is always possible that there may be changes in the key personnel presently operating the MIT Reactor and the possible availability of Dr. Lanning to fill in, even temporarily, could be a very important factor in maintaining a high level of competence at the reactor during its continued operation,” Theos J. Thompson wrote in a letter to the Atomic Energy Commission on Sept. 21, 1965
One modification, many changes
This was an invaluable decision to continue the MITR’s success as a nuclear research facility. In 1969 Thompson accepted a two-year term appointment as a U.S. atomic energy commissioner and requested Lanning to return to MIT to take his place during his temporary absence. Thompson initiated feasibility studies for a new MITR core design and believed Lanning was the most capable person to continue the task of seeing the MITR redesign to fruition.
Lanning returned to MIT in July 1969 with a faculty appointment to take over the subjects Thompson was teaching, in addition to being co-director of the MITR with Lincoln Clark Jr. during the redesign. Tragically, Thompson was killed in a plane accident in November 1970, just one week after Lanning and his team submitted the application for the redesign’s construction permit.
Thompson’s death meant his responsibilities were now Lanning’s on a permanent basis. Lanning continued to completion the redesign of the MITR, known today as the MITR-II. The redesign increased the neutron flux level by a factor of three without changing its operating power — expanding the reactor’s research capabilities and refreshing its status as a premier research facility.
Construction and startup tests for the MITR-II were completed in 1975 and the MITR-II went critical on Aug. 14, 1975. Management of the MITR-II was transferred the following year from the Nuclear Engineering Department to its own interdepartmental research center, the Nuclear Reactor Laboratory , where Lanning continued to use the MITR-II for research.
Beyond the redesign
In 1970, Lanning combined two reactor design courses he inherited and introduced a new course in which he had students apply their knowledge and critique the design and economic considerations of a reactor presented by a student in a prior term. He taught these courses through the late 1990s, in addition to leading new courses with other faculty for industry professionals on reactor safety.
Co-author of over 70 papers , many on the forefront of nuclear engineering, Lanning’s research included studies to improve the efficiency, cycle management, and design of nuclear fuel, as well as making reactors safer and more economical to operate.
Lanning was part of an ongoing research project team that introduced and demonstrated digital control and automation in nuclear reactor control mechanisms before any of the sort were found in reactors in the United States. Their research improved the regulatory barriers preventing commercial plants from replacing aging analog reactor control components with digital ones. The project also demonstrated that reactor operations would be more reliable, safe, and economical by introducing automation in certain reactor control systems. This led to the MITR being one of the first reactors in the United States licensed to operate using digital technology to control reactor power.
Lanning became professor emeritus in May 1989 and retired in 1994, but continued his passion for teaching through the late 1990s as a thesis advisor and reader. His legacy lives on in the still-operational MITR-II, with his former students following in his footsteps by working on fuel studies for the next version of the MITR core.
Lanning is predeceased by his wife of 60 years, Gloria Lanning, and is survived by his two children, a brother, and his many grandchildren .
Share this news article on:
Related links.
- MIT Nuclear Reactor Laboratory
- Department of Nuclear Science and Engineering
Related Topics
- Nuclear science and engineering
- Nuclear power and reactors
Related Articles
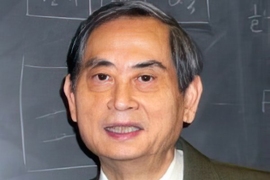
Professor Emeritus Sow-Hsin Chen, global expert in neutron science and devoted mentor, dies at 86
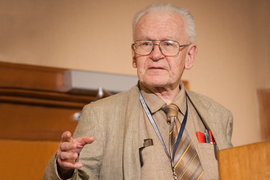
Professor Emeritus Michael Driscoll, leader in nuclear engineering and beloved mentor, dies at 86
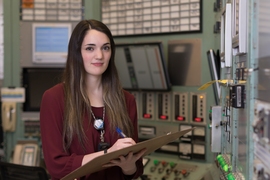
Sara Hauptman: Learning to operate a nuclear reactor
Retirement dinner honors 155 community members.
Previous item Next item
More MIT News
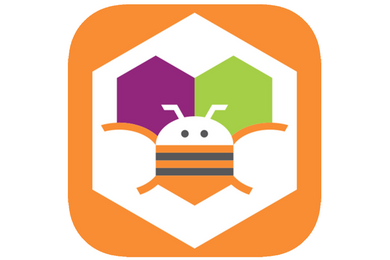
The power of App Inventor: Democratizing possibilities for mobile applications
Read full story →
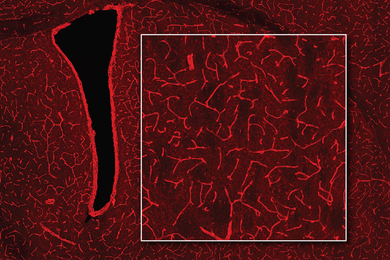
Using MRI, engineers have found a way to detect light deep in the brain
From steel engineering to ovarian tumor research
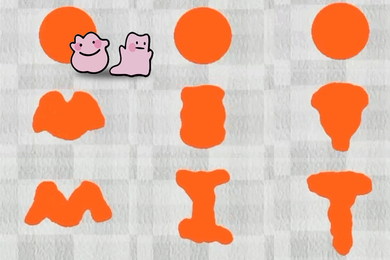
A better way to control shape-shifting soft robots
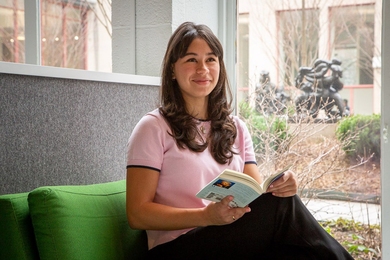
Discovering community and cultural connections
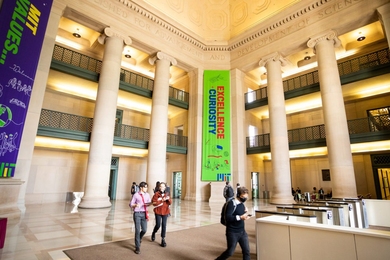
MIT Supply Chain Management Program earns top honors in three 2024 rankings
- More news on MIT News homepage →
Massachusetts Institute of Technology 77 Massachusetts Avenue, Cambridge, MA, USA
- Map (opens in new window)
- Events (opens in new window)
- People (opens in new window)
- Careers (opens in new window)
- Accessibility
- Social Media Hub
- MIT on Facebook
- MIT on YouTube
- MIT on Instagram

IMAGES
VIDEO
COMMENTS
Bioinformatics is a field of study that uses computation to extract knowledge from biological data. It includes the collection, storage, retrieval, manipulation and modelling of data for analysis ...
A recent study has found that the interest of researchers in these topics plateaued over after the early 2000s [1]. Besides the above mentioned hot topics, the following topics are considered demanding in bioinformatics. Cloud computing, big data, Hadoop. Machine learning. Artificial intelligence.
From one genome to many genomes: the evolution of computational approaches for pangenomics and metagenomics analysis. An innovative journal that provides a forum for new discoveries in bioinformatics. It focuses on how new tools and applications can bring insights to specific biological problems.
Bioinformatics researchers integrate and manage the vast amounts of biological data now being generated, including genomic data. ... Our Research Focus. ... project is a collaborative effort to address the need for consistent descriptions of gene products across databases. Mouse Phenome Database.
This is an editorial report of the supplements to BMC Bioinformatics that includes 6 papers selected from the BIOCOMP'19—The 2019 International Conference on Bioinformatics and Computational Biology. These articles reflect current trend and development in bioinformatics research.
Tips for a Successful Bioinformatics Project. Plan your project carefully and set clear objectives. Collaborate with experts in related fields. Stay updated with the latest bioinformatics ...
Undergraduate and Masters Research. General Information. There are plenty of opportunities for Bioinformatics research projects at UCLA. This program is designed to help interested students find research projects related to Bioinformatics across campus. Typically, these projects are for credit; in exceptional circumstances they may offer funding.
Abstract. Biological and biomedical research is increasingly conducted in large, interdisciplinary collaborations to address problems with significant societal impact, such as reducing antibiotic ...
Current Research Projects. 2019 Research Projects; 2018 Research Projects; 2017 Research Projects; 2016 Research Projects; 2015 Research Projects; ... These free, hands-on workshops are available to all Harvard affiliates and cover a variety of bioinformatics topics & related skills. The first workshop is a pre-requisite for the next three ...
The interdisciplinary nature of bioinformatics makes it an ideal framework to develop activities enabling enquiry-based learning. We describe here the development and implementation of a pilot project to use bioinformatics-based research activities in high schools, called "Bioinformatics@school." It includes web-based research projects that students can pursue alone or under teacher ...
In this article, we address the challenges—related to communication, good laboratory practice, and data handling—that may be encountered in core support facilities when providing bioinformatics support, drawing on our own experiences working as support bioinformaticians on multidisciplinary research projects.
MSc thesis: In the Bioinformatics group, we offer a wide range of MSc thesis projects, from applied bioinformatics to computational method development. Here is a list of available MSc thesis projects.Besides the fact that these topics can be pursued for a MSc thesis, they can also be pursued as part of a Research Practice.. BSc thesis: As a BSc student you will work as an apprentice alongside ...
When you want to immerse yourself into the core methodologies of bio-computation that actually operate within living cells, you might be interested about this paper and animations related to it [1].
Topic Selection. The initial topics were defined based on current developments in the health informatics field and an increasing number of published manuscripts between 2000 and 2021 (based on title-abstract-keyword screening in Scopus using the keywords "Health" AND "Informatics" AND "domain") in the respective subdomains (Figure 1 A).). After a first definition of the specific ...
The current field of neuroscience is increasingly using bioinformatics, which has provided new research avenues, thus enhancing our understanding of brain functions. Some well-known examples include high-throughput analysis of single-cell transcriptomics, comparative genomics, networks & neurophysiology, the latter encompassing neuronal modeling and high power computation. From focusing on a ...
In this video, we go over some of the main topics that will help you develop a research project using bioinformatics. For a detailed overview, please visit: ...
"Scientific community" refers to a group of people collaborating together on scientific-research-related activities who also share common goals, interests, and values. Such communities play a key role in many bioinformatics activities. Communities may be linked to a specific location or institute, or involve people working at many different institutions and locations. Education and ...
bioinformatics is used for within research labs and provides sever al resources for beginners to learn. how to code and perform bioinformatic tasks. Krutik Patel (Faculty of. Medical Sciences ...
During a research project, you learn how to plan and carry out a project from start to end. These courses are available only for students within the Master's programme in Bioinformatics at the Department of Biolog. You may choose to do a 7.5, 15 or 30 credits research project. You can carry out the project full time or part time, during the semester or in the summer.
Sample Grouping or individual as per experimental design, Group-wise OTU Clustering and abundance Report, OTU identification and taxonomic annotation Report (Sample Wise - Genius Level) and OTU Fasta file will be provided, Pie chart representation TOP 10 taxonomic classification; phylum to species-level. 5. SmallRNA Sequencing.
Apply Bioinformatics Science Projects (9 results) Apply Bioinformatics Science Projects. (9 results) Analyze health data to discover how genes can affect biological processes, specifically protein development. Search in an online database for the cause of the condition you are investigating or the best protein that will create better health.
Here is a list of project ideas based on Bioinformatics. Students belonging to third year or final year can use these projects as mini-projects as well as mega-projects. This list has been ...
Loïcka Baille's research focuses on developing remote sensing technologies to study and protect marine life. Her main project revolves around improving onboard whale detection technology to prevent vessel strikes, with a special focus on protecting North Atlantic right whales. Baille is also involved in an ongoing study of Emperor penguins.
In his faculty position, Lanning took responsibility for supervising lab subjects and research projects at the MITR, including the Heavy Water Lattice Project. This project supported the thesis work of more than 30 students doing experimental studies of sub-critical uranium fuel rods — including Lanning's own thesis.