Thank you for visiting nature.com. You are using a browser version with limited support for CSS. To obtain the best experience, we recommend you use a more up to date browser (or turn off compatibility mode in Internet Explorer). In the meantime, to ensure continued support, we are displaying the site without styles and JavaScript.
- View all journals
- My Account Login
- Explore content
- About the journal
- Publish with us
- Sign up for alerts
- Open access
- Published: 11 May 2023

Reassessing the econometric measurement of inequality and poverty: toward a cost-of-living approach
- Anson Au ORCID: orcid.org/0000-0002-8180-5104 1
Humanities and Social Sciences Communications volume 10 , Article number: 228 ( 2023 ) Cite this article
1629 Accesses
2 Citations
1 Altmetric
Metrics details
The econometric measurement of inequality and poverty in advanced capitalist economies has been preoccupied with aggregate measures of relative deprivation, namely, the Gini Index and a relative poverty rate, both of which are based on economic distances from the population median. Using the case of Hong Kong, this article demonstrates the limitations of relative measures: the Gini Index masks social mobility and the relative poverty line understates actual poverty. This article argues instead for a cost-of-living approach to measure poverty, where the poverty line is defined as the cost of essential goods and services. A cost-of-living approach produces a poverty line of HK$28,815 and attendant poverty rate of 44.47% in 2020, nearly double the poverty line of HK$13,450 and poverty rate of 23.6% according to the conventional relative measure of the poverty line set to 50% of median household income—capturing a shortfall of 551,400 poor households that have been overlooked by relative measures.
Similar content being viewed by others
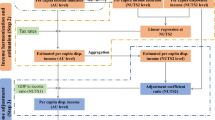
Harmonized disposable income dataset for Europe at subnational level
Mehdi Mikou, Améline Vallet & Céline Guivarch
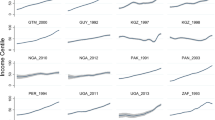
Systematic comparison of household income, consumption, and assets to measure health inequalities in low- and middle-income countries
Mathieu J. P. Poirier
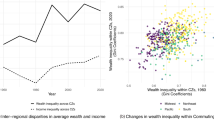
GEOWEALTH-US: Spatial wealth inequality data for the United States, 1960–2020
Joel Suss, Tom Kemeny & Dylan S. Connor
Introduction
The measurement of inequality and poverty in a society has direct implications for household wellbeing by determining the threshold for government program payouts like welfare and the size of payouts required, but the methodology for which these measures are determined has been contested since the 1970s (Atkinson, 1998 ; Gordon, 1972 ; Sen, 1973 , 1982 ).
The destabilization of the income distribution during this period led to a universal move to adopt the Gini Index as a gold standard measure for assessing inequality, which is a scaled measure of the sum of individual income differences from the median income in a population. This relative measure thus aims to depict inequality with an aggregate level distribution (Antonelli and Rehbein, 2018 ; DiPrete, 2007 ; Myles, 2003 ; Osberg, 2017 ).
Similar debates have emerged over the measurement of poverty, with the most recent consensus concluding that absolute measures, such as the World Bank’s ( 2021a , b ) famous US$1 to US$2 income per day threshold, are claimed to be better for developing nations by capturing absolute levels of material purchases deemed necessary for survival (Sen, 1982 ). However, absolute measures fail in accounting for the economic and social context within which needs arise. Such is the issue in the case of China, where, a recent study on the dibao , China’s welfare, identify that the rural dibao program excluded 87% of the poor and 82% of the non-poor in 2013 (Kakwani et al., 2019 ). This problem was sourced to the methodology for deciding poverty, which originally began as an attempt to determine absolute levels of needs, but eventually percolated into decentralized decision-making by village administrations that struggled to determine these levels (Kakwani et al., 2019 , p.7).
This issue surfaces in the understatement of poverty rates by official measures of poverty that rely on an arbitrary US$1 to US$2 a day poverty threshold (World Bank, 2021a , b ), despite successes in reducing poverty (Chen, 2008 ; Zhang et al., 2014 ). Turning their attention to the current dibao , Walker and Yang ( 2021 ) find that if China adopted the global standard for poverty that is based on relative measures—classifying one is poor if they are making less than 40 to 60% of the median income in the entire population—the poverty rate would shoot up to 8% to over 20%. Similarly, Wan et al. ( 2021 ) find that the same fast economic growth that explains declines in absolute poverty in China (based on the $1 to $2 a day poverty threshold) is also what explains a rising relative poverty rate.
Indeed, the global consensus for the measurement of poverty is gradually shifting to the adoption of relative measures akin to the consensus for Gini Index as a relative measure of inequality. The poverty threshold according to relative measurements of poverty, such as household incomes that fall below a certain percentage (40 to 60%) of the median income, are now widely considered to capture changes in household needs over time, especially in the case of developed economies or countries like those in the Asia-Pacific that have moved from low-income to middle-income status by GDP per capita. Such measures, according to Brady ( 2003 , 2019 ), are argued to capture needs because needs themselves are relative and contextualized within a given society to begin with, highly dependent on reference groups that individuals have within a society.
Recent political progressivism has sedimented this fascination with relative measurements, couched in slogans with popular political appeal that binarizes economic disenfranchisement in classes, like the “99%” poised against the “1%” in social movements such as Occupy Wall Street and newly emerging progressive agendas in the U.S. and China (Calhoun, 2013 ; Kakwani et al., 2022 ; Stroh, 2020 ; Van Gelder, 2011 ). Indeed, contemporary measurements of inequality by social scientists and the World Bank consistently measure it on an aggregate level by using the Gini Index (Liao, 2021 ; Oishi et al., 2011 ; Pickett and Wilkinson, 2015 ; Zagorski et al., 2014 ).
In conversation with this literature, this article contributes to the methodological study of inequality and poverty by demonstrating the limitations of relative measures, such as to fail to capture social mobility and understating actual poverty—and arguing instead for a cost-of-living approach to measurement. Qualitative and quantitative studies of the trauma of poverty demonstrate the myriad challenges faced exclusively by people who do not have enough to survive: including predisposition to mental health issues and disabilities from a lifetime of homelessness and eviction, cultural norms of discrimination, stigma, and shame on the basis of their class in access to social services, housing, education, and work, as well as exposure to illicit drug and violent activities as one of the few means of economic subsistence available (Beddoe and Keddell, 2016 ; Contreras, 2013 , 2017 ; Desmond, 2012 , 2016 ; Desmond and Kimbro, 2015 ; Desmond and Western, 2018 ; Desmond and Wilmers, 2019 ; Hansen et al., 2014 ; Sánchez-Jankowski, 2008 , p. 48; Shildrick, 2018 ).
Apart from its consequential or theoretical significance, a cost-of-living approach better accounts for inelasticity of certain material needs. Relative measures of poverty like 50% of the median income have been argued to capture the dependence of needs on reference groups in different social contexts, for what individuals are willing to spend or what they purchase may be dependent on what their communities or neighborhoods purchase (Brady, 2019 ), but this assumption erroneously overstates income and price elasticity of all needs. According to the National Bureau of Economic Research (2016), for instance, housing demand is actually income and price inelastic (Albouy et al., 2016 ). Rising relative rents, moreover, produce increases in real income inequality that are missed by relative measures of poverty and inequality. This finds resonance with Piketty’s ( 2014 ) observation that the value of land occupies a greater proportion of the economy because of this very same inelasticity in the demand for land, such that the price of housing has risen almost 40% more than other goods since 1970 in developed economies (Albouy et al., 2016 ).
This article addresses this limitation by using the case of Hong Kong to demonstrate the merits of a cost-of-living approach to measuring inequality and poverty over relative measures. Hong Kong is an urban city with a population of around 7 million people and an average GDP per capita is 49,800 USD as of 2021. Of its 3.67 million employed workers, according to the latest Census ( 2021a ), 1.102 million work in public administration, social and personal services (30.03% of the workforce), 862.4 million in financing, insurance, real estate, professional, and business services (23.5% of the workforce), 430.2 million in transportation, storage, information and communications (11.72% of the workforce), 516.4 million in retail, accommodation, and food services (14.06% of the workforce), 316.4 million in import/export trade and wholesale (8.62% of the workforce), 325.8 million in construction (8.88% of the workforce), and 94.3 million in manufacturing (2.57% of the workforce). Thus, as an advanced capitalist economy with an entirely service-based workforce, Hong Kong is an apposite case for comparing relative versus cost-of-living measurements of inequality and poverty in advanced capitalist economies at large, especially those in the Asia-Pacific where empirical evidence has been limited (Brady and Burton, 2016 ). Through a cost-of-living approach, the Gini Index and relative poverty are demonstrated to be insufficient in understanding within-population shifts in economic wellbeing and to understate the poverty rate among households.
Problems with relative measures of inequality and poverty
This section identifies the problems with relative measures of inequality and poverty, namely, the Gini Index and relative benchmarks of poverty as a proportion of the median income.
The Gini Index is one of the widest used measures of economic inequality, most of all in sociology. Devised by Gini in 1912, the Gini Index expresses a concentration ratio equal to the average distance between n quantities divided by double the arithmetic mean of a generic income distribution X . In a population of N individuals, i = 1,2…n, n ∈ N, n ≥ 3 with an income distribution of X = ( x 1 , x 2 … x i … x n ) where X ∈ R and x 1 ≤ x 2 ≤ … ≤ x n , an arithmetic mean of μ X , and x M is the individual with the median income:
G(X) is thus a scaled measure of all possible pairwise differences or individual diversity in income, through which it satisfies the six desirable properties of an inequality measure: continuity, additivity, linear homogeneity, translation invariance, symmetry, and anonymity (Ceriani and Verme, 2015 ). Configurations have since been made to the Gini Index, of which Ceriani and Verme ( 2012 ) identify thirteen. Such forms in which the Gini Index is expressed (and the individual functions underlying the different Ginis) consist of distances from the median, geometric mean, covariance, etc. (Anand, 1983 ; Gini, 1914 ; Sen, 1973 ). Distributions computed using Gini Indices, however, fundamentally rely on individual contributions based on endogenous values that conceptualize the distribution squarely as expressions of relative deprivation (Yitzhaki, 1979 ).
Attempts to broaden the application of the Gini Index have also yielded alternative configurations that decompose it at subgroup levels, such as when a population is classified into K number of groups for k = 1 to K , where G(X) is based on (a) the income difference between individual i in group k and other individuals j in a different group h , (b) the income difference between individual i and other individuals j in the same group k , such that n h is the size of the group h and n k is the size of group k , h ≠ k :
Nonetheless, these configurations converge on the same prototypical model of relative deprivation, whereby individual contributions to inequality are based on individual i diversity from other individuals j richer or poorer. As a result, this model and its configurations are ultimately poorly sensitive to changes in income and to whether pairwise differences are positive or negative (Alvaredo and Piketty, 2014 ; Atkinson et al., 2011 ; Ceriani and Vermea, 2015 ; Cowell and Ebert, 2004 ). The theoretical and empirical limitations of the Gini Index and relative deprivation thus rise to the fore.
Relative deprivation fails to capture material deprivation, or the ability to afford the cost of living, typically equated with the poverty line below which individuals within a population X are said to be living in poverty P in a given social unit often defined at the levels of cities, states or provinces, and nations.
Consider that in the Asia-Pacific (Table 1 ), the movement of the Gini Index is not in pace with the poverty rate: the Philippines exhibits a higher poverty rate than Hong Kong despite its markedly lower Gini Index.
The discrepancy between the Gini Index and the poverty rate is because changes to income—upward or downward—yield comparable distributions and, since the Gini measures scaled differences, they resultantly pass without detection in the Gini Index. Yet, it is in these changes that we glimpse powerful antecedents of poverty and social mobility in general. A Gini Index neglects, for instance, to identify broader patterns of material deprivation among households across time that would otherwise allow for subsequent analysis of their causes among externalities, such as the effects of inflation on lower-income households.
It follows that a Gini Index will fail to detect underlying shifts in economic wellbeing on upper and lower bounds so long as both ends experience changes that are relatively symmetrical. It also follows that derivations of the Gini Index over time will likely exhibit horizontal asymptotic tendencies once a society reaches a certain level of inequality. An examination of the Gini Indices of the ten societies with the highest Ginis and ten with the lowest illustrates this behavior (Fig. 1 ).
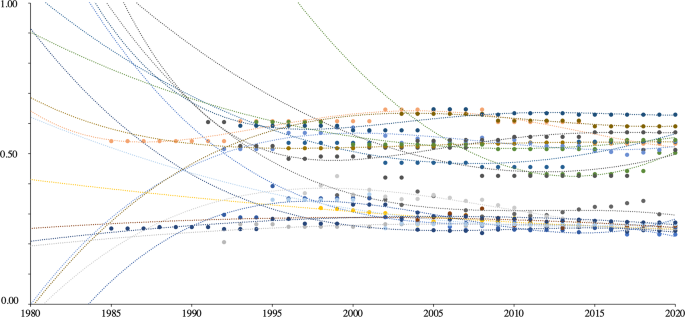
Source: Author’s calculations using data from the World Bank ( 2021a ).
For societies on the lower bounds, as time t approaches infinity, Gini Index G(X) approaches 0.2. For societies on the upper bounds, there exist several asymptotes as time t approaches infinity, but these are consistently placed at a Gini Index G(X) of about 0.5 and 0.6. The chief problem identified in these observations is that the Gini Index tends to stagnate, which erroneously suggests stalls in inequality, but for reasons endogenous to the way it is derived, rather than due to underlying shifts in economic means or pressures—casting doubt on the Gini’s efficacy as a meaningful measurement of economic wellbeing (and underlying expression of relative deprivation) over time.
The poverty rate is commonly calculated by the Organisation for Economic Co-operation and Development ( 2016 ) using 50% of median household income (other configurations have since been developed to push the rate to 30% in deciding the line of poverty) as a poverty line, roughly approximated as the following where z indicates individual income and u is median income in a population X :
Measuring poverty based on cost-of-living
The present article articulates a cost-of-living approach to reassess the poverty rate, rather than economic distances in income endogenous to a population. The poverty rates reported in Table 1 was based on (3). Yet, this benchmark is ultimately arbitrary and premised on the idea of income differences within the same population that resembles once more relative deprivation. As such, the poverty line based on (3) may fluctuate drastically and decrease in economic downturns because of a lower median income despite greater material deprivation as more people cannot afford basic goods and services.
The latest COVID-19 pandemic is a useful heuristic. In 2020, most economies worldwide suffered drops in Gross Domestic Product (and per capita) as travel and cross-border services shrivelled up, trade wound down, lockdowns sent cities and small and medium-sized enterprises shuttering down—and as a result, unemployment rates rose drastically. Poverty rate (3) thus understates the amount of actual material deprivation in a given society.
By contrast, benchmarking the poverty rate to cost of living captures far more meaningfully whether people can afford to survive and under what living conditions, particularly when income elasticities are unitary. Cost-of-living L in a population X can thus be calculated in two steps, where F is an index for changes in real prices of goods and services, C g is the cost of goods and Q g is the quantity of goods at time t , t = 0, 1… a , a ∈ N , n ≥ 3 and t = 0 is the base period:
(4) is effectively the Fisher Price Index that captures changes in real prices of goods and services, which is presently integrated into (6) a revised formula for the poverty rate P of a population X (or proportion of population X ) living below (5) the cost of living L(X) defined as the necessary amount Q g of goods C g for an individual i to consume in year t = a pro-rated to the base period t = 0, where I is an indicator function such that individuals are counted as poor ( I = 1) if individual income y i during the same period (between base period t = 0 and t = a) is below cost of living L(X) and individuals are counted as non-poor ( I = 0) if y i is above L(X) .
Equation ( 6 ) offers a methodologically flexible measure of material deprivation by leaving room for theoretical assumptions about the scope of study and population needs, namely interval t , the base period at which t = 0, and the amount of goods Q g needed to be consumed by individuals. This flexibility ultimately permits its application across societies, accounting for, rather than obscuring, differences by geographical contexts (Brady, 2003 ).
Re-examining inequality and poverty in Hong Kong
How is the economic wellbeing of Hong Kong residents? Let us consider the region’s household financial data over the past twenty-five years from 1996 to 2020. An aggregated Gini Index calculated using Eq. ( 1 ) produces a value within an inflexible range of about 0.52 to 0.54, effectively holding constant for the entire period (Fig. 2 ). Taken at face value, this means that inequality has not worsened for twenty-five years, bad as it may be. This is consistent with global trends of the Gini Index that show significant stagnation over time (Fig. 1 ).
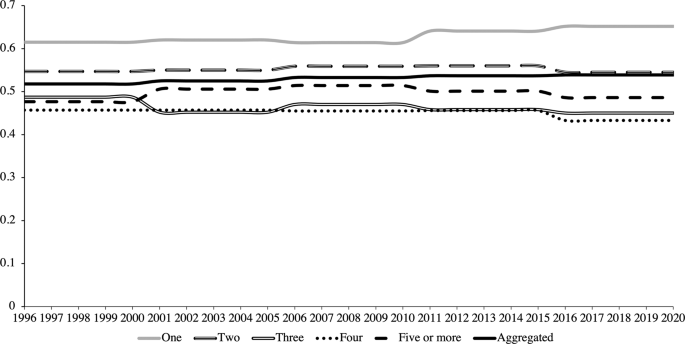
Source: Author’s calculations using data from the Legislative Council of Hong Kong ( 2001 ), Census and Statistics Department of Hong Kong ( 2021b ), and Social Indicators of Hong Kong ( 2022 ).
Applying Eq. ( 2 ) to decompose the Gini Index by groups, defined as households of different size, produces a marginal improvement (Fig. 2 ). All values moved beyond one standard deviation from the mean during this period, but the most significant moves in absolute terms were by households of one with a rise of 6.02% from a Gini of 0.615 to 0.652, households of three with a decline of 7.60% from a Gini of 0.487 to 0.45, and households of four with a decline of 5.25% from a Gini of 0.457 to 0.433, with the rest largely staying stagnant. Inequality appears to have gotten worse for households of one only, with the rest improving or staying within the same Gini brackets.
Though some stratification by household size is visible at a group level with Eq. ( 2 ), the Gini Index does not permit the visualization of the size or scope of such stratification or, relatedly, social mobility, which may be conflated with demographic patterns like changes in household sizes over time in general. Indeed, any attempt to observe social mobility out or into poverty is complicated by demographic shifts in household size.
Decomposing household income by household size in absolute numbers of individuals rather than as relative proportions in Fig. 3 , we observe nuances masked by the Gini Index. Households of one exhibited the greatest jump in inequality in Fig. 2 , but Fig. 3 shows us that this inequality is understated: we observe the greatest demographic growth in households of one (and two), and most of this growth was sequestered in the lowest income bracket (those earning HK$0 to HK$9,999 per month). More than a portrait of inequality, this sensitizes us to a growing issue of poverty .
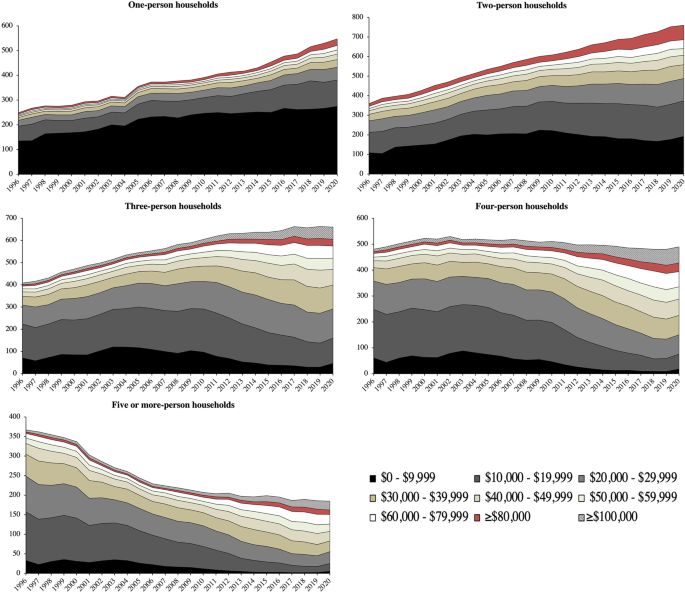
Sources: Author’s calculations based on data from the Census and Statistics Department of Hong Kong ( 2021b ).
Household sizes of two, which showed the greatest inertia in the Gini Index calculated with Eq. ( 2 ) in Fig. 2 , show growing inequality in Fig. 3 , as the absolute numbers of people in the highest (those making HK$80,000 or more per month) and lowest income brackets (HK$0 to HK$9,999 per month) have grown the most. This sensitizes us to the problem of the Gini Index as a scaled measure, where, even using configurations at a group level with Eq. ( 2 ), it is insensitive to social mobility and changes in income when upper and lower bounds exhibit symmetry in scalar changes.
Household sizes of three and four showed declines in inequality in Fig. 2 , but we observe in Fig. 2 that this owes to a steep decline in the number of households with the lowest income (HK$0 to HK$9,999 per month). In a similar vein, households of five or more persons were shown to stagnate in Fig. 2 , but this masks the greatest demographic collapse out of all household sizes. Examining economic means expressed in absolute numbers in Fig. 3 rather than relative scales in Fig. 3 thus warns us not to overinterpret declines or stagnation in the Gini as the equitable redistribution of economic means, but rather, as a trend in social mobility rooted in a dislocation between economic pressures and individual economic means. Reconciling these declines in the Gini Index with the large growth in the number of households of one and two persons, it is more plausible that a greater number of people are being siphoned into lower incomes over time and that those earning the lowest income have not been able to afford to get married or have children (and co-reside with them). This is also reflected in the general inverted relationship between household size and number of households over time in all households in Fig. 3 , signaling aggregate shifts in the general population from larger household sizes to smaller ones.
Underwritten in the limitations of the Gini Index (and adherent configurations, Eqs. ( 1 ) and ( 2 )) as its measure is the identification of the growing problem of—and need to examine—material deprivation. Adopting a cost-of-living approach thus leads us to evaluate the poverty rate using the standard measure of poverty as a proportion of median income, Eq. ( 3 ), and on the basis of real cost-of-living, Eq. ( 6 ).
Using Eq. ( 3 ), the poverty line and attendant poverty rate in Hong Kong are estimated in Fig. 4 . Based on government data, it is observed that the Hong Kong government has historically defined the poverty line to be 50% of median (monthly) household income for a household size equivalent of about 2.5 persons ( Q g = 2.5). Equation ( 3 ) has been configured accordingly. The poverty line is thus HK$13,450 in 2020 compared to HK$8,750 in 1996.
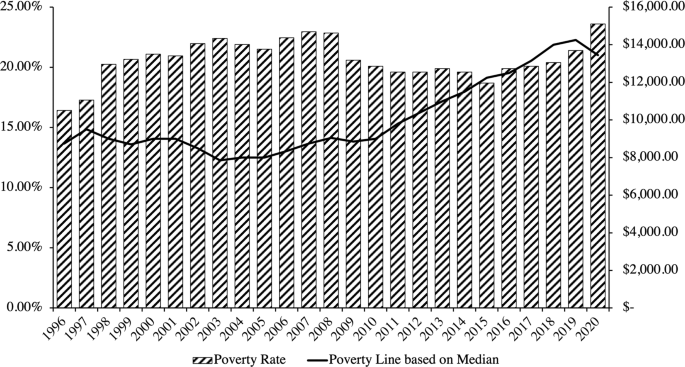
Source: Author’s calculations based on data from the Census and Statistics Department of Hong Kong ( 2021b ) and the Legislative Council of Hong Kong ( 2001 ).
Figure 4 reveals that the poverty rate is alarmingly high, hovering consistently around 20% for the better part of the past thirty years. Even still, however, we observe from Fig. 4 that the poverty rate calculated using Eq. ( 3 ) understates material deprivation because of its reliance on economic distances from the median as a relative—not absolute—determination of the poverty line.
To illustrate, let us consider four periods of economic crisis in Hong Kong: the 1998–1999 Asian Financial Crisis, the 2001–2003 recession, the 2008–2009 Financial Crisis, and the 2020 COVID-19 pandemic. These four crises inflicted the greatest hits to households’ economic means measured in losses of income and steep rises in the unemployment rate, coupled with rising Consumer Price Index (CPI) inflation (Table 2 ). It deserves noting that the unemployment rates for all four crises are higher than the mean, with three of the four rising to one standard deviation above the mean.
Since the poverty line is pegged to household median income, changes in the former are equivalent to those in the latter. From the comparisons between means and costs, we may further estimate whether a lag exists in the ability of the poverty line to capture all changes in cost-of-living for households. A positive difference between inflation and change in median household income will indicate a lag, where adjustments to the poverty line, equivalent to changes in median household income, fail to keep pace with changes in CPI inflation.
As Table 2 reveals, we witness lags in all four crises. Even in deflationary periods like in the 1998–1999 Asian Financial Crisis and the 2001–2003 recession that followed, changes in median household income, and therefore the poverty line, fail to capture rising growing economic pressures on a population that is doubly suffering from higher unemployment rates. We observe as much with declines in the poverty line across all crises: from HK$9,000 to HK$8,700 in the Asian Financial Crisis, from HK$9,000 to HK$7,850 in the 2001–2003 recession, from HK$9,050 to HK$8,850 in the Financial Crisis, and most recently from HK$14,250 to HK$13,450 in the COVID-19 pandemic.
In other words, Eq. ( 3 ) lowers the bar for who counts as poor in times when it should least do so, namely, during times of economic crisis when a larger proportion of households are out of work and simultaneously paying more for necessities. It excludes a number of households that would have been considered poor in previous years, misleading us to believe the poverty rate is lower than it actually is when the poverty line is pegged to the median, rather than cost-of-living.
Let us now reassess the poverty line and poverty rate based on cost-of-living, rather than the median. Let us keep the same assumptions of Eq. ( 3 ), using households of 2.5 persons ( Q g = 2.5) as our basic unit of analysis for determining the number of goods to be consumed and benchmarking the poverty line, supposing that t intervals is one-year intervals, and treating the year 1996 is the base period ( t = 0). With these assumptions in place, we can recalculate the cost of living with Eq. ( 5 ) using prices in nine categories from 1996 to 2020: food (including meat, fish, poultry, pork, bread, rice, fruit, vegetables, dairy products, drinks, sugar, flavorings, eating out), housing (including rent and property management company fees, as apartments are the most common type of residence in Hong Kong), clothing and footwear, durable goods (e.g., household appliances, furniture, etc.), miscellaneous goods (e.g., commodities that are non-durable, not clothing or footwear, and not food, such as jewellery, stationery, newspapers, etc.), transportation, miscellaneous services (e.g., health care, tutoring and education, banking, and other services). We thus arrive at F t=a = 144.1 and a default of F t=0 = 100 that reflect a cost of living L(X) of HK$28,815.08 in 2020 compared to HK$20,476.20 in 1996.
Figure 5 traces the trajectory of the poverty rate recalculated with Eq. ( 6 ) based on the cost of living. Informative patterns emerge. Unlike Fig. 4 , it is observed in Fig. 5 that the poverty rate has declined since 1996, rather than increased, but most salient is the distance in poverty rate and poverty line between the two charts—both appear in Fig. 5 to be multiples of what is reported in Fig. 3 . Where in the standard relative measure of the poverty line in Fig. 3 sits at HK$13,450 in 2020 compared to HK$8,750 in 1996 (based on 50% of median household monthly income), producing a poverty rate of 23.6% in 2020 compared to 16.43% in 1996, the poverty line in Fig. 5 is determined to be HK$28,815 in 2020 compared to HK$20,476.20 in 1996, producing a poverty rate of 44.47% in 2020 compared to 52.07% in 1996.
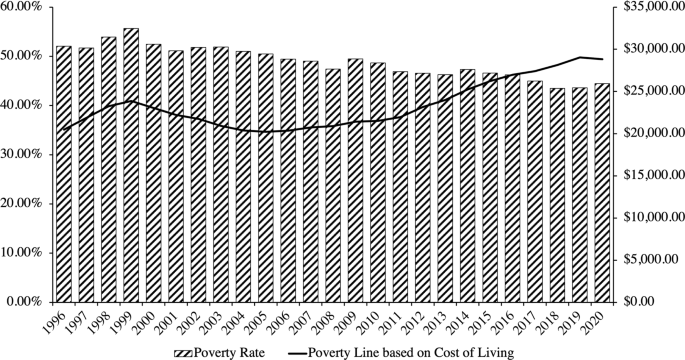
Figure 6 expresses the rift in poverty rates in absolute terms based on the number of poor households according to the two equations. Based on Eq. ( 3 ), relative measures of poverty identify the number of households below poverty line to be about 623,540 in 2020. Based on Eq. ( 6 ), however, it is identified that the number of households below the poverty line is nearly double at 1,174,930. This discrepancy represents a shortfall of 551,400 poor households whose means are below the cost of living that have gone unaccounted for in determinations of the poverty rate in relative terms like a proportion of the median in Eq. ( 3 ).
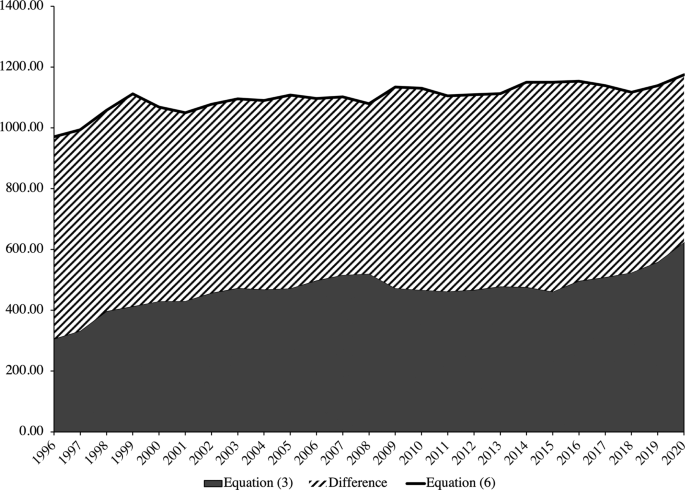
In the measurement of inequality, a consensus holds to adopt the Gini Index as a scaled relative measure of economic distances within a population (Liao, 2021 ). Conventional relative measurements of the poverty rate are similarly based on the sum of individual income diversity or differences from the median income in a population (Brady, 2019 ).
Testing these two relative measures in Hong Kong, this article has demonstrated that they fail to capture changes in economic wellbeing and understate poverty. The standard Gini Index at an aggregate level (Eq. ( 1 )) and configurations at group levels (Eq. ( 2 )) fail to capture, and even mask, tectonic shifts in household income, and as a result, household size. This finding adds to Blesch et al. ( 2022 ) recent observation that the Gini Index is lacklustre as a single-parameter measure that fails to distinguish inequality concentrated at lower and top income percentiles, a point echoed in this article’s breakdown of changes in incomes by household size in Fig. 1 . The breakdown of absolute income trends by household size illustrates the importance of what Osberg ( 2017 ) identifies as separating changes in income distribution and an index like the Gini that attempts to summarize this distribution.
In a similar vein, pegging the poverty to some proportion of the median as is convention (Eq. ( 3 )) is demonstrated to lag behind shifts in economic means and cost-of-living, particularly during times of economic crisis. This lag is not unusual, given the non-linear relationship identified between long-run inflation and income (inequality), where lower and higher inflation rates are most destabilizing for income distributions by causing the largest rises in income inequality (Monnin, 2014 ). Though the mechanisms through which this effect takes shape are unclear, it bears remarking that the present context of Hong Kong has demonstrated real wage growth of 0.5% per year, a significant illustration not only of inflation, but of the vulnerability of households and their attendant poverty rate to economic crises (Au, 2023 ).
This article adopts a cost-of-living approach in the measurement of poverty. Calculating the poverty line based on long-term changes in the real costs of goods and services (Eq. ( 5 )), this article demonstrates that relative measures of poverty, much like the Gini Index, underestimates the poverty rate by multiples, which translates into nearly half a million of poor households left unaccounted for. Measuring poverty in terms of cost-of-living (Eq. ( 6 )), by contrast, captures these differences and expresses poverty more accurately as households whose economic means fall below cost-of-living at a given time in society, a more accurate depiction of poverty than an arbitrary level of income based on what someone else earns, which may fall while cost-of-living rises.
This article thus contributes to ongoing debates about the relative versus absolute measurement of poverty and inequality by demonstrating the merits of an intermediate approach based on cost-of-living. Absolute measures are argued to overlook the effects of income distribution on welfare (Brady, 2003 ), whereas relative measures are claimed to overlook absolute needs for nutritional wellbeing and survival (Sen, 1983 , 2008 ).
A cost-of-living approach offers an alternative for this conundrum by determining a reference welfare level based on real costs, building an approach of basing the national poverty line on bundles of food requisite for nutritional survival in developing nations (Ravallion, 2012 ), yet extending it by encompassing a wider basket of essential goods and services as well as changes in their costs.
This approach gains credence from Ravallion’s ( 2020 ) observation that “existing poverty measures tend to opt for one of two very different assumptions, corresponding to the absolute and relative measures above: (a) that relative income does not matter to economic welfare or (b) that relative income is all that matters. Neither is plausible”. (p.168). The present approach echoes his skepticism in propounding the need for an alternative. But where Ravallion (Ravallion and Chen, 2019 ) moves on to develop an approach to account for both upward and downward relative comparisons in formulating a distribution-corrected mean income to benchmarking the poverty line, the present cost-of-living approach leans closer to Sen’s ( 1983 ) capabilities approach in accounting for material needs relevant to welfare.
Limitations and future research
In calculating the cost-of-living in Hong Kong, this article borrows the same assumption that the city’s government used to calculate its aggregate poverty rate (20%) on a relative basis, namely, it pegs its inputs to the needs of a household equivalent to 2.5 persons. The limitations of this article thus similarly share in those espoused by the government measure: as the distribution of households across different sizes changes over time, a unit of analysis larger or smaller than 2.5 persons may be required, generating different inputs (and poverty rate) as a result. Indeed, as even Ravallion ( 2020 ) bemoans, “any price index found in practice has some implicit welfare anchor, and the index value will (in general) vary as the reference welfare level varies… national consumer price indices are typically anchored to consumption bundles somewhere around the mean or median of the distribution of income” (p.170). This study, in sum, offers but a cross-sectional view of poverty.
Similarly, the measurement of poverty using a cost-of-living approach partially accounts for the fact that certain needs are inelastic (like property management fees), which is an improvement above relative measures, but may not fare better than relative measures in not fully factoring in an important fact: the adjustments to purchases that households make once their income falls below the poverty line (and how to measure these adjustments) remain an area of uncertainty and fertile grounds for future research. As economists have long recognized, consumers have an elasticity of substitution of products within a module, such as replacing bread with rice, even if needs themselves do not change (Handbury, 2019 ).
Moreover, though this study is based on Hong Kong, an advanced capitalist economy in the Asia-Pacific region, it is an urbanized context where the overwhelming majority of workers work in service-oriented professions. Future studies might seek to identify inequality and discrepancies in the poverty rate in advanced capitalist economies in the Asia-Pacific region where the labor market has a larger share of agricultural or rural workers.
Finally, this article opens dialog on another area for future research: investigating the links between inequality and poverty themselves. In the present article, though the link was between the two was not investigated, it was implied that inequality serves as but a sensitizing device for the deeper issue of poverty that has eluded conventional relative measures. This is akin to Brady’s argument ( 2019 ), for instance, that states it is not evident whether “poverty is simply a subset of status attainment or if it can be explained by broader theories of the income distribution” (p.1).
On a meta-level, Korom ( 2019 ) observes through a bibliometric citation network analysis of inequality research in sociology and economics that scholars overwhelmingly tend to study wealth inequalities between ethnic groups—but strikingly reveals a differentiation between poverty research and research on wealth inequality as separate research domains or clusters. In other words, despite the theoretical common ground between poverty and inequality, they empirically, and therefore policy-wise, remain islands (DiPrete and Fox-Williams, 2021 ; Goubin, 2018 ).
Data availability
The data generated during and/or analyzed during the current study are available from the Census and Statistics Department of Hong Kong.
Albouy D, Ehrlich G, Liu Y (2016) Housing demand, cost-of-living inequality, and the affordability crisis (No. w22816). National Bureau of Economic Research, Cambridge, MA
Book Google Scholar
Alvaredo F, Piketty T (2014) Measuring top incomes and inequality in the Middle East: Data limitations and illustration with the case of Egypt (CEPR Discussion Paper No. DP10068). Centre for Economic Policy Research, London
Google Scholar
Anand S (1983) Inequality and poverty in Malaysia: Measurement and decomposition. Oxford University Press, New York, NY
Antonelli G, Rehbein B (eds.) (2018) Inequality in economics and sociology: new perspectives. Routledge, New York, NY
Atkinson AB (1998) Poverty in Europe. Blackwell, Hoboken, NJ
Atkinson AB, Piketty T, Saez E (2011) Top incomes in the long run of history. J Econ Lit 49(1):3–71
Article Google Scholar
Au A (2023) Bourdieusian boundary-making, social networks, and capital conversion: inequality among International Degree-Holders in Hong Kong. Cult Sociol (Online First). https://doi.org/10.1177/17499755231157115
Beddoe L, Keddell E (2016) Informed outrage: tackling shame and stigma in poverty education in social work. Ethics Soc Welf 10(2):149–162
Blesch K, Hauser OP, Jachimowicz JM (2022) Measuring inequality beyond the Gini coefficient may clarify conflicting findings. Nat Hum Behav 6(11):1525–1536
Article PubMed PubMed Central Google Scholar
Brady D (2003) Rethinking the sociological measurement of poverty. Soc Forces 81(3):715–751
Brady D (2019) Theories of the Causes of Poverty. Ann Rev Sociol 45:155–175
Brady D, Burton L (eds.) (2016) The Oxford handbook of the social science of poverty. Oxford University Press, Oxford, United Kingdom
Calhoun C (2013) Occupy wall street in perspective. Br J Sociol 64(1):26–38
Article PubMed Google Scholar
Census and Statistics Department (2021a) Table E003: number of employed persons by industry and occupation. Census and Statistics Department, The Government of the Hong Kong Special Administrative Region, Hong Kong, https://www.censtatd.gov.hk/en/EIndexbySubject.html?scode=200&pcode=D5250003
Census and Statistics Department (2021b) Poverty situation. Census and Statistics Department, The Government of the Hong Kong Special Administrative Region, Hong Kong, People’s Republic of China, https://www.censtatd.gov.hk/en/scode461.html
Ceriani L, Verme P (2012) The origins of the Gini index: Extracts from Variabilità e Mutabilità (1912) by Corrado Gini. J Econ Inequal 10(3):421–443
Ceriani L, Verme P (2015) Individual diversity and the Gini decomposition. Soc Indicat Res 121(3):637–646
Chen S (2008) China is poorer than we thought, but no less successful in the fight against poverty (Vol. 4621). World Bank Publications, Geneva
Contreras R (2013) The stickup kids: Race, drugs, violence, and the American dream. University of California Press, Thousand Oaks, CA
Contreras R (2017) There’s no sunshine: Spatial anguish, deflections, and intersectionality in Compton and South Central. Environ Plann Soc Space 35(4):656–673
Cowell F, Ebert U (2004) Complaints and inequality. Soc Choice Welf 23:71–89
Article MathSciNet MATH Google Scholar
Desmond M (2012) Eviction and the reproduction of urban poverty. Am J Sociol 118(1):88–133
Desmond M (2016) Evicted: poverty and profit in the American city. Crown
Desmond M, Kimbro RT (2015) Eviction’s fallout: housing, hardship, and health. Soc Force 94(1):295–324
Desmond M, Western B (2018) Poverty in America: new directions and debates. Ann Rev Sociol 44:305–318
Desmond M, Wilmers N (2019) Do the poor pay more for housing? Exploitation, profit, and risk in rental markets. Am J Sociol 124(4):1090–1124
DiPrete TA (2007) What has sociology to contribute to the study of inequality trends? A historical and comparative perspective. Am Behav Sci 50(5):603–618
DiPrete TA, Fox-Williams BN (2021) The relevance of inequality research in sociology for inequality reduction. Socius 7:23780231211020199
Gini C (1914) Sulla Misura della Concentrazione e della Variabilità dei Caratteri. Atti del Reale Istituto Veneto di Scienze , Lettere ed Arti, LXXIII(II), 1203–1248
Gordon DM (1972) Theories of poverty and underemployment: orthodox, radical, and dual labor market perspectives. Lexington Books, Lanham, MD
Goubin S (2018) Is inequality a latent construct? An assessment of economic inequality indicators and their relation with social cohesion in Europe. Soc Indicat Res 136(1):21–40
Handbury J (2019) Are poor cities cheap for everyone? non-homotheticity and the cost of living across us cities (No. w26574). National Bureau of Economic Research, Cambridge, MA
MATH Google Scholar
Hansen H, Bourgois P, Drucker E (2014) Pathologizing poverty: New forms of diagnosis, disability, and structural stigma under welfare reform. Soc Sci Med 103:76–83
Kakwani N, Li S, Wang X, Zhu M (2019) Evaluating the effectiveness of the rural minimum living standard guarantee (Dibao) program in China. China Econ Rev 53:1–14
Kakwani N, Wang X, Xue N, Zhan P (2022) Growth and common prosperity in China. China World Econ 30(1):28–57
Korom P (2019) A bibliometric visualization of the economics and sociology of wealth inequality: a world apart? Scientometrics 118(3):849–868
Legislative Council of Hong Kong (2001) Household Income and Gini Coefficient Information Note. Legislative Council of Hong Kong, The Government of the Hong Kong Special Administrative Region, Hong Kong, China
Liao TF (2021) Income inequality, social comparison, and happiness in the United States. Socius 7:2378023120985648
Monnin P (2014) Inflation and income inequality in developed economies (2014/1). CEP Working Paper Series. Council on Economic Policies, Bern
Myles J (2003) Where have all the sociologists gone? Explaining economic inequality. Can J Sociol/Cahiers canadiens de sociologie 28(4):551–559
Oishi S, Kesebir S, Diener E (2011) Income inequality and happiness. Psychol Sci 22(9):1095–1100
Organization for Economic Co-operation and Development (2016) Terms of reference: OECD Project on the distribution of household incomes. Organization for Economic Co-operation and Development, Paris, France
Osberg L (2017) On the limitations of some current usages of the Gini Index. Rev Income Wealth 63(3):574–584
Philippine Statistics Authority (2021) Proportion of Poor Filipinos Registered at 23.7 Percentin the First Semester of 2021. Manila, Republic of the Philippines: Philippine Statistics Authority. Accessible online: https://psa.gov.ph/poverty-press-releases/nid/165535
Pickett KE, Wilkinson RG (2015) Income inequality and health: A causal review. Soc Sci Med 128:316–326
Piketty T (2014) Capital in the twenty-first century. Harvard University Press, Cambridge, MA
Ravallion M (2020) On measuring global poverty. Ann Rev Econ 12:167–188
Ravallion M, Chen S (2019) Global poverty measurement when relative income matters. J Public Econ 177:104046
Sánchez-Jankowski M (2008) Cracks in the pavement: social change and resilience in poor neighborhoods. University of California Press, Berkeley, CA
Sen AK (1973) On economic inequality. Clarendon Press, Oxford
Sen A (1982) Poverty and famines: an essay on entitlement and deprivation. Oxford University Press, Oxford
Sen A (1983) Poor, relatively speaking. Oxf Econ Paper 35(2):153–169
Sen A (2008) The idea of justice. J Hum Dev 9(3):331–342
Article MathSciNet Google Scholar
Shildrick T (2018) Lessons from Grenfell: poverty propaganda, stigma and class power. Sociol Rev 66(4):783–798
Social Indicators of Hong Kong (2022) Gini Coefficient. Social Indicators of Hong Kong, Hong Kong, China, https://www.socialindicators.org.hk/en/indicators/economy/11.6 Accessible online
Stroh DP (2020) Overcoming the systemic challenges of wealth inequality in the US. Found Rev 12(4):35–49
MathSciNet Google Scholar
World Bank (2021a) Gini Index. World Bank, Washington, DC, https://data.worldbank.org/indicator/SI.POV.GINI?locations=PH Accessible online
World Bank (2021b) Poverty & equity brief: India. World Bank, Washington, DC, https://databank.worldbank.org/data/download/poverty/987B9C90-CB9F-4D93-AE8C-750588BF00QA/SM2021/Global_POVEQ_IND.pdf Accessible online
Van Gelder S (Ed.) (2011) This changes everything: Occupy Wall Street and the 99% movement. Berrett-Koehler Publishers, Oakland, CA
Walker R, Yang L (2021) China’s move to measuring relative poverty: implications for social protection. ILO Working Paper 23. International Labour Organization
Wan G, Hu X, Liu W (2021) China’s poverty reduction miracle and relative poverty: focusing on the roles of growth and inequality. China Econ Rev 68:101643
Yitzhaki S (1979) Relative deprivation and the Gini coefficient. Q J Econ XCIII:321–324
Zagorski K, Evans MD, Kelley J, Piotrowska K (2014) Does national income inequality affect individuals’ quality of life in Europe? Inequality, happiness, finances, and health. Soc Indicat Res 117(3):1089–1110
Zhang C, Xu Q, Zhou X, Zhang X, Xie Y (2014) Are poverty rates underestimated in China? New evidence from four recent surveys. China Econ Rev 31:410–425
Download references
Acknowledgements
This research was funded by a Departmental Start-up Grant (No. P0040982) from the Department of Applied Social Sciences at the Hong Kong Polytechnic University.
Author information
Authors and affiliations.
Department of Applied Social Sciences, The Hong Kong Polytechnic University, 11 Yuk Choi Road, Hung Hom, Kowloon, Hong Kong
You can also search for this author in PubMed Google Scholar
Corresponding author
Correspondence to Anson Au .
Ethics declarations
Competing interests.
The author declares no competing interests.
Ethical approval
This article does not contain any studies with human participants performed by any of the authors.
Informed consent
Additional information.
Publisher’s note Springer Nature remains neutral with regard to jurisdictional claims in published maps and institutional affiliations.
Rights and permissions
Open Access This article is licensed under a Creative Commons Attribution 4.0 International License, which permits use, sharing, adaptation, distribution and reproduction in any medium or format, as long as you give appropriate credit to the original author(s) and the source, provide a link to the Creative Commons license, and indicate if changes were made. The images or other third party material in this article are included in the article’s Creative Commons license, unless indicated otherwise in a credit line to the material. If material is not included in the article’s Creative Commons license and your intended use is not permitted by statutory regulation or exceeds the permitted use, you will need to obtain permission directly from the copyright holder. To view a copy of this license, visit http://creativecommons.org/licenses/by/4.0/ .
Reprints and permissions
About this article
Cite this article.
Au, A. Reassessing the econometric measurement of inequality and poverty: toward a cost-of-living approach. Humanit Soc Sci Commun 10 , 228 (2023). https://doi.org/10.1057/s41599-023-01738-3
Download citation
Received : 30 November 2022
Accepted : 28 April 2023
Published : 11 May 2023
DOI : https://doi.org/10.1057/s41599-023-01738-3
Share this article
Anyone you share the following link with will be able to read this content:
Sorry, a shareable link is not currently available for this article.
Provided by the Springer Nature SharedIt content-sharing initiative
This article is cited by
How socio-economic inequalities cluster people with diabetes in malaysia: geographic evaluation of area disparities using a non-parameterized unsupervised learning method.
- Kurubaran Ganasegeran
- Mohd Rizal Abdul Manaf
- Muhammad Faid Mohd Rizal
Journal of Epidemiology and Global Health (2024)
Quick links
- Explore articles by subject
- Guide to authors
- Editorial policies

- Understanding Poverty

Poverty Reduction a Win-Win
Climate change adaptation and poverty reduction go hand in hand, a new World Bank book argues. So why not kill two birds with one stone?
Publication

Inequality in the Labor Market
The World Development Report of 2013 measures, perhaps for the first time, inequality of opportunity to labor market outcomes in a discrete setting. It focuses on Europe and Central Asia.
You have clicked on a link to a page that is not part of the beta version of the new worldbank.org. Before you leave, we’d love to get your feedback on your experience while you were here. Will you take two minutes to complete a brief survey that will help us to improve our website?
Feedback Survey
Thank you for agreeing to provide feedback on the new version of worldbank.org; your response will help us to improve our website.
Thank you for participating in this survey! Your feedback is very helpful to us as we work to improve the site functionality on worldbank.org.
- Original Article
- Open access
- Published: 02 November 2023
Job market polarization and American poverty
- Abu Bakkar Siddique ORCID: orcid.org/0000-0002-9964-7511 1
Journal for Labour Market Research volume 57 , Article number: 30 ( 2023 ) Cite this article
1786 Accesses
1 Citations
2 Altmetric
Metrics details
The article posits that the puzzles of stagnating poverty rates amidst high growth and declining unemployment in the United States can be substantially explained by polarized job markets characterized by job quality and job distribution. In recent decades, there has been an increased number of poor-quality jobs and an unequal distribution of jobs in the developed world, particularly in the United States. I have calculated measures of uneven job distribution indices that account for the distribution of jobs across households. A higher value of the uneven job distribution indices implies that there are relatively large numbers of households with multiple employed people and households with no employed people. Similarly, poor-quality jobs are those jobs that do not offer full-time work. Two-way fixed-effect models estimate that higher uneven job distribution across households worsens aggregated poverty at the state level. Similarly, good-quality jobs help households escape poverty, whereas poor-quality jobs do not. This paper suggests that eradicating poverty requires the government to direct labor market policies to be tailored more toward distributing jobs from individuals to households and altering bad jobs into good jobs, rather than merely creating more jobs in the economy. This paper contributes by elaborating on relations of employment and poverty, addressing employment quality and distribution, and providing empirical evidence.
1 Introduction
Poverty in the United States is measured by a threshold, and people fall into and escape poverty for many reasons. Many consider employment as the primary policy solution to all forms of poverty, accounting for both falling into and out of poverty (Middleton and Loumidis 2002 ; Saunders 2006 ; Krishna 2007 ). Footnote 1 In the recent pre-pandemic time, the United States reached its highest level of employment in 50 years, with low unemployment. By many metrics, the job market was doing well (Kelly 2019 ). However, poverty in America has remained stagnant for many decades (Desmond 2018 ). What kind of jobs are available? Do they not pay enough to live on? Are these jobs not equally distributed? Footnote 2
Although it may seem logical that higher employment rates would reduce poverty, as the income and consumption of poor people largely come from their work, the relationship between employment and poverty is not straightforward. While some scholars assume that increasing employment reduces poverty (Cantillon et al. 2003 ), others believe that creating more non-subsidized jobs may lead to a higher number of low-paid jobs, leaving more working people in poverty (Kalleberg 2009 , 2011 ). In other words, when employment growth occurs at the cost of wage reduction it does not eradicate poverty (Sloane and Theodossiou 1996 ; Gardiner and Millar 2006 ). Moreover, poverty reduction may not be realized if employment growth occurs in sectors that do not accommodate many poor people (Ravallion and Datt 2002 ; Satchi and Temple 2009 ) or in industries that require higher levels of skills (Loayza and Raddatz 2010 ) and capital (Siddique 2016 ). Furthermore, poor people cannot always afford to be unemployed, and they are not necessarily unemployed people (Visaria 1981 ; Saunders 2002 ). Despite this complexity, there is little research exploring the relationship between employment and poverty, leading to a lack of evidence-based policy actions in this area. Specifically, there is a need for scientific empirical evidence on the relationship between job distribution, job quality, and poverty, and this paper makes an important contribution in these fields.
Despite strong economic performances, poverty rates in the United States have remained stubbornly high, and past attempts to explain this phenomenon have fallen short (Hoover et al. 2004 ; Hoynes et al. 2006 ; Edelman 2013 ; Meyers 2014 ; Pacas and Rothwell 2020 ). In this paper, I argue that job market polarization is a major factor that perpetuates poverty, even during periods of high employment and low unemployment. When jobs are distributed unequally across households and are of poor quality, with no full-time work available, the benefits of job growth fail to reach those who need them the most. This means that the “trickle-down” economy may not work for majority of poor Americans, who face both poor job quality and uneven job distribution. As a result, job growth in a polarized job market does not benefit jobless households, and poverty remains stagnant. Recent data shows that employment-rich households are experiencing faster employment growth than employment-poor households (England 2017 ), exacerbating the problem. In a more equitable, non-polarized job market, the unemployment rate would be the same for both individuals and households (Gregg et al. 2010 ).
The United States has not also succeeded in reducing poverty despite high employment rates due to the poor-quality of available jobs. The job market in the United States has become increasingly bifurcated, which has significant implications for poverty reduction strategies and outcomes. This trend started in the 1980s as the service sector began to replace the manufacturing sector and technology started playing major roles in the labor market (Autor and Dorn 2013 ; Goos et al. 2014 ; Salvatori 2018 ). Since then, Americans have faced an increase in low-paying jobs with few hours, little job security, and no entitled benefits. These poor-quality jobs are prevalent across multiple industries and have impacted the daily lives of millions of Americans. Several studies have documented this trend, including Herzenberg et al. ( 2000 ), Desmond and Gershenson ( 2016 ), Kelly ( 2019 ), Newman ( 2009 ), and Kalleberg ( 2009 , 2011 ). Contrastingly, while some industries like technological offer attractive salaries, promotions, benefits, and even equity in the company, they struggle to find a sufficient pool of skilled applicants to fill their job openings (Goos and Manning 2003 ; Salvatori 2018 ; Kelly 2019 ), contributing to job market polarization. Therefore, economic prosperity alone cannot effectively reduce poverty if it does not generate an adequate number of good-quality jobs (Odhiambo 2011 ; Page and Shimeles 2015 ). Unfortunately, the majority of recently available jobs are low-paying, part-time contract positions that does not provide healthcare or other benefits. The rise of these poor-quality jobs contributes to the growth of the working poor and inequality, Footnote 3 which could be a potential explanation for the persistent high levels of poverty in the United States.
In this research paper, the impact of uneven job distribution and poor-quality jobs on poverty rates in the United States is examined. A longitudinal dataset was constructed by aggregating household-level data to the state-level to match with state-level variables, and a two-way fixed effect model was applied to estimate their effects. The results indicate that the eradication of poverty in the United States may depend on redistributing jobs from individuals to households and on improving quality of jobs. Rather than solely focusing on creating more jobs, policymakers should prioritize transforming existing poor-quality jobs into good-quality ones and ensuring their equitable distribution. It is important to note that creating more jobs is not discouraged, only the creation of poor-quality jobs. Moreover, the findings suggest that a high-growth economy and high employment rates are unlikely to alleviate poverty.
This paper makes a significant contribution by expanding our understanding about the intricate relationship between employment and poverty and presenting novel empirical evidence. Notably, this analysis introduces a fresh perspective by focusing on the United States context, which distinguishes it from previous research that primarily examined the United Kingdom and other European economies. In addition, it opens up avenues for further research into the potential implications of issues related to job distribution and job quality, not only on poverty but also on various aspects of socio-economic well-being.
The following section will delve into the intricate relationships between family dynamics, employment, and poverty. Data, measuring key variables, and empirical strategy will provide detailed insights into data, the metrics used for key variables of interest, and chosen empirical methodologies. Results: two-way fixed effect model will unveil the findings, and, finally, in Sect. 5, I will wrap up this paper with a conclusion.
2 Job distribution, job quality, and poverty
2.1 family is central to avoid poverty.
In most societies, the risk of poverty is unevenly distributed, affecting specific groups such as ethnic minorities, single parents, and people with disabilities more significantly than others. Unexpected events, such as illness, can also lead to poverty (Flaherty et al. 2004 ; Gardiner and Millar 2006 ). Vulnerable individuals often employ strategies like living with family members or relying on state transfer benefits and tax credits to prevent falling into poverty. Gardiner and Millar ( 2006 ) conducted a study in British society and found that over 30% of low-paid workers can escape poverty by depending on the income of other family members. More than 60% rely on the income of their partners and other adults in the household. Approximately 8% of low-paid workers manage to avoid poverty by working long hours to compensate for their low earnings, while around 13% achieve this by combining incomes from state transfers. Living with other individuals plays a crucial role in helping many low-income individuals mitigate the impact of poverty. Pooling together all sources of income is effective, as even a family member with relatively low earnings can improve the overall financial well-being of the household. It's important to note that a single individual earning a decent income has the potential to lift the entire family out of poverty.
Over the past four to five decades, the family structure in the United States has undergone significant transformations, marked by a notable decrease in marriage rates, a decline in the proportion of children born within marriages, and a rise in the number of children born outside of wedlock (Cancian and Haskins 2014 ; Thiede et al. 2017 ). Simultaneously, there has been a substantial increase in women entering the labor market, while many men, particularly those with lower levels of education, have faced diminishing employment opportunities (Cancian and Haskins 2014 ; Binder and Bound 2019 ). These shifts in family structure have had a considerable impact on poverty through their influence on labor market opportunities. Increased participation of women in the workforce may have a poverty-reducing effect if they entered the labor market to compensate for limited family income. Data from the United States Census and the American Community Survey show that families headed by single females with children have consistently experienced an average poverty rate of around 40% over the past four to five decades. In contrast, families headed by married couples with children have maintained a poverty rate of less than 8% during the same period. Moreover, the poverty rate for married couples without children has been even lower, averaging less than 4%. Conversely, families headed by single males and single females have experienced poverty rates higher than 15% on average (Cancian and Haskins 2014 ). Thus, families play a crucial role in shielding many individuals from falling into poverty.
When a single female gets married, the household's needs are likely to increase. However, this marriage also introduces a second earning adult to the household, potentially reducing the risk of poverty. Similarly, if she joins a joint family with another earner, similar benefits can arise. This phenomenon is known as economies of scale, where each additional person added to a household results in less than proportional increases in needs (Cancian and Haskins 2014 ; Reyes 2020 ). The current state of individual and household employment is influenced by various factors, including modernization, the prevalence of nuclear families, the feminization of labor markets, and an increasing number of individuals pursuing tertiary education (Corluy and Vandenbroucke 2017 ; Thiede et al. 2017 ). Considering these trends in family structure, I hypothesize that the unequal distribution of jobs among households may explain the persistent high levels of poverty in the United States. Surprisingly, there have been no studies to date that have specifically explored the distribution of employment across households and its associated consequences on poverty in the country.
2.2 Jobs quality, labor market policies, and poverty
The gap between good and bad jobs is widening in the United States, representing another dimension of job market polarization. The availability of good jobs that offer fringe benefits is declining, while the number of bad jobs without such benefits is increasing. In non-regulated and non-competitive labor markets, both good jobs and bad jobs can coexist. Acemoglu ( 2001 ) suggests that in a laissez-faire equilibrium, the labor market is biased towards poor-quality jobs due to a phenomenon known as "hold-up." According to his search model (Acemoglu 2001 ), the presence of diverse job creation costs results in differentiated compensation for similar workers. In this market, employers and employees share rents, thus breaking the relationship between wages and marginal productivity. Additionally, employers fail to internalize the externalities arising from rent-sharing, which could otherwise be addressed through market allocation. Capital-intensive firms that have made substantial sunk investments are compelled to negotiate and create significant positive pecuniary externalities for workers. Consequently, these firms tend to create a scarcity of good jobs while simultaneously generating an excess number of bad jobs.
There are also several policy factors that play a crucial role in determining the quality of jobs in the market. These factors may include but not limited to inadequate social security programs, such as unemployment benefits, and the absence of minimum wage laws. Both policies can potentially incentivize workers to wait for better job opportunities, consequently reducing firms' profits from creating low-quality jobs and shifting overall job compositions (Carter 1995 ; Acemoglu 1996 ). In the United States, due to the limited prevalence of social security programs, most workers cannot afford to remain unemployed while waiting for better job offers. This results in an oversupply of labor in the market, prompting firms to shift towards a higher proportion of poor-quality jobs. On the contrary, if workers have the protection of unemployment insurance, the cost of waiting for a better job would be less burdensome. Therefore, social protection programs can potentially reduce the labor supply by increasing reservation wages (Marinescu and Skandalis 2021 ).
Similarly, the absence of a minimum wage requirement is also contributing to the growth of poor-quality jobs. Firms find it more profitable to offer poor-quality jobs when there are no higher minimum wage laws in place. Conversely, setting a higher minimum wage in the economy would have compelled firms to pay higher wages for poor-quality jobs, making them less economically profitable and encouraging firms to improve their job compositions (Bulow and Summers 1986 ; Carter 1998 ).
Poor-quality work may not alleviate poverty but rather perpetuate it. To comprehend the implications for poverty, in addition to the economic theories discussed above, it is necessary to examine the policy changes that have promoted a flexible labor market over the last five decades. The Regulatory Flexibility Act (RFA) of 1980 was adopted on a bipartisan basis, reflecting liberal economic principles aimed at deregulating laws and enhancing business power with an aim to improve innovation and production. These flexible labor policies have encouraged the creation of low-quality jobs while reducing the number of high-quality jobs. Sectors that offer low-quality jobs experience high employee turnover (Albrecht and Vroman 1992 ; Carter 1998 ). During economic downturns, these positions can easily be terminated without incurring costs, only to be rehired when production needs increase (Kalleberg 2000 ; Van Arsdale 2013 ), offer no job security to these workers. In other words, the underlying logic of creating low-quality jobs is to pay only for the units of work performed by these positions, and thus, this policy change has direct implications for poverty.
Within these flexible labor law regimes, new third-party staffing firms (e.g., Kelly Services Inc., Robert Half, Toptal, etc.) have emerged to manage this flexible workforce, creating a triangular relationship and operating their businesses at the expense of labor wages (Van Arsdale 2013 ). Major corporations like Amazon, Microsoft, and Sheraton often outsource numerous positions to independent staffing companies, predominantly offering jobs with unpredictable schedules. Surprisingly, approximately 40% of hourly employees receive their work schedules only a week or less in advance (Desmond 2018 ). It is common to see cleaners, reception assistants, and security officers working at prominent corporations, yet these workers are not directly employed by these companies. Instead, they are engaged through independent contractors who take a share of their wages. These intermediary companies not only deduct a portion of the employees' wages offered by the host companies but also deny workers any opportunity for career advancement within the host company, regardless of their hard work. Consequently, many workers under these contracts do not even receive their full wages. By outsourcing these positions to independent contracting agencies, large organizations evade their responsibilities to provide healthcare and other security benefits.
In this triangular relationship, staffing firms negotiate wage and work conditions between employing firms and employees, often leaving employees with little to no voice. Consequently, employees frequently reject job offers, resulting in an increased pool of unemployed individuals, further enhancing labor market flexibility, and boosting company profit margins. The consequence of a flexible labor market is increased poverty, as a large pool of flexible labor enables staffing companies to exploit employees, leading to more individuals earning poverty-level wages and a disproportionate transfer of resources to businesses. The decline in regular employment by host companies and the growth in employment through these staffing firms are strategic policies employed by businesses to avoid the costs associated with adding people to their payrolls, such as health insurance, bonuses, and other human resource expenses, particularly during economic recessions (Van Arsdale 2013 ). The poverty implications of this changing employment era have not been thoroughly studied yet.
The growth of poor-quality jobs is also linked to various recent developments, including technological advancements, changes in work arrangements, the expansion of service sectors, and the decline of industrial employment. It is further associated with shifts in corporate governance and employer strategies (Kalleberg 2011 ). Moreover, it is intertwined with emerging trends such as privatization, marketization, and individualization (Keune 2013 ), along with the declining influence of trade unions (Farber and Levy 2000 ). Consequently, poverty reduction efforts have been stalled.
3 Data, measuring key variables, and empirical strategy
I utilized publicly available data in this study, primarily drawing from the American Community Survey (ACS). The ACS provides individual-level data with household and geographic codes, enabling precise estimates at the local administrative unit level and tracking long-term trends. This dataset is collected by the Census Bureau and is representative of the entire USA population, based on a 1% sample. To align with state-level macro-variables, the data was initially aggregated to the household level and subsequently to the state level. The household-level employment status data is derived from the ACS, while individual unemployment data at the state level is sourced from the Department of Labor and Training. Furthermore, the ACS data was instrumental in measuring households with good-quality jobs and poor-quality jobs. The ACS survey questionnaire includes multiple questions that inquire about the employment status of household members, facilitating the classification of households into full-time and part-time employment categories.
Other macroeconomic variables, such as GDP per capita and the estimated Theil inequality index, were acquired from the US Bureau of Economic Analysis (BEA). Data related to government expenditure and revenue were obtained from the National Association of State Budget Officers (NASBO) community. Human capital and educational attainment data were sourced from the US Census Bureau. Regarding poverty measures, I employed the official poverty measurement utilized by the Federal Government, which is based on the US Census Bureau's poverty threshold of $20,212 for a family consisting of two adults and one child in 2018. This poverty threshold has been adjusted for the number of children, meaning that households with more than one child have a higher poverty threshold. Table 1 in the paper presents the descriptive statistics of the data after aggregating it to the state level.
I aggregated individual and household-level data to the state level for several compelling reasons. Firstly, income is a flow variable that is commonly used to measure poverty, while employment / unemployment status represents the labor market conditions at a specific point in time, rendering it a stock variable. Consequently, if an individual is interviewed during a period of unemployment but remains employed for the rest of the year, their annual income may surpass the poverty line (Saunders 2002 ). Moreover, income is typically reported annually, whereas employment status is not regularly reported on an annual basis. Therefore, analyzing the impact of employment status on poverty without aggregation can lead to misleading results.
Secondly, employment status is usually analyzed at the individual level, whereas poverty measures consider the combined income of the entire family to determine the poverty level. As discussed earlier in this paper, an individual may have low or zero income but not meet the criteria for poverty according to Federal guidelines, as other family members may have higher earnings. Income within a household is shared, and it is the collective income that determines whether the individual or family qualifies for welfare benefits. Hence, measures of poverty and employment should ideally be aggregated at the family level over the entire year.
Thirdly, microdata sources like ACS exhibit variation over time but do not follow a panel format, which means they do not observe the same individual or household over an extended period. Without panel data, it is not possible to apply fixed-effect (FE) models, which allow for controlling observable and unobservable characteristics that remain constant over time (more details of empirical strategies are in Empirical strategies ). The two-way FE model is more suitable for statistical inference than the pooled cross-section model (Lechner et al. 2016 ). Lastly, public welfare expenditure is a crucial determinant of poverty. However, individual or household-level records in the ACS do not provide information about the benefits received from public welfare programs, such as cash assistance. Aggregating the data at the state level is necessary because states are the primary authorities responsible for disbursing these types of payments. Additionally, states play a crucial role in decision-making regarding most poverty reduction programs.
3.2 Measuring the index of uneven job distribution
The index of uneven job distribution indicates an increasing concentration of jobs at the individual level and a decreasing concentration of jobs at the household level. This index essentially reflects unequal job distribution between individuals and households, as opposed to the usual measures of job polarization in occupation, pay, and sector, as explained elsewhere (Goos and Manning 2003 ; Salvatori 2018 ; Jaimovich and Siu 2020 ). Footnote 4 In this paper, the index of uneven job distribution is measured using the method of Gregg & Wadsworth ( 2008 ), which discerns the discrepancy between individual-level joblessness and household-level joblessness. When a state's population consists of individuals 'i', the total number of households ‘H’ consists of individual household ‘h’, and person 'k' resides within household 'h', the total population 'P' can be expressed as:
When an individual person living in a household has no job, a binary outcome value of 1 ( \({n}_{ih}=1\) ) is assigned, and when an individual person living in a household has a job, a binary outcome value of 0 ( \({n}_{ih}=0\) ) is set. Therefore, the individual-level joblessness rate in the population will be:
Let's now consider household-level joblessness, where households can host both jobless individuals and individuals with a job. Such joblessness can now be grouped by households. When a household does not have any people with a job, a binary outcome value of 1 ( \({m}_{h}=1\) ) is assigned, and when a household has at least one person with a job, a binary value of 0 ( \({m}_{h}=0\) ) is set. Therefore, the household-level joblessness will be:
Here, 'm' essentially reflects the number of households with a value of 1 for \({m}_{h}\) weighted by the total number of households ' H '. A simple example will be highly useful here to understand the distinction between joblessness at the individual level and at the household level, and why this distinction should matter for poverty prevalence. Consider a small economy with only two households, each consisting of two people, for a total of four people. The economy offers only two jobs, leaving only two possible scenarios. In the first scenario, one person from each household is unemployed, denoted by \(N=[\left\{\mathrm{1,0}\right\}, \left\{\mathrm{1,0}\right\}]\) , with an associated joblessness rate of 50%. In the second scenario, both working individuals belong to the same household, leaving the other household with no employed individuals, denoted by \(N=[\left\{\mathrm{1,1}\right\}, \left\{\mathrm{0,0}\right\}]\) , with an associated joblessness rate of 50%. Despite both scenarios having the same unemployment rate, they have different rates of joblessness at the household level. In the first scenario, no households are jobless, whereas in the second scenario, 50% of households are jobless.
This illustrates how aggregated individual-level job statistics fail to reflect the actual distribution of jobs across households, much like how typical income distribution measures fail to capture the necessary details of income distribution. To address this, we can measure the degree of uneven job distribution across households by calculating the difference between the actual rate of jobless households and the rate predicted under a counterfactual scenario in which jobs are randomly distributed among the working-age population. This difference can offer insight into the extent to which households experience joblessness beyond what would be expected under random job distribution.
Assuming that all other factors remain constant, it is more likely for fewer individuals to be present in a household where all occupants are employed if the family structure is nuclear, as opposed to extended. This is because households are categorized based solely on their size, and as family sizes decrease, the number of households without any employed individuals is expected to increase. Consequently, the size of workless households can be attributed to a combination of the individual-level unemployment rate and the number of individuals in the household.
where \({uneven job distribution}_{it}\) represents the extent of uneven job distribution in state i for year t . Meanwhile, \({JL}_{it}\) denotes the fraction of households that do not have any employed individuals in state i for year t , regardless their size of households. \({JL}_{it}^{e}\) represents the expected rate of jobless households in state i for year t if jobs were randomly distributed. We can present a counterfactual rate of households without any employed individuals, denoted as \({\hat{J} }_{k}\) for households with k as follows:
Assuming that joblessness is randomly distributed, every individual adult in a household \(k\) has an equal chance of being unemployed. Consequently, the probability of observing an adult in a jobless household (counterfactual rate) should be the same as the observed unemployment rate at the individual level. Similarly, the likelihood of a household with two adults being jobless would be twice the individual unemployment rate (Gregg and Wadsworth 2008 ). To benchmark the probabilities of equal job distribution, we can consider it as having an intuitive appeal similar to the criterion of income equality used in the Lorenz curve. Finally, we can aggregate the counterfactual households with no employed individuals and weigh them by the population, ( \({s}_{k}\) ), of households of size k :
So, the uneven job distribution is the distinction between observed and counterfactual jobless households.
The measure of uneven job distribution discussed here does not have any normative implications. A higher index of uneven job distribution indicates a larger proportion of households are jobless and greater job distribution inequality. Since job numbers are generally limited, positive values in the index can be considered as reflecting ‘Matthew effects,’ wherein additional jobs are concentrated among households who already have employment, rather than being spread out more evenly across population, particularly households with all members are jobless. Footnote 5 Conversely, negative value of uneven job distribution index suggests that there are fewer jobless households than would be expected if jobs were randomly distributed, which can be seen as a form of solidarity. If the expected and observed rates of jobless households are identical, the index of uneven job distribution should have a value of zero.
We never know the counterfactual jobless household rate. While the individual unemployment rate can be a reasonable proxy for the counterfactual jobless households, this will still be an extremely conservative measure of uneven job distribution. This is because there are more jobs available than the number of households in the country. Consider a state with a population of 1 million; there will be a million households if each household only has one person. If the state's unemployment rate is 5%, then both the individual unemployment rate and household joblessness rate will also be 5%. Since job numbers are generally fixed at a point in time, a negative or positive uneven job distribution index will only emerge when two or more individuals form a family, and some of them are employed while others are not. If one household has multiple jobholders, it comes at the expense of another family that has no employed individuals. The value of the index of uneven job distribution increases as more households have no employed individuals while others have multiple jobholders.
3.3 Description of uneven job distribution measures and poverty with data from United States
Let’s consider the data provided by the United States Census Bureau for 2018. According to their report, the United States had a population of 326.68 million people, with 60% of them falling within the working-age bracket, totaling approximately 196.01 million individuals. With an average family size of 2.53, we can estimate that there were around 129.13 million households in the country. In comparison to the number of households, there were approximately 197.31 million available jobs in the country for the year 2018. If these jobs were randomly distributed, every household should ideally have at least one employed member (as shown in Table 2 ). Therefore, the percentage of workless households can serve as a measure of uneven job distribution, which, while still conservative, is less so than the measure expressed in Eq. ( 3 ). In this paper, I will examine the association between both uneven job distribution measures and the poverty rate. The less conservative uneven job distribution measure will be referred to as Uneven Job Distribution Index 1 (UJD-1), while the more conservative measure mentioned in Eq. ( 3 ) will be known as Uneven Job Distribution Index 2 (UJD-2).
With an average family size of 2.53, we can estimate that there were around 129.13 million households in the country. In comparison to the number of households, there were approximately 197.31 million available jobs in the country for the year 2018. If these jobs were randomly distributed, every household should ideally have at least one employed member (as shown in Table 2 ). Therefore, the percentage of workless households can serve as a measure of uneven job distribution, which, while still conservative, is less so than the measure expressed in Eq. ( 3 ).
In this paper, I will examine the association between both measures of uneven job distribution and the poverty rate. The less conservative measure of uneven job distribution will be referred to as Uneven Job Distribution Index 1 (UJD-1), while the more conservative measure mentioned in Eq. ( 3 ) will be known as Uneven Job Distribution Index 2 (UJD-2). Figures 1 and 2 provide valuable insights into job distribution measures at the national level as well as within and across states. Figure 1 demonstrates a noticeable divergence between the unemployment rate at the individual level and the prevalence of jobless households from 2008 to 2018. The state level heterogeneities in the same divergence trend are presented in the Appendix A.
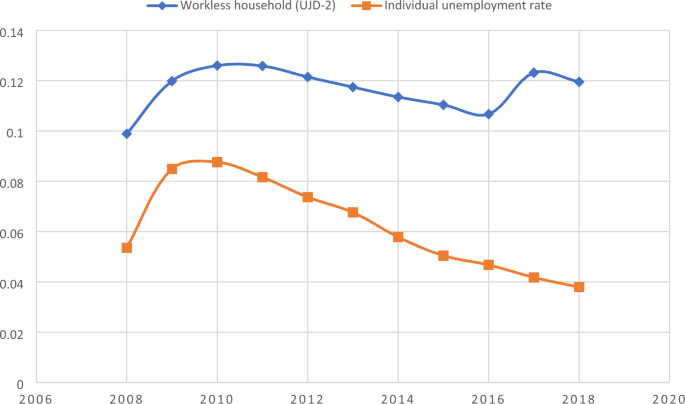
Workless households and individual unemployment rate in the United States. This was calculated using American Community Survey (ACS) and Bureau of Labor Statistics (BLS) data
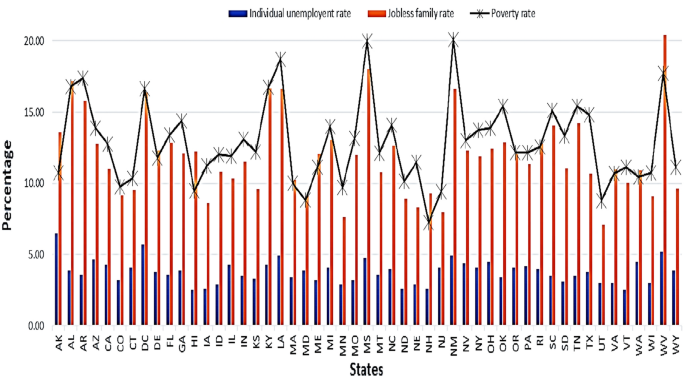
Employment and poverty across states for the year 2018. The data sources are ACS and BLS
The main concern highlighted by these illustrations is the increasing rate of workless households (UJD-2) in the United States despite a stable decrease in the individual-level unemployment rate. Consequently, there has been a growing disparity between the rates of unemployment for individuals and jobless households (UJD-2) in the country. This divergence indicates that job growth in the United States over the past few decades has not benefitted workless households. States with higher employment growth have primarily witnessed benefits for individuals or households with already employed members, resulting in a lack of improvement in job distributions at the household level and, in fact, worsening the situation. Similar trends of employment growth predominantly benefiting households with employed individuals have been observed not only in the United States but also in the UK, Netherlands, and other developed economies (Gregg and Wadsworth 1994 , 2003 ; Beer 2001 ; Cantillon et al. 2003 ; Corluy and Vandenbroucke 2017 ).
While the growing gap in employment distribution between households and individuals may pose various issues concerning labor market performance in the country, the rising rate of workless households (even if it is a stagnant one) carries significant implications for poverty reduction strategies. As mentioned earlier, in an ideal scenario with a normative world of random employment distribution, the unemployment rate at the individual level and the rate of joblessness in households should be identical, and more importantly, with zero joblessness at the household level in the United States. Therefore, the values presented in Fig. 1 represent the extent of uneven job distribution in the United States. Despite positive job market outcomes at the individual level, the situation at the household level has been deteriorating. In this paper, I propose the hypothesis that this higher index of uneven job distribution may be responsible for the increased poverty levels in the country.
Figure 2 showcases the percentage of workless families and individual unemployment rates in relation to the poverty rate across states for the year of 2018. It also highlights the disparity between individual-level unemployment and workless households across different states. Notably, the individual-level unemployment rate is significantly lower than the rate of workless households. Several states, including West Virginia, Mississippi, Alabama, Kentucky, New Mexico, Louisiana, and others, exhibit considerably higher index of uneven job distribution, indicating a pronounced discrepancy between individual-level unemployment and workless households. Intriguingly, these states with higher rates of jobless families are also characterized by higher poverty rates, as depicted in Fig. 2 . In contrast, the states of Utah, Minnesota, New Jersey, Nebraska, Iowa, North Dakota, Wisconsin, Maryland, Colorado, New Hampshire, Connecticut, and others show the lowest index of uneven job distribution. These states demonstrate a more balanced distribution of job opportunities, with a smaller gap between individual-level unemployment and workless households, and lower poverty rate.
3.4 Measure of job quality
Measuring job quality, particularly when identifying households with poor-quality jobs, presents complex challenges. While it's true that low-paying jobs are often categorized as poor-quality jobs, as conventionally defined in economics (Acemoglu 2001 ), this criterion alone doesn't provide the most comprehensive measure of job quality. Job quality is multifaceted (Findlay et al. 2013 ), and the International Labor Organization (2019) offers a more comprehensive definition of a high-quality job, encompassing factors such as higher pay, job security, safety, work-life balance, fairness in employment, social protections, and various other socio-economic considerations. Additionally, scholars like Stecy-Hildebrandt et al. ( 2019 ) and Adamson and Roper ( 2019 ) emphasize additional indicators, including fringe benefits, job security, and favorable income trajectories as characteristics of high-quality jobs. It's important to recognize that job quality is also subjective and influenced by individual perceptions (Clarke 2015 ). In some cases, even a low-paying job can be viewed as a high-quality job if it includes in-work benefits, job security, full-time employment, and other favorable aspects, particularly if it is perceived as such. Many employees prioritize factors like job security, as it can have a more substantial positive impact on their overall well-being than wages alone.
This paper defines a 'good job' as one held by a household with at least one full-time worker who commits to 35 h or more of work per week, and I refer to such households as having 'good jobs.' Conversely, it defines a 'bad job' when a household has only part-time worker(s) putting in less than 35 h of work per week and has no full-time worker, and I refer to such households as having 'bad jobs.' This classification of full-time and part-time employment status at the household level can serve as proxies for assessing job quality at the household level. Moreover, it is expected that part-time and full-time work status is associated with other indicators of job quality such as wages, and it provides suitable quantitative measurement for regression analysis in this paper. While it can be argued that some women and students voluntarily choose part-time positions to allocate more time to family responsibilities (Walsh 1999 ; Hill et al. 2004 ; Pech et al. 2021 ) and education, it's crucial to recognize here that the measurement used in this paper operates at the household level, where it is reasonable to expect that at least one member of the household should be willing to take on a full-time role, unless the household is a single-parent household or its member(s) require special care (e.g., disability).
Furthermore, there is strong evidence to suggest that a significant proportion of part-time jobs are involuntary, with individuals preferring full-time contracts if they were given the opportunity (Tilly 1991 , 2010 ; Kalleberg 2009 ; Kauhanen and Nätti 2015 ). Involuntary part-time jobs represent a form of underemployment, as these part-time workers are actively seeking full-time positions but have not been able to secure them. Notably, part-time workers constitute more than one-fifth of the total workforce in the United States, and their presence in the labor market has been steadily increasing since 1970 (Tilly 1991 ; Fullerton et al. 2020 ). This growth in involuntary part-time employment is primarily driven by employer demands for flexible scheduling, cost minimization, and their predictability of available labor forces, rather than worker’s preferences (Tilly 1991 , 2010 ; Kalleberg 2009 ).
Two significant theories, neoclassical and institutionalist, can potentially elucidate the issue of job composition in terms of part-time and full-time employment, particularly regarding involuntary part-time positions. The neoclassical approach can be extended in two ways—building a microeconomic foundation to analyze market constraints and addressing the lack of aggregate demand in the macroeconomic environment. Bulow and Summers ( 1986 ), who developed the "efficiency wage" model based on a microeconomic foundation, suggest that firms pay full-time workers higher wages than their marginal product, while part-time workers receive lower wages at the market rate. The higher pay for full-time workers is efficient because it disincentivizes them from shirking, leading to lower employee turnover and increased productivity. In contrast, part-time employees, who face low wages and high turnover, become less productive even though they may be identical to full-time employees in all other aspects. In such situations, involuntary part-timers may consider the loss of their job and associated income to be significantly more detrimental than accepting lower wages. Full-timers are also treated as insiders, while part-timers are seen as outsiders (Lindbeck and Snower 1986 ). Insiders have the power to threaten to leave, leading to higher costs for firms related to hiring and training new employees, but part-timers lack such bargaining power.
In macroeconomic contexts, involuntary part-time employment or underemployment emerges as a natural consequence. During economic downturns, part-time employment may be the preferred choice for employers, enabling them to retain skilled employees at reduced wages while reducing the workforce. For instance, the recent COVID-19 pandemic vividly exemplified that, as part-time workers were more susceptible to job loss, full-time workers were better equipped to retain their employment. In such economic downturns, workers often find that accepting lower wages is a more viable option than losing their jobs, particularly in the absence of unemployment insurance.
The institutionalist approach, while acknowledging the logic of neoclassical perspectives, underscores that part-time employment is distinguished not only by hours worked but also by various other attributes. Institutional theories contend that a fundamental shift in the organizational environment, including elements such as labor unions and policies, is necessary to transition between different job compositions rather than merely adjusting to economic variables within the same framework (Woodbury 1987 ; Tilly 2010 ). In contrast to the neoclassical approach, which assumes that market actors act rationally and that labor market outcomes are determined by the standard demand–supply model, the institutional approach recognizes that a range of labor market outcomes is possible and that these outcomes are shaped by socially imposed norms and traditions. Given the tradition of strong capitalism and a history of limited policy regulations in place in the United States, employers enjoy greater flexibility in crafting job configurations that maximize their profit margins compared to other welfare-oriented nations.
These part-time positions consistently come with a wage penalty (Hirsch 2005 ; Baffoe-Bonnie and Gyapong 2018 ; Gallego-Granados 2019 ) and provide limited opportunities for career advancement, resulting in high turnover rates (Tilly 1991 ; Sloane and Theodossiou 1996 ). Part-time work status also implies limited access to social protections due to shorter lengths of service (Stecy-Hildebrandt et al. 2019 ) and is generally associated with lower rates of unionization (Anderson et al. 2006 ). Research has also demonstrated that the prevalence of part-time jobs, primarily driven by neoliberal market restructuring, contributes to wage disparities across race (Wilson and Roscigno 2016 ) and gender (Fuller 2005 ). In addition to the reduced income potential associated with part-time positions, studies by McDonald et al. ( 2009 ) have raised several other concerns, including limitations on career progression, restricted access to high-status roles, increased workloads, challenging work environments, and related issues. For these various reasons, part-time positions are a fair representation of poor-quality jobs, while full-time positions signify good-quality jobs.
Hence, it is reasserted here that economic prosperity alone may not effectively alleviate poverty unless it results in a sufficient number of good-quality jobs with full-time contracts for households. An increase in the number of families with good-quality jobs is expected to play a pivotal role in poverty eradication in the United States. The combined remuneration package associated with full-time employment has the potential to lift a household above the poverty threshold. Conversely, households relying solely on part-time jobs are likely to contribute to an increase in poverty rates across the states. These household-level indicators of job quality are anticipated to have a direct and significant impact on the economic status of people living in poverty, surpassing the influence of broader macro-level determinants, such as overall economic growth and employment levels.
3.5 Empirical strategies
The identification strategy of this paper is to estimate Eqs. 4 and 5 using the two-way fixed effect (TWFE) method for a strong balanced panel data that spans from 2008 to 2018. With a large number of independent clusters of observations (i.e. states), the coefficient of our variable of interests can be consistently estimated using a TWFE regression specification, and by clustering standard errors the conclusion will be an asymptotically valid inference (Roth et al. 2023 ).
Where \({Y}_{it}\) represents the poverty rate, \({UJD}_{it}\) represents either the uneven job distribution index-1 or index-2, and \({JQ}_{it}\) represents either the measure of good-quality jobs or poor-quality jobs in state i for year t . \({X}_{it}\) is a vector of characteristics that vary over time at the state level, including GDP per capita, GDP growth rate, state population size, regional inequality, per capita tax revenue, intergovernmental transfers, population demographics (such as age groups, immigration/citizenship status, and human capital), and others. \({\varepsilon }_{it}\) is the random error term. By applying the two-way fixed effect method, I examine the within-state variation to estimate the impact of polarized job distribution and job quality on the poverty rate.
However, it is important to note that, given our observational data, this analysis does not intend to interpret the \(\beta\) coefficient as a strictly causal relationship between employment variables and the poverty rate outcome. The state fixed effect, \({\delta }_{i}\) , estimate accounts for both observable and unobservable time-invariant factors that may impact poverty rates, such as colonial history and geographic locations. The year fixed effect, \({\mu }_{t}\) , captures any unusual time trends, such as financial crises, that may also influence the poverty rate. However, the two-way fixed-effect model cannot address problems that may arise if employment distribution and quality and poverty are simultaneously related. For instance, it may not account for scenarios where higher poverty leads to either higher or lower uneven job distribution or job quality, thus failing to discern any such reverse effect. To tackle this issue, we would require an instrument that is strongly correlated with poverty but has no association with our employment variables, and then apply an instrumental variables regression approach. Unfortunately, our data lacks such an instrumental variable that fulfills both of these conditions for a good instrument. This concern regarding internal validity remains unaddressed in this paper.
Furthermore, while the two-way fixed-effect model accounts for observed and unobserved time-invariant factors, it does not consider omitted time-varying factors, such as union and firm density in the local area. These factors could not be controlled for due to the absence of long-panel data used in this paper. Similarly, measurement errors pose potential threats to estimating unbiased coefficients. For instance, using part-time and full-time working status at the household level as proxies for job quality may not accurately capture the true measures of job quality and may introduce bias into the estimates. If alternative measures of job quality in terms of pay and benefits were available, the results might differ from those presented in this paper, thus posing a threat to the external validity of these results in this paper.
4 Results: two-way fixed effect model
Figure 3 presents the scatterplot matrix depicting the relationships among the key variables of interest. Both the less conservative and more conservative measures of uneven job distribution are positively associated with the poverty rate. Conversely, there exists a strong negative relationship between poverty and good-quality jobs (households with full-time workers), while the relationship between poverty and poor-quality jobs (families with only part-time workers) is positive. All of these relationships confirm our earlier predictions in this paper that an unequal job distribution across households is a significant predictor of persistent poverty in the United States. Similarly, bad jobs also serve as a predictor of higher poverty, whereas good jobs act as a predictor of lower poverty in the country. While these scatterplots provide a visual representation of the basic strength, direction, and nature of the relationship between poverty and variables related to job distribution and quality, the following sections delve into the empirical findings obtained through the application of two-way fixed effect methods.
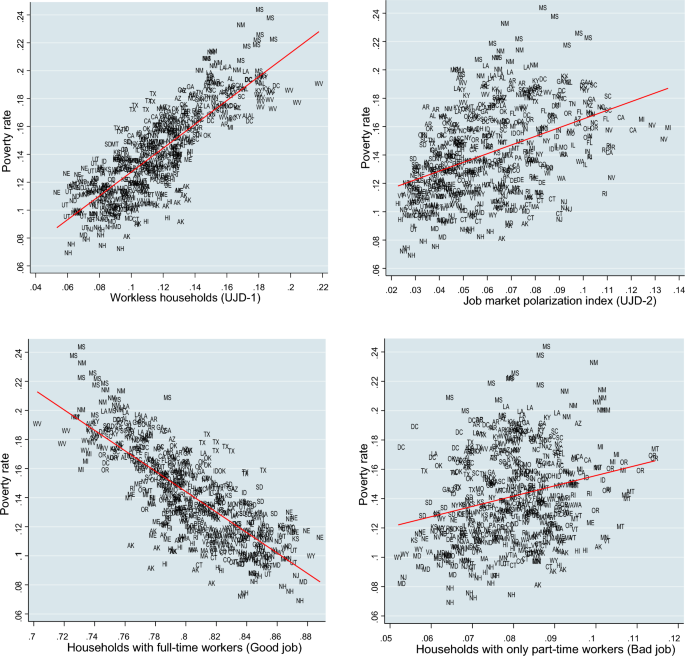
Scatterplot of uneven job distribution, job quality, and poverty
4.1 Uneven job distributions and poverty
Table 3 presents the two-way fixed effect estimates of the effect of uneven job distribution on the poverty rate. The poverty rate is measured as the average poverty across all races, based on the Federal poverty guideline at 100%. Uneven job distribution consists of two measures: the more conservative index-2 from Eq. ( 3 ) and the less conservative index-1, which represents the share of jobless households as discussed in section III. The estimates in Table 3 are presented as percentage point estimates for both job distribution indices. The results strongly support the prediction made earlier in this paper that higher job market polarization in the form of job distribution leads to a higher poverty.
Notably, the uneven job distribution index-1 has a significantly larger impact on the poverty rate compared to uneven job distribution index-2. The interpretation of these coefficients is straightforward. A one percentage point increase in uneven job distribution index-1 will result in approximately a 0.50 percentage point increase in the poverty rate. On the other hand, a one percentage point increase in uneven job distribution index-2 will lead to an approximately 0.25 percentage point increase in the poverty rate. This disparity in magnitudes between index-1 and index-2 can be attributed to the subtraction of unequal job distribution from index-1 to obtain index-2. This difference reinforces the hypothesis that as the level of uneven job distribution increases, the poverty rate also increases. In other words, this provides strong evidence that equal distribution of jobs is a necessary condition to reduce poverty in the United States. Furthermore, these results indicate that the higher employment levels in the United States did not benefit all families, especially low-income families. The findings persist in terms of both effect size and significance level across all model specifications.
The most vulnerable group of people in every society comprises those living in families where no one is employed (Gallie et al. 2000 ; Cantillon et al. 2003 ). Our results in this paper align with the findings from earlier literature. Even after controlling for a comprehensive set of variables as presented in Table 3 , the effect size remains large and statistically significant which is an alarming issue for the country. Förster (2000) reported raw figures indicating that the average poverty rate among households with a working-age head but only one employed member is approximately 36% across 16 OECD countries. In contrast, the corresponding figures are only about 13% for families with one employed household member and merely 3% for households with two employed members. It is important to note that the coefficient presented in this paper cannot be directly compared to the raw numbers reported in Förster's (2000) work. Nonetheless, our coefficients suggest that a ten-percentage point increase in jobless families (uneven job distribution index-1) leads to a substantial five-percentage point increase in poverty.
While factors such as the share of children, elderly individuals, non-white population, and non-professional occupations also contribute to a higher overall poverty rate in the USA, their magnitudes are considerably smaller compared to the job distribution measures. Moreover, variables negatively associated with poverty rates, such as GDP per capita, human capital, and public expenditures, also exhibit relatively small effects. Previous studies that excluded these job distribution measures in their analyses of poverty had less predictive power as a result. Appendix B presents a comparison of aggregated household-level employment (such as job distribution index) versus aggregated individual-level unemployment rate. The uneven job distribution index at the household level exhibits more than three times the predictive power to explain poverty compared to the individual unemployment rate.
A higher percentage of the non-white population and individuals outside the working age (such as children and older people) is positively associated with the poverty rate, which aligns with earlier literature (Bradbury et al. 2001 ; Hoover et al. 2004 ). This result suggests that a portion of children and individuals outside the working age lack the support of working-age individuals within their families. It is worth noting that the fraction of immigrants and non-white individuals has been increasing over the past few decades. Data indicates that recent immigrants, on average, have lower levels of education and fewer skillsets compared to native individuals, resulting in a higher proportion of immigrants earning lower incomes and living in poverty (Hoynes et al. 2006 ; Siddique et al. 2022 ). Alternatively, the influx of immigrants may reduce job market opportunities for native individuals. Consequently, if this argument holds, the overall association between the share of immigrants and the poverty rate will be positive, although the evidence in the literature is mixed (Llull 2017 ). Moreover, the increasing share of non-white and immigrant populations leads to greater ethnic diversity within states, which in turn contributes less to public income and resources (Siddique 2021 , 2022 ). This indirect effect may further hinder poverty reduction efforts.
On average, historically non-white populations face relative disadvantages in this country. Therefore, an increasing fraction of the non-white population can be seen as a predictor of higher poverty, indicating the ongoing disadvantages faced by non-white individuals in society (Siddique 2022 ). However, the statistical association between immigration status (citizenship) and poverty is not significant, except in model 3.2. This is because although recent immigrants experience higher poverty rates compared to earlier immigrant cohorts, their share in the overall population of the United States is relatively small (the non-citizen population is 4.91% as shown in Table 1 ) to significantly impact the state-level poverty rate. The growth rate among non-white children surpasses that of white children, and non-white children are more likely to experience poverty due to their parents' lower economic resources (Mordechay and Orfield 2017 ; Siddique 2022 ). Therefore, a higher share of non-white children may contribute to an overall increase in poverty. However, the share of non-white children is not statistically significant, suggesting that the effects may have been absorbed by the share of the non-white population as a whole.
Spatial variations and economic development can potentially play a role in determining poverty and inequality (Glasmeier 2002 ; Khan and Siddique 2021 ). As the spatial differences between states are likely to be fixed during our study period and are accounted for by fixed effects estimates, I also control for within-state spatial economic inequality, measured by Theil regional inequality, along with GDP per capita. The results show that higher GDP per capita is negatively associated with poverty and is statistically significant, while the Theil local inequality index is not statistically significant. The insignificant coefficient for regional inequality is small because regional inequality within states has remained relatively stable throughout the study period (Khan and Siddique 2021 ). Although a higher GDP per capita is negatively associated with the poverty rate, the relationship between the two variables is weak in terms of effect size. This finding is consistent with earlier literature in the United States and across countries (Adams 2004 ; Hoynes et al. 2006 ).
The proportion of non-management/professional jobs also serves as a predictor of higher poverty, although its impact is less consistent. On average, 62% of employment falls within this occupational category. In addition, I have included controls for various measures of human capital or educational attainment and government expenditure. Human capital has always played a crucial role in explaining economic growth and poverty. It directly influences employment and growth patterns by providing the skills necessary for the growth process, thereby impacting poverty (Gutierrez 2007). Controlling for human capital takes into account the reverse impact of poverty on employment. Limited human capital may prevent many low-income families from accessing good job opportunities. By including measures of human capital or educational attainment, we can capture the impact of poverty on employment, if any. Most educational achievements are negatively associated with the poverty rate, with the exception of those who have completed less than the 9th grade and the share of high school graduates or higher. Education below the 9th grade is likely insufficient in terms of human capital to prevent poverty. Increasing the percentage of high school graduates among individuals aged 25 years and above (who are likely to be part of the workforce) helps in poverty prevention, whereas the share of high school graduates among the overall population does not have the same effect, as many individuals in this group are not part of the labor force. Those who are not in the labor force do not utilize their human capital to earn income. Overall, the relationship between educational attainment and poverty aligns with the existing literature, which suggests that higher levels of education, such as a bachelor's degree or associate degree, help people escape poverty (Assari 2018 ).
Government taxes and transfers play a vital role as income sources for the poor. While a higher share of public expenditure is negatively associated with the poverty rate, a higher percentage of public revenues does not show the same relationship (models 3.5–6). The link between public spending and poverty is well-established in the literature (Hidalgo-Hidalgo and Iturbe-Ormaetxe 2018 ); however, there are debates regarding which types of public expenditures effectively help the poor escape poverty (Fan et al. 2000 ; Jung et al. 2009 ). Furthermore, I have included controls for two different measures related to welfare expenditures: the Temporary Assistance to Needy Families (TANF) program and other cash assistance. The TANF program does not have a significant impact on poverty reduction, whereas the other cash assistance program shows a significant negative effect on the poverty rate. Government transfers can have both direct and indirect consequences on family earnings. The immediate impact is that government transfers provide households with cash and other benefits, which directly affect income and poverty. However, there is an indirect effect as well, where households may adjust their behavior due to the availability of government transfers, potentially reducing their incentive to work and resulting in lower incomes (Schoeni and Blank 2000 ). Thus, the indirect impact may offset the direct effect. Moreover, estimating the immediate impact of government benefits on poverty can be challenging due to the various types of benefits and the definition of the poverty level used in this study. The TANF program, for example, provides cash benefits to low-income households with children. Assuming no behavioral changes due to TANF, it should directly increase the incomes of poor families. However, its impact on poverty reduction may be limited since TANF transfers phase out at income levels around the poverty line (Hoynes et al. 2006 ). Therefore, these estimates do not show any effects of TANF on the poverty rate.
4.2 Job quality and poverty
Table 4 presents the impact of job quality measures on the overall poverty rate at the state level. Good-quality jobs have a significant effect on poverty reduction, while poor-quality jobs have a significant opposite effect on poverty (increasing effect) across all model specifications in Table 4 . As previously defined, "good-quality jobs" refer to households with at least one full-time employee, while "poor-quality jobs" encompass households with only part-time employment. The model specifications in Table 4 are identical to those in Table 3 , except that here, I replaced the uneven job distribution index-1 and index-2 with measures of good-quality jobs and poor-quality jobs.
The interpretation of this result is simple: a one percentage point increase in the share of households with good-quality jobs reduces the poverty rate by 0.42 to 0.44 percentage points. On the other hand, a one percentage point increase in the share of households with poor-quality jobs increases the poverty rate by 0.35 to 0.38 percentage points. This evidence demonstrates that job quality matters for poverty reduction. The existence of a substantial share of poor-quality jobs in the economy, concentrated in households that have no other good jobs, is responsible for the higher persistent poverty rate in the country. In addition to uneven job distribution, as we have seen in the earlier section, job quality is another factor that can explain the persistent level of poverty in the United States. The significance level and size of coefficients are consistent, and the estimated model has high goodness of fit measures (R-square = 0.81 and 0.76 in models 4.5–6). After controlling for both state and year fixed effects and gradually including control variables, no inconsistencies in terms of the size of the coefficients and their significance levels have been noticed. These findings provide robust support for partial causal evidence that job quality plays a critical role in determining the poverty rate in the country: bad jobs increase poverty, and good jobs reduce poverty.
When people are working but still living below the poverty line, it is referred to as "poverty in work," as we have observed in the case of households with poor-quality jobs, which aligns with earlier evidence (Burkhauser and Finegan 1989 ). Due to the lack of extensive unemployment insurance, minimum wage protections, and the diminishing presence of trade unions in the USA, most poor individuals cannot afford to remain unemployed and wait for offers of good jobs. Consequently, they are compelled to accept these bad jobs regardless of their quality and level of compensation (Berry and Sabot 1978 ; Visaria 1981 ; Acemoglu 2001 ; Saunders 2002 ). The existence of bad jobs stems from employers being able to find an adequate labor supply to fill these positions. Therefore, the presence of bad jobs and higher poverty rates are closely intertwined. If these bad jobs persist in the labor market, it is unlikely that poverty will disappear from the economy.
Previous literature attempted to explain why the poverty rate remained persistent in the United States despite positive economic indicators (Hoover et al. 2004 ; Hoynes et al. 2006 ). However, they failed to adequately consider the role of employment quality and employment distribution, which are the primary sources of income for the poor. If jobs are not evenly distributed and predominantly consist of poor-quality positions, a rapidly growing economy is unlikely to help poor individuals escape poverty. A $1,000 increase in GDP per capita only reduces the poverty rate by 0.001 percentage points, which is minimal compared to the impact of uneven job distributions and job quality on poverty, as demonstrated in Tables 3 and 4 . These results suggest that the "trickle-down" economy does not effectively benefit the poor if employment, their main source of earnings, does not prioritize their well-being. In the United States, the role of uneven job distributions and job quality in determining poverty is more significant compared to other developed economies, as alternative means of support for the poor, such as transfers and redistribution, are relatively limited in this country (Gilens 2009 ; Garfinkel et al. 2010 ). Therefore, without sufficient redistribution programs, higher levels of uneven job distributions and the prevalence of low-quality jobs may explain why the income poverty rate remains high and persistent in the country, even during periods of robust economic growth and low unemployment.
5 Conclusions
The poverty rate in the United States has persistently remained high, posing a challenge to understand the underlying reasons. This paper aims to explore whether higher levels of uneven job distribution and the prevalence of poor-quality jobs contribute significantly to poverty in the country. The findings reveal that both uneven job distribution and poor-quality jobs play an important role in explaining poverty in the United States.
An increase of one percentage point in the uneven job distribution index—1, which represents workless households, leads to an almost 0.48 percentage point increase in the poverty rate. This suggests that for a state with an average poverty rate of 14 percent, a 1 percent increase in workless households will result in a corresponding rise of approximately 3.43 percent in the poverty rate. Similarly, a one percentage point increase in the share of households with poor-quality jobs results in a 0.35 percentage point increase in the poverty rate. This implies that in a state where the average poverty rate stands at 14 percent, a mere one percentage point rise in households with only part-time workers can potentially contribute to an increase in poverty by approximately 2.5 percent. In contrast, a mere one percentage point increment in the proportion of households with good-quality jobs results in a poverty reduction of 0.42 percentage points. Consequently, in a state with an average poverty rate of 14 percent, this translates to a significant decline in poverty by approximately 3 percent.
In comparison to other commonly studied variables in the literature, such as individual unemployment rate, GDP per capita, public expenditure, and human capital, which are expected to impact the poverty rate, the role of job distribution and job quality is significantly prominent and noteworthy. The evidence presented in this paper can serve as a valuable resource for public policy debates aimed at reducing poverty in developed economies. It sheds light on the reasons behind the stagnation in poverty reduction efforts in the United States. Both uneven job distribution and poor-quality jobs are structural problems that hinder progress in achieving poverty reduction targets. This is not to say that safety-net programs and other measures do not alleviate poverty—they likely lift millions of households above the poverty line each year. However, I find that the most effective anti-poverty solutions lie in the availability of good-quality jobs and their equitable distribution among households.
In the absence of policy guidance, the issue of uneven job distribution is likely to worsen in the future, potentially hindering the achievement of poverty reduction goals. Traditionally, when the first person from a family enters the labor market, it is more likely to be a male, unless the family is headed by a single mother. Subsequently, the second person from a family to enter the labor market is more likely to be a woman. When a woman becomes the second earner due to financial hardship, the family's economic situation improves, increasing their chances of surpassing the poverty threshold (Blackburn and Bloom 1994 ). However, in recent decades, a significant portion of the female labor force, particularly those married to well-paid men, has joined the workforce (Stier and Lewin 2002 ; Averett et al. 2021 ). There is a positive correlation between husbands' and wives' incomes (Averett et al. 2021 ). As female labor market opportunities expand, high-earning women tend to marry high-earning men, resulting in a rise in households with two high-earning individuals. Such structural forces play a role in determining employment opportunities, and as a result, the likelihood of both spouses in some families being unemployed may increase (De Graaf and Ultee 2000 ; Stier and Lewin 2002 ).
Therefore, it is crucial to establish public policy guidelines for the recruitment process that prioritize the common benefits over private benefits in order to reduce poverty in our society. One effective policy approach could be implementing a preference system similar to the veteran preference policy, where job applicants from workless families are entitled to preferences over applicants from households with already employed individuals in recruitment from competitive lists. Similar strategies should also be followed during firm’s downsizing times where employee retention offer should prioritize individual whose household does not have a second earning individual. This preference system can be implemented in the job market without sacrificing efficiency since candidates must still meet the minimum qualifications. It can be further strengthened by restricting job offers to employees' spouses, a practice that some institutions such as universities have recently adopted, although it overlooks the overall societal benefits. While achieving comprehensive job distribution efforts may pose challenges in the short term, even limited success would yield significant societal equity gains in the long-term.
Similarly, in the absence of a minimum wage, unemployment insurance, and trade unions, the proportion of poor-quality jobs will continue to rise and coexist with good-quality jobs. As a result, a high poverty rate will also persist. There is a significant disconnect between the booming labor market and the well-being of the people, particularly those at the bottom. The labor market is trapped in a cycle of bad jobs. The continuous rise of employment in gig economies may make the employment rate appear impressive, but without proper regulation and policies, the economy will keep producing more poor-quality jobs. This growth in poor-quality jobs is a byproduct of the massive scale development of the service sector, such as healthcare, leisure, hospitality, and restaurants, which predominantly hire people on a part-time basis and pay low wages. This trend also coincides with the declining manufacturing sector.
To reduce poverty, it is not only important to stop creating new poor-quality jobs, but also to replace the current poor-quality jobs with good-quality ones. Both direct and indirect policy guidance is necessary. The direct approach may include policy guidance by setting minimum work conditions and wages for all jobs in the market. Setting higher standards and a higher minimum wage would not only directly regulate job quality but also reduce incentives for firms to create more poor-quality jobs. Poor-quality jobs would be less beneficial for them compared to creating more good-quality jobs.
The indirect method should consist of increasing the coverage of unemployment insurance, investing in education to ensure equitable access to higher education for all, and allowing trade unions to function within each institution. Unemployment insurance should enable people to wait for better job offers instead of immediately accepting poor-quality jobs. It would also reduce the labor supply in the market, which would further push firms to raise pay and improve job quality. Similarly, increasing access to higher education is another way to create demand for good-quality jobs and reduce the supply of recipients of poor-quality jobs. This would leave firms with no choice but to produce more good-quality jobs. Highly skilled workers typically demand higher job quality than low-skilled workers (Cortés and Tessada 2011 ). Historically, unions have played a significant role in protecting workers' interests, and strengthening workers' unions can extend institutional regulations to represent worker interests and generate collective pressure to improve job quality (Simms 2017 ).
There are more full-time, good-quality jobs in the United States than the total number of households, as demonstrated in this paper. Therefore, their equitable distribution among families can play a significant role in eradicating poverty. While achieving a completely even distribution of jobs across households may not be immediately feasible due to structural constraints, combined and simultaneous efforts to allocate jobs from individuals to households and implement policies to enhance job quality would help alleviate poverty. Future research should explore methods to improve the distribution of jobs among households and transition from low-quality to high-quality jobs without sacrificing efficiency, as this paper did not adequately address these aspects, which fall beyond its scope.
Data are from the US Census Bureau, American Community Survey (ACS), World Development Indicators, and the Department of Labor.
Availability of data and materials
The associated data are publicly available, and the Stata code can be requested at [email protected] for reproducing and replicating the results.
Standard literature on poverty identified a wide range of factors that are responsible for poverty such as lack of education (Hofmarcher 2021 ), industrialization (Kimura and Chang 2017 ), technology adoption (Comin et al. 2010 ), redistribution (Jouini et al. 2018 ), and others. Read Ravallion ( 2012 ), McMillan ( 2016 ), and Rosenzweig ( 2012 ) for a more standard explanation of poverty.
In economics, this is called the productivity-pay gap when economic expansion does not broaden social uplift.
According to the Bureau of Labor Statistics, in 2016, there were about 7.6 million “working poor” who spent at least half the year either working or looking for employment.
For example, job polarization in occupation means higher growth of job in the highest-wage and lowest-wage occupations while wiping out the mid-waged occupational jobs (Goos and Manning 2003 ).
The Matthew effect is related to a statement from the Gospel of St Matthew—“For to all those who have, more will be given.” In recent times, this concept is used to present form of self-reinforcing inequality in income, wealth, political power, prestige, and others (Rigney 2010 ).
Acemoglu, D.: Good jobs versus bad jobs. J. Labor Econ. 19 , 1–21 (2001)
Article Google Scholar
Acemoglu D. (1996) Good jobs versus bad jobs: Theory and some evidence
Adams, R.H.: Economic growth, inequality and poverty: estimating the growth elasticity of poverty. World Dev. 32 , 1989–2014 (2004). https://doi.org/10.1016/j.worlddev.2004.08.006
Adamson, M., Roper, I.: ‘Good’ jobs and ‘bad’ jobs: contemplating job quality in different contexts. Work Employ Soc. 33 , 551–559 (2019). https://doi.org/10.1177/0950017019855510
Albrecht, J.W., Vroman, S.B.: Dual labor markets, efficiency wages, and search. J. Labor Econ. 10 , 438–461 (1992). https://doi.org/10.1086/298295
Anderson, J., Beaton, J., Laxer, K.: The union dimension: Mitigating precarious employment? precarious employ underst labour mark insecurity can. McGill-Queen’s University Press, Montreal (2006)
Google Scholar
Assari, S.: Parental education better helps white than black families escape poverty: national survey of children’s health. Economies 6 , 30 (2018). https://doi.org/10.3390/economies6020030
Autor, D.H., Dorn, D.: The growth of low-skill service jobs and the polarization of the US labor market. Am. Econ. Rev. 103 , 1553–1597 (2013)
Averett, S.L., Bansak, C., Smith, J.K.: Behind every high earning man is a conscientious woman: the impact of spousal personality on earnings and marriage. J. Fam. Econ. Issues 42 , 29–46 (2021). https://doi.org/10.1007/s10834-020-09692-x
Baffoe-Bonnie, J., Gyapong, A.O.: Definition of full-time and part-time employment, and distributional assumptions: the implications for the estimated full-time and part-time wage equations. Int. Rev. Appl. Econ. 32 , 259–279 (2018). https://doi.org/10.1080/02692171.2017.1340436
Beer PT (2001) Over werken in de postindustriële samenleving. SCP, Sociaal en Cultureel Planbureau
Berry, A., Sabot, R.H.: Labour market performance in developing countries: a survey. World Dev. 6 , 1199–1242 (1978). https://doi.org/10.1016/0305-750X(78)90011-6
Binder, A.J., Bound, J.: The declining labor market prospects of less-educated men. J. Econ. Perspect. 33 , 163–190 (2019). https://doi.org/10.1257/jep.33.2.163
Blackburn ML, Bloom DE (1994) Changes in the structure of family income inequality in the US and other industrialized nations during the 1980s. LIS Working Paper Series
Bradbury, B., Jenkins, S.P., Micklewright, J.: The dynamics of child poverty in industrialised countries. Cambridge University Press (2001)
Book Google Scholar
Bulow, J.I., Summers, L.H.: A theory of dual labor markets with application to industrial policy, discrimination, and keynesian unemployment. J. Labor Econ. 4 , 376–414 (1986). https://doi.org/10.1086/298116
Burkhauser, R.V., Finegan, T.A.: The minimum wage and the poor: the end of a relationship. J. Policy Anal. Manage. 8 , 53–71 (1989)
Cancian, M., Haskins, R.: Changes in family composition: implications for income, poverty, and public policy. Ann. Am. Acad. Pol. Soc. Sci. 654 , 31–47 (2014). https://doi.org/10.1177/0002716214525322
Cantillon, B., Marx, I., Van Den Bosch, K.: The puzzle of egalitarianism. the relationship between employment, wage inequality, social expenditure and poverty. Eur. J. Soc. Secur. 5 , 108–127 (2003). https://doi.org/10.1177/138826270300500203
Carter, T.J.: Efficiency wages: employment versus welfare. South. Econ. J. 62 (1), 116–125 (1995)
Carter, T.J.: Policy in a two-sector efficiency wage model: substituting good jobs for bad. J. Post. Keynes. Econ. 20 , 445–461 (1998). https://doi.org/10.1080/01603477.1998.11490163
Choodari-Oskooei, B., Royston, P., Parmar, M.K.B.: A simulation study of predictive ability measures in a survival model I: explained variation measures. Stat. Med. 31 , 2627–2643 (2012). https://doi.org/10.1002/sim.4242
Clarke, M.: To what extent a “bad” job? employee perceptions of job quality in community aged care. Empl. Relat. 37 (2), 192–208 (2015)
Comin, D., Easterly, W., Gong, E.: Was the wealth of nations determined in 1000 BC? Am. Econ. J. Macroecon. 2 , 65–97 (2010)
Corluy V, Vandenbroucke F (2017) Individual employment, household employment and risk of poverty in the EU. A decomposition analysis. Monit Soc Incl Eur Stat Books Eurostat Luxemb Publ Off Eur Union 2017
Cortés, P., Tessada, J.: Low-skilled immigration and the labor supply of highly skilled women. Am. Econ. J. Appl. Econ. 3 , 88–123 (2011). https://doi.org/10.1257/app.3.3.88
Desmond, M.: Americans want to believe jobs are the solution to poverty. They’re not. N. y. times. 11 , 11 (2018)
Desmond, M., Gershenson, C.: Housing and employment insecurity among the working poor. Soc. Probl. 63 , 46–67 (2016). https://doi.org/10.1093/socpro/spv025
Edelman, P.: So rich, so poor: why it’s so hard to end poverty in America. New Press, The (2013)
England, P.: Households, employment, and gender: a social, economic, and demographic view. Routledge (2017)
Fan, S., Hazell, P., Thorat, S.K.: Impact of public expenditure on poverty in rural India. Econ. Polit. Wkly. 35 , 3581–3588 (2000)
Farber, H.S., Levy, H.: Recent trends in employer-sponsored health insurance coverage: are bad jobs getting worse? J. Health Econ. 19 , 93–119 (2000). https://doi.org/10.1016/S0167-6296(99)00027-2
Findlay, P., Kalleberg, A.L., Warhurst, C.: The challenge of job quality. Hum. Relat. 66 , 441–451 (2013)
Flaherty, J., Veit-Wilson, J., Dornan, P.: Poverty: the Facts. CPAG, London (2004)
Förster MF, Pellizzari M (2000) Trends and driving factors in income distribution and poverty in the OECD area
Foster, F.D., Smith, T., Whaley, R.E.: Assessing goodness-of-fit of asset pricing models: the distribution of the maximal R2. J Finance 52 , 591–607 (1997). https://doi.org/10.1111/j.1540-6261.1997.tb04814.x
Fuller, S.: Public sector employment and gender wage inequalities in British Columbia: Assessing the effects of a shrinking public sector. Can. J. Sociol Can. Sociol. 1 , 405–439 (2005)
Fullerton, A.S., Dixon, J.C., McCollum, D.B.: The institutionalization of part-time work: cross-national differences in the relationship between part-time work and perceived insecurity. Soc. Sci. Res. 87 , 102402 (2020). https://doi.org/10.1016/j.ssresearch.2019.102402
Gallego-Granados, P.: The part-time wage gap across the wage distribution. Social Science Research Network, Rochester, NY (2019)
Gallie, D., Jacobs, S., Paugam, S.: Poverty and financial hardship among the unemployed. Welf Regimes Exp Unempl Eur. 2000 , 47–68 (2000)
Gardiner, K., Millar, J.: How low-paid employees avoid poverty: an analysis by family type and household structure. J. Soc. Policy 35 , 351–369 (2006)
Garfinkel, I., Rainwater, L., Smeeding, T.: Wealth and welfare states: Is America a laggard or leader? Oxford University Press (2010)
Gilens, M.: Why Americans hate welfare: race, media, and the politics of antipoverty policy. University of Chicago Press (2009)
Glasmeier, A.K.: One nation, pulling apart: the basis of persistent poverty in the USA. Prog. Hum. Geogr. 26 , 155–173 (2002)
Goos, M., Manning, A.: McJobs and MacJobs: the growing polarisation of jobs in the UK. Labour Mark New Labour State Work Br 2003 , 70–85 (2003)
Goos, M., Manning, A., Salomons, A.: Explaining job polarization: routine-biased technological change and offshoring. Am. Econ. Rev. 104 , 2509–2526 (2014)
De Graaf PM, Ultee WC (2000) United in employment, united in unemployment?: Employment and unemployment of couples in the European Union in 1994. In: Welfare regimes and the experience of unemployment in Europe. Oxford University Press, pp 265–285
Gregg P, Wadsworth J. (1994) More work in fewer households? National Institute of Economic and Social Research London
Gregg, P., Wadsworth, J.: Workless Households and the Recovery. In: Dickens, R., Gregg, P., Wadsworth, J. (eds.) The labour market under new labour: the state of working Britain 2003, pp. 32–39. Palgrave Macmillan UK, London (2003)
Chapter Google Scholar
Gregg, P., Wadsworth, J.: Two sides to every story: measuring polarization and inequality in the distribution of work. J. R. Stat. Soc. Ser. A Stat. Soc. 171 , 857–875 (2008)
Gregg, P., Scutella, R., Wadsworth, J.: Reconciling workless measures at the individual and household level. Theory and evidence from the United States, Britain, Germany. Spain and Australia. J Popul Econ 23 , 139–167 (2010)
Carlo, G.C.O., Paci, P.S., Pieter,: Does employment generation really matter for poverty reduction ? The World Bank, Washington, DC (2007)
Hagerty, M.R., Srinivasan, V.: Comparing the predictive powers of alternative multiple regression models. Psychometrika 56 , 77–85 (1991). https://doi.org/10.1007/BF02294587
Herzenberg, S.A., Alic, J.A., Wial, H.: New rules for a new economy: employment and opportunity in postindustrial America. Cornell University Press, New York (2000)
Hidalgo-Hidalgo, M., Iturbe-Ormaetxe, I.: Long-run effects of public expenditure on poverty. J. Econ. Inequal 16 , 1–22 (2018). https://doi.org/10.1007/s10888-017-9360-z
Hill, E.J., Märtinson, V.K., Ferris, M., Baker, R.Z.: Beyond the mommy track: the influence of new-concept part-time work for professional women on work and family. J. Fam. Econ. Issues 25 , 121–136 (2004). https://doi.org/10.1023/B:JEEI.0000016726.06264.91
Hirsch, B.T.: Why do part-time workers earn less? the role of worker and job skills. ILR Rev. 58 , 525–551 (2005). https://doi.org/10.1177/001979390505800401
Hofmarcher, T.: The effect of education on poverty: a European perspective. Econ. Educ. Rev. 83 , 102124 (2021). https://doi.org/10.1016/j.econedurev.2021.102124
Hoover, G.A., Formby, J.P., Kim, H.: Poverty, non-white poverty, and the sen index. Rev. Income Wealth 50 , 543–559 (2004). https://doi.org/10.1111/j.0034-6586.2004.00139.x
Hoynes, H.W., Page, M.E., Stevens, A.H.: Poverty in America: trends and explanations. J. Econ. Perspect. 20 , 47–68 (2006)
ILO (2019) Work for a Brighter Future: The Global Commission for the Future of Work. International Labour Organisation (ILO)
Jaimovich, N., Siu, H.E.: Job polarization and jobless recoveries. Rev. Econ. Stat. 102 , 129–147 (2020)
Jouini, N., Lustig, N., Moummi, A., Shimeles, A.: Fiscal policy, income redistribution, and poverty reduction: evidence from Tunisia. Rev. Income Wealth 64 , S225–S248 (2018). https://doi.org/10.1111/roiw.12372
Jung S, Cho S-H, Roberts RK (2009) Public Expenditure and Poverty Reduction in the Southern United States. In: AgEcon Search. https://ageconsearch.umn.edu/record/47145 . Accessed 1 Oct 2019
Kalleberg, A.L.: Nonstandard employment relations: Part-time, temporary and contract work. Annu. Rev. Sociol. 26 , 341–365 (2000)
Kalleberg, A.L.: Precarious work, insecure workers: employment relations in transition. Am. Sociol. Rev. 74 , 1–22 (2009). https://doi.org/10.1177/000312240907400101
Kalleberg, A.L.: Good jobs, bad jobs: the rise of polarized and precarious employment systems in the United States, 1970s–2000s. Russell Sage Foundation (2011)
Kauhanen, M., Nätti, J.: Involuntary temporary and part-time work, job quality and well-being at work. Soc. Indic. Res. 120 , 783–799 (2015). https://doi.org/10.1007/s11205-014-0617-7
Kelly J (2019) The Frightening Rise In Low-Quality, Low-Paying Jobs: Is This Really A Strong Job Market? Forbes
Keune, M.: Trade union responses to precarious work in seven European countries. Int. J. Labour Res. 5 , 59 (2013)
Khan, M.S., Siddique, A.B.: Spatial analysis of regional and income inequality in the United States. Economies 9 , 159 (2021). https://doi.org/10.3390/economies9040159
Kimura, F., Chang, M.S.: Industrialization and poverty reduction in East Asia: internal labor movements matter. J. Asian Econ. 48 , 23–37 (2017). https://doi.org/10.1016/j.asieco.2016.10.005
Krishna, A.: For reducing poverty faster: target reasons before people. World Dev. 35 , 1947–1960 (2007). https://doi.org/10.1016/j.worlddev.2006.12.003
Lechner, M., Rodriguez-Planas, N., Kranz, D.F.: Difference-in-difference estimation by FE and OLS when there is panel non-response. J. Appl. Stat. 43 , 2044–2052 (2016). https://doi.org/10.1080/02664763.2015.1126240
Lindbeck, A., Snower, D.J.: Wage setting, unemployment, and insider-outsider relations. Am. Econ. Rev. 76 , 235–239 (1986)
Llull, J.: The effect of immigration on wages: exploiting exogenous variation at the national level. J. Hum. Res. (2017). https://doi.org/10.3368/jhr.53.3.0315-7032R2
Loayza, N.V., Raddatz, C.: The composition of growth matters for poverty alleviation. J. Dev. Econ. 93 , 137–151 (2010)
Marinescu, I., Skandalis, D.: Unemployment insurance and job search behavior. Q. J. Econ. 136 , 887–931 (2021)
McDonald, P., Bradley, L., Brown, K.: ‘Full-time is a Given Here’: part-time versus full-time job quality*. Br. J. Manag. 20 , 143–157 (2009). https://doi.org/10.1111/j.1467-8551.2008.00560.x
McMillan, M.: Understanding African poverty over the Longue Durée: a review of Africa’s development in historical perspective. J Econ Lit 54 , 893–905 (2016). https://doi.org/10.1257/jel.20151293
Meyers, M.K.: Are we asking the right questions about poverty in America? Soc. Serv. Rev. 88 , 728–744 (2014). https://doi.org/10.1086/679331
Middleton S, Loumidis J (2002) Young people, poverty and part-time work. In: Hidden Hands. Routledge, pp 40–52
Mordechay, K., Orfield, G.: Demographic transformation in a policy vacuum: the changing face of u.s. metropolitan society and challenges for public schools. Educ Forum 81 , 193–203 (2017). https://doi.org/10.1080/00131725.2017.1280758
Newman, K.S.: No shame in my game: the working poor in the inner city. Vintage, New York (2009)
Odhiambo, N.M.: Growth, employment and poverty in south africa. in search of a trickle-down effect. J. Income Distrib. 20 , 49–62 (2011)
Pacas, J.D., Rothwell, D.W.: Why is poverty higher in rural America according to the supplemental poverty measure? an investigation of the geographic adjustment. Popul. Res. Policy Rev. 39 , 941–975 (2020). https://doi.org/10.1007/s11113-020-09612-9
Page, J., Shimeles, A.: Aid, employment and poverty reduction in Africa. Afr. Dev. Rev. 27 , 17–30 (2015). https://doi.org/10.1111/1467-8268.12136
Pech, C., Klainot-Hess, E., Norris, D.: Part-time by gender, not choice: the gender gap in involuntary part-time work. Sociol. Perspect. 64 , 280–300 (2021)
Ravallion, M.: Fighting poverty one experiment at a time: <em>poor economics: a radical rethinking of the way to fight global poverty</em>: review essay. J Econ Lit 50 , 103–114 (2012). https://doi.org/10.1257/jel.50.1.103
Ravallion, M., Datt, G.: Why has economic growth been more pro-poor in some states of India than others? J. Dev. Econ. 68 , 381–400 (2002)
Reyes, A.M.: Mitigating poverty through the formation of extended family households: race and ethnic differences. Soc. Probl. 67 , 782–799 (2020). https://doi.org/10.1093/socpro/spz046
Rigney D (2010) The Matthew Effect: How Advantage Begets Further Advantage. Columbia University Press
Rosenzweig, M.R.: Thinking small: <em>poor economics: a radical rethinking of the way to fight global poverty</em>: review essay. J. Econ. Lit. 50 , 115–127 (2012). https://doi.org/10.1257/jel.50.1.115
Roth, J., Sant’Anna PHC, Bilinski A, Poe J,: What’s trending in difference-in-differences? a synthesis of the recent econometrics literature. J. Econom. 235 , 2218–2244 (2023). https://doi.org/10.1016/j.jeconom.2023.03.008
Salvatori, A.: The anatomy of job polarisation in the UK. J. Labour Mark Res. 52 , 8 (2018). https://doi.org/10.1186/s12651-018-0242-z
Satchi, M., Temple, J.: Labor markets and productivity in developing countries. Rev. Econ. Dyn. 12 , 183–204 (2009). https://doi.org/10.1016/j.red.2008.09.001
Saunders, P.: The direct and indirect effects of unemployment on poverty and inequality. Aust. J. Labour Econ. 5 , 507 (2002)
Saunders P (2006) A perennial problem: Employment, joblessness and poverty. Social Policy Research Centre Sydney
Schoeni RF, Blank RM (2000) What has welfare reform accomplished? Impacts on welfare participation, employment, income, poverty, and family structure. National bureau of economic research
Siddique, A.B.: Comparative advantage defying development strategy and cross country poverty incidence. J. Econ. Dev. 41 , 45–78 (2016). https://doi.org/10.35866/caujed.2016.41.4.003
Siddique, A.B.: Does ethnic diversity hurt fiscal sustainability? Econ. Bull. 41 , 532–543 (2021)
Siddique AB (2022) White prototypicality threats, inequality in diversity, and collective goods provisions
Siddique, A.B., Haynes, K.E., Kulkarni, R., Li, M.-H.: Regional poverty and infection disease: early exploratory evidence from the COVID-19 pandemic. Ann. Reg. Sci. (2022). https://doi.org/10.1007/s00168-022-01109-x
Simms, M.: Unions and job quality in the UK: Extending interest representation within regulation institutions. Work. Occup. 44 , 47–67 (2017)
Sloane, P.J., Theodossiou, I.: Earnings mobility, family income and low pay. Econ. J. 106 , 657–666 (1996). https://doi.org/10.2307/2235573
Stecy-Hildebrandt, N., Fuller, S., Burns, A.: ‘Bad’jobs in a ‘Good’sector: examining the employment outcomes of temporary work in the Canadian public sector. Work Employ Soc. 33 , 560–579 (2019)
Stier, H., Lewin, A.C.: Does women’s employment reduce poverty? evidence from Israel. Work Employ Soc. 16 , 211–230 (2002). https://doi.org/10.1177/095001702400426811
Thiede, B.C., Kim, H., Slack, T.: Marriage, work, and racial inequalities in poverty: evidence from the United States. J. Marriage Fam. 79 , 1241–1257 (2017). https://doi.org/10.1111/jomf.12427
Tilly, C.: Reasons for the continuing growth of part-time employment. Mon. Labor Rev. 114 , 10–18 (1991)
Tilly, C.: Half a job: bad and good part-time jobs in a changing labor market. Temple University Press, Philadelphia (2010)
Van Arsdale, D.G.: The temporary work revolution: the shift from jobs that solve poverty to jobs that make poverty. Workingusa 16 , 87–112 (2013)
Visaria, P.: Poverty and unemployment in India: An analysis of recent evidence. World Dev. 9 , 277–300 (1981). https://doi.org/10.1016/0305-750X(81)90031-0
Walsh, J.: Myths and counter-myths: an analysis of part-time female employees and their orientations to work and working hours. Work Employ Soc. 13 , 179–203 (1999)
Wilson, G., Roscigno, V.J.: Public sector reform and racial occupational mobility. Work. Occup. 43 , 259–293 (2016)
Woodbury, S.A.: Power in the labor market: institutionalist approaches to labor problems. J. Econ. Issues 21 , 1781–1807 (1987). https://doi.org/10.1080/00213624.1987.11504722
Download references
Acknowledgements
I would like to express my gratitude to Drs. M. Kugler, K. Reinert, N. Koizumi, and S. Slavov for their invaluable guidance. Additionally, I extend my thanks to the two anonymous reviewers for their insightful and thorough referee reports. Special thanks to Prof. Christian Pfeifer, the Associate Editor of JLMR, for overseeing and managing the peer-review process for this manuscript and feedbacks. Your collective contributions have significantly enriched the quality of this work.
This research did not receive any financial support from any sources.
Author information
Authors and affiliations.
School of Public Administration, Florida Atlantic University, 777 Glades Road, Boca Raton, FL, 33431, USA
Abu Bakkar Siddique
You can also search for this author in PubMed Google Scholar
Contributions
All analysis and manuscript writing were done by ABS.
Corresponding author
Correspondence to Abu Bakkar Siddique .
Ethics declarations
Conflict of interests.
There are no conflicts of interest.
Additional information
Publisher's note.
Springer Nature remains neutral with regard to jurisdictional claims in published maps and institutional affiliations.
1.1 Appendix A
See Fig. 4 .
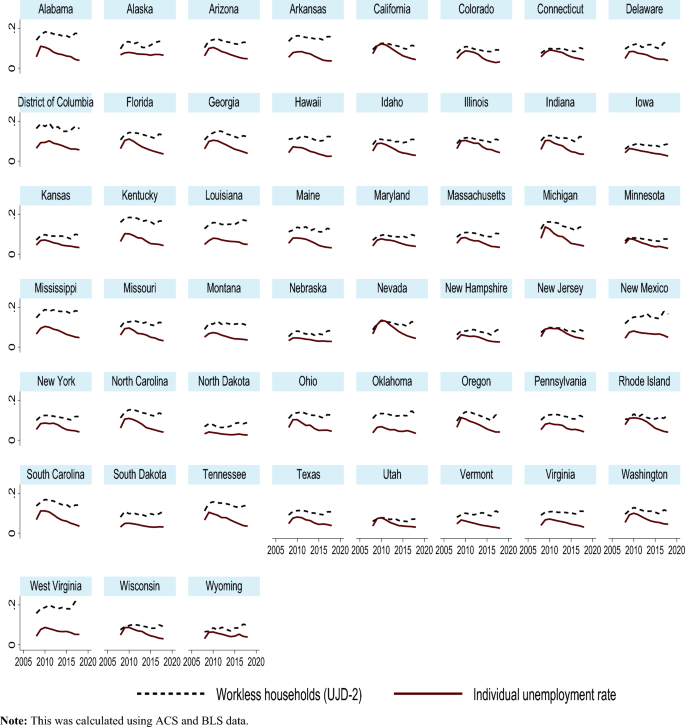
Workless households and individual unemployment rate by states. This was calculated using ACS and BLS data
1.2 Appendix B
Household-level versus aggregate-level measures.
This paper highlights an important issue regarding the significance of household-level employment measures compared to aggregate-level employment measures. To support this argument, a comparison is made between the predictive abilities of the job distribution index, job quality measure, and individual-level unemployment rate. Since the predictive power of two-way fixed effect estimates is largely driven by state and year fixed effects, I employ an ordinary least square model and compare their predictive power to offer suggestive evidence. In determining the statistical model's explanatory power, R-square is widely used and has been employed here (Hagerty and Srinivasan 1991 ; Foster et al. 1997 ; Choodari-Oskooei et al. 2012 ). Table 5 presents various estimates using the uneven job distribution index, job quality measures, and individual unemployment rate separately, as well as their estimates jointly. Remarkably, the uneven job distribution index, measured at the household level, exhibits more than three times the predictive power for poverty compared to the individual unemployment rate (R-square of 0.66 versus 0.19 in columns 5.1 to 5.2). Interestingly, including the individual unemployment rate alongside the uneven job distribution does not improve the R-square value. Additionally, it is important to note that good-quality jobs demonstrate higher explanatory power (R-square 0.60) as well, while poor-quality jobs have limited explanatory power. Therefore, the key takeaway from this analysis is that household-level employment distribution holds significantly greater explanatory power in understanding poverty than the commonly used economic indicator of the unemployment rate.
Rights and permissions
Open Access This article is licensed under a Creative Commons Attribution 4.0 International License, which permits use, sharing, adaptation, distribution and reproduction in any medium or format, as long as you give appropriate credit to the original author(s) and the source, provide a link to the Creative Commons licence, and indicate if changes were made. The images or other third party material in this article are included in the article's Creative Commons licence, unless indicated otherwise in a credit line to the material. If material is not included in the article's Creative Commons licence and your intended use is not permitted by statutory regulation or exceeds the permitted use, you will need to obtain permission directly from the copyright holder. To view a copy of this licence, visit http://creativecommons.org/licenses/by/4.0/ .
Reprints and permissions
About this article
Cite this article.
Siddique, A.B. Job market polarization and American poverty. J Labour Market Res 57 , 30 (2023). https://doi.org/10.1186/s12651-023-00356-5
Download citation
Received : 18 October 2022
Accepted : 16 October 2023
Published : 02 November 2023
DOI : https://doi.org/10.1186/s12651-023-00356-5
Share this article
Anyone you share the following link with will be able to read this content:
Sorry, a shareable link is not currently available for this article.
Provided by the Springer Nature SharedIt content-sharing initiative
- Job market polarization
- Job quality
- Job distribution
JEL Classification
Read our research on: Gun Policy | International Conflict | Election 2024
Regions & Countries
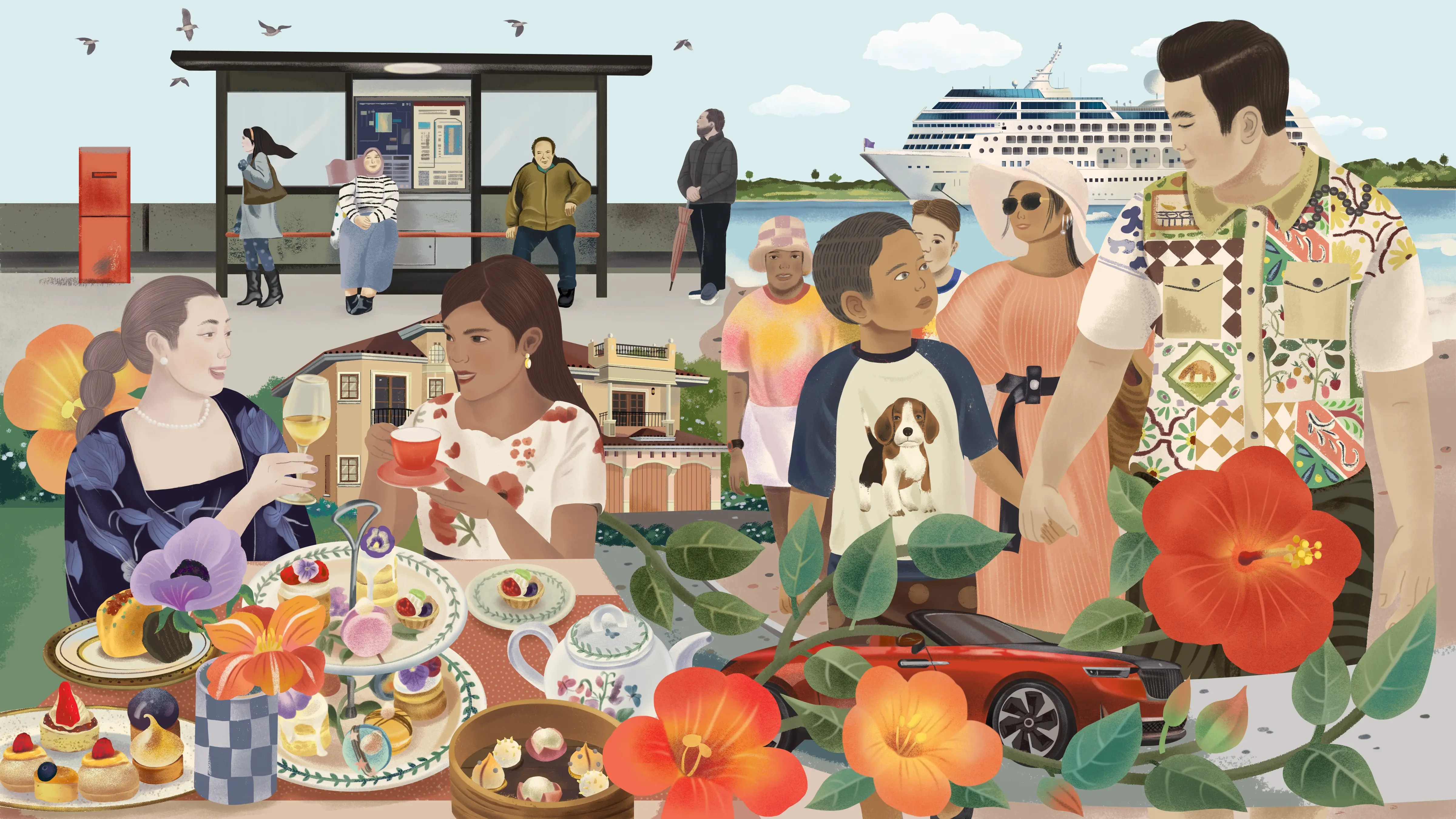
The Hardships and Dreams of Asian Americans Living in Poverty
Illustrations by Jing Li
Asian Americans are often portrayed as economically and educationally successful.
In reality, about one-in-ten Asian Americans live in poverty. Asian Americans also have the most income inequality of any major racial or ethnic group in the United States.
Without closely examining the diversity of Asian American experiences, it’s easy to miss the distinct stories of Asian Americans living with economic hardship.
To understand more about this population, Pew Research Center conducted 18 focus groups in 12 languages to explore the stories and experiences of Asian Americans living in poverty.
Table of Contents
Of the 24 million Asians living in the United States, about 2.3 million live in poverty . Many are working to overcome the economic hardships they encounter and achieve their American dream. But they face challenges along the way, from Asian immigrants grappling with language barriers to U.S.-born Asians navigating pathways to success.
In February 2023, Pew Research Center conducted 18 focus groups with adult participants from 11 Asian origin groups in different regions across the U.S. These are among the most likely Asian origin groups to experience economic hardship in the U.S. Focus groups included those whose approximate family income is at or below 140%-250% of the 2022 federal poverty line, depending on their location. Accompanying these focus group findings are results from a Pew Research Center survey about the hardships and dreams of Asians living in poverty, conducted from July 2022 to January 2023.
Some common themes that focus group participants shared include day-to-day financial difficulties, assumptions by others that they do not need help because they are Asian, and the importance of financial security in achieving the American dream.
Related: 1 in 10: Redefining the Asian American Dream (Short Film)
Focus groups also reveal that Asian Americans’ experiences with economic hardship differ by whether they were born in the U.S. or outside the country. Some immigrants not only experience difficulties making ends meet, but also face challenges that come with living in a new, unfamiliar country. These include learning English, navigating daily life in a new place and finding a stable job.
Even though U.S.-born Asians grew up in this country and speak English, they talk about the challenges of understanding what it takes to succeed in America. This includes getting the “right” education, getting access to the “right” knowledge and knowing the “right” people to succeed.
The findings in this data essay reveal what participants shared about their experiences with economic hardship, overcoming challenges, and their views of the American dream and social mobility in America.
The terms Asians and Asian Americans are used interchangeably throughout this data essay to refer to those who self-identify as Asian, either alone or in combination with other races or Hispanic identity.
The terms living in poverty, living near or below the federal poverty line and living with economic hardship are used interchangeably throughout this essay to refer to adults whose family income is close to or below the 2022 federal poverty line.
- For results on Asian adults from the focus groups, this refers to adults whose approximate family income is at or below 140%-250% of the federal poverty line. Thresholds varied by focus group recruitment locations to account for differences in the cost of living.
- For results on Asian adults from the survey , this refers to adults whose approximate family income falls at or below 100% of the federal poverty line.
- For data on the total U.S. Asian population from the U.S. Census Bureau , this refers to all Asian Americans whose family income is at or below 100% of the federal poverty line.
The terms federal poverty line and poverty line are used interchangeably to refer to the federal poverty guidelines published yearly by the U.S. Department of Health and Human Services.
The term U.S. born refers to people born in 50 U.S. states, the District of Columbia, Puerto Rico or other U.S. territories.
The term immigrant refers to people who were born outside the 50 U.S. states or the District of Columbia, Puerto Rico or other U.S. territories.
Asian Americans and financial struggles
Financial difficulties are part of many Asian Americans’ day-to-day lives, according to the 2022-23 survey. Asian adults were asked if they had experienced any of the following financial challenges in the past 12 months: gotten food from a food bank or a charitable organization, lost their health insurance, had problems paying for their rent or mortgage, had trouble paying for medical care for themselves or their family, had trouble paying their bills, or been unable to save money for emergencies.
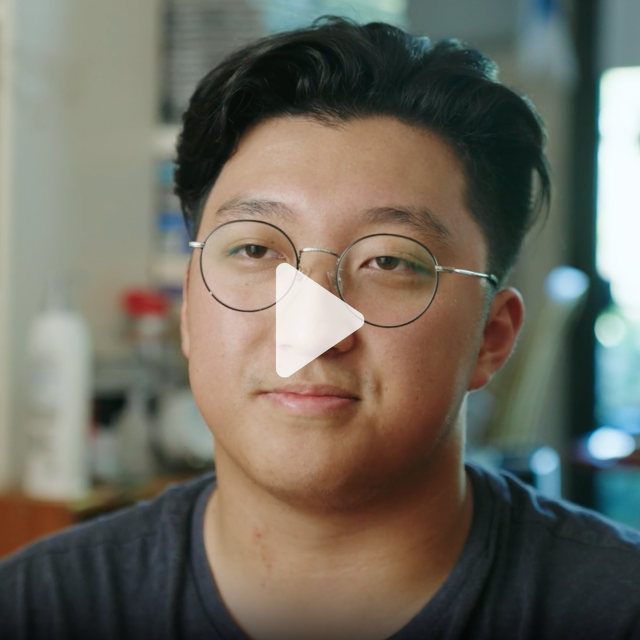
“It got really bad to the point where a simple bowl of rice, we weren’t even able to afford that. So there were times where a bowl of rice would be a meal for all three meals, or we just simply did not eat.” NOLAN , FILM PARTICIPANT
The most common financial difficulty experienced is being unable to save for emergencies. More than half of Asian adults living in poverty (57%) said this had happened to them. By comparison, fewer Asian adults living above the poverty line (40%) said this.
Note: “Asian adults living in poverty” refers to survey respondents whose approximate family income is at or below 100% of the federal poverty line. Share of respondents who didn’t offer an answer or answered “no” not shown.
Source: Survey of Asian American adults conducted July 5, 2022-Jan. 27, 2023. “The Hardships and Dreams of Asian Americans Living in Poverty”
Some focus group participants shared how challenging it was for them to save because of their earnings and their family needs. Participants also talked about the urgency they feel to save for their children and retirement:
“I feel a bit helpless [about my financial situation]. … I don’t want to be in debt. I have to save money to raise my kids, but I don’t have money to save.”
–Immigrant man of Korean origin in early 30s (translated from Korean)
“[I save money] to go to Pakistan. Because I have four children … I needed five or six tickets, in case my husband traveled with us, and it required a lot of money. We used to save for one whole year, and when we were back from Pakistan, we were usually empty-handed. Then the cycle started again.”
–Immigrant woman of Pakistani origin in late 40s (translated from Urdu)
“You’re not going to work forever. No one is going to work forever. You want to have savings … for your rent [or] in case of medical bills [if] something happens. [You] might as well [save for] some trips down the while when you [can] travel still. But you’re not going to be working at 80 years old, are you?”
–U.S.-born man of Chinese origin in early 40s
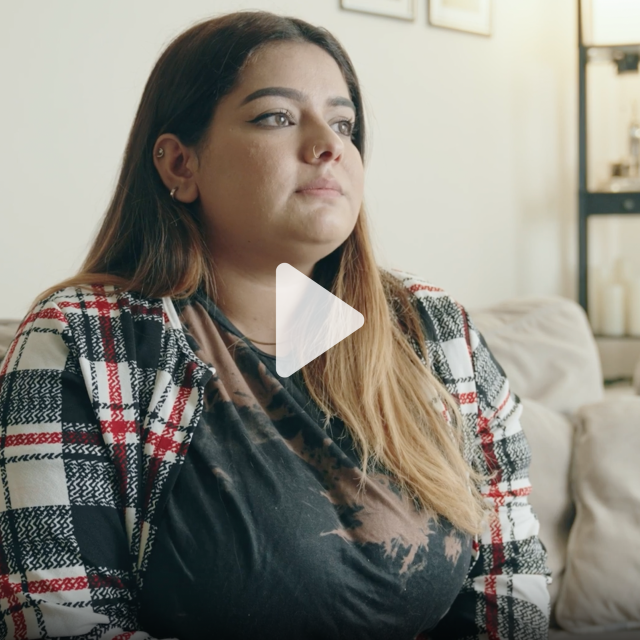
“We were all four of us in one apartment, four siblings, plus the parents, so that’s six people in a house, which was very, very cramped.” SABA , FILM PARTICIPANT
Other common difficulties for Asian Americans living near or below the poverty line include having trouble paying their bills (42%), needing to get food from a food bank or a charitable organization (38%) and having problems paying their rent or mortgage (33%), the survey found. Smaller shares of Asian adults living above the poverty line say they experienced difficulties paying their bills (17%), got food from a food bank or a charity (6%) or had trouble paying their rent or mortgage (11%).
These findings were echoed in our focus groups, where participants recalled the stress and tension their families felt when things like this happened to them:
“My dad lost his car a couple of times. There was this one time where I remember it was nighttime. All of a sudden, a cop comes over to our home [with another person]. … And my dad was forced to give up his car to this stranger … because, I don’t know, he wasn’t paying off the car or something. And it was very humiliating, and my brothers wanted to get physical with that person because he was acting very arrogantly. My dad was able to eventually pay back the car and somehow get it back. But there were many times when we might not have had a roof over our heads.”
–U.S.-born man of Pakistani origin in late 20s
Asian immigrants face challenges navigating life and employment in the U.S.
Immigrant and U.S.-born Asians experience economic hardship in different ways. Asian immigrants in the focus groups discussed how a lack of English proficiency, navigating transportation and getting a good job all shape their experiences with economic hardship.
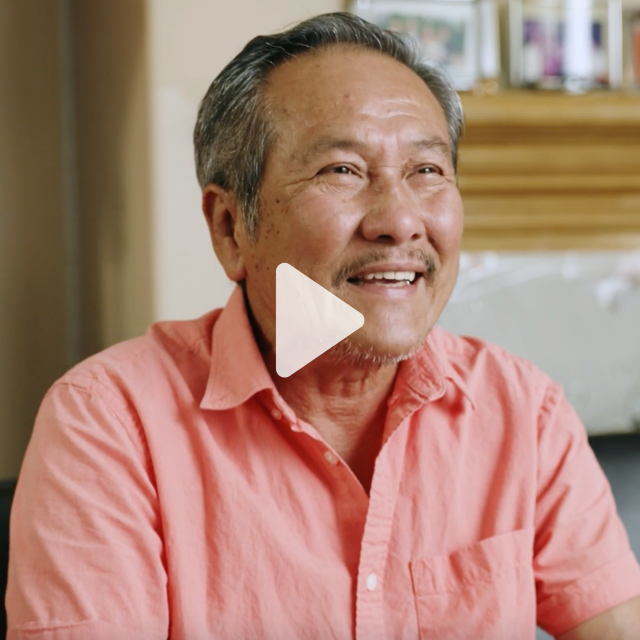
“I felt sad about life, didn’t know the language, didn’t know the roads. I had no friends, so I felt very sad.” PHONG , FILM PARTICIPANT (TRANSLATED FROM VIETNAMESE)
For example, not knowing English when they first arrived in the country created extra challenges when using local transportation systems and meeting basic daily life needs such as shopping for groceries:
“When we were very young, the most difficult thing we faced [after coming to the U.S.] was not being able to speak the language. Unless you lived in those times, you wouldn’t know. We didn’t know how to buy food. … We didn’t know the language and there was no interpreter available. … I didn’t know how to take the bus, I didn’t know where to go, or to which place they were taking me to school. When we were asked to go to the classroom, we didn’t know where to go. … There was no other way, because there was no communication.”
–Immigrant woman of Hmong origin in late 50s (translated from Hmong)
Language barriers also brought extra hurdles for Asian immigrants in the job market. Some focus group participants said it was hard to explain their skills to potential employers in English effectively, even if they had the relevant education or skills for the job and had learned English before they immigrated:
“After coming [to the U.S.], there were many problems to face, first … the language problem. We have read English … but we are not used to speaking. … We also had education … but since we can’t explain ourselves in English – what we can do, what we know … we are getting rejected [from jobs] as we cannot speak. … Another problem was that I had a child. My child was small. I could not go to work leaving him. At that time, my husband was working. He also had the same thing – he had education, but he could not get a good job because of the language. [As another participant] said, we had to work below the minimum wage.”
–Immigrant woman of Bangladeshi origin in late 30s (translated from Bengali)
Not wanting to be a burden influenced life choices of many U.S.-born participants
For many U.S.-born focus group participants, concerns about being a burden to their families shaped their childhoods and many of their life decisions:
“It’s difficult to talk to [my parents] because you grew up here and it’s just totally different from them growing up in Vietnam. … It’s the same like what [another participant] was saying, when you take off the burden to your parents, right? So I dropped out of college, just because I didn’t want them paying anymore. I just didn’t think that I was going to do or be anything in college, right? So I would rather work. So I started taking responsibility of my own and you start working really hard and you getting out of the house and helping them pay for bills.”
–U.S.-born man of Vietnamese origin in mid-40s
“My family’s struggling. Is education more important, [or] is working more important? I really felt that growing up because a lot of my friends, education – going to college and going to a techno school – wasn’t really on their radar, it wasn’t really something on their plan. I think talking to a lot of the folks and a lot of my friends during their time, they felt like they had to grow up to provide for their family or for you to find some type of income to kind of help their family. And so that really drove the direction of at least one of my friends, or a lot of my friends.”
–U.S.-born man of Hmong origin in mid-30s
Some U.S.-born focus group participants said that when reflecting on their childhoods, they could see the financial burden they had on their families in a way they did not realize as a child:
“At a certain point you become very aware of how much of a financial burden you are. You don’t ask for anything you want. Like, you don’t ask for prom. You don’t ask to join clubs. You don’t ask to go on field trips, things like that. You just know that it’s going to cause so much drain on your parents.”
–U.S.-born woman of Vietnamese origin in mid-20s
“[My parents] had like a lot of responsibilities, like … giving money back to their father, and then their sisters and brothers, helping them out back [in Pakistan]. … [My father] had to support us and then send money back constantly there. I didn’t know that until now, basically. … We would hardly see him. Maybe like on Sunday, we would see him a couple of hours. But it was on the weekdays, we would hardly see our father. He was always working.”
–U.S.-born woman of Pakistani origin in early 30s
Overcoming economic challenges
The survey found that when Asian adults living in poverty have needed help with bills, housing, food or seeking a job, about six-in-ten (61%) say they’ve turned to family or friends.
Some focus group participants mentioned that families and friends in their ethnic community were a great source of financial help. For others, the limited size of their ethnic community in the U.S. posed obstacles in obtaining assistance.
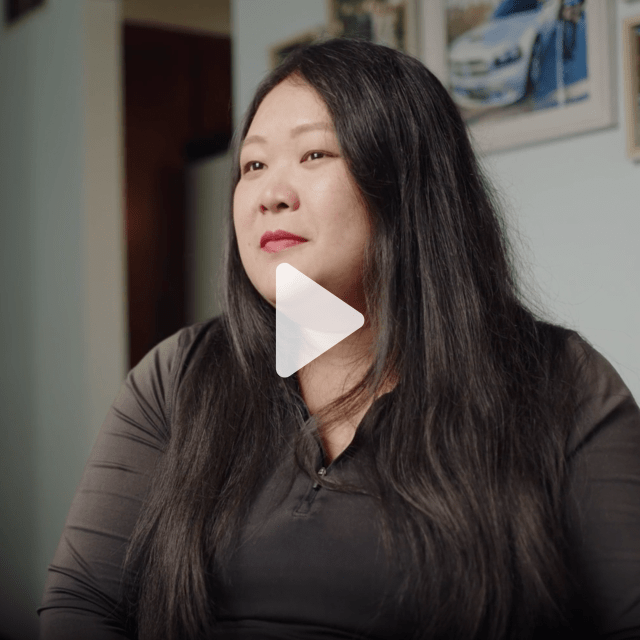
“My dad arrived in the U.S. when he was 26 years old, and I’m now 29 years old. … I have seven siblings and my parents who support me. And my parents didn’t have that, they didn’t have their parents to support them.” TANG , FILM PARTICIPANT
“It was very difficult during [my] study [at university]. … I had a scholarship, most of the part was scholarship; however, I had to pay something between $10,000 and $15,000 per semester. And I had to eat, I had to pay rent, I had to do everything. At the same time, there are many other things too, aren’t there? And there was always a stress about money. This semester is over now, how do I pay for the next? I had no clarity about what to do and not to do. In that situation, I approached those friends studying there or who came there a little earlier and were working to borrow money. … I [was] offered help by some friends and in finding a job and being helped for my needs.”
–Immigrant man of Nepalese origin in early 40s (translated from Nepali)
“We didn’t have a large Burmese community to ask for such help. It was not yet present. As we had no such community, when we had just arrived, we told close friends, got directions and went to ask for help.”
–Immigrant woman of Burmese origin in late 40s (translated from Burmese)
However, not all Asians living with economic hardship have asked for or received help. In the focus groups, participants shared why they or their families sometimes did not do so or felt hesitant. Fear of gossip and shame were mentioned multiple times:
“[I experienced financial difficulties after I first arrived in the U.S.] because I came here as a student. … It’s because I had to pay monthly rent and I paid for living expenses. I felt a little pressured when the monthly payment date approached. I had no choice but to ask my parents in Korea for money even as an adult, so I felt a sense of shame.”
–Immigrant woman of Korean origin in early 40s (translated from Korean)
“My cousin will [help me financially] without judgment. But, like, my aunt and elders – if it gets back to them [that I asked for help], it’s going to for sure come with judgment. And if I could figure it out myself, I will take the way without judgment.”
“To add on to what [another participant] said, if you go to the community [for help] or whatever, you know, by tomorrow everybody’s going to know it’s your problem.”
–U.S.-born woman of Pakistani origin in early 40s
Immigrants who came to the U.S. because of conflict are more familiar with government aid programs
Asian immigrants come to this country for a variety of reasons. In the focus groups, immigrant participants who came to the U.S. due to conflict or war in their origin countries referenced government assistance programs more often than those who came for other reasons.
This reflects a broader pattern among Asian immigrants overall: Those who came because of conflict or persecution have turned to federal, state or local governments for help with living expenses or employment more often than immigrants who came for economic or educational opportunities, according to the survey.
Focus group participants reflected on differences in the amount of government help available. Sometimes, they expressed a sense of unequal treatment:
“Vietnamese have this program where people got sponsored because of the war. So for other Asians, they feel that we are more privileged. Because from what I know, the Koreans and the Japanese, they must have money in order to come to America. As for us, we can come here through the refugee program, we can come here through the political program. They feel that we got more preferential treatment than other Asians in that regard.”
–Immigrant man of Vietnamese origin in early 40s (translated from Vietnamese)
“During the pandemic, I had to go through housing assistance and everything [to pay my rent]. Something like that with EBT [Electronic Benefits Transfer], how they send you stimulus checks. Korea doesn’t have any of that stuff.”
–U.S.-born woman of Korean origin in late 40s
“I think my community is relatively traditional. Because 20 years ago, we went straight to Chinatown fresh off the plane [after immigrating]. I still remember being in [the local] hospital, lots of social workers were there to help out, including with a medical insurance card, and applying for service, most importantly medical insurance. We all went to [the same] street. We relied on other Chinese people.”
–Immigrant man of Chinese origin in late 30s (translated from Mandarin)
Family ties contribute to increased awareness of government programs. For example, when asked how they learned about using government programs for help, some U.S.-born participants said:
“[I learned about the government programs from] my parents. I had to translate for them.”
–U.S.-born woman of Cambodian origin in mid-30s
“I was working at [a smoothie shop], and I was 17 and a half. … My college loan was like $50,000 [and I was] making $12.50 [an hour], how the hell am I supposed to be paying that month to month? Because my month-to-month was damn near $300, $500. My $12.50 an hour does not even cover for it, any of it, whatsoever. And, you know, me [having] been kicked out of home … I was living with my aunt. … I don’t want to burden her. So I had to go and ask her. She told me, ‘Hey, you should go and apply for food stamps.’”
–U.S.-born woman of Laotian origin in mid-30s
U.S.-born and immigrant focus group participants hold different views on education’s role in achieving a better future
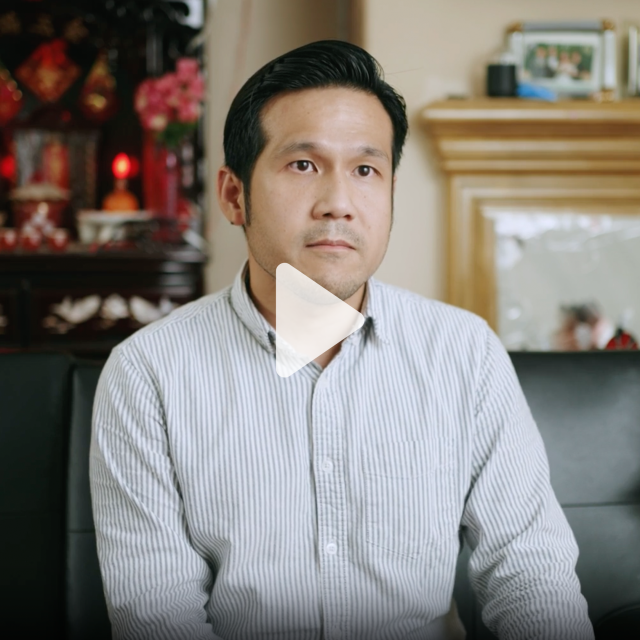
“My friend, he started out at internship … I was too naive. I was laughing at the time, like, ‘Man you spend your time? You took buses there every day? No pay?’ … I just didn’t know the big picture behind [it]. I wish I could plan for [it] just like how they did.” PHUOC , FILM PARTICIPANT
Reflecting on what could lead to success and achieving the American dream, focus group participants who were born in or grew up in the U.S. emphasized the value of getting connected to the “right” opportunities:
“[You don’t have] to go to school to be successful. I mean, they say there are people who are book smart and just people who are street smart, you know. [As long as you] grow up and you know the right people … networking on the right people to get into things. Or, you know, the right people to do the right things to get to where you want to be in life.”
–U.S.-born man of Hmong origin in late 20s
Other participants said it would have helped if their families had a deeper understanding of how the education system prepares them for good careers:
“I feel if my parents were educated and they could have guided me in the right direction [for college] – although, they tried their best. I’m not blaming them. But, you know, if I had someone of a more academic background who knew the system … I will try my best to help my daughter out in college or help her choose what her major is going to be. [My parents couldn’t provide] that kind of help that really helped me in choosing my major. … And so I think just the background that we come from was not the best – or not having the full grasp of this system. … Versus someone who’s had parents here for multiple years, and their parents are now telling them, like, ‘Hey, this is not the right decision for you. Try doing this. This will be better in the long run.’”
–U.S.-born man of Pakistani origin in early 30s
Some also said firsthand knowledge of how to invest and how the U.S. financial system works would have helped:
“[In] the newer generation, we have access to learn all the things we need to, right? [I watch videos] that talk about, like, ‘These are the things you need to do in order to be financially successful. You need to invest your money, get into stocks,’ and stuff like that. And I know that not even 1% of my Hmong community knows anything about that stuff. … So I think we can be more financially successful, including myself, if we were to look more deeply into those things.”
–U.S.-born woman of Hmong origin in late 20s
“If you’re educated and know how, like, let’s say investments work, if you know how that’s done and then you apply it actually going through [someone] like investors or even stockbrokers, then you’ll see the fruits of your labor, or at least experience that, as opposed to not even having the knowledge or even the experience to begin with.”
–U.S.-born man of Cambodian origin in mid-30s
Some participants shared that even when they have some knowledge of financial institutions, they feel the system is working against them:
“I think systematic racism [is a barrier to achieving the American dream]. … I mean, if you own a car, you got to get the bank to approve you. … And they charge people with, like, no credit the highest fee, the most percentage, which are a lot of the folks [like] us trying to achieve the American dream. And then we go to neighborhoods that have the highest crime rate, we also have the most premiums. … And so I think that, one, we’re paying a lot more with much less … the system [was] set up well before minorities, and I think we’re pretty much going to fall behind.”
Many focus group participants also see the value of education, especially a college one, in leading toward a better future and achieving the American dream:
“[When I think of the American dream, it means] if you work hard enough, you can succeed. … You can get an education or a higher education. Then you have so many choices here and exposure to so many ideas and concepts that you wouldn’t otherwise.”
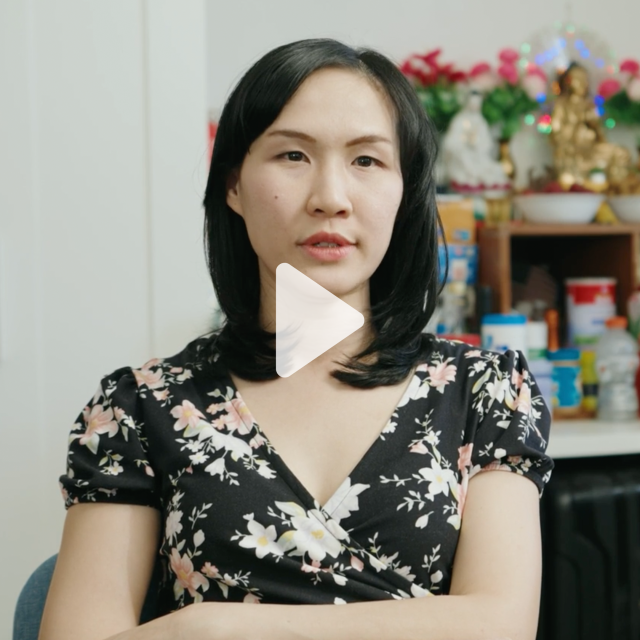
“The bachelor’s degree was important to me in the sense that I needed it so that I could apply for the jobs I wanted. … I guess it made things a bit easier.” THET , FILM PARTICIPANT (TRANSLATED FROM BURMESE)
But this sentiment resonated more with immigrant participants than those born in the U.S.:
“It is the education and the relevant knowledge I think that our Hmong people must have. We’ve been living in this country for the last 45 years. I think that to live in this country, it is very important for some people. I do not think everyone has a ‘lawyer’ or a ‘doctor’ in their house. If it happens, maybe we will reach our goal and the poverty will gradually disappear from our lives.”
–Immigrant woman of Hmong origin in mid-30s (translated from Hmong)
“I think if I obtain any degree, I would perhaps be able to do something.”

Assumptions about Asians hurt their chances of overcoming challenges
Participants shared that other people’s assumptions about Asians complicate their experience of living with economic hardship. Asians are often characterized as a “model minority” and portrayed as educationally and financially successful when compared with other groups.
Some participants shared how the assumption that all Asians are doing well hurt their ability to seek help:
“I have a daughter … she’s the only Asian in class. … Everybody tends to think, ‘She’s Asian; she’s so smart; her mommy has money. So you got to invite her to your birthday party because her mom is rich. [Her] mom will buy you a present.’ … I’m not rich, but because we’re Asian … she’s invited to all these parties.”
–U.S.-born woman of Hmong origin in early 30s
“What I can assume is that outside of our community, especially at the government level, [including] state level and central federal level here, we are missing out or not eligible for benefits. In their opinion, we are rich, no matter if we are working or not. [They may think] our stories may not be genuine. They may think we are making up a story [if we apply for benefits].”
Striving for the American dream
Freedom was a recurring theme in how focus group participants define their American dream. Two aspects were mentioned. The first was freedom from debt and stress over making ends meet, such as paying for everyday basic needs including rent and food. The second was the ability to make life choices freely without financial constraints, enabling them to live the life they aspire to.
Reaching the American dream
Half of Asians living near or below the federal poverty line say they believe they have achieved the American dream or are on their way to achieving it, the survey found. This includes 15% who say they have achieved it and 36% who say they are on their way. By comparison, among those living above the poverty line, 27% say they’ve achieved the American dream, and another 46% say they are on their way.
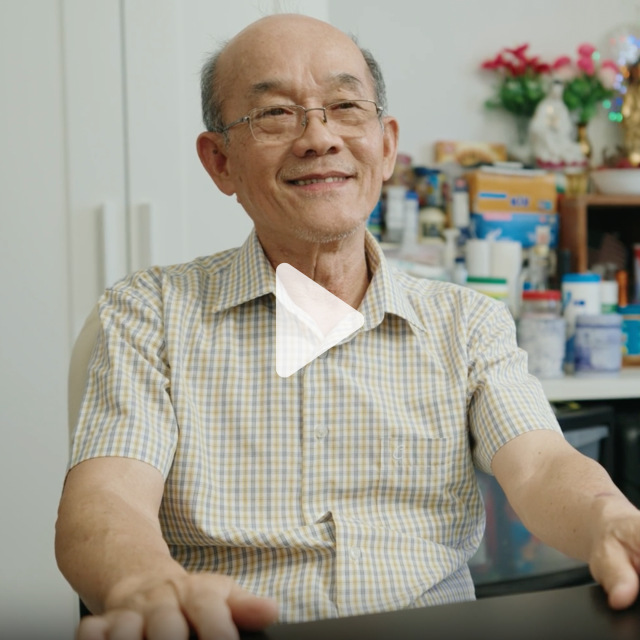
“Before I came to America, I had never heard of the American dream. … But because I was able to at least bring my son along, not only my life but also his education has improved significantly.” THEIN , FILM PARTICIPANT (TRANSLATED FROM BURMESE)
Among focus group participants, many were optimistic about reaching the American dream for themselves:
“[To me, the American dream is] the opportunity to come to America. I’ve learned a lot after reaching here. And I’ve been able to help my parents and relatives. Despite facing some troubles here, I’ve [provided them a] little financial assistance. I would’ve been unable to help them if I had been in Bhutan.”
–Immigrant woman of Bhutanese origin in late 40s (translated from Dzongkha)
Some participants were also hopeful that the next generation can achieve their American dream, even when they themselves are not there yet:
“When I think about the American dream, I look back at myself, because I belong to the first generation that came to this country. We all started very late. I know that this country will help you, but really it will not be easy for us. … What I think will help me to be happy is to ‘reach the American dream.’ If I can’t achieve it, then I will support my children so that they can reach the dream and I will be happy with them. I will give my children money to help them study.”
“If I can’t get [the American dream] for myself, it is okay. No matter how I am, I’ve already reached half of my life. But I’ve done as much as I can do for [my children], so my responsibility is done. If it’s their turn, I believe they will be able to do all that I couldn’t. I believe it.”
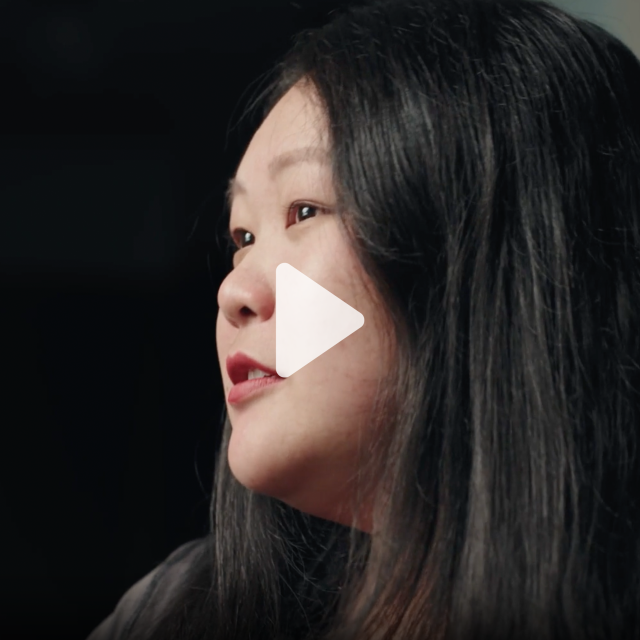
“I would like to own a home one day. And at this rate, and like many of my peers, that’s not a reachable goal right now. I don’t see it being a reachable goal for me for a very, very, very long time.” TANG , FILM PARTICIPANT
Still, the survey found that 47% of Asian adults living in poverty say the American dream is out of reach for them, higher than the share among those living above the poverty line (26%). Not all Asians living in poverty feel the same way about achieving the American dream, with U.S.-born Asians in the focus groups being less optimistic about reaching the American dream than immigrant Asians.
“In a certain era with the U.S. and the immigrants coming, the American dream [was] you come, you study, you do this, you can climb up the ladder, etc., etc. That was the big American dream. And I think there was a period where that was possible. Not any longer.”
Others also shared worries about their prospects of reaching the American dream because of different immigration histories and economic concerns such as inflation:
“I think I was conditioned to think too small to have the American dream. … Vietnamese Americans came over here at a very specific time. … There were Chinese Americans that came here like centuries ago, and they had the time to build generational wealth. We know that Vietnamese people came here in the ’70s. That’s not enough time to grow generational wealth.”
–U.S.-born woman of Vietnamese origin in late 20s
“I have kids. … They’re spoiled. … Now with inflation, houses are more expensive now [than 10, 20 years ago], right? Let’s say 20 years from now, when they buy a house, [the American dream] is going to be unachievable, you know what I mean? Like, unless they are a TikTok star or an entertainer or some kind. … [It’s] going to be tough.”
–U.S.-born man of Chinese origin in late 30s
Freedom from debt
For many participants, being debt-free is important to their vision of the American dream and promotes a life with more financial stability and independence:
“[If I could choose one dream in America, it would be to have] no debt. … When buying something, they always say, ‘Be careful, or you’ll be in debt.’ … And that is what got stuck in my throat.”
–Immigrant woman of Laotian origin in mid-30s (translated from Lao)
“[I haven’t achieved the American dream because I’m not] debt-free, you know, just trying to have extra money, instead of living paycheck to paycheck.”
“[My dream in America is] to be independent, for example, we always lived with the money of mom and dad. One is to be independent when you come here. Let me earn so much money that if I go to the store and buy something, I don’t even have to look at the price tag. That [is] my dream.”
–Immigrant woman of Nepalese origin in early 40s (translated from Nepali)
Participants shared that being debt-free also means having less stress and worry about making ends meet so that they can have extra resources and bandwidth to help their families:
“[The most important thing to achieving the American dream is] being debt-free and having real estate and income steadiness. … If you have rent income, you’re not trading in your time for money, so you have real estate. … You’re not stressing, you have time for your kids more, and your family. You’re probably a little bit happier.”
–Immigrant man of Cambodian origin in mid-20s
“The main thing is that I want to fully support my father and mother, and that I don’t have to worry about [how] I will support myself, or how I will pay my house rent. This is my number one.”
–Immigrant woman of Bangladeshi origin in late 20s (translated from Bengali)
For others, having a stable job is an important step to reaching the American dream:
“I want to have a job, and if I have a job, I’ll have money. I’m only working three and a half days a week right now, and I want to work more. I want more jobs the most, right now. I don’t need anything in America. Just a job.”
Freedom to dream
Focus group participants mentioned having the financial ability to not only meet their basic needs, but also pursue their dreams. Asians born in the U.S. mentioned the freedom to chase one’s aspirations without financial constraints more often than immigrants. Regardless of nativity, the ability to live the life they want is fundamental to many focus group participants’ definitions of the American dream:
“[When] everyone around you is immigrants and you’re all just trying to survive, the only thing you’re trained to think about is survival. But you’re not thinking about investment. Like, when you grow older and you start thinking, ‘Okay, I need to spend money to make money,’ that’s when you start thinking bigger. Yeah, I’m not just thinking about like having one home, I want 10 homes.”
“[Financial] stability is you have nothing but you could survive. [Financial] freedom is you have enough that you can do anything you want. That’s my financial freedom.”
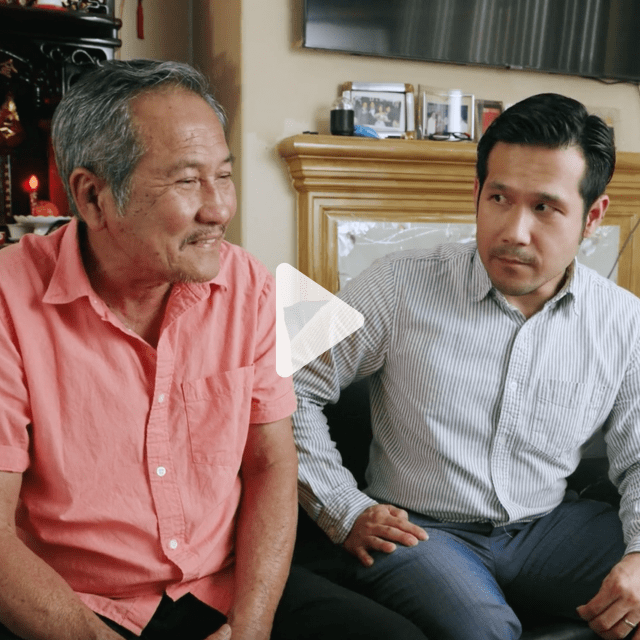
“As it was so hard at that time … what motivated you to keep going and work so hard?” “My strength, my mindset was I wanted to earn money so that my children could have a bright future.” PHUOC AND PHONG , FILM PARTICIPANTS (TRANSLATED FROM VIETNAMESE)
The American dream, to some focus group participants, is about more than financial achievements. Finding happiness and helping others, ultimately leading them to live the life they desire, are key parts of their American dream.
“I want to thank [another participant] for saying ‘self-actualization,’ because personally I think it’s really powerful to be able to know what you want. Because then you’ll know what kind of job you want, what kind of house you want, whether you want to be in politics or not. Like, loving yourself and understanding yourself to your core, then that will be the [deciding factor].”
–Immigrant man of Cambodian origin in early 40s
“I think for me [the American dream] is that there is a house for me, with no interest, I do not owe any loan, my parents could live there comfortably, their struggle is over, and also I have enough … to be able to do something for Pakistan later [in life], God willing.”
–Immigrant woman of Pakistani origin in mid-20s (translated from Urdu)
“[Some people define success as having] lots of money, kids, cars, right? But that’s not really … what I would consider success. Success is something that – does it make you happy? … Are you happy every day going to work? Does it make you happy? When you come home, are you happy?”
About this project
Pew Research Center designed these focus groups and survey questions to better understand the experiences of Asian Americans living with economic hardship. By including participants who are among the Asian origin groups most likely to experience poverty, the focus groups aimed to capture, in their own words, their experiences and challenges in America today. The discussions in these groups may or may not resonate with all Asians living in poverty in the United States.
The project is part of a broader research portfolio studying the diverse experiences of Asians living in the U.S.
Survey and demographic analysis of Asians living in poverty
For a comprehensive examination of Asian adults’ experiences with economic hardship from Pew Research Center’s 2022-23 survey of Asian Americans, as well as a demographic analysis of the U.S. Census Bureau’s 2022 American Community Survey, read “Key facts about Asian Americans living in poverty.”
Videos throughout this data essay illustrate what focus group participants discussed. Individuals recorded in these video clips did not participate in the focus groups but were selected based on similar demographic characteristics and thematically relevant stories.
Watch the short film related to the themes in the data essay.
Methodological note
This multi-method research project examines the many facets of living with economic hardship among Asian Americans today.
The qualitative analysis is based on 18 focus groups conducted in February 2023 in 12 languages with 144 participants across four locations. Recruited participants had an approximate family income that is at or below 140%-250% of the federal poverty line, depending on the location. More information about the focus group methodology and analysis can be found in the focus group methodology .
The survey analysis included in this data essay is based on 561 Asian adults living near or below the poverty line from Pew Research Center’s 2022-23 survey of Asian Americans, the largest nationally representative survey of Asian American adults of its kind to date, conducted in six languages. For more details, refer to the survey methodology . For questions used in this analysis, refer to the topline questionnaire .
Acknowledgments
Pew Research Center is a subsidiary of The Pew Charitable Trusts, its primary funder. The Center’s Asian American portfolio was funded by The Pew Charitable Trusts, with generous support from The Asian American Foundation; Chan Zuckerberg Initiative DAF, an advised fund of the Silicon Valley Community Foundation; the Robert Wood Johnson Foundation; the Henry Luce Foundation; the Doris Duke Foundation; The Wallace H. Coulter Foundation; The Dirk and Charlene Kabcenell Foundation; The Long Family Foundation; Lu-Hebert Fund; Gee Family Foundation; Joseph Cotchett; the Julian Abdey and Sabrina Moyle Charitable Fund; and Nanci Nishimura.
We would also like to thank the Leaders Forum for its thought leadership and valuable assistance in helping make this survey possible.
The strategic communications campaign used to promote the research was made possible with generous support from the Doris Duke Foundation.
This is a collaborative effort based on the input and analysis of a number of individuals and experts at Pew Research Center and outside experts.
- In this data essay, definitions of “living near or below the poverty line” and related terms differ between survey respondents and focus group participants. Refer to the terminology box for details. ↩
Sign up for our weekly newsletter
Fresh data delivered Saturday mornings
About Pew Research Center Pew Research Center is a nonpartisan fact tank that informs the public about the issues, attitudes and trends shaping the world. It conducts public opinion polling, demographic research, media content analysis and other empirical social science research. Pew Research Center does not take policy positions. It is a subsidiary of The Pew Charitable Trusts .

Poverty Measure
The Poverty Research Team is responsible for the development of the NYCgov Poverty Measure. The alternative NYC poverty measure, in comparison to the official U.S. measure of poverty, includes a threshold that accounts for the higher cost of housing in New York City. Additionally, it incorporates the value of programs intended to alleviate poverty; adjusting family incomes for benefits such as the Supplemental Nutritional Assistance Program (SNAP) and the Earned Income Tax Credit.
The work has received nationwide attention and contributed to development of the Federal Supplemental Poverty Measure.
The New York City Government Poverty Measure, 2020
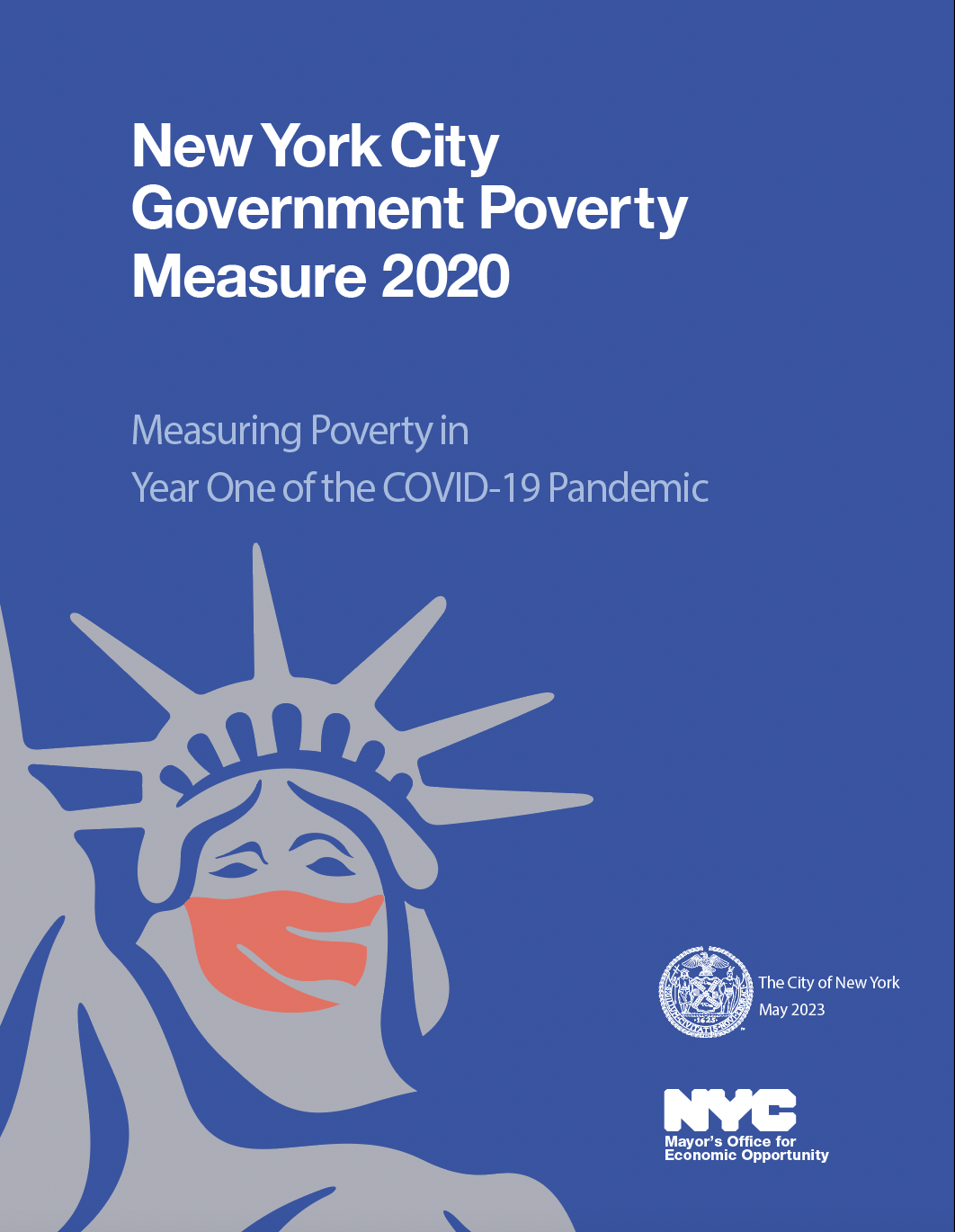
The latest NYC Opportunity annual report on poverty updates the NYCgov Poverty Measure for New York City in 2020. This year’s report, which covers the first year of Covid-19, provides less robust poverty data than past reports because of significant pandemic-related limitations in data collection in 2020.
The NYCgov poverty measure that this report contains each year is based on data from the U.S. Census Bureau’s annual American Community Survey (ACS) supplemented by city, state, and other national data. The 2020 ACS survey, however, was suspended for several months, and the overall response rate was lower, and uneven across demographic categories.
To compensate for these problems, the Census Bureau issued a set of “experimental” one-year data. Making cautious use of that data, this report finds that the poverty rate fell by 0.8 percentage points in 2020, a statistically significant drop that seems plausible in light of the temporary aid New York City households received. Because of the data problems, the trend’s trajectory (a notable decline in poverty) is more reliable than the actual rate.
Technical Notes Forthcoming
Past poverty reports and technical appendices.
CEO's first working paper on poverty in New York City, issued in August of 2008, contrasted poverty rates for 2006 derived from CEO's application of the NAS methodology against those based on the official method. The 2010 report focused on how and why poverty rates using our methodology have changed over time, using the one-year ACS samples for 2005 to 2008.
The CEO Poverty Measure

An official website of the United States government
The .gov means it’s official. Federal government websites often end in .gov or .mil. Before sharing sensitive information, make sure you’re on a federal government site.
The site is secure. The https:// ensures that you are connecting to the official website and that any information you provide is encrypted and transmitted securely.
- Publications
- Account settings
Preview improvements coming to the PMC website in October 2024. Learn More or Try it out now .
- Advanced Search
- Journal List
- HHS Author Manuscripts

Racial and Ethnic Inequality in Poverty and Affluence, 1959-2015
This paper examines patterns and trends in racial inequality in poverty and affluence over the 1959 to 2015 period. Analyzing data from decennial censuses and the American Community Survey, I find that that disparities have generally narrowed over the period. Nevertheless, considerable disparities remain, with whites least likely to be poor and Asians most likely to be affluent on the one hand, and blacks and American Indians much more likely to be poor and less likely to be affluent on the other—and Hispanics somewhat in between. Sociodemographic characteristics, such as education, family structure, and nativity explain some of the disparities—and an increasing proportion over the 1959 to 2015 period, indicative of the growing importance of disparities in human capital, the immigrant incorporation process, and the interaction between economic conditions and cultural shifts in attitudes towards marriage in explaining racial inequality in poverty and affluence. There also are still significant portions of the gaps that remain unexplained, especially for blacks and American Indians. The presence of this unexplained gap indicates that other factors are still at work in producing these disparities, though their effects have declined over time.
Few topics have received as much attention in recent years as the prevalence and nature of racial inequality in the United States. Racial inequality in policing and punishment, for example, was the impetus of the Black Lives Matter movement in 2013. The production and enormous success of the movie The Black Panther , released in 2018, highlighted previous inequality in representation in the movies and media. One of the reasons that these issues continue to resonate is that the material circumstances of the U.S. population historically varied–and continue to vary–by race and ethnicity. For example, in 1959, the first year of the official times series, over half of all blacks were poor, compared to fewer than 1 in 5 of whites. Poverty rates decreased for both groups during the economic boom of the 1960s, but since the early 1970s poverty has mainly fluctuated with the economic cycle, and considerable disparities remain, such that by 2015, 24 percent of blacks and 9 percent of non-Hispanic whites were poor ( U.S. Census Bureau 2016a ).
There is considerable variation in the incidence of poverty across other racial and ethnic groups as well. Poverty rates among American Indians are about as high as among African Americans, poverty rates among Hispanics are only modestly lower, while the poverty rate among Asians more closely resembles that of whites ( U.S. Census Bureau 2016a ). It is likely that a variety of factors help explain patterns across these different groups, including the prevalence of racial discrimination, a legacy of past inequality, the immigration process (principally for Asians and Hispanics), differences in human capital and other sociodemographic characteristics, and social and spatial isolation ( Iceland 2017 ).
Notably, while racial and ethnic disparities in poverty have been documented for some time, there has been considerably less analogous research on racial gaps in the prevalence of affluence , and how these gaps have changed over time. Understanding patterns of affluence are important because, as Richard Reeves (2017) has argued, affluent Americans have considerable control over many kinds of resources, not the least being community amenities and institutions, such as local public schools. Understanding patterns of affluence is all the more important given the growth of income inequality in the United States. Such inequality has been associated stagnant or declining living standards for those at the bottom of the income distribution along with large gains for those at the top ( Piketty 2014 ). Many thus argue we are living in an “age of extremes” ( Massey 1996 ). While we know that the poverty rates of many minority groups have been stagnant in recent years, we know relatively little about the extent to which these groups are part of the affluent class, and how this might have changed over time. Are patterns of poverty and affluence symmetrical, such that groups with higher poverty rates consistency have lower rates of affluence? There is reason to believe that this is not necessarily so, as studies have documented differences in income inequality within groups ( Kochhar and Cilluffo 2018 ).
This paper further investigates the extent to which individual and family-level characteristics help explain racial and ethnic differences in poverty and affluence, and how the influence of these characteristics might have changed over the 1959 to 2015 period. While other data would be needed to examine all of the deep structural roots of racial disparities in poverty and affluence, the analysis here will help ascertain the role of important sociodemographic correlates of poverty—such as educational attainment, family structure, and nativity—in explaining racial disparities. This will provide important information on whether, for example, educational disparities help drive differences among some groups, such as between whites and Hispanics, and whether the significance of these factors have grown or diminished over time. The study will also shed light on whether the factors have a symmetrical effect on poverty and affluence.
In short, this paper is guided by the following questions:
- What patterns and trends in poverty and affluence by race and ethnicity do we see over the 1959 to 2015 period?
- How has the relatively likelihood of poverty and affluence by race and ethnicity changed?
- To what extent do family- and individual-level characteristics help explain differences in the prevalence in poverty and affluence by race and ethnicity, and how have these associations changed over time?
To answer these questions, this study relies on data from the 1960 to 2000 decennial censuses and the 2010 and 2015 American Community Surveys. I calculate rates of poverty and affluence for whites, blacks, Hispanics, Asians, and American Indians. I then investigate, using logistic regressions, the relative likelihood of poverty and affluence by race and ethnicity, and finally, with a decomposition analysis, the extent to which individual- and family-level characteristics explain disparities at different points in time. In doing so, this study helps shed light on the evolving nature of racial and ethnic inequality in poverty and affluence in the United States in this era of profound demographic and economic change.
Overall trends in poverty and affluence by race and ethnicity
Racial and ethnic differences in the prevalence of poverty have been widely documented. Using the official U.S. poverty measure, in 2015, for example, the poverty rate of non-Hispanic whites was 9 percent, compared to 11 percent among Asians, 21 percent among Hispanics, 24 percent among blacks, and 26 percent among American Indians ( U.S. Census Bureau 2016a , 2016c ). There has been a modest narrowing of poverty differentials over time, as the non-Hispanic white poverty rate has inched up since the 1970s, while those of blacks, Hispanics, and Asians are a little lower—though among all groups there are considerable fluctuations in poverty with the economic cycle ( U.S. Census Bureau 2016a ).
Less has been written about the prevalence of affluence, and indeed there is no official government measure of affluence in the United States, nor a standard way to measure it in the literature. It is not uncommon for studies to examine people at different percentiles of the income distribution ( Piketty and Saez 2003 ; Murray 2012 ; Reeves 2017 ). Others have used absolute measures of affluence which are based on an unchanging benchmark over time, much like the official poverty measure ( Rank and Hirscl 2001 ; Danziger and Gottschalk 1995 ; Rothwell and Massey 2010 ). Danziger and Gottschalk (1995) document a significant increase in affluence over time.
The few studies that have looked at patterns of affluence by race and ethnicity find considerable disparities. Among these, Rank and Hirschl (2001) , using data from the Panel Study of Income Dynamics (PSID), take a life course approach to examine the probability of experience a year of affluence (defined as family incomes at least 10 times the poverty threshold) among whites and blacks. They find that only about 13 percent of blacks will experience at least one year of affluence over their lifetime, compared to 55 percent of whites. Their subsequent work confirmed that there was considerable differential in the likelihood of affluence by race ( Hirschl and Rank 2015 ).
Explanations for differences in poverty and affluence
There are a number of broad explanations of racial disparities in poverty and affluence. Among these are racial discrimination, the legacy of historical inequalities, differences in human, social, and cultural capital, immigration-related process (such as immigrant selection and generational incorporation), social and spatial isolation, and culture factors, among others (e.g., Alba and Nee 2003 ; Bourdieu 1977 ; Charles and Guryan 2008 ; Loury 1977 , 2002 ; Iceland 2017 ; Massey 2007 ; Massey and Denton 1993 ; Patterson and Fosse 2015 ; Wilson 1987 ). This analysis does not attempt to measure the impact of all of these; to do so would be beyond the scope possible with the data available and in the space of a journal article. Instead, using census data over a long period of time, I examine some of the important, more proximate, socio-demographic correlates of poverty, including education, family structure, and nativity status, among others. These have been shown to have significant associations with poverty, usually causally so, or at least as both a cause and reflection of poverty. Some of these also shed light on the broader perspectives described above, including human capital and immigration-related perspectives. These analyses will provide information on the extent to which these characteristics explain racial disparities, and the changes in their roles over time.
Below I begin with a discussion of the association between individual- and family-level factors and poverty and affluence. I then describe how they might help explain racial and ethnical differences in poverty and affluence over time. This is followed with a review of the previous empirical literature on these relationships, and I end the background section by detailing this study’s contribution to the literature.
The association of individual- and family-level characteristics with poverty and affluence
Among the most important individual- and family-level factors that may help explain racial and ethnic differentials in poverty and affluence are education, family structure, and nativity. I discuss these and additional factors in turn.
Education, a common marker of human capital, is strongly associated with poverty and affluence. Education helps people be more productive, and as such, more desirable to employers. Of secondary importance, attaining an educational degree also serves as a credential—a signal that an individual is meritorious and capable ( Becker 1994 ). Indicative of the importance of education, 5 percent of the population 25 and older with a BA or greater was poor in 2015, compared to 26 percent among those without a high school degree ( Proctor, Semega, and Kollar 2016 ).
Family structure is strongly associated with poverty (less work has been done on its association with affluence). For example, the poverty rate among married-couple families was 5 percent in 2015, compared to 28 percent among female-headed families ( U.S. Census Bureau 2016b ). Families with two adults are more likely two have two earners to help make ends meet. Single parents often face the challenge of finding and paying for childcare. Other factors may also help explain the higher poverty rates among single parents, such as lower average levels of education among these parents.
Nativity is also strongly associated with socioeconomic status. Immigrants tend to have higher rates of poverty than the native born, even within racial/ethnic groups ( Proctor, Semega, and Kollar 2016 ). Higher poverty rates among the foreign born stem from a variety of factors, as the foreign-born often have lower levels of education than the native-born, they might be less likely to translate their academic credentials into good jobs, they are more likely to have limited English language proficiency, and they may have less knowledge about job market and/or lack productive social capital ( Hoynes, Page, and Stevens 2006 ; Iceland 2013 ; Alba and Nee 2003 ).
Other individual- and family-related factors also help to explain poverty and affluence, and these are included as control variables in the analysis. For example, age is negatively associated with poverty, as earnings generally increase across the life course until retirement ( Rank and Hirschl 2001 ). Poverty rates also are higher in the South and nonmetropolitan areas than in other areas ( Thiede, Lichter, and Slack 2018 ). Women are more likely to experience poverty, and less likely to experience affluence, than men, for a variety of reasons, including lower levels of workforce attachment and discrimination in the labor market ( Blau, Ferber, and Winkler 1998 ; England 2010 ; Rank and Hirschl 2001 ).
How individual and family-level factors might help explain racial and ethnic differentials in poverty and affluence
Education might help explain racial and ethnic differential in poverty and affluence because education levels vary by race and ethnicity. Asians are the mostly likely to have a B.A. or higher degree, followed by whites, blacks, and Hispanics and American Indians (the last two groups have similar levels) ( U.S. Census Bureau 2016c ). Low levels of education among blacks and American Indians reflect historical inequalities, as well the poor quality of many schools in neighborhoods with a high proportion of blacks and American Indians—exacerbated by racial residential segregation ( Massey and Denton 1993 ; Snipp 1992 ; Snipp and Hirschman 2004 ).
One reason for high levels of education among Asians is the Asian immigrants are a very selective group, in that they have higher average levels of education than both people in their countries of origin and native-born Americans in the United States ( Sakamoto and Kim 2013 ; Lee and Zhou 2014 ). One reason for this selectivity is that a relatively high proportion of immigrants from Asia are admitted into the U.S. on the basis of their occupational skills. In contrast, a higher proportion of Hispanics enter because they already have kin living in the United States. Immigrants who enter via the occupational skills provisions have much higher levels of education on average that those who enter on the basis of the family reunification provisions, and in part as a result of this, Hispanic immigrants are much less positively selected on education ( Chiswick 1986 ; Feliciano 2005 ).
The association between family structure and poverty/affluence also likely helps explain racial disparities, given differences in marriage rates across groups: they are lowest among blacks, followed by American Indians, Hispanics, whites, and Asians, and differences generally widened over time ( Raley, Sweeney, and Wandra 2015 ). These difference in household living arrangements could reflect racial and ethnic differences in either the average value placed on marriage (a cultural argument) and/or the relative economic security of men and women (an economic argument). Research suggests that blacks and Hispanics are more supportive of single parenthood than whites and Asians, perhaps reflecting the greater occurrence of single parenthood among the former two groups ( Goldscheider and Kaufman 2006 ; Trent and South 1992 ). On the economic side, Black and Hispanic men have relatively low earnings compared to white and Asian men ( Sakamoto et al. 2000 ; Snipp and Cheung 2016 ). This study cannot distinguish between the cultural and economic argument, but can nevertheless shed light on how differences in family structure more generally affect disparities in poverty and affluence. An important caveat here is that family structure might not only affect poverty, but also be affected by it. In this way, past and current disparities in income by race and ethnicity contribute to differences in family structure, which in turn can further exacerbate poverty and affluence gaps.
The possible role of nativity in explaining racial and ethnic gaps is straight forward, and has been discussed at length in the literature (e.g., Sakamoto, Goyette, and Kim 2009 ; Duncan, Hotz, and Trejo 2006 ; Iceland 2017 ; Perlmann 2005 ; Snipp and Hirschman 2004 ). A significant percentage of Asians and Hispanics are immigrants, and immigrants tend to have lower socioeconomic outcomes than the native-born for reasons described above, so nativity might contribute to differentials in poverty and affluence among these groups.
Among other factors included in this analysis that might help explain racial and ethnic differentials in poverty and affluence, age is negatively associated with poverty and the age structure of racial groups differs—whites are the oldest group, followed by Asians, blacks and American Indians, Hispanics, and multiracial individuals ( Gao 2016 ). Since poverty rates also are higher in the South and nonmetropolitan areas than in other areas, to the extent that racial/ethnic groups are differentially distributed across these areas can affect disparities. American Indians, for example, are over-represented in nonmetropolitan areas ( Snipp and Sandefur 1988 ).
It is possible that racial and ethnic groups might experience different “returns” to the individual- and family-level factors that are examined in this analysis. For example, non-Hispanic whites might receive a greater return to education than non-Hispanic blacks. The decomposition method used here focuses on whether group attributes help explain differences in poverty and affluence, but in the Results section I also speak more briefly to the issue of whether differences in returns are important in explaining broad patterns of change.
Finally, it is important to note that disparities in poverty and affluence might be explained by factors not captured in the decennial census and ACS data. These unobserved factors could include, among others: discrimination, neighborhood conditions arising out racial and ethnic segregation (such as the variation in school quality across neighborhood and physical conditions such as environmental hazards), social capital, and culture and cultural capital. To the extent that disparities in these unobserved factors might have changed, we will see a change in the unaccounted for differences in poverty and affluence by race in the analyses.
Previous Empirical Findings
There are a number of studies that have focused on the effect of individual and family-level factors on racial and ethnic disparities in income and poverty, and especially with regards to average or median earnings (e.g., Farley 1996 ; Hirschman and Wong 1984 ; Sakamoto, Wu and Tzeng 2000 ; Sandefur and Scott 1983 ; Snipp and Cheung 2016 ). Among the most recent, Snipp and Cheung (2016) find that some racial and ethnic gaps in earnings have declined, especially between many Asian groups and whites. Asians are in fact often advantaged, but this can be explained mainly by education and regional clustering. Other studies provide mixed findings on the extent of Asian advantage/disadvantage in earnings, with some showing no disadvantage, but others with many controls showing a small disadvantage. Generally speaking, on the one hand, nativity helps explain some of the Asian disadvantage in earnings, but Asians also have higher earnings because they have higher levels of education ( Kim and Sakamoto 2010 ; Zeng and Xie 2004; see also Sakamoto, Goyette, and Kim 2009 ).
Snipp and Cheung (2016) find only a slight narrowing of gap in earnings between white and African American men, though observed characteristics play a larger role in explaining the gap over time. They attribute some of the unobserved gap to discrimination. The small narrowing of the gap and an increased role of observable characteristics is consistent with other studies, though there is some debate about the magnitude of the decline in black-white earnings and wage inequality ( Couch and Daly 2002 ; Farley 1984 ; Hirschman and Wong 1984 ; Sakamoto, Wu, and Tzeng 2000 ; Western and Pettit 2005 ). Differences in family structure have also been found to contribute to the black-white gap in poverty ( Lichter, Qian, and Crowly 2005 ; Thiede, Kim, and Slack 2017 ). However, the general association between family structure and poverty has weakened over time, as single parents are more likely to be employed than they used to ( Cancian and Reed 2008 ; Danziger and Gottschalk 1995 ; Iceland 2003 ; Sawhill 2006 ).
Snipp and Cheung (2016) also find no convergence in the earnings between Hispanics and whites (see also Sakamoto, Wu, and Tzeng 2000 ), with education playing an important role in explaining part of the gap. This is consistent with other studies showing that Hispanics remain disadvantaged relative to whites in terms of earnings and other socioeconomic outcomes; nativity and lower levels of educational also explain at least some, but not all of the Hispanic-white earnings gap ( Dávila, Mora, and Hales 2008 ; see Duncan and Trejo 2014 ).
Finally, the gap between whites and American Indians has decreased only slightly. Among American Indians, low levels of education are an important factor explaining high levels of poverty, as is spatial isolation in areas without many good jobs. A relatively high proportion of American Indian children live in single parent families as well ( Snipp and Sandefur 1988 ; Sarche and Spicer 2008 ; Sandefur and Liebler 1997 ; Snipp 2005 ).
Contributions of this study
This study contributes to the above literature in several ways. First, while general patterns and trends in poverty by race and ethnicity have been well documented, the analyses provide a careful accounting of the extent to which racial and ethnic gaps in poverty have narrowed, and some of group characteristics that help explain these gaps over a long period of time (more on this point below). Second, even less is known about patterns and trends in affluence. Some studies have indicated important differentials (e.g., Rank and Hirschl 2001 ), but none that I am aware of have tracked trends in affluence by race and ethnicity—and is done so here for the 1959 to 2015 period. As such, this analysis examines who occupies both tails of the income distribution . Doing so is timely given substantial increases in inequality since the 1970s, the accompanying hollowing out of the middle class, and the importance of understanding racial inequalities among both the most vulnerable members of society along with those who have substantial political, economic, and social power ( Reeves 2017 ).
Finally, using decomposition analyses, I examine the role of several individual- and family-level characteristics in explaining racial and ethnic differences in poverty and affluence. Many of these, such as education, family structure, and nativity, are thought to play important roles for different groups-- and their roles have likely have changed over time as the composition of racial and ethnic groups themselves have changed. No previous study has examined the effect of these factors at both the low and high ends of the income spectrum, so it is unknown whether they have similar effect on both. Thus, these analyses will yield a deeper understanding of racial and ethnic disparities in poverty and affluence from 1959 to 2015—a period of dramatic change in the social, economic, and demographic composition of the United States.
Data and Methods
The data for these analyses come from the 1960 to 2000 decennial censuses and the 2010 and 2015 American Community Survey (ACS), compiled and harmonized as part of the Integrated Public Use Microdata Series (IPUMS-USA) ( Ruggles et al. 2015 ). The analysis begins with the 1960 census (which collects information on respondents’ incomes in the previous calendar year), as 1959 marks the beginning of the official poverty time series. In addition, using the absolute measure of affluence described below, only a very small proportion of people were affluent in 1949 (2 percent), and this is all more the case for minority groups (1 percent or less for all groups), which also were demographically a small proportion of the total population in 1949 before growing considerable in subsequent decades. The sample includes people in the poverty universe, which excludes people living in institutionalized group quarters and unrelated individuals under the age of 15.
While I examine change over the entire 1959 to 2015 period, some of the analyses focus on changes between 1959 and 1979, and 1980 to the present. There are conceptual and practical reasons for this choice. Conceptually, the 1960s saw the passage of major Civil Rights legislation, with increased implementation into the 1970s, such as in the form of expanded school busing. The election of Ronald Reagan in 1980 saw the beginning of conservative retrenchment in several areas, including cuts in many government programs, as well as the acceleration of income inequality that began in the 1970s ( Danziger and Gottschalk 1995 ; Gottschalk and Danziger 2005 ). One important practical reason to split the analyses into these two time periods is the greater consistency in racial and ethnic groups definitions beginning in 1980, especially for Asians and Hispanics, as described in more detail below. Using a different cutoff year, such as 1989, would not affect the paper’s conclusions. Racial/ethnic gaps narrowed throughout the period, and the change in the role of, say, family structure in explaining black-white poverty differences, would be evident when using 1979 or 1989 as a midpoint year.
Measuring Poverty and Affluence
I use the official poverty measure in this analysis. Briefly, the official poverty measure has two components: poverty thresholds and the definition of family income that is compared to these thresholds. The thresholds remain the same over time, updated only for inflation. The thresholds vary by family size and number of children. In 2015, the poverty threshold for a family with two parents and two children was $24,036 ( Proctor, Semega, and Kollar 2016 ). A family and its members are considered poor if their income falls below the poverty threshold for a family of that size and composition.
Affluence is defined as family income-to-poverty ratios higher than five times the poverty threshold. For a family of two adults and two children, then, the threshold for affluence was $120,180 in 2015. While any threshold for poverty or affluence is inevitably somewhat arbitrary, this measure is reasonable in a couple of respects. In 2015, this dollar figure would place these families at a little below the 80 th percentile of income ($133,525) ( U.S. Census Bureau 2016d ), which is close to Reeve’s (2017) definition of the upper middle class. Secondly, if we were to choose a threshold much higher, only a very small percentage of the population would be affluent in the early years of this study, given overall increases in standards of living.
I also conducted the analyses with alternative measures of poverty and affluence. While these produce different point estimates of poverty and affluence, all of these yielded conclusions on racial and ethnic differentials similar to those presented here. Among the measures I used were relative poverty and affluence, defined as families with incomes in the bottom and top decile of the income distribution in each given year. Using the top decile as a measure of affluence is common in the literature (e.g., Piketty and Saez 2003 ). Since relative poverty measures are sometimes based on some fraction of income below the national median (as opposed to a bottom decile or quintile), I also conducted additional analyses with a relative poverty measure with a poverty threshold equal to one half the median household income in each year, as well as a relative affluence measure with an affluence threshold equal to twice the median household income in each year. These do not change the conclusions, and the results are shown in appendix tables and briefly discussed in the Sensitivity Analysis section below.
Race and ethnicity
I calculate poverty and affluence among the following mutually exclusive and exhaustive racial and ethnic groups: non-Hispanic whites, non-Hispanic blacks, non-Hispanic Asians, non-Hispanic American Indians, non-Hispanic other races, and Hispanics. There are a few data limitations when extending the analysis back to 1960 because of changes in the way data on race and ethnicity were collected by the U.S. Census Bureau. The categories for whites and blacks did not change much, so trends for these groups are the most reliable. In 1960 and 1970, there were response categories for only specific Asian groups, including Chinese, Japanese, Filipino, and Hawaiian (Korean was first used in 1970). In 1980 and thereafter, additional Asian groups were identified, plus a residual category for “other” Asian. Beginning in 2000, Pacific Islanders had a separate response category; to enhance comparability, I include all people who identify as Asian or Pacific Islander as Asian. Data on Hispanic origin were collected in a question that specifically asks respondents if they are of Hispanic origin or not (separate from the race question). This question was asked in a fairly consistent fashion beginning in 1980. In 1960 and 1970, the IPUMS imputes Hispanic origin using eight criteria based on Hispanic birthplace, parental birthplace, grandparental birthplace, Spanish surname, and/or family relationship to a person with one of these characteristics ( Gratton and Gutmann 2000 ).
With regard to other groups, the response category for American Indians did not change much over time. However, in recent decades, more people have reported being American Indian than would be expected given recorded patterns of fertility, mortality, and migration, indicating that it has become more common to assert an American Indian racial identity than in the past ( Liebler and Ortyl 2014 ; Snipp 1997 ). So patterns and trends in poverty and affluence among American Indians should be viewed with some caution. The “other race” category in the analysis needs to viewed with caution as well, as the Census Bureau used different procedures over time to classify respondents as “other race.” In addition, beginning in 2000, individuals could mark as many races as they pleased; these individuals are categorized as “other race” in the analysis. While people of “other” race are included in the accounting of the complete distribution of the poor and affluent populations, I do not focus on this group in many of the analyses.
I also conducted additional analyses where race groups are defined without reference to Hispanic origin (e.g., people who identified as white were categorized as such regardless of how they responded to the Hispanic origin question). The results of these analyses differed modestly in that racial and ethnic disparities narrowed by more using this approach than what is shown, mainly because whites who are Hispanic have a lower socioeconomic profile than non-Hispanic whites. Thus, the decline in disparities described below are more conservative estimates than the alternative approach.
The analyses focuses on differences poverty and affluence across panethnic racial and ethnic groups over time to because data are missing on a number of subgroups in earlier years, and some of the ethnic groups (e.g., Dominicans) were quite small through most of the study period. However, I include results on the largest Asian and Hispanic ethnic groups for 2015 in Appendix Tables A1 and andA2 A2 to show the extent to which differences in the likelihood of poverty and affluence reported for the panethnic groups are generalizable to subgroups. These results are described briefly in the results section.
Individual and Family Level Variables
I include a number of individual and family characteristics in the analysis, including gender, age, education, family structure, nativity, region, metropolitan status. Specifically, I compute four educational categories for the family householder: less than high school, high school only, some college, and Bachelor’s degree or more. Family structure is measure with variables for female-headed family, married-couple family, and other family type (such as a person living alone or with housemates). Variables are included for family size and number of children of the family householder. People are classified into four regions: Northeast, Midwest, South, and West. There is a dummy variable for whether a person lives in a metropolitan area. The analyses include age of the family householder, and a square term, as the association of age with poverty and affluence may be non-linear (e.g., a decline in affluence among the elderly).
Analytical Strategy
The analysis proceeds as follows. I begin with a descriptive look at patterns and trends in poverty and affluence by race and ethnicity from 1959 to 2015. These analyses will answer the first research question: How have patterns of poverty and affluence by race and ethnicity changed over time? This will be followed by logistic regressions to see how the likelihood of poverty and affluence across groups has changed over time including the sociodemographic characteristics described above. This analysis answers the second question posed: How has the relatively likelihood of poverty and affluence by race and ethnicity changed? These regressions will also show the relationship between the various control variables and poverty and affluence, which will be helpful for understanding results from the subsequent decomposition analysis.
Finally, to answer the third research question (to what extent do family- and individual-level characteristics help explain differences in the prevalence in poverty and affluence by race and ethnicity, and how have these associations changed over time?) I conduct a decomposition analysis using a variant of the well-known Blinder-Oaxaca decomposition for linear regression ( Blinder 1973 ; Oaxaca 1973 ) developed by Fairlie (2005) and Bauer and Sinning (2008) for nonlinear regression models. This decomposition method allows us to estimate the role of group characteristics in explaining differences in poverty and affluence by race in a given period versus what remains unexplained The analyses focus on three time periods: 1959, 1979, and 2015. The decomposition can be written as:
where Y ¯ A − Y ¯ B is the difference in the average probability of the outcome (poverty and affluence) between groups A and B, E βA (Y iA |X iA ) refers to the conditional expectation of Y iA and E βA (Y iB |X iB ) to the conditional expectation of Y iB evaluated at the parameter vector β A ( Bauer and Sinning 2006 ). Thus, the first two terms on the right-hand side of the equation provide an estimate of the impact of differences in the endowments (characteristics) of groups A and B on differences in the outcomes, while the last two terms reveal the effect of differences in coefficients on differences in the outcomes, which are treated as differences that cannot be explained by differences in the characteristics themselves. The contribution of specific characteristics is computed by using the Fairlie command in Stata ( Fairlie 2005 ; Fairlie and Robb 2007 ).
This decomposition approach shares the same potential issue of the original Blinder-Oaxaca decomposition in that results might be sensitive to the choice of the reference group. That is, in the equation above, Group A coefficients (i.e., from models that include only members of Group A) are applied to Group B means in the first two terms, and Group B means are used in the second two terms. An alternative would be to apply Group B coefficients (i.e., from models that include only members from Group B) to Group A means and use Group A means in the latter two terms. A more common approach, and the one used in the following analyses, is to use pooled regression coefficients (from a weighted sample that includes individuals of the two groups being compared) and apply them to means of both groups ( Neumark 1988 ; Oaxaca and Ransom 1994 ; Fairlie and Robb 2007 ). In sensitivity analyses, I find that using different reference group coefficients sometimes affects the magnitude of the effect of endowments, but it does not change this study’s conclusions. This issue is discussed in further detail at the end of the results section.
Descriptive findings
Figures 1 shows trends in poverty by race and ethnicity over the 1959 to 2015 period. The trends in Figure 1 are fairly widely known: poverty fell for all groups in the 1960s, but then fluctuated with the business cycle thereafter. Non-Hispanic whites have the lowest poverty rate (10.3 percent in 2015), followed by Asians (11.9 percent), Hispanics (22.5 percent), blacks (25.0 percent), and American Indians (26.1 percent). 1 Disparities in poverty tend to be larger in 1959 than in 2015, with significant narrowing of gaps during the 1960s. However, some narrowing has occurred in recent decades as well. For example, since 1980, the poverty rate for non-Hispanic whites drifted up from 8.7 percent to 10.3 percent in 2015. In contrast, the poverty rates for all other groups are lower in 2015 than 1980, though sometimes only slightly so.

Percent Poor by Race/Ethnicity and Year: 1959–2015
Figure 2 shows the percentage of the population that is affluent, by race and ethnicity, where families with incomes over five times the poverty line are considered affluent. Reflecting general increases in living standards, affluence rose for the total population and among all racial and ethnic groups. In 2015, Asians had the highest rate of affluence (35.8 percent), followed by whites (32.9 percent), blacks (14.4 percent), American Indians (13.3 percent), and Hispanics (12.1 percent). Thus, racial and ethnic disparities are large. The absolute gap in percentage of each group that is affluent rose over the period, though the relative differences narrowed substantially. For example, the absolute gap in affluence between whites and blacks was 18.5 percentage points in 2015, up from 6.2 percentage points in 1959. However, whites were 2.3 times more likely to be affluent than blacks in 2015, down from 9.9 times more likely in 1959, and even down modestly from 2.9 times more likely in 1979. Also of note, the gap between the percentage of Asians who were affluent compared with the percentage of whites who are affluent has grown since 2000, signifying a greater Asian advantage over this period.

Percent Affluent, Defined as Family Income Five Times the Poverty Line or Greater, by Race/Ethnicity and Year: 1959–2015
Multivariate Analysis
I examine the association between race/ethnicity and poverty and affluence over time with a series of logistic regressions. Table 1 shows the descriptive statistics for the independent variables in models. Overall, we see that the mean age of all groups increased over time, with whites having the oldest mean age. Education also increased among all groups. There was a decline in married-couple families for all groups over time, though there are some substantial differences in family structure across groups, with Asians the most likely to be living in married-couple families, followed by whites. Families became smaller over time, and there are substantial differences in the percent foreign-born across groups, with Asians having the highest percentage. The percentage of all groups living in metropolitan areas and in the South generally increased, though there are also some group differences by geography.
Descriptive Statistics, 1959–2015
Source: 1960 and 1980 decennial censuses and 2015 American Community Survey. Note: number of own children, educational attainment, foreign-born status, and age refer to the characteristics of the family householder.
Table 2 shows logistic regression results for the association between race/ethnicity and poverty in 1959, 1979, and 2015. It shows that all groups are more likely to be poor than whites in just about all years, with and without controls. In 2015, for example, that odds of being poor for blacks was 3.16 times that of whites when no controls are in the model. The odds were 1.31, 3.02, and 2.57, and 1.90 for Asians, American Indians, and Hispanics, respectively. The odds of being poor relative to whites generally declined over the period, though the declines for some groups were uneven. For example, the odds of being poor for blacks was 6.33 times that of whites in 1959, dropping to 4.36 in 1979, and finally to 3.16 times in 2015 when no controls are included. Among American Indians there was a continuous decline as well, though among Asians and Hispanics there was an increase between 1959 and 1979, followed by a decline. As noted before, the changes for Asians and Hispanics between 1959 and 1979 should be viewed with some caution, given changes in the definitions of these groups in this period.
Logistic Regressions for Poverty (Odds Ratios)
The control variables help explain some of the differences in the likelihood of poverty by race and ethnicity, but certainly not all. For example, the odds of being poor among blacks relative to whites falls from 3.16 with no controls to 1.71 with controls in 2015. For Hispanics it also drops, from 2.57 to 1.25, and for American Indians, from 3.02 to 1.96. These declines indicate that the observed variables in the model, such as education, age, and family structure, help explain some of the gap in poverty. As has been mentioned earlier, a caveat is that these are not meant to be wholly causal models. Some of the these variables can reflect poverty/affluence.
The control variables are associated with poverty in expected ways. Age has a negative association with poverty, men are less likely to be poor, as are people with higher levels of education. People living in female-headed families and other family types are substantially more likely to be poor than people in married couple families. Family size has a negative association with poverty, though more children are positively associated with poverty. Foreign-born people are more likely to be poor than the native-born in 1979 and 2015, and people living in metro areas are less likely to be poor. There are some regional differences in poverty, though the odds of being poor in the South relative to the Northeast declined over time.
Table 3 show results for affluence. Many of the patterns in this table are the same, but with a couple of important differences. In 1959, racial and ethnic differences were generally quite stark, as the odds ratios for blacks, Asians, American Indians, and Hispanics to be affluent relative to whites were only 0.09, 0.81, 0.17, and 0.26, respectively (without controls). The odds increased for all groups in 1979 (though the increase for Hispanics was slight), and there were further small increases for most groups in 2015, except for Hispanics, where the odds ratio remained at 0.29. In 2015, substantial disparities remain for most groups.
Logistic Regressions for Affluence (Odds Ratios)
One striking exception is that Asians reached parity with whites over the period. In 1979, with the odds of affluence for Asians was 1.01 times that of whites in models without controls. However, once controls were included in the model, the Asian advantage disappeared, and Asians were less likely to be affluent than whites (odds of 0.83), holding other characteristics constant. By 2015, however, the odds for Asians being affluent relative to whites increased to 1.10 times without controls, and differences were slight but associated with advantage (1.03) in models with the control variables. The control variables themselves are associated with affluence in expected ways, as the ones that were positively associated with poverty are for the most part negatively associated with affluence. Education stands out as having a very large association with affluence.
As described in the Data and Methods section, the analyses focus on differences in poverty and affluence across panethnic groups because of missing or sparse data for a number of groups in the earlier years of the study period. However, I include results for specific ethnic groups in 2015 in Appendix Tables A1 and andA2 A2 to show the extent to which results for panethnic groups are generalizable to specific ethnic groups. Table A1 , which focuses on Asians, indicates that three of the five largest Asian groups (Japanese, Filipinos, and Asian Indians) were less likely to poor than non-Hispanic whites in models without controls, though all except Filipinos were more likely to be poor once controls are included, mirroring the panethnic pattern. With regards to affluence, all specific groups except the Vietnamese were more likely to be affluent than whites in models without controls, and three of the groups remained more likely to be affluence in models with controls (Chinese, American Indians, and Japanese). Thus, there is clearly some variation across groups, though general conclusions derived from the panethnic models apply to most of the largest specific ethnic groups.
According to Table A2 , most of the conclusions about Hispanic poverty and affluence apply to the largest specific ethnic groups, with a couple of exceptions. All groups are more likely to be poor than whites in models with controls (except “other Central American”), and most are less likely to be affluent, with the exception of Cubans.
Decomposition analysis
Finally, the decomposition analysis examines whether there are particular individual- and family-level characteristics that explain groups differences in poverty and affluence, and how their effects have changed over time. Table 4 shows results for poverty. The first three rows confirm the descriptive statistics that poverty gaps between various minority groups and whites have generally narrowed over time. Figures in the next row indicate that over the course of the 1959 to 2015 period, the observed characteristics generally explain an increasing share of the poverty gap across groups. For example, the characteristics explains two thirds (67 percent) of the poverty gap between whites and blacks in 2015, up from about half (49 percent) in 1959. The decline in the proportion of the racial and ethnic disparities that cannot be explained by the variables in the models indicates that such unobservable factors play a smaller role than they used to. This could result if factors such as discrimination, neighborhood conditions, and other unobserved causes of disparities have become less important over time, while factors such as differences in human capital, family formation patterns, and factors associated with immigration have become more important.
Decompositions of Differences in Poverty, by Race and Year
Notes: all differences are statistically significant except for # of children for Asians in 1959 and for Hispanics in 2015, and region for Asians in 2015. NA: Not Applicable-- the differences in poverty are too small to be meaningfully explained.
With regard to the black-white poverty gap, differences in family structure, age, and educational attainment play the largest roles in explaining the gap (34, 16, and 15 percent, respectively in 2015), and the roles of each also increased over time. Among Asians, the gap in poverty was very small in 1959, such that the effect of any particular characteristics in explaining the difference would look very large (because the denominator in the calculation is the difference)—so these are not presented in the table. In 2015, observed characteristics explain 91 percent of the white-Asian poverty gap, with nativity explaining the largest portion (more than the entire net gap), followed by age. The table shows that family structure, living in metropolitan areas, and educational attainment are protective—if Asians more resembled whites in these characteristics, then the white-Asian poverty gap would have been even larger.
Among Hispanics, the percent the poverty gap explained by the observed characteristics was 92 percent in 2015 (up from just 31 percent in 1959), with education (46 percent), age (23 percent), and nativity (22 percent) explaining most of the white-Hispanic poverty gap. Thus, Hispanics resemble Asians in that immigration-related factors play an important role, but unlike Asians, education is not protective; rather, it contributes to their relatively high levels of poverty.
With regards to the American Indian-white poverty gap, observed characteristics explained 53 percent of the gap in poverty in 2015 (up from 42 percent in 1959), with, as for black-white gaps, differences in educational attainment, family structure, and age playing the largest roles (explaining 21, 12, and 10 percent of the gap, respectively).
In terms of the role of different factors across groups, we see that family structure played the largest role among blacks. Nativity and age were important among Asians and Hispanics. In addition, education also stood out as being particularly important for Hispanics, and multiple factors were important among American Indians.
Table 5 shows decomposition results when using affluence as the outcome. The patterns are in some ways similar to poverty: observed characteristics tend to explain a greater proportion of the affluence gaps over time, with some exceptions. Among blacks, family structure plays the most prominent role (explaining 32 percent of the gap in 2015), followed by education (25 percent). Interestingly, while the effect of family structure on poverty among African American did not change much between 1979 and 2015, its effect on affluence grew, as the negative association between single parenthood and affluence grew stronger over the period—perhaps due to increasing assortative mating ( McLanahan 2004 ). Among American Indians, education plays the largest role (33 percent in 2015), followed by family size (18 percent), family structure (15 percent) and metropolitan status (12 percent). With regards to metropolitan status, this is a function of people in nonmetropolitan areas considerably less likely to be affluent, and American Indians over-represented in nonmetropolitan areas.
Decompositions of Differences in Affluence, by Race and Year
Notes: all differences are statistically significant except for foreign born for blacks in 1979 and for American Indians in 1979; number of children for all groups in 2015. NA: Not Applicable-- the differences in affluence are too small to be meaningfully explained.
Education and nativity continue to play important roles among Asians and Hispanics. In particular, among Hispanics education plays the largest role (explaining 43 percent of the gap in 2015), followed by family size (21 percent) and nativity (13 percent). Among Asians, gaps again are small, so the decomposition shows relatively large effects of individual characteristics, especially in 1959 and 1979, when the results measured in percentage terms are not very useful. In 2015, Asians were advantaged over whites, with education, family structure, and metropolitan status contributing to their advantage, and family size and nativity working in the opposite direction. 2
Generally speaking, education tends to play a larger role in explaining racial differences in affluence than poverty, indicative of the particularly strong association between human capital and upward mobility. Conversely, nativity tends to be more important for understanding racial disparities in poverty than affluence.
Sensitivity Analyses
The use of alternative poverty and affluence measures.
I also conducted additional analyses with a relative poverty measure with a poverty threshold equal to one half the median household income in each year, as well as a relative affluence measure with an affluence threshold equal to twice the median household income in each year. These results are shown in Appendix Tables A3 and andA4, A4 , respectively. The results are similar to those shown in main decomposition tables for poverty and affluence ( Tables 4 and and5). 5 ). For example, in Appendix Table A3 we see that the difference in relative poverty between whites and blacks declined over the period, and characteristics explained a larger proportion of the difference in 2015 than 1959. The role of family structure increased from 1959 to 1979 and remained stable thereafter. Among Hispanics, the total difference explained by various characteristics also increased over time, and education in particular played a large role. The white-Asian difference in poverty is quite small in all years—to small to meaningfully decompose in 1979 and 2015.
With regards to relative affluence, we again see many similarities. The role of family structure in explaining white-black differences increased steadily over the time period, the Asian-white gap in affluence grew (signifying higher rates of affluence among Asians than whites over time), and education playing an important protective factor. Conversely, education once again plays an important role in the white-Hispanic gap in relative affluence. Among American Indians and whites, several factors remain important in explaining differences.
The Use of Alternative Reference Groups in the Decompositions
As described in the Data and Methods section, results of decompositions can be sensitive to the reference group chosen (i.e., whether one uses regression coefficients from models that contain members of Group A vs. Group B). The decomposition results shown above use regression coefficients from models that pool whites and the minority group members of interest. This section reviews results when using coefficients from models that contain whites only versus those that contain minority group members only.
Overall, the conclusions of this study remain the same regardless of the reference group chosen. Specifically, using a coefficients from a model including only one particular group (e.g., white vs. the minority group) does not consistently produce higher or lower estimates of the role of characteristics in explaining racial and ethnic differences. For example, using pooled regressions of blacks and whites produced an estimate that characteristics explained 67 percent of the white-black gap in poverty (shown in Table 4 ). Using whites as a reference group (i.e., models that include only whites) produced a modestly lower estimate of 57 percent ( Appendix Table A5 ), while using blacks as the reference group produced an estimate of 67 percent ( Appendix Table A6 ). Using pooled regressions produced an estimate that characteristics explained 67 percent of the white-black gap in affluence (shown in Table 5 ). Using whites as a reference group produced a similar estimate of 64 percent ( Appendix Table A7 ), while using blacks as the reference group produced a lower estimate of 55 percent ( Appendix Table A8 ). All of these figures are larger than the respective 1959 estimates, as also shown in the tables, supporting the conclusion that an increasing proportion of the black-white difference in poverty and affluence are explained by these observed characteristics, regardless of the reference group.
One of the reasons that the choice of the reference group has only a modest effect on results is that the independent variables, with some exceptions, tend to have similar associations with poverty and affluence across the racial and ethnic groups (and more so over time). For example, while the odds that a person in a female-headed family is affluent compared to a person in married-couple family in 2015 was 0.25 for the entire pooled sample ( Table 4 ), the odds for regressions that include, in turn, only non-Hispanic whites, non-Hispanic blacks, non-Hispanic Asians, non-Hispanic American Indians, and Hispanics, were 0.24, 0.24, 0.41, 0.19, and 0.24, respectively—a modest band of variability. Similarly, the odds that a person in family where the householder has a BA or more is affluent compared to a person in family where the householder does not have a high school diploma in 2015 was 13.20 for the entire pooled sample. In samples stratified by race, these odds were 11.92, 12.02, 13.40, 9.26, and 15.34 for non-Hispanic whites, non-Hispanic blacks, non-Hispanic Asians, non-Hispanic American Indians, and Hispanics, respectively. These difference are not trivial, but on the whole modest, as they are not great enough to affect the conclusions from the decomposition analysis.
Perhaps the most notable variation in the decomposition results when using different reference groups, is in Asian-white decomposition, where the role of nativity is consistently larger (in both the poor and affluent models) when using coefficients from models with Asians only than in models with whites only or the pooled equations. The reason for this is that association between nativity and poverty and affluence is stronger among Asians than for whites (or whites and Asians pooled). This is suggestive of a strong pattern of generational economic upward mobility among Asians. Among Hispanics, nativity actually plays a smaller role in the poverty decomposition when using coefficients from the Hispanic sample only than the pooled sample, though there is little difference in results by reference group when looking at the role of nativity in explaining affluence.
Conclusions
The goal of this analysis has been to document racial disparities in poverty and affluence from 1959 to 2015 and shed some light on the dynamics of these differences over the period. While trends in poverty have been fairly well documented, we know less about trends in affluence for all of the groups included here—whites, blacks, Asians, Hispanics, and American Indians. I examined changes in the relative likelihood of poverty and affluence by race and ethnicity and then used a decomposition analysis to examine the relative contribution of important sociodemographic correlates of poverty and affluence, including education, family structure, and nativity, and how their effects have changed over time.
The analyses indicate that racial disparities in poverty and affluence are generally large. However, disparities between minority groups and whites generally declined over the period. For example, poverty declined for all groups, but moderately more for minority groups than whites. Similarly, affluence increased substantially for all groups—indicative of rising living standards—but in relative terms more for minority groups than whites.
There is variation across groups. Blacks and American Indians tended to be the most disadvantaged groups, though the magnitude of disadvantage declined over the period, and there was only a little decline in the affluence gap after 1979. Hispanics were less likely to be poor than blacks and American Indians, but about as equally likely to be affluent. The white-Hispanic gap in poverty and affluence actually increased from 1959 to 1979, before declining slightly thereafter. Finally, while Asians continue to be more likely to be poor than whites, they reached parity with whites in affluence in 1979 and surpassed whites by 2015.
A key contribution of this paper lay in the results of the decomposition analyses, which examined which factors in particular were most important in explaining disparities. While a number of previous studies have documented differences in socioeconomic attainment between groups (e.g., Sakamoto and Kim 2013 , Snipp and Cheung 2016 ), they have not focused on the changing roles of groups characteristics in explaining change over time. The findings of this analysis indicates that the effect of these factors varied by group. Among Hispanics and Asians, education and nativity consistently were important factors. For Hispanics, education was particularly important for explaining disparities (explaining between 43 and 46 percent of the poverty and affluence gaps, respectively, in 2015), and we find that its role increased over time, especially with regards to poverty—a finding not widely appreciated in the literature. Among Asians, education was a “protective” factor—if Asians more resembled whites in terms of education, the disparities in poverty would have been larger. Nativity was important for both groups, though more so in explaining poverty differentials than those in affluence (another findings not as widely appreciated in the literature)—suggesting that being foreign born is associated with greater labor market challenges among low skill workers than those at the upper end—many who may have been admitted into the United States on the basis of their skills.
Thus, the analysis supports the notion that the effects of human capital differentials and the immigrant incorporation process are very important for understanding disparities among Hispanics and Asians ( Sakamoto and Kim 2013 ; Lee and Zhou 2014 ; Chiswick 1986 ; Feliciano 2005 ). Highly selective immigration from Asia likely helps explain good socioeconomic outcomes of Asians in the United States, combined with the large emphasis these immigrant parents place on schooling for their children ( Hsin and Xie 2014 ; Lee and Zhou 2014 ; Jiménez and Horowitz 2013 ). While Asian immigrants are positively selected on education, the same is not the case for Hispanics, especially immigrants from Mexico ( Chiswick 1986 ; Feliciano 2005 ). The undocumented status of many Latin American immigrants also slows the economic incorporation process, as such immigrants do not have access to many opportunities in the formal labor market, which would likely have a particularly strong effect on the likelihood of becoming affluent ( Brown 2007 ; Bean et al. 2015 ; Perlmann 2005 ). So to the extent to which immigration levels remain high and the selectivity patterns hold, we should continue to see divergent outcomes among Asians and Hispanics, even as generational progress slowly serves to narrow the gap between whites and Hispanics.
The decomposition analysis also showed that the effect of family structure grew in importance and became the most significant factor among blacks—not only for poverty, but also for affluence, explaining about a third of the disparity in poverty and affluence in 2015. While the impact of family structure on poverty grew mainly between 1959 and 1979, and remained stable thereafter, the effect of family structure on affluence increased further after 1979—a factor not appreciated in the existing literature on disparities in poverty and affluence, and indicative of the importance of comparing the factors that affect each of these outcomes. The patterns are likely a result of the slightly weakening correlation between family structure and poverty in recent decades, as indicated by the decline in poverty among female-householder families ( U.S. Census Bureau 2016b ; Cancian and Reed 2008 ; Baker 2015 ), though the strengthening correlation between family structure and affluence. The latter likely results from the increase in assortative mating by education, and the “diverging destinies” between families with well-educated two-parent families and others, and their contributions to racial inequality ( McLanahan 2004 ; McLanahan and Percheski 2008 ). The fact that family structure plays an important role is indicative of the importance of the interaction between changing economic conditions that have hindered the prospects of less-skilled men and increased opportunities for women, as well as changes in cultural attitudes that have reduced the stigma on single parenthood—both factors may have affected blacks and Hispanics more so than whites and Asians ( Cherlin 2004 , 2009 ; Smock and Greenland 2010 ; Thornton and Young-DeMarco 2001 ; Goldscheider and Kaufman 2006 ; Trent and South 1992 ; Sakamoto et al. 2000 ; Snipp and Cheung 2016 ). The continued increase in single-parenthood among white families ( Murray 2012 ), however, could over time narrow the contribution of family structure to racial and ethnic disparities in poverty and affluence in the future.
Among American Indians, no single factors plays a dominant role—several are important, including education (generally the most important), family structure, and, depending on the outcome, family size, age, or metropolitan status. Thus, it appears that cumulative disadvantages are important for American Indians, who are more likely to have lower levels of human capital, live in single parent families, and have a younger age structure and live in nonmetropolitan areas than other groups.
Finally, the implications of the existence of an unexplained gap for most groups—and its decline over time—are not clear cut, but suggestive. The presence of an unexplained difference is sometimes attributed to discrimination (e.g., Cancio, Evans, and Maume 1996 ; Snipp and Cheung 2016 ), since discrimination typically is not observed in survey data. However, it should be emphasized that there are other unobserved factors in the census data used, including neighborhood conditions, social capital, and cultural capital—all influenced by race-related factors, such as racial and ethnic segregation—that can also play a role. Nevertheless, the findings suggest that these types of factors played a smaller role in explaining racial and ethnic disparities in poverty and affluence over time. Instead, observed characteristics, such as educational attainment (a key indicator of human capital), nativity (indicative of the importance of the immigrant incorporation process), and family structure (indicative of the interaction between economic conditions and culture) played a larger role.
This study has a few limitations. The use of cross-sectional decennial and ACS data precludes making strong causal inferences about the effect of the variables of interest, such as family structure, on poverty. Family structure can be both a cause and reflection of poverty. Thus, this study mainly sheds light on the factors associated with poverty, and how differences in these characteristics across racial and ethnic groups might reflect and contribute to differences in the prevalence of poverty and affluence. This study is also limited to the indictors available in the decennial and ACS files. Ideally we would have a broader array of variables, such as wealth, or experiences of discrimination to further probe inequalities, but these are unavailable. As noted earlier, the definitions of some of the racial and ethnic groups studied also varied over time, especially among Asians and Hispanics prior to 1980, so conclusions that extend to before then need to be made with caution.
In summary, the findings suggest that there were moderate steps toward racial equality in poverty and affluence over the 1959 to 2015 period, consistent with notion that there has been a decline in the significance of race in shaping life chances ( Sakamoto, Wu, and Tzeng 2000 ; Wilson 1980 ). However, despite some narrowing of the racial gap and the general parity between whites and Asians, other large disparities remain, especially for blacks and American Indians. There are likely many causes for continued disparities among these groups, including racial discrimination in the labor market, which serves to reduce employment and wages. The increase in incarceration in the late 20 th century also served to reduce human capital and wages among black men in particular, and these show up in higher poverty rates and lower rates in affluence among black families ( Western and Pettit 2005 ). The legacy of historical inequalities may also play a role, as there is a fair amount of intergenerational transmission of socioeconomic status in the United States ( Duncan and Brooks-Gunn 1997 ; Isaacs, Sawhill, and Haskins 2008 ; Solon 1999 ). Differences in social and cultural capital, social and spatial isolation, and culture factors, may also help explain some of the differences ( Loury 1977 , 2002 ; Massey 2007 ; Massey and Denton 1993 ; Patterson and Fosse 2015 ; Wilson 1987 ). Thus, while gaps between groups have narrowed, considerable differences remain.
Acknowledgments
This research was supported by the National Institutes of Health, Population Research Institute Center Grant, R24HD041025.
Appendix Table A1.
Logistic Regressions of Poverty and Affluence among Detailed Asian Ethnic Groups, 2015
Appendix Table A2.
Logistic Regressions of Poverty and Affluence among Detailed Hispanic Ethnic Groups, 2015
Appendix Table A3.
Decompositions of Differences in Poverty, Using a Relative Poverty Measure, by Race and Year
Notes: the relative poverty measure uses a poverty threshold that equals one half the median household income. NA: Not Applicable-- the differences in poverty are too small to be meaningfully explained.
Appendix Table A4.
Decompositions of Differences in Affluence, Using a Relative Measure of Affluence, by Race and Year
Notes: the relative affluence measure uses a threshold that equals two times the median household income.
Appendix Table A5.
Decompositions of Differences in Poverty, by Race and Year, Using Whites as the Reference Group
NA: Not Applicable-- the differences in poverty are too small to be meaningfully explained.
Appendix Table A6.
Decompositions of Differences in Poverty, by Race and Year, Using the Minority Group as the Reference Group
Note: NA: Not Applicable-- the differences in poverty are too small to be meaningfully explained.
Appendix Table A7.
Decompositions of Differences in Affluence, by Race and Year, Using Whites as the Reference Group
NA: Not Applicable-- the differences in affluence are too small to be meaningfully explained.
Appendix Table A8.
Decompositions of Differences in Affluence, by Race and Year, Using the Minority Group as the Reference Group
1 Poverty rates using data from the American Community Survey are slightly higher than when using data from the Current Population Survey due to better coverage of income in the latter. Using ACS data likely does not introduce bias into the analysis on disparities since poverty rates are higher among all groups using ACS data.
2 The strong negative association between family size and affluence can be explained in large part by the fact that the thresholds for affluence increase with family size. For example, the threshold for affluence for a family with two adults and two children in 2015 was $120,180, while the threshold for affluence for a family with two adults and four children was $158,350.
- Alba Richard and Nee V 2003. Remaking the American mainstream: Assimilation and contemporary immigration . Cambridge: Harvard University Press. [ Google Scholar ]
- Baker Regina S. 2015. “ The Changing Association Among Marriage, Work, and Child Poverty in the United States, 1974–2010 .” Journal of Marriage and Family 77 : 1166–1178. [ Google Scholar ]
- Bauer Thomas K. and Sinning Mathias 2006. “ An Extension of the Blinder-Oaxaca Decomposition to Non-Linear Models .” RWI Discussion Papers , No. 49. [ Google Scholar ]
- Bean Frank D., Brown Susan K., and Bachmeier James D. 2015. Parents Without Papers: The Progress and Pitfalls of Mexican American Integration . New York: Russell Sage Foundation. [ Google Scholar ]
- Becker Gary S. 1994. Human Capital: A Theoretical and Empirical Analysis with Special Reference to Education , 3rd edition. Chicago: University of Chicago Press. [ Google Scholar ]
- Blau Francine D., Ferber Marianne A., and Winkler Anne E. 1998. The Economics of Women, Men, and Work , 3rd edition. Sadle River, NJ: Prentice Hall. [ Google Scholar ]
- Blinder Alan S. 1973. “ Wage discrimination: Reduced form and structural estimates .” Journal of Human Resources 8 : 436–455. [ Google Scholar ]
- Bourdieu Pierre 1977. "Cultural Reproduction and Social Reproduction" Pp. 487–511 in Power and Ideology in Education , edited by Karabel J and Halsey AH New York: Oxford University Press. [ Google Scholar ]
- Brown Susan K. 2007. “ Delayed Spatial Assimilation: Multigenerational Incorporation of the Mexican Origin Population in Los Angeles .” City & Community 6 , 3 : 193–209. [ Google Scholar ]
- Cancio A Silvia T Evans David, and Maume David J. Jr. 1996. “ Reconsidering the Declining Significance of Race: Racial Differences in Early Career Wages .” American Sociological Review 61 , 4 : 541–556. [ Google Scholar ]
- Cancian Maria and Reed Deborah 2008. “Family structure, childbearing, and parental employment: Implications for the level and trend in poverty” Institute for Research on Poverty , University of Wisconsin-Madison, Discussion Paper no. 1346–08. [ Google Scholar ]
- Couch Kenneth and Daly Mary C. 2002. “ Black-White Inequality in the 1990s: A Decade of Progress .” Economic Inquiry 40 , 1 : 31–41. [ Google Scholar ]
- Charles Kerwin Kofi and Guryan Jonathan 2008. “ Prejudice and Wages: An Empirical Assessment of Becker’s The Economics of Discrimination .” Journal of Political Economy 116 , 5 : 773–809. [ Google Scholar ]
- Cherlin Andrew J. 2004. “ The deinstitutionalization of American marriage .” Journal of Marriage and Family 66 : 848–861. [ Google Scholar ]
- Cherlin Andrew J. 2009. The Marriage-Go-Round: The State of Marriage and the Family in America Today . New York: Vintage Books. [ Google Scholar ]
- Chiswick Barry R. 1986. “ Is the New Immigration Less Skilled Than the Old? ” Journal of Labor Economics 4 , 2 : 168–192. [ PubMed ] [ Google Scholar ]
- Danziger Sheldon and Peter Gottschalk 1995. America Unequal . New York: Russell Sage Foundation. [ Google Scholar ]
- Dávila Alberto, Mora Marie T., and Hales Alma D. 2008. “Income, Earnings, and Poverty: A Portrait of Inequality Among Latinos/as in the United States” Pp. 181–195 In Latinas/os in the United States: Change the Face of America Edited by Rodríguez Havidán, Sáenz Rogelio, and Menjívar Cecilia Boston: Springer. [ Google Scholar ]
- Duncan Brian and Trejo Stephen J. 2014. “ Assessing the Socioeconomic Mobility and Integration of U.S. Immigrants and Their Descendants .” The ANNALS of the American Academy of Political Social Science 657 , 1 : 108–135. [ Google Scholar ]
- Duncan Brian, Hotz V. Joe, and Trejo Stephen J. 2006. “Hispanics in the U.S. Labor Market” Pp. 228–290 in Hispanics and the Future of America edited by Tienda Marta and Mitchell Faith Washington, DC: National Research Council. [ Google Scholar ]
- Duncan Greg J. and Brooks-Gunn Jeanne 1997. Consequences of Growing Up Poor . New York: Russell Sage Foundation. [ Google Scholar ]
- England Paula 2010. “ The Gender Revolution: Uneven and Stalled .” Gender and Society 24 , 2 : 149–166. [ Google Scholar ]
- Fairlie Robert W. 2005. “ An extension of the Blinder-Oaxaca decomposition technique to logit and probit models .” Journal of Economic and Social Measurement 30 : 305–316. [ Google Scholar ]
- Fairlie Robert W. and Robb Alicia M. 2007. " Why are Black-Owned Businesses Less Successful than White-Owned Businesses: The Role of Families, Inheritances, and Business Human Capital ." Journal of Labor Economics 25 2 : 289–323. [ Google Scholar ]
- Farley Reynolds 1984. Blacks and Whites. Narrowing the Gap? Cambridge: Harvard University Press. [ Google Scholar ]
- Farley Reynolds 1996. The New American Reality . New York: Russell Sage Foundation. [ Google Scholar ]
- Feliciano Cynthia 2005. “ Educational Selectivity in U.S. Immigration: How Do Immigrants Compare to Those Left Behind? ” Demography 42 , 1 : 131–152. [ PubMed ] [ Google Scholar ]
- Gao George 2016. “Biggest share of whites in U.S. are Boomers, but for minority groups it’s Millennials or younger.” Pew Research Center Fact Tank, New in the Numbers . Available www.pewresearch.org/fact-tank/2016/07/07/biggest-share-of-whites-in-u-sare-boomers-but-for-minority-groups-its-millennials-or-younger/ (accessed July 31, 2017).
- Goldscheider Frances and Kaufman Gayle 2006. “ Single Parenthood and the Double Standard .” Fathering 4 , 2 : 191–201, 203–208. [ Google Scholar ]
- Gottschalk Peter and Danziger Sheldon 2005. “ Inequality of Wage Rates, Earnings and Family Income in the United States, 1975–2002 .” Review of Income and Wealth 51 , 2 : 231–254. [ Google Scholar ]
- Gratton Brian and Gutmann Myron 2000. “ Hispanics in the United States, 1850–1990: Estimates of Population Size and National Origin " Historical Methods 33 :137–153. [ Google Scholar ]
- Hirschl Thomas A. and Rank Mark R. 2015. “ The Life Course Dynamics of Affluence .” PLoS One 10 , 1 : e0116370 10.1371/journal.pone.0116370. [ PMC free article ] [ PubMed ] [ CrossRef ] [ Google Scholar ]
- Charles Hirschman and Morrison G Wong M 1984. “ Socioeconomic gains of Asian Americans, blacks and Hispanics: 1960–1976 .” American Journal of Sociology 90 :584–607. [ Google Scholar ]
- Hsin Amy and Xie Yu 2014. “ Explaining Asian Americans’ academic advantage over whites .” Proceedings of the National Academy of Sciences of the United States of America 111 : 8416–8421. [ PMC free article ] [ PubMed ] [ Google Scholar ]
- Hoynes Hilary W., Page Marianne E., and Stevens Ann Huff 2006. “ Poverty in America: Trends and Explanations .” The Journal of Economic Perspectives 20 , 1 : 47–68. [ Google Scholar ]
- Iceland John 2003. “ Why poverty remains high: The role of income growth, economic inequality, and changes in family structure, 1949–1999 .” Demography 40 , 3 : 499–519. [ PubMed ] [ Google Scholar ]
- Iceland John 2013. Poverty in America: A Handbook , 3rd edition. Berkeley, CA: University of California Press. [ Google Scholar ]
- Iceland John 2017. Race and Ethnicity in America . Berkeley, CA: University of California Press. [ Google Scholar ]
- Isaacs Julia B., Sawhill Isabel V., and Haskins Ron 2008. Getting Ahead or Losing Ground: Economic Mobility in America . Washington, D.C.: The Brookings Institution. [ Google Scholar ]
- Jiménez Tomás R. and Horowitz Adam 2013. “ When White is Just Alright: How Immigrants Redefine Achievement and Reconfigure the Ethnoracial Hierarchy .” American Sociological Review 78 , 5 : 849–871. [ Google Scholar ]
- Kim ChangHwan and Sakamoto Arthur 2010. “ Have Asian American Men Achieved Labor Market Parity with White Men? ” American Sociological Review 75 , 6 : 934–957. [ Google Scholar ]
- Kochhar Rakesh and Cilluffo Anthony 2018. “Income Inequality in the U.S. Is Rising Most Rapidly Among Asians.” Pew Research Center report, Social & Demographic Trends, July 12 . Available at www.pewsocialtrends.org/2018/07/12/income-inequality-in-the-u-sis-rising-most-rapidly-among-asians/ (accessed November 2, 2018).
- Lee Jennifer and Zhou Min 2014. “ The Success Frame and Achievement Paradox: The Costs and Consequences for Asian Americans .” Race and Social Problems 6 : 38–55. [ Google Scholar ]
- Lichter Daniel T., Qian Zhenchao, and Crowley Martha L. 2005. “ Child Poverty Among Racial Minorities and Immigrants: Explaining Trends and Differentials .” Social Science Quarterly 86 : 1037–1059. [ Google Scholar ]
- Liebler Carolyn A. and Ortyl Timothy 2014. “ More Than One Million New American Indians in 2000: Who Are They? ” Demography 51 : 1101–1130. [ PMC free article ] [ PubMed ] [ Google Scholar ]
- Loury Glenn C. 1977. “A Dynamic Theory of Racial Income Differences In Women, Minorities, and Employment Discrimination , pp. 153–186, Wallace Phyllis A. and LaMond Anette M., eds. Lexington, MA: Heath. [ Google Scholar ]
- Loury Glenn C 2002. The Anatomy of Racial Inequality . Cambridge, MA: Harvard University Press. [ Google Scholar ]
- Massey Douglas S. 1996. “ The age of extremes: Concentrated affluence and poverty in the twenty-first century .” Demography 33 , 4 : 395–412. [ PubMed ] [ Google Scholar ]
- Massey Douglas S 2007. Categorically Unequal: The American Stratification System . New York: Russell Sage Foundation. [ Google Scholar ]
- Massey Douglas S. and Denton Nancy 1993. American Apartheid: Segregation and the Making of the Underclass . Cambridge: Harvard University Press. [ Google Scholar ]
- McLanahan Sara 2004. " Diverging destinies: How children are faring under the second demographic transition ." Demography 41 , 4 : 607–627. [ PubMed ] [ Google Scholar ]
- McLanahan Sara and Percheski Christine 2008. “ Family Structure and the Reproduction of Inequalities .” Annual Review of Sociology 34 : 257–276. [ Google Scholar ]
- Murray Charles 2012. Coming Apart: The State of White America, 1960–2010 . New York: Crown Forum. [ Google Scholar ]
- Neumark David 1988. “ Employers’ discriminatory behavior and the estimation of wage discrimination .” Journal of Human Resources 23 : 279–295. [ Google Scholar ]
- Oaxaca Ronald L. 1973. “ Male–female wage differentials in urban labor markets .” International Economic Review 14 : 693–709. [ Google Scholar ]
- Oaxaca Ronald L. and Ransom Michael R. 1994. “ On discrimination and the decomposition of wage differentials .” Journal of Econometrics 61 : 5–21. [ Google Scholar ]
- Patterson Orlando and Ethan Fosse (eds). 2015. The Cultural Matrix: Understanding Black Youth . Cambridge, MA: Harvard University Press. [ Google Scholar ]
- Perlmann Joel 2005. Italians Then, Mexicans Now: Immigrant Origins and Second-Generation Progress, 1980 to 2000 . New York: Russell Sage Foundation. [ Google Scholar ]
- Piketty Thomas 2014. Capital in the Twenty-first Century . Cambridge Massachusetts: The Belknap Press of Harvard University Press. [ Google Scholar ]
- Piketty Thomas and Saez Emmanuel 2003. “ Income Inequality in the United States .” The Quarterly Journal of Economics CXVIII , 1: 1–39. [ Google Scholar ]
- Proctor Bernadette D., Semega Jessica L., and Kollar Melissa A. 2016. “ Income and Poverty in the United States: 2015 .” U.S. Census Bureau, Current Population Survey report, P60256 . [ Google Scholar ]
- Kelly Raley, R., Sweeney Megan M., and Wondra Danielle 2015. “ The Growing Racial and Ethnic Divide in U.S. Marriage Patterns .” The Future of Children 25 , 2 : 89–109. [ PMC free article ] [ PubMed ] [ Google Scholar ]
- Rank Mark R. and Hirschl Thomas A. 2001. “ Rags or Riches? Estimating the Probabilities of Poverty and Affluence across the Adult American Life Span .” Social Science Quarterly 82 , 4 : 651–669. [ Google Scholar ]
- Reeves Richard V. 2017. Dream Hoarders: How the American Upper Middle Class is Leaving Everyone Else in the Dust, Why That Is a Problem, and What to Do about it . Washington, D.C.: The Brookings Institution. [ Google Scholar ]
- Rothwell Jonathan T. and Massey Douglas S. 2010. “ Density Zoning and Class Segregation in U.S. Metropolitan Areas .” Social Science Quarterly 91 , 5 : 1123–1143. [ PMC free article ] [ PubMed ] [ Google Scholar ]
- Ruggles Steven, Genadek Katie, Goeken Ronald, Grover Josiah, and Sobek Matthew 2015. Integrated Public Use Microdata Series: Version 6.0 [dataset]. Minneapolis: University of Minnesota; 10.18128/D010.V6.0. [ CrossRef ] [ Google Scholar ]
- Sakamoto Arthur, Wu Huei-Hsia, and Tzeng Jessie M. 2000. “ The Declining significance of race among American men during the latter half of the twentieth century .” Demography 37 , 1 : 41–51. [ PubMed ] [ Google Scholar ]
- Sakamoto Arthur, Goyette Kimberly A., and Kim ChangHwan 2009. “ Socioeconomic Attainments of Asian Americans .” Annual Review of Sociology 35 : 255–276. [ Google Scholar ]
- Sakamoto Arthur and Kim ChangHwan 2013. “The Economic Characteristics of Asian Americans in the 21st Century” In The Economics of Inequality, Poverty, and Discrimination in the 21st Century, Volume 1—Causes , edited by Rycroft Robert S. Santa Barbara, CA: Praeger. [ Google Scholar ]
- Sandefur Gary D. and Liebler Carolyn A. 1997. “The Demography of American Indian Families” P. 196–217 in Changing Numbers, Changing Needs: American Indian Demography and Public Health edited by Sandefur Gary D., Rindfuss Ronald R., and Cohen Barney Washington, D.C.: National Academy Press. [ PubMed ] [ Google Scholar ]
- Sandefur Gary D. and Scott. WJ 1983. " Minority Group Status and the Wages of Indian and Black Males ." Social Science Research 12 : 44–68. [ Google Scholar ]
- Sarche Michelle and Spicer Paul 2008. “ Poverty and Health Disparities for American Indian and Alaska Native Children .” ANNALS of the New York Academy of Sciences 1136 : 126–136. [ PMC free article ] [ PubMed ] [ Google Scholar ]
- Sawhill Isabel V. 2006. “ Teenage sex, pregnancy, and nonmarital births .” Gender Issues 23 : 48–59. [ Google Scholar ]
- Smock Pamela and Greenland Fiona Rose 2010. “ Diversity in Pathways to Parenthood: Patterns, Implications, and Emerging Research Directions .” Journal of Marriage and Family 72 , 3 : 576–593. [ Google Scholar ]
- Matthew Snipp, C. 1992. “ Sociological Perspectives on American Indians .” Annual Review of Sociology 18 : 351–371. [ Google Scholar ]
- Matthew Snipp, C. 1997. “ The Size and Distribution of the American Indian Population: Fertility, Mortality, Migration, and Residence .” Population Research and Policy Review 16 : 61–93. [ Google Scholar ]
- Matthew Snipp, C. 2005. “American Indian and Alaska Native Children: Results from the 2000 Census” Population Bulletin , Population Reference Bureau, August. [ Google Scholar ]
- Matthew Snipp, C. and Charles Hirschman 2004. “Assimilation in American Society: Occupational Achievement and Earnings for Ethnic Minorities in the United States, 1970 to 1990 . Research in Social Stratification and Mobility 22 : 93–117. [ Google Scholar ]
- Snipp C Matthew and Sandefur Gary D. 1988. “ Earnings of American Indians and Alaska Natives: the effects of residence and migration .” Social Forces 66 : 994–1008. [ Google Scholar ]
- Snipp Matthew C. and Cheung Sin Yi 2016. “ Changes in racial and gender inequality since 1970 .” The ANNALS of the American Academy of Political and Social Science 663 : 80–98. [ Google Scholar ]
- Solon Gary 1999. “Intergenerational mobility in the labor market” Pp. 1761–1800 in Handbook of labor economics edited by Ashenfelter Orley and Card David Amsterdam, The Netherlands: Elsevier. [ Google Scholar ]
- Thiede Brian C., Lichter Daniel T., and Slack Tim 2018. “ Working, but poor: The good life in rural America? ” Journal of Rural Studies 59 : 183–193. [ Google Scholar ]
- Thiede Brian C., Kim Hyojung, and Slack Tim 2017. “ Marriage, Work, and Racial Inequalities in Poverty: Evidence from the United States .” Journal of Marriage and Family 79 : 1241–1257. [ PMC free article ] [ PubMed ] [ Google Scholar ]
- Thornton Arland, and Linda Young-DeMarco 2001. “ Four Decades of Trends in Attitudes Toward Family Issues in the United States: The 1960s Through the 1990s .” Journal of Marriage and the Family 63 : 1009–1037. [ Google Scholar ]
- Trent Katherine and South Scott J. 1992. “ Sociodemographic Status, Parental Background, Childhood Family Structure, and Attitudes toward Family Formation .” Journal of Marriage and Family 54 , 2 : 427–439. [ Google Scholar ]
- U.S. Census Bureau. 2016a. “ Poverty Status of People by Family Relationship, Race, and Hispanic Origin: 1959 to 2015 .” Historical Poverty Tables- People, Table 2 . [ Google Scholar ]
- U.S. Census Bureau. 2016b. “ Table 4. Poverty Status of Families, by Type of Family, Presence of Related Children, Race, and Hispanic Origin: 1959 to 2015 .” Current Population Survey, Annual Social and Economic Supplements, Historical Income Inequality Tables . [ Google Scholar ]
- U.S. Census Bureau. 2016c. 2015 ACS 1-Year Estimates, downloaded from Social Explorer . Available at www.socialexplorer.com/explore/tables (accessed August 23, 2017).
- U.S. Census Bureau. 2016d. “ Table F-1. Income Limits for Each Fifth and Top 5 Percent of Families: 1947 to 2015 .” Current Population Survey, Annual Social and Economic Supplements, Historical Income Inequality Tables . [ Google Scholar ]
- Western Bruce and Pettit Becky 2005. “ Black-White Wage Inequality, Employment Rates, and Incarceration .” American Journal of Sociology 111 , 2 : 553–578. [ Google Scholar ]
- Wilson William Julius 1980. The Declining Significance of Race: Blacks and Changing American Institutions . Chicago: University of Chicago Press. [ Google Scholar ]
- Wilson William Julius 1987. The Truly Disadvantaged: The Inner City, the Underclass, and Public Policy . Chicago: University of Chicago Press. [ Google Scholar ]
Indian-Americans Have Lowest Poverty Rate Among Asian Americans: Research
Indian americans have a poverty rate of 6%, which is the lowest among all other asian groups living in the us, the study found..
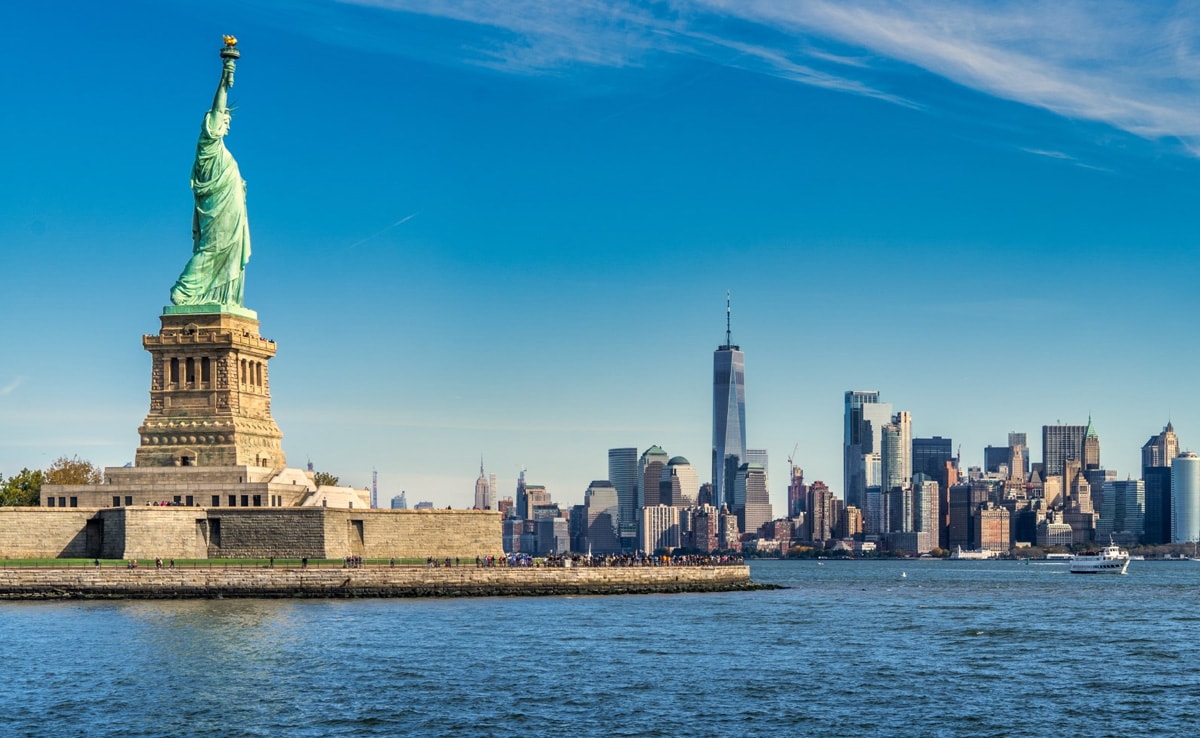
One out of every ten Asian Americans lives in poverty, as per the study. (Representative pic)
Indian-Americans are doing better than any other Asian-American group, according to a study by the Pew Research Center. For the research, experts studied the analysis of 18 focus groups conducted with 144 Asians living with economic hardships. They also studied the data of 561 Asian American adults whose approximate family income was at or below the 2022 federal poverty line. They found that over 2.3 million people with Asian roots living in the United States were living in poverty in 2022. The data also showed that one out of every ten Asian Americans lives in poverty.
According to the research , Indian-Americans have the lowest poverty rate among Asian Americans. They have a poverty rate of 6%, which is the lowest among all other Asian groups living in the US, the study found.
In contrast, Burmese and Hmong Americans were among the Asian-origin groups with the highest poverty rates. "Meanwhile, Filipinos (7%) and Indian Americans (6%) were among the groups with the lowest poverty rates," the research said.
The Pew Research Center also found that one out of every three Asian Americans aged 25 and older living in poverty has a bachelor's degree. By comparison, only 14% of non-Asians in poverty have the same level of education. "Asian Americans 25 and older with at least a bachelor's degree are still less likely to live in poverty (5%) than those with less formal education (13%)," it said.
Also read | Amid "Zombie Virus" Fears, 13 New Papillomaviruses Found In Antarctica
As per the study, around 6 out of 10 Asian Americans living in poverty were immigrants. And relatively few of these immigrants speak English proficiently. Among Asian immigrants aged 5 and older living below the poverty line, only 44% are proficient in English. By comparison, 61% of those immigrants above the poverty line speak English proficiently.
Promoted Listen to the latest songs, only on JioSaavn.com
According to a Pew Research Center survey conducted, nearly 8 out of 10 Asian adults living in poverty encountered financial difficulties in the past year. This contrasts with 48% of Asian adults above the poverty line who reported similar challenges.
"Roughly half of Asian Americans who live in poverty say the American dream is out of reach, but others say they have achieved it or are on their way to achieving it," as per the study.
Track Budget 2023 and get Latest News Live on NDTV.com.
Track Latest News Live on NDTV.com and get news updates from India and around the world .
Track Latest News and Election Results Coverage Live on NDTV.com and get news updates from India and around the world.
Watch Live News:

Interest Rate Volatility and Economic Growth in Nigeria: New Insight from the Quantile Autoregressive Distributed Lag (QARDL) Model
- Open access
- Published: 09 April 2024
Cite this article
You have full access to this open access article
- Godwin Olasehinde-Williams 1 , 2 ,
- Ruth Omotosho 3 &
- Festus Victor Bekun ORCID: orcid.org/0000-0003-4948-6905 4 , 5
This is a study on interest rate volatility, a crucial form of volatility which affects local and foreign investments in the real and financial sectors. Whether to prioritize interest rate stability to prevent distortions in the market mechanism or to prioritize other macroeconomic objectives while allowing interest rates to independently react to market forces is a key question for Nigeria’s apex monetary authority. Answering this question is the primary motivation for this research. This paper is an attempt to establish the effect of interest rate volatility on economic growth and further conclude on the suitability of the financial liberalization policy in Nigeria. To reach an evidence-based conclusion, the paper analyzes the relationship between interest rate volatility and economic growth in Nigeria for the period 1981–2020. The QARDL procedure was employed to establish the short-run and long-run quantile-specific impacts of interest rate volatility. As a final step, Granger causality tests are conducted to investigate the predictive powers of the variables. It is discovered from the econometric analysis that interest rate volatility adversely affects the economic performance of Nigeria in both the short run and long run. Consequently, full liberalization is not suitable for the economy. Moreover, we find that the short-run adverse growth effect of interest rate volatility is greater when the economy is already in a relatively weak state, whereas the long-run adverse growth effect is greater when the economy is already in a relatively strong position. The findings sufficiently prove that full interest rate liberalization is not Pareto efficient for Nigeria. Hence, greater supervision of the interest rate corridor system to reduce volatility in the rates and minimize chances of persistent upward or downward bias is advised. Study limitations and directions for further research are also provided.
Avoid common mistakes on your manuscript.
Introduction
Despite its status as Africa’s largest economy, Nigeria faces significant developmental challenges. A middle-income mixed economy with a growing market, Nigeria, is ranked the largest economy in Africa, the 27th largest economy in the world in terms of nominal GDP, and the 24th largest in purchasing power parity (Awe et al., 2023 ; Terwase et al., 2014 ). It is however an incontestable fact that the economy has grown weak over the decades in many respects. This is evident in the developmental challenges constantly being faced by the country. The 2022 multidimensional poverty index released by the National Bureau of Statistics reveals that about 63% of the populace live below the poverty line. It is thus an obvious reality that economic prosperity in Nigeria has been impeded by a myriad of factors. Particularly, the growing uncertainties of various forms plaguing the country is a cause for alarm.
For any developing economy on the right course, all influencers of national growth are topics of central discussion. Among multiple considerations of the various factors affecting the productivity and growth of any developing economy, the issue of the mechanism of interest rate, its variability, and trends through time has proven to deserve significant attention. Interest rate is the sum exacted on money given by a lender to a borrower and expressed as a proportion of the principal (Central Bank of Nigeria [CBN], 2016 ). This definition identifies it as the cost of borrowing from a lender. Another essential element in the definition is the recognition of the lender’s authority to charge a fee, also known as interest; for the creditor, the interest rate is the sum obtained for taking the risk to extend money to a borrower. Bergo ( 2003 ) describes it as compensation needed for placing capital at the disposal of others through savings. Interest rate can thus be seen from various perspectives. It can be seen as the cost of funds, the reward for savings, or the returns on investment of financial assets; whichever way, it is invariably an important economic price. As such, it is generally regarded as one factor that has a major impact on saving and investment within a country and, consequently, on economic growth. Typically, higher interest rates encourage savings as it leads to increased income. It however also raises the cost of capital, thus lowering a country’s rate of investment. Interest rate is therefore key to the transmission of monetary policy, and it is for this reason that it features in most financial and macroeconomic models (Das et al., 2014 ; Gil-Alana et al., 2017 ). Therefore, movements in interest rates, especially when unanticipated, are a major source of concern.
One common challenge plaguing Nigeria is the level of volatility often present in the financial system. Without a doubt, a robust financial system aids economic growth through the facilitation of savings and the channeling of funds from savers to investors. However, the challenges posed by the relatively high levels of instability in the system, especially with regard to sudden interest rate volatility, have the potential to adversely impact market efficiency (Istreffi & Mouabbi, 2017 ). Volatility creates inefficiencies in the financial system and consequently creates economic harm (Poterba, 2000 ). Di Giovanni and Levchenko ( 2006 ) emphasize that the adverse effects of volatility are even more pronounced in developing nations such as Nigeria when compared with developed nations.
As explained by Shunmugam and Hashim ( 2009 ), volatility in real interest rates impacts the entire economy either directly or indirectly. For instance, the statutory liquidity ratio portfolios and corporate bonds of financial institutions are directly susceptible to the risks posed by interest rate fluctuations. Institutional investors and mutual funds as well as the corporate sector are all also exposed to risks resulting from volatility in interest rates (Shunmugam & Hashim, 2009 ). Due to the strong interconnectivity between the financial sector, the corporate sector, and the overall economy, the adverse effects of interest rate volatility can quickly spread across the entire economy with dire consequences. Therefore, volatility in interest rate connotes a sense of uncertainty which adversely affects investment to the extent that investors lose confidence even in a currently low rate because of fear and expectations of a possible future rise which could pull down returns on investment. Istreffi and Mouabbi ( 2017 ) agree with this explanation that economies are adversely affected when uncertainty surrounds the future trajectory of interest rates, just as Donzwa et al. ( 2019 ) show that interest rate volatility spills over into other sectors of the economy.
For the aforementioned reasons, several researchers have advocated placing legal restrictions on interest rates to guard against market failures and information frictions that could be triggered by volatility (see Olasehinde-Williams & Özkan, 2022 ). On the other hand, it is also widely encouraged that interest rates should be left to the forces of demand and supply. It is claimed that the liberalization of the financial sector in this manner would improve economic growth (Ene et al., 2015 ; Mehran & Laurens, 1997 ). For instance, Ghironi and Ozhan ( 2020 ) present interest rate uncertainty as a useful tool for reducing ineffective capital inflows, as well as for modifying the structure of external accounts between marketable securities and foreign direct investment.
Thus, the best way of handling challenges triggered by sudden movements in interest rates has continued to generate debates in central bank policymaking (Olasehinde-Williams & Özkan, 2022 ). Nigeria’s apex bank, the CBN, through its monetary policy, power possesses significant influence on interest rates (Dabale & Jagero, 2013 ). Whether to prioritize interest rate stability to prevent distortions in the market mechanism or to prioritize other macroeconomic objectives while allowing interest rates to independently react to market forces is a key question for the CBN. Answering this question is the primary motivation for this paper.
Issues of interest rate volatility began to surface in Nigeria after the adoption of the liberalization policy in 1986. According to Kalejaiye et al. ( 2013 ), the application of the economic principle of liberalization began in the 1980s in Nigeria with the Structural Adjustment Program (SAP). At the commencement of this program, foreign trade and payment systems were liberalized, price controls and caps on interest rate were removed, and public sector enterprises were rationalized and restructured. All these efforts were aimed at increasing economic efficiency and placing the economy on a stable and durable growth path, especially in view of the problems encountered by Nigeria during the post-colonization era. However, economic growth has been epileptic, and there seems to be a relative insensitivity of the economy to the corrective liberalization policies. For instance, claims have been made that the interest rate liberalization policy in Nigeria has had no serious effect on the fund mobilization effort of commercial banks (Arikewuyo & Akingunola, 2019 ) or even on investment (Obinna, 2020 ).
A handful of studies have been carried out on interest rate volatility in Nigeria (see Dabale & Jagero, 2013 ; Ayopo et al., 2016 ; Omotunde & Nwokoma, 2016 ). Research has also been extensively done on Nigeria’s economic growth. However, so far, no study has attempted to merge these two critical issues. Considering the potential influence of interest rate volatility on overall productivity, findings about the growth effect of interest rate volatility provide policymakers with useful information. Overall, this paper augments the existing growth literature by determining the response of economic growth to interest rate volatility in a developing country such as Nigeria. The study further determines whether interest rate liberalization suits the Nigerian economy.
This paper contributes to existing literature in two unique ways. Firstly, it extends the empirical research on the interest rate-economic growth nexus by explicitly modeling interest rate volatility using the deviations of interest rate from trend components computed via the Hodrick–Prescott (HP) filter. Previous studies have mostly focused on inferring the growth effects of interest rate volatility indirectly from the growth impacts of interest rate. The ones that have actually estimated interest rate volatilities have done using ARCH/GARCH family models that are mainly useful for modeling volatility in high frequency data (see among others, Oseni & Nwosa, 2011 ; Bayracı & Ünal, 2014 ; Tian & Hamori, 2015 ). The method used in this study is better suited for modeling low-frequency data such as the annual data used in this study (see Grigoli & Mills, 2014 ). Secondly, this study takes into consideration the likelihood that various levels of interest rate volatility might not have similar effects on all levels of economic status, and the relationships might also vary from the short term to the long term. To this end, the novel Quantile Autoregressive Distributed Lag (QARDL) approach of Cho et al. ( 2015 ) is employed in modeling both short-run and long-run asymmetries between interest rate volatility and economic growth.
The remaining sections of this paper are organized as follows: the “ Literature Review ” section is the literature review, the “ Data and Methodology ” section describes the research methodology, the “ Results ” section reports the results, and the “ Conclusion and Policy Recommendations ” section concludes with a summary and policy recommendations.
Literature Review
Volatility in the macroeconomic space is generally caused by variability in fiscal and monetary policies (Shunmugam & Hashim, 2009 ). Volatility represents a measure of potential variations in economic variables captured through observed realizations over some historical period. It is thus commonly referred to as realized volatility and measured through standard deviations (Aizenman & Pinto, 2004 ; Lee et al., 2022 ). Thus, modeling volatility in macroeconomic variables involves establishing the deviations from equilibrium values in such variables (Aizenman & Pinto, 2004 ). Consequently, the effects of volatility in various economic variables have been examined this way in extant literature. Examples include the analysis of volatility in GDP growth rates and terms of trade by Acemoglu et al. ( 2003 ), the study on the investment-volatility dampening role of aid by Balcilar et al. ( 2022 ), the examination of the link between geopolitical threats and oil price volatility by Lee et al. ( 2021 ), the analysis of volatility in precious metals carried out by Khaskheli et al. ( 2022 ), and the study of volatility in house prices by Fan et al. ( 2022 ).
Specifically, interest rate volatility refers to the wide swings or changes in the rate of interest (Delebari & Didi, 2020 ). Volatility is usually experienced when liberalization policies are suspended. Interest rates may demonstrate “overshooting,” which arises from the upward drive compelled by inadequate fiscal and monetary policies; in this case, the perceived “volatility” is a normal dynamic adjustment in the marketplace (Glower, 1994 ). Studies have shown that interest rate volatility can affect the performance and monetary policy decisions of an economy through its effect on money demand (Githinji, 2015 ). Interest rate variations also influence consumer expenditure, household savings, and investment choices of enterprises (CBN, 2016 ). This causes fluctuations in the cost of borrowing and investment earnings. Rising interest rate volatility, especially when unanticipated, is also capable of lowering output (Evans, 1984 ; Friedman, 1982 ) and, as a consequence, raises unemployment (Dutkowsky, 1987 ). Naudé ( 1995 ) shows that the interest rate volatility that resulted from financial liberalization had a negative effect on the banking sectors of African countries. Patnaik and Shah ( 2004 ) report that interest rate volatility exposes banks to risks of equity capital loss. Olasehinde-Williams and Özkan ( 2022 ) find that uncertainties about interest rate generally aggravate investment volatility.
Studies linking interest rate and economic performance broadly fall into two categories (Olasehinde-Williams & Özkan, 2022 ). The first group of studies are those advocating the legal restriction of interest rates—financial repression. Financial repression is generally associated with the management of cross-border capital flows, a stronger relationship between the government and banks, interest rate caps, and relatively high reserve requirements (Reinhart, 2012 ). Arguments in support of restrictions of interest rates are mostly related to market failures and information frictions (see Stiglitz, 1989 , 1993 , 2000 ; Jafarov et al., 2019 ; Olasehinde-Williams & Özkan, 2022 ). Support for this position was widespread between the 1960s and 1980s; it has also regained prominence since the global economic crisis of the late 2000s (see Aloy et al., 2014 ; Reinhart & Sbrancia, 2015 ; Chari et al., 2020 ).
The second category comprises studies that support financial liberalization, which requires the government to play the role of an unbiased referee in order to ensure the smooth operation of the markets. Support for financial liberalization was kick-started by the theoretical arguments put forward by McKinnon ( 1973 ) and Shaw ( 1973 ). According to the authors, financial repression generates rates of return that are comparatively lower than what would be obtained in a competitive market, discouraging both savings and investment. They therefore consider financial liberalization a main stay for developing countries. Thornton ( 1991 ) further explains that the McKinnon-Shaw claim is that a repressed financial system interferes with development in the following ways: underdeveloped saving vehicles with negative or unstable returns, poor policies which discourage firms’ investments, and inefficient allocation of savings by the financial intermediaries. Ene et al. ( 2015 ) assert that liberalization of interest rate lays out an atmosphere for greater competitiveness in the mobilization of funds, an effective financial system and better performing organizations within the financial industry.
Many developing countries, Nigeria inclusive, have applied financial liberalization policies to strengthen the potency of monetary policy through greater reliance on market forces (Khan & Hassan, 1998 ). Succeeding the era of strict regulations and controls, liberalization featured in the Nigerian financial scene with the adoption of SAP in 1986. Ikhide ( 1998 ) reports that in 1987, all interest rate controls were eliminated; only the minimum rediscount rate was fixed to specify the preferred direction of the interest rate. In 1992, however, partial regulation was introduced, with financial institutions being compelled to keep a given level of spread between cost of funds and maximum lending rates. Controls were restored in 1994, but not without negative economic impacts. Hence, total liberalization was readopted in 1996 and has continued till date (Ene et al., 2015 ).
Data and Methodology
Model specification.
To observe the growth effect of interest rate volatility in the nation, a model is specified to capture the relationship that exists between the variables. Thus, gross domestic product (GDP) is the dependent variable, whereas interest rate volatility is the independent variable of interest. Taking a cue from theory and related research, exchange rate, inflation, investment, human capital, and population growth rate are included as control variables. Considering that these variables also influence economic growth, they are included as controls to overcome potential threats to valid inference. The functional relationship tested is given thus:
Here, gross domestic product (GDP) is shown as a function of interest rate volatility (ITRVOL), exchange rate (EXR), inflation (INF), investment (INV), human capital (HCAP), and population (POP).
Equation ( 1 ) is re-specified in an econometric form as follows:
where β 0 = intercept; β 1 , β 2 , β 3, β 4 , β 5 , β 6 = coefficients of the regressors; \(\mu\) = error term of the regression; \({{\text{LGDP}}}_{t}\) = logarithmic form of GDP; \({{\text{ITRVOL}}}_{t}=\mathrm{interest rate volatility}\) ; \({{\text{LEXR}}}_{t}\) = logarithmic form of exchange rate; \({{\text{INF}}}_{t}\) = inflation rate; \({{\text{LINV}}}_{t}\) = logarithmic form of investment; \({{\text{HCAP}}}_{t}\) = human capital; and \({{\text{POP}}}_{t}\) = population. It is worthy of note that variables that are not originally measured in percentages appear in logarithmic form for ease of interpretation and to eliminate potential heteroscedasticity in the data series.
Estimation Techniques
This study captures location-based (quantile) asymmetries in both short-term and long-term links between interest rate volatility and GDP in Nigeria, employing the QARDL model proposed by Cho et al. ( 2015 ). In utilizing the QARDL approach, the study assesses the stability of relationships across quantiles, offering a more flexible econometric framework to investigate the connection between interest rate volatility and GDP (see Lahiani, 2018 ; Jiang et al., 2021 ; Nawaz et al., 2021 ; Sadiq et al., 2021 ; Wu et al., 2021 ). In comparison to linear ARDL models, the QARDL model holds the advantage of introducing potential asymmetries in how GDP reacts to changes in interest rate volatility across different quantiles.
According to Shahbaz et al. ( 2018 ), methodologically, the QARDL model outperforms linear models on several grounds. Firstly, it accommodates locational asymmetry, where parameters may depend on the location of the dependent variable (GDP) within its conditional distribution. Secondly, the QARDL model concurrently addresses the long-term relationship between interest rate volatility and GDP, and the associated short-term dynamics across various quantiles of the conditional distribution of GDP. Thirdly, the method is able to identify the existence of quantile-varying cointegration coefficients over the short term which conventional techniques often miss (Xiao, 2009 ). Fourthly, the QARDL model also accommodates variations in the cointegrating coefficient over the innovation quantile, induced by shocks. Finally, rather than exogeneously define nonlinearity via a set threshold as is the case in the Nonlinear Autoregressive Distributed Lag (NARDL) model (Shin et al., 2014 ), QARDL uses a data-driven process. These attributes make QARDL a superior choice for more accurately modeling the nonlinear and asymmetric linkages between interest rate volatility and GDP in Nigeria. The technique is thus used to obtain both the short-run and long-run relationships between the variables of interest. To begin with, a traditional ARDL model is specified, as shown in Eq. ( 3 ).
Note the following: \({\varepsilon }_{t}\) is the error term measured as \({{\text{LGDP}}}_{t}-E\left[{{\text{LGDP}}}_{t}/{F}_{t-1}\right]\) . \({F}_{t-1}\) represents \(\delta\) which is the smallest field created via \(\left\{{{\text{ITRVOL}}}_{t}, {{\text{LEXR}}}_{t}, {{\text{INF}}}_{t}, {{\text{LINV}}}_{t},{{\text{LHCAP}}}_{t},{{\text{LPOP}}}_{t},{{\text{LGDP}}}_{t-1}, {{\text{ITRVOL}}}_{t=1}, {{\text{LEXR}}}_{t=1},{{\text{INF}}}_{t=1},{{\text{LINV}}}_{t=1}, {{\text{LHCAP}}}_{t=1},{{\text{LPOP}}}_{t=1}\dots \right\}\) . \(p, { q}_{1\dots ..},\mathrm{ and }{q}_{6}\) represent the lag orders selected through the Schwartz Bayesian Criterion (SBC).
Following Cho et al. ( 2015 ), Eq. ( 3 ) is extended into the basic QARDL ( p , q ) model to accommodate variations across quantiles as shown in Eq. ( 4 ):
Here, \({\varepsilon }_{t}(\gamma )\) equals \({{\text{LGDP}}}_{t}-{Q}_{{{\text{LGDP}}}_{t}}\left[\gamma /{F}_{t-1}\right]\) and \({Q}_{{{\text{LGDP}}}_{t}}\left[\gamma /{F}_{t-1}\right]\) refers to the \({\gamma }_{{\text{th}}}\) quantile of \({{\text{LGDP}}}_{t}\) conditional on the information set \({F}_{t-1}\) (see Kim & White, 2003 ).
Equation ( 5 ) is then specified to analyze the QARDL.
The parameters in Eq. ( 5 ) capture the short-run effects of the relationship between GDP and the regressors. The long-run effects are obtained by reworking Eq. ( 5 ) as follows:
where \(X\) is the vector of regressors \(\left({\text{ITRVOL}},\mathrm{ LEXR},\mathrm{ INF},\mathrm{ LINV},\mathrm{ LHCAP},\mathrm{ LPOP}\right).\)
To avoid serial correlation of the error term ( \({\varepsilon }_{t})\) , QARDL is further simplified as follows:
Finally, a QARDL-ECM model, which was estimated, is specified thus:
As a final step in the empirical analysis, Granger causality testing is performed to determine whether interest rate volatility is a significant predictor of Nigeria’s economic growth. This technique relies on the assumption that the past can cause the future (Odhiambo, 2009 ). A variable X t Granger causes another variable Y t , if the former contributes significantly to forecasting the latter. The technique involves the testing of the null hypothesis of no Granger causality from X t to Y t and vice versa through the estimation of the following equations:
The null hypothesis that X t does not Granger cause Y t is rejected if \({\alpha }_{12}\) exhibits joint significance. Similarly, the null hypothesis that Y t does not Granger cause X t is rejected if \({\alpha }_{22}\) exhibits joint significance (Granger, 1969 ). It is worthy of mention that the first differences of all non-stationary sequences are used for Granger causality testing, as the procedure requires stationarity in the data series.
Nature and Sources of Data
Due to data availability, annual time series data for each variable, over the period 1981–2020, is utilized. The chosen time frame is relevant to the study in that it spans both the eras before and after liberalization in the Nigerian economy. Similarly, it is a period long enough to provide reliable results that reflect the actual nature of the relationship between the variables of interest in relation to the Nigerian economy. Time series data are collected over time and can identify trends in the variables concerned. This work utilizes data series that are freely downloadable from https://www.dataworldbank.org ..
Data Description
Since GDP is a monetary measure of the market value of all the final goods and services produced in country within a given time period, it is often used as a single measure of a nation’s economic health. GDP is therefore often used as a measure of the dependent variable, economic growth (Abdulhakeem & Olasehinde-Williams, 2014 ; Balcilar et al., 2020 ; Coskuner & Olasehinde-Williams, 2017 ; Ntom Udemba et al., 2022 ). Interest rate volatility refers to variability in interest on loans and savings over time. It is the explanatory variable in the model whose impact on GDP the analysis seeks to estimate. Taking a cue from related research on volatility, its coefficient is expected to be negative, as increased volatility is most likely a deterrent to growth ( β 1 < 0) (see Ramey & Ramey, 1995 ; Aysan, 2007 ; Onwumere et al., 2012 ; Reyes-Heroles & Tenorio, 2017 ). Following Olasehinde-Williams ( 2021 ), volatility, as shown in Eq. ( 9 ), is constructed as the percentage change in the deviation of the absolute value of interest rate ( x t ) from its trend component calculated using the Hodrick–Prescott (HP) filter (τ x,t ).
where x t = interest rate at time t and τ = Hodrick-Prescott filter.
The exchange rate is an index which shows how valuable a currency is, relative to others. It has an impact on the GDP through its effect on international trade (importation and exportation); hence, it is included as one of the explanatory variables. Taking a cue from related research (see Chichi & Casmir, 2014 ; Ijirshar, 2019 ), its coefficient is expected to be positive ( β 2 > 0). Inflation is included as one of the controls, given the evident fact that it is one of the factors influencing Nigeria’s economic growth. The coefficient of this variable is expected to yield a negative sign ( β 3 < 0) (see Idris & Suleiman, 2019 ; Adaramola & Dada, 2020 ).
Investment refers to the process of acquiring an asset or an item with the understanding that it would help build future wealth. This variable is included as a control variable because its trend has an impact on GDP. Time series data on this variable is obtained as gross capital formation at constant prices. From economic theory, it is expected to positively influence GDP ( β 4 > 0) (see Jaiyeoba, 2015 ; Kalu & Mgbemena, 2015 ). Human capital is the economic value of labor’s skill, experience, and education, which increases productivity and efficiency in the process of production. Economic growth is expected to respond positively to an increased human capital ( β 5 > 0) (see Jaiyeoba, 2015 ; Ali et al., 2021 ). Population represents the number of residents within Nigeria, irrespective of legal status. It positively impacts GDP, provided the population also comes along with a skillful labor force; it could however exert a negative influence, if otherwise. Hence, the sign of its coefficient is indeterminate ( β 6 ≠ 0) (see Headey & Hodge, 2009 ).
Descriptive Analysis
Figure 1 presents the area plots of variables over the time frame covered by the research. As shown, GDP has consistently risen over time, hence its upward trend. Short declines are however visible around 2009 and 2015. Interest rate volatility (ITRVOL) is captured by the non-stationary movements visible in the second graph. The rate at which the naira exchanges for the dollar (EXR) has increased over the years. This is shown in the third graph by the upward movement of exchange rate all through the period covered in this study. With its peak in the 1990s, as presented in the fourth graph, inflation shows a non-stationary trend over the sampled period. Also, as can be seen from the fifth graph, investment (INV) dropped at the beginning of the period under study but began to rise gradually afterwards and has shown a gradual and steady increase over time. As for human capital (HCAP), its movement has been somewhat unstable. The trend line reveals that it was relatively high around 1985 but dropped to about 80% in 1990 and has adopted a non-stationary movement ever since with a mean value of about 93%. Population growth rate (POP) has also fluctuated over time, though with not very sharp spikes as seen in other variables modeled in this study.
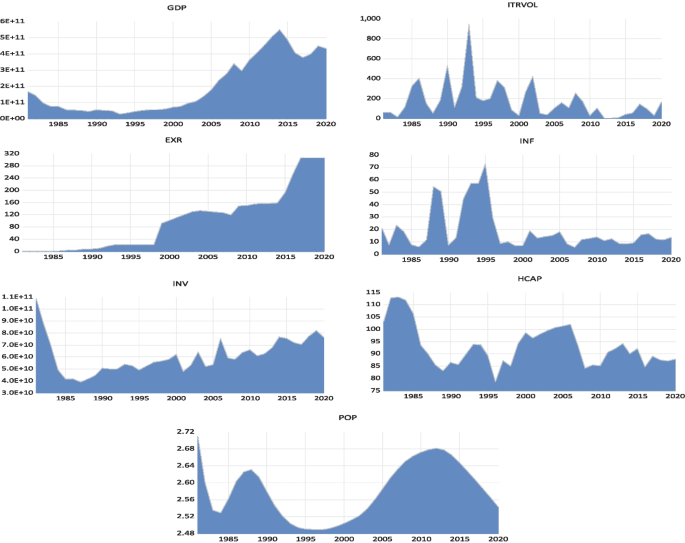
Trend analysis
Table 1 presents the summary statistics of the variables. For the sample period, it reveals the average values of the variables (mean), spread from the average value (standard deviation), the lowest recorded values (minimum), and the highest recorded values (maximum). It can be inferred from the Jacque-Berra statistics that exchange rate and population growth are normally distributed. On the other hand, GDP, interest rate volatility, inflation, investment, and human capital are not normally distributed as their significant P -values lead to the rejection of the null of normality.
Table 2 presents the correlation matrix, a surface description of the relationship that exists among the variables employed in this study. The purpose of this is to confirm that the relationships among the regressors are not very high to the extent of posing a problem of multicollinearity in the model. Each variable is treated as random or stochastic, and there is no distinction between the dependent or independent variables (Fig. 2 ).
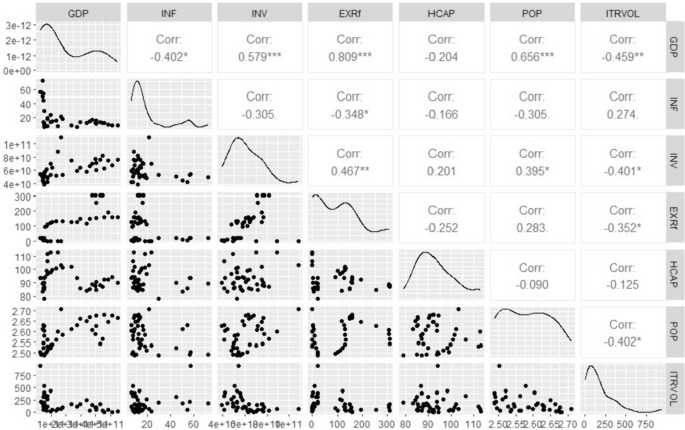
Correlation matrix
The correlation matrix suggests that GDP has statistically significant positive correlations with exchange rate, investment, and population growth rate. It however exhibits statistically significant negative relationships with interest rate volatility and inflation. The correlation between GDP and human capital is statistically insignificant. The degree of relationship among other variables is also explicitly revealed by the correlation matrix table. However, since the goal is to verify the absence of multicollinearity, the size of the correlation coefficients of the relationships among the regressors is observed. Following the rule of thumb that a correlation coefficient below 0.8 will not cause a multicollinearity issue, it is clearly seen that these variables can be safely used in the estimation since they all have coefficients less than 0.8.
Unit Root Testing
To avoid spurious regression results from non-stationary series, it is necessary to subject the variables to unit root testing. This research therefore conducts and reports the Phillip Perron (PP) unit root test results in Table 2 . The outcomes reveal the presence of unit root in all the variables except interest rate volatility, inflation, and investment. This means that only these variables are stationary at level ( I 0 ). All other variables turn out to be stationary only after first differencing ( I 1 ). The unit root results hence necessitate the use of a technique capable of estimating relationships between these ( I 0 ) and ( I 1 ) variables.
Presentation of Regression Results
The fact that the unit root tests reveal a mix of stationary and non-stationary variables provides another reason to employ the QARDL. Because QARDL is a variant of the traditional ARDL technique, it is able to accommodate a mix of ( I 0 ) and ( I 1 ) variables. The short-run and long-run QARDL estimates of the effect of interest rate volatility alongside other regressors on economic growth in Nigeria are reported in Table 3 .
To begin with, the quantile estimates of the error correction term (ECM coefficient) start with an 8.2% speed of adjustment at a very low quantile (0.1), increase to 14.5% at mid-quantile (0.5), and further increase to 21.3% at a very high quantile (0.9). Overall, while the ECM coefficients vary across quantiles, they remain significantly negative all through. This indicates that there is a convergence of the model back to long-run equilibrium after a short-run disequilibrium and therefore confirms the presence of cointegration among the variables.
The quantile estimates of interest rate volatility remain strictly negative in both the short run and the long run, confirming that interest rate volatility adversely affects the economic growth of Nigeria in the short to long term. This negative impact occurs because interest rate volatility destabilizes investment decisions of businesses, savers, and borrowers alike. It makes forecast of return on financial assets and investment projects unreliable, hence reducing the growth prospects of the economy. It also distorts the performance of the stock market. This finding confirms the a priori expectation and is in line with the works of Ramey and Ramey ( 1995 ), Aysan ( 2007 ), and Reyes-Heroles and Tenorio ( 2017 ), who observe that countries with higher volatility tend to be characterized by weaker growth. This negative relationship may also be indirectly inferred from the finding of Onwumere et al. ( 2012 ) that interest rate volatility lowers saving and investment in Nigeria.
Moreover, in the short run, the negative impact of interest rate volatility on economic growth monotonically decreases from low to middle quantiles, beyond which it remains relatively stable with minor fluctuations. On the other hand, in the long run, the adverse effect of interest rate volatility gradually increases from lower quantiles to higher quantiles till a decline becomes visible beyond the 0.8 quantile. These outcomes suggest that the short-run adverse growth effect of interest rate volatility is greater when the economy is already in a relatively weak state. The long-run adverse growth effect is however greater when the economy is already in a relatively strong position.
Concerning the control variables, exchange rate displays a statistically significant negative short-term growth effect and statistically significant positive long-term growth effect. There is an escalation of the negative short-run effect between 0.1 and 0.2 quantiles, a decline in the negative short-run effect between 0.2 and 0.4 quantiles, and a stable negative short-run effect beyond the 0.4 quantile. In the long run, a gradual decline in the positive impact of exchange rate is noticeable between 0.1 and 0.7 quantiles, beyond which an increase is recorded and then a further decline. This is an indication that currency devaluation spurs growth in the long run, even though it may initially cause a depressing effect. The long-term positive impact occurs because a weaker currency boosts economic growth by incentivizing exports. Devaluation of a currency can encourage domestic investment and production; this in turn encourages net exports, thus improving economic growth (Idris & Suleiman, 2019 ). On one hand, currency devaluation makes a nation’s money cheaper, thus rendering the nation’s goods more competitive in the global market and consequently boosting exports. On the other hand, it makes foreign products relatively more expensive and therefore lowers imports. Overall, it leads to an increase in net exports which is a component of GDP. The short-run negative impact provides some support for the argument by structural economists that weaker currency adversely affects economic growth. They argue that the restrictive effect of currency devaluation on imported inputs distorts the production structure of developing nations such as Nigeria (Bird & Rajan, 2004 ; Karahan, 2020 ). This claim is however found by our study to be true only in the short run.
Inflation exhibits statistically significant negative short-run and long-run effects on economic growth. The quantile-specific short-run coefficients show that the negative growth effect declines in the lower quantiles and becomes relatively constant beyond the 0.3 quantile. In the long run however, the coefficients remain relatively stable from the lower to middle quantiles, beyond which a steady increase in the size of the negative impact becomes visible. This indicates that rising price levels of productive inputs as well as finished products reduce the incentive-to-produce, as well as the purchasing power of firms and households respectively. This finding is in consonance with the outcomes of the studies by Idris and Suleiman ( 2019 ) and Adaramola and Dada ( 2020 ).
Investment has a positive effect on economic growth both in the short run and the long run. This finding is in line with economic theory (see Jaiyeoba, 2015 ; Kalu & Mgbemena, 2015 ). The positive short-run effect is however statistically insignificant beyond the 0.7 quantile, whereas the positive long-run effect is significant across all quantiles in the long term. Also, the sizes of the long-run coefficients are consistently larger than those of the short-run. This indicates that the impact of investment on economic growth is majorly a long-term phenomenon. Moreover, while the short-run coefficients do not show a clear pattern, the long-run coefficients increase from lower to middle quantiles and then decline at very high quantiles. This suggests that investment becomes less effective beyond a threshold of economic status.
Human capital, on the other hand, only has a statistically significant positive long-run impact on economic growth, indicating that human capital development drives economic growth. This conclusion reached on the basis of our results confirms the claims of Jaiyeoba ( 2015 ) and Ali et al. ( 2021 ). In addition, the long-term positive effect of human capital, on the average, gradually increases from lower to higher quantiles.
Finally, the results reveal that population growth positively impacts Nigeria’s economic growth in both the short run and the long run. The long-run coefficients are also consistently bigger than the short-run coefficients, indicating that the economic growth effect of population growth is more important in the long term. This finding confirms that population growth is capable of driving expansions in labor, products, and consumption in a manner that stimulates economic growth. A similar conclusion is also reached by Zhizhi and Owuda ( 2019 ). In addition, the short-run quantile coefficients increase between the low (0.1) and mid (0.3) quantiles and then gradually decreases. The long-run quantile coefficients decline from lower quantiles to higher quantiles. Predominantly, these results suggest that population growth has a bigger effect when the economy is relatively weak (Figs. 3 and 4 ).
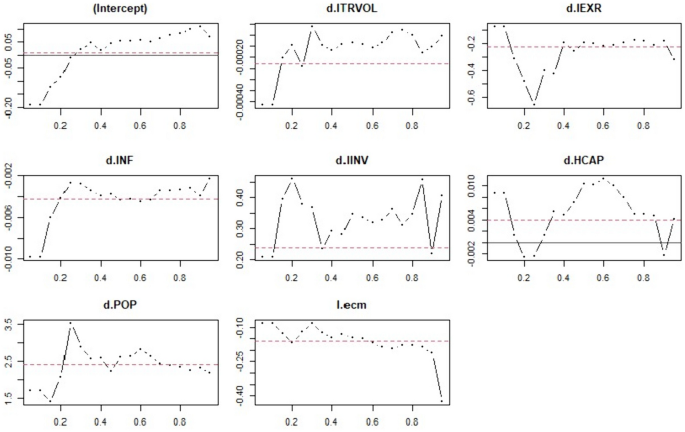
Time-series plots of the short-run QARDL estimates
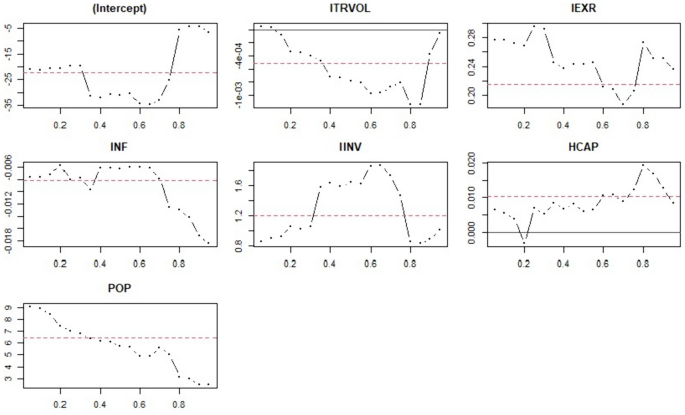
Table 4 contains the results of the Granger causality tests conducted as a means of further validating the authenticity of the estimated results. The causality test result shows that interest rate volatility Granger causes economic growth. This confirms the argument that investment volatility is a significant predictor of the economic growth of Nigeria. The test outcome further shows that other regressors asides investment and human capital also Granger cause economic growth in Nigeria over the period studied
Conclusion and Policy Recommendations
Concluding remarks.
This paper is an attempt to investigate the growth effect of interest rate volatility and further conclude on the suitability of liberalizing interest rates in Nigeria. To this end, empirical analyses of the link between interest rate volatility and the country’s GDP for the period 1981–2020 were carried out. The QARDL procedure was employed to establish the short-run and long-run quantile-specific impacts of interest rate volatility. As a final step, Granger causality tests were performed to investigate the predictive powers of the regressors. Based on the outcomes, it is concluded that interest rate volatility adversely affects the economic performance of Nigeria in both the short run and long run. Consequently, full liberalization is thus not suitable for the economy. Moreover, we find that the short-run adverse growth effect of interest rate volatility is greater when the economy is already in a relatively weak state, whereas the long-run adverse growth effect is greater when the economy is already in a relatively strong position.
Stronger regulation of the rates will lead to a stronger and more reliable economic system which can engender the growth of the Nigerian economy. Prioritization of interest rate stability will reduce the uncertainties caused by fluctuations in this rate and eliminate the detrimental impact of interest rate volatility on economic growth, as shown by this research. Not only will the internal fluctuations be minimized, the stability will also strengthen both local and foreign investors’ confidence in both real and financial sector investments.
This work has succeeded to a large extent in providing evidence-based research on how the growth of the Nigerian economy responds to interest rate volatility. Based on the results obtained and the realities faced by the economy, the following recommendations are made as policy directions to curb the current instability and encourage all-round growth and development. First, the study findings show that fully liberalizing the financial sector in a manner that permits interest rates to freely respond to market pressures is not Pareto efficient for Nigeria. Hence, it is advised that the CBN should prioritize greater supervision of the interest rate corridor system so as to reduce volatility in the rates and minimize chances of persistent upward or downward bias. Such supervision is needed, given the vulnerability and susceptibility of the economy to both internal and external shocks. In other words, the interest rate should not be left to the uncontrolled market forces but must be set by the monetary authority. This is because liberalizing interest rates makes it rise above binding ceilings sometimes; other times, it is forced down by increased pressure on margins or even reversing over time and not always in the same direction. To avoid these forms of wide swings, its movements must be closely monitored. Hence, the CBN must carefully watch all forms of interest rate movements which affect the economy.
Second, efforts to properly estimate the natural real interest rate should be prioritized by the CBN. Rates above it tends to depress economic growth, whereas rates below it tend to stimulate economic growth. As it is the interest rate that supports the economy at its optimum, wide unexpected deviations from it should be guarded against. Third, just like the natural real interest rate serves as a useful benchmark for achieving macroeconomic stability, the financial stability interest rate is also a useful benchmark for achieving financial stability. Thus, sudden unexpected deviations from the financial stability interest rate may induce instability in the financial system. The CBN is therefore also encouraged to pay close attention to this rate and introduce policies to guard against volatility in it. Overall, the CBN must carefully manage the interest rate. It must not be too high to discourage borrowing and not too low to discourage savings. As the economy expands and activities evolve, the rate can be revised and modified to suit the current economic situation.
There are some noteworthy study limitations. To begin with, the ARCH/GARCH family models are generally regarded as superior for modeling volatility. For instance, Hassani et al. ( 2020 ) demonstrate how these kinds of models are useful for modeling interest rate volatility. Unfortunately, the low frequency of the variables used for empirical analysis in this study makes the use of such models impracticable. It is therefore suggested that this topic be revisited for cases where higher frequency data is obtainable. Second, this work is centered on Nigeria alone. Therefore, the findings are not generalizable. Thus, as a direction for further research, panel studies of various country groupings may be conducted. This will help guide the direction of development policies toward financial stability in those specific country groupings. Also, a comparative study of the effect of interest rate volatility could be conducted between the regions of the world. This would be a global comparative analysis to further econometrically confirm how interest rate volatility impacts economic growth.
Data Availability
All data used for empirical analysis were sourced from the World Bank database available at http:dataworldbank.org .
Abdulhakeem, K. A., & Olasehinde-Williams, G. O. (2014). Governance and growth: Additional evidence from Africa. Political Science Review, 6 (1), 108–129.
Google Scholar
Acemoglu, D., Johnson, S., Robinson, J., & Thaicharoen, Y. (2003). Institutional causes, macroeconomic symptoms: Volatility, crises and growth. Journal of Monetary Economics, 50 (1), 49–123.
Article Google Scholar
Adaramola, A. O., & Dada, O. (2020). Impact of inflation on economic growth: Evidence from Nigeria. Investment Management and Financial Innovations, 17 (2), 1–13.
Aizenman, J., & Pinto, B. (2004). Managing volatility and crises: A practitioner’s guide overview.
Ali, M., Raza, S. A. A., Puah, C. H., & Samdani, S. (2021). How financial development and economic growth influence human capital in low-income countries. International Journal of Social Economics, 48 (10), 1393–1407.
Aloy, M., Dufrénot, G., & Péguin-Feissolle, A. (2014). Is financial repression a solution to reduce fiscal vulnerability? The example of France since the end of World War II. Applied Economics, 46 (6), 629–637.
Arikewuyo, K. A., & Akingunola, R. O. (2019). Impact of interest rate deregulation on fund mobilisation of deposit money banks in Nigeria. Izvestiya Journal of Varna University of Economics, 63 (2), 89–103.
Awe, O. O., Musa, A. P., & Sanusi, G. P. (2023). Revisiting economic diversification in Africa’s largest resource-rich nation: Empirical insights from unsupervised machine learning. Resources Policy, 82 , 103540.
Ayopo, B. A., Isola, L. A., & Olukayode, S. R. (2016). Stock market response to economic growth and interest rate volatility: Evidence from Nigeria. International Journal of Economics and Financial Issues, 6 (1), 354–360.
Aysan, A. F. (2007). The effects of volatility on growth and financial development through capital market imperfections. METU Studies in Development, 34 (1), 1.
Balcilar, M., Olasehinde-Williams, G., & Tokar, B. (2022). The investment volatility-dampening role of foreign aid in poor sub-Saharan African countries. The Journal of International Trade & Economic Development, 31 (5), 798–809.
Balcilar, M., Tokar, B., & Godwin, O. W. (2020). Examining the interactive growth effect of development aid and institutional quality in Sub-Saharan Africa. Journal of Development Effectiveness, 12 (4), 361–376.
Bayracı, S., & Ünal, G. (2014). Stochastic interest rate volatility modeling with a continuous-time GARCH (1, 1) model. Journal of Computational and Applied Mathematics, 259 , 464–473.
Bergo, J. (2003). The role of the interest rate in the economy. Central Bank of Norway BIS Review , 46 , 1–9. https://www.bis.org/review/r031104f.pdf
Bird, G., & Rajan, R. S. (2004). Does devaluation lead to economic recovery or contraction? Theory and policy with reference to Thailand. Journal of International Development: THe Journal of the Development Studies Association, 16 (2), 141–156.
Central Bank of Nigeria. (2016). Interest Rate (No. 3). CBN, Research Department. https://www.cbn.gov.ng/out/2017/rsd/cbn%20education%20in%20economics%20series%20no.%203%20interest%20rate.pdf
Chari, V. V., Dovis, A., & Kehoe, P. J. (2020). On the optimality of financial repression. Journal of Political Economy, 128 (2), 710–739.
Chichi, O. A., & Casmir, O. C. (2014). Exchange rate and the economic growth in Nigeria. International Journal of Management Sciences, 2 (2), 78–87.
Cho, J. S., Kim, T. H., & Shin, Y. (2015). Quantile cointegration in the autoregressive distributed-lag modeling framework. Journal of Econometrics, 188 (1), 281–300.
Coskuner, C., & Olasehinde-Williams, O. (2017). The impact of saving rates on economic growth in an open economy. International Journal of Economic Perspectives , 11 (4).
Dabale, W. P., & Jagero, N. (2013). Causes of interest rate volatility and its economic implications in Nigeria. International Journal of Academic Research in Accounting, Finance and Management Sciences, 3 (4), 27–32.
Das, S., Gupta, R., Kanda, P. T., Reid, M., Tipoy, C. K., & Zerihun, M. F. (2014). Real interest rate persistence in South Africa: Evidence and implications. Economic Change and Restructuring, 47 , 41–62.
Delebari, O. R. O., & Didi, E. (2020). GARCH modeling of interest rate in Nigeria from 1997 to 2017. International Journal of Innovative Mathematics, Statistics & Energy Policies, 8 (3), 53–68.
Donzwa, W., Gupta, R., & Wohar, M. E. (2019). Volatility spillovers between interest rates and equity markets of developed economies. Journal of Central Banking Theory and Practice, 8 (3), 39–50.
Dutkowsky, D. H. (1987). Unanticipated money growth, interest rate volatility, and unemployment in the United States. The Review of Economics and Statistics , 144–148.
Ene, E. E., Atong, A. S., & Ene, J. C. (2015). Effect of interest rates deregulation on the performance of deposit money banks in Nigeria. International Journal of Managerial Studies and Research , 3 (9), 164–176. https://www.arcjournals.org/pdfs/ijmsr/v3-i9/16.pdf
Evans, P. (1984). The effects on output of money growth and interest rate volatility in the United States. Journal of Political Economy, 92 (2), 204–222.
Fan, T., Khaskheli, A., Raza, S. A., & Shah, N. (2022). The role of economic policy uncertainty in forecasting housing prices volatility in developed economies: Evidence from a GARCH-MIDAS approach. International Journal of Housing Markets and Analysis , (ahead-of-print).
Friedman, B. M. (1982). Federal Reserve policy, interest rate volatility, and the US capital raising mechanism. Journal of Money, Credit and Banking, 14 (4), 721–745.
Ghironi, F., & Ozhan, K. (2020). Interest rate uncertainty as a policy tool. National Bureau of Economic Research Working Paper , 27084 , 1–38. https://doi.org/10.3386/w27084
Gil-Alana, L. A., Cunado, J., & Gupta, R. (2017). Evidence of persistence in US short and long-term interest rates. Journal of Policy Modeling, 39 (5), 775–789.
Di Giovanni, J., & Levchenko, A. A. (2006). Openness, volatility and the risk content of exports. In Conference The Growth and Welfare Effects of Macroeconomic Volatility by The World Bank, CEPR and CREI, Barcelona (pp. 17–18).
Githinji, A. N. (2015). The effect of interest rate volatility on money demand in Kenya. Faculty of Arts, Law, Social Sciences and Business Management, University of Nairobi , 1–34. http://hdl.handle.net/11295/93870
Glower, C. J. (1994). Interest rate deregulation: A brief survey of the policy issues and the Asian experience. Asian Development Bank . https://www.adb.org/sites/default/files/publication/28126/op009.pdf
Granger, C. W. (1969). Investigating causal relations by econometric models and cross-spectral methods. Econometrica: Journal of the Econometric Society , 424–438.
Grigoli, F., & Mills, Z. (2014). Institutions and public investment: An empirical analysis. Economics of Governance, 15 , 131–153.
Hassani, H., Yeganegi, M. R., Cuñado, J., & Gupta, R. (2020). Forecasting interest rate volatility of the United Kingdom: Evidence from over 150 years of data. Journal of Applied Statistics, 47 (6), 1128–1143.
Headey, D. D., & Hodge, A. (2009). The effect of population growth on economic growth: A meta-regression analysis of the macroeconomic literature. Population and Development Review, 35 (2), 221–248.
Idris, T. S., & Suleiman, S. (2019). Effect of inflation on economic growth in Nigeria: 1980–2017. Maiduguri Journal of Arts and Social Sciences, 18 (2), 33–48.
Ijirshar, V. U. (2019). Impact of trade openness on economic growth among ECOWAS countries: 1975–2017. CBN Journal of Applied Statistics (JAS), 10 (1), 4.
Ikhide, S. I. (1998). Financial sector reforms and monetary policy in Nigeria. IDS Working Paper , 68 , 2–46.
Istreffi, K., & Mouabbi, S. (2017). Interest rate uncertainty harms the economy. Banque de France . https://blocnotesdeleco.banque-france.fr/en/blog-entry/interest-rate-uncertainty-harms-economy
Jafarov, M. E., Maino, M. R., & Pani, M. M. (2019). Financial repression is knocking at the door, again . International Monetary Fund.
Jaiyeoba, S. V. (2015). Human capital investment and economic growth in Nigeria. African Research Review, 9 (1), 30–46.
Jiang, C., Zhang, Y., Kamran, H. W., & Afshan, S. (2021). Understanding the dynamics of the resource curse and financial development in China? A novel evidence based on QARDL model. Resources Policy, 72 , 102091.
Kalejaiye, P. O., Adebayo, K., & Lawal, O. (2013). Deregulation and privatization in Nigeria: The advantages and disadvantages so far. African Journal of Business Management, 7 (25), 2403.
Kalu, C. U., & Mgbemena, O. O. (2015). Domestic private investment and economic growth in Nigeria: Issues and further consideration. International Journal of Academic Research in Business and Social Sciences, 5 (2), 302–313.
Karahan, Ö. (2020). Influence of exchange rate on the economic growth in the Turkish economy. Financial Assets and Investing, 11 (1), 21–34.
Khan, A. H., & Hasan, L. (1998). Financial liberalization, savings, and economic development in Pakistan. Economic Development and Cultural Change, 46 (3), 581–597.
Khaskheli, A., Zhang, H., Raza, S. A., & Khan, K. A. (2022). Assessing the influence of news indicator on volatility of precious metals prices through GARCH-MIDAS model: A comparative study of pre and during COVID-19 period. Resources Policy, 79 , 102951.
Kim, T. H., & White, H. (2003). Estimation, inference, and specification testing for possibly misspecified quantile regression. In Maximum likelihood estimation of misspecified models: twenty years later (pp. 107–132). Emerald Group Publishing Limited.
Lahiani, A. (2018). Revisiting the growth-carbon dioxide emissions nexus in Pakistan. Environmental Science and Pollution Research, 25 , 35637–35645.
Lee, C. C., Olasehinde-Williams, G., & Akadiri, S. S. (2021). Are geopolitical threats powerful enough to predict global oil price volatility? Environmental Science and Pollution Research, 28 , 28720–28731.
Lee, C. C., Olasehinde-Williams, G. O., & Olanipekun, I. O. (2022). GDP volatility implication of tourism volatility in South Africa: A time-varying approach. Tourism Economics, 28 (2), 435–450.
McKinnon, R. I. (1973). Money and capital in economic development . Brookings Institution Press.
Mehran, H., & Laurens, B. (1997). Interest rates: An approach to liberalization. Finance and Development, 34 , 33–37.
Naudé, W. (1995). Financial liberalisation and interest rate risk management in Sub-Saharan Africa. University of Oxford . WPS/96–12, Centre for the Study of African Economies.
Nawaz, M. A., Hussain, M. S., Kamran, H. W., Ehsanullah, S., Maheen, R., & Shair, F. (2021). Trilemma association of energy consumption, carbon emission, and economic growth of BRICS and OECD regions: Quantile regression estimation. Environmental Science and Pollution Research, 28 , 16014–16028.
Ntom Udemba, E., Onyenegecha, I., & Olasehinde-Williams, G. (2022). Institutional transformation as an effective tool for reducing corruption and enhancing economic growth: A panel study of West African countries. Journal of Public Affairs, 22 (2), e2389.
Obinna, O. (2020). Impact of interest rate deregulation on investment growth in Nigeria. International Journal of Economics and Financial Issues, 10 (2), 170.
Odhiambo, N. M. (2009). Energy consumption and economic growth nexus in Tanzania: An ARDL bounds testing approach. Energy Policy, 37 (2), 617–622.
Olasehinde-Williams, G. (2021). Is US trade policy uncertainty powerful enough to predict global output volatility? The Journal of International Trade & Economic Development, 30 (1), 138–154.
Olasehinde-Williams, G., & Özkan, O. (2022). Is interest rate uncertainty a predictor of investment volatility? Evidence from the wild bootstrap likelihood ratio approach. Journal of Economics and Finance, 46 (3), 507–521.
Omotunde, R. A., & Nwokoma, N. I. (2016). Interest rate shocks and stock market volatility in Nigeria (1985–2014). West African Journal of Monetary and Economic Integration, 16 , 44–72.
Onwumere, J. U. J., Okore, O. A., & Ibe, I. G. (2012). The impact of interest rate liberalization on savings and investment: Evidence from Nigeria. Research Journal of Finance and Accounting, 3 (10), 130–136.
Oseni, I. O., & Nwosa, P. I. (2011). Stock market volatility and macroeconomic variables volatility in Nigeria: An exponential GARCH approach. Journal of Economics and Sustainable Development, 2 (10), 28–42.
Patnaik, I., & Shah, A. (2004). Interest rate volatility and risk in Indian banking (Vol. 4). International Monetary Fund.
Poterba, J. M. (2000). Stock market wealth and consumption. Journal of Economic Perspectives, 14 (2), 99–118.
Ramey, G., & Ramey, V. A. (1995). Cross-country evidence on the link between volatility and growth. The American Economic Review, 85 (5), 1138–1151.
Reinhart, C. M. (2012). The return of financial repression. Peterson Institute for International Economics, Financial Stability Review, 16 , 37–47.
Reinhart, C. M., & Sbrancia, M. B. (2015). The liquidation of government debt. Economic Policy, 30 (82), 291–333.
Reyes-Heroles, R., & Tenorio, G. (2017). Interest rate volatility and sudden stops: An empirical investigation. International Finance Discussion Paper , (1209).
Sadiq, M., Hsu, C. C., Zhang, Y., & Chien, F. (2021). COVID-19 fear and volatility index movements: Empirical insights from ASEAN stock markets. Environmental Science and Pollution Research, 28 , 67167–67184.
Shahbaz, M., Lahiani, A., Abosedra, S., & Hammoudeh, S. (2018). The role of globalization in energy consumption: A quantile cointegrating regression approach. Energy Economics, 71 , 161–170.
Shaw, E. S. (1973). Financial deepening in economic development . Oxford University Press.
Shin, Y., Yu, B., & Greenwood-Nimmo, M. (2014). Modelling asymmetric cointegration and dynamic multipliers in a nonlinear ARDL framework. Festschrift in honor of Peter Schmidt: Econometric methods and applications , 281–314.
Shunmugam, V., & Hashim, D. A. (2009). Volatility in interest rates: Its impact and management. Macroeconomics and Finance in Emerging Market Economies, 2 (2), 247–255.
Stiglitz, J. E. (1993). The role of the state in financial markets. The World Bank Economic Review, 7 (suppl_1), 19–52.
Stiglitz, J. E. (1989). Financial markets and development. Oxford Review of Economic Policy, 5 (4), 55–68.
Stiglitz, J. E. (2000). Capital market liberalization, economic growth, and instability. World Development, 28 (6), 1075–1086.
Terwase, I. T., Abdul-Talib, A. N., & Zengeni, K. T. (2014). Nigeria, Africa’s largest economy: International business perspective. International Journal of Management Sciences, 3 (7), 534–543.
Thornton, J. (1991). The financial repression paradigm: A survey of empirical research. Savings and Development, 15 (1), 5–18.
Tian, S., & Hamori, S. (2015). Modeling interest rate volatility: A realized GARCH approach. Journal of Banking & Finance, 61 , 158–171.
Wu, X., Sadiq, M., Chien, F., Ngo, Q. T., Nguyen, A. T., & Trinh, T. T. (2021). Testing role of green financing on climate change mitigation: Evidences from G7 and E7 countries. Environmental Science and Pollution Research, 28 , 66736–66750.
Xiao, Z. (2009). Quantile cointegrating regression. Journal of Econometrics, 150 (2), 248–260.
Zhizhi, M., & Owuda, R. L. (2019). The effects of population on economic growth in Nigeria. Available at SSRN 4146547 .
Download references
Open access funding provided by the Scientific and Technological Research Council of Türkiye (TÜBİTAK).
Author information
Authors and affiliations.
Department of Management Information Systems, Istanbul Ticaret University, Istanbul, Turkey
Godwin Olasehinde-Williams
Economics and Finance Application and Research Center, Istanbul Ticaret University, Istanbul, Turkey
Department of Economics, Faculty of Social Sciences, University of Ilorin, Ilorin, Nigeria
Ruth Omotosho
Faculty of Economics Administrative and Social Sciences, Istanbul Gelisim University, Istanbul, Turkey
Festus Victor Bekun
Adnan Kassar School of Business, Department of Economics, Lebanese American University, Beirut, Lebanon
You can also search for this author in PubMed Google Scholar
Corresponding author
Correspondence to Festus Victor Bekun .
Additional information
Publisher's note.
Springer Nature remains neutral with regard to jurisdictional claims in published maps and institutional affiliations.
Rights and permissions
Open Access This article is licensed under a Creative Commons Attribution 4.0 International License, which permits use, sharing, adaptation, distribution and reproduction in any medium or format, as long as you give appropriate credit to the original author(s) and the source, provide a link to the Creative Commons licence, and indicate if changes were made. The images or other third party material in this article are included in the article's Creative Commons licence, unless indicated otherwise in a credit line to the material. If material is not included in the article's Creative Commons licence and your intended use is not permitted by statutory regulation or exceeds the permitted use, you will need to obtain permission directly from the copyright holder. To view a copy of this licence, visit http://creativecommons.org/licenses/by/4.0/ .
Reprints and permissions
About this article
Olasehinde-Williams, G., Omotosho, R. & Bekun, F.V. Interest Rate Volatility and Economic Growth in Nigeria: New Insight from the Quantile Autoregressive Distributed Lag (QARDL) Model. J Knowl Econ (2024). https://doi.org/10.1007/s13132-024-01924-x
Download citation
Received : 12 September 2023
Accepted : 19 March 2024
Published : 09 April 2024
DOI : https://doi.org/10.1007/s13132-024-01924-x
Share this article
Anyone you share the following link with will be able to read this content:
Sorry, a shareable link is not currently available for this article.
Provided by the Springer Nature SharedIt content-sharing initiative
- Interest rate
- Liberalization
- Economic growth
- Find a journal
- Publish with us
- Track your research

IMAGES
COMMENTS
1. Introduction. Poverty "is one of the defining challenges of the 21st Century facing the world" (Gweshengwe et al., Citation 2020, p. 1).In 2019, about 1.3 billion people in 101 countries were living in poverty (United Nations Development Programme and Oxford Poverty and Human Development Initiative, Citation 2019).For this reason, the 2030 Global Agenda for Sustainable Development Goals ...
World Bank Policy Research Working Paper 8360. Washington, DC: World Bank. 10. ... Strong economic growth drove poverty rates down to 77 million, or 6% of the population, in 2019. India will ...
The World Bank uses the simple average of the regional poverty rate to impute the poverty rate for countries without enough data to estimate the poverty rate. 39. The population coverage for DAD method is 88.6% and 82.6% for CBN in 1990, while for 2015 coverage rates are 88.3% and 81.7% respectively.
Research and data on Poverty from Pew Research Center ... Burmese (19%) and Hmong Americans (17%) were among the Asian origin groups with the highest poverty rates in 2022. video | Mar 27, 2024. 1 in 10: Redefining the Asian American Dream (Short Film) ... United States, about 2.3 million live in poverty. This short film explores their diverse ...
The cross-national median represents "normal" or typical poverty for rich democracies. When the United States had a poverty rate near 18% in the mid-1980s, these other rich democracies had a far lower median of 7.7%. Over the entire period, the average median poverty rate in other rich democracies was only 8.8% versus 17% in the United States.
Research Institute of Sri Lanka, Lunuwila, 61150, Sri Lanka. Email: [email protected]. Abstract. In spite of the fact that there is some lucidity within the field of poverty with respect to the ...
Congressional Research Service https://crsreports.congress.gov R47354 . Congressional Research Service SUMMARY Poverty in the United States in 2021 ... For the official measure in 2021, no statistically significant change from 2020 was detected in the overall poverty rate—the
It expands on the work done by UN Women and the World Bank (2018) and Muñoz-Boudet et al. (2018) using updated data and additional analysis to explore the intersection between gender and poverty. The paper presents a stylized global view of poverty, specifically as it relates to sex and household composition.
Yet, recent theorizing and empirical research into the causes of global poverty reduction has mostly omitted demographic factors. This paper aims to fill this gap by testing for effects of demographic variables on poverty. Using time series data for 140 countries, we document a strong effect of lagged fertility on country-specific poverty rates.
The consequences of poverty and inequality for growth have long preoccupied academics and policy-makers. This paper revisits the inequality-growth and poverty-growth links. Using a panel of 158 countries between 1960 and 2010, we find that the correlation of growth with poverty is consistently negative: A 10 p.p. decrease in the headcount poverty rate is associated with a subsequent increase ...
A cost-of-living approach produces a poverty line of HK$28,815 and attendant poverty rate of 44.47% in 2020, nearly double the poverty line of HK$13,450 and poverty rate of 23.6% according to the ...
The U.S. has one of the highest rates of poverty in the developed world, despite its collective wealth, and the burden falls disproportionately on communities of color. ... A research agenda on issues affecting poor and minority women: a model for understanding their health needs. Women Health. (1987) 12:137-60. 10.1300/j013v12n03_08 [Google ...
Pater Saunders holds a Research Chair in Social Policy in the social Policy Research Centre at UNSW, where he served as Director from February 1978 to July 2007. He served as Director of the Social Policy Research Centre at UNSW from February 1987 until July 2007. He is an authority on poverty, income distribution and household needs and living standards.
Abstract. Poverty is commonly defined as a lack of economic resources that has negative social consequences, but surprisingly little is known about the importance of economic hardship for social outcomes. This article offers an empirical investigation into this issue. We apply panel data methods on longitudinal data from the Swedish Level-of ...
The World Development Report of 2013 measures, perhaps for the first time, inequality of opportunity to labor market outcomes in a discrete setting. It focuses on Europe and Central Asia. Latest research from the World Bank on Poverty, including reports, studies, publications, working papers and articles.
The nonelderly poverty rate declined from 1959-1969, but then rose from 10.7 percent in 1970 to 12.7 percent in 1980 and remained at 12.8 percent in ... Throughout the paper, we measure individual poverty rates (the alternative is to measure poverty rates among families) using the official Census Bureau definition. ...
low poverty rates?" s ing quantitative and qualitative data from all 0 states in the nited tates, made available in the icroase dataase (LeRoy 201), the researcher ... This research paper will use variable numer 4) OVERTY04, sourced from the States file, in order to operationally define the dependent variable, which is poverty ...
Poverty rates are disproportionately higher among most non-White populations. Compared to 8.2% of White Americans living in poverty, 26.8% of American Indian and Alaska Natives, 19.5% of Blacks, 17% of Hispanics and 8.1% of Asians are currently living in poverty. ... Support research on poverty and its relationship to health, education, and ...
The vast majority of prior research on poverty of place has relied upon data anchored to place of residence. Thus, poverty rates broadly reflect poverty as it exists at night. This bias toward nighttime statistics leaves us with an incomplete understanding of spatial inequalities because daytime poverty rates can differ markedly from nighttime ...
Poverty and mental health. The mental health of individuals is shaped by the social, environmental and economic conditions in which they are born, grow, work and age. 4 - 7 Poverty and deprivation are key determinants of children's social and behavioural development 8, 9 and adult mental health. 10 In Scotland, individuals living in the most ...
This paper offers a comprehensive analysis of poverty in India. It shows that no matter which of the two official . poverty lines is used, poverty has declined steadily in all states and for all social and religious groups. Accelerated growth between fiscal years 2004-2005 and 2009-2010 led to an accelerated decline in poverty rates. Moreover,
In this research paper, the impact of uneven job distribution and poor-quality jobs on poverty rates in the United States is examined. A longitudinal dataset was constructed by aggregating household-level data to the state-level to match with state-level variables, and a two-way fixed effect model was applied to estimate their effects.
The differences between high- and low-poverty schools are particularly striking. Most teachers from high-poverty schools say the academic performance (73%) and behavior (64%) of most students at their school are fair or poor. Much smaller shares of teachers from low-poverty schools say the same (27% for academic performance and 37% for behavior).
The survey analysis included in this data essay is based on 561 Asian adults living near or below the poverty line from Pew Research Center's 2022-23 survey of Asian Americans, the largest nationally representative survey of Asian American adults of its kind to date, conducted in six languages. For more details, refer to the survey methodology.
The Poverty Research Team is responsible for the development of the NYCgov Poverty Measure. The alternative NYC poverty measure, in comparison to the official U.S. measure of poverty, includes a threshold that accounts for the higher cost of housing in New York City. ... CEO's first working paper on poverty in New York City, issued in August of ...
This paper examines patterns and trends in racial inequality in poverty and affluence over the 1959 to 2015 period. ... Poverty rates among American Indians are about as high as among African ... and parental employment: Implications for the level and trend in poverty" Institute for Research on Poverty, University of Wisconsin-Madison ...
The Pew Research Center also found that one out of every three Asian Americans aged 25 and older living in poverty has a bachelor's degree. By comparison, only 14% of non-Asians in poverty have ...
This paper examines the relationship between trade and job quality, using productivity and wage rate data for export and non-export activities in a sample of 60 countries across all income levels and 45 sectors spanning the whole economy over 1995-2019.
Answering this question is the primary motivation for this research. This paper is an attempt to establish the effect of interest rate volatility on economic growth and further conclude on the suitability of the financial liberalization policy in Nigeria. ... The 2022 multidimensional poverty index released by the National Bureau of Statistics ...