Volume 23 Supplement 8

Selected articles from the 16th International Symposium on Bioinformatics Research and Applications (ISBRA-20): bioinformatics
- Open access
- Published: 30 September 2022
Visualizing the knowledge structure and evolution of bioinformatics
- Jiaqi Wang 1 ,
- Zeyu Li 1 &
- Jiawan Zhang 1
BMC Bioinformatics volume 23 , Article number: 404 ( 2022 ) Cite this article
2884 Accesses
3 Citations
4 Altmetric
Metrics details
Bioinformatics has gained much attention as a fast growing interdisciplinary field. Several attempts have been conducted to explore the field of bioinformatics by bibliometric analysis, however, such works did not elucidate the role of visualization in analysis, nor focus on the relationship between sub-topics of bioinformatics.
First, the hotspot of bioinformatics has moderately shifted from traditional molecular biology to omics research, and the computational method has also shifted from mathematical model to data mining and machine learning. Second, DNA-related topics are bridge topics in bioinformatics research. These topics gradually connect various sub-topics that are relatively independent at first. Third, only a small part of topics we have obtained involves a number of computational methods, and the other topics focus more on biological aspects. Fourth, the proportion of computing-related topics hit a trough in the 1980s. During this period, the use of traditional calculation methods such as mathematical model declined in a large proportion while the new calculation methods such as machine learning have not been applied in a large scale. This proportion began to increase gradually after the 1990s. Fifth, although the proportion of computing-related topics is only slightly higher than the original, the connection between other topics and computing-related topics has become closer, which means the support of computational methods is becoming increasingly important for the research of bioinformatics.
Conclusions
The results of our analysis imply that research on bioinformatics is becoming more diversified and the ranking of computational methods in bioinformatics research is also gradually improving.
Bioinformatics originated as a cross-disciplinary field because of the increasing need for computational solutions to research problems in biomedicine [ 1 ]. Since the field developed in leaps and bounds, researchers have shown an increasing interest in summing up the development and evolution of the entire discipline. However, the relationship and evolution of bioinformatics’s subtopics have unique characteristics due to its interdisciplinary nature. Most previous works failed to further explore this relationship and did not realize the great potential of visualization in exploring and displaying the evolution of this relationship.
Traditional bibliometric analysis
Bibliometrics is the application of mathematics and statistical methods to evaluate the literature in different disciplines. Patra et al. [ 2 ] analyzed the growth of the scientific literature in bioinformatics. They applied Bradford’s law which estimates the exponentially diminishing returns of searching for references in science journals and Lotka’s law which is used to describe the frequency of publication by authors in any given field [ 3 ] to identify core journals and analyze author’s productivity pattern. W Glänzel et al. [ 4 ] proposed a novel subject-delineation strategy to retrieve of the core literature in bioinformatics. They then analyzed the core literature with bibliometric analysis tools such as co-author citation analysis, national publication activity, citation impact etc. Song et al. [ 5 ] conducted a bibliometric analysis of bioinformatics by extracting citation data from PubMed Central full-text. They focused on evaluating the productivity and influence of bioinformatics. Four measures were used to identify productivity: most productive authors, most productive countries, most productive organizations, and most popular subject terms. Research impact was analyzed based on the measures of most cited papers, most cited authors, emerging stars, and leading organizations.
Text mining applied to bioinformatics bibliometrics
The development of text-mining techniques provides a new perspective for bibliometric analysis. Topic model is the most prevalent approach among techniques applied for bibliometric analysis. Latent Dirichlet Allocation(LDA) is one of the most popular models applied for bibliometric analysis. Song et al. [ 6 ] attempted to detect the knowledge structure of bioinformatics by applying LDA to a large set of bioinformatics full-text articles for topic model generation. Author-Conference-Topic (ACT) [ 7 ] model (an extension of LDA that can incorporate the paper, author, and conference into the topic distribution simultaneously.) was adopted by Heo et al. [ 8 ] to study the field of bioinformatics from the perspective of key phrases, authors, and journals. Heo analyzed the ACT Model results in each period to explore the development trend of bioinformatics
Traditional bibliometric analysis lacks thematic descriptions of bioinformatics subtopics, and the above-mentioned works filled that gap. However, LDA is a typical bag-of-words model that has two major weaknesses: it loses the ordering of the words and ignore their semantics. We choose another technical scheme: paragraph embedding [ 9 ], dimension reduction and clustering. Paragraph Embedding is an unsupervised framework that learns continuous distributed vector representations for pieces of texts which will take the ordering of words in to account. Its construction gives this algorithm the potential to overcome the weaknesses of bag-of-words models and allows us to capture more accurate features of documents to benefit document clustering. Thus, we can better map knowledge structure of bioinformatics.
Visualization applied to bioinformatics bibliometrics
Scholarly data visualization enables scientists to have a better way to represent the structure of data sets and reveal hidden patterns in the data [ 10 ]. However, most previous studies did not realize the benefit of using visualization for exploring knowledge structure of bioinformatics. Visual mapping intuitively shows the overall knowledge structure, research framework, and development trends of a discipline that is very helpful for researchers to rapidly comprehend the overall research status and hotspots [ 11 ]. Although basic charts are widely used to display changes in the number of literatures [ 12 ] or the number of citation count [ 6 ], those charts lack of description of the overall knowledge structure and interaction to explore more information. Therefore, the advantages of visualization are not fully utilized. Another visualization tool used in bibliometrics analysis is network, which is commonly used for co-analysis, such as co-author analysis [ 13 ], co-citation analysis [ 6 ] and term co-occurrence analysis [ 14 ]. This type of network focuses on only one specific relationship, such as co-author focus on relationships between scholars. These diverse network structures are difficult to combine for comparative analysis. Furthermore, when dealing with big data set, the structure of graph may look like a hairball which is incomprehensible for analysts.
In this study, we fully utilized the ability of text mining technology to extract abstract paradigms from massive data and the ability of visualization to display complex information. First, we revealed the distribution of topics in two-dimensional document space by drawing scientific maps of bioinformatics, and then checked the evolution of topic relationships with time filters. Finally, to further understand the interdisciplinary nature of bioinformatics, we combined themeriver with words co-occurrence network to elaborate the evolution of computing-related topics.
Compared with previous works, present study has the following contributions: (1) A science map of bioinformatics was drawn to depict the intersection and evolution of sub-topics of bioinformatics. (2) The interdisciplinary nature of bioinformatics was explored emphatically analyzing the evolution of computing-related topics. (3) The validity and necessity of visualization in analysis were pointed out and proved.
In this section, we will introduce the final visualization results. The goals of our visualization are twofold: (1) intuitively show the change in the popularity of different topics over time; and (2) clearly demonstrate the relationship between topics and how this relationship changes over time
Theme river
Goal 1 can be achieved by applying themeriver [ 15 ], where the color band represents clustering, and the width of the color band represents the number of articles in the clustering.
As shown in Fig. 1 , our theme river has two forms of expression. (a) The middle ordinate represents the actual number of documents, and the development of the origin of the overall discipline can be clearly seen. However, the number of documents is too far away from the origin stage because the development of the discipline has shown a violent growth trend in middle and late stages(From 72 papers in 1960 to more than 16,000 papers in 2010). Under such a quantitative gap, the category information from 1960 to 1990 is compressed by the scale, so that its internal category composition cannot be clearly seen in Fig. 1 a. Therefore, we designed the second form of expression. The ordinate no longer represents the true value but the proportion of sub-topics. The value range is from 0 to 1. From the Figure, we can clearly see that the hot topics also change over time. Moreover, some topics are slowly falling out of sight over time.
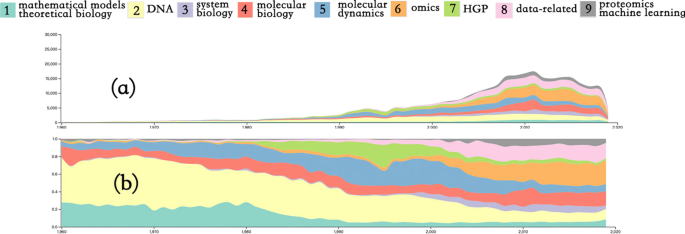
Themeriver of bioinformatics topics: Colors represent nine different topics; A focuses on quantitative changes; B reveals topic trends
Science map
Science map originated from a traditional scientific notation called “knowledge tree” [ 16 ]. According to this tree structure, knowledge is divided into branches, which are then merged into main disciplines, and further divided into molecular disciplines and different specialties. However, with the continuous increase in the speed of knowledge dissemination, the exchanges between disciplines have become more intense, and more interdisciplinary disciplines have emerged. The tree structure has been difficult to meet this demand for discipline description. We need to use a more vivid and intuitive way to show the structure of bioinformatics and the impact of exchanges between different disciplines on bioinformatics’ structure.
To show the relationship between bioinformatics papers in a two-dimensional space, we need to perform dimensionality reduction again on the document vectors obtained in the previous step. This time we still chose UMAP to reduce the vectors’ dimension to 2, which will enable us to project all vectors into two-dimensional space. We choose UMAP for two reasons: first, according to Espadoto et al. [ 17 ],UMAP performs well; second, it can support supervised/semi-supervised dimensionality reduction. To ensure that the data points that belong to two-dimensional space continue to maintain the structural information in the high-dimensional space, we first filtered the soft clustering results and selected those data labels whose category probability is greater than 0.9. We then used UMAP with those labels for semi-supervised dimensionality reduction. Finally, we projected the obtained vector into a two-dimensional space and presented it as a scatter plot shown in Fig. 2
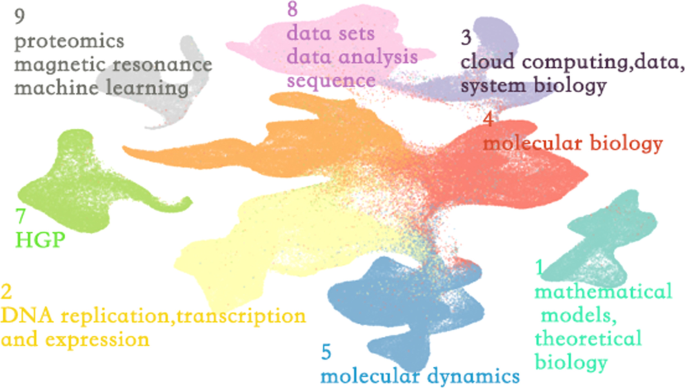
Scatterplot of bioinformatics
Each point represents a paper, and color codes the cluster it belongs to. However, when there is a larger number of points scatter plots may cause a misleading that the greater scope covered by an area, the more is the quantity of its papers. To eliminate this misleading and make the overall structure more visible, we implemented a contour line, where color depth is proportional to the density of papers, and line spacing is inversely proportional to the density gradient. The final science map of bioinformatics is shown in Fig. 3 . We also supported interactive operation to help in-depth analysis, The high-frequency MESH phrases of the papers in the region of interest can be obtained by selecting the region.
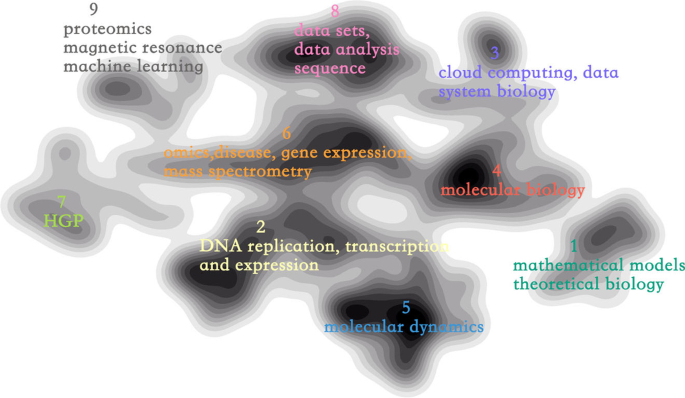
Knowledge structure of bioinformatics. Color opacity is proportional to the density of papers and the darker the color, the higher the density. Line spacing is inversely proportional to the density gradient. Descriptions for each cluster are summarized by TF-IDF
In this section, we analyzed the results from three different perspectives: knowledge structure of bioinformatics, evolution of knowledge structure, and evolution of computing-related topics.
Knowledge structure of bioinformatics
As shown in Fig. 1 b, the popularity of topic 1(mathematical models, theoretical biology) and topic 2(DNA replication, transcription and expression), which are the initial main topics, have gradually decreased since 1980; meanwhile, topic 4(molecular biology) and topic 5(Molecular dynamics) remain relatively stable with limited change. Topic 6(genomics and proteomics) and Topic 8(data-related) have developed rapidly after 2000 and became the main topics of bioinformatics. Topic 3(system biology) and Topic 9(proteomics) were developed around 2000; although they did not receive too much attention, they have maintained a small but stable growth since 2000. The mode of Topic 7(HGP: The Human Genome Project) is somewhat special. This topic has been growing steadily since it appeared in 1980, reaching a peak in about 1995, but began to decrease greatly after 2000. This phenomenon can be well explained by combining the start and end time of the human genome project (1990-2003).
Although themeriver can describe the changes in research hotspots, it cannot sketch the relationship between topics and thus cannot accomplish our goal 2. Thus, we need a science to map the whole knowledge structure of bioinformatics. By analyzing Fig. 3 , we obtain the following conclusions about the relationship between bioinformatics’ topics. (1) Topics 2, 4 and 6 are bridges connecting other topics. And they all deal with DNA indicating that DNA research is the ’skeleton’ of bioinformatics. (2) Among the topics distributed around the structure diagram, Topic 5 is related to Topic 2 and 4, while Topic 7 is mainly related to Topic 2 and 6. Topic 1 is relatively independent from other topics. (3) The three topics 3, 8 and 9 related to computing methods are all located at the top of the structural diagram. Topic 8 is at the center, which means that data are the core of computing-related topics of bioinformatics. (4) The closest non-computational topic to Topic 8 is Topic 6; hence, topic discussed in Topic 6 may use more methods of data science than other topics.
Evolution of knowledge structure
With the continuous development of bioinformatics, the relationship between topics is also changing. From 1960 to 2019, the change in the number of papers on different topics is shown in Fig. 1 a. We selected several time periods when the number of papers varied greatly.
At the beginning of the development of bioinformatics, the relationship between the main topics 1, 2, 4 and 5 was relatively weak. However, from 1980 to 2000, the 2,4,5 topics began to merge to a certain extent. We selected the fusion part interactively and examined at the high-frequency MESH phrases discussed in the merged papers. The top six phrases are: escherichia coli, amino acids, binding site, plasma membrane, binding sites, and signal transduction, which are all related to biological macromolecules (Fig. 4 ).
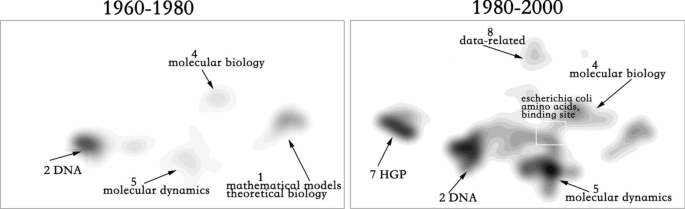
Evolution of bioinformatics from 1960 to 2000
From 2000 to 2005, the number of substructures of bioinformatics increased, but the upper and lower sides are relatively independent. Bridge topic 6(genomics and proteomics) has not become the main hot topic at that time, and has not produced more contact with Topic 3 and 8, but produced more fusion with topic 2, indicating that omics research began with DNA. The period from 2005 to 2010 is a period of vigorous development of bioinformatics. Topic 6, as a bridge topic and a hot topic, began to connect various parts (Fig. 5 ).
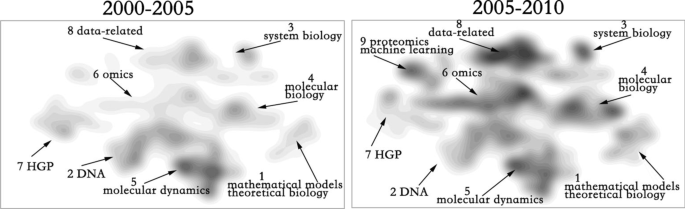
Evolution of bioinformatics from 2000 to 2010
After 2010, the hotspot began to move upward and the distance between Topic 6 and Topics 8,9 was shortened in this period. This finding indicates that omics research increasingly relied more on the support of computational methods (Fig. 6 ).
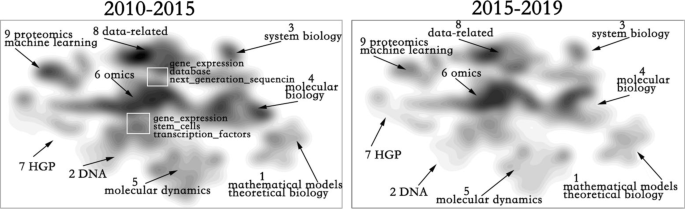
Evolution of bioinformatics from 2010 to 2019
Evolution of computing-related topics
Understanding the evolution of topics related to computation is crucial to understand the whole bioinformatics structure due to the special interdisciplinary nature of bioinformatics. We tried to answer two questions through visual analysis: (1) What is the proportion of computing-related topics in bioinformatics? Is this ratio stable? (2) What changes have taken place in the biological topics and computational methods involved in the evolution of these topics?
Goal 1 can be achieved by simply applying themeriver. We will mainly introduce the solution to Goal 2.
As shown in Fig. 7 , themeriver shows the change of the proportion of four computing-related topics and the word co-occurrence network elaborates the change of topics in each period. By default, the word co-occurrence network of all topics is displayed. Click the category label to hide other categories and explore the selected topic in depth. MESH phrases with high frequency will be displayed by default. Moving the mouse over the circle will display its corresponding MESH phrase. The terms related to computation are represented by stroked circles and marked with orange.
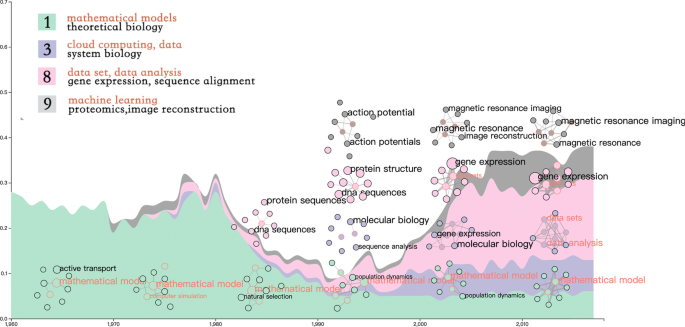
Evolution of computing-related topics. Themeriver shows the change in the proportion of papers and word co-occurrence network illustrates the change of topics in each period. Each circle represents a MESH phrase, and circle size represents the number of occurrences of the phrase
The proportion of papers that related to computation evidently changes. The proportion was close to 0.3 in the early stage of the development of bioinformatics, began to decline in the early 1980s, and only accounted for about 0.1 of the total papers in the early 1990s. The proportion then began to rise again in the middle and late 1990s, surpassing the initial proportion and occupying nearly half of the bioinformatics topics after 2005. During such changes, an obvious pattern is observed: although the proportion of computing-related topics is only a little higher than the original, the internal structure of those topics has undergone earth-shaking changes.
Through further analysis of the co-occurrence word network, we conclude the following. (1) Prior to 1980, Topic 1 occupied an absolute dominant position. The computational methods involved were mainly mathematical models, and computer simulation appeared after 1970. The main biological topics discussed during this period include: active transport, cell, etc. (2) The period of 1990-2000 was a turning point for major changes in the internal structure of computing-related topics. The overall proportion began to rise, but the popularity of Topic 1 remained the same; and Topic 8 gradually replaced Topic 1 to occupy the dominant position. No new computational method was found in Topic 8, but the discussion on sequence increased significantly. (3)The period of 2000 - 2010 is an era of data and genes. The popularity of topics 3 and 8 continues to rise, but the content of the discussion has undergone great changes. The computational topics are all centered on data, data analysis, and the popularity of gene expression is the highest in biological topics while discussions on sequences began to decrease. Topic 9 has also seen a significant increase, and the computational methods involved are mainly related to data; however, biological topics focus on magnetic resonance and images. (4) In 2010 - 2019, the overall structure has not changed, and the proportion of each topic has remained relatively stable, however, the contents they discussed are different from those before. During this period, more computing methods are involved, such as machine learning, data mining, text mining, cloud computing and so on. Thus the interaction between computing methods and bioinformatics becomes increasingly closer and closer.
As an interdisciplinary and fast-growing field of science, bibliometric analysis of bioinformatics has attracted the attention of many researchers. In this study, we collected 330192 bioinformatics papers and applied Doc2vec combined with clustering and dimension reduction technology to detect the knowledge structure of bioinformatics. And then we focus on the role of visual analysis in exploring this structure. Unlike previous works, we focus more on substructures’ relationship. The evolution process of computing-related topics was emphatically analyzed which is vital for understanding the interdisciplinary nature of bioinformatics. The results of our analysis imply that research on bioinformatics is becoming more diversified; the ranking of computational methods in bioinformatics research is also gradually improving. In the future, we plan to enrich and complete the knowledge structure diagram of bioinformatics by applying visualization to explore other aspects of bibliometric analysis, such as author analysis, organization analysis, citation analysis, etc.
In this section, we will introduce the overall procedure of the proposed approach for visualizing the knowledge structure of bioinformatics. Figure 8 shows the pipeline of our methods.
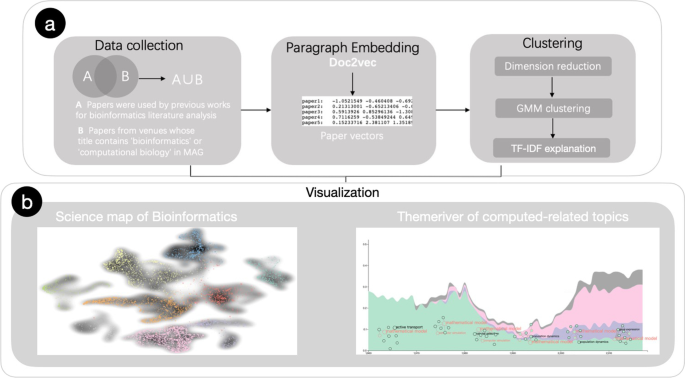
Pipeline of the proposed methods. a Shows the process of data collection and topic extraction. b Is our final visualization results. Science map shows the knowledge structure of bioinformatics and it can be filtered by time to show evolution.Themeriver shows the change in the proportion of papers and word cooccurrence network illustrates the change of topics in each period
Data collection and preprocessing
Data were obtained from Microsoft Academic Graph (MAG) [ 18 ]. MAG is a heterogeneous graph comprising more than 120 million publication entities and related authors, institutions, venues and fields of study. Papers in MAG that belongs to the union of two venue sets were analyzed: (1) 47 bioinformatics journals offered in Song [ 6 ], and 33 conferences used in Song [ 19 ]; (2) venues whose title contains ‘bioinformatics’ or ‘computational biology’ in MAG (“Appendix 1 and 2 ”). Corpus generation is mainly based on bioinformatics related venues. Papers of these venues have been strictly screened by review experts, thus they can better represent the knowledge structure of bioinformatics. This is also the reason why venue-filtered method is adopted in many previous works [ 5 , 6 , 13 , 19 ].
A total of 330,192 papers were analyzed in the following discussion. We then extracted the title and abstract of these papers from the MAG to form a corpus. Detecting phrases in the corpus is crucial because the semantics of these phrases change once they are split into words. So after removing the stop words, we used phrases in Medical Subject Headings(MESH) to annotate phrases in our corpus. These phrases used to analyze bioinformatics literatures in many previous studies [ 2 , 4 , 6 ] to detect phrases in the corpus.
Topic modeling
Paragraph embedding.
Paragraph embedding is an unsupervised framework that learns continuous distributed vector representations for documents. Semantic similarity between documents can be obtained through calculating cosine similarity between vectors. Paragraph embedding is implemented in the gensim package (called doc2vec in gensim), in which the representation vector of each word in the corpus can be obtained at the same time as the document vector by setting parameter ‘dm’ to 1, which is used to calculate the semantic similarity between words. The selection of parameters is based on the best practice given by Lau [ 20 ]: window size is equals to 5 and epoch is equals to 600;
To have a preliminary understanding of the knowledge structure of bioinformatics, we analyzed MESH term vectors first which is also better for subsequent document classification and interpretation. We used HDBSCAN [ 21 ], an outstanding soft clustering algorithm, to determine the clusters for MESH vectors. According to McInnes, HDBSCAN has the following characteristics compared to those parameter-sensitive algorithms: Performs DBSCAN over varying epsilon values and integrates the result to find a clustering that gives the best stability over epsilon. In practice this means that HDBSCAN returns a good clustering straight away with little or no parameter tuning. Phrases tend to be more specific than single word, so we chose to check on the top 10 most representative phrases in each category. Among nine clusters, only one cluster is related to computation, and the other clusters are all terms related to biology. Biology terms are mainly related to genes, proteins and sequences.
Clustering the document vectors can help us to obtain the topic distribution of bioinformatics and further understand the knowledge structure. Compared with LDA, our method can flexibly select the clustering method and the number of categories according to the clustering results after obtaining the vectors. After several rounds of attempts, we chose Gaussian Mixture Model (GMM). GMM is similar to K-Means, but it can be used as soft clustering, giving the probability of data points being assigned to a category.
To avoid high computational cost and remove noises of data, we used PCA to reduce the dimension of document vector from initial 300 to 50. To preserve data’s nonlinearity, we used the nonlinear dimensionality reduction algorithm UMAP [ 22 ] to further reduce the dimension of the document vector to 10. The parameters we used are as follows: n-neighbors is equal to 100 and dimension is equal to 10. These 10-dimensional vectors were used as the input of GMM. Basing on the clustering experience of MESH phrase with HDBSCAN, we selected the number of categories(the number of topics) to be 9. To explain the semantic meaning of each cluster, for each cluster, we used tf-idf algorithm to sort the words in the cluster, and selected the top 10 words as the descriptors of the cluster. Specifically, the tf-idf algorithm is as follows:
where \(n_{i}{j}\) is the count of word \(t_{i}\) in cluster \(d_{j}\) , \(\Sigma _{k}n_{k}{j}\) is the total count of all words in cluster \(d_{j}\) , | D | is the number of clusters and \(|j:t_{i} \in d_{j}|\) is the number of clusters containing \(t_{i}\) .
Results are shown in Table 1 . ‘compute’ means the words belongs to the category related to computation in the word vector classification, and ‘biology’ is the word in the eight other categories.
Co-occurrence word network
To further investigate bioinformatics topics related to computational methods, we combine topic clustering and co-occurrence words analysis to construct a co-occurrence word network. The calculation method is as follows:
The data set of each topic was divided into six time slices to show the changes in each topic over time. From 1960 to 2019, each time slice represents 10 years. We calculated the tf-idf value of MESH phrases in different time slices in each topic, and then divided top 10 phrases into computational phrases and biology phrases according to MESH classification results. A co-occurrence word network between computational phrases and biology phrases is constructed to illustrate this time slice.
Availability of data and materials
Data were obtained from Microsoft Academic Graph (MAG) : https://www.microsoft.com/en-us/research/project/microsoft-academic-graph/
Roos DS. Bioinformatics-trying to swim in a sea of data. Science. 2001;291(5507):1260–1.
Article CAS Google Scholar
Patra SK, Mishra S. Bibliometric study of bioinformatics literature. Scientometrics. 2006;67(3):477–89.
Chen Y-S, Leimkuhler FF. A relationship between Lotka’s law, Bradford’s law, and Zipf’s law. J Am Soc Inf Sci. 1986;37(5):307–14.
Article Google Scholar
Glänzel W, Janssens F, Thijs B. A comparative analysis of publication activity and citation impact based on the core literature in bioinformatics. Scientometrics. 2009;79(1):109–29.
Song M, Kim S, Zhang G, Ding Y, Chambers T. Productivity and influence in bioinformatics: a bibliometric analysis using pubmed central. J Am Soc Inf Sci. 2014;65(2):352–71.
Google Scholar
Song M, Kim SY. Detecting the knowledge structure of bioinformatics by mining full-text collections. Scientometrics. 2013;96(1):183–201.
Tang J, Zhang J, Yao L, Li J, Zhang L, Su Z. Arnetminer: extraction and mining of academic social networks. In: Proceedings of the 14th ACM SIGKDD international conference on knowledge discovery and data mining, 2008;990–998.
Heo GE, Kang KY, Song M, Lee JH. Analyzing the field of bioinformatics with the multi-faceted topic modeling technique. BMC Bioinform 2017;18(Suppl 7).
Le Q, Mikolov T. Distributed representations of sentences and documents. In: International conference on machine learning, 2014;1188–1196.
Liu J, Tang T, Wang W, Xu B, Kong X, Xia F. A survey of scholarly data visualization. IEEE Access. 2018;6:19205–21.
Gu D, Li J, Li X, Liang C. Visualizing the knowledge structure and evolution of big data research in healthcare informatics. Int J Med Inform. 2017;98:22–32.
Wu H, Wang M, Feng J, Pei Y. Research topic evolution in“bioinformatics”. In: 2010 4th international conference on bioinformatics and biomedical engineering, 2010;1–4.
Song M, Yang CC, Tang X. Detecting evolution of bioinformatics with a content and co-authorship analysis. Springerplus. 2013;2(1):186.
Liao H, Tang M, Luo L, Li C, Chiclana F, Zeng XJ. A bibliometric analysis and visualization of medical big data research. Sustainability (Switzerland). 2018;10(1):1–18.
Havre S, Hetzler B, Nowell L. Themeriver: visualizing theme changes over time. In: IEEE symposium on information visualization 2000. INFOVIS 2000. Proceedings, 2000;115–123.
Rafols I, Porter AL, Leydesdorff L. Science overlay maps: a new tool for research policy and library management. J Am Soc Inform Sci Technol. 2010;61(9):1871–87.
Espadoto M, Martins RM, Kerren A, Hirata NS, Telea AC. Towards a quantitative survey of dimension reduction techniques. IEEE Trans Visual Comput Graph 2019.
Wang K, Shen Z, Huang C, Wu C-H, Dong Y, Kanakia A. Microsoft academic graph: when experts are not enough. Quant Sci Stud. 2020;1(1):396–413.
Song M, Heo GE, Kim SY. Analyzing topic evolution in bioinformatics: investigation of dynamics of the field with conference data in DBLP. Scientometrics. 2014;101(1):397–428.
Lau JH, Baldwin T. An empirical evaluation of doc2vec with practical insights into document embedding generation 2016. arXiv preprint arXiv:1607.05368
McInnes L, Healy J, Astels S. hdbscan: Hierarchical density based clustering. J Open Source Softw. 2017;2(11):205.
McInnes L, Healy J, Melville J. Umap: Uniform manifold approximation and projection for dimension reduction 2018. arXiv preprint arXiv:1802.03426
Download references
Acknowledgements
Not applicable.
About this supplement
This article has been published as part of BMC Bioinformatics Volume 23 Supplement 8, 2022: Selected articles from the 16th International Symposium on Bioinformatics Research and Applications (ISBRA-20): bioinformatics. The full contents of the supplement are available online at https://bmcbioinformatics.biomedcentral.com/articles/supplements/volume-23-supplement-8 .
No funding.
Author information
Authors and affiliations.
College of Intelligence and Computing, Tianjin University, Tianjin, China
Jiaqi Wang, Zeyu Li & Jiawan Zhang
You can also search for this author in PubMed Google Scholar
Contributions
JW: Visualization, data analysis, Writing—Original Draft; ZL: Conceptualization, Methodology; JZ: Supervision, Writing—Review Editing; All authors have read and approved the final manuscript.
Corresponding authors
Correspondence to Jiaqi Wang , Zeyu Li or Jiawan Zhang .
Ethics declarations
Ethics approval and consent to participate, consent for publication, competing interests.
The authors declare that they have no competing interests.
Additional information
Publisher's note.
Springer Nature remains neutral with regard to jurisdictional claims in published maps and institutional affiliations.
Appendix 1: List of bioinformatics journals
Bmc bioinformatics.
BMC Genomics
PLoS Biology
Genome Biology
PLoS Genetics
PLoS Computational Biology
BMC Research Notes
- Bioinformatics
Molecular Systems Biology
BMC Systems Biology
Comparative and Functional Genomics
Bioinformation
Human Molecular Genetics
The Embo Journal
Cancer Informatics
Genome Medicine
Evolutionary Bioinformatics
Biochemistry
Algorithms for Molecular Biology
Eurasip Journal on Bioinformatics and Systems Biology
Journal of Molecular Biology
Molecular & Cellular Proteomics
Mammalian Genome
Source Code for Biology and Medicine
Biodata Mining
Journal of Computational Neuroscience
Journal of Proteome Research
Journal of Biomedical Semantics
Journal of Molecular Modeling
Bulletin of Mathematical Biology
Pharmacogenetics and Genomics
Statistical Methods in Medical Research
Neuroinformatics
Protein Science
Physiological Genomics
Trends in Genetics
Journal of Proteomics
Trends in Biochemical Sciences
Journal of Biotechnology
trends in Biotechnology
Journal of theoretical Biology
Journal of Integrative Bioinformatics
Computational Biology and Chemistry
International Journal of Data Mining and Bioinformatics
MOJ Proteomics & Bioinformatics
Journal of Proteomics & Bioinformatics
Network Modeling Analysis in Health Informatics and Bioinformatics
The Open Bioinformatics Journal
Advances in Bioinformatics
Dictionary of Bioinformatics and Computational Biology
IEEE ACM Transactions on Computational Biology and Bioinformatics
Current Bioinformatics
Bioinformatics and Biology Insights
biometrics and Bioinformatics
Journal of Clinical Bioinformatics
International Journal of Bioinformatics Research and Applications
International Journal of Computational Biology and Drug Design
International Journal of Bioscience Biochemistry and Bioinformatics
Applied Bioinformatics
International Journal of knowledge Discovery in Bioinformatics
Mathematical Biology and Bioinformatics
Journal of Bioinformatics and Computational Biology
trends in Bioinformatics
Journal of Computational Biology
briefings in Bioinformatics
biotechnologia Journal of Biotechnology Computational Biology and Bionanotechnology
IPSJ Transactions on Bioinformatics
China Journal of Bioinformatics
Genomics Proteomics & Bioinformatics
Appendix 2: List of bioinformatics conferences
B-interface
DNA computing
Brazilian symposium on bioinformatics
Data mining in bioinformatics
Computational intelligence methods for bioinformatics and biostatistics
Data and text mining in bioinformatics
International conference computational systems-biology and bioinformatics
Bioinformatics research and development
International conference on bioinformatics and biomedical engineering
International joint conferences on bioinformatics, systems biology and intelligent computing
International workshop on practical applications of computational biology and bioinformatics
International symposium health informatics and bioinformatics
Biocomputation, bioinformatics, and biomedical technologies
International conference bioscience, biochemistry and bioinformatics
Rights and permissions
Open Access This article is licensed under a Creative Commons Attribution 4.0 International License, which permits use, sharing, adaptation, distribution and reproduction in any medium or format, as long as you give appropriate credit to the original author(s) and the source, provide a link to the Creative Commons licence, and indicate if changes were made. The images or other third party material in this article are included in the article's Creative Commons licence, unless indicated otherwise in a credit line to the material. If material is not included in the article's Creative Commons licence and your intended use is not permitted by statutory regulation or exceeds the permitted use, you will need to obtain permission directly from the copyright holder. To view a copy of this licence, visit http://creativecommons.org/licenses/by/4.0/ . The Creative Commons Public Domain Dedication waiver ( http://creativecommons.org/publicdomain/zero/1.0/ ) applies to the data made available in this article, unless otherwise stated in a credit line to the data.
Reprints and permissions
About this article
Cite this article.
Wang, J., Li, Z. & Zhang, J. Visualizing the knowledge structure and evolution of bioinformatics. BMC Bioinformatics 23 (Suppl 8), 404 (2022). https://doi.org/10.1186/s12859-022-04948-9
Download citation
Received : 18 September 2022
Accepted : 19 September 2022
Published : 30 September 2022
DOI : https://doi.org/10.1186/s12859-022-04948-9
Share this article
Anyone you share the following link with will be able to read this content:
Sorry, a shareable link is not currently available for this article.
Provided by the Springer Nature SharedIt content-sharing initiative
- Visualization
- Knowledge structure
- Text-mining
ISSN: 1471-2105
- General enquiries: [email protected]
Loading metrics
Open Access
An Introduction to Programming for Bioscientists: A Python-Based Primer
Contributed equally to this work with: Berk Ekmekci, Charles E. McAnany
Affiliation Department of Chemistry, University of Virginia, Charlottesville, Virginia, United States of America
* E-mail: [email protected]
- Berk Ekmekci,
- Charles E. McAnany,
- Cameron Mura
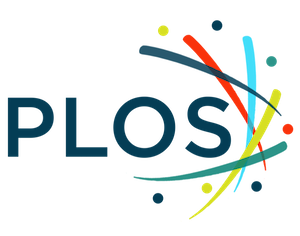
Published: June 7, 2016
- https://doi.org/10.1371/journal.pcbi.1004867
- Reader Comments
Computing has revolutionized the biological sciences over the past several decades, such that virtually all contemporary research in molecular biology, biochemistry, and other biosciences utilizes computer programs. The computational advances have come on many fronts, spurred by fundamental developments in hardware, software, and algorithms. These advances have influenced, and even engendered, a phenomenal array of bioscience fields, including molecular evolution and bioinformatics; genome-, proteome-, transcriptome- and metabolome-wide experimental studies; structural genomics; and atomistic simulations of cellular-scale molecular assemblies as large as ribosomes and intact viruses. In short, much of post-genomic biology is increasingly becoming a form of computational biology. The ability to design and write computer programs is among the most indispensable skills that a modern researcher can cultivate. Python has become a popular programming language in the biosciences, largely because (i) its straightforward semantics and clean syntax make it a readily accessible first language; (ii) it is expressive and well-suited to object-oriented programming, as well as other modern paradigms; and (iii) the many available libraries and third-party toolkits extend the functionality of the core language into virtually every biological domain (sequence and structure analyses, phylogenomics, workflow management systems, etc.). This primer offers a basic introduction to coding, via Python, and it includes concrete examples and exercises to illustrate the language’s usage and capabilities; the main text culminates with a final project in structural bioinformatics. A suite of Supplemental Chapters is also provided. Starting with basic concepts, such as that of a “variable,” the Chapters methodically advance the reader to the point of writing a graphical user interface to compute the Hamming distance between two DNA sequences.
Author Summary
Contemporary biology has largely become computational biology, whether it involves applying physical principles to simulate the motion of each atom in a piece of DNA, or using machine learning algorithms to integrate and mine “omics” data across whole cells (or even entire ecosystems). The ability to design algorithms and program computers, even at a novice level, may be the most indispensable skill that a modern researcher can cultivate. As with human languages, computational fluency is developed actively, not passively. This self-contained text, structured as a hybrid primer/tutorial, introduces any biologist—from college freshman to established senior scientist—to basic computing principles (control-flow, recursion, regular expressions, etc.) and the practicalities of programming and software design. We use the Python language because it now pervades virtually every domain of the biosciences, from sequence-based bioinformatics and molecular evolution to phylogenomics, systems biology, structural biology, and beyond. To introduce both coding (in general) and Python (in particular), we guide the reader via concrete examples and exercises. We also supply, as Supplemental Chapters, a few thousand lines of heavily-annotated, freely distributed source code for personal study.
Citation: Ekmekci B, McAnany CE, Mura C (2016) An Introduction to Programming for Bioscientists: A Python-Based Primer. PLoS Comput Biol 12(6): e1004867. https://doi.org/10.1371/journal.pcbi.1004867
Editor: Francis Ouellette, Ontario Institute for Cancer Research, CANADA
Copyright: © 2016 Ekmekci et al. This is an open access article distributed under the terms of the Creative Commons Attribution License , which permits unrestricted use, distribution, and reproduction in any medium, provided the original author and source are credited.
Funding: Portions of this work were supported by the University of Virginia, the Jeffress Memorial Trust (J-971), a UVa Harrison undergraduate research award (BE), NSF grant DUE-1044858 (CM), and NSF CAREER award MCB-1350957 (CM). The funders had no role in study design, data collection and analysis, decision to publish, or preparation of the manuscript.
Competing interests: The authors have declared that no competing interests exist.
Introduction
Motivation: big data and biology.
Datasets of unprecedented volume and heterogeneity are becoming the norm in science, and particularly in the biosciences. High-throughput experimental methodologies in genomics [ 1 ], proteomics [ 2 ], transcriptomics [ 3 ], metabolomics [ 4 ], and other “omics” [ 5 – 7 ] routinely yield vast stores of data on a system-wide scale. Growth in the quantity of data has been matched by an increase in heterogeneity: there is now great variability in the types of relevant data, including nucleic acid and protein sequences from large-scale sequencing projects, proteomic data and molecular interaction maps from microarray and chip experiments on entire organisms (and even ecosystems [ 8 – 10 ]), three-dimensional (3D) coordinate data from international structural genomics initiatives, petabytes of trajectory data from large-scale biomolecular simulations, and so on. In each of these areas, volumes of raw data are being generated at rates that dwarf the scale and exceed the scope of conventional data-processing and data-mining approaches.
The intense data-analysis needs of modern research projects feature at least three facets: data production , reduction/processing , and integration . Data production is largely driven by engineering and technological advances, such as commodity equipment for next-gen DNA sequencing [ 11 – 13 ] and robotics for structural genomics [ 14 , 15 ]. Data reduction requires efficient computational processing approaches, and data integration demands robust tools that can flexibly represent data ( abstractions ) so as to enable the detection of correlations and interdependencies (via, e.g., machine learning [ 16 ]). These facets are closely coupled: the rate at which raw data is now produced, e.g., in computing molecular dynamics (MD) trajectories [ 17 ], dictates the data storage, processing, and analysis needs. As a concrete example, the latest generation of highly-scalable, parallel MD codes can generate data more rapidly than they can be transferred via typical computer network backbones to local workstations for processing. Such demands have spurred the development of tools for “on-the-fly” trajectory analysis (e.g., [ 18 , 19 ]) as well as generic software toolkits for constructing parallel and distributed data-processing pipelines (e.g., [ 20 ] and S2 Text , §2). To appreciate the scale of the problem, note that calculation of all-atom MD trajectories over biologically-relevant timescales easily leads into petabyte-scale computing. Consider, for instance, a biomolecular simulation system of modest size, such as a 100-residue globular protein embedded in explicit water (corresponding to ≈10 5 particles), and with typical simulation parameters (32-bit precision, atomic coordinates written to disk, in binary format, for every ps of simulation time, etc.). Extending such a simulation to 10 µs duration—which may be at the low end of what is deemed biologically relevant for the system—would give an approximately 12-terabyte trajectory (≈10 5 particles × 3 coordinates/particle/frame × 10 7 frames × 4 bytes/coordinate = 12TB). To validate or otherwise follow-up predictions from a single trajectory, one might like to perform an additional suite of >10 such simulations, thus rapidly approaching the peta-scale.
Scenarios similar to the above example occur in other biological domains, too, at length-scales ranging from atomic to organismal. Atomistic MD simulations were mentioned above. At the molecular level of individual genes/proteins, an early step in characterizing a protein’s function and evolution might be to use sequence analysis methods to compare the protein sequence to every other known sequence, of which there are tens of millions [ 21 ]. Any form of 3D structural analysis will almost certainly involve the Protein Data Bank (PDB; [ 22 ]), which currently holds over 10 5 entries. At the cellular level, proteomics, transcriptomics, and various other “omics” areas (mentioned above) have been inextricably linked to high-throughput, big-data science since the inception of each of those fields. In genomics, the early bottleneck—DNA sequencing and raw data collection—was eventually supplanted by the problem of processing raw sequence data into derived (secondary) formats, from which point meaningful conclusions can be gleaned [ 23 ]. Enabled by the amount of data that can be rapidly generated, typical “omics” questions have become more subtle. For instance, simply assessing sequence similarity and conducting functional annotation of the open reading frames (ORFs) in a newly sequenced genome is no longer the end-goal; rather, one might now seek to derive networks of biomolecular functions from sparse, multi-dimensional datasets [ 24 ]. At the level of tissue systems, the modeling and simulation of inter-neuronal connections has developed into a new field of “connectomics” [ 25 , 26 ]. Finally, at the organismal and clinical level, the promise of personalized therapeutics hinges on the ability to analyze large, heterogeneous collections of data (e.g., [ 27 ]). As illustrated by these examples, all bioscientists would benefit from a basic understanding of the computational tools that are used daily to collect, process, represent, statistically manipulate, and otherwise analyze data. In every data-driven project, the overriding goal is to transform raw data into new biological principles and knowledge.
A New Kind of Scientist
Generating knowledge from large datasets is now recognized as a central challenge in science [ 28 ]. To succeed, each type of aforementioned data-analysis task hinges upon three things: greater computing power, improved computational methods, and computationally fluent scientists. Computing power is only marginally an issue: it lies outside the scope of most biological research projects, and the problem is often addressed by money and the acquisition of new hardware. In contrast, computational methods—improved algorithms, and the software engineering to implement the algorithms in high-quality codebases—are perpetual goals. To address the challenges, a new era of scientific training is required [ 29 – 32 ]. There is a dire need for biologists who can collect, structure, process/reduce, and analyze (both numerically and visually) large-scale datasets. The problems are more fundamental than, say, simply converting data files from one format to another (“data-wrangling”). Fortunately, the basics of the necessary computational techniques can be learned quickly. Two key pillars of computational fluency are (i) a working knowledge of some programming language and (ii) comprehension of core computer science principles (data structures, sort methods, etc.). All programming projects build upon the same set of basic principles, so a seemingly crude grasp of programming essentials will often suffice for one to understand the workings of very complex code; one can develop familiarity with more advanced topics (graph algorithms, computational geometry, numerical methods, etc.) as the need arises for particular research questions. Ideally, computational skills will begin to be developed during early scientific training. Recent educational studies have exposed the gap in life sciences and computer science knowledge among young scientists, and interdisciplinary education appears to be effective in helping bridge the gap [ 33 , 34 ].
Programming as the Way Forward
For many of the questions that arise in research, software tools have been designed. Some of these tools follow the Unix tradition to “make each program do one thing well” [ 35 ], while other programs have evolved into colossal applications that provide numerous sophisticated features, at the cost of accessibility and reliability. A small software tool that is designed to perform a simple task will, at some point, lack a feature that is necessary to analyze a particular type of dataset. A large program may provide the missing feature, but the program may be so complex that the user cannot readily master it, and the codebase may have become so unwieldy that it cannot be adapted to new projects without weeks of study. Guy Steele, a highly-regarded computer scientist, noted this principle in a lecture on programming language design [ 36 ]:
“ I should not design a small language, and I should not design a large one. I need to design a language that can grow. I need to plan ways in which it might grow—but I need, too, to leave some choices so that other persons can make those choices at a later time. ”
Programming languages provide just such a tool. Instead of supplying every conceivable feature, languages provide a small set of well-designed features and powerful tools to compose these features in new ways, using logical principles. Programming allows one to control every aspect of data analysis, and libraries provide commonly-used functionality and pre-made tools that the scientist can use for most tasks. A good library provides a simple interface for the user to perform routine tasks, but also allows the user to tweak and customize the behavior in any way desired (such code is said to be extensible ). The ability to compose programs into other programs is particularly valuable to the scientist. One program may be written to perform a particular statistical analysis, and another program may read in a data file from an experiment and then use the first program to perform the analysis. A third program might select certain datasets—each in its own file—and then call the second program for each chosen data file. In this way, the programs can serve as modules in a computational workflow.
On a related note, many software packages supply an application programming interface (API), which exposes some specific set of functionalities from the codebase without requiring the user/programmer to worry about the low-level implementation details. A well-written API enables users to combine already established codes in a modular fashion, thereby more efficiently creating customized new tools and pipelines for data processing and analysis.
A program that performs a useful task can (and, arguably, should [ 37 ]) be distributed to other scientists, who can then integrate it with their own code. Free software licenses facilitate this type of collaboration, and explicitly encourage individuals to enhance and share their programs [ 38 ]. This flexibility and ease of collaborating allows scientists to develop software relatively quickly, so they can spend more time integrating and mining, rather than simply processing, their data.
Data-processing workflows and pipelines that are designed for use with one particular program or software environment will eventually be incompatible with other software tools or workflow environments; such approaches are often described as being brittle . In contrast, algorithms and programming logic, together with robust and standards-compliant data-exchange formats, provide a completely universal solution that is portable between different tools. Simply stated, any problem that can be solved by a computer can be solved using any programming language [ 39 , 40 ]. The more feature-rich or high-level the language, the more concisely can a data-processing task be expressed using that language (the language is said to be expressive ). Many high-level languages (e.g., Python, Perl) are executed by an interpreter , which is a program that reads source code and does what the code says to do. Interpreted languages are not as numerically efficient as lower-level, compiled languages such as C or Fortran. The source code of a program in a compiled language must be converted to machine-specific instructions by a compiler, and those low-level machine code instructions ( binaries ) are executed directly by the hardware. Compiled code typically runs faster than interpreted code, but requires more work to program. High-level languages, such as Python or Perl, are often used to prototype ideas or to quickly combine modular tools (which may be written in a lower-level language) into “scripts”; for this reason they are also known as scripting languages . Very large programs often provide a scripting language for the user to run their own programs: Microsoft Office has the VBA scripting language, PyMOL [ 41 ] provides a Python interpreter, VMD [ 42 ] uses a Tcl interpreter for many tasks, and Coot [ 43 ] uses the Scheme language to provide an API to the end-user. The deep integration of high-level languages into packages such as PyMOL and VMD enables one to extend the functionality of these programs via both scripting commands (e.g., see PyMOL examples in [ 44 ]) and the creation of semi-standalone plugins (e.g., see the VMD plugin at [ 45 ]). While these tools supply interfaces to different programming languages, the fundamental concepts of programming are preserved in each case: a script written for PyMOL can be transliterated to a VMD script, and a closure in a Coot script is roughly equivalent to a closure in a Python script (see Supplemental Chapter 13 in S1 Text ). Because the logic underlying computer programming is universal, mastering one language will open the door to learning other languages with relative ease. As another major benefit, the algorithmic thinking involved in writing code to solve a problem will often lead to a deeper and more nuanced understanding of the scientific problem itself.
Why Python? (And Which Python?)
Python is the programming language used in this text because of its clear syntax [ 40 , 46 ], active developer community, free availability, extensive use in scientific communities such as bioinformatics, its role as a scripting language in major software suites, and the many freely available scientific libraries (e.g., BioPython [ 47 ]). Two of these characteristics are especially important for our purposes: (i) a clean syntax and straightforward semantics allow the student to focus on core programming concepts without the distraction of difficult syntactic forms, while (ii) the widespread adoption of Python has led to a vast base of scientific libraries and toolkits for more advanced programming projects [ 20 , 48 ]. As noted in the S2 Text (§1), several languages other than Python have also seen widespread use in the biosciences; see, e.g., [ 46 ] for a comparative analysis of some of these languages. As described by Hinsen [ 49 ], Python’s particularly rapid adoption in the sciences can be attributed to its powerful and versatile combination of features, including characteristics intrinsic to the language itself (e.g., expressiveness, a powerful object model) as well as extrinsic features (e.g., community libraries for numerical computing).
Two versions of Python are frequently encountered in scientific programming: Python 2 and Python 3. The differences between these are minor, and while this text uses Python 3 exclusively, most of the code we present will run under both versions of Python. Python 3 is being actively developed and new features are added regularly; Python 2 support continues mainly to serve existing (“legacy”) codes. New projects should use Python 3.
Role and Organization of This Text
This work, which has evolved from a modular “Programming for Bioscientists” tutorial series that has been offered at our institution, provides a self-contained, hands-on primer for general-purpose programming in the biosciences. Where possible, explanations are provided for key foundational concepts from computer science; more formal, and comprehensive, treatments can be found in several computer science texts [ 39 , 40 , 50 ] as well as bioinformatics titles, from both theoretical [ 16 , 51 ] and more practical [ 52 – 55 ] perspectives. Also, this work complements other practical Python primers [ 56 ], guides to getting started in bioinformatics (e.g., [ 57 , 58 ]), and more general educational resources for scientific programming [ 59 ].
Programming fundamentals, including variables, expressions, types, functions, and control flow and recursion, are introduced in the first half of the text (“Fundamentals of Programming”). The next major section (“Data Collections: Tuples, Lists, For Loops, and Dictionaries”) presents data structures for collections of items (lists, tuples, dictionaries) and more control flow (loops). Classes, methods, and other basics of object-oriented programming (OOP) are described in “Object-Oriented Programming in a Nutshell”. File management and input/output (I/O) is covered in “File Management and I/O”, and another practical (and fundamental) topic associated with data-processing—regular expressions for string parsing—is covered in “Regular Expressions for String Manipulations”. As an advanced topic, the text then describes how to use Python and Tkinter to create graphical user interfaces (GUIs) in “An Advanced Vignette: Creating Graphical User Interfaces with Tkinter”. Python’s role in general scientific computing is described as a topic for further exploration (“Python in General-Purpose Scientific Computing”), as is the role of software licensing (“Python and Software Licensing”) and project management via version control systems (“Managing Large Projects: Version Control Systems”). Exercises and examples occur throughout the text to concretely illustrate the language’s usage and capabilities. A final project (“Final Project: A Structural Bioinformatics Problem”) involves integrating several lessons from the text in order to address a structural bioinformatics question.
A collection of Supplemental Chapters ( S1 Text ) is also provided. The Chapters, which contain a few thousand lines of Python code, offer more detailed descriptions of much of the material in the main text. For instance, variables, functions and basic control flow are covered in Chapters 2, 3, and 5, respectively. Some topics are examined at greater depth, taking into account the interdependencies amongst topics—e.g., functions in Chapters 3, 7, and 13; lists, tuples, and other collections in Chapters 8, 9, and 10; OOP in Chapters 15 and 16. Finally, some topics that are either intermediate-level or otherwise not covered in the main text can be found in the Chapters, such as modules in Chapter 4 and lambda expressions in Chapter 13. The contents of the Chapters are summarized in Table 1 and in the S2 Text (§3, “Sample Python Chapters”).
- PPT PowerPoint slide
- PNG larger image
- TIFF original image
https://doi.org/10.1371/journal.pcbi.1004867.t001
Using This Text
This text and the Supplemental Chapters work like the lecture and lab components of a course, and they are designed to be used in tandem. For readers who are new to programming, we suggest reading a section of text, including working through any examples or exercises in that section, and then completing the corresponding Supplemental Chapters before moving on to the next section; such readers should also begin by looking at §3.1 in the S2 Text , which describes how to interact with the Python interpreter, both in the context of a Unix Shell and in an integrated development environment (IDE) such as IDLE. For bioscientists who are somewhat familiar with a programming language (Python or otherwise), we suggest reading this text for background information and to understand the conventions used in the field, followed by a study of the Supplemental Chapters to learn the syntax of Python. For those with a strong programming background, this text will provide useful information about the software and conventions that commonly appear in the biosciences; the Supplemental Chapters will be rather familiar in terms of algorithms and computer science fundamentals, while the biological examples and problems may be new for such readers.
Typographic Conventions
Blocks of code are typeset in monospace font, with keywords in bold and strings in italics. Output appears on its own line without a line number, as in the following example:
1 if ( True ):
2 print (" hello ")
Fundamentals of Programming
Variables and expressions.
The concept of a variable offers a natural starting point for programming. A variable is a name that can be set to represent, or “hold,” a specific value. This definition closely parallels that found in mathematics. For example, the simple algebraic statement x = 5 is interpreted mathematically as introducing the variable x and assigning it the value 5. When Python encounters that same statement, the interpreter generates a variable named x (literally, by allocating memory), and assigns the value 5 to the variable name. The parallels between variables in Python and those in arithmetic continue in the following example, which can be typed at the prompt in any Python shell (§3.1 of the S2 Text describes how to access a Python shell):
3 z = x + 2 * y
4 print (z)
As may be expected, the value of z is set equal to the sum of x and 2*y , or in this case 19. The print() function makes Python output some text (the argument ) to the screen; its name is a relic of early computing, when computers communicated with human users via ink-on-paper printouts. Beyond addition ( + ) and multiplication ( * ), Python can perform subtraction ( - ) and division ( / ) operations. Python is also natively capable (i.e., without add-on libraries) of other mathematical operations, including those summarized in Table 2 .
https://doi.org/10.1371/journal.pcbi.1004867.t002
1 import math
3 y = math.sin(x)
4 print (y)
0.8366556385360561
In the above program, the sine of 21 rad is calculated, stored in y , and printed to the screen as the code’s sole output. As in mathematics, an expression is formally defined as a unit of code that yields a value upon evaluation. As such, x + 2*y , 5 + 3 , sin(pi) , and even the number 5 alone, are examples of expressions (the final example is also known as a literal ). All variable definitions involve setting a variable name equal to an expression.
Python’s operator precedence rules mirror those in mathematics. For instance, 2+5*3 is interpreted as 2+(5*3) . Python supports some operations that are not often found in arithmetic, such as | and is ; a complete listing can be found in the official documentation [ 60 ]. Even complex expressions, like x+3>>1|y&4>=5 or 6 == z+ x) , are fully (unambiguously) resolved by Python’s operator precedence rules. However, few programmers would have the patience to determine the meaning of such an expression by simple inspection. Instead, when expressions become complex, it is almost always a good idea to use parentheses to explicitly clarify the order: (((x+3 >> 1) | y&4) >= 5) or (6 == (z + x)) .
The following block reveals an interesting deviation from the behavior of a variable as typically encountered in mathematics:
3 print (x)
Viewed algebraically, the first two statements define an inconsistent system of equations (one with no solution) and may seem nonsensical. However, in Python, lines 1–2 are a perfectly valid pair of statements. When run, the print statement will display 2 on the screen. This occurs because Python, like most other languages, takes the statement x = 2 to be a command to assign the value of 2 to x , ignoring any previous state of the variable x ; such variable assignment statements are often denoted with the typographic convention “ x ← 2”. Lines 1–2 above are instructions to the Python interpreter, rather than some system of equations with no solutions for the variable x . This example also touches upon the fact that a Python variable is purely a reference to an object such as the integer 5 (For now, take an object to simply be an addressable chunk of memory, meaning it can have a value and be referenced by a variable; objects are further described in the section on OOP.). This is a property of Python’s type system . Python is said to be dynamically typed , versus statically typed languages such as C. In statically typed languages, a program’s data (variable names) are bound to both an object and a type, and type checking is performed at compile-time; in contrast, variable names in a program written in a dynamically typed language are bound only to objects, and type checking is performed at run-time. An extensive treatment of this topic can be found in [ 61 ]. Dynamic typing is illustrated by the following example. (The pound sign, #, starts a comment ; Python ignores anything after a # sign, so in-line comments offer a useful mechanism for explaining and documenting one’s code.)
The above behavior results from the fact that, in Python, the notion of type (defined below) is attached to an object, not to any one of the potentially multiple names (variables) that reference that object. The first two lines illustrate that two or more variables can reference the same object (known as a shared reference ), which in this case is of type int . When y = x is executed, y points to the object x points to (the integer 1 ). When x is changed, y still points to that original integer object. Note that Python strings and integers are immutable , meaning they cannot be changed in-place. However, some other object types, such as lists (described below), are mutable. These aspects of the language can become rather subtle, and the various features of the variable/object relationship—shared references, object mutability, etc.—can give rise to complicated scenarios. Supplemental Chapter 8 ( S1 Text ) explores the Python memory model in more detail.
Statements and Types
A statement is a command that instructs the Python interpreter to do something. All expressions are statements, but a statement need not be an expression. For instance, a statement that, upon execution, causes a program to stop running would never return a value, so it cannot be an expression. Most broadly, statements are instructions, while expressions are combinations of symbols (variables, literals, operators, etc.) that evaluate to a particular value. This particular value might be numerical (e.g., 5 ), a string (e.g., 'foo' ), Boolean ( True / False ), or some other type. Further distinctions between expressions and statements can become esoteric, and are not pertinent to much of the practical programming done in the biosciences.
The type of an object determines how the interpreter will treat the object when it is used. Given the code x = 5 , we can say that “ x is a variable that refers to an object that is of type int ”. We may simplify this to say “ x is an int ”; while technically incorrect, that is a shorter and more natural phrase. When the Python interpreter encounters the expression x + y , if x and y are [variables that point to objects of type] int , then the interpreter would use the addition hardware on the computer to add them. If, on the other hand, x and y were of type str , then Python would join them together. If one is a str and one is an int , the Python interpreter would “raise an exception” and the program would crash. Thus far, each variable we have encountered has been an integer ( int ) type, a string ( str ), or, in the case of sin() ’s output, a real number stored to high precision (a float , for floating-point number). Strings and their constituent characters are among the most useful of Python’s built-in types. Strings are sequences of characters, such as any word in the English language. In Python, a character is simply a string of length one. Each character in a string has a corresponding index, starting from 0 and ranging to index n-1 for a string of n characters. Fig 1 diagrams the composition and some of the functionality of a string, and the following code-block demonstrates how to define and manipulate strings and characters:
1 x = " red "
2 y = " green "
3 z = " blue "
4 print (x + y + z)
redgreenblue
8 print (a + " " + b + " " + c)
The anatomy and basic behavior of Python strings are shown, as samples of actual code (left panel) and corresponding conceptual diagrams (right panel). The Python interpreter prompts for user input on lines beginning with >>> (leftmost edge), while a starting … denotes a continuation of the previous line; output lines are not prefixed by an initial character (e.g., the fourth line in this example). Strings are simply character array objects (of type str ), and a sample string-specific method ( replace ) is shown on line 3. As with ordinary lists, strings can be ‘sliced’ using the syntax shown here: the first list element to be included in the slice is indexed by start , and the last included element is at stop-1 , with an optional stride of size step (defaults to one). Concatenation, via the + operator, is the joining of whole strings or subsets of strings that are generated via slicing (as in this case). For clarity, the integer indices of the string positions are shown only in the forward (left to right) direction for mySnake1 and in the reverse direction for mySnake2 . These two strings are sliced and concatenated to yield the object newSnake ; note that slicing mySnake1 as [0:7] and not [0:6] means that a whitespace char is included between the two words in the resultant newSnake , thus obviating the need for further manipulations to insert whitespace (e.g., concatenations of the form word1+' '+word2 ).
https://doi.org/10.1371/journal.pcbi.1004867.g001
Here, three variables are created by assignment to three corresponding strings. The first print may seem unusual: the Python interpreter is instructed to “add” three strings; the interpreter joins them together in an operation known as concatenation . The second portion of code stores the character 'e' , as extracted from each of the first three strings, in the respective variables, a , b and c . Then, their content is printed, just as the first three strings were. Note that spacing is not implicitly handled by Python (or most languages) so as to produce human-readable text; therefore, quoted whitespace was explicitly included between the strings (line 8; see also the underscore characters, ‘_’, in Fig 1 ).
Exercise 1 : Write a program to convert a temperature in degrees Fahrenheit to degrees Celsius and Kelvin. The topic of user input has not been covered yet (to be addressed in the section on File Management and I/O), so begin with a variable that you pre-set to the initial temperature (in °F). Your code should convert the temperature to these other units and print it to the console.
A deep benefit of the programming approach to problem-solving is that computers enable mechanization of repetitive tasks, such as those associated with data-analysis workflows. This is true in biological research and beyond. To achieve automation, a discrete and well-defined component of the problem-solving logic is encapsulated as a function. A function is a block of code that expresses the solution to a small, standalone problem/task; quite literally, a function can be any block of code that is defined by the user as being a function. Other parts of a program can then call the function to perform its task and possibly return a solution. For instance, a function can be repetitively applied to a series of input values via looping constructs (described below) as part of a data-processing pipeline.
1 def myFun (a,b):
2 c = a + b
3 d = a − b
4 return c*d # NB: a return does not ' print ' anything on its own
5 x = myFun(1,3) + myFun(2,8) + myFun(-1,18)
6 print (x)
To see the utility of functions, consider how much code would be required to calculate x (line 5) in the absence of any calls to myFun . Note that discrete chunks of code, such as the body of a function, are delimited in Python via whitespace, not curly braces, {} , as in C or Perl. In Python, each level of indentation of the source code corresponds to a separate block of statements that group together in terms of program logic. The first line of above code illustrates the syntax to declare a function: a function definition begins with the keyword def , the following word names the function, and then the names within parentheses (separated by commas) define the arguments to the function. Finally, a colon terminates the function definition. (Default values of arguments can be specified as part of the function definition; e.g., writing line 1 as def myFun(a = 1,b = 3): would set default values of a and b .) The three statements after def myFun(a,b): are indented by some number of spaces (two, in this example), and so these three lines (2–4) constitute a block . In this block, lines 2–3 perform arithmetic operations on the arguments, and the final line of this function specifies the return value as the product of variables c and d . In effect, a return statement is what the function evaluates to when called, this return value taking the place of the original function call. It is also possible that a function returns nothing at all; e.g., a function might be intended to perform various manipulations and not necessarily return any output for downstream processing. For example, the following code defines (and then calls) a function that simply prints the values of three variables, without a return statement:
1 def readOut (a,b,c):
2 print (" Variable 1 is: ", a)
3 print (" Variable 2 is: ", b)
4 print (" Variable 3 is: ", c)
5 readOut(1,2,4)
Variable 1 is : 1
Variable 2 is : 2
Variable 3 is : 4
6 readOut(21,5553,3.33)
Variable 1 is : 21
Variable 2 is : 5553
Variable 3 is : 3.33
Code Organization and Scope
Beyond automation, structuring a program into functions also aids the modularity and interpretability of one’s code, and ultimately facilitates the debugging process—an important consideration in all programming projects, large or small.
Python functions can be nested ; that is, one function can be defined inside another. If a particular function is needed in only one place, it can be defined where it is needed and it will be unavailable elsewhere, where it would not be useful. Additionally, nested function definitions have access to the variables that are available when the nested function is defined. Supplemental Chapter 13 explores nested functions in greater detail. A function is an object in Python, just like a string or an integer. (Languages that allow function names to behave as objects are said to have “first-class functions.”) Therefore, a function can itself serve as an argument to another function, analogous to the mathematical composition of two functions, g ( f ( x )). This property of the language enables many interesting programming techniques, as explored in Supplemental Chapters 9 and 13.
https://doi.org/10.1371/journal.pcbi.1004867.g002
Well-established practices have evolved for structuring code in a logically organized (often hierarchical) and “clean” (lucid) manner, and comprehensive treatments of both practical and abstract topics are available in numerous texts. See, for instance, the practical guide Code Complete [ 64 ], the intermediate-level Design Patterns: Elements of Reusable Object-Oriented Software [ 65 ], and the classic (and more abstract) texts Structure and Interpretation of Computer Programs [ 39 ] and Algorithms [ 50 ]; a recent, and free, text in the latter class is Introduction to Computing [ 40 ]. Another important aspect of coding is closely related to the above: usage of brief, yet informative, names as identifiers for variables and function definitions. Even a mid-sized programming project can quickly grow to thousands of lines of code, employ hundreds of functions, and involve hundreds of variables. Though the fact that many variables will lie outside the scope of one another lessens the likelihood of undesirable references to ambiguous variable names, one should note that careless, inconsistent, or undisciplined nomenclature will confuse later efforts to understand a piece of code, for instance by a collaborator or, after some time, even the original programmer. Writing clear, well-defined and well-annotated code is an essential skill to develop. Table 3 outlines some suggested naming practices.
https://doi.org/10.1371/journal.pcbi.1004867.t003
Exercise 2 : Recall the temperature conversion program of Exercise 1. Now, write a function to perform the temperature conversion; this function should take one argument (the input temperature). To test your code, use the function to convert and print the output for some arbitrary temperatures of your choosing.
Control Flow: Conditionals
“Begin at the beginning,” the King said gravely, “and go on till you come to the end; then, stop.” —Lewis Carroll, Alice in Wonderland
Thus far, all of our sample code and exercises have featured a linear flow, with statements executed and values emitted in a predictable, deterministic manner. However, most scientific datasets are not amenable to analysis via a simple, predefined stream of instructions. For example, the initial data-processing stages in many types of experimental pipelines may entail the assignment of statistical confidence/reliability scores to the data, and then some form of decision-making logic might be applied to filter the data. Often, if a particular datum does not meet some statistical criterion and is considered a likely outlier, then a special task is performed; otherwise , another (default) route is taken. This branched if – then – else logic is a key decision-making component of virtually any algorithm, and it exemplifies the concept of control flow. The term control flow refers to the progression of logic as the Python interpreter traverses the code and the program “runs”—transitioning, as it runs, from one state to the next, choosing which statements are executed, iterating over a loop some number of times, and so on. (Loosely, the state can be taken as the line of code that is being executed, along with the collection of all variables, and their values, accessible to a running program at any instant; given the precise state, the next state of a deterministic program can be predicted with perfect precision.) The following code introduces the if statement:
1 from random import randint
2 a = randint(0,100) # get a random integer between 0 and 100 (inclusive)
3 if (a < 50):
4 print (" variable is less than 50 ")
6 print (" the variable is not less than 50 ")
variable is less than 50
In this example, a random integer between 0 and 100 is assigned to the variable a . (Though not applicable to randint , note that many sequence/list-related functions, such as range(a,b) , generate collections that start at the first argument and end just before the last argument. This is because the function range(a,b) produces b − a items starting at a ; with a default stepsize of one, this makes the endpoint b-1 .) Next, the if statement tests whether the variable is less than 50 . If that condition is unfulfilled, the block following else is executed. Syntactically, if is immediately followed by a test condition , and then a colon to denote the start of the if statement’s block ( Fig 3 illustrates the use of conditionals). Just as with functions, the further indentation on line 4 creates a block of statements that are executed together (here, the block has only one statement). Note that an if statement can be defined without a corresponding else block; in that case, Python simply continues executing the code that is indented by one less level (i.e., at the same indentation level as the if line). Also, Python offers a built-in elif keyword (a contraction of “else if”) that tests a subsequent conditional if and only if the first condition is not met. A series of elif statements can be used to achieve similar effects as the switch / case statement constructs found in C and in other languages (including Unix shell scripts) that are often encountered in bioinformatics.
https://doi.org/10.1371/journal.pcbi.1004867.g003
Now, consider the following extension to the preceding block of code. Is there any fundamental issue with it?
2 a = randint(0,100)
5 if (a > 50):
6 print (" variable is greater than 50 ")
8 print (" the variable must be 50 ")
variable is greater than 50
This code will function as expected for a = 50 , as well as values exceeding 50 . However, for a less than 50 , the print statements will be executed from both the less-than (line 4) and equal-to (line 8) comparisons. This erroneous behavior results because an else statement is bound solely to the if statement that it directly follows; in the above code-block, an elif would have been the appropriate keyword for line 5. This example also underscores the danger of assuming that lack of a certain condition (a False built-in Boolean type) necessarily implies the fulfillment of a second condition (a True ) for comparisons that seem, at least superficially, to be linked. In writing code with complicated streams of logic (conditionals and beyond), robust and somewhat redundant logical tests can be used to mitigate errors and unwanted behavior. A strategy for building streams of conditional statements into code, and for debugging existing codebases, involves (i) outlining the range of possible inputs (and their expected outputs), (ii) crafting the code itself, and then (iii) testing each possible type of input, carefully tracing the logical flow executed by the algorithm against what was originally anticipated. In step (iii), a careful examination of “edge cases” can help debug code and pinpoint errors or unexpected behavior. (In software engineering parlance, edge cases refer to extreme values of parameters, such as minima/maxima when considering ranges of numerical types. Recognition of edge-case behavior is useful, as a disproportionate share of errors occur near these cases; for instance, division by zero can crash a function if the denominator in each division operation that appears in the function is not carefully checked and handled appropriately. Though beyond the scope of this primer, note that Python supplies powerful error-reporting and exception-handling capabilities; see, for instance, Python Programming [ 66 ] for more information.) Supplemental Chapters 14 and 16 in S1 Text provide detailed examples of testing the behavior of code.
Exercise 3 : Recall the temperature-conversion program designed in Exercises 1 and 2. Now, rewrite this code such that it accepts two arguments: the initial temperature, and a letter designating the units of that temperature. Have the function convert the input temperature to the alternative scale. If the second argument is ‘C’ , convert the temperature to Fahrenheit, if that argument is ‘F’ , convert it to Celsius.
Integrating what has been described thus far, the following example demonstrates the power of control flow—not just to define computations in a structured/ordered manner, but also to solve real problems by devising an algorithm. In this example, we sort three randomly chosen integers:
2 def numberSort ():
3 a = randint(0,100)
4 b = randint(0,100)
5 c = randint(0,100)
6 # reminder: text following the pound sign is a comment in Python.
7 # begin sort; note the nested conditionals here
8 if ((a > b) and (a > c)):
9 largest = a
10 if (b > c):
11 second = b
12 third = c
13 else:
14 second = c
15 third = b
16 # a must not be largest
17 elif (b > c):
18 largest = b
19 if (c > a):
20 second = c
21 third = a
22 else:
23 second = a
24 third = c
25 # a and b are not largest, thus c must be
27 largest = c
28 if (b < a):
29 second = a
30 third = b
31 else:
32 second = b
33 third = a
34 # Python’s assert function can be used for sanity checks.
35 # If the argument to assert() is False, the program will crash.
36 assert (largest > second)
37 assert (second > third)
38 print (" Sorted: ", largest, ",", second, ",", third)
39 numberSort()
Sorted : 50, 47, 11
Control Flow: Repetition via While Loops
Whereas the if statement tests a condition exactly once and branches the code execution accordingly, the while statement instructs an enclosed block of code to repeat so long as the given condition (the continuation condition ) is satisfied. In fact, while can be considered as a repeated if . This is the simplest form of a loop, and is termed a while loop ( Fig 3 ). The condition check occurs once before entering the associated block; thus, Python’s while is a pre-test loop. (Some languages feature looping constructs wherein the condition check is performed after a first iteration; C’s do–while is an example of such a post-test loop. This is mentioned because looping constructs should be carefully examined when comparing source code in different languages.) If the condition is true, the block is executed and then the interpreter effectively jumps to the while statement that began the block. If the condition is false, the block is skipped and the interpreter jumps to the first statement after the block. The code below is a simple example of a while loop, used to generate a counter that prints each integer between 1 and 100 (inclusive):
1 counter = 1
2 while (counter <= 100):
3 print (counter)
4 counter = counter + 1
5 print (" done! ")
This code will begin with a variable, then print and increment it until its value is 101 , at which point the enclosing while loop ends and a final string (line 5) is printed. Crucially, one should verify that the loop termination condition can, in fact, be reached. If not—e.g., if the loop were specified as while(True): for some reason—then the loop would continue indefinitely, creating an infinite loop that would render the program unresponsive. (In many environments, such as a Unix shell, the keystroke Ctrl-c can be used as a keyboard interrupt to break out of the loop.)
Exercise 4 : With the above example as a starting point, write a function that chooses two randomly-generated integers between 0 and 100 , inclusive, and then prints all numbers between these two values, counting from the lower number to the upper number.
“In order to understand recursion, you must first understand recursion.” — Anonymous
1 def factorial (n):
2 assert (n > 0) # Crash on invalid input
3 if (n == 1):
4 return 1
6 return n * factorial(n-1)
A call to this factorial function will return 1 if the input is equal to one, and otherwise will return the input value multiplied by the factorial of that integer less one ( factorial(n-1) ). Note that this recursive implementation of the factorial perfectly matches its mathematical definition. This often holds true, and many mathematical operations on data are most easily expressed recursively. When the Python interpreter encounters the call to the factorial function within the function block itself (line 6), it generates a new instance of the function on the fly, while retaining the original function in memory (technically, these function instances occupy the runtime’s call stack ). Python places the current function call on hold in the call stack while the newly-called function is evaluated. This process continues until the base case is reached, at which point the function returns a value. Next, the previous function instance in the call stack resumes execution, calculates its result, and returns it. This process of traversing the call stack continues until the very first invocation has returned. At that point, the call stack is empty and the function evaluation has completed.
Expressing Problems Recursively
Defining recursion simply as a function calling itself misses some nuances of the recursive approach to problem-solving. Any difficult problem (e.g., f ( n ) = n !) that can be expressed as a simpler instance of the same problem (e.g., f ( n ) = n * f ( n − 1)) is amenable to a recursive solution. Only when the problem is trivially easy (1!, factorial(1) above) does the recursive solution give a direct (one-step) answer. Recursive approaches fundamentally differ from more iterative (also known as procedural ) strategies: Iterative constructs (loops) express the entire solution to a problem in more explicit form, whereas recursion repeatedly makes a problem simpler until it is trivial. Many data-processing functions are most naturally and compactly solved via recursion.
The recursive descent/ascent behavior described above is extremely powerful, and care is required to avoid pitfalls and frustration. For example, consider the following addition algorithm, which uses the equality operator ( == ) to test for the base case:
1 def badRecursiveAdder (x):
2 if (x == 1):
3 return x
5 return x + badRecursiveAdder(x−2)
This function does include a base case (lines 2–3), and at first glance may seem to act as expected, yielding a sequence of squares (1, 4, 9, 16…) for x = 1, 3, 5, 7,… Indeed, for odd x greater than 1 , the function will behave as anticipated. However, if the argument is negative or is an even number, the base case will never be reached (note that line 5 subtracts 2 ), causing the function call to simply hang, as would an infinite loop. (In this scenario, Python’s maximum recursion depth will be reached and the call stack will overflow.) Thus, in addition to defining the function’s base case, it is also crucial to confirm that all possible inputs will reach the base case. A valid recursive function must progress towards—and eventually reach—the base case with every call. More information on recursion can be found in Supplemental Chapter 7 in S1 Text , in Chapter 4 of [ 40 ], and in most computer science texts.
Exercise 6 : Many functions can be coded both recursively and iteratively (using loops), though often it will be clear that one approach is better suited to the given problem (the factorial is one such example). In this exercise, devise an iterative Python function to compute the factorial of a user-specified integer argument. As a bonus exercise, try coding the Fibonacci sequence in iterative form. Is this as straightforward as the recursive approach? Note that Supplemental Chapter 7 in the S1 Text might be useful here.
Data Collections: Tuples, Lists, For Loops, and Dictionaries
A staggering degree of algorithmic complexity is possible using only variables, functions, and control flow concepts. However, thus far, numbers and strings are the only data types that have been discussed. Such data types can be used to represent protein sequences (a string) and molecular masses (a floating point number), but actual scientific data are seldom so simple! The data from a mass spectrometry experiment are a list of intensities at various m / z values (the mass spectrum). Optical microscopy experiments yield thousands of images, each consisting of a large two-dimensional array of pixels, and each pixel has color information that one may wish to access [ 68 ]. A protein multiple sequence alignment can be considered as a two-dimensional array of characters drawn from a 21-letter alphabet (one letter per amino acid (AA) and a gap symbol), and a protein 3D structural alignment is even more complex. Phylogenetic trees consist of sets of species, individual proteins, or other taxonomic entities, organized as (typically) binary trees with branch weights that represent some metric of evolutionary distance. A trajectory from an MD or Brownian dynamics simulation is especially dense: Cartesian coordinates and velocities are specified for upwards of 10 6 atoms at >10 6 time-points (every ps in a μs-scale trajectory). As illustrated by these examples, real scientific data exhibit a level of complexity far beyond Python’s relatively simple built-in data types. Modern datasets are often quite heterogeneous, particularly in the biosciences [ 69 ], and therefore data abstraction and integration are often the major goals. The data challenges hold true at all levels, from individual RNA transcripts [ 70 ] to whole bacterial cells [ 71 ] to biomedical informatics [ 72 ].
In each of the above examples, the relevant data comprise a collection of entities, each of which, in turn, is of some simpler data type. This unifying principle offers a way forward. The term data structure refers to an object that stores data in a specifically organized (structured) manner, as defined by the programmer. Given an adequately well-specified/defined data structure, arbitrarily complex collections of data can be readily handled by Python, from a simple array of integers to a highly intricate, multi-dimensional, heterogeneous (mixed-type) data structure. Python offers several built-in sequence data structures, including strings, lists, and tuples.
A tuple (pronounced like “couple”) is simply an ordered sequence of objects, with essentially no restrictions as to the types of the objects. Thus, the tuple is especially useful in building data structures as higher-order collections. Data that are inherently sequential (e.g., time-series data recorded by an instrument) are naturally expressed as a tuple, as illustrated by the following syntactic form: myTuple = (0,1,3) . The tuple is surrounded by parentheses, and commas separate the individual elements. The empty tuple is denoted () , and a tuple of one element contains a comma after that element, e.g., (1,) ; the final comma lets Python distinguish between a tuple and a mathematical operation. That is, 2*(3+1) must not treat (3+1) as a tuple. A parenthesized expression is therefore not made into a tuple unless it contains commas. (The type function is a useful built-in function to probe an object’s type. At the Python interpreter, try the statements type((1)) and type((1,)) . How do the results differ?)
A tuple can contain any sort of object, including another tuple. For example, diverseTuple = (15.38,"someString",(0,1)) contains a floating-point number, a string, and another tuple. This versatility makes tuples an effective means of representing complex or heterogeneous data structures. Note that any component of a tuple can be referenced using the same notation used to index individual characters within a string; e.g., diverseTuple[0] gives 15.38 .
In general, data are optimally stored, analyzed, modified, and otherwise processed using data structures that reflect any underlying structure of the data itself. Thus, for example, two-dimensional datasets are most naturally stored as tuples of tuples. This abstraction can be taken to arbitrary depth, making tuples useful for storing arbitrarily complex data. For instance, tuples have been used to create generic tensor-like objects. These rich data structures have been used in developing new tools for the analysis of MD trajectories [ 18 ] and to represent biological sequence information as hierarchical, multidimensional entities that are amenable to further processing in Python [ 20 ].
As a concrete example, consider the problem of representing signal intensity data collected over time. If the data are sampled with perfect periodicity, say every second, then the information could be stored (most compactly) in a one-dimensional tuple, as a simple succession of intensities; the index of an element in the tuple maps to a time-point (index 0 corresponds to the measurement at time t 0 , index 1 is at time t 1 , etc.). What if the data were sampled unevenly in time? Then each datum could be represented as an ordered pair, ( t , I ( t )), of the intensity I at each time-point t ; the full time-series of measurements is then given by the sequence of 2-element tuples, like so:
Three notes concern the above code: (i) From this two-dimensional data structure, the syntax dataSet[i][j] retrieves the j th element from the i th tuple. (ii) Negative indices can be used as shorthand to index from the end of most collections (tuples, lists, etc.), as shown in Fig 1 ; thus, in the above example dataSet[-1] represents the same value as dataSet[4] . (iii) Recall that Python treats all lines of code that belong to the same block (or degree of indentation) as a single unit. In the example above, the first line alone is not a valid (closed) expression, and Python allows the expression to continue on to the next line; the lengthy dataSet expression was formatted as above in order to aid readability.
Once defined, a tuple cannot be altered; tuples are said to be immutable data structures. This rigidity can be helpful or restrictive, depending on the context and intended purpose. For instance, tuples are suitable for storing numerical constants, or for ordered collections that are generated once during execution and intended only for referencing thereafter (e.g., an input stream of raw data).
A mutable data structure is the Python list . This built-in sequence type allows for the addition, removal, and modification of elements. The syntactic form used to define lists resembles the definition of a tuple, except that the parentheses are replaced with square brackets, e.g. myList = [0, 1, 42, 78] . (A trailing comma is unnecessary in one-element lists, as [1] is unambiguously a list.) As suggested by the preceding line, the elements in a Python list are typically more homogeneous than might be found in a tuple: The statement myList2 = ['a',1] , which defines a list containing both string and numeric types, is technically valid, but myList2 = ['a','b'] or myList2 = [0, 1] would be more frequently encountered in practice. Note that myList[1] = 3.14 is a perfectly valid statement that can be applied to the already-defined object named myList (as long as myList already contains two or more elements), resulting in the modification of the second element in the list. Finally, note that myList[5] = 3.14 will raise an error, as the list defined above does not contain a sixth element. The index is said to be out of range , and a valid approach would be to append the value via myList.append(3.14) .
The foregoing description only scratches the surface of Python’s built-in data structures. Several functions and methods are available for lists, tuples, strings, and other built-in types. For lists, append , insert , and remove are examples of oft-used methods; the function len() returns the number of items in a sequence or collection, such as the length of a string or number of elements in a list. All of these “list methods” behave similarly as any other function—arguments are generally provided as input, some processing occurs, and values may be returned. (The OOP section, below, elaborates the relationship between functions and methods.)
Iteration with For Loops
Lists and tuples are examples of iterable types in Python, and the for loop is a useful construct in handling such objects. (Custom iterable types are introduced in Supplemental Chapter 17 in S1 Text .) A Python for loop iterates over a collection, which is a common operation in virtually all data-analysis workflows. Recall that a while loop requires a counter to track progress through the iteration, and this counter is tested against the continuation condition. In contrast, a for loop handles the count implicitly, given an argument that is an iterable object:
1 myData = [1.414, 2.718, 3.142, 4.669]
2 total = 0
3 for datum in myData:
4 # the next statement uses a compound assignment operator; in
5 # the addition assignment operator, a += b means a = a + b
6 total += datum
7 print (" added " + str (datum) + " to sum .")
8 # str makes a string from datum so we can concatenate with +.
added 1.414 to sum.
added 2.718 to sum.
added 3.142 to sum.
added 4.669 to sum.
9 print (total)
11.942999999999998
In the above loop, all elements in myData are of the same type (namely, floating-point numbers). This is not mandatory. For instance, the heterogeneous object myData = ['a','b',1,2] is iterable, and therefore it is a valid argument to a for loop (though not the above loop, as string and integer types cannot be mixed as operands to the + operator). The context dependence of the + symbol, meaning either numeric addition or a concatenation operator, depending on the arguments, is an example of operator overloading . (Together with dynamic typing, operator overloading helps make Python a highly expressive programming language.) In each iteration of the above loop, the variable datum is assigned each successive element in myData ; specifying this iterative task as a while loop is possible, but less straightforward. Finally, note the syntactic difference between Python’s for loops and the for(<initialize>; <condition>; <update>) {<body>} construct that is found in C, Perl, and other languages encountered in computational biology.
Exercise 7 : Consider the fermentation of glucose into ethanol: C 6 H 12 O 6 → 2C 2 H 5 OH + 2CO 2 . A fermentor is initially charged with 10,000 liters of feed solution and the rate of carbon dioxide production is measured by a sensor in moles/hour. At t = 10, 20, 30, 40, 50, 60, 70, and 80 hours, the CO 2 generation rates are 58.2, 65.2, 67.8, 65.4, 58.8, 49.6, 39.1, and 15.8 moles/hour respectively. Assuming that each reading represents the average CO 2 production rate over the previous ten hours, calculate the total amount of CO 2 generated and the final ethanol concentration in grams per liter. Note that Supplemental Chapters 6 and 9 might be useful here.
Exercise 8 : Write a program to compute the distance, d ( r 1 , r 2 ), between two arbitrary (user-specified) points, r 1 = ( x 1 , y 1 , z 1 ) and r 2 = ( x 2 , y 2 , z 2 ), in 3D space. Use the usual Euclidean distance between two points—the straight-line, “as the bird flies” distance. Other distance metrics, such as the Mahalanobis and Manhattan distances, often appear in computational biology too. With your code in hand, note the ease with which you can adjust your entire data-analysis workflow simply by modifying a few lines of code that correspond to the definition of the distance function. As a bonus exercise, generalize your code to read in a list of points and compute the total path length. Supplemental Chapters 6, 7, and 9 might be useful here.
Sets and Dictionaries
Further Data Structures: Trees and Beyond
Python’s built-in data structures are made for sequential data, and using them for other purposes can quickly become awkward. Consider the task of representing genealogy: an individual may have some number of children, and each child may have their own children, and so on. There is no straightforward way to represent this type of information as a list or tuple. A better approach would be to represent each organism as a tuple containing its children. Each of those elements would, in turn, be another tuple with children, and so on. A specific organism would be a node in this data structure, with a branch leading to each of its child nodes; an organism having no children is effectively a leaf . A node that is not the child of any other node would be the root of this tree. This intuitive description corresponds, in fact, to exactly the terminology used by computer scientists in describing trees [ 73 ]. Trees are pervasive in computer science. This document, for example, could be represented purely as a list of characters, but doing so neglects its underlying structure, which is that of a tree (sections, sub-sections, sub-sub-sections, …). The whole document is the root entity, each section is a node on a branch, each sub-section a branch from a section, and so on down through the paragraphs, sentences, words, and letters. A common and intuitive use of trees in bioinformatics is to represent phylogenetic relationships. However, trees are such a general data structure that they also find use, for instance, in computational geometry applications to biomolecules (e.g., to optimally partition data along different spatial dimensions [ 74 , 75 ]).
Trees are, by definition, (i) acyclic , meaning that following a branch from node i will never lead back to node i , and any node has exactly one parent; and (ii) directed , meaning that a node knows only about the nodes “below” it, not the ones “above” it. Relaxing these requirements gives a graph [ 76 ], which is an even more fundamental and universal data structure: A graph is a set of vertices that are connected by edges. Graphs can be subtle to work with and a number of clever algorithms are available to analyze them [ 77 ].
There are countless data structures available, and more are constantly being devised. Advanced examples range from the biologically-inspired neural network, which is essentially a graph wherein the vertices are linked into communication networks to emulate the neuronal layers in a brain [ 78 ], to very compact probabilistic data structures such as the Bloom filter [ 79 ], to self-balancing trees [ 80 ] that provide extremely fast insertion and removal of elements for performance-critical code, to copy-on-write B-trees that organize terabytes of information on hard drives [ 81 ].
Object-Oriented Programming in a Nutshell: Classes, Objects, Methods, and All That
Oop in theory: some basic principles.
Computer programs are characterized by two essential features [ 82 ]: (i) algorithms or, loosely, the “programming logic,” and (ii) data structures , or how data are represented within the program, whether certain components are manipulable, iterable, etc. The object-oriented programming (OOP) paradigm, to which Python is particularly well-suited, treats these two features of a program as inseparable. Several thorough treatments of OOP are available, including texts that are independent of any language [ 83 ] and books that specifically focus on OOP in Python [ 84 ]. The core ideas are explored in this section and in Supplemental Chapters 15 and 16 in S1 Text .
Most scientific data have some form of inherent structure, and this serves as a starting point in understanding OOP. For instance, the time-series example mentioned above is structured as a series of ordered pairs, ( t , I ( t )), an X-ray diffraction pattern consists of a collection of intensities that are indexed by integer triples ( h , k , l ), and so on. In general, the intrinsic structure of scientific data cannot be easily or efficiently described using one of Python’s standard data structures because those types (strings, lists, etc.) are far too simple and limited. Consider, for instance, the task of representing a protein 3D structure, where “representing” means storing all the information that one may wish to access and manipulate: AA sequence (residue types and numbers), the atoms comprising each residue, the spatial coordinates of each atom, whether a cysteine residue is disulfide-bonded or not, the protein’s function, the year the protein was discovered, a list of orthologs of known structure, and so on. What data structure might be capable of most naturally representing such an entity? A simple (generic) Python tuple or list is clearly insufficient.
For this problem, one could try to represent the protein as a single tuple, where the first element is a list of the sequence of residues, the second element is a string describing the protein’s function, the third element lists orthologs, etc. Somewhere within this top-level list, the coordinates of the C α atom of Alanine-42 might be represented as [x,y,z] , which is a simple list of length three. (The list is “simple” in the sense that its rank is one; the rank of a tuple or list is, loosely, the number of dimensions spanned by its rows, and in this case we have but one row.) In other words, our overall data-representation problem can be hierarchically decomposed into simpler sub-problems that are amenable to representation via Python’s built-in types. While valid, such a data structure will be difficult to use: The programmer will have to recall multiple arbitrary numbers (list and sub-list indices) in order to access anything, and extensions to this approach will only make it clumsier. Additionally, there are many functions that are meaningful only in the context of proteins, not all tuples. For example, we may need to compute the solvent-accessible surface areas of all residues in all β -strands for a list of proteins, but this operation would be nonsensical for a list of Supreme Court cases. Conversely, not all tuple methods would be relevant to this protein data structure, yet a function to find Court cases that reached a 5-4 decision along party lines would accept the protein as an argument. In other words, the tuple mentioned above has no clean way to make the necessary associations. It’s just a tuple.
OOP Terminology
This protein representation problem is elegantly solved via the OOP concepts of classes, objects, and methods. Briefly, an object is an instance of a data structure that contains members and methods. Members are data of potentially any type, including other objects. Unlike lists and tuples, where the elements are indexed by numbers starting from zero, the members of an object are given names, such as yearDiscovered . Methods are functions that (typically) make use of the members of the object. Methods perform operations that are related to the data in the object’s members. Objects are constructed from class definitions, which are blocks that define what most of the methods will be for an object. The examples in the 'OOP in Practice' section will help clarify this terminology. (Note that some languages require that all methods and members be specified in the class declaration, but Python allows duck punching , or adding members after declaring a class. Adding methods later is possible too, but uncommon. Some built-in types, such as int , do not support duck punching.)
During execution of an actual program, a specific object is created by calling the name of the class, as one would do for a function. The interpreter will set aside some memory for the object’s methods and members, and then call a method named __init__ , which initializes the object for use.
Classes can be created from previously defined classes. In such cases, all properties of the parent class are said to be inherited by the child class. The child class is termed a derived class , while the parent is described as a base class . For instance, a user-defined Biopolymer class may have derived classes named Protein and NucleicAcid , and may itself be derived from a more general Molecule base class. Class names often begin with a capital letter, while object names (i.e., variables) often start with a lowercase letter. Within a class definition, a leading underscore denotes member names that will be protected. Working examples and annotated descriptions of these concepts can be found, in the context of protein structural analysis, in ref [ 85 ].
The OOP paradigm suffuses the Python language: Every value is an object. For example, the statement foo = ‘bar’ instantiates a new object (of type str ) and binds the name foo to that object. All built-in string methods will be exposed for that object (e.g., foo.upper() returns ‘BAR’ ). Python’s built-in dir() function can be used to list all attributes and methods of an object, so dir(foo) will list all available attributes and valid methods on the variable foo . The statement dir(1) will show all the methods and members of an int (there are many!). This example also illustrates the conventional OOP dot-notation, object.attribute , which is used to access an object’s members, and to invoke its methods ( Fig 1 , left). For instance, protein1.residues[2].CA.x might give the x -coordinate of the C α atom of the third residue in protein1 as a floating-point number, and protein1.residues[5].ssbond(protein2.residues[6]) might be used to define a disulfide bond (the ssbond() method) between residue-6 of protein1 and residue-7 of protein2 . In this example, the residues member is a list or tuple of objects, and an item is retrieved from the collection using an index in brackets.
Benefits of OOP
By effectively compartmentalizing the programming logic and implicitly requiring a disciplined approach to data structures, the OOP paradigm offers several benefits. Chief among these are (i) clean data/code separation and bundling (i.e., modularization), (ii) code reusability, (iii) greater extensibility (derived classes can be created as needs become more specialized), and (iv) encapsulation into classes/objects provides a clearer interface for other programmers and users. Indeed, a generally good practice is to discourage end-users from directly accessing and modifying all of the members of an object. Instead, one can expose a limited and clean interface to the user, while the back-end functionality (which defines the class) remains safely under the control of the class’ author. As an example, custom getter and setter methods can be specified in the class definition itself, and these methods can be called in another user’s code in order to enable the safe and controlled access/modification of the object’s members. A setter can ‘sanity-check’ its input to verify that the values do not send the object into a nonsensical or broken state; e.g., specifying the string "ham" as the x -coordinate of an atom could be caught before program execution continues with a corrupted object. By forcing alterations and other interactions with an object to occur via a limited number of well-defined getters/setters, one can ensure that the integrity of the object’s data structure is preserved for downstream usage.
The OOP paradigm also solves the aforementioned problem wherein a protein implemented as a tuple had no good way to be associated with the appropriate functions—we could call Python’s built-in max() on a protein, which would be meaningless, or we could try to compute the isoelectric point of an arbitrary list (of Supreme Court cases), which would be similarly nonsensical. Using classes sidesteps these problems. If our Protein class does not define a max() method, then no attempt can be made to calculate its maximum. If it does define an isoelectricPoint() method, then that method can be applied only to an object of type Protein . For users/programmers, this is invaluable: If a class from a library has a particular method, one can be assured that that method will work with objects of that class.
OOP in Practice: Some Examples
Note the usage of self as the first argument in each method defined in the above code. The self keyword is necessary because when a method is invoked it must know which object to use. That is, an object instantiated from a class requires that methods on that object have some way to reference that particular instance of the class, versus other potential instances of that class. The self keyword provides such a “hook” to reference the specific object for which a method is called. Every method invocation for a given object, including even the initializer called __init__ , must pass it self (the current instance) as the first argument to the method; this subtlety is further described at [ 86 ] and [ 87 ]. A practical way to view the effect of self is that any occurrence of objName.methodName(arg1, arg2) effectively becomes methodName(objName, arg1, arg2) . This is one key deviation from the behavior of top-level functions, which exist outside of any class. When defining methods, usage of self provides an explicit way for the object itself to be provided as an argument (self-reference), and its disciplined usage will help minimize confusion about expected arguments.
To illustrate how objects may interact with one another, consider a class to represent a chemical’s atom:
Then, we can use this Atom class in constructing another class to represent molecules:
And, finally, the following code illustrates the construction of a diatomic molecule:
If the above code is run, for example, in an interactive Python session, then note that the aforementioned dir() function is an especially useful built-in tool for querying the properties of new classes and objects. For instance, issuing the statement dir(Molecule) will return detailed information about the Molecule class (including its available methods).
File Management and I/O
Scientific data are typically acquired, processed, stored, exchanged, and archived as computer files. As a means of input/output (I/O) communication, Python provides tools for reading, writing and otherwise manipulating files in various formats. Supplemental Chapter 11 in S1 Text focuses on file I/O in Python. Most simply, the Python interpreter allows command-line input and basic data output via the print() function. For real-time interaction with Python, the free IPython [ 89 ] system offers a shell that is both easy to use and uniquely powerful (e.g., it features tab completion and command history scrolling); see the S2 Text , §3 for more on interacting with Python. A more general approach to I/O, and a more robust (persistent) approach to data archival and exchange, is to use files for reading, writing, and processing data. Python handles file I/O via the creation of file objects, which are instantiated by calling the open function with the filename and access mode as its two arguments. The syntax is illustrated by fileObject = open("myName.pdb", mode = ‘r’) , which creates a new file object from a file named "myName.pdb" . This file will be only readable because the ‘r’ mode is specified; other valid modes include ‘w’ to allow writing and ‘a’ for appending. Depending on which mode is specified, different methods of the file object will be exposed for use. Table 4 describes mode types and the various methods of a File object.
https://doi.org/10.1371/journal.pcbi.1004867.t004
The following example opens a file named myDataFile.txt and reads the lines, en masse , into a list named listOfLines . (In this example, the variable readFile is also known as a “file handle,” as it references the file object.) As for all lists, this object is iterable and can be looped over in order to process the data.
1 readFile = open (" myDataFile.txt ", mode = ‘ r ’)
2 listOfLines = readFile.readlines()
3 # Process the lines. Simply dump the contents to the console:
4 for l in listOfLines:
5 print (l)
(The lines in the file will be printed)
6 readFile.close()
1 fp = open (‘ 1I8F.pdb ’, mode = ‘ r ’)
2 numHetatm = 0
3 for line in fp.readlines():
4 if ( len (line) > 6):
5 if (line[0:6] == " HETATM "):
6 numHetatm += 1
7 fp.close()
8 print (numHetatm)
Begin this exercise by choosing a FASTA protein sequence with more than 3000 AA residues. Then, write Python code to read in the sequence from the FASTA file and: (i) determine the relative frequencies of AAs that follow proline in the sequence; (ii) compare the distribution of AAs that follow proline to the distribution of AAs in the entire protein; and (iii) write these results to a human-readable file.
Regular Expressions for String Manipulations
In Python, a regex match es a string if the string starts with that regex. Python also provides a search function to locate a regex anywhere within a string. Returning to the notion that a regex “specifies a set of strings,” given some text the match es to a regex will be all strings that start with the regex, while the search hits will be all strings that contain the regex. For clarity, we will say that a regex find s a string if the string is completely described by the regex, with no trailing characters. (There is no find in Python but, for purposes of description here, it is useful to have a term to refer to a match without trailing characters.)
Beyond the central role of the regex in analyzing biological sequences, parsing datasets, etc., note that any effort spent learning Python regexes is highly transferable. In terms of general syntactic forms and functionality, regexes behave roughly similarly in Python and in many other mainstream languages (e.g., Perl, R), as well as in the shell scripts and command-line utilities (e.g., grep) found in the Unix family of operating systems (including all Linux distributions and Apple’s OS X).
Exercise 11 : Many human hereditary neurodegenerative disorders, such as Huntington’s disease (HD), are linked to anomalous expansions in the number of trinucleotide repeats in particular genes [ 94 ]. In HD, the pathological severity correlates with the number of (CAG) n repeats in exon-1 of the gene ( htt ) encoding the protein (huntingtin): More repeats means an earlier age of onset and a more rapid disease progression. The CAG codon specifies glutamine, and HD belongs to a broad class of polyglutamine (polyQ) diseases. Healthy (wild-type) variants of this gene feature n ≈ 6–35 tandem repeats, whereas n > 35 virtually assures the disease. For this exercise, write a Python regex that will locate any consecutive runs of (CAG) n >10 in an input DNA sequence. Because the codon CAA also encodes Q and has been found in long runs of CAGs, your regex should also allow interspersed CAAs. To extend this exercise, write code that uses your regex to count the number of CAG repeats (allow CAA too), and apply it to a publically-available genome sequence of your choosing (e.g., the NCBI GI code 588282786:1-585 is exon-1 from a human’s htt gene [accessible at http://1.usa.gov/1NjrDNJ ]).
An Advanced Vignette: Creating Graphical User Interfaces with Tkinter
Thus far, this primer has centered on Python programming as a tool for interacting with data and processing information. To illustrate an advanced topic, this section shifts the focus towards approaches for creating software that relies on user interaction, via the development of a graphical user interface (GUI; pronounced ‘gooey’). Text-based interfaces (e.g., the Python shell) have several distinct advantages over purely graphical interfaces, but such interfaces can be intimidating to the uninitiated. For this reason, many general users will prefer GUI-based software that permits options to be configured via graphical check boxes, radio buttons, pull-down menus and the like, versus text-based software that requires typing commands and editing configuration files. In Python, the tkinter package (pronounced ‘T-K-inter’) provides a set of tools to create GUIs. (Python 2.x calls this package Tkinter , with a capital T ; here, we use the Python 3.x notation.)
Tkinter programming has its own specialized vocabulary. Widgets are objects, such as text boxes, buttons and frames, that comprise the user interface. The root window is the widget that contains all other widgets. The root window is responsible for monitoring user interactions and informing the contained widgets to respond when the user triggers an interaction with them (called an event ). A frame is a widget that contains other widgets. Frames are used to group related widgets together, both in the code and on-screen. A geometry manager is a system that places widgets in a frame according to some style determined by the programmer. For example, the grid geometry manager arranges widgets on a grid, while the pack geometry manager places widgets in unoccupied space. Geometry managers are discussed at length in Supplemental Chapter 18 in S1 Text , which shows how intricate layouts can be generated.
The basic style of GUI programming fundamentally differs from the material presented thus far. The reason for this is that the programmer cannot predict what actions a user might perform, and, more importantly, in what order those actions will occur. As a result, GUI programming consists of placing a set of widgets on the screen and providing instructions that the widgets execute when a user interaction triggers an event. (Similar techniques are used, for instance, to create web interfaces and widgets in languages such as JavaScript.) Supplemental Chapter 19 ( S1 Text ) describes available techniques for providing functionality to widgets. Once the widgets are configured, the root window then awaits user input. A simple example follows:
1 from tkinter import Tk, Button
2 def buttonWindow ():
3 window = Tk()
4 def onClick ():
5 print (" Button clicked ")
6 btn = Button(window, text = " Sample Button ", command = onClick)
7 btn.pack()
8 window.mainloop()
Graphical widgets, such as text entry fields and check-boxes, receive data from the user, and must communicate that data within the program. To provide a conduit for this information, the programmer must provide a variable to the widget. When the value in the widget changes, the widget will update the variable and the program can read it. Conversely, when the program should change the data in a widget (e.g., to indicate the status of a real-time calculation), the programmer sets the value of the variable and the variable updates the value displayed on the widget. This roundabout tack is a result of differences in the architecture of Python and Tkinter—an integer in Python is represented differently than an integer in Tkinter, so reading the widget’s value directly would result in a nonsensical Python value. These variables are discussed in Supplemental Chapter 19 in S1 Text .
From a software engineering perspective, a drawback to graphical interfaces is that multiple GUIs cannot be readily composed into new programs. For instance, a GUI to display how a particular restriction enzyme will cleave a DNA sequence will not be practically useful in predicting the products of digesting thousands of sequences with the enzyme, even though some core component of the program (the key, non-GUI program logic) would be useful in automating that task. For this reason, GUI applications should be written in as modular a style as possible—one should be able to extract the useful functionality without interacting with the GUI-specific code. In the restriction enzyme example, an optimal solution would be to write the code that computes cleavage sites as a separate module, and then have the GUI code interact with the components of that module.
Python in General-purpose Scientific Computing: Numerical Efficiency, Libraries
In pursuing biological research, the computational tasks that arise will likely resemble problems that have already been solved, problems for which software libraries already exist. This occurs largely because of the interdisciplinary nature of biological research, wherein relatively well-established formalisms and algorithms from physics, computer science, and mathematics are applied to biological systems. For instance, (i) the simulated annealing method was developed as a physically-inspired approach to combinatorial optimization, and soon thereafter became a cornerstone in the refinement of biomolecular structures determined by NMR spectroscopy or X-ray crystallography [ 95 ]; (ii) dynamic programming was devised as an optimization approach in operations research, before becoming ubiquitous in sequence alignment algorithms and other areas of bioinformatics; and (iii) the Monte Carlo method, invented as a sampling approach in physics, underlies the algorithms used in problems ranging from protein structure prediction to phylogenetic tree estimation.
Each computational approach listed above can be implemented in Python. The language is well-suited to rapidly develop and prototype any algorithm, be it intended for a relatively lightweight problem or one that is more computationally intensive (see [ 96 ] for a text on general-purpose scientific computing in Python). When considering Python and other possible languages for a project, software development time must be balanced against a program’s execution time. These two factors are generally countervailing because of the inherent performance trade-offs between codes that are written in interpreted (high-level) versus compiled (lower-level) languages; ultimately, the computational demands of a problem will help guide the choice of language. In practice, the feasibility of a pure Python versus non-Python approach can be practically explored via numerical benchmarking. While Python enables rapid development, and is of sufficient computational speed for many bioinformatics problems, its performance simply cannot match the compiled languages that are traditionally used for high-performance computing applications (e.g., many MD integrators are written in C or Fortran). Nevertheless, Python codes are available for molecular simulations, parallel execution, and so on. Python’s popularity and utility in the biosciences can be attributed to its ease of use (expressiveness), its adequate numerical efficiency for many bioinformatics calculations, and the availability of numerous libraries that can be readily integrated into one’s Python code (and, conversely, one’s Python code can “hook” into the APIs of larger software tools, such as PyMOL). Finally, note that rapidly-developed Python software can be integrated with numerically efficient, high-performance code written in a low-level languages such as C, in an approach known as “mixed-language programming” [ 49 ].
Many additional libraries can be found at the official Python Package Index (PyPI; [ 102 ]), as well as myriad packages from unofficial third-party repositories. The BioPython project, mentioned above in the 'Why Python?' subsection, offers an integrated suite of tools for sequence- and structure-based bioinformatics, as well as phylogenetics, machine learning, and other feature sets. We survey the computational biology software landscape in the S2 Text (§2), including tools for structural bioinformatics, phylogenetics, omics-scale data-processing pipelines, and workflow management systems. Finally, note that Python code can be interfaced with other languages. For instance, current support is provided for low-level integration of Python and R [ 103 , 104 ], as well as C-extensions in Python (Cython; [ 105 , 106 ]). Such cross-language interfaces extend Python’s versatility and flexibility for computational problems at the intersection of multiple scientific domains, as often occurs in the biosciences.
Python and Software Licensing
Any discussion of libraries, modules, and extensions merits a brief note on the important role of licenses in scientific software development. As evidenced by the widespread utility of existing software libraries in modern research communities, the development work done by one scientist will almost certainly aid the research pursuits of others—either near-term or long-term, in subfields that might be near to one’s own or perhaps more distant (and unforeseen). Free software licenses promote the unfettered advance of scientific research by encouraging the open exchange, transparency, communicability, and reproducibility of research projects. To qualify as free software, a program must allow the user to view and change the source code (for any purpose), distribute the code to others, and distribute modified versions of the code to others. The Open Source Initiative provides alphabetized and categorized lists of licenses that comply, to various degrees, with the open-source definition [ 107 ]. As an example, the Python interpreter, itself, is under a free license. Software licensing is a major topic unto itself, and helpful primers are available on technical [ 38 ] and strategic [ 37 , 108 ] considerations in adopting one licensing scheme versus another. All of the content (code and comments) that is provided as Supplemental Chapters ( S1 Text ) is licensed under the GNU Affero General Public License (AGPL) version 3, which permits anyone to examine, edit, and distribute the source so long as any works using it are released under the same license.
Managing Large Projects: Version Control Systems
As a project grows, it becomes increasingly difficult—yet increasingly important—to be able to track changes in source code. A version control system (VCS) tracks changes to documents and facilitates the sharing of code among multiple individuals. In a distributed (as opposed to centralized) VCS, each developer has his own complete copy of the project, locally stored. Such a VCS supports the “committing,” “pulling,” “branching,” and “merging” of code. After making a change, the programmer commits the change to the VCS. The VCS stores a snapshot of the project, preserving the development history. If it is later discovered that a particular commit introduced a bug, one can easily revert the offending commit. Other developers who are working on the same project can pull from the author of the change (the most recent version, or any earlier snapshot). The VCS will incorporate the changes made by the author into the puller’s copy of the project. If a new feature will make the code temporarily unusable (until the feature is completely implemented), then that feature should be developed in a separate branch . Developers can switch between branches at will, and a commit made to one branch will not affect other branches. The master branch will still contain a working version of the program, and developers can still commit non-breaking changes to the master branch. Once the new feature is complete, the branches can be merged together. In a distributed VCS, each developer is, conceptually, a branch. When one developer pulls from others, this is equivalent to merging a branch from each developer. Git, Mercurial, and Darcs are common distributed VCS. In contrast, in a centralized VCS all commits are tracked in one central place (for both distributed and centralized VCS, this “place” is often a repository hosted in the cloud). When a developer makes a commit, it is pushed to every other developer (who is on the same branch). The essential behaviors—committing, branching, merging—are otherwise the same as for a distributed VCS. Examples of popular centralized VCSs include the Concurrent Versioning System (CVS) and Subversion.
While VCS are mainly designed to work with source code, they are not limited to this type of file. A VCS is useful in many situations where multiple people are collaborating on a single project, as it simplifies the task of combining, tracking, and otherwise reconciling the contributions of each person. In fact, this very document was developed using LaTeX and the Git VCS, enabling each author to work on the text in parallel. A helpful guide to Git and GitHub (a popular Git repository hosting service) was very recently published [ 109 ]; in addition to a general introduction to VCS, that guide offers extensive practical advice, such as what types of data/files are more or less ideal for version controlling.
Final Project: A Structural Bioinformatics Problem
Fluency in a programming language is developed actively, not passively. The exercises provided in this text have aimed to develop the reader’s command of basic features of the Python language. Most of these topics are covered more deeply in the Supplemental Chapters ( S1 Text ), which also include some advanced features of the language that lie beyond the scope of the main body of this primer. As a final exercise, a cumulative project is presented below. This project addresses a substantive scientific question, and its successful completion requires one to apply and integrate the skills from the foregoing exercises. Note that a project such as this—and really any project involving more than a few dozen lines of code—will benefit greatly from an initial planning phase. In this initial stage of software design, one should consider the basic functions, classes, algorithms, control flow, and overall code structure.
Data and algorithms are two pillars of modern biosciences. Data are acquired, filtered, and otherwise manipulated in preparation for further processing, and algorithms are applied in analyzing datasets so as to obtain results. In this way, computational workflows transform primary data into results that can, over time, become formulated into general principles and new knowledge. In the biosciences, modern scientific datasets are voluminous and heterogeneous. Thus, in developing and applying computational tools for data analysis, the two central goals are scalability , for handling the data-volume problem, and robust abstractions , for handling data heterogeneity and integration. These two challenges are particularly vexing in biology, and are exacerbated by the traditional lack of training in computational and quantitative methods in many biosciences curricula. Motivated by these factors, this primer has sought to introduce general principles of computer programming, at both basic and intermediate levels. The Python language was adopted for this purpose because of its broad prevalence and deep utility in the biosciences.
Supporting Information
S1 text. python chapters..
This suite of 19 Supplemental Chapters covers the essentials of programming. The Chapters are written in Python and guide the reader through the core concepts of programming, via numerous examples and explanations. The most recent versions of all materials are maintained at http://p4b.muralab.org . For purposes of self-study, solutions to the in-text exercises are also included.
https://doi.org/10.1371/journal.pcbi.1004867.s001
S2 Text. Supplemental text.
The supplemental text contains sections on: (i) Python as a general language for scientific computing, including the concepts of imperative and declarative languages, Python’s relationship to other languages, and a brief account of languages widely used in the biosciences; (ii) a structured guide to some of the available software packages in computational biology, with an emphasis on Python; and (iii) two sample Supplemental Chapters (one basic, one more advanced), along with a brief, practical introduction to the Python interpreter and integrated development environment (IDE) tools such as IDLE.
https://doi.org/10.1371/journal.pcbi.1004867.s002
Acknowledgments
We thank M. Cline, S. Coupe, S. Ehsan, D. Evans, R. Sood, and K. Stanek for critical reading and helpful feedback on the manuscript.
- View Article
- PubMed/NCBI
- Google Scholar
- 7. Gerstein Lab. “O M E S Table”;. Available from: http://bioinfo.mbb.yale.edu/what-is-it/omes/omes.html .
- 16. Baldi P, Brunak S. Bioinformatics: The Machine Learning Approach (2 nd Edition). A Bradford Book; 2001.
- 28. Rudin C, Dunson D, Irizarry R, Ji H, Laber E, Leek J, et al. Discovery with Data: Leveraging Statistics with Computer Science to Transform Science and Society. American Statistical Association; 2014. Available from: http://www.amstat.org/policy/pdfs/BigDataStatisticsJune2014.pdf .
- 29. Committee on a New Biology for the 21 st Century: Ensuring the United States Leads the Coming Biology Revolution and Board on Life Sciences and Division on Earth and Life Studies and National Research Council. A New Biology for the 21 st Century. National Academies Press; 2009.
- 39. Abelson H, Sussman GJ. Structure and Interpretation of Computer Programs (2 nd Edition). The MIT Press; 1996. Available from: http://mitpress.mit.edu/sicp/full-text/book/book.html .
- 40. Evans D. Introduction to Computing: Explorations in Language, Logic, and Machines. CreateSpace Independent Publishing Platform; 2011. Available from: http://www.computingbook.org .
- 41. The PyMOL Molecular Graphics System, Schrödinger, LLC;. Available from: http://pymol.org .
- 45. PBCTools Plugin, Version 2.7;. Available from: http://www.ks.uiuc.edu/Research/vmd/plugins/pbctools .
- 49. Hinsen K. High-Level Scientific Programming with Python. In: Proceedings of the International Conference on Computational Science-Part III. ICCS’02. London, UK, UK: Springer-Verlag; 2002. p. 691–700.
- 50. Cormen TH, Leiserson CE, Rivest RL, Stein C. Introduction to Algorithms (3 rd Edition). The MIT Press; 2009.
- 51. Jones NC, Pevzner PA. An Introduction to Bioinformatics Algorithms. The MIT Press; 2004.
- 52. Wünschiers R. Computational Biology: Unix/Linux, Data Processing and Programming. Springer-Verlag; 2004.
- 53. Model ML. Bioinformatics Programming Using Python: Practical Programming for Biological Data. O’Reilly Media; 2009.
- 54. Buffalo V. Bioinformatics Data Skills: Reproducible and Robust Research with Open Source Tools. O’Reilly Media; 2015.
- 55. Libeskind-Hadas R, Bush E. Computing for Biologists: Python Programming and Principles. Cambridge University Press; 2014.
- 59. Software Carpentry;. Accessed 2016-01-18. Available from: http://software-carpentry.org/ .
- 60. Expressions—Python 3.5.1 documentation; 2016. Accessed 2016-01-18. Available from: https://docs.python.org/3/reference/expressions.html#operator-precedence .
- 61. Pierce BC. Types and Programming Languages. The MIT Press; 2002.
- 63. More Control Flow Tools—Python 3.5.1 documentation; 2016. Accessed 2016-01-18. Available from: https://docs.python.org/3.5/tutorial/controlflow.html#keyword-arguments .
- 64. McConnell S. Code Complete: A Practical Handbook of Software Construction (2 nd Edition). Pearson Education; 2004.
- 65. Gamma E, Helm R, Johnson R, Vlissides J. Design Patterns: Elements of Reusable Object-oriented Software. Pearson Education; 1994.
- 66. Zelle J. Python Programming: An Introduction to Computer Science. 2 nd ed. Franklin, Beedle & Associates Inc.; 2010.
- 69. National Research Council (US) Committee on Frontiers at the Interface of Computing and Biology. On the Nature of Biological Data. In: Lin HS, Wooley JC, editors. Catalyzing Inquiry at the Interface of Computing and Biology. Washington, DC: The National Academies Press; 2005. Available from: http://www.ncbi.nlm.nih.gov/books/NBK25464 .
- 73. Wikipedia. Tree (data structure); 2016. Accessed 2016-01-18. Available from: https://en.wikipedia.org/wiki/Tree_%28data_structure%29 .
- 74. Scipy. scipy.spatial.KDTree—SciPy v0.14.0 Reference Guide; 2014. Accessed 2016-01-18. Available from: http://docs.scipy.org/doc/scipy-0.14.0/reference/generated/scipy.spatial.KDTree.html .
- 75. Wikipedia. k-d tree; 2016. Accessed 2016-01-18. Available from: https://en.wikipedia.org/wiki/K-d_tree .
- 76. Wikipedia. Graph (abstract data type); 2015. Accessed 2016-01-18. Available from: https://en.wikipedia.org/wiki/Graph_%28abstract_data_type%29 .
- 77. Hagberg AA, Schult DA, Swart PJ. Exploring network structure, dynamics, and function using NetworkX. In: Proceedings of the 7th Python in Science Conference (SciPy2008). Pasadena, CA USA; 2008. p. 11–15.
- 80. Moitzi M. bintrees 2.0.2; 2016. Accessed 2016-01-18. Available from: https://pypi.python.org/pypi/bintrees/2.0.2 .
- 82. Wirth N. Algorithms + Data Structures = Programs. Prentice-Hall Series in Automatic Computation. Prentice Hall; 1976.
- 83. Budd T. An Introduction to Object-Oriented Programming. 3 rd ed. Pearson; 2001.
- 84. Phillips D. Python 3 Object Oriented Programming. Packt Publishing; 2010.
- 86. The Self Variable in Python Explained;. Available from: http://pythontips.com/2013/08/07/the-self-variable-in-python-explained .
- 87. Why Explicit Self Has to Stay;. Available from: http://neopythonic.blogspot.com/2008/10/why-explicit-self-has-to-stay.html .
- 90. Python Data Analysis Library;. Available from: http://pandas.pydata.org/ .
- 91. Friedl JEF. Mastering Regular Expressions. O’Reilly Media; 2006.
- 92. Regexes on Stack Overflow;. Available from: http://stackoverflow.com/tags/regex/info .
- 93. Regex Tutorials, Examples and Reference;. Available from: http://www.regular-expressions.info .
- 96. Langtangen HP. A Primer on Scientific Programming with Python. Texts in Computational Science and Engineering. Springer; 2014.
- 97. Jones E, Oliphant T, Peterson P, et al. SciPy: Open-source Scientific Tools for Python; 2001-. [Online; accessed 2015-06-30]. Available from: http://www.scipy.org/ .
- 98. Scientific Computing Tools for Python;. Available from: http://www.scipy.org/about.html .
- 100. scikit-learn: machine learning in Python;. Available from: http://scikit-learn.org/ .
- 102. PyPI: The Python Package Index;. Available from: http://pypi.python.org .
- 104. rpy2, R in Python;. Available from: http://rpy.sourceforge.net .
- 106. Cython: C-extensions for Python;. Available from: http://cython.org .
- 107. Open Source Initiative: Licenses & Standards;. Available from: http://opensource.org/licenses .
Academia.edu no longer supports Internet Explorer.
To browse Academia.edu and the wider internet faster and more securely, please take a few seconds to upgrade your browser .
- We're Hiring!
- Help Center
Bioinformatics
- Most Cited Papers
- Most Downloaded Papers
- Newest Papers
- Save to Library
- Last »
- Computational Biology Follow Following
- Genomics Follow Following
- Protein Structure Prediction Follow Following
- Systems Biology Follow Following
- Molecular Dynamics Simulation Follow Following
- Next generation sequencing Follow Following
- Molecular Biology Follow Following
- Biomolecular Modeling Follow Following
- Cancer Biology Follow Following
- Gene expression and regulation Follow Following
Enter the email address you signed up with and we'll email you a reset link.
- Academia.edu Publishing
- We're Hiring!
- Help Center
- Find new research papers in:
- Health Sciences
- Earth Sciences
- Cognitive Science
- Mathematics
- Computer Science
- Academia ©2024
- Search Menu
- Advance articles
- High-Impact Collection
- Author Guidelines
- Call for Papers
- Why Publish with Us?
- Submission Site
- Open Access Policy
- Self-Archiving Policy
- About Genomics, Proteomics & Bioinformatics
- About the Beijing Institute of Genomics, Chinese Academy of Sciences / China National Center for Bioinformation and Genetics Society of China
- Editorial Board
- Advertising & Corporate Services
- Journals on Oxford Academic
- Books on Oxford Academic

Article Contents
Smartdb: an integrated database for exploring single-cell multi-omics data of reproductive medicine.
Equal contribution.
- Article contents
- Figures & tables
- Supplementary Data
Zekai Liu, Zhen Yuan, Yunlei Guo, Ruilin Wang, Yusheng Guan, Zhanglian Wang, Yunan Chen, Tianlu Wang, Meining Jiang, Shuhui Bian, SMARTdb: An Integrated Database for Exploring Single-cell Multi-omics Data of Reproductive Medicine, Genomics, Proteomics & Bioinformatics , 2024;, qzae005, https://doi.org/10.1093/gpbjnl/qzae005
- Permissions Icon Permissions
Single-cell multi-omics sequencing has greatly accelerated reproductive research in recent years, and the data are continually growing. However, utilizing these data resources is challenging for wet-lab researchers. A comprehensive platform for exploring single-cell multi-omics data related to reproduction is urgently needed. Here we introduce the single-cell multi-omics atlas of reproduction (SMARTdb), which is an integrative and user-friendly platform for exploring molecular dynamics of reproductive development, aging, and disease, covering multi-omics, multi-species, and multi-stage data. We have curated and analyzed single-cell transcriptome and epigenome data of over 2.0 million cells from 6 species across whole lifespan. A series of powerful functionalities are provided, such as “Query gene expression”, “DIY expression plot”, “DNA methylation plot”, and “Epigenome browser”. With SMARTdb, we found that the male germ-cell-specific expression pattern of RPL39L and RPL10L is conserved between human and other model animals. Moreover, DNA hypomethylation and open chromatin may regulate the specific expression pattern of RPL39L collectively in both male and female germ cells. In summary, SMARTdb is a powerful platform for convenient data mining and gaining novel insights into reproductive development, aging, and disease. SMARTdb is publicly available at https://smart-db.cn .
Email alerts
Citing articles via.
- About Genomics, Proteomics & Bioinformatics
- Recommend to your Library
- Advertising & Corporate Services
- Journals Career Network
Affiliations
- Online ISSN 2210-3244
- Print ISSN 1672-0229
- Copyright © 2024 Beijing Institute of Genomics, Chinese Academy of Sciences / China National Center for Bioinformation and Genetics Society of China
- About Oxford Academic
- Publish journals with us
- University press partners
- What we publish
- New features
- Open access
- Institutional account management
- Rights and permissions
- Get help with access
- Accessibility
- Advertising
- Media enquiries
- Oxford University Press
- Oxford Languages
- University of Oxford
Oxford University Press is a department of the University of Oxford. It furthers the University's objective of excellence in research, scholarship, and education by publishing worldwide
- Copyright © 2024 Oxford University Press
- Cookie settings
- Cookie policy
- Privacy policy
- Legal notice
This Feature Is Available To Subscribers Only
Sign In or Create an Account
This PDF is available to Subscribers Only
For full access to this pdf, sign in to an existing account, or purchase an annual subscription.
Thank you for visiting nature.com. You are using a browser version with limited support for CSS. To obtain the best experience, we recommend you use a more up to date browser (or turn off compatibility mode in Internet Explorer). In the meantime, to ensure continued support, we are displaying the site without styles and JavaScript.
- View all journals
- Explore content
- About the journal
- Publish with us
- Sign up for alerts
- Review Article
- Published: 08 November 2021
Nanopore sequencing technology, bioinformatics and applications
- Yunhao Wang 1 na1 ,
- Yue Zhao 1 , 2 na1 ,
- Audrey Bollas 1 na1 ,
- Yuru Wang 1 &
- Kin Fai Au ORCID: orcid.org/0000-0002-9222-4241 1 , 2
Nature Biotechnology volume 39 , pages 1348–1365 ( 2021 ) Cite this article
206k Accesses
440 Citations
181 Altmetric
Metrics details
- Bioinformatics
- Genome informatics
Rapid advances in nanopore technologies for sequencing single long DNA and RNA molecules have led to substantial improvements in accuracy, read length and throughput. These breakthroughs have required extensive development of experimental and bioinformatics methods to fully exploit nanopore long reads for investigations of genomes, transcriptomes, epigenomes and epitranscriptomes. Nanopore sequencing is being applied in genome assembly, full-length transcript detection and base modification detection and in more specialized areas, such as rapid clinical diagnoses and outbreak surveillance. Many opportunities remain for improving data quality and analytical approaches through the development of new nanopores, base-calling methods and experimental protocols tailored to particular applications.
Similar content being viewed by others
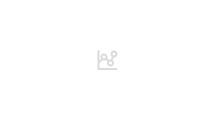
Bioinformatics of nanopore sequencing
Wojciech Makałowski & Victoria Shabardina
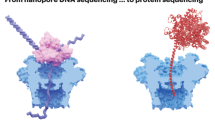
Nanopore DNA sequencing technologies and their applications towards single-molecule proteomics
Adam Dorey & Stefan Howorka
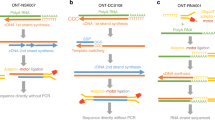
A comprehensive examination of Nanopore native RNA sequencing for characterization of complex transcriptomes
Charlotte Soneson, Yao Yao, … Shobbir Hussain
Nanopore sequencing technology and its applications in basic and applied research have undergone substantial growth since Oxford Nanopore Technologies (ONT) provided the first nanopore sequencer, MinION, in 2014 (refs. 1 , 2 ). The technology relies on a nanoscale protein pore, or ‘nanopore’, that serves as a biosensor and is embedded in an electrically resistant polymer membrane 1 , 3 (Fig. 1 ). In an electrolytic solution, a constant voltage is applied to produce an ionic current through the nanopore such that negatively charged single-stranded DNA or RNA molecules are driven through the nanopore from the negatively charged ‘ cis ’ side to the positively charged ‘ trans ’ side. Translocation speed is controlled by a motor protein that ratchets the nucleic acid molecule through the nanopore in a step-wise manner. Changes in the ionic current during translocation correspond to the nucleotide sequence present in the sensing region and are decoded using computational algorithms, allowing real-time sequencing of single molecules. In addition to controlling translocation speed, the motor protein has helicase activity, enabling double-stranded DNA or RNA–DNA duplexes to be unwound into single-stranded molecules that pass through the nanopore.
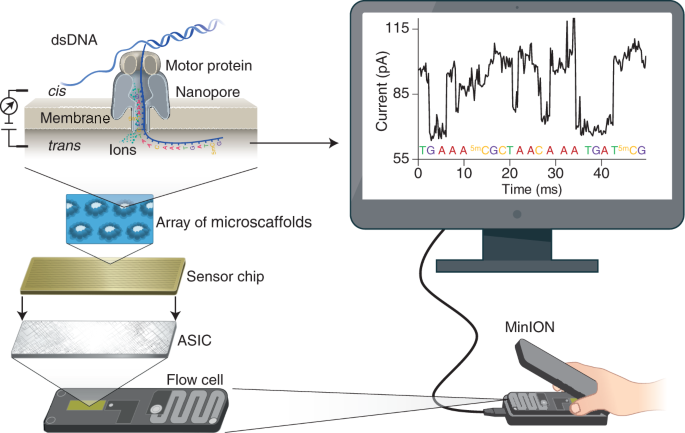
A MinION flow cell contains 512 channels with 4 nanopores in each channel, for a total of 2,048 nanopores used to sequence DNA or RNA. The wells are inserted into an electrically resistant polymer membrane supported by an array of microscaffolds connected to a sensor chip. Each channel associates with a separate electrode in the sensor chip and is controlled and measured individually by the application-specific integration circuit (ASIC). Ionic current passes through the nanopore because a constant voltage is applied across the membrane, where the trans side is positively charged. Under the control of a motor protein, a double-stranded DNA (dsDNA) molecule (or an RNA–DNA hybrid duplex) is first unwound, then single-stranded DNA or RNA with negative charge is ratcheted through the nanopore, driven by the voltage. As nucleotides pass through the nanopore, a characteristic current change is measured and is used to determine the corresponding nucleotide type at ~450 bases per s (R9.4 nanopore).
In this review, we first present an introduction to the technology development of nanopore sequencing and discuss improvements in the accuracy, read length and throughput of ONT data. Next, we describe the main bioinformatics methods applied to ONT data. We then review the major applications of nanopore sequencing in basic research, clinical studies and field research. We conclude by considering the limitations of the existing technologies and algorithms and directions for overcoming these limitations.
Technology development
Nanopore design.
The concept of nanopore sequencing emerged in the 1980s and was realized through a series of technical advances in both the nanopore and the associated motor protein 1 , 4 , 5 , 6 , 7 , 8 . α-Hemolysin, a membrane channel protein from Staphylococcus aureus with an internal diameter of ~1.4 nm to ~2.4 nm (refs. 1 , 9 ), was the first nanopore shown to detect recognizable ionic current blockades by both RNA and DNA homopolymers 10 , 11 , 12 . In a crucial step toward single-nucleotide-resolution nanopore sequencing, engineering of the wild-type α-hemolysin protein allowed the four DNA bases on oligonucleotide molecules to be distinguished, although complex sequences were not examined in these reports 13 , 14 , 15 . Similar results were achieved using another engineered nanopore, Mycobacterium smegmatis porin A (MspA) 16 , 17 , that has a similar channel diameter (~1.2 nm) 18 , 19 .
A key advance in improving the signal-to-noise ratio was the incorporation of processive enzymes to slow DNA translocation through the nanopore 20 , 21 , 22 . In particular, phi29 DNA polymerase was found to have superior performance in ratcheting DNA through the nanopore 23 , 24 . Indeed, this motor protein provided the last piece of the puzzle; in February 2012, two groups demonstrated processive recordings of ionic currents for single-stranded DNA molecules that could be resolved into signals from individual nucleotides by combining phi29 DNA polymerase and a nanopore (α-hemolysin 24 and MspA 25 ). In contrast to the previous DNA translocation tests that were poorly controlled 13 , 14 , 15 , 16 , 17 , the addition of the motor protein reduced the fluctuations in translocation kinetics, thus improving data quality. In the same month, ONT announced the first nanopore sequencing device, MinION 26 . ONT released the MinION to early users in 2014 and commercialized it in 2015 (ref. 2 ) (Fig. 2a ). There have been several other nanopore-based sequencing ventures, such as Genia Technologies’s nanotag-based real-time sequencing by synthesis (Nano-SBS) technology, NobleGen Biosciences’s optipore system and Quantum Biosystems’s sequencing by electronic tunneling (SBET) technology 27 , 28 . However, this review focuses on ONT technology as it has been used in most peer-reviewed studies of nanopore sequencing, data, analyses and applications.
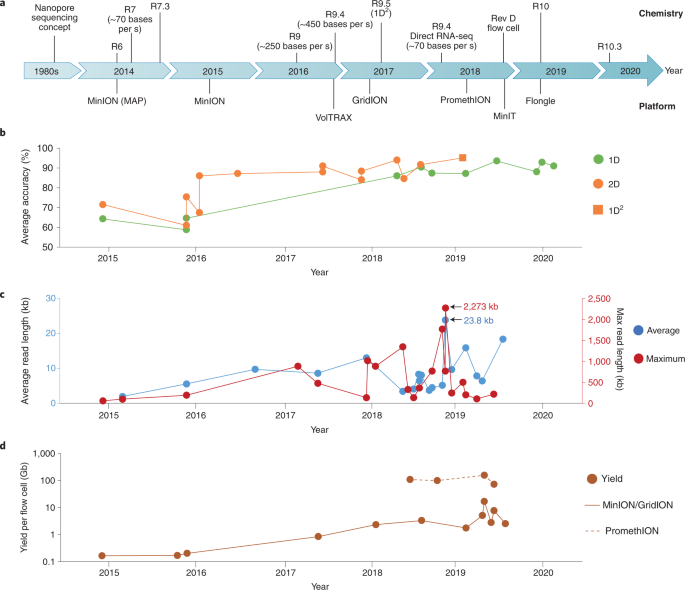
a , Timeline of the major chemistry and platform releases by ONT. b , Accuracy of 1D, 2D and 1D 2 reads. c , Average and maximum read lengths. Special efforts have been made in some studies to achieve ultralong read length. For example, by late 2019, the highest average sequencing length achieved has been 23.8 kilobases (kb) using a specific DNA extraction protocol 51 . The longest individual read is 2,273 kb, rescued by correcting an error in the software MinKNOW 49 . The DNA extraction and purification methods used in these independent studies are summarized in Supplementary Table 1 . Read lengths are reported for 1D reads. d , Yield per flow cell (in log 10 scale for y axis). Yields are reported for 1D reads. Data points shown in b (accuracy), c (read length) and d (yield) are from independent studies. Details for these data points are summarized in Supplementary Table 1 .
ONT has continually refined the nanopore and the motor protein, releasing eight versions of the system to date, including R6 (June 2014), R7 (July 2014), R7.3 (October 2014), R9 (May 2016), R9.4 (October 2016), R9.5 (May 2017), R10 (March 2019) and R10.3 (January 2020) (Fig. 2a ). The original or engineered proteins used in the R6, R7, R7.3, R10 and R10.3 nanopores have not been disclosed by the company to date. R9 achieved a notable increase in sequencing yield per unit of time and in sequencing accuracy (~87% (ref. 29 ) versus ~64% for R7 (ref. 30 )) by using the nanopore Curlin sigma S-dependent growth subunit G (CsgG) from Escherichia coli (Fig. 2b and Supplementary Table 1 ). This nanopore has a translocation rate of ~250 bases per s compared to ~70 bases per s for R7 (ref. 31 ). Subsequently, a mutant CsgG and a new motor enzyme (whose origin was not disclosed) were integrated into R9.4 to achieve higher sequencing accuracy (~85–94% as reported in refs. 32 , 33 , 34 , 35 , 36 ) and faster sequencing speeds (up to 450 bases per s). R9.5 was introduced to be compatible with the 1D 2 sequencing strategy, which measures a single DNA molecule twice (see below). However, the R9.4 and R9.5 have difficulty sequencing very long homopolymer runs because the current signal of CsgG is determined by approximately five consecutive nucleotides. The R10 and R10.3 nanopores have two sensing regions (also called reader heads) to aim for higher accuracy with homopolymers 37 , 38 , although independent studies are needed to assess this claim.
Additional strategies to improve accuracy
Beyond optimizing the nanopore and motor protein, several strategies have been developed to improve accuracy. Data quality can be improved by sequencing each dsDNA multiple times to generate a consensus sequence, similar to the ‘circular consensus sequencing’ strategy used in the other single-molecule long-read sequencing method from Pacific Biosciences (PacBio) 39 . Early versions of ONT sequencing used a 2D library preparation method to sequence each dsDNA molecule twice; the two strands of a dsDNA molecule are ligated together by a hairpin adapter, and a motor protein guides one strand (the ‘template’) through the nanopore, followed by the hairpin adapter and the second strand (the ‘complement’) 40 , 41 , 42 (Fig. 3d , left). After removing the hairpin sequence, the template and complement reads, called the 1D reads, are used to generate a consensus sequence, called the 2D read, of higher accuracy. Using the R9.4 nanopore as an example, the average accuracy of 2D reads is 94% versus 86% for 1D reads 33 (Fig. 2b ). In May 2017, ONT released the 1D 2 method together with the R9.5 nanopore; in this method, instead of being physically connected by a hairpin adapter, each strand is ligated separately to a special adapter (Fig. 3d , right). This special adapter provides a high probability (>60%) that the complement strand will immediately be captured by the same nanopore after the template strand, offering similar consensus sequence generation for dsDNA as the 2D library. The average accuracy of 1D 2 reads is up to 95% (R9.5 nanopore) 43 (Fig. 2b ). Unlike the 2D library, the complement strand in the 1D 2 library is not guaranteed to follow the template, resulting in imperfect consensus sequence generation. However, ONT no longer offers or supports the 2D and 1D 2 libraries. Currently, for DNA sequencing, ONT only supports the 1D method in which each strand of a dsDNA is ligated with an adapter and sequenced independently (Fig. 3d , middle).
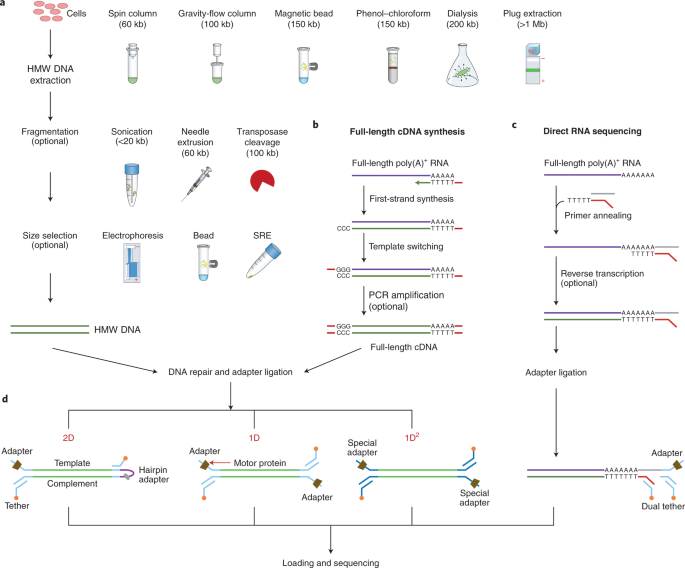
a , Special experimental techniques for ultralong genomic DNA sequencing, including HMW DNA extraction, fragmentation and size selection. b , Full-length cDNA synthesis for direct cDNA sequencing (without a PCR amplification step) and PCR-cDNA sequencing (with a PCR amplification step). c , Direct RNA-sequencing library preparation with or without a reverse transcription step, where only the RNA strand is ligated with an adapter and thus only the RNA strand is sequenced. d , Different library preparation strategies for DNA/cDNA sequencing, including 2D (where the template strand is sequenced, followed by a hairpin adapter and the complement strand), 1D (where each strand is ligated with an adapter and sequenced independently) and 1D 2 (where each strand is ligated with a special adapter such that there is a high probability that one strand will immediately be captured by the same nanopore following sequencing of the other strand of dsDNA); SRE, short read eliminator kit (Circulomics).
In parallel, accuracy has been improved through new base-calling algorithms, including many developed through independent research 32 , 44 (see below). Taking the R7.3 nanopore as an example, the 1D read accuracy was improved from 65% by hidden Markov model (HMM) 45 to 70% by Nanocall 46 and to 78% by DeepNano 47 .
Extending read length
Although the accuracy of ONT sequencing is relatively low, the read length provided by electrical detection has a very high upper bound because the method relies on the physical process of nucleic acid translocation 48 . Reads of up to 2.273 megabases (Mb) were demonstrated in 2018 (ref. 49 ). Thus, ONT read lengths depend crucially on the sizes of molecules in the sequencing library. Various approaches for extracting and purifying high-molecular-weight (HMW) DNA have been reported or applied to ONT sequencing, including spin columns (for example, Monarch Genomic DNA Purification kit, New England Biolabs), gravity-flow columns (for example, NucleoBond HMW DNA kit, Takara Bio), magnetic beads (for example, MagAttract HMW DNA kit, QIAGEN), phenol–chloroform, dialysis and plug extraction 50 (Fig. 3a ). HMW DNA can also be sheared to the desired size by sonication, needle extrusion or transposase cleavage (Fig. 3a ). However, overrepresented small fragments outside the desired size distribution may decrease sequencing yield because of higher efficiencies of both adapter ligation and translocation through nanopores than long fragments. To remove overrepresented small DNA fragments, various size selection methods (for example, the gel-based BluePippin system of Sage Science, magnetic beads and the Short Read Eliminator kit of Circulomics) have been used to obtain the desired data distribution and/or improve sequencing yield (Fig. 3a ).
With improvements in nanopore technology and library preparation protocols (Figs. 2a and 3a ), the maximum read length has increased from <800 kb in early 2017 to 2.273 Mb in 2018 (ref. 49 ) (Fig. 2c ). The average read length has increased from a few thousand bases at the initial release of MinION in 2014 to ~23 kb (ref. 51 ) in 2018 (Fig. 2c ), primarily due to improvements in HMW DNA extraction methods and size selection strategies. However, there is a trade-off between read length and yield; for example, the sequencing yield of the HMW genomic DNA library is relatively low.
Sequencing RNA
ONT devices have been adapted to directly sequence native RNA molecules 52 . The method requires special library preparation in which the primer is ligated to the 3′ end of native RNA, followed by direct ligation of the adapter without conventional reverse transcription (Fig. 3c ). Alternatively, a cDNA strand can be synthesized to obtain an RNA–cDNA hybrid duplex, followed by ligation of the adapter. The former strategy requires less sample manipulation and is quicker and thus is good for on-site applications, whereas the latter produces a more stable library for longer sequencing courses and therefore produces higher yields. In both cases, only the RNA strand passes through the nanopore, and therefore direct sequencing of RNA molecules does not generate a consensus sequence (for example, 2D or 1D 2 ). Compared to DNA sequencing, direct RNA sequencing is typically of lower average accuracy, around 83–86%, as reported by independent research 53 , 54 .
Like conventional RNA sequencing, ONT can be used to perform cDNA sequencing by utilizing existing full-length cDNA synthesis methods (for example, the SMARTer PCR cDNA Synthesis kit of Takara Bio and the TeloPrime Full-Length cDNA Amplification kit of Lexogen) followed by PCR amplification 42 , 55 (Fig. 3b ). ONT also offers a direct cDNA sequencing protocol without PCR amplification, in contrast to many existing cDNA sequencing methods. This approach avoids PCR amplification bias, but it requires a relatively large amount of input material and longer library preparation time, making it unsuitable for many clinical applications. A recent benchmarking study demonstrated that ONT sequencing of RNA, cDNA or PCR-cDNA for the identification and quantification of gene isoforms provides similar results 56 .
Increasing throughput
In addition to sequencing length and accuracy, throughput is another important consideration for ONT sequencing applications. To meet the needs of different project scales, ONT released several platforms (Box 1 ). The expected data output of a flow cell mainly depends on (1) the number of active nanopores, (2) DNA/RNA translocation speed through the nanopore and (3) running time.
Early MinION users reported typical yields of hundreds of megabases per flow cell, while current throughput has increased to ~10–15 gigabases (Gb) (Fig. 2d , solid line) for DNA sequencing through faster chemistry (increasing from ~30 bases per s by R6 nanopore to ~450 bases per s by R9.4 nanopore) and longer run times with the introduction of the Rev D ASIC chip. Subsequent devices, such as PromethION, run more flow cells with more nanopores per flow cell. An independent study reported a yield of 153 Gb from a single PromethION flow cell with an average sequencing speed of ~430 bases per s (ref. 57 ) (Fig. 2d , dashed line). By contrast, direct RNA sequencing currently produces about 1,000,000 reads (1–3 Gb) per MinION flow cell due in part to its relatively low sequencing speed (~70 bases per s).
Box 1 ONT devices
MinION is a flow cell containing 512 channels, with four nanopores per channel. Only one nanopore in each channel is measured at a time, allowing concurrent sequencing of up to 512 molecules.
GridION, for medium-scale projects, has five parallel MinION flow cells.
PromethION, a high-throughput device for large-scale projects, has 24 or 48 parallel flow cells (up to 3,000 channels per flow cell).
Flongle, for smaller projects, is a flow cell adapter for MinION or GridION with 126 channels.
VolTRAX is a programmable device for sample and library preparation.
MinIT is a data analysis device that eliminates the need for a computer to run MinION.
SmidgION is a smartphone-compatible device under development.
Data analysis
Bioinformatics analysis of ONT data has undergone continued improvement (Fig. 4 ). In addition to in-house data collection and specific data formats, many ONT-specific analyses focus on better utilizing the ionic current signal for purposes such as base calling, base modification detection and postassembly polishing. Other tools use long read length while accounting for high error rate. Many of these, such as tools for error correction, assembly and alignment, were developed for PacBio data but are also applicable to ONT data (Table 1 ).
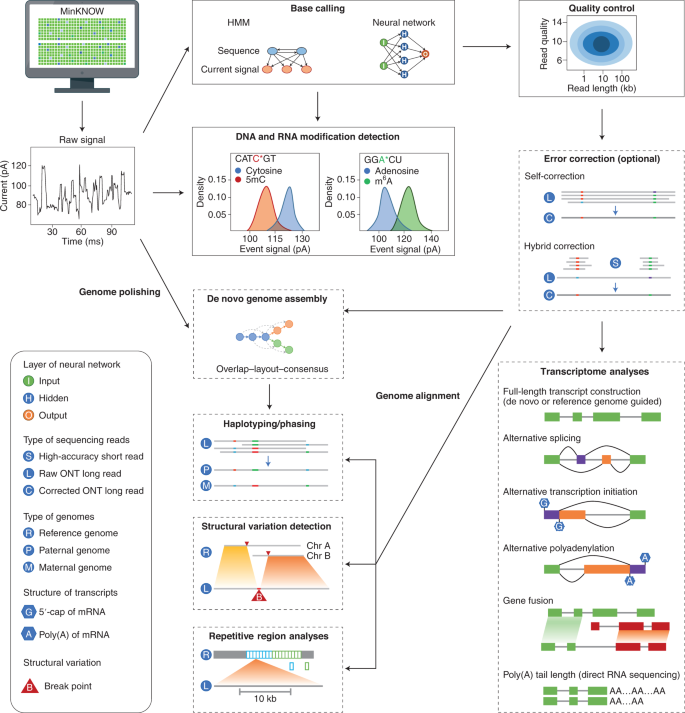
Typical bioinformatics analyses of ONT sequencing data, including the raw current data-specific approaches (for example, quality control, base calling and DNA/RNA modification detection), and error-prone long read-specific approaches (in dashed boxes; for example, error correction, de novo genome assembly, haplotyping/phasing, structural variation (SV) detection, repetitive region analyses and transcriptome analyses).
Because ONT devices do not require high-end computing resources or advanced skills for basic data processing, many laboratories can run data collection themselves. MinKNOW is the operating software used to control ONT devices by setting sequencing parameters and tracking samples (Fig. 4 , top left). MinKNOW also manages data acquisition and real-time analysis and performs local base calling and outputs the binary files in fast5 format to store both metadata and read information (for example, current measurement and read sequence if base calling is performed). The fast5 format organizes the multidimensional data in a nested manner, allowing the piece-wise access/extraction of information of interest without navigating through the whole dataset. Previous versions of MinKNOW output one fast5 file for each single read (named single-fast5), but later versions output one fast5 file for multiple reads (named multi-fast5) to meet the increasing throughput. Both fast5 and fastq files are output if the base-calling mode is applied during the sequencing experiment. In addition to official ONT tools (for example, ont_fast5_api software for format conversion between single-fast5 and multi-fast5 and data compression/decompression), several third-party software packages 40 , 58 , 59 , 60 , 61 , 62 have been developed for quality control, format conversion (for example, NanoR 63 for generating fastq files from fast5 files containing sequence information), data exploration and visualization of the raw ONT data (for example, Poretools 64 , NanoPack 65 and PyPore 66 ) and for after base-calling data analyses (for example, AlignQC 42 and BulkVis 49 ) (Fig. 4 , top right).
Base calling
Base calling, which decodes the current signal to the nucleotide sequence, is critical for data accuracy and detection of base modifications (Fig. 4 , top center). Overall, method development for base calling went through four stages 32 , 44 , 58 , 67 , 68 : (1) base calling from the segmented current data by HMM at the early stage and by recurrent neural network in late 2016, (2) base calling from raw current data in 2017, (3) using a flip–flop model for identifying individual nucleotides in 2018 and (4) training customized base-calling models in 2019. ONT developed new base callers as ‘technology demonstrator’ software (for example, Nanonet, Scrappie and Flappie), which were subsequently implemented into the officially available software packages (for example, Albacore and Guppy). Albacore development is now discontinued in favor of Guppy, which can also run on graphics processing units in addition to central processing units to accelerate base calling.
ONT devices take thousands of current measurements per second. Processive translocation of a DNA or RNA molecule leads to a characteristic current shift that is determined by multiple consecutive nucleotides (that is, k -mer) defined by the length of the nanopore sensing region 1 . The raw current measurement can be segmented based on current shift to capture individual signals from each k -mer. Each current segment contains multiple measurements, and the corresponding mean, variance and duration of the current measurements together make up the ‘event’ data. The dependence of event data on neighboring nucleotides is Markov chain-like, making HMM-based methods a natural match to decode current shifts to nucleotide sequence, such as early base callers (for example, cloud-based Metrichor by ONT and Nanocall 46 ). The subsequent Nanonet by ONT (implemented into Albacore) and DeepNano 47 implemented a recurrent neural network algorithm to improve base-calling accuracy by training a deep neural network to infer k -mers from the event data. In particular, Nanonet used a bidirectional method to include information from both upstream and downstream states on base calling.
However, information may be lost when converting raw current measurement into event data, potentially diminishing base-calling accuracy. Raw current data were first used for classifying ONT reads into specific species 69 . Later, ONT’s open-source base caller Scrappie (implemented into both Albacore and Guppy) and the third-party software Chiron 70 adopted neural networks to directly translate the raw current data into DNA sequence. Subsequently, ONT released the base caller Flappie, which uses a flip–flop model with a connectionist temporal classification decoding architecture and identifies individual bases instead of k -mers from raw current data. Furthermore, the software Causalcall uses a modified temporal convolutional network combined with a connectionist temporal classification decoder to model long-range sequence features 35 . In contrast to generalized base-calling models, ONT introduced Taiyaki (implemented into Guppy) to train customized (for example, application/species - specific) base-calling models by using language processing techniques to handle the high complexity and long-range dependencies of raw current data. Additionally, Taiyaki can train models for identifying modified bases (for example, 5-methylcytosine (5mC) or N 6 -methyladenine (6mA)) by adding a fifth output dimension. The R10 and R10.3 nanopores with two sensing regions may result in different signal features compared to previous raw current data, which will likely drive another wave of method development to improve data accuracy and base modification detection. To date, Guppy is the most widely used base caller because of its superiority in accuracy and speed 32 (Table 1 ).
Detecting DNA and RNA modifications
ONT enables the direct detection of some DNA and RNA modifications by distinguishing their current shifts from those of unmodified bases 52 , 71 , 72 , 73 , 74 (Fig. 4 , middle center), although the resolution varies from the bulk level to the single-molecule level. A handful of DNA and RNA modification detection tools have been developed over the years (Table 1 ). Nanoraw (integrated into the Tombo software package) was the first tool to identify the DNA modifications 5mC, 6mA and N 4 -methylcytosine (4mC) from ONT data 74 . Several other DNA modification detection tools followed, including Nanopolish (5mC) 75 , signalAlign (5mC, 5-hydroxymethylcytosine (5hmC) and 6mA) 71 , mCaller (5mC and 6mA) 76 , DeepMod (5mC and 6mA) 76 , DeepSignal (5mC and 6mA) 77 and NanoMod (5mC and 6mA) 78 . Nanpolish, Megalodon and DeepSignal were recently benchmarked and confirmed to have high accuracy for 5mC detection with single-nucleotide resolution at the single-molecule level 79 , 80 . Compared to PacBio, ONT performs better in detecting 5mC but has lower accuracy in detecting 6mA 68 , 75 , 81 .
The possibility of directly detecting N 6 -methyladenosine (m 6 A) modifications in RNA molecules was demonstrated using PacBio in 2012 (ref. 82 ), although few follow-up applications were published. Recently, ONT direct RNA sequencing has yielded robust data of reasonable quality, and several pilot studies have detected bulk-level RNA modifications by examining either error distribution profiles (for example, EpiNano (m 6 A) 73 and ELIGOS (m 6 A and 5-methoxyuridine (5moU)) 83 ) or current signals (for example, Tombo extension (m 6 A and m 5 C) 74 and MINES (m 6 A) 84 ). However, detection of RNA modifications with single-nucleotide resolution at the single-molecule level has yet to be demonstrated.
Error correction
Although the average accuracy of ONT sequencing is improving, certain subsets of reads or read fragments have very low accuracy, and the error rates of both 1D reads and 2D/1D 2 reads are still much higher than those of short reads generated by next-generation sequencing technologies. Thus, error correction is widely applied before many downstream analyses (for example, genome assembly and gene isoform identification), which can rescue reads for higher sensitivity (for example, mappability 85 ) and improve the quality of the results (for example, break point determination at single-nucleotide resolution 86 ). Two types of error correction algorithms are used 85 , 87 (Fig. 4 , middle right, and Table 1 ): ‘self-correction’ uses graph-based approaches to produce consensus sequences among different molecules from the same origins (for example, Canu 88 and LoRMA 89 ) in contrast to 2D and 1D 2 reads generated from the same molecules, and ‘hybrid correction’ uses high-accuracy short reads to correct long reads by alignment-based (for example, LSC 90 and Nanocorr 45 ), graph-based (for example, LorDEC 91 ) and dual alignment/graph-based algorithms (for example, HALC 92 ). Recently, two benchmark studies demonstrated that the existing hybrid error correction tools (for example, FMLRC 93 , LSC and LorDEC) together with sufficient short-read coverage can reduce the long-read error rate to a level (~1–4%) similar to that of short reads 85 , 87 , whereas self-correction reduces the error rate to ~3–6% (ref. 87 ), which may be due to non-random systematic errors in ONT data.
Aligners for error-prone long reads
Alignment tools have been developed to tackle the specific characteristics of error-prone long reads (Table 1 ). Very early aligners (for example, BLAST 94 ) were developed for small numbers of long reads (for example, Sanger sequencing data). More recently, there has been considerable growth in alignment methods for high-throughput accurate short reads (for example, Illumina sequencing data) in response to the growth in next-generation sequencing. Development of several error-prone long-read aligners was initially motivated by PacBio data, and they were also tested on ONT data. In 2016, the first aligner specifically for ONT reads, GraphMap, was developed 95 . GraphMap progressively refines candidate alignments to handle high error rates and uses fast graph transversal to align long reads with high speed and precision. Using a seed–chain–align procedure, minimap2 was developed to match increases in ONT read length beyond 100 kb (ref. 96 ). A recent benchmark paper revealed that minimap2 ran much faster than other long-read aligners (that is, LAST 97 , NGMLR 98 and GraphMap) without sacrificing the accuracy 99 . In addition, minimap2 can perform splice-aware alignment for ONT cDNA or direct RNA-sequencing reads.
In addition to minimap2, GMAP, published in 2005 (ref. 100 ), and a new mode of STAR, which was originally developed for short reads 101 , have been widely used in splice-aware alignment of error-prone transcriptome long reads to genomes. Other aligners have also been developed, such as Graphmap2 (ref. 102 ) and deSALT 103 , for ONT transcriptome data. Especially for ONT direct RNA-sequencing reads with dense base modifications, Graphmap2 has a higher alignment rate than minimap2 (ref. 104 ).
Hybrid sequencing
Many applications combine long reads and short reads in the bioinformatics analyses, termed hybrid sequencing. In contrast to hybrid correction of long reads for general purposes, many hybrid sequencing-based methods integrate long reads and short reads into the algorithms and pipeline designs to harness the strengths of both types of reads to address specific biological problems. The long-read length is well suited to identifying large-range genomic complexity with unambiguous alignments, whereas the high accuracy and high throughput of short reads is useful for characterizing local details (for example, splice site detection with single-nucleotide resolution) and improving quantitative analyses. For example, genome 105 , transcriptome 42 and metagenome 106 assemblies have shown superior performance with hybrid sequencing data compared to either error-prone long reads alone or high-accuracy short reads alone.
De novo genome assembly
Error-prone long reads have been used for de novo genome assembly. Assemblers (Table 1 ) such as Canu 88 and Miniasm 107 are based on the overlap–layout–consensus algorithm, which builds a graph by overlapping similar sequences and is robust to sequencing error 58 , 67 , 108 (Fig. 4 , middle center). To further remove errors, error correction of long reads and polishing of assembled draft genomes (that is, improving accuracy of consensus sequences using raw current data) are often performed before and after assembly, respectively. In addition to the genome-polishing software Nanopolish 109 , ONT released Medaka, a neural network-based method, aiming for improved accuracy and speed compared to Nanopolish (Table 1 ).
These approaches take into account not only general assembly performance but also certain specific aspects, such as complex genomic regions and computational intensity. For example, Flye improves genome assembly at long and highly repetitive regions by constructing an assembly graph from concatenated disjoint genomic segments 110 ; Miniasm uses all-versus-all read self-mapping for ultrafast assembly 107 , although postassembly polishing is necessary for higher accuracy. The recently developed assembler wtdbg2 runs much faster than other tools without sacrificing contiguity and accuracy 111 .
SVs and repetitive regions
When a reference genome is available, ONT data can be used to study sample-specific genomic details, including SVs and haplotypes, with much higher precision than other techniques. A few SV detection tools have been developed (for example, NanoSV 112 , Sniffles 98 , Picky 33 and NanoVar 113 ) (Fig. 4 , bottom center, and Table 1 ). Picky, in addition to detecting regular SVs, also reveals enriched short-span SVs (~300 bp) in repetitive regions, as long reads cover the entire region including the variations. Given that single long reads can encompass multiple variants, including both SNVs and SVs, it is possible to perform phasing of multiploid genomes as well as other haplotype-resolved analyses 112 , 114 , 115 with appropriate bioinformatics software, such as LongShot 116 for SNV detection and WhatsHap 117 for haplotyping/phasing.
Several tools have also been developed to investigate highly repetitive genomic regions by ONT sequencing, such as TLDR for identifying non-reference transposable elements 118 and TRiCoLOR for characterizing tandem repeats 119 (Table 1 ).
Transcriptome complexity
When used in transcriptome analyses, ONT reads can be clustered and assembled to reconstruct full-length gene isoforms or aligned to a reference genome to characterize complex transcriptional events 42 , 120 , 121 , 122 , 123 (Fig. 4 , bottom right). In particular, several transcript assemblers have been developed specifically for error-prone long reads, such as Traphlor 124 , FLAIR 123 , StringTie2 (ref. 125 ) and TALON 126 as well as several based on hybrid sequencing data (for example, IDP 127 ). In particular, IDP-denovo 128 and RATTLE 129 can perform de novo transcript assembly by long reads without a reference genome. More recently, ONT direct RNA sequencing has made transcriptome-wide investigation of native RNA molecules feasible 52 , 130 , 131 . However, development of corresponding bioinformatics tools, especially for quantitative analyses, remains inadequate.
Applications of nanopore sequencing
The long read length, portability and direct RNA sequencing capability of ONT devices have supported a diverse range of applications (Fig. 5 ). We review 11 applications that are the subject of the most publications since 2015.
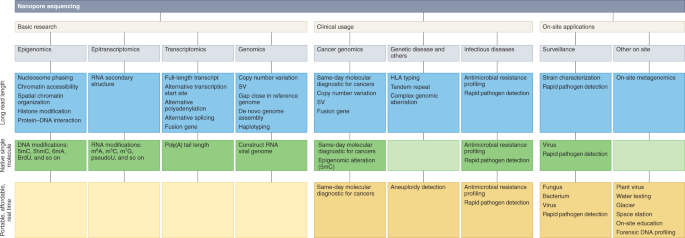
ONT sequencing applications are classified into three major groups (basic research, clinical usage and on-site applications) and are shown as a pie chart. The classifications are further categorized by specific topics, and the slice area is proportional to the number of publications (in log 2 scale). Some applications span two categories, such as SV detection and rapid pathogen detection. The applications are also organized by the corresponding strengths of ONT sequencing as three layers of the pie chart: (1) long read length, (2) native single molecule and (3) portable, affordable and real time. The width of each layer is proportional to the number of publications (in log 2 scale). Some applications that use all three strengths span all three layers (for example, antimicrobial resistance profiling). ‘Fungus’ includes Candida auris , ‘bacterium’ includes Salmonella , Neisseria meningitidis and Klebsiella pneumoniae and ‘virus’ includes severe acute respiratory syndrome coronavirus 2 (SARS-CoV-2), Ebola, Zika, Venezuelan equine encephalitis, yellow fever, Lassa fever and dengue; HLA, human leukocyte antigens.

Closing gaps in reference genomes
Genome assembly is one of the main uses of ONT sequencing (~30% of published ONT applications; Fig. 5 ). For species with available reference genomes, ONT long reads are useful for closing genome gaps, especially in the human genome. For example, ONT reads have been used to close 12 gaps (>50 kb for each gap) in the human reference genome and to measure the length of telomeric repeats 132 and also to assemble the centromeric region of the human Y chromosome 133 . Moreover, ONT enabled the first gapless telomere-to-telomere assembly of the human X chromosome, including reconstruction of a ~2.8 Mb centromeric satellite DNA array and closing of all remaining 29 gaps (totaling 1.1 Mb) 134 . The Telomere-to-Telomere Consortium reported the first complete human genome (T2T-CHM13) of the size 3.055 Gb (ref. 135 ).
The Caenorhabditis elegans reference genome has also been expanded by >2 Mb through accurate identification of repetitive regions using ONT long reads 136 . Similar progress has been achieved in other model organisms and closely related species (for example, Escherichia coli 109 , Saccharomyces cerevisiae 137 , Arabidopsis thaliana 138 and 15 Drosophila species 139 ) as well as in non-model organisms, including characterizing large tandem repeats in the bread wheat genome 140 and improving the continuity and completeness of the genome of Trypanosoma cruzi (the parasite causing Chagas disease) 141 .
Building new reference genomes
ONT long reads have been used extensively to assemble the initial reference genomes of many non-model organisms. For instance, ONT data alone were used to assemble the first genome of Rhizoctonia solani (a pathogenic fungal species that causes damping-off diseases in a wide range of crops) 142 , and hybrid sequencing data (ONT plus Illumina) were used to assemble the first draft genomes of Maccullochella peelii (Australia’s largest freshwater fish) 143 and Amphiprion ocellaris (the common clown fish) 144 . In more complicated cases, ONT long reads have been integrated with one or more other techniques (for example, Illumina short reads, PacBio long reads, 10x Genomics linked reads, optical mapping by Bionano Genomics and spatial distance by Hi-C) to assemble the initial reference genomes of many species, such as Maniola jurtina (the meadow brown butterfly, a model for ecological genetics) 145 , Varanus komodoensis (the largest extant monitor lizard) 146 , Pavo cristatus (the national bird of India) 147 , Panthera leo (the lion) 148 and Eumeta variegate (a bagworm moth that produces silk with potential in biomaterial design) 149 . In addition, ONT direct RNA sequencing has been used to construct RNA viral genomes while eliminating the need for the conventional reverse transcription step, including Mayaro virus 150 , Venezuelan equine encephalitis virus 150 , chikungunya virus 150 , Zika virus 150 , vesicular stomatitis Indiana virus 150 , Oropouche virus 150 , influenza A 53 and human coronavirus 86 . For small DNA/RNA viral genomes (for example, the 27-kb human coronavirus genome 86 ), the assembly process is not required given the long read length.
In the SARS-CoV-2 pandemic 151 , ONT sequencing was used to reconstruct full-length SARS-CoV-2 genome sequences via cDNA and direct RNA sequencing 152 , 153 , 154 , 155 , providing valuable information regarding the biology, evolution and pathogenicity of the virus.
The increasing yield, read length and accuracy of ONT data enable much more time- and cost-efficient genome assembly of all sizes of genomes, from bacteria of several megabases 109 , fruit fly 139 , 156 , fish 143 , 144 , 157 , blood clam 158 , banana 159 , cabbage 159 and walnut 160 , 161 , all of whose genomes are in the hundreds of megabases, as well as the Komodo dragon 146 , Steller sea lion 162 , lettuce ( https://nanoporetech.com/resource-centre/tip-iceberg-sequencing-lettuce-genome ) and giant sequoia 163 , with genomes of a few gigabases, to coast redwood ( https://www.savetheredwoods.org/project/redwood-genome-project/ ) and tulip ( https://nanoporetech.com/resource-centre/beauty-and-beast ), with genomes of 27–34 Gb. Only three PromethION flow cells were required to sequence the human genome, requiring <6 h for the computational assembly 164 .
Identifying large SVs
A powerful application of ONT long reads is to identify large SVs (especially from humans) in biomedical contexts, such as the breast cancer cell line HCC1187 (ref. 33 ), individuals with acute myeloid leukemia 113 , the construction of the first haplotype-resolved SV spectra for two individuals with congenital abnormalities 112 and the identification of 29,436 SVs from a Yoruban individual NA19240 (ref. 165 ).
Characterizing full-length transcriptomes and complex transcriptional events
A comprehensive examination of the feasibility of ONT cDNA sequencing (with R7 and R9 nanopores) in transcriptome analyses demonstrated its similar performance in gene isoform identification to PacBio long reads, both of which are superior to Illumina short reads 42 . With ONT data alone, there remain drawbacks in estimating gene/isoform abundance, detecting splice sites and mapping alternative polyadenylation sites, although recent improvements in accuracy and throughput have advanced these analyses. Nevertheless, ONT cDNA sequencing was also tested in individual B cells from mice 120 and humans 122 , 166 . Furthermore, ONT direct RNA sequencing has been used to measure the poly(A) tail length of native RNA molecules in humans 131 , C. elegans 167 , A. thaliana 168 and Locusta migratoria 169 , corroborating a negative correlation between poly(A) tail length and gene expression 167 , 168 . In addition, the full-length isoforms of human circular RNAs have been characterized by ONT sequencing following rolling circle amplification 170 , 171 .
Characterizing epigenetic marks
As early as 2013, independent reports demonstrated that methylated cytosines (5mC and 5hmC) in DNA could be distinguished from native cytosine by the characteristic current signals measured using the MspA nanopore 172 , 173 . Later, bioinformatics tools were developed to identify three kinds of DNA modifications (6mA, 5mC and 5hmC) from ONT data 71 , 75 . Recently, ONT was applied to characterize the methylomes from different biological samples, such as 6mA in a microbial reference community 174 as well as 5mC and 6mA in E. coli , Chlamydomonas reinhardtii and human genomes 76 .
Mapping DNA modifications using ONT sequencing in combination with exogenous methyltransferase treatment (inducing 5mC at GpC sites) led to the development of an experimental and bioinformatics approach, MeSMLR-seq, that maps nucleosome occupancy and chromatin accessibility at the single-molecule level and at long-range scale in S. cerevisiae 72 (Table 1 ). Later, another method, SMAC-seq adopted the same strategy with the additional exogenous modification 6mA to improve the resolution of mapping nucleosome occupancy and chromatin accessibility 175 . Similarly, multiple epigenetic features, including the endogenous 5mC methylome (at CpG sites), nucleosome occupancy and chromatin accessibility, can be simultaneously characterized on single long human DNA molecules by MeSMLR-seq (K.F.A., unpublished data, and ref. 176 ). Such epigenome analyses can be performed in a haplotype-resolved manner and thus will be informative for discovering allele-specific methylation linked to imprinted genes as well as for phasing genomic variants and chromatin states, even in heterogeneous cancer samples.
Similarly, several other methods have combined various biochemical techniques with ONT sequencing (Table 1 ). For example, the movement of DNA replication forks on single DNA molecules has been measured by detection of nucleotide analogs (for example, 5-bromodeoxyuridine (5-BrdU)) using ONT sequencing 177 , 178 , 179 , and the 3D chromatin organization in human cells has been analyzed by integrating a chromatin conformation capture technique and ONT sequencing to capture multiple loci in close spatial proximity by single reads 180 . Two other experimental assays, DiMeLo-seq 181 and BIND&MODIFY 182 , use ONT sequencing to map histone modifications (H3K9me3 and H3K27me3), a histone variant (CENP-A) and other specific protein–DNA interactions (for example, CTCF binding profile). They both construct a fusion protein of the adenosine methyltransferase and protein A to convert specific protein–DNA interactions to an artificial 6mA profile, which is subsequently detected by ONT sequencing.
Detecting RNA modifications
Compared to existing antibody-based approaches (which are usually followed by short-read sequencing), ONT direct RNA sequencing opens opportunities to directly identify RNA modifications (for example, m 6 A) and RNA editing (for example, inosine), which have critical biological functions. In 2018, distinct ionic current signals for unmodified and modified bases (for example, m 6 A and m 5 C) in ONT direct RNA-sequencing data were reported 52 . Since then, epitranscriptome analyses using ONT sequencing have progressed rapidly, including detection of 7-methylguanosine (m 7 G) and pseudouridine in 16S rRNAs of E. coli 183 , m 6 A in mRNAs of S. cerevisiae 73 and A. thaliana 168 and m 6 A 130 and pseudouridine 104 in human RNAs. Recent independent research (K.F.A., unpublished data, and refs. 184 , 185 ) has revealed that it is possible to probe RNA secondary structure using a combination of ONT direct RNA sequencing and artificial chemical modifications (Table 1 ). The dynamics of RNA metabolism were also analyzed by labeling nascent RNAs with base analogs (for example, 5-ethynyluridine 186 and 4-thiouridine 187 ) followed by ONT direct RNA sequencing (Table 1 ).
ONT sequencing has been applied to many cancer types, including leukemia 188 , 189 , 190 , 191 , 192 , breast 33 , 176 , 193 , brain 193 , colorectal 194 , pancreatic 195 and lung 196 cancers, to identify genomic variants of interests, especially large and complex ones. For example, ONT amplicon sequencing was used to identify TP53 mutations in 12 individuals with chronic lymphoblastic leukemia 188 . Likewise, MinION sequencing data revealed BCR- ABL1 kinase domain mutations in 19 individuals with chronic myeloid leukemia and 5 individuals with acute lymphoblastic leukemia with superior sensitivity and time efficiency compared to Sanger sequencing 189 . Additionally, ONT whole-genome sequencing was used to rapidly detect chromosomal translocations and precisely determine the breakpoints in an individual with acute myeloid leukemia 192 .
A combination of Cas9-assisted target enrichment and ONT sequencing has characterized a 200-kb region spanning the breast cancer susceptibility gene BRCA1 and its flanking regions despite a high repetitive sequence fraction (>50%) and large gene size (~80 kb) 197 . This study provided a template for the analysis of full variant profiles of disease-related genes.
The ability to directly detect DNA modifications using ONT data has enabled the simultaneous capture of genomic (that is, copy number variation) and epigenomic (that is, 5mC) alterations using only ONT data from brain tumor samples 193 . The whole workflow (from sample collection to bioinformatics results) was completed in a single day, delivering a multimodal and rapid molecular diagnostic for cancers. In addition, same-day detection of fusion genes in clinical specimens has also been demonstrated by MinION cDNA sequencing 198 .
Infectious disease
Because of its fast real-time sequencing capabilities and small size, MinION has been used for rapid pathogen detection, including diagnosis of bacterial meningitis 199 , bacterial lower respiratory tract infection 200 , infective endocarditis 201 , pneumonia 202 and infection in prosthetic joints 203 . In the example of bacterial meningitis, 16S amplicon sequencing took only 10 min using MinION to identify pathogenic bacteria in all six retrospective cases, making MinION particularly useful for the early administration of antibiotics through timely detection of bacterial infections 199 . Likewise, clinical diagnosis of bacterial lower respiratory tract infection using MinION was faster (6 h versus >2 d) and had higher sensitivity than existing culture-based ‘gold standard’ methods 200 .
In addition to pathogen detection, ONT sequencing can accelerate profiling antibiotic/antimicrobial resistance in bacteria and other microbes. For example, MinION was used to identify 51 acquired resistance genes directly from clinical urine samples (without culture) of 55 that were detected from cultivated bacteria using Illumina sequencing 204 , and a recent survey of resistance to colistin in 12,053 Salmonella strains used a combination of ONT, PacBio and Illumina data 205 . Indeed, ONT sequencing is useful for detecting specific species and strains (for example, virulent ones) from microbiome samples given the unambiguous mappability of longer reads, which provides accurate estimates of microbiome composition compared to the conventional studies relying on 16S rRNA and DNA amplicons 57 , 206 .
Genetic disease
ONT long reads have been applied to characterize complex genomic rearrangements in individuals with genetic disorders. For example, ONT sequencing of human genomes revealed that an expansion of tandem repeats in the ABCA7 gene was associated with an increased risk of Alzheimer’s disease 207 . ONT sequencing was also used to discover a new 3.8-Mb duplication in the intronic region of the F8 gene in an individual with hemophilia A 208 . Other examples cover a large range of diseases and conditions, including autism spectrum disorder 209 , Temple syndrome 210 , congenital abnormalities 112 , glycogen storage disease type Ia (ref. 211 ), intellectual disability and seizures 212 , epilepsy 213 , 214 , Parkinson’s disease 215 , Gaucher disease 215 , ataxia-pancytopenia syndrome and severe immune dysregulation 114 .
In another clinical application, human leukocyte antigen genotyping benefited from the improved accuracy of the R9.5 nanopore 216 , 217 , 218 . MinION enabled the detection of aneuploidy in prenatal and miscarriage samples in 4 h compared to 1–3 weeks with conventional techniques 219 .
Outbreak surveillance
The portable MinION device allows in-field and real-time genomic surveillance of emerging infectious diseases, aiding in phylogenetic and epidemiological investigations such as characterization of evolution rate, diagnostic targets, response to treatment and transmission rate. In April 2015, MinION devices were shipped to Guinea for real-time genomic surveillance of the ongoing Ebola outbreak. Only 15–60 min of sequencing per sample was required 220 . Likewise, a hospital outbreak of Salmonella was monitored with MinION, with positive cases identified within 2 h (ref. 221 ). MinION was also used to conduct genomic surveillance for Zika virus 222 , yellow fever virus 223 and dengue virus 224 outbreaks in Brazil.
With the increasing throughput of ONT sequencing, real-time surveillance has been applied to pathogens with larger genomes over the years, ranging from viruses of a few kilobases (for example, Ebola virus 220 , 18–19 kb; Zika virus 222 , 11 kb; Venezuelan equine encephalitis virus 225 , 11.4 kb; Lassa fever virus 226 , 10.4 kb and SARS-CoV-2 coronavirus 151 , 29.8 kb) to bacteria of several megabases (for example, Salmonella 221 , 5 Mb; N. meningitidis 227 , 2 Mb and K. pneumoniae 228 , 5.4 Mb) and to human fungal pathogens with genomes of >10 Mb (for example, Candida auris 229 , 12 Mb).
Other on-site applications
Portable ONT devices have also been used for on-site metagenomics research. MinION characterized pathogenic microbes, virulence genes and antimicrobial resistance markers in the polluted Little Bighorn River, Montana, United States 230 . MinION and MinIT devices were brought to farms in sub-Saharan Africa for early and rapid diagnosis (<3 h) of plant viruses and pests in cassava 231 . In forensic research, a portable strategy known as ‘MinION sketching’ was developed to identify human DNA with only 3 min of sequencing 232 , offering a rapid solution to cell authentication or contamination identification during cell or tissue culture.
The portability of the MinION system, which consists of the palm-sized MinION, mobile DNA extraction devices (for example, VolTRAX and Bento Lab) and real-time onboard base calling with Guppy and other offline bioinformatics tools, enables field research in scenarios where samples are hard to culture or store or where rapid genomic information is needed 233 . Examples include the International Space Station, future exploration of Mars and the Moon involving microgravity and high levels of ionizing radiation 69 , 234 , 235 , ships 236 , Greenland glaciers at subzero temperatures 237 , conservation work in the Madagascar forest 238 and educational outreach 238 .
Nanopore sequencing has enabled many biomedical studies by providing ultralong reads from single DNA/RNA molecules in real time. Nonetheless, current ONT sequencing techniques have several limitations, including relatively high error rates and the requirement for relatively high amounts of nucleic acid material. Overcoming these challenges will require further breakthroughs in nanopore technology, molecular experiments and bioinformatics software.
The principal concern in many applications is the error rate, which, at 6–15% for the R9.4 nanopore, is still much higher than that of Illumina short-read sequencing (0.1–1%). Despite substantial improvements in data accuracy over the past 7 years, there may be an intrinsic limit to 1D read accuracy. The sequencing of single molecules has a low signal-to-noise ratio, in contrast to bulk sequencing of molecules as in Illumina sequencing. Indeed, the same issue arises in the other single-molecule measurement techniques, such as Helicos, PacBio and BioNano Genomics. There is currently no theoretical estimation of this limit, but for reference, Helicos managed to reduce error rates to 4% (ref. 239 ). Future improvements in accuracy can be expected through optimization of molecule translocation ratcheting and, in particular, through engineering existing nanopores or discovering new ones. Indeed, many studies have been exploring new biological or non-biological nanopores with shorter sensing regions to achieve context-independent and high-quality raw signals. For example, graphene-based nanopores are capable of DNA sensing and have high durability and insulating capability in high ionic strength solutions 240 , 241 , 242 , where their thickness (~0.35 nm) is ideal for capturing single nucleotides 243 . Because such context-independent signals minimize the complex signal interference between adjacent modified bases, they could also make it possible to detect base modifications at single-molecule and single-nucleotide resolutions. Another approach for improving 1D read accuracy is to develop base-calling methods based on advanced computational techniques, such as deep learning.
Repetitive sequencing of the same molecule, for example, using 2D and 1D 2 reads, was helpful in improving accuracy. However, both of these approaches were limited in that each molecule could only be measured twice. By contrast, the R2C2 protocol involves the generation and sequencing of multiple copies of target molecules 122 . It may also be possible to increase data accuracy by recapturing DNA molecules into the same nanopore 244 or by using multilayer nanopores for multiple sequencing of each molecule.
Improved data accuracy would advance single-molecule omics studies. Haplotype-resolved genome assembly has been demonstrated for PacBio data 245 , which could likely be achieved using ONT sequencing. Methods are being developed to characterize epigenomic and epitranscriptomic events beyond base modifications at the single-molecule level, such as nucleosome occupancy and chromatin accessibility 72 , 175 , 176 and RNA secondary structure 184 , 185 . These approaches would allow investigation of the heterogeneity and dynamics of the epigenome and epitranscriptome as well as analysis of allele-specific and/or strand-specific epigenomic and epitranscriptomic phenomena. They would require specific experimental protocols (for example, identifying chromatin accessibility by detecting artificial 5mC footprints 72 , 175 , 176 ) rather than the simple generation of long reads.
Although the ultralong read length of ONT data remains its principal strength, further increases in read length would be beneficial, further facilitating genome assembly and the sequencing of difficult to analyze genomic regions (for example, eukaryotic centromeres and telomeres). Once read lengths reach a certain range, or even cover entire chromosomes, genome assembly would become trivial, requiring little computation and having superior completeness and accuracy. Personalized genome assembly would become widely available, and it would be possible to assemble the genomes of millions of species across the many Earth ecosystems. Obtaining megabase-scale or longer reads will require the development of HMW DNA extraction and size selection methods as well as protocols to maintain ultralong DNA fragments intact.
The other key experimental barrier to be addressed is the large amount of input DNA and RNA required for ONT sequencing, which is up to a few micrograms of DNA and hundreds of nanograms of RNA. PCR amplification of DNA is impractical for very long reads or impermissible for native DNA/RNA sequencing. Reducing the sample size requirement would make ONT sequencing useful for the many biomedical studies in which genetic material is limited. In parallel, ONT sequencing will benefit from the development of an end-to-end system. For example, the integration and automation of DNA/RNA extraction systems, sequencing library preparation and loading systems would allow users without specific training to generate ONT sequencing data. More robust and user-friendly bioinformatics software, such as cloud storage and computing and real-time analysis, will provide a further boost to ONT sequencing applications, ultimately moving the technology beyond the lab and into daily life.
Deamer, D., Akeson, M. & Branton, D. Three decades of nanopore sequencing. Nat. Biotechnol. 34 , 518–524 (2016).
Article CAS PubMed PubMed Central Google Scholar
Jain, M., Olsen, H. E., Paten, B. & Akeson, M. The Oxford Nanopore MinION: delivery of nanopore sequencing to the genomics community. Genome Biol. 17 , 239 (2016).
Article PubMed PubMed Central Google Scholar
van Dijk, E. L., Jaszczyszyn, Y., Naquin, D. & Thermes, C. The third revolution in sequencing technology. Trends Genet. 34 , 666–681 (2018).
Article PubMed Google Scholar
Yang, Y. et al. Advances in nanopore sequencing technology. J. Nanosci. Nanotechnol. 13 , 4521–4538 (2013).
Article CAS PubMed Google Scholar
Maitra, R. D., Kim, J. & Dunbar, W. B. Recent advances in nanopore sequencing. Electrophoresis 33 , 3418–3428 (2012).
Leggett, R. M. & Clark, M. D. A world of opportunities with nanopore sequencing. J. Exp. Bot. 68 , 5419–5429 (2017).
Noakes, M. T. et al. Increasing the accuracy of nanopore DNA sequencing using a time-varying cross membrane voltage. Nat. Biotechnol. 37 , 651–656 (2019).
Branton, D. et al. The potential and challenges of nanopore sequencing. Nat. Biotechnol. 26 , 1146–1153 (2008).
Song, L. et al. Structure of staphylococcal α-hemolysin, a heptameric transmembrane pore. Science 274 , 1859–1866 (1996).
Kasianowicz, J. J., Brandin, E., Branton, D. & Deamer, D. W. Characterization of individual polynucleotide molecules using a membrane channel. Proc. Natl Acad. Sci. USA 93 , 13770–13773 (1996).
Akeson, M., Branton, D., Kasianowicz, J. J., Brandin, E. & Deamer, D. W. Microsecond time-scale discrimination among polycytidylic acid, polyadenylic acid, and polyuridylic acid as homopolymers or as segments within single RNA molecules. Biophys. J. 77 , 3227–3233 (1999).
Meller, A., Nivon, L., Brandin, E., Golovchenko, J. & Branton, D. Rapid nanopore discrimination between single polynucleotide molecules. Proc. Natl Acad. Sci. USA 97 , 1079–1084 (2000).
Stoddart, D., Heron, A. J., Mikhailova, E., Maglia, G. & Bayley, H. Single-nucleotide discrimination in immobilized DNA oligonucleotides with a biological nanopore. Proc. Natl Acad. Sci. USA 106 , 7702–7707 (2009).
Stoddart, D. et al. Nucleobase recognition in ssDNA at the central constriction of the α-hemolysin pore. Nano Lett. 10 , 3633–3637 (2010).
Stoddart, D., Maglia, G., Mikhailova, E., Heron, A. J. & Bayley, H. Multiple base-recognition sites in a biological nanopore: two heads are better than one. Angew. Chem. Int. Ed. Engl. 49 , 556–559 (2010).
Butler, T. Z., Pavlenok, M., Derrington, I. M., Niederweis, M. & Gundlach, J. H. Single-molecule DNA detection with an engineered MspA protein nanopore. Proc. Natl Acad. Sci. USA 105 , 20647–20652 (2008).
Derrington, I. M. et al. Nanopore DNA sequencing with MspA. Proc. Natl Acad. Sci. USA 107 , 16060–16065 (2010).
Niederweis, M. et al. Cloning of the mspA gene encoding a porin from Mycobacterium smegmatis . Mol. Microbiol. 33 , 933–945 (1999).
Faller, M., Niederweis, M. & Schulz, G. E. The structure of a mycobacterial outer-membrane channel. Science 303 , 1189–1192 (2004).
Benner, S. et al. Sequence-specific detection of individual DNA polymerase complexes in real time using a nanopore. Nat. Nanotechnol. 2 , 718–724 (2007).
Hornblower, B. et al. Single-molecule analysis of DNA–protein complexes using nanopores. Nat. Methods 4 , 315–317 (2007).
Cockroft, S. L., Chu, J., Amorin, M. & Ghadiri, M. R. A single-molecule nanopore device detects DNA polymerase activity with single-nucleotide resolution. J. Am. Chem. Soc. 130 , 818–820 (2008).
Lieberman, K. R. et al. Processive replication of single DNA molecules in a nanopore catalyzed by phi29 DNA polymerase. J. Am. Chem. Soc. 132 , 17961–17972 (2010).
Cherf, G. M. et al. Automated forward and reverse ratcheting of DNA in a nanopore at 5-A precision. Nat. Biotechnol. 30 , 344–348 (2012).
Manrao, E. A. et al. Reading DNA at single-nucleotide resolution with a mutant MspA nanopore and phi29 DNA polymerase. Nat. Biotechnol. 30 , 349–353 (2012).
Mason, C. E. & Elemento, O. Faster sequencers, larger datasets, new challenges. Genome Biol. 13 , 314 (2012).
Wang, Y., Yang, Q. & Wang, Z. The evolution of nanopore sequencing. Front. Genet. 5 , 449 (2015).
Shi, W., Friedman, A. K. & Baker, L. A. Nanopore sensing. Anal. Chem. 89 , 157–188 (2017).
Minei, R., Hoshina, R. & Ogura, A. De novo assembly of middle-sized genome using MinION and Illumina sequencers. BMC Genomics 19 , 700 (2018).
Ashton, P. M. et al. MinION nanopore sequencing identifies the position and structure of a bacterial antibiotic resistance island. Nat. Biotechnol. 33 , 296–300 (2015).
Carter, J. M. & Hussain, S. Robust long-read native DNA sequencing using the ONT CsgG Nanopore system. Wellcome Open Res 2 , 23 (2017).
Wick, R. R., Judd, L. M. & Holt, K. E. Performance of neural network basecalling tools for Oxford Nanopore sequencing. Genome Biol. 20 , 129 (2019).
Gong, L. et al. Picky comprehensively detects high-resolution structural variants in nanopore long reads. Nat. Methods 15 , 455–460 (2018).
Brickwedde, A. et al. Structural, physiological and regulatory analysis of maltose transporter genes in Saccharomyces eubayanus CBS 12357 T . Front. Microbiol. 9 , 1786 (2018).
Zeng, J. et al. Causalcall: nanopore basecalling using a temporal convolutional network. Front. Genet. 10 , 1332 (2020).
Helmersen, K. & Aamot, H. V. DNA extraction of microbial DNA directly from infected tissue: an optimized protocol for use in nanopore sequencing. Sci. Rep. 10 , 2985 (2020).
Tytgat, O. et al. Nanopore sequencing of a forensic STR multiplex reveals loci suitable for single-contributor STR profiling. Genes 11 , 381 (2020).
Article CAS PubMed Central Google Scholar
Huang, Y. T., Liu, P. Y. & Shih, P. W. Homopolish: a method for the removal of systematic errors in nanopore sequencing by homologous polishing. Genome Biol. 22 , 95 (2021).
Rhoads, A. & Au, K. F. PacBio sequencing and its applications. Genomics Proteomics Bioinformatics 13 , 278–289 (2015).
Ip, C. L. C. et al. MinION Analysis and Reference Consortium: phase 1 data release and analysis. F1000Res 4 , 1075 (2015).
Jain, M. et al. MinION Analysis and Reference Consortium: phase 2 data release and analysis of R9.0 chemistry. F1000Res 6 , 760 (2017).
Weirather, J. L. et al. Comprehensive comparison of Pacific Biosciences and Oxford Nanopore Technologies and their applications to transcriptome analysis. F1000Res 6 , 100 (2017).
Seki, M. et al. Evaluation and application of RNA-seq by MinION. DNA Res. 26 , 55–65 (2019).
Rang, F. J., Kloosterman, W. P. & de Ridder, J. From squiggle to basepair: computational approaches for improving nanopore sequencing read accuracy. Genome Biol. 19 , 90 (2018).
Goodwin, S. et al. Oxford Nanopore sequencing, hybrid error correction, and de novo assembly of a eukaryotic genome. Genome Res. 25 , 1750–1756 (2015).
David, M., Dursi, L. J., Yao, D., Boutros, P. C. & Simpson, J. T. Nanocall: an open source basecaller for Oxford Nanopore sequencing data. Bioinformatics 33 , 49–55 (2017).
Boza, V., Brejova, B. & Vinar, T. DeepNano: deep recurrent neural networks for base calling in MinION nanopore reads. PLoS ONE 12 , e0178751 (2017).
Gong, L., Wong, C. H., Idol, J., Ngan, C. Y. & Wei, C. L. Ultra-long read sequencing for whole genomic DNA analysis. J. Vis. Exp . https://doi.org/10.3791/58954 (2019).
Payne, A., Holmes, N., Rakyan, V. & Loose, M. BulkVis: a graphical viewer for Oxford nanopore bulk FAST5 files. Bioinformatics 35 , 2193–2198 (2019).
Quick, J. & Loman, N. J. in Nanopore Sequencing: An Introduction Ch. 7 (World Scientific Press, 2019).
Deschamps, S. et al. A chromosome-scale assembly of the sorghum genome using nanopore sequencing and optical mapping. Nat. Commun. 9 , 4844 (2018).
Garalde, D. R. et al. Highly parallel direct RNA sequencing on an array of nanopores. Nat. Methods 15 , 201–206 (2018).
Keller, M. W. et al. Direct RNA sequencing of the coding complete influenza A virus genome. Sci. Rep. 8 , 14408 (2018).
Pitt, M. E. et al. Evaluating the genome and resistome of extensively drug-resistant Klebsiella pneumoniae using native DNA and RNA Nanopore sequencing. Gigascience 9 , giaa002 (2020).
Cartolano, M., Huettel, B., Hartwig, B., Reinhardt, R. & Schneeberger, K. cDNA library enrichment of full length transcripts for SMRT long read sequencing. PLoS ONE 11 , e0157779 (2016).
Chen, Y. et al. A systematic benchmark of Nanopore long read RNA sequencing for transcript level analysis in human cell lines. Preprint at bioRxiv https://doi.org/10.1101/2021.04.21.440736 (2021).
Nicholls, S. M., Quick, J. C., Tang, S. & Loman, N. J. Ultra-deep, long-read nanopore sequencing of mock microbial community standards. Gigascience 8 , giz043 (2019).
Magi, A., Semeraro, R., Mingrino, A., Giusti, B. & D’Aurizio, R. Nanopore sequencing data analysis: state of the art, applications and challenges. Brief. Bioinform. 19 , 1256–1272 (2018).
CAS PubMed Google Scholar
Cao, M. D., Ganesamoorthy, D., Cooper, M. A. & Coin, L. J. Realtime analysis and visualization of MinION sequencing data with npReader. Bioinformatics 32 , 764–766 (2016).
Watson, M. et al. poRe: an R package for the visualization and analysis of nanopore sequencing data. Bioinformatics 31 , 114–115 (2015).
Leggett, R. M., Heavens, D., Caccamo, M., Clark, M. D. & Davey, R. P. NanoOK: multi-reference alignment analysis of nanopore sequencing data, quality and error profiles. Bioinformatics 32 , 142–144 (2016).
Tarraga, J., Gallego, A., Arnau, V., Medina, I. & Dopazo, J. HPG pore: an efficient and scalable framework for nanopore sequencing data. BMC Bioinformatics 17 , 107 (2016).
Bolognini, D., Bartalucci, N., Mingrino, A., Vannucchi, A. M. & Magi, A. NanoR: a user-friendly R package to analyze and compare nanopore sequencing data. PLoS ONE 14 , e0216471 (2019).
Loman, N. J. & Quinlan, A. R. Poretools: a toolkit for analyzing nanopore sequence data. Bioinformatics 30 , 3399–3401 (2014).
De Coster, W., D’Hert, S., Schultz, D. T., Cruts, M. & Van Broeckhoven, C. NanoPack: visualizing and processing long-read sequencing data. Bioinformatics 34 , 2666–2669 (2018).
Semeraro, R. & Magi, A. PyPore: a python toolbox for nanopore sequencing data handling. Bioinformatics 35 , 4445–4447 (2019).
Senol Cali, D., Kim, J. S., Ghose, S., Alkan, C. & Mutlu, O. Nanopore sequencing technology and tools for genome assembly: computational analysis of the current state, bottlenecks and future directions. Brief. Bioinform. 20 , 1542–1559 (2019).
Amarasinghe, S. L. et al. Opportunities and challenges in long-read sequencing data analysis. Genome Biol. 21 , 30 (2020).
McIntyre, A. B. R. et al. Nanopore sequencing in microgravity. NPJ Microgravity 2 , 16035 (2016).
Teng, H. et al. Chiron: translating nanopore raw signal directly into nucleotide sequence using deep learning. Gigascience 7 , giy037 (2018).
Article PubMed Central Google Scholar
Rand, A. C. et al. Mapping DNA methylation with high-throughput nanopore sequencing. Nat. Methods 14 , 411–413 (2017).
Wang, Y. et al. Single-molecule long-read sequencing reveals the chromatin basis of gene expression. Genome Res. 29 , 1329–1342 (2019).
Liu, H. et al. Accurate detection of m 6 A RNA modifications in native RNA sequences. Nat. Commun. 10 , 4079 (2019).
Stoiber, M. H. et al. De novo identification of DNA modifications enabled by genome-guided nanopore signal processing. Preprint at bioRxiv https://doi.org/10.1101/094672 (2016).
Simpson, J. T. et al. Detecting DNA cytosine methylation using nanopore sequencing. Nat. Methods 14 , 407–410 (2017).
Liu, Q. et al. Detection of DNA base modifications by deep recurrent neural network on Oxford Nanopore sequencing data. Nat. Commun. 10 , 2449 (2019).
Ni, P. et al. DeepSignal: detecting DNA methylation state from Nanopore sequencing reads using deep-learning. Bioinformatics 35 , 4586–4595 (2019).
Liu, Q., Georgieva, D. C., Egli, D. & Wang, K. NanoMod: a computational tool to detect DNA modifications using Nanopore long-read sequencing data. BMC Genomics 20 , 78 (2019).
Yuen, Z. W. et al. Systematic benchmarking of tools for CpG methylation detection from nanopore sequencing. Nat. Commun. 12 , 3438 (2021).
Liu, Y. et al. DNA methylation-calling tools for Oxford Nanopore sequencing: a survey and human epigenome-wide evaluation. Genome Biol. 22 , 295 (2021).
Fang, G. et al. Genome-wide mapping of methylated adenine residues in pathogenic Escherichia coli using single-molecule real-time sequencing. Nat. Biotechnol. 30 , 1232–1239 (2012).
Saletore, Y. et al. The birth of the epitranscriptome: deciphering the function of RNA modifications. Genome Biol. 13 , 175 (2012).
Jenjaroenpun, P. et al. Decoding the epitranscriptional landscape from native RNA sequences. Nucleic Acids Res . 49 , e7 (2020).
Lorenz, D. A., Sathe, S., Einstein, J. M. & Yeo, G. W. Direct RNA sequencing enables m 6 A detection in endogenous transcript isoforms at base-specific resolution. RNA 26 , 19–28 (2020).
Fu, S., Wang, A. & Au, K. F. A comparative evaluation of hybrid error correction methods for error-prone long reads. Genome Biol. 20 , 26 (2019).
Viehweger, A. et al. Direct RNA nanopore sequencing of full-length coronavirus genomes provides novel insights into structural variants and enables modification analysis. Genome Res. 29 , 1545–1554 (2019).
Lima, L. et al. Comparative assessment of long-read error correction software applied to Nanopore RNA-sequencing data. Brief. Bioinform. 21 , 1164–1181 (2019).
Article Google Scholar
Koren, S. et al. Canu: scalable and accurate long-read assembly via adaptive k -mer weighting and repeat separation. Genome Res. 27 , 722–736 (2017).
Salmela, L., Walve, R., Rivals, E. & Ukkonen, E. Accurate self-correction of errors in long reads using de Bruijn graphs. Bioinformatics 33 , 799–806 (2017).
Au, K. F., Underwood, J. G., Lee, L. & Wong, W. H. Improving PacBio long read accuracy by short read alignment. PLoS ONE 7 , e46679 (2012).
Salmela, L. & Rivals, E. LoRDEC: accurate and efficient long read error correction. Bioinformatics 30 , 3506–3514 (2014).
Bao, E. & Lan, L. HALC: high throughput algorithm for long read error correction. BMC Bioinformatics 18 , 204 (2017).
Wang, J. R., Holt, J., McMillan, L. & Jones, C. D. FMLRC: hybrid long read error correction using an FM-index. BMC Bioinformatics 19 , 50 (2018).
Altschul, S. F., Gish, W., Miller, W., Myers, E. W. & Lipman, D. J. Basic local alignment search tool. J. Mol. Biol. 215 , 403–410 (1990).
Sovic, I. et al. Fast and sensitive mapping of nanopore sequencing reads with GraphMap. Nat. Commun. 7 , 11307 (2016).
Li, H. Minimap2: pairwise alignment for nucleotide sequences. Bioinformatics 34 , 3094–3100 (2018).
Kielbasa, S. M., Wan, R., Sato, K., Horton, P. & Frith, M. C. Adaptive seeds tame genomic sequence comparison. Genome Res. 21 , 487–493 (2011).
Sedlazeck, F. J. et al. Accurate detection of complex structural variations using single-molecule sequencing. Nat. Methods 15 , 461–468 (2018).
Zhou, A., Lin, T. & Xing, J. Evaluating nanopore sequencing data processing pipelines for structural variation identification. Genome Biol. 20 , 237 (2019).
Wu, T. D. & Watanabe, C. K. GMAP: a genomic mapping and alignment program for mRNA and EST sequences. Bioinformatics 21 , 1859–1875 (2005).
Dobin, A. et al. STAR: ultrafast universal RNA-seq aligner. Bioinformatics 29 , 15–21 (2013).
Marić, J., Sović, I., Križanović, K., Nagarajan, N. & Šikić, M. Graphmap2—splice-aware RNA-seq mapper for long reads. Preprint at bioRxiv https://doi.org/10.1101/720458 (2019).
Liu, B. et al. deSALT: fast and accurate long transcriptomic read alignment with de Bruijn graph-based index. Genome Biol. 20 , 274 (2019).
Begik, O. et al. Quantitative profiling of pseudouridylation dynamics in native RNAs with nanopore sequencing. Nat. Biotechnol . 39 , 1278–1291 (2021).
Giordano, F. et al. De novo yeast genome assemblies from MinION, PacBio and MiSeq platforms. Sci. Rep. 7 , 3935 (2017).
Bertrand, D. et al. Hybrid metagenomic assembly enables high-resolution analysis of resistance determinants and mobile elements in human microbiomes. Nat. Biotechnol. 37 , 937–944 (2019).
Li, H. Minimap and miniasm: fast mapping and de novo assembly for noisy long sequences. Bioinformatics 32 , 2103–2110 (2016).
de Lannoy, C., de Ridder, D. & Risse, J. The long reads ahead: de novo genome assembly using the MinION. F1000Res 6 , 1083 (2017).
PubMed PubMed Central Google Scholar
Loman, N. J., Quick, J. & Simpson, J. T. A complete bacterial genome assembled de novo using only nanopore sequencing data. Nat. Methods 12 , 733–735 (2015).
Kolmogorov, M., Yuan, J., Lin, Y. & Pevzner, P. A. Assembly of long, error-prone reads using repeat graphs. Nat. Biotechnol. 37 , 540–546 (2019).
Ruan, J. & Li, H. Fast and accurate long-read assembly with wtdbg2. Nat. Methods 17 , 155–158 (2020).
Cretu Stancu, M. et al. Mapping and phasing of structural variation in patient genomes using nanopore sequencing. Nat. Commun. 8 , 1326 (2017).
Tham, C. Y. et al. NanoVar: accurate characterization of patients’ genomic structural variants using low-depth nanopore sequencing. Genome Biol. 21 , 56 (2020).
Bowden, R. et al. Sequencing of human genomes with nanopore technology. Nat. Commun. 10 , 1869 (2019).
Chaisson, M. J. P. et al. Multi-platform discovery of haplotype-resolved structural variation in human genomes. Nat. Commun. 10 , 1784 (2019).
Edge, P. & Bansal, V. Longshot enables accurate variant calling in diploid genomes from single-molecule long read sequencing. Nat. Commun. 10 , 4660 (2019).
Schrinner, S. D. et al. Haplotype threading: accurate polyploid phasing from long reads. Genome Biol. 21 , 252 (2020).
Ewing, A. D. et al. Nanopore sequencing enables comprehensive transposable element epigenomic profiling. Mol. Cell 80 , 915–928 (2020).
Bolognini, D., Magi, A., Benes, V., Korbel, J. O. & Rausch, T. TRiCoLOR: tandem repeat profiling using whole-genome long-read sequencing data. Gigascience 9 , giaa101 (2020).
Byrne, A. et al. Nanopore long-read RNAseq reveals widespread transcriptional variation among the surface receptors of individual B cells. Nat. Commun. 8 , 16027 (2017).
Oikonomopoulos, S., Wang, Y. C., Djambazian, H., Badescu, D. & Ragoussis, J. Benchmarking of the Oxford Nanopore MinION sequencing for quantitative and qualitative assessment of cDNA populations. Sci. Rep. 6 , 31602 (2016).
Volden, R. et al. Improving nanopore read accuracy with the R2C2 method enables the sequencing of highly multiplexed full-length single-cell cDNA. Proc. Natl Acad. Sci. USA 115 , 9726–9731 (2018).
Tang, A. D. et al. Full-length transcript characterization of SF3B1 mutation in chronic lymphocytic leukemia reveals downregulation of retained introns. Nat. Commun. 11 , 1438 (2020).
Kuosmanen, A., Sobih, A., Rizzi, R., Mäkinen, V. & Tomescu, A. I. On using longer RNA-seq reads to improve transcript prediction accuracy. In Proc. 9th International Joint Conference on Biomedical Engineering Systems and Technologies 272–277 (BIOSTEC, 2016).
Kovaka, S. et al. Transcriptome assembly from long-read RNA-seq alignments with StringTie2. Genome Biol. 20 , 278 (2019).
Wyman, D. et al. A technology-agnostic long-read analysis pipeline for transcriptome discovery and quantification. Preprint at bioRxiv https://doi.org/10.1101/672931 (2020).
Au, K. F. et al. Characterization of the human ESC transcriptome by hybrid sequencing. Proc. Natl Acad. Sci. USA 110 , E4821–E4830 (2013).
Fu, S. et al. IDP-denovo: de novo transcriptome assembly and isoform annotation by hybrid sequencing. Bioinformatics 34 , 2168–2176 (2018).
de la Rubia, I. et al. Reference-free reconstruction and quantification of transcriptomes from Nanopore long-read sequencing. Preprint at bioRxiv https://doi.org/10.1101/2020.02.08.939942 (2021).
Workman, R. E. et al. Nanopore native RNA sequencing of a human poly(A) transcriptome. Nat. Methods 16 , 1297–1305 (2019).
Soneson, C. et al. A comprehensive examination of Nanopore native RNA sequencing for characterization of complex transcriptomes. Nat. Commun. 10 , 3359 (2019).
Jain, M. et al. Nanopore sequencing and assembly of a human genome with ultra-long reads. Nat. Biotechnol. 36 , 338–345 (2018).
Jain, M. et al. Linear assembly of a human centromere on the Y chromosome. Nat. Biotechnol. 36 , 321–323 (2018).
Miga, K. H. et al. Telomere-to-telomere assembly of a complete human X chromosome. Nature 585 , 79–84 (2020).
Nurk, S. et al. The complete sequence of a human genome. Preprint at bioRxiv https://doi.org/10.1101/2021.05.26.445798 (2021).
Tyson, J. R. et al. MinION-based long-read sequencing and assembly extends the Caenorhabditis elegans reference genome. Genome Res. 28 , 266–274 (2018).
Salazar, A. N. et al. Nanopore sequencing enables near-complete de novo assembly of Saccharomyces cerevisiae reference strain CEN.PK113-7D. FEMS Yeast Res. 17 , fox074 (2017).
Michael, T. P. et al. High contiguity Arabidopsis thaliana genome assembly with a single nanopore flow cell. Nat. Commun. 9 , 541 (2018).
Miller, D. E., Staber, C., Zeitlinger, J. & Hawley, R. S. Highly contiguous genome assemblies of 15 Drosophila species generated using nanopore sequencing. G3 8 , 3131–3141 (2018).
Kapustova, V. et al. The dark matter of large cereal genomes: long tandem repeats. Int. J. Mol. Sci. 20 , 2483 (2019).
Diaz-Viraque, F. et al. Nanopore sequencing significantly improves genome assembly of the protozoan parasite Trypanosoma cruzi . Genome Biol. Evol. 11 , 1952–1957 (2019).
Datema, E. et al. The megabase-sized fungal genome of Rhizoctonia solani assembled from nanopore reads only. Preprint at bioRxiv https://doi.org/10.1101/084772 (2016).
Austin, C. M. et al. De novo genome assembly and annotation of Australia’s largest freshwater fish, the Murray cod ( Maccullochella peelii ), from Illumina and Nanopore sequencing read. Gigascience 6 , 1–6 (2017).
Tan, M. H. et al. Finding Nemo: hybrid assembly with Oxford Nanopore and Illumina reads greatly improves the clownfish ( Amphiprion ocellaris ) genome assembly. Gigascience 7 , 1–6 (2018).
Singh, K. S. et al. De novo genome assembly of the Meadow Brown Butterfly, Maniola jurtina . G3 10 , 1477–1484 (2020).
Lind, A. L. et al. Genome of the Komodo dragon reveals adaptations in the cardiovascular and chemosensory systems of monitor lizards. Nat. Ecol. Evol. 3 , 1241–1252 (2019).
Dhar, R. et al. De novo assembly of the Indian blue peacock ( Pavo cristatus ) genome using Oxford Nanopore technology and Illumina sequencing. Gigascience 8 , giz038 (2019).
Armstrong, E. E. et al. Long live the king: chromosome-level assembly of the lion ( Panthera leo ) using linked-read, Hi-C, and long-read data. BMC Biol. 18 , 3 (2020).
Kono, N. et al. The bagworm genome reveals a unique fibroin gene that provides high tensile strength. Commun. Biol. 2 , 148 (2019).
Wongsurawat, T. et al. Rapid sequencing of multiple RNA viruses in their native form. Front. Microbiol. 10 , 260 (2019).
Lu, R. et al. Genomic characterisation and epidemiology of 2019 novel coronavirus: implications for virus origins and receptor binding. Lancet 395 , 565–574 (2020).
Kim, D. et al. The architecture of SARS-CoV-2 transcriptome. Cell 181 , 914–921 (2020).
Moore, S. C. et al. Amplicon based MinION sequencing of SARS-CoV-2 and metagenomic characterisation of nasopharyngeal swabs from patients with COVID-19. Preprint at medRxiv https://doi.org/10.1101/2020.03.05.20032011 (2020).
Taiaroa, G. et al. Direct RNA sequencing and early evolution of SARS-CoV-2. Preprint at bioRxiv https://doi.org/10.1101/2020.03.05.976167 (2020).
Wang, M. et al. Nanopore targeted sequencing for the accurate and comprehensive detection of SARS-CoV-2 and other respiratory viruses. Small 16 , e2002169 (2020).
Bayega, A. et al. De novo assembly of the olive fruit fly ( Bactrocera oleae ) genome with linked-reads and long-read technologies minimizes gaps and provides exceptional Y chromosome assembly. BMC Genomics 21 , 259 (2020).
Kadobianskyi, M., Schulze, L., Schuelke, M. & Judkewitz, B. Hybrid genome assembly and annotation of Danionella translucida . Sci. Data 6 , 156 (2019).
Bai, C. M. et al. Chromosomal-level assembly of the blood clam, Scapharca ( Anadara ) broughtonii , using long sequence reads and Hi-C. Gigascience 8 , giz067 (2019).
Belser, C. et al. Chromosome-scale assemblies of plant genomes using nanopore long reads and optical maps. Nat. Plants 4 , 879–887 (2018).
Marrano, A. et al. High-quality chromosome-scale assembly of the walnut ( Juglans regia L.) reference genome. Gigascience 9 , giaa050 (2020).
Ning, D. L. et al. Chromosomal-level assembly of Juglans sigillata genome using Nanopore, BioNano, and Hi-C analysis. Gigascience 9 , giaa006 (2020).
Kwan, H. H. et al. The genome of the Steller Sea Lion ( Eumetopias jubatus ). Genes 10 , 486 (2019).
Scott, A. D. et al. The giant sequoia genome and proliferation of disease resistance genes. Preprint at bioRxiv https://doi.org/10.1101/2020.03.17.995944 (2020).
Shafin, K. et al. Nanopore sequencing and the Shasta toolkit enable efficient de novo assembly of eleven human genomes. Nat. Biotechnol. 38 , 1044–1053 (2020).
De Coster, W. et al. Structural variants identified by Oxford Nanopore PromethION sequencing of the human genome. Genome Res. 29 , 1178–1187 (2019).
Singh, M. et al. High-throughput targeted long-read single cell sequencing reveals the clonal and transcriptional landscape of lymphocytes. Nat. Commun. 10 , 3120 (2019).
Roach, N. P. et al. The full-length transcriptome of C. elegans using direct RNA sequencing. Genome Res. 30 , 299–312 (2020).
Parker, M. T. et al. Nanopore direct RNA sequencing maps the complexity of Arabidopsis mRNA processing and m 6 A modification. eLife 9 , e49658 (2020).
Jiang, F. et al. Long-read direct RNA sequencing by 5′-cap capturing reveals the impact of Piwi on the widespread exonization of transposable elements in locusts. RNA Biol. 16 , 950–959 (2019).
Zhang, J. et al. Comprehensive profiling of circular RNAs with nanopore sequencing and CIRI-long. Nat. Biotechnol. 39 , 836–845 (2021).
Xin, R. et al. isoCirc catalogs full-length circular RNA isoforms in human transcriptomes. Nat. Commun. 12 , 266 (2021).
Laszlo, A. H. et al. Detection and mapping of 5-methylcytosine and 5-hydroxymethylcytosine with nanopore MspA. Proc. Natl Acad. Sci. USA 110 , 18904–18909 (2013).
Schreiber, J. et al. Error rates for nanopore discrimination among cytosine, methylcytosine, and hydroxymethylcytosine along individual DNA strands. Proc. Natl Acad. Sci. USA 110 , 18910–18915 (2013).
McIntyre, A. B. R. et al. Single-molecule sequencing detection of N 6 -methyladenine in microbial reference materials. Nat. Commun. 10 , 579 (2019).
Shipony, Z. et al. Long-range single-molecule mapping of chromatin accessibility in eukaryotes. Nat. Methods 17 , 319–327 (2020).
Lee, I. et al. Simultaneous profiling of chromatin accessibility and methylation on human cell lines with nanopore sequencing. Nat. Methods 17 , 1191–1199 (2020).
Georgieva, D., Liu, Q., Wang, K. & Egli, D. Detection of base analogs incorporated during DNA replication by nanopore sequencing. Nucleic Acids Res. 48 , e88 (2020).
Hennion, M. et al. Mapping DNA replication with nanopore sequencing. Preprint at bioRxiv https://doi.org/10.1101/426858 (2018).
Muller, C. A. et al. Capturing the dynamics of genome replication on individual ultra-long nanopore sequence reads. Nat. Methods 16 , 429–436 (2019).
Ulahannan, N. et al. Nanopore sequencing of DNA concatemers reveals higher-order features of chromatin structure. Preprint at bioRxiv https://doi.org/10.1101/833590 (2019).
Altemose, N. et al. DiMeLo-seq: a long-read, single-molecule method for mapping protein–DNA interactions genome-wide. Preprint at bioRxiv https://doi.org/10.1101/2021.07.06.451383 (2021).
Weng, Z. et al. Long-range single-molecule mapping of chromatin modification in eukaryotes. Preprint at bioRxiv https://doi.org/10.1101/2021.07.08.451578 (2021).
Smith, A. M., Jain, M., Mulroney, L., Garalde, D. R. & Akeson, M. Reading canonical and modified nucleobases in 16S ribosomal RNA using nanopore native RNA sequencing. PLoS ONE 14 , e0216709 (2019).
Aw, J. G. A. et al. Determination of isoform-specific RNA structure with nanopore long reads. Nat. Biotechnol. 39 , 336–346 (2020).
Stephenson, W. et al. Direct detection of RNA modifications and structure using single molecule nanopore sequencing. Preprint at bioRxiv https://doi.org/10.1101/2020.05.31.126763 (2020).
Maier, K. C., Gressel, S., Cramer, P. & Schwalb, B. Native molecule sequencing by nano-ID reveals synthesis and stability of RNA isoforms. Genome Res. 30 , 1332–1344 (2020).
Drexler, H. L., Choquet, K. & Churchman, L. S. Splicing kinetics and coordination revealed by direct nascent RNA sequencing through nanopores. Mol. Cell 77 , 985–998 (2020).
Minervini, C. F. et al. TP53 gene mutation analysis in chronic lymphocytic leukemia by nanopore MinION sequencing. Diagn. Pathol. 11 , 96 (2016).
Minervini, C. F. et al. Mutational analysis in BCR - ABL1 positive leukemia by deep sequencing based on nanopore MinION technology. Exp. Mol. Pathol. 103 , 33–37 (2017).
Orsini, P. et al. Design and MinION testing of a nanopore targeted gene sequencing panel for chronic lymphocytic leukemia. Sci. Rep. 8 , 11798 (2018).
Cumbo, C. et al. Genomic BCR - ABL1 breakpoint characterization by a multi-strategy approach for “personalized monitoring” of residual disease in chronic myeloid leukemia patients. Oncotarget 9 , 10978–10986 (2018).
Au, C. H. et al. Rapid detection of chromosomal translocation and precise breakpoint characterization in acute myeloid leukemia by nanopore long-read sequencing. Cancer Genet. 239 , 22–25 (2019).
Euskirchen, P. et al. Same-day genomic and epigenomic diagnosis of brain tumors using real-time nanopore sequencing. Acta Neuropathol. 134 , 691–703 (2017).
Pradhan, B. et al. Detection of subclonal L1 transductions in colorectal cancer by long-distance inverse-PCR and Nanopore sequencing. Sci. Rep. 7 , 14521 (2017).
Norris, A. L., Workman, R. E., Fan, Y., Eshleman, J. R. & Timp, W. Nanopore sequencing detects structural variants in cancer. Cancer Biol. Ther. 17 , 246–253 (2016).
Suzuki, A. et al. Sequencing and phasing cancer mutations in lung cancers using a long-read portable sequencer. DNA Res. 24 , 585–596 (2017).
Gabrieli, T. et al. Selective nanopore sequencing of human BRCA1 by Cas9-assisted targeting of chromosome segments (CATCH). Nucleic Acids Res. 46 , e87 (2018).
Jeck, W. R. et al. A nanopore sequencing-based assay for rapid detection of gene fusions. J. Mol. Diagn. 21 , 58–69 (2019).
Moon, J. et al. Rapid diagnosis of bacterial meningitis by nanopore 16S amplicon sequencing: a pilot study. Int. J. Med. Microbiol. 309 , 151338 (2019).
Charalampous, T. et al. Nanopore metagenomics enables rapid clinical diagnosis of bacterial lower respiratory infection. Nat. Biotechnol. 37 , 783–792 (2019).
Cheng, J. et al. Identification of pathogens in culture-negative infective endocarditis cases by metagenomic analysis. Ann. Clin. Microbiol Antimicrob. 17 , 43 (2018).
Gorrie, C. L. et al. Antimicrobial-resistant Klebsiella pneumoniae carriage and infection in specialized geriatric care wards linked to acquisition in the referring hospital. Clin. Infect. Dis. 67 , 161–170 (2018).
Sanderson, N. D. et al. Real-time analysis of nanopore-based metagenomic sequencing from infected orthopaedic devices. BMC Genomics 19 , 714 (2018).
Schmidt, K. et al. Identification of bacterial pathogens and antimicrobial resistance directly from clinical urines by nanopore-based metagenomic sequencing. J. Antimicrob. Chemother. 72 , 104–114 (2016).
Lu, X. et al. Epidemiologic and genomic insights on mcr-1 -harbouring Salmonella from diarrhoeal outpatients in Shanghai, China, 2006–2016. EBioMedicine 42 , 133–144 (2019).
Hu, Y., Fang, L., Nicholson, C. & Wang, K. Implications of error-prone long-read whole-genome shotgun sequencing on characterizing reference microbiomes. iScience 23 , 101223 (2020).
De Roeck, A. et al. NanoSatellite: accurate characterization of expanded tandem repeat length and sequence through whole genome long-read sequencing on PromethION. Genome Biol. 20 , 239 (2019).
Chatron, N. et al. Severe hemophilia A caused by an unbalanced chromosomal rearrangement identified using nanopore sequencing. J. Thromb. Haemost. 17 , 1097–1103 (2019).
Brandler, W. M. et al. Paternally inherited cis -regulatory structural variants are associated with autism. Science 360 , 327–331 (2018).
Carvalho, C. M. B. et al. Interchromosomal template-switching as a novel molecular mechanism for imprinting perturbations associated with Temple syndrome. Genome Med. 11 , 25 (2019).
Miao, H. et al. Long-read sequencing identified a causal structural variant in an exome-negative case and enabled preimplantation genetic diagnosis. Hereditas 155 , 32 (2018).
Dutta, U. R. et al. Breakpoint mapping of a novel de novo translocation t(X;20)(q11.1;p13) by positional cloning and long read sequencing. Genomics 111 , 1108–1114 (2019).
Ishiura, H. et al. Expansions of intronic TTTCA and TTTTA repeats in benign adult familial myoclonic epilepsy. Nat. Genet. 50 , 581–590 (2018).
Zeng, S. et al. Long-read sequencing identified intronic repeat expansions in SAMD12 from Chinese pedigrees affected with familial cortical myoclonic tremor with epilepsy. J. Med. Genet. 56 , 265–270 (2019).
Leija-Salazar, M. et al. Evaluation of the detection of GBA missense mutations and other variants using the Oxford Nanopore MinION. Mol. Genet Genom. Med 7 , e564 (2019).
Lang, K. et al. Full-length HLA class I genotyping with the MinION nanopore sequencer. Methods Mol. Biol. 1802 , 155–162 (2018).
Liu, C. et al. Accurate typing of human leukocyte antigen class I genes by Oxford Nanopore sequencing. J. Mol. Diagn. 20 , 428–435 (2018).
Duke, J. L. et al. Resolving MiSeq-generated ambiguities in HLA-DPB1 typing by using the Oxford Nanopore Technology. J. Mol. Diagn. 21 , 852–861 (2019).
Wei, S. & Williams, Z. Rapid short-read sequencing and aneuploidy detection using MinION nanopore technology. Genetics 202 , 37–44 (2016).
Quick, J. et al. Real-time, portable genome sequencing for Ebola surveillance. Nature 530 , 228–232 (2016).
Quick, J. et al. Rapid draft sequencing and real-time nanopore sequencing in a hospital outbreak of Salmonella . Genome Biol. 16 , 114 (2015).
Faria, N. R. et al. Establishment and cryptic transmission of Zika virus in Brazil and the Americas. Nature 546 , 406–410 (2017).
Faria, N. R. et al. Genomic and epidemiological monitoring of yellow fever virus transmission potential. Science 361 , 894–899 (2018).
de Jesus, J. G. et al. Genomic detection of a virus lineage replacement event of dengue virus serotype 2 in Brazil, 2019. Mem. Inst. Oswaldo Cruz 115 , e190423 (2020).
Russell, J. A. et al. Unbiased strain-typing of arbovirus directly from mosquitoes using nanopore sequencing: a field-forward biosurveillance protocol. Sci. Rep. 8 , 5417 (2018).
Kafetzopoulou, L. E. et al. Metagenomic sequencing at the epicenter of the Nigeria 2018 Lassa fever outbreak. Science 363 , 74–77 (2019).
Brynildsrud, O. B. et al. Acquisition of virulence genes by a carrier strain gave rise to the ongoing epidemics of meningococcal disease in West Africa. Proc. Natl Acad. Sci. USA 115 , 5510–5515 (2018).
Dong, N., Yang, X., Zhang, R., Chan, E. W. & Chen, S. Tracking microevolution events among ST11 carbapenemase-producing hypervirulent Klebsiella pneumoniae outbreak strains. Emerg. Microbes Infect. 7 , 146 (2018).
Rhodes, J. et al. Genomic epidemiology of the UK outbreak of the emerging human fungal pathogen Candida auris . Emerg. Microbes Infect. 7 , 43 (2018).
Hamner, S. et al. Metagenomic profiling of microbial pathogens in the Little Bighorn River, Montana. Int. J. Environ. Res. Public Health 16 , 1097 (2019).
Boykin, L. M. et al. Tree Lab: portable genomics for early detection of plant viruses and pests in sub-Saharan Africa. Genes 10 , 632 (2019).
Zaaijer, S. et al. Rapid re-identification of human samples using portable DNA sequencing. eLife 6 , e27798 (2017).
Runtuwene, L. R., Tuda, J. S. B., Mongan, A. E. & Suzuki, Y. On-site MinION sequencing. Adv. Exp. Med. Biol. 1129 , 143–150 (2019).
Sutton, M. A. et al. Radiation tolerance of nanopore sequencing technology for life detection on Mars and Europa. Sci. Rep. 9 , 5370 (2019).
Castro-Wallace, S. L. et al. Nanopore DNA sequencing and genome assembly on the International Space Station. Sci. Rep. 7 , 18022 (2017).
Ducluzeau, A., Lekanoff, R. M., Khalsa, N. S., Smith, H. H. & Drown, D. M. Introducing DNA sequencing to the next generation on a research vessel sailing the Bering Sea through a storm. Preprint at Preprints https://doi.org/10.20944/preprints201905.0113.v1 (2019).
Edwards, A. et al. In-field metagenome and 16S rRNA gene amplicon nanopore sequencing robustly characterize glacier microbiota. Preprint at bioRxiv https://doi.org/10.1101/073965 (2019).
Blanco, M. B. et al. Next-generation technologies applied to age-old challenges in Madagascar. Conserv. Genet. 21 , 785–793 (2020).
Pushkarev, D., Neff, N. F. & Quake, S. R. Single-molecule sequencing of an individual human genome. Nat. Biotechnol. 27 , 847–850 (2009).
Merchant, C. A. et al. DNA translocation through graphene nanopores. Nano Lett. 10 , 2915–2921 (2010).
Schneider, G. F. et al. DNA translocation through graphene nanopores. Nano Lett. 10 , 3163–3167 (2010).
Garaj, S. et al. Graphene as a subnanometre trans-electrode membrane. Nature 467 , 190–193 (2010).
Novoselov, K. S. et al. Electric field effect in atomically thin carbon films. Science 306 , 666–669 (2004).
Gershow, M. & Golovchenko, J. A. Recapturing and trapping single molecules with a solid-state nanopore. Nat. Nanotechnol. 2 , 775–779 (2007).
Seo, J. S. et al. De novo assembly and phasing of a Korean human genome. Nature 538 , 243–247 (2016).
Boza, V., Peresini, P., Brejova, B. & Vinar, T. DeepNano-blitz: a fast base caller for MinION nanopore sequencers. Bioinformatics 36 , 4191–4192 (2020).
Stoiber, M. & Brown, J. BasecRAWller: streaming nanopore basecalling directly from raw signal. Preprint at bioRxiv https://doi.org/10.1101/133058 (2017).
Wang, S., Li, Z., Yu, Y. & Gao, X. WaveNano: a signal-level nanopore base-caller via simultaneous prediction of nucleotide labels and move labels through bi-directional WaveNets. Quant. Biol. 6 , 359–368 (2018).
Article CAS Google Scholar
Miculinić, N., Ratković, M. & Šikić, M. MinCall-MinION end2end convolutional deep learning basecaller. Preprint at https://arxiv.org/abs/1904.10337 (2019).
Zhang, Y. et al. Nanopore basecalling from a perspective of instance segmentation. BMC Bioinformatics 21 , 136 (2020).
Lv, X., Chen, Z., Lu, Y. & Yang, Y. An end-to-end Oxford Nanopore basecaller using convolution-augmented transformer. 2020 IEEE Intl. Conf. Bioinformatics and Biomedicine (BIBM) 1 , 337–342 (2020).
Huang, N., Nie, F., Ni, P., Luo, F. & Wang, J. SACall: a neural network basecaller for Oxford Nanopore sequencing data based on self-attention mechanism. IEEE/ACM Trans. Comput. Biol. Bioinform . https://doi.org/10.1109/TCBB.2020.3039244 (2020).
Konishi, H., Yamaguchi, R., Yamaguchi, K., Furukawa, Y. & Imoto, S. Halcyon: an accurate basecaller exploiting an encoder-decoder model with monotonic attention. Bioinformatics 37 , 1211–1217 (2021).
Xu, Z. et al. Fast-Bonito: a faster basecaller for nanopore sequencing. Preprint at bioRxiv https://doi.org/10.1101/2020.10.08.318535 (2020).
Fukasawa, Y., Ermini, L., Wang, H., Carty, K. & Cheung, M. S. LongQC: a quality control tool for third generation sequencing long read data. G3 10 , 1193–1196 (2020).
Leger, A. & Leonardi, T. pycoQC, interactive quality control for Oxford Nanopore Sequencing. J. Open Source Softw. 4 , 1236 (2019).
Lanfear, R., Schalamun, M., Kainer, D., Wang, W. & Schwessinger, B. MinIONQC: fast and simple quality control for MinION sequencing data. Bioinformatics 35 , 523–525 (2019).
Yin, Z. et al. RabbitQC: high-speed scalable quality control for sequencing data. Bioinformatics 37 , 573–574 (2021).
Tardaguila, M. et al. SQANTI: extensive characterization of long-read transcript sequences for quality control in full-length transcriptome identification and quantification. Genome Res. 28 , 396–411 (2018).
Ferguson, J. M. & Smith, M. A. SquiggleKit: a toolkit for manipulating nanopore signal data. Bioinformatics 35 , 5372–5373 (2019).
Cheetham, S. W., Kindlova, M. & Ewing, A. D. Methylartist: tools for visualising modified bases from nanopore sequence data. Preprint at bioRxiv https://doi.org/10.1101/2021.07.22.453313 (2021).
Su, S. et al. NanoMethViz: an R/Bioconductor package for visualizing long-read methylation data. Preprint at bioRxiv https://doi.org/10.1101/2021.01.18.426757 (2021).
De Coster, W., Stovner, E. B. & Strazisar, M. Methplotlib: analysis of modified nucleotides from nanopore sequencing. Bioinformatics 36 , 3236–3238 (2020).
Pratanwanich, P. N. et al. Identification of differential RNA modifications from nanopore direct RNA sequencing with xPore. Nat. Biotechnol . https://doi.org/10.1038/s41587-021-00949-w (2021).
Leger, A. et al. RNA modifications detection by comparative Nanopore direct RNA sequencing. Preprint at bioRxiv https://doi.org/10.1101/843136 (2019).
Gao, Y. et al. Quantitative profiling of N 6 -methyladenosine at single-base resolution in stem-differentiating xylem of Populus trichocarpa using Nanopore direct RNA sequencing. Genome Biol. 22 , 22 (2021).
Parker, M. T., Barton, G. J. & Simpson, G. G. Yanocomp: robust prediction of m 6 A modifications in individual nanopore direct RNA reads. Preprint at bioRxiv https://doi.org/10.1101/2021.06.15.448494 (2021).
Price, A. M. et al. Direct RNA sequencing reveals m 6 A modifications on adenovirus RNA are necessary for efficient splicing. Nat. Commun. 11 , 6016 (2020).
Miclotte, G. et al. Jabba: hybrid error correction for long sequencing reads. Algorithms Mol. Biol. 11 , 10 (2016).
Lee, H. et al. Error correction and assembly complexity of single molecule sequencing reads. Preprint at bioRxiv https://doi.org/10.1101/006395 (2014).
Morisse, P., Lecroq, T. & Lefebvre, A. Hybrid correction of highly noisy long reads using a variable-order de Bruijn graph. Bioinformatics 34 , 4213–4222 (2018).
Madoui, M. A. et al. Genome assembly using Nanopore-guided long and error-free DNA reads. BMC Genomics 16 , 327 (2015).
Holley, G. et al. Ratatosk: hybrid error correction of long reads enables accurate variant calling and assembly. Genome Biol. 22 , 28 (2021).
Koren, S. et al. Hybrid error correction and de novo assembly of single-molecule sequencing reads. Nat. Biotechnol. 30 , 693–700 (2012).
Hackl, T., Hedrich, R., Schultz, J. & Forster, F. proovread: large-scale high-accuracy PacBio correction through iterative short read consensus. Bioinformatics 30 , 3004–3011 (2014).
Firtina, C., Bar-Joseph, Z., Alkan, C. & Cicek, A. E. Hercules: a profile HMM-based hybrid error correction algorithm for long reads. Nucleic Acids Res. 46 , e125 (2018).
Haghshenas, E., Hach, F., Sahinalp, S. C. & Chauve, C. CoLoRMap: correcting long reads by mapping short reads. Bioinformatics 32 , i545–i551 (2016).
Tischler, G. & Myers, E. W. Non hybrid long read consensus using local de Bruijn graph assembly. Preprint at bioRxiv https://doi.org/10.1101/106252 (2017).
Xiao, C. L. et al. MECAT: fast mapping, error correction, and de novo assembly for single-molecule sequencing reads. Nat. Methods 14 , 1072–1074 (2017).
Chin, C. S. et al. Nonhybrid, finished microbial genome assemblies from long-read SMRT sequencing data. Nat. Methods 10 , 563–569 (2013).
Bao, E., Xie, F., Song, C. & Song, D. FLAS: fast and high-throughput algorithm for PacBio long-read self-correction. Bioinformatics 35 , 3953–3960 (2019).
Nowoshilow, S. et al. The axolotl genome and the evolution of key tissue formation regulators. Nature 554 , 50–55 (2018).
Wang, L., Qu, L., Yang, L., Wang, Y. & Zhu, H. NanoReviser: an error-correction tool for nanopore sequencing based on a deep learning algorithm. Front. Genet. 11 , 900 (2020).
Broseus, L. et al. TALC: transcript-level aware long-read correction. Bioinformatics 36 , 5000–5006 (2020).
Sahlin, K. & Medvedev, P. Error correction enables use of Oxford Nanopore technology for reference-free transcriptome analysis. Nat. Commun. 12 , 2 (2021).
Li, H. & Durbin, R. Fast and accurate short read alignment with Burrows–Wheeler transform. Bioinformatics 25 , 1754–1760 (2009).
Ren, J. & Chaisson, M. J. P. lra: a long read aligner for sequences and contigs. PLoS Comput. Biol. 17 , e1009078 (2021).
Jain, C., Rhie, A., Hansen, N. F., Koren, S. & Phillippy, A. M. A long read mapping method for highly repetitive reference sequences. Preprint at bioRxiv https://doi.org/10.1101/2020.11.01.363887 (2020).
Jain, C., Koren, S., Dilthey, A., Phillippy, A. M. & Aluru, S. A fast adaptive algorithm for computing whole-genome homology maps. Bioinformatics 34 , i748–i756 (2018).
Amin, M. R., Skiena, S. & Schatz, M. C. NanoBLASTer: fast alignment and characterization of Oxford Nanopore single molecule sequencing reads. In 2016 IEEE 6th International Conference on Computational Advances in Bio and Medical Sciences 1–6 (ICCABS, 2016).
Yang, W. & Wang, L. Fast and accurate algorithms for mapping and aligning long reads. J. Comput. Biol. 28 , 789–803 (2021).
Rautiainen, M. & Marschall, T. GraphAligner: rapid and versatile sequence-to-graph alignment. Genome Biol. 21 , 253 (2020).
Wei, Z. G., Zhang, S. W. & Liu, F. smsMap: mapping single molecule sequencing reads by locating the alignment starting positions. BMC Bioinformatics 21 , 341 (2020).
Haghshenas, E., Sahinalp, S. C. & Hach, F. lordFAST: sensitive and fast alignment search tool for long noisy read sequencing data. Bioinformatics 35 , 20–27 (2019).
Chakraborty, A., Morgenstern, B. & Bandyopadhyay, S. S-conLSH: alignment-free gapped mapping of noisy long reads. BMC Bioinformatics 22 , 64 (2021).
Joshi, D., Mao, S., Kannan, S. & Diggavi, S. QAlign: aligning nanopore reads accurately using current-level modeling. Bioinformatics 37 , 625–633 (2021).
Boratyn, G. M., Thierry-Mieg, J., Thierry-Mieg, D., Busby, B. & Madden, T. L. Magic-BLAST, an accurate RNA-seq aligner for long and short reads. BMC Bioinformatics 20 , 405 (2019).
Hou, L. & Wang, Y. DEEP-LONG: a fast and accurate aligner for long RNA-seq. Preprint at Research Square https://doi.org/10.21203/rs.3.rs-79489/v1 (2020).
Sahlin, K. & Mäkinen, V. Accurate spliced alignment of long RNA sequencing reads. Bioinformatics https://doi.org/10.1093/bioinformatics/btab540 (2021).
Chin, C. S. et al. Phased diploid genome assembly with single-molecule real-time sequencing. Nat. Methods 13 , 1050–1054 (2016).
Vaser, R. & Šikić, M. Time- and memory-efficient genome assembly with Raven. Nat. Comput. Sci. 1 , 332–336 (2021).
Chin, C. S. & Khalak, A. Human genome assembly in 100 minutes. Preprint at bioRxiv https://doi.org/10.1101/705616 (2019).
Kamath, G. M., Shomorony, I., Xia, F., Courtade, T. A. & Tse, D. N. HINGE: long-read assembly achieves optimal repeat resolution. Genome Res. 27 , 747–756 (2017).
Jansen, H. J. et al. Rapid de novo assembly of the European eel genome from nanopore sequencing reads. Sci. Rep. 7 , 7213 (2017).
Chen, Y. et al. Efficient assembly of nanopore reads via highly accurate and intact error correction. Nat. Commun. 12 , 60 (2021).
Kolmogorov, M. et al. metaFlye: scalable long-read metagenome assembly using repeat graphs. Nat. Methods 17 , 1103–1110 (2020).
Cheng, H., Concepcion, G. T., Feng, X., Zhang, H. & Li, H. Haplotype-resolved de novo assembly using phased assembly graphs with hifiasm. Nat. Methods 18 , 170–175 (2021).
Vaser, R., Sovic, I., Nagarajan, N. & Sikic, M. Fast and accurate de novo genome assembly from long uncorrected reads. Genome Res. 27 , 737–746 (2017).
Huang, N. et al. NeuralPolish: a novel Nanopore polishing method based on alignment matrix construction and orthogonal Bi-GRU Networks. Bioinformatics 11 , btab354 (2021).
Google Scholar
Shafin, K. et al. Haplotype-aware variant calling enables high accuracy in nanopore long-reads using deep neural networks. Preprint at bioRxiv https://doi.org/10.1101/2021.03.04.433952 (2021).
Zimin, A. V. & Salzberg, S. L. The genome polishing tool POLCA makes fast and accurate corrections in genome assemblies. PLoS Comput. Biol. 16 , e1007981 (2020).
Heller, D. & Vingron, M. SVIM: structural variant identification using mapped long reads. Bioinformatics 35 , 2907–2915 (2019).
Cleal, K. & Baird, D. M. Dysgu: efficient structural variant calling using short or long reads. Preprint at bioRxiv https://doi.org/10.1101/2021.05.28.446147 (2021).
Leung, H. C. et al. SENSV: detecting structural variations with precise breakpoints using low-depth WGS data from a single Oxford Nanopore MinION flowcell. Preprint at bioRxiv https://doi.org/10.1101/2021.04.20.440583 (2021).
Jiang, T. et al. Long-read-based human genomic structural variation detection with cuteSV. Genome Biol. 21 , 189 (2020).
Feng, Z., Clemente, J. C., Wong, B. & Schadt, E. E. Detecting and phasing minor single-nucleotide variants from long-read sequencing data. Nat. Commun. 12 , 3032 (2021).
Popitsch, N., Preuner, S. & Lion, T. Nanopanel2 calls phased low-frequency variants in Nanopore panel sequencing data. Bioinformatics 16 , btab526 (2021).
Luo, R. et al. Exploring the limit of using a deep neural network on pileup data for germline variant calling. Nat. Mach. Intell. 2 , 220–227 (2020).
Edge, P., Bafna, V. & Bansal, V. HapCUT2: robust and accurate haplotype assembly for diverse sequencing technologies. Genome Res. 27 , 801–812 (2017).
Shaw, J. & Yu, Y. W. Practical probabilistic and graphical formulations of long-read polyploid haplotype phasing. Preprint at bioRxiv https://doi.org/10.1101/2020.11.06.371799 (2021).
Klasberg, S., Schmidt, A. H., Lange, V. & Schofl, G. DR2S: an integrated algorithm providing reference-grade haplotype sequences from heterozygous samples. BMC Bioinformatics 22 , 236 (2021).
Zhou, W. et al. Identification and characterization of occult human-specific LINE-1 insertions using long-read sequencing technology. Nucleic Acids Res. 48 , 1146–1163 (2020).
Giesselmann, P. et al. Analysis of short tandem repeat expansions and their methylation state with nanopore sequencing. Nat. Biotechnol. 37 , 1478–1481 (2019).
Marchet, C. et al. De novo clustering of long reads by gene from transcriptomics data. Nucleic Acids Res. 47 , e2 (2019).
Sahlin, K. & Medvedev, P. De novo clustering of long-read transcriptome data using a greedy, quality value-based algorithm. J. Comput. Biol. 27 , 472–484 (2020).
Tian, L. et al. Comprehensive characterization of single cell full-length isoforms in human and mouse with long-read sequencing. Preprint at bioRxiv https://doi.org/10.1101/2020.08.10.243543 (2020).
Hu, Y. et al. LIQA: long-read isoform quantification and analysis. Genome Biol. 22 , 182 (2021).
Rautiainen, M. et al. AERON: transcript quantification and gene-fusion detection using long reads. Preprint at bioRxiv https://doi.org/10.1101/2020.01.27.921338 (2020).
Weirather, J. L. et al. Characterization of fusion genes and the significantly expressed fusion isoforms in breast cancer by hybrid sequencing. Nucleic Acids Res. 43 , e116 (2015).
Davidson, N. M. et al. JAFFAL: detecting fusion genes with long read transcriptome sequencing. Preprint at bioRxiv https://doi.org/10.1101/2021.04.26.441398 (2021).
Liu, Q. et al. LongGF: computational algorithm and software tool for fast and accurate detection of gene fusions by long-read transcriptome sequencing. BMC Genomics 21 , 793 (2020).
Deonovic, B., Wang, Y., Weirather, J., Wang, X. J. & Au, K. F. IDP-ASE: haplotyping and quantifying allele-specific expression at the gene and gene isoform level by hybrid sequencing. Nucleic Acids Res. 45 , e32 (2017).
Glinos, D. A. et al. Transcriptome variation in human tissues revealed by long-read sequencing. Preprint at bioRxiv https://doi.org/10.1101/2021.01.22.427687 (2021).
Calus, S. T., Ijaz, U. Z. & Pinto, A. J. NanoAmpli-Seq: a workflow for amplicon sequencing for mixed microbial communities on the nanopore sequencing platform. Gigascience 7 , giy140 (2018).
Karst, S. M. et al. High-accuracy long-read amplicon sequences using unique molecular identifiers with Nanopore or PacBio sequencing. Nat. Methods 18 , 165–169 (2021).
Gilpatrick, T. et al. Targeted nanopore sequencing with Cas9-guided adapter ligation. Nat. Biotechnol. 38 , 433–438 (2020).
Cheetham, S. W. et al. Single-molecule simultaneous profiling of DNA methylation and DNA–protein interactions with Nanopore-DamID. Preprint at bioRxiv https://doi.org/10.1101/2021.08.09.455753 (2021).
Hennion, M. et al. FORK-seq: replication landscape of the Saccharomyces cerevisiae genome by nanopore sequencing. Genome Biol. 21 , 125 (2020).
Philpott, M. et al. Nanopore sequencing of single-cell transcriptomes with scCOLOR-seq. Nat. Biotechnol . https://doi.org/10.1038/s41587-021-00965-w (2021).
Gupta, I. et al. Single-cell isoform RNA sequencing characterizes isoforms in thousands of cerebellar cells. Nat. Biotechnol . 36 , 1197–1202 (2018).
Lebrigand, K., Magnone, V., Barbry, P. & Waldmann, R. High throughput error corrected Nanopore single cell transcriptome sequencing. Nat. Commun. 11 , 4025 (2020).
Bizuayehu, T. T., Labun, K., Jefimov, K. & Valen, E. Single molecule structure sequencing reveals RNA structural dependencies, breathing and ensembles. Preprint at bioRxiv https://doi.org/10.1101/2020.05.18.101402 (2020).
Drexler, H. L. et al. Revealing nascent RNA processing dynamics with nano-COP. Nat. Protoc. 16 , 1343–1375 (2021).
Download references
Acknowledgements
K.F.A., Yunhao Wang, Y.Z., A.B. and Yuru Wang are grateful for support from an institutional fund of the Department of Biomedical Informatics, The Ohio State University, and the National Institutes of Health (R01HG008759, R01HG011469 and R01GM136886). The authors apologize to colleagues whose studies were not cited due to length and reference constraints. The authors also apologize that the very latest research published during the publication process of this article was not included. We would like to thank K. Aschheim and G. Riddihough for critical reading and editing of the manuscript.
Author information
These authors contributed equally: Yunhao Wang, Yue Zhao, Audrey Bollas.
Authors and Affiliations
Department of Biomedical Informatics, The Ohio State University, Columbus, OH, USA
Yunhao Wang, Yue Zhao, Audrey Bollas, Yuru Wang & Kin Fai Au
Biomedical Informatics Shared Resources, The Ohio State University, Columbus, OH, USA
Yue Zhao & Kin Fai Au
You can also search for this author in PubMed Google Scholar
Contributions
K.F.A. designed the outline of the article. Yunhao Wang and A.B. collected information and prepared the materials for the ‘ Technology development ’ and ‘ Data analysis ’ sections. Y.Z. collected information and prepared the materials for the ‘ Applications of nanopore sequencing ’ section. K.F.A., Yunhao Wang, Y.Z. and A.B. wrote and revised the main text. Yuru Wang collected the references for the ‘ Applications of nanopore sequencing ’ section and prepared Fig. 1 .
Corresponding author
Correspondence to Kin Fai Au .
Ethics declarations
Competing interests.
K.F.A. was invited by ONT to present at the conference London Calling 2020.
Additional information
Publisher’s note Springer Nature remains neutral with regard to jurisdictional claims in published maps and institutional affiliations.
Supplementary information
Supplementary table.
Supplementary Table 1.
Rights and permissions
Reprints and permissions
About this article
Cite this article.
Wang, Y., Zhao, Y., Bollas, A. et al. Nanopore sequencing technology, bioinformatics and applications. Nat Biotechnol 39 , 1348–1365 (2021). https://doi.org/10.1038/s41587-021-01108-x
Download citation
Received : 09 December 2019
Accepted : 22 September 2021
Published : 08 November 2021
Issue Date : November 2021
DOI : https://doi.org/10.1038/s41587-021-01108-x
Share this article
Anyone you share the following link with will be able to read this content:
Sorry, a shareable link is not currently available for this article.
Provided by the Springer Nature SharedIt content-sharing initiative
This article is cited by
A comparison of methods for detecting dna methylation from long-read sequencing of human genomes.
- Brynja D. Sigurpalsdottir
- Olafur A. Stefansson
- Kari Stefansson
Genome Biology (2024)
Unraveling metagenomics through long-read sequencing: a comprehensive review
- Chankyung Kim
- Monnat Pongpanich
- Thantrira Porntaveetus
Journal of Translational Medicine (2024)
Genomic characterization, in vitro, and preclinical evaluation of two microencapsulated lytic phages VB_ST_E15 and VB_ST_SPNIS2 against clinical multidrug-resistant Salmonella serovars
- Reem A. Youssef
- Masarra M. Sakr
- Khaled M. Aboshanab
Annals of Clinical Microbiology and Antimicrobials (2024)
RUBICON: a framework for designing efficient deep learning-based genomic basecallers
- Gagandeep Singh
- Mohammed Alser
Understanding blaNDM-1 gene regulation in CRKP infections: toward novel antimicrobial strategies for hospital-acquired pneumonia
Molecular Medicine (2024)
Quick links
- Explore articles by subject
- Guide to authors
- Editorial policies
Sign up for the Nature Briefing: Translational Research newsletter — top stories in biotechnology, drug discovery and pharma.

This paper is in the following e-collection/theme issue:
Published on 17.4.2024 in Vol 26 (2024)
Service Quality and Residents’ Preferences for Facilitated Self-Service Fundus Disease Screening: Cross-Sectional Study
Authors of this article:

Original Paper
- Senlin Lin 1, 2, 3 * , MSc ;
- Yingyan Ma 1, 2, 3, 4 * , PhD ;
- Yanwei Jiang 5 * , MPH ;
- Wenwen Li 6 , PhD ;
- Yajun Peng 1, 2, 3 , BA ;
- Tao Yu 1, 2, 3 , BA ;
- Yi Xu 1, 2, 3 , MD ;
- Jianfeng Zhu 1, 2, 3 , MD ;
- Lina Lu 1, 2, 3 , MPH ;
- Haidong Zou 1, 2, 3, 4 , MD
1 Shanghai Eye Diseases Prevention &Treatment Center/ Shanghai Eye Hospital, School of Medicine, Tongji University, Shanghai, China
2 National Clinical Research Center for Eye Diseases, Shanghai, China
3 Shanghai Engineering Research Center of Precise Diagnosis and Treatment of Eye Diseases, Shanghai, China
4 Shanghai General Hospital, School of Medicine, Shanghai Jiao Tong University, Shanghai, China
5 Shanghai Hongkou Center for Disease Control and Prevention, Shanghai, China
6 School of Management, Fudan University, Shanghai, China
*these authors contributed equally
Corresponding Author:
Haidong Zou, MD
Shanghai Eye Diseases Prevention &Treatment Center/ Shanghai Eye Hospital
School of Medicine
Tongji University
No 1440, Hongqqiao Road
Shanghai, 200336
Phone: 86 02162539696
Email: [email protected]
Background: Fundus photography is the most important examination in eye disease screening. A facilitated self-service eye screening pattern based on the fully automatic fundus camera was developed in 2022 in Shanghai, China; it may help solve the problem of insufficient human resources in primary health care institutions. However, the service quality and residents’ preference for this new pattern are unclear.
Objective: This study aimed to compare the service quality and residents’ preferences between facilitated self-service eye screening and traditional manual screening and to explore the relationships between the screening service’s quality and residents’ preferences.
Methods: We conducted a cross-sectional study in Shanghai, China. Residents who underwent facilitated self-service fundus disease screening at one of the screening sites were assigned to the exposure group; those who were screened with a traditional fundus camera operated by an optometrist at an adjacent site comprised the control group. The primary outcome was the screening service quality, including effectiveness (image quality and screening efficiency), physiological discomfort, safety, convenience, and trustworthiness. The secondary outcome was the participants’ preferences. Differences in service quality and the participants’ preferences between the 2 groups were compared using chi-square tests separately. Subgroup analyses for exploring the relationships between the screening service’s quality and residents’ preference were conducted using generalized logit models.
Results: A total of 358 residents enrolled; among them, 176 (49.16%) were included in the exposure group and the remaining 182 (50.84%) in the control group. Residents’ basic characteristics were balanced between the 2 groups. There was no significant difference in service quality between the 2 groups (image quality pass rate: P =.79; average screening time: P =.57; no physiological discomfort rate: P =.92; safety rate: P =.78; convenience rate: P =.95; trustworthiness rate: P =.20). However, the proportion of participants who were willing to use the same technology for their next screening was significantly lower in the exposure group than in the control group ( P <.001). Subgroup analyses suggest that distrust in the facilitated self-service eye screening might increase the probability of refusal to undergo screening ( P =.02).
Conclusions: This study confirms that the facilitated self-service fundus disease screening pattern could achieve good service quality. However, it was difficult to reverse residents’ preferences for manual screening in a short period, especially when the original manual service was already excellent. Therefore, the digital transformation of health care must be cautious. We suggest that attention be paid to the residents’ individual needs. More efficient man-machine collaboration and personalized health management solutions based on large language models are both needed.
Introduction
Vision impairment and blindness are caused by a variety of eye diseases, including cataracts, glaucoma, uncorrected refractive error, age-related macular degeneration, diabetic retinopathy, and other eye diseases [ 1 ]. They not only reduce economic productivity but also harm the quality of life and increase mortality [ 2 - 6 ]. In 2020, an estimated 43.3 million individuals were blind, and 1.06 billion individuals aged 50 years and older had distance or near vision impairment [ 7 ]. With an increase in the aging population, the number of individuals affected by vision loss has increased substantially [ 1 ].
High-quality public health care for eye disease prevention, such as effective screening, can assist in eliminating approximately 57% of all blindness cases [ 8 ]. Digital technologies, such as telemedicine, 5G telecommunications, the Internet of Things, and artificial intelligence (AI), have provided the potential to improve the accessibility, availability, and productivity of existing resources and the overall efficiency of eye care services [ 9 , 10 ]. The use of digital technology not only reduces the cost of eye disease screening and improves its efficiency, but also assists residents living in remote areas to gain access to eye disease screening [ 11 - 13 ]. Therefore, an increasing number of countries (or regions) are attempting to establish eye screening systems based on digital technology [ 9 ].
Fundus photography is the most important examination in eye disease screening because the vast majority of diagnoses of blinding retinal diseases are based on fundus photographs. Diagnoses can be made by human experts or AI software. However, traditional fundus cameras must be operated by optometrists, who are usually in short supply in primary health care institutions when faced with the large demand for screening services.
Fortunately, the fully automatic fundus camera has been developed on the basis of digital technologies including AI, industrial automation, sensors, and voice navigation. It can automatically identify the person’s left and right eyes, search for pupils, adjust the lens position and shooting focus, and provide real-time voice feedback during the process, helping the residents to understand the current inspection steps clearly and cooperatively complete the inspection. Therefore, a facilitated self-service eye screening pattern has been newly established in 2022 in Shanghai, China.
However, evidence is inadequate about whether this new screening pattern performs well and whether the residents prefer it. Therefore, this cross-sectional study aims to compare the service quality and residents’ preferences of this new screening pattern with that of the traditional screening pattern. We aimed to (1) investigate whether the facilitated self-service eye screening can achieve service quality similar to that of traditional manual screening, (2) compare residents’ preferences between the facilitated self-service eye screening and traditional manual screening, and (3) explore the relationship between the screening service quality and residents’ preferences.
Study Setting
This study was conducted in Shanghai, China, in 2022. Since 2010, Shanghai has conducted an active community-based fundus disease telemedicine screening program. After 2018, an AI model was adopted ( Figure 1 ). At the end of 2021, the fully automatic fundus camera was adopted, and the facilitated self-service fundus disease screening pattern was established ( Figure 1 ). Within this new pattern, residents could perform fundus photography by themselves without professionals’ assistance ( Multimedia Appendix 1 ). The fundus images were sent to the cloud server center of the AI model, and the screening results were fed back immediately.

Study Design
We conducted a cross-sectional study at 2 adjacent screening sites. These 2 sites were expected to be very similar in terms of their socioeconomic and educational aspects since they were located next to each other. One site provided facilitated self-service fundus disease screening, and the residents who participated therein comprised the exposure group; the other site provided screening with a traditional fundus camera operated by an optometrist, and the residents who participated therein comprised the control group. All the adult residents could participant in our screening program, but their data were used for analysis only if they signed the informed consent form. Residents could opt out of the study at any time during the screening.
In the exposure group, the residents were assessed using an updated version of the nonmydriatic fundus camera Kestrel 3100m (Shanghai Top View Industrial Co Ltd) with a self-service module. In the process of fundus photography, the residents pressed the “Start” button by themselves. All checking steps (including focusing, shooting, and image quality review) were undertaken automatically by the fundus camera ( Figure 2 ). Screening data were transmitted to the AI algorithm on a cloud-based server center through the telemedicine platform, and the screening results were fed back immediately. Residents were fully informed that the assessment was fully automated and not performed by the optometrist.

In the control group, the residents were assessed using the basic version of the same nonmydriatic fundus camera. The optical components were identical to those in the exposure group but without the self-service module. In the process of fundus photography, all steps were carried out by the optometrist (including focusing, shooting, and image quality review). Screening data were transmitted to the AI algorithm on a cloud-based server center through the telemedicine platform, and the screening results were fed back immediately. Residents were also fully informed.
Measures and Outcomes
The primary outcome was the screening service’s quality. Based on the World Health Organization’s recommendations for the evaluation of AI-based medical devices [ 14 ] and the European Union’s Assessment List for Trustworthy Artificial Intelligence [ 15 ], 5 dimensions were selected to reflect the service quality of eye disease screening: effectiveness, physiological discomfort, safety, convenience, and trustworthiness.
Furthermore, effectiveness was based on 2 indicators: image quality and screening efficiency. A staff member recorded the time required for each resident to take fundus photographs (excluding the time taken for diagnosis) at the screening site. Then, a professional ophthalmologist evaluated the quality of each fundus photograph after the on-site experiment. The ophthalmologist was blinded to the grouping of participants. Image quality was assessed on the basis of the image quality pass rate, expressed as the number of eyes with high-quality fundus images per 100 eyes. Screening efficiency was assessed on the basis of the average screening time, expressed as the mean of the time required for each resident to take fundus photographs.
To assess physiological discomfort, safety, convenience, and trustworthiness of screening services, residents were asked to finish a questionnaire just after they received the screening results. A 5-point Likert scale was adopted for each dimension, from the best to the worst, except for the physiological discomfort ( Multimedia Appendix 2 ). A no physiological discomfort rate was expressed as the number of residents who chose the “There is no physiological discomfort during the screening” per 100 individuals in each group. Safety rate is expressed as the number of residents who chose “The screening is very safe” or “The screening is safe” per 100 individuals in each group. Convenience rate is expressed as the number of residents who chose “The screening is very convenient” or “The screening is convenient” per 100 individuals in each group. The trustworthiness rate is expressed as the number of residents who chose “The screening result is very trustworthy” or “The screening result is trustworthy” per 100 individuals in each group.
The secondary outcome was the preference rate, expressed as the number of residents who were willing to use the same technology for their next screening per 100 individuals. In detail, in the exposure group, the preference rate was expressed as the number of the residents who preferred facilitated self-service eye screening per 100 individuals, while in the control group, it was expressed as the number of residents who preferred traditional manual screening per 100 individuals.
To understand the residents’ preference, a video displaying the processes of both facilitated self-service eye screening and traditional manual screening was shown to the residents. Then, the following question was asked: “At your next eye disease screening, you can choose either facilitated self-service eye screening or traditional manual screening. Which one do you prefer?” A total of 4 alternatives were set: “Prefer traditional manual screening,” “Prefer facilitated self-service eye screening,” “Both are acceptable,” and “Neither is acceptable (Refusal of screening).” Each resident could choose only 1 option, which best reflected their preference.
Sample Size
The rule of events per variable was used for sample size estimation. In this study, 2 logit models were established for the 2 groups separately, each containing 8 independent variables. We set 10 events per variable in general. According to a previous study [ 16 ], when the decision-making process had high uncertainty, the proportion of individuals who preferred the algorithms was about 50%. This led us to arrive at a sample size of 160 (8 variables multiplied by 10 events each, with 50% of individuals potentially preferring facilitated screening [ie, 50% of 8×10]) for each group.
Every dimension of the screening service quality and the preference rate were calculated separately. Chi-square and t tests were used to test whether the service quality or the residents’ preferences differed between the 2 groups. A total of 7 hypotheses were tested, as shown in Textbox 1 .
- H1: image quality pass rate exposure group ≠ image quality pass rate control group H0: image quality pass rate exposure group =image quality pass rate control group
- H1: screening time exposure group ≠screening time control group H0: screening time exposure group =screening time control group
- H1: no discomfort rate exposure group ≠no discomfort rate control group H0: no discomfort rate exposure group = no discomfort rate control group
- H1: safety rate exposure group ≠safety rate control group H0: safety rate exposure group = safety rate control group
- H1: convenience rate exposure group ≠convenience rate control group H0: convenience rate exposure group = convenience rate control group
- H1: trustworthiness rate exposure group ≠trustworthiness rate control group H0: trustworthiness rate exposure group = trustworthiness rate control group
- H1: preference rate exposure group ≠preference rate control group H0: preference rate exposure group = preference rate control group
If any of the hypotheses among hypotheses 1-6 ( Textbox 1 ) were significant, it indicated that the service quality was different between facilitated self-service eye screening and traditional manual screening. If hypothesis 7 was significant, it meant that the residents’ preference for facilitated self-service eye screening was different from that for traditional manual screening.
Additionally, subgroup analyses in the exposure and control groups were conducted to explore the relationships between the screening service quality and the residents’ preferences, using generalized logit models. The option “Prefer facilitated self-service eye screening” was used as the reference level for the dependent variable in the models. The independent variables included age, sex, image quality, screening efficiency, physiological discomfort, safety, convenience, and trustworthiness. All statistics were performed using SAS (version 9.4; SAS Institute).
Ethical Considerations
The study adhered to the ethical principles of the Declaration of Helsinki and was approved by the Shanghai General Hospital Ethics Committee (2022SQ272). All participants provided written informed consent before participating in this study. The study data were anonymous, and no identification of individual participants in any images of the manuscript or supplementary material is possible.
Participants’ Characteristics
A total of 358 residents enrolled; among them, 176 (49.16%) were in the exposure group and the remaining 182 (50.84%) were in the control group. Residents’ basic characteristics were balanced between the 2 groups. The mean age was 65.05 (SD 12.28) years for the exposure group and 63.96 (SD 13.06) years for the control group; however, this difference was nonsignificant ( P =.42). The proportion of women was 67.05% (n=118) for the exposure group and 62.09% (n=113) for the control group; this difference was also nonsignificant between the 2 groups ( P =.33).
Screening Service Quality
In the exposure group, high-quality fundus images were obtained for 268 out of 352 eyes (image quality pass rate=76.14%; Figure 3 ). The average screening time was 81.03 (SD 36.98) seconds ( Figure 3 ). In the control group, high-quality fundus images were obtained for 274 out of 364 eyes (image quality pass rate=75.27%; Figure 3 ). The average screening time was 78.22 (SD 54.01) seconds ( Figure 3 ). There was no significant difference in the image quality pass rate ( χ 2 1 =0.07, P =.79) and average screening time ( t 321.01 =–0.58 [Welch–Satterthwaite–adjusted df ], P =.56) between the 2 groups ( Figure 3 ).

For the other dimensions, detailed information is shown in Figure 3 . There were no significant differences between any of these rates between the 2 groups (no physiological discomfort rate: χ 2 1 =0.01, P =.92; safety rate: χ 2 1 =0.08, P =.78; convenience rate: χ 2 1 =0.004, P =.95; trustworthiness rate: χ 2 1 =1.63, P =.20).
Residents’ Preferences
In the exposure group, 120 (68.18%) residents preferred traditional manual screening, 19 (10.80%) preferred facilitated self-service eye screening, 19 (10.80%) preferred both, and the remaining 18 (10.23%) preferred neither. In the control group, 123 (67.58%) residents preferred traditional manual screening, 14 (7.69%) preferred facilitated self-service eye screening, 20 (10.99%) preferred both, and the remaining 25 (13.74%) preferred neither.
The proportion of residents who chose the category “Prefer facilitated self-service eye screening” in the exposure group was significantly lower than that of residents who chose the category “Prefer traditional manual screening” in the control group ( χ 2 1 =120.57, P <.001; Figure 3 ).
Subgroup Analyses
In the exposure group, 4 generalized logit models were generated ( Table 1 ). Regarding the effectiveness of facilitated self-service eye screening, neither the image quality nor the screening time had an impact on the residents’ preferences. Regarding the other dimensions for facilitated self-service eye screening service quality, models 3 and 4 demonstrated that distrust in the results of facilitated self-service eye screening might decrease the probability of preferring this screening service and increase the probability of preferring neither of the 2 screening services.
a Age and gender were adjusted in model 1. Age, gender, image quality, and screening efficiency were adjusted in model 2. Age, gender, physiological discomfort, safety, convenience, and trustworthiness were adjusted in model 3. Age, gender, image quality, screening efficiency, physiological discomfort, safety, convenience, and trustworthiness were adjusted in model 4.
b In the exposure group, distrust in the results of facilitated self-service eye screening might decrease the probability of preferring this screening service and increase the probability of preferring neither the traditional nor the facilitated self-service screening services.
c Not available.
In the control group, another 4 generalized logit models were generated ( Table 2 ). Men were more likely to choose a preference both screening services. The probability of preferring manual screening might increase with age, as long as the probability of preferring facilitated self-service eye screening decreased. Regarding the effectiveness of traditional manual screening, neither the image quality pass rate nor the screening time had an impact on the residents’ preferences. For the other dimensions of the quality of traditional manual screening, models 7 and 8 showed that if the residents feel unsafe about traditional manual screening, their preference for traditional manual screening might decrease, and they might turn to facilitated self-service eye screening.
a Age and gender were adjusted in model 5. Age, gender, image quality, and screening efficiency were adjusted in model 6. Age, gender, physiological discomfort, safety, convenience, and trustworthiness were adjusted in model 7. Age, gender, image quality, screening efficiency, physiological discomfort, safety, convenience, and trustworthiness were adjusted in model 8.
b In the control group, if the residents feel unsafe about traditional manual screening, their preference for traditional manual screening might decrease, and they might turn to facilitated self-service eye screening.
A new fundus disease screening pattern was established using the fully automatic fundus camera without any manual intervention. Our findings suggest that facilitated self-service eye screening can achieve a service quality similar to that of traditional manual screening. The study further evaluated the residents’ preferences and associated factors for the newly established self-service fundus disease screening. Our study found that the residents’ preference for facilitated self-service eye screening is significantly less than that for traditional manual screening. This implies that the association between the service quality of the screening technology and residents’ preferences was weak, suggesting that aversion to the algorithm might exist. In addition, the subgroup analyses suggest that even the high quality of facilitated self-service eye screening cannot increase the residents’ preference for this new screening pattern. Worse still, distrust in the results of this new pattern may lead to lower usage of eye disease screening services as a whole. To the best of our knowledge, this study is one of the first to evaluate service quality and residents’ preferences for facilitated self-service fundus disease screening.
Previous studies have suggested that people significantly prefer manual services to algorithms in the field of medicine [ 16 - 18 ]. Individuals have an aversion to algorithms underlying digital technology, especially when they see errors in the algorithm’s functioning [ 18 ]. The preference for algorithms does not increase even if the residents are told that the algorithm outperforms human doctors [ 19 , 20 ]. Our results confirm that fundus image quality in the exposure group is similar to that in the control group in our study, and both are similar to or even better than those reported in previous studies [ 21 , 22 ]. However, the preference for facilitated self-service fundus disease screening is significantly less than that for traditional manual screening. One possible explanation is that uniqueness neglect—a concern that algorithm providers are less able than human providers to account for residents’ (or patients’) unique characteristics and circumstances—drives consumer resistance to digital medical technology [ 23 ]. Therefore, personalized health management solutions based on large language models should be developed urgently [ 24 ] to meet the residents’ individual demands. In addition, a survey of population preferences for medical AI indicated that the most important factor for the public is that physicians are ultimately responsible for diagnosis and treatment planning [ 25 ]. As a result, man-machine collaboration, such as human supervision, is still necessary [ 26 ], especially in the early stages of digital transformation to help residents understand and accept the digital technologies.
Furthermore, our study suggests that distrust in the results of facilitated self-service fundus disease screening may cause residents to abandon eye disease screening, irrespective of whether it is provided using this new screening pattern or via the traditional manual screening pattern. This is critical to digital transformation in medicine. This implies that if the digital technology does not perform well, residents will not only be averse to the digital technology itself but also be more likely to abandon health care services as a whole. Digital transformation is a fundamental change to the health care delivery system. This implies that it can self-disrupt its ability to question the practices and production models of existing health care services. As a result, it may become incompatible with the existing models, processes, activities, and even cultures [ 27 ]. Therefore, it is important to assess whether the adoption of digital technologies contributes to health system objectives in an optimal manner, and this assessment should be carried out at the level of health services but not at the level of digital transformation [ 28 ].
The most prominent limitation of our study is that it was conducted only in Shanghai, China. Because of the sound health care system in Shanghai, residents have already received high-quality eye disease screening services before the adoption of the facilitated self-service eye screening pattern. Consequently, residents are bound to demand more from this new pattern. This situation is quite different from that in lower-income regions. Digital technology was adapted in poverty-stricken areas to build an eye care system, but it did not replace the original system that is based on manually delivered services [ 13 ]. Therefore, the framing effect may be weak [ 29 ], and there is little practical value in comparing digital technology and manual services in these regions. Second, our study is an observational study and blind grouping was not practical due to the special characteristics of fundus examination. However, we have attempted to use blind processing whenever possible. For instance, ophthalmologists’ evaluation of image quality was conducted in a blinded manner. Third, the manner in which we inquired about residents’ preferences might affect the results. For example, participants in the exposure group generally have experience with manual screening, but those in the control group may not have had enough experience with facilitated screening despite having been shown a video. This might make the participants in the control group more likely to choose manual screening because the new technology was unfamiliar. Finally, individual-level socioeconomic factors or educational level were not recorded, so we cannot rule out the influence of these factors on residents’ preferences.
In summary, this study confirms that the facilitated self-service fundus disease screening pattern could achieve high service quality. The preference of the residents for this new mode, however, was not ideal. It was difficult to reverse residents’ preference for manual screening in a short period, especially when the original manual service was already excellent. Therefore, the digital transformation of health care must proceed with caution. We suggest that attention be paid to the residents’ individual needs. Although more efficient man-machine collaboration is necessary to help the public understand and accept new technologies, personalized health management solutions based on large language models are required.
Acknowledgments
This study was funded by the Shanghai Public Health Three-Year Action Plan (GWVI-11.1-30, GWVI-11.1-22), Science and Technology Commission of Shanghai Municipality (20DZ1100200 and 23ZR1481000), Shanghai Municipal Health Commission (2022HP61, 2022YQ051, and 20234Y0062), Shanghai First People's Hospital featured research projects (CCTR-2022C08) and Medical Research Program of Hongkou District Health Commission (Hongwei2202-07).
Data Availability
Data are available from the corresponding author upon reasonable request.
Authors' Contributions
SL, YM, and YJ contributed to the conceptualization and design of the study. SL, YM, YJ, YP, TY, and YX collected the data. SL and YM analyzed the data. SL, YM, and YJ drafted the manuscript. WL, YX, JZ, LL, and HZ extensively revised the manuscript. All authors read and approved the final manuscript submitted.
Conflicts of Interest
None declared.
Video of the non-mydriatic fundus camera Kestrel-3100m with the self-service module.
Questions for screening service quality.
- GBD 2019 BlindnessVision Impairment Collaborators, Vision Loss Expert Group of the Global Burden of Disease Study. Causes of blindness and vision impairment in 2020 and trends over 30 years, and prevalence of avoidable blindness in relation to VISION 2020: the Right to Sight: an analysis for the Global Burden of Disease Study. Lancet Glob Health. Feb 2021;9(2):e144-e160. [ FREE Full text ] [ CrossRef ] [ Medline ]
- Marques AP, Ramke J, Cairns J, Butt T, Zhang JH, Muirhead D, et al. Global economic productivity losses from vision impairment and blindness. EClinicalMedicine. May 2021;35:100852. [ FREE Full text ] [ CrossRef ] [ Medline ]
- Jan C, Li S, Kang M, Liu L, Li H, Jin L, et al. Association of visual acuity with educational outcomes: a prospective cohort study. Br J Ophthalmol. Nov 18, 2019;103(11):1666-1671. [ CrossRef ] [ Medline ]
- Chai YX, Gan ATL, Fenwick EK, Sui AY, Tan BKJ, Quek DQY, et al. Relationship between vision impairment and employment. Br J Ophthalmol. Mar 16, 2023;107(3):361-366. [ CrossRef ] [ Medline ]
- Nayeni M, Dang A, Mao AJ, Malvankar-Mehta MS. Quality of life of low vision patients: a systematic review and meta-analysis. Can J Ophthalmol. Jun 2021;56(3):151-157. [ CrossRef ] [ Medline ]
- Wang L, Zhu Z, Scheetz J, He M. Visual impairment and ten-year mortality: the Liwan Eye Study. Eye (Lond). Aug 19, 2021;35(8):2173-2179. [ FREE Full text ] [ CrossRef ] [ Medline ]
- GBD 2019 BlindnessVision Impairment Collaborators, Vision Loss Expert Group of the Global Burden of Disease Study. Trends in prevalence of blindness and distance and near vision impairment over 30 years: an analysis for the Global Burden of Disease Study. Lancet Glob Health. Feb 2021;9(2):e130-e143. [ FREE Full text ] [ CrossRef ] [ Medline ]
- Cheng C, Wang N, Wong TY, Congdon N, He M, Wang YX, et al. Vision Loss Expert Group of the Global Burden of Disease Study. Prevalence and causes of vision loss in East Asia in 2015: magnitude, temporal trends and projections. Br J Ophthalmol. May 28, 2020;104(5):616-622. [ CrossRef ] [ Medline ]
- Li JO, Liu H, Ting DS, Jeon S, Chan RP, Kim JE, et al. Digital technology, tele-medicine and artificial intelligence in ophthalmology: a global perspective. Prog Retin Eye Res. May 2021;82:100900. [ FREE Full text ] [ CrossRef ] [ Medline ]
- Ting DSW, Pasquale LR, Peng L, Campbell JP, Lee AY, Raman R, et al. Artificial intelligence and deep learning in ophthalmology. Br J Ophthalmol. Feb 25, 2019;103(2):167-175. [ FREE Full text ] [ CrossRef ] [ Medline ]
- Xie Y, Nguyen QD, Hamzah H, Lim G, Bellemo V, Gunasekeran DV, et al. Artificial intelligence for teleophthalmology-based diabetic retinopathy screening in a national programme: an economic analysis modelling study. Lancet Digit Health. May 2020;2(5):e240-e249. [ CrossRef ]
- Tang J, Liang Y, O'Neill C, Kee F, Jiang J, Congdon N. Cost-effectiveness and cost-utility of population-based glaucoma screening in China: a decision-analytic Markov model. Lancet Glob Health. Jul 2019;7(7):e968-e978. [ CrossRef ]
- Xiao X, Xue L, Ye L, Li H, He Y. Health care cost and benefits of artificial intelligence-assisted population-based glaucoma screening for the elderly in remote areas of China: a cost-offset analysis. BMC Public Health. Jun 04, 2021;21(1):1065. [ FREE Full text ] [ CrossRef ] [ Medline ]
- Generating Evidence for Artificial Intelligence Based Medical Devices: A Framework for Training Validation and Evaluation. World Health Organization. URL: https://www.who.int/publications/i/item/9789240038462 [accessed 2024-03-27]
- The Assessment List for Trustworthy Artificial Intelligence. URL: https://altai.insight-centre.org/ [accessed 2024-03-27]
- Dietvorst BJ, Bharti S. People reject algorithms in uncertain decision domains because they have diminishing sensitivity to forecasting error. Psychol Sci. Oct 11, 2020;31(10):1302-1314. [ CrossRef ] [ Medline ]
- DeCamp M, Tilburt JC. Why we cannot trust artificial intelligence in medicine. Lancet Digit Health. Dec 2019;1(8):e390. [ CrossRef ]
- Frank D, Elbæk CT, Børsting CK, Mitkidis P, Otterbring T, Borau S. Drivers and social implications of artificial intelligence adoption in healthcare during the COVID-19 pandemic. PLoS One. Nov 22, 2021;16(11):e0259928. [ FREE Full text ] [ CrossRef ] [ Medline ]
- Juravle G, Boudouraki A, Terziyska M, Rezlescu C. Trust in artificial intelligence for medical diagnoses. Prog Brain Res. 2020;253:263-282. [ CrossRef ] [ Medline ]
- Liu X, Faes L, Kale AU, Wagner SK, Fu DJ, Bruynseels A, et al. A comparison of deep learning performance against health-care professionals in detecting diseases from medical imaging: a systematic review and meta-analysis. Lancet Digit Health. Oct 2019;1(6):e271-e297. [ CrossRef ]
- Scanlon PH, Foy C, Malhotra R, Aldington SJ. The influence of age, duration of diabetes, cataract, and pupil size on image quality in digital photographic retinal screening. Diabetes Care. Oct 2005;28(10):2448-2453. [ FREE Full text ] [ CrossRef ] [ Medline ]
- Cen L, Ji J, Lin J, Ju S, Lin H, Li T, et al. Automatic detection of 39 fundus diseases and conditions in retinal photographs using deep neural networks. Nat Commun. Aug 10, 2021;12(1):4828. [ FREE Full text ] [ CrossRef ] [ Medline ]
- Longoni C, Bonezzi A, Morewedge C. Resistance to medical artificial intelligence. J Consum Res. 2019;46:650. [ CrossRef ]
- Huang AS, Hirabayashi K, Barna L, Parikh D, Pasquale LR. Assessment of a Large Language Model's Responses to Questions and Cases About Glaucoma and Retina Management. JAMA Ophthalmol. Feb 22, 2024. [ FREE Full text ] [ CrossRef ] [ Medline ]
- Ploug T, Sundby A, Moeslund TB, Holm S. Population preferences for performance and explainability of artificial intelligence in health care: choice-based conjoint survey. J Med Internet Res. Dec 13, 2021;23(12):e26611. [ FREE Full text ] [ CrossRef ] [ Medline ]
- Young AT, Amara D, Bhattacharya A, Wei ML. Patient and general public attitudes towards clinical artificial intelligence: a mixed methods systematic review. Lancet Digit Health. Sep 2021;3(9):e599-e611. [ CrossRef ]
- Alami H, Gagnon M, Fortin J. Digital health and the challenge of health systems transformation. Mhealth. Aug 08, 2017;3:31-31. [ FREE Full text ] [ CrossRef ] [ Medline ]
- Ricciardi W, Pita Barros P, Bourek A, Brouwer W, Kelsey T, Lehtonen L, et al. Expert Panel on Effective Ways of Investing in Health (EXPH). How to govern the digital transformation of health services. Eur J Public Health. Oct 01, 2019;29(Supplement_3):7-12. [ FREE Full text ] [ CrossRef ] [ Medline ]
- Khan WU, Shachak A, Seto E. Understanding decision-making in the adoption of digital health technology: the role of behavioral economics' prospect theory. J Med Internet Res. Feb 07, 2022;24(2):e32714. [ FREE Full text ] [ CrossRef ] [ Medline ]
Abbreviations
Edited by A Mavragani; submitted 06.01.23; peer-reviewed by B Li, A Bate, CW Pan; comments to author 13.09.23; revised version received 15.10.23; accepted 12.03.24; published 17.04.24.
©Senlin Lin, Yingyan Ma, Yanwei Jiang, Wenwen Li, Yajun Peng, Tao Yu, Yi Xu, Jianfeng Zhu, Lina Lu, Haidong Zou. Originally published in the Journal of Medical Internet Research (https://www.jmir.org), 17.04.2024.
This is an open-access article distributed under the terms of the Creative Commons Attribution License (https://creativecommons.org/licenses/by/4.0/), which permits unrestricted use, distribution, and reproduction in any medium, provided the original work, first published in the Journal of Medical Internet Research, is properly cited. The complete bibliographic information, a link to the original publication on https://www.jmir.org/, as well as this copyright and license information must be included.
Help | Advanced Search
Computer Science > Computation and Language
Title: leave no context behind: efficient infinite context transformers with infini-attention.
Abstract: This work introduces an efficient method to scale Transformer-based Large Language Models (LLMs) to infinitely long inputs with bounded memory and computation. A key component in our proposed approach is a new attention technique dubbed Infini-attention. The Infini-attention incorporates a compressive memory into the vanilla attention mechanism and builds in both masked local attention and long-term linear attention mechanisms in a single Transformer block. We demonstrate the effectiveness of our approach on long-context language modeling benchmarks, 1M sequence length passkey context block retrieval and 500K length book summarization tasks with 1B and 8B LLMs. Our approach introduces minimal bounded memory parameters and enables fast streaming inference for LLMs.
Submission history
Access paper:.
- HTML (experimental)
- Other Formats
References & Citations
- Google Scholar
- Semantic Scholar
BibTeX formatted citation

Bibliographic and Citation Tools
Code, data and media associated with this article, recommenders and search tools.
- Institution
arXivLabs: experimental projects with community collaborators
arXivLabs is a framework that allows collaborators to develop and share new arXiv features directly on our website.
Both individuals and organizations that work with arXivLabs have embraced and accepted our values of openness, community, excellence, and user data privacy. arXiv is committed to these values and only works with partners that adhere to them.
Have an idea for a project that will add value for arXiv's community? Learn more about arXivLabs .

An official website of the United States government
The .gov means it’s official. Federal government websites often end in .gov or .mil. Before sharing sensitive information, make sure you’re on a federal government site.
The site is secure. The https:// ensures that you are connecting to the official website and that any information you provide is encrypted and transmitted securely.
- Publications
- Account settings
Preview improvements coming to the PMC website in October 2024. Learn More or Try it out now .
- Advanced Search
- Journal List
- HHS Author Manuscripts

Statistical Contributions to Bioinformatics: Design, Modeling, Structure Learning, and Integration
Jeffrey s. morris.
1 Department of Biostatistics, The University of Texas M.D. Anderson Cancer Center, Houston, Texas, USA
Veerabhadran Baladandayuthapani
The advent of high-throughput multi-platform genomics technologies providing whole-genome molecular summaries of biological samples has revolutionalized biomedical research. These technologiees yield highly structured big data, whose analysis poses significant quantitative challenges. The field of Bioinformatics has emerged to deal with these challenges, and is comprised of many quantitative and biological scientists working together to effectively process these data and extract the treasure trove of information they contain. Statisticians, with their deep understanding of variability and uncertainty quantification, play a key role in these efforts. In this article, we attempt to summarize some of the key contributions of statisticians to bioinformatics, focusing on four areas: (1) experimental design and reproducibility, (2) preprocessing and feature extraction, (3) unified modeling, and (4) structure learning and integration. In each of these areas, we highlight some key contributions and try to elucidate the key statistical principles underlying these methods and approaches. Our goals are to demonstrate major ways in which statisticians have contributed to bioinformatics, encourage statisticians to get involved early in methods development as new technologies emerge, and to stimulate future methodological work based on the statistical principles elucidated in this article and utilizing all availble information to uncover new biological insights.
1 Introduction
Rapid technological advances accompanied by a steep decline in experimental costs have led to a proliferation of genomic data across many scientific disciplines and virtually all disease areas. These include high-throughout technologies that can profile genomes, transcriptomes, proteomes and metabolomes at a comprehensive and detailed resolution unimaginable only a couple of decades ago ( Schuster, 2008 ) – a summary of some of the key technologies is presented in Section 2 and Figure 1 . This has led to data generation at an unprecedented scale in various formats, structure and sizes ( Bild et al., 2014 ; Hamid et al., 2009 ) – raising a plethora of analytic and computational challenges. The general term Bioinformatics refers to a multidisciplinary field involving computational biologists, computer scientists, mathematical modelers, systems biologists, and statisticians exploring different facets of the data ranging from storing, retrieving, organizing and subsequent analysis of biological data. Given the myriad challenges posed by this complex field, bioinformatics is necessarily interdisciplinary in nature, as it is not feasible for any single researcher in themselves to possess all clinical, biological, computational, data management, mathematical modeling, and statistical knowledge and skills necessary to optimally discover and validate the vast scientific knowledge contained in the outputs from these technologies.

Illustration of Types of Multi-platform Genomics Data and Their Interrelationships
Statisticians have a unique perspective and skill set that places them at the center of this process. One of the key attributes that sets statisticians apart from other quantitative scientists is their understanding of variability and uncertainty quantification. These are essential considerations in building reproducible methods for biological discovery and validation, especially for complex, high-dimensional data as encountered in genomics. Statisticians are “data scientists” who understand the profound effect of sampling design decisions on downstream analysis, potential propagation of errors from multi-step processing algorithms, and the potential loss of information from overly reductionistic feature extraction approaches. They are experts in inferential reasoning, which equips them to recognize the importance of multiple testing adjustment to avoid reporting spurious results as discoveries, and to properly design algorithms to search high-dimensional spaces and build predictive models while obtaining accurate measures of their predictive accuracy.
While statisticians have been involved in many aspects of bioinformatics, they have been hesitant to get heavily involved in other aspects. For example, many statisticians are primarily interested in end-stage modeling after all of the data already been collected and preprocessed. Statistical expertise in the experimental design and low-level processing stages are equally if not more important than end-stage modeling, since errors and inefficiencies in these steps propagate into subsequent analyses, and can preclude the possibility of making valid discoveries and scientific conclusions even with the best constructed end-stage modeling strategies. This has resulted in a missed opportunity for the statistical community to play a larger leadership role in bioinformatics that in many cases has been instead assumed by other quantitative scientists and computational biologists, and congruously, a missed opportunity for biologists as well, to more efficiently learn true reproducible biological insights from their data.
With statisticians being a little slow to get involved on the front lines of bioinformatics, many basic and advanced statistical principles have been underutilized in the collection and modeling of bioinformatics data. As a result, we see far too many studies with non-replicated false positive results from confounded experimental designs or improper training-validation procedures, even in high-profile journals. Driven by the computational challenges of high dimensionality and out of convenience, many commonly used standard analysis approaches are reductionistic (not modeling the entire data set), ad hoc and algorithmic (not model-based), stepwise and piecemeal (not integrating information together in a statistically efficient way or propagating uncertainty through to the final analysis). Failure to use all of the information in the data increases the risk of missed discoveries. Greater involvement by the statistical community can help improve the efficiency and reproducibility of genomics research.
In spite of missed opportunities, there have been substantial efforts and success stories where (sometimes advanced) statistical tools have been developed for these data, leading to improved results and deep scientific contributions. The goals of this article are to highlight how statistical modeling has and can make a difference in bioinformatics, elucidate the key underlying statistical principles that are relevant in many areas of bioinformatics, and stimulate future methodological development. While drawing some general conclusions, we organize the core of this paper around four key areas:
- Experimental design and reproducible research (Section 3)
- Improved preprocessing and feature extraction (Section 4)
- Flexible and unified modeling (Section 5)
- Structure learning and integration (Section 6)
In this article, we do not attempt to exhaustively summarize the work that has been done, but instead attempt to illustrate contributions and highlight the motivating statistical principles. For each area, we will present case examples, including some high profile research that has significantly impacted bioinformatics practice as well as other works (including some of our own) that illustrate some of the key statistical principles even if not as impactful. Through these examples we will extract and highlight some of the key underlying statistical principles, including randomization and blocking, denoising, borrowing strength across correlated measurements, and unified modeling. Our hope is that the elucidation of these principles will help guide and stimulate future methodological development and increase the role and impact of statistics in bioinformatics.
2 Background
As alluded to above, the advent of high-throughput molecular technologies has revolutionized biomedical science. In this section, we overview some basic data structures generated by molecular platforms at different resolution levels, including mRNA-based (transcriptomics), DNA-based (genomics), protein-based (proteomics), as well as epigenetic factors (e.g. methylation). The key underlying principle is that the biological behavior of cells, normal or diseased, is regulated by molecular processes, and different aspects of these processes are measured by these various platforms.
The basic information flow across the various resolution levels starts with a gene encoded by DNA to messenger RNA (mRNA) by a biological process called transcription and mRNA to protein by a process called translation . This basic process can be regulated and altered through epigenetic processes such as DNA methylation that help regulate transcription, post translational modification of histone proteins within the chromatin structures encasing the DNA, or by micro RNAs (miRNAs) that degrade targeted mRNA. It is through these molecular activities that biological processes are regulated and phenotypes within organisms are determined. A simplified model illustrating these interrelationships is given by Mallick et al. (2009) .
More complicated relationships and feedback loops are being discovered and work off of this fundamental information flow. A schematic of the various data types and some of their interrelationsips is provided in Figure 1 .
To provide a backdrop for our discussion, we briefly overview each of these molecular resolution levels and explain some of the specific data structures generated by the corresponding assays. To make the article accessible to quantitative/statistical readers we eschew some of the biological/technical details and refer the readers to specific references in each section.
2.1 Transcriptomics
Early work in measuring gene mRNA expression data were based on a “one-gene-at-time” process using hybridization based methods ( Gillespie and Spiegelman, 1965 ) such as Northern Blots ( Alwine et al., 1977 ) and Reverse transcription polymerase chain reaction (RT-PCR) experiments. Broadly, the purpose of these low-throughput experiments was to measure the size and abundance of the RNA transcribed for an individual gene using cellular RNA extraction procedures applied to multiple cells from a organism or sample. These experiments were typically time-consuming and involved selection of individual genes to assay expression and were mostly used for hypothesis driven endeavors.
The advent of microarray-based technologies in the mid-1990’s then automated these techniques to simultaneously measure expressions of thousands of genes in parallel. This shifted gene expression analyses from mostly hypothesis driven endeavors to hypothesis generating ones that involve an unbiased exploration of the expression patterns of the entire transcriptome.
The major types of arrays can be predominantly classified into three main categories (see Seidel (2008) for detailed review). The first reported works in microarrays involved spotted microarrays developed at Stanford University ( Schena et al., 1995 ; Shalon et al., 1996 ; Schena et al., 1998 ). Broadly, the process involves printing libraries of PCR products or long oligonucleotide sequences from a set of genes onto glass slides via robotics and then estimating the gene expression intensities through fluorescent tags (see Brown and Botstein (1999) for a detailed review). Other institutions developed laboratories for printing their own spotted microarrays, which had variable data quality given the challenge of reproducible manufacture of the arrays. Affymetrix was the among the first company to standardize the production of microarrays, becoming the most established and widely-used commercial platform for measure high-throughput gene expression data. Their arrays consist of 25-mer oligo-nucleotides synthesized on a glass chip ( Pease et al., 1994 ). As opposed to the single sequence of probes used in spotted microarrays, Affymetrix uses a set of probes to measure and summarize expression of the genes. Subsequently, other companies, including Illumina, Agilent and Nimblegen, have produced microarrays involving in situ synthesis, with each using a different type and length of oligonucleotide as well as photo-chemical process for measurement of gene expression ( Blanchard et al., 1996 ). As described in the next section, the development of more efficient and cost-effective sequencing technologies has led to the use of next generation sequencing (NGS) technologies applied to RNA, RNAseq, as the preferred mode of measuring gene expression.
While each technology has its own characteristics and caveats (see Section 4.1), the basic read outs contain expression level estimates for thousands on genes on a per-sample basis. This has been used for discovery of the relative fold change in disease versus normal tissues ( Alizadeh et al., 2000 ) and among different disease tissue types ( Ramaswamy et al., 2001 ). Moreover, these technologies have been used to discover molecular signatures that can differentiate subtypes within a given disease that are molecularly distinct ( Bhattacharjee et al., 2001 ; DeRisi et al., 1997 ; Eisen et al., 1998 ; Guinney et al., 2015 ). Clinical applications include but are not limited to development of diagnostic and prognostic indicators and signatures ( Cardoso et al., 2008 ; Bueno-de Mesquita et al., 2007 ; Bonato et al., 2011 ).
2.2 Genomics
Taking a step back, DNA-based assays measure genomic events at the DNA level before transcription. Relevant DNA alterations include natural variability in germline genotype, or the DNA sequence, across individuals that sometimes affect biological function and disease risk, as well as germline or somatic genomic aberrations including various types of mutations, including substitutions, insertions, deletions and translocations, as well as broader changes in the genome including loss of entire chromosomes or parts of a chromosome or loss of heterozygosity (LOH), involving the loss of one of two distinct alleles originally possessed by the cell.
Diploid organisms such as humans have two copies of each autosome (i.e. non-sex chromosomes), but many diseases are associated with aberration in the number of DNA copies in a cell, especially cancer ( Pinkel and Albertson, 2005 ). Most diseases acquire DNA copy number changes manifesting as entire chromosomal changes, segment-wise changes in the chromosome, or modification of the DNA folding structure. Such cytogenetic modifications during the life of the patient can result in disease initiation and progression by mechanisms wherein disease-suppression genes are lost or silenced, or promoter genes that encourage disease progression are amplified. The detection of these regions of aberration has the potential to impact the basic knowledge and treatment of many types of diseases and can play a role in the discovery and development of molecular-based personalized therapies.
In early years, cytogeneticists were limited to visually examining whole genomes with a microscope, a technique known as karyotyping or chromosome analysis . In mid-70’s and 80’s the development and application of molecular diagnostic methods such as Southern blots, polymerase chain reaction (PCR) and flourescence in situ hybirdization (FISH) allowed clinical researchers to make many important advances in genetics, including clinical cytogenetics. However these techniques have several limitations. First, they are very time-consuming and labor-intensive, and only a limited number and regions of the chromosome can be tested simultaneously. Further, because the probes are targeted to specific chromosome regions, the analysis requires prior knowledge of an abnormality and was of limited use for screening complex karyotypes. More recently scientists have developed techniques that integrate aspects of both traditional and molecular cytogenetic techniques called chromosomal micorarrays ( Vissers et al., 2010 ). These high-throughput high-resolution microarrays have allowed researchers to diagnose numerous subtle genome-wide chromosomal abnormalities that were previously undetectable and find many cytogenetic abnormalities in part or all of a single gene. Such information is useful for biologists to detect new genetic disorders and also provide better understanding of the pathogenetic mechanisms of many chromosomal aberrations.
Broadly, there are two types of chromosomal microarrays: array-based Comparative genomic hybridization (aCGH arrays) and single nucleotide polymorphism microarrays (SNP arrays). CGH-based methods were developed to survey DNA copy number variations across a whole genome in a single experiment ( Kallioniemi et al., 1992 )With CGH, differentially labeled test (e.g., tumor) and reference (e.g., normal individual) genomic DNAs are co-hybridized to normal metaphase chromosomes, and fluorescence ratios along the length of chromosomes provide a cytogenetic representation of the relative DNA copy number variation. Chromosomal CGH resolution is limited to 10–20 Mb, hence any aberration smaller than that will not be detected. Array-based comparative genomic hybridization (aCGH) is a subsequent modification of CGH that provided greater resolution by using microarrays of DNA fragments rather than metaphase chromosomes ( Pinkel et al., 1998 ; Snijders et al., 2001 ). These arrays can be generated with different types of DNA preparations. One method uses bacterial artificial chromosomes (BACs), each of which consists of a 100- to 200-kilobase DNA segment. Other arrays are based on complimentary DNA (cDNA, Pollack et al. (1999) ) or oligonucleotide fragments ( Lucito et al., 2000 ). As in CGH analysis, the resultant map of gains and losses is obtained by calculating fluorescence ratios measured via image analysis tools.
SNP arrays are one of the most common types of high-resolution chromosomal microarrays ( Mei et al., 2000 ). SNPs, or single nucleotide polymorphisms, are single nucleotides in the genome in which variability across individuals or across paired chromosomes has been observed. Researchers have already identified more than 50 million SNPs in the human genome. SNP arrays take advantage of hybridization of strands of DNA derived from samples, each with hundreds of thousands of probes representing unique nucleotide sequences. As with aCGH, SNP-based microarrays quantitatively determine relative copy number for a region within a single genome. Platform-specific specialized software packages are used to align the SNPs to chromosomal locations, generating genome-wide DNA profiles of copy number alterations and allelic frequencies that can then be interrogated to answer various scientific and clinical questions.
Note that unlike aCGH arrays, SNP arrays have the advantage of detecting both copy number alterations as well as LOH events given the allelic fractions, typically referred to as the B-allele frequencies ( Beroukhim et al., 2006 ). They also provide genotypic information for the SNPs, which when considered across multiple SNPs can be used to study haplotypes. SNP array analysis of germline samples have been extensively used in genome-wide association studies (GWAS) to find genetic markers associated with various disease of interest. We refer the reader to Yau and Holmes (2009) for nice review of the data elements obtained via SNP arrays.
The initial human genome project involved a complete sequencing of a human genome, which took 13 years (1990–2003) and cost roughly $3 billion. Over the past decade, great improvements have been made in the hardware and software undergirding sequencing, leading to next generation sequencing (NGS) that can now be used to sequence an entire human genome in less than a day for a cost of about $1000. This sequencing data obtained by applying NGS to DNA, DNAseq, can be used to completely characterize genotypes in GWAS studies, and to characterize genetic mutations for diseased tissue such as cancer tumors. Many types of mutations can be characterized, including point mutations, insertions, deletions, and translocations. DNAseq can also be used to estimate copy number variation and LOH throughout the genome. Cost and time of sequencing is largely determined by depth of sequencing, e.g. with 30× depth indicating that we expect to get at least 30 counts of each genomic location. When focus is on common mutational variants and copy number determination, low depth sequencing (8x–10x) may be sufficient, but much higher depth is required if rare variants are to be detected. Also, at times targeted sequencing is done to focus on specific parts of the genome, e.g. whole exome sequencing for which the gene coding regions only are sequenced.
2.3 Proteomics
Proteomic technologies allow direct quantification of protein expression as well as post-translational events. Although much more difficult to study than DNA or RNA because their abundance levels span many orders of magnitude, it is important to study proteins as these play a functional role in cellular processes and numerous studies have found that mRNA expression and protein abundance often correlate poorly with each other. Here, we will briefly overview several important proteomic technologies that involve estimating absolute or relative abundance levels, including low to moderate-throughput assays that can be used to study small numbers of pre-specified proteins and high-throughput methods that can survey a larger slice of the proteome.
Low to moderate-throughput proteomic assays
Traditional low-throughput protein assays include immunohistochemistry (IHC), Western blotting and enzyme-linked immunosorbent assay (ELISA). Although IHC is a very powerful technique for the detection of protein expression and location, it is critically limited in statistical analyses by its non- to semi-quantitative nature. Western blotting can also provide important information, but due to its requirement for relatively large amounts of protein, it is difficult to use when comprehensively assessing large-scale proteomic investigations, and also is semi-quantitative in nature. The ELISA method provides quantitative analysis, but is similarly limited by requirements of relatively high amounts of specimen and by the high cost of analyzing large pools of specimens.
To overcome these limitations, Reverse-phase protein arrays (RPPA) have been developed to provide quantitative, high-throughput, time- and cost-efficient analysis of small to moderate number of proteins (dozens to hundreds) using small amounts of biological material ( Tibes et al., 2006 ). In RPPA analyses, proteins are isolated from the biological specimens such as cell lines, tumors, or serum using standard laboratory-based methods. The protein concentrations are then determined for the samples and subsequently, serial 2-fold dilutions prepared from each sample are then arrayed on a glass slide. Each slide is then probed with an antibody that recognizes a specific protein epitope, that reflects the activation status of the protein. A visible signal is then generated through the use of a signal amplification system and staining. The signal reflects the relative amount of that epitope in each spot on the slide. The arrays are then scanned and the resulting images are analyzed with an imaging software (MicroVigene, VigeneTech Inc., Carlisle, MA) that can be used to quantify protein abundance for each protein for each sample. We refer the reader to Paweletz et al. (2001) and Hennessy et al. (2010) for more biological and technical details and Baladandayuthapani et al. (2014) for quantitative details concerning RPPAs.
High throughput proteomic assays
While RPPAs are useful for studying pre-specified panels of proteins, at times researchers would like to assess proteomic content and abundance on a larger and unbiased scale using high-throughput technologies. 2D gel electrophoresis was developed in the 1970’s ( O’Farrell, 1975 ), and has served as the primary workhorse for high-throughput expression proteomics. 2DGE physically separates the proteomic content of a biological sample on a polyacrimide gel based on isoelectric point (pH) and molecular mass, which is then scanned. The resulting gel image is characterized by hundreds or thousands of spots each corresponding to proteins present in the sample that are analyzed to assess protein differences across samples. Because the spots on the gel contain actual physical proteins, the proteomic identity of a spot can be determined by cutting it out of the gel and further analyzing it using protein identification techniques like tandem mass spectrometry (see below). A variant of 2DGE that can potentially lead to more accurate relative abundance measurements is 2D difference gel electrophoresis (DIGE, Karp and Lilley (2005) ), which involves labeling two samples with two different dyes, loading them onto the same gel, and then scanning the gel twice using different lasers that differentially pick up on the two dyes. This can be used in paired designs to find proteins with differential abundance between two conditions, or in more general designs a common reference material can be used on the second channel as an internal reference factor.
Recently, 2DGE has fallen out of fashion for high-throughput proteomics, at least partly because of the lack of automatic, efficient, and effective methods to analyze the gel images. Mass spectrometry (MS) approaches have gained prominence in its place. Mass spectrometry methods survey the proteomic content of a biological sample by measuring the mass-per-unit charge (m/z) ratio of charged particles. Various technologies exist, which vary in terms of the approach used to generate the ions (e.g. MALDI, matrix-assisted laser desorption and ionization, and ESI, electrospray ionization) and to separate the proteins based on their molecular mass (e.g. TOF, time of flight, QIT, quadruple ion trap, FT-ICR, Fourier-transform ion cyclotron resonance). In each case, the separated ions are detected and assembled into a mass spectrum, a spiky function that measures abundance of particles over a series of time points, which can subsequently be mapped to m/z values. While commonly used for protein identification, relative protein abundances can also be assessed and compared between groups via quantitative analysis of the spectra.
Given the large number of proteins present in a sample at varying abundance levels spanning many orders of magnitudes, it is not possible to survey all proteins in a single spectrum. Liquid chromatography (LC) is combined with mass spectrometry to survey a larger slice of the proteome. In LC-MS, proteins are digested and separated in an LC column based on the gradient of some chosen factor (e.g. hydrophobicity). Over a series of elution times, the set of separated proteins are then fed into a MS analyzer to produce a spectrum. This technique effectively separates the proteins based on two factors (e.g. hydrophobicity and m/z), and can be visualized as “image data” with “spots” consisting of m/z peaks over a series of elution times corresponding to particular proteins. Commonly, a second MS step is done to produce protein identifications and peptide counts for a subset of peaks at each elution time, in which case the approach is called LC-MS/MS. While taking a long time to run, these techniques show promise for broad proteomic characterization of a biological sample.
2.4 Epigenetics
Although the central dogma of genetics is that DNA is transcribed into mRNA which is then translated into proteins, this process is regulated and can be altered by many other molecular processes that affect gene expression but are not directly related to the genetic code. The study of these processes is called epigenetics , and includes processes such as methylation, histone modification, transcription factor binding, and micro RNA (miRNA) expression.
One of the major and most studied epigenetic factors is methylation, whereby a methyl group is added to DNA at a CpG site in which a cytosine is connected to a guanine by a phosphodiester bond. This methylation can alter gene expression, for example by repressing transcription especially when located near the promoter region of the gene, but methylation at other locations can also affect gene expression in various ways. Methylation can be modified by environmental factors, and modifications are usually inherited through mitosis and sometimes even meiosis, so can permanently alter gene expression and is an important component of many diseases, including cancer.
One common approach to measure methylation is to use sodium bisulfite conversion, in which sodium bisulfite added to DNA fragments that converts unmethylated cytosine into uracil, allowing the estimation of a beta value measuring the percent methylation at a given CpG site. This technique can be used on individual CpGs, or has been used to generate methylation arrays capable of surveying the entire genome. In 2009, Illumina introduced a 27k array ( Bibikova et al., 2009 ) that measured methylation at 27,578 CpG sites from 14,495 genes, with roughly two CpG per genes. This array focused on promoter regions, including CpG islands, genomic regions containing a high frequency of CpG sites ( Bird et al., 1987 ). Early methylation research focused on CpG islands, which were thought to be the most important regulatory regions. However, a team led by biostatistician Rafael Irizarry ( Irizarry et al., 2009 ) (1317 citations, Google Scholar) discovered by empirical analyses of a broader array of CpG sites in the genome that most methylation alterations that separate different types of tissues and characterize differences between normal tissue and cancer do not occur on these CpG islands, but in sequences up to 2kb distant from CpG islands, which they term CpG shores . This discovery fueled further development of tools to more broadly survey methylation across the genome, first with Illumina devleoping a 450k array ( Bibikova et al., 2011 ) that included 487,557 CpGs from many different locations in the genome, and whole genome bisulfite sequencing (WGBS) ( Lister et al., 2009 ) which uses bisulfite conversion and NGS to obtain beta values for every CpG in the genome.
Some other epigenetic factors include histone modification, transcription factor binding, and miRNA expression. DNA is contained within chromatin structures in which DNA wraps around histone proteins forming nucleosomes. These histones can be modified by processes such as acetylation, phosphorylation, methylation, and deimination, that can affect DNA expression ( Bannister and Kouzarides, 2011 ). While known to be important, the full functional characterization of these modifications is still being discovered. Histone modification status can be measured genome-wide using chromatin immunoprecipitation (ChIP) ( Collas, 2010 ). Transcription is typically initiated by the binding of a protein known as a transcription factor to a binding site close to the 5′ end of the gene, and the study of which transcription factors affect which genes has important functional implications. ChIP-seq is a modern tool that combines chromatin immunoprecipitation with sequencing to find binding sites for transcription factors. MicroRNAs (miRNA) are short, single-stranded fragments of non-coding RNA that are involved in gene expression regulation. Typically, a miRNA functions by binding to a target sequence and degrading a set of target mRNA, inhibiting translation. miRNA can be measured like other mRNA, including real-time quantitative PCR, microarrays, and RNAseq.
2.5 Data structures, characteristics and modeling challenges
The data structures emanating from these high-throughput technologies have various explicit and implicit structured dependencies , some caused by underlying biological factors and others technically induced by experimental design – which can have profound implications in downstream modeling. We attempt to characterize some major modes of these dependencies. Transcriptomic and proteomic data typically generate large scale multivariate data with large number of variables (genes/proteins), typically much higher orders than the sample size – the large p , small n situation, which is a common thread in nearly all of the technologies described above. Copy number and methylation typically generate profiles, indexed by genomic location, hence inherently exhibit serial or spatial correlations which can be both short and long range. In addition, the data structures can be on vastly different scales, continuous (e.g. protein/gene expression), discrete (e.g. copy number states), count data (e.g. RNA sequencing) and measurements on bounded intervals (e.g. methylation status ∈ (0, 1)).
Furthermore, the underlying biological principles induce a natural higher order organization to these variables – such as grouping based on common biological functions of the genes/proteins and complex regulatory signaling and mechanistic interactions between them. These can induce dependencies in the data across genes/proteins/genomic locations. This raises modeling and inferential challenges that requires the appropriate level of sophistication, ranging from pre-processing to downstream modeling, that we detail in the following sections.
3 Experimental Design, Reproducible Research, and Forensic Statistics
The importance of statistical input into experimental design has been known for some time. In the early 1930’s, R.A. Fisher famously said, “ To call in the statistician after the experiment is done may be no more than asking him to perform a post-mortem examination: he may be able to say what the experiment died of. ” This is even more true for modern experiments involving highly sensitive technologies generating complex high dimensional data. The involvement of statisticians in the design phase can make fundamental contributions to science by helping ensure reproducible research.
Reproducibility and replicability of results are key elements of scientific research. The field of multi-platform genomics has been plagued with lack of reproducibility ( Ioannidis et al., 2009 ; Begley and Ellis, 2012 ), much of which can be explained by two primary factors: (1) sensitivity of the technologies to varying experimental conditions or sample processing and (2) the inherent complexity of the data leading to poorly documented and sometimes flawed analytical workflows and a neglect to do due diligence in exploratory data analysis. In this section, we will summarize two case studies in which re-analyses of data from seminal publication by statisticians revealed erroneous results, and effectively served as the type of post-mortem analysis mentioned by Fisher that could be called forensic statistics . We will describe how these efforts illustrate the importance of applying fundamental statistical principles of experimental design and exploratory data analysis ( Tukey, 1977 ) to multi-platform genomics, and have provided an impetus to major scientific journals and federal agencies to establish policies to ensure greater reproducibility of research, especially for high-throughput multi-platform genomics data.
3.1 OvaCheck ™ : Proteomic Blood Test for Ovarian Cancer
In 2002, a paper in the The Lancet ( Petricoin et al., 2002 ) reported a blood test based on proteomics mass-spectrometry could be used to detect ovarian cancer with near 100% sensitivity and specificity. If true, this could revolutionize the management of ovarian cancer, as this lethal disease is typically detected in later stages when treatments are generally ineffective, and at the time there were no reliable screening techniques for early detection of this disease. This generated a great deal of interest in the medical community, and the researchers developed a commercial blood test, OvaCheck ™ , that was to become available to women nationwide in early 2004.
This also generated a great deal of interest at M.D. Anderson Cancer Center, as many cancer researchers wanted to try this approach for early detection of other cancers and came to the Biostatistics department for help planning these studies. With no previous experience with mass spectrometry data, Kevin Coombes, Keith Baggerly, and Jeffrey Morris set out to understand these data so we could assist our collaborators on doing similar studies. Fortunately, the authors of the seminal Lancet paper made their data publicly available, so we downloaded it with the intention of familiarizing ourselves with the data and figuring out how to analyze it. However, instead we ended up uncovering some serious questions about the data and the veracity of the published results.
In the initial paper, they had blood serum from 100 healthy subjects, 100 ovarian cancer patients, and 16 patients with benign ovarian cysts. They split off 50 healthy subjects and 50 ovarian cancer subjects and trained a classifier using proteomic features from the mass spectrometry, and then for validation, they applied to the additional samples and correctly classified all 50 cancers as cancers, 47/50 of the normals as normal, and remarkably 16/16 of the benign cysts as neither cancer nor normal. These data were obtained from running the samples on a Ciphergen H4 ProteinChip array, and then the samples were rerun on a Ciphergen WCX2 ProteinChip array, which binds a different subset of the proteome, and both of these data sets were posted on the web. One of the first things we did after downloading these data was plot a heatmap of each of them, see Figure 2 . Based on this heatmap, it became evident that the benign cysts were very different from both cancers and normals, and that the benign cyst mass spectra from the first data set looked much like the WCX2 array data from the second study. Thus, it appeared that the benign cyst samples in Petricoin et al. (2002) were in fact run on a different Ciphergen chip, and their correct classification as neither cancer nor normal was driven by this technical artifact, not biology. This was disturbing, as the article stated that “positives and controls were run concurrently, intermingled on the same chip and multiple chips,” and surprisingly, no one had caught this error before our analysis. This demonstrates the importance of exploratory data analysis ( Tukey, 1977 ).

Heatmap of Ovarian Cancer Data: Heatmap of mass spectra from 216 samples in Petricoin et al. (2002) run on Ciphergen H4 ProteinChip (top) and Ciphergen WCX2 (bottom).
Data from another follow-up study by this group was also posted on the web. This data set contained spectra from running the Ciphergen WCX2 ProteinChip array on 91 healthy subjects and 162 subjects with ovarian cancer, independent from the original data set. Near-perfect classification was also reported for these data, although the mass spectrometry protein features reported were different from those reported in Petricoin et al. (2002) . We performed numerous analyses that demonstrated that both data sets seemed to discriminate cancer from normal when cross validation was used for evaluation, but the classifiers derived from one data set did not discriminate for the other data set ( Baggerly et al., 2004b , 2005a , b ). A simulation study ( Baggerly et al., 2005b ) revealed that the spectra in the second data set had pervasive differences between cancer and normal, which should not be the case since we expect that biological proteomic differences should be characterized by a limited number of specific peaks, not the entire spectrum. In spite of the authors originally asserting that the cases and controls were co-mingled on the arrays for these data ( Petricoin et al. (2004) , appeared in print as commentary to Sorace and Zhan (2003) ), they later acknowledged that in fact “case and control samples were run in separate batches on separate days” ( Liotta et al., 2005 ), which would explain such pervasive differences. We also confirmed such confounding of run order and case/control status in another follow up study by this group ( Baggerly et al., 2004a ). Such confounding can hard-code spurious signals between cases and controls, and is a major source of irreproducible results in science.
A month after publication of Baggerly et al. (2004b) , the FDA sent a letter to the company to hold off on marketing Ovacheck ™ , and six months later they notified them of the need to conduct further validation studies, studies which never were able to reproduce the initial spectacular results. The involvement of statisticians in this type of forensic statistical analysis revealed that the initial remarkable results were spurious artifacts of severe design flaws in these studies and prevented an ineffective diagnostic from going to market.
3.2 Duke Scandal: Predicting Response to Cancer Therapy
Potti et al. (2006) introduced a new strategy for using information from microarrays run on the NCI60 cell lines to build predictive signatures to determine which patients are likely to respond to which cancer therapy. Their strategy was to use drug panels to select the 10 most sensitive and 10 most resistant cell lines for a given therapy, train a classifier using the publicly available microarray data for these cell lines, and then apply this model to patient microarray data to obtain a prediction of response. They reported remarkable success of this approach for a number of common chemotherapy agents, including docetaxel, doxorubicin, paclitaxel, 5-FU, cyclophosphamide, etoposide, and topotecan, and some combination therapies.
This work generated a lot of excitement in the medical community, and was named one of the “Top 6 Genetic Stories of 2006” ( Discover, 2007 ). These authors successfully repeated this strategy in other settings, including cisplatin and pemetrexed ( Hsu et al., 2007 ), several combination therapies in breast cancer ( Bonnefoi et al., 2007 ), and temozolomide ( Augustin et al., 2009 ), with over 15 papers published on this approach over a three year period and clinical trials commenced to prospectively test them. The researchers, university, and other collaborators started up a company that could market this as a potential clinical decision making tool. Despite the excitement generated by these apparent successes, no other groups were able to get this strategy to work, in spite of its sole reliance on publicly available microarray data and cell lines that anyone could have obtained. Perhaps this should have been a warning sign that these results were not all they appeared to be.
Again in the backdrop of this hype, M.D. Anderson investigators wanted to utilize this strategy and so reached out to faculty in the Department of Bioinformatics and Computational Biology for assistance in designing these studies. Keith Baggerly and Kevin Coombes set out to understand these studies so they could replicate them in other settings. However, poor documentation turned this effort into an intensive post-facto reconstruction, and another case of forensic statistics.
Their analysis, which ultimately took 1000s of hours, uncovered a slew of data handling and processing errors including scrambling of gene and group (sensitive/resistant) labels, design confounding, inclusion of genes of unknown origin, and figure duplication. One common error was the swapping of sensitive/resistant labels in the training data, leading to signatures that would in fact propose treatments that patients are least likely to benefit from. There also were numerous irregularities in labeling of the test set, with some mislabeled and others used multiple times in the same analysis, which led to inaccurate reports of the methods’ performance. One key study included four key signature genes whose origins are unknown, as they were clearly not produced by the software and two were not even on the microarrays used for the NCI60 data set. One figure in a later publication duplicated the figure from an earlier publication with a completely different treatment. In one case, the authors asserted that they were blinded to the clinical response in the test data ( The Cancer Letter , October 2, 2009), but collaborators who sent the data disputed this assertion and instead claimed that the study was not blinded and further they could not replicate the authors’ findings themselves ( The Cancer Letter , October 23, 2009). Baggerly and Coombes had numerous interactions with the senior authors, Potti and Nevins, but they were unable or unwilling to address the most serious of these issues and eventually stopped responding. Baggerly and Coombes (2010) reported these irregularities in an Annals of Applied Statistics paper, showed that their attempts to follow the reported procedures resulted in predictive results no better than random chance, and strongly urged that clinical trials that had commenced to test these signatures be suspended until these irregularities are rectified.
Shortly after publication of this paper, three clinical trials based on these studies were suspended and a fourth terminated ( The Cancer Letter , October 9 and 23, 2009), and Duke University convened a panel of outside experts to investigate this research and the original results. Surprisingly, this panel determined that the concerns were unfounded and the trials were restarted. Apparently, the panel was never shown the full list of irregularities raised by Baggerly and Coombes, but only a hand-picked subset that were more easily addressible. It appeared that these concerns were falling on deaf ears and this work was going to be allowed to continue, until it was discovered that one of the senior authors on the work, Anil Potti, had falsified information on his vitae. This provided impetus to review the work more carefully, and Baggerly and Coombes’ concerns were verified, and led to strong suggestions of data manipulation and research misconduct. All of this resulted in the ultimate shutdown of all of these trials, a large court settlement from Duke University to families of patients who participated in these trials, and the tarnishing of the reputation of many researchers associated with them. This saga received a great deal of coverage in the national and international media, with 60 Minutes doing a story on it ( https://archive.org/details/KPIX2012021303000060Minutes ) in 2012 that featured Baggerly and Coombes. Once again, a group of statisticians performing forensic statistics uncovered flaws in a high profile study, in this case exposing serious data provenance and integrity issues, and sparing patients exposure to ineffective clinical devices based on the spurious results.
3.3 Lessons Learned: Statistics and Reproducibility
These case studies highlight the perils of research based on complex, high-dimensional data, and the crucial role good statistical practice plays in reproducible research. The first case study highlights the importance of exploratory data analysis and sound experimental design. Many researchers neglect to examine basic graphical summaries of their data prior to analysis, presumably because of their high dimensionality and complexity. The false positive results reported in the ovarian cancer study ( Petricoin et al., 2002 ) would have never seen the light of day had the investigators simply looked at heat maps of the raw spectra plotted above that took less than five minutes to produce. Tukey (1977) highlights the importance of good exploratory data analysis in statistics, and this is not any less true in the world of big data. The first case study demonstrated the hazards of neglecting this aspect of statistical analysis.
Second, it is tempting to use convenience designs in running large scale genomics and proteomics studies since their assays feature complex, multi-step laboratory procedures. However, the great biological sensitivity that makes these assays so desirable for research can also make them highly sensitive to variability in experimental conditions or sample handling. Thus, it is crucial to think carefully about how the experiment is run, with special care taken to avoid confounding the factors of interest with technical factors. This confounding of case control status with run order was the fatal flaw leading to the false positive results in the first case study. This means that sample handling needs to be consistent across cases and controls, and one must be careful to not confound case and control status with run order. Randomized block designs should be used whenever possible, and diagnostics such as cluster and principal component analyses should be used to examine potential effects of batch and other technical factors. These principles are fundamental to the field of statistics, yet in many cases underutilized in genomics, indicating there is a greater need for statistical involvement in the research process to prevent the need for forensic statistical analyses.
The second case study highlighted the importance of careful documentation of analytical steps in sufficient detail so that the results in the publication can be reproduced given the raw data. This documentation should include any preprocessing steps, gene selection, model training, and model validation procedures. The lack of such documentation made it necessary for Baggerly and Coombes to spend 1000s of hours to reconstruct what was done. In most cases, such efforts are not feasible, and thus there could be many other pivotal studies with spurious or otherwise erroneous results that are allowed to stand, and countless resources spent trying to replicate or build on these results, and in some cases patient treatment decisions being based upon them. Many journals have introduced guidelines requiring a greater level of data sharing and documentation of design and analytical details in the supplementary materials. These high profile re-analyses by statisticians have contributed to a greater level of awareness of these crucial issues, and statisticians have been taking leadership roles in helping funding agencies and journals to construct policies that contribute to greater transparency and reproducibility in research ( Peng, 2009 ; Stodden et al., 2013 ; Collins and Tabak, 2014 ; Fuentes, 2016 ; Hofner et al., 2016 )
3.4 Proper Validation and Multiple Testing Adjustment
The second case study also highlighted the importance of proper validation of predictive models. In that case study, there were fundamental problems involving data provenance and blinding irregularities, but in many other cases models built from high-dimensional genomics data are not properly validated for other reasons. Given a large number of potential predictors, it is very easy in high dimensional settings to arrive at a model with excellent or even perfect predictive accuracy for the training data. Thus, it is especially important to properly validate these predictive models to ensure their predictive performance is not biased as a result of overfitting the training data. This can be done by splitting a single data set into training and validation data through cross validation. However, as seen in the first case study, this cannot overcome technical artifacts that might be hard-wired into the entire data set, and so it is far preferable to validate using a second independent data set whenever available. In either case, it is important to ensure all gene selection and modeling decisions are made using the training data alone. A common erroneous practice is to use combined training-validation data set for some modeling decisions such as gene selection, and then only truly validate the parameter estimation step. Since it is the gene selection and not the parameter estimation that typically introduces the most variability into the modeling, this practice can lead to strongly biased predictive accuracy assessments.
A related aspect of reproducible research for high-dimensional genomics and proteomics data in which statisticians have played a crucial role is multiple testing adjustment. In the early days of microarrays, researchers would flag genes as “differentially expressed” based on non-statistical measures like fold-change that ignore within-group variability, or after applying independent statistical tests to 1000s or more of genes while using a standard 0.05-level significance level, leading to high false discovery rates. Early work by statisticians emphasized the importance of using statistical tests and to adjust for multiple testing. For example, Dudoit et al. (2002) presented various methods that strongly controlled family-wise error rate (FWER), including Bonferroni and also step-down strategies that accounted for intergene correlation and thus were less conservative. These initial solutions, however, were not so well received by the biological community because of the low power resulting from their strict experimentwise error rate criteria. Against this backdrop, researchers turned to use of false discovery rate (FDR), a concept introduced by Benjamini and Hochberg (1995) that controls the proportion of false discoveries rather than the probability of at least one false discovery. Researchers broadly deemed this a more appropriate statistical criterion for discovery involving high-dimensional genomics and proteomics data. The statistical community has developed a whole set of tools for FDR analyses, including seminal frequentist methods ( Storey, 2002 , 2003 ; Efron, 2004 ) as well as some Bayesian methods ( Newton et al., 2004 ; Muller et al., 2004 ; Morris et al., 2008b ) for multiple testing adjustment. Statisticians have been able to successfully communicate the necessity of accounting for multiple testing to the broader genomics and proteomics communities, which has to some degree helped mitigate the publishing of false positive discoveries.
4 Improved Preprocessing and Feature Extraction
Several processing steps need to be applied to the raw data generated by the genomics platforms described in Section 2 before they are ready for downstream statistical analysis. While the particulars are technology-specific, there are several general considerations that apply to nearly all technologies. These include additive correction for background signal, multiplicative normalization to account for factors such as variability in amount of biological material loaded or contrast on an optical scanner, and registration of the data so cognate features are aligned across replicates. Batch correction can be a major consideration when samples are collected or processed over a period of time or at different locations. If these steps are not performed well, then technical artifacts can creep into the data and make it difficult to extract biological information from them no matter how well thought out the downstream statistical analysis plan. While many statisticians may consider these preprocessing issues to be low-level “data cleaning” and not fundamental research problems, they are quantitatively challenging, and of primary importance to molecular biology. In a number of cases statisticians have devised preprocessing tools that have made strong contributions to the field. We highlight some of these contributions in this section.
The data generated by many of the high throughput assays described above are complex and high dimensional, and in many cases can be characterized as highly structured functional and image data. A book by Ramsay and Silverman (1997) popularized the concept of functional data analysis , which involves treating functional objects as single entities rather than just a collection of discrete data points. These functional objects can be simple smooth curves on one-dimensional Euclidean domains, or can be more complex objects with local features, and potentially defined on higher dimensional domains or non-Euclidean manifolds, as many types of modern data including various types of high-throughput genomics. One strategy for modeling complex functional data is to simplify the data using a feature extraction approach , which is a two-step approach whereby first, statistical summaries believed to capture the key biological information in the data are computed, and then second, these summaries are modeled using standard statistical analysis tools.
This is the predominant analytical strategy for nearly all of the high throughput assays described in Section 2. Examples include aggregation of information across multiple probes or genomic locations to produce gene expression summaries, performing peak or spot detection in proteomics to obtain counts or relative protein abundance measurements, or segmenting regions of the genome believed to have common copy number values.
Feature extraction can be considered to be another aspect of “preprocessing”, and as for other preprocessing problems, the statistical community at large has been a little slow to get involved in providing solutions in spite of its clearly quantitative nature. If done well, feature extraction can be an efficient strategy to reduce dimensionality, simplify the data, and focus inference on quantities that are most readily biologically interpretable. However, it is essential that it be done effectively and efficiently, since any biological information in the raw data not contained in the extracted summaries are lost to subsequent analysis. Feature extraction approaches can be much more effective when they are based upon key statistical principles including regularization and unified modeling, which lead to greater efficiency by borrowing strength (i.e. combining information) across subjects, replicates, or genomic regions that are similar to each other. The methods summarized below include feature extraction methods devised by statisticians that either explicitly or implicitly utilize these fundamental principles.
4.1 Statistical Methods for Microarray Preprocessing: Loess Normalization, dChip and RMA
The early 2000’s were characterized by increasing use of microarrays to measure genome-wide gene expression, first on custom cDNA-based spotted arrays and then automated oligonucleotide arrays made by Affymetrix. Empirical investigations of these data revealed various sources of technical variability that sometimes were strong enough to dominate biological variability, including dye bias on spotted arrays, variable probe binding affinities, and general array-specific effects that made combining information across arrays challenging. A number of statisticians rose to these challenges and developed statistical normalization tools that set the field and became the standard tools used by nearly all molecular biologists, as can be seen by the corresponding papers’ high citation counts.
Spotted microarrays typically measured gene expression for pairs of samples, with one sample’s intensities measured by a Cy3 (green) dye and the other measured by a Cy5 (red) dye. Empirical analyses revealed a dye bias, with Cy3 yielding systematically higher values than Cy5 for the same mRNA abundance, yet this relationship did not appear to be linear across the dynamic range. To adjust for this factor, Dudoit et al. (2002) (1730 citations, Google Scholar) developed a robust local linear nonparametric smoother that could be applied to each array to adjust out this dye effect. This strategy became standard practice, and these types of loess smoothers became a standard normalization tool for all kinds of gene expression data, including non-paired oligonucleotide arrays for which each sample was normalized either to a reference sample ( Li and Wong, 2001a ) (1115 citations, Google Scholar) or to all others using a pairwise approach ( Bolstad et al., 2003 ) (6124 citations, Google Scholar). This strategy may be the most impactful practical application of the nonparametric smoothing methods developed in the statistical literature starting in the 1980’s.
Affymetrix was the first company to mass produce oligonucleotide microarrays, which allowed any investigator to survey whole genome gene expression whether or not their university had its own core facility. To gain more reliable estimates of gene expression than cDNA spotted arrays that typically contained a single probe, Affymetrix included 11–20 probes of 25 base pairs of length for each gene since statistical principles suggested that the averaging over probes should increase efficiency. Recognizing that not all probes have the same binding affinity, for each probe ( perfect match PM ) a second mismatch probe (MM) was added for which the 13th base pair was switched. In the software shipped with their arrays, they quantified gene expression by taking a simple average of the differences of PM –MM for all probes for a given array, a method they called AvDiff .
The empirical analyses by careful statisticians revealed some problems not handled by this approach, including heteroscedasticity across probes with more abundant probes having greater variability, the presence of outlying probes, samples, or individual observations, and differential binding affinities for different probes that were not adequately handled by the mismatch probes. First, Li and Wong (2001b) (3384 citations, Google Scholar) developed a method Model-Based Expression Index ( MBEI ) distributed as part of the dCHIP software package that used a statistical model to adjust for probe-specific binding affinities, which were estimated by borrowing strength across multiple arrays. For a given sample i and gene, let PM ij and MM ij be the measured perfect match and mismatch expression for probe j , their model was PM ij − MM ij = θ i ϕ j + ε ij , ε ij ~ N (0 , σ 2 ) whereby θ i was the gene expression for sample i and ϕ j the binding affinity effect for probe j with ∑ j ϕ j 2 = J with J is the number of probes for the gene. This model was fitted using alternating least squares applied after performing a loess-based normalization to a reference array, and using an iterative outlier filtering algorithm to remove outlying probes, arrays, and observations, with missing data principles allowing the estimation of the gene expression values θ i . This method was shown to effectively extend the effective lower detection limit for gene expression.
In their package Robust Multiarray Analysis ( RMA ), Irizarry et al. (2003a) (4161 citations, Google Scholar) improved upon this approach by modeling the log transformed gene expressions, which adjusted for the heteroscedasticity in the data and allowed the fitting of the multiplicative probe affinities in a linear model framework. For robustness they fit their model using robust linear filtering instead of least squares, and adjusted for additive background through transformation and explored array-specific normalization using various approaches ( Bolstad et al., 2003 ), with pairwise nonparametric loess and quantile normalization strategies seeming to work best. They compared their approach with AvDiff and MBEI , and found their approach had significant advantages ( Irizarry et al., 2003b ) in terms of bias, variance, model fit, and detection of known differential expression from spike-in experiments.
These statistical model-based microarray preprocessing packages have become the status quo for preprocessing and are still widely used today.
4.2 Peak Detection for Proteomics
Feature extraction is the predominant strategy for analyzing high throughput proteomics data. For mass spectrometry (MS) data, feature extraction involves detecting peaks on the spectra and then obtaining semi-quantitative measures for each peak by either computing the area under the peak or taking the maximum peak intensity. For 2DGE data, feature extraction involves detecting spots on the gel images, and then quantifying each spot by either taking the volume under the spot within defined spot boundaries or taking the maximum intensity within the spot region. For LC-MS data, feature extraction is sometimes done by summing counts of peptides that map to a given protein. Typically, there is some signal-to-noise (S/N) threshold that must be exceeded for a region to be selected as a feature. For simplicity, we refer to all proteomic objects as “spectra” and all proteomic features as “peaks” for the remainder of this section, although the principles apply across these technologies.
A number of different feature extraction approaches are available in the current literature and in commercial software packages. Until recently, most methods performed detection on individual spectra or gel images, and then matched results across individuals to produce an n × p matrix of quantifications for p proteins for each of n individuals in the sample. This approach has a number of key weaknesses. It leads to missing data when a given peak does not have a corresponding detected peak for all spectra, and leads to many types of errors, including peak detection errors, peak matching errors, and peak boundary estimation errors that all worsen considerably as the number of spectra increases. These problems are partially responsible for limiting the impact of high-throughput proteomics on biomedical science ( Clark and Gutstein, 2008 ).
The fundamental problem with this strategy is that it uses a piecemeal approach that does not efficiently integrate the information present in the data. Alignment is only done after peak or spot detection, so does not make use of the spatial information in the raw spectra or gels that might lead to improved registration. Peak detection is only done on individual spectra, while ignoring information about whether there appears to be a corresponding peak present in other replicate spectra. If a given potential feature is apparent but near the S/N threshold, knowledge of whether there appears to be a peak at this location in other spectra may help inform the decision of whether it should be flagged as a feature or noise. The detection of peak boundaries from individual spectra also ignores information from other spectra that could be used to refine the boundaries for those features.
Against this backdrop, we developed peak detection methods for MS data ( Cromwell , Coombes et al. (2005 ); Morris et al. (2005) , 312 and 324 citations, Google Scholar) and spot detection algorithms for 2DGE data ( Pinnacle , Morris et al. (2008a )) that utilize fundamental statistical principles to more efficiently borrow strength within and between spectra, and thus produce substantially improved results. First, spectra are registered to each other in a way that borrows information spatially through their local smoothness properties ( Dowsey et al., 2008 ). Second, rather than detecting peaks on individual spectra, peak detection is performed on the average spectrum, computed by taking the point-wise average across the registered spectra. As demonstrated by Morris et al. (2005) and Morris et al. (2008a) , this approach leads to more accurate peak detection, since the averaging reduces the noise by a factor of n while reinforcing signals present on many individual spectra, which leads to increased signal-to-noise ratios for peaks present in many spectra. Since this approach effectively borrows strength across spectra, the detection accuracy actually increases as the analysis includes more spectra. This is in contrast to standard approaches for which larger numbers of spectra produce increasing propagation of errors (and I have heard many proteomic researchers give this as a reason for running small, and thus underpowered, studies with few samples). Third, wavelet thresholding is used to adaptively denoise the mean spectrum, and its adaptive properties allow the removal of many spurious peaks while retaining the dominant ones. Fourth, peaks are quantified for each individual spectrum by taking the local maximum within a neighborhood of the peak location on the mean spectrum. This ensures there is no missing data, and as shown by Morris et al. (2008a , 2010) , this estimation of peak intensity using a local maximum precludes the need to estimate peak boundaries, which leads to more precise and reliable peak quantifications.
These papers have been highly cited and software made freely available for implementing the corresponding approaches. Also, since the publishing of these papers, the commercial software packages for preprocessing proteomics data have incorporated many of these principles and as a result improved their performance.
4.3 Segmentation of DNA copy number data
As alluded to in Section 2.2, array CGH (aCGH) based methods provide a high-resolution view of the DNA-based copy number changes across the whole genome. The resulting data consist of log fluorescence intensity ratios of test to reference samples for specific markers along with the genomic/chromosomal co-ordinates. In an idealized scenario where all of the cells in a disease sample have the same genomic alterations and are uncontaminated by normal cells, the log-ratios would assume specific discrete values e.g. for normal probes, log 2 (2/2) = 0; single copy losses, log 2 (1/2) = −1; single copy gains, log 2 (3/2) = 0.58 etc. In this idealized situation, all copy number alterations could be promptly observed from the data – obviating the need for statistical techniques. However, in real applications in disease areas, the log-ratios differ considerably from these expected values for various technical and biological reasons. DNA copy number data are characterized by high noise levels that add random measurement errors to the observations. Also, the DNA material assessed is not completely homogeneous, as there is typically considerable genomic variabilty across individual disease cells and there also may be contamination with neighboring normal cells. This heterogeneity implies that we actually measure a composite copy number estimate across a mixture of cell types, which tends to attenuate the ratios toward zero. Finally, and most importantly, these genetic aberrations occur in contiguous spatial regions of the chromosome that often cover multiple markers and can extend up to whole chromosome arms or chromosomes.
In one of the seminal works towards analyzing such data, Olshen et al. (2004) proposed a statistically principled method called circular binary segmentation (CBS) that provides natural way to segment a chromosome into contiguous regions and bypasses parametric modeling of the data. The fundamental novelty of CBS is that, first, it naturally accounts for the genomic ordering of the markers and adaptively determines what segments share common values and thus adaptively borrow strength from nearby markers. This “local” averaging not only reduces noise but also increases precision in detecting the genomic break-points. CBS is freely available as an R-package (DNAcopy) and is widely used in numerous papers as evidenced by close to 1400 citations for the original article ( Olshen et al., 2004 ) – as a starting point for analysis of copy number data.
4.4 Integrated Extraction of Genotype and Copy Number
In contrast to aCGH arrays, genome-wide single nucleotide polymorphism (SNP) genotyping platforms or DNA sequencing provide provide simultaneous information on both genotypes (e.g allele-specific frequencies) and copy number variants, CNVs (e.g. logR ratios). This allows for a “generalized” genotyping of both SNPs and CNVs simultaneously on a common sample set, with advantages in terms of cost and unified analysis ( Yau and Holmes, 2009 ).
The segmentation methods described in Section 4.3 are useful to detect copy number changes but fail to account for genotype information or the allele-specific information such as B-Allele frequencies that are typically also provided by such arrays. These two metrics are inherently correlated due to the increased number of genotypes available with increasing copy number, and vice-versa. The idea of generalized genotyping is to flexibly model SNP genotyping data in a way that fully exploits the available information by simultaneously modeling structural changes in both the log ratios and the B-allele frequencies. By working in this original two-dimensional feature space, both the distribution and dependency can be used for better copy number inference and detection. This strategy has subsequently been used by a number of recent algorithms ( Colella et al., 2007 ; Wang et al., 2007 ). In particular, Colella et al. (2007) propose an objective Bayes Hidden-Markov Model which effectively borrows strength between neighboring SNPs by explicitly incorporating distance information as well the genotype information via B-allele frequencies. This framework provides probabilistic quantification of copy number state classifications and significantly improves the accuracy of segmental identification and mapping relative to existing analytical tools that do not explicitly borrow strength between inherent correlated metrics.
4.5 Supercurve for Protein Quantification in RPPA
As introduced in Section 2.3, Reverse-phase protein arrays (RPPA) have been developed to provide quantitative, high-throughput, time- and cost-efficient analysis of proteins and antibodies. Similar to other high-throughput array-based technologies, RPPA data generation undergoes a series of preprocessing steps before a formal downstream analysis analysis. The three main preprocessing steps are background subtraction, quantification and normalization ( Neeley et al., 2009 ; Zhang et al., 2009 ). Background correction involves subtraction of baseline or non-specific signals from the foreground intensities. Once the raw intensities from the RPPA slides have been adjusted for background and other spatial trends, the next preprocessing step is to quantify/estimate the concentration of each protein and sample based on the underlying assumption that the intensity of a given spot on the array is proportional to the amount of protein.
The resulting data consists of a series of dilution series for each RPPA slide, so as to ensure at least one spot from the series in the linear range of expression. As with any standard dilution assays, the expression patterns typically follow a sigmoidal curve i.e. highly diluted spots will often have little protein beyond the background level and conversely, undiluted spots will often have much higher protein levels, with saturation occurring as protein levels get beyond a certain point. The key analytical challenge in protein quantification is to appropriately use the information provided by the entire dilution series to estimate the relative protein abundance for each sample.
A variety of methods have been proposed to estimate the protein concentration. Initial methods performed protein quantification one-sample-at-a-time. Neeley et al. (2009) proposed a joint sample method that aggregates information from all samples on an array to estimate the protein concentration. In essence, it allows borrowing strength across all samples such that all samples on a given array contribute to the overall serial dilution curve. They propose a joint estimation model based on a three-parameter logistic curve and estimating the parameters pooling all the information on an array to estimate global parameters. This joint method has several advantages over naive single sample approaches. First, since each slide is probed with a single antibody targeting that protein, the protein expression of the different samples should share common chemical and hybridization profiles. Second, all of the samples can provide information about the baseline and saturation level, as well as the rate of signal increase at each dilution point. Third, estimating parameters using pooled data can yield more accurate estimates with smaller variances and joint estimation can yield estimates with more dynamic range ( Neeley et al., 2009 ). An R package, SuperCurve, developed to use with this joint estimation method is available at http://bioinformatics.mdanderson.org/Software/OOMPA – and often serves as the starting point of any RPPA data analyses.
5 Flexible and Unified Modeling
As discussed in Section 5, feature extraction is the predominant analytical strategy for high-throughput genomics and proteomics data. Feature extraction works well when the extracted features contain all of the scientific information in the data, but otherwise may miss key insights since any information not contained in the extracted features is lost to subsequent analysis. Methods that model the raw data in their entirety have potential to capture insights missed by feature extraction approaches. One prevalent way to model the raw data is to use an elementwise modeling approach, whereby individual elements in the raw data are modeled independently of each other. Some examples of this strategy would be to independently model RNA probes for expression data, CpG sites for methylation data, individual spectral locations for MS proteomics data, and SNPs for DNA-based data. This strategy has the advantage of modeling all of the data and being straightforward to implement, with one able to apply any desired statistical model in parallel to the different elements of the object. However, this strategy ignores the correlation structure among the elements, which has several statistical disadvantages. While unbiased, it leads to inefficient estimators, suboptimal inference, and can exacerbate the inherent multiple testing problem.
In recent years, advances in statistical modeling have led to a growing set of tools available to build flexible statistical models. This includes the development of various basis function modeling approaches including splines, various types of wavelets, empirical basis functions like principal components or independent components, and radial basis functions. Other advances include the development of penalized likelihood approaches to induce sparsity and regularize the fitting of models in high dimensional spaces, and the parallel development in the Bayesian community of prior distributions that induce sparsity such as spike-slab, Bayesian Lasso, Normal-Gamma, Horseshoe, Generalized Double Pareto, and Dirichlet-Laplace priors, plus Bayesian nonparametric priors to flexibly estimate distributions. Hierarchical models have become fundamental tools in Bayesian modeling, and are able to capture various levels of structure and variability in complex, high-dimensional data. These tools can be combined together to build flexible methods that can capture the structure of complex data generated by modern technologies, take this structure into account in a unified fashion, and provide inferential results for many important questions of interest. Fast computational methods including various types of stochastic EM algorithms and approximate Bayesian computational approaches like variational Bayes have been developed and enable the development of flexible methods that are fast enough to fit to high-dimensional data.
Flexible modeling can bridge the gap between the extremes of reductionistic feature extraction approaches that can miss information contained in the data and elementwise modeling approaches that model all of the data but sacrifice efficiency and inferential accuracy by ignoring relationships in the data. In addition to gaining efficiency by accounting for various types of correlation structures inherent to the data, these models also can have inferential advantages, yielding inference accounting for all sources of variability in the data, and potentially adjusting for multiple testing. These benefits are best realized by models deemed to realistically capture structure in the data, at least empirically, so care should be taken to assess model fit when using flexible modeling approaches.
Below we describe a few methods that attempt to flexibly model high-dimensional genomics data while avoiding feature extraction, and were demonstrated to capture biological information missed by some commonly-used feature extraction approaches. While not commonly used in practice for high-throughput genomics data, we believe flexible modeling strategies like these are promising, and should be further pursued and explored by statistical researchers for complex biomedical data like these.
5.1 Flexible Modeling by Functional Regression Methods
One useful class of flexible modeling methods is functional regression , which involves regression analyses for which either the response, predictor, or both are functions or images. This can be applied to genome-wide data including methylation and copy number data by modeling the data as a function of the chromosomal locus, or to proteomics data by modeling mass spectra as spiky functions of m/z values or 2DGE images or LC-MS profiles as image data, which can be viewed as functional data on a two-dimensional domain. To detect differentially expressed regions of the functions, these functions can be modeled as responses and regressed on outcomes of interest, e.g. case vs. control. In these regression models, the regression coefficients are themselves functions defined on the same space as the responses, and so after model fitting differential expression can be assessed by determining for which functional locations the coefficients differ significantly from zero.
As described in Morris (2015) , one of the hallmarks of functional regression is to use basis function representations (e.g. splines, wavelets, principal components) and either L1 or L2 penalization to smooth or regularize the resulting functional coefficients. This use of basis function modeling induces a borrowing of strength across nearby measurements within the function which in turn leads to improved efficiency in estimation and inference over elementwise modeling approaches.
Many functional regression methods in existing literature are designed for simple, smooth functions on 1D Euclidean domains, so may not be suitable for high-throughput genomics data. However, we have developed a series of Bayesian methods for functional response regression based on a functional mixed model framework ( Morris and Carroll, 2006 ; Morris et al., 2008b , 2011 ; Morris, 2012 ; Zhu et al., 2012 ; Zhang et al., 2016 ; Meyer et al., 2016 ) that are designed for complex, high-dimensional data like these. The functional mixed model is simply a functional response regression model with additional random effect function terms that can be used to account for between-function correlation induced by the experimental design, e.g. for cluster-sampled or longitudinally observed functions, such that it generalizes linear mixed models to the functional response setting.
Our approach for fitting these data is to first represent the observed functions with a lossless or near-lossless basis representation, fit a basis-space version of the functional mixed model using a Markov Chain Monte Carlo (MCMC), and then project these posterior samples back to the original functional space for Bayesian inference to find differentially expressed regions of the function, which can be done while controlling FDR ( Morris et al., 2008b ) or experimentwise error rate ( Meyer et al., 2016 ). Prior distributions are placed on the basis-space regression coefficients that induce the type of L1 or L2 penalization behavior that leads to appropriately smoothed/regularized functional coefficients. While any lossless or near lossless basis can be used, much of our work has utilized wavelet bases that are well-suited for capturing local features like peaks, spots and change-points that characterize many types of genomics and proteomics data.
This modeling strategy combines together basis function modeling, mixed models or hierarchical models, and sparsity priors to produce a flexible yet scalable model for complex, high dimensional data that has many positive statistical characteristics. The smoothing of the functional regression coefficients induces a borrowing of strength across functional regions that leads to more efficient estimators. The manner of the basis-space modeling induces intra-functional correlation in the residual errors, which is automatically accounted for in the estimation of functional coefficients leading to more efficient estimates and more accurate inferences. The fully Bayesian model propagates all uncertainties into the final posterior inference, which can adjust for multiple testing using either an FDR or experimentwise error rate based approach.
Finding Differentially Expressed Proteins
Morris et al. (2008b) applied this strategy to MS proteomics data and Morris (2012) compared results with those obtained by Cromwell , a feature extraction based approach that detects and quantifies peaks present in the spectra. We found that the functional regression based approach was able to find approximately double the number of differentially expressed proteomic regions, including all of those found by the feature extraction approach and many others, some of which had no corresponding peak detected. Liao et al. (2013) and Liao et al. (2014) demonstrated that this strategy also works and finds protein differences missed by feature extraction approaches for LC-MS data.
Morris et al. (2011) extended this strategy to image data, and applied it to 2DGE data and Morris (2012) compared results with those obtained by Pinnacle , a feature extraction based approach that detects and quantifies spots present in the gels. We found that the functional regression based method was able to find nearly all of the results found by the feature extraction based approach, plus approximately 50% more. Many of these novel results were missed by the spot-based approach because of the problem of co-migrating proteins. In 2DGE, the proteins are not perfectly resolved, and so many times a single visual spot contains a convolution of multiple proteins. While spot-based methods will tend to treat this region as a single spot, the functional regression based method is able to detect a differentially expressed protein that is only represented in part of that spot.
For all of these proteomic applications, the basis-space modeling appeared to capture the complex structure of the data well, as data simulated from the functional model look just like real spectra and gels.
Finding Differentially Methylated Regions
Early studies of methylation focused on CpG islands , genomic regions containing high frequency of CpG sites, which contain cytosine and guanine connected by a phosphodiester bond. They frequently occur in the promoter region of genes, and have been thought to be the most relevant methylation sites to study. However, recent findings have resulted in a rethinking of this belief. Irizarry et al. (2009) demonstrated that most methylation alterations in colon cancer were not reflected in the CpG island locations, but in regions some distance from the CpG islands, which they coined CpG Shores . This was learned by studying DNA methylation on a genome-wide scale, not just restricting to CpG island sites. This suggests that traditional approaches that focus on specific genomic regions such as CpG islands (i.e. a feature extraction approach) are likely to miss important findings and that genome-wide studies of DNA methylation are preferred. This discovery has led to the development of methylation arrays that sample a broader array of CpG sites along the genome, and now to bisulfite sequencing approaches that can survey all CpG sites across the entire genome ( Lister et al., 2009 ).
The collection of genome-wide methylation data raises issues of modeling. In practice, many researchers out of convenience model each CpG site independently (e.g., ( Barfield et al., 2012 ; Touleimat and Tost, 2012 ), for example detecting differentially methylated regions (DMRs) as CpG sites with mean methylation levels differing significantly across different conditions. However, given that methylation levels of nearby CpG sites tend to be similar ( Leek et al., 2010 ), this elementwise modeling approach is inefficient as it ignores the correlation structure in the data. Jaffe et al. (2012) use post hoc loess smoothing to account for correlation in the data and gain further efficiency, and Lee and Morris (2015) apply Bayesian functional mixed models to detect DMRs. Through simulations and real examples, Lee and Morris (2015) show that the borrowing of strength from the basis function modeling inherent to the functional regression method leads to clearly higher power and lower FDR for discovering DMRs than elementwise methods that model CpG sites independently and ignore their inherent correlation.
5.2 Unified Segmentation Models for Copy Number Data
As mentioned above, one of the hallmarks of genetic variations in cancer is genomic instability of cancerous cells that are manifested as copy number changes across the genome that can be measured using high-resolution and high-throughput assays such as aCGH and SNP arrays (see Section 2.2 for details). The resulting data consist of log fluorescence ratios as a function of the genomic location and provide a cytogenetic representation of the relative DNA copy number variation. Analysis of such data typically involves estimating the underlying copy number state at each genomic location and segmenting regions of the chromosome with similar copy number states. Modeling and inferential challenges of such data include (1) high-dimensionality of the datasets, (2) existence of serial correlations along the genome and (3) multiple assays from a common pool of subjects.
Most methods proceed by modeling a single sample/array at a time, and thus fail to borrow strength across multiple samples to infer shared regions of copy number aberrations ( Olshen et al., 2004 ; Tibshirani and Wang, 2008 ). Baladandayuthapani et al. (2010) proposed a hierarchical Bayesian approach to address these challenges based on the characterizations of the copy number profiles as functional data i.e. log-ratios as a function of the genomic location – that allows efficient borrowing of strength both within and across arrays to model such data. This approach is based on a multilevel functional mixed effects model that flexibly models not only within subject variability but also allows us to conduct population-level inference to obtain segments of shared copy number changes. The unified Bayesian model uses piece-wise constant functions with random segmentations for the functions that allows determination of optimal segmental rearrangements from the data and more importantly allow incorporation of biological knowledge in the calling of the states of the segments via a hierarchical prior. Applying this method to a well-studied lung cancer dataset, we found many shared regions/genes of interest that are associated with disease progression that were missed by competing simpler approaches. Regions of shared aberrations are of particular importance in cancer genomics – these are key bits of information that can be used to determine subtypes of disease and in to design personalized therapies based on molecular markers, which is one of the most important problems in cancer research today.
6 Structure Learning and Integration
The sheer volume and information-rich nature of the data generated by various high-throughput technologies has pushed the envelope of analytical tools needed to analyze such data. As alluded to in the previous sections, the experimental procedures producing these data and underlying biological principles undergirding them induce a natural higher order organization and structure to these data elements that ideally should be accounted for or included in any downstream modeling endeavors. For example, this includes correlations across genes present in common biological pathways and relationships among measurements from different technological platforms that each contain different biological information based the their molecular resolution level (e.g. DNA, RNA, protein).
These structures are fundamentally ignored by the prevailing piecemeal, multi-step procedures commonly used in practice, presumably out of convenience and lack of available methods. By failing to integrate information effectively, these strategies potentially sacrifice statistical power for making discoveries, and by not propagating inherent correlations throughout inference, they fail to yield optimal inference. By expanding the frontiers of statistical modeling to be able to account for more of this structure, the statistical community has opportunities right now to produce next-generation tools that more efficiently integrate information together, and as a result do a better job of extracting the biological information contained in these rich data.
While by far the most common analytical approaches used are ad hoc multi-step algorithmic approaches, model-based approaches if developed to carefully and accurately account for the underlying structure in the data enjoy several inherent advantages. First, they allow full probabilistic formulation of the data generating process. Second, they allow coherent borrowing strength among heterogenous mixed-scale data sources through appropriate parameterizations. Third, they allow specific inferential questions to be answered through explicit parameterizations. Fourth, they produce uncertainty quantifications and admit natural multiplicity controls. To illustrate these principles, we describe two broad modeling approaches here: structure learning and multi-platform integration.
6.1 Structure Learning
It is well-established that genes/proteins function in co-ordination within organized modules such as functional or cell signaling pathways or networks ( Boehm and Hahn, 2011 ), for example in cancer to promote or inhibit tumor development. These genes and their corresponding pathways form common modules or networks that regulate various cellular functions. Thus the estimation and incorporation of such modules and networks and their modeling constituents are of great interest for characterizing and understanding the biological mechanisms behind disease development and progression, especially cancer.
Moreover, in the last several years, multiple public and commercial databases have been curated to store the vast amounts of biological knowledge such as signaling, metabolic or regulatory pathways. In general, a gene class is defined as a collection of genes defined to be biologically associated given a biological reference based on scientific literature, transcription factor database, expert opinion, or empirical and theoretical evidence. A few of these inlcude Gene Ontology (GO) ( Ashburner et al., 2000 ), Kyoto Encyclopedia of Genes and Genomes (KEGG) ( Kanehisa and Goto, 2000 ), MetaCyc ( Krieger et al., 2004 ), Reactome KnowledgeBase ( Joshi-Tope et al., 2005 ), Invitrogen (iPath, www.invitrogen.com ) and Cell Signaling Technology (CST) Pathway ( www.cellsignal.com ). From a statistical viewpoint, models that incorporate these pathway/network structures and combine this outside biological information across genes have been shown to have more statistical power in addition to providing more refined biological interpretations. Following are a few examples.
Gene Set analyses
Broadly, gene set analyses refers set of methods and procedures for integrating the observed experimental data with available agene set information within various scientific contexts ( Newton and Wang, 2015 ). Two broad categories of such methods as reviewed by Newton and Wang include uniset methods , that focus on methods for gene sets considered one at a time, ando multi-set methods that simultaneously model all of the sets as a unified collection.
A widely used uniset method that relates pathways to a set of experimental data on genes is called gene set enrichment analysis (GSEA, Subramanian et al. (2005) ). Briefly, given a list of genes that show some level of significant activity (e.g. differential expression, fold change etc), GSEA computes an “enrichment score” to reflect the degree to which a predefined pathway (using the databases above) is over-represented and then can be used to obtain ranked lists. These procedures are useful starting points to summarize gene expression changes for known biological processes. However, most uniset methods suffer from two drawbacks/challenges as outlined by Newton and Wang. The first is that the set size affects testing power due to imbalance between null and alternate hypothesis ( Newton et al., 2007 ). This imbalance affects how one prioritizes or ranks pertinent gene sets in a given analysis. The second pertains to overlap among different gene sets in their membership, called pleiotropy, which may lead to spurious gene set associations ( Bauer et al., 2010 ; Newton et al., 2012 ).
Multi-set methods inlcuding many model-based methods can alleviate these issues to a certain degree. Examples of multi-set methods include model-based gene set analysis (MGSA) ( Bauer et al., 2010 , 2011 ), and multifunctional analysis (MFA) ( Wang et al., 2015 ). Briefly, the major features of model-based statistical approaches are that they bypass the size as well as overlap problems via explicit representations of the gene-level data using latent representations. These multiset methods often involve relatively sophisticated computations and optimizations, and they have demonstrated improved performance over their heuristic/algorithmic uniset counterparts (see Newton and Wang (2015 ) for further details).
Network and Graphical Models
Graphs and networks provide a natural way of representing the dependency structure among variables. Increasingly, network data are being generated from many scientific areas, especially biology, where large-scale protein-protein interaction and gene regulatory networks are now routinely available. The key scientific hypothesis underpinning the statistical approaches is to look at the system of variables (genes/proteins) as a whole rather than individual elements to understand their implicit dependencies. There are typically two key modeling and inferential challenges that underlie these endeavors. The first is to construct the graph/network based on observed data and second is to use the knowledge to guide models in supervised (e.g regression) and unsupervised (e.g. clustering) settings. These challenges are further accentuated by the fact that typically in many settings the variables far exceed the sample size (a.k.a big n , small p problem).
Graphical models are statistical models that use a graph-based representation to compactly describe probabilistic relationships between the variables, typically genes or proteins. There are two main approaches to estimate the graphs/networks – undirected networks and directed networks, which further incorporate directionality between the edges. In an undirected setting, perhaps the most ubiquitous models are Gaussian graphical models (GGMs) ( Cox and Wermuth, 1996 ) for which the conditional dependencies are encoded by the non-zero entries in the concentration or precision matrix, which is the inverse of covariance matrix of the data. These models provide representations of the conditional independence structure of the multivariate distribution – to develop and infer gene/protein networks. Estimation and application of GGMs have seen a surge in recent years, especially for high-dimensional genomic settings( Meinshausen and Bühlmann, 2006 ; Friedman et al., 2008 ; Dobra et al., 2004 ; Ni et al., 2016 ). Most of these methods have been widely used in high-dimensional bioinformatics settings, where the primary objective is to induce sparsity based on shrinkage and model selection – which can lead to better edge detection with more statistical power and lower false discovery rates. This usually serves as a first step in filtering for empirical structure supported by the data for further downstream experimental validations.
In contrast to the undirected setting, probabilistic network-based approaches, such as Directed Acyclic Graphs (DAG) or Bayesian networks (BN) aim to search through the space of all the possible topological network arrangements while incorporating certain constraints such as directionality ( Chen et al., 2006 ; Myllymäki et al., 2002 ; Werhli et al., 2006 ). This has the potential to discover potential casual mechanisms between genes that are not typically accorded by other more naive methods. Various DAG-based methods have been proposed in the literature for use in a variety of contexts. Friedman et al. (2000) developed DAGs from gene expression data using a bootstrap-based approach. Li, Yang, and Xing (2006) constructed DAG-based gene regulatory networks from expression microarray data using linear appraoches. Stingo et al. (2010) proposed a DAG-based model to infer microRNA regulatory networks. Recently, Ni et al. (2015) developed an efficient Bayesian method for discovering non-linear edge structures in DAG models which allows the functional form of the relationships between nodes to be non-parametrically determined by the data.
In regression settings, there is a growing set of literature containing methods for conducting variable/feature selection using structured covariates lying on a known graph. These developments mirror the growing recognition that incorporation of supplementary biological information in the analysis of genomic data can be instrumental for improving inference ( Pan et al., 2010 ). Such developments have been aided by a proliferation of genomic databases storing pathway and gene-gene interaction information ( Stingo et al., 2011 ; Li and Zhang, 2010 ; Shen et al., 2012 ), and different procedures have been proposed to incorporate available prior information in building structured penalties in a regression model for gene grouping and selection. Park et al. (2007) attempted to incorporate Gene Ontology pathway information to predict survival time. Some examples of Bayesian regression and variable selection approaches for graph structured covariates include Stingo et al. (2011) and Li and Zhang (2010) . Recently, estimation and computational approaches have been developed the generalize graphical model estimation for multi-platform data to infer more integrated networks for get a holistic view of the dependencies ( Ha et al., 2015 ; Ni et al., 2014 , 2016 ). All of these examples demonstrate that the incorporation of external biological information into the modeling leads to better variable selection properties than the more commonly used approaches that ignore such information, and thus borrowing strength from existing resources can lead to more efficient and refined analyses.
6.2 Integromics
A rather nascent but burgeoning field is the field of “integromics” – integrative analysis of multi-platform genomics data. Initial studies in genomics relying on single platform analyses (mostly gene expression- and protein- based) have discovered multiple candidate “druggable” targets especially in cancer such as KRAS mutation in colon and lung cancer ( Capon et al., 1982 ) and BRAF in colorectal, thyroid, and melanoma cancers ( Davies et al., 2002 ). However, it is believed that integrating data across multiple molecular platforms has the potential to discover more co-ordinated changes on a global level ( Chin et al., 2011 ). Integromics espouses the philosophy that a disease is driven by numerous molecular/genetic alterations and the interactions between them, with each type of alteration likely to provide a unique but complementary view of disease progression. This offers a more holistic view of the genomic landscape of a given disease with increased power and lower false discovery rates in detecting important biomarkers ( Tyekucheva et al., 2011 ; Wang et al., 2013 ), and translating to substantially improved understanding, clinical management and treatment.
The integration of data across diverse platforms has sound biological justifications because of the natural interplay among diverse genomic features. Looking across platforms, attributes at the epigenetic and DNA level such as methylation and copy number variation can affect mRNA expression, which in turn is known to influence clinical outcomes such as progression times and stage of disease through proteins and subsequent post-translational modifications. Figure 1 illustrates some of these inter-platform relationships. Within-platform interactions arise from pathway-based dependencies as well as dependencies based on chromosomal/genomic location. We review some of the recent developments in this area, mostly in the context of cancer, since its one of most well-characterized disease-system at different molecular levels. Large scale co-ordinated efforts in cancer include worldwide consortiums such as the International Cancer Genome Consortium (ICGC; icgc.org) and The Cancer Genome Atlas (TCGA; cancergenome.nih.gov), which have collated data over multiple types of cancer on diverse molecular platforms. This has led to proliferation of statistical, bioinformatics and data mining efforts to collectively analyze and model the large volume of data.
Statistically, there are multiple types of data integration methods depending on the scientific question of interest, and the taxonomy can be classified into three broad categories ( Kristensen et al., 2014 ). The first class of methods deal with understanding mechanistic relationships between different molecular platforms, with the main objective being to delineate cross-platform interactions such as DNA-mRNA, mRNA-protein etc. The second class of methods involves the identification of latent groups of patients or genes using the multi-platform molecular data and can be cast as either a classification (supervised) or clustering (unsupervised) problems. Finally, the third class of methods deals with prediction of an outcome or phenotype (e.g. survival/stage, treatment outcomes) for prospective patients. Some methods focus on one of these categories while others simultaneously consider multiple ones.
Early attempts at integromics involved the sequential analysis of the data from the different platforms in order to understand the biological evolution of disease as opposed to predicting clinical outcome ( Fridlyand et al., 2006 ; Tomioka et al., 2008 ; Qin, 2008 ). Briefly, data obtained from one platform are analyzed along with the clinical outcome data, and then a second data platform is subsequently used to clarify or confirm the results obtained from the first platform. For example, Qin (2008) showed that microRNA expression can be used to sort tumors from normal tissues regardless of tumor type. The study then analyzed the relationship between the candidate target genes for the cancer-related microRNAs and mRNA expression and disease status.
More recently, model-based methods have been proposed for which data from multiple platforms are combined into one statistical model. Model-based methods have the advantage of incorporating structural assumptions directly into model-building and several inferential questions can be framed based on appropriate parameterizations. Lanckriet et al. (2004) propose a two stage approach, first computing a kernel representation for data in each platform and subsequently combining kernels across platforms in a classification model. Mo et al. (2013) and Shen et al. (2013) proposed a clustering model “iCluster”, which uses a joint latent variable model to cluster samples into tumor subtypes. Through applications to breast and lung cancer data, iCluster identified potential novel tumor subtypes Similarly, Lock et al. (2013) proposed an additive decomposition of variation approach consisting of low-rank approximations capturing joint variation across and within platforms, while using orthogonality constraints to ensure that patterns within and across platforms are unrelated. Tyekucheva et al. (2011) proposed a logistic regression model regressing a clinical outcome on covariates across multiple platforms.
iBAG: Integrative Bayesian analysis of genomics data
Recently Wang et al. (2013) introduced integrative Bayesian analysis of genomics data (iBAG), a unified framework for integrating information across genomic, transcriptomic and epigenemic data as well clinical outcomes. iBAG uses a two-component hierarchical model construction: a mechanistic model to infer direct effects of different platforms on gene expression, and a clinical model that uses this information to associate with a relevant clinical outcome (e.g. survival times). The mechanistic model takes into account the biological relationships between platforms by modeling mRNA gene expression as a linear or nonlinear function of its upstream regulators and decomposes a given gene’s expression into separate components that are regulated by each upstream platform and a residual component that accounts for expression effectors not included in the model. This serves a bi-fold objective: first, it captures the mechanistic dependencies among different platforms modulating the expression and second, serves as a denoising step for the expression before correlating them with the clinical outcomes. Subsequently, the clinical component hierarchically learns from the mechanistic model by incorporating the platform-specific genomic components and the clinical factors (age, stage, demographics) into one model including multiple genes to find “optimal” integrative signatures associated with the clinical outcomes.
The authors demonstrated that this modeling framework, by statistically borrowing strength across different data sources, allows: (a) better delineation of biological mechanisms between different platforms; (b) increased power to detect important biomarkers of disease progression and (c) increased prediction accuracy for clinical outcomes. This framework has been further generalized to multiple platforms ( Jennings et al., 2013 ), incorporating non-linear dependencies ( Jennings et al., 2016 ), and is in the process of being extended to incorporate pathway-based dependencies and integrate radiology-based imaging data.
These methods exemplify how integrative statistical models can be used to borrow strength from disparate data sources in order to perform more refined anlayses that have potential to provide additional insights into the biological mechanisms governing the disease processes that might be missed by the prevalent piecemeal approaches.
7 Conclusions and Future Directions
Statisticians have played a prominent role in bioinformatics, helping develop rigorous design and analysis tools for researchers to use to extract meanginful biological information from the rich treasure trove of multi-platform genomics data. Their deep understanding of the scientific process as well as variability and uncertainty has uniquely equipped them to serve a fundamental role in this venture.
In this paper, we have attempted to summarize this contribution, focusing on four key areas of experimental design and reproducibilty, preprocessing, unified modeling, and structure learning and integration. There has been considerable high-impact work done in these areas, and the success and benefit of these statistician-derived methods is driven by the key statistical concepts motivating and underlying them.
One of the key statistical concepts is that unified models that borrow strength across related elements enjoy statistical benefits over piecemeal approaches, leading to more efficient estimation, improved prediction, and greater sensitivity and lower false discovery rates for making discoveries. This borrowing of strength can occur across samples, across measurements within an object (e.g. across probes, spectral locations, genomic locations, or genes in common pathway), across data types, and between data and biological knowledge in the literature. In this paper, we see this concept at work in peak detection on the mean spectrum, incorporation of copy number and B-allele frequency to determine copy number estimates, borrowing of strength across samples to estimate underlying protein abundances, borrowing strength across samples to identify shared genomic copy number aberrations, incorporating pathway information into models, or integrating across platforms using DAGs or hierarchical models that model their natural interrelationships.
This principle is also at work in flexible modeling approaches that borrow strength across nearby observations in functional or image data using basis function modeling and regularization priors, a strategy that has been applied to MS, 2DGE, copy number, and methylation data. The concept of regularization is used when smoothing functional data in normalization of microarrays, when penalizing regression coefficients in high-dimensional regression models, when denoising spectra before performing peak detection, and when segmenting DNA copy number data.
By applying these principles, we can continue to develop efficient methods that can strongly impact the field of bioinformatics moving forward. New technologies are continually being developed and introduced at a rapid rate, and there are many new challenges these data will bring. Our hope is that statisticians will be involved on the front lines of methods development for these technologies as they are introduced, and that we are involved in all aspects of the science including design, preprocessing, and end-stage analysis, not just end-stage analysis.
We acknowledge that some of the genomic platforms featured in this paper comprise older technologies that have been mostly supplanted by newer ones. However, our experience is that while new technologies always bring some new challenges, many of the quantitative issues remain the same. Thus, methods and approaches developed on older platforms have some translational importance to the new ones, at least in terms of key issues and the underlying principles behind effective solutions to them. Our hope is that by elucidating key statistical principles driving some of these methods, we will help stimulate future researchers in finding effective solutions to future challenges.
There are a number of areas where more work is clearly needed and future developments are possible. One key area is in integrative analysis. This field is really just getting started and the scientific community is in dire need of new methods for integrating information across multiple platforms to gain more holistic insights into the underlying molecular biology. These methods must balance statistical rigor in building connections, computational efficiency to scale up to big data settings, and interpretability of results so our collaborators can make sense of them. Also, given the extensive efforts in the biological research community to build up knowledge resources that are freely available online, such the recent large-scale federal efforts for unified databases especially in cancer e.g. NCI Genomic Data Commons (GDC, Grossman et al. (2016 )). Hence, the statistical community needs to find better ways to incorporate this information into the modeling, which can lead to improved predictions and discoveries as well as enhanced interpretability of the results. Given the interdepencies underlying genetic processes, pathway information is one of the most important types of information that we need to better incorporate.
Biology and medicine have moved to a place where big data are becoming ubiquitous in research and even clinical practice. This provides great opportunitiees for the statistical community to play a fundamental role in pushing the science forward, as we equip other scientists with the tools they need to extract the valuable information they contain.
Acknowledgments
This work has been supported by grants from the National Cancer Institute (R01-CA178744, P30-CA016672, R01-CA160736, R01-CA194391) and the National Science Foundation (1550088, 1463233).
- Alizadeh AA, Eisen MB, Davis RE, Ma C, Lossos IS, Rosenwald A, Boldrick JC, Sabet H, Tran T, Yu X, et al. Distinct types of diffuse large b-cell lymphoma identified by gene expression profiling. Nature. 2000; 403 (6769):503–511. [ PubMed ] [ Google Scholar ]
- Alwine JC, Kemp DJ, Stark GR. Method for detection of specific rnas in agarose gels by transfer to diazobenzyloxymethyl-paper and hybridization with dna probes. Proceedings of the National Academy of Sciences. 1977; 74 (12):5350–5354. [ PMC free article ] [ PubMed ] [ Google Scholar ]
- Ashburner M, Ball CA, Blake JA, Botstein D, Butler H, Cherry JM, Davis AP, Dolinski K, Dwight SS, Eppig JT, et al. Gene ontology: tool for the unification of biology. Nature Genetics. 2000; 25 (1):25–29. [ PMC free article ] [ PubMed ] [ Google Scholar ]
- Augustin CK, Yoo JS, Potti A, Yoshimoto Y, Zipfel PA, Friedman HS, Nevens JR, Ali-Osman F, Tyler DS. Genomic and molecular profiling predicts response to temozolomide in melanoma. Clinical Cancer Research. 2009; 15 :502–510. [ PubMed ] [ Google Scholar ]
- Baggerly KA, Coombes KR. Deriving chemosensitivity from cell lines: Forensic bioinformatics and reproducible research in high-throughput biology. The Annals of Applied Statistics. 2010; 3 (4):1309–1334. [ Google Scholar ]
- Baggerly KA, Edmonson SR, Morris JS, Coombes KR. High-resolution serum proteomic patterns for ovarian cancer detection. Endocrine-Related Cancer. 2004a; 11 (4):583–584. [ PubMed ] [ Google Scholar ]
- Baggerly KA, Morris JS, Coombes KR. Reproducibility of selditof protein patterns in serum: Comparing data sets from different experiments. Bioinformatics. 2004b; 20 :777–785. [ PubMed ] [ Google Scholar ]
- Baggerly KA, Coombes KR, Morris JS. Bias, randomization, and ovarian proteomic data: A reply to ?producers and consumers. Cancer Informatics. 2005a; 1 (1):9–14. [ PMC free article ] [ PubMed ] [ Google Scholar ]
- Baggerly KA, Morris JS, Edmonson SR, Coombes KR. Signal in noise: Evaluating reported reproducibility of serum proteomic tests for ovarian cancer. Journal of the National Cancer Institute. 2005b; 97 (4):307–309. [ PubMed ] [ Google Scholar ]
- Baladandayuthapani V, Ji Y, Talluri R, Nieto-Barajas LE, Morris JS. Bayesian random segmentation models to identify shared copy number aberrations for array cgh data. Journal of the American Statistical Association. 2010; 105 (492):1358–1375. [ PMC free article ] [ PubMed ] [ Google Scholar ]
- Baladandayuthapani V, Talluri R, Ji Y, Coombes KR, Lu Y, Hennessy BT, Davies MA, Mallick BK. Bayesian sparse graphical models for classification with application to protein expression data. The Annals of Applied Statistics. 2014; 8 (3):1443. [ PMC free article ] [ PubMed ] [ Google Scholar ]
- Bannister AJ, Kouzarides T. Regulation of chromatin by histone modifications. Cell Research. 2011; 21 (3):381–395. [ PMC free article ] [ PubMed ] [ Google Scholar ]
- Barfield RT, Kilaru V, Smith AK, Conneely KN. CpGassoc: an R function for analysis of DNA methylation microarray data. Bioinformatics. 2012; 28 (9):1280–1281. [ PMC free article ] [ PubMed ] [ Google Scholar ]
- Bauer S, Gagneur J, Robinson PN. Going bayesian: model-based gene set analysis of genome-scale data. Nucleic Acids Research. 2010:gkq045. [ PMC free article ] [ PubMed ] [ Google Scholar ]
- Bauer S, Robinson PN, Gagneur J. Model-based gene set analysis for bioconductor. Bioinformatics. 2011; 27 (13):1882–1883. [ PMC free article ] [ PubMed ] [ Google Scholar ]
- Begley CG, Ellis L. Drug development: Raise standards for preclinical cancer research. Nature. 2012; 483 :531–533. [ PubMed ] [ Google Scholar ]
- Benjamini Y, Hochberg Y. Controlling the false discovery rate: A practical and powerful approach to multiple testing. JRSS-B. 1995; 57 :289–300. [ Google Scholar ]
- Beroukhim R, Lin M, Park Y, Hao K, Zhao X, Garraway LA, Fox EA, Hochberg EP, Mellinghoff IK, Hofer MD, et al. Inferring loss-of-heterozygosity from unpaired tumors using high-density oligonucleotide snp arrays. PLoS Comput Biol. 2006; 2 (5):e41. [ PMC free article ] [ PubMed ] [ Google Scholar ]
- Bhattacharjee A, Richards WG, Staunton J, Li C, Monti S, Vasa P, Ladd C, Beheshti J, Bueno R, Gillette M, et al. Classification of human lung carcinomas by mrna expression profiling reveals distinct adenocarcinoma subclasses. Proceedings of the National Academy of Sciences. 2001; 98 (24):13790–13795. [ PMC free article ] [ PubMed ] [ Google Scholar ]
- Bibikova JL, Barnes B, Saedinia-Melnyk S, Zhou L, Shen R, Gunderson KL. Genome-wide dna methylation profiling using infinium assay. Epigenetics. 2009; 1 :177–200. [ PubMed ] [ Google Scholar ]
- Bibikova M, Barnes B, Tsan C, Ho V, Klotzle B, Le JM, Delano D, Zhang L, Schroth GP, Gunderson KL, Fan JB, Shen R. High density dna methylation array with single cpg site resolution. Genomics. 2011; 98 (4):288–295. [ PubMed ] [ Google Scholar ]
- Bild AH, Chang JT, Johnson WE, Piccolo SR. A field guide to genomics research. PLoS Biol. 2014; 12 (1):e1001744. [ PMC free article ] [ PubMed ] [ Google Scholar ]
- Bird AP, Taggart MH, Nicholls RD, Higgs DR. Non-methylated cpg-rich islands at the human alpha-globin locus: implications for evolution of the alpha-globin pseudogene. The EMBO journal. 1987; 6 (4):999–1004. [ PMC free article ] [ PubMed ] [ Google Scholar ]
- Blanchard A, Kaiser R, Hood L. High-density oligonucleotide arrays. Biosensors and bioelectronics. 1996; 11 (6):687–690. [ Google Scholar ]
- Boehm JS, Hahn WC. Towards systematic functional characterization of cancer genomes. Nature Reviews Genetics. 2011; 12 (7):487–498. [ PubMed ] [ Google Scholar ]
- Bolstad BM, Irizarry RA, Ashard M, Speed TP. A comparison of normlization methods for high density oligonucleotide array data based on variance and bias. Bioinformatics. 2003; 19 (2):185–193. [ PubMed ] [ Google Scholar ]
- Bonato V, Baladandayuthapani V, Broom BM, Sulman EP, Aldape KD, Do KA. Bayesian ensemble methods for survival prediction in gene expression data. Bioinformatics. 2011; 27 (3):359–367. [ PMC free article ] [ PubMed ] [ Google Scholar ]
- Bonnefoi H, Potti A, Delorenzi M, Mauriac L, Campone M, Tubianahulin M, Petit T, Rouanet P, Jassem J, Blot E, Becette V, Farmer P, Andre S, Acharya CR, Mukherjee S, Cameron D, Bergh J, Nevins JR, Iggo RD. Validation of gene signatures that predict the response of breast cancer to neoadjuvant chemotherapyy: A substudy of the eortc 10994/big 00-01 clinical trial. Lancet Oncology. 2007; 8 :1071–1078. [ PubMed ] [ Google Scholar ]
- Brown PO, Botstein D. Exploring the new world of the genome with dna microarrays. Nature Genetics. 1999; 21 :33–37. [ PubMed ] [ Google Scholar ]
- Bueno-de Mesquita JM, van Harten WH, Retel VP, van’t Veer LJ, van Dam FS, Karsenberg K, Douma KF, van Tinteren H, Peterse JL, Wesseling J, et al. Use of 70-gene signature to predict prognosis of patients with nodenegative breast cancer: a prospective community-based feasibility study (raster) The Lancet Oncology. 2007; 8 (12):1079–1087. [ PubMed ] [ Google Scholar ]
- Capon DJ, Seeburg PH, McGrath JP, Hayflick JS, Edman U, Levinson AD, Goeddel DV. Activation of ki-ras2 gene in human colon and lung carcinomas by two different point mutations. Nature. 1982; 304 (5926):507–513. [ PubMed ] [ Google Scholar ]
- Cardoso F, Van’t Veer L, Rutgers E, Loi S, Mook S, Piccart-Gebhart MJ. Clinical application of the 70-gene profile: the mindact trial. Journal of Clinical Oncology. 2008; 26 (5):729–735. [ PubMed ] [ Google Scholar ]
- Chen X, Chen M, Ning K. Bnarray: an r package for constructing gene regulatory networks from microarray data by using bayesian network. Bioinformatics. 2006; 22 (23):2952–2954. [ PubMed ] [ Google Scholar ]
- Chin L, Hahn WC, Getz G, Meyerson M. Making sense of cancer genomic data. Genes & Development. 2011; 25 (6):534–555. [ PMC free article ] [ PubMed ] [ Google Scholar ]
- Clark BN, Gutstein HB. The myth of automated, high-throughput two-dimensional gel analysis. Proteomics. 2008; 8 :1197–1203. [ PubMed ] [ Google Scholar ]
- Colella S, Yau C, Taylor JM, Mirza G, Butler H, Clouston P, Bassett AS, Seller A, Holmes CC, Ragoussis J. QuantiSNP: an Objective Bayes Hidden-Markov Model to detect and accurately map copy number variation using SNP genotyping data. Nucleic Acids Res. 2007; 35 (6):2013–2025. [ PMC free article ] [ PubMed ] [ Google Scholar ]
- Collas P. The current state of chromatin immunoprecipitation. Molecular Biotechnology. 2010; 45 (1):87–100. [ PubMed ] [ Google Scholar ]
- Collins FS, Tabak LA. Policy: Nih plans to enhance reproducibility. Nature. 2014; 505 (7485):612–613. [ PMC free article ] [ PubMed ] [ Google Scholar ]
- Coombes KR, Tsavachidis S, Morris JS, Baggerly KA, Hung MC, Kuerer HM. Improved peak detection and quantification of mass spectrometry data acquired from surface-enhanced laser desorption and ionization by denoising spectra with the undecimated discrete wavelet transform. Proteomics. 2005; 5 :4107–4117. [ PubMed ] [ Google Scholar ]
- Cox DR, Wermuth N. Multivariate dependencies: Models, analysis and interpretation. Vol. 67. CRC Press; 1996. [ Google Scholar ]
- Davies H, Bignell GR, Cox C, Stephens P, Edkins S, Clegg S, Teague J, Woffendin H, Garnett MJ, Bottomley W, et al. Mutations of the braf gene in human cancer. Nature. 2002; 417 (6892):949–954. [ PubMed ] [ Google Scholar ]
- DeRisi JL, Iyer VR, Brown PO. Exploring the metabolic and genetic control of gene expression on a genomic scale. Science. 1997; 278 (5338):680–686. [ PubMed ] [ Google Scholar ]
- Discover. Discover. 2007. Jan, The top 6 genetics stories of 2006. [ Google Scholar ]
- Dobra A, Hans C, Jones B, Nevins JR, Yao G, West M. Sparse graphical models for exploring gene expression data. Journal of Multivariate Analysis. 2004; 90 (1):196– 212. doi: 10.1016/j.jmva.2004.02.009. URL http://www.sciencedirect.com/science/article/B6WK9-4C604WK-1/2/9a861453b1df438db4cff4e718f94246 . Special Issue on Multivariate Methods in Genomic Data Analysis. [ CrossRef ] [ Google Scholar ]
- Dowsey A, Dunn M, Yang G. Automated image alignment for 2d gel electrophoresis in a high-throughput proteomics pipeline. Bioinformatics. 2008; 24 :950–957. [ PubMed ] [ Google Scholar ]
- Dudoit S, Yang YH, Callow MJ, Speed TP. Statistical methods for identifying differentially expressed genes in replicated cdna microarray experiments. Statistica Sinica. 2002; 12 :111–139. [ Google Scholar ]
- Efron B. Large-scale simultaneous hypothesis testing: the choice of a null hypothesis. Journal of the American Statistical Association. 2004; 99 :96–104. [ Google Scholar ]
- Eisen MB, Spellman PT, Brown PO, Botstein D. Cluster analysis and display of genome-wide expression patterns. Proceedings of the National Academy of Sciences. 1998; 95 (25):14863–14868. [ PMC free article ] [ PubMed ] [ Google Scholar ]
- Fridlyand J, Snijders AM, Ylstra B, Li H, Olshen A, Segraves R, Dairkee S, Tokuyasu T, Ljung BM, Jain AN, et al. Breast tumor copy number aberration phenotypes and genomic instability. BMC cancer. 2006; 6 (1):1. [ PMC free article ] [ PubMed ] [ Google Scholar ]
- Friedman J, Hastie T, Tibshirani R. Sparse inverse covariance estimation with the graphical lasso. Biostatistics. 2008; 9 (3):432–441. doi: 10.1093/biostatistics/kxm045. http://biostatistics.oxfordjournals.org/cgi/content/abstract/9/3/432 . [ PMC free article ] [ PubMed ] [ CrossRef ] [ Google Scholar ]
- Friedman N, Linial M, Nachman I, Pe’er D. Using Bayesian networks to analyze expression data. Journal of Computational Biology. 2000; 7 (3–4):601–620. [ PubMed ] [ Google Scholar ]
- Fuentes M. Reproducible research in jasa. AmStat News. 2016 Jul 1 [ Google Scholar ]
- Gillespie D, Spiegelman S. A quantitative assay for dna-rna hybrids with dna immobilized on a membrane. Journal of Molecular Biology. 1965; 12 (3):829–842. [ PubMed ] [ Google Scholar ]
- Grossman RL, Heath AP, Ferretti V, Varmus HE, Lowy DR, Kibbe WA, Staudt LM. Toward a shared vision for cancer genomic data. New England Journal of Medicine. 2016; 375 (12):1109–1112. doi: 10.1056/NEJMp1607591. URL http://dx.doi.org/10.1056/NEJMp1607591 . [ PMC free article ] [ PubMed ] [ CrossRef ] [ Google Scholar ]
- Guinney J, Dienstmann R, Wang X, de Reynies A, Schlicker A, Soneson C, Marisa L, Roepman P, Nyamundanda G, Angelino P, Bot B, Morris J, Simon I, Gerster S, Fessler E, de Sousa A, Melo F, Missiaglia E, Ramay H, Barras D, Homicsko K, Maru D, Manyam G, Broom B, Boige V, Laderas T, Salazar R, Gray J, Tabernero J, Bernards R, Friend S, Laurent-Puig P, Medema J, Sadanandam A, Wessels L, Delorenzi M, Kopetz S, Vermeulen L, Tejpar S. The consensus molecular subtypes of colorectal cancer. Nature Medicine. 2015; 21 (11):1350–1356. [ PMC free article ] [ PubMed ] [ Google Scholar ]
- Ha MJ, Baladandayuthapani V, Do KA. Dingo: differential network analysis in genomics. Bioinformatics. 2015; 31 (21):3413–3420. [ PMC free article ] [ PubMed ] [ Google Scholar ]
- Hamid JS, Hu P, Roslin NM, Ling V, Greenwood CM, Beyene J. Data integration in genetics and genomics: methods and challenges. Human Genomics and Proteomics. 2009; 1 (1) [ PMC free article ] [ PubMed ] [ Google Scholar ]
- Hennessy B, Lu Y, Gonzalez-Angulo A, Carey M, Myhre S, Ju Z, Davies M, Liu W, Coombes K, Meric-Bernstam F, et al. A technical assessment of the utility of reverse phase protein arrays for the study of the functional proteome in non-microdissected human breast cancers. Clinical Proteomics. 2010; 6 (4):129–151. [ PMC free article ] [ PubMed ] [ Google Scholar ]
- Hofner B, Schmid M, Edler L. Reproducible research in statistics: A review and guidelines for the biometrical journal. Biometrical Journal. 2016; 58 (2):416–427. [ PubMed ] [ Google Scholar ]
- Hsu DS, Balakumaran BS, Acharya CR, Vlahovic V, Walters KS, Garman K, Anders C, Riedel RF, Lancaster J, Harpole D, Dressman HK, Nevins JR, Febbo PG, Potti A. Pharmacogenomic strategies provide a rational approach to the treatment of cisplatin-resistant patients with advanced cancer. Journal of Clinical Oncology. 2007; 25 :4350–4357. [ PubMed ] [ Google Scholar ]
- Ioannidis JP, Allison DB, Ball CA, Coulibaly I, Cui X, Culhane AC, Falchi M, Furlanello C, Game L, Jurman G, Mangion J, Mehta T, Nitzberg M, Page GP, Petretto E, Van Noort V. Repeatability of published microarray gene expression analyses. Nature Genetics. 2009; 41 :149–155. [ PubMed ] [ Google Scholar ]
- Irizarry RA, Bolstad BM, Collin F, Cope LM, Hobbs B, Speed TP. Summaries of affymetrix genechip probe level data. Nucleic Acids Research. 2003a; 31 (4):e15. [ PMC free article ] [ PubMed ] [ Google Scholar ]
- Irizarry RA, Hobbs B, Collin F, Beazer-Barday YD, Antonelli KJ, Scherf U, Speed TP. Exploration, normalization, and summaries of high density oligonucleotide array probe level data. Biostatistics. 2003b; 4 (2):249–264. [ PubMed ] [ Google Scholar ]
- Irizarry RA, Ladd-Acosta CL, Wen B, Wu Z, Montano C, Onyango P, Cui H, Gabo K, Rongione M, Webster M, Ji H, Potash JB, Sabunciyan S, Feinberg AP. The human colon cancer methylome shows similar hypo- and hypermethylation at conserved tissue-specific spg island shores. Nature Genetics. 2009; 41 :178–186. [ PMC free article ] [ PubMed ] [ Google Scholar ]
- Jaffe AE, Murakami P, Lee H, Leek JT, Fallin MD, Feinberg AP, Irizarry RA. Bump hunting to identify differentially methylated regions in epigenetic epidemiology studies. International Journal of Epidemiology. 2012; 41 (1):200–209. [ PMC free article ] [ PubMed ] [ Google Scholar ]
- Jennings EM, Morris JS, Manyam GC, Carroll RJ, Baladandayuthapani V. Bayesian models for flexible integrative analysis of multi-platform genomic data 2016 [ Google Scholar ]
- Jennings EM, Morris JS, Carroll RJ, Manyam G, Baladandayuthapani V. Bayesian methods for expression-based integration of various types of genomics data. EURASIP J. Bioinformatics and Systems Biology. 2013; 2013 :13. [ PMC free article ] [ PubMed ] [ Google Scholar ]
- Joshi-Tope G, Gillespie M, Vastrik I, D’Eustachio P, Schmidt E, de Bono B, Jassal B, Gopinath G, Wu G, Matthews L, et al. Reactome: a knowledgebase of biological pathways. Nucleic Acids Research. 2005; 33 (suppl 1):D428–D432. [ PMC free article ] [ PubMed ] [ Google Scholar ]
- Kallioniemi A, Kallioniemi OP, Sudar D, Rutovitz D, Gray JW, Waldman F, Pinkel D. Comparative genomic hybridization for molecular cytogenetic analysis of solid tumors. Science. 1992; 258 (5083):818–821. [ PubMed ] [ Google Scholar ]
- Kanehisa M, Goto S. Kegg: kyoto encyclopedia of genes and genomes. Nucleic Acids Research. 2000; 28 (1):27–30. [ PMC free article ] [ PubMed ] [ Google Scholar ]
- Karp NA, Lilley KS. Maximizing sensitivity for detecting changes in protein expression: Experimental design using minimal cydyes. Proteomics. 2005; 5 :3105–3115. [ PubMed ] [ Google Scholar ]
- Krieger CJ, Zhang P, Mueller LA, Wang A, Paley S, Arnaud M, Pick J, Rhee SY, Karp PD. Metacyc: a multiorganism database of metabolic pathways and enzymes. Nucleic Acids Research. 2004; 32 (suppl 1):D438–D442. [ PMC free article ] [ PubMed ] [ Google Scholar ]
- Kristensen VN, Lingjærde OC, Russnes HG, Vollan HKM, Frigessi A, Børresen-Dale AL. Principles and methods of integrative genomic analyses in cancer. Nature Reviews Cancer. 2014; 14 (5):299–313. [ PubMed ] [ Google Scholar ]
- Lanckriet GR, De Bie T, Cristianini N, Jordan MI, Noble WS. A statistical framework for genomic data fusion. Bioinformatics. 2004; 20 (16):2626–2635. [ PubMed ] [ Google Scholar ]
- Lee W, Morris J. Identification of differentially methylated loci using wavelet-based functional mixed models. Bioinformatics 2015 [ PMC free article ] [ PubMed ] [ Google Scholar ]
- Leek JT, Scharpf RB, Bravo HC, Simcha D, Langmead B, Johnson WE, Geman D, Baggerly K, Irizarry RA. Tackling the widespread and critical impact of batch effects in high-throughput data. Nature Reviews Genetics. 2010; 11 (10):733–739. [ PMC free article ] [ PubMed ] [ Google Scholar ]
- Li C, Wong W. Model-based anlysis of oligonucleotide arrays: model validation, design issues, and standard error approxiation. Genome Biology. 2001a; 2 (8):RESEARCH 0032. [ PMC free article ] [ PubMed ] [ Google Scholar ]
- Li C, Wong W. Model-based analysis of oligonucleotide arrays: expression index computation and outlier detection. Proceedings of the National Academy of Science (USA) 2001b; 98 :31–36. [ PMC free article ] [ PubMed ] [ Google Scholar ]
- Li F, Zhang NR. Bayesian variable selection in structured high-dimensional covariate spaces with applications in genomics. Journal of the American Statistical Association. 2010; 105 (491):1202–1214. [ Google Scholar ]
- Li F, Yang Y, Xing E. Inferring regulatory networks using a hierarchical Bayesian graphical Gaussian model. Carnegie Mellon University, School of Computer Science, Machine Learning Department; 2006. [ Google Scholar ]
- Liao H, Moschidis E, Riba-Garcia I, Zhang I, Unwin R, Morris J, Graham J, Dowsey A. A new paradigm for clinical biomarker discovery and screening with mass spectrometry based on biomedical image analysis principles. IEEE International Symposium on Biomedical Imaging 2014 [ Google Scholar ]
- Liao L, Moschidis E, Riba-Garcia I, Unwin R, Dunn W, Morris J, Graham J, Dowsey A. A workflow for novel image-based differential analysis of lc-ms experiments. Proceedings of 61st ASMS Conference on Mass Spectrometry and Allied Topics.2013. [ Google Scholar ]
- Liotta LA, Lowenthal M, Mehta A, Conrades TP, Veenstra TD, Fishman DA, Petricoin EF., III Importance of communication between producers and consumers of publicly available experimental data. Journal of the National Cancer Institute. 2005; 97 (4):310–314. [ PubMed ] [ Google Scholar ]
- Lister R, Pelizzola M, Dowen RH, Hawkins RD, Hon G, Tonti-Filippini J, Nery JR, Lee L, Ye Z, Ngo QM, Edsall L, Antosiewicz-Bourget J, Stewart R, Ruotti V, Millar AH, Thomson JA, Ren B, Ecker JR. Human dna methylomes at base resolution show widespread epigenomic differences. Nature. 2009; 462 :315–322. [ PMC free article ] [ PubMed ] [ Google Scholar ]
- Lock EF, Hoadley KA, Marron JS, Nobel AB. Joint and individual variation explained (jive) for integrated analysis of multiple data types. The Annals of Applied Statistics. 2013; 7 (1):523. [ PMC free article ] [ PubMed ] [ Google Scholar ]
- Lucito R, West J, Reiner A, Alexander J, Esposito D, Mishra B, Powers S, Norton L, Wigler M. Detecting gene copy number fluctuations in tumor cells by microarray analysis of genomic representations. Genome Research. 2000; 10 (11):1726–1736. [ PMC free article ] [ PubMed ] [ Google Scholar ]
- Mallick BK, Gold DL, Baladandayuthapani V. Front Matter. Wiley Online Library; 2009. [ Google Scholar ]
- Mei R, Galipeau PC, Prass C, Berno A, Ghandour G, Patil N, Wolff RK, Chee MS, Reid BJ, Lockhart DJ. Genome-wide detection of allelic imbalance using human snps and high-density dna arrays. Genome Research. 2000; 10 (8):1126–1137. [ PMC free article ] [ PubMed ] [ Google Scholar ]
- Meinshausen N, Bühlmann P. High-dimensional graphs and variable selection with the lasso. The Annals of Statistics. 2006; 34 (3):1436–1462. URL http://www.jstor.org/stable/25463463 . [ Google Scholar ]
- Meyer M, Coull B, Versace F, Cinciripini P, Morris J. Bayesian function-on-function regression for multi-level functional data. Biometrics. 2016; 71 (3):563–574. [ PMC free article ] [ PubMed ] [ Google Scholar ]
- Mo Q, Wang S, Seshan VE, Olshen AB, Schultz N, Sander C, Powers RS, Ladanyi M, Shen R. Pattern discovery and cancer gene identification in integrated cancer genomic data. Proceedings of the National Academy of Sciences. 2013; 110 (11):4245–4250. [ PMC free article ] [ PubMed ] [ Google Scholar ]
- Morris JS, Coombes K, Kooman J, Baggerly K, Kobayashi R. Feature extraction and quantification for mass spectrometry data in biomedical applications using the mean spectrum. Bioinformatics. 2005; 21 :1764–1775. [ PubMed ] [ Google Scholar ]
- Morris JS, Clark B, Gutstein H. Pinnacle: A fast, automatic and accurate method for detecting and quantifying protein spots in 2-dimensional gel electrophoresis data. Bioinformatics. 2008a; 24 :529–536. [ PMC free article ] [ PubMed ] [ Google Scholar ]
- Morris JS, Clark BN, Wei W, Gutstein HB. Evaluating the performance of new approaches to spot quantification and differential expression in 2-dimensional gel electrophoresis studies. Journal of Proteome Research. 2010; 9 (1):595–604. [ PMC free article ] [ PubMed ] [ Google Scholar ]
- Morris JS, Brown PJ, Herrick RC, Baggerly KA, Coombes KR. Bayesian analysis of mass spectrometry data using wavelet-based functional mixed models. Biometrics. 2008b; 12 :479–489. [ PMC free article ] [ PubMed ] [ Google Scholar ]
- Morris J. Statistical methods for proteomic biomarker discovery using feature extraction or functional data analysis approaches. Statistics and its Interface. 2012; 5 (1):117–136. [ PMC free article ] [ PubMed ] [ Google Scholar ]
- Morris J. Functional regression. Annual Review of Statistics and its Application. 2015; 2 :321–359. [ Google Scholar ]
- Morris J, Carroll R. Wavelet-based functional mixed models. J R Statist Soc B. 2006; 68 (2):179–199. [ PMC free article ] [ PubMed ] [ Google Scholar ]
- Morris J, Baladandayuthapani V, Herrick R, Sanna P, Gutstein H. Automated analysis of quantitative image data using isomorphic functional mixed models, with application to proteomics data. The Annals of Applied Statistics. 2011; 5 :894–923. [ PMC free article ] [ PubMed ] [ Google Scholar ]
- Muller P, Parmigiani G, Robert C, Rousseau J. Optimal sample size for multiple testing: The case of gene expression microarrays. Journal of the American Statistical Association. 2004; 99 (468):990–1001. [ Google Scholar ]
- Myllymäki P, Silander T, Tirri H, Uronen P. B-course: A web-based tool for bayesian and causal data analysis. International Journal on Artificial Intelligence Tools. 2002; 11 (03):369–387. [ Google Scholar ]
- Neeley ES, Kornblau SM, Coombes KR, Baggerly KA. Variable slope normalization of reverse phase protein arrays. Bioinformatics. 2009; 25 (11):1384–1389. doi: 10.1093/bioinformatics/btp174. URL http://bioinformatics.oxfordjournals.org/cgi/content/abstract/25/11/1384 . [ PMC free article ] [ PubMed ] [ CrossRef ] [ Google Scholar ]
- Newton MA, Wang Z. Multiset statistics for gene set analysis. Annual Review of Statistics and its Application. 2015; 2 :95–111. [ PMC free article ] [ PubMed ] [ Google Scholar ]
- Newton MA, Noueiry A, Sarker D, Ahlquist P. Detecting differential gene expression with a semiparametric hierarchical mixture model. Biostatistics. 2004; 5 (2):155–176. [ PubMed ] [ Google Scholar ]
- Newton MA, Quintana FA, Den Boon JA, Sengupta S, Ahlquist P. Random-set methods identify distinct aspects of the enrichment signal in gene-set analysis. The Annals of Applied Statistics. 2007:85–106. [ Google Scholar ]
- Newton MA, He Q, Kendziorski C. A model-based analysis to infer the functional content of a gene list. Statistical Applications in Genetics and Molecular Biology. 2012; 11 (2) [ PMC free article ] [ PubMed ] [ Google Scholar ]
- Ni Y, Stingo FC, Baladandayuthapani V. Integrative Bayesian network analysis of genomic data. Cancer Informatics. 2014; 13 (Suppl 2):39. [ PMC free article ] [ PubMed ] [ Google Scholar ]
- Ni Y, Stingo FC, Baladandayuthapani V. Bayesian nonlinear model selection for gene regulatory networks. Biometrics. 2015; 71 (3):585–595. [ PMC free article ] [ PubMed ] [ Google Scholar ]
- Ni Y, Stingo FC, Baladandayuthapani V. Sparse multi-dimensional graphical models: A unified Bayesian framework. Journal of the American Statistical Association. 2016 (to appear) [ Google Scholar ]
- O’Farrell PH. High-resolution two-dimensional electrophoresis of proteins. Journal of Biological Chemistry. 1975; 250 :4007–4021. [ PMC free article ] [ PubMed ] [ Google Scholar ]
- Olshen AB, Venkatraman E, Lucito R, Wigler M. Circular binary segmentation for the analysis of array-based dna copy number data. Biostatistics. 2004; 5 (4):557–572. [ PubMed ] [ Google Scholar ]
- Pan W, Xie B, Shen X. Incorporating predictor network in penalized regression with application to microarray data. Biometrics. 2010; 66 (2):474–484. [ PMC free article ] [ PubMed ] [ Google Scholar ]
- Park MY, Hastie T, Tibshirani R. Averaged gene expressions for regression. Biostatistics. 2007; 8 (2):212–227. [ PubMed ] [ Google Scholar ]
- Paweletz C, Charboneau L, Bichsel V, Simone N, Chen T, Gillespie J, Emmert-Buck M, Roth M, Petricoin E, Liotta L. Reverse phase protein microarrays which capture disease progression show activation of prosurvival pathways at the cancer invasion front. Oncogene. 2001; 20 (16):1981–1989. [ PubMed ] [ Google Scholar ]
- Pease AC, Solas D, Sullivan EJ, Cronin MT, Holmes CP, Fodor S. Light-generated oligonucleotide arrays for rapid dna sequence analysis. Proceedings of the National Academy of Sciences. 1994; 91 (11):5022–5026. [ PMC free article ] [ PubMed ] [ Google Scholar ]
- Peng R. Reproduble research and Biostatistics . Biostatistics. 2009; 10 (3):405–408. [ PubMed ] [ Google Scholar ]
- Petricoin EFI, Fishman DA, Conrads TP, Veenstra TD, Liotta LA. Proteomic pattern diagnostics: Producers and consumers in the era of correlative science. comment on sorace and zhan. BMC Bioinformatics 2004 [ Google Scholar ]
- Petricoin EFI, Ardekani AM, Hitt BA, Levine PJ, Fusaro VA, Steinberg SM, Mils GB, Simone C, Fishman DA, Kohn EC, Liotta LA. Use of proteomic patterns in serum to identify ovarian cancer. The Lancet. 2002; 359 :572–577. [ PubMed ] [ Google Scholar ]
- Pinkel D, Albertson DG. Array comparative genomic hybridization and its applications in cancer. Nature Genetics. 2005; 37 :S11–S17. [ PubMed ] [ Google Scholar ]
- Pinkel D, Segraves R, Sudar D, Clark S, Poole I, Kowbel D, Collins C, Kuo WL, Chen C, Zhai Y, et al. High resolution analysis of dna copy number variation using comparative genomic hybridization to microarrays. Nature Genetics. 1998; 20 (2):207–211. [ PubMed ] [ Google Scholar ]
- Pollack JR, Perou CM, Alizadeh AA, Eisen MB, Pergamenschikov A, Williams CF, Jeffrey SS, Botstein D, Brown PO. Genome-wide analysis of dna copy-number changes using cdna microarrays. Nature Genetics. 1999; 23 (1):41–46. [ PubMed ] [ Google Scholar ]
- Potti A, Dressman HK, Bild A, Riedel RF, Chan G, Sayer R, Cragun J, Cottrill H, Kelley MJ, Petersen R, Harpole D, Marks J, Berchuck A, Ginsburg GS, Febbo P, Lancaster J, Nevins JR. Genomic signatures to guide the use of chemotherapeutics. Nature Medicine. 2006; 12 :1294–1300. [ PubMed ] [ Google Scholar ]
- Qin L-X. An integrative analysis of microrna and mrna expression-a case study. Cancer Informatics. 2008:6. [ PMC free article ] [ PubMed ] [ Google Scholar ]
- Ramaswamy S, Tamayo P, Rifkin R, Mukherjee S, Yeang CH, Angelo M, Ladd C, Reich M, Latulippe E, Mesirov JP, et al. Multiclass cancer diagnosis using tumor gene expression signatures. Proceedings of the National Academy of Sciences. 2001; 98 (26):15149–15154. [ PMC free article ] [ PubMed ] [ Google Scholar ]
- Ramsay J, Silverman B. Functional Data Analysis. Springer-Verlag; New York: 1997. [ Google Scholar ]
- Schena M, Shalon D, Davis RW, Brown PO. Quantitative monitoring of gene expression patterns with a complementary dna microarray. Science. 1995; 270 (5235):467–470. [ PubMed ] [ Google Scholar ]
- Schena M, Heller RA, Theriault TP, Konrad K, Lachenmeier E, Davis RW. Microarrays: biotechnology’s discovery platform for functional genomics. Trends in Biotechnology. 1998; 16 (7):301–306. [ PubMed ] [ Google Scholar ]
- Schuster SC. Next-generation sequencing transforms today’s biology. Nature Methods. 2008; 5 (1):16–18. [ PubMed ] [ Google Scholar ]
- Seidel C. Introduction to DNA microarrays. Analysis of Microarray Data: A Network-based Approach. 2008; 1 :1. [ Google Scholar ]
- Shalon D, Smith SJ, Brown PO. A dna microarray system for analyzing complex dna samples using two-color fluorescent probe hybridization. Genome Research. 1996; 6 (7):639–645. [ PubMed ] [ Google Scholar ]
- Shen R, Wang S, Mo Q. Sparse integrative clustering of multiple omics data sets. The Annals of Applied Statistics. 2013; 7 (1):269. [ PMC free article ] [ PubMed ] [ Google Scholar ]
- Shen X, Huang HC, Pan W. Simultaneous supervised clustering and feature selection over a graph. Biometrika. 2012; 99 (4):899–914. [ PMC free article ] [ PubMed ] [ Google Scholar ]
- Snijders AM, Nowak N, Segraves R, Blackwood S, Brown N, Conroy J, Hamilton G, Hindle AK, Huey B, Kimura K, et al. Assembly of microarrays for genome-wide measurement of dna copy number. Nature Genetics. 2001; 29 (3):263–264. [ PubMed ] [ Google Scholar ]
- Sorace J, Zhan M. A data review and re-assessment of ovarian cancer serum proteomic profiling. BMC Bioinformatics. 2003:4. [ PMC free article ] [ PubMed ] [ Google Scholar ]
- Stingo FC, Chen YA, Vannucci M, Barrier M, Mirkes PE. A Bayesian graphical modeling approach to microRNA regulatory network inference. The Annals of Applied Statistics. 2010; 4 (4):2024–2048. [ PMC free article ] [ PubMed ] [ Google Scholar ]
- Stingo FC, Chen YA, Tadesse MG, Vannucci M. Incorporating biological information into linear models: A bayesian approach to the selection of pathways and genes. The Annals of Applied Statistics. 2011; 5 (3) [ PMC free article ] [ PubMed ] [ Google Scholar ]
- Stodden V, Guo P, Ma Z. Toward reproducible computational research: An empirical analysis of data and code policy adoption by journals. PLOS One. 2013; 8 (6):e67111. [ PMC free article ] [ PubMed ] [ Google Scholar ]
- Storey JD. A direct approach to false discovery rate. JRSS-B. 2002; 64 :479–498. [ Google Scholar ]
- Storey JD. A positive false discovery rate: a bayesian interpretation and the q-value. Annals of Statistics. 2003; 31 :2013–2035. [ Google Scholar ]
- Subramanian A, Tamayo P, Mootha VK, Mukherjee S, Ebert BL, Gillette MA, Paulovich A, Pomeroy SL, Golub TR, Lander ES, et al. Gene set enrichment analysis: a knowledge-based approach for interpreting genome-wide expression profiles. Proceedings of the National Academy of Sciences. 2005; 102 (43):15545–15550. [ PMC free article ] [ PubMed ] [ Google Scholar ]
- Tibes R, Qiu Y, Lu Y, Hennessy B, Andreeff M, Mills GB, Kornblau SM. Reverse phase protein array: validation of a novel proteomic technology and utility for analysis of primary leukemia specimens and hematopoietic stem cells. Molecular Cancer Therapeutics. 2006; 5 (10):2512–2521. [ PubMed ] [ Google Scholar ]
- Tibshirani R, Wang P. Spatial smoothing and hot spot detection for cgh data using the fused lasso. Biostatistics. 2008; 9 (1):18–29. [ PubMed ] [ Google Scholar ]
- Tomioka N, Oba S, Ohira M, Misra A, Fridlyand J, Ishii S, Nakamura Y, Isogai E, Hirata T, Yoshida Y, et al. Novel risk stratification of patients with neuroblastoma by genomic signature, which is independent of molecular signature. Oncogene. 2008; 27 (4):441–449. [ PubMed ] [ Google Scholar ]
- Touleimat N, Tost J. Complete pipeline for infinium human methylation 450K BeadChip data processing using subset quantile normalization for accurate DNA methylation estimation. Epigenomics. 2012; 4 (3):325–341. [ PubMed ] [ Google Scholar ]
- Tukey JW. Exploratory Data Analysis. Addison-Wesley Publishing Company; 1977. [ Google Scholar ]
- Tyekucheva S, Marchionni L, Karchin R, Parmigiani G. Integrating diverse genomic data using gene sets. Genome Biology. 2011; 12 (10):1–14. [ PMC free article ] [ PubMed ] [ Google Scholar ]
- Vissers LE, de Vries BB, Veltman JA. Genomic microarrays in mental retardation: from copy number variation to gene, from research to diagnosis. Journal of Medical Genetics. 2010; 47 (5):289–297. [ PubMed ] [ Google Scholar ]
- Wang K, Li M, Hadley D, Liu R, Glessner J, Grant SF, Hakonarson H, Bucan M. PennCNV: an integrated hidden Markov model designed for high-resolution copy number variation detection in whole-genome SNP genotyping data. Genome Research. 2007; 17 (11):1665–1674. [ PMC free article ] [ PubMed ] [ Google Scholar ]
- Wang W, Baladandayuthapani V, Morris JS, Broom BM, Manyam G, Do KA. ibag: integrative bayesian analysis of high-dimensional multiplatform genomics data. Bioinformatics. 2013; 29 (2):149–159. [ PMC free article ] [ PubMed ] [ Google Scholar ]
- Wang Z, He Q, Larget B, Newton MA, et al. A multi-functional analyzer uses parameter constraints to improve the efficiency of model-based geneset analysis. The Annals of Applied Statistics. 2015; 9 (1):225–246. [ Google Scholar ]
- Werhli AV, Grzegorczyk M, Husmeier D. Comparative evaluation of reverse engineering gene regulatory networks with relevance networks, graphical gaussian models and bayesian networks. Bioinformatics. 2006; 22 (20):2523–2531. [ PubMed ] [ Google Scholar ]
- Yau C, Holmes C. Cnv discovery using snp genotyping arrays. Cytogenetic and Genome Research. 2009; 123 (1–4):307–312. [ PubMed ] [ Google Scholar ]
- Zhang L, Baladandayuthapani V, Baggerly K, Czerniak B, Morris J. Functional car models for spatially correlation high-dimensional functional data. Journal of the American Statistical Association. 2016 to appear. [ PMC free article ] [ PubMed ] [ Google Scholar ]
- Zhang L, Wei Q, Mao L, Liu W, Mills GB, Coombes K. Serial dilution curve: a new method for analysis of reverse phase protein array data. Bioinformatics. 2009; 25 (5):650–654. doi: 10.1093/bioinformatics/btn663. URL http://bioinformatics.oxfordjournals.org/cgi/content/abstract/25/5/650 . [ PMC free article ] [ PubMed ] [ CrossRef ] [ Google Scholar ]
- Zhu H, Brown P, Morris J. Robust classification of functional and quantitative image data using functional mixed models. Biometrics. 2012; 68 :1260–1268. [ PMC free article ] [ PubMed ] [ Google Scholar ]

IMAGES
VIDEO
COMMENTS
Bioinformatics is a field of study that uses computation to extract knowledge from biological data. It includes the collection, storage, retrieval, manipulation and modelling of data for analysis ...
Designing and delivering bioinformatics project-based learning in East Africa. The Eastern Africa Network for Bioinformatics Training (EANBiT) has matured through continuous evaluation, feedback, and codesign. ... Neuroscience research in Drosophila is benefiting from large-scale connectomics efforts using electron microscopy (EM) to reveal all ...
This is an editorial report of the supplements to BMC Bioinformatics that includes 6 papers selected from the BIOCOMP'19—The 2019 International Conference on Bioinformatics and Computational Biology. These articles reflect current trend and development in bioinformatics research. ... network based on the 407 differential expressed genes ...
Bioinformatics is an official journal of the International Society for Computational Biology, the leading professional society for computational biology and bioinformatics. Members of the society receive a 15% discount on article processing charges when publishing Open Access in the journal. Read papers from the ISCB. Find out more.
Bioinformatics and Biology Insights is an open access, peer-reviewed journal that considers articles on bioinformatics methods and their applications, which must pertain to biological insights. All papers should be easily amenable to biologists and, as such, help bridge the gap between theories and applications. View full journal description
This is an editorial report of the supplements to BMC Bioinformatics that includes 6 papers selected from the BIOCOMP'19—The 2019 International Conference on Bioinformatics and Computational Biology. These articles reflect current trend and development in bioinformatics research. Keywords: Bioinformatics, Biomarkers, Human disease, Microbiome.
The results of our analysis imply that research on bioinformatics is becoming more diversified and the ranking of computational methods in bioinformatics research is also gradually improving. ... most productive organizations, and most popular subject terms. Research impact was analyzed based on the measures of most cited papers, most cited ...
Bioinformatics is defined as the application of tools of computation and analysis to the capture and interpretation of biological data. It is an interdisciplinary field, which harnesses computer science, mathematics, physics, and biology (fig. . 1 ). Bioinformatics is essential for management of data in modern biology and medicine.
Author Summary Contemporary biology has largely become computational biology, whether it involves applying physical principles to simulate the motion of each atom in a piece of DNA, or using machine learning algorithms to integrate and mine "omics" data across whole cells (or even entire ecosystems). The ability to design algorithms and program computers, even at a novice level, may be the ...
This paper provides a comprehensive review of the current state of cloud-based bioinformatics applications and big data (as summarized in Table 1, Table 2) to explore the potential role of cloud computing in this field. The paper makes three significant contributions: firstly, it diagnoses the use of cloud computing in bioinformatics; secondly ...
Interdisciplinary research: Big science at the table. Researchers are adopting the tools of bioinformatics and pharmaceuticals to study and interpret the ever-growing body of data on the interplay ...
The 13 computational research and training needs investigated in the NSF survey. Computational research and training needs of NSF DBS investigators. Research needs. Training and support needs. Publish data to the community. Data management and metadata. Sufficient data storage. Bioinformatics and data analysis. Share data with colleagues.
High Performance Computing, Big Data Analytics and Integration for Multi-Omics Biomedical Data. An innovative journal that provides a forum for new discoveries in bioinformatics. It focuses on how new tools and applications can bring insights to specific biological problems.
Inspired by the theory and applications of spaced seeds, we propose a graph representation of genome sequences called gapped pattern graph. These graphs can be transformed through a Graph Convolutional Network to form lower-dimensional embeddings for downstream tasks. On the basis of the gapped pattern graphs, we implemented a neural network ...
View Bioinformatics Research Papers on Academia.edu for free. Skip to main content ... 50 children aged 7-12 with combined form of AD/HD were randomly divided into two groups based on gender blocks: one received melatonin (3 or 6 mg based on weight) combined with ritalin (1mg/kg) and the other took placebo combined with ritalin (1mg/kg) in a ...
The R programming language is approaching its 30th birthday, and in the last three decades it has achieved a prominent role in statistics, bioinformatics, and data science in general. It currently ranks among the top 10 most popular languages worldwide, and its community has produced tens of thousands of extensions and packages, with scopes ...
BIOINFORMATICS: AN OVERVIEW FOR CANCER RESEARCH. Mamta Chowdhary *1, Dr. Asha R ani 1, Jyoti Parkash 2, Mohd Shahn az 2, and Dhruv Dev 2. 1 Dolphin (PG) college of Life Sciences, Chuni Kalan, Dist ...
Single-cell multi-omics sequencing has greatly accelerated reproductive research in recent years, and the data are continually growing. However, utilizing these data resources is challenging for wet-lab researchers.
Background: Although patients have easy access to their electronic health records and laboratory test result data through patient portals, laboratory test results are often confusing and hard to understand. Many patients turn to web-based forums or question-and-answer (Q&A) sites to seek advice from their peers. The quality of answers from social Q&A sites on health-related questions ...
A recent benchmark paper ... Compared to existing antibody-based ... and real-time onboard base calling with Guppy and other offline bioinformatics tools, enables field research in ...
Meanwhile, analytical methods based on bioinformatics are also well developed in many aspects of plant genomic research including comparative genomic analysis, phylogenomics and evolutionary analysis, and genome-wide association study. ... is the real challenge for plant genome research. This review paper focuses on challenges and opportunities ...
Background: Fundus photography is the most important examination in eye disease screening. A facilitated self-service eye screening pattern based on the fully automatic fundus camera was developed in 2022 in Shanghai, China; it may help solve the problem of insufficient human resources in primary health care institutions. However, the service quality and residents' preference for this new ...
Leave No Context Behind: Efficient Infinite Context Transformers with Infini-attention. This work introduces an efficient method to scale Transformer-based Large Language Models (LLMs) to infinitely long inputs with bounded memory and computation. A key component in our proposed approach is a new attention technique dubbed Infini-attention.
We focused our search on the most relevant journals for bioinformatics and translational informatics with electronic publication dates on or after January 1, 2021. The journals surveyed for best papers are as follows: Journal of the American Medical Informatics Association (JAMIA), Journal of Biomedical Informatics (JBI), PLoS Computational ...
Shortly after publication of this paper, three clinical trials based on these studies were suspended and a fourth terminated (The Cancer Letter, October 9 and 23, 2009), and Duke University convened a panel of outside experts to investigate this research and the original results. Surprisingly, this panel determined that the concerns were ...