Thank you for visiting nature.com. You are using a browser version with limited support for CSS. To obtain the best experience, we recommend you use a more up to date browser (or turn off compatibility mode in Internet Explorer). In the meantime, to ensure continued support, we are displaying the site without styles and JavaScript.
- View all journals
- Explore content
- About the journal
- Publish with us
- Sign up for alerts
- Open access
- Published: 29 April 2024

Problematic social media use mediates the effect of cyberbullying victimisation on psychosomatic complaints in adolescents
- Prince Peprah 1 , 2 ,
- Michael Safo Oduro 3 ,
- Godfred Atta-Osei 4 ,
- Isaac Yeboah Addo 5 , 6 ,
- Anthony Kwame Morgan 7 &
- Razak M. Gyasi 8 , 9
Scientific Reports volume 14 , Article number: 9773 ( 2024 ) Cite this article
1246 Accesses
1 Citations
1 Altmetric
Metrics details
- Public health
- Risk factors
Adolescent psychosomatic complaints remain a public health issue globally. Studies suggest that cyberbullying victimisation, particularly on social media, could heighten the risk of psychosomatic complaints. However, the mechanisms underlying the associations between cyberbullying victimisation and psychosomatic complaints remain unclear. This cross-cultural study examines the mediating effect of problematic social media use (PSMU) on the association between cyberbullying victimisation and psychosomatic complaints among adolescents in high income countries. We analysed data on adolescents aged 11–16.5 years (weighted N = 142,298) in 35 countries participating in the 2018 Health Behaviour in School-aged Children (HBSC) study. Path analysis using bootstrapping technique tested the hypothesised mediating role of PSMU. Results from the sequential binary mixed effects logit models showed that adolescents who were victims of cyberbullying were 2.39 times significantly more likely to report psychosomatic complaints than those who never experienced cyberbullying (AOR = 2.39; 95%CI = 2.29, 2.49). PSMU partially mediated the association between cyberbullying victimisation and psychosomatic complaints accounting for 12% ( \(\beta\) = 0.01162, 95%CI = 0.0110, 0.0120) of the total effect. Additional analysis revealed a moderation effect of PSMU on the association between cyberbullying victimisation and psychosomatic complaints. Our findings suggest that while cyberbullying victimisation substantially influences psychosomatic complaints, the association is partially explained by PSMU. Policy and public health interventions for cyberbullying-related psychosomatic complaints in adolescents should target safe social media use.
Similar content being viewed by others
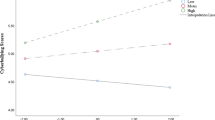
Cyberbullying and cybervictimization on digital media platforms: the role of demographic variables and parental mediation strategies
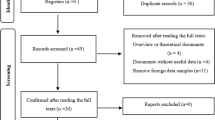
The relationship between childhood trauma and cyberbullying: a meta-analysis of mainland Chinese adolescents and young adults
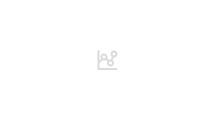
Problematic situations related to social media use and competencies to prevent them: results of a Delphi study
Introduction.
Adolescence is noted to be a critical developmental stage, with many problems, including loneliness 1 , poor friendships, an adverse class climate, school pressure 2 , suicidal ideation and attempts, and psychosomatic complaints 3 . Psychosomatic complaint is a combination of physical ailments (i.e., headaches, stomach aches, fatigue, and muscle pain) caused or exacerbated by psychological factors such as stress, irritability, anxiety, or emotional distress 4 , 5 . Psychosomatic complaints are common among adolescents, and recent estimates indicate that the global prevalence of psychosomatic complaints ranges between 10 and 50% 6 . Also, an increase in self-reported psychosomatic complaints and related mental health complaints have been reported in adolescents from high-income countries 7 , 8 . The high prevalence of psychosomatic complaints is of concern as psychosomatic complaints have severe implications for multiple detrimental health outcomes, healthcare expenditure, and quality of life of young people 9 . Thus, it is of utmost importance to identify the proximate risk factors for psychosomatic complaints among young people to aid in developing targeted interventions to reduce the incidence of psychosomatic complaints, mainly in high-income countries.
While extant research has identified risk factors for psychosomatic complaints, including malnutrition, low physical activity, and poor parental guidance 10 , 11 , 12 , one understudied but potentially important risk factor is cyberbullying victimisation. Cyberbullying victimisation is an internet-based aggressive and intentional act of continually threatening, harassing, or embarrassing individuals who cannot defend themselves using electronic contact forms such as emails, text messages, images, and videos 13 , 14 . Indeed, being typical of interpersonal interactions, cyberbullying victimisation has shown a rising trend, particularly during adolescence 15 . International literature has shown the prevalence of cyberbullying victimisation to be between 12 and 72% among young people 14 , 16 . It may be hypothesised that cyberbullying victimisation potentially increases the risk of psychosomatic complaints through factors such as problematic social media use (PSMU) 17 , 18 . However, studies are needed to identify whether and the extent to which such factors mediate the potential association of cyberbullying victimisation with psychosomatic complaints among young people.
Given this background, the present study aimed to investigate the association between cyberbullying victmisation and psychosomatic complaints in 142,298 young people aged 11–16.5 years from 35 high-income countries. A further aim was to quantify how PSMU mediates the association between cyberbullying victimisation and psychosomatic complaints.
Cyberbullying victimisation and adolescents’ psychosomatic complaints
Research has consistently shown that cyberbullying victimisation significantly impacts adolescents’ mental health 19 . For example, Kowalski and Limber 20 found that cyberbullying victimisation is associated with increased levels of depression, anxiety, and social anxiety, as well as psychosomatic complaints, such as fatigue and muscle tension. Further, studies have shown that cyberbullying victimisation and perpetration can lead to a variety of physical, social, and mental health issues, including substance abuse and suicidal thoughts and attempts 21 , 22 , 23 , 24 . Furthermore, cyberbullying victimisation is strongly associated with suicidal thoughts and attempts, regardless of demographic factors like gender or age 21 , 25 . These findings underscore the urgent need for interventions that address the mental health consequences of cyberbullying, particularly for adolescents, who are most vulnerable to its harmful effects. The findings also suggest that cyberbullying might be a potential underlying predictor of higher psychosomatic disorders among adolescents. This present study, therefore, hypothesises that H1: there is a statistically significant association between cyberbullying victimisation (X) and psychosomatic complaints (Y) (total effect).
The role of adolescents’ PSMU
Problematic Social Media Use (PSMU), a subtype of problematic internet use, refers to the uncontrolled, compulsive or excessive engagement with social media platforms such as Facebook and Twitter, characterised by addictive behaviours like mood alteration, withdrawal symptoms, and interpersonal conflicts. This pattern of social media usage can result in functional impairments and adverse outcomes 26 . Scholars and professionals have shown great concern about the length of time adolescents spend on social media. Studies have observed that (early) adolescence could be a crucial and sensitive developmental stage in which adolescent users might be unable to avoid the harmful impacts of social media use 27 . According to current research, PSMU may increase adolescents’ exposure to cyberbullying victimisation, which can have severe consequences for their mental health 28 , 29 , 30 . Similarly, an association between PSMU and physical/somatic problems, as well as somatic disorders, has been established in many studies 31 , 32 . Hanprathet et al. 33 demonstrated the negative impact of problematic Facebook use on general health, including somatic symptoms, anxiety, insomnia, depression, and social dysfunction. According to Cerutti et al. 34 , adolescents with problematic social media usage have more somatic symptoms, such as stomach pain, headaches, sore muscles, and poor energy, than their counterparts. Hence, inadequate sleep may be associated with PSMU, harming both perceived physical and mental health 35 , 36 . Again, supporting the above evidence, the relationship between PSMU, well-being, and psychological issues have been highlighted in meta-analytic research and systematic reviews 27 , 31 , 37 , 38 . Thus, this study proposes the following hypothesis: H2: there is a specific indirect effect of cyberbullying victimisation (X) on psychosomatic complaints (Y) through PSMU (M1) (indirect effect a 1 b 1 ).
Study, sample, and procedures
This study used data from the 2018 Health Behaviour in School-aged Children (HBSC) survey conducted in 35 countries and regions across Europe and Canada during the 2017–2018 academic year 39 . The HBSC research team/network is an international alliance of researchers collaborating on a cross-national survey of school students. The HBSC collects data every four years on 11-, 13- and 15- year-old adolescent boys’ and girls’ health and well-being, social environments, and health behaviours. The sampling procedure for the 2018 survey followed international guidelines 40 , 41 . A systematic sampling method was used to identify schools in each region from the complete list of both public and private schools. Participants were recruited through a cluster sampling approach, using the school class as the primary sampling unit 42 . Some countries oversampled subpopulations (e.g., by geography and ethnicity), and standardised weights were created to ensure representativeness of the population of 11, 13, and 15 years 43 . Questionnaires were translated based on a standard procedure to allow comparability between the participating countries. Our analysis used data from 35 countries and regions with complete data on cyberbullying victimisation, PSMU, and psychosomatic complaints. The study complies with ethical standards in each country and follows ethical guidelines for research and data protection from the World Health Organisation and the Organisation for Economic Co-operation and Development. Depending on the country, active or passive consent was sought from parents or legal guardians and students which was checked by teachers to participate in the study. The survey was conducted anonymously and participation in the study was voluntary for schools and students. Schools, children and adolescents could refuse to participate or withdraw their consent until the day of the survey. Moreover, all participating students were free to cease filling out the questionnaire at any moment, or to answer only selected questions. More detailed information on the methodology of the HBSC study including ethics and data protection can be found elsewhere 44 , 45 .
Outcome variable: psychosomatic complaints
Psychosomatic complaints was assessed by one collective item asking students how often they had experienced the following complaints over the past six months: headache, stomach aches, feeling low, irritability or bad mood, feeling nervous, dizziness, abdominal pain, sleep difficulty, and backache. Response options included: about every day, more than once a week, about every week, about every month, and rarely or never. This scale has sufficient test–retest reliability and validity 46 , good internal consistency (Cronbach’s a = 0.82) 47 , and has been applied in several multiple country analyses 48 , 49 . The scale is predictive of emotional problems and suicidal ideation in adolescents 50 , 51 . For our analysis, the scale was dichotomised with two or more complaints several times a week or daily coded as having psychosomatic complaints 47 , 49 .
Exposure variable: Cyberbullying victimisation
Cyberbullying victimisation is the exposure variable in this study. Thus, the exposure variable pertains to only being a victim of cyberbullying and does not include perpetration of cyberbullying. Students were first asked to read and understand a short definition of cyberbullying victimisation. They were then asked how often they were bullied over the past two months (e.g., someone sending mean instant messages, emails, or text messages about you; wall postings; creating a website making fun of you; posting unflattering or inappropriate pictures of you online without your permission or sharing them with others). Responses included: “ I have not been cyberbullied”, “once or twice”, “two or three times a month”, “about once a week”, and “several times a week”. These were dichotomised into “never" or “once or more". This measure of bullying victimisation has been validated across multiple cultural settings 43 , 52 , 53 , 54 .
Mediating variable
Problematic social media use (PSMU) was assessed with the Social Media Disorder Scale (Cronbach’s a = 0.89) 55 . The scale contains nine dichotomous (yes/no) items describing addiction-like symptoms, including preoccupation with social media, dissatisfaction about lack of time for social media, feeling bad when not using social media, trying but failing to spend less time using social media, neglecting other duties to use social media, frequent arguments over social media, lying to parents or friends about social media use, using social media to escape from negative feelings, and having a severe conflict with family over social media use. In this study, the endorsement of six or more items indicated PSMU as evidence suggests that a threshold of six or more is an indicative of PSMU 54 , 56 . This scale has been used across cultural contexts 43 , 52 , 54 .
Informed by previous studies 43 , 54 , 57 , the analysis controlled for theoretically relevant confounders, including sex (male/female) and age. Family affluence/socio-economic class was assessed using the Relative Family Affluence Scale, a validated six-item measure of material assets in the home, such as the number of vehicles, bedroom sharing, computer ownership, bathrooms at home, dishwashers at home, and family vacations) 56 , 58 . Finally, parental and peer support were measured using an eight item-measure 59 . Responses were recorded on a 7-point Likert scale (ranging from 0 indicating very strongly disagree to 6 indicating very strongly agree).
Statistical analysis
Region-specific descriptive statistics were calculated to describe the sample. Next, Pearson’s Chi-squared association test with Yates’ continuity correction was performed to examine plausible associations between psychosomatic complaints and other categorical study variables. Also, to account for the regional clustering or unobserved heterogeneity observed in the analytic sample, sequential mixed effect binary logit models with the inclusion of a random intercept were fitted to further examine the associations between psychosomatic complaints and cyberbullying victimisation as well as other considered covariates. Furthermore, a parallel mediator model was fitted to evaluate the specified hypothesis and understand the potential mechanism linking cyberbullying victimisation and psychosomatic complaints. More specifically, cyberbullying victimisation (X) was modelled to directly influence psychosomatic complaints (Y) and indirectly via PSMU (M). Since core variables were binary, paths could be estimated with a sequence of three logit equations: 60 , 61
where, \({i}_{1}\) , \({i}_{2}\) , and \({i}_{3}\) represent the intercept in the respective equations. The path coefficient, c, in Eq. ( 1 ) represents the total effect of predictor X on outcome Y . In Eq. ( 2 ), the path coefficient a denotes the effect of predictor X on the mediator M . Also, the c' parameter in Eq. ( 3 ) represents the direct effect of the predictor X on the response Y , adjusting for the mediator M . Lastly, the path coefficient b coefficient in Eq. ( 3 ) represents the indirect effect of the mediator M on the outcome Y , when adjusting for the predictor X . These logit models provide effect estimates on the log-odds scale, and thus can be transformed into odds ratios. Each model was adjusted for the potential confounding variables.
All statistical analyses were performed using R Software (v4.1.2; R Core Team 2021) with \(\alpha\) = 0.05 as the significance level. More specifically, the package “mediation” in R 62 was used for the mediation analysis to estimate direct, indirect, and total effects. Inference is based on a non-parametric, 95% bias-corrected and accelerated (BCa) bootstrapped confidence interval 63 , 64 . Bootstrapping for indirect effects was set at 1000 samples, and once the 95% bootstrapped CI of the mediation effects did not include zero (0), it was deemed statistically significant. We also conducted further analysis by including an interaction between cyberbullying victimisation and PSMU to obtain insights analogous to the mediation model.
Ethics approval and consent to participate
The research was exclusively based on data sourced from the World Bank, which adheres to rigorous ethical standards in its data collection processes. Therefore, no separate ethical approval was sought or deemed necessary. Ethical approval was not required for this study since the data used for this study are secondary data. Necessary permissions and survey data were obtained from the World Bank. The World Bank data collection process upheld ethical standards and relevant guidelines in the research process including informed consent from all subjects and/or their legal guardian(s).
Preliminary analyses
The final analytic sample comprised complete information on 142,298 adolescents from 35 high-income countries (Table 1 ). The median age of the sample was 13.6 years. Most participants resided in Wales (6.26%) and the Czech Republic (6.16%). Notably, the prevalence of cyberbullying victimisation was 26.2%, and the majority (53%) were females. As observed in Table 2 , 84.6% of the participants self-reported high levels of psychosomatic complaints. Furthermore, among the participants who experienced PSMU, about 81.16% reported high levels of psychosomatic complaints. About 84.47% of the participants indicated receiving parental and peer support (see Table 2 ).
Main analyses
Results from the sequential binary mixed effects logit model are shown in Table 3 . In the first step, we included only cyberbullying victimisation in the model. We found that cyberbullying victims were 2.430 times more likely to report psychosomatic complaints than those who were not cyberbullied (OR = 2.430; 95%CI = 2.330, 2.530). The second step included sex, PSMU, parental and peer support, and family affluence as covariates. We found that cyber bullying victims were 2.390 times significantly more likely to report psychosomatic complaints than those who never experienced cyberbullying (AOR = 2.390; 95%CI = 2.29, 2.49). Additionally, the third model, which is an additional analysis involved the inclusion of an interaction between and cyberbullying victimisation and PSMU. The results showed that PSMU moderates the association between cyberbullying victimisation and psychosomatic complaints. Adolescents who were cyberbullied but did not report PSMU had reduced odds of psychosomatic complaints compared to those with PSMU (AOR = 1.220; 95%CI = 1.110–1.350). Furthermore, a caterpillar plot of empirical Bayes residuals of the models for the random intercept, region/country is obtained and shown in Fig. 1 . This represents individual effects for each country and offers additional insights into the extent of psychosomatic complaints heterogeneity across different countries. The plots visually demonstrates that regional variation for psychosomatic complaints does exist.
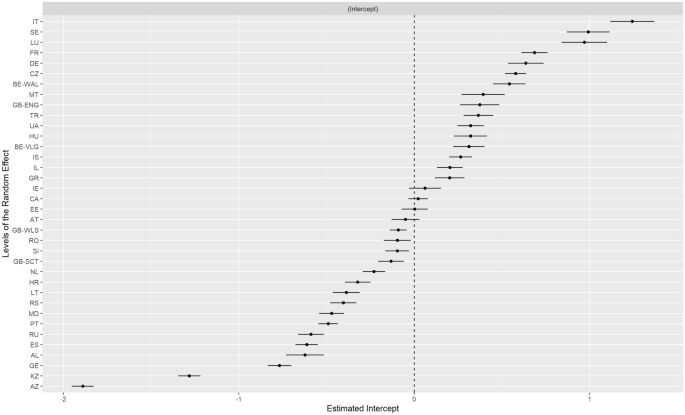
A caterpillar plot of empirical Bayes residuals of the models for the random intercept, region/country. This represents individual effects for each region/country. Region or country abbreviations in the figure are as follows: [AL] Albania, [AZ] Azerbaijan, [AT] Austria, [BE-VLG] Vlaamse Gewest (Belgium), [BE-WAL] Wallone, Région (Belgium), [CA] Canada, [CZ] Czech Republic, [DE] Germany, [EE] Estonia, [CA] Canada, [ES] Spain, [FR] France, [GB-ENG] England, [GB-SCT] Scotland, [GB-WLS] Wales, [GE] Georgia, [GR] Greece, [HR] Croatia, [HU] Hungary, [IE] Ireland, [IL] Israel, [IS] Iceland, [IT] Italy, [KZ] Kazakhstan, [LT] Lithuania, [LU] Luxembourg, [MD] Moldova, [MT] Malta, [NL] Netherlands, [PT] Portugal, [RO] Romania, [RS] Serbia, [RU] Russia, [SE] Sweden, [SI] Slovenia, [TR] Turkey, [LU] Luxembourg and [UA] Ukraine.
Figure 2 shows the adjusted parallel mediation results. The effect of cyberbullying victimisation on psychosomatic complaints was significantly mediated by PSMU. The paths from cyberbullying victimisation to PSMU (a: \(\beta\) =0.648, p < 0.001), PSMU to psychosomatic complaints (b: \(\beta\) =0.889, p < 0.001), and that of cyberbullying victimisation to 0.8069 (c′: \(\beta\) =0.051, p < 0.001) were also statistically significant.
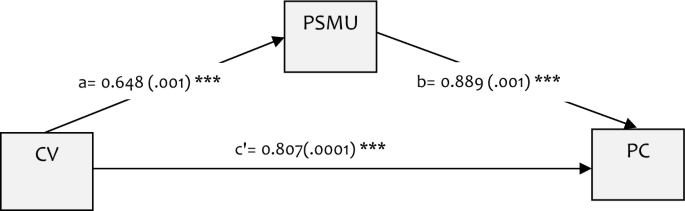
A parallel mediation model of the influence of PSMU on the association between Cyberbullying Victimisation and Psychosomatic Complaints. a = path coefficient of the effect of exposure on the mediator. b = path coefficient of the effect of the mediator on the outcome. c’ = path coefficient of the direct effect of the exposure on outcome. CV, cyberbullying victimisation. PC, psychosomatic complaints.
Bootstrapping test of mediating effects
The total, direct, and indirect effects of the mediation model based on nonparametric bootstrap are presented in Table 4 . We observe that the estimated CI did not include zero (0) for any effects. This observation suggests a statistically significant indirect effect of cyberbullying victimisation on psychosomatic complaints via PSMU ( \(\beta\) = 0.01162, 95%CI = 0.0110, 0.0120), yielding 12% of the total effect.
Key findings
This cross-cultural study examined the direct and indirect associations of cyberbullying victimisation with psychosomatic complaints via PSMU among adolescents. The results showed that cyberbullying victimisation independently influenced the experience of psychosomatic complaints. Specifically, adolescents who were victims of cyberbullying were more than two times more likely to report psychosomatic complaints. Crucially, our mediation analyses indicated that PSMU explain approximately 12% of the association between cyberbullying victimisation and psychosomatic complaints. In a further analysis, PSMU moderated the association between cyberbullying victimisation and psychosomatic complaints. This study is the first to examine the direct and indirect associations between cyberbullying victimisation and psychosomatic complaints through PSMU in adolescents across multiple high-income countries.
Interpretation of the findings
Our results confirmed the first hypothesis that there is a statistically significant direct association between cyberbullying victimisation and psychosomatic complaints. Thus, we found that cyberbullying independently directly affected the adolescents' experience of psychosomatic complaints. Previous studies have mainly focused on the direct effect of traditional face-to-face bullying on psychosomatic complaints 20 , 65 or compared the impact of traditional face-to-face bullying to cyberbullying concerning mental health 19 , 66 , 67 , 68 , 69 . A systematic review of traditional bullying and cyberbullying victimisation offers a comprehensive synthesis of the consequences of cyberbullying on adolescent health 19 . Another review suggested that cyberbullying threatened adolescents’ well-being and underscored many studies that have demonstrated effective relationships between adolescents’ involvement in cyberbullying and adverse health outcomes 70 . Other population-based cross-sectional studies have similarly shown that victims of cyberbullying experience significant psychological distress and feelings of isolation, which can further exacerbate their physical and mental health challenges 22 , 71 , 72 . The present study builds on the previously published literature by highlighting the effect of cyberbullying victimisation on adolescent psychosomatic complaints and the extent to which the association is mediated by PSMU.
Consistent with the second hypothesis, we found that PSMU mediated about 12% of the association between cyberbullying victimisation and psychosomatic complaints in this sample. While studies on the mediational role of PSMU in the relationship between cyberbullying victimisation and psychosomatic complaints are limited, evidence shows significant interplay among PSMU, cyberbullying victimisation, and psychosomatic complaints. For example, a study of over 58,000 young people in Italy found that PSMU was associated with increased levels of multiple somatic and psychological symptoms, such as anxiety and depression. 73 Another study of 1707 adolescents in Sweden found that cyberbullying victimisation was associated with increased depressive symptoms and the lowest level of subjective well-being 74 .
Other possible mediators of the cyberbullying victimisation-psychosomatic complaints association may include low self-esteem, negative body image, emotion regulation difficulties, social support, and personality traits such as neuroticism and impulsivity 20 , 67 , 72 , 75 , 76 . For example, Schneider et al. 75 have shown that emotional distress could increase psychosomatic symptoms such as headaches, stomach aches, and muscle tension. In addition, social isolation can lead to social withdrawal and a decreased sense of belonging 78 , 79 . Therefore, it is essential to explore these variables further and develop effective interventions and prevention strategies to address these interrelated factors and reduce their negative impact on adolescent health and well-being.
In a further analysis, the results show that PSMU does not only mediate but also moderate the association between cyberbullying victimisation and psychosomatic complaints among adolescents. Specifically, cyberbullied adolescents with no report of PSMU had reduced likelihoods of experiencing psychosomatic complaints compared to those with PSMU. This result is interesting and could be due to several factors. First, individuals with PSMU may already be experiencing heightened levels of psychological distress due to their excessive social media use, making them more vulnerable to the negative effects of cyberbullying 80 , 81 , 82 . For instance, excessive time spent on social media, particularly in activities such as comparing oneself to others or seeking validation through likes and comments, has been linked to increased psychological distress 83 , 84 . Conversely, the finding that cyberbullied adolescents without PSMU had reduced likelihoods of experiencing psychosomatic complaints compared to those with PSMU suggests a protective effect of lower social media use. Adolescents who are not excessively engaged with social media may have fewer opportunities for exposure to cyberbullying and may also have healthier coping strategies in place to deal with any instances of online victimisation 43 , 85 , 86 .
The results suggest that professionals in the fields of education, counselling, and healthcare should prioritise addressing the issue of cyberbullying victimisation when assessing the physical and psychological health of adolescents. Evidently, adolescents who experience cyberbullying require support. Thus, proactive measures are essential, and support could be provided by multiple professional communities that serve adolescents and young people in society, such as educational, behavioural health, and medical professionals. Sensitive inquiry regarding cyberbullying experiences is necessary when addressing adolescent health issues such as depression, substance use, suicidal ideation, and somatic concerns 19 . Our findings underscore the need for comprehensive, school-based programs focused on cyberbullying victimisation prevention and intervention.
Strengths and limitations
The study's main strength lies in the use of a large sample size representing multiple countries in high income countries. This large sample size improved the representativeness and veracity of our findings. The complex research approach helps advance our understanding of the interrelationships between cyberbullying victimisation, PSMU, and psychosomatic complaints among adolescents. However, the study has its limitations. First, the cross-sectional design does not allow directionality and causal inferences. Second, retrospective self-reporting for the critical study variables could lead to recall and social desirability biases. Third, the presence of residual and unobserved confounders, despite adjusting for some covariates, can be considered a limitation of this study. Further research is needed to confirm these findings and better understand how PSMU mediates the relationship between cyberbullying victimisation and psychosomatic complaints.
Conclusions
This study has provided essential insights into the interrelationships between cyberbullying victimisation, PSMU, and psychosomatic complaints among adolescents in high income countries. The findings suggest that cyberbullying is directly associated with psychosomatic complaints and that PSMU significantly and partially mediates this association. This study also highlights the importance of addressing cyberbullying victimisation and its negative impact on adolescent health and emphasises the need to address PSMU. Overall, the study underscores the importance of promoting healthy online behaviour and providing appropriate support for adolescents who experience cyberbullying victimisation. Further studies will benefit from longitudinal data to confirm our findings.
Data availability
The data that support the findings of this study are available from the World Bank, but restrictions apply to the availability of these data, which were used under license for the current study and so are not publicly available. Data are, however, available from the corresponding author ([email protected]) upon reasonable request and with permission of the World Bank.
Lyyra, N., Välimaa, R. & Tynjälä, J. Loneliness and subjective health complaints among school-aged children. Scand. J. Public Health 46 (20), 87–93. https://doi.org/10.1177/1403494817743901 (2018).
Article PubMed Google Scholar
Ottova, V. et al. The role of individual-and macro-level social determinants on young adolescents’ psychosomatic complaints. J. Early. 32 (1), 126–158. https://doi.org/10.1177/0272431611419510 (2012).
Article Google Scholar
Heinz, A., Catunda, C., van Duin, C. & Willems, H. Suicide prevention: Using the number of health complaints as an indirect alternative for screening suicidal adolescents. J. Affect. Disord. 260 , 61–66. https://doi.org/10.1016/j.jad.2019.08.025 (2020).
Högberg, B., Strandh, M. & Hagquist, C. Gender and secular trends in adolescent mental health over 24 years–the role of school-related stress. Soc. Cci Med. 250 , 112890. https://doi.org/10.1016/j.socscimed.2020.112890 (2020).
Hagquist, C., Due, P., Torsheim, T. & Välimaa, R. Cross-country comparisons of trends in adolescent psychosomatic symptoms—a Rasch analysis of HBSC data from four Nordic countries. Health Qual. Life Outcomes 17 (1), 1–13. https://doi.org/10.1186/s12955-019-1097-x (2019).
Shorey, S., Ng, E. D. & Wong, C. H. Global prevalence of depression and elevated depressive symptoms among adolescents: A systematic review and meta-analysis. Br. J. Clin. Psychol. 61 (2), 287–305. https://doi.org/10.1111/bjc.12333 (2022).
Potrebny, T. et al. Health complaints among adolescents in Norway: A twenty-year perspective on trends. PloS one 14 (1), e0210509. https://doi.org/10.1371/journal.pone.0210509 (2019).
Article CAS PubMed PubMed Central Google Scholar
van Geelen, S. M. & Hagquist, C. Are the time trends in adolescent psychosomatic problems related to functional impairment in daily life? A 23-year study among 20,000 15–16 year olds in Sweden. J. Psychol. Res. 87 , 50–56. https://doi.org/10.1016/j.jpsychores.2016.06.003 (2016).
Swedish Association of Local Authorities and Regions and Ministry of Health and Social Affairs. Insatser inom området psykisk hälsa och suicidprevention. Överenskommelse mellan staten och Sveriges Kommuner och Regioner (SKR). Swedish Association of Local Authorities and Regions and Ministry of Health and Social Affairs. Stokholm, Sweden: 2021–2022. https://skr.se/skr/halsasjukvard/utvecklingavverksamhet/psykiskhalsa/overenskommelsepsykiskhalsa.234.html (2022).
Brooks, S. J., Feldman, I., Schiöth, H. B. & Titova, O. E. Important gender differences in psychosomatic and school-related complaints in relation to adolescent weight status. Sci. Rep. 11 (1), 14147. https://doi.org/10.1038/s41598-021-93761-0 (2021).
Article ADS CAS PubMed PubMed Central Google Scholar
Whitehead, R. et al. Trends in adolescent overweight perception and its association with psychosomatic health 2002–2014: Evidence from 33 countries. J. Adol. Health 60 (2), 204–211. https://doi.org/10.1016/j.jadohealth.2016.09.029 (2017).
Nilsen, W., Karevold, E., Røysamb, E., Gustavson, K. & Mathiesen, K. S. Social skills and depressive symptoms across adolescence: Social support as a mediator in girls versus boys. J. Adol. 36 (1), 11–20. https://doi.org/10.1016/j.adolescence.2012.08.005 (2013).
Englander, E., Donnerstein, E., Kowalski, R., Lin, C. A. & Parti, K. Defining cyberbullying. Pediatric 140 (S2), 148–151. https://doi.org/10.1542/peds.2016-1758U (2017).
Chan, H. C. O. & Wong, D. S. Traditional school bullying and cyberbullying in Chinese societies: Prevalence and a review of the whole-school intervention approach. Aggress. Viol. Behav. 23 , 98–108. https://doi.org/10.1016/j.avb.2015.05.010 (2015).
Griffiths, M. D., Kuss, D. J. & Demetrovics, Z. Social networking addiction: An overview of preliminary findings. Behav Addict. 2014 , 119–141. https://doi.org/10.1016/B978-0-12-407724-9.00006-9 (2014).
Athanasiou, K. et al. Cross-national aspects of cyberbullying victimization among 14–17-year-old adolescents across seven European countries. BMC Public Health 18 , 1–15. https://doi.org/10.1186/s12889-018-5682-4 (2018).
Nagata, J. M. et al. Cyberbullying and Sleep Disturbance among Early Adolescents in the US. Acad. Pediatr. 23 (6), 1220–1225. https://doi.org/10.1016/j.acap.2022.12.007 (2022).
Fahy, A. E. et al. Longitudinal associations between cyberbullying involvement and adolescent mental health. J. Ado.l Health 59 (5), 502–509. https://doi.org/10.1016/j.jadohealth.2016.06.006 (2016).
Zych, I., Ortega-Ruiz, R. & Del Rey, R. Systematic review of theoretical studies on bullying and cyberbullying: Facts, knowledge, prevention, and intervention. Aggress. Viol. Behav. 23 , 1–21. https://doi.org/10.1016/j.avb.2015.10.001 (2015).
Kowalski, R. M. & Limber, S. P. Psychological, physical, and academic correlates of cyberbullying and traditional bullying. J. Adol. Health 53 (1), S13–S20. https://doi.org/10.1016/j.jadohealth.2012.09.018 (2013).
Van Geel, M., Vedder, P. & Tanilon, J. Relationship between peer victimization, cyberbullying, and suicide in children and adolescents: A meta-analysis. JAMA Pediatr. 168 (5), 435–442. https://doi.org/10.1001/jamapediatrics.2013.4143 (2014).
Article CAS PubMed Google Scholar
Albdour, M., Hong, J. S., Lewin, L. & Yarandi, H. The impact of cyberbullying on physical and psychological health of Arab American adolescents. J. Immig. Minor. Health 21 , 706–715. https://doi.org/10.1007/s10903-018-00850-w (2019).
Yoon, Y. et al. Association of cyberbullying involvement with subsequent substance use among adolescents. J. Adol. Health 65 (5), 613–620. https://doi.org/10.1016/j.jadohealth.2019.05.006 (2019).
Yuchang, J., Junyi, L., Junxiu, A., Jing, W. & Mingcheng, H. The differential victimization associated with depression and anxiety in cross-cultural perspective: A meta-analysis. Trauma Viol. Abuse 20 (4), 560–573. https://doi.org/10.1177/1524838017726426 (2019).
Gini, G. & Espelage, D. L. Peer victimization, cyberbullying, and suicide risk in children and adolescents. Jama 312 (5), 545–546. https://doi.org/10.1001/jama.2014.3212 (2014).
Tullett-Prado, D., Doley, J. R., Zarate, D., Gomez, R. & Stavropoulos, V. Conceptualising social media addiction: A longitudinal network analysis of social media addiction symptoms and their relationships with psychological distress in a community sample of adults. BMC Psychol. 23 (1), 1–27. https://doi.org/10.1186/s12888-023-04985-5 (2023).
Keles, B., McCrae, N. & Grealish, A. A systematic review: The influence of social media on depression, anxiety and psychological distress in adolescents. Int. J. Adol Youth 25 (1), 79–93. https://doi.org/10.1080/02673843.2019.1590851 (2020).
O’reilly, M. et al. Is social media bad for mental health and wellbeing? Exploring the perspectives of adolescents. Clin. Child Psychol. Psych. 23 (4), 601–613. https://doi.org/10.1177/1359104518775154 (2018).
Marino, C., Gini, G., Angelini, F., Vieno, A. & Spada, M. M. Social norms and e-motions in problematic social media use among adolescents. Addict. Behav. Rep. 11 , 100250. https://doi.org/10.1016/j.abrep.2020.100250 (2020).
Article PubMed PubMed Central Google Scholar
Sedgwick, R., Epstein, S., Dutta, R. & Ougrin, D. Social media, internet use and suicide attempts in adolescents. Curr. Opin. Psychol. 32 (6), 534. https://doi.org/10.1097/YCO.0000000000000547 (2019).
Marino, C., Hirst, C. M., Murray, C., Vieno, A. & Spada, M. M. Positive mental health as a predictor of problematic internet and Facebook use in adolescents and young adults. J. Happ. Stud. 19 , 2009–2022. https://doi.org/10.1007/s10902-017-9908-4 (2018).
Sarmiento, I. G. et al. How does social media use relate to adolescents’ internalizing symptoms? Conclusions from a systematic narrative review. Adol. Res. Rev. 5 , 381–404. https://doi.org/10.1007/s40894-018-0095-2 (2020).
Hanprathet, N., Manwong, M., Khumsri, J. M. S., Yingyeun, R. & Phanasathit, M. Facebook addiction and its relationship with mental health among Thai high school students. J. Med. Assoc. Thailand 98 , 81–90 (2015).
Google Scholar
Cerutti, R. et al. Sleep disturbances partially mediate the association between problematic internet use and somatic symptomatology in adolescence. Curr. Psychol. 40 , 4581–4589. https://doi.org/10.1007/s12144-019-00414-7 (2021).
Van Den Eijnden, R., Koning, I., Doornwaard, S., Van Gurp, F. & Ter Bogt, T. The impact of heavy and disordered use of games and social media on adolescents’ psychological, social, and school functioning. J. Behav. Addit. 7 (3), 697–706. https://doi.org/10.1556/2006.7.2018.65 (2018).
Andreassen, C. S. & Pallesen, S. Social network site addiction-an overview. Curr. Pharma Des. 20 (25), 4053–4061. https://doi.org/10.2174/13816128113199990616 (2014).
Article CAS Google Scholar
Andreassen, C. S. Online social network site addiction: A comprehensive review. Curr. Addit Rep. 2 (2), 175–184. https://doi.org/10.1007/s40429-015-0056-9 (2015).
Best, P., Manktelow, R. & Taylor, B. Online communication, social media and adolescent wellbeing: A systematic narrative review. Child. Youth Serv. Rev. 41 , 27–36. https://doi.org/10.1016/j.childyouth.2014.03.001 (2014).
Boer, M. et al. Adolescents’ intense and problematic social media use and their well-being in 29 countries. J. Adol. Health 66 (6), S89–S99. https://doi.org/10.1016/j.jadohealth.2020.02.014 (2020).
Inchley, J. et al . Adolescent alcohol-related behaviours: Trends and inequalities in the WHO European Region, 2002–2014: Observations from the Health Behaviour in School-aged Children (HBSC) WHO collaborative cross-national study. World Health Organization. Regional Office for Europe (2018). https://apps.who.int/iris/handle/10665/342239 .
Moor, I. et al. The 2017/18 Health Behaviour in School-aged Children (HBSC) study–mthodology of the World Health Organization’s child and adolescent health study. J. Health Monitor. 5 (3), 88. https://doi.org/10.25646/6904 (2020).
Nardone, P. et al. Dietary habits among Italian adolescents and their relation to socio-demographic characteristics. Ann. Istit. Super. Sanita 56 (4), 504–513. https://doi.org/10.4415/ANN_20_04_15 (2020).
Craig, W. et al. Social media use and cyber-bullying: A cross-national analysis of young people in 42 countries. J. Adol. Health 66 (6), S100–S108. https://doi.org/10.1016/j.jadohealth.2020.03.006 (2020).
Moor, I. et al. The 2017/18 Health Behaviour in School-aged Children (HBSC) study–methodology of the World Health Organization’s child and adolescent health study. J. Health Monitor. 5 (3), 88 (2020).
Inchley, J., Currie, D., Cosma, A. & Samdal, O. Health Behaviour in School-Aged Children (HBSC) Study Protocol: Background, Methodology and Mandatory Items for the 2017/18 Survey ; CAHRU: St Andrews, UK (2018).
Haugland, S. & Wold, B. Subjective health complaints in adolescence—reliability and validity of survey methods. J. Adol. 24 (5), 611–624. https://doi.org/10.1006/jado.2000.0393 (2001).
Khan, A., Khan, S. R. & Lee, E. Y. Association between lifestyle behaviours and mental health of adolescents: Evidence from the Canadian HBSC Surveys, 2002–2014. Int. J. Environ. Res. Public Health 19 (11), 6899. https://doi.org/10.3390/ijerph19116899 (2022).
Högberg, B., Strandh, M., Johansson, K. & Petersen, S. Trends in adolescent psychosomatic complaints: A quantile regression analysis of Swedish HBSC data 1985–2017. Scand. J. Public Health 2022 , 21094497. https://doi.org/10.1177/14034948221094497 (2022).
Bjereld, Y., Augustine, L., Turner, R., Löfstedt, P. & Ng, K. The association between self-reported psychosomatic complaints and bullying victimisation and disability among adolescents in Finland and Sweden. Scand. J. Public Health 2022 , 1089769. https://doi.org/10.1177/14034948221089769 (2022).
Heinz, A., van Duin, C., Kern, M. R., Catunda, C. & Willems, H. Trends from 2006–2018 in Health, Health Behaviour, Health Outcomes and Social Context of Adolescents in Luxembourg . University of Luxembourg (2020). http://hdl.habndle.net/10993/42571 .
Gariepy, G., McKinnon, B., Sentenac, M. & Elgar, F. J. Validity and reliability of a brief symptom checklist to measure psychological health in school-aged children. Child Indic. Res. 9 , 471–484. https://doi.org/10.1007/s12187-015-9326-2 (2016).
Biswas, T. et al. Variation in the prevalence of different forms of bullying victimisation among adolescents and their associations with family, peer and school connectedness: A population-based study in 40 lower and middle income to high-income countries (LMIC-HICs). J. Child. Adol. Trauma 2022 , 1–11. https://doi.org/10.1007/s40653-022-00451-8 (2022).
Sasson, H., Tur-Sinai, A., Dvir, K. & Harel-Fisch, Y. The role of parents and peers in cyberbullying perpetration: Comparison among Arab and Jewish and youth in Israel. Child Indic. Res. 2022 , 1–21. https://doi.org/10.1007/s12187-022-09986-6 (2022).
Marengo, N. et al. Cyberbullying and problematic social media use: An insight into the positive role of social support in adolescents—data from the Health Behaviour in School-aged Children study in Italy. Public Health 199 , 46–50. https://doi.org/10.1016/j.puhe.2021.08.010 (2021).
Van den Eijnden, R. J. J. M., Lemmens, J. & Valkenburg, P. The social media disorder scale: Validity and psychometric properties. Comp. Hum. Behav. 61 (August), 478487. https://doi.org/10.1016/j.chb.2016.03.038 (2016).
Borraccino, A. et al. Problematic social media use and cyber aggression in Italian adolescents: The remarkable role of social support. Int. J. Environ. Res. Public Health 19 (15), 9763. https://doi.org/10.3390/ijerph19159763 (2022).
Hamre, R., Smith, O. R. F., Samdal, O. & Haug, E. Gaming behaviors and the association with sleep duration, social jetlag, and difficulties falling asleep among Norwegian adolescents. Int. J. Environ. Res. Public Health 19 (3), 1765. https://doi.org/10.3390/ijerph19031765 (2022).
Currie, C. et al. Researching health inequalities in adolescents: The development of the Health Behaviour in School-Aged Children (HBSC) family affluence scale. Soc. Sci Med. 66 (6), 1429–1436. https://doi.org/10.1016/j.socscimed.2007.11.024 (2008).
Zimet, G. D., Powell, S. S., Farley, G. K., Werkman, S. & Berkoff, K. A. Psychometric characteristics of the multidimensional scale of perceived social support. J. Person. Assess. 55 (3–4), 610–617. https://doi.org/10.1080/00223891.1990.9674095 (1990).
MacKinnon, D. P., Lockwood, C. M., Brown, C. H., Wang, W. & Hoffman, J. M. The intermediate endpoint effect in logistic and probit regression. Clin. Trial 4 (5), 499–513. https://doi.org/10.1177/1740774507083434 (2007).
Rijnhart, J. J., Valente, M. J., Smyth, H. L. & MacKinnon, D. P. Statistical mediation analysis for models with a binary mediator and a binary outcome: The differences between causal and traditional mediation analysis. Prevent. Sci. 2021 , 1–11. https://doi.org/10.1007/s11121-021-01308-6 (2021).
Tingley D, Yamamoto T, Hirose K, Keele L, Imai K, Yamamoto MT. Package ‘mediation’. Computer software manual. 2019 Sep 13:175-84.
DiCiccio, T. J. & Efron, B. Bootstrap confidence intervals. Stat. Sci. 11 (3), 189–228. https://doi.org/10.1214/ss/1032280214 (1996).
Article MathSciNet Google Scholar
Preacher, K. J. & Hayes, A. F. Asymptotic and resampling strategies for assessing and comparing indirect effects in multiple mediator models. Behav. Res. Method 40 (3), 879–891. https://doi.org/10.3758/BRM.40.3.879 (2008).
Tomşa, R., Jenaro, C., Campbell, M. & Neacşu, D. Student’s experiences with traditional bullying and cyberbullying: Findings from a Romanian sample. Procedia-Soc. Behav. Sci. 78 , 586–590. https://doi.org/10.1016/j.sbspro.2013.04.356 (2013).
Baier, D., Hong, J. S., Kliem, S. & Bergmann, M. C. Consequences of bullying on adolescents’ mental health in Germany: Comparing face-to-face bullying and cyberbullying. J. Child Fam. Stud. 28 , 2347–2357. https://doi.org/10.1007/s10826-018-1181-6 (2019).
Beckman, L., Hagquist, C. & Hellström, L. Does the association with psychosomatic health problems differ between cyberbullying and traditional bullying?. Emot. Behav. Differ. 17 (3–4), 421–434. https://doi.org/10.1080/13632752.2012.704228 (2012).
Lazuras, L., Barkoukis, V. & Tsorbatzoudis, H. Face-to-face bullying and cyberbullying in adolescents: Trans-contextual effects and role overlap. Tech. Soc. 48 , 97–101. https://doi.org/10.1016/j.techsoc.2016.12.001 (2017).
Li, J., Sidibe, A. M., Shen, X. & Hesketh, T. Incidence, risk factors and psychosomatic symptoms for traditional bullying and cyberbullying in Chinese adolescents. Child. Youth Serv. Rev. 107 , 104511. https://doi.org/10.1016/j.childyouth.2019.104511 (2019).
Nixon, C. L. Current perspectives: The impact of cyberbullying on adolescent health. Adol. Health Med. Therapy 2014 , 143–158. https://doi.org/10.2147/AHMT.S36456 (2014).
Olenik-Shemesh, D., Heiman, T. & Eden, S. Cyberbullying victimisation in adolescence: Relationships with loneliness and depressive mood. Emot. Behav. Differ. 17 (3–4), 361–374. https://doi.org/10.1080/13632752.2012.704227 (2012).
Sourander, A. et al. Psychosocial risk factors associated with cyberbullying among adolescents: A population-based study. Arch. Gener. Psychiatry 67 (7), 720–728. https://doi.org/10.1001/archgenpsychiatry.2010.79 (2010).
Claudia, M. et al. Problematic social media use: Associations with health complaints among adolescents. Ann. Istit. Super. Sanità 56 (4), 514–521. https://doi.org/10.4415/ANN_20_04_16 (2020).
Hellfeldt, K., López-Romero, L. & Andershed, H. Cyberbullying and psychological well-being in young adolescence: The potential protective mediation effects of social support from family, friends, and teachers. Int.. J. Environ. Res. Public Health 17 (1), 45. https://doi.org/10.3390/ijerph17010045 (2020).
Gini, G. & Pozzoli, T. Bullied children and psychosomatic problems: A meta-analysis. Pediatrics 132 (4), 720–729. https://doi.org/10.1542/peds.2013-0614 (2013).
Landstedt, E. & Persson, S. Bullying, cyberbullying, and mental health in young people. Scand. J. Public Health 42 (4), 393–399. https://doi.org/10.1177/1403494814525 (2014).
Schneider, S. K., Odonnell, L., Stueve, A. & Coulter, R. W. Cyberbullying, school bullying, and psychological distress: A regional census of high school students. Am. J. Public Health 102 (1), 171–177. https://doi.org/10.2105/AJPH.2011.300308 (2012).
Brighi, A., Guarini, A., Melotti, G., Galli, S. & Genta, M. L. Predictors of victimisation across direct bullying, indirect bullying and cyberbullying. Emot. Behav. Differ. 17 (3–4), 375–388. https://doi.org/10.1080/13632752.2012.704684 (2012).
Cowie, H. Cyberbullying and its impact on young people’s emotional health and well-being. The Psychia 37 (5), 167–170. https://doi.org/10.1192/pb.bp.112.040840 (2013).
Berryman, C., Ferguson, C. J. & Negy, C. Social media use and mental health among young adults. Psych. Q. 89 , 307–314. https://doi.org/10.1007/s11126-017-9535-6 (2018).
Verduyn, P., Ybarra, O., Résibois, M., Jonides, J. & Kross, E. Do social network sites enhance or undermine subjective well-being? A critical review. Soc. Issue Policy Rev. 11 (1), 274–302. https://doi.org/10.1542/peds.2007-0693 (2017).
Vogel, E. A., Rose, J. P., Okdie, B. M., Eckles, K. & Franz, B. Who compares and despairs? The effect of social comparison orientation on social media use and its outcomes. Person. Individ. Differ. 86 , 249–256. https://doi.org/10.1016/j.paid.2015.06.026 (2015).
Keles, B., McCrae, N. & Grealish, A. A systematic review: The influence of social media on depression, anxiety and psychological distress in adolescents. Int. J. Adol. Youth 25 (1), 79–93. https://doi.org/10.1080/02673843.2019.1590851 (2020).
Boer, M. et al. Adolescents’ intense and problematic social media use and their well-being in 29 countries. J. Adol. Health 66 (6), 89–99. https://doi.org/10.1016/j.jadohealth.2020.02.014 (2020).
McHugh, B. C., Wisniewski, P., Rosson, M. B. & Carroll, J. M. When social media traumatizes teens: The roles of online risk exposure, coping, and post-traumatic stress. Int. Res. 28 (5), 1169–1188. https://doi.org/10.1108/IntR-02-2017-0077 (2018).
Trnka, R., Martínková, Z. & Tavel, P. An integrative review of coping related to problematic computer use in adolescence. Int. J. Public Health 61 , 317–327. https://doi.org/10.1007/s00038-015-0693-8 (2016).
Chen, L., Ho, S. S. & Lwin, M. O. A meta-analysis of factors predicting cyberbullying perpetration and victimization: From the social cognitive and media effects approach. New Media Soc. 19 (8), 1194–1213. https://doi.org/10.1177/1461444816634037 (2017).
Download references
Acknowledgements
We thank the 2017/2018 HBSC survey team/network, the coordinator and the Data Bank Manager for granting us access to the datasets. We duly acknowledge all school children who participated in the surveys.
Author information
Authors and affiliations.
Social Policy Research Centre, University of New South Wales, Sydney, Australia
Prince Peprah
Centre for Primary Health Care and Equity, University of New South Wales, Sydney, Australia
Pfizer Research and Development, PSSM Data Sciences, Pfizer, Inc., Connecticut, USA
Michael Safo Oduro
Centre for Disability and Rehabilitation Studies, Kwame Nkrumah University of Science and Technology, Kumasi, Ghana
Godfred Atta-Osei
Centre for Social Research in Health, University of New South Wales, Sydney, Australia
Isaac Yeboah Addo
Concord Clinical School, University of Sydney, Sydney, Australia
Department of Planning, Kwame Nkrumah University of Science and Technology, Kumasi, Ghana
Anthony Kwame Morgan
African Population and Health Research Center, Nairobi, Kenya
Razak M. Gyasi
National Centre for Naturopathic Medicine, Faculty of Health, Southern Cross University, Lismore, NSW, Australia
You can also search for this author in PubMed Google Scholar
Contributions
PP: conceptualization, methodology and writing; MOS: conceptualization, statistical methodology and analysis, and writing; GA-O: conceptualization and writing; IA: methodology and writing; RMG: conceptualization and writing. AKM: writing. All the authors reviewed and to the publication of this paper.
Corresponding author
Correspondence to Anthony Kwame Morgan .
Ethics declarations
Competing interests.
The authors declare no competing interests.
Additional information
Publisher's note.
Springer Nature remains neutral with regard to jurisdictional claims in published maps and institutional affiliations.
Rights and permissions
Open Access This article is licensed under a Creative Commons Attribution 4.0 International License, which permits use, sharing, adaptation, distribution and reproduction in any medium or format, as long as you give appropriate credit to the original author(s) and the source, provide a link to the Creative Commons licence, and indicate if changes were made. The images or other third party material in this article are included in the article's Creative Commons licence, unless indicated otherwise in a credit line to the material. If material is not included in the article's Creative Commons licence and your intended use is not permitted by statutory regulation or exceeds the permitted use, you will need to obtain permission directly from the copyright holder. To view a copy of this licence, visit http://creativecommons.org/licenses/by/4.0/ .
Reprints and permissions
About this article
Cite this article.
Peprah, P., Oduro, M.S., Atta-Osei, G. et al. Problematic social media use mediates the effect of cyberbullying victimisation on psychosomatic complaints in adolescents. Sci Rep 14 , 9773 (2024). https://doi.org/10.1038/s41598-024-59509-2
Download citation
Received : 27 November 2023
Accepted : 11 April 2024
Published : 29 April 2024
DOI : https://doi.org/10.1038/s41598-024-59509-2
Share this article
Anyone you share the following link with will be able to read this content:
Sorry, a shareable link is not currently available for this article.
Provided by the Springer Nature SharedIt content-sharing initiative
- Adolescent well-being
- Psychosomatic complaints
- Cyberbullying victimisation
- Sleep quality
- Social media use
By submitting a comment you agree to abide by our Terms and Community Guidelines . If you find something abusive or that does not comply with our terms or guidelines please flag it as inappropriate.
Quick links
- Explore articles by subject
- Guide to authors
- Editorial policies
Sign up for the Nature Briefing newsletter — what matters in science, free to your inbox daily.

Information
- Author Services
Initiatives
You are accessing a machine-readable page. In order to be human-readable, please install an RSS reader.
All articles published by MDPI are made immediately available worldwide under an open access license. No special permission is required to reuse all or part of the article published by MDPI, including figures and tables. For articles published under an open access Creative Common CC BY license, any part of the article may be reused without permission provided that the original article is clearly cited. For more information, please refer to https://www.mdpi.com/openaccess .
Feature papers represent the most advanced research with significant potential for high impact in the field. A Feature Paper should be a substantial original Article that involves several techniques or approaches, provides an outlook for future research directions and describes possible research applications.
Feature papers are submitted upon individual invitation or recommendation by the scientific editors and must receive positive feedback from the reviewers.
Editor’s Choice articles are based on recommendations by the scientific editors of MDPI journals from around the world. Editors select a small number of articles recently published in the journal that they believe will be particularly interesting to readers, or important in the respective research area. The aim is to provide a snapshot of some of the most exciting work published in the various research areas of the journal.
Original Submission Date Received: .
- Active Journals
- Find a Journal
- Proceedings Series
- For Authors
- For Reviewers
- For Editors
- For Librarians
- For Publishers
- For Societies
- For Conference Organizers
- Open Access Policy
- Institutional Open Access Program
- Special Issues Guidelines
- Editorial Process
- Research and Publication Ethics
- Article Processing Charges
- Testimonials
- Preprints.org
- SciProfiles
- Encyclopedia
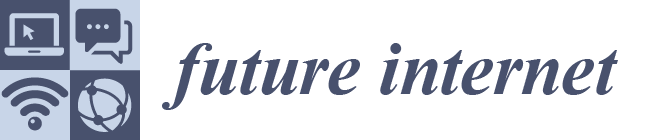
Article Menu
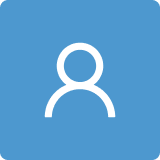
- Subscribe SciFeed
- Recommended Articles
- Google Scholar
- on Google Scholar
- Table of Contents
Find support for a specific problem in the support section of our website.
Please let us know what you think of our products and services.
Visit our dedicated information section to learn more about MDPI.
JSmol Viewer
A review on deep-learning-based cyberbullying detection.

1. Introduction
- We present a DL-based cyberbullying defense ecosystem with the help of a taxonomy. We also discuss data representation, models and frameworks for DL techniques.
- We compare several RNN, CNN, attention, and their fusion-based cyberbullying detection studies in the existing literature.
- We analyze several text and image datasets extracted from social media and virtual platforms related to cyberbullying detection.
- We identify the challenges and open issues related to cyberbullying.
2. Related Works
3. methodology, 4. data representation techniques, 4.1. text data representation, 4.1.1. one-hot encoding, 4.1.2. tf-idf, 4.1.3. word2vec, 4.1.4. glove, 4.1.5. elmo, 4.1.6. fasttext, 4.1.7. bert, 4.1.8. efficacy of various embeddings for detecting cyberbullying, 4.2. image data representation, 4.2.1. cognitive image representation, 4.2.2. bsp representation, 4.2.3. bio-inspired model representation, 4.2.4. mps representation, 4.2.5. deep neural networks-based image representation [ 83 ], 4.2.6. optical character recognition (ocr), 5. deep-learning-based models, 5.1. deep neural network (dnn), 5.2. boltzmann machines (bms), 5.3. deep belief network (dbn), 5.4. deep autoencoder (dae), 5.5. generative adversarial network (gan), 5.6. recurrent neural network (rnn), 5.7. long short-term memory.
- ⊗ —element wise multiplication;
- ⊕ —element wise addition;
- C t = current cell memory ;
- C t − 1 = previous cell memory ;
- o t = output gate ;
- f t = forget gate ;
- σ = sigmoid function ;
- w , b = weight vectors ;
- h t − 1 = previous cell output ;
- x t = input vector ;
- h t = current cell output .
5.8. Convolutional Neural Network (CNN)
5.9. hybrid models (lstm-cnn, cnn-lstm), 5.10. attention-based model, 5.10.1. transformers, 5.10.2. bert (bidirectional encoder representations from transformers), 5.10.3. hierarchical attention networks (han), 5.10.4. convolutional neural networks with attention (cnn-att), 5.10.5. long short-term memory networks with attention (lstm-att), 5.10.6. gated recurrent units with attention (gru-att).
DL Models | Used in Cyberbullying Applications | Area of Applications | Limitations |
---|---|---|---|
Deep Neural Network (DNN) [ ] | Chats and Tweets [ ], Social networks’ text and image [ ] | Speech Recognition, Image recognition and the natural language processing | Requires large amount of data, expensive to train, and issues of overfitting |
Boltzmann Machines (BMs) [ ] | Offline content [ ], Image content [ ], Arabic content [ ] | Emotion recognition from thermal images, estimation of music similarity, extracting the structure of explored data | Training is challenging, and weight adjustment is hard |
Deep Belief Networks (DBN) [ ] | Arabic content [ ], Social media text [ ], Social media image [ ] | Image classification, natural language understanding, speech recognition to audio classification | Expensive to train because of the complex data models, huge data is required, and needs classifiers to grasp the output |
Deep Autoencoder (DAE) [ ] | Chats and Tweets [ ], Social media content [ ] | Image search and data compression, dimensionality reduction, image denoising | The bottleneck layer is too narrow, lossy, and requires large amount of data |
Generative Adversarial Networks (GAN) [ ] | Web-application for detecting cyberbullying [ ] | Improve astronomical images, gravitational lens simulation for dark matter exploration, excellent low resolution, generate realistic images and cartoon characters | Non-convergence, mode collapse, and diminished gradient |
Recurrent Neural Networks (RNN) [ ] | Social Commentary [ ], Cyberbert: Bert for cyber- bullying identification [ ], Identification and classification from social media [ ] | Image captioning, time-series analysis, natural language processing, handwriting recognition, and machine translation | The gradation disappears and the problem explodes, difficult to train, and unable to handle very long sequences when tanh or ReLU is used as the activation function |
Long Short-Term Memory (LSTM) [ ] | Social media content [ , , ], Wikipedia, Twitter, Formspring and YouTube [ ], CyberBERT [ ], Bangla text [ ], Indonesian language [ ], Twitter [ , ] | Time-series prediction, speech recognition, music composition, and pharmaceutical development | Training takes time, training requires more memory, easy to overfit, and Dropouts are much more difficult to implement in LSTMs |
Bidirectional LSTM (Bi-LSTM) | Social media content [ , , , , ], Visual contents [ ], Wikipedia, Twitter, Formspring and YouTube [ ], CyberBERT [ ], Bangla text [ ], Indonesian language [ ], Text and emoji data [ ], Facebook [ ], Twitter[ , ] | Text classification, speech recognition, and forecasting models | Costly as double LSTM cells are used, takes longer to train, and easy to overfit |
Convolutional Neural Networks (CNN) [ ] | Social media content [ , , , , , , ], Visual contents [ ], Twitter [ , , , , , , ], Formspring.me [ , ], Facebook [ ], Chats [ ], YouTube and Wikipedia [ ] | Image processing, and object detection | Significantly slower due to an operation such as maxpooling, large datasets are required to process, and train neural networks [ ] |
Radial Basis Function Networks (RBFNs) [ ] | Youtube content [ ], Formspring.me, MySpace, and YouTube content [ ] | Classification, regression and time-series prediction | Classification is slow because every node in the hidden layer needs to compute the RBF function |
Multilayer Perceptrons (MLPs) | Text and emoji data [ ] | Speech recognition, image-recognition, and machine translation | As it is fully connected, there are too many parameters, each node is connected to another node in a very dense network, which creates redundancy and inefficiency |
Self-Organizing Maps (SOMs) [ ] | Social media content [ ] | Data visualization for high dimensional data | Requires sufficient neuron weight to cluster inputs [ ] |
Restricted Boltzmann Machines (RBMs) [ ] | Turkish social media contents [ ], Arabic content [ ] | Dimensionality reduction, classification, regression, feature learning, topic modeling, and collaborative filtering | Training is more difficult because it is difficult to calculate the energy gradient function, the CD-k algorithm used in RBM is not as well known as the backpropagation algorithm, weight adjustment |
Gated Recurrent Units (GRU) [ ] | Social Commentary [ ], Facebook and Twitter aggressive speech [ ], Bangla text [ ], Formspring.me, MySpace and YouTube content [ ] | Sequence learning, Solved Vanishing–Exploding gradients problem | Slow convergence and low learning efficiency |
Attention-based model [ ] | Twitter bullied text identification [ ], social media text analysis [ ], online textual harassment detection [ ], contextual textual bullies [ ], Instagram bullied text identification [ ], Abusive Bangla Comment detection [ ], Trait-based bullying detection [ ] | The method provides a simple and efficient architecture with a fixed length vector to pay attention of a sentence’s high-level meaning | The model requires more weight parameters, which results in a longer training time |
5.11. Performance Comparison of DL Models in Cyberbullying Detection
6. dl in cyberbullying detection, 7. deep learning frameworks, applicability of different dl frameworks, 8. datasets for experiments, 9. challenges, open issues, and future trends, 9.1. issues in dl.
- Require a large amount of dataset: Large volumes of labeled data are required for DL. For example, the creation of self-driving cars involves millions of photos and hundreds of hours of video [ 198 ]. It is commonly known that data preparation consumes 80–90% of the time spent on ML development. Furthermore, even the strongest DL algorithms will struggle to function without good data and present weak performance to handle biased and unclean data during model training [ 199 ].
- High computational power: DL takes a lot of computational power. The parallel design of high-performance GPUs is ideal for DL. When used in conjunction with clusters or cloud computing, this allows development teams to cut DL network time for training from weeks to hours or less [ 198 ].
- Reasoning of prediction unexplainable: DL result prediction follows the Black-Box testing approach. Thus, it is not capable of making any explainable predictions. Since DL’s hidden weight and activation are non-interpretable, its predictions are considered as non-explainable [ 200 ].
- Security issue: Preventing the DL models from security attacks is the biggest challenge nowadays. Based on the occurring time, there are two types of security attacks. One is poisoning attack, which occurs during the training period, and another one is evasion attack, which occurs during interference (after training). By corrupting the data with malicious examples, poisoning attacks compromise the training process. On the other hand, evasion attacks use adversarial examples to confuse the entire classification process [ 201 ].
- Models are not adaptive: In the present world, data are very dynamic. Data are changing due to various factors, which may be constantly changing, such as location, time, and many other factors. However, DL models are built using a defined set, which is called the training dataset. Later, the performance of the model is measured by the data, which also comes from the same distribution of the training data, and eventually, the model performs well. Later, the same model may start performing poorly due to the changing the characteristics of the data, which are not entirely different, but have some variations from the training data. This is difficult to manage in DL to retrain the old models.
9.2. Challenges in Cyberbullying detection
- Cultural diversity for cyberbullying: Language is one of the important parts of the culture of a nation. Since cyberbullying has become a common problem among different nations, we may not expect a good prediction model by using a dataset of one nation and testing over the dataset of another culturally varied nation.
- Language challenge: Capturing context and analyzing the sentiment from different types of sentences is a difficult task and challenging work for cyberbullying detection. For example, “The image that you have sent so irritated me and I would rather not contact with you any longer!” is not easy to detect as cyberbullying without investigating from a rationale factor, albeit that model shows negative sentiment [ 26 ].
- Dataset challenge: Retrieving data from social media is not an easy task, as it relates to private information. Moreover, social media sites do not share user data publicly. Due to these issues, it is hard to gather quality data from social sites, which causes the lack of quality data to improve learning. Another challenging task is to annotate or label the data because they require a domain expert to label the corpus [ 202 ].
- Data representation challenge: Setting up an effective cyberbullying-detection system is difficult due to the need for human interaction and the nature of cyberbullying. Furthermore, the nature of cyberbullying is challenging to identify in the cyberbullying detection problem. The vast majority of the exploratory works directly identified bullying words in social media. However, separating content-based features have their own difficulties. For the absence of appropriate information, the performance of the model might decay [ 203 ].
- Natural Language Processing (NLP) challenges: The biggest challenge in natural language processing is understanding the meaning of the text. The relevant task is to build the right vocabulary, link the various components of the vocabulary, establish context, and extract semantic meaning from the data [ 204 ]. Misspelling and ambiguous expressions are other challenges that are very difficult to solve for the machine.
- Reusability of pre-trained model for sentiment analysis and cyberbullying: Although cyberbullying detection and sentiment analysis are related tasks, these two tasks have significant differences from each other; therefore, the pre-trained model of one task is likely to be difficult to use to predict another task. Sentiment analysis involves determining the overall emotional tone of a text, where the sentence is positive, negative, or neutral. On the contrary, cyberbullying detection involves identifying specific patterns of harmful words. Yet, there are some sentiment analysis approaches that can be used to identify cyberbullying. Atoum et al. [ 205 ] proposed an approach for detecting cyberbullying using sentiment analysis techniques. Nahar et al. [ 206 ] presented a novel method for identifying online bullying on social media sites from sentiment analysis. Dani et al. [ 207 ] presented a novel framework for supervised learning that uses sentiment analysis to identify cyberbullying. Overall, while sentiment analysis models may be helpful for cyberbullying detection, they cannot be directly reused without significant modifications and additional training. Cyberbullying detection (i.e., yes/no classes) largely needs to identify negative words, which are used to harass a person, while sentiment analysis has three different classes (i.e., negative, positive, and neutral) where negative patterns are part of the problem. In this case, positive and neutral categories are also dominant class labels. Since the nature of the outputs is different in two different problems, we cannot completely reuse one pre-trained model for other cases.
9.3. Future Trends
- Multilingual and multimedia content: In current times, social media and other virtual platforms are widely used among different levels of users in terms of age group, culture, language, taste, education, etc. Since social media is a vital platform for propagating cyber harassment, users may use multilingual and multimedia content; therefore, we may put more attention on building efficient cyberbullying detection systems for multilingual and multimedia content.
- Cyberbullying detection-specific word embedding: In recent times, researchers are introducing different domain specific word-embedding techniques, because these platforms produce accurate results for relevant sets of vocabularies. For example, Med-BERT is used for health-domain-based BERT-aware embedding systems. In this connection, researchers may propose a specialized word-embedding system for cyberbullying detection problems.
- Cyberbullying detection in SMS and email: Users are concerned with combating cyberbullying problems, which largely propagate through social media platforms. However, future researchers may put more attention on investigating Short Message Service (SMS)- and email-based cyberbullying detection methods.
- Cyberbullying impact on mental health: Cyberbullying may leave a long-term impact on the mental status of an individual. Some may take a life-threatening step or commit self-injury to curb the severity of the harassment and take death for granted. Therefore, mental health researchers can consider this issue as a timely topic and introduce different methods to fight against cyber harassment.
- Use of cutting-edge deep learning: With the advancement of deep-learning-based methods, we may introduce more subtle and delicate techniques to detect cyberbullying problems. For example, stacked and multi-channel CNN or Bi-LSTM-based cyberbullying-based frameworks or their advanced version or hybridization of these models may produce more sophisticated solutions to counter the problems.
10. Conclusions
Author contributions, data availability statement, conflicts of interest.
- Feinberg, T.; Robey, N. Cyberbullying. Educ. Dig. 2009 , 74 , 26. [ Google Scholar ]
- Marwa, T.; Salima, O.; Souham, M. Deep learning for online harassment detection in tweets. In Proceedings of the 2018 3rd International Conference on Pattern Analysis and Intelligent Systems (PAIS), Tebessa, Algeria, 24–25 October 2018; IEEE: Piscataway, NJ, USA, 2018; pp. 1–5. [ Google Scholar ]
- Nikolaou, D. Does cyberbullying impact youth suicidal behaviors? J. Health Econ. 2017 , 56 , 30–46. [ Google Scholar ] [ CrossRef ] [ PubMed ]
- Brailovskaia, J.; Teismann, T.; Margraf, J. Cyberbullying, positive mental health and suicide ideation/behavior. Psychiatry Res. 2018 , 267 , 240–242. [ Google Scholar ] [ CrossRef ] [ PubMed ]
- Lu, N.; Wu, G.; Zhang, Z.; Zheng, Y.; Ren, Y.; Choo, K.K.R. Cyberbullying detection in social media text based on character-level convolutional neural network with shortcuts. Concurr. Comput. Pract. Exp. 2020 , 32 , e5627. [ Google Scholar ] [ CrossRef ]
- Paul, S.; Saha, S.; Hasanuzzaman, M. Identification of cyberbullying: A deep learning based multimodal approach. Multimed. Tools Appl. 2020 , 81 , 26989–27008. [ Google Scholar ] [ CrossRef ]
- Buan, T.A.; Ramachandra, R. Automated cyberbullying detection in social media using an svm activated stacked convolution lstm network. In Proceedings of the 2020 the 4th International Conference on Compute and Data Analysis, Silicon Valley, CA, USA, 9–12 March 2020; pp. 170–174. [ Google Scholar ]
- Cho, K.; Van Merriënboer, B.; Gulcehre, C.; Bahdanau, D.; Bougares, F.; Schwenk, H.; Bengio, Y. Learning phrase representations using RNN encoder-decoder for statistical machine translation. arXiv 2014 , arXiv:1406.1078. [ Google Scholar ]
- Gers, F.A.; Schmidhuber, J.; Cummins, F. Learning to forget: Continual prediction with LSTM. Neural Comput. 2000 , 12 , 2451–2471. [ Google Scholar ] [ CrossRef ]
- Huang, Z.; Xu, W.; Yu, K. Bidirectional LSTM-CRF models for sequence tagging. arXiv 2015 , arXiv:1508.01991. [ Google Scholar ]
- Caroppo, A.; Leone, A.; Siciliano, P. Comparison between deep learning models and traditional machine learning approaches for facial expression recognition in ageing adults. J. Comput. Sci. Technol. 2020 , 35 , 1127–1146. [ Google Scholar ] [ CrossRef ]
- Yilmaz, A.; Demircali, A.A.; Kocaman, S.; Uvet, H. Comparison of Deep Learning and Traditional Machine Learning Techniques for Classification of Pap Smear Images. arXiv 2020 , arXiv:2009.06366. [ Google Scholar ]
- Finizola, J.S.; Targino, J.M.; Teodoro, F.G.S.; Moraes Lima, C.A.d. A comparative study between deep learning and traditional machine learning techniques for facial biometric recognition. In Proceedings of the Ibero-American Conference on Artificial Intelligence, Trujillo, Peru, 13–16 November 2018; Springer: Berlin/Heidelberg, Germany, 2018; pp. 217–228. [ Google Scholar ]
- Banerjee, V.; Telavane, J.; Gaikwad, P.; Vartak, P. Detection of cyberbullying using Deep Neural Network. In Proceedings of the 2019 5th International Conference on Advanced Computing & Communication Systems (ICACCS), Coimbatore, India, 15–16 March 2019; IEEE: PIscataway, NJ, USA, 2019; pp. 604–607. [ Google Scholar ]
- Kamath, C.N.; Bukhari, S.S.; Dengel, A. Comparative study between traditional machine learning and deep learning approaches for text classification. In Proceedings of the ACM Symposium on Document Engineering 2018, Halifax, NS, Canada, 28–31 August 2018; pp. 1–11. [ Google Scholar ]
- Wang, P.; Fan, E.; Wang, P. Comparative analysis of image classification algorithms based on traditional machine learning and deep learning. Pattern Recognit. Lett. 2021 , 141 , 61–67. [ Google Scholar ] [ CrossRef ]
- Naufal, M.F.; Kusuma, S.F.; Prayuska, Z.A.; Yoshua, A.A.; Lauwoto, Y.A.; Dinata, N.S.; Sugiarto, D. Comparative Analysis of Image Classification Algorithms for Face Mask Detection. J. Inf. Syst. Eng. Bus. Intell. 2021 , 7 , 56–66. [ Google Scholar ] [ CrossRef ]
- Ahmed, M.T.; Rahman, M.; Nur, S.; Islam, A.; Das, D. Deployment of machine learning and deep learning algorithms in detecting cyberbullying in bangla and romanized bangla text: A comparative study. In Proceedings of the 2021 International Conference on Advances in Electrical, Computing, Communication and Sustainable Technologies (ICAECT), Bhilai, India, 19–20 February 2021; IEEE: PIscataway, NJ, USA, 2021; pp. 1–10. [ Google Scholar ]
- Rezvani, N.; Beheshti, A.; Tabebordbar, A. Linking textual and contextual features for intelligent cyberbullying detection in social media. In Proceedings of the 18th International Conference on Advances in Mobile Computing & Multimedia, Chiang Mai, Thailand, 30 November–2 December 2020; pp. 3–10. [ Google Scholar ]
- Al-Ajlan, M.A.; Ykhlef, M. Deep learning algorithm for cyberbullying detection. Int. J. Adv. Comput. Sci. Appl 2018 , 9 , 199–205. [ Google Scholar ] [ CrossRef ]
- Iwendi, C.; Srivastava, G.; Khan, S.; Maddikunta, P.K.R. Cyberbullying detection solutions based on deep learning architectures. Multimed. Syst. 2020 , 1–14. [ Google Scholar ] [ CrossRef ]
- Paul, S.; Saha, S. CyberBERT: BERT for cyberbullying identification. Multimed. Syst. 2022 , 28 , 1897–1904. [ Google Scholar ] [ CrossRef ]
- Akhter, M.P.; Jiangbin, Z.; Naqvi, I.R.; AbdelMajeed, M.; Zia, T. Abusive language detection from social media comments using conventional machine learning and deep learning approaches. Multimed. Syst. 2022 , 28 , 1925–1940. [ Google Scholar ] [ CrossRef ]
- Picon, A.; Alvarez-Gila, A.; Irusta, U.; Echazarra, J. Why deep learning performs better than classical machine learning? Dyna Ing. Ind. 2020 , 95 , 119–122. [ Google Scholar ] [ CrossRef ]
- Dadvar, M.; Eckert, K. Cyberbullying detection in social networks using deep learning based models; a reproducibility study. arXiv 2018 , arXiv:1812.08046. [ Google Scholar ]
- Salawu, S.; He, Y.; Lumsden, J. Approaches to automated detection of cyberbullying: A survey. IEEE Trans. Affect. Comput. 2017 , 11 , 3–24. [ Google Scholar ] [ CrossRef ]
- Rosa, H.; Pereira, N.; Ribeiro, R.; Ferreira, P.C.; Carvalho, J.P.; Oliveira, S.; Coheur, L.; Paulino, P.; Simão, A.V.; Trancoso, I. Automatic cyberbullying detection: A systematic review. Comput. Hum. Behav. 2019 , 93 , 333–345. [ Google Scholar ] [ CrossRef ]
- Kim, S.; Razi, A.; Stringhini, G.; Wisniewski, P.J.; De Choudhury, M. A Human-Centered Systematic Literature Review of Cyberbullying Detection Algorithms. Proc. ACM Hum.-Comput. Interact. 2021 , 5 , 1–34. [ Google Scholar ] [ CrossRef ]
- Elsafoury, F.; Katsigiannis, S.; Pervez, Z.; Ramzan, N. When the Timeline Meets the Pipeline: A Survey on Automated Cyberbullying Detection. IEEE Access 2021 , 9 , 103541–103563. [ Google Scholar ] [ CrossRef ]
- Haidar, B.; Chamoun, M.; Yamout, F. Cyberbullying detection: A survey on multilingual techniques. In Proceedings of the 2016 European Modelling Symposium (EMS), Pisa, Italy, 28–30 November 2016; pp. 165–171. [ Google Scholar ]
- Al-Garadi, M.A.; Hussain, M.R.; Khan, N.; Murtaza, G.; Nweke, H.F.; Ali, I.; Mujtaba, G.; Chiroma, H.; Khattak, H.A.; Gani, A. Predicting Cyberbullying on Social Media in the Big Data Era Using Machine Learning Algorithms: Review of Literature and Open Challenges. IEEE Access 2019 , 7 , 70701–70718. [ Google Scholar ] [ CrossRef ]
- Mikolov, T.; Chen, K.; Corrado, G.; Dean, J. Efficient estimation of word representations in vector space. arXiv 2013 , arXiv:1301.3781. [ Google Scholar ]
- Mikolov, T.; Sutskever, I.; Chen, K.; Corrado, G.S.; Dean, J. Distributed representations of words and phrases and their compositionality. Adv. Neural Inf. Process. Syst. 2013 , 26 . [ Google Scholar ]
- Pennington, J.; Socher, R.; Manning, C.D. Glove: Global vectors for word representation. In Proceedings of the 2014 Conference on Empirical Methods in Natural Language Processing (EMNLP), Doha, Qatar, 25–29 October 2014; pp. 1532–1543. [ Google Scholar ]
- Peters, M.E.; Neumann, M.; Iyyer, M.; Gardner, M.; Clark, C.; Lee, K.; Zettlemoyer, L. Deep contextualized word representations. arXiv 2018 , arXiv:1802.05365. [ Google Scholar ] [ CrossRef ]
- Joulin, A.; Grave, E.; Bojanowski, P.; Mikolov, T. Bag of tricks for efficient text classification. arXiv 2016 , arXiv:1607.01759. [ Google Scholar ]
- Devlin, J.; Chang, M.W.; Lee, K.; Toutanova, K. Bert: Pre-training of deep bidirectional transformers for language understanding. arXiv 2018 , arXiv:1810.04805. [ Google Scholar ]
- Ramos, J. Using tf-idf to determine word relevance in document queries. In Proceedings of the First Instructional Conference on Machine Learning ; Rutgers University: Piscataway, NJ, USA, 2003; Volume 242, pp. 29–48. [ Google Scholar ]
- Ackley, D.H.; Hinton, G.E.; Sejnowski, T.J. A learning algorithm for Boltzmann machines. Cogn. Sci. 1985 , 9 , 147–169. [ Google Scholar ] [ CrossRef ]
- Hinton, G.E. Deep belief networks. Scholarpedia 2009 , 4 , 5947. [ Google Scholar ] [ CrossRef ]
- Baldi, P. Autoencoders, unsupervised learning, and deep architectures. In Proceedings of the ICML Workshop on Unsupervised and Transfer Learning, Bellevue, WA, USA, 2 July 2011; pp. 37–49. [ Google Scholar ]
- Goodfellow, I.; Pouget-Abadie, J.; Mirza, M.; Xu, B.; Warde-Farley, D.; Ozair, S.; Courville, A.; Bengio, Y. Generative adversarial nets. Adv. Neural Inf. Process. Syst. 2014 , 27 . [ Google Scholar ]
- Albawi, S.; Mohammed, T.A.; Al-Zawi, S. Understanding of a convolutional neural network. In Proceedings of the 2017 International Conference on Engineering and Technology (ICET), Antalya, Turkey, 21–23 August 2017; pp. 1–6. [ Google Scholar ]
- Mikolov, T.; Karafiát, M.; Burget, L.; Cernockỳ, J.; Khudanpur, S. Recurrent neural network based language model. In Proceedings of the Interspeech, Makuhari, Japan, 26–30 September 2010; Volume 2, pp. 1045–1048. [ Google Scholar ]
- Al-Harigy, L.M.; Al-Nuaim, H.A.; Moradpoor, N.; Tan, Z. Building toward Automated Cyberbullying Detection: A Comparative Analysis. Comput. Intell. Neurosci. 2022 , 2022 , 4794227. [ Google Scholar ] [ CrossRef ] [ PubMed ]
- Riadi, I.; Widiandana, P. Mobile Forensics for Cyberbullying Detection using Term Frequency-Inverse Document Frequency (TF-IDF). J. Eng. Sci. Technol. 2019 , 5 , 68–76. [ Google Scholar ] [ CrossRef ]
- Rahman, S.; Talukder, K.H.; Mithila, S.K. An Empirical Study to Detect Cyberbullying with TF-IDF and Machine Learning Algorithms. In Proceedings of the 2021 International Conference on Electronics, Communications and Information Technology (ICECIT), Khulna, Bangladesh, 14–16 September 2021; pp. 1–4. [ Google Scholar ]
- Liu, Y.; Ott, M.; Goyal, N.; Du, J.; Joshi, M.; Chen, D.; Levy, O.; Lewis, M.; Zettlemoyer, L.; Stoyanov, V. Roberta: A robustly optimized bert pretraining approach. arXiv 2019 , arXiv:1907.11692. [ Google Scholar ]
- Yani, M.A.A.; Maharani, W. Analyzing Cyberbullying Negative Content on Twitter Social Media with the RoBERTa Method. JINAV J. Inf. Vis. 2023 , 4 . [ Google Scholar ]
- Lan, Z.; Chen, M.; Goodman, S.; Gimpel, K.; Sharma, P.; Soricut, R. Albert: A lite bert for self-supervised learning of language representations. arXiv 2019 , arXiv:1909.11942. [ Google Scholar ]
- Tripathy, J.K.; Chakkaravarthy, S.S.; Satapathy, S.C.; Sahoo, M.; Vaidehi, V. ALBERT-based fine-tuning model for cyberbullying analysis. Multimed. Syst. 2022 , 28 , 1941–1949. [ Google Scholar ] [ CrossRef ]
- Clark, K.; Luong, M.T.; Le, Q.V.; Manning, C.D. Electra: Pre-training text encoders as discriminators rather than generators. arXiv 2020 , arXiv:2003.10555. [ Google Scholar ]
- Sanh, V.; Debut, L.; Chaumond, J.; Wolf, T. DistilBERT, a distilled version of BERT: Smaller, faster, cheaper and lighter. arXiv 2019 , arXiv:1910.01108. [ Google Scholar ]
- Harbaoui, A.; Benaissa, A.R. Cost-Sensitive PLM-based Approach for Arabic and English Cyberbullying Classification. Available at Research Square. 2023. Available online: https://www.researchsquare.com/article/rs-2524732/v1 (accessed on 6 April 2023).
- Sokolová, Z.; Staš, J.; Juhár, J. Review of Recent Trends in the Detection of Hate Speech and Offensive Language on Social Media. Acta Electrotech. Inform. 2022 , 22 , 18–24. [ Google Scholar ]
- Warke, O.; Jose, J.M.; Breitsohl, J. Utilising Twitter Metadata for Hate Classification. In Proceedings of the Advances in Information Retrieval: 45th European Conference on Information Retrieval, ECIR 2023, Dublin, Ireland, 2–6 April 2023; Proceedings, Part II. Springer: Berlin/Heidelberg, Germany, 2023; pp. 676–684. [ Google Scholar ]
- Sun, Z.; Yu, H.; Song, X.; Liu, R.; Yang, Y.; Zhou, D. Mobilebert: A compact task-agnostic bert for resource-limited devices. arXiv 2020 , arXiv:2004.02984. [ Google Scholar ]
- Mazari, A.C.; Boudoukhani, N.; Djeffal, A. BERT-based ensemble learning for multi-aspect hate speech detection. Clust. Comput. 2023 , 1–15. [ Google Scholar ] [ CrossRef ]
- Coban, O.; Ozel, S.A.; Inan, A. Detection and cross-domain evaluation of cyberbullying in Facebook activity contents for Turkish. In ACM Transactions on Asian and Low-Resource Language Information Processing ; Association for Computing Machinery: New York, NY, USA, 2023; Volume 22. [ Google Scholar ]
- Mozafari, M.; Farahbakhsh, R.; Crespi, N. A BERT-based transfer learning approach for hate speech detection in online social media. In Proceedings of the Complex Networks and Their Applications VIII: Volume 1 Proceedings of the Eighth International Conference on Complex Networks and Their Applications COMPLEX NETWORKS 2019, Lisbon, Portugal, 10–12 December 2020; Springer: Berlin/Heidelberg, Germany, 2020; pp. 928–940. [ Google Scholar ]
- Feng, Z.; Su, J.; Cao, J. BHF: BERT-based Hierarchical Attention Fusion Network for Cyberbullying Remarks Detection. In Proceedings of the 2022 5th International Conference on Machine Learning and Natural Language Processing, Hangzhou, China, 23–25 September 2022; pp. 1–7. [ Google Scholar ]
- Ishaq, A.; Malik, K.M.; Zafar, A. Hatespeechbert: Retraining Bert for Automatic Hate Speechdetection. Available online: https://papers.ssrn.com/sol3/papers.cfm?abstract_id=4329716 (accessed on 5 April 2023).
- Gada, M.; Damania, K.; Sankhe, S. Cyberbullying Detection using LSTM-CNN architecture and its applications. In Proceedings of the 2021 International Conference on Computer Communication and Informatics (ICCCI), Coimbatore, India, 27–29 January 2021; pp. 1–6. [ Google Scholar ]
- Anindyati, L.; Purwarianti, A.; Nursanti, A. Optimizing deep learning for detection cyberbullying text in indonesian language. In Proceedings of the 2019 International Conference of Advanced Informatics: Concepts, Theory and Applications (ICAICTA), Yogyakarta, Indonesia, 20–21 September 2019; pp. 1–5. [ Google Scholar ]
- Al-Hashedi, M.; Soon, L.K.; Goh, H.N. Cyberbullying detection using deep learning and word embeddings: An empirical study. In Proceedings of the 2019 2nd International Conference on Computational Intelligence and Intelligent Systems, Bangkok Thailand, 23–25 November 2019; pp. 17–21. [ Google Scholar ]
- Bu, S.J.; Cho, S.B. A hybrid deep learning system of CNN and LRCN to detect cyberbullying from SNS comments. In Proceedings of the International Conference on Hybrid Artificial Intelligence Systems, Oviedo, Spain, 20–22 June 2018; Springer: Berlin/Heidelberg, Germany, 2018; pp. 561–572. [ Google Scholar ]
- Al-Ajlan, M.A.; Ykhlef, M. Optimized twitter cyberbullying detection based on deep learning. In Proceedings of the 2018 21st Saudi Computer Society National Computer Conference (NCC), Riyadh, Saudi Arabia, 25–26 April 2018; pp. 1–5. [ Google Scholar ]
- Agrawal, S.; Awekar, A. Deep learning for detecting cyberbullying across multiple social media platforms. In Proceedings of the European Conference on Information Retrieval, Grenoble, France, 26–29 March 2018; Springer: Berlin/Heidelberg, Germany, 2018; pp. 141–153. [ Google Scholar ]
- Azumah, S.W.; Elsayed, N.; ElSayed, Z.; Ozer, M. Cyberbullying in Text Content Detection: An Analytical Review. Available online: https://arxiv.org/pdf/2303.10502.pdf (accessed on 5 April 2023).
- Bhatt, J. Using Hybrid Deep Learning and Word Embedding Based Approach for Advance Cyberbullying Detection. Ph.D Thesis, National College of Ireland, Dublin, Ireland, 2020. [ Google Scholar ]
- Kumar, A.; Sachdeva, N. A Bi-GRU with attention and CapsNet hybrid model for cyberbullying detection on social media. World Wide Web 2022 , 25 , 1537–1550. [ Google Scholar ] [ CrossRef ]
- Wang, K.; Cui, Y.; Hu, J.; Zhang, Y.; Zhao, W.; Feng, L. Cyberbullying detection, based on the fasttext and word similarity schemes. ACM Trans. Asian Low-Resour. Lang. Inf. Process. (TALLIP) 2020 , 20 , 1–15. [ Google Scholar ] [ CrossRef ]
- Yadav, J.; Kumar, D.; Chauhan, D. Cyberbullying detection using pre-trained BERT model. In Proceedings of the 2020 International Conference on Electronics and Sustainable Communication Systems (ICESC), Coimbatore, India, 2–4 July 2020; pp. 1096–1100. [ Google Scholar ]
- Haidar, B.; Chamoun, M.; Serhrouchni, A. Arabic cyberbullying detection: Using deep learning. In Proceedings of the 2018 7th International Conference on Computer and Communication Engineering (ICCCE), Kuala Lumpur, Malaysia, 19–20 September 2018; pp. 284–289. [ Google Scholar ]
- Aldhyani, T.H.; Al-Adhaileh, M.H.; Alsubari, S.N. Cyberbullying identification system based deep learning algorithms. Electronics 2022 , 11 , 3273. [ Google Scholar ] [ CrossRef ]
- Pericherla, S.; Ilavarasan, E. Performance analysis of word embeddings for cyberbullying detection. In Proceedings of the IOP Conference Series: Materials Science and Engineering, Sanya, China, 12–14 November 2021; IOP Publishing: Bristol, UK, 2021; Volume 1085, p. 012008. [ Google Scholar ]
- Eronen, J.; Ptaszynski, M.; Masui, F. Comparing Performance of Different Linguistically Backed Word Embeddings for Cyberbullying Detection. arXiv 2022 , arXiv:2206.01950. [ Google Scholar ]
- Alhloul, A.; Alam, A. Bullying Tweets Detection Using CNN-Attention. Int. J. Cybern. Inform. (IJCI) 2023 , 12 , 65–78. [ Google Scholar ] [ CrossRef ]
- Kountchev, R.; Rubin, S.; Milanova, M.; Todorov, V.; Kountcheva, R. Cognitive image representation based on spectrum pyramid decomposition. In Proceedings of the WSEAS International Conference on Mathematical Methods and Computational Techniques in Electrical Engineering (MMACTEE), Athens, Greece, 29–31 December 2008; pp. 230–235. [ Google Scholar ]
- Sudirman, S.; Qiu, G. Colour image representation using BSP Tree. Proc. CGIP 2000 , 1–4. Available online: http://www.cs.nott.ac.uk/~pszqiu/Online/CGIP2000.pdf (accessed on 5 April 2023).
- Wei, H.; Zuo, Q.; Lang, B. A bio-inspired model for image representation and image analysis. In Proceedings of the 2011 IEEE 23rd International Conference on Tools with Artificial Intelligence, Boca Raton, FL, USA, 7–9 November 2011; pp. 409–413. [ Google Scholar ]
- Xue, X.; Wu, X. Directly operable image representation of multiscale primal sketch. IEEE Trans. Multimed. 2005 , 7 , 805–816. [ Google Scholar ]
- Gao, S.; Duan, L.; Tsang, I.W. DEFEATnet—A deep conventional image representation for image classification. IEEE Trans. Circuits Syst. Video Technol. 2015 , 26 , 494–505. [ Google Scholar ] [ CrossRef ]
- Mori, S.; Suen, C.Y.; Yamamoto, K. Historical review of OCR research and development. Proc. IEEE 1992 , 80 , 1029–1058. [ Google Scholar ] [ CrossRef ]
- Pradheep, T.; Sheeba, J.; Yogeshwaran, T.; Pradeep Devaneyan, S. Automatic Multi Model Cyber Bullying Detection from Social Networks. In Proceedings of the International Conference on Intelligent Computing Systems (ICICS 2017), Irbid, Jordan, 15–16 September 2017; Sona College of Technology: Tamil Nadu, Idia, 2017. [ Google Scholar ]
- Sheeba, J.; Devaneyan, S.P. Impulsive intermodal cyber bullying recognition from public nets. Int. J. Adv. Res. Comput. Sci. 2018 , 9 . [ Google Scholar ]
- Kumari, K.; Singh, J.P.; Dwivedi, Y.K.; Rana, N.P. toward Cyberbullying-free social media in smart cities: A unified multi-modal approach. Soft Comput. 2020 , 24 , 11059–11070. [ Google Scholar ] [ CrossRef ]
- Verge, T. Instagram’s Bullying Comment Filter Hides Mean Comments. 2018. Available online: https://www.theverge.com/2018/5/1/17307980/instagram-bullying-comment-filter-machine-learning (accessed on 8 March 2023).
- Facebook. Inside Feed: Fighting Abuse. 2018. Available online: https://about.fb.com/news/2018/05/inside-feed-fighting-abuse/ (accessed on 8 March 2023).
- Gao, X.; Han, Y.; Tang, Q.; Liu, Z.; Ma, J. Chinese cyberbullying detection with OCR and deep learning. J. Intell. Fuzzy Syst. 2021 , 40 , 4731–4743. [ Google Scholar ]
- Borah, N.; Borah, P. Detecting cyberbullying on social media using OCR and machine learning. In Proceedings of the 4th International Conference on Intelligent Computing and Control Systems (ICICCS 2020), Madurai, India, 13–15 May 2020; pp. 300–305. [ Google Scholar ]
- Schmidhuber, J. Deep learning in neural networks: An overview. Neural Netw. 2015 , 61 , 85–117. [ Google Scholar ] [ CrossRef ]
- Srivastava, N.; Hinton, G.; Krizhevsky, A.; Sutskever, I.; Salakhutdinov, R. Dropout: A simple way to prevent neural networks from overfitting. J. Mach. Learn. Res. 2014 , 15 , 1929–1958. [ Google Scholar ]
- Prechelt, L. Early stopping-but when? In Neural Networks: Tricks of the Trade ; Springer: Berlin/Heidelberg, Germany, 1998; pp. 55–69. [ Google Scholar ]
- Das, H.S.; Roy, P. A deep dive into deep learning techniques for solving spoken language identification problems. In Intelligent Speech Signal Processing ; Elsevier: Amsterdam, The Netherlands, 2019; pp. 81–100. [ Google Scholar ]
- Hinton, G.E. A practical guide to training restricted Boltzmann machines. In Neural networks: Tricks of the Trade ; Springer: Berlin/Heidelberg, Germany, 2012; pp. 599–619. [ Google Scholar ]
- Salakhutdinov, R.; Hinton, G. Deep Boltzmann Machines. In Proceedings of the Twelth International Conference on Artificial Intelligence and Statistics, Clearwater Beach, FL, USA, 16–18 April 2009; van Dyk, D., Welling, M., Eds.; Proceedings of Machine Learning Research. PMLR, Hilton Clearwater Beach Resort: Clearwater, FL, USA, 2009; Volume 5, pp. 448–455. [ Google Scholar ]
- Courville, A.; Bergstra, J.; Bengio, Y. A spike and slab restricted Boltzmann machine. In Proceedings of the Fourteenth International Conference on Artificial Intelligence and Statistics, Fort Lauderdale, FL, USA, 11–13 April 2011; JMLR Workshop and Conference Proceedings. pp. 233–241. [ Google Scholar ]
- Hinton, G.E. Boltzmann machine. Scholarpedia 2007 , 2 , 1668. [ Google Scholar ] [ CrossRef ]
- Bengio, Y.; Lamblin, P.; Popovici, D.; Larochelle, H. Greedy layer-wise training of deep networks. Adv. Neural Inf. Process. Syst. 2006 , 19 . [ Google Scholar ]
- Li, C.; Wang, Y.; Zhang, X.; Gao, H.; Yang, Y.; Wang, J. Deep belief network for spectral–spatial classification of hyperspectral remote sensor data. Sensors 2019 , 19 , 204. [ Google Scholar ] [ CrossRef ]
- Salakhutdinov, R.; Hinton, G. Semantic hashing. Int. J. Approx. Reason. 2009 , 50 , 969–978. [ Google Scholar ] [ CrossRef ]
- Goodfellow, I.; Bengio, Y.; Courville, A. Deep Learning ; MIT Press: Cambridge, MA, USA, 2016. [ Google Scholar ]
- Hinton, G.E.; Salakhutdinov, R.R. Reducing the dimensionality of data with neural networks. Science 2006 , 313 , 504–507. [ Google Scholar ] [ CrossRef ]
- Vachhani, B.; Bhat, C.; Das, B.; Kopparapu, S.K. Deep Autoencoder Based Speech Features for Improved Dysarthric Speech Recognition. In Proceedings of the Interspeech, Stockholm, Sweden, 20–24 August 2017; pp. 1854–1858. [ Google Scholar ]
- Donahue, J.; Krähenbühl, P.; Darrell, T. Adversarial feature learning. arXiv 2016 , arXiv:1605.09782. [ Google Scholar ]
- Makhzani, A.; Shlens, J.; Jaitly, N.; Goodfellow, I.; Frey, B. Adversarial autoencoders. arXiv 2015 , arXiv:1511.05644. [ Google Scholar ]
- Feng, J.; Feng, X.; Chen, J.; Cao, X.; Zhang, X.; Jiao, L.; Yu, T. Generative adversarial networks based on collaborative learning and attention mechanism for hyperspectral image classification. Remote Sens. 2020 , 12 , 1149. [ Google Scholar ] [ CrossRef ]
- Hochreiter, S.; Schmidhuber, J. Long short-term memory. Neural Comput. 1997 , 9 , 1735–1780. [ Google Scholar ] [ CrossRef ] [ PubMed ]
- Hopfield, J.J. Hopfield network. Scholarpedia 2007 , 2 , 1977. [ Google Scholar ] [ CrossRef ]
- Chung, J.; Gulcehre, C.; Cho, K.; Bengio, Y. Empirical evaluation of gated recurrent neural networks on sequence modeling. arXiv 2014 , arXiv:1412.3555. [ Google Scholar ]
- Rezvani, N.; Beheshti, A. toward Attention-Based Context-Boosted Cyberbullying Detection in social media. J. Data Intell. 2021 , 2 , 418–433. [ Google Scholar ] [ CrossRef ]
- Pericherla, S.; Ilavarasan, E. Transformer network-based word embeddings approach for autonomous cyberbullying detection. Int. J. Intell. Unmanned Syst. 2021; ahead-of-print . [ Google Scholar ]
- Ahmed, T.; Ivan, S.; Kabir, M.; Mahmud, H.; Hasan, K. Performance analysis of transformer-based architectures and their ensembles to detect trait-based cyberbullying. Soc. Netw. Anal. Min. 2022 , 12 , 99. [ Google Scholar ] [ CrossRef ]
- Alotaibi, M.; Alotaibi, B.; Razaque, A. A multichannel deep learning framework for cyberbullying detection on social media. Electronics 2021 , 10 , 2664. [ Google Scholar ] [ CrossRef ]
- Yafooz, W.M.; Al-Dhaqm, A.; Alsaeedi, A. Detecting Kids Cyberbullying Using Transfer Learning Approach: Transformer Fine-Tuning Models. In Kids Cybersecurity Using Computational Intelligence Techniques ; Springer International Publishing: Berlin, Germany, 2023. [ Google Scholar ]
- Guo, X.; Anjum, U.; Zhan, J. Cyberbully Detection Using BERT with Augmented Texts. In Proceedings of the 2022 IEEE International Conference on Big Data (Big Data), Osaka, Japan, 17–20 December 2022; pp. 1246–1253. [ Google Scholar ]
- Cheng, L.; Guo, R.; Silva, Y.; Hall, D.; Liu, H. Hierarchical attention networks for cyberbullying detection on the instagram social network. In Proceedings of the 2019 SIAM International Conference on Data Mining, Calgary, AB, Canada, 2–4 May 2019; pp. 235–243. [ Google Scholar ]
- Cheng, L.; Guo, R.; Silva, Y.N.; Hall, D.; Liu, H. Modeling temporal patterns of cyberbullying detection with hierarchical attention networks. ACM/IMS Trans. Data Sci. 2021 , 2 , 1–23. [ Google Scholar ] [ CrossRef ]
- Sreelakshmi, K.; Premjith, B.; Soman, K.P. Detection of Hate Speech Text in Hindi-English Code-mixed Data. Procedia Comput. Sci. 2020 , 171 , 737–744. [ Google Scholar ] [ CrossRef ]
- Aurpa, T.T.; Sadik, R.; Ahmed, M.S. Abusive Bangla comments detection on Facebook using transformer-based deep learning models. Soc. Netw. Anal. Min. 2022 , 12 , 24. [ Google Scholar ] [ CrossRef ]
- Khan, S.; Fazil, M.; Sejwal, V.K.; Alshara, M.A.; Alotaibi, R.M.; Kamal, A.; Baig, A.R. BiCHAT: BiLSTM with deep CNN and hierarchical attention for hate speech detection. J. King Saud Univ.-Comput. Inf. Sci. 2022 , 34 , 4335–4344. [ Google Scholar ] [ CrossRef ]
- Fang, Y.; Yang, S.; Zhao, B.; Huang, C. Cyberbullying detection in social networks using Bi-gru with self-attention mechanism. Information 2021 , 12 , 171. [ Google Scholar ] [ CrossRef ]
- Maity, K.; Kumar, A.; Saha, S. A Multitask Multimodal Framework for Sentiment and Emotion-Aided Cyberbullying Detection. IEEE Internet Comput. 2022 , 26 , 68–78. [ Google Scholar ] [ CrossRef ]
- Jang, B.; Kim, M.; Harerimana, G.; Kang, S.u.; Kim, J.W. Bi-LSTM model to increase accuracy in text classification: Combining Word2vec CNN and attention mechanism. Appl. Sci. 2020 , 10 , 5841. [ Google Scholar ] [ CrossRef ]
- Jiang, M.; Liang, Y.; Feng, X.; Fan, X.; Pei, Z.; Xue, Y.; Guan, R. Text classification based on deep belief network and softmax regression. Neural Comput. Appl. 2018 , 29 , 61–70. [ Google Scholar ] [ CrossRef ]
- Goroshin, R.; LeCun, Y. Saturating auto-encoders. arXiv 2013 , arXiv:1301.3577. [ Google Scholar ]
- Dadvar, M.; Eckert, K. Cyberbullying detection in social networks using deep learning based models. In Proceedings of the International Conference on Big Data Analytics and Knowledge Discovery, Bratislava, Slovakia, 14–17 September 2020; Springer: Berlin/Heidelberg, Germany, 2020; pp. 245–255. [ Google Scholar ]
- Chandra, N.; Khatri, S.K.; Som, S. Cyberbullying detection using recursive neural network through offline repository. In Proceedings of the 2018 7th International Conference on Reliability, Infocom Technologies and Optimization (Trends and Future Directions) (ICRITO), Nordia, India, 29–31 August 2018; pp. 748–754. [ Google Scholar ]
- Elmezain, M.; Malki, A.; Ibrahim, G.; El-Sayed, A. Hybrid Deep Learning Model–Based Prediction of Images Related to Cyberbullying. Int. J. Appl. Math. Comput. Sci. 2022 , 32 , 323–334. [ Google Scholar ]
- Chandrasekaran, S.; Singh Pundir, A.K.; Lingaiah, T.B. Deep Learning Approaches for Cyberbullying Detection and Classification on Social Media. Comput. Intell. Neurosci. 2022 , 2022 , 2163458. [ Google Scholar ]
- Kumar, A.; Sachdeva, N. Cyberbullying detection on social multimedia using soft computing techniques: A meta-analysis. Multimed. Tools Appl. 2019 , 78 , 23973–24010. [ Google Scholar ] [ CrossRef ]
- Sripada, N.K.; Sirikonda, S.; Areefa; Leena, G.; Sriabhinay, K. Web-application for detecting cyber bullying using machine learning approach. Proc. AIP Conf. Proc. 2022 , 2418 , 020082. [ Google Scholar ]
- Agarwal, A.; Chivukula, A.S.; Bhuyan, M.H.; Jan, T.; Narayan, B.; Prasad, M. Identification and classification of cyberbullying posts: A recurrent neural network approach using under-sampling and class weighting. In Proceedings of the International Conference on Neural Information Processing, New Delhi, India, 22–26 November 2021; Springer: Berlin/Heidelberg, Germany, 2020; pp. 113–120. [ Google Scholar ]
- Luo, Y.; Zhang, X.; Hua, J.; Shen, W. Multi-featured Cyberbullying Detection Based on Deep Learning. In Proceedings of the 2021 16th International Conference on Computer Science & Education (ICCSE), Lancaster, UK, 17–21 August 2021; pp. 746–751. [ Google Scholar ]
- Golem, V.; Karan, M.; Šnajder, J. Combining shallow and deep learning for aggressive text detection. In Proceedings of the First Workshop on Trolling, Aggression and Cyberbullying (TRAC-2018), Santa Fe, NM, USA, 25 August 2018; pp. 188–198. [ Google Scholar ]
- Zhang, X.; Tong, J.; Vishwamitra, N.; Whittaker, E.; Mazer, J.P.; Kowalski, R.; Hu, H.; Luo, F.; Macbeth, J.; Dillon, E. Cyberbullying detection with a pronunciation based convolutional neural network. In Proceedings of the 2016 15th IEEE International Conference on Machine Learning and Applications (ICMLA), Anaheim, CA, USA, 18–20 December 2016; pp. 740–745. [ Google Scholar ]
- O’Shea, K.; Nash, R. An introduction to convolutional neural networks. arXiv 2015 , arXiv:1511.08458. [ Google Scholar ]
- Orr, M.J. Introduction to Radial Basis Function Networks ; Technical Report; Center for Cognitive Science, University of Edinburgh: Edinburgh, UK, 1996. [ Google Scholar ]
- Çürük, E.; Acı, Ç.; Eşsiz, E.S. The effects of attribute selection in artificial neural network based classifiers on cyberbullying detection. In Proceedings of the 2018 3rd International Conference on Computer Science and Engineering (UBMK), Sarajevo, Bosnia and Herzegovina, 20–23 September 2018; pp. 6–11. [ Google Scholar ]
- Çiğdem, A.; Çürük, E.; Eşsiz, E.S. Automatic detection of cyberbullying in formspring. me, Myspace and YouTube social networks. Turk. J. Eng. 2019 , 3 , 168–178. [ Google Scholar ]
- Ritter, H.; Martinetz, T.; Schulten, K. Neural Computation and Self-Organizing Maps: An Introduction ; Addison-Wesley Reading: Boston, MA, USA, 1992. [ Google Scholar ]
- Desai, A.; Kalaskar, S.; Kumbhar, O.; Dhumal, R. Cyber Bullying Detection on Social Media using Machine Learning. In Proceedings of the ITM Web of Conferences, Navi Mumbai, India, 14–15 July 2021; EDP Sciences: Ulis, France, 2021; Volume 40, p. 03038. [ Google Scholar ]
- Miljković, D. Brief review of self-organizing maps. In Proceedings of the 2017 40th International Convention on Information and Communication Technology, Electronics and Microelectronics (MIPRO), Opatija, Croatia, 22–26 May 2017; pp. 1061–1066. [ Google Scholar ]
- Bozyiğit, A.; Utku, S.; Nasiboğlu, E. Cyberbullying detection by using artificial neural network models. In Proceedings of the 2019 4th International Conference on Computer Science and Engineering (UBMK), Samsun, Turkey, 11–15 September 2019; pp. 520–524. [ Google Scholar ]
- Cho, K.; Van Merriënboer, B.; Bahdanau, D.; Bengio, Y. On the properties of neural machine translation: Encoder-decoder approaches. arXiv 2014 , arXiv:1409.1259. [ Google Scholar ]
- de Santana Correia, A.; Colombini, E.L. Attention, please! A survey of neural attention models in deep learning. Artif. Intell. Rev. 2022 , 55 , 6037–6124. [ Google Scholar ] [ CrossRef ]
- Zhang, A.; Li, B.; Wan, S.; Wang, K. Cyberbullying detection with birnn and attention mechanism. In Proceedings of the Machine Learning and Intelligent Communications: 4th International Conference, MLICOM 2019, Nanjing, China, 24–25 August 2019; Springer: Berlin/Heidelberg, Germany, 2019; pp. 623–635. [ Google Scholar ]
- Raj, C.; Agarwal, A.; Bharathy, G.; Narayan, B.; Prasad, M. Cyberbullying detection: Hybrid models based on machine learning and natural language processing techniques. Electronics 2021 , 10 , 2810. [ Google Scholar ] [ CrossRef ]
- Bharti, S.; Yadav, A.K.; Kumar, M.; Yadav, D. Cyberbullying detection from tweets using deep learning. Kybernetes 2021 , 51 , 2695–2711. [ Google Scholar ] [ CrossRef ]
- Murshed, B.A.H.; Abawajy, J.; Mallappa, S.; Saif, M.A.N.; Al-Ariki, H.D.E. DEA-RNN: A hybrid deep learning approach for cyberbullying detection in Twitter social media platform. IEEE Access 2022 , 10 , 25857–25871. [ Google Scholar ] [ CrossRef ]
- Raj, M.; Singh, S.; Solanki, K.; Selvanambi, R. An application to detect cyberbullying using machine learning and deep learning techniques. SN Comput. Sci. 2022 , 3 , 401. [ Google Scholar ] [ CrossRef ] [ PubMed ]
- Beniwal, R.; Maurya, A. Toxic comment classification using hybrid deep learning model. In Proceedings of the Sustainable Communication Networks and Application: Proceedings of ICSCN 2020, Erode, India, 6–7 August 2020; Springer: Berlin/Heidelberg, Germany, 2021; pp. 461–473. [ Google Scholar ]
- Singh, N.K.; Singh, P.; Chand, S. Deep Learning based Methods for Cyberbullying Detection on Social Media. In Proceedings of the 2022 International Conference on Computing, Communication, and Intelligent Systems (ICCCIS), Greater Noida, India, 4–5 November 2022; pp. 521–525. [ Google Scholar ]
- Pang, B.; Nijkamp, E.; Wu, Y.N. Deep learning with tensorflow: A review. J. Educ. Behav. Stat. 2020 , 45 , 227–248. [ Google Scholar ] [ CrossRef ]
- Borkan, D.; Dixon, L.; Sorensen, J.; Thain, N.; Vasserman, L. Nuanced metrics for measuring unintended bias with real data for text classification. In Proceedings of the Companion Proceedings of the 2019 World Wide Web Conference, San Francisco, CA, USA, 13–17 May 2019; pp. 491–500. [ Google Scholar ]
- Huang, X.; Xing, L.; Dernoncourt, F.; Paul, M.J. Multilingual twitter corpus and baselines for evaluating demographic bias in hate speech recognition. arXiv 2020 , arXiv:2002.10361. [ Google Scholar ]
- Wulczyn, E.; Thain, N.; Dixon, L. Ex machina: Personal attacks seen at scale. In Proceedings of the 26th International Conference on World Wide Web, Perth, Australia, 3–7 April 2017; pp. 1391–1399. [ Google Scholar ]
- Kennedy, B.; Atari, M.; Davani, A.M.; Yeh, L.; Omrani, A.; Kim, Y.; Coombs, K.; Havaldar, S.; Portillo-Wightman, G.; Gonzalez, E.; et al. Introducing the Gab Hate Corpus: Defining and applying hate-based rhetoric to social media posts at scale. Lang. Resour. Eval. 2022 , 56 , 79–108. [ Google Scholar ] [ CrossRef ]
- Gencoglu, O. Cyberbullying detection with fairness constraints. IEEE Internet Comput. 2020 , 25 , 20–29. [ Google Scholar ] [ CrossRef ]
- Gulli, A.; Pal, S. Deep Learning with Keras ; Packt Publishing Ltd.: Birmingham, UK, 2017. [ Google Scholar ]
- Kargutkar, S.; Chitre, V. Implementation of Cyberbullying Detection Using Machine Learning Techniques. Int. J. Res. Appl. Sci. Eng. Technol. 2021 , 9 , 290–294. [ Google Scholar ] [ CrossRef ]
- Roy, P.K.; Mali, F.U. Cyberbullying detection using deep transfer learning. Complex Intell. Syst. 2022 , 8 , 5449–5467. [ Google Scholar ] [ CrossRef ]
- Jain, V.; Kumar, V.; Pal, V.; Vishwakarma, D.K. Detection of cyberbullying on social media using machine learning. In Proceedings of the 2021 5th International Conference on Computing Methodologies and Communication (ICCMC), Erode, India, 8–10 April 2021; pp. 1091–1096. [ Google Scholar ]
- Stevens, E.; Antiga, L.; Viehmann, T. Deep Learning with PyTorch ; Manning Publications: Shelter Island, NY, USA, 2020. [ Google Scholar ]
- Pramanick, S.; Sharma, S.; Dimitrov, D.; Akhtar, M.S.; Nakov, P.; Chakraborty, T. MOMENTA: A multimodal framework for detecting harmful memes and their targets. arXiv 2021 , arXiv:2109.05184. [ Google Scholar ]
- Sharma, S.; Akhtar, M.; Nakov, P.; Chakraborty, T. DISARM: Detecting the victims targeted by harmful memes. arXiv 2022 , arXiv:2205.05738. [ Google Scholar ]
- Vishwamitra, N.; Hu, H.; Luo, F.; Cheng, L. toward understanding and detecting cyberbullying in real-world images. In Proceedings of the 2020 19th IEEE International Conference on Machine Learning and Applications (ICMLA), Online, 14–17 December 2020. [ Google Scholar ]
- Brownlee, J. Deep Learning with Python: Develop Deep Learning Models on Theano and 1678 Tensor Flow Using Keras ; Machine Learning Mastery: Vermont, VIC, Australia, 2016. [ Google Scholar ]
- Sintaha, M.; Satter, S.B.; Zawad, N.; Swarnaker, C.; Hassan, A. Cyberbullying Detection Using Sentiment Analysis in Social Media. Ph.D Thesis, BRAC University, Dhaka, Bangladesh, 2016. [ Google Scholar ]
- Kumar, A.; Sachdeva, N. Multimodal cyberbullying detection using capsule network with dynamic routing and deep convolutional neural network. Multimed. Syst. 2021 , 28 , 2043–2052. [ Google Scholar ] [ CrossRef ]
- Kumar, A.; Sachdeva, N. Multi-input integrative learning using Deep Neural Networks and 1684 transfer learning for cyberbullying detection in real-time code-mix data. Multimed. Syst. 2022 , 28 , 2027–2041. [ Google Scholar ] [ CrossRef ]
- Jia, Y.; Shelhamer, E.; Donahue, J.; Karayev, S.; Long, J.; Girshick, R.; Guadarrama, S.; Darrell, T. Caffe: Convolutional architecture for fast feature embedding. In Proceedings of the 22nd ACM International Conference on Multimedia, Orlando, FL, USA, 3–7 November 2014; pp. 675–678. [ Google Scholar ]
- Tokui, S.; Okuta, R.; Akiba, T.; Niitani, Y.; Ogawa, T.; Saito, S.; Suzuki, S.; Uenishi, K.; Vogel, B.; Yamazaki Vincent, H. Chainer: A Deep Learning Framework for Accelerating the Research Cycle. In Proceedings of the 25th ACM SIGKDD International Conference on Knowledge Discovery & Data Mining, Anchorage, AK, USA, 4–8 August 2019; ACM: NewYork, NY, USA, 2019; pp. 2002–2011. [ Google Scholar ]
- Parvat, A.; Chavan, J.; Kadam, S.; Dev, S.; Pathak, V. A survey of deep-learning frameworks. In Proceedings of the 2017 International Conference on Inventive Systems and Control (ICISC), Coimbatore, India, 19–20 January 2017; pp. 1–7. [ Google Scholar ]
- Neubig, G.; Dyer, C.; Goldberg, Y.; Matthews, A.; Ammar, W.; Anastasopoulos, A.; Ballesteros, M.; Chiang, D.; Clothiaux, D.; Cohn, T.; et al. Dynet: The dynamic neural network toolkit. arXiv 2017 , arXiv:1701.03980. [ Google Scholar ]
- Hodnett, M.; Wiley, J.F. R Deep Learning Essentials: A Step-by-Step Guide to Building Deep Learning Models Using TensorFlow, Keras, and MXNet ; Packt Publishing Ltd.: Birmingham, UK, 2018. [ Google Scholar ]
- Zaheri, S.; Leath, J.; Stroud, D. Toxic comment classification. SMU Data Sci. Rev. 2020 , 3 , 13. [ Google Scholar ]
- Dieleman, S.; Schlüter, J.; Raffel, C.; Olson, E.; Sønderby, S.K.; Nouri, D. Lasagne: First Release ; Zenodo: Geneva, Switzerland, 2015. [ Google Scholar ]
- Candel, A.; Parmar, V.; LeDell, E.; Arora, A. Deep learning with H2O ; H2O ai Inc.: Mountain View, CA, USA, 2016; pp. 1–21. [ Google Scholar ]
- Tong, Z.; Du, N.; Song, X.; Wang, X. Study on MindSpore Deep Learning Framework. In Proceedings of the 2021 17th International Conference on Computational Intelligence and Security (CIS), Chengdu, China, 19–22 November 2020; pp. 183–186. [ Google Scholar ]
- Zhao, Z.; Gao, M.; Luo, F.; Zhang, Y.; Xiong, Q. LSHWE: Improving similarity-based word embedding with locality sensitive hashing for cyberbullying detection. In Proceedings of the 2020 International Joint Conference on Neural Networks (IJCNN), Glasgow, UK, 19–24 July 2020; pp. 1–8. [ Google Scholar ]
- Ahmed, M.F.; Mahmud, Z.; Biash, Z.T.; Ryen, A.A.N.; Hossain, A.; Ashraf, F.B. Cyberbullying detection using Deep Neural Network from social media comments in bangla language. arXiv 2021 , arXiv:2106.04506. [ Google Scholar ]
- Dewani, A.; Memon, M.A.; Bhatti, S. Cyberbullying detection: Advanced preprocessing techniques & deep learning architecture for Roman Urdu data. J. Big Data 2021 , 8 , 160. [ Google Scholar ]
- Paszke, A.; Gross, S.; Massa, F.; Lerer, A.; Bradbury, J.; Chanan, G.; Killeen, T.; Lin, Z.; Gimelshein, N.; Antiga, L.; et al. Pytorch: An imperative style, high-performance deep learning library. Adv. Neural Inf. Process. Syst. 2019 , 32 . [ Google Scholar ]
- Collobert, R.; Bengio, S.; Mariéthoz, J. Torch: A modular Machine Learning Software Library ; Technical Report; Idiap: Maldini, Switzerland, 2002. [ Google Scholar ]
- Patange, T.; Singh, J.; Thorve, A.; Somaraj, Y.; Vyawahare, M. Detection of Cyberhectoring on Instagram. In Proceedings of the Proceedings 2019: Conference on Technologies for Future Cities (CTFC), Pillai College of Engineering, New Panvel, India, 8–9 February 2019. [ Google Scholar ]
- Shahane, P.; Gore, D. Detection of Fake Profiles on Twitter using Random Forest & Deep Convolutional Neural Network. Int. J. Manag. Technol. Eng. 2019 , 9 , 3663–3667. [ Google Scholar ]
- Bhaskaran, J.; Kamath, A.; Paul, S. DISCo: Detecting Insults in Social Commentary. 2017. Available online: http://cs229.stanford.edu/proj2017/final-reports/5242067.pdf (accessed on 5 April 2023).
- Waseem, Z.; Hovy, D. Hateful symbols or hateful people? predictive features for hate speech detection on twitter. In Proceedings of the NAACL Student Research Workshop, San Diego, CA, USA, 13–15 June 2016; pp. 88–93. [ Google Scholar ]
- Kasture, A.S. A predictive Model to Detect Online Cyberbullying. Ph.D Thesis, Auckland University of Technology, Auckland, New Zealand, 2015. [ Google Scholar ]
- Davidson, T.; Warmsley, D.; Macy, M.; Weber, I. Automated hate speech detection and the problem of offensive language. In Proceedings of the International AAAI Conference on Web and Social Media, Montreal, QC, Canada, 15–18 May 2017; Volume 11, pp. 512–515. [ Google Scholar ]
- Dadvar, M.; Trieschnigg, D.; Jong, F.d. Experts and machines against bullies: A hybrid approach to detect cyberbullies. In Proceedings of the Canadian conference on artificial intelligence, Montreal, QC, USA, 6–9 May 2014; Springer: Berlin/Heidelberg, Germany, 2014; pp. 275–281. [ Google Scholar ]
- Toxic Comment Classification Challenge. Available online: https://www.kaggle.com/competitions/jigsaw-toxic-comment-classification-challenge/data (accessed on 5 April 2023).
- Data and Code for the Study of Bullying. Available online: https://research.cs.wisc.edu/bullying/data.html (accessed on 5 April 2023).
- Rafiq, R.I.; Hosseinmardi, H.; Han, R.; Lv, Q.; Mishra, S.; Mattson, S.A. Careful what you share in six seconds: Detecting cyberbullying instances in Vine. In Proceedings of the 2015 IEEE/ACM International Conference on Advances in Social Networks Analysis and Mining (ASONAM), Paris, France, 25–28 August 2015; pp. 617–622. [ Google Scholar ]
- Rafiq, R.I.; Hosseinmardi, H.; Mattson, S.A.; Han, R.; Lv, Q.; Mishra, S. Analysis and detection of labeled cyberbullying instances in Vine, a video-based social network. Soc. Netw. Anal. Min. 2016 , 6 , 88. [ Google Scholar ] [ CrossRef ]
- Zohuri, B.; Moghaddam, M. Deep learning limitations and flaws. Mod. Approaches Mater. Sci 2020 , 2 , 241–250. [ Google Scholar ] [ CrossRef ]
- Whang, S.; Lee, J.G. Data Collection and Quality Challenges for Deep Learning. In Proceedings of the VLDB Endowment, Online, 31 August–4 September 2020; VLDB Endowment: Los Angeles, CA, USA, 2020; Volume 13. [ Google Scholar ]
- Pramod, A.; Naicker, H.S.; Tyagi, A.K. Machine learning and deep learning: Open issues and future research directions for the next 10 years. Computational Analysis and Deep Learning for Medical Care: Principles, Methods, and Applications ; John Wiley & Sons, Ltd.: Chichester, UK, 2021; pp. 463–490. [ Google Scholar ]
- Bae, H.; Jang, J.; Jung, D.; Jang, H.; Ha, H.; Lee, H.; Yoon, S. Security and privacy issues in deep learning. arXiv 2018 , arXiv:1807.11655. [ Google Scholar ]
- Raisi, E.; Huang, B. Cyberbullying identification using participant-vocabulary consistency. arXiv 2016 , arXiv:1606.08084. [ Google Scholar ]
- Ali, W.N.H.W.; Mohd, M.; Fauzi, F. Cyberbullying detection: An overview. In Proceedings of the 2018 Cyber Resilience Conference (CRC), Putrajaya, Malaysia, 13–15 November 2018; pp. 1–3. [ Google Scholar ]
- Weischedel, R.M.; Bates, M. Challenges in Natural Language Processing ; Cambridge University Press: Cambridge, UK, 2006. [ Google Scholar ]
- Atoum, J.O. Cyberbullying Detection Through Sentiment Analysis. In Proceedings of the 2020 International Conference on Computational Science and Computational Intelligence (CSCI), Las Vegas, NV, USA, 16–18 December 2020; pp. 292–297. [ Google Scholar ]
- Nahar, V.; Unankard, S.; Li, X.; Pang, C. Sentiment analysis for effective detection of cyber bullying. In Proceedings of the Web Technologies and Applications: 14th Asia-Pacific Web Conference, APWeb 2012, Kunming, China, 11–13 April 2012; Proceedings 14. Springer: Berlin/Heidelberg, Germany, 2012; pp. 767–774. [ Google Scholar ]
- Dani, H.; Li, J.; Liu, H. Sentiment informed cyberbullying detection in social media. In Proceedings of the Machine Learning and Knowledge Discovery in Databases: European Conference, ECML PKDD 2017, Skopje, Macedonia, 18–22 September 2017; Proceedings, Part I 10. Springer: Berlin/Heidelberg, Germany, 2017; pp. 52–67. [ Google Scholar ]
Click here to enlarge figure
Reference | Deep Learning Models | Method. | Taxnom. | Data Represent. Tech. | Framewrk. | Dataset (Pub. Avail.) | Discussion in Challenges and Future Trends | |||||
---|---|---|---|---|---|---|---|---|---|---|---|---|
Application in Cyberbullying | Strength and Limitation | Text | Img. | Cultural Diversity | Data Represent. | Multimedia and Multilingual Content | Impact on Mental Health | |||||
[ ] | ✘ | ✘ | ✘ | ✘ | ✓ | ✘ | N/A | ✘ | ✘ | ✘ | ✘ | ✘ |
[ ] | ✓ | ✓ | ✓ | ✘ | ✓ | ✘ | ✘ | ✓ | ✘ | ✘ | ✘ | ✓ |
[ ] | ✘ | ✘ | ✓ | ✘ | ✓ | ✓ | N/A | ✓ | ✘ | ✘ | ✘ | ✘ |
[ ] | ✘ | ✘ | ✓ | ✘ | ✓ | ✘ | N/A | ✓ | ✓ | ✓ | ✓ | ✘ |
[ ] | ✓ | ✘ | ✓ | ✘ | ✓ | ✘ | ✘ | ✓ | ✘ | ✓ | ✓ | ✘ |
[ ] | ✓ | ✘ | ✓ | ✘ | ✓ | ✓ | ✘ | ✘ | ✘ | ✘ | ✘ | ✘ |
[ ] | ✓ | ✘ | ✓ | ✘ | ✓ | ✓ | ✘ | ✘ | ✘ | ✘ | ✘ | ✓ |
Ours | ✓ | ✓ | ✓ | ✓ | ✓ | ✓ | ✓ | ✓ | ✓ | ✓ | ✓ | ✓ |
Reference | Collection Sources | Keywords | Timeline | Initial Paper Count | Final Paper Count |
---|---|---|---|---|---|
[ ] | - | - | - | - | |
[ ] | Scopus, the ACM Digital Library, and the IEEE Xplore digital library | Cyberbully or cyberbullying detection, detecting cyberbully or cyberbullying, electronic or online bullying detection, detecting electronic or online bullying, cyberbullying prevention tool, cyberbullying prevention software, cyberbullying software, anti cyberbu- llying detecting electronic or online harassment | 2008–2016 | 89 | 46 |
[ ] | Google Scholar, Research Gate, ACM Digital Library, Arxiv, Scopus, Mendeley | - | 2011–2018 | 71 | 22 |
[ ] | Scopus, Clarivate Analytics’ Web of Science, DBLP Computer Science Bibliography, ACM Digital Library, ScienceDirect, SpringerLink, and IEEE Xplore, Qatar University’s digital library | Cyberbullying, aggressive behavior, big data, and cyberbullying models | - | - | |
[ ] | Google Scholar, IEEE Xplore, Science Direct, ACM Digital Library and Wiley online databases | Cyberbullying detection | 2008–2020 | 106 | 65 |
[ ] | The ACM Digital Library, IEEE Xplore Digital Library, and Springer Link databases | Cyberbullying detection, Cyberbullying detection algorithm | 2010–2020 | 118 | 56 |
[ ] | Google Scholar, IEEE, Springer, ACM, and others | Abuse, offensive or hate speech, sarcasm, and irony | 2012–2020 | 70 | 45 |
Ours | IEEE Xplore, ScienceDirect, ACM Digital Library, Wiley, Springer Link, Taylor & Francis, MDPI, etc. | Cyberbullying and deep learning, cyberbullying detection, cyberharassment and deep learning, social media and cyberbullying, deep fake and cyberbullying | 2017–Jan 2023 | 1331 | 63 |
Word-Embedding Technique | Context Sensitive Embedding | ML Based | RNN Based | Transformer Based | Pretrained | Used in Cyberbullying Application |
---|---|---|---|---|---|---|
One-hot Embedding | No | No | No | No | No | YouTube Bengali text [ ] |
TF-IDF | No | No | No | No | No | Chinese Weibo dataset and English tweets [ ], Twitter English text [ ], YouTube Bengali text [ ] |
Word2Vec | No | Yes | No | No | Yes | Twitter Indonesian text [ ], Twitter English text [ , ], Social media text [ , ] |
GloVe | No | Yes | No | No | Yes | Twitter English text [ , , ], Formspring, Twitter, and Wikipedia posts [ , ], YouTube English text [ ], Social media text [ ] |
ELMo | Yes | Yes | Yes | No | Yes | Social media text [ ], Formspring English text [ , , ], MySpace English text [ , ] |
fastText | No | Yes | No | No | Yes | Formspring English text [ ], Social media text [ ] |
BERT | Yes | Yes | No | Yes | Yes | Arabic Social media text [ ], Formspring, Twitter, Wikipedia English posts [ ] |
Study | Dataset | Hybrid Model | Experimental Models | Best Performing Model | Performance Metrics |
---|---|---|---|---|---|
Raj et al. [ ] | Wikipedia Attack Dataset | No | LSTM, Bi-LSTM, GRU, Bi-GRU | Bi-GRU | Accuracy: 96.98%, F1 Score: 98.56% |
Raj et al. [ ] | Wikipedia Web Toxicity Dataset | No | LSTM, Bi-LSTM, GRU, Bi-GRU | Bi-LSTM | Accuracy: 96.5%, F1 Score: 98.69% |
Bharti et al. [ ] | Tweets | No | Bi-LSTM | Bi-LSTM | Accuracy: 92.60%, Precision: 96.60%, F1 Score: 94.20% |
Iwendi et al. [ ] | DISCo dataset | No | Bi-LSTM, GRU, LSTM, RNN | Bi-LSTM | Accuracy: 82.18% |
Agarwal et al. [ ] | Wikipedia dataset | No | Bi-LSTM with attention layers | Bi-LSTM with attention layers | Precision: 89%, Recall: 86%, F1 Score: 88% |
Singh et al. [ ] | Twitter dataset | No | LSTM, GRU, traditional ML algorithms | GRU | F1 Score: 92% |
Alotaibi et al. [ ] | Twitter comments | Yes | Transformer block, Bi-GRU, CNN | Proposed model | Accuracy: 88% |
Bu et al. [ ] | SNS comments | Yes | CNN, LRCN | Proposed model | AUC-ROC score: 88.54%, Accuracy: 87.22% |
Murshed et al. [ ] | Twitter dataset | Yes | Bi-LSTM, RNN, DEA-RNN (proposed model) | DEA-RNN | Accuracy: 90.45%, Precision: 89.52%, Recall: 88.98%, F1 Score: 89.25% |
Raj et al. [ ] | Real-time posts on Twitter | Yes | CNN + Bi-GRU, Bi-LSTM + Bi-GRU, CNN + Bi-LSTM (proposed model) | Proposed model | Accuracy: 95% |
Beniwal et al. [ ] | Toxic Comment Classification Challenge | Yes | CNN + Bi-GRU | Proposed model | Accuracy: 98.39%, F1 Score: 79.91% |
References | Theme | Major Contributions | Future Research Directions |
---|---|---|---|
[ , , ] | Improvement of DL models | These studies show improvement of cyberbullying detection by using CNN, LSTM, and BiGRUA-CNN models. These models show enhancement of the classification problem by adjusting activation function, weight regularization, and dropout configuration. | ]. ]. ]. |
[ , , ] | Performance optimization of the models | Studies applied char-CNN, BiGRU, and transformer models. They largely optimize weights, number of layers, combination of models during cyberbullying detection in social media discourse. | ] ] ] |
[ , , ] | Improving data capability | LSTM-CNN and RNN-based models have been applied in text, randomized and wikipedia datasets. The authors proposed several techniques to improve the capacity of the dataset. | ]. ]. ] ]. ]. |
Frameworks | Strengths | Limitations | Supported DL Algorithms | Used in Cyberbullying |
---|---|---|---|---|
TensorFlow | Wide range of models including CNN, RNN, GAN, Transformer, etc. [ ] | Chats and Tweets [ ], Bangla Text [ ], Offline Content [ ], Social Media text analysis [ ], Comments and Toxicity [ ], Multilingual Tweets and Hate speech [ ], Wikipedia talk page [ ], Post of Social Network platform Gab [ , ] | ||
Keras | Wide range of models including CNN, RNN, GAN, Transformer, etc. [ ] | Twitter [ , , , ], Bully, Sentiment, Emotion and Sarcasm from Twitter and Reddit [ ], Social media content[ , , ], Twitter and Wikipedia [ ], Chats and Tweets [ ], Wikipedia, Twitter, Formspring and YouTube [ ], Social networks’ text and image [ ], online textual harassment [ ] | ||
Torch/ PyTorch | Majority of the DL Models including CNN, RNN, GAN, Transformer, etc. [ ] | Social Network platform Gab [ ], Twitter, Wikipedia, Formspring [ ], Harmful meme of COVID-19 [ ], Memes of US politics [ ], Image from online [ ], Cyberbert: BERT for cyberbullying identification [ ], Social media content [ ] | ||
Theano | Majority of the DL Models [ ] | Twitter and Formspring.me [ ], Twitter [ , ], Comments and posts from YouTube, Instagram and Twitter [ ], Twitter and Facebook [ ], Social media image [ ], Online textual harassment [ ] | ||
Caffe | Initially designed for CNNs [ ] | No Works Found | ||
Chainer | For CNNs, Dynamic Computational Graph [ ] | No Works Found | ||
Deep- Learning4j | DL models that are used in NLP tasks [ ] | No Works Found | ||
DyNet | RNNs [ ] | No Works Found | ||
MXNet | CNNs, RNNs, GANs [ ] | Wikipedia talk pages [ ] | ||
Lasagne | Feed-Forward Networs such as CNNs, Recurrent Networks including LSTM, and any combination thereof [ ]. | No Works Found | ||
H O | Variety of DL models including CNNs RNNs [ ]. | No Works Found | ||
Google JAX | Variety of DL models including CNNs and autoaggressive models. | No Works Found | ||
Mind- Spore | CNNs and RNNs with a focus on distributed training and image processing [ ]. | No Works Found |
Dataset | DL Architectures | Major Tasks |
---|---|---|
Impermium [ ] | Bi-LSTM, GRU, LSTM, and RNN | Intimidation detection on social media platforms [ ] |
Formspring (a Q&A forum) | Single Linear Neural Network Layer and Transformer | Cyberbullying detection [ ] |
CNN, LSTM, Bi-LSTM, Bi-LSTM with Attention | Systematically analyzes cyberbullying detection [ ] | |
PCNN | Handle the difficulty of noise and distortion in social media postings and messages in detecting cyberbullying [ ] | |
Wikipedia | Single Linear NN, Transformer [ ], MLP [ ] | Cyberbullying detection [ , , ] |
CNN, LSTM, Bi-LSTM, Bi-LSTM with Attention [ ] | Systematically analyzes cyberbullying detection | |
Twitter [ ] | CNN, LSTM, Bi-LSTM, Bi-LSTM with Attention [ ] | Systematically analyzes cyberbullying detection [ ] |
Char-CNNS | Cyberbullying detection [ ] | |
Twitter [ ] | PCNN | Handling the difficulty of noise and distortion in social media postings and messages in detecting cyberbullying [ ] |
Twitter (combination of 3 datasets) [ , , ] | Bi-GRU, Transformer Block, and CNN | Detecting Aggressive Behavior |
Twitter (Indonesian Language) [ ] | LSTM, Bi-LSTM, and CNN | Cyberbullying Detection |
YouTube [ ] | Bi-LSTM with attention | Cyberbullying Detection [ ] |
Bangla and Romanized Bangla [ ] | CNN, LSTM, Bi-LSTM, and GRU | Comparative analysis [ ] |
Toxic Comment Classification challenge [ ] | LSTM-CNN [ ] | Cyberbullying Detection [ ] |
The bullying traces dataset [ ] | SVM activated stacked convolution LSTM network [ ] | |
Vine [ , ] | ResidualBiLSTM-RCNN | Cyberbullying Detection [ ] |
The statements, opinions and data contained in all publications are solely those of the individual author(s) and contributor(s) and not of MDPI and/or the editor(s). MDPI and/or the editor(s) disclaim responsibility for any injury to people or property resulting from any ideas, methods, instructions or products referred to in the content. |
Share and Cite
Hasan, M.T.; Hossain, M.A.E.; Mukta, M.S.H.; Akter, A.; Ahmed, M.; Islam, S. A Review on Deep-Learning-Based Cyberbullying Detection. Future Internet 2023 , 15 , 179. https://doi.org/10.3390/fi15050179
Hasan MT, Hossain MAE, Mukta MSH, Akter A, Ahmed M, Islam S. A Review on Deep-Learning-Based Cyberbullying Detection. Future Internet . 2023; 15(5):179. https://doi.org/10.3390/fi15050179
Hasan, Md. Tarek, Md. Al Emran Hossain, Md. Saddam Hossain Mukta, Arifa Akter, Mohiuddin Ahmed, and Salekul Islam. 2023. "A Review on Deep-Learning-Based Cyberbullying Detection" Future Internet 15, no. 5: 179. https://doi.org/10.3390/fi15050179
Article Metrics
Article access statistics, further information, mdpi initiatives, follow mdpi.
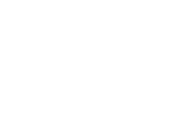
Subscribe to receive issue release notifications and newsletters from MDPI journals
Prevalence of Cyber Bullying on Social Media: A Review
- December 2022
- 26(4):58-66

- Gautam Buddha University

Discover the world's research
- 25+ million members
- 160+ million publication pages
- 2.3+ billion citations

- SPAN J PSYCHOL

- B S Shivashankar
- Recruit researchers
- Join for free
- Login Email Tip: Most researchers use their institutional email address as their ResearchGate login Password Forgot password? Keep me logged in Log in or Continue with Google Welcome back! Please log in. Email · Hint Tip: Most researchers use their institutional email address as their ResearchGate login Password Forgot password? Keep me logged in Log in or Continue with Google No account? Sign up

An official website of the United States government
The .gov means it’s official. Federal government websites often end in .gov or .mil. Before sharing sensitive information, make sure you’re on a federal government site.
The site is secure. The https:// ensures that you are connecting to the official website and that any information you provide is encrypted and transmitted securely.
- Publications
- Account settings
Preview improvements coming to the PMC website in October 2024. Learn More or Try it out now .
- Advanced Search
- Journal List
- v.5(3); 2019 Mar

Cyberbullying and its influence on academic, social, and emotional development of undergraduate students
This study investigated the influence of cyberbullying on the academic, social, and emotional development of undergraduate students. It's objective is to provides additional data and understanding of the influence of cyberbullying on various variables affecting undergraduate students. The survey sample consisted of 638 Israeli undergraduate students. The data were collected using the Revised Cyber Bullying Survey, which evaluates the frequency and media used to perpetrate cyberbullying, and the College Adjustment Scales, which evaluate three aspects of development in college students. It was found that 57% of the students had experienced cyberbullying at least once or twice through different types of media. Three variables were found to have significant influences on the research variables: gender, religion and sexual preferences. Correlation analyses were conducted and confirmed significant relationships between cyberbullying, mainly through instant messaging, and the academic, social and emotional development of undergraduate students. Instant messaging (IM) was found to be the most common means of cyberbullying among the students.
The main conclusions are that although cyberbullying existence has been proven, studies of cyberbullying among undergraduate students have not been fully developed. This particular population needs special attention in future research. The results of this study indicate that cyberbullying has an influence on the academic, social, and emotional development of undergraduate students. Additional Implications of the findings are discussed.
1. Introduction
Cyberbullying is defined as the electronic posting of mean-spirited messages about a person (such as a student) often done anonymously ( Merriam-Webster, 2017 ). Most of the investigations of cyberbullying have been conducted with students in elementary, middle and high school who were between 9 and 18 years old. Those studies focused on examining the prevalence and frequency of cyberbullying. Using “cyberbullying” and “higher-education” as key words in Google scholar (January, 2019) (all in title) yields only twenty one articles. In 2009, 2012 and 2013 one article appeared each year, since 2014 each year there were few publications. Of these articles only seven relates to effect of cyberbullying on the students, thus a gap in the literature exists in that it only minimally reports on studies involving undergraduate students. Given their relationship and access to technology, it is likely that cyberbullying occurs frequently among undergraduates. The purpose of this study is to examine the frequency and media used to perpetrate cyberbullying, as well as the relationship that it has with the academic, social and emotional development of undergraduate students.
Undergraduate students use the Internet for a wide variety of purposes. Those purposes include recreation, such as communicating in online groups or playing games; academics, such as doing assignments, researching scholarships or completing online applications; and practical, such as preparing for job interviews by researching companies. Students also use the Internet for social communication with increasing frequency.
The literature suggests that cyberbullied victims generally manifest psychological problems such as depression, loneliness, low self-esteem, school phobias and social anxiety ( Grene, 2003 ; Juvonen et al., 2003 ; Akcil, 2018 ). Moreover, research findings have shown that cyberbullying causes emotional and physiological damage to defenseless victims ( Akbulut and Eristi, 2011 ) as well as psychosocial difficulties including behavior problems ( Ybarra and Mitchell, 2007 ), drinking alcohol ( Selkie et al., 2015 ), smoking, depression, and low commitment to academics ( Ybarra and Mitchell, 2007 ).
Under great emotional stress, victims of cyberbullying are unable to concentrate on their studies, and thus their academic progress is adversely affected ( Akcil, 2018 ). Since the victims are often hurt psychologically, the depressive effect of cyberbullying prevents students from excelling in their studies ( Faryadi, 2011 ). The overall presence of cyberbullying victimization among undergraduate college students was found to be significantly related to the experience of anxiety, depression, substance abuse, low self-esteem, interpersonal problems, family tensions and academic underperformance ( Beebe, 2010 ).
1.1. Cyberbullying and internet
The Internet has been the most useful technology of modern times, which has enabled entirely new forms of social interaction, activities, and organizing. This has been possible thanks to its basic features such as widespread usability and access. However, it also causes undesirable behaviors that are offensive or threatening to others, such as cyberbullying. This is a relatively new phenomenon.
According to Belsey (2006, p.1) , “Cyberbullying involves the use of information and communication technologies such as e-mail, cell-phone and pager text messages, instant messaging, defamatory personal web sites, blogs, online games and defamatory online personal polling web sites, to support deliberate, repeated, and hostile behavior by an individual or group that is intended to harm others.” Characteristics like anonymity, accessibility to electronic communication, and rapid audience spread, result in a limitless number of individuals that can be affected by cyberbullying.
Different studies suggest that undergraduate students' use of the Internet is more significant and frequent than any other demographic group. A 2014 survey of 1006 participants in the U.S. conducted by the Pew Research Center revealed that 97% of young adults aged from 18 to 29 years use the Internet, email, or access the Internet via a mobile device. Among them, 91% were college students.
1.2. Mediums to perpetrate cyberbullying
The most frequent and common media within which cyberbullying can occur are:
Electronic mail (email): a method of exchanging digital messages from an author to one or more recipients.
Instant messaging: a type of online chat that offers real-time text transmission between two parties.
Chat rooms: a real-time online interaction with strangers with a shared interest or other similar connection.
Text messaging (SMS): the act of composing and sending a brief electronic message between two or more mobile phones.
Social networking sites: a platform to build social networks or social relations among people who share interests, activities, backgrounds or real-life connections.
Web sites : a platform that provides service for personal, commercial, or government purpose.
Studies indicate that undergraduate students are cyberbullied most frequently through email, and least often in chat rooms ( Beebe, 2010 ). Other studies suggest that instant messaging is the most common electronic medium used to perpetrate cyberbullying ( Kowalski et al., 2018 ).
1.3. Types of cyberbullying
Watts et al. (2017) Describe 7 types of cyberbullying: flaming, online harassment, cyberstalking, denigration, masquerading, trickery and outing, and exclusion. Flaming involves sending angry, rude, or vulgar messages via text or email about a person either to that person privately or to an online group.
Harassment involves repeatedly sending offensive messages, and cyberstalking moves harassment online, with the offender sending threatening messages to his or her victim. Denigration occurs when the cyberbully sends untrue or hurtful messages about a person to others. Masquerading takes elements of harassment and denigration where the cyberbully pretends to be someone else and sends or posts threatening or harmful information about one person to other people. Trickery and outing occur when the cyberbully tricks an individual into providing embarrassing, private, or sensitive information and posts or sends the information for others to view. Exclusion is deliberately leaving individuals out of an online group, thereby automatically stigmatizing the excluded individuals.
Additional types of cyberbullying are: Fraping - where a person accesses the victim's social media account and impersonates them in an attempt to be funny or to ruin their reputation. Dissing - share or post cruel information online to ruin one's reputation or friendships with others. Trolling - is insulting an individual online to provoke them enough to get a response. Catfishing - steals one's online identity to re-creates social networking profiles for deceptive purposes. Such as signing up for services in the victim's name so that the victim receives emails or other offers for potentially embarrassing things such as gay-rights newsletters or incontinence treatment. Phishing - a tactic that requires tricking, persuading or manipulating the target into revealing personal and/or financial information about themselves and/or their loved ones. Stalking – Online stalking when a person shares her personal information publicly through social networking websites. With this information, stalkers can send them personal messages, send mysterious gifts to someone's home address and more. Blackmail – Anonymous e-mails, phone-calls and private messages are often done to a person who bear secrets. Photographs & video - Threaten to share them publicly unless the victim complies with a particular demand; Distribute them via text or email, making it impossible for the victim to control who sees the picture; Publish the pictures on the Internet for anyone to view. Shunning - persistently avoid, ignore, or reject someone mainly from participating in social networks. Sexting - send sexually explicit photographs or messages via mobile phone.
1.4. Prevalence of cyberbullying
Previous studies have found that cyberbullying incidents among college students can range from 9% to 34% ( Baldasare et al., 2012 ).
Beebe (2010) conducted a study with 202 college students in United States. Results indicated that 50.7% of the undergraduate students represented in the sample reported experiencing cyberbullying victimization once or twice during their time in college. Additionally, 36.3% reported cyberbullying victimization on a monthly basis while in college. According to Dılmaç (2009) , 22.5% of 666 students at Selcuk University in Turkey reported cyberbullying another person at least once and 55.35% reported being a victim of cyberbullying at least once in their lifetimes. In a study of 131 students from seven undergraduate classes in United States, 11% of the respondents indicated having experienced cyberbullying at the university ( Walker et al., 2011 ). Of those, Facebook (64%), cell phones (43%) and instant messaging (43%) were the most frequent technologies used. Students indicated that 50% of the cyberbullies were classmates, 57% were individuals outside of the university, and 43% did not know who was cyberbullying them.
Data from the last two years (2017–18) is similar to the above. A research, of 187 undergraduate students matriculated at a large U.S. Northeastern metropolitan Roman Catholic university ( Webber and Ovedovitz, 2018 ), found that 4.3% indicated that they were victims of cyberbullying at the university level and a total of 7.5% students acknowledged having participated in bullying at that level while A survey (N = 338) at a large midwestern university conducted by Varghese and Pistole (2017) , showed that frequency counts indicated that 15.1% undergraduate students were cyberbully victims during college, and 8.0% were cyberbully offenders during college.
A study of 201 students from sixteen different colleges across the United States found a prevalence rate of 85.2% for college students who reported being victims of cyberbullying out of the total 201 responses recorded. This ranged from only occasional incidents to almost daily experiences with cyberbullying victimization ( Poole, 2017 ).
In A research of international students, 20.7% reported that they have been cyberbullied in the last 30 days once to many times ( Akcil, 2018 ).
1.5. Psychological impact of cyberbullying
Cyberbullying literature suggests that victims generally manifest psychological problems such as depression, anxiety, loneliness, low self-esteem, social exclusion, school phobias and poor academic performance ( DeHue et al., 2008 ; Juvonen and Gross, 2008 ; Kowalski and Limber, 2007 ; Grene, 2003 ; Juvonen et al., 2003 ; Rivituso, 2012 ; Varghese and Pistole, 2017 ; Na, 2014 ; Akcil, 2018 ), low self-esteem, family problems, school violence and delinquent behavior ( Webber and Ovedovitz, 2018 ), which brings them to experience suicidal thoughts as a means of escaping the torture ( Ghadampour et al., 2017 ).
Moreover, research findings have shown that cyberbullying causes emotional and physiological damage to defenseless victims ( Faryadi, 2011 ) as well as psychosocial problems including inappropriate behaviors, drinking alcohol, smoking, depression and low commitment to academics ( Walker et al., 2011 ).
The victims of cyberbullying, under great emotional stress, are unable to concentrate on their studies, and thus their academic progress is adversely affected ( Faryadi, 2011 ). Since the victims are often hurt psychologically, the depressive effect of cyberbullying prevents students from excelling in their studies ( Faryadi, 2011 ).
In a Malaysian university study with 365 first year students, the majority of the participants (85%) interviewed indicated that cyberbullying affected their academic performance, specifically their grades ( Faryadi, 2011 ). Also, 85% of the respondents agreed that bullying caused a devastating impact on students' emotions and equally caused unimaginable psychological problems among the victims. Heiman and Olenik-Shemesh (2018) report that for students with learning disabilities, predictors of cybervictimization were low social support, low self-perception, and being female, whereas for students without learning disabilities, the predictors were low social support, low well-being, and low body perception.
1.6. Academic, social, and emotional development of undergraduate students
The transition to academic institutions is marked by complex challenges in emotional, social, and academic adjustment ( Gerdes and Mallinckrodt, 1994 ; Parker et al., 2004 ).
The adaptation to a new environment is an important factor in academic performance and future achievement. Undergraduate students are not only developing academically and intellectually, they are also establishing and maintaining personal relationships, developing an identity, deciding about a career and lifestyle, and maintaining personal health and wellness. Many students are interacting with people from diverse backgrounds who hold different values and making new friends. Some are also adapting to living away from home for the very first time ( Inkelas et al., 2007 ).
The concept of academic development involves not only academic abilities, but motivational factors, and institutional commitment. Motivation to learn, taking actions to meet academic demands, a clear sense of purpose, and general satisfaction with the academic environment are also important components of the academic field ( Lau, 2003 ).
A second dimension, the social field, may be as important as academic factors. Writers have emphasized integration into the social environment as a crucial element in commitment to a particular academic institution ( Tinto, 1975 ). Becoming integrated into the social life of college, forming a support network, and managing new social freedoms are some important elements of social development. Crises in the social field include conflict in a living situation, starting or maintaining relationships, interpersonal conflicts, family issues, and financial issues ( McGrath, 2005 ), which are manifested as feelings of loneliness ( Clark et al., 2015 ).
In the emotional field, students commonly question their relationships, direction in life, and self-worth ( Rey et al., 2011 ). A balanced personality is one which is emotionally adjusted. Emotional adjustment is essential for creating a sound personality. physical, intellectual mental and esthetical adjustments are possible when emotional adjustment is made ( Ziapour et al., 2018 ). Inner disorders may result from questions about identity and can sometimes lead to personal crises ( Gerdes and Mallinckrodt, 1994 ). Emotional problems may be manifested as global psychological distress, somatic distress, anxiety, low self-esteem, or depression. Impediments to success in emotional development include depression and anxiety, stress, substance abuse, and relationship problems ( Beebe, 2010 ).
The current study is designed to address two research questions: (1) does cyberbullying affect college students' emotional state, as measured by the nine factors of the College Adjustment Scales ( Anton and Reed, 1991 ); (2) which mode of cyberbullying most affects students' emotional state?
2.1. Research settings and participants
The present study is set in Israeli higher education colleges. These, function as: (1) institutions offering undergraduate programs in a limited number of disciplinary fields (mainly the social sciences), (2) centers for training studies (i.e.: teacher training curricula), as well as (3) as creators of access to higher education. The general student population is heterogeneous, coming from the Western Galilee. In this study, 638 Israeli undergraduate students participated. The sample is a representative of the population of the Western galilee in Israel. The sample was 76% female, 70% single, 51% Jewish, 27% Arabs, 7% Druze, and 15% other ethnicity. On the dimension of religiosity, 47% were secular, 37% traditional, 12% religious, 0.5% very religious, and 3.5% other. On the dimension of sexual orientation, 71% were straight women, 23.5% straight men, 4% bisexual, 1% lesbians, and 0.5% gay males (note: according to the Williams Institute, approximately 4% of the population in the US are LGBT, [ Gates, 2011 ], while 6% of the EU population are LGBT, [ Dalia, 2016 ]).
2.2. Instrumentation
Two instruments were used to collect data: The Revised Cyber Bullying Survey (RCBS), with a Cronbach's alpha ranging from .74 to .91 ( Kowalski and Limber, 2007 ), designed to measure incidence, frequency and medium used to perpetrate cyberbullying. The survey is a 32-item questionnaire. The frequency was investigated using a 5-item scale with anchors ranging from ‘it has never happened to me’ to ‘several times a week’. Five different media were explored: email, instant messaging, chat room, text messaging, and social networking sites. Each medium was examined with the same six questions related to cases of cyberbullying (see Table 1 ).
Description of the Revised Cyber Bullying Survey (RCBS) variables.
Means of cyberbullying | N | Minimum | Maximum | Mean | SD | Reliability |
---|---|---|---|---|---|---|
Chat | 610 | .00 | 24 | .48 | 1.64 | 0.87 |
Social networking | 635 | .00 | 20 | .95 | 1.93 | 0.85 |
SMS | 631 | .00 | 12 | .78 | 1.53 | 0.80 |
Instant messages | 634 | .00 | 13 | .96 | 1.81 | 0.81 |
637 | .00 | 11 | .41 | 1.05 | 0.68 | |
Valid N (listwise) | 608 |
Note: the theoretical range is between zero to twenty-four.
Table 1 shows the five variables that composed the RCBS questionnaire (all of the variables are composed of 6 statements). The results indicate that the levels of all the variables is very low, which means that the respondents experienced cyberbullying once or twice. The internal consistency reliability estimate based on the current sample suggested that most of the variables have an adequate to high level of reliability, with a Cronbach's alpha of 0.68–0.87.
The College Adjustment Scales (CAS) ( Anton and Reed, 1991 ), evaluated the academic, social, and emotional development of college students. Values were standardized and validated for use with college students. The validity for each subscale ranged from .64 to .80, noting high correlations among scales. Reliability of the scales ranged from .80 to .92, with a mean of .86. The instrument included 128 items, divided into 10 scales: anxiety, depression, suicidal ideation, substance abuse, self-esteem problems, interpersonal problems, family problems, academic problems, career problems, and regular activities (see Table 2 ). Students responded to each item using a four-point scale.
Description of CAS variables.
Variables | N | Minimum | Maximum | Mean | SD | Reliability |
---|---|---|---|---|---|---|
Academic problems | 634 | 28 | 73 | 47.87 | 8.87 | 0.77 |
Anxiety | 633 | 30 | 78 | 51.17 | 9.57 | 0.88 |
Career problems | 632 | 36 | 80 | 55.47 | 8.63 | 0.87 |
Depression | 633 | 27 | 78 | 53.27 | 9.14 | 0.81 |
Family problems | 633 | 32 | 74 | 44.61 | 11.19 | 0.72 |
Interpersonal problems | 633 | 29 | 77 | 52.51 | 8.38 | 0.72 |
Regular activities | 624 | 27 | 78 | 57.10 | 8.80 | 0.69 |
Self-esteem problems | 633 | 22 | 74 | 50.31 | 9.19 | 0.76 |
Substance abuse | 633 | 39 | 75 | 49.72 | 8.45 | 0.78 |
Suicidal ideation | 633 | 44 | 76 | 51.92 | 9.63 | 0.87 |
Valid N (listwise) | 624 |
Anxiety: A measure of clinical anxiety, focusing on common affective, cognitive, and physiological symptoms.
Depression: A measure of clinical depression, focusing on common affective, cognitive, and physiological symptoms.
Suicidal Ideation: A measure of the extent of recent ideation reflecting suicide, including thoughts of suicide, hopelessness, and resignation.
Substance Abuse: A measure of the extent of disruption in interpersonal, social, academic, and vocational functioning as a result of substance use and abuse.
Self-esteem Problems: A measure of global self-esteem which taps negative self-evaluations and dissatisfaction with personal achievement.
Interpersonal Problems: A measure of the extent of problems in relating to others in the campus environment.
Family Problems: A measure of difficulties experienced in relationships with family members.
Academic Problems: A measure of the extent of problems related to academic performance.
Career Problems: A measure of the extent of problems related to career choice.
Participants also responded to a demographic questionnaire that included items on gender, birth year, marital status, ethnicity, and sexual orientation. As sexual orientation is a major cause for bullying ( Pollock, 2006 ; Cahill and Makadon, 2014 ), it was included in the background information.
Convenience sampling and purposive sampling were used for this study. Surveys with written instructions were administered in classrooms, libraries and online via Google Docs at the end of the semester.
The surveys were translated to Hebrew and back translated four times until sufficient translation was achieved. The research was approved by the Western Galilee College Research and Ethic Committee.
A sizeable percentage, 57.4% (366), of the respondents reported being cyber bullied at least once and 3.4% (22) reported being cyber bullied at least once a week. The types of bullies can be seen in Fig. 1 .

Types of bullies.
Three variables were found to have significant influences on the research variables: (1) gender (see Table 3 ); (2) religion (see Table 4 ); and (3) sexual preferences (see Table 5 ).
Results of independent t-tests for research variables by gender.
M | SD | t | ||
---|---|---|---|---|
Depression | Male | 51.82 | 8.08 | 1.99 |
Female | 53.63 | 9.37 | ||
Regular activities | Male | 55.66 | 8.82 | 2.05 |
Female | 57.47 | 8.77 | ||
Self-esteem problems | Male | 48.79 | 9.19 | 2.08 |
Female | 50.68 | 9.16 | ||
Suicidal ideation | Male | 50.10 | 8.91 | 2.48 |
Female | 52.34 | 9.74 |
Note: n male = 127, n female = 510, *p < .05.
Results of independent t-tests for research variables by level of religion.
M | SD | T | ||
---|---|---|---|---|
Depression | Secular | 52.07 | 8.97 | 3.08 |
Religious | 54.30 | 9.17 | ||
Family problemýs | Secular | 43.60 | 11.16 | 2.09 |
Religious | 45.46 | 11.16 | ||
Interpersonal problems | Secular | 51.77 | 8.80 | 2.04 |
Religious | 53.14 | 7.97 | ||
Suicidal ideation | Secular | 50.13 | 8.85 | 4.42 |
Religious | 53.44 | 10.00 |
Note: n religious = 345, n secular = 293, ∗ p < .05, ∗∗ p < .01, ∗∗∗ p < .001.
Results of independent t-tests for research variables by sexual preference.
M | SD | t | ||
---|---|---|---|---|
Anxiety | Heterosexual | 50.92 | 9.63 | 2.41 |
Other | 54.60 | 8.12 | ||
Depression | Heterosexual | 52.88 | 8.90 | 4.14 |
Other | 58.86 | 10.59 | ||
Family problems | Heterosexual | 44.11 | 10.94 | 4.20 |
Other | 51.52 | 12.42 | ||
Interpersonal problems | Heterosexual | 52.26 | 8.31 | 2.80 |
Other | 56.00 | 8.80 | ||
Self-esteem problems | Heterosexual | 50.07 | 9.14 | 2.44 |
Other | 53.64 | 9.28 | ||
Substance abuse | Heterosexual | 49.34 | 8.19 | 3.48 |
Other | 54.98 | 10.27 | ||
Suicidal ideation | Heterosexual | 51.33 | 9.34 | 5.88 |
Other | 60.14 | 9.89 |
Note: n heterosexual = 596, n other = 42, ∗ p < .05, ∗∗ p < .01, ∗∗∗ p < .001.
Independent t-tests between the CAS variables and gender show significant differences between females and males (see Table 3 ).
Independent t-tests between the CAS variables and level of religiosity show significant differences between secular and religious persons, i.e., observant believers (see Table 4 ).
Independent t-tests between the CAS variables and sexual preference show significant differences between heterosexual individuals and others (see Table 5 ).
The research population was divided into three age groups having five year intervals. One respondent who was 14 years old was removed from the population.
For the variable “career problems” it was found that there was a significant difference between the 26–30 year age group [p < .05, F(2,5815) = 3.49, M = 56.55] and the 31–35 (M = 56.07) as well as the 20–25 (M = 54.58) age groups.
For the variable "depression" it was found that there was a significant difference between the 20–25 year age group [p < .05, F(2,5815) = 3.84, M = 54.56] and the 31–35 (M = 51.61) as well as the 26–30 (M = 52.83) age groups.
For the variable “interpersonal problems” it was found that there was a significant difference between the 20–25 year age group [p < .06, F(2,5815) = 3.84, M = 53.85] and the 31–35 (M = 51.29) as well as the 26–30 (M = 52.19) age groups.
For the variable “suicidal ideation” it was found that there was a significant difference between the 20–25 year age group [p < .06, F(2,5815) = 3.84, M = 55.45] and the 31–35 (M = 49.71) as well as the 26–30 (M = 50.13) age groups (see Table 6 ).
Results of one way Anova for research variables by age.
Age Group | M | SD | F | |
---|---|---|---|---|
Career problems | 20–25 | 54.58 | 7.97 | 3.49 |
26–30 | 56.55 | 8.36 | ||
31–35 | 56.07 | 9.29 | ||
Depression | 20–25 | 54.56 | 10.08 | 3.84 |
26–30 | 52.83 | 8.62 | ||
31–35 | 51.61 | 8.14 | ||
Interpersonal problems | 20–25 | 53.58 | 8.23 | 2.87 |
26–30 | 52.19 | 8.42 | ||
31–35 | 51.29 | 8.06 | ||
Suicidal ideation | 20–25 | 55.45 | 10.48 | 22.79 |
26–30 | 50.13 | 8.67 | ||
31–35 | 49.71 | 8.58 |
Note: n 20-25 = 216, n 26-30 = 287, n 31-35 = 82, ∗ p < .05, ∗∗ p < .01, ∗∗∗ p < .001.
To confirm that there was no effect among the independent variables, a Pearson correlation analysis of cyberbullying with CAS variables was run. As the correlations between the independent variables are weak, no multicollinearity between them was noted (see Table 7 ).
Pearson correlation of cyberbullying with CAS variables.
CAS Variables | Cyberbullying | ||||
---|---|---|---|---|---|
IM | Chat | SMS | Social Network | ||
Academic problems | 0.018 | 0.196*** | 0.079 | 0.141*** | 0.189*** |
Anxiety | 0.042 | 0.216*** | 0.080* | 0.159*** | 0.194*** |
Career problems | -0.007 | 0.089 | -0.08 | 0.079 | 0.057 |
Depression | 0.064 | 0.210*** | 0.122** | 0.102* | 0.172*** |
Family problems | 0.142*** | 0.227*** | 0.081* | 0.132** | 0.156*** |
Interpersonal problems | 0.054 | 0.150*** | 0.094 | 0.040 | 0.110** |
Regular activities | -0.121** | -0.014 | 0.005- | -0.015 | 0.003 |
Self-esteem | 0.041 | 0.229*** | 0.124** | 0.171*** | 0.208*** |
Substance abuse | 0.150*** | 0.235*** | 0.184*** | 0.161*** | 0.174*** |
Suicidal ideation | 0.130** | 0.230*** | 0.148*** | 0.093* | 0.130** |
Note: n = 638, ∼ p < .06, ∗ p < .05, ∗∗ p < .01, ∗∗∗ p < .001.
Regression analyses on the effect of the cyberbullying variables on the CAS variables (see Fig. 2 ) show that an increase in cyberbullying by social networking and IM increases the academic problems variable. The model explained 6.1% of the variance (F (13,585) = 2.94, p < .001) and shows an increase in the suicidal ideation variable. There is also a marginal effect of cyberbullying by SMS on suicidal ideation, revealing that an increase in cyberbullying by SMS causes a decrease in suicidal ideation. The explained variance of the model is 24.8% (F (11,584) = 14.80, p < .001). Higher cyberbullying by social networking results in an increase in the anxiety variable. The explained variance of the model is 8.8% (F (13,584) = 4.32, p < .001). An increase in cyberbullying by chat and IM shows an increase in the substance abuse variable. The model explains 13% of the variance (F (13,584) = 6.71, p < .001). Increasing cyberbullying by social networking and IM increases the self-esteem problems variable. The explained variance of the model is 9% (F (13,584) = 4.43, p < .001). An increase of cyberbullying by email increases the problems students have with regular activities. The explained variance of the model is 5.2% (F (13,575) = 2.44, p < .01). Heightened cyberbullying by social networking and IM increases students' interpersonal problems. There is also an effect of cyberbullying by IM on suicidal ideation, such that an increase in cyberbullying by IM causes a decrease in interpersonal problems. The explained variance of the model is 8% (F (13,584) = 3.89, p < .001). An increase in cyberbullying by SMS decreases the family problems variable. The explained variance of the model is 11.4% (F (13,584) = 5.76, p < .001). And finally, heightened cyberbullying by IM and social networking decreases the depression variable. The variance explained by the model is 11.9% (F (13,584) = 6.04, p < .001).

The influence of academic cyberbullying variables on the CAS variables.
4. Discussion
The objective of this study was to fill an existing gap in the literature regarding the influence of cyberbullying on the academic, social, and emotional development of undergraduate students.
As has been presented, cyberbullying continues to be a disturbing trend not only among adolescents but also undergraduate students. Cyberbullying exists in colleges and universities, and it has an influence on the development of students. Fifty seven percent of the undergraduate students who participated in this study had experienced cyberbullying at least once during their time in college. As previous studies have found that cyberbullying incidents among college students can range from 9% to 50% ( Baldasare et al., 2012 ; Beebe, 2010 ) it seems that 57% is high. Considering the effect of smartphone abundance on one hand and on the other the increasing use of online services and activities by young-adults can explain that percentage.
Considering the effect of such an encounter on the academic, social and emotional development of undergraduate students, policy makers face a formidable task to address the relevant issues and to take corrective action as Myers and Cowie (2017) point out that due to the fact that universities are in the business of education, it is a fine balancing act between addressing the problem, in this case cyberbullying, and maintaining a duty of care to both the victim and the perpetrator to ensure they get their degrees. There is a clear tension for university authorities between acknowledging that university students are independent young adults, each responsible for his or her own actions, on one hand, and providing supervision and monitoring to ensure students' safety in educational and leisure contexts.
Although there are increasing reports on connections between cyberbullying and social-networks (see: Gahagan et al., 2016 ), sending SMS or MMS messages through Internet gateways ensures anonymity, thus indirectly supporting cyberbullying. A lot of websites require only login or a phone number that can also be made up ( Gálik et al., 2018 ) which can explain the fact that instant-messaging (IM) was found to be the most common means of cyberbullying among undergraduate students with a negative influence on academic, family, and emotional development (depression, anxiety, and suicidal ideation). A possible interpretation of the higher frequency of cyberbullying through IM may be that young adults have a need to be connected.
This medium allows for being online in ‘real time’ with many peers or groups. With the possibility of remaining anonymous (by creating an avatar – a fake profile) and the possibility of exposing private information that remains recorded, students who use instant messaging become easy targets for cyberbullying. IM apps such as WhatsApp are extremely popular as they allow messages, photos, videos, and recordings to be shared and spread widely and in real time.
Students use the Internet as a medium and use it with great frequency in their everyday lives. As more aspects of students' lives and daily affairs are conducted online, coupled with the fact that excessive use may have consequences, it is important for researchers and academic policy makers to study the phenomenon of cyberbullying more deeply.
Sexual orientation is also a significant factor that increases the risk of victimization. Similarly, Rivers (2016) documented the rising incidence of homophobic and transphobic bullying at university and argues strongly for universities to be more active in promoting tolerance and inclusion on campus. It is worth noting that relationships and sexual orientation probably play a huge role in bullying among university students due to their age and the fact that the majority of students are away from home and experiencing different forms of relationships for the first time. Faucher et al. (2014) actually found that same sex cyberbullying was more common at university level than at school. Nonetheless, the research is just not there yet to make firm conclusions.
Finally, cyberbullying is not only an adolescent issue. Although its existence has been proven, studies of cyberbullying among undergraduate students have not been fully developed. This particular population needs special attention in future research.
The results of this study indicate that cyberbullying has an influence on the academic, social, and emotional development of undergraduate students.
In the academic field, findings revealed a statistically significant correlation between cyberbullying perpetrated by email and academic problems. Relationships between academic problems and cyberbullying perpetrated by other media were not found. This suggests that cyberbullying through instant messaging, chat room, text messaging, and social networking sites, have not influenced academic abilities, motivation to learn, and general satisfaction with the academic environment. However, cyberbullying perpetrated by email has an influence on academics, perhaps because of the high use of this medium among undergraduate students.
With regard to career problems, correlations with cyberbullying were not found. This indicates that cyberbullying has no influence on career problems, perhaps because these kinds of problems are related to future career inspirations, and not to the day-to-day aspects of a student's life.
In the social field, it was found that interpersonal problems such as integration into the social environment, forming a support network, and managing new social freedoms, were related to cyberbullying via social networking sites. This finding is consistent with the high use of social networking sites, the purpose of the medium, and the reported episodes of cyberbullying in that medium.
Family problems were also related to cyberbullying perpetrated by all kinds of media. This may indicate that as cyberbullying through the use of email, instant messaging, chat rooms, text messaging, and social networking sites increases, so do family problems. This could be due to the strong influence that cyberbullying generates in all the frameworks of students, including their families.
Finally, in the emotional field, correlations between cyberbullying perpetrated by all kinds of media and substance abuse were found. This may indicate that as cyberbullying through the use of email, instant messaging, chat rooms, text messaging, and social networking sites increases, so does substance abuse. This is important because cyberbullying may be another risk factor for increasing the probability of substance abuse.
Depression and suicidal ideation were significantly related to the same media – email instant messaging and chat cyberbullying – suggesting that depression may lead to a decision of suicide as a solution to the problem. Previous findings support the above that being an undergraduate student – a victim of cyberbullying emerges as an additional risk factor for the development of depressive symptoms ( Myers and Cowie, 2017 ). Also Selkie et al. (2015) reported among 265 female college students, being engaged in cyberbullying as bullies, victims, or both led to higher rates of depression and alcohol use.
Relationships between anxiety and cyberbullying, through all the media, were not found although Schenk and Fremouw (2012) found that college student victims of cyberbullying scored higher than matched controls on measures of depression, anxiety, phobic anxiety, and paranoia. This may be because it was demonstrated that anxiety is one of the most common reported mental health problems in all undergraduate students, cyberbullied or not.
Self-esteem problems were significantly related to cyberbullying via instant messaging, social networking sites, and text messaging. This may suggest that as cyberbullying through instant messaging, social networking sites, and text messaging increases, so do self-esteem problems. This is an important finding, given that these were the media with more reported episodes of cyberbullying.
5. Conclusions
This findings of this study revealed that cyberbullying exists in colleges and universities, and it has an influence on the academic, social, and emotional development of undergraduate students.
It was shown that cyberbullying is perpetrated through multiple electronic media such as email, instant messaging, chat rooms, text messaging, and social networking sites. Also, it was demonstrated that students exposed to cyberbullying experience academic problems, interpersonal problems, family problems, depression, substance abuse, suicidal ideation, and self-esteem problems.
Students have exhibited clear preferences towards using the Internet as a medium and utilize it with great frequency in their everyday lives. As more and more aspects of students' lives are conducted online, and with the knowledge that excessive use may have consequences for them, it is important to study the phenomenon of cyberbullying more deeply.
Because college students are preparing to enter the workforce, and several studies have indicated a trend of cyberbullying behavior and victimization throughout a person's lifetime ( Watts et al., 2017 ), the concern is these young adults are bringing these attitudes into the workplace.
Finally, cyberbullying is not only an adolescent issue. Given that studies of cyberbullying among undergraduate students are not fully developed, although existence of the phenomenon is proven, we conclude that the college and university population needs special attention in future areas of research. As it has been indicated by Peled et al. (2012) that firm policy in regard to academic cheating reduces its occurrence, colleges should draw clear guidelines to deal with the problem of cyberbullying, part of it should be a safe and if needed anonymous report system as well as clear punishing policy for perpetrators.
As there's very little research on the effect of cyberbullying on undergraduates students, especially in light of the availability of hand held devices (mainly smartphones) and the dependence on the internet for basically every and any activity, the additional data provided in this research adds to the understanding of the effect of cyberbullying on the welfare of undergraduate students.
Declarations
Author contribution statement.
Yehuda Peled: Conceived and designed the experiments; Performed the experiments; Analyzed and interpreted the data; Contributed reagents, materials, analysis tools or data; Wrote the paper.
Funding statement
This research did not receive any specific grant from funding agencies in the public, commercial, or not-for-profit sectors.
Competing interest statement
The authors declare no conflict of interest.
Additional information
No additional information is available for this paper.
- Akbulut Y., Eristi B. Cyberbullying and victimization among Turkish university students. Australas. J. Educ. Technol. 2011; 27 (7):1155–1170. [ Google Scholar ]
- Akcil S. Kent State University; 2018. Cyberbullying-Victimization, Acculturative Stress, and Depression Among International College Students. Doctoral dissertation. [ Google Scholar ]
- Anton D.W., Reed R.J. Psychological Assessment Resources, Inc; 1991. College Adjustment Scales. [ Google Scholar ]
- Baldasare A., Bauman S., Goldman L., Robie A. Cyberbullying: voices of college students. Cutting Edge Technol. Higher Educ. 2012; 5 :127–155. https://studentaffairs.arizona.edu/assessment/documents/CyberbullyChapterFinal.pdf Retrieved from. [ Google Scholar ]
- Beebe J.E. University of Northern Colorado; 2010. The Prevalence of Cyber Bullying Victimization and its Relationship to Academic, Social, and Emotional Adjustment Among College Students. https://eric.ed.gov/?id=ED517400 ProQuest LLC, Ph.D. Dissertation. [ Google Scholar ]
- Belsey B. 2006. Cyber Bullying: an Emerging Threat to “Always on” Generation. http://www.cyberbullying.ca/pdf/Cyberbullying_Article_by_Bill_Belsey.pdf From. [ Google Scholar ]
- Cahill S., Makadon H. Sexual orientation and gender identity data collection in clinical settings and in electronic health records: a key to ending LGBT health disparities. LGBT Health. 2014; 1 (1):34–41. [ PubMed ] [ Google Scholar ]
- Clark D.M.T., Loxton N.J., Tobin S.J. Declining loneliness over time: evidence from American colleges and high schools. Pers. Soc. Psychol. Bull. 2015; 41 (1):78–89. [ PubMed ] [ Google Scholar ]
- Dalia–Global consumer understanding . 2016. Counting the LGBT Population: 6% of Europeans Identify as LGBT. https://daliaresearch.com/counting-the-lgbt-population-6-of-europeans-identify-as-lgbt/ [ Google Scholar ]
- DeHue F., Bolman C., Völlink T. Cyberbullying: youngsters' experiences and parental perception. Cyberpsychol. Behav. 2008; 11 (2):217–223. [ PubMed ] [ Google Scholar ]
- Dılmaç B. Psychological needs as a predictor of cyber bullying: a preliminary report on college students. Educ. Sci. Theor. Pract. 2009; 9 (3):1307–1325. [ Google Scholar ]
- Faryadi Q. Cyber bullying and academic performance. Int. J. Comput. Eng. Res. 2011; 1 (1):2250–3005. [ Google Scholar ]
- Faucher C., Jackson M., Cassidy W. Cyberbullying among university students: gendered experiences, impacts, and perspectives. Educ. Res. Int. 2014 [ Google Scholar ]
- Gálik S., Hladíková V., Pavlák L. Cyberbullying and opportunities for its prevention. Media Lit. Acad. Res. 2018; 1 (1):6–17. [ Google Scholar ]
- Gahagan K., Vaterlaus J.M., Frost L.R. College student cyberbullying on social networking sites: conceptualization, prevalence, and perceived bystander responsibility. Comput. Hum. Behav. 2016; 55 :1097–1105. [ Google Scholar ]
- Gates G.J. The Williams Institute, UCLA School of Law; 2011. How many People Are Lesbian, Gay, Bisexual and Transgender? https://escholarship.org/uc/item/09h684x2 [ Google Scholar ]
- Gerdes H., Mallinckrodt B. Emotional, social, and academic adjustment of college students: a longitudinal study of retention. J. Couns. Dev. 1994; 72 (3):281–288. [ Google Scholar ]
- Ghadampour F., Shafiei M., Heidarirad H. Relationships among cyberbullying, psychological vulnerability and suicidal thoughts in female and male students. J. Res. Psychol. Health. 2017; 11 :28–40. [ Google Scholar ]
- Grene M.B. Counselling and climate change as treatment modalities for bullying in school. Int. J. Adv. Couns. 2003; 25 (4):293–302. [ Google Scholar ]
- Heiman T., Olenik Shemesh D. Predictors of cyber-victimization of higher-education students with and without learning disabilities. J. Youth Stud. 2018:1–18. [ Google Scholar ]
- Inkelas K.K., Daver Z.E., Vogt K.E., Leonard J.B. Living–learning programs and first-generation college students’ academic and social transition to college. Res. High. Educ. 2007; 48 (4):403–434. [ Google Scholar ]
- Juvonen J., Graham S., Shuster M.A. Bullying among young adolescents: the strong, the weak, and the troubled. Paediatrics. 2003; 112 (6):1231–1237. [ PubMed ] [ Google Scholar ]
- Juvonen J., Gross E.F. Extending the school grounds?—bullying experiences in cyberspace. J. Sch. Health. 2008; 78 (9):496–505. [ PubMed ] [ Google Scholar ]
- Kowalski R.M., Limber S.P. Electronic bullying among middle school children. J. Adolesc. Health. 2007; 41 :S22–S30. [ PubMed ] [ Google Scholar ]
- Kowalski R., Limber S.P., McCord A. A developmental approach to cyberbullying: prevalence and protective factors. Aggress. Violent Behav. 2018 [ Google Scholar ]
- Lau L.K. Institutional factors affecting student retention. Education. 2003; 124 (1):126–137. [ Google Scholar ]
- McGrath S. 2005. The Multiple Contexts of Vocational Education and Training in Southern Africa. Vocational Education and Training in Southern Africa: a Comparative Study; pp. 1–8. http://hdl.handle.net/20.500.11910/7250 URI: [ Google Scholar ]
- Merriam-Webster . 2017. On-line Dictionary. https://www.merriam-webster.com/dictionary/cyberbullying [ Google Scholar ]
- Myers C.A., Cowie H. Bullying at university: the social and legal contexts of cyberbullying among university students. J. Cross Cult. Psychol. 2017; 48 (8):1172–1182. [ Google Scholar ]
- Na H. 2014. The Effects of Cyberbullying Victimization on Psychological Adjustments Among College Students. https://dspace-prod.lib.uic.edu/bitstream/handle/10027/11288/Na_Hyunjoo.pdf?sequence=1&isAllowed=y Doctoral dissertation. [ Google Scholar ]
- Parker J.D., Summerfeldt L.J., Hogan M.J., Majeski S.A. Emotional intelligence and academic success: examining the transition from high school to university. Pers. Indiv. Differ. 2004; 36 (1):163–172. [ Google Scholar ]
- Peled Y., Barczyk C., Sarid M. Institutional Characteristics and faculty perceptions of academic dishonesty. Educ. Pract. Theor. 2012; 34 (2):61–79. [ Google Scholar ]
- Pollock S.L. Counsellor roles in dealing with bullies and their LGBT victims. Middle Sch. J. 2006; 38 (2):29–36. [ Google Scholar ]
- Poole S.P. Vol. 115. 2017. The Experience of Victimization as the Result of Cyberbullying Among College Students: A Study of Demographics, Self-Esteem, and Locus of Control. http://scholarworks.sfasu.edu/etds/115 Electronic Theses and Dissertations. [ Google Scholar ]
- Rey L., Extremera N., Pena M. Perceived emotional intelligence, self-esteem and life satisfaction in adolescents. Psychosoc. Interv. 2011; 20 (2) [ Google Scholar ]
- Rivers I. Homophobic and transphobic bullying in universities. In: Cowie H., Myers C.-A., editors. Bullying Among university Students. Routledge; London, England: 2016. pp. 48–60. [ Google Scholar ]
- Rivituso G. 2012. Cyberbullying: an Exploration of the Lived Experiences and the Psychological Impact of Victimization Among College Students an Interpretive Phenomenological Analysis. Education Doctoral Theses. Paper 21. [ Google Scholar ]
- Selkie E.M., Kota R., Chan Y.F., Moreno M. Cyberbullying, depression, and problem alcohol use in female college students: a multisite study. Cyberpsychol. Behav. Soc. Netw. 2015; 18 (2):79–86. [ PMC free article ] [ PubMed ] [ Google Scholar ]
- Schenk A.M., Fremouw W.J. Prevalence, psychological impact, and coping of cyberbully victims among college students. J. Sch. Violence. 2012; 11 :21–37. [ Google Scholar ]
- Tinto V. Dropout from higher education: a theoretical synthesis of recent research. Rev. Educ. Res. 1975; 45 (1):89–125. [ Google Scholar ]
- Varghese M.E., Pistole M.C. College student cyberbullying: self-esteem, depression, loneliness, and attachment. J. Coll. Couns. 2017; 20 (1):7–21. [ Google Scholar ]
- Walker C.M., Sockman B.J., Koehn S. An exploratory study of cyberbullying with undergraduate university students. TechTrends. 2011; 55 (2):31–38. [ Google Scholar ]
- Watts L.K., Wagner J., Velasquez B., Behrens P.I. Cyberbullying in higher education: a literature review. Comput. Hum. Behav. 2017; 69 :268–274. [ Google Scholar ]
- Webber M.A., Ovedovitz A.C. Cyberbullying among college students: a look at its prevalence at a U.S. Catholic University. Int. J. Educ. Methodol. 2018; 4 (2):101–107. [ Google Scholar ]
- Ybarra M.L., Mitchell K.J. Prevalence and frequency of internet harassment instigation: implications for adolescent health. J. Adolesc. Health. 2007; 41 :189–195. [ PubMed ] [ Google Scholar ]
- Ziapour A., Khatony A., Jafari F., Kianipour N. Correlation of personality traits with happiness among university students. J. Clin. Diagn. Res. 2018; 12 (4) [ Google Scholar ]
Academia.edu no longer supports Internet Explorer.
To browse Academia.edu and the wider internet faster and more securely, please take a few seconds to upgrade your browser .
Enter the email address you signed up with and we'll email you a reset link.
- We're Hiring!
- Help Center
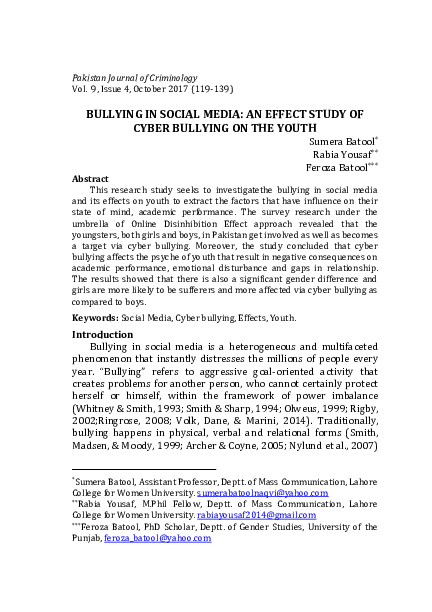
BULLYING IN SOCIAL MEDIA: AN EFFECT STUDY OF CYBER BULLYING ON THE YOUTH

2017, Pakistan Journal of Criminology
This research study seeks to investigatethe bullying in social media and its effects on youth to extract the factors that have influence on their state of mind, academic performance. The survey research under the umbrella of Online Disinhibition Effect approach revealed that the youngsters, both girls and boys, in Pakistan get involved as well as becomes a target via cyber bullying. Moreover, the study concluded that cyber bullying affects the psyche of youth that result in negative consequences on academic performance, emotional disturbance and gaps in relationship. The results showed that there is also a significant gender difference and girls are more likely to be sufferers and more affected via cyber bullying as compared to boys.
Related Papers
Aiman Khursheed
The purpose of this study was to examine the effects of cyber bullying on girls of University of Sindh, Jamshoro. There are many victims who were facing many problems due to extra and frequent use of Internet. Mostly girls have been targeted in the field of social media. The study focused only the girl students of university of Sindh, Jamshoro. Study showed that how cybercrimes effects on a girl’s students life, for this selection of respondents was very important, researcher conduct survey with 100 girl students from faculty of social sciences, University of Sindh, Jamshoro. The researcher after analyzing data found out that the girl students always use social media for communication purpose, and also interested in educating themselves by using of social media applications regarding harassment and bullying. The study has concluded that majority of the girl students believe that social media has created problems in their daily life. Further, study found that majority of the girls wa...
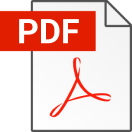
Global Regional Review
sobia altaf
While forms of traditional bullying have been declining over the past two decades, cyber bullying has emerged as a modern form of bullying in recent times. Previous research studies have not been developed much on the issue of cyber bullying. The current study reviews the relevant research studies as the excessive usage of social media and by young people and different themes have been carried out from the help of the existing literature. The present study has synthesised current literature on: i) prevalence of cyber bullying among adolescents ii) huge level of cyber bullying occurrences in educational institutions iii) people who are more prone to be the victims and perpetrators iv) effects of cyber bullying on adolescence v) preventive measures for the wellbeing of Pakistani adolescents. In this article, the researcher reviews the literature related to cyber bullying conducted between the years from 2000 to 2019.
International Journal of Social Science and Human Research
Tabassum Fatima
Bullying on various social media platforms is a new societal issue that has gotten a lot of attention from academics. It has been recognized as a serious health issue among adolescents. Cyber bullying can take many different forms. It doesn't always imply breaking into someone's accounts or pretending to be someone else. It also includes making disparaging remarks about someone or circulating rumors in order to slander them. Because everyone is engrossed in social media, it is quite easy for anyone to abuse their access. The present study is an attempt to analyze various aspects of bullying on social media among senior secondary school students. The study has also taken into consideration the type of school (government and private) and gender variables which was carried out on the sample of 305 senior secondary school adolescents of Aligarh district. The study has revealed mixed results which have been interpreted with some suggestive measures.
Dr. Majid Sadeeq
Most of the research on cyber bullying available on the internet is of foreign origin. Research studies on this constant on Indian population are required. Thus calls for a narrative review of the available literature in foreign as well as Indian context. This present study tried to meet this requirement by presenting a narrative review on cyber bullying construct literature. Electronic data bases like Eric, Proquest, google scholar, J-gate, Eric were used as the data sources. Literature in the form of research articles and reviews articles were searched the words with the exact key words “cyber bullying” and “Indian” in the title was selected for study and literature on related key words was strictly ignored. The literature included research articles citing the first recorded case of bullying to certain latest finding in this subject in recent times. The study found that the use of technology is in rise in Indian, with the Indian youth spending at least an hour or two on the social networking sites being venerable to cyber bullying and suffering in due course owing to poor awareness and bad implementation ofcyber laws.
IRA-International Journal of Management & Social Sciences (ISSN 2455-2267)
Sulakshana Khatoniyar
International Journal of Innovative Research and Development
Usman Saadu
IOSR Journals
This paper attempted to explore the impact of cyber-bullying on the females. This study was conducted at Kushtia district in Bangladesh from June 2020 to February 2021. 60 females were selected from two upazillas, Kushtia Sadar and Kumarkhali (30 from Kushtia Sadar Upazilla and 30 from Kumarkhali Upazilla). Questionnaire and in-depth individual interview were used as the instruments for collecting quantitative and qualitative data adopting a mixed method approach (Both quantitative and qualitative). Quantitative data were collected from 60 females aged 15-25 years through a set of questionnaire and qualitative data of this study were collected from 6 females through in-depth face to face interview. Articles, journals and research papers were the sources of secondary data of this study. Quantitative data were first coded and analyzed, and then, qualitative data analysis was done through content analysis. After interpretation of data, the findings of this study were presented in tables and charts using numbers and percentages. The study revealed that cyber-bullying had negative impacts on the females.
EUROASIA SUMMIT (Congress on Scientific Researches and Recent Trends-8th)
Ceren Çubukçu Çerasi
The developments in mobile technologies and the spread of 4G and 5G internet infrastructures increase internet usage day by day. Especially in early 2020, due to the COVID-19 pandemic, which affected the whole world, the transfer of face-to-face activities to the online environment has accelerated the widespread use of the internet. Despite the risk of COVID-19, face-to-face education has started to be done online on e-learning platforms. Thus, almost all of the students, from preschool level to higher education level, started to spend more time on the internet. This situation has also increased the possibility of students encountering negative behaviors and threats in the internet environment. In this study, the cyber bullying status of university students was investigated and it was examined whether there was a relationship between students' cyber bullying levels and various variables such as gender, age and family income. As a result of the research, it was determined that the students' level of cyber bullying in general was low. In addition, it was concluded that the cyber bullying status of the students did not differ statistically according to gender, family income, internet and social media usage. In future studies, the situation of being a cyber-bully or cyber victim of university students from different regions can be investigated according to their faculties and departments.
New Horizons
Inayatullah Magsi
This research was carried out to explore how female university students suffer from cyber bullying within their campuses. The data for this study was collected from 120 female students at four universities in Sindh province of Pakistan. The results show that the female students were threatened and blackmailed frequently in the university campuses. While, 45 percent of the students did not disclose such incidents to their families because of the fear of being considered immoral. Therefore, young women prefer to suffer in silence, which not only discourages the students to use cyber spaces freely, but also disturbs their academic life. Furthermore, the findings unveiled that the female students not only lacked trust in the law enforcement agencies, but were also ignorant to the current laws against cyber harassment. Therefore, it is suggested that the universities should organize awareness campaigns as well as introduce a separate body to prevent cyber stalking of young women at the country level.
JKKNIU Journal of Social Science
Sadik Shuvo
The number of people using social networking sites is increasing day by day. Girls are also using social networking sites as like as their male counterparts. Especially the youth and educated females are using the social networking sites to communicate with their friends and other people they need. It is seen, frequently girls accused that they are being bullied or victimized of cyber bullying through the social networking sites. Though the problem is being acute no remarkable study and government actions have been taken on this issue. This article is looking for the answer of some questions regarding bullying on social networking sites. The purpose of the study is to know the type of bullying, type of stalkers, reasons, impact of bullying and preventive actions to be protected on social networking sites. Findings of the study address the objective chronologically. To finalize the study data has been collected from both primary and secondary sources. Primary data has been collected from only female students about their experience regarding cyber bullying.
Loading Preview
Sorry, preview is currently unavailable. You can download the paper by clicking the button above.
RELATED PAPERS
Social Work Chronicle
Publishing India Group
BJSTR Angela Roy
Editor IJIRMF
AMAN CHAUHAN
Aminuddin Hashemi
IJIP Journal
EDITOR IASET
IrisPublishers LLC
iris publishers
Procedia - Social and Behavioral Sciences
Ozgur Erdur
Mohinder Singh
Conference of the International Journal of Arts & Sciences
Rattiya Deenamjued , Waiphot Kulachai
hadir elmahdy
Md. Mostafizur Rahman
International Journal Of Computational Engineering Research 2011
Dr. Qais Faryadi
International Journal of Academic Research in Progressive Education and Development
DR MOHAMMAD FAHMI ABDUL HAMID
Marija Nešić
Norhartini Zubir
Çağla Tahtakale
Technology in Society
Sumera Saleem
Jurnal Aplikasi IPTEK Indonesia
azzah haura
Research, Society and Development
Marcel Pereira Pordeus
Communication Today
Shalini sharma
accounts ziraf
Psychological Studies
Humaira Jami
RELATED TOPICS
- We're Hiring!
- Help Center
- Find new research papers in:
- Health Sciences
- Earth Sciences
- Cognitive Science
- Mathematics
- Computer Science
- Academia ©2024
SYSTEMATIC REVIEW article
Cyberbullying among adolescents and children: a comprehensive review of the global situation, risk factors, and preventive measures.

- 1 School of Political Science and Public Administration, Wuhan University, Wuhan, China
- 2 School of Medicine and Health Management, Tongji Medical College, Huazhong University of Science and Technology, Wuhan, China
- 3 College of Engineering, Design and Physical Sciences, Brunel University London, Uxbridge, United Kingdom
Background: Cyberbullying is well-recognized as a severe public health issue which affects both adolescents and children. Most extant studies have focused on national and regional effects of cyberbullying, with few examining the global perspective of cyberbullying. This systematic review comprehensively examines the global situation, risk factors, and preventive measures taken worldwide to fight cyberbullying among adolescents and children.
Methods: A systematic review of available literature was completed following PRISMA guidelines using the search themes “cyberbullying” and “adolescent or children”; the time frame was from January 1st, 2015 to December 31st, 2019. Eight academic databases pertaining to public health, and communication and psychology were consulted, namely: Web of Science, Science Direct, PubMed, Google Scholar, ProQuest, Communication & Mass Media Complete, CINAHL, and PsycArticles. Additional records identified through other sources included the references of reviews and two websites, Cyberbullying Research Center and United Nations Children's Fund. A total of 63 studies out of 2070 were included in our final review focusing on cyberbullying prevalence and risk factors.
Results: The prevalence rates of cyberbullying preparation ranged from 6.0 to 46.3%, while the rates of cyberbullying victimization ranged from 13.99 to 57.5%, based on 63 references. Verbal violence was the most common type of cyberbullying. Fourteen risk factors and three protective factors were revealed in this study. At the personal level, variables associated with cyberbullying including age, gender, online behavior, race, health condition, past experience of victimization, and impulsiveness were reviewed as risk factors. Likewise, at the situational level, parent-child relationship, interpersonal relationships, and geographical location were also reviewed in relation to cyberbullying. As for protective factors, empathy and emotional intelligence, parent-child relationship, and school climate were frequently mentioned.
Conclusion: The prevalence rate of cyberbullying has increased significantly in the observed 5-year period, and it is imperative that researchers from low and middle income countries focus sufficient attention on cyberbullying of children and adolescents. Despite a lack of scientific intervention research on cyberbullying, the review also identified several promising strategies for its prevention from the perspectives of youths, parents and schools. More research on cyberbullying is needed, especially on the issue of cross-national cyberbullying. International cooperation, multi-pronged and systematic approaches are highly encouraged to deal with cyberbullying.
Introduction
Childhood and adolescence are not only periods of growth, but also of emerging risk taking. Young people during these periods are particularly vulnerable and cannot fully understand the connection between behaviors and consequences ( 1 ). With peer pressures, the heat of passion, children and adolescents usually perform worse than adults when people are required to maintain self-discipline to achieve good results in unfamiliar situations. Impulsiveness, sensation seeking, thrill seeking, and other individual differences cause adolescents to risk rejecting standardized risk interventions ( 2 ).
About one-third of Internet users in the world are children and adolescents under the age of 18 ( 3 ). Digital technology provide a new form of interpersonal communication ( 4 ). However, surveys and news reports also show another picture in the Internet Age. The dark side of young people's internet usage is that they may bully or suffer from others' bullying in cyberspace. This behavior is also acknowledged as cyberbullying ( 5 ). Based on Olweus's definition, cyberbullying is usually regarded as bullying implemented through electronic media ( 6 , 7 ). Specifically, cyberbullying among children and adolescents can be summarized as the intentional and repeated harm from one or more peers that occurs in cyberspace caused by the use of computers, smartphones and other devices ( 4 , 8 – 12 ). In recent years, new forms of cyberbullying behaviors have emerged, such as cyberstalking and online dating abuse ( 13 – 15 ).
Although cyberbullying is still a relatively new field of research, cyberbullying among adolescents is considered to be a serious public health issue that is closely related to adolescents' behavior, mental health and development ( 16 , 17 ). The increasing rate of Internet adoption worldwide and the popularity of social media platforms among the young people have worsened this situation with most children and adolescents experiencing cyberbullying or online victimization during their lives. The confines of space and time are alleviated for bullies in virtual environments, creating new venues for cyberbullying with no geographical boundaries ( 6 ). Cyberbullying exerts negative effects on many aspects of young people's lives, including personal privacy invasion and psychological disorders. The influence of cyberbullying may be worse than traditional bullying as perpetrators can act anonymously and connect easily with children and adolescents at any time ( 18 ). In comparison with traditional victims, those bullied online show greater levels of depression, anxiety and loneliness ( 19 ). Self-esteem problems and school absenteeism have also proven to be related to cyberbullying ( 20 ).
Due to changes in use and behavioral patterns among the youth on social media, the manifestations and risk factors of cyberbullying have faced significant transformation. Further, as the boundaries of cyberbullying are not limited by geography, cyberbullying may not be a problem contained within a single country. In this sense, cyberbullying is a global problem and tackling it requires greater international collaboration. The adverse effects caused by cyberbullying, including reduced safety, lower educational attainment, poorer mental health and greater unhappiness, led UNICEF to state that “no child is absolutely safe in the digital world” ( 3 ).
Extant research has examined the prevalence and risk factors of cyberbullying to unravel the complexity of cyberbullying across different countries and their corresponding causes. However, due to variations in cyberbullying measurement and methodologies, no consistent conclusions have been drawn ( 21 ). Studies into inconsistencies in prevalence rates of cyberbullying, measured in the same country during the same time period, occur frequently. Selkie et al. systematically reviewed cyberbullying among American middle and high school students aged 10–19 years old in 2015, and revealed that the prevalence of cyberbullying victimization ranged from 3 to 72%, while perpetration ranged from 1 to 41% ( 22 ). Risk and protective factors have also been broadly studied, but confirmation is still needed of those factors which have more significant effects on cyberbullying among young people. Clarification of these issues would be useful to allow further research to recognize cyberbullying more accurately.
This review aims to extend prior contributions and provide a comprehensive review of cyberbullying of children and adolescents from a global perspective, with the focus being on prevalence, associated risk factors and protective factors across countries. It is necessary to provide a global panorama based on research syntheses to fill the gaps in knowledge on this topic.
Search Strategies
This study strictly employed Preferred Reporting Items for Systematic Reviews and Meta-Analyses (PRISMA) guidelines. We consulted eight academic databases pertaining to public health, and communication and psychology, namely: Web of Science, Science Direct, PubMed, Google Scholar, ProQuest, Communication & Mass Media Complete, CINAHL, and PsycArticles. Additional records identified through other sources included the references of reviews and two websites, Cyberbullying Research Center and United Nations Children's Fund. With regard to the duration of our review, since most studies on cyberbullying arose around 2015 ( 9 , 21 ), this study highlights the complementary aspects of the available information about cyberbullying during the recent 5 year period from January 1st, 2015 to December 31st, 2019.
One researcher extracted keywords and two researchers proposed modifications. We used two sets of subject terms to review articles, “cyberbullying” and “child OR adolescent.” Some keywords that refer to cyberbullying behaviors and young people are also included, such as threat, harass, intimidate, abuse, insult, humiliate, condemn, isolate, embarrass, forgery, slander, flame, stalk, manhunt, as well as teen, youth, young people and student. The search formula is (cyberbullying OR cyber-bullying OR cyber-aggression OR ((cyber OR online OR electronic OR Internet) AND (bully * OR aggres * OR violence OR perpetrat * OR victim * OR threat * OR harass * OR intimidat * OR * OR insult * OR humiliate * OR condemn * OR isolate * OR embarrass * OR forgery OR slander * OR flame OR stalk * OR manhunt))) AND (adolescen * OR child OR children OR teen? OR teenager? OR youth? OR “young people” OR “elementary school student * ” OR “middle school student * ” OR “high school student * ”). The main search approach is title search. Search strategies varied according to the database consulted, and we did not limit the type of literature for inclusion. Journals, conference papers and dissertations are all available.
Specifically, the inclusion criteria for our study were as follows: (a). reported or evaluated the prevalence and possible risk factors associated with cyberbullying, (b). respondents were students under the age of 18 or in primary, junior or senior high schools, and (c). studies were written in English. Exclusion criteria were: (a). respondents came from specific groups, such as clinical samples, children with disabilities, sexual minorities, specific ethnic groups, specific faith groups or samples with cross-national background, (b). review studies, qualitative studies, conceptual studies, book reviews, news reports or abstracts of meetings, and (c). studies focused solely on preventive measures that were usually meta-analytic and qualitative in nature. Figure 1 presents the details of the employed screening process, showing that a total of 63 studies out of 2070 were included in our final review.
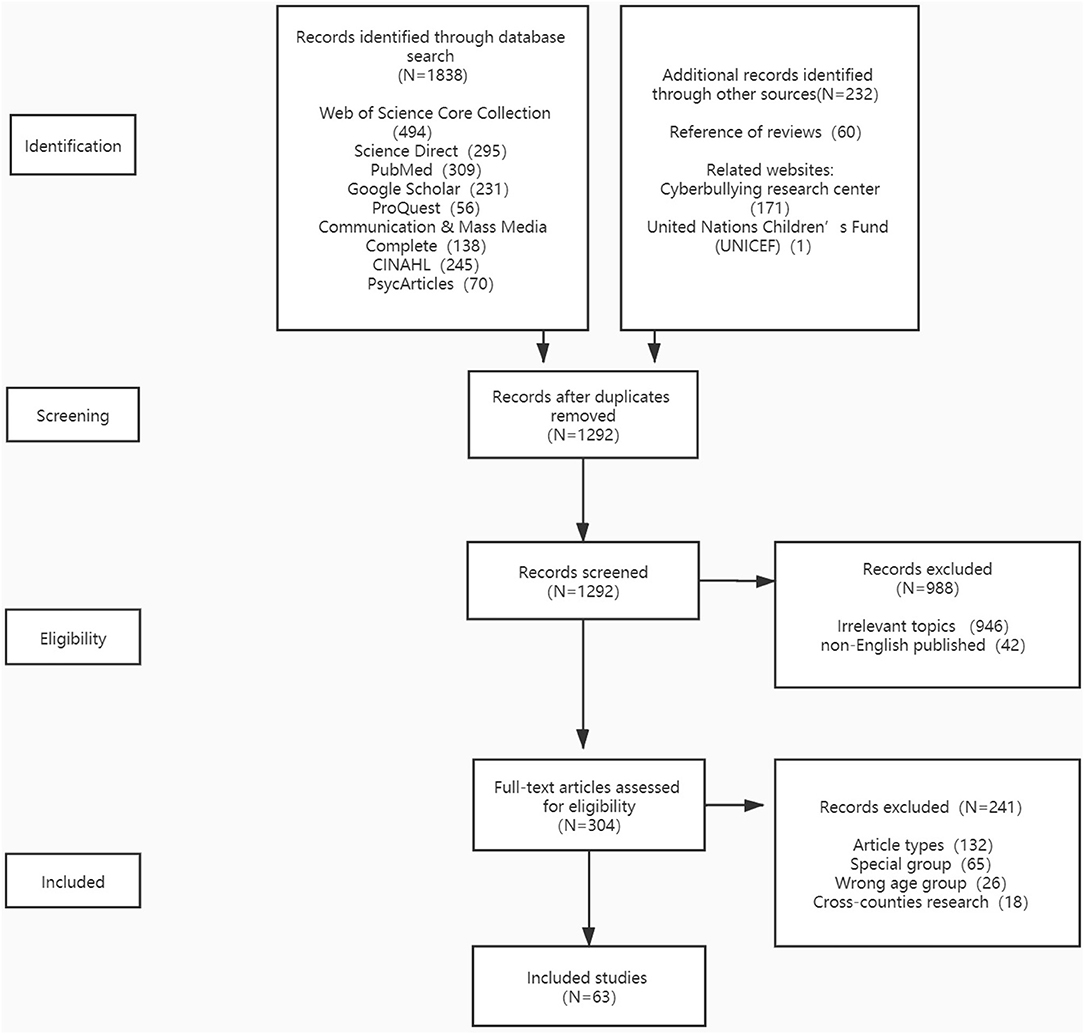
Figure 1 . PRISMA flow chart diagram showing the process of study selection for inclusion in the systematic review on children and adolescents cyberbullying.
Meta-analysis was not conducted as the limited research published within the 5 years revealed little research which reported odds ratio. On the other hand, due to the inconsistency of concepts, measuring instruments and recall periods, considerable variation could be found in research quality ( 23 ). Meta-analysis is not a preferred method.
Coding Scheme
For coding, we created a comprehensive code scheme to include the characteristics. For cyberbullying, we coded five types proposed by Willard ( 24 – 26 ), which included verbal violence, group violence, visual violence, impersonating and account forgery, and other behaviors. Among them, verbal violence is considered one of the most common types of cyberbullying and refers to the behavior of offensive responses, insults, mocking, threats, slander, and harassment. Group violence is associated with preventing others from joining certain groups or isolating others, forcing others to leave the group. Visual violence relates to the release and sharing of embarrassing photos and information without the owners' consent. Impersonating and account forgery refers to identity theft, stealing passwords, violating accounts and the creation of fake accounts to fraudulently present the behavior of others. Other behaviors include disclosure of privacy, sexual harassment, and cyberstalking. To comprehensively examine cyberbullying, we coded cyberbullying behaviors from both the perspectives of cyberbullying perpetrators and victims, if mentioned in the studies.
In relation to risk factors, we drew insights from the general aggression model, which contributes to the understanding of personal and situational factors in the cyberbullying of children and adolescents. We chose the general aggression model because (a) it contains more situational factors than other models (e.g., social ecological models) - such as school climate ( 9 ), and (b) we believe that the general aggression model is more suitable for helping researchers conduct a systematic review of cyberbullying risk and protective factors. This model provides a comprehensive framework that integrates domain specific theories of aggression, and has been widely applied in cyberbullying research ( 27 ). For instance, Kowalski and colleagues proposed a cyberbullying encounter through the general aggression model to understand the formation and development process of youth cyberbullying related to both victimization and perpetration ( 9 ). Victims and perpetrators enter the cyberbullying encounter with various individual characteristics, experiences, attitudes, desires, personalities, and motives that intersect to determine the course of the interaction. Correspondingly, the antecedents pertaining to cyberbullying are divided into two broad categories, personal factors and situational factors. Personal factors refer to individual characteristics, such as gender, age, motivation, personality, psychological states, socioeconomic status and technology use, values and perceptions, and other maladaptive behaviors. Situational factors focus on the provocation/support, parental involvement, school climate, and perceived anonymity. Consequently, our coders related to risk factors consisting of personal factors and situational factors from the perspectives of both cyberbullying perpetrators and victims.
We extracted information relating to individual papers and sample characteristics, including authors, year of publication, country, article type, sampling procedures, sample characteristics, measures of cyberbullying, and prevalence and risk factors from both cyberbullying perpetration and victimization perspectives. The key words extraction and coding work were performed twice by two trained research assistants in health informatics. The consistency test results are as follows: the Kappa value with “personal factors” was 0.932, and the Kappa value with “situational factors” was 0.807. The result shows that the coding consistency was high enough and acceptable. Disagreements were resolved through discussion with other authors.
Quality Assessment of Studies
The quality assessment of the studies is based on the recommended tool for assessing risk of bias, Cochrane Collaboration. This quality assessment tool focused on seven items: random sequence generation, allocation concealment, blinding of participants and personnel, blinding of outcome assessment, incomplete outcome data, selective reporting, and other sources of bias ( 28 ). We assessed each item as “low risk,” “high risk,” and “unclear” for included studies. A study is considered of “high quality” when it meets three or more “low risk” requirements. When one or more main flaw of a study may affect the research results, the study is considered as “low quality.” When a lack of information leads to a difficult judgement, the quality is considered to be “unclear.” Please refer to Appendix 1 for more details.
This comprehensive systematic review comprised a total of 63 studies. Appendices 2 , 3 show the descriptive information of the studies included. Among them, 58 (92%) studies measured two or more cyberbullying behavior types. The sample sizes of the youths range from several hundred to tens of thousands, with one thousand to five thousand being the most common. As for study distribution, the United States of America, Spain and China were most frequently mentioned. Table 1 presents the detail.
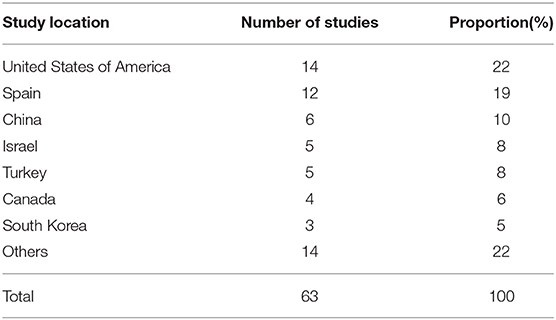
Table 1 . Descriptive information of studies included (2015–2019).
Prevalence of Global Cyberbullying
Prevalence across countries.
Among the 63 studies included, 22 studies reported on cyberbullying prevalence and 20 studies reported on prevalence from victimization and perpetration perspectives, respectively. Among the 20 studies, 11 national studies indicated that the prevalence of cyberbullying victimization and cyberbullying perpetration ranged from 14.6 to 52.2% and 6.3 to 32%, respectively. These studies were conducted in the United States of America ( N = 4) ( 29 – 32 ), South Korea ( N = 3) ( 33 – 35 ), Singapore ( N = 1) ( 36 ), Malaysia ( N = 1) ( 37 ), Israel ( N = 1) ( 38 ), and Canada ( N = 1) ( 39 ). Only one of these 11 national studies is from an upper middle income country, and the rest are from highincome countries identified by the World Bank ( 40 ). By combining regional and community-level studies, the prevalence of cyberbullying victimization and cyberbullying perpetration ranged from 13.99 to 57.5% and 6.0 to 46.3%, respectively. Spain reported the highest prevalence of cyberbullying victimization (57.5%) ( 41 ), followed by Malaysia (52.2%) ( 37 ), Israel (45%) ( 42 ), and China (44.5%) ( 43 ). The lowest reported victim rates were observed in Canada (13.99%) and South Korea (14.6%) ( 34 , 39 ). The reported prevalence of cyberbullying victimization in the United States of America ranged from 15.5 to 31.4% ( 29 , 44 ), while in Israel, rates ranged from 30 to 45% ( 26 , 42 ). In China, rates ranged from 6 to 46.3% with the country showing the highest prevalence of cyberbullying perpetration (46.30%) ( 15 , 43 , 45 , 46 ). Canadian and South Korean studies reported the lowest prevalence of cyberbullying perpetration at 7.99 and 6.3%, respectively ( 34 , 39 ).
A total of 10 studies were assessed as high quality studies. Among them, six studies came from high income countries, including Canada, Germany, Italy, Portugal, and South Korea ( 13 , 34 , 39 , 46 – 48 ). Three studies were from upper middle income countries, including Malaysia and China ( 37 , 43 ) and one from a lower middle income country, Nigeria ( 49 ). Figures 2 , 3 describe the prevalence of cyberbullying victimization and perpetration respectively among high quality studies.
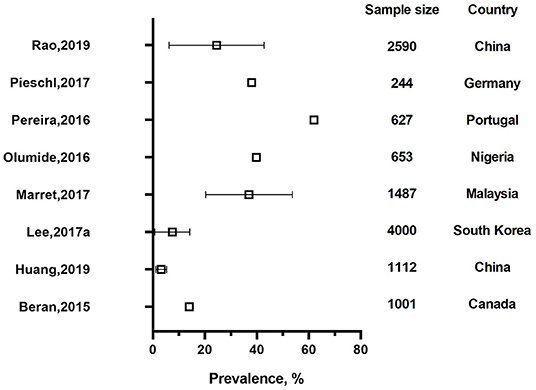
Figure 2 . The prevalence of cyberbullying victimization of high quality studies.
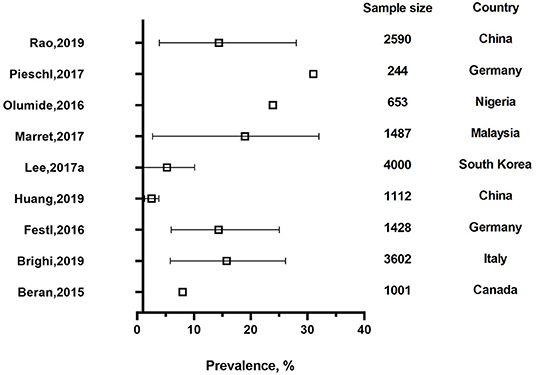
Figure 3 . The prevalence of cyberbullying perpetration of high quality studies.
Prevalence of Various Cyberbullying Behaviors
For the prevalence of cyberbullying victimization and perpetration, the data were reported in 18 and 14 studies, respectively. Figure 4 shows the distribution characteristics of the estimated value of prevalence of different cyberbullying behaviors with box plots. The longer the box, the greater the degree of variation of the numerical data and vice versa. The rate of victimization and crime of verbal violence, as well as the rate of victimization of other behaviors, such as cyberstalking and digital dating abuse, has a large degree of variation. Among the four specified types of cyberbullying behaviors, verbal violence was regarded as the most commonly reported behaviors in both perpetration and victimization rates, with a wide range of prevalence, ranging from 5 to 18%. Fewer studies reported the prevalence data for visual violence and group violence. Studies also showed that the prevalence of impersonation and account forgery were within a comparatively small scale. Specific results were as follows.
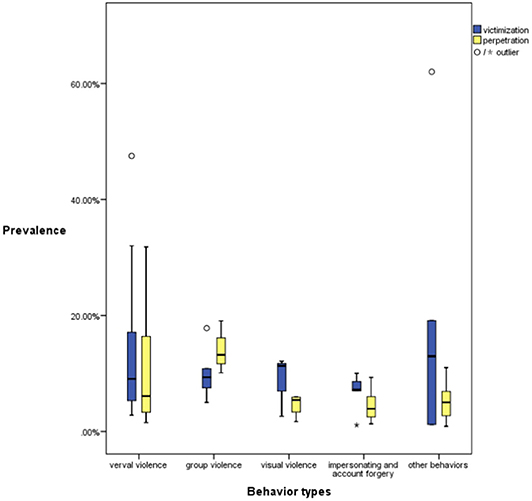
Figure 4 . Cyberbullying prevalence across types (2015–2019).
Verbal Violence
A total of 13 studies reported verbal violence prevalence data ( 15 , 26 , 34 , 37 – 39 , 42 , 43 , 47 , 48 , 50 , 51 ). Ten studies reported the prevalence of verbal violence victimization ranging from 2.8 to 47.5%, while seven studies claimed perpetration prevalence ranging from 1.5 to 31.8%. Malaysia reported the highest prevalence of verbal violence victimization (47.5%) ( 37 ), followed by China (32%) ( 43 ). China reported that the prevalence of verbal violence victimization ranged from 5.1 to 32% ( 15 , 43 ). Israel reported that the prevalence of verbal violence victimization ranged from 3.4 to 18% ( 26 , 38 , 42 ). For perpetration rate, Malaysia reported the highest level at 31.8% ( 37 ), while a study for Spain reported the lowest, ranging from 3.2 to 6.4% ( 51 ).
Group Violence
The prevalence of group violence victimization was explored within 4 studies and ranged from 5 to 17.8% ( 26 , 34 , 42 , 43 ), while perpetration prevalence was reported in three studies, ranging from 10.1 to 19.07% ( 34 , 43 , 47 ). An Israeli study suggested that 9.8% of respondents had been excluded from the Internet, while 8.9% had been refused entry to a group or team ( 26 ). A study in South Korea argued that the perpetration prevalence of group violence was 10.1% ( 34 ), while a study in Italy reported that the rate of online group violence against others was 19.07% ( 47 ).
Visual Violence
The prevalence of visual violence victimization was explored within three studies and ranged from 2.6 to 12.1% ( 26 , 34 , 43 ), while the perpetration prevalence reported in four studies ranged from 1.7 to 6% ( 34 , 43 , 47 , 48 ). For victimization prevalence, a South Korean study found that 12.1% of respondents reported that their personal information was leaked online ( 34 ). An Israel study reported that the prevalence of outing the picture was 2.6% ( 26 ). For perpetration prevalence, a South Korean study found that 1.7% of respondents had reported that they had disclosed someone's personal information online ( 34 ). A German study reported that 6% of respondents had written a message (e.g., an email) to somebody using a fake identity ( 48 ).

Impersonating and Account Forgery
Four studies reported on the victimization prevalence of impersonating and account forgery, ranging from 1.1 to 10% ( 15 , 42 , 43 ), while five studies reported on perpetration prevalence, with the range being from 1.3 to 9.31% ( 15 , 43 , 47 , 48 , 51 ). In a Spanish study, 10% of respondents reported that their accounts had been infringed by others or that they could not access their account due to stolen passwords. In contrast, 4.5% of respondents reported that they had infringed other people's accounts or stolen passwords, with 2.5% stating that they had forged other people's accounts ( 51 ). An Israeli study reported that the prevalence of being impersonated was 7% ( 42 ), while in China, a study reported this to be 8.6% ( 43 ). Another study from China found that 1.1% of respondents had been impersonated to send dating-for-money messages ( 15 ).
Other Behaviors
The prevalence of disclosure of privacy, sexual harassment, and cyberstalking were also explored by scholars. Six studies reported the victimization prevalence of other cyberbullying behaviors ( 13 , 15 , 34 , 37 , 42 , 43 ), and four studies reported on perpetration prevalence ( 34 , 37 , 43 , 48 ). A study in China found that 1.2% of respondents reported that their privacy had been compromised without permission due to disputes ( 15 ). A study from China reported the prevalence of cyberstalking victimization was 11.9% ( 43 ), while a Portuguese study reported that this was 62% ( 13 ). In terms of perpetration prevalence, a Malaysian study reported 2.7% for sexual harassment ( 37 ).
Risk and Protective Factors of Cyberbullying
In terms of the risk factors associated with cyberbullying among children and adolescents, this comprehensive review highlighted both personal and situational factors. Personal factors referred to age, gender, online behavior, race, health conditions, past experiences of victimization, and impulsiveness, while situational factors consisted of parent-child relationship, interpersonal relationships, and geographical location. In addition, protective factors against cyberbullying included: empathy and emotional intelligence, parent-child relationship, and school climate. Table 2 shows the risk and protective factors for child and adolescent cyberbullying.
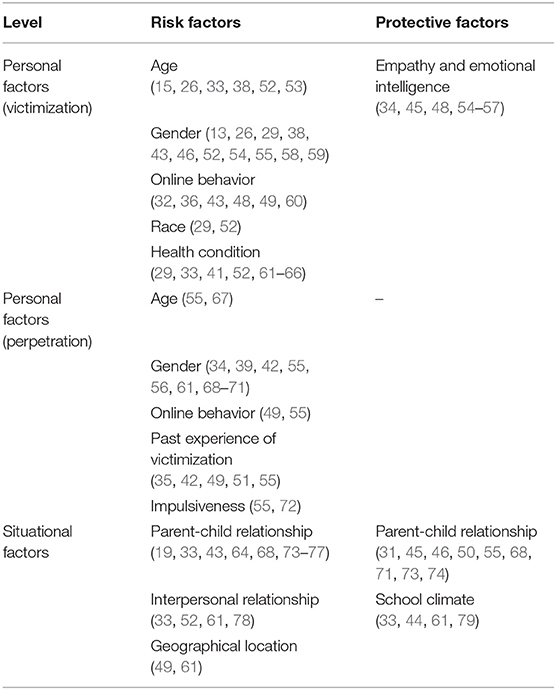
Table 2 . Risk and protective factors of cyberbullying among children and adolescents.
In terms of the risk factors associated with cyberbullying victimization at the personal level, many studies evidenced that females were more likely to be cyberbullied than males ( 13 , 26 , 29 , 38 , 43 , 52 , 54 , 55 , 58 ). Meanwhile, adolescents with mental health problems ( 61 ), such as depression ( 33 , 62 ), borderline personality disorder ( 63 ), eating disorders ( 41 ), sleep deprivation ( 56 ), and suicidal thoughts and suicide plans ( 64 ), were more likely to be associated with cyberbullying victimization. As for Internet usage, researchers agreed that youth victims were probably those that spent more time online than their counterparts ( 32 , 36 , 43 , 45 , 48 , 49 , 60 ). For situational risk factors, some studies have proven the relationship between cyberbullying victims and parental abuse, parental neglect, family dysfunction, inadequate monitoring, and parents' inconsistency in mediation, as well as communication issues ( 33 , 64 , 68 , 73 ). In terms of geographical location, some studies have reported that youths residing in city locations are more likely to be victims of cyberbullying than their peers from suburban areas ( 61 ).
Regarding the risk factors of cyberbullying perpetration at the personal level, it is generally believed that older teenagers, especially those aged over 15 years, are at greater risk of becoming cyberbullying perpetrators ( 55 , 67 ). When considering prior cyberbullying experiences, evidence showed that individuals who had experienced cyberbullying or face-to-face bullying tended to be aggressors in cyberbullying ( 35 , 42 , 49 , 51 , 55 ); in addition, the relationship between impulsiveness and cyberbullying perpetration was also explored by several pioneering scholars ( 55 , 72 , 80 ). The situational factors highlight the role of parents and teachers in cyberbullying experiences. For example, over-control and authoritarian parenting styles, as well as inharmonious teacher-student relationships ( 61 ) are perceived to lead to cyberbullying behaviors ( 74 , 75 ). In terms of differences in geographical locations, students residing in cities have a higher rate of online harassment than students living in more rural locations ( 49 ).
In terms of the protective factors in child and adolescent cyberbullying, scholars have focused on youths who have limited experiences of cyberbullying. At the personal level, high emotional intelligence, an ability for emotional self-control and empathy, such as cognitive empathy ability ( 44 , 55 ), were associated with lower rates of cyberbullying ( 57 ). At the situational level, a parent's role is seen as critical. For example, intimate parent-child relationships ( 46 ) and open active communication ( 19 ) were demonstrated to be related to lower experiences of cyberbullying and perpetration. Some scholars argued that parental supervision and monitoring of children's online activities can reduce their tendency to participate in some negative activities associated with cyberbullying ( 31 , 46 , 73 ). They further claimed that an authoritative parental style protects youths against cyberbullying ( 43 ). Conversely, another string of studies evidenced that parents' supervision of Internet usage was meaningless ( 45 ). In addition to conflicting roles of parental supervision, researchers have also looked into the role of schools, and posited that positive school climates contribute to less cyberbullying experiences ( 61 , 79 ).
Some risk factors may be protective factors under another condition. Some studies suggest that parental aggressive communication is related to severe cyberbullying victims, while open communication is a potential protective factor ( 19 ). Parental neglect, parental abuse, parental inconsistency in supervision of adolescents' online behavior, and family dysfunction are related to the direct or indirect harm of cyberbullying ( 33 , 68 ). Parental participation, a good parental-children relationship, communication and dialogue can enhance children's school adaptability and prevent cyberbullying behaviors ( 31 , 74 ). When parental monitoring reaches a balance between control and openness, it could become a protective factor against cyberbullying, and it could be a risk factor, if parental monitoring is too low or over-controlled ( 47 ).
Despite frequent discussion about the risk factors associated with cyberbullying among children and adolescents, some are still deemed controversial factors, such as age, race, gender, and the frequency of suffering on the internet. For cyberbullying victims, some studies claim that older teenagers are more vulnerable to cyberbullying ( 15 , 38 , 52 , 53 ), while other studies found conflicting results ( 26 , 33 ). As for student race, Alhajji et al. argued that non-white students were less likely to report cyberbullying ( 29 ), while Morin et al. observed no significant correlation between race and cyberbullying ( 52 ). For cyberbullying perpetration, Alvarez-Garcia found that gender differences may have indirect effects on cyberbullying perpetration ( 55 ), while others disagreed ( 42 , 61 , 68 – 70 ). Specifically, some studies revealed that males were more likely to become cyberbullying perpetrators ( 34 , 39 , 56 ), while Khurana et al. presented an opposite point of view, proposing that females were more likely to attack others ( 71 ). In terms of time spent on the Internet, some claimed that students who frequently surf the Internet had a higher chance of becoming perpetrators ( 49 ), while others stated that there was no clear and direct association between Internet usage and cyberbullying perpetration ( 55 ).
In addition to personal and situational factors, scholars have also explored other specific factors pertaining to cyberbullying risk and protection. For instance, mindfulness and depression were found to be significantly related to cyber perpetration ( 76 ), while eating disorder psychopathology in adolescents was associated with cyber victimization ( 41 ). For males who were familiar with their victims, such as family members, friends and acquaintances, they were more likely to be cyberstalking perpetrators than females or strangers, while pursuing desired closer relationships ( 13 ). In the school context, a lower social likability in class was identified as an indirect factor for cyberbullying ( 48 ).
This comprehensive review has established that the prevalence of global childhood and adolescent victimization from cyberbullying ranges from 13.99 to 57.5%, and that the perpetration prevalence ranges from 6.0 to 46.3%. Across the studies included in our research, verbal violence is observed as one of the most common acts of cyberbullying, including verbal offensive responses, insults, mocking, threats, slander, and harassment. The victimization prevalence of verbal violence is reported to be between 5 and 47.5%, and the perpetration prevalence is between 3.2 and 26.1%. Personal factors, such as gender, frequent use of social media platforms, depression, borderline personality disorder, eating disorders, sleep deprivation, and suicidal tendencies, were generally considered to be related to becoming a cyberbullying victim. Personal factors, such as high school students, past experiences, impulse, improperly controlled family education, poor teacher-student relationships, and the urban environment, were considered risk factors for cyberbullying perpetration. Situational factors, including parental abuse and neglect, improper monitoring, communication barriers between parents and children, as well as the urban environment, were also seen to potentially contribute to higher risks of both cyberbullying victimization and perpetration.
Increasing Prevalence of Global Cyberbullying With Changing Social Media Landscape and Measurement Alterations
This comprehensive review suggests that global cyberbullying rates, in terms of victimization and perpetration, were on the rise during the 5 year period, from 2015 to 2019. For example, in an earlier study conducted by Modecki et al. the average cyberbullying involvement rate was 15% ( 81 ). Similar observations were made by Hamm et al. who found that the median rates of youth having experienced bullying or who had bullied others online, was 23 and 15.2%, respectively ( 82 ). However, our systematic review summarized global children and adolescents cyberbullying in the last 5 years and revealed an average cyberbullying perpetration rate of 25.03%, ranging from 6.0 to 46.3%, while the average victimization was 33.08%, ranging from 13.99 to 57.5%. The underlying reason for increases may be attributed to the rapid changing landscape of social media and, in recent years, the drastic increase in Internet penetration rates. With the rise in Internet access, youths have greater opportunities to participate in online activities, provided by emerging social media platforms.
Although our review aims to provide a broader picture of cyberbullying, it is well-noted in extant research that difficulties exist in accurately estimating variations in prevalence in different countries ( 23 , 83 ). Many reasons exist to explain this. The first largely relates poor or unclear definition of the term cyberbullying; this hinders the determination of cyberbullying victimization and perpetration ( 84 ). Although traditional bullying behavior is well-defined, the definition cannot directly be applied to the virtual environment due to the complexity in changing online interactions. Without consensus on definitions, measurement and cyberbullying types may vary noticeably ( 83 , 85 ). Secondly, the estimation of prevalence of cyberbullying is heavily affected by research methods, such as recall period (lifetime, last year, last 6 months, last month, or last week etc.), demographic characteristics of the survey sample (age, gender, race, etc.), perspectives of cyberbullying experiences (victims, perpetrators, or both victim and perpetrator), and instruments (scales, study-specific questions) ( 23 , 84 , 86 ). The variety in research tools and instruments used to assess the prevalence of cyberbullying can cause confusion on this issue ( 84 ). Thirdly, variations in economic development, cultural backgrounds, human values, internet penetration rates, and frequency of using social media may lead to different conclusions across countries ( 87 ).
Acknowledging the Conflicting Role of the Identified Risk Factors With More Research Needed to Establish the Causality
Although this review has identified many personal and situational factors associated with cyberbullying, the majority of studies adopted a cross-sectional design and failed to reveal the causality ( 21 ). Nevertheless, knowledge on these correlational relationships provide valuable insights for understanding and preventing cyberbullying incidents. In terms of gender differences, females are believed to be at a higher risk of cyberbullying victimization compared to males. Two reasons may help to explain this. First, the preferred violence behaviors between two genders. females prefer indirect harassment, such as the spreading of rumors, while males tend toward direct bullying (e.g., assault) ( 29 ) and second, the cultural factors. From the traditional gender perspective, females tended to perceive a greater risk of communicating with others on the Internet, while males were more reluctant to express fear, vulnerability and insecurity when asked about their cyberbullying experiences ( 46 ). Females were more intolerant when experiencing cyberstalking and were more likely to report victimization experiences than males ( 13 ). Meanwhile, many researchers suggested that females are frequent users of emerging digital communication platforms, which increases their risk of unpleasant interpersonal contact and violence. From the perspective of cultural norms and masculinity, the reporting of cyberbullying is also widely acknowledged ( 37 ). For example, in addition, engaging in online activities is also regarded as a critical predictor for cyberbullying victimization. Enabled by the Internet, youths can easily find potential victims and start harassment at any time ( 49 ). Participating in online activities directly increases the chance of experiencing cyberbullying victimization and the possibility of becoming a victim ( 36 , 45 ). As for age, earlier involvement on social media and instant messaging tools may increase the chances of experiencing cyberbullying. For example, in Spain, these tools cannot be used without parental permission before the age of 14 ( 55 ). Besides, senior students were more likely to be more impulsive and less sympathetic. They may portray more aggressive and anti-social behaviors ( 55 , 72 ); hence senior students and students with higher impulsivity were usually more likely to become cyberbullying perpetrators.
Past experiences of victimization and family-related factors are another risk for cyberbullying crime. As for past experiences, one possible explanation is that young people who had experienced online or traditional school bullying may commit cyberbullying using e-mails, instant messages, and text messages for revenge, self-protection, or improving their social status ( 35 , 42 , 49 , 55 ). In becoming a cyberbullying perpetrator, the student may feel more powerful and superior, externalizing angry feelings and relieving the feelings of helplessness and sadness produced by past victimization experiences ( 51 ). As for family related factors, parenting styles are proven to be highly correlated to cyberbullying. In authoritative families, parents focus on rational behavioral control with clear rules and a high component of supervision and parental warmth, which have beneficial effects on children's lifestyles ( 43 ). Conversely, in indulgent families, children's behaviors are not heavily restricted and parents guide and encourage their children to adapt to society. The characteristics of this indulgent style, including parental support, positive communication, low imposition, and emotional expressiveness, possibly contribute to more parent-child trust and less misunderstanding ( 75 ). The protective role of warmth/affection and appropriate supervision, which are common features of authoritative or indulgent parenting styles, mitigate youth engagement in cyberbullying. On the contrary, authoritarian and neglectful styles, whether with excessive or insufficient control, are both proven to be risk factors for being a target of cyberbullying ( 33 , 76 ). In terms of geographical location, although several studies found that children residing in urban areas were more likely to be cyberbullying victims than those living in rural or suburban areas, we cannot draw a quick conclusion here, since whether this difference attributes to macro-level differences, such as community safety or socioeconomic status, or micro-level differences, such as teacher intervention in the classroom, courses provided, teacher-student ratio, is unclear across studies ( 61 ). An alternative explanation for this is the higher internet usage rate in urban areas ( 49 ).
Regarding health conditions, especially mental health, some scholars believe that young people with health problems are more likely to be identified as victims than people without health problems. They perceive health condition as a risk factor for cyberbullying ( 61 , 63 ). On the other hand, another group of scholars believe that cyberbullying has an important impact on the mental health of adolescents which can cause psychological distress consequences, such as post-traumatic stress mental disorder, depression, suicidal ideation, and drug abuse ( 70 , 87 ). It is highly possible that mental health could be risk factors, consequences of cyberbullying or both. Mental health cannot be used as standards, requirements, or decisive responses in cyberbullying research ( 13 ).
The Joint Effort Between Youth, Parents, Schools, and Communities to Form a Cyberbullying-Free Environment
This comprehensive review suggests that protecting children and adolescents from cyberbullying requires joint efforts between individuals, parents, schools, and communities, to form a cyberbullying-free environment. For individuals, young people are expected to improve their digital technology capabilities, especially in the use of social media platforms and instant messaging tools ( 55 ). To reduce the number of cyberbullying perpetrators, it is necessary to cultivate emotional self-regulation ability through appropriate emotional management training. Moreover, teachers, counselors, and parents are required to be armed with sufficient knowledge of emotional management and to develop emotional management capabilities and skills. In this way, they can be alert to the aggressive or angry emotions expressed by young people, and help them mediate any negative emotions ( 45 ), and avoid further anti-social behaviors ( 57 ).
For parents, styles of parenting involving a high level of parental involvement, care and support, are desirable in reducing the possibility of children's engagement in cyberbullying ( 74 , 75 ). If difficulties are encountered, open communication can contribute to enhancing the sense of security ( 73 ). In this vein, parents should be aware of the importance of caring, communicating and supervising their children, and participate actively in their children's lives ( 71 ). In order to keep a balance between control and openness ( 47 ), parents can engage in unbiased open communication with their children, and reach an agreement on the usage of computers and smart phones ( 34 , 35 , 55 ). Similarly, it is of vital importance to establish a positive communication channel with children ( 19 ).
For schools, a higher priority is needed to create a safe and positive campus environment, providing students with learning opportunities and ensuring that every student is treated equally. With a youth-friendly environment, students are able to focus more on their academic performance and develop a strong sense of belonging to the school ( 79 ). For countries recognizing collectivist cultural values, such as China and India, emphasizing peer attachment and a sense of collectivism can reduce the risk of cyberbullying perpetration and victimization ( 78 ). Besides, schools can cooperate with mental health agencies and neighboring communities to develop preventive programs, such as extracurricular activities and training ( 44 , 53 , 62 ). Specifically, school-based preventive measures against cyberbullying are expected to be sensitive to the characteristics of young people at different ages, and the intersection of race and school diversity ( 29 , 76 ). It is recommended that school policies that aim to embrace diversity and embody mutual respect among students are created ( 26 ). Considering the high prevalence of cyberbullying and a series of serious consequences, it is suggested that intervention against cyberbullying starts from an early stage, at about 10 years old ( 54 ). Schools can organize seminars to strengthen communication between teachers and students so that they can better understand the needs of students ( 61 ). In addition, schools should encourage cyberbullying victims to seek help and provide students with opportunities to report cyberbullying behaviors, such as creating online anonymous calls.
Conclusions and Limitations
The comprehensive study has reviewed related research on children and adolescents cyberbullying across different countries and regions, providing a positive understanding of the current situation of cyberbullying. The number of studies on cyberbullying has surged in the last 5 years, especially those related to risk factors and protective factors of cyberbullying. However, research on effective prevention is insufficient and evaluation of policy tools for cyberbullying intervention is a nascent research field. Our comprehensive review concludes with possible strategies for cyberbullying prevention, including personal emotion management, digital ability training, policy applicability, and interpersonal skills. We highlight the important role of parental control in cyberbullying prevention. As for the role of parental control, it depends on whether children believe their parents are capable of adequately supporting them, rather than simply interfering in their lives, restricting their online behavior, and controlling or removing their devices ( 50 ). In general, cyberbullying is on the rise, with the effectiveness of interventions to meet this problem still requiring further development and exploration ( 83 ).
Considering the overlaps between cyberbullying and traditional offline bullying, future research can explore the unique risk and protective factors that are distinguishable from traditional bullying ( 86 ). To further reveal the variations, researchers can compare the outcomes of interventions conducted in cyberbullying and traditional bullying preventions simultaneously, and the same interventions only targeting cyberbullying ( 88 ). In addition, cyberbullying also reflects a series of other social issues, such as personal privacy and security, public opinion monitoring, multinational perpetration and group crimes. To address this problem, efforts from multiple disciplines and novel analytical methods in the digital era are required. As the Internet provides enormous opportunities to connect young people from all over the world, cyberbullying perpetrators may come from transnational networks. Hence, cyberbullying of children and adolescents, involving multiple countries, is worth further attention.
Our study has several limitations. First, national representative studies are scarce, while few studies from middle and low income countries were included in our research due to language restrictions. Many of the studies included were conducted in schools, communities, provinces, and cities in high income countries. Meanwhile, our review only focused on victimization and perpetration. Future studies should consider more perspectives, such as bystanders and those with the dual identity of victim/perpetrator, to comprehensively analyze the risk and protective factors of cyberbullying.
Data Availability Statement
The original contributions presented in the study are included in the article/ Supplementary Material , further inquiries can be directed to the corresponding author/s.
Author Contributions
SH, CZ, RE, and WZ conceived the study and developed the design. WZ analyzed the result and supervised the study. CZ and SH wrote the first draft. All authors contributed to the article and approved the submitted version.
Conflict of Interest
The authors declare that the research was conducted in the absence of any commercial or financial relationships that could be construed as a potential conflict of interest.
Supplementary Material
The Supplementary Material for this article can be found online at: https://www.frontiersin.org/articles/10.3389/fpubh.2021.634909/full#supplementary-material
1. Ang RP. Adolescent cyberbullying: a review of characteristics, prevention and intervention strategies. Aggress Violent Behav. (2015) 25:35–42. doi: 10.1016/j.avb.2015.07.011
CrossRef Full Text | Google Scholar
2. Reyna VF, Farley F. Risk and rationality in adolescent decision making: implications for theory, practice, and public policy. Psychol Sci Public Interest. (2006) 7:1–44. doi: 10.1111/j.1529-1006.2006.00026.x
PubMed Abstract | CrossRef Full Text | Google Scholar
3. UNICEF ed. Children in a Digital World . New York, NY: UNICEF (2017).
Google Scholar
4. Thomas HJ, Connor JP, Scott JG. Integrating traditional bullying and cyberbullying: challenges of definition and measurement in adolescents - a review. Educ Psychol Rev. (2015) 27:135–52. doi: 10.1007/s10648-014-9261-7
5. Baldry AC, Farrington DP, Sorrentino A. “Am I at risk of cyberbullying”? A narrative review and conceptual framework for research on risk of cyberbullying and cybervictimization: the risk and needs assessment approach. Aggress Violent Behav. (2015) 23:36–51. doi: 10.1016/j.avb.2015.05.014
6. Olweus D. Bullying at School: What We Know and What We Can Do . Oxford; Cambridge, MA: Blackwell (1993).
PubMed Abstract | Google Scholar
7. Dooley JJ, Pyzalski J, Cross D. Cyberbullying versus face-to-face bullying: a theoretical and conceptual review. J Psychol. (2009) 217:182–8. doi: 10.1027/0044-3409.217.4.182
8. Smith PK, Mahdavi J, Carvalho M, Fisher S, Russell S, Tippett N. Cyberbullying: its nature and impact in secondary school pupils. J Child Psychol Psychiatry. (2008) 49:376–85. doi: 10.1111/j.1469-7610.2007.01846.x
9. Kowalski RM, Giumetti GW, Schroeder AN, Lattanner MR. Bullying in the digital age: a critical review and meta-analysis of cyberbullying research among youth. Psychol Bull. (2014) 140:1073–137. doi: 10.1037/a0035618
10. León Vicente I. Cybervictimization by cyberbullying: children at risk and children as risk (dissertation). University of the Basque Country, Leioa, Spain (2016).
11. Hinduja S, Patchin JW. Identification, Prevention, and Response. (2020).
12. Jadambaa A, Thomas HJ, Scott JG, Graves N, Brain D, Pacella R. Prevalence of traditional bullying and cyberbullying among children and adolescents in Australia: a systematic review and meta-analysis. Aust N Z J Psychiatry. (2019) 53:878–88. doi: 10.1177/0004867419846393
13. Pereira F, Matos M. Cyber-stalking victimization: what predicts fear among Portuguese adolescents? Eur J Crim Policy Res. (2016) 22:253–70. doi: 10.1007/s10610-015-9285-7
14. Reed LA, Ward LM, Tolman RM, Lippman JR, Seabrook RC. The association between stereotypical gender and dating beliefs and digital dating abuse perpetration in adolescent dating relationships. J Interpers Violence . (2018). doi: 10.1177/0886260518801933
15. Huang CL, Yang SC, Hsieh LS. The cyberbullying behavior of Taiwanese adolescents in an online gaming environment. Children Youth Serv Rev. (2019) 106:104461. doi: 10.1016/j.childyouth.2019.104461
16. Raskauskas J, Huynh A. The process of coping with cyberbullying: a systematic review. Aggress Violent Behav. (2015) 23:118–25. doi: 10.1016/j.avb.2015.05.019
17. Bradshaw J, Crous G, Rees G, Turner N. Comparing children's experiences of schools-based bullying across countries. Children Youth Serv Rev. (2017) 80:171–80. doi: 10.1016/j.childyouth.2017.06.060
18. Hutson E, Kelly S, Militello LK. Systematic review of cyberbullying interventions for youth and parents with implications for evidence-based practice. Worldviews Evid Based Nurs. (2018) 15:72–9. doi: 10.1111/wvn.12257
19. Larranaga E, Yubero S, Ovejero A, Navarro R. Loneliness, parent-child communication and cyberbullying victimization among Spanish youths. Comp Hum Behav. (2016) 65:1–8. doi: 10.1016/j.chb.2016.08.015
20. van Geel M, Vedder P, Tanilon J. Relationship between peer victimization, cyberbullying, and suicide in children and adolescents: a meta-analysis. JAMA Pediatr. (2014) 168:435–42. doi: 10.1001/jamapediatrics.2013.4143
21. Zych I, Ortega-Ruiz R, Del Rey R. Systematic review of theoretical studies on bullying and cyberbullying: facts, knowledge, prevention, and intervention. Aggress Violent Behav. (2015) 23:1–21. doi: 10.1016/j.avb.2015.10.001
22. Selkie EM, Kota R, Chan Y-F, Moreno M. Cyberbullying, depression, and problem alcohol use in female college students: a multisite study. Cyberpsychol Behav Soc Netw. (2015) 18:79–86. doi: 10.1089/cyber.2014.0371
23. Brochado S, Soares S, Fraga S. A scoping review on studies of cyberbullying prevalence among adolescents. Trauma Violence Abus. (2017) 18:523–31. doi: 10.1177/1524838016641668
24. Nocentini A, Calmaestra J, Schultze-Krumbholz A, Scheithauer H, Ortega R, Menesini E. Cyberbullying: labels, behaviours and definition in three European Countries. Aust J Guid Couns. (2010) 20:129–42. doi: 10.1375/ajgc.20.2.129
25. Willard NE. Cyberbullying and Cyberthreats: Responding to the Challenge of Online Social Aggression, Threats, and Distress . Champaign: Research Press (2007).
26. Aizenkot D, Kashy-Rosenbaum G. Cyberbullying victimization in whatsapp classmate groups among Israeli Elementary, Middle, and High School Students. J Interpers Violence . (2019). doi: 10.1177/0886260519842860
27. Anderson CA, Bushman BJ. Human aggression. Ann Rev Psychol. (2002) 53:27–51. doi: 10.1146/annurev.psych.53.100901.135231
28. Higgins JP, Green S. Cochrane Handbook for Systematic Reviews of Interventions . (2011). Available online at: http://handbook-5-1.cochrane.org/ (accessed January 17, 2021).
29. Alhajji M, Bass S, Dai T. Cyberbullying, mental health, and violence in adolescents and associations with sex and race: data from the 2015 youth risk behavior survey. Global Pediatr Health . (2019) 6:2333794X19868887. doi: 10.1177/2333794X19868887
30. Grinshteyn E, Yang YT. The association between electronic bullying and school absenteeism among high school students in the United States. J School Health. (2017) 87:142–9. doi: 10.1111/josh.12476
31. Mesch GS. Parent-child connections on social networking sites and cyberbullying. Youth Soc. (2018) 50:1145–62. doi: 10.1177/0044118X16659685
32. Sam J, Wisniewski P, Xu H, Rosson MB, Carroll JM. How are social capital and parental mediation associated with cyberbullying and cybervictimization among youth in the United States? In: Stephanidis C, editor. HCI International 2017 – Posters' Extended Abstracts Communications in Computer Information Science Cham: Springer International Publishing. p. 638–644.
33. Hong JS, Kim DH, Thornberg R, Kang JH, Morgan JT. Correlates of direct and indirect forms of cyberbullying victimization involving South Korean adolescents: an ecological perspective. Comput Hum Behav. (2018) 87:327–36. doi: 10.1016/j.chb.2018.06.010
34. Lee C, Shin N. Prevalence of cyberbullying and predictors of cyberbullying perpetration among Korean adolescents. Comp Hum Behav. (2017) 68:352–8. doi: 10.1016/j.chb.2016.11.047
35. You S, Lim SA. Longitudinal predictors of cyberbullying perpetration: evidence from Korean middle school students. Person Ind Differ. (2016) 89:172–6. doi: 10.1016/j.paid.2015.10.019
36. Holt TJ, Fitzgerald S, Bossler AM, Chee G, Ng E. Assessing the risk factors of cyber and mobile phone bullying victimization in a nationally representative sample of Singapore Youth. Int J Offend Ther Comp Criminol. (2016) 60:598–615. doi: 10.1177/0306624X14554852
37. Marret MJ, Choo WY. Factors associated with online victimisation among Malaysian adolescents who use social networking sites: a cross-sectional study. BMJ Open. (2017) 7:e014959. doi: 10.1136/bmjopen-2016-014959
38. Tesler R, Nissanholtz-Gannot R, Zigdon A, Harel-Fisch Y. The association of cyber-bullying and adolescents in religious and secular schools in Israel. J Relig Health. (2019) 58:2095–109. doi: 10.1007/s10943-019-00938-z
39. Beran T, Mishna F, McInroy LB, Shariff S. Children's experiences of cyberbullying: a Canadian National Study. Child School. (2015) 37:207–14. doi: 10.1093/cs/cdv024
40. World Bank Country and Lending Groups – World Bank Data Help Desk. Available online at: https://datahelpdesk.worldbank.org/knowledgebase/articles/906519-world-bank-country-and-lending-groups [accessed January 25, 2021).
41. Marco JH, Tormo-Irun P. Cyber victimization is associated with eating disorder psychopathology in adolescents. Front Psychol. (2018) 9:987. doi: 10.3389/fpsyg.2018.00987
42. Olenik-Shemesh D, Heiman T. Cyberbullying victimization in adolescents as related to body esteem, social support, and social self-efficacy. J Genet Psychol. (2017) 178:28–43. doi: 10.1080/00221325.2016.1195331
43. Rao J, Wang H, Pang M, Yang J, Zhang J, Ye Y, et al. Cyberbullying perpetration and victimisation among junior and senior high school students in Guangzhou, China. Inj Prev. (2019) 25:13–9. doi: 10.1136/injuryprev-2016-042210
44. Lee C. Weak Commitment to School, Deviant Peers, and Cyberbullying Victimization-Strain in Adolescent Cyberbullying . (2017). Available online at: https://search.proquest.com/pqdtglobal/docview/1937915933/abstract/9D85437600564444PQ/30 (accessed June 17, 2020).
45. Lin MT. Risk factors associated with cyberbullying victimization and perpetration among Taiwan children (dissertation). The University of Texas, Austin, TX, United States (2019).
46. Pieschl S, Porsch T. The complex relationship between cyberbullying and trust. Int J Dev Sci. (2017) 11:9–17. doi: 10.3233/DEV-160208
47. Brighi A, Menin D, Skrzypiec G, Guarini A. Young, bullying, and connected. Common pathways to cyberbullying and problematic internet use in adolescence. Front Psychol. (2019) 10:1467. doi: 10.3389/fpsyg.2019.01467
48. Festl R. Perpetrators on the internet: analyzing individual and structural explanation factors of cyberbullying in school context. Comp Hum Behav. (2016) 59:237–48. doi: 10.1016/j.chb.2016.02.017
49. Olumide AO, Adams P, Amodu OK. Prevalence and correlates of the perpetration of cyberbullying among in-school adolescents in Oyo State, Nigeria. Int J Adolesc Med Health. (2016) 28:183–91. doi: 10.1515/ijamh-2015-0009
50. Baldry AC, Sorrentino A, Farrington DP. Cyberbullying and cybervictimization versus parental supervision, monitoring and control of adolescents' online activities. Child Youth Serv Rev. (2019) 96:302–7. doi: 10.1016/j.childyouth.2018.11.058
51. Garaigordobil M. Cyberbullying in adolescents and youth in the Basque Country: prevalence of cybervictims, cyberaggressors, and cyberobservers. J Youth Stud. (2015) 18:569–82. doi: 10.1080/13676261.2014.992324
52. Morin HK, Bradshaw CP, Kush JM. Adjustment outcomes of victims of cyberbullying: the role of personal and contextual factors. J School Psychol. (2018) 70:74–88. doi: 10.1016/j.jsp.2018.07.002
53. Baraldsnes D. The prevalence of cyberbullying and the views of 5-12 grade pupils and teachers on cyberbullying prevention in Lithuanian Schools. Uinv J Educ Res. (2015) 3:949–59. doi: 10.13189/ujer.2015.031201
54. Razjouyan K, Mobarake AH, Sadr SS, Ardestani SMS, Yaseri M. The relationship between emotional intelligence and the different roles in cyberbullying among high school students in Tehran. Iran J Psychiatry Behav Sci. (2018) 12:UNSP e11560. doi: 10.5812/ijpbs.11560
55. Alvarez-Garcia D, Carlos Nunez J, Garcia T, Barreiro-Collazo A. Individual, family, and community predictors of cyber-aggression among adolescents. Eur J Psychol Appl Legal Context. (2018) 10:79–88. doi: 10.5093/ejpalc2018a8
56. Horzum MB, Ayas T, Randler C, Dusunceli B. The effects of empathy and circadian preference on cyberbullying of adolescents in Turkey. Biol Rhythm Res . (2019). doi: 10.1080/09291016.2019.1603839
57. Carmen Martinez-Monteagudo M, Delgado B, Manuel Garcia-Fernandez J, Rubio E. Cyberbullying, aggressiveness, and emotional intelligence in adolescence. Int J Environ Res Public Health. (2019) 16:5079. doi: 10.3390/ijerph16245079
58. Sasson H, Mesch G. The role of parental mediation and peer norms on the likelihood of cyberbullying. J Genet Psychol. (2017) 178:15–27. doi: 10.1080/00221325.2016.1195330
59. Wang X, Lei L, Liu D, Hu H. Moderating effects of moral reasoning and gender on the relation between moral disengagement and cyberbullying in adolescents. Person Ind Differ. (2016) 98:244–9. doi: 10.1016/j.paid.2016.04.056
60. Simsek N, Sahin D, Evli M. Internet addiction, cyberbullying, and victimization relationship in adolescents a sample from Turkey. J Addict Nurs. (2019) 30:201–10. doi: 10.1097/JAN.0000000000000296
61. McQuillan BE. Ecological Factors Associated with Middle School Students' Experiences of Cyberbullying . (2016). Available online at: https://search.proquest.com/pqdtglobal/docview/1794167537/abstract/9D85437600564444PQ/4 (accessed June 17, 2020).
62. Rose CA, Tynes BM. Longitudinal associations between cybervictimization and mental health among U.S. adolescents. J Adolesc Health. (2015) 57:305–12. doi: 10.1016/j.jadohealth.2015.05.002
63. Stockdale LA, Coyne SM, Nelson DA, Erickson DH. Borderline personality disorder features, jealousy, and cyberbullying in adolescence. Pers Individ Differ. (2015) 83:148–53. doi: 10.1016/j.paid.2015.04.003
64. Chen Q, Lo Camilla KM, Yuhong Z, Anne C, Ling CK, Patrick I. Family poly-victimization and cyberbullying among adolescents in a Chinese school sample. Child Abuse Negl. (2018) 77:180–7. doi: 10.1016/j.chiabu.2018.01.015
65. Landoll RR, La Greca AM, Lai BS, Chan SF, Herge WM. Cyber victimization by peers: prospective associations with adolescent social anxiety and depressive symptoms. J Adolesc. (2015) 42:77–86. doi: 10.1016/j.adolescence.2015.04.002
66. Iranzo B, Buelga S, Cava M-J, Ortega-Baron J. Cyberbullying, psychosocial adjustment, and suicidal ideation in adolescence. Psychosoc Interv. (2019) 28:75–81. doi: 10.5093/pi2019a5
67. Buelga S, Cava MJ, Musitu G, Torralba E. Cyberbullying aggressors among Spanish secondary education students: an exploratory study. Interact Tech Smart Ed. (2015) 12:100–15. doi: 10.1108/ITSE-08-2014-0025
68. Katz I, Lemish D, Cohen R, Arden A. When parents are inconsistent: parenting style and adolescents' involvement in cyberbullying. J Adolesc. (2019) 74:1–2. doi: 10.1016/j.adolescence.2019.04.006
69. Cénat JM, Blais M, Lavoie F, Caron P-O, Hébert M. Cyberbullying victimization and substance use among Quebec high schools students: the mediating role of psychological distress. Comp Hum Behav. (2018) 89:207–12. doi: 10.1016/j.chb.2018.08.014
70. Hoareau N, Bages C, Allaire M, Guerrien A. The role of psychopathic traits and moral disengagement in cyberbullying among adolescents. Crim Behav Ment Health. (2019) 29:321–31. doi: 10.1002/cbm.2135
71. Khurana A, Bleakley A, Jordan A, Romer D. The protective effects of parental monitoring and internet restriction on adolescents' risk of online harassment. J Youth Adolesc. (2015) 44:1039–47. doi: 10.1007/s10964-014-0242-4
72. Martínez I, Murgui S, Garcia OF, Garcia F. Parenting in the digital era: protective and risk parenting styles for traditional bullying and cyberbullying victimization. Comp Hum Behav. (2019) 90:84–92. doi: 10.1016/j.chb.2018.08.036
73. Yusuf S, Salleh H, Bahaman A, Shamsul M, Ramli N, Ramli AN, et al. Parental attachment and cyberbullying experiences among Malaysian children. Pertanika J Scholarly Res Rev . (2018) 4:67–80.
74. Martinez-Ferrer B, Leon-Moreno C, Musitu-Ferrer D, Romero-Abrio A, Callejas-Jeronimo JE, Musitu-Ochoa G. Parental socialization, school adjustment and cyber-aggression among adolescents. Int J Environ Res Public Health. (2019) 16:4005. doi: 10.3390/ijerph16204005
75. Moreno–Ruiz D, Martínez–Ferrer B, García–Bacete F. Parenting styles, cyberaggression, and cybervictimization among adolescents. Comp Hum Behav. (2019) 93:252–9. doi: 10.1016/j.chb.2018.12.031
76. Ho SS, Chen L, Ng APY. Comparing cyberbullying perpetration on social media between primary and secondary school students. Comp Educ. (2017) 109:74–84. doi: 10.1016/j.compedu.2017.02.004
77. Gómez-Ortiz O, Romera EM, Ortega-Ruiz R, Del Rey R. Parenting practices as risk or preventive factors for adolescent involvement in cyberbullying: contribution of children and parent gender. Int J Environ Res Public Health. (2018) 15:2664. doi: 10.3390/ijerph15122664
78. Wright MF, Kamble SV, Soudi SP. Indian adolescents' cyber aggression involvement and cultural values: the moderation of peer attachment. Sch Psychol Int. (2015) 36:410–27. doi: 10.1177/0143034315584696
79. Holfeld B, Leadbeater BJ. Concurrent and longitudinal associations between early adolescents' experiences of school climate and cyber victimization. Comput Hum Behav. (2017) 76:321–8. doi: 10.1016/j.chb.2017.07.037
80. Álvarez-García D, Núñez JC, González-Castro P, Rodríguez C, Cerezo R. The effect of parental control on cyber-victimization in adolescence: the mediating role of impulsivity and high-risk behaviors. Front Psychol. (2019) 10:1159. doi: 10.3389/fpsyg.2019.01159
81. Modecki KL, Minchin J, Harbaugh AG, Guerra NG, Runions KC. Bullying prevalence across contexts: a meta-analysis measuring cyber and traditional bullying. J Adolesc Health. (2014) 55:602–11. doi: 10.1016/j.jadohealth.2014.06.007
82. Hamm MP, Newton AS, Chisholm A, Shulhan J, Milne A, Sundar P, et al. Prevalence and effect of cyberbullying on children and young people: a scoping review of social media studies. JAMA Pediatr. (2015) 169:770. doi: 10.1001/jamapediatrics.2015.0944
83. Gaffney H, Farrington DP, Espelage DL, Ttofi MM. Are cyberbullying intervention and prevention programs effective? A systematic and meta-analytical review. Aggress Violent Behav. (2019) 45:134–53. doi: 10.1016/j.avb.2018.07.002
84. Selkie EM, Fales JL, Moreno MA. Cyberbullying prevalence among US middle and high school-aged adolescents: a systematic review and quality assessment. J Adolesc Health. (2016) 58:125–33. doi: 10.1016/j.jadohealth.2015.09.026
85. Ybarra ML, Boyd D, Korchmaros JD, Oppenheim J. Defining and measuring cyberbullying within the larger context of bullying victimization. J Adolesc Health. (2012) 51:53–8. doi: 10.1016/j.jadohealth.2011.12.031
86. Kowalski RM, Limber SP, McCord A. A developmental approach to cyberbullying: prevalence and protective factors. Aggress Violent Behav. (2019) 45:20–32. doi: 10.1016/j.avb.2018.02.009
87. Dilmac B, Yurt E, Aydin M, Kasarci I. Predictive relationship between humane values of adolescents cyberbullying and cyberbullying sensibility. Electron J Res Educ Psychol. (2016) 14:3–22. doi: 10.14204/ejrep.38.14123
88. Reed KP, Cooper RL, Nugent WR, Russell K. Cyberbullying: a literature review of its relationship to adolescent depression and current intervention strategies. J Hum Behav Soc Environ. (2016) 26:37–45. doi: 10.1080/10911359.2015.1059165
Keywords: cyberbullying, children, adolescents, globalization, risk factors, preventive measures
Citation: Zhu C, Huang S, Evans R and Zhang W (2021) Cyberbullying Among Adolescents and Children: A Comprehensive Review of the Global Situation, Risk Factors, and Preventive Measures. Front. Public Health 9:634909. doi: 10.3389/fpubh.2021.634909
Received: 29 November 2020; Accepted: 10 February 2021; Published: 11 March 2021.
Reviewed by:
Copyright © 2021 Zhu, Huang, Evans and Zhang. This is an open-access article distributed under the terms of the Creative Commons Attribution License (CC BY) . The use, distribution or reproduction in other forums is permitted, provided the original author(s) and the copyright owner(s) are credited and that the original publication in this journal is cited, in accordance with accepted academic practice. No use, distribution or reproduction is permitted which does not comply with these terms.
*Correspondence: Wei Zhang, weizhanghust@hust.edu.cn
† These authors have contributed equally to this work and share first authorship
Disclaimer: All claims expressed in this article are solely those of the authors and do not necessarily represent those of their affiliated organizations, or those of the publisher, the editors and the reviewers. Any product that may be evaluated in this article or claim that may be made by its manufacturer is not guaranteed or endorsed by the publisher.
A Survey About the Cyberbullying Problem on Social Media by Using Machine Learning Approaches
- Conference paper
- First Online: 21 February 2021
- Cite this conference paper
- Carlo Sansone ORCID: orcid.org/0000-0002-8176-6950 16 &
- Giancarlo Sperlí ORCID: orcid.org/0000-0003-4033-3777 16
Part of the book series: Lecture Notes in Computer Science ((LNIP,volume 12667))
Included in the following conference series:
- International Conference on Pattern Recognition
2001 Accesses
The exponential growth of connected devices (i.e. laptops, smartphones or tablets) has radically changed communications means, also making it faster and impersonal by using On-line Social Networks and Instant messaging through several apps. In this paper we discuss about the cyberbullying problem, focusing on the analysis of the state-of-the-art approaches that can be classified in four different tasks ( Binary Classification , Role Identification , Severity Score Computation and Incident prediction ). In particular, the first task aims to predict if a particular action is aggressive or not based on the analysis of different features. In turn, the second and the third task investigate the cyberbullying problem by identifying users’ role in the exchanged message or assigning a severity score to a given users or session respectively. Nevertheless, information heterogeneity, due to different multimedia contents (i.e. text, emojis, stickers or gifs), and the use of datasets, which are typically unlabeled or manually labelled, create continuous challenges in addressing the cyberbullying problem.
This is a preview of subscription content, log in via an institution to check access.
Access this chapter
Subscribe and save.
- Get 10 units per month
- Download Article/Chapter or eBook
- 1 Unit = 1 Article or 1 Chapter
- Cancel anytime
- Available as PDF
- Read on any device
- Instant download
- Own it forever
- Available as EPUB and PDF
- Compact, lightweight edition
- Dispatched in 3 to 5 business days
- Free shipping worldwide - see info
Tax calculation will be finalised at checkout
Purchases are for personal use only
Institutional subscriptions
Similar content being viewed by others
A Survey on Detection of Cyberbullying in Social Media Using Machine Learning Techniques
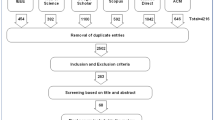
Cyberbullying detection and machine learning: a systematic literature review
Cyberbullying Instilled in Social Media
https://facebook.com/ .
https://ask.fm/ .
http://www.bullyingstatistics.org/category/bullying-statistics .
http://websites.psychology.uwa.edu.au/school/MRCDatabase/mrc2.html .
https://www.kaggle.com/swetaagrawal/formspring-data-for-cyberbullying-detection .
https://www.chatcoder.com/drupal/index.php .
http://www.cucybersafety.org/home/cyberbullying-detection-project/dataset .
Balakrishnan, V., Khan, S., Arabnia, H.R.: Improving cyberbullying detection using Twitter users’ psychological features and machine learning. Comput. Secur. 101710 (2020)
Google Scholar
Calvo-Morata, A., Rotaru, D.C., Alonso-Fernández, C., Freire, M., Martínez-Ortiz, I., Fernández-Manjón, B.: Validation of a cyberbullying serious game using game analytics. IEEE Trans. Learn. Technol. (2018)
Chatzakou, D., et al.: Detecting cyberbullying and cyberaggression in social media. ACM Trans. Web (TWEB) 13 (3), 1–51 (2019)
Article Google Scholar
Cheng, L., Guo, R., Silva, Y., Hall, D., Liu, H.: Hierarchical attention networks for cyberbullying detection on the Instagram social network. In: Proceedings of the 2019 SIAM International Conference on Data Mining, pp. 235–243. SIAM (2019)
Cheng, L., Li, J., Silva, Y.N., Hall, D.L., Liu, H.: XBully: cyberbullying detection within a multi-modal context. In: Proceedings of the Twelfth ACM International Conference on Web Search and Data Mining, pp. 339–347 (2019)
Di Capua, M., Di Nardo, E., Petrosino, A.: Unsupervised cyber bullying detection in social networks. In: 2016 23rd International Conference on Pattern Recognition (ICPR), pp. 432–437, December 2016. https://doi.org/10.1109/ICPR.2016.7899672
Dinakar, K., Jones, B., Havasi, C., Lieberman, H., Picard, R.: Common sense reasoning for detection, prevention, and mitigation of cyberbullying. ACM Trans. Interact. Intell. Syst. (TiiS) 2 (3), 18 (2012)
Farag, N., El-Seoud, S.A., McKee, G., Hassan, G.: Bullying hurts: a survey on non-supervised techniques for cyber-bullying detection. In: Proceedings of the 2019 8th International Conference on Software and Information Engineering, pp. 85–90 (2019)
Farley, S., Coyne, I., D’Cruz, P.: Cyberbullying at work: understanding the influence of technology (2018)
Hosseinmardi, H., Ghasemianlangroodi, A., Han, R., Lv, Q., Mishra, S.: Towards understanding cyberbullying behavior in a semi-anonymous social network. In: 2014 IEEE/ACM International Conference on Advances in Social Networks Analysis and Mining (ASONAM 2014), pp. 244–252. IEEE (2014)
Hosseinmardi, H., et al.: A comparison of common users across Instagram and Ask.fm to better understand cyberbullying. In: 2014 IEEE Fourth International Conference on Big Data and Cloud Computing, pp. 355–362. IEEE (2014)
Hosseinmardi, H., Mattson, S.A., Rafiq, R.I., Han, R., Lv, Q., Mishra, S.: Detection of cyberbullying incidents on the Instagram social network. In: Association for the Advancement of Artificial Intelligence (2015)
Hosseinmardi, H., Rafiq, R.I., Han, R., Lv, Q., Mishra, S.: Prediction of cyberbullying incidents in a media-based social network. In: 2016 IEEE/ACM International Conference on Advances in Social Networks Analysis and Mining (ASONAM), pp. 186–192. IEEE (2016)
Juvonen, J., Gross, E.F.: Extending the school grounds?-bullying experiences in cyberspace. J. Sch. Health 78 (9), 496–505 (2008)
Katzer, C., Fetchenhauer, D., Belschak, F.: Cyberbullying: who are the victims? A comparison of victimization in internet chatrooms and victimization in school. J. Media Psychol. 21 (1), 25–36 (2009)
Kowalski, R.M., Toth, A., Morgan, M.: Bullying and cyberbullying in adulthood and the workplace. J. Soc. Psychol. 158 (1), 64–81 (2018)
Kshetri, N., Voas, J.: Thoughts on cyberbullying. Computer 52 (4), 64–68 (2019)
Kumar, A., Sachdeva, N.: Cyberbullying detection on social multimedia using soft computing techniques: a meta-analysis. Multimed. Tools Appl. 78 (17), 23973–24010 (2019). https://doi.org/10.1007/s11042-019-7234-z
Kumari, K., Singh, J.P., Dwivedi, Y.K., Rana, N.P.: Towards cyberbullying-free social media in smart cities: a unified multi-modal approach. Soft Comput. 1–12 (2019)
Li, Q.: New bottle but old wine: a research of cyberbullying in schools. Comput. Hum. Behav. 23 (4), 1777–1791 (2007)
Newall, M.: Cyberbullying: A Global Advisor Survey. Ipsos (2018)
Patchin, J.W., Hinduja, S.: Bullies move beyond the schoolyard: a preliminary look at cyberbullying. Youth Violence Juvenile Justice 4 (2), 148–169 (2006)
Potha, N., Maragoudakis, M.: Time series forecasting in cyberbullying data. In: Iliadis, L., Jayne, C. (eds.) EANN 2015. CCIS, vol. 517, pp. 289–303. Springer, Cham (2015). https://doi.org/10.1007/978-3-319-23983-5_27
Chapter Google Scholar
Potha, N., Maragoudakis, M., Lyras, D.: A biology-inspired, data mining framework for extracting patterns in sexual cyberbullying data. Knowl.-Based Syst. 96 , 134–155 (2016)
Raisi, E., Huang, B.: Cyberbullying detection with weakly supervised machine learning. In: Proceedings of the 2017 IEEE/ACM International Conference on Advances in Social Networks Analysis and Mining 2017, pp. 409–416 (2017)
Raisi, E., Huang, B.: Weakly supervised cyberbullying detection using co-trained ensembles of embedding models. In: 2018 IEEE/ACM International Conference on Advances in Social Networks Analysis and Mining (ASONAM), pp. 479–486. IEEE (2018)
Rosa, H., Matos, D., Ribeiro, R., Coheur, L., Carvalho, J.P.: A “deeper” look at detecting cyberbullying in social networks. In: 2018 International Joint Conference on Neural Networks (IJCNN), pp. 1–8, July 2018. https://doi.org/10.1109/IJCNN.2018.8489211
Salawu, S., He, Y., Lumsden, J.: Approaches to automated detection of cyberbullying: a survey. IEEE Trans. Affect. Comput. (2017)
Schwartz, H.A., et al.: Personality, gender, and age in the language of social media: the open-vocabulary approach. PLoS ONE 8 (9), e73791 (2013)
Singh, V.K., Hofenbitzer, C.: Fairness across network positions in cyberbullying detection algorithms. In: Proceedings of the 2019 IEEE/ACM International Conference on Advances in Social Networks Analysis and Mining, pp. 557–559 (2019)
Squicciarini, A., Rajtmajer, S., Liu, Y., Griffin, C.: Identification and characterization of cyberbullying dynamics in an online social network. In: Proceedings of the 2015 IEEE/ACM International Conference on Advances in Social Networks Analysis and Mining 2015, pp. 280–285 (2015)
Tomkins, S., Getoor, L., Chen, Y., Zhang, Y.: A socio-linguistic model for cyberbullying detection. In: 2018 IEEE/ACM International Conference on Advances in Social Networks Analysis and Mining (ASONAM), pp. 53–60. IEEE (2018)
Yao, M., Chelmis, C., Zois, D.: Cyberbullying detection on Instagram with optimal online feature selection. In: 2018 IEEE/ACM International Conference on Advances in Social Networks Analysis and Mining (ASONAM), pp. 401–408, August 2018. https://doi.org/10.1109/ASONAM.2018.8508329
Yao, M., Chelmis, C., Zois, D.S.: Cyberbullying ends here: towards robust detection of cyberbullying in social media. In: The World Wide Web Conference, pp. 3427–3433 (2019)
Zhao, R., Mao, K.: Cyberbullying detection based on semantic-enhanced marginalized denoising auto-encoder. IEEE Trans. Affect. Comput. 8 (3), 328–339 (2016)
Zhong, H., et al.: Content-driven detection of cyberbullying on the Instagram social network. In: Proceedings of the Twenty-Fifth International Joint Conference on Artificial Intelligence, pp. 3952–3958 (2016)
Zych, I., Ortega-Ruiz, R., Del Rey, R.: Systematic review of theoretical studies on bullying and cyberbullying: facts, knowledge, prevention, and intervention. Aggress. Violent Behav. 23 , 1–21 (2015)
Download references
Acknowledgement
This work is supported by the Italian Ministry of Education, University and Research (MIUR) within the PRIN2017 - BullyBuster - A framework for bullying and cyberbullying action detection by computer vision and artificial intelligence methods and algorithms (CUP: F74I19000370001).
Author information
Authors and affiliations.
Department of Information Technology and Electrical Engineering (DIETI), University of Naples “Federico II”, Via Claudio 21, 80125, Naples, Italy
Carlo Sansone & Giancarlo Sperlí
You can also search for this author in PubMed Google Scholar
Corresponding author
Correspondence to Giancarlo Sperlí .
Editor information
Editors and affiliations.
Dipartimento di Ingegneria dell’Informazione, University of Firenze, Firenze, Italy
Alberto Del Bimbo
Dipartimento di Ingegneria “Enzo Ferrari”, Università di Modena e Reggio Emilia, Modena, Italy
Rita Cucchiara
Department of Computer Science, Boston University, Boston, MA, USA
Stan Sclaroff
Dipartimento di Matematica e Informatica, University of Catania, Catania, Italy
Giovanni Maria Farinella
Cloud & AI, JD.COM, Beijing, China
Marco Bertini
Computational Sciences Department, National Institute of Astrophysics, Optics and Electronics (INAOE), Tonantzintla, Puebla, Mexico
Hugo Jair Escalante
Roberto Vezzani
Rights and permissions
Reprints and permissions
Copyright information
© 2021 Springer Nature Switzerland AG
About this paper
Cite this paper.
Sansone, C., Sperlí, G. (2021). A Survey About the Cyberbullying Problem on Social Media by Using Machine Learning Approaches. In: Del Bimbo, A., et al. Pattern Recognition. ICPR International Workshops and Challenges. ICPR 2021. Lecture Notes in Computer Science(), vol 12667. Springer, Cham. https://doi.org/10.1007/978-3-030-68787-8_48
Download citation
DOI : https://doi.org/10.1007/978-3-030-68787-8_48
Published : 21 February 2021
Publisher Name : Springer, Cham
Print ISBN : 978-3-030-68786-1
Online ISBN : 978-3-030-68787-8
eBook Packages : Computer Science Computer Science (R0)
Share this paper
Anyone you share the following link with will be able to read this content:
Sorry, a shareable link is not currently available for this article.
Provided by the Springer Nature SharedIt content-sharing initiative
- Publish with us
Policies and ethics
Societies and partnerships
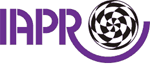
- Find a journal
- Track your research
- Open access
- Published: 22 December 2021
Cyberbullying detection: advanced preprocessing techniques & deep learning architecture for Roman Urdu data
- Amirita Dewani ORCID: orcid.org/0000-0002-3816-3644 1 ,
- Mohsin Ali Memon ORCID: orcid.org/0000-0003-2638-4252 1 &
- Sania Bhatti ORCID: orcid.org/0000-0002-0887-8083 1
Journal of Big Data volume 8 , Article number: 160 ( 2021 ) Cite this article
11k Accesses
33 Citations
1 Altmetric
Metrics details
Social media have become a very viable medium for communication, collaboration, exchange of information, knowledge, and ideas. However, due to anonymity preservation, the incidents of hate speech and cyberbullying have been diversified across the globe. This intimidating problem has recently sought the attention of researchers and scholars worldwide and studies have been undertaken to formulate solution strategies for automatic detection of cyberaggression and hate speech, varying from machine learning models with vast features to more complex deep neural network models and different SN platforms. However, the existing research is directed towards mature languages and highlights a huge gap in newly embraced resource poor languages. One such language that has been recently adopted worldwide and more specifically by south Asian countries for communication on social media is Roman Urdu i-e Urdu language written using Roman scripting. To address this research gap, we have performed extensive preprocessing on Roman Urdu microtext. This typically involves formation of Roman Urdu slang- phrase dictionary and mapping slangs after tokenization. We have also eliminated cyberbullying domain specific stop words for dimensionality reduction of corpus. The unstructured data were further processed to handle encoded text formats and metadata/non-linguistic features. Furthermore, we performed extensive experiments by implementing RNN-LSTM, RNN-BiLSTM and CNN models varying epochs executions, model layers and tuning hyperparameters to analyze and uncover cyberbullying textual patterns in Roman Urdu. The efficiency and performance of models were evaluated using different metrics to present the comparative analysis. Results highlight that RNN-LSTM and RNN-BiLSTM performed best and achieved validation accuracy of 85.5 and 85% whereas F1 score was 0.7 and 0.67 respectively over aggression class.
Introduction
Cyberbullying (aka hate speech, cyberaggression and toxic speech) is a critical social problem plaguing today’s Internet users typically youth and lead to severe consequences like low self-esteem, anxiety, depression, hopelessness and in some cases causes lack of motivation to be alive, ultimately resulting in death of a victim [ 1 ]. Cyberbullying incidents can occur via various modalities. For example, it can take the form of sharing/ posting offensive video content or uploading violent images or sharing the pictures without permission of the owner etc. However, cyberbullying via textual content is far more common [ 2 ]. In Pakistan, the usage of internet, smartphones and social media has increasingly become prevalent these days and the very frequent users are youngsters. According to a report, more than 65% of all the users lie between 18 and 29, and typically women are more susceptible and unprotected. People often use offensive language, use hate speech, and become aggressive to bully celebrities, leaders, women and an individual [ 3 ]. In Pakistan, victims have reported life disturbing and annoying experiences and most of the victims are educated youngsters (age group of 21–30 years) [ 4 ]. The traffic in cyberspace has escalated significantly during covid-19 pandemic. A report “COVID 19 and Cyber Harassment”, released by DRF in 2020 highlights a great rise in the number of cyberbullying and harassment cases during the pandemic. The complaints registered with DRF’s Cyber Harassment Helpline were surged by 189% [ 5 ].
Recently, Roman Urdu language has been a contemporary trend and a viable language for communication on different social networking platforms. Urdu is national and official language of Pakistan and predominant among most communities across different regions. A survey statistic in [ 6 ] affirms that 300 million people are speaking Urdu language and approximately 11 million speakers are in Pakistan from which maximum users switched to Roman Urdu language for the textual communication, typically on social media. It is linguistically rich and morphologically complex language [ 7 ]. Roman Urdu language is highly variant with respect to word structures, writing styles, irregularities, and grammatical compositions. It is deficit of standard lexicon and available resources and hence become extremely challenging when performing NLP tasks.
An elaboration of script of Urdu instances and Roman Urdu is given in Table 1 . Instances highlighted are describing anti-social behavior.
This paper addresses toxicity/cyberbullying detection problem in Roman Urdu language using deep learning techniques and advanced preprocessing methods including usage of lexicons/resource that are typically developed to accomplish this work. Intricacies in analyzing the structure and patterns behind these typical aggressive behaviors, typically in a newly adopted language, and forming it as a comprehensive computational task is very complicated. The major contributions of this study are formation of a slang and contraction mapping procedure along with slang lexicon for Roman Urdu language and development of hybrid deep neural network models to capture complex aggression and bullying patterns.
The rest of the paper is organized as follows: Review of existing literature is presented in " Related Work " Section. " Problem statement " Section states research gap and gives formal definition of the addressed problem. " Methodology " Section describes the steps of research methodology and techniques and models used for the experimentations. Advanced preprocessing steps applied on Roman Urdu data are elaborated in " Data Preprocessing on Roman Urdu microtext " section. Implementation of proposed model architecture and hyperparameter settings are discussed in " Experimental Setup " section. " Results and Discussion " Section highlights and discusses study results and finally " Conlusion " Section concludes the research work and provides future research directions.
Related work
Due to the accretion of social media communication and adverse effects arising from its darker side on users, the field of automatic cyberbullying detection has become an emerging and evolving research trend [ 8 ]. Research work in [ 9 ] presents cyberbullying detection algorithm for textual data in English language. It is considered as one of the pioneers and highly cited research. They divided the task in text-classification sub problems related to sensitive topics and collected 4500 textual comments on controversial YouTube videos. This study implemented Naive Bayes, SVM and J48 binary and multiclass classifiers using general and specific feature sets. Study contributed in [ 10 ] applied deep learning architectures on Kaggle dataset and conducted experimental analysis to determine the effectiveness and performance of deep learning algorithms LSTM, BiLSTM, RNN and GRU in detecting antisocial behavior. Authors in [ 11 ] extracted data from four platforms i-e Twitter, YouTube, Wikipedia, and Reddit for developing an online hate classifier in English language using different classification techniques. Research carried out in [ 12 ] developed an automated approach to detect toxicity and unethical behavior in online communication using word embeddings and varying neural network layers. They suggested that LSTM layers and mimicked word embedding can uncover such behavior with good accuracy level.
Few of the studies in recent years has been contributed by researchers on other languages apart from English. Research work in [ 13 ] is unique and has gathered textual data from Instagram and twitter in Turkish language. They have implemented Naïve Bayes Multinomial, SVM, KNN and decision trees for cyberbullying detection along with Chi-square and information gain (IG) for feature selection. Work accomplished in [ 14 ] also addresses the problem of cyberaggression in Turkish language. The work extends comparison of different machine learning algorithms and found optimal results using Light Gradient Boosting Model. Van Hee, Cynthia, et al. in [ 15 ] proposed cyberbullying detection scheme for Dutch language. This is the first study on Dutch social media. Data was collected from ASKfm where users can ask and answer questions. The research uses default parameter settings for un-optimized linear kernel SVM based on n-grams and keyword system to identify bullying traces. F1 score for Dutch language was 61%. Problem of Arabic language cyberbullying detection was addressed and accomplished in [ 16 ]. This study used Dataiku DSS and WEKA for ML tasks. The data was scrapped from facebook and twitter. The study concluded that even though the detection approach was not comparable with the other studies in English language but overall Naive Bayes and SVM yield reasonable performance. Research work in [ 17 ] by Gomez-Adorno, Helena, et al. proposed automatic aggression detection for Spanish tweets. Several types of n-grams and linguistically motivated patterns were used but the best run could only achieve F1 score of 42.85%. Studies presented in [ 18 , 19 , 20 ] are based on automatic detection of cyberbullying content in German language. Research conducted in [ 18 ] proposed an approach based on SVM, CNN and ensemble model using unigram, bigrams and character N-grams for categorizing offensive tweets in German language. Research presented in [ 21 ] attempted for the very first time to identify bullying traces in Indonesian language. Association Rule mining and FP growth text mining were used to identify trends for bullying patterns in Jakarta and Surabaya cities using social media text. This baseline study on Indonesian language was further extended by Nurrahmi, Hani et al. in [ 22 ]. Study in [ 23 ] made first attempt to develop a corpus of code-mixed data considering Hindi and English language. They proposed a scheme for hate speech detection using N-grams and lexical features. An ensemble approach by combining the predictions of Convolutional Neural Network (CNN) and SVM algorithms were used for identifying such patterns. The weighted F1 score for Hindi language ranged between 0.37 and 0.55 for different experiments [ 24 ]. In the year 2019, Association for computational linguistics initiated the project for automatic detection of cyberbullying in Polish language [ 25 ]. Research conducted in [ 26 ] attempted to uncover cyberbullying patterns in Bengali language implementing passive aggressive, SVM and logistic regression. The optimum accuracy achieved was 78.1%. Recently, work contributed in [ 27 ] presented first study in Roman Urdu using lexicon based approach. The dataset was highly skewed comprising of only 2.2% toxic data. As according to [ 28 ], biased sampling and measurement errors are highly prone to classification errors when working on such datasets. Moreover, pattern detection based on predefined bullying and non-bullying lexicons were shortcomings of this study.
For automated detection of complex cyberbullying patterns, studies contributed by different scholars employ supervised, unsupervised, hybrid and deep learning models, vast feature engineering techniques, corpora, and social media platforms. However, the existing literature is mainly oriented towards unstructured data in English language. Some recent studies and projects have been initiated in other languages as discussed previously. To the best of our knowledge and literature review, no detailed work has been contributed in Roman Urdu to systematically analyze cyberbullying detection phenomenon using advanced preprocessing techniques (involving the usage of Roman Urdu resources) and deep learning approaches under different configurations.
Problem statement
The escalated usage of social networking sites and freedom of speech has given optimal ground to individuals across all demographics for cyberbullying and cyberaggression. This leaves drastic and noticeable impacts on behavior of a victim, ranging from disturbance in emotional wellbeing and isolation from society to more severe and deadly consequences [ 29 ]. Automatic Cyberbullying detection has remained very challenging task since social media content is in natural language and is usually posted in unstructured free-text form leaving behind the language norms, rules, and standards. Evidently, there exists a substantial number of research studies which primarily focus on discovering cyberbullying textual patterns over diverse social media platforms as discussed previously in literature review section. However, most of the detection schemes and automated approaches formulated are for resource-rich and mature languages spoken worldwide. Roman Urdu is typically spoken in South Asia and is a highly resource deficient language. Hence this research puts novel efforts to propose data pre-processing techniques on Roman Urdu scripting and develop deep learning-based hybrid models for automated cyberbullying detection in Roman Urdu language. The outcomes of this study, if implemented, will assist cybercrime centers and investigation agencies for monitoring social media contents and in making cyberspace secure and safer place for all segments of society.
Methodology
The research methodology is depicted in Fig. 1 .
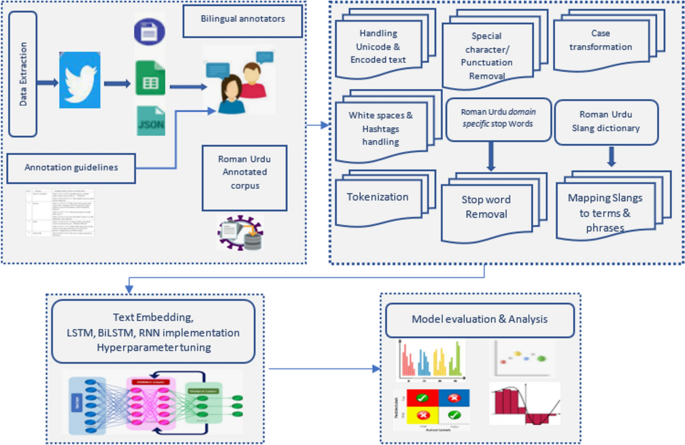
Proposed research methodology
The development of hate speech/cyberbullying corpus with minor skew and automated development of domain specific roman Urdu stop words is published in our previous work [ 30 ]. The work details formation of computational linguist resources. Further steps of methodology are discussed in subsequent sections. The Deep Neural Network (DNN) based techniques and models used for the experimentations are detailed below.
Model description
Recurrent neural networks (rnn).
RNN [ 31 ] has been applied in literature for successive time series applications with temporal dependencies. An unfolded RNN can handle processing of current data by utilizing past data. Meanwhile, RNN has the issue of training long-term dependencies. This has been addressed by one of the RNN variant.
Long short-term memory networks (LSTM)
LSTM has been employed as an advanced version of RNN network. It resolves the shortcoming of RNN by applying memory cells also known as hidden layer units. Memory cells are controlled through three gates named as: input gate, output gate and forget gate. They have the self-connections which store the temporal state of network [ 31 ]. Input and output gates address and control the flow of information from memory cell input and output to rest of the network. The forget gate, usually called as a remember vector, transfers the information with higher weights from previous neuron to the next neuron. The forget gate is added to the memory cell. The information resides in memory depending upon the high activation results; the information will be stored in memory cell iff the input unit has high activation. However, the information will be transferred to next neuron if the output unit has high activation. Otherwise, input information with high weights resides in memory cell [ 31 ].
Mathematically, LSTM network can be described as [ 32 ]:
where W h ∈ R m × d and U h ∈ R m × m indicates weight matrices, x t denotes the current word embedding, b h ∈ R m refers to bias term, whereas f(x ) is a non-linear function.
LSTM has more complex architecture including hidden states and tends to remember information for either short or long term. The hidden state [ 33 ] of LSTM is computed as follows:
where f t denotes the forget gate, i t refers to the input gate, c t denotes the cell state, o t is the output gate, h t is the regular hidden state, σ indicates sigmoid function, and ◦ is the Hadamard product.
Bidirectional Long short-term memory networks (BiLSTM)
In the traditional recurrent neural network model and LSTM model, the propagation of information is only in forward direction. This results in computation of an output vector only based on the current input at time t and the output of the previous unit. The back propagation of information in network is achieved by merging two bidirectional recurrent neural network (BiRNN) and LSTM units, one for forward direction and one for backward direction. This helps in capturing contextual information and enhances learning ability [ 34 ].
In bidirectional LSTM, outputs of two LSTM networks are stacked together. The first LSTM is a regular sequence starting from the starting of the paragraph, while the second LSTM is a standard sequence, and the series of inputs are fed in the opposite order. The first hidden state is denoted by ht forward whereas second LSTM unit’s hidden state is denoted by ht backward . After processing data, the final state ht Bilstm is computed by concatenating the two hidden states as given in Eq. 3 .
where ⊕ denotes a concatenation operator.
Convolutional neural networks (CNN)
Convolution neural networks (aka CNN), originally incorporated for image processing tasks, have become very efficacious in different NLP and text classification applications. The network identifies correlations and patterns of data via their feature maps. Information about local structure of data is extracted by applying multiple filters with different dimensions [ 35 ].
Data preprocessing on Roman Urdu microtext
Big social media data in Roman Urdu language is inconsistent, incomplete, or precise, missing in certain behaviors or trends, and is likely to incorporate many errors. Roman Urdu users highly deviate language norms while communicating on social media. Hence data preprocessing is immensely significant. Some major data preprocessing steps applied on Roman Urdu microtext are detailed below.
Handling Unicode and encoded text formats
Unicode scheme provides every character in natural language text a unique code from 0 to 0 × 10FFFF. The uncleaned Roman Urdu data comprised of special symbols, emojis, and other typical stray characters represented using Unicode. We used Unicode transformation type 8 encoding to convert the data. This data was converted and handled using re and string modules in python.
Text cleaning
Text cleaning is essential step to eliminate or at least reduce noise from Microtext. This step comprised of case transformation, removal of punctuations and URLs, elimination of additional white spaces, exclusion of hashtags, digits & special character removal and removal of metadata/non-linguistic features.
Tokenization
Tokenization is immensely essential phase of text processing. It is the process of generating tokens by splitting textual content into words, phrases, or other meaningful parts. It is generally a form of text segmentation [ 36 ]. Tokenization was performed using Keras tokenizer to prepare the text for implementing deep learning networks.
Filtering stop words
Stop words are non-semantic division of text in natural language. The necessity that stop words should be eliminated from text is that they make the text higher dimensional with redundant features which are less significant for analysts. Removing stop words reduces the dimensionality of term space [ 37 ]. Development of domain specific stop words in Roman Urdu language automatically using statistical techniques and bilingual experts’ input, comprising of 173 words is detailed in our previous work [ 30 ]. Insignificant Roman Urdu words were typically articles such as ek (ایک), conjunctions and pronouns such as tum (تم), tumhara (تمھارا), us (اُس), wo/who (وہ), usko (اُسکو), preposition such as main (میں), pe (پے), par (پر), demonstratives such as ye (یے), inko (انکو), yahan(یہاں), and interrogatives such as kahan (کہاں), kab (کب), kisko (کس کو), kiski (کس کی) etc. Stop words were removed from Roman Urdu corpus, leaving behind the index terms which are important.
Mapping slangs and contractions
Existing libraries, APIs and toolkits in python language primarily support preprocessing functions for English and other mature languages. They can be partially used for Roman Urdu language. Moreover, most of the communication in Roman Urdu comprises of bully terms being used as slangs. High dimensional textual data also suppress significant features. Hence contraction mapping is mandatory for dimensionality reduction and to capture complex bullying patterns. Currently, Pycontractions Library [ 38 ] only supports English contraction mapping process. To address this problem, the study developed data slang mapping process. To map slangs to original terms and phrases in Roman Urdu language, we created Slang lexicon in Roman Urdu (SLRU) which also included Roman Urdu abuses and offensive terms used as a norm by Roman Urdu users. SLRU is in the form of a dictionary. It comprises of the key: value pairs, where key is the slang and value is its equivalent Roman Urdu phrase/term such as “AFIK”: “Jahan tak mujhay pata hai”, ASAP: “Jitna jaldi ho sakay”, “tbh”: “Sach main” and so on. The process of slang mapping is detailed in Fig. 2 .
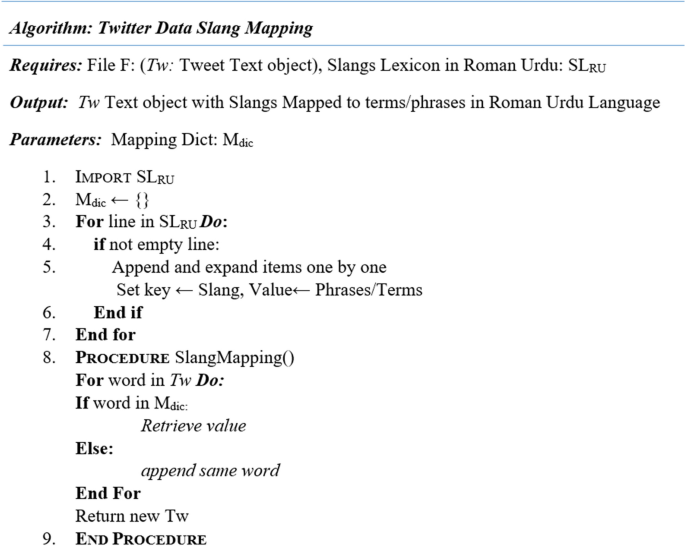
Mapping process for slangs in Roman Urdu
The results of mapping process are highlighted in Fig. 3 .

Mapping on Roman Urdu Data
Experimental setup
This section discusses implementation of proposed neural network architecture and all hyper-parameter settings. All the experiments were performed on 11 Gen, core i7, 4 cores, 8 logical microprocessors, with 2.8 GHz processor speed, 256 GB Solid State Drive and python version 3.8, 64 bits.
Proposed model implementation and hyper-parameter settings
All models were implemented and trained in Keras; a high-level neural network API that works with open-source machine learning framework called TensorFlow [ 39 ]. All the implementation was accomplished using PyCharm. The optimal parameters and results were achieved through repeated experimentations.
Data was split into training and testing datasets. The data split was 0.8 for training and 0.2 for testing i-e 80% of data instances were used for training and 20% were holdout for testing and validation purpose. The sets were made using shuffled array. This allows model to learn over different data instances. Moreover, it helps to uncover reliability of model and consistency of results over repeated executions. Random state is also generated using numpy.random [ 40 ] for random sampling during splitting of data to ensure reproducible splits.
Textual input data must be integer encoded. In RNN-LSTM architecture, a sequential model was created. Initially an Embedding layer was added to the network and textual Roman Urdu data was provided as an input. Embedding layer embedded high dimensional text data in low dimensional vector space for generating dense vector representation of data. Embedding was formed using 2000 features and 128 embed dimensions. The experiment was initially executed on 20 epochs and 50 batch size. The batch size was based on the fact that model was having single lstm layer, and comparatively took lower training and validation time per step. The execution time for each epoch was approximately 10 ms. SpatialDropout1D was used with rate of 0.3. It helped to regularize the activations and maintain effective learning rate of the model. For updating network weights iteratively, this work uses binary cross entropy loss function and Adam optimizer. Sigmoid activation function was also implemented. It is denoted by f(x) and is defined as:
The Spatial Dropout layer was implemented instead of a simple Dropout. The major reason being was to retain the context of textual data established by neighboring words. Dropping random words (except for stop words, which were already removed during preprocessing step) can highly affect the context of uttered sentences and ultimately the performance of model. We incorporated two hidden dense layers denoted by D 1 and D 2 . The output of each hidden layer was computed to get the final output for cyberbullying text detection.
Keras tokenizer was used to accomplish pre-tokenization of all the data required for the implementation of RNN-biLSTM model. We created a sequential model with Embedding layer having 2000 maximum features. Subsequently a biLSTM layer comprising of two LSTM layers, one to read sequence in forward direction and other in backward direction, each with 64 units was added. Hidden layer (H 1 ) was formed using sigmoid activation function. For down sampling the feature maps, Dropout layer was added with 0.2 dropout rate. Moreover, we used 128 batch size to utilize low to moderate computational resources while still not slowing down the training process. Batch size highlights number of samples processed by model before updating of internal parameters. To combat overfitting, we added second dropout layer with rate of 0.25. Adam optimization was used with learning rate of 0.01 since batch size was not too small. For this model, we used binary cross entropy loss function. As the Epochs increase, the generalization ability of the model improves. However, too many epochs also lead to the problem of overfitting. The model was executed over different number of Epochs and average execution time for each epoch was 13 to 15 ms. The performance of model stabilized over 20 Epochs, above which the improvement was almost negligible.
In CNN model, initially the sentence was transformed into matrix where each row of matrix represented word vectors representation of data. We used 1000 features and 32 dimensions. Two convolutional filters were applied with 8 and 16 filters and 3 kernel size. Each filter was used to perform one dimensional convolution on word embeddings. Both Layers were 1D in nature. We set two dropout layers with dropout rate of 0.25 to improve generalization ability of developed model. Hidden layers with Relu and sigmoid activation functions were used. To extract most salient and prominent features, global maximum pooling layers were used with pool size = 2. Flatten layer was created after convolutional layers to flatten the output of the previous layer to a single long feature vector. The experiment was simulated over different Epochs. However, results got stable at 30 epochs.
Results and discussion
Empirical evaluation of cyberbullying detection scheme performance in Roman Urdu and experimental setups is accomplished via accuracy, precision, recall, and f1 measure metrics.
All the implemented models were executed several times over number of epochs to get consistency in evaluation parameters until it was a minor difference of ± 0.1. The results for LSTM are depicted in Fig. 4 . To ensure results validity and reliability, for a comparatively less skewed dataset, F1 measure (i-e a harmonic mean of precision and recall) is used as an evaluation metric. Furthermore, we have also reported macro and weighted average scores across all the classes. The evaluation results of RNN-LSTM are given in Fig. 4 .
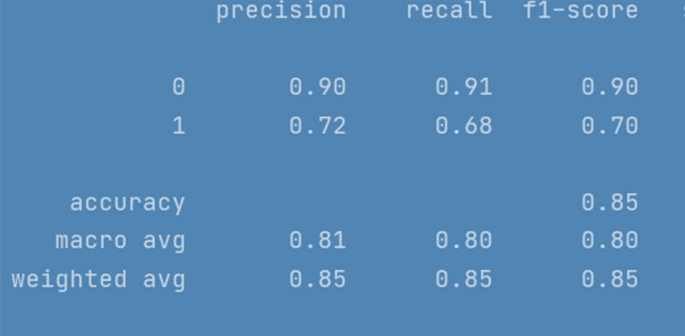
RNN-LSTM evaluation Results
F1 score for RNN-LSTM over cyberbullying class was only 70%, however for non-cyberbullying class, score was 90%. We observed that nearly all the instances of majority class of non-cyber bullying are correctly classified by this model. The experimental simulation depicting model accuracy and validation accuracy during training and validation phases, before and after stabilization of evaluation parameters is represented in Figs. 5 and 6 respectively.
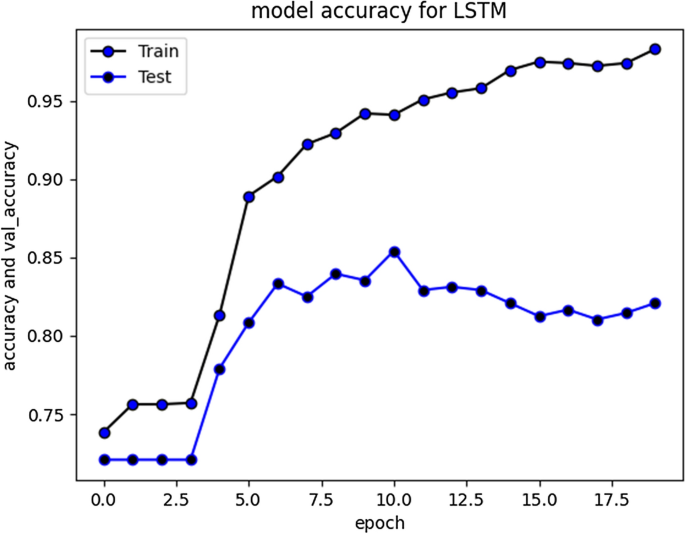
RNN-LSTM Model accuracy graph for 20 epochs
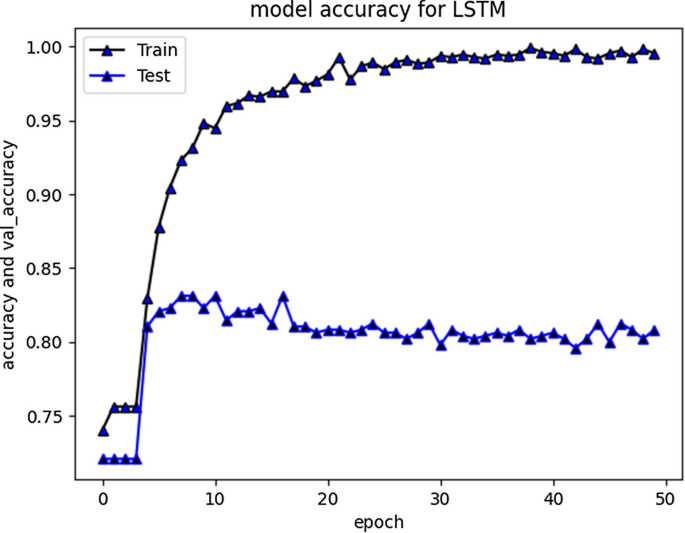
RNN-LSTM Model accuracy graph for 50 epochs
The accuracy improved over subsequent epochs. However, after 20 epochs it got stabilized. The average accuracy produced by this model was 93.5% during training and 85.5% during validation. Overall curve variation is indicating that no overfitting problem arise. The model loss during training and validation loss during testing over 20 and 50 epochs is shown in Figs. 7 and 8 respectively. The cross-entropy loss considered during configuration over different epochs converged well, thus indicating optimal model performance.
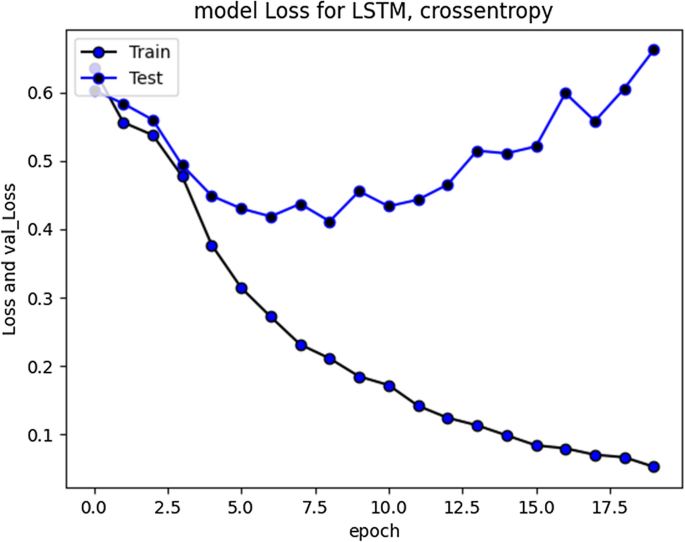
RNN-LSTM Model loss plot- Binary Cross entropy for 20 epochs
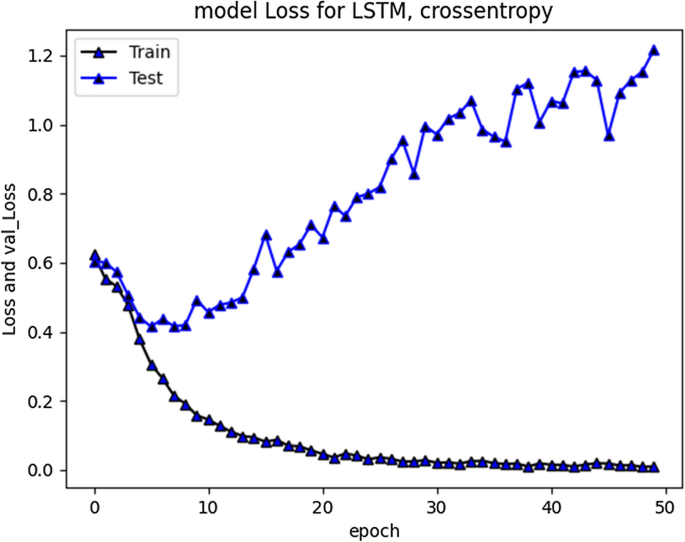
RNN-LSTM Model loss plot- Binary Cross entropy for 50 epochs
The evaluation results of RNN-BILSTM model over 20 epochs are given in Fig. 9 .
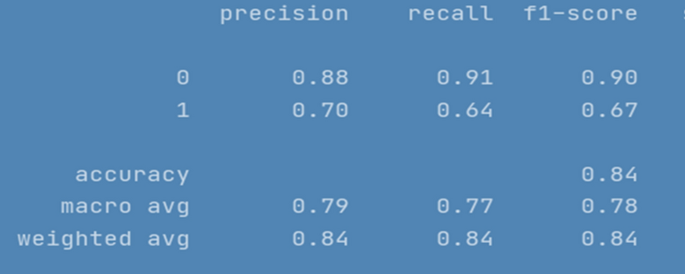
RNN-BiLSTM evaluation Results
RNN-LSTM also performed reasonably well for cyberbullying detection task on Roman Urdu data. F1 score for non-cyberbullying content prediction was 90% whereas for cyberbullying content, the score was 67% only. This indicates that model erroneously classified/misclassified some of the aggressive class instances and TN rate was at average. Figs. 10 and 11 are depicting model accuracy and validation accuracy for RNN-BiLSTM.
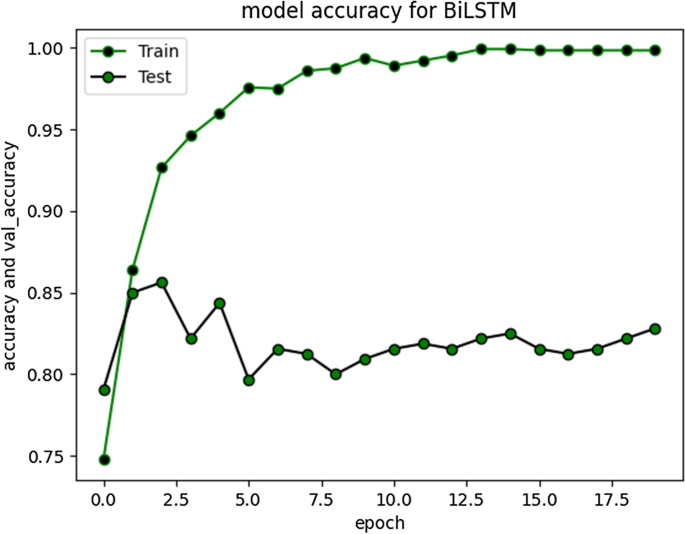
RNN-biLSTM Model accuracy plot for 20 epochs
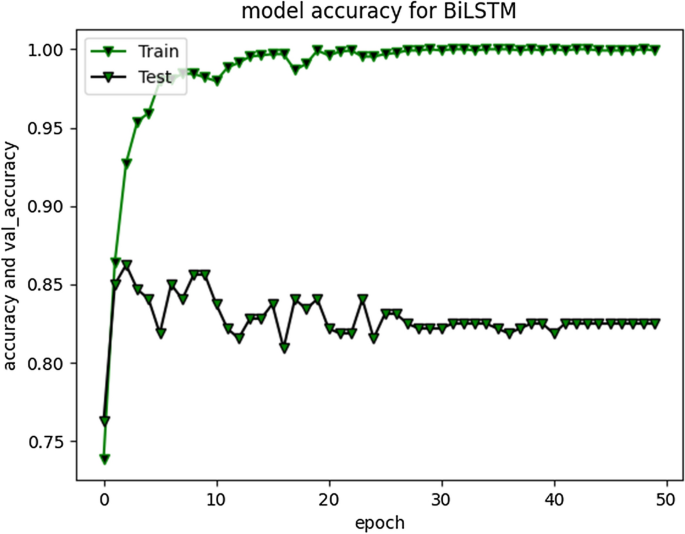
RNN-biLSTM Model accuracy plot for 50 epochs
The accuracy improved highly during training process up to 20 epochs. Overall average accuracy was 97% in training and 85% on validation set. 20% of the data was used for as a validation set, as stated earlier. During experimentation, we identified that accuracy of our model is not improving after a specific point i-e after 20 Epochs. The trivial variations can be clearly visualized from the graph in Fig. 9 . Model loss and validation loss during training and testing process for RNN-BiLSTM over 20 and 50 epochs is given in Figs. 12 and 13 respectively.
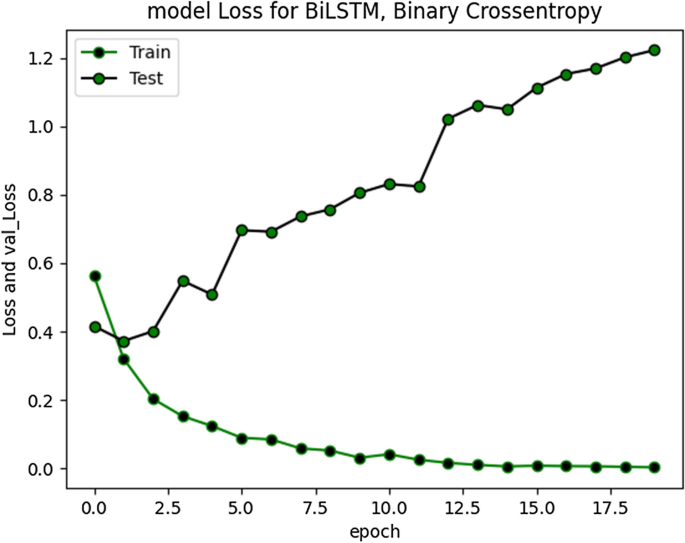
RNN-BiLSTM Model loss plot- Binary Cross entropy for 20 epochs
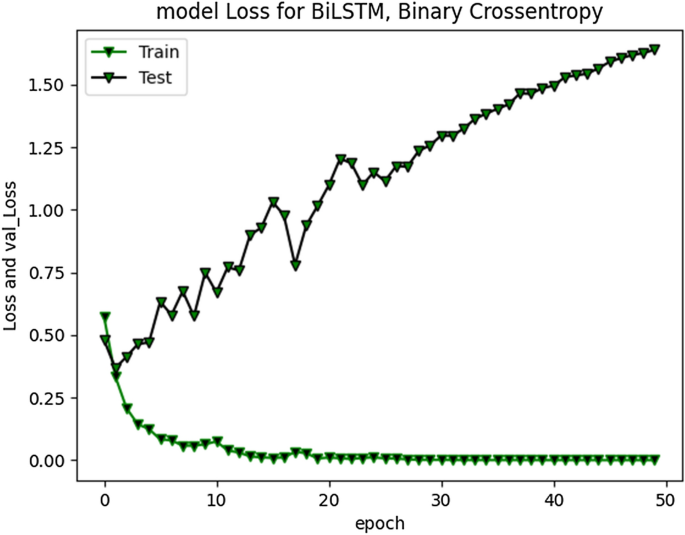
RNN-BiLSTM Model loss plot- Binary Cross entropy for 50 epochs
The cross-entropy loss was minimal (approximately 1.2), indicating good prediction capability of developed model.
Figure 14 represents the evaluation results for CNN model.
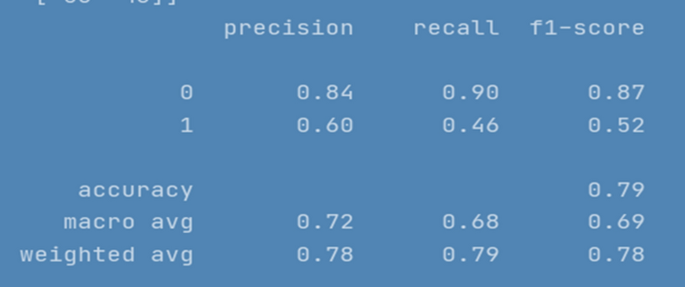
CNN model evaluation Results
CNN performed well for prediction of non-cyberbullying content, providing F1 score of 87%. However, model did not yield good efficiency for categorizing cyberbullying class, producing f1-score of 52%. The repeated experiments performed for CNN showed continuous improvements up to 30 Epochs. Figure 15 depicts model accuracy and validation accuracy. The experimental simulation over 50 epochs only shown minor improvements as represented in Fig. 16 . The average execution time for Epoch was 9 ms each. The training accuracy of 98% was achieved over different executions whereas model produced 85% validation accuracy.
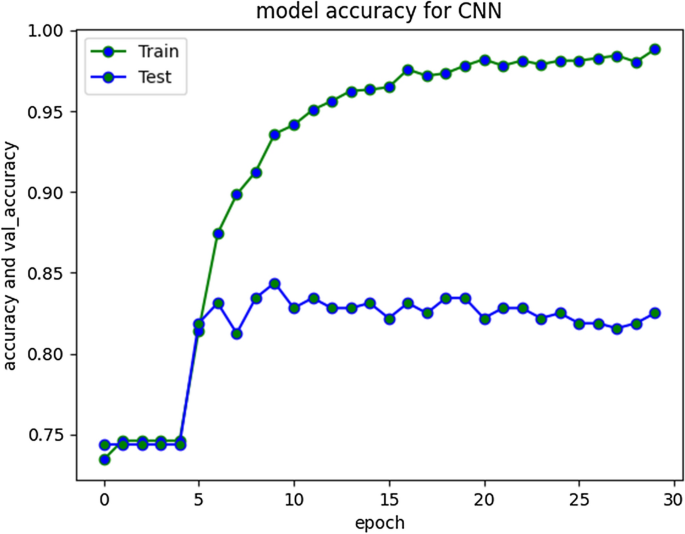
CNN Model accuracy plot for 30 epochs
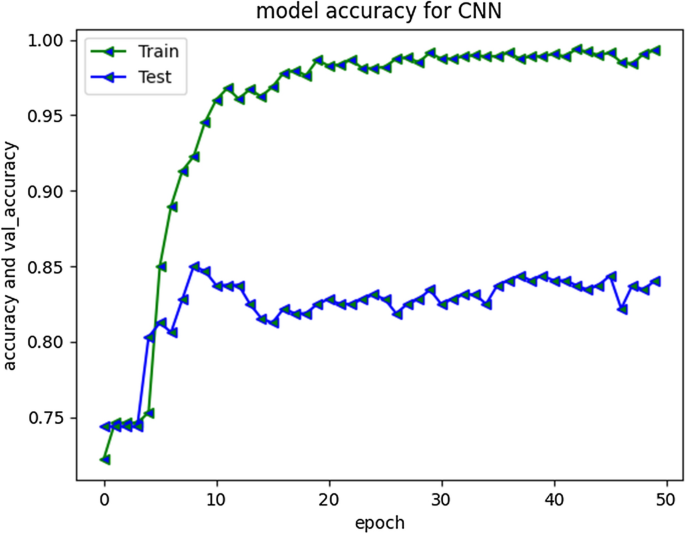
CNN Model accuracy plot for 50 epochs
CNN model loss and validation loss results at 30 and 50 epochs are presented in Fig. 17 and 18 respectively. The loss was minimal during training and converged. During validation the loss increased and diverged indicating only moderate performance over unseen instances typically from aggressive class.
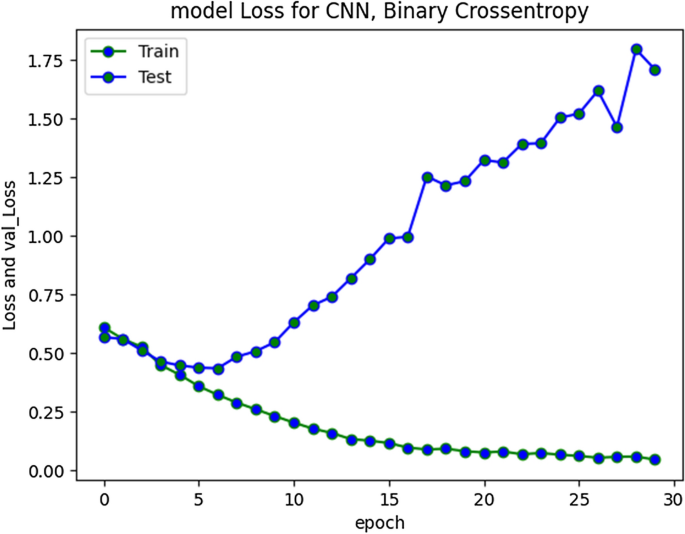
CNN Model loss plot- Binary Cross entropy for 30 epochs
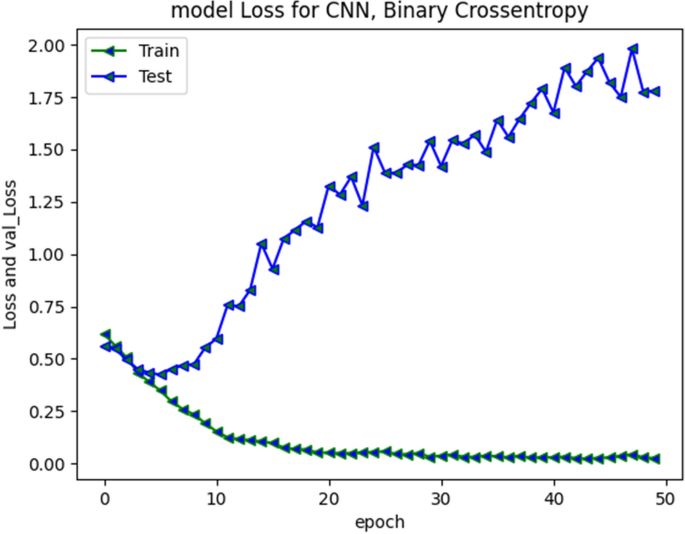
CNN Model loss plot- Binary Cross entropy for 50 epochs
The compiled model results indicating evaluation measures at stabilized epochs are depicted in Table 2 .
Cyberbullying has become an alarming social threat for today’s youth and has recently gained huge attention from research community. This research has addressed the problem of cyberbullying detection in Roman Urdu Language. Since Roman Urdu is highly resource deficient language, having different writing patterns, word structures, and irregularities thus making this work a challenging task. In this work we have presented advanced preprocessing techniques mainly a slang mapping mechanism, domain specific stop word removal, handling encoded formats and formulation of deep learning architecture to detect cyberbullying patterns in Roman Urdu language. We created experiments with vast parameters to build optimal classifier for cyberbullying tweets. The results highlighted that RNN-LSTM and RNN-BiLSTM with concatenation of forward and backward units provided better performance in 20 Epochs as compared to CNN. The existing work can be extended in numerous ways. The future studies can focus on development of ensemble models to uncover harassing and hate speech patterns. Moreover, the incorporation of context-specific features and handling of morphological variations might produce better results.
Availability of data and materials
The used raw dataset in this research is not publicly available. The data that support the findings of this research work are available from the corresponding author, on valid request due to privacy and ethical restrictions.
Abbreviations
Recurrent neural network
Long-short term memory
Bidirectional long-short term memory
Convolutional neural network
Social networking
Right to left
Left to right
True negative
Hellfeldt K, López-Romero L, Andershed H. Cyberbullying and psychological well-being in young adolescence: the potential protective mediation effects of social support from family, friends, and teachers. Int J Environ Res Public Health. 2020;17(1):45.
Article Google Scholar
Dadvar M. Experts and machines united against cyberbullying [PhD thesis]. University of Twente. 2014.
Magsi H, Agha N, Magsi I. Understanding cyber bullying in Pakistani context: causes and effects on young female university students in Sindh province. New Horiz. 2017;11(1):103.
Google Scholar
Qureshi SF, Abbasi M, Shahzad M. Cyber harassment and women of Pakistan: analysis of female victimization. J Bus Soc Rev Emerg Econ. 2020;6(2):503–10.
S. Irfan Ahmed, Cyber bullying doubles during pandemic. https://www.thenews.com.pk/tns/detail/671918-cyber-bullying-doubles-during-pandemic . Accessed 24 Aug 2020.
Shahroz M, Mushtaq MF, Mehmood A, Ullah S, Choi GS. RUTUT: roman Urdu to Urdu translator based on character substitution rules and unicode mapping. IEEE Access. 2020;8:189823–41.
Mehmood F, Ghani MU, Ibrahim MA, Shahzadi R, Mahmood W, Asim MN. A precisely xtreme-multi channel hybrid approach for roman urdu sentiment analysis. IEEE Access. 2020;8:192740–59.
Alotaibi M, Alotaibi B, Razaque A. A multichannel deep learning framework for cyberbullying detection on social media. Electronics. 2021;10(21):2664.
Dinakar K, Reichart R, Lieberman H. Modeling the detection of textual cyberbullying. In: 5th international AAAI conference on weblogs and social media. 2011.
Iwendi C, Srivastava G, Khan S, Maddikunta PKR. Cyberbullying detection solutions based on deep learning architectures. Multimed Syst. 2020. https://doi.org/10.1007/s00530-020-00701-5 .
Salminen J, Hopf M, Chowdhury SA, Jung S, Almerekhi H, Jansen BJ. Developing an online hate classifier for multiple social media platforms. Hum Cent Comput Inf Sci. 2020;10(1):1–34.
Dessì D, Recupero DR, Sack H. An assessment of deep learning models and word embeddings for toxicity detection within online textual comments. Electronics. 2021;10(7):779.
S. A. Özel, E. Saraç, S. Akdemir, and H. Aksu, Detection of cyberbullying on social media messages in Turkish, In: 2017 International Conference on Computer Science and Engineering (UBMK), 2017, pp. 366–370.
E. C. Ates, E. Bostanci, and M. S. Guzel, Comparative Performance of Machine Learning Algorithms in Cyberbullying Detection: Using Turkish Language Preprocessing Techniques, arXiv Prepr. arXiv2101.12718, 2021.
Van Hee C, et al. Automatic detection of cyberbullying in social media text. PLoS ONE. 2018;13(10):e0203794.
Haidar B, Chamoun M, Serhrouchni A. A multilingual system for cyberbullying detection: Arabic content detection using machine learning. Adv Sci Technol Eng Syst J. 2017;2(6):275–84.
Gómez-Adorno H, Bel-Enguix G, Sierra G, Sánchez O, Quezada D. A machine learning approach for detecting aggressive tweets in Spanish, In: IberEval@ SEPLN. 2018. pp. 102–107.
X. Bai, F. Merenda, C. Zaghi, T. Caselli, and M. Nissim, RuG at GermEval: Detecting Offensive Speech in German Social Media, in 14th Conference on Natural Language Processing KONVENS 2018, 2018, p. 63.
B. Birkeneder, J. Mitrovic, J. Niemeier, L. Teubert, and S. Handschuh, upInf—Offensive Language Detection in German Tweets, In: Proceedings of the GermEval 2018 Workshop, 2018, pp. 71–78.
J. M. Schneider, R. Roller, P. Bourgonje, S. Hegele, and G. Rehm, Towards the Automatic Classification of Offensive Language and Related Phenomena in German Tweets, In: 14th Conference on Natural Language Processing KONVENS 2018, 2018, p. 95.
H. Margono, X. Yi, and G. K. Raikundalia, Mining Indonesian cyber bullying patterns in social networks, In: Proceedings of the Thirty-Seventh Australasian Computer Science Conference-Volume 147, 2014, pp. 115–124.
H. Nurrahmi and D. Nurjanah, Indonesian Twitter Cyberbullying Detection using Text Classification and User Credibility, In: 2018 International Conference on Information and Communications Technology (ICOIACT), 2018, pp. 543–548.
A. Bohra, D. Vijay, V. Singh, S. S. Akhtar, and M. Shrivastava, A Dataset of Hindi-English Code-Mixed Social Media Text for Hate Speech Detection, In: Proceedings of the Second Workshop on Computational Modeling of People’s Opinions, Personality, and Emotions in Social Media, 2018, pp. 36–41.
A. Roy, P. Kapil, K. Basak, and A. Ekbal, An ensemble approach for aggression identification in english and hindi text, In: Proceedings of the First Workshop on Trolling, Aggression and Cyberbullying (TRAC-2018), 2018, pp. 66–73.
Association for Computational Linguistics. https://www.aclweb.org/portal/content/deadline-extension-first-task-automatic-cyberbullying-detection-polish-language . Accessed 09 May 2019.
Ghosh R, Nowal S, Manju G. Social media cyberbullying detection using machine learning in bengali language. Int J Eng Res Technol. 2021. https://doi.org/10.1109/ICECE.2018.8636797 .
Talpur KR, Yuhaniz SS, Sjarif NNBA, Ali B. Cyberbullying detection in Roman Urdu language using lexicon based approach. J Crit Rev. 2020;7(16):834–48. https://doi.org/10.31838/jcr.07.16.109 .
J. Brownlee, Imbalanced Classification, December 23, 2019. https://machinelearningmastery.com . Accessed 10 May 2021.
Arif M. A systematic review of machine learning algorithms in cyberbullying detection: future directions and challenges. J Inf Secur Cybercrimes Res. 2021;4(1):1–26.
A. Dewani, M. Ali Memon, and S. Bhatti, Development of Computational Linguistic Resources for Automated Detection of Textual Cyberbullying Threats in Roman Urdu Language, 3C TIC. Cuad. Desarro. Apl. a las TIC, 101–121., p. 17, 2021.
Shahid F, Zameer A, Muneeb M. Predictions for COVID-19 with deep learning models of LSTM, GRU and Bi-LSTM. Chaos Solitons Fractals. 2020;140:110212.
M. Cliche, “BB_twtr at SemEval-2017 task 4: Twitter sentiment analysis with CNNs and LSTMs,” arXiv Prepr. arXiv1704.06125, 2017.
W. Zaremba, I. Sutskever, and O. Vinyals, Recurrent neural network regularization, arXiv Prepr. arXiv1409.2329, 2014.
Xu G, Meng Y, Qiu X, Yu Z, Wu X. Sentiment analysis of comment texts based on BiLSTM. Ieee Access. 2019;7:51522–32.
S. Minaee, E. Azimi, and A. Abdolrashidi, Deep-sentiment: Sentiment analysis using ensemble of cnn and bi-lstm models, arXiv Prepr. arXiv1904.04206, 2019.
Uysal AK, Gunal S. The impact of preprocessing on text classification. Inf Process Manag. 2014;50(1):104–12.
Vijayarani S, Ilamathi MJ, Nithya M. Preprocessing techniques for text mining-an overview. Int J Comput Sci Commun Networks. 2015;5(1):7–16.
Pycontractions 2.0.1. https://pypi.org/project/pycontractions/ . Accessed 17 Nov 2021.
API Documentation. https://www.tensorflow.org/api_docs . Accessed 21 Oct 2020.
NumPy. https://numpy.org/ . Accessed 18 Nov 2021.
Download references
Acknowledgements
We would like to thank Institute of Information and Communication Technology, Mehran University of Engineering & Technology, for providing resources and funding, necessary to accomplish this research work.
This research has been performed at Institute of Information and Communication Technology, Mehran University of Engineering and Technology, Pakistan and is fully funded under MUET funds for postgraduate students.
Author information
Authors and affiliations.
Institute of Information and Communication Technologies, Department of Software Engineering, Mehran University of Engineering & Technology, Jamshoro, Sindh, Pakistan
Amirita Dewani, Mohsin Ali Memon & Sania Bhatti
You can also search for this author in PubMed Google Scholar
Contributions
Corresponding author is the main contributor of this work from conception, drafting, algorithms development and implementation to analysis of results. Other authors are research supervisors who provided valuable guidance for work and content improvement. All authors read and approved the final manuscript.
Corresponding author
Correspondence to Amirita Dewani .
Ethics declarations
Ethics approval and consent to participate.
Not applicable.
Consent for publication
Competing interests.
The authors declare that they have no competing or conflicts of interest to report regarding the present study.
Additional information
Publisher's note.
Springer Nature remains neutral with regard to jurisdictional claims in published maps and institutional affiliations.
Rights and permissions
Open Access This article is licensed under a Creative Commons Attribution 4.0 International License, which permits use, sharing, adaptation, distribution and reproduction in any medium or format, as long as you give appropriate credit to the original author(s) and the source, provide a link to the Creative Commons licence, and indicate if changes were made. The images or other third party material in this article are included in the article's Creative Commons licence, unless indicated otherwise in a credit line to the material. If material is not included in the article's Creative Commons licence and your intended use is not permitted by statutory regulation or exceeds the permitted use, you will need to obtain permission directly from the copyright holder. To view a copy of this licence, visit http://creativecommons.org/licenses/by/4.0/ .
Reprints and permissions
About this article
Cite this article.
Dewani, A., Memon, M.A. & Bhatti, S. Cyberbullying detection: advanced preprocessing techniques & deep learning architecture for Roman Urdu data. J Big Data 8 , 160 (2021). https://doi.org/10.1186/s40537-021-00550-7
Download citation
Received : 03 September 2021
Accepted : 10 December 2021
Published : 22 December 2021
DOI : https://doi.org/10.1186/s40537-021-00550-7
Share this article
Anyone you share the following link with will be able to read this content:
Sorry, a shareable link is not currently available for this article.
Provided by the Springer Nature SharedIt content-sharing initiative
- Advanced preprocessing
- Deep learning
- Hate speech detection
- Cyberbullying
Numbers, Facts and Trends Shaping Your World
Read our research on:
Full Topic List
Regions & Countries
- Publications
- Our Methods
- Short Reads
- Tools & Resources
Read Our Research On:
- Teens and Cyberbullying 2022
Nearly half of U.S. teens have been bullied or harassed online, with physical appearance being seen as a relatively common reason why. Older teen girls are especially likely to report being targeted by online abuse overall and because of their appearance
Table of contents.
- Acknowledgments
- Methodology
Pew Research Center conducted this study to better understand teens’ experiences with and views on bullying and harassment online. For this analysis, we surveyed 1,316 U.S. teens. The survey was conducted online by Ipsos from April 14 to May 4, 2022.
This research was reviewed and approved by an external institutional review board (IRB), Advarra, which is an independent committee of experts that specializes in helping to protect the rights of research participants.
Ipsos recruited the teens via their parents who were a part of its KnowledgePanel , a probability-based web panel recruited primarily through national, random sampling of residential addresses. The survey is weighted to be representative of U.S. teens ages 13 to 17 who live with parents by age, gender, race, ethnicity, household income and other categories.
Here are the questions used for this report , along with responses, and its methodology .
While bullying existed long before the internet, the rise of smartphones and social media has brought a new and more public arena into play for this aggressive behavior.
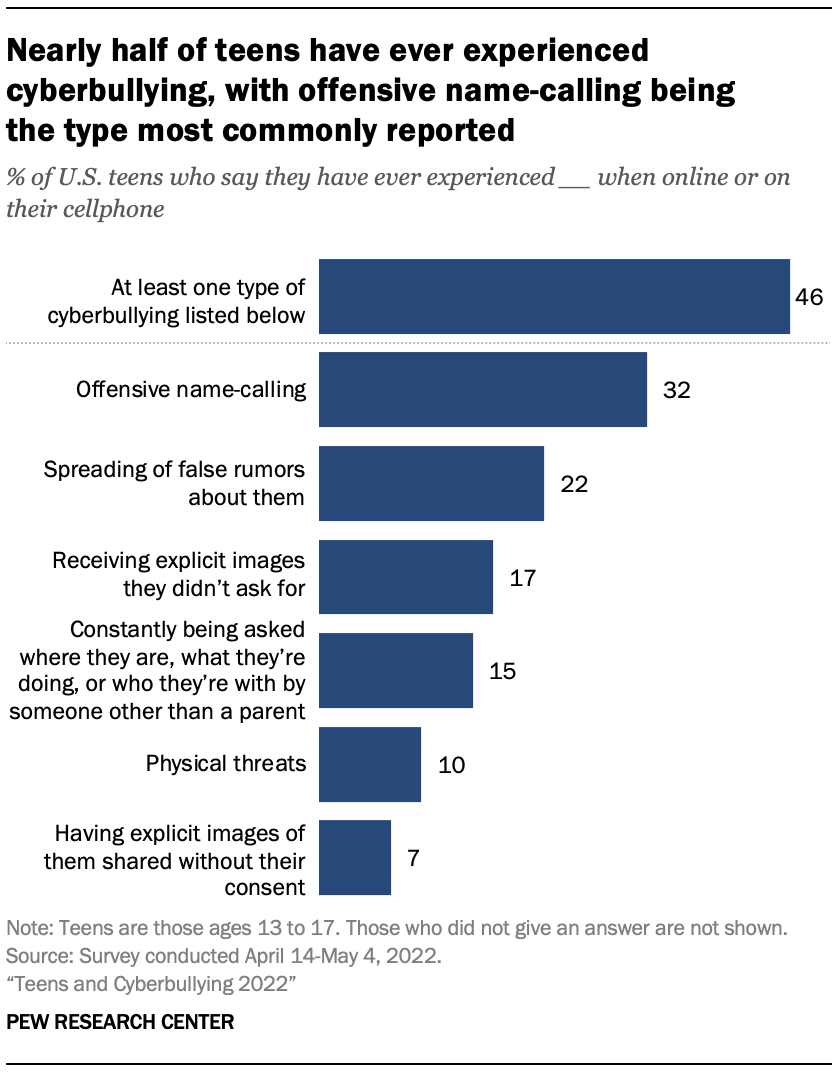
Nearly half of U.S. teens ages 13 to 17 (46%) report ever experiencing at least one of six cyberbullying behaviors asked about in a Pew Research Center survey conducted April 14-May 4, 2022. 1
The most commonly reported behavior in this survey is name-calling, with 32% of teens saying they have been called an offensive name online or on their cellphone. Smaller shares say they have had false rumors spread about them online (22%) or have been sent explicit images they didn’t ask for (17%).
Some 15% of teens say they have experienced someone other than a parent constantly asking them where they are, what they’re doing or who they’re with, while 10% say they have been physically threatened and 7% of teens say they have had explicit images of them shared without their consent.
In total, 28% of teens have experienced multiple types of cyberbullying.
Defining cyberbullying in this report
This report measures cyberbullying of teens using six distinct behaviors:
- Offensive name-calling
- Spreading of false rumors about them
- Receiving explicit images they didn’t ask for
- Physical threats
- Constantly being asked where they are, what they’re doing, or who they’re with by someone other than a parent
- Having explicit images of them shared without their consent
Teens who indicate they have personally experienced any of these behaviors online or while using their cellphone are considered targets of cyberbullying in this report. The terms “cyberbullying” and “online harassment” are used interchangeably throughout this report.
Age and gender are related to teens’ cyberbullying experiences, with older teen girls being especially likely to face this abuse
Teens’ experiences with online harassment vary by age. Some 49% of 15- to 17-year-olds have experienced at least one of the six online behaviors, compared with 42% of those ages 13 to 14. While similar shares of older and younger teens report being the target of name-calling or rumor spreading, older teens are more likely than their younger counterparts (22% vs. 11%) to say someone has sent them explicit images they didn’t ask for, an act sometimes referred to as cyberflashing ; had someone share explicit images of them without their consent, in what is also known as revenge porn (8% vs. 4%); or been the target of persistent questioning about their whereabouts and activities (17% vs. 12%).
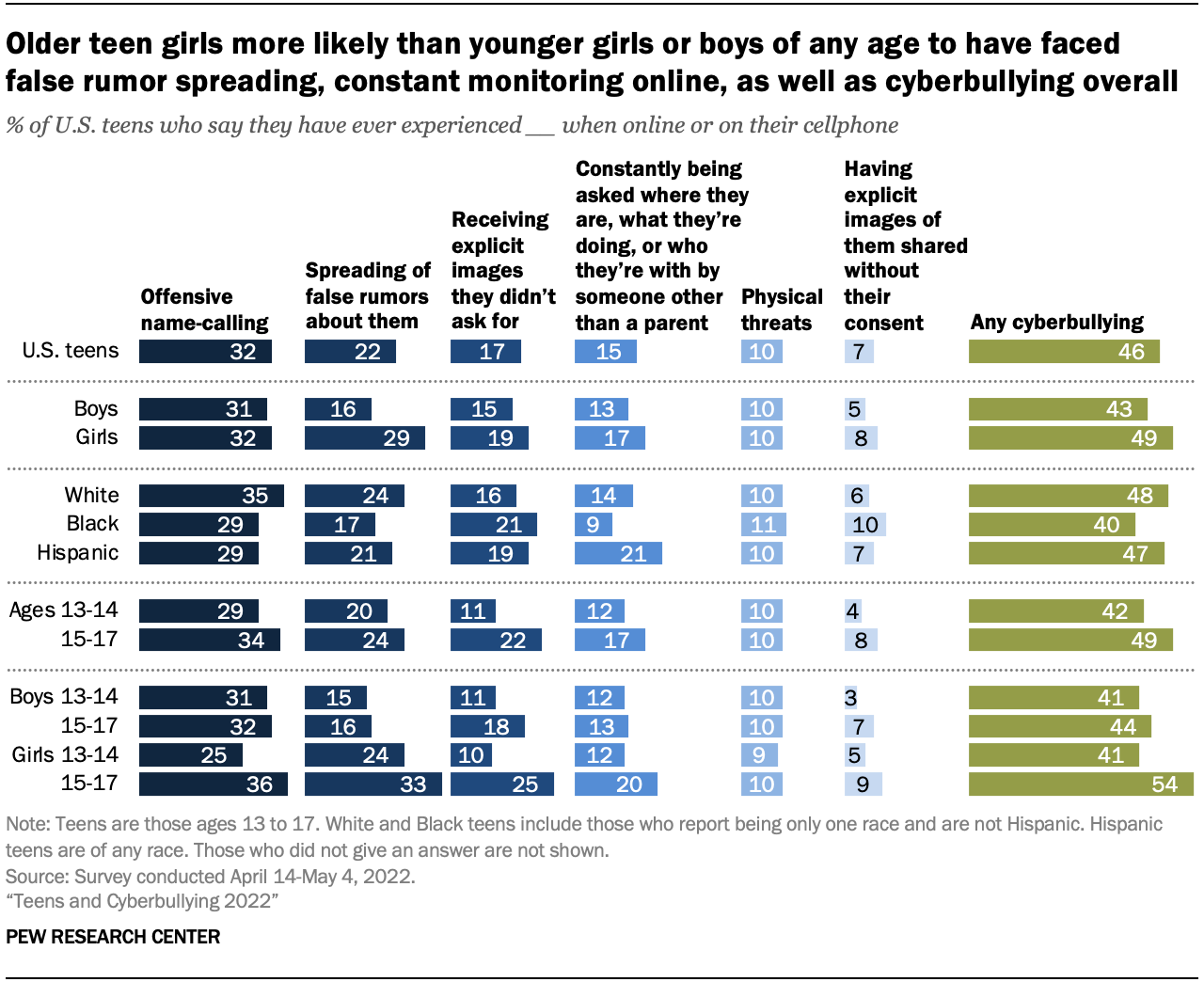
While there is no gender difference in having ever experienced online abuse, teen girls are more likely than teen boys to say false rumors have been spread about them. But further differences are seen when looking at age and gender together: 15- to 17-year-old girls stand out for being particularly likely to have faced any cyberbullying, compared with younger teen girls and teen boys of any age. Some 54% of girls ages 15 to 17 have experienced at least one of the six cyberbullying behaviors, while 44% of 15- to 17-year-old boys and 41% of boys and girls ages 13 to 14 say the same. These older teen girls are also more likely than younger teen girls and teen boys of any age to report being the target of false rumors and constant monitoring by someone other than a parent.
White, Black and Hispanic teens do not statistically differ in having ever been harassed online, but specific types of online attacks are more prevalent among certain groups. 2 For example, White teens are more likely to report being targeted by false rumors than Black teens. Hispanic teens are more likely than White or Black teens to say they have been asked constantly where they are, what they’re doing or who they’re with by someone other than a parent.
There are also differences by household income when it comes to physical threats. Teens who are from households making less than $30,000 annually are twice as likely as teens living in households making $75,000 or more a year to say they have been physically threatened online (16% vs. 8%).
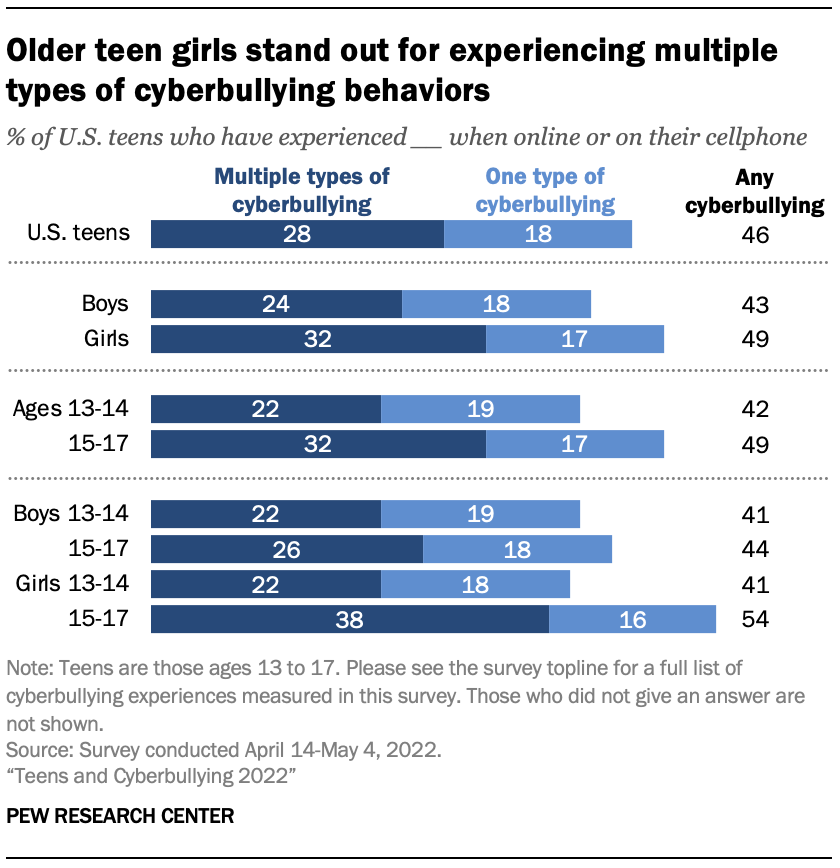
Beyond those differences related to specific harassing behaviors, older teen girls are particularly likely to say they experience multiple types of online harassment. Some 32% of teen girls have experienced two or more types of online harassment asked about in this survey, while 24% of teen boys say the same. And 15- to 17-year-olds are more likely than 13- to 14-year-olds to have been the target of multiple types of cyberbullying (32% vs. 22%).
These differences are largely driven by older teen girls: 38% of teen girls ages 15 to 17 have experienced at least two of the harassing behaviors asked about in this survey, while roughly a quarter of younger teen girls and teen boys of any age say the same.
Beyond demographic differences, being the target of these behaviors and facing multiple types of these behaviors also vary by the amount of time youth spend online. Teens who say they are online almost constantly are not only more likely to have ever been harassed online than those who report being online less often (53% vs 40%), but are also more likely to have faced multiple forms of online abuse (37% vs. 21%).
These are some of the findings from a Pew Research Center online survey of 1,316 U.S. teens conducted from April 14 to May 4, 2022.
Black teens are about twice as likely as Hispanic or White teens to say they think their race or ethnicity made them a target of online abuse
There are numerous reasons why a teen may be targeted with online abuse. This survey asked youth if they believed their physical appearance, gender, race or ethnicity, sexual orientation or political views were a factor in them being the target of abusive behavior online.
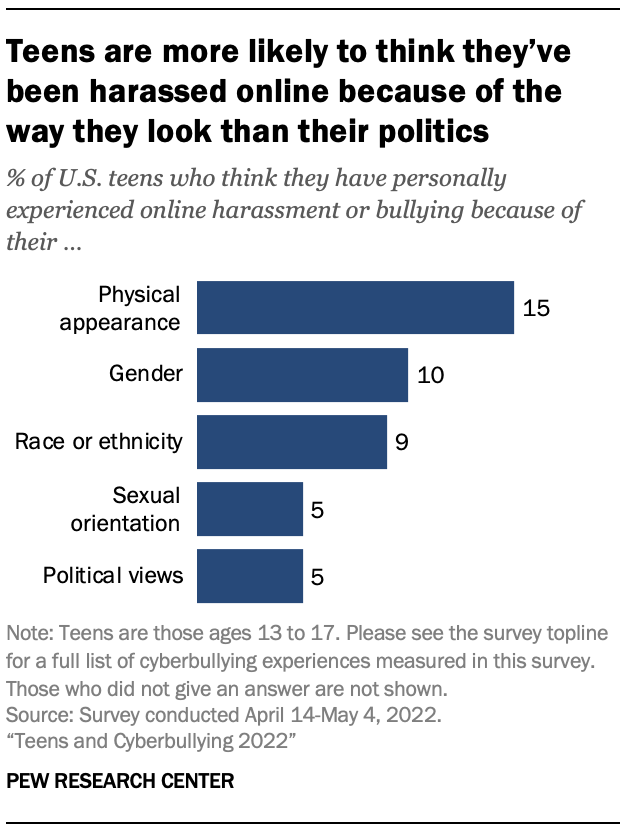
Teens are most likely to say their physical appearance made them the target of cyberbullying. Some 15% of all teens think they were cyberbullied because of their appearance.
About one-in-ten teens say they were targeted because of their gender (10%) or their race or ethnicity (9%). Teens less commonly report being harassed for their sexual orientation or their political views – just 5% each.
Looking at these numbers in a different way, 31% of teens who have personally experienced online harassment or bullying think they were targeted because of their physical appearance. About one-in-five cyberbullied teens say they were targeted due to their gender (22%) or their racial or ethnic background (20%). And roughly one-in-ten affected teens point to their sexual orientation (12%) or their political views (11%) as a reason why they were targeted with harassment or bullying online.
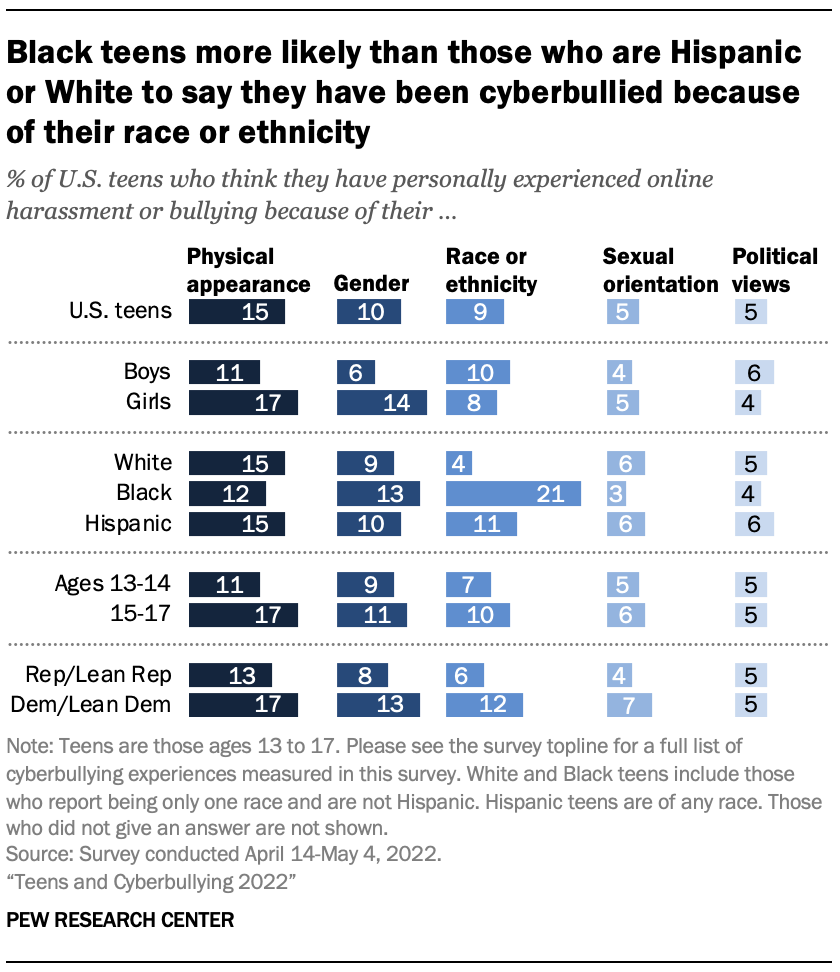
The reasons teens cite for why they were targeted for cyberbullying are largely similar across major demographic groups, but there are a few key differences. For example, teen girls overall are more likely than teen boys to say they have been cyberbullied because of their physical appearance (17% vs. 11%) or their gender (14% vs. 6%). Older teens are also more likely to say they have been harassed online because of their appearance: 17% of 15- to 17-year-olds have experienced cyberbullying because of their physical appearance, compared with 11% of teens ages 13 to 14.
Older teen girls are particularly likely to think they have been harassed online because of their physical appearance: 21% of all 15- to 17-year-old girls think they have been targeted for this reason. This compares with about one-in-ten younger teen girls or teen boys, regardless of age, who think they have been cyberbullied because of their appearance.
A teen’s racial or ethnic background relates to whether they report having been targeted for cyberbullying because of race or ethnicity. Some 21% of Black teens report being made a target because of their race or ethnicity, compared with 11% of Hispanic teens and an even smaller share of White teens (4%).
There are no partisan differences in teens being targeted for their political views, with 5% of those who identify as either Democratic or Republican – including those who lean toward each party – saying they think their political views contributed to them being cyberbullied.
Black or Hispanic teens are more likely than White teens to say cyberbullying is a major problem for people their age
In addition to measuring teens’ own personal experiences with cyberbullying, the survey also sought to understand young people’s views about online harassment more generally.
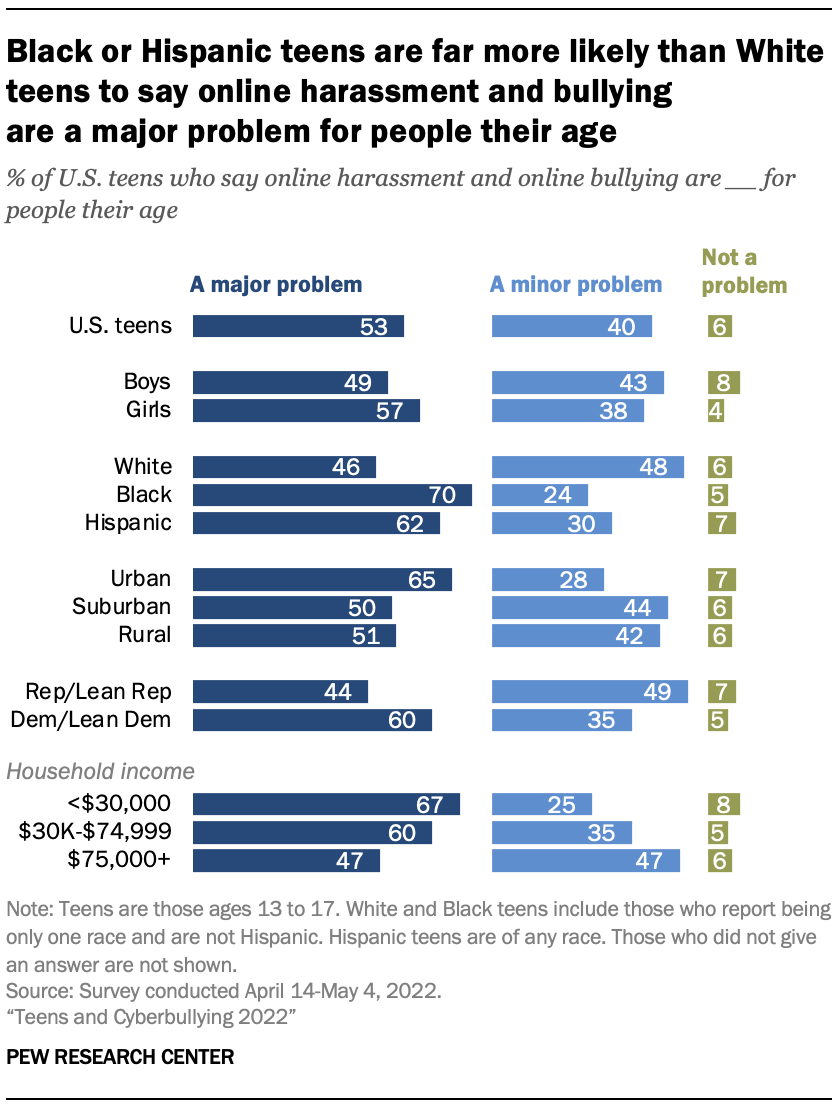
The vast majority of teens say online harassment and online bullying are a problem for people their age, with 53% saying they are a major problem. Just 6% of teens think they are not a problem.
Certain demographic groups stand out for how much of a problem they say cyberbullying is. Seven-in-ten Black teens and 62% of Hispanic teens say online harassment and bullying are a major problem for people their age, compared with 46% of White teens. Teens from households making under $75,000 a year are similarly inclined to call this type of harassment a major problem, with 62% making this claim, compared with 47% of teens from more affluent homes. Teen girls are also more likely than boys to view cyberbullying as a major problem.
Views also vary by community type. Some 65% of teens living in urban areas say online harassment and bullying are a major problem for people their age, compared with about half of suburban and rural teens.
Partisan differences appear as well: Six-in-ten Democratic teens say this is a major problem for people their age, compared with 44% of Republican teens saying this.
Roughly three-quarters of teens or more think elected officials and social media sites aren’t adequately addressing online abuse
In recent years, there have been several initiatives and programs aimed at curtailing bad behavior online, but teens by and large view some of those behind these efforts – including social media companies and politicians – in a decidedly negative light.
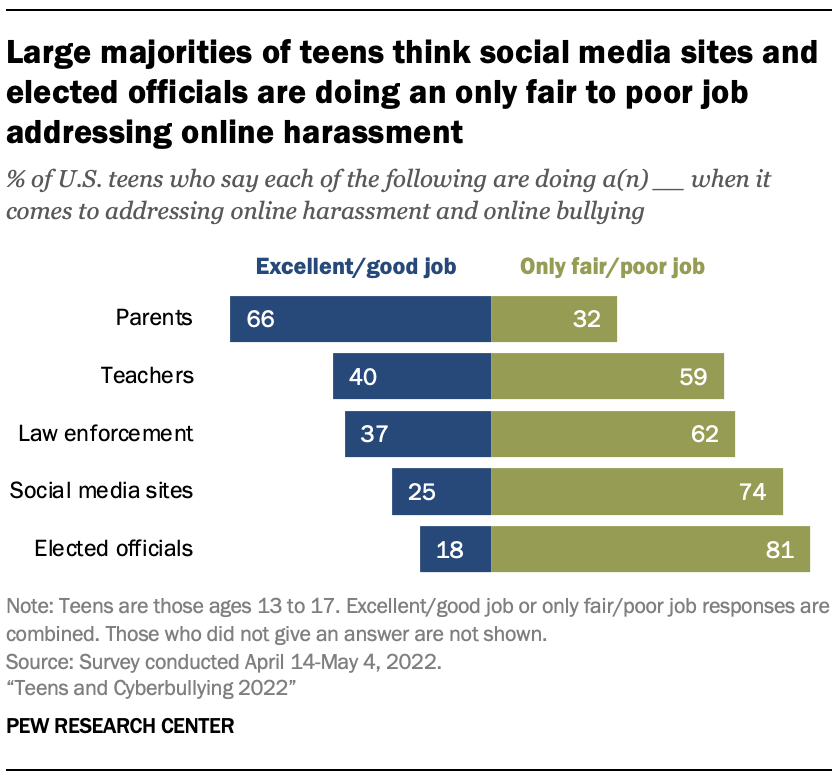
According to teens, parents are doing the best of the five groups asked about in terms of addressing online harassment and online bullying, with 66% of teens saying parents are doing at least a good job, including one-in-five saying it is an excellent job. Roughly four-in-ten teens report thinking teachers (40%) or law enforcement (37%) are doing a good or excellent job addressing online abuse. A quarter of teens say social media sites are doing at least a good job addressing online harassment and cyberbullying, and just 18% say the same of elected officials. In fact, 44% of teens say elected officials have done a poor job addressing online harassment and online bullying.
Teens who have been cyberbullied are more critical of how various groups have addressed online bullying than those who haven’t
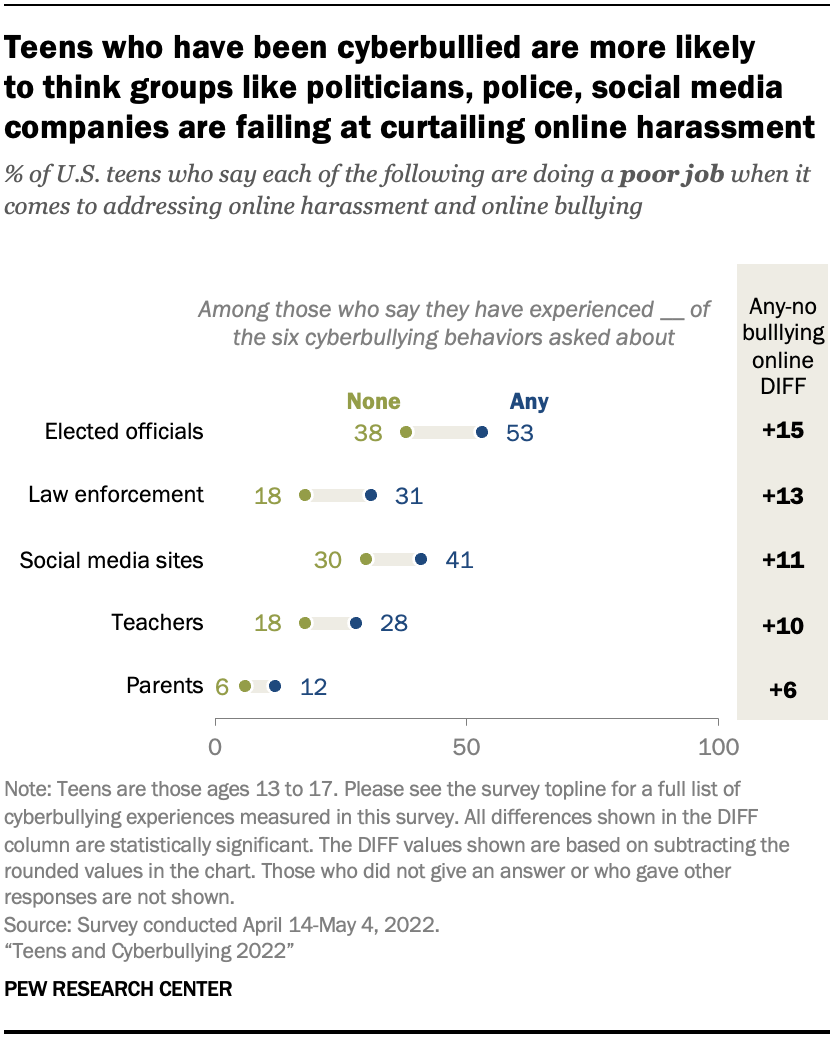
Teens who have experienced harassment or bullying online have a very different perspective on how various groups have been handling cyberbullying compared with those who have not faced this type of abuse. Some 53% of teens who have been cyberbullied say elected officials have done a poor job when it comes to addressing online harassment and online bullying, while 38% who have not undergone these experiences say the same (a 15 percentage point gap). Double-digit differences also appear between teens who have and have not been cyberbullied in their views on how law enforcement, social media sites and teachers have addressed online abuse, with teens who have been harassed or bullied online being more critical of each of these three groups. These harassed teens are also twice as likely as their peers who report no abuse to say parents have done a poor job of combatting online harassment and bullying.
Aside from these differences based on personal experience with cyberbullying, only a few differences are seen across major demographic groups. For example, Black teens express greater cynicism than White teens about how law enforcement has fared in this space: 33% of Black teens say law enforcement is doing a poor job when it comes to addressing online harassment and online bullying; 21% of White teens say the same. Hispanic teens (25%) do not differ from either group on this question.
Large majorities of teens believe permanent bans from social media and criminal charges can help reduce harassment on the platforms
Teens have varying views about possible actions that could help to curb the amount of online harassment youth encounter on social media.
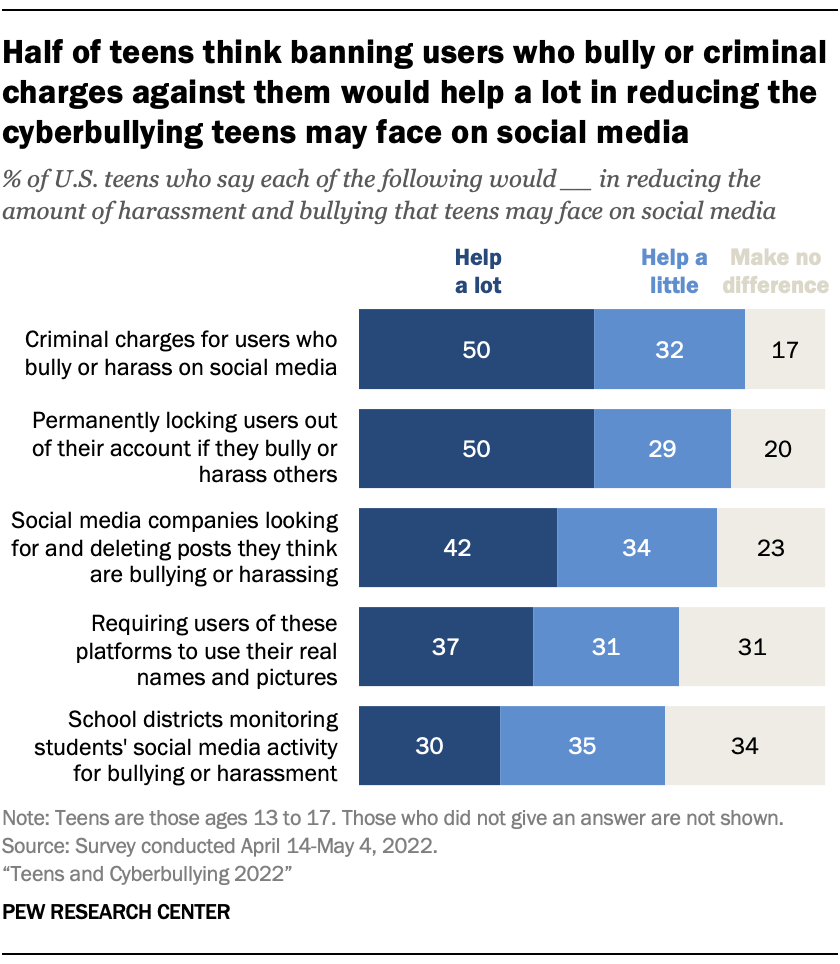
While a majority of teens say each of five possible solutions asked about in the survey would at least help a little, certain measures are viewed as being more effective than others.
Teens see the most benefit in criminal charges for users who bully or harass on social media or permanently locking these users out of their account. Half of teens say each of these options would help a lot in reducing the amount of harassment and bullying teens may face on social media sites.
About four-in-ten teens think that if social media companies looked for and deleted posts they think are bullying or harassing (42%) or if users of these platforms were required to use their real names and pictures (37%) it would help a lot in addressing these issues. The idea of forcing people to use their real name while online has long existed and been heavily debated: Proponents see it as a way to hold bad actors accountable and keep online conversations more civil , while detractors believe it would do little to solve harassment and could even worsen it .
Three-in-ten teens say school districts monitoring students’ social media activity for bullying or harassment would help a lot. Some school districts already use digital monitoring software to help them identify worrying student behavior on school-owned devices , social media and other online platforms . However, these programs have been met with criticism regarding privacy issues , mixed results and whether they do more harm than good .
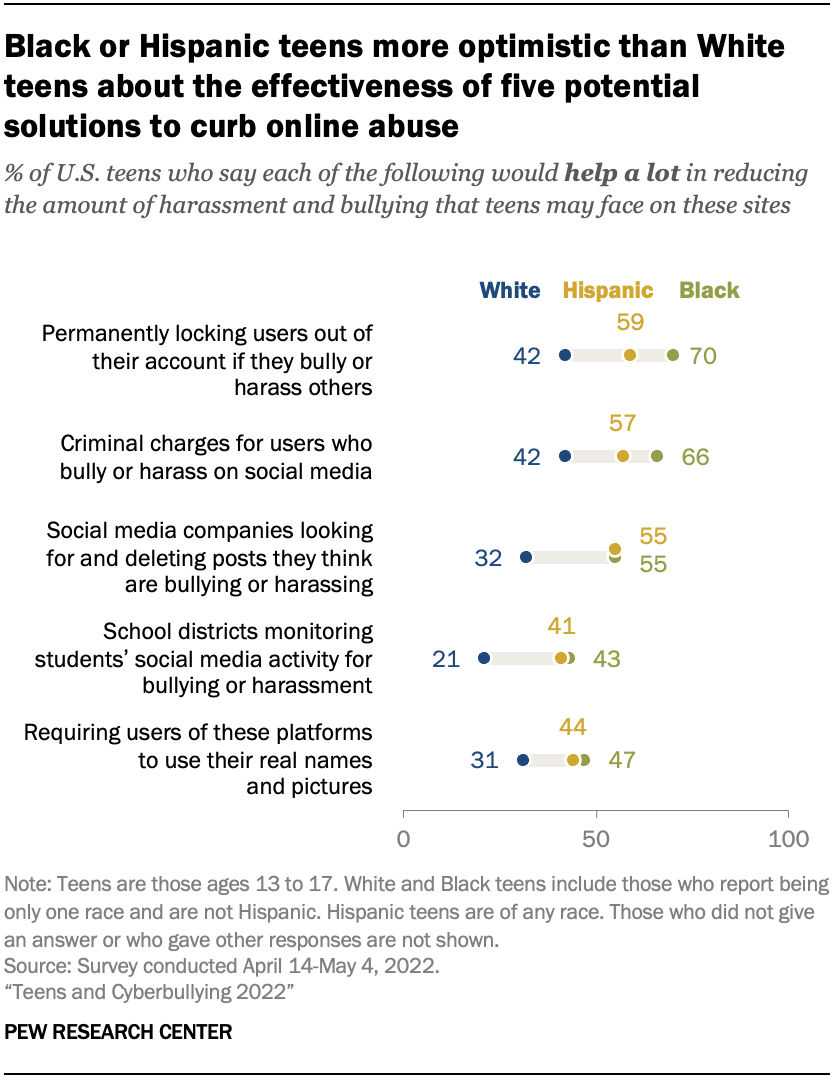
Having personally experienced online harassment is unrelated to a teen’s view on whether these potential measures would help a lot in reducing these types of adverse experiences on social media. Views do vary widely by a teen’s racial or ethnic background, however.
Black or Hispanic teens are consistently more optimistic than White teens about the effectiveness of each of these measures.
Majorities of both Black and Hispanic teens say permanently locking users out of their account if they bully or harass others or criminal charges for users who bully or harass on social media would help a lot, while about four-in-ten White teens express each view.
In the case of permanent bans, Black teens further stand out from their Hispanic peers: Seven-in-ten say this would help a lot, followed by 59% of Hispanic teens and 42% of White teens.
- It is important to note that there are various ways researchers measure youths’ experiences with cyberbullying and online harassment. As a result, there may be a range of estimates for how many teens report having these experiences. In addition, since the Center last polled on this topic in 2018, there have been changes in how the surveys were conducted and how the questions were asked. For instance, the 2018 survey asked about bullying by listing a number of possible behaviors and asking respondents to “check all that apply.” This survey asked teens to answer “yes” or “no” to each item individually. Due to these changes, direct comparisons cannot be made across the two surveys. ↩
- There were not enough Asian American teen respondents in the sample to be broken out into a separate analysis. As always, their responses are incorporated into the general population figures throughout the report. ↩
Sign up for our weekly newsletter
Fresh data delivery Saturday mornings
Sign up for The Briefing
Weekly updates on the world of news & information
- Online Harassment & Bullying
- Teens & Tech
- Teens & Youth
Teens and Video Games Today
How teens and parents approach screen time, teens, social media and technology 2023, teens and social media: key findings from pew research center surveys, gun deaths among u.s. children and teens rose 50% in two years, most popular, report materials.
901 E St. NW, Suite 300 Washington, DC 20004 USA (+1) 202-419-4300 | Main (+1) 202-857-8562 | Fax (+1) 202-419-4372 | Media Inquiries
Research Topics
- Email Newsletters
ABOUT PEW RESEARCH CENTER Pew Research Center is a nonpartisan fact tank that informs the public about the issues, attitudes and trends shaping the world. It conducts public opinion polling, demographic research, media content analysis and other empirical social science research. Pew Research Center does not take policy positions. It is a subsidiary of The Pew Charitable Trusts .
© 2024 Pew Research Center
- INNOVATION FESTIVAL
- Capital One
08-06-2024 DESIGN
Even Taylor Swift isn’t immune to the dark side of social media
Arguably, platforms breed bullying: People are more likely to engage in cruel behavior online than they would face-to-face.
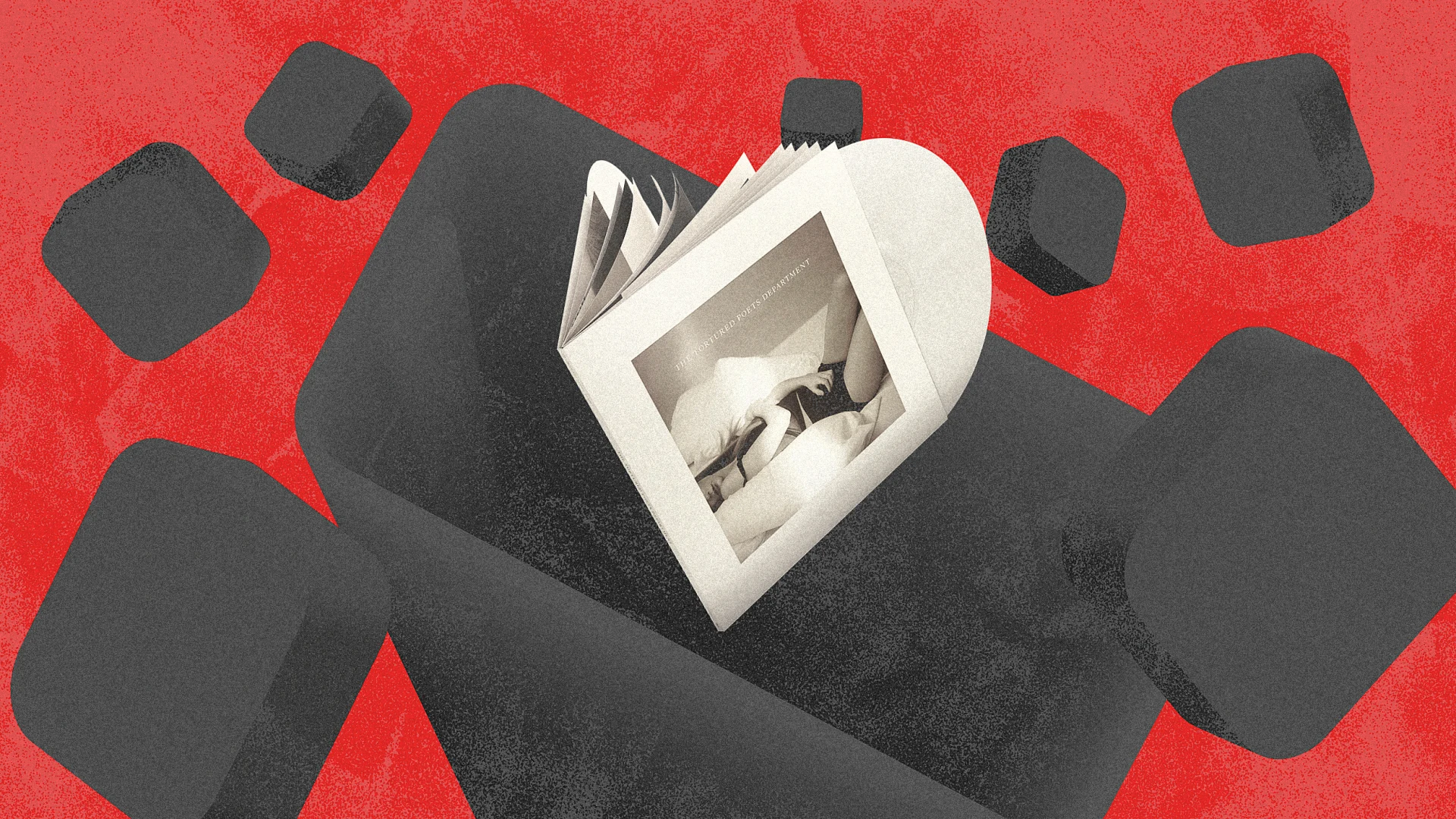
[Illustration: FC]
BY The Conversation 4 minute read
As an expert in consumer behavior, I recently edited a book about how social media affects mental health .
I’m also a big fan of Taylor Swift .
So when I listened to Swift’s latest album, The Tortured Poets Department , I couldn’t help but notice parallels to the research that I’ve been studying for the past decade.
It might seem like an outlandish comparison. What can the bestselling album of 2024 have to do with research into the dark side of social media?
But bear with me: Taylor Swift lives in the same social media-saturated universe as the rest of us. That may be why the melancholic themes of her album resonate with so many people.
With young people out of school for the summer and spending free time on social media, now is a time to put on some tunes and think about mental health and what is called “consumer well-being” in the transformative consumer research area of scholarship.
Here are three Taylor-made takeaways that shed light on some of the themes in my latest edited book, The Darker Side of Social Media: Consumer Psychology and Mental Health .
Lesson 1: Modern life through the social media lens can get you down
If you’ve been feeling out of sorts lately, you’re hardly alone: Anxiety and depression can be exacerbated by overuse of social media, research summarized in Chapter 1 shows. And social media use is on the rise.
The average American teenager spends nearly five hours every day scrolling TikTok, Instagram and the like, polling shows, while adults clock more than two hours a day on social media. Such could be compulsive social media use and overall overuse.
Digital life can simulate addiction and sometimes manifest as a distinct form of anxiety called “disconnection anxiety,” researchers Line Lervik-Olsen, Bob Fennis, and Tor Wallin Andreassen note in their book chapter on compulsive social media use. This can breed feelings of depression— a mood that recurs throughout The Tortured Poets Department .
Oftentimes, depression goes hand in hand with feelings of loneliness. Social media has, in some ways, made people feel even lonelier—nearly four in five Americans say that social media has made social divisions worse , according to Pew Research. In our book chapter, my graduate student Betül Dayan and I consider the prevalence of loneliness in the digital world.
The pandemic showed the world that social media relationships can’t replace physical company. Even celebrities with hundreds of millions of followers simply want someone to be with. In the song “The Prophecy,” Swift sings of loneliness and wanting someone who simply enjoys her presence:
Don’t want money/ Just someone who wants my company ( “The Prophecy” )
Lesson 2: Comparisons will make you miserable
Social media is a breeding ground for comparisons . And since people tend to portray idealized versions of themselves on social media—rather than their authentic selves—these comparisons are often false or skewed . Research has shown that people on social media tend to make “upward comparisons,” judging themselves relative to people they find inspiring. Social media can breed false comparisons, as what someone is aspiring to may not be authentic.
This can lead to what researchers call a “negative self-discrepancy”—a sense of disappointment with one’s failure to meet a personal ideal. As researchers Ashesh Mukherjee and Arani Roy note in their book chapter , social media makes people more dissatisfied with their own sense of control, intelligence and power. This, in turn, can worsen stress and anxiety.
The theme of comparisons comes through loud and clear in the song “ The Tortured Poets Department ,” in which Swift castigates a partner with literary pretensions—and herself for dating him. Swift may be the most rich, famous and successful pop star on the planet, but comparing yourself with even more heroic figures is sure to make anyone feel worse:
You’re not Dylan Thomas, I’m not Patti Smith. This ain’t the Chelsea Hotel, we’re modern idiots. ( “The Tortured Poets Department” )
Lesson 3: Bullying isn’t a minor problem
In today’s social media-focused world, bullying has transitioned to online platforms. And arguably, platforms breed bullying: People are more likely to engage in cruel behavior online than they would face-to-face.
Policymakers increasingly recognize bullying as an important political concern. In their book chapter, researchers Madison Brown, Kate Pounders, and Gary Wilcox have examined laws intended to fight bullying.
One such effort, the Kids Online Safety Act , which among other things would require online platforms to take steps to address cyberbullying , recently passed the U.S. Senate.
Lawmakers aren’t the only ones taking bullying seriously. In her latest album, Swift refers to bullies in her own life as vipers who “disgrace her good name” and who say insults that stick with her for a long time. Themes of reputation and bullying have run throughout Swift’s entire body of work—hardly surprising for someone who has lived such a public life , both online and off.
I’ll tell you something ’bout my good name. It’s mine alone to disgrace. I don’t cater to all these vipers dressed in empath’s clothing. ( “But Daddy I Love Him” )
It is not known whether overall social media use or overuse alone causes some of these outcomes, but our research does demonstrate that in many ways there’s a darker side to social media when it comes to consumer well-being—even for celebrities. So if you’re going to see the Eras Tour in Europe this summer , you might want to leave your phone back at the hotel.
Angeline Close Scheinbaum is an associate professor of marketing at Clemson University .
This article is republished from The Conversation under a Creative Commons license. Read the original article .
Apply to the Most Innovative Companies Awards and be recognized as an organization driving the world forward through innovation. Early-rate deadline: Friday, August 23.
ABOUT THE AUTHOR
The Conversation is a nonprofit, independent news organization dedicated to unlocking the knowledge of experts for the public good. More
Explore Topics
- social media
- Taylor Swift
- Tech Trump’s campaign claims their emails were hacked and Iran is to blame
- Tech Elon Musk is set to interview Trump on X
- Tech How the FTC will make it easier for you to click ‘unsubscribe’
- News Truth Social may be a barometer for Trump’s presidential campaign—and stock is down after a $16 million loss
- News Blink Fitness bankruptcy: Why the discount gym owned by Equinox Holdings just filed for Chapter 11
- News Disneyland and Disney World expansion plans: Every new theme park attraction just announced
- Design LA 2028: How the city is preparing for its third Olympics
- Design Why you should look down—not up—when you’re near a skyscraper
- Design Why TikTok’s ‘Underconsumption Core’ trend won’t die
- Work Life Tech failures like the Crowdstrike outage are the new normal. Here’s how leaders can prep for them
- Work Life This is exactly how Project 2025 plans to gut DEI
- Work Life 5 strategies to avoid mid-year burnout this summer

IMAGES
COMMENTS
There was a steady increase in the number of cyberbullying studies published during the 3-year review period: 1 each in 2013 and 2014 (4.5%, respectively), 7 in 2014 (31.8%), and 11 in 2015 (50%). Appendix A summarizes the 22 papers that were reviewed. There was a general consensus that cyberbullying only affects youths.
1. Introduction. Cyberbullying is an emerging societal issue in the digital era [1, 2].The Cyberbullying Research Centre [3] conducted a nationwide survey of 5700 adolescents in the US and found that 33.8 % of the respondents had been cyberbullied and 11.5 % had cyberbullied others.While cyberbullying occurs in different online channels and platforms, social networking sites (SNSs) are fertile ...
Due to changes in use and behavioral patterns among the youth on social media, the manifestations and risk factors of cyberbullying have faced significant transformation. Further, as the boundaries of cyberbullying are not limited by geography, cyberbullying may not be a problem contained within a single country.
The increased use of social media by teenagers, has led to cyberbullying becoming a major issue. Cyberbullying is the use of information and communication technology to harass and harm in a ...
Marengo, N. et al. Cyberbullying and problematic social media use: An insight into the positive role of social support in adolescents—data from the Health Behaviour in School-aged Children study ...
Prevention and intervention. Cyberbullying via social media and well-being. Since 2004, there has been increased research attention on cyberbullying, with a particular focus on predictors and outcomes of victimization and perpetration. Outcomes have included measures of well-being, such as self-esteem, depression, and social support [1].
Most of the social media today prohibit the use of abusive and insulting comments. These ways are not efficient as it’s time consuming to monitor or to inspect all the reported posts daily. Many researchers in our field introduced many approaches to detect cyberbullying in social media which will be discussed in the next section. 2.
In spite of these difficulties, the fact remains that cyberbullying is a serious problem confronting adolescents today, requiring further research to better understand its nature and how it may impact our youth. ... Craig W, et al. Social media use and cyber-bullying: a cross-national analysis of young people in 42 countries. J Adolesc Health ...
cyberbullying, in which individuals or groups of individuals use the media to inflict emotional distress on. other individuals (Bocij 2004). According to a rece nt study of 743 teenager s and ...
Abstract and Figures. Cyberbullying on social networking sites is an emerging societal issue that has drawn significant scholarly attention. The purpose of this study is to consolidate the ...
The rise in research work focusing on detection of cyberbullying incidents on social media platforms particularly reflect how dire cyberbullying consequences are, regardless of age, gender or location. This paper examines scholarly publications (i.e., 2011-2022) on cyberbullying detection using machine learning through a systematic literature review approach. Specifically, articles were ...
Defining verbal, social, physical, and cyberbullying victimization. Bullying occurs when someone takes an adverse action against another that inflicts intentional harm or discomfort (Olweus, Citation 1994).The method of delivery, however, can substantially vary from slapping, name-calling, exclusion from groups, or even harassment/embarrassment on social media.
In this paper we analyze the state-of-the-art techniques to deal with cyber-bullying problem, focusing on several automated tasks and also considering data crawled from social media. In particular, we classified the approaches proposed so far in four categories according to addressed tasks, ranging from cyberbully-
Cyberbullying detection in SMS and email: Users are concerned with combating cyberbullying problems, which largely propagate through social media platforms. However, future researchers may put more attention on investigating Short Message Service (SMS)- and email-based cyberbullying detection methods.
Recent research studies have revealed that cyberbullying and online harassment are considerable problems for users of social media platforms, especially young people. A 2016 report of the Cyberbullying Research Centre indicates that 33.8% of middle-and high-school students aged between 13 and 17 are at some point subject to being victims of ...
Data were collected from 200 students in the UAE. 91% of the study sample confirmed the existence of acts of cyberbullying on social media with Instagram (55.5%) and Facebook (38%) in the lead.
Abstract. Cyberbullying is an important issue for our society and has a major negative effect on the victims, that can be highly damaging due to the frequency and high propagation provided by Information Technologies. Therefore, the early detection of cyberbullying in social networks becomes crucial to mitigate the impact on the victims.
The survey sample consisted of 638 Israeli undergraduate students. The data were collected using the Revised Cyber Bullying Survey, which evaluates the frequency and media used to perpetrate cyberbullying, and the College Adjustment Scales, which evaluate three aspects of development in college students.
Pakistan Journal of Criminology Vol. 9, Issue 4, October 2017 (119-139) BULLYING IN SOCIAL MEDIA: AN EFFECT STUDY OF CYBER BULLYING ON THE YOUTH Sumera Batool Rabia Yousaf Feroza Batool Abstract This research study seeks to investigatethe bullying in social media and its effects on youth to extract the factors that have influence on their state ...
Due to changes in use and behavioral patterns among the youth on social media, the manifestations and risk factors of cyberbullying have faced significant transformation. Further, as the boundaries of cyberbullying are not limited by geography, cyberbullying may not be a problem contained within a single country.
In this paper we analyze the state-of-the-art techniques to deal with cyberbullying problem, focusing on several automated tasks and also considering data crawled from social media. In particular, we classified the approaches proposed so far in four categories according to addressed tasks, ranging from cyberbullying prediction until to incident ...
Social media have become a very viable medium for communication, collaboration, exchange of information, knowledge, and ideas. However, due to anonymity preservation, the incidents of hate speech and cyberbullying have been diversified across the globe. This intimidating problem has recently sought the attention of researchers and scholars worldwide and studies have been undertaken to ...
While bullying existed long before the internet, the rise of smartphones and social media has brought a new and more public arena into play for this aggressive behavior.. Nearly half of U.S. teens ages 13 to 17 (46%) report ever experiencing at least one of six cyberbullying behaviors asked about in a Pew Research Center survey conducted April 14-May 4, 2022. 1
Lesson 3: Bullying isn't a minor problem. In today's social media-focused world, bullying has transitioned to online platforms. And arguably, platforms breed bullying: ...