- Privacy Policy

Home » Questionnaire – Definition, Types, and Examples

Questionnaire – Definition, Types, and Examples
Table of Contents

Questionnaire
Definition:
A Questionnaire is a research tool or survey instrument that consists of a set of questions or prompts designed to gather information from individuals or groups of people.
It is a standardized way of collecting data from a large number of people by asking them a series of questions related to a specific topic or research objective. The questions may be open-ended or closed-ended, and the responses can be quantitative or qualitative. Questionnaires are widely used in research, marketing, social sciences, healthcare, and many other fields to collect data and insights from a target population.
History of Questionnaire
The history of questionnaires can be traced back to the ancient Greeks, who used questionnaires as a means of assessing public opinion. However, the modern history of questionnaires began in the late 19th century with the rise of social surveys.
The first social survey was conducted in the United States in 1874 by Francis A. Walker, who used a questionnaire to collect data on labor conditions. In the early 20th century, questionnaires became a popular tool for conducting social research, particularly in the fields of sociology and psychology.
One of the most influential figures in the development of the questionnaire was the psychologist Raymond Cattell, who in the 1940s and 1950s developed the personality questionnaire, a standardized instrument for measuring personality traits. Cattell’s work helped establish the questionnaire as a key tool in personality research.
In the 1960s and 1970s, the use of questionnaires expanded into other fields, including market research, public opinion polling, and health surveys. With the rise of computer technology, questionnaires became easier and more cost-effective to administer, leading to their widespread use in research and business settings.
Today, questionnaires are used in a wide range of settings, including academic research, business, healthcare, and government. They continue to evolve as a research tool, with advances in computer technology and data analysis techniques making it easier to collect and analyze data from large numbers of participants.
Types of Questionnaire
Types of Questionnaires are as follows:
Structured Questionnaire
This type of questionnaire has a fixed format with predetermined questions that the respondent must answer. The questions are usually closed-ended, which means that the respondent must select a response from a list of options.
Unstructured Questionnaire
An unstructured questionnaire does not have a fixed format or predetermined questions. Instead, the interviewer or researcher can ask open-ended questions to the respondent and let them provide their own answers.
Open-ended Questionnaire
An open-ended questionnaire allows the respondent to answer the question in their own words, without any pre-determined response options. The questions usually start with phrases like “how,” “why,” or “what,” and encourage the respondent to provide more detailed and personalized answers.
Close-ended Questionnaire
In a closed-ended questionnaire, the respondent is given a set of predetermined response options to choose from. This type of questionnaire is easier to analyze and summarize, but may not provide as much insight into the respondent’s opinions or attitudes.
Mixed Questionnaire
A mixed questionnaire is a combination of open-ended and closed-ended questions. This type of questionnaire allows for more flexibility in terms of the questions that can be asked, and can provide both quantitative and qualitative data.
Pictorial Questionnaire:
In a pictorial questionnaire, instead of using words to ask questions, the questions are presented in the form of pictures, diagrams or images. This can be particularly useful for respondents who have low literacy skills, or for situations where language barriers exist. Pictorial questionnaires can also be useful in cross-cultural research where respondents may come from different language backgrounds.
Types of Questions in Questionnaire
The types of Questions in Questionnaire are as follows:
Multiple Choice Questions
These questions have several options for participants to choose from. They are useful for getting quantitative data and can be used to collect demographic information.
- a. Red b . Blue c. Green d . Yellow
Rating Scale Questions
These questions ask participants to rate something on a scale (e.g. from 1 to 10). They are useful for measuring attitudes and opinions.
- On a scale of 1 to 10, how likely are you to recommend this product to a friend?
Open-Ended Questions
These questions allow participants to answer in their own words and provide more in-depth and detailed responses. They are useful for getting qualitative data.
- What do you think are the biggest challenges facing your community?
Likert Scale Questions
These questions ask participants to rate how much they agree or disagree with a statement. They are useful for measuring attitudes and opinions.
How strongly do you agree or disagree with the following statement:
“I enjoy exercising regularly.”
- a . Strongly Agree
- c . Neither Agree nor Disagree
- d . Disagree
- e . Strongly Disagree
Demographic Questions
These questions ask about the participant’s personal information such as age, gender, ethnicity, education level, etc. They are useful for segmenting the data and analyzing results by demographic groups.
- What is your age?
Yes/No Questions
These questions only have two options: Yes or No. They are useful for getting simple, straightforward answers to a specific question.
Have you ever traveled outside of your home country?
Ranking Questions
These questions ask participants to rank several items in order of preference or importance. They are useful for measuring priorities or preferences.
Please rank the following factors in order of importance when choosing a restaurant:
- a. Quality of Food
- c. Ambiance
- d. Location
Matrix Questions
These questions present a matrix or grid of options that participants can choose from. They are useful for getting data on multiple variables at once.
The product is easy to use | ||||
The product meets my needs | ||||
The product is affordable |
Dichotomous Questions
These questions present two options that are opposite or contradictory. They are useful for measuring binary or polarized attitudes.
Do you support the death penalty?
How to Make a Questionnaire
Step-by-Step Guide for Making a Questionnaire:
- Define your research objectives: Before you start creating questions, you need to define the purpose of your questionnaire and what you hope to achieve from the data you collect.
- Choose the appropriate question types: Based on your research objectives, choose the appropriate question types to collect the data you need. Refer to the types of questions mentioned earlier for guidance.
- Develop questions: Develop clear and concise questions that are easy for participants to understand. Avoid leading or biased questions that might influence the responses.
- Organize questions: Organize questions in a logical and coherent order, starting with demographic questions followed by general questions, and ending with specific or sensitive questions.
- Pilot the questionnaire : Test your questionnaire on a small group of participants to identify any flaws or issues with the questions or the format.
- Refine the questionnaire : Based on feedback from the pilot, refine and revise the questionnaire as necessary to ensure that it is valid and reliable.
- Distribute the questionnaire: Distribute the questionnaire to your target audience using a method that is appropriate for your research objectives, such as online surveys, email, or paper surveys.
- Collect and analyze data: Collect the completed questionnaires and analyze the data using appropriate statistical methods. Draw conclusions from the data and use them to inform decision-making or further research.
- Report findings: Present your findings in a clear and concise report, including a summary of the research objectives, methodology, key findings, and recommendations.
Questionnaire Administration Modes
There are several modes of questionnaire administration. The choice of mode depends on the research objectives, sample size, and available resources. Some common modes of administration include:
- Self-administered paper questionnaires: Participants complete the questionnaire on paper, either in person or by mail. This mode is relatively low cost and easy to administer, but it may result in lower response rates and greater potential for errors in data entry.
- Online questionnaires: Participants complete the questionnaire on a website or through email. This mode is convenient for both researchers and participants, as it allows for fast and easy data collection. However, it may be subject to issues such as low response rates, lack of internet access, and potential for fraudulent responses.
- Telephone surveys: Trained interviewers administer the questionnaire over the phone. This mode allows for a large sample size and can result in higher response rates, but it is also more expensive and time-consuming than other modes.
- Face-to-face interviews : Trained interviewers administer the questionnaire in person. This mode allows for a high degree of control over the survey environment and can result in higher response rates, but it is also more expensive and time-consuming than other modes.
- Mixed-mode surveys: Researchers use a combination of two or more modes to administer the questionnaire, such as using online questionnaires for initial screening and following up with telephone interviews for more detailed information. This mode can help overcome some of the limitations of individual modes, but it requires careful planning and coordination.
Example of Questionnaire
Title of the Survey: Customer Satisfaction Survey
Introduction:
We appreciate your business and would like to ensure that we are meeting your needs. Please take a few minutes to complete this survey so that we can better understand your experience with our products and services. Your feedback is important to us and will help us improve our offerings.
Instructions:
Please read each question carefully and select the response that best reflects your experience. If you have any additional comments or suggestions, please feel free to include them in the space provided at the end of the survey.
1. How satisfied are you with our product quality?
- Very satisfied
- Somewhat satisfied
- Somewhat dissatisfied
- Very dissatisfied
2. How satisfied are you with our customer service?
3. How satisfied are you with the price of our products?
4. How likely are you to recommend our products to others?
- Very likely
- Somewhat likely
- Somewhat unlikely
- Very unlikely
5. How easy was it to find the information you were looking for on our website?
- Somewhat easy
- Somewhat difficult
- Very difficult
6. How satisfied are you with the overall experience of using our products and services?
7. Is there anything that you would like to see us improve upon or change in the future?
…………………………………………………………………………………………………………………………..
Conclusion:
Thank you for taking the time to complete this survey. Your feedback is valuable to us and will help us improve our products and services. If you have any further comments or concerns, please do not hesitate to contact us.
Applications of Questionnaire
Some common applications of questionnaires include:
- Research : Questionnaires are commonly used in research to gather information from participants about their attitudes, opinions, behaviors, and experiences. This information can then be analyzed and used to draw conclusions and make inferences.
- Healthcare : In healthcare, questionnaires can be used to gather information about patients’ medical history, symptoms, and lifestyle habits. This information can help healthcare professionals diagnose and treat medical conditions more effectively.
- Marketing : Questionnaires are commonly used in marketing to gather information about consumers’ preferences, buying habits, and opinions on products and services. This information can help businesses develop and market products more effectively.
- Human Resources: Questionnaires are used in human resources to gather information from job applicants, employees, and managers about job satisfaction, performance, and workplace culture. This information can help organizations improve their hiring practices, employee retention, and organizational culture.
- Education : Questionnaires are used in education to gather information from students, teachers, and parents about their perceptions of the educational experience. This information can help educators identify areas for improvement and develop more effective teaching strategies.
Purpose of Questionnaire
Some common purposes of questionnaires include:
- To collect information on attitudes, opinions, and beliefs: Questionnaires can be used to gather information on people’s attitudes, opinions, and beliefs on a particular topic. For example, a questionnaire can be used to gather information on people’s opinions about a particular political issue.
- To collect demographic information: Questionnaires can be used to collect demographic information such as age, gender, income, education level, and occupation. This information can be used to analyze trends and patterns in the data.
- To measure behaviors or experiences: Questionnaires can be used to gather information on behaviors or experiences such as health-related behaviors or experiences, job satisfaction, or customer satisfaction.
- To evaluate programs or interventions: Questionnaires can be used to evaluate the effectiveness of programs or interventions by gathering information on participants’ experiences, opinions, and behaviors.
- To gather information for research: Questionnaires can be used to gather data for research purposes on a variety of topics.
When to use Questionnaire
Here are some situations when questionnaires might be used:
- When you want to collect data from a large number of people: Questionnaires are useful when you want to collect data from a large number of people. They can be distributed to a wide audience and can be completed at the respondent’s convenience.
- When you want to collect data on specific topics: Questionnaires are useful when you want to collect data on specific topics or research questions. They can be designed to ask specific questions and can be used to gather quantitative data that can be analyzed statistically.
- When you want to compare responses across groups: Questionnaires are useful when you want to compare responses across different groups of people. For example, you might want to compare responses from men and women, or from people of different ages or educational backgrounds.
- When you want to collect data anonymously: Questionnaires can be useful when you want to collect data anonymously. Respondents can complete the questionnaire without fear of judgment or repercussions, which can lead to more honest and accurate responses.
- When you want to save time and resources: Questionnaires can be more efficient and cost-effective than other methods of data collection such as interviews or focus groups. They can be completed quickly and easily, and can be analyzed using software to save time and resources.
Characteristics of Questionnaire
Here are some of the characteristics of questionnaires:
- Standardization : Questionnaires are standardized tools that ask the same questions in the same order to all respondents. This ensures that all respondents are answering the same questions and that the responses can be compared and analyzed.
- Objectivity : Questionnaires are designed to be objective, meaning that they do not contain leading questions or bias that could influence the respondent’s answers.
- Predefined responses: Questionnaires typically provide predefined response options for the respondents to choose from, which helps to standardize the responses and make them easier to analyze.
- Quantitative data: Questionnaires are designed to collect quantitative data, meaning that they provide numerical or categorical data that can be analyzed using statistical methods.
- Convenience : Questionnaires are convenient for both the researcher and the respondents. They can be distributed and completed at the respondent’s convenience and can be easily administered to a large number of people.
- Anonymity : Questionnaires can be anonymous, which can encourage respondents to answer more honestly and provide more accurate data.
- Reliability : Questionnaires are designed to be reliable, meaning that they produce consistent results when administered multiple times to the same group of people.
- Validity : Questionnaires are designed to be valid, meaning that they measure what they are intended to measure and are not influenced by other factors.
Advantage of Questionnaire
Some Advantage of Questionnaire are as follows:
- Standardization: Questionnaires allow researchers to ask the same questions to all participants in a standardized manner. This helps ensure consistency in the data collected and eliminates potential bias that might arise if questions were asked differently to different participants.
- Efficiency: Questionnaires can be administered to a large number of people at once, making them an efficient way to collect data from a large sample.
- Anonymity: Participants can remain anonymous when completing a questionnaire, which may make them more likely to answer honestly and openly.
- Cost-effective: Questionnaires can be relatively inexpensive to administer compared to other research methods, such as interviews or focus groups.
- Objectivity: Because questionnaires are typically designed to collect quantitative data, they can be analyzed objectively without the influence of the researcher’s subjective interpretation.
- Flexibility: Questionnaires can be adapted to a wide range of research questions and can be used in various settings, including online surveys, mail surveys, or in-person interviews.
Limitations of Questionnaire
Limitations of Questionnaire are as follows:
- Limited depth: Questionnaires are typically designed to collect quantitative data, which may not provide a complete understanding of the topic being studied. Questionnaires may miss important details and nuances that could be captured through other research methods, such as interviews or observations.
- R esponse bias: Participants may not always answer questions truthfully or accurately, either because they do not remember or because they want to present themselves in a particular way. This can lead to response bias, which can affect the validity and reliability of the data collected.
- Limited flexibility: While questionnaires can be adapted to a wide range of research questions, they may not be suitable for all types of research. For example, they may not be appropriate for studying complex phenomena or for exploring participants’ experiences and perceptions in-depth.
- Limited context: Questionnaires typically do not provide a rich contextual understanding of the topic being studied. They may not capture the broader social, cultural, or historical factors that may influence participants’ responses.
- Limited control : Researchers may not have control over how participants complete the questionnaire, which can lead to variations in response quality or consistency.
About the author
Muhammad Hassan
Researcher, Academic Writer, Web developer
You may also like

Experimental Design – Types, Methods, Guide

Explanatory Research – Types, Methods, Guide

Focus Groups – Steps, Examples and Guide

Textual Analysis – Types, Examples and Guide

Quasi-Experimental Research Design – Types...

Applied Research – Types, Methods and Examples

How to Design Effective Research Questionnaires for Robust Findings
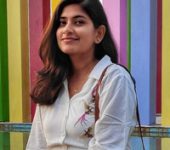
As a staple in data collection, questionnaires help uncover robust and reliable findings that can transform industries, shape policies, and revolutionize understanding. Whether you are exploring societal trends or delving into scientific phenomena, the effectiveness of your research questionnaire can make or break your findings.
In this article, we aim to understand the core purpose of questionnaires, exploring how they serve as essential tools for gathering systematic data, both qualitative and quantitative, from diverse respondents. Read on as we explore the key elements that make up a winning questionnaire, the art of framing questions which are both compelling and rigorous, and the careful balance between simplicity and depth.
Table of Contents
The Role of Questionnaires in Research
So, what is a questionnaire? A questionnaire is a structured set of questions designed to collect information, opinions, attitudes, or behaviors from respondents. It is one of the most commonly used data collection methods in research. Moreover, questionnaires can be used in various research fields, including social sciences, market research, healthcare, education, and psychology. Their adaptability makes them suitable for investigating diverse research questions.
Questionnaire and survey are two terms often used interchangeably, but they have distinct meanings in the context of research. A survey refers to the broader process of data collection that may involve various methods. A survey can encompass different data collection techniques, such as interviews , focus groups, observations, and yes, questionnaires.
Pros and Cons of Using Questionnaires in Research:
While questionnaires offer numerous advantages in research, they also come with some disadvantages that researchers must be aware of and address appropriately. Careful questionnaire design, validation, and consideration of potential biases can help mitigate these disadvantages and enhance the effectiveness of using questionnaires as a data collection method.
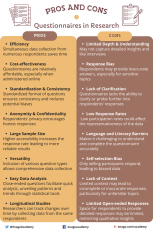
Structured vs Unstructured Questionnaires
Structured questionnaire:.
A structured questionnaire consists of questions with predefined response options. Respondents are presented with a fixed set of choices and are required to select from those options. The questions in a structured questionnaire are designed to elicit specific and quantifiable responses. Structured questionnaires are particularly useful for collecting quantitative data and are often employed in surveys and studies where standardized and comparable data are necessary.
Advantages of Structured Questionnaires:
- Easy to analyze and interpret: The fixed response options facilitate straightforward data analysis and comparison across respondents.
- Efficient for large-scale data collection: Structured questionnaires are time-efficient, allowing researchers to collect data from a large number of respondents.
- Reduces response bias: The predefined response options minimize potential response bias and maintain consistency in data collection.
Limitations of Structured Questionnaires:
- Lack of depth: Structured questionnaires may not capture in-depth insights or nuances as respondents are limited to pre-defined response choices. Hence, they may not reveal the reasons behind respondents’ choices, limiting the understanding of their perspectives.
- Limited flexibility: The fixed response options may not cover all potential responses, therefore, potentially restricting respondents’ answers.
Unstructured Questionnaire:
An unstructured questionnaire consists of questions that allow respondents to provide detailed and unrestricted responses. Unlike structured questionnaires, there are no predefined response options, giving respondents the freedom to express their thoughts in their own words. Furthermore, unstructured questionnaires are valuable for collecting qualitative data and obtaining in-depth insights into respondents’ experiences, opinions, or feelings.
Advantages of Unstructured Questionnaires:
- Rich qualitative data: Unstructured questionnaires yield detailed and comprehensive qualitative data, providing valuable and novel insights into respondents’ perspectives.
- Flexibility in responses: Respondents have the freedom to express themselves in their own words. Hence, allowing for a wide range of responses.
Limitations of Unstructured Questionnaires:
- Time-consuming analysis: Analyzing open-ended responses can be time-consuming, since, each response requires careful reading and interpretation.
- Subjectivity in interpretation: The analysis of open-ended responses may be subjective, as researchers interpret and categorize responses based on their judgment.
- May require smaller sample size: Due to the depth of responses, researchers may need a smaller sample size for comprehensive analysis, making generalizations more challenging.
Types of Questions in a Questionnaire
In a questionnaire, researchers typically use the following most common types of questions to gather a variety of information from respondents:
1. Open-Ended Questions:
These questions allow respondents to provide detailed and unrestricted responses in their own words. Open-ended questions are valuable for gathering qualitative data and in-depth insights.
Example: What suggestions do you have for improving our product?
2. Multiple-Choice Questions
Respondents choose one answer from a list of provided options. This type of question is suitable for gathering categorical data or preferences.
Example: Which of the following social media/academic networking platforms do you use to promote your research?
- ResearchGate
- Academia.edu
3. Dichotomous Questions
Respondents choose between two options, typically “yes” or “no”, “true” or “false”, or “agree” or “disagree”.
Example: Have you ever published in open access journals before?
4. Scaling Questions
These questions, also known as rating scale questions, use a predefined scale that allows respondents to rate or rank their level of agreement, satisfaction, importance, or other subjective assessments. These scales help researchers quantify subjective data and make comparisons across respondents.
There are several types of scaling techniques used in scaling questions:
i. Likert Scale:
The Likert scale is one of the most common scaling techniques. It presents respondents with a series of statements and asks them to rate their level of agreement or disagreement using a range of options, typically from “strongly agree” to “strongly disagree”.For example: Please indicate your level of agreement with the statement: “The content presented in the webinar was relevant and aligned with the advertised topic.”
- Strongly Agree
- Strongly Disagree
ii. Semantic Differential Scale:
The semantic differential scale measures respondents’ perceptions or attitudes towards an item using opposite adjectives or bipolar words. Respondents rate the item on a scale between the two opposites. For example:
- Easy —— Difficult
- Satisfied —— Unsatisfied
- Very likely —— Very unlikely
iii. Numerical Rating Scale:
This scale requires respondents to provide a numerical rating on a predefined scale. It can be a simple 1 to 5 or 1 to 10 scale, where higher numbers indicate higher agreement, satisfaction, or importance.
iv. Ranking Questions:
Respondents rank items in order of preference or importance. Ranking questions help identify preferences or priorities.
Example: Please rank the following features of our app in order of importance (1 = Most Important, 5 = Least Important):
- User Interface
- Functionality
- Customer Support
By using a mix of question types, researchers can gather both quantitative and qualitative data, providing a comprehensive understanding of the research topic and enabling meaningful analysis and interpretation of the results. The choice of question types depends on the research objectives , the desired depth of information, and the data analysis requirements.
Methods of Administering Questionnaires
There are several methods for administering questionnaires, and the choice of method depends on factors such as the target population, research objectives , convenience, and resources available. Here are some common methods of administering questionnaires:
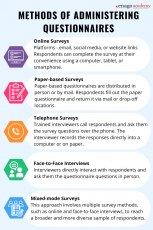
Each method has its advantages and limitations. Online surveys offer convenience and a large reach, but they may be limited to individuals with internet access. Face-to-face interviews allow for in-depth responses but can be time-consuming and costly. Telephone surveys have broad reach but may be limited by declining response rates. Researchers should choose the method that best suits their research objectives, target population, and available resources to ensure successful data collection.
How to Design a Questionnaire
Designing a good questionnaire is crucial for gathering accurate and meaningful data that aligns with your research objectives. Here are essential steps and tips to create a well-designed questionnaire:
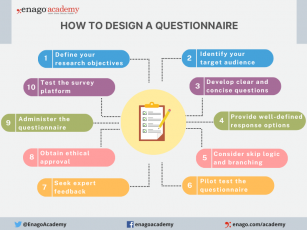
1. Define Your Research Objectives : Clearly outline the purpose and specific information you aim to gather through the questionnaire.
2. Identify Your Target Audience : Understand respondents’ characteristics and tailor the questionnaire accordingly.
3. Develop the Questions :
- Write Clear and Concise Questions
- Avoid Leading or Biasing Questions
- Sequence Questions Logically
- Group Related Questions
- Include Demographic Questions
4. Provide Well-defined Response Options : Offer exhaustive response choices for closed-ended questions.
5. Consider Skip Logic and Branching : Customize the questionnaire based on previous answers.
6. Pilot Test the Questionnaire : Identify and address issues through a pilot study .
7. Seek Expert Feedback : Validate the questionnaire with subject matter experts.
8. Obtain Ethical Approval : Comply with ethical guidelines , obtain consent, and ensure confidentiality before administering the questionnaire.
9. Administer the Questionnaire : Choose the right mode and provide clear instructions.
10. Test the Survey Platform : Ensure compatibility and usability for online surveys.
By following these steps and paying attention to questionnaire design principles, you can create a well-structured and effective questionnaire that gathers reliable data and helps you achieve your research objectives.
Characteristics of a Good Questionnaire
A good questionnaire possesses several essential elements that contribute to its effectiveness. Furthermore, these characteristics ensure that the questionnaire is well-designed, easy to understand, and capable of providing valuable insights. Here are some key characteristics of a good questionnaire:
1. Clarity and Simplicity : Questions should be clear, concise, and unambiguous. Avoid using complex language or technical terms that may confuse respondents. Simple and straightforward questions ensure that respondents interpret them consistently.
2. Relevance and Focus : Each question should directly relate to the research objectives and contribute to answering the research questions. Consequently, avoid including extraneous or irrelevant questions that could lead to data clutter.
3. Mix of Question Types : Utilize a mix of question types, including open-ended, Likert scale, and multiple-choice questions. This variety allows for both qualitative and quantitative data collections .
4. Validity and Reliability : Ensure the questionnaire measures what it intends to measure (validity) and produces consistent results upon repeated administration (reliability). Validation should be conducted through expert review and previous research.
5. Appropriate Length : Keep the questionnaire’s length appropriate and manageable to avoid respondent fatigue or dropouts. Long questionnaires may result in incomplete or rushed responses.
6. Clear Instructions : Include clear instructions at the beginning of the questionnaire to guide respondents on how to complete it. Explain any technical terms, formats, or concepts if necessary.
7. User-Friendly Format : Design the questionnaire to be visually appealing and user-friendly. Use consistent formatting, adequate spacing, and a logical page layout.
8. Data Validation and Cleaning : Incorporate validation checks to ensure data accuracy and reliability. Consider mechanisms to detect and correct inconsistent or missing responses during data cleaning.
By incorporating these characteristics, researchers can create a questionnaire that maximizes data quality, minimizes response bias, and provides valuable insights for their research.
In the pursuit of advancing research and gaining meaningful insights, investing time and effort into designing effective questionnaires is a crucial step. A well-designed questionnaire is more than a mere set of questions; it is a masterpiece of precision and ingenuity. Each question plays a vital role in shaping the narrative of our research, guiding us through the labyrinth of data to meaningful conclusions. Indeed, a well-designed questionnaire serves as a powerful tool for unlocking valuable insights and generating robust findings that impact society positively.
Have you ever designed a research questionnaire? Reflect on your experience and share your insights with researchers globally through Enago Academy’s Open Blogging Platform . Join our diverse community of 1000K+ researchers and authors to exchange ideas, strategies, and best practices, and together, let’s shape the future of data collection and maximize the impact of questionnaires in the ever-evolving landscape of research.
Frequently Asked Questions
A research questionnaire is a structured tool used to gather data from participants in a systematic manner. It consists of a series of carefully crafted questions designed to collect specific information related to a research study.
Questionnaires play a pivotal role in both quantitative and qualitative research, enabling researchers to collect insights, opinions, attitudes, or behaviors from respondents. This aids in hypothesis testing, understanding, and informed decision-making, ensuring consistency, efficiency, and facilitating comparisons.
Questionnaires are a versatile tool employed in various research designs to gather data efficiently and comprehensively. They find extensive use in both quantitative and qualitative research methodologies, making them a fundamental component of research across disciplines. Some research designs that commonly utilize questionnaires include: a) Cross-Sectional Studies b) Longitudinal Studies c) Descriptive Research d) Correlational Studies e) Causal-Comparative Studies f) Experimental Research g) Survey Research h) Case Studies i) Exploratory Research
A survey is a comprehensive data collection method that can include various techniques like interviews and observations. A questionnaire is a specific set of structured questions within a survey designed to gather standardized responses. While a survey is a broader approach, a questionnaire is a focused tool for collecting specific data.
The choice of questionnaire type depends on the research objectives, the type of data required, and the preferences of respondents. Some common types include: • Structured Questionnaires: These questionnaires consist of predefined, closed-ended questions with fixed response options. They are easy to analyze and suitable for quantitative research. • Semi-Structured Questionnaires: These questionnaires combine closed-ended questions with open-ended ones. They offer more flexibility for respondents to provide detailed explanations. • Unstructured Questionnaires: These questionnaires contain open-ended questions only, allowing respondents to express their thoughts and opinions freely. They are commonly used in qualitative research.
Following these steps ensures effective questionnaire administration for reliable data collection: • Choose a Method: Decide on online, face-to-face, mail, or phone administration. • Online Surveys: Use platforms like SurveyMonkey • Pilot Test: Test on a small group before full deployment • Clear Instructions: Provide concise guidelines • Follow-Up: Send reminders if needed

Thank you, Riya. This is quite helpful. As discussed, response bias is one of the disadvantages in the use of questionnaires. One way to help limit this can be to use scenario based questions. These type of questions may help the respondents to be more reflective and active in the process.
Thank you, Dear Riya. This is quite helpful.
Great insights there Doc
Rate this article Cancel Reply
Your email address will not be published.
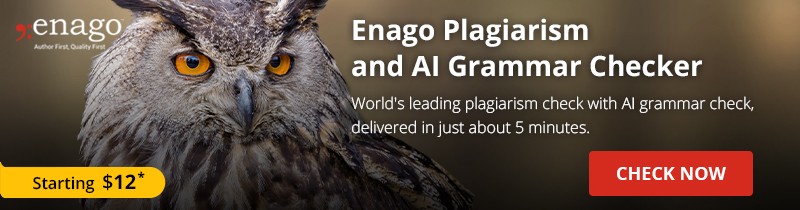
Enago Academy's Most Popular Articles
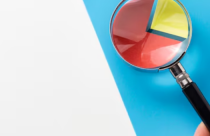
- Promoting Research
Graphical Abstracts Vs. Infographics: Best practices for using visual illustrations for increased research impact
Dr. Sarah Chen stared at her computer screen, her eyes staring at her recently published…
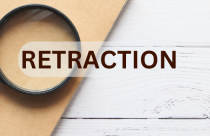
- Publishing Research
10 Tips to Prevent Research Papers From Being Retracted
Research paper retractions represent a critical event in the scientific community. When a published article…
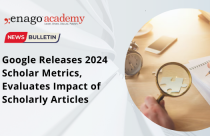
- Industry News
Google Releases 2024 Scholar Metrics, Evaluates Impact of Scholarly Articles
Google has released its 2024 Scholar Metrics, assessing scholarly articles from 2019 to 2023. This…
![questionnaire in research paper What is Academic Integrity and How to Uphold it [FREE CHECKLIST]](https://www.enago.com/academy/wp-content/uploads/2024/05/FeatureImages-59-210x136.png)
Ensuring Academic Integrity and Transparency in Academic Research: A comprehensive checklist for researchers
Academic integrity is the foundation upon which the credibility and value of scientific findings are…
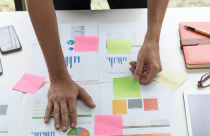
- Reporting Research
How to Optimize Your Research Process: A step-by-step guide
For researchers across disciplines, the path to uncovering novel findings and insights is often filled…
Choosing the Right Analytical Approach: Thematic analysis vs. content analysis for…
Comparing Cross Sectional and Longitudinal Studies: 5 steps for choosing the right…
Research Recommendations – Guiding policy-makers for evidence-based decision making

Sign-up to read more
Subscribe for free to get unrestricted access to all our resources on research writing and academic publishing including:
- 2000+ blog articles
- 50+ Webinars
- 10+ Expert podcasts
- 50+ Infographics
- 10+ Checklists
- Research Guides
We hate spam too. We promise to protect your privacy and never spam you.
Academia.edu no longer supports Internet Explorer.
To browse Academia.edu and the wider internet faster and more securely, please take a few seconds to upgrade your browser .
Enter the email address you signed up with and we'll email you a reset link.
- We're Hiring!
- Help Center
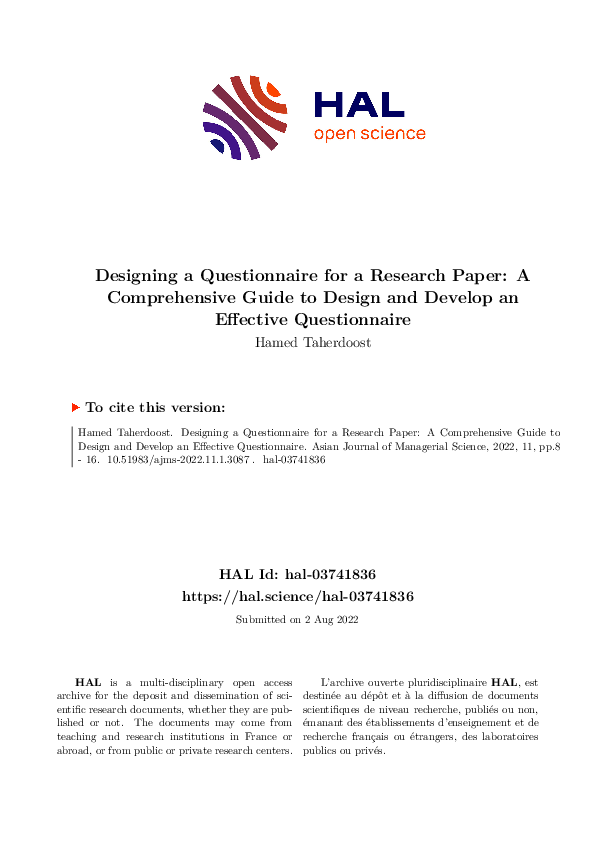
Designing a Questionnaire for a Research Paper: A Comprehensive Guide to Design and Develop an Effective Questionnaire

A questionnaire is an important instrument in a research study to help the researcher collect relevant data regarding the research topic. It is significant to ensure that the design of the questionnaire is arranged to minimize errors. However, researchers commonly face challenges in designing an effective questionnaire including its content, appearance and usage that leads to inappropriate and biased findings in a study. This paper aims to review the main steps to design a questionnaire introducing the process that starts with defining the information required for a study, then continues with the identification of the type of survey and types of questions, writing questions and building the construct of the questionnaire. It also develops the demand to pre-test the questionnaire and finalizing the questionnaire to conduct the survey.
Related Papers
Innovations in Measuring and Evaluating Scientific Information
Rayees Farooq
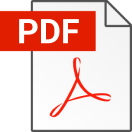
Indian Journal of Anaesthesia
Narayana Yaddanapudi
Edrine Wanyama
Questionnaire construction has overtime evolved with consistency and rarely, it has been skipped in the world’s researches. Questionnaires form the basis for which most pieces of information can be obtained. In the very light, response rates to questions and accuracy of data findings are possible through the use of questionnaire usage. Where a questionnaire is poorly constructed, one faces the risk of missing out vital information which could be forming the basis for research. This paper discusses the relevance and importance of questionnaire construction in data collection and research. An attempt is made to show questionnaire usage in social research and other research processes. Ultimately, questionnaire construction is considered just as important as any other research process used while collecting data. Some key recommendations that could make questionnaire usage in research better are also briefly considered.
Trisha Greenhalgh
Abla BENBELLAL
International Dental & Medical Journal of Advanced Research - VOLUME 2015
Saumya Dubey
Ebenezer Consultan
International Journal of Market Research
Petra Lietz
Some consider responding to survey questions as a sophisticated cognitive process whereby respondents go through, often iterative, steps to process the information provided to them by questions and response options. Others focus more on the interplay between questions and answers as a complex communication process between researchers and respondents, their assumptions, expectations and perceptions. In this article, cognitive and communication research is reviewed that has tested the impact of different question and answer alternatives on the responses obtained. This leads to evidence-based recommendations for market researchers, who frequently have to make decisions regarding various aspects of questionnaire design such as question length and order, question wording, as well as the optimal number of response options and the desirability or otherwise of a ‘don't know’ option or a middle alternative.
Journal of Family Planning and Reproductive Health Care
Gill Wakley
Daniela Garcia
Loading Preview
Sorry, preview is currently unavailable. You can download the paper by clicking the button above.
RELATED PAPERS
De Wet Schutte
Lyberg/Survey
Norbert Schwarz
International Statistical Review
massimo borelli
Health Information & Libraries Journal
Andrew Booth
Nursing Times Research
michael kirk-smith
Petra Boynton
Market Research Society. Journal.
Zenodo (CERN European Organization for Nuclear Research)
Mohamad Adam Bujang
Medical Teacher
Anthony Artino
Jiayi Zhang
Sallie Newell
Health technology assessment (Winchester, England)
Lois Thomas , Claire Bamford , E. McColl
Burcu Akhun
International Journal of Assessment Tools in Education
Betty Adams
Jason Harlacher
Clinical Nurse Specialist
Sandra Siedlecki
Otolaryngology–Head and Neck Surgery
Randal Paniello
Nipuni Rangika
André Pereira
Biometrics & Biostatistics International Journal
Prof. Dr. İlker Etikan
International Journal of Management, Technology, and Social Sciences (IJMTS)
Srinivas Publication , Sreeramana Aithal
Zurina Saaya
Amanda Hunn
Anco Hundepool
RELATED TOPICS
- We're Hiring!
- Help Center
- Find new research papers in:
- Health Sciences
- Earth Sciences
- Cognitive Science
- Mathematics
- Computer Science
- Academia ©2024

An official website of the United States government
The .gov means it’s official. Federal government websites often end in .gov or .mil. Before sharing sensitive information, make sure you’re on a federal government site.
The site is secure. The https:// ensures that you are connecting to the official website and that any information you provide is encrypted and transmitted securely.
- Publications
- Account settings
- My Bibliography
- Collections
- Citation manager
Save citation to file
Email citation, add to collections.
- Create a new collection
- Add to an existing collection
Add to My Bibliography
Your saved search, create a file for external citation management software, your rss feed.
- Search in PubMed
- Search in NLM Catalog
- Add to Search
How to Design and Validate A Questionnaire: A Guide
Affiliations.
- 1 Department of Pharmacology, Government Institute of Medical Sciences, Greater Noida, Uttar Pradesh, 201310, India.
- 2 Department of Pharmacology, AIIMS, Jodhpur, Rajasthan, 342005, India.
- 3 Department of Gynaecology and Obstetrics, AIIMS Jodhpur, Rajasthan, India.
- PMID: 30084336
- DOI: 10.2174/1574884713666180807151328
Background: A questionnaire is a commonly used data collection method and is a very crucial part of the research. However, designing a questionnaire can be a daunting task for postgraduate students.
Methods: This manuscript illustrates the various steps required in questionnaire designing and provides an insight into the essentials of questionnaire construction and validation. Data from a questionnaire should be able to comprehend the objectives of the study; else it may lead to wrong interpretation or bias, decreased power of study and inability to generalize the study results.
Conclusion: Since it is equally important to verify the usefulness of the designed questionnaire, the article briefly describes the process of psychometric evaluation of a questionnaire.
Keywords: Questionnaire validation; WOMAC; pragmatic research; psychometric assessment; questionnaire designing; validity..
Copyright© Bentham Science Publishers; For any queries, please email at [email protected].
PubMed Disclaimer
Similar articles
- Psychometric properties of the Farsi version of Attitudes to Aging Questionnaire in Iranian older adults. Rejeh N, Heravi-Karimooi M, Vaismoradi M, Griffiths P, Nikkhah M, Bahrami T. Rejeh N, et al. Clin Interv Aging. 2017 Sep 22;12:1531-1542. doi: 10.2147/CIA.S139321. eCollection 2017. Clin Interv Aging. 2017. PMID: 29026291 Free PMC article.
- Validation of the spanish version of the Roland-Morris questionnaire. Kovacs FM, Llobera J, Gil Del Real MT, Abraira V, Gestoso M, Fernández C, Primaria Group KA. Kovacs FM, et al. Spine (Phila Pa 1976). 2002 Mar 1;27(5):538-42. doi: 10.1097/00007632-200203010-00016. Spine (Phila Pa 1976). 2002. PMID: 11880841
- The Nordic Musculoskeletal Questionnaire: cross-cultural adaptation into Turkish assessing its psychometric properties. Kahraman T, Genç A, Göz E. Kahraman T, et al. Disabil Rehabil. 2016 Oct;38(21):2153-60. doi: 10.3109/09638288.2015.1114034. Epub 2016 Jan 4. Disabil Rehabil. 2016. PMID: 26726840
- The assessment of fatigue: a practical guide for clinicians and researchers. Dittner AJ, Wessely SC, Brown RG. Dittner AJ, et al. J Psychosom Res. 2004 Feb;56(2):157-70. doi: 10.1016/S0022-3999(03)00371-4. J Psychosom Res. 2004. PMID: 15016573 Review.
- Selection and use of content experts for instrument development. Grant JS, Davis LL. Grant JS, et al. Res Nurs Health. 1997 Jun;20(3):269-74. doi: 10.1002/(sici)1098-240x(199706)20:3 3.0.co;2-g. Res Nurs Health. 1997. PMID: 9179180 Review.
- Oral care of intubated patients, challenging task of ICU nurses: a survey of knowledge, attitudes and practices. Asadi N, Jahanimoghadam F. Asadi N, et al. BMC Oral Health. 2024 Aug 10;24(1):925. doi: 10.1186/s12903-024-04652-5. BMC Oral Health. 2024. PMID: 39127638 Free PMC article.
- The Moderation Effect of Approach Motivation Between Schizotypy and Creative Ideational Behavior. Wang L, Pei Y, Zhu Y, Long H, Pang W. Wang L, et al. Psychol Res Behav Manag. 2024 May 9;17:1947-1960. doi: 10.2147/PRBM.S441013. eCollection 2024. Psychol Res Behav Manag. 2024. PMID: 38742225 Free PMC article.
- Effects of Wildfire Events on California Radiation Oncology Clinics and Patients. Lichter KE, Baniel CC, Do I, Medhat Y, Avula V, Nogueira LM, Bates JE, Paulsson A, Malik N, Hiatt RA, Yom SS, Mohamad O. Lichter KE, et al. Adv Radiat Oncol. 2023 Oct 22;9(3):101395. doi: 10.1016/j.adro.2023.101395. eCollection 2024 Mar. Adv Radiat Oncol. 2023. PMID: 38304108 Free PMC article.
- The German version of the mHealth App Usability Questionnaire (GER-MAUQ): Translation and validation study in patients with cardiovascular disease. Tacke T, Nohl-Deryk P, Lingwal N, Reimer LM, Starnecker F, Güthlin C, Gerlach FM, Schunkert H, Jonas S, Müller A. Tacke T, et al. Digit Health. 2024 Jan 31;10:20552076231225168. doi: 10.1177/20552076231225168. eCollection 2024 Jan-Dec. Digit Health. 2024. PMID: 38303970 Free PMC article.
- Enhancing Human Papillomavirus Vaccination Rates through Better Knowledge? Insights from a Survey among German Medical Students. Aksoy C, Reimold P, Schumann A, Schneidewind L, Karschuck P, Flegar L, Leitsmann M, Heers H, Huber J, Zacharis A, Ihrig A. Aksoy C, et al. Urol Int. 2024;108(2):153-158. doi: 10.1159/000536257. Epub 2024 Jan 19. Urol Int. 2024. PMID: 38246131 Free PMC article.
Publication types
- Search in MeSH
LinkOut - more resources
Full text sources.
- Bentham Science Publishers Ltd.
- Ingenta plc
Other Literature Sources
- scite Smart Citations
- Citation Manager
NCBI Literature Resources
MeSH PMC Bookshelf Disclaimer
The PubMed wordmark and PubMed logo are registered trademarks of the U.S. Department of Health and Human Services (HHS). Unauthorized use of these marks is strictly prohibited.
- PRO Courses Guides New Tech Help Pro Expert Videos About wikiHow Pro Upgrade Sign In
- EDIT Edit this Article
- EXPLORE Tech Help Pro About Us Random Article Quizzes Request a New Article Community Dashboard This Or That Game Happiness Hub Popular Categories Arts and Entertainment Artwork Books Movies Computers and Electronics Computers Phone Skills Technology Hacks Health Men's Health Mental Health Women's Health Relationships Dating Love Relationship Issues Hobbies and Crafts Crafts Drawing Games Education & Communication Communication Skills Personal Development Studying Personal Care and Style Fashion Hair Care Personal Hygiene Youth Personal Care School Stuff Dating All Categories Arts and Entertainment Finance and Business Home and Garden Relationship Quizzes Cars & Other Vehicles Food and Entertaining Personal Care and Style Sports and Fitness Computers and Electronics Health Pets and Animals Travel Education & Communication Hobbies and Crafts Philosophy and Religion Work World Family Life Holidays and Traditions Relationships Youth
- Browse Articles
- Learn Something New
- Quizzes Hot
- Happiness Hub
- This Or That Game
- Train Your Brain
- Explore More
- Support wikiHow
- About wikiHow
- Log in / Sign up
- Education and Communications
How to Develop a Questionnaire for Research
Last Updated: July 21, 2024 Fact Checked
This article was co-authored by Alexander Ruiz, M.Ed. . Alexander Ruiz is an Educational Consultant and the Educational Director of Link Educational Institute, a tutoring business based in Claremont, California that provides customizable educational plans, subject and test prep tutoring, and college application consulting. With over a decade and a half of experience in the education industry, Alexander coaches students to increase their self-awareness and emotional intelligence while achieving skills and the goal of achieving skills and higher education. He holds a BA in Psychology from Florida International University and an MA in Education from Georgia Southern University. There are 12 references cited in this article, which can be found at the bottom of the page. This article has been fact-checked, ensuring the accuracy of any cited facts and confirming the authority of its sources. This article has been viewed 593,374 times.
A questionnaire is a technique for collecting data in which a respondent provides answers to a series of questions. [1] X Research source To develop a questionnaire that will collect the data you want takes effort and time. However, by taking a step-by-step approach to questionnaire development, you can come up with an effective means to collect data that will answer your unique research question.
Designing Your Questionnaire

- Come up with a research question. It can be one question or several, but this should be the focal point of your questionnaire.
- Develop one or several hypotheses that you want to test. The questions that you include on your questionnaire should be aimed at systematically testing these hypotheses.

- Dichotomous question: this is a question that will generally be a “yes/no” question, but may also be an “agree/disagree” question. It is the quickest and simplest question to analyze, but is not a highly sensitive measure.
- Open-ended questions: these questions allow the respondent to respond in their own words. They can be useful for gaining insight into the feelings of the respondent, but can be a challenge when it comes to analysis of data. It is recommended to use open-ended questions to address the issue of “why.” [2] X Research source
- Multiple choice questions: these questions consist of three or more mutually-exclusive categories and ask for a single answer or several answers. [3] X Research source Multiple choice questions allow for easy analysis of results, but may not give the respondent the answer they want.
- Rank-order (or ordinal) scale questions: this type of question asks your respondent to rank items or choose items in a particular order from a set. For example, it might ask your respondents to order five things from least to most important. These types of questions forces discrimination among alternatives, but does not address the issue of why the respondent made these discriminations. [4] X Research source
- Rating scale questions: these questions allow the respondent to assess a particular issue based on a given dimension. You can provide a scale that gives an equal number of positive and negative choices, for example, ranging from “strongly agree” to “strongly disagree.” [5] X Research source These questions are very flexible, but also do not answer the question “why.”

- Write questions that are succinct and simple. You should not be writing complex statements or using technical jargon, as it will only confuse your respondents and lead to incorrect responses.
- Ask only one question at a time. This will help avoid confusion
- Asking questions such as these usually require you to anonymize or encrypt the demographic data you collect.
- Determine if you will include an answer such as “I don’t know” or “Not applicable to me.” While these can give your respondents a way of not answering certain questions, providing these options can also lead to missing data, which can be problematic during data analysis.
- Put the most important questions at the beginning of your questionnaire. This can help you gather important data even if you sense that your respondents may be becoming distracted by the end of the questionnaire.

- Only include questions that are directly useful to your research question. [8] X Trustworthy Source Food and Agricultural Organization of the United Nations Specialized agency of the United Nations responsible for leading international efforts to end world hunger and improve nutrition Go to source A questionnaire is not an opportunity to collect all kinds of information about your respondents.
- Avoid asking redundant questions. This will frustrate those who are taking your questionnaire.

- Consider if you want your questionnaire to collect information from both men and women. Some studies will only survey one sex.
- Consider including a range of ages in your target demographic. For example, you can consider young adult to be 18-29 years old, adults to be 30-54 years old, and mature adults to be 55+. Providing the an age range will help you get more respondents than limiting yourself to a specific age.
- Consider what else would make a person a target for your questionnaire. Do they need to drive a car? Do they need to have health insurance? Do they need to have a child under 3? Make sure you are very clear about this before you distribute your questionnaire.

- Consider an anonymous questionnaire. You may not want to ask for names on your questionnaire. This is one step you can take to prevent privacy, however it is often possible to figure out a respondent’s identity using other demographic information (such as age, physical features, or zipcode).
- Consider de-identifying the identity of your respondents. Give each questionnaire (and thus, each respondent) a unique number or word, and only refer to them using that new identifier. Shred any personal information that can be used to determine identity.
- Remember that you do not need to collect much demographic information to be able to identify someone. People may be wary to provide this information, so you may get more respondents by asking less demographic questions (if it is possible for your questionnaire).
- Make sure you destroy all identifying information after your study is complete.
Writing your questionnaire

- My name is Jack Smith and I am one of the creators of this questionnaire. I am part of the Department of Psychology at the University of Michigan, where I am focusing in developing cognition in infants.
- I’m Kelly Smith, a 3rd year undergraduate student at the University of New Mexico. This questionnaire is part of my final exam in statistics.
- My name is Steve Johnson, and I’m a marketing analyst for The Best Company. I’ve been working on questionnaire development to determine attitudes surrounding drug use in Canada for several years.

- I am collecting data regarding the attitudes surrounding gun control. This information is being collected for my Anthropology 101 class at the University of Maryland.
- This questionnaire will ask you 15 questions about your eating and exercise habits. We are attempting to make a correlation between healthy eating, frequency of exercise, and incidence of cancer in mature adults.
- This questionnaire will ask you about your recent experiences with international air travel. There will be three sections of questions that will ask you to recount your recent trips and your feelings surrounding these trips, as well as your travel plans for the future. We are looking to understand how a person’s feelings surrounding air travel impact their future plans.

- Beware that if you are collecting information for a university or for publication, you may need to check in with your institution’s Institutional Review Board (IRB) for permission before beginning. Most research universities have a dedicated IRB staff, and their information can usually be found on the school’s website.
- Remember that transparency is best. It is important to be honest about what will happen with the data you collect.
- Include an informed consent for if necessary. Note that you cannot guarantee confidentiality, but you will make all reasonable attempts to ensure that you protect their information. [11] X Research source

- Time yourself taking the survey. Then consider that it will take some people longer than you, and some people less time than you.
- Provide a time range instead of a specific time. For example, it’s better to say that a survey will take between 15 and 30 minutes than to say it will take 15 minutes and have some respondents quit halfway through.
- Use this as a reason to keep your survey concise! You will feel much better asking people to take a 20 minute survey than you will asking them to take a 3 hour one.

- Incentives can attract the wrong kind of respondent. You don’t want to incorporate responses from people who rush through your questionnaire just to get the reward at the end. This is a danger of offering an incentive. [12] X Research source
- Incentives can encourage people to respond to your survey who might not have responded without a reward. This is a situation in which incentives can help you reach your target number of respondents. [13] X Research source
- Consider the strategy used by SurveyMonkey. Instead of directly paying respondents to take their surveys, they offer 50 cents to the charity of their choice when a respondent fills out a survey. They feel that this lessens the chances that a respondent will fill out a questionnaire out of pure self-interest. [14] X Research source
- Consider entering each respondent in to a drawing for a prize if they complete the questionnaire. You can offer a 25$ gift card to a restaurant, or a new iPod, or a ticket to a movie. This makes it less tempting just to respond to your questionnaire for the incentive alone, but still offers the chance of a pleasant reward.

- Always proof read. Check for spelling, grammar, and punctuation errors.
- Include a title. This is a good way for your respondents to understand the focus of the survey as quickly as possible.
- Thank your respondents. Thank them for taking the time and effort to complete your survey.
Distributing Your Questionnaire

- Was the questionnaire easy to understand? Were there any questions that confused you?
- Was the questionnaire easy to access? (Especially important if your questionnaire is online).
- Do you feel the questionnaire was worth your time?
- Were you comfortable answering the questions asked?
- Are there any improvements you would make to the questionnaire?

- Use an online site, such as SurveyMonkey.com. This site allows you to write your own questionnaire with their survey builder, and provides additional options such as the option to buy a target audience and use their analytics to analyze your data. [18] X Research source
- Consider using the mail. If you mail your survey, always make sure you include a self-addressed stamped envelope so that the respondent can easily mail their responses back. Make sure that your questionnaire will fit inside a standard business envelope.
- Conduct face-to-face interviews. This can be a good way to ensure that you are reaching your target demographic and can reduce missing information in your questionnaires, as it is more difficult for a respondent to avoid answering a question when you ask it directly.
- Try using the telephone. While this can be a more time-effective way to collect your data, it can be difficult to get people to respond to telephone questionnaires.

- Make your deadline reasonable. Giving respondents up to 2 weeks to answer should be more than sufficient. Anything longer and you risk your respondents forgetting about your questionnaire.
- Consider providing a reminder. A week before the deadline is a good time to provide a gentle reminder about returning the questionnaire. Include a replacement of the questionnaire in case it has been misplaced by your respondent.
Community Q&A

You Might Also Like

- ↑ https://www.questionpro.com/blog/what-is-a-questionnaire/
- ↑ https://www.hotjar.com/blog/open-ended-questions/
- ↑ https://www.questionpro.com/a/showArticle.do?articleID=survey-questions
- ↑ https://surveysparrow.com/blog/ranking-questions-examples/
- ↑ https://www.lumoa.me/blog/rating-scale/
- ↑ http://www.sciencebuddies.org/science-fair-projects/project_ideas/Soc_survey.shtml
- ↑ http://www.fao.org/docrep/W3241E/w3241e05.htm
- ↑ http://managementhelp.org/businessresearch/questionaires.htm
- ↑ https://www.surveymonkey.com/mp/survey-rewards/
- ↑ http://www.ideafit.com/fitness-library/how-to-develop-a-questionnaire
- ↑ https://www.surveymonkey.com/mp/take-a-tour/?ut_source=header
About This Article

To develop a questionnaire for research, identify the main objective of your research to act as the focal point for the questionnaire. Then, choose the type of questions that you want to include, and come up with succinct, straightforward questions to gather the information that you need to answer your questions. Keep your questionnaire as short as possible, and identify a target demographic who you would like to answer the questions. Remember to make the questionnaires as anonymous as possible to protect the integrity of the person answering the questions! For tips on writing out your questions and distributing the questionnaire, keep reading! Did this summary help you? Yes No
- Send fan mail to authors
Reader Success Stories

Abdul Bari Khan
Nov 11, 2020
Did this article help you?

Jul 25, 2023

Iman Ilhusadi
Nov 26, 2016

Jaydeepa Das
Aug 21, 2018

Atefeh Abdollahi
Jan 3, 2017

Featured Articles

Trending Articles

Watch Articles

- Terms of Use
- Privacy Policy
- Do Not Sell or Share My Info
- Not Selling Info
Get all the best how-tos!
Sign up for wikiHow's weekly email newsletter
- Skip to main content
- Skip to primary sidebar
- Skip to footer
- QuestionPro

- Solutions Industries Gaming Automotive Sports and events Education Government Travel & Hospitality Financial Services Healthcare Cannabis Technology Use Case AskWhy Communities Audience Contactless surveys Mobile LivePolls Member Experience GDPR Positive People Science 360 Feedback Surveys
- Resources Blog eBooks Survey Templates Case Studies Training Help center

Home Surveys Questionnaire
21 Questionnaire Templates: Examples and Samples
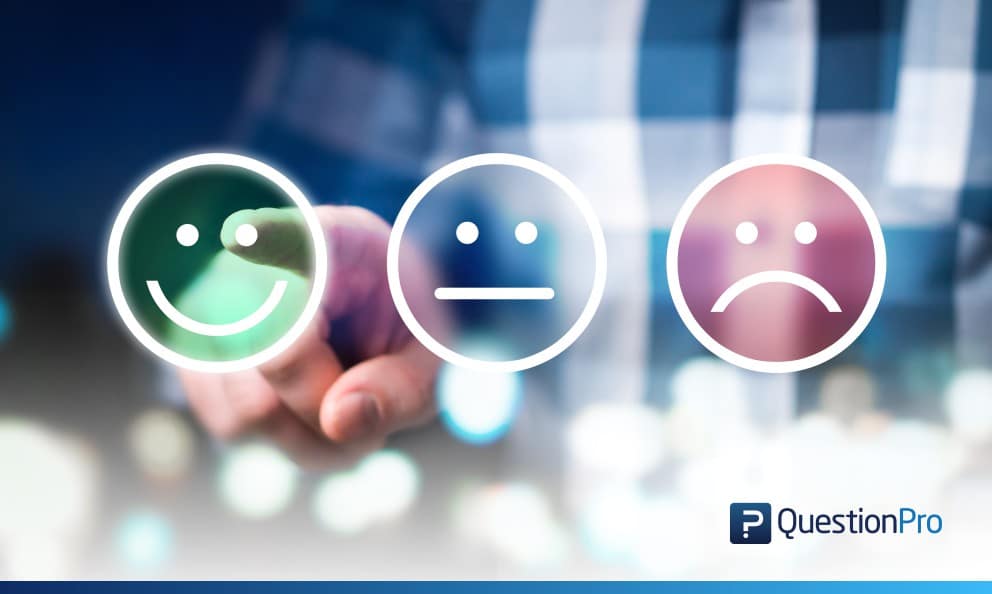
Questionnaire: Definition
A questionnaire is defined a market research instrument that consists of questions or prompts to elicit and collect responses from a sample of respondents. A questionnaire is typically a mix of open-ended questions and close-ended questions ; the latter allowing for respondents to enlist their views in detail.
A questionnaire can be used in both, qualitative market research as well as quantitative market research with the use of different types of questions .
LEARN ABOUT: Open-Ended Questions
Types of Questionnaires
We have learnt that a questionnaire could either be structured or free-flow. To explain this better:
- Structured Questionnaires: A structured questionnaires helps collect quantitative data . In this case, the questionnaire is designed in a way that it collects very specific type of information. It can be used to initiate a formal enquiry on collect data to prove or disprove a prior hypothesis.
- Unstructured Questionnaires: An unstructured questionnaire collects qualitative data . The questionnaire in this case has a basic structure and some branching questions but nothing that limits the responses of a respondent. The questions are more open-ended.
LEARN ABOUT: Structured Question
Types of Questions used in a Questionnaire
A questionnaire can consist of many types of questions . Some of the commonly and widely used question types though, are:
- Open-Ended Questions: One of the commonly used question type in questionnaire is an open-ended question . These questions help collect in-depth data from a respondent as there is a huge scope to respond in detail.
- Dichotomous Questions: The dichotomous question is a “yes/no” close-ended question . This question is generally used in case of the need of basic validation. It is the easiest question type in a questionnaire.
- Multiple-Choice Questions: An easy to administer and respond to, question type in a questionnaire is the multiple-choice question . These questions are close-ended questions with either a single select multiple choice question or a multiple select multiple choice question. Each multiple choice question consists of an incomplete stem (question), right answer or answers, close alternatives, distractors and incorrect answers. Depending on the objective of the research, a mix of the above option types can be used.
- Net Promoter Score (NPS) Question: Another commonly used question type in a questionnaire is the Net Promoter Score (NPS) Question where one single question collects data on the referencability of the research topic in question.
- Scaling Questions: Scaling questions are widely used in a questionnaire as they make responding to the questionnaire, very easy. These questions are based on the principles of the 4 measurement scales – nominal, ordinal, interval and ratio .
Questionnaires help enterprises collect valuable data to help them make well-informed business decisions. There are powerful tools available in the market that allows using multiple question types, ready to use survey format templates, robust analytics, and many more features to conduct comprehensive market research.
LEARN ABOUT: course evaluation survey examples
For example, an enterprise wants to conduct market research to understand what pricing would be best for their new product to capture a higher market share. In such a case, a questionnaire for competitor analysis can be sent to the targeted audience using a powerful market research survey software which can help the enterprise conduct 360 market research that will enable them to make strategic business decisions.
Now that we have learned what a questionnaire is and its use in market research , some examples and samples of widely used questionnaire templates on the QuestionPro platform are as below:
LEARN ABOUT: Speaker evaluation form
Customer Questionnaire Templates: Examples and Samples
QuestionPro specializes in end-to-end Customer Questionnaire Templates that can be used to evaluate a customer journey right from indulging with a brand to the continued use and referenceability of the brand. These templates form excellent samples to form your own questionnaire and begin testing your customer satisfaction and experience based on customer feedback.
LEARN ABOUT: Structured Questionnaire
USE THIS FREE TEMPLATE
Employee & Human Resource (HR) Questionnaire Templates: Examples and Samples
QuestionPro has built a huge repository of employee questionnaires and HR questionnaires that can be readily deployed to collect feedback from the workforce on an organization on multiple parameters like employee satisfaction, benefits evaluation, manager evaluation , exit formalities etc. These templates provide a holistic overview of collecting actionable data from employees.
Community Questionnaire Templates: Examples and Samples
The QuestionPro repository of community questionnaires helps collect varied data on all community aspects. This template library includes popular questionnaires such as community service, demographic questionnaires, psychographic questionnaires, personal questionnaires and much more.
Academic Evaluation Questionnaire Templates: Examples and Samples
Another vastly used section of QuestionPro questionnaire templates are the academic evaluation questionnaires . These questionnaires are crafted to collect in-depth data about academic institutions and the quality of teaching provided, extra-curricular activities etc and also feedback about other educational activities.
MORE LIKE THIS
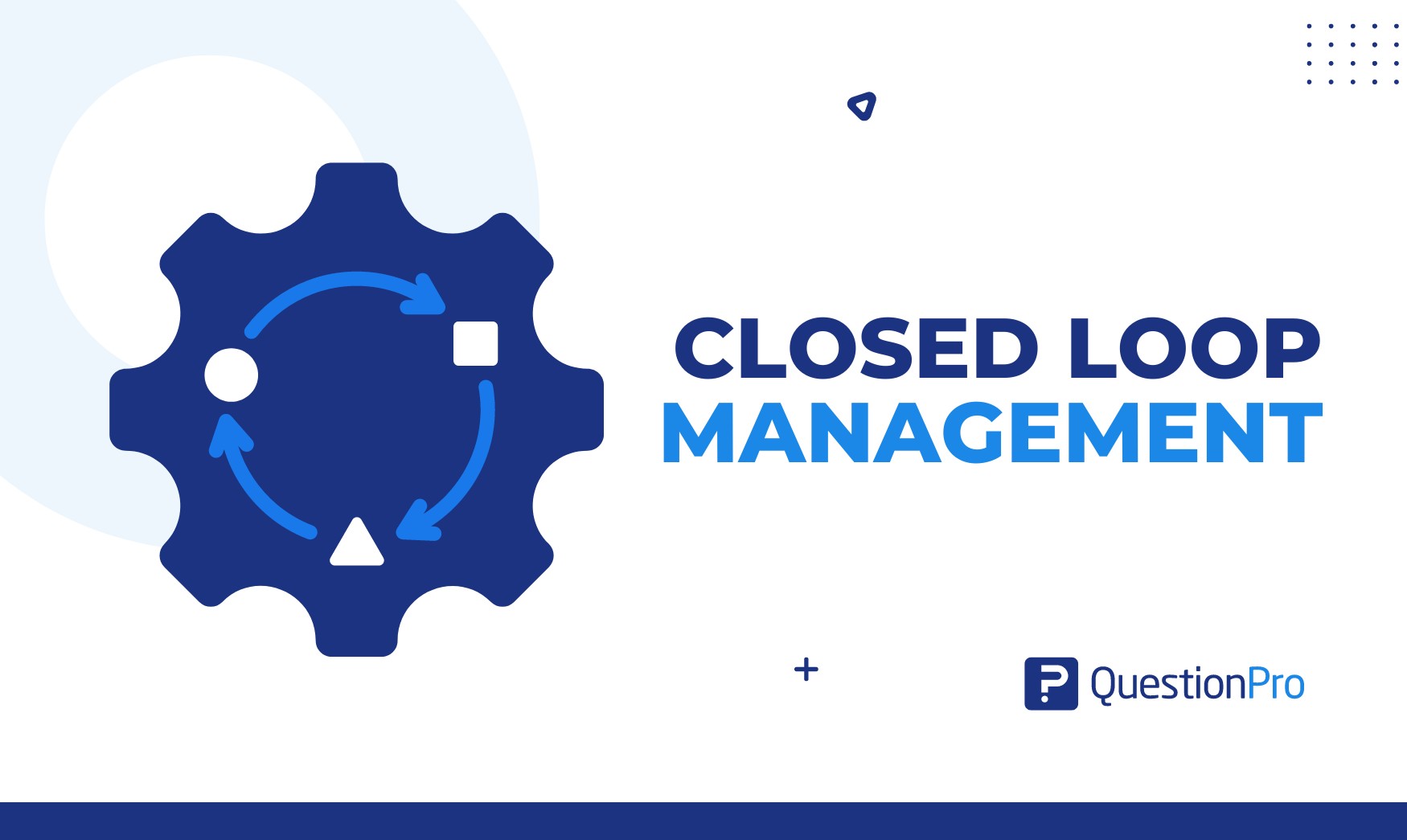
Closed-Loop Management: The Key to Customer Centricity
Sep 3, 2024
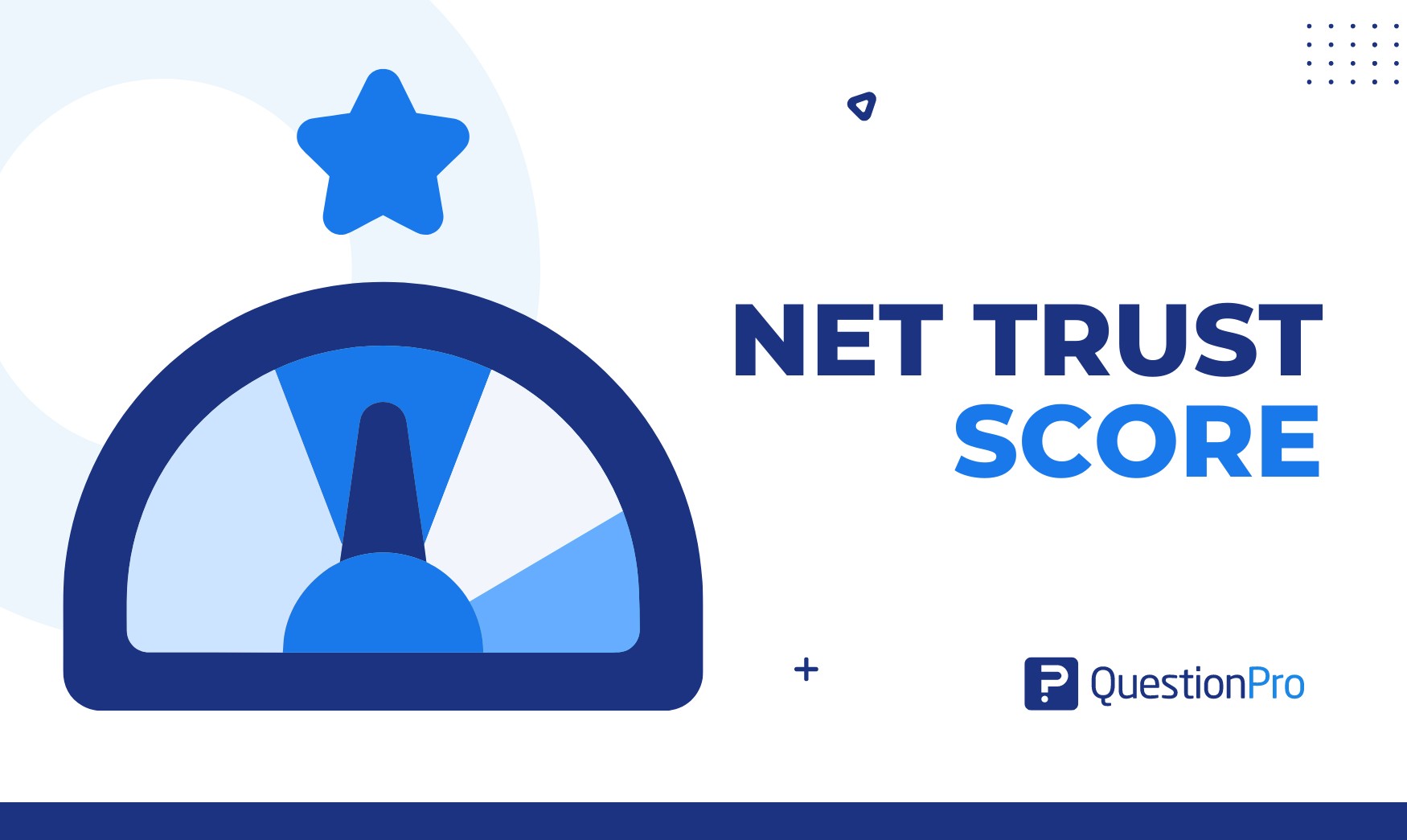
Net Trust Score: Tool for Measuring Trust in Organization
Sep 2, 2024
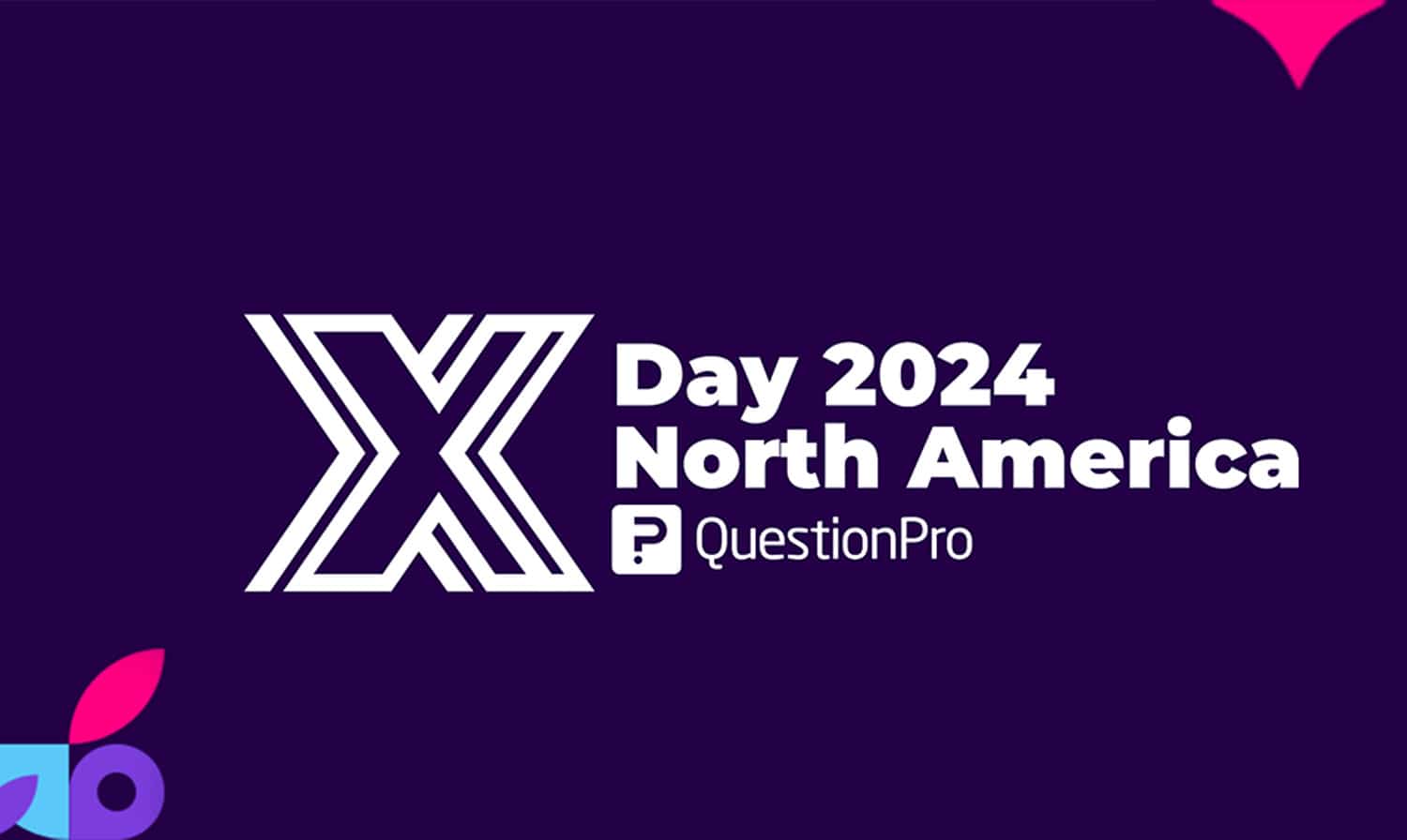
Why You Should Attend XDAY 2024
Aug 30, 2024
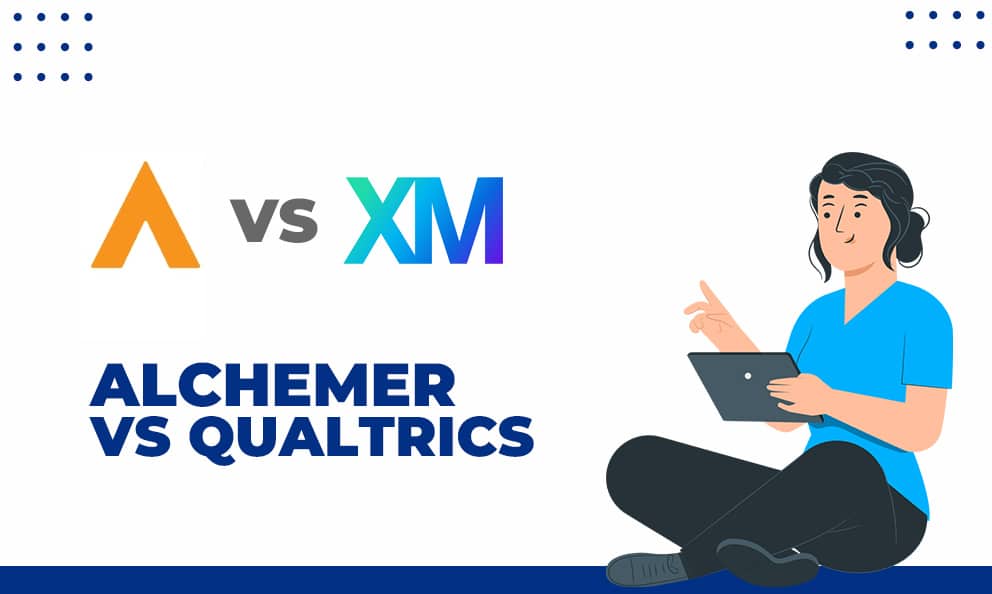
Alchemer vs Qualtrics: Find out which one you should choose
Other categories.
- Academic Research
- Artificial Intelligence
- Assessments
- Brand Awareness
- Case Studies
- Communities
- Consumer Insights
- Customer effort score
- Customer Engagement
- Customer Experience
- Customer Loyalty
- Customer Research
- Customer Satisfaction
- Employee Benefits
- Employee Engagement
- Employee Retention
- Friday Five
- General Data Protection Regulation
- Insights Hub
- Life@QuestionPro
- Market Research
- Mobile diaries
- Mobile Surveys
- New Features
- Online Communities
- Question Types
- Questionnaire
- QuestionPro Products
- Release Notes
- Research Tools and Apps
- Revenue at Risk
- Survey Templates
- Training Tips
- Tuesday CX Thoughts (TCXT)
- Uncategorized
- What’s Coming Up
- Workforce Intelligence
- Utility Menu

Harvard University Program on Survey Research
- Questionnaire Design Tip Sheet
This PSR Tip Sheet provides some basic tips about how to write good survey questions and design a good survey questionnaire.
40 KB |
PSR Resources
- Managing and Manipulating Survey Data: A Beginners Guide
- Finding and Hiring Survey Contractors
- How to Frame and Explain the Survey Data Used in a Thesis
- Overview of Cognitive Testing and Questionnaire Evaluation
- Sampling, Coverage, and Nonresponse Tip Sheet
- Introduction to Surveys for Honors Thesis Writers
- PSR Introduction to the Survey Process
- Related Centers/Programs at Harvard
- General Survey Reference
- Institutional Review Boards
- Select Funding Opportunities
- Survey Analysis Software
- Professional Standards
- Professional Organizations
- Major Public Polls
- Survey Data Collections
- Major Longitudinal Surveys
- Other Links
Have a language expert improve your writing
Run a free plagiarism check in 10 minutes, automatically generate references for free.
- Knowledge Base
- Methodology
- Questionnaire Design | Methods, Question Types & Examples
Questionnaire Design | Methods, Question Types & Examples
Published on 6 May 2022 by Pritha Bhandari . Revised on 10 October 2022.
A questionnaire is a list of questions or items used to gather data from respondents about their attitudes, experiences, or opinions. Questionnaires can be used to collect quantitative and/or qualitative information.
Questionnaires are commonly used in market research as well as in the social and health sciences. For example, a company may ask for feedback about a recent customer service experience, or psychology researchers may investigate health risk perceptions using questionnaires.
Table of contents
Questionnaires vs surveys, questionnaire methods, open-ended vs closed-ended questions, question wording, question order, step-by-step guide to design, frequently asked questions about questionnaire design.
A survey is a research method where you collect and analyse data from a group of people. A questionnaire is a specific tool or instrument for collecting the data.
Designing a questionnaire means creating valid and reliable questions that address your research objectives, placing them in a useful order, and selecting an appropriate method for administration.
But designing a questionnaire is only one component of survey research. Survey research also involves defining the population you’re interested in, choosing an appropriate sampling method , administering questionnaires, data cleaning and analysis, and interpretation.
Sampling is important in survey research because you’ll often aim to generalise your results to the population. Gather data from a sample that represents the range of views in the population for externally valid results. There will always be some differences between the population and the sample, but minimising these will help you avoid sampling bias .
Prevent plagiarism, run a free check.
Questionnaires can be self-administered or researcher-administered . Self-administered questionnaires are more common because they are easy to implement and inexpensive, but researcher-administered questionnaires allow deeper insights.
Self-administered questionnaires
Self-administered questionnaires can be delivered online or in paper-and-pen formats, in person or by post. All questions are standardised so that all respondents receive the same questions with identical wording.
Self-administered questionnaires can be:
- Cost-effective
- Easy to administer for small and large groups
- Anonymous and suitable for sensitive topics
But they may also be:
- Unsuitable for people with limited literacy or verbal skills
- Susceptible to a nonreponse bias (most people invited may not complete the questionnaire)
- Biased towards people who volunteer because impersonal survey requests often go ignored
Researcher-administered questionnaires
Researcher-administered questionnaires are interviews that take place by phone, in person, or online between researchers and respondents.
Researcher-administered questionnaires can:
- Help you ensure the respondents are representative of your target audience
- Allow clarifications of ambiguous or unclear questions and answers
- Have high response rates because it’s harder to refuse an interview when personal attention is given to respondents
But researcher-administered questionnaires can be limiting in terms of resources. They are:
- Costly and time-consuming to perform
- More difficult to analyse if you have qualitative responses
- Likely to contain experimenter bias or demand characteristics
- Likely to encourage social desirability bias in responses because of a lack of anonymity
Your questionnaire can include open-ended or closed-ended questions, or a combination of both.
Using closed-ended questions limits your responses, while open-ended questions enable a broad range of answers. You’ll need to balance these considerations with your available time and resources.
Closed-ended questions
Closed-ended, or restricted-choice, questions offer respondents a fixed set of choices to select from. Closed-ended questions are best for collecting data on categorical or quantitative variables.
Categorical variables can be nominal or ordinal. Quantitative variables can be interval or ratio. Understanding the type of variable and level of measurement means you can perform appropriate statistical analyses for generalisable results.
Examples of closed-ended questions for different variables
Nominal variables include categories that can’t be ranked, such as race or ethnicity. This includes binary or dichotomous categories.
It’s best to include categories that cover all possible answers and are mutually exclusive. There should be no overlap between response items.
In binary or dichotomous questions, you’ll give respondents only two options to choose from.
White Black or African American American Indian or Alaska Native Asian Native Hawaiian or Other Pacific Islander
Ordinal variables include categories that can be ranked. Consider how wide or narrow a range you’ll include in your response items, and their relevance to your respondents.
Likert-type questions collect ordinal data using rating scales with five or seven points.
When you have four or more Likert-type questions, you can treat the composite data as quantitative data on an interval scale . Intelligence tests, psychological scales, and personality inventories use multiple Likert-type questions to collect interval data.
With interval or ratio data, you can apply strong statistical hypothesis tests to address your research aims.
Pros and cons of closed-ended questions
Well-designed closed-ended questions are easy to understand and can be answered quickly. However, you might still miss important answers that are relevant to respondents. An incomplete set of response items may force some respondents to pick the closest alternative to their true answer. These types of questions may also miss out on valuable detail.
To solve these problems, you can make questions partially closed-ended, and include an open-ended option where respondents can fill in their own answer.
Open-ended questions
Open-ended, or long-form, questions allow respondents to give answers in their own words. Because there are no restrictions on their choices, respondents can answer in ways that researchers may not have otherwise considered. For example, respondents may want to answer ‘multiracial’ for the question on race rather than selecting from a restricted list.
- How do you feel about open science?
- How would you describe your personality?
- In your opinion, what is the biggest obstacle to productivity in remote work?
Open-ended questions have a few downsides.
They require more time and effort from respondents, which may deter them from completing the questionnaire.
For researchers, understanding and summarising responses to these questions can take a lot of time and resources. You’ll need to develop a systematic coding scheme to categorise answers, and you may also need to involve other researchers in data analysis for high reliability .
Question wording can influence your respondents’ answers, especially if the language is unclear, ambiguous, or biased. Good questions need to be understood by all respondents in the same way ( reliable ) and measure exactly what you’re interested in ( valid ).
Use clear language
You should design questions with your target audience in mind. Consider their familiarity with your questionnaire topics and language and tailor your questions to them.
For readability and clarity, avoid jargon or overly complex language. Don’t use double negatives because they can be harder to understand.
Use balanced framing
Respondents often answer in different ways depending on the question framing. Positive frames are interpreted as more neutral than negative frames and may encourage more socially desirable answers.
Positive frame | Negative frame |
---|---|
Should protests of pandemic-related restrictions be allowed? | Should protests of pandemic-related restrictions be forbidden? |
Use a mix of both positive and negative frames to avoid bias , and ensure that your question wording is balanced wherever possible.
Unbalanced questions focus on only one side of an argument. Respondents may be less likely to oppose the question if it is framed in a particular direction. It’s best practice to provide a counterargument within the question as well.
Unbalanced | Balanced |
---|---|
Do you favour …? | Do you favour or oppose …? |
Do you agree that …? | Do you agree or disagree that …? |
Avoid leading questions
Leading questions guide respondents towards answering in specific ways, even if that’s not how they truly feel, by explicitly or implicitly providing them with extra information.
It’s best to keep your questions short and specific to your topic of interest.
- The average daily work commute in the US takes 54.2 minutes and costs $29 per day. Since 2020, working from home has saved many employees time and money. Do you favour flexible work-from-home policies even after it’s safe to return to offices?
- Experts agree that a well-balanced diet provides sufficient vitamins and minerals, and multivitamins and supplements are not necessary or effective. Do you agree or disagree that multivitamins are helpful for balanced nutrition?
Keep your questions focused
Ask about only one idea at a time and avoid double-barrelled questions. Double-barrelled questions ask about more than one item at a time, which can confuse respondents.
This question could be difficult to answer for respondents who feel strongly about the right to clean drinking water but not high-speed internet. They might only answer about the topic they feel passionate about or provide a neutral answer instead – but neither of these options capture their true answers.
Instead, you should ask two separate questions to gauge respondents’ opinions.
Strongly Agree Agree Undecided Disagree Strongly Disagree
Do you agree or disagree that the government should be responsible for providing high-speed internet to everyone?
You can organise the questions logically, with a clear progression from simple to complex. Alternatively, you can randomise the question order between respondents.
Logical flow
Using a logical flow to your question order means starting with simple questions, such as behavioural or opinion questions, and ending with more complex, sensitive, or controversial questions.
The question order that you use can significantly affect the responses by priming them in specific directions. Question order effects, or context effects, occur when earlier questions influence the responses to later questions, reducing the validity of your questionnaire.
While demographic questions are usually unaffected by order effects, questions about opinions and attitudes are more susceptible to them.
- How knowledgeable are you about Joe Biden’s executive orders in his first 100 days?
- Are you satisfied or dissatisfied with the way Joe Biden is managing the economy?
- Do you approve or disapprove of the way Joe Biden is handling his job as president?
It’s important to minimise order effects because they can be a source of systematic error or bias in your study.
Randomisation
Randomisation involves presenting individual respondents with the same questionnaire but with different question orders.
When you use randomisation, order effects will be minimised in your dataset. But a randomised order may also make it harder for respondents to process your questionnaire. Some questions may need more cognitive effort, while others are easier to answer, so a random order could require more time or mental capacity for respondents to switch between questions.
Follow this step-by-step guide to design your questionnaire.
Step 1: Define your goals and objectives
The first step of designing a questionnaire is determining your aims.
- What topics or experiences are you studying?
- What specifically do you want to find out?
- Is a self-report questionnaire an appropriate tool for investigating this topic?
Once you’ve specified your research aims, you can operationalise your variables of interest into questionnaire items. Operationalising concepts means turning them from abstract ideas into concrete measurements. Every question needs to address a defined need and have a clear purpose.

Step 2: Use questions that are suitable for your sample
Create appropriate questions by taking the perspective of your respondents. Consider their language proficiency and available time and energy when designing your questionnaire.
- Are the respondents familiar with the language and terms used in your questions?
- Would any of the questions insult, confuse, or embarrass them?
- Do the response items for any closed-ended questions capture all possible answers?
- Are the response items mutually exclusive?
- Do the respondents have time to respond to open-ended questions?
Consider all possible options for responses to closed-ended questions. From a respondent’s perspective, a lack of response options reflecting their point of view or true answer may make them feel alienated or excluded. In turn, they’ll become disengaged or inattentive to the rest of the questionnaire.
Step 3: Decide on your questionnaire length and question order
Once you have your questions, make sure that the length and order of your questions are appropriate for your sample.
If respondents are not being incentivised or compensated, keep your questionnaire short and easy to answer. Otherwise, your sample may be biased with only highly motivated respondents completing the questionnaire.
Decide on your question order based on your aims and resources. Use a logical flow if your respondents have limited time or if you cannot randomise questions. Randomising questions helps you avoid bias, but it can take more complex statistical analysis to interpret your data.
Step 4: Pretest your questionnaire
When you have a complete list of questions, you’ll need to pretest it to make sure what you’re asking is always clear and unambiguous. Pretesting helps you catch any errors or points of confusion before performing your study.
Ask friends, classmates, or members of your target audience to complete your questionnaire using the same method you’ll use for your research. Find out if any questions were particularly difficult to answer or if the directions were unclear or inconsistent, and make changes as necessary.
If you have the resources, running a pilot study will help you test the validity and reliability of your questionnaire. A pilot study is a practice run of the full study, and it includes sampling, data collection , and analysis.
You can find out whether your procedures are unfeasible or susceptible to bias and make changes in time, but you can’t test a hypothesis with this type of study because it’s usually statistically underpowered .
A questionnaire is a data collection tool or instrument, while a survey is an overarching research method that involves collecting and analysing data from people using questionnaires.
Closed-ended, or restricted-choice, questions offer respondents a fixed set of choices to select from. These questions are easier to answer quickly.
Open-ended or long-form questions allow respondents to answer in their own words. Because there are no restrictions on their choices, respondents can answer in ways that researchers may not have otherwise considered.
A Likert scale is a rating scale that quantitatively assesses opinions, attitudes, or behaviours. It is made up of four or more questions that measure a single attitude or trait when response scores are combined.
To use a Likert scale in a survey , you present participants with Likert-type questions or statements, and a continuum of items, usually with five or seven possible responses, to capture their degree of agreement.
You can organise the questions logically, with a clear progression from simple to complex, or randomly between respondents. A logical flow helps respondents process the questionnaire easier and quicker, but it may lead to bias. Randomisation can minimise the bias from order effects.
Questionnaires can be self-administered or researcher-administered.
Researcher-administered questionnaires are interviews that take place by phone, in person, or online between researchers and respondents. You can gain deeper insights by clarifying questions for respondents or asking follow-up questions.
Cite this Scribbr article
If you want to cite this source, you can copy and paste the citation or click the ‘Cite this Scribbr article’ button to automatically add the citation to our free Reference Generator.
Bhandari, P. (2022, October 10). Questionnaire Design | Methods, Question Types & Examples. Scribbr. Retrieved 3 September 2024, from https://www.scribbr.co.uk/research-methods/questionnaire-design/
Is this article helpful?
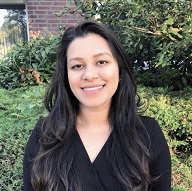
Pritha Bhandari
Other students also liked, doing survey research | a step-by-step guide & examples, what is a likert scale | guide & examples, reliability vs validity in research | differences, types & examples.
We Trust in Human Precision
20,000+ Professional Language Experts Ready to Help. Expertise in a variety of Niches.
API Solutions
- API Pricing
- Cost estimate
- Customer loyalty program
- Educational Discount
- Non-Profit Discount
- Green Initiative Discount1
Value-Driven Pricing
Unmatched expertise at affordable rates tailored for your needs. Our services empower you to boost your productivity.

- Special Discounts
- Enterprise transcription solutions
- Enterprise translation solutions
- Transcription/Caption API
- AI Transcription Proofreading API
Trusted by Global Leaders
GoTranscript is the chosen service for top media organizations, universities, and Fortune 50 companies.
GoTranscript
One of the Largest Online Transcription and Translation Agencies in the World. Founded in 2005.
Speaker 1: In many of the college classes that rely a lot on writing, you may hear your instructors refer to something that's known as the research question, which can be kind of vague and frustrating if it's not explained very well. What is it? Why do I need it? And where do I get one? This video is going to tackle all of these questions, but first, let's break down the definition of research question. It's a clear, focused, concise, complex, and arguable question around which you center your research. Or it's just a thing that's meant to frustrate and confuse students throughout college writing. But hopefully after this video, research questions will be a little less confusing and frustrating. So why do I need a research question? Well, a research question helps you keep your research focused and on track. If you've ever had one of those experiences where you waited for the last minute to write your paper and you just all of a sudden started typing out your writing and a few hours later you read back over it and you realize, oh, this doesn't make sense, there's no clear focus, there's no clear line of thought, well, a research question will help you avoid that. But a research question is also very important because the answer to this question will actually turn into your thesis statement or the main argument of your paper. So it's important to make sure that your research question is strong. So how do I do that? Well, I'm going to walk you through a few steps that have helped me as I come up with research questions for my own writing. The first one is to find an issue that interests you. No matter what class you're in, try to find a way to connect that class to something that you're already interested in. Say you're in a psych class but you want to be a vet. Well, you could look at how pets affect our psychological health. Maybe you're a women's studies major and you're in a computer science class and you want to know why there aren't more women in technology-related fields. Or maybe you're an early childhood education major and you're in a nutrition class. Well, you could explore childhood obesity and how to avoid it. Step two, explore this issue. Just do a quick Google search. For the purpose of this video, I'm actually going to look at the issue of women and how few of them are actually in computer-related fields. This is an issue that I'm really interested in. So if I do a quick search on Google with these terms, I come across as my first hit a Wikipedia article, which I can't use to cite in my paper as a credible source, but it is a great place to start for ideas. And in this article, I found this really interesting quote that tells me that even though teenage girls are using computers at the same rate as teenage guys, they're still much less likely to consider a degree in a technology-related field. Well, I want to know why that is. So I start asking questions about it. I start asking, well, is it important for women to pursue computer-related jobs and why? Why are there so few girls with computer-related degrees? How can we encourage girls to be more involved in computer technology? And who else cares about this issue? Why is it important? Step four, start refining and focusing my question. Just because I have a research question doesn't necessarily mean it's a good question. So we're going to go through a couple of bad questions and talk about how to make them better. Let's start with this question. When did the first woman graduate with a degree in computer science? This isn't such a great question because there's really only one answer to this. There is no way to argue or defend or explore this question very well. A better question would be when, during their college career, do girls usually drop out of computer science programs, and how can we prevent this from happening? This is a question that I can actually explore and then take a stance or position on and then defend. Another bad question is, why do girls hate computers? Well, there's several reasons why this is a bad question. One of them is it's pretty general. It's blanketly stating that all girls hate computers, which isn't necessarily true. There's also really no way to explore or actually defend a feeling. You can't really tell me why girls hate computers. This isn't a question I can actually research. A better question is, why are girls dropping out of computer science programs at higher rates than guys? This is an issue I can dig into. I can come to an opinion on and then defend. So as you start coming up with and exploring research questions of your own, here are a few closing rules of thumbs to remember. One, avoid yes or no questions. Ask questions that might have multiple answers or opinions. This leads us to question two. If you don't ask yes or no questions, you'll start coming up with questions that require you to explain or defend your answer. They'll make you take a stance, which is what you're looking to do in college papers. And then finally, three, ask a question that can be tackled within your page limit. Don't pick a question that is so broad that you find yourself going on and on and on and overreaching your page limit. Find something that's manageable and that's small enough that you can actually answer in the page limit that you're given by your instructors. Now follow these rules, follow these guidelines, and hopefully coming up with research questions The next time you have to do this, it'll be a little simpler and a little less frustrating.

Developing Surveys on Questionable Research Practices: Four Challenging Design Problems
- Open access
- Published: 02 September 2024
Cite this article
You have full access to this open access article
- Christian Berggren ORCID: orcid.org/0000-0002-4233-5138 1 ,
- Bengt Gerdin ORCID: orcid.org/0000-0001-8360-5387 2 &
- Solmaz Filiz Karabag ORCID: orcid.org/0000-0002-3863-1073 1 , 3
2 Altmetric
The exposure of scientific scandals and the increase of dubious research practices have generated a stream of studies on Questionable Research Practices (QRPs), such as failure to acknowledge co-authors, selective presentation of findings, or removal of data not supporting desired outcomes. In contrast to high-profile fraud cases, QRPs can be investigated using quantitative, survey-based methods. However, several design issues remain to be solved. This paper starts with a review of four problems in the QRP research: the problem of precision and prevalence, the problem of social desirability bias, the problem of incomplete coverage, and the problem of controversiality, sensitivity and missing responses. Various ways to handle these problems are discussed based on a case study of the design of a large, cross-field QRP survey in the social and medical sciences in Sweden. The paper describes the key steps in the design process, including technical and cognitive testing and repeated test versions to arrive at reliable survey items on the prevalence of QRPs and hypothesized associated factors in the organizational and normative environments. Partial solutions to the four problems are assessed, unresolved issues are discussed, and tradeoffs that resist simple solutions are articulated. The paper ends with a call for systematic comparisons of survey designs and item quality to build a much-needed cumulative knowledge trajectory in the field of integrity studies.
Similar content being viewed by others
Lies, Damned Lies, and Crafty Questionnaire Design
Design, Run, and Interpret Survey-Based Research in the Fields of Academic Integrity and Misconduct
Explore related subjects.
- Medical Ethics
Avoid common mistakes on your manuscript.
Introduction
The public revelations of research fraud and non-replicable findings (Berggren & Karabag, 2019 ; Levelt et al., 2012 ; Nosek et al., 2022 ) have created a lively interest in studying research integrity. Most studies in this field tend to focus on questionable research practices, QRPs, rather than blatant fraud, which is less common and hard to study with rigorous methods (Butler et al., 2017 ). Despite the significant contributions of this research about the incidence of QRPs in various countries and contexts, several issues still need to be addressed regarding the challenges of designing precise and valid survey instruments and achieving satisfactory response rates in this sensitive area. While studies in management (Hinkin, 1998 ; Lietz, 2010 ), behavioral sciences, psychology (Breakwell et al., 2020 ), sociology (Brenner, 2020 ), and education (Hill et al., 2022 ) have provided guidelines to design surveys, they rarely discuss how to develop, test, and use surveys targeting sensitive and controversial issues such as organizational or individual corruption (Lin & Yu, 2020 ), fraud (Lawlor et al., 2021 ), and misconduct. The aim of this study is to contribute to a systematic discussion of challenges facing survey designers in these areas and, by way of a detailed case study, highlight alternative ways to increase participation and reliability of surveys focusing on questionable research practices, scientific norms, and organizational climate.
The following section starts with a literature-based review of four important problems:
the lack of conceptual consensus and precise measurements,
the problem of social desirability bias.
the difficulty of covering both quantitative and qualitative research fields.
the problem of controversiality and sensitivity.
Section 3 presents an in-depth case study of developing and implementing a survey on QRPs in the social and medical sciences in Sweden 2018–2021, designed to target these problems. Its first results were presented in this journal (Karabag et al., 2024 ). The section also describes the development process and the survey content and highlights the general design challenges. Section 4 returns to the four problems by discussing partial solutions, difficult tradeoffs, and remaining issues.
Four Design Problems in the Study of Questionable Research Practices
Extant QRP studies have generated an impressive body of knowledge regarding the occurrence and complexities of questionable practices, their increasing trend in several academic fields, and the difficulty of mitigating them with conventional interventions such as ethics courses and espousal of integrity policies (Gopalakrishna et al., 2022 ; Karabag et al., 2024 ; Necker, 2014 ). However, investigations on the prevalence of QRPs have so far lacked systematic problem analysis. Below, four main problems are discussed.
The Problem of Conceptual Clarity and Measurement Precision
Studies of QRP prevalence in the literature exhibit high levels of questionable behaviors but also considerable variation in their estimates. This is illustrated in the examples below:
“42% hade collected more data after inspecting whether results were statistically significant… and 51% had reported an unexpected finding as though it had been hypothesized from the start (HARKing)”( Fraser et al., 2018 , p. 1) , “51 , 3% of respondents engaging frequently in at least one QRP” ( Gopalakrishna et al., 2022 , p. 1) , “…one third of the researchers stated that for the express purpose of supporting hypotheses with statistical significance they engaged in post hoc exclusion of data” ( Banks et al., 2016 , p. 10).
On a general level, QRPs constitute deviations from the responsible conduct of research, that are not severe enough to be defined as fraud and fabrication (Steneck, 2006 ). Within these borders, there is no conceptual consensus regarding specific forms of QRPs (Bruton et al., 2020 ; Xie et al., 2021 ). This has resulted in a considerable variation in prevalence estimates (Agnoli et al., 2017 ; Artino et al. Jr, 2019 ; Fiedler & Schwarz, 2016 ). Many studies emphasize the role of intentionality, implying a purpose to support a specific assertion with biased evidence (Banks et al., 2016 ). This tends to be backed by reports of malpractices in quantitative research, such as p-hacking or HARKing, where unexpected findings or results from an exploratory analysis are reported as having been predicted from the start (Andrade, 2021 ). Other QRP studies, however, build on another, often implicit conceptual definition and include practices that could instead be defined as sloppy or under-resourced research, e.g. insufficient attention to equipment, deficient supervision of junior co-workers, inadequate note-keeping of the research process, or use of inappropriate research designs (Gopalakrishna et al., 2022 ). Alternatively, those studies include behaviors such as “Fashion-determined choice of research topic”, “Instrumental and marketable approach”, and “Overselling methods, data or results” (Ravn & Sørensen, 2021 , p. 30; Vermeulen & Hartmann, 2015 ) which may be opportunistic or survivalist but not necessarily involve intentions to mislead.
To shed light on the prevalence of QRPs in different environments, the first step is to conceptualize and delimit the practices to be considered. The next step is to operationalize the conceptual approach into useful indicators and, if needed, to reformulate and reword the indicators into unambiguous, easily understood items (Hinkin, 1995 , 1998 ). The importance of careful item design has been demonstrated by Fiedler and Schwarz ( 2016 ). They show how the perceived QRP prevalence changes by adding specifications to well-known QRP items. Such specifications include: “ failing to report all dependent measures that are relevant for a finding ”, “ selectively reporting studies related to a specific finding that ‘’worked’ ” (Fiedler & Schwarz, 2016 , p. 46, italics in original ), or “collecting more data after seeing whether results were significant in order to render non-significant results significant ” (Fiedler & Schwarz, 2016 , p. 49, italics in original ). These specifications demonstrate the importance of precision in item design, the need for item tests before applications in a large-scale survey, and as the case study in Sect. 3 indicates, the value of statistically analyzing the selected items post-implementation.
The Problem of Social Desirability
Case studies of publicly exposed scientific misconduct have the advantage of explicitness and possible triangulation of sources (Berggren & Karabag, 2019 ; Huistra & Paul, 2022 ). Opinions may be contradictory, but researchers/investigators may often approach a variety of stakeholders and compare oral statements with documents and other sources (Berggren & Karabag, 2019 ). By contrast, quantitative studies of QRPs need to rely on non-public sources in the form of statements and appraisals of survey respondents for the dependent variables and for potentially associated factors such as publication pressure, job insecurity, or competitive climate.
Many QRP surveys use items that target the respondents’ personal attitudes and preferences regarding the dependent variables, indicating QRP prevalence, as well as the explanatory variables. This has the advantage that the respondents presumably know their own preferences and practices. A significant disadvantage, however, concerns social desirability, which in this context means the tendency of respondents to portray themselves, sometimes inadvertently, in more positive ways than justified by their behavior. The extent of this problem was indicated in a meta-study by Fanelli ( 2009 ), which demonstrated major differences between answers to sensitive survey questions that targeted the respondents’ own behavior and questions that focused on the behavior of their colleagues. In the case study below, the pros and cons of the latter indirect approaches are analyzed.
The Problem of Covering Both Quantitative and Qualitative Research
Studies of QRP prevalence are dominated by quantitative research approaches, where there exists a common understanding of the meaning of facts, proper procedures and scientific evidence. Several research fields, also in the social and medical sciences, include qualitative approaches — case studies, interpretive inquiries, or discourse analysis — where assessments of ‘truth’ and ‘evidence’ may be different or more complex to evaluate.
This does not mean that all qualitative endeavors are equal or that deceit—such as presenting fabricated interview quotes or referring to non-existent protocols —is accepted. However, while there are defined criteria for reporting qualitative research, such as the Consolidated Criteria for Reporting Qualitative Research (COREQ) (Tong et al., 2007 ) or the Standards for Reporting Qualitative Research (SRQR checklist) (O’Brien et al., 2014 ), the field of qualitative research encompasses a wide range of different approaches. This includes comparative case studies that offer detailed evidence to support their claims—such as the differences between British and Japanese factories (Dore, 1973 /2011)—as well as discourse analyses and interpretive studies, where the concept of ‘evidence’ is more fluid and hard to apply. The generative richness of the analysis is a key component of their quality (Flick, 2013 ). This intra-field variation makes it hard to pin down and agree upon general QRP items to capture such behaviors in qualitative research. Some researchers have tried to interpret and report qualitative research by means of quantified methods (Ravn & Sørensen, 2021 ), but so far, these attempts constitute a marginal phenomenon. Consequently, the challenges of measuring the prevalence of QRPs (or similar issues) in the variegated field of qualitative research remain largely unexplored.
The Problem of Institutional Controversiality and Personal Sensitivity
Science and academia depend on public trust for funding and executing research. This makes investigations of questionable behaviors a controversial issue for universities and may lead to institutional refusal/non-response. This resistance was experienced by the designers of a large-scale survey of norms and practices in the Dutch academia when several universities decided not to take part, referring to the potential danger of negative publicity (de Vrieze, 2021 ). A Flemish survey on academic careers encountered similar participation problems (Aubert Bonn & Pinxten, 2019 ). Another study on universities’ willingness to solicit whistleblowers for participation revealed that university officers, managers, and lawyers tend to feel obligated to protect their institution’s reputation (Byrn et al., 2016 ). Such institutional actors may resist participation to avoid the exposure of potentially negative information about their institutions and management practices, which might damage the university’s brand (Byrn et al., 2016 ; Downes, 2017 ).
QRP surveys involve sensitive and potentially intrusive questions also from a respondent’s personal perspective that can lead to a reluctance to participate and non-response behavior (Roberts & John, 2014 ; Tourangeau & Yan, 2007 ). Studies show that willingness to participate declines for surveys covering sensitive issues such as misconduct, crime, and corruption, compared to less sensitive ones like leisure activities (cf. Tourangeau et al., 2010 ). The method of survey administration—whether face-to-face, over the phone, via the web, or paper-based—can influence the perceived sensitivity and response rate (Siewert & Udani, 2016 ; Szolnoki & Hoffmann, 2013 ). In the case study below, the survey did not require any institutional support. Instead, the designers focused on minimizing the individual sensitivity problem by avoiding questions about the respondents’ personal practices. To manage this, they concentrated on their colleagues’ behaviors (see Sect. 4.2). Even if a respondent agrees to participate, they may not answer the QRP items due to insufficient knowledge about her colleagues’ practices or a lack of motivation to answer critical questions about their colleagues’ practices (Beatty & Herrmann, 2002 ; Yan & Curtin, 2010 ). Additionally, a significant time gap between observing specific QRPs in the respondent’s research environment and receiving the survey may make it difficult to recall and accurately respond to the questions. Such issues may also result in non-response problems.
Addressing the Problems: Case Study of a Cross-Field QRP Survey – Design Process, Survey Content, Design Challenges
This section presents a case study of the way these four problems were addressed in a cross-field survey intended to capture QRP prevalence and associated factors across the social and medical sciences in Sweden. The account is based on the authors’ intensive involvement in the design and analysis of the survey, including the technical and cognitive testing, and post-implementation analysis of item quality, missing responses, and open respondent comments. The theoretical background and the substantive results of the study are presented in a separate paper (Karabag et al., 2024 ). Method and language experts at Statistics Sweden, a government agency responsible for public statistics in Sweden, supported the testing procedures, the stratified respondent sampling and administered the survey roll-out.
The Survey Design Process – Repeated Testing and Prototyping
The design process included four steps of testing, revising, and prototyping, which allowed the researchers to iteratively improve the survey and plan the roll-out.
Step 1: Development of the Baseline Survey
This step involved searching the literature and creating a list of alternative constructs concerning the key concepts in the planned survey. Based on the study’s aim, the first and third authors compared these constructs and examined how they had been itemized in the literature. After two rounds of discussions, they agreed on construct formulations and relevant ways to measure them, rephrased items if deemed necessary, and designed new items in areas where the extant literature did not provide any guidance. In this way, Survey Version 1 was compiled.
Step 2: Pre-Testing by Means of a Large Convenience Sample
In the second step, this survey version was reviewed by two experts in organizational behavior at Linköping University. This review led to minor adjustments and the creation of Survey Version 2 , which was used for a major pretest. The aim was both to check the quality of individual items and to garner enough responses for a factor analysis that could be used to build a preliminary theoretical model. This dual aim required a larger sample than suggested in the literature on pretesting (Perneger et al., 2015 ). At the same time, it was essential to minimize the contamination of the planned target population in Sweden. To accomplish this, the authors used their access to a community of organization scholars to administer Survey Version 2 to 200 European management researchers.
This mass pre-testing yielded 163 responses. The data were used to form preliminary factor structures and test a structural equation model. Feedback from a few of the respondents highlighted conceptual issues and duplicated questions. Survey Version 3 was developed and prepared for detailed pretesting based on this feedback.
Step 3: Focused Pre-Testing and Technical Assessment
This step focused on the pre-testing and technical assessment. The participants in this step’s pretesting were ten researchers (six in the social sciences and four in the medical sciences) at five Swedish universities: Linköping, Uppsala, Gothenburg, Gävle, and Stockholm School of Economics. Five of those researchers mainly used qualitative research methods, two used both qualitative and quantitative methods, and three used quantitative methods. In addition, Statistics Sweden conducted a technical assessment of the survey items, focusing on wording, sequence, and response options. Footnote 1 Based on feedback from the ten pretest participants and the Statistics Sweden assessment, Survey Version 4 was developed, translated into Swedish, and reviewed by two researchers with expertise in research ethics and scientific misconduct.
It should be highlighted that Swedish academia is predominantly bilingual. While most researchers have Swedish as their mother tongue, many are more proficient in English, and a minority have limited or no knowledge of Swedish. During the design process, the two language versions were compared item by item and slightly adjusted by skilled bilingual researchers. This task was relatively straightforward since most items and concepts were derived from previously published literature in English. Notably, the Swedish versions of key terms and concepts have long been utilized within Swedish academia (see for example Berggren, 2016 ; Hasselberg, 2012 ). To secure translation quality, the language was controlled by a language expert at Statistics Sweden.
Step 4: Cognitive Interviews by Survey and Measurement Experts
Next, cognitive interviews (Willis, 2004 ) were organized with eight researchers from the social and medical sciences and conducted by an expert from Statistics Sweden (Wallenborg Likidis, 2019 ). The participants included four women and four men, ranging in age from 30 to 60. They were two doctoral students, two lecturers, and four professors, representing five different universities and colleges. Additionally, two participants had a non-Nordic background. To ensure confidentiality, no connections are provided between these characteristics and the individual participants.
An effort was made to achieve a distribution of gender, age, subject, employment, and institution. Four social science researchers primarily used qualitative research methods, while the remaining four employed qualitative and quantitative methods. Additionally, four respondents completed the Swedish version of the survey, and four completed the English version.
The respondents completed the survey in the presence of a methods expert from Statistics Sweden, who observed their entire response process. The expert noted spontaneous reactions and recorded instances where respondents hesitated or struggled to understand an item. After the survey, the expert conducted a structured interview with all eight participants, addressing details in each section of the survey, including the missive for recruiting respondents. Some respondents provided oral feedback while reading the cover letter and answering the questions, while others offered feedback during the subsequent interview.
During the cognitive interview process, the methods expert continuously communicated suggestions for improvements to the design team. A detailed test protocol confirmed that most items were sufficiently strong, although a few required minor modifications. The research team then finalized Survey Version 5 , which included both English and Swedish versions (for the complete survey, see Supplementary Material S1).
Although the test successfully captured a diverse range of participants, it would have been desirable to conduct additional tests of the English survey with more non-Nordic participants; as it stands, only one such test was conducted. Despite the participants’ different approaches to completing the survey, the estimated time to complete it was approximately 15–20 min. No significant time difference was observed between completing the survey in Swedish and English.
Design Challenges – the Dearth of an Item-Specific Public Quality Discussion
The design decision to employ survey items from the relevant literature as much as possible was motivated by a desire to increase comparability with previous studies of questionable research practices. However, this approach came with several challenges. Survey-based studies of QRPs rely on the respondents’ subjective assessments, with no possibility to compare the answers with other sources. Thus, an open discussion of survey problems would be highly valuable. However, although published studies usually present the items used in the surveys, there is seldom any analysis of the problems and tradeoffs involved when using a particular type of item or response format and meager information about item validity. Few studies, for example, contain any analysis that clarifies which items that measured the targeted variables with sufficient precision and which items that failed to do so.
Another challenge when using existing survey studies is the lack of information regarding the respondents’ free-text comments about the survey’s content and quality. This could be because the survey did not contain any open questions or because the authors of the report could not statistically analyze the answers. As seen below, however, open respondent feedback on a questionnaire involving sensitive or controversial aspects may provide important feedback regarding problems that did not surface during the pretest process, which by necessity targets much smaller samples.
Survey Content
The survey started with questions about the respondent’s current employment and research environment. It ended with background questions on the respondents’ positions and the extent of their research activity, plus space for open comments about the survey. The core content of the survey consisted of sections on the organizational climate (15 items), scientific norms (13 items), good and questionable research practices (16 items), perceptions of fairness in the academic system (4 items), motivation for conducting research (8 items), ethics training and policies (5 items); and questions on the quality of the research environment and the respondent’s perceived job security.
Sample and Response Rate
All researchers, teachers, and Ph.D. students employed at Swedish universities are registered by Statistics Sweden. To ensure balanced representation and perspectives from both large universities and smaller university colleges, the institutions were divided into three strata based on the number of researchers, teachers, and Ph.D. students: more than 1,000 individuals (7 universities and university colleges), 500–999 individuals (3 institutions), and fewer than 500 individuals (29 institutions). From these strata, Statistics Sweden randomly sampled 35%, 45%, and 50% of the relevant employees, resulting in a sample of 10,047 individuals. After coverage analysis and exclusion of wrongly included, 9,626 individuals remained.
The selected individuals received a personal postal letter with a missive in both English and Swedish informing them about the project and the survey and notifying them that they could respond on paper or online. The online version provided the option to answer in either English or Swedish. The paper version was available only in English to reduce the cost of production and posting. The missive provided the recipients with comprehensive information about the study and what their involvement would entail. It emphasized the voluntary character of participation and their right to withdraw from the survey at any time, adding: “If you do not want to answer the questions , we kindly ask you to contact us. Then you will not receive any reminders.” Sixty-three individuals used this decline option. In line with standard Statistics Sweden procedures, survey completion implied an agreement to participation and to the publication of anonymized results and indicated participants’ understanding of the terms provided (Duncan & Cheng, 2021 ). An email address was provided for respondents to request study outputs or for any other reason. The survey was open for data collection for two months, during which two reminders were sent to non-responders who had not opted out.
Once Statistics Sweden had collected the answers, they were anonymized and used to generate data files delivered to the authors. Statistics Sweden also provided anonymized information about age, gender, and type of employment of each respondent in the dataset delivered to the researchers. Of the targeted individuals, 3,295 responded, amounting to an overall response rate of 34.2%. An analysis of missing value patterns revealed that 290 of the respondents either lacked data for an entire factor or had too many missing values dispersed over several survey sections. After removing these 290 responses, we used SPSS algorithms (IBM-SPSS Statistics 27) to analyze the remaining missing values, which were randomly distributed and constituted less than 5% of the data. These values were replaced using the program’s imputation program (Madley-Dowd et al., 2019 ). The final dataset consisted of 3,005 individuals, evenly distributed between female and male respondents (53,5% vs. 46,5%) and medical and social scientists (51,3% vs. 48,5%). An overview of the sample and the response rate is provided in Table 1 , which can also be found in (Karabag et al., 2024 ). As shown in Table 1 , the proportion of male and female respondents, as well as the proportion of respondents from medical and social science, and the age distribution of the respondents compared well with the original selection frame from Statistics Sweden.
Revisiting the Four Problems. Partial Solutions and Remaining Issues
Managing the precision problem - the value of factor analyses.
As noted above, the lack of conceptual consensus and standard ways to measure QRPs has resulted in a huge variation in estimated prevalence. In the case studied here, the purpose was to investigate deviations from research integrity and not low-quality research in general. This conceptual focus implied that selected survey items regarding QRP should build on the core aspect of intention, as suggested by Banks et al. ( 2016 , p. 323): “design, analytic, or reporting practices that have been questioned because of the potential for the practice to be employed with the purpose of presenting biased evidence in favor of an assertion”. After scrutinizing the literature, five items were selected as general indicators of QRP, irrespective of the research approach (see Table 2 ).
An analysis of the survey responses indicated that the general QRP indicators worked well in terms of understandability and precision. Considering the sensitive nature of the items, features that typically yield very high rates of missing data (Fanelli, 2009 ; Tourangeau & Yan, 2007 ), our missing rates of 11–21% must be considered modest. In addition, there were a few critical comments on the item formulation in the open response section at the end of the survey (see below).
Regarding the explanatory (independent) variables, the survey was inspired by studies showing the importance of the organizational climate and the normative environment within academia (Anderson et al., 2010 ). Organizational climate can be measured in several ways; the studied survey focused on items related to a collegial versus a competitive climate. The analysis of the normative environment was inspired by the classical norms of science articulated by Robert Merton in his CUDOS framework: communism (communalism), universalism, disinterestedness, and organized skepticism (Merton, 1942 /1973). This framework has been extensively discussed and challenged but remains a key reference (Anderson et al., 2010 ; Chalmers & Glasziou, 2009 ; Kim & Kim, 2018 ; Macfarlane & Cheng, 2008 ). Moreover, we were inspired by the late work of Merton on the ambivalence and ambiguities of scientists (Merton, 1942 /1973), and the counter norms suggested by Mitroff ( 1974 ). Thus, the survey involved a composite set of items to capture the contradictory normative environment in academia: classical norms as well as their counter norms.
To reduce the problems of social desirability bias and personal sensitivity, the survey design avoided items about the respondent’s personal adherence to explicit ideals, which are common in many surveys (Gopalakrishna et al., 2022 ). Instead, the studied survey focused on the normative preferences and attitudes within the respondent’s environment. This necessitated the identification, selection, and refinement of 3–4 items for each potentially relevant norm/counter-norm. The selection process was used in previous studies of norm subscription in various research communities (Anderson et al., 2007 ; Braxton, 1993 ; Bray & von Storch, 2017 ). For the norm “skepticism”, we consulted studies in the accounting literature of the three key elements of professional skepticism: questioning mind, suspension of judgment and search for knowledge (Hurtt, 2010 ).
The first analytical step after receiving the completed survey set from Statistics Sweden was to conduct a set of factor analyses to assess the quality and validity of the survey items related to the normative environment and the organizational climate. These analyses suggested three clearly identifiable factors related to the normative environment: (1) a counter norm factor combining Mitroff’s particularism and dogmatism (‘Biasedness’ in the further analysis), and two Mertonian factors: (2) Skepticism and (3) Openness, a variant of Merton’s Communalism (see Table 3 ). A fourth Merton factor, Disinterestedness, could not be identified in our analysis.
The analytical process for organizational climate involved reducing the number of items from 15 to 11 (see Table 4 ). Here, the factor analysis suggested two clearly identifiable factors, one related to collegiality and the other related to competition (see Table 4 ). Overall, the factor analyses suggested that the design efforts had paid off in terms of high item quality, robust factor loadings, and a very limited need to remove any items.
In a parallel step, the open comments were assessed as an indication of how the study was perceived by the respondents (see Table 5 ). Of the 3005 respondents, 622 provided comprehensible comments, and many of them were extensive. 187 comments were related to the respondents’ own employment/role, 120 were related to the respondents’ working conditions and research environment, and 98 were related to the academic environment and atmosphere. Problems in knowing details of collegial practices were mentioned in 82 comments.
Reducing Desirability Bias - the Challenge of Nonresponse
It is well established that studies on topics where the respondent has anything embarrassing or sensitive to report suffer from more missing responses than studies on neutral subjects and that respondents may edit the information they provide on sensitive topics (Tourangeau & Yan, 2007 ). Such a social desirability bias is applicable for QRP studies which explicitly target the respondents’ personal attitudes and behaviors. To reduce this problem, the studied survey applied a non-self-format focusing on the behaviors and preferences of the respondents’ colleagues. Relevant survey items from published studies were rephrased from self-format designs to non-self-questions about practices in the respondent’s environment, using the format: “In my research environment, colleagues…” followed by a five-step incremental response format from “(1) never” to “(5) always”. In a similar way the survey avoided “should”-statements about ideal normative values: “Scientists and scholars should critically examine…”. Instead, the survey used items intended to indicate the revealed preferences in the respondent’s normative environment regarding universalism versus particularism or openness versus secrecy.
As indicated by Fanelli ( 2009 ), these redesign efforts probably reduced the social desirability bias significantly. At the same time, however, the redesign seemed to increase a problem not discussed by Fanelli ( 2009 ): an increased uncertainty problem related to the respondents’ difficulties of knowing the practices of their colleagues in questionable areas. This issue was indicated by the open comment at the end of the studied survey, where 13% of the 622 respondents pointed out that they lacked sufficient knowledge about the behavior of their colleagues to answer the QRP questions (see Table 5 ). One respondent wrote:
“It’s difficult to answer questions about ‘colleagues in my research area’ because I don’t have an insight into their research practices; I can only make informed guesses and generalizations. Therefore, I am forced to answer ‘don’t know’ to a lot of questions”.
Regarding the questions on general QRPs, the rate of missing responses varied between 11% and 21%. As for the questions targeting specific QRP practices in quantitative and qualitative research, the rate of missing responses ranged from 38 to 49%. Unfortunately, the non-response alternative to these questions (“Don’t know/not relevant”) combined the two issues: the lack of knowledge and the lack of relevance. Thus, we don’t know what part of the missing responses related to a non-presence of the specific research approach in the respondent’s environment and what part signaled a lack of knowledge about collegial practices in this environment.
Measuring QRPs in Qualitative Research - the Limited Role of Pretests
Studies of QRP prevalence focus on quantitative research approaches, where there exists a common understanding of the interpretation of scientific evidence, clearly recommended procedures, and established QRP items related to compliance with these procedures. In the heterogenous field of qualitative research, there are several established standards for reporting the research (O’Brien et al., 2014 ; Tong et al., 2007 ), but, as noted above, hardly any commonly accepted survey items that capture behaviors that fulfill the criteria for QRPs. As a result, the studied survey project designed such items from the start during the survey development process. After technical and cognitive tests, four items were selected. See Table 6 .
Despite the series of pretests, however, the first two of these items met severe criticism from a few respondents in the survey’s open commentary section. Here, qualitative researchers argued that the items were unduly influenced by the truth claims in quantitative studies, whereas their research dealt with interpretation and discourse analysis. Thus, they rejected the items regarding selective usage of respondents and of interview quotes as indicators of questionable practices:
“The alternative regarding using quotes is a bit misleading. Supporting your results by quotes is a way to strengthen credibility in a qualitative method….” “The question about dubious practices is off target for us, who work with interpretation rather than solid truths. You can present new interpretations, but normally that does not imply that previous ‘findings’ should be considered incorrect.” “The questions regarding qualitative research were somewhat irrelevant. Often this research is not guided by a given hypothesis, and researchers may use a convenient sample without this resulting in lower quality.”
One comment focused on other problems related to qualitative research:
“Several questions do not quite capture the ethical dilemmas we wrestle with. For example , is the issue of dishonesty and ‘inaccuracies’ a little misplaced for us who work with interpretation? …At the same time , we have a lot of ethical discussions , which , for example , deal with power relations between researchers and ‘researched’ , participant observation/informal contacts and informed consent (rather than patients participating in a study)”.
Unfortunately, the survey received these comments and criticism only after the full-scale rollout and not during the pretest rounds. Thus, we had no chance to replace the contested items with other formulations or contemplate a differentiation of the subsection to target specific types of qualitative research with appropriate questions. Instead, we had to limit the post-roll-out survey analysis to the last two items in Table 6 , although they captured devious behaviors rather than gray zone practices.
Why then was this criticism of QRP items related to qualitative research not exposed in the pretest phase? This is a relevant question, also for future survey designers. An intuitive answer could be that the research team only involved quantitative researchers. However, as highlighted above, the pretest participants varied in their research methods: some exclusively used qualitative methods, others employed mixed methods, and some utilized quantitative methods. This diversity suggests that the selection of test participants was appropriate. Moreover, all three members of the research team had experience of both quantitative and qualitative studies. However, as discussed above, the field of qualitative research involves several different types of research, with different goals and methods – from detailed case studies grounded in original empirical fieldwork to participant observations of complex organizational phenomena to discursive re-interpretations of previous studies. Of the 3,005 respondents who answered the survey in a satisfactory way, only 16 respondents, or 0,5%, had any critical comments about the QRP items related to qualitative research. A failure to capture the objections from such a small proportion in a pretest phase is hardly surprising. The general problem could be compared with the challenge of detecting negative side-effects in drug development. Although the pharmaceutical firms conduct large-scale tests of candidate drugs before government approval, doctors nevertheless detect new side-effects when the medicine is rolled out to significantly more people than the test populations – and report these less frequent problems in the additional drug information (Galeano et al., 2020 ; McNeil et al., 2010 ).
In the social sciences, the purpose of pre-testing is to identify problems related to ambiguities and bias in item formulation and survey format and initiate a search for relevant solutions. A pre-test on a small, selected subsample cannot guarantee that all respondent problems during the full-scale data collection will be detected. The pretest aims to reduce errors to acceptable levels and ensure that the respondents will understand the language and terminology chosen. Pretesting in survey development is also essential to help the researchers to assess the overall flow and structure of the survey, and to make necessary adjustments to enhance respondent engagement and data quality (Ikart, 2019 ; Presser & Blair, 1994 ).
In our view, more pretests would hardly solve the epistemological challenge of formulating generally acceptable QRP items for qualitative research. The open comments studied here suggest that there is no one-size-fits-all solution. If this is right, the problem should rather be reformulated to a question of identifying different strands of qualitative research with diverse views of integrity and evidence which need to be measured with different measures. To address this challenge in a comprehensive way, however, goes far beyond the current study.
Controversiality and Collegial sensitivity - the Challenge of Predicting Nonresponse
Studies of research integrity, questionable research practices, and misconduct in science tend to be organizationally controversial and personally sensitive. If university leaders are asked to support such studies, there is a considerable risk that the answer will be negative. In the case studied here, the survey roll-out was not dependent on any active organizational participation since Statistics Sweden possessed all relevant respondent information in-house. This, we assumed, would take the controversiality problem off the agenda. Our belief was supported by the non-existent complaints regarding a potential negativity bias from the pretest participants. Instead, the problem surfaced when the survey was rolled out, and all the respondents contemplated the survey. The open comment section at the end of the survey provided insights into this reception.
Many respondents provided positive feedback, reflected in 30 different comments such as:
“Thank you for doing this survey. I really hope it will lead to changes because it is needed”. “This is an important survey. However , there are conflicting norms , such as those you cite in the survey , /concerning/ for example , data protection. How are researchers supposed to be open when we cannot share data for re-analysis?” “I am glad that the problems with egoism and non-collegiality are addressed in this manner ”.
Several of them asked for more critical questions regarding power, self-interest, and leadership:
“What I lack in the survey were items regarding academic leadership. Otherwise, I am happy that someone is doing research on these issues”. “A good survey but needs to be complemented with questions regarding researchers who put their commercial interests above research and exploit academic grants for commercial purposes”.
A small minority criticized the survey for being overly negative towards academia:
“A major part of the survey feels very negative and /conveys/ the impression that you have a strong pre-understanding of academia as a horrible environments”. “Some of the questions are uncomfortable and downright suggestive. Why such a negative attitude towards research?” “The questions have a tendency to make us /the respondents/ informers. An unpleasant feeling when you are supposed to lay information against your university”. “Many questions are hard to answer, and I feel that they measure my degree of suspicion against my closest colleagues and their motivation … Several questions I did not want to answer since they contain a negative interpretation of behaviors which I don’t consider as automatically negative”.
A few of these respondents stated that they abstained from answering some of the ‘negative questions’, since they did not want to report on or slander their colleagues. The general impact is hard to assess. Only 20% of the respondents offered open survey comments, and only seven argued that questions were “negative”. The small number explains why the issue of negativity did not show up during the testing process. However, a perceived sense of negativity may have affected the willingness to answer among more respondents than those who provided free test comments.
Conclusion - The Needs for a Cumulative Knowledge Trajectory in Integrity Studies
In the broad field of research integrity studies, investigations of QRPs in different contexts and countries play an important role. The comparability of the results, however, depends on the conceptual focus of the survey design and the quality of the survey items. This paper starts with a discussion of four common problems in QRP research: the problems of precision, social desirability, incomplete coverage, and organizational controversiality and sensitivity. This is followed by a case study of how these problems were addressed in a detailed survey design process. An assessment of the solutions employed in the studied survey design reveals progress as well as unresolved issues.
Overall, the paper shows that the problem and challenges of precision could be effectively managed through explicit conceptual definitions and careful item design.
The problem of social desirability bias was probably reduced by means of a non-self-response format referring to preferences and behaviors among colleagues instead of personal behaviors. However, an investigation of open respondent comments indicated that the reduced risk of social bias came at the expense of higher uncertainty due to the respondents’ lack of insight in the concrete practices of their colleagues.
The problem of incomplete coverage of QRPs in qualitative research, the authors initially linked to “the lack of standard items” to capture QRPs in qualitative studies. Open comments at the end of the survey, however, suggested that the lack of such standards would not be easily managed by the design of new items. Rather, it seems to be an epistemological challenge related to the multifarious nature of the qualitative research field, where the understanding of ‘evidence’ is unproblematic in some qualitative sub-fields but contested in others. This conjecture and other possible explanations will hopefully be addressed in forthcoming epistemological and empirical studies.
Regarding the problem of controversiality and sensitivity, previous studies show that QRP research is a controversial and sensitive area for academic executives and university brand managers. The case study discussed here indicates that this is a sensitive subject also for rank-and-file researchers who may hesitate to answer, even when the questions do not target the respondents’ own practices but the practices and preferences of their colleagues. Future survey designers may need to engage in framing, presenting, and balancing sensitive items to reduce respondent suspicions and minimize the rate of missing responses. Reflections on the case indicate that this is doable but requires thoughtful design, as well as repeated tests, including feedback from a broad selection of prospective participants.
In conclusion, the paper suggests that more resources should be spent on the systematic evaluation of different survey designs and item formulations. In the long term, such investments in method development will yield a higher proportion of robust and comparable studies. This would mitigate the problems discussed here and contribute to the creation of a much-needed cumulative knowledge trajectory in research integrity studies.
An issue not covered here is that surveys, however finely developed, only give quantitative information about patterns, behaviors, and structures. An understanding of underlying thoughts and perspectives requires other procedures. Thus, methods that integrate and triangulate qualitative and quantitative data —known as mixed methods (Karabag & Berggren, 2016 ; Ordu & Yılmaz, 2024 ; Smajic et al., 2022 )— may give a deeper and more complete picture of the phenomenon of QRP.
Data Availability
The data supporting the findings of this study are available from the corresponding author, upon reasonable request.
Wallenborg Likidis ( 2019 ). Academic norms and scientific attitudes: Metrology Review of a survey for doctoral students , researchers and academic teachers (In Swedish: Akademiska normer och vetenskapliga förhallningssätt. Mätteknisk granskning av en enkät till doktorander , forskare och akademiska lärare) . Prod.nr. 8,942,146, Statistics Sweden, Örebro.
Agnoli, F., Wicherts, J. M., Veldkamp, C. L., Albiero, P., & Cubelli, R. (2017). Questionable research practices among Italian research psychologists. PLoS One , 12(3), e0172792.
Anderson, M. S., Ronning, E. A., De Vries, R., & Martinson, B. C. (2007). The perverse effects of competition on scientists’ work and relationships. Science and Engineering Ethics , 13 , 437–461.
Article Google Scholar
Anderson, M. S., Ronning, E. A., Devries, R., & Martinson, B. C. (2010). Extending the Mertonian norms: Scientists’ subscription to norms of Research. The Journal of Higher Education , 81 (3), 366–393. https://doi.org/10.1353/jhe.0.0095
Andrade, C. (2021). HARKing, cherry-picking, p-hacking, fishing expeditions, and data dredging and mining as questionable research practices. The Journal of Clinical Psychiatry , 82 (1), 25941.
ArtinoJr, A. R., Driessen, E. W., & Maggio, L. A. (2019). Ethical shades of gray: International frequency of scientific misconduct and questionable research practices in health professions education. Academic Medicine , 94 (1), 76–84.
Aubert Bonn, N., & Pinxten, W. (2019). A decade of empirical research on research integrity: What have we (not) looked at? Journal of Empirical Research on Human Research Ethics , 14 (4), 338–352.
Banks, G. C., O’Boyle Jr, E. H., Pollack, J. M., White, C. D., Batchelor, J. H., Whelpley, C. E., & Adkins, C. L. (2016). Questions about questionable research practices in the field of management: A guest commentary. Journal of Management , 42 (1), 5–20.
Beatty, P., & Herrmann, D. (2002). To answer or not to answer: Decision processes related to survey item nonresponse. Survey Nonresponse , 71 , 86.
Google Scholar
Berggren, C. (2016). Scientific Publishing: History, practice, and ethics (in Swedish: Vetenskaplig Publicering: Historik, Praktik Och Etik) . Studentlitteratur AB.
Berggren, C., & Karabag, S. F. (2019). Scientific misconduct at an elite medical institute: The role of competing institutional logics and fragmented control. Research Policy , 48 (2), 428–443. https://doi.org/10.1016/j.respol.2018.03.020
Braxton, J. M. (1993). Deviancy from the norms of science: The effects of anomie and alienation in the academic profession. Research in Higher Education , 54 (2), 213–228. https://www.jstor.org/stable/40196105
Bray, D., & von Storch, H. (2017). The normative orientations of climate scientists. Science and Engineering Ethics , 23 (5), 1351–1367.
Breakwell, G. M., Wright, D. B., & Barnett, J. (2020). Research questions, design, strategy and choice of methods. Research Methods in Psychology , 1–30.
Brenner, P. S. (2020). Why survey methodology needs sociology and why sociology needs survey methodology: Introduction to understanding survey methodology: Sociological theory and applications. In Understanding survey methodology: Sociological theory and applications (pp. 1–11). https://doi.org/10.1007/978-3-030-47256-6_1
Bruton, S. V., Medlin, M., Brown, M., & Sacco, D. F. (2020). Personal motivations and systemic incentives: Scientists on questionable research practices. Science and Engineering Ethics , 26 (3), 1531–1547.
Butler, N., Delaney, H., & Spoelstra, S. (2017). The gray zone: Questionable research practices in the business school. Academy of Management Learning & Education , 16 (1), 94–109.
Byrn, M. J., Redman, B. K., & Merz, J. F. (2016). A pilot study of universities’ willingness to solicit whistleblowers for participation in a study. AJOB Empirical Bioethics , 7 (4), 260–264.
Chalmers, I., & Glasziou, P. (2009). Avoidable waste in the production and reporting of research evidence. The Lancet , 374 (9683), 86–89.
de Vrieze, J. (2021). Large survey finds questionable research practices are common. Science . https://doi.org/10.1126/science.373.6552.265
Dore, R. P. (1973/2011). British Factory Japanese Factory: The origins of National Diversity in Industrial Relations, with a New Afterword . University of California Press/Routledge.
Downes, M. (2017). University scandal, reputation and governance. International Journal for Educational Integrity , 13 , 1–20.
Duncan, L. J., & Cheng, K. F. (2021). Public perception of NHS general practice during the first six months of the COVID-19 pandemic in England. F1000Research , 10 .
Fanelli, D. (2009). How many scientists fabricate and falsify research? A systematic review and meta-analysis of survey data. PLoS One , 4(5), e5738.
Fiedler, K., & Schwarz, N. (2016). Questionable research practices revisited. Social Psychological and Personality Science , 7 (1), 45–52.
Flick, U. (2013). The SAGE Handbook of Qualitative Data Analysis . sage.
Fraser, H., Parker, T., Nakagawa, S., Barnett, A., & Fidler, F. (2018). Questionable research practices in ecology and evolution. PLoS One , 13(7), e0200303.
Galeano, D., Li, S., Gerstein, M., & Paccanaro, A. (2020). Predicting the frequencies of drug side effects. Nature Communications , 11 (1), 4575.
Gopalakrishna, G., Ter Riet, G., Vink, G., Stoop, I., Wicherts, J. M., & Bouter, L. M. (2022). Prevalence of questionable research practices, research misconduct and their potential explanatory factors: A survey among academic researchers in the Netherlands. PLoS One , 17 (2), e0263023.
Hasselberg, Y. (2012). Science as Work: Norms and Work Organization in Commodified Science (in Swedish: Vetenskap Som arbete: Normer och arbetsorganisation i den kommodifierade vetenskapen) . Gidlunds förlag.
Hill, J., Ogle, K., Gottlieb, M., Santen, S. A., & ArtinoJr, A. R. (2022). Educator’s blueprint: a how-to guide for collecting validity evidence in survey‐based research. AEM Education and Training , 6(6), e10835.
Hinkin, T. R. (1995). A review of scale development practices in the study of organizations. Journal of Management , 21 (5), 967–988.
Hinkin, T. R. (1998). A brief tutorial on the development of measures for use in survey questionnaires. Organizational Research Methods , 1 (1), 104–121.
Huistra, P., & Paul, H. (2022). Systemic explanations of scientific misconduct: Provoked by spectacular cases of norm violation? Journal of Academic Ethics , 20 (1), 51–65.
Hurtt, R. K. (2010). Development of a scale to measure professional skepticism. Auditing: A Journal of Practice & Theory , 29 (1), 149–171.
Ikart, E. M. (2019). Survey questionnaire survey pretesting method: An evaluation of survey questionnaire via expert reviews technique. Asian Journal of Social Science Studies , 4 (2), 1.
Karabag, S. F., & Berggren, C. (2016). Misconduct, marginality and editorial practices in management, business and economics journals. PLoS One , 11 (7), e0159492. https://doi.org/10.1371/journal.pone.0159492
Karabag, S. F., Berggren, C., Pielaszkiewicz, J., & Gerdin, B. (2024). Minimizing questionable research practices–the role of norms, counter norms, and micro-organizational ethics discussion. Journal of Academic Ethics , 1–27. https://doi.org/10.1007/s10805-024-09520-z
Kim, S. Y., & Kim, Y. (2018). The ethos of Science and its correlates: An empirical analysis of scientists’ endorsement of Mertonian norms. Science Technology and Society , 23 (1), 1–24. https://doi.org/10.1177/0971721817744438
Lawlor, J., Thomas, C., Guhin, A. T., Kenyon, K., Lerner, M. D., Consortium, U., & Drahota, A. (2021). Suspicious and fraudulent online survey participation: Introducing the REAL framework. Methodological Innovations , 14 (3), 20597991211050467.
Levelt, W. J., Drenth, P., & Noort, E. (2012). Flawed science: The fraudulent research practices of social psychologist Diederik Stapel (in Dutch: Falende wetenschap: De frauduleuze onderzoekspraktijken van social-psycholoog Diederik Stapel) . Commissioned by the Tilburg University, University of Amsterdam and the University of Groningen. https://doi.org/http://hdl.handle.net/11858/00-001M-0000-0010-258A-9
Lietz, P. (2010). Research into questionnaire design: A summary of the literature. International Journal of Market Research , 52 (2), 249–272.
Lin, M. W., & Yu, C. (2020). Can corruption be measured? Comparing global versus local perceptions of corruption in East and Southeast Asia. In Regional comparisons in comparative policy analysis studies (pp. 90–107). Routledge.
Macfarlane, B., & Cheng, M. (2008). Communism, universalism and disinterestedness: Re-examining contemporary support among academics for Merton’s scientific norms. Journal of Academic Ethics , 6 , 67–78.
Madley-Dowd, P., Hughes, R., Tilling, K., & Heron, J. (2019). The proportion of missing data should not be used to guide decisions on multiple imputation. Journal of Clinical Epidemiology , 110 , 63–73.
McNeil, J. J., Piccenna, L., Ronaldson, K., & Ioannides-Demos, L. L. (2010). The value of patient-centred registries in phase IV drug surveillance. Pharmaceutical Medicine , 24 , 281–288.
Merton, R. K. (1942/1973). The normative structure of science. In The sociology of science: Theoretical and empirical investigations . The University of Chicago Press.
Mitroff, I. I. (1974). Norms and counter-norms in a select group of the Apollo Moon scientists: A case study of the ambivalence of scientists. American Sociological Review , 39 (4), 579–595. https://doi.org/10.2307/2094423
Necker, S. (2014). Scientific misbehavior in economics. Research Policy , 43 (10), 1747–1759. https://doi.org/10.1016/j.respol.2014.05.002
Nosek, B. A., Hardwicke, T. E., Moshontz, H., Allard, A., Corker, K. S., Dreber, A., & Nuijten, M. B. (2022). Replicability, robustness, and reproducibility in psychological science. Annual Review of Psychology , 73 (1), 719–748.
O’Brien, B. C., Harris, I. B., Beckman, T. J., Reed, D. A., & Cook, D. A. (2014). Standards for reporting qualitative research: A synthesis of recommendations. Academic Medicine , 89 (9). https://journals.lww.com/academicmedicine/fulltext/2014/09000/standards_for_reporting_qualitative_research__a.21.aspx
Ordu, Y., & Yılmaz, S. (2024). Examining the impact of dramatization simulation on nursing students’ ethical attitudes: A mixed-method study. Journal of Academic Ethics , 1–13.
Perneger, T. V., Courvoisier, D. S., Hudelson, P. M., & Gayet-Ageron, A. (2015). Sample size for pre-tests of questionnaires. Quality of life Research , 24 , 147–151.
Presser, S., & Blair, J. (1994). Survey pretesting: Do different methods produce different results? Sociological Methodology , 73–104.
Ravn, T., & Sørensen, M. P. (2021). Exploring the gray area: Similarities and differences in questionable research practices (QRPs) across main areas of research. Science and Engineering Ethics , 27 (4), 40.
Roberts, D. L., & John, F. A. S. (2014). Estimating the prevalence of researcher misconduct: a study of UK academics within biological sciences. PeerJ , 2 , e562.
Siewert, W., & Udani, A. (2016). Missouri municipal ethics survey: Do ethics measures work at the municipal level? Public Integrity , 18 (3), 269–289.
Smajic, E., Avdic, D., Pasic, A., Prcic, A., & Stancic, M. (2022). Mixed methodology of scientific research in healthcare. Acta Informatica Medica , 30 (1), 57–60. https://doi.org/10.5455/aim.2022.30.57-60
Steneck, N. H. (2006). Fostering integrity in research: Definitions, current knowledge, and future directions. Science and Engineering Ethics , 12 , 53–74.
Szolnoki, G., & Hoffmann, D. (2013). Online, face-to-face and telephone surveys—comparing different sampling methods in wine consumer research. Wine Economics and Policy , 2 (2), 57–66.
Tong, A., Sainsbury, P., & Craig, J. (2007). Consolidated criteria for reporting qualitative research (COREQ): A 32-item checklist for interviews and focus groups. International Journal for Quality in Health Care , 19 (6), 349–357. https://doi.org/10.1093/intqhc/mzm042
Tourangeau, R., & Yan, T. (2007). Sensitive questions in surveys. Psychological Bulletin , 133 (5), 859.
Tourangeau, R., Groves, R. M., & Redline, C. D. (2010). Sensitive topics and reluctant respondents: Demonstrating a link between nonresponse bias and measurement error. Public Opinion Quarterly , 74 (3), 413–432.
Vermeulen, I., & Hartmann, T. (2015). Questionable research and publication practices in communication science. Communication Methods and Measures , 9 (4), 189–192.
Wallenborg Likidis, J. (2019). Academic norms and scientific attitudes: Metrology review of a survey for doctoral students, researchers and academic teachers (In Swedish: Akademiska normer och vetenskapliga förhallningssätt. Mätteknisk granskning av en enkät till doktorander, forskare och akademiska lärare) . Prod.nr. 8942146, Statistics Sweden, Örebro.
Willis, G. B. (2004). Cognitive interviewing: A tool for improving questionnaire design . Sage Publications.
Xie, Y., Wang, K., & Kong, Y. (2021). Prevalence of research misconduct and questionable research practices: A systematic review and meta-analysis. Science and Engineering Ethics , 27 (4), 41.
Yan, T., & Curtin, R. (2010). The relation between unit nonresponse and item nonresponse: A response continuum perspective. International Journal of Public Opinion Research , 22 (4), 535–551.
Download references
Acknowledgements
We thank Jennica Wallenborg Likidis, Statistics Sweden, for providing expert support in the survey design. We are grateful to colleagues Ingrid Johansson Mignon, Cecilia Enberg, Anna Dreber Almenberg, Andrea Fried, Sara Liin, Mariano Salazar, Lars Bengtsson, Harriet Wallberg, Karl Wennberg, and Thomas Magnusson, who joined the pretest or cognitive tests. We also thank Ksenia Onufrey, Peter Hedström, Jan-Ingvar Jönsson, Richard Öhrvall, Kerstin Sahlin, and David Ludvigsson for constructive comments or suggestions.
Open access funding provided by Linköping University. Swedish Forte: Research Council for Health, Working Life and Welfare ( https://www.vr.se/swecris?#/project/2018-00321_Forte ) Grant No. 2018-00321.
Open access funding provided by Linköping University.
Author information
Authors and affiliations.
Department of Management and Engineering [IEI], Linköping University, Linköping, SE-581 83, Sweden
Christian Berggren & Solmaz Filiz Karabag
Department of Surgical Sciences, Uppsala University, Uppsala University Hospital, entrance 70, Uppsala, SE-751 85, Sweden
Bengt Gerdin
Department of Civil and Industrial Engineering, Uppsala University, Box 169, Uppsala, SE-751 04, Sweden
Solmaz Filiz Karabag
You can also search for this author in PubMed Google Scholar
Contributions
Conceptualization: CB. Survey Design: SFK, CB, Methodology: SFK, BG, CB. Visualization: SFK, BG. Funding acquisition: SFK. Project administration and management: SFK. Writing – original draft: CB. Writing – review & editing: CB, BG, SFK. Approval of the final manuscript: SFK, BG, CB.
Corresponding author
Correspondence to Solmaz Filiz Karabag .
Ethics declarations
Ethics approval and consent to participate.
The Swedish Act concerning the Ethical Review of Research Involving Humans (2003:460) defines the type of studies which requires an ethics approval. In line with the General Data Protection Regulation (EU 2016/67), the act is applicable for studies that collect personal data that reveal racial or ethnic origin, political opinions, trade union membership, religious or philosophical beliefs, or health and sexual orientation. The present study does not involve any of the above, why no formal ethical permit was required. The ethical aspects of the project and its compliance with the guidelines of the Swedish Research Council (2017) were also part of the review process at the project’s public funding agency Forte.
Competing Interests
The authors declare that they have no competing interests.
Supporting Information
The complete case study survey of social and medical science researchers in Sweden 2020.
Additional information
Publisher’s note.
Springer Nature remains neutral with regard to jurisdictional claims in published maps and institutional affiliations.
Rights and permissions
Open Access This article is licensed under a Creative Commons Attribution 4.0 International License, which permits use, sharing, adaptation, distribution and reproduction in any medium or format, as long as you give appropriate credit to the original author(s) and the source, provide a link to the Creative Commons licence, and indicate if changes were made. The images or other third party material in this article are included in the article’s Creative Commons licence, unless indicated otherwise in a credit line to the material. If material is not included in the article’s Creative Commons licence and your intended use is not permitted by statutory regulation or exceeds the permitted use, you will need to obtain permission directly from the copyright holder. To view a copy of this licence, visit http://creativecommons.org/licenses/by/4.0/ .
Reprints and permissions
About this article
Berggren, C., Gerdin, B. & Karabag, S.F. Developing Surveys on Questionable Research Practices: Four Challenging Design Problems. J Acad Ethics (2024). https://doi.org/10.1007/s10805-024-09565-0
Download citation
Accepted : 23 August 2024
Published : 02 September 2024
DOI : https://doi.org/10.1007/s10805-024-09565-0
Share this article
Anyone you share the following link with will be able to read this content:
Sorry, a shareable link is not currently available for this article.
Provided by the Springer Nature SharedIt content-sharing initiative
- Questionable Research Practices
- Normative Environment
- Organizational Climate
- Survey Development
- Design Problems
- Problem of Incomplete Coverage
- Survey Design Process
- Baseline Survey
- Pre-testing
- Technical Assessment
- Cognitive Interviews
- Social Desirability
- Sensitivity
- Organizational Controversiality
- Challenge of Nonresponse
- Qualitative Research
- Quantitative Research
- Find a journal
- Publish with us
- Track your research
Have a language expert improve your writing
Run a free plagiarism check in 10 minutes, generate accurate citations for free.
- Knowledge Base
- Starting the research process
- Writing Strong Research Questions | Criteria & Examples
Writing Strong Research Questions | Criteria & Examples
Published on October 26, 2022 by Shona McCombes . Revised on November 21, 2023.
A research question pinpoints exactly what you want to find out in your work. A good research question is essential to guide your research paper , dissertation , or thesis .
All research questions should be:
- Focused on a single problem or issue
- Researchable using primary and/or secondary sources
- Feasible to answer within the timeframe and practical constraints
- Specific enough to answer thoroughly
- Complex enough to develop the answer over the space of a paper or thesis
- Relevant to your field of study and/or society more broadly
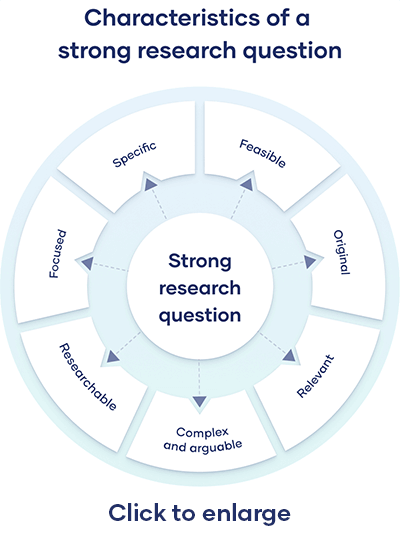
Table of contents
How to write a research question, what makes a strong research question, using sub-questions to strengthen your main research question, research questions quiz, other interesting articles, frequently asked questions about research questions.
You can follow these steps to develop a strong research question:
- Choose your topic
- Do some preliminary reading about the current state of the field
- Narrow your focus to a specific niche
- Identify the research problem that you will address
The way you frame your question depends on what your research aims to achieve. The table below shows some examples of how you might formulate questions for different purposes.
Research question formulations | |
---|---|
Describing and exploring | |
Explaining and testing | |
Evaluating and acting | is X |
Using your research problem to develop your research question
Example research problem | Example research question(s) |
---|---|
Teachers at the school do not have the skills to recognize or properly guide gifted children in the classroom. | What practical techniques can teachers use to better identify and guide gifted children? |
Young people increasingly engage in the “gig economy,” rather than traditional full-time employment. However, it is unclear why they choose to do so. | What are the main factors influencing young people’s decisions to engage in the gig economy? |
Note that while most research questions can be answered with various types of research , the way you frame your question should help determine your choices.
Prevent plagiarism. Run a free check.
Research questions anchor your whole project, so it’s important to spend some time refining them. The criteria below can help you evaluate the strength of your research question.
Focused and researchable
Criteria | Explanation |
---|---|
Focused on a single topic | Your central research question should work together with your research problem to keep your work focused. If you have multiple questions, they should all clearly tie back to your central aim. |
Answerable using | Your question must be answerable using and/or , or by reading scholarly sources on the to develop your argument. If such data is impossible to access, you likely need to rethink your question. |
Not based on value judgements | Avoid subjective words like , , and . These do not give clear criteria for answering the question. |
Feasible and specific
Criteria | Explanation |
---|---|
Answerable within practical constraints | Make sure you have enough time and resources to do all research required to answer your question. If it seems you will not be able to gain access to the data you need, consider narrowing down your question to be more specific. |
Uses specific, well-defined concepts | All the terms you use in the research question should have clear meanings. Avoid vague language, jargon, and too-broad ideas. |
Does not demand a conclusive solution, policy, or course of action | Research is about informing, not instructing. Even if your project is focused on a practical problem, it should aim to improve understanding rather than demand a ready-made solution. If ready-made solutions are necessary, consider conducting instead. Action research is a research method that aims to simultaneously investigate an issue as it is solved. In other words, as its name suggests, action research conducts research and takes action at the same time. |
Complex and arguable
Criteria | Explanation |
---|---|
Cannot be answered with or | Closed-ended, / questions are too simple to work as good research questions—they don’t provide enough for robust investigation and discussion. |
Cannot be answered with easily-found facts | If you can answer the question through a single Google search, book, or article, it is probably not complex enough. A good research question requires original data, synthesis of multiple sources, and original interpretation and argumentation prior to providing an answer. |
Relevant and original
Criteria | Explanation |
---|---|
Addresses a relevant problem | Your research question should be developed based on initial reading around your . It should focus on addressing a problem or gap in the existing knowledge in your field or discipline. |
Contributes to a timely social or academic debate | The question should aim to contribute to an existing and current debate in your field or in society at large. It should produce knowledge that future researchers or practitioners can later build on. |
Has not already been answered | You don’t have to ask something that nobody has ever thought of before, but your question should have some aspect of originality. For example, you can focus on a specific location, or explore a new angle. |
Chances are that your main research question likely can’t be answered all at once. That’s why sub-questions are important: they allow you to answer your main question in a step-by-step manner.
Good sub-questions should be:
- Less complex than the main question
- Focused only on 1 type of research
- Presented in a logical order
Here are a few examples of descriptive and framing questions:
- Descriptive: According to current government arguments, how should a European bank tax be implemented?
- Descriptive: Which countries have a bank tax/levy on financial transactions?
- Framing: How should a bank tax/levy on financial transactions look at a European level?
Keep in mind that sub-questions are by no means mandatory. They should only be asked if you need the findings to answer your main question. If your main question is simple enough to stand on its own, it’s okay to skip the sub-question part. As a rule of thumb, the more complex your subject, the more sub-questions you’ll need.
Try to limit yourself to 4 or 5 sub-questions, maximum. If you feel you need more than this, it may be indication that your main research question is not sufficiently specific. In this case, it’s is better to revisit your problem statement and try to tighten your main question up.
Here's why students love Scribbr's proofreading services
Discover proofreading & editing
If you want to know more about the research process , methodology , research bias , or statistics , make sure to check out some of our other articles with explanations and examples.
Methodology
- Sampling methods
- Simple random sampling
- Stratified sampling
- Cluster sampling
- Likert scales
- Reproducibility
Statistics
- Null hypothesis
- Statistical power
- Probability distribution
- Effect size
- Poisson distribution
Research bias
- Optimism bias
- Cognitive bias
- Implicit bias
- Hawthorne effect
- Anchoring bias
- Explicit bias
The way you present your research problem in your introduction varies depending on the nature of your research paper . A research paper that presents a sustained argument will usually encapsulate this argument in a thesis statement .
A research paper designed to present the results of empirical research tends to present a research question that it seeks to answer. It may also include a hypothesis —a prediction that will be confirmed or disproved by your research.
As you cannot possibly read every source related to your topic, it’s important to evaluate sources to assess their relevance. Use preliminary evaluation to determine whether a source is worth examining in more depth.
This involves:
- Reading abstracts , prefaces, introductions , and conclusions
- Looking at the table of contents to determine the scope of the work
- Consulting the index for key terms or the names of important scholars
A research hypothesis is your proposed answer to your research question. The research hypothesis usually includes an explanation (“ x affects y because …”).
A statistical hypothesis, on the other hand, is a mathematical statement about a population parameter. Statistical hypotheses always come in pairs: the null and alternative hypotheses . In a well-designed study , the statistical hypotheses correspond logically to the research hypothesis.
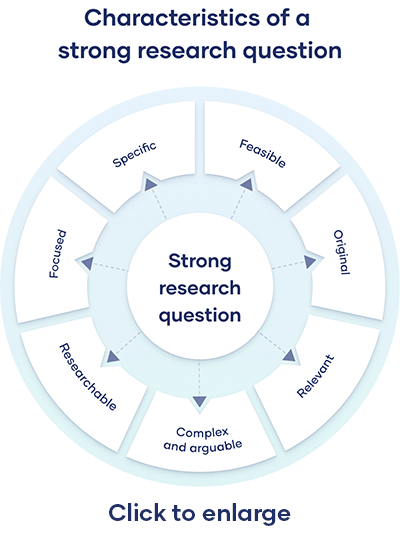
Formulating a main research question can be a difficult task. Overall, your question should contribute to solving the problem that you have defined in your problem statement .
However, it should also fulfill criteria in three main areas:
- Researchability
- Feasibility and specificity
- Relevance and originality
Cite this Scribbr article
If you want to cite this source, you can copy and paste the citation or click the “Cite this Scribbr article” button to automatically add the citation to our free Citation Generator.
McCombes, S. (2023, November 21). Writing Strong Research Questions | Criteria & Examples. Scribbr. Retrieved September 3, 2024, from https://www.scribbr.com/research-process/research-questions/
Is this article helpful?
Shona McCombes
Other students also liked, how to define a research problem | ideas & examples, how to write a problem statement | guide & examples, 10 research question examples to guide your research project, get unlimited documents corrected.
✔ Free APA citation check included ✔ Unlimited document corrections ✔ Specialized in correcting academic texts

An official website of the United States government
The .gov means it’s official. Federal government websites often end in .gov or .mil. Before sharing sensitive information, make sure you’re on a federal government site.
The site is secure. The https:// ensures that you are connecting to the official website and that any information you provide is encrypted and transmitted securely.
- Publications
- Account settings
Preview improvements coming to the PMC website in October 2024. Learn More or Try it out now .
- Advanced Search
- Journal List
- v.328(7452); 2004 Jun 5
Hands-on guide to questionnaire research
Administering, analysing, and reporting your questionnaire, petra m boynton.
1 Department of Primary Care and Population Sciences, University College London, London N19 5LW [email protected]
Associated Data
Short abstract.
Understanding your study group is key to getting a good response to a questionnaire; dealing with the resulting mass of data is another challenge
The first step in producing good questionnaire research is getting the right questionnaire. 1 However, even the best questionnaire will not get adequate results if it is not used properly. This article outlines how to pilot your questionnaire, distribute and administer it; and get it returned, analysed, and written up for publication. It is intended to supplement published guidance on questionnaire research, three quarters of which focuses on content and design. 2
Questionnaires tend to fail because participants don't understand them, can't complete them, get bored or offended by them, or dislike how they look. Although friends and colleagues can help check spelling, grammar, and layout, they cannot reliably predict the emotional reactions or comprehension difficulties of other groups. Whether you have constructed your own questionnaire or are using an existing instrument, always pilot it on participants who are representative of your definitive sample. You need to build in protected time for this phase and get approval from an ethics committee. 3
During piloting, take detailed notes on how participants react to both the general format of your instrument and the specific questions. How long do people take to complete it? Do any questions need to be repeated or explained? How do participants indicate that they have arrived at an answer? Do they show confusion or surprise at a particular response—if so, why? Short, abrupt questions may unintentionally provoke short, abrupt answers. Piloting will provide a guide for rephrasing questions to invite a richer response (box 1). 1).

Box 1: Patient preference is preferable
I worked on a sexual health study where we initially planned to present the questionnaire on a computer, since we had read people were supposedly more comfortable “talking” to a computer. Although this seemed to be the case in practices with middle class patients, we struggled to recruit in practices where participants were less familiar with computers. Their reasons for refusal were not linked to the topic of the research, but because they saw our laptops as something they might break, could make them look foolish, or would feed directly to the internet (which was inextricably linked to computers in some people's minds). We found offering a choice between completing the questionnaire on paper or the laptop computer greatly increased response rates.
Planning data collection
You should be aware of the relevant data protection legislation (for United Kingdom see www.informationcommissioner.gov.uk ) and ensure that you follow internal codes of practice for your institution—for example, obtaining and completing a form from your data protection officer. Do not include names, addresses, or other identifying markers within your electronic database, except for a participant number linked to a securely kept manual file.
The piloting phase should include planning and testing a strategy for getting your questionnaire out and back—for example, who you have invited to complete it (the sampling frame), who has agreed to do so (the response rate), who you've had usable returns from (the completion rate), and whether and when you needed to send a reminder letter. If you are employing researchers to deliver and collect the questionnaire it's important they know exactly how to do this. 4
Administrative errors can hamper the progress of your research. Real examples include researchers giving the questionnaire to wrong participants (for example, a questionnaire aimed at men given to women); incomplete instructions on how to fill in the questionnaire (for example, participants did not know whether to tick one or several items); postal surveys in which the questionnaire was missing from the envelope; and a study of over 3000 participants in which the questionnaire was sent out with no return address.
Administering your questionnaire
The choice of how to administer a questionnaire is too often made on convenience or cost grounds (see table A on bmj.com ). Scientific and ethical considerations should include:
- The needs and preferences of participants, who should understand what is required of them; remain interested and cooperative throughout completion; be asked the right questions and have their responses recorded accurately; and receive appropriate support during and after completing the questionnaire
- The skills and resources available to your research team
- The nature of your study—for example, short term feasibility projects, clinical trials, or large scale surveys.
Maximising your response rate
Sending out hundreds of questionnaires is a thankless task, and it is sometimes hard to pay attention to the many minor details that combine to raise response and completion rates. Extensive evidence exists on best practice (box 2), and principal investigators should ensure that they provide their staff with the necessary time and resources to follow it. Note, however, that it is better to collect fewer questionnaires with good quality responses than high numbers of questionnaires that are inaccurate or incomplete. The third article in this series discusses how to maximise response rates from groups that are hard to research. 15
Accounting for those who refuse to participate
Survey research tends to focus on people who have completed the study. Yet those who don't participate are equally important scientifically, and their details should also be recorded (remember to seek ethical approval for this). 4 , 16 , 17
Box 2: Factors shown to increase response rates
- The questionnaire is clearly designed and has a simple layout 5
- It offers participants incentives or prizes in return for completion 6
- It has been thoroughly piloted and tested 5
- Participants are notified about the study in advance with a personalised invitation 7
- The aim of study and means of completing the questionnaire are clearly explained 8 , 9
- A researcher is available to answer questions and collect the completed questionnaire 10
- If using a postal questionnaire, a stamped addressed envelope is included 7
- The participant feels they are a stakeholder in the study 11
- Questions are phrased in a way that holds the participant's attention 11
- Questionnaire has clear focus and purpose and is kept concise 7 , 8 , 11
- The questionnaire is appealing to look at, 12 as is the researcher 13
- If appropriate, the questionnaire is delivered electronically 14
One way of reducing refusal and non-completion rates is to set strict exclusion criteria at the start of your research. For example, for practical reasons many studies exclude participants who are unable to read or write in the language of the questionnaire and those with certain physical and mental disabilities that might interfere with their ability to give informed consent, cooperate with the researcher, or understand the questions asked. However, research that systematically excludes hard to reach groups is increasingly seen as unethical, and you may need to build additional strategies and resources into your study protocol at the outset. 15 Keep a record of all participants that fit the different exclusion categories (see bmj.com ).
Collecting data on non-participants will also allow you to monitor the research process. For example, you may find that certain researchers seem to have a higher proportion of participants refusing, and if so you should work with those individuals to improve the way they introduce the research or seek consent. In addition, if early refusals are found to be unusually high, you might need to rethink your overall approach. 10
Entering, checking, and cleaning data
Novice researchers often assume that once they have selected, designed, and distributed their questionnaire, their work is largely complete. In reality, entering, checking, and cleaning the data account for much of the workload. Some principles for keeping quantitative data clean are listed on bmj.com .
Even if a specialist team sets up the database(s), all researchers should be taught how to enter, clean, code, and back up the data, and the system for doing this should be universally agreed and understood. Agree on the statistical package you wish to use (such as SPSS, Stata, EpiInfo, Excel, or Access) and decide on a coding system before anyone starts work on the dataset.
It is good practice to enter data into an electronic database as the study progresses rather than face a mountain of processing at the end. The project manager should normally take responsibility for coordinating and overseeing this process and for ensuring that all researchers know what their role is with data management. These and other management tasks are time consuming and must be built into the study protocol and budget. Include data entry and coding in any pilot study to get an estimate of the time required and potential problems to troubleshoot.
Analysing your data
You should be able to predict the type of analysis required for your different questionnaire items at the planning stage of your study by considering the structure of each item and the likely distribution of responses (box 3). 1 Table B on bmj.com shows some examples of data analysis methods for different types of responses. 18 , 19 w1
Writing up and reporting
Once you have completed your data analysis, you will need to think creatively about the clearest and most parsimonious way to report and present your findings. You will almost certainly find that you have too much data to fit into a standard journal article, dissertation, or research report, so deciding what to include and omit is crucial. Take statistical advice from the outset of your research. This can keep you focused on the hypothesis or question you are testing and the important results from your study (and therefore what tables and graphs to present).
Box 3: Nasty surprise from a simple questionnaire
Moshe selected a standardised measure on emotional wellbeing to use in his research, which looked easy to complete and participants answered readily. When he came to analysing his data, he discovered that rather than scoring each response directly as indicated on the questionnaire, a complicated computer algorithm had to be created, and he was stumped. He found a statistician to help with the recoding, and realised that for future studies it might be an idea to check both the measure and its scoring system before selecting it.
Box 4: An unexpected result
Priti, a specialist registrar in hepatology, completed an attitude questionnaire in patients having liver transplantation and those who were still waiting for a donor. She expected to find that those who had received a new liver would be happier than those awaiting a donor. However, the morale scale used in her questionnaire showed that the transplantation group did not have significantly better morale scores. Priti felt that this negative finding was worth further investigation.
Methods section
The methods section should give details of your exclusion criteria and discuss their implications for the transferability of your findings. Data on refusals and unsuitable participants should also be presented and discussed, preferably using a recruitment diagram. w2 Finally, state and justify the statistical or qualitative analyses used. 18 , 19 w2
Results section
When compiling the results section you should return to your original research question and set out the findings that addressed this. In other words, make sure your results are hypothesis driven. Do not be afraid to report non-significant results, which in reality are often as important as significant results—for example, if participants did not experience anxiety in a particular situation (box 4). Don't analyse and report on every question within your questionnaire
Choose the most statistically appropriate and visually appealing format for graphs ( table ). w3 Label graphs and their axes adequately and include meaningful titles for tables and diagrams. Refer your reader to any tables or graphs within your text, and highlight the main findings.
Examples of ways of presenting data and when to use them
Data table | If you need to produce something that is simple and quick and that has a low publication cost for journals. If you want to make data accessible to the interested reader for further manipulations | Do not use if you want to make your work look visually appealing. Too many tables can weigh down the results section and obscure the really key results. The reader is forced to work too hard and may give up reading your report |
Bar chart | If you need to convey changes and differences, particularly between groups (eg how men and women differed in their views on an exercise programme for recovering heart attack patients) | If your data are linear and each item is related to the previous then you should use a (line) graph. Bar charts treat data as though they are separate groups not continuous variables |
Scatter graph | Mostly used for displaying correlations or regressions (eg association between number of cigarettes smoked and reduced lung capacity) | If your data are based on groups or aggregated outcomes rather than individual scores |
Pie chart | Used for simple summaries of data, particularly if a small number of choices were provided | As with bar charts, avoid if you want to present linear or relational data |
Line graph | Where the points on the graph are logically linked, usually in time (eg scores on quality of life and emotional wellbeing measures taken monthly over six months) | If your data were not linked over time, repetition, etc it is inappropriate to suggest a linear relation by presenting findings in this format |
If you have used open ended questions within your questionnaire, do not cherry pick quotes for your results section. You need to outline what main themes emerged, and use quotes as necessary to illustrate the themes and supplement your quantitative findings.
Discussion section
The discussion should refer back to the results section and suggest what the main findings mean. You should acknowledge the limitations of your study and couch the discussion in the light of these. For example, if your response rate was low, you may need to recommend further studies to confirm your preliminary results. Your conclusions must not go beyond the scope of your study—for example, if you have done a small, parochial study do not suggest changes in national policy. You should also discuss any questions your participants persistently refused to answer or answered in a way you didn't expect.
Taking account of psychological and social influences
Questionnaire research (and indeed science in general) can never be completely objective. Researchers and participants are all human beings with psychological, emotional, and social needs. Too often, we fail to take these factors into account when planning, undertaking, and analysing our work. A questionnaire means something different to participants and researchers. w4 Researchers want data (with a view to publications, promotion, academic recognition, and further grant income). Junior research staff and administrators, especially if poorly trained and supervised, may be put under pressure, leading to critical errors in piloting (for example, piloting on friends rather than the target group), sampling (for example, drifting towards convenience rather than random samples) and in the distribution, collection, and coding of questionnaires. 15 Staff employed to assist with a questionnaire study may not be familiar with all the tasks required to make it a success and may be unaware that covering up their ignorance or skill deficits will make the entire study unsound.
Summary points
Piloting is essential to check the questionnaire works in the study group and identify administrative and analytical problems
The method of administration should be determined by scientific considerations not just costs
Entering, checking, and cleaning data should be done as the study progresses
Don't try to include all the results when reporting studies
Do include exclusion criteria and data on non-respondents
Research participants, on the other hand, may be motivated to complete a questionnaire through interest, boredom, a desire to help others (particularly true in health studies), because they feel pressurised to do so, through loneliness, or for an unconscious ulterior motive (“pleasing the doctor”). All of these introduce potential biases into the recruitment and data collection process.
Supplementary Material
This is the second in a series of three articles edited by Trisha Greenhalgh
I thank Alicia O'Cathain, Trish Greenhalgh, Jill Russell, Geoff Wong, Marcia Rigby, Sara Shaw, Fraser Macfarlane, and Will Callaghan for their helpful feedback on earlier versions of this paper and Gary Wood for advice on statistics and analysis.
PMB has taught research methods in a primary care setting for the past 13 years, specialising in practical approaches and using the experiences and concerns of researchers and participants as the basis of learning. This series of papers arose directly from questions asked about real questionnaire studies. To address these questions she and Trisha Greenhalgh explored a wide range of sources from the psychological and health services research literature.
Competing interests: None declared.
Thank you for visiting nature.com. You are using a browser version with limited support for CSS. To obtain the best experience, we recommend you use a more up to date browser (or turn off compatibility mode in Internet Explorer). In the meantime, to ensure continued support, we are displaying the site without styles and JavaScript.
- View all journals
- Explore content
- About the journal
- Publish with us
- Sign up for alerts
- Open access
- Published: 31 August 2024
Knowledge mapping and evolution of research on older adults’ technology acceptance: a bibliometric study from 2013 to 2023
- Xianru Shang ORCID: orcid.org/0009-0000-8906-3216 1 ,
- Zijian Liu 1 ,
- Chen Gong 1 ,
- Zhigang Hu 1 ,
- Yuexuan Wu 1 &
- Chengliang Wang ORCID: orcid.org/0000-0003-2208-3508 2
Humanities and Social Sciences Communications volume 11 , Article number: 1115 ( 2024 ) Cite this article
Metrics details
- Science, technology and society
The rapid expansion of information technology and the intensification of population aging are two prominent features of contemporary societal development. Investigating older adults’ acceptance and use of technology is key to facilitating their integration into an information-driven society. Given this context, the technology acceptance of older adults has emerged as a prioritized research topic, attracting widespread attention in the academic community. However, existing research remains fragmented and lacks a systematic framework. To address this gap, we employed bibliometric methods, utilizing the Web of Science Core Collection to conduct a comprehensive review of literature on older adults’ technology acceptance from 2013 to 2023. Utilizing VOSviewer and CiteSpace for data assessment and visualization, we created knowledge mappings of research on older adults’ technology acceptance. Our study employed multidimensional methods such as co-occurrence analysis, clustering, and burst analysis to: (1) reveal research dynamics, key journals, and domains in this field; (2) identify leading countries, their collaborative networks, and core research institutions and authors; (3) recognize the foundational knowledge system centered on theoretical model deepening, emerging technology applications, and research methods and evaluation, uncovering seminal literature and observing a shift from early theoretical and influential factor analyses to empirical studies focusing on individual factors and emerging technologies; (4) moreover, current research hotspots are primarily in the areas of factors influencing technology adoption, human-robot interaction experiences, mobile health management, and aging-in-place technology, highlighting the evolutionary context and quality distribution of research themes. Finally, we recommend that future research should deeply explore improvements in theoretical models, long-term usage, and user experience evaluation. Overall, this study presents a clear framework of existing research in the field of older adults’ technology acceptance, providing an important reference for future theoretical exploration and innovative applications.
Similar content being viewed by others
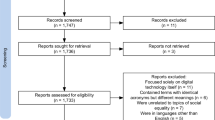
Research progress and intellectual structure of design for digital equity (DDE): A bibliometric analysis based on citespace
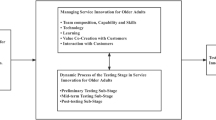
Exploring the role of interaction in older-adult service innovation: insights from the testing stage
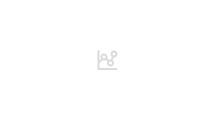
Smart device interest, perceived usefulness, and preferences in rural Alabama seniors
Introduction.
In contemporary society, the rapid development of information technology has been intricately intertwined with the intensifying trend of population aging. According to the latest United Nations forecast, by 2050, the global population aged 65 and above is expected to reach 1.6 billion, representing about 16% of the total global population (UN 2023 ). Given the significant challenges of global aging, there is increasing evidence that emerging technologies have significant potential to maintain health and independence for older adults in their home and healthcare environments (Barnard et al. 2013 ; Soar 2010 ; Vancea and Solé-Casals 2016 ). This includes, but is not limited to, enhancing residential safety with smart home technologies (Touqeer et al. 2021 ; Wang et al. 2022 ), improving living independence through wearable technologies (Perez et al. 2023 ), and increasing medical accessibility via telehealth services (Kruse et al. 2020 ). Technological innovations are redefining the lifestyles of older adults, encouraging a shift from passive to active participation (González et al. 2012 ; Mostaghel 2016 ). Nevertheless, the effective application and dissemination of technology still depends on user acceptance and usage intentions (Naseri et al. 2023 ; Wang et al. 2023a ; Xia et al. 2024 ; Yu et al. 2023 ). Particularly, older adults face numerous challenges in accepting and using new technologies. These challenges include not only physical and cognitive limitations but also a lack of technological experience, along with the influences of social and economic factors (Valk et al. 2018 ; Wilson et al. 2021 ).
User acceptance of technology is a significant focus within information systems (IS) research (Dai et al. 2024 ), with several models developed to explain and predict user behavior towards technology usage, including the Technology Acceptance Model (TAM) (Davis 1989 ), TAM2, TAM3, and the Unified Theory of Acceptance and Use of Technology (UTAUT) (Venkatesh et al. 2003 ). Older adults, as a group with unique needs, exhibit different behavioral patterns during technology acceptance than other user groups, and these uniquenesses include changes in cognitive abilities, as well as motivations, attitudes, and perceptions of the use of new technologies (Chen and Chan 2011 ). The continual expansion of technology introduces considerable challenges for older adults, rendering the understanding of their technology acceptance a research priority. Thus, conducting in-depth research into older adults’ acceptance of technology is critically important for enhancing their integration into the information society and improving their quality of life through technological advancements.
Reviewing relevant literature to identify research gaps helps further solidify the theoretical foundation of the research topic. However, many existing literature reviews primarily focus on the factors influencing older adults’ acceptance or intentions to use technology. For instance, Ma et al. ( 2021 ) conducted a comprehensive analysis of the determinants of older adults’ behavioral intentions to use technology; Liu et al. ( 2022 ) categorized key variables in studies of older adults’ technology acceptance, noting a shift in focus towards social and emotional factors; Yap et al. ( 2022 ) identified seven categories of antecedents affecting older adults’ use of technology from an analysis of 26 articles, including technological, psychological, social, personal, cost, behavioral, and environmental factors; Schroeder et al. ( 2023 ) extracted 119 influencing factors from 59 articles and further categorized these into six themes covering demographics, health status, and emotional awareness. Additionally, some studies focus on the application of specific technologies, such as Ferguson et al. ( 2021 ), who explored barriers and facilitators to older adults using wearable devices for heart monitoring, and He et al. ( 2022 ) and Baer et al. ( 2022 ), who each conducted in-depth investigations into the acceptance of social assistive robots and mobile nutrition and fitness apps, respectively. In summary, current literature reviews on older adults’ technology acceptance exhibit certain limitations. Due to the interdisciplinary nature and complex knowledge structure of this field, traditional literature reviews often rely on qualitative analysis, based on literature analysis and periodic summaries, which lack sufficient objectivity and comprehensiveness. Additionally, systematic research is relatively limited, lacking a macroscopic description of the research trajectory from a holistic perspective. Over the past decade, research on older adults’ technology acceptance has experienced rapid growth, with a significant increase in literature, necessitating the adoption of new methods to review and examine the developmental trends in this field (Chen 2006 ; Van Eck and Waltman 2010 ). Bibliometric analysis, as an effective quantitative research method, analyzes published literature through visualization, offering a viable approach to extracting patterns and insights from a large volume of papers, and has been widely applied in numerous scientific research fields (Achuthan et al. 2023 ; Liu and Duffy 2023 ). Therefore, this study will employ bibliometric methods to systematically analyze research articles related to older adults’ technology acceptance published in the Web of Science Core Collection from 2013 to 2023, aiming to understand the core issues and evolutionary trends in the field, and to provide valuable references for future related research. Specifically, this study aims to explore and answer the following questions:
RQ1: What are the research dynamics in the field of older adults’ technology acceptance over the past decade? What are the main academic journals and fields that publish studies related to older adults’ technology acceptance?
RQ2: How is the productivity in older adults’ technology acceptance research distributed among countries, institutions, and authors?
RQ3: What are the knowledge base and seminal literature in older adults’ technology acceptance research? How has the research theme progressed?
RQ4: What are the current hot topics and their evolutionary trajectories in older adults’ technology acceptance research? How is the quality of research distributed?
Methodology and materials
Research method.
In recent years, bibliometrics has become one of the crucial methods for analyzing literature reviews and is widely used in disciplinary and industrial intelligence analysis (Jing et al. 2023 ; Lin and Yu 2024a ; Wang et al. 2024a ; Xu et al. 2021 ). Bibliometric software facilitates the visualization analysis of extensive literature data, intuitively displaying the network relationships and evolutionary processes between knowledge units, and revealing the underlying knowledge structure and potential information (Chen et al. 2024 ; López-Robles et al. 2018 ; Wang et al. 2024c ). This method provides new insights into the current status and trends of specific research areas, along with quantitative evidence, thereby enhancing the objectivity and scientific validity of the research conclusions (Chen et al. 2023 ; Geng et al. 2024 ). VOSviewer and CiteSpace are two widely used bibliometric software tools in academia (Pan et al. 2018 ), recognized for their robust functionalities based on the JAVA platform. Although each has its unique features, combining these two software tools effectively constructs mapping relationships between literature knowledge units and clearly displays the macrostructure of the knowledge domains. Particularly, VOSviewer, with its excellent graphical representation capabilities, serves as an ideal tool for handling large datasets and precisely identifying the focal points and hotspots of research topics. Therefore, this study utilizes VOSviewer (version 1.6.19) and CiteSpace (version 6.1.R6), combined with in-depth literature analysis, to comprehensively examine and interpret the research theme of older adults’ technology acceptance through an integrated application of quantitative and qualitative methods.
Data source
Web of Science is a comprehensively recognized database in academia, featuring literature that has undergone rigorous peer review and editorial scrutiny (Lin and Yu 2024b ; Mongeon and Paul-Hus 2016 ; Pranckutė 2021 ). This study utilizes the Web of Science Core Collection as its data source, specifically including three major citation indices: Science Citation Index Expanded (SCIE), Social Sciences Citation Index (SSCI), and Arts & Humanities Citation Index (A&HCI). These indices encompass high-quality research literature in the fields of science, social sciences, and arts and humanities, ensuring the comprehensiveness and reliability of the data. We combined “older adults” with “technology acceptance” through thematic search, with the specific search strategy being: TS = (elder OR elderly OR aging OR ageing OR senile OR senior OR old people OR “older adult*”) AND TS = (“technology acceptance” OR “user acceptance” OR “consumer acceptance”). The time span of literature search is from 2013 to 2023, with the types limited to “Article” and “Review” and the language to “English”. Additionally, the search was completed by October 27, 2023, to avoid data discrepancies caused by database updates. The initial search yielded 764 journal articles. Given that searches often retrieve articles that are superficially relevant but actually non-compliant, manual screening post-search was essential to ensure the relevance of the literature (Chen et al. 2024 ). Through manual screening, articles significantly deviating from the research theme were eliminated and rigorously reviewed. Ultimately, this study obtained 500 valid sample articles from the Web of Science Core Collection. The complete PRISMA screening process is illustrated in Fig. 1 .
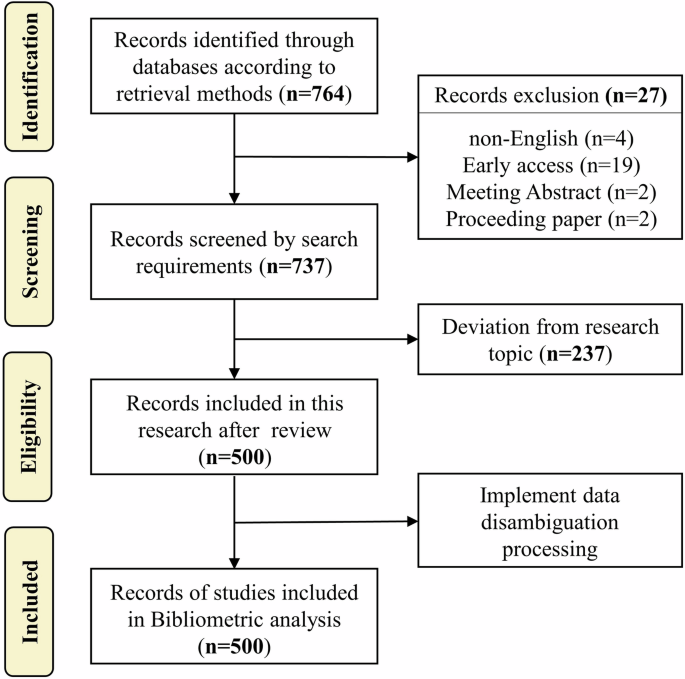
Presentation of the data culling process in detail.
Data standardization
Raw data exported from databases often contain multiple expressions of the same terminology (Nguyen and Hallinger 2020 ). To ensure the accuracy and consistency of data, it is necessary to standardize the raw data (Strotmann and Zhao 2012 ). This study follows the data standardization process proposed by Taskin and Al ( 2019 ), mainly executing the following operations:
(1) Standardization of author and institution names is conducted to address different name expressions for the same author. For instance, “Chan, Alan Hoi Shou” and “Chan, Alan H. S.” are considered the same author, and distinct authors with the same name are differentiated by adding identifiers. Diverse forms of institutional names are unified to address variations caused by name changes or abbreviations, such as standardizing “FRANKFURT UNIV APPL SCI” and “Frankfurt University of Applied Sciences,” as well as “Chinese University of Hong Kong” and “University of Hong Kong” to consistent names.
(2) Different expressions of journal names are unified. For example, “International Journal of Human-Computer Interaction” and “Int J Hum Comput Interact” are standardized to a single name. This ensures consistency in journal names and prevents misclassification of literature due to differing journal names. Additionally, it involves checking if the journals have undergone name changes in the past decade to prevent any impact on the analysis due to such changes.
(3) Keywords data are cleansed by removing words that do not directly pertain to specific research content (e.g., people, review), merging synonyms (e.g., “UX” and “User Experience,” “aging-in-place” and “aging in place”), and standardizing plural forms of keywords (e.g., “assistive technologies” and “assistive technology,” “social robots” and “social robot”). This reduces redundant information in knowledge mapping.
Bibliometric results and analysis
Distribution power (rq1), literature descriptive statistical analysis.
Table 1 presents a detailed descriptive statistical overview of the literature in the field of older adults’ technology acceptance. After deduplication using the CiteSpace software, this study confirmed a valid sample size of 500 articles. Authored by 1839 researchers, the documents encompass 792 research institutions across 54 countries and are published in 217 different academic journals. As of the search cutoff date, these articles have accumulated 13,829 citations, with an annual average of 1156 citations, and an average of 27.66 citations per article. The h-index, a composite metric of quantity and quality of scientific output (Kamrani et al. 2021 ), reached 60 in this study.
Trends in publications and disciplinary distribution
The number of publications and citations are significant indicators of the research field’s development, reflecting its continuity, attention, and impact (Ale Ebrahim et al. 2014 ). The ranking of annual publications and citations in the field of older adults’ technology acceptance studies is presented chronologically in Fig. 2A . The figure shows a clear upward trend in the amount of literature in this field. Between 2013 and 2017, the number of publications increased slowly and decreased in 2018. However, in 2019, the number of publications increased rapidly to 52 and reached a peak of 108 in 2022, which is 6.75 times higher than in 2013. In 2022, the frequency of document citations reached its highest point with 3466 citations, reflecting the widespread recognition and citation of research in this field. Moreover, the curve of the annual number of publications fits a quadratic function, with a goodness-of-fit R 2 of 0.9661, indicating that the number of future publications is expected to increase even more rapidly.
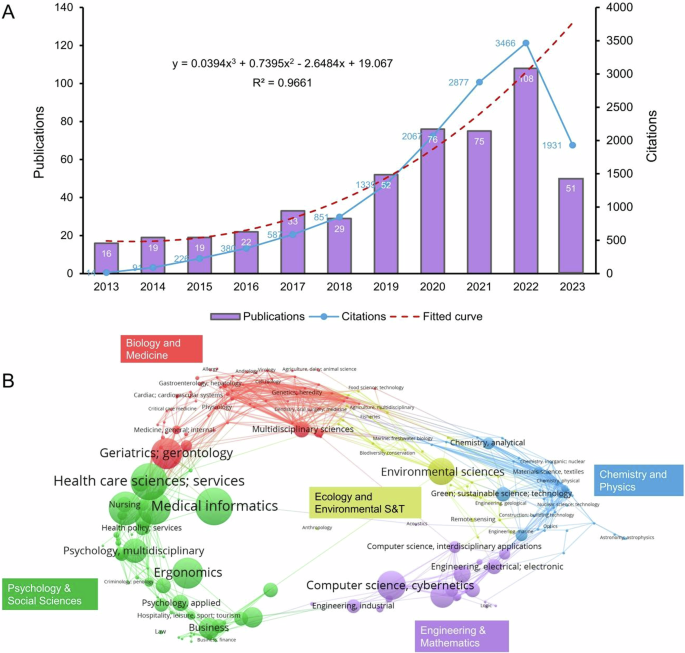
A Trends in trends in annual publications and citations (2013–2023). B Overlay analysis of the distribution of discipline fields.
Figure 2B shows that research on older adults’ technology acceptance involves the integration of multidisciplinary knowledge. According to Web of Science Categories, these 500 articles are distributed across 85 different disciplines. We have tabulated the top ten disciplines by publication volume (Table 2 ), which include Medical Informatics (75 articles, 15.00%), Health Care Sciences & Services (71 articles, 14.20%), Gerontology (61 articles, 12.20%), Public Environmental & Occupational Health (57 articles, 11.40%), and Geriatrics & Gerontology (52 articles, 10.40%), among others. The high output in these disciplines reflects the concentrated global academic interest in this comprehensive research topic. Additionally, interdisciplinary research approaches provide diverse perspectives and a solid theoretical foundation for studies on older adults’ technology acceptance, also paving the way for new research directions.
Knowledge flow analysis
A dual-map overlay is a CiteSpace map superimposed on top of a base map, which shows the interrelationships between journals in different domains, representing the publication and citation activities in each domain (Chen and Leydesdorff 2014 ). The overlay map reveals the link between the citing domain (on the left side) and the cited domain (on the right side), reflecting the knowledge flow of the discipline at the journal level (Leydesdorff and Rafols 2012 ). We utilize the in-built Z-score algorithm of the software to cluster the graph, as shown in Fig. 3 .
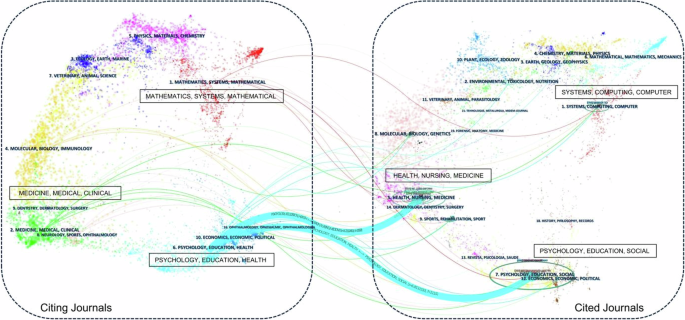
The left side shows the citing journal, and the right side shows the cited journal.
Figure 3 shows the distribution of citing journals clusters for older adults’ technology acceptance on the left side, while the right side refers to the main cited journals clusters. Two knowledge flow citation trajectories were obtained; they are presented by the color of the cited regions, and the thickness of these trajectories is proportional to the Z-score scaled frequency of citations (Chen et al. 2014 ). Within the cited regions, the most popular fields with the most records covered are “HEALTH, NURSING, MEDICINE” and “PSYCHOLOGY, EDUCATION, SOCIAL”, and the elliptical aspect ratio of these two fields stands out. Fields have prominent elliptical aspect ratios, highlighting their significant influence on older adults’ technology acceptance research. Additionally, the major citation trajectories originate in these two areas and progress to the frontier research area of “PSYCHOLOGY, EDUCATION, HEALTH”. It is worth noting that the citation trajectory from “PSYCHOLOGY, EDUCATION, SOCIAL” has a significant Z-value (z = 6.81), emphasizing the significance and impact of this development path. In the future, “MATHEMATICS, SYSTEMS, MATHEMATICAL”, “MOLECULAR, BIOLOGY, IMMUNOLOGY”, and “NEUROLOGY, SPORTS, OPHTHALMOLOGY” may become emerging fields. The fields of “MEDICINE, MEDICAL, CLINICAL” may be emerging areas of cutting-edge research.
Main research journals analysis
Table 3 provides statistics for the top ten journals by publication volume in the field of older adults’ technology acceptance. Together, these journals have published 137 articles, accounting for 27.40% of the total publications, indicating that there is no highly concentrated core group of journals in this field, with publications being relatively dispersed. Notably, Computers in Human Behavior , Journal of Medical Internet Research , and International Journal of Human-Computer Interaction each lead with 15 publications. In terms of citation metrics, International Journal of Medical Informatics and Computers in Human Behavior stand out significantly, with the former accumulating a total of 1,904 citations, averaging 211.56 citations per article, and the latter totaling 1,449 citations, with an average of 96.60 citations per article. These figures emphasize the academic authority and widespread impact of these journals within the research field.
Research power (RQ2)
Countries and collaborations analysis.
The analysis revealed the global research pattern for country distribution and collaboration (Chen et al. 2019 ). Figure 4A shows the network of national collaborations on older adults’ technology acceptance research. The size of the bubbles represents the amount of publications in each country, while the thickness of the connecting lines expresses the closeness of the collaboration among countries. Generally, this research subject has received extensive international attention, with China and the USA publishing far more than any other countries. China has established notable research collaborations with the USA, UK and Malaysia in this field, while other countries have collaborations, but the closeness is relatively low and scattered. Figure 4B shows the annual publication volume dynamics of the top ten countries in terms of total publications. Since 2017, China has consistently increased its annual publications, while the USA has remained relatively stable. In 2019, the volume of publications in each country increased significantly, this was largely due to the global outbreak of the COVID-19 pandemic, which has led to increased reliance on information technology among the elderly for medical consultations, online socialization, and health management (Sinha et al. 2021 ). This phenomenon has led to research advances in technology acceptance among older adults in various countries. Table 4 shows that the top ten countries account for 93.20% of the total cumulative number of publications, with each country having published more than 20 papers. Among these ten countries, all of them except China are developed countries, indicating that the research field of older adults’ technology acceptance has received general attention from developed countries. Currently, China and the USA were the leading countries in terms of publications with 111 and 104 respectively, accounting for 22.20% and 20.80%. The UK, Germany, Italy, and the Netherlands also made significant contributions. The USA and China ranked first and second in terms of the number of citations, while the Netherlands had the highest average citations, indicating the high impact and quality of its research. The UK has shown outstanding performance in international cooperation, while the USA highlights its significant academic influence in this field with the highest h-index value.
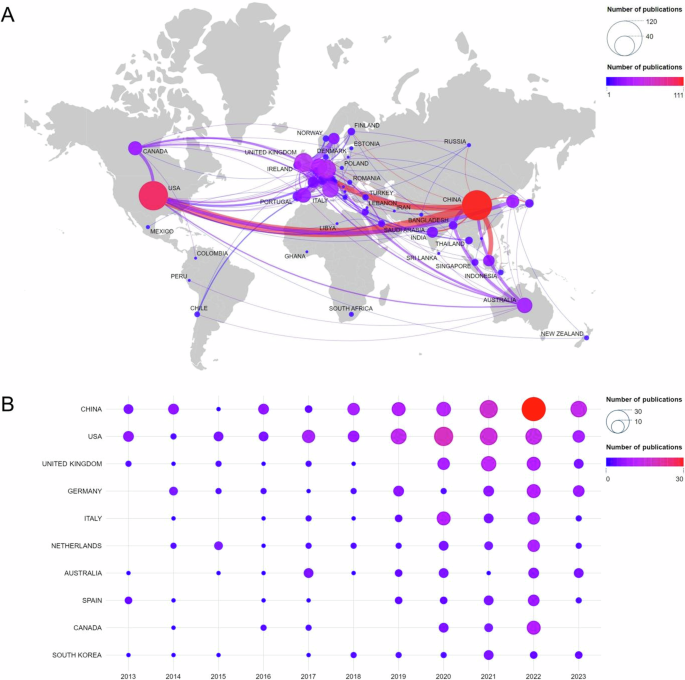
A National collaboration network. B Annual volume of publications in the top 10 countries.
Institutions and authors analysis
Analyzing the number of publications and citations can reveal an institution’s or author’s research strength and influence in a particular research area (Kwiek 2021 ). Tables 5 and 6 show the statistics of the institutions and authors whose publication counts are in the top ten, respectively. As shown in Table 5 , higher education institutions hold the main position in this research field. Among the top ten institutions, City University of Hong Kong and The University of Hong Kong from China lead with 14 and 9 publications, respectively. City University of Hong Kong has the highest h-index, highlighting its significant influence in the field. It is worth noting that Tilburg University in the Netherlands is not among the top five in terms of publications, but the high average citation count (130.14) of its literature demonstrates the high quality of its research.
After analyzing the authors’ output using Price’s Law (Redner 1998 ), the highest number of publications among the authors counted ( n = 10) defines a publication threshold of 3 for core authors in this research area. As a result of quantitative screening, a total of 63 core authors were identified. Table 6 shows that Chen from Zhejiang University, China, Ziefle from RWTH Aachen University, Germany, and Rogers from Macquarie University, Australia, were the top three authors in terms of the number of publications, with 10, 9, and 8 articles, respectively. In terms of average citation rate, Peek and Wouters, both scholars from the Netherlands, have significantly higher rates than other scholars, with 183.2 and 152.67 respectively. This suggests that their research is of high quality and widely recognized. Additionally, Chen and Rogers have high h-indices in this field.
Knowledge base and theme progress (RQ3)
Research knowledge base.
Co-citation relationships occur when two documents are cited together (Zhang and Zhu 2022 ). Co-citation mapping uses references as nodes to represent the knowledge base of a subject area (Min et al. 2021). Figure 5A illustrates co-occurrence mapping in older adults’ technology acceptance research, where larger nodes signify higher co-citation frequencies. Co-citation cluster analysis can be used to explore knowledge structure and research boundaries (Hota et al. 2020 ; Shiau et al. 2023 ). The co-citation clustering mapping of older adults’ technology acceptance research literature (Fig. 5B ) shows that the Q value of the clustering result is 0.8129 (>0.3), and the average value of the weight S is 0.9391 (>0.7), indicating that the clusters are uniformly distributed with a significant and credible structure. This further proves that the boundaries of the research field are clear and there is significant differentiation in the field. The figure features 18 cluster labels, each associated with thematic color blocks corresponding to different time slices. Highlighted emerging research themes include #2 Smart Home Technology, #7 Social Live, and #10 Customer Service. Furthermore, the clustering labels extracted are primarily classified into three categories: theoretical model deepening, emerging technology applications, research methods and evaluation, as detailed in Table 7 .
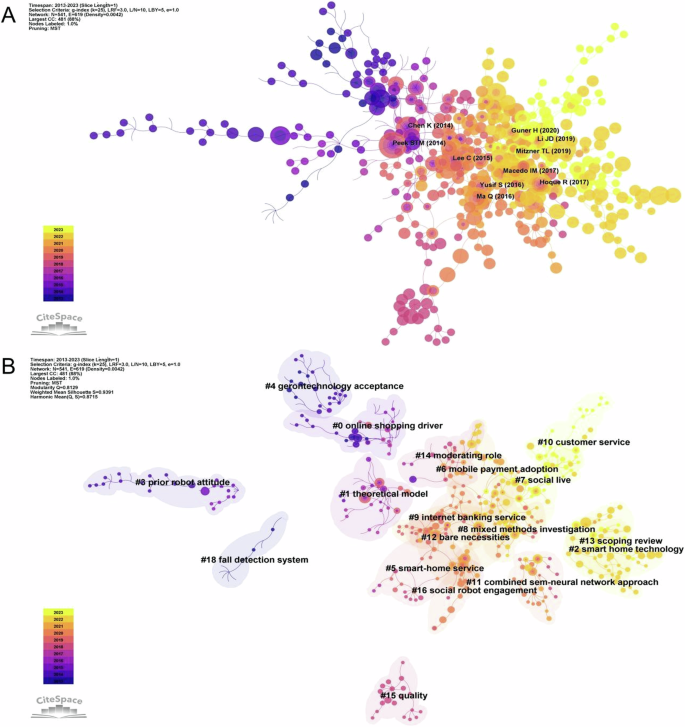
A Co-citation analysis of references. B Clustering network analysis of references.
Seminal literature analysis
The top ten nodes in terms of co-citation frequency were selected for further analysis. Table 8 displays the corresponding node information. Studies were categorized into four main groups based on content analysis. (1) Research focusing on specific technology usage by older adults includes studies by Peek et al. ( 2014 ), Ma et al. ( 2016 ), Hoque and Sorwar ( 2017 ), and Li et al. ( 2019 ), who investigated the factors influencing the use of e-technology, smartphones, mHealth, and smart wearables, respectively. (2) Concerning the development of theoretical models of technology acceptance, Chen and Chan ( 2014 ) introduced the Senior Technology Acceptance Model (STAM), and Macedo ( 2017 ) analyzed the predictive power of UTAUT2 in explaining older adults’ intentional behaviors and information technology usage. (3) In exploring older adults’ information technology adoption and behavior, Lee and Coughlin ( 2015 ) emphasized that the adoption of technology by older adults is a multifactorial process that includes performance, price, value, usability, affordability, accessibility, technical support, social support, emotion, independence, experience, and confidence. Yusif et al. ( 2016 ) conducted a literature review examining the key barriers affecting older adults’ adoption of assistive technology, including factors such as privacy, trust, functionality/added value, cost, and stigma. (4) From the perspective of research into older adults’ technology acceptance, Mitzner et al. ( 2019 ) assessed the long-term usage of computer systems designed for the elderly, whereas Guner and Acarturk ( 2020 ) compared information technology usage and acceptance between older and younger adults. The breadth and prevalence of this literature make it a vital reference for researchers in the field, also providing new perspectives and inspiration for future research directions.
Research thematic progress
Burst citation is a node of literature that guides the sudden change in dosage, which usually represents a prominent development or major change in a particular field, with innovative and forward-looking qualities. By analyzing the emergent literature, it is often easy to understand the dynamics of the subject area, mapping the emerging thematic change (Chen et al. 2022 ). Figure 6 shows the burst citation mapping in the field of older adults’ technology acceptance research, with burst citations represented by red nodes (Fig. 6A ). For the ten papers with the highest burst intensity (Fig. 6B ), this study will conduct further analysis in conjunction with literature review.
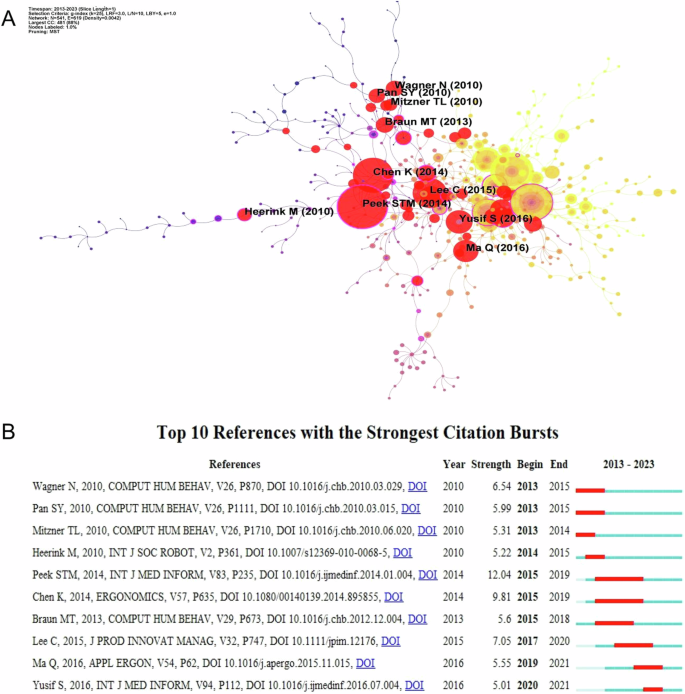
A Burst detection of co-citation. B The top 10 references with the strongest citation bursts.
As shown in Fig. 6 , Mitzner et al. ( 2010 ) broke the stereotype that older adults are fearful of technology, found that they actually have positive attitudes toward technology, and emphasized the centrality of ease of use and usefulness in the process of technology acceptance. This finding provides an important foundation for subsequent research. During the same period, Wagner et al. ( 2010 ) conducted theory-deepening and applied research on technology acceptance among older adults. The research focused on older adults’ interactions with computers from the perspective of Social Cognitive Theory (SCT). This expanded the understanding of technology acceptance, particularly regarding the relationship between behavior, environment, and other SCT elements. In addition, Pan and Jordan-Marsh ( 2010 ) extended the TAM to examine the interactions among predictors of perceived usefulness, perceived ease of use, subjective norm, and convenience conditions when older adults use the Internet, taking into account the moderating roles of gender and age. Heerink et al. ( 2010 ) adapted and extended the UTAUT, constructed a technology acceptance model specifically designed for older users’ acceptance of assistive social agents, and validated it using controlled experiments and longitudinal data, explaining intention to use by combining functional assessment and social interaction variables.
Then the research theme shifted to an in-depth analysis of the factors influencing technology acceptance among older adults. Two papers with high burst strengths emerged during this period: Peek et al. ( 2014 ) (Strength = 12.04), Chen and Chan ( 2014 ) (Strength = 9.81). Through a systematic literature review and empirical study, Peek STM and Chen K, among others, identified multidimensional factors that influence older adults’ technology acceptance. Peek et al. ( 2014 ) analyzed literature on the acceptance of in-home care technology among older adults and identified six factors that influence their acceptance: concerns about technology, expected benefits, technology needs, technology alternatives, social influences, and older adult characteristics, with a focus on differences between pre- and post-implementation factors. Chen and Chan ( 2014 ) constructed the STAM by administering a questionnaire to 1012 older adults and adding eight important factors, including technology anxiety, self-efficacy, cognitive ability, and physical function, based on the TAM. This enriches the theoretical foundation of the field. In addition, Braun ( 2013 ) highlighted the role of perceived usefulness, trust in social networks, and frequency of Internet use in older adults’ use of social networks, while ease of use and social pressure were not significant influences. These findings contribute to the study of older adults’ technology acceptance within specific technology application domains.
Recent research has focused on empirical studies of personal factors and emerging technologies. Ma et al. ( 2016 ) identified key personal factors affecting smartphone acceptance among older adults through structured questionnaires and face-to-face interviews with 120 participants. The study found that cost, self-satisfaction, and convenience were important factors influencing perceived usefulness and ease of use. This study offers empirical evidence to comprehend the main factors that drive smartphone acceptance among Chinese older adults. Additionally, Yusif et al. ( 2016 ) presented an overview of the obstacles that hinder older adults’ acceptance of assistive technologies, focusing on privacy, trust, and functionality.
In summary, research on older adults’ technology acceptance has shifted from early theoretical deepening and analysis of influencing factors to empirical studies in the areas of personal factors and emerging technologies, which have greatly enriched the theoretical basis of older adults’ technology acceptance and provided practical guidance for the design of emerging technology products.
Research hotspots, evolutionary trends, and quality distribution (RQ4)
Core keywords analysis.
Keywords concise the main idea and core of the literature, and are a refined summary of the research content (Huang et al. 2021 ). In CiteSpace, nodes with a centrality value greater than 0.1 are considered to be critical nodes. Analyzing keywords with high frequency and centrality helps to visualize the hot topics in the research field (Park et al. 2018 ). The merged keywords were imported into CiteSpace, and the top 10 keywords were counted and sorted by frequency and centrality respectively, as shown in Table 9 . The results show that the keyword “TAM” has the highest frequency (92), followed by “UTAUT” (24), which reflects that the in-depth study of the existing technology acceptance model and its theoretical expansion occupy a central position in research related to older adults’ technology acceptance. Furthermore, the terms ‘assistive technology’ and ‘virtual reality’ are both high-frequency and high-centrality terms (frequency = 17, centrality = 0.10), indicating that the research on assistive technology and virtual reality for older adults is the focus of current academic attention.
Research hotspots analysis
Using VOSviewer for keyword co-occurrence analysis organizes keywords into groups or clusters based on their intrinsic connections and frequencies, clearly highlighting the research field’s hot topics. The connectivity among keywords reveals correlations between different topics. To ensure accuracy, the analysis only considered the authors’ keywords. Subsequently, the keywords were filtered by setting the keyword frequency to 5 to obtain the keyword clustering map of the research on older adults’ technology acceptance research keyword clustering mapping (Fig. 7 ), combined with the keyword co-occurrence clustering network (Fig. 7A ) and the corresponding density situation (Fig. 7B ) to make a detailed analysis of the following four groups of clustered themes.
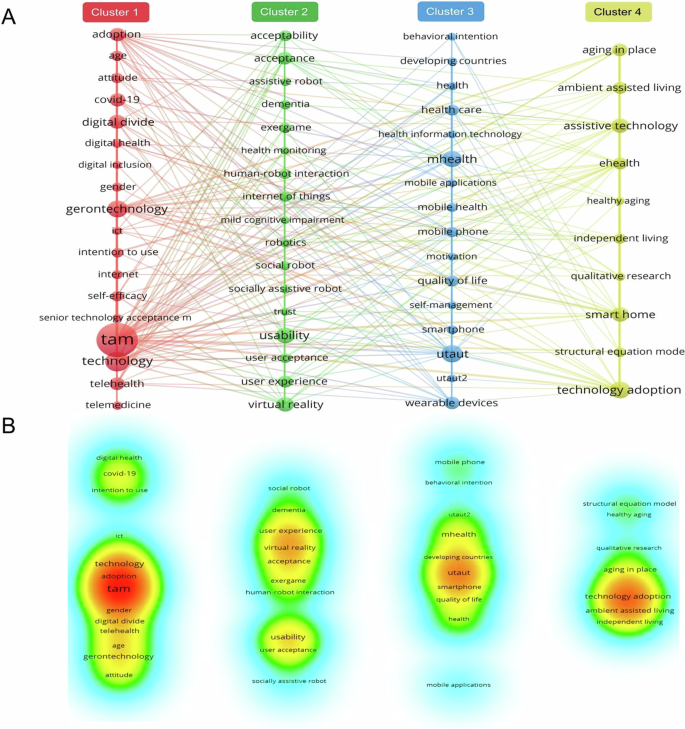
A Co-occurrence clustering network. B Keyword density.
Cluster #1—Research on the factors influencing technology adoption among older adults is a prominent topic, covering age, gender, self-efficacy, attitude, and and intention to use (Berkowsky et al. 2017 ; Wang et al. 2017 ). It also examined older adults’ attitudes towards and acceptance of digital health technologies (Ahmad and Mozelius, 2022 ). Moreover, the COVID-19 pandemic, significantly impacting older adults’ technology attitudes and usage, has underscored the study’s importance and urgency. Therefore, it is crucial to conduct in-depth studies on how older adults accept, adopt, and effectively use new technologies, to address their needs and help them overcome the digital divide within digital inclusion. This will improve their quality of life and healthcare experiences.
Cluster #2—Research focuses on how older adults interact with assistive technologies, especially assistive robots and health monitoring devices, emphasizing trust, usability, and user experience as crucial factors (Halim et al. 2022 ). Moreover, health monitoring technologies effectively track and manage health issues common in older adults, like dementia and mild cognitive impairment (Lussier et al. 2018 ; Piau et al. 2019 ). Interactive exercise games and virtual reality have been deployed to encourage more physical and cognitive engagement among older adults (Campo-Prieto et al. 2021 ). Personalized and innovative technology significantly enhances older adults’ participation, improving their health and well-being.
Cluster #3—Optimizing health management for older adults using mobile technology. With the development of mobile health (mHealth) and health information technology, mobile applications, smartphones, and smart wearable devices have become effective tools to help older users better manage chronic conditions, conduct real-time health monitoring, and even receive telehealth services (Dupuis and Tsotsos 2018 ; Olmedo-Aguirre et al. 2022 ; Kim et al. 2014 ). Additionally, these technologies can mitigate the problem of healthcare resource inequality, especially in developing countries. Older adults’ acceptance and use of these technologies are significantly influenced by their behavioral intentions, motivational factors, and self-management skills. These internal motivational factors, along with external factors, jointly affect older adults’ performance in health management and quality of life.
Cluster #4—Research on technology-assisted home care for older adults is gaining popularity. Environmentally assisted living enhances older adults’ independence and comfort at home, offering essential support and security. This has a crucial impact on promoting healthy aging (Friesen et al. 2016 ; Wahlroos et al. 2023 ). The smart home is a core application in this field, providing a range of solutions that facilitate independent living for the elderly in a highly integrated and user-friendly manner. This fulfills different dimensions of living and health needs (Majumder et al. 2017 ). Moreover, eHealth offers accurate and personalized health management and healthcare services for older adults (Delmastro et al. 2018 ), ensuring their needs are met at home. Research in this field often employs qualitative methods and structural equation modeling to fully understand older adults’ needs and experiences at home and analyze factors influencing technology adoption.
Evolutionary trends analysis
To gain a deeper understanding of the evolutionary trends in research hotspots within the field of older adults’ technology acceptance, we conducted a statistical analysis of the average appearance times of keywords, using CiteSpace to generate the time-zone evolution mapping (Fig. 8 ) and burst keywords. The time-zone mapping visually displays the evolution of keywords over time, intuitively reflecting the frequency and initial appearance of keywords in research, commonly used to identify trends in research topics (Jing et al. 2024a ; Kumar et al. 2021 ). Table 10 lists the top 15 keywords by burst strength, with the red sections indicating high-frequency citations and their burst strength in specific years. These burst keywords reveal the focus and trends of research themes over different periods (Kleinberg 2002 ). Combining insights from the time-zone mapping and burst keywords provides more objective and accurate research insights (Wang et al. 2023b ).
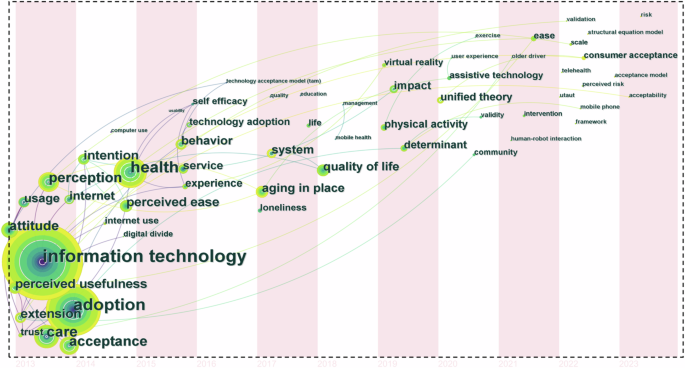
Reflecting the frequency and time of first appearance of keywords in the study.
An integrated analysis of Fig. 8 and Table 10 shows that early research on older adults’ technology acceptance primarily focused on factors such as perceived usefulness, ease of use, and attitudes towards information technology, including their use of computers and the internet (Pan and Jordan-Marsh 2010 ), as well as differences in technology use between older adults and other age groups (Guner and Acarturk 2020 ). Subsequently, the research focus expanded to improving the quality of life for older adults, exploring how technology can optimize health management and enhance the possibility of independent living, emphasizing the significant role of technology in improving the quality of life for the elderly. With ongoing technological advancements, recent research has shifted towards areas such as “virtual reality,” “telehealth,” and “human-robot interaction,” with a focus on the user experience of older adults (Halim et al. 2022 ). The appearance of keywords such as “physical activity” and “exercise” highlights the value of technology in promoting physical activity and health among older adults. This phase of research tends to make cutting-edge technology genuinely serve the practical needs of older adults, achieving its widespread application in daily life. Additionally, research has focused on expanding and quantifying theoretical models of older adults’ technology acceptance, involving keywords such as “perceived risk”, “validation” and “UTAUT”.
In summary, from 2013 to 2023, the field of older adults’ technology acceptance has evolved from initial explorations of influencing factors, to comprehensive enhancements in quality of life and health management, and further to the application and deepening of theoretical models and cutting-edge technologies. This research not only reflects the diversity and complexity of the field but also demonstrates a comprehensive and in-depth understanding of older adults’ interactions with technology across various life scenarios and needs.
Research quality distribution
To reveal the distribution of research quality in the field of older adults’ technology acceptance, a strategic diagram analysis is employed to calculate and illustrate the internal development and interrelationships among various research themes (Xie et al. 2020 ). The strategic diagram uses Centrality as the X-axis and Density as the Y-axis to divide into four quadrants, where the X-axis represents the strength of the connection between thematic clusters and other themes, with higher values indicating a central position in the research field; the Y-axis indicates the level of development within the thematic clusters, with higher values denoting a more mature and widely recognized field (Li and Zhou 2020 ).
Through cluster analysis and manual verification, this study categorized 61 core keywords (Frequency ≥5) into 11 thematic clusters. Subsequently, based on the keywords covered by each thematic cluster, the research themes and their directions for each cluster were summarized (Table 11 ), and the centrality and density coordinates for each cluster were precisely calculated (Table 12 ). Finally, a strategic diagram of the older adults’ technology acceptance research field was constructed (Fig. 9 ). Based on the distribution of thematic clusters across the quadrants in the strategic diagram, the structure and developmental trends of the field were interpreted.
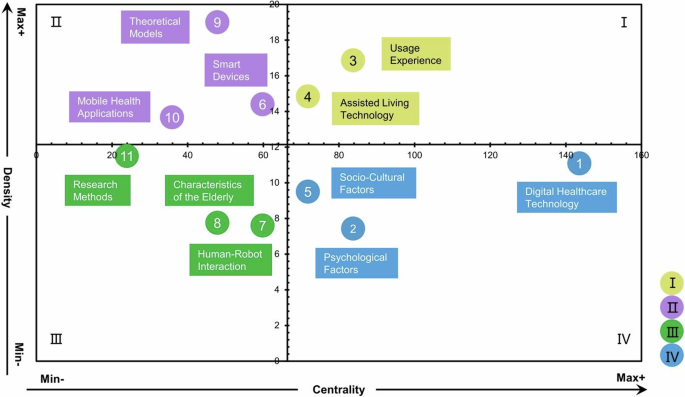
Classification and visualization of theme clusters based on density and centrality.
As illustrated in Fig. 9 , (1) the theme clusters of #3 Usage Experience and #4 Assisted Living Technology are in the first quadrant, characterized by high centrality and density. Their internal cohesion and close links with other themes indicate their mature development, systematic research content or directions have been formed, and they have a significant influence on other themes. These themes play a central role in the field of older adults’ technology acceptance and have promising prospects. (2) The theme clusters of #6 Smart Devices, #9 Theoretical Models, and #10 Mobile Health Applications are in the second quadrant, with higher density but lower centrality. These themes have strong internal connections but weaker external links, indicating that these three themes have received widespread attention from researchers and have been the subject of related research, but more as self-contained systems and exhibit independence. Therefore, future research should further explore in-depth cooperation and cross-application with other themes. (3) The theme clusters of #7 Human-Robot Interaction, #8 Characteristics of the Elderly, and #11 Research Methods are in the third quadrant, with lower centrality and density. These themes are loosely connected internally and have weak links with others, indicating their developmental immaturity. Compared to other topics, they belong to the lower attention edge and niche themes, and there is a need for further investigation. (4) The theme clusters of #1 Digital Healthcare Technology, #2 Psychological Factors, and #5 Socio-Cultural Factors are located in the fourth quadrant, with high centrality but low density. Although closely associated with other research themes, the internal cohesion within these clusters is relatively weak. This suggests that while these themes are closely linked to other research areas, their own development remains underdeveloped, indicating a core immaturity. Nevertheless, these themes are crucial within the research domain of elderly technology acceptance and possess significant potential for future exploration.
Discussion on distribution power (RQ1)
Over the past decade, academic interest and influence in the area of older adults’ technology acceptance have significantly increased. This trend is evidenced by a quantitative analysis of publication and citation volumes, particularly noticeable in 2019 and 2022, where there was a substantial rise in both metrics. The rise is closely linked to the widespread adoption of emerging technologies such as smart homes, wearable devices, and telemedicine among older adults. While these technologies have enhanced their quality of life, they also pose numerous challenges, sparking extensive research into their acceptance, usage behaviors, and influencing factors among the older adults (Pirzada et al. 2022 ; Garcia Reyes et al. 2023 ). Furthermore, the COVID-19 pandemic led to a surge in technology demand among older adults, especially in areas like medical consultation, online socialization, and health management, further highlighting the importance and challenges of technology. Health risks and social isolation have compelled older adults to rely on technology for daily activities, accelerating its adoption and application within this demographic. This phenomenon has made technology acceptance a critical issue, driving societal and academic focus on the study of technology acceptance among older adults.
The flow of knowledge at the level of high-output disciplines and journals, along with the primary publishing outlets, indicates the highly interdisciplinary nature of research into older adults’ technology acceptance. This reflects the complexity and breadth of issues related to older adults’ technology acceptance, necessitating the integration of multidisciplinary knowledge and approaches. Currently, research is primarily focused on medical health and human-computer interaction, demonstrating academic interest in improving health and quality of life for older adults and addressing the urgent needs related to their interactions with technology. In the field of medical health, research aims to provide advanced and innovative healthcare technologies and services to meet the challenges of an aging population while improving the quality of life for older adults (Abdi et al. 2020 ; Wilson et al. 2021 ). In the field of human-computer interaction, research is focused on developing smarter and more user-friendly interaction models to meet the needs of older adults in the digital age, enabling them to actively participate in social activities and enjoy a higher quality of life (Sayago, 2019 ). These studies are crucial for addressing the challenges faced by aging societies, providing increased support and opportunities for the health, welfare, and social participation of older adults.
Discussion on research power (RQ2)
This study analyzes leading countries and collaboration networks, core institutions and authors, revealing the global research landscape and distribution of research strength in the field of older adults’ technology acceptance, and presents quantitative data on global research trends. From the analysis of country distribution and collaborations, China and the USA hold dominant positions in this field, with developed countries like the UK, Germany, Italy, and the Netherlands also excelling in international cooperation and research influence. The significant investment in technological research and the focus on the technological needs of older adults by many developed countries reflect their rapidly aging societies, policy support, and resource allocation.
China is the only developing country that has become a major contributor in this field, indicating its growing research capabilities and high priority given to aging societies and technological innovation. Additionally, China has close collaborations with countries such as USA, the UK, and Malaysia, driven not only by technological research needs but also by shared challenges and complementarities in aging issues among these nations. For instance, the UK has extensive experience in social welfare and aging research, providing valuable theoretical guidance and practical experience. International collaborations, aimed at addressing the challenges of aging, integrate the strengths of various countries, advancing in-depth and widespread development in the research of technology acceptance among older adults.
At the institutional and author level, City University of Hong Kong leads in publication volume, with research teams led by Chan and Chen demonstrating significant academic activity and contributions. Their research primarily focuses on older adults’ acceptance and usage behaviors of various technologies, including smartphones, smart wearables, and social robots (Chen et al. 2015 ; Li et al. 2019 ; Ma et al. 2016 ). These studies, targeting specific needs and product characteristics of older adults, have developed new models of technology acceptance based on existing frameworks, enhancing the integration of these technologies into their daily lives and laying a foundation for further advancements in the field. Although Tilburg University has a smaller publication output, it holds significant influence in the field of older adults’ technology acceptance. Particularly, the high citation rate of Peek’s studies highlights their excellence in research. Peek extensively explored older adults’ acceptance and usage of home care technologies, revealing the complexity and dynamics of their technology use behaviors. His research spans from identifying systemic influencing factors (Peek et al. 2014 ; Peek et al. 2016 ), emphasizing familial impacts (Luijkx et al. 2015 ), to constructing comprehensive models (Peek et al. 2017 ), and examining the dynamics of long-term usage (Peek et al. 2019 ), fully reflecting the evolving technology landscape and the changing needs of older adults. Additionally, the ongoing contributions of researchers like Ziefle, Rogers, and Wouters in the field of older adults’ technology acceptance demonstrate their research influence and leadership. These researchers have significantly enriched the knowledge base in this area with their diverse perspectives. For instance, Ziefle has uncovered the complex attitudes of older adults towards technology usage, especially the trade-offs between privacy and security, and how different types of activities affect their privacy needs (Maidhof et al. 2023 ; Mujirishvili et al. 2023 ; Schomakers and Ziefle 2023 ; Wilkowska et al. 2022 ), reflecting a deep exploration and ongoing innovation in the field of older adults’ technology acceptance.
Discussion on knowledge base and thematic progress (RQ3)
Through co-citation analysis and systematic review of seminal literature, this study reveals the knowledge foundation and thematic progress in the field of older adults’ technology acceptance. Co-citation networks and cluster analyses illustrate the structural themes of the research, delineating the differentiation and boundaries within this field. Additionally, burst detection analysis offers a valuable perspective for understanding the thematic evolution in the field of technology acceptance among older adults. The development and innovation of theoretical models are foundational to this research. Researchers enhance the explanatory power of constructed models by deepening and expanding existing technology acceptance theories to address theoretical limitations. For instance, Heerink et al. ( 2010 ) modified and expanded the UTAUT model by integrating functional assessment and social interaction variables to create the almere model. This model significantly enhances the ability to explain the intentions of older users in utilizing assistive social agents and improves the explanation of actual usage behaviors. Additionally, Chen and Chan ( 2014 ) extended the TAM to include age-related health and capability features of older adults, creating the STAM, which substantially improves predictions of older adults’ technology usage behaviors. Personal attributes, health and capability features, and facilitating conditions have a direct impact on technology acceptance. These factors more effectively predict older adults’ technology usage behaviors than traditional attitudinal factors.
With the advancement of technology and the application of emerging technologies, new research topics have emerged, increasingly focusing on older adults’ acceptance and use of these technologies. Prior to this, the study by Mitzner et al. ( 2010 ) challenged the stereotype of older adults’ conservative attitudes towards technology, highlighting the central roles of usability and usefulness in the technology acceptance process. This discovery laid an important foundation for subsequent research. Research fields such as “smart home technology,” “social life,” and “customer service” are emerging, indicating a shift in focus towards the practical and social applications of technology in older adults’ lives. Research not only focuses on the technology itself but also on how these technologies integrate into older adults’ daily lives and how they can improve the quality of life through technology. For instance, studies such as those by Ma et al. ( 2016 ), Hoque and Sorwar ( 2017 ), and Li et al. ( 2019 ) have explored factors influencing older adults’ use of smartphones, mHealth, and smart wearable devices.
Furthermore, the diversification of research methodologies and innovation in evaluation techniques, such as the use of mixed methods, structural equation modeling (SEM), and neural network (NN) approaches, have enhanced the rigor and reliability of the findings, enabling more precise identification of the factors and mechanisms influencing technology acceptance. Talukder et al. ( 2020 ) employed an effective multimethodological strategy by integrating SEM and NN to leverage the complementary strengths of both approaches, thus overcoming their individual limitations and more accurately analyzing and predicting older adults’ acceptance of wearable health technologies (WHT). SEM is utilized to assess the determinants’ impact on the adoption of WHT, while neural network models validate SEM outcomes and predict the significance of key determinants. This combined approach not only boosts the models’ reliability and explanatory power but also provides a nuanced understanding of the motivations and barriers behind older adults’ acceptance of WHT, offering deep research insights.
Overall, co-citation analysis of the literature in the field of older adults’ technology acceptance has uncovered deeper theoretical modeling and empirical studies on emerging technologies, while emphasizing the importance of research methodological and evaluation innovations in understanding complex social science issues. These findings are crucial for guiding the design and marketing strategies of future technology products, especially in the rapidly growing market of older adults.
Discussion on research hotspots and evolutionary trends (RQ4)
By analyzing core keywords, we can gain deep insights into the hot topics, evolutionary trends, and quality distribution of research in the field of older adults’ technology acceptance. The frequent occurrence of the keywords “TAM” and “UTAUT” indicates that the applicability and theoretical extension of existing technology acceptance models among older adults remain a focal point in academia. This phenomenon underscores the enduring influence of the studies by Davis ( 1989 ) and Venkatesh et al. ( 2003 ), whose models provide a robust theoretical framework for explaining and predicting older adults’ acceptance and usage of emerging technologies. With the widespread application of artificial intelligence (AI) and big data technologies, these theoretical models have incorporated new variables such as perceived risk, trust, and privacy issues (Amin et al. 2024 ; Chen et al. 2024 ; Jing et al. 2024b ; Seibert et al. 2021 ; Wang et al. 2024b ), advancing the theoretical depth and empirical research in this field.
Keyword co-occurrence cluster analysis has revealed multiple research hotspots in the field, including factors influencing technology adoption, interactive experiences between older adults and assistive technologies, the application of mobile health technology in health management, and technology-assisted home care. These studies primarily focus on enhancing the quality of life and health management of older adults through emerging technologies, particularly in the areas of ambient assisted living, smart health monitoring, and intelligent medical care. In these domains, the role of AI technology is increasingly significant (Qian et al. 2021 ; Ho 2020 ). With the evolution of next-generation information technologies, AI is increasingly integrated into elder care systems, offering intelligent, efficient, and personalized service solutions by analyzing the lifestyles and health conditions of older adults. This integration aims to enhance older adults’ quality of life in aspects such as health monitoring and alerts, rehabilitation assistance, daily health management, and emotional support (Lee et al. 2023 ). A survey indicates that 83% of older adults prefer AI-driven solutions when selecting smart products, demonstrating the increasing acceptance of AI in elder care (Zhao and Li 2024 ). Integrating AI into elder care presents both opportunities and challenges, particularly in terms of user acceptance, trust, and long-term usage effects, which warrant further exploration (Mhlanga 2023 ). These studies will help better understand the profound impact of AI technology on the lifestyles of older adults and provide critical references for optimizing AI-driven elder care services.
The Time-zone evolution mapping and burst keyword analysis further reveal the evolutionary trends of research hotspots. Early studies focused on basic technology acceptance models and user perceptions, later expanding to include quality of life and health management. In recent years, research has increasingly focused on cutting-edge technologies such as virtual reality, telehealth, and human-robot interaction, with a concurrent emphasis on the user experience of older adults. This evolutionary process demonstrates a deepening shift from theoretical models to practical applications, underscoring the significant role of technology in enhancing the quality of life for older adults. Furthermore, the strategic coordinate mapping analysis clearly demonstrates the development and mutual influence of different research themes. High centrality and density in the themes of Usage Experience and Assisted Living Technology indicate their mature research status and significant impact on other themes. The themes of Smart Devices, Theoretical Models, and Mobile Health Applications demonstrate self-contained research trends. The themes of Human-Robot Interaction, Characteristics of the Elderly, and Research Methods are not yet mature, but they hold potential for development. Themes of Digital Healthcare Technology, Psychological Factors, and Socio-Cultural Factors are closely related to other themes, displaying core immaturity but significant potential.
In summary, the research hotspots in the field of older adults’ technology acceptance are diverse and dynamic, demonstrating the academic community’s profound understanding of how older adults interact with technology across various life contexts and needs. Under the influence of AI and big data, research should continue to focus on the application of emerging technologies among older adults, exploring in depth how they adapt to and effectively use these technologies. This not only enhances the quality of life and healthcare experiences for older adults but also drives ongoing innovation and development in this field.
Research agenda
Based on the above research findings, to further understand and promote technology acceptance and usage among older adults, we recommend future studies focus on refining theoretical models, exploring long-term usage, and assessing user experience in the following detailed aspects:
Refinement and validation of specific technology acceptance models for older adults: Future research should focus on developing and validating technology acceptance models based on individual characteristics, particularly considering variations in technology acceptance among older adults across different educational levels and cultural backgrounds. This includes factors such as age, gender, educational background, and cultural differences. Additionally, research should examine how well specific technologies, such as wearable devices and mobile health applications, meet the needs of older adults. Building on existing theoretical models, this research should integrate insights from multiple disciplines such as psychology, sociology, design, and engineering through interdisciplinary collaboration to create more accurate and comprehensive models, which should then be validated in relevant contexts.
Deepening the exploration of the relationship between long-term technology use and quality of life among older adults: The acceptance and use of technology by users is a complex and dynamic process (Seuwou et al. 2016 ). Existing research predominantly focuses on older adults’ initial acceptance or short-term use of new technologies; however, the impact of long-term use on their quality of life and health is more significant. Future research should focus on the evolution of older adults’ experiences and needs during long-term technology usage, and the enduring effects of technology on their social interactions, mental health, and life satisfaction. Through longitudinal studies and qualitative analysis, this research reveals the specific needs and challenges of older adults in long-term technology use, providing a basis for developing technologies and strategies that better meet their requirements. This understanding aids in comprehensively assessing the impact of technology on older adults’ quality of life and guiding the optimization and improvement of technological products.
Evaluating the Importance of User Experience in Research on Older Adults’ Technology Acceptance: Understanding the mechanisms of information technology acceptance and use is central to human-computer interaction research. Although technology acceptance models and user experience models differ in objectives, they share many potential intersections. Technology acceptance research focuses on structured prediction and assessment, while user experience research concentrates on interpreting design impacts and new frameworks. Integrating user experience to assess older adults’ acceptance of technology products and systems is crucial (Codfrey et al. 2022 ; Wang et al. 2019 ), particularly for older users, where specific product designs should emphasize practicality and usability (Fisk et al. 2020 ). Researchers need to explore innovative age-appropriate design methods to enhance older adults’ usage experience. This includes studying older users’ actual usage preferences and behaviors, optimizing user interfaces, and interaction designs. Integrating feedback from older adults to tailor products to their needs can further promote their acceptance and continued use of technology products.
Conclusions
This study conducted a systematic review of the literature on older adults’ technology acceptance over the past decade through bibliometric analysis, focusing on the distribution power, research power, knowledge base and theme progress, research hotspots, evolutionary trends, and quality distribution. Using a combination of quantitative and qualitative methods, this study has reached the following conclusions:
Technology acceptance among older adults has become a hot topic in the international academic community, involving the integration of knowledge across multiple disciplines, including Medical Informatics, Health Care Sciences Services, and Ergonomics. In terms of journals, “PSYCHOLOGY, EDUCATION, HEALTH” represents a leading field, with key publications including Computers in Human Behavior , Journal of Medical Internet Research , and International Journal of Human-Computer Interaction . These journals possess significant academic authority and extensive influence in the field.
Research on technology acceptance among older adults is particularly active in developed countries, with China and USA publishing significantly more than other nations. The Netherlands leads in high average citation rates, indicating the depth and impact of its research. Meanwhile, the UK stands out in terms of international collaboration. At the institutional level, City University of Hong Kong and The University of Hong Kong in China are in leading positions. Tilburg University in the Netherlands demonstrates exceptional research quality through its high average citation count. At the author level, Chen from China has the highest number of publications, while Peek from the Netherlands has the highest average citation count.
Co-citation analysis of references indicates that the knowledge base in this field is divided into three main categories: theoretical model deepening, emerging technology applications, and research methods and evaluation. Seminal literature focuses on four areas: specific technology use by older adults, expansion of theoretical models of technology acceptance, information technology adoption behavior, and research perspectives. Research themes have evolved from initial theoretical deepening and analysis of influencing factors to empirical studies on individual factors and emerging technologies.
Keyword analysis indicates that TAM and UTAUT are the most frequently occurring terms, while “assistive technology” and “virtual reality” are focal points with high frequency and centrality. Keyword clustering analysis reveals that research hotspots are concentrated on the influencing factors of technology adoption, human-robot interaction experiences, mobile health management, and technology for aging in place. Time-zone evolution mapping and burst keyword analysis have revealed the research evolution from preliminary exploration of influencing factors, to enhancements in quality of life and health management, and onto advanced technology applications and deepening of theoretical models. Furthermore, analysis of research quality distribution indicates that Usage Experience and Assisted Living Technology have become core topics, while Smart Devices, Theoretical Models, and Mobile Health Applications point towards future research directions.
Through this study, we have systematically reviewed the dynamics, core issues, and evolutionary trends in the field of older adults’ technology acceptance, constructing a comprehensive Knowledge Mapping of the domain and presenting a clear framework of existing research. This not only lays the foundation for subsequent theoretical discussions and innovative applications in the field but also provides an important reference for relevant scholars.
Limitations
To our knowledge, this is the first bibliometric analysis concerning technology acceptance among older adults, and we adhered strictly to bibliometric standards throughout our research. However, this study relies on the Web of Science Core Collection, and while its authority and breadth are widely recognized, this choice may have missed relevant literature published in other significant databases such as PubMed, Scopus, and Google Scholar, potentially overlooking some critical academic contributions. Moreover, given that our analysis was confined to literature in English, it may not reflect studies published in other languages, somewhat limiting the global representativeness of our data sample.
It is noteworthy that with the rapid development of AI technology, its increasingly widespread application in elderly care services is significantly transforming traditional care models. AI is profoundly altering the lifestyles of the elderly, from health monitoring and smart diagnostics to intelligent home systems and personalized care, significantly enhancing their quality of life and health care standards. The potential for AI technology within the elderly population is immense, and research in this area is rapidly expanding. However, due to the restrictive nature of the search terms used in this study, it did not fully cover research in this critical area, particularly in addressing key issues such as trust, privacy, and ethics.
Consequently, future research should not only expand data sources, incorporating multilingual and multidatabase literature, but also particularly focus on exploring older adults’ acceptance of AI technology and its applications, in order to construct a more comprehensive academic landscape of older adults’ technology acceptance, thereby enriching and extending the knowledge system and academic trends in this field.
Data availability
The datasets analyzed during the current study are available in the Dataverse repository: https://doi.org/10.7910/DVN/6K0GJH .
Abdi S, de Witte L, Hawley M (2020) Emerging technologies with potential care and support applications for older people: review of gray literature. JMIR Aging 3(2):e17286. https://doi.org/10.2196/17286
Article PubMed PubMed Central Google Scholar
Achuthan K, Nair VK, Kowalski R, Ramanathan S, Raman R (2023) Cyberbullying research—Alignment to sustainable development and impact of COVID-19: Bibliometrics and science mapping analysis. Comput Human Behav 140:107566. https://doi.org/10.1016/j.chb.2022.107566
Article Google Scholar
Ahmad A, Mozelius P (2022) Human-Computer Interaction for Older Adults: a Literature Review on Technology Acceptance of eHealth Systems. J Eng Res Sci 1(4):119–126. https://doi.org/10.55708/js0104014
Ale Ebrahim N, Salehi H, Embi MA, Habibi F, Gholizadeh H, Motahar SM (2014) Visibility and citation impact. Int Educ Stud 7(4):120–125. https://doi.org/10.5539/ies.v7n4p120
Amin MS, Johnson VL, Prybutok V, Koh CE (2024) An investigation into factors affecting the willingness to disclose personal health information when using AI-enabled caregiver robots. Ind Manag Data Syst 124(4):1677–1699. https://doi.org/10.1108/IMDS-09-2023-0608
Baer NR, Vietzke J, Schenk L (2022) Middle-aged and older adults’ acceptance of mobile nutrition and fitness apps: a systematic mixed studies review. PLoS One 17(12):e0278879. https://doi.org/10.1371/journal.pone.0278879
Barnard Y, Bradley MD, Hodgson F, Lloyd AD (2013) Learning to use new technologies by older adults: Perceived difficulties, experimentation behaviour and usability. Comput Human Behav 29(4):1715–1724. https://doi.org/10.1016/j.chb.2013.02.006
Berkowsky RW, Sharit J, Czaja SJ (2017) Factors predicting decisions about technology adoption among older adults. Innov Aging 3(1):igy002. https://doi.org/10.1093/geroni/igy002
Braun MT (2013) Obstacles to social networking website use among older adults. Comput Human Behav 29(3):673–680. https://doi.org/10.1016/j.chb.2012.12.004
Article MathSciNet Google Scholar
Campo-Prieto P, Rodríguez-Fuentes G, Cancela-Carral JM (2021) Immersive virtual reality exergame promotes the practice of physical activity in older people: An opportunity during COVID-19. Multimodal Technol Interact 5(9):52. https://doi.org/10.3390/mti5090052
Chen C (2006) CiteSpace II: Detecting and visualizing emerging trends and transient patterns in scientific literature. J Am Soc Inf Sci Technol 57(3):359–377. https://doi.org/10.1002/asi.20317
Chen C, Dubin R, Kim MC (2014) Emerging trends and new developments in regenerative medicine: a scientometric update (2000–2014). Expert Opin Biol Ther 14(9):1295–1317. https://doi.org/10.1517/14712598.2014.920813
Article PubMed Google Scholar
Chen C, Leydesdorff L (2014) Patterns of connections and movements in dual‐map overlays: A new method of publication portfolio analysis. J Assoc Inf Sci Technol 65(2):334–351. https://doi.org/10.1002/asi.22968
Chen J, Wang C, Tang Y (2022) Knowledge mapping of volunteer motivation: A bibliometric analysis and cross-cultural comparative study. Front Psychol 13:883150. https://doi.org/10.3389/fpsyg.2022.883150
Chen JY, Liu YD, Dai J, Wang CL (2023) Development and status of moral education research: Visual analysis based on knowledge graph. Front Psychol 13:1079955. https://doi.org/10.3389/fpsyg.2022.1079955
Chen K, Chan AH (2011) A review of technology acceptance by older adults. Gerontechnology 10(1):1–12. https://doi.org/10.4017/gt.2011.10.01.006.00
Chen K, Chan AH (2014) Gerontechnology acceptance by elderly Hong Kong Chinese: a senior technology acceptance model (STAM). Ergonomics 57(5):635–652. https://doi.org/10.1080/00140139.2014.895855
Chen K, Zhang Y, Fu X (2019) International research collaboration: An emerging domain of innovation studies? Res Policy 48(1):149–168. https://doi.org/10.1016/j.respol.2018.08.005
Chen X, Hu Z, Wang C (2024) Empowering education development through AIGC: A systematic literature review. Educ Inf Technol 1–53. https://doi.org/10.1007/s10639-024-12549-7
Chen Y, Chen CM, Liu ZY, Hu ZG, Wang XW (2015) The methodology function of CiteSpace mapping knowledge domains. Stud Sci Sci 33(2):242–253. https://doi.org/10.16192/j.cnki.1003-2053.2015.02.009
Codfrey GS, Baharum A, Zain NHM, Omar M, Deris FD (2022) User Experience in Product Design and Development: Perspectives and Strategies. Math Stat Eng Appl 71(2):257–262. https://doi.org/10.17762/msea.v71i2.83
Dai J, Zhang X, Wang CL (2024) A meta-analysis of learners’ continuance intention toward online education platforms. Educ Inf Technol 1–36. https://doi.org/10.1007/s10639-024-12654-7
Davis FD (1989) Perceived usefulness, perceived ease of use, and user acceptance of information technology. MIS Q 13(3):319–340. https://doi.org/10.2307/249008
Delmastro F, Dolciotti C, Palumbo F, Magrini M, Di Martino F, La Rosa D, Barcaro U (2018) Long-term care: how to improve the quality of life with mobile and e-health services. In 2018 14th International Conference on Wireless and Mobile Computing, Networking and Communications (WiMob), pp. 12–19. IEEE. https://doi.org/10.1109/WiMOB.2018.8589157
Dupuis K, Tsotsos LE (2018) Technology for remote health monitoring in an older population: a role for mobile devices. Multimodal Technol Interact 2(3):43. https://doi.org/10.3390/mti2030043
Ferguson C, Hickman LD, Turkmani S, Breen P, Gargiulo G, Inglis SC (2021) Wearables only work on patients that wear them”: Barriers and facilitators to the adoption of wearable cardiac monitoring technologies. Cardiovasc Digit Health J 2(2):137–147. https://doi.org/10.1016/j.cvdhj.2021.02.001
Fisk AD, Czaja SJ, Rogers WA, Charness N, Sharit J (2020) Designing for older adults: Principles and creative human factors approaches. CRC Press. https://doi.org/10.1201/9781420080681
Friesen S, Brémault-Phillips S, Rudrum L, Rogers LG (2016) Environmental design that supports healthy aging: Evaluating a new supportive living facility. J Hous Elderly 30(1):18–34. https://doi.org/10.1080/02763893.2015.1129380
Garcia Reyes EP, Kelly R, Buchanan G, Waycott J (2023) Understanding Older Adults’ Experiences With Technologies for Health Self-management: Interview Study. JMIR Aging 6:e43197. https://doi.org/10.2196/43197
Geng Z, Wang J, Liu J, Miao J (2024) Bibliometric analysis of the development, current status, and trends in adult degenerative scoliosis research: A systematic review from 1998 to 2023. J Pain Res 17:153–169. https://doi.org/10.2147/JPR.S437575
González A, Ramírez MP, Viadel V (2012) Attitudes of the elderly toward information and communications technologies. Educ Gerontol 38(9):585–594. https://doi.org/10.1080/03601277.2011.595314
Guner H, Acarturk C (2020) The use and acceptance of ICT by senior citizens: a comparison of technology acceptance model (TAM) for elderly and young adults. Univ Access Inf Soc 19(2):311–330. https://doi.org/10.1007/s10209-018-0642-4
Halim I, Saptari A, Perumal PA, Abdullah Z, Abdullah S, Muhammad MN (2022) A Review on Usability and User Experience of Assistive Social Robots for Older Persons. Int J Integr Eng 14(6):102–124. https://penerbit.uthm.edu.my/ojs/index.php/ijie/article/view/8566
He Y, He Q, Liu Q (2022) Technology acceptance in socially assistive robots: Scoping review of models, measurement, and influencing factors. J Healthc Eng 2022(1):6334732. https://doi.org/10.1155/2022/6334732
Heerink M, Kröse B, Evers V, Wielinga B (2010) Assessing acceptance of assistive social agent technology by older adults: the almere model. Int J Soc Robot 2:361–375. https://doi.org/10.1007/s12369-010-0068-5
Ho A (2020) Are we ready for artificial intelligence health monitoring in elder care? BMC Geriatr 20(1):358. https://doi.org/10.1186/s12877-020-01764-9
Hoque R, Sorwar G (2017) Understanding factors influencing the adoption of mHealth by the elderly: An extension of the UTAUT model. Int J Med Inform 101:75–84. https://doi.org/10.1016/j.ijmedinf.2017.02.002
Hota PK, Subramanian B, Narayanamurthy G (2020) Mapping the intellectual structure of social entrepreneurship research: A citation/co-citation analysis. J Bus Ethics 166(1):89–114. https://doi.org/10.1007/s10551-019-04129-4
Huang R, Yan P, Yang X (2021) Knowledge map visualization of technology hotspots and development trends in China’s textile manufacturing industry. IET Collab Intell Manuf 3(3):243–251. https://doi.org/10.1049/cim2.12024
Article ADS Google Scholar
Jing Y, Wang C, Chen Y, Wang H, Yu T, Shadiev R (2023) Bibliometric mapping techniques in educational technology research: A systematic literature review. Educ Inf Technol 1–29. https://doi.org/10.1007/s10639-023-12178-6
Jing YH, Wang CL, Chen ZY, Shen SS, Shadiev R (2024a) A Bibliometric Analysis of Studies on Technology-Supported Learning Environments: Hotopics and Frontier Evolution. J Comput Assist Learn 1–16. https://doi.org/10.1111/jcal.12934
Jing YH, Wang HM, Chen XJ, Wang CL (2024b) What factors will affect the effectiveness of using ChatGPT to solve programming problems? A quasi-experimental study. Humanit Soc Sci Commun 11:319. https://doi.org/10.1057/s41599-024-02751-w
Kamrani P, Dorsch I, Stock WG (2021) Do researchers know what the h-index is? And how do they estimate its importance? Scientometrics 126(7):5489–5508. https://doi.org/10.1007/s11192-021-03968-1
Kim HS, Lee KH, Kim H, Kim JH (2014) Using mobile phones in healthcare management for the elderly. Maturitas 79(4):381–388. https://doi.org/10.1016/j.maturitas.2014.08.013
Article MathSciNet PubMed Google Scholar
Kleinberg J (2002) Bursty and hierarchical structure in streams. In Proceedings of the eighth ACM SIGKDD international conference on Knowledge discovery and data mining, pp. 91–101. https://doi.org/10.1145/775047.775061
Kruse C, Fohn J, Wilson N, Patlan EN, Zipp S, Mileski M (2020) Utilization barriers and medical outcomes commensurate with the use of telehealth among older adults: systematic review. JMIR Med Inform 8(8):e20359. https://doi.org/10.2196/20359
Kumar S, Lim WM, Pandey N, Christopher Westland J (2021) 20 years of electronic commerce research. Electron Commer Res 21:1–40. https://doi.org/10.1007/s10660-021-09464-1
Kwiek M (2021) What large-scale publication and citation data tell us about international research collaboration in Europe: Changing national patterns in global contexts. Stud High Educ 46(12):2629–2649. https://doi.org/10.1080/03075079.2020.1749254
Lee C, Coughlin JF (2015) PERSPECTIVE: Older adults’ adoption of technology: an integrated approach to identifying determinants and barriers. J Prod Innov Manag 32(5):747–759. https://doi.org/10.1111/jpim.12176
Lee CH, Wang C, Fan X, Li F, Chen CH (2023) Artificial intelligence-enabled digital transformation in elderly healthcare field: scoping review. Adv Eng Inform 55:101874. https://doi.org/10.1016/j.aei.2023.101874
Leydesdorff L, Rafols I (2012) Interactive overlays: A new method for generating global journal maps from Web-of-Science data. J Informetr 6(2):318–332. https://doi.org/10.1016/j.joi.2011.11.003
Li J, Ma Q, Chan AH, Man S (2019) Health monitoring through wearable technologies for older adults: Smart wearables acceptance model. Appl Ergon 75:162–169. https://doi.org/10.1016/j.apergo.2018.10.006
Article ADS PubMed Google Scholar
Li X, Zhou D (2020) Product design requirement information visualization approach for intelligent manufacturing services. China Mech Eng 31(07):871, http://www.cmemo.org.cn/EN/Y2020/V31/I07/871
Google Scholar
Lin Y, Yu Z (2024a) An integrated bibliometric analysis and systematic review modelling students’ technostress in higher education. Behav Inf Technol 1–25. https://doi.org/10.1080/0144929X.2024.2332458
Lin Y, Yu Z (2024b) A bibliometric analysis of artificial intelligence chatbots in educational contexts. Interact Technol Smart Educ 21(2):189–213. https://doi.org/10.1108/ITSE-12-2022-0165
Liu L, Duffy VG (2023) Exploring the future development of Artificial Intelligence (AI) applications in chatbots: a bibliometric analysis. Int J Soc Robot 15(5):703–716. https://doi.org/10.1007/s12369-022-00956-0
Liu R, Li X, Chu J (2022) Evolution of applied variables in the research on technology acceptance of the elderly. In: International Conference on Human-Computer Interaction, Cham: Springer International Publishing, pp 500–520. https://doi.org/10.1007/978-3-031-05581-23_5
Luijkx K, Peek S, Wouters E (2015) “Grandma, you should do it—It’s cool” Older Adults and the Role of Family Members in Their Acceptance of Technology. Int J Environ Res Public Health 12(12):15470–15485. https://doi.org/10.3390/ijerph121214999
Lussier M, Lavoie M, Giroux S, Consel C, Guay M, Macoir J, Bier N (2018) Early detection of mild cognitive impairment with in-home monitoring sensor technologies using functional measures: a systematic review. IEEE J Biomed Health Inform 23(2):838–847. https://doi.org/10.1109/JBHI.2018.2834317
López-Robles JR, Otegi-Olaso JR, Porto Gomez I, Gamboa-Rosales NK, Gamboa-Rosales H, Robles-Berumen H (2018) Bibliometric network analysis to identify the intellectual structure and evolution of the big data research field. In: International Conference on Intelligent Data Engineering and Automated Learning, Cham: Springer International Publishing, pp 113–120. https://doi.org/10.1007/978-3-030-03496-2_13
Ma Q, Chan AH, Chen K (2016) Personal and other factors affecting acceptance of smartphone technology by older Chinese adults. Appl Ergon 54:62–71. https://doi.org/10.1016/j.apergo.2015.11.015
Ma Q, Chan AHS, Teh PL (2021) Insights into Older Adults’ Technology Acceptance through Meta-Analysis. Int J Hum-Comput Interact 37(11):1049–1062. https://doi.org/10.1080/10447318.2020.1865005
Macedo IM (2017) Predicting the acceptance and use of information and communication technology by older adults: An empirical examination of the revised UTAUT2. Comput Human Behav 75:935–948. https://doi.org/10.1016/j.chb.2017.06.013
Maidhof C, Offermann J, Ziefle M (2023) Eyes on privacy: acceptance of video-based AAL impacted by activities being filmed. Front Public Health 11:1186944. https://doi.org/10.3389/fpubh.2023.1186944
Majumder S, Aghayi E, Noferesti M, Memarzadeh-Tehran H, Mondal T, Pang Z, Deen MJ (2017) Smart homes for elderly healthcare—Recent advances and research challenges. Sensors 17(11):2496. https://doi.org/10.3390/s17112496
Article ADS PubMed PubMed Central Google Scholar
Mhlanga D (2023) Artificial Intelligence in elderly care: Navigating ethical and responsible AI adoption for seniors. Available at SSRN 4675564. 4675564 min) Identifying citation patterns of scientific breakthroughs: A perspective of dynamic citation process. Inf Process Manag 58(1):102428. https://doi.org/10.1016/j.ipm.2020.102428
Mitzner TL, Boron JB, Fausset CB, Adams AE, Charness N, Czaja SJ, Sharit J (2010) Older adults talk technology: Technology usage and attitudes. Comput Human Behav 26(6):1710–1721. https://doi.org/10.1016/j.chb.2010.06.020
Mitzner TL, Savla J, Boot WR, Sharit J, Charness N, Czaja SJ, Rogers WA (2019) Technology adoption by older adults: Findings from the PRISM trial. Gerontologist 59(1):34–44. https://doi.org/10.1093/geront/gny113
Mongeon P, Paul-Hus A (2016) The journal coverage of Web of Science and Scopus: a comparative analysis. Scientometrics 106:213–228. https://doi.org/10.1007/s11192-015-1765-5
Mostaghel R (2016) Innovation and technology for the elderly: Systematic literature review. J Bus Res 69(11):4896–4900. https://doi.org/10.1016/j.jbusres.2016.04.049
Mujirishvili T, Maidhof C, Florez-Revuelta F, Ziefle M, Richart-Martinez M, Cabrero-García J (2023) Acceptance and privacy perceptions toward video-based active and assisted living technologies: Scoping review. J Med Internet Res 25:e45297. https://doi.org/10.2196/45297
Naseri RNN, Azis SN, Abas N (2023) A Review of Technology Acceptance and Adoption Models in Consumer Study. FIRM J Manage Stud 8(2):188–199. https://doi.org/10.33021/firm.v8i2.4536
Nguyen UP, Hallinger P (2020) Assessing the distinctive contributions of Simulation & Gaming to the literature, 1970–2019: A bibliometric review. Simul Gaming 51(6):744–769. https://doi.org/10.1177/1046878120941569
Olmedo-Aguirre JO, Reyes-Campos J, Alor-Hernández G, Machorro-Cano I, Rodríguez-Mazahua L, Sánchez-Cervantes JL (2022) Remote healthcare for elderly people using wearables: A review. Biosensors 12(2):73. https://doi.org/10.3390/bios12020073
Pan S, Jordan-Marsh M (2010) Internet use intention and adoption among Chinese older adults: From the expanded technology acceptance model perspective. Comput Human Behav 26(5):1111–1119. https://doi.org/10.1016/j.chb.2010.03.015
Pan X, Yan E, Cui M, Hua W (2018) Examining the usage, citation, and diffusion patterns of bibliometric map software: A comparative study of three tools. J Informetr 12(2):481–493. https://doi.org/10.1016/j.joi.2018.03.005
Park JS, Kim NR, Han EJ (2018) Analysis of trends in science and technology using keyword network analysis. J Korea Ind Inf Syst Res 23(2):63–73. https://doi.org/10.9723/jksiis.2018.23.2.063
Peek ST, Luijkx KG, Rijnaard MD, Nieboer ME, Van Der Voort CS, Aarts S, Wouters EJ (2016) Older adults’ reasons for using technology while aging in place. Gerontology 62(2):226–237. https://doi.org/10.1159/000430949
Peek ST, Luijkx KG, Vrijhoef HJ, Nieboer ME, Aarts S, van der Voort CS, Wouters EJ (2017) Origins and consequences of technology acquirement by independent-living seniors: Towards an integrative model. BMC Geriatr 17:1–18. https://doi.org/10.1186/s12877-017-0582-5
Peek ST, Wouters EJ, Van Hoof J, Luijkx KG, Boeije HR, Vrijhoef HJ (2014) Factors influencing acceptance of technology for aging in place: a systematic review. Int J Med Inform 83(4):235–248. https://doi.org/10.1016/j.ijmedinf.2014.01.004
Peek STM, Luijkx KG, Vrijhoef HJM, Nieboer ME, Aarts S, Van Der Voort CS, Wouters EJM (2019) Understanding changes and stability in the long-term use of technologies by seniors who are aging in place: a dynamical framework. BMC Geriatr 19:1–13. https://doi.org/10.1186/s12877-019-1241-9
Perez AJ, Siddiqui F, Zeadally S, Lane D (2023) A review of IoT systems to enable independence for the elderly and disabled individuals. Internet Things 21:100653. https://doi.org/10.1016/j.iot.2022.100653
Piau A, Wild K, Mattek N, Kaye J (2019) Current state of digital biomarker technologies for real-life, home-based monitoring of cognitive function for mild cognitive impairment to mild Alzheimer disease and implications for clinical care: systematic review. J Med Internet Res 21(8):e12785. https://doi.org/10.2196/12785
Pirzada P, Wilde A, Doherty GH, Harris-Birtill D (2022) Ethics and acceptance of smart homes for older adults. Inform Health Soc Care 47(1):10–37. https://doi.org/10.1080/17538157.2021.1923500
Pranckutė R (2021) Web of Science (WoS) and Scopus: The titans of bibliographic information in today’s academic world. Publications 9(1):12. https://doi.org/10.3390/publications9010012
Qian K, Zhang Z, Yamamoto Y, Schuller BW (2021) Artificial intelligence internet of things for the elderly: From assisted living to health-care monitoring. IEEE Signal Process Mag 38(4):78–88. https://doi.org/10.1109/MSP.2021.3057298
Redner S (1998) How popular is your paper? An empirical study of the citation distribution. Eur Phys J B-Condens Matter Complex Syst 4(2):131–134. https://doi.org/10.1007/s100510050359
Sayago S (ed.) (2019) Perspectives on human-computer interaction research with older people. Switzerland: Springer International Publishing. https://doi.org/10.1007/978-3-030-06076-3
Schomakers EM, Ziefle M (2023) Privacy vs. security: trade-offs in the acceptance of smart technologies for aging-in-place. Int J Hum Comput Interact 39(5):1043–1058. https://doi.org/10.1080/10447318.2022.2078463
Schroeder T, Dodds L, Georgiou A, Gewald H, Siette J (2023) Older adults and new technology: Mapping review of the factors associated with older adults’ intention to adopt digital technologies. JMIR Aging 6(1):e44564. https://doi.org/10.2196/44564
Seibert K, Domhoff D, Bruch D, Schulte-Althoff M, Fürstenau D, Biessmann F, Wolf-Ostermann K (2021) Application scenarios for artificial intelligence in nursing care: rapid review. J Med Internet Res 23(11):e26522. https://doi.org/10.2196/26522
Seuwou P, Banissi E, Ubakanma G (2016) User acceptance of information technology: A critical review of technology acceptance models and the decision to invest in Information Security. In: Global Security, Safety and Sustainability-The Security Challenges of the Connected World: 11th International Conference, ICGS3 2017, London, UK, January 18-20, 2017, Proceedings 11:230-251. Springer International Publishing. https://doi.org/10.1007/978-3-319-51064-4_19
Shiau WL, Wang X, Zheng F (2023) What are the trend and core knowledge of information security? A citation and co-citation analysis. Inf Manag 60(3):103774. https://doi.org/10.1016/j.im.2023.103774
Sinha S, Verma A, Tiwari P (2021) Technology: Saving and enriching life during COVID-19. Front Psychol 12:647681. https://doi.org/10.3389/fpsyg.2021.647681
Soar J (2010) The potential of information and communication technologies to support ageing and independent living. Ann Telecommun 65:479–483. https://doi.org/10.1007/s12243-010-0167-1
Strotmann A, Zhao D (2012) Author name disambiguation: What difference does it make in author‐based citation analysis? J Am Soc Inf Sci Technol 63(9):1820–1833. https://doi.org/10.1002/asi.22695
Talukder MS, Sorwar G, Bao Y, Ahmed JU, Palash MAS (2020) Predicting antecedents of wearable healthcare technology acceptance by elderly: A combined SEM-Neural Network approach. Technol Forecast Soc Change 150:119793. https://doi.org/10.1016/j.techfore.2019.119793
Taskin Z, Al U (2019) Natural language processing applications in library and information science. Online Inf Rev 43(4):676–690. https://doi.org/10.1108/oir-07-2018-0217
Touqeer H, Zaman S, Amin R, Hussain M, Al-Turjman F, Bilal M (2021) Smart home security: challenges, issues and solutions at different IoT layers. J Supercomput 77(12):14053–14089. https://doi.org/10.1007/s11227-021-03825-1
United Nations Department of Economic and Social Affairs (2023) World population ageing 2023: Highlights. https://www.un.org/zh/193220
Valk CAL, Lu Y, Randriambelonoro M, Jessen J (2018) Designing for technology acceptance of wearable and mobile technologies for senior citizen users. In: 21st DMI: Academic Design Management Conference (ADMC 2018), Design Management Institute, pp 1361–1373. https://www.dmi.org/page/ADMC2018
Van Eck N, Waltman L (2010) Software survey: VOSviewer, a computer program for bibliometric mapping. Scientometrics 84(2):523–538. https://doi.org/10.1007/s11192-009-0146-3
Vancea M, Solé-Casals J (2016) Population aging in the European Information Societies: towards a comprehensive research agenda in eHealth innovations for elderly. Aging Dis 7(4):526. https://doi.org/10.14336/AD.2015.1214
Venkatesh V, Morris MG, Davis GB, Davis FD (2003) User acceptance of information technology: Toward a unified view. MIS Q 27(3):425–478. https://doi.org/10.2307/30036540
Wagner N, Hassanein K, Head M (2010) Computer use by older adults: A multi-disciplinary review. Comput Human Behav 26(5):870–882. https://doi.org/10.1016/j.chb.2010.03.029
Wahlroos N, Narsakka N, Stolt M, Suhonen R (2023) Physical environment maintaining independence and self-management of older people in long-term care settings—An integrative literature review. J Aging Environ 37(3):295–313. https://doi.org/10.1080/26892618.2022.2092927
Wang CL, Chen XJ, Yu T, Liu YD, Jing YH (2024a) Education reform and change driven by digital technology: a bibliometric study from a global perspective. Humanit Soc Sci Commun 11(1):1–17. https://doi.org/10.1057/s41599-024-02717-y
Wang CL, Dai J, Zhu KK, Yu T, Gu XQ (2023a) Understanding the Continuance Intention of College Students Toward New E-learning Spaces Based on an Integrated Model of the TAM and TTF. Int J Hum-comput Int 1–14. https://doi.org/10.1080/10447318.2023.2291609
Wang CL, Wang HM, Li YY, Dai J, Gu XQ, Yu T (2024b) Factors Influencing University Students’ Behavioral Intention to Use Generative Artificial Intelligence: Integrating the Theory of Planned Behavior and AI Literacy. Int J Hum-comput Int 1–23. https://doi.org/10.1080/10447318.2024.2383033
Wang J, Zhao W, Zhang Z, Liu X, Xie T, Wang L, Zhang Y (2024c) A journey of challenges and victories: a bibliometric worldview of nanomedicine since the 21st century. Adv Mater 36(15):2308915. https://doi.org/10.1002/adma.202308915
Wang J, Chen Y, Huo S, Mai L, Jia F (2023b) Research hotspots and trends of social robot interaction design: A bibliometric analysis. Sensors 23(23):9369. https://doi.org/10.3390/s23239369
Wang KH, Chen G, Chen HG (2017) A model of technology adoption by older adults. Soc Behav Personal 45(4):563–572. https://doi.org/10.2224/sbp.5778
Wang S, Bolling K, Mao W, Reichstadt J, Jeste D, Kim HC, Nebeker C (2019) Technology to Support Aging in Place: Older Adults’ Perspectives. Healthcare 7(2):60. https://doi.org/10.3390/healthcare7020060
Wang Z, Liu D, Sun Y, Pang X, Sun P, Lin F, Ren K (2022) A survey on IoT-enabled home automation systems: Attacks and defenses. IEEE Commun Surv Tutor 24(4):2292–2328. https://doi.org/10.1109/COMST.2022.3201557
Wilkowska W, Offermann J, Spinsante S, Poli A, Ziefle M (2022) Analyzing technology acceptance and perception of privacy in ambient assisted living for using sensor-based technologies. PloS One 17(7):e0269642. https://doi.org/10.1371/journal.pone.0269642
Wilson J, Heinsch M, Betts D, Booth D, Kay-Lambkin F (2021) Barriers and facilitators to the use of e-health by older adults: a scoping review. BMC Public Health 21:1–12. https://doi.org/10.1186/s12889-021-11623-w
Xia YQ, Deng YL, Tao XY, Zhang SN, Wang CL (2024) Digital art exhibitions and psychological well-being in Chinese Generation Z: An analysis based on the S-O-R framework. Humanit Soc Sci Commun 11:266. https://doi.org/10.1057/s41599-024-02718-x
Xie H, Zhang Y, Duan K (2020) Evolutionary overview of urban expansion based on bibliometric analysis in Web of Science from 1990 to 2019. Habitat Int 95:102100. https://doi.org/10.1016/j.habitatint.2019.10210
Xu Z, Ge Z, Wang X, Skare M (2021) Bibliometric analysis of technology adoption literature published from 1997 to 2020. Technol Forecast Soc Change 170:120896. https://doi.org/10.1016/j.techfore.2021.120896
Yap YY, Tan SH, Choon SW (2022) Elderly’s intention to use technologies: a systematic literature review. Heliyon 8(1). https://doi.org/10.1016/j.heliyon.2022.e08765
Yu T, Dai J, Wang CL (2023) Adoption of blended learning: Chinese university students’ perspectives. Humanit Soc Sci Commun 10:390. https://doi.org/10.1057/s41599-023-01904-7
Yusif S, Soar J, Hafeez-Baig A (2016) Older people, assistive technologies, and the barriers to adoption: A systematic review. Int J Med Inform 94:112–116. https://doi.org/10.1016/j.ijmedinf.2016.07.004
Zhang J, Zhu L (2022) Citation recommendation using semantic representation of cited papers’ relations and content. Expert Syst Appl 187:115826. https://doi.org/10.1016/j.eswa.2021.115826
Zhao Y, Li J (2024) Opportunities and challenges of integrating artificial intelligence in China’s elderly care services. Sci Rep 14(1):9254. https://doi.org/10.1038/s41598-024-60067-w
Article ADS MathSciNet PubMed PubMed Central Google Scholar
Download references
Acknowledgements
This research was supported by the Social Science Foundation of Shaanxi Province in China (Grant No. 2023J014).
Author information
Authors and affiliations.
School of Art and Design, Shaanxi University of Science and Technology, Xi’an, China
Xianru Shang, Zijian Liu, Chen Gong, Zhigang Hu & Yuexuan Wu
Department of Education Information Technology, Faculty of Education, East China Normal University, Shanghai, China
Chengliang Wang
You can also search for this author in PubMed Google Scholar
Contributions
Conceptualization, XS, YW, CW; methodology, XS, ZL, CG, CW; software, XS, CG, YW; writing-original draft preparation, XS, CW; writing-review and editing, XS, CG, ZH, CW; supervision, ZL, ZH, CW; project administration, ZL, ZH, CW; funding acquisition, XS, CG. All authors read and approved the final manuscript. All authors have read and approved the re-submission of the manuscript.
Corresponding author
Correspondence to Chengliang Wang .
Ethics declarations
Competing interests.
The authors declare no competing interests.
Ethical approval
Ethical approval was not required as the study did not involve human participants.
Informed consent
Informed consent was not required as the study did not involve human participants.
Additional information
Publisher’s note Springer Nature remains neutral with regard to jurisdictional claims in published maps and institutional affiliations.
Rights and permissions
Open Access This article is licensed under a Creative Commons Attribution-NonCommercial-NoDerivatives 4.0 International License, which permits any non-commercial use, sharing, distribution and reproduction in any medium or format, as long as you give appropriate credit to the original author(s) and the source, provide a link to the Creative Commons licence, and indicate if you modified the licensed material. You do not have permission under this licence to share adapted material derived from this article or parts of it. The images or other third party material in this article are included in the article’s Creative Commons licence, unless indicated otherwise in a credit line to the material. If material is not included in the article’s Creative Commons licence and your intended use is not permitted by statutory regulation or exceeds the permitted use, you will need to obtain permission directly from the copyright holder. To view a copy of this licence, visit http://creativecommons.org/licenses/by-nc-nd/4.0/ .
Reprints and permissions
About this article
Cite this article.
Shang, X., Liu, Z., Gong, C. et al. Knowledge mapping and evolution of research on older adults’ technology acceptance: a bibliometric study from 2013 to 2023. Humanit Soc Sci Commun 11 , 1115 (2024). https://doi.org/10.1057/s41599-024-03658-2
Download citation
Received : 20 June 2024
Accepted : 21 August 2024
Published : 31 August 2024
DOI : https://doi.org/10.1057/s41599-024-03658-2
Share this article
Anyone you share the following link with will be able to read this content:
Sorry, a shareable link is not currently available for this article.
Provided by the Springer Nature SharedIt content-sharing initiative
Quick links
- Explore articles by subject
- Guide to authors
- Editorial policies

- Library of Congress
- Research Guides
- Main Reading Room
Harriet Tubman: A Resource Guide
Introduction.
- Digital Resources
- External Websites
- Print Bibliography
Digital Collections : Ask a Librarian
Have a question? Need assistance? Use our online form to ask a librarian for help.
Chat with a librarian , Monday through Friday, 12-4pm Eastern Time (except Federal Holidays).
Author: Angela McMillian, Digital Reference Specialist, Researcher & Reference Services
Created: March 1, 2019
Last Updated: December 14, 2022
The digital collections of the Library of Congress contain a wide variety of material associated with Harriet Tubman. Over the course of 10 years, and at great personal risk, Tubman led hundreds of slaves to freedom along the Underground Railroad, a secret network of safe houses where runaway slaves could stay on their journey north to freedom. She later became a leader in the abolitionist movement, and during the Civil War she was a spy for the federal forces in South Carolina as well as a nurse.
This guide compiles links to digital materials related to Harriet Tubman such as documents, books, and images that are available from the Library of Congress. In addition, it provides links to recommended external websites focusing on Harriet Tubman and a bibliography containing selected works for both general and younger readers.

Benjamin F. Powelson, photographer. Portrait of Harriet Tubman. 1868 or 1869. Library of Congress Prints and Photographs Division.

Harriet Tubman, full-length portrait, seated in chair, facing front, probably at her home in Auburn, New York. 1911. Library of Congress Prints and Photographs Division.

Harvey B. Lindsley, photographer. Portrait of Harriet Tubman. Between ca. 1871 and 1876. Library of Congress Prints and Photographs Division.
- Next: Digital Resources >>
- Last Updated: Apr 17, 2024 2:36 PM
- URL: https://guides.loc.gov/harriet-tubman

COMMENTS
Questionnaires vs. surveys. A survey is a research method where you collect and analyze data from a group of people. A questionnaire is a specific tool or instrument for collecting the data.. Designing a questionnaire means creating valid and reliable questions that address your research objectives, placing them in a useful order, and selecting an appropriate method for administration.
The paper outlines a systematic approach to the research and writing process, covering key stages such as topic selection, preliminary research, formulating research questions, developing thesis ...
However, the quality and accuracy of data collected using a questionnaire depend on how it is designed, used, and validated. In this two-part series, we discuss how to design (part 1) and how to use and validate (part 2) a research questionnaire. It is important to emphasize that questionnaires seek to gather information from other people and ...
Self-administered paper questionnaires: Participants complete the questionnaire on paper, either in person or by mail. This mode is relatively low cost and easy to administer, but it may result in lower response rates and greater potential for errors in data entry. ... Research: Questionnaires are commonly used in research to gather information ...
Designing a Questionnaire for a Research Paper: A Comprehensive Guide to Design and Develop an Effective Questionnaire . Hamed Taherdoost . University Canada West, Vancouver, Canada . E-mail: [email protected] . Abstract - A questionnaire is an important instrument in a research study to help the researcher collect relevant data
The great popularity with questionnaires is they provide a "quick fix" for research methodology. No single method has been so abused. 1 Questionnaires offer an objective means of collecting information about people's knowledge, beliefs, attitudes, and behaviour. 2,3 Do our patients like our opening hours? What do teenagers think of a local antidrugs campaign and has it changed their attitudes?
10. Test the Survey Platform: Ensure compatibility and usability for online surveys. By following these steps and paying attention to questionnaire design principles, you can create a well-structured and effective questionnaire that gathers reliable data and helps you achieve your research objectives.
AJMS Vol.11 No.1 January-June 2022 10 Designing a Questionnaire for a Research Paper: A Comprehensive Guide to Design and Develop an Effective Questionnaire The main scaling types are as the following. commonly chosen between 5 to 7-point as this range is easily rescaled which can help to facilitate the comparisons. It can also be noted as a ...
Background: A questionnaire is a commonly used data collection method and is a very crucial part of the research. However, designing a questionnaire can be a daunting task for postgraduate students. Methods: This manuscript illustrates the various steps required in questionnaire designing and provides an insight into the essentials of questionnaire construction and validation.
1. Early questions should be easy and pleasant to answer, and should build rapport between the respondent and the researcher. 2. Questions at the very beginning of a questionnaire should explicitly address the topic of the survey, as it was described to the respondent prior to the interview. 3. Questions on the same topic should be grouped ...
Come up with a research question. It can be one question or several, but this should be the focal point of your questionnaire. Develop one or several hypotheses that you want to test. The questions that you include on your questionnaire should be aimed at systematically testing these hypotheses. 2.
Moreover, the quality of a survey is greatly dependent on the design of the questionnaire used. This editorial briefly outlines the process of development of a questionnaire in the context of the three survey-based studies published in this issue of the journal.[1,2,3] A questionnaire appears to be just a simple list of questions to the naive.
10 Research Question Examples to Guide your Research Project. Published on October 30, 2022 by Shona McCombes.Revised on October 19, 2023. The research question is one of the most important parts of your research paper, thesis or dissertation.It's important to spend some time assessing and refining your question before you get started.
A questionnaire is defined a market research instrument that consists of questions or prompts to elicit and collect responses from a sample of respondents. This article enlists 21 questionnaire templates along with samples and examples. It also describes the different types of questionnaires and the question types that are used in these questionnaires.
Guides to Survey Research. Managing and Manipulating Survey Data: A Beginners Guide; Finding and Hiring Survey Contractors; How to Frame and Explain the Survey Data Used in a Thesis; Overview of Cognitive Testing and Questionnaire Evaluation; Questionnaire Design Tip Sheet; Sampling, Coverage, and Nonresponse Tip Sheet; PSR Survey Toolbox
First, in terms of data collection, this study was obtained using a questionnaire method, that is, participants' self-report, which is the most common and popular method for quantitative research ...
Survey research uses a list of questions to collect data about a group of people. You can conduct surveys online, by mail, or in person. FAQ ... Sending out a paper survey by mail is a common method of gathering demographic information (for example, in a government census of the population).
Questionnaires are frequently used in quantitative marketing research and social research. A questionnaire is a series of questions asked to individuals to obtain statistically useful information ...
Survey research means collecting information about a group of people by asking them questions and analysing the results. To conduct an effective survey, follow these six steps: Determine who will participate in the survey. Decide the type of survey (mail, online, or in-person) Design the survey questions and layout. Distribute the survey.
Thus, the questionnaire-based research was criticized by many in the past for being a soft science. The scale construction is also not a part of most of the graduate and postgraduate training. ... Greenlaw C, Brown-Welty S. A comparison of web-based and paper-based survey methods: Testing assumptions of survey mode and response cost. Eval Rev ...
Questionnaires vs surveys. A survey is a research method where you collect and analyse data from a group of people. A questionnaire is a specific tool or instrument for collecting the data.. Designing a questionnaire means creating valid and reliable questions that address your research objectives, placing them in a useful order, and selecting an appropriate method for administration.
Learn the importance of research questions in college writing, how to create strong ones, and tips to keep your research focused and on track. ... which I can't use to cite in my paper as a credible source, but it is a great place to start for ideas. And in this article, I found this really interesting quote that tells me that even though ...
Survey research is sometimes regarded skeptically as a box-ticking exercise that eschews the nuances of people's perceptions and experiences. But precisely because the format is so constraining, developing a survey can stimulate constructive confrontation with theoretical challenges. If a survey includes the question "why did you migrate ...
The exposure of scientific scandals and the increase of dubious research practices have generated a stream of studies on Questionable Research Practices (QRPs), such as failure to acknowledge co-authors, selective presentation of findings, or removal of data not supporting desired outcomes. In contrast to high-profile fraud cases, QRPs can be investigated using quantitative, survey-based ...
A good research question is essential to guide your research paper, dissertation, or thesis. All research questions should be: Focused on a single problem or issue. Researchable using primary and/or secondary sources. Feasible to answer within the timeframe and practical constraints. Specific enough to answer thoroughly.
PMB has taught research methods in a primary care setting for the past 13 years, specialising in practical approaches and using the experiences and concerns of researchers and participants as the basis of learning. This series of papers arose directly from questions asked about real questionnaire studies.
The rapid expansion of information technology and the intensification of population aging are two prominent features of contemporary societal development. Investigating older adults' acceptance ...
The digital collections of the Library of Congress contain a wide variety of material associated with Harriet Tubman. Over the course of 10 years, and at great personal risk, Tubman led hundreds of slaves to freedom along the Underground Railroad, a secret network of safe houses where runaway slaves could stay on their journey north to freedom.
An online survey was completed by 162 primary caregivers of children and youth with Down syndrome. ... care from family and friends (62.8%), assistive technology (58.3%), and floortime (55.6%). Future research should focus on understanding the process of treatment selection by caregivers of children with Down syndrome and develop accessible ...