- Submit News
- Purchase Webinar Listing

ArchShowcase
- Submit New Showcase
- Submit New Event
- Submit New Video
- Submit New YouTube Video
- AECCafe Media Kit
- Banner Ad Specifications
- eMail Blast Specifications
Back to 'AECCafe Blogs'
Subscribe to aeccafe.

Submit Your Project
Recent posts.
- Houbu Ancient Village Cafe Post Station Suzhou, Jiangsu , China by Parallect Design
- Departamento R&J,Monterrey, Mexico by López Duplan Arquitectos
- Tongrentang Health Live Broadcast Base , by WUUX Architecture Design Studio
- City of London Insurance Company Offices, LondonUK by Align Design and Architecture
- Financial Investment Company Headquarters, San Francisco, California, USA By TEF Design
- Entries RSS
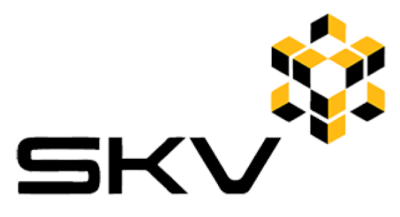
- Turnkey Solutions
- General Contracting
- SKV 360 Virtual Tours
- Become a partner
Workspace Exuding Luxury through utility : The New Workspace for Droom
Case study 1.
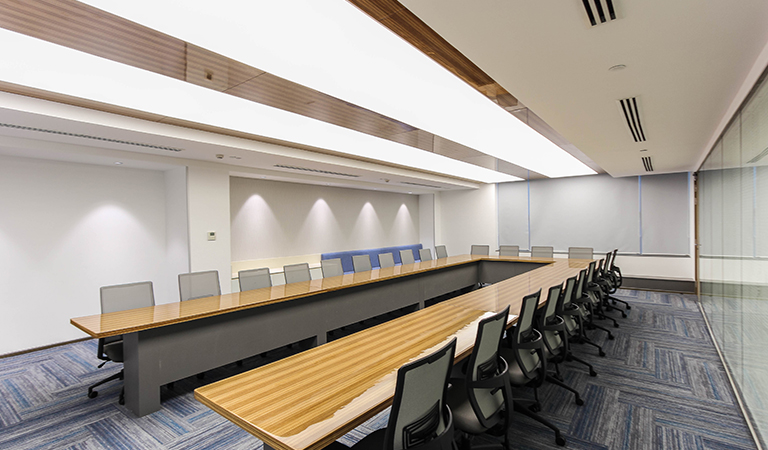
The office space of a company can play an integral role in building the right relationship with its employees, partners, and clients. Integrating the superstructures of ideology and thought process into everyday workings, it can become a tool for the company to increase productivity, further innovation, and build culture. The same reflects in the workspace
Workspace Aesthetics: The Future Of Happy Workplace , A case study on successful execution for the new workspace for Saif Partners
Case study 2.
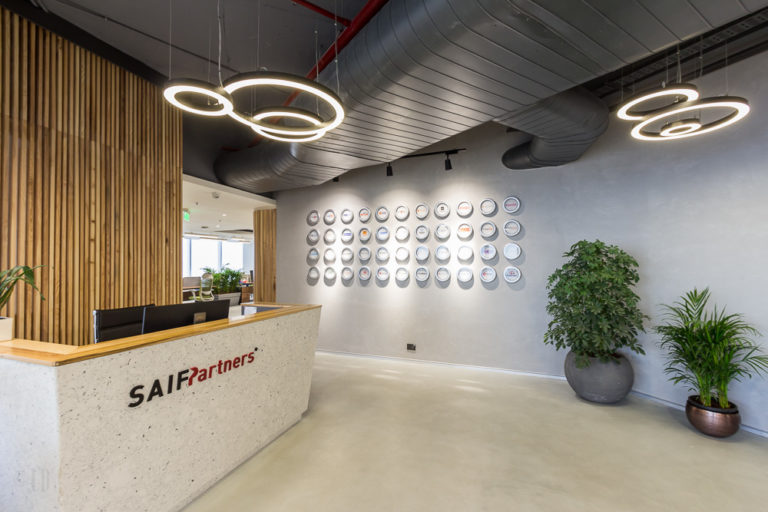
Set up in true grandeur of luxury and ethos, this workplace is a masterpiece in versatility. The office of Saif Partners, located in the vicinity of Gurugram, is designed by Alka Vernekar, Meraki Studio & executed by SKV (Studiokon Ventures Pvt. Ltd.). The workspace is dominated by natural sunlight entering into the glass facade that
Decoding the Ideal Work-space for Millennials! A case study on the successful completion of the DMI Finance new office in Delhi
Case study 3.
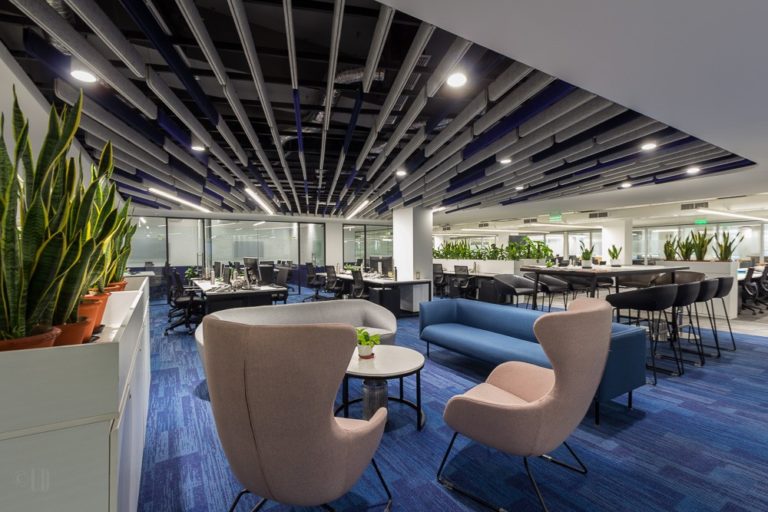
Work-space design is nowadays intended to foster employers’ relationships and engagements. After all, it is the only place where people spend most of their time and hence, it becomes influential if the space around them motivates and comforts them amidst all the stress of meetings and work deadlines.Nestled in one of the most commercially well-developing
Workspace for DCM
Case study 4.
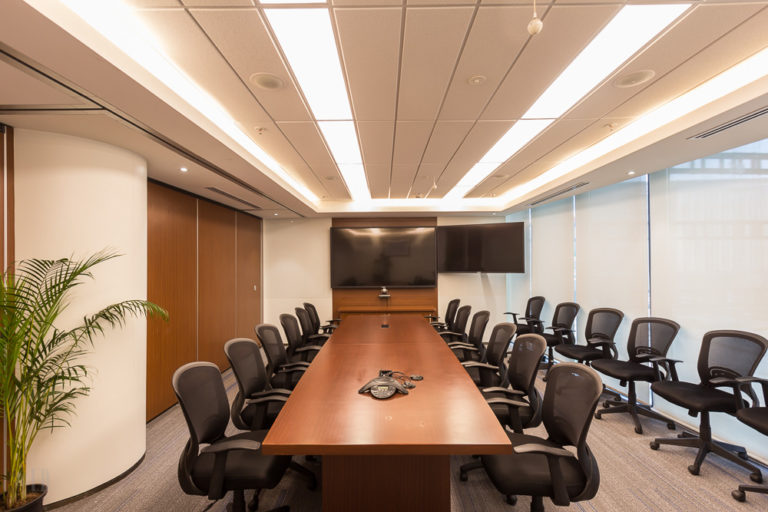
Nestled in the heart of Aerocity, New Delhi, DCM is integrated into three separate working spaces, two of which are located in separate blocks. he primary office space, named as WorldMark 1 is a 14500 Square feet leisure-workspace, aimed to accommodate workers looking for a well-designed, in style workplace that could offer a bespoke experience.
Get A Call x
chat with us
- Login / Register
- You are here: India
‘The office complex in New Delhi by Raj Rewal appears as a fortress in the landscape’
19 May 1995 By Paul Joubert India
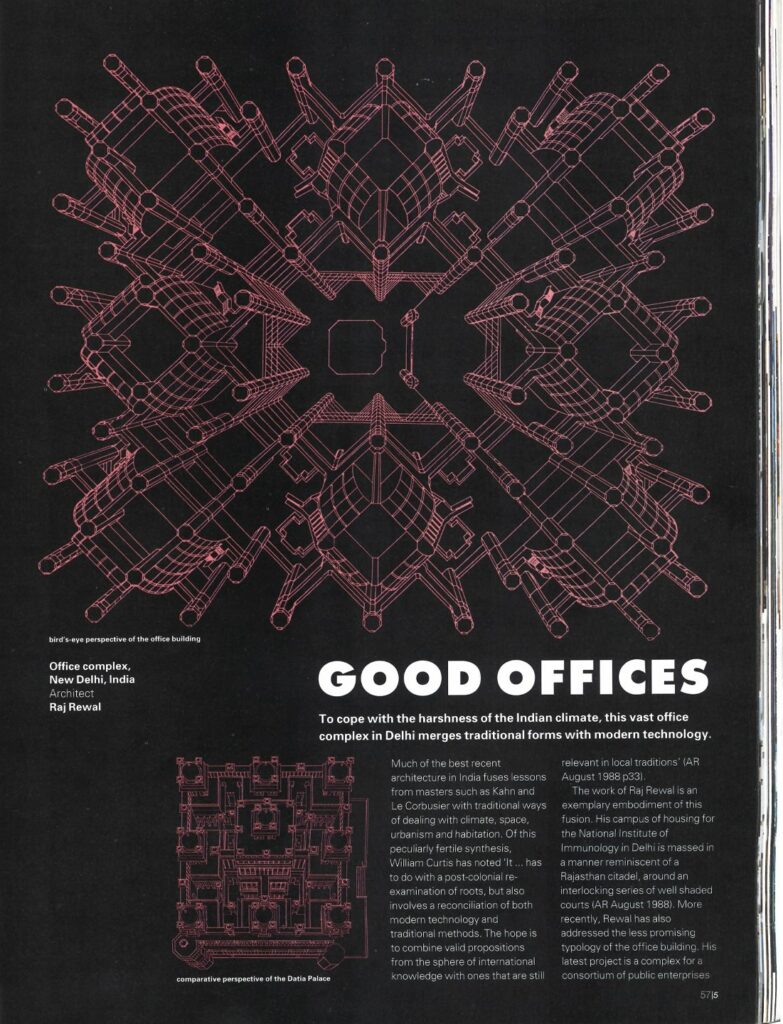
To cope with the harshness of the Indian climate, this vast office complex in Delhi merges traditional forms with modern technology.
Originally published in AR May 1995, this piece was republished online in May 2016
Much of the best recent architecture in India fuses essons from masters such as Kahn and Le Corbusier with traditional ways of dealing with climate, space, urbanism and habitation. Of this peculiarity fertile synthesis, William Curtis has noted it… has to do with a post-colonian re-examination of roots, but also involves a reconciliation of both modern technology and traditional methods. The hope is to combine valid propositions from the sphere of international knowledge with ones that are still relevant in local traditions (AR August 1988 p33).
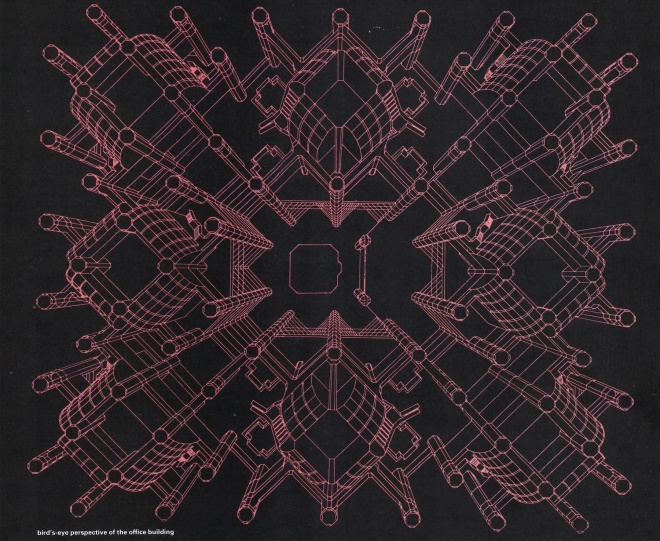
The work of Raj Rewal is an exemplary embodiment of this fusion. His campus of housing for National Institute of Inmunology in Delhi is massed in a manner reminiscent of a Rajasthan citadel, around an interlocking series of well shaded courts (AR August 1988). More recently, Rewal has also addressed the less promising typology of the office building. His latest project is a complex for a consortium of public enterprises that require the usual working and administrative spaces, but also share common facilities such as an auditorium, seminar rooms and a restaurant. The site is in a newly redeveloped office zone for government organisations on the outskirts of South Delhi. Barely a quarter of a mile away are historic sites containing the Hamayun Tomb and the Khankhanan.
‘The influence of historic Indian structures is also apparent in the umbrella-like viewing towers’
Planning stipulations in respect of height and size of floor plates established the precedent of a densely built form, that Rewal has courageously strived to humanize in various ways. The great amorphous mass of office accommodation (housing an astonishing 7000 workers) has been subdivided into a more manageable composition of eight blocks. These interlock around a central courtyard - a traditional Indian device - that provides diffuse natural light and ventilation. Although part of a much greater whole, each block is regarded as an individual administrative and social unit, so that workers can identify with their particular territory. Blocks are arranged around a central circulation and services core. At the top of each block is a cafeteria enclosed by segments of barrelvault. Intended to provide a welcome respite for workers, these roof-top eyries are approached through open roof gardens, and afford splendid views of the nearby monuments.
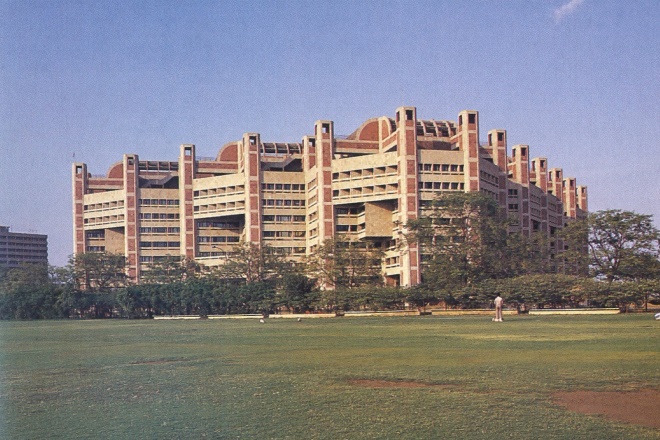
The influence of historic Indian structures (such as the palace of Datia) is also apparent in the umbrella-like viewing towers or chatris that articulate the roofline. Yet although the building sits like a fortress in the landscape, it has a rich and complex inner realm, offering the potential for fruitful colonisation by its inhabitants. Particular attention has been paid to ways in which the building form can temper the unremitting harshness of the climate. On the external perimeter, the upper floors overhang to create deep shadows. Throughout, windows are deeply recessed to shield the interior from the sun’s glare and give the building an almost sculptural articulation.
‘With its complex vocabulary of interacting courts the entire ensemble exhibits all the density and diversity of a traditional city’
On the courtyard side, the floors are recessed at different levels to create a series of stepped, protected terraces.The structural system is based on a basic module of a 12 m square slab supported at each corner by a cluster of four columns. The column clusters (which contain services and air-conditioning ducts) are expressed on the facade in the manner of structural minarets. Each cluster terminates in a chatri and the contrasting pink and beige bands of masonry recall ancient Mogul geometries. With its complex vocabulary of interacting courts, galleries, terraces and platforms, the entire ensemble exhibits all the density and diversity of a traditional city yet this prodigious anthill of activity is resolutely ordered around humane and civilizing principles.
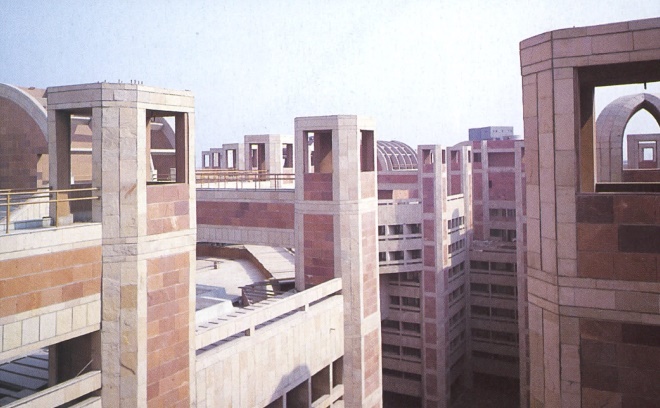
Since 1896, The Architectural Review has scoured the globe for architecture that challenges and inspires. Buildings old and new are chosen as prisms through which arguments and broader narratives are constructed. In their fearless storytelling, independent critical voices explore the forces that shape the homes, cities and places we inhabit.
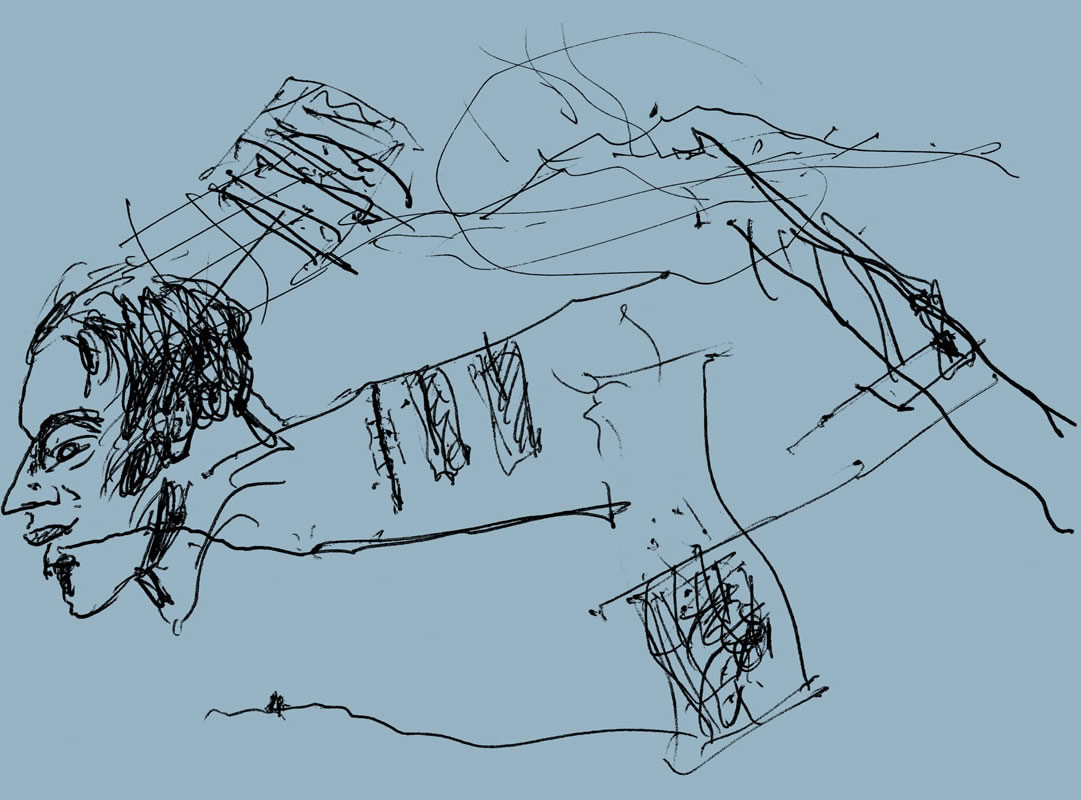
Join the conversation online

Examples of Climate responsive architecture in Indian cities

Nature is facing the brunt of human actions. The consequences of said actions have given rise to problems that affect the world of design either directly or indirectly. Climate change is one such issue. It is not a foreign concept as it is one of the greatest challenges faced by human society. Architects themselves have begun focusing on creating structures that have a lesser impact on the environment. Climate responsive architecture is one such design practice that functions in adherence to the climate of the location it is in. Its application in design reflects the weather conditions of the location the structure is built in and reduces its dependence on artificial energy.
India is home to a variety of climatic regions and has its own share of energy-efficient and climate responsive designs. The climate of India can be segregated into 6 climatic zones: Hot and Dry, Warm and Humid, Moderate, Cold and Sunny, Cold and Cloudy, and Composite. Based on the climatic zones, architecture and design elements have gone through various modifications to create comfortable spaces.
Here are a few interesting examples of climate-responsive architecture that is found in Indian cities.
1. Solar Passive Hostel, University of Jodhpur (Hot and Dry)
The structure was part of the research project undertaken by the Centre of Energy Studies, IIT Delhi. Designed by architect Vinod Gupta, the building was an attempt to test as well as exhibit methods that would provide thermal comfort in the hot and dry climate of Rajasthan. The building houses 14 double room suites as accommodation for married students. The suites are split between the first and ground floor, which is partially sunk into the ground to take advantage of the natural insulation of earth.
Stonemasonry, the local material was used for the walls as it helps balance out temperature variations. The roof was treated with small inverted terracotta pots to combat the summer heat gain. A wind tower that connected both floors supplied cool air to the units and helped aid ventilation by expelling hot air during the evenings and night. The design makes use of favorable orientation, massing, external finishes and elements such as deep sunshades to create a structure that not only conserves energy but also makes use of passive methods to the best of its ability.

2. Silent Valley, Kalasa (Warm and Humid)
Designed by architect K. Jaisim, the Silent Valley Resort is an eco-friendly holiday home that consists of several cottages that were constructed with a herbal theme. The resort fuses modern demands with ecological balance by creating a sustainable habitat with a minimum footprint. The cottages adopt circular plans as well as forms, which helps reduce solar gains from the building envelope and windows.
The usage of materials like solid mud blocks, timber, and Mangalore tiles as well as the position of the cottage i.e partially sunk into the ground further help in aiding energy efficiency. The resort merges with the natural landscape and adopts sustainable measures such as water harvesting, biomass, energy-intensive spaces, etc.

3. TERI SRC Building, Bangalore (Moderate)
The Energy and Resources Institute was designed by architect Sanjay Mohe. Set in a location with a moderate climate, the design depicts an interplay of the five elements of nature i.e. air, water, earth, sky, and fire (sun) with the built form to attain aural, thermal, and visual comfort. The simplest measure taken to tackle the climatic conditions was the orientation of the building, which is along the east-west axis.
While the building opens up towards the northern wall and takes advantage of glare-free light, the south walls allow the breeze to flow over the building. To minimize heat gain from the roof, insulation techniques that consisted of filler slabs and roof gardens were applied. The fenestrations and integration of atriums were designed after detailed studies to minimize the usage of artificial lighting in a day. The air chimneys help enhance the convection currents in the building. An efficient rainwater system also helps preserve water, which in turn is used for landscaping as well as in the toilets.

4. Degree College and Hill Council Complex, Leh (Cold and Sunny)
Designed by Arvind Kishan & Kunal Jain, the institution is built on a rectangular site that is along the north-south direction and is surrounded by snow-capped ranges on all sides. The individual structures are oriented in various directions and treated specifically based on their orientations. However, the buildings on the north side of the complex have thick walls to minimize heat loss and those on the south side maximize heating and daylight distribution.
The openings have been maximized to tap into natural light sources and the double glazing helps control loss of heat without condensation. Ventilation is achieved through a connective loop activated by buoyancy and it is also coupled with cross ventilation through the eastern-western fenestration. The usage of Trombe walls, glass, and insulation on the roof allows the building to attain internal thermal comfort based on the conditions outside.

5. Residence of Mohini Mullick, Nainital (Cold and Cloudy)
The residence is an example of traditional hill architecture and maximizes solar energy to meet its operational needs. The structure is load-bearing in nature and has a timber-framed roof. While the compact shape of the cottage reduces heat loss, the living spaces on the south maximize solar gain. The walls are made of thick random rubble. The roof system is clad with local stone and insulated with rock wool.

6. Monama House, Hyderabad (Composite)
The underlying ideology behind the design was to generate minimum environmental impact. Due to the high solar radiation and ambient temperature in the summer, the windows on the west wall were made smaller or replaced with other solutions. The openings are oriented specifically to attain continuous ventilation after careful consideration of pressure differences and wind direction.
The open plan allows full movement of air throughout with little to no internal resistance. Evaporative cooling is attained with a system that consists of a water pond and a fan. The structure also reduces energy load whenever possible, and reverts to renewable sources to attain energy efficiency.

With a notebook and pen in her bag and an arsenal of questions in her mind, Arathi Biju has always had a keen interest in telling a story. Currently pursuing her degree in architecture, she has always been a strong advocate of expression be it through art, architecture or words.

20 Innovative Rising Indian Architectural Firms

Places to visit in Beirut for the Travelling Architect
Related posts.

Luxury Housing and Sustainable Materials

The Interplay of Form and Function: Exploring Artistic Elements in Architectural Design

Building Connections: The Integral Role of Architecture in Human Existence and Community

Visionary Horizons: A Girl’s Journey through the Future of Architecture in 2060

Cities: A Chronicle in Concrete and Steel

Sustainable Design Practices for Mixed-Use Public Spaces
- Architectural Community
- Architectural Facts
- RTF Architectural Reviews
- Architectural styles
- City and Architecture
- Fun & Architecture
- History of Architecture
- Design Studio Portfolios
- Designing for typologies
- RTF Design Inspiration
- Architecture News
- Career Advice
- Case Studies
- Construction & Materials
- Covid and Architecture
- Interior Design
- Know Your Architects
- Landscape Architecture
- Materials & Construction
- Product Design
- RTF Fresh Perspectives
- Sustainable Architecture
- Top Architects
- Travel and Architecture
- Rethinking The Future Awards 2022
- RTF Awards 2021 | Results
- GADA 2021 | Results
- RTF Awards 2020 | Results
- ACD Awards 2020 | Results
- GADA 2019 | Results
- ACD Awards 2018 | Results
- GADA 2018 | Results
- RTF Awards 2017 | Results
- RTF Sustainability Awards 2017 | Results
- RTF Sustainability Awards 2016 | Results
- RTF Sustainability Awards 2015 | Results
- RTF Awards 2014 | Results
- RTF Architectural Visualization Competition 2020 – Results
- Architectural Photography Competition 2020 – Results
- Designer’s Days of Quarantine Contest – Results
- Urban Sketching Competition May 2020 – Results
- RTF Essay Writing Competition April 2020 – Results
- Architectural Photography Competition 2019 – Finalists
- The Ultimate Thesis Guide
- Introduction to Landscape Architecture
- Perfect Guide to Architecting Your Career
- How to Design Architecture Portfolio
- How to Design Streets
- Introduction to Urban Design
- Introduction to Product Design
- Complete Guide to Dissertation Writing
- Introduction to Skyscraper Design
- Educational
- Hospitality
- Institutional
- Office Buildings
- Public Building
- Residential
- Sports & Recreation
- Temporary Structure
- Commercial Interior Design
- Corporate Interior Design
- Healthcare Interior Design
- Hospitality Interior Design
- Residential Interior Design
- Sustainability
- Transportation
- Urban Design
- Host your Course with RTF
- Architectural Writing Training Programme | WFH
- Editorial Internship | In-office
- Graphic Design Internship
- Research Internship | WFH
- Research Internship | New Delhi
- RTF | About RTF
- Submit Your Story
Looking for Job/ Internship?
Rtf will connect you with right design studios.

Additional study space available; impeded access to KSL: May 3, 2024
To the Case Western Reserve community,
We are aware that access to Kelvin Smith Library may be impeded, especially for those who use wheelchairs and other assistive devices. To address these concerns as well as provide an additional quiet study space, we are opening Adelbert Gym from 6 p.m. to 6 a.m. daily. The university has set up study areas and enhanced WiFi connectivity in this air-conditioned space. Like all buildings on campus, it will require a CWRU ID to enter.
As a reminder, yesterday we adjusted hours to provide alternate study options, with the closure of Tinkham Veale University Center and Thwing Center after 6 p.m.:
- Leutner Commons’ Division of Student Affairs room and Lower-Level Fireside Lounge will be open 24/7, beginning Friday.
- In Fribley Commons, Elephant Step Inn’s late-night seating area and second floor lounges will have 24/7 access, beginning Friday.
- The Biomedical Research Building cafeteria will remain open until midnight.
- Shuttles will be available throughout the week, 7 a.m. to 11 p.m., to transport to and from Samson Pavilion. As always, Safe Ride is available until 3 a.m. each night.
I appreciate that these interim safety measures may be inconvenient in the midst of final exams, but the safety of our community must be our highest priority.
Eric W. Kaler President
Deep learning for prediction of energy consumption: an applied use case in an office building
- Open access
- Published: 30 April 2024
Cite this article
You have full access to this open access article
- Roberto Morcillo-Jimenez ORCID: orcid.org/0000-0002-8771-5636 1 ,
- Jesús Mesa 1 ,
- Juan Gómez-Romero 1 ,
- M. Amparo Vila 1 &
- Maria J. Martin-Bautista 1
256 Accesses
1 Altmetric
Explore all metrics
Non-residential buildings are responsible for more than a third of global energy consumption. Estimating building energy consumption is the first step towards identifying inefficiencies and optimizing energy management policies. This paper presents a study of Deep Learning techniques for time series analysis applied to building energy prediction with real environments. We collected multisource sensor data from an actual office building under normal operating conditions, pre-processed them, and performed a comprehensive evaluation of the accuracy of feed-forward and recurrent neural networks to predict energy consumption. The results show that memory-based architectures (LSTMs) perform better than stateless ones (MLPs) even without data aggregation (CNNs), although the lack of ample usable data in this type of problem avoids making the most of recent techniques such as sequence-to-sequence (Seq2Seq).
Graphical abstract
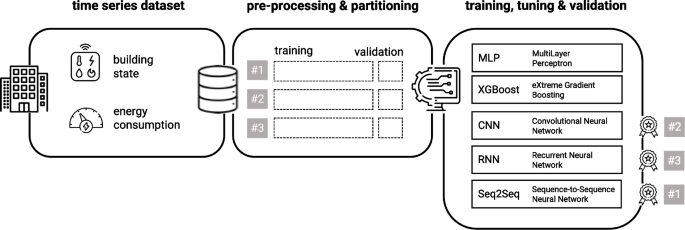
Similar content being viewed by others
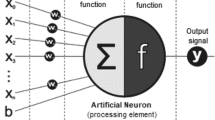
Deep Learning: A Comprehensive Overview on Techniques, Taxonomy, Applications and Research Directions
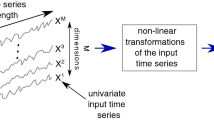
Deep learning for time series classification: a review
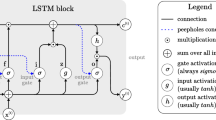
A review on the long short-term memory model
Avoid common mistakes on your manuscript.
1 Introduction
The current unstable situation in Europe has led to a decrease in the amount of energy supplied to the European continent, causing an exorbitant increase in prices across the continent. It is therefore necessary to reduce energy consumption in general, to prevent inflation from increasing further and the energy crisis from continuing to grow. Our work focuses on increasing energy efficiency in office buildings, as this is one of the most energy intensive areas due to the number of hours they are in operation. Reducing energy consumption by controlling consumption through algorithmic artificial intelligence techniques is one of the goals of our society in the coming years and our case study.
According to [ 26 ], residential, office, and industrial buildings account for 20-40% of global energy consumption. In 2010 in the USA, buildings energy consumption accounted for 41% of their total energy consumption, with 75% of this energy coming from fossil fuels [ 17 ]. Meanwhile, in 2012 in the European Union, buildings consumed approximately 40% of the total energy used [ 17 ]. More than two-thirds of the energy consumed by buildings goes to heating systems (37%), water heating (12%), air conditioning (10%) and lighting (9%). Several case studies have shown that the operational phase is the most energy-consuming stage of the buildings life cycle, accounting for 90% in conventional buildings and 50% in low-energy buildings [ 29 ].
In the current context in Europe, it is important to reduce energy consumption due to the high prices being achieved in the various energy markets. It is not surprising that interest in improving the energy efficiency of buildings has increased. According to [ 6 ] and [ 4 ], this interest is driven by three factors: rising energy prices, increasingly restrictive environmental regulations and increased environmental awareness among citizens. All over the world, public policies are being developed to increase this efficiency, as reflected in the Unsustainable Development Goals and the European Green Deal.
One of the first steps towards improving building energy efficiency is studying how consumption happens and knowing the factors that significantly impact it. In particular, predicting energy consumption from historical data allows building operators and managers to anticipate peaks in energy demand, modify building uses to shift this demand and plan equipment operation appropriately. Likewise, accurate consumption prediction allows assessing the improvement in building performance when making improvements or implementing new energy policies by comparing the actual consumption with the estimated consumption (or baseline). Data Science has emerged as an effective tool to address these objectives [ 21 ].
In the literature, there can be found numerous proposals for estimating energy consumption in buildings throughout time series prediction. Traditionally, these approaches were based on numerical regression or moving average models, which have limitations regarding multivariate series and series with changing trends. In contrast, modern machine learning methods based on neural networks have shown more effective in those scenarios [ 34 ]. However, the application of these techniques is often hampered by the noisy and incomplete nature of building energy data in real environments [ 9 ], which results in a gap between theoretical and practical works.
The rationale behind this work is to perform a comprehensive evaluation of neural network methods in a real-world scenario and to draw conclusions for practical application in similar contexts. More specifically, this paper studies the accuracy of several methods for heating consumption prediction, namely XGBoost, MLPs, RNNs, CNNs and Seq2Seq. The need and impact of preprocessing, which is applied to remove noisy and missing values and for data reduction, is also discussed.
The dataset was collected in the ICPE office building located in Bucharest, one of the pilot buildings considered in the Energy IN TIME Footnote 1 . Our starting hypothesis is that modern neural network techniques improve the performance of other approaches, and within them, memory-based architectures (RNNs, Seq2Seq) are superior. The results show that the hypothesis holds, despite the risk of overfitting these techniques when applied to not very large datasets.
The remainder of this paper is structured as follows. We first provide a review of related works on prediction of building energy consumption (Section 2 ). Next, we describe the data used in the study (Section 3 ), the methodology (Section 4 ), and the experimentation (Section 5 ). At the end of the paper conclusions and directions for future research work (Section 6 ) will be exposed.
2 Related work
Energy consumption forecasting models can be differentiated into categories based on their respective energy end-uses, such as cooling, heating, space heating, primary, natural gas, electricity, and steam load consumption [ 35 ]. Regarding the application of the models, [ 33 ] identified two primary categories: (1) model-based control, demand response, and optimization of energy consumption in buildings; and (2) design and modernization of building parameters, including energy planning and assessing the impact of buildings on climate change.
Also in [ 33 ], numerous factors impacting energy consumption were also identified, mainly the number and type of buildings under consideration. The temporal horizon of the prediction and the resolution of the sensor data are also relevant. Remarkably, natural time-based groupings (i.e., hours, days, months, and years) were proved superior in [ 35 ] to the more generic short-, medium-, and long-term ranking schemes proposed in [ 1 ].
Many data-driven techniques have proved effective for estimating building energy consumption, ranging from classical statistical regression to modern deep learning architectures. Regarding the former, [ 15 ] evaluated four models incorporating exogenous inputs, specifically autoregressive moving average models with exogenous inputs (ARMAX). [ 10 ] proposed a system utilizing a seasonal autoregressive integrated moving average model (SARIMA) and a least squares support vector regression model based on firefly metaheuristic algorithms (MetaFA-LSSVR). The prediction system yielded highly accurate and reliable day-ahead predictions of building energy consumption, with an overall error rate of 1.18%.
Another interesting study is [ 19 ], which investigated two stochastic models for short-term time series prediction of energy consumption, namely the conditional constrained Boltzmann machine (CRBM) and the factored conditional constrained Boltzmann machine (FCRBM). In the comparison, the results showed that the FCRBM outperformed the artificial neural network, support vector machine, recurrent neural networks and CRBM. The work was extended to include a deep belief network with automated feature extraction for the short-term building energy modeling process [ 20 ]. Other relevant work applying classical learning techniques is [ 24 ], which used a decision tree method (C4.5). In addition to obtaining accurate results, this algorithm was able to identify the factors contributing to building energy use. A sophisticated regression tree algorithm (Chi-Square Automatic Interaction Automatic Detector) was used in [ 11 ] to predict short-term heating and cooling load.
Raza and Khosravi [ 28 ] provided a comprehensive review of artificial intelligence-based load demand forecasting techniques for smart grids and buildings. The authors explored various machine learning algorithms used in load forecasting, including artificial neural networks, fuzzy logic, genetic algorithms, and support vector regression. [ 3 ] also reviewed the applications of artificial neural networks (ANNs) and support vector machines (SVMs) for building electrical energy consumption forecasting. The authors compared the performance of ANNs and SVMs with traditional statistical methods used in energy forecasting. [ 30 ] explored the different ML algorithms used in the field, including artificial neural networks, support vector regression, decision trees, and clustering methods. They also discussed the challenges associated with accurate data acquisition and modelling and the limitations of different ML algorithms. More recently, [ 14 ] emphasized that applying machine learning and statistical analysis techniques can lead to significant energy savings and cost reductions. [ 37 ] examined the advantages and limitations of different ML algorithms, including artificial neural networks, decision trees, and support vector machines, and explore their application in different building load prediction scenarios. [ 27 ] proposed using deep recurrent neural networks (DRNNs) for predicting heating, ventilation, and air conditioning (HVAC) loads in commercial buildings. The authors describde the architecture and training process of the DRNN model, which included a combination of convolutional neural networks (CNNs) and long short-term memory (LSTM) layers.
Finally, other works showed the importance of preprocessing in building energy forecasting, e.g., data cleaning and feature selection For instance, [ 2 ] reviewed the current development of machine learning (ML) techniques for predicting building energy consumption and discussed the challenges associated with data acquisition, feature selection, and model validation.
Table 1 summarises the related works mentioned in this section.
Our data was collected from the ICPE building (Institute of Technologies for Sustainable Development) in Bucarest (Romania). This is a three-building, each one divided into areas. For the experiments, we defined a pilot zone through a transversely cut of the building covering three areas (D1, D2, D5/2) of the three floors (see Figs. 1 , 2 ).
The building is equipped with sensors to measure room temperatures and meters measuring electricity, heating, and water consumption in distinct zones of the building. We focused on predicting heating meter values, given the higher contribution of this subsystem to the total energy consumption. Due to the low temperatures in these countries, buildings are powered by a system called district heating, which is used to prevent pipes from freezing outside, and then energy is consumed inside the building to raise the temperature of the water before it is distributed to the radiators inside.
We considered as prediction targets the three heating meters that respectively cover the area D1, D2 and D5/2 from buildings. However, we found a problem with area D2 data was not available because of errors in sensors, we only selected zone D1 and zone D5/2 of our building. The variables that we select for the D1 zone of our building are H-F123-D1, where all the heating consumption of the D1 zone in the floor 1, 2, and 3 are grouped, and for the D5/2 zone are H-F123-D5/2W and H-F123-D5/2E, where the heating consumption are grouped as the previous one, both for the West zone and for the East zone. We have other external variables that are related to our building, these variables are the outdoor temperature and the building occupancy, which are crucial for our experiments.
We collected data in 2017 before the implementation of the new control system. We focused on heating, and only the cold season (January, February and March) was considered in the experimentation. We re-sampled all variables at 15-minute intervals and calculated the cumulative consumption values. As a result, we obtained a data set with 8640 samples. The dataset is not publicly available but an excerpt can be obtained on demand.
4 Methodology
The methodological approach of the experiments followed the workflow of the Data Science process applied to energy data presented in [ 21 ]. As shown in Fig. 3 , we retrieved the data through an API REST and carried out data preprocessing, including outliers removal, missing value imputation, normalization and feature selection. Afterwards, we continued splitting these data by months to apply the learning algorithms and get the best prediction models for each period. Next, we describe how data is partitioned, as well as the parameters used to train the models and the evaluation metrics.
4.1 Definition of the prediction problem and data partition
The problem we aim to solve is to predict the energy usage of a building for the next 12 hours. We need to partition the original dataset into training and validation samples in chronological order to train our model. Given the different energy consumption patterns at the beginning and the end of the data collection period, we decided to split the full dataset into smaller chunks (namely, subproblems) and then make the training and validation partitions within each one. This implies that we have a different model trained specifically for each subproblem, which must be conveniently selected during the test phase to calculate the predictions depending on the date of the observations.
To perform the splits, we applied time series decomposition to analyze the evolution of the energy consumption variables and identify potential cut points, focusing on trend change. This study, which is explained below, showed that we could safely split the data in months since the time series have consistent behaviour in each of these periods. Furthermore, a preliminary analysis of the stability of the training, in terms of errors obtained with slightly different splits, showed that the differences were not significant. While this is a rough approximation of a more fine-grained splitting, it has the advantage of facilitating the selection of the model to be applied for prediction. It remains for future work to apply a more sophisticated time series splitting algorithm [ 18 ] and a more comprehensive analysis of the impact of the splitting.
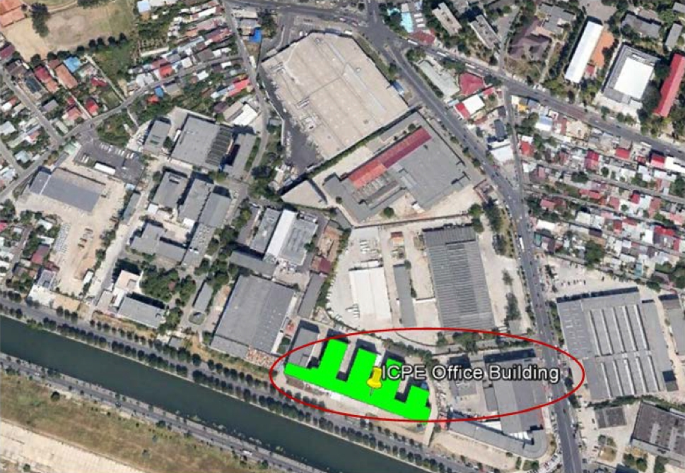
Aerial view
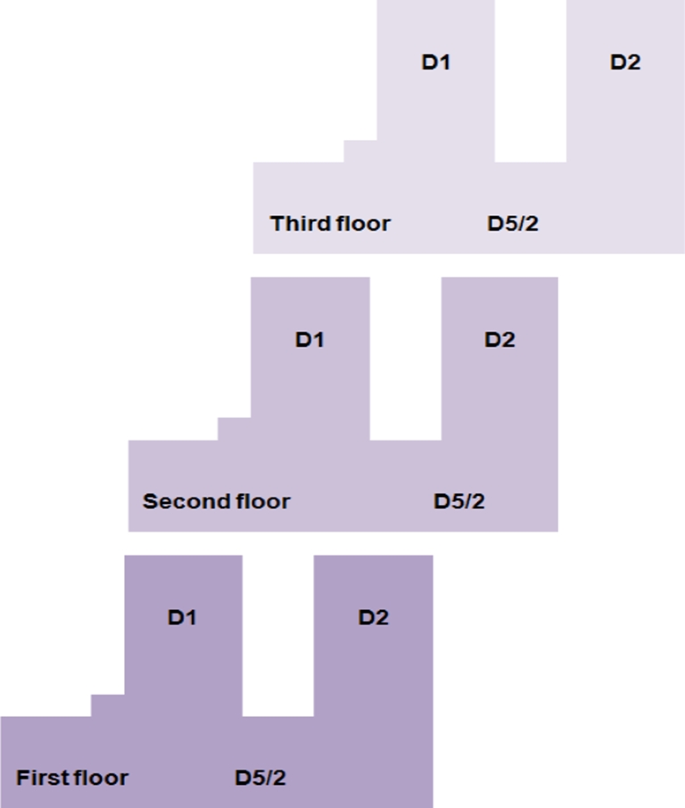
The analysis of the trend change to assess the monthly splitting was based on the decomposition of the time series with STL technique (Seasonal-Trend decomposition using LOESS), which has been previously used for energy demand time series [ 25 ]. Figure 4 depicts the decomposition of the heating consumption variables into three components: trend, i.e., long-term evolution of values; seasonality, i.e., repetitions in fixed periods; and irregularity, i.e., random patterns that remain after removing the other two components. It can be seen that there is a clear pattern of increasingly high consumption in January, followed by a steady decline in consumption in February and a plateau in March. The irregularity component throughout the whole series remains quite stable, meaning that the trend and season components capture quite well the changes in the series. Therefore, we proceeded to split the dataset in two parts, training and validation. Specifically, the selected training samples were 70% of each month’s observations (e.g., from 2016-01-05 00:15:00 until 2016-01-21 23:45:00 in January), while the remaining values were used for validation.
4.2 Methods
The methods used to build the predictions models are XGBoost [ 8 ] and a selection of neural networks including CNNs [ 7 ], RNNs [ 31 ], MLPs [ 32 ] and Seq2Seq [ 12 ]. The results with Seq2Seq suggested that other techniques, such as transformers, would not be very useful given the relatively small size of each partition, in line with the recent literature [ 36 ].
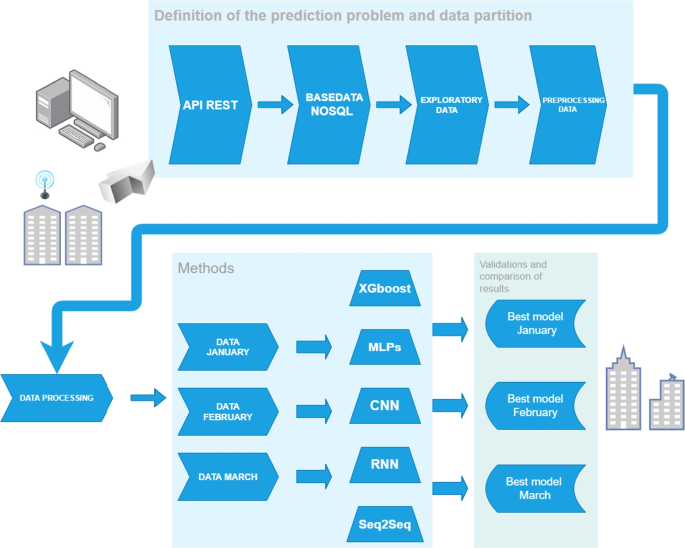
Methodology
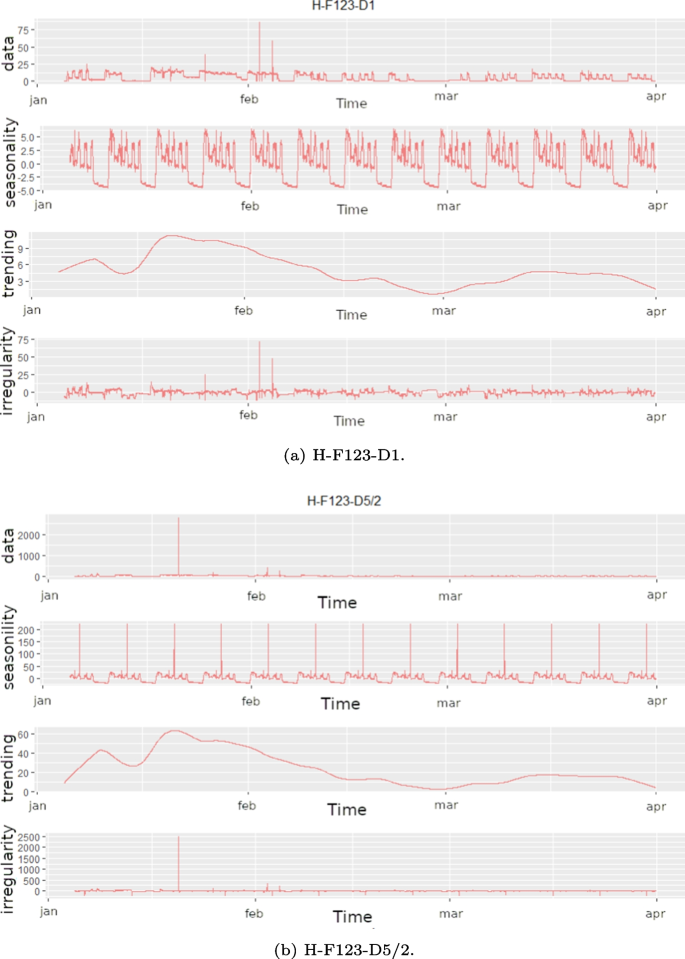
Decomposition of the heating consumption variables of plants 1, 2, and 3 in the pilot zone of the ICPE building
Table 2 summarises the configuration of the models and the hyperparameters probed with each technique. For XGBoost, we reflect the number of trees, maximum depth, and learning rate. For CNN, we describe the number of convolutional layers, max pooling 1D, and the number of filters. For MLP, RNN and Seq2Seq, the table lists the number of hidden layers, dropout layers, and layer size. The best configurations obtained with the training data are highlighted in section 5.2 .
4.3 Metrics
To validate and compare the different results of our experiments, we must define the error metrics to be minimized. Since we have a regression problem, we use MAE (Mean Absolute Error), which aggregates at m the absolute difference between the predictions and the actual values of delay data points:
We also use the normalized MAE, namely NMAE, which averages the error for a batch of size N .
5 Experiments and results
This section shows the results of the experiments after data preprocessing and model training and validation with the algorithms mentioned above: decision trees, XGBoost, and neural networks (MLPs, CNNs, RNNs and Seq2Seq). The implementation of the preprocessing and the prediction algorithms was developed with TSxtend [ 23 ], our open source library for batch analysis of sensor data.

5.1 Preprocessing and data preparation
In our experiment, we used processing data techniques such as aggregation, data modification and removal, data transformation, outliers detection, missing values detection, normalization and feature selection. We discarded heating consumption with null values to discard rows with no heating consumption. Then, we eliminated the variables with extreme values and finally joined the variables measuring the same consumption type on the same floor.
The variables in the collected dataset store aggregated values, e.g., summing up new values at each instant. The transformation of these variables was done with the differences between each instant of time, thus obtaining the actual consumption of each variable at each instant of time. These variables were used as inputs for the execution of our prediction algorithms.
In the subsequent step, we employed techniques to detect and remove outliers for each consumption variable. We calculated percentiles for each variable and searched for values outside this range. Such values were substituted with more conventional values (mean, median, etc...). This approach, however, leads to a loss of information. Fortunately, the number of outliers in our case is limited.
We had in our dataset missing values in February when the system did not gather data, for which we applied missing values imputation algorithms. Particularly, we used Interp to fill gaps by interpolation of known values. Interpolation techniques are utilized to fill gaps by interpolating known values, as mentioned in work by [ 22 ]. Specifically, this approach involves the utilization of Interp, a commonly employed software for performing interpolation. This methodology aims to accurately estimate values within a given range based on the available data. The interpolation process involves estimating each data point’s value by considering its neighbouring data points. Applying this technique makes the resulting dataset complete and can be analyzed more effectively for the intended purpose. Figure 5 depicts data after imputation for three variables.
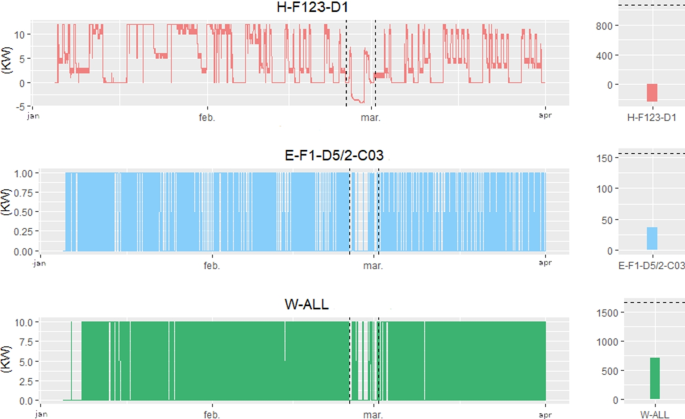
Imputation of missing values ((end February) with Interpolate for variables Energy Zone D1, E-F1-D5/2-C03 and W-ALL
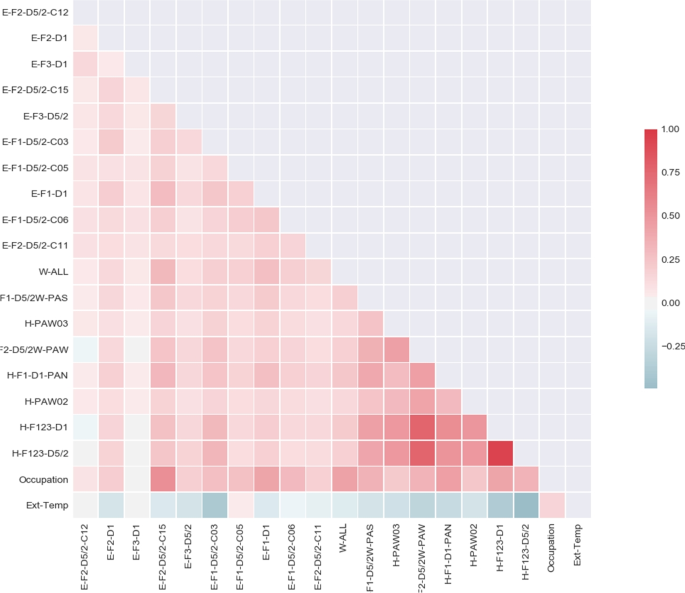
Heatmap showing correlations between variables
Then, we selected the most relevant variables for predicting energy consumption as Energy Zone D1 and Energy Zone D5/2. We calculated the cross-correlation between pairs of variables and grouped them by levels according to their correlation to the prediction target. Figure 6 indicates few dependencies between the electrical sensors. There is a high correlation between the heating consumption variables, while water consumption does not correlate. In the case of the occupancy variables, there are more correlations, but it is not high because the values are estimated. We can also observe energy consumption when the building is empty.
Then we used XGBoost algorithm for the assessment of variable importance. In Fig. 7 , we can see that the most relevant variables for energy consumption are occupancy, outside temperature and heating consumption (Energy Zone D1 and Energy Zone D5/2). With this information, we performed the selection of variables, being the most important ones (not surprisingly) Outside Temperature, Occupation, Energy Zone D5/2 and Energy Zone D1.
The last step in order to preprocess data is to normalize the dataset. To do this, we calculated the mean and standard deviation of each variable, and then transformed the values to the range [0, 1].
5.2 Results and discussion
We experimented with the January, February and March data using the selected algorithms. We applied grid search to obtain the best configurations and hyperparameter, yielding the values depicted in Table 3 . We can observe that the best configurations are similar for each subproblem.
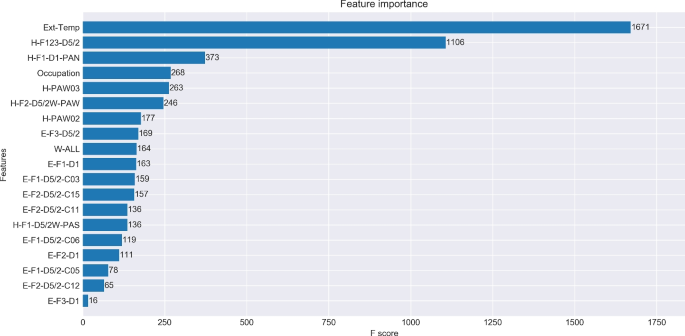
Ranking of variables by importance with XGBoost
The validation errors of the best models are shown in Tables 4 and 5 , while Figs. 8 , 9 , 10 , 11 , 12 , and 13 depict the predicted vs the real values. In January, the algorithm that offered the best results was Seq2Seq, which achieved a validation NMAE of 0.21 for the prediction of consumption in zone D1 and 0.20 for zone D5/2, significantly improving the performance of the other models. The best model for February in both zones was CNNs. Overfitting was lower than that with other algorithms, at the cost of having worse validation metrics —the model tends to ignore or lessen the consumption peaks, as it can be seen in the chart. A second reason to explain these results is that we had many more missing values to impute in this period. The best method for March was RNNs, with an NMAE of 0.29 in both zones, almost twice better than MLP and XGBoost. Overall, we confirmed the ability of memory-based models (Seq2Seq and RNN) to extract characteristics from the input time series and learn the predictions in more diverse scenarios, while CNNs were slightly better when there was a uniform trend and fewer data. In all cases, the best models’ MAE was around 5 kW, which is small enough for this type of applications.
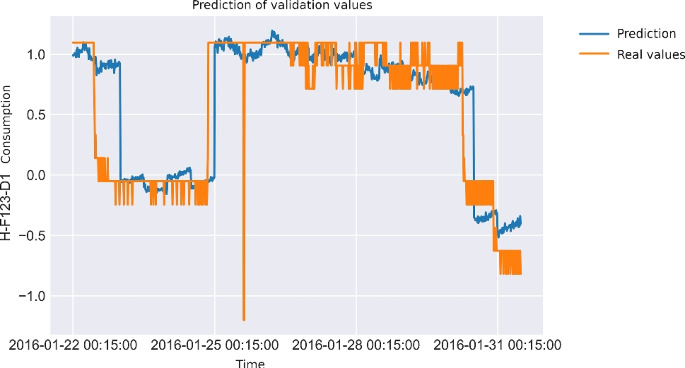
Prediction of the Validation Values in January with Seq2Seq Energy Zone D1
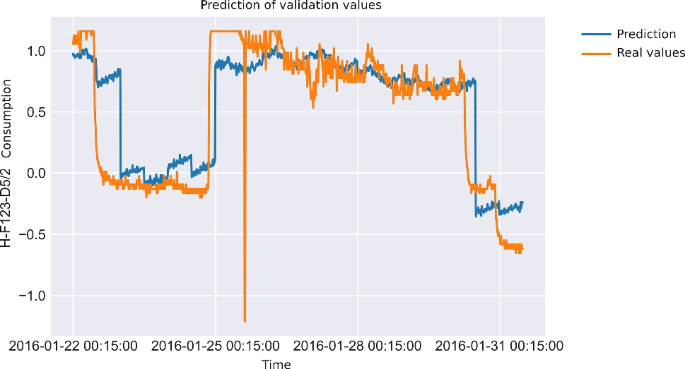
Prediction of the Validation Values in January with Seq2Seq Energy Zone D5/2
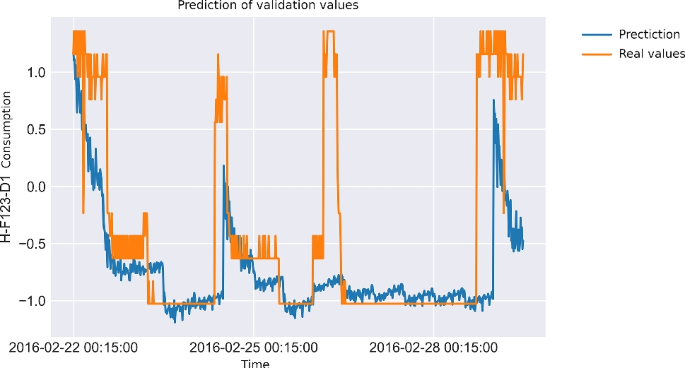
Prediction of the Validation Values in February with CNNs Energy Zone D1
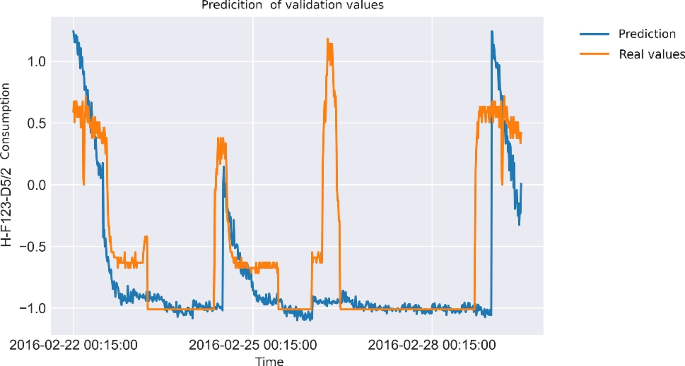
Prediction of the Validation Values in February with CNNs Energy Zone D5/2
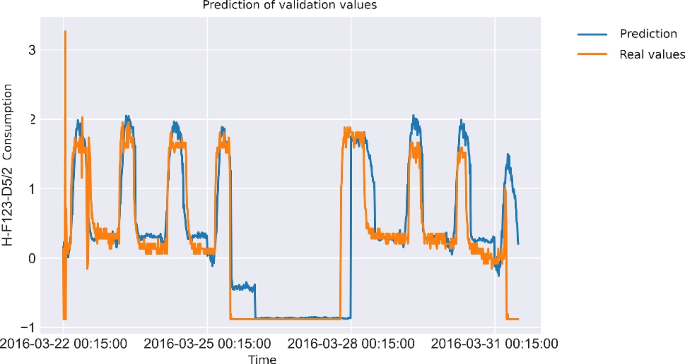
Prediction of the Validation Values in March with RNNs Energy Zone D1
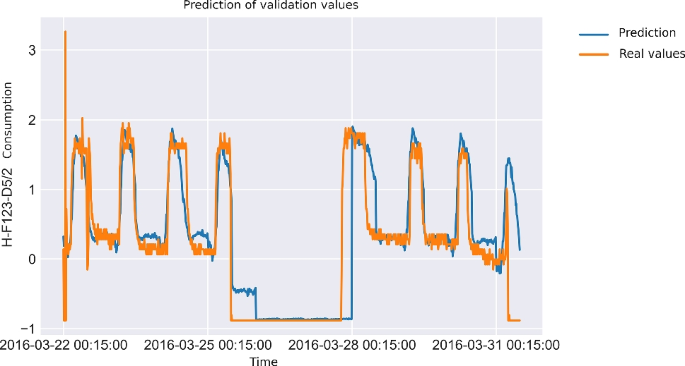
Prediction of the Validation Values in March with RNNs Energy Zone D5/2
Regarding the drawback of having limited data for training and validation, it resulted in that the more complex models did not learn the prediction of heating consumption as well as it could be expected. Hence, we suggest that more sophisticated techniques (Seq2Seq, but also transformer-based architectures) might not be necessary in this kind of problems or under similar circumstances. Instead, RNNs or CNNs with proper data pre-processing could be precise enough and less prone to overfitting.
6 Conclusions and future work
Concluding our discussion, we can see how the XGBoost model shows worse results, as XGBoost does not extract the dependencies between the variables it receives as input to perform the energy consumption prediction. With MLP models we have shown that they do not provide better results than other models because they do not allow to follow a chronological order in our dataset. With respect to the use of RNNs on our dataset, we have been able to reduce the NMAE error function by half, as these algorithms remember the information by processing the data in chronological order. Furthermore, we have applied Seq2Seq, which has allowed us to observe that, like RNNs, they obtain good results in general but do not perform very well on datasets with too many missing values (i.e., smaller in size). Finally, convolution networks (CNNs) have been found to perform better than RNNs and Seq2Seq algorithms on sections of the dataset with a large number of missing values and steady trends.
One of the problems we have in this study has been the limited amount and quality of data, since we have only worked with the ICPE building sensors for three months including many missing values. In the future we can take the ICPE building sensors from other years to have a more extensive training and validation. Another option may be to generate artificial values with building simulation models. We can also use recurrent neural network models using attention mechanisms to improve synthetic data generation and missing values imputation [ 5 ]. Additionally, peak changes could be addressed with noise reduction techniques to smooth abrupt oscillations, e.g., as the filter proposed in [ 16 ].
Energy IN TIME was an European project running in 2013-2017. The aim of the project was to implement a model-predictive control system to improve the energy efficiency of non-residential buildings [ 13 ].
Ahmad T, Chen H (2018) Short and medium-term forecasting of cooling and heating load demand in building environment with data-mining based approaches. Energy Build 166:460–476. https://doi.org/10.1016/J.ENBUILD.2018.01.066
Article Google Scholar
Ahmad T, Chen H, Guo Y et al (2018) A comprehensive overview on the data driven and large scale based approaches for forecasting of building energy demand: A review. Energy Build 165:301–320. https://doi.org/10.1016/j.enbuild.2018.01.017
Arpanahi G, Javadi M (2018) A review on applications of artificial neural networks and support vector machines for building electrical energy consumption forecasting. Renew Sustain Energy Rev 82:1814–1832. https://doi.org/10.1016/j.rser.2014.01.069
Benedetti M (2015) A proposal for energy services classification including a product service systems perspective. Procedia CIRP 30:251–256. https://doi.org/10.1016/j.procir.2015.02.121
Bülte C, Kleinebrahm M, Yilmaz HÜ et al (2023) Multivariate time series imputation for energy data using neural networks. Energy and AI 13:100,239. https://doi.org/10.1016/j.egyai.2023.100239 , https://www.sciencedirect.com/science/article/pii/S2666546823000113
Chau C, Leung T, Ng W (2015) A review on life cycle assessment, life cycle energy assessment and life cycle carbon emissions assessment on buildings. Appl Energy 143:395–413. https://doi.org/10.1016/j.apenergy.2015.01.023
Chauhan R, Ghanshala KK, Joshi RC (2018) Convolutional Neural Network (CNN) for Image Detection and Recognition. ICSCCC 2018 - 1st International Conference on Secure Cyber Computing and Communications pp 278–282. https://doi.org/10.1109/ICSCCC.2018.8703316
Chen T, Guestrin C (2016) Xgboost: A scalable tree boosting system pp 785–794. https://doi.org/10.1145/2939672.2939785
Chen W, Zhou K, Yang S et al (2017) Data quality of electricity consumption data in a smart grid environment. Renew Sustain Energy Rev 75:98–105. https://doi.org/10.1016/j.rser.2016.10.054
Chou JS, Ngo NT (2016) Time series analytics using sliding window metaheuristic optimization-based machine learning system for identifying building energy consumption patterns. Appl Energy 177:751–770. https://doi.org/10.1016/J.APENERGY.2016.05.074
Ezan MA, Uçan ON, Kalfa M (2017) Predicting short-term building heating and cooling load using regression tree algorithm. J Build Perform Simul 10(5):487–502. https://doi.org/10.1080/19401493.2016.1202888
Gong G (2019) Research on short-term load prediction based on Seq2Seq model. Energies 12:3199. https://doi.org/10.3390/en12163199
Gómez-Romero J, Fernández-Basso CJ, Cambronero MV et al (2019) A probabilistic algorithm for predictive control with full-complexity models in non-residential buildings. IEEE Access 7:38,748–38,765. https://doi.org/10.1109/ACCESS.2019.2906311
Khalil M, McGough AS, Pourmirza Z et al (2022) Machine learning, deep learning and statistical analysis for forecasting building energy consumption - a systematic review. Eng Appl Artif Intell 115(105):287. https://doi.org/10.1016/j.engappai.2022.105287
Lago J, Marcjasz G, De Schutter B et al (2021) Forecasting day-ahead electricity prices: A review of state-of-the-art algorithms, best practices and an open-access benchmark. Appl Energy 293(116):983. https://doi.org/10.1016/j.apenergy.2021.116983
Ma W, Wang W, Wu X et al (2019) Control strategy of a hybrid energy storage system to smooth photovoltaic power fluctuations considering photovoltaic output power curtailment. Sustain 11(5). https://doi.org/10.3390/su11051324 , https://www.mdpi.com/2071-1050/11/5/1324
Marino DL, Amarasinghe K, Manic M (2016) Building energy load forecasting using deep neural networks pp 7046–7051. https://doi.org/10.1109/IECON.2016.7793413
Micheletti A, Aletti G, Ferrandi G et al (2020) A weighted \(\chi ^2\) test to detect the presence of a major change point in non-stationary markov chains. Stat Methods Appl 29(4):899–912. https://doi.org/10.1007/s10260-020-00510-0 , https://doi.org/10.1007/s10260-020-00510-0
Mocanu E, Nguyen PH, Gibescu M et al (2016) Deep learning for estimating building energy consumption. Sustain Energy, Grids Netw 6:91–99. https://doi.org/10.1016/j.segan.2016.02.005
Mocanu E, Nguyen PH, Kling WL et al (2016) Unsupervised energy prediction in a smart grid context using reinforcement cross-building transfer learning. Energy and Buildings 116:646–655. https://doi.org/10.1016/J.ENBUILD.2016.01.030
Molina-Solana M, Ros M, Ruiz MD et al (2017) Data science for building energy management: A review. Renew Sustain Energy Rev 70:598–609. https://doi.org/10.1016/j.rser.2016.11.132
Montero-Manso P, Hyndman RJ (2021) Principles and algorithms for forecasting groups of time series: Locality and globality. Int J Forecast 37(4):1632–1653. https://doi.org/10.1016/j.ijforecast.2021.03.004
Morcillo-Jimenez R, Gutiérrez-Batista K, Gómez-Romero J (2023) Tsxtend: A tool for batch analysis of temporal sensor data. Energies 16(4). https://doi.org/10.3390/en16041581 , https://www.mdpi.com/1996-1073/16/4/1581
Pachauri N, Ahn CW (2022) Regression tree ensemble learning-based prediction of the heating and cooling loads of residential buildings. Build Simul 15(11):2003–2017. https://doi.org/10.1007/s12273-022-0908-x
Phinikarides A, Makrides G, Zinsser B et al (2015) Analysis of photovoltaic system performance time series: Seasonality and performance loss. Renew Energy 77:51–63. https://doi.org/10.1016/j.renene.2014.11.091 , https://www.sciencedirect.com/science/article/pii/S0960148114008222
Pérez-Lombard L, Ortiz J, Pout C (2008) A review on buildings energy consumption information. Energy Build 40(3):394–398. https://doi.org/10.1016/j.enbuild.2007.03.007
Rahman A, Srikumar V, Smith AD (2018) Predicting electricity consumption for commercial and residential buildings using deep recurrent neural networks. Appl Energy 212:372–385. https://doi.org/10.1016/j.apenergy.2017.12.051 , https://www.sciencedirect.com/science/article/pii/S0306261917317658
Raza MQ, Khosravi A (2015) A review on artificial intelligence based load demand forecasting techniques for smart grid and buildings. Renew Sustain Energy Rev 50:1352–1372. https://doi.org/10.1016/j.rser.2015.04.065
Schmidt M, Åhlund C (2018) Smart buildings as cyber-physical systems: Data-driven predictive control strategies for energy efficiency. Renew Sustain Energy Rev 90:742–756. https://doi.org/10.1016/j.rser.2018.04.013
Seyedzadeh S, Rahimian FP, Glesk I et al (2018) Machine learning for estimation of building energy consumption and performance: a review. Vis Eng 6:1–20. https://doi.org/10.1186/s40327-018-0064-7
Sherstinsky A (2020) Fundamentals of recurrent neural network (RNN) and long short-term memory (LSTM) network. Physica D: Nonlinear Phenom 404(132):306. https://doi.org/10.1016/j.physd.2019.132306
Taud H, Mas J (2018) Multilayer perceptron (MLP) pp 451–455. https://doi.org/10.1007/978-3-319-60801-3_27
Tien PW, Wei S, Darkwa J et al (2022) Machine learning and deep learning methods for enhancing building energy efficiency and indoor environmental quality - a review. Energy AI 10:100,198. https://doi.org/10.1016/j.egyai.2022.100198 , https://www.sciencedirect.com/science/article/pii/S2666546822000441
Torres JF (2021) Deep learning for time series forecasting: A survey. Big Data 9:3–21. https://doi.org/10.1089/big.2020.0159
Wang Z, Srinivasan RS (2016) A review of artificial intelligence based building energy prediction with a focus on ensemble prediction models. pp 3438–3448. https://doi.org/10.1109/WSC.2015.7408504
Zeng A, Chen M, Zhang L et al (2023) Are transformers effective for time series forecasting?
Zhang L, Wen J, Li Y et al (2021) A review of machine learning in building load prediction. Appl Energy. https://doi.org/10.1016/j.apenergy.2021.116452
Download references
Acknowledgements
This work was funded by the European Union NextGenerationEU/PRTR through the IA4TES project (MIA.2021.M04.0008); by MICIU/AEI/10.13039/501100011033 through the SINERGY project (PID2021.125537NA.I00); by ERDF/Junta de Andalucía through the D3S project (P21.00247); by the FEDER programme 2014-2020 (B-TIC-145-UGR18 and P18-RT-1765); and by the European Union (Energy IN TIME EeB.NMP.2013-4, No. 608981).
Funding for open access publishing: Universidad de Granada/CBUA.
Author information
Authors and affiliations.
Department of Computer Science and Artificial Intelligence, Escuela Técnica Superior de Ingenierías Informática y de Telecomunicación, Universidad de Granada, Granada, 18071, Spain
Roberto Morcillo-Jimenez, Jesús Mesa, Juan Gómez-Romero, M. Amparo Vila & Maria J. Martin-Bautista
You can also search for this author in PubMed Google Scholar
Corresponding author
Correspondence to Roberto Morcillo-Jimenez .
Additional information
Publisher's note.
Springer Nature remains neutral with regard to jurisdictional claims in published maps and institutional affiliations.
Rights and permissions
Open Access This article is licensed under a Creative Commons Attribution 4.0 International License, which permits use, sharing, adaptation, distribution and reproduction in any medium or format, as long as you give appropriate credit to the original author(s) and the source, provide a link to the Creative Commons licence, and indicate if changes were made. The images or other third party material in this article are included in the article’s Creative Commons licence, unless indicated otherwise in a credit line to the material. If material is not included in the article’s Creative Commons licence and your intended use is not permitted by statutory regulation or exceeds the permitted use, you will need to obtain permission directly from the copyright holder. To view a copy of this licence, visit http://creativecommons.org/licenses/by/4.0/ .
Reprints and permissions
About this article
Morcillo-Jimenez, R., Mesa, J., Gómez-Romero, J. et al. Deep learning for prediction of energy consumption: an applied use case in an office building. Appl Intell (2024). https://doi.org/10.1007/s10489-024-05451-9
Download citation
Accepted : 07 April 2024
Published : 30 April 2024
DOI : https://doi.org/10.1007/s10489-024-05451-9
Share this article
Anyone you share the following link with will be able to read this content:
Sorry, a shareable link is not currently available for this article.
Provided by the Springer Nature SharedIt content-sharing initiative
- Energy consumption forecasting
- Time series
- Deep learning
- Multilayer perceptron
- Recurrent neural networks
- Convolutional neural networks
- Sequence to sequence networks
- Find a journal
- Publish with us
- Track your research
Accessibility Links
- Skip to content
- Skip to search IOPscience
- Skip to Journals list
- Accessibility help
- Accessibility Help
Click here to close this panel.
Purpose-led Publishing is a coalition of three not-for-profit publishers in the field of physical sciences: AIP Publishing, the American Physical Society and IOP Publishing.
Together, as publishers that will always put purpose above profit, we have defined a set of industry standards that underpin high-quality, ethical scholarly communications.
We are proudly declaring that science is our only shareholder.
Comparison of Conventional and Precast Half Slab Work Methods (Case Study: Japfa Office Building Daan – Mogot)
Putri Arumsari 1 and Brilliant Palagian 1
Published under licence by IOP Publishing Ltd IOP Conference Series: Earth and Environmental Science , Volume 1324 , 7th International Conference on Eco Engineering Development 2023 08/11/2023 - 08/11/2023 Online Citation Putri Arumsari and Brilliant Palagian 2024 IOP Conf. Ser.: Earth Environ. Sci. 1324 012012 DOI 10.1088/1755-1315/1324/1/012012
Article metrics
20 Total downloads
Share this article
Author e-mails.
Author affiliations
1 Civil Engineering Department, Faculty of Engineering, Bina Nusantara University, Jakarta, Indonesia 11480
Buy this article in print
In the construction of vertical buildings, the implementation method used affects the productivity of construction. The development of construction system innovation in Indonesia which continues to grow has led to a new system, one of which is the precast system. Precast systems have been proven to be able to increase productivity in terms of construction time and costs, as well as the value of waste costs, which results in little from conventional methods. The main objective of this study was to compare the efficiency of the method of implementing floor slabs in the field by comparing the variables of field implementation, costs, time, quality control, and material waste costs of the conventional and precast half - slab methods. The analysis was carried out using the unit price is based on the Jakarta Worker's Wage Unit Price 40 year XXVII - 2021 edition. The index coefficient (conventional) refers to the Minister for Public Works and Human Settlements Regulation No. 1 of 2022, and the index coefficient (precast half slab) refers to SNI 7832:2012. Scheduling was performed using Microsoft Projects. The results show that the precast half - slab method is more efficient than the conventional method.
Export citation and abstract BibTeX RIS
Content from this work may be used under the terms of the Creative Commons Attribution 3.0 licence . Any further distribution of this work must maintain attribution to the author(s) and the title of the work, journal citation and DOI.
- Case Studies
- India Case Studies
A Decrease font size. A Reset font size. A Increase font size.
Atal Akshay Urja Bhawan

Detailed Case Study
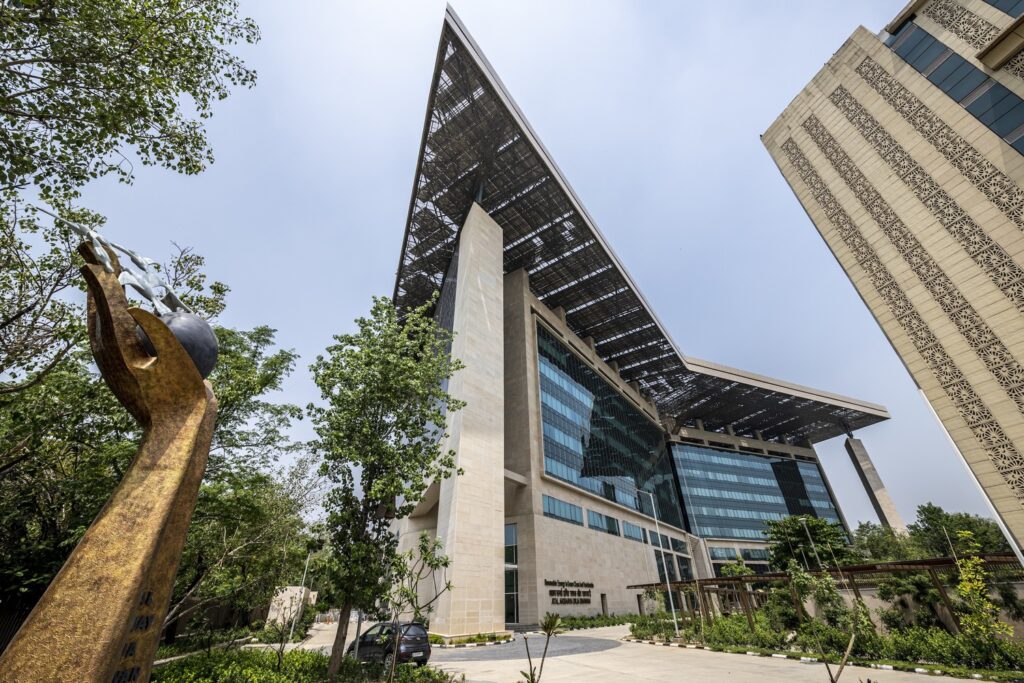
Badriya Jum’A Masjid
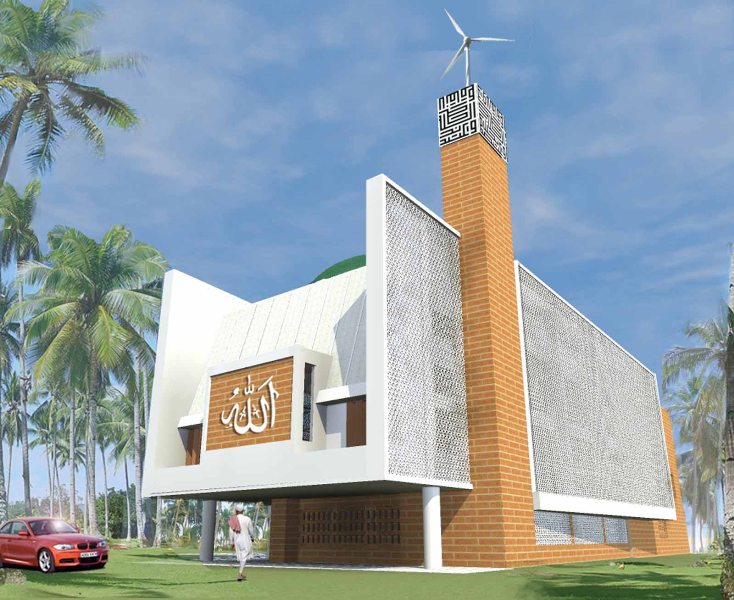
Vaibhav Global Limited, Jaipur
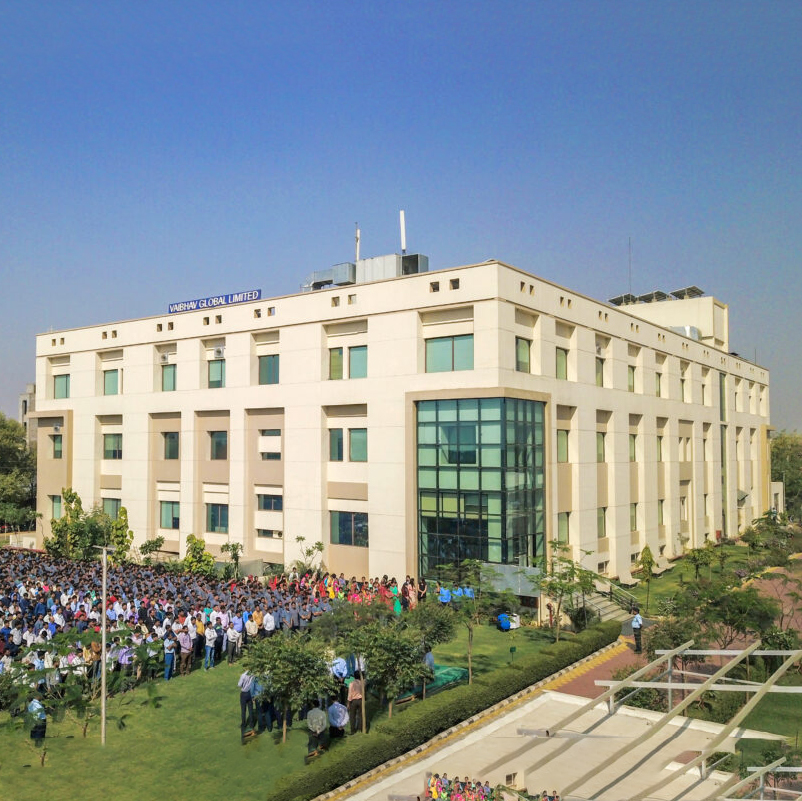
Bhawar Residence, Chennai
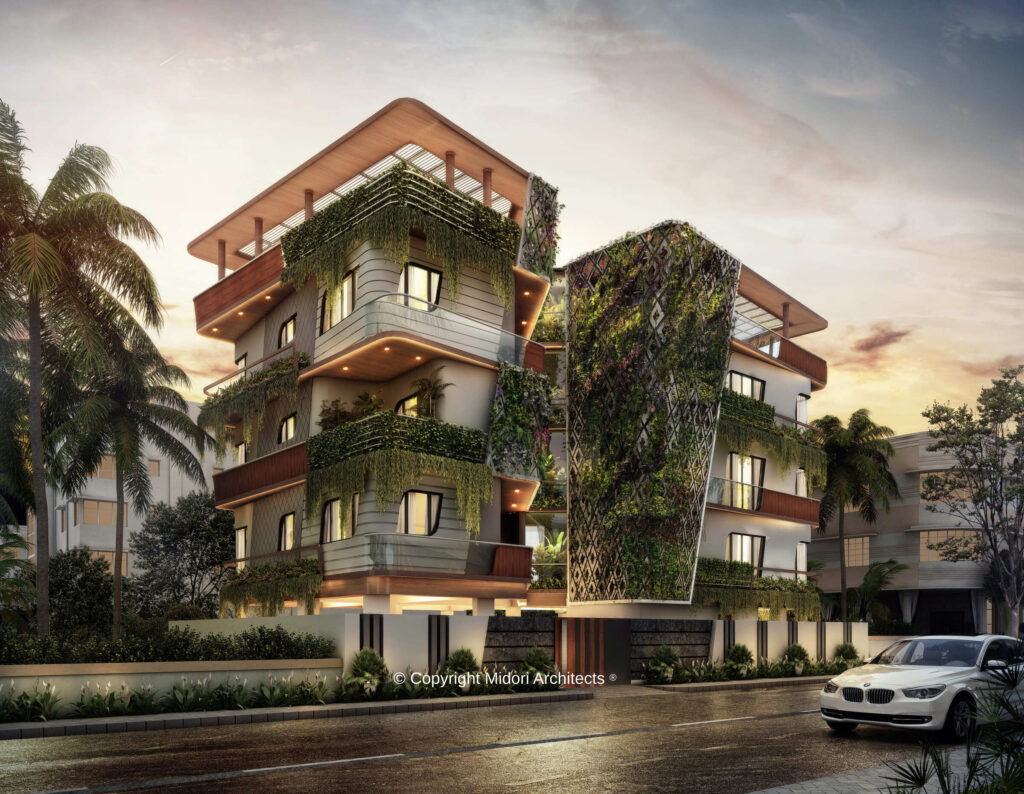
SIERRA’s eFACiLiTY® Green Office Building, Coimbatore
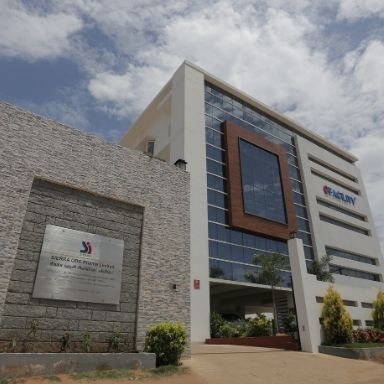
Lodsi Community Project for Forest Essentials, Uttarakhand
More information
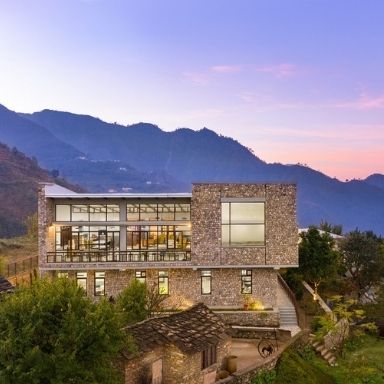
IIT Jodhpur, Rajasthan
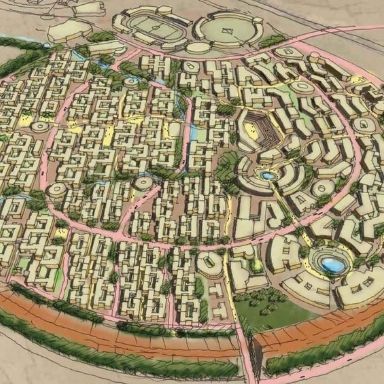
St. Andrews Boys Hostel Block, Gurugram
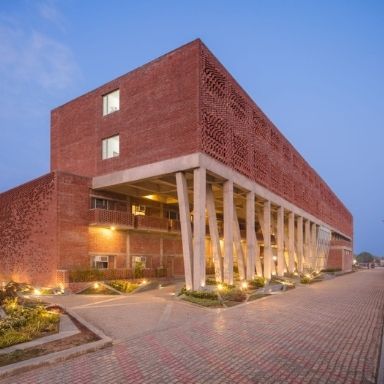
St. Andrews Girls Hostel Block, Gurugram
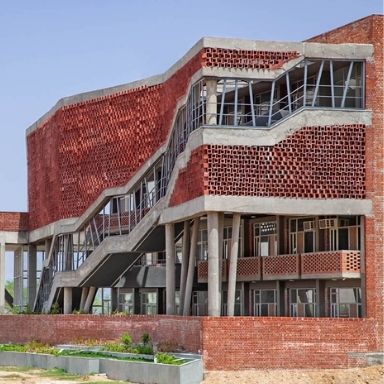
Rajkumari Ratnavati Girls’ School, Rajasthan
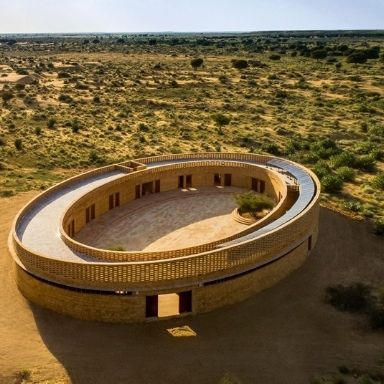
Kalpana Housing
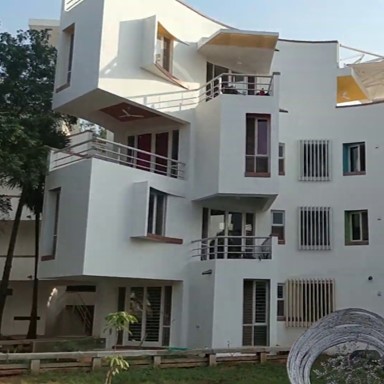
Jaquar Headquarters
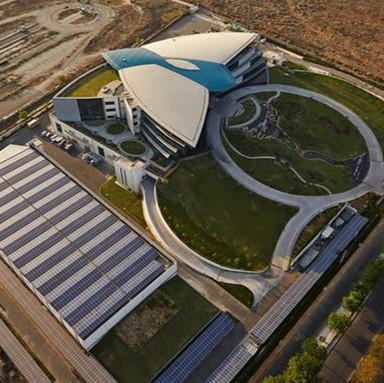
Humanscapes
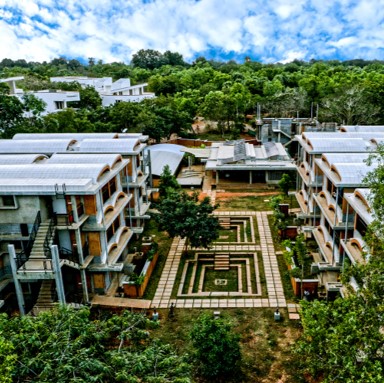
Avasara Academy
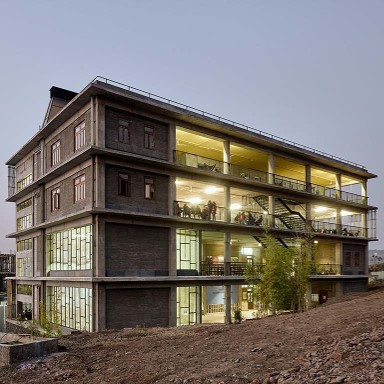
Infosys – Pocharam Campus
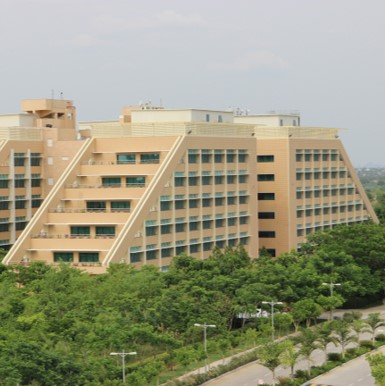
Indian Green Business Centre (IGBC)
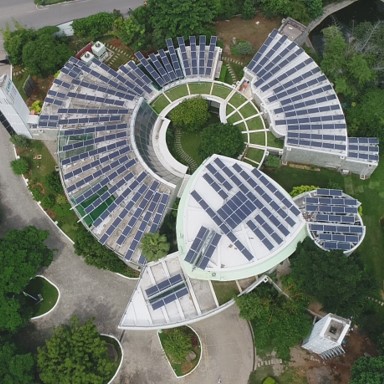
Godrej Plant 13 Annexe
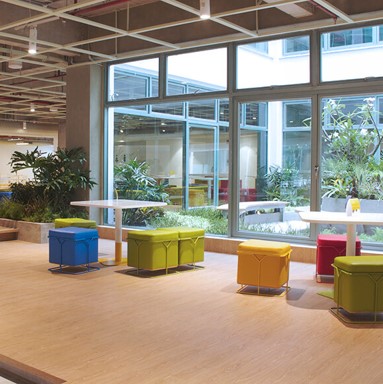
CEPT, A Living Laboratory
Renewable Energy Integration 50 % roof covered with 27 kW PV panels tilted at 23º facing south for on-site generation equivalent to 70kWh/m 2 /yr.
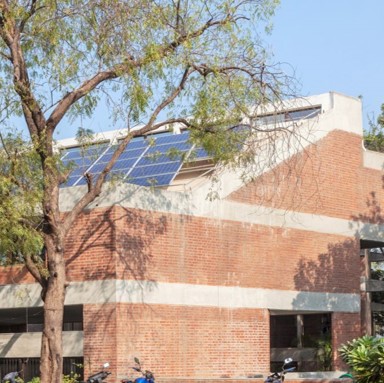
Indira Paryavaran Bhawan, MoEF
Renewable Energy Integration 930 kW PV panels with a total area of 4650m 2 for on-site generation, tilted at 23º facing south to generate equivalent to 70kWh/m 2 /yr
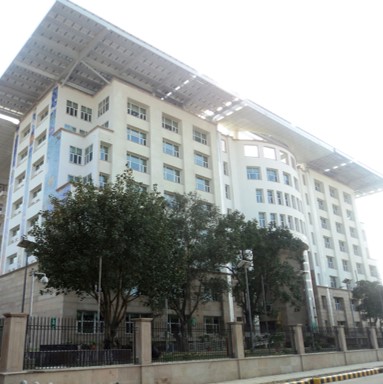
Akshay Urja Bhawan, HAREDA, Panchkula, Haryana
Renewable Energy Technology Building Integrated Photovoltaic(BIPV) System with 42.50 kW capacity to supply total annual energy consumption.
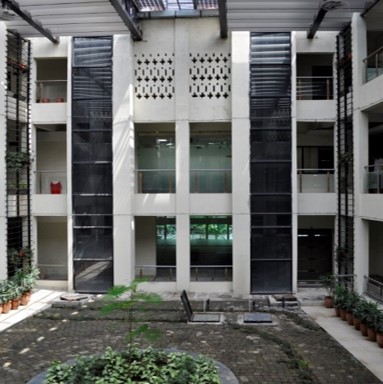
Eco Commercial Building (ECB) Bayer Material Science
Renewable Energy Technology Draws 100% of its energy from roof-top PV plant. 57 kW solar PV generates 88.9 MWh/yr. Excess energy fed to other buildings at site.
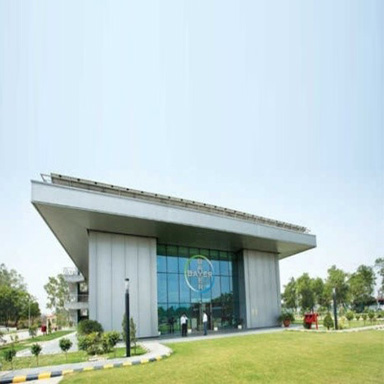
Malankara Tea Plantation
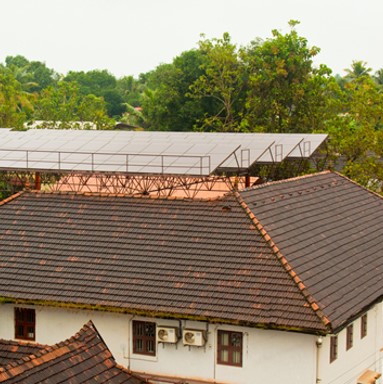
GRIDCO Bhubaneswar
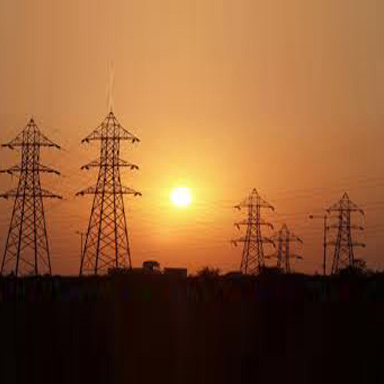
Sun Carrier Omega NZEB
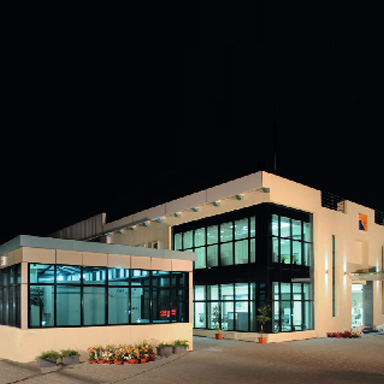
Case-Studies
- other regions
- nalanda university [PACE-D pilot]
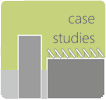
Privacy Policy
- Hispanoamérica
- Work at ArchDaily
- Terms of Use
- Privacy Policy
- Cookie Policy
Office buildings
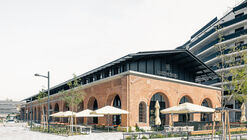
GOH Conversion Gösserhalle / AllesWirdGut Architektur
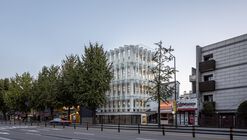
West Glow Office and Retail Building / Society of Architecture
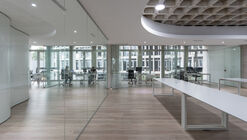
Orange Obras Civiles Offices / Agustín Lozada
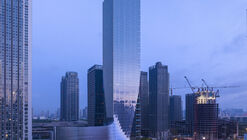
Foshan Entrepreneur Building / CCDI
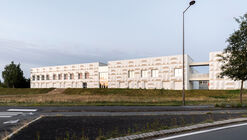
Technocampus Acoustique / ars. architectes urbanistes associés
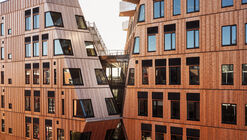
Vertikal Nydalen / Snøhetta
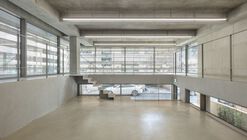
Fa-brick / Society of Architecture
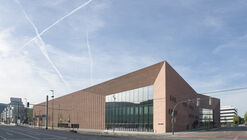
Heidelberg Congress Center / Degelo Architekten
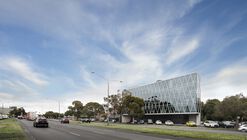
Spink St. Office Building / Peter Ryan Architects
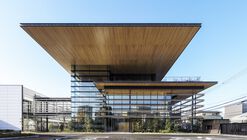
T-LINKS / TAISEI DESIGN Planners Architects & Engineers
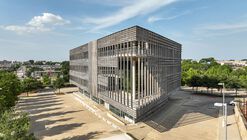
Shady Brook Office Building / Cunningham Architects
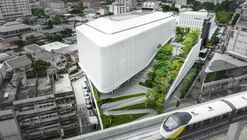
The Headquarters of Council of Engineers / AATTN8A
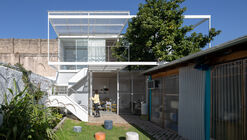
Mutan Project / Morsa Taller + Emilia Pascarelli
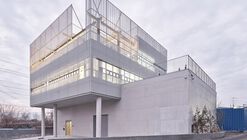
C-PROJECT Commercial and Office Building / GAGAHOHO Architects
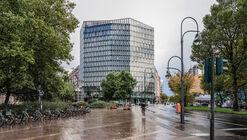
Budapester Straße Berlin / Grüntuch Ernst Architects
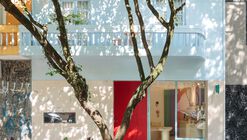
MR Atelier and Store Flex / Gema Arquitetura
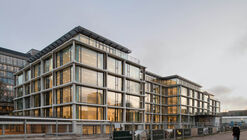
PI59 Offices / V8 Architects
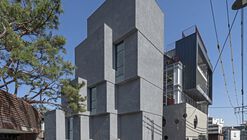
Chrome Company HQ Building / JYA-RCHITECTS
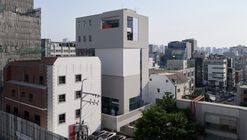
Chai Communication Office Building / ARCHITECTS BAN + Fluxys Lab
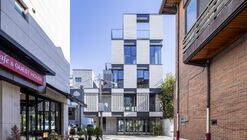
Intec Office Building / ARCHITECTS BAN
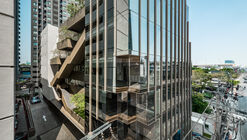
Ritratana Office Building / Urban Praxis
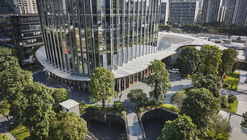
Star River Headquarters / Skidmore, Owings & Merrill
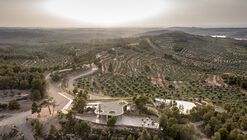
El Trull CV Oil Mill / Alventosa Morell Arquitectes
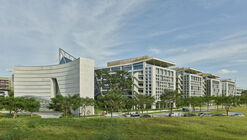
Surbana Jurong Campus / Safdie Architects

IMAGES
VIDEO
COMMENTS
Discover the latest office buildings in India, designed by renowned architects and firms. Browse through photos, plans, and details of these innovative projects.
Projects Built Projects Selected Projects Offices Office buildings Ahmedabad India Published on March 22, 2023 Cite: "Zydus Headquarters / Morphogenesis" 22 Mar 2023.
44 kWh/m 2 /yr. Introduction. Indira Paryavaran Bhawan, the new office building for Ministry of Environment and Forest (MoEF) sets is a radical change from a conventional building design. The project team put special emphasis on strategies for reducing energy demand by providing adequate natural light, shading, landscape to reduce ambient ...
October 5th, 2020 by Sumit Singhal. Article source: Sanjay Puri Architects. Cantilevered cuboid volumes create a sculptural office building in Chennai, India. Located in the old business district of Chennai in South India, the plot had multiple challenges to be overcome. With a very narrow access from an arterial city road and no road frontage ...
12. Coal India Limited Office Building Entrance_© wypages.com. The Coal India Limited Office Building is located in Kolkata with 25159 sqm by Raj Rewal Associates & Urban Design Consultants. The project was completed in 2014 with a 4-star GRIHA rating. The energy Consumption is claimed to have been 37.9% less than the GRIHA benchmarks.
The Unnati Office Building is the regional headquarter (North) for Gainwell Commosales Pvt. Ltd., part of a larger 5 acre campus. It is the first building in India to be certified Platinum under LEED v4 BD+C : NC rating in 2018. The building performs 59% better than a conventional office building in the region, and 40% of the building energy ...
Case Study 1. The office space of a company can play an integral role in building the right relationship with its employees, partners, and clients. Integrating the superstructures of ideology and thought process into everyday workings, it can become a tool for the company to increase productivity, further innovation, and build culture.
The work of Raj Rewal is an exemplary embodiment of this fusion. His campus of housing for National Institute of Inmunology in Delhi is massed in a manner reminiscent of a Rajasthan citadel, around an interlocking series of well shaded courts (AR August 1988). More recently, Rewal has also addressed the less promising typology of the office ...
The commercial complex (2B+G+8+R) has a built-up area of 275000 sq. ft. Team Consistent achieved a 20% reduction in power requirement, and the service spaces, were designed in 3.5% of the entire built-up area. Cost-effective Design. Cost-effectiveness was a key criterion for the design. Hence, Team CECPL suggested various options to ensure the ...
Here are 10 of the most inspiring greenest building in India. 1. Rajiv Gandhi International Airport, Hyderabad | Greenest Building in India. The Rajiv Gandhi airport, commissioned in 2008, is one of the leading examples of highly environmentally sensitive infrastructure design and management in India. Spread over an area of 2223 hectares, the ...
Case Study : TCI headquarters by AB Lall architect. A prototype for the modern city office. A climate responsive solution based on the courtyard principle. The building achieves a high level of energy efficiency at practically no extra cost. All materials are indigenous and attain a new aesthetic in this contemporary form.
3.1 Building 1 Building 1 is the office building for one of the Central Ministry, Government of India. The eight-storey office building with three basements is approximately 31,400 m2 and is in Jor Bagh, New Delhi. It is a new building completed in 2013, and occupied in 2014, designed primarily to house open-plan offices with meeting rooms.
case study sustainibility indian office highrise. May 2, 2015 • Download as PPTX, PDF •. 12 likes • 5,805 views. Anurag Biswas. case study of sustainibility measures for office buildings in india. Design. Slideshow view. Download now. case study sustainibility indian office highrise - Download as a PDF or view online for free.
SIERRA'S eFACiLiTY® Green Office Building. Coimbatore, Tamil Nadu. Summary. SIERRA's eFACiLiTY® building is a software development centre in Coimbatore, India. The building is one of the most innovative smart building as it leverages its cutting-edge latest-tech building management systems, controls, and automation to reduce its carbon ...
Here are a few interesting examples of climate-responsive architecture that is found in Indian cities. 1. Solar Passive Hostel, University of Jodhpur (Hot and Dry) The structure was part of the research project undertaken by the Centre of Energy Studies, IIT Delhi. Designed by architect Vinod Gupta, the building was an attempt to test as well ...
Case Study: GBCI India Corporate Head Office
To the Case Western Reserve community, We are aware that access to Kelvin Smith Library may be impeded, especially for those who use wheelchairs and other assistive devices. To address these concerns as well as provide an additional quiet study space, we are opening Adelbert Gym from 6 p.m. to 6 a.m. daily. The university has set up study areas and enhanced WiFi connectivity in this air ...
NZEBs Case Studies. Case studies of energy efficient and net zero energy buildings with a focus on India, provide important insights to the feasibility of the concept. Considering the concept is still in its nascent stage in India, it is very useful to study how architects and building owners have gone about setting net-zero and energy ...
Abstract Non-residential buildings are responsible for more than a third of global energy consumption. Estimating building energy consumption is the first step towards identifying inefficiencies and optimizing energy management policies. This paper presents a study of Deep Learning techniques for time series analysis applied to building energy prediction with real environments. We collected ...
Comparison of Conventional and Precast Half Slab Work Methods (Case Study: Japfa Office Building Daan - Mogot), Putri Arumsari, Brilliant Palagian ... The main objective of this study was to compare the efficiency of the method of implementing floor slabs in the field by comparing the variables of field implementation, costs, time, quality ...
9,565 m 2. Grid Connectivity. Grid connected. EPI. 44 kWh/m 2 /yr. Renewable Energy Integration 930 kW PV panels with a total area of 4650m 2 for on-site generation, tilted at 23º facing south to generate equivalent to 70kWh/m 2 /yr. Detailed Case Study. Akshay Urja Bhawan, HAREDA, Panchkula, Haryana. Location.
Ten Impact Grants have been awarded following a call for applications jointly issued by TDR and WHO's Regional Office for South-East Asia (SEARO), who have a common interest in strengthening the capacity for health research in low- and middle-income countries. The call reflects a commitment to support implementation research that informs the delivery of high-quality and cost-efficient health ...
Ritratana Office Building / Urban Praxis Star River Headquarters / Skidmore, Owings & Merrill El Trull CV Oil Mill / Alventosa Morell Arquitectes Surbana Jurong Campus / Safdie Architects ...