

Sampling Methods & Strategies 101
Everything you need to know (including examples)
By: Derek Jansen (MBA) | Expert Reviewed By: Kerryn Warren (PhD) | January 2023
If you’re new to research, sooner or later you’re bound to wander into the intimidating world of sampling methods and strategies. If you find yourself on this page, chances are you’re feeling a little overwhelmed or confused. Fear not – in this post we’ll unpack sampling in straightforward language , along with loads of examples .
Overview: Sampling Methods & Strategies
- What is sampling in a research context?
- The two overarching approaches
Simple random sampling
Stratified random sampling, cluster sampling, systematic sampling, purposive sampling, convenience sampling, snowball sampling.
- How to choose the right sampling method
What (exactly) is sampling?
At the simplest level, sampling (within a research context) is the process of selecting a subset of participants from a larger group . For example, if your research involved assessing US consumers’ perceptions about a particular brand of laundry detergent, you wouldn’t be able to collect data from every single person that uses laundry detergent (good luck with that!) – but you could potentially collect data from a smaller subset of this group.
In technical terms, the larger group is referred to as the population , and the subset (the group you’ll actually engage with in your research) is called the sample . Put another way, you can look at the population as a full cake and the sample as a single slice of that cake. In an ideal world, you’d want your sample to be perfectly representative of the population, as that would allow you to generalise your findings to the entire population. In other words, you’d want to cut a perfect cross-sectional slice of cake, such that the slice reflects every layer of the cake in perfect proportion.
Achieving a truly representative sample is, unfortunately, a little trickier than slicing a cake, as there are many practical challenges and obstacles to achieving this in a real-world setting. Thankfully though, you don’t always need to have a perfectly representative sample – it all depends on the specific research aims of each study – so don’t stress yourself out about that just yet!
With the concept of sampling broadly defined, let’s look at the different approaches to sampling to get a better understanding of what it all looks like in practice.
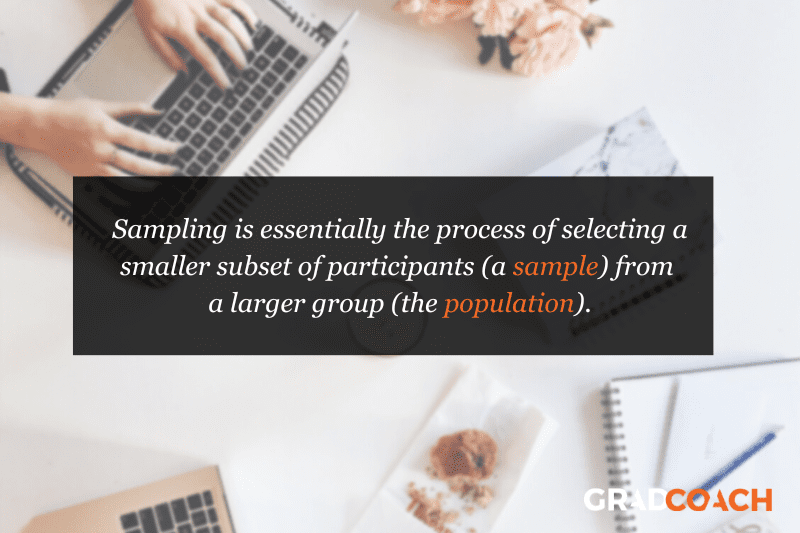
The two overarching sampling approaches
At the highest level, there are two approaches to sampling: probability sampling and non-probability sampling . Within each of these, there are a variety of sampling methods , which we’ll explore a little later.
Probability sampling involves selecting participants (or any unit of interest) on a statistically random basis , which is why it’s also called “random sampling”. In other words, the selection of each individual participant is based on a pre-determined process (not the discretion of the researcher). As a result, this approach achieves a random sample.
Probability-based sampling methods are most commonly used in quantitative research , especially when it’s important to achieve a representative sample that allows the researcher to generalise their findings.
Non-probability sampling , on the other hand, refers to sampling methods in which the selection of participants is not statistically random . In other words, the selection of individual participants is based on the discretion and judgment of the researcher, rather than on a pre-determined process.
Non-probability sampling methods are commonly used in qualitative research , where the richness and depth of the data are more important than the generalisability of the findings.
If that all sounds a little too conceptual and fluffy, don’t worry. Let’s take a look at some actual sampling methods to make it more tangible.
Need a helping hand?
Probability-based sampling methods
First, we’ll look at four common probability-based (random) sampling methods:
Importantly, this is not a comprehensive list of all the probability sampling methods – these are just four of the most common ones. So, if you’re interested in adopting a probability-based sampling approach, be sure to explore all the options.
Simple random sampling involves selecting participants in a completely random fashion , where each participant has an equal chance of being selected. Basically, this sampling method is the equivalent of pulling names out of a hat , except that you can do it digitally. For example, if you had a list of 500 people, you could use a random number generator to draw a list of 50 numbers (each number, reflecting a participant) and then use that dataset as your sample.
Thanks to its simplicity, simple random sampling is easy to implement , and as a consequence, is typically quite cheap and efficient . Given that the selection process is completely random, the results can be generalised fairly reliably. However, this also means it can hide the impact of large subgroups within the data, which can result in minority subgroups having little representation in the results – if any at all. To address this, one needs to take a slightly different approach, which we’ll look at next.
Stratified random sampling is similar to simple random sampling, but it kicks things up a notch. As the name suggests, stratified sampling involves selecting participants randomly , but from within certain pre-defined subgroups (i.e., strata) that share a common trait . For example, you might divide the population into strata based on gender, ethnicity, age range or level of education, and then select randomly from each group.
The benefit of this sampling method is that it gives you more control over the impact of large subgroups (strata) within the population. For example, if a population comprises 80% males and 20% females, you may want to “balance” this skew out by selecting a random sample from an equal number of males and females. This would, of course, reduce the representativeness of the sample, but it would allow you to identify differences between subgroups. So, depending on your research aims, the stratified approach could work well.
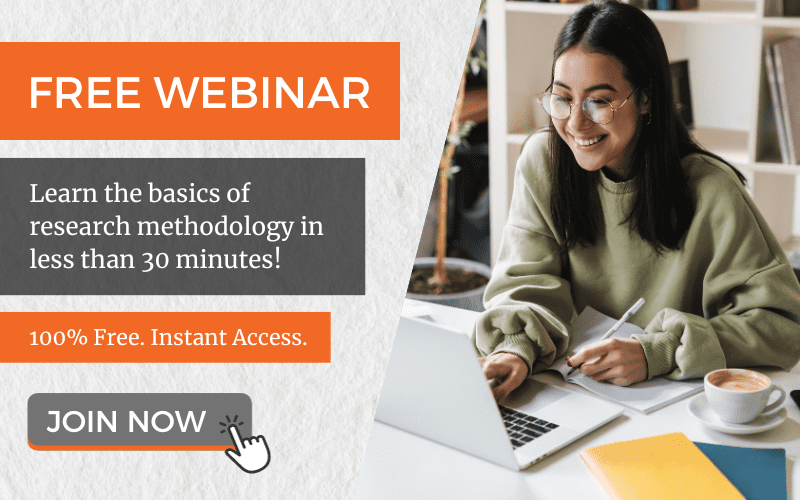
Next on the list is cluster sampling. As the name suggests, this sampling method involves sampling from naturally occurring, mutually exclusive clusters within a population – for example, area codes within a city or cities within a country. Once the clusters are defined, a set of clusters are randomly selected and then a set of participants are randomly selected from each cluster.
Now, you’re probably wondering, “how is cluster sampling different from stratified random sampling?”. Well, let’s look at the previous example where each cluster reflects an area code in a given city.
With cluster sampling, you would collect data from clusters of participants in a handful of area codes (let’s say 5 neighbourhoods). Conversely, with stratified random sampling, you would need to collect data from all over the city (i.e., many more neighbourhoods). You’d still achieve the same sample size either way (let’s say 200 people, for example), but with stratified sampling, you’d need to do a lot more running around, as participants would be scattered across a vast geographic area. As a result, cluster sampling is often the more practical and economical option.
If that all sounds a little mind-bending, you can use the following general rule of thumb. If a population is relatively homogeneous , cluster sampling will often be adequate. Conversely, if a population is quite heterogeneous (i.e., diverse), stratified sampling will generally be more appropriate.
The last probability sampling method we’ll look at is systematic sampling. This method simply involves selecting participants at a set interval , starting from a random point .
For example, if you have a list of students that reflects the population of a university, you could systematically sample that population by selecting participants at an interval of 8 . In other words, you would randomly select a starting point – let’s say student number 40 – followed by student 48, 56, 64, etc.
What’s important with systematic sampling is that the population list you select from needs to be randomly ordered . If there are underlying patterns in the list (for example, if the list is ordered by gender, IQ, age, etc.), this will result in a non-random sample, which would defeat the purpose of adopting this sampling method. Of course, you could safeguard against this by “shuffling” your population list using a random number generator or similar tool.
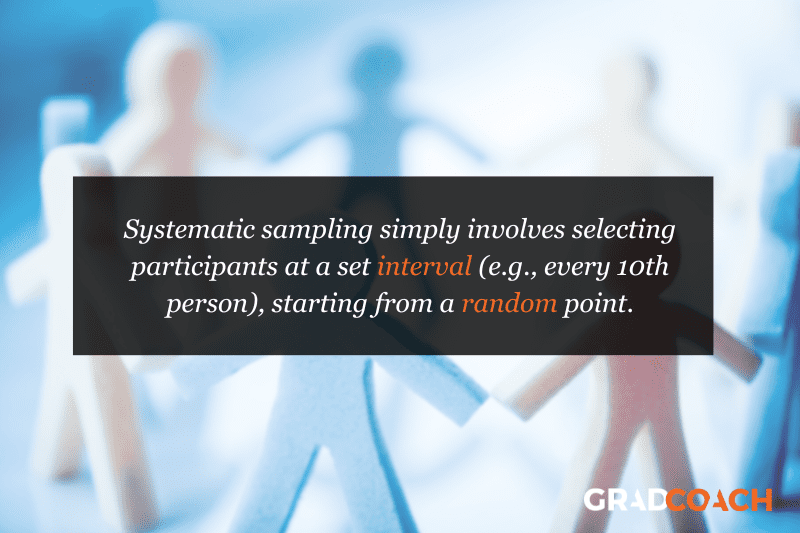
Non-probability-based sampling methods
Right, now that we’ve looked at a few probability-based sampling methods, let’s look at three non-probability methods :
Again, this is not an exhaustive list of all possible sampling methods, so be sure to explore further if you’re interested in adopting a non-probability sampling approach.
First up, we’ve got purposive sampling – also known as judgment , selective or subjective sampling. Again, the name provides some clues, as this method involves the researcher selecting participants using his or her own judgement , based on the purpose of the study (i.e., the research aims).
For example, suppose your research aims were to understand the perceptions of hyper-loyal customers of a particular retail store. In that case, you could use your judgement to engage with frequent shoppers, as well as rare or occasional shoppers, to understand what judgements drive the two behavioural extremes .
Purposive sampling is often used in studies where the aim is to gather information from a small population (especially rare or hard-to-find populations), as it allows the researcher to target specific individuals who have unique knowledge or experience . Naturally, this sampling method is quite prone to researcher bias and judgement error, and it’s unlikely to produce generalisable results, so it’s best suited to studies where the aim is to go deep rather than broad .
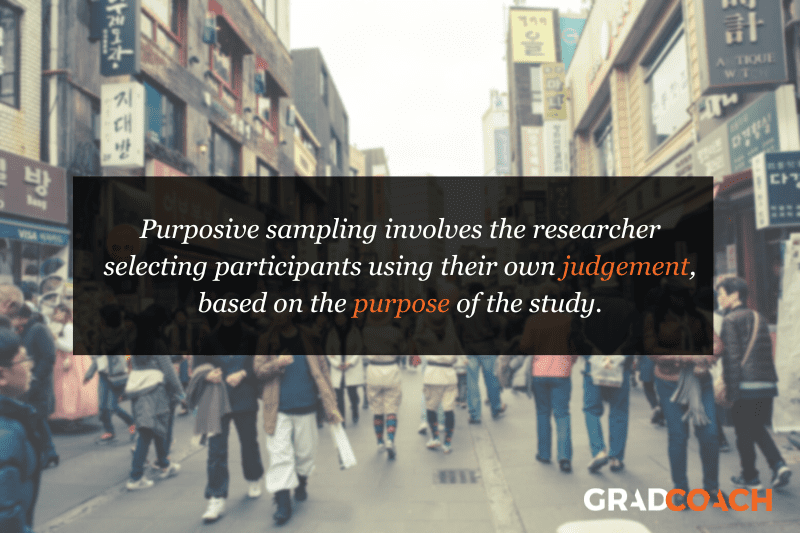
Next up, we have convenience sampling. As the name suggests, with this method, participants are selected based on their availability or accessibility . In other words, the sample is selected based on how convenient it is for the researcher to access it, as opposed to using a defined and objective process.
Naturally, convenience sampling provides a quick and easy way to gather data, as the sample is selected based on the individuals who are readily available or willing to participate. This makes it an attractive option if you’re particularly tight on resources and/or time. However, as you’d expect, this sampling method is unlikely to produce a representative sample and will of course be vulnerable to researcher bias , so it’s important to approach it with caution.
Last but not least, we have the snowball sampling method. This method relies on referrals from initial participants to recruit additional participants. In other words, the initial subjects form the first (small) snowball and each additional subject recruited through referral is added to the snowball, making it larger as it rolls along .
Snowball sampling is often used in research contexts where it’s difficult to identify and access a particular population. For example, people with a rare medical condition or members of an exclusive group. It can also be useful in cases where the research topic is sensitive or taboo and people are unlikely to open up unless they’re referred by someone they trust.
Simply put, snowball sampling is ideal for research that involves reaching hard-to-access populations . But, keep in mind that, once again, it’s a sampling method that’s highly prone to researcher bias and is unlikely to produce a representative sample. So, make sure that it aligns with your research aims and questions before adopting this method.
How to choose a sampling method
Now that we’ve looked at a few popular sampling methods (both probability and non-probability based), the obvious question is, “ how do I choose the right sampling method for my study?”. When selecting a sampling method for your research project, you’ll need to consider two important factors: your research aims and your resources .
As with all research design and methodology choices, your sampling approach needs to be guided by and aligned with your research aims, objectives and research questions – in other words, your golden thread. Specifically, you need to consider whether your research aims are primarily concerned with producing generalisable findings (in which case, you’ll likely opt for a probability-based sampling method) or with achieving rich , deep insights (in which case, a non-probability-based approach could be more practical). Typically, quantitative studies lean toward the former, while qualitative studies aim for the latter, so be sure to consider your broader methodology as well.
The second factor you need to consider is your resources and, more generally, the practical constraints at play. If, for example, you have easy, free access to a large sample at your workplace or university and a healthy budget to help you attract participants, that will open up multiple options in terms of sampling methods. Conversely, if you’re cash-strapped, short on time and don’t have unfettered access to your population of interest, you may be restricted to convenience or referral-based methods.
In short, be ready for trade-offs – you won’t always be able to utilise the “perfect” sampling method for your study, and that’s okay. Much like all the other methodological choices you’ll make as part of your study, you’ll often need to compromise and accept practical trade-offs when it comes to sampling. Don’t let this get you down though – as long as your sampling choice is well explained and justified, and the limitations of your approach are clearly articulated, you’ll be on the right track.
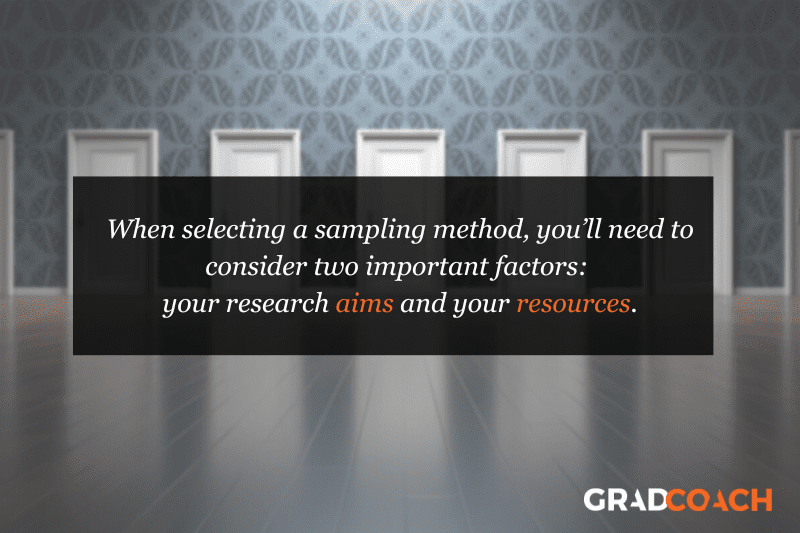
Let’s recap…
In this post, we’ve covered the basics of sampling within the context of a typical research project.
- Sampling refers to the process of defining a subgroup (sample) from the larger group of interest (population).
- The two overarching approaches to sampling are probability sampling (random) and non-probability sampling .
- Common probability-based sampling methods include simple random sampling, stratified random sampling, cluster sampling and systematic sampling.
- Common non-probability-based sampling methods include purposive sampling, convenience sampling and snowball sampling.
- When choosing a sampling method, you need to consider your research aims , objectives and questions, as well as your resources and other practical constraints .
If you’d like to see an example of a sampling strategy in action, be sure to check out our research methodology chapter sample .
Last but not least, if you need hands-on help with your sampling (or any other aspect of your research), take a look at our 1-on-1 coaching service , where we guide you through each step of the research process, at your own pace.
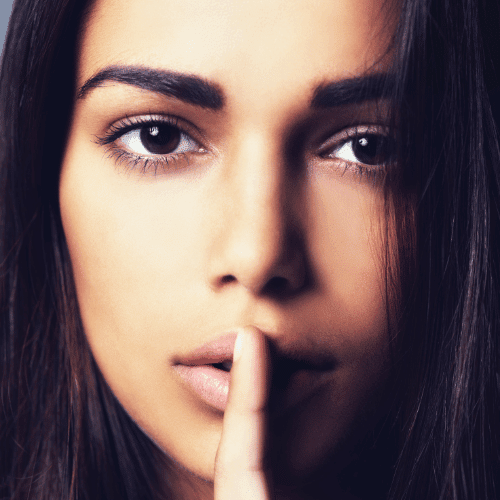
Psst... there’s more!
This post was based on one of our popular Research Bootcamps . If you're working on a research project, you'll definitely want to check this out ...
You Might Also Like:
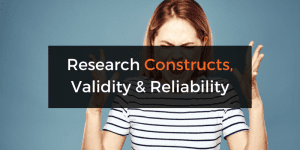
Excellent and helpful. Best site to get a full understanding of Research methodology. I’m nolonger as “clueless “..😉
Excellent and helpful for junior researcher!
Grad Coach tutorials are excellent – I recommend them to everyone doing research. I will be working with a sample of imprisoned women and now have a much clearer idea concerning sampling. Thank you to all at Grad Coach for generously sharing your expertise with students.
Submit a Comment Cancel reply
Your email address will not be published. Required fields are marked *
Save my name, email, and website in this browser for the next time I comment.
- Print Friendly
Have a language expert improve your writing
Run a free plagiarism check in 10 minutes, automatically generate references for free.
- Knowledge Base
- Methodology
- Sampling Methods | Types, Techniques, & Examples
Sampling Methods | Types, Techniques, & Examples
Published on 3 May 2022 by Shona McCombes . Revised on 10 October 2022.
When you conduct research about a group of people, it’s rarely possible to collect data from every person in that group. Instead, you select a sample. The sample is the group of individuals who will actually participate in the research.
To draw valid conclusions from your results, you have to carefully decide how you will select a sample that is representative of the group as a whole. There are two types of sampling methods:
- Probability sampling involves random selection, allowing you to make strong statistical inferences about the whole group. It minimises the risk of selection bias .
- Non-probability sampling involves non-random selection based on convenience or other criteria, allowing you to easily collect data.
You should clearly explain how you selected your sample in the methodology section of your paper or thesis.
Table of contents
Population vs sample, probability sampling methods, non-probability sampling methods, frequently asked questions about sampling.
First, you need to understand the difference between a population and a sample , and identify the target population of your research.
- The population is the entire group that you want to draw conclusions about.
- The sample is the specific group of individuals that you will collect data from.
The population can be defined in terms of geographical location, age, income, and many other characteristics.
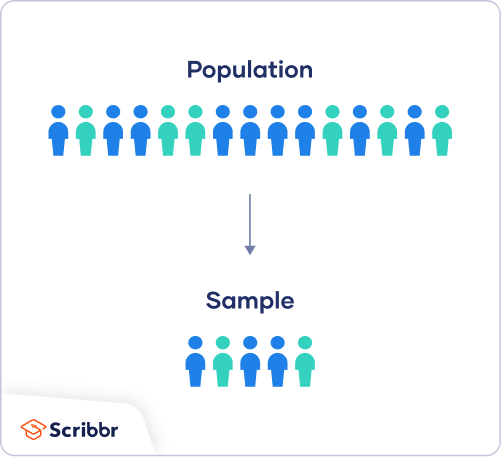
It is important to carefully define your target population according to the purpose and practicalities of your project.
If the population is very large, demographically mixed, and geographically dispersed, it might be difficult to gain access to a representative sample.
Sampling frame
The sampling frame is the actual list of individuals that the sample will be drawn from. Ideally, it should include the entire target population (and nobody who is not part of that population).
You are doing research on working conditions at Company X. Your population is all 1,000 employees of the company. Your sampling frame is the company’s HR database, which lists the names and contact details of every employee.
Sample size
The number of individuals you should include in your sample depends on various factors, including the size and variability of the population and your research design. There are different sample size calculators and formulas depending on what you want to achieve with statistical analysis .
Prevent plagiarism, run a free check.
Probability sampling means that every member of the population has a chance of being selected. It is mainly used in quantitative research . If you want to produce results that are representative of the whole population, probability sampling techniques are the most valid choice.
There are four main types of probability sample.
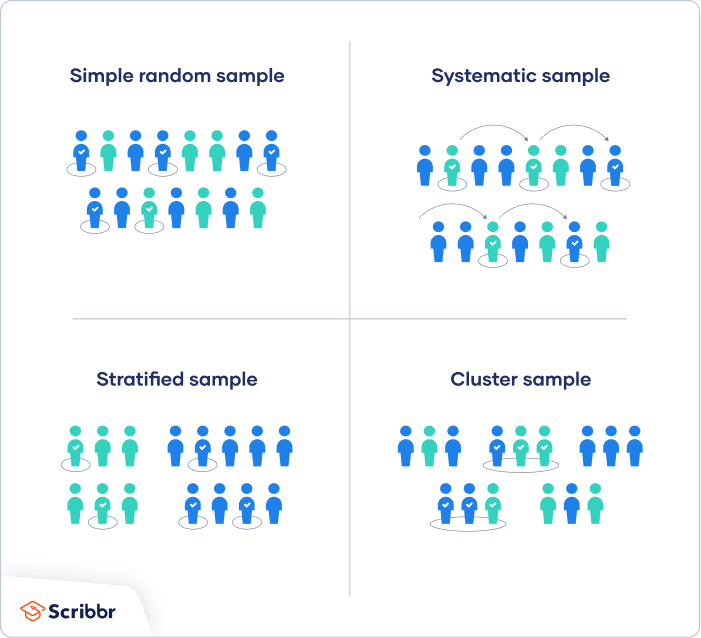
1. Simple random sampling
In a simple random sample , every member of the population has an equal chance of being selected. Your sampling frame should include the whole population.
To conduct this type of sampling, you can use tools like random number generators or other techniques that are based entirely on chance.
You want to select a simple random sample of 100 employees of Company X. You assign a number to every employee in the company database from 1 to 1000, and use a random number generator to select 100 numbers.
2. Systematic sampling
Systematic sampling is similar to simple random sampling, but it is usually slightly easier to conduct. Every member of the population is listed with a number, but instead of randomly generating numbers, individuals are chosen at regular intervals.
All employees of the company are listed in alphabetical order. From the first 10 numbers, you randomly select a starting point: number 6. From number 6 onwards, every 10th person on the list is selected (6, 16, 26, 36, and so on), and you end up with a sample of 100 people.
If you use this technique, it is important to make sure that there is no hidden pattern in the list that might skew the sample. For example, if the HR database groups employees by team, and team members are listed in order of seniority, there is a risk that your interval might skip over people in junior roles, resulting in a sample that is skewed towards senior employees.
3. Stratified sampling
Stratified sampling involves dividing the population into subpopulations that may differ in important ways. It allows you draw more precise conclusions by ensuring that every subgroup is properly represented in the sample.
To use this sampling method, you divide the population into subgroups (called strata) based on the relevant characteristic (e.g., gender, age range, income bracket, job role).
Based on the overall proportions of the population, you calculate how many people should be sampled from each subgroup. Then you use random or systematic sampling to select a sample from each subgroup.
The company has 800 female employees and 200 male employees. You want to ensure that the sample reflects the gender balance of the company, so you sort the population into two strata based on gender. Then you use random sampling on each group, selecting 80 women and 20 men, which gives you a representative sample of 100 people.
4. Cluster sampling
Cluster sampling also involves dividing the population into subgroups, but each subgroup should have similar characteristics to the whole sample. Instead of sampling individuals from each subgroup, you randomly select entire subgroups.
If it is practically possible, you might include every individual from each sampled cluster. If the clusters themselves are large, you can also sample individuals from within each cluster using one of the techniques above. This is called multistage sampling .
This method is good for dealing with large and dispersed populations, but there is more risk of error in the sample, as there could be substantial differences between clusters. It’s difficult to guarantee that the sampled clusters are really representative of the whole population.
The company has offices in 10 cities across the country (all with roughly the same number of employees in similar roles). You don’t have the capacity to travel to every office to collect your data, so you use random sampling to select 3 offices – these are your clusters.
In a non-probability sample , individuals are selected based on non-random criteria, and not every individual has a chance of being included.
This type of sample is easier and cheaper to access, but it has a higher risk of sampling bias . That means the inferences you can make about the population are weaker than with probability samples, and your conclusions may be more limited. If you use a non-probability sample, you should still aim to make it as representative of the population as possible.
Non-probability sampling techniques are often used in exploratory and qualitative research . In these types of research, the aim is not to test a hypothesis about a broad population, but to develop an initial understanding of a small or under-researched population.
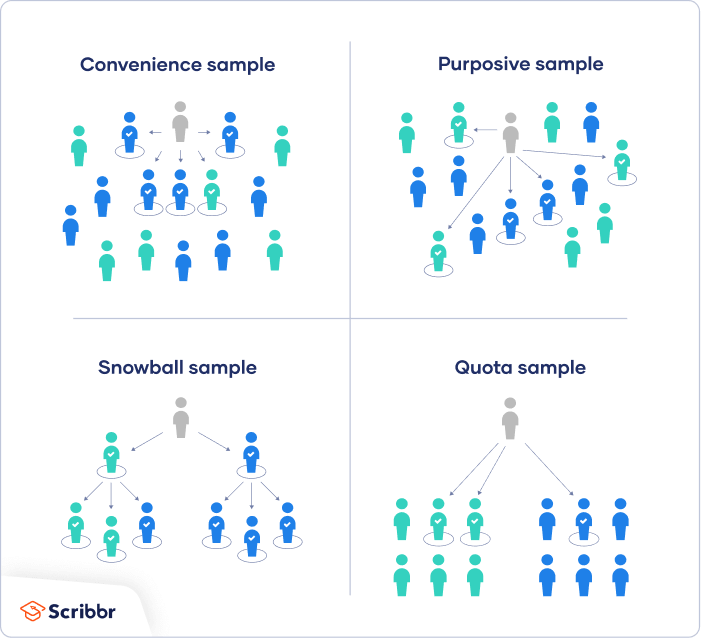
1. Convenience sampling
A convenience sample simply includes the individuals who happen to be most accessible to the researcher.
This is an easy and inexpensive way to gather initial data, but there is no way to tell if the sample is representative of the population, so it can’t produce generalisable results.
You are researching opinions about student support services in your university, so after each of your classes, you ask your fellow students to complete a survey on the topic. This is a convenient way to gather data, but as you only surveyed students taking the same classes as you at the same level, the sample is not representative of all the students at your university.
2. Voluntary response sampling
Similar to a convenience sample, a voluntary response sample is mainly based on ease of access. Instead of the researcher choosing participants and directly contacting them, people volunteer themselves (e.g., by responding to a public online survey).
Voluntary response samples are always at least somewhat biased, as some people will inherently be more likely to volunteer than others.
You send out the survey to all students at your university and many students decide to complete it. This can certainly give you some insight into the topic, but the people who responded are more likely to be those who have strong opinions about the student support services, so you can’t be sure that their opinions are representative of all students.
3. Purposive sampling
Purposive sampling , also known as judgement sampling, involves the researcher using their expertise to select a sample that is most useful to the purposes of the research.
It is often used in qualitative research , where the researcher wants to gain detailed knowledge about a specific phenomenon rather than make statistical inferences, or where the population is very small and specific. An effective purposive sample must have clear criteria and rationale for inclusion.
You want to know more about the opinions and experiences of students with a disability at your university, so you purposely select a number of students with different support needs in order to gather a varied range of data on their experiences with student services.
4. Snowball sampling
If the population is hard to access, snowball sampling can be used to recruit participants via other participants. The number of people you have access to ‘snowballs’ as you get in contact with more people.
You are researching experiences of homelessness in your city. Since there is no list of all homeless people in the city, probability sampling isn’t possible. You meet one person who agrees to participate in the research, and she puts you in contact with other homeless people she knows in the area.
A sample is a subset of individuals from a larger population. Sampling means selecting the group that you will actually collect data from in your research.
For example, if you are researching the opinions of students in your university, you could survey a sample of 100 students.
Statistical sampling allows you to test a hypothesis about the characteristics of a population. There are various sampling methods you can use to ensure that your sample is representative of the population as a whole.
Samples are used to make inferences about populations . Samples are easier to collect data from because they are practical, cost-effective, convenient, and manageable.
Probability sampling means that every member of the target population has a known chance of being included in the sample.
Probability sampling methods include simple random sampling , systematic sampling , stratified sampling , and cluster sampling .
In non-probability sampling , the sample is selected based on non-random criteria, and not every member of the population has a chance of being included.
Common non-probability sampling methods include convenience sampling , voluntary response sampling, purposive sampling , snowball sampling , and quota sampling .
Sampling bias occurs when some members of a population are systematically more likely to be selected in a sample than others.
Cite this Scribbr article
If you want to cite this source, you can copy and paste the citation or click the ‘Cite this Scribbr article’ button to automatically add the citation to our free Reference Generator.
McCombes, S. (2022, October 10). Sampling Methods | Types, Techniques, & Examples. Scribbr. Retrieved 22 April 2024, from https://www.scribbr.co.uk/research-methods/sampling/
Is this article helpful?
Shona McCombes
Other students also liked, what is quantitative research | definition & methods, a quick guide to experimental design | 5 steps & examples, controlled experiments | methods & examples of control.
An overview of sampling methods
Last updated
27 February 2023
Reviewed by
Cathy Heath
When researching perceptions or attributes of a product, service, or people, you have two options:
Survey every person in your chosen group (the target market, or population), collate your responses, and reach your conclusions.
Select a smaller group from within your target market and use their answers to represent everyone. This option is sampling .
Sampling saves you time and money. When you use the sampling method, the whole population being studied is called the sampling frame .
The sample you choose should represent your target market, or the sampling frame, well enough to do one of the following:
Generalize your findings across the sampling frame and use them as though you had surveyed everyone
Use the findings to decide on your next step, which might involve more in-depth sampling
Make research less tedious
Dovetail streamlines research to help you uncover and share actionable insights
How was sampling developed?
Valery Glivenko and Francesco Cantelli, two mathematicians studying probability theory in the early 1900s, devised the sampling method. Their research showed that a properly chosen sample of people would reflect the larger group’s status, opinions, decisions, and decision-making steps.
They proved you don't need to survey the entire target market, thereby saving the rest of us a lot of time and money.
- Why is sampling important?
We’ve already touched on the fact that sampling saves you time and money. When you get reliable results quickly, you can act on them sooner. And the money you save can pay for something else.
It’s often easier to survey a sample than a whole population. Sample inferences can be more reliable than those you get from a very large group because you can choose your samples carefully and scientifically.
Sampling is also useful because it is often impossible to survey the entire population. You probably have no choice but to collect only a sample in the first place.
Because you’re working with fewer people, you can collect richer data, which makes your research more accurate. You can:
Ask more questions
Go into more detail
Seek opinions instead of just collecting facts
Observe user behaviors
Double-check your findings if you need to
In short, sampling works! Let's take a look at the most common sampling methods.
- Types of sampling methods
There are two main sampling methods: probability sampling and non-probability sampling. These can be further refined, which we'll cover shortly. You can then decide which approach best suits your research project.
Probability sampling method
Probability sampling is used in quantitative research , so it provides data on the survey topic in terms of numbers. Probability relates to mathematics, hence the name ‘quantitative research’. Subjects are asked questions like:
How many boxes of candy do you buy at one time?
How often do you shop for candy?
How much would you pay for a box of candy?
This method is also called random sampling because everyone in the target market has an equal chance of being chosen for the survey. It is designed to reduce sampling error for the most important variables. You should, therefore, get results that fairly reflect the larger population.
Non-probability sampling method
In this method, not everyone has an equal chance of being part of the sample. It's usually easier (and cheaper) to select people for the sample group. You choose people who are more likely to be involved in or know more about the topic you’re researching.
Non-probability sampling is used for qualitative research. Qualitative data is generated by questions like:
Where do you usually shop for candy (supermarket, gas station, etc.?)
Which candy brand do you usually buy?
Why do you like that brand?
- Probability sampling methods
Here are five ways of doing probability sampling:
Simple random sampling (basic probability sampling)
Systematic sampling
Stratified sampling.
Cluster sampling
Multi-stage sampling
Simple random sampling.
There are three basic steps to simple random sampling:
Choose your sampling frame.
Decide on your sample size. Make sure it is large enough to give you reliable data.
Randomly choose your sample participants.
You could put all their names in a hat, shake the hat to mix the names, and pull out however many names you want in your sample (without looking!)
You could be more scientific by giving each participant a number and then using a random number generator program to choose the numbers.
Instead of choosing names or numbers, you decide beforehand on a selection method. For example, collect all the names in your sampling frame and start at, for example, the fifth person on the list, then choose every fourth name or every tenth name. Alternatively, you could choose everyone whose last name begins with randomly-selected initials, such as A, G, or W.
Choose your system of selecting names, and away you go.
This is a more sophisticated way to choose your sample. You break the sampling frame down into important subgroups or strata . Then, decide how many you want in your sample, and choose an equal number (or a proportionate number) from each subgroup.
For example, you want to survey how many people in a geographic area buy candy, so you compile a list of everyone in that area. You then break that list down into, for example, males and females, then into pre-teens, teenagers, young adults, senior citizens, etc. who are male or female.
So, if there are 1,000 young male adults and 2,000 young female adults in the whole sampling frame, you may want to choose 100 males and 200 females to keep the proportions balanced. You then choose the individual survey participants through the systematic sampling method.
Clustered sampling
This method is used when you want to subdivide a sample into smaller groups or clusters that are geographically or organizationally related.
Let’s say you’re doing quantitative research into candy sales. You could choose your sample participants from urban, suburban, or rural populations. This would give you three geographic clusters from which to select your participants.
This is a more refined way of doing cluster sampling. Let’s say you have your urban cluster, which is your primary sampling unit. You can subdivide this into a secondary sampling unit, say, participants who typically buy their candy in supermarkets. You could then further subdivide this group into your ultimate sampling unit. Finally, you select the actual survey participants from this unit.
- Uses of probability sampling
Probability sampling has three main advantages:
It helps minimizes the likelihood of sampling bias. How you choose your sample determines the quality of your results. Probability sampling gives you an unbiased, randomly selected sample of your target market.
It allows you to create representative samples and subgroups within a sample out of a large or diverse target market.
It lets you use sophisticated statistical methods to select as close to perfect samples as possible.
- Non-probability sampling methods
To recap, with non-probability sampling, you choose people for your sample in a non-random way, so not everyone in your sampling frame has an equal chance of being chosen. Your research findings, therefore, may not be as representative overall as probability sampling, but you may not want them to be.
Sampling bias is not a concern if all potential survey participants share similar traits. For example, you may want to specifically focus on young male adults who spend more than others on candy. In addition, it is usually a cheaper and quicker method because you don't have to work out a complex selection system that represents the entire population in that community.
Researchers do need to be mindful of carefully considering the strengths and limitations of each method before selecting a sampling technique.
Non-probability sampling is best for exploratory research , such as at the beginning of a research project.
There are five main types of non-probability sampling methods:
Convenience sampling
Purposive sampling, voluntary response sampling, snowball sampling, quota sampling.
The strategy of convenience sampling is to choose your sample quickly and efficiently, using the least effort, usually to save money.
Let's say you want to survey the opinions of 100 millennials about a particular topic. You could send out a questionnaire over the social media platforms millennials use. Ask respondents to confirm their birth year at the top of their response sheet and, when you have your 100 responses, begin your analysis. Or you could visit restaurants and bars where millennials spend their evenings and sign people up.
A drawback of convenience sampling is that it may not yield results that apply to a broader population.
This method relies on your judgment to choose the most likely sample to deliver the most useful results. You must know enough about the survey goals and the sampling frame to choose the most appropriate sample respondents.
Your knowledge and experience save you time because you know your ideal sample candidates, so you should get high-quality results.
This method is similar to convenience sampling, but it is based on potential sample members volunteering rather than you looking for people.
You make it known you want to do a survey on a particular topic for a particular reason and wait until enough people volunteer. Then you give them the questionnaire or arrange interviews to ask your questions directly.
Snowball sampling involves asking selected participants to refer others who may qualify for the survey. This method is best used when there is no sampling frame available. It is also useful when the researcher doesn’t know much about the target population.
Let's say you want to research a niche topic that involves people who may be difficult to locate. For our candy example, this could be young males who buy a lot of candy, go rock climbing during the day, and watch adventure movies at night. You ask each participant to name others they know who do the same things, so you can contact them. As you make contact with more people, your sample 'snowballs' until you have all the names you need.
This sampling method involves collecting the specific number of units (quotas) from your predetermined subpopulations. Quota sampling is a way of ensuring that your sample accurately represents the sampling frame.
- Uses of non-probability sampling
You can use non-probability sampling when you:
Want to do a quick test to see if a more detailed and sophisticated survey may be worthwhile
Want to explore an idea to see if it 'has legs'
Launch a pilot study
Do some initial qualitative research
Have little time or money available (half a loaf is better than no bread at all)
Want to see if the initial results will help you justify a longer, more detailed, and more expensive research project
- The main types of sampling bias, and how to avoid them
Sampling bias can fog or limit your research results. This will have an impact when you generalize your results across the whole target market. The two main causes of sampling bias are faulty research design and poor data collection or recording. They can affect probability and non-probability sampling.
Faulty research
If a surveyor chooses participants inappropriately, the results will not reflect the population as a whole.
A famous example is the 1948 presidential race. A telephone survey was conducted to see which candidate had more support. The problem with the research design was that, in 1948, most people with telephones were wealthy, and their opinions were very different from voters as a whole. The research implied Dewey would win, but it was Truman who became president.
Poor data collection or recording
This problem speaks for itself. The survey may be well structured, the sample groups appropriate, the questions clear and easy to understand, and the cluster sizes appropriate. But if surveyors check the wrong boxes when they get an answer or if the entire subgroup results are lost, the survey results will be biased.
How do you minimize bias in sampling?
To get results you can rely on, you must:
Know enough about your target market
Choose one or more sample surveys to cover the whole target market properly
Choose enough people in each sample so your results mirror your target market
Have content validity . This means the content of your questions must be direct and efficiently worded. If it isn’t, the viability of your survey could be questioned. That would also be a waste of time and money, so make the wording of your questions your top focus.
If using probability sampling, make sure your sampling frame includes everyone it should and that your random sampling selection process includes the right proportion of the subgroups
If using non-probability sampling, focus on fairness, equality, and completeness in identifying your samples and subgroups. Then balance those criteria against simple convenience or other relevant factors.
What are the five types of sampling bias?
Self-selection bias. If you mass-mail questionnaires to everyone in the sample, you’re more likely to get results from people with extrovert or activist personalities and not from introverts or pragmatists. So if your convenience sampling focuses on getting your quota responses quickly, it may be skewed.
Non-response bias. Unhappy customers, stressed-out employees, or other sub-groups may not want to cooperate or they may pull out early.
Undercoverage bias. If your survey is done, say, via email or social media platforms, it will miss people without internet access, such as those living in rural areas, the elderly, or lower-income groups.
Survivorship bias. Unsuccessful people are less likely to take part. Another example may be a researcher excluding results that don’t support the overall goal. If the CEO wants to tell the shareholders about a successful product or project at the AGM, some less positive survey results may go “missing” (to take an extreme example.) The result is that your data will reflect an overly optimistic representation of the truth.
Pre-screening bias. If the researcher, whose experience and knowledge are being used to pre-select respondents in a judgmental sampling, focuses more on convenience than judgment, the results may be compromised.
How do you minimize sampling bias?
Focus on the bullet points in the next section and:
Make survey questionnaires as direct, easy, short, and available as possible, so participants are more likely to complete them accurately and send them back
Follow up with the people who have been selected but have not returned their responses
Ignore any pressure that may produce bias
- How do you decide on the type of sampling to use?
Use the ideas you've gleaned from this article to give yourself a platform, then choose the best method to meet your goals while staying within your time and cost limits.
If it isn't obvious which method you should choose, use this strategy:
Clarify your research goals
Clarify how accurate your research results must be to reach your goals
Evaluate your goals against time and budget
List the two or three most obvious sampling methods that will work for you
Confirm the availability of your resources (researchers, computer time, etc.)
Compare each of the possible methods with your goals, accuracy, precision, resource, time, and cost constraints
Make your decision
- The takeaway
Effective market research is the basis of successful marketing, advertising, and future productivity. By selecting the most appropriate sampling methods, you will collect the most useful market data and make the most effective decisions.
Get started today
Go from raw data to valuable insights with a flexible research platform
Editor’s picks
Last updated: 21 December 2023
Last updated: 16 December 2023
Last updated: 6 October 2023
Last updated: 25 November 2023
Last updated: 12 May 2023
Last updated: 15 February 2024
Last updated: 11 March 2024
Last updated: 12 December 2023
Last updated: 18 May 2023
Last updated: 6 March 2024
Last updated: 10 April 2023
Last updated: 20 December 2023
Latest articles
Related topics, log in or sign up.
Get started for free
Sampling Methods In Reseach: Types, Techniques, & Examples
Saul Mcleod, PhD
Editor-in-Chief for Simply Psychology
BSc (Hons) Psychology, MRes, PhD, University of Manchester
Saul Mcleod, PhD., is a qualified psychology teacher with over 18 years of experience in further and higher education. He has been published in peer-reviewed journals, including the Journal of Clinical Psychology.
Learn about our Editorial Process
Olivia Guy-Evans, MSc
Associate Editor for Simply Psychology
BSc (Hons) Psychology, MSc Psychology of Education
Olivia Guy-Evans is a writer and associate editor for Simply Psychology. She has previously worked in healthcare and educational sectors.
On This Page:
Sampling methods in psychology refer to strategies used to select a subset of individuals (a sample) from a larger population, to study and draw inferences about the entire population. Common methods include random sampling, stratified sampling, cluster sampling, and convenience sampling. Proper sampling ensures representative, generalizable, and valid research results.
- Sampling : the process of selecting a representative group from the population under study.
- Target population : the total group of individuals from which the sample might be drawn.
- Sample: a subset of individuals selected from a larger population for study or investigation. Those included in the sample are termed “participants.”
- Generalizability : the ability to apply research findings from a sample to the broader target population, contingent on the sample being representative of that population.
For instance, if the advert for volunteers is published in the New York Times, this limits how much the study’s findings can be generalized to the whole population, because NYT readers may not represent the entire population in certain respects (e.g., politically, socio-economically).
The Purpose of Sampling
We are interested in learning about large groups of people with something in common in psychological research. We call the group interested in studying our “target population.”
In some types of research, the target population might be as broad as all humans. Still, in other types of research, the target population might be a smaller group, such as teenagers, preschool children, or people who misuse drugs.
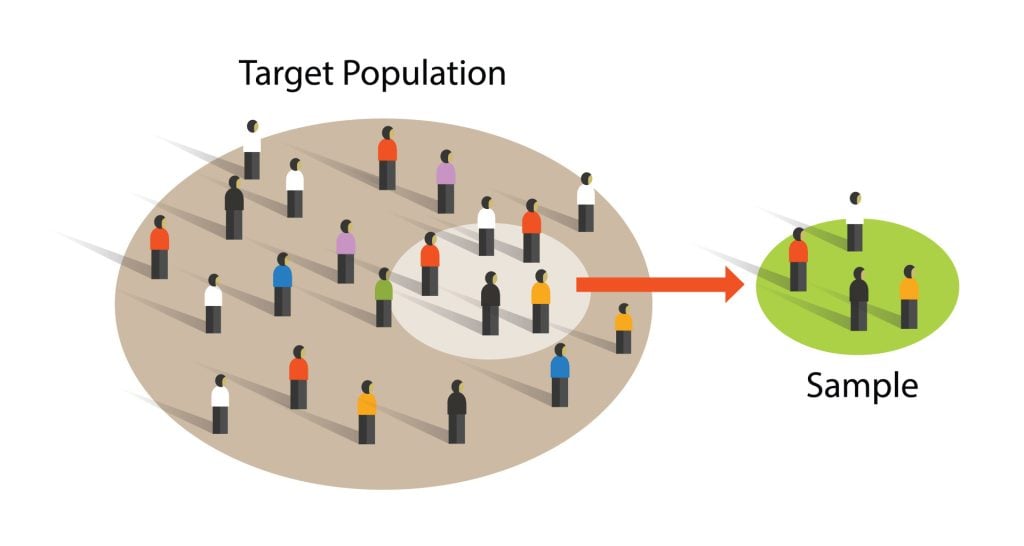
Studying every person in a target population is more or less impossible. Hence, psychologists select a sample or sub-group of the population that is likely to be representative of the target population we are interested in.
This is important because we want to generalize from the sample to the target population. The more representative the sample, the more confident the researcher can be that the results can be generalized to the target population.
One of the problems that can occur when selecting a sample from a target population is sampling bias. Sampling bias refers to situations where the sample does not reflect the characteristics of the target population.
Many psychology studies have a biased sample because they have used an opportunity sample that comprises university students as their participants (e.g., Asch ).
OK, so you’ve thought up this brilliant psychological study and designed it perfectly. But who will you try it out on, and how will you select your participants?
There are various sampling methods. The one chosen will depend on a number of factors (such as time, money, etc.).
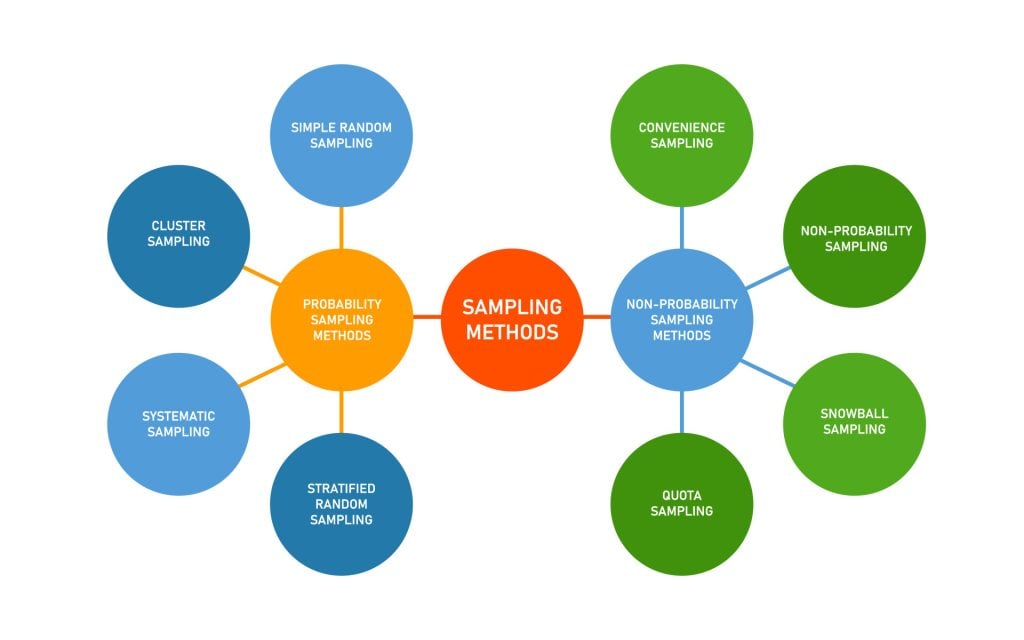
Random Sampling
Random sampling is a type of probability sampling where everyone in the entire target population has an equal chance of being selected.
This is similar to the national lottery. If the “population” is everyone who bought a lottery ticket, then everyone has an equal chance of winning the lottery (assuming they all have one ticket each).
Random samples require naming or numbering the target population and then using some raffle method to choose those to make up the sample. Random samples are the best method of selecting your sample from the population of interest.
- The advantages are that your sample should represent the target population and eliminate sampling bias.
- The disadvantage is that it is very difficult to achieve (i.e., time, effort, and money).
Stratified Sampling
During stratified sampling , the researcher identifies the different types of people that make up the target population and works out the proportions needed for the sample to be representative.
A list is made of each variable (e.g., IQ, gender, etc.) that might have an effect on the research. For example, if we are interested in the money spent on books by undergraduates, then the main subject studied may be an important variable.
For example, students studying English Literature may spend more money on books than engineering students, so if we use a large percentage of English students or engineering students, our results will not be accurate.
We have to determine the relative percentage of each group at a university, e.g., Engineering 10%, Social Sciences 15%, English 20%, Sciences 25%, Languages 10%, Law 5%, and Medicine 15%. The sample must then contain all these groups in the same proportion as the target population (university students).
- The disadvantage of stratified sampling is that gathering such a sample would be extremely time-consuming and difficult to do. This method is rarely used in Psychology.
- However, the advantage is that the sample should be highly representative of the target population, and therefore we can generalize from the results obtained.
Opportunity Sampling
Opportunity sampling is a method in which participants are chosen based on their ease of availability and proximity to the researcher, rather than using random or systematic criteria. It’s a type of convenience sampling .
An opportunity sample is obtained by asking members of the population of interest if they would participate in your research. An example would be selecting a sample of students from those coming out of the library.
- This is a quick and easy way of choosing participants (advantage)
- It may not provide a representative sample and could be biased (disadvantage).
Systematic Sampling
Systematic sampling is a method where every nth individual is selected from a list or sequence to form a sample, ensuring even and regular intervals between chosen subjects.
Participants are systematically selected (i.e., orderly/logical) from the target population, like every nth participant on a list of names.
To take a systematic sample, you list all the population members and then decide upon a sample you would like. By dividing the number of people in the population by the number of people you want in your sample, you get a number we will call n.
If you take every nth name, you will get a systematic sample of the correct size. If, for example, you wanted to sample 150 children from a school of 1,500, you would take every 10th name.
- The advantage of this method is that it should provide a representative sample.
Sample size
The sample size is a critical factor in determining the reliability and validity of a study’s findings. While increasing the sample size can enhance the generalizability of results, it’s also essential to balance practical considerations, such as resource constraints and diminishing returns from ever-larger samples.
Reliability and Validity
Reliability refers to the consistency and reproducibility of research findings across different occasions, researchers, or instruments. A small sample size may lead to inconsistent results due to increased susceptibility to random error or the influence of outliers. In contrast, a larger sample minimizes these errors, promoting more reliable results.
Validity pertains to the accuracy and truthfulness of research findings. For a study to be valid, it should accurately measure what it intends to do. A small, unrepresentative sample can compromise external validity, meaning the results don’t generalize well to the larger population. A larger sample captures more variability, ensuring that specific subgroups or anomalies don’t overly influence results.
Practical Considerations
Resource Constraints : Larger samples demand more time, money, and resources. Data collection becomes more extensive, data analysis more complex, and logistics more challenging.
Diminishing Returns : While increasing the sample size generally leads to improved accuracy and precision, there’s a point where adding more participants yields only marginal benefits. For instance, going from 50 to 500 participants might significantly boost a study’s robustness, but jumping from 10,000 to 10,500 might not offer a comparable advantage, especially considering the added costs.

- En español – ExME
- Em português – EME
What are sampling methods and how do you choose the best one?
Posted on 18th November 2020 by Mohamed Khalifa
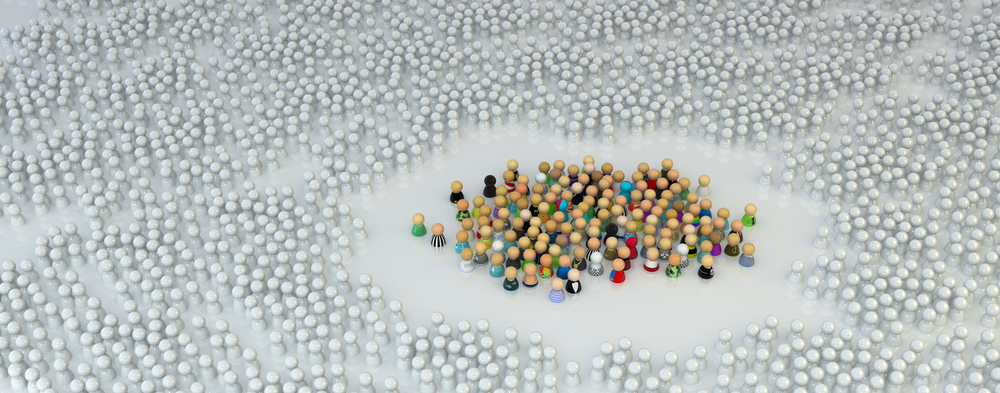
This tutorial will introduce sampling methods and potential sampling errors to avoid when conducting medical research.
Introduction to sampling methods
Examples of different sampling methods, choosing the best sampling method.
It is important to understand why we sample the population; for example, studies are built to investigate the relationships between risk factors and disease. In other words, we want to find out if this is a true association, while still aiming for the minimum risk for errors such as: chance, bias or confounding .
However, it would not be feasible to experiment on the whole population, we would need to take a good sample and aim to reduce the risk of having errors by proper sampling technique.
What is a sampling frame?
A sampling frame is a record of the target population containing all participants of interest. In other words, it is a list from which we can extract a sample.
What makes a good sample?
A good sample should be a representative subset of the population we are interested in studying, therefore, with each participant having equal chance of being randomly selected into the study.
We could choose a sampling method based on whether we want to account for sampling bias; a random sampling method is often preferred over a non-random method for this reason. Random sampling examples include: simple, systematic, stratified, and cluster sampling. Non-random sampling methods are liable to bias, and common examples include: convenience, purposive, snowballing, and quota sampling. For the purposes of this blog we will be focusing on random sampling methods .
Example: We want to conduct an experimental trial in a small population such as: employees in a company, or students in a college. We include everyone in a list and use a random number generator to select the participants
Advantages: Generalisable results possible, random sampling, the sampling frame is the whole population, every participant has an equal probability of being selected
Disadvantages: Less precise than stratified method, less representative than the systematic method
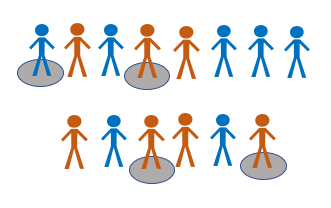
Example: Every nth patient entering the out-patient clinic is selected and included in our sample
Advantages: More feasible than simple or stratified methods, sampling frame is not always required
Disadvantages: Generalisability may decrease if baseline characteristics repeat across every nth participant
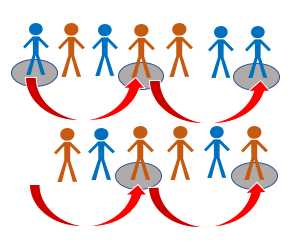
Example: We have a big population (a city) and we want to ensure representativeness of all groups with a pre-determined characteristic such as: age groups, ethnic origin, and gender
Advantages: Inclusive of strata (subgroups), reliable and generalisable results
Disadvantages: Does not work well with multiple variables
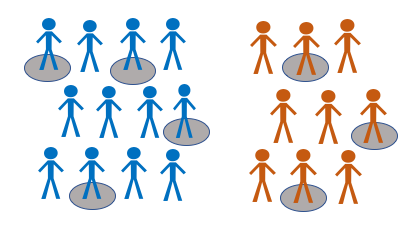
Example: 10 schools have the same number of students across the county. We can randomly select 3 out of 10 schools as our clusters
Advantages: Readily doable with most budgets, does not require a sampling frame
Disadvantages: Results may not be reliable nor generalisable
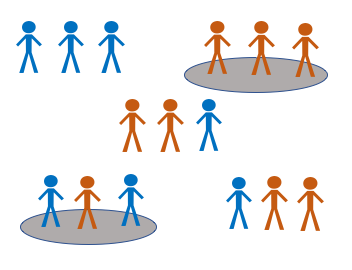
How can you identify sampling errors?
Non-random selection increases the probability of sampling (selection) bias if the sample does not represent the population we want to study. We could avoid this by random sampling and ensuring representativeness of our sample with regards to sample size.
An inadequate sample size decreases the confidence in our results as we may think there is no significant difference when actually there is. This type two error results from having a small sample size, or from participants dropping out of the sample.
In medical research of disease, if we select people with certain diseases while strictly excluding participants with other co-morbidities, we run the risk of diagnostic purity bias where important sub-groups of the population are not represented.
Furthermore, measurement bias may occur during re-collection of risk factors by participants (recall bias) or assessment of outcome where people who live longer are associated with treatment success, when in fact people who died were not included in the sample or data analysis (survivors bias).
By following the steps below we could choose the best sampling method for our study in an orderly fashion.
Research objectiveness
Firstly, a refined research question and goal would help us define our population of interest. If our calculated sample size is small then it would be easier to get a random sample. If, however, the sample size is large, then we should check if our budget and resources can handle a random sampling method.
Sampling frame availability
Secondly, we need to check for availability of a sampling frame (Simple), if not, could we make a list of our own (Stratified). If neither option is possible, we could still use other random sampling methods, for instance, systematic or cluster sampling.
Study design
Moreover, we could consider the prevalence of the topic (exposure or outcome) in the population, and what would be the suitable study design. In addition, checking if our target population is widely varied in its baseline characteristics. For example, a population with large ethnic subgroups could best be studied using a stratified sampling method.
Random sampling
Finally, the best sampling method is always the one that could best answer our research question while also allowing for others to make use of our results (generalisability of results). When we cannot afford a random sampling method, we can always choose from the non-random sampling methods.
To sum up, we now understand that choosing between random or non-random sampling methods is multifactorial. We might often be tempted to choose a convenience sample from the start, but that would not only decrease precision of our results, and would make us miss out on producing research that is more robust and reliable.
References (pdf)
Mohamed Khalifa
Leave a reply cancel reply.
Your email address will not be published. Required fields are marked *
Save my name, email, and website in this browser for the next time I comment.
No Comments on What are sampling methods and how do you choose the best one?
Thank you for this overview. A concise approach for research.
really helps! am an ecology student preparing to write my lab report for sampling.
I learned a lot to the given presentation.. It’s very comprehensive… Thanks for sharing…
Very informative and useful for my study. Thank you
Oversimplified info on sampling methods. Probabilistic of the sampling and sampling of samples by chance does rest solely on the random methods. Factors such as the random visits or presentation of the potential participants at clinics or sites could be sufficiently random in nature and should be used for the sake of efficiency and feasibility. Nevertheless, this approach has to be taken only after careful thoughts. Representativeness of the study samples have to be checked at the end or during reporting by comparing it to the published larger studies or register of some kind in/from the local population.
Thank you so much Mr.mohamed very useful and informative article
Subscribe to our newsletter
You will receive our monthly newsletter and free access to Trip Premium.
Related Articles
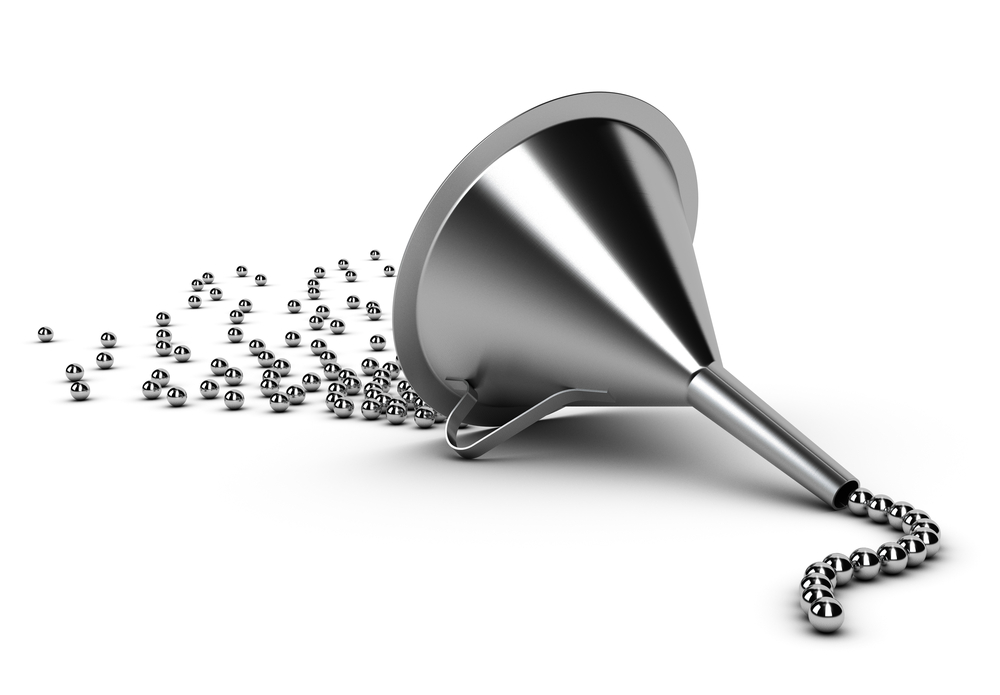
How to read a funnel plot
This blog introduces you to funnel plots, guiding you through how to read them and what may cause them to look asymmetrical.
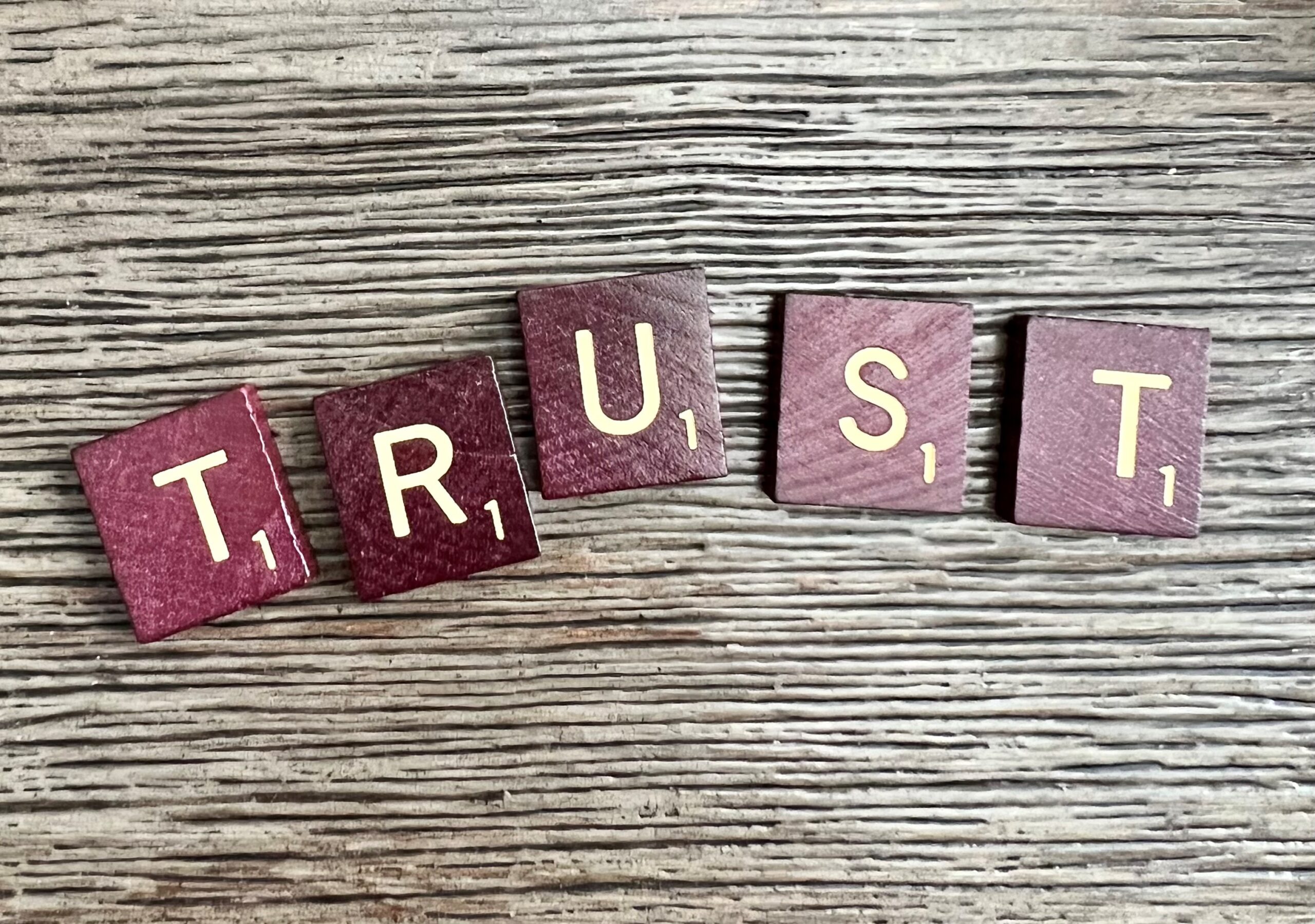
Internal and external validity: what are they and how do they differ?
Is this study valid? Can I trust this study’s methods and design? Can I apply the results of this study to other contexts? Learn more about internal and external validity in research to help you answer these questions when you next look at a paper.
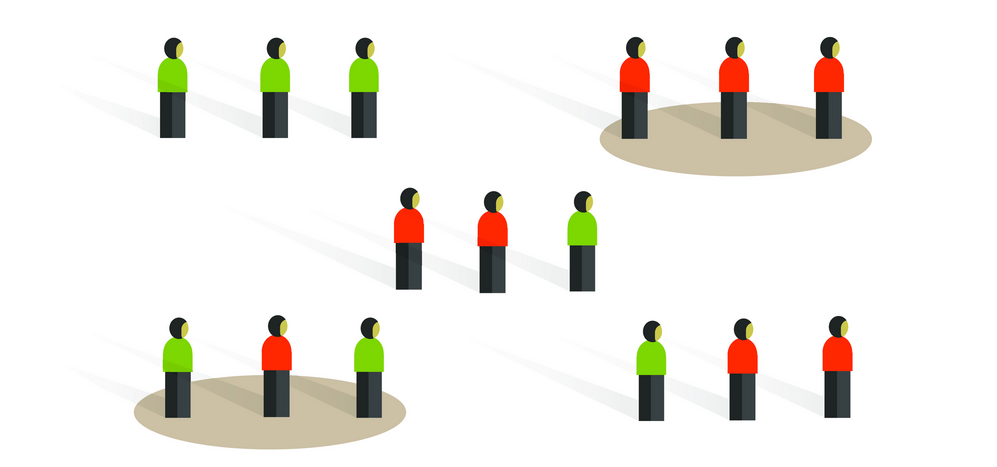
Cluster Randomized Trials: Concepts
This blog summarizes the concepts of cluster randomization, and the logistical and statistical considerations while designing a cluster randomized controlled trial.
- Subject List
- Take a Tour
- For Authors
- Subscriber Services
- Publications
- African American Studies
- African Studies
- American Literature
- Anthropology
- Architecture Planning and Preservation
- Art History
- Atlantic History
- Biblical Studies
- British and Irish Literature
- Childhood Studies
- Chinese Studies
- Cinema and Media Studies
- Communication
- Criminology
- Environmental Science
- Evolutionary Biology
- International Law
- International Relations
- Islamic Studies
- Jewish Studies
- Latin American Studies
- Latino Studies
- Linguistics
- Literary and Critical Theory
- Medieval Studies
- Military History
- Political Science
- Public Health
- Renaissance and Reformation
- Social Work
- Urban Studies
- Victorian Literature
- Browse All Subjects
How to Subscribe
- Free Trials
In This Article Expand or collapse the "in this article" section Qualitative, Quantitative, and Mixed Methods Research Sampling Strategies
Introduction.
- Sampling Strategies
- Sample Size
- Qualitative Design Considerations
- Discipline Specific and Special Considerations
- Sampling Strategies Unique to Mixed Methods Designs
Related Articles Expand or collapse the "related articles" section about
About related articles close popup.
Lorem Ipsum Sit Dolor Amet
Vestibulum ante ipsum primis in faucibus orci luctus et ultrices posuere cubilia Curae; Aliquam ligula odio, euismod ut aliquam et, vestibulum nec risus. Nulla viverra, arcu et iaculis consequat, justo diam ornare tellus, semper ultrices tellus nunc eu tellus.
- Mixed Methods Research
- Qualitative Research Design
- Quantitative Research Designs in Educational Research
Other Subject Areas
Forthcoming articles expand or collapse the "forthcoming articles" section.
- English as an International Language for Academic Publishing
- Girls' Education in the Developing World
- History of Education in Europe
- Find more forthcoming articles...
- Export Citations
- Share This Facebook LinkedIn Twitter
Qualitative, Quantitative, and Mixed Methods Research Sampling Strategies by Timothy C. Guetterman LAST REVIEWED: 26 February 2020 LAST MODIFIED: 26 February 2020 DOI: 10.1093/obo/9780199756810-0241
Sampling is a critical, often overlooked aspect of the research process. The importance of sampling extends to the ability to draw accurate inferences, and it is an integral part of qualitative guidelines across research methods. Sampling considerations are important in quantitative and qualitative research when considering a target population and when drawing a sample that will either allow us to generalize (i.e., quantitatively) or go into sufficient depth (i.e., qualitatively). While quantitative research is generally concerned with probability-based approaches, qualitative research typically uses nonprobability purposeful sampling approaches. Scholars generally focus on two major sampling topics: sampling strategies and sample sizes. Or simply, researchers should think about who to include and how many; both of these concerns are key. Mixed methods studies have both qualitative and quantitative sampling considerations. However, mixed methods studies also have unique considerations based on the relationship of quantitative and qualitative research within the study.
Sampling in Qualitative Research
Sampling in qualitative research may be divided into two major areas: overall sampling strategies and issues around sample size. Sampling strategies refers to the process of sampling and how to design a sampling. Qualitative sampling typically follows a nonprobability-based approach, such as purposive or purposeful sampling where participants or other units of analysis are selected intentionally for their ability to provide information to address research questions. Sample size refers to how many participants or other units are needed to address research questions. The methodological literature about sampling tends to fall into these two broad categories, though some articles, chapters, and books cover both concepts. Others have connected sampling to the type of qualitative design that is employed. Additionally, researchers might consider discipline specific sampling issues as much research does tend to operate within disciplinary views and constraints. Scholars in many disciplines have examined sampling around specific topics, research problems, or disciplines and provide guidance to making sampling decisions, such as appropriate strategies and sample size.
back to top
Users without a subscription are not able to see the full content on this page. Please subscribe or login .
Oxford Bibliographies Online is available by subscription and perpetual access to institutions. For more information or to contact an Oxford Sales Representative click here .
- About Education »
- Meet the Editorial Board »
- Academic Achievement
- Academic Audit for Universities
- Academic Freedom and Tenure in the United States
- Action Research in Education
- Adjuncts in Higher Education in the United States
- Administrator Preparation
- Adolescence
- Advanced Placement and International Baccalaureate Courses
- Advocacy and Activism in Early Childhood
- African American Racial Identity and Learning
- Alaska Native Education
- Alternative Certification Programs for Educators
- Alternative Schools
- American Indian Education
- Animals in Environmental Education
- Art Education
- Artificial Intelligence and Learning
- Assessing School Leader Effectiveness
- Assessment, Behavioral
- Assessment, Educational
- Assessment in Early Childhood Education
- Assistive Technology
- Augmented Reality in Education
- Beginning-Teacher Induction
- Bilingual Education and Bilingualism
- Black Undergraduate Women: Critical Race and Gender Perspe...
- Blended Learning
- Case Study in Education Research
- Changing Professional and Academic Identities
- Character Education
- Children’s and Young Adult Literature
- Children's Beliefs about Intelligence
- Children's Rights in Early Childhood Education
- Citizenship Education
- Civic and Social Engagement of Higher Education
- Classroom Learning Environments: Assessing and Investigati...
- Classroom Management
- Coherent Instructional Systems at the School and School Sy...
- College Admissions in the United States
- College Athletics in the United States
- Community Relations
- Comparative Education
- Computer-Assisted Language Learning
- Computer-Based Testing
- Conceptualizing, Measuring, and Evaluating Improvement Net...
- Continuous Improvement and "High Leverage" Educational Pro...
- Counseling in Schools
- Critical Approaches to Gender in Higher Education
- Critical Perspectives on Educational Innovation and Improv...
- Critical Race Theory
- Crossborder and Transnational Higher Education
- Cross-National Research on Continuous Improvement
- Cross-Sector Research on Continuous Learning and Improveme...
- Cultural Diversity in Early Childhood Education
- Culturally Responsive Leadership
- Culturally Responsive Pedagogies
- Culturally Responsive Teacher Education in the United Stat...
- Curriculum Design
- Data Collection in Educational Research
- Data-driven Decision Making in the United States
- Deaf Education
- Desegregation and Integration
- Design Thinking and the Learning Sciences: Theoretical, Pr...
- Development, Moral
- Dialogic Pedagogy
- Digital Age Teacher, The
- Digital Citizenship
- Digital Divides
- Disabilities
- Distance Learning
- Distributed Leadership
- Doctoral Education and Training
- Early Childhood Education and Care (ECEC) in Denmark
- Early Childhood Education and Development in Mexico
- Early Childhood Education in Aotearoa New Zealand
- Early Childhood Education in Australia
- Early Childhood Education in China
- Early Childhood Education in Europe
- Early Childhood Education in Sub-Saharan Africa
- Early Childhood Education in Sweden
- Early Childhood Education Pedagogy
- Early Childhood Education Policy
- Early Childhood Education, The Arts in
- Early Childhood Mathematics
- Early Childhood Science
- Early Childhood Teacher Education
- Early Childhood Teachers in Aotearoa New Zealand
- Early Years Professionalism and Professionalization Polici...
- Economics of Education
- Education For Children with Autism
- Education for Sustainable Development
- Education Leadership, Empirical Perspectives in
- Education of Native Hawaiian Students
- Education Reform and School Change
- Educational Statistics for Longitudinal Research
- Educator Partnerships with Parents and Families with a Foc...
- Emotional and Affective Issues in Environmental and Sustai...
- Emotional and Behavioral Disorders
- Environmental and Science Education: Overlaps and Issues
- Environmental Education
- Environmental Education in Brazil
- Epistemic Beliefs
- Equity and Improvement: Engaging Communities in Educationa...
- Equity, Ethnicity, Diversity, and Excellence in Education
- Ethical Research with Young Children
- Ethics and Education
- Ethics of Teaching
- Ethnic Studies
- Evidence-Based Communication Assessment and Intervention
- Family and Community Partnerships in Education
- Family Day Care
- Federal Government Programs and Issues
- Feminization of Labor in Academia
- Finance, Education
- Financial Aid
- Formative Assessment
- Future-Focused Education
- Gender and Achievement
- Gender and Alternative Education
- Gender, Power and Politics in the Academy
- Gender-Based Violence on University Campuses
- Gifted Education
- Global Mindedness and Global Citizenship Education
- Global University Rankings
- Governance, Education
- Grounded Theory
- Growth of Effective Mental Health Services in Schools in t...
- Higher Education and Globalization
- Higher Education and the Developing World
- Higher Education Faculty Characteristics and Trends in the...
- Higher Education Finance
- Higher Education Governance
- Higher Education Graduate Outcomes and Destinations
- Higher Education in Africa
- Higher Education in China
- Higher Education in Latin America
- Higher Education in the United States, Historical Evolutio...
- Higher Education, International Issues in
- Higher Education Management
- Higher Education Policy
- Higher Education Research
- Higher Education Student Assessment
- High-stakes Testing
- History of Early Childhood Education in the United States
- History of Education in the United States
- History of Technology Integration in Education
- Homeschooling
- Inclusion in Early Childhood: Difference, Disability, and ...
- Inclusive Education
- Indigenous Education in a Global Context
- Indigenous Learning Environments
- Indigenous Students in Higher Education in the United Stat...
- Infant and Toddler Pedagogy
- Inservice Teacher Education
- Integrating Art across the Curriculum
- Intelligence
- Intensive Interventions for Children and Adolescents with ...
- International Perspectives on Academic Freedom
- Intersectionality and Education
- Knowledge Development in Early Childhood
- Leadership Development, Coaching and Feedback for
- Leadership in Early Childhood Education
- Leadership Training with an Emphasis on the United States
- Learning Analytics in Higher Education
- Learning Difficulties
- Learning, Lifelong
- Learning, Multimedia
- Learning Strategies
- Legal Matters and Education Law
- LGBT Youth in Schools
- Linguistic Diversity
- Linguistically Inclusive Pedagogy
- Literacy Development and Language Acquisition
- Literature Reviews
- Mathematics Identity
- Mathematics Instruction and Interventions for Students wit...
- Mathematics Teacher Education
- Measurement for Improvement in Education
- Measurement in Education in the United States
- Meta-Analysis and Research Synthesis in Education
- Methodological Approaches for Impact Evaluation in Educati...
- Methodologies for Conducting Education Research
- Mindfulness, Learning, and Education
- Motherscholars
- Multiliteracies in Early Childhood Education
- Multiple Documents Literacy: Theory, Research, and Applica...
- Multivariate Research Methodology
- Museums, Education, and Curriculum
- Music Education
- Narrative Research in Education
- Native American Studies
- Nonformal and Informal Environmental Education
- Note-Taking
- Numeracy Education
- One-to-One Technology in the K-12 Classroom
- Online Education
- Open Education
- Organizing for Continuous Improvement in Education
- Organizing Schools for the Inclusion of Students with Disa...
- Outdoor Play and Learning
- Outdoor Play and Learning in Early Childhood Education
- Pedagogical Leadership
- Pedagogy of Teacher Education, A
- Performance Objectives and Measurement
- Performance-based Research Assessment in Higher Education
- Performance-based Research Funding
- Phenomenology in Educational Research
- Philosophy of Education
- Physical Education
- Podcasts in Education
- Policy Context of United States Educational Innovation and...
- Politics of Education
- Portable Technology Use in Special Education Programs and ...
- Post-humanism and Environmental Education
- Pre-Service Teacher Education
- Problem Solving
- Productivity and Higher Education
- Professional Development
- Professional Learning Communities
- Program Evaluation
- Programs and Services for Students with Emotional or Behav...
- Psychology Learning and Teaching
- Psychometric Issues in the Assessment of English Language ...
- Qualitative Data Analysis Techniques
- Qualitative, Quantitative, and Mixed Methods Research Samp...
- Queering the English Language Arts (ELA) Writing Classroom
- Race and Affirmative Action in Higher Education
- Reading Education
- Refugee and New Immigrant Learners
- Relational and Developmental Trauma and Schools
- Relational Pedagogies in Early Childhood Education
- Reliability in Educational Assessments
- Religion in Elementary and Secondary Education in the Unit...
- Researcher Development and Skills Training within the Cont...
- Research-Practice Partnerships in Education within the Uni...
- Response to Intervention
- Restorative Practices
- Risky Play in Early Childhood Education
- Scale and Sustainability of Education Innovation and Impro...
- Scaling Up Research-based Educational Practices
- School Accreditation
- School Choice
- School Culture
- School District Budgeting and Financial Management in the ...
- School Improvement through Inclusive Education
- School Reform
- Schools, Private and Independent
- School-Wide Positive Behavior Support
- Science Education
- Secondary to Postsecondary Transition Issues
- Self-Regulated Learning
- Self-Study of Teacher Education Practices
- Service-Learning
- Severe Disabilities
- Single Salary Schedule
- Single-sex Education
- Single-Subject Research Design
- Social Context of Education
- Social Justice
- Social Network Analysis
- Social Pedagogy
- Social Science and Education Research
- Social Studies Education
- Sociology of Education
- Standards-Based Education
- Statistical Assumptions
- Student Access, Equity, and Diversity in Higher Education
- Student Assignment Policy
- Student Engagement in Tertiary Education
- Student Learning, Development, Engagement, and Motivation ...
- Student Participation
- Student Voice in Teacher Development
- Sustainability Education in Early Childhood Education
- Sustainability in Early Childhood Education
- Sustainability in Higher Education
- Teacher Beliefs and Epistemologies
- Teacher Collaboration in School Improvement
- Teacher Evaluation and Teacher Effectiveness
- Teacher Preparation
- Teacher Training and Development
- Teacher Unions and Associations
- Teacher-Student Relationships
- Teaching Critical Thinking
- Technologies, Teaching, and Learning in Higher Education
- Technology Education in Early Childhood
- Technology, Educational
- Technology-based Assessment
- The Bologna Process
- The Regulation of Standards in Higher Education
- Theories of Educational Leadership
- Three Conceptions of Literacy: Media, Narrative, and Gamin...
- Tracking and Detracking
- Traditions of Quality Improvement in Education
- Transformative Learning
- Transitions in Early Childhood Education
- Tribally Controlled Colleges and Universities in the Unite...
- Understanding the Psycho-Social Dimensions of Schools and ...
- University Faculty Roles and Responsibilities in the Unite...
- Using Ethnography in Educational Research
- Value of Higher Education for Students and Other Stakehold...
- Virtual Learning Environments
- Vocational and Technical Education
- Wellness and Well-Being in Education
- Women's and Gender Studies
- Young Children and Spirituality
- Young Children's Learning Dispositions
- Young Children's Working Theories
- Privacy Policy
- Cookie Policy
- Legal Notice
- Accessibility
Powered by:
- [66.249.64.20|185.80.149.115]
- 185.80.149.115
Root out friction in every digital experience, super-charge conversion rates, and optimize digital self-service
Uncover insights from any interaction, deliver AI-powered agent coaching, and reduce cost to serve
Increase revenue and loyalty with real-time insights and recommendations delivered to teams on the ground
Know how your people feel and empower managers to improve employee engagement, productivity, and retention
Take action in the moments that matter most along the employee journey and drive bottom line growth
Whatever they’re are saying, wherever they’re saying it, know exactly what’s going on with your people
Get faster, richer insights with qual and quant tools that make powerful market research available to everyone
Run concept tests, pricing studies, prototyping + more with fast, powerful studies designed by UX research experts
Track your brand performance 24/7 and act quickly to respond to opportunities and challenges in your market
Explore the platform powering Experience Management
- Free Account
- For Digital
- For Customer Care
- For Human Resources
- For Researchers
- Financial Services
- All Industries
Popular Use Cases
- Customer Experience
- Employee Experience
- Employee Exit Interviews
- Net Promoter Score
- Voice of Customer
- Customer Success Hub
- Product Documentation
- Training & Certification
- XM Institute
- Popular Resources
- Customer Stories
- Market Research
- Artificial Intelligence
- Partnerships
- Marketplace
The annual gathering of the experience leaders at the world’s iconic brands building breakthrough business results, live in Salt Lake City.
- English/AU & NZ
- Español/Europa
- Español/América Latina
- Português Brasileiro
- REQUEST DEMO
- Experience Management
- Sampling Methods
Try Qualtrics for free
Sampling methods, types & techniques.
15 min read Your comprehensive guide to the different sampling methods available to researchers – and how to know which is right for your research.
Author: Will Webster
What is sampling?
In survey research, sampling is the process of using a subset of a population to represent the whole population. To help illustrate this further, let’s look at data sampling methods with examples below.
Let’s say you wanted to do some research on everyone in North America. To ask every person would be almost impossible. Even if everyone said “yes”, carrying out a survey across different states, in different languages and timezones, and then collecting and processing all the results , would take a long time and be very costly.
Sampling allows large-scale research to be carried out with a more realistic cost and time-frame because it uses a smaller number of individuals in the population with representative characteristics to stand in for the whole.
However, when you decide to sample, you take on a new task. You have to decide who is part of your sample list and how to choose the people who will best represent the whole population. How you go about that is what the practice of sampling is all about.
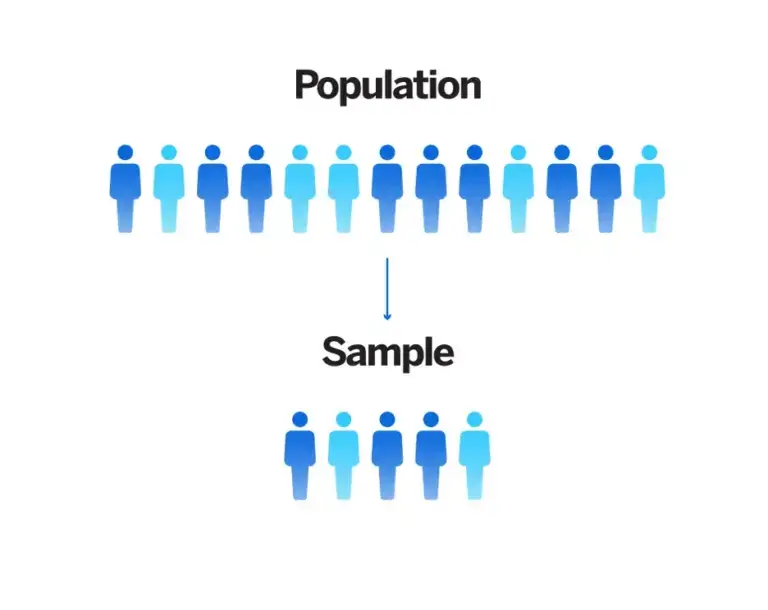
Sampling definitions
- Population: The total number of people or things you are interested in
- Sample: A smaller number within your population that will represent the whole
- Sampling: The process and method of selecting your sample
Free eBook: 2024 Market Research Trends
Why is sampling important?
Although the idea of sampling is easiest to understand when you think about a very large population, it makes sense to use sampling methods in research studies of all types and sizes. After all, if you can reduce the effort and cost of doing a study, why wouldn’t you? And because sampling allows you to research larger target populations using the same resources as you would smaller ones, it dramatically opens up the possibilities for research.
Sampling is a little like having gears on a car or bicycle. Instead of always turning a set of wheels of a specific size and being constrained by their physical properties, it allows you to translate your effort to the wheels via the different gears, so you’re effectively choosing bigger or smaller wheels depending on the terrain you’re on and how much work you’re able to do.
Sampling allows you to “gear” your research so you’re less limited by the constraints of cost, time, and complexity that come with different population sizes.
It allows us to do things like carrying out exit polls during elections, map the spread and effects rates of epidemics across geographical areas, and carry out nationwide census research that provides a snapshot of society and culture.
Types of sampling
Sampling strategies in research vary widely across different disciplines and research areas, and from study to study.
There are two major types of sampling methods: probability and non-probability sampling.
- Probability sampling , also known as random sampling , is a kind of sample selection where randomization is used instead of deliberate choice. Each member of the population has a known, non-zero chance of being selected.
- Non-probability sampling techniques are where the researcher deliberately picks items or individuals for the sample based on non-random factors such as convenience, geographic availability, or costs.
As we delve into these categories, it’s essential to understand the nuances and applications of each method to ensure that the chosen sampling strategy aligns with the research goals.
Probability sampling methods
There’s a wide range of probability sampling methods to explore and consider. Here are some of the best-known options.
1. Simple random sampling
With simple random sampling , every element in the population has an equal chance of being selected as part of the sample. It’s something like picking a name out of a hat. Simple random sampling can be done by anonymizing the population – e.g. by assigning each item or person in the population a number and then picking numbers at random.
Pros: Simple random sampling is easy to do and cheap. Designed to ensure that every member of the population has an equal chance of being selected, it reduces the risk of bias compared to non-random sampling.
Cons: It offers no control for the researcher and may lead to unrepresentative groupings being picked by chance.
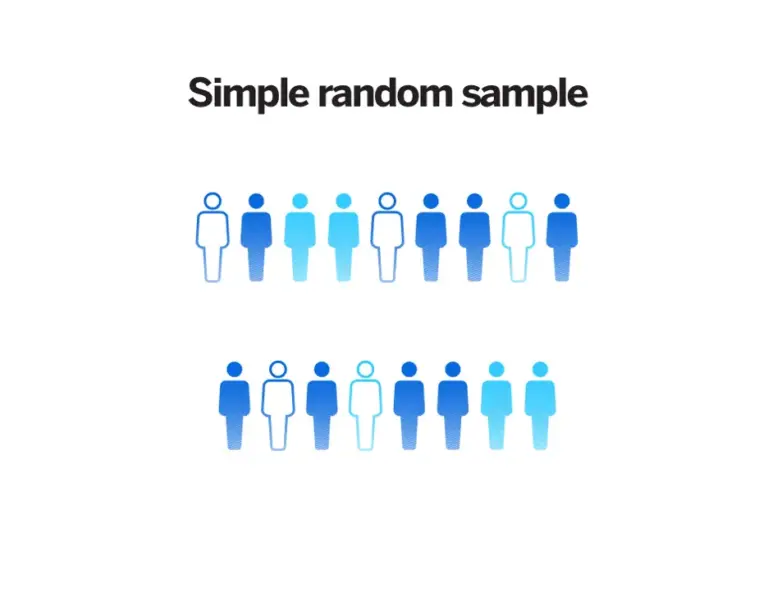
2. Systematic sampling
With systematic sampling the random selection only applies to the first item chosen. A rule then applies so that every nth item or person after that is picked.
Best practice is to sort your list in a random way to ensure that selections won’t be accidentally clustered together. This is commonly achieved using a random number generator. If that’s not available you might order your list alphabetically by first name and then pick every fifth name to eliminate bias, for example.
Next, you need to decide your sampling interval – for example, if your sample will be 10% of your full list, your sampling interval is one in 10 – and pick a random start between one and 10 – for example three. This means you would start with person number three on your list and pick every tenth person.
Pros: Systematic sampling is efficient and straightforward, especially when dealing with populations that have a clear order. It ensures a uniform selection across the population.
Cons: There’s a potential risk of introducing bias if there’s an unrecognized pattern in the population that aligns with the sampling interval.
3. Stratified sampling
Stratified sampling involves random selection within predefined groups. It’s a useful method for researchers wanting to determine what aspects of a sample are highly correlated with what’s being measured. They can then decide how to subdivide (stratify) it in a way that makes sense for the research.
For example, you want to measure the height of students at a college where 80% of students are female and 20% are male. We know that gender is highly correlated with height, and if we took a simple random sample of 200 students (out of the 2,000 who attend the college), we could by chance get 200 females and not one male. This would bias our results and we would underestimate the height of students overall. Instead, we could stratify by gender and make sure that 20% of our sample (40 students) are male and 80% (160 students) are female.
Pros: Stratified sampling enhances the representation of all identified subgroups within a population, leading to more accurate results in heterogeneous populations.
Cons: This method requires accurate knowledge about the population’s stratification, and its design and execution can be more intricate than other methods.
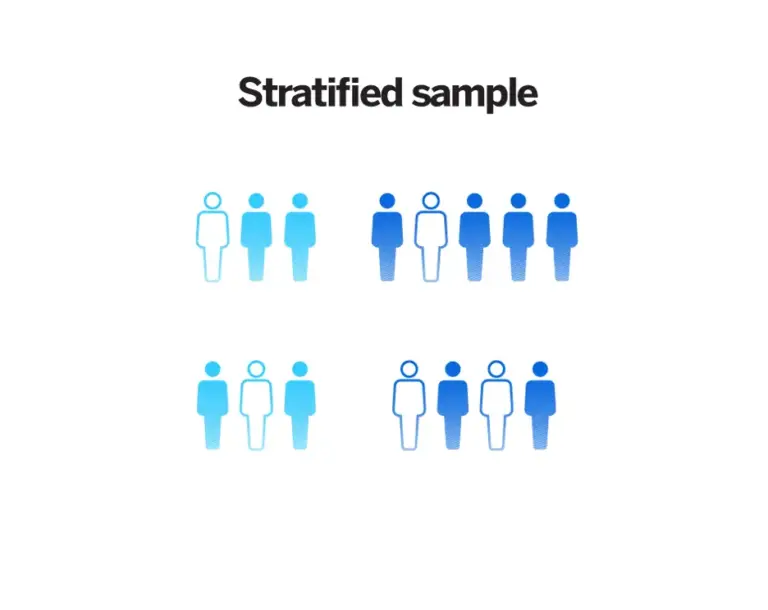
4. Cluster sampling
With cluster sampling, groups rather than individual units of the target population are selected at random for the sample. These might be pre-existing groups, such as people in certain zip codes or students belonging to an academic year.
Cluster sampling can be done by selecting the entire cluster, or in the case of two-stage cluster sampling, by randomly selecting the cluster itself, then selecting at random again within the cluster.
Pros: Cluster sampling is economically beneficial and logistically easier when dealing with vast and geographically dispersed populations.
Cons: Due to potential similarities within clusters, this method can introduce a greater sampling error compared to other methods.
Non-probability sampling methods
The non-probability sampling methodology doesn’t offer the same bias-removal benefits as probability sampling, but there are times when these types of sampling are chosen for expediency or simplicity. Here are some forms of non-probability sampling and how they work.
1. Convenience sampling
People or elements in a sample are selected on the basis of their accessibility and availability. If you are doing a research survey and you work at a university, for example, a convenience sample might consist of students or co-workers who happen to be on campus with open schedules who are willing to take your questionnaire .
This kind of sample can have value, especially if it’s done as an early or preliminary step, but significant bias will be introduced.
Pros: Convenience sampling is the most straightforward method, requiring minimal planning, making it quick to implement.
Cons: Due to its non-random nature, the method is highly susceptible to biases, and the results are often lacking in their application to the real world.
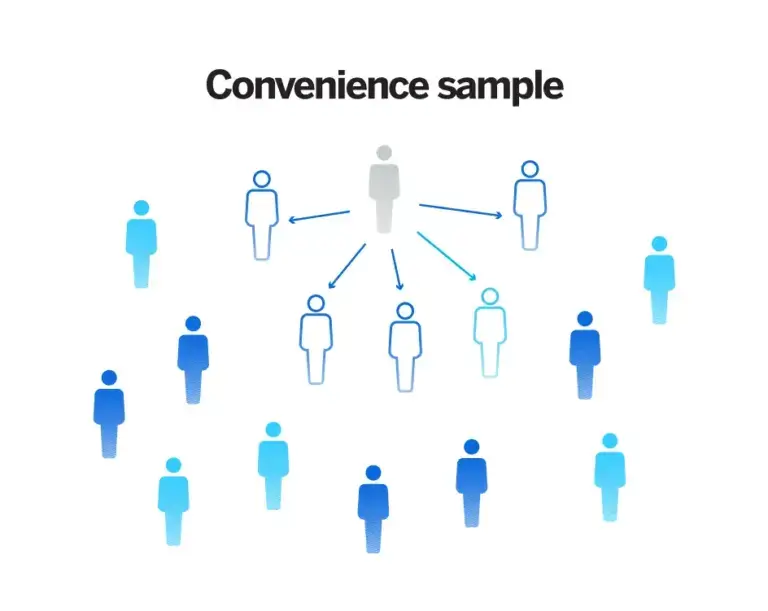
2. Quota sampling
Like the probability-based stratified sampling method, this approach aims to achieve a spread across the target population by specifying who should be recruited for a survey according to certain groups or criteria.
For example, your quota might include a certain number of males and a certain number of females. Alternatively, you might want your samples to be at a specific income level or in certain age brackets or ethnic groups.
Pros: Quota sampling ensures certain subgroups are adequately represented, making it great for when random sampling isn’t feasible but representation is necessary.
Cons: The selection within each quota is non-random and researchers’ discretion can influence the representation, which both strongly increase the risk of bias.
3. Purposive sampling
Participants for the sample are chosen consciously by researchers based on their knowledge and understanding of the research question at hand or their goals.
Also known as judgment sampling, this technique is unlikely to result in a representative sample , but it is a quick and fairly easy way to get a range of results or responses.
Pros: Purposive sampling targets specific criteria or characteristics, making it ideal for studies that require specialized participants or specific conditions.
Cons: It’s highly subjective and based on researchers’ judgment, which can introduce biases and limit the study’s real-world application.
4. Snowball or referral sampling
With this approach, people recruited to be part of a sample are asked to invite those they know to take part, who are then asked to invite their friends and family and so on. The participation radiates through a community of connected individuals like a snowball rolling downhill.
Pros: Especially useful for hard-to-reach or secretive populations, snowball sampling is effective for certain niche studies.
Cons: The method can introduce bias due to the reliance on participant referrals, and the choice of initial seeds can significantly influence the final sample.
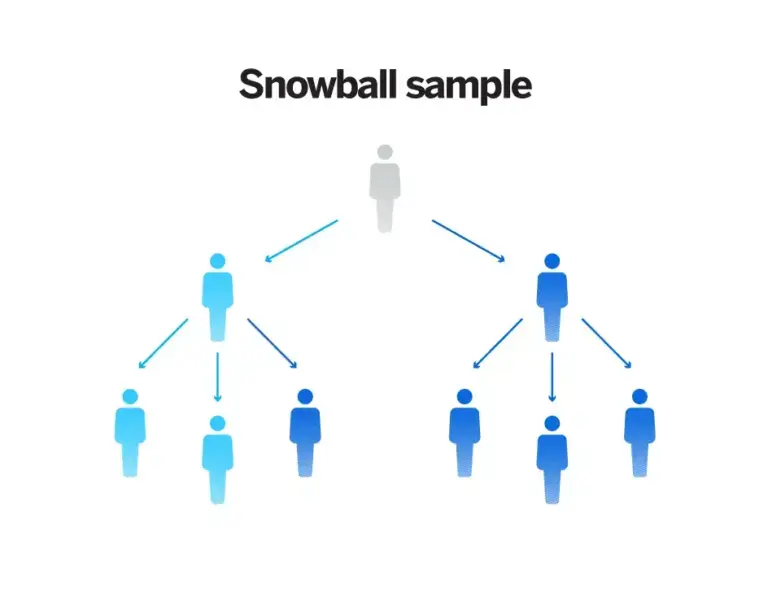
What type of sampling should I use?
Choosing the right sampling method is a pivotal aspect of any research process, but it can be a stumbling block for many.
Here’s a structured approach to guide your decision.
1) Define your research goals
If you aim to get a general sense of a larger group, simple random or stratified sampling could be your best bet. For focused insights or studying unique communities, snowball or purposive sampling might be more suitable.
2) Assess the nature of your population
The nature of the group you’re studying can guide your method. For a diverse group with different categories, stratified sampling can ensure all segments are covered. If they’re widely spread geographically , cluster sampling becomes useful. If they’re arranged in a certain sequence or order, systematic sampling might be effective.
3) Consider your constraints
Your available time, budget and ease of accessing participants matter. Convenience or quota sampling can be practical for quicker studies, but they come with some trade-offs. If reaching everyone in your desired group is challenging, snowball or purposive sampling can be more feasible.
4) Determine the reach of your findings
Decide if you want your findings to represent a much broader group. For a wider representation, methods that include everyone fairly (like probability sampling ) are a good option. For specialized insights into specific groups, non-probability sampling methods can be more suitable.
5) Get feedback
Before fully committing, discuss your chosen method with others in your field and consider a test run.
Avoid or reduce sampling errors and bias
Using a sample is a kind of short-cut. If you could ask every single person in a population to take part in your study and have each of them reply, you’d have a highly accurate (and very labor-intensive) project on your hands.
But since that’s not realistic, sampling offers a “good-enough” solution that sacrifices some accuracy for the sake of practicality and ease. How much accuracy you lose out on depends on how well you control for sampling error, non-sampling error, and bias in your survey design . Our blog post helps you to steer clear of some of these issues.
How to choose the correct sample size
Finding the best sample size for your target population is something you’ll need to do again and again, as it’s different for every study.
To make life easier, we’ve provided a sample size calculator . To use it, you need to know your:
- Population size
- Confidence level
- Margin of error (confidence interval)
If any of those terms are unfamiliar, have a look at our blog post on determining sample size for details of what they mean and how to find them.
Unlock the insights of yesterday to shape tomorrow
In the ever-evolving business landscape, relying on the most recent market research is paramount. Reflecting on 2022, brands and businesses can harness crucial insights to outmaneuver challenges and seize opportunities.
Equip yourself with this knowledge by exploring Qualtrics’ ‘2022 Market Research Global Trends’ report.
Delve into this comprehensive study to unearth:
- How businesses made sense of tricky situations in 2022
- Tips that really helped improve research results
- Steps to take your findings and put them into action

Related resources
How to determine sample size 12 min read, selection bias 11 min read, systematic random sampling 15 min read, convenience sampling 18 min read, probability sampling 8 min read, non-probability sampling 17 min read, stratified random sampling 12 min read, request demo.
Ready to learn more about Qualtrics?
If you're seeing this message, it means we're having trouble loading external resources on our website.
If you're behind a web filter, please make sure that the domains *.kastatic.org and *.kasandbox.org are unblocked.
To log in and use all the features of Khan Academy, please enable JavaScript in your browser.
Statistics and probability
Course: statistics and probability > unit 6.
- Picking fairly
- Using probability to make fair decisions
- Techniques for generating a simple random sample
- Simple random samples
- Techniques for random sampling and avoiding bias
- Sampling methods
Sampling methods review
- Samples and surveys
What are sampling methods?
Bad ways to sample.
- (Choice A) Convenience sampling A Convenience sampling
- (Choice B) Voluntary response sampling B Voluntary response sampling
Good ways to sample
- (Choice A) Simple random sampling A Simple random sampling
- (Choice B) Stratified random sampling B Stratified random sampling
- (Choice C) Cluster random sampling C Cluster random sampling
- (Choice D) Systematic random sampling D Systematic random sampling
Want to join the conversation?
- Upvote Button navigates to signup page
- Downvote Button navigates to signup page
- Flag Button navigates to signup page

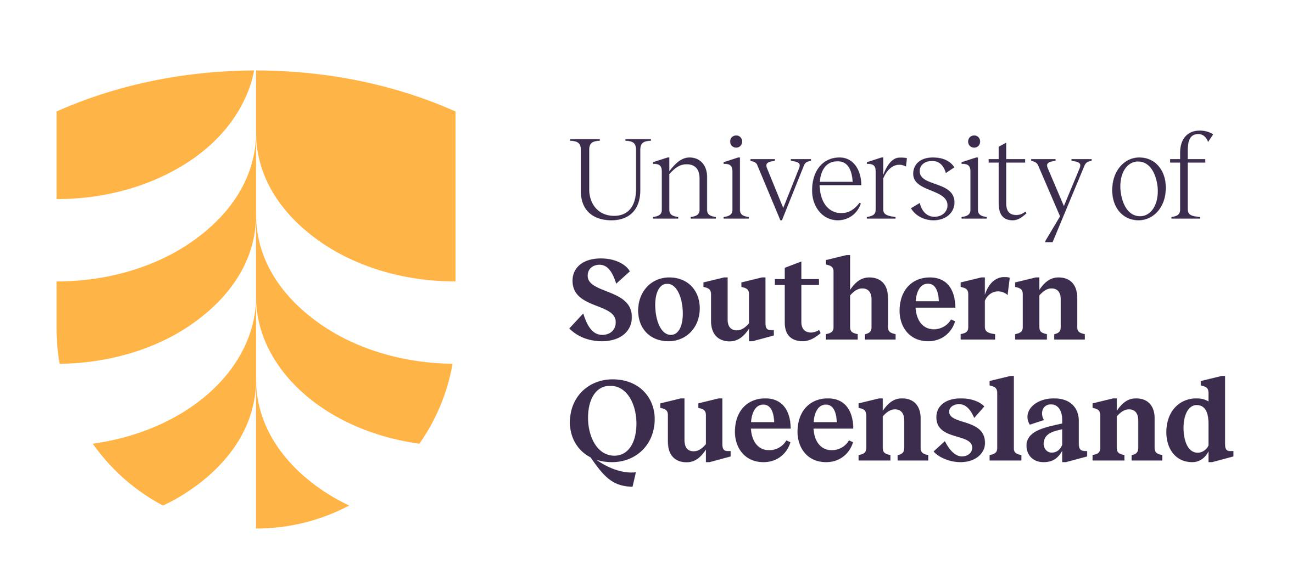
Want to create or adapt books like this? Learn more about how Pressbooks supports open publishing practices.
Sampling is the statistical process of selecting a subset—called a ‘sample’—of a population of interest for the purpose of making observations and statistical inferences about that population. Social science research is generally about inferring patterns of behaviours within specific populations. We cannot study entire populations because of feasibility and cost constraints, and hence, we must select a representative sample from the population of interest for observation and analysis. It is extremely important to choose a sample that is truly representative of the population so that the inferences derived from the sample can be generalised back to the population of interest. Improper and biased sampling is the primary reason for the often divergent and erroneous inferences reported in opinion polls and exit polls conducted by different polling groups such as CNN/Gallup Poll, ABC, and CBS, prior to every US Presidential election.
The sampling process
As Figure 8.1 shows, the sampling process comprises of several stages. The first stage is defining the target population. A population can be defined as all people or items ( unit of analysis ) with the characteristics that one wishes to study. The unit of analysis may be a person, group, organisation, country, object, or any other entity that you wish to draw scientific inferences about. Sometimes the population is obvious. For example, if a manufacturer wants to determine whether finished goods manufactured at a production line meet certain quality requirements or must be scrapped and reworked, then the population consists of the entire set of finished goods manufactured at that production facility. At other times, the target population may be a little harder to understand. If you wish to identify the primary drivers of academic learning among high school students, then what is your target population: high school students, their teachers, school principals, or parents? The right answer in this case is high school students, because you are interested in their performance, not the performance of their teachers, parents, or schools. Likewise, if you wish to analyse the behaviour of roulette wheels to identify biased wheels, your population of interest is not different observations from a single roulette wheel, but different roulette wheels (i.e., their behaviour over an infinite set of wheels).
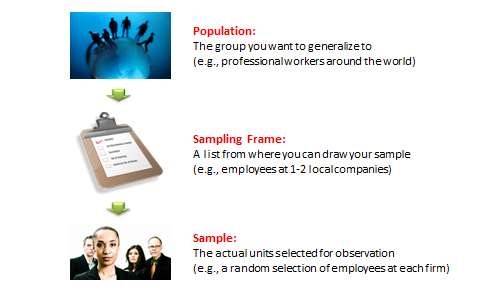
The second step in the sampling process is to choose a sampling frame . This is an accessible section of the target population—usually a list with contact information—from where a sample can be drawn. If your target population is professional employees at work, because you cannot access all professional employees around the world, a more realistic sampling frame will be employee lists of one or two local companies that are willing to participate in your study. If your target population is organisations, then the Fortune 500 list of firms or the Standard & Poor’s (S&P) list of firms registered with the New York Stock exchange may be acceptable sampling frames.
Note that sampling frames may not entirely be representative of the population at large, and if so, inferences derived by such a sample may not be generalisable to the population. For instance, if your target population is organisational employees at large (e.g., you wish to study employee self-esteem in this population) and your sampling frame is employees at automotive companies in the American Midwest, findings from such groups may not even be generalisable to the American workforce at large, let alone the global workplace. This is because the American auto industry has been under severe competitive pressures for the last 50 years and has seen numerous episodes of reorganisation and downsizing, possibly resulting in low employee morale and self-esteem. Furthermore, the majority of the American workforce is employed in service industries or in small businesses, and not in automotive industry. Hence, a sample of American auto industry employees is not particularly representative of the American workforce. Likewise, the Fortune 500 list includes the 500 largest American enterprises, which is not representative of all American firms, most of which are medium or small sized firms rather than large firms, and is therefore, a biased sampling frame. In contrast, the S&P list will allow you to select large, medium, and/or small companies, depending on whether you use the S&P LargeCap, MidCap, or SmallCap lists, but includes publicly traded firms (and not private firms) and is hence still biased. Also note that the population from which a sample is drawn may not necessarily be the same as the population about which we actually want information. For example, if a researcher wants to examine the success rate of a new ‘quit smoking’ program, then the target population is the universe of smokers who had access to this program, which may be an unknown population. Hence, the researcher may sample patients arriving at a local medical facility for smoking cessation treatment, some of whom may not have had exposure to this particular ‘quit smoking’ program, in which case, the sampling frame does not correspond to the population of interest.
The last step in sampling is choosing a sample from the sampling frame using a well-defined sampling technique. Sampling techniques can be grouped into two broad categories: probability (random) sampling and non-probability sampling. Probability sampling is ideal if generalisability of results is important for your study, but there may be unique circumstances where non-probability sampling can also be justified. These techniques are discussed in the next two sections.
Probability sampling
Probability sampling is a technique in which every unit in the population has a chance (non-zero probability) of being selected in the sample, and this chance can be accurately determined. Sample statistics thus produced, such as sample mean or standard deviation, are unbiased estimates of population parameters, as long as the sampled units are weighted according to their probability of selection. All probability sampling have two attributes in common: every unit in the population has a known non-zero probability of being sampled, and the sampling procedure involves random selection at some point. The different types of probability sampling techniques include:

Stratified sampling. In stratified sampling, the sampling frame is divided into homogeneous and non-overlapping subgroups (called ‘strata’), and a simple random sample is drawn within each subgroup. In the previous example of selecting 200 firms from a list of 1,000 firms, you can start by categorising the firms based on their size as large (more than 500 employees), medium (between 50 and 500 employees), and small (less than 50 employees). You can then randomly select 67 firms from each subgroup to make up your sample of 200 firms. However, since there are many more small firms in a sampling frame than large firms, having an equal number of small, medium, and large firms will make the sample less representative of the population (i.e., biased in favour of large firms that are fewer in number in the target population). This is called non-proportional stratified sampling because the proportion of the sample within each subgroup does not reflect the proportions in the sampling frame—or the population of interest—and the smaller subgroup (large-sized firms) is oversampled . An alternative technique will be to select subgroup samples in proportion to their size in the population. For instance, if there are 100 large firms, 300 mid-sized firms, and 600 small firms, you can sample 20 firms from the ‘large’ group, 60 from the ‘medium’ group and 120 from the ‘small’ group. In this case, the proportional distribution of firms in the population is retained in the sample, and hence this technique is called proportional stratified sampling. Note that the non-proportional approach is particularly effective in representing small subgroups, such as large-sized firms, and is not necessarily less representative of the population compared to the proportional approach, as long as the findings of the non-proportional approach are weighted in accordance to a subgroup’s proportion in the overall population.
Cluster sampling. If you have a population dispersed over a wide geographic region, it may not be feasible to conduct a simple random sampling of the entire population. In such case, it may be reasonable to divide the population into ‘clusters’—usually along geographic boundaries—randomly sample a few clusters, and measure all units within that cluster. For instance, if you wish to sample city governments in the state of New York, rather than travel all over the state to interview key city officials (as you may have to do with a simple random sample), you can cluster these governments based on their counties, randomly select a set of three counties, and then interview officials from every office in those counties. However, depending on between-cluster differences, the variability of sample estimates in a cluster sample will generally be higher than that of a simple random sample, and hence the results are less generalisable to the population than those obtained from simple random samples.
Matched-pairs sampling. Sometimes, researchers may want to compare two subgroups within one population based on a specific criterion. For instance, why are some firms consistently more profitable than other firms? To conduct such a study, you would have to categorise a sampling frame of firms into ‘high profitable’ firms and ‘low profitable firms’ based on gross margins, earnings per share, or some other measure of profitability. You would then select a simple random sample of firms in one subgroup, and match each firm in this group with a firm in the second subgroup, based on its size, industry segment, and/or other matching criteria. Now, you have two matched samples of high-profitability and low-profitability firms that you can study in greater detail. Matched-pairs sampling techniques are often an ideal way of understanding bipolar differences between different subgroups within a given population.
Multi-stage sampling. The probability sampling techniques described previously are all examples of single-stage sampling techniques. Depending on your sampling needs, you may combine these single-stage techniques to conduct multi-stage sampling. For instance, you can stratify a list of businesses based on firm size, and then conduct systematic sampling within each stratum. This is a two-stage combination of stratified and systematic sampling. Likewise, you can start with a cluster of school districts in the state of New York, and within each cluster, select a simple random sample of schools. Within each school, you can select a simple random sample of grade levels, and within each grade level, you can select a simple random sample of students for study. In this case, you have a four-stage sampling process consisting of cluster and simple random sampling.
Non-probability sampling
Non-probability sampling is a sampling technique in which some units of the population have zero chance of selection or where the probability of selection cannot be accurately determined. Typically, units are selected based on certain non-random criteria, such as quota or convenience. Because selection is non-random, non-probability sampling does not allow the estimation of sampling errors, and may be subjected to a sampling bias. Therefore, information from a sample cannot be generalised back to the population. Types of non-probability sampling techniques include:
Convenience sampling. Also called accidental or opportunity sampling, this is a technique in which a sample is drawn from that part of the population that is close to hand, readily available, or convenient. For instance, if you stand outside a shopping centre and hand out questionnaire surveys to people or interview them as they walk in, the sample of respondents you will obtain will be a convenience sample. This is a non-probability sample because you are systematically excluding all people who shop at other shopping centres. The opinions that you would get from your chosen sample may reflect the unique characteristics of this shopping centre such as the nature of its stores (e.g., high end-stores will attract a more affluent demographic), the demographic profile of its patrons, or its location (e.g., a shopping centre close to a university will attract primarily university students with unique purchasing habits), and therefore may not be representative of the opinions of the shopper population at large. Hence, the scientific generalisability of such observations will be very limited. Other examples of convenience sampling are sampling students registered in a certain class or sampling patients arriving at a certain medical clinic. This type of sampling is most useful for pilot testing, where the goal is instrument testing or measurement validation rather than obtaining generalisable inferences.
Quota sampling. In this technique, the population is segmented into mutually exclusive subgroups (just as in stratified sampling), and then a non-random set of observations is chosen from each subgroup to meet a predefined quota. In proportional quota sampling , the proportion of respondents in each subgroup should match that of the population. For instance, if the American population consists of 70 per cent Caucasians, 15 per cent Hispanic-Americans, and 13 per cent African-Americans, and you wish to understand their voting preferences in an sample of 98 people, you can stand outside a shopping centre and ask people their voting preferences. But you will have to stop asking Hispanic-looking people when you have 15 responses from that subgroup (or African-Americans when you have 13 responses) even as you continue sampling other ethnic groups, so that the ethnic composition of your sample matches that of the general American population.
Non-proportional quota sampling is less restrictive in that you do not have to achieve a proportional representation, but perhaps meet a minimum size in each subgroup. In this case, you may decide to have 50 respondents from each of the three ethnic subgroups (Caucasians, Hispanic-Americans, and African-Americans), and stop when your quota for each subgroup is reached. Neither type of quota sampling will be representative of the American population, since depending on whether your study was conducted in a shopping centre in New York or Kansas, your results may be entirely different. The non-proportional technique is even less representative of the population, but may be useful in that it allows capturing the opinions of small and under-represented groups through oversampling.
Expert sampling. This is a technique where respondents are chosen in a non-random manner based on their expertise on the phenomenon being studied. For instance, in order to understand the impacts of a new governmental policy such as the Sarbanes-Oxley Act, you can sample a group of corporate accountants who are familiar with this Act. The advantage of this approach is that since experts tend to be more familiar with the subject matter than non-experts, opinions from a sample of experts are more credible than a sample that includes both experts and non-experts, although the findings are still not generalisable to the overall population at large.
Snowball sampling. In snowball sampling, you start by identifying a few respondents that match the criteria for inclusion in your study, and then ask them to recommend others they know who also meet your selection criteria. For instance, if you wish to survey computer network administrators and you know of only one or two such people, you can start with them and ask them to recommend others who also work in network administration. Although this method hardly leads to representative samples, it may sometimes be the only way to reach hard-to-reach populations or when no sampling frame is available.
Statistics of sampling
In the preceding sections, we introduced terms such as population parameter, sample statistic, and sampling bias. In this section, we will try to understand what these terms mean and how they are related to each other.
When you measure a certain observation from a given unit, such as a person’s response to a Likert-scaled item, that observation is called a response (see Figure 8.2). In other words, a response is a measurement value provided by a sampled unit. Each respondent will give you different responses to different items in an instrument. Responses from different respondents to the same item or observation can be graphed into a frequency distribution based on their frequency of occurrences. For a large number of responses in a sample, this frequency distribution tends to resemble a bell-shaped curve called a normal distribution , which can be used to estimate overall characteristics of the entire sample, such as sample mean (average of all observations in a sample) or standard deviation (variability or spread of observations in a sample). These sample estimates are called sample statistics (a ‘statistic’ is a value that is estimated from observed data). Populations also have means and standard deviations that could be obtained if we could sample the entire population. However, since the entire population can never be sampled, population characteristics are always unknown, and are called population parameters (and not ‘statistic’ because they are not statistically estimated from data). Sample statistics may differ from population parameters if the sample is not perfectly representative of the population. The difference between the two is called sampling error . Theoretically, if we could gradually increase the sample size so that the sample approaches closer and closer to the population, then sampling error will decrease and a sample statistic will increasingly approximate the corresponding population parameter.
If a sample is truly representative of the population, then the estimated sample statistics should be identical to the corresponding theoretical population parameters. How do we know if the sample statistics are at least reasonably close to the population parameters? Here, we need to understand the concept of a sampling distribution . Imagine that you took three different random samples from a given population, as shown in Figure 8.3, and for each sample, you derived sample statistics such as sample mean and standard deviation. If each random sample was truly representative of the population, then your three sample means from the three random samples will be identical—and equal to the population parameter—and the variability in sample means will be zero. But this is extremely unlikely, given that each random sample will likely constitute a different subset of the population, and hence, their means may be slightly different from each other. However, you can take these three sample means and plot a frequency histogram of sample means. If the number of such samples increases from three to 10 to 100, the frequency histogram becomes a sampling distribution. Hence, a sampling distribution is a frequency distribution of a sample statistic (like sample mean) from a set of samples , while the commonly referenced frequency distribution is the distribution of a response (observation) from a single sample . Just like a frequency distribution, the sampling distribution will also tend to have more sample statistics clustered around the mean (which presumably is an estimate of a population parameter), with fewer values scattered around the mean. With an infinitely large number of samples, this distribution will approach a normal distribution. The variability or spread of a sample statistic in a sampling distribution (i.e., the standard deviation of a sampling statistic) is called its standard error . In contrast, the term standard deviation is reserved for variability of an observed response from a single sample.
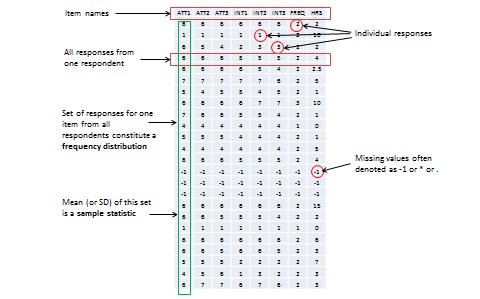
Social Science Research: Principles, Methods and Practices (Revised edition) Copyright © 2019 by Anol Bhattacherjee is licensed under a Creative Commons Attribution-NonCommercial-ShareAlike 4.0 International License , except where otherwise noted.
Share This Book

An official website of the United States government
The .gov means it’s official. Federal government websites often end in .gov or .mil. Before sharing sensitive information, make sure you’re on a federal government site.
The site is secure. The https:// ensures that you are connecting to the official website and that any information you provide is encrypted and transmitted securely.
- Publications
- Account settings
Preview improvements coming to the PMC website in October 2024. Learn More or Try it out now .
- Advanced Search
- Journal List
- An Bras Dermatol
- v.91(3); May-Jun 2016
Sampling: how to select participants in my research study? *
Jeovany martínez-mesa.
1 Faculdade Meridional (IMED) - Passo Fundo (RS), Brazil.
David Alejandro González-Chica
2 University of Adelaide - Adelaide, Australia.
Rodrigo Pereira Duquia
3 Universidade Federal de Ciências da Saúde de Porto Alegre (UFCSPA) - Porto Alegre (RS), Brazil.
Renan Rangel Bonamigo
João luiz bastos.
4 Universidade Federal de Santa Catarina (UFSC) - Florianópolis (RS), Brazil.
In this paper, the basic elements related to the selection of participants for a health research are discussed. Sample representativeness, sample frame, types of sampling, as well as the impact that non-respondents may have on results of a study are described. The whole discussion is supported by practical examples to facilitate the reader's understanding.
To introduce readers to issues related to sampling.
INTRODUCTION
The essential topics related to the selection of participants for a health research are: 1) whether to work with samples or include the whole reference population in the study (census); 2) the sample basis; 3) the sampling process and 4) the potential effects nonrespondents might have on study results. We will refer to each of these aspects with theoretical and practical examples for better understanding in the sections that follow.
TO SAMPLE OR NOT TO SAMPLE
In a previous paper, we discussed the necessary parameters on which to estimate the sample size. 1 We define sample as a finite part or subset of participants drawn from the target population. In turn, the target population corresponds to the entire set of subjects whose characteristics are of interest to the research team. Based on results obtained from a sample, researchers may draw their conclusions about the target population with a certain level of confidence, following a process called statistical inference. When the sample contains fewer individuals than the minimum necessary, but the representativeness is preserved, statistical inference may be compromised in terms of precision (prevalence studies) and/or statistical power to detect the associations of interest. 1 On the other hand, samples without representativeness may not be a reliable source to draw conclusions about the reference population (i.e., statistical inference is not deemed possible), even if the sample size reaches the required number of participants. Lack of representativeness can occur as a result of flawed selection procedures (sampling bias) or when the probability of refusal/non-participation in the study is related to the object of research (nonresponse bias). 1 , 2
Although most studies are performed using samples, whether or not they represent any target population, census-based estimates should be preferred whenever possible. 3 , 4 For instance, if all cases of melanoma are available on a national or regional database, and information on the potential risk factors are also available, it would be preferable to conduct a census instead of investigating a sample.
However, there are several theoretical and practical reasons that prevent us from carrying out census-based surveys, including:
- Ethical issues: it is unethical to include a greater number of individuals than that effectively required;
- Budgetary limitations: the high costs of a census survey often limits its use as a strategy to select participants for a study;
- Logistics: censuses often impose great challenges in terms of required staff, equipment, etc. to conduct the study;
- Time restrictions: the amount of time needed to plan and conduct a census-based survey may be excessive; and,
- Unknown target population size: if the study objective is to investigate the presence of premalignant skin lesions in illicit drugs users, lack of information on all existing users makes it impossible to conduct a census-based study.
All these reasons explain why samples are more frequently used. However, researchers must be aware that sample results can be affected by the random error (or sampling error). 3 To exemplify this concept, we will consider a research study aiming to estimate the prevalence of premalignant skin lesions (outcome) among individuals >18 years residing in a specific city (target population). The city has a total population of 4,000 adults, but the investigator decided to collect data on a representative sample of 400 participants, detecting an 8% prevalence of premalignant skin lesions. A week later, the researcher selects another sample of 400 participants from the same target population to confirm the results, but this time observes a 12% prevalence of premalignant skin lesions. Based on these findings, is it possible to assume that the prevalence of lesions increased from the first to the second week? The answer is probably not. Each time we select a new sample, it is very likely to obtain a different result. These fluctuations are attributed to the "random error." They occur because individuals composing different samples are not the same, even though they were selected from the same target population. Therefore, the parameters of interest may vary randomly from one sample to another. Despite this fluctuation, if it were possible to obtain 100 different samples of the same population, approximately 95 of them would provide prevalence estimates very close to the real estimate in the target population - the value that we would observe if we investigated all the 4,000 adults residing in the city. Thus, during the sample size estimation the investigator must specify in advance the highest or maximum acceptable random error value in the study. Most population-based studies use a random error ranging from 2 to 5 percentage points. Nevertheless, the researcher should be aware that the smaller the random error considered in the study, the larger the required sample size. 1
SAMPLE FRAME
The sample frame is the group of individuals that can be selected from the target population given the sampling process used in the study. For example, to identify cases of cutaneous melanoma the researcher may consider to utilize as sample frame the national cancer registry system or the anatomopathological records of skin biopsies. Given that the sample may represent only a portion of the target population, the researcher needs to examine carefully whether the selected sample frame fits the study objectives or hypotheses, and especially if there are strategies to overcome the sample frame limitations (see Chart 1 for examples and possible limitations).
Examples of sample frames and potential limitations as regards representativeness
Sampling can be defined as the process through which individuals or sampling units are selected from the sample frame. The sampling strategy needs to be specified in advance, given that the sampling method may affect the sample size estimation. 1 , 5 Without a rigorous sampling plan the estimates derived from the study may be biased (selection bias). 3
TYPES OF SAMPLING
In figure 1 , we depict a summary of the main sampling types. There are two major sampling types: probabilistic and nonprobabilistic.

Sampling types used in scientific studies
NONPROBABILISTIC SAMPLING
In the context of nonprobabilistic sampling, the likelihood of selecting some individuals from the target population is null. This type of sampling does not render a representative sample; therefore, the observed results are usually not generalizable to the target population. Still, unrepresentative samples may be useful for some specific research objectives, and may help answer particular research questions, as well as contribute to the generation of new hypotheses. 4 The different types of nonprobabilistic sampling are detailed below.
Convenience sampling : the participants are consecutively selected in order of apperance according to their convenient accessibility (also known as consecutive sampling). The sampling process comes to an end when the total amount of participants (sample saturation) and/or the time limit (time saturation) are reached. Randomized clinical trials are usually based on convenience sampling. After sampling, participants are usually randomly allocated to the intervention or control group (randomization). 3 Although randomization is a probabilistic process to obtain two comparable groups (treatment and control), the samples used in these studies are generally not representative of the target population.
Purposive sampling: this is used when a diverse sample is necessary or the opinion of experts in a particular field is the topic of interest. This technique was used in the study by Roubille et al, in which recommendations for the treatment of comorbidities in patients with rheumatoid arthritis, psoriasis, and psoriatic arthritis were made based on the opinion of a group of experts. 6
Quota sampling: according to this sampling technique, the population is first classified by characteristics such as gender, age, etc. Subsequently, sampling units are selected to complete each quota. For example, in the study by Larkin et al., the combination of vemurafenib and cobimetinib versus placebo was tested in patients with locally-advanced melanoma, stage IIIC or IV, with BRAF mutation. 7 The study recruited 495 patients from 135 health centers located in several countries. In this type of study, each center has a "quota" of patients.
"Snowball" sampling : in this case, the researcher selects an initial group of individuals. Then, these participants indicate other potential members with similar characteristics to take part in the study. This is frequently used in studies investigating special populations, for example, those including illicit drugs users, as was the case of the study by Gonçalves et al, which assessed 27 users of cocaine and crack in combination with marijuana. 8
PROBABILISTIC SAMPLING
In the context of probabilistic sampling, all units of the target population have a nonzero probability to take part in the study. If all participants are equally likely to be selected in the study, equiprobabilistic sampling is being used, and the odds of being selected by the research team may be expressed by the formula: P=1/N, where P equals the probability of taking part in the study and N corresponds to the size of the target population. The main types of probabilistic sampling are described below.
Simple random sampling: in this case, we have a full list of sample units or participants (sample basis), and we randomly select individuals using a table of random numbers. An example is the study by Pimenta et al, in which the authors obtained a listing from the Health Department of all elderly enrolled in the Family Health Strategy and, by simple random sampling, selected a sample of 449 participants. 9
Systematic random sampling: in this case, participants are selected from fixed intervals previously defined from a ranked list of participants. For example, in the study of Kelbore et al, children who were assisted at the Pediatric Dermatology Service were selected to evaluate factors associated with atopic dermatitis, selecting always the second child by consulting order. 10
Stratified sampling: in this type of sampling, the target population is first divided into separate strata. Then, samples are selected within each stratum, either through simple or systematic sampling. The total number of individuals to be selected in each stratum can be fixed or proportional to the size of each stratum. Each individual may be equally likely to be selected to participate in the study. However, the fixed method usually involves the use of sampling weights in the statistical analysis (inverse of the probability of selection or 1/P). An example is the study conducted in South Australia to investigate factors associated with vitamin D deficiency in preschool children. Using the national census as the sample frame, households were randomly selected in each stratum and all children in the age group of interest identified in the selected houses were investigated. 11
Cluster sampling: in this type of probabilistic sampling, groups such as health facilities, schools, etc., are sampled. In the above-mentioned study, the selection of households is an example of cluster sampling. 11
Complex or multi-stage sampling: This probabilistic sampling method combines different strategies in the selection of the sample units. An example is the study of Duquia et al. to assess the prevalence and factors associated with the use of sunscreen in adults. The sampling process included two stages. 12 Using the 2000 Brazilian demographic census as sampling frame, all 404 census tracts from Pelotas (Southern Brazil) were listed in ascending order of family income. A sample of 120 tracts were systematically selected (first sampling stage units). In the second stage, 12 households in each of these census tract (second sampling stage units) were systematically drawn. All adult residents in these households were included in the study (third sampling stage units). All these stages have to be considered in the statistical analysis to provide correct estimates.
NONRESPONDENTS
Frequently, sample sizes are increased by 10% to compensate for potential nonresponses (refusals/losses). 1 Let us imagine that in a study to assess the prevalence of premalignant skin lesions there is a higher percentage of nonrespondents among men (10%) than among women (1%). If the highest percentage of nonresponse occurs because these men are not at home during the scheduled visits, and these participants are more likely to be exposed to the sun, the number of skin lesions will be underestimated. For this reason, it is strongly recommended to collect and describe some basic characteristics of nonrespondents (sex, age, etc.) so they can be compared to the respondents to evaluate whether the results may have been affected by this systematic error.
Often, in study protocols, refusal to participate or sign the informed consent is considered an "exclusion criteria". However, this is not correct, as these individuals are eligible for the study and need to be reported as "nonrespondents".
SAMPLING METHOD ACCORDING TO THE TYPE OF STUDY
In general, clinical trials aim to obtain a homogeneous sample which is not necessarily representative of any target population. Clinical trials often recruit those participants who are most likely to benefit from the intervention. 3 Thus, the more strict criteria for inclusion and exclusion of subjects in clinical trials often make it difficult to locate participants: after verification of the eligibility criteria, just one out of ten possible candidates will enter the study. Therefore, clinical trials usually show limitations to generalize the results to the entire population of patients with the disease, but only to those with similar characteristics to the sample included in the study. These peculiarities in clinical trials justify the necessity of conducting a multicenter and/or global studiesto accelerate the recruitment rate and to reach, in a shorter time, the number of patients required for the study. 13
In turn, in observational studies to build a solid sampling plan is important because of the great heterogeneity usually observed in the target population. Therefore, this heterogeneity has to be also reflected in the sample. A cross-sectional population-based study aiming to assess disease estimates or identify risk factors often uses complex probabilistic sampling, because the sample representativeness is crucial. However, in a case-control study, we face the challenge of selecting two different samples for the same study. One sample is formed by the cases, which are identified based on the diagnosis of the disease of interest. The other consists of controls, which need to be representative of the population that originated the cases. Improper selection of control individuals may introduce selection bias in the results. Thus, the concern with representativeness in this type of study is established based on the relationship between cases and controls (comparability).
In cohort studies, individuals are recruited based on the exposure (exposed and unexposed subjects), and they are followed over time to evaluate the occurrence of the outcome of interest. At baseline, the sample can be selected from a representative sample (population-based cohort studies) or a non-representative sample. However, in the successive follow-ups of the cohort member, study participants must be a representative sample of those included in the baseline. 14 , 15 In this type of study, losses over time may cause follow-up bias.
Researchers need to decide during the planning stage of the study if they will work with the entire target population or a sample. Working with a sample involves different steps, including sample size estimation, identification of the sample frame, and selection of the sampling method to be adopted.
Financial Support: None.
* Study performed at Faculdade Meridional - Escola de Medicina (IMED) - Passo Fundo (RS), Brazil.
- Privacy Policy

Home » Research Methodology – Types, Examples and writing Guide
Research Methodology – Types, Examples and writing Guide
Table of Contents

Research Methodology
Definition:
Research Methodology refers to the systematic and scientific approach used to conduct research, investigate problems, and gather data and information for a specific purpose. It involves the techniques and procedures used to identify, collect , analyze , and interpret data to answer research questions or solve research problems . Moreover, They are philosophical and theoretical frameworks that guide the research process.
Structure of Research Methodology
Research methodology formats can vary depending on the specific requirements of the research project, but the following is a basic example of a structure for a research methodology section:
I. Introduction
- Provide an overview of the research problem and the need for a research methodology section
- Outline the main research questions and objectives
II. Research Design
- Explain the research design chosen and why it is appropriate for the research question(s) and objectives
- Discuss any alternative research designs considered and why they were not chosen
- Describe the research setting and participants (if applicable)
III. Data Collection Methods
- Describe the methods used to collect data (e.g., surveys, interviews, observations)
- Explain how the data collection methods were chosen and why they are appropriate for the research question(s) and objectives
- Detail any procedures or instruments used for data collection
IV. Data Analysis Methods
- Describe the methods used to analyze the data (e.g., statistical analysis, content analysis )
- Explain how the data analysis methods were chosen and why they are appropriate for the research question(s) and objectives
- Detail any procedures or software used for data analysis
V. Ethical Considerations
- Discuss any ethical issues that may arise from the research and how they were addressed
- Explain how informed consent was obtained (if applicable)
- Detail any measures taken to ensure confidentiality and anonymity
VI. Limitations
- Identify any potential limitations of the research methodology and how they may impact the results and conclusions
VII. Conclusion
- Summarize the key aspects of the research methodology section
- Explain how the research methodology addresses the research question(s) and objectives
Research Methodology Types
Types of Research Methodology are as follows:
Quantitative Research Methodology
This is a research methodology that involves the collection and analysis of numerical data using statistical methods. This type of research is often used to study cause-and-effect relationships and to make predictions.
Qualitative Research Methodology
This is a research methodology that involves the collection and analysis of non-numerical data such as words, images, and observations. This type of research is often used to explore complex phenomena, to gain an in-depth understanding of a particular topic, and to generate hypotheses.
Mixed-Methods Research Methodology
This is a research methodology that combines elements of both quantitative and qualitative research. This approach can be particularly useful for studies that aim to explore complex phenomena and to provide a more comprehensive understanding of a particular topic.
Case Study Research Methodology
This is a research methodology that involves in-depth examination of a single case or a small number of cases. Case studies are often used in psychology, sociology, and anthropology to gain a detailed understanding of a particular individual or group.
Action Research Methodology
This is a research methodology that involves a collaborative process between researchers and practitioners to identify and solve real-world problems. Action research is often used in education, healthcare, and social work.
Experimental Research Methodology
This is a research methodology that involves the manipulation of one or more independent variables to observe their effects on a dependent variable. Experimental research is often used to study cause-and-effect relationships and to make predictions.
Survey Research Methodology
This is a research methodology that involves the collection of data from a sample of individuals using questionnaires or interviews. Survey research is often used to study attitudes, opinions, and behaviors.
Grounded Theory Research Methodology
This is a research methodology that involves the development of theories based on the data collected during the research process. Grounded theory is often used in sociology and anthropology to generate theories about social phenomena.
Research Methodology Example
An Example of Research Methodology could be the following:
Research Methodology for Investigating the Effectiveness of Cognitive Behavioral Therapy in Reducing Symptoms of Depression in Adults
Introduction:
The aim of this research is to investigate the effectiveness of cognitive-behavioral therapy (CBT) in reducing symptoms of depression in adults. To achieve this objective, a randomized controlled trial (RCT) will be conducted using a mixed-methods approach.
Research Design:
The study will follow a pre-test and post-test design with two groups: an experimental group receiving CBT and a control group receiving no intervention. The study will also include a qualitative component, in which semi-structured interviews will be conducted with a subset of participants to explore their experiences of receiving CBT.
Participants:
Participants will be recruited from community mental health clinics in the local area. The sample will consist of 100 adults aged 18-65 years old who meet the diagnostic criteria for major depressive disorder. Participants will be randomly assigned to either the experimental group or the control group.
Intervention :
The experimental group will receive 12 weekly sessions of CBT, each lasting 60 minutes. The intervention will be delivered by licensed mental health professionals who have been trained in CBT. The control group will receive no intervention during the study period.
Data Collection:
Quantitative data will be collected through the use of standardized measures such as the Beck Depression Inventory-II (BDI-II) and the Generalized Anxiety Disorder-7 (GAD-7). Data will be collected at baseline, immediately after the intervention, and at a 3-month follow-up. Qualitative data will be collected through semi-structured interviews with a subset of participants from the experimental group. The interviews will be conducted at the end of the intervention period, and will explore participants’ experiences of receiving CBT.
Data Analysis:
Quantitative data will be analyzed using descriptive statistics, t-tests, and mixed-model analyses of variance (ANOVA) to assess the effectiveness of the intervention. Qualitative data will be analyzed using thematic analysis to identify common themes and patterns in participants’ experiences of receiving CBT.
Ethical Considerations:
This study will comply with ethical guidelines for research involving human subjects. Participants will provide informed consent before participating in the study, and their privacy and confidentiality will be protected throughout the study. Any adverse events or reactions will be reported and managed appropriately.
Data Management:
All data collected will be kept confidential and stored securely using password-protected databases. Identifying information will be removed from qualitative data transcripts to ensure participants’ anonymity.
Limitations:
One potential limitation of this study is that it only focuses on one type of psychotherapy, CBT, and may not generalize to other types of therapy or interventions. Another limitation is that the study will only include participants from community mental health clinics, which may not be representative of the general population.
Conclusion:
This research aims to investigate the effectiveness of CBT in reducing symptoms of depression in adults. By using a randomized controlled trial and a mixed-methods approach, the study will provide valuable insights into the mechanisms underlying the relationship between CBT and depression. The results of this study will have important implications for the development of effective treatments for depression in clinical settings.
How to Write Research Methodology
Writing a research methodology involves explaining the methods and techniques you used to conduct research, collect data, and analyze results. It’s an essential section of any research paper or thesis, as it helps readers understand the validity and reliability of your findings. Here are the steps to write a research methodology:
- Start by explaining your research question: Begin the methodology section by restating your research question and explaining why it’s important. This helps readers understand the purpose of your research and the rationale behind your methods.
- Describe your research design: Explain the overall approach you used to conduct research. This could be a qualitative or quantitative research design, experimental or non-experimental, case study or survey, etc. Discuss the advantages and limitations of the chosen design.
- Discuss your sample: Describe the participants or subjects you included in your study. Include details such as their demographics, sampling method, sample size, and any exclusion criteria used.
- Describe your data collection methods : Explain how you collected data from your participants. This could include surveys, interviews, observations, questionnaires, or experiments. Include details on how you obtained informed consent, how you administered the tools, and how you minimized the risk of bias.
- Explain your data analysis techniques: Describe the methods you used to analyze the data you collected. This could include statistical analysis, content analysis, thematic analysis, or discourse analysis. Explain how you dealt with missing data, outliers, and any other issues that arose during the analysis.
- Discuss the validity and reliability of your research : Explain how you ensured the validity and reliability of your study. This could include measures such as triangulation, member checking, peer review, or inter-coder reliability.
- Acknowledge any limitations of your research: Discuss any limitations of your study, including any potential threats to validity or generalizability. This helps readers understand the scope of your findings and how they might apply to other contexts.
- Provide a summary: End the methodology section by summarizing the methods and techniques you used to conduct your research. This provides a clear overview of your research methodology and helps readers understand the process you followed to arrive at your findings.
When to Write Research Methodology
Research methodology is typically written after the research proposal has been approved and before the actual research is conducted. It should be written prior to data collection and analysis, as it provides a clear roadmap for the research project.
The research methodology is an important section of any research paper or thesis, as it describes the methods and procedures that will be used to conduct the research. It should include details about the research design, data collection methods, data analysis techniques, and any ethical considerations.
The methodology should be written in a clear and concise manner, and it should be based on established research practices and standards. It is important to provide enough detail so that the reader can understand how the research was conducted and evaluate the validity of the results.
Applications of Research Methodology
Here are some of the applications of research methodology:
- To identify the research problem: Research methodology is used to identify the research problem, which is the first step in conducting any research.
- To design the research: Research methodology helps in designing the research by selecting the appropriate research method, research design, and sampling technique.
- To collect data: Research methodology provides a systematic approach to collect data from primary and secondary sources.
- To analyze data: Research methodology helps in analyzing the collected data using various statistical and non-statistical techniques.
- To test hypotheses: Research methodology provides a framework for testing hypotheses and drawing conclusions based on the analysis of data.
- To generalize findings: Research methodology helps in generalizing the findings of the research to the target population.
- To develop theories : Research methodology is used to develop new theories and modify existing theories based on the findings of the research.
- To evaluate programs and policies : Research methodology is used to evaluate the effectiveness of programs and policies by collecting data and analyzing it.
- To improve decision-making: Research methodology helps in making informed decisions by providing reliable and valid data.
Purpose of Research Methodology
Research methodology serves several important purposes, including:
- To guide the research process: Research methodology provides a systematic framework for conducting research. It helps researchers to plan their research, define their research questions, and select appropriate methods and techniques for collecting and analyzing data.
- To ensure research quality: Research methodology helps researchers to ensure that their research is rigorous, reliable, and valid. It provides guidelines for minimizing bias and error in data collection and analysis, and for ensuring that research findings are accurate and trustworthy.
- To replicate research: Research methodology provides a clear and detailed account of the research process, making it possible for other researchers to replicate the study and verify its findings.
- To advance knowledge: Research methodology enables researchers to generate new knowledge and to contribute to the body of knowledge in their field. It provides a means for testing hypotheses, exploring new ideas, and discovering new insights.
- To inform decision-making: Research methodology provides evidence-based information that can inform policy and decision-making in a variety of fields, including medicine, public health, education, and business.
Advantages of Research Methodology
Research methodology has several advantages that make it a valuable tool for conducting research in various fields. Here are some of the key advantages of research methodology:
- Systematic and structured approach : Research methodology provides a systematic and structured approach to conducting research, which ensures that the research is conducted in a rigorous and comprehensive manner.
- Objectivity : Research methodology aims to ensure objectivity in the research process, which means that the research findings are based on evidence and not influenced by personal bias or subjective opinions.
- Replicability : Research methodology ensures that research can be replicated by other researchers, which is essential for validating research findings and ensuring their accuracy.
- Reliability : Research methodology aims to ensure that the research findings are reliable, which means that they are consistent and can be depended upon.
- Validity : Research methodology ensures that the research findings are valid, which means that they accurately reflect the research question or hypothesis being tested.
- Efficiency : Research methodology provides a structured and efficient way of conducting research, which helps to save time and resources.
- Flexibility : Research methodology allows researchers to choose the most appropriate research methods and techniques based on the research question, data availability, and other relevant factors.
- Scope for innovation: Research methodology provides scope for innovation and creativity in designing research studies and developing new research techniques.
Research Methodology Vs Research Methods
About the author.
Muhammad Hassan
Researcher, Academic Writer, Web developer
You may also like

How to Cite Research Paper – All Formats and...

Data Collection – Methods Types and Examples

Delimitations in Research – Types, Examples and...

Research Paper Format – Types, Examples and...

Research Process – Steps, Examples and Tips

Research Design – Types, Methods and Examples
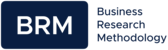
Cluster Sampling
Cluster sampling (also known as one-stage cluster sampling) is a technique in which clusters of participants representing the population are identified and included in the sample [1] . This is a popular method in conducting marketing researches.
The main aim of cluster sampling can be specified as cost reduction and increasing the levels of efficiency of sampling. This specific technique can also be applied in integration with multi-stage sampling .
A major difference between cluster and stratified sampling relates to the fact that in cluster sampling a cluster is perceived as a sampling unit, whereas in stratified sampling only specific elements of strata are accepted as sampling unit.
Accordingly, in cluster sampling a complete list of clusters represent the sampling frame. Then, a few clusters are chosen randomly as the source of primary data.
Cluster sampling process can be single stage or multistage. In single stage sampling, all members of selected clusters are included in the study, whereas in multistage sampling additional sampling methods are used to choose certain individuals within selected clusters.
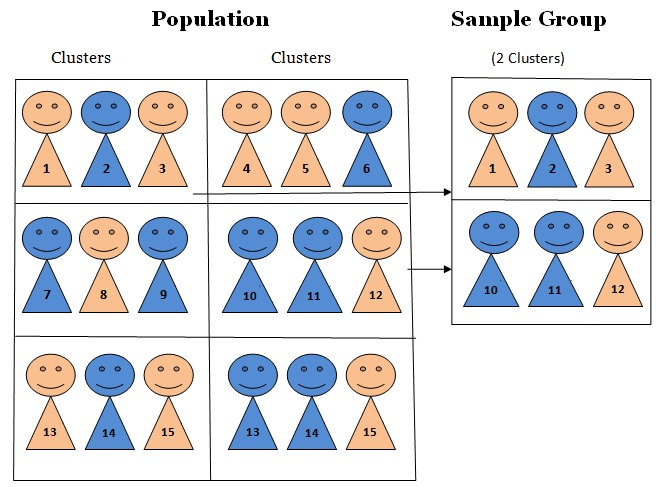
Area or geographical sampling can be specified as the most popular version of cluster sampling. Specifically, a specific area can be divided into clusters and primary data can be collected from each cluster to represent the viewpoint of the whole area.
The pattern of cluster analysis depends on comparative size of separate clusters. If there are no major differences between sizes of clusters, then analysis can be facilitated by combining clusters. Alternatively, if there are vast differences in sizes of clusters, probability sampling proportionate to sample size can be applied to conduct the analysis.
Application of Cluster Sampling: an Example
Imagine you want to evaluate consumer spending on various modes of transportation in Greater London. Since Greater London is a large area, we need to sample from only 6 boroughs out of total 32 boroughs it comprises.
There are following five stages for the application of cluster sampling for this research:
1. Choosing target audience and sample size . The target audience for such a study is Greater London and sample size includes all the people living in Greater London.
2. Dividing population into clusters . Population in each cluster should be diverse and potential characteristics of the entire population should be represented in each cluster. Overlap between clusters should not exist, i.e. same people should not belong to more than one clusters. The Greater London consists of 32 boroughs. Each borough meets requirements above to be considered as a cluster. Accordingly, the area can be divided into 32 clusters with each cluster representing a borough.
3. Marking each cluster with a unique number . We can easily number each borough from 1 to 32.
4. Choosing a sample of clusters applying probability sampling . Usingsystematic random sampling (or any other probability sampling), we can choose 6 boroughs from the total 32 boroughs. It can be argued that these 6 boroughs can be considered as mini-representation of the entire Greater London. Households residing in 6 boroughs will represent samples for the study.
5. Choosing individual households to be included in the study. For this research we would be using multistage, rather than single stage cluster sampling. Accordingly, rather than using all households within selected 6 boroughs, we will choose certain households residing in these boroughs using probability sampling method such as systematic or stratified.
Advantages of Cluster Sampling
- It is the most time-efficient and cost-efficient probability design for large geographical areas
- This method is easy to be used from practicality point of view
- Larger sample size can be used due to increased level of accessibility of perspective sample group members
Disadvantages of Cluster Sampling
- Requires group-level information to be known
- Commonly has higher sampling error than othersampling techniques
- Cluster sampling may fail to reflect the diversity in the sampling frame
My e-book, The Ultimate Guide to Writing a Dissertation in Business Studies: a step by step approach contains a detailed, yet simple explanation of sampling methods . The e-book explains all stages of the research process starting from the selection of the research area to writing personal reflection. Important elements of dissertations such as research philosophy , research approach , research design , methods of data collection and data analysis are explained in this e-book in simple words.
John Dudovskiy
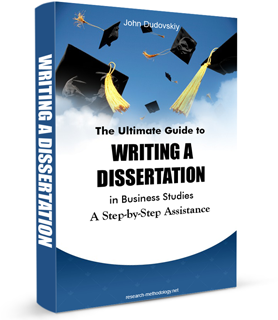
[1] Jackson, S.L. (2011) “Research Methods and Statistics: A Critical Approach” 4 th edition, Cengage Learning
Numbers, Facts and Trends Shaping Your World
Read our research on:
Full Topic List
Regions & Countries
- Publications
- Our Methods
- Short Reads
- Tools & Resources
Read Our Research On:
U.S. Surveys
Pew Research Center has deep roots in U.S. public opinion research. Launched initially as a project focused primarily on U.S. policy and politics in the early 1990s, the Center has grown over time to study a wide range of topics vital to explaining America to itself and to the world. Our hallmarks: a rigorous approach to methodological quality, complete transparency as to our methods, and a commitment to exploring and evaluating ongoing developments in data collection. Learn more about how we conduct our domestic surveys here .
The American Trends Panel
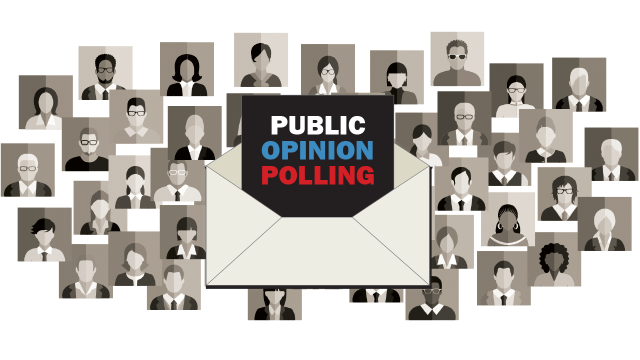
Try our email course on polling
Want to know more about polling? Take your knowledge to the next level with a short email mini-course from Pew Research Center. Sign up now .
From the 1980s until relatively recently, most national polling organizations conducted surveys by telephone, relying on live interviewers to call randomly selected Americans across the country. Then came the internet. While it took survey researchers some time to adapt to the idea of online surveys, a quick look at the public polls on an issue like presidential approval reveals a landscape now dominated by online polls rather than phone polls.
Most of our U.S. surveys are conducted on the American Trends Panel (ATP), Pew Research Center’s national survey panel of over 10,000 randomly selected U.S. adults. ATP participants are recruited offline using random sampling from the U.S. Postal Service’s residential address file. Survey length is capped at 15 minutes, and respondents are reimbursed for their time. Respondents complete the surveys online using smartphones, tablets or desktop devices. We provide tablets and data plans to adults without home internet. Learn more about how people in the U.S. take Pew Research Center surveys.
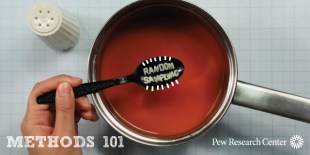
Methods 101
Our video series helps explain the fundamental concepts of survey research including random sampling , question wording , mode effects , non probability surveys and how polling is done around. the world.
The Center also conducts custom surveys of special populations (e.g., Muslim Americans , Jewish Americans , Black Americans , Hispanic Americans , teenagers ) that are not readily studied using national, general population sampling. The Center’s survey research is sometimes paired with demographic or organic data to provide new insights. In addition to our U.S. survey research, you can also read more details on our international survey research , our demographic research and our data science methods.
Our survey researchers are committed to contributing to the larger community of survey research professionals, and are active in AAPOR and is a charter member of the American Association of Public Opinion Research (AAPOR) Transparency Initiative .
Frequently asked questions about surveys
- Why am I never asked to take a poll?
- Can I volunteer to be polled?
- Why should I participate in surveys?
- What good are polls?
- Do pollsters have a code of ethics? If so, what is in the code?
- How are your surveys different from market research?
- Do you survey Asian Americans?
- How are people selected for your polls?
- Do people lie to pollsters?
- Do people really have opinions on all of those questions?
- How can I tell a high-quality poll from a lower-quality one?
Reports on the state of polling
- Key Things to Know about Election Polling in the United States
- A Field Guide to Polling: 2020 Edition
- Confronting 2016 and 2020 Polling Limitations
- What 2020’s Election Poll Errors Tell Us About the Accuracy of Issue Polling
- Q&A: After misses in 2016 and 2020, does polling need to be fixed again? What our survey experts say
- Understanding how 2020 election polls performed and what it might mean for other kinds of survey work
- Can We Still Trust Polls?
- Political Polls and the 2016 Election
- Flashpoints in Polling: 2016
Sign up for our Methods newsletter
The latest on survey methods, data science and more, delivered quarterly.
OTHER RESEARCH METHODS
Sign up for our weekly newsletter.
Fresh data delivered Saturday mornings
1615 L St. NW, Suite 800 Washington, DC 20036 USA (+1) 202-419-4300 | Main (+1) 202-857-8562 | Fax (+1) 202-419-4372 | Media Inquiries
Research Topics
- Age & Generations
- Coronavirus (COVID-19)
- Economy & Work
- Family & Relationships
- Gender & LGBTQ
- Immigration & Migration
- International Affairs
- Internet & Technology
- Methodological Research
- News Habits & Media
- Non-U.S. Governments
- Other Topics
- Politics & Policy
- Race & Ethnicity
- Email Newsletters
ABOUT PEW RESEARCH CENTER Pew Research Center is a nonpartisan fact tank that informs the public about the issues, attitudes and trends shaping the world. It conducts public opinion polling, demographic research, media content analysis and other empirical social science research. Pew Research Center does not take policy positions. It is a subsidiary of The Pew Charitable Trusts .
Copyright 2024 Pew Research Center
Terms & Conditions
Privacy Policy
Cookie Settings
Reprints, Permissions & Use Policy
Certification of the total element mass fractions in UME EnvCRM 03 soil sample via a joint research project
- Practitioner's Report
- Published: 23 April 2024
Cite this article
- Alper Isleyen 1 ,
- Suleyman Z. Can 1 ,
- Oktay Cankur 1 ,
- Murat Tunc 1 ,
- Jochen Vogl 2 ,
- Maren Koenig 2 ,
- Milena Horvat 3 ,
- Radojko Jacimovic 3 ,
- Tea Zuliani 3 ,
- Vesna Fajon 3 ,
- Aida Jotanovic 4 ,
- Luka Gaževic 5 ,
- Milena Milosevic 5 ,
- Maria Ochsenkuehn–Petropoulou 6 ,
- Fotis Tsopelas 6 ,
- Theopisti Lymberopoulou 6 ,
- Lamprini-Areti Tsakanika 6 ,
- Olga Serifi 6 ,
- Klaus M. Ochsenkuehn 6 ,
- Ewa Bulska 7 ,
- Anna Tomiak 7 ,
- Eliza Kurek 7 ,
- Zehra Cakılbahçe 1 ,
- Gokhan Aktas 1 ,
- Hatice Altuntas 1 ,
- Elif Basaran 1 ,
- Barıs Kısacık 1 &
- Zeynep Gumus 1
Soil certified reference material (CRM), UME EnvCRM 03 was produced by a collaborative approach among national metrology institutes, designated institutes and university research laboratories within the scope of the EMPIR project: Matrix Reference Materials for Environmental Analysis. This paper presents the sampling and processing methodology, homogeneity, stability, characterization campaign, the assignment of property values and their associated uncertainties in compliance with ISO 17034:2016. The material processing methodology involves blending a natural soil sample with a contaminated soil sample obtained by spiking elemental solutions for 8 elements (Cd, Co, Cu, Hg, Ni, Pb, Sb and Zn) to reach the level of warning risk monitoring values specified for metals and metalloids of soils in Europe. Comparative homogeneity and stability test data were obtained by two different institutes, ensuring the reliability and back up of the data. The certified values and associated expanded uncertainties for the total mass fractions of thirteen elements (As, Cd, Co, Cr, Cu, Fe, Hg, Mn, Ni, Pb, Sb, V and Zn) are established. The developed CRM can be used for the development and validation of measurement procedures for the determination of the total mass fractions of elements in soil and also for quality control/assurance purposes. The developed CRM is the first example of a soil material originating from Türkiye.
This is a preview of subscription content, log in via an institution to check access.
Access this article
Price includes VAT (Russian Federation)
Instant access to the full article PDF.
Rent this article via DeepDyve
Institutional subscriptions
Matrix reference materials for environmental analysis EURAMET project page. https://www.euramet.org/research-innovation/search-research-projects/details/project/matrix-reference-materials-for-environmental-analysis
ISO 17034 (2016) General requirements for the competence of reference materials producers
Vassileva E, Azemard S, Mandjukov P (2017) Certification for trace elements and methyl mercury mass fractions in IAEA-456 marine sediment sample Accred. Qual Assur 23:29–37
Google Scholar
Mackey EA, Christopher SJ, Lindstrom RM, Long SE, Marlow AF, Murphy KE, Paul RL, Popelka-Filcoff RS, Rabb SA, Sieber JR, Spatz RO, Tomlin BE, Wood LJ, Yu LL, Zeisler R, Yen JH, Wilson SA, Adams MG, Brown ZA, Lamothe PL, Taggart JE, Jones C, Nebelsick J (2010) NIST special publication 260–172, certification of three NIST renewal soil standard reference materials for element content: SRM 2709a San Joaquin Soil, SRM 2710a Montana Soil I, and SRM 2711a Montana Soil II
Birgersson-Liebich A, Venelinov T, Santoro A, Held A (2010) Certification report, the certification of the mass fraction of the total content and the aqua regia extractable content of As, Cd, Co, Cr, Cu, Mn, Ni, Pb and Zn in Loam soil certified reference material ERM ® -CC141
Scharf H, Lück D, Bremser W (2006) Bericht zur Zertifizierung der gesamtgehalte und der mit königswasser extrahierbaren gehalte der elemente As, Cd, Co, Cr, Cu, Hg, Mn, Ni, Pb und Zn in einer bodenprobe Zertifiziertes Referenzmaterial BAM-U110
Griepink B, Muntau H, Vercoutere K (1994) Final report, certification of the total contents (mass fractions) of Cd, Co, Cu, Pb, Mn, Hg and Ni and the aqua regia soluble contents (mass fractions) of Cd, Pb, Ni and Zn in a light sandy soil CRM 142R
Semenkov IN, Koroleva TV (2019) International environmental legislation on the content of chemical elements in soils: guidelines and schemes. Eurasian Soil Sci 52(10):1289–1297
Article CAS Google Scholar
Carlon C (Ed.) (2007) Derivation methods of soil screening values in Europe. A review and evaluation of national procedures towards harmonization. European Commission Joint Research Centre, Ispra
Karaca A, Türkmen C, Arcak S, Haktanır K, Topçuoğlu B, Yıldız H (2009) The determination of the effect of Cayirhan coal-fired power plant emission on heavy metals and sulphur contents of regional soils. Ankara Üniversitesi Çevrebilimleri Dergisi 1(1):25–41
Article Google Scholar
Lamberty A, Schimmel H, Pauwels J (1998) The study of the stability of reference materials by isochronous measurements. Fres J Anal Chem 360:359–361
ISO Guide 35 (2017) Reference materials — guidance for characterization and assessment of homogeneity and stability
Linsinger TPJ, Pauwels J, Van der Veen AMH, Schimmel H, Lamberty A (2001) Homogeneity and stability of reference materials. Accred Qual Assur 6:20–25
Certificate of the Reference Material UME EnvCRM 03-Soil. https://rm.ume.tubitak.gov.tr/sertifika/ume_crm_envcrm03_certificate.pdf
International vocabulary of metrology - basic and general concepts and associated terms, 3rd ed (VIM 3). Available from https://www.bipm.org or as ISO/IEC guide 99-12:2007
ISO/TC 334 Position Paper (2023) The need for assessment of commutability of reference materials. https://committee.iso.org/files/live/sites/tc334/files/ISO-TC334_Commutability_document_2023-03.pdf
Download references
Acknowledgements
The work of this study is part of the project 14RPT03-EnvCRM, which was funded within the framework of the EMPIR. The EMPIR initiative is co-funded by the European Union’s Horizon 2020 research and innovation programme and the EMPIR Participating States. Authors thank to TUBITAK UME intern trainees Esma Eroğlu, Büşra Bıyıklı, Onur Uygun, H. Merve Kırbaş for their dedicated work during the processing of the soil material and Doğan Meriç for the logistics and supply of the soil material. We dedicate this article to the memory of Prof. Osman Yavuz Ataman, a doyen of analytical chemistry, who encouraged and directed us in producing reference materials.
European Metrology Programme for Innovation and Research, 14RPT03-EnvCRM.
Author information
Authors and affiliations.
TÜBİTAK UME-Ulusal Metroloji Enstitüsü, Kocaeli, Türkiye
Alper Isleyen, Suleyman Z. Can, Oktay Cankur, Murat Tunc, Zehra Cakılbahçe, Gokhan Aktas, Hatice Altuntas, Elif Basaran, Barıs Kısacık & Zeynep Gumus
BAM-Bundesanstalt für Materialforschung und –prüfung, Berlin, Germany
Jochen Vogl & Maren Koenig
IJS-Institute Jozef Stefan, Ljubljana, Slovenia
Milena Horvat, Radojko Jacimovic, Tea Zuliani & Vesna Fajon
IMBIH- Institute of Metrology, Sarajevo, Bosnia and Herzegovina
Aida Jotanovic
Directorate of Measures and Precious Metals, MoE-DMDM- Ministry of Economy, Beograde, Serbia
Luka Gaževic & Milena Milosevic
NTUA-National Technical University of Athens, Athens, Greece
Maria Ochsenkuehn–Petropoulou, Fotis Tsopelas, Theopisti Lymberopoulou, Lamprini-Areti Tsakanika, Olga Serifi & Klaus M. Ochsenkuehn
UWAR-University of Warsaw, Warsaw, Poland
Ewa Bulska, Anna Tomiak & Eliza Kurek
You can also search for this author in PubMed Google Scholar
Contributions
A.I. wrote the main manuscript text. AI., Z.C., G.A., H.A., E.B., B.K., Z.G. contributed to the material processing of the soil CRM. S.Z.C., O.C., M.T., J.V., M.K., M.H., R.J., T.Z., V.F., A.J., L.G., M.M., M.O-P., F.T., T.L., L-A.T., O.S.,K.M.O., E.B., A.T., E.K. contributed to the analysis and data evaluation. All authors reviewed the manuscript.
Corresponding author
Correspondence to Alper Isleyen .
Ethics declarations
Conflict of interest.
The authors declare no competing interests.
Additional information
Publisher's note.
Springer Nature remains neutral with regard to jurisdictional claims in published maps and institutional affiliations.
Supplementary Information
Below is the link to the electronic supplementary material.
Supplementary file1 (DOCX 2407 KB)
Rights and permissions.
Reprints and permissions
About this article
Isleyen, A., Can, S.Z., Cankur, O. et al. Certification of the total element mass fractions in UME EnvCRM 03 soil sample via a joint research project. Accred Qual Assur (2024). https://doi.org/10.1007/s00769-024-01597-8
Download citation
Received : 26 December 2023
Accepted : 02 April 2024
Published : 23 April 2024
DOI : https://doi.org/10.1007/s00769-024-01597-8
Share this article
Anyone you share the following link with will be able to read this content:
Sorry, a shareable link is not currently available for this article.
Provided by the Springer Nature SharedIt content-sharing initiative
- Total element content
- Certification
- Environmental pollution monitoring
- Find a journal
- Publish with us
- Track your research
- Open access
- Published: 19 April 2024
Asparagine reduces the risk of schizophrenia: a bidirectional two-sample mendelian randomization study of aspartate, asparagine and schizophrenia
- Huang-Hui Liu 1 , 2 na1 ,
- Yao Gao 1 , 2 na1 ,
- Dan Xu 1 , 2 ,
- Xin-Zhe Du 1 , 2 ,
- Si-Meng Wei 1 , 2 ,
- Jian-Zhen Hu 1 , 2 ,
- Yong Xu 1 , 2 &
- Liu Sha 1 , 2
BMC Psychiatry volume 24 , Article number: 299 ( 2024 ) Cite this article
296 Accesses
1 Altmetric
Metrics details
Despite ongoing research, the underlying causes of schizophrenia remain unclear. Aspartate and asparagine, essential amino acids, have been linked to schizophrenia in recent studies, but their causal relationship is still unclear. This study used a bidirectional two-sample Mendelian randomization (MR) method to explore the causal relationship between aspartate and asparagine with schizophrenia.
This study employed summary data from genome-wide association studies (GWAS) conducted on European populations to examine the correlation between aspartate and asparagine with schizophrenia. In order to investigate the causal effects of aspartate and asparagine on schizophrenia, this study conducted a two-sample bidirectional MR analysis using genetic factors as instrumental variables.
No causal relationship was found between aspartate and schizophrenia, with an odds ratio (OR) of 1.221 (95%CI: 0.483–3.088, P -value = 0.674). Reverse MR analysis also indicated that no causal effects were found between schizophrenia and aspartate, with an OR of 0.999 (95%CI: 0.987–1.010, P -value = 0.841). There is a negative causal relationship between asparagine and schizophrenia, with an OR of 0.485 (95%CI: 0.262-0.900, P -value = 0.020). Reverse MR analysis indicates that there is no causal effect between schizophrenia and asparagine, with an OR of 1.005(95%CI: 0.999–1.011, P -value = 0.132).
This study suggests that there may be a potential risk reduction for schizophrenia with increased levels of asparagine, while also indicating the absence of a causal link between elevated or diminished levels of asparagine in individuals diagnosed with schizophrenia. There is no potential causal relationship between aspartate and schizophrenia, whether prospective or reverse MR. However, it is important to note that these associations necessitate additional research for further validation.
Peer Review reports
Introduction
Schizophrenia is a serious psychiatric illness that affects 0.5 -1% of the global population [ 1 ]. The burden of mental illness was estimated to account for 7% of all diseases worldwide in 2016, and nearly 20% of all years lived with disability [ 2 ]. The characteristics of schizophrenia are positive symptoms, negative symptoms, and cognitive symptoms, which are often severe functional impairments and significant social maladaptations for patients suffering from schizophrenia [ 3 ]. It is still unclear what causes schizophrenia and what the pathogenesis is. There are a number of hypotheses based on neurochemical mechanisms, including dopaminergic and glutamatergic systems [ 4 ]. Although schizophrenia research has made significant advances in the past, further insight into its mechanisms and causes is still needed.
Association genetics research and genome-wide association studies have successfully identified more than 24 candidate genes that serve as molecular biomarkers for the susceptibility to treatment- refractory schizophrenia (TRS). It is worth noting that some proteins in these genes are related to glutamate transfer, especially the N-methyl-D-aspartate receptor (NMDAR) [ 5 ]. It is thought that NMDARs are important for neural plasticity, which is the ability of the brain itself to adapt to new environments. With age, NMDAR function usually declines, which may lead to decreased plasticity, leading to learning and memory problems. Consequently, the manifestation of cognitive deficits observed in diverse pathologies, including Alzheimer’s disease, amyotrophic lateral sclerosis, Huntington’s disease, Parkinson’s disease, schizophrenia, and major depression, can be attributed to the dysfunction of NMDAR [ 4 ]. There are two enantiomers of aspartate (Asp): L and D [ 6 ]. In the brain, D-aspartate (D-Asp) stimulates glutamate receptors and dopaminergic neurons through its direct NMDAR agonist action [ 7 ]. According to the glutamate theory of Sch, glutamate NMDAR dysfunction is a primary contributor to the development of this psychiatric disorder and TRS [ 8 ]. It has been shown in two autopsy studies that D-Asp of prefrontal cortex neurons in patients with schizophrenia are significantly reduced, which is related to an increased expression of D-Asp oxidase [ 9 ] or an increased activity of D-Asp oxidase [ 10 ]. Several studies in animal models and humans have shown that D-amino acids, particularly D-Ser and D-Asp [ 11 ], are able to modulate several NMDAR-dependent processes, including synaptic plasticity, brain development, cognition and brain ageing [ 12 ]. In addition, D-Asp is synthesized in hippocampal and prefrontal cortex neurons, which play an important role in the development of schizophrenia [ 13 ]. It has been reported that the precursor substance of asparagine (Asn), aspartate, activates the N-methyl-D-aspartate receptor [ 14 ]. Asparagine is essential for the survival of all cells [ 15 ], and it was decreased in schizophrenia patients [ 16 ]. Asparagine can cause metabolic disorders of alanine, aspartate, and glutamic acid, leading to dysfunction of the glutamine-glutamate cycle and further affecting it Gamma-Aminobutyric Acid(GABA) level [ 17 ].It is widely understood that the imbalance of GABA levels and NMDAR plays a crucial role in the pathogenesis of schizophrenia, causing neurotoxic effects, synaptic dysfunction, and cognitive impairments [ 18 ].Schizophrenic patients exhibited significantly higher levels of serum aspartate, glutamate, isoleucine, histidine and tyrosine and significantly lower concentrations of serum asparagine, tryptophan and serine [ 19 ]. Other studies have also shown that schizophrenics have higher levels of asparagine, phenylalanine, and cystine, and lower ratios of tyrosine, tryptophan, and tryptophan to competing amino acids, compared to healthy individuals [ 20 ]. Aspartate and asparagine’s association with schizophrenia is not fully understood, and their causal relationship remains unclear.
The MR method is a method that uses Mendelian independence principle to infer causality, which uses genetic variation to study the impact of exposure on outcomes. By using this approach, confounding factors in general research are overcome, and causal reasoning is provided on a reasonable temporal basis [ 21 ]. The instrumental variables for genetic variation that are chosen must adhere to three primary hypotheses: the correlation hypothesis, which posits a robust correlation between single nucleotide polymorphisms (SNPs) and exposure factors; the independence hypothesis, which asserts that SNPs are not affected by various confounding factors; the exclusivity hypothesis, which maintains that SNPs solely influence outcomes through on exposure factors. In a recent study, Mendelian randomization was used to reveal a causal connection between thyroid function and schizophrenia [ 22 ]. According to another Mendelian randomization study, physical activity is causally related to schizophrenia [ 23 ]. Therefore, this study used Mendelian randomization method to explore the causal effects of aspartate on schizophrenia and asparagine on schizophrenia.
To elucidate the causal effects of aspartate and asparagine on schizophrenia. This study used bidirectional MR analysis. In the prospective analysis of MR, the exposure factors under consideration were aspartate and asparagine, while the outcome of interest was the risk of schizophrenia. On the contrary, in the reverse MR analysis, schizophrenia was utilized as the exposure factor, with aspartate and asparagine being chosen as the outcomes.
Materials and methods
Obtaining data sources, select genetic tools closely related to aspartate or asparagine.
In this research, publicly accessible GWAS summary statistical datasets from the MR basic platform were utilized. These datasets consisted of 7721 individuals of European ancestry [ 24 ] for the exposure phenotype instrumental variable of aspartate, and 7761 individuals of European ancestry [ 24 ] for the exposure phenotype instrumental variable of asparagine.
Select genetic tools closely related to schizophrenia
Data from the MR basic platform was used in this study for GWAS summary statistics, which included 77,096 individuals of European ancestry [ 5 ], as instrumental variables related to schizophrenia exposure phenotype.
Obtaining result data
The publicly accessible GWAS summary statistical dataset for schizophrenia was utilized on the MR basic platform, with a sample size of 77096. Additionally, the summary level data for aspartate and asparagine were obtained from the publicly available GWAS summary dataset on the MR basic platform, with sample sizes of 7721 and 7761, respectively, serving as outcome variables.
Instrumental variable filtering
Eliminating linkage disequilibrium.
The selection criteria for identifying exposure related SNPs from the aggregated data of GWAS include: (1) Reaching a significance level that meets the threshold for whole genome research, expressed as P -value < 5 * 10 − 6 [ 25 ]; (2) Ensure the independence of the selected SNPs and eliminate linkage disequilibrium SNPs ( r 2 < 0.001, window size of 10000KB) [ 26 ]; (3) There are corresponding data related to the research results in the GWAS summary data.
Eliminating weak instruments
To evaluate whether the instrumental variables selected for this MR study have weak values, we calculated the F-statistic. If the F-value is greater than 10, it indicates that there are no weak instruments in this study, indicating the reliability of the study. Using the formula F =[(N-K-1)/K] × [R 2 /(1-R 2 )], where N denotes the sample size pertaining to the exposure factor, K signifies the count of instrumental variables, and R 2 denotes the proportion of variations in the exposure factor that can be elucidated by the instrumental variables.
The final instrumental variable obtained
As a result of removing linkage disequilibrium and weak instrumental variables, finally, 3 SNPs related to aspartate and 24 SNPs related to asparagine were selected for MR analysis.
Bidirectional MR analysis
Research design.
Figure 1 presents a comprehensive depiction of the overarching design employed in the MR analysis undertaken in this study. We ascertained SNPs exhibiting robust correlation with the target exposure through analysis of publicly available published data, subsequently investigating the existence of a causal association between these SNPs and the corresponding outcomes. This study conducted two bidirectional MR analyses, one prospective and reverse MR on the causal relationship between aspartate and schizophrenia, and the other prospective and reverse MR on the causal relationship between asparagine and schizophrenia.
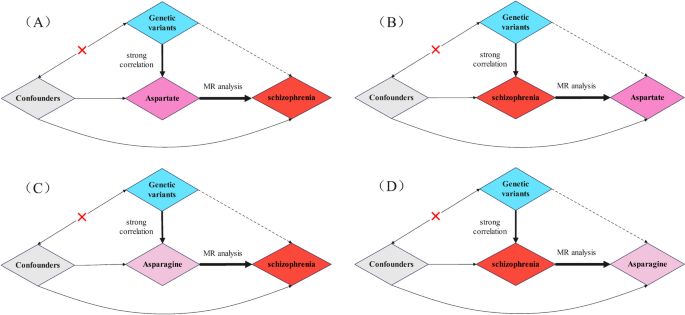
A MR analysis of aspartate and schizophrenia (located in the upper left corner). B MR analysis of schizophrenia and aspartate (located in the upper right corner). C MR analysis of asparagine and schizophrenia (located in the lower left corner). D MR analysis of schizophrenia and asparagine (located in the lower right corner)
Statistical analysis
Weighted median, weighted mode, MR Egger, and inverse variance weighting (IVW) were used to conduct a MR study. The primary research findings were derived from the results obtained through IVW, the results of sensitivity analysis using other methods to estimate causal effects are considered. Statistical significance was determined if the P -value was less than 0.05. To enhance the interpretation of the findings, this study converted the beta values obtained in to OR, accompanied by the calculation of a 95% confidence interval (CI).
Test for directional horizontal pleiotropy
This study used MR Egger intercept to test horizontal pleiotropy. If the P -value is greater than 0.05, it indicates that there is no horizontal pleiotropy in this study, meaning that instrumental variables can only regulate outcome variables through exposure factors.
Results of bidirectional MR analysis of aspartate and schizophrenia
Analysis results of aspartate and schizophrenia.
In prospective MR analysis, this study set aspartate as the exposure factor and schizophrenia as the outcome. We used 3 SNPs significantly associated with aspartate screened across the entire genome. The instrumental variables exhibited F-values exceeding 10, signifying the absence of weak instruments and thereby affirming the robustness of our findings. Through MR analysis (Fig. 2 A), we assessed the individual influence of each SNP locus on schizophrenia. The results of the IVW method indicate that no causal effect was found between aspartate and schizophrenia, with an OR of 1.221 (95%CI: 0.483–3.088, P -value = 0.674).
In addition, the analyses conducted using the weighted mode and weighted median methods yielded similar results, indicating the absence of a causal association between aspartate and schizophrenia. Furthermore, the MR Egger analysis demonstrated no statistically significant disparity in effectiveness between aspartate and schizophrenia, as evidenced by a P -value greater than 0.05 (Table 1 ; Fig. 2 B).
In order to test the reliability of the research results, this study used MR Egger intercept analysis to examine horizontal pleiotropy, and the result was P -value = 0.579 > 0.05, indicating the absence of level pleiotropy. Furthermore, a leave-one-out test was conducted to demonstrate that no single SNP had a substantial impact on the stability of the results, indicating that this study has considerable stability (Fig. 2 C). Accordingly, the MR analysis results demonstrate the conclusion that aspartate and schizophrenia do not exhibit a causal relationship.
Analysis results of schizophrenia and aspartate
Different from prospective MR studies, in reverse MR studies, schizophrenia was set as an exposure factor and aspartate was set as the outcome. Through MR analysis (Fig. 2 D), we assessed the individual influence of each SNP locus on aspartate .The results of the IVW method indicate that there is no causal effect between schizophrenia and aspartate, with an OR of 0.999(95%CI: 0.987–1.010, P -value = 0.841). Similarly, the weighted mode, weighted median methods also failed to demonstrate a causal link between schizophrenia and aspartate. Additionally, the MR Egger analysis did not reveal any statistically significant difference in effectiveness between schizophrenia and aspartate ( P -value > 0.05) (Table 1 and Fig . 2 E).
The MR Egger intercept was used to test horizontal pleiotropy, and the result was P -value = 0.226 > 0.05, proving that this study is not affected by horizontal pleiotropy. Furthermore, a leave-one-out test revealed that no individual SNP significantly influenced the robustness of the findings (Fig. 2 F).
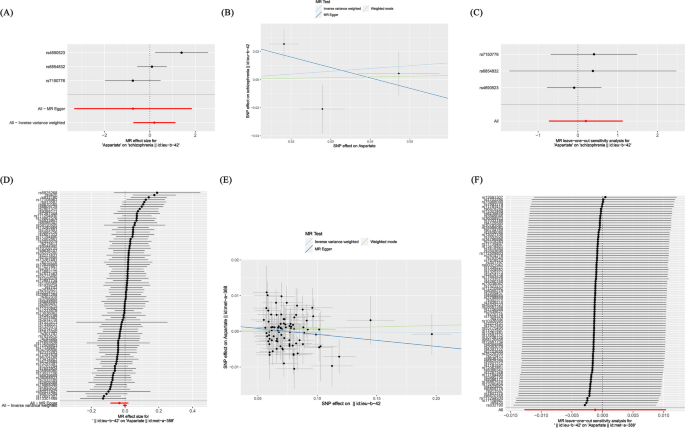
Depicts the causal association between aspartate and schizophrenia through diverse statistical analyses, as well as the causal association between schizophrenia and aspartate through diverse statistical analyses. A The forest plot of aspartate related SNPs and schizophrenia analysis results, with the red line showing the MR Egger test and IVW method. B Scatter plot of the analysis results of aspartate and schizophrenia, with the slope indicating the strength of the causal relationship. C Leave-one-out test of research results on aspartate and schizophrenia. D The forest plot of schizophrenia related SNPs and aspartate analysis results, with the red line showing the MR Egger test and IVW method. E Scatter plot of the analysis results of schizophrenia and aspartate, with the slope indicating the strength of the causal relationship. F Leave-one-out test of research results on schizophrenia and aspartate
Results of bidirectional MR analysis of asparagine and schizophrenia
Analysis results of asparagine and schizophrenia.
In prospective MR studies, we used asparagine as an exposure factor and schizophrenia as a result to investigate the potential causal relationship between them. Through a rigorous screening process, we identified 24 genome-wide significant SNPs associated with asparagine. In addition, the instrumental variable F values all exceeded 10, indicating that this study was not affected by weak instruments, thus proving the stability of the results. This study conducted MR analysis to evaluate the impact of all SNP loci on schizophrenia. (Fig. 3 A). According to the results of IVW, a causal relationship was found between asparagine and schizophrenia, and the relationship is negatively correlated, with an OR of 0.485 (95%CI: 0.262-0.900, P -value = 0.020).
The weighted median results also showed a causal relationship between asparagine and schizophrenia, and it was negatively correlated. In the weighted mode method, asparagine and schizophrenia did not have a causal relationship, while in the MR Egger method, there was no statistically significant difference in efficacy between them ( P -value > 0.05) (Table 1 ; Fig. 3 B).
In order to examine the horizontal pleiotropy, the MR Egger intercept was applied, and P -value = 0.768 > 0.05 result proves that this study is not affected by horizontal pleiotropy Furthermore, a leave-one-out test was conducted to demonstrate that no individual SNP had a substantial impact on the stability of the results, indicating that this study has good stability. (Fig. 3 C). Therefore, MR analysis shows that asparagine is inversely proportional to schizophrenia.
Analysis results of schizophrenia and asparagine
In reverse MR analysis, schizophrenia is considered an exposure factor, and asparagine is considered the result, studying the causal effects of schizophrenia and asparagine. Through MR analysis (Fig. 3 D), we assessed the individual influence of each SNP locus on s asparagine. The IVW method results indicated no potential causal relationship between schizophrenia and asparagine, with an OR of 1.005(95%CI: 0.999–1.011, P -value = 0.132). The research results of weighted mode method and weighted median method did not find a causal effects of schizophrenia and asparagine. Additionally, the MR Egger analysis did not reveal any statistically significant difference in effectiveness between schizophrenia and asparagine ( P -value > 0.05) (Table 1 ; Fig. 3 E).
In order to examine the horizontal pleiotropy, the MR Egger intercept was applied, and the result was P -value = 0.474 > 0.05, proving that this study is not affected by horizontal pleiotropy. Furthermore, a leave-one-out test was conducted to demonstrate that no individual SNP had a substantial impact on the stability of the results, indicating that this study has good stability (Fig. 3 F).
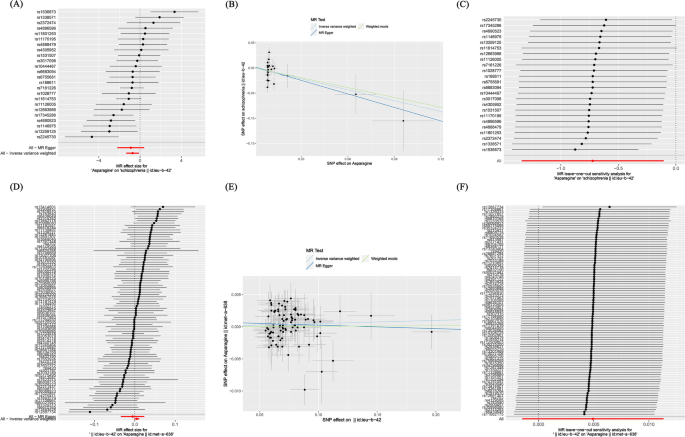
Depicts the causal association between asparagine and schizophrenia through diverse statistical analyses, as well as the causal association between schizophrenia and asparagine through diverse statistical analyses. A The forest plot of asparagine related SNPs and schizophrenia analysis results, with the red line showing the MR Egger test and IVW method. B Scatter plot of the analysis results of asparagine and schizophrenia, with the slope indicating the strength of the causal relationship. C Leave-one-out test of research results on asparagine and schizophrenia. D The forest plot of schizophrenia related SNPs and asparagine analysis results, with the red line showing the MR Egger test and IVW method. E Scatter plot of the analysis results of schizophrenia and asparagine, with the slope indicating the strength of the causal relationship. F Leave-one-out test of research results on schizophrenia and asparagine
In this study, the MR analysis results after sensitivity analysis suggested a causal relationship between asparagine and schizophrenia, which was negatively correlated. However, the reverse MR analysis did not reveal any potential relationship between schizophrenia and asparagine, no potential causal relationship between aspartate and schizophrenia was found in both prospective and reverse MR analyses (Fig. 4 ).
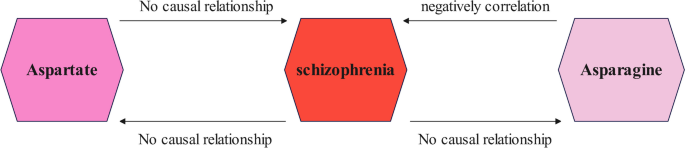
Summary of results from bidirectional two-sample MR study
The levels of asparagine in schizophrenia patients decrease, according to studies [ 16 ]. Based on the findings of the Madis Parksepp research team, a continuous five-year administration of antipsychotic drugs (AP) has been observed to induce significant metabolic changes in individuals diagnosed with schizophrenia. Significantly, the concentrations of asparagine, glutamine (Gln), methionine, ornithine, and taurine have experienced a substantial rise, whereas aspartate, glutamate (Glu), and alpha-aminoadipic acid(α-AAA) levels have demonstrated a notable decline. Olanzapine (OLZ) treatment resulted in significantly lower levels of Asn compared to control mice [ 27 ]. Asn and Asp play significant roles in various biological processes within the human body, such as participating in glycoprotein synthesis and contributing to brain functionality. It is worth noting that the ammonia produced in brain tissue needs to have a rapid excretion pathway in the brain. Asn plays a crucial role in regulating cellular function within neural tissues through metabolic control. This amino acid is synthesized by the combination of Asp and ammonia, facilitated by the enzyme asparagine synthase. Additionally, the brain effectively manages ammonia elimination by producing glutamine Gln and Asn. This may be an explanation for the significant increase in Asn and Gln levels (as well as a decrease in Asp and Glu levels) during 5 years of illness and after receiving AP treatment [ 28 ]. The study by Marie Luise Rao’s team compared unmedicated schizophrenic patients, healthy individuals and patients receiving antipsychotic treatment. Unmedicated schizophrenics had higher levels of asparagine, citrulline, phenylalanine, and cysteine, while the ratios of tyrosine, tryptophan, and tryptophan to competing amino acids were significantly lower than in healthy individuals [ 29 ].
The findings of our study demonstrate an inverse association between asparagine levels and the susceptibility to schizophrenia, suggesting that asparagine may serve as a protective factor against the development of this psychiatric disorder. However, we did not find a causal relationship between schizophrenia and asparagine. Consequently, additional investigation and scholarly discourse are warranted to gain a comprehensive understanding of this complex association.
Two different autopsy studies measured D-ASP levels in two different brain samples from patients with schizophrenia and a control group [ 14 ]. The first study, which utilized a limited sample size (7–10 subjects per diagnosis), demonstrated a reduction in D-ASP levels within the prefrontal cortex (PFC) postmortem among individuals diagnosed with schizophrenia, amounting to approximately 101%. This decrease was found to be correlated with a notable elevation in D-aspartate oxidase (DDO) mRNA levels within the same cerebral region [ 30 ]. In addition, the second study was conducted on a large sample size (20 subjects/diagnosis/brain regions). The findings of this study indicated a noteworthy decrease of approximately 30% in D-ASP selectivity within the dorsal lateral PFC (DLPFC) of individuals diagnosed with schizophrenia, when compared to corresponding brain regions of individuals without schizophrenia. However, no significant reduction in D-ASP was observed in the hippocampus of patients with schizophrenia. The decrease in D-Asp content was associated with a significant increase (about 25%) in DDO enzyme activity in the DLPFC of schizophrenia patients. This observation highlights the existence of a dysfunctional metabolic process in DDO activity levels in the brains of schizophrenia patients [ 31 ].
Numerous preclinical investigations have demonstrated the influence of D-Asp on various phenotypes reliant on NMDAR, which are linked to schizophrenia. After administering D-Asp to D-Asp oxidase gene knockout mice, the abnormal neuronal pre-pulse inhibition induced by psychoactive drugs such as MK-801 and amphetamine was significantly reduced by the sustained increase in D-Asp [ 32 ]. According to a review, free amino acids, specifically D-Asp and D-Ser (D-serine), have been identified as highly effective and safe nutrients for promoting mental well-being. These amino acids not only serve as integral components of the central nervous system’s structural proteins, but also play a vital role in maintaining optimal functioning of the central nervous system. This is due to their essential role in regulating neurotransmitter levels, including dopamine, norepinephrine, serotonin, and others. For many patients with schizophrenia, a most persistent and effective improvement therapy may be supplementing amino acids, which can improve the expected therapeutic effect of AP and alleviate positive and negative symptoms of schizophrenia [ 33 ].
Numerous studies have demonstrated a plausible correlation between aspartate and schizophrenia; however, our prospective and reverse MR investigations have failed to establish a causal link between aspartate and schizophrenia. This discrepancy may be attributed to the indirect influence of aspartate on the central nervous system through the stimulation of NMDAR, necessitating further investigation to elucidate the direct relationship between aspartate and schizophrenia.
This study used a bidirectional two-sample MR analysis method to explore the causal relationship between aspartate and asparagine with schizophrenia, as well as its inverse relationship [ 34 ]. The utilization of MR analysis presents numerous benefits in the determination of causality [ 35 ]. Notably, the random allocation of alleles to gametes within this method permits the assumption of no correlation between instrumental variables and confounding factors. Consequently, this approach effectively alleviates bias stemming from confounding factors during the inference of causality. Furthermore, the study’s utilization of a substantial sample size in the GWAS summary data engenders a heightened level of confidence in the obtained results [ 36 ]. Consequently, this investigation not only advances the existing body of research on the relationship between aspartate and asparagine with schizophrenia, but also contributed to clinical treatment decisions for patients with schizophrenia.
Nevertheless, this study possesses certain limitations, as it solely relies on populations of European ancestry for both exposure and results. Consequently, it remains uncertain whether these findings can be replicated among non-European races, necessitating further investigation. In addition, in this study, whether the effects of aspartate and asparagine on schizophrenia vary by gender or age cannot be evaluated, and stratified MR analysis should be performed. Additional experimental research is imperative for a comprehensive understanding of the underlying biological mechanisms connecting aspartate and asparagine with schizophrenia.
In summary, our MR analysis found a negative correlation between asparagine and schizophrenia, indicating that asparagine reduces the risk of schizophrenia. However, there is no potential causal relationship between schizophrenia and asparagine. This study provides new ideas for the early detection of schizophrenia in the clinical setting and offers new insights into the etiology and pathogenesis of schizophrenia. Nonetheless, additional research is required to elucidate the potential mechanisms that underlie the association between aspartate and asparagine with schizophrenia.
Availability of data and materials
The datasets generated and analysed during the current study are available in the GWAS repository. https://gwas.mrcieu.ac.uk/datasets/met-a-388/ , https://gwas.mrcieu.ac.uk/datasets/met-a-638/ , https://gwas.mrcieu.ac.uk/datasets/ieu-b-42/ .
Charlson FJ, Ferrari AJ, Santomauro DF, Diminic S, Stockings E, Scott JG, McGrath JJ, Whiteford HA. Global epidemiology and burden of schizophrenia: findings from the global burden of disease study 2016. Schizophr Bull. 2018;44(6):1195–203.
Article PubMed PubMed Central Google Scholar
Rehm J, Shield KD. Global burden of disease and the impact of mental and addictive disorders. Curr Psychiatry Rep. 2019;21(2):10.
Article PubMed Google Scholar
Vita A, Minelli A, Barlati S, Deste G, Giacopuzzi E, Valsecchi P, Turrina C, Gennarelli M. Treatment-resistant Schizophrenia: genetic and neuroimaging correlates. Front Pharmacol. 2019;10:402.
Article CAS PubMed PubMed Central Google Scholar
Adell A. Brain NMDA receptors in schizophrenia and depression. Biomolecules. 2020;10(6):947.
Biological insights from 108 schizophrenia-associated genetic loci. Nature. 2014;511(7510):421–7.
Abdulbagi M, Wang L, Siddig O, Di B, Li B. D-Amino acids and D-amino acid-containing peptides: potential disease biomarkers and therapeutic targets? Biomolecules. 2021;11(11):1716.
Krashia P, Ledonne A, Nobili A, Cordella A, Errico F, Usiello A, D’Amelio M, Mercuri NB, Guatteo E, Carunchio I. Persistent elevation of D-Aspartate enhances NMDA receptor-mediated responses in mouse substantia Nigra pars compacta dopamine neurons. Neuropharmacology. 2016;103:69–78.
Article CAS PubMed Google Scholar
Kantrowitz JT, Epstein ML, Lee M, Lehrfeld N, Nolan KA, Shope C, Petkova E, Silipo G, Javitt DC. Improvement in mismatch negativity generation during d-serine treatment in schizophrenia: correlation with symptoms. Schizophr Res. 2018;191:70–9.
Elkis H, Buckley PF. Treatment-resistant Schizophrenia. Psychiatr Clin North Am. 2016;39(2):239–65.
Dunlop DS, Neidle A, McHale D, Dunlop DM, Lajtha A. The presence of free D-aspartic acid in rodents and man. Biochem Biophys Res Commun. 1986;141(1):27–32.
de Bartolomeis A, Vellucci L, Austin MC, De Simone G, Barone A. Rational and translational implications of D-Amino acids for treatment-resistant Schizophrenia: from neurobiology to the clinics. Biomolecules. 2022;12(7):909.
Taniguchi K, Sawamura H, Ikeda Y, Tsuji A, Kitagishi Y, Matsuda S. D-amino acids as a biomarker in schizophrenia. Diseases. 2022;10(1):9.
Singh SP, Singh V. Meta-analysis of the efficacy of adjunctive NMDA receptor modulators in chronic schizophrenia. CNS Drugs. 2011;25(10):859–85.
Errico F, Napolitano F, Squillace M, Vitucci D, Blasi G, de Bartolomeis A, Bertolino A, D’Aniello A, Usiello A. Decreased levels of D-aspartate and NMDA in the prefrontal cortex and striatum of patients with schizophrenia. J Psychiatr Res. 2013;47(10):1432–7.
Rousseau J, Gagné V, Labuda M, Beaubois C, Sinnett D, Laverdière C, Moghrabi A, Sallan SE, Silverman LB, Neuberg D, et al. ATF5 polymorphisms influence ATF function and response to treatment in children with childhood acute lymphoblastic leukemia. Blood. 2011;118(22):5883–90.
Liu L, Zhao J, Chen Y, Feng R. Metabolomics strategy assisted by transcriptomics analysis to identify biomarkers associated with schizophrenia. Anal Chim Acta. 2020;1140:18–29.
Cao B, Wang D, Brietzke E, McIntyre RS, Pan Z, Cha D, Rosenblat JD, Zuckerman H, Liu Y, Xie Q, et al. Characterizing amino-acid biosignatures amongst individuals with schizophrenia: a case-control study. Amino Acids. 2018;50(8):1013–23.
Olthof BMJ, Gartside SE, Rees A. Puncta of neuronal nitric oxide synthase (nNOS) mediate NMDA receptor signaling in the Auditory Midbrain. J Neuroscience: Official J Soc Neurosci. 2019;39(5):876–87.
Article CAS Google Scholar
Tortorella A, Monteleone P, Fabrazzo M, Viggiano A, De Luca L, Maj M. Plasma concentrations of amino acids in chronic schizophrenics treated with clozapine. Neuropsychobiology. 2001;44(4):167–71.
Rao ML, Strebel B, Gross G, Huber G. Serum amino acid profiles and dopamine in schizophrenic patients and healthy subjects: window to the brain? Amino Acids. 1992;2(1–2):111–8.
Davey Smith G, Ebrahim S. What can mendelian randomisation tell us about modifiable behavioural and environmental exposures? BMJ (Clinical Res ed). 2005;330(7499):1076–9.
Article Google Scholar
Freuer D, Meisinger C. Causal link between thyroid function and schizophrenia: a two-sample mendelian randomization study. Eur J Epidemiol. 2023;38(10):1081–8.
Papiol S, Schmitt A, Maurus I, Rossner MJ, Schulze TG, Falkai P. Association between Physical Activity and Schizophrenia: results of a 2-Sample mendelian randomization analysis. JAMA Psychiat. 2021;78(4):441–4.
Shin SY, Fauman EB, Petersen AK, Krumsiek J, Santos R, Huang J, Arnold M, Erte I, Forgetta V, Yang TP, et al. An atlas of genetic influences on human blood metabolites. Nat Genet. 2014;46(6):543–50.
Zhao JV, Kwok MK, Schooling CM. Effect of glutamate and aspartate on ischemic heart disease, blood pressure, and diabetes: a mendelian randomization study. Am J Clin Nutr. 2019;109(4):1197–206.
Zhou K, Zhu L, Chen N, Huang G, Feng G, Wu Q, Wei X, Gou X. Causal associations between schizophrenia and cancers risk: a mendelian randomization study. Front Oncol. 2023;13:1258015.
Zapata RC, Rosenthal SB, Fisch K, Dao K, Jain M, Osborn O. Metabolomic profiles associated with a mouse model of antipsychotic-induced food intake and weight gain. Sci Rep. 2020;10(1):18581.
Parksepp M, Leppik L, Koch K, Uppin K, Kangro R, Haring L, Vasar E, Zilmer M. Metabolomics approach revealed robust changes in amino acid and biogenic amine signatures in patients with schizophrenia in the early course of the disease. Sci Rep. 2020;10(1):13983.
Rao ML, Gross G, Strebel B, Bräunig P, Huber G, Klosterkötter J. Serum amino acids, central monoamines, and hormones in drug-naive, drug-free, and neuroleptic-treated schizophrenic patients and healthy subjects. Psychiatry Res. 1990;34(3):243–57.
Errico F, D’Argenio V, Sforazzini F, Iasevoli F, Squillace M, Guerri G, Napolitano F, Angrisano T, Di Maio A, Keller S, et al. A role for D-aspartate oxidase in schizophrenia and in schizophrenia-related symptoms induced by phencyclidine in mice. Transl Psychiatry. 2015;5(2):e512.
Nuzzo T, Sacchi S, Errico F, Keller S, Palumbo O, Florio E, Punzo D, Napolitano F, Copetti M, Carella M, et al. Decreased free d-aspartate levels are linked to enhanced d-aspartate oxidase activity in the dorsolateral prefrontal cortex of schizophrenia patients. NPJ Schizophr. 2017;3:16.
Errico F, Rossi S, Napolitano F, Catuogno V, Topo E, Fisone G, D’Aniello A, Centonze D, Usiello A. D-aspartate prevents corticostriatal long-term depression and attenuates schizophrenia-like symptoms induced by amphetamine and MK-801. J Neurosci. 2008;28(41):10404–14.
Nasyrova RF, Khasanova AK, Altynbekov KS, Asadullin AR, Markina EA, Gayduk AJ, Shipulin GA, Petrova MM, Shnayder NA. The role of D-Serine and D-aspartate in the pathogenesis and therapy of treatment-resistant schizophrenia. Nutrients. 2022;14(23):5142.
Hao D, Liu C. Deepening insights into food and medicine continuum within the context of pharmacophylogeny. Chin Herb Med. 2023;15(1):1–2.
PubMed Google Scholar
Zhang Y. Awareness and ability of paradigm shift are needed for research on dominant diseases of TCM. Chin Herb Med. 2023;15(4):475.
CAS PubMed PubMed Central Google Scholar
Chen J. Essential role of medicine and food homology in health and wellness. Chin Herb Med. 2023;15(3):347–8.
PubMed PubMed Central Google Scholar
Download references
This work was supported by the National Natural Science Foundation of China (82271546, 82301725, 81971601); National Key Research and Development Program of China (2023YFC2506201); Key Project of Science and Technology Innovation 2030 of China (2021ZD0201800, 2021ZD0201805); China Postdoctoral Science Foundation (2023M732155); Fundamental Research Program of Shanxi Province (202203021211018, 202203021212028, 202203021212038). Research Project Supported by Shanxi Scholarship Council of China (2022 − 190); Scientific Research Plan of Shanxi Health Commission (2020081, 2020SYS03,2021RC24); Shanxi Provincial Administration of Traditional Chinese Medicine (2023ZYYC2034), Scientific and Technological Innovation Programs of Higher Education Institutions in Shanxi (2022L132); Shanxi Medical University School-level Doctoral Initiation Fund Project (XD2102); Youth Project of First Hospital of Shanxi Medical University (YQ2203); Doctor Fund Project of Shanxi Medical University in Shanxi Province (SD2216); Shanxi Science and Technology Innovation Talent Team (202304051001049); 136 Medical Rejuvenation Project of Shanxi Province, China; STI2030-Major Projects-2021ZD0200700. Key laboratory of Health Commission of Shanxi Province (2020SYS03);
Author information
Huang-Hui Liu and Yao Gao contributed equally to this work.
Authors and Affiliations
Department of Psychiatry, First Hospital/First Clinical Medical College of Shanxi Medical University, NO.85 Jiefang Nan Road, Taiyuan, China
Huang-Hui Liu, Yao Gao, Dan Xu, Xin-Zhe Du, Si-Meng Wei, Jian-Zhen Hu, Yong Xu & Liu Sha
Shanxi Key Laboratory of Artificial Intelligence Assisted Diagnosis and Treatment for Mental Disorder, First Hospital of Shanxi Medical University, Taiyuan, China
You can also search for this author in PubMed Google Scholar
Contributions
Huang-Hui Liu and Yao Gao provided the concept and designed the study. Huang-Hui Liu and Yao Gao conducted the analyses and wrote the manuscript. Dan Xu, Huang-Hui Liu and Yao Gao participated in data collection. Xin-Zhe Du, Si-Meng Wei and Jian-Zhen Hu participated in the analysis of the data. Liu Sha, Yong Xu and Yao Gao revised and proof-read the manuscript. All authors contributed to the article and approved the submitted version.
Corresponding authors
Correspondence to Yong Xu or Liu Sha .
Ethics declarations
Ethics approval and consent to participate.
Not applicable’ for that section.
Consent for publication
Competing interests.
The authors declare no competing interests.
Additional information
Publisher’s note.
Springer Nature remains neutral with regard to jurisdictional claims in published maps and institutional affiliations.
Rights and permissions
Open Access This article is licensed under a Creative Commons Attribution 4.0 International License, which permits use, sharing, adaptation, distribution and reproduction in any medium or format, as long as you give appropriate credit to the original author(s) and the source, provide a link to the Creative Commons licence, and indicate if changes were made. The images or other third party material in this article are included in the article's Creative Commons licence, unless indicated otherwise in a credit line to the material. If material is not included in the article's Creative Commons licence and your intended use is not permitted by statutory regulation or exceeds the permitted use, you will need to obtain permission directly from the copyright holder. To view a copy of this licence, visit http://creativecommons.org/licenses/by/4.0/ . The Creative Commons Public Domain Dedication waiver ( http://creativecommons.org/publicdomain/zero/1.0/ ) applies to the data made available in this article, unless otherwise stated in a credit line to the data.
Reprints and permissions
About this article
Cite this article.
Liu, HH., Gao, Y., Xu, D. et al. Asparagine reduces the risk of schizophrenia: a bidirectional two-sample mendelian randomization study of aspartate, asparagine and schizophrenia. BMC Psychiatry 24 , 299 (2024). https://doi.org/10.1186/s12888-024-05765-5
Download citation
Received : 20 February 2024
Accepted : 15 April 2024
Published : 19 April 2024
DOI : https://doi.org/10.1186/s12888-024-05765-5
Share this article
Anyone you share the following link with will be able to read this content:
Sorry, a shareable link is not currently available for this article.
Provided by the Springer Nature SharedIt content-sharing initiative
- Schizophrenia
- Mendelian randomization
BMC Psychiatry
ISSN: 1471-244X
- Submission enquiries: [email protected]
- General enquiries: [email protected]

IMAGES
VIDEO
COMMENTS
Sampling methods are crucial for conducting reliable research. In this article, you will learn about the types, techniques and examples of sampling methods, and how to choose the best one for your study. Scribbr also offers free tools and guides for other aspects of academic writing, such as citation, bibliography, and fallacy.
Simple random sampling. Simple random sampling involves selecting participants in a completely random fashion, where each participant has an equal chance of being selected.Basically, this sampling method is the equivalent of pulling names out of a hat, except that you can do it digitally.For example, if you had a list of 500 people, you could use a random number generator to draw a list of 50 ...
Probability sampling methods. Probability sampling means that every member of the population has a chance of being selected. It is mainly used in quantitative research. If you want to produce results that are representative of the whole population, probability sampling techniques are the most valid choice.
Researchers do need to be mindful of carefully considering the strengths and limitations of each method before selecting a sampling technique. Non-probability sampling is best for exploratory research, such as at the beginning of a research project. There are five main types of non-probability sampling methods: Convenience sampling. Purposive ...
Sampling methods in psychology refer to strategies used to select a subset of individuals (a sample) from a larger population, to study and draw inferences about the entire population. Common methods include random sampling, stratified sampling, cluster sampling, and convenience sampling. Proper sampling ensures representative, generalizable, and valid research results.
For example, a population with large ethnic subgroups could best be studied using a stratified sampling method. Random sampling. Finally, the best sampling method is always the one that could best answer our research question while also allowing for others to make use of our results (generalisability of results). When we cannot afford a random ...
Understand sampling methods in research, from simple random sampling to stratified, systematic, and cluster sampling. Learn how these sampling techniques boost data accuracy and representation, ensuring robust, reliable results. Check this article to learn about the different sampling method techniques, types and examples.
Abstract. Knowledge of sampling methods is essential to design quality research. Critical questions are provided to help researchers choose a sampling method. This article reviews probability and non-probability sampling methods, lists and defines specific sampling techniques, and provides pros and cons for consideration.
The importance of sampling extends to the ability to draw accurate inferences, and it is an integral part of qualitative guidelines across research methods. Sampling considerations are important in quantitative and qualitative research when considering a target population and when drawing a sample that will either allow us to generalize (i.e ...
The sampling method will depend on the research question. For instance, the researcher may want to understand an issue in greater detail for one particular population rather than worry about the ' generalizability' of these results. In such a scenario, the researcher may want to use ' purposive sampling' for the study. ...
Sampling methods refer to the techniques used to select a subset of individuals or units from a larger population for the purpose of conducting statistical analysis or research. Sampling is an essential part of the Research because it allows researchers to draw conclusions about a population without having to collect data from every member of ...
There are two major types of sampling methods: probability and non-probability sampling. Probability sampling, also known as random sampling, is a kind of sample selection where randomization is used instead of deliberate choice. Each member of the population has a known, non-zero chance of being selected.
A sample is the subset of the population that you actually measure, test, or evaluate and base your results. Sampling methods are how you obtain your sample. Before beginning your study, carefully define the population because your results apply to the target population. You can define your population as narrowly as necessary to meet the needs ...
Cluster sampling- she puts 50 into random groups of 5 so we get 10 groups then randomly selects 5 of them and interviews everyone in those groups --> 25 people are asked. 2. Stratified sampling- she puts 50 into categories: high achieving smart kids, decently achieving kids, mediumly achieving kids, lower poorer achieving kids and clueless ...
Non-probability sampling method. Convenience sampling. Although it is a non-probability sampling method, it is the most applicable and widely used method in clinical research. In this method, the investigators enroll subjects according to their availability and accessibility. Therefore, this method is quick, inexpensive, and convenient.
Sampling is the statistical process of selecting a subset—called a 'sample'—of a population of interest for the purpose of making observations and statistical inferences about that population. Social science research is generally about inferring patterns of behaviours within specific populations. We cannot study entire populations because of feasibility and cost constraints, and hence ...
Purposive sampling was the approach utilized to gather the sample data on this research. To be more precise, purposive sampling was used to choose the firms that are pertinent to the research [32 ...
Sampling can be defined as the process through which individuals or sampling units are selected from the sample frame. The sampling strategy needs to be specified in advance, given that the sampling method may affect the sample size estimation. 1,5 Without a rigorous sampling plan the estimates derived from the study may be biased (selection ...
Research methodology formats can vary depending on the specific requirements of the research project, but the following is a basic example of a structure for a research methodology section: ... To design the research: Research methodology helps in designing the research by selecting the appropriate research method, research design, and sampling ...
Cluster sampling (also known as one-stage cluster sampling) is a technique in which clusters of participants representing the population are identified and included in the sample[1]. This is a popular method in conducting marketing researches. The main aim of cluster sampling can be specified as ...
Pew Research Center has deep roots in U.S. public opinion research. Launched initially as a project focused primarily on U.S. policy and politics in the early 1990s, the Center has grown over time to study a wide range of topics vital to explaining America to itself and to the world.Our hallmarks: a rigorous approach to methodological quality, complete transparency as to our methods, and a ...
use of statistical sampling in research credit cases,1 the methodology set forth in IRM 42(18) has been approved by an outside expert on statistical sampling. Thus, the Service is confident that its sampling methodology set forth in the Internal Revenue 1 Although there is no case law addressing the validity of the Statistical Sampling
Soil certified reference material (CRM), UME EnvCRM 03 was produced by a collaborative approach among national metrology institutes, designated institutes and university research laboratories within the scope of the EMPIR project: Matrix Reference Materials for Environmental Analysis. This paper presents the sampling and processing methodology, homogeneity, stability, characterization campaign ...
Despite ongoing research, the underlying causes of schizophrenia remain unclear. Aspartate and asparagine, essential amino acids, have been linked to schizophrenia in recent studies, but their causal relationship is still unclear. This study used a bidirectional two-sample Mendelian randomization (MR) method to explore the causal relationship between aspartate and asparagine with schizophrenia.