We will keep fighting for all libraries - stand with us!

Internet Archive Audio
- This Just In
- Grateful Dead
- Old Time Radio
- 78 RPMs and Cylinder Recordings
- Audio Books & Poetry
- Computers, Technology and Science
- Music, Arts & Culture
- News & Public Affairs
- Spirituality & Religion
- Radio News Archive
- Flickr Commons
- Occupy Wall Street Flickr
- NASA Images
- Solar System Collection
- Ames Research Center
- All Software
- Old School Emulation
- MS-DOS Games
- Historical Software
- Classic PC Games
- Software Library
- Kodi Archive and Support File
- Vintage Software
- CD-ROM Software
- CD-ROM Software Library
- Software Sites
- Tucows Software Library
- Shareware CD-ROMs
- Software Capsules Compilation
- CD-ROM Images
- ZX Spectrum
- DOOM Level CD

- Smithsonian Libraries
- FEDLINK (US)
- Lincoln Collection
- American Libraries
- Canadian Libraries
- Universal Library
- Project Gutenberg
- Children's Library
- Biodiversity Heritage Library
- Books by Language
- Additional Collections
- Prelinger Archives
- Democracy Now!
- Occupy Wall Street
- TV NSA Clip Library
- Animation & Cartoons
- Arts & Music
- Computers & Technology
- Cultural & Academic Films
- Ephemeral Films
- Sports Videos
- Videogame Videos
- Youth Media
Search the history of over 866 billion web pages on the Internet.
Mobile Apps
- Wayback Machine (iOS)
- Wayback Machine (Android)
Browser Extensions
Archive-it subscription.
- Explore the Collections
- Build Collections
Save Page Now
Capture a web page as it appears now for use as a trusted citation in the future.
Please enter a valid web address
- Donate Donate icon An illustration of a heart shape
Practical data analysis : case studies in business statistics
Bookreader item preview, share or embed this item, flag this item for.
- Graphic Violence
- Explicit Sexual Content
- Hate Speech
- Misinformation/Disinformation
- Marketing/Phishing/Advertising
- Misleading/Inaccurate/Missing Metadata
plus-circle Add Review comment Reviews
209 Previews
3 Favorites
DOWNLOAD OPTIONS
No suitable files to display here.
PDF access not available for this item.
IN COLLECTIONS
Uploaded by station16.cebu on October 22, 2020
SIMILAR ITEMS (based on metadata)
10 Real World Data Science Case Studies Projects with Example
Top 10 Data Science Case Studies Projects with Examples and Solutions in Python to inspire your data science learning in 2023.
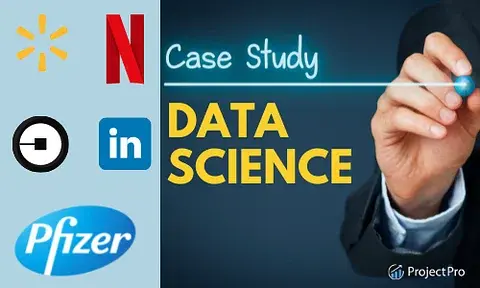
BelData science has been a trending buzzword in recent times. With wide applications in various sectors like healthcare , education, retail, transportation, media, and banking -data science applications are at the core of pretty much every industry out there. The possibilities are endless: analysis of frauds in the finance sector or the personalization of recommendations on eCommerce businesses. We have developed ten exciting data science case studies to explain how data science is leveraged across various industries to make smarter decisions and develop innovative personalized products tailored to specific customers.

Walmart Sales Forecasting Data Science Project
Downloadable solution code | Explanatory videos | Tech Support
Table of Contents
Data science case studies in retail , data science case study examples in entertainment industry , data analytics case study examples in travel industry , case studies for data analytics in social media , real world data science projects in healthcare, data analytics case studies in oil and gas, what is a case study in data science, how do you prepare a data science case study, 10 most interesting data science case studies with examples.
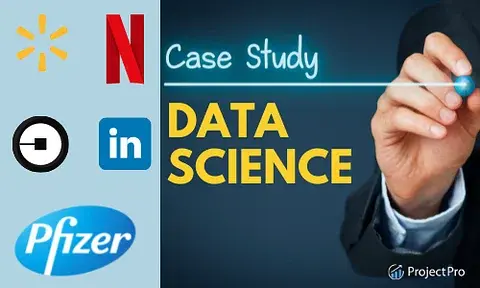
So, without much ado, let's get started with data science business case studies !
With humble beginnings as a simple discount retailer, today, Walmart operates in 10,500 stores and clubs in 24 countries and eCommerce websites, employing around 2.2 million people around the globe. For the fiscal year ended January 31, 2021, Walmart's total revenue was $559 billion showing a growth of $35 billion with the expansion of the eCommerce sector. Walmart is a data-driven company that works on the principle of 'Everyday low cost' for its consumers. To achieve this goal, they heavily depend on the advances of their data science and analytics department for research and development, also known as Walmart Labs. Walmart is home to the world's largest private cloud, which can manage 2.5 petabytes of data every hour! To analyze this humongous amount of data, Walmart has created 'Data Café,' a state-of-the-art analytics hub located within its Bentonville, Arkansas headquarters. The Walmart Labs team heavily invests in building and managing technologies like cloud, data, DevOps , infrastructure, and security.
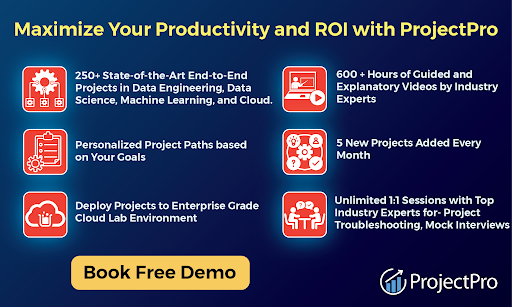
Walmart is experiencing massive digital growth as the world's largest retailer . Walmart has been leveraging Big data and advances in data science to build solutions to enhance, optimize and customize the shopping experience and serve their customers in a better way. At Walmart Labs, data scientists are focused on creating data-driven solutions that power the efficiency and effectiveness of complex supply chain management processes. Here are some of the applications of data science at Walmart:
i) Personalized Customer Shopping Experience
Walmart analyses customer preferences and shopping patterns to optimize the stocking and displaying of merchandise in their stores. Analysis of Big data also helps them understand new item sales, make decisions on discontinuing products, and the performance of brands.
ii) Order Sourcing and On-Time Delivery Promise
Millions of customers view items on Walmart.com, and Walmart provides each customer a real-time estimated delivery date for the items purchased. Walmart runs a backend algorithm that estimates this based on the distance between the customer and the fulfillment center, inventory levels, and shipping methods available. The supply chain management system determines the optimum fulfillment center based on distance and inventory levels for every order. It also has to decide on the shipping method to minimize transportation costs while meeting the promised delivery date.
Here's what valued users are saying about ProjectPro
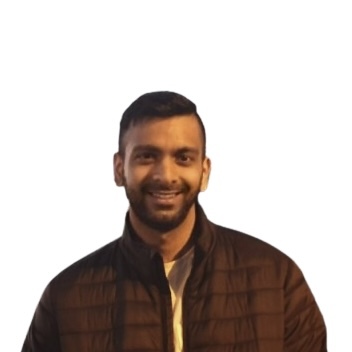
Gautam Vermani
Data Consultant at Confidential
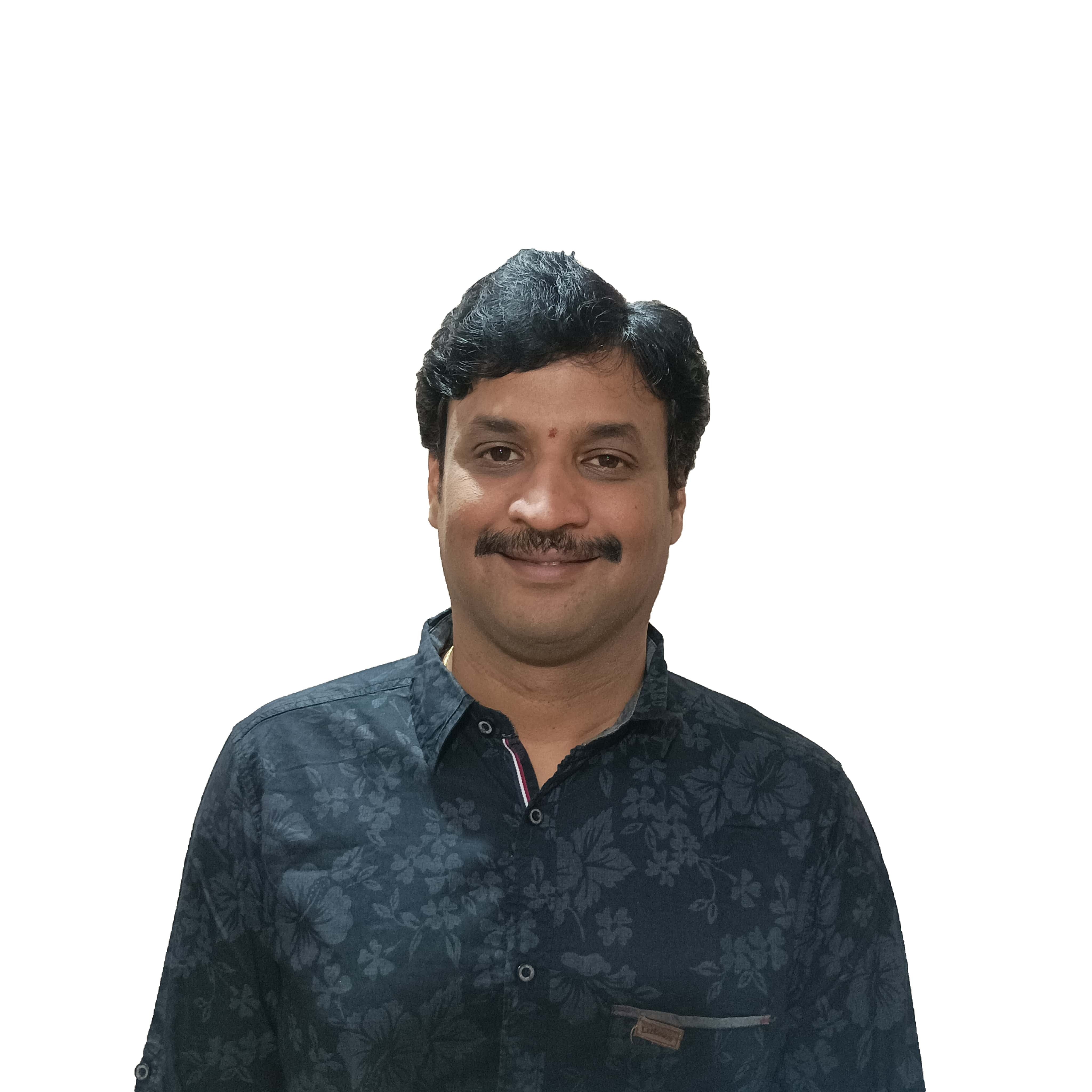
Anand Kumpatla
Sr Data Scientist @ Doubleslash Software Solutions Pvt Ltd
Not sure what you are looking for?
iii) Packing Optimization
Also known as Box recommendation is a daily occurrence in the shipping of items in retail and eCommerce business. When items of an order or multiple orders for the same customer are ready for packing, Walmart has developed a recommender system that picks the best-sized box which holds all the ordered items with the least in-box space wastage within a fixed amount of time. This Bin Packing problem is a classic NP-Hard problem familiar to data scientists .
Whenever items of an order or multiple orders placed by the same customer are picked from the shelf and are ready for packing, the box recommendation system determines the best-sized box to hold all the ordered items with a minimum of in-box space wasted. This problem is known as the Bin Packing Problem, another classic NP-Hard problem familiar to data scientists.
Here is a link to a sales prediction data science case study to help you understand the applications of Data Science in the real world. Walmart Sales Forecasting Project uses historical sales data for 45 Walmart stores located in different regions. Each store contains many departments, and you must build a model to project the sales for each department in each store. This data science case study aims to create a predictive model to predict the sales of each product. You can also try your hands-on Inventory Demand Forecasting Data Science Project to develop a machine learning model to forecast inventory demand accurately based on historical sales data.
Get Closer To Your Dream of Becoming a Data Scientist with 70+ Solved End-to-End ML Projects
Amazon is an American multinational technology-based company based in Seattle, USA. It started as an online bookseller, but today it focuses on eCommerce, cloud computing , digital streaming, and artificial intelligence . It hosts an estimate of 1,000,000,000 gigabytes of data across more than 1,400,000 servers. Through its constant innovation in data science and big data Amazon is always ahead in understanding its customers. Here are a few data analytics case study examples at Amazon:
i) Recommendation Systems
Data science models help amazon understand the customers' needs and recommend them to them before the customer searches for a product; this model uses collaborative filtering. Amazon uses 152 million customer purchases data to help users to decide on products to be purchased. The company generates 35% of its annual sales using the Recommendation based systems (RBS) method.
Here is a Recommender System Project to help you build a recommendation system using collaborative filtering.
ii) Retail Price Optimization
Amazon product prices are optimized based on a predictive model that determines the best price so that the users do not refuse to buy it based on price. The model carefully determines the optimal prices considering the customers' likelihood of purchasing the product and thinks the price will affect the customers' future buying patterns. Price for a product is determined according to your activity on the website, competitors' pricing, product availability, item preferences, order history, expected profit margin, and other factors.
Check Out this Retail Price Optimization Project to build a Dynamic Pricing Model.
iii) Fraud Detection
Being a significant eCommerce business, Amazon remains at high risk of retail fraud. As a preemptive measure, the company collects historical and real-time data for every order. It uses Machine learning algorithms to find transactions with a higher probability of being fraudulent. This proactive measure has helped the company restrict clients with an excessive number of returns of products.
You can look at this Credit Card Fraud Detection Project to implement a fraud detection model to classify fraudulent credit card transactions.
New Projects
Let us explore data analytics case study examples in the entertainment indusry.
Ace Your Next Job Interview with Mock Interviews from Experts to Improve Your Skills and Boost Confidence!
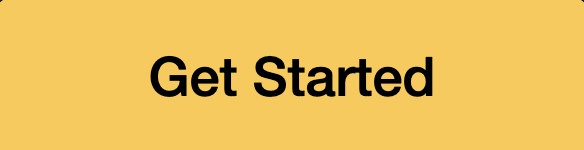
Netflix started as a DVD rental service in 1997 and then has expanded into the streaming business. Headquartered in Los Gatos, California, Netflix is the largest content streaming company in the world. Currently, Netflix has over 208 million paid subscribers worldwide, and with thousands of smart devices which are presently streaming supported, Netflix has around 3 billion hours watched every month. The secret to this massive growth and popularity of Netflix is its advanced use of data analytics and recommendation systems to provide personalized and relevant content recommendations to its users. The data is collected over 100 billion events every day. Here are a few examples of data analysis case studies applied at Netflix :
i) Personalized Recommendation System
Netflix uses over 1300 recommendation clusters based on consumer viewing preferences to provide a personalized experience. Some of the data that Netflix collects from its users include Viewing time, platform searches for keywords, Metadata related to content abandonment, such as content pause time, rewind, rewatched. Using this data, Netflix can predict what a viewer is likely to watch and give a personalized watchlist to a user. Some of the algorithms used by the Netflix recommendation system are Personalized video Ranking, Trending now ranker, and the Continue watching now ranker.
ii) Content Development using Data Analytics
Netflix uses data science to analyze the behavior and patterns of its user to recognize themes and categories that the masses prefer to watch. This data is used to produce shows like The umbrella academy, and Orange Is the New Black, and the Queen's Gambit. These shows seem like a huge risk but are significantly based on data analytics using parameters, which assured Netflix that they would succeed with its audience. Data analytics is helping Netflix come up with content that their viewers want to watch even before they know they want to watch it.
iii) Marketing Analytics for Campaigns
Netflix uses data analytics to find the right time to launch shows and ad campaigns to have maximum impact on the target audience. Marketing analytics helps come up with different trailers and thumbnails for other groups of viewers. For example, the House of Cards Season 5 trailer with a giant American flag was launched during the American presidential elections, as it would resonate well with the audience.
Here is a Customer Segmentation Project using association rule mining to understand the primary grouping of customers based on various parameters.
Get FREE Access to Machine Learning Example Codes for Data Cleaning , Data Munging, and Data Visualization
In a world where Purchasing music is a thing of the past and streaming music is a current trend, Spotify has emerged as one of the most popular streaming platforms. With 320 million monthly users, around 4 billion playlists, and approximately 2 million podcasts, Spotify leads the pack among well-known streaming platforms like Apple Music, Wynk, Songza, amazon music, etc. The success of Spotify has mainly depended on data analytics. By analyzing massive volumes of listener data, Spotify provides real-time and personalized services to its listeners. Most of Spotify's revenue comes from paid premium subscriptions. Here are some of the examples of case study on data analytics used by Spotify to provide enhanced services to its listeners:
i) Personalization of Content using Recommendation Systems
Spotify uses Bart or Bayesian Additive Regression Trees to generate music recommendations to its listeners in real-time. Bart ignores any song a user listens to for less than 30 seconds. The model is retrained every day to provide updated recommendations. A new Patent granted to Spotify for an AI application is used to identify a user's musical tastes based on audio signals, gender, age, accent to make better music recommendations.
Spotify creates daily playlists for its listeners, based on the taste profiles called 'Daily Mixes,' which have songs the user has added to their playlists or created by the artists that the user has included in their playlists. It also includes new artists and songs that the user might be unfamiliar with but might improve the playlist. Similar to it is the weekly 'Release Radar' playlists that have newly released artists' songs that the listener follows or has liked before.
ii) Targetted marketing through Customer Segmentation
With user data for enhancing personalized song recommendations, Spotify uses this massive dataset for targeted ad campaigns and personalized service recommendations for its users. Spotify uses ML models to analyze the listener's behavior and group them based on music preferences, age, gender, ethnicity, etc. These insights help them create ad campaigns for a specific target audience. One of their well-known ad campaigns was the meme-inspired ads for potential target customers, which was a huge success globally.
iii) CNN's for Classification of Songs and Audio Tracks
Spotify builds audio models to evaluate the songs and tracks, which helps develop better playlists and recommendations for its users. These allow Spotify to filter new tracks based on their lyrics and rhythms and recommend them to users like similar tracks ( collaborative filtering). Spotify also uses NLP ( Natural language processing) to scan articles and blogs to analyze the words used to describe songs and artists. These analytical insights can help group and identify similar artists and songs and leverage them to build playlists.
Here is a Music Recommender System Project for you to start learning. We have listed another music recommendations dataset for you to use for your projects: Dataset1 . You can use this dataset of Spotify metadata to classify songs based on artists, mood, liveliness. Plot histograms, heatmaps to get a better understanding of the dataset. Use classification algorithms like logistic regression, SVM, and Principal component analysis to generate valuable insights from the dataset.
Explore Categories
Below you will find case studies for data analytics in the travel and tourism industry.
Airbnb was born in 2007 in San Francisco and has since grown to 4 million Hosts and 5.6 million listings worldwide who have welcomed more than 1 billion guest arrivals in almost every country across the globe. Airbnb is active in every country on the planet except for Iran, Sudan, Syria, and North Korea. That is around 97.95% of the world. Using data as a voice of their customers, Airbnb uses the large volume of customer reviews, host inputs to understand trends across communities, rate user experiences, and uses these analytics to make informed decisions to build a better business model. The data scientists at Airbnb are developing exciting new solutions to boost the business and find the best mapping for its customers and hosts. Airbnb data servers serve approximately 10 million requests a day and process around one million search queries. Data is the voice of customers at AirBnB and offers personalized services by creating a perfect match between the guests and hosts for a supreme customer experience.
i) Recommendation Systems and Search Ranking Algorithms
Airbnb helps people find 'local experiences' in a place with the help of search algorithms that make searches and listings precise. Airbnb uses a 'listing quality score' to find homes based on the proximity to the searched location and uses previous guest reviews. Airbnb uses deep neural networks to build models that take the guest's earlier stays into account and area information to find a perfect match. The search algorithms are optimized based on guest and host preferences, rankings, pricing, and availability to understand users’ needs and provide the best match possible.
ii) Natural Language Processing for Review Analysis
Airbnb characterizes data as the voice of its customers. The customer and host reviews give a direct insight into the experience. The star ratings alone cannot be an excellent way to understand it quantitatively. Hence Airbnb uses natural language processing to understand reviews and the sentiments behind them. The NLP models are developed using Convolutional neural networks .
Practice this Sentiment Analysis Project for analyzing product reviews to understand the basic concepts of natural language processing.
iii) Smart Pricing using Predictive Analytics
The Airbnb hosts community uses the service as a supplementary income. The vacation homes and guest houses rented to customers provide for rising local community earnings as Airbnb guests stay 2.4 times longer and spend approximately 2.3 times the money compared to a hotel guest. The profits are a significant positive impact on the local neighborhood community. Airbnb uses predictive analytics to predict the prices of the listings and help the hosts set a competitive and optimal price. The overall profitability of the Airbnb host depends on factors like the time invested by the host and responsiveness to changing demands for different seasons. The factors that impact the real-time smart pricing are the location of the listing, proximity to transport options, season, and amenities available in the neighborhood of the listing.
Here is a Price Prediction Project to help you understand the concept of predictive analysis which is widely common in case studies for data analytics.
Uber is the biggest global taxi service provider. As of December 2018, Uber has 91 million monthly active consumers and 3.8 million drivers. Uber completes 14 million trips each day. Uber uses data analytics and big data-driven technologies to optimize their business processes and provide enhanced customer service. The Data Science team at uber has been exploring futuristic technologies to provide better service constantly. Machine learning and data analytics help Uber make data-driven decisions that enable benefits like ride-sharing, dynamic price surges, better customer support, and demand forecasting. Here are some of the real world data science projects used by uber:
i) Dynamic Pricing for Price Surges and Demand Forecasting
Uber prices change at peak hours based on demand. Uber uses surge pricing to encourage more cab drivers to sign up with the company, to meet the demand from the passengers. When the prices increase, the driver and the passenger are both informed about the surge in price. Uber uses a predictive model for price surging called the 'Geosurge' ( patented). It is based on the demand for the ride and the location.
ii) One-Click Chat
Uber has developed a Machine learning and natural language processing solution called one-click chat or OCC for coordination between drivers and users. This feature anticipates responses for commonly asked questions, making it easy for the drivers to respond to customer messages. Drivers can reply with the clock of just one button. One-Click chat is developed on Uber's machine learning platform Michelangelo to perform NLP on rider chat messages and generate appropriate responses to them.
iii) Customer Retention
Failure to meet the customer demand for cabs could lead to users opting for other services. Uber uses machine learning models to bridge this demand-supply gap. By using prediction models to predict the demand in any location, uber retains its customers. Uber also uses a tier-based reward system, which segments customers into different levels based on usage. The higher level the user achieves, the better are the perks. Uber also provides personalized destination suggestions based on the history of the user and their frequently traveled destinations.
You can take a look at this Python Chatbot Project and build a simple chatbot application to understand better the techniques used for natural language processing. You can also practice the working of a demand forecasting model with this project using time series analysis. You can look at this project which uses time series forecasting and clustering on a dataset containing geospatial data for forecasting customer demand for ola rides.
Explore More Data Science and Machine Learning Projects for Practice. Fast-Track Your Career Transition with ProjectPro
7) LinkedIn
LinkedIn is the largest professional social networking site with nearly 800 million members in more than 200 countries worldwide. Almost 40% of the users access LinkedIn daily, clocking around 1 billion interactions per month. The data science team at LinkedIn works with this massive pool of data to generate insights to build strategies, apply algorithms and statistical inferences to optimize engineering solutions, and help the company achieve its goals. Here are some of the real world data science projects at LinkedIn:
i) LinkedIn Recruiter Implement Search Algorithms and Recommendation Systems
LinkedIn Recruiter helps recruiters build and manage a talent pool to optimize the chances of hiring candidates successfully. This sophisticated product works on search and recommendation engines. The LinkedIn recruiter handles complex queries and filters on a constantly growing large dataset. The results delivered have to be relevant and specific. The initial search model was based on linear regression but was eventually upgraded to Gradient Boosted decision trees to include non-linear correlations in the dataset. In addition to these models, the LinkedIn recruiter also uses the Generalized Linear Mix model to improve the results of prediction problems to give personalized results.
ii) Recommendation Systems Personalized for News Feed
The LinkedIn news feed is the heart and soul of the professional community. A member's newsfeed is a place to discover conversations among connections, career news, posts, suggestions, photos, and videos. Every time a member visits LinkedIn, machine learning algorithms identify the best exchanges to be displayed on the feed by sorting through posts and ranking the most relevant results on top. The algorithms help LinkedIn understand member preferences and help provide personalized news feeds. The algorithms used include logistic regression, gradient boosted decision trees and neural networks for recommendation systems.
iii) CNN's to Detect Inappropriate Content
To provide a professional space where people can trust and express themselves professionally in a safe community has been a critical goal at LinkedIn. LinkedIn has heavily invested in building solutions to detect fake accounts and abusive behavior on their platform. Any form of spam, harassment, inappropriate content is immediately flagged and taken down. These can range from profanity to advertisements for illegal services. LinkedIn uses a Convolutional neural networks based machine learning model. This classifier trains on a training dataset containing accounts labeled as either "inappropriate" or "appropriate." The inappropriate list consists of accounts having content from "blocklisted" phrases or words and a small portion of manually reviewed accounts reported by the user community.
Here is a Text Classification Project to help you understand NLP basics for text classification. You can find a news recommendation system dataset to help you build a personalized news recommender system. You can also use this dataset to build a classifier using logistic regression, Naive Bayes, or Neural networks to classify toxic comments.
Get confident to build end-to-end projects
Access to a curated library of 250+ end-to-end industry projects with solution code, videos and tech support.
Pfizer is a multinational pharmaceutical company headquartered in New York, USA. One of the largest pharmaceutical companies globally known for developing a wide range of medicines and vaccines in disciplines like immunology, oncology, cardiology, and neurology. Pfizer became a household name in 2010 when it was the first to have a COVID-19 vaccine with FDA. In early November 2021, The CDC has approved the Pfizer vaccine for kids aged 5 to 11. Pfizer has been using machine learning and artificial intelligence to develop drugs and streamline trials, which played a massive role in developing and deploying the COVID-19 vaccine. Here are a few data analytics case studies by Pfizer :
i) Identifying Patients for Clinical Trials
Artificial intelligence and machine learning are used to streamline and optimize clinical trials to increase their efficiency. Natural language processing and exploratory data analysis of patient records can help identify suitable patients for clinical trials. These can help identify patients with distinct symptoms. These can help examine interactions of potential trial members' specific biomarkers, predict drug interactions and side effects which can help avoid complications. Pfizer's AI implementation helped rapidly identify signals within the noise of millions of data points across their 44,000-candidate COVID-19 clinical trial.
ii) Supply Chain and Manufacturing
Data science and machine learning techniques help pharmaceutical companies better forecast demand for vaccines and drugs and distribute them efficiently. Machine learning models can help identify efficient supply systems by automating and optimizing the production steps. These will help supply drugs customized to small pools of patients in specific gene pools. Pfizer uses Machine learning to predict the maintenance cost of equipment used. Predictive maintenance using AI is the next big step for Pharmaceutical companies to reduce costs.
iii) Drug Development
Computer simulations of proteins, and tests of their interactions, and yield analysis help researchers develop and test drugs more efficiently. In 2016 Watson Health and Pfizer announced a collaboration to utilize IBM Watson for Drug Discovery to help accelerate Pfizer's research in immuno-oncology, an approach to cancer treatment that uses the body's immune system to help fight cancer. Deep learning models have been used recently for bioactivity and synthesis prediction for drugs and vaccines in addition to molecular design. Deep learning has been a revolutionary technique for drug discovery as it factors everything from new applications of medications to possible toxic reactions which can save millions in drug trials.
You can create a Machine learning model to predict molecular activity to help design medicine using this dataset . You may build a CNN or a Deep neural network for this data analyst case study project.
Access Data Science and Machine Learning Project Code Examples
9) Shell Data Analyst Case Study Project
Shell is a global group of energy and petrochemical companies with over 80,000 employees in around 70 countries. Shell uses advanced technologies and innovations to help build a sustainable energy future. Shell is going through a significant transition as the world needs more and cleaner energy solutions to be a clean energy company by 2050. It requires substantial changes in the way in which energy is used. Digital technologies, including AI and Machine Learning, play an essential role in this transformation. These include efficient exploration and energy production, more reliable manufacturing, more nimble trading, and a personalized customer experience. Using AI in various phases of the organization will help achieve this goal and stay competitive in the market. Here are a few data analytics case studies in the petrochemical industry:
i) Precision Drilling
Shell is involved in the processing mining oil and gas supply, ranging from mining hydrocarbons to refining the fuel to retailing them to customers. Recently Shell has included reinforcement learning to control the drilling equipment used in mining. Reinforcement learning works on a reward-based system based on the outcome of the AI model. The algorithm is designed to guide the drills as they move through the surface, based on the historical data from drilling records. It includes information such as the size of drill bits, temperatures, pressures, and knowledge of the seismic activity. This model helps the human operator understand the environment better, leading to better and faster results will minor damage to machinery used.
ii) Efficient Charging Terminals
Due to climate changes, governments have encouraged people to switch to electric vehicles to reduce carbon dioxide emissions. However, the lack of public charging terminals has deterred people from switching to electric cars. Shell uses AI to monitor and predict the demand for terminals to provide efficient supply. Multiple vehicles charging from a single terminal may create a considerable grid load, and predictions on demand can help make this process more efficient.
iii) Monitoring Service and Charging Stations
Another Shell initiative trialed in Thailand and Singapore is the use of computer vision cameras, which can think and understand to watch out for potentially hazardous activities like lighting cigarettes in the vicinity of the pumps while refueling. The model is built to process the content of the captured images and label and classify it. The algorithm can then alert the staff and hence reduce the risk of fires. You can further train the model to detect rash driving or thefts in the future.
Here is a project to help you understand multiclass image classification. You can use the Hourly Energy Consumption Dataset to build an energy consumption prediction model. You can use time series with XGBoost to develop your model.
10) Zomato Case Study on Data Analytics
Zomato was founded in 2010 and is currently one of the most well-known food tech companies. Zomato offers services like restaurant discovery, home delivery, online table reservation, online payments for dining, etc. Zomato partners with restaurants to provide tools to acquire more customers while also providing delivery services and easy procurement of ingredients and kitchen supplies. Currently, Zomato has over 2 lakh restaurant partners and around 1 lakh delivery partners. Zomato has closed over ten crore delivery orders as of date. Zomato uses ML and AI to boost their business growth, with the massive amount of data collected over the years from food orders and user consumption patterns. Here are a few examples of data analyst case study project developed by the data scientists at Zomato:
i) Personalized Recommendation System for Homepage
Zomato uses data analytics to create personalized homepages for its users. Zomato uses data science to provide order personalization, like giving recommendations to the customers for specific cuisines, locations, prices, brands, etc. Restaurant recommendations are made based on a customer's past purchases, browsing history, and what other similar customers in the vicinity are ordering. This personalized recommendation system has led to a 15% improvement in order conversions and click-through rates for Zomato.
You can use the Restaurant Recommendation Dataset to build a restaurant recommendation system to predict what restaurants customers are most likely to order from, given the customer location, restaurant information, and customer order history.
ii) Analyzing Customer Sentiment
Zomato uses Natural language processing and Machine learning to understand customer sentiments using social media posts and customer reviews. These help the company gauge the inclination of its customer base towards the brand. Deep learning models analyze the sentiments of various brand mentions on social networking sites like Twitter, Instagram, Linked In, and Facebook. These analytics give insights to the company, which helps build the brand and understand the target audience.
iii) Predicting Food Preparation Time (FPT)
Food delivery time is an essential variable in the estimated delivery time of the order placed by the customer using Zomato. The food preparation time depends on numerous factors like the number of dishes ordered, time of the day, footfall in the restaurant, day of the week, etc. Accurate prediction of the food preparation time can help make a better prediction of the Estimated delivery time, which will help delivery partners less likely to breach it. Zomato uses a Bidirectional LSTM-based deep learning model that considers all these features and provides food preparation time for each order in real-time.
Data scientists are companies' secret weapons when analyzing customer sentiments and behavior and leveraging it to drive conversion, loyalty, and profits. These 10 data science case studies projects with examples and solutions show you how various organizations use data science technologies to succeed and be at the top of their field! To summarize, Data Science has not only accelerated the performance of companies but has also made it possible to manage & sustain their performance with ease.
FAQs on Data Analysis Case Studies
A case study in data science is an in-depth analysis of a real-world problem using data-driven approaches. It involves collecting, cleaning, and analyzing data to extract insights and solve challenges, offering practical insights into how data science techniques can address complex issues across various industries.
To create a data science case study, identify a relevant problem, define objectives, and gather suitable data. Clean and preprocess data, perform exploratory data analysis, and apply appropriate algorithms for analysis. Summarize findings, visualize results, and provide actionable recommendations, showcasing the problem-solving potential of data science techniques.
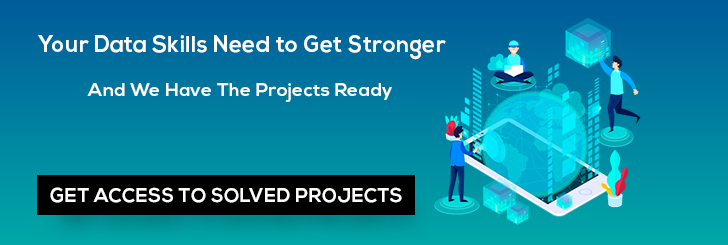
About the Author

ProjectPro is the only online platform designed to help professionals gain practical, hands-on experience in big data, data engineering, data science, and machine learning related technologies. Having over 270+ reusable project templates in data science and big data with step-by-step walkthroughs,

© 2024
© 2024 Iconiq Inc.
Privacy policy
User policy
Write for ProjectPro

Published: 5 April 2024 Contributors: Tim Mucci, Cole Stryker
Big data analytics refers to the systematic processing and analysis of large amounts of data and complex data sets, known as big data, to extract valuable insights. Big data analytics allows for the uncovering of trends, patterns and correlations in large amounts of raw data to help analysts make data-informed decisions. This process allows organizations to leverage the exponentially growing data generated from diverse sources, including internet-of-things (IoT) sensors, social media, financial transactions and smart devices to derive actionable intelligence through advanced analytic techniques.
In the early 2000s, advances in software and hardware capabilities made it possible for organizations to collect and handle large amounts of unstructured data. With this explosion of useful data, open-source communities developed big data frameworks to store and process this data. These frameworks are used for distributed storage and processing of large data sets across a network of computers. Along with additional tools and libraries, big data frameworks can be used for:
- Predictive modeling by incorporating artificial intelligence (AI) and statistical algorithms
- Statistical analysis for in-depth data exploration and to uncover hidden patterns
- What-if analysis to simulate different scenarios and explore potential outcomes
- Processing diverse data sets, including structured, semi-structured and unstructured data from various sources.
Four main data analysis methods – descriptive, diagnostic, predictive and prescriptive – are used to uncover insights and patterns within an organization's data. These methods facilitate a deeper understanding of market trends, customer preferences and other important business metrics.
IBM named a Leader in the 2024 Gartner® Magic Quadrant™ for Augmented Data Quality Solutions.
Structured vs unstructured data
What is data management?
The main difference between big data analytics and traditional data analytics is the type of data handled and the tools used to analyze it. Traditional analytics deals with structured data, typically stored in relational databases . This type of database helps ensure that data is well-organized and easy for a computer to understand. Traditional data analytics relies on statistical methods and tools like structured query language (SQL) for querying databases.
Big data analytics involves massive amounts of data in various formats, including structured, semi-structured and unstructured data. The complexity of this data requires more sophisticated analysis techniques. Big data analytics employs advanced techniques like machine learning and data mining to extract information from complex data sets. It often requires distributed processing systems like Hadoop to manage the sheer volume of data.
These are the four methods of data analysis at work within big data:
The "what happened" stage of data analysis. Here, the focus is on summarizing and describing past data to understand its basic characteristics.
The “why it happened” stage. By delving deep into the data, diagnostic analysis identifies the root patterns and trends observed in descriptive analytics.
The “what will happen” stage. It uses historical data, statistical modeling and machine learning to forecast trends.
Describes the “what to do” stage, which goes beyond prediction to provide recommendations for optimizing future actions based on insights derived from all previous.
The following dimensions highlight the core challenges and opportunities inherent in big data analytics.
The sheer volume of data generated today, from social media feeds, IoT devices, transaction records and more, presents a significant challenge. Traditional data storage and processing solutions are often inadequate to handle this scale efficiently. Big data technologies and cloud-based storage solutions enable organizations to store and manage these vast data sets cost-effectively, protecting valuable data from being discarded due to storage limitations.
Data is being produced at unprecedented speeds, from real-time social media updates to high-frequency stock trading records. The velocity at which data flows into organizations requires robust processing capabilities to capture, process and deliver accurate analysis in near real-time. Stream processing frameworks and in-memory data processing are designed to handle these rapid data streams and balance supply with demand.
Today's data comes in many formats, from structured to numeric data in traditional databases to unstructured text, video and images from diverse sources like social media and video surveillance. This variety demans flexible data management systems to handle and integrate disparate data types for comprehensive analysis. NoSQL databases , data lakes and schema -on-read technologies provide the necessary flexibility to accommodate the diverse nature of big data.
Data reliability and accuracy are critical, as decisions based on inaccurate or incomplete data can lead to negative outcomes. Veracity refers to the data's trustworthiness, encompassing data quality, noise and anomaly detection issues. Techniques and tools for data cleaning, validation and verification are integral to ensuring the integrity of big data, enabling organizations to make better decisions based on reliable information.
Big data analytics aims to extract actionable insights that offer tangible value. This involves turning vast data sets into meaningful information that can inform strategic decisions, uncover new opportunities and drive innovation. Advanced analytics, machine learning and AI are key to unlocking the value contained within big data, transforming raw data into strategic assets.
Data professionals, analysts, scientists and statisticians prepare and process data in a data lakehouse, which combines the performance of a data lakehouse with the flexibility of a data lake to clean data and ensure its quality. The process of turning raw data into valuable insights encompasses several key stages:
- Collect data: The first step involves gathering data, which can be a mix of structured and unstructured forms from myriad sources like cloud, mobile applications and IoT sensors. This step is where organizations adapt their data collection strategies and integrate data from varied sources into central repositories like a data lake, which can automatically assign metadata for better manageability and accessibility.
- Process data: After being collected, data must be systematically organized, extracted, transformed and then loaded into a storage system to ensure accurate analytical outcomes. Processing involves converting raw data into a format that is usable for analysis, which might involve aggregating data from different sources, converting data types or organizing data into structure formats. Given the exponential growth of available data, this stage can be challenging. Processing strategies may vary between batch processing, which handles large data volumes over extended periods and stream processing, which deals with smaller real-time data batches.
- Clean data: Regardless of size, data must be cleaned to ensure quality and relevance. Cleaning data involves formatting it correctly, removing duplicates and eliminating irrelevant entries. Clean data prevents the corruption of output and safeguard’s reliability and accuracy.
- Analyze data: Advanced analytics, such as data mining, predictive analytics, machine learning and deep learning, are employed to sift through the processed and cleaned data. These methods allow users to discover patterns, relationships and trends within the data, providing a solid foundation for informed decision-making.
Under the Analyze umbrella, there are potentially many technologies at work, including data mining, which is used to identify patterns and relationships within large data sets; predictive analytics, which forecasts future trends and opportunities; and deep learning , which mimics human learning patterns to uncover more abstract ideas.
Deep learning uses an artificial neural network with multiple layers to model complex patterns in data. Unlike traditional machine learning algorithms, deep learning learns from images, sound and text without manual help. For big data analytics, this powerful capability means the volume and complexity of data is not an issue.
Natural language processing (NLP) models allow machines to understand, interpret and generate human language. Within big data analytics, NLP extracts insights from massive unstructured text data generated across an organization and beyond.
Structured Data
Structured data refers to highly organized information that is easily searchable and typically stored in relational databases or spreadsheets. It adheres to a rigid schema, meaning each data element is clearly defined and accessible in a fixed field within a record or file. Examples of structured data include:
- Customer names and addresses in a customer relationship management (CRM) system
- Transactional data in financial records, such as sales figures and account balances
- Employee data in human resources databases, including job titles and salaries
Structured data's main advantage is its simplicity for entry, search and analysis, often using straightforward database queries like SQL. However, the rapidly expanding universe of big data means that structured data represents a relatively small portion of the total data available to organizations.
Unstructured Data
Unstructured data lacks a pre-defined data model, making it more difficult to collect, process and analyze. It comprises the majority of data generated today, and includes formats such as:
- Textual content from documents, emails and social media posts
- Multimedia content, including images, audio files and videos
- Data from IoT devices, which can include a mix of sensor data, log files and time-series data
The primary challenge with unstructured data is its complexity and lack of uniformity, requiring more sophisticated methods for indexing, searching and analyzing. NLP, machine learning and advanced analytics platforms are often employed to extract meaningful insights from unstructured data.
Semi-structured data
Semi-structured data occupies the middle ground between structured and unstructured data. While it does not reside in a relational database, it contains tags or other markers to separate semantic elements and enforce hierarchies of records and fields within the data. Examples include:
- JSON (JavaScript Object Notation) and XML (eXtensible Markup Language) files, which are commonly used for web data interchange
- Email, where the data has a standardized format (e.g., headers, subject, body) but the content within each section is unstructured
- NoSQL databases, can store and manage semi-structured data more efficiently than traditional relational databases
Semi-structured data is more flexible than structured data but easier to analyze than unstructured data, providing a balance that is particularly useful in web applications and data integration tasks.
Ensuring data quality and integrity, integrating disparate data sources, protecting data privacy and security and finding the right talent to analyze and interpret data can present challenges to organizations looking to leverage their extensive data volumes. What follows are the benefits organizations can realize once they see success with big data analytics:
Real-time intelligence
One of the standout advantages of big data analytics is the capacity to provide real-time intelligence. Organizations can analyze vast amounts of data as it is generated from myriad sources and in various formats. Real-time insight allows businesses to make quick decisions, respond to market changes instantaneously and identify and act on opportunities as they arise.
Better-informed decisions
With big data analytics, organizations can uncover previously hidden trends, patterns and correlations. A deeper understanding equips leaders and decision-makers with the information needed to strategize effectively, enhancing business decision-making in supply chain management, e-commerce, operations and overall strategic direction.
Cost savings
Big data analytics drives cost savings by identifying business process efficiencies and optimizations. Organizations can pinpoint wasteful expenditures by analyzing large datasets, streamlining operations and enhancing productivity. Moreover, predictive analytics can forecast future trends, allowing companies to allocate resources more efficiently and avoid costly missteps.
Better customer engagement
Understanding customer needs, behaviors and sentiments is crucial for successful engagement and big data analytics provides the tools to achieve this understanding. Companies gain insights into consumer preferences and tailor their marketing strategies by analyzing customer data.
Optimized risk management strategies
Big data analytics enhances an organization's ability to manage risk by providing the tools to identify, assess and address threats in real time. Predictive analytics can foresee potential dangers before they materialize, allowing companies to devise preemptive strategies.
As organizations across industries seek to leverage data to drive decision-making, improve operational efficiencies and enhance customer experiences, the demand for skilled professionals in big data analytics has surged. Here are some prominent career paths that utilize big data analytics:
Data scientist
Data scientists analyze complex digital data to assist businesses in making decisions. Using their data science training and advanced analytics technologies, including machine learning and predictive modeling, they uncover hidden insights in data.
Data analyst
Data analysts turn data into information and information into insights. They use statistical techniques to analyze and extract meaningful trends from data sets, often to inform business strategy and decisions.
Data engineer
Data engineers prepare, process and manage big data infrastructure and tools. They also develop, maintain, test and evaluate data solutions within organizations, often working with massive datasets to assist in analytics projects.
Machine learning engineer
Machine learning engineers focus on designing and implementing machine learning applications. They develop sophisticated algorithms that learn from and make predictions on data.
Business intelligence analyst
Business intelligence (BI) analysts help businesses make data-driven decisions by analyzing data to produce actionable insights. They often use BI tools to convert data into easy-to-understand reports and visualizations for business stakeholders.
Data visualization specialist
These specialists focus on the visual representation of data. They create data visualizations that help end users understand the significance of data by placing it in a visual context.
Data architect
Data architects design, create, deploy and manage an organization's data architecture. They define how data is stored, consumed, integrated and managed by different data entities and IT systems.
IBM and Cloudera have partnered to create an industry-leading, enterprise-grade big data framework distribution plus a variety of cloud services and products — all designed to achieve faster analytics at scale.
IBM Db2 Database on IBM Cloud Pak for Data combines a proven, AI-infused, enterprise-ready data management system with an integrated data and AI platform built on the security-rich, scalable Red Hat OpenShift foundation.
IBM Big Replicate is an enterprise-class data replication software platform that keeps data consistent in a distributed environment, on-premises and in the hybrid cloud, including SQL and NoSQL databases.
A data warehouse is a system that aggregates data from different sources into a single, central, consistent data store to support data analysis, data mining, artificial intelligence and machine learning.
Business intelligence gives organizations the ability to get answers they can understand. Instead of using best guesses, they can base decisions on what their business data is telling them — whether it relates to production, supply chain, customers or market trends.
Cloud computing is the on-demand access of physical or virtual servers, data storage, networking capabilities, application development tools, software, AI analytic tools and more—over the internet with pay-per-use pricing. The cloud computing model offers customers flexibility and scalability compared to traditional infrastructure.
Purpose-built data-driven architecture helps support business intelligence across the organization. IBM analytics solutions allow organizations to simplify raw data access, provide end-to-end data management and empower business users with AI-driven self-service analytics to predict outcomes.

Log in using your username and password
- Search More Search for this keyword Advanced search
- Latest content
- BMJ Journals More You are viewing from: Google Indexer
You are here
- Volume 13, Issue 2
- When do medical operators choose to use, or not use, video in emergency calls? A case study
- Article Text
- Article info
- Citation Tools
- Rapid Responses
- Article metrics

- http://orcid.org/0000-0002-9390-4310 Astrid Karina V Harring 1 ,
- Siri Idland 1 , 2 ,
- Janne Dugstad 3
- 1 Department for Prehospital Education and Research , Oslo Metropolitan University , Oslo , Norway
- 2 Division of Prehospital Services , Oslo University Hospital , Oslo , Norway
- 3 Centre for Health and Technology, Faculty of Health and Social Sciences , University of South-Eastern Norway , Drammen , Norway
- Correspondence to Astrid Karina V Harring; astridka{at}oslomet.no
Background An evaluation report for a pilot project on the use of video in medical emergency calls between the caller and medical operator indicates that video is only used in 4% of phone calls to the emergency medical communication centre (EMCC). Furthermore, the report found that in half of these cases, the use of video did not alter the assessment made by the medical operator at the EMCC.
We aimed to describe the reasons for when and why medical operators choose to use or not use video in emergency calls.
Method The study was conducted in a Norwegian EMCC, employing a thematic analysis of notes from medical operators responding to emergency calls regarding the use of video.
Result Informants reported 19 cases where video was used and 46 cases where it was not used. When video was used, three main themes appeared: ‘unclear situation or patient condition’, ‘visible problem’ and ‘children’. When video was not used the following themes emerged: ‘cannot be executed/technical problems’, ‘does not follow instructions’, ‘perceived as unnecessary’. Video was mostly used in cases where the medical operators were uncertain about the situation or the patients’ conditions.
Conclusion The results indicate that medical operators were selective in choosing when to use video. In cases where operators employed video, it provided a better understanding of the situation, potentially enhancing the basis for decision-making.
- Telemedicine
- Decision support, clinical
- Prehospital care
Data availability statement
Data are available on reasonable request.
This is an open access article distributed in accordance with the Creative Commons Attribution Non Commercial (CC BY-NC 4.0) license, which permits others to distribute, remix, adapt, build upon this work non-commercially, and license their derivative works on different terms, provided the original work is properly cited, appropriate credit is given, any changes made indicated, and the use is non-commercial. See: http://creativecommons.org/licenses/by-nc/4.0/ .
https://doi.org/10.1136/bmjoq-2024-002751
Statistics from Altmetric.com
Request permissions.
If you wish to reuse any or all of this article please use the link below which will take you to the Copyright Clearance Center’s RightsLink service. You will be able to get a quick price and instant permission to reuse the content in many different ways.
WHAT IS ALREADY KNOWN ON THIS TOPIC
There has been an increasing interest in, and rapid implementation of video communication during emergency calls. However, the use remains limited, despite studies indicating that video frequently influences the assessments made by operators.
Most of the previous studies have been based on simulated situations or primarily focused on cases where video was used in specific situations such as assessing the quality of cardiopulmonary resuscitation, secondary triage or guiding callers in first aid measures, providing a somewhat one-sided view of the situation.
WHAT THIS STUDY ADDS
This is one of the first studies to describe the reason why medical operators choose to use video or not.
The results demonstrate that operators are selective in choosing when to use video, as it is used primarily in cases where operators are uncertain about the situation or the patient’s condition.
HOW THIS STUDY MIGHT AFFECT RESEARCH, PRACTICE OR POLICY
Despite few respondents, the findings and method can form the basis for the development of future studies.
When operators opted for video, they indicated that it provided better situational understanding and strengthened the decision-making.
In Norway, when someone dials the medical emergency number 1-1-3, they are connected to the nearest emergency medical communication centre (EMCC). 1 The calls are answered by medical operators, who are nurses, paramedics or emergency medical technicians. Based on the reported symptoms, the operator provides necessary guidance while dispatching one or more ambulances and, if needed, a doctor or air ambulance, depending on the severity of the situation. 2 If there is no immediate threat to life or health, the emergency call is transferred to the patient’s corresponding emergency primary care centre for further follow-up.
Through simulated calls, Tuden et al 3 found that telenurses attempt to visualise what the caller is experiencing. Video recordings revealed instances where nurses touched their own arm or leg to understand the potential problem, attempting to create a mental ‘image’ of the situation. Yet a Norwegian pilot project in 2019 demonstrated that video only was used in 4% of phone calls at the EMCCs and in 1% of the calls to the emergency primary care centres. 4 Since then, the use of video calls between operators and callers during medical emergency calls has proven to be a valuable tool, 5 6 and it has been found that in approximately half of the cases, it influences the assessment made by the operator. 4 6 An emergency operations centre in the UK introduced video triage during the COVID-19 pandemic as a supplement to their traditional 999-telephone triage. 7 Doctors experienced that it provided a better examination of the patient than just a telephone call, thus improving the decision-making. Patients who received video consultations were also satisfied with this solution, and they had a 10% lower rate of recontact with the healthcare system within 24 hours compared with those who received assistance only over the phone. 7 The benefit of telemedicine in rural and remote facilities is recently studied, providing further insights into its use for secondary triage and clinical advice to ensure that patients are referred and transported to the appropriate level of care. 8 Video has also proven to be suitable for assessing the quality of cardiopulmonary resuscitation 9–11 and to improve guidance for the caller in first aid measures such as stopping bleeding or placing an unconscious person in the recovery position. 5 However, many of the previous studies were based on simulated situations 9–11 or primarily focused on the cases where video was used. 4 5 7 11 Thus, they provide a somewhat one-sided view of the situation.
Using a qualitative approach, we sought to describe the reasons for when and why operators chose to use, or not use video, and thereby gaining new insights into this emerging field.
Case description
The case study was performed in cooperation with Oslo EMCC. This is the largest in Norway, serving a population of approximately 1.5 million inhabitants, coordinating between 70 and 90 units in its area. The EMCC manages critical incidents and acute medical situations and coordinates urgent and planned transfers 2 and is responsible for all air ambulance operations in the South-Eastern Health region. Video has been available at Oslo EMCC since June 2020, as an additional support tool during medical emergency calls. 4 5 It was fully up to the operator’s discretion to consider if video may provide significant information to determine the appropriate response or resource, or to provide better advice or guidance.
No downloads, applications or special software or hardware were needed to activate the video, neither for the EMCC nor for the caller. However, the mobile phone had to be a ‘smartphone’ with a camera, Wi-Fi or 3–5G network access and a web browser. The EMCC operator logged on to the video solution in a web browser, at the start of their shift using a two-step sign-in. If the operator initiated video, the caller was asked for consent and instructed to activate the speaker. A link was sent to the caller by SMS (text) message, and when accepted, the one-way video started. The operator could switch between front and back cameras on the caller’s phone and could end the video streaming without ending the call. If the caller did not accept camera sharing, had a low battery or the phone was in power-save mode, video would not commence, and further instructions were required from the operator to help resolve the issue.
Data collection
Data collection took place during selected shifts at Oslo EMCC from October to November 2021, with one of the authors present during these shifts. Whereas the research had a case study design, Oslo EMCC considered this as an internal quality improvement initiative, approved by the departmental leader. Medical operators at the EMCC received written information about the study. Participation was voluntary and based on consent. It was emphasised that the EMCC was in a demanding situation and that data collection should not compromise the operators’ capacity or response time.
Participants were provided with a sheet containing two columns, one for cases where video was used and another for cases where video was not used. They were free to choose which and how many calls to include. In the included emergency calls, they noted whether video was used and the reasons why or why not they used video in the emergency call. The use of notes was chosen to ensure that it would not be time-intensive or resource-intensive for the operator. Operators were also entrusted to write themselves, preventing alterations in wording or potential misinterpretation of the raw material. The information was anonymous throughout the processing. It was explicitly stated that personally identifiable information should not be reproduced, such as the patient or caller’s name, date of birth/social security number, address or any other information about the nature of the incident that could make it identifiable. Hence, a table describing the callers or patients’ age, gender, demographics, etc is not provided in this article.
No sensitive nor identifiable data were recorded, such as the specific form’s date/shift or information that could identify participating colleagues. Therefore, the study falls outside the scope of requiring a formal privacy application. 12 13
The study was conducted through thematic analysis 14 of notes written by medical operators. All data material was gathered in an Excel document, with one sheet for ‘video not used’ and another for ‘video used.’ The codes formed primary patterns (codes) that created subthemes and overarching themes ( table 1 ).
- View inline
Examples of the analysis of cases where video was not used, focusing on the subtheme ‘invisible’ condition
A total of four different informants submitted a total of six forms, reporting 19 cases where video was used and 46 cases where it was not used. All the informants were women with a minimum of 2 years of experience as medical operators.
Situations where the informants used video were categorised into three overarching themes. The themes were ‘unclear situation or patient condition,’ ‘visible problem’ and ‘children.’ Similarly, situations where video was not used in the emergency call were also categorised. The themes were ‘cannot be executed/technical problems,’ ‘does not follow instructions,’ ‘perceived as unnecessary.’ Furthermore, the overarching themes were divided into subthemes ( tables 2 and 3 ).
Situations where video was used
Situations where video was not used.
Video tended to be used when the operator reported that the situation or patient condition was unclear ( table 2 ). In situations involving an intoxicated caller and/or patient, there was significant uncertainty and difficulty in accurately describing the problem.
Quote: ‘Drunk, bleeding, lying outside. Too dark to see much’.
Furthermore, the use of video seemed to be reliant on the operator’s expectation that the symptom would be observed visually. In two cases, video was used to assess the skin in cases of suspected blood clots in the legs, and once for a chronic wound. In these cases, it was explicitly noted that video was very useful.
Quote: ‘Clarify signs of arterial thrombus, DVT [deep venous thrombosis], erysipelas, etc. Very useful!’
Regarding children, operators often experienced the situations as unclear, especially for the youngest children. Video was used to determine the amount of bleeding from cuts and injuries, as well as non-traumatic bleeding such as nosebleeds and bloody vomiting. None reported the use of video on children with injuries, only in one accident.
Quote: ‘Child in accident. To assess condition and pain of a 9-year-old with stomach pain after being hit by the scooter handlebar in the stomach’.
Included in the category of ‘injury’ are fractures, wounds and cuts, but the distinction between injuries and accidents was not entirely clear. Accidents recurred in all three overarching themes and included serious patient injuries, as well as the use of video to assess the extent of the accident or the accident scene. Furthermore, the transition between consciousness and overall condition was somewhat unclear; patients waking up when addressed encompassed both reduced consciousness and reduced overall condition.
Pain was indicated to be ‘visible’, even though pain as a phenomenon is both invisible and subjective.
Sometimes video could not be conducted, either physically or technically ( table 3 ).
Quote: ‘The caller has left the scene’.
In many cases, video was not used because the operator perceived it as ‘unnecessary’. These would be the instances where they report to ‘already have a good understanding’ or that there is ‘nothing to see’. Other times the operators stated that the situation was ‘urgent regardless’ as in the case of chest pain, cerebral events or when vital measurements had been taken, and they assessed that video would not change the outcome. Several mentioned not using video when healthcare professionals or the police were the callers.
Quote: ‘Calling from the police station due to a seizure. Choosing to trust the police and to be considerate of the others at the location’.
In some instances, they stated that video could have been useful, as in events in public places, but they wanted to spare the patient or avoid the time delay associated with video. A combination of physical and cognitive barriers also led to the decision not to attempt video, as in cases of advanced age, various disabilities or emotional distress.
Quote: ‘Caller (mother) is panicking’.
In this study, we sought to describe the reasons for when and why operators chose to use, or not use video. We found that the operators were selective when they used video, using it in situations where they were uncertain about the situation or the patient’s condition.
Paradoxically, some conditions, where the caller and/or patient was intoxicated, were identified both as a situation where video was used and a situation where video was not used. Tuden et al 3 studied how nurses, through telephone communication, made decisions and employed decision support systems. In some instances, the nurse mentioned that they recognised the situation from previous experiences, and therefore, acted spontaneously. Such recognition-based decisions are common in complex, time-constrained situations where decision-makers possess a high level of expertise, such as in emergency medicine, 15 similar to the setting in the current study. However, when the problem or solution was not apparent, Tuden et al 3 noticed that the nurse would sometimes pause, review the decision support system, or contemplate potential issues or conditions affecting the patient without explicitly discussing this ‘uncertainty’ with the caller. We found the operators’ self-perceived level of uncertainty with the situation to be the deciding factor of whether to initiate video, rather the condition itself. This might be why some conditions are found in both tables, such as an intoxicated caller and/or patient, that sometimes it is the reason for not using video, and at other times, it is the main reason for considering video necessary, depending on the situation.
If video is primarily used when one is uncertain, there is no wonder that previous studies have indicated that video altered the assessment and the response in approximately half of the cases. 4 6 This potential reduction in overestimation or underestimation of the severity was also reported in a qualitative study of EMCC operators’ experiences with video, where some of the operators believed that video could contribute to better resource utilisation. 5 Thus, it would be tempting to develop a guideline to increase the use of video. However, this would be challenging to implement as it seems that was the operators’ subjective need that triggered the decision to use video and not any specific symptom or condition.
In the pilot report, ‘unconscious adult,’ ‘injuries’ and ‘unclear problem’ were recurring situations where EMCC- operators reported using video. 4 This was in accordance with our findings ( table 2 ), and it seems to suggest that the callers were perceived to particularly overestimate bleeding amount and injuries, and thus, operators found great utility in video. According to Idland et al , 5 several operators perceived video as a reassurance for their own decisions. This is consistent with our findings, where operators felt that video confirmed that the patient’s severity matched what they had perceived during the phone call. It was not ‘unnecessary’ or ‘useless’; on the contrary, it strengthened the quality of the assessment. Furthermore, operators emphasised that it became easier to provide advice and guidance to the caller, as mentioned in the pilot report. 4 These instances would, therefore, be reflected as instances where the operators’ assessment would be deemed ‘unchanged’, even though the operator found video useful. 4
According to the Danish study, unconscious patients were one of the largest patient groups where EMCC operators chose to use video. 6 Consciousness was also noted in the pilot report 4 as a frequent reason for using video, while in our study, it was explicitly mentioned only once that consciousness was assessed. There might be under-reporting of the use of video for consciousness assessment in our study, or it could be that this was done so automatically when using video that operators did not consciously think about it. The same pattern was observed in the assessment of respiration using video. Medical operators are accustomed to hearing breathing sounds over the phone, and it is likely that the conscious or unconscious assessment was made before they choose to start the video or not.
Similarly, video was reported to be used for some conditions such as fever and pain while for other conditions like dizziness, it was not used. When a patient has a high fever there are visual clues such as flushed skin and one can assess if the general condition is reduced. What then is a paradox is that a patient with prominent vertigo also could have observable signs, often appearing uncomfortable, pale and cold sweating. As mentioned, pain was indicated to be ‘visible’ and something that could be objectively assessed, even though pain as a phenomenon is both invisible and subjective. We understand the operators to mean that pain expressed physically could be objectively assessed, allowing them to form an opinion about whether the patient was affected by pain and to what degree, whether it was mild, moderate or severe pain that was being expressed. It is also likely referred to as a way of gaining situational understanding. It should be noted that the depth and scope of the data material are limited, and it is pertinent to question how aware operators are of the position of power they possess, being able to overrule callers.
We also found it interesting that no cases indicated that video was used when the caller was healthcare professional or a police officer. This is understood as the operator feeling that they trusted the caller to provide sufficient information, and that video would not add anything. However, when taking the results from the vCare project 8 regarding clinical advice, referral and retrieval into account, it seems that there is potential for a new and expanded use for the video solution at the EMCC, that needs to be investigated further.
Time was a common factor mentioned. This aligns with the findings of Lin et al 10 that the use of video creates an undefined time delay and that one therefore must assess the benefit against the potential time loss. In many cases, the medical operators stated that the situation was ‘urgent regardless,’ as in the case of chest pain, cerebral events, where vital measurements, such as pulse, blood pressure, had been taken, and they assessed that video would not change the outcome. Implicitly, this suggests that they were confident in their decision, and that they did not want to spend ‘unnecessary’ time on video. Linderoth et al 6 expand on this, for example, when there was only a short time until the arrival of the ambulance or to avoid a delay in answering emergency calls.
Several challenges regarding videocalls have been identified. 11 In the data material, operators attempted to use video without success in only five cases. This is lower than Bell et al 7 who found that video was not feasible in 40% of cases. One possible reason could be that operators made a possibly unconscious, selection when choosing to offer the use of video, as it is fully up to the operator to decide whether to use video or not. In contrast, most other studies required that there were two bystanders on site. 11 There were no cases where language problems were the reason for using video, but in several cases, it was cited as a reason not to use it. This could be interpreted as the process of sharing video is more challenging to convey than the incident itself. There were also no recorded cases where video was used for substances other than alcohol or when the main issue was related to psychiatry.
Strengths and limitations
This is one of the first studies to investigate the reasons why medical operators choose to use video or not. The study was originally undertaken as an internal quality improvement initiative, using a simple study design of text analysis of notes. Despite few respondents, the findings and the method can serve as a basis for the development of future studies. For instance, focus group interviews might provide deeper insights into organisational aspects of the use of video than the current study design.
The results indicate that operators were selective in their use of video. Video was offered to the caller when operators were uncertain, either about the situation or the patient’s condition. In almost all cases where video was used, the issue was visible in some form. Technical issues or challenges from the caller’s side caused video calls to fail in some instances while in other situations, operators deemed it unnecessary. When operators used video, it enhanced their situational understanding, facilitated recognition of the situation, thereby strengthening the decision-making.
Ethics statements
Patient consent for publication.
Not applicable.
Ethics approval
Ethics approval was not needed for this study as the study was completely anonymous and did not collect data from interventions or invasive procedures on human beings, nor personal health data. Participation was voluntary.
- Harring AKV ,
- Blinkenberg J ,
- Brattebø G , et al
- Kjærvoll HK ,
- Andersson L-J ,
- Bakkelund KEN , et al
- Borycki EM ,
- Kushniruk AW
- Iversen E ,
- Linderoth G ,
- Lippert F ,
- Østergaard D , et al
- Pilbery R ,
- Connell R , et al
- McKenna E , et al
- Bielski K ,
- Böttiger BW ,
- Pruc M , et al
- Chiang W-C ,
- Hsieh M-J , et al
- Renza M , et al
- Shippey B ,
- Rutherford G
Contributors AKVH: conception, design, literature, data collection, data analysis, interpretation of data, drafting/revising the manuscript and acts as guarantor. SI: literature, data analysis, interpretation of data, drafting/revising the manuscript. JD: conception, design, data analysis, interpretation of data, drafting/revising the manuscript. All authors have given their approval for the submitted manuscript version.
Funding The APC was covered by OsloMet's Publication Fund.
Competing interests None declared.
Patient and public involvement Patients and/or the public were not involved in the design, or conduct, or reporting, or dissemination plans of this research.
Provenance and peer review Not commissioned; externally peer reviewed.
Read the full text or download the PDF:

A .gov website belongs to an official government organization in the United States.
A lock ( ) or https:// means you've safely connected to the .gov website. Share sensitive information only on official, secure websites.
- About Adverse Childhood Experiences
- Risk and Protective Factors
- Program: Essentials for Childhood: Preventing Adverse Childhood Experiences through Data to Action
- Adverse childhood experiences can have long-term impacts on health, opportunity and well-being.
- Adverse childhood experiences are common and some groups experience them more than others.
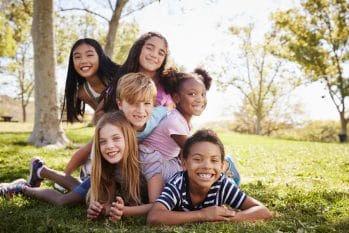
What are adverse childhood experiences?
Adverse childhood experiences, or ACEs, are potentially traumatic events that occur in childhood (0-17 years). Examples include: 1
- Experiencing violence, abuse, or neglect.
- Witnessing violence in the home or community.
- Having a family member attempt or die by suicide.
Also included are aspects of the child’s environment that can undermine their sense of safety, stability, and bonding. Examples can include growing up in a household with: 1
- Substance use problems.
- Mental health problems.
- Instability due to parental separation.
- Instability due to household members being in jail or prison.
The examples above are not a complete list of adverse experiences. Many other traumatic experiences could impact health and well-being. This can include not having enough food to eat, experiencing homelessness or unstable housing, or experiencing discrimination. 2 3 4 5 6
Quick facts and stats
ACEs are common. About 64% of adults in the United States reported they had experienced at least one type of ACE before age 18. Nearly one in six (17.3%) adults reported they had experienced four or more types of ACEs. 7
Preventing ACEs could potentially reduce many health conditions. Estimates show up to 1.9 million heart disease cases and 21 million depression cases potentially could have been avoided by preventing ACEs. 1
Some people are at greater risk of experiencing one or more ACEs than others. While all children are at risk of ACEs, numerous studies show inequities in such experiences. These inequalities are linked to the historical, social, and economic environments in which some families live. 5 6 ACEs were highest among females, non-Hispanic American Indian or Alaska Native adults, and adults who are unemployed or unable to work. 7
ACEs are costly. ACEs-related health consequences cost an estimated economic burden of $748 billion annually in Bermuda, Canada, and the United States. 8
ACEs can have lasting effects on health and well-being in childhood and life opportunities well into adulthood. 9 Life opportunities include things like education and job potential. These experiences can increase the risks of injury, sexually transmitted infections, and involvement in sex trafficking. They can also increase risks for maternal and child health problems including teen pregnancy, pregnancy complications, and fetal death. Also included are a range of chronic diseases and leading causes of death, such as cancer, diabetes, heart disease, and suicide. 1 10 11 12 13 14 15 16 17
ACEs and associated social determinants of health, such as living in under-resourced or racially segregated neighborhoods, can cause toxic stress. Toxic stress, or extended or prolonged stress, from ACEs can negatively affect children’s brain development, immune systems, and stress-response systems. These changes can affect children’s attention, decision-making, and learning. 18
Children growing up with toxic stress may have difficulty forming healthy and stable relationships. They may also have unstable work histories as adults and struggle with finances, jobs, and depression throughout life. 18 These effects can also be passed on to their own children. 19 20 21 Some children may face further exposure to toxic stress from historical and ongoing traumas. These historical and ongoing traumas refer to experiences of racial discrimination or the impacts of poverty resulting from limited educational and economic opportunities. 1 6
Adverse childhood experiences can be prevented. Certain factors may increase or decrease the risk of experiencing adverse childhood experiences.
Preventing adverse childhood experiences requires understanding and addressing the factors that put people at risk for or protect them from violence.
Creating safe, stable, nurturing relationships and environments for all children can prevent ACEs and help all children reach their full potential. We all have a role to play.
- Merrick MT, Ford DC, Ports KA, et al. Vital Signs: Estimated Proportion of Adult Health Problems Attributable to Adverse Childhood Experiences and Implications for Prevention — 25 States, 2015–2017. MMWR Morb Mortal Wkly Rep 2019;68:999-1005. DOI: http://dx.doi.org/10.15585/mmwr.mm6844e1 .
- Cain KS, Meyer SC, Cummer E, Patel KK, Casacchia NJ, Montez K, Palakshappa D, Brown CL. Association of Food Insecurity with Mental Health Outcomes in Parents and Children. Science Direct. 2022; 22:7; 1105-1114. DOI: https://doi.org/10.1016/j.acap.2022.04.010 .
- Smith-Grant J, Kilmer G, Brener N, Robin L, Underwood M. Risk Behaviors and Experiences Among Youth Experiencing Homelessness—Youth Risk Behavior Survey, 23 U.S. States and 11 Local School Districts. Journal of Community Health. 2022; 47: 324-333.
- Experiencing discrimination: Early Childhood Adversity, Toxic Stress, and the Impacts of Racism on the Foundations of Health | Annual Review of Public Health ( annualreviews.org).
- Sedlak A, Mettenburg J, Basena M, et al. Fourth national incidence study of child abuse and neglect (NIS-4): Report to Congress. Executive Summary. Washington, DC: U.S. Department of Health an Human Services, Administration for Children and Families.; 2010.
- Font S, Maguire-Jack K. Pathways from childhood abuse and other adversities to adult health risks: The role of adult socioeconomic conditions. Child Abuse Negl. 2016;51:390-399.
- Swedo EA, Aslam MV, Dahlberg LL, et al. Prevalence of Adverse Childhood Experiences Among U.S. Adults — Behavioral Risk Factor Surveillance System, 2011–2020. MMWR Morb Mortal Wkly Rep 2023;72:707–715. DOI: http://dx.doi.org/10.15585/mmwr.mm7226a2 .
- Bellis, MA, et al. Life Course Health Consequences and Associated Annual Costs of Adverse Childhood Experiences Across Europe and North America: A Systematic Review and Meta-Analysis. Lancet Public Health 2019.
- Adverse Childhood Experiences During the COVID-19 Pandemic and Associations with Poor Mental Health and Suicidal Behaviors Among High School Students — Adolescent Behaviors and Experiences Survey, United States, January–June 2021 | MMWR
- Hillis SD, Anda RF, Dube SR, Felitti VJ, Marchbanks PA, Marks JS. The association between adverse childhood experiences and adolescent pregnancy, long-term psychosocial consequences, and fetal death. Pediatrics. 2004 Feb;113(2):320-7.
- Miller ES, Fleming O, Ekpe EE, Grobman WA, Heard-Garris N. Association Between Adverse Childhood Experiences and Adverse Pregnancy Outcomes. Obstetrics & Gynecology . 2021;138(5):770-776. https://doi.org/10.1097/AOG.0000000000004570 .
- Sulaiman S, Premji SS, Tavangar F, et al. Total Adverse Childhood Experiences and Preterm Birth: A Systematic Review. Matern Child Health J . 2021;25(10):1581-1594. https://doi.org/10.1007/s10995-021-03176-6 .
- Ciciolla L, Shreffler KM, Tiemeyer S. Maternal Childhood Adversity as a Risk for Perinatal Complications and NICU Hospitalization. Journal of Pediatric Psychology . 2021;46(7):801-813. https://doi.org/10.1093/jpepsy/jsab027 .
- Mersky JP, Lee CP. Adverse childhood experiences and poor birth outcomes in a diverse, low-income sample. BMC pregnancy and childbirth. 2019;19(1). https://doi.org/10.1186/s12884-019-2560-8.
- Reid JA, Baglivio MT, Piquero AR, Greenwald MA, Epps N. No youth left behind to human trafficking: Exploring profiles of risk. American journal of orthopsychiatry. 2019;89(6):704.
- Diamond-Welch B, Kosloski AE. Adverse childhood experiences and propensity to participate in the commercialized sex market. Child Abuse & Neglect. 2020 Jun 1;104:104468.
- Shonkoff, J. P., Garner, A. S., Committee on Psychosocial Aspects of Child and Family Health, Committee on Early Childhood, Adoption, and Dependent Care, & Section on Developmental and Behavioral Pediatrics (2012). The lifelong effects of early childhood adversity and toxic stress. Pediatrics, 129(1), e232–e246. https://doi.org/10.1542/peds.2011-2663
- Narayan AJ, Kalstabakken AW, Labella MH, Nerenberg LS, Monn AR, Masten AS. Intergenerational continuity of adverse childhood experiences in homeless families: unpacking exposure to maltreatment versus family dysfunction. Am J Orthopsych. 2017;87(1):3. https://doi.org/10.1037/ort0000133.
- Schofield TJ, Donnellan MB, Merrick MT, Ports KA, Klevens J, Leeb R. Intergenerational continuity in adverse childhood experiences and rural community environments. Am J Public Health. 2018;108(9):1148-1152. https://doi.org/10.2105/AJPH.2018.304598.
- Schofield TJ, Lee RD, Merrick MT. Safe, stable, nurturing relationships as a moderator of intergenerational continuity of child maltreatment: a meta-analysis. J Adolesc Health. 2013;53(4 Suppl):S32-38. https://doi.org/10.1016/j.jadohealth.2013.05.004 .
Adverse Childhood Experiences (ACEs)
ACEs can have a tremendous impact on lifelong health and opportunity. CDC works to understand ACEs and prevent them.

IMAGES
VIDEO
COMMENTS
For case study analysis, one of the most desirable techniques is to use a pattern-matching logic. Such a logic (Trochim, 1989) compares an empiri-cally based pattern with a predicted one (or with several alternative predic-tions). If the patterns coincide, the results can help a case study to strengthen its internal validity. If the case study ...
propose an approach to the analysis of case study data by logically linking the data to a series of propositions and then interpreting the subsequent information. Like the Yin (1994) strategy, the Miles and Huberman (1994) process of analysis of case study data, although quite detailed, may still be insufficient to guide the novice researcher.
Qualitative case study methodology is an appropriate strategy for exploring phenomena such as lived experiences, events, and the contexts in which they occur (Houghton et al. 2014;Miles and ...
To conclude, there are two main objectives of this study. First is to provide a step-by-step guideline to research students for conducting case study. Second, an analysis of authors' multiple case studies is presented in order to provide an application of step-by-step guideline. This article has been divided into two sections.
question and to create an illustrative data analysis - and the domain expertise needed. As a result, case studies based on realistic challenges, not toy examples, are scarce. To address this, we developed the Open Case Studies (opencasestudies.org) project, which offers a new statistical and data science education case study model.
How to Analyze a Case Study Adapted from Ellet, W. (2007). The case study handbook. Boston, MA: Harvard Business School. A business case simulates a real situation and has three characteristics: 1. a significant issue, 2. enough information to reach a reasonable conclusion, 3. no stated conclusion. A case may include 1. irrelevant information 2.
McMaster University, West Hamilton, Ontario, Canada. Qualitative case study methodology prov ides tools for researchers to study. complex phenomena within their contexts. When the approach is ...
Data Analysis Case Studies The case studies were selected primarily to showcase a wide breadth of analytical methods, and are not meant to repre-sent a complete picture of the data analysis landscape. In some instances, the results were published in peer-reviewed journals or presented at conferences. In each case, we pro-vide the:
Practical data analysis : case studies in business statistics Bookreader Item Preview ... Pdf_module_version 0.0.20 Ppi 300 Rcs_key 24143 Republisher_date 20201024173236 Republisher_operator [email protected] Republisher_time 376 Scandate ...
A case study in data science is an in-depth analysis of a real-world problem using data-driven approaches. It involves collecting, cleaning, and analyzing data to extract insights and solve challenges, offering practical insights into how data science techniques can address complex issues across various industries.
Data Analytics for Smart Manufacturing: A Case Study. DOI: 10.5220/0008116203920399 In Proceedings of the 8th International Conference on Data Science, T echnology and Applications (DAT A 2019 ...
1. Describe when the case study approach is the most appropriate qualitative research method. 2. Outline the components of a case study research method. 3. Discuss data coding and analysis and how categories and themes are developed. 4. Identify considerations for reporting the findings of case study research.
Revised on November 20, 2023. A case study is a detailed study of a specific subject, such as a person, group, place, event, organization, or phenomenon. Case studies are commonly used in social, educational, clinical, and business research. A case study research design usually involves qualitative methods, but quantitative methods are ...
However, the qualitative data analysis process for multiple case studies is a multi-step process that can be challenging for doctoral researchers. This article thus outlines the qualitative data analysis process for a doctoral-level multiple case study in management and leadership, including conducting descriptive coding and cross-case ...
The scope of study is often determined by project budget constraints. Data Analysis: Analyzing Data - Case StudiesAccountability Modules. Data Analysis: Analyzing Data - Case Studies - 2Texas State Auditor's Office, Methodology Manual, rev. 5/95. Design the case study, taking care to select the most relevant event(s) for examination.
The main difference between big data analytics and traditional data analytics is the type of data handled and the tools used to analyze it. Traditional analytics deals with structured data, typically stored in relational databases.This type of database helps ensure that data is well-organized and easy for a computer to understand.
Background An evaluation report for a pilot project on the use of video in medical emergency calls between the caller and medical operator indicates that video is only used in 4% of phone calls to the emergency medical communication centre (EMCC). Furthermore, the report found that in half of these cases, the use of video did not alter the assessment made by the medical operator at the EMCC ...
1. Case study is a research strategy, and not just a method/technique/process of data collection. 2. A case study involves a detailed study of the concerned unit of analysis within its natural setting. A de-contextualised study has no relevance in a case study research. 3. Since an in-depth study is conducted, a case study research allows the
Solar photovoltaic (PV) systems are becoming increasingly popular because they offer a sustainable and cost-effective solution for generating electricity. PV panels are the most critical components of PV systems as they convert solar energy into electric energy. Therefore, analyzing their reliability, risk, safety, and degradation is crucial to ensuring continuous electricity generation based ...
that can help pave the way to the researcher's interpretive judgements and improve the ir quality. By using this paper, novice researchers will be able to reflect more carefully on the ...
Outcomes. ACEs can have lasting effects on health and well-being in childhood and life opportunities well into adulthood. 9 Life opportunities include things like education and job potential. These experiences can increase the risks of injury, sexually transmitted infections, and involvement in sex trafficking.