Information
- Author Services

Initiatives
You are accessing a machine-readable page. In order to be human-readable, please install an RSS reader.
All articles published by MDPI are made immediately available worldwide under an open access license. No special permission is required to reuse all or part of the article published by MDPI, including figures and tables. For articles published under an open access Creative Common CC BY license, any part of the article may be reused without permission provided that the original article is clearly cited. For more information, please refer to https://www.mdpi.com/openaccess .
Feature papers represent the most advanced research with significant potential for high impact in the field. A Feature Paper should be a substantial original Article that involves several techniques or approaches, provides an outlook for future research directions and describes possible research applications.
Feature papers are submitted upon individual invitation or recommendation by the scientific editors and must receive positive feedback from the reviewers.
Editor’s Choice articles are based on recommendations by the scientific editors of MDPI journals from around the world. Editors select a small number of articles recently published in the journal that they believe will be particularly interesting to readers, or important in the respective research area. The aim is to provide a snapshot of some of the most exciting work published in the various research areas of the journal.
Original Submission Date Received: .
- Active Journals
- Find a Journal
- Proceedings Series
- For Authors
- For Reviewers
- For Editors
- For Librarians
- For Publishers
- For Societies
- For Conference Organizers
- Open Access Policy
- Institutional Open Access Program
- Special Issues Guidelines
- Editorial Process
- Research and Publication Ethics
- Article Processing Charges
- Testimonials
- Preprints.org
- SciProfiles
- Encyclopedia
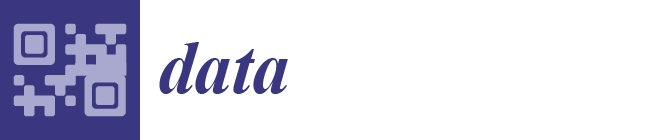
Article Menu
- Subscribe SciFeed
- Recommended Articles
- Google Scholar
- on Google Scholar
- Table of Contents
Find support for a specific problem in the support section of our website.
Please let us know what you think of our products and services.
Visit our dedicated information section to learn more about MDPI.
JSmol Viewer
An interdisciplinary review of camera image collection and analysis techniques, with considerations for environmental conservation social science.
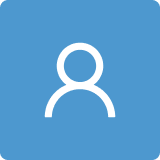
1. Introduction
2. interdisciplinary learning, 3. camera usage as a research method, 3.1. methods are discipline specific and discipline transcending, 3.2. methods have evolved in diversity and complexity, 4. research questions.
- In what contexts have cameras been used in general?
- In what contexts have cameras been used in ECSS?
- What are common image collection techniques for image data?
- What are common analysis techniques for image data?
5. Materials and Methods
6.1. contexts of camera use in general, 6.2. contexts of camera use in ecss, 6.3. common data collection techniques, 6.4. common image analysis techniques, 7. discussion, 8. camera usage, 9. image analysis, 10. limitations, 11. future research, 12. conclusions, supplementary materials, author contributions, conflicts of interest.
- Al-Rousan, T.; Masad, E.; Tutumluer, E.; Pan, T. Evaluation of image analysis techniques for quantifying aggregate shape characteristics. Constr. Build. Mater. 2007 , 21 , 978–990. [ Google Scholar ] [ CrossRef ]
- Anderson, K.; Gaston, K.J. Lightweight unmanned aerial vehicles will revolutionize spatial ecology. Front. Ecol. Environ. 2013 , 11 , 138–146. [ Google Scholar ] [ CrossRef ] [ Green Version ]
- Cox, M. A basic guide for empirical environmental social science. Ecol. Soc. 2015 , 20 . [ Google Scholar ] [ CrossRef ]
- Hazen, D.; Puri, R.; Ramchandran, K. Multi-camera video resolution enhancement by fusion of spatial disparity and temporal motion fields. In Proceedings of the Fourth IEEE International Conference on Computer Vision Systems (ICVS’06), New York, NY, USA, 4–7 January 2006; p. 38. [ Google Scholar ] [ CrossRef ]
- Mansilla, V.B. Interdisciplinary learning: A cognitive-epistemological foundation. In The Oxford handbook of Interdisciplinarity , 2nd ed.; Frodeman, R., Ed.; Oxford University Press: Oxford, UK, 2017. [ Google Scholar ] [ CrossRef ] [ Green Version ]
- Spelt, E.J.H.; Biemans, H.J.A.; Tobi, H.; Luning, P.A.; Mulder, M. Teaching and learning in interdisciplinary higher education: A systematic review. Educ. Psychol. Rev. 2009 , 21 , 365. [ Google Scholar ] [ CrossRef ] [ Green Version ]
- Liu, J.-S.; Huang, T.-K. A project mediation approach to interdisciplinary learning. In Proceedings of the Fifth IEEE International Conference on Advanced Learning Technologies (ICALT’05), Kaohsiung, Taiwan, 5–8 July 2005; pp. 54–58. [ Google Scholar ] [ CrossRef ]
- Johnson, D.T.; Neal, L.; Vantassel-Baska, B.J. Science curriculum review: Evaluating materials for high-ability learners. Gift. Child Q. 1995 , 39 , 36–44. [ Google Scholar ] [ CrossRef ]
- Haigh, W.; Rehfeld, D. Integration of secondary mathematics and science methods courses: A model. Sch. Sci. Math. 1995 , 95 , 240. [ Google Scholar ] [ CrossRef ]
- Alden, D.S.; Laxton, R.; Patzer, G.; Howard, L. Establishing cross-disciplinary marketing education. J. Mark. Educ. 1991 , 13 , 25–30. [ Google Scholar ] [ CrossRef ]
- Dimitropoulos, G.; Hacker, P. Learning and the law: Improving behavioral regulation from an international and comparative perspective. J. Law Policy 2016 , 25 , 473–548. [ Google Scholar ]
- Kucera, K.; Harrison, L.M.; Cappello, M.; Modis, Y. Ancylostoma ceylanicum excretory–secretory protein 2 adopts a netrin-like fold and defines a novel family of nematode proteins. J. Mol. Biol. 2011 , 408 , 9–17. [ Google Scholar ] [ CrossRef ] [ Green Version ]
- Menzie, C.; Ryther, J.; Boyer, L.; Germano, J.; Rhodes, D. Remote methods of mapping seafloor topography, sediment type, bedforms, and benthic biology. OCEANS 1982 , 82 , 1046–1051. [ Google Scholar ] [ CrossRef ]
- Schuckman, K.; Raber, G.T.; Jensen, J.R.; Schill, S. Creation of digital terrain models using an adaptive Lidar vegetation point removal process. Photogramm. Eng. Remote Sens. 2002 , 68 , 1307–1314. [ Google Scholar ]
- An, F.-P. Pedestrian re-recognition algorithm based on optimization deep learning-sequence memory model. Complexity 2019 , 2019 , 1. [ Google Scholar ] [ CrossRef ] [ Green Version ]
- Su, C.; Zhang, S.; Xing, J.; Gao, W.; Tian, Q. Deep attributes driven multi-camera person re-identification. In Computer Vision—ECCV 2016 ; Lecture Notes in Computer Science; Springer: Cham, Switzerland, 2016; Volume 9906. [ Google Scholar ] [ CrossRef ] [ Green Version ]
- Marion, J.L. A review and synthesis of recreation ecology research supporting carrying capacity and visitor use management decisionmaking. J. For. 2016 , 114 , 339–351. [ Google Scholar ] [ CrossRef ]
- Peterson, B.; Brownlee, M.; Sharp, R.; Cribbs, T. Visitor Use and Associated Thresholds at Buffalo National River. In Fulfillment of Cooperative Agreement No. P16AC00194 ; Technical report submitted to the U.S. National Park Service; Clemson University: Clemson, SC, USA, 2018. [ Google Scholar ]
- Schmid Mast, M.; Gatica-Perez, D.; Frauendorfer, D.; Nguyen, L.; Choudhury, T. Social sensing for psychology: Automated interpersonal behavior assessment. Curr. Dir. Psychol. Sci. 2015 , 24 , 154–160. [ Google Scholar ] [ CrossRef ]
- Kharrazi, M.; Sencar, H.T.; Memon, N. Blind source camera identification. In Proceedings of the 2004 International Conference on Image Processing, ICIP ’04, Singapore, 24–27 October 2004; Volume 1, pp. 709–712. [ Google Scholar ] [ CrossRef ]
- Huang, A.S.; Bachrach, A.; Henry, P.; Krainin, M.; Maturana, D.; Fox, D.; Roy, N. Visual odometry and mapping for autonomous flight using an RGB-D Camera. In Robotics Research ; Christensen, H.I., Khatib, O., Eds.; Springer: Berlin/Heidelberg, Germany, 2017; Volume 100, pp. 235–252. [ Google Scholar ] [ CrossRef ]
- Bente, G. Facilities for the graphical computer simulation of head and body movements. Behav. Res. Methods Instrum. Comput. 1989 , 21 , 455–462. [ Google Scholar ] [ CrossRef ]
- Alvar, S.R.; Bajić, I.V. MV-YOLO: Motion vector-aided tracking by semantic object detection. arXiv 2018 , arXiv:1805.00107. [ Google Scholar ]
- Staab, J. Applying Computer Vision for Monitoring Visitor Numbers—A Geographical Approach. Master’s Thesis, University of Wurzburg, Heidelberg, Germany, 2017. Available online: https://www.researchgate.net/publication/320948063_Applying_Computer_Vision_for_Monitoring_Visitor_Numbers_-_A_Geographical_Approach (accessed on 7 June 2020).
- Chouinard, B.; Scott, K.; Cusack, R. Using automatic face analysis to score infant behaviour from video collected online. Infant Behav. Dev. 2019 , 54 , 1–12. [ Google Scholar ] [ CrossRef ]
- Fraser, C.S. Digital camera self-calibration. ISPRS J. Photogramm. Remote Sens. 1997 , 52 , 149–159. [ Google Scholar ] [ CrossRef ]
- Tatsuno, K. Current trends in digital cameras and camera-phones. Sci. Technol. Q. Rev. 2006 , 18 , 35–44. [ Google Scholar ]
- English, F.W. The utility of the camera in qualitative inquiry. Educ. Res. 1988 , 17 , 8–15. [ Google Scholar ] [ CrossRef ]
- Park, J.-I.; Yagi, N.; Enami, K.; Aizawa, K.; Hatori, M. Estimation of camera parameters from image sequence for model-based video coding. IEEE Trans. Circuits Syst. Video Technol. 1994 , 4 , 288–296. [ Google Scholar ] [ CrossRef ]
- Velloso, E.; Bulling, A.; Gellersen, H. AutoBAP: Automatic coding of body action and posture units from wearable sensors. In Proceedings of the 2013 Humaine Association Conference on Affective Computing and Intelligent Interaction, Geneva, Switzerland, 2–5 September 2013; pp. 135–140. [ Google Scholar ] [ CrossRef ] [ Green Version ]
- Rust, C. How artistic inquiry can inform interdisciplinary research. Int. J. Des. 2007 , 1 , 69–76. [ Google Scholar ]
- Zhao, W.; Chellappa, R.; Phillips, P.J.; Rosenfeld, A. Face recognition: A literature survey. ACM Comput. Surv. 2003 , 35 , 399–459. [ Google Scholar ] [ CrossRef ]
- Blaschke, T. Object based image analysis for remote sensing. ISPRS J. Photogramm. Remote Sens. 2010 , 65 , 2–16. [ Google Scholar ] [ CrossRef ] [ Green Version ]
- Pal, N.R.; Pal, S.K. A review of image segmentation techniques. Pattern Recognit. 1993 , 26 , 1277–1294. [ Google Scholar ] [ CrossRef ]
- Lu, D.; Weng, Q. A survey of image classification methods and techniques for improving classification performance. Int. J. Remote Sens. 2007 , 28 , 823–870. [ Google Scholar ] [ CrossRef ]
- Muller, H.; Michoux, N.; Bandon, D.; Geissbuhler, A. A review of content-based image retrieval systems in medical applications—Clinical benefits and future directions. Int. J. Med. Inform. 2004 , 73 , 1–23. [ Google Scholar ] [ CrossRef ]
- Kelly, P.; Marshall, S.J.; Badland, H.; Kerr, J.; Oliver, M.; Doherty, A.R.; Foster, C. An ethical framework for automated, wearable cameras in health behavior research. Am. J. Prev. Med. 2013 , 44 , 314–319. [ Google Scholar ] [ CrossRef ]
- Meek, P.D.; Ballard, G.; Claridge, A.; Kays, R.; Moseby, K.; O’Brien, T.; Townsend, S. Recommended guiding principles for reporting on camera trapping research. Biodivers. Conserv. 2014 , 23 , 2321–2343. [ Google Scholar ] [ CrossRef ]
- Pickering, C.M.; Byrne, J. The benefits of publishing systematic quantitative literature reviews for PhD candidates and other early career researchers. High. Educ. Res. Dev. 2014 , 33 , 534–548. [ Google Scholar ] [ CrossRef ] [ Green Version ]
- Moher, D.; Liberati, A.; Tetzlaff, J.; Altman, D.G.; PRISMA Group. Preferred reporting items for systematic reviews and meta-analyses: The PRISMA Statement. PLoS Med. 2009 , 6 , e1000097. [ Google Scholar ] [ CrossRef ] [ PubMed ] [ Green Version ]
- Burton, A.C.; Neilson, E.; Moreira, D.; Ladle, A.; Steenweg, R.; Fisher, J.T.; Boutin, S. Wildlife camera trapping: A review and recommendations for linking surveys to ecological processes. J. Appl. Ecol. 2015 , 52 , 675–685. [ Google Scholar ] [ CrossRef ]
- Rovero, F.; Marshall, A.R. Camera trapping photographic rate as an index of density in forest ungulates. J. Appl. Ecol. 2009 , 46 , 1011–1017. [ Google Scholar ] [ CrossRef ] [ Green Version ]
- Scotson, L.; Johnston, L.R.; Iannarilli, F.; Wearn, O.R.; Mohd-Azlan, J.; Wong, W.M.; Frechette, J. Best practices and software for the management and sharing of camera trap data for small and large scales studies. Remote Sens. Ecol. Conserv. 2017 , 3 , 158–172. [ Google Scholar ] [ CrossRef ]
- Trolliet, F.; Vermeulen, C.; Huynen, M.C.; Hambuckers, A. Use of camera traps for wildlife studies: A review. Biotechnologie Agronomie Société et Environnement 2014 , 18 , 446–454. [ Google Scholar ]
- Saldana, J. The Coding Manual for Qualitative Researchers , 2nd ed.; Sage Publishing: Los Angeles, CA, USA, 2013. [ Google Scholar ]
- Balomenou, N.; Garrod, B. Photographs in tourism research: Prejudice, power, performance, and participant-generated images. Tour. Manag. 2019 , 70 , 201–217. [ Google Scholar ] [ CrossRef ]
- Rose, J.; Spencer, C. Immaterial labour in spaces of leisure: Producing biopolitical subjectivities through Facebook. Leis. Stud. 2016 , 35 , 809–826. [ Google Scholar ] [ CrossRef ]
Click here to enlarge figure
Agriculture | Biology/Microbiology | Botany/Plant Science | Computer Science/Programming | Engineering and Technology | Environmental Biophysical Sciences | Environmental Conservation Social Science | Food Science | Forestry | Geography | Marine Science | Medicine/Health Science | Other * | Psychology | Urban Studies | Total | |
---|---|---|---|---|---|---|---|---|---|---|---|---|---|---|---|---|
Article | 96 | 100 | 84 | 58 | 68 | 69 | 72 | 100 | 80 | 32 | 40 | 79 | 89 | 100 | 60 | 75 |
Conference Proceedings | 0 | 0 | 0 | 0 | 0 | 0 | 4 | 0 | 0 | 0 | 0 | 0 | 0 | 0 | 20 | 1 |
Dissertation/Thesis | 2 | 0 | 11 | 33 | 24 | 19 | 24 | 0 | 16 | 68 | 20 | 11 | 5 | 0 | 20 | 19 |
Report | 2 | 0 | 5 | 10 | 8 | 12 | 0 | 0 | 4 | 0 | 40 | 11 | 5 | 0 | 0 | 5 |
1985–1989 | 0 | 0 | 0 | 0 | 0 | 0 | 0 | 0 | 0 | 0 | 0 | 11 | 0 | 0 | 0 | 0 |
1990–1994 | 0 | 0 | 0 | 0 | 0 | 0 | 0 | 0 | 0 | 0 | 0 | 0 | 0 | 0 | 0 | 0 |
1995–1999 | 2 | 0 | 5 | 0 | 2 | 4 | 1 | 0 | 12 | 0 | 0 | 0 | 0 | 0 | 0 | 2 |
2000–2004 | 5 | 0 | 11 | 3 | 0 | 4 | 5 | 0 | 16 | 11 | 20 | 0 | 5 | 0 | 0 | 5 |
2005–2009 | 14 | 88 | 21 | 8 | 20 | 27 | 10 | 36 | 24 | 32 | 0 | 32 | 0 | 17 | 0 | 19 |
2010–2014 | 39 | 0 | 37 | 0 | 3 | 23 | 27 | 14 | 20 | 37 | 0 | 21 | 26 | 33 | 40 | 21 |
2015–2019 | 41 | 13 | 26 | 90 | 75 | 42 | 57 | 50 | 28 | 21 | 80 | 37 | 68 | 50 | 60 | 53 |
Africa | 0 | 0 | 0 | 8 | 0 | 4 | 7 | 0 | 0 | 0 | 0 | 0 | 0 | 0 | 0 | 3 |
Asia | 11 | 0 | 26 | 25 | 34 | 12 | 15 | 21 | 32 | 16 | 20 | 16 | 11 | 0 | 20 | 18 |
Australia/Oceania | 4 | 0 | 11 | 0 | 8 | 8 | 11 | 7 | 4 | 0 | 20 | 5 | 5 | 0 | 0 | 7 |
Europe | 33 | 38 | 11 | 28 | 22 | 19 | 22 | 7 | 24 | 5 | 0 | 21 | 32 | 17 | 0 | 21 |
North America | 18 | 63 | 37 | 38 | 32 | 50 | 32 | 29 | 36 | 74 | 20 | 53 | 26 | 67 | 80 | 37 |
South America | 7 | 0 | 0 | 0 | 2 | 0 | 5 | 36 | 0 | 0 | 0 | 0 | 5 | 0 | 0 | 4 |
International | 7 | 0 | 0 | 3 | 0 | 4 | 7 | 0 | 0 | 5 | 40 | 0 | 5 | 17 | 0 | 4 |
Not Mentioned | 20 | 0 | 16 | 0 | 2 | 4 | 1 | 0 | 4 | 0 | 0 | 5 | 16 | 0 | 0 | 5 |
Agriculture | Biology/Microbiology | Botany/Plant Science | Computer Science/Programming | Engineering and Technology | Environmental Biophysical Sciences | Environmental Conservation Social Science | Food Science | Forestry | Geography | Marine Science | Medicine/Health Science | Other * | Psychology | Urban Studies | Total | |
---|---|---|---|---|---|---|---|---|---|---|---|---|---|---|---|---|
Aircraft | 38 | 13 | 27 | 5 | 16 | 21 | 19 | 14 | 29 | 38 | 0 | 0 | 12 | 0 | 0 | 18 |
Computer | 3 | 0 | 7 | 25 | 23 | 8 | 3 | 7 | 0 | 0 | 0 | 6 | 6 | 0 | 0 | 8 |
Indoor lab equipment | 16 | 75 | 33 | 13 | 30 | 4 | 0 | 43 | 14 | 0 | 0 | 78 | 35 | 33 | 0 | 20 |
Outdoor fixed | 38 | 13 | 33 | 35 | 14 | 29 | 60 | 29 | 48 | 6 | 50 | 0 | 12 | 17 | 0 | 34 |
Participatory | 0 | 0 | 0 | 15 | 2 | 8 | 5 | 0 | 5 | 0 | 0 | 0 | 18 | 17 | 25 | 5 |
Satellite | 0 | 0 | 0 | 0 | 2 | 21 | 3 | 0 | 5 | 56 | 25 | 0 | 6 | 0 | 75 | 6 |
Vehicle | 3 | 0 | 0 | 5 | 7 | 4 | 0 | 0 | 0 | 0 | 0 | 6 | 0 | 0 | 0 | 2 |
Watercraft | 0 | 0 | 0 | 0 | 0 | 4 | 6 | 0 | 0 | 0 | 25 | 0 | 0 | 0 | 0 | 2 |
Wearable | 3 | 0 | 0 | 3 | 7 | 0 | 5 | 7 | 0 | 0 | 0 | 11 | 12 | 33 | 0 | 4 |
Alternate/Modified | 6 | 21 | 19 | 2 | 14 | 3 | 5 | 29 | 11 | 8 | 0 | 8 | 10 | 0 | 0 | 9 |
Fixed/Mounted | 45 | 57 | 48 | 59 | 36 | 43 | 50 | 43 | 36 | 23 | 67 | 50 | 29 | 60 | 0 | 46 |
Held/Worn | 6 | 14 | 5 | 17 | 26 | 13 | 15 | 19 | 18 | 0 | 0 | 19 | 33 | 20 | 0 | 17 |
Moving | 27 | 0 | 14 | 7 | 17 | 33 | 22 | 10 | 29 | 69 | 33 | 8 | 14 | 0 | 100 | 19 |
Multiple | 3 | 0 | 5 | 2 | 0 | 0 | 2 | 0 | 4 | 0 | 0 | 4 | 5 | 0 | 0 | 2 |
Security/Surveillance | 12 | 7 | 10 | 14 | 7 | 7 | 6 | 0 | 4 | 0 | 0 | 12 | 10 | 20 | 0 | 7 |
Automated | 22 | 0 | 0 | 55 | 55 | 0 | 10 | 29 | 17 | 57 | 33 | 25 | 29 | 50 | 0 | 32 |
Geospatial | 22 | 0 | 33 | 5 | 9 | 50 | 27 | 0 | 33 | 43 | 67 | 0 | 0 | 0 | 71 | 20 |
LiDAR | 11 | 0 | 0 | 5 | 0 | 0 | 3 | 0 | 0 | 0 | 0 | 0 | 0 | 0 | 0 | 3 |
Manual | 22 | 0 | 33 | 10 | 18 | 50 | 50 | 57 | 33 | 0 | 0 | 75 | 29 | 50 | 14 | 29 |
Mixed methods | 22 | 0 | 33 | 25 | 18 | 0 | 10 | 14 | 17 | 0 | 0 | 0 | 43 | 0 | 14 | 16 |
Share and Cite
Little, C.L.; Perry, E.E.; Fefer, J.P.; Brownlee, M.T.J.; Sharp, R.L. An Interdisciplinary Review of Camera Image Collection and Analysis Techniques, with Considerations for Environmental Conservation Social Science. Data 2020 , 5 , 51. https://doi.org/10.3390/data5020051
Little CL, Perry EE, Fefer JP, Brownlee MTJ, Sharp RL. An Interdisciplinary Review of Camera Image Collection and Analysis Techniques, with Considerations for Environmental Conservation Social Science. Data . 2020; 5(2):51. https://doi.org/10.3390/data5020051
Little, Coleman L., Elizabeth E. Perry, Jessica P. Fefer, Matthew T. J. Brownlee, and Ryan L. Sharp. 2020. "An Interdisciplinary Review of Camera Image Collection and Analysis Techniques, with Considerations for Environmental Conservation Social Science" Data 5, no. 2: 51. https://doi.org/10.3390/data5020051
Article Metrics
Article access statistics, supplementary material.
PDF-Document (PDF, 467 KiB)
Further Information
Mdpi initiatives, follow mdpi.
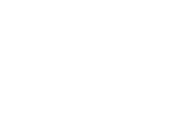
Subscribe to receive issue release notifications and newsletters from MDPI journals
- DOI: 10.18494/sam.2018.1785
- Corpus ID: 251276771
Autofocus System and Evaluation Methodologies: A Literature Review
- Yupeng Zhang , Liyan Liu , +5 authors T. Ueda
- Published 2018
- Engineering, Computer Science
Figures from this paper
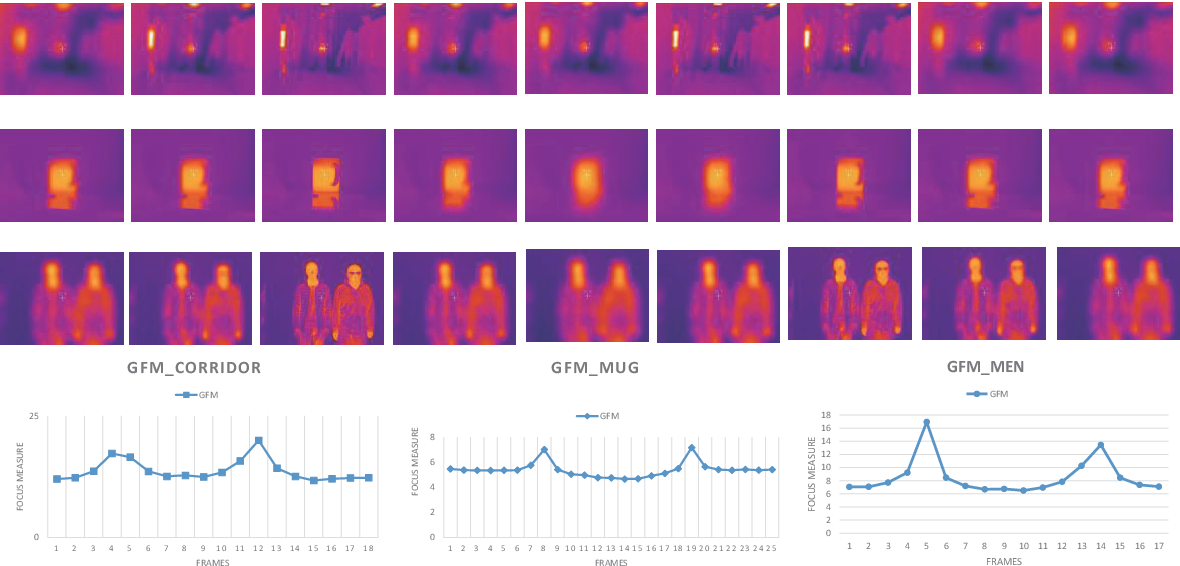
29 Citations
Passive autofocusing system for a thermal camera, design of wide angle and large aperture optical system with inner focus for compact system camera applications, dual-camera high magnification surveillance system with non-delay gaze control and always-in-focus function in indoor scenes, high-magnification object tracking with ultra-fast view adjustment and continuous autofocus based on dynamic-range focal sweep, a study on the image based auto-focus method considering jittering of airborne eo/ir, small zoom mismatch adjustment method for dual-band fusion imaging system based on edge-gradient normalized mutual information, can liquid lenses increase depth of field in head mounted video see-through devices, autofocusing imaging based on electrically controlled liquid-crystal microlens array, robust fastener detection based on force and vision algorithms in robotic (un)screwing applications, learning to autofocus based on gradient boosting machine for optical microscopy, 7 references, managing gigabytes: compressing and indexing documents and images, is&t / spie electronic imaging, related papers.
Showing 1 through 3 of 0 Related Papers
IEEE Account
- Change Username/Password
- Update Address
Purchase Details
- Payment Options
- Order History
- View Purchased Documents
Profile Information
- Communications Preferences
- Profession and Education
- Technical Interests
- US & Canada: +1 800 678 4333
- Worldwide: +1 732 981 0060
- Contact & Support
- About IEEE Xplore
- Accessibility
- Terms of Use
- Nondiscrimination Policy
- Privacy & Opting Out of Cookies
A not-for-profit organization, IEEE is the world's largest technical professional organization dedicated to advancing technology for the benefit of humanity. © Copyright 2024 IEEE - All rights reserved. Use of this web site signifies your agreement to the terms and conditions.
- Survey paper
- Open access
- Published: 06 June 2019
Intelligent video surveillance: a review through deep learning techniques for crowd analysis
- G. Sreenu ORCID: orcid.org/0000-0002-2298-9177 1 &
- M. A. Saleem Durai 1
Journal of Big Data volume 6 , Article number: 48 ( 2019 ) Cite this article
73k Accesses
265 Citations
12 Altmetric
Metrics details
Big data applications are consuming most of the space in industry and research area. Among the widespread examples of big data, the role of video streams from CCTV cameras is equally important as other sources like social media data, sensor data, agriculture data, medical data and data evolved from space research. Surveillance videos have a major contribution in unstructured big data. CCTV cameras are implemented in all places where security having much importance. Manual surveillance seems tedious and time consuming. Security can be defined in different terms in different contexts like theft identification, violence detection, chances of explosion etc. In crowded public places the term security covers almost all type of abnormal events. Among them violence detection is difficult to handle since it involves group activity. The anomalous or abnormal activity analysis in a crowd video scene is very difficult due to several real world constraints. The paper includes a deep rooted survey which starts from object recognition, action recognition, crowd analysis and finally violence detection in a crowd environment. Majority of the papers reviewed in this survey are based on deep learning technique. Various deep learning methods are compared in terms of their algorithms and models. The main focus of this survey is application of deep learning techniques in detecting the exact count, involved persons and the happened activity in a large crowd at all climate conditions. Paper discusses the underlying deep learning implementation technology involved in various crowd video analysis methods. Real time processing, an important issue which is yet to be explored more in this field is also considered. Not many methods are there in handling all these issues simultaneously. The issues recognized in existing methods are identified and summarized. Also future direction is given to reduce the obstacles identified. The survey provides a bibliographic summary of papers from ScienceDirect, IEEE Xplore and ACM digital library.
Bibliographic Summary of papers in different digital repositories
Bibliographic summary about published papers under the area “Surveillance video analysis through deep learning” in digital repositories like ScienceDirect, IEEExplore and ACM are graphically demonstrated.
ScienceDirect
SceinceDirect lists around 1851 papers. Figure 1 demonstrates the year wise statistics.
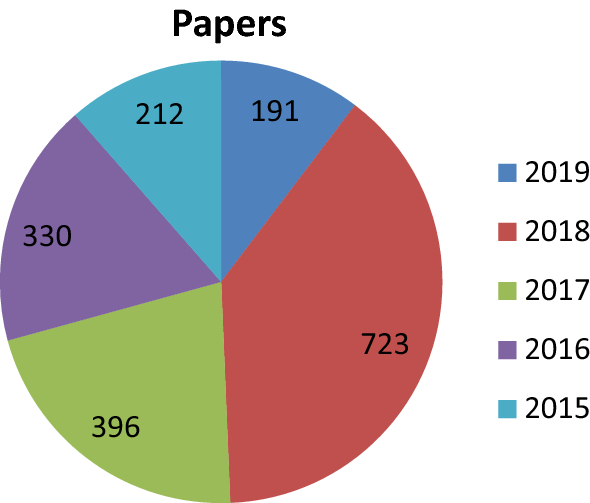
Year wise paper statistics of “surveillance video analysis by deep learning”, in ScienceDirect
Table 1 list title of 25 papers published under same area.
Table 2 gives the list of journals in ScienceDirect where above mentioned papers are published.
Keywords always indicate the main disciplines of the paper. An analysis is conducted through keywords used in published papers. Table 3 list the frequency of most frequently used keywords.
ACM digital library includes 20,975 papers in the given area. The table below includes most recently published surveillance video analysis papers under deep learning field. Table 4 lists the details of published papers in the area.
IEEE Xplore
Table 5 shows details of published papers in the given area in IEEEXplore digital library.
Violence detection among crowd
The above survey presents the topic surveillance video analysis as a general topic. By going more deeper into the area more focus is given to violence detection in crowd behavior analysis.
Table 6 lists papers specific to “violence detection in crowd behavior” from above mentioned three journals.
Introduction
Artificial intelligence paves the way for computers to think like human. Machine learning makes the way more even by adding training and learning components. The availability of huge dataset and high performance computers lead the light to deep learning concept, which extract automatically features or the factors of variation that distinguishes objects from one another. Among the various data sources which contribute to terabytes of big data, video surveillance data is having much social relevance in today’s world. The widespread availability of surveillance data from cameras installed in residential areas, industrial plants, educational institutions and commercial firms contribute towards private data while the cameras placed in public places such as city centers, public conveyances and religious places contribute to public data.
Analysis of surveillance videos involves a series of modules like object recognition, action recognition and classification of identified actions into categories like anomalous or normal. This survey giving specific focus on solutions based on deep learning architectures. Among the various architectures in deep learning, commonly used models for surveillance analysis are CNN, auto-encoders and their combination. The paper Video surveillance systems-current status and future trends [ 14 ] compares 20 papers published recently in the area of surveillance video analysis. The paper begins with identifying the main outcomes of video analysis. Application areas where surveillance cameras are unavoidable are discussed. Current status and trends in video analysis are revealed through literature review. Finally the vital points which need more consideration in near future are explicitly stated.
Surveillance video analysis: relevance in present world
The main objectives identified which illustrate the relevance of the topic are listed out below.
Continuous monitoring of videos is difficult and tiresome for humans.
Intelligent surveillance video analysis is a solution to laborious human task.
Intelligence should be visible in all real world scenarios.
Maximum accuracy is needed in object identification and action recognition.
Tasks like crowd analysis are still needs lot of improvement.
Time taken for response generation is highly important in real world situation.
Prediction of certain movement or action or violence is highly useful in emergency situation like stampede.
Availability of huge data in video forms.
The majority of papers covered for this survey give importance to object recognition and action detection. Some papers are using procedures similar to a binary classification that whether action is anomalous or not anomalous. Methods for Crowd analysis and violence detection are also included. Application areas identified are included in the next section.
Application areas identified
The contexts identified are listed as application areas. Major part in existing work provides solutions specifically based on the context.
Traffic signals and main junctions
Residential areas
Crowd pulling meetings
Festivals as part of religious institutions
Inside office buildings
Among the listed contexts crowd analysis is the most difficult part. All type of actions, behavior and movement are needed to be identified.
Surveillance video data as Big Data
Big video data have evolved in the form of increasing number of public cameras situated towards public places. A huge amount of networked public cameras are positioned around worldwide. A heavy data stream is generated from public surveillance cameras that are creatively exploitable for capturing behaviors. Considering the huge amount of data that can be documented over time, a vital scenario is facility for data warehousing and data analysis. Only one high definition video camera can produce around 10 GB of data per day [ 87 ].
The space needed for storing large amount of surveillance videos for long time is difficult to allot. Instead of having data, it will be useful to have the analysis result. That will result in reduced storage space. Deep learning techniques are involved with two main components; training and learning. Both can be achieved with highest accuracy through huge amount of data.
Main advantages of training with huge amount of data are listed below. It’s possible to adapt variety in data representation and also it can be divided into training and testing equally. Various data sets available for analysis are listed below. The dataset not only includes video sequences but also frames. The analysis part mainly includes analysis of frames which were extracted from videos. So dataset including images are also useful.
The datasets widely used for various kinds of application implementation are listed in below Table 7 . The list is not specific to a particular application though it is specified against an application.
Methods identified/reviewed other than deep learning
Methods identified are mainly classified into two categories which are either based on deep learning or not based on deep learning. This section is reviewing methods other than deep learning.
SVAS deals with automatic recognition and deduction of complex events. The event detection procedure consists of mainly two levels, low level and high level. As a result of low level analysis people and objects are detected. The results obtained from low level are used for high level analysis that is event detection. The architecture proposed in the model includes five main modules. The five sections are
Event model learning
Action model learning
Action detection
Complex event model learning
Complex event detection
Interval-based spatio-temporal model (IBSTM) is the proposed model and is a hybrid event model. Other than this methods like Threshold models, Bayesian Networks, Bag of actions and Highly cohesive intervals and Markov logic networks are used.
SVAS method can be improved to deal with moving camera and multi camera data set. Further enhancements are needed in dealing with complex events specifically in areas like calibration and noise elimination.
Multiple anomalous activity detection in videos [ 88 ] is a rule based system. The features are identified as motion patterns. Detection of anomalous events are done either by training the system or by following dominant set property.
The concept of dominant set where events are detected as normal based on dominant behavior and anomalous events are decided based on less dominant behavior. The advantage of rule based system is that easy to recognize new events by modifying some rules. The main steps involved in a recognition system are
Pre processing
Feature extraction
Object tracking
Behavior understanding
As a preprocessing system video segmentation is used. Background modeling is implemented through Gaussian Mixture Model (GMM). For object recognition external rules are required. The system is implemented in Matlab 2014. The areas were more concentration further needed are doubtful activities and situations where multiple object overlapping happens.
Mining anomalous events against frequent sequences in surveillance videos from commercial environments [ 89 ] focus on abnormal events linked with frequent chain of events. The main result in identifying such events is early deployment of resources in particular areas. The implementation part is done using Matlab, Inputs are already noticed events and identified frequent series of events. The main investigation under this method is to recognize events which are implausible to chase given sequential pattern by fulfilling the user identified parameters.
The method is giving more focus on event level analysis and it will be interesting if pay attention at entity level and action level. But at the same time going in such granular level make the process costly.
Video feature descriptor combining motion and appearance cues with length invariant characteristics [ 90 ] is a feature descriptor. Many trajectory based methods have been used in abundant installations. But those methods have to face problems related with occlusions. As a solution to that, feature descriptor using optical flow based method.
As per the algorithm the training set is divided into snippet set. From each set images are extracted and then optical flow are calculated. The covariance is calculated from optical flow. One class SVM is used for learning samples. For testing also same procedure is performed.
The model can be extended in future by handling local abnormal event detection through proposed feature which is related with objectness method.
Multiple Hierarchical Dirichlet processes for anomaly detection in Traffic [ 91 ] is mainly for understanding the situation in real world traffic. The anomalies are mainly due to global patterns instead of local patterns. That include entire frame. Concept of super pixel is included. Super pixels are grouped into regions of interest. Optical flow based method is used for calculating motion in each super pixel. Points of interest are then taken out in active super pixel. Those interested points are then tracked by Kanade–Lucas–Tomasi (KLT) tracker.
The method is better the handle videos involving complex patterns with less cost. But not mentioning about videos taken in rainy season and bad weather conditions.
Intelligent video surveillance beyond robust background modeling [ 92 ] handle complex environment with sudden illumination changes. Also the method will reduce false alerts. Mainly two components are there. IDS and PSD are the two components.
First stage intruder detection system will detect object. Classifier will verify the result and identify scenes causing problems. Then in second stage problematic scene descriptor will handle positives generated from IDS. Global features are used to avoid false positives from IDS.
Though the method deals with complex scenes, it does not mentioning about bad weather conditions.
Towards abnormal trajectory and event detection in video surveillance [ 93 ] works like an integrated pipeline. Existing methods either use trajectory based approaches or pixel based approaches. But this proposal incorporates both methods. Proposal include components like
Object and group tracking
Grid based analysis
Trajectory filtering
Abnormal behavior detection using actions descriptors
The method can identify abnormal behavior in both individual and groups. The method can be enhanced by adapting it to work in real time environment.
RIMOC: a feature to discriminate unstructured motions: application to violence detection for video surveillance [ 94 ]. There is no unique definition for violent behaviors. Those kind of behaviors show large variances in body poses. The method works by taking the eigen values of histograms of optical flow.
The input video undergoes dense sampling. Local spatio temporal volumes are created around each sampled point. Those frames of STV are coded as histograms of optical flow. Eigen values are computed from this frame. The papers already published in surveillance area span across a large set. Among them methods which are unique in either implementation method or the application for which it is proposed are listed in the below Table 8 .
The methods already described and listed are able to perform following steps
Object detection
Object discrimination
Action recognition
But these methods are not so efficient in selecting good features in general. The lag identified in methods was absence of automatic feature identification. That issue can be solved by applying concepts of deep learning.
The evolution of artificial intelligence from rule based system to automatic feature identification passes machine learning, representation learning and finally deep learning.
Real-time processing in video analysis
Real time Violence Detection Framework for Football Stadium comprising of Big Data Analysis and deep learning through Bidirectional LSTM [ 103 ] predicts violent behavior of crowd in real time. The real time processing speed is achieved through SPARK frame work. The model architecture includes Apache spark framework, spark streaming, Histogram of oriented Gradients function and bidirectional LSTM. The model takes stream of videos from diverse sources as input. The videos are converted in the form of non overlapping frames. Features are extracted from this group of frames through HOG FUNCTION. The images are manually modeled into different groups. The BDLSTM is trained through all these models. The SPARK framework handles the streaming data in a micro batch mode. Two kinds of processing are there like stream and batch processing.
Intelligent video surveillance for real-time detection of suicide attempts [ 104 ] is an effort to prevent suicide by hanging in prisons. The method uses depth streams offered by an RGB-D camera. The body joints’ points are analyzed to represent suicidal behavior.
Spatio-temporal texture modeling for real-time crowd anomaly detection [ 105 ]. Spatio temporal texture is a combination of spatio temporal slices and spatio temporal volumes. The information present in these slices are abstracted through wavelet transforms. A Gaussian approximation model is applied to texture patterns to distinguish normal behaviors from abnormal behaviors.
Deep learning models in surveillance
Deep convolutional framework for abnormal behavior detection in a smart surveillance system [ 106 ] includes three sections.
Human subject detection and discrimination
A posture classification module
An abnormal behavior detection module
The models used for above three sections are, Correspondingly
You only look once (YOLO) network
Long short-term memory (LSTM)
For object discrimination Kalman filter based object entity discrimination algorithm is used. Posture classification study recognizes 10 types of poses. RNN uses back propagation through time (BPTT) to update weight.
The main issue identified in the method is that similar activities like pointing and punching are difficult to distinguish.
Detecting Anomalous events in videos by learning deep representations of appearance and motion [ 107 ] proposes a new model named as AMDN. The model automatically learns feature representations. The model uses stacked de-noising auto encoders for learning appearance and motion features separately and jointly. After learning, multiple one class SVM’s are trained. These SVM predict anomaly score of each input. Later these scores are combined and detect abnormal event. A double fusion framework is used. The computational overhead in testing time is too high for real time processing.
A study of deep convolutional auto encoders for anomaly detection in videos [ 12 ] proposes a structure that is a mixture of auto encoders and CNN. An auto encoder includes an encoder part and decoder part. The encoder part includes convolutional and pooling layers, the decoding part include de convolutional and unpool layers. The architecture allows a combination of low level frames withs high level appearance and motion features. Anomaly scores are represented through reconstruction errors.
Going deeper with convolutions [ 108 ] suggests improvements over traditional neural network. Fully connected layers are replaced by sparse ones by adding sparsity into architecture. The paper suggests for dimensionality reduction which help to reduce the increasing demand for computational resources. Computing reductions happens with 1 × 1 convolutions before reaching 5 × 5 convolutions. The method is not mentioning about the execution time. Along with that not able to make conclusion about the crowd size that the method can handle successfully.
Deep learning for visual understanding: a review [ 109 ], reviewing the fundamental models in deep learning. Models and technique described were CNN, RBM, Autoencoder and Sparse coding. The paper also mention the drawbacks of deep learning models such as people were not able to understand the underlying theory very well.
Deep learning methods other than the ones discussed above are listed in the following Table 9 .
The methods reviewed in above sections are good in automatic feature generation. All methods are good in handling individual entity and group entities with limited size.
Majority of problems in real world arises among crowd. Above mentioned methods are not effective in handling crowd scenes. Next section will review intelligent methods for analyzing crowd video scenes.
Review in the field of crowd analysis
The review include methods which are having deep learning background and methods which are not having that background.
Spatial temporal convolutional neural networks for anomaly detection and localization in crowded scenes [ 114 ] shows the problem related with crowd analysis is challenging because of the following reasons
Large number of pedestrians
Close proximity
Volatility of individual appearance
Frequent partial occlusions
Irregular motion pattern in crowd
Dangerous activities like crowd panic
Frame level and pixel level detection
The paper suggests optical flow based solution. The CNN is having eight layers. Training is based on BVLC caffe. Random initialization of parameters is done and system is trained through stochastic gradient descent based back propagation. The implementation part is done by considering four different datasets like UCSD, UMN, Subway and finally U-turn. The details of implementation regarding UCSD includes frame level and pixel level criterion. Frame level criterion concentrates on temporal domain and pixel level criterion considers both spatiial and temporal domain. Different metrics to evaluate performance includes EER (Equal Error Rate) and Detection Rate (DR).
Online real time crowd behavior detection in video sequences [ 115 ] suggests FSCB, behavior detection through feature tracking and image segmentation. The procedure involves following steps
Feature detection and temporal filtering
Image segmentation and blob extraction
Activity detection
Activity map
Activity analysis
The main advantage is no need of training stage for this method. The method is quantitatively analyzed through ROC curve generation. The computational speed is evaluated through frame rate. The data set considered for experiments include UMN, PETS2009, AGORASET and Rome Marathon.
Deep learning for scene independent crowd analysis [ 82 ] proposes a scene independent method which include following procedures
Crowd segmentation and detection
Crowd tracking
Crowd counting
Pedestrian travelling time estimation
Crowd attribute recognition
Crowd behavior analysis
Abnormality detection in a crowd
Attribute recognition is done thorugh a slicing CNN. By using a 2D CNN model learn appearance features then represent it as a cuboid. In the cuboid three temporal filters are identified. Then a classifier is applied on concatenated feature vector extracted from cuboid. Crowd counting and crowd density estimation is treated as a regression problem. Crowd attribute recognition is applied on WWW Crowd dataset. Evaluation metrics used are AUC and AP.
The analysis of High Density Crowds in videos [ 80 ] describes methods like data driven crowd analysis and density aware tracking. Data driven analysis learn crowd motion patterns from large collection of crowd videos through an off line manner. Learned pattern can be applied or transferred in applications. The solution includes a two step procedure. Global crowded scene matching and local crowd patch matching. Figure 2 illustrates the two step procedure.
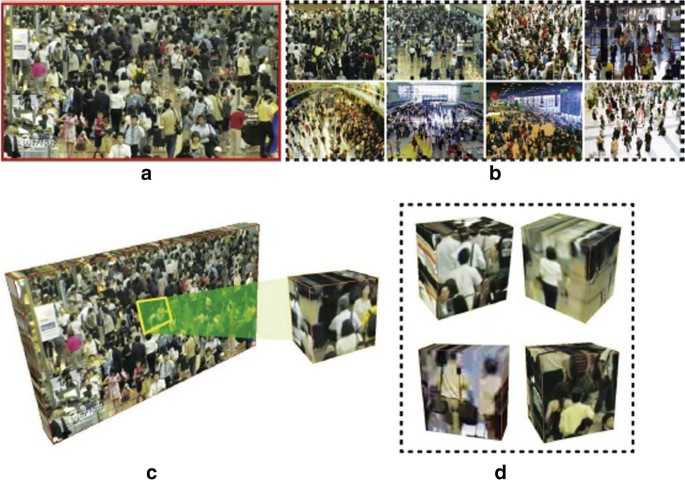
a Test video, b results of global matching, c a query crowd patch, d matching crowd patches [ 80 ]
The database selected for experimental evaluation includes 520 unique videos with 720 × 480 resolutions. The main evaluation is to track unusual and unexpected actions of individuals in a crowd. Through experiments it is proven that data driven tracking is better than batch mode tracking. Density based person detection and tracking include steps like baseline detector, geometric filtering and tracking using density aware detector.
A review on classifying abnormal behavior in crowd scene [ 77 ] mainly demonstrates four key approaches such as Hidden Markov Model (HMM), GMM, optical flow and STT. GMM itself is enhanced with different techniques to capture abnormal behaviours. The enhanced versions of GMM are
GMM and Markov random field
Gaussian poisson mixture model and
GMM and support vector machine
GMM architecture includes components like local descriptor, global descriptor, classifiers and finally a fusion strategy. The distinction between normal and and abnormal behaviour is evaluated based on Mahalanobis distance method. GMM–MRF model mainly divided into two sections where first section identifies motion pttern through GMM and crowd context modelling is done through MRF. GPMM adds one extra feture such as count of occurrence of observed behaviour. Also EM is used for training at later stage of GPMM. GMM–SVM incorporate features such as crowd collectiveness, crowd density, crowd conflict etc. for abnormality detection.
HMM has also variants like
HM and OSVMs
Hidden Markov Model is a density aware detection method used to detect motion based abnormality. The method generates foreground mask and perspective mask through ORB detector. GM-HMM involves four major steps. First step GMBM is used for identifying foreground pixels and further lead to development of blobs generation. In second stage PCA–HOG and motion HOG are used for feature extraction. The third stage applies k means clustering to separately cluster features generated through PCA–HOG and motion–HOG. In final stage HMM processes continuous information of moving target through the application of GM. In SLT-HMM short local trajectories are used along with HMM to achieve better localization of moving objects. MOHMM uses KLT in first phase to generate trajectories and clustering is applied on them. Second phase uses MOHMM to represent the trajectories to define usual and unusual frames. OSVM uses kernel functions to solve the nonlinearity problem by mapping high dimensional features in to a linear space by using kernel function.
In optical flow based method the enhancements made are categorized into following techniques such as HOFH, HOFME, HMOFP and MOFE.
In HOFH video frames are divided into several same size patches. Then optical flows are extracted. It is divided into eight directions. Then expectation and variance features are used to calculate optical flow between frames. HOFME descriptor is used at the final stage of abnormal behaviour detection. As the first step frame difference is calculated then extraction of optical flow pattern and finally spatio temporal description using HOFME is completed. HMOFP Extract optical flow from each frame and divided into patches. The optical flows are segmented into number of bins. Maximum amplitude flows are concatenated to form global HMOFP. MOFE method convert frames into blobs and optical flow in all the blobs are extracted. These optical flow are then clustered into different groups. In STT, crowd tracking and abnormal behaviour detection is done through combing spatial and temporal dimensions of features.
Crowd behaviour analysis from fixed and moving cameras [ 78 ] covers topics like microscopic and macroscopic crowd modeling, crowd behavior and crowd density analysis and datasets for crowd behavior analysis. Large crowds are handled through macroscopic approaches. Here agents are handled as a whole. In microscopic approaches agents are handled individually. Motion information to represent crowd can be collected through fixed and moving cameras. CNN based methods like end-to-end deep CNN, Hydra-CNN architecture, switching CNN, cascade CNN architecture, 3D CNN and spatio temporal CNN are discussed for crowd behaviour analysis. Different datasets useful specifically for crowd behaviour analysis are also described in the chapter. The metrics used are MOTA (multiple person tracker accuracy) and MOTP (multiple person tracker precision). These metrics consider multi target scenarios usually present in crowd scenes. The dataset used for experimental evaluation consists of UCSD, Violent-flows, CUHK, UCF50, Rodriguez’s, The mall and finally the worldExpo’s dataset.
Zero-shot crowd behavior recognition [ 79 ] suggests recognizers with no or little training data. The basic idea behind the approach is attribute-context cooccurrence. Prediction of behavioural attribute is done based on their relationship with known attributes. The method encompass different steps like probabilistic zero shot prediction. The method calculates the conditional probability of known to original appropriate attribute relation. The second step includes learning attribute relatedness from Text Corpora and Context learning from visual co-occurrence. Figure 3 shows the illustration of results.
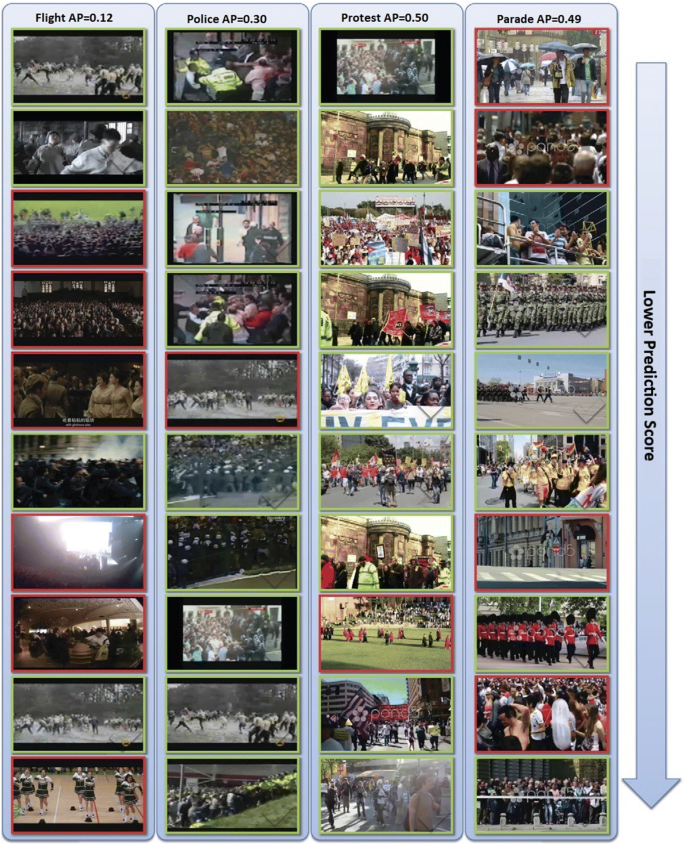
Demonstration of crowd videos ranked in accordance with prediction values [ 79 ]
Computer vision based crowd disaster avoidance system: a survey [ 81 ] covers different perspectives of crowd scene analysis such as number of cameras employed and target of interest. Along with that crowd behavior analysis, people count, crowd density estimation, person re identification, crowd evacuation, and forensic analysis on crowd disaster and computations on crowd analysis. A brief summary about benchmarked datasets are also given.
Fast Face Detection in Violent Video Scenes [ 83 ] suggests an architecture with three steps such as violent scene detector, a normalization algorithm and finally a face detector. ViF descriptor along with Horn–Schunck is used for violent scene detection, used as optical flow algorithm. Normalization procedure includes gamma intensity correction, difference Gauss, Local Histogram Coincidence and Local Normal Distribution. Face detection involve mainly two stages. First stage is segmenting regions of skin and the second stage check each component of face.
Rejecting Motion Outliers for Efficient Crowd Anomaly Detection [ 54 ] provides a solution which consists of two phases. Feature extraction and anomaly classification. Feature extraction is based on flow. Different steps involved in the pipeline are input video is divided into frames, frames are divided into super pixels, extracting histogram for each super pixel, aggregating histograms spatially and finally concatenation of combined histograms from consecutive frames for taking out final feature. Anomaly can be detected through existing classification algorithms. The implementation is done through UCSD dataset. Two subsets with resolution 158 × 238 and 240 × 360 are present. The normal behavior was used to train k means and KUGDA. The normal and abnormal behavior is used to train linear SVM. The hardware part includes Artix 7 xc7a200t FPGA from Xilinx, Xilinx IST and XPower Analyzer.
Deep Metric Learning for Crowdedness Regression [ 84 ] includes deep network model where learning of features and distance measurements are done concurrently. Metric learning is used to study a fine distance measurement. The proposed model is implemented through Tensorflow package. Rectified linear unit is used as an activation function. The training method applied is gradient descent. Performance is evaluated through mean squared error and mean absolute error. The WorldExpo dataset and the Shanghai Tech dataset are used for experimental evaluation.
A Deep Spatiotemporal Perspective for Understanding Crowd Behavior [ 61 ] is a combination of convolution layer and long short-term memory. Spatial informations are captured through convolution layer and temporal motion dynamics are confined through LSTM. The method forecasts the pedestrian path, estimate the destination and finally categorize the behavior of individuals according to motion pattern. Path forecasting technique includes two stacked ConvLSTM layers by 128 hidden states. Kernel of ConvLSTM size is 3 × 3, with a stride of 1 and zeropadding. Model takes up a single convolution layer with a 1 × 1 kernel size. Crowd behavior classification is achieved through a combination of three layers namely an average spatial pooling layer, a fully connected layer and a softmax layer.
Crowded Scene Understanding by Deeply Learned Volumetric Slices [ 85 ] suggests a deep model and different fusion approaches. The architecture involves convolution layers, global sum pooling layer and fully connected layers. Slice fusion and weight sharing schemes are required by the architecture. A new multitask learning deep model is projected to equally study motion features and appearance features and successfully join them. A new concept of crowd motion channels are designed as input to the model. The motion channel analyzes the temporal progress of contents in crowd videos. The motion channels are stirred by temporal slices that clearly demonstrate the temporal growth of contents in crowd videos. In addition, we also conduct wide-ranging evaluations by multiple deep structures with various data fusion and weights sharing schemes to find out temporal features. The network is configured with convlutional layer, pooling layer and fully connected layer with activation functions such as rectified linear unit and sigmoid function. Three different kinds of slice fusion techniques are applied to measure the efficiency of proposed input channels.
Crowd Scene Understanding from Video A survey [ 86 ] mainly deals with crowd counting. Different approaches for crowd counting are categorized into six. Pixel level analysis, texture level analysis, object level analysis, line counting, density mapping and joint detection and counting. Edge features are analyzed through pixel level analysis. Image patches are analysed through texture level analysis. Object level analysis is more accurate compared to pixel and texture analysis. The method identifies individual subjects in a scene. Line counting is used to take the count of people crossed a particular line.
Table 10 will discuss some more crowd analysis methods.
Results observed from the survey and future directions
The accuracy analysis conducted for some of the above discussed methods based on various evaluation criteria like AUC, precision and recall are discussed below.
Rejecting Motion Outliers for Efficient Crowd Anomaly Detection [ 54 ] compare different methods as shown in Fig. 4 . KUGDA is a classifier proposed in Rejecting Motion Outliers for Efficient Crowd Anomaly Detection [ 54 ].
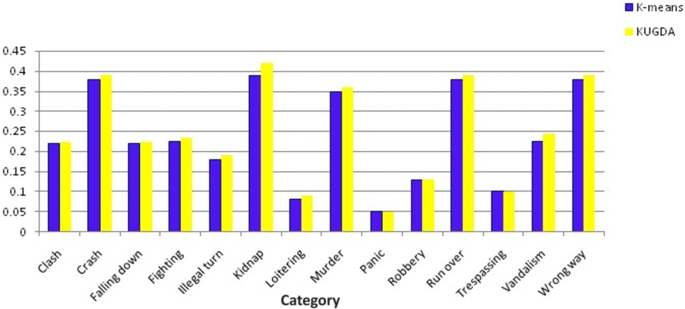
Comparing KUGDA with K-means [ 54 ]
Fast Face Detection in Violent Video Scenes [ 83 ] uses a ViF descriptor for violence scene detection. Figure 5 shows the evaluation of an SVM classifier using ROC curve.
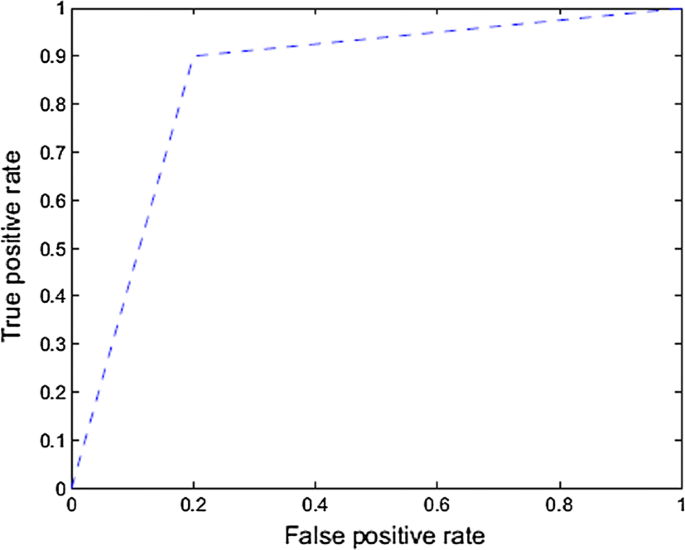
Receiver operating characteristics of a classifier with ViF descriptor [ 83 ]
Figure 6 represents a comparison of detection performance which is conducted by different methods [ 80 ]. The comparison shows the improvement of density aware detector over other methods.
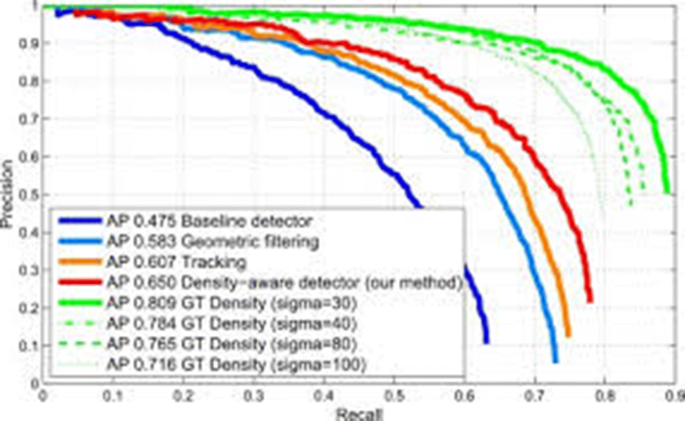
Comparing detection performance of density aware detector with different methods [ 80 ]
As an analysis of existing methods the following shortcomings were identified. Real world problems are having following objectives like
Time complexity
Bad weather conditions
Real world dynamics
Overlapping of objects
Existing methods were handling the problems separately. No method handles all the objectives as features in a single proposal.
To handle effective intelligent crowd video analysis in real time the method should be able to provide solutions to all these problems. Traditional methods are not able to generate efficient economic solution in a time bounded manner.
The availability of high performance computational resource like GPU allows implementation of deep learning based solutions for fast processing of big data. Existing deep learning architectures or models can be combined by including good features and removing unwanted features.
The paper reviews intelligent surveillance video analysis techniques. Reviewed papers cover wide variety of applications. The techniques, tools and dataset identified were listed in form of tables. Survey begins with video surveillance analysis in general perspective, and then finally moves towards crowd analysis. Crowd analysis is difficult in such a way that crowd size is large and dynamic in real world scenarios. Identifying each entity and their behavior is a difficult task. Methods analyzing crowd behavior were discussed. The issues identified in existing methods were listed as future directions to provide efficient solution.
Abbreviations
Surveillance Video Analysis System
Interval-Based Spatio-Temporal Model
Kanade–Lucas–Tomasi
Gaussian Mixture Model
Support Vector Machine
Deep activation-based attribute learning
Hidden Markov Model
You only look once
Long short-term memory
Area under the curve
Violent flow descriptor
Kardas K, Cicekli NK. SVAS: surveillance video analysis system. Expert Syst Appl. 2017;89:343–61.
Article Google Scholar
Wang Y, Shuai Y, Zhu Y, Zhang J. An P Jointly learning perceptually heterogeneous features for blind 3D video quality assessment. Neurocomputing. 2019;332:298–304 (ISSN 0925-2312) .
Tzelepis C, Galanopoulos D, Mezaris V, Patras I. Learning to detect video events from zero or very few video examples. Image Vis Comput. 2016;53:35–44 (ISSN 0262-8856) .
Fakhar B, Kanan HR, Behrad A. Learning an event-oriented and discriminative dictionary based on an adaptive label-consistent K-SVD method for event detection in soccer videos. J Vis Commun Image Represent. 2018;55:489–503 (ISSN 1047-3203) .
Luo X, Li H, Cao D, Yu Y, Yang X, Huang T. Towards efficient and objective work sampling: recognizing workers’ activities in site surveillance videos with two-stream convolutional networks. Autom Constr. 2018;94:360–70 (ISSN 0926-5805) .
Wang D, Tang J, Zhu W, Li H, Xin J, He D. Dairy goat detection based on Faster R-CNN from surveillance video. Comput Electron Agric. 2018;154:443–9 (ISSN 0168-1699) .
Shao L, Cai Z, Liu L, Lu K. Performance evaluation of deep feature learning for RGB-D image/video classification. Inf Sci. 2017;385:266–83 (ISSN 0020-0255) .
Ahmed SA, Dogra DP, Kar S, Roy PP. Surveillance scene representation and trajectory abnormality detection using aggregation of multiple concepts. Expert Syst Appl. 2018;101:43–55 (ISSN 0957-4174) .
Arunnehru J, Chamundeeswari G, Prasanna Bharathi S. Human action recognition using 3D convolutional neural networks with 3D motion cuboids in surveillance videos. Procedia Comput Sci. 2018;133:471–7 (ISSN 1877-0509) .
Guraya FF, Cheikh FA. Neural networks based visual attention model for surveillance videos. Neurocomputing. 2015;149(Part C):1348–59 (ISSN 0925-2312) .
Pathak AR, Pandey M, Rautaray S. Application of deep learning for object detection. Procedia Comput Sci. 2018;132:1706–17 (ISSN 1877-0509) .
Ribeiro M, Lazzaretti AE, Lopes HS. A study of deep convolutional auto-encoders for anomaly detection in videos. Pattern Recogn Lett. 2018;105:13–22.
Huang W, Ding H, Chen G. A novel deep multi-channel residual networks-based metric learning method for moving human localization in video surveillance. Signal Process. 2018;142:104–13 (ISSN 0165-1684) .
Tsakanikas V, Dagiuklas T. Video surveillance systems-current status and future trends. Comput Electr Eng. In press, corrected proof, Available online 14 November 2017.
Wang Y, Zhang D, Liu Y, Dai B, Lee LH. Enhancing transportation systems via deep learning: a survey. Transport Res Part C Emerg Technol. 2018. https://doi.org/10.1016/j.trc.2018.12.004 (ISSN 0968-090X) .
Huang H, Xu Y, Huang Y, Yang Q, Zhou Z. Pedestrian tracking by learning deep features. J Vis Commun Image Represent. 2018;57:172–5 (ISSN 1047-3203) .
Yuan Y, Zhao Y, Wang Q. Action recognition using spatial-optical data organization and sequential learning framework. Neurocomputing. 2018;315:221–33 (ISSN 0925-2312) .
Perez M, Avila S, Moreira D, Moraes D, Testoni V, Valle E, Goldenstein S, Rocha A. Video pornography detection through deep learning techniques and motion information. Neurocomputing. 2017;230:279–93 (ISSN 0925-2312) .
Pang S, del Coz JJ, Yu Z, Luaces O, Díez J. Deep learning to frame objects for visual target tracking. Eng Appl Artif Intell. 2017;65:406–20 (ISSN 0952-1976) .
Wei X, Du J, Liang M, Ye L. Boosting deep attribute learning via support vector regression for fast moving crowd counting. Pattern Recogn Lett. 2017. https://doi.org/10.1016/j.patrec.2017.12.002 .
Xu M, Fang H, Lv P, Cui L, Zhang S, Zhou B. D-stc: deep learning with spatio-temporal constraints for train drivers detection from videos. Pattern Recogn Lett. 2017. https://doi.org/10.1016/j.patrec.2017.09.040 (ISSN 0167-8655) .
Hassan MM, Uddin MZ, Mohamed A, Almogren A. A robust human activity recognition system using smartphone sensors and deep learning. Future Gener Comput Syst. 2018;81:307–13 (ISSN 0167-739X) .
Wu G, Lu W, Gao G, Zhao C, Liu J. Regional deep learning model for visual tracking. Neurocomputing. 2016;175:310–23 (ISSN 0925-2312) .
Nasir M, Muhammad K, Lloret J, Sangaiah AK, Sajjad M. Fog computing enabled cost-effective distributed summarization of surveillance videos for smart cities. J Parallel Comput. 2018. https://doi.org/10.1016/j.jpdc.2018.11.004 (ISSN 0743-7315) .
Najva N, Bijoy KE. SIFT and tensor based object detection and classification in videos using deep neural networks. Procedia Comput Sci. 2016;93:351–8 (ISSN 1877-0509) .
Yu Z, Li T, Yu N, Pan Y, Chen H, Liu B. Reconstruction of hidden representation for Robust feature extraction. ACM Trans Intell Syst Technol. 2019;10(2):18.
Mammadli R, Wolf F, Jannesari A. The art of getting deep neural networks in shape. ACM Trans Archit Code Optim. 2019;15:62.
Zhou T, Tucker R, Flynn J, Fyffe G, Snavely N. Stereo magnification: learning view synthesis using multiplane images. ACM Trans Graph. 2018;37:65
Google Scholar
Fan Z, Song X, Xia T, Jiang R, Shibasaki R, Sakuramachi R. Online Deep Ensemble Learning for Predicting Citywide Human Mobility. Proc ACM Interact Mob Wearable Ubiquitous Technol. 2018;2:105.
Hanocka R, Fish N, Wang Z, Giryes R, Fleishman S, Cohen-Or D. ALIGNet: partial-shape agnostic alignment via unsupervised learning. ACM Trans Graph. 2018;38:1.
Xu M, Qian F, Mei Q, Huang K, Liu X. DeepType: on-device deep learning for input personalization service with minimal privacy concern. Proc ACM Interact Mob Wearable Ubiquitous Technol. 2018;2:197.
Potok TE, Schuman C, Young S, Patton R, Spedalieri F, Liu J, Yao KT, Rose G, Chakma G. A study of complex deep learning networks on high-performance, neuromorphic, and quantum computers. J Emerg Technol Comput Syst. 2018;14:19.
Pouyanfar S, Sadiq S, Yan Y, Tian H, Tao Y, Reyes MP, Shyu ML, Chen SC, Iyengar SS. A survey on deep learning: algorithms, techniques, and applications. ACM Comput Surv. 2018;51:92.
Tian Y, Lee GH, He H, Hsu CY, Katabi D. RF-based fall monitoring using convolutional neural networks. Proc ACM Interact Mob Wearable Ubiquitous Technol. 2018;2:137.
Roy P, Song SL, Krishnamoorthy S, Vishnu A, Sengupta D, Liu X. NUMA-Caffe: NUMA-aware deep learning neural networks. ACM Trans Archit Code Optim. 2018;15:24.
Lovering C, Lu A, Nguyen C, Nguyen H, Hurley D, Agu E. Fact or fiction. Proc ACM Hum-Comput Interact. 2018;2:111.
Ben-Hamu H, Maron H, Kezurer I, Avineri G, Lipman Y. Multi-chart generative surface modeling. ACM Trans Graph. 2018;37:215
Ge W, Gong B, Yu Y. Image super-resolution via deterministic-stochastic synthesis and local statistical rectification. ACM Trans Graph. 2018;37:260
Hedman P, Philip J, Price T, Frahm JM, Drettakis G, Brostow G. Deep blending for free-viewpoint image-based rendering. ACM Trans Graph. 2018;37:257
Sundararajan K, Woodard DL. Deep learning for biometrics: a survey. ACM Comput Surv. 2018;51:65.
Kim H, Kim T, Kim J, Kim JJ. Deep neural network optimized to resistive memory with nonlinear current–voltage characteristics. J Emerg Technol Comput Syst. 2018;14:15.
Wang C, Yang H, Bartz C, Meinel C. Image captioning with deep bidirectional LSTMs and multi-task learning. ACM Trans Multimedia Comput Commun Appl. 2018;14:40.
Yao S, Zhao Y, Shao H, Zhang A, Zhang C, Li S, Abdelzaher T. RDeepSense: Reliable Deep Mobile Computing Models with Uncertainty Estimations. Proc ACM Interact Mob Wearable Ubiquitous Technol. 2018;1:173.
Liu D, Cui W, Jin K, Guo Y, Qu H. DeepTracker: visualizing the training process of convolutional neural networks. ACM Trans Intell Syst Technol. 2018;10:6.
Yi L, Huang H, Liu D, Kalogerakis E, Su H, Guibas L. Deep part induction from articulated object pairs. ACM Trans Graph. 2018. https://doi.org/10.1145/3272127.3275027 .
Zhao N, Cao Y, Lau RW. What characterizes personalities of graphic designs? ACM Trans Graph. 2018;37:116.
Tan J, Wan X, Liu H, Xiao J. QuoteRec: toward quote recommendation for writing. ACM Trans Inf Syst. 2018;36:34.
Qu Y, Fang B, Zhang W, Tang R, Niu M, Guo H, Yu Y, He X. Product-based neural networks for user response prediction over multi-field categorical data. ACM Trans Inf Syst. 2018;37:5.
Yin K, Huang H, Cohen-Or D, Zhang H. P2P-NET: bidirectional point displacement net for shape transform. ACM Trans Graph. 2018;37:152.
Yao S, Zhao Y, Shao H, Zhang C, Zhang A, Hu S, Liu D, Liu S, Su L, Abdelzaher T. SenseGAN: enabling deep learning for internet of things with a semi-supervised framework. Proc ACM Interact Mob Wearable Ubiquitous Technol. 2018;2:144.
Saito S, Hu L, Ma C, Ibayashi H, Luo L, Li H. 3D hair synthesis using volumetric variational autoencoders. ACM Trans Graph. 2018. https://doi.org/10.1145/3272127.3275019 .
Chen A, Wu M, Zhang Y, Li N, Lu J, Gao S, Yu J. Deep surface light fields. Proc ACM Comput Graph Interact Tech. 2018;1:14.
Chu W, Xue H, Yao C, Cai D. Sparse coding guided spatiotemporal feature learning for abnormal event detection in large videos. IEEE Trans Multimedia. 2019;21(1):246–55.
Khan MUK, Park H, Kyung C. Rejecting motion outliers for efficient crowd anomaly detection. IEEE Trans Inf Forensics Secur. 2019;14(2):541–56.
Tao D, Guo Y, Yu B, Pang J, Yu Z. Deep multi-view feature learning for person re-identification. IEEE Trans Circuits Syst Video Technol. 2018;28(10):2657–66.
Zhang D, Wu W, Cheng H, Zhang R, Dong Z, Cai Z. Image-to-video person re-identification with temporally memorized similarity learning. IEEE Trans Circuits Syst Video Technol. 2018;28(10):2622–32.
Serrano I, Deniz O, Espinosa-Aranda JL, Bueno G. Fight recognition in video using hough forests and 2D convolutional neural network. IEEE Trans Image Process. 2018;27(10):4787–97. https://doi.org/10.1109/tip.2018.2845742 .
Article MathSciNet MATH Google Scholar
Li Y, Li X, Zhang Y, Liu M, Wang W. Anomalous sound detection using deep audio representation and a blstm network for audio surveillance of roads. IEEE Access. 2018;6:58043–55.
Muhammad K, Ahmad J, Mehmood I, Rho S, Baik SW. Convolutional neural networks based fire detection in surveillance videos. IEEE Access. 2018;6:18174–83.
Ullah A, Ahmad J, Muhammad K, Sajjad M, Baik SW. Action recognition in video sequences using deep bi-directional LSTM with CNN features. IEEE Access. 2018;6:1155–66.
Li Y. A deep spatiotemporal perspective for understanding crowd behavior. IEEE Trans Multimedia. 2018;20(12):3289–97.
Pamula T. Road traffic conditions classification based on multilevel filtering of image content using convolutional neural networks. IEEE Intell Transp Syst Mag. 2018;10(3):11–21.
Vandersmissen B, et al. indoor person identification using a low-power FMCW radar. IEEE Trans Geosci Remote Sens. 2018;56(7):3941–52.
Min W, Yao L, Lin Z, Liu L. Support vector machine approach to fall recognition based on simplified expression of human skeleton action and fast detection of start key frame using torso angle. IET Comput Vision. 2018;12(8):1133–40.
Perwaiz N, Fraz MM, Shahzad M. Person re-identification using hybrid representation reinforced by metric learning. IEEE Access. 2018;6:77334–49.
Olague G, Hernández DE, Clemente E, Chan-Ley M. Evolving head tracking routines with brain programming. IEEE Access. 2018;6:26254–70.
Dilawari A, Khan MUG, Farooq A, Rehman Z, Rho S, Mehmood I. Natural language description of video streams using task-specific feature encoding. IEEE Access. 2018;6:16639–45.
Zeng D, Zhu M. Background subtraction using multiscale fully convolutional network. IEEE Access. 2018;6:16010–21.
Goswami G, Vatsa M, Singh R. Face verification via learned representation on feature-rich video frames. IEEE Trans Inf Forensics Secur. 2017;12(7):1686–98.
Keçeli AS, Kaya A. Violent activity detection with transfer learning method. Electron Lett. 2017;53(15):1047–8.
Lu W, et al. Unsupervised sequential outlier detection with deep architectures. IEEE Trans Image Process. 2017;26(9):4321–30.
Feizi A. High-level feature extraction for classification and person re-identification. IEEE Sens J. 2017;17(21):7064–73.
Lee Y, Chen S, Hwang J, Hung Y. An ensemble of invariant features for person reidentification. IEEE Trans Circuits Syst Video Technol. 2017;27(3):470–83.
Uddin MZ, Khaksar W, Torresen J. Facial expression recognition using salient features and convolutional neural network. IEEE Access. 2017;5:26146–61.
Mukherjee SS, Robertson NM. Deep head pose: Gaze-direction estimation in multimodal video. IEEE Trans Multimedia. 2015;17(11):2094–107.
Hayat M, Bennamoun M, An S. Deep reconstruction models for image set classification. IEEE Trans Pattern Anal Mach Intell. 2015;37(4):713–27.
Afiq AA, Zakariya MA, Saad MN, Nurfarzana AA, Khir MHM, Fadzil AF, Jale A, Gunawan W, Izuddin ZAA, Faizari M. A review on classifying abnormal behavior in crowd scene. J Vis Commun Image Represent. 2019;58:285–303.
Bour P, Cribelier E, Argyriou V. Chapter 14—Crowd behavior analysis from fixed and moving cameras. In: Computer vision and pattern recognition, multimodal behavior analysis in the wild. Cambridge: Academic Press; 2019. pp. 289–322.
Chapter Google Scholar
Xu X, Gong S, Hospedales TM. Chapter 15—Zero-shot crowd behavior recognition. In: Group and crowd behavior for computer vision. Cambridge: Academic Press; 2017:341–369.
Rodriguez M, Sivic J, Laptev I. Chapter 5—The analysis of high density crowds in videos. In: Group and crowd behavior for computer vision. Cambridge: Academic Press. 2017. pp. 89–113.
Yogameena B, Nagananthini C. Computer vision based crowd disaster avoidance system: a survey. Int J Disaster Risk Reduct. 2017;22:95–129.
Wang X, Loy CC. Chapter 10—Deep learning for scene-independent crowd analysis. In: Group and crowd behavior for computer vision. Cambridge: Academic Press; 2017. pp. 209–52.
Arceda VM, Fabián KF, Laura PL, Tito JR, Cáceres JG. Fast face detection in violent video scenes. Electron Notes Theor Comput Sci. 2016;329:5–26.
Wang Q, Wan J, Yuan Y. Deep metric learning for crowdedness regression. IEEE Trans Circuits Syst Video Technol. 2018;28(10):2633–43.
Shao J, Loy CC, Kang K, Wang X. Crowded scene understanding by deeply learned volumetric slices. IEEE Trans Circuits Syst Video Technol. 2017;27(3):613–23.
Grant JM, Flynn PJ. Crowd scene understanding from video: a survey. ACM Trans Multimedia Comput Commun Appl. 2017;13(2):19.
Tay L, Jebb AT, Woo SE. Video capture of human behaviors: toward a Big Data approach. Curr Opin Behav Sci. 2017;18:17–22 (ISSN 2352-1546) .
Chaudhary S, Khan MA, Bhatnagar C. Multiple anomalous activity detection in videos. Procedia Comput Sci. 2018;125:336–45.
Anwar F, Petrounias I, Morris T, Kodogiannis V. Mining anomalous events against frequent sequences in surveillance videos from commercial environments. Expert Syst Appl. 2012;39(4):4511–31.
Wang T, Qiao M, Chen Y, Chen J, Snoussi H. Video feature descriptor combining motion and appearance cues with length-invariant characteristics. Optik. 2018;157:1143–54.
Kaltsa V, Briassouli A, Kompatsiaris I, Strintzis MG. Multiple Hierarchical Dirichlet Processes for anomaly detection in traffic. Comput Vis Image Underst. 2018;169:28–39.
Cermeño E, Pérez A, Sigüenza JA. Intelligent video surveillance beyond robust background modeling. Expert Syst Appl. 2018;91:138–49.
Coşar S, Donatiello G, Bogorny V, Garate C, Alvares LO, Brémond F. Toward abnormal trajectory and event detection in video surveillance. IEEE Trans Circuits Syst Video Technol. 2017;27(3):683–95.
Ribeiro PC, Audigier R, Pham QC. Romaric Audigier, Quoc Cuong Pham, RIMOC, a feature to discriminate unstructured motions: application to violence detection for video-surveillance. Comput Vis Image Underst. 2016;144:121–43.
Şaykol E, Güdükbay U, Ulusoy Ö. Scenario-based query processing for video-surveillance archives. Eng Appl Artif Intell. 2010;23(3):331–45.
Castanon G, Jodoin PM, Saligrama V, Caron A. Activity retrieval in large surveillance videos. In: Academic Press library in signal processing. Vol. 4. London: Elsevier; 2014.
Cheng HY, Hwang JN. Integrated video object tracking with applications in trajectory-based event detection. J Vis Commun Image Represent. 2011;22(7):673–85.
Hong X, Huang Y, Ma W, Varadarajan S, Miller P, Liu W, Romero MJ, del Rincon JM, Zhou H. Evidential event inference in transport video surveillance. Comput Vis Image Underst. 2016;144:276–97.
Wang T, Qiao M, Deng Y, Zhou Y, Wang H, Lyu Q, Snoussi H. Abnormal event detection based on analysis of movement information of video sequence. Optik. 2018;152:50–60.
Ullah H, Altamimi AB, Uzair M, Ullah M. Anomalous entities detection and localization in pedestrian flows. Neurocomputing. 2018;290:74–86.
Roy D, Mohan CK. Snatch theft detection in unconstrained surveillance videos using action attribute modelling. Pattern Recogn Lett. 2018;108:56–61.
Lee WK, Leong CF, Lai WK, Leow LK, Yap TH. ArchCam: real time expert system for suspicious behaviour detection in ATM site. Expert Syst Appl. 2018;109:12–24.
Dinesh Jackson Samuel R, Fenil E, Manogaran G, Vivekananda GN, Thanjaivadivel T, Jeeva S, Ahilan A. Real time violence detection framework for football stadium comprising of big data analysis and deep learning through bidirectional LSTM. Comput Netw. 2019;151:191–200 (ISSN 1389-1286) .
Bouachir W, Gouiaa R, Li B, Noumeir R. Intelligent video surveillance for real-time detection of suicide attempts. Pattern Recogn Lett. 2018;110:1–7 (ISSN 0167-8655) .
Wang J, Xu Z. Spatio-temporal texture modelling for real-time crowd anomaly detection. Comput Vis Image Underst. 2016;144:177–87 (ISSN 1077-3142) .
Ko KE, Sim KB. Deep convolutional framework for abnormal behavior detection in a smart surveillance system. Eng Appl Artif Intell. 2018;67:226–34.
Dan X, Yan Y, Ricci E, Sebe N. Detecting anomalous events in videos by learning deep representations of appearance and motion. Comput Vis Image Underst. 2017;156:117–27.
Szegedy C, Liu W, Jia Y, Sermanet P, Reed S, Anguelov D, Erhan D, Vanhoucke V, Rabinovich A. Going deeper with convolutions. In: 2015 IEEE conference on computer vision and pattern recognition (CVPR). 2015.
Guo Y, Liu Y, Oerlemans A, Lao S, Lew MS. Deep learning for visual understanding: a review. Neurocomputing. 2016;187(26):27–48.
Babaee M, Dinh DT, Rigoll G. A deep convolutional neural network for video sequence background subtraction. Pattern Recogn. 2018;76:635–49.
Xue H, Liu Y, Cai D, He X. Tracking people in RGBD videos using deep learning and motion clues. Neurocomputing. 2016;204:70–6.
Dong Z, Jing C, Pei M, Jia Y. Deep CNN based binary hash video representations for face retrieval. Pattern Recogn. 2018;81:357–69.
Zhang C, Tian Y, Guo X, Liu J. DAAL: deep activation-based attribute learning for action recognition in depth videos. Comput Vis Image Underst. 2018;167:37–49.
Zhou S, Shen W, Zeng D, Fang M, Zhang Z. Spatial–temporal convolutional neural networks for anomaly detection and localization in crowded scenes. Signal Process Image Commun. 2016;47:358–68.
Pennisi A, Bloisi DD, Iocchi L. Online real-time crowd behavior detection in video sequences. Comput Vis Image Underst. 2016;144:166–76.
Feliciani C, Nishinari K. Measurement of congestion and intrinsic risk in pedestrian crowds. Transp Res Part C Emerg Technol. 2018;91:124–55.
Wang X, He X, Wu X, Xie C, Li Y. A classification method based on streak flow for abnormal crowd behaviors. Optik Int J Light Electron Optics. 2016;127(4):2386–92.
Kumar S, Datta D, Singh SK, Sangaiah AK. An intelligent decision computing paradigm for crowd monitoring in the smart city. J Parallel Distrib Comput. 2018;118(2):344–58.
Feng Y, Yuan Y, Lu X. Learning deep event models for crowd anomaly detection. Neurocomputing. 2017;219:548–56.
Download references
Acknowledgements
Not applicable.
Author information
Authors and affiliations.
VIT, Vellore, 632014, Tamil Nadu, India
G. Sreenu & M. A. Saleem Durai
You can also search for this author in PubMed Google Scholar
Contributions
GS and MASD selected and analyzed different papers for getting more in depth view about current scenarios of the problem and its solutions. Both authors read and approved the final manuscript.
Corresponding author
Correspondence to G. Sreenu .
Ethics declarations
Competing interests.
The authors declare that they have no competing interests.
Availability of data and materials
Data sharing is not applicable to this article as no datasets were generated or analysed during the current study.
Additional information
Publisher's note.
Springer Nature remains neutral with regard to jurisdictional claims in published maps and institutional affiliations.
Rights and permissions
Open Access This article is distributed under the terms of the Creative Commons Attribution 4.0 International License ( http://creativecommons.org/licenses/by/4.0/ ), which permits unrestricted use, distribution, and reproduction in any medium, provided you give appropriate credit to the original author(s) and the source, provide a link to the Creative Commons license, and indicate if changes were made.
Reprints and permissions

About this article
Cite this article.
Sreenu, G., Saleem Durai, M.A. Intelligent video surveillance: a review through deep learning techniques for crowd analysis. J Big Data 6 , 48 (2019). https://doi.org/10.1186/s40537-019-0212-5
Download citation
Received : 07 December 2018
Accepted : 28 May 2019
Published : 06 June 2019
DOI : https://doi.org/10.1186/s40537-019-0212-5
Share this article
Anyone you share the following link with will be able to read this content:
Sorry, a shareable link is not currently available for this article.
Provided by the Springer Nature SharedIt content-sharing initiative
- Video surveillance
- Deep learning
- Crowd analysis

Onboard Camera Applications for Buses (2016)
Chapter: chapter two literature review.
Below is the uncorrected machine-read text of this chapter, intended to provide our own search engines and external engines with highly rich, chapter-representative searchable text of each book. Because it is UNCORRECTED material, please consider the following text as a useful but insufficient proxy for the authoritative book pages.
5 CHAPTER TWO LITERATURE REVIEW John F. Kennedy School of Government, Harvard Univer- sity, testified before the Commonwealth of Massachusetts on âMass Transit Security After the London Bombings,â and the vulnerability of public transit to terrorist attacks. He stated the following: According to the Congressional Research Service, fully one-third of terrorist attacks worldwide target transportation systems, and public transit is the most frequent transportation target. Analysis of more than 22,000 terrorist incidents from 1968 through 2004 indicate that attacks on land-based transportation targets, including mass transit, have the highest casualty rates of any type of terrorist attack. On average, attacks against such systems created more than two-and-a-half times the casualties per incident as attacks on aviation targets. In terms of fatalities, attacks on surface transportation are among the deadliest, ranking behind attacks on aviation and nearly equaling fatality rates of attacks on religious and tourist targets. (Prieto 2005) Prieto explained how there are a number of low-cost ini- tiatives that transit agencies could pursue to improve secu- rity, including visibility of security, public awareness, and assessments of vulnerabilities. He also stated that after the Madrid bombings in 2004, transit agencies recommended cameras and other surveillance equipment as part of their security measures; however, the expense could pose obsta- cles. Other measures included interoperable communications systems, training, canine teams, and capital improvements to infrastructure. Security is a key motivator for agencies to install video surveillance on their bus fleets. As described in a 2013 arti- cle from Security Today, widespread use of video surveil- lance has resulted from terrorist attacks throughout the years (Notbohm 2013). Within hours after the London bombings in 2005, the terrorists were arrestedâlargely because they were seen on camera entering and exiting the London Under- ground. Transit bombings such as those in Madrid (2004) and London have served to demonstrate the way transit systems can be vulnerable to terrorist attacks (Notbohm 2013). As a result, the federally funded Transit Security Grant Program invested $250 million in mass transit security. These grants help augment and improve surveillance systems, providing funding for new and improved surveillance technology that has proved to have better visual clarity as well as have the ability to obtain audio recordings of injuries, altercations, traffic incidents, and so forth. Currently, clear digital camera images are available in real time and can be transferred wirelessly and automatically to transit agency management and security, just as passen- gers have real-time transit information available to them on mobile devices. While onboard electronic surveillance tech- nology on buses has become widespread and commonplace over the past decade, this has not always been the case. The transit agencies surveyed did not start using cameras on buses regularly until 10 to 15 years ago. What led up to its widespread use and what problems are agencies still facing? In 2001, TRB published TCRP Synthesis 38: Electronic Surveillance Technology on Transit Vehicles. The report described the practice and use of onboard surveillance technologies that were designed to address both safety and security issues at public transit agencies at that time. Costs, maintenance requirements, and liability and privacy concerns were a barrier of implementation. Liability and privacy concerns have been trumped by security concerns since 9/11. Since 2001 and 9/11, the federal government and transit agencies around the country have been focusing on secu- rity and emergency preparedness. In the 2011 Mass Transit article, â10 Years Later: How Has Transit Security Changed Since 9/11?â APTAâs director of operations for safety and security programs, Greg Hull, stated, âPrior to 9/11 what we had in the industry were calmer approaches toward security and policing.â APTA had worked with FTA to develop com- mon approaches toward system safety, which included secu- rity and emergency preparedness; however, within hours of the attacks, senior management at transit systems and the FTA convened to discuss what needed to be put in place and what resources were available (Kaiser 2011). âAmong the first things that were undertaken were financial resources being put into place by the FTA for vulnerability assessments to aid transit systems in refining their security plans and tightening up any aspects that needed to be addressed.â APTA Director of Operations for Safety and Security Programs As time went on, there were more attacks, some specific to public transit. In 2005, Daniel B. Prieto, research director of the Homeland Security Partnership Initiative and fellow at the Belfer Center for Science and International Affairs at the
6 As camera applications continue to prevail, transit agen- cies have found themselves grappling with issues such as funding, legal battles, and union disputes; however, they have also reaped financial benefits resulting from the cameras. Funding for surveillance equipment is not reliable and the practices are not standard. Among the findings in TCRP Synthesis 90: Video Surveillance Uses by Rail Transit Agen- cies (Moses Schulz and Gilbert 2011), which documented the use of electronic video surveillance technology, ⢠Reliable funding sources are necessary to assist agen- cies in making more effective use of available grants to upgrade security systems; ⢠Policies tend to vary on how images are accessed; and ⢠Publicizing successful applications of video surveil- lance may result in diversifying funding sources for systems installation and upgrading. As video surveillance is growing, TriMet in Oregon is currently in arbitration with its bus operatorâs union for installing cameras focused on the operators. Before 2010, TriMet was opposed to installing cameras until a customer recorded with his mobile phone a bus operator reading a Kindle while driving the bus. The video went viral and the operator was fired. By 2012, all new buses had eight cam- eras, with one specifically focused on the operator. The purpose of the camera focused on the operator is to prevent crimes and assaults committed against the driver, to further improve customer satisfaction, and to clarify false reports. Currently, TriMet is going to arbitration over whether the cameras can roll when the bus operator is on breaks or not driving (Rose 2014). Electronic surveillance has reportedly saved South- eastern Pennsylvania Transportation Authority (SEPTA) $11 million in claims. Payouts made by the transit agency dropped by $11 million as well as an additional 5% in fis- cal year 2013. In addition to the payout rate going down, the numbers of claims and lawsuits have also declined. Video surveillance provides the images plaintiffs will see in court. Lawyers who would typically sue SEPTA, con- sidered an easier claim to collect on, now call first. The technology has changed their mentality. As of today, 75% of SEPTAâs buses are equipped with cameras, and in 2016, all of their bus fleets will be equipped with video surveil- lance (Fisher 2014). Massachusetts Bay Transportation Authority (MBTA) uses video surveillance to assist with legal claims and improve security. They secured a grant from the Department of Homeland Security to improve the security of its fleets. The MBTA credits the short time they have recently had to spend on closing cases to the new surveillance technology. Customer feedback surveys indicate they feel safer, which is invaluable (Clarke 2014).
TRB's Transit Cooperative Research Program (TCRP) Synthesis 123: Onboard Camera Applications for Buses explores the current technologies, research, and opportunities for use. The report also provides examples of how surveillance systems are used to improve operations, safety, security, training, and customer satisfaction.
READ FREE ONLINE
Welcome to OpenBook!
You're looking at OpenBook, NAP.edu's online reading room since 1999. Based on feedback from you, our users, we've made some improvements that make it easier than ever to read thousands of publications on our website.
Do you want to take a quick tour of the OpenBook's features?
Show this book's table of contents , where you can jump to any chapter by name.
...or use these buttons to go back to the previous chapter or skip to the next one.
Jump up to the previous page or down to the next one. Also, you can type in a page number and press Enter to go directly to that page in the book.
To search the entire text of this book, type in your search term here and press Enter .
Share a link to this book page on your preferred social network or via email.
View our suggested citation for this chapter.
Ready to take your reading offline? Click here to buy this book in print or download it as a free PDF, if available.
Get Email Updates
Do you enjoy reading reports from the Academies online for free ? Sign up for email notifications and we'll let you know about new publications in your areas of interest when they're released.
Advertisement
A literature review on 360-degree video as an educational tool: towards design guidelines
- Published: 24 June 2022
- Volume 10 , pages 325–375, ( 2023 )
Cite this article
- Marie Evens ORCID: orcid.org/0000-0001-8906-2723 1 ,
- Michaël Empsen 1 &
- Wouter Hustinx 1
1731 Accesses
18 Citations
3 Altmetric
Explore all metrics
Due to its possibility to create immersive learning experiences through simulated realism, virtual reality (VR) is a promising tool for educational purposes. A more user-friendly, realistic and affordable version of VR is 360° video, recording real-world footage with omnidirectional or multi-camera systems. 360° videos are slowly finding their way into educational research and practice, but a strong research basis is lacking. The current review describes the designs of educational 360° videos in existing empirical studies, and specifically searches for design guidelines for 360° videos. Browsing four databases, we compiled a dataset of 26 empirical educational studies on 360° video. The articles were carefully analysed focussing on two research questions: (1) How are 360° videos and 360° video lessons designed in empirical educational research on 360° video? and (2) What conclusions about the effectivity of the design of 360° video lessons can be drawn from empirical educational research on 360° video? Regarding RQ1, the studies in the dataset fit within three kinds of video content categories: environments, situations and demonstrations. We distinguished between videos with and without (interactive or non-interactive) add-ons. We found that 360° videos are most often used in higher education (healthcare and teacher education), have a duration of five to 15 min and are watched by means of head-mounted displays. Concerning RQ2, 360° videos are beneficial for the development of several dependent variables, such as mastery of learning content, attitudes, immersion and presence. Based on the reviewed papers we put up a list of design guidelines for educational 360° videos.
This is a preview of subscription content, log in via an institution to check access.
Access this article
Subscribe and save.
- Get 10 units per month
- Download Article/Chapter or eBook
- 1 Unit = 1 Article or 1 Chapter
- Cancel anytime
Price includes VAT (Russian Federation)
Instant access to the full article PDF.
Rent this article via DeepDyve
Institutional subscriptions
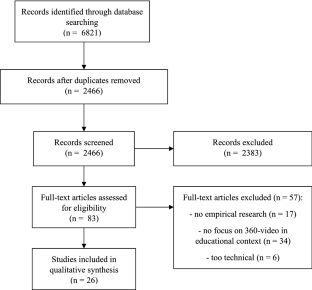
adapted from Moher et al., 2009 )
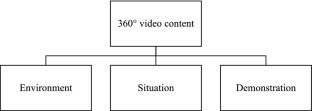
Similar content being viewed by others
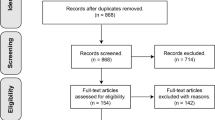
Educational 360-Degree Videos in Virtual Reality: a Scoping Review of the Emerging Research

360° videos in education – A systematic literature review on application areas and future potentials
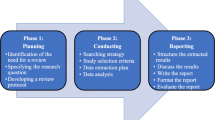
Exploring the trends of educational virtual reality games: a systematic review of empirical studies
Explore related subjects.
- Artificial Intelligence
- Digital Education and Educational Technology
Aguayo, C., Cochrane, T., & Narayan, V. (2017). Key themes in mobile learning: Prospects for learner-generated learning through AR and VR. Australasian Journal of Educational Technology , 33 (6), 27–40. https://doi.org/10.14742/ajet.3671
Baric, D., Havârneanu, G. M., & Mairean, C. (2020). Attitudes of learner drivers toward safety at level crossings: Do they change after a 360° video-based educational intervention? Transportation Research Part F, 69 , 335–348. https://doi.org/10.1016/j.trf.2020.01.018
Article Google Scholar
Barreda-Angeles, M., Serra-Blasco, M., Trepat, E., Pereda-Baños, A., Pàmias, M., Palao, D., Goldberg, X., & Cardoner, N. (2021). Development and experimental validation of a dataset of 360◦-videos for facilitating school-based bullying prevention programs. Computers & Education . https://doi.org/10.1016/j.compedu.2020.104065
Bembenutty, H. (2011). Introduction: Self-regulation of learning in post-secondary education. In H. Bembenutty (Ed.), Self-regulated Learning (pp. 2–8). Jossey-Bass.
Google Scholar
Boda, P. A., & Brown, B. (2020). Designing for relationality in virtual reality: Context-specific learning as a primer for content relevancy. Journal of Science Education and Technology, 29 , 691–702. https://doi.org/10.1007/s10956-020-09849-1
Brown, A., & Green, T. (2016). Virtual reality: Low-cost tools and resources for the classroom. Tech Trends, 60 , 517–519. https://doi.org/10.1007/s11528-016-0102-z
Buchman, S., & Henderson, D. (2019). Interprofessional empathy and communication competency development in healthcare professions’ curriculum through immersive virtual reality experiences. Journal of Interprofessional Education & Practice, 15 , 127–130. https://doi.org/10.1016/j.xjep.2019.03.010
Calvert, J., & Abadia, R. (2020). Impact of immersing university and high school students in educational linear narratives using virtual reality technology. Computers & Education . https://doi.org/10.1016/j.compedu.2020.104005
Chang, E., Kim, H. T., & Yoo, B. (2020). Virtual reality sickness: A review of causes and measurements. International Journal of Human-Computer Interaction, 36 (17), 1658–1682. https://doi.org/10.1080/10447318.2020.1778351
Chi, M. T. H., Feltovich, P. J., & Glaser, R. (1979). Categorization and representation of physics problems by experts and novices. Cognitive Science, 5 , 121–152. https://doi.org/10.1207/s15516709cog0502_2
Clark, R. E., Kirschner, P. A., & Sweller, J. (2012). Putting students on the path to learning: The case for fully guided instruction. American Educator, 36 , 6–11. https://eric.ed.gov/?id=EJ971752
Eiris, R., Gheisari, M., & Emaeili, B. (2020a). Desktop-based safety training using 360-degree panorama and static virtual reality techniques: A comparative experimental study. Automation in Construction . https://doi.org/10.1016/j.autcon.2019.102969
Eiris, R., Jain, A., Gheisari, M., & Wehle, A. (2020b). Safety immersive storytelling using narrated 360-degree panoramas: A fall hazard training within the electrical trade context. Safety Science . https://doi.org/10.1016/j.ssci.2020.104703
Ferdig, R. E., & Kosko, K. W. (2020). Implementing 360 video to increase immersion, perceptual capacity, and teacher noticing. TechTrends, 64 , 849–859. https://doi.org/10.1007/s11528-020-00522-3
Feurstein, M. S. (2018). Towards an Integration of 360-Degree Video in Higher Education. Workflow, challenges and scenarios . Proceedings of DeLFI Workshops 2018 co-located with 16th e-Learning Conference of the German Computer Society (DeLFI 2018). Frankfurt, Germany. http://ceur-ws.org/Vol-2250/WS_VRAR_paper3.pdf
Fukuta, J., Gill, N., Rooney, R., Coombs, A., & Murphy, D. (2020). Use of 360° video for a virtual operating theatre orientation for medical students. Journal of Surgical Education, 78 , 391–393. https://doi.org/10.1016/j.jsurg.2020.08.014
Gold, B., & Windscheid, J. (2020). Observing 360-degree classroom videos—effects of video type on presence, emotions, workload, classroom observations, and ratings of teaching quality. Computers & Education . https://doi.org/10.1016/j.compedu.2020.103960
Hallberg, S., Hirsto, L., & Kaasinen, J. (2020). Experiences and outcomes of craft skill learning with a 360° virtual learning environment and a head-mounted display. Heliyon . https://doi.org/10.1016/j.heliyon.2020.e04705
Hamilton, D., McKechnie, J., Edgerton, E., & Wilson, C. (2021). Immersive virtual reality as a pedagogical tool in education: A systematic literature review of quantitative learning outcomes and experimental design. Journal of Computers in Education, 8 (1), 1–32. https://doi.org/10.1007/s40692-020-00169-2
Harrington, C. M., Kavanagh, D. O., Wright Ballester, G., Wright Ballester, A., Dicker, P., Traynor, O., Hill, A., & Tierney, S. (2017). 360° operative videos: A randomised cross-over study evaluating attentiveness and information retention. Journal of Surgical Education, 75 , 993–1000. https://doi.org/10.1016/j.jsurg.2017.10.010
Herault, R. C., Lincke, A., Milrad, M., Forsgärde, E., & Elmqvist, C. (2018). Using 360-degrees interactive videos in patient trauma treatment education: Design, development and evaluation aspects. Smart Learning Environments . https://doi.org/10.1186/s40561-018-0074-x
Hodgson, P., Lee, V. W. Y., Chan, J. C. S., Fong, A., Tang, C. S. Y., Chan, L., & Wong, C. (2019). Immersive virtual reality (IVR) in higher education: Development and implementation. In M. C. T. Dieck & T. Jung (Eds.), Augmented Reality and Virtual Reality: The Power of AR and VR for Business (pp. 161–173). Springer.
Chapter Google Scholar
Jensen, L., & Konradsen, F. (2018). A review of the use of virtual reality head-mounted displays in education and training. Education and Information Technologies, 23 , 1515–1529. https://doi.org/10.1007/s10639-017-9676-0
Kavanagh, S., Luxton-Reilly, A., Wüensche, B., & Plimmer, B. (2017). A systematic review of virtual reality in education. Themes in Science & Technology Education, 10 (2), 85–119. https://eric.ed.gov/?id=EJ1165633
Lazow, M. A., Real, F. J., Ollberding, N. J., Davis, D., Cruse, B., & Klein, M. D. (2018). Modernizing training on social determinants of health: A virtual neighborhood tour is noninferior to an in-person experience. Academic Pediatrics, 18 (6), 720–722. https://doi.org/10.1016/j.acap.2018.04.007
Lee, S. H., Sergueeva, K., Catangui, M., & Kandaurova, M. (2017). Assessing Google Cardboard virtual reality as a content delivery system in business classrooms. Journal of Education for Business, 92 (4), 153–160. https://doi.org/10.1080/08832323.2017.1308308
Luo, H., Li, G., Feng, Q., Yang, Y., & Zuo, M. (2021). Virtual reality in K-12 and higher education: A systematic review of the literature from 2000 to 2019. Journal of Computer Assisted Learning, 2021 , 1–15. https://doi.org/10.1111/jcal.12538
Mayer, R. E. (2011). Applying the science of learning to multimedia instruction. Psychology of Learning and Motivation, 55 , 77–108. https://doi.org/10.1016/B978-0-12-387691-1.00003-X
McKenzie, S., Rough, J., Spence, A., & Patterson, N. (2019). Virtually there: The potential, process and problems of using 360° video in the classroom. Issues in Informing. Science and Information Technology, 16 , 211–219. https://doi.org/10.28945/4318
Moher, D., Liberati, A., Tetzlaff, J., & Altman, D. G. (2009). Preferred reporting items for systematic reviews and meta-analyses: The PRISMA statement. PLoS Med, 6 (7), e1000097. https://doi.org/10.1371/journal.pmed1000097
Nortvig, A.-M., Petersen, A. K., Helsinghof, H., & Braender, B. (2020). Digital expansions of physical learning spaces in practice-based subjects—blended learning in art and craft & design in teacher education. Computers & Education . https://doi.org/10.1016/j.compedu.2020.104020
Paalimäki-Paakki, K., Virtanen, M., Henner, A., Nieminen, M. T., & Kääriäinen, M. (2020). Patients’, radiographers’ and radiography students’ experiences of 360° virtual counselling environment for the coronary computed tomography angiography: A qualitative study. Radiography, 27 (2), 381–388. https://doi.org/10.1016/j.radi.2020.09.019
Riva, G., Mantovani, F., Capideville, C. S., Preziosa, A., Morganti, F., Villani, D., Gaggioli, A., Botella, C., & Alcañiz, M. (2007). Affective interactions using virtual reality: The link between presence and emotions. CyberPsychology & Behavior, 10 , 45–56. https://doi.org/10.1089/cpb.2006.9993
Ros, M., Debien, B., Cyteval, C., Molinari, N., Gatto, F., & Lonjon, N. (2020). Applying an immersive tutorial in virtual reality to learning a new technique. Neuro-Chirurgie, 66 , 212–218. https://doi.org/10.1016/j.neuchi.2020.05.006
Rupp, M. A., Odette, K. L., Kozachuk, J., Michaelis, J. R., Smither, J. A., & McConnell, D. S. (2019). Investigating learning outcomes and subjective experiences in 360-degree videos. Computers & Education, 128 , 256–268. https://doi.org/10.1016/j.compedu.2018.09.015
Scargle, J. D. (1999). Publication bias (the ‘file-drawer problem’) in scientific inference. Proceedings of the Sturrock Symposium , Stanford University, March 1999.
Shephard, K. (2003). Questioning, promoting and evaluating the use of streaming video to support student learning. British Journal of Educational Technology, 34 (3), 295–308. https://doi.org/10.1111/1467-8535.00328
Slater, M., Lotto, B., Arnold, M. M., & Sanchez-Vives, M. V. (2009). How we experience immersive virtual environments: The concept of presence and its measurement. Anuario De Psicologia, 40 (2), 193–210.
Snelson, C., & Hsu, Y.-C. (2020). Educational 360-degree videos in virtual reality: A scoping review of the emerging research. TechTrends, 64 , 404–412. https://doi.org/10.1007/s11528-019-004774-3
Theelen, H., van den Beemt, A., & den Brok, P. (2020). Developing preservice teachers’ interpersonal knowledge with 360-degree videos in teacher education. Teaching and Teacher Education . https://doi.org/10.1016/j.tate.2019.102992
Violante, M. G., Vezzetti, E., & Piazzolla, P. (2019). Interactive virtual technologies in engineering education: Why not 360° videos? International Journal on Interactive Design and Manufacturing, 13 , 729–742. https://doi.org/10.1007/s12008-019-00553-y
Walshe, N., & Driver, P. (2019). Developing reflective trainee teacher practice with 360-degree video. Teaching and Teacher Education, 78 , 97–105. https://doi.org/10.1016/j.tate.2018.11.009
Yoganathan, S., Finch, D. A., Parking, E., & Pollard, J. (2018). 360° virtual reality video for the acquisition of knot tying skills: A randomised controlled trial. International Journal of Surgery, 54 , 24–27. https://doi.org/10.1016/j.ijsu.2018.04.002
Download references
Author information
Authors and affiliations.
Center for Digital Learning, PXL University of Applied Sciences and Arts, Elfde-Liniestraat 24, 3500, Hasselt, Belgium
Marie Evens, Michaël Empsen & Wouter Hustinx
You can also search for this author in PubMed Google Scholar
Corresponding author
Correspondence to Marie Evens .
Additional information
Publisher's note.
Springer Nature remains neutral with regard to jurisdictional claims in published maps and institutional affiliations.
See Appendix Table
Rights and permissions
Reprints and permissions
About this article
Evens, M., Empsen, M. & Hustinx, W. A literature review on 360-degree video as an educational tool: towards design guidelines. J. Comput. Educ. 10 , 325–375 (2023). https://doi.org/10.1007/s40692-022-00233-z
Download citation
Received : 24 December 2021
Revised : 23 March 2022
Accepted : 30 May 2022
Published : 24 June 2022
Issue Date : June 2023
DOI : https://doi.org/10.1007/s40692-022-00233-z
Share this article
Anyone you share the following link with will be able to read this content:
Sorry, a shareable link is not currently available for this article.
Provided by the Springer Nature SharedIt content-sharing initiative
- 360-degree video
- Virtual reality
- Systematic review
- Instructional design
- Educational technology
- Find a journal
- Publish with us
- Track your research
Have a language expert improve your writing
Run a free plagiarism check in 10 minutes, generate accurate citations for free.
- Knowledge Base
Methodology
- How to Write a Literature Review | Guide, Examples, & Templates
How to Write a Literature Review | Guide, Examples, & Templates
Published on January 2, 2023 by Shona McCombes . Revised on September 11, 2023.
What is a literature review? A literature review is a survey of scholarly sources on a specific topic. It provides an overview of current knowledge, allowing you to identify relevant theories, methods, and gaps in the existing research that you can later apply to your paper, thesis, or dissertation topic .
There are five key steps to writing a literature review:
- Search for relevant literature
- Evaluate sources
- Identify themes, debates, and gaps
- Outline the structure
- Write your literature review
A good literature review doesn’t just summarize sources—it analyzes, synthesizes , and critically evaluates to give a clear picture of the state of knowledge on the subject.
Instantly correct all language mistakes in your text
Upload your document to correct all your mistakes in minutes
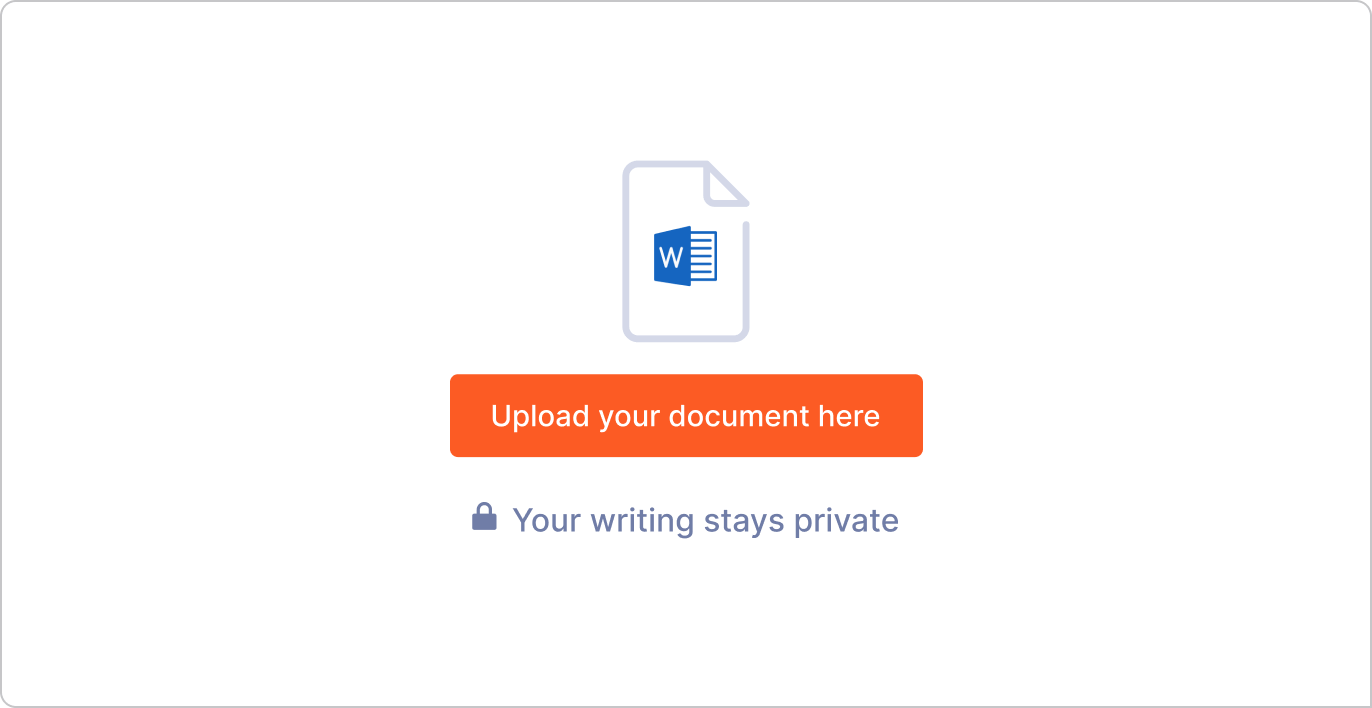
Table of contents
What is the purpose of a literature review, examples of literature reviews, step 1 – search for relevant literature, step 2 – evaluate and select sources, step 3 – identify themes, debates, and gaps, step 4 – outline your literature review’s structure, step 5 – write your literature review, free lecture slides, other interesting articles, frequently asked questions, introduction.
- Quick Run-through
- Step 1 & 2
When you write a thesis , dissertation , or research paper , you will likely have to conduct a literature review to situate your research within existing knowledge. The literature review gives you a chance to:
- Demonstrate your familiarity with the topic and its scholarly context
- Develop a theoretical framework and methodology for your research
- Position your work in relation to other researchers and theorists
- Show how your research addresses a gap or contributes to a debate
- Evaluate the current state of research and demonstrate your knowledge of the scholarly debates around your topic.
Writing literature reviews is a particularly important skill if you want to apply for graduate school or pursue a career in research. We’ve written a step-by-step guide that you can follow below.
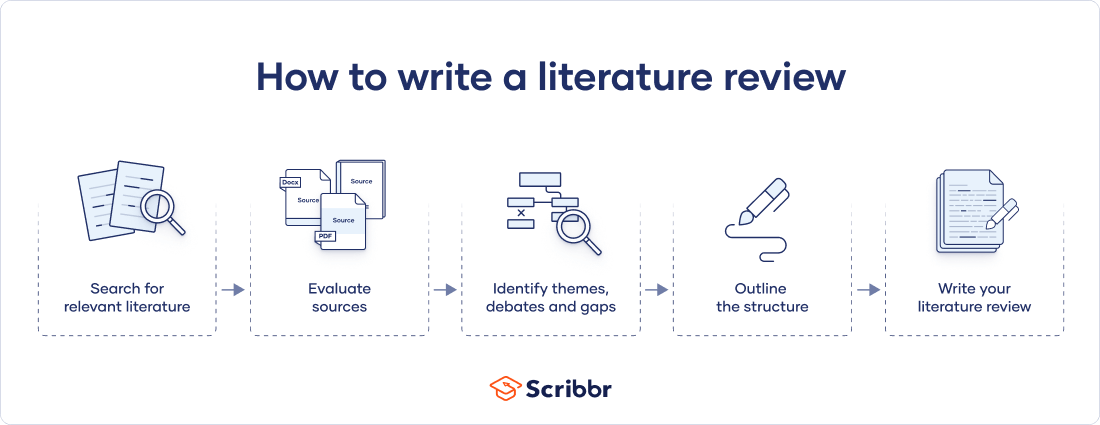
Don't submit your assignments before you do this
The academic proofreading tool has been trained on 1000s of academic texts. Making it the most accurate and reliable proofreading tool for students. Free citation check included.
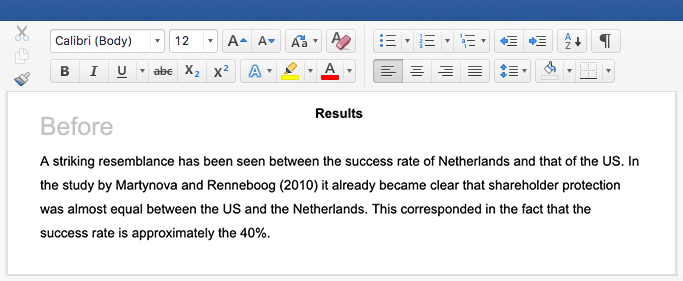
Try for free
Writing literature reviews can be quite challenging! A good starting point could be to look at some examples, depending on what kind of literature review you’d like to write.
- Example literature review #1: “Why Do People Migrate? A Review of the Theoretical Literature” ( Theoretical literature review about the development of economic migration theory from the 1950s to today.)
- Example literature review #2: “Literature review as a research methodology: An overview and guidelines” ( Methodological literature review about interdisciplinary knowledge acquisition and production.)
- Example literature review #3: “The Use of Technology in English Language Learning: A Literature Review” ( Thematic literature review about the effects of technology on language acquisition.)
- Example literature review #4: “Learners’ Listening Comprehension Difficulties in English Language Learning: A Literature Review” ( Chronological literature review about how the concept of listening skills has changed over time.)
You can also check out our templates with literature review examples and sample outlines at the links below.
Download Word doc Download Google doc
Before you begin searching for literature, you need a clearly defined topic .
If you are writing the literature review section of a dissertation or research paper, you will search for literature related to your research problem and questions .
Make a list of keywords
Start by creating a list of keywords related to your research question. Include each of the key concepts or variables you’re interested in, and list any synonyms and related terms. You can add to this list as you discover new keywords in the process of your literature search.
- Social media, Facebook, Instagram, Twitter, Snapchat, TikTok
- Body image, self-perception, self-esteem, mental health
- Generation Z, teenagers, adolescents, youth
Search for relevant sources
Use your keywords to begin searching for sources. Some useful databases to search for journals and articles include:
- Your university’s library catalogue
- Google Scholar
- Project Muse (humanities and social sciences)
- Medline (life sciences and biomedicine)
- EconLit (economics)
- Inspec (physics, engineering and computer science)
You can also use boolean operators to help narrow down your search.
Make sure to read the abstract to find out whether an article is relevant to your question. When you find a useful book or article, you can check the bibliography to find other relevant sources.
You likely won’t be able to read absolutely everything that has been written on your topic, so it will be necessary to evaluate which sources are most relevant to your research question.
For each publication, ask yourself:
- What question or problem is the author addressing?
- What are the key concepts and how are they defined?
- What are the key theories, models, and methods?
- Does the research use established frameworks or take an innovative approach?
- What are the results and conclusions of the study?
- How does the publication relate to other literature in the field? Does it confirm, add to, or challenge established knowledge?
- What are the strengths and weaknesses of the research?
Make sure the sources you use are credible , and make sure you read any landmark studies and major theories in your field of research.
You can use our template to summarize and evaluate sources you’re thinking about using. Click on either button below to download.
Take notes and cite your sources
As you read, you should also begin the writing process. Take notes that you can later incorporate into the text of your literature review.
It is important to keep track of your sources with citations to avoid plagiarism . It can be helpful to make an annotated bibliography , where you compile full citation information and write a paragraph of summary and analysis for each source. This helps you remember what you read and saves time later in the process.
To begin organizing your literature review’s argument and structure, be sure you understand the connections and relationships between the sources you’ve read. Based on your reading and notes, you can look for:
- Trends and patterns (in theory, method or results): do certain approaches become more or less popular over time?
- Themes: what questions or concepts recur across the literature?
- Debates, conflicts and contradictions: where do sources disagree?
- Pivotal publications: are there any influential theories or studies that changed the direction of the field?
- Gaps: what is missing from the literature? Are there weaknesses that need to be addressed?
This step will help you work out the structure of your literature review and (if applicable) show how your own research will contribute to existing knowledge.
- Most research has focused on young women.
- There is an increasing interest in the visual aspects of social media.
- But there is still a lack of robust research on highly visual platforms like Instagram and Snapchat—this is a gap that you could address in your own research.
There are various approaches to organizing the body of a literature review. Depending on the length of your literature review, you can combine several of these strategies (for example, your overall structure might be thematic, but each theme is discussed chronologically).
Chronological
The simplest approach is to trace the development of the topic over time. However, if you choose this strategy, be careful to avoid simply listing and summarizing sources in order.
Try to analyze patterns, turning points and key debates that have shaped the direction of the field. Give your interpretation of how and why certain developments occurred.
If you have found some recurring central themes, you can organize your literature review into subsections that address different aspects of the topic.
For example, if you are reviewing literature about inequalities in migrant health outcomes, key themes might include healthcare policy, language barriers, cultural attitudes, legal status, and economic access.
Methodological
If you draw your sources from different disciplines or fields that use a variety of research methods , you might want to compare the results and conclusions that emerge from different approaches. For example:
- Look at what results have emerged in qualitative versus quantitative research
- Discuss how the topic has been approached by empirical versus theoretical scholarship
- Divide the literature into sociological, historical, and cultural sources
Theoretical
A literature review is often the foundation for a theoretical framework . You can use it to discuss various theories, models, and definitions of key concepts.
You might argue for the relevance of a specific theoretical approach, or combine various theoretical concepts to create a framework for your research.
Like any other academic text , your literature review should have an introduction , a main body, and a conclusion . What you include in each depends on the objective of your literature review.
The introduction should clearly establish the focus and purpose of the literature review.
Depending on the length of your literature review, you might want to divide the body into subsections. You can use a subheading for each theme, time period, or methodological approach.
As you write, you can follow these tips:
- Summarize and synthesize: give an overview of the main points of each source and combine them into a coherent whole
- Analyze and interpret: don’t just paraphrase other researchers — add your own interpretations where possible, discussing the significance of findings in relation to the literature as a whole
- Critically evaluate: mention the strengths and weaknesses of your sources
- Write in well-structured paragraphs: use transition words and topic sentences to draw connections, comparisons and contrasts
In the conclusion, you should summarize the key findings you have taken from the literature and emphasize their significance.
When you’ve finished writing and revising your literature review, don’t forget to proofread thoroughly before submitting. Not a language expert? Check out Scribbr’s professional proofreading services !
This article has been adapted into lecture slides that you can use to teach your students about writing a literature review.
Scribbr slides are free to use, customize, and distribute for educational purposes.
Open Google Slides Download PowerPoint
If you want to know more about the research process , methodology , research bias , or statistics , make sure to check out some of our other articles with explanations and examples.
- Sampling methods
- Simple random sampling
- Stratified sampling
- Cluster sampling
- Likert scales
- Reproducibility
Statistics
- Null hypothesis
- Statistical power
- Probability distribution
- Effect size
- Poisson distribution
Research bias
- Optimism bias
- Cognitive bias
- Implicit bias
- Hawthorne effect
- Anchoring bias
- Explicit bias
A literature review is a survey of scholarly sources (such as books, journal articles, and theses) related to a specific topic or research question .
It is often written as part of a thesis, dissertation , or research paper , in order to situate your work in relation to existing knowledge.
There are several reasons to conduct a literature review at the beginning of a research project:
- To familiarize yourself with the current state of knowledge on your topic
- To ensure that you’re not just repeating what others have already done
- To identify gaps in knowledge and unresolved problems that your research can address
- To develop your theoretical framework and methodology
- To provide an overview of the key findings and debates on the topic
Writing the literature review shows your reader how your work relates to existing research and what new insights it will contribute.
The literature review usually comes near the beginning of your thesis or dissertation . After the introduction , it grounds your research in a scholarly field and leads directly to your theoretical framework or methodology .
A literature review is a survey of credible sources on a topic, often used in dissertations , theses, and research papers . Literature reviews give an overview of knowledge on a subject, helping you identify relevant theories and methods, as well as gaps in existing research. Literature reviews are set up similarly to other academic texts , with an introduction , a main body, and a conclusion .
An annotated bibliography is a list of source references that has a short description (called an annotation ) for each of the sources. It is often assigned as part of the research process for a paper .
Cite this Scribbr article
If you want to cite this source, you can copy and paste the citation or click the “Cite this Scribbr article” button to automatically add the citation to our free Citation Generator.
McCombes, S. (2023, September 11). How to Write a Literature Review | Guide, Examples, & Templates. Scribbr. Retrieved September 9, 2024, from https://www.scribbr.com/dissertation/literature-review/
Is this article helpful?
Shona McCombes
Other students also liked, what is a theoretical framework | guide to organizing, what is a research methodology | steps & tips, how to write a research proposal | examples & templates, "i thought ai proofreading was useless but..".
I've been using Scribbr for years now and I know it's a service that won't disappoint. It does a good job spotting mistakes”
A narrative review on the use of camera traps and machine learning in wildlife research
- December 2022
- Columella Journal of Agricultural and Environmental Sciences 9(2):47-69
- CC BY-NC-ND 4.0

- Hungarian University of Agriculture and Life Sciences

- Hungarian University of Agriculture and Life Sciences (MATE)
Abstract and Figures

Discover the world's research
- 25+ million members
- 160+ million publication pages
- 2.3+ billion citations

- Ashlea Dunn

- Henri Decoeur

- Ioannis Alexiou

- Benjamin Kellenberger

- Tanya Berger-Wolf
- Stephanie M. Bilodeau
- Austin W. H. Schwartz
- Miles Silman
- Elgiriyage M. K. de Silva

- Kottahachchi K. D. A. K. Indrajith

- Alexis Racelis
- Matthew Duggan
- Melissa F. Groleau

- Recruit researchers
- Join for free
- Login Email Tip: Most researchers use their institutional email address as their ResearchGate login Password Forgot password? Keep me logged in Log in or Continue with Google Welcome back! Please log in. Email · Hint Tip: Most researchers use their institutional email address as their ResearchGate login Password Forgot password? Keep me logged in Log in or Continue with Google No account? Sign up
Academia.edu no longer supports Internet Explorer.
To browse Academia.edu and the wider internet faster and more securely, please take a few seconds to upgrade your browser .
Enter the email address you signed up with and we'll email you a reset link.
- We're Hiring!
- Help Center
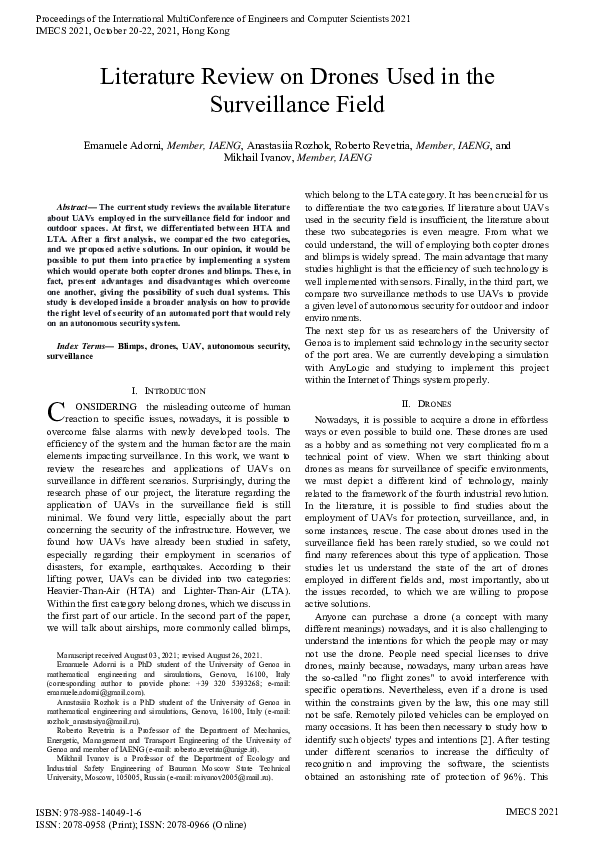
Literature Review on Drones Used in the Surveillance Field

The current study reviews the available literature about UAVs employed in the surveillance field for indoor and outdoor spaces. At first, we differentiated between HTA and LTA. After a first analysis, we compared the two categories, and we proposed active solutions. In our opinion, it would be possible to put them into practice by implementing a system which would operate both copter drones and blimps. These, in fact, present advantages and disadvantages which overcome one another, giving the possibility of such dual systems. This study is developed inside a broader analysis on how to provide the right level of security of an automated port that would rely on an autonomous security system.
Related Papers
Transport Systems Telematics: 10th Conference TST 2010
Zdzislaw Sliwa
Drones started to be very popular and visible in the last two decades. Those more and more sophisticated, cheaper in production and exploitation technologically advances air and land based platforms are entering new domains of people's life very progressively. At the present it is not so easy to fully predict to what purposes drones could be used in the future. We are usually consider them as unmanned platforms to be used by armed forces or national security related services to enhance their capabilities to create safe and secure environment. Nowadays we see new solutions and applications everyday. It is assessed that drones utilization is an opportunity to be exploited for both civilian purposes and their growing presence in airspace is unstoppable process. The variety of application will grow extremely quickly as of new technological discoveries and innovative approach of designers. The growing density in the air is causing the urgent need to enhance legal coverage to avoid incidents as those are a danger now and that aspect will be more serious in the future. The paper is composed of two major parts. The first is focusing on current and future applications of drones and the second one is discussing dangers toward using unmanned aerial vehicles requiring legal solutions national and globally. The purpose of the paper to present drones applications as an advantage but also a risk for national and private security along with requirements for formalizing their utilization within legal frameworks..
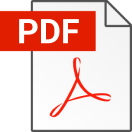
International Journal of Advanced Computer Science and Applications
Faheem mushtaq
Recent advancements in drone technology are opening new opportunities and applications in various fields of life especially in the form of small drones. However, these advancements are also causing new challenges in terms of security, adaptability, and consistency. This research discusses the drone technology, area of usages, citizen multi-objective uses, drones security, protection, and secrecy apprehensions, drone current intimidations and susceptibilities, existing approaches for drone cyber-security methods, security threats to drones and data sources for current literature review. Small drones are proving to be a new opportunity for the civil and military industries. The small drones are suffering from architectural issues and the definition of security and safety issues. The rapid growth of the Internet of Things (IoT) opens new dimensions for drone technology but posing new threats as well. The tiny flying intelligent devices are challenging for the security and privacy of da...
International Journal of Maritime Crime and Security
Jacek Mieżaniec
An unmanned aerial vehicle is a flying apparatus that does not require a crewmember on board to complete a task. Its piloting is done by means of indirect control. UAVs also have the ability to perform autonomous flight on a pre-programmed flight path. The purpose of the study was to use the unmanned aircraft “Fly Eye” by WB Electronic used by the Border Guard to patrol the state border in 103 TRA zone on the section of the Republic of Poland with the Kaliningrad District of the Russian Federation. The TRA zones constitute a specially designated area for the use of the Border Guard and the army, in order to carry out flights there beyond the scope of the pattern for training purposes and to patrol the state border. The article also contains information of the Border Guard formation and the characteristics of the unmanned aerial vehicle “FlyEye”. In order to ensure the safety of the UAV flight, the test was carried out in the TRA zone, which is used for special use for the Border Gua...
Periodica Polytechnica Transportation Engineering
Bianka Károly
The problems related to the integration of the unmanned aerial vehicles into national airspaces is one of the main topics that the scientific researchers are dealing with these days. Despite that, none of them are investigating the UAVs from the surveillance point of view. For that reason, highlighting the problems that have to be dealt with and assessing them through a holistic approach is the aim of the paper. Therefore, the paper takes the different stakeholders view and identify the key factors that have to be taken into account. The authors propose the surveillance technologies that can be considered and evaluated them along the revealed factors through a global view. The results can be applied as a base for the further research in UAVs surveillance domain.
Roberto Revetria
This article represents an effort to investigate the unmanned aerial vehicle (UAV) or ‘drone’ as patrolum service, bringing together its diverse forms, current and projected uses and legal challenges it raises, with a view to its large-scale capabilities for searching and identifying people. The relatively tight focus of contemporary recognition systems allows the use of this technology for searching of individuals. Now the degree of surveillance coverage in time and space that the UAV allows is having important effects, drones are increasingly being used in vast array of civilian and governmental situations. Application of drone’s usage in public places increases the chance that individuals will come under scrutiny from faraway aircraft without knowing it; there is apprehension in the use of domestic drones due to several safety and privacy concerns. After the introduction, the paper describes the overall features of propagation of drone usage from military to civil purposes; then,...
Andrzej Bujak
Drones started to be very popular and visible in the last two decades. Those more and more sophisticated, cheaper in production and exploitation technologically advances air and land based platforms are entering new domains of people's life very progressively. At the present it is not so easy to fully predict to what purposes drones could be used in the future. We are usually consider them as unmanned platforms to be used by armed forces or national security related services to enhance their capabilities to create safe and secure environment. Nowadays we see new solutions and applications everyday. It is assessed that drones utilization is an opportunity to be exploited for both civilian purposes and their growing presence in airspace is unstoppable process. The variety of application will grow extremely quickly as of new technological discoveries and innovative approach of designers. The growing density in the air is causing the urgent need to enhance legal coverage to avoid in...
Gordon Ononiwu
This work presents the design and construction of an unmanned aerial vehicle (UAV), to be used as an autonomous guided security system for civilian applications in areas such as surveillance and gathering. The UAV was designed to be powered by a DC battery and to carry a maximum payload of 1.9kg. It is equipped with a microcontroller, actuators and sensors which enable it acquire information from its environment, respond to sensory inputs and move accordingly desired destination. The hexacopter when tested after its construction met some of its design specifications such as weight, communication, correct calibration of motors, imaging. All the objectives for stable flight wer achieved due to the difficulty in implementing a controller to balance the system. More work in this area to improve on the PID controller in other to achieve stable flight.
International Journal on Cybernetics & Informatics
Jose Silvestre S . Silva , Verónica Mendes Pinto
The objective of this work is to support the continuous development and modernization of the Gendarmerie Forces, analyzing the use of Drones in the police activities of the Portuguese and Spanish Gendarmerie forces, examining the extent to which the implementation and expansion of these resources represent an advantage for the police service and operations of the Gendarmerie. Due to the great changes taking place in the world, it is crucial to rethink state security. The convergence of internal and external threats, together with the increase in the feeling of insecurity on a global scale, are emerging factors that require different strategies of the security forces, especially with regard to the support tools used. Thus, there was a need to analyze the legal panorama of the Drones used in police, military and customs missions, verifying their classification as state aircraft, verifying the current doctrine (civil, military and police), taking into account the analysis of these scenarios. To this end, a methodology based on the inductive method was adopted, which made it possible to generalize the data collected through the analysis of data on the Spanish Gendarmerie force, appreciating their characteristics and use, with the aim of comparing the modus operandi with the Portuguese Gendarmerie force.
Loading Preview
Sorry, preview is currently unavailable. You can download the paper by clicking the button above.
RELATED PAPERS
International Journal of Computer Applications
Fred Ondieki
International Journal of Business Analytics & Intelligence
Publishing India Group
2017 IEEE/AIAA 36th Digital Avionics Systems Conference (DASC)
Keith Mayes
Máté Gajdos
IJARTET Journal
ACM Transactions on Cyber-Physical Systems
Riham AlTawy
Abayomi O Agbeyangi , Adam B Ọlọ́runlómẹrúẹ̀, Ph.D
2019 22nd Euromicro Conference on Digital System Design (DSD)
Reda Nouacer
Journal of Civil & Environmental Engineering
Jacek Doskocz
Security and Defence Quarterly
IMPLEMENTATION OF UNMANNED AERIAL VEHICLE (DRONE) SURVEILLANCE FOR VIDEO DATA ACQUISITION
Abdullahi Muhammed Gulak
Zvonimir Ivanovic
Internet of Things
James Sluss
John Rey Lirag
International Journal Of engineering and Computer Science (IJECS)
Iulisloi Zacarias
ENGINEERING. TECHOLOGIES. EDUCATION. SECURITY. '19 Proceedings Volume 3. Security
János Csengeri
IEEE Access
Mohammed Gismalla
Sumant Kumar Pandey
Andrea Gilli
George Eftychidis
Abubakar Bala
Unmanned/Unattended Sensors and Sensor Networks V
T. Landers , Robert Huck
Vishal Arora
- We're Hiring!
- Help Center
- Find new research papers in:
- Health Sciences
- Earth Sciences
- Cognitive Science
- Mathematics
- Computer Science
- Academia ©2024

IMAGES
VIDEO
COMMENTS
employing camera methods, we present a systematic literature review of camera use and image. Data 2020, 5, 51; doi:10.3390 / data5020051 www.mdpi.com / journal / data. Data 2020, 5, 51 2 of 19.
This systematic review examines the current evidence base for efficacy of body-worn video and the current case for implementation. Five articles were identified as pertinent to this review from a search of five electronic databases, with a further six articles of grey literature included.
Camera-based data collection and image analysis are integral methods in many research disciplines. However, few studies are specifically dedicated to trends in these methods or opportunities for interdisciplinary learning. In this systematic literature review, we analyze published sources (n = 391) to synthesize camera use patterns and image collection and analysis techniques across research ...
Research Summary. In this article, we provide the most comprehensive narrative review to date of the research evidence base for body-worn cameras (BWCs). Seventy empirical studies of BWCs were examined covering the impact of cameras on officer behavior, officer perceptions, citizen behavior, citizen perceptions, police investigations, and ...
The thesis explores the new perception and photographic uses of smartphone cameras in photography in 2018. The target group of the study are adults from the age of 20 and above. The research was conducted using literature review and online questionnaire. Photography is a form of art that involves capturing images and showcasing them to the ...
A literature review about the existing video systems. ... Cameras can be classified into two categories: simple cameras and night vision cameras.The first category operates in daylight when the space is lit up, the second category operates day and night, thus taking into consideration the illumination conditions. ...
This article serves as a literature review of the state of the art of the AF system in both visible light digital cameras and thermal infrared cameras. The autofocus (AF) system has gained popularity in over 40 years. Embedded in a camera, it can bring the best focused image to the viewer within a few seconds, which frees users from focusing manually.
This paper provides a comprehensive and systematic review on the literature from various video surveillance system studies published from 2010 through 2019. Within a selected study extraction process, 220 journal-based publications were identified and analyzed to illustrate the research trends, datasets, methods, and frameworks used in the ...
The paper begins with identifying the main outcomes of video analysis. Application areas where surveillance cameras are unavoidable are discussed. Current status and trends in video analysis are revealed through literature review. Finally the vital points which need more consideration in near future are explicitly stated.
Suggested Citation: "CHAPTER TWO Literature Review." National Academies of Sciences, Engineering, and Medicine. 2016. Onboard Camera Applications for Buses. Washington, DC: The National Academies Press. doi: 10.17226/23554. ×. Save. Cancel. Below is the uncorrected machine-read text of this chapter, intended to provide our own search engines ...
In 2014 the results of the first large scale study of the impact of officer body worn cameras, or. BWCs have on policing was published. This study, in Rialto California, found that BWCs. reduced complaints against Rialto officers by approximately 90% and reductions in the use of. force by officers by approximately 50%.
Thermal influence on cameras - literature review. Photogrammetric methods have been proved to be particularly useful in engineering where high precision measurements are required (Kölbl, 1976, Rieke-Zapp and Nearing, 2005, Luhmann, 2010). Multiple factors can have an impact on photogrammetric accuracy. First of all, a higher resolution of ...
define "smart camera", or "intelligent camera", as an embedded vision system that. is capable of extracting application-specific information from the captured images. and generating ...
CCTV: Literature Review and Bibliography. Wade Deisman, PhD. 2003. Faced with the demand that they develop more efficacious security measures and find more cost-effective crime prevention strategies, law enforcement agencies around the globe are, now more than ever, turning toward technological systems to enhance operational capacities, extend ...
Due to its possibility to create immersive learning experiences through simulated realism, virtual reality (VR) is a promising tool for educational purposes. A more user-friendly, realistic and affordable version of VR is 360° video, recording real-world footage with omnidirectional or multi-camera systems. 360° videos are slowly finding their way into educational research and practice, but ...
Research Summary We report on the findings of an updated systematic review and meta‐analysis of the effects of closed‐circuit television (CCTV) surveillance cameras on crime. The findings show ...
Literature reviews establish the foundation of academic inquires. However, in the planning field, we lack rigorous systematic reviews. In this article, through a systematic search on the methodology of literature review, we categorize a typology of literature reviews, discuss steps in conducting a systematic literature review, and provide suggestions on how to enhance rigor in literature ...
Examples of literature reviews. Step 1 - Search for relevant literature. Step 2 - Evaluate and select sources. Step 3 - Identify themes, debates, and gaps. Step 4 - Outline your literature review's structure. Step 5 - Write your literature review.
CCTV: Literature Review and Bibliography. CCTV: Lit erat ure Revie w and Bi bliogr aphy. by. Wade Deisman. University of Ottawa. Research and Evaluation B ranc h. Commun ity, Co ntr act a nd Ab ...
Literature Review and Annotated Bibliography . By Joan M. Ratz and Shannon J. Conk . Open-File Report 2010-1306 . ... camera use covered in the literature review include: camera options, image retrieval, system maintenance and monitoring, time to assemble, power source, light source, camera mount, frequency of ...
In the literature, it is possible to find studies about the employment of UAVs for protection, surveillance, and, in some instances, rescue. The case about drones used in the surveillance field has been rarely studied, so we could not find many references about this type of application. Those studies let us understand the state of the art of ...
A summary is provided on the use of both camera traps and machine learning and the main challenges that come with it by performing a general literature review. Remote cameras can be used in a ...
The current study reviews the available literature about UAVs employed in the surveillance field for indoor and outdoor spaces. At first, we differentiated between HTA and LTA. After a first analysis, we compared the two categories, and we proposed active solutions. In our opinion, it would be possible to put them into practice by implementing ...