Jump to navigation


Cochrane Training
Chapter 3: defining the criteria for including studies and how they will be grouped for the synthesis.
Joanne E McKenzie, Sue E Brennan, Rebecca E Ryan, Hilary J Thomson, Renea V Johnston, James Thomas
Key Points:
- The scope of a review is defined by the types of population (participants), types of interventions (and comparisons), and the types of outcomes that are of interest. The acronym PICO (population, interventions, comparators and outcomes) helps to serve as a reminder of these.
- The population, intervention and comparison components of the question, with the additional specification of types of study that will be included, form the basis of the pre-specified eligibility criteria for the review. It is rare to use outcomes as eligibility criteria: studies should be included irrespective of whether they report outcome data, but may legitimately be excluded if they do not measure outcomes of interest, or if they explicitly aim to prevent a particular outcome.
- Cochrane Reviews should include all outcomes that are likely to be meaningful and not include trivial outcomes. Critical and important outcomes should be limited in number and include adverse as well as beneficial outcomes.
- Review authors should plan at the protocol stage how the different populations, interventions, outcomes and study designs within the scope of the review will be grouped for analysis.
Cite this chapter as: McKenzie JE, Brennan SE, Ryan RE, Thomson HJ, Johnston RV, Thomas J. Chapter 3: Defining the criteria for including studies and how they will be grouped for the synthesis. In: Higgins JPT, Thomas J, Chandler J, Cumpston M, Li T, Page MJ, Welch VA (editors). Cochrane Handbook for Systematic Reviews of Interventions version 6.4 (updated August 2023). Cochrane, 2023. Available from www.training.cochrane.org/handbook .
3.1 Introduction
One of the features that distinguishes a systematic review from a narrative review is that systematic review authors should pre-specify criteria for including and excluding studies in the review (eligibility criteria, see MECIR Box 3.2.a ).
When developing the protocol, one of the first steps is to determine the elements of the review question (including the population, intervention(s), comparator(s) and outcomes, or PICO elements) and how the intervention, in the specified population, produces the expected outcomes (see Chapter 2, Section 2.5.1 and Chapter 17, Section 17.2.1 ). Eligibility criteria are based on the PICO elements of the review question plus a specification of the types of studies that have addressed these questions. The population, interventions and comparators in the review question usually translate directly into eligibility criteria for the review, though this is not always a straightforward process and requires a thoughtful approach, as this chapter shows. Outcomes usually are not part of the criteria for including studies, and a Cochrane Review would typically seek all sufficiently rigorous studies (most commonly randomized trials) of a particular comparison of interventions in a particular population of participants, irrespective of the outcomes measured or reported. It should be noted that some reviews do legitimately restrict eligibility to specific outcomes. For example, the same intervention may be studied in the same population for different purposes; or a review may specifically address the adverse effects of an intervention used for several conditions (see Chapter 19 ).
Eligibility criteria do not exist in isolation, but should be specified with the synthesis of the studies they describe in mind. This will involve making plans for how to group variants of the PICO elements for synthesis. This chapter describes the processes by which the structure of the synthesis can be mapped out at the beginning of the review, and the interplay between the review question, considerations for the analysis and their operationalization in terms of eligibility criteria. Decisions about which studies to include (and exclude), and how they will be combined in the review’s synthesis, should be documented and justified in the review protocol.
A distinction between three different stages in the review at which the PICO construct might be used is helpful for understanding the decisions that need to be made. In Chapter 2, Section 2.3 , we introduced the ideas of a review PICO (on which eligibility of studies is based), the PICO for each synthesis (defining the question that each specific synthesis aims to answer) and the PICO of the included studies (what was actually investigated in the included studies). In this chapter, we focus on the review PICO and the PICO for each synthesis as a basis for specifying which studies should be included in the review and planning its syntheses. These PICOs should relate clearly and directly to the questions or hypotheses that are posed when the review is formulated (see Chapter 2 ) and will involve specifying the population in question, and a set of comparisons between the intervention groups.
An integral part of the process of setting up the review is to specify which characteristics of the interventions (e.g. individual compounds of a drug), populations (e.g. acute and chronic conditions), outcomes (e.g. different depression measurement scales) and study designs, will be grouped together. Such decisions should be made independent of knowing which studies will be included and the methods of synthesis that will be used (e.g. meta-analysis). There may be a need to modify the comparisons and even add new ones at the review stage in light of the data that are collected. For example, important variations in the intervention may be discovered only after data are collected, or modifying the comparison may facilitate the possibility of synthesis when only one or few studies meet the comparison PICO. Planning for the latter scenario at the protocol stage may lead to less post-hoc decision making ( Chapter 2, Section 2.5.3 ) and, of course, any changes made during the conduct of the review should be recorded and documented in the final report.
3.2 Articulating the review and comparison PICO
3.2.1 defining types of participants: which people and populations.
The criteria for considering types of people included in studies in a review should be sufficiently broad to encompass the likely diversity of studies and the likely scenarios in which the interventions will be used, but sufficiently narrow to ensure that a meaningful answer can be obtained when studies are considered together; they should be specified in advance (see MECIR Box 3.2.a ). As discussed in Chapter 2, Section 2.3.1 , the degree of breadth will vary, depending on the question being asked and the analytical approach to be employed. A range of evidence may inform the choice of population characteristics to examine, including theoretical considerations, evidence from other interventions that have a similar mechanism of action, and in vitro or animal studies. Consideration should be given to whether the population characteristic is at the level of the participant (e.g. age, severity of disease) or the study (e.g. care setting, geographical location), since this has implications for grouping studies and for the method of synthesis ( Chapter 10, Section 10.11.5 ). It is often helpful to consider the types of people that are of interest in three steps.
MECIR Box 3.2.a Relevant expectations for conduct of intervention reviews
First, the diseases or conditions of interest should be defined using explicit criteria for establishing their presence (or absence). Criteria that will force the unnecessary exclusion of studies should be avoided. For example, diagnostic criteria that were developed more recently – which may be viewed as the current gold standard for diagnosing the condition of interest – will not have been used in earlier studies. Expensive or recent diagnostic tests may not be available in many countries or settings, and time-consuming tests may not be practical in routine healthcare settings.
Second, the broad population and setting of interest should be defined . This involves deciding whether a specific population group is within scope, determined by factors such as age, sex, race, educational status or the presence of a particular condition such as angina or shortness of breath. Interest may focus on a particular setting such as a community, hospital, nursing home, chronic care institution, or outpatient setting. Box 3.2.a outlines some factors to consider when developing population criteria.
Whichever criteria are used for defining the population and setting of interest, it is common to encounter studies that only partially overlap with the review’s population. For example, in a review focusing on children, a cut-point of less than 16 years might be desirable, but studies may be identified with participants aged from 12 to 18. Unless the study reports separate data from the eligible section of the population (in which case data from the eligible participants can be included in the review), review authors will need a strategy for dealing with these studies (see MECIR Box 3.2.a ). This will involve balancing concerns about reduced applicability by including participants who do not meet the eligibility criteria, against the loss of data when studies are excluded. Arbitrary rules (such as including a study if more than 80% of the participants are under 16) will not be practical if detailed information is not available from the study. A less stringent rule, such as ‘the majority of participants are under 16’ may be sufficient. Although there is a risk of review authors’ biases affecting post-hoc inclusion decisions (which is why many authors endeavour to pre-specify these rules), this may be outweighed by a common-sense strategy in which eligibility decisions keep faith with the objectives of the review rather than with arbitrary rules. Difficult decisions should be documented in the review, checked with the advisory group (if available, see Chapter 1 ), and sensitivity analyses can assess the impact of these decisions on the review’s findings (see Chapter 10, Section 10.14 and MECIR Box 3.2.b ).
Box 3.2.a Factors to consider when developing criteria for ‘Types of participants’
MECIR Box 3.2.b Relevant expectations for conduct of intervention reviews
Third, there should be consideration of whether there are population characteristics that might be expected to modify the size of the intervention effects (e.g. different severities of heart failure). Identifying subpopulations may be important for implementation of the intervention. If relevant subpopulations are identified, two courses of action are possible: limiting the scope of the review to exclude certain subpopulations; or maintaining the breadth of the review and addressing subpopulations in the analysis.
Restricting the review with respect to specific population characteristics or settings should be based on a sound rationale. It is important that Cochrane Reviews are globally relevant, so the rationale for the exclusion of studies based on population characteristics should be justified. For example, focusing a review of the effectiveness of mammographic screening on women between 40 and 50 years old may be justified based on biological plausibility, previously published systematic reviews and existing controversy. On the other hand, focusing a review on a particular subgroup of people on the basis of their age, sex or ethnicity simply because of personal interests, when there is no underlying biologic or sociological justification for doing so, should be avoided, as these reviews will be less useful to decision makers and readers of the review.
Maintaining the breadth of the review may be best when it is uncertain whether there are important differences in effects among various subgroups of people, since this allows investigation of these differences (see Chapter 10, Section 10.11.5 ). Review authors may combine the results from different subpopulations in the same synthesis, examining whether a given subdivision explains variation (heterogeneity) among the intervention effects. Alternatively, the results may be synthesized in separate comparisons representing different subpopulations. Splitting by subpopulation risks there being too few studies to yield a useful synthesis (see Table 3.2.a and Chapter 2, Section 2.3.2 ). Consideration needs to be given to the subgroup analysis method, particularly for population characteristics measured at the participant level (see Chapter 10 and Chapter 26 , Fisher et al 2017). All subgroup analyses should ideally be planned a priori and stated as a secondary objective in the protocol, and not driven by the availability of data.
In practice, it may be difficult to assign included studies to defined subpopulations because of missing information about the population characteristic, variability in how the population characteristic is measured across studies (e.g. variation in the method used to define the severity of heart failure), or because the study does not wholly fall within (or report the results separately by) the defined subpopulation. The latter issue mainly applies for participant characteristics but can also arise for settings or geographic locations where these vary within studies. Review authors should consider planning for these scenarios (see example reviews Hetrick et al 2012, Safi et al 2017; Table 3.2.b , column 3).
Table 3.2.a Examples of population attributes and characteristics
3.2.2 Defining interventions and how they will be grouped
In some reviews, predefining the intervention ( MECIR Box 3.2.c ) may be straightforward. For example, in a review of the effect of a given anticoagulant on deep vein thrombosis, the intervention can be defined precisely. A more complicated definition might be required for a multi-component intervention composed of dietary advice, training and support groups to reduce rates of obesity in a given population.
The inherent complexity present when defining an intervention often comes to light when considering how it is thought to achieve its intended effect and whether the effect is likely to differ when variants of the intervention are used. In the first example, the anticoagulant warfarin is thought to reduce blood clots by blocking an enzyme that depends on vitamin K to generate clotting factors. In the second, the behavioural intervention is thought to increase individuals’ self-efficacy in their ability to prepare healthy food. In both examples, we cannot assume that all forms of the intervention will work in the same way. When defining drug interventions, such as anticoagulants, factors such as the drug preparation, route of administration, dose, duration, and frequency should be considered. For multi-component interventions (such as interventions to reduce rates of obesity), the common or core features of the interventions must be defined, so that the review authors can clearly differentiate them from other interventions not included in the review.
MECIR Box 3.2.c Relevant expectations for conduct of intervention reviews
In general, it is useful to consider exactly what is delivered, who delivers it, how it is delivered, where it is delivered, when and how much is delivered, and whether the intervention can be adapted or tailored , and to consider this for each type of intervention included in the review (see the TIDieR checklist (Hoffmann et al 2014)). As argued in Chapter 17 , separating interventions into ‘simple’ and ‘complex’ is a false dichotomy; all interventions can be complex in some ways. The critical issue for review authors is to identify the most important factors to be considered in a specific review. Box 3.2.b outlines some factors to consider when developing broad criteria for the ‘Types of interventions’ (and comparisons).
Box 3.2.b Factors to consider when developing criteria for ‘Types of interventions’
Once interventions eligible for the review have been broadly defined, decisions should be made about how variants of the intervention will be handled in the synthesis. Differences in intervention characteristics across studies occur in all reviews. If these reflect minor differences in the form of the intervention used in practice (such as small differences in the duration or content of brief alcohol counselling interventions), then an overall synthesis can provide useful information for decision makers. Where differences in intervention characteristics are more substantial (such as delivery of brief alcohol counselling by nurses versus doctors), and are expected to have a substantial impact on the size of intervention effects, these differences should be examined in the synthesis. What constitutes an important difference requires judgement, but in general differences that alter decisions about how an intervention is implemented or whether the intervention is used or not are likely to be important. In such circumstances, review authors should consider specifying separate groups (or subgroups) to examine in their synthesis.
Clearly defined intervention groups serve two main purposes in the synthesis. First, the way in which interventions are grouped for synthesis (meta-analysis or other synthesis) is likely to influence review findings. Careful planning of intervention groups makes best use of the available data, avoids decisions that are influenced by study findings (which may introduce bias), and produces a review focused on questions relevant to decision makers. Second, the intervention groups specified in a protocol provide a standardized terminology for describing the interventions throughout the review, overcoming the varied descriptions used by study authors (e.g. where different labels are used for the same intervention, or similar labels used for different techniques) (Michie et al 2013). This standardization enables comparison and synthesis of information about intervention characteristics across studies (common characteristics and differences) and provides a consistent language for reporting that supports interpretation of review findings.
Table 3.2.b outlines a process for planning intervention groups as a basis for/precursor to synthesis, and the decision points and considerations at each step. The table is intended to guide, rather than to be prescriptive and, although it is presented as a sequence of steps, the process is likely to be iterative, and some steps may be done concurrently or in a different sequence. The process aims to minimize data-driven approaches that can arise once review authors have knowledge of the findings of the included studies. It also includes principles for developing a flexible plan that maximizes the potential to synthesize in circumstances where there are few studies, many variants of an intervention, or where the variants are difficult to anticipate. In all stages, review authors should consider how to categorize studies whose reports contain insufficient detail.
Table 3.2.b A process for planning intervention groups for synthesis
3.2.3 Defining which comparisons will be made
When articulating the PICO for each synthesis, defining the intervention groups alone is not sufficient for complete specification of the planned syntheses. The next step is to define the comparisons that will be made between the intervention groups. Setting aside for a moment more complex analyses such as network meta-analyses, which can simultaneously compare many groups ( Chapter 11 ), standard meta-analysis ( Chapter 10 ) aims to draw conclusions about the comparative effects of two groups at a time (i.e. which of two intervention groups is more effective?). These comparisons form the basis for the syntheses that will be undertaken if data are available. Cochrane Reviews sometimes include one comparison, but most often include multiple comparisons. Three commonly identified types of comparisons include the following (Davey et al 2011).
- newer generation antidepressants versus placebo (Hetrick et al 2012); and
- vertebroplasty for osteoporotic vertebral compression fractures versus placebo (sham procedure) (Buchbinder et al 2018).
- chemotherapy or targeted therapy plus best supportive care (BSC) versus BSC for palliative treatment of esophageal and gastroesophageal-junction carcinoma (Janmaat et al 2017); and
- personalized care planning versus usual care for people with long-term conditions (Coulter et al 2015).
- early (commenced at less than two weeks of age) versus late (two weeks of age or more) parenteral zinc supplementation in term and preterm infants (Taylor et al 2017);
- high intensity versus low intensity physical activity or exercise in people with hip or knee osteoarthritis (Regnaux et al 2015);
- multimedia education versus other education for consumers about prescribed and over the counter medications (Ciciriello et al 2013).
The first two types of comparisons aim to establish the effectiveness of an intervention, while the last aims to compare the effectiveness of two interventions. However, the distinction between the placebo and control is often arbitrary, since any differences in the care provided between trials with a control arm and those with a placebo arm may be unimportant , especially where ‘usual care’ is provided to both. Therefore, placebo and control groups may be determined to be similar enough to be combined for synthesis.
In reviews including multiple intervention groups, many comparisons are possible. In some of these reviews, authors seek to synthesize evidence on the comparative effectiveness of all their included interventions, including where there may be only indirect comparison of some interventions across the included studies ( Chapter 11, Section 11.2.1 ). However, in many reviews including multiple intervention groups, a limited subset of the possible comparisons will be selected. The chosen subset of comparisons should address the most important clinical and research questions. For example, if an established intervention (or dose of an intervention) is used in practice, then the synthesis would ideally compare novel or alternative interventions to this established intervention, and not, for example, to no intervention.
3.2.3.1 Dealing with co-interventions
Planning is needed for the special case where the same supplementary intervention is delivered to both the intervention and comparator groups. A supplementary intervention is an additional intervention delivered alongside the intervention of interest, such as massage in a review examining the effects of aromatherapy (i.e. aromatherapy plus massage versus massage alone). In many cases, the supplementary intervention will be unimportant and can be ignored. In other situations, the effect of the intervention of interest may differ according to whether participants receive the supplementary therapy. For example, the effect of aromatherapy among people who receive a massage may differ from the effect of the aromatherapy given alone. This will be the case if the intervention of interest interacts with the supplementary intervention leading to larger (synergistic) or smaller (dysynergistic/antagonistic) effects than the intervention of interest alone (Squires et al 2013). While qualitative interactions are rare (where the effect of the intervention is in the opposite direction when combined with the supplementary intervention), it is possible that there will be more variation in the intervention effects (heterogeneity) when supplementary interventions are involved, and it is important to plan for this. Approaches for dealing with this in the statistical synthesis may include fitting a random-effects meta-analysis model that encompasses heterogeneity ( Chapter 10, Section 10.10.4 ), or investigating whether the intervention effect is modified by the addition of the supplementary intervention through subgroup analysis ( Chapter 10, Section 10.11.2 ).
3.2.4 Selecting, prioritizing and grouping review outcomes
3.2.4.1 selecting review outcomes.
Broad outcome domains are decided at the time of setting up the review PICO (see Chapter 2 ). Once the broad domains are agreed, further specification is required to define the domains to facilitate reporting and synthesis (i.e. the PICO for comparison) (see Chapter 2, Section 2.3 ). The process for specifying and grouping outcomes largely parallels that used for specifying intervention groups.
Reporting of outcomes should rarely determine study eligibility for a review. In particular, studies should not be excluded because they do not report results of an outcome they may have measured, or provide ‘no usable data’ ( MECIR Box 3.2.d ). This is essential to avoid bias arising from selective reporting of findings by the study authors (see Chapter 13 ). However, in some circumstances, the measurement of certain outcomes may be a study eligibility criterion. This may be the case, for example, when the review addresses the potential for an intervention to prevent a particular outcome, or when the review addresses a specific purpose of an intervention that can be used in the same population for different purposes (such as hormone replacement therapy, or aspirin).
MECIR Box 3.2.d Relevant expectations for conduct of intervention reviews
In general, systematic reviews should aim to include outcomes that are likely to be meaningful to the intended users and recipients of the reviewed evidence. This may include clinicians, patients (consumers), the general public, administrators and policy makers. Outcomes may include survival (mortality), clinical events (e.g. strokes or myocardial infarction), behavioural outcomes (e.g. changes in diet, use of services), patient-reported outcomes (e.g. symptoms, quality of life), adverse events, burdens (e.g. demands on caregivers, frequency of tests, restrictions on lifestyle) and economic outcomes (e.g. cost and resource use). It is critical that outcomes used to assess adverse effects as well as outcomes used to assess beneficial effects are among those addressed by a review (see Chapter 19 ).
Outcomes that are trivial or meaningless to decision makers should not be included in Cochrane Reviews. Inclusion of outcomes that are of little or no importance risks overwhelming and potentially misleading readers. Interim or surrogate outcomes measures, such as laboratory results or radiologic results (e.g. loss of bone mineral content as a surrogate for fractures in hormone replacement therapy), while potentially helpful in explaining effects or determining intervention integrity (see Chapter 5, Section 5.3.4.1 ), can also be misleading since they may not predict clinically important outcomes accurately. Many interventions reduce the risk for a surrogate outcome but have no effect or have harmful effects on clinically relevant outcomes, and some interventions have no effect on surrogate measures but improve clinical outcomes.
Various sources can be used to develop a list of relevant outcomes, including input from consumers and advisory groups (see Chapter 2 ), the clinical experiences of the review authors, and evidence from the literature (including qualitative research about outcomes important to those affected (see Chapter 21 )). A further driver of outcome selection is consideration of outcomes used in related reviews. Harmonization of outcomes across reviews addressing related questions facilitates broader evidence synthesis questions being addressed through the use of Overviews of reviews (see Chapter V ).
Outcomes considered to be meaningful, and therefore addressed in a review, may not have been reported in the primary studies. For example, quality of life is an important outcome, perhaps the most important outcome, for people considering whether or not to use chemotherapy for advanced cancer, even if the available studies are found to report only survival (see Chapter 18 ). A further example arises with timing of the outcome measurement, where time points determined as clinically meaningful in a review are not measured in the primary studies. Including and discussing all important outcomes in a review will highlight gaps in the primary research and encourage researchers to address these gaps in future studies.
3.2.4.2 Prioritizing review outcomes
Once a full list of relevant outcomes has been compiled for the review, authors should prioritize the outcomes and select the outcomes of most relevance to the review question. The GRADE approach to assessing the certainty of evidence (see Chapter 14 ) suggests that review authors separate outcomes into those that are ‘critical’, ‘important’ and ‘not important’ for decision making.
The critical outcomes are the essential outcomes for decision making, and are those that would form the basis of a ‘Summary of findings’ table or other summary versions of the review, such as the Abstract or Plain Language Summary. ‘Summary of findings’ tables provide key information about the amount of evidence for important comparisons and outcomes, the quality of the evidence and the magnitude of effect (see Chapter 14, Section 14.1 ). There should be no more than seven outcomes included in a ‘Summary of findings’ table, and those outcomes that will be included in summaries should be specified at the protocol stage. They should generally not include surrogate or interim outcomes. They should not be chosen on the basis of any anticipated or observed magnitude of effect, or because they are likely to have been addressed in the studies to be reviewed. Box 3.2.c summarizes the principal factors to consider when selecting and prioritizing review outcomes.
Box 3.2.c Factors to consider when selecting and prioritizing review outcomes
3.2.4.3 Defining and grouping outcomes for synthesis
Table 3.2.c outlines a process for planning for the diversity in outcome measurement that may be encountered in the studies included in a review and which can complicate, and sometimes prevent, synthesis. Research has repeatedly documented inconsistency in the outcomes measured across trials in the same clinical areas (Harrison et al 2016, Williamson et al 2017). This inconsistency occurs across all aspects of outcome measurement, including the broad domains considered, the outcomes measured, the way these outcomes are labelled and defined, and the methods and timing of measurement. For example, a review of outcome measures used in 563 studies of interventions for dementia and mild cognitive impairment found that 321 unique measurement methods were used for 1278 assessments of cognitive outcomes (Harrison et al 2016). Initiatives like COMET ( Core Outcome Measures in Effectiveness Trials ) aim to encourage standardization of outcome measurement across trials (Williamson et al 2017), but these initiatives are comparatively new and review authors will inevitably encounter diversity in outcomes across studies.
The process begins by describing the scope of each outcome domain in sufficient detail to enable outcomes from included studies to be categorized ( Table 3.2.c Step 1). This step may be straightforward in areas for which core outcome sets (or equivalent systems) exist ( Table 3.2.c Step 2). The methods and timing of outcome measurement also need to be specified, giving consideration to how differences across studies will be handled ( Table 3.2.c Steps 3 and 4). Subsequent steps consider options for dealing with studies that report multiple measures within an outcome domain ( Table 3.2.c Step 5), planning how outcome domains will be used in synthesis ( Table 3.2.c Step 6), and building in contingencies to maximize potential to synthesize ( Table 3.2.c Step 7).
Table 3.2.c A process for planning outcome groups for synthesis
3.3 Determining which study designs to include
Some study designs are more appropriate than others for answering particular questions. Authors need to consider a priori what study designs are likely to provide reliable data with which to address the objectives of their review ( MECIR Box 3.3.a ). Sections 3.3.1 and 3.3.2 cover randomized and non-randomized designs for assessing treatment effects; Chapter 17, Section 17.2.5 discusses other study designs in the context of addressing intervention complexity.
MECIR Box 3.3.a Relevant expectations for conduct of intervention reviews
3.3.1 Including randomized trials
Because Cochrane Reviews address questions about the effects of health care, they focus primarily on randomized trials and randomized trials should be included if they are feasible for the interventions of interest ( MECIR Box 3.3.b ). Randomization is the only way to prevent systematic differences between baseline characteristics of participants in different intervention groups in terms of both known and unknown (or unmeasured) confounders (see Chapter 8 ), and claims about cause and effect can be based on their findings with far more confidence than almost any other type of study. For clinical interventions, deciding who receives an intervention and who does not is influenced by many factors, including prognostic factors. Empirical evidence suggests that, on average, non-randomized studies produce effect estimates that indicate more extreme benefits of the effects of health care than randomized trials. However, the extent, and even the direction, of the bias is difficult to predict. These issues are discussed at length in Chapter 24 , which provides guidance on when it might be appropriate to include non-randomized studies in a Cochrane Review.
Practical considerations also motivate the restriction of many Cochrane Reviews to randomized trials. In recent decades there has been considerable investment internationally in establishing infrastructure to index and identify randomized trials. Cochrane has contributed to these efforts, including building up and maintaining a database of randomized trials, developing search filters to aid their identification, working with MEDLINE to improve tagging and identification of randomized trials, and using machine learning and crowdsourcing to reduce author workload in identifying randomized trials ( Chapter 4, Section 4.6.6.2 ). The same scale of organizational investment has not (yet) been matched for the identification of other types of studies. Consequently, identifying and including other types of studies may require additional efforts to identify studies and to keep the review up to date, and might increase the risk that the result of the review will be influenced by publication bias. This issue and other bias-related issues that are important to consider when defining types of studies are discussed in detail in Chapter 7 and Chapter 13 .
Specific aspects of study design and conduct should be considered when defining eligibility criteria, even if the review is restricted to randomized trials. For example, whether cluster-randomized trials ( Chapter 23, Section 23.1 ) and crossover trials ( Chapter 23, Section 23.2 ) are eligible, as well as other criteria for eligibility such as use of a placebo comparison group, evaluation of outcomes blinded to allocation sequence, or a minimum period of follow-up. There will always be a trade-off between restrictive study design criteria (which might result in the inclusion of studies that are at low risk of bias, but very few in number) and more liberal design criteria (which might result in the inclusion of more studies, but at a higher risk of bias). Furthermore, excessively broad criteria might result in the inclusion of misleading evidence. If, for example, interest focuses on whether a therapy improves survival in patients with a chronic condition, it might be inappropriate to look at studies of very short duration, except to make explicit the point that they cannot address the question of interest.
MECIR Box 3.3.b Relevant expectations for conduct of intervention reviews
3.3.2 Including non-randomized studies
The decision of whether non-randomized studies (and what type) will be included is decided alongside the formulation of the review PICO. The main drivers that may lead to the inclusion of non-randomized studies include: (i) when randomized trials are unable to address the effects of the intervention on harm and long-term outcomes or in specific populations or settings; or (ii) for interventions that cannot be randomized (e.g. policy change introduced in a single or small number of jurisdictions) (see Chapter 24 ). Cochrane, in collaboration with others, has developed guidance for review authors to support their decision about when to look for and include non-randomized studies (Schünemann et al 2013).
Non-randomized designs have the commonality of not using randomization to allocate units to comparison groups, but their different design features mean that they are variable in their susceptibility to bias. Eligibility criteria should be based on explicit study design features, and not the study labels applied by the primary researchers (e.g. case-control, cohort), which are often used inconsistently (Reeves et al 2017; see Chapter 24 ).
When non-randomized studies are included, review authors should consider how the studies will be grouped and used in the synthesis. The Cochrane Non-randomized Studies Methods Group taxonomy of design features (see Chapter 24 ) may provide a basis for grouping together studies that are expected to have similar inferential strength and for providing a consistent language for describing the study design.
Once decisions have been made about grouping study designs, planning of how these will be used in the synthesis is required. Review authors need to decide whether it is useful to synthesize results from non-randomized studies and, if so, whether results from randomized trials and non-randomized studies should be included in the same synthesis (for the purpose of examining whether study design explains heterogeneity among the intervention effects), or whether the effects should be synthesized in separate comparisons (Valentine and Thompson 2013). Decisions should be made for each of the different types of non-randomized studies under consideration. Review authors might anticipate increased heterogeneity when non-randomized studies are synthesized, and adoption of a meta-analysis model that encompasses heterogeneity is wise (Valentine and Thompson 2013) (such as a random effects model, see Chapter 10, Section 10.10.4 ). For further discussion of non-randomized studies, see Chapter 24 .
3.4 Eligibility based on publication status and language
Chapter 4 contains detailed guidance on how to identify studies from a range of sources including, but not limited to, those in peer-reviewed journals. In general, a strategy to include studies reported in all types of publication will reduce bias ( Chapter 7 ). There would need to be a compelling argument for the exclusion of studies on the basis of their publication status ( MECIR Box 3.4.a ), including unpublished studies, partially published studies, and studies published in ‘grey’ literature sources. Given the additional challenge in obtaining unpublished studies, it is possible that any unpublished studies identified in a given review may be an unrepresentative subset of all the unpublished studies in existence. However, the bias this introduces is of less concern than the bias introduced by excluding all unpublished studies, given what is known about the impact of reporting biases (see Chapter 13 on bias due to missing studies, and Chapter 4, Section 4.3 for a more detailed discussion of searching for unpublished and grey literature).
Likewise, while searching for, and analysing, studies in any language can be extremely resource-intensive, review authors should consider carefully the implications for bias (and equity, see Chapter 16 ) if they restrict eligible studies to those published in one specific language (usually English). See Chapter 4, Section 4.4.5 , for further discussion of language and other restrictions while searching.
MECIR Box 3.4.a Relevant expectations for conduct of intervention reviews
3.5 Chapter information
Authors: Joanne E McKenzie, Sue E Brennan, Rebecca E Ryan, Hilary J Thomson, Renea V Johnston, James Thomas
Acknowledgements: This chapter builds on earlier versions of the Handbook . In particular, Version 5, Chapter 5 , edited by Denise O’Connor, Sally Green and Julian Higgins.
Funding: JEM is supported by an Australian National Health and Medical Research Council (NHMRC) Career Development Fellowship (1143429). SEB and RER’s positions are supported by the NHMRC Cochrane Collaboration Funding Program. HJT is funded by the UK Medical Research Council (MC_UU_12017-13 and MC_UU_12017-15) and Scottish Government Chief Scientist Office (SPHSU13 and SPHSU15). RVJ’s position is supported by the NHMRC Cochrane Collaboration Funding Program and Cabrini Institute. JT is supported by the National Institute for Health Research (NIHR) Collaboration for Leadership in Applied Health Research and Care North Thames at Barts Health NHS Trust. The views expressed are those of the author(s) and not necessarily those of the NHS, the NIHR or the Department of Health.
3.6 References
Bailey JV, Murray E, Rait G, Mercer CH, Morris RW, Peacock R, Cassell J, Nazareth I. Interactive computer-based interventions for sexual health promotion. Cochrane Database of Systematic Reviews 2010; 9 : CD006483.
Bender R, Bunce C, Clarke M, Gates S, Lange S, Pace NL, Thorlund K. Attention should be given to multiplicity issues in systematic reviews. Journal of Clinical Epidemiology 2008; 61 : 857–865.
Buchbinder R, Johnston RV, Rischin KJ, Homik J, Jones CA, Golmohammadi K, Kallmes DF. Percutaneous vertebroplasty for osteoporotic vertebral compression fracture. Cochrane Database of Systematic Reviews 2018; 4 : CD006349.
Caldwell DM, Welton NJ. Approaches for synthesising complex mental health interventions in meta-analysis. Evidence-Based Mental Health 2016; 19 : 16–21.
Chamberlain C, O’Mara-Eves A, Porter J, Coleman T, Perlen S, Thomas J, McKenzie J. Psychosocial interventions for supporting women to stop smoking in pregnancy. Cochrane Database of Systematic Reviews 2017; 2 : CD001055.
Ciciriello S, Johnston RV, Osborne RH, Wicks I, deKroo T, Clerehan R, O’Neill C, Buchbinder R. Multimedia educational interventions for consumers about prescribed and over-the-counter medications. Cochrane Database of Systematic Reviews 2013; 4 : CD008416.
Cochrane Consumers & Communication Group. Outcomes of Interest to the Cochrane Consumers & Communication Group: taxonomy. http://cccrg.cochrane.org/ .
COnsensus-based Standards for the selection of health Measurement INstruments (COSMIN) initiative. COSMIN database of systematic reviews of outcome measurement instruments. https://database.cosmin.nl/ .
Coulter A, Entwistle VA, Eccles A, Ryan S, Shepperd S, Perera R. Personalised care planning for adults with chronic or long-term health conditions. Cochrane Database of Systematic Reviews 2015; 3 : CD010523.
Davey J, Turner RM, Clarke MJ, Higgins JPT. Characteristics of meta-analyses and their component studies in the Cochrane Database of Systematic Reviews: a cross-sectional, descriptive analysis. BMC Medical Research Methodology 2011; 11 : 160.
Desroches S, Lapointe A, Ratte S, Gravel K, Legare F, Turcotte S. Interventions to enhance adherence to dietary advice for preventing and managing chronic diseases in adults. Cochrane Database of Systematic Reviews 2013; 2 : CD008722.
Deyo RA, Dworkin SF, Amtmann D, Andersson G, Borenstein D, Carragee E, Carrino J, Chou R, Cook K, DeLitto A, Goertz C, Khalsa P, Loeser J, Mackey S, Panagis J, Rainville J, Tosteson T, Turk D, Von Korff M, Weiner DK. Report of the NIH Task Force on research standards for chronic low back pain. Journal of Pain 2014; 15 : 569–585.
Dodd S, Clarke M, Becker L, Mavergames C, Fish R, Williamson PR. A taxonomy has been developed for outcomes in medical research to help improve knowledge discovery. Journal of Clinical Epidemiology 2018; 96 : 84–92.
Fisher DJ, Carpenter JR, Morris TP, Freeman SC, Tierney JF. Meta-analytical methods to identify who benefits most from treatments: daft, deluded, or deft approach? BMJ 2017; 356 : j573.
Fransen M, McConnell S, Harmer AR, Van der Esch M, Simic M, Bennell KL. Exercise for osteoarthritis of the knee. Cochrane Database of Systematic Reviews 2015; 1 : CD004376.
Guise JM, Chang C, Viswanathan M, Glick S, Treadwell J, Umscheid CA. Systematic reviews of complex multicomponent health care interventions. Report No. 14-EHC003-EF . Rockville, MD: Agency for Healthcare Research and Quality; 2014.
Harrison JK, Noel-Storr AH, Demeyere N, Reynish EL, Quinn TJ. Outcomes measures in a decade of dementia and mild cognitive impairment trials. Alzheimer’s Research and Therapy 2016; 8 : 48.
Hedges LV, Tipton E, Johnson M, C. Robust variance estimation in meta-regression with dependent effect size estimates. Research Synthesis Methods 2010; 1 : 39–65.
Hetrick SE, McKenzie JE, Cox GR, Simmons MB, Merry SN. Newer generation antidepressants for depressive disorders in children and adolescents. Cochrane Database of Systematic Reviews 2012; 11 : CD004851.
Higgins JPT, López-López JA, Becker BJ, Davies SR, Dawson S, Grimshaw JM, McGuinness LA, Moore THM, Rehfuess E, Thomas J, Caldwell DM. Synthesizing quantitative evidence in systematic reviews of complex health interventions. BMJ Global Health 2019; 4 : e000858.
Hoffmann T, Glasziou P, Barbour V, Macdonald H. Better reporting of interventions: template for intervention description and replication (TIDieR) checklist and guide. BMJ 2014; 1687 : 1-13.
Hollands GJ, Shemilt I, Marteau TM, Jebb SA, Lewis HB, Wei Y, Higgins JPT, Ogilvie D. Portion, package or tableware size for changing selection and consumption of food, alcohol and tobacco. Cochrane Database of Systematic Reviews 2015; 9 : CD011045.
Howe TE, Shea B, Dawson LJ, Downie F, Murray A, Ross C, Harbour RT, Caldwell LM, Creed G. Exercise for preventing and treating osteoporosis in postmenopausal women. Cochrane Database of Systematic Reviews 2011; 7 : CD000333.
ICHOM. The International Consortium for Health Outcomes Measurement 2018. http://www.ichom.org/ .
IPDAS. International Patient Decision Aid Standards Collaboration (IPDAS) standards. www.ipdas.ohri.ca .
Ivers N, Jamtvedt G, Flottorp S, Young JM, Odgaard-Jensen J, French SD, O’Brien MA, Johansen M, Grimshaw J, Oxman AD. Audit and feedback: effects on professional practice and healthcare outcomes. Cochrane Database of Systematic Reviews 2012; 6 : CD000259.
Janmaat VT, Steyerberg EW, van der Gaast A, Mathijssen RH, Bruno MJ, Peppelenbosch MP, Kuipers EJ, Spaander MC. Palliative chemotherapy and targeted therapies for esophageal and gastroesophageal junction cancer. Cochrane Database of Systematic Reviews 2017; 11 : CD004063.
Kendrick D, Kumar A, Carpenter H, Zijlstra GAR, Skelton DA, Cook JR, Stevens Z, Belcher CM, Haworth D, Gawler SJ, Gage H, Masud T, Bowling A, Pearl M, Morris RW, Iliffe S, Delbaere K. Exercise for reducing fear of falling in older people living in the community. Cochrane Database of Systematic Reviews 2014; 11 : CD009848.
Kirkham JJ, Gargon E, Clarke M, Williamson PR. Can a core outcome set improve the quality of systematic reviews? A survey of the Co-ordinating Editors of Cochrane Review Groups. Trials 2013; 14 : 21.
Konstantopoulos S. Fixed effects and variance components estimation in three-level meta-analysis. Research Synthesis Methods 2011; 2 : 61–76.
Lamb SE, Becker C, Gillespie LD, Smith JL, Finnegan S, Potter R, Pfeiffer K. Reporting of complex interventions in clinical trials: development of a taxonomy to classify and describe fall-prevention interventions. Trials 2011; 12 : 125.
Lewin S, Hendry M, Chandler J, Oxman AD, Michie S, Shepperd S, Reeves BC, Tugwell P, Hannes K, Rehfuess EA, Welch V, Mckenzie JE, Burford B, Petkovic J, Anderson LM, Harris J, Noyes J. Assessing the complexity of interventions within systematic reviews: development, content and use of a new tool (iCAT_SR). BMC Medical Research Methodology 2017; 17 : 76.
López-López JA, Page MJ, Lipsey MW, Higgins JPT. Dealing with multiplicity of effect sizes in systematic reviews and meta-analyses. Research Synthesis Methods 2018; 9 : 336–351.
Mavridis D, Salanti G. A practical introduction to multivariate meta-analysis. Statistical Methods in Medical Research 2013; 22 : 133–158.
Michie S, van Stralen M, West R. The Behaviour Change Wheel: a new method for characterising and designing behaviour change interventions. Implementation Science 2011; 6 : 42.
Michie S, Richardson M, Johnston M, Abraham C, Francis J, Hardeman W, Eccles MP, Cane J, Wood CE. The behavior change technique taxonomy (v1) of 93 hierarchically clustered techniques: building an international consensus for the reporting of behavior change interventions. Annals of Behavioral Medicine 2013; 46 : 81–95.
Moraes VY, Lenza M, Tamaoki MJ, Faloppa F, Belloti JC. Platelet-rich therapies for musculoskeletal soft tissue injuries. Cochrane Database of Systematic Reviews 2014; 4 : CD010071.
O'Neill J, Tabish H, Welch V, Petticrew M, Pottie K, Clarke M, Evans T, Pardo Pardo J, Waters E, White H, Tugwell P. Applying an equity lens to interventions: using PROGRESS ensures consideration of socially stratifying factors to illuminate inequities in health. Journal of Clinical Epidemiology 2014; 67 : 56–64.
Pompoli A, Furukawa TA, Imai H, Tajika A, Efthimiou O, Salanti G. Psychological therapies for panic disorder with or without agoraphobia in adults: a network meta-analysis. Cochrane Database of Systematic Reviews 2016; 4 : CD011004.
Pompoli A, Furukawa TA, Efthimiou O, Imai H, Tajika A, Salanti G. Dismantling cognitive-behaviour therapy for panic disorder: a systematic review and component network meta-analysis. Psychological Medicine 2018; 48 : 1–9.
Reeves BC, Wells GA, Waddington H. Quasi-experimental study designs series-paper 5: a checklist for classifying studies evaluating the effects on health interventions – a taxonomy without labels. Journal of Clinical Epidemiology 2017; 89 : 30–42.
Regnaux J-P, Lefevre-Colau M-M, Trinquart L, Nguyen C, Boutron I, Brosseau L, Ravaud P. High-intensity versus low-intensity physical activity or exercise in people with hip or knee osteoarthritis. Cochrane Database of Systematic Reviews 2015; 10 : CD010203.
Richards SH, Anderson L, Jenkinson CE, Whalley B, Rees K, Davies P, Bennett P, Liu Z, West R, Thompson DR, Taylor RS. Psychological interventions for coronary heart disease. Cochrane Database of Systematic Reviews 2017; 4 : CD002902.
Safi S, Korang SK, Nielsen EE, Sethi NJ, Feinberg J, Gluud C, Jakobsen JC. Beta-blockers for heart failure. Cochrane Database of Systematic Reviews 2017; 12 : CD012897.
Santesso N, Carrasco-Labra A, Brignardello-Petersen R. Hip protectors for preventing hip fractures in older people. Cochrane Database of Systematic Reviews 2014; 3 : CD001255.
Shepherd E, Gomersall JC, Tieu J, Han S, Crowther CA, Middleton P. Combined diet and exercise interventions for preventing gestational diabetes mellitus. Cochrane Database of Systematic Reviews 2017; 11 : CD010443.
Squires J, Valentine J, Grimshaw J. Systematic reviews of complex interventions: framing the review question. Journal of Clinical Epidemiology 2013; 66 : 1215–1222.
Stacey D, Légaré F, Lewis K, Barry MJ, Bennett CL, Eden KB, Holmes-Rovner M, Llewellyn-Thomas H, Lyddiatt A, Thomson R, Trevena L. Decision aids for people facing health treatment or screening decisions. Cochrane Database of Systematic Reviews 2017; 4 : CD001431.
Stroke Unit Trialists Collaboration. Organised inpatient (stroke unit) care for stroke. Cochrane Database of Systematic Reviews 2013; 9 : CD000197.
Taylor AJ, Jones LJ, Osborn DA. Zinc supplementation of parenteral nutrition in newborn infants. Cochrane Database of Systematic Reviews 2017; 2 : CD012561.
Valentine JC, Thompson SG. Issues relating to confounding and meta-analysis when including non-randomized studies in systematic reviews on the effects of interventions. Research Synthesis Methods 2013; 4 : 26–35.
Vaona A, Banzi R, Kwag KH, Rigon G, Cereda D, Pecoraro V, Tramacere I, Moja L. E-learning for health professionals. Cochrane Database of Systematic Reviews 2018; 1 : CD011736.
Verheyden GSAF, Weerdesteyn V, Pickering RM, Kunkel D, Lennon S, Geurts ACH, Ashburn A. Interventions for preventing falls in people after stroke. Cochrane Database of Systematic Reviews 2013; 5 : CD008728.
Weisz JR, Kuppens S, Ng MY, Eckshtain D, Ugueto AM, Vaughn-Coaxum R, Jensen-Doss A, Hawley KM, Krumholz Marchette LS, Chu BC, Weersing VR, Fordwood SR. What five decades of research tells us about the effects of youth psychological therapy: a multilevel meta-analysis and implications for science and practice. American Psychologist 2017; 72 : 79–117.
Welch V, Petkovic J, Simeon R, Presseau J, Gagnon D, Hossain A, Pardo Pardo J, Pottie K, Rader T, Sokolovski A, Yoganathan M, Tugwell P, DesMeules M. Interactive social media interventions for health behaviour change, health outcomes, and health equity in the adult population. Cochrane Database of Systematic Reviews 2018; 2 : CD012932.
Welton NJ, Caldwell DM, Adamopoulos E, Vedhara K. Mixed treatment comparison meta-analysis of complex interventions: psychological interventions in coronary heart disease. American Journal of Epidemiology 2009; 169 : 1158–1165.
Williamson PR, Altman DG, Bagley H, Barnes KL, Blazeby JM, Brookes ST, Clarke M, Gargon E, Gorst S, Harman N, Kirkham JJ, McNair A, Prinsen CAC, Schmitt J, Terwee CB, Young B. The COMET Handbook: version 1.0. Trials 2017; 18 : 280.
For permission to re-use material from the Handbook (either academic or commercial), please see here for full details.

Evidence-Based Practice (EBP)
- The EBP Process
- Forming a Clinical Question
- Inclusion & Exclusion Criteria
- Acquiring Evidence
- Appraising the Quality of the Evidence
- Writing a Literature Review
- Finding Psychological Tests & Assessment Instruments
Selection Criteria

Inclusion and exclusion criteria are determined after formulating the research question but usually before the search is conducted (although preliminary scoping searches may need to be undertaken to determine appropriate criteria). It may be helpful to determine the inclusion criteria and exclusion criteria for each PICO component.
Be aware that you may introduce bias into the final review if these are not used thoughtfully.
Inclusion and exclusion are two sides of the same coin, so—depending on your perspective—a single database filter can be said to either include or exclude. For instance, if articles must be published within the last 3 years, that is inclusion. If articles cannot be more than 3 years old, that is exclusion.
The most straightforward way to include or exclude results is to use database limiters (filters), usually found on the left side of the search results page.
Inclusion Criteria
Inclusion criteria are the elements of an article that must be present in order for it to be eligible for inclusion in a literature review. Some examples are:
- Included studies must have compared certain treatments
- Included studies must be a certain type (e.g., only Randomized Controlled Trials)
- Included studies must be located in a certain geographic area
- Included studies must have been published in the last 5 years
Exclusion Criteria
Exclusion criteria are the elements of an article that disqualify the study from inclusion in a literature review. Some examples are:
- Study used an observational design
- Study used a qualitative methodology
- Study was published more than 5 years ago
- Study was published in a language other than English
- << Previous: Forming a Clinical Question
- Next: Acquiring Evidence >>
- Last Updated: May 16, 2024 2:44 PM
- URL: https://libguides.umsl.edu/ebp

Systematic Reviews
- The Research Question
- Inclusion and Exclusion Criteria
- Original Studies
- Translating
- Deduplication
- Project Management Tools
- Useful Resources
- What is not a systematic review?
Inclusion/Exclusion Criteria
Inclusion criteria.
Identify the criteria that will be used to determine which research studies will be included. The inclusion and exclusion criteria must be decided before you start the review. Inclusion criteria is everything a study must have to be included. Exclusion criteria are the factors that would make a study ineligible to be included. Criteria that should be considered include:
Type of studies: It is important to select articles with an appropriate study design for the research question. Dates for the studies and a timeline of the problem/issue being examined may need to be identified.
Type of participants: Identify the target population characteristics. It is important to define the target population's age, sex/gender, diagnosis, as well as any other relevant factors.
Types of intervention: Describe the intervention being investigated. Consider whether to include interventions carried out globally or just in the United States. Eligibility criteria for interventions should include things such as the dose, delivery method, and duration of the investigated intervention. The interventions that are to be excluded may also need to be described here.
Types of outcome measures: Outcome measures usually refer to measurable outcomes or ‘clinical changes in health’. For example, these could include body structures and functions like pain and fatigue, activities as in functional abilities, and participation or quality of life questionnaires.
Read Chapter 3 of the Cochrane Handbook
Exclusion criteria.
A balance of specific inclusion and exclusion criteria is paramount. For some systematic reviews, there may already be a large pre-existing body of literature. The search strategy may retrieve thousands of results that must be screened. Having explicit exclusion criteria from the beginning allows those conducting the screening process, an efficient workflow. For the final product there should be a section in the review dedicated to 'Characteristics of excluded studies.' It is important to summarize why studies were excluded, especially if to a reader the study would appear to be eligible for the systematic review.
For example, a team is conducting a systematic review regarding intervention options for the treatment of opioid addiction. The research team may want to exclude studies that also involve alcohol addiction to isolate the conditions for treatment interventions solely for opioid addiction.
- << Previous: Planning and Protocols
- Next: Searching >>
- Last Updated: Oct 12, 2023 12:30 PM
- URL: https://libguides.sph.uth.tmc.edu/SystematicReviews
Human Subjects Protection
You are here, protocol design - inclusion and exclusion criteria.
Investigators must include in their protocols a thorough description of the study population. The first priority is that the subject population have the attributes that will make it possible to accomplish the purpose of the research. The investigator must specify inclusion and exclusion criteria for participation in a study. Inclusion criteria are characteristics that the prospective subjects must have if they are to be included in the study. Exclusion criteria are those characteristics that disqualify prospective subjects from inclusion in the study. Inclusion and exclusion criteria may include factors such as age, gender, race, ethnicity, type and stage of disease, the subject’s previous treatment history, and the presence or absence (as in the case of the “healthy” or “control” subject) of other medical, psychosocial, or emotional conditions. Healthy, or control, subjects may be defined as those individuals who are free of certain specified attributes of non-health. Additional information on screening potential subjects for attributes of non-health is available in the Specific Guidance on Special Issues section of this module . Defining inclusion and exclusion criteria increases the likelihood of producing reliable and reproducible results, minimizes the likelihood of harm to the subjects, and guards against exploitation of vulnerable persons.
An example of inclusion criteria for a study of chemotherapy of breast cancer subjects might be postmenopausal women between the ages of 45 and 75 who have been diagnosed with Stage II breast cancer. An exclusion criterion for this study might be abnormal renal function tests, if the combination of study drugs includes one or more that is nephrotoxic. In this case it would be required to specify which tests of renal function are to be performed to evaluate renal function and the threshold values that would disqualify the prospective subject (e.g., serum creatinine above 1.9 mg/dl). Social science research generally does not have defined exclusion criteria. Rather, ethnographic research usually defines the community of interest and seeks to include the broadest sampling of individuals who meet the inclusion criteria. For example, in an ethnographic study of Amazonian communities and their use of forest products, individuals living near the Amazonian forest would be included. Within these communities everyone would be eligible to participate.
Furthermore, the investigator must be prepared to provide a rationale in case one or more of the inclusion or exclusion criteria is questioned. The investigator should review the inclusion and exclusion criteria and decide if any group(s) is inappropriately excluded. If the justification for the exclusion of this group is not reasonable with regard to the risks, benefits, and purpose of the research, then this group should be included. In the breast cancer study example discussed above, there would be no justification for the exclusion of minority women, but there is justification for the exclusion of men (based upon the target population of the research.) As noted in this example, the requirement that groups not be excluded without adequate justification does not mean that it is never appropriate for exclusion criteria to be based on race, gender, or age. For example, some ethnographic research, by definition, may be limited to a certain ethnic group. To assess the rationale for selecting certain subject groups, the investigator needs to review information on the distribution of the health condition in the general population. Research that has the potential to benefit men, women, and children or different races should target a population of subjects that is diverse enough to distinguish differing effects, risks, and benefits. No group or individual subject should be excluded without a scientifically sound reason or a requirement of the regulations to do so.
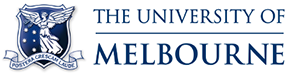
Systematic Reviews for Health Sciences and Medicine
- Systematic Reviews
- The research question
- Common search errors
- Search translation
- Managing results
- Inclusion and exclusion criteria
- Critical appraisal
- Updating a Review
- Resources by Review Stage
Inclusion and Exclusion Criteria
Inclusion and exclusion criteria set the boundaries for the systematic review. They are determined after setting the research question usually before the search is conducted, however scoping searches may need to be undertaken to determine appropriate criteria. Many different factors can be used as inclusion or exclusion criteria. Information about the inclusion and exclusion criteria is usually recorded as a paragraph or table within the methods section of the systematic review. It may also be necessary to give the definitions, and source of the definition, used for particular concepts in the research question (e.g. adolescence, depression).

Other inclusion/exclusion criteria can include the sample size, method of sampling or availability of a relevant comparison group in the study. Where a single study is reported across multiple papers the findings from the papers may be merged or only the latest data may be included.
- << Previous: Managing results
- Next: Critical appraisal >>
- Last Updated: Apr 17, 2024 12:42 PM
- URL: https://unimelb.libguides.com/sysrev
Europe PMC requires Javascript to function effectively.
Either your web browser doesn't support Javascript or it is currently turned off. In the latter case, please turn on Javascript support in your web browser and reload this page.
Search life-sciences literature (44,081,976 articles, preprints and more)
- Free full text
- Citations & impact
- Similar Articles
Inclusion and exclusion criteria in research studies: definitions and why they matter.
Author information, affiliations.
- Patino CM 1
- Ferreira JC 1
ORCIDs linked to this article
- Patino CM | 0000-0001-5742-2157
- Ferreira JC | 0000-0001-6548-1384
Jornal Brasileiro de Pneumologia : Publicacao Oficial da Sociedade Brasileira de Pneumologia e Tisilogia , 01 Apr 2018 , 44(2): 84 Language: por https://doi.org/10.1590/s1806-37562018000000088 PMID: 29791550 PMCID: PMC6044655
Abstract
Free full text , inclusion and exclusion criteria in research studies: definitions and why they matter, critérios de inclusão e exclusão em estudos de pesquisa: definições e por que eles importam, cecilia maria patino.
1 . Methods in Epidemiologic, Clinical, and Operations Research-MECOR-program, American Thoracic Society/Asociación Latinoamericana del Tórax, Montevideo, Uruguay.
2 . Department of Preventive Medicine, Keck School of Medicine, University of Southern California, Los Angeles, CA, USA.
Juliana Carvalho Ferreira
3 . Divisão de Pneumologia, Instituto do Coração, Hospital das Clínicas, Faculdade de Medicina, Universidade de São Paulo, São Paulo (SP) Brasil.
- PRACTICAL SCENARIO
A cross-sectional multicenter study evaluated self-reported adherence to inhaled therapies among patients with COPD in Latin America. 1 Inclusion and exclusion criteria for the study are shown in Chart 1 . The authors found that self-reported adherence was low in 20% of the patients, intermediate in 29%, and high in 51%; and that poor adherence was associated with more exacerbations in the past year, a lower smoking history, and a lower level of education. The authors concluded that suboptimal adherence to inhaled therapies among COPD patients was common and that interventions to improve adherence are warranted.
Establishing inclusion and exclusion criteria for study participants is a standard, required practice when designing high-quality research protocols. Inclusion criteria are defined as the key features of the target population that the investigators will use to answer their research question. 2 Typical inclusion criteria include demographic, clinical, and geographic characteristics. In contrast, exclusion criteria are defined as features of the potential study participants who meet the inclusion criteria but present with additional characteristics that could interfere with the success of the study or increase their risk for an unfavorable outcome. Common exclusion criteria include characteristics of eligible individuals that make them highly likely to be lost to follow-up, miss scheduled appointments to collect data, provide inaccurate data, have comorbidities that could bias the results of the study, or increase their risk for adverse events (most relevant in studies testing interventions).
It is very important that investigators not only define the appropriate inclusion and exclusion criteria when designing a study but also evaluate how those decisions will impact the external validity of the results of the study. Common errors regarding inclusion and exclusion criteria include the following: using the same variable to define both inclusion and exclusion criteria (for example, in a study including only men, listing being a female as an exclusion criterion); selecting variables as inclusion criteria that are not related to answering the research question; and not describing key variables in the inclusion criteria that are needed to make a statement about the external validity of the study results.
- IMPACT OF THE INCLUSION AND EXCLUSION CRITERIA ON THE EXTERNAL VALIDITY OF THE STUDY
In our example, the investigators described the inclusion criteria related to demographic characteristics (age ≥ 40 years of age and male or female gender), clinical characteristics (diagnosis of COPD, stable disease, outpatient, and current or former smoker); and exclusion criteria related to comorbidities that could bias the results (sleep apnea, other chronic respiratory diseases, and acute or chronic conditions that could limit the ability of the patient to participate in the study). On the basis of these inclusion and exclusion criteria, we can make a judgment regarding their impact on the external validity of the results. Making those judgments requires in-depth knowledge of the area of research, as well as of in what direction each criterion could affect the external validity of the study. As an example, the authors excluded patients with comorbidities, and it is therefore possible that the levels of nonadherence reported would not be generalizable to COPD patients with comorbidities, who most likely would show higher levels of nonadherence due to their more complex medication regimens.
- REFERÊNCIAS
Full text links
Read article at publisher's site: https://doi.org/10.1590/s1806-37562018000000088
Citations & impact
Impact metrics, citations of article over time, alternative metrics.

Article citations
Route-t1d: a behavioral intervention to promote optimal continuous glucose monitor use among racially minoritized youth with type 1 diabetes: design and development..
Straton E , Bryant BL , Kang L , Wang C , Barber J , Perkins A , Gallant L , Marks B , Agarwal S , Majidi S , Monaghan M , Streisand R
Contemp Clin Trials , 140:107493, 07 Mar 2024
Cited by: 0 articles | PMID: 38460913
Long Non-coding RNA X-Inactive Specific Transcript Promotes Esophageal Squamous Cell Carcinoma Progression via the MicroRNA 34a/Zinc Finger E-box-Binding Homeobox 1 Pathway.
Guo B , He M , Ma M , Tian Z , Jin J , Tian G
Dig Dis Sci , 69(4):1169-1181, 16 Feb 2024
Cited by: 0 articles | PMID: 38366093 | PMCID: PMC11026218
Study protocol for the family empowerment program: a randomized waitlist-controlled trial to evaluate the effectiveness of online Community Reinforcement and Family Training (CRAFT) on the wellbeing of family members with a relative experiencing substance dependence and mental illness.
Allan J , Snowdon N , Thapa S , Ahmed KY
BMC Psychiatry , 24(1):43, 10 Jan 2024
Cited by: 0 articles | PMID: 38200508 | PMCID: PMC10782775
Appraising systematic reviews: a comprehensive guide to ensuring validity and reliability.
Shaheen N , Shaheen A , Ramadan A , Hefnawy MT , Ramadan A , Ibrahim IA , Hassanein ME , Ashour ME , Flouty O
Front Res Metr Anal , 8:1268045, 21 Dec 2023
Cited by: 2 articles | PMID: 38179256 | PMCID: PMC10764628
Management of COPD and Comorbidities in COPD patients by Dispensing Pharmaceutical Care following Global Initiative for chronic Obstructive Lung Disease-Guidelines (GOLD guidelines 2020): A study protocol for a Prospective Randomized Clinical Trial.
Kanwal H , Khan S , Eldesoky GE , Mushtaq S , Khan A
Heliyon , 9(11):e21539, 25 Oct 2023
Cited by: 0 articles | PMID: 37942165 | PMCID: PMC10628705
Similar Articles
To arrive at the top five similar articles we use a word-weighted algorithm to compare words from the Title and Abstract of each citation.
The generalizability of clinical and experimental tobacco research: Do exclusion criteria impact men and women differently?
Spinella TC , Perry RN , Schlagintweit HE , Barrett SP
J Psychopharmacol , 34(5):584-585, 22 Jan 2020
Cited by: 0 articles | PMID: 31965900
Exclusion criteria in treatment research on alcohol, tobacco and illicit drug use disorders: A review and critical analysis.
Moberg CA , Humphreys K
Drug Alcohol Rev , 36(3):378-388, 21 Jun 2016
Cited by: 12 articles | PMID: 27324921
The European Union Directive on Clinical Research: present status of implementation in EU member states' legislations with regard to the incompetent patient.
Lemaire F , Bion J , Blanco J , Damas P , Druml C , Falke K , Kesecioglu J , Larsson A , Mancebo J , Matamis D , Pesenti A , Pimentel J , Ranieri M , ESICM Task Force on Legislation Affecting Clinical Research in the Critically Ill Patient
Intensive Care Med , 31(3):476-479, 15 Feb 2005
Cited by: 50 articles | PMID: 15711974
Impact of patient selection in various study designs: identifying potential bias in clinical results.
Berbano EP , Baxi N
South Med J , 105(3):149-155, 01 Mar 2012
Cited by: 4 articles | PMID: 22392211
Standardized patient method in tuberculosis research.
Grace GA , Devaleenal DB
Natl Med J India , 30(4):210-211, 01 Jul 2017
Cited by: 0 articles | PMID: 29162755
Europe PMC is part of the ELIXIR infrastructure
Log in using your username and password
- Search More Search for this keyword Advanced search
- Latest content
- For authors
- Browse by collection
- BMJ Journals More You are viewing from: Google Indexer
You are here
- Volume 10, Issue 11
- Ensuring that COVID-19 research is inclusive: guidance from the NIHR INCLUDE project
- Article Text
- Article info
- Citation Tools
- Rapid Responses
- Article metrics

- http://orcid.org/0000-0002-1967-0990 Miles D Witham 1 , 2 ,
- Eleanor Anderson 2 ,
- http://orcid.org/0000-0001-7472-953X Camille B Carroll 3 ,
- Paul M Dark 4 ,
- Kim Down 2 ,
- Alistair S Hall 5 ,
- Joanna Knee 6 ,
- Eamonn R Maher 7 ,
- Rebecca H Maier 8 , 9 ,
- Gail A Mountain 10 ,
- Gary Nestor 2 ,
- John T O'Brien 11 ,
- Laurie Oliva 12 ,
- James Wason 9 , 13 ,
- Lynn Rochester 2 , 14
- On behalf of the NIHR CRN INCLUDE Steering Group
- 1 NIHR Newcastle Biomedical Research Centre , Newcastle University , Newcastle upon Tyne , UK
- 2 NIHR Clinical Research Network Cluster E , Newcastle University , Newcastle upon Tyne , Tyne and Wear , UK
- 3 Faculty of Health , University of Plymouth , Plymouth , Devon , UK
- 4 NIHR Manchester Biomedical Research Centre , The University of Manchester and Northern Care Alliance NHS Group , Manchester , UK
- 5 Cardiology Department , Leeds General Infirmary Department of Cardiology , Leeds , West Yorkshire , UK
- 6 NIHR Clinical Research Network Coordinating Centre , University of Leeds , Leeds , West Yorkshire , UK
- 7 Department of Medical Genetics , University of Cambridge and NIHR Cambridge Biomedical Research Centre , Cambridge , UK
- 8 Newcastle Clinical Trials Unit , Newcastle University , Newcastle upon Tyne , Tyne and Wear , UK
- 9 Population Health Sciences Institute , Newcastle University , Newcastle upon Tyne , Tyne and Wear , UK
- 10 Centre for Applied Dementia Studies , University of Bradford , Bradford , West Yorkshire , UK
- 11 Department of Psychiatry , University of Cambridge , Cambridge , Cambridgeshire , UK
- 12 NIHR Clinical Research Network Coordinating Centre , Guy's and St Thomas' NHS Foundation Trust , London , UK
- 13 MRC Biostatistics Unit , University of Cambridge , Cambridge , Cambridgeshire , UK
- 14 Brain and Movement Group, Translational Clinical Research Institute , Newcastle University , Newcastle upon Tyne , Tyne and Wear , UK
- Correspondence to Professor Miles D Witham; miles.witham{at}newcastle.ac.uk
Objective To provide guidance to researchers, funders, regulators and study delivery teams to ensure that research on COVID-19 is inclusive, particularly of groups disproportionately affected by COVID-19 and who may have been historically under-served by research.
Summary of key points Groups who are disproportionately affected by COVID-19 include (but are not limited to) older people, people with multiple long-term conditions, people with disabilities, people from Black, Asian and Ethnic minority groups, people living with obesity, people who are socioeconomically deprived and people living in care homes. All these groups are under-served by clinical research, and there is an urgent need to rectify this if COVID-19 research is to deliver relevant evidence for these groups who are most in need. We provide a framework and checklists for addressing key issues when designing and delivering inclusive COVID-19 research, based on the National Institute for Health Research INnovations in CLinical trial design and delivery for the UnDEr-served project roadmap. Strong community engagement, codevelopment and prioritisation of research questions and interventions are essential. Under-served groups should be represented on funding panels and ethics committees, who should insist on the removal of barriers to participation. Exclusion criteria should be kept to a minimum; intervention delivery and outcome measurement should be simple, flexible and tailored to the needs of different groups, and local advice on the best way to reach and engage with under-served communities should be taken by study delivery teams. Data on characteristics that allow identification of under-served groups must be collected, analyses should include these data to enable subgroup comparisons and results should be shared with under-served groups at an early stage.
Conclusion Inclusive COVID-19 research is a necessity, not a luxury, if research is to benefit all the communities it seeks to serve. It requires close engagement with under-served groups and attention to aspects of study topic, design, delivery, analysis and dissemination across the research life cycle.
- statistics & research methods
- public health
This is an open access article distributed in accordance with the Creative Commons Attribution 4.0 Unported (CC BY 4.0) license, which permits others to copy, redistribute, remix, transform and build upon this work for any purpose, provided the original work is properly cited, a link to the licence is given, and indication of whether changes were made. See: https://creativecommons.org/licenses/by/4.0/ .
https://doi.org/10.1136/bmjopen-2020-043634
Statistics from Altmetric.com
Request permissions.
If you wish to reuse any or all of this article please use the link below which will take you to the Copyright Clearance Center’s RightsLink service. You will be able to get a quick price and instant permission to reuse the content in many different ways.
Strengths and limitations of this study
We provide detailed guidance across the research life cycle—a whole-systems approach to improve inclusion in COVID-19 research.
We base the guidance on the robustly developed INnovations in CLinical trial design and delivery for the UnDEr-served roadmap for improving inclusion in research.
The novelty of COVID-19 research precludes our recommendations being based on evidence directly derived from studies with people with COVID-19.
Introduction
COVID-19 is challenging health systems globally and has triggered an unprecedented research response. 1 Across the world, teams are designing and delivering studies encompassing a range of COVID-19 research including epidemiology, disease surveillance, disease consequences, early and late phase clinical trials. Early evidence suggests that some segments of the population are at high risk either of contracting COVID-19 or of more severe consequences of COVID-19 including hospitalisation and death. 2 3 Some of these groups have not been well reached by traditional research design and delivery mechanisms, 4 and there is growing concern that some of the groups most vulnerable to the impact of COVID-19 are under-represented in research studies. 5
This guidance is designed to facilitate best practice in the design, funding, approval, regulation and delivery of research on COVID-19 and is a product of the ongoing National Institute for Health Research (NIHR) INnovations in CLinical trial design and delivery for the UnDEr-served (INCLUDE) project which seeks to improve research for under-served groups; the guidance has been made available by the NIHR website. 6
What is an under-served group?
There is no single definition of an under-served group in research; the definition is context-dependent and will vary depending on the population, disease and intervention being studied. 7 However, if a group is enrolled in a study at lower rates than one sees in the population affected by the disease, this is evidence that the group is likely to be under-served. Reasons for a group being under-served may include protocol design exclusions, an intervention that is unsuitable for that group or because research delivery does not enable them to participate in practice.
Who is at risk of contracting COVID-19?
Our knowledge of who is more or less likely to be infected with COVID-19 (as opposed to suffering severe consequences of infection) is limited at present. However, individuals with a high degree of contact with those who are infected, including healthcare workers, retail staff, transport staff and other key workers with face-to-face roles, are likely to be at high risk. Other groups in which risk appears elevated are patients who spend prolonged periods of time in close proximity to other patients (eg, patients attending hospital-based haemodialysis 8 ) and those in care homes 9 ; emerging evidence suggests that once COVID-19 starts to affect a care home, it can spread very rapidly through the care home population of both residents and staff.
Who is at risk of severe consequences of COVID-19?
Emerging data suggest that several groups are at a higher risk of death or critical care unit admission from COVID-19 infection. 2 3 10 These groups include, but are not limited to, older people (particularly those aged 70 and over); men; people living with obesity; people with disabilities, people living with multiple long-term conditions; care home residents and others living with frailty; Black, Asian and minority ethnic (BAME) groups; and people with immunosuppression due to medication, systemic illness or malnutrition.
What groups may be under-served by healthcare and research systems with respect to COVID-19?
This is also likely to be context-specific, but examples include the following.
Older people and others who are self-isolating (who may not be able to access healthcare easily) on government advice.
Those who may not be prioritised for hospital admission or critical care unit admission due to perceived limited life expectancy or a low chance of benefitting from such interventions.
Those who lack digital literacy or access to digital technologies.
People with cognitive problems including dementia, especially those living alone, who may not be able to understand and/or adhere to the symptom reporting and testing needed for diagnosis.
People with disabilities, who may not be able to access information or testing facilities and may struggle to access healthcare and care support during periods of lockdown. 11
Socioeconomically deprived groups, who are likely to have less resilience to the economic shocks precipitated by the COVID-19 pandemic response.
People without a car or who live in rural areas (who may find it difficult to access testing facilities that require attendees to come in a car).
In addition, thought needs to be given to the fear that many of the population currently have of leaving their immediate environment, entering a hospital or other healthcare facility. This may disproportionately affect certain segments of the population (eg, those with anxiety disorders or who perceive themselves to be especially vulnerable).
How can under-served groups be more successfully included in COVID-19 research?
The INCLUDE project contains a roadmap outlining the potential points of intervention to improve the inclusion of under-served groups ( figure 1 ) and a structure that may be helpful in guiding teams considering how to focus their efforts. Ensuring that effective training for researchers, funders, delivery teams and regulators is key, as is ensuring that regulation is proportionate and does not place barriers in the way of including under-served groups. Identifying members of under-served groups and using their knowledge is critical to successful delivery of research, as is building on existing resources that have been created to facilitate research for specific under-served groups such as older people 12 or people from BAME communities. 13
- Download figure
- Open in new tab
- Download powerpoint
The INCLUDE roadmap (reproduced from National Institute for Health Research [ 6 ] under a creative commons attribution 4.0 international license).
Recommendations from the include project for COVID-19 research
Below, we set out a series of recommendations across the research life cycle for how under-served groups can be included more successfully in COVID-19 research.
Research priority setting
Representatives from under-served groups should be consulted at the earliest possible time to agree a set of national research priorities for COVID-19 research. A rapid James Lind Alliance project (a priority-setting process co-produced by researchers, patients and a range of other stakeholders) would be one possible way to achieve this, but opportunities also exist to ensure representation on COVID-19 funding committees and prioritisation committees.
Strategic priorities for funding bodies
Strategic priorities for research funding bodies (eg, government funders and charities) should reflect the views of under-served groups from any national or international research priority exercise, as well as reflecting current scientific paradigms and national health and social care system imperatives.
Patient and public representation should be in place on all COVID-19-related funding and prioritisation panels, and this patient and public representation should encompass representatives from groups who are at risk of being under-served by COVID-19 research.
Research investigator questions
Specific research questions to be answered should be codeveloped by research teams, funders, government and patient and public representatives including people from under-served groups.
Initial design and funding
Inclusion criteria for research studies should be kept to the minimum necessary to ensure patient safety and criteria likely to exclude broad sectors of populations should be avoided. In particular, we recommend that studies do not overtly or inadvertently exclude participants on the basis of age, sex, ethnicity, geography, socioeconomic status, multimorbidity, disability, cognitive impairment, body mass index or place of residence unless studies specifically aim to generate data for groups that have to date been under-served by COVID-19 research.
Interventions should be designed and selected to be usable by a wide range of participants, including those from under-served groups. If this is not possible, then serious consideration should be given to abandoning the intervention on the grounds that it will not be broadly applicable in practice. Exceptions to this are interventions designed to target a specific biological pathway identified by disease severity or other disease characteristics (ie, stratified or precision medicine approaches), or interventions designed specifically to target under-served groups where current approaches are not sufficiently effective.
Outcomes should be selected to be relevant to a broad range of participants; outcomes should be simple and should place minimal burden on both participants and research teams. Outcomes should be deliverable under pandemic conditions (eg, when wearing full personal protective equipment (PPE) in overloaded hospitals). Outcomes that can be collected from routinely collected clinical data or participant-reported outcomes that have the flexibility to be collected by telephone, by video link, by remote devices (eg, accelerometers or other Bluetooth-enabled devices 14 ) or by web-based/SMS interfaces should be preferred, ensuring that such platforms can be used by participants with a range of sensory and motor disabilities.
When outcomes must be collected face to face, these should be able to be collected in a range of different environments including the participant’s own home, not just in hospital wards or clinics. Outcomes should, where possible, be able to be assessed by a range of staff with no or minimal training to ensure that studies are resilient against staff illness or redeployment in future pandemic waves. Research teams should build the costs of hardware, software, accommodations for people with disability and adequate time to support these outcome collection methods into their proposals.
Researchers should design study protocols to allow recruitment through as wide a range of routes as possible. Without good scientific reason, recruitment should not be limited to hospitals, primary care practices or care institutions, but should be enabled through all of these routes and others including through community social hubs and organisations, via adverts, web-based signup, verbally over the telephone, in response to SMS and traditional mail, and other routes appropriate to reach particular under-served groups. The emergence of new community networks to support isolated and vulnerable people during the pandemic provides new opportunities for contacting and engaging under-served groups in research. Key issues to consider when designing COVID-19 studies are given in box 1 .
Funders can apply the checklist given later in this document (see box 2 ) to assess whether research proposals have been developed in conjunction with under-served groups and that proposals are designed and will be delivered in a way likely to be able to recruit under-served groups successfully in COVID-19 research.
Funders should ensure that additional funds (above and beyond the amount of money traditionally viewed as adequate to support recruitment and retention) are available to research teams to support the successful recruitment of under-served groups. This may require funds for home visiting, transportation (in many cases by car or taxi, not public transport), PPE, more screening per participant recruited, longer study visits, provision of translators or translated materials.
A checklist to guide researchers in designing inclusive COVID-19 research
Have I engaged with representatives of under-served groups in designing my study question and protocol?
Have I avoided inclusion/exclusion criteria likely to lead to exclusion of under-served groups? (eg, age limits, excluding multimorbidities, body mass index limits)
Is my study intervention suitable for, and acceptable to, under-served groups? (eg, avoiding gelatine capsules on medication)
Are my outcomes validated and relevant to a broad range of patients in the populations that my research seeks to serve? (eg, cognition assessment tools in translated versions)
Have I avoided study burden that is likely to make it more difficult for some groups to participate? (eg, frequent study visits)
Have I provided a range of recruitment pathways that give flexibility in how participants are sought and contacted? (eg, not confining recruitment to drive-in testing centres, allowing recruitment from care homes)
Have I provided study information in a format that is accessible to under-served groups? (eg, translations of study information, simplified study information, information written with a reading age of 9 or below, written in large font size, screen reader compatible)
Have I enabled consent to be obtained in a way that is flexible and tailored to the needs of different groups? (eg, EConsent for those isolating, assent or supported decision making from relatives for those with cognitive impairment). Have I involved carers and assentees in the design of the assent process for studies where consent cannot be obtained from the participant?
Will my study still be deliverable in the event of a second pandemic wave, with a possible increase in lockdown restrictions and study staff redeployment?
A checklist to guide funders and reviewers in assessing inclusiveness of COVID-19 research
Does the topic of the study reflect the priorities elicited by consultation with under-served groups?
Have representatives from under-served groups been engaged in the design of the study, and in what way?
Does the target population for the study (as defined by the inclusion and exclusion criteria) reflect those who are at risk of contracting COVID-19 or who are at increased risk of the adverse consequences of COVID-19?
Have the investigators taken steps to ensure that these potentially under-served groups will be included in the study with appropriate mitigation of any risks?
Are there unnecessary or unjustifiable exclusion criteria (eg, old age, sex, ethnicity, obesity, cognitive impairment, multimorbidity) or research methods that will act as barriers to inclusion of under-served groups at high risk of COVID-19 infection and its adverse consequences?
Is the intervention designed and delivered in a way that is acceptable and feasible to a broad range of people most at risk from COVID-19 infection?
Are the study outcomes easy to measure, and can they be measured in a range of different environments? Will they still be able to be measured during the peak of a pandemic wave or during population lockdown?
Does the study target a specific under-served group? If so, is adequate justification for this given, and is the strategy proposed to target the group likely to succeed?
Final study design
Research Ethics Committees should pay particular attention to inclusion and exclusion criteria, routes of recruitment, information materials, consent processes (including the needs of those without capacity) and whether under-served groups have been engaged in study design. Where study designs seem likely to fail to include sufficient people from under-served groups, ethics committees should robustly call attention to this and ask research teams to modify their proposals accordingly even if doing so leads to short delays in approval of projects. Key issues to consider are listed in box 2 .
Sponsor organisations should apply similar scrutiny to research proposals at the design stage and insist on changes where the design makes the exclusion of under-served groups likely.
Sponsor organisations and Research Ethics Committees should both welcome and encourage novel and efficient methods of approaching, consenting, recruiting and retaining participants in research studies. Many proposals will be very different from those that such organisations are used to seeing, but this should be viewed as an opportunity, not a reason to fall back on traditional (and often ineffective) methods of study conduct.
Study delivery
Local research delivery teams should seek local advice on how to reach and engage with under-served groups in their areas. In many cases, this will entail getting advice from members of specific ethnic communities, or those with lived experience of particular population sectors (eg, those living in care homes, homeless people). In selected cases, this may extend to using members of under-served communities to deliver research. Key issues to consider are listed in box 3 .
Training for research delivery staff (local investigators, research nurses and other research team members) should be delivered to raise awareness of the need to recruit inclusively, to highlight particular under-served groups and to provide the generic skills required to engage with specific under-served groups.
Local research teams should configure themselves to be able to recruit in environments other than standard inpatient and outpatient secondary care departments. This should include conducting study procedures in participants’ own homes, care homes, conducting study procedures remotely (eg, via telephone and video links) and taking care to accommodate the needs of those with a range of disabilities. Linking with neighbouring healthcare research organisations will enable cross-cover of research staff in the event of staff sickness or of one organisation becoming overloaded by clinical work in future pandemic waves.
For trials of medicinal products, dispensing arrangements for oral medications should be put in place to enable the delivery of medications directly to participants’ homes or local pharmacy rather than needing to collect medication from a research facility. This approach will help to build resilience against future restrictions on movement or closure of workplaces.
Local research support agencies (such as the UK NIHR local Clinical Research Networks and hospital Trusts) should provide local research teams with the equipment and resources that they need to deliver research in non-traditional settings. This may include transportation, contracts and working practice procedures enabling work in participants’ homes, provision of COVID-19 testing and PPE in line with national guidance, IT hardware and software to enable video links, EConsent and electronic case record form use while roaming, translation services and sufficient time to conduct study visits in non-traditional settings.
Local research support agencies should put in place systems to monitor local recruitment of under-served groups to each study. Combining these local data across studies will enable study teams to adapt their recruitment strategies at an early point, for instance, switching place of recruitment, oversampling particular under-served groups or changing how study teams approach participants.
A checklist to guide research delivery teams in delivering inclusive COVID-19 research
Have we engaged with local representatives from under-served groups to understand how best to approach, recruit and retain participants to this study locally?
Are our staff trained to approach and recruit people from under-served groups? (eg, those living in care homes). If not, what training is required (eg, age inclusion training, disability inclusion training) and how will we deliver this?
Do our staff have the correct equipment to deliver the study to under-served groups (eg, portable testing and blood taking kit, car transport and PPE to conduce home visits, contracts and lone working policies to support home working, video and phone consulting systems to enable remote follow-up, accommodations to support the inclusion of people with disabilities)?
Do we have staff with particular skills or background who would be well suited to engage with under-served groups (eg, speak the same language, live in the same area, experience working in a particular healthcare sector)?
Do we have processes in place locally to monitor whether we are reaching people from under-served groups—and if not, why not?
If recruiting people from under-served groups is taking more time or resources, is the extra time and resource being made available, either from the funder or from local resources?
Study closure and analysis
Investigators and sponsors should develop plans for how pauses or discontinuation of study activity due to future pandemic waves will be managed (ideally to at least enable the study follow-up to continue), and how these plans will be communicated to participants.
Study reports and analysis plans should report the proportion of relevant under-served groups and compare these proportions to those found in the general population with COVID-19 illness.
Study analysis plans should include adequately powered prespecified subgroup analyses for key under-served groups, both to explore differences within studies and to enable later pooling of results from different under-served groups.
Impact, dissemination and engagement
All studies should devise a comprehensive dissemination and engagement plan, and representatives from under-served groups should be part of the team drawing up these plans.
Specific engagement plans for different under-served groups should be drawn up to enable appropriate tailoring of messages to different groups, in a way that best encourages feedback, debate and engagement within different groups.
At a time when research on COVID-19 is being designed and delivered at an extraordinary speed, it may seem that the urgency of the situation obviates the need to fully engage under-served groups in the design and delivery of COVID-19 research. We argue however that doing so is not a luxury but a necessity and failure to engage represents an enormous wasted opportunity. 15 It will hamper scientific progress and potentially lead to the deployment of ineffective or harmful diagnostics and therapeutics to large sections of the population. This in turn will lead to worse health outcomes for under-served groups, and healthcare resources being wasted. Fully engaging under-served groups and enabling their inclusion in COVID-19 research is the only way that we will be able to understand the health impacts of COVID-19 in these most vulnerable groups and thus mitigate the impact of COVID-19 on health and society in an equitable way.
- Ramanan M ,
- Rooplalsingh R , et al
- International Severe Acute Respiratory and Emerging Infections Consortium (ISARIC) consortium
- Williamson EJ ,
- Walker AJ ,
- Bhaskaran K , et al
- Cherubini A , et al
- Treweek S ,
- Forouhi NG ,
- Narayan KMV , et al
- National Institute for Health Research
- Witham MD ,
- Anderson E ,
- Carroll C , et al
- Prendecki M ,
- Dhutia A , et al
- Graham NSN ,
- Junghans C ,
- Downes R , et al
- Intensive Care National Audit and Research Centre (ICNARC)
- Bright T , et al
- McMurdo MET ,
- Roberts H ,
- Parker S , et al
- NIHR CLAHRC East Midlands Centre for BME Health
- Rolinski M ,
- McNaney R , et al
- Glasziou PP ,
- Sanders S ,
Twitter @OlderTrialsProf
Contributors MW: conceptualisation, wrote first draft, critical revision and editing, approval of final version. EA, CBC, PD, KD, ASH, JK, EM, RM, GM, GN, JO, LO, JW and LR: conceptualisation, critical revision and editing, approval of final version.
Funding This work was undertaken as part of the INCLUDE project, which is commissioned and funded by the UK National Institute for Health Research Clinical Research Network Coordinating Centre.
Competing interests None declared.
Patient consent for publication Not required.
Provenance and peer review Not commissioned; externally peer reviewed.
Read the full text or download the PDF:
Reporting standards for literature searches and report inclusion criteria: making research syntheses more transparent and easy to replicate
Affiliation.
- 1 Duke University, Psychology and Neuroscience, Durham, North Carolina, USA.
- PMID: 26035472
- DOI: 10.1002/jrsm.1127
A complete description of the literature search, including the criteria used for the inclusion of reports after they have been located, used in a research synthesis or meta-analysis is critical if subsequent researchers are to accurately evaluate and reproduce a synthesis' methods and results. Based on previous guidelines and new suggestions, we present a set of focused and detailed standards for reporting the methods used in a literature search. The guidelines cover five search strategies: reference database searches, journal and bibliography searches, searches of the reference lists of reports, citation searches, and direct contact searches. First, we bring together all the unique recommendations made in existing guidelines for research synthesis. Second, we identify gaps in reporting standards for search strategies. Third, we address these gaps by providing new reporting recommendations. Our hope is to facilitate successful evaluation and replication of research synthesis results.
Keywords: guidelines; literature search; meta‐analysis; research synthesis.
Copyright © 2014 John Wiley & Sons, Ltd.
Publication types
- Research Support, Non-U.S. Gov't
- Biostatistics / methods
- Databases as Topic / statistics & numerical data
- Guidelines as Topic / standards
- Information Storage and Retrieval / standards*
- Information Storage and Retrieval / statistics & numerical data
- Meta-Analysis as Topic*
- Periodicals as Topic / statistics & numerical data
Criteria for Good Qualitative Research: A Comprehensive Review
- Regular Article
- Open access
- Published: 18 September 2021
- Volume 31 , pages 679–689, ( 2022 )
Cite this article
You have full access to this open access article
- Drishti Yadav ORCID: orcid.org/0000-0002-2974-0323 1
83k Accesses
27 Citations
72 Altmetric
Explore all metrics
This review aims to synthesize a published set of evaluative criteria for good qualitative research. The aim is to shed light on existing standards for assessing the rigor of qualitative research encompassing a range of epistemological and ontological standpoints. Using a systematic search strategy, published journal articles that deliberate criteria for rigorous research were identified. Then, references of relevant articles were surveyed to find noteworthy, distinct, and well-defined pointers to good qualitative research. This review presents an investigative assessment of the pivotal features in qualitative research that can permit the readers to pass judgment on its quality and to condemn it as good research when objectively and adequately utilized. Overall, this review underlines the crux of qualitative research and accentuates the necessity to evaluate such research by the very tenets of its being. It also offers some prospects and recommendations to improve the quality of qualitative research. Based on the findings of this review, it is concluded that quality criteria are the aftereffect of socio-institutional procedures and existing paradigmatic conducts. Owing to the paradigmatic diversity of qualitative research, a single and specific set of quality criteria is neither feasible nor anticipated. Since qualitative research is not a cohesive discipline, researchers need to educate and familiarize themselves with applicable norms and decisive factors to evaluate qualitative research from within its theoretical and methodological framework of origin.
Similar content being viewed by others

Good Qualitative Research: Opening up the Debate
Beyond qualitative/quantitative structuralism: the positivist qualitative research and the paradigmatic disclaimer.
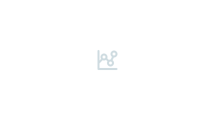
What is Qualitative in Research
Avoid common mistakes on your manuscript.
Introduction
“… It is important to regularly dialogue about what makes for good qualitative research” (Tracy, 2010 , p. 837)
To decide what represents good qualitative research is highly debatable. There are numerous methods that are contained within qualitative research and that are established on diverse philosophical perspectives. Bryman et al., ( 2008 , p. 262) suggest that “It is widely assumed that whereas quality criteria for quantitative research are well‐known and widely agreed, this is not the case for qualitative research.” Hence, the question “how to evaluate the quality of qualitative research” has been continuously debated. There are many areas of science and technology wherein these debates on the assessment of qualitative research have taken place. Examples include various areas of psychology: general psychology (Madill et al., 2000 ); counseling psychology (Morrow, 2005 ); and clinical psychology (Barker & Pistrang, 2005 ), and other disciplines of social sciences: social policy (Bryman et al., 2008 ); health research (Sparkes, 2001 ); business and management research (Johnson et al., 2006 ); information systems (Klein & Myers, 1999 ); and environmental studies (Reid & Gough, 2000 ). In the literature, these debates are enthused by the impression that the blanket application of criteria for good qualitative research developed around the positivist paradigm is improper. Such debates are based on the wide range of philosophical backgrounds within which qualitative research is conducted (e.g., Sandberg, 2000 ; Schwandt, 1996 ). The existence of methodological diversity led to the formulation of different sets of criteria applicable to qualitative research.
Among qualitative researchers, the dilemma of governing the measures to assess the quality of research is not a new phenomenon, especially when the virtuous triad of objectivity, reliability, and validity (Spencer et al., 2004 ) are not adequate. Occasionally, the criteria of quantitative research are used to evaluate qualitative research (Cohen & Crabtree, 2008 ; Lather, 2004 ). Indeed, Howe ( 2004 ) claims that the prevailing paradigm in educational research is scientifically based experimental research. Hypotheses and conjectures about the preeminence of quantitative research can weaken the worth and usefulness of qualitative research by neglecting the prominence of harmonizing match for purpose on research paradigm, the epistemological stance of the researcher, and the choice of methodology. Researchers have been reprimanded concerning this in “paradigmatic controversies, contradictions, and emerging confluences” (Lincoln & Guba, 2000 ).
In general, qualitative research tends to come from a very different paradigmatic stance and intrinsically demands distinctive and out-of-the-ordinary criteria for evaluating good research and varieties of research contributions that can be made. This review attempts to present a series of evaluative criteria for qualitative researchers, arguing that their choice of criteria needs to be compatible with the unique nature of the research in question (its methodology, aims, and assumptions). This review aims to assist researchers in identifying some of the indispensable features or markers of high-quality qualitative research. In a nutshell, the purpose of this systematic literature review is to analyze the existing knowledge on high-quality qualitative research and to verify the existence of research studies dealing with the critical assessment of qualitative research based on the concept of diverse paradigmatic stances. Contrary to the existing reviews, this review also suggests some critical directions to follow to improve the quality of qualitative research in different epistemological and ontological perspectives. This review is also intended to provide guidelines for the acceleration of future developments and dialogues among qualitative researchers in the context of assessing the qualitative research.
The rest of this review article is structured in the following fashion: Sect. Methods describes the method followed for performing this review. Section Criteria for Evaluating Qualitative Studies provides a comprehensive description of the criteria for evaluating qualitative studies. This section is followed by a summary of the strategies to improve the quality of qualitative research in Sect. Improving Quality: Strategies . Section How to Assess the Quality of the Research Findings? provides details on how to assess the quality of the research findings. After that, some of the quality checklists (as tools to evaluate quality) are discussed in Sect. Quality Checklists: Tools for Assessing the Quality . At last, the review ends with the concluding remarks presented in Sect. Conclusions, Future Directions and Outlook . Some prospects in qualitative research for enhancing its quality and usefulness in the social and techno-scientific research community are also presented in Sect. Conclusions, Future Directions and Outlook .
For this review, a comprehensive literature search was performed from many databases using generic search terms such as Qualitative Research , Criteria , etc . The following databases were chosen for the literature search based on the high number of results: IEEE Explore, ScienceDirect, PubMed, Google Scholar, and Web of Science. The following keywords (and their combinations using Boolean connectives OR/AND) were adopted for the literature search: qualitative research, criteria, quality, assessment, and validity. The synonyms for these keywords were collected and arranged in a logical structure (see Table 1 ). All publications in journals and conference proceedings later than 1950 till 2021 were considered for the search. Other articles extracted from the references of the papers identified in the electronic search were also included. A large number of publications on qualitative research were retrieved during the initial screening. Hence, to include the searches with the main focus on criteria for good qualitative research, an inclusion criterion was utilized in the search string.
From the selected databases, the search retrieved a total of 765 publications. Then, the duplicate records were removed. After that, based on the title and abstract, the remaining 426 publications were screened for their relevance by using the following inclusion and exclusion criteria (see Table 2 ). Publications focusing on evaluation criteria for good qualitative research were included, whereas those works which delivered theoretical concepts on qualitative research were excluded. Based on the screening and eligibility, 45 research articles were identified that offered explicit criteria for evaluating the quality of qualitative research and were found to be relevant to this review.
Figure 1 illustrates the complete review process in the form of PRISMA flow diagram. PRISMA, i.e., “preferred reporting items for systematic reviews and meta-analyses” is employed in systematic reviews to refine the quality of reporting.
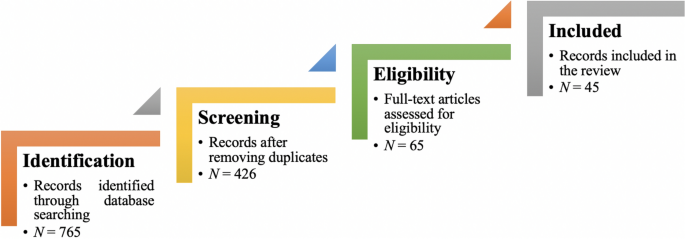
PRISMA flow diagram illustrating the search and inclusion process. N represents the number of records
Criteria for Evaluating Qualitative Studies
Fundamental criteria: general research quality.
Various researchers have put forward criteria for evaluating qualitative research, which have been summarized in Table 3 . Also, the criteria outlined in Table 4 effectively deliver the various approaches to evaluate and assess the quality of qualitative work. The entries in Table 4 are based on Tracy’s “Eight big‐tent criteria for excellent qualitative research” (Tracy, 2010 ). Tracy argues that high-quality qualitative work should formulate criteria focusing on the worthiness, relevance, timeliness, significance, morality, and practicality of the research topic, and the ethical stance of the research itself. Researchers have also suggested a series of questions as guiding principles to assess the quality of a qualitative study (Mays & Pope, 2020 ). Nassaji ( 2020 ) argues that good qualitative research should be robust, well informed, and thoroughly documented.
Qualitative Research: Interpretive Paradigms
All qualitative researchers follow highly abstract principles which bring together beliefs about ontology, epistemology, and methodology. These beliefs govern how the researcher perceives and acts. The net, which encompasses the researcher’s epistemological, ontological, and methodological premises, is referred to as a paradigm, or an interpretive structure, a “Basic set of beliefs that guides action” (Guba, 1990 ). Four major interpretive paradigms structure the qualitative research: positivist and postpositivist, constructivist interpretive, critical (Marxist, emancipatory), and feminist poststructural. The complexity of these four abstract paradigms increases at the level of concrete, specific interpretive communities. Table 5 presents these paradigms and their assumptions, including their criteria for evaluating research, and the typical form that an interpretive or theoretical statement assumes in each paradigm. Moreover, for evaluating qualitative research, quantitative conceptualizations of reliability and validity are proven to be incompatible (Horsburgh, 2003 ). In addition, a series of questions have been put forward in the literature to assist a reviewer (who is proficient in qualitative methods) for meticulous assessment and endorsement of qualitative research (Morse, 2003 ). Hammersley ( 2007 ) also suggests that guiding principles for qualitative research are advantageous, but methodological pluralism should not be simply acknowledged for all qualitative approaches. Seale ( 1999 ) also points out the significance of methodological cognizance in research studies.
Table 5 reflects that criteria for assessing the quality of qualitative research are the aftermath of socio-institutional practices and existing paradigmatic standpoints. Owing to the paradigmatic diversity of qualitative research, a single set of quality criteria is neither possible nor desirable. Hence, the researchers must be reflexive about the criteria they use in the various roles they play within their research community.
Improving Quality: Strategies
Another critical question is “How can the qualitative researchers ensure that the abovementioned quality criteria can be met?” Lincoln and Guba ( 1986 ) delineated several strategies to intensify each criteria of trustworthiness. Other researchers (Merriam & Tisdell, 2016 ; Shenton, 2004 ) also presented such strategies. A brief description of these strategies is shown in Table 6 .
It is worth mentioning that generalizability is also an integral part of qualitative research (Hays & McKibben, 2021 ). In general, the guiding principle pertaining to generalizability speaks about inducing and comprehending knowledge to synthesize interpretive components of an underlying context. Table 7 summarizes the main metasynthesis steps required to ascertain generalizability in qualitative research.
Figure 2 reflects the crucial components of a conceptual framework and their contribution to decisions regarding research design, implementation, and applications of results to future thinking, study, and practice (Johnson et al., 2020 ). The synergy and interrelationship of these components signifies their role to different stances of a qualitative research study.
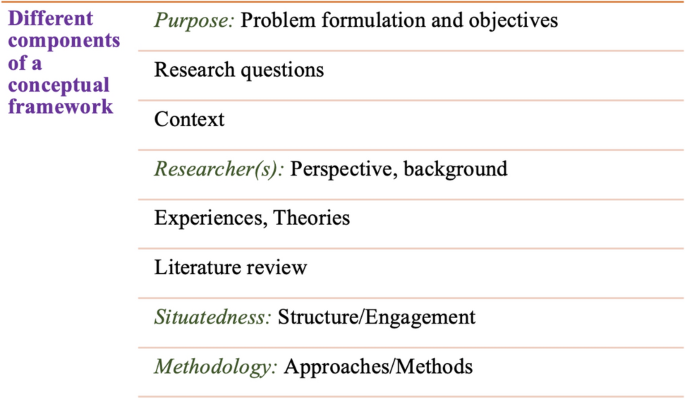
Essential elements of a conceptual framework
In a nutshell, to assess the rationale of a study, its conceptual framework and research question(s), quality criteria must take account of the following: lucid context for the problem statement in the introduction; well-articulated research problems and questions; precise conceptual framework; distinct research purpose; and clear presentation and investigation of the paradigms. These criteria would expedite the quality of qualitative research.
How to Assess the Quality of the Research Findings?
The inclusion of quotes or similar research data enhances the confirmability in the write-up of the findings. The use of expressions (for instance, “80% of all respondents agreed that” or “only one of the interviewees mentioned that”) may also quantify qualitative findings (Stenfors et al., 2020 ). On the other hand, the persuasive reason for “why this may not help in intensifying the research” has also been provided (Monrouxe & Rees, 2020 ). Further, the Discussion and Conclusion sections of an article also prove robust markers of high-quality qualitative research, as elucidated in Table 8 .
Quality Checklists: Tools for Assessing the Quality
Numerous checklists are available to speed up the assessment of the quality of qualitative research. However, if used uncritically and recklessly concerning the research context, these checklists may be counterproductive. I recommend that such lists and guiding principles may assist in pinpointing the markers of high-quality qualitative research. However, considering enormous variations in the authors’ theoretical and philosophical contexts, I would emphasize that high dependability on such checklists may say little about whether the findings can be applied in your setting. A combination of such checklists might be appropriate for novice researchers. Some of these checklists are listed below:
The most commonly used framework is Consolidated Criteria for Reporting Qualitative Research (COREQ) (Tong et al., 2007 ). This framework is recommended by some journals to be followed by the authors during article submission.
Standards for Reporting Qualitative Research (SRQR) is another checklist that has been created particularly for medical education (O’Brien et al., 2014 ).
Also, Tracy ( 2010 ) and Critical Appraisal Skills Programme (CASP, 2021 ) offer criteria for qualitative research relevant across methods and approaches.
Further, researchers have also outlined different criteria as hallmarks of high-quality qualitative research. For instance, the “Road Trip Checklist” (Epp & Otnes, 2021 ) provides a quick reference to specific questions to address different elements of high-quality qualitative research.
Conclusions, Future Directions, and Outlook
This work presents a broad review of the criteria for good qualitative research. In addition, this article presents an exploratory analysis of the essential elements in qualitative research that can enable the readers of qualitative work to judge it as good research when objectively and adequately utilized. In this review, some of the essential markers that indicate high-quality qualitative research have been highlighted. I scope them narrowly to achieve rigor in qualitative research and note that they do not completely cover the broader considerations necessary for high-quality research. This review points out that a universal and versatile one-size-fits-all guideline for evaluating the quality of qualitative research does not exist. In other words, this review also emphasizes the non-existence of a set of common guidelines among qualitative researchers. In unison, this review reinforces that each qualitative approach should be treated uniquely on account of its own distinctive features for different epistemological and disciplinary positions. Owing to the sensitivity of the worth of qualitative research towards the specific context and the type of paradigmatic stance, researchers should themselves analyze what approaches can be and must be tailored to ensemble the distinct characteristics of the phenomenon under investigation. Although this article does not assert to put forward a magic bullet and to provide a one-stop solution for dealing with dilemmas about how, why, or whether to evaluate the “goodness” of qualitative research, it offers a platform to assist the researchers in improving their qualitative studies. This work provides an assembly of concerns to reflect on, a series of questions to ask, and multiple sets of criteria to look at, when attempting to determine the quality of qualitative research. Overall, this review underlines the crux of qualitative research and accentuates the need to evaluate such research by the very tenets of its being. Bringing together the vital arguments and delineating the requirements that good qualitative research should satisfy, this review strives to equip the researchers as well as reviewers to make well-versed judgment about the worth and significance of the qualitative research under scrutiny. In a nutshell, a comprehensive portrayal of the research process (from the context of research to the research objectives, research questions and design, speculative foundations, and from approaches of collecting data to analyzing the results, to deriving inferences) frequently proliferates the quality of a qualitative research.
Prospects : A Road Ahead for Qualitative Research
Irrefutably, qualitative research is a vivacious and evolving discipline wherein different epistemological and disciplinary positions have their own characteristics and importance. In addition, not surprisingly, owing to the sprouting and varied features of qualitative research, no consensus has been pulled off till date. Researchers have reflected various concerns and proposed several recommendations for editors and reviewers on conducting reviews of critical qualitative research (Levitt et al., 2021 ; McGinley et al., 2021 ). Following are some prospects and a few recommendations put forward towards the maturation of qualitative research and its quality evaluation:
In general, most of the manuscript and grant reviewers are not qualitative experts. Hence, it is more likely that they would prefer to adopt a broad set of criteria. However, researchers and reviewers need to keep in mind that it is inappropriate to utilize the same approaches and conducts among all qualitative research. Therefore, future work needs to focus on educating researchers and reviewers about the criteria to evaluate qualitative research from within the suitable theoretical and methodological context.
There is an urgent need to refurbish and augment critical assessment of some well-known and widely accepted tools (including checklists such as COREQ, SRQR) to interrogate their applicability on different aspects (along with their epistemological ramifications).
Efforts should be made towards creating more space for creativity, experimentation, and a dialogue between the diverse traditions of qualitative research. This would potentially help to avoid the enforcement of one's own set of quality criteria on the work carried out by others.
Moreover, journal reviewers need to be aware of various methodological practices and philosophical debates.
It is pivotal to highlight the expressions and considerations of qualitative researchers and bring them into a more open and transparent dialogue about assessing qualitative research in techno-scientific, academic, sociocultural, and political rooms.
Frequent debates on the use of evaluative criteria are required to solve some potentially resolved issues (including the applicability of a single set of criteria in multi-disciplinary aspects). Such debates would not only benefit the group of qualitative researchers themselves, but primarily assist in augmenting the well-being and vivacity of the entire discipline.
To conclude, I speculate that the criteria, and my perspective, may transfer to other methods, approaches, and contexts. I hope that they spark dialog and debate – about criteria for excellent qualitative research and the underpinnings of the discipline more broadly – and, therefore, help improve the quality of a qualitative study. Further, I anticipate that this review will assist the researchers to contemplate on the quality of their own research, to substantiate research design and help the reviewers to review qualitative research for journals. On a final note, I pinpoint the need to formulate a framework (encompassing the prerequisites of a qualitative study) by the cohesive efforts of qualitative researchers of different disciplines with different theoretic-paradigmatic origins. I believe that tailoring such a framework (of guiding principles) paves the way for qualitative researchers to consolidate the status of qualitative research in the wide-ranging open science debate. Dialogue on this issue across different approaches is crucial for the impending prospects of socio-techno-educational research.
Amin, M. E. K., Nørgaard, L. S., Cavaco, A. M., Witry, M. J., Hillman, L., Cernasev, A., & Desselle, S. P. (2020). Establishing trustworthiness and authenticity in qualitative pharmacy research. Research in Social and Administrative Pharmacy, 16 (10), 1472–1482.
Article Google Scholar
Barker, C., & Pistrang, N. (2005). Quality criteria under methodological pluralism: Implications for conducting and evaluating research. American Journal of Community Psychology, 35 (3–4), 201–212.
Bryman, A., Becker, S., & Sempik, J. (2008). Quality criteria for quantitative, qualitative and mixed methods research: A view from social policy. International Journal of Social Research Methodology, 11 (4), 261–276.
Caelli, K., Ray, L., & Mill, J. (2003). ‘Clear as mud’: Toward greater clarity in generic qualitative research. International Journal of Qualitative Methods, 2 (2), 1–13.
CASP (2021). CASP checklists. Retrieved May 2021 from https://casp-uk.net/casp-tools-checklists/
Cohen, D. J., & Crabtree, B. F. (2008). Evaluative criteria for qualitative research in health care: Controversies and recommendations. The Annals of Family Medicine, 6 (4), 331–339.
Denzin, N. K., & Lincoln, Y. S. (2005). Introduction: The discipline and practice of qualitative research. In N. K. Denzin & Y. S. Lincoln (Eds.), The sage handbook of qualitative research (pp. 1–32). Sage Publications Ltd.
Google Scholar
Elliott, R., Fischer, C. T., & Rennie, D. L. (1999). Evolving guidelines for publication of qualitative research studies in psychology and related fields. British Journal of Clinical Psychology, 38 (3), 215–229.
Epp, A. M., & Otnes, C. C. (2021). High-quality qualitative research: Getting into gear. Journal of Service Research . https://doi.org/10.1177/1094670520961445
Guba, E. G. (1990). The paradigm dialog. In Alternative paradigms conference, mar, 1989, Indiana u, school of education, San Francisco, ca, us . Sage Publications, Inc.
Hammersley, M. (2007). The issue of quality in qualitative research. International Journal of Research and Method in Education, 30 (3), 287–305.
Haven, T. L., Errington, T. M., Gleditsch, K. S., van Grootel, L., Jacobs, A. M., Kern, F. G., & Mokkink, L. B. (2020). Preregistering qualitative research: A Delphi study. International Journal of Qualitative Methods, 19 , 1609406920976417.
Hays, D. G., & McKibben, W. B. (2021). Promoting rigorous research: Generalizability and qualitative research. Journal of Counseling and Development, 99 (2), 178–188.
Horsburgh, D. (2003). Evaluation of qualitative research. Journal of Clinical Nursing, 12 (2), 307–312.
Howe, K. R. (2004). A critique of experimentalism. Qualitative Inquiry, 10 (1), 42–46.
Johnson, J. L., Adkins, D., & Chauvin, S. (2020). A review of the quality indicators of rigor in qualitative research. American Journal of Pharmaceutical Education, 84 (1), 7120.
Johnson, P., Buehring, A., Cassell, C., & Symon, G. (2006). Evaluating qualitative management research: Towards a contingent criteriology. International Journal of Management Reviews, 8 (3), 131–156.
Klein, H. K., & Myers, M. D. (1999). A set of principles for conducting and evaluating interpretive field studies in information systems. MIS Quarterly, 23 (1), 67–93.
Lather, P. (2004). This is your father’s paradigm: Government intrusion and the case of qualitative research in education. Qualitative Inquiry, 10 (1), 15–34.
Levitt, H. M., Morrill, Z., Collins, K. M., & Rizo, J. L. (2021). The methodological integrity of critical qualitative research: Principles to support design and research review. Journal of Counseling Psychology, 68 (3), 357.
Lincoln, Y. S., & Guba, E. G. (1986). But is it rigorous? Trustworthiness and authenticity in naturalistic evaluation. New Directions for Program Evaluation, 1986 (30), 73–84.
Lincoln, Y. S., & Guba, E. G. (2000). Paradigmatic controversies, contradictions and emerging confluences. In N. K. Denzin & Y. S. Lincoln (Eds.), Handbook of qualitative research (2nd ed., pp. 163–188). Sage Publications.
Madill, A., Jordan, A., & Shirley, C. (2000). Objectivity and reliability in qualitative analysis: Realist, contextualist and radical constructionist epistemologies. British Journal of Psychology, 91 (1), 1–20.
Mays, N., & Pope, C. (2020). Quality in qualitative research. Qualitative Research in Health Care . https://doi.org/10.1002/9781119410867.ch15
McGinley, S., Wei, W., Zhang, L., & Zheng, Y. (2021). The state of qualitative research in hospitality: A 5-year review 2014 to 2019. Cornell Hospitality Quarterly, 62 (1), 8–20.
Merriam, S., & Tisdell, E. (2016). Qualitative research: A guide to design and implementation. San Francisco, US.
Meyer, M., & Dykes, J. (2019). Criteria for rigor in visualization design study. IEEE Transactions on Visualization and Computer Graphics, 26 (1), 87–97.
Monrouxe, L. V., & Rees, C. E. (2020). When I say… quantification in qualitative research. Medical Education, 54 (3), 186–187.
Morrow, S. L. (2005). Quality and trustworthiness in qualitative research in counseling psychology. Journal of Counseling Psychology, 52 (2), 250.
Morse, J. M. (2003). A review committee’s guide for evaluating qualitative proposals. Qualitative Health Research, 13 (6), 833–851.
Nassaji, H. (2020). Good qualitative research. Language Teaching Research, 24 (4), 427–431.
O’Brien, B. C., Harris, I. B., Beckman, T. J., Reed, D. A., & Cook, D. A. (2014). Standards for reporting qualitative research: A synthesis of recommendations. Academic Medicine, 89 (9), 1245–1251.
O’Connor, C., & Joffe, H. (2020). Intercoder reliability in qualitative research: Debates and practical guidelines. International Journal of Qualitative Methods, 19 , 1609406919899220.
Reid, A., & Gough, S. (2000). Guidelines for reporting and evaluating qualitative research: What are the alternatives? Environmental Education Research, 6 (1), 59–91.
Rocco, T. S. (2010). Criteria for evaluating qualitative studies. Human Resource Development International . https://doi.org/10.1080/13678868.2010.501959
Sandberg, J. (2000). Understanding human competence at work: An interpretative approach. Academy of Management Journal, 43 (1), 9–25.
Schwandt, T. A. (1996). Farewell to criteriology. Qualitative Inquiry, 2 (1), 58–72.
Seale, C. (1999). Quality in qualitative research. Qualitative Inquiry, 5 (4), 465–478.
Shenton, A. K. (2004). Strategies for ensuring trustworthiness in qualitative research projects. Education for Information, 22 (2), 63–75.
Sparkes, A. C. (2001). Myth 94: Qualitative health researchers will agree about validity. Qualitative Health Research, 11 (4), 538–552.
Spencer, L., Ritchie, J., Lewis, J., & Dillon, L. (2004). Quality in qualitative evaluation: A framework for assessing research evidence.
Stenfors, T., Kajamaa, A., & Bennett, D. (2020). How to assess the quality of qualitative research. The Clinical Teacher, 17 (6), 596–599.
Taylor, E. W., Beck, J., & Ainsworth, E. (2001). Publishing qualitative adult education research: A peer review perspective. Studies in the Education of Adults, 33 (2), 163–179.
Tong, A., Sainsbury, P., & Craig, J. (2007). Consolidated criteria for reporting qualitative research (COREQ): A 32-item checklist for interviews and focus groups. International Journal for Quality in Health Care, 19 (6), 349–357.
Tracy, S. J. (2010). Qualitative quality: Eight “big-tent” criteria for excellent qualitative research. Qualitative Inquiry, 16 (10), 837–851.
Download references
Open access funding provided by TU Wien (TUW).
Author information
Authors and affiliations.
Faculty of Informatics, Technische Universität Wien, 1040, Vienna, Austria
Drishti Yadav
You can also search for this author in PubMed Google Scholar
Corresponding author
Correspondence to Drishti Yadav .
Ethics declarations
Conflict of interest.
The author declares no conflict of interest.
Additional information
Publisher's note.
Springer Nature remains neutral with regard to jurisdictional claims in published maps and institutional affiliations.
Rights and permissions
Open Access This article is licensed under a Creative Commons Attribution 4.0 International License, which permits use, sharing, adaptation, distribution and reproduction in any medium or format, as long as you give appropriate credit to the original author(s) and the source, provide a link to the Creative Commons licence, and indicate if changes were made. The images or other third party material in this article are included in the article's Creative Commons licence, unless indicated otherwise in a credit line to the material. If material is not included in the article's Creative Commons licence and your intended use is not permitted by statutory regulation or exceeds the permitted use, you will need to obtain permission directly from the copyright holder. To view a copy of this licence, visit http://creativecommons.org/licenses/by/4.0/ .
Reprints and permissions
About this article
Yadav, D. Criteria for Good Qualitative Research: A Comprehensive Review. Asia-Pacific Edu Res 31 , 679–689 (2022). https://doi.org/10.1007/s40299-021-00619-0
Download citation
Accepted : 28 August 2021
Published : 18 September 2021
Issue Date : December 2022
DOI : https://doi.org/10.1007/s40299-021-00619-0
Share this article
Anyone you share the following link with will be able to read this content:
Sorry, a shareable link is not currently available for this article.
Provided by the Springer Nature SharedIt content-sharing initiative
- Qualitative research
- Evaluative criteria
- Find a journal
- Publish with us
- Track your research
- Open access
- Published: 16 May 2024
Promoting equality, diversity and inclusion in research and funding: reflections from a digital manufacturing research network
- Oliver J. Fisher 1 ,
- Debra Fearnshaw ORCID: orcid.org/0000-0002-6498-9888 2 ,
- Nicholas J. Watson 3 ,
- Peter Green 4 ,
- Fiona Charnley 5 ,
- Duncan McFarlane 6 &
- Sarah Sharples 2
Research Integrity and Peer Review volume 9 , Article number: 5 ( 2024 ) Cite this article
146 Accesses
Metrics details
Equal, diverse, and inclusive teams lead to higher productivity, creativity, and greater problem-solving ability resulting in more impactful research. However, there is a gap between equality, diversity, and inclusion (EDI) research and practices to create an inclusive research culture. Research networks are vital to the research ecosystem, creating valuable opportunities for researchers to develop their partnerships with both academics and industrialists, progress their careers, and enable new areas of scientific discovery. A feature of a network is the provision of funding to support feasibility studies – an opportunity to develop new concepts or ideas, as well as to ‘fail fast’ in a supportive environment. The work of networks can address inequalities through equitable allocation of funding and proactive consideration of inclusion in all of their activities.
This study proposes a strategy to embed EDI within research network activities and funding review processes. This paper evaluates 21 planned mitigations introduced to address known inequalities within research events and how funding is awarded. EDI data were collected from researchers engaging in a digital manufacturing network activities and funding calls to measure the impact of the proposed method.
Quantitative analysis indicates that the network’s approach was successful in creating a more ethnically diverse network, engaging with early career researchers, and supporting researchers with care responsibilities. However, more work is required to create a gender balance across the network activities and ensure the representation of academics who declare a disability. Preliminary findings suggest the network’s anonymous funding review process has helped address inequalities in funding award rates for women and those with care responsibilities, more data are required to validate these observations and understand the impact of different interventions individually and in combination.
Conclusions
In summary, this study offers compelling evidence regarding the efficacy of a research network's approach in advancing EDI within research and funding. The network hopes that these findings will inform broader efforts to promote EDI in research and funding and that researchers, funders, and other stakeholders will be encouraged to adopt evidence-based strategies for advancing this important goal.
Peer Review reports
Introduction
Achieving equality, diversity, and inclusion (EDI) is an underpinning contributor to human rights, civilisation and society-wide responsibility [ 1 ]. Furthermore, promoting and embedding EDI within research environments is essential to make the advancements required to meet today’s research challenges [ 2 ]. This is evidenced by equal, diverse and inclusive teams leading to higher productivity, creativity and greater problem-solving ability [ 3 ], which increases the scientific impact of research outputs and researchers [ 4 ]. However, there remains a gap between EDI research and the everyday implementation of inclusive practices to achieve change [ 5 ]. This paper presents and reflects on the EDI measures trialled by the UK Engineering and Physical Sciences Research Council (EPSRC) funded digital manufacturing research network, Connected Everything (grant number: EP/S036113/1) [ 6 ]. The EPSRC is a UK research council that funds engineering and physical sciences research. By sharing these reflections, this work aims to contribute to the wider effort of creating an inclusive research culture. The perceptions of equality, diversity, and inclusion may vary among individuals. For the scope of this study, the following definitions are adopted:
Equality: Equality is about ensuring that every individual has an equal opportunity to make the most of their lives and talents. No one should have poorer life chances because of the way they were born, where they come from, what they believe, or whether they have a disability.
Diversity: Diversity concerns understanding that each individual is unique, recognising our differences, and exploring these differences in a safe, positive, and nurturing way to value each other as individuals.
Inclusion: Inclusion is an effort and practice in which groups or individuals with different backgrounds are culturally and socially accepted, welcomed and treated equally. This concerns treating each person as an individual, making them feel valued, and supported and being respectful of who they are.
Research networks have varied goals, but a common purpose is to create new interdisciplinary research communities, by fostering interactions between researchers and appropriate scientific, technological and industrial groups. These networks aim to offer valuable career progression opportunities for researchers, through access to research funding, forming academic and industrial collaborations at network events, personal and professional development, and research dissemination. However, feedback from a 2021 survey of 19 UK research networks, suggests that these research networks are not always diverse, and whilst on the face of it they seem inclusive, they are perceived as less inclusive by minority groups (including non-males, those with disabilities, and ethnic minority respondents) [ 7 ]. The exclusivity of these networks further exacerbates the inequality within the academic community as it prevents certain groups from being able to engage with all aspects of network activities.
Research investigating the causes of inequality and exclusivity has identified several suggestions to make research culture more inclusive, including improving diverse representation within event programmes and panels [ 8 , 9 ]; ensuring events are accessible to all [ 10 ]; providing personalised resources and training to build capacity and increase engagement [ 11 ]; educating institutions and funders to understand and address the barriers to research [ 12 ]; and increasing diversity in peer review and funding panels [ 13 ]. Universities, research institutions and research funding bodies are increasingly taking responsibility to ensure the health of the research and innovation system and to foster inclusion. For example, the EPSRC has set out their own ‘Expectation for EDI’ to promote the formation of a diverse and inclusive research culture [ 14 ]. To drive change, there is an emphasis on the importance of measuring diversity and links to measured outcomes to benchmark future studies on how interventions affect diversity [ 5 ]. Further, collecting and sharing EDI data can also drive aspirations, provide a target for actions, and allow institutions to consider common issues. However, there is a lack of available data regarding the impact of EDI practices on diversity that presents an obstacle, impeding the realisation of these benefits and hampering progress in addressing common issues and fostering diversity and inclusion [ 5 ].
Funding acquisition is important to an academic’s career progression, yet funding may often be awarded in ways that feel unequal and/or non-transparent. The importance of funding in academic career progression means that, if credit for obtaining funding is not recognised appropriately, careers can be damaged, and, as a result of the lack of recognition for those who have been involved in successful research, funding bodies may not have a complete picture of the research community, and are unable to deliver the best value for money [ 15 ]. Awarding funding is often a key research network activity and an area where networks can have a positive impact on the wider research community. It is therefore important that practices are established to embed EDI consideration within the funding process and to ensure that network funding is awarded without bias. Recommendations from the literature to make the funding award process fairer include: ensuring a diverse funding panel; funders instituting reviewer anti-bias training; anonymous review; and/or automatic adjustments to correct for known biases [ 16 ]. In the UK, the government organisation UK Research and Innovation (UKRI), tasked with overseeing research and innovation funding, has pledged to publish data to enhance transparency. This initiative aims to furnish an evidence base for designing interventions and evaluating their efficacy. While the data show some positive signs (e.g., the award rates for male and female PI applicants were equal at 29% in 2020–21), Ottoline Leyser (UKRI Chief Executive) highlights the ‘persistent pernicious disparities for under-represented groups in applying for and winning research funding’ [ 17 ]. This suggests that a more radical approach to rethinking the traditional funding review process may be required.
This paper describes the approach taken by the ‘Connected Everything’ EPSRC-funded Network to embed EDI in all aspects of its research funding process, and evaluates the impact of this ambition, leading to recommendations for embedding EDI in research funding allocation.
Connected everything’s equality diversity and inclusion strategy
Connected Everything aims to create a multidisciplinary community of researchers and industrialists to address key challenges associated with the future of digital manufacturing. The network is managed by an investigator team who are responsible for the strategic planning and, working with the network manager, to oversee the delivery of key activities. The network was first funded between 2016–2019 (grant number: EP/P001246/1) and was awarded a second grant (grant number: EP/S036113/1). The network activities are based around three goals: building partnerships, developing leadership and accelerating impact.
The Connected Everything network represents a broad range of disciplines, including manufacturing, computer science, cybersecurity, engineering, human factors, business, sociology, innovation and design. Some of the subject areas, such as Computer Science and Engineering, tend to be male-dominated (e.g., in 2021/22, a total of 185,42 higher education student enrolments in engineering & technology subjects was broken down as 20.5% Female and 79.5% Male [ 18 ]). The networks also face challenges in terms of accessibility for people with care responsibilities and disabilities. In 2019, Connected Everything committed to embedding EDI in all its network activities and published a guiding principle and goals for improving EDI (see Additional file 1 ). When designing the processes to deliver the second iteration of Connected Everything, the team identified several sources of potential bias/exclusion which have the potential to impact engagement with the network. Based on these identified factors, a series of mitigation interventions were implemented and are outlined in Table 1 .
Connected everything anonymous review process
A key Connected Everything activity is the funding of feasibility studies to enable cross-disciplinary, foresight, speculative and risky early-stage research, with a focus on low technology-readiness levels. Awards are made via a short, written application followed by a pitch to a multidisciplinary diverse panel including representatives from industry. Six- to twelve-month-long projects are funded to a maximum value of £60,000.
The current peer-review process used by funders may reveal the applicants’ identities to the reviewer. This can introduce dilemmas to the reviewer regarding (a) deciding whether to rely exclusively on information present within the application or search for additional information about the applicants and (b) whether or not to account for institutional prestige [ 34 ]. Knowing an applicant’s identity can bias the assessment of the proposal, but by focusing the assessment on the science rather than the researcher, equality is more frequently achieved between award rates (i.e., the proportion of successful applications) [ 15 ]. To progress Connected Everything’s commitment to EDI, the project team created a 2-stage review process, where the applicants’ identity was kept anonymous during the peer review stage. This anonymous process, which is outlined in Fig. 1 , was created for the feasibility study funding calls in 2019 and used for subsequent funding calls.
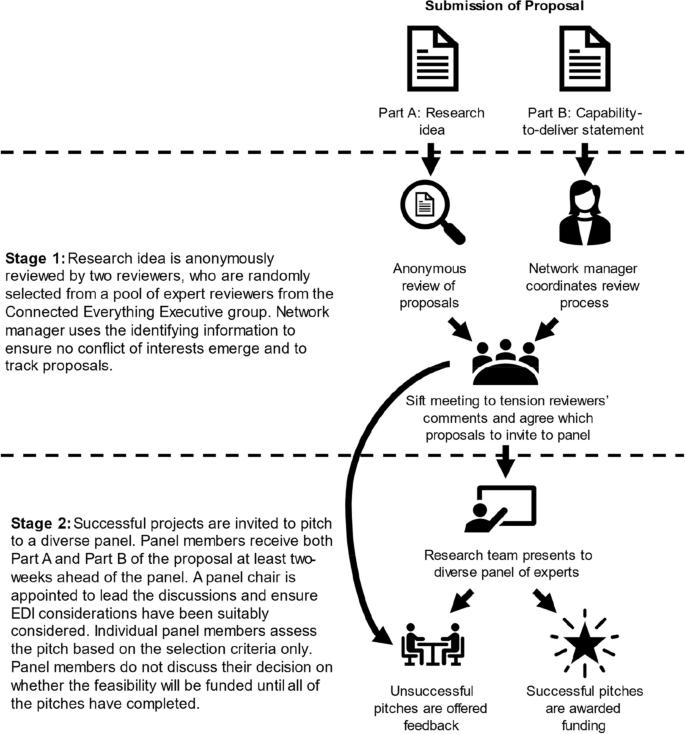
Connected Everything’s anonymous review process [EDI: Equality, diversity, and inclusion]
To facilitate the anonymous review process, the proposal was submitted in two parts: part A the research idea and part B the capability-to-deliver statement. All proposals were first anonymously reviewed by a random selection of two members from the Connected Everything executive group, which is a diverse group of digital manufacturing experts and peers from academia, industry and research institutions that provide guidance and leadership on Connected Everything activities. The reviewers rated the proposals against the selection criteria (see Additional file 1 , Table 1) and provided overall comments alongside a recommendation on whether or not the applicant should be invited to the panel pitch. This information was summarised and shared with a moderation sift panel, made up of a minimum of two Connected Everything investigators and a minimum of one member of the executive group, that tensioned the reviewers’ comments (i.e. comments and evaluations provided by the peer reviewers are carefully considered and weighed against each other) and ultimately decided which proposals to invite to the panel. This tension process included using the identifying information to ensure the applicants did have the capability to deliver the project. If this remained unclear, the applicants were asked to confirm expertise in an area the moderation sift panel thought was key or asked to bring in additional expertise to the project team during the panel pitch.
During stage two the applicants were invited to pitch their research idea to a panel of experts who were selected to reflect the diversity of the community. The proposals, including applicants’ identities, were shared with the panel at least two weeks ahead of the panel. Individual panel members completed a summary sheet at the end of the pitch session to record how well the proposal met the selection criteria (see Additional file 1 , Table 1). Panel members did not discuss their funding decision until all the pitches had been completed. A panel chair oversaw the process but did not declare their opinion on a specific feasibility study unless the panel could not agree on an outcome. The panel and panel chair were reminded to consider ways to manage their unconscious bias during the selection process.
Due to the positive response received regarding the anonymous review process, Connected Everything extended its use when reviewing other funded activities. As these awards were for smaller grant values (~ £5,000), it was decided that no panel pitch was required, and the researcher’s identity was kept anonymous for the entire process.
Data collection and analysis methods
Data collection.
Equality, diversity and inclusion data were voluntarily collected from applicants for Connected Everything research funding and from participants who won scholarships to attend Connected Everything funded activities. Responses to the EDI data requests were collected from nine Connected Everything coordinated activities between 2019 and 2022. Data requests were sent after the applicant had applied for Connected Everything funding or had attended a Connected Everything funded activity. All data requests were completed voluntarily, with reassurance given that completion of the data requested in no way affected their application. In total 260 responses were received, of which the three feasibility study calls comprised 56.2% of the total responses received. Overall, there was a 73.8% response rate.
To understand the diversity of participants engaging with Connected Everything activities and funding, the data requests asked for details of specific diversity characteristics: gender, transgender, disability, ethnicity, age, and care responsibilities. Although sex and gender are terms that are often used interchangeably, they are two different concepts. To clarify, the definitions used by the UK government describe sex as a set of biological attributes that is generally limited to male or female, and typically attributed to individuals at birth. In contrast, gender identity is a social construction related to behaviours and attributes, and is self-determined based on a person’s internal perception, identification and experience. Transgender is a term used to describe people whose gender identity is not the same as the sex they were registered at birth. Respondents were first asked to identify their gender and then whether their gender was different from their birth sex.
For this study, respondents were asked to (voluntarily) self-declare whether they consider themselves to be disabled or not. Ethnicity within the data requests was based on the 2011 census classification system. When reporting ethnicity data, this study followed the AdvanceHE example to aggregate the census categories into six groups to enable benchmarking against the available academic ethnicity data. AdvanceHE is a UK charity that works to improve the higher education system for staff, students and society. However, it was acknowledged that there were limitations with this grouping, including the assumption that minority ethnic staff or students are a homogenous group [ 16 ]. Therefore, this study made sure to breakdown these groups during the discussion of the results. The six groups are:
Asian: Asian/Asian British: Indian, Pakistani, Bangladeshi, and any other Asian background;
Black: Black/African/Caribbean/Black British: African, Caribbean, and any other Black/African/Caribbean background;
Other ethnic backgrounds, including Arab.
White: all white ethnic groups.
Benchmarking data
Published data from the Higher Education Statistics Agency [ 26 ] (a UK organisation responsible for collecting, analysing, and disseminating data related to higher education institutions and students), UKRI funding data [ 19 , 35 ] and 2011 census data [ 36 ] were used to benchmark the EDI data collected within this study. The responses to the data collected were compared to the engineering and technology cluster of academic disciplines, as this is most represented by Connected Everything’s main funded EPSRC. The Higher Education Statistics Agency defines the engineering and technology cluster as including the following subject areas: general engineering; chemical engineering; mineral, metallurgy & materials engineering; civil engineering; electrical, electronic & computer engineering; mechanical, aero & production engineering and; IT, systems sciences & computer software engineering [ 37 ].
When assessing the equality in funding award rates, previous studies have focused on analysing the success rates of only the principal investigators [ 15 , 16 , 38 ]; however, Connected Everything recognised that writing research proposals is a collaborative task, so requested diversity data from the whole research team. The average of the last six years of published principal investigator and co-investigator diversity data for UKRI and EPSRC funding awards (2015–2021) was used to benchmark the Connected Everything funding data [ 35 ]. The UKRI and EPSRC funding review process includes a peer review stage followed by panel pitch and assessment stage; however, the applicant's track record is assessed during the peer review stage, unlike the Connected Everything review process.
The data collected have been used to evaluate the success of the planned migrations to address EDI factors affecting the higher education research ecosystem, as outlined in Table 1 (" Connected Everything’s Equality Diversity and Inclusion Strategy " Section).
Dominance of small number of research-intensive universities receiving funding from network
The dominance of a small number of research-intensive universities receiving funding from a network can have implications for the field of research, including: the unequal distribution of resources; a lack of diversity of research, limited collaboration opportunities; and impact on innovation and progress. Analysis of published EPSRC funding data between 2015 and 2021 [ 19 ], shows that the funding has been predominately (74.1%, 95% CI [71.%, 76.9%] out of £3.98 billion) awarded to Russell Group universities. The Russell Group is a self-selected association of 24 research-intensive universities (out of the 174 universities) in the UK, established in 1994. Evaluation of the universities that received Connected Everything feasibility study funding between 2016–2019, shows that Connected Everything awarded just over half (54.6%, 95% CI [25.1%, 84.0%] out of 11 awards) to Russell Group universities. Figure 2 shows that the Connected Everything funding awarded to Russell Group universities reduced to 44.4%, 95% CI [12.0%, 76.9%] of 9 awards between 2019–2022.
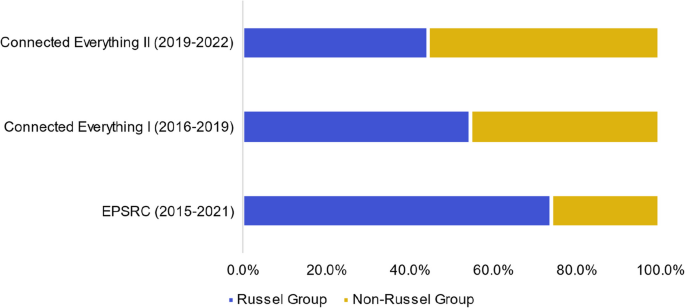
A comparison of funding awarded by EPSRC (total = £3.98 billion) across Russell Group universities and non-Russell Group universities, alongside the allocations for Connected Everything I (total = £660 k) and Connected Everything II (total = £540 k)
Dominance of successful applications from men
The percentage point difference between the award rates of researchers who identified as female, those who declare a disability, or identified as ethnic minority applicants and carers and their respective counterparts have been plotted in Fig. 3 . Bars to the right of the axis mean that the award rate of the female/declared-disability/ethnic-minority/carer applicants is greater than that of male/non- disability/white/not carer applicants.
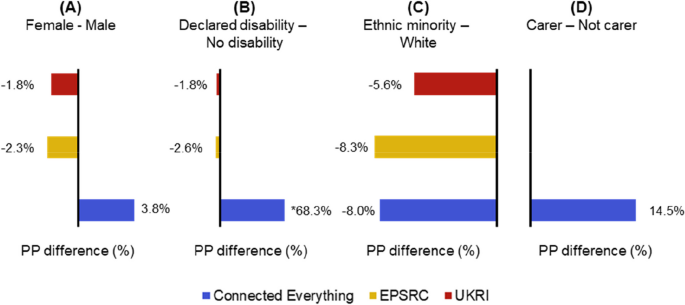
Percentage point (PP) differences in award rate by funding provider for gender, disability status, ethnicity and care responsibilities (data not collected by UKRI and EPSRC [ 35 ]). The total number of applicants for each funder are as follows: Connected Everything = 146, EPSRC = 37,960, and UKRI = 140,135. *The numbers of applicants were too small (< 5) to enable a meaningful discussion
Figure 3 (A) shows that between 2015 and 2021 research team applicants who identified as male had a higher award rate than those who identified as female when applying for EPSRC and wider UKRI research council funding. Connected Everything funding applicants who identified as female achieved a higher award rate (19.4%, 95% CI [6.5%, 32.4%] out of 146) compared to male applicants (15.6%, 95% CI [8.8%, 22.4%] out of 146). These data suggest that biases have been reduced by the Connected Everything review process and other mitigation strategies (e.g., visible gender diversity in panel pitch members and publishing CE principal and goals to demonstrate commitment to equality and fairness). This finding aligns with an earlier study that found gender bias during the peer review process, resulting in female investigators receiving less favourable evaluations than their male counterparts [ 15 ].
Over-representation of people identifying as male in engineering and technology academic community
Figure 4 shows the response to the gender question, with 24.2%, 95% CI [19.0%, 29.4%] of 260 responses identifying as female. This aligns with the average for the engineering and technology cluster (21.4%, 95% CI [20.9%, 21.9%] female of 27,740 academic staff), which includes subject areas representative of our main funder, EPSRC [ 22 ]. We also sought to understand the representation of transgender researchers within the network. However, following the rounding policy outlined by UK Government statistics policies and procedures [ 39 ], the number of responses that identified as a different sex to birth was too low (< 5) to enable a meaningful discussion.
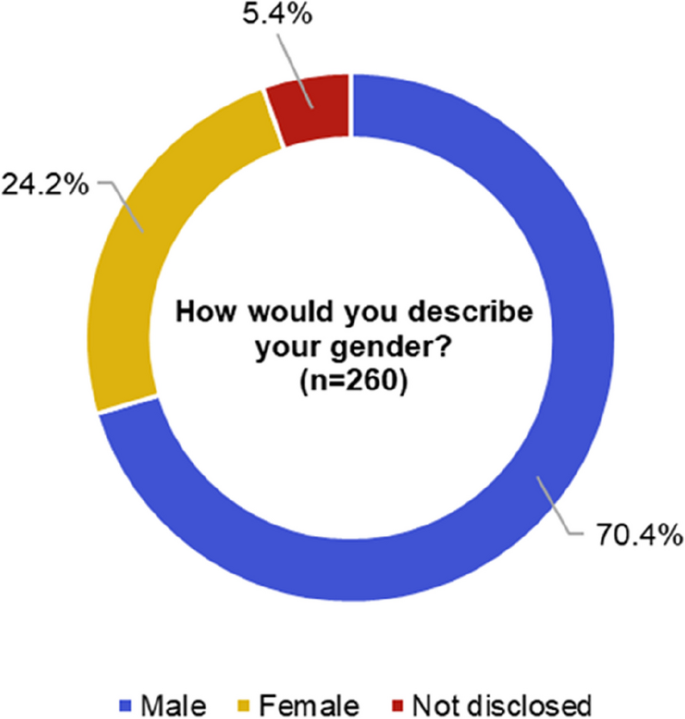
Gender question responses from a total of 260 respondents
Dominance of successful applications from white academics
Figure 3 (C) shows that researchers with a minority ethnicity consistently have a lower award rate than white researchers when applying for EPSRC and UKRI funding. Similarly, the results in Fig. 3 (C) indicate that white researchers are more successful (8.0% percentage point, 95% CI [-8.6%, 24.6%]) when applying for Connected Everything funding. These results indicate that more measures should be implemented to support the ethnic minority researchers applying for Connected Everything funding, as well as sense checking there is no unconscious bias in any of the Connected Everything funding processes. The breakdown of the ethnicity diversity of applicants at different stages of the Connected Everything review process (i.e. all applications, applicants invited to panel pitch and awarded feasibility studies) has been plotted in Fig. 5 to help identify where more support is needed. Figure 5 shows an increase in the proportion of white researchers from 54%, 95% CI [45.4%, 61.8%] of all 146 applicants to 66%, 95% CI [52.8%, 79.1%] of the 50 researchers invited to the panel pitch. This suggests that stage 1 of the Connected Everything review process (anonymous review of written applications) may favour white applicants and/or introduce unconscious bias into the process.
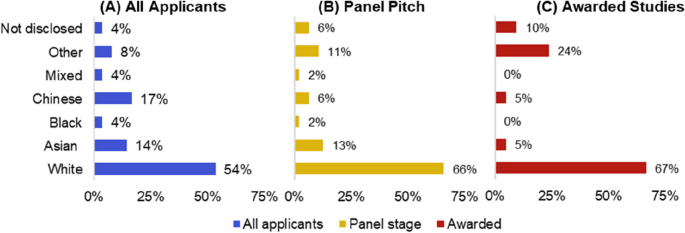
Ethnicity questions responses from different stages during the Connected Everything anonymous review process. The total number of applicants is 146, with 50 at the panel stage and 23 ultimately awarded
Under-representation of those from black or minority ethnic backgrounds
Connected Everything appears to have a wide range of ethnic diversity, as shown in Fig. 6 . The ethnicities Asian (18.3%, 95% CI [13.6%, 23.0%]), Black (5.1%, 95% CI [2.4%, 7.7%]), Chinese (12.5%, 95% CI [8.4%, 16.5%]), mixed (3.5%, 95% CI [1.3%, 5.7%]) and other (7.8%, 95% CI [4.5%, 11.1%]) have a higher representation among the 260 individuals engaging with network’s activities, in contrast to both the engineering and technology academic community and the wider UK population. When separating these groups into the original ethnic diversity answers, it becomes apparent that there is no engagement with ‘Black or Black British: Caribbean’, ‘Mixed: White and Black Caribbean’ or ‘Mixed: White and Asian’ researchers within Connected Everything activities. The lack of engagement with researchers from a Caribbean heritage is systemic of a lack of representation within the UK research landscape [ 25 ].
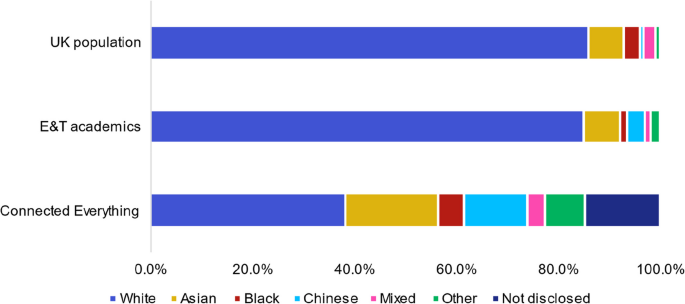
Ethnicity question responses from a total of 260 respondents compared to distribution of the 13,085 UK engineering and technology (E&T) academic staff [ 22 ] and 56 million people recorded in the UK 2011 census data [ 36 ]
Under-representation of disabilities, chronic conditions, invisible illnesses and neurodiversity in funded activities and events.
Figure 7 (A) shows that 5.7%, 95% CI [2.4%, 8.9%] of 194 responses declared a disability. This is higher than the average of engineering and technology academics that identify as disabled (3.4%, 95% CI [3.2%, 3.7%] of 27,730 academics). Between Jan-March 2022, 9.0 million people of working age (16–64) within the UK were identified as disabled by the Office for National Statistics [ 40 ], which is 21% of the working age population [ 27 ]. Considering these statistics, there is a stark under-representation of disabilities, chronic conditions, invisible illnesses and neurodiversity amongst engineering and technology academic staff and those engaging in Connected Everything activities.
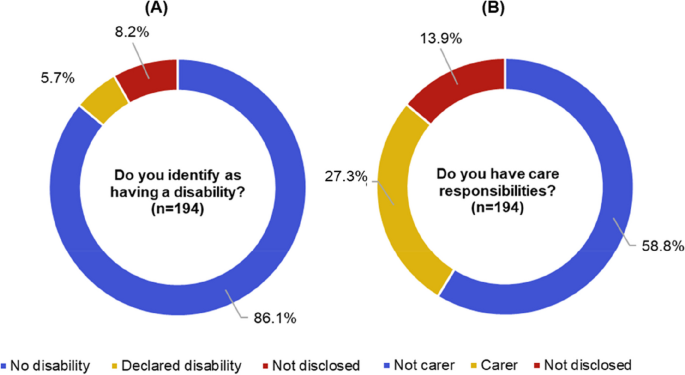
Responses to A Disability and B Care responsibilities questions colected from a total of 194 respondents
Between 2015 and 2020 academics that declared a disability have been less successful than academics without a disability in attracting UKRI and EPSRC funding, as shown in Fig. 3 (B). While Fig. 3 (B) shows that those who declare a disability have a higher Connected Everything funding award rate, the number of applicants who declared a disability was too small (< 5) to enable a meaningful discussion regarding this result.
Under-representation of those with care responsibilities in funded activities and events
In response to the care responsibilities question, Fig. 7 (B) shows that 27.3%, 95% CI [21.1%, 33.6%] of 194 respondents identified as carers, which is higher than the 6% of adults estimated to be providing informal care across the UK in a UK Government survey of the 2020/2021 financial year [ 41 ]. However, the ‘informal care’ definition used by the 2021 survey includes unpaid care to a friend or family member needing support, perhaps due to illness, older age, disability, a mental health condition or addiction [ 41 ]. The Connected Everything survey included care responsibilities across the spectrum of care that includes partners, children, other relatives, pets, friends and kin. It is important to consider a wide spectrum of care responsibilities, as key academic events, such as conferences, have previously been demonstrably exclusionary sites for academics with care responsibilities [ 42 ]. Breakdown analysis of the responses to care responsibilities by gender in Fig. 8 reveals that 37.8%, 95% CI [25.3%, 50.3%] of 58 women respondents reported care responsibilities, compared to 22.6%, 95% CI [61.1%, 76.7%] of 136 men respondents. Our findings reinforce similar studies that conclude the burden of care falls disproportionately on female academics [ 43 ].
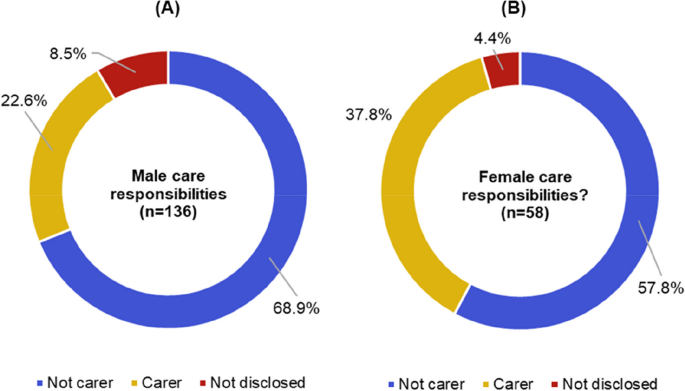
Responses to care responsibilities when grouped by A 136 males and B 58 females
Figure 3 (D) shows that researchers with careering responsibilities applying for Connected Everything funding have a higher award rate than those researchers applying without care responsibilities. These results suggest that the Connected Everything review process is supportive of researchers with care responsibilities, who have faced barriers in other areas of academia.
Reduced opportunities for ECRs
Early-career researchers (ECRs) represent the transition stage between starting a PhD and senior academic positions. EPSRC defines an ECR as someone who is either within eight years of their PhD award, or equivalent professional training or within six years of their first academic appointment [ 44 ]. These periods exclude any career break, for example, due to family care; health reasons; and reasons related to COVID-19 such as home schooling or increased teaching load. The median age for starting a PhD in the UK is 24 to 25, while PhDs usually last between three and four years [ 45 ]. Therefore, these data would imply that the EPSRC median age of ECRs is between 27 and 37 years. It should be noted, however, that this definition is not ideal and excludes ECRs who may have started their research career later in life.
Connected Everything aims to support ECRs via measures that include mentoring support, workshops, summer schools and podcasts. Figure 9 shows a greater representation of researchers engaging with Connected Everything activities that are aged between 30–44 (62.4%, 95% CI [55.6%, 69.2%] of 194 respondents) when compared to the wider engineering and technology academic community (43.7%, 95% CI [43.1%, 44.3%] of 27,780 academics) and UK population (26.9%, 95% CI [26.9%, 26.9%]).
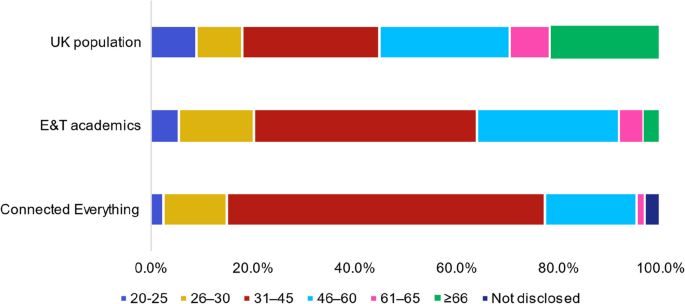
Age question responses from a total of 194 respondents compared to distribution of the 27,780 UK engineering and technology (E&T) academic staff [ 22 ] and 56 million people recorded in the UK 2011 census data [ 36 ]
High competition for funding has a greater impact on ECRs
Figure 10 shows that the largest age bracket applying for and winning Connected Everything funding is 31–45, whereas 72%, CI 95% [70.1%, 74.5%] of 12,075 researchers awarded EPSRC grants between 2015 and 2021 were 40 years or older. These results suggest that measures introduced by Connected Everything has been successful at providing funding opportunities for researchers who are likely to be early-mid career stage.
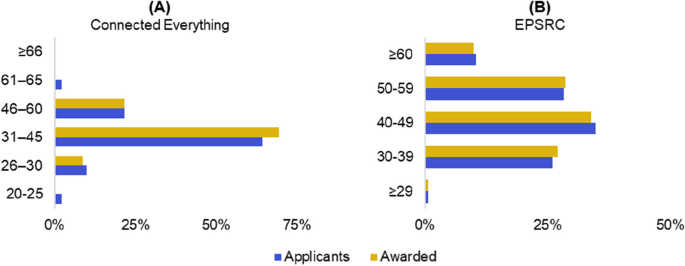
Age of researchers at applicant and awarded funding stages for A Connected Everything between 2019–2022 (total of 146 applicants and 23 awarded) and B EPSRC funding between 2015–2021 [ 35 ] (total of 35,780 applicants and 12,075 awarded)
The results of this paper provide insights into the impact that Connected Everything’s planned mitigations have had on promoting equality, diversity, and inclusion (EDI) in research and funding. Collecting EDI data from individuals who engage with network activities and apply for research funding enabled an evaluation of whether these mitigations have been successful in achieving the intended outcomes outlined at the start of the study, as summarised in Table 2 .
The results in Table 2 indicate that Connected Everything’s approach to EDI has helped achieve the intended outcome to improve representation of women, ECRs, those with a declared disability and black/minority ethnic backgrounds engaging with network events when compared to the engineering and technology academic community. In addition, the network has helped raise awareness of the high presence of researchers with care responsibilities at network events, which can help to track progress towards making future events inclusive and accessible towards these carers. The data highlights two areas for improvement: (1) ensuring a gender balance; and (2) increasing representation of those with declared disabilities. Both these discrepancies are indicative of the wider imbalances and underrepresentation of these groups in the engineering and technology academic community [ 26 ], yet represent areas where networks can strive to make a difference. Possible strategies include: using targeted outreach; promoting greater representation of these groups in event speakers; and going further to create a welcoming and inclusive environment. One barrier that can disproportionately affect women researchers is the need to balance care responsibilities with attending network events [ 46 ]. This was reflected in the Connected Everything data that reported 37.8%, 95% CI [25.3%, 50.3%] of women engaging with network activities had care responsibilities, compared to 22.6%, 95% CI [61.1%, 76.7%] of men. Providing accommodations such as on-site childcare, flexible scheduling, or virtual attendance options can therefore help to promote inclusivity and allow more women researchers to attend.
Only 5.7%, 95% CI [2.4%, 8.9%] of responses engaging with Connected Everything declared a disability, which is higher than the engineering and technology academic community (3.4%, 95% CI [3.2%, 3.7%]) [ 26 ], but unrepresentative of the wider UK population. It has been suggested that academics can be uncomfortable when declaring disabilities because scholarly contributions and institutional citizenship are so prized that they feel they cannot be honest about their issues or health concerns and keep them secret [ 47 ]. In research networks, it is important to be mindful of this hidden group within higher education and ensure that measures are put in place to make the network’s activities inclusive to all. Future considerations for accommodations to improve research events inclusivity include: improving physical accessibility of events; providing assistive technology such as screen readers, audio descriptions, and captioning can help individuals with visual or hearing impairments to access and participate; providing sign language interpreters; offering flexible scheduling options; and the provision of quiet rooms, written materials in accessible formats, and support staff trained to work with individuals with cognitive disabilities.
Connected Everything introduced measures (e.g., anonymised reviewing process, Q&A sessions before funding calls, inclusive design of panel pitch) to help address inequalities in how funding is awarded. Table 2 shows success in reducing the dominance of researchers who identify as male and research-intensive universities in winning research funding and that researchers with care responsibilities were more successful at winning funding than those without care responsibilities. The data revealed that the proposed measures were unable to address the inequality in award rates between white and ethnic minority researchers, which is an area to look to improve. The inequality appears to occur during the anonymous review stage, with a greater proportion of white researchers being invited to panel. Recommendations to make the review process fairer include: ensuring greater diversity of reviewers; reviewer anti-bias training; and automatic adjustments to correct for known biases in writing style [ 16 , 32 ].
When reflecting on the development of a strategy to embed EDI throughout the network, Connected Everything has learned several key lessons that may benefit other networks undergoing a similar activity. These include:
EDI is never ‘done’: There is a constant need to review approaches to EDI to ensure they remain relevant to the network community. Connected Everything could review its principles to include the concept of justice in its approach to diversity and inclusion. The concept of justice concerning EDI refers to the removal of systematic barriers that stop fair and equitable distribution of resources and opportunities among all members of society, regardless of their individual characteristics or backgrounds. The principles and subsequent actions could be reviewed against the EDI expectations [ 14 ], paying particular attention to areas where barriers may still be present. For example, shifting from welcoming people into existing structures and culture to creating new structures and culture together, with specific emphasis on decision or advisory mechanisms within the network. This activity could lend itself to focusing more on tailored support to overcome barriers, thus achieving equity, if it is not within the control of the network to remove the barrier itself (justice).
Widen diversity categories: By collecting data on a broad range of characteristics, we can identify and address disparities and biases that might otherwise be overlooked. A weakness of this dataset is that ignores the experience of those with intersectional identities, across race, ethnicity, gender, class, disability and/ or LGBTQI. The Wellcome Trust noted how little was known about the socio-economic background of scientists and researchers [ 48 ].
Collect data on whole research teams: For the first two calls for feasibility study funding, Connected Everything only asked the Principal Investigator to voluntarily provide their data. We realised that this was a limited approach and, in the third call, asked for the data regarding the whole research team to be shared anonymously. Furthermore, we do not currently measure the diversity of our event speakers, panellists or reviewers. Collecting these data in the future will help to ensure the network is accountable and will ensure that all groups are represented during our activities and in the funding decision-making process.
High response rate: Previous surveys measuring network diversity (e.g., [ 7 ]) have struggled to get responses when surveying their memberships; whereas, this study achieved a response rate of 73.8%. We attribute this high response rate to sending EDI data requests on the point of contact with the network (e.g., on submitting funding proposals or after attending network events), rather than trying to survey the entire network membership at anyone point in time.
Improve administration: The administration associated with collecting EDI data requires a commitment to transparency, inclusivity, and continuous improvement. For example, during the first feasibility funding call, Connected Everything made it clear that the review process would be anonymous, but the application form was not in separate documents. This made anonymising the application forms extremely time-consuming. For the subsequent calls, separate documents were created – Part A for identifying information (Principal Investigator contact details, Project Team and Industry collaborators) and Part B for the research idea.
Accepting that this can be uncomfortable: Trying to improve EDI can be uncomfortable because it often requires challenging our assumptions, biases, and existing systems and structures. However, it is essential if we want to make real progress towards equity and inclusivity. Creating processes to support embedding EDI takes time and Connected Everything has found it is rare to get it right the first time. Connected Everything is sharing its learning as widely as possible both to support others in their approaches and continue our learning as we reflect on how to continually improve, even when it is challenging.
Enabling individual engagement with EDI: During this work, Connected Everything recognised that methods for engaging with such EDI issues in research design and delivery are lacking. Connected Everything, with support from the Future Food Beacon of Excellence at the University of Nottingham, set out to develop a card-based tool [ 49 ] to help researchers and stakeholders identify questions around how their work may promote equity and increase inclusion or have a negative impact towards one or more protected groups and how this can be overcome. The results of this have been shared at conference presentations [ 50 ] and will be published later.
While this study provides insights into how EDI can be improved in research network activities and funding processes, it is essential to acknowledge several limitations that may impact the interpretation of the findings.
Sample size and generalisability: A total of 260 responses were received, which may not be representative of our overall network of 500 + members. Nevertheless, this data provides a sense of the current diversity engaging in Connected Everything activities and funding opportunities, which we can compare with other available data to steer action to further diversify the network.
Handling of missing data: Out of the 260 responses, 66 data points were missing for questions regarding age, disability, and caring responsibilities. These questions were mistakenly omitted from a Connected Everything summer school survey, contributing to 62 missing data points. While we assumed the remainer of missing data to be at random during analysis, it's important to acknowledge it could be related to other factors, potentially introducing bias into our results.
Emphasis on quantitative data: The study relies on using quantitative data to evaluate the impact of the EDI measures introduced by Connected Everything. However, relying solely on quantitative metrics may overlook nuanced aspects of EDI that cannot be easily quantified. For example, EDI encompasses multifaceted issues influenced by historical, cultural, and contextual factors. These nuances may not be fully captured by numbers alone. In addition, some EDI efforts may not yield immediate measurable outcomes but still contribute to a more inclusive environment.
Diversity and inclusion are not synonymous: The study proposes 21 measures to contribute towards creating an equal, diverse and inclusive research culture and collects diversity data to measure the impact of these measures. However, while diversity is simpler to monitor, increasing diversity alone does not guarantee equality or inclusion. Even with diverse research groups, individuals from underrepresented groups may still face barriers, microaggressions, or exclusion.
Balancing anonymity and rigour in grant reviews:The proposed anonymous review process proposed by Connected Everything removes personal and organisational details from the research ideas under reviewer evaluation. However, there exists a possibility that a reviewer could discern the identity of the grant applicant based on the research idea. Reviewers are expected to be subject matter experts in the field relevant to the grant proposal they are evaluating. Given the specialised nature of scientific research, it is conceivable that a well-known applicant could be identified through the specifics of the work, the methodologies employed, and even the writing style.
Expanding gender identity options: A limitation of this study emerged from the restricted gender options (male, female, other, prefer not to say) provided to respondents when answering the gender identity question. This limitation reflects the context of data collection in 2018, a time when diversity monitoring guidance was still limited. As our understanding of gender identity evolves beyond binary definitions, future data collection efforts should embrace a more expansive and inclusive approach, recognising the diverse spectrum of gender identities.
In conclusion, this study provides evidence of the effectiveness of a research network's approach to promoting equality, diversity, and inclusion (EDI) in research and funding. By collecting EDI data from individuals who engage with network activities and apply for research funding, this study has shown that the network's initiatives have had a positive impact on representation and fairness in the funding process. Specifically, the analysis reveals that the network is successful at engaging with ECRs, and those with care responsibilities and has a diverse range of ethnicities represented at Connected Everything events. Additionally, the network activities have a more equal gender balance and greater representation of researchers with disabilities when compared to the engineering and technology academic community, though there is still an underrepresentation of these groups compared to the national population.
Connected Everything introduced measures to help address inequalities in how funding is awarded. The measures introduced helped reduce the dominance of researchers who identified as male and research-intensive universities in winning research funding. Additionally, researchers with care responsibilities were more successful at winning funding than those without care responsibilities. However, inequality persisted with white researchers achieving higher award rates than those from ethnic minority backgrounds. Recommendations to make the review process fairer include: ensuring greater diversity of reviewers; reviewer anti-bias training; and automatic adjustments to correct for known biases in writing style.
Connected Everything’s approach to embedding EDI in network activities has already been shared widely with other EPSRC-funded networks and Hubs (e.g. the UKRI Circular Economy Hub and the UK Acoustics Network Plus). The network hopes that these findings will inform broader efforts to promote EDI in research and funding and that researchers, funders, and other stakeholders will be encouraged to adopt evidence-based strategies for advancing this important goal.
Availability of data and materials
The data collected was anonymously, however, it may be possible to identify an individual by combining specific records of the data request form data. Therefore, the study data has been presented in aggregate form to protect the confidential of individuals and the data utilised in this study cannot be made openly accessible due to ethical obligations to protect the privacy and confidentiality of the data providers.
Abbreviations
Early career researcher
Equality, diversity and inclusion
Engineering physical sciences research council
UK research and innovation
Xuan J, Ocone R. The equality, diversity and inclusion in energy and AI: call for actions. Energy AI. 2022;8:100152.
Article Google Scholar
Guyan K, Oloyede FD. Equality, diversity and inclusion in research and innovation: UK review. Advance HE; 2019. https://www.ukri.org/wp-content/uploads/2020/10/UKRI-020920-EDI-EvidenceReviewUK.pdf .
Cooke A, Kemeny T. Cities, immigrant diversity, and complex problem solving. Res Policy. 2017;46:1175–85.
AlShebli BK, Rahwan T, Woon WL. The preeminence of ethnic diversity in scientific collaboration. Nat Commun. 2018;9:5163.
Gagnon S, Augustin T, Cukier W. Interplay for change in equality, diversity and inclusion studies: Hum Relations. Epub ahead of print 23 April 2021. https://doi.org/10.1177/00187267211002239 .
Everything C. https://connectedeverything.ac.uk/ . Accessed 27 Feb (2023).
Chandler-Wilde S, Kanza S, Fisher O, Fearnshaw D, Jones E. Reflections on an EDI Survey of UK-Government-Funded Research Networks in the UK. In: The 51st International Congress and Exposition on Noise Control Engineering. St. Albans: Institute of Acoustics; 2022. p. 9.0–940.
Google Scholar
Prathivadi Bhayankaram K, Prathivadi Bhayankaram N. Conference panels: do they reflect the diversity of the NHS workforce? BMJ Lead 2022;6:57 LP – 59.
Goodman SW, Pepinsky TB. Gender representation and strategies for panel diversity: Lessons from the APSA Annual Meeting. PS Polit Sci Polit 2019;52:669–676.
Olsen J, Griffiths M, Soorenian A, et al. Reporting from the margins: disabled academics reflections on higher education. Scand J Disabil Res. 2020;22:265–74.
Baldie D, Dickson CAW, Sixsmith J. Building an Inclusive Research Culture. In: Knowledge, Innovation, and Impact. 2021, pp. 149–157.
Sato S, Gygax PM, Randall J, et al. The leaky pipeline in research grant peer review and funding decisions: challenges and future directions. High Educ 2020 821. 2020;82:145–62.
Recio-Saucedo A, Crane K, Meadmore K, et al. What works for peer review and decision-making in research funding: a realist synthesis. Res Integr Peer Rev. 2022;2022 71:7: 1–28.
EPSRC. Expectations for equality, diversity and inclusion – UKRI, https://www.ukri.org/about-us/epsrc/our-policies-and-standards/equality-diversity-and-inclusion/expectations-for-equality-diversity-and-inclusion/ (2022, Accessed 26 Apr 2022).
Witteman HO, Hendricks M, Straus S, et al. Are gender gaps due to evaluations of the applicant or the science? A natural experiment at a national funding agency. Lancet. 2019;393:531–40.
Li YL, Bretscher H, Oliver R, et al. Racism, equity and inclusion in research funding. Sci Parliam. 2020;76:17–9.
UKRI publishes latest diversity. data for research funding – UKRI, https://www.ukri.org/news/ukri-publishes-latest-diversity-data-for-research-funding/ (Accessed 28 July 2022).
Higher Education Statistics Agency. What do HE students study? https://www.hesa.ac.uk/data-and-analysis/students/what-study (2023, Accessed 25 March 2023).
UKRI. Competitive funding decisions, https://www.ukri.org/what-we-offer/what-we-have-funded/competitive-funding-decisions / (2023, Accessed 2 April 2023).
Santos G, Van Phu SD. Gender and academic rank in the UK. Sustain. 2019;11:3171.
Jebsen JM, Nicoll Baines K, Oliver RA, et al. Dismantling barriers faced by women in STEM. Nat Chem. 2022;14:1203–6.
Advance HE. Equality in higher education: staff statistical report 2021 | Advance HE, https://www.advance-he.ac.uk/knowledge-hub/equality-higher-education-statistical-report-2021 (28 October 2021, Accessed 26 April 2022).
EngineeringUK. Engineering in Higher Education, https://www.engineeringuk.com/media/318874/engineering-in-higher-education_report_engineeringuk_march23_fv.pdf (2023, Accessed 25 March 2023).
Bhopal K. Academics of colour in elite universities in the UK and the USA: the ‘unspoken system of exclusion’. Stud High Educ. 2022;47:2127–37.
Williams P, Bath S, Arday J et al. The Broken Pieline: Barriers to Black PhD Students Accessing Research Council Funding . 2019.
HESA. Who’s working in HE? Personal characteristics, https://www.hesa.ac.uk/data-and-analysis/staff/working-in-he/characteristics (2023, Accessed 1 April 2023).
Office for National Statistics. Principal projection - UK population in age groups, https://www.ons.gov.uk/peoplepopulationandcommunity/populationandmigration/populationprojections/datasets/tablea21principalprojectionukpopulationinagegroups (2022, Accessed 3 August 2022).
HESA. Who’s studying in HE? Personal characteristics, https://www.hesa.ac.uk/data-and-analysis/students/whos-in-he/characteristics (2023, Accessed 1 April 2023).
Herman E, Nicholas D, Watkinson A et al. The impact of the pandemic on early career researchers: what we already know from the internationally published literature. Prof la Inf ; 30. Epub ahead of print 11 March 2021. https://doi.org/10.3145/epi.2021.mar.08 .
Moreau M-P, Robertson M. ‘Care-free at the top’? Exploring the experiences of senior academic staff who are caregivers, https://srhe.ac.uk/wp-content/uploads/2020/03/Moreau-Robertson-SRHE-Research-Report.pdf (2019).
Shillington AM, Gehlert S, Nurius PS, et al. COVID-19 and long-term impacts on tenure-line careers. J Soc Social Work Res. 2020;11:499–507.
de Winde CM, Sarabipour S, Carignano H et al. Towards inclusive funding practices for early career researchers. J Sci Policy Gov; 18. Epub ahead of print 24 March 2021. https://doi.org/10.38126/JSPG180105 .
Trust W. Grant funding data report 2018/19, https://wellcome.org/sites/default/files/grant-funding-data-2018-2019.pdf (2020).
Vallée-Tourangeau G, Wheelock A, Vandrevala T, et al. Peer reviewers’ dilemmas: a qualitative exploration of decisional conflict in the evaluation of grant applications in the medical humanities and social sciences. Humanit Soc Sci Commun. 2022;2022 91:9: 1–11.
Diversity data – UKRI. https://www.ukri.org/what-we-offer/supporting-healthy-research-and-innovation-culture/equality-diversity-and-inclusion/diversity-data/ (accessed 30 September 2022).
2011 Census - Office for National Statistics. https://www.ons.gov.uk/census/2011census (Accessed 2 August 2022).
Cost centres. (2012/13 onwards) | HESA, https://www.hesa.ac.uk/support/documentation/cost-centres/2012-13-onwards (Accessed 28 July 2022).
Viner N, Powell P, Green R. Institutionalized biases in the award of research grants: a preliminary analysis revisiting the principle of accumulative advantage. Res Policy. 2004;33:443–54.
ofqual. Rounding policy - GOV.UK, https://www.gov.uk/government/publications/ofquals-statistics-policies-and-procedures/rounding-policy (2023, Accessed 2 April 2023).
Office for National Statistics. Labour market status of disabled people, https://www.ons.gov.uk/employmentandlabourmarket/peopleinwork/employmentandemployeetypes/datasets/labourmarketstatusofdisabledpeoplea08 (2022, Accessed 3 August 2022).
Family Resources Survey. financial year 2020 to 2021 - GOV.UK, https://www.gov.uk/government/statistics/family-resources-survey-financial-year-2020-to-2021 (Accessed 10 Aug 2022).
Henderson E. Academics in two places at once: (not) managing caring responsibilities at conferences. 2018, p. 218.
Jolly S, Griffith KA, DeCastro R, et al. Gender differences in time spent on parenting and domestic responsibilities by high-achieving young physician-researchers. Ann Intern Med. 2014;160:344–53.
UKRI. Early career researchers, https://www.ukri.org/what-we-offer/developing-people-and-skills/esrc/early-career-researchers/ (2022, Accessed 2 April 2023).
Cornell B. PhD Life: The UK student experience , www.hepi.ac.uk (2019, Accessed 2 April 2023).
Kibbe MR, Kapadia MR. Underrepresentation of women at academic medical conferences—manels must stop. JAMA Netw Open 2020; 3:e2018676–e2018676.
Brown N, Leigh J. Ableism in academia: where are the disabled and ill academics? 2018; 33: 985–989. https://doi.org/10.1080/0968759920181455627
Bridge Group. Diversity in Grant Awarding and Recruitment at Wellcome Summary Report. 2017.
Peter Craigon O, Fisher D, Fearnshaw et al. VERSION 1 - The Equality Diversity and Inclusion cards. Epub ahead of print 2022. https://doi.org/10.6084/m9.figshare.21222212.v3 .
Connected Everything II. EDI ideation cards for research - YouTube, https://www.youtube.com/watch?v=GdJjL6AaBbc&ab_channel=ConnectedEverythingII (2022, Accessed 7 June 2023).
Download references
Acknowledgements
The authors would like to acknowledge the support Engineering and Physical Sciences Research Council (EPSRC) [grant number EP/S036113/1], Connected Everything II: Accelerating Digital Manufacturing Research Collaboration and Innovation. The authors would also like to gratefully acknowledge the Connected Everything Executive Group for their contribution towards developing Connected Everything’s equality, diversity and inclusion strategy.
This work was supported by the Engineering and Physical Sciences Research Council (EPSRC) [grant number EP/S036113/1].
Author information
Authors and affiliations.
Food, Water, Waste Research Group, Faculty of Engineering, University of Nottingham, University Park, Nottingham, UK
Oliver J. Fisher
Human Factors Research Group, Faculty of Engineering, University of Nottingham, University Park, Nottingham, UK
Debra Fearnshaw & Sarah Sharples
School of Food Science and Nutrition, University of Leeds, Leeds, UK
Nicholas J. Watson
School of Engineering, University of Liverpool, Liverpool, UK
Peter Green
Centre for Circular Economy, University of Exeter, Exeter, UK
Fiona Charnley
Institute for Manufacturing, University of Cambridge, Cambridge, UK
Duncan McFarlane
You can also search for this author in PubMed Google Scholar
Contributions
OJF analysed and interpreted the data, and was the lead author in writing and revising the manuscript. DF led the data acquisition and supported the interpretation of the data. DF was also a major contributor to the design of the equality diversity and inclusion (EDI) strategy proposed in this work. NJW supported the design of the EDI strategy and was a major contributor in reviewing and revising the manuscript. PG supported the design of the EDI strategy, and was a major contributor in reviewing and revising the manuscript. FC supported the design of the EDI strategy and the interpretation of the data. DM supported the design of the EDI strategy. SS led the development EDI strategy proposed in this work, and was a major contributor in data interpretation and reviewing and revising the manuscript. All authors read and approved the final manuscript.
Corresponding author
Correspondence to Debra Fearnshaw .
Ethics declarations
Ethics approval and consent to participate.
Research was considered exempt from requiring ethical approval as is uses completely anonymous surveys results that are routinely collected as part of the administration of the network plus and informed consent was obtained at the time of original data collection.
Consent for publication
Not applicable.
Competing interests
The authors declare that they have no competing interests.
Additional information
Publisher’s note.
Springer Nature remains neutral with regard to jurisdictional claims in published maps and institutional affiliations.
Supplementary Information
Supplementary material 1., rights and permissions.
Open Access This article is licensed under a Creative Commons Attribution 4.0 International License, which permits use, sharing, adaptation, distribution and reproduction in any medium or format, as long as you give appropriate credit to the original author(s) and the source, provide a link to the Creative Commons licence, and indicate if changes were made. The images or other third party material in this article are included in the article's Creative Commons licence, unless indicated otherwise in a credit line to the material. If material is not included in the article's Creative Commons licence and your intended use is not permitted by statutory regulation or exceeds the permitted use, you will need to obtain permission directly from the copyright holder. To view a copy of this licence, visit http://creativecommons.org/licenses/by/4.0/ . The Creative Commons Public Domain Dedication waiver ( http://creativecommons.org/publicdomain/zero/1.0/ ) applies to the data made available in this article, unless otherwise stated in a credit line to the data.
Reprints and permissions
About this article
Cite this article.
Fisher, O.J., Fearnshaw, D., Watson, N.J. et al. Promoting equality, diversity and inclusion in research and funding: reflections from a digital manufacturing research network. Res Integr Peer Rev 9 , 5 (2024). https://doi.org/10.1186/s41073-024-00144-w
Download citation
Received : 12 October 2023
Accepted : 09 April 2024
Published : 16 May 2024
DOI : https://doi.org/10.1186/s41073-024-00144-w
Share this article
Anyone you share the following link with will be able to read this content:
Sorry, a shareable link is not currently available for this article.
Provided by the Springer Nature SharedIt content-sharing initiative
- Research integrity
- Network policy
- Funding reviewing
- EDI interventions
Research Integrity and Peer Review
ISSN: 2058-8615
- Submission enquiries: [email protected]
- General enquiries: [email protected]

An official website of the United States government
The .gov means it’s official. Federal government websites often end in .gov or .mil. Before sharing sensitive information, make sure you’re on a federal government site.
The site is secure. The https:// ensures that you are connecting to the official website and that any information you provide is encrypted and transmitted securely.
- Publications
- Account settings
Preview improvements coming to the PMC website in October 2024. Learn More or Try it out now .
- Advanced Search
- Journal List
- Scientific Reports
- PMC10725886

Implementation of inclusion and exclusion criteria in clinical studies in OHDSI ATLAS software
Romina blasini.
1 Institute of Medical Informatics, Justus Liebig University, Giessen, Germany
Kornelia Marta Buchowicz
2 Faculty of Health Sciences, University of Applied Sciences, Giessen, Germany
Henning Schneider
Birgit samans, keywan sohrabi, associated data.
All data generated or analyzed during this study are included in this published article and its supplementary information files.
Clinical trials are essential parts of a medical study process, but studies are often cancelled due to a lack of participants. Clinical Trial Recruitment Support Systems are systems that help to increase the number of participants by seeking more suitable subjects. The software ATLAS (developed by Observational Health Data Sciences and Informatics) can support the launch of a clinical trial by building cohorts of patients who fulfill certain criteria. The correct use of medical classification systems aiming at clearly defined inclusion and exclusion criteria in the studies is an important pillar of this software. The aim of this investigation was to determine whether ATLAS can be used in a Clinical Trial Recruitment Support System to portray the eligibility criteria of clinical studies. Our analysis considered the number of criteria feasible for integration with ATLAS and identified its strengths and weaknesses. Additionally, we investigated whether nonrepresentable criteria were associated with the utilized terminology systems. We analyzed ATLAS using 223 objective eligibility criteria from 30 randomly selected trials conducted in the last 10 years. In the next step, we selected appropriate ICD, OPS, LOINC, or ATC codes to feed the software. We classified each criterion and study based on its implementation capability in the software, ensuring a clear and logical progression of information. Based on our observations, 51% of the analyzed inclusion criteria were fully implemented in ATLAS. Within our selected example set, 10% of the studies were classified as fully portrayable, and 73% were portrayed to some extent. Additionally, we conducted an evaluation of the software regarding its technical limitations and interaction with medical classification systems. To improve and expand the scope of criteria within a cohort definition in a practical setting, it is recommended to work closely with personnel involved in the study to define the criteria precisely and to carefully select terminology systems. The chosen criteria should be combined according to the specific setting. Additional work is needed to specify the significance and amount of the extracted criteria.
Introduction
The field of medicine is eternally linked to study goals to discover more about the human body in an effective and positively influential way. The ultimate aim of a medical study is always to improve patient care and therapeutic standards throughout clinical trials when applied. To observe the influence of new interventions and prevention strategies in terms of safety and efficiency, a certain number of participants are recruited according to some specified criteria. Such a recruitment process is always a challenging and time-consuming stage of the medical study pipeline, resulting in a large body of cancelled or incomplete studies 1 – 3 .
Eligibility criteria (EC) are established at the outset of each clinical trial to describe the shared characteristics of participating individuals. Patient cohorts are typically identified and recruited by clinical or primary care personnel who query clinical systems manually for eligible patients. This process is costly and time-intensive, constituting an important component of the clinical research study pipeline. Therefore, utilizing digital tools can substantially enhance subject recruitment and decrease associated expenses and necessary labor resources 4 .
Clinical Trial Recruitment Support Systems (CTRSSs), also known as patient recruitment systems (PRSs), can bolster patient inclusion in clinical trials by automatically analyzing eligibility criteria based on electronic health records 4 – 6 . Although these systems are currently increasingly integrated into many medical research projects, most study centers do not tend to use such digital tools for patient recruitment 7 .
The primary challenge is the need for technical staff and equipment to execute implementation and supportive tasks for the CTRSS. One possible solution is to deploy a dedicated system that functions across multiple platforms or to incorporate the CTRSS into existing research systems. In addition to the existing CTRSS or commercial solutions developed as part of different projects, several open-source tools, such as the Observational Medical Outcomes Partnership Common Data Model (OMOP CDM) developed by Observational Health Data Sciences and Informatics (OHDSI) or Informatics for Integrating Biology and the Beside (i2b2) established by the National Institutes of Health (NIH), are used in multisite research projects. Both systems are based on the idea of using standard terminologies within data repositories; however, they are not limited to a particular one. In addition, data can be extracted and harmonized from different sources, so they may be utilized in different medical contexts and locations. On the user interface of these systems, it is possible to define criteria, which are used to filter the data and therefore search for certain patient cohorts 8 , 9 .
In a previous work by Reinecke et al., they described an architecture for a CTRSS that technically integrates OMOP CDM as the data basis and ATLAS as a graphical user interface for formalizing eligibility criteria 10 , 11 .
Observational health data sciences and informatics (OHDSI)
The OMOP CDM is part of a whole tool suite created and maintained by OHDSI as a collaboration among 150 organizations worldwide that collect and process health care data. OHDSI offers a wide range of open-source tools to support various data analytics use cases on observational patient-level data, all of which interact with databases using the Common Data Model 8 , 12 .
Inspired by OHDSI, developers created the open-source web-based software application known as ATLAS. Its primary function is to serve as a user interface for the OMOP CDM and to facilitate observational analysis by extracting patient data from clinical practice for generating real-world evidence. The software enables the definition of cohorts, selection of analysis configurations, and application of appropriate codes to tag diseases. Additionally, it allows for easy project sharing among researchers 8 , 10 .
ATLAS can also be used for subject recruitment by formalizing the eligibility criteria of a trial into the corresponding cohort 10 . The definition of trials in ATLAS works with two main designs: cohorts and concepts. Concepts are individual codes that belong to a terminology system integrated in the OMOP CDM. They can be grouped into concept sets. A concept set contains several concepts from the standardized vocabulary in combination with logical indicators. The set allows the user to specify related concepts that should be included or excluded in the vocabulary hierarchy. Cohorts are a collection of individuals searched for by a particular query based on specific criteria, so all of the trial eligibility criteria are part of the cohort definition 8 .
Terminology systems
The application of terminology systems forms an essential fundament when using digital tools because they are unambiguous compared to natural language, which minimizes the risk for misunderstandings or nonmatching subjects. A terminology system offers a comprehensive and standardized definition of terms utilized in a specific field and the associated linguistic expressions, including synonymous terms 13 , 14 . The OMOP Common Data Model (CDM) requires that data ingestion adhere to vocabularies, which are essentially terminology systems embedded within the OMOP CDM. Standard vocabularies, such as Logical Observation Identifiers Names and Codes (LOINC) or Systematized Nomenclature of Medicine Clinical Terms (SNOMED CT), are supported by default in OMOP CDM. Furthermore, it is feasible to incorporate additional nonstandard vocabularies. Usually, all codes of these nonstandard vocabularies are mapped to one or more codes of the standard vocabularies 8 , 15 , 16 .
Several analyses have been conducted to investigate the use of digital systems based on international terminologies 17 , 18 . For example, Chondrogiannis et al. investigated the use of a Clinical Data Interchange Standard Consortium (CDISC)-based model in conjunction with a patient-centered model to structure eligibility criteria for clinical studies. International classification systems, such as the International Classification of Diseases (ICD), formed the basis for this project. They found that the majority of the criteria could be represented in the system 19 .
When conducting digital searches for potential clinical trial participants in local hospitals, researchers use the patients' electronic health record (EHR) data that are already available. The success of this search depends on the terminology system utilized for documentation within the hospital staff's workflow.
Some studies have integrated eligibility criteria with OMOP CDM. Automated tools such as criteria2query implement natural language processing (NLP) to formalize eligibility criteria into ATLAS cohort definitions. The latter method is effective when working with standard OMOP vocabularies. Although more studies have been conducted on this issue, there remains a gap between theory and practical implementation of eligibility criteria using specific terminology systems in software 17 , 20 . Therefore, further analysis should be conducted regarding this matter to explore the hidden potentials behind the different software and coding systems. This study considered the possibility of applying the inclusion and exclusion criteria of different clinical trials in ATLAS as a proof of concept.
The objective of this study was to investigate the representation of eligibility criteria in ATLAS. Furthermore, this study aimed to identify the obstacles in the implementation of the aforementioned criteria. This also included the technical difficulties in the implementation process as well as the qualitative drawbacks. The secondary care data were connected to data available in the EHRs of the patients. Therefore, we decided to define the search parameters with the terminology systems that were already locally in use. We selected terminology systems that are widely used in Germany. First, the German modifications of the standard classification systems ICD, Tenth Revision (ICD-10-GM) and the International Classification of Procedures in Medicine (ICPM) named the Operation and Procedure Code (OPS) in Version 2021 were used. These systems are utilized for billing in all German hospitals and are therefore widely used. Additionally, the Anatomical Therapeutic Chemical classification (ATC) Version 2021 was selected for the medications, and LOINC Version 2.71 was selected as the coding system for laboratory results.
As a secondary objective, we wished to determine to what extent and which impact the selection of terminology systems had on the results. Therefore, we considered whether implementation would be possible with the help of SNOMED CT. We used SNOMED CT version 2021-01-31.
This research focused on classification systems and standards, as well as dealing with the mapping of inclusion and exclusion criteria generally and in ATLAS specifically. Therefore, this work might be interesting for experts involved in subject recruitment phases while applying digital solutions in this area.
Study design
To complement our research, we randomly chose 30 studies across various medical disciplines. To select these studies, we searched on the platform "ClinicalTrials.gov", one of the largest registries of clinical trials including approximately 425,817 studies from 222 different countries 21 . The selected studies had to have been started within the past 10 years, resulting in 114 studies, among which 30 studies were chosen randomly.
To assess the feasibility of implementing eligibility criteria in ATLAS, we compiled a table documenting 331 criteria from 30 studies. We then categorized these criteria based on Luo et al.'s semantic categories, excluding redundancies 22 .
Procedure in ATLAS
To start the criteria introduction procedure in ATLAS, we selected an appropriate terminology system with fitting codes for each eligibility criterion, as shown in Fig. 1 . The suitable codes were identified using official online catalogs 15 , 23 – 25 .

Procedure of portraying a trial in ATLAS software.
If no appropriate codes were found in these catalogs, SNOMED CT was used in the analysis to check for an alternative way to introduce or embed an approval criterion 16 .
In the next step, the eligibility criteria were implemented in ATLAS software. We used ATLAS Version 2.9.1 in combination with OMOP CDM 5.3.1. For this purpose, the corresponding vocabulary for the chosen terminology systems was utilized in the most recent version: ICD-10-GM, OPS, LOINC, ATC and SNOMED CT from the official Vocabulary Set of OHDSI 26 . In ATLAS, all fitting codes describing a single eligibility criterion are summarized in one concept set, which bundles one or more concepts. A selection criterion corresponds to a concept set, which consists of one or several codes covered by single concepts. This is necessary because a single criterion can be composed of one to hundreds of codes 27 . For example, type I diabetes mellitus can have different characteristics, which can be marked with different ICD codes. Codes for these disease individualities create one concept set. In comparison, hemophilia A could be created as a concept set using a single code, as there is only one ICD-GM-10 code for this specific disease (Fig. 2 ). If there was uncertainty regarding an eligibility criterion or related codes, medical personnel were consulted. Afterward, a separate concept set had to be created for each eligibility criterion, although no concept sets were created for demographic data such as age or sex. The aforementioned criteria were entered directly during a later process within a cohort by means of the corresponding functions available in ATLAS.

Comparison of concept sets between type I diabetes mellitus (left) and hemophilia A (right).
Once all the concept sets required for a study were established, cohorts that included all the inclusion and exclusion criteria for a study had to be defined. To outline a cohort, it was first necessary to define an initial event, which was a main criterion and was used for the preselection of subjects. All individuals who met the requirements of the initial event comprised the main cohort of patients. To set further constraints, it was possible to set other eligibility criteria to obtain more specified results. We selected the disease under study of the trial as an initial event for this analysis. This information can be found on ClinicalTrials.gov under “Conditions”. For example, if a study has the eligibility criteria of type I diabetes mellitus, age under 18 years and elevated body mass index (BMI), we would define diabetes mellitus as the initial event. All patients with type I diabetes compose the main cohort. The criteria “age” and “BMI” would be added as further eligibility criteria.
Categorization of eligibility criteria and studies
Of all 331 unique eligibility criteria, 108 criteria were excluded because of missing relevance. These criteria were those that have no relevance at this stage of recruitment and do not exist prior to a face-to-face interview with a study physician, such as the availability of a consent form. Only after identification of a patient who fulfils certain characteristics (such as the presence of a disease) using the electronic tool, a study physician interviews the potential subject regarding, for example, medical history, study procedure, language skills, and informed consent. For this reason, these criteria cannot be analyzed in the process of electronic patient screening.
To categorize the eligibility criteria, we analyzed the remaining 223 suitability criteria and classified them in terms of their implementation in ATLAS into the following categories: implementable, partially implementable, and not implementable (Table (Table1). 1 ). If a criterion were only partially or not implementable, we would also add short notes expressing our decision.
Eligibility criteria categories.
After weighting the studies with respect to their feasibility, four categories were established: fully portrayable, portrayable, partially portrayable, and not portrayable (see Table Table2 2 ).
Explanation of categories in the overall evaluation of the feasibility of measures taken in ATLAS.
Classification of partially/not implementable criteria
Finally, we performed a secondary analysis to discover the main difficulties in the introduction or implementation of eligibility criteria in the software ATLAS. Therefore, all reasons and arguments were considered to justify the categorization and the implementation issues of the eligibility criteria.
Quality assurance
This study was divided into three main steps, which were conducted by two authors with a background in medical informatics. This study began with a training phase, during which both individuals independently rated and categorized the same study in ATLAS. Afterward, they discussed their results until a consensus was reached. This step was repeated until both authors independently categorized the study without collusion. In the next step, one individual performed all mapping and categorizing for all trials. Any criteria that were partially or ambiguously depicted were discussed with the other individual. Furthermore, the second author randomly checked selected trials.
Ethical considerations
No ethical approval was needed.
The described categorization resulted in an implementation of 30 cohorts with one initial event per cohort and an additional 223 eligibility criteria. To implement all eligibility criteria and initial events, we built 129 different concept sets, including 11.20 concepts on average. Each cohort included an average of 5.33 concept sets with 59.73 concepts in total. The whole table of eligibility criteria and categorization can be found in Appendix 1 .
Evaluation of eligibility criteria
Of all 223 criteria, 35.19% (n = 82) were focused on a patient’s health status, 21.46% (n = 50) pertained to treatment or health care, 16.31% (n = 38) to diagnostic or laboratory tests, 10.73% (n = 25) to demographics, 0.43% (n = 1) to ethical considerations, and 3% (n = 7) addressed lifestyle choices. Additionally, 8.97% (n = 20) addressed more than one of the semantic categories and were therefore categorized as “Various”.
According to our observations, 51.12% (n = 114) of all eligibility criteria identified in the studies could be mapped in ATLAS and so were classified as implementable (see Table Table1). 1 ). This set corresponded with the eligibility criteria referring to clearly defined diseases, procedures, or drugs with the corresponding ICD, OPS, ATC or LOINC codes. Additionally, 21.11% (n = 47) of the criteria were defined as partially implementable, and 27.80% (n = 62) could not be implemented in ATLAS at all.
Evaluation of studies
We grouped the selected 30 studies after the ICD-10 chapters of their primary addressed health issue. This information was taken from their official trial description on ClinicalTrials.gov. The distribution of this grouping can be found in Table Table3 3 .
Distribution of the primary addressed health issues of the selected trials.
Based on our evaluation, in 10% of the studies (n = 3), all criteria could be fully mapped, so these studies were classified as fully portrayable (see Table Table2) 2 ) The latter set included a few simple and clearly defined criteria, such as a well-defined disease, forming a group of subjects which could be filtered by one or two additional criteria, like age or sex. In these cases, the disease was introduced into ATLAS using the appropriate codes and as an initial event.
Additionally, 73.33% of the studies (n = 22) were classified as portrayable, where at least one of the eligibility criteria was only partially or not implementable according to the rules in Table Table2. 2 . However, the initial event could be fully implemented as a concept set using ICD, OPS, LOINC or ATC. In 16.67% (n = 5) of studies, it was not possible to fully implement the initial event, and these were assigned to the partially portrayable category. Finally, none of the studies were classified as not portrayable.
Partially implementable criteria
After analyzing the justifications corresponding to the criteria that could not be introduced into ATLAS, we categorized them into four groups described in Table Table4 4 .
Categories of partially implementable criteria.
According to the results, 36.17% (n = 17) of the partially implementable criteria belonged to the associated class due to technical reasons, with different causes. For example, in some studies, age was indicated in years, but in others, such as pediatric studies dealing with infants, age was indicated in months. Some criteria also needed a cause-and-effect relationship. In such criteria, information regarding whether the patient is involved in cross-studies is required, which cannot be extracted from an OMOP data model.
Among the remaining criteria, 27.66% (n = 13) could not be fully implemented due to missing information. Those criteria mainly refer to “norm” or “stable” values even if there are no defined norm quantities valid in all countries or locations but different standards from site to site. The latter set includes criteria that refer to the groups of medicaments, diagnosis, procedures, or laboratory results, which are not globally defined.
A total of 17.02% (n = 8) of criteria were too specific, and no terminology system was found to have a specific corresponding code, except for the codes that also included a group of diseases/procedures/laboratory results/medications. Finally, 19.15% (n = 9) of eligible criteria were classified with SNOMED CT. All these results are shown in Fig. 3 .

Number of eligibility criteria and implementability with respect to the reasons for categorization.
Nonimplementable criteria
To categorize the criteria that were not implementable, we selected the same categories, without the category corresponding to the wrong terminology system. As shown in Fig. 3 , in 51.61% (n = 32) of the nonimplementable criteria, missing information was the problem. A total of 27.42% (n = 17) of the criteria were too specific, and 20.97% (n = 13) were not implementable due to technical reasons.
Semantic criteria categories
Figure 4 shows the portraiture of ATLAS in relation to the semantic criteria categories. The different semantic categories of the inclusion criteria can be portrayed to varying degrees in ATLAS. For instance, criteria under the category "Disease, Symptom, and Sign" can be portrayed up to 60% in ATLAS, while other categories are either not portrayable or to a lesser degree.

Eligibility criteria categorized with the semantic categories of Luo et al. 22 with their degree of implementability in ATLAS. The underlying data can be found in Appendix 2 .
Technical limitations of ATLAS
ATLAS software allows for the mapping of medical criteria using various options, ensuring flexibility. Nevertheless, the software's user interface is complex, making intuitive use of it challenging, even for experienced personnel. Before commencing research, an extensive familiarization phase is needed. Additionally, individual customizations are necessary to input intricate or nested study criteria. Scores are frequently not available as a standalone code. Analyzed scores generally comprise a number of subitems, such as diseases or laboratory values, with each being assigned a value based on the severity of its impact. As a result, each aspect of a score is initially treated as a distinct criterion that must be included in the system as separate concept sets, a significant undertaking in complex-scale studies.
ATLAS has the possibility to input basic demographic data in an easy way and without assigning a concept, but these data are limited to age and sex. Other basic information, such as height and weight, must be input similar to other observations. For this purpose, the appropriate LOINC code has to be identified, created as a concept set, and time constraints might have to be set when only the latest data on height and weight are searched. At this point, this information can be integrated into the cohort.
Additionally, temporal information, such as the starting point of an event or a treatment, can only be introduced as an absolute date or relative to the time of the initial event, not as relative time information such as “six months ago”. Therefore, the selection criteria for a cohort regarding the period must be updated on a daily basis.
The utilized terminology systems
Since ATLAS software is based on the involvement of terminology systems, this analysis highlights the importance of the choice of terminology systems and thus the availability of codes contained in the terminology system. The ICD-10-GM, ATC and LOINC are international classification systems widely used in German clinics in hospital information systems; therefore, they are easy to access and use if hospital data are queried. Additionally, OPS is a terminology system used only in Germany for billing code procedures 28 .
The medical terminology within SNOMED CT provides considerably more detailed diagnostics than ICD-10. Not only does SNOMED CT include diagnoses, but it also incorporates medical terms that are interrelated. In contrast, the ICD-10 is based on a hierarchically organized structure of diagnoses and is employed in over 140 countries with varying adaptations 29 . In Germany, the use of the ICD-10-GM is mandatory for billing in hospitals and primary care settings. For that reason, it is more widely utilized in patient care systems, providing ease of access. Additionally, medical personnel are accustomed to working with ICD-10-GM codes and are familiar with their strengths and weaknesses.
Nevertheless, it is important to examine the usefulness and intended audience before asserting that certain terminologies are better suited for a specific purpose. Since the ICD-10 was developed for epidemiological and billing purposes, it takes a different approach than the SNOMED CT, which is suited for the integration of eHealth applications. Utilizing a combination of coding methods, such as combining ICD and SNOMED CT, can also prove advantageous 30 .
In addition to the 51% of the terms that could be mapped by the ICD-10-GM, ATC, LOINC and OPS*, further criteria could be mapped by using SNOMED CT* 8 . Nine criteria were not possible to map by the chosen terminology systems but with the system SNOMED CT. Thus, including all SNOMED CT codes in criteria mapping can increase the coverage rate to 55% 30 .
However, there were many eligibility criteria that could not be mapped by means of chosen classification systems (neither by the ICD-10-GM, OPS, ATC, or LOINC nor by SNOMED CT). * In certain cases, a specific manifestation of a disease, procedure, or laboratory value was searched for, but only the basic information without the specifics could be entered into the system, which was represented by eight partially implementable criteria. Some criteria could not be implemented at all because the level of specification was too high (17 of the nonportrayable criteria). This is because clinical trials are often designed to gain knowledge about novel or underresearched diseases. The codes for these conditions do not yet exist in the evaluated terminology systems, which makes it difficult or impossible to introduce some selection criteria into ATLAS. To enhance this metric, incorporating additional terminology systems such as OrphaCodes, which cater to rare diseases, may be beneficial 31 .
We discovered three criteria that could only be mapped utilizing OrphaCodes, although the OMOP system had not yet integrated OrphaCodes’ terminology system at the time of analysis 32 .
Another example of this is clinical research in oncology. The study by Verwej et al. showed that oncology is characterized by constant progress due to the continuous discovery of novel and specific gene mutations in cancer screening and care 33 This aspect was also present in this project because specific gene mutations were identified among the partially or nonimplementable eligibility criteria, and these mutations cannot be represented in ATLAS due to the current state of research.
According to our observations, we can conclude that formulated eligibility criteria have a great impact on the use of correct terms, but on the other hand, for specialized criteria, no fitting codes are available. Additionally, it has been shown that the choice and correct use of medical classification systems, such as the definition of inclusion and exclusion criteria, is an important basis for working with ATLAS. Therefore, there is a need for the correct use of terminology systems and standardization, as well as continual adaptations to medical progress, which researchers, physicians, and others involved in health care need to strive for.
In this study, the effectiveness and suitability of the individual classification systems were not analyzed. Therefore, we could not draw conclusions about the usability of some terminologies in ATLAS.
*These statements refer to the versions described in Section “ Observational health data sciences and informatics (OHDSI) ”.
Process of cohort definition
The tool criteria2query (first version) can assist in generating cohort definitions based on standard concepts, but its ability to actively select specific vocabularies for cohort definition generation is limited. As the definition of an ATLAS cohort heavily relies on engaged terminology systems, these tools may not be suitable for use in the presence of other supported vocabularies 34 – 37 .
Criteria2query provides a considerable advantage, as it automatically assigns concepts or concept sets to criteria and maps the logic of links between individual concepts. This feature saves time in defining cohorts 34 . While the tool supports nonstandard vocabularies, using it becomes more complicated when assigning special concepts through the tool. It may even require external tools to find the appropriate codes. Hence, we abstained from utilizing criteria2query for our analysis.
Categorization of criteria
Our results show a relatively poor coverage rate of eligibility criteria in ATLAS. Nevertheless, ATLAS is widely used in the international community to perform medical research. The number of research studies conducted with the help of OMOP CDM and ATLAS has also increased in the last five years 38 .
International research projects utilizing the OMOP CDM rely on ATLAS to establish patient cohorts and assess them based on various patient-related variables. This is performed to acquire new medical knowledge. The patient cohort's eligibility criteria have been adapted and precisely formulated for use on OMOP CDM and the current data fields. Eligibility criteria for clinical trials are not intended for technical use but rather serve as guidelines for researchers with extensive knowledge of the medical field relevant to the study. Thus, they tend to be imprecise, as our results demonstrated. Additionally, eligibility criteria are not computer-readable and are sometimes complex 39 . Another factor contributing to the low coverage rate is the fact that some eligibility criteria are too specialized to be depicted by ATLAS or described by any of the provided terminology systems. The primary reason is that eligibility criteria are defined for use by study physicians or personnel and often afford room for individual professional judgment. However, these criteria are not universally defined, and each person may have a slightly different interpretation of, for example, "stable laboratory values." Nonetheless, an accurate and unambiguous description of the criteria is crucial for a deep understanding of this process. Without a precise description of criteria, it is not possible to implement them accurately.
Limitations of ATLAS
Training is needed to fully understand the ATLAS software. Additionally, there may be a need for making individual adaptations in some situations to introduce complex or nested datasets, such as scores. These issues highlight usability problems with ATLAS, which have been confirmed in other studies as well. In 2021, Schüttler et al. performed a qualitative exploratory study followed by a web-based usability test to evaluate three research tools. They found that ATLAS provides significantly more features than Integrating Biology and the Bedside (i2b2) developed by the National Institutes of Health or Sample Locator by the German Biobank Alliance, along with a well-designed interface. However, they found numerous usability issues arising and can be attributed to the various functions and diverse approaches for introducing selection criteria 40 .
ATLAS was primarily developed for researchers and experts who frequently formulate intricate cohort inquiries. Consequently, it is crucial to offer them a range of selection and input options, even if it results in increased operational expenses 40 .
This can present difficulties, particularly when employed in a CTRSS, as study personnel often lack the necessary time and technical expertise. Since becoming familiar with the software may be a time commitment for all study personnel, it is prudent to designate only a few personnel as ATLAS specialists for the team. For example, the European Health Data and Evidence Network (EHDEN) offers a variety of training sessions that may prove useful for this endeavor 41 . It may be helpful for study personnel to assist with implementing eligibility criteria in ATLAS by utilizing criteria2query.
It is worth mentioning that an inaccurate implementation of eligibility criteria in ATLAS can lead to less precise subject recommendations. If the entry of studies into ATLAS is intended to lead to suitable subject recruitment, the involvement of specialized study physicians or study personnel in the process of cohort definition will be helpful.
Digital subject recruitment
OMOP CDM and ATLAS, as products of OHDSI, have been developed to support multi-institutional research projects. In this study, we intended to test the possibility of using OMOP CDM and ATLAS not only to perform analyses of the existing datasets but also to implement the eligibility criteria of clinical trials. This makes it suitable as a digital tool for recruiting candidates for clinical studies. The implementation of trial eligibility criteria in ATLAS cohorts is more complex than a 1:1 translation: approximately half of the eligibility criteria could not be fully implemented in ATLAS.
Forty-five of the analyzed criteria could only be partially mapped or not mapped at all in ATLAS due to missing descriptions of the criterion. The lack of such an understanding results in criteria not being introduced into the system or being incorrectly implemented, and subjects are often incorrectly excluded from studies. The precise formulation of subject characteristics is initially needed for clinical research, so well-chosen criteria are crucial for the feasibility and internal validity of studies 42 . In terms of ATLAS, carefully chosen and precisely formulated eligibility criteria form the basis for being able to use the software correctly and to exploit its potential functionalities. To obtain better results in the process of formalizing eligibility criteria for an automated search, it is helpful to collaborate tightly with the study personnel so that imprecise criteria can be refined by professionals. In addition, the use of correct and standard medical language is important so that the meaning of the criteria can be better understood. If everyone follows and understands given standards, such as vocabulary or terminologies, this will positively impact the use of systems for patient recruitment 43 . Subsequently, all these criteria and therefore more trials can be implemented in ATLAS.
As stated previously, numerous inclusion and exclusion criteria cannot be implemented in ATLAS due to insufficient information in the descriptions. Therefore, a secondary analysis should be conducted, which includes the cohort definitions alongside medical experts from the relevant clinical department. We aim to raise the rate of implementable eligibility criteria by 20%.
We aim to assess the applicability of all eligibility criteria specified in the official trial descriptions for searching real-world data. We assume that exclusion rates for certain criteria may substantially differ, and thus, we endeavor to identify the screening criteria that are most important and those that are negligible.
Although clinical studies are vital for medical research, the lack of a sufficient number of participants often leads to their failure. It is a major challenge to identify eligible candidates for clinical trials. OMOP CDM, when combined with ATLAS, can be utilized to search for subjects in principle. However, we found that mapping inclusion criteria in ATLAS works seamlessly for only approximately half of the criteria. Reasons for this are often that the specified criteria are not clearly defined and for this reason cannot be fully implemented. Unclear and undefined criteria can result in the failure to implement approximately 20% of all inclusion criteria. Effective collaboration with study personnel to map out these criteria can lead to the replacement of imprecise information with unambiguous criteria, leading to substantially higher coverage rates.
Another reason criteria may not be fully implemented is that some require querying highly specialized diagnostic characteristics, laboratory values, medications, or other patient features. SNOMED CT or OrphaCodes may enable the mapping of additional criteria in certain cases. Employing other terminology systems may also prove beneficial depending on the local setting.
Finally, some of the criteria (13%, n = 30) could not be fully implemented due to the circumstances of ATLAS. Here, whether the criteria can be entered in a simpler form that is possible to implement must be evaluated in cooperation with the study staff. However, it is sometimes possible to use similar criteria that are valid for preselection. In addition, the study personnel can prioritize the relevance of different criteria, as some of the criteria are more important for a selection of candidates than others.
It is unclear at this time whether there is a prioritization of inclusion criteria that must be considered when implementing study criteria in ATLAS. It is possible that the nonimplementable criteria may also be of less relevance for the search for subjects. On the other hand, it is also conceivable that precisely these criteria that could not be mapped are particularly important. Further work is planned to examine this connection and to be able to make a more precise statement about how well ATLAS works in a real-world setting for study recruitment.
Supplementary Information
Acknowledgements.
We are thankful to Dr. rer. nat. Seyed Amir Hossein Tabatabaei for reviewing this work and offering useful advice.
Abbreviations
Author contributions.
R.B. and K.M.B. wrote the original texts of this work. R.B. and K.S. did the conceptual work of this study. K.M.B. analyzed all trials and criteria, as well as implemented and categorized the criteria. H.S. and B.S. were involved in the conceptual work of this article. All authors read and approved the final manuscript.
Open Access funding enabled and organized by Projekt DEAL. This work is part of the project MIRACUM, funded by the German Ministry of Education and Research (FKZ 01ZZ1801D).
Data availability
Competing interests.
The authors declare no competing interests.
Publisher's note
Springer Nature remains neutral with regard to jurisdictional claims in published maps and institutional affiliations.
The online version contains supplementary material available at 10.1038/s41598-023-49560-w.

IMAGES
VIDEO
COMMENTS
What are inclusion criteria? Inclusion criteria comprise the characteristics or attributes that prospective research participants must have in order to be included in the study. Common inclusion criteria can be demographic, clinical, or geographic in nature. Example: Inclusion criteria You are running a clinical trial for a new treatment for individuals with chronic heart failure.
PRACTICAL SCENARIO. A cross-sectional multicenter study evaluated self-reported adherence to inhaled therapies among patients with COPD in Latin America. 1 Inclusion and exclusion criteria for the study are shown in Chart 1.The authors found that self-reported adherence was low in 20% of the patients, intermediate in 29%, and high in 51%; and that poor adherence was associated with more ...
Establishing inclusion and exclusion criteria for study participants is a standard, required practice when designing high-quality research protocols. Defining inclusion and exclusion criteria increases the likelihood of producing reliable and reproducible results, minimizes the likelihood of harm to the subjects, and guards against exploitation ...
Content-specific research literature and expertise should inform this step. ... Minimum criteria for inclusion of a measure may include: ... Khalsa P, Loeser J, Mackey S, Panagis J, Rainville J, Tosteson T, Turk D, Von Korff M, Weiner DK. Report of the NIH Task Force on research standards for chronic low back pain. Journal of Pain 2014; 15: 569 ...
You may want to think about criteria that will be used to select articles for your literature review based on your research question. These are commonly known as inclusion criteria and exclusion criteria, and they set the boundaries for the literature review.. Inclusion and exclusion criteria are determined after formulating the research question but usually before the search is conducted ...
Explanation: Specifying the criteria used to decide what evidence was eligible or ineligible in sufficient detail should enable readers to understand the scope of the review and verify inclusion decisions. 35 The PICO framework is commonly used to structure the reporting of eligibility criteria for reviews of interventions. 36 In addition to ...
Inclusion criteria for the population(s) of interest should be defined in terms of relevant demographic variables, disease variables (i.e., variations in diagnostic criteria, ... This report is based on research conducted by the Oregon Health & Science University, McMaster University, and Southern California Evidence-based Practice Centers ...
Well-constructed, clear, and explicit inclusion and exclusion criteria assist decision making consistency. In this chapter, I focus on inclusion and exclusion criteria, explain their benefits, provide guidelines on their construction and use, and illustrate with examples from sport, exercise, and physical activity research. Download chapter PDF.
A complete description of the literature search, including the criteria used for the inclusion of reports after they have been located, ... defining this as "using the reference lists at the end of research reports to locate other reports that might be relevant to a search" (p. 64) and the forward search, or citation analysis, as a ...
searches and report inclusion criteria: making research syntheses more transparent and easy to replicate‡ Kayla M. Atkinson, Alison C. Koenka, Carmen E. Sanchez, Hannah Moshontz and Harris Cooper*† A complete description of the literature search, including the criteria used for the inclusion of reports after
A complete description of the literature search, including the criteria used for the inclusion of reports after they have been located, used in a research synthesis or meta‐analysis is critical if subsequent researchers are to accurately evaluate and reproduce a synthesis' methods and results. Based on previous guidelines and new suggestions, we present a set of focused and detailed ...
Inclusion/exclusion criteria. In clinical research, specific group of relatively homogeneous patient population needs to be selected. Inclusion and exclusion criteria define who can be included or excluded from the study sample. The inclusion criteria identify the study population in a consistent, reliable, uniform and objective manner.
Inclusion criteria is everything a study must have to be included. Exclusion criteria are the factors that would make a study ineligible to be included. Criteria that should be considered include: Type of studies: It is important to select articles with an appropriate study design for the research question. Dates for the studies and a timeline ...
Inclusion and exclusion criteria may include factors such as age, gender, race, ethnicity, type and stage of disease, the subject's previous treatment history, and the presence or absence (as in the case of the "healthy" or "control" subject) of other medical, psychosocial, or emotional conditions. Healthy, or control, subjects may be ...
Overly restrictive inclusion and exclusion criteria may limit study accrual, patient access to trials, and generalizability of the study intervention [].As regulatory approval is based on data from the enrolled study population, unnecessarily stringent eligibility criteria result in studies that fail to reflect the heterogeneity of the patient population who might benefit from the study ...
Inclusion and exclusion criteria are developed after a research question is finalized but before a search is carried out. ... Empty reviews are when no studies meet the inclusion criteria for a SR. Empty reviews are more likely to subject to publication bias, however, they are important in identifying gaps in the literature. ... Slyer, Jason T ...
Inclusion and exclusion criteria set the boundaries for the systematic review. They are determined after setting the research question usually before the search is conducted, however scoping searches may need to be undertaken to determine appropriate criteria. Many different factors can be used as inclusion or exclusion criteria.
The present research made a commitment to accessibility by only seeking two inclusion criteria—that participants be 18 years or over; and self-identify as deafblind, Deafblind or a person with dual sensory loss or impairment. The latter distinction was important because not all view hearing and vision loss as an impairment or disability.
A cross-sectional multicenter study evaluated self-reported adherence to inhaled therapies among patients with COPD in Latin America. 1 Inclusion and exclusion criteria for the study are shown in Chart 1. The authors found that self-reported adherence was low in 20% of the patients, intermediate in 29%, and high in 51%; and that poor adherence ...
Inclusion criteria for research studies should be kept to the minimum necessary to ensure patient safety and criteria likely to exclude broad sectors of populations should be avoided. ... Study reports and analysis plans should report the proportion of relevant under-served groups and compare these proportions to those found in the general ...
A complete description of the literature search, including the criteria used for the inclusion of reports after they have been located, used in a research synthesis or meta-analysis is critical if subsequent researchers are to accurately evaluate and reproduce a synthesis' methods and results. Based …
From the selected databases, the search retrieved a total of 765 publications. Then, the duplicate records were removed. After that, based on the title and abstract, the remaining 426 publications were screened for their relevance by using the following inclusion and exclusion criteria (see Table 2).Publications focusing on evaluation criteria for good qualitative research were included ...
Achieving equality, diversity, and inclusion (EDI) is an underpinning contributor to human rights, civilisation and society-wide responsibility [].Furthermore, promoting and embedding EDI within research environments is essential to make the advancements required to meet today's research challenges [].This is evidenced by equal, diverse and inclusive teams leading to higher productivity ...
This set corresponded with the eligibility criteria referring to clearly defined diseases, procedures, or drugs with the corresponding ICD, OPS, ATC or LOINC codes. Additionally, 21.11% (n = 47) of the criteria were defined as partially implementable, and 27.80% (n = 62) could not be implemented in ATLAS at all.