Study Design 101: Meta-Analysis
- Case Report
- Case Control Study
- Cohort Study
- Randomized Controlled Trial
- Practice Guideline
- Systematic Review

Meta-Analysis
- Helpful Formulas
- Finding Specific Study Types
A subset of systematic reviews; a method for systematically combining pertinent qualitative and quantitative study data from several selected studies to develop a single conclusion that has greater statistical power. This conclusion is statistically stronger than the analysis of any single study, due to increased numbers of subjects, greater diversity among subjects, or accumulated effects and results.
Meta-analysis would be used for the following purposes:
- To establish statistical significance with studies that have conflicting results
- To develop a more correct estimate of effect magnitude
- To provide a more complex analysis of harms, safety data, and benefits
- To examine subgroups with individual numbers that are not statistically significant
If the individual studies utilized randomized controlled trials (RCT), combining several selected RCT results would be the highest-level of evidence on the evidence hierarchy, followed by systematic reviews, which analyze all available studies on a topic.
- Greater statistical power
- Confirmatory data analysis
- Greater ability to extrapolate to general population affected
- Considered an evidence-based resource
Disadvantages
- Difficult and time consuming to identify appropriate studies
- Not all studies provide adequate data for inclusion and analysis
- Requires advanced statistical techniques
- Heterogeneity of study populations
Design pitfalls to look out for
The studies pooled for review should be similar in type (i.e. all randomized controlled trials).
Are the studies being reviewed all the same type of study or are they a mixture of different types?
The analysis should include published and unpublished results to avoid publication bias.
Does the meta-analysis include any appropriate relevant studies that may have had negative outcomes?
Fictitious Example
Do individuals who wear sunscreen have fewer cases of melanoma than those who do not wear sunscreen? A MEDLINE search was conducted using the terms melanoma, sunscreening agents, and zinc oxide, resulting in 8 randomized controlled studies, each with between 100 and 120 subjects. All of the studies showed a positive effect between wearing sunscreen and reducing the likelihood of melanoma. The subjects from all eight studies (total: 860 subjects) were pooled and statistically analyzed to determine the effect of the relationship between wearing sunscreen and melanoma. This meta-analysis showed a 50% reduction in melanoma diagnosis among sunscreen-wearers.
Real-life Examples
Goyal, A., Elminawy, M., Kerezoudis, P., Lu, V., Yolcu, Y., Alvi, M., & Bydon, M. (2019). Impact of obesity on outcomes following lumbar spine surgery: A systematic review and meta-analysis. Clinical Neurology and Neurosurgery, 177 , 27-36. https://doi.org/10.1016/j.clineuro.2018.12.012
This meta-analysis was interested in determining whether obesity affects the outcome of spinal surgery. Some previous studies have shown higher perioperative morbidity in patients with obesity while other studies have not shown this effect. This study looked at surgical outcomes including "blood loss, operative time, length of stay, complication and reoperation rates and functional outcomes" between patients with and without obesity. A meta-analysis of 32 studies (23,415 patients) was conducted. There were no significant differences for patients undergoing minimally invasive surgery, but patients with obesity who had open surgery had experienced higher blood loss and longer operative times (not clinically meaningful) as well as higher complication and reoperation rates. Further research is needed to explore this issue in patients with morbid obesity.
Nakamura, A., van Der Waerden, J., Melchior, M., Bolze, C., El-Khoury, F., & Pryor, L. (2019). Physical activity during pregnancy and postpartum depression: Systematic review and meta-analysis. Journal of Affective Disorders, 246 , 29-41. https://doi.org/10.1016/j.jad.2018.12.009
This meta-analysis explored whether physical activity during pregnancy prevents postpartum depression. Seventeen studies were included (93,676 women) and analysis showed a "significant reduction in postpartum depression scores in women who were physically active during their pregnancies when compared with inactive women." Possible limitations or moderators of this effect include intensity and frequency of physical activity, type of physical activity, and timepoint in pregnancy (e.g. trimester).
Related Terms
A document often written by a panel that provides a comprehensive review of all relevant studies on a particular clinical or health-related topic/question.
Publication Bias
A phenomenon in which studies with positive results have a better chance of being published, are published earlier, and are published in journals with higher impact factors. Therefore, conclusions based exclusively on published studies can be misleading.
Now test yourself!
1. A Meta-Analysis pools together the sample populations from different studies, such as Randomized Controlled Trials, into one statistical analysis and treats them as one large sample population with one conclusion.
a) True b) False
2. One potential design pitfall of Meta-Analyses that is important to pay attention to is:
a) Whether it is evidence-based. b) If the authors combined studies with conflicting results. c) If the authors appropriately combined studies so they did not compare apples and oranges. d) If the authors used only quantitative data.
Evidence Pyramid - Navigation
- Meta- Analysis
- Case Reports
- << Previous: Systematic Review
- Next: Helpful Formulas >>

- Last Updated: Sep 25, 2023 10:59 AM
- URL: https://guides.himmelfarb.gwu.edu/studydesign101

- Himmelfarb Intranet
- Privacy Notice
- Terms of Use
- GW is committed to digital accessibility. If you experience a barrier that affects your ability to access content on this page, let us know via the Accessibility Feedback Form .
- Himmelfarb Health Sciences Library
- 2300 Eye St., NW, Washington, DC 20037
- Phone: (202) 994-2850
- [email protected]
- https://himmelfarb.gwu.edu

Meta-analysis
Reviewed by Psychology Today Staff
Meta-analysis is an objective examination of published data from many studies of the same research topic identified through a literature search. Through the use of rigorous statistical methods, it can reveal patterns hidden in individual studies and can yield conclusions that have a high degree of reliability. It is a method of analysis that is especially useful for gaining an understanding of complex phenomena when independent studies have produced conflicting findings.
Meta-analysis provides much of the underpinning for evidence-based medicine. It is particularly helpful in identifying risk factors for a disorder, diagnostic criteria, and the effects of treatments on specific populations of people, as well as quantifying the size of the effects. Meta-analysis is well-suited to understanding the complexities of human behavior.
- How Does It Differ From Other Studies?
- When Is It Used?
- What Are Some Important Things Revealed by Meta-analysis?
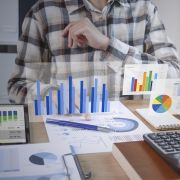
There are well-established scientific criteria for selecting studies for meta-analysis. Usually, meta-analysis is conducted on the gold standard of scientific research—randomized, controlled, double-blind trials. In addition, published guidelines not only describe standards for the inclusion of studies to be analyzed but also rank the quality of different types of studies. For example, cohort studies are likely to provide more reliable information than case reports.
Through statistical methods applied to the original data collected in the included studies, meta-analysis can account for and overcome many differences in the way the studies were conducted, such as the populations studied, how interventions were administered, and what outcomes were assessed and how. Meta-analyses, and the questions they are attempting to answer, are typically specified and registered with a scientific organization, and, with the protocols and methods openly described and reviewed independently by outside investigators, the research process is highly transparent.
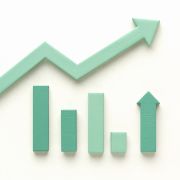
Meta-analysis is often used to validate observed phenomena, determine the conditions under which effects occur, and get enough clarity in clinical decision-making to indicate a course of therapeutic action when individual studies have produced disparate findings. In reviewing the aggregate results of well-controlled studies meeting criteria for inclusion, meta-analysis can also reveal which research questions, test conditions, and research methods yield the most reliable results, not only providing findings of immediate clinical utility but furthering science.
The technique can be used to answer social and behavioral questions large and small. For example, to clarify whether or not having more options makes it harder for people to settle on any one item, a meta-analysis of over 53 conflicting studies on the phenomenon was conducted. The meta-analysis revealed that choice overload exists—but only under certain conditions. You will have difficulty selecting a TV show to watch from the massive array of possibilities, for example, if the shows differ from each other in multiple ways or if you don’t have any strong preferences when you finally get to sit down in front of the TV.
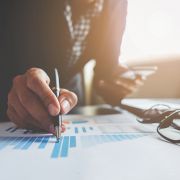
A meta-analysis conducted in 2000, for example, answered the question of whether physically attractive people have “better” personalities . Among other traits, they prove to be more extroverted and have more social skills than others. Another meta-analysis, in 2014, showed strong ties between physical attractiveness as rated by others and having good mental and physical health. The effects on such personality factors as extraversion are too small to reliably show up in individual studies but real enough to be detected in the aggregate number of study participants. Together, the studies validate hypotheses put forth by evolutionary psychologists that physical attractiveness is important in mate selection because it is a reliable cue of health and, likely, fertility.
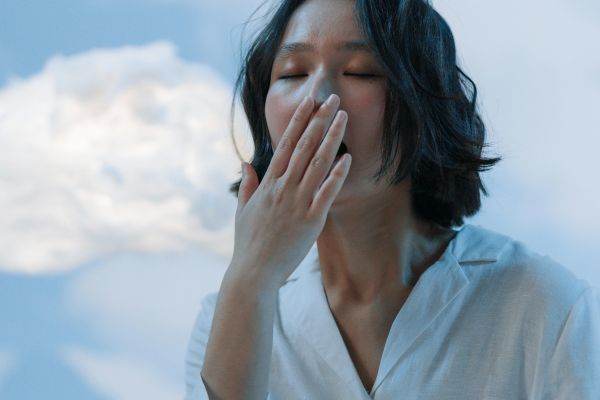
A recent review provides compelling evidence that arts engagement significantly reduces cognitive decline and enhances the quality of life among healthy older adults.
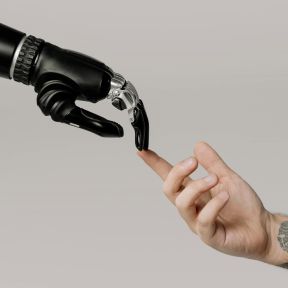
Personal Perspective: Mental healthcare AI is evolving beyond administrative roles. By automating routine tasks, therapists can spend sessions focusing on human interactions.
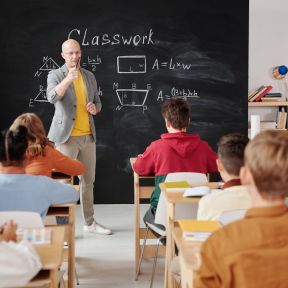
Investing in building a positive classroom climate holds benefits for students and teachers alike.
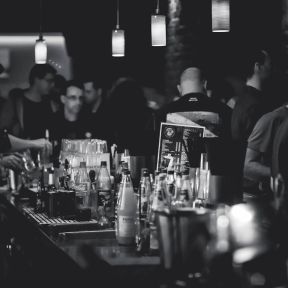
Mistakenly blaming cancer-causing chemicals and radiation for most cancers lets us avoid the simple lifestyle changes that could protect us from cancer far more.
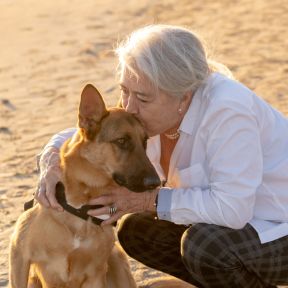
According to astronomer Carl Sagan, "Extraordinary claims require extraordinary evidence." Does the claim that pet owners live longer pass the extraordinary evidence requirement?
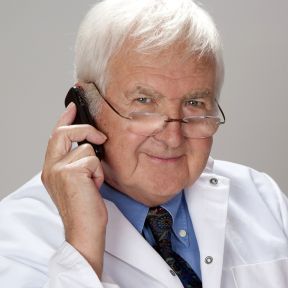
People, including leading politicians, are working later in life than ever before. Luckily, social science suggests that aging does not get in the way of job performance.
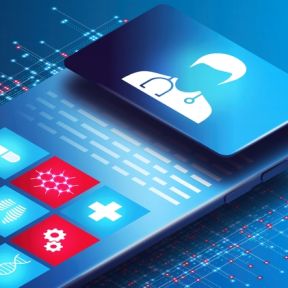
The healthcare industry is regulated to ensure patient safety, efficacy of treatments, and ethical practices. Why aren't these standards applied to mental health apps?
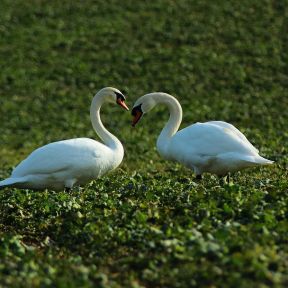
Being able to forgive others makes you more resilient. You can learn to let go of anger and bitterness.
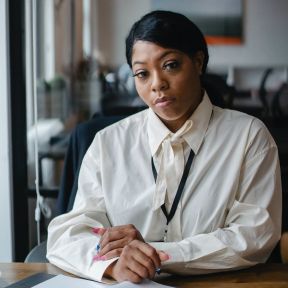
Discover how recent findings reveal a more promising outlook on the accuracy of performance appraisals.
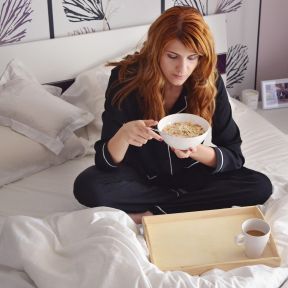
We all love the thrill of experiencing something new. However, recent research can help us understand the powerful appeal of the familiar.
- Find a Therapist
- Find a Treatment Center
- Find a Psychiatrist
- Find a Support Group
- Find Online Therapy
- United States
- Brooklyn, NY
- Chicago, IL
- Houston, TX
- Los Angeles, CA
- New York, NY
- Portland, OR
- San Diego, CA
- San Francisco, CA
- Seattle, WA
- Washington, DC
- Asperger's
- Bipolar Disorder
- Chronic Pain
- Eating Disorders
- Passive Aggression
- Personality
- Goal Setting
- Positive Psychology
- Stopping Smoking
- Low Sexual Desire
- Relationships
- Child Development
- Self Tests NEW
- Therapy Center
- Diagnosis Dictionary
- Types of Therapy
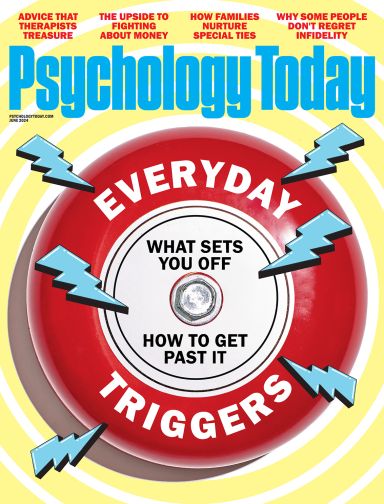
At any moment, someone’s aggravating behavior or our own bad luck can set us off on an emotional spiral that threatens to derail our entire day. Here’s how we can face our triggers with less reactivity so that we can get on with our lives.
- Emotional Intelligence
- Gaslighting
- Affective Forecasting
- Neuroscience
- How it works
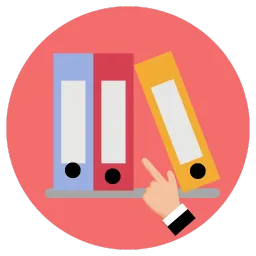
Meta-Analysis – Guide with Definition, Steps & Examples
Published by Owen Ingram at April 26th, 2023 , Revised On April 26, 2023
“A meta-analysis is a formal, epidemiological, quantitative study design that uses statistical methods to generalise the findings of the selected independent studies. “
Meta-analysis and systematic review are the two most authentic strategies in research. When researchers start looking for the best available evidence concerning their research work, they are advised to begin from the top of the evidence pyramid. The evidence available in the form of meta-analysis or systematic reviews addressing important questions is significant in academics because it informs decision-making.
What is Meta-Analysis
Meta-analysis estimates the absolute effect of individual independent research studies by systematically synthesising or merging the results. Meta-analysis isn’t only about achieving a wider population by combining several smaller studies. It involves systematic methods to evaluate the inconsistencies in participants, variability (also known as heterogeneity), and findings to check how sensitive their findings are to the selected systematic review protocol.
When Should you Conduct a Meta-Analysis?
Meta-analysis has become a widely-used research method in medical sciences and other fields of work for several reasons. The technique involves summarising the results of independent systematic review studies.
The Cochrane Handbook explains that “an important step in a systematic review is the thoughtful consideration of whether it is appropriate to combine the numerical results of all, or perhaps some, of the studies. Such a meta-analysis yields an overall statistic (together with its confidence interval) that summarizes the effectiveness of an experimental intervention compared with a comparator intervention” (section 10.2).
A researcher or a practitioner should choose meta-analysis when the following outcomes are desirable.
For generating new hypotheses or ending controversies resulting from different research studies. Quantifying and evaluating the variable results and identifying the extent of conflict in literature through meta-analysis is possible.
To find research gaps left unfilled and address questions not posed by individual studies. Primary research studies involve specific types of participants and interventions. A review of these studies with variable characteristics and methodologies can allow the researcher to gauge the consistency of findings across a wider range of participants and interventions. With the help of meta-analysis, the reasons for differences in the effect can also be explored.
To provide convincing evidence. Estimating the effects with a larger sample size and interventions can provide convincing evidence. Many academic studies are based on a very small dataset, so the estimated intervention effects in isolation are not fully reliable.
Elements of a Meta-Analysis
Deeks et al. (2019), Haidilch (2010), and Grant & Booth (2009) explored the characteristics, strengths, and weaknesses of conducting the meta-analysis. They are briefly explained below.
Characteristics:
- A systematic review must be completed before conducting the meta-analysis because it provides a summary of the findings of the individual studies synthesised.
- You can only conduct a meta-analysis by synthesising studies in a systematic review.
- The studies selected for statistical analysis for the purpose of meta-analysis should be similar in terms of comparison, intervention, and population.
Strengths:
- A meta-analysis takes place after the systematic review. The end product is a comprehensive quantitative analysis that is complicated but reliable.
- It gives more value and weightage to existing studies that do not hold practical value on their own.
- Policy-makers and academicians cannot base their decisions on individual research studies. Meta-analysis provides them with a complex and solid analysis of evidence to make informed decisions.
Criticisms:
- The meta-analysis uses studies exploring similar topics. Finding similar studies for the meta-analysis can be challenging.
- When and if biases in the individual studies or those related to reporting and specific research methodologies are involved, the meta-analysis results could be misleading.
Steps of Conducting the Meta-Analysis
The process of conducting the meta-analysis has remained a topic of debate among researchers and scientists. However, the following 5-step process is widely accepted.
Step 1: Research Question
The first step in conducting clinical research involves identifying a research question and proposing a hypothesis . The potential clinical significance of the research question is then explained, and the study design and analytical plan are justified.
Step 2: Systematic Review
The purpose of a systematic review (SR) is to address a research question by identifying all relevant studies that meet the required quality standards for inclusion. While established journals typically serve as the primary source for identified studies, it is important to also consider unpublished data to avoid publication bias or the exclusion of studies with negative results.
While some meta-analyses may limit their focus to randomized controlled trials (RCTs) for the sake of obtaining the highest quality evidence, other experimental and quasi-experimental studies may be included if they meet the specific inclusion/exclusion criteria established for the review.
Step 3: Data Extraction
After selecting studies for the meta-analysis, researchers extract summary data or outcomes, as well as sample sizes and measures of data variability for both intervention and control groups. The choice of outcome measures depends on the research question and the type of study, and may include numerical or categorical measures.
For instance, numerical means may be used to report differences in scores on a questionnaire or changes in a measurement, such as blood pressure. In contrast, risk measures like odds ratios (OR) or relative risks (RR) are typically used to report differences in the probability of belonging to one category or another, such as vaginal birth versus cesarean birth.
Step 4: Standardisation and Weighting Studies
After gathering all the required data, the fourth step involves computing suitable summary measures from each study for further examination. These measures are typically referred to as Effect Sizes and indicate the difference in average scores between the control and intervention groups. For instance, it could be the variation in blood pressure changes between study participants who used drug X and those who used a placebo.
Since the units of measurement often differ across the included studies, standardization is necessary to create comparable effect size estimates. Standardization is accomplished by determining, for each study, the average score for the intervention group, subtracting the average score for the control group, and dividing the result by the relevant measure of variability in that dataset.
In some cases, the results of certain studies must carry more significance than others. Larger studies, as measured by their sample sizes, are deemed to produce more precise estimates of effect size than smaller studies. Additionally, studies with less variability in data, such as smaller standard deviation or narrower confidence intervals, are typically regarded as higher quality in study design. A weighting statistic that aims to incorporate both of these factors, known as inverse variance, is commonly employed.
Step 5: Absolute Effect Estimation
The ultimate step in conducting a meta-analysis is to choose and utilize an appropriate model for comparing Effect Sizes among diverse studies. Two popular models for this purpose are the Fixed Effects and Random Effects models. The Fixed Effects model relies on the premise that each study is evaluating a common treatment effect, implying that all studies would have estimated the same Effect Size if sample variability were equal across all studies.
Conversely, the Random Effects model posits that the true treatment effects in individual studies may vary from each other, and endeavors to consider this additional source of interstudy variation in Effect Sizes. The existence and magnitude of this latter variability is usually evaluated within the meta-analysis through a test for ‘heterogeneity.’
Forest Plot
The results of a meta-analysis are often visually presented using a “Forest Plot”. This type of plot displays, for each study, included in the analysis, a horizontal line that indicates the standardized Effect Size estimate and 95% confidence interval for the risk ratio used. Figure A provides an example of a hypothetical Forest Plot in which drug X reduces the risk of death in all three studies.
However, the first study was larger than the other two, and as a result, the estimates for the smaller studies were not statistically significant. This is indicated by the lines emanating from their boxes, including the value of 1. The size of the boxes represents the relative weights assigned to each study by the meta-analysis. The combined estimate of the drug’s effect, represented by the diamond, provides a more precise estimate of the drug’s effect, with the diamond indicating both the combined risk ratio estimate and the 95% confidence interval limits.
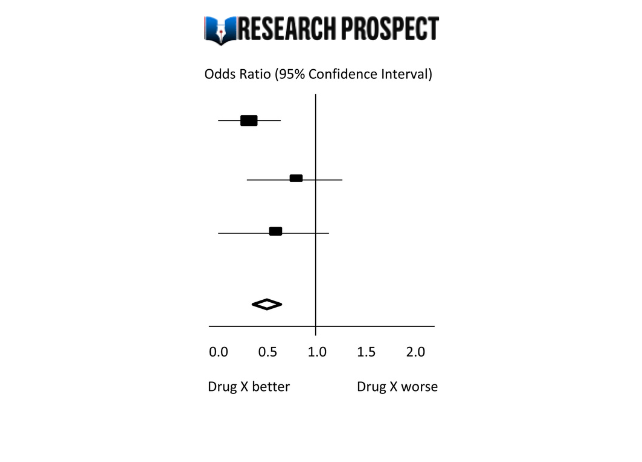
Figure-A: Hypothetical Forest Plot
Relevance to Practice and Research
Evidence Based Nursing commentaries often include recently published systematic reviews and meta-analyses, as they can provide new insights and strengthen recommendations for effective healthcare practices. Additionally, they can identify gaps or limitations in current evidence and guide future research directions.
The quality of the data available for synthesis is a critical factor in the strength of conclusions drawn from meta-analyses, and this is influenced by the quality of individual studies and the systematic review itself. However, meta-analysis cannot overcome issues related to underpowered or poorly designed studies.
Therefore, clinicians may still encounter situations where the evidence is weak or uncertain, and where higher-quality research is required to improve clinical decision-making. While such findings can be frustrating, they remain important for informing practice and highlighting the need for further research to fill gaps in the evidence base.
Methods and Assumptions in Meta-Analysis
Ensuring the credibility of findings is imperative in all types of research, including meta-analyses. To validate the outcomes of a meta-analysis, the researcher must confirm that the research techniques used were accurate in measuring the intended variables. Typically, researchers establish the validity of a meta-analysis by testing the outcomes for homogeneity or the degree of similarity between the results of the combined studies.
Homogeneity is preferred in meta-analyses as it allows the data to be combined without needing adjustments to suit the study’s requirements. To determine homogeneity, researchers assess heterogeneity, the opposite of homogeneity. Two widely used statistical methods for evaluating heterogeneity in research results are Cochran’s-Q and I-Square, also known as I-2 Index.
Difference Between Meta-Analysis and Systematic Reviews
Meta-analysis and systematic reviews are both research methods used to synthesise evidence from multiple studies on a particular topic. However, there are some key differences between the two.
Systematic reviews involve a comprehensive and structured approach to identifying, selecting, and critically appraising all available evidence relevant to a specific research question. This process involves searching multiple databases, screening the identified studies for relevance and quality, and summarizing the findings in a narrative report.
Meta-analysis, on the other hand, involves using statistical methods to combine and analyze the data from multiple studies, with the aim of producing a quantitative summary of the overall effect size. Meta-analysis requires the studies to be similar enough in terms of their design, methodology, and outcome measures to allow for meaningful comparison and analysis.
Therefore, systematic reviews are broader in scope and summarize the findings of all studies on a topic, while meta-analyses are more focused on producing a quantitative estimate of the effect size of an intervention across multiple studies that meet certain criteria. In some cases, a systematic review may be conducted without a meta-analysis if the studies are too diverse or the quality of the data is not sufficient to allow for statistical pooling.
Software Packages For Meta-Analysis
Meta-analysis can be done through software packages, including free and paid options. One of the most commonly used software packages for meta-analysis is RevMan by the Cochrane Collaboration.
Assessing the Quality of Meta-Analysis
Assessing the quality of a meta-analysis involves evaluating the methods used to conduct the analysis and the quality of the studies included. Here are some key factors to consider:
- Study selection: The studies included in the meta-analysis should be relevant to the research question and meet predetermined criteria for quality.
- Search strategy: The search strategy should be comprehensive and transparent, including databases and search terms used to identify relevant studies.
- Study quality assessment: The quality of included studies should be assessed using appropriate tools, and this assessment should be reported in the meta-analysis.
- Data extraction: The data extraction process should be systematic and clearly reported, including any discrepancies that arose.
- Analysis methods: The meta-analysis should use appropriate statistical methods to combine the results of the included studies, and these methods should be transparently reported.
- Publication bias: The potential for publication bias should be assessed and reported in the meta-analysis, including any efforts to identify and include unpublished studies.
- Interpretation of results: The results should be interpreted in the context of the study limitations and the overall quality of the evidence.
- Sensitivity analysis: Sensitivity analysis should be conducted to evaluate the impact of study quality, inclusion criteria, and other factors on the overall results.
Overall, a high-quality meta-analysis should be transparent in its methods and clearly report the included studies’ limitations and the evidence’s overall quality.
Hire an Expert Writer
Orders completed by our expert writers are
- Formally drafted in an academic style
- Free Amendments and 100% Plagiarism Free – or your money back!
- 100% Confidential and Timely Delivery!
- Free anti-plagiarism report
- Appreciated by thousands of clients. Check client reviews
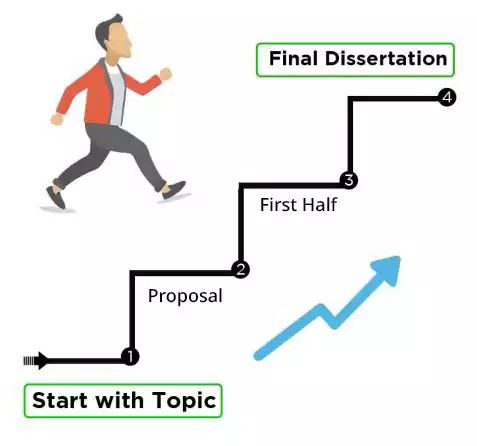
Examples of Meta-Analysis
- STANLEY T.D. et JARRELL S.B. (1989), « Meta-regression analysis : a quantitative method of literature surveys », Journal of Economics Surveys, vol. 3, n°2, pp. 161-170.
- DATTA D.K., PINCHES G.E. et NARAYANAN V.K. (1992), « Factors influencing wealth creation from mergers and acquisitions : a meta-analysis », Strategic Management Journal, Vol. 13, pp. 67-84.
- GLASS G. (1983), « Synthesising empirical research : Meta-analysis » in S.A. Ward and L.J. Reed (Eds), Knowledge structure and use : Implications for synthesis and interpretation, Philadelphia : Temple University Press.
- WOLF F.M. (1986), Meta-analysis : Quantitative methods for research synthesis, Sage University Paper n°59.
- HUNTER J.E., SCHMIDT F.L. et JACKSON G.B. (1982), « Meta-analysis : cumulating research findings across studies », Beverly Hills, CA : Sage.
Frequently Asked Questions
What is a meta-analysis in research.
Meta-analysis is a statistical method used to combine results from multiple studies on a specific topic. By pooling data from various sources, meta-analysis can provide a more precise estimate of the effect size of a treatment or intervention and identify areas for future research.
Why is meta-analysis important?
Meta-analysis is important because it combines and summarizes results from multiple studies to provide a more precise and reliable estimate of the effect of a treatment or intervention. This helps clinicians and policymakers make evidence-based decisions and identify areas for further research.
What is an example of a meta-analysis?
A meta-analysis of studies evaluating physical exercise’s effect on depression in adults is an example. Researchers gathered data from 49 studies involving a total of 2669 participants. The studies used different types of exercise and measures of depression, which made it difficult to compare the results.
Through meta-analysis, the researchers calculated an overall effect size and determined that exercise was associated with a statistically significant reduction in depression symptoms. The study also identified that moderate-intensity aerobic exercise, performed three to five times per week, was the most effective. The meta-analysis provided a more comprehensive understanding of the impact of exercise on depression than any single study could provide.
What is the definition of meta-analysis in clinical research?
Meta-analysis in clinical research is a statistical technique that combines data from multiple independent studies on a particular topic to generate a summary or “meta” estimate of the effect of a particular intervention or exposure.
This type of analysis allows researchers to synthesise the results of multiple studies, potentially increasing the statistical power and providing more precise estimates of treatment effects. Meta-analyses are commonly used in clinical research to evaluate the effectiveness and safety of medical interventions and to inform clinical practice guidelines.
Is meta-analysis qualitative or quantitative?
Meta-analysis is a quantitative method used to combine and analyze data from multiple studies. It involves the statistical synthesis of results from individual studies to obtain a pooled estimate of the effect size of a particular intervention or treatment. Therefore, meta-analysis is considered a quantitative approach to research synthesis.
You May Also Like
A confounding variable can potentially affect both the suspected cause and the suspected effect. Here is all you need to know about accounting for confounding variables in research.
Textual analysis is the method of analysing and understanding the text. We need to look carefully at the text to identify the writer’s context and message.
Baffled by the concept of reliability and validity? Reliability refers to the consistency of measurement. Validity refers to the accuracy of measurement.
USEFUL LINKS
LEARNING RESOURCES
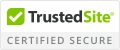
COMPANY DETAILS
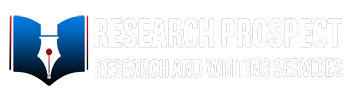
- How It Works

Introduction to Meta-Analysis: A Guide for the Novice
- Experimental Psychology
- Methodology
- Statistical Analysis
Free Meta-Analysis Software and Macros
MetaXL (Version 2.0)
RevMan (Version 5.3)
Meta-Analysis Macros for SAS, SPSS, and Stata
Opposing theories and disparate findings populate the field of psychology; scientists must interpret the results of any single study in the context of its limitations. Meta-analysis is a robust tool that can help researchers overcome these challenges by assimilating data across studies identified through a literature review. In other words, rather than surveying participants, a meta-analysis surveys studies. The goal is to calculate the direction and/or magnitude of an effect across all relevant studies, both published and unpublished. Despite the utility of this statistical technique, it can intimidate a beginner who has no formal training in the approach. However, any motivated researcher with a statistics background can complete a meta-analysis. This article provides an overview of the main steps of basic meta-analysis.
Meta-analysis has many strengths. First, meta-analysis provides an organized approach for handling a large number of studies. Second, the process is systematic and documented in great detail, which allows readers to evaluate the researchers’ decisions and conclusions. Third, meta-analysis allows researchers to examine an effect within a collection of studies in a more sophisticated manner than a qualitative summary.
However, meta-analysis also involves numerous challenges. First, it consumes a great deal of time and requires a great deal of effort. Second, meta-analysis has been criticized for aggregating studies that are too different (i.e., mixing “apples and oranges”). Third, some scientists argue that the objective coding procedure used in meta-analysis ignores the context of each individual study, such as its methodological rigor. Fourth, when a researcher includes low-quality studies in a meta-analysis, the limitations of these studies impact the mean effect size (i.e., “garbage in, garbage out”). As long as researchers are aware of these issues and consider the potential influence of these limitations on their findings, meta-analysis can serve as a powerful and informative approach to help us draw conclusions from a large literature base.
Identifying the Right Question
Similar to any research study, a meta-analysis begins with a research question. Meta-analysis can be used in any situation where the goal is to summarize quantitative findings from empirical studies. It can be used to examine different types of effects, including prevalence rates (e.g., percentage of rape survivors with depression), growth rates (e.g., changes in depression from pretreatment to posttreatment), group differences (e.g., comparison of treatment and placebo groups on depression), and associations between variables (e.g., correlation between depression and self-esteem). To select the effect metric, researchers should consider the statistical form of the results in the literature. Any given meta-analysis can focus on only one metric at a time. While selecting a research question, researchers should think about the size of the literature base and select a manageable topic. At the same time, they should make sure the number of existing studies is large enough to warrant a meta-analysis.
Determining Eligibility Criteria
After choosing a relevant question, researchers should then identify and explicitly state the types of studies to be included. These criteria ensure that the studies overlap enough in topic and methodology that it makes sense to combine them. The inclusion and exclusion criteria depend on the specific research question and characteristics of the literature. First, researchers can specify relevant participant characteristics, such as age or gender. Second, researchers can identify the key variables that must be included in the study. Third, the language, date range, and types (e.g., peer-reviewed journal articles) of studies should be specified. Fourth, pertinent study characteristics, such as experimental design, can be defined. Eligibility criteria should be clearly documented and relevant to the research question. Specifying the eligibility criteria prior to conducting the literature search allows the researcher to perform a more targeted search and reduces the number of irrelevant studies. Eligibility criteria can also be revised later, because the researcher may become aware of unforeseen issues during the literature search stage.
Conducting a Literature Search and Review
The next step is to identify, retrieve, and review published and unpublished studies. The goal is to be exhaustive; however, being too broad can result in an overwhelming number of studies to review.
Online databases, such as PsycINFO and PubMed, compile millions of searchable records, including peer-reviewed journals, books, and dissertations. In addition, through these electronic databases, researchers can access the full text of many of the records. It is important that researchers carefully choose search terms and databases, because these decisions impact the breadth of the review. Researchers who aren’t familiar with the research topic should consult with an expert.
Additional ways to identify studies include searching conference proceedings, examining reference lists of relevant studies, and directly contacting researchers. After the literature search is completed, researchers must evaluate each study for inclusion using the eligibility criteria. At least a subset of the studies should be reviewed by two individuals (i.e., double coded) to serve as a reliability check. It is vital that researchers keep meticulous records of this process; for publication, a flow diagram is typically required to depict the search and results. Researchers should allow adequate time, because this step can be quite time consuming.
Calculating Effect Size
Next, researchers calculate an effect size for each eligible study. The effect size is the key component of a meta-analysis because it encodes the results in a numeric value that can then be aggregated. Examples of commonly used effect size metrics include Cohen’s d (i.e., group differences) and Pearson’s r (i.e., association between two variables). The effect size metric is based on the statistical form of the results in the literature and the research question. Because studies that include more participants provide more accurate estimates of an effect than those that include fewer participants, it is important to also calculate the precision of the effect size (e.g., standard error).
Meta-analysis software guides researchers through the calculation process by requesting the necessary information for the specified effect size metric. I have identified some potentially useful resources and programs below. Although meta-analysis software makes effect size calculations simple, it is good practice for researchers to understand what computations are being used.
The effect size and precision of each individual study are aggregated into a summary statistic, which can be done with meta-analysis software. Researchers should confirm that the effect sizes are independent of each other (i.e., no overlap in participants). Additionally, researchers must select either a fixed effects model (i.e., assumes all studies share one true effect size) or a random effects model (i.e., assumes the true effect size varies among studies). The random effects model is typically preferred when the studies have been conducted using different methodologies. Depending on the software, additional specifications or adjustments may be possible.
During analysis, the effect sizes of the included studies are weighted by their precision (e.g., inverse of the sampling error variance) and the mean is calculated. The mean effect size represents the direction and/or magnitude of the effect summarized across all eligible studies. This statistic is typically accompanied by an estimate of its precision (e.g., confidence interval) and p -value representing statistical significance. Forest plots are a common way of displaying meta-analysis results.
Depending on the situation, follow-up analyses may be advised. Researchers can quantify heterogeneity (e.g., Q, t 2 , I 2 ), which is a measure of the variation among the effect sizes of included studies. Moderator variables, such as the quality of the studies or age of participants, may be included to examine sources of heterogeneity. Because published studies may be biased towards significant effects, it is important to evaluate the impact of publication bias (e.g., funnel plot, Rosenthal’s Fail-safe N ). Sensitivity analysis can indicate how the results of the meta-analysis would change if one study were excluded from the analysis.
If properly conducted and clearly documented, meta-analyses often make significant contributions to a specific field of study and therefore stand a good chance of being published in a top-tier journal. The biggest obstacle for most researchers who attempt meta-analysis for the first time is the amount of work and organization required for proper execution, rather than their level of statistical knowledge.
Recommended Resources
Borenstein, M., Hedges, L. V., Higgins, J. P., & Rothstein, H. R. (2009). Introduction to meta-analysis . Hoboken, NJ: Wiley.
Cooper, H., Hedges, L., & Valentine, J. (2009). The handbook of research synthesis and meta-analysis (2nd ed.). New York, NY: Russell Sage Foundation.
Lipsey, M. W., & Wilson, D. B. (2001). Practical meta-analysis . Thousand Oaks, California: Sage Publications.
Rothstein, H. R., Sutton, A. J., & Borenstein, M. (2005). Publication bias in meta-analysis: Prevention, assessment, and adjustments . Hoboken, NJ: Wiley.
It is nice to see the software we developed (MetaXL) being mentioned. However, the reason we developed the software and made publicly available for free is that we disagree with an important statement in the review. This statement is “researchers must select either a fixed effects model (i.e., assumes all studies share one true effect size) or a random effects model (i.e., assumes the true effect size varies among studies)”. We developed MetaXL because we think that the random effects model is seriously flawed and should be abandoned. We implemented in MetaXL two additional models, the Inverse Variance heterogeneity model and the Quality Effects model, both meant to be used in case of heterogeneity. More details are in the User Guide, available from the Epigear website.
Thank you very much! The article really helped me to start understanding what meta-analysis is about
thank you for sharing this article; it is very helpful.But I am still confused about how to remove quickly duplicates papers without wasting time if we more than 10 000 papers?
Not being one to blow my own horn all the time, but I would like to suggest that you may want to take a look at a web based application I wrote that conducts a Hunter-Schmidt type meta-analysis. The Meta-Analysis is very easy to use and corrects for sampling and error variance due to reliability. It also exports the results in excel format. You can also export the dataset effect sizes (r, d, and z), sample sizes and reliability information in excel as well.
http://www.lyonsmorris.com/lyons/MaCalc/index.cfm
APS regularly opens certain online articles for discussion on our website. Effective February 2021, you must be a logged-in APS member to post comments. By posting a comment, you agree to our Community Guidelines and the display of your profile information, including your name and affiliation. Any opinions, findings, conclusions, or recommendations present in article comments are those of the writers and do not necessarily reflect the views of APS or the article’s author. For more information, please see our Community Guidelines .
Please login with your APS account to comment.
About the Author
Laura C. Wilson is an Assistant Professor in the Psychology Department at the University of Mary Washington. She earned a PhD in Clinical Psychology from Virginia Tech and MA in General/Experimental Psychology from The College of William & Mary. Her main area of expertise is post-trauma functioning, particularly in survivors of sexual violence or mass trauma (e.g., terrorism, mass shootings, combat). She also has interest in predictors of violence and aggression, including psychophysiological and personality factors.

Careers Up Close: Joel Anderson on Gender and Sexual Prejudices, the Freedoms of Academic Research, and the Importance of Collaboration
Joel Anderson, a senior research fellow at both Australian Catholic University and La Trobe University, researches group processes, with a specific interest on prejudice, stigma, and stereotypes.

Experimental Methods Are Not Neutral Tools
Ana Sofia Morais and Ralph Hertwig explain how experimental psychologists have painted too negative a picture of human rationality, and how their pessimism is rooted in a seemingly mundane detail: methodological choices.
APS Fellows Elected to SEP
In addition, an APS Rising Star receives the society’s Early Investigator Award.
Privacy Overview
What is Meta-Analysis? Definition, Research & Examples
Appinio Research · 01.02.2024 · 39min read
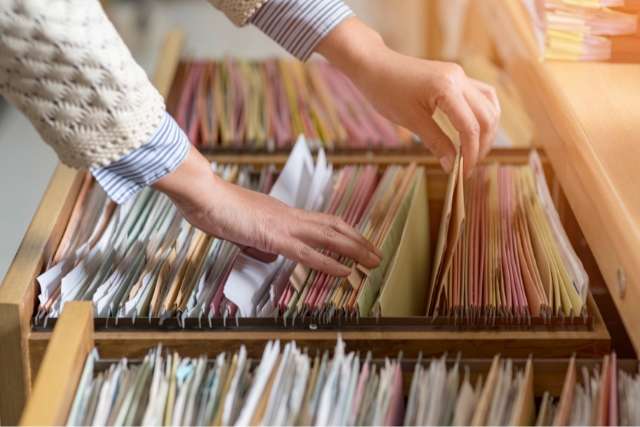
Are you looking to harness the power of data and uncover meaningful insights from a multitude of research studies? In a world overflowing with information, meta-analysis emerges as a guiding light, offering a systematic and quantitative approach to distilling knowledge from a sea of research.
This guide will demystify the art and science of meta-analysis, walking you through the process, from defining your research question to interpreting the results. Whether you're an academic researcher, a policymaker, or a curious mind eager to explore the depths of data, this guide will equip you with the tools and understanding needed to undertake robust and impactful meta-analyses.
What is a Meta Analysis?
Meta-analysis is a quantitative research method that involves the systematic synthesis and statistical analysis of data from multiple individual studies on a particular topic or research question. It aims to provide a comprehensive and robust summary of existing evidence by pooling the results of these studies, often leading to more precise and generalizable conclusions.
The primary purpose of meta-analysis is to:
- Quantify Effect Sizes: Determine the magnitude and direction of an effect or relationship across studies.
- Evaluate Consistency: Assess the consistency of findings among studies and identify sources of heterogeneity.
- Enhance Statistical Power: Increase the statistical power to detect significant effects by combining data from multiple studies.
- Generalize Results: Provide more generalizable results by analyzing a more extensive and diverse sample of participants or contexts.
- Examine Subgroup Effects: Explore whether the effect varies across different subgroups or study characteristics.
Importance of Meta-Analysis
Meta-analysis plays a crucial role in scientific research and evidence-based decision-making. Here are key reasons why meta-analysis is highly valuable:
- Enhanced Precision: By pooling data from multiple studies, meta-analysis provides a more precise estimate of the effect size, reducing the impact of random variation.
- Increased Statistical Power: The combination of numerous studies enhances statistical power, making it easier to detect small but meaningful effects.
- Resolution of Inconsistencies: Meta-analysis can help resolve conflicting findings in the literature by systematically analyzing and synthesizing evidence.
- Identification of Moderators: It allows for the identification of factors that may moderate the effect, helping to understand when and for whom interventions or treatments are most effective.
- Evidence-Based Decision-Making: Policymakers, clinicians, and researchers use meta-analysis to inform evidence-based decision-making, leading to more informed choices in healthcare , education, and other fields.
- Efficient Use of Resources: Meta-analysis can guide future research by identifying gaps in knowledge, reducing duplication of efforts, and directing resources to areas with the most significant potential impact.
Types of Research Questions Addressed
Meta-analysis can address a wide range of research questions across various disciplines. Some common types of research questions that meta-analysis can tackle include:
- Treatment Efficacy: Does a specific medical treatment, therapy, or intervention have a significant impact on patient outcomes or symptoms?
- Intervention Effectiveness: How effective are educational programs, training methods, or interventions in improving learning outcomes or skills?
- Risk Factors and Associations: What are the associations between specific risk factors, such as smoking or diet, and the likelihood of developing certain diseases or conditions?
- Impact of Policies: What is the effect of government policies, regulations, or interventions on social, economic, or environmental outcomes?
- Psychological Constructs: How do psychological constructs, such as self-esteem, anxiety, or motivation, influence behavior or mental health outcomes?
- Comparative Effectiveness: Which of two or more competing interventions or treatments is more effective for a particular condition or population?
- Dose-Response Relationships: Is there a dose-response relationship between exposure to a substance or treatment and the likelihood or severity of an outcome?
Meta-analysis is a versatile tool that can provide valuable insights into a wide array of research questions, making it an indispensable method in evidence synthesis and knowledge advancement.
Meta-Analysis vs. Systematic Review
In evidence synthesis and research aggregation, meta-analysis and systematic reviews are two commonly used methods, each serving distinct purposes while sharing some similarities. Let's explore the differences and similarities between these two approaches.
Meta-Analysis
- Purpose: Meta-analysis is a statistical technique used to combine and analyze quantitative data from multiple individual studies that address the same research question. The primary aim of meta-analysis is to provide a single summary effect size that quantifies the magnitude and direction of an effect or relationship across studies.
- Data Synthesis: In meta-analysis, researchers extract and analyze numerical data, such as means, standard deviations, correlation coefficients, or odds ratios, from each study. These effect size estimates are then combined using statistical methods to generate an overall effect size and associated confidence interval.
- Quantitative: Meta-analysis is inherently quantitative, focusing on numerical data and statistical analyses to derive a single effect size estimate.
- Main Outcome: The main outcome of a meta-analysis is the summary effect size, which provides a quantitative estimate of the research question's answer.
Systematic Review
- Purpose: A systematic review is a comprehensive and structured overview of the available evidence on a specific research question. While systematic reviews may include meta-analysis, their primary goal is to provide a thorough and unbiased summary of the existing literature.
- Data Synthesis: Systematic reviews involve a meticulous process of literature search, study selection, data extraction, and quality assessment. Researchers may narratively synthesize the findings, providing a qualitative summary of the evidence.
- Qualitative: Systematic reviews are often qualitative in nature, summarizing and synthesizing findings in a narrative format. They do not always involve statistical analysis .
- Main Outcome: The primary outcome of a systematic review is a comprehensive narrative summary of the existing evidence. While some systematic reviews include meta-analyses, not all do so.
Key Differences
- Nature of Data: Meta-analysis primarily deals with quantitative data and statistical analysis , while systematic reviews encompass both quantitative and qualitative data, often presenting findings in a narrative format.
- Focus on Effect Size: Meta-analysis focuses on deriving a single, quantitative effect size estimate, whereas systematic reviews emphasize providing a comprehensive overview of the literature, including study characteristics, methodologies, and key findings.
- Synthesis Approach: Meta-analysis is a quantitative synthesis method, while systematic reviews may use both quantitative and qualitative synthesis approaches.
Commonalities
- Structured Process: Both meta-analyses and systematic reviews follow a structured and systematic process for literature search, study selection, data extraction, and quality assessment.
- Evidence-Based: Both approaches aim to provide evidence-based answers to specific research questions, offering valuable insights for decision-making in various fields.
- Transparency: Both meta-analyses and systematic reviews prioritize transparency and rigor in their methodologies to minimize bias and enhance the reliability of their findings.
While meta-analysis and systematic reviews share the overarching goal of synthesizing research evidence, they differ in their approach and main outcomes. Meta-analysis is quantitative, focusing on effect sizes, while systematic reviews provide comprehensive overviews, utilizing both quantitative and qualitative data to summarize the literature. Depending on the research question and available data, one or both of these methods may be employed to provide valuable insights for evidence-based decision-making.
How to Conduct a Meta-Analysis?
Planning a meta-analysis is a critical phase that lays the groundwork for a successful and meaningful study. We will explore each component of the planning process in more detail, ensuring you have a solid foundation before diving into data analysis.
How to Formulate Research Questions?
Your research questions are the guiding compass of your meta-analysis. They should be precise and tailored to the topic you're investigating. To craft effective research questions:
- Clearly Define the Problem: Start by identifying the specific problem or topic you want to address through meta-analysis.
- Specify Key Variables: Determine the essential variables or factors you'll examine in the included studies.
- Frame Hypotheses: If applicable, create clear hypotheses that your meta-analysis will test.
For example, if you're studying the impact of a specific intervention on patient outcomes, your research question might be: "What is the effect of Intervention X on Patient Outcome Y in published clinical trials?"
Eligibility Criteria
Eligibility criteria define the boundaries of your meta-analysis. By establishing clear criteria, you ensure that the studies you include are relevant and contribute to your research objectives. Key considerations for eligibility criteria include:
- Study Types: Decide which types of studies will be considered (e.g., randomized controlled trials, cohort studies, case-control studies).
- Publication Time Frame: Specify the publication date range for included studies.
- Language: Determine whether studies in languages other than your primary language will be included.
- Geographic Region: If relevant, define any geographic restrictions.
Your eligibility criteria should strike a balance between inclusivity and relevance. Excluding certain studies based on valid criteria ensures the quality and relevance of the data you analyze.
Search Strategy
A robust search strategy is fundamental to identifying all relevant studies. To create an effective search strategy:
- Select Databases: Choose appropriate databases that cover your research area (e.g., PubMed, Scopus, Web of Science).
- Keywords and Search Terms: Develop a comprehensive list of relevant keywords and search terms related to your research questions.
- Search Filters: Utilize search filters and Boolean operators (AND, OR) to refine your search queries.
- Manual Searches: Consider conducting hand-searches of key journals and reviewing the reference lists of relevant studies for additional sources.
Remember that the goal is to cast a wide net while maintaining precision to capture all relevant studies.
Data Extraction
Data extraction is the process of systematically collecting information from each selected study. It involves retrieving key data points, including:
- Study Characteristics: Author(s), publication year, study design, sample size, duration, and location.
- Outcome Data: Effect sizes, standard errors, confidence intervals, p-values, and any other relevant statistics.
- Methodological Details: Information on study quality, risk of bias, and potential sources of heterogeneity.
Creating a standardized data extraction form is essential to ensure consistency and accuracy throughout this phase. Spreadsheet software, such as Microsoft Excel, is commonly used for data extraction.
Quality Assessment
Assessing the quality of included studies is crucial to determine their reliability and potential impact on your meta-analysis. Various quality assessment tools and checklists are available, depending on the study design. Some commonly used tools include:
- Newcastle-Ottawa Scale: Used for assessing the quality of non-randomized studies (e.g., cohort, case-control studies).
- Cochrane Risk of Bias Tool: Designed for evaluating randomized controlled trials.
Quality assessment typically involves evaluating aspects such as study design, sample size, data collection methods , and potential biases. This step helps you weigh the contribution of each study to the overall analysis.
How to Conduct a Literature Review?
Conducting a thorough literature review is a critical step in the meta-analysis process. We will explore the essential components of a literature review, from designing a comprehensive search strategy to establishing clear inclusion and exclusion criteria and, finally, the study selection process.
Comprehensive Search
To ensure the success of your meta-analysis, it's imperative to cast a wide net when searching for relevant studies. A comprehensive search strategy involves:
- Selecting Relevant Databases: Identify databases that cover your research area comprehensively, such as PubMed, Scopus, Web of Science, or specialized databases specific to your field.
- Creating a Keyword List: Develop a list of relevant keywords and search terms related to your research questions. Think broadly and consider synonyms, acronyms, and variations.
- Using Boolean Operators: Utilize Boolean operators (AND, OR) to combine keywords effectively and refine your search.
- Applying Filters: Employ search filters (e.g., publication date range, study type) to narrow down results based on your eligibility criteria.
Remember that the goal is to leave no relevant stone unturned, as missing key studies can introduce bias into your meta-analysis.
Inclusion and Exclusion Criteria
Clearly defined inclusion and exclusion criteria are the gatekeepers of your meta-analysis. These criteria ensure that the studies you include meet your research objectives and maintain the quality of your analysis. Consider the following factors when establishing criteria:
- Study Types: Determine which types of studies are eligible for inclusion (e.g., randomized controlled trials, observational studies, case reports).
- Publication Time Frame: Specify the time frame within which studies must have been published.
- Language: Decide whether studies in languages other than your primary language will be included or excluded.
- Geographic Region: If applicable, define any geographic restrictions.
- Relevance to Research Questions: Ensure that selected studies align with your research questions and objectives.
Your inclusion and exclusion criteria should strike a balance between inclusivity and relevance. Rigorous criteria help maintain the quality and applicability of the studies included in your meta-analysis.
Study Selection Process
The study selection process involves systematically screening and evaluating each potential study to determine whether it meets your predefined inclusion criteria. Here's a step-by-step guide:
- Screen Titles and Abstracts: Begin by reviewing the titles and abstracts of the retrieved studies. Exclude studies that clearly do not meet your inclusion criteria.
- Full-Text Assessment: Assess the full text of potentially relevant studies to confirm their eligibility. Pay attention to study design, sample size, and other specific criteria.
- Data Extraction: For studies that meet your criteria, extract the necessary data, including study characteristics, effect sizes, and other relevant information.
- Record Exclusions: Keep a record of the reasons for excluding studies. This transparency is crucial for the reproducibility of your meta-analysis.
- Resolve Discrepancies: If multiple reviewers are involved, resolve any disagreements through discussion or a third-party arbitrator.
Maintaining a clear and organized record of your study selection process is essential for transparency and reproducibility. Software tools like EndNote or Covidence can facilitate the screening and data extraction process.
By following these systematic steps in conducting a literature review, you ensure that your meta-analysis is built on a solid foundation of relevant and high-quality studies.
Data Extraction and Management
As you progress in your meta-analysis journey, the data extraction and management phase becomes paramount. We will delve deeper into the critical aspects of this phase, including the data collection process, data coding and transformation, and how to handle missing data effectively.
Data Collection Process
The data collection process is the heart of your meta-analysis, where you systematically extract essential information from each selected study. To ensure accuracy and consistency:
- Create a Data Extraction Form: Develop a standardized data extraction form that includes all the necessary fields for collecting relevant data. This form should align with your research questions and inclusion criteria.
- Data Extractors: Assign one or more reviewers to extract data from the selected studies. Ensure they are familiar with the form and the specific data points to collect.
- Double-Check Accuracy: Implement a verification process where a second reviewer cross-checks a random sample of data extractions to identify discrepancies or errors.
- Extract All Relevant Information: Collect data on study characteristics, participant demographics, outcome measures, effect sizes, confidence intervals, and any additional information required for your analysis.
- Maintain Consistency: Use clear guidelines and definitions for data extraction to ensure uniformity across studies.
To optimize your data collection process and streamline the extraction and management of crucial information, consider leveraging innovative solutions like Appinio . With Appinio, you can effortlessly collect real-time consumer insights, ensuring your meta-analysis benefits from the latest data trends and user perspectives. Ready to learn more? Book a demo today and unlock a world of data-driven possibilities!

Get a free demo and see the Appinio platform in action!
Data Coding and Transformation
After data collection, you may need to code and transform the extracted data to ensure uniformity and compatibility across studies. This process involves:
- Coding Categorical Variables: If studies report data differently, code categorical variables consistently . For example, ensure that categories like "male" and "female" are coded consistently across studies.
- Standardizing Units of Measurement: Convert all measurements to a common unit if studies use different measurement units. For instance, if one study reports height in inches and another in centimeters, standardize to one unit for comparability.
- Calculating Effect Sizes: Calculate effect sizes and their standard errors or variances if they are not directly reported in the studies. Common effect size measures include Cohen's d, odds ratio (OR), and hazard ratio (HR).
- Data Transformation: Transform data if necessary to meet assumptions of statistical tests. Common transformations include log transformation for skewed data or arcsine transformation for proportions.
- Heterogeneity Adjustment: Consider using transformation methods to address heterogeneity among studies, such as applying the Freeman-Tukey double arcsine transformation for proportions.
The goal of data coding and transformation is to make sure that data from different studies are compatible and can be effectively synthesized during the analysis phase. Spreadsheet software like Excel or statistical software like R can be used for these tasks.
Handling Missing Data
Missing data is a common challenge in meta-analysis, and how you handle it can impact the validity and precision of your results. Strategies for handling missing data include:
- Contact Authors: If feasible, contact the authors of the original studies to request missing data or clarifications.
- Imputation: Consider using appropriate imputation methods to estimate missing values, but exercise caution and report the imputation methods used.
- Sensitivity Analysis: Conduct sensitivity analyses to assess the impact of missing data on your results by comparing the main analysis to alternative scenarios.
Remember that transparency in reporting how you handled missing data is crucial for the credibility of your meta-analysis.
By following these steps in data extraction and management, you will ensure the integrity and reliability of your meta-analysis dataset.
Meta-Analysis Example
Meta-analysis is a versatile research method that can be applied to various fields and disciplines, providing valuable insights by synthesizing existing evidence.
Example 1: Analyzing the Impact of Advertising Campaigns on Sales
Background: A market research agency is tasked with assessing the effectiveness of advertising campaigns on sales outcomes for a range of consumer products. They have access to multiple studies and reports conducted by different companies, each analyzing the impact of advertising on sales revenue.
Meta-Analysis Approach:
- Study Selection: Identify relevant studies that meet specific inclusion criteria, such as the type of advertising campaign (e.g., TV commercials, social media ads), the products examined, and the sales metrics assessed.
- Data Extraction: Collect data from each study, including details about the advertising campaign (e.g., budget, duration), sales data (e.g., revenue, units sold), and any reported effect sizes or correlations.
- Effect Size Calculation: Calculate effect sizes (e.g., correlation coefficients) based on the data provided in each study, quantifying the strength and direction of the relationship between advertising and sales.
- Data Synthesis: Employ meta-analysis techniques to combine the effect sizes from the selected studies. Compute a summary effect size and its confidence interval to estimate the overall impact of advertising on sales.
- Publication Bias Assessment: Use funnel plots and statistical tests to assess the potential presence of publication bias, ensuring that the meta-analysis results are not unduly influenced by selective reporting.
Findings: Through meta-analysis, the market research agency discovers that advertising campaigns have a statistically significant and positive impact on sales across various product categories. The findings provide evidence for the effectiveness of advertising efforts and assist companies in making data-driven decisions regarding their marketing strategies.
These examples illustrate how meta-analysis can be applied in diverse domains, from tech startups seeking to optimize user engagement to market research agencies evaluating the impact of advertising campaigns. By systematically synthesizing existing evidence, meta-analysis empowers decision-makers with valuable insights for informed choices and evidence-based strategies.
How to Assess Study Quality and Bias?
Ensuring the quality and reliability of the studies included in your meta-analysis is essential for drawing accurate conclusions. We'll show you how you can assess study quality using specific tools, evaluate potential bias, and address publication bias.
Quality Assessment Tools
Quality assessment tools provide structured frameworks for evaluating the methodological rigor of each included study. The choice of tool depends on the study design. Here are some commonly used quality assessment tools:
For Randomized Controlled Trials (RCTs):
- Cochrane Risk of Bias Tool: This tool assesses the risk of bias in RCTs based on six domains: random sequence generation, allocation concealment, blinding of participants and personnel, blinding of outcome assessment, incomplete outcome data, and selective reporting.
- Jadad Scale: A simpler tool specifically for RCTs, the Jadad Scale focuses on randomization, blinding, and the handling of withdrawals and dropouts.
For Observational Studies:
- Newcastle-Ottawa Scale (NOS): The NOS assesses the quality of cohort and case-control studies based on three categories: selection, comparability, and outcome.
- ROBINS-I: Designed for non-randomized studies of interventions, the Risk of Bias in Non-randomized Studies of Interventions tool evaluates bias in domains such as confounding, selection bias, and measurement bias.
- MINORS: The Methodological Index for Non-Randomized Studies (MINORS) assesses non-comparative studies and includes items related to study design, reporting, and statistical analysis.
Bias Assessment
Evaluating potential sources of bias is crucial to understanding the limitations of the included studies. Common sources of bias include:
- Selection Bias: Occurs when the selection of participants is not random or representative of the target population.
- Performance Bias: Arises when participants or researchers are aware of the treatment or intervention status, potentially influencing outcomes.
- Detection Bias: Occurs when outcome assessors are not blinded to the treatment groups.
- Attrition Bias: Results from incomplete data or differential loss to follow-up between treatment groups.
- Reporting Bias: Involves selective reporting of outcomes, where only positive or statistically significant results are published.
To assess bias, reviewers often use the quality assessment tools mentioned earlier, which include domains related to bias, or they may specifically address bias concerns in the narrative synthesis.
We'll move on to the core of meta-analysis: data synthesis. We'll explore different effect size measures, fixed-effect versus random-effects models, and techniques for assessing and addressing heterogeneity among studies.
Data Synthesis
Now that you've gathered data from multiple studies and assessed their quality, it's time to synthesize this information effectively.
Effect Size Measures
Effect size measures quantify the magnitude of the relationship or difference you're investigating in your meta-analysis. The choice of effect size measure depends on your research question and the type of data provided by the included studies. Here are some commonly used effect size measures:
Continuous Outcome Data:
- Cohen's d: Measures the standardized mean difference between two groups. It's suitable for continuous outcome variables.
- Hedges' g: Similar to Cohen's d but incorporates a correction factor for small sample sizes.
Binary Outcome Data:
- Odds Ratio (OR): Used for dichotomous outcomes, such as success/failure or presence/absence.
- Risk Ratio (RR): Similar to OR but used when the outcome is relatively common.
Time-to-Event Data:
- Hazard Ratio (HR): Used in survival analysis to assess the risk of an event occurring over time.
- Risk Difference (RD): Measures the absolute difference in event rates between two groups.
Selecting the appropriate effect size measure depends on the nature of your data and the research question. When effect sizes are not directly reported in the studies, you may need to calculate them using available data, such as means, standard deviations, and sample sizes.
Formula for Cohen's d:
d = (Mean of Group A - Mean of Group B) / Pooled Standard Deviation
Fixed-Effect vs. Random-Effects Models
In meta-analysis, you can choose between fixed-effect and random-effects models to combine the results of individual studies:
Fixed-Effect Model:
- Assumes that all included studies share a common true effect size.
- Accounts for only within-study variability ( sampling error ).
- Appropriate when studies are very similar or when there's minimal heterogeneity.
Random-Effects Model:
- Acknowledges that there may be variability in effect sizes across studies.
- Accounts for both within-study variability (sampling error) and between-study variability (real differences between studies).
- More conservative and applicable when there's substantial heterogeneity.
The choice between these models should be guided by the degree of heterogeneity observed among the included studies. If heterogeneity is significant, the random-effects model is often preferred, as it provides a more robust estimate of the overall effect.
Forest Plots
Forest plots are graphical representations commonly used in meta-analysis to display the results of individual studies along with the combined summary estimate. Key components of a forest plot include:
- Vertical Line: Represents the null effect (e.g., no difference or no effect).
- Horizontal Lines: Represent the confidence intervals for each study's effect size estimate.
- Diamond or Square: Represents the summary effect size estimate, with its width indicating the confidence interval around the summary estimate.
- Study Names: Listed on the left side of the plot, identifying each study.
Forest plots help visualize the distribution of effect sizes across studies and provide insights into the consistency and direction of the findings.
Heterogeneity Assessment
Heterogeneity refers to the variability in effect sizes among the included studies. It's important to assess and understand heterogeneity as it can impact the interpretation of your meta-analysis results. Standard methods for assessing heterogeneity include:
- Cochran's Q Test: A statistical test that assesses whether there is significant heterogeneity among the effect sizes of the included studies.
- I² Statistic: A measure that quantifies the proportion of total variation in effect sizes that is due to heterogeneity. I² values range from 0% to 100%, with higher values indicating greater heterogeneity.
Assessing heterogeneity is crucial because it informs your choice of meta-analysis model (fixed-effect vs. random-effects) and whether subgroup analyses or sensitivity analyses are warranted to explore potential sources of heterogeneity.
How to Interpret Meta-Analysis Results?
With the data synthesis complete, it's time to make sense of the results of your meta-analysis.
Meta-Analytic Summary
The meta-analytic summary is the culmination of your efforts in data synthesis. It provides a consolidated estimate of the effect size and its confidence interval, combining the results of all included studies. To interpret the meta-analytic summary effectively:
- Effect Size Estimate: Understand the primary effect size estimate, such as Cohen's d, odds ratio, or hazard ratio, and its associated confidence interval.
- Significance: Determine whether the summary effect size is statistically significant. This is indicated when the confidence interval does not include the null value (e.g., 0 for Cohen's d or 1 for odds ratio).
- Magnitude: Assess the magnitude of the effect size. Is it large, moderate, or small, and what are the practical implications of this magnitude?
- Direction: Consider the direction of the effect. Is it in the hypothesized direction, or does it contradict the expected outcome?
- Clinical or Practical Significance: Reflect on the clinical or practical significance of the findings. Does the effect size have real-world implications?
- Consistency: Evaluate the consistency of the findings across studies. Are most studies in agreement with the summary effect size estimate, or are there outliers?
Subgroup Analyses
Subgroup analyses allow you to explore whether the effect size varies across different subgroups of studies or participants. This can help identify potential sources of heterogeneity or assess whether the intervention's effect differs based on specific characteristics. Steps for conducting subgroup analyses:
- Define Subgroups: Clearly define the subgroups you want to investigate based on relevant study characteristics (e.g., age groups, study design , intervention type).
- Analyze Subgroups: Calculate separate summary effect sizes for each subgroup and compare them to the overall summary effect.
- Assess Heterogeneity: Evaluate whether subgroup differences are statistically significant. If so, this suggests that the effect size varies significantly among subgroups.
- Interpretation: Interpret the subgroup findings in the context of your research question. Are there meaningful differences in the effect across subgroups? What might explain these differences?
Subgroup analyses can provide valuable insights into the factors influencing the overall effect size and help tailor recommendations for specific populations or conditions.
Sensitivity Analyses
Sensitivity analyses are conducted to assess the robustness of your meta-analysis results by exploring how different choices or assumptions might affect the findings. Common sensitivity analyses include:
- Exclusion of Low-Quality Studies: Repeating the meta-analysis after excluding studies with low quality or a high risk of bias.
- Changing Effect Size Measure: Re-running the analysis using a different effect size measure to assess whether the choice of measure significantly impacts the results.
- Publication Bias Adjustment: Applying methods like the trim-and-fill procedure to adjust for potential publication bias.
- Subsample Analysis: Analyzing a subset of studies based on specific criteria or characteristics to investigate their impact on the summary effect.
Sensitivity analyses help assess the robustness and reliability of your meta-analysis results, providing a more comprehensive understanding of the potential influence of various factors.
Reporting and Publication
The final stages of your meta-analysis involve preparing your findings for publication.
Manuscript Preparation
When preparing your meta-analysis manuscript, consider the following:
- Structured Format: Organize your manuscript following a structured format, including sections such as introduction, methods, results, discussion, and conclusions.
- Clarity and Conciseness: Write your findings clearly and concisely, avoiding jargon or overly technical language. Use tables and figures to enhance clarity.
- Transparent Methods: Provide detailed descriptions of your methods, including eligibility criteria, search strategy, data extraction, and statistical analysis.
- Incorporate Tables and Figures: Present your meta-analysis results using tables and forest plots to visually convey key findings.
- Interpretation: Interpret the implications of your findings, discussing the clinical or practical significance and limitations.
Transparent Reporting Guidelines
Adhering to transparent reporting guidelines ensures that your meta-analysis is transparent, reproducible, and credible. Some widely recognized guidelines include:
- PRISMA (Preferred Reporting Items for Systematic Reviews and Meta-Analyses): PRISMA provides a checklist and flow diagram for reporting systematic reviews and meta-analyses, enhancing transparency and rigor.
- MOOSE (Meta-analysis of Observational Studies in Epidemiology): MOOSE guidelines are designed for meta-analyses of observational studies and provide a framework for transparent reporting.
- ROBINS-I: If your meta-analysis involves non-randomized studies, follow the Risk Of Bias In Non-randomized Studies of Interventions guidelines for reporting.
Adhering to these guidelines ensures that your meta-analysis is transparent, reproducible, and credible. It enhances the quality of your research and aids readers and reviewers in assessing the rigor of your study.
PRISMA Statement
The PRISMA statement is a valuable resource for conducting and reporting systematic reviews and meta-analyses. Key elements of PRISMA include:
- Title: Clearly indicate that your paper is a systematic review or meta-analysis.
- Structured Abstract: Provide a structured summary of your study, including objectives, methods, results, and conclusions.
- Transparent Reporting: Follow the PRISMA checklist, which covers items such as the rationale, eligibility criteria, search strategy, data extraction, and risk of bias assessment.
- Flow Diagram: Include a flow diagram illustrating the study selection process.
By adhering to the PRISMA statement, you enhance the transparency and credibility of your meta-analysis, facilitating its acceptance for publication and aiding readers in evaluating the quality of your research.
Conclusion for Meta-Analysis
Meta-analysis is a powerful tool that allows you to combine and analyze data from multiple studies to find meaningful patterns and make informed decisions. It helps you see the bigger picture and draw more accurate conclusions than individual studies alone. Whether you're in healthcare, education, business, or any other field, the principles of meta-analysis can be applied to enhance your research and decision-making processes. Remember that conducting a successful meta-analysis requires careful planning, attention to detail, and transparency in reporting. By following the steps outlined in this guide, you can embark on your own meta-analysis journey with confidence, contributing to the advancement of knowledge and evidence-based practices in your area of interest.
How to Elevate Your Meta-Analysis With Real-Time Insights?
Introducing Appinio , the real-time market research platform that brings a new level of excitement to your meta-analysis journey. With Appinio, you can seamlessly collect your own market research data in minutes, empowering your meta-analysis with fresh, real-time consumer insights.
Here's why Appinio is your ideal partner for efficient data collection:
- From Questions to Insights in Minutes: Appinio's lightning-fast platform ensures you get the answers you need when you need them, accelerating your meta-analysis process.
- No Research PhD Required: Our intuitive platform is designed for everyone, eliminating the need for specialized research skills and putting the power of data collection in your hands.
- Global Reach, Minimal Time: With an average field time of less than 23 minutes for 1,000 respondents and access to over 90 countries, you can define precise target groups and gather data swiftly.
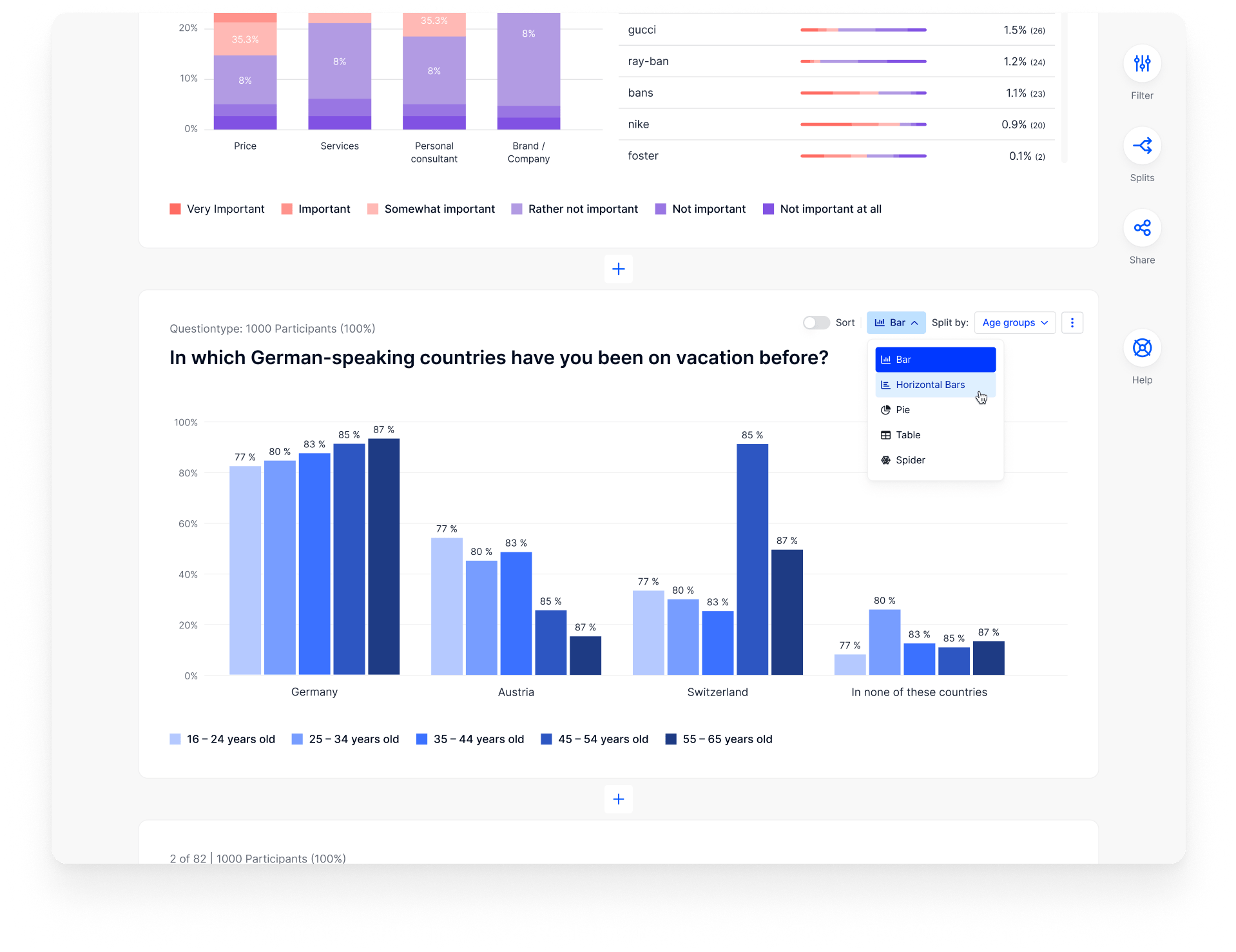
Get free access to the platform!
Join the loop 💌
Be the first to hear about new updates, product news, and data insights. We'll send it all straight to your inbox.
Get the latest market research news straight to your inbox! 💌
Wait, there's more
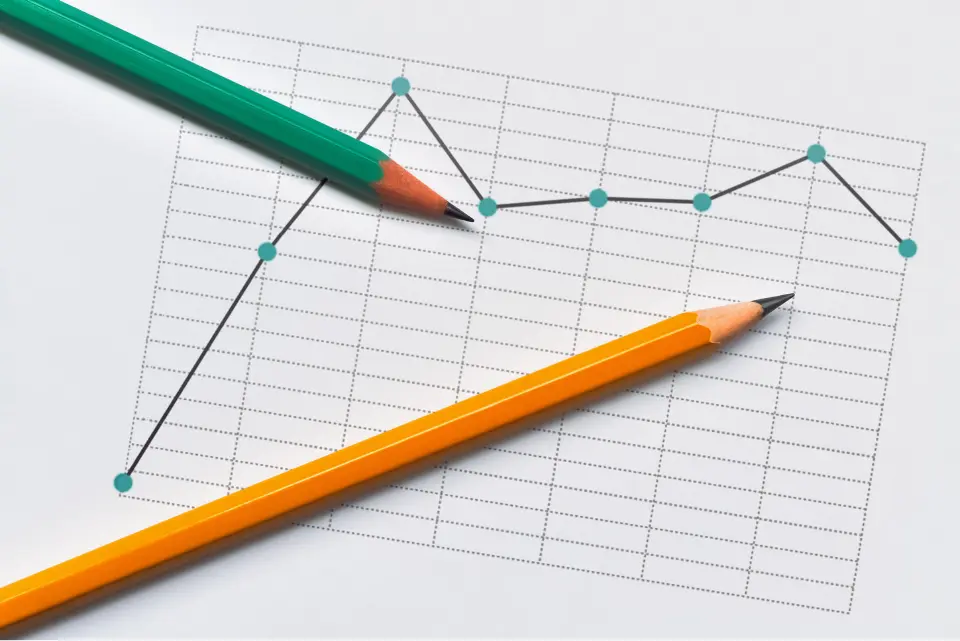
16.05.2024 | 30min read
Time Series Analysis: Definition, Types, Techniques, Examples
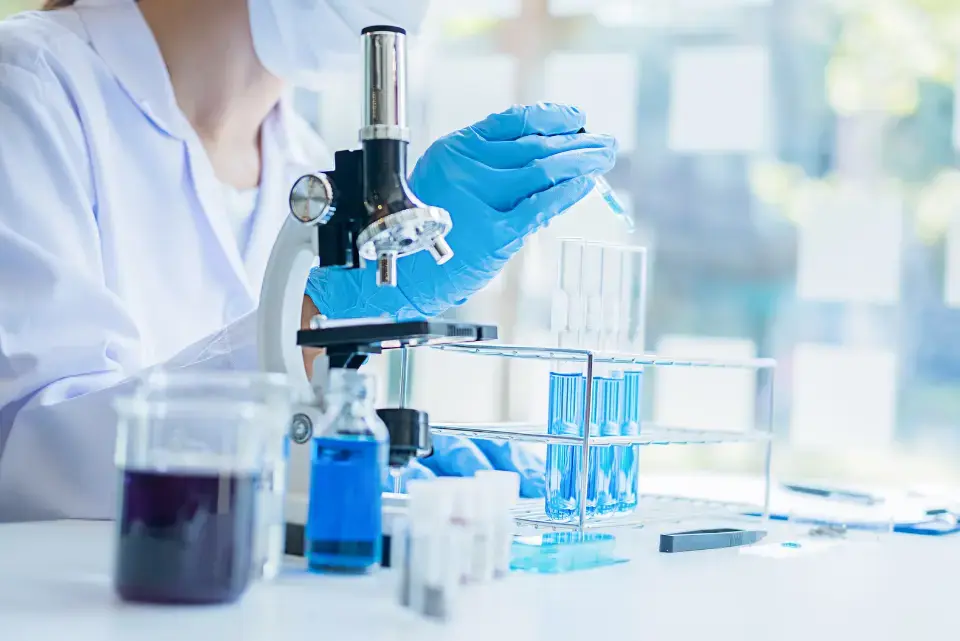
14.05.2024 | 31min read
Experimental Research: Definition, Types, Design, Examples
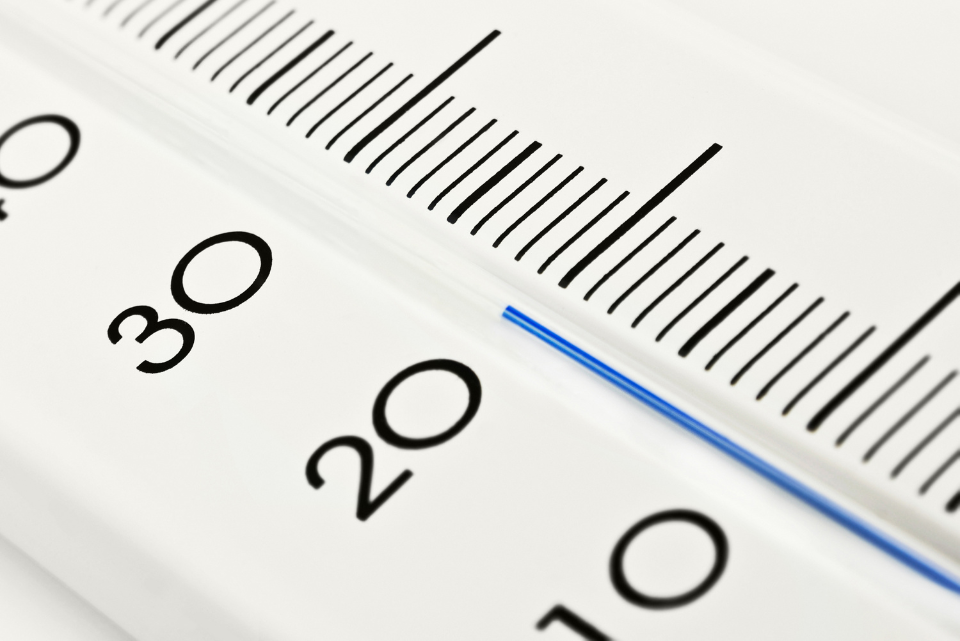
07.05.2024 | 29min read
Interval Scale: Definition, Characteristics, Examples
Understanding the Basics of Meta-Analysis and How to Read a Forest Plot: As Simple as It Gets
Affiliation.
- 1 Department of Clinical Psychopharmacology and Neurotoxicology, National Institute of Mental Health and Neurosciences, Bangalore, India. [email protected].
- PMID: 33027562
- DOI: 10.4088/JCP.20f13698
The results of research on a specific question differ across studies, some to a small extent and some to a large extent. Meta-analysis is a way to statistically combine and summarize the results of different studies so as to obtain a pooled or summary estimate that may better represent what is true in the population. Meta-analysis can be conducted for a variety of statistics, including means, mean differences, standardized mean differences, proportions, differences in proportions, relative risks, odds ratios, and others. The results of meta-analysis are presented in forest plots. This article explains why meta-analysis may be necessary, how a systematic review is conducted to identify studies for meta-analysis, and how to interpret the various elements in a forest plot. Brief discussions are provided about important concepts relevant to meta-analysis, including heterogeneity, subgroup analyses, sensitivity analyses, fixed effect and random effects meta-analyses, and the detection of publication bias. Other procedures briefly explained include meta-regression analysis, pooled analysis, individual participant data meta-analysis, and network meta-analysis. The limitations of meta-analysis are also discussed.
© Copyright 2020 Physicians Postgraduate Press, Inc.
- Data Interpretation, Statistical*
- Meta-Analysis as Topic*
- Publication Bias
- Regression Analysis
- Statistics as Topic
- Systematic Reviews as Topic
Log in using your username and password
- Search More Search for this keyword Advanced search
- Latest content
- Current issue
- Write for Us
- BMJ Journals More You are viewing from: Google Indexer
You are here
- Volume 16, Issue 1

What is meta-analysis?
- Article Text
- Article info
- Citation Tools
- Rapid Responses
- Article metrics

- Allison Shorten 1 ,
- Brett Shorten 2
- 1 School of Nursing , Yale University , New Haven, Connecticut , USA
- 2 Informed Health Choices Trust, Wollongong, New South Wales, Australia
- Correspondence to : Dr Allison Shorten Yale University School of Nursing, 100 Church Street South, PO Box 9740, New Haven, CT 06536, USA; allison.shorten{at}yale.edu
https://doi.org/10.1136/eb-2012-101118
Statistics from Altmetric.com
Request permissions.
If you wish to reuse any or all of this article please use the link below which will take you to the Copyright Clearance Center’s RightsLink service. You will be able to get a quick price and instant permission to reuse the content in many different ways.
When clinicians begin their search for the best available evidence to inform decision-making, they are usually directed to the top of the ‘evidence pyramid’ to find out whether a systematic review and meta-analysis have been conducted. The Cochrane Library 1 is fast filling with systematic reviews and meta-analyses that aim to answer important clinical questions and provide the most reliable evidence to inform practice and research. So what is meta-analysis and how can it contribute to practice?
The Five-step process
There is debate about the best practice for meta-analysis, however there are five common steps.
Step 1: the research question
A clinical research question is identified and a hypothesis proposed. The likely clinical significance is explained and the study design and analytical plan are justified.
Step 2: systematic review
A systematic review (SR) is specifically designed to address the research question and conducted to identify all studies considered to be both relevant and of sufficiently good quality to warrant inclusion. Often, only studies published in established journals are identified, but identification of ‘unpublished’ data is important to avoid ‘publication bias’ or exclusion of studies with negative findings. 4 Some meta-analyses only consider randomised control trials (RCTs) in the quest for highest quality evidence. Other types of ‘experimental’ and ‘quasi-experimental’ studies may be included if they satisfy the defined inclusion/exclusion criteria.
Step 3: data extraction
Once studies are selected for inclusion in the meta-analysis, summary data or outcomes are extracted from each study. In addition, sample sizes and measures of data variability for both intervention and control groups are required. Depending on the study and the research question, outcome measures could include numerical measures or categorical measures. For example, differences in scores on a questionnaire or differences in a measurement level such as blood pressure would be reported as a numerical mean. However, differences in the likelihood of being in one category versus another (eg, vaginal birth versus cesarean birth) are usually reported in terms of risk measures such as OR or relative risk (RR).
Step 4: standardisation and weighting studies
Having assembled all the necessary data, the fourth step is to calculate appropriate summary measures from each study for further analysis. These measures are usually called Effect Sizes and represent the difference in average scores between intervention and control groups. For example, the difference in change in blood pressure between study participants who used drug X compared with participants who used a placebo. Since units of measurement typically vary across included studies, they usually need to be ‘standardised’ in order to produce comparable estimates of this effect. When different outcome measures are used, such as when researchers use different tests, standardisation is imperative. Standardisation is achieved by taking, for each study, the mean score for the intervention group, subtracting the mean for the control group and dividing this result by the appropriate measure of variability in that data set.
The results of some studies need to carry more weight than others. Larger studies (as measured by sample sizes) are thought to produce more precise effect size estimates than smaller studies. Second, studies with less data variability, for example, smaller SD or narrower CIs are often regarded as ‘better quality’ in study design. A weighting statistic that seeks to incorporate both these factors, known as inverse variance , is commonly used.
Step 5: final estimates of effect
The final stage is to select and apply an appropriate model to compare Effect Sizes across different studies. The most common models used are Fixed Effects and Random Effects models. Fixed Effects models are based on the ‘assumption that every study is evaluating a common treatment effect’. 5 This means that the assumption is that all studies would estimate the same Effect Size were it not for different levels of sample variability across different studies. In contrast, the Random Effects model ‘assumes that the true treatment effects in the individual studies may be different from each other’. 5 and attempts to allow for this additional source of interstudy variation in Effect Sizes . Whether this latter source of variability is likely to be important is often assessed within the meta-analysis by testing for ‘heterogeneity’.
Forest plot
The final estimates from a meta-analysis are often graphically reported in the form of a ‘Forest Plot’.
In the hypothetical Forest Plot shown in figure 1 , for each study, a horizontal line indicates the standardised Effect Size estimate (the rectangular box in the centre of each line) and 95% CI for the risk ratio used. For each of the studies, drug X reduced the risk of death (the risk ratio is less than 1.0). However, the first study was larger than the other two (the size of the boxes represents the relative weights calculated by the meta-analysis). Perhaps, because of this, the estimates for the two smaller studies were not statistically significant (the lines emanating from their boxes include the value of 1). When all the three studies were combined in the meta-analysis, as represented by the diamond, we get a more precise estimate of the effect of the drug, where the diamond represents both the combined risk ratio estimate and the limits of the 95% CI.
- Download figure
- Open in new tab
- Download powerpoint
Hypothetical Forest Plot
Relevance to practice and research
Many Evidence Based Nursing commentaries feature recently published systematic review and meta-analysis because they not only bring new insight or strength to recommendations about the most effective healthcare practices but they also identify where future research should be directed to bridge the gaps or limitations in current evidence. The strength of conclusions from meta-analysis largely depends on the quality of the data available for synthesis. This reflects the quality of individual studies and the systematic review. Meta-analysis does not magically resolve the problem of underpowered or poorly designed studies and clinicians can be frustrated to find that even when a meta-analysis has been conducted, all that the researchers can conclude is that the evidence is weak, there is uncertainty about the effects of treatment and that higher quality research is needed to better inform practice. This is still an important finding and can inform our practice and challenge us to fill the evidence gaps with better quality research in the future.
- ↵ The Cochrane Library . http://www.thecochranelibrary.com/view/0/index.html (accessed 23 Oct 2012).
- Davey Smith G
- Davey Smoth G
- Higgins JPT ,
Competing interests None.
Read the full text or download the PDF:
Cochrane UK is now closed
Due to changes in NIHR funding in evidence synthesis, Cochrane UK is now closed. We are so grateful to all our contributors and supporters.
If you would like to get involved with Cochrane, please visit the Join Cochrane pages
To learn more about Cochrane, visit Cochrane's homepage
If you would like to propose a topic for a Cochrane Review, please see our instructions for authors
If you have any queries, please contact Cochrane Support
Cochrane UK
Meta-analysis: what, why, and how.
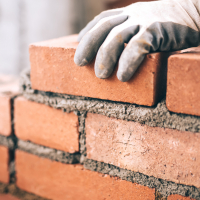
This is an excerpt from a blog originally published on Students 4 Best Evidence
What is a meta-analysis?
Meta-analysis is a statistical technique for combining data from multiple studies on a particular topic.
Meta-analyses play a fundamental role in evidence-based healthcare. Compared to other study designs (such as randomized controlled trials or cohort studies), the meta-analysis comes in at the top of the evidence-based medicine pyramid. This is a pyramid which enables us to weigh up the different levels of evidence available to us. As we go up the pyramid, each level of evidence is less subject to bias than the level below it. Therefore, meta-analyses can be seen as the pinnacle of evidence-based medicine (1).
Meta-analyses began to appear as a leading part of research in the late 70s. Since then, they have become a common way for synthesizing evidence and summarizing the results of individual studies (2).
Read the full article here
Thank you for visiting nature.com. You are using a browser version with limited support for CSS. To obtain the best experience, we recommend you use a more up to date browser (or turn off compatibility mode in Internet Explorer). In the meantime, to ensure continued support, we are displaying the site without styles and JavaScript.
- View all journals
- Explore content
- About the journal
- Publish with us
- Sign up for alerts
- Published: 08 May 2024
A meta-analysis on global change drivers and the risk of infectious disease
- Michael B. Mahon ORCID: orcid.org/0000-0002-9436-2998 1 , 2 na1 ,
- Alexandra Sack 1 , 3 na1 ,
- O. Alejandro Aleuy 1 ,
- Carly Barbera 1 ,
- Ethan Brown ORCID: orcid.org/0000-0003-0827-4906 1 ,
- Heather Buelow ORCID: orcid.org/0000-0003-3535-4151 1 ,
- David J. Civitello 4 ,
- Jeremy M. Cohen ORCID: orcid.org/0000-0001-9611-9150 5 ,
- Luz A. de Wit ORCID: orcid.org/0000-0002-3045-4017 1 ,
- Meghan Forstchen 1 , 3 ,
- Fletcher W. Halliday 6 ,
- Patrick Heffernan 1 ,
- Sarah A. Knutie 7 ,
- Alexis Korotasz 1 ,
- Joanna G. Larson ORCID: orcid.org/0000-0002-1401-7837 1 ,
- Samantha L. Rumschlag ORCID: orcid.org/0000-0003-3125-8402 1 , 2 ,
- Emily Selland ORCID: orcid.org/0000-0002-4527-297X 1 , 3 ,
- Alexander Shepack 1 ,
- Nitin Vincent ORCID: orcid.org/0000-0002-8593-1116 1 &
- Jason R. Rohr ORCID: orcid.org/0000-0001-8285-4912 1 , 2 , 3 na1
Nature ( 2024 ) Cite this article
5275 Accesses
600 Altmetric
Metrics details
- Infectious diseases
Anthropogenic change is contributing to the rise in emerging infectious diseases, which are significantly correlated with socioeconomic, environmental and ecological factors 1 . Studies have shown that infectious disease risk is modified by changes to biodiversity 2 , 3 , 4 , 5 , 6 , climate change 7 , 8 , 9 , 10 , 11 , chemical pollution 12 , 13 , 14 , landscape transformations 15 , 16 , 17 , 18 , 19 , 20 and species introductions 21 . However, it remains unclear which global change drivers most increase disease and under what contexts. Here we amassed a dataset from the literature that contains 2,938 observations of infectious disease responses to global change drivers across 1,497 host–parasite combinations, including plant, animal and human hosts. We found that biodiversity loss, chemical pollution, climate change and introduced species are associated with increases in disease-related end points or harm, whereas urbanization is associated with decreases in disease end points. Natural biodiversity gradients, deforestation and forest fragmentation are comparatively unimportant or idiosyncratic as drivers of disease. Overall, these results are consistent across human and non-human diseases. Nevertheless, context-dependent effects of the global change drivers on disease were found to be common. The findings uncovered by this meta-analysis should help target disease management and surveillance efforts towards global change drivers that increase disease. Specifically, reducing greenhouse gas emissions, managing ecosystem health, and preventing biological invasions and biodiversity loss could help to reduce the burden of plant, animal and human diseases, especially when coupled with improvements to social and economic determinants of health.
This is a preview of subscription content, access via your institution
Access options
Access Nature and 54 other Nature Portfolio journals
Get Nature+, our best-value online-access subscription
24,99 € / 30 days
cancel any time
Subscribe to this journal
Receive 51 print issues and online access
185,98 € per year
only 3,65 € per issue
Buy this article
- Purchase on Springer Link
- Instant access to full article PDF
Prices may be subject to local taxes which are calculated during checkout
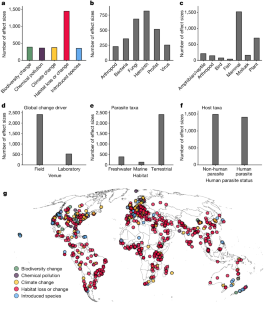
Similar content being viewed by others
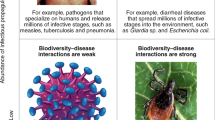
Towards common ground in the biodiversity–disease debate
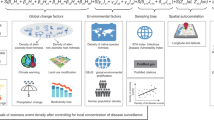
Biological invasions facilitate zoonotic disease emergences
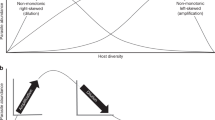
Measuring the shape of the biodiversity-disease relationship across systems reveals new findings and key gaps
Data availability.
All the data for this Article have been deposited at Zenodo ( https://doi.org/10.5281/zenodo.8169979 ) 52 and GitHub ( https://github.com/mahonmb/GCDofDisease ) 53 .
Code availability
All the code for this Article has been deposited at Zenodo ( https://doi.org/10.5281/zenodo.8169979 ) 52 and GitHub ( https://github.com/mahonmb/GCDofDisease ) 53 . R markdown is provided in Supplementary Data 1 .
Jones, K. E. et al. Global trends in emerging infectious diseases. Nature 451 , 990–994 (2008).
Article ADS CAS PubMed PubMed Central Google Scholar
Civitello, D. J. et al. Biodiversity inhibits parasites: broad evidence for the dilution effect. Proc. Natl Acad. Sci USA 112 , 8667–8671 (2015).
Halliday, F. W., Rohr, J. R. & Laine, A.-L. Biodiversity loss underlies the dilution effect of biodiversity. Ecol. Lett. 23 , 1611–1622 (2020).
Article PubMed PubMed Central Google Scholar
Rohr, J. R. et al. Towards common ground in the biodiversity–disease debate. Nat. Ecol. Evol. 4 , 24–33 (2020).
Article PubMed Google Scholar
Johnson, P. T. J., Ostfeld, R. S. & Keesing, F. Frontiers in research on biodiversity and disease. Ecol. Lett. 18 , 1119–1133 (2015).
Keesing, F. et al. Impacts of biodiversity on the emergence and transmission of infectious diseases. Nature 468 , 647–652 (2010).
Cohen, J. M., Sauer, E. L., Santiago, O., Spencer, S. & Rohr, J. R. Divergent impacts of warming weather on wildlife disease risk across climates. Science 370 , eabb1702 (2020).
Article CAS PubMed PubMed Central Google Scholar
Rohr, J. R. et al. Frontiers in climate change-disease research. Trends Ecol. Evol. 26 , 270–277 (2011).
Altizer, S., Ostfeld, R. S., Johnson, P. T. J., Kutz, S. & Harvell, C. D. Climate change and infectious diseases: from evidence to a predictive framework. Science 341 , 514–519 (2013).
Article ADS CAS PubMed Google Scholar
Rohr, J. R. & Cohen, J. M. Understanding how temperature shifts could impact infectious disease. PLoS Biol. 18 , e3000938 (2020).
Carlson, C. J. et al. Climate change increases cross-species viral transmission risk. Nature 607 , 555–562 (2022).
Halstead, N. T. et al. Agrochemicals increase risk of human schistosomiasis by supporting higher densities of intermediate hosts. Nat. Commun. 9 , 837 (2018).
Article ADS PubMed PubMed Central Google Scholar
Martin, L. B., Hopkins, W. A., Mydlarz, L. D. & Rohr, J. R. The effects of anthropogenic global changes on immune functions and disease resistance. Ann. N. Y. Acad. Sci. 1195 , 129–148 (2010).
Rumschlag, S. L. et al. Effects of pesticides on exposure and susceptibility to parasites can be generalised to pesticide class and type in aquatic communities. Ecol. Lett. 22 , 962–972 (2019).
Allan, B. F., Keesing, F. & Ostfeld, R. S. Effect of forest fragmentation on Lyme disease risk. Conserv. Biol. 17 , 267–272 (2003).
Article Google Scholar
Brearley, G. et al. Wildlife disease prevalence in human‐modified landscapes. Biol. Rev. 88 , 427–442 (2013).
Rohr, J. R. et al. Emerging human infectious diseases and the links to global food production. Nat. Sustain. 2 , 445–456 (2019).
Bradley, C. A. & Altizer, S. Urbanization and the ecology of wildlife diseases. Trends Ecol. Evol. 22 , 95–102 (2007).
Allen, T. et al. Global hotspots and correlates of emerging zoonotic diseases. Nat. Commun. 8 , 1124 (2017).
Sokolow, S. H. et al. Ecological and socioeconomic factors associated with the human burden of environmentally mediated pathogens: a global analysis. Lancet Planet. Health 6 , e870–e879 (2022).
Young, H. S., Parker, I. M., Gilbert, G. S., Guerra, A. S. & Nunn, C. L. Introduced species, disease ecology, and biodiversity–disease relationships. Trends Ecol. Evol. 32 , 41–54 (2017).
Barouki, R. et al. The COVID-19 pandemic and global environmental change: emerging research needs. Environ. Int. 146 , 106272 (2021).
Article CAS PubMed Google Scholar
Nova, N., Athni, T. S., Childs, M. L., Mandle, L. & Mordecai, E. A. Global change and emerging infectious diseases. Ann. Rev. Resour. Econ. 14 , 333–354 (2021).
Zhang, L. et al. Biological invasions facilitate zoonotic disease emergences. Nat. Commun. 13 , 1762 (2022).
Olival, K. J. et al. Host and viral traits predict zoonotic spillover from mammals. Nature 546 , 646–650 (2017).
Guth, S. et al. Bats host the most virulent—but not the most dangerous—zoonotic viruses. Proc. Natl Acad. Sci. USA 119 , e2113628119 (2022).
Nelson, G. C. et al. in Ecosystems and Human Well-Being (Millennium Ecosystem Assessment) Vol. 2 (eds Rola, A. et al) Ch. 7, 172–222 (Island Press, 2005).
Read, A. F., Graham, A. L. & Raberg, L. Animal defenses against infectious agents: is damage control more important than pathogen control? PLoS Biol. 6 , 2638–2641 (2008).
Article CAS Google Scholar
Medzhitov, R., Schneider, D. S. & Soares, M. P. Disease tolerance as a defense strategy. Science 335 , 936–941 (2012).
Torchin, M. E. & Mitchell, C. E. Parasites, pathogens, and invasions by plants and animals. Front. Ecol. Environ. 2 , 183–190 (2004).
Bellay, S., de Oliveira, E. F., Almeida-Neto, M. & Takemoto, R. M. Ectoparasites are more vulnerable to host extinction than co-occurring endoparasites: evidence from metazoan parasites of freshwater and marine fishes. Hydrobiologia 847 , 2873–2882 (2020).
Scheffer, M. Critical Transitions in Nature and Society Vol. 16 (Princeton Univ. Press, 2020).
Rohr, J. R. et al. A planetary health innovation for disease, food and water challenges in Africa. Nature 619 , 782–787 (2023).
Reaser, J. K., Witt, A., Tabor, G. M., Hudson, P. J. & Plowright, R. K. Ecological countermeasures for preventing zoonotic disease outbreaks: when ecological restoration is a human health imperative. Restor. Ecol. 29 , e13357 (2021).
Hopkins, S. R. et al. Evidence gaps and diversity among potential win–win solutions for conservation and human infectious disease control. Lancet Planet. Health 6 , e694–e705 (2022).
Mitchell, C. E. & Power, A. G. Release of invasive plants from fungal and viral pathogens. Nature 421 , 625–627 (2003).
Chamberlain, S. A. & Szöcs, E. taxize: taxonomic search and retrieval in R. F1000Research 2 , 191 (2013).
Newman, M. Fundamentals of Ecotoxicology (CRC Press/Taylor & Francis Group, 2010).
Rohatgi, A. WebPlotDigitizer v.4.5 (2021); automeris.io/WebPlotDigitizer .
Lüdecke, D. esc: effect size computation for meta analysis (version 0.5.1). Zenodo https://doi.org/10.5281/zenodo.1249218 (2019).
Lipsey, M. W. & Wilson, D. B. Practical Meta-Analysis (SAGE, 2001).
R Core Team. R: A Language and Environment for Statistical Computing Vol. 2022 (R Foundation for Statistical Computing, 2020); www.R-project.org/ .
Viechtbauer, W. Conducting meta-analyses in R with the metafor package. J. Stat. Softw. 36 , 1–48 (2010).
Pustejovsky, J. E. & Tipton, E. Meta-analysis with robust variance estimation: Expanding the range of working models. Prev. Sci. 23 , 425–438 (2022).
Lenth, R. emmeans: estimated marginal means, aka least-squares means. R package v.1.5.1 (2020).
Bartoń, K. MuMIn: multi-modal inference. Model selection and model averaging based on information criteria (AICc and alike) (2019).
Burnham, K. P. & Anderson, D. R. Multimodel inference: understanding AIC and BIC in model selection. Sociol. Methods Res. 33 , 261–304 (2004).
Article MathSciNet Google Scholar
Marks‐Anglin, A. & Chen, Y. A historical review of publication bias. Res. Synth. Methods 11 , 725–742 (2020).
Nakagawa, S. et al. Methods for testing publication bias in ecological and evolutionary meta‐analyses. Methods Ecol. Evol. 13 , 4–21 (2022).
Gurevitch, J., Koricheva, J., Nakagawa, S. & Stewart, G. Meta-analysis and the science of research synthesis. Nature 555 , 175–182 (2018).
Bates, D., Mächler, M., Bolker, B. & Walker, S. Fitting linear mixed-effects models using lme4. J. Stat. Softw. 67 , 1–48 (2015).
Mahon, M. B. et al. Data and code for ‘A meta-analysis on global change drivers and the risk of infectious disease’. Zenodo https://doi.org/10.5281/zenodo.8169979 (2024).
Mahon, M. B. et al. Data and code for ‘A meta-analysis on global change drivers and the risk of infectious disease’. GitHub github.com/mahonmb/GCDofDisease (2024).
Download references
Acknowledgements
We thank C. Mitchell for contributing data on enemy release; L. Albert and B. Shayhorn for assisting with data collection; J. Gurevitch, M. Lajeunesse and G. Stewart for providing comments on an earlier version of this manuscript; and C. Carlson and two anonymous reviewers for improving this paper. This research was supported by grants from the National Science Foundation (DEB-2109293, DEB-2017785, DEB-1518681, IOS-1754868), National Institutes of Health (R01TW010286) and US Department of Agriculture (2021-38420-34065) to J.R.R.; a US Geological Survey Powell grant to J.R.R. and S.L.R.; University of Connecticut Start-up funds to S.A.K.; grants from the National Science Foundation (IOS-1755002) and National Institutes of Health (R01 AI150774) to D.J.C.; and an Ambizione grant (PZ00P3_202027) from the Swiss National Science Foundation to F.W.H. The funders had no role in study design, data collection and analysis, decision to publish or preparation of the manuscript.
Author information
These authors contributed equally: Michael B. Mahon, Alexandra Sack, Jason R. Rohr
Authors and Affiliations
Department of Biological Sciences, University of Notre Dame, Notre Dame, IN, USA
Michael B. Mahon, Alexandra Sack, O. Alejandro Aleuy, Carly Barbera, Ethan Brown, Heather Buelow, Luz A. de Wit, Meghan Forstchen, Patrick Heffernan, Alexis Korotasz, Joanna G. Larson, Samantha L. Rumschlag, Emily Selland, Alexander Shepack, Nitin Vincent & Jason R. Rohr
Environmental Change Initiative, University of Notre Dame, Notre Dame, IN, USA
Michael B. Mahon, Samantha L. Rumschlag & Jason R. Rohr
Eck Institute of Global Health, University of Notre Dame, Notre Dame, IN, USA
Alexandra Sack, Meghan Forstchen, Emily Selland & Jason R. Rohr
Department of Biology, Emory University, Atlanta, GA, USA
David J. Civitello
Department of Ecology and Evolutionary Biology, Yale University, New Haven, CT, USA
Jeremy M. Cohen
Department of Botany and Plant Pathology, Oregon State University, Corvallis, OR, USA
Fletcher W. Halliday
Department of Ecology and Evolutionary Biology, Institute for Systems Genomics, University of Connecticut, Storrs, CT, USA
Sarah A. Knutie
You can also search for this author in PubMed Google Scholar
Contributions
J.R.R. conceptualized the study. All of the authors contributed to the methodology. All of the authors contributed to investigation. Visualization was performed by M.B.M. The initial study list and related information were compiled by D.J.C., J.M.C., F.W.H., S.A.K., S.L.R. and J.R.R. Data extraction was performed by M.B.M., A.S., O.A.A., C.B., E.B., H.B., L.A.d.W., M.F., P.H., A.K., J.G.L., E.S., A.S. and N.V. Data were checked for accuracy by M.B.M. and A.S. Analyses were performed by M.B.M. and J.R.R. Funding was acquired by D.J.C., J.R.R., S.A.K. and S.L.R. Project administration was done by J.R.R. J.R.R. supervised the study. J.R.R. and M.B.M. wrote the original draft. All of the authors reviewed and edited the manuscript. J.R.R. and M.B.M. responded to reviewers.
Corresponding author
Correspondence to Jason R. Rohr .
Ethics declarations
Competing interests.
The authors declare no competing interests.
Peer review
Peer review information.
Nature thanks Colin Carlson and the other, anonymous, reviewer(s) for their contribution to the peer review of this work. Peer reviewer reports are available.
Additional information
Publisher’s note Springer Nature remains neutral with regard to jurisdictional claims in published maps and institutional affiliations.
Extended data figures and tables
Extended data fig. 1 prisma flowchart..
The PRISMA flow diagram of the search and selection of studies included in this meta-analysis. Note that 77 studies came from the Halliday et al. 3 database on biodiversity change.
Extended Data Fig. 2 Summary of the number of studies (A-F) and parasite taxa (G-L) in the infectious disease database across ecological contexts.
The contexts are global change driver ( A , G ), parasite taxa ( B , H ), host taxa ( C , I ), experimental venue ( D , J ), study habitat ( E , K ), and human parasite status ( F , L ).
Extended Data Fig. 3 Summary of the number of effect sizes (A-I), studies (J-R), and parasite taxa (S-a) in the infectious disease database for various parasite and host contexts.
Shown are parasite type ( A , J , S ), host thermy ( B , K , T ), vector status ( C , L , U ), vector-borne status ( D , M , V ), parasite transmission ( E , N , W ), free living stages ( F , O , X ), host (e.g. disease, host growth, host survival) or parasite (e.g. parasite abundance, prevalence, fecundity) endpoint ( G , P , Y ), micro- vs macroparasite ( H , Q , Z ), and zoonotic status ( I , R , a ).
Extended Data Fig. 4 The effects of global change drivers and subsequent subcategories on disease responses with Log Response Ratio instead of Hedge’s g.
Here, Log Response Ratio shows similar trends to that of Hedge’s g presented in the main text. The displayed points represent the mean predicted values (with 95% confidence intervals) from a meta-analytical model with separate random intercepts for study. Points that do not share letters are significantly different from one another (p < 0.05) based on a two-sided Tukey’s posthoc multiple comparison test with adjustment for multiple comparisons. See Table S 3 for pairwise comparison results. Effects of the five common global change drivers ( A ) have the same directionality, similar magnitude, and significance as those presented in Fig. 2 . Global change driver effects are significant when confidence intervals do not overlap with zero and explicitly tested with two-tailed t-test (indicated by asterisks; t 80.62 = 2.16, p = 0.034 for CP; t 71.42 = 2.10, p = 0.039 for CC; t 131.79 = −3.52, p < 0.001 for HLC; t 61.9 = 2.10, p = 0.040 for IS). The subcategories ( B ) also show similar patterns as those presented in Fig. 3 . Subcategories are significant when confidence intervals do not overlap with zero and were explicitly tested with two-tailed one sample t-test (t 30.52 = 2.17, p = 0.038 for CO 2 ; t 40.03 = 4.64, p < 0.001 for Enemy Release; t 47.45 = 2.18, p = 0.034 for Mean Temperature; t 110.81 = −4.05, p < 0.001 for Urbanization); all other subcategories have p > 0.20. Note that effect size and study numbers are lower here than in Figs. 3 and 4 , because log response ratios cannot be calculated for studies that provide coefficients (e.g., odds ratio) rather than raw data; as such, all observations within BC did not have associated RR values. Despite strong differences in sample size, patterns are consistent across effect sizes, and therefore, we can be confident that the results presented in the main text are not biased because of effect size selection.
Extended Data Fig. 5 Average standard errors of the effect sizes (A) and sample sizes per effect size (B) for each of the five global change drivers.
The displayed points represent the mean predicted values (with 95% confidence intervals) from the generalized linear mixed effects models with separate random intercepts for study (Gaussian distribution for standard error model, A ; Poisson distribution for sample size model, B ). Points that do not share letters are significantly different from one another (p < 0.05) based on a two-sided Tukey’s posthoc multiple comparison test with adjustment for multiple comparisons. Sample sizes (number of studies, n, and effect sizes, k) for each driver are as follows: n = 77, k = 392 for BC; n = 124, k = 364 for CP; n = 202, k = 380 for CC; n = 517, k = 1449 for HLC; n = 96, k = 355 for IS.
Extended Data Fig. 6 Forest plots of effect sizes, associated variances, and relative weights (A), Funnel plots (B), and Egger’s Test plots (C) for each of the five global change drivers and leave-one-out publication bias analyses (D).
In panel A , points are the individual effect sizes (Hedge’s G), error bars are standard errors of the effect size, and size of the points is the relative weight of the observation in the model, with larger points representing observations with higher weight in the model. Sample sizes are provided for each effect size in the meta-analytic database. Effect sizes were plotted in a random order. Egger’s tests indicated significant asymmetries (p < 0.05) in Biodiversity Change (worst asymmetry – likely not bias, just real effect of positive relationship between diversity and disease), Climate Change – (weak asymmetry, again likely not bias, climate change generally increases disease), and Introduced Species (relatively weak asymmetry – unclear whether this is a bias, may be driven by some outliers). No significant asymmetries (p > 0.05) were found in Chemical Pollution and Habitat Loss/Change, suggesting negligible publication bias in reported disease responses across these global change drivers ( B , C ). Egger’s test included publication year as moderator but found no significant relationship between Hedge’s g and publication year (p > 0.05) implying no temporal bias in effect size magnitude or direction. In panel D , the horizontal red lines denote the grand mean and SE of Hedge’s g and (g = 0.1009, SE = 0.0338). Grey points and error bars indicate the Hedge’s g and SEs, respectively, using the leave-one-out method (grand mean is recalculated after a given study is removed from dataset). While the removal of certain studies resulted in values that differed from the grand mean, all estimated Hedge’s g values fell well within the standard error of the grand mean. This sensitivity analysis indicates that our results were robust to the iterative exclusion of individual studies.
Extended Data Fig. 7 The effects of habitat loss/change on disease depend on parasite taxa and land use conversion contexts.
A) Enemy type influences the magnitude of the effect of urbanization on disease: helminths, protists, and arthropods were all negatively associated with urbanization, whereas viruses were non-significantly positively associated with urbanization. B) Reference (control) land use type influences the magnitude of the effect of urbanization on disease: disease was reduced in urban settings compared to rural and peri-urban settings, whereas there were no differences in disease along urbanization gradients or between urban and natural settings. C) The effect of forest fragmentation depends on whether a large/continuous habitat patch is compared to a small patch or whether disease it is measured along an increasing fragmentation gradient (Z = −2.828, p = 0.005). Conversely, the effect of deforestation on disease does not depend on whether the habitat has been destroyed and allowed to regrow (e.g., clearcutting, second growth forests, etc.) or whether it has been replaced with agriculture (e.g., row crop, agroforestry, livestock grazing; Z = 1.809, p = 0.0705). The displayed points represent the mean predicted values (with 95% confidence intervals) from a metafor model where the response variable was a Hedge’s g (representing the effect on an infectious disease endpoint relative to control), study was treated as a random effect, and the independent variables included enemy type (A), reference land use type (B), or land use conversion type (C). Data for (A) and (B) were only those studies that were within the “urbanization” subcategory; data for (C) were only those studies that were within the “deforestation” and “forest fragmentation” subcategories. Sample sizes (number of studies, n, and effect sizes, k) in (A) for each enemy are n = 48, k = 98 for Virus; n = 193, k = 343 for Protist; n = 159, k = 490 for Helminth; n = 10, k = 24 for Fungi; n = 103, k = 223 for Bacteria; and n = 30, k = 73 for Arthropod. Sample sizes in (B) for each reference land use type are n = 391, k = 1073 for Rural; n = 29, k = 74 for Peri-urban; n = 33, k = 83 for Natural; and n = 24, k = 58 for Urban Gradient. Sample sizes in (C) for each land use conversion type are n = 7, k = 47 for Continuous Gradient; n = 16, k = 44 for High/Low Fragmentation; n = 11, k = 27 for Clearcut/Regrowth; and n = 21, k = 43 for Agriculture.
Extended Data Fig. 8 The effects of common global change drivers on mean infectious disease responses in the literature depends on whether the endpoint is the host or parasite; whether the parasite is a vector, is vector-borne, has a complex or direct life cycle, or is a macroparasite; whether the host is an ectotherm or endotherm; or the venue and habitat in which the study was conducted.
A ) Parasite endpoints. B ) Vector-borne status. C ) Parasite transmission route. D ) Parasite size. E ) Venue. F ) Habitat. G ) Host thermy. H ) Parasite type (ecto- or endoparasite). See Table S 2 for number of studies and effect sizes across ecological contexts and global change drivers. See Table S 3 for pairwise comparison results. The displayed points represent the mean predicted values (with 95% confidence intervals) from a metafor model where the response variable was a Hedge’s g (representing the effect on an infectious disease endpoint relative to control), study was treated as a random effect, and the independent variables included the main effects and an interaction between global change driver and the focal independent variable (whether the endpoint measured was a host or parasite, whether the parasite is vector-borne, has a complex or direct life cycle, is a macroparasite, whether the study was conducted in the field or lab, habitat, the host is ectothermic, or the parasite is an ectoparasite).
Extended Data Fig. 9 The effects of five common global change drivers on mean infectious disease responses in the literature only occasionally depend on location, host taxon, and parasite taxon.
A ) Continent in which the field study occurred. Lack of replication in chemical pollution precluded us from including South America, Australia, and Africa in this analysis. B ) Host taxa. C ) Enemy taxa. See Table S 2 for number of studies and effect sizes across ecological contexts and global change drivers. See Table S 3 for pairwise comparison results. The displayed points represent the mean predicted values (with 95% confidence intervals) from a metafor model where the response variable was a Hedge’s g (representing the effect on an infectious disease endpoint relative to control), study was treated as a random effect, and the independent variables included the main effects and an interaction between global change driver and continent, host taxon, and enemy taxon.
Extended Data Fig. 10 The effects of human vs. non-human endpoints for the zoonotic disease subset of database and wild vs. domesticated animal endpoints for the non-human animal subset of database are consistent across global change drivers.
(A) Zoonotic disease responses measured on human hosts responded less positively (closer to zero when positive, further from zero when negative) than those measured on non-human (animal) hosts (Z = 2.306, p = 0.021). Note, IS studies were removed because of missing cells. (B) Disease responses measured on domestic animal hosts responded less positively (closer to zero when positive, further from zero when negative) than those measured on wild animal hosts (Z = 2.636, p = 0.008). These results were consistent across global change drivers (i.e., no significant interaction between endpoint and global change driver). As many of the global change drivers increase zoonotic parasites in non-human animals and all parasites in wild animals, this may suggest that anthropogenic change might increase the occurrence of parasite spillover from animals to humans and thus also pandemic risk. The displayed points represent the mean predicted values (with 95% confidence intervals) from a metafor model where the response variable was a Hedge’s g (representing the effect on an infectious disease endpoint relative to control), study was treated as a random effect, and the independent variable of global change driver and human/non-human hosts. Data for (A) were only those diseases that are considered “zoonotic”; data for (B) were only those endpoints that were measured on non-human animals. Sample sizes in (A) for zoonotic disease measured on human endpoints across global change drivers are n = 3, k = 17 for BC; n = 2, k = 6 for CP; n = 25, k = 39 for CC; and n = 175, k = 331 for HLC. Sample sizes in (A) for zoonotic disease measured on non-human endpoints across global change drivers are n = 25, k = 52 for BC; n = 2, k = 3 for CP; n = 18, k = 29 for CC; n = 126, k = 289 for HLC. Sample sizes in (B) for wild animal endpoints across global change drivers are n = 28, k = 69 for BC; n = 21, k = 44 for CP; n = 50, k = 89 for CC; n = 121, k = 360 for HLC; and n = 29, k = 45 for IS. Sample sizes in (B) for domesticated animal endpoints across global change drivers are n = 2, k = 4 for BC; n = 4, k = 11 for CP; n = 7, k = 20 for CC; n = 78, k = 197 for HLC; and n = 1, k = 2 for IS.
Supplementary information
Supplementary information.
Supplementary Discussion, Supplementary References and Supplementary Tables 1–3.
Reporting Summary
Peer review file, supplementary data 1.
R markdown code and output associated with this paper.
Supplementary Table 4
EcoEvo PRISMA checklist.
Rights and permissions
Springer Nature or its licensor (e.g. a society or other partner) holds exclusive rights to this article under a publishing agreement with the author(s) or other rightsholder(s); author self-archiving of the accepted manuscript version of this article is solely governed by the terms of such publishing agreement and applicable law.
Reprints and permissions
About this article
Cite this article.
Mahon, M.B., Sack, A., Aleuy, O.A. et al. A meta-analysis on global change drivers and the risk of infectious disease. Nature (2024). https://doi.org/10.1038/s41586-024-07380-6
Download citation
Received : 02 August 2022
Accepted : 03 April 2024
Published : 08 May 2024
DOI : https://doi.org/10.1038/s41586-024-07380-6
Share this article
Anyone you share the following link with will be able to read this content:
Sorry, a shareable link is not currently available for this article.
Provided by the Springer Nature SharedIt content-sharing initiative
By submitting a comment you agree to abide by our Terms and Community Guidelines . If you find something abusive or that does not comply with our terms or guidelines please flag it as inappropriate.
Quick links
- Explore articles by subject
- Guide to authors
- Editorial policies
Sign up for the Nature Briefing: Anthropocene newsletter — what matters in anthropocene research, free to your inbox weekly.

Sleep Loss and Emotion: A Systematic Review and Meta-Analysis
Saul Mcleod, PhD
Editor-in-Chief for Simply Psychology
BSc (Hons) Psychology, MRes, PhD, University of Manchester
Saul Mcleod, PhD., is a qualified psychology teacher with over 18 years of experience in further and higher education. He has been published in peer-reviewed journals, including the Journal of Clinical Psychology.
Learn about our Editorial Process
Olivia Guy-Evans, MSc
Associate Editor for Simply Psychology
BSc (Hons) Psychology, MSc Psychology of Education
Olivia Guy-Evans is a writer and associate editor for Simply Psychology. She has previously worked in healthcare and educational sectors.
Sleep and emotion are fundamentally intertwined, both being universal human experiences that profoundly shape our daily functioning and well-being. Our emotional states influence every facet of our lives, from our physical health and cognitive performance to our social relationships and overall life satisfaction. Similarly, sleep plays a vital role in regulating our emotional processing , reactivity, and mood.
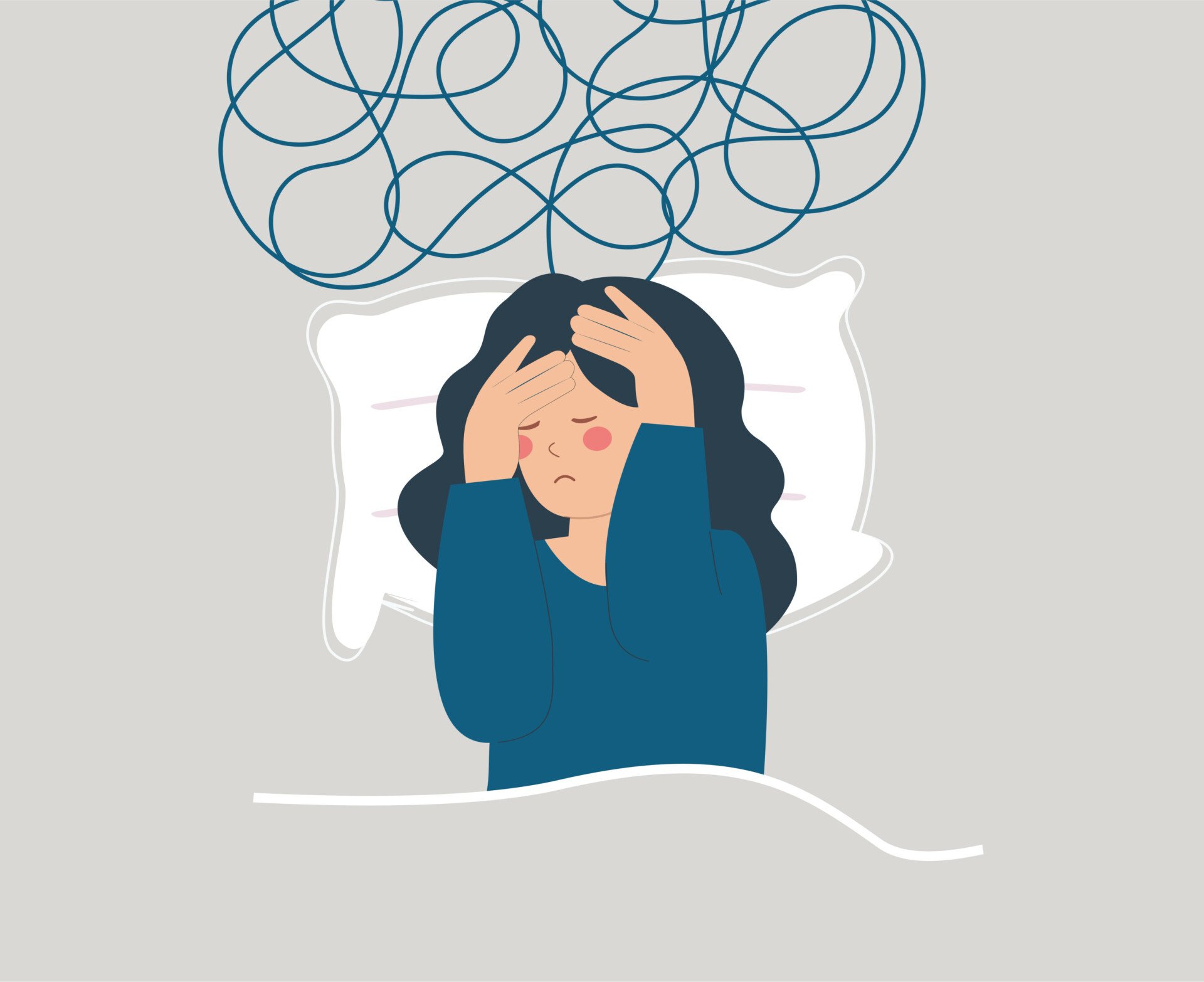
- This meta-analysis synthesized over 50 years of experimental research on sleep loss and emotion, examining the effects of sleep deprivation, sleep restriction, and sleep fragmentation on various emotional outcomes.
- Sleep loss resulted in reduced positive affect (SMD = -0.27 to -1.14), increased anxiety symptoms (SMD = 0.57-0.63), and blunted arousal in response to emotional stimuli (SMD = -0.20 to -0.53). Findings for negative affect, emotional valence, and depressive symptoms varied based on the type of sleep loss.
- Nonlinear dose-response effects were found for the amount of sleep loss on some emotional outcomes. Losing REM sleep had a stronger effect on unpleasant emotional reactivity compared to losing slow-wave sleep.
- While the research sheds light on the detrimental effects of sleep loss on emotions, it has some limitations such as relying predominantly on young adult samples and potential expectancy effects.
- The pervasiveness of insufficient sleep worldwide makes this an important public health issue with significant implications for emotional well-being and risk for psychiatric disorders.
Sleep loss is common in modern society, with large segments of the population regularly failing to obtain adequate sleep (Hafner et al., 2017).
Poor sleep is known to catalyze the development of emotional difficulties and affective disorders across the lifespan (Goldstein & Walker, 2014; Gregory et al., 2009).
While an increasing number of experimental studies have demonstrated the adverse effects of sleep loss on emotion in recent decades (Palmer & Alfano, 2017; Tempesta et al., 2018), findings have been mixed and a comprehensive quantitative synthesis was needed to integrate results across the heterogeneous research designs and consolidate conclusions.
This meta-analysis aimed to quantify the effects of various forms of experimental sleep loss (deprivation, restriction, fragmentation) on multiple aspects of emotional experience and elucidate factors that may influence these effects.
The researchers conducted a preregistered systematic review and meta-analysis following PRISMA guidelines.
They searched PubMed/MEDLINE, PsychINFO, and Web of Science databases for experimental studies examining the effects of sleep deprivation, sleep restriction, or sleep fragmentation on positive affect, negative affect, mood disturbance, emotional reactivity, anxiety symptoms and/or depressive symptoms in healthy populations.
Additional unpublished data was sought via listservs and contacting authors. Inclusion criteria required studies to have healthy samples, an experimental sleep loss manipulation, an adequate control/baseline condition, and an emotion-related dependent variable.
Two independent coders screened and extracted data from eligible studies.
Search strategy and terms
The search criteria included human studies mentioning experimental sleep manipulations and any emotion-related outcomes in the title/abstract. There were no restrictions on language, location or date.
Inclusion and exclusion criteria
Included studies had to 1) use healthy samples without psychiatric, sleep or medical disorders that impact sleep/emotions, 2) experimentally manipulate nighttime sleep deprivation, restriction or fragmentation, 3) have an adequate control/baseline condition, 4) assess an emotion-related dependent variable after the sleep manipulation.
Studies were excluded if they used specialized samples, nap/circadian protocols, or had an intervention prior to the emotional assessment that could affect outcomes.
Statistical measures
Standardized mean differences (Hedges’ g) were calculated for each eligible outcome. Multivariate multilevel random effects models were used to estimate overall effects for each sleep loss type and emotional outcome, accounting for non-independent effect sizes.
Mixed effects models examined potential moderators. Heterogeneity, outliers, and indices of publication bias were assessed.
The meta-analysis included 154 studies (N=5,717) yielding 1,338 effect sizes.
For sleep deprivation (k=599), significant effects were found for reduced positive affect (SMD=-0.86), increased negative affect (SMD=0.37), mood disturbance (SMD=0.71), blunted arousal (SMD=-0.53), and anxiety (SMD=0.63). Nonlinear dose-response effects showed negative affect, mood disturbance and anxiety peaking at 30-60 hours of wakefulness.
For sleep restriction (k=483), effects were significant for reduced positive affect (SMD=-0.56), increased negative affect (SMD=0.20), mood disturbance (SMD=0.56), greater unpleasantness (SMD=0.23), blunted arousal (SMD=-0.20), anxiety (SMD=0.57) and depression (SMD=0.46). Nonlinear dose-response effects found the largest deficits for positive affect and unpleasantness around 4 hours of sleep.
Sleep fragmentation (k=256) significantly reduced positive affect (SMD=-0.40) and blunted arousal (SMD=-0.36). There were no significant effects on negative affect, mood, or valence.
Some moderating effects of age, sex, and study characteristics emerged, though not consistently across outcomes. Importantly, losing REM sleep had a stronger effect than losing slow-wave sleep on ratings of unpleasantness to emotional stimuli.
This meta-analysis provides the most comprehensive picture to date of how sleep loss impacts human emotions.
It demonstrates that multiple aspects of emotional functioning are significantly altered by sleep deprivation, restriction, and fragmentation.
The most robust effects across all three types of sleep loss were found for reductions in positive affect, suggesting that inadequate sleep may be particularly detrimental for experiences and expressions of positive emotions.
This could have important mental health implications given the role of positive emotionality in psychological well-being and resilience (Fredrickson, 2001). The blunting of emotional arousal also seen after sleep loss may reflect impairments in top-down emotional processing.
In contrast, sleep loss effects on negative affect were smaller and less consistent across studies. The evolutionary importance of negative emotions for signaling threats and promoting survival could make negative affective responses more resistant to the effects of sleep loss compared to positive affect.
However, anxiety symptoms were consistently increased by sleep loss, indicating heightened feelings of apprehension and worry. The dose-response findings suggest these detrimental effects on negative affect and anxiety are exacerbated by more extreme sleep deprivation.
An intriguing finding was that losing REM sleep impacted ratings of emotional stimuli more than losing slow-wave sleep. This aligns with theories proposing a key role of REM sleep in emotional memory consolidation and maintaining emotional reactivity (Walker & van der Helm, 2009).
More targeted research comparing the effects of selective REM and slow-wave sleep deprivation on emotional reactivity and regulation is needed.
While only a small number of studies included children or older adults, some moderating effects of age did emerge, with sleep loss having stronger effects on mood disturbances in older individuals. Examining sleep-emotion dynamics in developmental and lifespan contexts is an important future direction.
Sex differences were also found for some outcomes, but not consistently, highlighting the need for more studies powered to detect potential gender differences in emotional vulnerability to sleep loss.
Overall, these findings underscore the consequences of insufficient sleep for affective experience and functioning. They provide an important foundation for further investigating the mechanisms linking sleep and emotion and developing interventions to mitigate the risks of sleep loss for emotional health.
- Preregistration of the study design and analytical plan
- Adherence to PRISMA guidelines for transparent reporting
- Comprehensive search strategy across multiple databases
- Inclusion of unpublished data to mitigate publication bias
- Rigorous coding procedures with two independent coders
- Examination of multiple types of sleep loss and emotional outcomes
- Advanced statistical methods accounting for non-independent effect sizes
- Assessment of heterogeneity, outliers, and publication bias indices
Limitations
- Most studies used young adult samples, limiting generalizability to other ages
- There was a lack of geographical diversity, with studies predominantly from Western countries
- Masking participants to sleep loss conditions is not possible, so expectancy effects may have influenced results
- Some analyses for specific emotional outcomes or sleep types had a small number of studies
- Variability in emotional assessments and sleep manipulation procedures across studies
Implications
The finding that even relatively modest amounts of sleep loss can have significant negative repercussions for emotional well-being has important real-world implications.
With up to one-third of the general adult population reporting insufficient sleep (Liu et al., 2016), a substantial proportion of people may be at heightened risk for emotional difficulties and affective disorders as a result of inadequate sleep.
This makes sleep a critical target for public health interventions aimed at promoting mental health.
The differential impacts of REM versus slow-wave sleep loss on emotional reactivity also have clinical relevance, suggesting sleep stage-specific interventions may be warranted for certain emotional issues.
The nonlinear dose-response effects for several outcomes indicate that sleeping less than 4-5 hours and/or being continuously awake for over 24 hours may represent particularly dangerous thresholds for emotional health.
Organizations and occupations where sleep loss is common (e.g., military, healthcare, shift work) need to be aware of the risks to emotional well-being and implement strategies to mitigate these effects.
Public policies regulating work hours and school start times should prioritize sleep to reduce adverse emotional consequences at the population level.
With the high rates of insufficient sleep among adolescents (Basch et al., 2014) and emerging mood disorders during this developmental period, optimizing sleep could be an important avenue for youth mental health promotion.
More broadly, initiatives to increase public awareness about the importance of sleep for emotional health, address barriers to adequate sleep, and promote evidence-based sleep hygiene practices have the potential to make a substantial and much-needed impact on psychological well-being and public health.
The current findings underscore the affective benefits of prioritizing sleep and the dangers of sacrificing it.
Primary reference
Palmer, C. A., Bower, J. L., Cho, K. W., Clementi, M. A., Lau, S., Oosterhoff, B., & Alfano, C. A. (2024). Sleep loss and emotion: A systematic review and meta-analysis of over 50 years of experimental research. Psychological Bulletin, 150 (4), 440–463. https://doi.org/10.1037/bul0000410
Other references
Basch, C. E., Basch, C. H., Ruggles, K. V., & Rajan, S. (2014). Prevalence of sleep duration on an average school night among 4 nationally representative successive samples of American high school students, 2007–2013. Preventing Chronic Disease, 11 , Article 140383. https://doi.org/10.5888/pcd11.140383
Fredrickson, B. L. (2001). The role of positive emotions in positive psychology. The broaden-and-build theory of positive emotions. American Psychologist, 56 (3), 218–226. https://doi.org/10.1037/0003-066X.56.3.218
Goldstein, A. N., & Walker, M. P. (2014). The role of sleep in emotional brain function. Annual Review of Clinical Psychology, 10, 679-708. https://doi.org/10.1146/annurev-clinpsy-032813-153716
Gregory, A. M., Rijsdijk, F. V., Lau, J. Y., Dahl, R. E., & Eley, T. C. (2009). The direction of longitudinal associations between sleep problems and depression symptoms: a study of twins aged 8 and 10 years. Sleep, 32 (2), 189-199. https://doi.org/10.1093/sleep/32.2.189
Hafner, M., Stepanek, M., Taylor, J., Troxel, W. M., & van Stolk, C. (2017). Why sleep matters—the economic costs of insufficient sleep: a cross-country comparative analysis. Rand Health Quarterly, 6 (4), 11. https://doi.org/10.7249/RB9962
Liu, Y., Wheaton, A. G., Chapman, D. P., Cunningham, T. J., Lu, H., & Croft, J. B. (2016). Prevalence of healthy sleep duration among adults — United States, 2014. MMWR. Morbidity and Mortality Weekly Report, 65 (6), 137–141. https://doi.org/10.15585/mmwr.mm6506a1
Palmer, C. A., & Alfano, C. A. (2017). Sleep and emotion regulation: An organizing, integrative review. Sleep Medicine Reviews, 31, 6-16. https://doi.org/10.1016/j.smrv.2015.12.006
Tempesta, D., Socci, V., De Gennaro, L., & Ferrara, M. (2018). Sleep and emotional processing. Sleep Medicine Reviews, 40, 183-195. https://doi.org/10.1016/j.smrv.2017.12.005
Walker, M. P., & van der Helm, E. (2009). Overnight therapy? The role of sleep in emotional brain processing. Psychological Bulletin, 135 (5), 731–748. https://doi.org/10.1037/a0016570
Keep Learning
- How does this meta-analysis advance our understanding of the relationship between sleep and emotion compared to previous individual studies? What are the advantages of synthesizing results across multiple studies?
- The strongest effects of sleep loss were found for positive affect. Why might inadequate sleep be particularly detrimental for positive emotions from an evolutionary or neurobiological perspective? What are the potential mental health implications of this finding?
- The study found some moderating effects of age on the sleep loss-emotion relationship. How might the effects of sleep loss on emotional functioning differ across the lifespan from childhood to older adulthood? What developmental factors could influence this?
- Losing REM sleep impacted emotional reactivity to unpleasant stimuli more than losing slow-wave sleep. What are the potential mechanisms that could explain this finding? How does it relate to theories about the role of REM sleep in emotional memory processing?
- Given the pervasiveness of insufficient sleep in the population, what public health strategies or policies could help mitigate the negative emotional consequences of sleep loss at a societal level? How could this research inform interventions for at-risk groups?
- What are some of the limitations of experimental sleep research for understanding real-world emotional functioning? How well do these controlled laboratory studies generalize to chronic partial sleep loss as it’s experienced in daily life?
- Where should sleep and emotion research go from here? What are the most pressing unanswered questions or promising future directions based on the current state of the science? What types of studies or methodologies are needed to advance the field?
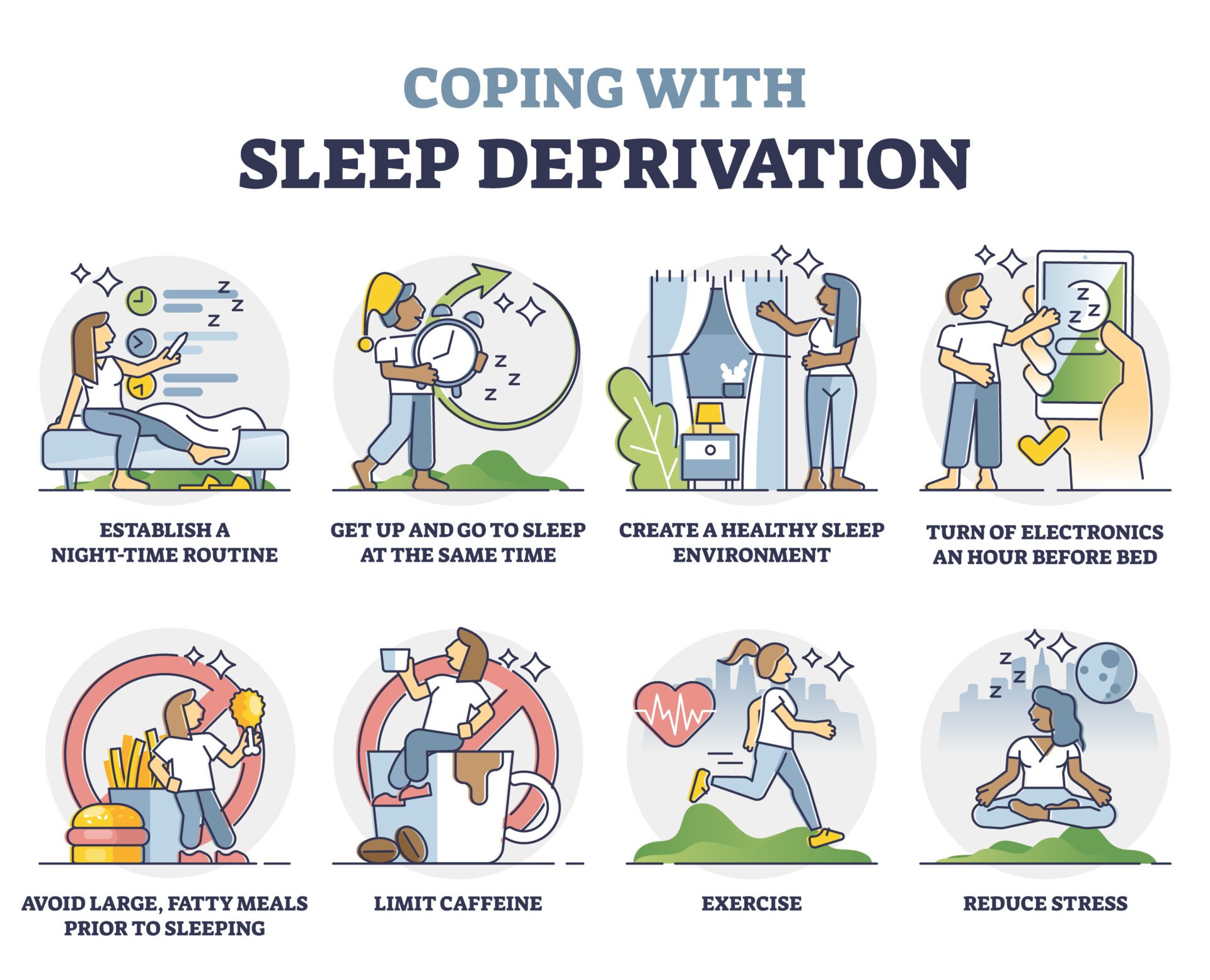
Related Articles
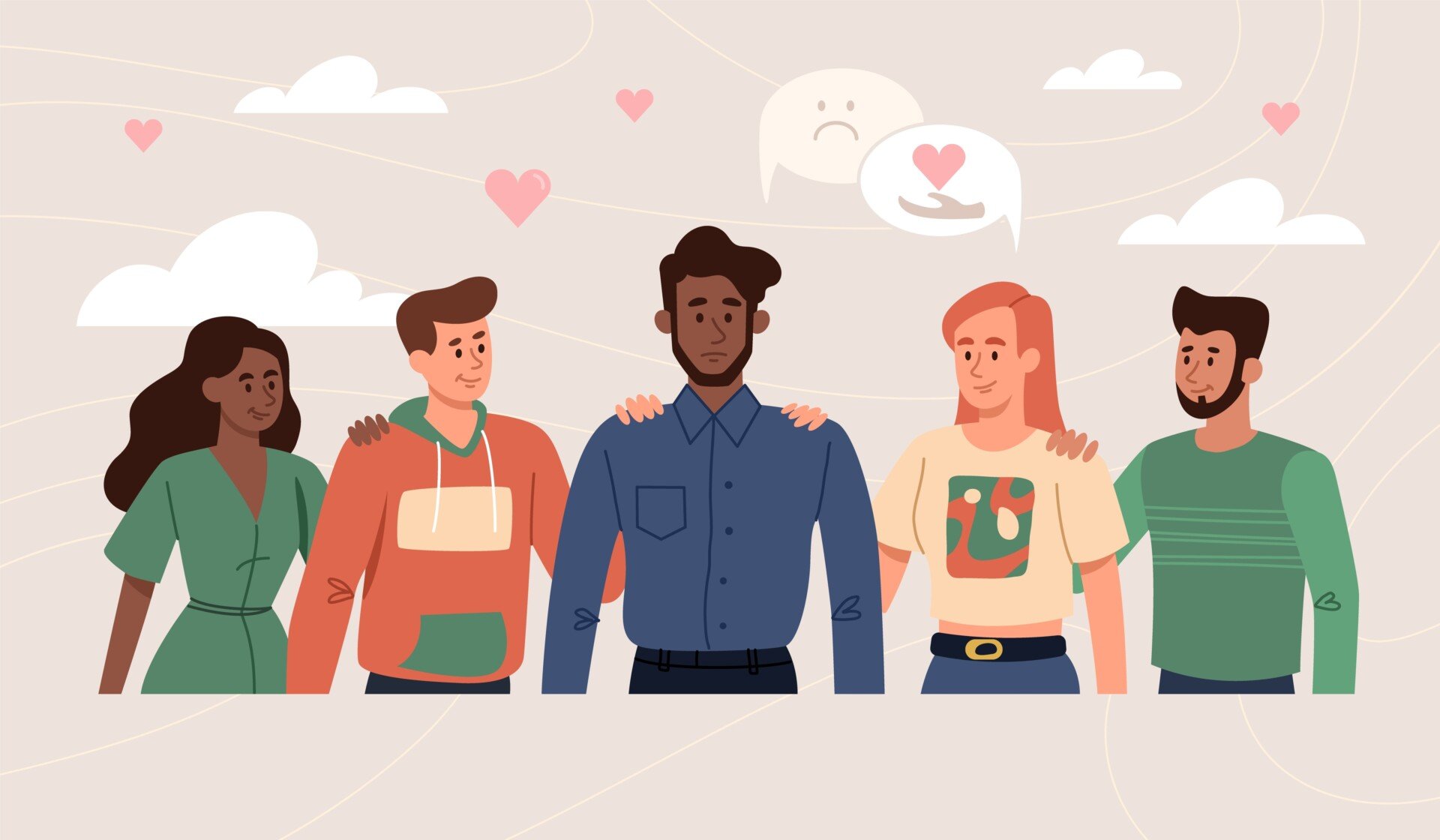
Clinical Psychology
A Study Of Social Anxiety And Perceived Social Support
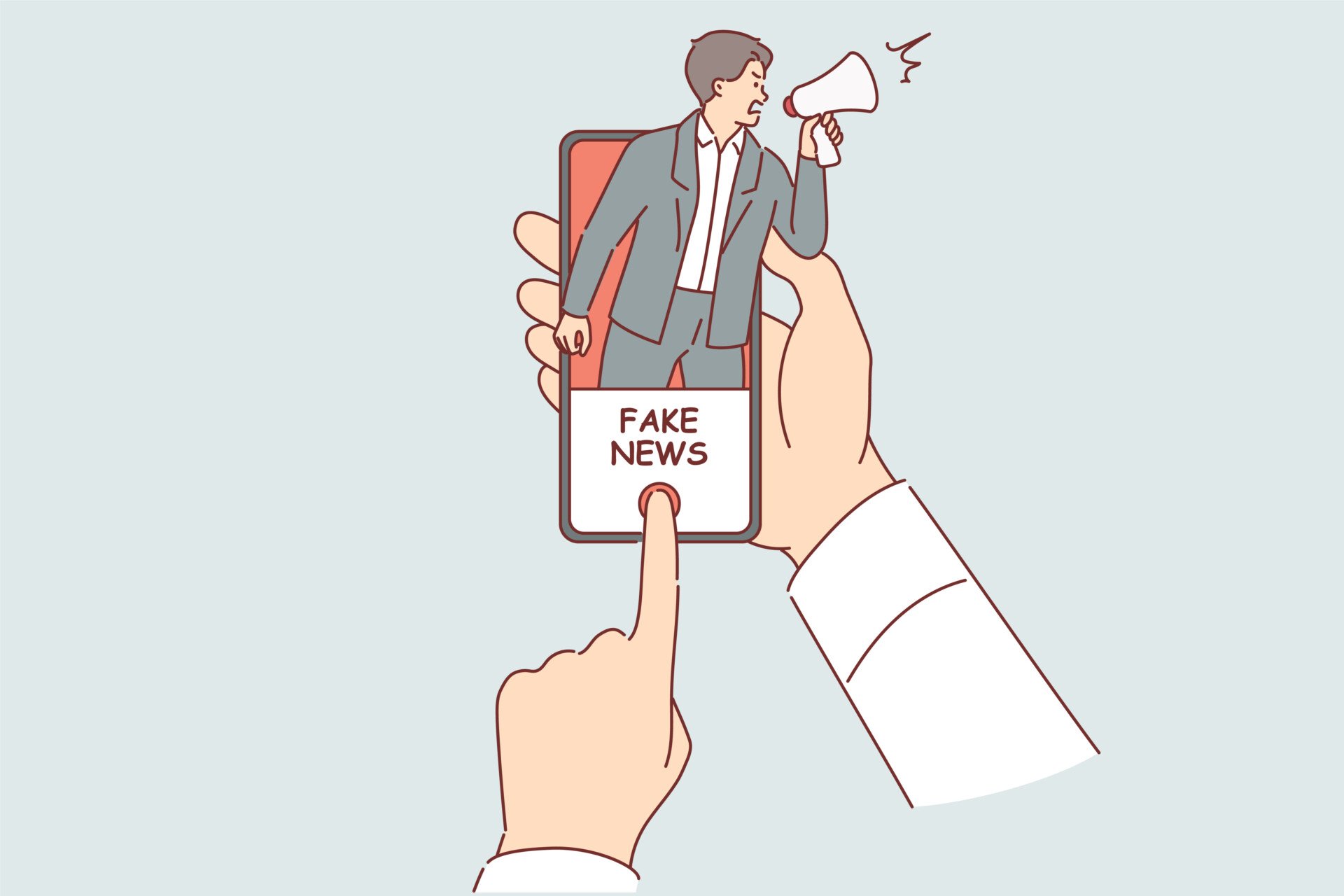
Psychological Impact Of Health Misinformation: A Systematic Review
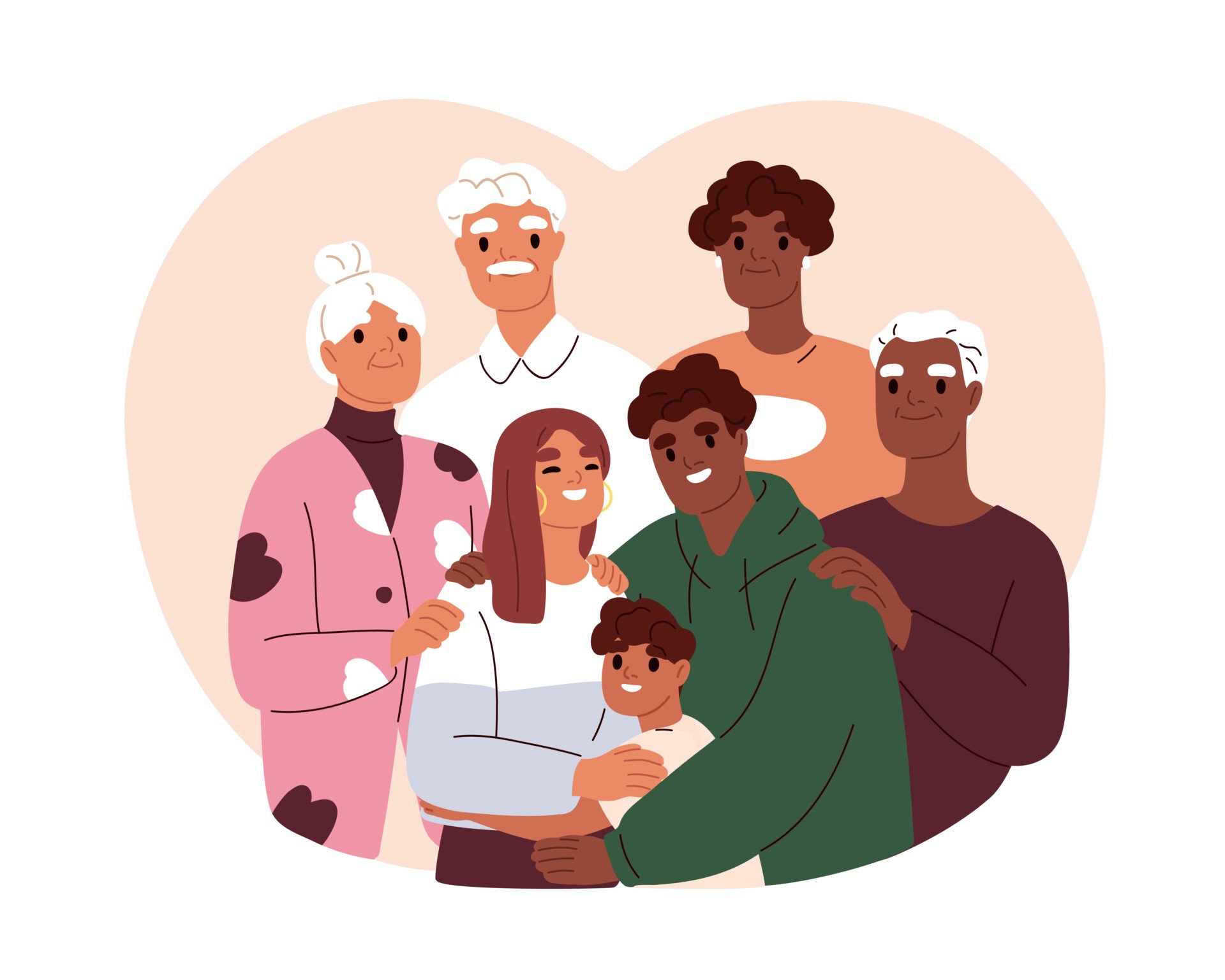
Family History Of Autism And ADHD Vary With Recruitment Approach And SES
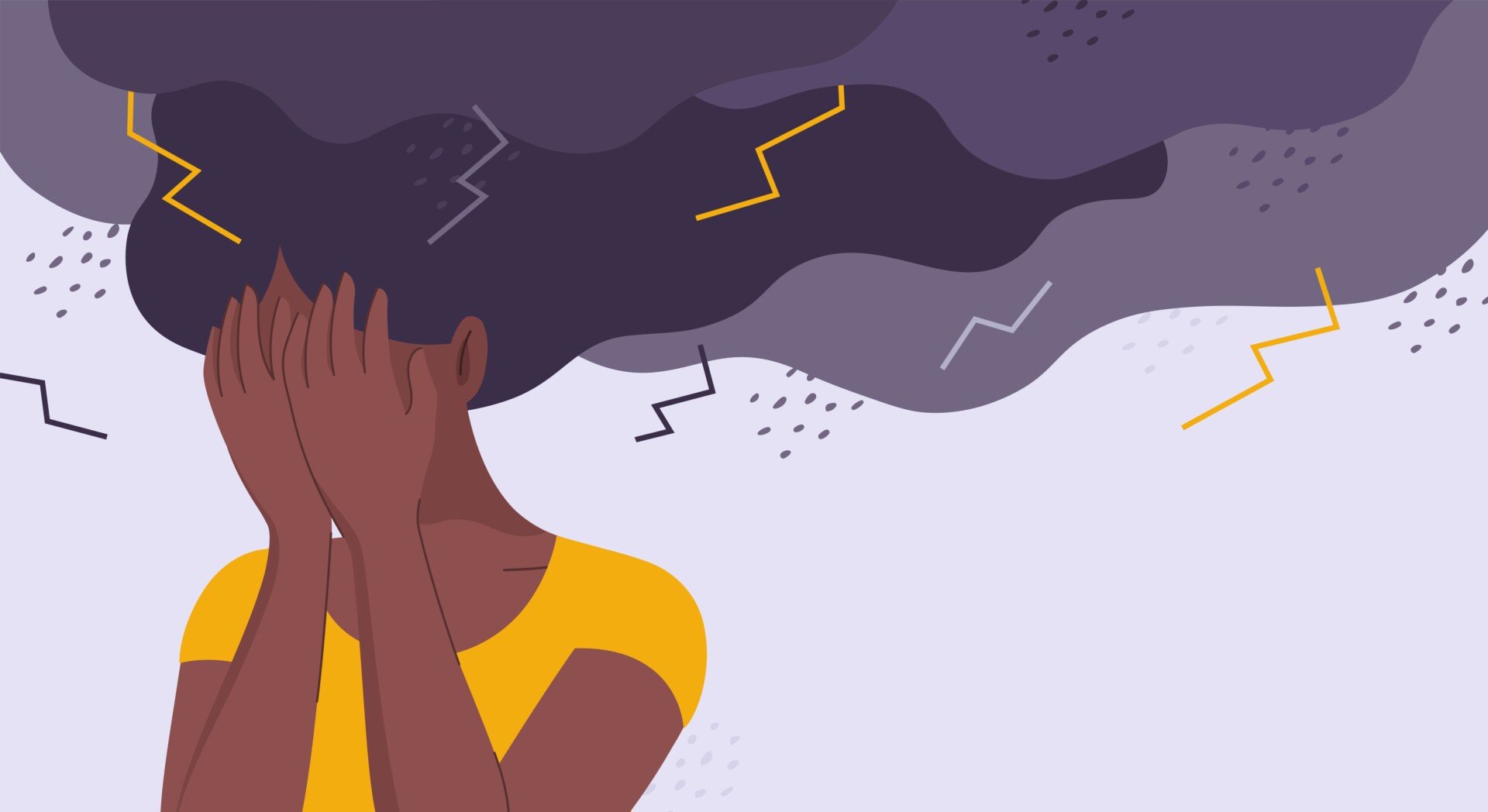
Measuring And Validating Autistic Burnout
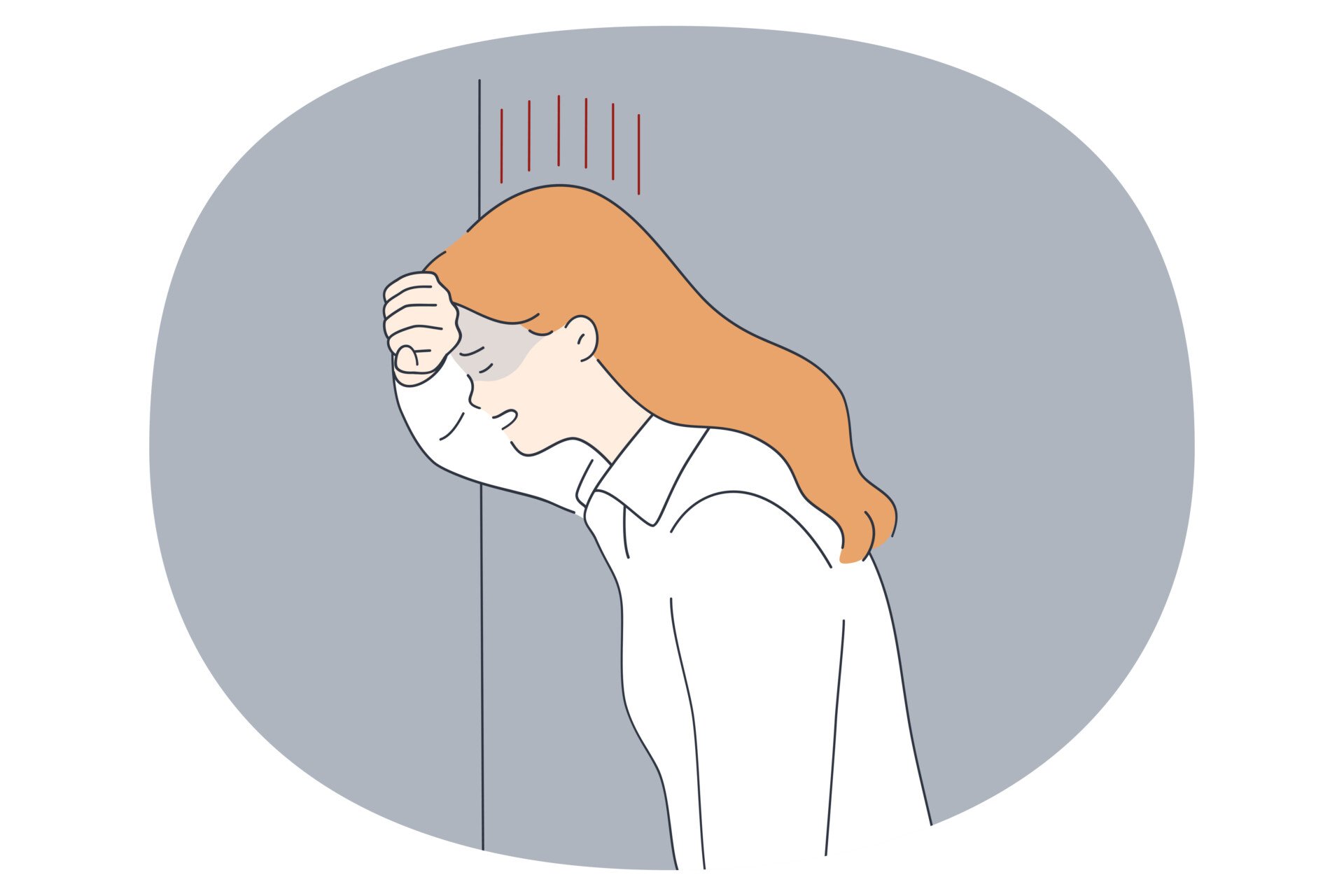
A Systematic Review of Grief and Depression in Adults

Anxiety in Early Adolescents During the Covid-19 Pandemic
- Search Menu
- Advance articles
- Author Interviews
- Research Curations
- Author Guidelines
- Open Access
- Submission Site
- Why Submit?
- About Journal of Consumer Research
- Editorial Board
- Advertising and Corporate Services
- Self-Archiving Policy
- Dispatch Dates
- Journals on Oxford Academic
- Books on Oxford Academic

Article Contents
Measuring knowledge amount, progress, and research trends, future research agenda, data collection statement, author notes.
- < Previous
How Much Have We Learned about Consumer Research? A Meta-Meta-Analysis

- Article contents
- Figures & tables
- Supplementary Data
Martin Eisend, Gratiana Pol, Dominika Niewiadomska, Joseph Riley, Rick Wedgeworth, How Much Have We Learned about Consumer Research? A Meta-Meta-Analysis, Journal of Consumer Research , Volume 51, Issue 1, June 2024, Pages 180–190, https://doi.org/10.1093/jcr/ucad062
- Permissions Icon Permissions
This meta-meta-analysis study quantifies the development of scientific knowledge in consumer research by summarizing the findings of 222 meta-analyses that together include 2481 meta-analytic effect sizes. The results provide an overview of how much we know and how knowledge has developed in consumer research over time. By explaining 7.8% variance ( r = 0.28) in consumer-relevant dependent variables, the findings show that consumer research, a comparatively young discipline, is relatively effective at knowledge development compared to other disciplines. Furthermore, the accumulation of knowledge is significantly increasing, suggesting that our discipline is still in the growing phase of its life cycle and generating continuously improving explanations of consumer-related phenomena. The development of knowledge varies across consumer-relevant dependent variables, with strong explanations for relationships but significantly weaker ones for memory , affect , and attitudes . Moreover, the knowledge synthesized in meta-analyses is fairly—though not fully—representative of the content of primary research on consumers overall. The findings convey a future research agenda by identifying under-researched areas, advising on the selection of dependent variables, providing indicators for the expected contributions of future studies, suggesting implications for career strategies of consumer researchers, and discussing explanations for the observed knowledge growth effects.
The 50-year anniversary of the Journal of Consumer Research ( JCR ) is a sign of a matured field of inquiry that has accumulated substantial knowledge about consumers, as evidenced by the thousands of published consumer research studies. Several scholars have taken stock of the status of the discipline, painting a big picture of what we have learned about consumers, and how impactful existing research has been. Some scholars agree on a successful history of investigation ( Cohen and Wilkie 2022 ). For instance, using text-mining and citation analysis of all articles published in JCR , Wang et al. (2015) show that social identity research has been flourishing and that consumer culture articles are heavily cited. A bibliometric analysis by Baumgartner (2010) identifies influential research articles and reveals that only few shooting stars exist, while many articles have a long and steady or even accelerating impact, providing little evidence of obsolescence of the field. Other scholars paint a more differentiated picture. Simonson et al. (2001) describe a growing emphasis on substantive phenomena, originality, and theory development over practical applications, while also identifying hot and cold topics and a fragmentation into subareas. MacInnis et al. (2020) highlight the relatively narrow impact of the field and the lack of research significance. These exemplars of big-picture approaches to consumer research all focus on what is done and appreciated by scholars . However, they do not address the crucial questions of what and how much is known .
We do not know how much empirical knowledge—in terms of the variance explained in consumer responses—we have accumulated, how this knowledge differs across consumer variables, and how it compares to knowledge accumulation in other fields. Moreover, as knowledge from primary research gets synthesized and further developed via secondary research studies, it is vital to verify whether such studies are representative of the content of primary research on consumers overall, and where disconnects between primary and secondary research may lie. Answering those questions is important, as they allow researchers to objectively assess the maturity level and knowledge growth trajectory of consumer research, benchmark it against that of related fields, and identify promising future research avenues.
The current study answers the questions of: (1) how much we know about consumers (that is, how well we have explained the variance observed in different consumer responses), (2) how this explained variance varies across (a) time and (b) key research constructs (i.e., dependent variables), (3) how this variance compares to that observed in other research fields, and (4) how well the knowledge synthesized in secondary research is aligned with primary research on consumers. We answer the first three questions by quantitatively measuring knowledge using the meta-analytic effect sizes extracted from all 222 meta-analyses published so far in consumer research. We focus on meta-analyses (and their derived product, meta-meta-analyses), as they represent the “highest level of evidence” in empirical research ( Ioannidis 2017 ), whose benefits include: providing robust conclusions about the size of cause–effect relationships ( Chan and Arvey 2012 ), helping to uncovering explanations for inconsistent findings ( Grewal, Puccinelli, and Monroe 2018 ), and potentially contributing toward alleviating the replication crisis in science ( Ones, Viswesvaran, and Schmidt 2017 ; Sharpe and Poets 2020 ). We answer the fourth question by comparing research trends in meta-analyses (i.e., changes in research topic volume before and after 2014, the year associated with JCR ’s 40th anniversary) with recent research trends observed in primary research ( Wang et al. 2015 ).
In recent years, the number of meta-analyses in marketing and consumer research has increased exponentially ( Grewal et al. 2018 )—a phenomenon consistent with the proliferation of meta-analyses in the behavioral and life sciences ( Ioannidis 2017 ). In view of this expanding body of research, the present work complements the burgeoning practice of evaluating knowledge accumulation in a specific behavioral field via a meta-meta-analytical approach that summarizes all meta-analyses conducted in that field ( Nuijten et al. 2020 ; Siegel et al. 2022 ). Our findings contribute to the consumer research field by quantitatively measuring its knowledge development, comparing it to that of neighboring fields, and investigating the alignment in trends between primary and meta-analytical research topics. The findings differ across dependent variables and research topics, which helps to identify under-researched yet promising topics for future research and funding initiatives. The findings also illustrate what effect sizes are considered normal and should be exceeded in future studies, for such studies to provide substantial research contributions. This knowledge in turn helps researchers, reviewers, and readers better evaluate the merits of future research. Ultimately, as effect sizes are linked to scientific contributions and recognition in the academic community, the findings provide insights for successful career strategies of consumer research scholars.
Knowledge Amount and Progress Measurement
Successful scientific knowledge development rests on the explanatory power of research statements (i.e., hypotheses and theories) that link constructs to one another ( Lehmann 1996 ). Explanatory power is empirically assessed via the effect size , which provides evidence for how strongly two variables are related to or depend on each other—in other words, whether and how well a research question has been answered ( Chan and Arvey 2012 ). Effect sizes indicate the value of scientific explanations and the usefulness of scientific hypotheses and theories: the more variance is explained, the more useful and relevant the underlying theory, and the more valuable the knowledge generated by that theory ( Aguinis et al. 2011 ). Effect sizes also have practical relevance in applied behavioral research, as acting on theories supported by small effects produces results that are likely trivial ( Combs 2010 ). The value of scientific knowledge further depends on the generalization potential of the corresponding effect—that is, being able to identify patterns that are likely to recur in future situations ( Lehmann 1996 ). Because a meta-analysis relies on large samples and repeated tests, averages out sampling error deviations from correct values, and corrects mean values for biases caused by measurement error and other artifacts ( Schmidt 1992 ), the meta-analytic effect size serves as a quantifiable and generalizable measure of the merit of scientific explanations and the value of scientific knowledge ( Aguinis et al. 2011 ). Moreover, summarizing all meta-analytical effect sizes across a particular field—via a so-called meta-meta-analysis ( Ioannidis 2017 ; Schmidt and Oh 2013 )—provides a quantitative, big-picture overview of the amount of knowledge accumulation in that field. A meta-meta-analysis is a straightforward generalization of first-order meta-analytic methods which integrates mean effect sizes across multiple meta-analyses, while modeling the between-meta-analysis variance ( Schmidt and Oh 2013 ).
Changes in observed effect sizes over time can be used to assess how scientific knowledge develops ( Eisend 2015 ), whereby positive changes signal increases in the scope, depth, or precision of a scientific paradigm ( Chan and Arvey 2012 ). Based on a discussion of different science philosophers’ views on the trajectory of scientific progress, Eisend (2015) suggests three knowledge development models: continuous growth , where additional empirical research increases the scope of explanations; discontinuous growth , where strong research contributions occur especially in the beginning of a research program or certain topics exhibit patterns of exhaustion over time; and stasis , where researchers do not build on one another’s work, as no research paradigm is widely accepted and the research environment is selecting problems unsystematically. The three models can be identified by effect size variations over time that follow a linear trend or an exponential growth curve (continuous growth model), a quadratic curve (discontinuous growth), or remain unchanged (stasis).
Measuring Alignment between Primary and Meta-Analytical Research Trends
A meta-analysis is typically conducted when a topic reaches maturity (i.e., research findings around that topic reach a volume and level of complexity or dispersion large enough that evidence synthesis is needed to shed light on the true strength or nature of the observed effects, Paul and Barari 2022 ). As such, the volume of meta-analyses on a topic should closely track the research volume for that topic (albeit with a time delay). We examine the extent to which recent research trends observed in primary studies on consumers line up with research trends observed in meta-analytical research, to identify how representative meta-analysis research is of the consumer research field as a whole, and where re-alignment opportunities lie.
Meta-Analysis Dataset
To investigate the amount and progress of knowledge development in consumer research, we perform a meta-meta-analysis that follows the procedure employed in marketing research ( Eisend 2015 ) and related fields ( Richard, Bond, and Stokes-Zoota 2003 ; Siegel et al. 2022 ; Stanley, Carter, and Doucouliagos 2018 ). To locate all meta-analyses published in consumer research until the end of 2021, we performed the following steps: (1) retrieved all relevant meta-analyses from a prior meta-meta-analysis ( Eisend 2015 ); (2) searched all relevant electronic databases (e.g., ABI/INFORM , EBSCO , INFORMS PubsOnLine ) and Google Scholar , using the keywords “metaanaly*,” “meta-analy*,” “quantitative review,” “synthesis,” and “generalization,” combined with “consumer;” and (3) systematically searched journals that were major outlets of meta-analyses. To be included, a paper had to (1) be a meta-analysis and (2) qualify as a consumer research study. All meta-analytic effect sizes that have as dependent variable a construct that measures a consumer response (i.e., a consumer-related state, evaluation, or behavior) were included. Web appendix A provides full details on the inclusion and exclusion criteria used, including the operationalization of consumer research topic . We chose to focus on dependent variables as the main unit of categorization because dependent variables are considered the central constructs in behavioral research studies ( Larsen et al. 2021 ).
To achieve the broadest generalization, the most highly aggregated meta-analytic effect sizes were selected. If a meta-analysis combined findings from primary studies into several meta-analytic effect sizes rather than a single one, we coded all of those effect sizes. On average, a meta-analysis provides 11.13 effect sizes (median = 6, with 25% of the meta-analyses providing only one effect size). We observed that the number of meta-analytic effect sizes extracted from each meta-analysis is highly correlated with the number of studies included in a meta-analysis, indicating that the number of effect sizes that a meta-analysis contributes is not the effect of randomness or inconsistent dependent variable coding (see web appendix A for further details). We retrieved data from meta-analyses published until 2012 from the dataset reported in Eisend (2015) , which we updated and recoded when necessary. Data from the meta-analyses published after 2012 were coded by a total of 5 coders, who also provided quality checks and oversight. The coding was done using the Cognetto (Hyperthesis) Meta-Extractor ( https://cognetto.ai/meta ), an artificial intelligence (AI)-enabled, interactive data extraction tool for meta-analyses and systematic reviews that allows data coding directly on top of PDF documents, automatically detects and extracts key elements of research papers, organizes the coded data, and links it back to its source location in the text, facilitating fast and accurate data extraction and rapid quality checks.
The correlation coefficient was chosen as the meta-analytic effect size that captures the explained variance in a relationship between two variables. The final dataset includes 2,481 meta-analytic effect sizes extracted from 222 meta-analyses published by the end of 2021 (see web appendix B for the full list). Of the 222 meta-analyses, 93 were included in Eisend (2015) . The meta-analyses include around 14,000 primary studies (62.9 studies per meta-analysis) 1 and more than 100,000 primary effect sizes. Based on the 121 meta-analyses that reported the sample sizes of the included primary studies, we calculated that, on average, a meta-analysis includes data from 58,475 consumers. Assuming roughly the same sample size for the remaining meta-analyses, the full dataset of 222 meta-analyses covers an overall sample size of more than 20 million consumers. Absolute values of the correlation coefficient were coded because we are interested in the size of the effect rather than its direction. 2
Effect Sizes and Dependent Variables
For each effect size, we assigned the dependent variable to a conceptual category. The categories were developed based on a review of the consumer research literature and inspection of the dependent variables in our set of collected meta-analyses. The assignment of each dependent variable to its corresponding category was done via a rule-based computer classification model, which produced a 90.6% agreement rate with a human coder (see table 1 and web appendix C for the categorization details).
META-META-ANALYTIC EFFECT SIZES PER DV CATEGORY AND THE FUNCTIONAL FORMS OF THE RELATIONSHIP BETWEEN EFFECT SIZE AND TIME
Note: All mean r s are significant at p < .01.
Effect Sizes and Time
We assume that the time variable explains effect sizes as a function of consumer research progression over time. To this end, we used a variable that reflects the time when the knowledge included in a meta-analysis was generated. This variable was calculated from the average publication year of the studies included in a meta-analysis, where available. On average, the mean is located after 66% of the time has passed since the publication of the oldest study. If we could not retrieve the full list of studies, the mean value was computed based on the time difference between the publication year of the oldest and the most recently published study of the meta-analysis. 3 To explore the best-fitting model(s) of scientific progress, we estimated different functional forms: a linear function (for continuous, linear progress), a logistic or growth function (for continuous, non-linear progress), and a quadratic function (for discontinuous progress, see Eisend 2015 ). A non-significant effect denotes a static progress model. In cases where more than one model was significant, the additional explained variance of each model was tested against the explained variance of the significant linear model as the base model, to determine the model with the highest explanatory power.
Primary and Meta-Analytical Research Trends
To measure trends in primary research on consumers, we used Wang et al.’s (2015) historical analysis of the content of JCR articles. Published on the occasion of JCR ’s 40th anniversary, 4 the analysis employs a Latent Dirichlet Allocation-based topic modeling procedure to group JCR article abstracts into 16 topics investigated in consumer research. Using these 16 topics, along with Wang et al.’s (2015) list of representative terms for each topic, we trained two coders to manually assign the abstracts of the 222 meta-analyses to each topic. Since each abstract can be represented by a mixture of topics ( Wang et al. 2015 ), coders could categorize an abstract to up to three topics (see web appendix H for an overview of the topics and the categorization criteria used). Inter-rater reliability for each topic (Cohen’s kappa) was sufficiently high (0.81), and differences were resolved through discussions. To determine how well trends in meta-analysis research line up with trends in primary research in consumer behavior, our analysis compares, for each topic, the change in meta-analysis research volume before and after 2014 (where 2014 represents the cutoff point for Wang et al.’s [2015] analysis). We then contrast the observed changes in meta-analysis research volume against the changes identified or predicted by Wang et al. (2015) . Importantly, since the overall number of meta-analyses in consumer research has been exponentially increasing over the years, examining research volume in terms of number of published meta-analyses may not reveal true trends, as publication numbers would be trending up across all topics. Hence, a more informative metric for research volume is the proportion of all meta-analysis publications that is represented by each meta-analysis topic. We therefore examine changes in the proportional representation of each topic.
Overall Effect Sizes
The observed mean effect size (i.e., the meta-meta-analytic effect size, corresponding to the correlation coefficient Pearson’s r ) is 0.28. This effect size indicates 7.8% explained variance (0.28 2 ), leaves 92.2% variance unaccounted for, and counts as a medium-sized effect ( Gignac and Szodorai 2016 ).
Effect Size Variations by Dependent Variable Category
The meta-meta-analytic effect sizes differ based on the dependent variable used, as indicated in table 1 . These differences are substantial, with effect sizes ranging from large to medium to small. The effects for relationship strength (16.5% explained variance) and satisfaction and social behaviors (both 13.2%) stand out as being large in size. They are also significantly stronger ( p < .05) than the effects for the remaining dependent variable categories (except for trust , involvement , choice/decision , willingness-to-pay , and attention ; see web appendix D for all statistical difference tests). Small-sized effects include, among others, effects for attitudes and processing . Among those, the weakest effects are for affect and memor y (4.0% and 4.2%). The differences between the largest and smallest effects are notable, as the largest effect (for relationship strength ) explains more than four times the variance explained by the weakest one (for affect ). Also notable is the finding that effect sizes related to attitudes , one of the most frequently used dependent variables in consumer research (second, in our list of effect frequencies, only to cognitions and behaviors ), are substantially lower ( r = 0.22) compared to effect sizes for conceptually related constructs such as choice or satisfaction . Table 1 reports not only the raw mean but also the median, the mean based on multiple effect sizes from a meta-analysis that were averaged before integration, and a multi-level mean value that accounts for dependencies of multiple effects. The analytical approach is described in more detail in web appendix E , alongside further robustness tests. The comparison of the different mean computation procedures shows that for all effect sizes and for all but two dependent variables the deviation from the raw mean r is less than 0.05. These findings suggest that the results are quite robust, even for variables that are based on sparse data (i.e., less than 10 meta-analyses).
Effect Size Variations over Time
Table 1 additionally provides the results of the curve estimation procedure, with time as the independent variable and effect size as the dependent variable (see detailed results in web appendix F ). The overall relationship between time and all effect sizes is significant and described by a linear and positive trend, suggesting a pattern of continuous knowledge growth. Trend variations, nevertheless, occur across dependent variables. We notice a positive linear trend for attitudes , behavior/intentions , and purchase-related behaviors , a positive growth curve for relationship strength and trust , a negative linear trend for cognitions , and a static (non-significant) trend for all remaining dependent variables. 5 Web appendix G depicts the fitted values of the significant relationships ( p < .1).
Web appendix I shows an overview of the distribution and trends of meta-analyses by research topic and indicates how well those trends align with primary research trends. We find that, for meta-analyses published before 2014, the most frequently researched topics include Persuasion (21%), Advertising (17%), Satisfying Customers (16%), Methodological Issues , Buying Process , and Self-Control and Goals (all at 9%). For meta-analyses published in or after 2014, the most frequently researched topics include Satisfying Customers (29%), Advertising (13%), Persuasion (14%), Social Identity and Influence (12%), and Buying Process (10%). When comparing the changes in meta-analysis research volume distribution before and after 2014 (using χ 2 tests) against the trends predicted by Wang et al. (2015) , we observe a substantial overlap between primary and meta-analytical research trends. For example, consistent with Wang et al. (2015) , there is a significant decline in the share of meta-analysis research on Persuasion and Methodological Issues , a significant increase in the share of Emotional Decision-Making research, and a static development for Contextual Effects. Discrepancies also exist. For Advertising , while primary research volume was on a clearly declining trajectory by 2014, the share of meta-analysis research shows no evidence of decline. For Satisfying Customers , despite speculations that primary research volume may have peaked by 2014, the share of meta-analysis research continued increasing, to the point where it represents the most frequently researched topic after 2014 (29%), with a research volume more than twice as large as the next most frequently researched topic ( Advertising , with 13%). Additionally, while Self-Control and Goals and Social Identity and Influence were expected to deliver healthy streams of consumer research past 2014, the share of meta-analysis research for those topics shows a static development.
The findings show how much is known in consumer research, how this knowledge has progressed over time, and how well its synthesis aligns with the content of primary studies. They provide not only a big picture of the development and current status of a dynamic research field but also a research agenda with implications for knowledge progress.
Distinction from Eisend’s (2015) Meta-Meta-Analysis of Marketing Research
Ninety-three out of the 222 meta-analyses in this article were also included in Eisend’s (2015) meta-meta-analysis of marketing research. When compared to Eisend (2015) , the present investigation offers additional contributions in both methodology and findings. First, our results show an overall effect size of 0.28, which is larger than the 0.24 effect size obtained by Eisend (2015) , suggesting that consumer research is better able to explain its phenomena than the broader area of marketing. Second, while Eisend (2015) finds that marketing research up to 2012 displays knowledge growth but at a decreasing rate, the consumer research knowledge growth trajectory until 2021 is linear and steady, indicating that the field has not yet matured as much as marketing, hence still offering plenty of room for new contributions. The reasons for the observed distinction between consumer and marketing research results are both substantial and methodological. Substantially, consumer research benefits from a constant provision of more varied and innovative research topics that are inspired by a strong interdisciplinary approach, by interactions between different research areas and disciplines, and by fewer challenges brought on by increasing specialization ( Eisend 2015 ; MacInnis and Folkes 2010 ). Compared to the broader area of marketing, consumer research gets published in several journal outlets, which—despite some specializations—still seem to reach the whole research community. As for methodological explanations of knowledge progress, it appears that the advancement of rigorous methods has strengthened the effects obtained in consumer research. This finding is in line with the improvements observed in behavioral research over the recent decades due to the implementation of stronger experimental controls ( Cohen and Wilkie 2022 ). Consumer research hence appears more successful at knowledge development than marketing research overall, which suggests that consumer researchers can capitalize on some of the key characteristics of our field to further accelerate knowledge generation. For example, important knowledge contributions may arise from the exploration of interdisciplinary, boundary-breaking consumer research topics ( MacInnis et al. 2020 ), such as timely investigations at the intersection of consumer and computer science—an area currently being transformed by the adoption of generative AI technologies into mass consumption practices. Finally, the present investigation further extends Eisend’s (2015) approach methodologically, as it breaks findings down per dependent variables, thereby shedding light on individual constructs’ contributions to knowledge development in consumer research and illustrating how the careful, strategic selection of study variables can be key to researchers successfully explaining consumer phenomena of interest.
Contributions
First, by explaining 7.8% variance in the dependent variables, the results offer an objective, standardized measure of how much we have learned in consumer research. While this variance may initially appear small, it allows us to determine how our field’s knowledge development compares to that of other fields, as shown in table 2 . In other behavioral fields—whether across a broad swath of the behavioral sciences (particularly psychology, 3.6%), in specific sub-disciplines such as memory, intelligence, or individual differences research (from 4% to 6.8%), or in applied fields such as organizational psychology (6.8%)—the explained variance is below the level observed in consumer research. The explained variance differences are small, but given the range from 3.6% to 7.8%, a 1% advantage in consumer research is relevant. This represents an encouraging finding, as it suggests that consumer research, a comparatively young discipline, is relatively effective at explaining its phenomena compared to neighboring disciplines. Consumer research also compares favorably to a more remote field such as medical (clinical) research, where effect sizes range from 0.13 (for dichotomous) to 0.15 (for continuous dependent variables).
COMPARISON OF EFFECT SIZES WITH OTHER DISCIPLINES
Second, the findings show that knowledge accumulation in consumer research has been steadily growing and is improving the explanations of phenomena related to consumers, suggesting that the field has still not reached the peak of its knowledge life cycle. This result is notable, because other behavioral and psychological science research indicates either the absence of a time effect ( Schäfer and Schwarz 2019 ) or a downright decline in effect sizes over time in well-established fields such as intelligence research ( Gong and Jiao 2019 ; Pietschnig et al. 2019 ). This insight, combined with the favorable effect size comparison to other disciplines, paints consumer research as a field characterized by dynamism and relatively powerful findings. The effect size increase in consumer research—especially when compared to marketing—can be explained both substantially and methodologically, as discussed in the previous section comparing this investigation to Eisend (2015) .
Third, knowledge development in consumer research varies across dependent variable categories. The field excels at explaining relationship building and maintaining, which is in line with a shift toward relationships in marketing research ( Palmatier et al. 2006 ). The variation in effect sizes across dependent variables suggests that relationship strength is linked closest to behavior and furthest to processing. Less successful explanations refer to variables such as affect , memory , or attitudes . The finding that effect sizes for choice and satisfaction are larger than those for either attitudes or behavior/intentions (in general) suggests that classic hierarchical models (e.g., awareness to attitude to intention [purchase]) may not operate as strongly as previously thought in our field. The observed small effect sizes could also be due to the heterogeneity of measures used for assessing such constructs. For example, affect , cognitions , and behavior/intentions (general) represent combinations of different individual constructs and measures, which could explain their lower effect size estimates compared to more homogenous or standardized constructs like satisfaction , choice , or willingness-to-pay . Of course, the low effect sizes could indicate that those constructs are more difficult to capture by the current measures and manipulations. Finally, knowledge progress also varies across dependent variable categories: relationship variables show an increase in knowledge accumulation, while cognitions even display a decrease. What stands out are purchase and behavioral intentions : although the effects are only medium-sized, their development over time shows an increase, which could be explained by a stronger focus on measuring and explaining managerially relevant variables.
The insights from this meta-meta-analysis provide several substantive, theoretical, and methodological opportunities for future consumer research (points 1–4) and implications for meta-analysis research (point 5).
Mismatch and Its Implications
Assuming that meta-analytic research provides a representative picture of research in a field, 6 the current findings suggest areas of mismatch between research activities and explanatory power in primary research. The constructs that have been investigated the most (i.e., in the largest number of meta-analyses) as dependent variables (e.g., cognitions , attitudes , purchase ) do not provide high explanatory power, while some with high explanatory power are investigated less frequently (e.g., involvement , choice ). As larger effect sizes promise more reliable and replicable effects, the observed mismatch suggests that methodological shifts such as focusing more on incentivized choice rather than attitudes could be fruitful, provided that the theoretical insights are comparable and useful for the field.
Dependent Variable Selection and Career Considerations
Both small and large effect sizes present opportunities for researchers, particularly junior scholars who are starting out their careers. A large effect size means that an effect can more easily be uncovered or replicated. Hence, when the goal is to determine whether an initial research hypothesis should be rejected or not, researchers may want to supplement the dependent variables most frequently examined in consumer research ( attitudes , purchase intentions ) with variables associated with larger effect sizes ( choice , willingness-to-pay , satisfaction ). Alternatively, if a study calls for the use of a dependent variable associated with small effect sizes (e.g., attitudes ), researchers may want to increase the sample size to ensure that the study is adequately powered. We recognize that the former suggestion is not without controversy, in light of the practice of “fishing” for significant effects being discouraged in scientific research. Yet, our results show that not all dependent variables are created equally, as some are significantly better than others at reflecting the impact of various predictors. Hence, we believe that the strategic inclusion of a battery of different dependent variables in a study is warranted, provided that the study authors report all the dependent variables and the effect size-based rationale for their inclusion in the results section.
At the same time, small effect sizes present an opportunity for further theoretical, methodological, or programmatic advances around a given topic. In the case of post-purchase behaviors , for example, the small effect sizes observed likely signal that the topic has not yet reached maturity and is a promising candidate for further research initiatives and funding programs. Small effect sizes suggest the need to develop more precise and nuanced theories of the consumption phenomenon being studied or to explore alternative or complementary methods for studying a phenomenon, for example, by combining different methods or using more sensitive measures. The field could particularly benefit from using consistent constructs and measures to avoid construct fragmentation (i.e., situations where several conceptualizations coexist with no clear shared understanding of the construct among consumer researchers), since such fragmentation hampers knowledge accumulation (as illustrated by the development of the involvement construct; Bergkvist and Eisend 2021 ; Bosco 2022 ).
The findings also provide insights for career considerations of scholars and their strategies in a competitive academic market, as academic career success strongly depends on the contribution one makes to the field. Given that high effect sizes increase a scientific paper’s likelihood to be published in a leading academic journal and its subsequent citation volume ( Eisend and Tarrahi 2014 ) and hence act as a predictor for both research contribution and recognition by the scientific community, the current findings illustrate how the choice of dependent variables can influence a study’s contribution. The findings reveal varying knowledge life cycles and show which variables and thus topics still promise knowledge growth in the future (e.g., relationship-related variables). Junior researchers can use the present findings as a tool for guiding research topics and study design selections for primary research studies.
Minimum Amount of Knowledge Required for Future Research Contributions
The effect sizes observed for different dependent variables can serve as indicators for the amount of knowledge currently produced that is considered “normal” for primary research. They can provide guidance for reviewers and authors regarding the minimum amount of knowledge a future study should provide or exceed, so as to offer a substantial research contribution. For instance, the effect size of 0.32 for attention could serve as an approximation for the expected contribution of new attention-focused consumer studies. Similarly, knowing that the average effect size for satisfaction is about twice as large as for affect could help ensure a fairer evaluation of a study that may report a significant effect for satisfaction, but not for affect.
Need for Further Probing of the Mechanisms Underlying Effect Size Increases
Our findings require further explanations regarding knowledge development in consumer research. While they point toward higher effect sizes over time, the exact reasons for such increases can benefit from in-depth probing. In general, larger meta-analytical effect sizes can be achieved via (1) increasing the precision of a paradigm (using better construct operationalization and measurement), (2) broadening the scope of a paradigm (by extrapolating to other domains), or (3) building consensus in a field (by creating feedback loops between initial and subsequent results) ( Chan and Arvey 2012 ). Hence, larger effect sizes may imply not only larger amounts of knowledge but also “better” knowledge—that is, more confidence in the documented knowledge. For the increases we observed, a plausible explanation involves methodological advances such as stronger manipulations in experiments, better controls of other variables, or the use of dependent variables that are more sensitive to manipulations (in response to journals’ demand for stronger effects that are linked to small p -values). Effects might increase due to publication bias, though our analysis does not support this explanation. 7
Further research can shed light on the exact factors driving the observed effect size increases. Moreover, the finding that effect sizes have been increasing for attitudes , behavioral intentions , and purchase-related behaviors , as well as for trust and relationship strength , but decreasing for cognitions , can also benefit from further examination. It is particularly noteworthy that cognitions—which cover the largest share (23%) of all effect sizes across consumer meta-analyses—are characterized by both low and decreasing effect sizes. Has our field reached maturity when it comes to explaining what drives consumers’ cognitions? Alternatively, as the number and type of cognitions studied in our field keep increasing, the development and adoption of validated measures for such cognitions may not have kept up. If our community has difficulty reaching measurement consensus for the increasingly large number of consumer cognitions being assessed, this could explain why such cognitions show less knowledge accumulation progress compared to other, less heterogeneous constructs ( Bergkvist and Eisend 2021 ). Future research can explore why the pattern for cognitions deviates from that of other major constructs, and which cognitive responses could benefit the most from additional consensus-building measure development efforts.
Opportunities for Future Meta-Analytical Research
Our findings indicate that the trends observed in meta-analysis research are relatively representative of those observed in primary research, suggesting that evidence synthesis in consumer research follows a fairly balanced and rigorous pattern. Nevertheless, an imbalance exists for certain topics, creating opportunities for further meta-analytical research. Notably, meta-analyses on Satisfying Consumers and Advertising appear over-represented compared to primary research on those topics, suggesting saturation on the meta-analytical front, while research on consumers’ Self-control and Goals and Social Identity and Influence appears under-represented, suggesting promising opportunities for additional meta-analytical work. We would also like to point out the relative scarcity of meta-analytical research that measures willingness-to-pay , which was investigated in a single meta-analysis in our dataset, despite its managerial importance.
In summary, the present investigation paints an optimistic picture of the knowledge we have accumulated in consumer research. At the same time, it identifies important research gaps and helps ensure that the next wave of primary and meta-analytic research is well-equipped to provide significant contributions to the consumer research literature.
For meta-analyses published after 2012, the systematic retrieval round took place between April 2021 and September 2021 and was followed by cross-checks for the meta-analyses retrieved from Eisend (2015) , that is, meta-analyses published by 2012. Another round of retrieval for meta-analyses published until December 2021 was performed in March 2022. The data were collected by the first, third, and fourth authors. The data were coded by the second, third, fourth, and fifth authors with the help of two student assistants, from June 2021 to March 2022 and from July to November 2022. The data were analyzed by the first and second authors from February to April 2022 and from September to November 2022. The data are currently stored at ResearchBox.
Martin Eisend ( [email protected] ) is a professor of marketing at the European University Viadrina, Frankfurt (Oder), Germany, and an adjunct professor of marketing at the Copenhagen Business School, Copenhagen, Denmark.
Gratiana Pol ( [email protected] ) is CEO and Co-Founder of Hyperthesis (Cognetto), Sherman Oaks, CA, USA.
Dominika Niewiadomska ( [email protected] ) is a PhD candidate at the European University Viadrina, Frankfurt (Oder), Germany.
Joseph Riley ( [email protected] ) is a PhD candidate at the European University Viadrina, Frankfurt (Oder), Germany.
Rick Wedgeworth ( [email protected] ) is CTO and Co-Founder of Cognetto, Sherman Oaks, CA, USA.
The authors would like to thank everyone who contributed, directly or indirectly, to the development of the software used for annotating the papers included in this meta-analysis, particularly Abishek Borah, whose help was invaluable in getting the software development funded, Roy Nijhof, Luciano Silvi, and Jude Calvillo. Supplementary materials are included in the web appendix accompanying the online version of this article.
We checked for study overlap (i.e., studies that were included in more than one meta-analysis) in meta-analyses for which we could retrieve the study list. We found that 16% of the meta-analyses include only unique, non-overlapping studies and 50% show an overlap of less than 16%. The relationship between the percentage of overlapping studies and the mean effect size of a meta-analysis is not significant ( r = 0.12, p = .11, n = 188). Because our analysis is largely descriptive, the overlap of studies should cause no problem, as the overlap affects only the standard errors and confidence intervals and causes no biases in averages or mean values ( Stanley et al. 2018 ).
Around 10% of the meta-analytic effect sizes were negative and were converted into positive ones (see web appendix E for the exact figures of negative effect sizes). Similar to Eisend (2015) , we found that attenuation-corrected estimates are larger than unattenuated ones (0.31 vs. 0.28, F (1, 479) = 18.11, p < .001), because the correction factor increases unattenuated effect sizes. The average ratio of unattenuated to attenuated effect sizes is 0.89, which was used to correct the estimates from meta-analyses that provide attenuation-corrected estimates (i.e., the estimates were multiplied by 0.89). We found no difference between unweighted and weighted mean values (0.28 vs. 0.27, F (1, 479) = .31, p = .57) and thus did not correct them.
For 24 meta-analyses, neither the study list nor information on the timeframe could be retrieved, and they were therefore excluded from the analysis of temporal developments.
While extrapolating the results from JCR articles to the entirety of the consumer research field is not without limitations, we nevertheless consider JCR , with its “big tent” approach to research, to be representative enough of consumer research developments and trends ( Inman et al. 2018 ) to warrant such extrapolation.
We find a positive relationship between time and the number of studies in a meta-analysis ( r = 0.26, p < .01), suggesting that the overall positive trend results could reflect learning as expressed by the progressively larger number of studies included in consumer research meta-analyses. Thus, we added the number of studies to the models in table 2 and found it to be a significant predictor in several cases. However, the significant effects of time remain unchanged, supporting the robustness of our results.
We explored the assumption that the distribution of dependent variables examined across meta-analyses is representative of those variables’ distribution across primary research studies. To do so, we first separated meta-analyses into two categories: (1) those that focused on a specific dependent variable (either alongside a specified predictor or alongside all predictors examined in relation to that dependent variable) and (2) those that focused on a specific predictor variable and hence captured all available dependent variables examined in the literature in relation to that predictor. We assume that, when taken as a whole, the latter category (which covers 33% of all meta-analyses) provides a representative coverage of the dependent variables examined across primary research. We then compared the latter category of meta-analyses against the full dataset of meta-analyses and observed that the dependent variables have comparable distributions across the two datasets, particularly when it comes to the most and least frequently researched variables. This implies that the distribution of dependent variables examined across all meta-analyses can be considered largely representative of those variables’ distribution across primary research studies. The supporting data for this analysis are provided in web appendix J .
While we find that, as expected, the percentage of unpublished papers in a meta-analysis is negatively related to the magnitude of the effect sizes in a meta-analysis ( r = −0.15, p < .01), this percentage is not related to the average publication year of studies in a meta-analysis ( r = 0.02, p = .51). When controlling the relationship between effect size and time for the percentage of unpublished studies, the relationship becomes weaker compared to the finding in web appendix I but remains significant ( r = 0.05, p = .04). At the same time, we find a highly encouraging change in the application of publication bias tools in consumer research over time, though the use of such tools is not related to effect sizes: We correlated the year variable with several dummy variables indicating whether publication bias tools were used in meta-analyses and found an increase in the general reporting of a publication bias analysis ( r = 0.41, p < .01), the comparison of effect sizes by publication source ( r = 0.16, p = .03), the use of publication source as a moderator in meta-regression ( r = 0.15, p = .03), trim-and-fill analysis ( r = 0.13, p = .08), reporting of funnel plots ( r = 0.29, p < .01), fail safe N ( r = 0.20, p < .01), and the application of other publication bias analysis methods ( r = 0.20, p < .01), but we did not find any relationship between use of publication bias techniques and meta-analytical results.
Aguinis Herman , Dalton Dan R. , Bosco Frank A. , Pierce Charles A. , Dalton Catherine M. ( 2011 ), “ Meta-Analytic Choices and Judgment Calls: Implications for Theory Building and Testing, Obtained Effect Sizes, and Scholarly Impact ,” Journal of Management , 37 ( 1 ), 5 – 38 .
Google Scholar
Baumgartner Hans ( 2010 ), “ Bibliometric Reflections on the History of Consumer Research ,” Journal of Consumer Psychology , 20 ( 3 ), 233 – 8 .
Bergkvist Lars , Eisend Martin ( 2021 ), “ The Dynamic Nature of Marketing Constructs ,” Journal of the Academy of Marketing Science , 49 ( 3 ), 521 – 41 .
Bosco Frank A. ( 2022 ), “ Accumulating Knowledge in the Organizational Sciences ,” Annual Review of Organizational Psychology and Organizational Behavior , 9 ( 1 ), 441 – 64 .
Chan Meow Lan Evelyn , Arvey Richard D. ( 2012 ), “ Meta-Analysis and the Development of Knowledge ,” Perspectives on Psychological Science , 7 ( 1 ), 79 – 92 .
Cohen Joel B. , Wilkie Willam L. ( 2022 ), “Consumer Psychology: Evolving Goals and Research Orientations,” in APA Handbook of Consumer Psychology , ed. Lynn R. Kahle, Tina M. Lowrey, and Joel Huber, Washington, DC : American Psychological Association, 3-45 .
Google Preview
Combs James G. ( 2010 ), “ Big Samples and Small Effects: Let's Not Trade Relevance and Rigor for Power ,” Academy of Management Journal , 53 ( 1 ), 9 – 13 .
Eisend Martin ( 2015 ), “ Have We Progressed Marketing Knowledge? A Meta-Meta-Analysis of Effect Sizes in Marketing Research ,” Journal of Marketing , 79 ( 3 ), 23 – 40 .
Eisend Martin , Tarrahi Farid ( 2014 ), “ Meta-Analysis Selection Bias in Marketing Research ,” International Journal of Research in Marketing , 31 ( 3 ), 317 – 26 .
Gignac Gilles E. , Szodorai Eva T. ( 2016 ), “ Effect Size Guidelines for Individual Differences Researchers ,” Personality and Individual Differences , 102 , 74 – 8 .
Gong Zhun , Jiao Xinian ( 2019 ), “ Are Effect Sizes in Emotional Intelligence Field Declining? A Meta-Meta Analysis ,” Frontiers in Psychology , 10 , 1655 .
Grewal Dhruv , Puccinelli Nancy , Monroe Kent ( 2018 ), “ Meta-Analysis: Integrating Accumulated Knowledge ,” Journal of the Academy of Marketing Science , 46 ( 1 ), 9 – 30 .
Inman Jeffrey J. , Campbell Margaret C. , Kirmani Amna , Price Linda L. ( 2018 ), “ Our Vision for the Journal of Consumer Research: It’s All about the Consumer ,” Journal of Consumer Research , 44 ( 5 ), 955 – 9 .
IntHout Joanna , Ioannidis John P. A. , Borm George F. , Goeman Jelle J. ( 2015 ), “ Small Studies Are More Heterogeneous Than Large Ones: A Meta-Meta-Analysis ,” Journal of Clinical Epidemiology , 68 ( 8 ), 860 – 9 .
Ioannidis John ( 2017 ), “ Next-Generation Systematic Reviews: Prospective Meta-Analysis, Individual-Level Data, Networks and Umbrella Reviews ,” British Journal of Sports Medicine , 51 ( 20 ), 1456 – 8 .
Larsen Kai R. , Ramsay Lauren J. , Godinho Cristina A. , Gershuny Victoria , Hovorka Dirk S. ( 2021 ), “ IC-Behavior: An Interdisciplinary Taxonomy of Behaviors ,” PloS One , 16 ( 9 ), e0252003 .
Lehmann Donald R. ( 1996 ), “Knowledge Generalization and the Convention of Consumer Research: A Study in Inconsistency,” in Advances in Consumer Research , ed. Corfman K. , Lynch J. , Vol. 23 . Provo, UT : Association for Consumer Research, 1–23 .
MacInnis Deborah J. , Folkes Valerie S. ( 2010 ), “ The Disciplinary Status of Consumer Behavior: A Sociology of Science Perspective on Key Controversies ,” Journal of Consumer Research , 36 ( 6 ), 899 – 914 .
MacInnis Deborah J. , Morwitz Vicki G. , Botti Simona , Hoffman Donna L. , Kozinets Robert V. , Lehmann Donald R. , Lynch John G. Jr. , Pechmann Cornelia ( 2020 ), “ Creating Boundary-Breaking, Marketing-Relevant Consumer Research ,” Journal of Marketing , 84 ( 2 ), 1 – 23 .
Nuijten Michèle B. , van Assen Marcel A. L. M. , Augusteijn Hilde E. M. , Crompvoets Elise A. V. , Wicherts Jelte M. ( 2020 ), “ Effect Sizes, Power, and Biases in Intelligence Research: A Meta-Meta-Analysis ,” Journal of Intelligence , 8 ( 4 ), 36 .
Ones Denis S. , Viswesvaran Chockalingam , Schmidt Frank L. ( 2017 ), “ Realizing the Full Potential of Psychometric Meta-Analysis for a Cumulative Science and Practice of Human Resource Management ,” Human Resource Management Review , 27 ( 1 ), 201 – 15 .
Palmatier Robert W. , Dant Rajiv P. , Grewal Dhruv , Evans Kenneth R. ( 2006 ), “ Factors Influencing the Effectiveness of Relationship Marketing: A Meta-Analysis ,” Journal of Marketing , 70 ( 4 ), 136 – 53 .
Paul Justin , Barari Mojtaba ( 2022 ), “ Meta-Analysis and Traditional Systematic Reviews—What, Why, When, Where, and How? ,” Psychology & Marketing , 39 ( 6 ), 1099 – 115 .
Pietschnig Jacob , Siegel Magdalena , Eder Junia Sophia Nur , Gittler Georg ( 2019 ), “ Effect Declines Are Systematic, Strong, and Ubiquitous: A Meta-Meta-Analysis of the Decline Effect in Intelligence Research ,” Frontiers in Psychology , 10 , 2874 .
Richard F. D. , Bond Charles F. , Stokes-Zoota Juli J. ( 2003 ), “ One Hundred Years of Social Psychology Quantitatively Described ,” Review of General Psychology , 7 ( 4 ), 331 – 63 .
Rubio-Aparicio María , Marín-Martínez Fulgenico , Sánchez-Meca Julio , López-López José A. ( 2018 ), “ A Methodological Review of Meta-Analyses of the Effectiveness of Clinical Psychology Treatments ,” Behavior Research Methods , 50 ( 5 ), 2057 – 73 .
Schäfer Thomas , Schwarz Marcus A. ( 2019 ), “ The Meaningfulness of Effect Sizes in Psychological Research: Differences between Sub-Disciplines and the Impact of Potential Biases ,” Frontiers in Psychology , 10 , 813 .
Schmidt Frank L. ( 1992 ), “ What Do Data Really Mean? ” American Psychologist , 47 ( 10 ), 1173 – 81 .
Schmidt Frank L. , Oh In-Sue ( 2013 ), “ Methods for Second Order Meta-Analysis and Illustrative Applications ,” Organizational Behavior and Human Decision Processes , 121 ( 2 ), 204 – 18 .
Sharpe Donald , Poets Sarena ( 2020 ), “ Meta-Analysis as a Response to the Replication Crisis ,” Canadian Psychology / Psychologie Canadienne , 61 ( 4 ), 377 – 87 .
Siegel Magdalena , Eder Junia Sophia Nur , Wicherts Jelte M. , Pietschnig Jakob ( 2022 ), “ Times Are Changing, Bias Isn't: A Meta-Meta-Analysis on Publication Bias Detection Practices, Prevalence Rates, and Predictors ,” The Journal of Applied Psychology , 107 ( 11 ), 2013 – 39 .
Simonson Itamar , Carmon Ziv , Dhar Ravi , Drolet Aimee , Nowlis Stephen ( 2001 ), “ Consumer Research: Search of Identity ,” Annual Review of Psychology , 52 , 249 – 75 .
Stanley T. D. , Carter Evan C. , Doucouliagos Hristos ( 2018 ), “ What Meta-Analyses Reveal about the Replicability of Psychological Research ,” Psychological Bulletin , 144 ( 12 ), 1325 – 46 .
Wang Xin , Bendle Neil T. , Mai Feng , Cotte June ( 2015 ), “ The Journal of Consumer Research at 40: A Historical Analysis ,” Journal of Consumer Research , 42 ( 1 ), 5 – 18 .
Supplementary data
Email alerts, citing articles via.
- Recommend to your Library
Affiliations
- Online ISSN 1537-5277
- Print ISSN 0093-5301
- Copyright © 2024 Journal of Consumer Research Inc.
- About Oxford Academic
- Publish journals with us
- University press partners
- What we publish
- New features
- Open access
- Institutional account management
- Rights and permissions
- Get help with access
- Accessibility
- Advertising
- Media enquiries
- Oxford University Press
- Oxford Languages
- University of Oxford
Oxford University Press is a department of the University of Oxford. It furthers the University's objective of excellence in research, scholarship, and education by publishing worldwide
- Copyright © 2024 Oxford University Press
- Cookie settings
- Cookie policy
- Privacy policy
- Legal notice
This Feature Is Available To Subscribers Only
Sign In or Create an Account
This PDF is available to Subscribers Only
For full access to this pdf, sign in to an existing account, or purchase an annual subscription.
- Introduction
- Conclusions
- Article Information
Dark blue boxes indicate RRs, and horizontal bars indicate 95% CIs. Sizes of dark blue boxes are proportional to the inverse variance. The light blue diamond indicates the pooled RR estimate and 95% CI in the random-effects model meta-analysis. RRs are maximally adjusted estimates as reported by studies (see eTable 1 in Supplement 1 for adjustment variables). Badr et al 32 for RR of all-cause death and Postigo et al 28 for RR of MACE were crude estimates calculated by this study’s authors based on number of participants and number of events reported for patients living with HIV and control groups. The definition of MACE for Shitole et al 18 and Postigo et al 28 was death or cardiovascular admissions.
RRs are shown for recurrent acute coronary syndrome (ACS) (A), heart failure (HF) admission (B), cardiovascular (CV) death (C), and restenosis (D). Dark blue boxes indicate RRs, and horizontal bars indicate 95% CIs. Sizes of dark blue boxes are proportional to the inverse variance. The light blue diamond indicates the pooled RR estimate and 95% CI in the random-effects model meta-analysis.
eTable 1. Additional Patient Characteristics by Study for Patients Living With HIV and Patients in Control Groups
eTable 2. Comparison of Patient Characteristics Between Patients Living With HIV and Patients in Control Groups
eTable 3. Clinical Outcomes, Relative Risks, and Adjustment Variables by Study
eTable 4. Sensitivity Analysis of Pooled Relative Risks Calculated Using Knapp-Hartung Method for Random-Effects Model Meta-Analysis
eTable 5. Quality Assessment of Included Studies With Newcastle-Ottawa Scale
eFigure 1. Study Flow Sheet
eFigure 2. Pooled Relative Risks for Patients Living With HIV vs Patients in Control Groups for TLR and TVR
eFigure 3. Pooled Unadjusted Relative Risks for Patients Living With HIV vs Patients in Control Groups for All-Cause Mortality, MACE, and Recurrent ACS
eFigure 4. Funnel Plot of Relative Risks for All-Cause Mortality and MACE
eMethods. Detailed Description of Statistical Analysis
Data Sharing Statement
See More About
Sign up for emails based on your interests, select your interests.
Customize your JAMA Network experience by selecting one or more topics from the list below.
- Academic Medicine
- Acid Base, Electrolytes, Fluids
- Allergy and Clinical Immunology
- American Indian or Alaska Natives
- Anesthesiology
- Anticoagulation
- Art and Images in Psychiatry
- Artificial Intelligence
- Assisted Reproduction
- Bleeding and Transfusion
- Caring for the Critically Ill Patient
- Challenges in Clinical Electrocardiography
- Climate and Health
- Climate Change
- Clinical Challenge
- Clinical Decision Support
- Clinical Implications of Basic Neuroscience
- Clinical Pharmacy and Pharmacology
- Complementary and Alternative Medicine
- Consensus Statements
- Coronavirus (COVID-19)
- Critical Care Medicine
- Cultural Competency
- Dental Medicine
- Dermatology
- Diabetes and Endocrinology
- Diagnostic Test Interpretation
- Drug Development
- Electronic Health Records
- Emergency Medicine
- End of Life, Hospice, Palliative Care
- Environmental Health
- Equity, Diversity, and Inclusion
- Facial Plastic Surgery
- Gastroenterology and Hepatology
- Genetics and Genomics
- Genomics and Precision Health
- Global Health
- Guide to Statistics and Methods
- Hair Disorders
- Health Care Delivery Models
- Health Care Economics, Insurance, Payment
- Health Care Quality
- Health Care Reform
- Health Care Safety
- Health Care Workforce
- Health Disparities
- Health Inequities
- Health Policy
- Health Systems Science
- History of Medicine
- Hypertension
- Images in Neurology
- Implementation Science
- Infectious Diseases
- Innovations in Health Care Delivery
- JAMA Infographic
- Law and Medicine
- Leading Change
- Less is More
- LGBTQIA Medicine
- Lifestyle Behaviors
- Medical Coding
- Medical Devices and Equipment
- Medical Education
- Medical Education and Training
- Medical Journals and Publishing
- Mobile Health and Telemedicine
- Narrative Medicine
- Neuroscience and Psychiatry
- Notable Notes
- Nutrition, Obesity, Exercise
- Obstetrics and Gynecology
- Occupational Health
- Ophthalmology
- Orthopedics
- Otolaryngology
- Pain Medicine
- Palliative Care
- Pathology and Laboratory Medicine
- Patient Care
- Patient Information
- Performance Improvement
- Performance Measures
- Perioperative Care and Consultation
- Pharmacoeconomics
- Pharmacoepidemiology
- Pharmacogenetics
- Pharmacy and Clinical Pharmacology
- Physical Medicine and Rehabilitation
- Physical Therapy
- Physician Leadership
- Population Health
- Primary Care
- Professional Well-being
- Professionalism
- Psychiatry and Behavioral Health
- Public Health
- Pulmonary Medicine
- Regulatory Agencies
- Reproductive Health
- Research, Methods, Statistics
- Resuscitation
- Rheumatology
- Risk Management
- Scientific Discovery and the Future of Medicine
- Shared Decision Making and Communication
- Sleep Medicine
- Sports Medicine
- Stem Cell Transplantation
- Substance Use and Addiction Medicine
- Surgical Innovation
- Surgical Pearls
- Teachable Moment
- Technology and Finance
- The Art of JAMA
- The Arts and Medicine
- The Rational Clinical Examination
- Tobacco and e-Cigarettes
- Translational Medicine
- Trauma and Injury
- Treatment Adherence
- Ultrasonography
- Users' Guide to the Medical Literature
- Vaccination
- Venous Thromboembolism
- Veterans Health
- Women's Health
- Workflow and Process
- Wound Care, Infection, Healing
Get the latest research based on your areas of interest.
Others also liked.
- Download PDF
- X Facebook More LinkedIn
Haji M , Capilupi M , Kwok M, et al. Clinical Outcomes After Acute Coronary Syndromes or Revascularization Among People Living With HIV : A Systematic Review and Meta-Analysis . JAMA Netw Open. 2024;7(5):e2411159. doi:10.1001/jamanetworkopen.2024.11159
Manage citations:
© 2024
- Permissions
Clinical Outcomes After Acute Coronary Syndromes or Revascularization Among People Living With HIV : A Systematic Review and Meta-Analysis
- 1 Department of Medicine, Alpert Medical School of Brown University, Providence, Rhode Island
- 2 Department of Medicine, Washington University School of Medicine in St Louis, St Louis, Missouri
- 3 Department of Medicine, Duke Global Health Institute and Duke Clinical Research Institute, Duke University, Durham, North Carolina
- 4 Global Health Institute, University of Washington, Seattle
- 5 Infectious Disease Section, Michael E. DeBakey VA Medical Center, Houston, Texas
- 6 Department of Medicine, Baylor College of Medicine, Houston, Texas
- 7 Pharmacy Service, Michael E. DeBakey VA Medical Center, Houston, Texas
- 8 Center of Innovation, Providence VA Medical Center, Providence, Rhode Island
- 9 Evidence Synthesis Program Center, Providence VA Health Care System, Providence, Rhode Island
- 10 Department of Medicine, Providence VA Medical Center, Providence, Rhode Island
- 11 Department of Pharmacy, University of Rhode Island, Providence
- 12 Department of Health Services, Policy and Practice, Brown University, Providence, Rhode Island
Question What are the postdischarge outcomes for patients living with HIV after acute coronary syndromes or coronary revascularization?
Findings In this systematic review and meta-analysis of 15 studies involving 9499 patients living with HIV and 1 531 117 patients without HIV, patients living with HIV had a higher risk of all-cause mortality, major adverse cardiovascular events, recurrent acute coronary syndromes, and admission for heart failure after the index event, despite being approximately 11 years younger at the time of the event. Patients living with HIV were more likely to be current smokers and engage in illicit drug use and had higher triglyceride and lower high-density lipoprotein cholesterol levels than those without HIV.
Meaning This analysis highlights the need for attention toward secondary prevention strategies to address poor outcomes of cardiovascular disease among patients living with HIV.
Importance Clinical outcomes after acute coronary syndromes (ACS) or percutaneous coronary interventions (PCIs) in people living with HIV have not been characterized in sufficient detail, and extant data have not been synthesized adequately.
Objective To better characterize clinical outcomes and postdischarge treatment of patients living with HIV after ACS or PCIs compared with patients in an HIV-negative control group.
Data Sources Ovid MEDLINE, Embase, and Web of Science were searched for all available longitudinal studies of patients living with HIV after ACS or PCIs from inception until August 2023.
Study Selection Included studies met the following criteria: patients living with HIV and HIV-negative comparator group included, patients presenting with ACS or undergoing PCI included, and longitudinal follow-up data collected after the initial event.
Data Extraction and Synthesis Data extraction was performed following the Preferred Reporting Items for Systematic Reviews and Meta-Analysis (PRISMA) statement. Clinical outcome data were pooled using a random-effects model meta-analysis.
Main Outcome and Measures The following clinical outcomes were studied: all-cause mortality, major adverse cardiovascular events, cardiovascular death, recurrent ACS, stroke, new heart failure, total lesion revascularization, and total vessel revascularization. The maximally adjusted relative risk (RR) of clinical outcomes on follow-up comparing patients living with HIV with patients in control groups was taken as the main outcome measure.
Results A total of 15 studies including 9499 patients living with HIV (pooled proportion [range], 76.4% [64.3%-100%] male; pooled mean [range] age, 56.2 [47.0-63.0] years) and 1 531 117 patients without HIV in a control group (pooled proportion [range], 61.7% [59.7%-100%] male; pooled mean [range] age, 67.7 [42.0-69.4] years) were included; both populations were predominantly male, but patients living with HIV were younger by approximately 11 years. Patients living with HIV were also significantly more likely to be current smokers (pooled proportion [range], 59.1% [24.0%-75.0%] smokers vs 42.8% [26.0%-64.1%] smokers) and engage in illicit drug use (pooled proportion [range], 31.2% [2.0%-33.7%] drug use vs 6.8% [0%-11.5%] drug use) and had higher triglyceride (pooled mean [range], 233 [167-268] vs 171 [148-220] mg/dL) and lower high-density lipoprotein-cholesterol (pooled mean [range], 40 [26-43] vs 46 [29-46] mg/dL) levels. Populations with and without HIV were followed up for a pooled mean (range) of 16.2 (3.0-60.8) months and 11.9 (3.0-60.8) months, respectively. On postdischarge follow-up, patients living with HIV had lower prevalence of statin (pooled proportion [range], 53.3% [45.8%-96.1%] vs 59.9% [58.4%-99.0%]) and β-blocker (pooled proportion [range], 54.0% [51.3%-90.0%] vs 60.6% [59.6%-93.6%]) prescriptions compared with those in the control group, but these differences were not statistically significant. There was a significantly increased risk among patients living with HIV vs those without HIV for all-cause mortality (RR, 1.64; 95% CI, 1.32-2.04), major adverse cardiovascular events (RR, 1.11; 95% CI, 1.01-1.22), recurrent ACS (RR, 1.83; 95% CI, 1.12-2.97), and admissions for new heart failure (RR, 3.39; 95% CI, 1.73-6.62).
Conclusions and Relevance These findings suggest the need for attention toward secondary prevention strategies to address poor outcomes of cardiovascular disease among patients living with HIV.
The widespread use of effective antiretroviral therapies (ARTs) has led to increased survivorship among people living with HIV. Therefore, people living with HIV are experiencing an increased prevalence of age-related disease, such as cardiovascular disease (CVD). 1 , 2 The increase in CVD in this population has been attributed to multiple factors, including increasing age, the increase in burden of traditional CVD factors and psychosocial risk factors, the long-term metabolic effects of ART, and the low-grade immune activation of chronic HIV. 1 , 3 - 8
Epidemiological studies have shown that compared with populations without HIV, people living with HIV have a higher risk of coronary artery disease, acute coronary syndromes (ACS), and heart failure, with onset at younger ages. 4 , 9 - 12 Given this earlier emergence of CVD among people living with HIV, there has been significant attention and evidence generated for primary prevention strategies involving statins. 13 , 14 In conjunction with these studies, characterization of longitudinal CVD outcomes is important to identify strategies for secondary prevention and further improve survivorship among people living with HIV. Studies on clinical outcomes after ACS and percutaneous coronary interventions (PCIs) among patients living with HIV have shown higher rates of recurrent coronary disease and mortality compared with patients in HIV-negative control groups. 11 , 15 - 17 However, this association has not been characterized in sufficient detail in current literature, and extant data have not been adequately synthesized. We conducted a systematic review and meta-analysis of longitudinal studies of patients living with HIV after ACS or PCIs to better characterize clinical outcomes and postdischarge treatment compared with patients in HIV-negative control groups.
We report this systematic review and meta-analysis according to the Preferred Reporting Items for Systematic Reviews and Meta-analyses ( PRISMA ) reporting guideline. This study was not preregistered. Please see the eMethods in Supplement 1 for a detailed description of methods used in this meta-analysis, as recommended by the International Committee of Medical Journal Editors.
We searched Ovid MEDLINE, Embase, and Web of Science for all available articles from inception to August 2023 for the key terms coronary artery disease , myocardial infarction , non-fatal myocardial infarction , acute coronary syndrome , revascularization , percutaneous coronary intervention , and secondary prevention . We also reviewed references of relevant articles.
Articles were screened by 2 reviewers (M.H. and M.C.) by title and abstract and later by full text. We included studies if they fulfilled the following criteria: patients living with HIV and a comparator group of patients without HIV (control group) included, patients with obstructive coronary artery disease presenting with ACS or undergoing revascularization through PCI included, and longitudinal follow-up data on clinical outcomes after initial event collected. We initially also searched for studies that discussed outcomes after stroke and peripheral artery disease.
We extracted the following data where available using standardized forms: study characteristics, baseline demographics (ie, age, sex, and race and ethnicity) and other characteristics (ie, underlying comorbidities, revascularization strategies, and postdischarge medications) of HIV-positive and HIV-negative control populations, HIV-specific characteristics (use of ART, CD4 count, and viral load), number of events by group and hazard ratios (HRs) of clinical outcomes (ie, all-cause mortality, major adverse cardiovascular events [MACE], cardiovascular death, recurrent ACS, stroke, total lesion revascularization, total vessel revascularization, and admission for heart failure). We extracted maximally adjusted HRs where available, as well as unadjusted (crude) or minimally adjusted HRs for clinical outcomes. We captured data on race and ethnicity to help assess the full scope of diversity among patients living with HIV and how applicable our data may be within the global population of people living with HIV. Race and ethnicity were self-reported in the study by Shitole et al. 18 In the other studies reporting this information, data were obtained from review of medical records, including electronic health records. Reported race and ethnicity categories included African American, American Indian, Asian, Hispanic, Pacific Islander, White, and other. We primarily report aggregated data for Black, White, and Hispanic populations only given that there were limited data available on other races and ethnicities.
We combined summary study characteristics (eg, mean age, percentage male and female, percentage Black and White, and percentage Hispanic) across studies using study sizes as analytical weights to provide estimates of pooled means or percentages. The δ and P values comparing summary study-level characteristics (means or prevalences pooled across studies) between HIV-positive and HIV-negative groups were calculated from a linear regression model of each variable on HIV status weighted by the number of participants for each study (ie, a fixed-effects meta-regression). When HRs were not reported, we calculated crude risk ratios from the number of events in each group. In 2 studies, 15 , 19 data were reported as odds ratios. We pooled HRs of clinical outcomes across studies using a random-effects model meta-analysis, estimating between-study heterogeneity using the DerSimonian-Laird method. 20 As a sensitivity analysis, we also estimated between-study heterogeneity using the residual maximum likelihood method and calculated variances ( P values and CIs) of pooled relative risk (RR) estimates using modifications proposed by Knapp and Hartung. 21 For the purpose of the meta-analysis, we considered odds ratios, risk ratios, and HRs as equivalent measures of RR.
We assessed between-study heterogeneity using the Cochran Q statistic and I 2 statistic, which estimates the percentage of total variation across studies due to true between-study difference rather than chance. 22 , 23 We did not explore heterogeneity further owing to the limited numbers of studies available for most comparisons.
The quality of included studies was assessed using the Newcastle-Ottawa Scale for cohort studies. 24 We visually inspected funnel plots to assess the risk of publication bias. We also performed the Egger test for small study bias, although this was limited by the small number of studies that were generally available for investigated outcomes. Where there were P values trending toward small study bias, we performed trim and fill analyses to help assess the impact of the bias on pooled estimates (even if Egger test P values did not reach statistical significance). A 2-sided P value less than .05 was considered statistically significant. For the meta-analysis of RRs, we report point estimates and 95% CIs. All analyses were performed using Stata software statistical software version 15 (StataCorp).
An initial search yielded 3263 studies, which were screened using titles, abstracts, and full texts. Studies reviewing patient outcomes after diagnoses and interventions of peripheral artery disease and stroke were limited, reporting mainly in-hospital outcomes, short-term follow-up, or results without non-HIV comparator groups, and were not further considered in this meta-analysis. We identified 15 studies 11 , 15 , 16 , 18 , 25 - 35 of post-ACS or revascularization outcomes from 2003 to 2023 that met inclusion criteria (eFigure 1 in Supplement 1 ). Of identified studies, 2 were abstracts. 30 , 31 All were retrospective cohort studies except for 3 prospective studies ( Table 1 ). 11 , 26 , 30
Details of patient characteristics and outcomes by study are presented in Table 1 and eTable 1 in Supplement 1 . A total of 9499 patients living with HIV (pooled proportion [range], 76.4% [64.3%-100%] male; pooled mean [range] age, 56.2 [47.0-63.0] years; pooled proportion [range], 10.1% [95% CI, 7.0%-62.5%] Black; 8.1% [95% CI, 0.4%-54.6%] Hispanic, and 13.1% [95% CI, 7.2%-64.0%] White) and 1 531 117 patients in control groups without HIV (pooled proportion [range], 61.7% [59.7%-100%] male; pooled mean [range] age, 67.7 [42.0-69.4] years; pooled proportion [range], 3.3% [95% CI, 2.5%-21.4%] Black, 3.6% [95% CI, 0.7%-36.3%] Hispanic, and 21.1% [95% CI, 14.3%-68.0%] White) who experienced ACS or underwent coronary revascularization were included in the meta-analysis. Summary baseline characteristics of study participants and comparisons of patients living with HIV with patients in control groups are presented in Table 2 and eTable 2 in Supplement 1 . The mean age of patients living with HIV was 11.1 years (95% CI, 6.2-16.0 years) less than that of patients in HIV-negative control groups ( P < .001). HIV-positive and control populations were similarly male dominant. Patients living with HIV were statistically significantly more likely to be current smokers (pooled proportion [range], 59.1% [24.0%-75.0%] smokers vs 42.8% [26.0%-64.1%] smokers; P < .001) and engage in illicit drug use (pooled proportion [range], 31.2% [2.0%-33.7%] drug use vs 6.8% [0%-11.5%] drug use; P < .001) and had significantly higher pooled mean (range) triglyceride (233 [167-268] vs 171 [148-220] mg/dL; P = .01) and lower pooled mean (range) high-density lipoprotein cholesterol (40 [26-43] vs 46 [29-46] mg/dL; P = .03) levels. (To convert triglycerides and cholesterol to millimoles per liter, multiply by 0.0113 and 0.0259, respectively.) There were similar proportions of patients with diabetes, hypertension, and a family history of coronary artery disease in the 2 groups ( Table 2 ; eTable 2 in Supplement 1 ).
Patients with HIV had been diagnosed with HIV for a pooled mean (range) of 11.2 (8.5-12.0) years. From 9 studies 11 , 16 , 18 , 26 , 28 , 29 , 31 , 34 , 35 that provided these data, a pooled proportion (range) of 75.2% (50.0%-94.1%) of patients living with HIV were receiving ART and 47.6% (25.0%-85.6%) had previously received protease inhibitor therapy. The pooled mean (range) CD4 count was 377 (318-462) cells/mm 3 among patients living with HIV, and most of these patients (pooled proportion [range], 77.8% [63.3%-94.6%]) had a viral load less of than 200 copies per mL ( Table 2 ).
Among 13 studies 11 , 15 , 16 , 18 , 25 - 29 , 31 - 34 that reported data on ACS, patients living with HIV and those in control groups presented similarly with ST-segment elevation myocardial infarction, non–ST-segment elevation myocardial infarction, and unstable angina. Additionally, the groups received PCIs or coronary artery bypass graft surgery at similar proportions. After revascularization, pooled mean (range) left ventricular ejection fraction values were similar between groups (49.4% [44.0%-55.4%] vs 50.9% [48.0%-54.8%]). On postdischarge follow up, patients living with HIV had a lower proportion (range) of statin (53.3% [45.8%-96.1%] vs 59.9% [58.4%-99.0%]) and β-blocker (54.0% [51.3%-90.0%] vs 60.6% [59.6%-93.6%]) prescription compared with patients in control groups, but these differences were not statistically significant ( Table 2 ; eTable 2 in Supplement 1 ).
Over a pooled mean (range) follow-up of a mean of 16.2 (3.0-60.8) months after ACS or revascularization, patients living with HIV had a significantly higher adjusted risk of all-cause mortality (pooled adjusted RR, 1.64; 95% CI, 1.32-2.04), MACE (RR, 1.11; 95% CI, 1.01-1.22), recurrent ACS (RR, 1.83; 95% CI, 1.12-2.97), and heart failure readmission (RR, 3.39; 95% CI, 1.73-6.62) ( Figure 1 ), as well as restenosis (RR, 2.40; 95% CI, 1.13-5.09) ( Figure 2 ) compared with patients in HIV-negative control groups (pooled mean [range] follow-up, 11.9 [3.0-60.8] months). For CV death, total vessel revascularization, and total lesion revascularization, pooled HRs showed no significantly higher risk among patients living with HIV compared with patients in control groups (eFigure 2 in Supplement 1 ). RRs of clinical outcomes and adjustment variables included in multivariate models that were reported by each study are presented in eTable 3 in Supplement 1 . Sensitivity analyses specifying an alternative method for the random-effects model yielded comparable results (eTable 4 in Supplement 1 ). In a separate subsidiary analysis, there was no association between HIV status and risk of post–ACS or PCI mortality, recurrent ACS, or MACE outcomes in the unadjusted (minimally adjusted in some studies) model (eFigure 3 in Supplement 1 ).
There was generally low heterogeneity across studies for most outcomes ( Figure 1 and Figure 2 ). Visual inspection of the funnel plot for publication bias assessment and Egger tests did not suggest the presence of significant publication bias (eFigure 4 in Supplement 1 ). For the all-cause mortality outcome, the Egger test for bias was borderline, and so we performed trim and fill analysis; this yielded similar results (RR, 1.61; 95% CI, 1.30-2.00). Included studies were of moderate to high quality based on the Newcastle-Ottawa Scale, indicating a low to moderate risk of bias (eTable 5 in Supplement 1 ).
We performed a literature-based systematic review and meta-analysis of 15 studies of longitudinal clinical outcomes after ACS or revascularization from 2003 to 2023, comprising a total of 9499 patients living with HIV and 1 531 117 patients without HIV in control groups. We found that patients living with HIV were younger and had a higher risk of all-cause mortality, MACE, recurrent ACS, and heart failure after the index event. We also noted lower rates of statin and β-blocker prescription after discharge among patients living with HIV. Overall, these findings highlight the need to develop and implement strategies for secondary prevention of CVD among patients living with HIV.
The increased mortality, recurrence of ACS, and heart failure admissions among patients living with HIV may be attributed to increased traditional CVD risk factors, psychosocial factors, HIV-related chronic inflammation, and long-term effects of ART. 11 , 16 These factors are equally difficult to control after an initial coronary event. 19 , 35 , 36 The study by Boccara et al 11 from 2020 compared its findings with those of their first, 2011 study 37 and noted an increased rate of recurrence of ACS in patients living with HIV; the authors also noted persistent smoking and chronic inflammation as factors associated with some of the greatest increases in risk for recurrent disease. This further reinforces the need for a multifaceted approach to secondary prevention.
Of note, our study found suboptimal statin prescription in patients living with HIV after ACS or revascularization, which is consistent with results of other retrospective studies. 11 , 18 , 19 , 26 , 28 , 38 - 42 These findings and those of the Evaluating the Use of Pitavastatin to Reduce the Risk of Cardiovascular Disease in HIV-Infected Adults (REPRIEVE) trial, 14 which demonstrated the benefits of pitavastatin for primary prevention of atherosclerotic cardiovascular disease among patients living with HIV, highlight the need for a concerted effort to improve guideline-directed statin prescription and adherence among these patients. 43 Additionally, the higher prevalence of smoking and higher triglyceride levels we found among patients living with HIV highlight areas for optimization, with the goal of improving secondary prevention of atherosclerotic cardiovascular disease. Differences in statin and β-blocker prescriptions on follow-up were not statistically significant, although patients living with HIV had numerically lower percentages for both outcomes.
Our pooled estimates for postdischarge antiplatelet therapy are influenced by the study from Parks et al, 33 which defined antiplatelet use as a filled prescription for clopidogrel, ticagrelor, prasugrel, or ticlopidine and as a retrospective observational study, could not reliably exclude patients with type 2 myocardial infarctions who would not typically qualify for these therapies. In that study’s sensitivity analyses of patients who received coronary angiography, percentages of patients with postdischarge antiplatelet therapies were significantly higher. We performed an analysis of aggregate postdischarge antiplatelet therapy rates excluding data from Parks et al, 33 and aggregate data for postdischarge antiplatelet therapy was much higher.
Few studies reported race or ethnicity of participants, leading to overall low aggregate percentages of White and Black patients living with HIV in our analysis, which is not representative of the global population of these patients. Race and ethnicity in most studies were obtained from review of electronic health records, except in the study by Shitole et al, 18 in which race and ethnicity were self-reported. The analysis of race and ethnicity was skewed by 2 studies; in 1 study, 44 most of the population’s race and ethnicity was unknown, and in the other study, 19 the population was mainly Hispanic. Likewise, the percentage of patients who underwent PCIs was lower than expected for a typical population presenting with ACS. This was also contributed by the Parks et al study, 33 which included patients with type 2 myocardial infarctions, who were not candidates for PCIs in their analysis.
Most studies in our analysis included patients receiving ART with low viral loads and CD4 counts greater than 200 cells/mm 3 , indicating patients with good control of their HIV disease, who are representative of people living with HIV in the current era. 1 , 4 , 7 , 45 We found 8 studies 11 , 16 , 26 - 28 , 31 , 34 , 35 that reported use of protease inhibitors among approximately 50% of patients living with HIV (47.6%). Protease inhibitors are known to have metabolic effects associated with CVD, presenting a plausible explanation for the difference in hypertriglyceridemia between patients living with HIV and patients without HIV in our study. 46 Modern ART regimens have transitioned away from the use of protease inhibitors and now include integrase inhibitors. 7 Conflicting data have emerged around the possible association of integrase inhibitors with increased incidence of CVD. 47 , 48 Therefore, further research on long-term outcomes associated with ART will be essential to primary and secondary prevention of CVD among patients living with HIV.
The period after ACS or PCI provides additional opportunity to introduce aggressive interventions to improve CVD risk factors in patients living with HIV, and these interventions may involve multidisciplinary teams. Ensuring access to and engagement of cardiologists for patients living with HIV will be important to improve outcomes, especially among underrepresented racial and ethnic minorities. 49 Input from pharmacists can also help with optimal selection of statin types, other lipid-lowering agents, and dosages to avoid drug interactions and drug-related adverse effects and maximize adherence to these therapies. Additionally, input from addiction medicine specialists and psychologists can help address underlying mental health disorders (eg, depression and anxiety) and behavioral risk factors (eg, smoking, alcohol use, and cocaine use). In our study, patients living with HIV were more likely to be smokers and engage in illicit drug use, similar to contemporary studies that also show that these behaviors are associated with an overall increased mortality in patients living with HIV despite adequate control of their underlying infection. 50 Likewise, assistance from social workers can help to mitigate social determinants associated with diet and the ability to afford crucial medications. 36 , 51 - 53 Addressing this latter aspect is critically important to improve secondary outcomes of CVD in patients living with HIV because despite increased prescription rates for cardioprotective medications, patients living with HIV have been found to be less likely to fill these medications. 38 , 42 , 52 A multifaceted or multidisciplinary intervention to address psychosocial barriers to cardiovascular care may have the potential to limit mortality and morbidity after ACS or PCI for patients living with HIV.
The findings of this meta-analysis should be considered in context of several limitations. First, given that this was a literature based meta-analysis of aggregate published data, we were unable to compare the association between HIV status and CVD outcomes by clinically important subgroup, such as age, race and ethnicity, or sex. Second, the degree of adjustment for confounders in RR estimates is limited to what is reported in individual studies, is not consistent across studies, and may be inadequate overall. For instance, very few studies accounted for HIV-specific characteristics. However, the goal of the meta-analysis was to understand the difference in secondary CVD outcomes stratified by HIV status regardless of factors that may be contributing to them. We also performed a comparison between maximally adjusted and unadjusted or minimally adjusted RRs to provide further insight into the association. Our analysis showed that there was no association between HIV status and post-ACS or -PCI mortality, recurrent ACS, or MACE outcomes in the unadjusted model. This is likely due to the reverse confounding effect of age given that patients living with HIV were significantly younger than patients in control groups, with a difference of 11 years in pooled mean age across studies. Third, most studies included in this review evaluated patients living with HIV who lived in high-income countries, which may limit generalizability to the global population of patients living with HIV. Fourth, we were not able to perform subgroup analyses of patients who had ACS and were treated medically vs PCI, as well as those who received PCI for stable coronary disease, because these data were not reported separately. Future assessment of outcomes within these subgroups would be important for preventative efforts. Fifth, we were unable to identify timelines for prescription of or adherence to ART or cardioprotective medications based on these aggregate data. Understanding these trends will also be an important focus for secondary prevention in future studies.
In this literature based systematic review and meta-analysis of longitudinal studies from 2000 to 2023, we found that patients living with HIV were significantly younger than patients in control groups. Patients living with HIV had a significantly higher risk of all-cause mortality, MACE, recurrent ACS, and admission for heart failure after the index event compared with patients in control groups.
Patients living with HIV were also significantly more likely to be current smokers and engage in illicit drug use and had higher triglyceride levels at baseline. As more data emerge for primary prevention, this analysis highlights the need for optimization of secondary prevention strategies to address poor outcomes of CVD among patients living with HIV. Future studies can focus on assessing the role of aggressive interventions, including use of multidisciplinary teams to target important risk factors and improve prescription of and adherence to cardioprotective medications among patients living with HIV after ACS or PCI.
Accepted for Publication: March 7, 2024.
Published: May 14, 2024. doi:10.1001/jamanetworkopen.2024.11159
Open Access: This is an open access article distributed under the terms of the CC-BY License . © 2024 Haji M et al. JAMA Network Open .
Corresponding Author: Sebhat Erqou, MD, PhD, Department of Medicine, Providence VA Medical Center, 830 Chalkstone Ave, Providence, RI 02908 ( [email protected] ).
Author Contributions: Drs Haji and Erqou had full access to all of the data in the study and take responsibility for the integrity of the data and the accuracy of the data analysis.
Concept and design: Haji, Ashong, Richard, Wu, Erqou.
Acquisition, analysis, or interpretation of data: Haji, Capilupi, Kwok, Ibrahim, Bloomfield, Longenecker, Rodriguez-Barradas, Jutkowitz, Taveira, Sullivan, Rudolph, Wu, Erqou.
Drafting of the manuscript: Haji, Capilupi, Kwok, Taveira, Erqou.
Critical review of the manuscript for important intellectual content: Capilupi, Kwok, Ibrahim, Bloomfield, Longenecker, Rodriguez-Barradas, Ashong, Jutkowitz, Taveira, Richard, Sullivan, Rudolph, Wu.
Statistical analysis: Kwok, Erqou.
Obtained funding: Erqou.
Administrative, technical, or material support: Haji, Capilupi, Kwok, Jutkowitz, Sullivan, Rudolph, Wu.
Supervision: Bloomfield, Taveira, Richard, Rudolph, Wu, Erqou.
Conflict of Interest Disclosures: Dr Longenecker reported receiving personal fees from Theratechnologies advisory board outside the submitted work. Dr Jutkowitz reported receiving grants from the Department of Veterans Affairs, Veterans Health Administration, Health Services Research and Development during the conduct of the study. Dr Rudolph reported receiving grants from the National Institute on Aging during the conduct of the study. No other disclosures were reported.
Funding/Support: This study was supported by a VISN 1 Career Development Award from the Department of Veterans Affairs, Veterans Health Administration, to Dr Erqou. Dr Erqou was also funded by the Center for Aids Research, Rhode Island Foundation, and Lifespan Cardiovascular Institute. Drs Sullivan, Rudolph, and Wu were funded by grants CIN 13-419 and C19-20-213 from the VA Health Services Research and Development Center of Innovation in Long Term Services and Supports.
Role of the Funder/Sponsor: The funders had no role in the design and conduct of the study; collection, management, analysis, and interpretation of the data; preparation, review, or approval of the manuscript; and decision to submit the manuscript for publication.
Disclaimer: The views expressed in this article are those of the authors and do not necessarily reflect the position or policy of the Department of Veterans Affairs or the US government.
Data Sharing Statement: See Supplement 2 .
- Register for email alerts with links to free full-text articles
- Access PDFs of free articles
- Manage your interests
- Save searches and receive search alerts
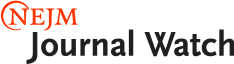
Wait! Try this Special Offer
Subscribe now and save 15%.
- Facebook . opens in new tab
- Twitter . opens in new tab
- LinkedIn . opens in new tab
- Open Athens/Shibboleth
- Activate Print Subscription
- Create Account
- Renew subscription
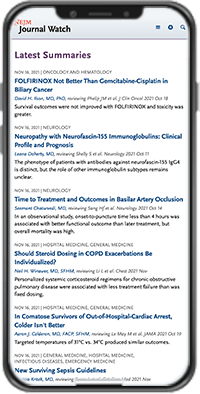
Stay Informed, with Concise, Evidence-Based Information
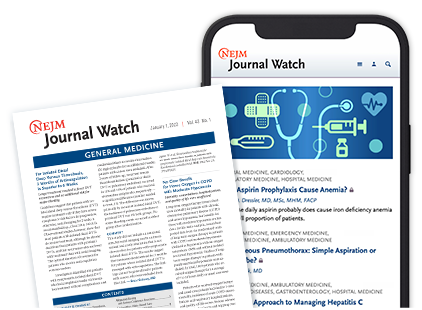
Renew today to continue your uninterrupted access to NEJM Journal Watch.
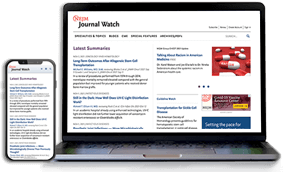
Stay Informed
Subscribe Or Renew
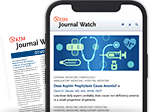
Sign into your account
Forgot Password? Login via Athens or your Institution
Already a print subscriber? Activate your online access.
- SUMMARY AND COMMENT |
- General Medicine
- Ambulatory Medicine
- May 14, 2024
What Is the Incidence of Statin-Induced Diabetes?
Allan S. Brett, MD , reviewing Cholesterol Treatment Trialists' (CTT) Collaboration. Lancet Diabetes Endocrinol 2024 May Gerstein HC and Pigeyre M. Lancet Diabetes Endocrinol 2024 May
A meta-analysis suggests that patients who take high-dose statins have about 1% higher risk annually for newly diagnosed diabetes.
Statins are known to raise risk for diabetes modestly. In this meta-analysis of individual participant data from 23 randomized statin trials, researchers estimated the incidence of this adverse effect. Findings were as follows:
The incidence of new-onset diabetes was only slightly (but statistically significantly) higher with low-to-moderate–intensity statins compared with placebo (1.3% vs. 1.2% annually).
In contrast to the small effect with low-to-moderate–intensity statin, risk for new-onset diabetes was more substantial with high-intensity statins compared with placebo (4.8% vs. 3.5% annually).
New-onset diabetes was diagnosed more often in patients with baseline glycosylated hemoglobin (HbA 1c ) in the prediabetes range than in patients with lower HbA 1c .
In part, variability in incidence of diabetes across trials reflected how often HbA 1c was measured during follow-up.
Among patients with known diabetes at baseline, glycemia worsened slightly with statin therapy compared with placebo.
Statins have a dose-dependent effect on glycemia; in a small proportion of cases, that effect will result in a new diagnosis of diabetes. The authors conclude that any small increase in diabetes-related cardiovascular risk is “greatly outweighed” by the overall risk-reducing benefit of statin therapy. That's certainly true for secondary prevention and for primary prevention in people at high risk. But it might not be true for primary prevention in patients with borderline indications for statin therapy. An editorial by researchers from McMaster University in Canada provides a balanced perspective on these matters.
Cholesterol Treatment Trialists' (CTT) Collaboration. Effects of statin therapy on diagnoses of new-onset diabetes and worsening glycaemia in large-scale randomised blinded statin trials: An individual participant data meta-analysis. Lancet Diabetes Endocrinol 2024 May; 12:306. ( https://doi.org/10.1016/S2213-8587(24)00040-8 )
Gerstein HC and Pigeyre M. How clinically relevant is statin-induced diabetes? Lancet Diabetes Endocrinol 2024 May; 12:286. ( https://doi.org/10.1016/S2213-8587(24)00059-7 )
Facebook | Twitter | LinkedIn | Email | Copy URL
- Disclosures
Disclosures for Allan S. Brett, MD, at time of publication
Topics diabetes lipid management, latest in general medicine, glp-1 agonists and retained gastric contents at endoscopy: an update.
Allan S. Brett, MD Judith Staub, MD
Still Double-Cover for Community-Acquired Pneumonia
Daniel D. Dressler, MD, MSc, MHM, FACP
Observations from ID and Beyond: Just in Time for Mother's Day, Some Admiration and Gratitude
Antihypertensive medication initiation and fracture risk in nursing home residents.
Paul S. Mueller, MD, MPH, FACP
- May 9, 2024
Do GLP-1 Agonists Cause Severe Depression?
Abigail Zuger, MD
A Specific Bacterial Subspecies Is Linked to Colorectal Cancer
Anthony L. Komaroff, MD
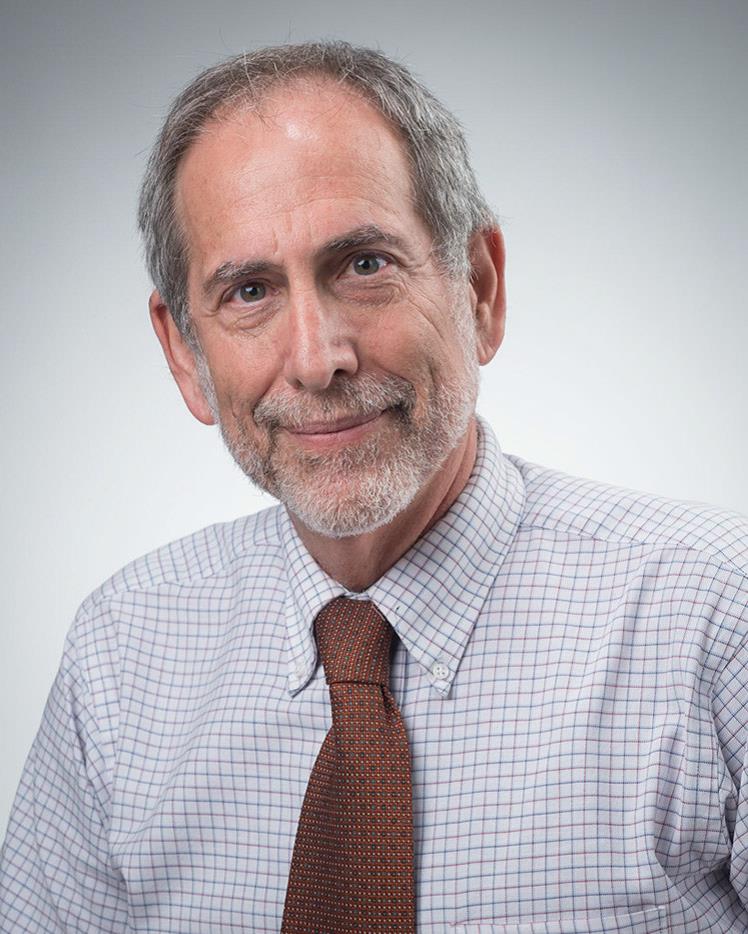
Allan S. Brett, MD
Editor-in-Chief
NEJM Journal Watch NEJM Journal Watch General Medicine
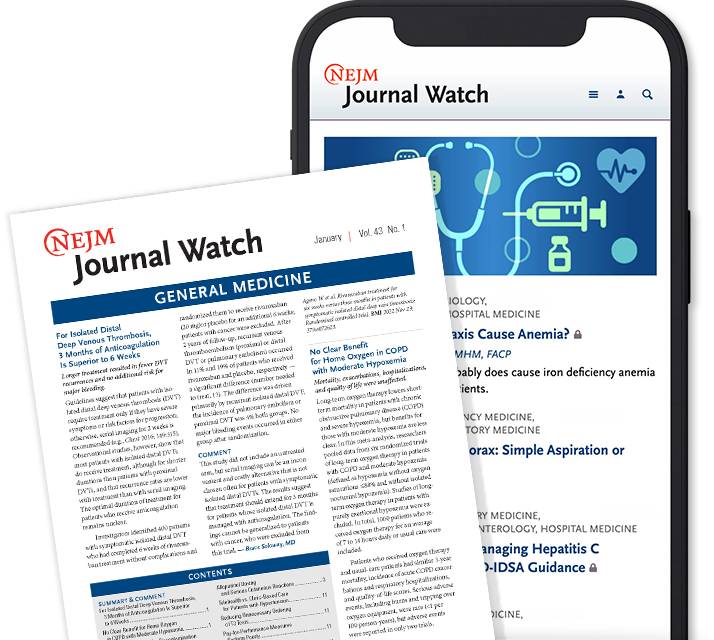
Plus 2 free gifts
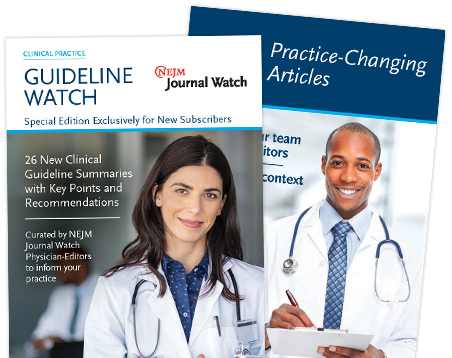

An official website of the United States government
The .gov means it’s official. Federal government websites often end in .gov or .mil. Before sharing sensitive information, make sure you’re on a federal government site.
The site is secure. The https:// ensures that you are connecting to the official website and that any information you provide is encrypted and transmitted securely.
- Publications
- Account settings
Preview improvements coming to the PMC website in October 2024. Learn More or Try it out now .
- Advanced Search
- Journal List
- Hippokratia
- v.14(Suppl 1); 2010 Dec
Meta-analysis in medical research
The objectives of this paper are to provide an introduction to meta-analysis and to discuss the rationale for this type of research and other general considerations. Methods used to produce a rigorous meta-analysis are highlighted and some aspects of presentation and interpretation of meta-analysis are discussed.
Meta-analysis is a quantitative, formal, epidemiological study design used to systematically assess previous research studies to derive conclusions about that body of research. Outcomes from a meta-analysis may include a more precise estimate of the effect of treatment or risk factor for disease, or other outcomes, than any individual study contributing to the pooled analysis. The examination of variability or heterogeneity in study results is also a critical outcome. The benefits of meta-analysis include a consolidated and quantitative review of a large, and often complex, sometimes apparently conflicting, body of literature. The specification of the outcome and hypotheses that are tested is critical to the conduct of meta-analyses, as is a sensitive literature search. A failure to identify the majority of existing studies can lead to erroneous conclusions; however, there are methods of examining data to identify the potential for studies to be missing; for example, by the use of funnel plots. Rigorously conducted meta-analyses are useful tools in evidence-based medicine. The need to integrate findings from many studies ensures that meta-analytic research is desirable and the large body of research now generated makes the conduct of this research feasible.
Important medical questions are typically studied more than once, often by different research teams in different locations. In many instances, the results of these multiple small studies of an issue are diverse and conflicting, which makes the clinical decision-making difficult. The need to arrive at decisions affecting clinical practise fostered the momentum toward "evidence-based medicine" 1 – 2 . Evidence-based medicine may be defined as the systematic, quantitative, preferentially experimental approach to obtaining and using medical information. Therefore, meta-analysis, a statistical procedure that integrates the results of several independent studies, plays a central role in evidence-based medicine. In fact, in the hierarchy of evidence ( Figure 1 ), where clinical evidence is ranked according to the strength of the freedom from various biases that beset medical research, meta-analyses are in the top. In contrast, animal research, laboratory studies, case series and case reports have little clinical value as proof, hence being in the bottom.

Meta-analysis did not begin to appear regularly in the medical literature until the late 1970s but since then a plethora of meta-analyses have emerged and the growth is exponential over time ( Figure 2 ) 3 . Moreover, it has been shown that meta-analyses are the most frequently cited form of clinical research 4 . The merits and perils of the somewhat mysterious procedure of meta-analysis, however, continue to be debated in the medical community 5 – 8 . The objectives of this paper are to introduce meta-analysis and to discuss the rationale for this type of research and other general considerations.

Meta-Analysis and Systematic Review
Glass first defined meta-analysis in the social science literature as "The statistical analysis of a large collection of analysis results from individual studies for the purpose of integrating the findings" 9 . Meta-analysis is a quantitative, formal, epidemiological study design used to systematically assess the results of previous research to derive conclusions about that body of research. Typically, but not necessarily, the study is based on randomized, controlled clinical trials. Outcomes from a meta-analysis may include a more precise estimate of the effect of treatment or risk factor for disease, or other outcomes, than any individual study contributing to the pooled analysis. Identifying sources of variation in responses; that is, examining heterogeneity of a group of studies, and generalizability of responses can lead to more effective treatments or modifications of management. Examination of heterogeneity is perhaps the most important task in meta-analysis. The Cochrane collaboration has been a long-standing, rigorous, and innovative leader in developing methods in the field 10 . Major contributions include the development of protocols that provide structure for literature search methods, and new and extended analytic and diagnostic methods for evaluating the output of meta-analyses. Use of the methods outlined in the handbook should provide a consistent approach to the conduct of meta-analysis. Moreover, a useful guide to improve reporting of systematic reviews and meta-analyses is the PRISMA (Preferred Reporting Items for Systematic reviews and Meta-analyses) statement that replaced the QUOROM (QUality Of Reporting of Meta-analyses) statement 11 – 13 .
Meta-analyses are a subset of systematic review. A systematic review attempts to collate empirical evidence that fits prespecified eligibility criteria to answer a specific research question. The key characteristics of a systematic review are a clearly stated set of objectives with predefined eligibility criteria for studies; an explicit, reproducible methodology; a systematic search that attempts to identify all studies that meet the eligibility criteria; an assessment of the validity of the findings of the included studies (e.g., through the assessment of risk of bias); and a systematic presentation and synthesis of the attributes and findings from the studies used. Systematic methods are used to minimize bias, thus providing more reliable findings from which conclusions can be drawn and decisions made than traditional review methods 14 , 15 . Systematic reviews need not contain a meta-analysisthere are times when it is not appropriate or possible; however, many systematic reviews contain meta-analyses 16 .
The inclusion of observational medical studies in meta-analyses led to considerable debate over the validity of meta-analytical approaches, as there was necessarily a concern that the observational studies were likely to be subject to unidentified sources of confounding and risk modification 17 . Pooling such findings may not lead to more certain outcomes. Moreover, an empirical study showed that in meta-analyses were both randomized and non-randomized was included, nonrandomized studies tended to show larger treatment effects 18 .
Meta-analyses are conducted to assess the strength of evidence present on a disease and treatment. One aim is to determine whether an effect exists; another aim is to determine whether the effect is positive or negative and, ideally, to obtain a single summary estimate of the effect. The results of a meta-analysis can improve precision of estimates of effect, answer questions not posed by the individual studies, settle controversies arising from apparently conflicting studies, and generate new hypotheses. In particular, the examination of heterogeneity is vital to the development of new hypotheses.
Individual or Aggregated Data
The majority of meta-analyses are based on a series of studies to produce a point estimate of an effect and measures of the precision of that estimate. However, methods have been developed for the meta-analyses to be conducted on data obtained from original trials 19 , 20 . This approach may be considered the "gold standard" in metaanalysis because it offers advantages over analyses using aggregated data, including a greater ability to validate the quality of data and to conduct appropriate statistical analysis. Further, it is easier to explore differences in effect across subgroups within the study population than with aggregated data. The use of standardized individual-level information may help to avoid the problems encountered in meta-analyses of prognostic factors 21 , 22 . It is the best way to obtain a more global picture of the natural history and predictors of risk for major outcomes, such as in scleroderma 23 – 26 .This approach relies on cooperation between researchers who conducted the relevant studies. Researchers who are aware of the potential to contribute or conduct these studies will provide and obtain additional benefits by careful maintenance of original databases and making these available for future studies.
Literature Search
A sound meta-analysis is characterized by a thorough and disciplined literature search. A clear definition of hypotheses to be investigated provides the framework for such an investigation. According to the PRISMA statement, an explicit statement of questions being addressed with reference to participants, interventions, comparisons, outcomes and study design (PICOS) should be provided 11 , 12 . It is important to obtain all relevant studies, because loss of studies can lead to bias in the study. Typically, published papers and abstracts are identified by a computerized literature search of electronic databases that can include PubMed ( www.ncbi.nlm.nih.gov./entrez/query.fcgi ), ScienceDirect ( www.sciencedirect.com ), Scirus ( www.scirus.com/srsapp ), ISI Web of Knowledge ( http://www.isiwebofknowledge.com ), Google Scholar ( http://scholar.google.com ) and CENTRAL (Cochrane Central Register of Controlled Trials, http://www.mrw.interscience.wiley.com/cochrane/cochrane_clcentral_articles_fs.htm ). PRISMA statement recommends that a full electronic search strategy for at least one major database to be presented 12 . Database searches should be augmented with hand searches of library resources for relevant papers, books, abstracts, and conference proceedings. Crosschecking of references, citations in review papers, and communication with scientists who have been working in the relevant field are important methods used to provide a comprehensive search. Communication with pharmaceutical companies manufacturing and distributing test products can be appropriate for studies examining the use of pharmaceutical interventions.
It is not feasible to find absolutely every relevant study on a subject. Some or even many studies may not be published, and those that are might not be indexed in computer-searchable databases. Useful sources for unpublished trials are the clinical trials registers, such as the National Library of Medicine's ClinicalTrials.gov Website. The reviews should attempt to be sensitive; that is, find as many studies as possible, to minimize bias and be efficient. It may be appropriate to frame a hypothesis that considers the time over which a study is conducted or to target a particular subpopulation. The decision whether to include unpublished studies is difficult. Although language of publication can provide a difficulty, it is important to overcome this difficulty, provided that the populations studied are relevant to the hypothesis being tested.
Inclusion or Exclusion Criteria and Potential for Bias
Studies are chosen for meta-analysis based on inclusion criteria. If there is more than one hypothesis to be tested, separate selection criteria should be defined for each hypothesis. Inclusion criteria are ideally defined at the stage of initial development of the study protocol. The rationale for the criteria for study selection used should be clearly stated.
One important potential source of bias in meta-analysis is the loss of trials and subjects. Ideally, all randomized subjects in all studies satisfy all of the trial selection criteria, comply with all the trial procedures, and provide complete data. Under these conditions, an "intention-totreat" analysis is straightforward to implement; that is, statistical analysis is conducted on all subjects that are enrolled in a study rather than those that complete all stages of study considered desirable. Some empirical studies had shown that certain methodological characteristics, such as poor concealment of treatment allocation or no blinding in studies exaggerate treatment effects 27 . Therefore, it is important to critically appraise the quality of studies in order to assess the risk of bias.
The study design, including details of the method of randomization of subjects to treatment groups, criteria for eligibility in the study, blinding, method of assessing the outcome, and handling of protocol deviations are important features defining study quality. When studies are excluded from a meta-analysis, reasons for exclusion should be provided for each excluded study. Usually, more than one assessor decides independently which studies to include or exclude, together with a well-defined checklist and a procedure that is followed when the assessors disagree. Two people familiar with the study topic perform the quality assessment for each study, independently. This is followed by a consensus meeting to discuss the studies excluded or included. Practically, the blinding of reviewers from details of a study such as authorship and journal source is difficult.
Before assessing study quality, a quality assessment protocol and data forms should be developed. The goal of this process is to reduce the risk of bias in the estimate of effect. Quality scores that summarize multiple components into a single number exist but are misleading and unhelpful 28 . Rather, investigators should use individual components of quality assessment and describe trials that do not meet the specified quality standards and probably assess the effect on the overall results by excluding them, as part of the sensitivity analyses.
Further, not all studies are completed, because of protocol failure, treatment failure, or other factors. Nonetheless, missing subjects and studies can provide important evidence. It is desirable to obtain data from all relevant randomized trials, so that the most appropriate analysis can be undertaken. Previous studies have discussed the significance of missing trials to the interpretation of intervention studies in medicine 29 , 30 . Journal editors and reviewers need to be aware of the existing bias toward publishing positive findings and ensure that papers that publish negative or even failed trials be published, as long as these meet the quality guidelines for publication.
There are occasions when authors of the selected papers have chosen different outcome criteria for their main analysis. In practice, it may be necessary to revise the inclusion criteria for a meta-analysis after reviewing all of the studies found through the search strategy. Variation in studies reflects the type of study design used, type and application of experimental and control therapies, whether or not the study was published, and, if published, subjected to peer review, and the definition used for the outcome of interest. There are no standardized criteria for inclusion of studies in meta-analysis. Universal criteria are not appropriate, however, because meta-analysis can be applied to a broad spectrum of topics. Published data in journal papers should also be cross-checked with conference papers to avoid repetition in presented data.
Clearly, unpublished studies are not found by searching the literature. It is possible that published studies are systemically different from unpublished studies; for example, positive trial findings may be more likely to be published. Therefore, a meta-analysis based on literature search results alone may lead to publication bias.
Efforts to minimize this potential bias include working from the references in published studies, searching computerized databases of unpublished material, and investigating other sources of information including conference proceedings, graduate dissertations and clinical trial registers.
Statistical analysis
The most common measures of effect used for dichotomous data are the risk ratio (also called relative risk) and the odds ratio. The dominant method used for continuous data are standardized mean difference (SMD) estimation. Methods used in meta-analysis for post hoc analysis of findings are relatively specific to meta-analysis and include heterogeneity analysis, sensitivity analysis, and evaluation of publication bias.
All methods used should allow for the weighting of studies. The concept of weighting reflects the value of the evidence of any particular study. Usually, studies are weighted according to the inverse of their variance 31 . It is important to recognize that smaller studies, therefore, usually contribute less to the estimates of overall effect. However, well-conducted studies with tight control of measurement variation and sources of confounding contribute more to estimates of overall effect than a study of identical size less well conducted.
One of the foremost decisions to be made when conducting a meta-analysis is whether to use a fixed-effects or a random-effects model. A fixed-effects model is based on the assumption that the sole source of variation in observed outcomes is that occurring within the study; that is, the effect expected from each study is the same. Consequently, it is assumed that the models are homogeneous; there are no differences in the underlying study population, no differences in subject selection criteria, and treatments are applied the same way 32 . Fixed-effect methods used for dichotomous data include most often the Mantel-Haenzel method 33 and the Peto method 34 (only for odds ratios).
Random-effects models have an underlying assumption that a distribution of effects exists, resulting in heterogeneity among study results, known as τ2. Consequently, as software has improved, random-effects models that require greater computing power have become more frequently conducted. This is desirable because the strong assumption that the effect of interest is the same in all studies is frequently untenable. Moreover, the fixed effects model is not appropriate when statistical heterogeneity (τ2) is present in the results of studies in the meta-analysis. In the random-effects model, studies are weighted with the inverse of their variance and the heterogeneity parameter. Therefore, it is usually a more conservative approach with wider confidence intervals than the fixed-effects model where the studies are weighted only with the inverse of their variance. The most commonly used random-effects method is the DerSimonian and Laird method 35 . Furthermore, it is suggested that comparing the fixed-effects and random-effect models developed as this process can yield insights to the data 36 .
Heterogeneity
Arguably, the greatest benefit of conducting metaanalysis is to examine sources of heterogeneity, if present, among studies. If heterogeneity is present, the summary measure must be interpreted with caution 37 . When heterogeneity is present, one should question whether and how to generalize the results. Understanding sources of heterogeneity will lead to more effective targeting of prevention and treatment strategies and will result in new research topics being identified. Part of the strategy in conducting a meta-analysis is to identify factors that may be significant determinants of subpopulation analysis or covariates that may be appropriate to explore in all studies.
To understand the nature of variability in studies, it is important to distinguish between different sources of heterogeneity. Variability in the participants, interventions, and outcomes studied has been described as clinical diversity, and variability in study design and risk of bias has been described as methodological diversity 10 . Variability in the intervention effects being evaluated among the different studies is known as statistical heterogeneity and is a consequence of clinical or methodological diversity, or both, among the studies. Statistical heterogeneity manifests itself in the observed intervention effects varying by more than the differences expected among studies that would be attributable to random error alone. Usually, in the literature, statistical heterogeneity is simply referred to as heterogeneity.
Clinical variation will cause heterogeneity if the intervention effect is modified by the factors that vary across studies; most obviously, the specific interventions or participant characteristics that are often reflected in different levels of risk in the control group when the outcome is dichotomous. In other words, the true intervention effect will differ for different studies. Differences between studies in terms of methods used, such as use of blinding or differences between studies in the definition or measurement of outcomes, may lead to differences in observed effects. Significant statistical heterogeneity arising from differences in methods used or differences in outcome assessments suggests that the studies are not all estimating the same effect, but does not necessarily suggest that the true intervention effect varies. In particular, heterogeneity associated solely with methodological diversity indicates that studies suffer from different degrees of bias. Empirical evidence suggests that some aspects of design can affect the result of clinical trials, although this may not always be the case.
The scope of a meta-analysis will largely determine the extent to which studies included in a review are diverse. Meta-analysis should be conducted when a group of studies is sufficiently homogeneous in terms of subjects involved, interventions, and outcomes to provide a meaningful summary. However, it is often appropriate to take a broader perspective in a meta-analysis than in a single clinical trial. Combining studies that differ substantially in design and other factors can yield a meaningless summary result, but the evaluation of reasons for the heterogeneity among studies can be very insightful. It may be argued that these studies are of intrinsic interest on their own, even though it is not appropriate to produce a single summary estimate of effect.
Variation among k trials is usually assessed using Cochran's Q statistic, a chi-squared (χ 2 ) test of heterogeneity with k-1 degrees of freedom. This test has relatively poor power to detect heterogeneity among small numbers of trials; consequently, an α-level of 0.10 is used to test hypotheses 38 , 39 .
Heterogeneity of results among trials is better quantified using the inconsistency index I 2 , which describes the percentage of total variation across studies 40 . Uncertainty intervals for I 2 (dependent on Q and k) are calculated using the method described by Higgins and Thompson 41 . Negative values of I 2 are put equal to zero, consequently I 2 lies between 0 and 100%. A value >75% may be considered substantial heterogeneity 41 . This statistic is less influenced by the number of trials compared with other methods used to estimate the heterogeneity and provides a logical and readily interpretable metric but it still can be unstable when only a few studies are combined 42 .
Given that there are several potential sources of heterogeneity in the data, several steps should be considered in the investigation of the causes. Although random-effects models are appropriate, it may be still very desirable to examine the data to identify sources of heterogeneity and to take steps to produce models that have a lower level of heterogeneity, if appropriate. Further, if the studies examined are highly heterogeneous, it may be not appropriate to present an overall summary estimate, even when random effects models are used. As Petiti notes 43 , statistical analysis alone will not make contradictory studies agree; critically, however, one should use common sense in decision-making. Despite heterogeneity in responses, if all studies had a positive point direction and the pooled confidence interval did not include zero, it would not be logical to conclude that there was not a positive effect, provided that sufficient studies and subject numbers were present. The appropriateness of the point estimate of the effect is much more in question.
Some of the ways to investigate the reasons for heterogeneity; are subgroup analysis and meta-regression. The subgroup analysis approach, a variation on those described above, groups categories of subjects (e.g., by age, sex) to compare effect sizes. The meta-regression approach uses regression analysis to determine the influence of selected variables (the independent variables) on the effect size (the dependent variable). In a meta-regresregression, studies are regarded as if they were individual patients, but their effects are properly weighted to account for their different variances 44 .
Sensitivity analyses have also been used to examine the effects of studies identified as being aberrant concerning conduct or result, or being highly influential in the analysis. Recently, another method has been proposed that reduces the weight of studies that are outliers in meta-analyses 45 . All of these methods for examining heterogeneity have merit, and the variety of methods available reflects the importance of this activity.
Presentation of results
A useful graph, presented in the PRISMA statement 11 , is the four-phase flow diagram ( Figure 3 ).

This flow-diagram depicts the flow of information through the different phases of a systematic review or meta-analysis. It maps out the number of records identified, included and excluded, and the reasons for exclusions. The results of meta-analyses are often presented in a forest plot, where each study is shown with its effect size and the corresponding 95% confidence interval ( Figure 4 ).

The pooled effect and 95% confidence interval is shown in the bottom in the same line with "Overall". In the right panel of Figure 4 , the cumulative meta-analysis is graphically displayed, where data are entered successively, typically in the order of their chronological appearance 46 , 47 . Such cumulative meta-analysis can retrospectively identify the point in time when a treatment effect first reached conventional levels of significance. Cumulative meta-analysis is a compelling way to examine trends in the evolution of the summary-effect size, and to assess the impact of a specific study on the overall conclusions 46 . The figure shows that many studies were performed long after cumulative meta-analysis would have shown a significant beneficial effect of antibiotic prophylaxis in colon surgery.
Biases in meta-analysis
Although the intent of a meta-analysis is to find and assess all studies meeting the inclusion criteria, it is not always possible to obtain these. A critical concern is the papers that may have been missed. There is good reason to be concerned about this potential loss because studies with significant, positive results (positive studies) are more likely to be published and, in the case of interventions with a commercial value, to be promoted, than studies with non-significant or "negative" results (negative studies). Studies that produce a positive result, especially large studies, are more likely to have been published and, conversely, there has been a reluctance to publish small studies that have non-significant results. Further, publication bias is not solely the responsibility of editorial policy as there is reluctance among researchers to publish results that were either uninteresting or are not randomized 48 . There are, however, problems with simply including all studies that have failed to meet peer-review standards. All methods of retrospectively dealing with bias in studies are imperfect.
It is important to examine the results of each meta-analysis for evidence of publication bias. An estimation of likely size of the publication bias in the review and an approach to dealing with the bias is inherent to the conduct of many meta-analyses. Several methods have been developed to provide an assessment of publication bias; the most commonly used is the funnel plot. The funnel plot provides a graphical evaluation of the potential for bias and was developed by Light and Pillemer 49 and discussed in detail by Egger and colleagues 50 , 51 . A funnel plot is a scatterplot of treatment effect against a measure of study size. If publication bias is not present, the plot is expected to have a symmetric inverted funnel shape, as shown in Figure 5A .

In a study in which there is no publication bias, larger studies (i.e., have lower standard error) tend to cluster closely to the point estimate. As studies become less precise, such as in smaller trials (i.e., have a higher standard error), the results of the studies can be expected to be more variable and are scattered to both sides of the more precise larger studies. Figure 5A shows that the smaller, less precise studies are, indeed, scattered to both sides of the point estimate of effect and that these seem to be symmetrical, as an inverted funnel-plot, showing no evidence of publication bias. In contrast to Figure 5A , Figure 5B shows evidence of publication bias. There is evidence of the possibility that studies using smaller numbers of subjects and showing an decrease in effect size (lower odds ratio) were not published.
Asymmetry of funnel plots is not solely attributable to publication bias, but may also result from clinical heterogeneity among studies. Sources of clinical heterogeneity include differences in control or exposure of subjects to confounders or effect modifiers, or methodological heterogeneity between studies; for example, a failure to conceal treatment allocation. There are several statistical tests for detecting funnel plot asymmetry; for example, Eggers linear regression test 50 , and Begg's rank correlation test 52 but these do not have considerable power and are rarely used. However, the funnel plot is not without problems. If high precision studies really are different than low precision studies with respect to effect size (e.g., due different populations examined) a funnel plot may give a wrong impression of publication bias 53 . The appearance of the funnel plot plot can change quite dramatically depending on the scale on the y-axis - whether it is the inverse square error or the trial size 54 .
Other types of biases in meta-analysis include the time lag bias, selective reporting bias and the language bias. The time lag bias arises from the published studies, when those with striking results are published earlier than those with non-significant findings 55 . Moreover, it has been shown that positive studies with high early accrual of patients are published sooner than negative trials with low early accrual 56 . However, missing studies, either due to publication bias or time-lag bias may increasingly be identified from trials registries.
The selective reporting bias exists when published articles have incomplete or inadequate reporting. Empirical studies have shown that this bias is widespread and of considerable importance when published studies were compared with their study protocols 29 , 30 . Furthermore, recent evidence suggests that selective reporting might be an issue in safety outcomes and the reporting of harms in clinical trials is still suboptimal 57 . Therefore, it might not be possible to use quantitative objective evidence for harms in performing meta-analyses and making therapeutic decisions.
Excluding clinical trials reported in languages other than English from meta-analyses may introduce the language bias and reduce the precision of combined estimates of treatment effects. Trials with statistically significant results have been shown to be published in English 58 . In contrast, a later more extensive investigation showed that trials published in languages other than English tend to be of lower quality and produce more favourable treatment effects than trials published in English and concluded that excluding non-English language trials has generally only modest effects on summary treatment effect estimates but the effect is difficult to predict for individual meta-analyses 59 .
Evolution of meta-analyses
The classical meta-analysis compares two treatments while network meta-analysis (or multiple treatment metaanalysis) can provide estimates of treatment efficacy of multiple treatment regimens, even when direct comparisons are unavailable by indirect comparisons 60 . An example of a network analysis would be the following. An initial trial compares drug A to drug B. A different trial studying the same patient population compares drug B to drug C. Assume that drug A is found to be superior to drug B in the first trial. Assume drug B is found to be equivalent to drug C in a second trial. Network analysis then, allows one to potentially say statistically that drug A is also superior to drug C for this particular patient population. (Since drug A is better than drug B, and drug B is equivalent to drug C, then drug A is also better to drug C even though it was not directly tested against drug C.)
Meta-analysis can also be used to summarize the performance of diagnostic and prognostic tests. However, studies that evaluate the accuracy of tests have a unique design requiring different criteria to appropriately assess the quality of studies and the potential for bias. Additionally, each study reports a pair of related summary statistics (for example, sensitivity and specificity) rather than a single statistic (such as a risk ratio) and hence requires different statistical methods to pool the results of the studies 61 . Various techniques to summarize results from diagnostic and prognostic test results have been proposed 62 – 64 . Furthermore, there are many methodologies for advanced meta-analysis that have been developed to address specific concerns, such as multivariate meta-analysis 65 – 67 , and special types of meta-analysis in genetics 68 but will not be discussed here.
Meta-analysis is no longer a novelty in medicine. Numerous meta-analyses have been conducted for the same medical topic by different researchers. Recently, there is a trend to combine the results of different meta-analyses, known as a meta-epidemiological study, to assess the risk of bias 79 , 70 .
Conclusions
The traditional basis of medical practice has been changed by the use of randomized, blinded, multicenter clinical trials and meta-analysis, leading to the widely used term "evidence-based medicine". Leaders in initiating this change have been the Cochrane Collaboration who have produced guidelines for conducting systematic reviews and meta-analyses 10 and recently the PRISMA statement, a helpful resource to improve reporting of systematic reviews and meta-analyses has been released 11 . Moreover, standards by which to conduct and report meta-analyses of observational studies have been published to improve the quality of reporting 71 .
Meta-analysis of randomized clinical trials is not an infallible tool, however, and several examples exist of meta-analyses which were later contradicted by single large randomized controlled trials, and of meta-analyses addressing the same issue which have reached opposite conclusions 72 . A recent example, was the controversy between a meta-analysis of 42 studies 73 and the subsequent publication of the large-scale trial (RECORD trial) that did not support the cardiovascular risk of rosiglitazone 74 . However, the reason for this controversy was explained by the numerous methodological flaws found both in the meta-analysis and the large clinical trial 75 .
No single study, whether meta-analytic or not, will provide the definitive understanding of responses to treatment, diagnostic tests, or risk factors influencing disease. Despite this limitation, meta-analytic approaches have demonstrable benefits in addressing the limitations of study size, can include diverse populations, provide the opportunity to evaluate new hypotheses, and are more valuable than any single study contributing to the analysis. The conduct of the studies is critical to the value of a meta-analysis and the methods used need to be as rigorous as any other study conducted.

IMAGES
VIDEO
COMMENTS
2.1 Step 1: defining the research question. The first step in conducting a meta-analysis, as with any other empirical study, is the definition of the research question. Most importantly, the research question determines the realm of constructs to be considered or the type of interventions whose effects shall be analyzed.
It is easy to confuse systematic reviews and meta-analyses. A systematic review is an objective, reproducible method to find answers to a certain research question, by collecting all available studies related to that question and reviewing and analyzing their results. A meta-analysis differs from a systematic review in that it uses statistical ...
Meta-analysis is the statistical combination of the results of multiple studies addressing a similar research question. An important part of this method involves computing an effect size across all of the studies; this involves extracting effect sizes and variance measures from various studies.
Meta-analysis refers to the statistical analysis of the data from independent primary studies focused on the same question, which aims to generate a quantitative estimate of the studied phenomenon, for example, the effectiveness of the intervention (Gopalakrishnan and Ganeshkumar, 2013). In clinical research, systematic reviews and meta ...
Meta-analysis would be used for the following purposes: To establish statistical significance with studies that have conflicting results. To develop a more correct estimate of effect magnitude. To provide a more complex analysis of harms, safety data, and benefits. To examine subgroups with individual numbers that are not statistically significant.
Meta-analysis is an objective examination of published data from many studies of the same research topic identified through a literature search. Through the use of rigorous statistical methods, it ...
Tip 1: Know the type of outcome than. There are differences in a forest plot depending on the type of outcomes. For a continuous outcome, the mean, standard deviation and number of patients are ...
Meta-analysis is the quantitative, scientific synthesis of research results. Since the term and modern approaches to research synthesis were first introduced in the 1970s, meta-analysis has had a ...
Meta-analysis is a central method for knowledge accumulation in many scien-tic elds (Aguinis et al. 2011c; Kepes et al. 2013). Similar to a narrative review, it serves as a synopsis of a research question or eld. However, going beyond a narra-tive summary of key ndings, a meta-analysis adds value in providing a quantitative
Additional statistical research should include study of the impact of outliers on the meta-analysis and the potential insight that they could provide into a research question . Statistically valid methods to combine data across studies of varying quality and design, including data from case-control studies, will enable metaanalysts to maximize ...
Definition. "A meta-analysis is a formal, epidemiological, quantitative study design that uses statistical methods to generalise the findings of the selected independent studies. Meta-analysis and systematic review are the two most authentic strategies in research. When researchers start looking for the best available evidence concerning ...
Meta-analysis is a robust tool that can help researchers overcome these challenges by assimilating data across studies identified through a literature review. In other words, rather than surveying participants, a meta-analysis surveys studies. The goal is to calculate the direction and/or magnitude of an effect across all relevant studies, both ...
Abstract. Meta-analysis is widely accepted as the preferred method to synthesize research findings in various disciplines. This paper provides an introduction to when and how to conduct a meta-analysis. Several practical questions, such as advantages of meta-analysis over conventional narrative review and the number of studies required for a ...
Meta-analysis is a quantitative research method that involves the systematic synthesis and statistical analysis of data from multiple individual studies on a particular topic or research question. It aims to provide a comprehensive and robust summary of existing evidence by pooling the results of these studies, often leading to more precise and ...
Meta-analysis is a way to statistically combine and summarize the results of different studies so as to obtain a pooled or summary estimate that may better represent what is true … The results of research on a specific question differ across studies, some to a small extent and some to a large extent. Meta-analysis is a way to statistically ...
What is meta-analysis? Meta-analysis is a research process used to systematically synthesise or merge the findings of single, independent studies, using statistical methods to calculate an overall or 'absolute' effect.2 Meta-analysis does not simply pool data from smaller studies to achieve a larger sample size. Analysts use well recognised, systematic methods to account for differences in ...
Meta-analysis is a research method for systematically combining and synthesizing findings from multiple quantitative studies in a research domain. Despite its importance, most literature evaluating meta-analyses are based on data analysis and statistical discussions. This paper takes a holistic view, comparing meta-analyses to traditional ...
meta-analysis, in statistics, approach to synthesizing the results of separate but related studies. In general, meta-analysis involves the systematic identification, evaluation, statistical synthesis, and interpretation of results from multiple studies. It is useful particularly when studies on the same or a similar subject or problem present ...
Meta‐analyses, which summarize all eligible evidence and quantitatively synthesize individual results on a specific clinical question, have become the best available evidence for informing clinical practice and are increasingly important in medical research. This article has described the basic concept, common methods, principles, steps ...
Meta-analyses play a fundamental role in evidence-based healthcare. Compared to other study designs (such as randomized controlled trials or cohort studies), the meta-analysis comes in at the top of the evidence-based medicine pyramid. This is a pyramid which enables us to weigh up the different levels of evidence available to us.
Main. Emerging infectious diseases are on the rise, often originate from wildlife, and are significantly correlated with socioeconomic, environmental and ecological factors 1. As a consequence ...
This meta-analysis aimed to quantify the effects of various forms of experimental sleep loss (deprivation, restriction, fragmentation) on multiple aspects of emotional experience and elucidate factors that may influence these effects. Methods. The researchers conducted a preregistered systematic review and meta-analysis following PRISMA guidelines.
Job satisfaction has long been discussed as an important factor determining individual behavior at work. To what extent this relationship is also evident in the teaching profession is especially relevant given the manifold job tasks and tremendous responsibility teachers bear for the development of their students. From a theoretical perspective, teachers' job satisfaction should be ...
Our findings indicate that the trends observed in meta-analysis research are relatively representative of those observed in primary research, suggesting that evidence synthesis in consumer research follows a fairly balanced and rigorous pattern. Nevertheless, an imbalance exists for certain topics, creating opportunities for further meta ...
Meta-analysis would be used for the following purposes: To establish statistical significance with studies that have conflicting results. To develop a more correct estimate of effect magnitude. To provide a more complex analysis of harms, safety data, and benefits. To examine subgroups with individual numbers that are not statistically significant.
Systematic review and meta-analysis is a way of summarizing research evidence, which is generally the best form of evidence, and hence positioned at the top of the hierarchy of evidence. Systematic reviews can be very useful decision-making tools for primary care/family physicians.
The findings of this meta-analysis should be considered in context of several limitations. First, given that this was a literature based meta-analysis of aggregate published data, we were unable to compare the association between HIV status and CVD outcomes by clinically important subgroup, such as age, race and ethnicity, or sex.
A meta-analysis suggests that patients who take high-dose statins have about 1% higher risk annually for newly diagnosed diabetes. Statins are known to raise risk for diabetes modestly. In this meta-analysis of individual participant data from 23 randomized statin trials, researchers estimated the incidence of this adverse effect.
Meta-Analysis and Systematic Review. Glass first defined meta-analysis in the social science literature as "The statistical analysis of a large collection of analysis results from individual studies for the purpose of integrating the findings" 9.Meta-analysis is a quantitative, formal, epidemiological study design used to systematically assess the results of previous research to derive ...
Birds are used as bioindicators of environmental mercury (Hg) contamination, and toxicity reference values are needed for injury assessments. We conducted a comprehensive review, summarized data from 168 studies, performed a series of Bayesian hierarchical meta-analyses, and developed new toxicity reference values for the effects of methylmercury (MeHg) on birds using a benchmark dose analysis fra