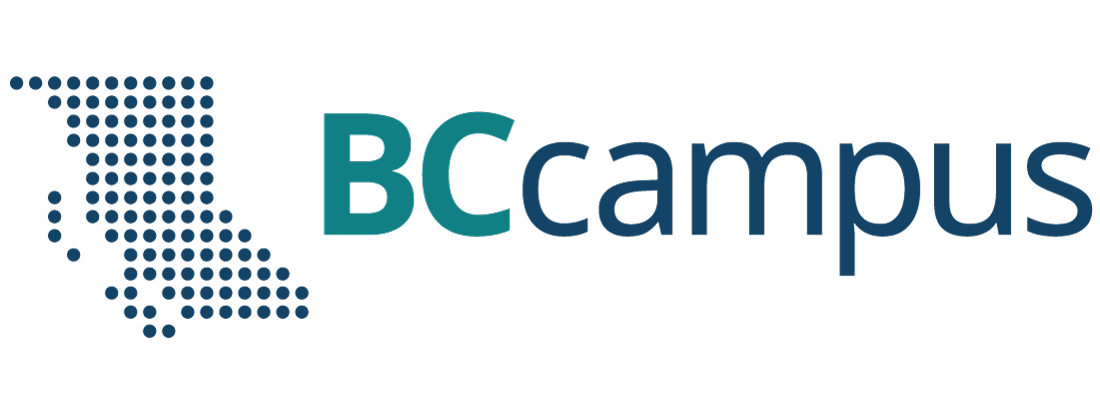
Want to create or adapt books like this? Learn more about how Pressbooks supports open publishing practices.
Chapter 3: Developing a Research Question

3.5 Quantitative, Qualitative, & Mixed Methods Research Approaches
Generally speaking, qualitative and quantitative approaches are the most common methods utilized by researchers. While these two approaches are often presented as a dichotomy, in reality it is much more complicated. Certainly, there are researchers who fall on the more extreme ends of these two approaches, however most recognize the advantages and usefulness of combining both methods (mixed methods). In the following sections we look at quantitative, qualitative, and mixed methodological approaches to undertaking research. Table 2.3 synthesizes the differences between quantitative and qualitative research approaches.
Quantitative Research Approaches
A quantitative approach to research is probably the most familiar approach for the typical research student studying at the introductory level. Arising from the natural sciences, e.g., chemistry and biology), the quantitative approach is framed by the belief that there is one reality or truth that simply requires discovering, known as realism. Therefore, asking the “right” questions is key. Further, this perspective favours observable causes and effects and is therefore outcome-oriented. Typically, aggregate data is used to see patterns and “truth” about the phenomenon under study. True understanding is determined by the ability to predict the phenomenon.
Qualitative Research Approaches
On the other side of research approaches is the qualitative approach. This is generally considered to be the opposite of the quantitative approach. Qualitative researchers are considered phenomenologists, or human-centred researchers. Any research must account for the humanness, i.e., that they have thoughts, feelings, and experiences that they interpret of the participants. Instead of a realist perspective suggesting one reality or truth, qualitative researchers tend to favour the constructionist perspective: knowledge is created, not discovered, and there are multiple realities based on someone’s perspective. Specifically, a researcher needs to understand why, how and to whom a phenomenon applies. These aspects are usually unobservable since they are the thoughts, feelings and experiences of the person. Most importantly, they are a function of their perception of those things rather than what the outside researcher interprets them to be. As a result, there is no such thing as a neutral or objective outsider, as in the quantitative approach. Rather, the approach is generally process-oriented. True understanding, rather than information based on prediction, is based on understanding action and on the interpretive meaning of that action.
Tests hypotheses that the researcher generates | Discovers and encapsulates meanings once the researcher becomes immersed in the data. |
Concepts are in the form of distinct variables. | Concepts tend to be in the form of themes, motifs, generalizations, and taxonomies. However, the objective is still to generate concepts. |
Measures are systematically created before data collection and are standardized as far as possible; e.g. measures of job satisfaction | Measures are more specific and may be specific to the individual setting or researcher; e.g. a specific scheme of values. |
Data are in the form of numbers from precise measurement | Data are in the form of words from documents, observations, and transcripts. However, quantification is still used in qualitative research |
Theory is largely causal and is deductive. | Theory can be causal or non-causal and is often inductive |
Procedures are standard, and replication is assumed. | Research procedures are particular and replication is difficult. |
Analysis proceeds by using statistics, tables, or charts and discussing how they relate to hypotheses. | Analysis proceeds by extracting themes or generalizations from evidence and organizing data to present a coherent, consistent picture. These generalizations can then be used to generate hypotheses |
Note: Researchers in emergency and safety professions are increasingly turning toward qualitative methods. Here is an interesting peer paper related to qualitative research in emergency care (two parts).
Qualitative Research in Emergency Care Part I: Research Principles and Common Applications by Choo, Garro, Ranney, Meisel, and Guthrie (2015)
Interview-based Qualitative Research in Emergency Care Part II: Data Collection, Analysis and Results Reporting
Research Methods for the Social Sciences: An Introduction Copyright © 2020 by Valerie Sheppard is licensed under a Creative Commons Attribution-NonCommercial-ShareAlike 4.0 International License , except where otherwise noted.
Share This Book

How To Choose Your Research Methodology
Qualitative vs quantitative vs mixed methods.
By: Derek Jansen (MBA). Expert Reviewed By: Dr Eunice Rautenbach | June 2021
Without a doubt, one of the most common questions we receive at Grad Coach is “ How do I choose the right methodology for my research? ”. It’s easy to see why – with so many options on the research design table, it’s easy to get intimidated, especially with all the complex lingo!
In this post, we’ll explain the three overarching types of research – qualitative, quantitative and mixed methods – and how you can go about choosing the best methodological approach for your research.
Overview: Choosing Your Methodology
Understanding the options – Qualitative research – Quantitative research – Mixed methods-based research
Choosing a research methodology – Nature of the research – Research area norms – Practicalities
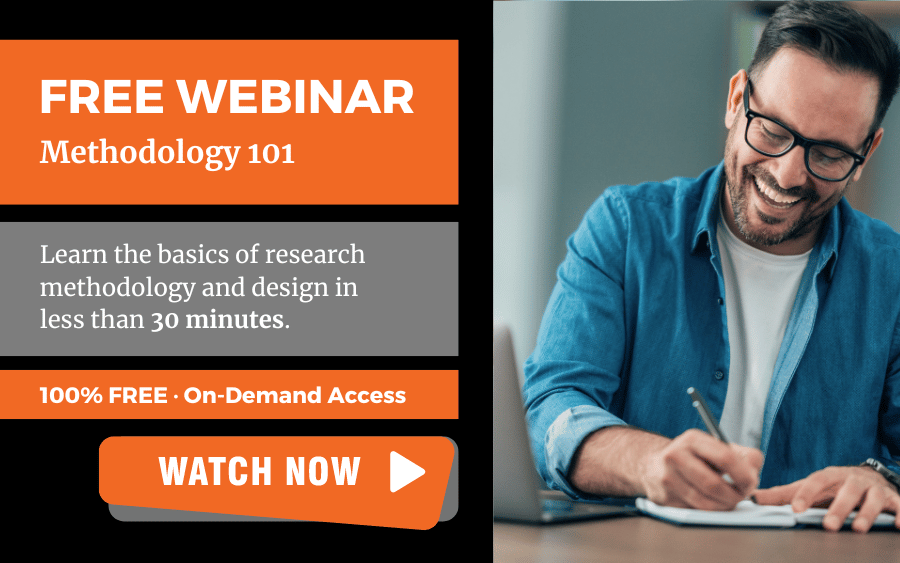
1. Understanding the options
Before we jump into the question of how to choose a research methodology, it’s useful to take a step back to understand the three overarching types of research – qualitative , quantitative and mixed methods -based research. Each of these options takes a different methodological approach.
Qualitative research utilises data that is not numbers-based. In other words, qualitative research focuses on words , descriptions , concepts or ideas – while quantitative research makes use of numbers and statistics. Qualitative research investigates the “softer side” of things to explore and describe, while quantitative research focuses on the “hard numbers”, to measure differences between variables and the relationships between them.
Importantly, qualitative research methods are typically used to explore and gain a deeper understanding of the complexity of a situation – to draw a rich picture . In contrast to this, quantitative methods are usually used to confirm or test hypotheses . In other words, they have distinctly different purposes. The table below highlights a few of the key differences between qualitative and quantitative research – you can learn more about the differences here.
- Uses an inductive approach
- Is used to build theories
- Takes a subjective approach
- Adopts an open and flexible approach
- The researcher is close to the respondents
- Interviews and focus groups are oftentimes used to collect word-based data.
- Generally, draws on small sample sizes
- Uses qualitative data analysis techniques (e.g. content analysis , thematic analysis , etc)
- Uses a deductive approach
- Is used to test theories
- Takes an objective approach
- Adopts a closed, highly planned approach
- The research is disconnected from respondents
- Surveys or laboratory equipment are often used to collect number-based data.
- Generally, requires large sample sizes
- Uses statistical analysis techniques to make sense of the data
Mixed methods -based research, as you’d expect, attempts to bring these two types of research together, drawing on both qualitative and quantitative data. Quite often, mixed methods-based studies will use qualitative research to explore a situation and develop a potential model of understanding (this is called a conceptual framework), and then go on to use quantitative methods to test that model empirically.
In other words, while qualitative and quantitative methods (and the philosophies that underpin them) are completely different, they are not at odds with each other. It’s not a competition of qualitative vs quantitative. On the contrary, they can be used together to develop a high-quality piece of research. Of course, this is easier said than done, so we usually recommend that first-time researchers stick to a single approach , unless the nature of their study truly warrants a mixed-methods approach.
The key takeaway here, and the reason we started by looking at the three options, is that it’s important to understand that each methodological approach has a different purpose – for example, to explore and understand situations (qualitative), to test and measure (quantitative) or to do both. They’re not simply alternative tools for the same job.
Right – now that we’ve got that out of the way, let’s look at how you can go about choosing the right methodology for your research.
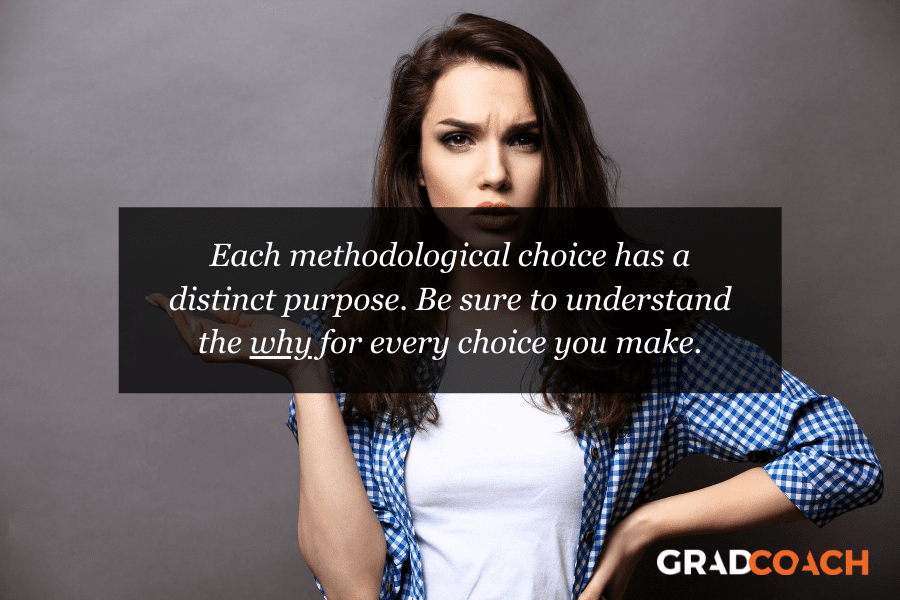
2. How to choose a research methodology
To choose the right research methodology for your dissertation or thesis, you need to consider three important factors . Based on these three factors, you can decide on your overarching approach – qualitative, quantitative or mixed methods. Once you’ve made that decision, you can flesh out the finer details of your methodology, such as the sampling , data collection methods and analysis techniques (we discuss these separately in other posts ).
The three factors you need to consider are:
- The nature of your research aims, objectives and research questions
- The methodological approaches taken in the existing literature
- Practicalities and constraints
Let’s take a look at each of these.
Factor #1: The nature of your research
As I mentioned earlier, each type of research (and therefore, research methodology), whether qualitative, quantitative or mixed, has a different purpose and helps solve a different type of question. So, it’s logical that the key deciding factor in terms of which research methodology you adopt is the nature of your research aims, objectives and research questions .
But, what types of research exist?
Broadly speaking, research can fall into one of three categories:
- Exploratory – getting a better understanding of an issue and potentially developing a theory regarding it
- Confirmatory – confirming a potential theory or hypothesis by testing it empirically
- A mix of both – building a potential theory or hypothesis and then testing it
As a rule of thumb, exploratory research tends to adopt a qualitative approach , whereas confirmatory research tends to use quantitative methods . This isn’t set in stone, but it’s a very useful heuristic. Naturally then, research that combines a mix of both, or is seeking to develop a theory from the ground up and then test that theory, would utilize a mixed-methods approach.
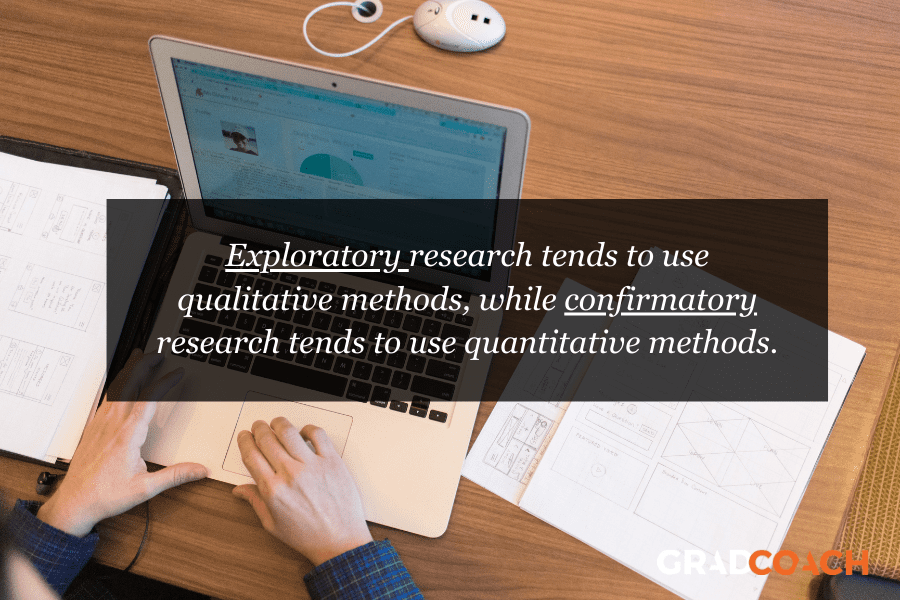
Let’s look at an example in action.
If your research aims were to understand the perspectives of war veterans regarding certain political matters, you’d likely adopt a qualitative methodology, making use of interviews to collect data and one or more qualitative data analysis methods to make sense of the data.
If, on the other hand, your research aims involved testing a set of hypotheses regarding the link between political leaning and income levels, you’d likely adopt a quantitative methodology, using numbers-based data from a survey to measure the links between variables and/or constructs .
So, the first (and most important thing) thing you need to consider when deciding which methodological approach to use for your research project is the nature of your research aims , objectives and research questions. Specifically, you need to assess whether your research leans in an exploratory or confirmatory direction or involves a mix of both.
The importance of achieving solid alignment between these three factors and your methodology can’t be overstated. If they’re misaligned, you’re going to be forcing a square peg into a round hole. In other words, you’ll be using the wrong tool for the job, and your research will become a disjointed mess.
If your research is a mix of both exploratory and confirmatory, but you have a tight word count limit, you may need to consider trimming down the scope a little and focusing on one or the other. One methodology executed well has a far better chance of earning marks than a poorly executed mixed methods approach. So, don’t try to be a hero, unless there is a very strong underpinning logic.
Need a helping hand?
Factor #2: The disciplinary norms
Choosing the right methodology for your research also involves looking at the approaches used by other researchers in the field, and studies with similar research aims and objectives to yours. Oftentimes, within a discipline, there is a common methodological approach (or set of approaches) used in studies. While this doesn’t mean you should follow the herd “just because”, you should at least consider these approaches and evaluate their merit within your context.
A major benefit of reviewing the research methodologies used by similar studies in your field is that you can often piggyback on the data collection techniques that other (more experienced) researchers have developed. For example, if you’re undertaking a quantitative study, you can often find tried and tested survey scales with high Cronbach’s alphas. These are usually included in the appendices of journal articles, so you don’t even have to contact the original authors. By using these, you’ll save a lot of time and ensure that your study stands on the proverbial “shoulders of giants” by using high-quality measurement instruments .
Of course, when reviewing existing literature, keep point #1 front of mind. In other words, your methodology needs to align with your research aims, objectives and questions. Don’t fall into the trap of adopting the methodological “norm” of other studies just because it’s popular. Only adopt that which is relevant to your research.
Factor #3: Practicalities
When choosing a research methodology, there will always be a tension between doing what’s theoretically best (i.e., the most scientifically rigorous research design ) and doing what’s practical , given your constraints . This is the nature of doing research and there are always trade-offs, as with anything else.
But what constraints, you ask?
When you’re evaluating your methodological options, you need to consider the following constraints:
- Data access
- Equipment and software
- Your knowledge and skills
Let’s look at each of these.
Constraint #1: Data access
The first practical constraint you need to consider is your access to data . If you’re going to be undertaking primary research , you need to think critically about the sample of respondents you realistically have access to. For example, if you plan to use in-person interviews , you need to ask yourself how many people you’ll need to interview, whether they’ll be agreeable to being interviewed, where they’re located, and so on.
If you’re wanting to undertake a quantitative approach using surveys to collect data, you’ll need to consider how many responses you’ll require to achieve statistically significant results. For many statistical tests, a sample of a few hundred respondents is typically needed to develop convincing conclusions.
So, think carefully about what data you’ll need access to, how much data you’ll need and how you’ll collect it. The last thing you want is to spend a huge amount of time on your research only to find that you can’t get access to the required data.
Constraint #2: Time
The next constraint is time. If you’re undertaking research as part of a PhD, you may have a fairly open-ended time limit, but this is unlikely to be the case for undergrad and Masters-level projects. So, pay attention to your timeline, as the data collection and analysis components of different methodologies have a major impact on time requirements . Also, keep in mind that these stages of the research often take a lot longer than originally anticipated.
Another practical implication of time limits is that it will directly impact which time horizon you can use – i.e. longitudinal vs cross-sectional . For example, if you’ve got a 6-month limit for your entire research project, it’s quite unlikely that you’ll be able to adopt a longitudinal time horizon.
Constraint #3: Money
As with so many things, money is another important constraint you’ll need to consider when deciding on your research methodology. While some research designs will cost near zero to execute, others may require a substantial budget .
Some of the costs that may arise include:
- Software costs – e.g. survey hosting services, analysis software, etc.
- Promotion costs – e.g. advertising a survey to attract respondents
- Incentive costs – e.g. providing a prize or cash payment incentive to attract respondents
- Equipment rental costs – e.g. recording equipment, lab equipment, etc.
- Travel costs
- Food & beverages
These are just a handful of costs that can creep into your research budget. Like most projects, the actual costs tend to be higher than the estimates, so be sure to err on the conservative side and expect the unexpected. It’s critically important that you’re honest with yourself about these costs, or you could end up getting stuck midway through your project because you’ve run out of money.
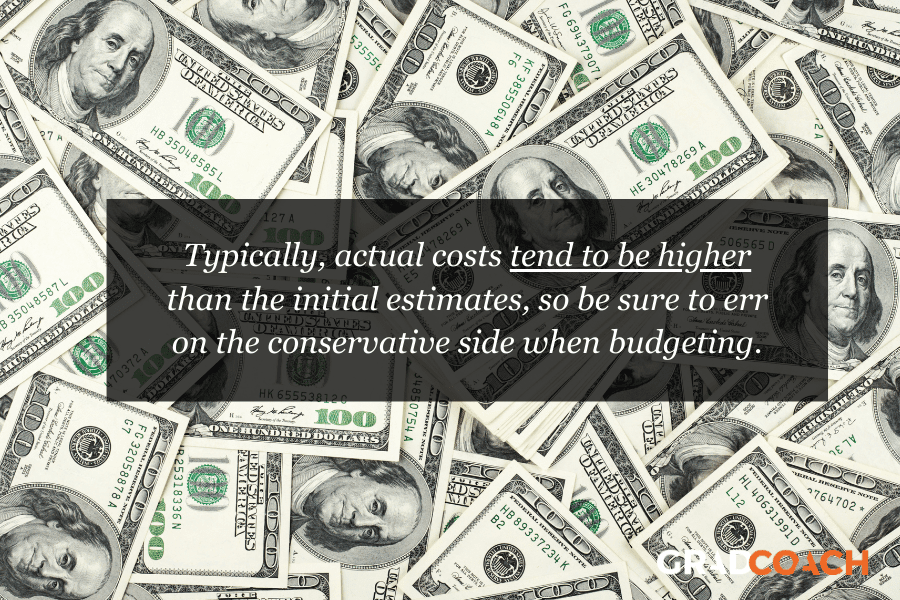
Constraint #4: Equipment & software
Another practical consideration is the hardware and/or software you’ll need in order to undertake your research. Of course, this variable will depend on the type of data you’re collecting and analysing. For example, you may need lab equipment to analyse substances, or you may need specific analysis software to analyse statistical data. So, be sure to think about what hardware and/or software you’ll need for each potential methodological approach, and whether you have access to these.
Constraint #5: Your knowledge and skillset
The final practical constraint is a big one. Naturally, the research process involves a lot of learning and development along the way, so you will accrue knowledge and skills as you progress. However, when considering your methodological options, you should still consider your current position on the ladder.
Some of the questions you should ask yourself are:
- Am I more of a “numbers person” or a “words person”?
- How much do I know about the analysis methods I’ll potentially use (e.g. statistical analysis)?
- How much do I know about the software and/or hardware that I’ll potentially use?
- How excited am I to learn new research skills and gain new knowledge?
- How much time do I have to learn the things I need to learn?
Answering these questions honestly will provide you with another set of criteria against which you can evaluate the research methodology options you’ve shortlisted.
So, as you can see, there is a wide range of practicalities and constraints that you need to take into account when you’re deciding on a research methodology. These practicalities create a tension between the “ideal” methodology and the methodology that you can realistically pull off. This is perfectly normal, and it’s your job to find the option that presents the best set of trade-offs.
Recap: Choosing a methodology
In this post, we’ve discussed how to go about choosing a research methodology. The three major deciding factors we looked at were:
- Exploratory
- Confirmatory
- Combination
- Research area norms
- Hardware and software
- Your knowledge and skillset
If you have any questions, feel free to leave a comment below. If you’d like a helping hand with your research methodology, check out our 1-on-1 research coaching service , or book a free consultation with a friendly Grad Coach.
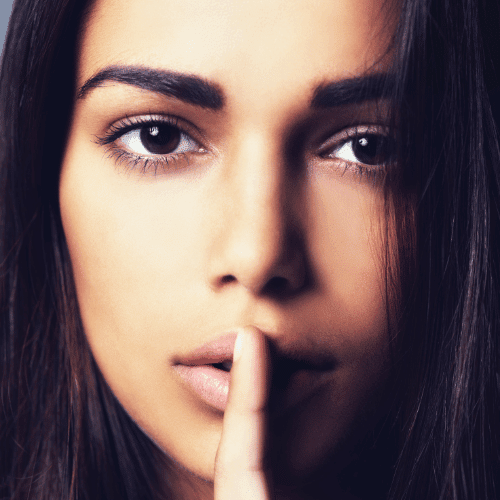
Psst... there’s more!
This post was based on one of our popular Research Bootcamps . If you're working on a research project, you'll definitely want to check this out ...
Very useful and informative especially for beginners
Nice article! I’m a beginner in the field of cybersecurity research. I am a Telecom and Network Engineer and Also aiming for PhD scholarship.
I find the article very informative especially for my decitation it has been helpful and an eye opener.
Hi I am Anna ,
I am a PHD candidate in the area of cyber security, maybe we can link up
The Examples shows by you, for sure they are really direct me and others to knows and practices the Research Design and prepration.
I found the post very informative and practical.
I struggle so much with designs of the research for sure!
I’m the process of constructing my research design and I want to know if the data analysis I plan to present in my thesis defense proposal possibly change especially after I gathered the data already.
Thank you so much this site is such a life saver. How I wish 1-1 coaching is available in our country but sadly it’s not.
Submit a Comment Cancel reply
Your email address will not be published. Required fields are marked *
Save my name, email, and website in this browser for the next time I comment.
- Print Friendly

An official website of the United States government
The .gov means it’s official. Federal government websites often end in .gov or .mil. Before sharing sensitive information, make sure you’re on a federal government site.
The site is secure. The https:// ensures that you are connecting to the official website and that any information you provide is encrypted and transmitted securely.
- Publications
- Account settings
The PMC website is updating on October 15, 2024. Learn More or Try it out now .
- Advanced Search
- Journal List
- Acta Inform Med
- v.30(1); 2022 Mar
Mixed Methodology of Scientific Research in Healthcare
Emina smajic.
1 Agram Polyclinic, Sarajevo, Bosnia and Herzegovina
Dijana Avdic
2 Faculty of Health Studies, Sarajevo, Bosnia and Herzegovina
Aleksandra Pasic
3 Clinical Biochemistry with Immunology, Sarajevo, Clinical Center University of Sarajevo, Bosnia and Herzegovina
Alden Prcic
4 General Hospital “Prim dr. Abdulah Nakas”, Sarajevo, Bosnia and Herzegovina
Maja Stancic
Background:.
Scientific research is usually classified as quantitative or qualitative. However, methodologists are increasingly emphasizing the integration of qualitative and quantitative data as the center of mixed methods (mix methodologies). Mixed research method implies the use of different research methods, ie. quantitative and qualitative methods in one study.
The aim of this review paper is to present the purpose of using a mixed methodology in health research.
The relevant articles were searched from online data sources including PubMed and Google Scholar.
This approach to the use of mixed methods creates opportunities for a deeper study of various problems. The purpose of using mixed research methods is to obtain valid answers to research questions, however the researcher may still have different reasons or purposes for which he wants to strengthen the research study and its conclusions by applying mixed methods. The use of mixed scientific methodology is widely used in the field of health outcomes and should not be limited to a closed list of possible methodological options.
Conclusion:
Recently, there has been an increase in the number of scientific studies in healthcare that use mixed research methods. The advantage of applying this scientific method is that through the triangulation of data obtained by different (quantitative / qualitative) approaches, we get a deeper and more complete picture of the phenomenon in health care that we observe.
1. BACKGROUND
In health science research, there is a priority to develop new methodologies to improve the quality and scientific strength of data leading to an extraordinary increase in methodological diversity. This diversity reflects the nature of public health problems, such as differences between populations, age groups, ethnic groups and cultures, poor adherence to treatments considered effective, behavioral factors contributing to disability and health, and translational needs for health research. Diversity also signals a growing acceptance of qualitative and social science research, the formation of interdisciplinary research teams, and the use of multilevel approaches to research complex health issues such as patient attitudes and cultural and social models of disease and health (1) .
2. OBJECTIVE
The retrieved articles were reviewed by the authors and the results are presented along with the relevant discussion
4.1. MIXED METHODOLOGY
Scientific research is usually classified as quantitative or qualitative. However, methodologists are increasingly emphasizing the integration of qualitative and quantitative data as the center of mixed methods (mix methodologies). Integration is a deliberate process by which the researcher combines quantitative and qualitative approaches in the study. Quantitative and qualitative data then become interdependent in solving questions and hypotheses. Mixed research method implies the use of different research methods, quantitative and qualitative methods in one study ( 2 , 3 ). Research on mixed methods should be distinguished from multi-method research (method-combination) in which either multiple qualitative approaches or only multiple quantitative approaches are combined (4) .

The most accepted definition of a mixed research method is that it is a research in which a researcher or team of researchers combines elements of a qualitative and quantitative approach to research (use of qualitative and quantitative perspectives, data collection, analysis, inference techniques) to understand and support research. As we see in the definition, the use of both quantitative and qualitative methods in a single study (or series of related studies) is crucial, unlike the use of combined methods that combine two or more quantitative or two or more qualitative research methods ( 2 , 4 ).
The basic premise of using mixed research methods is that some research issues can be addressed more comprehensively than using either quantitative or qualitative methods. The issues that benefit most from the design of mixed methods tend to be broad and complex, with multiple aspects that each can have. Mixed research methods can exploit the strengths and weaknesses of both approaches and can be particularly useful when addressing complex, multifaceted issues such as health service interventions and living with chronic diseases (2) . This approach to the use of mixed methods creates opportunities for a deeper study of various problems (5) .
4.2. PURPOSE OF USING MIXED RESEARCH
The purpose of using mixed research methods is to obtain valid answers to research questions, however the researcher may still have different reasons or purposes for which he wants to strengthen the research study and its conclusions by applying mixed methods. The purpose classification of mixed research methods was first introduced in 1989 by Greene, Caracelli, and Graham, based on an analysis of published studies of mixed methods. This classification is still used and we have a total of five “purposes” for why a mixed methodology is used in research (4) . Classification of the purpose of using mixed methodology:
- Complementarity. Using data obtained from one method to illustrate the results of another method.
- Development. Using the results of one method to develop or inform about the use of another method.
- Initiation. We can use the results of different methods to search for areas of non-compliance in certain areas in order to create new insights.
- Expansion. Examining different aspects of a research question, with each aspect justifying different methods.
- Triangulation. Use of data obtained by both methods to support the findings (2) .
In the last 28 years, this classification has been supplemented by several other authors. So in 2006 Bryman compiled a list of more specific rationales for the use or purpose of mixed research methods. Bryman’s classification decomposes the categorization of Greene et al. on several aspects and adds a number of additional aspects (3) .

Bryman’s addition to the classification of Greene et al.
Credibility. It refers to suggestions that the application of both methodological approaches improves the integrity of the results.
Context. Refers to cases where the combination is justified in terms of qualitative research that provides contextual understanding, along with generalized, externally valid results, or broad relationships between the variables identified by the survey.
Illustration. It refers to the use of qualitative data to illustrate quantitative findings, often referred to as putting “meat on the bone” “dry” quantitative findings.
Usefulness or improvement of usefulness of results. It refers to the suggestion, which is more likely to be highlighted among articles with an applied focus, that combining the two approaches will be more useful for practitioners.
Confirmation and discovery. It involves the use of qualitative data to generate hypotheses and the use of quantitative research to test them within a single project.
Diversity of views. This includes two slightly different explanations–namely, combining the perspective of researchers and participants through quantitative and qualitative research, and discovering the relationship between variables through quantitative research, while revealing meanings among research participants through qualitative research (3) .
4.3. RESEARCH DESIGN
Research designs are procedures for collecting, analyzing, interpreting, and reporting data in research studies. They represent different models for doing research, and these models have distinct names and procedures associated with them (6) .
The four major types of mixed methods designs are the Triangulation Design, the Embedded Design, the Explanatory Design, and the Exploratory Design (6) .
4.4. HEALTH APPLICATION
There is a wide range of methods used to collect both quantitative and qualitative data. And the research question and the necessary data are the main determinants of the methods used. To a lesser extent, the choice of methods may be influenced by feasibility. Method priority refers to the emphasis on each method in the study. For example, a study may be predominantly quantitative with a small qualitative component or vice versa. Alternatively, both quantitative and qualitative methods and data may be equally weighted. The emphasis on each methodological component of the study will be driven mainly by the research question, research team skills and feasibility. Finally, researchers must decide when each method will be used in research (2) . By analyzing the research methods and research designs used, Bryman suggests that on the quantitative side, structured interview and questionnaire research within cross-sectional design predominates, while on the qualitative side, semi-structured interviews within cross-sectional design predominate (7) . A key feature of mixed-method research is its methodological pluralism, which often results in research that provides broader perspectives than those offered by monomethodal designs (8) .
The use of mixed scientific methodology is widely used in the field of health outcomes and should not be limited to a closed list of possible methodological options, but should be seen as a framework for a specific research issue to be addressed using quantitative and qualitative components (data and / or methods) , when quantitative and qualitative components are articulated intentionally and prospectively in a well-defined, pre-specified research design and as a framework for meta-inference (9) .
The importance of applying mixed research methods can be reflected in the trend of “measuring / analysing what is important” for patients and the treatment outcomes reported by patients are increasingly used in clinical care and research. However, a recent review of studies documenting the development of outcome measures reported by patients highlights that only 11% of them were developed actually asking patients which outcomes are important to them. This emphasizes the importance of applying mixed qualitative and quantitative methods in health research to ensure a focus on the priorities identified by the patient, scientific rigor, and improved patient outcomes (9) . Mixed methods are also an increasingly accepted approach used to investigate organizational phenomena in health care (10) .
The application of the mix methodology is considered a significant contribution to health science. By combining quantitative and qualitative data in the same study, health researchers can reap the benefits of each approach while minimizing their disadvantages. In practice, this endeavor facilitates research by health science researchers on the complex and multifactorial nature of human health and disease. Researchers using the mixed method approach for the first time can easily feel overwhelmed by uncertainty about the philosophical foundations of the method, as well as the multitude of typologies of mixed method research. Although further research and debate are warranted, health researchers seeking solutions to real problems are increasingly embracing pragmatism as a paradigm of choice (11) .
Author’s contribution:
All authors were involved in preparation of this article. Final proofreading was made by first author.
Financial support and sponsorship:
Conflicts of interest:.
There are no conflicts of interest.
Have a language expert improve your writing
Run a free plagiarism check in 10 minutes, generate accurate citations for free.
- Knowledge Base
Methodology
- Qualitative vs. Quantitative Research | Differences, Examples & Methods
Qualitative vs. Quantitative Research | Differences, Examples & Methods
Published on April 12, 2019 by Raimo Streefkerk . Revised on June 22, 2023.
When collecting and analyzing data, quantitative research deals with numbers and statistics, while qualitative research deals with words and meanings. Both are important for gaining different kinds of knowledge.
Common quantitative methods include experiments, observations recorded as numbers, and surveys with closed-ended questions.
Quantitative research is at risk for research biases including information bias , omitted variable bias , sampling bias , or selection bias . Qualitative research Qualitative research is expressed in words . It is used to understand concepts, thoughts or experiences. This type of research enables you to gather in-depth insights on topics that are not well understood.
Common qualitative methods include interviews with open-ended questions, observations described in words, and literature reviews that explore concepts and theories.
Table of contents
The differences between quantitative and qualitative research, data collection methods, when to use qualitative vs. quantitative research, how to analyze qualitative and quantitative data, other interesting articles, frequently asked questions about qualitative and quantitative research.
Quantitative and qualitative research use different research methods to collect and analyze data, and they allow you to answer different kinds of research questions.
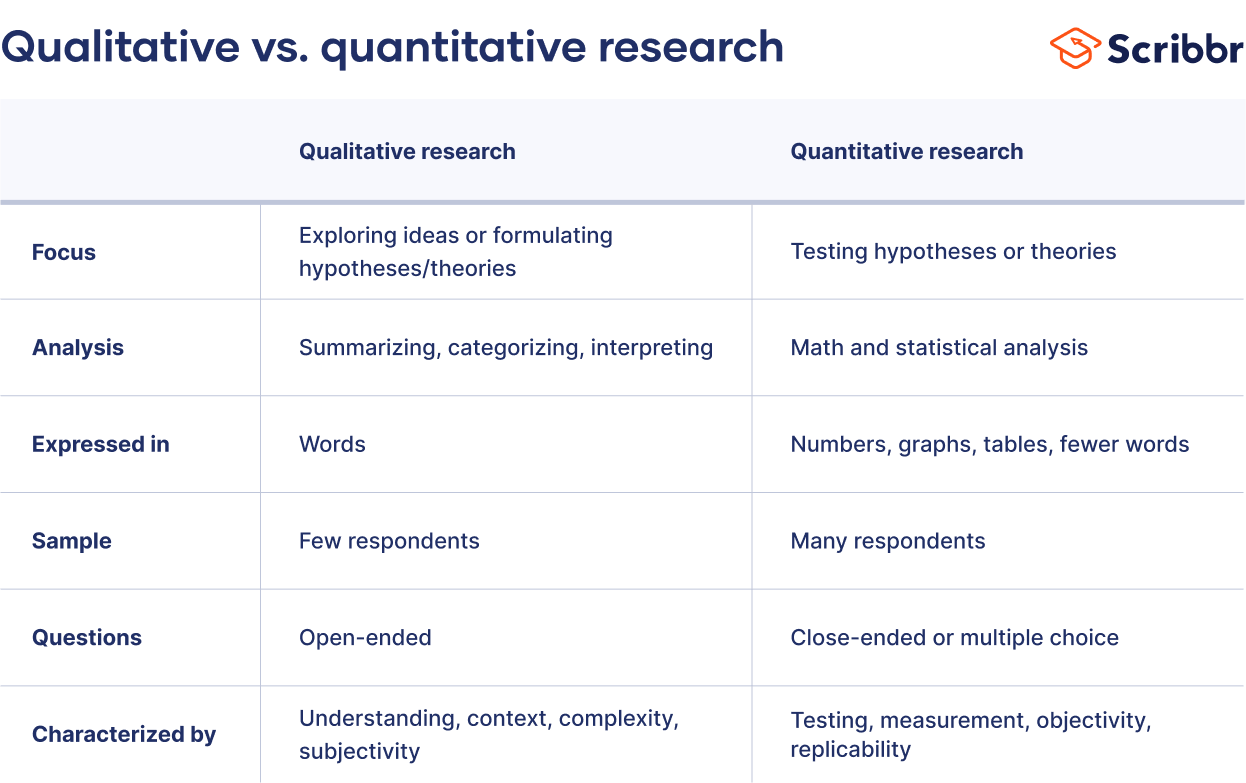
Quantitative and qualitative data can be collected using various methods. It is important to use a data collection method that will help answer your research question(s).
Many data collection methods can be either qualitative or quantitative. For example, in surveys, observational studies or case studies , your data can be represented as numbers (e.g., using rating scales or counting frequencies) or as words (e.g., with open-ended questions or descriptions of what you observe).
However, some methods are more commonly used in one type or the other.
Quantitative data collection methods
- Surveys : List of closed or multiple choice questions that is distributed to a sample (online, in person, or over the phone).
- Experiments : Situation in which different types of variables are controlled and manipulated to establish cause-and-effect relationships.
- Observations : Observing subjects in a natural environment where variables can’t be controlled.
Qualitative data collection methods
- Interviews : Asking open-ended questions verbally to respondents.
- Focus groups : Discussion among a group of people about a topic to gather opinions that can be used for further research.
- Ethnography : Participating in a community or organization for an extended period of time to closely observe culture and behavior.
- Literature review : Survey of published works by other authors.
A rule of thumb for deciding whether to use qualitative or quantitative data is:
- Use quantitative research if you want to confirm or test something (a theory or hypothesis )
- Use qualitative research if you want to understand something (concepts, thoughts, experiences)
For most research topics you can choose a qualitative, quantitative or mixed methods approach . Which type you choose depends on, among other things, whether you’re taking an inductive vs. deductive research approach ; your research question(s) ; whether you’re doing experimental , correlational , or descriptive research ; and practical considerations such as time, money, availability of data, and access to respondents.
Quantitative research approach
You survey 300 students at your university and ask them questions such as: “on a scale from 1-5, how satisfied are your with your professors?”
You can perform statistical analysis on the data and draw conclusions such as: “on average students rated their professors 4.4”.
Qualitative research approach
You conduct in-depth interviews with 15 students and ask them open-ended questions such as: “How satisfied are you with your studies?”, “What is the most positive aspect of your study program?” and “What can be done to improve the study program?”
Based on the answers you get you can ask follow-up questions to clarify things. You transcribe all interviews using transcription software and try to find commonalities and patterns.
Mixed methods approach
You conduct interviews to find out how satisfied students are with their studies. Through open-ended questions you learn things you never thought about before and gain new insights. Later, you use a survey to test these insights on a larger scale.
It’s also possible to start with a survey to find out the overall trends, followed by interviews to better understand the reasons behind the trends.
Qualitative or quantitative data by itself can’t prove or demonstrate anything, but has to be analyzed to show its meaning in relation to the research questions. The method of analysis differs for each type of data.
Analyzing quantitative data
Quantitative data is based on numbers. Simple math or more advanced statistical analysis is used to discover commonalities or patterns in the data. The results are often reported in graphs and tables.
Applications such as Excel, SPSS, or R can be used to calculate things like:
- Average scores ( means )
- The number of times a particular answer was given
- The correlation or causation between two or more variables
- The reliability and validity of the results
Analyzing qualitative data
Qualitative data is more difficult to analyze than quantitative data. It consists of text, images or videos instead of numbers.
Some common approaches to analyzing qualitative data include:
- Qualitative content analysis : Tracking the occurrence, position and meaning of words or phrases
- Thematic analysis : Closely examining the data to identify the main themes and patterns
- Discourse analysis : Studying how communication works in social contexts
If you want to know more about statistics , methodology , or research bias , make sure to check out some of our other articles with explanations and examples.
- Chi square goodness of fit test
- Degrees of freedom
- Null hypothesis
- Discourse analysis
- Control groups
- Mixed methods research
- Non-probability sampling
- Quantitative research
- Inclusion and exclusion criteria
Research bias
- Rosenthal effect
- Implicit bias
- Cognitive bias
- Selection bias
- Negativity bias
- Status quo bias
Quantitative research deals with numbers and statistics, while qualitative research deals with words and meanings.
Quantitative methods allow you to systematically measure variables and test hypotheses . Qualitative methods allow you to explore concepts and experiences in more detail.
In mixed methods research , you use both qualitative and quantitative data collection and analysis methods to answer your research question .
The research methods you use depend on the type of data you need to answer your research question .
- If you want to measure something or test a hypothesis , use quantitative methods . If you want to explore ideas, thoughts and meanings, use qualitative methods .
- If you want to analyze a large amount of readily-available data, use secondary data. If you want data specific to your purposes with control over how it is generated, collect primary data.
- If you want to establish cause-and-effect relationships between variables , use experimental methods. If you want to understand the characteristics of a research subject, use descriptive methods.
Data collection is the systematic process by which observations or measurements are gathered in research. It is used in many different contexts by academics, governments, businesses, and other organizations.
There are various approaches to qualitative data analysis , but they all share five steps in common:
- Prepare and organize your data.
- Review and explore your data.
- Develop a data coding system.
- Assign codes to the data.
- Identify recurring themes.
The specifics of each step depend on the focus of the analysis. Some common approaches include textual analysis , thematic analysis , and discourse analysis .
A research project is an academic, scientific, or professional undertaking to answer a research question . Research projects can take many forms, such as qualitative or quantitative , descriptive , longitudinal , experimental , or correlational . What kind of research approach you choose will depend on your topic.
Cite this Scribbr article
If you want to cite this source, you can copy and paste the citation or click the “Cite this Scribbr article” button to automatically add the citation to our free Citation Generator.
Streefkerk, R. (2023, June 22). Qualitative vs. Quantitative Research | Differences, Examples & Methods. Scribbr. Retrieved September 16, 2024, from https://www.scribbr.com/methodology/qualitative-quantitative-research/
Is this article helpful?
Raimo Streefkerk
Other students also liked, what is quantitative research | definition, uses & methods, what is qualitative research | methods & examples, mixed methods research | definition, guide & examples, what is your plagiarism score.
- - Google Chrome
Intended for healthcare professionals
- My email alerts
- BMA member login
- Username * Password * Forgot your log in details? Need to activate BMA Member Log In Log in via OpenAthens Log in via your institution

Search form
- Advanced search
- Search responses
- Search blogs
- Three techniques for...
Three techniques for integrating data in mixed methods studies
- Related content
- Peer review
- Alicia O’Cathain , professor 1 ,
- Elizabeth Murphy , professor 2 ,
- Jon Nicholl , professor 1
- 1 Medical Care Research Unit, School of Health and Related Research, University of Sheffield, Sheffield S1 4DA, UK
- 2 University of Leicester, Leicester, UK
- Correspondence to: A O’Cathain a.ocathain{at}sheffield.ac.uk
- Accepted 8 June 2010
Techniques designed to combine the results of qualitative and quantitative studies can provide researchers with more knowledge than separate analysis
Health researchers are increasingly using designs that combine qualitative and quantitative methods, and this is often called mixed methods research. 1 Integration—the interaction or conversation between the qualitative and quantitative components of a study—is an important aspect of mixed methods research, and, indeed, is essential to some definitions. 2 Recent empirical studies of mixed methods research in health show, however, a lack of integration between components, 3 4 which limits the amount of knowledge that these types of studies generate. Without integration, the knowledge yield is equivalent to that from a qualitative study and a quantitative study undertaken independently, rather than achieving a “whole greater than the sum of the parts.” 5
Barriers to integration have been identified in both health and social research. 6 7 One barrier is the absence of formal education in mixed methods research. Fortunately, literature is rapidly expanding to fill this educational gap, including descriptions of how to integrate data and findings from qualitative and quantitative methods. 8 9 In this article we outline three techniques that may help health researchers to integrate data or findings in their mixed methods studies and show how these might enhance knowledge generated from this approach.
Triangulation protocol
Researchers will often use qualitative and quantitative methods to examine different aspects of an overall research question. For example, they might use a randomised controlled trial to assess the effectiveness of a healthcare intervention and semistructured interviews with patients and health professionals to consider the way in which the intervention was used in the real world. Alternatively, they might use a survey of service users to measure satisfaction with a service and focus groups to explore views of care in more depth. Data are collected and analysed separately for each component to produce two sets of findings. Researchers will then attempt to combine these findings, sometimes calling this process triangulation. The term triangulation can be confusing because it has two meanings. 10 It can be used to describe corroboration between two sets of findings or to describe a process of studying a problem using different methods to gain a more complete picture. The latter meaning is commonly used in mixed methods research and is the meaning used here.
The process of triangulating findings from different methods takes place at the interpretation stage of a study when both data sets have been analysed separately (figure ⇓ ). Several techniques have been described for triangulating findings. They require researchers to list the findings from each component of a study on the same page and consider where findings from each method agree (convergence), offer complementary information on the same issue (complementarity), or appear to contradict each other (discrepancy or dissonance). 11 12 13 Explicitly looking for disagreements between findings from different methods is an important part of this process. Disagreement is not a sign that something is wrong with a study. Exploration of any apparent “inter-method discrepancy” may lead to a better understanding of the research question, 14 and a range of approaches have been used within health services research to explore inter-method discrepancy. 15
Point of application for three techniques for integrating data in mixed methods research
- Download figure
- Open in new tab
- Download powerpoint
The most detailed description of how to carry out triangulation is the triangulation protocol, 11 which although developed for multiple qualitative methods, is relevant to mixed methods studies. This technique involves producing a “convergence coding matrix” to display findings emerging from each component of a study on the same page. This is followed by consideration of where there is agreement, partial agreement, silence, or dissonance between findings from different components. This technique for triangulation is the only one to include silence—where a theme or finding arises from one data set and not another. Silence might be expected because of the strengths of different methods to examine different aspects of a phenomenon, but surprise silences might also arise that help to increase understanding or lead to further investigations.
The triangulation protocol moves researchers from thinking about the findings related to each method, to what Farmer and colleagues call meta-themes that cut across the findings from different methods. 11 They show a worked example of triangulation protocol, but we could find no other published example. However, similar principles were used in an iterative mixed methods study to understand patient and carer satisfaction with a new primary angioplasty service. 16 Researchers conducted semistructured interviews with 16 users and carers to explore their experiences and views of the new service. These were used to develop a questionnaire for a survey of 595 patients (and 418 of their carers) receiving either the new service or usual care. Finally, 17 of the patients who expressed dissatisfaction with aftercare and rehabilitation were followed up to explore this further in semistructured interviews. A shift of thinking to meta-themes led the researchers away from reporting the findings from the interviews, survey, and follow-up interviews sequentially to consider the meta-themes of speed and efficiency, convenience of care, and discharge and after care. The survey identified that a higher percentage of carers of patients using the new service rated the convenience of visiting the hospital as poor than those using usual care. The interviews supported this concern about the new service, but also identified that the weight carers gave to this concern was low in the context of their family member’s life being saved.
Morgan describes this move as the “third effort” because it occurs after analysis of the qualitative and the quantitative components. 17 It requires time and energy that must be planned into the study timetable. It is also useful to consider who will carry out the integration process. Farmer and colleagues require two researchers to work together during triangulation, which can be particularly important in mixed methods studies if different researchers take responsibility for the qualitative and quantitative components. 11
Following a thread
Moran-Ellis and colleagues describe a different technique for integrating the findings from the qualitative and quantitative components of a study, called following a thread. 18 They state that this takes place at the analysis stage of the research process (figure ⇑ ). It begins with an initial analysis of each component to identify key themes and questions requiring further exploration. Then the researchers select a question or theme from one component and follow it across the other components—they call this the thread. The authors do not specify steps in this technique but offer a visual model for working between datasets. An approach similar to this has been undertaken in health services research, although the researchers did not label it as such, probably because the technique has not been used frequently in the literature (box)
An example of following a thread 19
Adamson and colleagues explored the effect of patient views on the appropriate use of services and help seeking using a survey of people registered at a general practice and semistructured interviews. The qualitative (22 interviews) and quantitative components (survey with 911 respondents) took place concurrently.
The researchers describe what they call an iterative or cyclical approach to analysis. Firstly, the preliminary findings from the interviews generated a hypothesis for testing in the survey data. A key theme from the interviews concerned the self rationing of services as a responsible way of using scarce health care. This theme was then explored in the survey data by testing the hypothesis that people’s views of the appropriate use of services would explain their help seeking behaviour. However, there was no support for this hypothesis in the quantitative analysis because the half of survey respondents who felt that health services were used inappropriately were as likely to report help seeking for a series of symptoms presented in standardised vignettes as were respondents who thought that services were not used inappropriately. The researchers then followed the thread back to the interview data to help interpret this finding.
After further analysis of the interview data the researchers understood that people considered the help seeking of other people to be inappropriate, rather than their own. They also noted that feeling anxious about symptoms was considered to be a good justification for seeking care. The researchers followed this thread back into the survey data and tested whether anxiety levels about the symptoms in the standardised vignettes predicted help seeking behaviour. This second hypothesis was supported by the survey data. Following a thread led the researchers to conclude that patients who seek health care for seemingly minor problems have exceeded their thresholds for the trade-off between not using services inappropriately and any anxiety caused by their symptoms.
Mixed methods matrix
A unique aspect of some mixed methods studies is the availability of both qualitative and quantitative data on the same cases. Data from the qualitative and quantitative components can be integrated at the analysis stage of a mixed methods study (figure ⇑ ). For example, in-depth interviews might be carried out with a sample of survey respondents, creating a subset of cases for which there is both a completed questionnaire and a transcript. Cases may be individuals, groups, organisations, or geographical areas. 9 All the data collected on a single case can be studied together, focusing attention on cases, rather than variables or themes, within a study. The data can be examined in detail for each case—for example, comparing people’s responses to a questionnaire with their interview transcript. Alternatively, data on each case can be summarised and displayed in a matrix 8 9 20 along the lines of Miles and Huberman’s meta-matrix. 21 Within a mixed methods matrix, the rows represent the cases for which there is both qualitative and quantitative data, and the columns display different data collected on each case. This allows researchers to pay attention to surprises and paradoxes between types of data on a single case and then look for patterns across all cases 20 in a qualitative cross case analysis. 21
We used a mixed methods matrix to study the relation between types of team working and the extent of integration in mixed methods studies in health services research (table ⇓ ). 22 Quantitative data were extracted from the proposals, reports, and peer reviewed publications of 75 mixed methods studies, and these were analysed to describe the proportion of studies with integrated outputs such as mixed methods journal articles. Two key variables in the quantitative component were whether the study was assessed as attempting to integrate qualitative or quantitative data or findings and the type of publications produced. We conducted qualitative interviews with 20 researchers who had worked on some of these studies to explore how mixed methods research was practised, including how the team worked together.
Example of a mixed methods matrix for a study exploring the relationship between types of teams and integration between qualitative and quantitative components of studies* 22
- View inline
The shared cases between the qualitative and quantitative components were 21 mixed methods studies (because one interviewee had worked on two studies in the quantitative component). A matrix was formed with each of the 21 studies as a row. The first column of the matrix contained the study identification, the second column indicated whether integration had occurred in that project, and the third column the score for integration of publications emerging from the study. The rows were then ordered to show the most integrated cases first. This ordering of rows helped us to see patterns across rows.
The next columns were themes from the qualitative interview with a researcher from that project. For example, the first theme was about the expertise in qualitative research within the team and whether the interviewee reported this as adequate for the study. The matrix was then used in the context of the qualitative analysis to explore the issues that affected integration. In particular, it helped to identify negative cases (when someone in the analysis doesn’t fit with the conclusions the analysis is coming to) within the qualitative analysis to facilitate understanding. Interviewees reported the need for experienced qualitative researchers on mixed methods studies to ensure that the qualitative component was published, yet two cases showed that this was neither necessary nor sufficient. This pushed us to explore other factors in a research team that helped generate outputs, and integrated outputs, from a mixed methods study.
Themes from a qualitative study can be summarised to the point where they are coded into quantitative data. In the matrix (table ⇑ ), the interviewee’s perception of the adequacy of qualitative expertise on the team could have been coded as adequate=1 or not=2. This is called “quantitising” of qualitative data 23 ; coded data can then be analysed with data from the quantitative component. This technique has been used to great effect in healthcare research to identify the discrepancy between health improvement assessed using quantitative measures and with in-depth interviews in a randomised controlled trial. 24
We have presented three techniques for integration in mixed methods research in the hope that they will inspire researchers to explore what can be learnt from bringing together data from the qualitative and quantitative components of their studies. Using these techniques may give the process of integration credibility rather than leaving researchers feeling that they have “made things up.” It may also encourage researchers to describe their approaches to integration, allowing them to be transparent and helping them to develop, critique, and improve on these techniques. Most importantly, we believe it may help researchers to generate further understanding from their research.
We have presented integration as unproblematic, but it is not. It may be easier for single researchers to use these techniques than a large research team. Large teams will need to pay attention to team dynamics, considering who will take responsibility for integration and who will be taking part in the process. In addition, we have taken a technical stance here rather than paying attention to different philosophical beliefs that may shape approaches to integration. We consider that these techniques would work in the context of a pragmatic or subtle realist stance adopted by some mixed methods researchers. 25 Finally, it is important to remember that these techniques are aids to integration and are helpful only when applied with expertise.
Summary points
Health researchers are increasingly using designs which combine qualitative and quantitative methods
However, there is often lack of integration between methods
Three techniques are described that can help researchers to integrate data from different components of a study: triangulation protocol, following a thread, and the mixed methods matrix
Use of these methods will allow researchers to learn more from the information they have collected
Cite this as: BMJ 2010;341:c4587
Funding: Medical Research Council grant reference G106/1116
Competing interests: All authors have completed the unified competing interest form at www.icmje.org/coi_disclosure.pdf (available on request from the corresponding author) and declare financial support for the submitted work from the Medical Research Council; no financial relationships with commercial entities that might have an interest in the submitted work; no spouses, partners, or children with relationships with commercial entities that might have an interest in the submitted work; and no non-financial interests that may be relevant to the submitted work.
Contributors: AOC wrote the paper. JN and EM contributed to drafts and all authors agreed the final version. AOC is guarantor.
Provenance and peer review: Not commissioned; externally peer reviewed.
- ↵ Lingard L, Albert M, Levinson W. Grounded theory, mixed methods and action research. BMJ 2008 ; 337 : a567 . OpenUrl FREE Full Text
- ↵ Creswell JW, Fetters MD, Ivankova NV. Designing a mixed methods study in primary care. Ann Fam Med 2004 ; 2 : 7 -12. OpenUrl Abstract / FREE Full Text
- ↵ Lewin S, Glenton C, Oxman AD. Use of qualitative methods alongside randomised controlled trials of complex healthcare interventions: methodological study. BMJ 2009 ; 339 : b3496 . OpenUrl Abstract / FREE Full Text
- ↵ O’Cathain A, Murphy E, Nicholl J. Integration and publications as indicators of ‘yield’ from mixed methods studies. J Mix Methods Res 2007 ; 1 : 147 -63. OpenUrl CrossRef Web of Science
- ↵ Barbour RS. The case for combining qualitative and quantitative approaches in health services research. J Health Serv Res Policy 1999 ; 4 : 39 -43. OpenUrl PubMed
- ↵ O’Cathain A, Nicholl J, Murphy E. Structural issues affecting mixed methods studies in health research: a qualitative study. BMC Med Res Methodol 2009 ; 9 : 82 . OpenUrl CrossRef PubMed
- ↵ Bryman A. Barriers to integrating quantitative and qualitative research. J Mix Methods Res 2007 ; 1 : 8 -22. OpenUrl CrossRef
- ↵ Creswell JW, Plano-Clark V. Designing and conducting mixed methods research . Sage, 2007 .
- ↵ Bazeley P. Analysing mixed methods data. In: Andrew S, Halcomb EJ, eds. Mixed methods research for nursing and the health sciences . Wiley-Blackwell, 2009 :84-118.
- ↵ Sandelowski M. Triangles and crystals: on the geometry of qualitative research. Res Nurs Health 1995 ; 18 : 569 -74. OpenUrl CrossRef PubMed Web of Science
- ↵ Farmer T, Robinson K, Elliott SJ, Eyles J. Developing and implementing a triangulation protocol for qualitative health research. Qual Health Res 2006 ; 16 : 377 -94. OpenUrl Abstract / FREE Full Text
- ↵ Foster RL. Addressing the epistemologic and practical issues in multimethod research: a procedure for conceptual triangulation. Adv Nurs Sci 1997 ; 20 : 1 -12. OpenUrl PubMed
- ↵ Erzerberger C, Prein G. Triangulation: validity and empirically based hypothesis construction. Qual Quant 1997 ; 31 : 141 -54. OpenUrl CrossRef Web of Science
- ↵ Fielding NG, Fielding JL. Linking data . Sage, 1986 .
- ↵ Moffatt S, White M, Mackintosh J, Howel D. Using quantitative and qualitative data in health services research—what happens when mixed method findings conflict? BMC Health Serv Res 2006 ; 6 : 28 . OpenUrl CrossRef PubMed
- ↵ Sampson FC, O’Cathain A, Goodacre S. Is primary angioplasty an acceptable alternative to thrombolysis? Quantitative and qualitative study of patient and carer satisfaction. Health Expectations (forthcoming).
- ↵ Morgan DL. Practical strategies for combining qualitative and quantitative methods: applications to health research. Qual Health Res 1998 ; 8 : 362 -76. OpenUrl Abstract / FREE Full Text
- ↵ Moran-Ellis J, Alexander VD, Cronin A, Dickinson M, Fielding J, Sleney J, et al. Triangulation and integration: processes, claims and implications. Qualitative Research 2006 ; 6 : 45 -59. OpenUrl Abstract / FREE Full Text
- ↵ Adamson J, Ben-Shlomo Y, Chaturvedi N, Donovan J. Exploring the impact of patient views on ‘appropriate’ use of services and help seeking: a mixed method study. Br J Gen Pract 2009 ; 59 : 496 -502. OpenUrl Web of Science
- ↵ Wendler MC. Triangulation using a meta-matrix. J Adv Nurs 2001 ; 35 : 521 -5. OpenUrl CrossRef PubMed Web of Science
- ↵ Miles M, Huberman A. Qualitative data analysis: an expanded sourcebook . Sage, 1994 .
- ↵ O’Cathain A, Murphy E, Nicholl J. Multidisciplinary, interdisciplinary or dysfunctional? Team working in mixed methods research. Qual Health Res 2008 ; 18 : 1574 -85. OpenUrl Abstract / FREE Full Text
- ↵ Sandelowski M. Combining qualitative and quantitative sampling, data collection, and analysis techniques in mixed-method studies. Res Nurs Health 2000 ; 23 : 246 -55. OpenUrl CrossRef PubMed Web of Science
- ↵ Campbell R, Quilty B, Dieppe P. Discrepancies between patients’ assessments of outcome: qualitative study nested within a randomised controlled trial. BMJ 2003 ; 326 : 252 -3. OpenUrl FREE Full Text
- ↵ Mays N, Pope C. Assessing quality in qualitative research. BMJ 2000 ; 320 : 50 -2. OpenUrl FREE Full Text
|
Quantitative, Qualitative, and Mixed-Methods Research: Home
Quantitative, qualitative, and mixed-methods research.
Depending on the philosophy of the researcher, the nature of the data, and how it is collected, behavioral science can be classified into qualitative, quantitative, or mixed methods research. Below are descriptions of each method.
Quantitative Research
Collects numerical data, such as frequencies or scores to focus on cause-and-effect relationships among variables
Variables and research methodologies are defined in advance by theories and hypotheses derived from other theories. These remain unchanged throughout the research process.
The researcher tries to achieve objectivity by distancing himself or herself from the research, not allowing himself or herself to be emotionally involved.
The researcher mostly studies research in artificial or less than its natural setting, and manipulates behavior as opposed to studying the behavior in its natural context.
The researcher tries to maintain internal validity and focuses on average behavior or thoughts of people in a population
Qualitative Research
Where researchers collect non-numerical information, such as descriptions of behavioral phenomena, how people experience or interpret events, and/or answers to participants' open-ended responses.
The researcher's variables andmethods used come from the researcher's experiences and can be modified as the research progresses.
The researcher is involved and his or her experiences are valuable as well as the participants' experiences.
The researcher studies behavior as it naturally happens in the natural context.
The researcher tries to maximize ecological validity.
The researcher focuses on similarities and differences in experiences and how people interpret them.
Mixed-Methods Research
Involves both quantitative and qualitative components.
The researcher specifies in advance the types of information necessary to accomplish the study's goals.
The researcher needs to carefully consider the order in which the data types will be collected and the selection criteria for participants in the various parts of the study (e.g., which people will participate in the qualitative assessment if a sub-selection of participants will be involved).
Involves development (where the researcher uses one method to inform data collection or analysis with another method) initiation (where unexpected results change protocol in the other method), corroboration (where consistency is evaluated and compared between methods), and elaboration (where one method is used to expand on the results of the other method).
Whitley, B. E. & Kite, M. E. (2013). Principles of research in behavioral science (3rd ed.). Routledge.
- Last Updated: Sep 2, 2020 12:29 PM
- URL: https://library.divinemercy.edu/research-types
Instant insights, infinite possibilities
- What is mixed methods research?
Last updated
20 February 2023
Reviewed by
Miroslav Damyanov
Short on time? Get an AI generated summary of this article instead
By blending both quantitative and qualitative data, mixed methods research allows for a more thorough exploration of a research question. It can answer complex research queries that cannot be solved with either qualitative or quantitative research .
Analyze your mixed methods research
Dovetail streamlines analysis to help you uncover and share actionable insights
Mixed methods research combines the elements of two types of research: quantitative and qualitative.
Quantitative data is collected through the use of surveys and experiments, for example, containing numerical measures such as ages, scores, and percentages.
Qualitative data involves non-numerical measures like beliefs, motivations, attitudes, and experiences, often derived through interviews and focus group research to gain a deeper understanding of a research question or phenomenon.
Mixed methods research is often used in the behavioral, health, and social sciences, as it allows for the collection of numerical and non-numerical data.
- When to use mixed methods research
Mixed methods research is a great choice when quantitative or qualitative data alone will not sufficiently answer a research question. By collecting and analyzing both quantitative and qualitative data in the same study, you can draw more meaningful conclusions.
There are several reasons why mixed methods research can be beneficial, including generalizability, contextualization, and credibility.
For example, let's say you are conducting a survey about consumer preferences for a certain product. You could collect only quantitative data, such as how many people prefer each product and their demographics. Or you could supplement your quantitative data with qualitative data, such as interviews and focus groups , to get a better sense of why people prefer one product over another.
It is important to note that mixed methods research does not only mean collecting both types of data. Rather, it also requires carefully considering the relationship between the two and method flexibility.
You may find differing or even conflicting results by combining quantitative and qualitative data . It is up to the researcher to then carefully analyze the results and consider them in the context of the research question to draw meaningful conclusions.
When designing a mixed methods study, it is important to consider your research approach, research questions, and available data. Think about how you can use different techniques to integrate the data to provide an answer to your research question.
- Mixed methods research design
A mixed methods research design is an approach to collecting and analyzing both qualitative and quantitative data in a single study.
Mixed methods designs allow for method flexibility and can provide differing and even conflicting results. Examples of mixed methods research designs include convergent parallel, explanatory sequential, and exploratory sequential.
By integrating data from both quantitative and qualitative sources, researchers can gain valuable insights into their research topic . For example, a study looking into the impact of technology on learning could use surveys to measure quantitative data on students' use of technology in the classroom. At the same time, interviews or focus groups can provide qualitative data on students' experiences and opinions.
- Types of mixed method research designs
Researchers often struggle to put mixed methods research into practice, as it is challenging and can lead to research bias. Although mixed methods research can reveal differences or conflicting results between studies, it can also offer method flexibility.
Designing a mixed methods study can be broken down into four types: convergent parallel, embedded, explanatory sequential, and exploratory sequential.
Convergent parallel
The convergent parallel design is when data collection and analysis of both quantitative and qualitative data occur simultaneously and are analyzed separately. This design aims to create mutually exclusive sets of data that inform each other.
For example, you might interview people who live in a certain neighborhood while also conducting a survey of the same people to determine their satisfaction with the area.
Embedded design
The embedded design is when the quantitative and qualitative data are collected simultaneously, but the qualitative data is embedded within the quantitative data. This design is best used when you want to focus on the quantitative data but still need to understand how the qualitative data further explains it.
For instance, you may survey students about their opinions of an online learning platform and conduct individual interviews to gain further insight into their responses.
Explanatory sequential design
In an explanatory sequential design, quantitative data is collected first, followed by qualitative data. This design is used when you want to further explain a set of quantitative data with additional qualitative information.
An example of this would be if you surveyed employees at a company about their satisfaction with their job and then conducted interviews to gain more information about why they responded the way they did.
Exploratory sequential design
The exploratory sequential design collects qualitative data first, followed by quantitative data. This type of mixed methods research is used when the goal is to explore a topic before collecting any quantitative data.
An example of this could be studying how parents interact with their children by conducting interviews and then using a survey to further explore and measure these interactions.
Integrating data in mixed methods studies can be challenging, but it can be done successfully with careful planning.
No matter which type of design you choose, understanding and applying these principles can help you draw meaningful conclusions from your research.
- Strengths of mixed methods research
Mixed methods research designs combine the strengths of qualitative and quantitative data, deepening and enriching qualitative results with quantitative data and validating quantitative findings with qualitative data. This method offers more flexibility in designing research, combining theory generation and hypothesis testing, and being less tied to disciplines and established research paradigms.
Take the example of a study examining the impact of exercise on mental health. Mixed methods research would allow for a comprehensive look at the issue from different angles.
Researchers could begin by collecting quantitative data through surveys to get an overall view of the participants' levels of physical activity and mental health. Qualitative interviews would follow this to explore the underlying dynamics of participants' experiences of exercise, physical activity, and mental health in greater detail.
Through a mixed methods approach, researchers could more easily compare and contrast their results to better understand the phenomenon as a whole.
Additionally, mixed methods research is useful when there are conflicting or differing results in different studies. By combining both quantitative and qualitative data, mixed methods research can offer insights into why those differences exist.
For example, if a quantitative survey yields one result while a qualitative interview yields another, mixed methods research can help identify what factors influence these differences by integrating data from both sources.
Overall, mixed methods research designs offer a range of advantages for studying complex phenomena. They can provide insight into different elements of a phenomenon in ways that are not possible with either qualitative or quantitative data alone. Additionally, they allow researchers to integrate data from multiple sources to gain a deeper understanding of the phenomenon in question.
- Challenges of mixed methods research
Mixed methods research is labor-intensive and often requires interdisciplinary teams of researchers to collaborate. It also has the potential to cost more than conducting a stand alone qualitative or quantitative study .
Interpreting the results of mixed methods research can be tricky, as it can involve conflicting or differing results. Researchers must find ways to systematically compare the results from different sources and methods to avoid bias.
For example, imagine a situation where a team of researchers has employed an explanatory sequential design for their mixed methods study. After collecting data from both the quantitative and qualitative stages, the team finds that the two sets of data provide differing results. This could be challenging for the team, as they must now decide how to effectively integrate the two types of data in order to reach meaningful conclusions. The team would need to identify method flexibility and be strategic when integrating data in order to draw meaningful conclusions from the conflicting results.
- Advanced frameworks in mixed methods research
Mixed methods research offers powerful tools for investigating complex processes and systems, such as in health and healthcare.
Besides the three basic mixed method designs—exploratory sequential, explanatory sequential, and convergent parallel—you can use one of the four advanced frameworks to extend mixed methods research designs. These include multistage, intervention, case study , and participatory.
This framework mixes qualitative and quantitative data collection methods in stages to gather a more nuanced view of the research question. An example of this is a study that first has an online survey to collect initial data and is followed by in-depth interviews to gain further insights.
Intervention
This design involves collecting quantitative data and then taking action, usually in the form of an intervention or intervention program. An example of this could be a research team who collects data from a group of participants, evaluates it, and then implements an intervention program based on their findings .
This utilizes both qualitative and quantitative research methods to analyze a single case. The researcher will examine the specific case in detail to understand the factors influencing it. An example of this could be a study of a specific business organization to understand the organizational dynamics and culture within the organization.
Participatory
This type of research focuses on the involvement of participants in the research process. It involves the active participation of participants in formulating and developing research questions, data collection, and analysis.
An example of this could be a study that involves forming focus groups with participants who actively develop the research questions and then provide feedback during the data collection and analysis stages.
The flexibility of mixed methods research designs means that researchers can choose any combination of the four frameworks outlined above and other methodologies , such as convergent parallel, explanatory sequential, and exploratory sequential, to suit their particular needs.
Through this method's flexibility, researchers can gain multiple perspectives and uncover differing or even conflicting results when integrating data.
When it comes to integration at the methods level, there are four approaches.
Connecting involves collecting both qualitative and quantitative data during different phases of the research.
Building involves the collection of both quantitative and qualitative data within a single phase.
Merging involves the concurrent collection of both qualitative and quantitative data.
Embedding involves including qualitative data within a quantitative study or vice versa.
- Techniques for integrating data in mixed method studies
Integrating data is an important step in mixed methods research designs. It allows researchers to gain further understanding from their research and gives credibility to the integration process. There are three main techniques for integrating data in mixed methods studies: triangulation protocol, following a thread, and the mixed methods matrix.
Triangulation protocol
This integration method combines different methods with differing or conflicting results to generate one unified answer.
For example, if a researcher wanted to know what type of music teenagers enjoy listening to, they might employ a survey of 1,000 teenagers as well as five focus group interviews to investigate this. The results might differ; the survey may find that rap is the most popular genre, whereas the focus groups may suggest rock music is more widely listened to.
The researcher can then use the triangulation protocol to come up with a unified answer—such as that both rap and rock music are popular genres for teenage listeners.
Following a thread
This is another method of integration where the researcher follows the same theme or idea from one method of data collection to the next.
A research design that follows a thread starts by collecting quantitative data on a specific issue, followed by collecting qualitative data to explain the results. This allows whoever is conducting the research to detect any conflicting information and further look into the conflicting information to understand what is really going on.
For example, a researcher who used this research method might collect quantitative data about how satisfied employees are with their jobs at a certain company, followed by qualitative interviews to investigate why job satisfaction levels are low. They could then use the results to explore any conflicting or differing results, allowing them to gain a deeper understanding of job satisfaction at the company.
By following a thread, the researcher can explore various research topics related to the original issue and gain a more comprehensive view of the issue.
Mixed methods matrix
This technique is a visual representation of the different types of mixed methods research designs and the order in which they should be implemented. It enables researchers to quickly assess their research design and adjust it as needed.
The matrix consists of four boxes with four different types of mixed methods research designs: convergent parallel, explanatory sequential, exploratory sequential, and method flexibility.
For example, imagine a researcher who wanted to understand why people don't exercise regularly. To answer this question, they could use a convergent parallel design, collecting both quantitative (e.g., survey responses) and qualitative (e.g., interviews) data simultaneously.
If the researcher found conflicting results, they could switch to an explanatory sequential design and collect quantitative data first, then follow up with qualitative data if needed. This way, the researcher can make adjustments based on their findings and integrate their data more effectively.
Mixed methods research is a powerful tool for understanding complex research topics. Using qualitative and quantitative data in one study allows researchers to understand their subject more deeply.
Mixed methods research designs such as convergent parallel, explanatory sequential, and exploratory sequential provide method flexibility, enabling researchers to collect both types of data while avoiding the limitations of either approach alone.
However, it's important to remember that mixed methods research can produce differing or even conflicting results, so it's important to be aware of the potential pitfalls and take steps to ensure that data is being correctly integrated. If used effectively, mixed methods research can offer valuable insight into topics that would otherwise remain largely unexplored.
What is an example of mixed methods research?
An example of mixed methods research is a study that combines quantitative and qualitative data. This type of research uses surveys, interviews, and observations to collect data from multiple sources.
Which sampling method is best for mixed methods?
It depends on the research objectives, but a few methods are often used in mixed methods research designs. These include snowball sampling, convenience sampling, and purposive sampling. Each method has its own advantages and disadvantages.
What is the difference between mixed methods and multiple methods?
Mixed methods research combines quantitative and qualitative data in a single study. Multiple methods involve collecting data from different sources, such as surveys and interviews, but not necessarily combining them into one analysis. Mixed methods offer greater flexibility but can lead to differing or conflicting results when integrating data.
Should you be using a customer insights hub?
Do you want to discover previous research faster?
Do you share your research findings with others?
Do you analyze research data?
Start for free today, add your research, and get to key insights faster
Editor’s picks
Last updated: 18 April 2023
Last updated: 27 February 2023
Last updated: 22 August 2024
Last updated: 5 February 2023
Last updated: 16 August 2024
Last updated: 9 March 2023
Last updated: 30 April 2024
Last updated: 12 December 2023
Last updated: 11 March 2024
Last updated: 4 July 2024
Last updated: 6 March 2024
Last updated: 5 March 2024
Last updated: 13 May 2024
Latest articles
Related topics, .css-je19u9{-webkit-align-items:flex-end;-webkit-box-align:flex-end;-ms-flex-align:flex-end;align-items:flex-end;display:-webkit-box;display:-webkit-flex;display:-ms-flexbox;display:flex;-webkit-flex-direction:row;-ms-flex-direction:row;flex-direction:row;-webkit-box-flex-wrap:wrap;-webkit-flex-wrap:wrap;-ms-flex-wrap:wrap;flex-wrap:wrap;-webkit-box-pack:center;-ms-flex-pack:center;-webkit-justify-content:center;justify-content:center;row-gap:0;text-align:center;max-width:671px;}@media (max-width: 1079px){.css-je19u9{max-width:400px;}.css-je19u9>span{white-space:pre;}}@media (max-width: 799px){.css-je19u9{max-width:400px;}.css-je19u9>span{white-space:pre;}} decide what to .css-1kiodld{max-height:56px;display:-webkit-box;display:-webkit-flex;display:-ms-flexbox;display:flex;-webkit-align-items:center;-webkit-box-align:center;-ms-flex-align:center;align-items:center;}@media (max-width: 1079px){.css-1kiodld{display:none;}} build next, decide what to build next, log in or sign up.
Get started for free
- News & Highlights

- Publications and Documents
- Education in C/T Science
- Browse Our Courses
- C/T Research Academy
- K12 Investigator Training
- Harvard Catalyst On-Demand
- SMART IRB Reliance Request
- Biostatistics Consulting
- Regulatory Support
- Pilot Funding
- Informatics Program
- Community Engagement
- Diversity Inclusion
- Research Enrollment and Diversity
- Harvard Catalyst Profiles
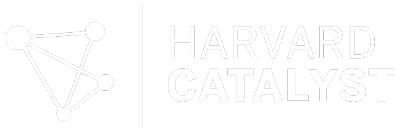
Community Engagement Program
Supporting bi-directional community engagement to improve the relevance, quality, and impact of research.
- Getting Started
- Resources for Community Engaged Implementation Science
- Resources for Equity in Research
- Community-Engaged Student Practice Placement
- Maternal Health Equity
- Youth Mental Health
- Leadership and Membership
- Past Members
- Study Review Rubric
- Community Ambassador Initiative
- Implementation Science Working Group
- Past Webinars & Podcasts
- Policy Atlas
- Community Advisory Board
For more information:
Mixed methods research.
According to the National Institutes of Health , mixed methods strategically integrates or combines rigorous quantitative and qualitative research methods to draw on the strengths of each. Mixed method approaches allow researchers to use a diversity of methods, combining inductive and deductive thinking, and offsetting limitations of exclusively quantitative and qualitative research through a complementary approach that maximizes strengths of each data type and facilitates a more comprehensive understanding of health issues and potential resolutions.¹ Mixed methods may be employed to produce a robust description and interpretation of the data, make quantitative results more understandable, or understand broader applicability of small-sample qualitative findings.

Integration
This refers to the ways in which qualitative and quantitative research activities are brought together to achieve greater insight. Mixed methods is not simply having quantitative and qualitative data available or analyzing and presenting data findings separately. The integration process can occur during data collection, analysis, or in the presentation of results.
¹ NIH Office of Behavioral and Social Sciences Research: Best Practices for Mixed Methods Research in the Health Sciences
Basic Mixed Methods Research Designs

View image description . Figure adapted from Creswell, J. W. (2014). A concise introduction to mixed methods research. SAGE Publications.
Five Key Questions for Getting Started
- What do you want to know?
- What will be the detailed quantitative, qualitative, and mixed methods research questions that you hope to address?
- What quantitative and qualitative data will you collect and analyze?
- Which rigorous methods will you use to collect data and/or engage stakeholders?
- How will you integrate the data in a way that allows you to address the first question?
Rationale for Using Mixed Methods
- Obtain different, multiple perspectives: validation
- Build comprehensive understanding
- Explain statistical results in more depth
- Have better contextualized measures
- Track the process of program or intervention
- Study patient-centered outcomes and stakeholder engagement
Sample Mixed Methods Research Study
The EQUALITY study used an exploratory sequential design to identify the optimal patient-centered approach to collect sexual orientation data in the emergency department.
Qualitative Data Collection and Analysis : Semi-structured interviews with patients of different sexual orientation, age, race/ethnicity, as well as healthcare professionals of different roles, age, and race/ethnicity.
Builds Into : Themes identified in the interviews were used to develop questions for the national survey.
Quantitative Data Collection and Analysis : Representative national survey of patients and healthcare professionals on the topic of reporting gender identity and sexual orientation in healthcare.
Other Resources:
Introduction to Mixed Methods Research : Harvard Catalyst’s eight-week online course offers an opportunity for investigators who want to understand and apply a mixed methods approach to their research.
Best Practices for Mixed Methods Research in the Health Sciences [PDF] : This guide provides a detailed overview of mixed methods designs, best practices, and application to various types of grants and projects.
Mixed Methods Research Training Program for the Health Sciences (MMRTP ): Selected scholars for this summer training program, hosted by Johns Hopkins’ Bloomberg School of Public Health, have access to webinars, resources, a retreat to discuss their research project with expert faculty, and are matched with mixed methods consultants for ongoing support.
Michigan Mixed Methods : University of Michigan Mixed Methods program offers a variety of resources, including short web videos and recommended reading.
To use a mixed methods approach, you may want to first brush up on your qualitative skills. Below are a few helpful resources specific to qualitative research:
- Qualitative Research Guidelines Project : A comprehensive guide for designing, writing, reviewing and reporting qualitative research.
- Fundamentals of Qualitative Research Methods – What is Qualitative Research : A six-module web video series covering essential topics in qualitative research, including what is qualitative research and how to use the most common methods, in-depth interviews, and focus groups.
View PDF of the above information.
Academia.edu no longer supports Internet Explorer.
To browse Academia.edu and the wider internet faster and more securely, please take a few seconds to upgrade your browser .
Enter the email address you signed up with and we'll email you a reset link.
- We're Hiring!
- Help Center
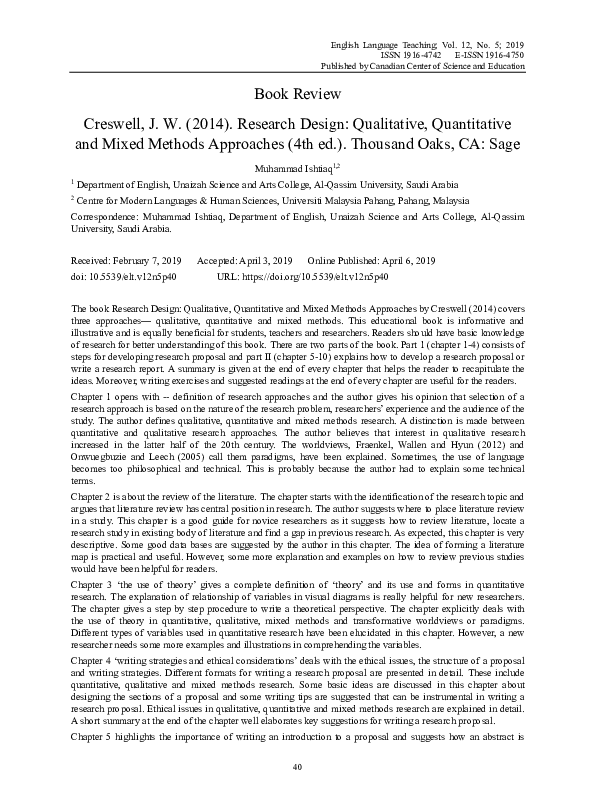
Creswell, J. W. (2014). Research Design: Qualitative, Quantitative and Mixed Methods Approaches (4th ed.). Thousand Oaks, CA: Sage

The book Research Design: Qualitative, Quantitative and Mixed Methods Approaches by Creswell (2014) covers three approaches-qualitative, quantitative and mixed methods. This educational book is informative and illustrative and is equally beneficial for students, teachers and researchers. Readers should have basic knowledge of research for better understanding of this book. There are two parts of the book. Part 1 (chapter 1-4) consists of steps for developing research proposal and part II (chapter 5-10) explains how to develop a research proposal or write a research report. A summary is given at the end of every chapter that helps the reader to recapitulate the ideas. Moreover, writing exercises and suggested readings at the end of every chapter are useful for the readers. Chapter 1 opens with-definition of research approaches and the author gives his opinion that selection of a research approach is based on the nature of the research problem, researchers' experience and the audience of the study. The author defines qualitative, quantitative and mixed methods research. A distinction is made between quantitative and qualitative research approaches. The author believes that interest in qualitative research increased in the latter half of the 20th century. The worldviews, Fraenkel, Wallen and Hyun (2012) and Onwuegbuzie and Leech (2005) call them paradigms, have been explained. Sometimes, the use of language becomes too philosophical and technical. This is probably because the author had to explain some technical terms.
Related Papers
Daniel Ortiz
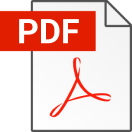
Research Design: Qualitative, Quantitative and Mixed Methods
Dr. Esra Öztürk Çalık
Conducting a well-established research requires deep knowledge about the research designs. Doing research can be likened to jumping into the sea which may transform into a huge ocean if the researcher is not experienced. As a PhD candidate and a novice researcher, I believe that the book "Research Design: Qualitative, Quantitative and Mixed Methods Approaches" by J.W. Creswell is a true reference guide for novice researchers since it is the most comprehensive and informative source with its reader-friendly structure.
International Journal of Social Sciences Interdisciplinary Research
Prashant Astalin
rhoda taller
Yan-yi Chang
John W. Creswell was previously a professor in educational psychology in the University of Nebraska–Lincoln. He moved to the University of Michigan in 2015 as a professor in the Department of Family Medicine. He has published many articles and close to 27 books on mixed methods. Professor Creswell is also one of the founding members of the Journal of Mixed Methods Research. He was a Fulbright scholar in South Africa in 2008 and Thailand in 2012. In 2011, he served as a visiting professor in the School of Public Health of Harvard University. In 2014, he became the Chairman of the Mixed Methods International Research Association. Professor Creswell has a personal website called “Mixed Methods Research” at http://johnwcreswell.com/. The site contains the information about his background, his own blog, consulting works and published books. He also posted replies questions from academic researchers and practitioners in the blog.
Chisomo Mgunda
Gloria Thakane Leutle
Richard Baskas, Ed.D. Candidate
Nurul Gasmi
To understand educational research, you now have the map (the steps that exist in the process of research) and the different paths you can take (quantitative and qualitative). Now we will explore some distinguishing features along the qualitative research design. These features are the research designs you can use to collect, analyze, and interpret data using quantitative and qualitative research. Some of the research designs may be familiar; others may be new, such as how these paths can converge with two designs called mixed methods research and action research. The discussion of designs will provide a more advanced understanding of educational research on your journey.
Loading Preview
Sorry, preview is currently unavailable. You can download the paper by clicking the button above.
RELATED PAPERS
IRJET Journal
The SAGE Handbook of Applied Social Research Methods
Global Academic Journal of Linguistics and Literature
Dr. Lok R A J Sharma
IJIP Journal
Education for Health: Change in Learning & Practice
Kelly Devers
Proceedings of the Sixth International Conference on Technological Ecosystems for Enhancing Multiculturality
ANA MARIA PINTO-LLORENTE
International Journal of Academic Multidisciplinary Research (IJAMR
Ramatu Ussif
Kathryn Pole
iosrjournals.org
Presented at Academic Business World International Conference. Nashville, Tennessee. May, 2007
Greg L Lowhorn
Changsong Wang
Mid-Western Educational Researcher
Mixed Methods Research for Improved Scientific Study
Mette Baran
Bostley Asenahabi
Studies in Comparative International Development
Gerardo Munck
International Research Journal of MMC
Umesh Raj Aryal
Villia Jefremovas
Seda Khadimally
shiroashi fullbuster
Nova Southeastern University
Addisalem Tadesse
Sarah Baloyi
koek Comfort
Dhea Savella Segui
RELATED TOPICS
- We're Hiring!
- Help Center
- Find new research papers in:
- Health Sciences
- Earth Sciences
- Cognitive Science
- Mathematics
- Computer Science
- Academia ©2024
Psychological resilience and valued living in difficult times: mixed method research in cultural context
- Published: 12 September 2024
Cite this article
- Feyza Topçu ORCID: orcid.org/0000-0002-5853-2670 1 &
- Mehmet Dinç ORCID: orcid.org/0000-0001-9544-8999 1
2 Altmetric
Valued living involves aligning one’s actions with one’s personal values, which can contribute to mental strength and psychological resilience. The present study aimed to examine the importance of living a valued life in maintaining mental strength, particularly during challenging times. In addition, it identifies the culturally relevant values that strengthen adults. Our study used both quantitative and qualitative methods. In the quantitative phase, 493 adults completed the following scales Valued Living Questionnaire (VLQ), Connor-Davidson Resilience Scale Short Form (CD-RISC-10), and Depression, Anxiety, and Stress Scale (DASS-21). We calculated Pearson’s product-moment correlation coefficient and used structural equation modeling (SEM) to conduct the path analysis. For the qualitative phase, we recruited 15 participants from a larger quantitative sample based on their scores obtained on administered scales and conducted in-depth interviews along with researcher’s journal and memos. Based on our quantitative findings, this study indicates that the concept of valued living serves as a mediating factor between psychological resilience and mental health outcomes. In our qualitative analysis, we identified certain values (e.g., acceptance, love, and goodwill) that serve to empower adults during challenging periods. The findings of this study are deemed to serve as a reference for the formulation and implementation of interventions intended to strengthen psychological resilience, from the viewpoints of both mental health specialists and psychological counselors.
This is a preview of subscription content, log in via an institution to check access.
Access this article
Subscribe and save.
- Get 10 units per month
- Download Article/Chapter or eBook
- 1 Unit = 1 Article or 1 Chapter
- Cancel anytime
Price includes VAT (Russian Federation)
Instant access to the full article PDF.
Rent this article via DeepDyve
Institutional subscriptions
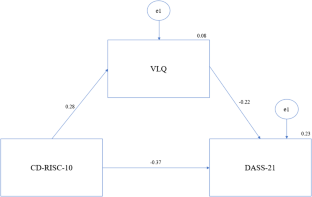
Similar content being viewed by others
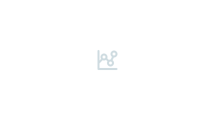
Role of Values and Resilience in Well-Being among Individuals Affected by the Fukushima Disaster
Valued living in daily experience: relations with mindfulness, meaning, psychological flexibility, and stressors.
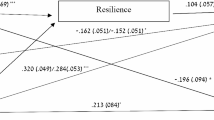
Is Resilience the Bridge Connecting Social and Family Factors to Mental Well-Being and Life Satisfaction?
Explore related subjects.
- Artificial Intelligence
Data availability
The data sets used in the study can be provided upon request to the corresponding author.
Ağırkan, M., & Kağan, M. (2017). Üniversite Öğrencilerinin Değer Yönelimleri Ile Psikolojik Dayanıklılık Düzeyleri Arasındaki İlişki. Erzincan Üniversitesi Eğitim Fakültesi Dergisi , 19 (3), 225–245. https://doi.org/10.17556/erziefd.335063
Article Google Scholar
Ahmadi, N., Ahmadi, F., Erbil, P., & Cetrez, Ö. A. (2016). Religious meaning-making coping in Turkey: A study among cancer patients. Illness Crisis & Loss , 27 (3), 190–208.
Ahmadi, F., Erbil, P., Ahmadi, N., & Cetrez, Ö. A. (2019). Religion, culture and meaning-making coping: A study among cancer patients in Turkey. Journal of Religion and Health , 58 , 1115–1124.
Article PubMed Google Scholar
Boer, D., & Fischer, R. (2013). How and when do personal values guide our attitudes and sociality? Explaining cross-cultural variability in attitude-value linkages. Psychological Bulletin , 139 (5), 1113–1147. https://doi.org/10.1037/a0031347
Bojanowska, A., & Piotrowski, K. (2021). Two levels of personality: Temperament and values and their effects on hedonic and eudaimonic well-being. Current Psychology , 40 , 1185–1193. https://doi.org/10.1007/s12144-018-0043-0
Campbell-Sills, L., & Stein, M. B. (2007). Psychometric analysis and refinement of the Connor–Davidson Resilience Scale (CD-RISC): Validation of a 10-item measure of resilience. Journal of Traumatic Stress: Official Publication of the International Society for Traumatic Stress Studies , 20 (6), 1019–1028. https://doi.org/10.1002/jts.20271
Carvalho, S. A., Xavier, A., Gillanders, D., Pinto-Gouveia, J., & Castilho, P. (2021). Rumination and valued living in women with chronic pain: How they relate to the link between mindfulness and depressive symptoms. Current Psychology , 40 , 1411–1419. https://doi.org/10.1007/s12144-018-0052-z
Ceary, C. D., Donahue, J. J., & Shaffer, K. (2019). The strength of pursuing your values: Valued living as a path to resilience among college students. Stress and Health , 35 (4), 532–541. https://doi.org/10.1002/smi.2886
Çekici, F., Aydın-Sünbül, Z., Malkoç, A., Aslan-Gördesli, M., & Arslan, R. (2018). Değer Odaklı Yaşam Ölçeği: Türk kültürüne uyarlama, geçerlik ve güvenirlik çalışması. Electronic Turkish Studies , 13 (19), 459–471. https://doi.org/10.7827/TurkishStudies.14094
Cénat, J. M., Kouamou, L. N., Farahi, S. M. M. M., Darius, W. P., Dalexis, R. D., Charles, M., & Kogan, C. S. (2022). Perceived racial discrimination, psychosomatic symptoms, and resilience among black individuals in Canada: A moderated mediation model. Journal of Psychosomatic Research , 163 , 111053. https://doi.org/10.1016/j.jpsychores.2022.111053
Chow, K. M., Tang, W. K. F., Chan, W. H. C., Sit, W. H. J., Choi, K. C., & Chan, S. (2018). Resilience and well-being of university nursing students in Hong Kong: A cross-sectional study. BMC Medical Education , 18 (1), 1–8. https://doi.org/10.1186/s12909-018-1119-0
Connor, K. M., & Davidson, J. R. T. (2003). Development of a new resilience scale: The Connor-Davidson Resilience Scale (CD-RISC). Depression and Anxiety , 18 , 76–82. https://doi.org/10.1002/da.10113
Cooley, S. J., Quinton, M. L., Holland, M. J. G., Parry, B. J., & Cumming, J. (2019). The experiences of homeless youth when using strengths profiling to identify their character strengths. Frontiers in Psychology , 10 , 1–16. https://doi.org/10.3389/fpsyg.2019.02036
Corbin, J., & Strauss, A. (2015). Basics of qualitative research: Techniques and procedures for developing grounded theory (4th ed.). SAGE.
Google Scholar
Creswell, J. W., & Plano-Clark, V. L. (2007). Designing and conducting mixed methods research (1th ed.). SAGE.
Creswell, J. W., & Plano-Clark, V. L. (2011). Designing and conducting mixed methods research (2nd ed.). SAGE.
Creswell, J. W., & Plano-Clark, V. L. (2018). Designing and conducting mixed methods research (3rd ed.). SAGE.
Cross, S. E. (1995). Self-construals, coping, and stress in cross-cultural adaptation. Journal of Cross-cultural Psychology , 26 (6), 673–697.
Deng, J., Zhou, R., & Yan, L. (2019). The relationship between conscience and subjective well-being among junior high school students: Mediator role of social support. Advances in Psychology , 9 (7), 1230–1239. https://doi.org/10.12677/ap.2019.97151
Eldem, E. (2013). Istanbul as a cosmopolitan city. In A. Quayson, & G. Daswani (Eds.), A companion to diaspora and transnationalism (pp. 212–230). Blackwell.
Chapter Google Scholar
EVS/WVS. (2022). European Values Study and World Values Survey: Joint EVS/WVS 2017–2022 Dataset (Joint EVS/WVS). JD Systems Institute & WVSA. Dataset Version 4.0.0. https://www.worldvaluessurvey.org/WVSEVSjoint2017.jsp
Färber, F., & Rosendahl, J. (2018). The association between resilience and mental health in the somatically ill: A systematic review and meta-analysis. Deutsches Ärzteblatt International , 115 (38), 621–627. https://doi.org/10.3238/arztebl.2018.0621
Article PubMed PubMed Central Google Scholar
Farnsworth, J. K., Drescher, K. D., Evans, W., & Walser, R. D. (2017). A functional approach to understanding and treating military-related moral injury. Journal of Contextual Behavioral Science , 6 (4), 391–397. https://doi.org/10.1016/j.jcbs.2017.07.003
Fischer, A. H., & Manstead, A. S. R. (2000). The relation between gender and emotions in different cultures. In A. Fischer (Ed.), Gender and emotion: Social psychological perspectives (pp. 71–94). Cambridge University Press.
Fredrickson, B. L. (2001). The role of positive emotions in positive psychology: The broaden-and-build theory of positive emotions. American Psychologist , 56 (3), 218. https://doi.org/10.1037//0003-066x.56.3.218
Gentili, C., Rickardsson, J., Zetterqvist, V., Simons, L. E., Lekander, M., & Wicksell, R. K. (2019). Psychological flexibility as a resilience factor in individuals with chronic pain. Frontiers in Psychology , 10 , 1–11. https://doi.org/10.3389/fpsyg.2019.02016
Greene, J. C. (2007). Mixed methods in social inquiry . Jossey-Bass.
Hall, N. A., Everson, A. T., Billingsley, M. R., & Miller, M. B. (2021). Moral injury, mental health and behavioural health outcomes: A systematic review of the literature. Clinical Psychology & Psychotherapy , 29 (1), 92–110. https://doi.org/10.1002/cpp.2607
Hanel, P. H., Maio, G. R., Soares, A. K., Vione, K. C., de Holanda Coelho, G. L., Gouveia, V. V., & Manstead, A. S. (2018). Cross-cultural differences and similarities in human value instantiation. Frontiers in Psychology , 9 , 366179. https://doi.org/10.3389/fpsyg.2018.00849
Hayes, S. C. (2005). Get out of your mind and into your life: The new acceptance and commitment therapy . New Harbinger.
Hofstede, G., Hofstede, G. J., & Minkov, M. (2010). Cultures and organizations: Software of the mind (3rd ed.). Mc Graw-Hill.
Howell Smith, M. C., Babchuk, W. A., Stevens, J., Garrett, A. L., Wang, S. C., & Guetterman, T. C. (2020). Modeling the use of mixed methods–grounded theory: Developing scales for a new measurement model. Journal of Mixed Methods Research , 14 (2), 184–206. https://doi.org/10.1177/155868981987259
Kaya, F., & Odacı, H. (2021). Connor-Davidson Psikolojik Sağlamlık ölçeği kısa Formu: Türkçe’ye uyarlama, geçerlik ve güvenirlik çalışması. HAYEF: Journal of Education , 17 (2), 38–54. https://doi.org/10.5152/hayef.2021.20029
Kim, H. Y. (2013). Statistical notes for clinical researchers: Assessing normal distribution (2) using skewness and kurtosis. Restorative Dentistry & Endodontics , 38 (1), 52–54. https://doi.org/10.5395/rde.2013.38.1.52
Kuhar, M., & Zager Kocjan, G. (2022). Adverse childhood experiences and somatic symptoms in adulthood: A moderated mediation effects of disturbed self-organization and resilient coping. Psychological Trauma: Theory Research Practice and Policy , 14 (8), 1288–1298. https://doi.org/10.1037/tra0001040
Kuzulugil, Ş. (2010). A classification of Turkish cultural values . ICSS 2010 Proceedings.
Lazarus, R. S. (2000). Toward better research on stress and coping. American Psychological Association , 55 (6), 665–673. https://doi.org/10.1037/0003-066X.55.6.665
Lijadi, A. A. (2019). W hat are universally accepted human values that define ‘a good life’? Historical perspective of value theory. IIASA Working Paper . IIASA.
Lipowski, Z. J. (1988). Somatization: The concept and its clinical application. American Journal of Psychiatry , 145 (11), 1358–1368.
Lovibond, P. F., & Lovibond, S. H. (1995). The structure of negative emotional states: Comparison of the Depression Anxiety Stress Scales (DASS) with the Beck Depression and anxiety inventories. Behaviour Research and Therapy, 33 (3), 335–343.
Maercker, A., Zhang, X. C., Gao, Z., Kochetkov, Y., Lu, S., Sang, Z., & Margraf, J. (2015). Personal value orientations as mediated predictors of mental health: A three-culture study of Chinese, Russian, and German university students. International Journal of Clinical and Health Psychology , 15 (1), 8–17. https://doi.org/10.1016/j.ijchp.2014.06.001
Martin, F., & Oliver, T. (2019). Behavioral activation for children and adolescents: A systematic review of progress and promise. European Child & Adolescent Psychiatry , 28 , 427–441. https://doi.org/10.1007/s00787-018-1126-z
Masten, A. S. (2014). Ordinary magic: Resilience in development . Guilford.
Miller, J. G. (1997). Cultural conceptions of duty. In D. Munro, J. E. Schumaker, & S. C. Carr (Eds.), Motivation and culture (pp. 178–192). Routledge.
Miller, A. N., & Orsillo, S. M. (2020). Values, acceptance, and belongingess in graduate school: Perspectives from underrepresented minority students. Journal of Contextual Behavioral Science , 15 , 197–206. https://doi.org/10.1016/j.jcbs.2020.01.002
Motti-Stefanidi, F. (2018). Resilience among immigrant youth: The role of culture, development and acculturation. Developmental Review , 50 , 99–109. https://doi.org/10.1016/j.dr.2018.04.002
Ostermann, M., Huffziger, S., Kleindienst, N., Mata, J., Schmahl, C., Beierlein, C., & Lyssenko, L. (2017). Realization of personal values predicts mental health and satisfaction with life in a German population. Journal of Social and Clinical Psychology , 36 (8), 651–674. https://doi.org/10.1521/jscp.2017.36.8.651
Özcan, B., & Bulus, M. (2022). Protective factors associated with academic resilience of adolescents in individualist and collectivist cultures: Evidence from PISA 2018 large scale assessment. Current Psychology , 41 (4), 1740–1756. https://doi.org/10.1007/s12144-022-02944-z
Özgen, N. (2015). Demographic development and some cultural characteristics of Anatolian Circassians. In E. Efe, M. Ayışığı, Ö. Düzbakar, & M. Arslan (Eds.), Turkey at the Beginning of 21st Century: Past and present (pp. 394–422). St. Kliment Ohridski University Press Sofia.
Patterson, J. H., Collins, L., & Abbott, G. (2004). A study of teacher resilience in urban schools. Journal of Instructional Psychology , 31 (1), 3–11.
Peterson, C., & Seligman, M. E. (2004). Character strengths and virtues: A handbook and classification . Oxford University Press.
Plumb, J. C., Stewart, I., Dahl, J., & Lundgren, T. (2009). In search of meaning: Values in modern clinical behavior analysis. The Behavior Analyst , 32 , 85–103. https://doi.org/10.1007/BF03392177
Ran, L., Wang, W., Ai, M., Kong, Y., Chen, J., & Kuang, L. (2020). Psychological resilience, depression, anxiety, and somatization symptoms in response to COVID-19: A study of the general population in China at the peak of its epidemic. Social Science & Medicine , 262 , 113261. https://doi.org/10.1016/j.socscimed.2020.113261
Ruiz, F. J., Suárez-Falcón, J. C., Segura‐Vargas, M. A., & ve Gil‐Luciano, B. (2021). Psychometric properties of the Spanish version of the Valuing Questionnaire in Colombian clinical and nonclinical samples. Journal of Clinical Psychology , 78 (2), 233–248. https://doi.org/10.1002/jclp.23212
Sahare, P., & Kotnala, A. (2022). Effectiveness of spiritual augmented psychotherapy on resilience and conscience on juvenile delinquents. Yoga Mimamsa , 54 (2), 56–61. https://doi.org/10.4103/ym.ym_124_22
Schwartz, S. H. (2012). An overview of the Schwartz theory of basic values. Online Readings in Psychology and Culture , 2 (1), 2307–0919. https://doi.org/10.9707/2307-0919.1116
Sebre, S., Miltuze, A., & Bite, I. (2014). Resilience in relation to self-direction values and anxious/depressed symptoms across the life span. Poster session presentation at the meeting of the 16th European Conference on Developmental Pscyhology, Switzerland .
Shim, M., Johnson, B., Bradt, J., & Gasson, S. (2021). A mixed methods–grounded theory design for producing more refined theoretical models. Journal of Mixed Methods Research , 15 (1), 61–86. https://doi.org/10.1177/1558689820932311
Siegmann, P., Teismann, T., Fritsch, N., Forkmann, T., Glaesmer, H., Zhang, X. C., & Margraf, J. (2018). Resilience to suicide ideation: A cross-cultural test of the buffering hypothesis. Clinical Psychology & Psychotherapy , 25 (1), e1–e9. https://doi.org/10.1002/cpp.2118
Skevington, S. M., & The WHOQOL SRPB Group. (2020). Is culture ımportant to the relationship between quality of life and resilience? Global ımplications for preparing communities for environmental and health disasters. Frontiers in Psychology , 11 , 1492. https://doi.org/10.3389/fpsyg.2020.01492
Tajul Ariffin, A. H., Khaiyom, A., J. H., & Md Rosli, A. N. (2022). Islam, Iman, and Ihsan: The role of religiosity on quality of life and mental health of muslim undergraduate students. IIUM Medical Journal Malaysia , 21 (3), 146–154. https://doi.org/10.31436/imjm.v21i3
Tanyu, M., Spier, E., Pulizzi, S., Rooney, M., Sorenson, I., & Fernandez, J. (2020). Improving education outcomes for students who have experienced trauma and/or adversity. OECD Education Working Papers , 242 . https://doi.org/10.1787/54d45980-en
Teddlie, C., & Tashakkori, A. (2009). Foundations of mixed methods research: Integrating quantitative and qualitative approaches in the social and behavioral sciences . Sage.
Triandis, H. C. (2001). Individualism-collectivism and personality. Journal of Personality , 69 (6), 907–924. https://doi.org/10.1111/1467-6494.696169
Tunç, H., Morris, P. G., Kyranides, M. N., McArdle, A., McConachie, D., & Williams, J. (2023). The relationships between valued living and depression and anxiety: A systematic review, meta -analysis, and metaregression. Journal of Contextual Behavioral Science , 28 , 102–126. https://doi.org/10.1016/j.jcbs.2023.02.004
Ungar, M., Liebenberg, L., & Brown, M. (2005). The International resilience project. In M. Ungar (Eds.), Handbook for working with children and youth: Pathways to resilience across cultures and contexts (pp. 211–229). Sage.
Ungar, M., & Theron, L. (2020). Resilience and mental health: How multisystemic processes contribute to positive outcomes. The Lancet Psychiatry, 7 (5), 441–448. https://doi.org/10.1016/S2215-0366(19)30434-1
Ungar, M., Theron, L., Murphy, K., & Jefferies, P. (2021). Researching multisystemic resilience: A sample methodology. Frontiers in Psychology , 11 , 607994. https://doi.org/10.3389/fpsyg.2020.607994
Williams, K. E., Ciarrochi, J., & Heaven, P. C. (2015). Relationships between valued act ion and well-being across the transit ion from high school to early adulthood. The Journal of Positive Psychology , 10 (2), 127–140. https://doi.org/10.1080/17439760.2014.920404
Wilson, K. G., & Murrell, A. R. (2004). Values work in acceptance and commitment therapy: Setting a course for behavioral treatment. In S. C. Hayes, V. M. Folette, & M. Linehan (Eds.), Mindfulness and acceptance: Expanding the cognitive behavioral tradition (Vol. 151, p. 120). Guilford Press.
World Population Review (2024). Religion by country 2024 . Retrieved July 11, 2024, from https://worldpopulationreview.com/country-rankings/religion-by-country
Wright, M. O. D., Masten, A. S., & Narayan, A. J. (2013). Resilience processes in development: Four waves of research on positive adaptation in the context of adversity. In S. Goldstein, & R. B. Brooks (Eds.), Handbook of Resilience in Children (pp. 15–37). Springer.
Xie, Q., & Wong, D. F. K. (2020). Culturally sensitive conceptualization of resilience: A multidimensional model of Chinese resilience. Transcultural Psychiatry , 58 (3), 323–334. https://doi.org/10.1177/1363461520951306
Yang, Y. (2020). Daily stressor, daily resilience, and daily somatization: The role of trait aggression. Personality and Individual Differences , 165 , 110141. https://doi.org/10.1016/j.paid.2020.110141
Yaşaroğlu, C. (2013). Türkiye’deki hayat bilgisi ile singapur’daki vatandaşlık ve ahlak eğitimi derslerinin değerler eğitimi bağlamında karşılaştırılması. Journal of Turkish Studies , 8 (8), 1453–1461.
Yeh, C. J., Arora, A. K., & Wu, K. A. (2006). A new theoretical model of collectivistic coping. In P. T. P. Wong, & L. C. J. Wong (Eds.), Handbook of multicultural perspectives on stress and coping (pp. 55–72). Springer.
Yıldırım, A., Boysan, M., & Kefeli, M. C. (2018). Psychometric properties of the Turkish version of the Depression anxiety stress Scale-21 (DASS-21). British Journal of Guidance & Counselling , 46 (5), 582–595. https://doi.org/10.1080/03069885.2018.1442558
Yılmaz, G. (2019). Being immigrant in a Conflict-Ridden Society. In G. Yılmaz, İ. D. Karatepe, & T. Tören (Eds.), Integration through Exploitation: Syrians in Turkey (pp. 11–127). Rainer Hampp.
Zümbül, S. (2021). Coping: A holistic review. İnönü University Journal of the Faculty of Education , 22 (1), 109–143. https://doi.org/10.17679/inuefd.688844
Download references
Acknowledgements
We thank all participating for their effort.
This research is not supported by any side.
Author information
Authors and affiliations.
Department of Psychology, Faculty of Economic Administrative and Social Sciences, Hasan Kalyoncu University, Havalimanı Yolu Üzeri 8. Km, Şahinbey/Gaziantep, Turkey
Feyza Topçu & Mehmet Dinç
You can also search for this author in PubMed Google Scholar
Contributions
All authors contributed to the study conception and design. Material preparation, data collection and analysis were performed by [Feyza Topçu]. The first draft of the manuscript was written by [Feyza Topçu], [Mehmet Dinç] and all authors commented on previous versions of the manuscript. All authors read and approved the final manuscript.
Corresponding authors
Correspondence to Feyza Topçu or Mehmet Dinç .
Ethics declarations
Ethics approval and consent to participate.
This study was performed in line with the principles of the Declaration of Helsinki. The present study was approved by the Hasan Kalyoncu University Graduate Education Institute Ethics Committee.
Informed consent
Informed consent was obtained from all individual participants included in the study.
Consent to publish
Competing interests.
The authors have no conflicts of interest to declare that are relevant to the content of this article.
Additional information
Publisher’s note.
Springer Nature remains neutral with regard to jurisdictional claims in published maps and institutional affiliations.
Electronic Supplementary Material
Below is the link to the electronic supplementary material.
Supplementary Material (DOCX 15.4 KB)
Rights and permissions.
Springer Nature or its licensor (e.g. a society or other partner) holds exclusive rights to this article under a publishing agreement with the author(s) or other rightsholder(s); author self-archiving of the accepted manuscript version of this article is solely governed by the terms of such publishing agreement and applicable law.
Reprints and permissions
About this article
Topçu, F., Dinç, M. Psychological resilience and valued living in difficult times: mixed method research in cultural context. Curr Psychol (2024). https://doi.org/10.1007/s12144-024-06552-x
Download citation
Accepted : 11 August 2024
Published : 12 September 2024
DOI : https://doi.org/10.1007/s12144-024-06552-x
Share this article
Anyone you share the following link with will be able to read this content:
Sorry, a shareable link is not currently available for this article.
Provided by the Springer Nature SharedIt content-sharing initiative
- Valued living
- Psychological resilience
- Mental health
- Find a journal
- Publish with us
- Track your research
Krieger School of Arts & Sciences Undergraduate Research, Scholarly & Creative Activity
Pursuing social sciences research: quantitative, qualitative, and mixed-methods, october 23 @ 5:00 pm – 6:00 pm.
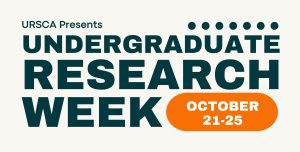
s part of URSCA’s Undergraduate Research Week (October 21-25), this panel discussion will explore how undergraduates can get involved in social science research in all its many forms. Panelists include current Woodrow Wilson Fellows and other URSCA-funded undergraduate researchers, as well as URSCA staff, who will offer guidance on getting started and on applying for funding.
Register on HopkinsGroups !
- Google Calendar
- Outlook 365
- Outlook Live
Event Navigation
- « Virtual Panel: Creative Arts Projects as Undergraduate Research
- Virtual Info Session: Navigating IRB as an Undergraduate »
- Open access
- Published: 16 September 2024
Artificial intelligence (AI) -integrated educational applications and college students’ creativity and academic emotions: students and teachers’ perceptions and attitudes
- Haozhuo Lin 1 &
- Qiu Chen 2
BMC Psychology volume 12 , Article number: 487 ( 2024 ) Cite this article
Metrics details
Integrating Artificial Intelligence (AI) in educational applications is becoming increasingly prevalent, bringing opportunities and challenges to the learning environment. While AI applications have the potential to enhance structured learning, they may also significantly impact students’ creativity and academic emotions.
This study aims to explore the effects of AI-integrated educational applications on college students’ creativity and academic emotions from the perspectives of both students and teachers. It also assessed undergraduate students’ and faculty’s attitudes to AI-integrated applications.
Methodology
A mixed-method research design was used. In the first phase, a qualitative research approach was employed, utilizing theoretical sampling to select informants. Data were collected through in-depth interviews with undergraduate students and university lecturers to gain comprehensive insights into their experiences and perceptions. A scale was developed, validated, and administered to 120 students and faculty in the quantitative phase. Descriptive statistics was used to analyze the data.
The study revealed that AI applications often impose rigid frameworks that constrain creative thinking and innovation, leading to emotional disengagement due to AI interactions’ repetitive and impersonal nature. Additionally, constant AI assessments heightened performance anxiety, and technical frustrations disrupted the learning process. Conversely, AI applications stimulated creativity by introducing new ideas and problem-solving techniques, enhanced engagement through interactive elements, provided personalized feedback, and supported emotional well-being with gamified elements and constant availability. Quantitative data also verified that teachers and students have positive attitudes toward the benefits and challenges of these applications.
Conclusions
AI integration in educational applications has a dual-edged impact on college students’ creativity and academic emotions. While there are notable benefits in stimulating creativity and enhancing engagement, significant challenges such as creativity constraints, emotional disengagement, and performance anxiety must be addressed. Balancing these factors requires thoughtful implementation and continuous evaluation to optimize the role of AI in education.
Peer Review reports
Introduction
Artificial Intelligence (AI) represents a subdivision of computer science that employs algorithms and machine learning techniques to emulate or mimic human intelligence [ 1 ]. AI is categorized into three types: narrow AI, general AI, and artificial superintelligence. Narrow AI, the most prevalent and developed form of AI to date, is highly goal-oriented and utilizes machine learning techniques to accomplish specific objectives or tasks, such as image and facial recognition or virtual assistants like Siri and Alexa. General AI, also known as deep AI, possesses capabilities comparable to human intelligence, including understanding the needs and emotions of other intelligent beings. In contrast, artificial superintelligence surpasses human capabilities in all respects, resembling portrayals of AI in science fiction that exceed human intelligence [ 2 ].
In the educational context, the development of AI is likely to remain within the scope of narrow AI. Current educational technologies encompass speech semantic recognition, image recognition, augmented reality/virtual reality, machine learning, brain neuroscience, quantum computing, and blockchain. These technologies are increasingly being integrated into classrooms. Many AI-based educational products are being implemented in K-12 education [ 3 ]. Research indicates that AI technology in education has been applied in at least ten areas: automatic grading systems, interval reminders, teacher feedback, virtual teachers, personalized learning, adaptive learning, augmented reality/virtual reality, precise reading, intelligent campuses, and distance learning [ 3 ]. The Artificial Intelligence in Education (AIED) community focuses on developing systems as effective as one-on-one human tutoring [ 4 ]. Significant advancements toward this goal have been made over the past 25 years. However, prioritizing the human tutor or teacher as the benchmark, AIED practices typically involve students working with computers to solve step-based problems centred on domain-specific knowledge in subjects such as science and mathematics [ 5 ]. This approach needs to fully account for recent educational practices and theory developments, including emphasizing 21st-century competencies. The 21st-century competency approach to education highlights the importance of general skills and competencies such as creativity. Modern classrooms aim to incorporate authentic practices using real-world problems in collaborative learning environments. To remain relevant and enhance its impact, the field of AIED must adapt to these evolving educational paradigms.
The impact of AI applications on various aspects of education has garnered significant attention in recent years. While research has delved into its effects on different variables, one area deserving deeper exploration is its influence on students’ creativity [ 6 , 7 , 8 , 9 , 10 , 11 , 12 , 13 , 14 , 15 ]. Creativity is a multifaceted construct crucial for problem-solving, innovation, and adaptability in an ever-evolving society. Traditional educational paradigms often need help to fully nurture and assess creativity due to their structured nature and emphasis on standardized assessments. However, AI-integrated educational applications possess the potential to revolutionize this landscape [ 6 , 7 , 8 , 9 , 10 , 11 , 12 , 13 , 14 , 15 ].
AI applications can provide personalized learning experiences tailored to students’ unique cognitive profiles, preferences, and learning styles. By offering adaptive feedback, generating diverse learning materials, and facilitating interactive learning environments, AI can foster a conducive atmosphere for creativity to flourish. Through algorithms that analyze students’ performance, identify patterns, and suggest novel approaches, AI empowers learners to explore unconventional solutions, think critically, and engage in creative problem-solving processes [ 16 , 17 , 18 , 19 , 20 , 21 , 22 ].
Moreover, AI technologies can facilitate collaborative and interdisciplinary learning experiences, exposing students to diverse perspectives, ideas, and methodologies. Virtual reality simulations, augmented reality tools, and intelligent tutoring systems can immerse students in interactive learning environments where they can experiment, innovate, and co-create content. By transcending the constraints of physical classrooms and textbooks, AI-enabled platforms offer limitless possibilities for creative expression and exploration [ 23 , 24 , 25 , 26 , 27 , 28 , 29 , 30 ].
Furthermore, AI’s ability to curate and recommend relevant resources from vast repositories of educational content enhances students’ exposure to diverse sources of inspiration and knowledge. By leveraging natural language processing algorithms, sentiment analysis, and recommendation systems, AI can identify content aligned with students’ interests, passions, and learning objectives, nurturing intrinsic motivation and curiosity-driven exploration [ 31 , 32 , 33 ]. In addition to creativity, another crucial aspect of the educational experience that AI-integrated applications may influence is academic emotions. These are the emotions experienced by students and educators in educational settings. These emotions are directly linked to academic activities like learning, teaching, studying, and taking exams. They can be positive (e.g., enjoyment, pride, and hope) or negative (e.g., anxiety, frustration, and boredom) and significantly impact motivation, learning strategies, cognitive resources, and academic performance [ 34 ]. Academic emotions encompass a spectrum of affective states, including motivation, engagement, anxiety, boredom, and satisfaction, significantly impacting students’ learning outcomes, perseverance, and overall well-being. Traditional educational approaches often overlook the complex interplay between cognitive processes and emotional experiences, resulting in suboptimal learning environments and outcomes [ 1 , 2 , 3 , 4 , 5 , 35 ].
However, AI technologies offer unprecedented opportunities to monitor, analyze, and respond to students’ academic emotions in real time [ 4 ]. By employing affective computing techniques, sentiment analysis algorithms, and facial recognition technology, AI can detect subtle cues indicative of students’ emotional states and adjust learning experiences accordingly [ 1 ]. For instance, adaptive tutoring systems can dynamically adapt to the difficulty level of tasks, provide scaffolding support, or offer motivational prompts based on students’ emotional responses and performance metrics [ 5 ]. Moreover, AI-integrated learning platforms can incorporate gamification elements, immersive storytelling, and personalized avatars to enhance students’ emotional engagement and investment in learning activities [ 4 ]. By fostering a supportive and inclusive learning environment that acknowledges and addresses students’ diverse emotional needs, AI can promote positive academic emotions, such as curiosity, excitement, and self-efficacy, while mitigating negative ones, such as frustration, anxiety, and disengagement.
Furthermore, AI-driven analytics and data visualization tools empower educators to gain deeper insights into students’ emotional trajectories, identify at-risk individuals, and implement timely interventions. By harnessing predictive analytics and machine learning algorithms, educators can anticipate students’ emotional responses to various instructional strategies, anticipate potential challenges, and proactively implement personalized interventions to foster resilience, motivation, and emotional well-being. In line with the existing gap, the following research questions were raised:
How do teachers and students perceive the challenges of using AI applications in the students’ creativity and academic emotions?
How do teachers and students perceive the merits of using AI applications in the students’ creativity and academic emotions?
What are the teachers and students’ attitudes to AI-integrated educational applications?
Artificial intelligence and higher education
21st-century higher education is rapidly changing due to globalization, technological advancements, and student demographics [ 16 ]. Online learning platforms have become widely accessible, enabling universities to offer fully online courses and degree programs, expanding access to education and providing flexibility in learning [ 17 ]. The growing diversity of the educational field, with students from various backgrounds, highlights the significance of global citizenship and intercultural understanding. Universities are playing a significant role in promoting innovation and research as technology advancements pick up speed [ 18 ], encouraging industry-academia cooperation and placing a focus on commercialization and entrepreneurship. The emphasis is shifting toward skills-based learning patterns for practical, career-focused skills, as evidenced by recent recruitment trends favouring graduates with particular skills [ 19 ].
To enhance the quality of higher education, the industry is exploring various strategies to meet stakeholders’ requirements [ 20 ]. Artificial intelligence (AI) integration is one particularly hopeful solution [ 21 ]. As technology advances, artificial intelligence (AI) in education has enormous potential to change the teaching and learning environment [ 22 ]. AI is significantly improving the quality of higher education in several ways [ 23 ]. Artificial intelligence (AI)--powered learning strategies evaluate students’ performance, pinpoint their advantages and disadvantages and offer individualized learning experiences. With the help of this strategy, students can acquire knowledge and produce more valuable results in the real world [ 24 ].
Chatbots, virtual assistants, and adaptive learning systems are examples of AI-based technology providing immersive and exciting learning environments while actively enabling students to investigate complicated ideas [ 25 ]. Artificial intelligence (AI) helps with assessment and feedback by helping with assignment grading, tracking student participation, giving quicker and more accurate feedback, and freeing up teachers’ time for other teaching responsibilities [ 26 ]. Chatbots with artificial intelligence (AI) provide quick, individualized support by evaluating student data to identify individuals who may be at risk and enabling early interventions for academic success—various AI applications and platforms, including Bit. AI, Mendeley, Turnitin, elinik. Io and Coursera tools support higher education research by analyzing large datasets, generating insights, and identifying patterns challenging for human researchers to detect [ 27 ]. We expect even more cutting-edge AI applications in education due to continued technological advancement, giving students individualized, engaging, and productive learning experiences [ 28 ].
The exciting development of AI dramatically improves both the effectiveness and engagement of instructors in postsecondary education. Adopting AI helps educators free up time for more meaningful activities by automating administrative duties like tracking attendance and grading assignments [ 29 ]. Additionally, AI helps educators pinpoint areas in which they can grow by offering individualized opportunities for professional development [ 30 ]. Solutions to enduring problems in modern higher education are needed, such as limited inclusivity and unequal access [ 31 ]. Traditional teaching methods often fail to engage students with diverse learning preferences, hindering active participation and critical thinking skills [ 32 ]. The inability of conventional assessment techniques to capture thorough understanding makes using AI necessary. AI algorithms analyze individual learning patterns, tailor coursework, and predict at-risk students, enabling timely interventions [ 33 ]. Content delivery is revolutionized by AI-driven systems that adjust to students’ learning styles, pace, and knowledge gaps.
In conclusion, adopting AI in higher education empowers the system by addressing challenges and enhancing the quality of education. Ongoing research aims to understand faculty members’ awareness of AI’s applicability and impact on learning experiences, work engagement, and productivity in higher education. This research provides insights for institutional policymakers to facilitate the adoption of new technologies and overcome specific challenges. Despite the increasing integration of technology and artificial intelligence (AI) in education, there is a notable gap in understanding how AI-empowered technology educational apps specifically influence undergraduate students’ academic emotions and test anxiety. While various studies have explored the general impact of technology on education and student emotions, there is a need for focused research on the unique effects of AI-powered educational apps. Understanding the dynamics between these technologies and students’ emotional experiences can provide valuable insights into the efficacy of AI applications in promoting positive emotions and reducing test anxiety.
AI applications and the students’ creativity
Students should be aware of AI’s potential to bolster their creativity and learning processes. Modern educational methodologies prioritize problem-solving approaches, underscoring the significance of nurturing children’s creative thinking abilities. However, extensive research corroborates the existence of a decline in creativity among younger individuals across various disciplines [ 6 , 7 ]. One explanation for this decline is attributed to the overly structured nature of school curricula and a shortage of play-based learning activities within educational frameworks [ 8 ].
Emerging research indicates how AI can enhance skills commonly associated with creativity, such as curiosity [ 9 ], perseverance, and attentiveness [ 10 ]. The potential of AI to support creativity is also under investigation. Kafai and Burke assert that AI in education aims to foster problem-solving and creativity skills through collaborative interactions with AI systems rather than solely focusing on knowledge acquisition within specific domains [ 11 ]. They suggest that AI can facilitate the unfolding of creativity, thus being intertwined with the creative process. Additionally, Ryu and Han examine Korean educators’ perceptions of AI in education, noting that experienced teachers acknowledge AI’s potential to enhance creativity [ 12 ]. Hence, AI in education could address concerns related to the decline of creativity, particularly by emphasizing the creative process. This may aid in enhancing students’ creative thinking abilities and comfort level with utilizing AI, thereby adequately preparing them for the contemporary workforce [ 13 , 14 , 15 ].
To effectively merge AI and creativity, it is imperative to gain a deeper understanding of how students perceive the relationship between these concepts. Situating AI within prevailing creativity theories, such as the 4 C model of creativity, can further enrich this understanding.
Creativity and AI in an educational setting can be analyzed through the lens of the 4 C model [ 8 ]. Mini-Q, or ‘personal creativity,’ encapsulates creativity’s subjective and developmental facets. Mini-X pertains to individualized creative discoveries that may not be recognized as such by others. For instance, a slight variation on a well-known recipe could exemplify mini-c creativity. Little-c, also known as ‘everyday creativity,’ refers to creative outputs acknowledged by others, like inventing a new recipe. Pro-c, or ‘professional creativity,’ involves becoming an expert in a particular field or discipline, akin to the chef Gordon Ramsay. Big-C, or ‘legendary creativity,’ epitomizes eminent creativity that leaves a lasting legacy, as seen in figures like August Escoffier, who revolutionized the culinary landscape [ 15 ].
AI can support creativity at the pro-c and potentially Big-C levels by extending expertise in specific domains. However, its role in fostering mini-c and little-c contributions is less apparent, as the focus in these levels lies more on the process of self-discovery than on the creative output itself. Therefore, it is crucial to understand when and where AI is most beneficial, particularly in delineating the narrow domains where AI is most apt for educational purposes and how it can encourage mini-c and little-c contributions. This study aims to explore students’ perceptions of AI and creativity and the interplay between the two.
Studies on academic emotions
Lei and Cui [ 36 ] defined academic emotions as students’ emotional experiences related to the academic processes of teaching and learning, including enjoyment, hopelessness, boredom, anxiety, anger, and pride. Based on arousal and enjoyment concepts, academic emotions have been divided into four categories: positive low-arousal, negative low-arousal, and negative high-arousal [ 37 ]. It is also argued that achievement emotions include prospective emotions, such as fear of failure, and retrospective emotions, e.g., shame, which learners experience after they receive feedback on their achievements.
Academic accomplishment serves as a commonly employed criterion for evaluating the effectiveness of educational systems, teachers, schools, and the success or failure of students. Consequently, scholars in this field have conducted empirical investigations to explore the causal link between students’ academic emotions and academic achievements, as evidenced by a body of practical studies [ 38 ]. However, the findings from these studies could be more consistent. In general, there is an anticipation that positive emotions will forecast favorable outcomes in academic settings, including high grades and commendable performance in both local and large-scale educational assessments [ 39 , 40 ]. Conversely, it is hypothesized that negative emotions will correlate with adverse consequences, such as lower grades and compromised performance in classroom activities and standardized examinations [ 41 ].
Results of the meta-analysis study undertaken by Lei and Cui [ 36 ] developed the Chinese version of the Academic Emotions Questionnaire, which was employed to evaluate the academic emotions of adolescents. Academic emotions have been linked to various variables, including cognitive activity, learning motivation, and strategies. Lei and Cui’s [ 36 ] meta-analysis study revealed positive correlations between positive high-arousal, positive low-arousal, and academic achievement and negative correlations between negative high-arousal, negative low-arousal, and academic achievement. The study suggested that factors such as the student’s age, regional location, and gender could moderate the effects of epistemic cognition on academic achievement.
Positive correlations between positive high-arousal, positive low-arousal, and academic achievement and negative correlations between negative high-arousal, negative low arousal, and academic achievement. The authors suggested that the student’s age, regional location, and gender moderated the effects of epistemic cognition on academic achievement [ 42 ].
Currently, domestically and internationally scholars are directing their attention towards analyzing academic emotions in distance learners, resulting in noteworthy research outcomes [ 43 ]. Research conducted by Thelwall et al. [ 44 ] delved into the impact of screen time on emotion regulation and student performance. The study involved over 400 children over four years, examining their usage of smartphones and tablets. The research analyzed the correlation between these behaviours, emotions, and academic performance, concurrently evaluating students’ abilities and educational achievements. Similarly [ 45 ], investigated the influence of early childhood emotions on academic preparation and social-emotional issues. Emotion regulation, identified as the process of managing emotional arousal and expression, plays a crucial role in determining children’s adaptation to the school environment.
Building on the perspectives of the previously mentioned scholars, Sakulwichitsintu [ 46 ] integrated connectionist learning theory to devise an innovative distance education model. This model introduced educational content that was aligned with emotional education objectives and implemented the Mu class teaching mode, establishing a distance learning community and humanized network courses to address emotional shortcomings in the distance education process. Ensuring effectiveness, Pekrun et al. [ 35 ] developed a hybrid reality virtual intelligent classroom system incorporating television broadcasting and interactive space technology to create a networked teaching environment. Teachers utilized diverse techniques, including video, audio, and text, to foster engagement and enhance communication between educators and students during the network teaching phase.
In addition to the earlier scholars, Fang et al. [ 47 ] introduced an emotion recognition algorithm based on facial expression scale-invariant feature transformation. This algorithm captures the facial expressions of distance learners, employing SIFT feature extraction and expression recognition to address emotional gaps in the learning phase of distance education. Simultaneously, Méndez López [ 48 ] developed a learner emotion prediction model for an intelligent learning environment utilizing a fuzzy cognitive map. This model facilitated the extraction and prediction of distance learners’ emotional states, allowing real-time adjustments to the teaching approach based on predicted emotions. Huang and Bo [ 49 ] contributed to the field by introducing the distance learner emotion self-assessment scale, defining essential emotion variables, and establishing an early warning model.
Drawing inspiration from the valuable contributions of the scholars mentioned earlier, Zembylas [ 50 ] examined the online academic emotions experienced by adults. This investigation involved the analysis of diverse influencing factors and the exploration of an environmental factor model within the online learning community, specifically focusing on academic emotional tendencies. Building upon the insights derived from these scholars, our objective is to delve into the academic emotions of distance learners. We plan to achieve this through the analysis of online learning behaviour data, with the anticipation of uncovering meaningful findings in this domain.
This study used mixed-method research (qualitative-quantitative). The following sections describe each phase.
Qualitative method
Sampling and design.
This study employs a qualitative research design to explore the impact of AI-integrated educational applications on undergraduate students’ creativity and academic emotions from the perspectives of both students and university faculties. The research was conducted at Wenzhou University, leveraging theoretical sampling to ensure a comprehensive understanding of the phenomena under investigation. The informants were selected using theoretical sampling, a technique where participants are chosen based on their potential to contribute to the development of emerging theories, ensuring that the sample is rich in information pertinent to the research questions. A total of 23 participants were included in the study, comprising 15 students and eight teachers. The decision to interview these specific numbers was driven by the principle of data saturation, which refers to the point at which no new information or themes are observed in the data. Data saturation was achieved after interviewing the 15th student and the 8th teacher, indicating that the sample size was sufficient to capture the full range of perspectives necessary for the research. The criterion for including the participants in the study was their familiarity with the components of AI. AI-integrated educational applications. These components include Adaptive Learning Systems, Intelligent Tutoring Systems (ITS), Natural Language Processing (NLP) applications, AI-enhanced collaborative Learning Platforms, and Predictive Analytics.
To evaluate the impact of AI-integrated educational applications on students’ creativity and academic emotions, we focused on several key components of AI applied to educational processes. These components include Adaptive Learning Systems, which personalize learning experiences by adjusting content and pace based on individual student performance and preferences, enhancing creativity through personalized challenges and immediate feedback. Intelligent Tutoring Systems (ITS) offer personalized tutoring and feedback, fostering creative problem-solving skills and reducing negative emotions such as anxiety and frustration. Natural Language Processing (NLP) applications facilitate interaction between computers and humans using natural language, enhancing creativity through brainstorming sessions and interactive writing assistance while monitoring changes in academic emotions. AI-enhanced collaborative Learning Platforms support and enhance collaborative learning experiences with features like intelligent grouping, real-time feedback, and automated moderation, impacting group creativity and collective emotional states. Predictive Analytics analyze data to predict student performance, engagement, and emotional states, informing instructional decisions and personalized interventions to enhance creativity and mitigate negative academic emotions.
Data collection
Data collection was carried out through semi-structured interviews, a method well-suited to qualitative research. This method allows for in-depth exploration of participants’ experiences and perceptions while providing some level of structure to ensure that all relevant topics are covered. The semi-structured format includes predefined questions but also allows for flexibility in probing deeper into interesting or unexpected responses.
Interviews were conducted in a quiet and comfortable setting within the university premises to ensure participants felt at ease, thereby facilitating open and honest communication. Each interview lasted approximately 45 to 60 min. For the student participants, the interview questions focused on their experiences using AI-integrated educational applications, perceived impacts on their creativity, and any changes in their academic emotions (e.g., motivation, anxiety, enjoyment). Teacher participants were asked about their observations of students’ engagement and creativity, as well as their own experiences and attitudes towards integrating AI applications in their teaching practices.
Before the interviews, informed consent was obtained from all participants, ensuring they were aware of the study’s purpose, their rights to confidentiality, and their freedom to withdraw from the study at any point without any repercussions. The interviews were audio-recorded with participants’ permission to ensure accurate data capture and were later transcribed verbatim for analysis.
Data analysis
The data analysis process began with the transcription of the audio-recorded interviews, followed by a thorough reading of the transcripts to gain an initial understanding of the data. Thematic analysis was employed to identify, analyze, and report patterns (themes) within the data. This method is particularly effective in qualitative research as it provides a systematic approach to handling large volumes of text and can reveal complex patterns in participants’ narratives.
The thematic analysis was conducted in several steps. First, open coding was performed, where the transcripts were examined line-by-line, and initial codes were generated to capture significant statements and ideas. These codes were then grouped into broader categories based on similarities and relationships. For instance, codes related to students’ enhanced engagement and creativity when using AI applications were grouped under a category labelled “positive impacts on creativity.” Next, the categories were reviewed and refined into overarching themes. This involved constant comparison within and between the data to ensure the themes accurately represented the participants’ perspectives. Themes were then defined and named, providing a clear and concise description of each theme’s essence. Open themes were then classified into two main categories: Challenges and Merits of AI-integrated applications.
Research quality
To ensure research quality, several rigorous steps were undertaken. The transcription of audio-recorded interviews was done verbatim to preserve the original meaning and nuances, maintaining data integrity. Researchers immersed themselves in the data by reading the transcripts multiple times, allowing for a deep understanding. Thematic analysis was systematically employed to identify, analyze, and report patterns, facilitating the uncovering of complex themes. Open coding involved line-by-line examination and initial coding to capture significant statements and ideas, ensuring comprehensive data consideration. Codes were then grouped into broader categories, organizing data meaningfully and aiding in the identification of overarching themes.
Peer debriefing sessions with colleagues provided external validation, enhancing credibility by identifying potential biases and ensuring balanced interpretations. Triangulation was used to confirm consistency and validity by comparing data from multiple sources, reinforcing the reliability of the themes. Detailed documentation of the analytical process ensured transparency and created an audit trail, allowing verification of the research steps and findings. Finally, researchers engaged in reflexivity, continuously reflecting on potential biases to ensure objectivity and accurate representation of participants’ voices, further contributing to the study’s reliability.
Quantitative method
The quantitative phase explored teachers’ and students’ attitudes towards AI applications in education. The sample consisted of 120 undergraduate students and 30 teachers. Participants were selected using a convenience sampling method, ensuring a diverse representation of experiences and perspectives within the educational environment.
Participants were asked to complete a survey that included statements related to the perceived challenges and benefits of AI applications in education. The survey featured a series of Likert scale questions where respondents indicated their level of agreement with each statement on a scale of 1 to 5, where one represented “Strongly Disagree,” 2 represented “Disagree,” 3 represented “Neutral,” 4 represented “Agree,” and five represented “Strongly Agree. The construct validity. It was estimated using exploratory factor analysis, and the items were reduced to factors: challenges and merits. All items had loading factors above 0.70, indicating that the scale enjoyed acceptable construct validity. The reliability of the scale was estimated using Cronbach’s alpha. The internal consistency of the factors of the scale were 0.85 and 0.89, respectively, and the reliability of the total scale was 0.90, which verifies the reliability of the scale (See Appendix).
The survey was divided into two sections: Constraints of AI Applications and Merits of AI Applications. The Constraints section included statements about creativity constraints, emotional disengagement, performance anxiety, technical frustration, over-reliance on AI, the digital divide, and ethical concerns. The Merits section included statements about stimulated creativity, increased engagement, personalized feedback, emotional support, collaborative creativity, accessible learning resources, and enhanced academic emotions.
Data were collected through an online survey platform, ensuring anonymity and confidentiality for all respondents. Descriptive statistics, specifically percentages, were used to summarize the responses. The rate of respondents in each agreement category (Strongly Disagree, Disagree, Neutral, Agree, Strongly Agree) was calculated for each statement. The results were then tabulated separately for teachers and students to identify any significant differences or similarities in their perceptions. This approach provided a clear overview of the collective attitudes of both groups towards AI applications in education, facilitating a detailed comparative analysis. Finally, the findings were interpreted to understand the broader implications of these attitudes on the integration of AI in educational settings. This comprehensive methodology ensured that the study captured a wide range of perspectives, providing valuable insights into how AI is perceived in the context of teaching and learning.
Qualitative findings
The interviews with participants were analyzed, resulting in two selective codes: Challenges and Merits. Each code consists of seven main themes related to students’ creativity and academic emotions. Below, each theme is explained in detail and followed by quotations from both students and teachers to exemplify these findings.
Challenges of AI-applications
Interviews with the informants were thematically analyzed, and different themes were extracted. The interviews highlighted challenges of AI applications in education, including creativity constraints, emotional disengagement, performance anxiety, technical frustration, over-reliance on AI, the digital divide, and ethical concerns. These issues affect students’ creativity, engagement, stress levels, and equitable access to technology. Each sub-theme is explained as follows.
Creativity constraints
The first challenge identified was creativity constraints. Participants noted that some AI applications impose rigid frameworks and lack the flexibility needed to foster creative thinking. These constraints can hinder students’ ability to think outside the box and explore innovative solutions. The following quotations exemplify this finding:
Student 1: “Sometimes the AI applications don’t allow much room for creativity because they follow a strict format.” Teacher 2: “I’ve noticed that some students feel boxed in by the structure imposed by the AI, hindering their creative expression.”
Emotional disengagement
Another challenge was emotional disengagement. The repetitive nature of AI interactions and the absence of a human touch were found to diminish emotional connection and motivation among students. This lack of engagement can detract from the overall learning experience. The following quotations exemplify this finding:
Student 10: “Interacting with AI can get monotonous, and I miss the personal interaction with my teachers.” Teacher 8: “There’s a certain emotional warmth in human interactions that AI can’t replicate, which some students really miss.”
Performance anxiety
Performance anxiety was a significant challenge, with students experiencing heightened stress due to constant monitoring and frequent AI assessments. This pressure can make students more fearful of making mistakes, impacting their academic emotions negatively. The following quotations exemplify this finding:
Student: “The AI assessments are so frequent that I constantly feel pressured to perform well, which makes me anxious.” Teacher: “I’ve observed that some students become overly anxious about their performance because they know the AI is always evaluating them.”
Technical frustration
Technical frustration was a common issue, with frequent glitches and difficult-to-navigate interfaces disrupting the learning process and causing frustration among students. This negatively impacted their creativity and emotional state. The following quotations exemplify this finding:
Student 8: “When the app glitches, it disrupts my workflow and frustrates me, killing my creative vibe.” Teacher 6: “Technical problems often leave students frustrated, which can stifle their creativity and motivation.”
Over-reliance on AI
Over-reliance on AI applications was another challenge, leading to reduced critical thinking and self-initiative among students. This dependency can hinder the development of essential problem-solving skills. The following quotations exemplify this finding:
Student 11: “I sometimes rely too much on the AI for answers instead of trying to figure things out myself.” Teacher 9: “There’s a danger that students may become too dependent on AI, which can hinder their ability to think critically and independently.”
Digital divide
The digital divide posed a significant challenge, with inequitable access to technology and varying levels of technological literacy affecting students’ ability to engage fully and creatively. This disparity can exacerbate existing educational inequalities. The following quotations exemplify this finding:
Student 12: “Not everyone has the same access to the necessary technology, which can be limiting for those who don’t.” Teacher 4: “Students with limited tech skills or access are at a disadvantage, impacting their ability to participate fully and creatively.”
Ethical concerns
Participants raised ethical concerns about biases in AI algorithms and the ethical use of AI in education. These concerns are related to fairness and equity in academic evaluations and the potential for AI to perpetuate existing biases. The following quotations exemplify this finding:
Student: “I’m concerned that the AI might have biases that affect how it evaluates my work.” Teacher: “There are significant ethical questions about how AI is used and whether it treats all students fairly, which can impact their academic emotions and creativity.”
Teachers and students’ perceptions of the merits of AI-applications
Teachers and students believe that AI-integrated educational applications stimulate creativity, increase engagement, provide personalized feedback, offer emotional support, facilitate collaborative creativity, and make learning resources more accessible. These benefits enhance students’ academic emotions and foster innovative approaches to learning, as illustrated by student and teacher testimonials. Each of these themes is explained and exemplified in detail as follows.
Stimulated creativity
On the positive side, AI applications were found to stimulate creativity by presenting new ideas and enhancing problem-solving skills. This allowed students to explore innovative approaches to learning. The following quotations exemplify this finding:
Student 6: “The AI applications introduce me to new ideas that I wouldn’t have thought of on my own, boosting my creativity.” Teacher 8: “I’ve seen students come up with innovative solutions and creative projects thanks to the AI applications.”
Increased engagement
Increased engagement was another significant benefit, with the interactive nature of AI applications making learning more enjoyable and keeping students motivated. This positive engagement enhanced both creativity and academic emotions. The following quotations exemplify this finding:
Student 9: “The interactive features make learning more enjoyable and keep me engaged.” Teacher 5: “Students are more engaged and seem to enjoy the learning process more when using AI applications.”
Personalized feedback
Personalized feedback provided by AI applications offered tailored guidance and immediate responses, helping students improve their work and boosting their confidence. This customised approach supported their creative and emotional development. The following quotations exemplify this finding:
Student 5: “The AI gives me personalized feedback that really helps me understand where I can improve.” Teacher 3: “The immediate, tailored feedback from AI applications helps students feel more confident and supported in their learning.”
Emotional support
AI applications also provide emotional support by reducing anxiety through their constant availability and increasing motivation with gamified elements and positive reinforcement. This support helped maintain a positive emotional state conducive to learning. The following quotations exemplify this finding:
Student 9: “The AI apps reduce my anxiety by being available whenever I need help, and the gamified elements keep me motivated.” Teacher 6: “Students seem less anxious and more motivated when they use AI applications that provide continuous support and positive feedback.”
Collaborative creativity
Collaborative creativity was facilitated by AI, which supported group projects and peer interactions, fostering a sense of community and collective problem-solving. This collaborative environment enhanced creative outcomes. The following quotations exemplify this finding:
Student 13: “AI applications make group projects easier and more creative by allowing us to collaborate effectively.” Teacher 9: “The AI applications encourage peer interaction and collaboration, leading to more creative and well-rounded projects.”
Accessible learning resources
The accessibility of a wide range of learning resources through AI applications supported continuous learning and inspired creativity. Students could explore diverse materials anytime, enhancing their educational experience. The following quotations exemplify this finding:
Student 8: “Having access to a wide range of resources anytime I need them inspires me to be more creative in my studies.” Teacher 7: “The vast array of resources available through AI applications encourages students to explore topics more deeply and creatively.”
Enhanced academic emotions
Finally, AI applications enhance academic emotions by creating positive learning experiences and building emotional resilience through adaptive learning paths and supportive environments. This improvement in emotional well-being positively influenced students’ academic performance. The following quotations exemplify this finding:
Student 4: “The AI apps make learning a more positive experience, which helps me stay emotionally resilient.” Teacher 5: “I’ve seen students develop greater emotional resilience and have more positive learning experiences with the support of AI applications.”
These findings illustrate a nuanced view of AI-integrated educational applications, highlighting both the challenges and benefits in terms of students’ creativity and academic emotions. While there are significant obstacles to overcome, the potential for enhancing creativity and emotional well-being is substantial.
Quantitative findings
To present teachers’ and students’ attitudes towards AI applications in education, we used descriptive statistics to summarize their responses to the statements provided. Tables 1 and 2 include the percentage of respondents in each category of agreement (Strongly Disagree, Disagree, Neutral, Agree, Strongly Agree) for teachers and students, respectively.
Both groups were concerned about AI applications imposing rigid frameworks that could hinder creative thinking, with 25% of both teachers and students agreeing and 15% strongly agreeing. A similar percentage disagreed, with 20% of teachers and 25% of students, while 10% of teachers and 15% of students strongly disagreed. Teachers were more neutral, with 30% compared to 20% of students.
Emotional disengagement due to AI was also a concern, with 35% of both teachers and students agreeing that AI interactions lack a personal touch. An additional 20% of teachers and 15% of students strongly agreed. Neutral responses were common, with 25% of teachers and 20% of students, while fewer disagreed (15% of teachers and 20% of students) or strongly disagreed (5% of teachers and 10% of students).
Performance anxiety caused by frequent AI assessments was another shared concern, with 25% of teachers and 20% of students agreeing and 15% of teachers and 20% of students strongly agreeing. Neutral responses were common, with 20% of teachers and 15% of students, while 25% of both teachers and students disagreed and 15% of teachers and 20% of students strongly disagreed.
Both teachers and students expressed concern over technical issues in AI applications that could disrupt the learning process. A quarter (25%) of each group agreed with this sentiment, while 15% strongly agreed. Neutral responses were quite common, with 30% of teachers and 25% of students expressing no strong opinion. A smaller proportion of participants disagreed (20% of both groups) or strongly disagreed (10% of teachers and 15% of students). There was also a shared recognition among both groups about the potential drawbacks of excessive reliance on AI, as 35% of teachers and 30% of students agreed that AI could diminish critical thinking and self-initiative, with 20% of teachers and 15% of students strongly agreeing. Neutral responses were frequent (25% for both groups), while a minority disagreed (15% of teachers and 20% of students) or strongly disagreed (5% of teachers and 10% of students).
Both groups similarly acknowledged the impact of the digital divide, with 30% of teachers and 25% of students agreeing, and 20% of both groups strongly agree. Neutral responses were common (20% for both groups), while a smaller number disagreed (20% of teachers and 15% of students) or strongly disagreed (10% of teachers and 15% of students). Ethical concerns regarding biases in AI algorithms were also similarly perceived. Agreement was noted among 30% of teachers and students, with 15% strongly agreeing. Neutral responses were pretty common (25% of teachers and 30% of students), and fewer respondents disagreed (20% of teachers and 15% of students) or strongly disagreed (10% from each group).
Both teachers and students had a favourable view of AI’s capacity to enhance problem-solving skills and creativity. 40% of both groups agreed with this perspective, and a notable number strongly agreed (25% of teachers and 20% of students). Neutral responses were less frequent (20% of teachers and 25% of students), while disagreement was relatively uncommon (10% from each group), as was strong disagreement (5% from each group). Furthermore, both groups acknowledged that AI could increase the enjoyment of learning, with 30% of teachers and 35% of students agreeing and 20% from each group strongly agreeing. Neutral responses were moderate (25% of teachers and 20% of students), while fewer participants disagreed (15% from both groups) or strongly disagreed (10% from each group).
The benefits of AI in providing personalized feedback were highly recognized, with 35% of teachers and students agreeing and a substantial proportion strongly agreeing (30% of teachers and 35% of students). Neutral responses were moderate (20% of teachers and 15% of students), while fewer respondents disagreed (10% from each group) or strongly disagreed (5% from each group). AI’s role in reducing anxiety through constant availability was similarly viewed, with 25% of teachers and 30% of students agreeing and 15% from each group strongly agreeing. Neutral responses were moderate (25% from both groups), with some disagreement (20% of teachers and 15% of students) and strong disagreement (15% of teachers and 10% of students).
Both groups positively perceived AI’s facilitation of group projects, with 35% of teachers and students agreeing and 25% from each group strongly agreeing. Neutral responses were common (25% of teachers and 20% of students), with fewer participants disagreeing (10% of teachers and 15% of students) or strongly disagreeing (5% from each group). The accessibility of a wide range of learning resources through AI was highly valued, with 35% of teachers and students agreeing and a notable portion strongly agreeing (30% of teachers and 25% of students). Neutral responses were moderate (20% of teachers and 25% of students), while fewer disagreed (10% from each group) or strongly disagreed (5% from each group). Lastly, both groups acknowledged AI’s role in fostering positive learning experiences, with 30% of teachers and students agreeing and 20% strongly agreeing. Neutral responses were moderate (25% from each group), while fewer participants disagreed (15% from both groups) or strongly disagreed (10% from each group).
The integration of AI in educational applications presents several significant challenges that impact students’ creativity and academic emotions. One major issue is the creativity constraints imposed by AI applications. Specifically, the rigid frameworks and lack of flexibility in some applications limit students’ ability to think creatively and explore innovative solutions. This finding aligns with previous research indicating that while AI can facilitate structured learning, it can also stifle creative thinking by enforcing rigid paths [ 51 , 52 ]. Moreover, another significant challenge is emotional disengagement. The repetitive nature of AI interactions and the lack of a human touch can lead to emotional detachment, reducing students’ motivation and engagement. This phenomenon is supported by studies showing that human interaction plays a crucial role in maintaining student engagement and emotional connection [ 53 , 54 ].
Additionally, technical frustration due to frequent glitches and complicated interfaces further hampers the learning experience. This frustration can disrupt creative processes and negatively affect academic emotions [ 55 ]. This issue is highlighted by research showing that technical difficulties are a common barrier to effective AI implementation in education [ 56 ].
Another concern is the over-reliance on AI applications, which can reduce critical thinking and self-initiative among students. This dependency can hinder the development of essential problem-solving skills. Zhai et al. [ 56 ] emphasized the importance of balancing AI use with opportunities for independent thought and critical reasoning.
The digital divide remains a significant challenge, with inequitable access to technology and varying levels of technological literacy among students creating disparities. This issue is well-documented, with recent studies highlighting how unequal access to digital applications can exacerbate existing educational inequalities [ 57 ].
Lastly, ethical concerns regarding biases in AI algorithms and the ethical use of AI in education were prominent. Participants worried about the fairness and equity of AI evaluations, consistent with findings from Bogina et al. [ 58 ], who discussed the potential for AI to perpetuate existing biases and inequalities in educational settings.
Despite these challenges, the integration of AI in educational applications also presents numerous merits that positively impact students’ creativity and academic emotions. One significant benefit is the stimulation of creativity. AI applications can introduce new ideas and enhance problem-solving skills, fostering innovative approaches to learning. This finding is supported by studies showing that AI can provide diverse perspectives and problem-solving techniques that stimulate creative thinking [ 59 , 60 ]. Additionally, increased engagement is another notable merit, with AI’s interactive nature making learning more enjoyable and motivating for students. This enhanced engagement is consistent with research indicating that interactive AI applications can significantly boost student motivation and participation [ 61 ]. Moreover, personalized feedback provided by AI applications offers tailored guidance and immediate responses, helping students improve their work and boosting their confidence. This personalized approach is crucial for supporting students’ creative and emotional development, as noted by Chang et al. [ 62 ], who found that personalized AI feedback enhances learning outcomes and student confidence.
Furthermore, emotional support is another significant benefit, with AI applications reducing anxiety through their constant availability and increasing motivation with gamified elements and positive reinforcement. Studies have shown that such support mechanisms are effective in maintaining a positive emotional state conducive to learning [ 63 ]. In addition, collaborative creativity facilitated by AI applications supports group projects and peer interactions, fostering a sense of community and collective problem-solving. This collaborative environment aligns with findings from Graesser et al. [ 64 ], who emphasized the role of technology in enhancing collaborative learning and creativity.
The provision of accessible learning resources by AI applications supports continuous learning and inspires creativity by allowing students to explore diverse materials anytime. This accessibility is crucial for fostering an inclusive learning environment, as highlighted by Yenduri et al. [ 65 ], who noted that diverse and readily available resources enhance educational equity and creativity. Finally, enhanced academic emotions resulting from AI integration create positive learning experiences and build emotional resilience. Adaptive learning paths and supportive environments provided by AI applications contribute to improved emotional well-being and academic performance. This is supported by research indicating that adaptive learning technologies positively impact student emotions and resilience [ 5 , 6 , 7 , 8 , 9 ].
The integration of AI in education has elicited varied responses from both teachers and students, reflecting a complex interplay of benefits and challenges. One prominent concern is the potential for AI applications to impose rigid frameworks that may stifle creativity. This apprehension aligns with the notion that while AI can provide structured guidance, it may also limit the spontaneous and divergent thinking essential for creative processes. This balance between structure and freedom is critical, as noted in the literature on educational methodologies and creativity development [ 1 , 2 , 3 ].
Emotional disengagement emerges as another significant issue, with both groups expressing that AI interactions often lack the personal touch necessary for effective learning experiences. The importance of human elements in education is well-documented, with studies emphasizing the role of personal connection in fostering student engagement and motivation [ 4 , 5 ]. This emotional component is vital, as AI systems, despite their capabilities, may only partially replicate the nuanced and empathetic interactions provided by human educators [ 6 , 7 ].
Performance anxiety due to frequent AI assessments is another shared concern. AI’s ability to provide continuous and immediate feedback can be a double-edged sword, potentially leading to increased stress and anxiety among students. This is consistent with findings that highlight the psychological impact of constant monitoring and assessment, which can detract from the learning experience and affect student well-being [ 8 , 9 ].
Technical issues associated with AI applications also pose significant challenges. Both teachers and students have reported frustrations with technical glitches disrupting the learning process. These disruptions can hinder the seamless integration of AI into educational environments, underscoring the need for robust and reliable technology infrastructure [ 10 , 11 ].
Despite these concerns, both groups recognize the benefits of AI, particularly in enhancing creativity and engagement. AI’s ability to stimulate problem-solving skills and foster creativity is acknowledged as a significant advantage. This aligns with research suggesting that AI can catalyze creative thinking by providing novel applications and approaches to problem-solving [ 12 , 13 , 14 ]. Additionally, the literature supports AI’s potential to increase student engagement through interactive and personalized learning experiences [ 15 , 16 ].
The role of AI in providing personalized feedback is highly valued, with both teachers and students appreciating its capacity to tailor educational experiences to individual needs. Customised learning, facilitated by AI, can address diverse learning styles and paces, thereby enhancing educational outcomes [ 17 , 18 ]. This personalization is crucial in meeting the unique needs of each student, fostering a more inclusive and effective learning environment [ 19 , 20 ].
AI’s contribution to collaborative creativity and accessible learning resources is also positively viewed. AI’s ability to facilitate group projects and provide a wide range of learning materials supports collaborative learning and resource accessibility, which are essential components of a modern educational framework [ 21 , 22 , 23 ]. Moreover, the enhancement of academic emotions through AI-driven learning experiences highlights AI’s potential to create positive and engaging educational environments [ 24 , 25 ].
In conclusion, the attitudes of teachers and students towards AI in education reflect a balanced perspective that acknowledges both its limitations and advantages. While there are valid concerns about emotional disengagement, ethical issues, and performance anxiety, the benefits of enhanced creativity, engagement, and personalized feedback cannot be overlooked. This underscores the need for thoughtful and strategic integration of AI in educational settings, ensuring that its deployment maximizes benefits while mitigating potential drawbacks. As AI continues to evolve, ongoing research and dialogue will be essential in navigating its role in education and optimizing its impact on teaching and learning [ 26 , 27 , 28 ].
Conclusions and implications
The integration of AI in educational applications presents a complex landscape characterized by significant challenges and notable benefits impacting students’ creativity and academic emotions. On the downside, AI applications often impose rigid frameworks that constrain creative thinking and innovation, echoing previous research on the stifling effects of structured learning paths. Emotional disengagement is another critical issue, as the repetitive and impersonal nature of AI interactions can diminish student motivation and engagement. This phenomenon underscores the importance of human interaction for maintaining emotional connections in learning. Additionally, the constant monitoring and assessments by AI applications heighten performance anxiety, negatively affecting student well-being. Technical frustrations due to frequent glitches and complex interfaces further disrupt the learning process. At the same time, an over-reliance on AI can reduce critical thinking and self-initiative, hindering essential problem-solving skills. The digital divide exacerbates educational disparities, highlighting the need for equitable access to technology. Ethical concerns about biases in AI algorithms also raise questions about fairness and equity in educational evaluations.
Conversely, AI integration offers substantial benefits, including the stimulation of creativity and enhanced engagement. AI applications can introduce new ideas and improve problem-solving skills, fostering innovative learning approaches. Their interactive nature makes learning more enjoyable and motivating, significantly boosting student participation. Personalized feedback from AI applications offers tailored guidance and immediate responses, helping students improve their work and build confidence. AI applications also provide emotional support, reducing anxiety through constant availability and enhancing motivation with gamified elements and positive reinforcement. They facilitate collaborative creativity, fostering a sense of community and collective problem-solving. Additionally, AI applications offer accessible learning resources, supporting continuous learning and inspiring creativity, which is crucial for educational equity. Adaptive learning paths and supportive environments provided by AI applications improve emotional well-being and academic performance, fostering positive learning experiences and building emotional resilience. Balancing these benefits with the challenges requires thoughtful implementation and continuous evaluation to optimize AI’s role in education.
Limitations and suggestions for further studies
Despite the merits and rich data, this study has some limitations which need to be mentioned. Firstly, the exclusive use of interviews for data collection limits the breadth of perspectives gathered. Interviews may reflect individual viewpoints rather than broader trends or consensus among participants. Additionally, the absence of focus groups in data collection further restricts the depth of insights obtained, as group dynamics and interactions that could reveal shared experiences or divergent opinions are not explored. Moreover, the study lacks detailed demographic information about participants, such as their majors, teaching experience (for teachers), or other relevant characteristics. This omission must include a nuanced understanding of how these factors might influence perceptions of AI-integrated educational applications.
Furthermore, the study’s small sample size raises concerns about the generalizability of findings. With a limited number of participants, the variability in perceptions and attitudes towards AI in education may need to be adequately captured. Additionally, a comparative analysis between teachers’ and students’ perceptions and attitudes needs to be conducted to uncover potential differences or similarities that could provide richer insights into the impact of AI on educational experiences from both perspectives.
Suggestions for future research include employing mixed-methods approaches that combine interviews with other qualitative methods, such as focus groups. This would allow for a more comprehensive exploration of diverse perspectives and enable researchers to triangulate findings for greater validity. Moreover, expanding the sample size and ensuring diversity among participants in terms of academic disciplines, teaching experience, and student backgrounds could provide a more robust basis for generalizing findings. Additionally, conducting comparative analyses between different stakeholder groups (e.g., teachers vs. students) would deepen understanding of how AI-integrated educational applications affect various participants differently. Finally, longitudinal studies could track changes in perceptions and attitudes over time as AI technologies in education continue to evolve, offering insights into the long-term impacts and adaptations within educational settings. These methodological enhancements and research directions would contribute to a more comprehensive understanding of the complex interactions between AI technology and educational practices.
Data availability
Data is provided within the manuscript or supplementary information files.
Helm JM, Swiergosz AM, Haeberle HS, Karnuta JM, Schaffer JL, Krebs VE, Spitzer AI, Ramkumar PN. Machine learning and artificial intelligence: definitions, applications, and future directions. Curr Rev Musculoskelet Med. 2020;13:69–76. https://doi.org/10.1007/s12178-020-09620-7 .
Article PubMed PubMed Central Google Scholar
Hassani H, Silva ES, Unger S, TajMazinani M, Mac Feely S. Artificial Intelligence (AI) or Intelligence Augmentation (IA): What Is the Future? AI. 2020;1:143–155. https://doi.org/10.3390/ai1020011
Yufeia L, Salehb S, Jiahuic H, Syed Mohamad Syed S. Review of the application of Artificial Intelligence in Education. Int J Innov Create Change. 2020;12:548–62.
Google Scholar
VanLehn K. The relative effectiveness of human tutoring, intelligent tutoring systems, and other tutoring systems. Educ Psychol. 2011;46:197–221.
Article Google Scholar
Trilling B, Fadel C. 21st Century skills: learning for life in our Times. San Francisco: Wiley; 2009.
Torrance EP. A longitudinal examination of the fourth-grade slump in creativity. Gifted Child Q. 1968;12:195–9.
Tubb AL, Cropley DH, Marrone RL, Patston T, Kaufman JC. The development of mathematical creativity across high school: increasing, decreasing, or both? Think Skills Creativity. 2020;35:100634.
Alves-Oliveira P, Arriaga P, Paiva A, Hoffman G, Design. Yolo, a robot for creativity: A co-design study with children. In: Proceedings of the and Children; June 27–30, 2017; Stanford, CA, USA. ACM; 2017:423–429.
Gordon G, Breazeal C, Engel S. Can children catch curiosity from a social robot? In: Proceedings of the Tenth Annual ACM/IEEE International Conference on Human-Robot Interaction; March 2–5, 2015; Portland, OR, USA. ACM; 2015:91–98.
Belpaeme T, Kennedy J, Ramachandran A, Scassellati B, Tanaka F. Social robots for education: a review. Sci Robot. 2018;3.
Kafai YB, Burke Q. Connected code: why children need to learn programming. Cambridge: MIT Press; 2014.
Book Google Scholar
Ryu M, Han S. The educational perception on artificial intelligence by elementary school teachers. J Korean Assoc Inf Educ. 2018;22:317–24.
Kaufman JC, Beghetto RA. Beyond big and little: the four C model of creativity. Rev Gen Psychol. 2009;13:1–12.
Beghetto RA, Kaufman JC, Hatcher R. Applying creativity research to cooking. J Creat Behav. 2016;50:171–7.
Marrone R, Taddeo V, Hill G. Creativity and Artificial Intelligence—A Student Perspective. J Intell. 2022;10(3):65. https://doi.org/10.3390/jintelligence10030065 .
Johnson L, Becker A, Estrada S, V., Freeman A. NMC Horizon Report: 2015 higher Education Edition. Austin, Texas: The New Media Consortium; 2015.
Allen IE, Seaman J. Digital Learning Compass: Distance Education Enrollment Report 2017. Babson Survey Research Group, e-Literate, and WCET; 2017.
Banks JA. Cultural Diversity and Education: foundations, curriculum, and teaching. Routledge; 2015.
Etzkowitz H, Leydesdorff L. The dynamics of innovation: from National systems and Mode 2 to a Triple Helix of university–industry–government relations. Res Policy. 2000;29(2):109–23.
Carnevale AP, Smith N, Strohl J. Recovery: Job Growth and Education requirements through 2020. Georgetown University Center on Education and the Workforce; 2013.
Altbach PG, Reisberg L, Rumbley LE. (2009). Trends in Global Higher Education: Tracking an Academic Revolution . A report prepared for the UNESCO 2009 World Conference on Higher Education.
Luckin R, Holmes W, Griffiths M, Forcier LB. Intelligence unleashed: an argument for AI in Education. Pearson; 2016.
Cope B, Kalantzis M. Interpreting evidence-of-learning: Educational research in the era of big data. Open Rev Educational Res. 2015;2(1):218–39.
Roll I, Wylie R. Evolution and revolution in artificial intelligence in education. Int J Artif Intell Educ. 2016;26(2):582–99.
Siemens G, Long P. Penetrating the fog: Analytics in learning and education. EDUCAUSE Rev. 2011;46(5):30–2.
Warschauer M. Technology and social inclusion: rethinking the digital divide. MIT Press; 2004.
Barocas S, Hardt M. Fairness and Abstraction in Sociotechnical Systems. IEEE Data Eng Bull. 2019;42(3):56–68.
Amornkitpinyo T, Yoosomboon S, Sopapradit S, Amornkitpinyo P. The structural equation model of actual use of Cloud Learning for Higher Education students in the 21st Century. J e-Learn Knowl Soc. 2021;17(1):72–80. https://doi.org/10.20368/1971-8829/1135300 .
Koçak O, Koçak ÖE, Younis MZ. The psychological consequences of COVID-19 fear and the moderator effects of individuals’ underlying illness and witnessing infected friends and family. Int J Environ Res Public Health. 2021;18(4):1836. https://doi.org/10.3390/ijerph18041836 .
Khan S, Zaman SI, Rais M. Measuring student satisfaction through overall quality at business schools: a structural equation modelling: student satisfaction and quality of education. South Asian J Soc Rev. 2022;1(2):34–55.
Chedrawi C, Howayeck P, Tarhini A. CSR and legitimacy in higher education accreditation programs, an isomorphic approach of Lebanese business schools. Qual Assur Educ. 2019;27(1):70–81. https://doi.org/10.1108/QAE-04-2018-0053 .
Hu J. The challenge of Traditional Teaching Approach: a study on the path to Improve Classroom Teaching Effectiveness based on secondary School Students’ psychology. Lecture Notes Educ Psychol Public Media. 2024;50(1):213–9. https://doi.org/10.54254/2753-7048/50/20240945 .
Ali SS, Choi BJ. State-of-the-art artificial intelligence techniques for distributed smart grids: a review. Electronics. 2020;9(6):1030. https://doi.org/10.3390/electronics9061030 .
Derry SJ. Learning strategies for acquiring useful knowledge. Dimensions of thinking and cognitive instruction. Routledge; 2013. pp. 347–79.
Pekrun R, Goetz T, Titz W, Perry RP. Academic emotions in students’ self-regulated learning and achievement: a program of qualitative and quantitative research. Educ Psychol. 2002;37(2):91–105.
Lei H, Cui Y. Effects of academic emotions on achievement among mainland Chinese students: a meta-analysis. Soc Behav Pers. 2016;44(9):1541–54. https://doi.org/10.2224/sbp.2016.44.9.1541 .
Artino AR, Jones KD. Exploring the complex relations between achievement emotions and self-regulated learning behaviours in online learning. Internet High Educ. 2012;15(3):170–5. https://doi.org/10.1016/j.iheduc.2012.01.006 .
Cocoradă E. Achievement emotions and performance among university students. Bull Transilv Univ Braşov. 2016;9(2–Suppl):119–28.
Lam UF, Chen WW, Zhang J, Liang T. It feels good to learn where I belong: School belonging, academic emotions, and academic achievement in adolescents. School Psychol Int. 2015;36:393–409. https://doi.org/10.1177/0143034316680410 .
Villavicencio FT. Critical thinking, negative academic emotions, and achievement: a meditational analysis. Asia-Pac Educ Res. 2011;20:118–26.
Shen B, Wang Y, Yang Y, Yu X. Relationships between Chinese university EFL learners’ academic emotions and self-regulated learning strategies: a structural equation modelling analysis. Front Psychol. 2021;12:629892. https://doi.org/10.3389/fpsyg.2021.629892 .
Alam MM, Rayhan MI, Rahman MA, Imran S. The impact of teachers’ emotion regulation, students’ learning strategies, and academic emotions on students’ learning outcomes in Bangladesh. Int J Educ Pract. 2021;9(2):306–21.
Kahu ER, Stephens C, Zepke N, Leach L. Space and time to engage: mature-aged distance students’ learning experiences. High Educ Res Dev. 2013;32(5):791–804.
Thelwall M, Buckley K, Paltoglou G. Sentiment in Twitter events. J Am Soc Inf Sci Technol. 2011;62(2):406–18.
Graziano PA, Reavis RD, Keane SP, Calkins SD. The role of emotion regulation and children’s early academic success. J Sch Psychol. 2007;45(1):3–19. https://doi.org/10.1016/j.jsp.2006.09.002 . PMID: 21179384; PMCID: PMC3004175.
Sakulwichitsintu S. Mobile technology–An innovative instructional design model in distance education. Int J Interact Mob Technol. 2023;17(7).
Fang B, Li X, Han G, He J. Facial expression recognition in educational research from the perspective of machine learning: a systematic review. IEEE Access. 2023.
Méndez López MG. Emotion and language learning: An exploration of experience and motivation in a Mexican university context [doctoral dissertation]. University of Nottingham; 2011.
Huang Y, Bo D. Emotion classification and achievement of students in distance learning based on the knowledge state model. Sustainability. 2023;15(3):2367.
Zembylas M. Adult learners’ emotions in online learning. Distance Educ. 2008;29(1):71–87.
Saputra I, Astuti M, Sayuti M, Kusumastuti D. Integration of artificial intelligence in education: opportunities, challenges, threats and obstacles. A literature review. Indones J Comput Sci. 2023;12(4).
Marrone R, Taddeo V, Hill G. Creativity and artificial intelligence—A student perspective. J Intell. 2022;10(3):65.
Lavinder KW. Staff experiences with the organizational departure of chief student affairs officers: An interpretative phenomenological analysis [doctoral dissertation]. Northeastern University; 2021.
Pekrun R, Linnenbrink-Garcia L. Academic emotions and student engagement. Handbook of Research on Student Engagement. Boston, MA: Springer US; 2012. pp. 259–82.
Chapter Google Scholar
Welter VDE, Becker LB, Großschedl J. Helping learners become their own teachers: the beneficial impact of trained concept-mapping-strategy use on metacognitive regulation in learning. Educ Sci. 2022;12(5):325.
Zhai C, Wibowo S, Li LD. The effects of over-reliance on AI dialogue systems on students’ cognitive abilities: a systematic review. Smart Learn Environ. 2024;11(1):28.
Kelly MA. Bridging digital and cultural divides: TPCK for equity of access to technology. Handbook of Technological Pedagogical Content Knowledge (TPCK) for educators. Routledge; 2014. pp. 41–68.
Bogina V, Hartman A, Kuflik T, Shulner-Tal A. Educating software and AI stakeholders about algorithmic fairness, accountability, transparency and ethics. Int J Artif Intell Educ. 2022;1–26.
Urmeneta A, Romero M. Creative applications of artificial intelligence in education. Springer Nature; 2024.
Li G, Zarei MA, Alibakhshi G, Labbafi A. Teachers and educators’ experiences and perceptions of artificial-powered interventions for autism groups. BMC Psychol. 2024;12(1):199.
Huang AY, Lu OH, Yang SJ. Effects of artificial intelligence–enabled personalized recommendations on learners’ learning engagement, motivation, and outcomes in a flipped classroom. Comput Educ. 2023;194:104684.
Chang DH, Lin MPC, Hajian S, Wang QQ. Educational design principles of using AI chatbot that supports self-regulated learning in education: goal setting, feedback, and personalization. Sustainability. 2023;15(17):12921.
Li L, Gow ADI, Zhou J. The role of positive emotions in education: a neuroscience perspective. Mind Brain Educ. 2020;14(3):220–34.
Graesser AC, Fiore SM, Greiff S, Andrews-Todd J, Foltz PW, Hesse FW. Advancing the science of collaborative problem-solving. Psychol Sci Public Interest. 2018;19(2):59–92.
Article PubMed Google Scholar
Yenduri G, Kaluri R, Rajput DS, Lakshmanna K, Gadekallu TR, Mahmud M, Brown DJ. From assistive technologies to metaverse—technologies in inclusive higher education for students with specific learning difficulties: a review. IEEE Access. 2023;11:64907–27.
Download references
Acknowledgements
The author would like to thank all the participants.
2024 Higher Education Research Project (KT2024176), ZAHE, the Zhejiang Provincial Association of Higher Education.
Author information
Authors and affiliations.
School of Marxism, Wenzhou University of Technology, Wenzhou, Zhejiang, 32500, China
Haozhuo Lin
School of Humanities, Wenzhou University, Wenzhou, Zhejiang, 32500, China
You can also search for this author in PubMed Google Scholar
Contributions
HL and QC designed the study. HL and QC collected the data. HL and QC analyzed and interpreted the data. HL and QC drafted the manuscript. HL and QC proofread the paper. HL and QC agreed to be accountable and verified the submitted version.
Corresponding author
Correspondence to Qiu Chen .
Ethics declarations
Ethics approval and consent to participate.
The Institutional Review Board of Wenzhou approved this study and issued a letter indicating that it had no side effects on the participants. All experiments and methods were carried out in accordance with relevant guidelines and regulations. Informed consent was obtained from all subjects.
Consent for publication
Not applicable .
Competing interests
The authors declare no competing interests.
Additional information
Publisher’s note.
Springer Nature remains neutral with regard to jurisdictional claims in published maps and institutional affiliations.
Electronic supplementary material
Below is the link to the electronic supplementary material.
Supplementary Material 1
Rights and permissions.
Open Access This article is licensed under a Creative Commons Attribution 4.0 International License, which permits use, sharing, adaptation, distribution and reproduction in any medium or format, as long as you give appropriate credit to the original author(s) and the source, provide a link to the Creative Commons licence, and indicate if changes were made. The images or other third party material in this article are included in the article’s Creative Commons licence, unless indicated otherwise in a credit line to the material. If material is not included in the article’s Creative Commons licence and your intended use is not permitted by statutory regulation or exceeds the permitted use, you will need to obtain permission directly from the copyright holder. To view a copy of this licence, visit http://creativecommons.org/licenses/by/4.0/ .
Reprints and permissions
About this article
Cite this article.
Lin, H., Chen, Q. Artificial intelligence (AI) -integrated educational applications and college students’ creativity and academic emotions: students and teachers’ perceptions and attitudes. BMC Psychol 12 , 487 (2024). https://doi.org/10.1186/s40359-024-01979-0
Download citation
Received : 20 May 2024
Accepted : 03 September 2024
Published : 16 September 2024
DOI : https://doi.org/10.1186/s40359-024-01979-0
Share this article
Anyone you share the following link with will be able to read this content:
Sorry, a shareable link is not currently available for this article.
Provided by the Springer Nature SharedIt content-sharing initiative
- Artificial intelligence
- Academic emotions
- AI-empowered applications
- Chinese students
BMC Psychology
ISSN: 2050-7283
- General enquiries: [email protected]

IMAGES
VIDEO
COMMENTS
A quantitative approach to research is probably the most familiar approach for the typical research student studying at the introductory level. Arising from the natural sciences, e.g., chemistry and biology), the quantitative approach is framed by the belief that there is one reality or truth that simply requires discovering, known as realism.
The weighting, or priority, of the qualitative and quantitative data in a mixed methods study is dependent upon various factors including; the aims of the study and whether the purpose is, for example, to contextualise quantitative data using qualitative data or to use qualitative data to inform a larger quantitative approach such as a survey.
Mixed methods research combines elements of quantitative research and qualitative research in order to answer your research question. Mixed methods can help you gain a more complete picture than a standalone quantitative or qualitative study, as it integrates benefits of both methods. Mixed methods research is often used in the behavioral ...
Design/methodology/approach: A mixed-methods approach was used, which involves collecting and analyzing quantitative and qualitative data to understand the research problem comprehensively.
1. Understanding the options. Before we jump into the question of how to choose a research methodology, it's useful to take a step back to understand the three overarching types of research - qualitative, quantitative and mixed methods -based research. Each of these options takes a different methodological approach.
Mixed methods approaches allows researchers to use a diversity of methods, combining inductive and deductive thinking, and offsetting limitations of exclusively quantitative and qualitative research through a complementary approach that maximizes strengths of each data type and facilitates a more comprehensive understanding of health issues and ...
Mixed methods research (MMR) has become increasingly popular over the last 25 years (Creswell, 2015).However, collecting qualitative and quantitative data was commonplace in many social sciences throughout the first 60 years of the 20th Century. During the 1980's, MMR re-emerged as a distinct approach, inducing a second wave of popularity (Creswell, 2015; Guest, 2013; Johnson, Onwuegbuzie ...
The use of mixed scientific methodology is widely used in the field of health outcomes and should not be limited to a closed list of possible methodological options, but should be seen as a framework for a specific research issue to be addressed using quantitative and qualitative components (data and / or methods) , when quantitative and ...
Choosing between qualitative, quantitative, and mixed methods designs can be quite challenging. It's like picking the right tool for the job. Each approach has its own strengths and limitations. Qualitative research allows you to dig deep, while quantitative research lets you crunch numbers and make broader generalizations.
When collecting and analyzing data, quantitative research deals with numbers and statistics, while qualitative research deals with words and meanings. Both are important for gaining different kinds of knowledge. Quantitative research. Quantitative research is expressed in numbers and graphs. It is used to test or confirm theories and assumptions.
Historically, views on the appropriateness of quantitative and qualitative research methods have become polarised and captured by the notion of a 'paradigm war' (Ukpabi et al. 2014). In a mixed methods approach there is no inherent conflict, with quantitative and qualitative research methods able to make their own distinctive contribution.
This review examines John W. Creswell and David Creswell's sixth edition, which covers the most popular research methods, offering readers a comprehensive understanding and practical guidance in qualitative, quantitative, and mixed methods. The review includes observations on existing drawbacks, gaps, and ideas on potential areas for improvement in the book. The book is an excellent entry ...
The mixed methods research definition is a design that utilizes both quantitative (numeric) and qualitative (descriptive) research elements to gain a conclusion for a study. A mixed methods study ...
Techniques designed to combine the results of qualitative and quantitative studies can provide researchers with more knowledge than separate analysis Health researchers are increasingly using designs that combine qualitative and quantitative methods, and this is often called mixed methods research.1 Integration—the interaction or conversation between the qualitative and quantitative ...
Quantitative, Qualitative, and Mixed-Methods Research. Depending on the philosophy of the researcher, the nature of the data, and how it is collected, behavioral science can be classified into qualitative, quantitative, or mixed methods research. Below are descriptions of each method.
A mixed methods research design is an approach to collecting and analyzing both qualitative and quantitative data in a single study. Mixed methods designs allow for method flexibility and can provide differing and even conflicting results. Examples of mixed methods research designs include convergent parallel, explanatory sequential, and exploratory sequential.
The interaction of quantitative and qualitative methodology in the mixed methods research (MMR) is analysed in a context of distinctions of the meanings between the terms typically used for description, combination, and integration. The interaction in the phases that precede the results, and their interpretation is considered.
A mixed method combines quantitative and qualitative methods in the same study. in order to get a full understanding of the phenomenon under study. Thus a mixed. research method usually results in ...
The use of mixed methods to study complex social phenomenon goes back to the mid 19th century where most investigators started using both qualitative and quantitative approaches in single studies (Maxwell, 2016).For instance, in 1898, DuBois engaged in field work to obtain data while studying 8000 inhabitants of a slum in Philadelphia, using in-depth house-to-house interviews, a phenomenon ...
Mixed methods means that you collect both quantitative and qualitative data and analyse both together to answer your question. For instance, say you wanted to know whether parents' feelings about reading with their children affected children's reading scores. You could interview parents, sort them into groups (e.g. confident, mixed
Mixed Methods Research. According to the National Institutes of Health, mixed methods strategically integrates or combines rigorous quantitative and qualitative research methods to draw on the strengths of each.Mixed method approaches allow researchers to use a diversity of methods, combining inductive and deductive thinking, and offsetting limitations of exclusively quantitative and ...
Summary. Research Approaches: Health and social care professionals use qualitative, quantitative, and mixed methods research to gather insights, measure variables, and understand complex behaviours.Each approach serves a unique purpose based on the research question. Methodologies Explained: Various methodologies like descriptive, analytical, exploratory, empirical, and applied research help ...
These include quantitative, qualitative and mixed methods research. Some basic ideas are discussed in this chapter about designing the sections of a proposal and some writing tips are suggested that can be instrumental in writing a research proposal. Ethical issues in qualitative, quantitative and mixed methods research are explained in detail.
Mixed methods research methodology is a valuable approach that combines quantitative and qualitative methods to gain a deep understanding of complex research problems (Creswell & Plano-Clark, 2007). In this study, we focused on the cultural values that contribute to individuals' mental health during challenging times.
Wyman 350 s part of URSCA's Undergraduate Research Week (October 21-25), this panel discussion will explore how undergraduates can get involved in social science research in all its many forms. Panelists include current Woodrow Wilson Fellows and other URSCA-funded undergraduate researchers, as well as URSCA staff, who will offer guidance on getting started and on...
Qualitative research is a type of research that aims to gather and analyse non-numerical (descriptive) data in order to gain an understanding of individuals' social reality, including understanding their attitudes, beliefs, and motivation.This type of research typically involves in-depth interviews, focus groups, or field observations in order to collect data that is rich in detail and context.
The mixed methods framework allows us to advance in the ways of integration between qualitative and quantitative elements, taking advantage of the proven possibilities of systematic observation, which we can consider mixed method in itself, and which provides rigor and flexibility in the study of the communicative flow in sport.
A mixed-method research design was used. In the first phase, a qualitative research approach was employed, utilizing theoretical sampling to select informants. Data were collected through in-depth interviews with undergraduate students and university lecturers to gain comprehensive insights into their experiences and perceptions.
Since 2015, he has been an adjunct professor of family medicine at the University of Michigan and co-director of the Michigan Mixed Methods Research and Scholarship Program. He was formerly a professor of educational psychology at the University of Nebraska-Lincoln, where he held the Clifton Endowed Professor Chair.He was a founding co-editor of the Journal of Mixed Methods Research, and was ...