- USC Libraries
- Research Guides

Organizing Your Social Sciences Research Paper
- Quantitative Methods
- Purpose of Guide
- Design Flaws to Avoid
- Independent and Dependent Variables
- Glossary of Research Terms
- Reading Research Effectively
- Narrowing a Topic Idea
- Broadening a Topic Idea
- Extending the Timeliness of a Topic Idea
- Academic Writing Style
- Applying Critical Thinking
- Choosing a Title
- Making an Outline
- Paragraph Development
- Research Process Video Series
- Executive Summary
- The C.A.R.S. Model
- Background Information
- The Research Problem/Question
- Theoretical Framework
- Citation Tracking
- Content Alert Services
- Evaluating Sources
- Primary Sources
- Secondary Sources
- Tiertiary Sources
- Scholarly vs. Popular Publications
- Qualitative Methods
- Insiderness
- Using Non-Textual Elements
- Limitations of the Study
- Common Grammar Mistakes
- Writing Concisely
- Avoiding Plagiarism
- Footnotes or Endnotes?
- Further Readings
- Generative AI and Writing
- USC Libraries Tutorials and Other Guides
- Bibliography
Quantitative methods emphasize objective measurements and the statistical, mathematical, or numerical analysis of data collected through polls, questionnaires, and surveys, or by manipulating pre-existing statistical data using computational techniques . Quantitative research focuses on gathering numerical data and generalizing it across groups of people or to explain a particular phenomenon.
Babbie, Earl R. The Practice of Social Research . 12th ed. Belmont, CA: Wadsworth Cengage, 2010; Muijs, Daniel. Doing Quantitative Research in Education with SPSS . 2nd edition. London: SAGE Publications, 2010.
Need Help Locating Statistics?
Resources for locating data and statistics can be found here:
Statistics & Data Research Guide
Characteristics of Quantitative Research
Your goal in conducting quantitative research study is to determine the relationship between one thing [an independent variable] and another [a dependent or outcome variable] within a population. Quantitative research designs are either descriptive [subjects usually measured once] or experimental [subjects measured before and after a treatment]. A descriptive study establishes only associations between variables; an experimental study establishes causality.
Quantitative research deals in numbers, logic, and an objective stance. Quantitative research focuses on numeric and unchanging data and detailed, convergent reasoning rather than divergent reasoning [i.e., the generation of a variety of ideas about a research problem in a spontaneous, free-flowing manner].
Its main characteristics are :
- The data is usually gathered using structured research instruments.
- The results are based on larger sample sizes that are representative of the population.
- The research study can usually be replicated or repeated, given its high reliability.
- Researcher has a clearly defined research question to which objective answers are sought.
- All aspects of the study are carefully designed before data is collected.
- Data are in the form of numbers and statistics, often arranged in tables, charts, figures, or other non-textual forms.
- Project can be used to generalize concepts more widely, predict future results, or investigate causal relationships.
- Researcher uses tools, such as questionnaires or computer software, to collect numerical data.
The overarching aim of a quantitative research study is to classify features, count them, and construct statistical models in an attempt to explain what is observed.
Things to keep in mind when reporting the results of a study using quantitative methods :
- Explain the data collected and their statistical treatment as well as all relevant results in relation to the research problem you are investigating. Interpretation of results is not appropriate in this section.
- Report unanticipated events that occurred during your data collection. Explain how the actual analysis differs from the planned analysis. Explain your handling of missing data and why any missing data does not undermine the validity of your analysis.
- Explain the techniques you used to "clean" your data set.
- Choose a minimally sufficient statistical procedure ; provide a rationale for its use and a reference for it. Specify any computer programs used.
- Describe the assumptions for each procedure and the steps you took to ensure that they were not violated.
- When using inferential statistics , provide the descriptive statistics, confidence intervals, and sample sizes for each variable as well as the value of the test statistic, its direction, the degrees of freedom, and the significance level [report the actual p value].
- Avoid inferring causality , particularly in nonrandomized designs or without further experimentation.
- Use tables to provide exact values ; use figures to convey global effects. Keep figures small in size; include graphic representations of confidence intervals whenever possible.
- Always tell the reader what to look for in tables and figures .
NOTE: When using pre-existing statistical data gathered and made available by anyone other than yourself [e.g., government agency], you still must report on the methods that were used to gather the data and describe any missing data that exists and, if there is any, provide a clear explanation why the missing data does not undermine the validity of your final analysis.
Babbie, Earl R. The Practice of Social Research . 12th ed. Belmont, CA: Wadsworth Cengage, 2010; Brians, Craig Leonard et al. Empirical Political Analysis: Quantitative and Qualitative Research Methods . 8th ed. Boston, MA: Longman, 2011; McNabb, David E. Research Methods in Public Administration and Nonprofit Management: Quantitative and Qualitative Approaches . 2nd ed. Armonk, NY: M.E. Sharpe, 2008; Quantitative Research Methods. Writing@CSU. Colorado State University; Singh, Kultar. Quantitative Social Research Methods . Los Angeles, CA: Sage, 2007.
Basic Research Design for Quantitative Studies
Before designing a quantitative research study, you must decide whether it will be descriptive or experimental because this will dictate how you gather, analyze, and interpret the results. A descriptive study is governed by the following rules: subjects are generally measured once; the intention is to only establish associations between variables; and, the study may include a sample population of hundreds or thousands of subjects to ensure that a valid estimate of a generalized relationship between variables has been obtained. An experimental design includes subjects measured before and after a particular treatment, the sample population may be very small and purposefully chosen, and it is intended to establish causality between variables. Introduction The introduction to a quantitative study is usually written in the present tense and from the third person point of view. It covers the following information:
- Identifies the research problem -- as with any academic study, you must state clearly and concisely the research problem being investigated.
- Reviews the literature -- review scholarship on the topic, synthesizing key themes and, if necessary, noting studies that have used similar methods of inquiry and analysis. Note where key gaps exist and how your study helps to fill these gaps or clarifies existing knowledge.
- Describes the theoretical framework -- provide an outline of the theory or hypothesis underpinning your study. If necessary, define unfamiliar or complex terms, concepts, or ideas and provide the appropriate background information to place the research problem in proper context [e.g., historical, cultural, economic, etc.].
Methodology The methods section of a quantitative study should describe how each objective of your study will be achieved. Be sure to provide enough detail to enable the reader can make an informed assessment of the methods being used to obtain results associated with the research problem. The methods section should be presented in the past tense.
- Study population and sampling -- where did the data come from; how robust is it; note where gaps exist or what was excluded. Note the procedures used for their selection;
- Data collection – describe the tools and methods used to collect information and identify the variables being measured; describe the methods used to obtain the data; and, note if the data was pre-existing [i.e., government data] or you gathered it yourself. If you gathered it yourself, describe what type of instrument you used and why. Note that no data set is perfect--describe any limitations in methods of gathering data.
- Data analysis -- describe the procedures for processing and analyzing the data. If appropriate, describe the specific instruments of analysis used to study each research objective, including mathematical techniques and the type of computer software used to manipulate the data.
Results The finding of your study should be written objectively and in a succinct and precise format. In quantitative studies, it is common to use graphs, tables, charts, and other non-textual elements to help the reader understand the data. Make sure that non-textual elements do not stand in isolation from the text but are being used to supplement the overall description of the results and to help clarify key points being made. Further information about how to effectively present data using charts and graphs can be found here .
- Statistical analysis -- how did you analyze the data? What were the key findings from the data? The findings should be present in a logical, sequential order. Describe but do not interpret these trends or negative results; save that for the discussion section. The results should be presented in the past tense.
Discussion Discussions should be analytic, logical, and comprehensive. The discussion should meld together your findings in relation to those identified in the literature review, and placed within the context of the theoretical framework underpinning the study. The discussion should be presented in the present tense.
- Interpretation of results -- reiterate the research problem being investigated and compare and contrast the findings with the research questions underlying the study. Did they affirm predicted outcomes or did the data refute it?
- Description of trends, comparison of groups, or relationships among variables -- describe any trends that emerged from your analysis and explain all unanticipated and statistical insignificant findings.
- Discussion of implications – what is the meaning of your results? Highlight key findings based on the overall results and note findings that you believe are important. How have the results helped fill gaps in understanding the research problem?
- Limitations -- describe any limitations or unavoidable bias in your study and, if necessary, note why these limitations did not inhibit effective interpretation of the results.
Conclusion End your study by to summarizing the topic and provide a final comment and assessment of the study.
- Summary of findings – synthesize the answers to your research questions. Do not report any statistical data here; just provide a narrative summary of the key findings and describe what was learned that you did not know before conducting the study.
- Recommendations – if appropriate to the aim of the assignment, tie key findings with policy recommendations or actions to be taken in practice.
- Future research – note the need for future research linked to your study’s limitations or to any remaining gaps in the literature that were not addressed in your study.
Black, Thomas R. Doing Quantitative Research in the Social Sciences: An Integrated Approach to Research Design, Measurement and Statistics . London: Sage, 1999; Gay,L. R. and Peter Airasain. Educational Research: Competencies for Analysis and Applications . 7th edition. Upper Saddle River, NJ: Merril Prentice Hall, 2003; Hector, Anestine. An Overview of Quantitative Research in Composition and TESOL . Department of English, Indiana University of Pennsylvania; Hopkins, Will G. “Quantitative Research Design.” Sportscience 4, 1 (2000); "A Strategy for Writing Up Research Results. The Structure, Format, Content, and Style of a Journal-Style Scientific Paper." Department of Biology. Bates College; Nenty, H. Johnson. "Writing a Quantitative Research Thesis." International Journal of Educational Science 1 (2009): 19-32; Ouyang, Ronghua (John). Basic Inquiry of Quantitative Research . Kennesaw State University.
Strengths of Using Quantitative Methods
Quantitative researchers try to recognize and isolate specific variables contained within the study framework, seek correlation, relationships and causality, and attempt to control the environment in which the data is collected to avoid the risk of variables, other than the one being studied, accounting for the relationships identified.
Among the specific strengths of using quantitative methods to study social science research problems:
- Allows for a broader study, involving a greater number of subjects, and enhancing the generalization of the results;
- Allows for greater objectivity and accuracy of results. Generally, quantitative methods are designed to provide summaries of data that support generalizations about the phenomenon under study. In order to accomplish this, quantitative research usually involves few variables and many cases, and employs prescribed procedures to ensure validity and reliability;
- Applying well established standards means that the research can be replicated, and then analyzed and compared with similar studies;
- You can summarize vast sources of information and make comparisons across categories and over time; and,
- Personal bias can be avoided by keeping a 'distance' from participating subjects and using accepted computational techniques .
Babbie, Earl R. The Practice of Social Research . 12th ed. Belmont, CA: Wadsworth Cengage, 2010; Brians, Craig Leonard et al. Empirical Political Analysis: Quantitative and Qualitative Research Methods . 8th ed. Boston, MA: Longman, 2011; McNabb, David E. Research Methods in Public Administration and Nonprofit Management: Quantitative and Qualitative Approaches . 2nd ed. Armonk, NY: M.E. Sharpe, 2008; Singh, Kultar. Quantitative Social Research Methods . Los Angeles, CA: Sage, 2007.
Limitations of Using Quantitative Methods
Quantitative methods presume to have an objective approach to studying research problems, where data is controlled and measured, to address the accumulation of facts, and to determine the causes of behavior. As a consequence, the results of quantitative research may be statistically significant but are often humanly insignificant.
Some specific limitations associated with using quantitative methods to study research problems in the social sciences include:
- Quantitative data is more efficient and able to test hypotheses, but may miss contextual detail;
- Uses a static and rigid approach and so employs an inflexible process of discovery;
- The development of standard questions by researchers can lead to "structural bias" and false representation, where the data actually reflects the view of the researcher instead of the participating subject;
- Results provide less detail on behavior, attitudes, and motivation;
- Researcher may collect a much narrower and sometimes superficial dataset;
- Results are limited as they provide numerical descriptions rather than detailed narrative and generally provide less elaborate accounts of human perception;
- The research is often carried out in an unnatural, artificial environment so that a level of control can be applied to the exercise. This level of control might not normally be in place in the real world thus yielding "laboratory results" as opposed to "real world results"; and,
- Preset answers will not necessarily reflect how people really feel about a subject and, in some cases, might just be the closest match to the preconceived hypothesis.
Research Tip
Finding Examples of How to Apply Different Types of Research Methods
SAGE publications is a major publisher of studies about how to design and conduct research in the social and behavioral sciences. Their SAGE Research Methods Online and Cases database includes contents from books, articles, encyclopedias, handbooks, and videos covering social science research design and methods including the complete Little Green Book Series of Quantitative Applications in the Social Sciences and the Little Blue Book Series of Qualitative Research techniques. The database also includes case studies outlining the research methods used in real research projects. This is an excellent source for finding definitions of key terms and descriptions of research design and practice, techniques of data gathering, analysis, and reporting, and information about theories of research [e.g., grounded theory]. The database covers both qualitative and quantitative research methods as well as mixed methods approaches to conducting research.
SAGE Research Methods Online and Cases
- << Previous: Qualitative Methods
- Next: Insiderness >>
- Last Updated: Sep 4, 2024 9:40 AM
- URL: https://libguides.usc.edu/writingguide
General Problem Solving
Problem solving and estimating, learning outcomes.
- Identify and apply a solution pathway for multi-step problems
Problem solving is best approached by first starting at the end: identifying exactly what you are looking for. From there, you then work backwards, asking “what information and procedures will I need to find this?” Very few interesting questions can be answered in one mathematical step; often times you will need to chain together a solution pathway , a series of steps that will allow you to answer the question.
Problem Solving Process
- Identify the question you’re trying to answer.
- Work backwards, identifying the information you will need and the relationships you will use to answer that question.
- Continue working backwards, creating a solution pathway.
- If you are missing necessary information, look it up or estimate it. If you have unnecessary information, ignore it.
- Solve the problem, following your solution pathway.
In most problems we work, we will be approximating a solution, because we will not have perfect information. We will begin with a few examples where we will be able to approximate the solution using basic knowledge from our lives.
Recall: operations on Fractions
When simplifying algebraic expressions, we may sometimes need to add, subtract, simplify, multiply, or divide fractions. It is important to be able to do these operations on the fractions without converting them to decimals.
- [latex]\dfrac{a}{b}\cdot\dfrac{c}{d} = \dfrac {ac}{bd}[/latex]
- [latex]\dfrac{a}{b}\div\dfrac{c}{d}=\dfrac{a}{b}\cdot\dfrac{d}{c}=\dfrac{ad}{bc}[/latex]
- [latex]\dfrac{24}{32}=\dfrac{2\cdot2\cdot2\cdot3}{2\cdot2\cdot2\cdot2\cdot2}=\dfrac{3}{2\cdot2}=\dfrac{3}{4}[/latex]
- [latex]\dfrac{a}{b}\pm\dfrac{c}{d} = \dfrac{ad \pm bc}{bd}[/latex]
In the first example, we will need to think about time scales, we are asked to find how many times a heart beats in a year, but usually we measure heart rate in beats per minute.
How many times does your heart beat in a year?
This question is asking for the rate of heart beats per year. Since a year is a long time to measure heart beats for, if we knew the rate of heart beats per minute, we could scale that quantity up to a year. So the information we need to answer this question is heart beats per minute. This is something you can easily measure by counting your pulse while watching a clock for a minute.
Suppose you count 80 beats in a minute. To convert this to beats per year:
[latex]\displaystyle\frac{80\text{ beats}}{1\text{ minute}}\cdot\frac{60\text{ minutes}}{1\text{ hour}}\cdot\frac{24\text{ hours}}{1\text{ day}}\cdot\frac{365\text{ days}}{1\text{ year}}=42,048,000\text{ beats per year}[/latex]
The technique that helped us solve the last problem was to get the number of heartbeats in a minute translated into the number of heartbeats in a year. Converting units from one to another, like minutes to years is a common tool for solving problems.
In the next example, we show how to infer the thickness of something too small to measure with every-day tools. Before precision instruments were widely available, scientists and engineers had to get creative with ways to measure either very small or very large things. Imagine how early astronomers inferred the distance to stars, or the circumference of the earth.
How thick is a single sheet of paper? How much does it weigh?
While you might have a sheet of paper handy, trying to measure it would be tricky. Instead we might imagine a stack of paper, and then scale the thickness and weight to a single sheet. If you’ve ever bought paper for a printer or copier, you probably bought a ream, which contains 500 sheets. We could estimate that a ream of paper is about 2 inches thick and weighs about 5 pounds. Scaling these down,
[latex]\displaystyle\frac{2\text{ inches}}{\text{ream}}\cdot\frac{1\text{ ream}}{500\text{ pages}}=0.004\text{ inches per sheet}[/latex]
[latex]\displaystyle\frac{5\text{ pounds}}{\text{ream}}\cdot\frac{1\text{ ream}}{500\text{ pages}}=0.01\text{ pounds per sheet, or }=0.16\text{ ounces per sheet.}[/latex]
The first two example questions in this set are examined in more detail here.
We can infer a measurement by using scaling. If 500 sheets of paper is two inches thick, then we could use proportional reasoning to infer the thickness of one sheet of paper.
In the next example, we use proportional reasoning to determine how many calories are in a mini muffin when you are given the amount of calories for a regular sized muffin.
A recipe for zucchini muffins states that it yields 12 muffins, with 250 calories per muffin. You instead decide to make mini-muffins, and the recipe yields 20 muffins. If you eat 4, how many calories will you consume?
There are several possible solution pathways to answer this question. We will explore one.
To answer the question of how many calories 4 mini-muffins will contain, we would want to know the number of calories in each mini-muffin. To find the calories in each mini-muffin, we could first find the total calories for the entire recipe, then divide it by the number of mini-muffins produced. To find the total calories for the recipe, we could multiply the calories per standard muffin by the number per muffin. Notice that this produces a multi-step solution pathway. It is often easier to solve a problem in small steps, rather than trying to find a way to jump directly from the given information to the solution.
We can now execute our plan:
[latex]\displaystyle{12}\text{ muffins}\cdot\frac{250\text{ calories}}{\text{muffin}}=3000\text{ calories for the whole recipe}[/latex]
[latex]\displaystyle\frac{3000\text{ calories}}{20\text{ mini-muffins}}=\text{ gives }150\text{ calories per mini-muffin}[/latex]
[latex]\displaystyle4\text{ mini-muffins}\cdot\frac{150\text{ calories}}{\text{mini-muffin}}=\text{totals }600\text{ calories consumed.}[/latex]
View the following video for more about the zucchini muffin problem.
We have found that ratios are very helpful when we know some information but it is not in the right units, or parts to answer our question. Making comparisons mathematically often involves using ratios and proportions. In the next examples we will
You need to replace the boards on your deck. About how much will the materials cost?
There are two approaches we could take to this problem: 1) estimate the number of boards we will need and find the cost per board, or 2) estimate the area of the deck and find the approximate cost per square foot for deck boards. We will take the latter approach.
For this solution pathway, we will be able to answer the question if we know the cost per square foot for decking boards and the square footage of the deck. To find the cost per square foot for decking boards, we could compute the area of a single board, and divide it into the cost for that board. We can compute the square footage of the deck using geometric formulas. So first we need information: the dimensions of the deck, and the cost and dimensions of a single deck board.
Suppose that a rectangular deck measures 16 ft by 24 ft, for a total area of 384 ft 2 .
From a visit to the local home store, you find that an 8 foot by 4 inch cedar deck board costs about $7.50. The area of this board, doing the necessary conversion from inches to feet, is:
[latex]\displaystyle{8}\text{ feet}\cdot4\text{ inches}\cdot\frac{1\text{ foot}}{12\text{ inches}}=2.667\text{ft}^2{.}[/latex] The cost per square foot is then [latex]\displaystyle\frac{\$7.50}{2.667\text{ft}^2}=\$2.8125\text{ per ft}^2{.}[/latex]
This will allow us to estimate the material cost for the whole 384 ft 2 deck
[latex]\displaystyle\$384\text{ft}^2\cdot\frac{\$2.8125}{\text{ft}^2}=\$1080\text{ total cost.}[/latex]
Of course, this cost estimate assumes that there is no waste, which is rarely the case. It is common to add at least 10% to the cost estimate to account for waste.
This example is worked through in the following video.
Is it worth buying a Hyundai Sonata hybrid instead the regular Hyundai Sonata?
To make this decision, we must first decide what our basis for comparison will be. For the purposes of this example, we’ll focus on fuel and purchase costs, but environmental impacts and maintenance costs are other factors a buyer might consider.
It might be interesting to compare the cost of gas to run both cars for a year. To determine this, we will need to know the miles per gallon both cars get, as well as the number of miles we expect to drive in a year. From that information, we can find the number of gallons required from a year. Using the price of gas per gallon, we can find the running cost.
From Hyundai’s website, the 2013 Sonata will get 24 miles per gallon (mpg) in the city, and 35 mpg on the highway. The hybrid will get 35 mpg in the city, and 40 mpg on the highway.
An average driver drives about 12,000 miles a year. Suppose that you expect to drive about 75% of that in the city, so 9,000 city miles a year, and 3,000 highway miles a year.
We can then find the number of gallons each car would require for the year.
Sonata: [latex]\displaystyle{9000}\text{ city miles}\cdot\frac{1\text{ gallon}}{24\text{ city miles}}+3000\text{ highway miles}\cdot\frac{1\text{ gallon}}{35\text{ highway miles}}=460.7\text{ gallons}[/latex]
Hybrid: [latex]\displaystyle{9000}\text{ city miles}\cdot\frac{1\text{ gallon}}{35\text{ city miles}}+3000\text{ highway miles}\cdot\frac{1\text{ gallon}}{40\text{ highway miles}}=332.1\text{ gallons}[/latex]
If gas in your area averages about $3.50 per gallon, we can use that to find the running cost:
Sonata: [latex]\displaystyle{460.7}\text{ gallons}\cdot\frac{\$3.50}{\text{gallon}}=\$1612.45[/latex]
Hybrid: [latex]\displaystyle{332.1}\text{ gallons}\cdot\frac{\$3.50}{\text{gallon}}=\$1162.35[/latex]
The hybrid will save $450.10 a year. The gas costs for the hybrid are about [latex]\displaystyle\frac{\$450.10}{\$1612.45}[/latex] = 0.279 = 27.9% lower than the costs for the standard Sonata.
While both the absolute and relative comparisons are useful here, they still make it hard to answer the original question, since “is it worth it” implies there is some tradeoff for the gas savings. Indeed, the hybrid Sonata costs about $25,850, compared to the base model for the regular Sonata, at $20,895.
To better answer the “is it worth it” question, we might explore how long it will take the gas savings to make up for the additional initial cost. The hybrid costs $4965 more. With gas savings of $451.10 a year, it will take about 11 years for the gas savings to make up for the higher initial costs.
We can conclude that if you expect to own the car 11 years, the hybrid is indeed worth it. If you plan to own the car for less than 11 years, it may still be worth it, since the resale value of the hybrid may be higher, or for other non-monetary reasons. This is a case where math can help guide your decision, but it can’t make it for you.
This question pulls together all the skills discussed previously on this page, as the video demonstration illustrates.
- Revision and Adaptation. Provided by : Lumen Learning. License : CC BY: Attribution
- Problem Solving. Authored by : David Lippman. Located at : http://www.opentextbookstore.com/mathinsociety/ . Project : Math in Society. License : CC BY-SA: Attribution-ShareAlike
- Estimating with imperfect information. Authored by : OCLPhase2's channel. Located at : https://youtu.be/xF5BNEr0gjo . License : CC BY: Attribution
- Multistep proportions / problem solving process. Authored by : OCLPhase2's channel. Located at : https://youtu.be/NVCwFO-w2z4 . License : CC BY: Attribution
- Estimating the cost of a deck. Authored by : OCLPhase2's channel. Located at : https://youtu.be/adPGfeTy-Pc . License : CC BY: Attribution
- Guiding decision using math: Sonata vs Hybrid. Authored by : OCLPhase2's channel. Located at : https://youtu.be/HXmc-EkOYJE . License : CC BY: Attribution
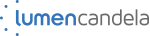
Privacy Policy
10 Quantitative Skills and How to Develop Them
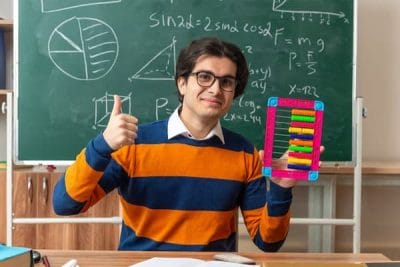
- Updated December 25, 2023
- Published August 8, 2023
Are you looking to learn more about Quantitative skills? In this article, we discuss Quantitative skills in more detail and give you tips about how you can develop and improve them.
What are Quantitative skills?
Quantitative skills refer to the ability to work with numerical data, perform mathematical calculations, and analyze information using quantitative methods. These skills are crucial in various fields, including but not limited to science, engineering, finance, economics, data science, and social sciences. Here are some key aspects of quantitative skills:
Mathematics
Data analysis.
- Critical Thinking
Modeling and Simulation
Problem solving, computer programming, financial analysis, economics and econometrics, research and surveys, data visualization.
Developing and honing quantitative skills can greatly enhance your problem-solving abilities and increase marketability across various industries and academic disciplines.
Top 10 Quantitative Skills
Below we discuss the top 10 Quantitative skills. Each skill is discussed in more detail, and we will also give you tips on improving them.
Mathematics is a fundamental quantitative skill that forms the bedrock of various disciplines and problem-solving processes. It encompasses various mathematical concepts, including arithmetic, algebra, calculus, geometry, and statistics. With a solid understanding of mathematics, you can work with numerical data, make accurate calculations, and analyze complex patterns and relationships.
How to Improve Mathematics
Improving your mathematical skills involves consistent practice and a growth mindset. Revisiting basic concepts, such as arithmetic operations and algebraic equations, to build a strong foundation. As you progress, delve into more advanced topics like calculus and statistics to understand quantitative analysis better. Embrace challenges and problem-solving exercises to enhance your critical thinking abilities, and seek out resources like textbooks, online courses, or tutorials to reinforce your knowledge.
Practical application is essential for strengthening your mathematical skills. Engage in real-world problems and projects that require quantitative analysis. Work with data sets, interpret graphs, and tackle mathematical modeling tasks. Collaborate with others and seek feedback to gain different perspectives and learn new approaches to problem-solving. The more you immerse yourself in mathematical applications, the more proficient and confident you will become in handling quantitative challenges across various fields. Remember, with determination and perseverance. You can continually improve your mathematical skills and unlock a world of opportunities in the data-driven landscape.
Data analysis is a vital quantitative skill that involves collecting, cleaning, organizing, and interpreting data to extract valuable insights and make informed decisions. It encompasses various techniques, including statistical methods, data visualization, and data mining. Mastering data analysis empowers you to uncover patterns, trends, and correlations within datasets, enabling you to draw meaningful conclusions and address complex problems.
How to Improve Data Analysis
To improve your data analysis skills, familiarize yourself with various data manipulation and cleaning techniques. Learn how to handle missing data, remove outliers, and transform data into a usable format. Next, dive into statistical concepts such as hypothesis testing, regression analysis, and descriptive statistics. Understanding these methods will help you draw accurate conclusions from data and support your decision-making process.
Practice is key to honing your data analysis skills. Seek out real-world datasets and work on projects that require data analysis. Engage in data-driven research, participate in data analysis competitions, or collaborate on data projects with others. Leveraging data analysis tools and software like Python, R, or Excel will also aid in gaining hands-on experience. Continuously challenge yourself to tackle increasingly complex datasets and problems, and seek feedback from peers or mentors to refine your analytical techniques. By combining theoretical knowledge with practical experience, you will become a proficient data analyst, capable of extracting valuable insights from data and driving evidence-based decision-making in diverse domains.
Critical thinking is a foundational quantitative skill that involves analyzing, evaluating, and synthesizing information objectively to make reasoned decisions and solve problems effectively. It encompasses logical reasoning, questioning assumptions, and considering different perspectives. Mastering critical thinking empowers you to approach complex issues with a clear and open mind, make well-informed choices, and overcome challenges more efficiently.
How to Improve Critical Thinking
To improve your critical thinking skills, start by practicing active reading and engaging with diverse sources of information. Question the author’s arguments, identify biases, and assess the validity of the evidence presented. Cultivate a habit of seeking alternative viewpoints to broaden your understanding of complex topics and strengthen your ability to evaluate arguments objectively.
Engaging in thought-provoking discussions and debates can also sharpen your critical thinking skills. Participate in group discussions or join forums where ideas are exchanged and challenged. Defend your viewpoints logically and be receptive to constructive criticism. Through this process, you’ll develop the ability to analyze different perspectives and refine your own arguments.
Additionally, solve puzzles, riddles, and brain-teasers regularly to enhance problem-solving abilities. These activities stimulate your mind and encourage creative thinking, essential in critical thinking. Embrace intellectual curiosity, be open to learning from various disciplines, and continuously question assumptions and conclusions. By consistently practicing critical thinking, you’ll become more adept at making informed decisions, solving complex problems, and navigating the challenges of a data-rich world.
Related : Quantitative Analyst vs. Data Scientist – What’s The Difference?
Modeling and simulation is a powerful quantitative skill that involves creating mathematical or computational models to represent real-world systems and processes. These models help you understand and analyze complex phenomena, make predictions, and simulate different scenarios to gain insights into how the system behaves under various conditions. Mastering modeling and simulation empower you to solve complex problems, optimize processes, and make data-driven decisions in diverse fields.
How to Improve Modeling and Simulation
To improve your modeling and simulation skills, start by gaining a strong foundation in mathematics, especially in calculus, differential equations, and linear algebra. These mathematical concepts are the building blocks of many modeling techniques. Familiarize yourself with relevant software and programming languages like Python, MATLAB, or simulation-specific tools. Practice implementing models and simulations with real data to understand how they apply to specific situations and improve your technical proficiency.
Study and analyze existing models and simulations in your area of interest. By examining how experts have approached similar problems, you can learn valuable insights and adapt their approaches to your own work. Engage in projects that require creating models and simulations and challenge yourself to develop innovative ways to represent complex systems. Collaborate with professionals in your field or join simulation-focused communities to share knowledge and receive feedback on your work. With dedication and continuous learning, you can enhance your modeling and simulation skills and contribute to cutting-edge research and problem-solving in various domains.
Problem-solving is a fundamental quantitative skill that involves the ability to approach challenges methodically, analyze them, and devise effective solutions. It encompasses critical thinking, data analysis, and decision-making to tackle complex issues across various domains. Mastering problem-solving empowers you to identify problems, break them down into manageable parts, and apply quantitative and qualitative methods to reach well-reasoned conclusions.
How to Improve Problem-Solving
To improve your problem-solving skills, embrace a growth mindset and view challenges as opportunities to learn and grow. Analyze problems systematically by breaking them into smaller components and understanding the relationships between them. Practice active brainstorming to generate multiple solutions and evaluate each option’s feasibility and potential outcomes.
Foster collaboration and seek diverse perspectives by discussing problems with colleagues or mentors. Working in teams can provide valuable insights and different problem-solving approaches. Continuously seek opportunities to apply your problem-solving skills in academic studies, professional work, or personal projects. Embrace failures as learning experiences and use feedback to refine your problem-solving strategies. As you encounter new problems, keep track of your approach, document the steps you take, and reflect on the effectiveness of your solutions. Over time, your problem-solving skills will strengthen, and you will become a resourceful and confident solver of complex quantitative challenges.
Related : Problem-Solving Interview Questions & Answers
Computer programming is a crucial quantitative skill that involves writing instructions in programming languages to create software, applications, and algorithms. It allows you to automate tasks, manipulate data, and implement complex quantitative models. Mastering computer programming empowers you to turn ideas into reality and leverage the power of technology to solve a wide range of quantitative problems.
How to Improve Computer Programming
To improve your computer programming skills, select a programming language that aligns with your goals and interests. Popular languages like Python, R, or Java offer robust capabilities for quantitative tasks. Begin with the basics, such as learning syntax, variables, and control structures. As you gain confidence, progress to more advanced topics like functions, object-oriented programming, and data structures.
Engage in hands-on projects to apply your programming skills. Work on real-world problems, tackle coding challenges and develop small applications or scripts. Collaborate with others in coding communities or join open-source projects to gain exposure to different coding styles and problem-solving approaches. Seek feedback from peers or mentors to improve your code quality and efficiency. Embrace continuous learning by exploring online tutorials, coding boot camps, or advanced courses in your chosen programming language. As you persistently practice and refine your programming abilities, you’ll become adept at using this quantitative skill to create innovative solutions and contribute to various quantitative domains.
Financial analysis is a vital quantitative skill that involves examining financial data, statements, and economic trends to evaluate the financial health and performance of individuals, companies, or organizations. It encompasses skills like ratio analysis, cash flow analysis, and risk assessment. Mastering financial analysis empowers you to make informed investment decisions, assess business profitability, and manage financial risks effectively.
How to Improve Financial Analysis
To improve your financial analysis skills, familiarize yourself with financial statements like balance sheets, income statements, and cash flow statements. Learn how to interpret these documents and extract meaningful information about a company’s financial position and performance. Practice calculating and interpreting financial ratios to assess a business’s liquidity, profitability, and leverage.
Stay updated on economic and financial market trends to understand their impact on financial analysis. Follow news and market reports and analyze how economic indicators influence financial data. Engage in case studies and financial modeling exercises to simulate real-world scenarios and strengthen your analytical abilities. Seek internships or work opportunities in finance-related roles to gain practical experience and exposure to financial analysis in a professional setting. Seek feedback from experienced financial analysts and mentors to refine your skills and build confidence in your financial analysis capabilities. With dedication and continuous learning, you can become a proficient financial analyst capable of providing valuable insights and recommendations in the dynamic world of finance.
Economics and econometrics are valuable quantitative skills that study economic systems, behavior, and trends. Furthermore, Economics deals with understanding how individuals, businesses, and governments make choices to allocate resources to satisfy their needs and wants. Econometrics involves applying statistical and mathematical methods to economic data to develop and test economic models. Mastering economics and econometrics empower you to analyze economic phenomena, forecast trends, and evaluate policy impacts.
How to Improve Economics and Econometrics
To improve your skills in economics and econometrics, start by building a strong foundation in economic principles, theories, and concepts. Understand the fundamental factors influencing supply and demand, market structures, and economic growth. As you progress, familiarize yourself with statistical techniques commonly used in econometrics, such as regression analysis, time-series analysis, and hypothesis testing.
Engage in economic research and data analysis projects to gain hands-on experience. Utilize economic databases, access publicly available economic data, and practice applying econometric methods to analyze the data. Consider taking specialized courses or pursuing advanced degrees in economics or econometrics to deepen your knowledge and expertise. Collaborate with professors, researchers, or peers to receive feedback on your work and exchange ideas. Embrace interdisciplinary approaches by integrating knowledge from related fields such as finance, international relations, or environmental studies. By continuously challenging yourself to apply economic principles and econometric methods to real-world problems, you’ll become a skilled economist capable of contributing valuable to economic research and policy analysis.
These are essential quantitative skills for gathering and analyzing academic, business, or social data. Research involves designing studies, formulating hypotheses, and collecting data through various methods such as surveys, experiments, or observations. Surveys are specific data collection tools that involve asking a targeted group of individuals questions to gather information about their opinions, behaviors, or preferences. Mastering research and surveys empower you to obtain valuable insights, draw meaningful conclusions, and contribute to evidence-based decision-making.
How to Improve Research and Surveys
To improve your skills in research and surveys, start by learning about research methodologies and survey design. Understand the different types of research approaches, sampling techniques, and data collection methods. Practice creating survey questionnaires that are clear, unbiased, and effectively capture the information you need. Consider using online survey platforms to distribute surveys and analyze the responses efficiently.
Emphasize the importance of ethics in research and surveys. Familiarize yourself with ethical guidelines for conducting research involving human subjects, ensuring confidentiality, and obtaining informed consent. Participate in research projects or volunteer to assist with surveys to gain practical experience. Collaborate with experienced researchers or survey specialists to learn from their expertise and receive feedback on your own work. Continuously review and improve your research and survey techniques based on feedback and evolving best practices. By refining your skills and adhering to rigorous research standards, you’ll become a proficient researcher capable of conducting insightful studies and providing valuable contributions to your field of interest.
Data visualization is a crucial quantitative skill that involves presenting data in graphical or visual formats to convey complex information in a clear and intuitive manner. It encompasses various visualization techniques such as charts, graphs, maps, and infographics. Mastering data visualization empowers you to communicate data-driven insights effectively, enabling others to understand trends, patterns, and relationships within datasets more easily.
How to Improve Data Visualization
To improve your data visualization skills, start by understanding the principles of effective data visualization. Learn about different types of charts and graphs and when to use each to best represent your data. Practice using data visualization tools like Tableau, Excel, or Python libraries (e.g., Matplotlib, Seaborn) to create compelling visualizations. Experiment with different color schemes, fonts, and design elements to enhance the visual appeal and clarity of your visualizations.
Seek inspiration from existing data visualization examples and data-driven stories. Analyze how other professionals and data journalists present complex information visually and learn from their techniques. Participate in data visualization challenges or competitions to challenge yourself and receive feedback from a broader audience. Collaborate with peers or mentors in data-related fields to exchange ideas and insights. By continuously practicing data visualization and incorporating feedback into your work, you’ll develop the skills to create impactful visualizations that effectively communicate data insights and aid decision-making in diverse domains.
Quantitative Skills Conclusion
In conclusion, developing quantitative skills is paramount in today’s data-driven world. Whether you are a student, a professional, or an aspiring researcher, honing these skills can significantly enhance your problem-solving abilities and boost your career prospects. Working with numbers, analyzing data, and making informed decisions based on quantitative evidence is highly valued across various fields and industries.
Improving these skills requires dedication, practice, and a growth mindset. Embrace challenges and seek opportunities to apply quantitative techniques in your academic or professional projects. Use online courses, tutorials, and resources to reinforce your knowledge and learn new methodologies. Collaborate with others to gain different perspectives and approaches to problem-solving. Seek feedback from mentors or experts to refine your techniques and continue to grow.
Related posts:
- 10 Life Skills Coach Skills and How to Develop Them
- Quantitative Analyst vs. Data Scientist – What’s The Difference?
- 10 Oratory Skills and How To Develop Them
- 10 Mechanical Skills and How to Develop Them
- 10 Integrity Skills and How to Develop Them
Rate this article
Your page rank:
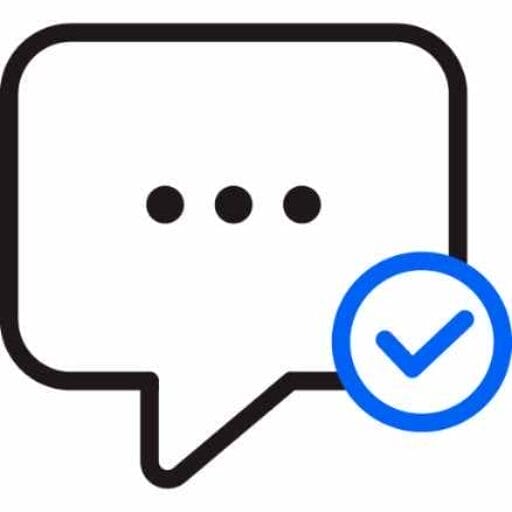
MegaInterview Company Career Coach
Step into the world of Megainterview.com, where our dedicated team of career experts, job interview trainers, and seasoned career coaches collaborates to empower individuals on their professional journeys. With decades of combined experience across diverse HR fields, our team is committed to fostering positive and impactful career development.
You may also be interested in:
70 condolence messages for coworker, 10 nail technician skills and how to develop them, 10 creative writing skills and how to develop them, 10 financial management skills and how to develop them, interview categories.
- Interview Questions
- Cover Letter
- Interview Tips
Megainterview/Contact
- Career Interview Questions
- Write For Megainterview!
- Editorial Policy
- Privacy Policy / GDPR
- Terms & Conditions
- Contact: [email protected]
Sign-up for our newsletter
🤝 We’ll never spam you or sell your data
Popular Topics
- Accomplishments
- Adaptability
- Career Change
- Career Goals
- Communication
- Conflict Resolution
- Creative Thinking
- Cultural Fit
- Customer Service
- Entry-Level & No Experience
- Growth Potential
- Honesty & Integrity
- Job Satisfaction
- Negotiation Skills
- Performance Based
- Phone Interview
- Problem-Solving
- Questions to Ask the Interviewer
- Salary & Benefits
- Situational & Scenario-Based
- Stress Management
- Time Management & Prioritization
- Uncomfortable
- Work Experience
Popular Articles
- What Is The Most Challenging Project You Have Worked On?
- Tell Me About a Time You Had to Deal With a Difficult Customer
- What Have You Done To Improve Yourself In The Past Year?
- Interview Question: How Do You Deal With Tight Deadlines?
- Describe a Time You Demonstrated Leadership
- Tell Me About a Time When You Took Action to Resolve a Problem
- Job Interview Questions About Working in Fast-Paced Environments
- Job Interview: What Areas Need Improvement? (+ Answers)
- Tell Me About a Time You Were On a Team Project That Failed
- Tell Me About a Time You Managed an Important Project
Our mission is to
Help you get hired.
Hofplein 20
3032 AC, Rotterdam, the Netherlands
Turn interviews into offers
Every other Tuesday, get our Chief Coach’s best job-seeking and interviewing tips to land your dream job. 5-minute read.
Quantitative Methods
- Living reference work entry
- First Online: 11 June 2021
- Cite this living reference work entry
- Juwel Rana 2 , 3 , 4 ,
- Patricia Luna Gutierrez 5 &
- John C. Oldroyd 6
648 Accesses
2 Citations
Quantitative analysis ; Quantitative research methods ; Study design
Quantitative method is the collection and analysis of numerical data to answer scientific research questions. Quantitative method is used to summarize, average, find patterns, make predictions, and test causal associations as well as generalizing results to wider populations. It allows us to quantify effect sizes, determine the strength of associations, rank priorities, and weigh the strength of evidence of effectiveness.
Introduction
This entry aims to introduce the most common ways to use numbers and statistics to describe variables, establish relationships among variables, and build numerical understanding of a topic. In general, the quantitative research process uses a deductive approach (Neuman 2014 ; Leavy 2017 ), extrapolating from a particular case to the general situation (Babones 2016 ).
In practical ways, quantitative methods are an approach to studying a research topic. In research, the...
This is a preview of subscription content, log in via an institution to check access.
Access this chapter
Institutional subscriptions
Babones S (2016) Interpretive quantitative methods for the social sciences. Sociology. https://doi.org/10.1177/0038038515583637
Balnaves M, Caputi P (2001) Introduction to quantitative research methods: an investigative approach. Sage, London
Book Google Scholar
Brenner PS (2020) Understanding survey methodology: sociological theory and applications. Springer, Boston
Google Scholar
Creswell JW (2014) Research design: qualitative, quantitative, and mixed methods approaches. Sage, London
Leavy P (2017) Research design. The Gilford Press, New York
Mertens W, Pugliese A, Recker J (2018) Quantitative data analysis, research methods: information, systems, and contexts: second edition. https://doi.org/10.1016/B978-0-08-102220-7.00018-2
Neuman LW (2014) Social research methods: qualitative and quantitative approaches. Pearson Education Limited, Edinburgh
Treiman DJ (2009) Quantitative data analysis: doing social research to test ideas. Jossey-Bass, San Francisco
Download references
Author information
Authors and affiliations.
Department of Public Health, School of Health and Life Sciences, North South University, Dhaka, Bangladesh
Department of Biostatistics and Epidemiology, School of Health and Health Sciences, University of Massachusetts Amherst, MA, USA
Department of Research and Innovation, South Asia Institute for Social Transformation (SAIST), Dhaka, Bangladesh
Independent Researcher, Masatepe, Nicaragua
Patricia Luna Gutierrez
School of Behavioral and Health Sciences, Australian Catholic University, Fitzroy, VIC, Australia
John C. Oldroyd
You can also search for this author in PubMed Google Scholar
Corresponding author
Correspondence to Juwel Rana .
Editor information
Editors and affiliations.
Florida Atlantic University, Boca Raton, FL, USA
Ali Farazmand
Rights and permissions
Reprints and permissions
Copyright information
© 2021 Springer Nature Switzerland AG
About this entry
Cite this entry.
Rana, J., Gutierrez, P.L., Oldroyd, J.C. (2021). Quantitative Methods. In: Farazmand, A. (eds) Global Encyclopedia of Public Administration, Public Policy, and Governance. Springer, Cham. https://doi.org/10.1007/978-3-319-31816-5_460-1
Download citation
DOI : https://doi.org/10.1007/978-3-319-31816-5_460-1
Received : 31 January 2021
Accepted : 14 February 2021
Published : 11 June 2021
Publisher Name : Springer, Cham
Print ISBN : 978-3-319-31816-5
Online ISBN : 978-3-319-31816-5
eBook Packages : Springer Reference Economics and Finance Reference Module Humanities and Social Sciences Reference Module Business, Economics and Social Sciences
- Publish with us
Policies and ethics
- Find a journal
- Track your research
Have a language expert improve your writing
Run a free plagiarism check in 10 minutes, generate accurate citations for free.
- Knowledge Base
Methodology
- What Is Quantitative Research? | Definition, Uses & Methods
What Is Quantitative Research? | Definition, Uses & Methods
Published on June 12, 2020 by Pritha Bhandari . Revised on June 22, 2023.
Quantitative research is the process of collecting and analyzing numerical data. It can be used to find patterns and averages, make predictions, test causal relationships, and generalize results to wider populations.
Quantitative research is the opposite of qualitative research , which involves collecting and analyzing non-numerical data (e.g., text, video, or audio).
Quantitative research is widely used in the natural and social sciences: biology, chemistry, psychology, economics, sociology, marketing, etc.
- What is the demographic makeup of Singapore in 2020?
- How has the average temperature changed globally over the last century?
- Does environmental pollution affect the prevalence of honey bees?
- Does working from home increase productivity for people with long commutes?
Table of contents
Quantitative research methods, quantitative data analysis, advantages of quantitative research, disadvantages of quantitative research, other interesting articles, frequently asked questions about quantitative research.
You can use quantitative research methods for descriptive, correlational or experimental research.
- In descriptive research , you simply seek an overall summary of your study variables.
- In correlational research , you investigate relationships between your study variables.
- In experimental research , you systematically examine whether there is a cause-and-effect relationship between variables.
Correlational and experimental research can both be used to formally test hypotheses , or predictions, using statistics. The results may be generalized to broader populations based on the sampling method used.
To collect quantitative data, you will often need to use operational definitions that translate abstract concepts (e.g., mood) into observable and quantifiable measures (e.g., self-ratings of feelings and energy levels).
Research method | How to use | Example |
---|---|---|
Control or manipulate an to measure its effect on a dependent variable. | To test whether an intervention can reduce procrastination in college students, you give equal-sized groups either a procrastination intervention or a comparable task. You compare self-ratings of procrastination behaviors between the groups after the intervention. | |
Ask questions of a group of people in-person, over-the-phone or online. | You distribute with rating scales to first-year international college students to investigate their experiences of culture shock. | |
(Systematic) observation | Identify a behavior or occurrence of interest and monitor it in its natural setting. | To study college classroom participation, you sit in on classes to observe them, counting and recording the prevalence of active and passive behaviors by students from different backgrounds. |
Secondary research | Collect data that has been gathered for other purposes e.g., national surveys or historical records. | To assess whether attitudes towards climate change have changed since the 1980s, you collect relevant questionnaire data from widely available . |
Note that quantitative research is at risk for certain research biases , including information bias , omitted variable bias , sampling bias , or selection bias . Be sure that you’re aware of potential biases as you collect and analyze your data to prevent them from impacting your work too much.
Receive feedback on language, structure, and formatting
Professional editors proofread and edit your paper by focusing on:
- Academic style
- Vague sentences
- Style consistency
See an example
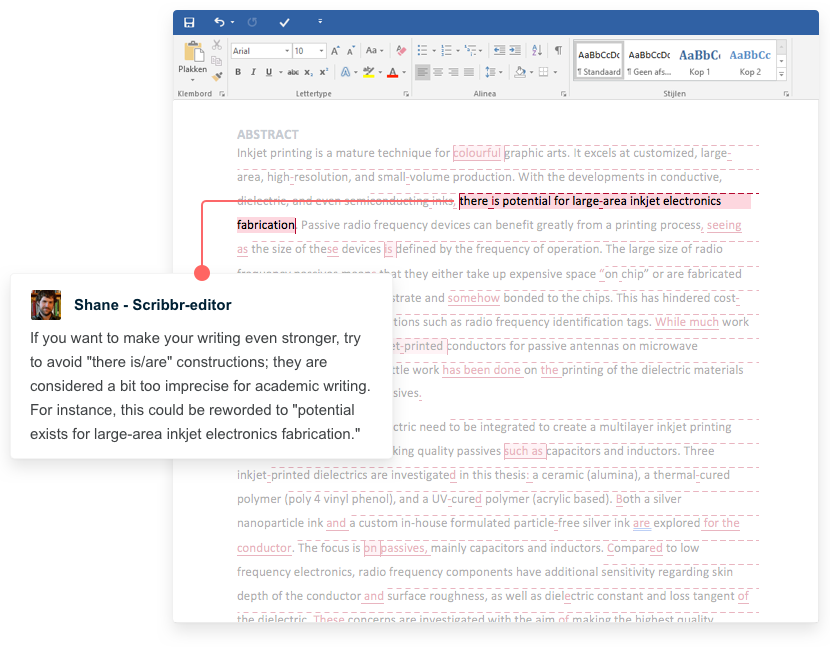
Once data is collected, you may need to process it before it can be analyzed. For example, survey and test data may need to be transformed from words to numbers. Then, you can use statistical analysis to answer your research questions .
Descriptive statistics will give you a summary of your data and include measures of averages and variability. You can also use graphs, scatter plots and frequency tables to visualize your data and check for any trends or outliers.
Using inferential statistics , you can make predictions or generalizations based on your data. You can test your hypothesis or use your sample data to estimate the population parameter .
First, you use descriptive statistics to get a summary of the data. You find the mean (average) and the mode (most frequent rating) of procrastination of the two groups, and plot the data to see if there are any outliers.
You can also assess the reliability and validity of your data collection methods to indicate how consistently and accurately your methods actually measured what you wanted them to.
Quantitative research is often used to standardize data collection and generalize findings . Strengths of this approach include:
- Replication
Repeating the study is possible because of standardized data collection protocols and tangible definitions of abstract concepts.
- Direct comparisons of results
The study can be reproduced in other cultural settings, times or with different groups of participants. Results can be compared statistically.
- Large samples
Data from large samples can be processed and analyzed using reliable and consistent procedures through quantitative data analysis.
- Hypothesis testing
Using formalized and established hypothesis testing procedures means that you have to carefully consider and report your research variables, predictions, data collection and testing methods before coming to a conclusion.
Despite the benefits of quantitative research, it is sometimes inadequate in explaining complex research topics. Its limitations include:
- Superficiality
Using precise and restrictive operational definitions may inadequately represent complex concepts. For example, the concept of mood may be represented with just a number in quantitative research, but explained with elaboration in qualitative research.
- Narrow focus
Predetermined variables and measurement procedures can mean that you ignore other relevant observations.
- Structural bias
Despite standardized procedures, structural biases can still affect quantitative research. Missing data , imprecise measurements or inappropriate sampling methods are biases that can lead to the wrong conclusions.
- Lack of context
Quantitative research often uses unnatural settings like laboratories or fails to consider historical and cultural contexts that may affect data collection and results.
Here's why students love Scribbr's proofreading services
Discover proofreading & editing
If you want to know more about statistics , methodology , or research bias , make sure to check out some of our other articles with explanations and examples.
- Chi square goodness of fit test
- Degrees of freedom
- Null hypothesis
- Discourse analysis
- Control groups
- Mixed methods research
- Non-probability sampling
- Inclusion and exclusion criteria
Research bias
- Rosenthal effect
- Implicit bias
- Cognitive bias
- Selection bias
- Negativity bias
- Status quo bias
Quantitative research deals with numbers and statistics, while qualitative research deals with words and meanings.
Quantitative methods allow you to systematically measure variables and test hypotheses . Qualitative methods allow you to explore concepts and experiences in more detail.
In mixed methods research , you use both qualitative and quantitative data collection and analysis methods to answer your research question .
Data collection is the systematic process by which observations or measurements are gathered in research. It is used in many different contexts by academics, governments, businesses, and other organizations.
Operationalization means turning abstract conceptual ideas into measurable observations.
For example, the concept of social anxiety isn’t directly observable, but it can be operationally defined in terms of self-rating scores, behavioral avoidance of crowded places, or physical anxiety symptoms in social situations.
Before collecting data , it’s important to consider how you will operationalize the variables that you want to measure.
Reliability and validity are both about how well a method measures something:
- Reliability refers to the consistency of a measure (whether the results can be reproduced under the same conditions).
- Validity refers to the accuracy of a measure (whether the results really do represent what they are supposed to measure).
If you are doing experimental research, you also have to consider the internal and external validity of your experiment.
Hypothesis testing is a formal procedure for investigating our ideas about the world using statistics. It is used by scientists to test specific predictions, called hypotheses , by calculating how likely it is that a pattern or relationship between variables could have arisen by chance.
Cite this Scribbr article
If you want to cite this source, you can copy and paste the citation or click the “Cite this Scribbr article” button to automatically add the citation to our free Citation Generator.
Bhandari, P. (2023, June 22). What Is Quantitative Research? | Definition, Uses & Methods. Scribbr. Retrieved September 9, 2024, from https://www.scribbr.com/methodology/quantitative-research/
Is this article helpful?
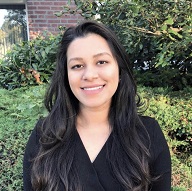
Pritha Bhandari
Other students also liked, descriptive statistics | definitions, types, examples, inferential statistics | an easy introduction & examples, get unlimited documents corrected.
✔ Free APA citation check included ✔ Unlimited document corrections ✔ Specialized in correcting academic texts
Breadcrumbs Section. Click here to navigate to respective pages.

Quantitative Methods for Second Language Research
DOI link for Quantitative Methods for Second Language Research
Get Citation
Quantitative Methods for Second Language Research introduces approaches to and techniques for quantitative data analysis in second language research, with a primary focus on second language learning and assessment research. It takes a conceptual, problem-solving approach by emphasizing the understanding of statistical theory and its application to research problems while paying less attention to the mathematical side of statistical analysis. The text discusses a range of common statistical analysis techniques, presented and illustrated through applications of the IBM Statistical Package for Social Sciences (SPSS) program. These include tools for descriptive analysis (e.g., means and percentages) as well as inferential analysis (e.g., correlational analysis, t -tests, and analysis of variance [ANOVA]). The text provides conceptual explanations of quantitative methods through the use of examples, cases, and published studies in the field. In addition, a companion website to the book hosts slides, review exercises, and answer keys for each chapter as well as SPSS files. Practical and lucid, this book is the ideal resource for data analysis for graduate students and researchers in applied linguistics.
TABLE OF CONTENTS
Chapter 1 | 13 pages, quantification, chapter 2 | 14 pages, introduction to spss, chapter 3 | 16 pages, descriptive statistics, chapter 4 | 16 pages, descriptive statistics in spss, chapter 5 | 21 pages, correlational analysis, chapter 6 | 11 pages, basics of inferential statistics, chapter 7 | 14 pages, chapter 8 | 11 pages, mann-whitney u and wilcoxon signed-rank tests, chapter 9 | 18 pages, one-way analysis of variance (anova), chapter 10 | 19 pages, analysis of covariance (ancova), chapter 11 | 12 pages, repeated-measures anova, chapter 12 | 16 pages, two-way mixed-design anova, chapter 13 | 18 pages, chi-square test, chapter 14 | 19 pages, multiple regression, chapter 15 | 27 pages, reliability analysis, chapter | 4 pages.
- Privacy Policy
- Terms & Conditions
- Cookie Policy
- Taylor & Francis Online
- Taylor & Francis Group
- Students/Researchers
- Librarians/Institutions
Connect with us
Registered in England & Wales No. 3099067 5 Howick Place | London | SW1P 1WG © 2024 Informa UK Limited
What is Quantitative Analysis?
Quantitative analysis techniques, applications of quantitative analysis in the business sector, the bottom line, related readings, quantitative analysis.
The collection and evaluation of measurable and verifiable financial data to understand the behavior and performance of a business
Quantitative analysis is the process of collecting and evaluating measurable and verifiable data such as revenues, market share, and wages in order to understand the behavior and performance of a business. In the past, business owners and company directors relied heavily on their experience and instinct when making decisions. However, with data technology, quantitative analysis is now considered a better approach to making informed decisions.
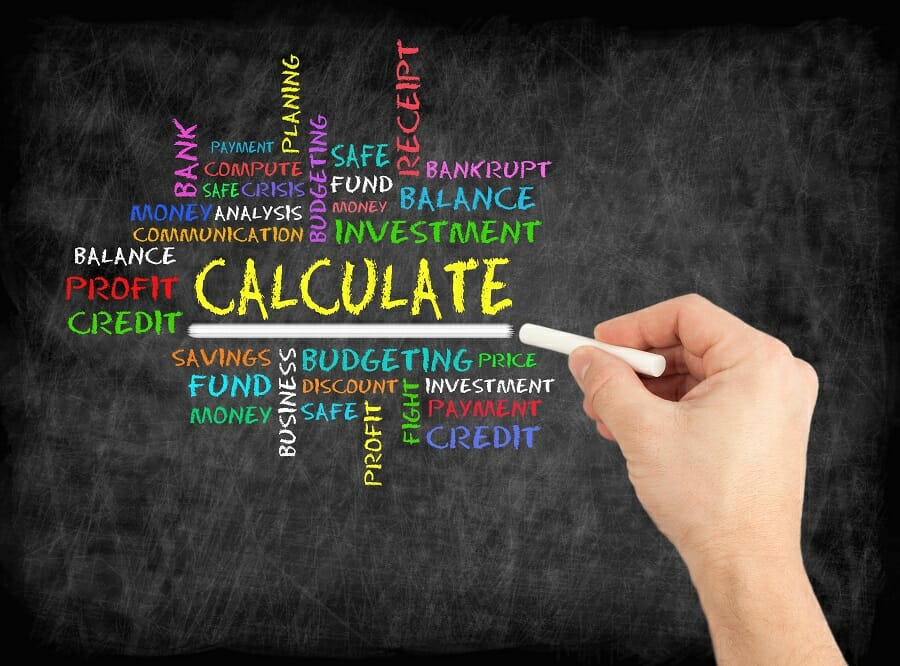
A quantitative analyst’s main task is to present a given hypothetical situation in terms of numerical values. Quantitative analysis helps in evaluating performance, assessing financial instruments, and making predictions. It encompasses three main techniques of measuring data: regression analysis , linear programming, and data mining.
1. Regression Analysis
Regression analysis is a common technique that is not only employed by business owners but also by statisticians and economists. It involves using statistical equations to predict or estimate the impact of one variable on another. For instance, regression analysis can determine how interest rates affect consumers’ behavior regarding asset investment. One other core application of regression analysis is establishing the effect of education and work experience on employees’ annual earnings.
In the business sector, owners can use regression analysis to determine the impact of advertising expenses on business profits. Using this approach, a business owner can establish a positive or negative correlation between two variables.
2. Linear Programming
Most companies occasionally encounter a shortage of resources such as facility space, production machinery , and labor. In such situations, company managers must find ways to allocate resources effectively. Linear programming is a quantitative method that determines how to achieve such an optimal solution. It is also used to determine how a company can make optimal profits and reduce its operating costs, subject to a given set of constraints, such as labor.
3. Data Mining
Data mining is a combination of computer programming skills and statistical methods. The popularity of data mining continues to grow in parallel with the increase in the quantity and size of available data sets. Data mining techniques are used to evaluate very large sets of data to find patterns or correlations concealed within them.
Business owners are often forced to make decisions under conditions of uncertainty. Luckily, quantitative techniques enable them to make the best estimates and thus minimize the risks associated with a particular decision. Ideally, quantitative models provide company owners with a better understanding of information to enable them to make the best possible decisions.
Project Management
One area where quantitative analysis is considered an indispensable tool is in project management . As mentioned earlier, quantitative methods are used to find the best ways of allocating resources, especially if these resources are scarce. Projects are then scheduled based on the availability of certain resources.
Production Planning
Quantitative analysis also helps individuals to make informed product-planning decisions. Let’s say a company finds it challenging to estimate the size and location of a new production facility. Quantitative analysis can be employed to assess different proposals for costs, timing, and location. With effective product planning and scheduling, companies will be more able to meet their customers’ needs while maximizing their profits.
Every business needs a proper marketing strategy. However, setting a budget for the marketing department can be tricky, especially if its objectives are not set. With the right quantitative method, marketers can easily set the required budget and allocate media purchases. The decisions can be based on data obtained from marketing campaigns.
The accounting department of a business also relies heavily on quantitative analysis. Accounting personnel uses different quantitative data and methods, such as the discounted cash flow model , to estimate the value of an investment. Products can also be evaluated based on the costs of producing them and the profits they generate.

Purchase and Inventory
One of the greatest challenges that businesses face is being able to predict the demand for a product or service. However, with quantitative techniques, companies can be guided on just how many materials they need to purchase, the level of inventory to maintain, and the costs they’re likely to incur when shipping and storing finished goods.
Quantitative analysis is the use of mathematical and statistical techniques to assess the performance of a business. Before the advent of quantitative analysis, many company directors based their decisions on experience and gut. Business owners can now use quantitative methods to predict trends, determine the allocation of resources, and manage projects.
Quantitative techniques are also used to evaluate investments. In such a way, organizations can determine the best assets to invest in and the best time to do so. Some of the quantitative analysis methods include regression analysis, linear programming, and data mining.
Thank you for reading CFI’s guide to quantitative analysis. To keep learning and advancing your career, the following CFI resources will be helpful:
- Correlation
- Consumer Surplus Formula
- Quantitative Trading
- Econometrics
- See all data science resources
- Share this article
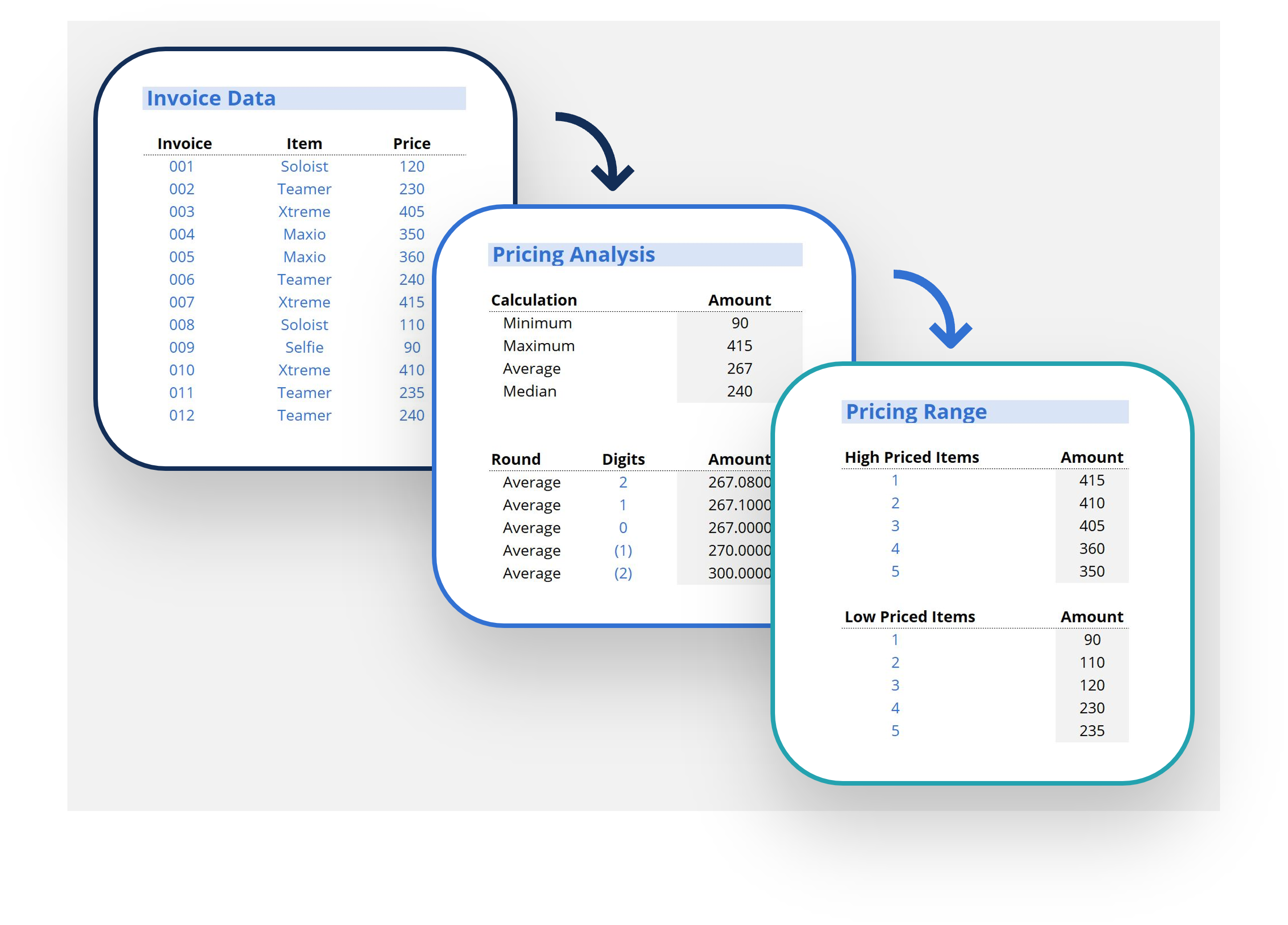
Create a free account to unlock this Template
Access and download collection of free Templates to help power your productivity and performance.
Already have an account? Log in
Supercharge your skills with Premium Templates
Take your learning and productivity to the next level with our Premium Templates.
Upgrading to a paid membership gives you access to our extensive collection of plug-and-play Templates designed to power your performance—as well as CFI's full course catalog and accredited Certification Programs.
Already have a Self-Study or Full-Immersion membership? Log in
Access Exclusive Templates
Gain unlimited access to more than 250 productivity Templates, CFI's full course catalog and accredited Certification Programs, hundreds of resources, expert reviews and support, the chance to work with real-world finance and research tools, and more.
Already have a Full-Immersion membership? Log in
- Utility Menu

66765bdee9aa52c20d12f4e18d6c81dd
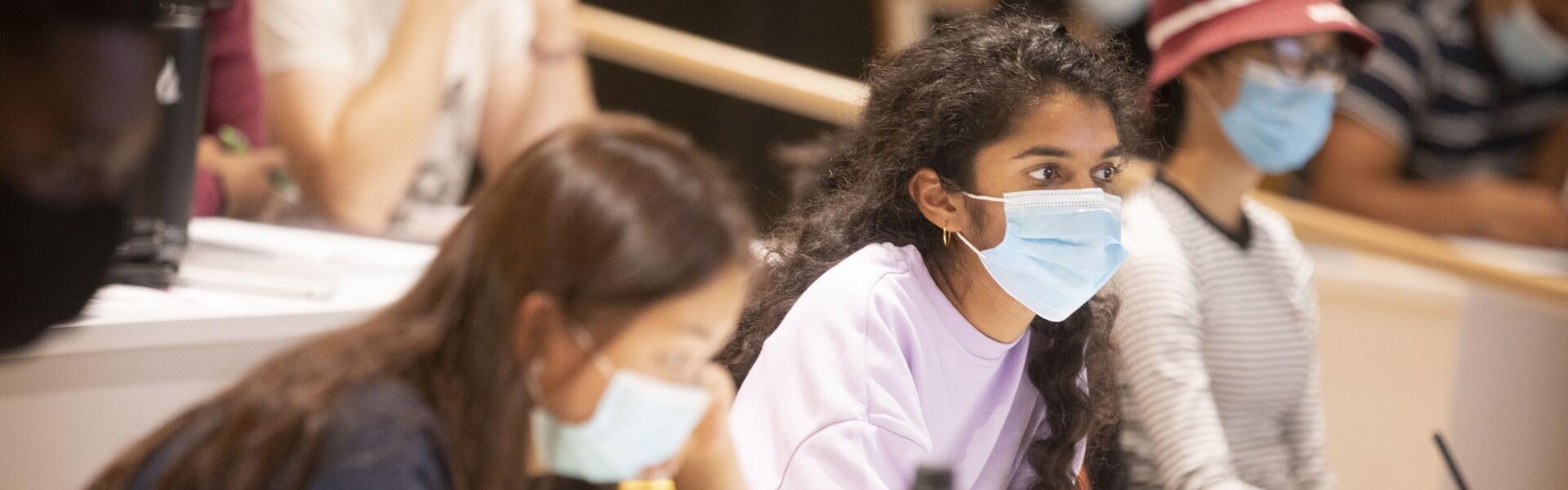
The following courses fulfill the Quantitative Reasoning with Data (QRD) requirement
Find courses.
- Applied Computation (2) Apply Applied Computation filter
- Applied Mathematics (9) Apply Applied Mathematics filter
- Applied Physics (1) Apply Applied Physics filter
- Astronomy (1) Apply Astronomy filter
- Computer Science (6) Apply Computer Science filter
- Earth & Planetary Sciences (5) Apply Earth & Planetary Sciences filter
- Economics (4) Apply Economics filter
- Engineering Sciences (3) Apply Engineering Sciences filter
- Environmental Science & Engineering (4) Apply Environmental Science & Engineering filter
- Government (8) Apply Government filter
- Life Sciences (1) Apply Life Sciences filter
- Linguistics (1) Apply Linguistics filter
- Mathematics (9) Apply Mathematics filter
- Molecular & Cellular Biology (3) Apply Molecular & Cellular Biology filter
- Physics (8) Apply Physics filter
- Psychology (1) Apply Psychology filter
- Sociology (1) Apply Sociology filter
- Statistics (15) Apply Statistics filter
- Arts & Humanities (1) Apply Arts & Humanities filter
- Engineering & Applied Sciences (25) Apply Engineering & Applied Sciences filter
- Science (43) Apply Science filter
- Social Science (14) Apply Social Science filter
Prerequisites/Recommended preparation
- AP Calculus AB (1) Apply AP Calculus AB filter
- APCOMP 209A/COMPSCI 109A/STAT 109A (5) Apply APCOMP 209A/COMPSCI 109A/STAT 109A filter
- APCOMP 209b/COMPSCI 109B/STAT 109B (2) Apply APCOMP 209b/COMPSCI 109B/STAT 109B filter
- APMTH 10 (2) Apply APMTH 10 filter
- APMTH 21A (5) Apply APMTH 21A filter
- APMTH 21B (3) Apply APMTH 21B filter
- APMTH 22A (5) Apply APMTH 22A filter
- APMTH 22B (2) Apply APMTH 22B filter
- APPHY 50A (4) Apply APPHY 50A filter
- APPHY 50B (1) Apply APPHY 50B filter
- COMPSCI 181 (1) Apply COMPSCI 181 filter
- COMPSCI 50 (7) Apply COMPSCI 50 filter
- COMPSCI 51 (1) Apply COMPSCI 51 filter
- COMPSCI 61 (1) Apply COMPSCI 61 filter
- ECON 20 (1) Apply ECON 20 filter
- GOV 50 (3) Apply GOV 50 filter
- GOV 51 (1) Apply GOV 51 filter
- LIFESCI 1A (1) Apply LIFESCI 1A filter
- LIFESCI 1B (1) Apply LIFESCI 1B filter
- MATH 18A (1) Apply MATH 18A filter
- MATH 19A (2) Apply MATH 19A filter
- MATH 1A (2) Apply MATH 1A filter
- MATH 1B (16) Apply MATH 1B filter
- MATH 21A (14) Apply MATH 21A filter
- MATH 21B (14) Apply MATH 21B filter
- MATH 22A (2) Apply MATH 22A filter
- MATH 22B (1) Apply MATH 22B filter
- MATH MA (1) Apply MATH MA filter
- MATH MB (1) Apply MATH MB filter
- None (15) Apply None filter
- PHYSCI 12A (6) Apply PHYSCI 12A filter
- PHYSCI 12B (2) Apply PHYSCI 12B filter
- PHYSCI 2 (1) Apply PHYSCI 2 filter
- PHYSCI 3 (1) Apply PHYSCI 3 filter
- PHYSICS 143A (1) Apply PHYSICS 143A filter
- PHYSICS 143B (1) Apply PHYSICS 143B filter
- PHYSICS 15A (6) Apply PHYSICS 15A filter
- PHYSICS 15B (2) Apply PHYSICS 15B filter
- PHYSICS 191 (1) Apply PHYSICS 191 filter
- PHYSICS 247 (1) Apply PHYSICS 247 filter
- PSY 1 (1) Apply PSY 1 filter
- STAT 100 (1) Apply STAT 100 filter
- STAT 100 (3) Apply STAT 100 filter
- STAT 104 (1) Apply STAT 104 filter
- STAT 110 (11) Apply STAT 110 filter
- STAT 111 (6) Apply STAT 111 filter
- STAT 139 (5) Apply STAT 139 filter
- Fall 2024 (42) Apply Fall 2024 filter
- Spring 2025 (36) Apply Spring 2025 filter
Search Courses
Apcomp 209a: data science 1: introduction to data science, semester: , offered: .
APCOMP 209A/CS 109A/STAT 109A is an interdisciplinary course that relies on the data science process; the course equips students with computational, mathematical, and statistical methods for analyzing data, making inferences, and predicting outcomes, covering subject matter including tech, medicine, and pop culture. The course emphasizes not only theoretical understanding but also the practical application of these methods to real-world datasets, helping students recognize the value and impact of data in diverse fields. While no strict prerequisites are required, a fundamental...
APCOMP 209B: Data Science 2: Advanced Topics in Data Science
APCOMP 209B/COMPSCI 109B/STAT 109B is a direct continuation of APCOMP 209A/COMPSCI 109A/STAT 109A that will cover topics such as smoothing and additive models, unsupervised learning, Bayesian analysis, deep learning including convolutional neural networks, recurrent neural networks, language models, generative models and deep reinforcement learning. The course aligns with the QRD criteria by teaching methods for analyzing data, drawing statistical inferences, and making predictions applied to real data sets. Prerequisites: APCOMP 209A/COMPSCI 109A/STAT 109A.
APMTH 101: Statistical Inference for Scientists and Engineers
APMTH 101 is an introductory statistics course for students in engineering and the sciences that covers probability, statistical inference, and regression modeling. Students are expected to diligently read prior to class, such that sessions can be weighted towards performing in-class problem solving and lecturing can be reserved for the nuanced points of the material. A comprehensive understanding of multivariable calculus is required, however computational software (MATLAB) is leveraged to dedicate more attention on the application of statistical methods.
APMTH 10: Computing with Python for Scientists and Engineers
Students will learn to code in Python to solve mathematical and statistical problems in science and engineering, using real data such as epidemiological data for predicting the spread of the SARS-Cov-2 virus, financial data for risk assessment, and climate time series for calculating global trends. Students will learn to analyze and fit real data, calculate and propagate uncertainty, extract parameter values from data, and explore how the sampling of data affects inferences and prediction, while being aware of numerical approximation errors, overfitting, and model limitations. Students...
APMTH 120: Applied Linear Algebra and Big Data
APM120 teaches basic big-data analysis methods, all demonstrated using real-world examples, that based on student feedback are very often then used by students in their summer internships/career. Many methods for analyzing data are covered, and there is an emphasis throughout on motivating these methods with real problems and real data, in both lectures and homework assignments. Prerequisites: Math 21a and 21b or equivalent; and CS50 or APM10, or equivalent.
APMTH 205: Advanced Scientific Computing: Numerical Methods
This course covers the mathematical foundations of well-established numerical algorithms that are used for both modeling and data analysis. The algorithms will be explored through a range of real-world examples drawn from a variety of fields. Requires familiarity with linear algebra and calculus, as well as some programming experience at the level of COMPSCI 50 and above.
APMTH 207: Advanced Scientific Computing: Stochastic Methods for Data Analysis, Inference and Optimization
This class presents methodologies to model, analyze and optimize, physical systems using stochastic processes. We use data to develop and evaluate models and in turn algorithms that link models with data. We discuss advantages aan limitations of these processes and link them to computational thinking. Course prerequisites include a good level of probabilities and statistics, programming skills, as well as knowledge of partial differential equations.
APMTH 22A: Solving and Optimizing
This course covers concepts from linear algebra and multivariate calculus with a view towards data analysis, computation, and optimization. The course covers both the underlying mathematical theory as well as the practical tools needed for application to real world data. Students should already be comfortable with the material from Math 1ab.
APMTH 22B: Integrating and Approximating
This course teaches multivariable and vector calculus, supplemented with numerical methods. The concepts and techniques taught in this class form the basis of many data analysis algorithms. We will use real data to illustrate some of the concepts taught in class, and explore some of these algorithms.
APMTH 231: Decision Theory
APMTH 231 teaches statistical inference and estimation from a signal processing perspective. The courses aims to teach students a) to think about probabilistic models of data, b) how to develop estimation and inference algorithms, and c) how to apply it to real data. The course emphasizes the entire pipeline from writing a model, estimating its parameters and performing inference utilizing sports data, neuroscience data, geyser eruption data and other sources. The course also teaches students how to assess the goodness of fit of models to data, diagnostic tools to detect lack of fit, and...
APMTH 50: Introduction to Applied Mathematics
This course provides an introduction to the problems and issues of applied mathematics, focusing on areas where mathematical ideas have had a major impact on diverse fields of human inquiry. The course is organized around two-week topics drawn from a variety of fields, and involves reading classic mathematical papers in each topic. We will build mathematical and statistical models of real life problems such as spread of disease and option pricing, use computational methods to calculate predictions of our models, and test these predictions on real data. Mathematics 1b is a prerequisite,...
APPHY 50A: Physics as a Foundation for Science and Engineering, Part I
The AP50A/B sequence is a team- and project-based introduction to physics designed for engineering and physics concentrators focusing on the application of physics to real-world problems. It is equivalent in content and rigor to a standard calculus-based introductory physics course sequence (A: mechanics and waves; B: electromagnetism and optics). Through, hands-on and collaborative learning activities you will hone your scientific reasoning, estimation, and open-ended problem-solving skills. In addition, the activities in the course are designed to help you grow your capacity for self-...
ASTRON 2: Celestial Navigation
Students have described Celestial Navigation as “the most hands-on course at Harvard.” Teaching is almost entirely experienced in a laboratory setting with constant day and night climbs to the roof for data collection and culminating with a day on the water navigating eastward until Boston sinks below the horizon. Using a quantitative approach, we will construct and use maps to inform us, build quantitative models to predict how objects in the sky move, and explore the development of instruments and methods that resonant in current technologies...
COMPSCI 1090A: Data Science 1: Introduction to Data Science
Compsci 1090b: data science 2: advanced topics in data science, compsci 1810: machine learning.
CS 181 provides a broad and rigorous introduction to machine learning, probabilistic reasoning and decision making in uncertain environments. Students will derive the mathematical underpinnings for many common methods, work computationally, applying machine learning to challenges with real data, and give consideration to the ethical implications of machine learning. The material is aimed at an advanced undergraduate level and students should be comfortable with writing non-trivial programs, have a background in probability theory, and familiarity with calculus and linear algebra.
COMPSCI 1: Great Ideas in Computer Science
COMPSCI 1 is a broad introduction to some of the most important principles and practices of functional and object-oriented software development using a methodology that places a high value on programs that are easy to read, maintain and modify. Most of the programming will be done using Python, through which fundamental data structures and their algorithms will be investigated. Lectures and problem sets include visualization techniques using real-world data sets, linear regression, variance, and both formal and empirical analysis of execution time. This course is designed for...
COMPSCI 32: Computational Thinking and Problem Solving
COMPSCI 32 is an introduction to computational thinking, useful concepts in the field of computer science, and the art of computer programming using Python. Each class meeting and assignment focuses on learning to use computers to gain insights about the world around us and solve real-world problems, often using real-world data. The material assumes no prior experience in computer science or computer programming.
COMPSCI 50: Introduction to Computer Science
CS50 is an introduction to the intellectual enterprises of computer science and the art of programming. CS50 introduces students to the study of information: how do you represent it and with what methods can you process it? No prerequisites.
E-PSCI 100: The Missing MATLAB Course: A Practical Intro to Programming and Data Analysis
This hands-on course aims to empower students for solving problems using computer programming. This involves learning the programming language (syntaxes) but more importantly, how to break down a problem into small pieces that can individually be solved easily. A significant part of this course will be spent on solving problems and discussing various approaches with which they can be analyzed. No previous experience in computer programming is necessary but students must be comfortable with vector/matrix calculations.
E-PSCI 101: Global Warming Science 101
E-PSCI/ESE 101 teaches climate change science from a quantitative perspective, so that students can understand the exact mechanisms behind changes often discussed in the news, as well as the mathematical or statistical methods used to analyze them. Many methods for analyzing climate data and simple climate modeling are covered, and the students will be involved in in-class climate data analysis during every lecture. Prerequisites: Basic calculus and ordinary differential equations, as covered for example by Math 1b, Math 19a, Math 21b. Basic programming experience is assumed (not...
E-PSCI 102: Data Analysis and Statistical Inference in the Earth and Environmental Sciences
Students will become familiar with techniques in statistical inference, deterministic and stochastic models of data, regression, time series analysis, bootstrap and Markov Chain Monte Carlo methods, and receive a brief introduction to machine learning. The course emphasizes hands-on learning: all examples we use are real data sets drawn from current research in the Earth, atmospheric, and planetary sciences. Familiarity with single variable calculus is required while multivariable calculus is recommended, but not required.
E-PSCI 131: Introduction to Physical Oceanography and Climate
EPS/ESE131 teaches ocean physics from a quantitative perspective, so that students can understand the dynamics behind ocean circulation and waves and their role in climate and climate change. The course is math-based, and involves analyzing ocean data, and simple ocean and climate models; the students will be involved in in-class small-group collaborative work during lectures, developing and deriving the physical and mathematical foundations of ocean dynamics and complementing lecture materials. Basic calculus and ordinary differential equations as covered for example by Math 21b, and...
E-PSCI 139: Paleoclimate as Prologue
In this course we will quantitatively assess past events in Earth's history involving temperature, precipitation, and sea level, and leverage these past phenomena to inform about future changes in climate. Topics include inferring temperature from instrumental, dendrochronological, ice-core, and marine proxy records over the Medieval Warm Period, Little Ice Age, and post-industrial epochs; exploring variations in sea level recorded by tide gages and coral records over the Holocene and Last Interglacial; assessing precipitation variability using modern instrumental records and late-...
ECON 1123: Introduction to Econometrics
This course introduces state-of-the-art methods for answering important public policy questions, such as quantifying the causal effect of incarceration on recidivism or measuring the causal effect of unemployment insurance on unemployment durations. Students will learn how to develop, evaluate, and implement their own research designs to answer these types of questions and quantify uncertainty associated with such estimates. The class concludes with time series forecasting and financial econometrics. Most students will have taken one prior course in statistics or probability theory, such...
ECON 1126: Quantitative Methods in Economics
ECON 1126 is an advanced econometrics class that focuses on the analysis of empirical quantitative models that are commonly used in applied economics. We focus first on studying the mathematical and statistical foundations of using linear models. This includes approximating conditional expectations, forming predictions, studying omitted variables bias, and most importantly analyzing requirements for causal interpretations. The class uses real world data as a vehicle to illustrate the theoretical concepts. Applications include the demand and supply model of simultaneous causality,...
ECON 50: Using Big Data to Solve Economic and Social Problems
This course will show how "big data" can be used to understand and address some of the most important social and economic problems of our time. In empirical projects and weekly labs, students will work with real data to learn how the methods discussed in the course can be implemented in practice. The course will give students an introduction to frontier research and policy applications in economics and social science in a non-technical manner that does not require prior coursework in economics or statistics, making it suitable both for students exploring economics for the first time, as...
ECON 50A: Using Big Data to Solve Economic and Social Problems with Laboratory Component
Eng-sci 120: introduction to the mechanics of solids.
ES 120 teaches how to design simple mechanical structures to ensure that they satisfy given load requirements. Students learn mathematical methods for making prediction about how these structures deform and fail under load, and use these methods to analyze and test real structures. The course requires a good understanding of the mechanics of rigid bodies, elementary linear algebra, and multivariable calculus.
ENG-SCI 20R: The Physics of Sports
Students will focus on analyzing the dynamics of a specific sport/ physical activity through a project that they develop. this course teaches the application of the laws of physics to understand the world of athletics. emphasis will be placed on analyzing and drawing conclusions from the messy data produced by inertial measurement units (imus) and reconciling these data with video analysis. there are no specific prerequisites for the course however math 21a is recommended..
- Skip to main content
- Skip to primary sidebar
- Skip to footer
Additional menu

The 5 steps of the solving problem process
August 17, 2023 by MindManager Blog
Whether you run a business, manage a team, or work in an industry where change is the norm, it may feel like something is always going wrong. Thankfully, becoming proficient in the problem solving process can alleviate a great deal of the stress that business issues can create.
Understanding the right way to solve problems not only takes the guesswork out of how to deal with difficult, unexpected, or complex situations, it can lead to more effective long-term solutions.
In this article, we’ll walk you through the 5 steps of problem solving, and help you explore a few examples of problem solving scenarios where you can see the problem solving process in action before putting it to work.
Understanding the problem solving process
When something isn’t working, it’s important to understand what’s at the root of the problem so you can fix it and prevent it from happening again. That’s why resolving difficult or complex issues works best when you apply proven business problem solving tools and techniques – from soft skills, to software.
The problem solving process typically includes:
- Pinpointing what’s broken by gathering data and consulting with team members.
- Figuring out why it’s not working by mapping out and troubleshooting the problem.
- Deciding on the most effective way to fix it by brainstorming and then implementing a solution.
While skills like active listening, collaboration, and leadership play an important role in problem solving, tools like visual mapping software make it easier to define and share problem solving objectives, play out various solutions, and even put the best fit to work.
Before you can take your first step toward solving a problem, you need to have a clear idea of what the issue is and the outcome you want to achieve by resolving it.
For example, if your company currently manufactures 50 widgets a day, but you’ve started processing orders for 75 widgets a day, you could simply say you have a production deficit.
However, the problem solving process will prove far more valuable if you define the start and end point by clarifying that production is running short by 25 widgets a day, and you need to increase daily production by 50%.
Once you know where you’re at and where you need to end up, these five steps will take you from Point A to Point B:
- Figure out what’s causing the problem . You may need to gather knowledge and evaluate input from different documents, departments, and personnel to isolate the factors that are contributing to your problem. Knowledge visualization software like MindManager can help.
- Come up with a few viable solutions . Since hitting on exactly the right solution – right away – can be tough, brainstorming with your team and mapping out various scenarios is the best way to move forward. If your first strategy doesn’t pan out, you’ll have others on tap you can turn to.
- Choose the best option . Decision-making skills, and software that lets you lay out process relationships, priorities, and criteria, are invaluable for selecting the most promising solution. Whether it’s you or someone higher up making that choice, it should include weighing costs, time commitments, and any implementation hurdles.
- Put your chosen solution to work . Before implementing your fix of choice, you should make key personnel aware of changes that might affect their daily workflow, and set up benchmarks that will make it easy to see if your solution is working.
- Evaluate your outcome . Now comes the moment of truth: did the solution you implemented solve your problem? Do your benchmarks show you achieved the outcome you wanted? If so, congratulations! If not, you’ll need to tweak your solution to meet your problem solving goal.
In practice, you might not hit a home-run with every solution you execute. But the beauty of a repeatable process like problem solving is that you can carry out steps 4 and 5 again by drawing from the brainstorm options you documented during step 2.
Examples of problem solving scenarios
The best way to get a sense of how the problem solving process works before you try it for yourself is to work through some simple scenarios.
Here are three examples of how you can apply business problem solving techniques to common workplace challenges.
Scenario #1: Manufacturing
Building on our original manufacturing example, you determine that your company is consistently short producing 25 widgets a day and needs to increase daily production by 50%.
Since you’d like to gather data and input from both your manufacturing and sales order departments, you schedule a brainstorming session to discover the root cause of the shortage.
After examining four key production areas – machines, materials, methods, and management – you determine the cause of the problem: the material used to manufacture your widgets can only be fed into your equipment once the machinery warms up to a specific temperature for the day.
Your team comes up with three possible solutions.
- Leave your machinery running 24 hours so it’s always at temperature.
- Invest in equipment that heats up faster.
- Find an alternate material for your widgets.
After weighing the expense of the first two solutions, and conducting some online research, you decide that switching to a comparable but less expensive material that can be worked at a lower temperature is your best option.
You implement your plan, monitor your widget quality and output over the following week, and declare your solution a success when daily production increases by 100%.
Scenario #2: Service Delivery
Business training is booming and you’ve had to onboard new staff over the past month. Now you learn that several clients have expressed concern about the quality of your recent training sessions.
After speaking with both clients and staff, you discover there are actually two distinct factors contributing to your quality problem:
- The additional conference room you’ve leased to accommodate your expanding training sessions has terrible acoustics
- The AV equipment you’ve purchased to accommodate your expanding workforce is on back-order – and your new hires have been making do without
You could look for a new conference room or re-schedule upcoming training sessions until after your new equipment arrives. But your team collaboratively determines that the best way to mitigate both issues at once is by temporarily renting the high-quality sound and visual system they need.
Using benchmarks that include several weeks of feedback from session attendees, and random session spot-checks you conduct personally, you conclude the solution has worked.
Scenario #3: Marketing
You’ve invested heavily in product marketing, but still can’t meet your sales goals. Specifically, you missed your revenue target by 30% last year and would like to meet that same target this year.
After collecting and examining reams of information from your sales and accounting departments, you sit down with your marketing team to figure out what’s hindering your success in the marketplace.
Determining that your product isn’t competitively priced, you map out two viable solutions.
- Hire a third-party specialist to conduct a detailed market analysis.
- Drop the price of your product to undercut competitors.
Since you’re in a hurry for results, you decide to immediately reduce the price of your product and market it accordingly.
When revenue figures for the following quarter show sales have declined even further – and marketing surveys show potential customers are doubting the quality of your product – you revert back to your original pricing, revisit your problem solving process, and implement the market analysis solution instead.
With the valuable information you gain, you finally arrive at just the right product price for your target market and sales begin to pick up. Although you miss your revenue target again this year, you meet it by the second quarter of the following year.
Kickstart your collaborative brainstorming sessions and try MindManager for free today !
Ready to take the next step?
MindManager helps boost collaboration and productivity among remote and hybrid teams to achieve better results, faster.

Why choose MindManager?
MindManager® helps individuals, teams, and enterprises bring greater clarity and structure to plans, projects, and processes. It provides visual productivity tools and mind mapping software to help take you and your organization to where you want to be.
Explore MindManager
Application of Quantitative Methods on problem solving
- Conference: International Scientific conference EUROBRAND

- Inventis Clinical Trial Management

- West University of Timisoara
Abstract and Figures

Discover the world's research
- 25+ million members
- 160+ million publication pages
- 2.3+ billion citations

- J B Lawrence
- S Krcevinac
- D Joksimovic
- Recruit researchers
- Join for free
- Login Email Tip: Most researchers use their institutional email address as their ResearchGate login Password Forgot password? Keep me logged in Log in or Continue with Google Welcome back! Please log in. Email · Hint Tip: Most researchers use their institutional email address as their ResearchGate login Password Forgot password? Keep me logged in Log in or Continue with Google No account? Sign up

1st Edition
Quantitative Methods for Second Language Research A Problem-Solving Approach
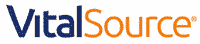
- Taylor & Francis eBooks (Institutional Purchase) Opens in new tab or window
Description
Quantitative Methods for Second Language Research introduces approaches to and techniques for quantitative data analysis in second language research, with a primary focus on second language learning and assessment research. It takes a conceptual, problem-solving approach by emphasizing the understanding of statistical theory and its application to research problems while paying less attention to the mathematical side of statistical analysis. The text discusses a range of common statistical analysis techniques, presented and illustrated through applications of the IBM Statistical Package for Social Sciences (SPSS) program. These include tools for descriptive analysis (e.g., means and percentages) as well as inferential analysis (e.g., correlational analysis, t -tests, and analysis of variance [ANOVA]). The text provides conceptual explanations of quantitative methods through the use of examples, cases, and published studies in the field. In addition, a companion website to the book hosts slides, review exercises, and answer keys for each chapter as well as SPSS files. Practical and lucid, this book is the ideal resource for data analysis for graduate students and researchers in applied linguistics.
Table of Contents
Carsten Roever is Associate Professor in Applied Linguistics in the School of Languages and Linguistics at the University of Melbourne, Australia. Aek Phakiti is Associate Professor in TESOL in the Sydney School of Education and Social Work at the University of Sydney, Australia.
Critics' Reviews
"This book affords beginning applied linguists an enjoyable journey through all important inferential tests to regression analysis. With their clear style, a wealth of language learning and testing examples, and online SPSS exercises, the authors make it easy to become statistics savvy and learn to love statistics. A fantastic resource!" Lourdes Ortega, Georgetown University, USA " Quantitative Methods for Second Language Research cuts through the mathematical jargon of statistical analysis and teaches researchers the necessary skills to carry out quantitative studies in applied linguistics. With real case studies and a problem-solving approach, it is a must-have resource for those who are embarking on quantitative research for the first time." Heath Rose, The University of Oxford, UK "In each of their coverage of twelve commonly used parametric and non-parametric statistical procedures, the authors discuss assumptions, significance testing, effect sizes, and SPSS examples. The straightforward writing style, practical problem-solving approach, and companion website make this book accessible and useful for beginners and veteran quantitative researchers alike." James Dean Brown, The University of Hawai’i at Manoa, USA
About VitalSource eBooks
VitalSource is a leading provider of eBooks.
- Access your materials anywhere, at anytime.
- Customer preferences like text size, font type, page color and more.
- Take annotations in line as you read.
Multiple eBook Copies
This eBook is already in your shopping cart. If you would like to replace it with a different purchasing option please remove the current eBook option from your cart.
Book Preview

The country you have selected will result in the following:
- Product pricing will be adjusted to match the corresponding currency.
- The title Perception will be removed from your cart because it is not available in this region.
Our systems are now restored following recent technical disruption, and we’re working hard to catch up on publishing. We apologise for the inconvenience caused. Find out more: https://www.cambridge.org/universitypress/about-us/news-and-blogs/cambridge-university-press-publishing-update-following-technical-disruption
We use cookies to distinguish you from other users and to provide you with a better experience on our websites. Close this message to accept cookies or find out how to manage your cookie settings .
Login Alert

- > Problem Solving in Organizations
- > Qualitative research methods
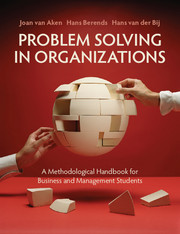
Book contents
- Frontmatter
- Part I Fundamentals
- Part II The Problem Solving Project
- Part III Methods
- 11 Qualitative research methods
- 12 Searching and using scholarly literature
- Chapter 13 Quality criteria for research
- Part IV Case Material
- Author index
11 - Qualitative research methods
from Part III - Methods
Qualitative versus quantitative
The literature on methodology distinguishes between qualitative and quantitative research methods. The term ‘qualitative’ does not refer to the quality of methods. Qualitative methods are those that are oriented towards the discovery of qualities of things – that is, the properties of objects, phenomena, situations, people, meanings and events. In contrast, quantitative methods are oriented towards the number or amount of these qualities. This chapter discusses a number of qualitative research methods that can be used in FPS projects. For quantitative research methods, we refer the reader to textbooks on social science methodology, such as those by Cooper and Schindler (2010) and Hair et al . (2005).
Imagine a marketing manager who would like to know the opinions of potential customers about a television commercial. He/she could ask some respondents to talk freely about their feelings with regard to the commercial, their associations, what they like about it and what they do not, and so on. Such a study would be qualitative in nature, since it aims to uncover the characteristics of people, in this case their attitude towards the commercial. The same marketing manager could also use a standardized questionnaire and ask respondents to express the degree to which they understand and like the commercial on a five-point scale. Such a study would be quantitative in nature, since it measures the amount of a particular property.
Access options
Save book to kindle.
To save this book to your Kindle, first ensure [email protected] is added to your Approved Personal Document E-mail List under your Personal Document Settings on the Manage Your Content and Devices page of your Amazon account. Then enter the ‘name’ part of your Kindle email address below. Find out more about saving to your Kindle .
Note you can select to save to either the @free.kindle.com or @kindle.com variations. ‘@free.kindle.com’ emails are free but can only be saved to your device when it is connected to wi-fi. ‘@kindle.com’ emails can be delivered even when you are not connected to wi-fi, but note that service fees apply.
Find out more about the Kindle Personal Document Service .
- Qualitative research methods
- Joan van Aken , Technische Universiteit Eindhoven, The Netherlands , Hans Berends , Technische Universiteit Eindhoven, The Netherlands , Hans van der Bij , Rijksuniversiteit Groningen, The Netherlands
- Book: Problem Solving in Organizations
- Online publication: 05 August 2012
- Chapter DOI: https://doi.org/10.1017/CBO9781139094351.015
Save book to Dropbox
To save content items to your account, please confirm that you agree to abide by our usage policies. If this is the first time you use this feature, you will be asked to authorise Cambridge Core to connect with your account. Find out more about saving content to Dropbox .
Save book to Google Drive
To save content items to your account, please confirm that you agree to abide by our usage policies. If this is the first time you use this feature, you will be asked to authorise Cambridge Core to connect with your account. Find out more about saving content to Google Drive .

COMMENTS
What is an example of quantitative reasoning? An example of quantitative reasoning would be one of George Polya 's steps to problem solving, developing a plan.
Quantitative research deals in numbers, logic, and an objective stance. Quantitative research focuses on numeric and unchanging data and detailed, convergent reasoning rather than divergent reasoning [i.e., the generation of a variety of ideas about a research problem in a spontaneous, free-flowing manner]. Its main characteristics are:
It concludes by describing the function of quantitative decision-making methods and by recommending an approach for using decision-making tools to gauge options and solve problems while ensuring that soft issues are incorporated into the process.
But as far as I'm concerned, realizing how the mind constructs knowledge and understanding in a problem-solving task is an empowering notion. Alan Schoenfeld, mathematician and math- education specialist, has identified four aspects of the mental process of problem-solving that are essential: Resources, Heuristics, Control, and Belief.
Problem solving is best approached by first starting at the end: identifying exactly what you are looking for. From there, you then work backwards, asking "what information and procedures will I need to find this?" Very few interesting questions can be answered in one mathematical step; often times you will need to chain together a solution pathway, a series of steps that will allow you to ...
Mastering problem-solving empowers you to identify problems, break them down into manageable parts, and apply quantitative and qualitative methods to reach well-reasoned conclusions.
Quantitative method is used to summarize, average, find patterns, make predictions, and test causal associations as well as generalizing results to wider populations. It allows us to quantify effect sizes, determine the strength of associations, rank priorities, and weigh the strength of evidence of effectiveness.
ES322 EXAMPLE QUANTITATIVE PROBLEM SOLVING TECHNIQUES WITH SOLUTIONS Primer on Solving Quantitative Style Word Problems in Physical Science and Geology Study Technique: Follow the step-by-step problem solving method outlined below; review the attached example problems and solutions; practice repetitively and compare your answers to key.
Quantitative research methods You can use quantitative research methods for descriptive, correlational or experimental research. In descriptive research, you simply seek an overall summary of your study variables. In correlational research, you investigate relationships between your study variables.
Questions in the Quantitative Reasoning measure of the GRE® General Test ask you to model and solve problems using quantitative, or mathematical, methods. Generally, there are three basic steps in solving a mathematics problem: • Step 1: Understand the problem. • Step 2: Carry out a strategy for solving the problem.
Figure 2. The decision making process. We can define quantitative techniques like mathematical and statistical models which are describing a diverse array of variables' relationship, and they are designed to assist managers with management problem-solving and decision making.
ABSTRACT. Quantitative Methods for Second Language Research introduces approaches to and techniques for quantitative data analysis in second language research, with a primary focus on second language learning and assessment research. It takes a conceptual, problem-solving approach by emphasizing the understanding of statistical theory and its ...
Methodology The methodology of the course is based on what is known as Operations Research, a science that offer to the decision maker different quantitative methodologies in order to make decisions. The objective of the course is to learn the fundamental concepts, the quantitative models, up to date solution techniques in problem solving and complex decision making. During the course we will ...
Quantitative analysis is the use of mathematical and statistical techniques to assess the performance of a business. Before the advent of quantitative analysis, many company directors based their decisions on experience and gut. Business owners can now use quantitative methods to predict trends, determine the allocation of resources, and manage ...
Decision Making with Quantitative Tools Quantitative techniques help a manager improve the overall quality of decision making. These techniques are most commonly used in the rational/logical decision model, but they can apply in any of the other models as well. Among the most common techniques are decision trees, payback analysis, and simulations.
Students are expected to diligently read prior to class, such that sessions can be weighted towards performing in-class problem solving and lecturing can be reserved for the nuanced points of the material.
Though qualitative and quantitative research methods lies on separate continuum, they all aimed at identifying educational problems using different approach. This study critically examined the usefulness of both qualitative and quantitative approaches in researching problem-solving ability in science education curriculum.
The quantitative approach to decision-making isolates optimal decisions using statistics to analyze the potential outcomes. Learn the methods of decision trees, network analysis, simulation models ...
In this article, we'll walk you through the 5 steps of problem solving, and help you explore a few examples of problem solving scenarios where you can see the problem solving process in action before putting it to work.
Within scope of this paper there are presented quantitative methods-linear programming applied on problems defined by case study of Calgary Desk Company. In this paper is presented situation of ...
Description. Quantitative Methods for Second Language Research introduces approaches to and techniques for quantitative data analysis in second language research, with a primary focus on second language learning and assessment research. It takes a conceptual, problem-solving approach by emphasizing the understanding of statistical theory and ...
This chapter discusses a number of qualitative research methods that can be used in FPS projects. For quantitative research methods, we refer the reader to textbooks on social science methodology, such as those by Cooper and Schindler (2010) and Hair et al. (2005).