Criteria for Good Qualitative Research: A Comprehensive Review
- Regular Article
- Open access
- Published: 18 September 2021
- Volume 31 , pages 679–689, ( 2022 )

Cite this article
You have full access to this open access article
- Drishti Yadav ORCID: orcid.org/0000-0002-2974-0323 1
102k Accesses
47 Citations
70 Altmetric
Explore all metrics
This review aims to synthesize a published set of evaluative criteria for good qualitative research. The aim is to shed light on existing standards for assessing the rigor of qualitative research encompassing a range of epistemological and ontological standpoints. Using a systematic search strategy, published journal articles that deliberate criteria for rigorous research were identified. Then, references of relevant articles were surveyed to find noteworthy, distinct, and well-defined pointers to good qualitative research. This review presents an investigative assessment of the pivotal features in qualitative research that can permit the readers to pass judgment on its quality and to condemn it as good research when objectively and adequately utilized. Overall, this review underlines the crux of qualitative research and accentuates the necessity to evaluate such research by the very tenets of its being. It also offers some prospects and recommendations to improve the quality of qualitative research. Based on the findings of this review, it is concluded that quality criteria are the aftereffect of socio-institutional procedures and existing paradigmatic conducts. Owing to the paradigmatic diversity of qualitative research, a single and specific set of quality criteria is neither feasible nor anticipated. Since qualitative research is not a cohesive discipline, researchers need to educate and familiarize themselves with applicable norms and decisive factors to evaluate qualitative research from within its theoretical and methodological framework of origin.
Similar content being viewed by others
Good Qualitative Research: Opening up the Debate
Beyond qualitative/quantitative structuralism: the positivist qualitative research and the paradigmatic disclaimer.
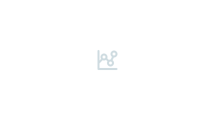
What is Qualitative in Research
Avoid common mistakes on your manuscript.
Introduction
“… It is important to regularly dialogue about what makes for good qualitative research” (Tracy, 2010 , p. 837)
To decide what represents good qualitative research is highly debatable. There are numerous methods that are contained within qualitative research and that are established on diverse philosophical perspectives. Bryman et al., ( 2008 , p. 262) suggest that “It is widely assumed that whereas quality criteria for quantitative research are well‐known and widely agreed, this is not the case for qualitative research.” Hence, the question “how to evaluate the quality of qualitative research” has been continuously debated. There are many areas of science and technology wherein these debates on the assessment of qualitative research have taken place. Examples include various areas of psychology: general psychology (Madill et al., 2000 ); counseling psychology (Morrow, 2005 ); and clinical psychology (Barker & Pistrang, 2005 ), and other disciplines of social sciences: social policy (Bryman et al., 2008 ); health research (Sparkes, 2001 ); business and management research (Johnson et al., 2006 ); information systems (Klein & Myers, 1999 ); and environmental studies (Reid & Gough, 2000 ). In the literature, these debates are enthused by the impression that the blanket application of criteria for good qualitative research developed around the positivist paradigm is improper. Such debates are based on the wide range of philosophical backgrounds within which qualitative research is conducted (e.g., Sandberg, 2000 ; Schwandt, 1996 ). The existence of methodological diversity led to the formulation of different sets of criteria applicable to qualitative research.
Among qualitative researchers, the dilemma of governing the measures to assess the quality of research is not a new phenomenon, especially when the virtuous triad of objectivity, reliability, and validity (Spencer et al., 2004 ) are not adequate. Occasionally, the criteria of quantitative research are used to evaluate qualitative research (Cohen & Crabtree, 2008 ; Lather, 2004 ). Indeed, Howe ( 2004 ) claims that the prevailing paradigm in educational research is scientifically based experimental research. Hypotheses and conjectures about the preeminence of quantitative research can weaken the worth and usefulness of qualitative research by neglecting the prominence of harmonizing match for purpose on research paradigm, the epistemological stance of the researcher, and the choice of methodology. Researchers have been reprimanded concerning this in “paradigmatic controversies, contradictions, and emerging confluences” (Lincoln & Guba, 2000 ).
In general, qualitative research tends to come from a very different paradigmatic stance and intrinsically demands distinctive and out-of-the-ordinary criteria for evaluating good research and varieties of research contributions that can be made. This review attempts to present a series of evaluative criteria for qualitative researchers, arguing that their choice of criteria needs to be compatible with the unique nature of the research in question (its methodology, aims, and assumptions). This review aims to assist researchers in identifying some of the indispensable features or markers of high-quality qualitative research. In a nutshell, the purpose of this systematic literature review is to analyze the existing knowledge on high-quality qualitative research and to verify the existence of research studies dealing with the critical assessment of qualitative research based on the concept of diverse paradigmatic stances. Contrary to the existing reviews, this review also suggests some critical directions to follow to improve the quality of qualitative research in different epistemological and ontological perspectives. This review is also intended to provide guidelines for the acceleration of future developments and dialogues among qualitative researchers in the context of assessing the qualitative research.
The rest of this review article is structured in the following fashion: Sect. Methods describes the method followed for performing this review. Section Criteria for Evaluating Qualitative Studies provides a comprehensive description of the criteria for evaluating qualitative studies. This section is followed by a summary of the strategies to improve the quality of qualitative research in Sect. Improving Quality: Strategies . Section How to Assess the Quality of the Research Findings? provides details on how to assess the quality of the research findings. After that, some of the quality checklists (as tools to evaluate quality) are discussed in Sect. Quality Checklists: Tools for Assessing the Quality . At last, the review ends with the concluding remarks presented in Sect. Conclusions, Future Directions and Outlook . Some prospects in qualitative research for enhancing its quality and usefulness in the social and techno-scientific research community are also presented in Sect. Conclusions, Future Directions and Outlook .
For this review, a comprehensive literature search was performed from many databases using generic search terms such as Qualitative Research , Criteria , etc . The following databases were chosen for the literature search based on the high number of results: IEEE Explore, ScienceDirect, PubMed, Google Scholar, and Web of Science. The following keywords (and their combinations using Boolean connectives OR/AND) were adopted for the literature search: qualitative research, criteria, quality, assessment, and validity. The synonyms for these keywords were collected and arranged in a logical structure (see Table 1 ). All publications in journals and conference proceedings later than 1950 till 2021 were considered for the search. Other articles extracted from the references of the papers identified in the electronic search were also included. A large number of publications on qualitative research were retrieved during the initial screening. Hence, to include the searches with the main focus on criteria for good qualitative research, an inclusion criterion was utilized in the search string.
From the selected databases, the search retrieved a total of 765 publications. Then, the duplicate records were removed. After that, based on the title and abstract, the remaining 426 publications were screened for their relevance by using the following inclusion and exclusion criteria (see Table 2 ). Publications focusing on evaluation criteria for good qualitative research were included, whereas those works which delivered theoretical concepts on qualitative research were excluded. Based on the screening and eligibility, 45 research articles were identified that offered explicit criteria for evaluating the quality of qualitative research and were found to be relevant to this review.
Figure 1 illustrates the complete review process in the form of PRISMA flow diagram. PRISMA, i.e., “preferred reporting items for systematic reviews and meta-analyses” is employed in systematic reviews to refine the quality of reporting.
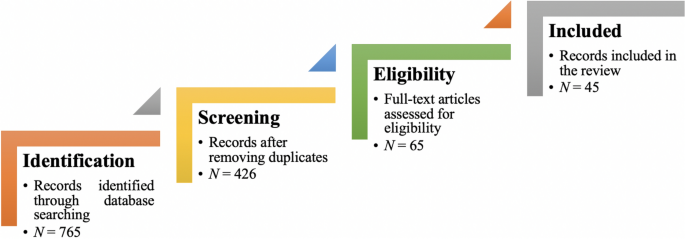
PRISMA flow diagram illustrating the search and inclusion process. N represents the number of records
Criteria for Evaluating Qualitative Studies
Fundamental criteria: general research quality.
Various researchers have put forward criteria for evaluating qualitative research, which have been summarized in Table 3 . Also, the criteria outlined in Table 4 effectively deliver the various approaches to evaluate and assess the quality of qualitative work. The entries in Table 4 are based on Tracy’s “Eight big‐tent criteria for excellent qualitative research” (Tracy, 2010 ). Tracy argues that high-quality qualitative work should formulate criteria focusing on the worthiness, relevance, timeliness, significance, morality, and practicality of the research topic, and the ethical stance of the research itself. Researchers have also suggested a series of questions as guiding principles to assess the quality of a qualitative study (Mays & Pope, 2020 ). Nassaji ( 2020 ) argues that good qualitative research should be robust, well informed, and thoroughly documented.
Qualitative Research: Interpretive Paradigms
All qualitative researchers follow highly abstract principles which bring together beliefs about ontology, epistemology, and methodology. These beliefs govern how the researcher perceives and acts. The net, which encompasses the researcher’s epistemological, ontological, and methodological premises, is referred to as a paradigm, or an interpretive structure, a “Basic set of beliefs that guides action” (Guba, 1990 ). Four major interpretive paradigms structure the qualitative research: positivist and postpositivist, constructivist interpretive, critical (Marxist, emancipatory), and feminist poststructural. The complexity of these four abstract paradigms increases at the level of concrete, specific interpretive communities. Table 5 presents these paradigms and their assumptions, including their criteria for evaluating research, and the typical form that an interpretive or theoretical statement assumes in each paradigm. Moreover, for evaluating qualitative research, quantitative conceptualizations of reliability and validity are proven to be incompatible (Horsburgh, 2003 ). In addition, a series of questions have been put forward in the literature to assist a reviewer (who is proficient in qualitative methods) for meticulous assessment and endorsement of qualitative research (Morse, 2003 ). Hammersley ( 2007 ) also suggests that guiding principles for qualitative research are advantageous, but methodological pluralism should not be simply acknowledged for all qualitative approaches. Seale ( 1999 ) also points out the significance of methodological cognizance in research studies.
Table 5 reflects that criteria for assessing the quality of qualitative research are the aftermath of socio-institutional practices and existing paradigmatic standpoints. Owing to the paradigmatic diversity of qualitative research, a single set of quality criteria is neither possible nor desirable. Hence, the researchers must be reflexive about the criteria they use in the various roles they play within their research community.
Improving Quality: Strategies
Another critical question is “How can the qualitative researchers ensure that the abovementioned quality criteria can be met?” Lincoln and Guba ( 1986 ) delineated several strategies to intensify each criteria of trustworthiness. Other researchers (Merriam & Tisdell, 2016 ; Shenton, 2004 ) also presented such strategies. A brief description of these strategies is shown in Table 6 .
It is worth mentioning that generalizability is also an integral part of qualitative research (Hays & McKibben, 2021 ). In general, the guiding principle pertaining to generalizability speaks about inducing and comprehending knowledge to synthesize interpretive components of an underlying context. Table 7 summarizes the main metasynthesis steps required to ascertain generalizability in qualitative research.
Figure 2 reflects the crucial components of a conceptual framework and their contribution to decisions regarding research design, implementation, and applications of results to future thinking, study, and practice (Johnson et al., 2020 ). The synergy and interrelationship of these components signifies their role to different stances of a qualitative research study.
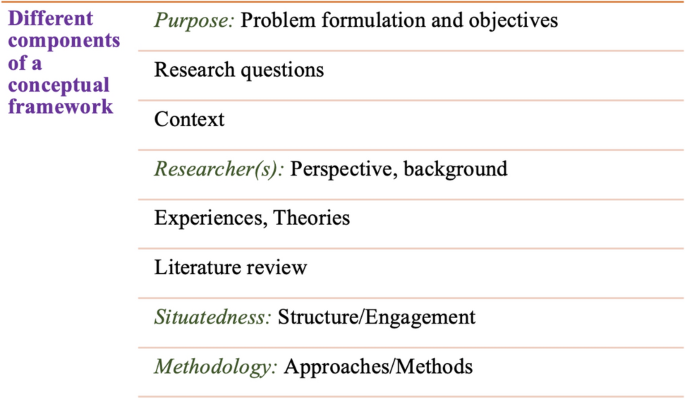
Essential elements of a conceptual framework
In a nutshell, to assess the rationale of a study, its conceptual framework and research question(s), quality criteria must take account of the following: lucid context for the problem statement in the introduction; well-articulated research problems and questions; precise conceptual framework; distinct research purpose; and clear presentation and investigation of the paradigms. These criteria would expedite the quality of qualitative research.
How to Assess the Quality of the Research Findings?
The inclusion of quotes or similar research data enhances the confirmability in the write-up of the findings. The use of expressions (for instance, “80% of all respondents agreed that” or “only one of the interviewees mentioned that”) may also quantify qualitative findings (Stenfors et al., 2020 ). On the other hand, the persuasive reason for “why this may not help in intensifying the research” has also been provided (Monrouxe & Rees, 2020 ). Further, the Discussion and Conclusion sections of an article also prove robust markers of high-quality qualitative research, as elucidated in Table 8 .
Quality Checklists: Tools for Assessing the Quality
Numerous checklists are available to speed up the assessment of the quality of qualitative research. However, if used uncritically and recklessly concerning the research context, these checklists may be counterproductive. I recommend that such lists and guiding principles may assist in pinpointing the markers of high-quality qualitative research. However, considering enormous variations in the authors’ theoretical and philosophical contexts, I would emphasize that high dependability on such checklists may say little about whether the findings can be applied in your setting. A combination of such checklists might be appropriate for novice researchers. Some of these checklists are listed below:
The most commonly used framework is Consolidated Criteria for Reporting Qualitative Research (COREQ) (Tong et al., 2007 ). This framework is recommended by some journals to be followed by the authors during article submission.
Standards for Reporting Qualitative Research (SRQR) is another checklist that has been created particularly for medical education (O’Brien et al., 2014 ).
Also, Tracy ( 2010 ) and Critical Appraisal Skills Programme (CASP, 2021 ) offer criteria for qualitative research relevant across methods and approaches.
Further, researchers have also outlined different criteria as hallmarks of high-quality qualitative research. For instance, the “Road Trip Checklist” (Epp & Otnes, 2021 ) provides a quick reference to specific questions to address different elements of high-quality qualitative research.
Conclusions, Future Directions, and Outlook
This work presents a broad review of the criteria for good qualitative research. In addition, this article presents an exploratory analysis of the essential elements in qualitative research that can enable the readers of qualitative work to judge it as good research when objectively and adequately utilized. In this review, some of the essential markers that indicate high-quality qualitative research have been highlighted. I scope them narrowly to achieve rigor in qualitative research and note that they do not completely cover the broader considerations necessary for high-quality research. This review points out that a universal and versatile one-size-fits-all guideline for evaluating the quality of qualitative research does not exist. In other words, this review also emphasizes the non-existence of a set of common guidelines among qualitative researchers. In unison, this review reinforces that each qualitative approach should be treated uniquely on account of its own distinctive features for different epistemological and disciplinary positions. Owing to the sensitivity of the worth of qualitative research towards the specific context and the type of paradigmatic stance, researchers should themselves analyze what approaches can be and must be tailored to ensemble the distinct characteristics of the phenomenon under investigation. Although this article does not assert to put forward a magic bullet and to provide a one-stop solution for dealing with dilemmas about how, why, or whether to evaluate the “goodness” of qualitative research, it offers a platform to assist the researchers in improving their qualitative studies. This work provides an assembly of concerns to reflect on, a series of questions to ask, and multiple sets of criteria to look at, when attempting to determine the quality of qualitative research. Overall, this review underlines the crux of qualitative research and accentuates the need to evaluate such research by the very tenets of its being. Bringing together the vital arguments and delineating the requirements that good qualitative research should satisfy, this review strives to equip the researchers as well as reviewers to make well-versed judgment about the worth and significance of the qualitative research under scrutiny. In a nutshell, a comprehensive portrayal of the research process (from the context of research to the research objectives, research questions and design, speculative foundations, and from approaches of collecting data to analyzing the results, to deriving inferences) frequently proliferates the quality of a qualitative research.
Prospects : A Road Ahead for Qualitative Research
Irrefutably, qualitative research is a vivacious and evolving discipline wherein different epistemological and disciplinary positions have their own characteristics and importance. In addition, not surprisingly, owing to the sprouting and varied features of qualitative research, no consensus has been pulled off till date. Researchers have reflected various concerns and proposed several recommendations for editors and reviewers on conducting reviews of critical qualitative research (Levitt et al., 2021 ; McGinley et al., 2021 ). Following are some prospects and a few recommendations put forward towards the maturation of qualitative research and its quality evaluation:
In general, most of the manuscript and grant reviewers are not qualitative experts. Hence, it is more likely that they would prefer to adopt a broad set of criteria. However, researchers and reviewers need to keep in mind that it is inappropriate to utilize the same approaches and conducts among all qualitative research. Therefore, future work needs to focus on educating researchers and reviewers about the criteria to evaluate qualitative research from within the suitable theoretical and methodological context.
There is an urgent need to refurbish and augment critical assessment of some well-known and widely accepted tools (including checklists such as COREQ, SRQR) to interrogate their applicability on different aspects (along with their epistemological ramifications).
Efforts should be made towards creating more space for creativity, experimentation, and a dialogue between the diverse traditions of qualitative research. This would potentially help to avoid the enforcement of one's own set of quality criteria on the work carried out by others.
Moreover, journal reviewers need to be aware of various methodological practices and philosophical debates.
It is pivotal to highlight the expressions and considerations of qualitative researchers and bring them into a more open and transparent dialogue about assessing qualitative research in techno-scientific, academic, sociocultural, and political rooms.
Frequent debates on the use of evaluative criteria are required to solve some potentially resolved issues (including the applicability of a single set of criteria in multi-disciplinary aspects). Such debates would not only benefit the group of qualitative researchers themselves, but primarily assist in augmenting the well-being and vivacity of the entire discipline.
To conclude, I speculate that the criteria, and my perspective, may transfer to other methods, approaches, and contexts. I hope that they spark dialog and debate – about criteria for excellent qualitative research and the underpinnings of the discipline more broadly – and, therefore, help improve the quality of a qualitative study. Further, I anticipate that this review will assist the researchers to contemplate on the quality of their own research, to substantiate research design and help the reviewers to review qualitative research for journals. On a final note, I pinpoint the need to formulate a framework (encompassing the prerequisites of a qualitative study) by the cohesive efforts of qualitative researchers of different disciplines with different theoretic-paradigmatic origins. I believe that tailoring such a framework (of guiding principles) paves the way for qualitative researchers to consolidate the status of qualitative research in the wide-ranging open science debate. Dialogue on this issue across different approaches is crucial for the impending prospects of socio-techno-educational research.
Amin, M. E. K., Nørgaard, L. S., Cavaco, A. M., Witry, M. J., Hillman, L., Cernasev, A., & Desselle, S. P. (2020). Establishing trustworthiness and authenticity in qualitative pharmacy research. Research in Social and Administrative Pharmacy, 16 (10), 1472–1482.
Article Google Scholar
Barker, C., & Pistrang, N. (2005). Quality criteria under methodological pluralism: Implications for conducting and evaluating research. American Journal of Community Psychology, 35 (3–4), 201–212.
Bryman, A., Becker, S., & Sempik, J. (2008). Quality criteria for quantitative, qualitative and mixed methods research: A view from social policy. International Journal of Social Research Methodology, 11 (4), 261–276.
Caelli, K., Ray, L., & Mill, J. (2003). ‘Clear as mud’: Toward greater clarity in generic qualitative research. International Journal of Qualitative Methods, 2 (2), 1–13.
CASP (2021). CASP checklists. Retrieved May 2021 from https://casp-uk.net/casp-tools-checklists/
Cohen, D. J., & Crabtree, B. F. (2008). Evaluative criteria for qualitative research in health care: Controversies and recommendations. The Annals of Family Medicine, 6 (4), 331–339.
Denzin, N. K., & Lincoln, Y. S. (2005). Introduction: The discipline and practice of qualitative research. In N. K. Denzin & Y. S. Lincoln (Eds.), The sage handbook of qualitative research (pp. 1–32). Sage Publications Ltd.
Google Scholar
Elliott, R., Fischer, C. T., & Rennie, D. L. (1999). Evolving guidelines for publication of qualitative research studies in psychology and related fields. British Journal of Clinical Psychology, 38 (3), 215–229.
Epp, A. M., & Otnes, C. C. (2021). High-quality qualitative research: Getting into gear. Journal of Service Research . https://doi.org/10.1177/1094670520961445
Guba, E. G. (1990). The paradigm dialog. In Alternative paradigms conference, mar, 1989, Indiana u, school of education, San Francisco, ca, us . Sage Publications, Inc.
Hammersley, M. (2007). The issue of quality in qualitative research. International Journal of Research and Method in Education, 30 (3), 287–305.
Haven, T. L., Errington, T. M., Gleditsch, K. S., van Grootel, L., Jacobs, A. M., Kern, F. G., & Mokkink, L. B. (2020). Preregistering qualitative research: A Delphi study. International Journal of Qualitative Methods, 19 , 1609406920976417.
Hays, D. G., & McKibben, W. B. (2021). Promoting rigorous research: Generalizability and qualitative research. Journal of Counseling and Development, 99 (2), 178–188.
Horsburgh, D. (2003). Evaluation of qualitative research. Journal of Clinical Nursing, 12 (2), 307–312.
Howe, K. R. (2004). A critique of experimentalism. Qualitative Inquiry, 10 (1), 42–46.
Johnson, J. L., Adkins, D., & Chauvin, S. (2020). A review of the quality indicators of rigor in qualitative research. American Journal of Pharmaceutical Education, 84 (1), 7120.
Johnson, P., Buehring, A., Cassell, C., & Symon, G. (2006). Evaluating qualitative management research: Towards a contingent criteriology. International Journal of Management Reviews, 8 (3), 131–156.
Klein, H. K., & Myers, M. D. (1999). A set of principles for conducting and evaluating interpretive field studies in information systems. MIS Quarterly, 23 (1), 67–93.
Lather, P. (2004). This is your father’s paradigm: Government intrusion and the case of qualitative research in education. Qualitative Inquiry, 10 (1), 15–34.
Levitt, H. M., Morrill, Z., Collins, K. M., & Rizo, J. L. (2021). The methodological integrity of critical qualitative research: Principles to support design and research review. Journal of Counseling Psychology, 68 (3), 357.
Lincoln, Y. S., & Guba, E. G. (1986). But is it rigorous? Trustworthiness and authenticity in naturalistic evaluation. New Directions for Program Evaluation, 1986 (30), 73–84.
Lincoln, Y. S., & Guba, E. G. (2000). Paradigmatic controversies, contradictions and emerging confluences. In N. K. Denzin & Y. S. Lincoln (Eds.), Handbook of qualitative research (2nd ed., pp. 163–188). Sage Publications.
Madill, A., Jordan, A., & Shirley, C. (2000). Objectivity and reliability in qualitative analysis: Realist, contextualist and radical constructionist epistemologies. British Journal of Psychology, 91 (1), 1–20.
Mays, N., & Pope, C. (2020). Quality in qualitative research. Qualitative Research in Health Care . https://doi.org/10.1002/9781119410867.ch15
McGinley, S., Wei, W., Zhang, L., & Zheng, Y. (2021). The state of qualitative research in hospitality: A 5-year review 2014 to 2019. Cornell Hospitality Quarterly, 62 (1), 8–20.
Merriam, S., & Tisdell, E. (2016). Qualitative research: A guide to design and implementation. San Francisco, US.
Meyer, M., & Dykes, J. (2019). Criteria for rigor in visualization design study. IEEE Transactions on Visualization and Computer Graphics, 26 (1), 87–97.
Monrouxe, L. V., & Rees, C. E. (2020). When I say… quantification in qualitative research. Medical Education, 54 (3), 186–187.
Morrow, S. L. (2005). Quality and trustworthiness in qualitative research in counseling psychology. Journal of Counseling Psychology, 52 (2), 250.
Morse, J. M. (2003). A review committee’s guide for evaluating qualitative proposals. Qualitative Health Research, 13 (6), 833–851.
Nassaji, H. (2020). Good qualitative research. Language Teaching Research, 24 (4), 427–431.
O’Brien, B. C., Harris, I. B., Beckman, T. J., Reed, D. A., & Cook, D. A. (2014). Standards for reporting qualitative research: A synthesis of recommendations. Academic Medicine, 89 (9), 1245–1251.
O’Connor, C., & Joffe, H. (2020). Intercoder reliability in qualitative research: Debates and practical guidelines. International Journal of Qualitative Methods, 19 , 1609406919899220.
Reid, A., & Gough, S. (2000). Guidelines for reporting and evaluating qualitative research: What are the alternatives? Environmental Education Research, 6 (1), 59–91.
Rocco, T. S. (2010). Criteria for evaluating qualitative studies. Human Resource Development International . https://doi.org/10.1080/13678868.2010.501959
Sandberg, J. (2000). Understanding human competence at work: An interpretative approach. Academy of Management Journal, 43 (1), 9–25.
Schwandt, T. A. (1996). Farewell to criteriology. Qualitative Inquiry, 2 (1), 58–72.
Seale, C. (1999). Quality in qualitative research. Qualitative Inquiry, 5 (4), 465–478.
Shenton, A. K. (2004). Strategies for ensuring trustworthiness in qualitative research projects. Education for Information, 22 (2), 63–75.
Sparkes, A. C. (2001). Myth 94: Qualitative health researchers will agree about validity. Qualitative Health Research, 11 (4), 538–552.
Spencer, L., Ritchie, J., Lewis, J., & Dillon, L. (2004). Quality in qualitative evaluation: A framework for assessing research evidence.
Stenfors, T., Kajamaa, A., & Bennett, D. (2020). How to assess the quality of qualitative research. The Clinical Teacher, 17 (6), 596–599.
Taylor, E. W., Beck, J., & Ainsworth, E. (2001). Publishing qualitative adult education research: A peer review perspective. Studies in the Education of Adults, 33 (2), 163–179.
Tong, A., Sainsbury, P., & Craig, J. (2007). Consolidated criteria for reporting qualitative research (COREQ): A 32-item checklist for interviews and focus groups. International Journal for Quality in Health Care, 19 (6), 349–357.
Tracy, S. J. (2010). Qualitative quality: Eight “big-tent” criteria for excellent qualitative research. Qualitative Inquiry, 16 (10), 837–851.
Download references
Open access funding provided by TU Wien (TUW).
Author information
Authors and affiliations.
Faculty of Informatics, Technische Universität Wien, 1040, Vienna, Austria
Drishti Yadav
You can also search for this author in PubMed Google Scholar
Corresponding author
Correspondence to Drishti Yadav .
Ethics declarations
Conflict of interest.
The author declares no conflict of interest.
Additional information
Publisher's note.
Springer Nature remains neutral with regard to jurisdictional claims in published maps and institutional affiliations.
Rights and permissions
Open Access This article is licensed under a Creative Commons Attribution 4.0 International License, which permits use, sharing, adaptation, distribution and reproduction in any medium or format, as long as you give appropriate credit to the original author(s) and the source, provide a link to the Creative Commons licence, and indicate if changes were made. The images or other third party material in this article are included in the article's Creative Commons licence, unless indicated otherwise in a credit line to the material. If material is not included in the article's Creative Commons licence and your intended use is not permitted by statutory regulation or exceeds the permitted use, you will need to obtain permission directly from the copyright holder. To view a copy of this licence, visit http://creativecommons.org/licenses/by/4.0/ .
Reprints and permissions
About this article
Yadav, D. Criteria for Good Qualitative Research: A Comprehensive Review. Asia-Pacific Edu Res 31 , 679–689 (2022). https://doi.org/10.1007/s40299-021-00619-0
Download citation
Accepted : 28 August 2021
Published : 18 September 2021
Issue Date : December 2022
DOI : https://doi.org/10.1007/s40299-021-00619-0
Share this article
Anyone you share the following link with will be able to read this content:
Sorry, a shareable link is not currently available for this article.
Provided by the Springer Nature SharedIt content-sharing initiative
- Qualitative research
- Evaluative criteria
- Find a journal
- Publish with us
- Track your research

Essentials of Descriptive-Interpretive Qualitative Research
Available formats, also available from.
- Table of contents
- Contributor bios
- Book details
- Additional Resources
The brief, practical texts in the Essentials of Qualitative Methods series introduce social science and psychology researchers to key approaches to capturing phenomena not easily measured quantitatively, offering exciting, nimble opportunities to gather in-depth qualitative data.
This book offers a no-nonsense, step-by-step approach to qualitative research in psychology and related fields, presenting principles for using a generic approach to descriptive-interpretive qualitative research. Based on more than 50 years of combined experience doing qualitative research on psychotherapy, the authors offer an overarching framework of best research practices common to a wide range of approaches.
About the Essentials of Qualitative Methods book series
Even for experienced researchers, selecting and correctly applying the right method can be challenging. In this groundbreaking series, leading experts in qualitative methods provide clear, crisp, and comprehensive descriptions of their approach, including its methodological integrity, and its benefits and limitations.
Each book includes numerous examples to enable readers to quickly and thoroughly grasp how to leverage these valuable methods.
Series Foreword by Clara E. Hill and Sarah Knox
- Why a Generic Descriptive-Interpretive Approach to Qualitative Research?
- Designing the Study
- Data Collection
- A Framework of Key Modes of Qualitative Data Analysis
- Writing the Manuscript
- Methodological Integrity
- Summary and Conclusions
Appendix. Example Studies
Robert Elliott, PhD, is professor of counselling at the University of Strathclyde. He received his doctorate in clinical psychology from the University of California, Los Angeles, and is professor emeritus of psychology at the University of Toledo (Ohio). He has spent most of his career as a psychotherapy researcher trying out and inventing different research methods.
He is co-author of Facilitating Emotional Change (1993), Learning Process-Experiential Psychotherapy (2004), Research Methods in Clinical Psychology (3rd ed., 2015), as well as more than 170 journal articles and book chapters.
He is past president of the Society for Psychotherapy Research and previously co-edited the journals Psychotherapy Research and Person-Centered and Experiential Psychotherapies .
Ladislav Timulak, PhD, is an associate professor at Trinity College Dublin, Ireland. He is course director of the Doctorate in Counselling Psychology course. Ladislav (or Laco for short; read Latso) is involved in the training of counselling psychologists and various psychotherapy trainings in Ireland and internationally. Laco is both an academic and a practitioner.
He is interested in research methodology and psychotherapy research, particularly the development of emotion-focused therapy. He has written six books, over 80 peer-reviewed papers, and various chapters in both his native language, Slovak, and in English.
He serves on various editorial boards and in the past served as a co-editor of Counselling Psychology Quarterly .
Sign up for the upcoming webinars presented by the series authors walking you through the basics of their approach.
29 Conceptualization in qualitative research
Chapter outline
- 15.1 Alternative paradigms: Interpretivism, critical paradigm, and pragmatism
15.2 Multiparadigmatic research: An example
15.3 idiographic causal relationships, 15.4 qualitative research questions.
Now let’s change things up! In the previous chapters, we explored steps to create and carry out a quantitative research study. Quantitative studies are great when we want to summarize or test relationships between ideas using numbers and the power of statistics. However, qualitative research offers us a different and equally important tool. Sometimes the aim of research projects is to explore meaning and lived experience. Instead of trying to arrive at generalizable conclusions for all people, some research projects establish a deep, authentic description of a specific time, place, and group of people.
Qualitative research relies on the power of human expression through words, pictures, movies, performance and other artifacts that represent these things. All of these tell stories about the human experience and we want to learn from them and have them be represented in our research. Generally speaking, qualitative research is about the gathering up of these stories, breaking them into pieces so we can examine the ideas that make them up, and putting them back together in a way that allows us to tell a common or shared story that responds to our research question. To do that, we need to discuss the assumptions underlying social science.
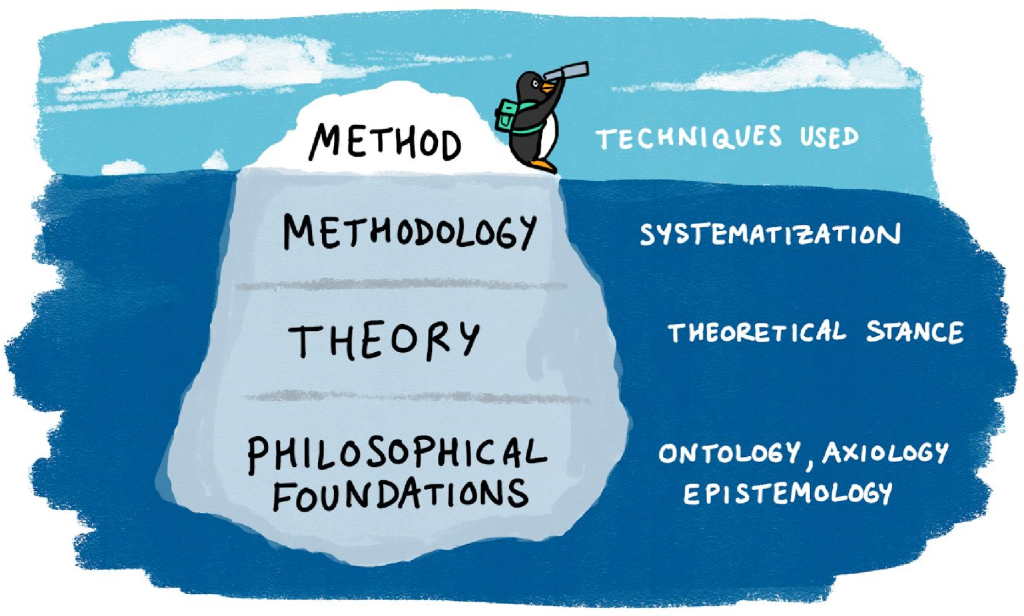
17.1 Alternative paradigms: Interpretivism, critical, and pragmatism
Learning objectives.
Students will be able to…
- Distinguish between the assumptions of positivism, interpretivism, critical, and pragmatist research paradigms.
- Use paradigm to describe how scientific thought changes over time.
In Chapter 10, we reviewed the assumptions that underly post-positivism (abbreviated hereafter as positivism for brevity). Quantitative methods are most often the choice for positivist research questions because they conform to these assumptions. Qualitative methods can conform to these assumptions; however, they are limited in their generalizability.
Kivunja & Kuyini (2017) [1] describe the essential features of positivism as:
- A belief that theory is universal and law-like generalizations can be made across contexts
- The assumption that context is not important
- The belief that truth or knowledge is ‘out there to be discovered’ by research
- The belief that cause and effect are distinguishable and analytically separable
- The belief that results of inquiry can be quantified
- The belief that theory can be used to predict and to control outcomes
- The belief that research should follow the scientific method of investigation
- Rests on formulation and testing of hypotheses
- Employs empirical or analytical approaches
- Pursues an objective search for facts
- Believes in ability to observe knowledge
- The researcher’s ultimate aim is to establish a comprehensive universal theory, to account for human and social behavior
- Application of the scientific method
Because positivism is the dominant social science research paradigm, it can be easy to ignore or be confused by research that does not use these assumptions. We covered in Chapter 10 the table reprinted below when discussing the assumptions underlying positivistic social science.
As you consider your research project, keep these philosophical assumptions in mind. They are useful shortcuts to understanding the deeper ideas and assumptions behind the construction of knowledge. The purpose of exploring these philosophical assumptions isn’t to find out which is true and which is false. Instead, the goal is to identify the assumptions that fit with how you think about your research question. Choosing a paradigm helps you make those assumptions explicit.
Ontology: assumptions about what is real | |
Epistemology: assumptions about how we come to know what is real |
|
Assumptions about the researcher |
|
Assumptions about human action |
|
Assumptions about the social world | |
Assumptions about the purpose of research |
|
Before we explore alternative paradigms, it’s important for us to review what paradigms are.
How do scientific ideas change over time?
Much like your ideas develop over time as you learn more, so does the body of scientific knowledge. Kuhn’s (1962) [2] The Structure of Scientific Revolutions is one of the most influential works on the philosophy of science, and is credited with introducing the idea of competing paradigms (or “disciplinary matrices”) in research. Kuhn investigated the way that scientific practices evolve over time, arguing that we don’t have a simple progression from “less knowledge” to “more knowledge” because the way that we approach inquiry is changing over time. This can happen gradually, but the process results in moments of change where our understanding of a phenomenon changes more radically (such as in the transition from Newtonian to Einsteinian physics; or from Lamarckian to Darwinian theories of evolution). For a social work practice example, Fleuridas & Krafcik (2019) [3] trace the development of the “four forces” of psychotherapy , from psychodynamics to behaviorism to humanism as well as the competition among emerging perspectives to establish itself as the fourth force to guide psychotherapeutic practice. But how did the problems in one paradigm inspire new paradigms? Kuhn presents us with a way of understanding the history of scientific development across all topics and disciplines.
As you can see in this video from Matthew J. Brown (CC-BY), there are four stages in the cycle of science in Kuhn’s approach. Firstly, a pre-paradigmatic state where competing approaches share no consensus. Secondly, the “normal” state where there is wide acceptance of a particular set of methods and assumptions. Thirdly, a state of crisis where anomalies that cannot be solved within the existing paradigm emerge and competing theories to address them follow. Fourthly, a revolutionary phase where some new paradigmatic approach becomes dominant and supplants the old. Shnieder (2009) [4] suggests that the Kuhnian phases are characterized by different kinds of scientific activity.
Newer approaches often build upon rather than replace older ones, but they also overlap and can exist within a state of competition. Scientists working within a particular paradigm often share methods, assumptions and values. In addition to supporting specific methods, research paradigms also influence things like the ambition and nature of research, the researcher-participant relationship and how the role of the researcher is understood.
Paradigm vs. theory
The terms ‘ paradigm ‘ and ‘ theory ‘ are often used interchangeably in social science. There is not a consensus among social scientists as to whether these are identical or distinct concepts. With that said, in this text, we will make a clear distinction between the two ideas because thinking about each concept separately is more useful for our purposes.
We define paradigm a set of common philosophical (ontological, epistemological, and axiological) assumptions that inform research. The four paradigms we describe in this section refer to patterns in how groups of researchers resolve philosophical questions. Some assumptions naturally make sense together, and paradigms grow out of researchers with shared assumptions about what is important and how to study it. Paradigms are like “analytic lenses” and a provide framework on top of which we can build theoretical and empirical knowledge (Kuhn, 1962). [5] Consider this video of an interview with world-famous physicist Richard Feynman in which he explains why “when you explain a ‘why,’ you have to be in some framework that you allow something to be true. Otherwise, you are perpetually asking why.” In order to answer basic physics question like “what is happening when two magnets attract?” or a social work research question like “what is the impact of this therapeutic intervention on depression,” you must understand the assumptions you are making about social science and the social world. Paradigmatic assumptions about objective and subjective truth support methodological choices like whether to conduct interviews or send out surveys, for example.
While paradigms are broad philosophical assumptions, theory is more specific, and refers to a set of concepts and relationships scientists use to explain the social world. Theories are more concrete, while paradigms are more abstract. Look back to Figure 7.1 at the beginning of this chapter. Theory helps you identify the concepts and relationships that align with your paradigmatic understanding of the problem. Moreover, theory informs how you will measure the concepts in your research question and the design of your project.
For both theories and paradigms, Kuhn’s observation of scientific paradigms, crises, and revolutions is instructive for understanding the history of science. Researchers inherit institutions, norms, and ideas that are marked by the battlegrounds of theoretical and paradigmatic debates that stretch back hundreds of years. We have necessarily simplified this history into four paradigms: positivism, interpretivism, critical, and pragmatism. Our framework and explanation are inspired by the framework of Guba and Lincoln (1990) [6] and Burrell and Morgan (1979). [7] while also incorporating pragmatism as a way of resolving paradigmatic questions. Most of social work research and theory can be classified as belonging to one of these four paradigms, though this classification system represents only one of many useful approaches to analyzing social science research paradigms.
Building on our discussion in section 7.1 on objective vs. subjective epistemologies and ontologies, we will start with the difference between positivism and interpretivism. Afterward, we will link our discussion of axiology in section 7.2 with the critical paradigm. Finally, we will situate pragmatism as a way to resolve paradigmatic questions strategically. The difference between positivism and interpretivism is a good place to start, since the critical paradigm and pragmatism build on their philosophical insights.
It’s important to think of paradigms less as distinct categories and more as a spectrum along which projects might fall. For example, some projects may be somewhat positivist, somewhat interpretivist, and a little critical. No project fits perfectly into one paradigm. Additionally, there is no paradigm that is more correct than the other. Each paradigm uses assumptions that are logically consistent, and when combined, are a useful approach to understanding the social world using science. The purpose of this section is to acquaint you with what research projects in each paradigm look like and how they are grounded in philosophical assumptions about social science.
You should read this section to situate yourself in terms of what paradigm feels most “at home” to both you as a person and to your project. You may find, as I have, that your research projects are more conventional and less radical than what feels most like home to you, personally. In a research project, however, students should start with their working question rather than their heart. Use the paradigm that fits with your question the best, rather than which paradigm you think fits you the best.
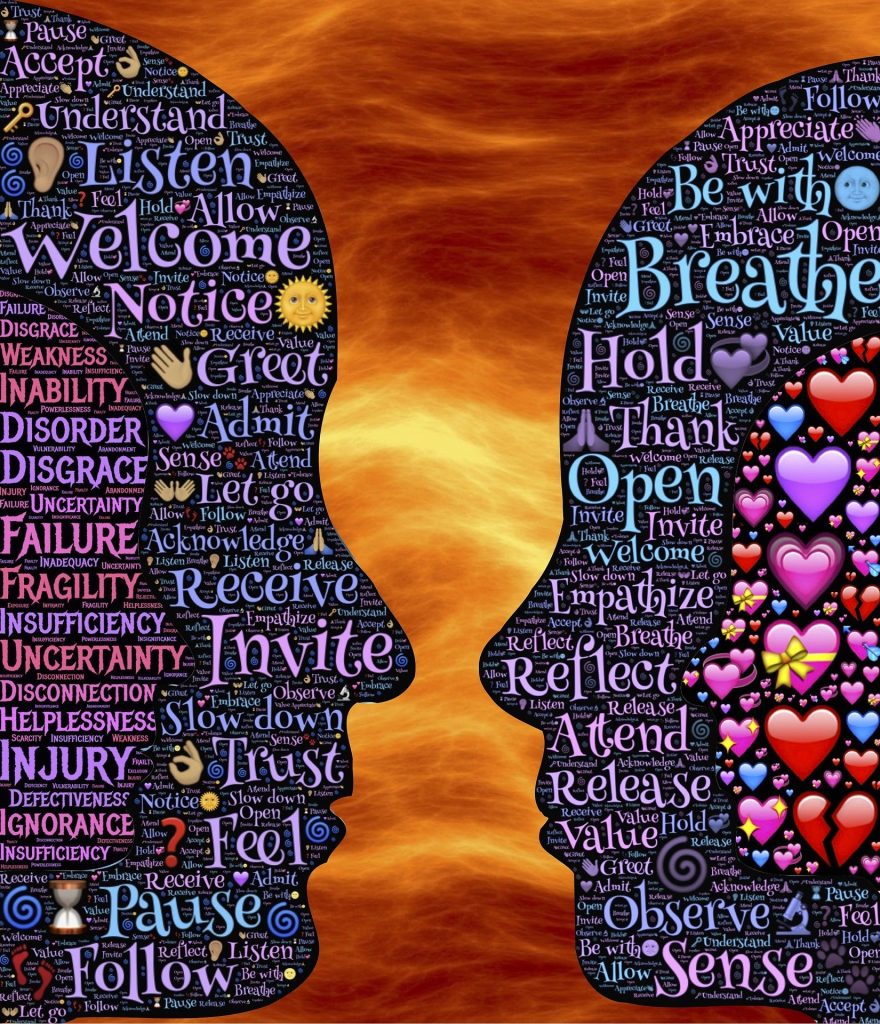
Interpretivism: Researcher as “empathizer”
Positivism is focused on generalizable truth. Interpretivism , by contrast, develops from the idea that we want to understand the truths of individuals, how they interpret and experience the world, their thought processes, and the social structures that emerge from sharing those interpretations through language and behavior. The process of interpretation (or social construction) is guided by the empathy of the researcher to understand the meaning behind what other people say.
Historically, interpretivism grew out of a specific critique of positivism: that knowledge in the human and social sciences cannot conform to the model of natural science because there are features of human experience that cannot objectively be “known”. The tools we use to understand objects that have no self-awareness may not be well-attuned to subjective experiences like emotions, understandings, values, feelings, socio-cultural factors, historical influences, and other meaningful aspects of social life. Instead of finding a single generalizable “truth,” the interpretivist researcher aims to generate understanding and often adopts a relativist position.
While positivists seek “the truth,” the social constructionist framework argues that “truth” varies. Truth differs based on who you ask, and people change what they believe is true based on social interactions. These subjective truths also exist within social and historical contexts, and our understanding of truth varies across communities and time periods. This is because we, according to this paradigm, create reality ourselves through our social interactions and our interpretations of those interactions. Key to the interpretivist perspective is the idea that social context and interaction frame our realities.
Researchers operating within this framework take keen interest in how people come to socially agree, or disagree, about what is real and true. Consider how people, depending on their social and geographical context, ascribe different meanings to certain hand gestures. When a person raises their middle finger, those of us in Western cultures will probably think that this person isn’t very happy (not to mention the person at whom the middle finger is being directed!). In other societies around the world, a thumbs-up gesture, rather than a middle finger, signifies discontent (Wong, 2007). [8] The fact that these hand gestures have different meanings across cultures aptly demonstrates that those meanings are socially and collectively constructed. What, then, is the “truth” of the middle finger or thumbs up? As we’ve seen in this section, the truth depends on the intention of the person making the gesture, the interpretation of the person receiving it, and the social context in which the action occurred.
Qualitative methods are preferred as ways to investigate these phenomena. Data collected might be unstructured (or “messy”) and correspondingly a range of techniques for approaching data collection have been developed. Interpretivism acknowledges that it is impossible to remove cultural and individual influence from research, often instead making a virtue of the positionality of the researcher and the socio-cultural context of a study.
One common objection positivists levy against interpretivists is that interpretivism tends to emphasize the subjective over the objective. If the starting point for an investigation is that we can’t fully and objectively know the world, how can we do research into this without everything being a matter of opinion? For the positivist, this risk for confirmation bias as well as invalid and unreliable measures makes interpretivist research unscientific. Clearly, we disagree with this assessment, and you should, too. Positivism and interpretivism have different ontologies and epistemologies with contrasting notions of rigor and validity (for more information on assumptions about measurement, see Chapter 11 for quantitative validity and reliability and Chapter 20 for qualitative rigor). Nevertheless, both paradigms apply the values and concepts of the scientific method through systematic investigation of the social world, even if their assumptions lead them to do so in different ways. Interpretivist research often embraces a relativist epistemology, bringing together different perspectives in search of a trustworthy and authentic understanding or narrative.
Kivunja & Kuyini (2017) [9] describe the essential features of interpretivism as:
- The belief that truths are multiple and socially constructed
- The acceptance that there is inevitable interaction between the researcher and his or her research participants
- The acceptance that context is vital for knowledge and knowing
- The belief that knowledge can be value laden and the researcher’s values need to be made explicit
- The need to understand specific cases and contexts rather deriving universal laws that apply to everyone, everywhere.
- The belief that causes and effects are mutually interdependent, and that causality may be circular or contradictory
- The belief that contextual factors need to be taken into consideration in any systematic pursuit of understanding
One important clarification: it’s important to think of the interpretivist perspective as not just about individual interpretations but the social life of interpretations. While individuals may construct their own realities, groups—from a small one such as a married couple to large ones such as nations—often agree on notions of what is true and what “is” and what “is not.” In other words, the meanings that we construct have power beyond the individuals who create them. Therefore, the ways that people and communities act based on such meanings is of as much interest to interpretivists as how they were created in the first place. Theories like social constructionism, phenomenology, and symbolic interactionism are often used in concert with interpretivism.
Is interpretivism right for your project?
An interpretivist orientation to research is appropriate when your working question asks about subjective truths. The cause-and-effect relationships that interpretivist studies produce are specific to the time and place in which the study happened, rather than a generalizable objective truth. More pragmatically, if you picture yourself having a conversation with participants like an interview or focus group, then interpretivism is likely going to be a major influence for your study.
Positivists critique the interpretivist paradigm as non-scientific. They view the interpretivist focus on subjectivity and values as sources of bias. Positivists and interpretivists differ on the degree to which social phenomena are like natural phenomena. Positivists believe that the assumptions of the social sciences and natural sciences are the same, while interpretivists strongly believe that social sciences differ from the natural sciences because their subjects are social creatures.
Similarly, the critical paradigm finds fault with the interpretivist focus on the status quo rather than social change. Although interpretivists often proceed from a feminist or other standpoint theory, the focus is less on liberation than on understanding the present from multiple perspectives. Other critical theorists may object to the consensus orientation of interpretivist research. By searching for commonalities between people’s stories, they may erase the uniqueness of each individual’s story. For example, while interpretivists may arrive at a consensus definition of what the experience of “coming out” is like for people who identify as lesbian, gay, bisexual, transgender, or queer, it cannot represent the diversity of each person’s unique “coming out” experience and what it meant to them. For example, see Rosario and colleagues’ (2009) [10] critique the literature on lesbians “coming out” because previous studies did not addressing how appearing, behaving, or identifying as a butch or femme impacted the experience of “coming out” for lesbians.
- From your literature search, identify an empirical article that uses qualitative methods to answer a research question similar to your working question or about your research topic.
- Review the assumptions of the interpretivist research paradigm.
- Discuss in a few sentences how the author’s conclusions are based on some of these paradigmatic assumptions. How might a researcher operating from a different paradigm (like positivism or the critical paradigm) critique the conclusions of this study?
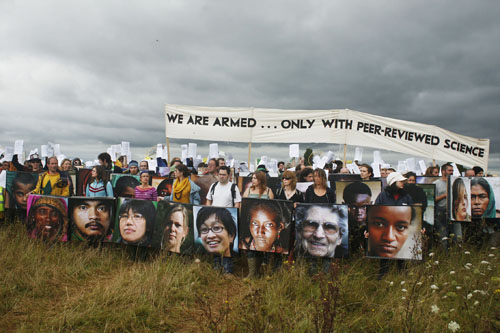
Critical paradigm: Researcher as “activist”
As we’ve discussed a bit in the preceding sections, the critical paradigm focuses on power, inequality, and social change. Although some rather diverse perspectives are included here, the critical paradigm, in general, includes ideas developed by early social theorists, such as Max Horkheimer (Calhoun et al., 2007), [11] and later works developed by feminist scholars, such as Nancy Fraser (1989). [12] Unlike the positivist paradigm, the critical paradigm assumes that social science can never be truly objective or value-free. Furthermore, this paradigm operates from the perspective that scientific investigation should be conducted with the express goal of social change. Researchers in the critical paradigm foreground axiology, positionality and values . In contrast with the detached, “objective” observations associated with the positivist researcher, critical approaches make explicit the intention for research to act as a transformative or emancipatory force within and beyond the study.
Researchers in the critical paradigm might start with the knowledge that systems are biased against certain groups, such as women or ethnic minorities, building upon previous theory and empirical data. Moreover, their research projects are designed not only to collect data, but to impact the participants as well as the systems being studied. The critical paradigm applies its study of power and inequality to change those power imbalances as part of the research process itself. If this sounds familiar to you, you may remember hearing similar ideas when discussing social conflict theory in your human behavior in the social environment (HBSE) class. [13] Because of this focus on social change, the critical paradigm is a natural home for social work research. However, we fall far short of adopting this approach widely in our profession’s research efforts.
Is the critical paradigm right for your project?
Every social work research project impacts social justice in some way. What distinguishes critical research is how it integrates an analysis of power into the research process itself. Critical research is appropriate for projects that are activist in orientation. For example, critical research projects should have working questions that explicitly seek to raise the consciousness of an oppressed group or collaborate equitably with community members and clients to addresses issues of concern. Because of their transformative potential, critical research projects can be incredibly rewarding to complete. However, partnerships take a long time to develop and social change can evolve slowly on an issue, making critical research projects a more challenging fit for student research projects which must be completed under a tight deadline with few resources.
Positivists critique the critical paradigm on multiple fronts. First and foremost, the focus on oppression and values as part of the research process is seen as likely to bias the research process, most problematically, towards confirmation bias. If you start out with the assumption that oppression exists and must be dealt with, then you are likely to find that regardless of whether it is truly there or not. Similarly, positivists may fault critical researchers for focusing on how the world should be, rather than how it truly is . In this, they may focus too much on theoretical and abstract inquiry and less on traditional experimentation and empirical inquiry. Finally, the goal of social transformation is seen as inherently unscientific, as science is not a political practice.
Interpretivists often find common cause with critical researchers. Feminist studies, for example, may explore the perspectives of women while centering gender-based oppression as part of the research process. In interpretivist research, the focus is less on radical change as part of the research process and more on small, incremental changes based on the results and conclusions drawn from the research project. Additionally, some critical researchers’ focus on individuality of experience is in stark contrast to the consensus-orientation of interpretivists. Interpretivists seek to understand people’s true selves. Some critical theorists argue that people have multiple selves or no self at all.
- From your literature search, identify an article relevant to your working question or broad research topic that uses a critical perspective. You should look for articles where the authors are clear that they are applying a critical approach to research like feminism, anti-racism, Marxism and critical theory, decolonization, anti-oppressive practice, or other social justice-focused theoretical perspectives. To target your search further, include keywords in your queries to research methods commonly used in the critical paradigm like participatory action research and community-based participatory research. If you have trouble identifying an article for this exercise, consult your professor for some help. These articles may be more challenging to find, but reviewing one is necessary to get a feel for what research in this paradigm is like.
- Review the assumptions of the critical research paradigm.
- Discuss in a few sentences how the author’s conclusions are based on some of these paradigmatic assumptions. How might a researcher operating from different assumptions (like values-neutrality or researcher as neutral and unbiased) critique the conclusions of this study?
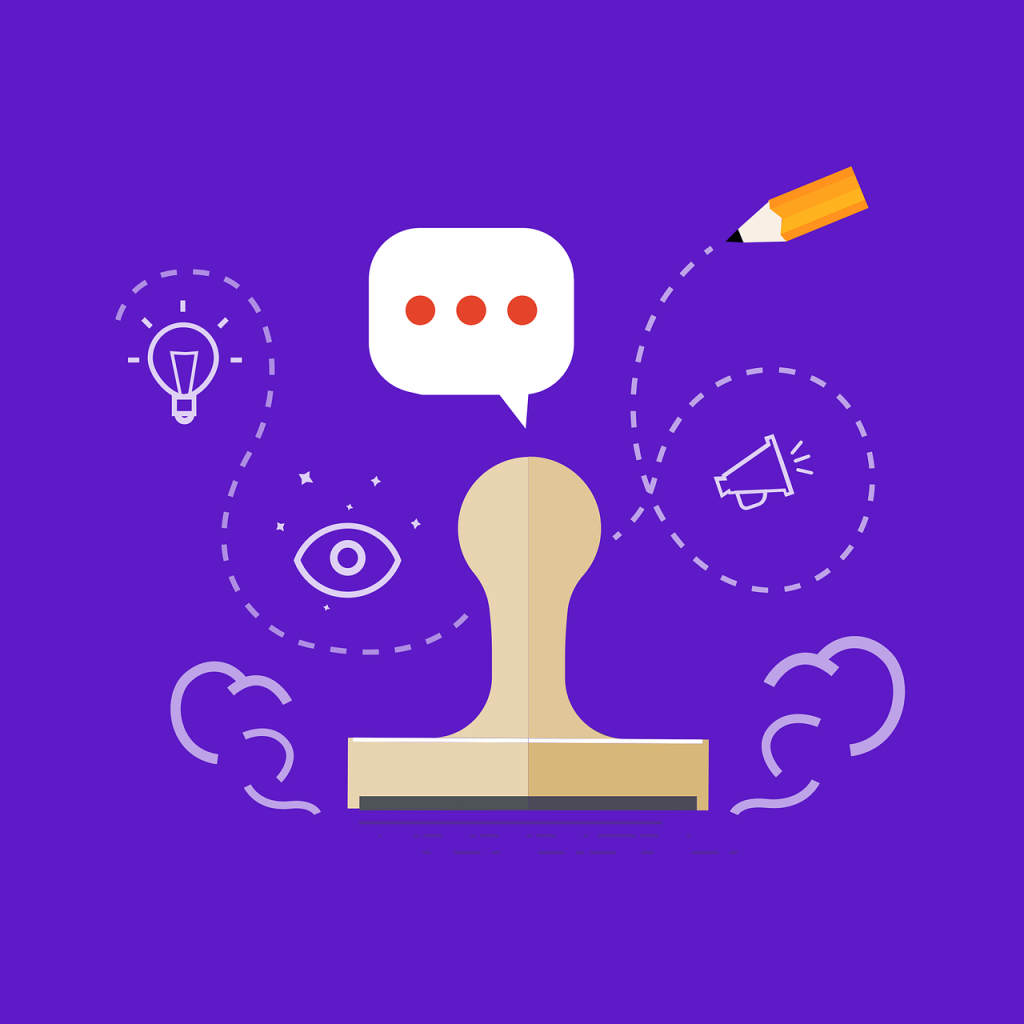
Pragmatism: Researcher as “strategist”
“Essentially, all models are wrong but some are useful.” (Box, 1976) [14]
Pragmatism is a research paradigm that suspends questions of philosophical ‘truth’ and focuses more on how different philosophies, theories, and methods can be used strategically to provide a multidimensional view of a topic. Researchers employing pragmatism will mix elements of positivist, interpretivist, and critical research depending on the purpose of a particular project and the practical constraints faced by the researcher and their research context. We favor this approach for student projects because it avoids getting bogged down in choosing the “right” paradigm and instead focuses on the assumptions that help you answer your question, given the limitations of your research context. Student research projects are completed quickly and moving in the direction of pragmatism can be a route to successfully completing a project. Your project is a representation of what you think is feasible, ethical, and important enough for you to study.
The crucial consideration for the pragmatist is whether the outcomes of research have any real-world application, rather than whether they are “true.” The methods, theories, and philosophies chosen by pragmatic researchers are guided by their working question. There are no distinctively pragmatic research methods since this approach is about making judicious use whichever methods fit best with the problem under investigation. Pragmatic approaches may be less likely to prioritize ontological, epistemological or axiological consistency when combining different research methods. Instead, the emphasis is on solving a pressing problem and adapting to the limitations and opportunities in the researchers’ context.
Adopt a multi-paradigmatic perspective
Believe it or not, there is a long literature of acrimonious conflict between scientists from positivist, interpretivist, and critical camps (see Heineman-Pieper et al., 2002 [15] for a longer discussion). Pragmatism is an old idea, but it is appealing precisely because it attempts to resolve the problem of multiple incompatible philosophical assumptions in social science. To a pragmatist, there is no “correct” paradigm. All paradigms rely on assumptions about the social world that are the subject of philosophical debate. Each paradigm is an incomplete understanding of the world, and it requires a scientific community using all of them to gain a comprehensive view of the social world. This multi-paradigmatic perspective is a unique gift of social work research, as our emphasis on empathy and social change makes us more critical of positivism, the dominant paradigm in social science.
We offered the metaphors of expert, empathizer, activist, and strategist for each paradigm. It’s important not to take these labels too seriously. For example, some may view that scientists should be experts or that activists are biased and unscientific. Nevertheless, we hope that these metaphors give you a sense of what it feels like to conduct research within each paradigm.
One of the unique aspects of paradigmatic thinking is that often where you think you are most at home may actually be the opposite of where your research project is. For example, in my graduate and doctoral education, I thought I was a critical researcher. In fact, I thought I was a radical researcher focused on social change and transformation. Yet, often times when I sit down to conceptualize and start a research project, I find myself squarely in the positivist paradigm, thinking through neat cause-and-effect relationships that can be mathematically measured. There is nothing wrong with that! Your task for your research project is to find the paradigm that best matches your research question. Think through what you really want to study and how you think about the topic, then use assumptions of that paradigm to guide your inquiry.
Another important lesson is that no research project fits perfectly in one paradigm or another. Instead, there is a spectrum along which studies are, to varying degrees, interpretivist, positivist, and critical. For example, all social work research is a bit activist in that our research projects are designed to inform action for change on behalf of clients and systems. However, some projects will focus on the conclusions and implications of projects informing social change (i.e., positivist and interpretivist projects) while others will partner with community members and design research projects collaboratively in a way that leads to social change (i.e. critical projects). In section 7.5, we will describe a pragmatic approach to research design guided by your paradigmatic and theoretical framework.
Key Takeaways
- Social work research falls, to some degree, in each of the four paradigms: positivism, interpretivism, critical, and pragmatist.
- Adopting a pragmatic, multi-paradigmatic approach to research makes sense for student researchers, as it directs students to use the philosophical assumptions and methodological approaches that best match their research question and research context.
- Research in all paradigms is necessary to come to a comprehensive understanding of a topic, and social workers must be able to understand and apply knowledge from each research paradigm.
- Describe which paradigm best fits your perspective on the world and which best fits with your project.
- Identify any similarities and differences in your personal assumptions and the assumption your research project relies upon. For example, are you a more critical and radical thinker but have chosen a more “expert” role for yourself in your research project?
Learners will be able to…
- Apply the assumptions of each paradigm to your project
- Summarize what aspects of your project stem from positivist, interpretivist, or critical assumptions
In the previous sections, we reviewed the major paradigms and theories in social work research. In this section, we will provide an example of how to apply theory and paradigm in research. This process is depicted in Figure 7.2 below with some quick summary questions for each stage. Some questions in the figure below have example answers like designs (i.e., experimental, survey) and data analysis approaches (i.e., discourse analysis). These examples are arbitrary. There are a lot of options that are not listed. So, don’t feel like you have to memorize them or use them in your study.
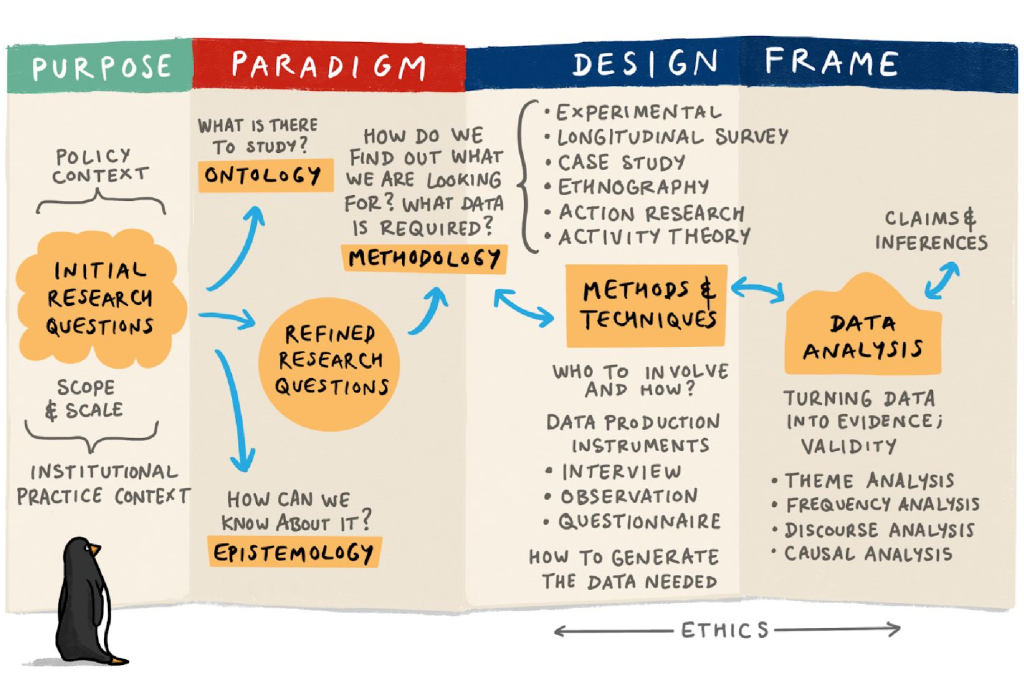
This diagram (taken from an archived Open University (UK) course entitled E89 - Educational Inquiry ) shows one way to visualize the research design process. While research is far from linear, in general, this is how research projects progress sequentially. Researchers begin with a working question, and through engaging with the literature, develop and refine those questions into research questions (a process we will finalize in Chapter 9 ). But in order to get to the part where you gather your sample, measure your participants, and analyze your data, you need to start with paradigm. Based on your work in section 7.3, you should have a sense of which paradigm or paradigms are best suited to answering your question. The approach taken will often reflect the nature of the research question; the kind of data it is possible to collect; and work previously done in the area under consideration. When evaluating paradigm and theory, it is important to look at what other authors have done previously and the framework used by studies that are similar to the one you are thinking of conducting.
Once you situate your project in a research paradigm, it becomes possible to start making concrete choices about methods. Depending on the project, this will involve choices about things like:
- What is my final research question?
- What are the key variables and concepts under investigation, and how will I measure them?
- How do I find a representative sample of people who experience the topic I’m studying?
- What design is most appropriate for my research question?
- How will I collect and analyze data?
- How do I determine whether my results describe real patterns in the world or are the result of bias or error?
The data collection phase can begin once these decisions are made. It can be very tempting to start collecting data as soon as possible in the research process as this gives a sense of progress. However, it is usually worth getting things exactly right before collecting data as an error found in your approach further down the line can be harder to correct or recalibrate around.
Designing a study using paradigm and theory: An example
Paradigm and theory have the potential to turn some people off since there is a lot of abstract terminology and thinking about real-world social work practice contexts. In this section, I’ll use an example from my own research, and I hope it will illustrate a few things. First, it will show that paradigms are really just philosophical statements about things you already understand and think about normally. It will also show that no project neatly sits in one paradigm and that a social work researcher should use whichever paradigm or combination of paradigms suit their question the best. Finally, I hope it is one example of how to be a pragmatist and strategically use the strengths of different theories and paradigms to answering a research question. We will pick up the discussion of mixed methods in the next chapter.
Thinking as an expert: Positivism
In my undergraduate research methods class, I used an open textbook much like this one and wanted to study whether it improved student learning. You can read a copy of the article we wrote on based on our study . We’ll learn more about the specifics of experiments and evaluation research in Chapter 13 , but you know enough to understand what evaluating an intervention might look like. My first thought was to conduct an experiment, which placed me firmly within the positivist or “expert” paradigm.
Experiments focus on isolating the relationship between cause and effect. For my study, this meant studying an open textbook (the cause, or intervention) and final grades (the effect, or outcome). Notice that my position as “expert” lets me assume many things in this process. First, it assumes that I can distill the many dimensions of student learning into one number—the final grade. Second, as the “expert,” I’ve determined what the intervention is: indeed, I created the book I was studying, and applied a theory from experts in the field that explains how and why it should impact student learning.
Theory is part of applying all paradigms, but I’ll discuss its impact within positivism first. Theories grounded in positivism help explain why one thing causes another. More specifically, these theories isolate a causal relationship between two (or more) concepts while holding constant the effects of other variables that might confound the relationship between the key variables. That is why experimental design is so common in positivist research. The researcher isolates the environment from anything that might impact or bias the cause and effect relationship they want to investigate.
But in order for one thing to lead to change in something else, there must be some logical, rational reason why it would do so. In open education, there are a few hypotheses (though no full-fledged theories) on why students might perform better using open textbooks. The most common is the access hypothesis , which states that students who cannot afford expensive textbooks or wouldn’t buy them anyway can access open textbooks because they are free, which will improve their grades. It’s important to note that I held this theory prior to starting the experiment, as in positivist research you spell out your hypotheses in advance and design an experiment to support or refute that hypothesis.
Notice that the hypothesis here applies not only to the people in my experiment, but to any student in higher education. Positivism seeks generalizable truth, or what is true for everyone. The results of my study should provide evidence that anyone who uses an open textbook would achieve similar outcomes. Of course, there were a number of limitations as it was difficult to tightly control the study. I could not randomly assign students or prevent them from sharing resources with one another, for example. So, while this study had many positivist elements, it was far from a perfect positivist study because I was forced to adapt to the pragmatic limitations of my research context (e.g., I cannot randomly assign students to classes) that made it difficult to establish an objective, generalizable truth.
Thinking like an empathizer: Interpretivism
One of the things that did not sit right with me about the study was the reliance on final grades to signify everything that was going on with students. I added another quantitative measure that measured research knowledge, but this was still too simplistic. I wanted to understand how students used the book and what they thought about it. I could create survey questions that ask about these things, but to get at the subjective truths here, I thought it best to use focus groups in which students would talk to one another with a researcher moderating the discussion and guiding it using predetermined questions. You will learn more about focus groups in Chapter 18 .
Researchers spoke with small groups of students during the last class of the semester. They prompted people to talk about aspects of the textbook they liked and didn’t like, compare it to textbooks from other classes, describe how they used it, and so forth. It was this focus on understanding and subjective experience that brought us into the interpretivist paradigm. Alongside other researchers, I created the focus group questions but encouraged researchers who moderated the focus groups to allow the conversation to flow organically.
We originally started out with the assumption, for which there is support in the literature, that students would be angry with the high-cost textbook that we used prior to the free one, and this cost shock might play a role in students’ negative attitudes about research. But unlike the hypotheses in positivism, these are merely a place to start and are open to revision throughout the research process. This is because the researchers are not the experts, the participants are! Just like your clients are the experts on their lives, so were the students in my study. Our job as researchers was to create a group in which they would reveal their informed thoughts about the issue, coming to consensus around a few key themes.
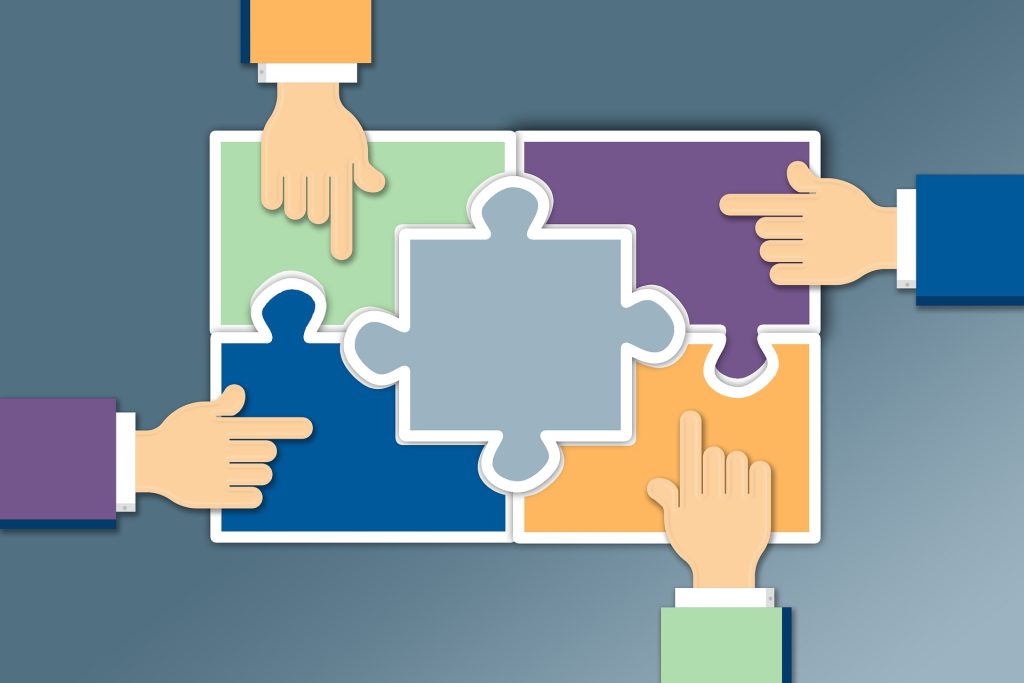
When we initially analyzed the focus groups, we uncovered themes that seemed to fit the data. But the overall picture was murky. How were themes related to each other? And how could we distill these themes and relationships into something meaningful? We went back to the data again. We could do this because there isn’t one truth, as in positivism, but multiple truths and multiple ways of interpreting the data. When we looked again, we focused on some of the effects of having a textbook customized to the course. It was that customization process that helped make the language more approachable, engaging, and relevant to social work practice.
Ultimately, our data revealed differences in how students perceived a free textbook versus a free textbook that is customized to the class. When we went to interpret this finding, the remix hypothesis of open textbook was helpful in understanding that relationship. It states that the more faculty incorporate editing and creating into the course, the better student learning will be. Our study helped flesh out that theory by discussing the customization process and how students made sense of a customized resource.
In this way, theoretical analysis operates differently in interpretivist research. While positivist research tests existing theories, interpretivist research creates theories based on the stories of research participants. However, it is difficult to say if this theory was totally emergent in the dataset or if my prior knowledge of the remix hypothesis influenced my thinking about the data. Interpretivist researchers are encouraged to put a box around their prior experiences and beliefs, acknowledging them, but trying to approach the data with fresh eyes. Interpretivists know that this is never perfectly possible, though, as we are always influenced by our previous experiences when interpreting data and conducting scientific research projects.
Thinking like an activist: Critical
Although adding focus groups helped ease my concern about reducing student learning down to just final grades by providing a more rich set of conversations to analyze. However, my role as researcher and “expert” was still an important part of the analysis. As someone who has been out of school for a while, and indeed has taught this course for years, I have lost touch with what it is like to be a student taking research methods for the first time. How could I accurately interpret or understand what students were saying? Perhaps I would overlook things that reflected poorly on my teaching or my book. I brought other faculty researchers on board to help me analyze the data, but this still didn’t feel like enough.
By luck, an undergraduate student approached me about wanting to work together on a research project. I asked her if she would like to collaborate on evaluating the textbook with me. Over the next year, she assisted me with conceptualizing the project, creating research questions, as well as conducting and analyzing the focus groups. Not only would she provide an “insider” perspective on coding the data, steeped in her lived experience as a student, but she would serve as a check on my power through the process.
Including people from the group you are measuring as part of your research team is a common component of critical research. Ultimately, critical theorists would find my study to be inadequate in many ways. I still developed the research question, created the intervention, and wrote up the results for publication, which privileges my voice and role as “expert.” Instead, critical theorists would emphasize the role of students (community members) in identifying research questions, choosing the best intervention to used, and so forth. But collaborating with students as part of a research team did address some of the power imbalances in the research process.
Critical research projects also aim to have an impact on the people and systems involved in research. No students or researchers had profound personal realizations as a result of my study, nor did it lessen the impact of oppressive structures in society. I can claim some small victory that my department switched to using my textbook after the study was complete (changing a system), though this was likely the result of factors other than the study (my advocacy for open textbooks).
Social work research is almost always designed to create change for people or systems. To that end, every social work project is at least somewhat critical. However, the additional steps of conducting research with people rather than on people reveal a depth to the critical paradigm. By bringing students on board the research team, study had student perspectives represented in conceptualization, data collection, and analysis. That said, there was much to critique about this study from a critical perspective. I retained a lot of the power in the research process, and students did not have the ability to determine the research question or purpose of the project. For example, students might likely have said that textbook costs and the quality of their research methods textbook were less important than student debt, racism, or other potential issues experienced by students in my class. Instead of a ground-up research process based in community engagement, my research included some important participation by students on project created and led by faculty.
Conceptualization is an iterative process
I hope this conversation was useful in applying paradigms to a research project. While my example discusses education research, the same would apply for social work research about social welfare programs, clinical interventions, or other topics. Paradigm and theory are covered at the beginning of the conceptualization of your project because these assumptions will structure the rest of your project. Each of the research steps that occur after this chapter (e.g., forming a question, choosing a design) rely upon philosophical and theoretical assumptions. As you continue conceptualizing your project over the next few weeks, you may find yourself shifting between paradigms. That is normal, as conceptualization is not a linear process. As you move through the next steps of conceptualizing and designing a project, you’ll find philosophies and theories that best match how you want to study your topic.
Viewing theoretical and empirical arguments through this lens is one of the true gifts of the social work approach to research. The multi-paradigmatic perspective is a hallmark of social work research and one that helps us contribute something unique on research teams and in practice.
- Multi-paradigmatic research is a distinguishing hallmark of social work research. Understanding the limitations and strengths of each paradigm will help you justify your research approach and strategically choose elements from one or more paradigms to answer your question.
- Paradigmatic assumptions help you understand the “blind spots” in your research project and how to adjust and address these areas. Keep in mind, it is not necessary to address all of your blind spots, as all projects have limitations.
- Sketch out which paradigm applies best to your project. Second, building on your answer to the exercise in section 7.3, identify how the theory you chose and the paradigm in which you find yourself are consistent or are in conflict with one another. For example, if you are using systems theory in a positivist framework, you might talk about how they both rely on a deterministic approach to human behavior with a focus on the status-quo and social order.
- Define and provide an example of an idiographic causal explanation
- Differentiate between idiographic and nomothetic causal relationships
- Link idiographic and nomothetic causal relationships with the process of theory building and theory testing
- Describe how idiographic and nomothetic causal explanations can be complementary
As we transition away from positivism, it is important to highlight the assumptions it makes about the scientific process–the hypothetico-deductive method, sometimes referred to as the research circle.
The hypothetico-deductive method
The primary way that researchers in the positivist paradigm use theories is sometimes called the hypothetico-deductive method (although this term is much more likely to be used by philosophers of science than by scientists themselves). Researchers choose an existing theory. Then, they make a prediction about some new phenomenon that should be observed if the theory is correct. Again, this prediction is called a hypothesis. The researchers then conduct an empirical study to test the hypothesis. Finally, they reevaluate the theory in light of the new results and revise it if necessary.
This process is usually conceptualized as a cycle because the researchers can then derive a new hypothesis from the revised theory, conduct a new empirical study to test the hypothesis, and so on. As Figure 8.8 shows, this approach meshes nicely with the process of conducting a research project—creating a more detailed model of “theoretically motivated” or “theory-driven” research. Together, they form a model of theoretically motivated research.
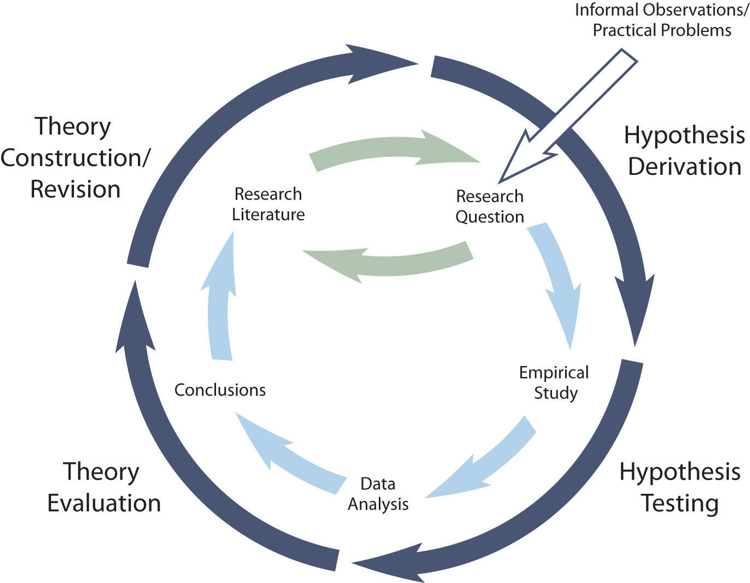
Keep in mind the hypothetico-deductive method is only one way of using social theory to inform social science research. It starts with describing one or more existing theories, deriving a hypothesis from one of those theories, testing your hypothesis in a new study, and finally reevaluating the theory based on the results data analyses. This format works well when there is an existing theory that addresses the research question—especially if the resulting hypothesis is surprising or conflicts with a hypothesis derived from a different theory.
But what if your research question is more interpretive? What if it is less about theory-testing and more about theory-building? This is what our next chapter covers: the process of inductively deriving theory from people’s stories and experiences. This process looks different than that depicted in Figure 8.8. It still starts with your research question and answering that question by conducting a research study. But instead of testing a hypothesis you created based on a theory, you will create a theory of your own that explain the data you collected. This format works well for qualitative research questions and for research questions that existing theories do not address.
Inductive reasoning is most commonly found in studies using qualitative methods, such as focus groups and interviews. Because inductive reasoning involves the creation of a new theory, researchers need very nuanced data on how the key concepts in their working question operate in the real world. Qualitative data is often drawn from lengthy interactions and observations with the individuals and phenomena under examination. For this reason, inductive reasoning is most often associated with qualitative methods, though it is used in both quantitative and qualitative research.
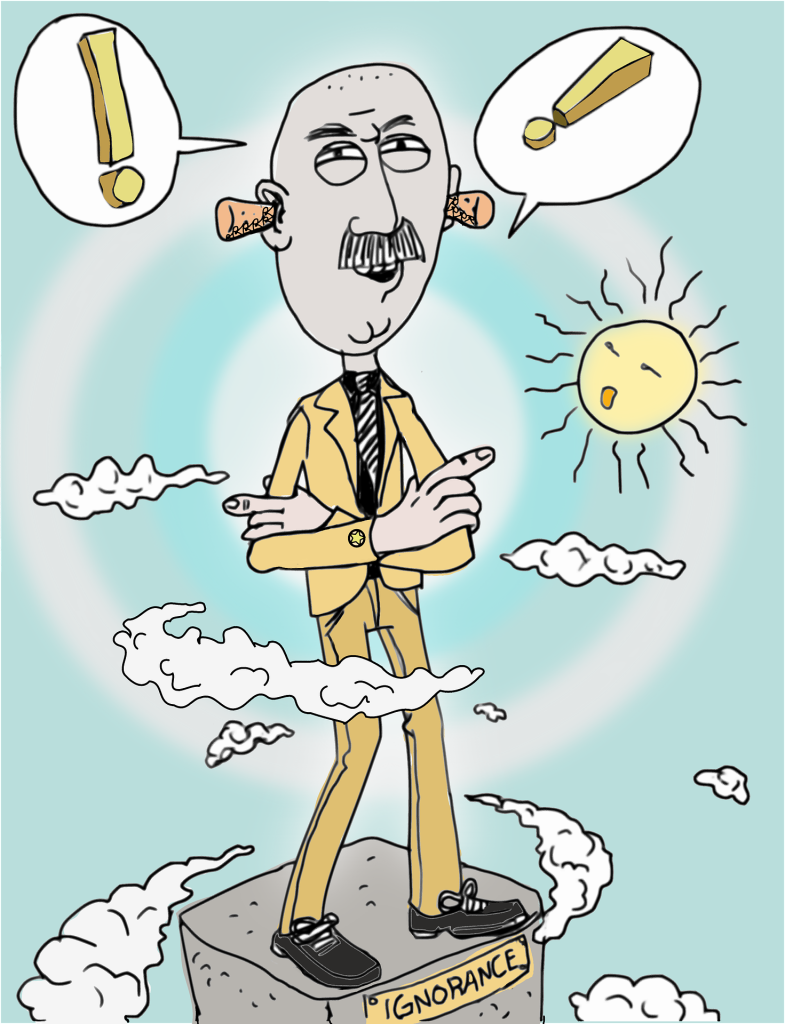
Whose truth does science establish?
Social work is concerned with the “isms” of oppression (ableism, ageism, cissexism, classism, heterosexism, racism, sexism, etc.), and so our approach to science must reconcile its history as both a tool of oppression and its exclusion of oppressed groups. Science grew out of the Enlightenment, a philosophical movement which applied reason and empirical analysis to understanding the world. While the Enlightenment brought forth tremendous achievements, the critiques of Marxian, feminist, and other critical theorists complicated the Enlightenment understanding of science. For this section, I will focus on feminist critiques of science, building upon an entry in the Stanford Encyclopedia of Philosophy (Crasnow, 2020). [16]
In its original formulation, science was an individualistic endeavor. As we learned in Chapter 1 , a basic statement of the scientific method is that a researcher studies existing theories on a topic, formulates a hypothesis about what might be true, and either confirms or disconfirms their hypothesis through experiment and rigorous observation. Over time, our theories become more accurate in their predictions and more comprehensive in their conclusions. Scientists put aside their preconceptions, look at the data, and build their theories based on objective rationality.
Yet, this cannot be perfectly true. Scientists are human, after all. As a profession historically dominated by white men, scientists have dismissed women and other minorities as being psychologically unfit for the scientific profession. While attitudes have improved, science, technology, engineering, mathematics (STEM) and related fields remain dominated by white men (Grogan, 2019). [17] Biases can persist in social work theory and research when social scientists do not have similar experiences to the populations they study.
Gender bias can influence the research questions scientists choose to answer. Feminist critiques of medical science drew attention to women’s health issues, spurring research and changing standards of care. The focus on domestic violence in the empirical literature can also be seen as a result of feminist critique. Thus, critical theory helps us critique what is on the agenda for science. If science is to answer important questions, it must speak to the concerns of all people. Through the democratization in access to scientific knowledge and the means to produce it, science becomes a sister process of social development and social justice.
The goal of a diverse and participatory scientific community lies in contrast to much of what we understand to be “proper” scientific knowledge. Many of the older, classic social science theories were developed based on research which observed males or from university students in the United States or other Western nations. How these observations were made, what questions were asked, and how the data were interpreted were shaped by the same oppressive forces that existed in broader society, a process that continues into the present. In psychology, the concept of hysteria or hysterical women was believed to be caused by a wandering womb (Tasca et al., 2012). [18] Even today, there are gender biases in diagnoses of histrionic personality disorder and racial biases in psychotic disorders (Klonsky et al., 2002) [19] because the theories underlying them were created in a sexist and racist culture. In these ways, science can reinforce the truth of the white Western male perspective.
Finally, it is important to note that social science research is often conducted on populations rather than with populations. Historically, this has often meant Western men traveling to other countries and seeking to understand other cultures through a Western lens. Lacking cultural humility and failing to engage stakeholders, ethnocentric research of this sort has led to the view of non-Western cultures as inferior. Moreover, the use of these populations as research subjects rather than co-equal participants in the research process privileges the researcher’s knowledge over that from other groups or cultures. Researchers working with indigenous cultures, in particular, had a destructive habit of conducting research for a short time and then leaving, without regard for the impact their study had on the population. These critiques of Western science aim to decolonize social science and dismantle the racist ideas the oppress indigenous and non-Western peoples through research (Smith, 2013). [20]
The central concept in feminist, anti-racist, and decolonization critiques (among other critical frames) is epistemic injustice. Epistemic injustice happens when someone is treated unfairly in their capacity to know something or describe their experience of the world. As described by Fricker (2011), [21] the injustice emerges from the dismissal of knowledge from oppressed groups, discrimination against oppressed groups in scientific communities, and the resulting gap between what scientists can make sense of from their experience and the experiences of people with less power who have lived experience of the topic. We recommend this video from Edinburgh Law School which applies epistemic injustice to studying public health emergencies, disabilities, and refugee services .
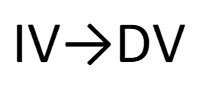
Positivism relies on nomothetic causality, or the idea that “one event, behavior, or belief will result in the occurrence of another, subsequent event, behavior, or belief.” Then, we described one kind of causality: a simple cause-and-effect relationship supported by existing theory and research on the topic, also known as a nomothetic causal relationship. But what if there is not a lot of literature on your topic? What if your question is more exploratory than explanatory? Then, you need a different kind of causal explanation, one that accounts for the complexity of human interactions.
How can we build causal relationships if we are just describing or exploring a topic? Recall the definitions of exploratory research , descriptive research , and explanatory research from Chapter 2. Wouldn’t we need to do explanatory research to build any kind of causal explanation? Explanatory research attempts to establish nomothetic causal relationships: an independent variable is demonstrated to cause change in a dependent variable. Exploratory and descriptive qualitative research contains some causal relationships, but they are actually descriptions of the causal relationships established by the study participants.
What do idiographic causal explanations look like?
An idiographic causal relationship tries to identify the many, interrelated causes that account for the phenomenon the researcher is investigating. So, if idiographic causal explanations do not look like Figure 8.5, 8.6, or 8.7 what do they look like? Instead of saying “x causes y,” your participants will describe their experiences with “x,” which they will tell you was caused and influenced by a variety of other factors, as interpreted through their unique perspective, time, and environment. As we stated before, idiographic causal explanations are messy. Your job as a social science researcher is to accurately describe the patterns in what your participants tell you.
Let’s think about this using an example. If I asked you why you decided to become a social worker, what might you say? For me, I would say that I wanted to be a mental health clinician since I was in high school. I was interested in how people thought, and I was privileged enough to have psychology courses at my local high school. I thought I wanted to be a psychologist, but at my second internship in my undergraduate program, my supervisors advised me to become a social worker because the license provided greater authority for independent practice and flexibility for career change. Once I found out social workers were like psychologists who also raised trouble about social justice, I was hooked.
That’s not a simple explanation at all! But it’s definitely a causal explanation. It is my individual, subjective truth of a complex process. If we were to ask multiple social workers the same question, we might find out that many social workers begin their careers based on factors like personal experience with a disability or social injustice, positive experiences with social workers, or a desire to help others. No one factor is the “most important factor,” like with nomothetic causal relationships. Instead, a complex web of factors, contingent on context, emerge when you interpret what people tell you about their lives.
Understanding “why?”
In creating an idiographic explanation, you are still asking “why?” But the answer is going to be more complex. Those complexities are described in Table 8.1 as well as this short video comparing nomothetic and idiographic relationships .
Nomothetic causal relationships | Idiographic causal relationships | |
---|---|---|
Paradigm | Positivist | Interpretivist |
Purpose of research | Prediction & generalization | Understanding & particularity |
Reasoning | Deductive | Inductive |
Purpose of research | Explanatory | Exploratory or descriptive |
Research methods | Quantitative | Qualitative |
Causality | Simple: cause and effect | Complex: context-dependent, sometimes circular or contradictory |
Role of theory | Theory testing | Theory building |
Remember our question from the last section, “Are you trying to generalize or nah?” If you answered nah (or no, like a normal person), you are trying to establish an idiographic causal explanation. The purpose of that explanation isn’t to predict the future or generalize to larger populations, but to describe the here-and-now as it is experienced by individuals within small groups and communities. Idiographic explanations are focused less on what is generally experienced by all people but more on the particularities of what specific individuals in a unique time and place experience.
Researchers seeking idiographic causal relationships are not trying to generalize or predict, so they have no need to reduce phenomena to mathematics. In fact, only examining things that can be counted can rob a causal relationship of its meaning and context. Instead, the goal of idiographic causal relationships is understanding, rather than prediction. Idiographic causal relationships are formed by interpreting people’s stories and experiences. Usually, these are expressed through words. Not all qualitative studies use word data, as some can use interpretations of visual or performance art. However, the vast majority of qualitative studies do use word data, like the transcripts from interviews and focus groups or documents like journal entries or meeting notes. Your participants are the experts on their lives—much like in social work practice—and as in practice, people’s experiences are embedded in their cultural, historical, and environmental context.
Idiographic causal explanations are powerful because they can describe the complicated and interconnected nature of human life. Nomothetic causal explanations, by comparison, are simplistic. Think about if someone asked you why you wanted to be a social worker. Your story might include a couple of vignettes from your education and early employment. It might include personal experience with the social welfare system or family traditions. Maybe you decided on a whim to enroll in a social work course during your graduate program. The impact of each of these events on your career is unique to you.
Idiographic causal explanations are concerned with individual stories, their idiosyncrasies, and the patterns that emerge when you collect and analyze multiple people’s stories. This is the inductive reasoning we discussed at the beginning of this chapter. Often, idiographic causal explanations begin by collecting a lot of qualitative data, whether though interviews, focus groups, or looking at available documents or cultural artifacts. Next, the researcher looks for patterns in the data and arrives at a tentative theory for how the key ideas in people’s stories are causally related.
Unlike nomothetic causal relationships, there are no formal criteria (e.g., covariation) for establishing causality in idiographic causal relationships. In fact, some criteria like temporality and nonspuriousness may be violated. For example, if an adolescent client says, “It’s hard for me to tell whether my depression began before my drinking, but both got worse when I was expelled from my first high school,” they are recognizing that it may not so simple that one thing causes another. Sometimes, there is a reciprocal relationship where one variable (depression) impacts another (alcohol abuse), which then feeds back into the first variable (depression) and into other variables as well (school). Other criteria, such as covariation and plausibility, still make sense, as the relationships you highlight as part of your idiographic causal explanation should still be plausible and its elements should vary together.
Theory building and theory testing
As we learned in the previous section, nomothetic causal explanations are created by researchers applying deductive reasoning to their topic and creating hypotheses using social science theories. Much of what we think of as social science is based on this hypothetico-deductive method, but this leaves out the other half of the equation. Where do theories come from? Are they all just revisions of one another? How do any new ideas enter social science?
Through inductive reasoning and idiographic causal explanations!
Let’s consider a social work example. If you plan to study domestic and sexual violence, you will likely encounter the Power and Control Wheel, also known as the Duluth Model (Figure 8.9). The wheel is a model designed to depict the process of domestic violence. The wheel was developed based on qualitative focus groups conducted by sexual and domestic violence advocates in Duluth, MN. This video explains more about the Duluth Model of domestic abuse.
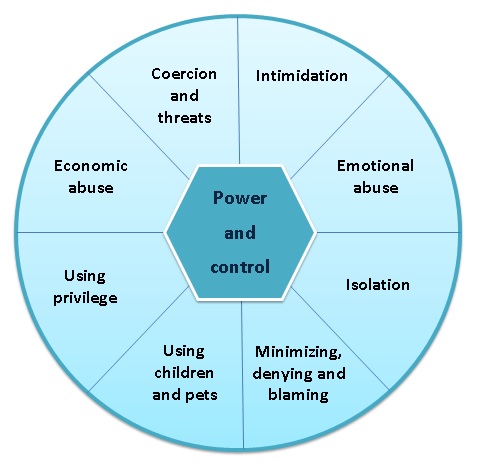
The Power and Control Wheel is an example of what an idiographic causal relationship looks like. By contrast, look back at the previous section’s Figure 8.5, 8.6, and 8.7 on nomothetic causal relationships between independent and dependent variables. See how much more complex idiographic causal explanations are?! They are complex, but not difficult to understand. At the center of domestic abuse is power and control, and while not every abuser would say that is what they were doing, that is the understanding of the survivors who informed this theoretical model. Their power and control is maintained through a variety of abusive tactics from social isolation to use of privilege to avoid consequences.
What about the role of hypotheses in idiographic causal explanations? In nomothetic causal explanations, researchers create hypotheses using existing theory and then test them for accuracy. Hypotheses in idiographic causality are much more tentative, and are probably best considered as “hunches” about what they think might be true. Importantly, they might indicate the researcher’s prior knowledge and biases before the project begins, but the goal of idiographic research is to let your participants guide you rather than existing social work knowledge. Continuing with our Duluth Model example, advocates likely had some tentative hypotheses about what was important in a relationship with domestic violence. After all, they worked with this population for years prior to the creation of the model. However, it was the stories of the participants in these focus groups that led the Power and Control Wheel explanation for domestic abuse.
As qualitative inquiry unfolds, hypotheses and hunches are likely to emerge and shift as researchers learn from what their participants share. Because the participants are the experts in idiographic causal relationships, a researcher should be open to emerging topics and shift their research questions and hypotheses accordingly. This is in contrast to hypotheses in quantitative research, which remain constant throughout the study and are shown to be true or false.
Over time, as more qualitative studies are done and patterns emerge across different studies and locations, more sophisticated theories emerge that explain phenomena across multiple contexts. Once a theory is developed from qualitative studies, a quantitative researcher can seek to test that theory. For example, a quantitative researcher may hypothesize that men who hold traditional gender roles are more likely to engage in domestic violence. That would make sense based on the Power and Control Wheel model, as the category of “using male privilege” speaks to this relationship. In this way, qualitatively-derived theory can inspire a hypothesis for a quantitative research project, as we will explore in the next section.
Complementary approaches
If idiographic and nomothetic still seem like obscure philosophy terms, let’s consider another example. Imagine you are working for a community-based non-profit agency serving people with disabilities. You are putting together a report to lobby the state government for additional funding for community support programs. As part of that lobbying, you are likely to rely on both nomothetic and idiographic causal relationships.
If you looked at nomothetic causal relationships, you might learn how previous studies have shown that, in general, community-based programs like yours are linked with better health and employment outcomes for people with disabilities. Nomothetic causal explanations seek to establish that community-based programs are better for everyone with disabilities, including people in your community.
If you looked at idiographic causal explanations, you would use stories and experiences of people in community-based programs. These individual stories are full of detail about the lived experience of being in a community-based program. You might use one story from a client in your lobbying campaign, so policymakers can understand the lived experience of what it’s like to be a person with a disability in this program. For example, a client who said “I feel at home when I’m at this agency because they treat me like a family member,” or “this is the agency that helped me get my first paycheck,” can communicate richer, more complex causal relationships.
Neither kind of causal explanation is better than the other. A decision to seek idiographic causal explanations means that you will attempt to explain or describe your phenomenon exhaustively, attending to cultural context and subjective interpretations. A decision to seek nomothetic causal explanations, on the other hand, means that you will try to explain what is true for everyone and predict what will be true in the future. In short, idiographic explanations have greater depth, and nomothetic explanations have greater breadth.
Most importantly, social workers understand the value of both approaches to understanding the social world. A social worker helping a client with substance abuse issues seeks idiographic explanations when they ask about that client’s life story, investigate their unique physical environment, or probe how their family relationships. At the same time, a social worker also uses nomothetic explanations to guide their interventions. Nomothetic explanations may help guide them to minimize risk factors and maximize protective factors or use an evidence-based therapy, relying on knowledge about what in general helps people with substance abuse issues.
So, which approach speaks to you? Are you interested in learning about (a) a few people’s experiences in a great deal of depth, or (b) a lot of people’s experiences more superficially, while also hoping your findings can be generalized to a greater number of people? The answer to this question will drive your research question and project. These approaches provide different types of information and both types are valuable.
- Idiographic causal explanations focus on subjectivity, context, and meaning.
- Idiographic causal explanations are best suited to exploratory research questions and qualitative methods.
- Idiographic causal explanations are used to create new theories in social science.
- Explore the literature on the theory you identified in section 8.1.
- Read about the origins of your theory. Who developed it and from what data?
- See if you can find a figure like Figure 8.9 in an article or book chapter that depicts the key concepts in your theory and how those concepts are related to one another causally. Write out a short statement on the causal relationships contained in the figure.
- List the key terms associated with qualitative research questions
- Distinguish between qualitative and quantitative research questions
Qualitative research questions differ from quantitative research questions. Because qualitative research questions seek to explore or describe phenomena, not provide a neat nomothetic explanation, they are often more general and openly worded. They may include only one concept, though many include more than one. Instead of asking how one variable causes changes in another, we are instead trying to understand the experiences , understandings , and meanings that people have about the concepts in our research question. These keywords often make an appearance in qualitative research questions.
Let’s work through an example from our last section. In Table 9.1, a student asked, “What is the relationship between sexual orientation or gender identity and homelessness for late adolescents in foster care?” In this question, it is pretty clear that the student believes that adolescents in foster care who identify as LGBTQ+ may be at greater risk for homelessness. This is a nomothetic causal relationship—LGBTQ+ status causes changes in homelessness.
However, what if the student were less interested in predicting homelessness based on LGBTQ+ status and more interested in understanding the stories of foster care youth who identify as LGBTQ+ and may be at risk for homelessness? In that case, the researcher would be building an idiographic causal explanation . The youths whom the researcher interviews may share stories of how their foster families, caseworkers, and others treated them. They may share stories about how they thought of their own sexuality or gender identity and how it changed over time. They may have different ideas about what it means to transition out of foster care.
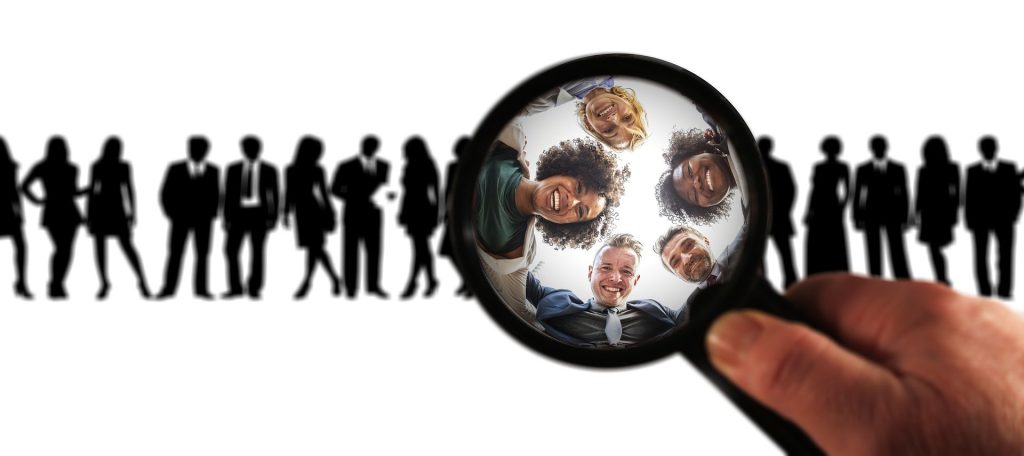
Because qualitative questions usually center on idiographic causal relationships, they look different than quantitative questions. Table 9.3 below takes the final research questions from Table 9.1 and adapts them for qualitative research. The guidelines for research questions previously described in this chapter still apply, but there are some new elements to qualitative research questions that are not present in quantitative questions.
- Qualitative research questions often ask about lived experience, personal experience, understanding, meaning, and stories.
- Qualitative research questions may be more general and less specific.
- Qualitative research questions may also contain only one variable, rather than asking about relationships between multiple variables.
How does witnessing domestic violence impact a child’s romantic relationships in adulthood? | How do people who witness domestic violence understand its effects on their current relationships? |
What is the relationship between sexual orientation or gender identity and homelessness for late adolescents in foster care? | What is the experience of identifying as LGBTQ+ in the foster care system? |
How does income inequality affect ambivalence in high-density urban areas? | What does racial ambivalence mean to residents of an urban neighborhood with high income inequality? |
How does race impact rates of mental health diagnosis for children in foster care? | How do African-Americans experience seeking help for mental health concerns? |
Qualitative research questions have one final feature that distinguishes them from quantitative research questions: they can change over the course of a study. Qualitative research is a reflexive process, one in which the researcher adapts their approach based on what participants say and do. The researcher must constantly evaluate whether their question is important and relevant to the participants. As the researcher gains information from participants, it is normal for the focus of the inquiry to shift.
For example, a qualitative researcher may want to study how a new truancy rule impacts youth at risk of expulsion. However, after interviewing some of the youth in their community, a researcher might find that the rule is actually irrelevant to their behavior and thoughts. Instead, their participants will direct the discussion to their frustration with the school administrators or the lack of job opportunities in the area. This is a natural part of qualitative research, and it is normal for research questions and hypothesis to evolve based on information gleaned from participants.
However, this reflexivity and openness unacceptable in quantitative research for good reasons. Researchers using quantitative methods are testing a hypothesis, and if they could revise that hypothesis to match what they found, they could never be wrong! Indeed, an important component of open science and reproducability is the preregistration of a researcher’s hypotheses and data analysis plan in a central repository that can be verified and replicated by reviewers and other researchers. This interactive graphic from 538 shows how an unscrupulous research could come up with a hypothesis and theoretical explanation after collecting data by hunting for a combination of factors that results in a statistically significant relationship. This is an excellent example of how the positivist assumptions behind quantitative research and intepretivist assumptions behind qualitative research result in different approaches to social science.
- Qualitative research questions often contain words or phrases like “lived experience,” “personal experience,” “understanding,” “meaning,” and “stories.”
- Qualitative research questions can change and evolve over the course of the study.
- Using the guidance in this chapter, write a qualitative research question. You may want to use some of the keywords mentioned above.
- Kivuna, C. & Kuyini, A. B. (2017). Understanding and applying research paradigms in educational contexts. International Journal of Higher Education, 6 (5), 26-41. https://eric.ed.gov/?id=EJ1154775 ↵
- Kuhn, T. (1962). The structure of scientific revolutions . Chicago: University of Chicago Press. ↵
- Fleuridas, C., & Krafcik, D. (2019). Beyond four forces: The evolution of psychotherapy. Sage Open , 9 (1), 2158244018824492. ↵
- Shneider, A. M. (2009). Four stages of a scientific discipline; four types of scientist. Trends in Biochemical Sciences 34 (5), 217-233. https://doi.org/10.1016/j.tibs.2009.02.00 ↵
- Burrell, G. & Morgan, G. (1979). Sociological paradigms and organizational analysis . Routledge. Guba, E. (ed.) (1990). The paradigm dialog . SAGE. ↵
- Routledge. Guba, E. (ed.) (1990). The paradigm dialog . SAGE. ↵
- Burrell, G. & Morgan, G. (1979). Sociological paradigms and organizational analysis . Here is a summary of Burrell & Morgan from Babson College , and our classification collapses radical humanism and radical structuralism into the critical paradigm, following Guba and Lincoln's three-paradigm framework. We feel this approach is more parsimonious and easier for students to understand on an introductory level. ↵
- For more about how the meanings of hand gestures vary by region, you might read the following blog entry: Wong, W. (2007). The top 10 hand gestures you’d better get right . Retrieved from: http://www.languagetrainers.co.uk/blog/2007/09/24/top-10-hand-gestures ↵
- Rosario, M., Schrimshaw, E. W., Hunter, J., & Levy-Warren, A. (2009). The coming-out process of young lesbian and bisexual women: Are there butch/femme differences in sexual identity development?. Archives of sexual behavior , 38 (1), 34-49. ↵
- Calhoun, C., Gerteis, J., Moody, J., Pfaff, S., & Virk, I. (Eds.). (2007). Classical sociological theory (2nd ed.). Malden, MA: Blackwell. ↵
- Fraser, N. (1989). Unruly practices: Power, discourse, and gender in contemporary social theory . Minneapolis, MN: University of Minnesota Press. ↵
- Here are links to two HBSE open textbooks, if you are unfamiliar with social work theories and would like more background. https://uark.pressbooks.pub/hbse1/ and https://uark.pressbooks.pub/humanbehaviorandthesocialenvironment2/ ↵
- Box, G. E. P.. (1976). Science and statistics. Journal of the American Statistical Association, 71 (356), 791. ↵
- Heineman-Pieper, J., Tyson, K., & Pieper, M. H. (2002). Doing good science without sacrificing good values: Why the heuristic paradigm is the best choice for social work. Families in Society , 83 (1), 15-28. ↵
- Crasnow, S. (2020). Feminist perspectives on science. In E. N. Zalta (ed.), The Stanford Encyclopedia of Philosophy (Winter 2020 Edition). Retrieved from: https://plato.stanford.edu/entries/feminist-science/ ↵
- Grogan, K.E. (2019) How the entire scientific community can confront gender bias in the workplace. Nature Ecology & Evolution, 3 , 3–6. doi:10.1038/s41559-018-0747-4 ↵
- Tasca, C., Rapetti, M., Carta, M. G., & Fadda, B. (2012). Women and hysteria in the history of mental health. Clinical practice and epidemiology in mental health: Clinical practice & epidemiology in mental health , 8 , 110-119. ↵
- Klonsky, E. D., Jane, J. S., Turkheimer, E., & Oltmanns, T. F. (2002). Gender role and personality disorders. Journal of personality disorders , 16 (5), 464-476. ↵
- Smith, L. T. (2013). Decolonizing methodologies: Research and indigenous peoples . Zed Books Ltd. ↵
- Fricker, M. (2011). Epistemic injustice: Power and the ethics of knowing . Oxford University Press. ↵
The highest level of measurement. Denoted by mutually exclusive categories, a hierarchy (order), values can be added, subtracted, multiplied, and divided, and the presence of an absolute zero.
a paradigm based on the idea that social context and interaction frame our realities
a paradigm in social science research focused on power, inequality, and social change
a research paradigm that suspends questions of philosophical ‘truth’ and focuses more on how different philosophies, theories, and methods can be used strategically to resolve a problem or question within the researcher's unique context
A cyclical process of theory development, starting with an observed phenomenon, then developing or using a theory to make a specific prediction of what should happen if that theory is correct, testing that prediction, refining the theory in light of the findings, and using that refined theory to develop new hypotheses, and so on.
when someone is treated unfairly in their capacity to know something or describe their experience of the world
conducted during the early stages of a project, usually when a researcher wants to test the feasibility of conducting a more extensive study or if the topic has not been studied in the past
research that describes or defines a particular phenomenon
explains why particular phenomena work in the way that they do; answers “why” questions
attempts to explain or describe your phenomenon exhaustively, based on the subjective understandings of your participants
"Assuming that the null hypothesis is true and the study is repeated an infinite number times by drawing random samples from the same populations(s), less than 5% of these results will be more extreme than the current result" (Cassidy et al., 2019, p. 233).
Scientific Inquiry in Social Work (2nd Edition) Copyright © 2020 by Matthew DeCarlo, Cory Cummings, and Kate Agnelli is licensed under a Creative Commons Attribution-NonCommercial-ShareAlike 4.0 International License , except where otherwise noted.
Share This Book
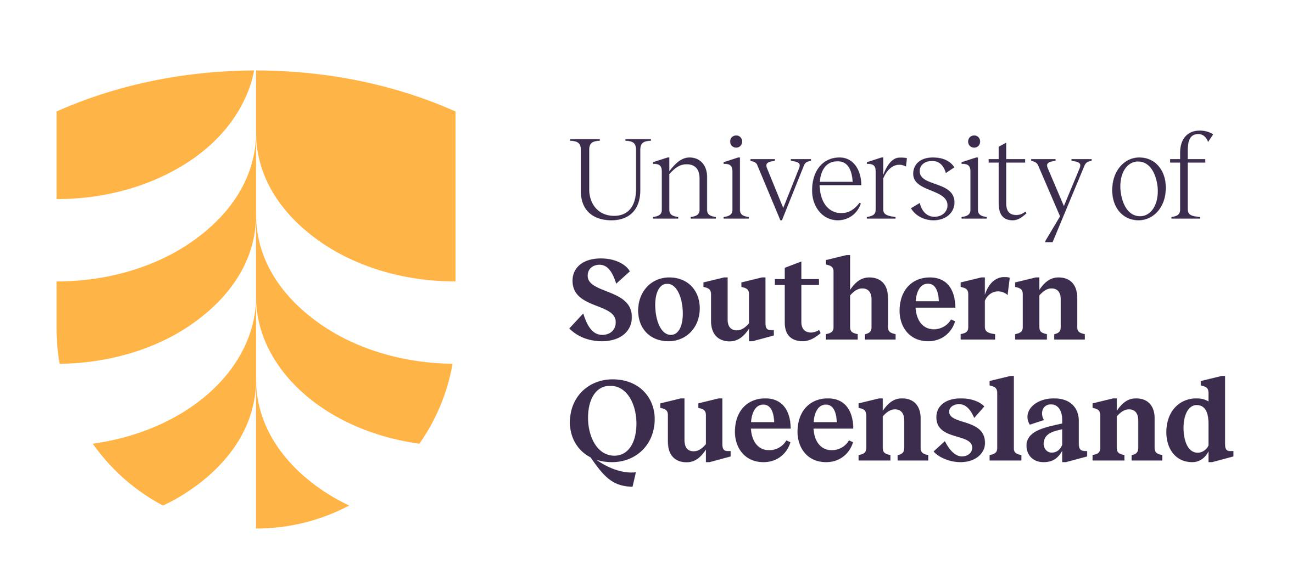
Want to create or adapt books like this? Learn more about how Pressbooks supports open publishing practices.
12 Interpretive research
Chapter 11 introduced interpretive research—or more specifically, interpretive case research. This chapter will explore other kinds of interpretive research. Recall that positivist or deductive methods—such as laboratory experiments and survey research—are those that are specifically intended for theory (or hypotheses) testing. Interpretive or inductive methods—such as action research and ethnography—one the other hand, are intended for theory building. Unlike a positivist method, where the researcher tests existing theoretical postulates using empirical data, in interpretive methods, the researcher tries to derive a theory about the phenomenon of interest from the existing observed data.
The term ‘interpretive research’ is often used loosely and synonymously with ‘qualitative research’, although the two concepts are quite different. Interpretive research is a research paradigm (see Chapter 3) that is based on the assumption that social reality is not singular or objective. Rather, it is shaped by human experiences and social contexts (ontology), and is therefore best studied within its sociohistoric context by reconciling the subjective interpretations of its various participants (epistemology). Because interpretive researchers view social reality as being embedded within—and therefore impossible to abstract from—their social settings, they ‘interpret’ the reality though a ‘sense-making’ process rather than a hypothesis testing process. This is in contrast to the positivist or functionalist paradigm that assumes that the reality is relatively independent of the context, can be abstracted from their contexts, and studied in a decomposable functional manner using objective techniques such as standardised measures. Whether a researcher should pursue interpretive or positivist research depends on paradigmatic considerations about the nature of the phenomenon under consideration and the best way to study it.
However, qualitative versus quantitative research refers to empirical or data-oriented considerations about the type of data to collect and how to analyse it. Qualitative research relies mostly on non-numeric data, such as interviews and observations, in contrast to quantitative research which employs numeric data such as scores and metrics. Hence, qualitative research is not amenable to statistical procedures such as regression analysis, but is coded using techniques like content analysis. Sometimes, coded qualitative data is tabulated quantitatively as frequencies of codes, but this data is not statistically analysed. Many puritan interpretive researchers reject this coding approach as a futile effort to seek consensus or objectivity in a social phenomenon which is essentially subjective.
Although interpretive research tends to rely heavily on qualitative data, quantitative data may add more precision and clearer understanding of the phenomenon of interest than qualitative data. For example, Eisenhardt (1989), [1] in her interpretive study of decision-making in high-velocity firms (discussed in the previous chapter on case research), collected numeric data on how long it took each firm to make certain strategic decisions—which ranged from approximately six weeks to 18 months—how many decision alternatives were considered for each decision, and surveyed her respondents to capture their perceptions of organisational conflict. Such numeric data helped her clearly distinguish the high-speed decision-making firms from the low-speed decision-makers without relying on respondents’ subjective perceptions, which then allowed her to examine the number of decision alternatives considered by and the extent of conflict in high-speed versus low-speed firms. Interpretive research should attempt to collect both qualitative and quantitative data pertaining to the phenomenon of interest, and so should positivist research as well. Joint use of qualitative and quantitative data—often called ‘mixed-mode design’—may lead to unique insights, and is therefore highly prized in the scientific community.
Interpretive research came into existence in the early nineteenth century—long before positivist techniques were developed—and has its roots in anthropology, sociology, psychology, linguistics, and semiotics. Many positivist researchers view interpretive research as erroneous and biased, given the subjective nature of the qualitative data collection and interpretation process employed in such research. However, since the 1970s, many positivist techniques’ failure to generate interesting insights or new knowledge has resulted in a resurgence of interest in interpretive research—albeit with exacting methods and stringent criteria to ensure the reliability and validity of interpretive inferences.
Distinctions from positivist research
In addition to the fundamental paradigmatic differences in ontological and epistemological assumptions discussed above, interpretive and positivist research differ in several other ways. First, interpretive research employs a theoretical sampling strategy, where study sites, respondents, or cases are selected based on theoretical considerations such as whether they fit the phenomenon being studied (e.g., sustainable practices can only be studied in organisations that have implemented sustainable practices), whether they possess certain characteristics that make them uniquely suited for the study (e.g., a study of the drivers of firm innovations should include some firms that are high innovators and some that are low innovators, in order to draw contrast between these firms), and so forth. In contrast, positivist research employs random sampling —or a variation of this technique—in which cases are chosen randomly from a population for the purpose of generalisability. Hence, convenience samples and small samples are considered acceptable in interpretive research—as long as they fit the nature and purpose of the study—but not in positivist research.
Second, the role of the researcher receives critical attention in interpretive research. In some methods such as ethnography, action research, and participant observation, the researcher is considered part of the social phenomenon, and their specific role and involvement in the research process must be made clear during data analysis. In other methods, such as case research, the researcher must take a ’neutral’ or unbiased stance during the data collection and analysis processes, and ensure that their personal biases or preconceptions do not taint the nature of subjective inferences derived from interpretive research. In positivist research, however, the researcher is considered to be external to and independent of the research context, and is not presumed to bias the data collection and analytic procedures.
Third, interpretive analysis is holistic and contextual, rather than being reductionist and isolationist. Interpretive interpretations tend to focus on language, signs, and meanings from the perspective of the participants involved in the social phenomenon, in contrast to statistical techniques that are employed heavily in positivist research. Rigor in interpretive research is viewed in terms of systematic and transparent approaches to data collection and analysis, rather than statistical benchmarks for construct validity or significance testing.
Lastly, data collection and analysis can proceed simultaneously and iteratively in interpretive research. For instance, the researcher may conduct an interview and code it before proceeding to the next interview. Simultaneous analysis helps the researcher correct potential flaws in the interview protocol or adjust it to capture the phenomenon of interest better. The researcher may even change their original research question if they realise that their original research questions are unlikely to generate new or useful insights. This is a valuable—but often understated—benefit of interpretive research, and is not available in positivist research, where the research project cannot be modified or changed once the data collection has started without redoing the entire project from the start.
Benefits and challenges of interpretive research
Interpretive research has several unique advantages. First, it is well-suited for exploring hidden reasons behind complex, interrelated, or multifaceted social processes—such as inter-firm relationships or inter-office politics—where quantitative evidence may be biased, inaccurate, or otherwise difficult to obtain. Second, it is often helpful for theory construction in areas with no or insufficient a priori theory. Third, it is also appropriate for studying context-specific, unique, or idiosyncratic events or processes. Fourth, interpretive research can also help uncover interesting and relevant research questions and issues for follow-up research.
At the same time, interpretive research also has its own set of challenges. First, this type of research tends to be more time and resource intensive than positivist research in data collection and analytic efforts. Too little data can lead to false or premature assumptions, while too much data may not be effectively processed by the researcher. Second, interpretive research requires well-trained researchers who are capable of seeing and interpreting complex social phenomenon from the perspectives of the embedded participants, and reconciling the diverse perspectives of these participants, without injecting their personal biases or preconceptions into their inferences. Third, all participants or data sources may not be equally credible, unbiased, or knowledgeable about the phenomenon of interest, or may have undisclosed political agendas which may lead to misleading or false impressions. Inadequate trust between the researcher and participants may hinder full and honest self-representation by participants, and such trust building takes time. It is the job of the interpretive researcher to ‘see through the smoke’ (i.e., hidden or biased agendas) and understand the true nature of the problem. Fourth, given the heavily contextualised nature of inferences drawn from interpretive research, such inferences do not lend themselves well to replicability or generalisability. Finally, interpretive research may sometimes fail to answer the research questions of interest or predict future behaviours.
Characteristics of interpretive research
All interpretive research must adhere to a common set of principles, as described below.
Naturalistic inquiry: Social phenomena must be studied within their natural setting.
Because interpretive research assumes that social phenomena are situated within—and cannot be isolated from—their social context, interpretations of such phenomena must be grounded within their sociohistorical context. This implies that contextual variables should be observed and considered in seeking explanations of a phenomenon of interest, even though context sensitivity may limit the generalisability of inferences.
Researcher as instrument: Researchers are often embedded within the social context that they are studying, and are considered part of the data collection instrument in that they must use their observational skills, their trust with the participants, and their ability to extract the correct information. Further, their personal insights, knowledge, and experiences of the social context are critical to accurately interpreting the phenomenon of interest. At the same time, researchers must be fully aware of their personal biases and preconceptions, and not let such biases interfere with their ability to present a fair and accurate portrayal of the phenomenon.
Interpretive analysis: Observations must be interpreted through the eyes of the participants embedded in the social context. Interpretation must occur at two levels. The first level involves viewing or experiencing the phenomenon from the subjective perspectives of the social participants. The second level is to understand the meaning of the participants’ experiences in order to provide a ‘thick description’ or a rich narrative story of the phenomenon of interest that can communicate why participants acted the way they did.
Use of expressive language: Documenting the verbal and non-verbal language of participants and the analysis of such language are integral components of interpretive analysis. The study must ensure that the story is viewed through the eyes of a person, and not a machine, and must depict the emotions and experiences of that person, so that readers can understand and relate to that person. Use of imageries, metaphors, sarcasm, and other figures of speech are very common in interpretive analysis.
Temporal nature: Interpretive research is often not concerned with searching for specific answers, but with understanding or ‘making sense of’ a dynamic social process as it unfolds over time. Hence, such research requires the researcher to immerse themself in the study site for an extended period of time in order to capture the entire evolution of the phenomenon of interest.
Hermeneutic circle: Interpretive interpretation is an iterative process of moving back and forth from pieces of observations (text), to the entirety of the social phenomenon (context), to reconcile their apparent discord, and to construct a theory that is consistent with the diverse subjective viewpoints and experiences of the embedded participants. Such iterations between the understanding/meaning of a phenomenon and observations must continue until ‘theoretical saturation’ is reached, whereby any additional iteration does not yield any more insight into the phenomenon of interest.
Interpretive data collection
Data is collected in interpretive research using a variety of techniques. The most frequently used technique is interviews (face-to-face, telephone, or focus groups). Interview types and strategies are discussed in detail in Chapter 9. A second technique is observation . Observational techniques include direct observation , where the researcher is a neutral and passive external observer, and is not involved in the phenomenon of interest (as in case research), and participant observation , where the researcher is an active participant in the phenomenon, and their input or mere presence influence the phenomenon being studied (as in action research). A third technique is documentation , where external and internal documents—such as memos, emails, annual reports, financial statements, newspaper articles, or websites—may be used to cast further insight into the phenomenon of interest or to corroborate other forms of evidence.
Interpretive research designs
Case research . As discussed in the previous chapter, case research is an intensive longitudinal study of a phenomenon at one or more research sites for the purpose of deriving detailed, contextualised inferences, and understanding the dynamic process underlying a phenomenon of interest. Case research is a unique research design in that it can be used in an interpretive manner to build theories, or in a positivist manner to test theories. The previous chapter on case research discusses both techniques in depth and provides illustrative exemplars. Furthermore, the case researcher is a neutral observer (direct observation) in the social setting, rather than an active participant (participant observation). As with any other interpretive approach, drawing meaningful inferences from case research depends heavily on the observational skills and integrative abilities of the researcher.
Action research . Action research is a qualitative but positivist research design aimed at theory testing rather than theory building. This is an interactive design that assumes that complex social phenomena are best understood by introducing changes, interventions, or ‘actions’ into those phenomena, and observing the outcomes of such actions on the phenomena of interest. In this method, the researcher is usually a consultant or an organisational member embedded into a social context —such as an organisation—who initiates an action in response to a social problem, and examines how their action influences the phenomenon, while also learning and generating insights about the relationship between the action and the phenomenon. Examples of actions may include organisational change programs—such as the introduction of new organisational processes, procedures, people, or technology or the replacement of old ones—initiated with the goal of improving an organisation’s performance or profitability. The researcher’s choice of actions must be based on theory, which should explain why and how such actions may bring forth the desired social change. The theory is validated by the extent to which the chosen action is successful in remedying the targeted problem. Simultaneous problem-solving and insight generation are the central feature that distinguishes action research from other research methods (which may not involve problem solving), and from consulting (which may not involve insight generation). Hence, action research is an excellent method for bridging research and practice.
There are several variations of the action research method. The most popular of these methods is participatory action research , designed by Susman and Evered (1978). [2] This method follows an action research cycle consisting of five phases: diagnosing, action-planning, action-taking, evaluating, and learning (see Figure 12.1). Diagnosing involves identifying and defining a problem in its social context. Action-planning involves identifying and evaluating alternative solutions to the problem, and deciding on a future course of action based on theoretical rationale. Action-taking is the implementation of the planned course of action. The evaluation stage examines the extent to which the initiated action is successful in resolving the original problem—i.e., whether theorised effects are indeed realised in practice. In the learning phase, the experiences and feedback from action evaluation are used to generate insights about the problem and suggest future modifications or improvements to the action. Based on action evaluation and learning, the action may be modified or adjusted to address the problem better, and the action research cycle is repeated with the modified action sequence. It is suggested that the entire action research cycle be traversed at least twice so that learning from the first cycle can be implemented in the second cycle. The primary mode of data collection is participant observation, although other techniques such as interviews and documentary evidence may be used to corroborate the researcher’s observations.
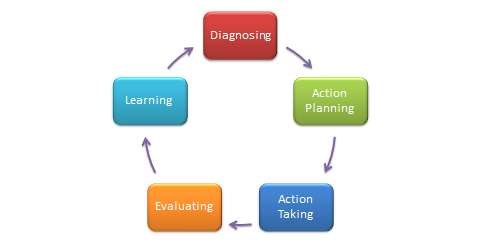
Ethnography . The ethnographic research method—derived largely from the field of anthropology—emphasises studying a phenomenon within the context of its culture. The researcher must be deeply immersed in the social culture over an extended period of time—usually eight months to two years—and should engage, observe, and record the daily life of the studied culture and its social participants within their natural setting. The primary mode of data collection is participant observation, and data analysis involves a ‘sense-making’ approach. In addition, the researcher must take extensive field notes, and narrate her experience in descriptive detail so that readers may experience the same culture as the researcher. In this method, the researcher has two roles: rely on her unique knowledge and engagement to generate insights (theory), and convince the scientific community of the transsituational nature of the studied phenomenon.
The classic example of ethnographic research is Jane Goodall’s study of primate behaviours. While living with chimpanzees in their natural habitat at Gombe National Park in Tanzania, she observed their behaviours, interacted with them, and shared their lives. During that process, she learnt and chronicled how chimpanzees seek food and shelter, how they socialise with each other, their communication patterns, their mating behaviours, and so forth. A more contemporary example of ethnographic research is Myra Bluebond-Langer’s (1996) [3] study of decision-making in families with children suffering from life-threatening illnesses, and the physical, psychological, environmental, ethical, legal, and cultural issues that influence such decision-making. The researcher followed the experiences of approximately 80 children with incurable illnesses and their families for a period of over two years. Data collection involved participant observation and formal/informal conversations with children, their parents and relatives, and healthcare providers to document their lived experience.
Phenomenology. Phenomenology is a research method that emphasises the study of conscious experiences as a way of understanding the reality around us. It is based on the ideas of early twentieth century German philosopher, Edmund Husserl, who believed that human experience is the source of all knowledge. Phenomenology is concerned with the systematic reflection and analysis of phenomena associated with conscious experiences such as human judgment, perceptions, and actions. Its goal is (appreciating and describing social reality from the diverse subjective perspectives of the participants involved, and understanding the symbolic meanings (‘deep structure’) underlying these subjective experiences. Phenomenological inquiry requires that researchers eliminate any prior assumptions and personal biases, empathise with the participant’s situation, and tune into existential dimensions of that situation so that they can fully understand the deep structures that drive the conscious thinking, feeling, and behaviour of the studied participants.
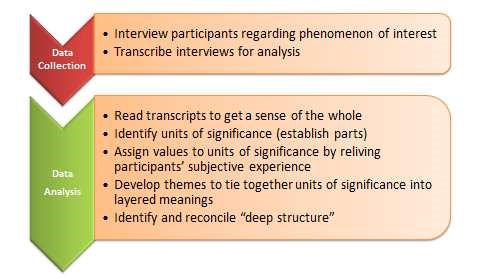
Some researchers view phenomenology as a philosophy rather than as a research method. In response to this criticism, Giorgi and Giorgi (2003) [4] developed an existential phenomenological research method to guide studies in this area. This method, illustrated in Figure 12.2, can be grouped into data collection and data analysis phases. In the data collection phase, participants embedded in a social phenomenon are interviewed to capture their subjective experiences and perspectives regarding the phenomenon under investigation. Examples of questions that may be asked include ‘Can you describe a typical day?’ or ‘Can you describe that particular incident in more detail?’. These interviews are recorded and transcribed for further analysis. During data analysis , the researcher reads the transcripts to: get a sense of the whole, and establish ‘units of significance’ that can faithfully represent participants’ subjective experiences. Examples of such units of significance are concepts such as ‘felt-space’ and ‘felt-time’, which are then used to document participants’ psychological experiences. For instance, did participants feel safe, free, trapped, or joyous when experiencing a phenomenon (‘felt-space’)? Did they feel that their experience was pressured, slow, or discontinuous (‘felt-time’)? Phenomenological analysis should take into account the participants’ temporal landscape (i.e., their sense of past, present, and future), and the researcher must transpose his/herself in an imaginary sense into the participant’s situation (i.e., temporarily live the participant’s life). The participants’ lived experience is described in the form of a narrative or using emergent themes. The analysis then delves into these themes to identify multiple layers of meaning while retaining the fragility and ambiguity of subjects’ lived experiences.
Rigor in interpretive research
While positivist research employs a ‘reductionist’ approach by simplifying social reality into parsimonious theories and laws, interpretive research attempts to interpret social reality through the subjective viewpoints of the embedded participants within the context where the reality is situated. These interpretations are heavily contextualised, and are naturally less generalisable to other contexts. However, because interpretive analysis is subjective and sensitive to the experiences and insight of the embedded researcher, it is often considered less rigorous by many positivist (functionalist) researchers. Because interpretive research is based on a different set of ontological and epistemological assumptions about social phenomena than positivist research, the positivist notions of rigor—such as reliability, internal validity, and generalisability—do not apply in a similar manner. However, Lincoln and Guba (1985) [5] provide an alternative set of criteria that can be used to judge the rigor of interpretive research.
Dependability. Interpretive research can be viewed as dependable or authentic if two researchers assessing the same phenomenon, using the same set of evidence, independently arrive at the same conclusions, or the same researcher, observing the same or a similar phenomenon at different times arrives at similar conclusions. This concept is similar to that of reliability in positivist research, with agreement between two independent researchers being similar to the notion of inter-rater reliability, and agreement between two observations of the same phenomenon by the same researcher akin to test-retest reliability. To ensure dependability, interpretive researchers must provide adequate details about their phenomenon of interest and the social context in which it is embedded, so as to allow readers to independently authenticate their interpretive inferences.
Credibility. Interpretive research can be considered credible if readers find its inferences to be believable. This concept is akin to that of internal validity in functionalistic research. The credibility of interpretive research can be improved by providing evidence of the researcher’s extended engagement in the field, by demonstrating data triangulation across subjects or data collection techniques, and by maintaining meticulous data management and analytic procedures—such as verbatim transcription of interviews, accurate records of contacts and interviews—and clear notes on theoretical and methodological decisions, that can allow an independent audit of data collection and analysis if needed.
Confirmability. Confirmability refers to the extent to which the findings reported in interpretive research can be independently confirmed by others—typically, participants. This is similar to the notion of objectivity in functionalistic research. Since interpretive research rejects the notion of an objective reality, confirmability is demonstrated in terms of ‘intersubjectivity’—i.e., if the study’s participants agree with the inferences derived by the researcher. For instance, if a study’s participants generally agree with the inferences drawn by a researcher about a phenomenon of interest—based on a review of the research paper or report—then the findings can be viewed as confirmable.
Transferability. Transferability in interpretive research refers to the extent to which the findings can be generalised to other settings. This idea is similar to that of external validity in functionalistic research. The researcher must provide rich, detailed descriptions of the research context (‘thick description’) and thoroughly describe the structures, assumptions, and processes revealed from the data so that readers can independently assess whether and to what extent the reported findings are transferable to other settings.
- Eisenhardt, K. M. (1989). Making fast strategic decisions in high-velocity environments. Academy of Management Journal , 32(3), 543–576. ↵
- Susman, G. I. and Evered, R. D. (1978) An assessment of the scientific merits of action research. Administrative Science Quarterly , 23, 582–603. ↵
- Bluebond-Langer, M. (1996). In the shadow of illness: Parents and siblings of the chronically ill child . Princeton, NJ: Princeton University Press. ↵
- Giorgi, A., & Giorgi, B. (2003). Phenomenology. In J. A. Smith (ed.), Qualitative psychology: A practical guide to research methods (pp. 25–50). London: Sage Publications ↵
- Lincoln, Y. S., & Guba, E. G. (1985). Naturalistic inquiry . Beverly Hills: Sage Publications. ↵
Social Science Research: Principles, Methods and Practices (Revised edition) Copyright © 2019 by Anol Bhattacherjee is licensed under a Creative Commons Attribution-NonCommercial-ShareAlike 4.0 International License , except where otherwise noted.
Share This Book
Chapter 2. Philosophical Assumptions and Interpretive Frameworks
Total Page: 16
File Type: pdf , Size: 1020Kb
- Abstract and Figures
- Public Full-text
- Jackson: Choosing a Methodology: Philosophical Underpinning JACKSON: CHOOSING A METHODOLOGY: PHILOSOPHICAL UNDERPINNING Choosing a Methodology: Philosophical Practitioner Research Underpinning In Higher Education Copyright © 2013 University of Cumbria Vol 7 (1) pages 49-62 Elizabeth Jackson University of Cumbria [email protected] Abstract As a university lecturer, I find that a frequent question raised by Masters students concerns the methodology chosen for research and the rationale required in dissertations. This paper unpicks some of the philosophical coherence that can inform choices to be made regarding methodology and a well-thought out rationale that can add to the rigour of a research project. It considers the conceptual framework for research including the ontological and epistemological perspectives that are pertinent in choosing a methodology and subsequently the methods to be used. The discussion is exemplified using a concrete example of a research project in order to contextualise theory within practice. Key words Ontology; epistemology; positionality; relationality; methodology; method. Introduction This paper arises from work with students writing Masters dissertations who frequently express confusion and doubt about how appropriate methodology is chosen for research. It will be argued here that consideration of philosophical underpinning can be crucial for both shaping research design and for explaining approaches taken in order to support credibility of research outcomes. It is beneficial, within the unique context of the research, for the researcher to carefully [Show full text]
- Introduction to Philosophy. Social Studies--Language Arts: 6414.16. INSTITUTION Dade County Public Schools, Miami, Fla DOCUMENT RESUME ED 086 604 SO 006 822 AUTHOR Norris, Jack A., Jr. TITLE Introduction to Philosophy. Social Studies--Language Arts: 6414.16. INSTITUTION Dade County Public Schools, Miami, Fla. PUB DATE 72 NOTE 20p.; Authorized Course of Instruction for the Quinmester Program EDRS PRICE MF-$0.65 HC-$3.29 DESCRIPTORS Course Objectives; Curriculum Guides; Grade 10; Grade 11; Grade 12; *Language Arts; Learnin4 Activities; *Logic; Non Western Civilization; *Philosophy; Resource Guides; Secondary Grades; *Social Studies; *Social Studies Units; Western Civilization IDENTIFIERS *Quinmester Program ABSTRACT Western and non - western philosophers and their ideas are introduced to 10th through 12th grade students in this general social studies Quinmester course designed to be used as a preparation for in-depth study of the various schools of philosophical thought. By acquainting students with the questions and categories of philosophy, a point of departure for further study is developed. Through suggested learning activities the meaning of philosopky is defined. The Socratic, deductive, inductive, intuitive and eclectic approaches to philosophical thought are examined, as are three general areas of philosophy, metaphysics, epistemology,and axiology. Logical reasoning is applied to major philosophical questions. This course is arranged, as are other quinmester courses, with sections on broad goals, course content, activities, and materials. A related document is ED 071 937.(KSM) FILMED FROM BEST AVAILABLE COPY U S DEPARTMENT EDUCATION OF HEALTH. NAT10N41 [Show full text]
- Qualitative Research Encyclopedia of Leadership ______________________________________________________________________________ Edited by G. Goethals, G. Sorenson, J. MacGregor Copyright © 2004 SAGE Publications London, Thousand Oaks CA, New Delhi www.sagepublications.com A R T I C L E Qualitative Research Sonia Ospina Robert F. Wagner Graduate School of Public Service New York University QUALITATIVE RESEARCH Leadership scholars seeking to answer questions about culture and meaning have found experimental and quantitative methods to be insufficient on their own in explaining the phenomenon they wish to study. As a result, qualitative research has gained momentum as a mode of inquiry. This trend has roots in the development of the New Leadership School, (Conger, 1999; Hunt, 1999), on the recent emergence of an approach to leadership that views it as a relational phenomenon (Fletcher, 2002), and on the increased recognition of the strengths of qualitative inquiry generally. Shank (2002) defines qualitative research as “a form of systematic empirical inquiry into meaning” (p. 5). By systematic he means “planned, ordered and public”, following rules agreed upon by members of the qualitative research community. By empirical, he means that this type of inquiry is grounded in the world of experience. Inquiry into meaning says researchers try to understand how others make sense of their experience. Denzin and Lincoln (2000) claim that qualitative research involves an interpretive and naturalistic approach: “This means that qualitative researchers study things in their [Show full text]
- Study of Ontology, Epistemology and Axiology on Management The Second International Conference on Entrepreneurship STUDY OF ONTOLOGY, EPISTEMOLOGY AND AXIOLOGY ON MANAGEMENT Rahmat Setiawan1 Airlangga University, Surabaya INDONESIA Email: [email protected] ABSTRACT There is still a difference of opinion among experts in the field of management of what is meant by management, namely whether the management is a science, an art or a profession. In addition, the management theory and studies have also experienced rapid growth, especially until the 19th century until the present. These developments have given rise to various groups of schools of thought about the management, which is a group of classical management perspective, a group of behavior management perspective, and a group of quantitative management perspective. Therefore, it is necessary to study on the development of management in terms of the philosophy of science perspective. By doing this assessment, management will be studied ontological, epistemological and axiological. Ontologically, management is the science, art and profession of work done through others. Material object is a behavior management work done through others. In management development, ontologically most experts view reality of social management in management as something objective, not subjective. Epistemologically, in management development, the approach most widely used by management experts is a deductive approach. However, the trend also shows that the inductive approach is also widely used lately. In axiological, largely through the efforts of study and research in the development of management is not value-free because the paradigm used by most bear management experts in developing management are positivist or functionalist paradigm. However, in applying the results of research to take a policy, then the leader of the company must still pay attention to the values of ethics and humanity. [Show full text]
- Formal Axiology and the Philosophy of Social Science; Esp., Political Science Formal Axiology and the Philosophy of Social Science; esp., Political Science Introduction In the 22 centuries from Aristotle to Galileo, man’s way of life, and knowledge of nature, changed very little compared to the explosion of invention and discovery in the mere four centuries since Galileo. According to philosopher of science, R.S. Hartman, Galileo empowered humanity to make such progress when he “created the empirico-mathematical world picture;” that is, the worldview of natural science.1 During the Scientific Revolution, the European sense of reality was transformed from a dream-like condition under the control of “God’s Will,” and which only He could fully understand, to sets of processes which could be understood and explained in precise mathematical formulas. Natural philosophy, prior to Galileo, offered “explanations” of natural phenomena, but without much precision. For example, Aristotle defined “movement” as “the transition from potentiality to actuality.” This was accepted and studied for centuries. But Galileo re-defined “motion,” so that it became mathematically measurable. Rather than the vagaries of “realizing potential,” Galileo offered the formula V=s/t. He showed that by measuring the space (s) traversed by an object, and the time (t) it took, a precise measurement of speed, or velocity (V), could be calculated. Now motion was much less a mystery. Hartman notes that “Galileo’s formula led to a multitude of consequences; [eventually including] the systems of Newton and Einstein.”2 Galileo thus changed the way of thinking about nature from vague philosophical speculation to a method applying precise formal analysis and explanation. That shift in the way of thinking made possible all that followed. [Show full text]
- Mixed Methods Research Approaches:Warrant Consideration Phenomena in Themethodological Thirdmovementon the Humanities Sciences IOSR Journal Of Humanities And Social Science (IOSR-JHSS) Volume 20, Issue 11, Ver. II (Nov. 2015) PP 21-28 e-ISSN: 2279-0837, p-ISSN: 2279-0845. www.iosrjournals.org Mixed Methods Research Approaches:Warrant Consideration Phenomena in theMethodological ThirdMovementon the Humanities Sciences Kamal koohi Assistant Professor of Institute ofSocial Research, University of Tabriz Abstract:Today, Dramatic changes and transformations has happened in theories sociology similar to other areas.We have seen in recent sociological theories emerging paradigms of integrated. Social research methods are not exempt from this rule. Simplification of complex social problems cannot be easily by a deterministic selection approach to both qualitative and quantitative methods. Therefore, since the condition of today's postmodern discourse of diversity technique, Selection mixed research approach is a methodological necessity in the social sciences. Today, the simultaneous use of both quantitative and qualitative methods is justified.As mentioned, the mixed researchapproach qualitative and quantitative methods are combined by each other.The main objective of this paper is to introduce integrated research approach and review of advantage and disadvantage mentioned method. It is expected that using this approach contribute to overcome the shortcomings of positivistic hard and soft humanistic Blumer. Because the main idea of mixed researchapproach is to combine of qualitative and quantitative approaches,more appropriate and comprehensive understanding is obtained of topic. Keywords:Mixed ResearchApproach, Third Movement ofMethodological, Qualitative Method and Quantitative Method. I. Introduction In the present age, significant changes has occurred in sociological theory and paradigm as a researcher thought and action guidance ( the entire process of research ). [Show full text]
- Philosophical Approaches to Qualitative Research Loyola University Chicago Loyola eCommons School of Social Work: Faculty Publications and Other Works Faculty Publications 2014 Philosophical Approaches to Qualitative Research Julia Pryce [email protected] Renée Spencer Jill Walsh Follow this and additional works at: https://ecommons.luc.edu/socialwork_facpubs Part of the Social Work Commons Recommended Citation Pryce, Julia; Spencer, Renée; and Walsh, Jill. Philosophical Approaches to Qualitative Research. The Oxford Handbook of Qualitative Research Methods, , : 81-98, 2014. Retrieved from Loyola eCommons, School of Social Work: Faculty Publications and Other Works, http://dx.doi.org/10.1093/oxfordhb/ 9780199811755.001.0001 This Book Chapter is brought to you for free and open access by the Faculty Publications at Loyola eCommons. It has been accepted for inclusion in School of Social Work: Faculty Publications and Other Works by an authorized administrator of Loyola eCommons. For more information, please contact [email protected] . This work is licensed under a Creative Commons Attribution-Noncommercial-No Derivative Works 3.0 License. © Oxford University Press, 2014. CHAPTER Philosophical Approaches to 5 Qualitative Research Renée Spencer, Julia M. Pryce, and Jill Walsh Abstract This chapter reviews some of the major overarching philosophical approaches to qualitative inquiry and includes some historical background for each. Taking a “big picture” view, the chapter discusses post-positivism, constructivism, critical theory, feminism, and queer theory and offers a brief history of these [Show full text]
- Population Axiology Population axiology Hilary Greaves This is the pre-peer reviewed version of this article. The final version is forthcoming in Philosophy Compass; please cite the published version. This ar- ticle may be used for non-commercial purposes in accordance with Wiley Terms and Conditions for Self-Archiving. Abstract Population axiology is the study of the conditions under which one state of affairs is better than another, when the states of affairs in ques- tion may differ over the numbers and the identities of the persons who ever live. Extant theories include totalism, averagism, variable value theories, critical level theories, and \person-affecting” theories. Each of these the- ories is open to objections that are at least prima facie serious. A series of impossibility theorems shows that this is no coincidence: it can be proved, for various sets of prima facie intuitively compelling desiderata, that no axiology can simultaneously satisfy all the desiderata on the list. One's choice of population axiology appears to be a choice of which intuition one is least unwilling to give up. 1 Population ethics and population axiology: The basic questions In many decision situations, at least in expectation, an agent's decision has no effect on the numbers and identities of persons born. For those situations, fixed-population ethics is adequate. But in many other decision situations, this condition does not hold. Should one have an additional child? How should life-saving resources be prioritised between the young (who might go on to have children) and the old (who are past reproductive age)? How much should one do to prevent climate change from reducing the number of persons the Earth is able to sustain in the future? Should one fund condom distribution in the developing world? In all these cases, one's actions can affect both who is born and how many people are (ever) born. [Show full text]
- International Journal of Quantitative and Qualitative Research Methods International Journal of Quantitative and Qualitative Research Methods Vol.8, No.3, pp.1-23, September 2020 Published by ECRTD-UK ISSN 2056-3620(Print), ISSN 2056-3639(Online) CIRCULAR REASONING FOR THE EVOLUTION OF RESEARCH THROUGH A STRATEGIC CONSTRUCTION OF RESEARCH METHODOLOGIES Dongmyung Park 1 Dyson School of Design Engineering Imperial College London South Kensington London SW7 2DB United Kingdom e-mail: [email protected] Fadzli Irwan Bahrudin Department of Applied Art & Design Kulliyyah of Architecture & Environmental Design International Islamic University Malaysia Selangor 53100 Malaysia e-mail: [email protected] Ji Han Division of Industrial Design University of Liverpool Brownlow Hill Liverpool L69 3GH United Kingdom e-mail: [email protected] ABSTRACT: In a research process, the inductive and deductive reasoning approach has shortcomings in terms of validity and applicability, respectively. Also, the objective-oriented reasoning approach can output findings with some of the two limitations. That meaning, the reasoning approaches do have flaws as a means of methodically and reliably answering research questions. Hence, they are often coupled together and formed a strong basis for an expansion of knowledge. However, academic discourse on best practice in selecting multiple reasonings for a research project is limited. This paper highlights the concept of a circular reasoning process of which a reasoning approach is complemented with one another for robust research findings. Through a strategic sequence of research methodologies, the circular reasoning process enables the capitalisation of strengths and compensation of weaknesses of inductive, deductive, and objective-oriented reasoning. Notably, an extensive cycle of the circular reasoning process would provide a strong foundation for embarking into new research, as well as expanding current research. [Show full text]
- Axiological Futurism View metadata, citation and similar papers at core.ac.uk brought to you by CORE provided by PhilPapers Axiological Futurism The Systematic Study of the Future of Human Values John Danaher, Senior Lecturer NUI Galway [email protected] Abstract: Human values seem to vary across time and space. What implications does this have for the future of human value? Will our human and (perhaps) post-human offspring have very different values from our own? Can we study the future of human values in an insightful and systematic way? This article makes three contributions to the debate about the future of human values. First, it argues that the systematic study of future values is both necessary in and of itself and an important complement to other future-oriented inquiries. Second, it sets out a methodology and a set of methods for undertaking this study. Third, it gives a practical illustration of what this ‘axiological futurism’ might look like by developing a model of the axiological possibility space that humans are likely to navigate over the coming decades. 1. Introduction Axiological change is a constant feature of human history. When we look back to the moral values of our ancestors, we cannot help but notice that they differed from our own. Our grandparents, for example, are quite likely to have harboured moral beliefs that would count as prejudiced and bigoted by modern standards; and we are quite likely to harbour moral beliefs that they would find abhorrent. As we go further back in time, the changes become even more pronounced (Pleasants 2018; Appiah 2010; Buchanan 2004; Pinker 2011). [Show full text]
- The Positivist Qualitative Research and the Paradigmatic Disclaimer 1 Positivist Qualitative Research Beyond qualitative/quantitative structuralism: The positivist qualitative research and the paradigmatic disclaimer Izhak Berkovich Department of Education and Psychology, The Open University of Israel, Ra`anana, Israel Abstract Scholarly discourse concerning the distinction between qualitative and quantitative approach often takes on a binary character. This structuralism undermines the legitimacy of positivist qualitative research, a unique method frequently used in social science research. In the present essay, the author argues that positivist qualitative research should be recognized as a unique form of qualitative research. The essay focuses on three issues: (a) the paradigmatic roots of positivist qualitative research, (b) the components of positivist qualitative research as an empirical research approach, including a typology for mapping various manifestations of partially and fully positivist qualitative research, and (c) incorporating a paradigmatic disclaimer section in articles to improve the quality of qualitative research, positivist and non- positivist alike. Recognizing positivist qualitative research as a distinct and legitimate type can improve qualitative studies in social science. Keywords paradigm; paradigmatic disclaimer; positivism, positivist qualitative research; qualitative research Forthcoming in Quality and Quantity. The final publication is available at link.springer.com via https://doi.org/10.1007/s11135-017-0607-3 2 Positivist Qualitative Research 1 Introduction The use of qualitative research in social science has a long and distinguished history (Denzin and Lincoln 2003). The field of social science underwent a "qualitative research revolution" in the 1970s and 1980s (Donmoyer and Galloway 2009, p. 7). In social science and education, qualitative research (and researchers), once disregarded and marginalized by hegemonic quantitative research (and researchers), has become a mainstream research approach (Lichtman 2013, p. [Show full text]
- A Systematic Framework for Exploring Worldviews and Its Generalization As a Multi-Purpose Inquiry Framework systems Article A Systematic Framework for Exploring Worldviews and Its Generalization as a Multi-Purpose Inquiry Framework David Rousseau 1,2,3,4,* and Julie Billingham 5,6 1 Centre for Systems Philosophy, Surrey KTI5 1EL, UK 2 Centre for Systems Studies, University of Hull, Kingston-on-Hull HU6 7RX, UK 3 Alister Hardy Research Centre, University of Wales TSD, Lampeter SA48 7ED, UK 4 Bertalanffy Center for the Study of Systems Science, 1040 Vienna, Austria 5 Centre for Systems Philosophy, Surrey KTI5 1EL, UK; [email protected] 6 Salesforce, Inc., San Francisco, CA 94105, USA * Correspondence: [email protected] ; Tel.: +44-(0)-7714-677-687 Received: 31 March 2018; Accepted: 5 July 2018; Published: 10 July 2018 Abstract: Systems science methodologies do not have a consistent way of working with worldviews, even though determining stakeholder perspectives is central to systems thinking. In this paper, we propose a comprehensive “Worldview Inquiry Framework” that can be used across methodologies to govern the process of eliciting, documenting, and comparing the worldviews of stakeholders. We discuss the systemicity of worldviews and explain how this can help practitioners to find the roots of stakeholders’ disagreements about value judgements. We then generalize the structure of the Worldview Inquiry Framework to produce a “General Inquiry Framework” that can be used to govern an inquiry process in other contexts. We show that the presented Worldview Inquiry Framework is a special case of this General Inquiry Framework and show how the General Inquiry Framework can be tailored for other contexts such as problem solving, product design, and fundamental research. [Show full text]
- Systematic review
- Open access
- Published: 11 September 2024
Evaluation of research co-design in health: a systematic overview of reviews and development of a framework
- Sanne Peters ORCID: orcid.org/0000-0001-6235-1752 1 ,
- Lisa Guccione 2 , 3 ,
- Jill Francis 1 , 2 , 3 , 4 ,
- Stephanie Best 1 , 2 , 3 , 5 ,
- Emma Tavender 6 , 7 ,
- Janet Curran 8 , 9 ,
- Katie Davies 10 ,
- Stephanie Rowe 1 , 8 ,
- Victoria J. Palmer 11 &
- Marlena Klaic 1
Implementation Science volume 19 , Article number: 63 ( 2024 ) Cite this article
13 Altmetric
Metrics details
Co-design with consumers and healthcare professionals is widely used in applied health research. While this approach appears to be ethically the right thing to do, a rigorous evaluation of its process and impact is frequently missing. Evaluation of research co-design is important to identify areas of improvement in the methods and processes, as well as to determine whether research co-design leads to better outcomes. We aimed to build on current literature to develop a framework to assist researchers with the evaluation of co-design processes and impacts.
A multifaceted, iterative approach, including three steps, was undertaken to develop a Co-design Evaluation Framework: 1) A systematic overview of reviews; 2) Stakeholder panel meetings to discuss and debate findings from the overview of reviews and 3) Consensus meeting with stakeholder panel. The systematic overview of reviews included relevant papers published between 2000 and 2022. OVID (Medline, Embase, PsycINFO), EBSCOhost (Cinahl) and the Cochrane Database of Systematic reviews were searched for papers that reported co-design evaluation or outcomes in health research. Extracted data was inductively analysed and evaluation themes were identified. Review findings were presented to a stakeholder panel, including consumers, healthcare professionals and researchers, to interpret and critique. A consensus meeting, including a nominal group technique, was applied to agree upon the Co-design Evaluation Framework.
A total of 51 reviews were included in the systematic overview of reviews. Fifteen evaluation themes were identified and grouped into the following seven clusters: People (within co-design group), group processes, research processes, co-design context, people (outside co-design group), system and sustainment. If evaluation methods were mentioned, they mainly included qualitative data, informal consumer feedback and researchers’ reflections. The Co-Design Evaluation Framework used a tree metaphor to represent the processes and people in the co-design group (below-ground), underpinning system- and people-level outcomes beyond the co-design group (above-ground). To evaluate research co-design, researchers may wish to consider any or all components in the tree.
Conclusions
The Co-Design Evaluation Framework has been collaboratively developed with various stakeholders to be used prospectively (planning for evaluation), concurrently (making adjustments during the co-design process) and retrospectively (reviewing past co-design efforts to inform future activities).
Peer Review reports
Contributions to the literature
While stakeholder engagement in research seems ethically the right thing to do, a rigorous evaluation of its process and outcomes is frequently missing.
Fifteen evaluation themes were identified in the literature, of which research process , cognitive and emotional factors were the most frequently reported.
The Co-design Evaluation Framework can assist researchers with research co-design evaluation and provide guidance regarding what and when to evaluate.
The framework can be used prospectively, concurrently, and retrospectively to make improvements to existing and future research co-design projects.
Introduction
Lots of money is wasted in health research that does not lead to meaningful benefits for end-users, such as healthcare professionals and consumers [ 1 , 2 , 3 ]. One contributor to this waste is that research often focusses on questions and outcomes that are of limited importance to end-users [ 4 , 5 ]. Engaging relevant people in research co-design has increased in order to respond to this issue. There is a lack of consensus in the literature on the definition and processes involved in undertaking a co-design approach. For the purposes of this review, we define research co-design as meaningful end-user engagement that occurs across any stage of the research process , from the research planning phase to dissemination of research findings [ 6 ]. Meaningful end-user engagement refers to an explicit and measurable responsibility, such as contributing to writing a study proposal [ 6 ]. The variety of research co-design methods can be seen as a continuum ranging from limited involvement, such as consulting with end-users, to the much higher effort research approaches in which end-users and researchers aim for equal decision-making power and responsibility across the entire research process [ 6 ]. Irrespective of the intensity of involvement, it is generally recommended that a co-design approach should be based on several important principles such as equity, inclusion and shared ownership [ 7 ].
Over time, increasing attention has been given to research co-design [ 6 , 8 ]. Funding bodies encourage its use and it is recommended in the updated UK MRC framework on developing and evaluating complex interventions [ 9 ]. End-user engagement has an Equator reporting checklist [ 10 ] and related work has been reported by key organisations, such as the James Lind Alliance in the UK ( www.jla.nihr.ac.uk ), Patient Centered Outcomes Research Institute in the US ( www.pcori.org ) and Canadian Institutes of Health Research ( https://cihrirsc.gc.ca/e/41592.html ). In addition, peer reviewed publications involving co-design have risen from 173 per year in 2000 to 2617 in 2022 (PubMed), suggesting a growing importance in research activities.
Engaging end-users in the health research process is arguably the right thing to do, but the processes and outcomes of co-design have rarely been evaluated in a rigorous way [ 6 ]. Existing anecdotal evidence suggests that research co-design can benefit researchers, end-users and lead to more robust research processes [ 11 , 12 , 13 , 14 , 15 , 16 , 17 , 18 , 19 ]. Both researchers and end-users have reported positive experiences of engaging in the co-design process. Potential benefits include a better understanding of community needs, more applicable research questions, designs and materials and improved trust between the researchers and end-users. Several reviews on conducting research co-design have concluded that co-design can be feasible, though predominantly used in the early phases of research, for example formulating research questions and developing a study protocol [ 6 , 11 , 12 , 13 , 14 , 15 , 16 , 17 , 18 , 19 ]. However, these reviews highlighted that engagement of end-users in the research process required extra time and funding and had the risk of becoming tokenistic [ 6 , 11 , 12 , 13 , 14 , 15 , 16 , 17 , 18 , 19 ].
The use of resources in co-design studies might need to be justified to the funder as well as its impacts. A rigorous evaluation of research co-design processes and outcomes is needed to identify areas of potential improvement and to determine the impact of research co-design. Several overviews of reviews on research co-design have been published but with no or limited focus on evaluation [ 20 , 21 , 22 , 23 ]. Moreover, current literature provides little guidance around how and what to evaluate, and which outcomes are key.
This study thus had two aims:
To conduct a systematic overview of reviews to identify evaluation methods and process and outcome variables reported in the published health research co-design literature.
To develop a framework to assist researchers with the evaluation of co-design processes and impacts.
This project used a multifaceted, iterative approach to develop a Co-design Evaluation Framework. It consisted of the following steps: 1) A systematic overview of reviews; 2) Stakeholder panel meetings to discuss and debate findings from the overview of reviews and 3) Consensus meeting with stakeholder panel. The reporting checklist for overviews of reviews was applied in Additional file 1 [ 24 ].
Step 1: A systematic overview of reviews
We conducted a systematic overview of reviews [ 25 ], reviewing literature reviews rather than primary studies, to investigate the following question: What is known in the published literature about the evaluation of research co-design in health research? The protocol of our systematic overview of reviews was published in the PROSPERO database (CRD42022355338).
Sub questions:
What has been co-designed and what were the objectives of the co-design process?
Who was involved and what was the level of involvement?
What methods were used to evaluate the co-design processes and outcomes?
What was evaluated (outcome and process measures) and at what timepoint (for example concurrently, or after, the co-design process)?
Was a co-design evaluation framework used to guide evaluation?
Search strategy
We searched OVID (Medline, Embase, PsycINFO), EBSCOhost (Cinahl) and the Cochrane Database of Systematic reviews on the 11th of October 2022 for literature reviews that reported co-design evaluation or outcomes in health research. The search strategy was based on previous reviews on co-design [ 6 , 14 , 26 ] and refined with the assistance of a research librarian and the research team (search terms in Additional file 2). Papers published from January 2000 to September 2022 were identified and retrieved by one author (SP).
Study selection
Database records were imported into EndNote X9 (The EndNote Team, Philadelphia, 2013) and duplicates removed. We managed the study selection process in the software program Covidence (Veritas Health Innovation, Melbourne, Australia). Two independent reviewers (SP, MK or LG) screened the titles and abstracts of all studies against the eligibility criteria (Table 1 ). Discrepancies were resolved through discussion or with a third reviewer (either SP, MK or LG, depending on which 2 reviewers disagreed). If there was insufficient information in the abstract to decide about eligibility, the paper was retained to the full-text screening phase. Full-text versions of studies not excluded at the title and abstract screening phase were retrieved and independently screened by two reviewers (SP, MK or LG) against eligibility criteria. Disagreements were resolved through discussion, or with a third reviewer, and recorded in Covidence.
Data extraction of included papers was conducted by one of three reviewers (SP, MK or LG). A second reviewer checked a random sample of 20% of all extracted data (LG or SP). Disagreements were resolved through regular discussion. Data were extracted using an excel spreadsheet developed by the research team and included review characteristics (such as references, type of review, number of included studies, review aim), details about the co-design process (such as who was involved in the co-design, which topics the co-design focused on, what research phase(s) the co-design covered, in which research phase the co-design took place and what the end-users’ level of involvement was) and details about the co-design evaluation (what outcomes were reported, methods of data collection, who the participants of the evaluation were, the timepoint of evaluation, whether an evaluation framework was used or developed and conclusions about co-design evaluation).
Types of end-users’ involvement were categorised into four groups based on the categories proposed by Hughes et al. (2018): 1. Targeted consultation; 2. Embedded consultation; 3. Collaboration and co-production and 4. User-led research, see Table 2 .
Data extraction and analysis took place in three iterative phases (Fig. 1 ), with each phase containing one third of the included studies. Each phase of data extraction and analysis was followed by stakeholder panel meetings (see step 2 below). This stepwise approach enabled a form of triangulation wherein themes that emerged through each phase were discussed with the stakeholder panel and incorporated both retrospectively (re-coding data in the prior phase) and prospectively (coding new data in the next phase).
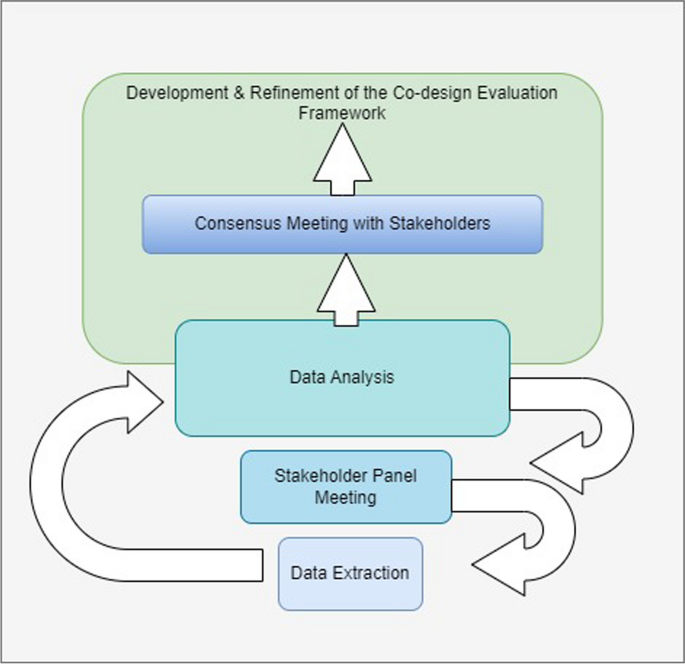
Iterative phases in the process of the Co-design evaluation framework development
All reported outcomes of research co-design in the first phase (one third of all data) were inductively coded into themes, according to the principles of thematic analysis [ 28 ]. Two researchers (SP and MK) double coded 10% of all data and reached consensus through discussion. Given that consensus was high, one researcher (SP) continued the coding while having frequent discussions and reviews within the research team. In phase 2 (also one third of all data), deductive coding was based on the themes identified in the first round. Data of the first phase were re-coded, if new codes emerged during the stakeholder panel meeting. The same process took place for the third phase.
Step 2: Stakeholder panel meetings to discuss and debate findings from the overview of reviews
Results from step 1 were presented to the stakeholder panel to interpret and critique the review findings. The panel consisted of ten people, including a mix of consumers, healthcare professionals and researchers. Stakeholders were selected for their experience or expertise in research co-design. The number of meetings was not pre-determined, rather, it was informed by the outcomes from step 1. The number of stakeholders in each meeting ranged from six to ten.
A core group from the broader stakeholder panel (SP, MK, LG, JF) with a breadth of research experience and methodological expertise discussed the themes arising from both steps 1 and 2 and considered various ways of presenting them. Multiple design options were considered and preliminary frameworks were developed. Following discussion with the stakeholder panel, it was agreed that the evaluation themes could be grouped into several clusters to make the framework more comprehensible. The grouping of evaluation themes into clusters was informed by reported proposed associations between evaluation themes in the literature as well as the stakeholder panel’s co-design experience and expertise. Evaluation themes as well as clusters were agreed upon during the stakeholder panel meetings.
Step 3: Consensus meeting with stakeholder panel
The consensus meeting included the same stakeholder panel as in step 2. The meeting was informed by a modified Nominal Group Technique (NGT). The NGT is a structured process for obtaining information and reaching consensus with a target group who have some association or experience with the topic [ 29 ]. Various adaptations of the NGT have been used and additional pre-meeting information has been suggested to enable more time for participants to consider their contribution to the topic [ 30 ]. The modified NGT utilised in this study contained the following: (i) identification of group members to include experts with depth and diverse experiences. They were purposively identified at the start of this study for their expertise or experience in research co-design and included: a patient consumer, a clinician, three clinician researchers and six researchers with backgrounds in behavioural sciences, psychology, education, applied ethics and participatory design. All authors on this paper were invited by e-mail to attend an online meeting; (ii) provision of information prior to the group meeting included findings of the overview of reviews, a draft framework and objectives of the meeting. Five authors with extensive research co-design experience were asked to prepare a case example of one of their co-design projects for sharing at the group meeting. The intention of this exercise was to discuss the fit between a real-world example and the proposed framework; (iii) hybrid meeting facilitated by two researchers (SP & JF) who have experience in facilitating consensus meetings. Following presentation of the meeting materials, including the preliminary framework, group members were invited to silently consider the preliminary framework and generate ideas and critiques; iv) participants sharing their ideas and critiques; v) clarification process where group members shared their co-design example project and discussed the fit with components of the initial framework, and vi) silent voting and/or agreement on the framework via a personal email to one of the researchers (SP).
Step 1: Systematic overview of reviews
The database searches identified a total of 8912 papers. After removing 3016 duplicates and screening 5896 titles and abstracts, 148 full texts were sought for retrieval. Sixteen were not retrieved as they were not available in English ( n = 2) or full-text was not available ( n = 14). Of the remaining 132 papers assessed for eligibility, 81 were excluded. The final number of papers included in this overview of reviews was 51 (See Fig. 2 ).
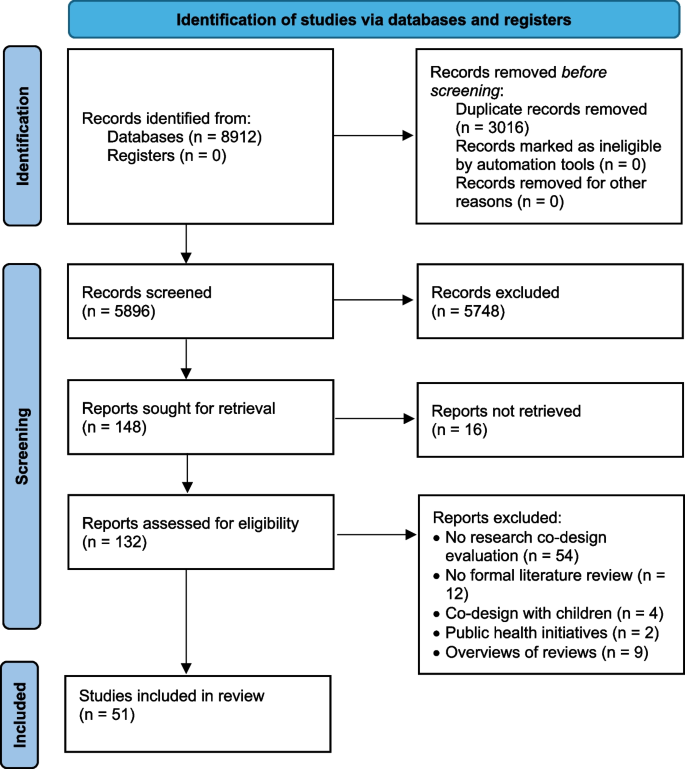
PRISMA flow chart (based on [ 31 ]) of overview of reviews
Characteristics of the included studies
Of the 51 included reviews [ 11 , 12 , 14 , 32 , 33 , 34 , 35 , 36 , 37 , 38 , 39 , 40 , 41 , 42 , 43 , 44 , 45 , 46 , 47 , 48 , 49 , 50 , 51 , 52 , 53 , 54 , 55 , 56 , 57 , 58 , 59 , 60 , 61 , 62 , 63 , 64 , 65 , 66 , 67 , 68 , 69 , 70 , 71 , 72 , 73 , 74 , 75 , 76 , 77 , 78 , 79 ], 17 were systematic reviews, 12 were scoping reviews, 14 did not report the type or method of review, three were narrative reviews, two were qualitative evidence synthesis, another two were a structured literature search and one was a realist review. The number of studies included in the reviews ranged from 7 to 260. Nineteen reviews focused on co-design with specific populations, for example older people, people with intellectual disabilities, people living with dementia and 32 reviews included co-design with a range of end-users. The co-design focused in most cases on a mix of topics ( n = 31). Some reviews were specifically about one clinical topic, for example critical care or dementia. In ten cases, the clinical topics were not reported. Co-design took place during multiple research phases. Thirty-six reviews covered co-design in agenda/priority setting, 36 in study design, 30 in data collection, 25 in data analysis and 27 in dissemination. With regards to the research translation continuum, most of the co-design was reported in practice and community-based research ( n = 32), three reviews were conducted in basic research and 11 in human research. The types of end-users’ involvement in co-design ranged from targeted consultation ( n = 14) to embedded consultation ( n = 20), collaboration and co-production ( n = 14) to end-user- led research ( n = 6), including papers covering multiple types of involvement. Seventeen papers did not report the types of involvement. The reported co-design included a variety of time commitments, from a minimum of a one-off 60-min meeting to multiple meetings over multiple years. Twenty-seven reviews did not report details about the end-users’ types of involvement.
Identified evaluation themes
Fifteen evaluation themes were identified and were arranged into two higher level groups: 1. within the co-design team and 2. broader than co-design team (Table 3 ). The themes related to the first group (within the co-design team) included: Structure and composition of the co-design group, contextual enablers/barriers, interrelationships between group members, decision making process, emotional factors, cognitive factors, value proposition, level/ quality of engagement, research process, health outcomes for co-design group and sustainment of the co-design team or activities. The themes within the second group (broader than co-design team) included: Healthcare professional-level outcomes, healthcare system level outcomes, organisational level outcomes and patient and community outcomes.
The research process was the most frequently reported evaluation theme in the reviews ( n = 44, 86% of reviews), followed by cognitive factors ( n = 35, 69%) and emotional factors ( n = 34, 67%) (Table 4 ). Due to variability in reporting practices, it was not possible to specify the number of primary studies that reported specific evaluation themes. Evaluation methods for the themes were not reported in the majority of reviews ( n = 43, 84%). If evaluation methods were mentioned, they were mainly based on qualitative data, including interviews, focus groups, field notes, document reviews and observations (see overview with references in Additional file 3). Survey data was mentioned in three reviews. Many reviews reported informal evaluation based on participant experiences (e.g. informal feedback), reflection meetings, narrative reflections and authors’ hypotheses (Additional file 3). The timing of the evaluation was only mentioned in two papers: 1. Before and after the co-design activities and 2. Post co-design activities. One paper suggested that continuous evaluation might be helpful to improve the co-design process (Additional file 3).
The systematic overview of reviews found that some authors reported proposed positive associations between evaluation themes (Table 5 ). The most frequently reported proposed association was between level/quality of engagement and emotional factors ( n = 5, 10%). However, these proposed associations did not seem to have any empirical evidence and evaluation methods were not reported.
All evaluation themes were grouped into the following clusters (Table 6 ): People (within co-design group), group processes, research processes, co-design context, people (outside co-design group), system and sustainment.
Only one paper reported the evaluation in connection to the research phases (Agenda/priority setting, study design, data collection, data analysis and dissemination). This paper reported the following outcomes for the following research phases [ 58 ]:
Agenda/priority setting: Research process; Level/quality of engagement; Cognitive factors; Attributes of the co-design group; Interrelationships between group members; Sustainment of the co-design team or activities; Patient and community outcomes.
Study design: Attributes of the co-design group; Interrelationships between group members; Level/quality of engagement; Cognitive factors; Emotional factors; Research process.
The various research phases in which consumers could be involved, as well as the clusters of evaluation themes, informed the design of the co-design evaluation framework.
Two main options were voted on and discussed within the stakeholder panel. The two main options can be found in Additional file 4. Draft 2 was the prefered option as it was perceived as more dynamic than draft 1, representing a clearer interplay between the two contexts. The stakeholder panel suggested a few edits to the draft, such as the inclusion of bi-directional arrows in the tree trunk and a vertical arrow from underground to above ground with the label ‘impact’.
The final version of the Co-design Evaluation framework is presented in Fig. 3 .
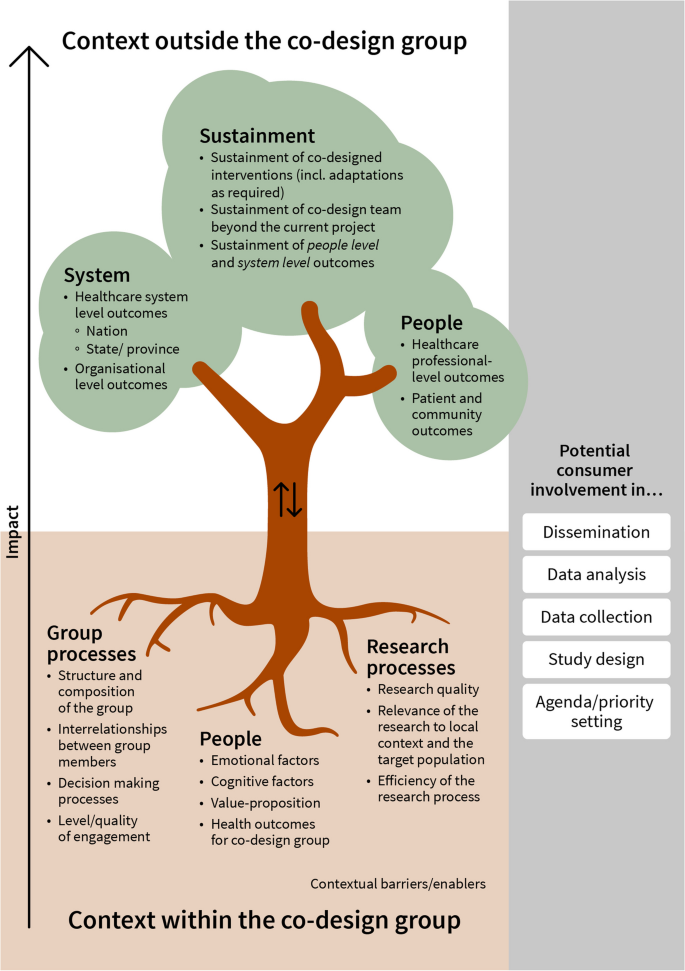
Research Co-design evaluation framework
Figure 3 presents co-design evaluation as the below-ground and above-ground structures of a tree. The tree metaphor presents the processes and people in the co-design group (below-ground) as the basis for system- and people-level outcomes beyond the co-design group (above-ground). To evaluate research co-design, researchers may wish to consider any or all components in this Figure. These evaluation components relate to the methods, processes, and outcomes of consumer involvement in research.
The context within the co-design group (the roots of the tree) consists of the people, group processes and research processes, with various evaluation themes (dot points) related to them, as well as contextual barriers and enablers that relate to situational aspects that might enable or hinder consumer engagement. The context outside the co-design group, i.e., the wider community (the branches and leaves of the tree), comprises people who were not involved in the research co-design process, the system-level and sustainment-related outcomes. These above ground groups are potential beneficiaries or targets of the co-design activities.
The arrows in the middle of the trunk represent the potential mutual influence of the two contexts, suggesting that an iterative approach to evaluation might be beneficial. For example, when deciding the composition of the co-design group, it may be important to have an appropriate representation of the people most impacted by the problem issue or topic at hand. Or, if a co-designed healthcare intervention does not achieve the desired outcomes in the wider context, the co-design group might consider potential ways to improve the intervention or how it was delivered. Evaluation of a research co-design process might start with the foundations (the roots of the tree) and progress to above ground (the tree grows and might develop fruit). Yet, depending on the aim of the evaluation, a focus on one of the two contexts, either below or above ground, might be appropriate.
Which, and how many, components are appropriate to evaluate depends on the nature of the co-design approach and the key questions of the evaluation. For example, if a co-design approach is used in the very early stages of a research program, perhaps to identify priorities or to articulate a research question, then 'below' the ground components are key. While a randomised study comparing the effects of a co-designed intervention versus a researcher-designed intervention might only consider 'above' the ground components.
The white boxes on the right-hand side of Fig. 3 indicate the research phases, from agenda/priority setting to dissemination, in which consumers can and should be involved. This co-design evaluation framework may be applied at any phase of the research process or applied iteratively with a view to improving future co-design activities.
This systematic overview of reviews aimed to build on current literature and develop a framework to assist researchers with the evaluation of research co-design. Fifty-one included reviews reported on fifteen evaluation themes, which were grouped into the following clusters: People (within co-design group), group processes, research processes, co-design context, people (outside co-design group), system and sustainment. Most reviews did not report measurement methods for the evaluation themes. If methods were mentioned, they mostly included qualitative data, informal consumer feedback and researchers’ reflections. This finding strengthens our argument that a framework may be helpful in supporting methodologically robust studies to assess co-design processes and impacts. The Co-Design Evaluation Framework has adopted a tree metaphor. It presents the processes and people in the co-design group (below-ground) as the underpinning system- and people-level outcomes beyond the co-design group (above-ground). To evaluate stakeholder involvement in research, researchers may wish to consider any or all components in the tree. Which, and how many, components are appropriate to evaluate depends on the nature of the co-design approach and the key questions that stakeholders aim to address. Nonetheless, it will be important that evaluations delineate what parts of the research project have incorporated a co-design approach.
The Equator reporting checklist for Research Co-Design, GRIPP2, provides researchers with a series of concepts that should be considered and reported on when incorporating patient and public involvement in research [ 10 ]. These concepts include, but are not limited to, methods of involving patients and the public in research and intensity of engagement. The Co-Design Evaluation Framework is not intended as a replacement for the GRIPP2, rather, it can be used prospectively to inform development of the co-design project or retropsectively to inform completion of the GRIPP2. Table 7 provides hypothetical examples of research questions that co-design evaluation projects might address. The framework could be used at multiple points within co-design projects, including prospectively (planning for evaluation before the co-design process has started), concurrently ( incorporating improvements during the co-design process) and retrospectively (reviewing past co-design efforts to inform future projects).
Our systematic overview of reviews identified multiple evaluation themes. Some of these overlapped with reported values associated with public involvement in research [ 80 ], community engagement measures [ 15 ] and reported impacts of patient and public involvement in research, as described by others [ 16 , 81 , 82 ]. The added value of our systematic overview of reviews is that we went beyond a list of items and took it one step further by looking at evaluation themes, potential associations between evaluation themes, clusters of evaluation themes and ultimately developed a framework to assist others with research co-design evaluation.
Some reviews in our overview of reviews proposed potential associations between evaluation themes. Yet, these proposed associations were not empirically tested. One of the included studies [ 58 ] proposed conditions and mechanisms involved in co-design processes and outcomes related to diabetes research. Although it is a promising starting point, this should be further explored. A realist evaluation including other research topics and other approaches, such as the use of logic models, which was also recognised in the updated MRC framework [ 9 ], might help to build on explorations of included mechanisms of action [ 83 ] and give insight into how core ingredients contribute to certain co-design processes and outcomes. As recognised by others [ 6 , 84 ], the reporting practice of research co-design in the literature could be improved as details about context, mechanisms and expected outcomes are frequently missing. This will also help us to gain a better understanding of what works for whom, why, how and in which circumstances.
The lack of a consistent definition of co-design makes it challenging to identify and synthesise the literature, as recognised by others [ 6 ]. Given that there are so many different terms used in the literature, there is a risk that we might have missed some relevant papers in our overview of reviews. Nevertheless, we tried to capture as many as possible synonyms of co-design in our search terms. The absence of quality assessment of included studies in our overview of reviews can be seen as a limitation. However, our overview of reviews did not aim to assess existing literature on the co-design process, but rather focused on what to evaluate, how and when. We did note whether the reported evaluation themes were based on empirical evidence or authors’ opinions. Primary studies reported in the included reviews were not individually reviewed as this was outside the scope of this paper. A strength in our methods was the cyclical process undertaken between steps 1 and 2. Analysis of the data extracted from the overview was refined over three phases following rigorous discussions with a diverse and experienced stakeholder panel. It was a strength of our project that a mix of stakeholders were involved, including consumers, healthcare professionals and researchers.
Stakeholders are frequently engaged in research but if research co-design processes and outcomes are not evaluated, there will be limited learning from past experiences. Evaluation is essential to make refinements during existing projects and improve future co-design activities. It is also critical for ensuring commitments to the underpinning values of c-odesign are embedded within activities.
A systematic review of all primary studies within the included reviews of this overview of reviews, would allow greater depth relating to the practicalities of how to evaluate certain themes. It would lead to a better understanding of existing measures and methods and which evaluation areas need further development. Future research should also focus on whether co-design leads to better outcomes than no co-design (only researcher-driven research). To our knowledge, this has not been explored yet. Moreover, future research could gain better insight into the mechanisms of change within co-design and explore potential associations between evaluation themes for example, those proposed in the included reviews between level/quality of engagement and emotional factors.
We followed a systematic, iterative approach to develop a Co-Design Evaluation Framework that can be applied to various phases of the research co-design process. Testing of the utility of the framework is an important next step. We propose that the framework could be used at multiple points within co-design projects, including prospectively (planning for evaluation before the co-design process has started), concurrently (to incorporate improvements during the co-design process) and retrospectively (reviewing past co-design efforts to inform future projects).
Availability of data and materials
All data generated during this study are included either within the text or as a supplementary file.
Abbreviations
Medical Research Council
Guidance for Reporting Involvement of Patients and the Public
Chalmers I, Glasziou P. Systematic reviews and research waste. Lancet. 2016;387(10014):122–3.
Article PubMed Google Scholar
Chalmers I, Glasziou P. Avoidable waste in the production and reporting of research evidence. Lancet. 2009;374(9683):86–9.
Glasziou P, Altman DG, Bossuyt P, Boutron I, Clarke M, Julious S, et al. Reducing waste from incomplete or unusable reports of biomedical research. Lancet. 2014;383(9913):267–76.
Ioannidis JP. Why Most Clinical Research Is Not Useful. PLoS Med. 2016;13(6):e1002049.
Article PubMed PubMed Central Google Scholar
Oliver S. Patient involvement in setting research agendas. Eur J Gastroenterol Hepatol. 2006;18(9):935–8.
Slattery P, Saeri AK, Bragge P. Research co-design in health: a rapid overview of reviews. Health Res Policy Syst. 2020;18(1):17.
Ní Shé É, Harrison R. Mitigating unintended consequences of co-design in health care. Health Expect. 2021;24(5):1551–6.
Peters S, Sukumar K, Blanchard S, Ramasamy A, Malinowski J, Ginex P, et al. Trends in guideline implementation: an updated scoping review. Implement Sci. 2022;17:50.
Skivington K, Matthews L, Simpson SA, Craig P, Baird J, Blazeby JM, et al. A new framework for developing and evaluating complex interventions: update of Medical Research Council guidance. BMJ. 2021;374:n2061.
Staniszewska S, Brett J, Simera I, Seers K, Mockford C, Goodlad S, et al. GRIPP2 reporting checklists: tools to improve reporting of patient and public involvement in research. BMJ. 2017;358:j3453.
Article CAS PubMed PubMed Central Google Scholar
Domecq JP, Prutsky G, Elraiyah T, Wang Z, Nabhan M, Shippee N, et al. Patient engagement in research: a systematic review. BMC Health Serv Res. 2014;14:89.
Manafo E, Petermann L, Mason-Lai P, Vandall-Walker V. Patient engagement in Canada: a scoping review of the “how” and “what” of patient engagement in health research. Health Res Policy Syst. 2018;16(1):5.
Fergusson D, Monfaredi Z, Pussegoda K, Garritty C, Lyddiatt A, Shea B, et al. The prevalence of patient engagement in published trials: a systematic review. Res Involv Engagem. 2018;4:17.
Vat LE, Finlay T, Jan Schuitmaker-Warnaar T, Fahy N, Robinson P, Boudes M, et al. Evaluating the “return on patient engagement initiatives” in medicines research and development: A literature review. Health Expect. 2020;23(1):5–18.
Luger TM, Hamilton AB, True G. Measuring Community-Engaged Research Contexts, Processes, and Outcomes: A Mapping Review. Milbank Q. 2020;98(2):493–553.
Modigh A, Sampaio F, Moberg L, Fredriksson M. The impact of patient and public involvement in health research versus healthcare: A scoping review of reviews. Health Policy. 2021;125(9):1208–21.
Clavel N, Paquette J, Dumez V, Del Grande C, Ghadiri DPS, Pomey MP, et al. Patient engagement in care: A scoping review of recently validated tools assessing patients’ and healthcare professionals’ preferences and experience. Health Expect. 2021;24(6):1924–35.
Newman B, Joseph K, Chauhan A, Seale H, Li J, Manias E, et al. Do patient engagement interventions work for all patients? A systematic review and realist synthesis of interventions to enhance patient safety. Health Expect. 2021;24:1905 No Pagination Specified.
Lowe D, Ryan R, Schonfeld L, Merner B, Walsh L, Graham-Wisener L, et al. Effects of consumers and health providers working in partnership on health services planning, delivery and evaluation. Cochrane Database Syst Rev. 2021;9:CD013373.
PubMed Google Scholar
Price A, Albarqouni L, Kirkpatrick J, Clarke M, Liew SM, Roberts N, et al. Patient and public involvement in the design of clinical trials: An overview of systematic reviews. J Eval Clin Pract. 2018;24(1):240–53.
Sarrami-Foroushani P, Travaglia J, Debono D, Braithwaite J. Implementing strategies in consumer and community engagement in health care: results of a large-scale, scoping meta-review. BMC Health Serv Res. 2014;14:402.
Abrams R, Park S, Wong G, Rastogi J, Boylan A-M, Tierney S, et al. Lost in reviews: Looking for the involvement of stakeholders, patients, public and other non-researcher contributors in realist reviews. Research Synthesis Methods. 2021;12(2):239–47.
Zych MM, Berta WB, Gagliardi AR. Conceptualising the initiation of researcher and research user partnerships: a meta-narrative review. Health Res Policy Syst. 2020;18(1):24.
Gates M, Gates A, Pieper D, Fernandes RM, Tricco AC, Moher D, et al. Reporting guideline for overviews of reviews of healthcare interventions: development of the PRIOR statement. BMJ. 2022;378:e070849.
Pollock A, Campbell P, Brunton G, Hunt H, Estcourt L. Selecting and implementing overview methods: implications from five exemplar overviews. Syst Rev. 2017;6(1):145.
Greenhalgh T, Hinton L, Finlay T, Macfarlane A, Fahy N, Clyde B, et al. Frameworks for supporting patient and public involvement in research: Systematic review and co-design pilot. Health Expectations: An International Journal of Public Participation in Health Care & Health Policy. 2019;22(4):785–801.
Article Google Scholar
Hughes M, Duffy C. Public involvement in health and social sciences research: A concept analysis. Health Expectations: An International Journal of Public Participation in Health Care & Health Policy. 2018;21(6):1183–90.
Braun V, Clarke V. Using thematic analysis in psychology. Qual Res Psychol. 2008;3(2):77–101.
Waggoner J, Carline JD, Durning SJ. Is There a Consensus on Consensus Methodology? Descriptions and Recommendations for Future Consensus Research. Acad Med. 2016;91(5):663–8.
Harvey N, Holmes CA. Nominal group technique: an effective method for obtaining group consensus. Int J Nurs Pract. 2012;18(2):188–94.
Page MJ, McKenzie JE, Bossuyt PM, Boutron I, Hoffmann TC, Mulrow CD, et al. The PRISMA 2020 statement: an updated guideline for reporting systematic reviews. BMJ. 2021;372:n71.
Baldwin JN, Napier S, Neville S, Clair VAWS. Impacts of older people’s patient and public involvement in health and social care research: a systematic review. Age Ageing. 2018;47(6):801–9.
Bench S, Eassom E, Poursanidou K. The nature and extent of service user involvement in critical care research and quality improvement: A scoping review of the literature. Int J Consum Stud. 2018;42(2):217–31.
Bethell J, Commisso E, Rostad HM, Puts M, Babineau J, Grinbergs-Saull A, et al. Patient engagement in research related to dementia: a scoping review. Dementia. 2018;17(8):944–75.
Brett J, Staniszewska S, Mockford C, Herron-Marx S, Hughes J, Tysall C, et al. A systematic review of the impact of patient and public involvement on service users, researchers and communities. The Patient: Patient-Centered Outcomes Research. 2014;7(4):387–95.
Di Lorito C, Birt L, Poland F, Csipke E, Gove D, Diaz-Ponce A, et al. A synthesis of the evidence on peer research with potentially vulnerable adults: how this relates to dementia. Int J Geriatr Psychiatry. 2017;32(1):58–67.
Di Lorito C, Bosco A, Birt L, Hassiotis A. Co-research with adults with intellectual disability: A systematic review. J Appl Res Intellect Disabil. 2018;31(5):669–86.
Fox G, Fergusson DA, Daham Z, Youssef M, Foster M, Poole E, et al. Patient engagement in preclinical laboratory research: A scoping review. EBioMedicine. 2021;70:103484.
Frankena TK, Naaldenberg J, Cardol M, Linehan C, van Schrojenstein Lantman-de Valk H. Active involvement of people with intellectual disabilities in health research - a structured literature review. Res Dev Disabil. 2015;45–46:271–83.
Fudge N, Wolfe CD, McKevitt C. Involving older people in health research. Age Ageing. 2007;36(5):492–500.
Article CAS PubMed Google Scholar
George AS, Mehra V, Scott K, Sriram V. Community participation in health systems research: A systematic review assessing the state of research, the nature of interventions involved and the features of engagement with communities. PLoS One. 2015;10(10):ArtID e0141091.
Legare F, Boivin A, van der Weijden T, Pakenham C, Burgers J, Legare J, et al. Patient and public involvement in clinical practice guidelines: A knowledge synthesis of existing programs. Med Decis Making. 2011;31(6):E45–74.
McCarron TL, Clement F, Rasiah J, Moran C, Moffat K, Gonzalez A, et al. Patients as partners in health research: a scoping review. Health Expect. 2021;24:1378 No Pagination Specified.
Miller J, Knott V, Wilson C, Roder D. A review of community engagement in cancer control studies among indigenous people of Australia, New Zealand, Canada and the USA. Eur J Cancer Care. 2012;21(3):283–95.
Article CAS Google Scholar
Shen S, Doyle-Thomas KAR, Beesley L, Karmali A, Williams L, Tanel N, et al. How and why should we engage parents as co-researchers in health research? A scoping review of current practices. Health Expect. 2017;20(4):543–54.
Velvin G, Hartman T, Bathen T. Patient involvement in rare diseases research: a scoping review of the literature and mixed method evaluation of Norwegian researchers’ experiences and perceptions. Orphanet J Rare Dis. 2022;17(1):212.
Wiles LK, Kay D, Luker JA, Worley A, Austin J, Ball A, et al. Consumer engagement in health care policy, research and services: A systematic review and meta-analysis of methods and effects. PLoS One. 2022;17:e0261808.no pagination
Cook N, Siddiqi N, Twiddy M, Kenyon R. Patient and public involvement in health research in low and middle-income countries: a systematic review. BMJ Open. 2019;9(5):e026514.
Chambers E, Gardiner C, Thompson J, Seymour J. Patient and carer involvement in palliative care research: An integrative qualitative evidence synthesis review. Palliat Med. 2019;33(8):969–84.
Brett J, Staniszewska S, Mockford C, Herron-Marx S, Hughes J, Tysall C, et al. Mapping the impact of patient and public involvement on health and social care research: a systematic review. Health Expect. 2014;17(5):637–50.
Boaz A, Hanney S, Jones T, Soper B. Does the engagement of clinicians and organisations in research improve healthcare performance: a three-stage review. BMJ Open. 2015;5(12):e009415.
Boivin A, L’Esperance A, Gauvin FP, Dumez V, Macaulay AC, Lehoux P, et al. Patient and public engagement in research and health system decision making: A systematic review of evaluation tools. Health Expect. 2018;21(6):1075–84.
Anderst A, Conroy K, Fairbrother G, Hallam L, McPhail A, Taylor V. Engaging consumers in health research: a narrative review. Aust Health Rev. 2020;44(5):806–13.
Arnstein L, Wadsworth AC, Yamamoto BA, Stephens R, Sehmi K, Jones R, et al. Patient involvement in preparing health research peer-reviewed publications or results summaries: a systematic review and evidence-based recommendations. Res Involv Engagem. 2020;6:34.
Becerril-Montekio V, Garcia-Bello LA, Torres-Pereda P, Alcalde-Rabanal J, Reveiz L, Langlois EV. Collaboration between health system decision makers and professional researchers to coproduce knowledge, a scoping review. Int J Health Plann Manage. 2022;28:28.
Google Scholar
Bird M, Ouellette C, Whitmore C, Li L, Nair K, McGillion MH, et al. Preparing for patient partnership: A scoping review of patient partner engagement and evaluation in research. Health Expect. 2020;23:523 No Pagination Specified.
Dawson S, Campbell SM, Giles SJ, Morris RL, Cheraghi-Sohi S. Black and minority ethnic group involvement in health and social care research: A systematic review. Health Expect. 2018;21(1):3–22.
Harris J, Haltbakk J, Dunning T, Austrheim G, Kirkevold M, Johnson M, et al. How patient and community involvement in diabetes research influences health outcomes: A realist review. Health Expectations: An International Journal of Public Participation in Health Care & Health Policy. 2019;22(5):907–20.
Hubbard G, Kidd L, Donaghy E. Involving people affected by cancer in research: a review of literature. Eur J Cancer Care. 2008;17(3):233–44.
Hubbard G, Kidd L, Donaghy E, McDonald C, Kearney N. A review of literature about involving people affected by cancer in research, policy and planning and practice. Patient Educ Couns. 2007;65(1):21–33.
Jones EL, Williams-Yesson BA, Hackett RC, Staniszewska SH, Evans D, Francis NK. Quality of reporting on patient and public involvement within surgical research: a systematic review. Ann Surg. 2015;261(2):243–50.
Drahota A, Meza RD, Brikho B, Naaf M, Estabillo JA, Gomez ED, et al. Community-Academic Partnerships: A Systematic Review of the State of the Literature and Recommendations for Future Research. Milbank Q. 2016;94(1):163–214.
Forsythe LP, Szydlowski V, Murad MH, Ip S, Wang Z, Elraiyah TA, et al. A systematic review of approaches for engaging patients for research on rare diseases. J Gen Intern Med. 2014;29(Suppl 3):788–800.
Article PubMed Central Google Scholar
Lander J, Hainz T, Hirschberg I, Strech D. Current practice of public involvement activities in biomedical research and innovation: a systematic qualitative review. PLoS One [Electronic Resource]. 2014;9(12):e113274.
Lee DJ, Avulova S, Conwill R, Barocas DA. Patient engagement in the design and execution of urologic oncology research. Urol Oncol. 2017;35(9):552–8.
Malterud K, Elvbakken KT. Patients participating as co-researchers in health research: A systematic review of outcomes and experiences. Scandinavian Journal of Public Health. 2020;48(6):617–28.
Miah J, Dawes P, Edwards S, Leroi I, Starling B, Parsons S. Patient and public involvement in dementia research in the European Union: a scoping review. BMC geriatr. 2019;19(1):220.
Nilsen ES, Myrhaug HT, Johansen M, Oliver S, Oxman AD. Methods of consumer involvement in developing healthcare policy and research, clinical practice guidelines and patient information material. Cochrane Database Syst Rev. 2006;2006(3):CD004563.
PubMed PubMed Central Google Scholar
Oliver S, Clarke-Jones L, Rees R, Milne R, Buchanan P, Gabbay J, et al. Involving consumers in research and development agenda setting for the NHS: developing an evidence-based approach. Health Technol Assess. 2004;8(15):1-148 III-IV.
Orlowski SK, Lawn S, Venning A, Winsall M, Jones GM, Wyld K, et al. Participatory Research as One Piece of the Puzzle: A Systematic Review of Consumer Involvement in Design of Technology-Based Youth Mental Health and Well-Being Interventions. JMIR Hum Factors. 2015;2(2):e12.
Pii KH, Schou LH, Piil K, Jarden M. Current trends in patient and public involvement in cancer research: A systematic review. Health Expectations: An International Journal of Public Participation in Health Care & Health Policy. 2019;22(1):3–20.
Sandoval JA, Lucero J, Oetzel J, Avila M, Belone L, Mau M, et al. Process and outcome constructs for evaluating community-based participatory research projects: a matrix of existing measures. Health Educ Res. 2012;27(4):680–90.
Sangill C, Buus N, Hybholt L, Berring LL. Service user’s actual involvement in mental health research practices: A scoping review. Int J Ment Health Nurs. 2019;28(4):798–815.
Schelven F, Boeije H, Marien V, Rademakers J. Patient and public involvement of young people with a chronic condition in projects in health and social care: A scoping review. Health Expect. 2020;23:789 No Pagination Specified.
Schilling I, Gerhardus A. Methods for Involving Older People in Health Research-A Review of the Literature. International Journal of Environmental Research & Public Health [Electronic Resource]. 2017;14(12):29.
Shippee ND, Domecq Garces JP, Prutsky Lopez GJ, Wang Z, Elraiyah TA, Nabhan M, et al. Patient and service user engagement in research: a systematic review and synthesized framework. Health Expect. 2015;18(5):1151–66.
Vaughn LM, Jacquez F, Lindquist-Grantz R, Parsons A, Melink K. Immigrants as research partners: A review of immigrants in community-based participatory research (CBPR). J Immigr Minor Health. 2017;19(6):1457–68.
Walmsley J, Strnadova I, Johnson K. The added value of inclusive research. J Appl Res Intellect Disabil. 2018;31(5):751–9.
Weschke S, Franzen DL, Sierawska AK, Bonde LS, Strech D. Schorr SG. Reporting of patient involvement: A mixed-methods analysis of current practice in health research publications. medRxiv; 2022. p. 21.
Gradinger F, Britten N, Wyatt K, Froggatt K, Gibson A, Jacoby A, et al. Values associated with public involvement in health and social care research: A narrative review. Health Expectations: An International Journal of Public Participation in Health Care & Health Policy. 2015;18(5):661–75.
Hoekstra F, Mrklas KJ, Khan M, McKay RC, Vis-Dunbar M, Sibley KM, et al. A review of reviews on principles, strategies, outcomes and impacts of research partnerships approaches: A first step in synthesising the research partnership literature. Health Res Pol Syst. 2020;18(1):51 no pagination.
Stallings SC, Boyer AP, Joosten YA, Novak LL, Richmond A, Vaughn YC, et al. A taxonomy of impacts on clinical and translational research from community stakeholder engagement. Health Expect. 2019;22(4):731–42.
Grindell C, Coates E, Croot L, O’Cathain A. The use of co-production, co-design and co-creation to mobilise knowledge in the management of health conditions: a systematic review. BMC Health Serv Res. 2022;22(1):877.
Staley K. “Is it worth doing?” Measuring the impact of patient and public involvement in research. Res Involv Engagem. 2015;1:6.
Download references
Acknowledgements
The authors would like to thank the graphic designers, Jenni Quinn and Kevin Calthorpe, for their work on Fig. 3 .
Not applicable.
Author information
Authors and affiliations.
School of Health Sciences, The University of Melbourne, Melbourne, Australia
Sanne Peters, Jill Francis, Stephanie Best, Stephanie Rowe & Marlena Klaic
Department of Health Services Research, Peter MacCallum Cancer Centre, Melbourne, Australia
Lisa Guccione, Jill Francis & Stephanie Best
Sir Peter MacCallum Department of Oncology, Faculty of Medicine, Dentistry and Health Sciences, The University of Melbourne, Melbourne, Australia
Centre for Implementation Research, Ottawa Hospital Research Institute, Ottawa, Canada
Jill Francis
Victorian Comprehensive Cancer Centre, Melbourne, VIC, Australia
Stephanie Best
Emergency Research, Murdoch Children’s Research Institute, Melbourne, Australia
Emma Tavender
Department of Critical Care, The University of Melbourne , Melbourne, Australia
School of Nursing, Faculty of Health, Ottawa, Canada
Janet Curran & Stephanie Rowe
Emergency Medicine, Faculty of Medicine, Ottawa, Canada
Janet Curran
Neurological Rehabilitation Group Mount Waverley, Mount Waverley, Australia
Katie Davies
The ALIVE National Centre for Mental Health Research Translation, The University of Melbourne, Melbourne, Australia
Victoria J. Palmer
You can also search for this author in PubMed Google Scholar
Contributions
SP coordinated the authorship team, completed the systematic literature searches, synthesis of data, framework design and substantial writing. MK and LG were the second reviewers for the systematic overview of reviews. MK, LG and JF assisted with framework design. SP, LG, JF, SB, ET, JC, KD, SR, VP and MK participated in the stakeholder meetings and the consensus process. All authors commented on drafts and approved the final submitted version of the manuscript.
Corresponding author
Correspondence to Sanne Peters .
Ethics declarations
Ethics approval and consent to participate, consent for publication, competing interests.
The authors declare that they have no competing interests.
Additional information
Publisher’s note.
Springer Nature remains neutral with regard to jurisdictional claims in published maps and institutional affiliations.
Supplementary Information
Supplementary material 1., supplementary material 2., supplementary material 3., supplementary material 4., rights and permissions.
Open Access This article is licensed under a Creative Commons Attribution 4.0 International License, which permits use, sharing, adaptation, distribution and reproduction in any medium or format, as long as you give appropriate credit to the original author(s) and the source, provide a link to the Creative Commons licence, and indicate if changes were made. The images or other third party material in this article are included in the article's Creative Commons licence, unless indicated otherwise in a credit line to the material. If material is not included in the article's Creative Commons licence and your intended use is not permitted by statutory regulation or exceeds the permitted use, you will need to obtain permission directly from the copyright holder. To view a copy of this licence, visit http://creativecommons.org/licenses/by/4.0/ .
Reprints and permissions
About this article
Cite this article.
Peters, S., Guccione, L., Francis, J. et al. Evaluation of research co-design in health: a systematic overview of reviews and development of a framework. Implementation Sci 19 , 63 (2024). https://doi.org/10.1186/s13012-024-01394-4
Download citation
Received : 01 April 2024
Accepted : 31 August 2024
Published : 11 September 2024
DOI : https://doi.org/10.1186/s13012-024-01394-4
Share this article
Anyone you share the following link with will be able to read this content:
Sorry, a shareable link is not currently available for this article.
Provided by the Springer Nature SharedIt content-sharing initiative
- Research co-design
- Stakeholder involvement
- End-user engagement
- Consumer participation
- Outcome measures
Implementation Science
ISSN: 1748-5908
- Submission enquiries: Access here and click Contact Us
- General enquiries: [email protected]
Log in using your username and password
- Search More Search for this keyword Advanced search
- Latest content
- For authors
- Browse by collection
- BMJ Journals
You are here
- Volume 14, Issue 9
- Barriers and facilitators to implementing imaging-based diagnostic artificial intelligence-assisted decision-making software in hospitals in China: a qualitative study using the updated Consolidated Framework for Implementation Research
- Article Text
- Article info
- Citation Tools
- Rapid Responses
- Article metrics

- http://orcid.org/0000-0002-2349-8775 Xiwen Liao 1 , 2 ,
- Chen Yao 1 , 2 ,
- http://orcid.org/0000-0002-4991-0158 Feifei Jin 3 , 4 ,
- Jun Zhang 5 ,
- Larry Liu 6 , 7
- 1 Peking University First Hospital , Beijing , China
- 2 Clinical Research Institute, Institute of Advanced Clinical Medicine , Peking University , Beijing , China
- 3 Trauma Medicine Center , Peking University People's Hospital , Beijing , China
- 4 Key Laboratory of Trauma treatment and Neural Regeneration, Peking University , Ministry of Education , Beijing , China
- 5 MSD R&D (China) Co., Ltd , Beijing , China
- 6 Merck & Co Inc , Rahway , New Jersey , USA
- 7 Weill Cornell Medical College , New York City , New York , USA
- Correspondence to Chen Yao; yaochen.pucri{at}foxmail.com
Objectives To identify the barriers and facilitators to the successful implementation of imaging-based diagnostic artificial intelligence (AI)-assisted decision-making software in China, using the updated Consolidated Framework for Implementation Research (CFIR) as a theoretical basis to develop strategies that promote effective implementation.
Design This qualitative study involved semistructured interviews with key stakeholders from both clinical settings and industry. Interview guide development, coding, analysis and reporting of findings were thoroughly informed by the updated CFIR.
Setting Four healthcare institutions in Beijing and Shanghai and two vendors of AI-assisted decision-making software for lung nodules detection and diabetic retinopathy screening were selected based on purposive sampling.
Participants A total of 23 healthcare practitioners, 6 hospital informatics specialists, 4 hospital administrators and 7 vendors of the selected AI-assisted decision-making software were included in the study.
Results Within the 5 CFIR domains, 10 constructs were identified as barriers, 8 as facilitators and 3 as both barriers and facilitators. Major barriers included unsatisfactory clinical performance (Innovation); lack of collaborative network between primary and tertiary hospitals, lack of information security measures and certification (outer setting); suboptimal data quality, misalignment between software functions and goals of healthcare institutions (inner setting); unmet clinical needs (individuals). Key facilitators were strong empirical evidence of effectiveness, improved clinical efficiency (innovation); national guidelines related to AI, deployment of AI software in peer hospitals (outer setting); integration of AI software into existing hospital systems (inner setting) and involvement of clinicians (implementation process).
Conclusions The study findings contributed to the ongoing exploration of AI integration in healthcare from the perspective of China, emphasising the need for a comprehensive approach considering both innovation-specific factors and the broader organisational and contextual dynamics. As China and other developing countries continue to advance in adopting AI technologies, the derived insights could further inform healthcare practitioners, industry stakeholders and policy-makers, guiding policies and practices that promote the successful implementation of imaging-based diagnostic AI-assisted decision-making software in healthcare for optimal patient care.
- Clinical Decision-Making
- Implementation Science
- Information technology
Data availability statement
Data are available on reasonable request. Study protocol and interview transcripts are available on request by contacting the corresponding author.
This is an open access article distributed in accordance with the Creative Commons Attribution Non Commercial (CC BY-NC 4.0) license, which permits others to distribute, remix, adapt, build upon this work non-commercially, and license their derivative works on different terms, provided the original work is properly cited, appropriate credit is given, any changes made indicated, and the use is non-commercial. See: http://creativecommons.org/licenses/by-nc/4.0/ .
https://doi.org/10.1136/bmjopen-2024-084398
Statistics from Altmetric.com
Request permissions.
If you wish to reuse any or all of this article please use the link below which will take you to the Copyright Clearance Center’s RightsLink service. You will be able to get a quick price and instant permission to reuse the content in many different ways.
STRENGTHS AND LIMITATIONS OF THIS STUDY
Used the updated Consolidated Framework for Implementation Research to systematically identify barriers and facilitators.
Conducted semistructured interviews with a wide range of key stakeholders, both from clinical settings and industry.
Potential generalisability limitations due to purposive sampling of artificial intelligence software and the cluster of healthcare institutions and study participants in big cities.
The inclusion of perspectives from patients should be addressed in future research.

Introduction
Clinical decision-making (CDM) is a challenging and complex process. 1 Effective and informed CDM requires a delicate balance between the best available evidence, environmental and organisational factors, knowledge of the patient and comprehensive professional capabilities, such as clinical skills and experiences. 2 3 However, the ability of healthcare professionals to make such decision is often restricted by the dynamic and uncertain nature of clinical practices. 3 In response, decision-making tools have been developed to enhance and streamline CDM for optimal healthcare outcomes. Traditional decision-making tools heavily depend on computerised clinical knowledge bases, supporting CDM by matching individual patient data with the knowledge base to provide patient-specific assessments or recommendations. 4 5 However, relying solely on knowledge-based tools has become insufficient to fulfil the growing need for accessible, efficient and personalised healthcare services, due to its inherent limitations such as time-consuming processes, disruptions in routine clinical workflow and challenges in constructing complex queries. 6 7
Non-knowledge-based decision support tools, on the other hand, harness artificial intelligence (AI) algorithms to analyse large and complex datasets and learn continuously for more accurate and individualised recommendations. 5 8 AI technology has been rapidly advancing since 2000, unleashing substantial potential to revolutionise the conventional CDM process and driving a fundamental shift in healthcare paradigm. 9 The development and extensive growth of clinical real-world data (RWD) have made integrating AI technology into the healthcare sector a priority for both the healthcare industry and regulatory agencies.
The US Food and Drug Administration (FDA) has well recognised the use of AI techniques combined with clinical RWD for both drug and medical device development. In drug development, the FDA has reported a marked increase in the number of drug and biological application submissions with AI components across different stages of life cycle. 10 AI algorithms have been actively integrated into biomarker discovery, eligible population identification and prescreening, clinical drug repurposing, and adverse event (AE) detection, ranging from drug discovery and premarket clinical studies to postmarket safety surveillance. 11 Particularly, the number of studies on AE detection with the use of natural language processing increased from 1 between 2005 and 2018 to 32 between 2017 and 2020. 11
In addition to the pharmaceutical industry, AI medical devices, whether intended for decision-making or other purposes, have experienced rapid development between 2000 and 2018. 12 During this period, the growing sophistication of imaging medical supplies, such as CT scanners, has led to an exponential increase in the volume of high-dimensional imaging data. This surge has gradually shifted the focus of AI medical devices towards imaging-based diagnostic decision-making software, making lung nodule detection and diabetic retinopathy screening popular areas of research. 13 These AI systems, classified as software as medical devices, are designed to support diagnostic decision-making using clinical RWD, particularly imaging data generated by medical devices, leveraging AI technology to perform functions independently of hardware medical devices. 14–16 Notably, the FDA’s approval of the first imaging-based AI-assisted medical device for detecting diabetes-related eye diseases in 2018 marked major progress towards the implementation of imaging-based diagnostic AI-assisted decision-making software. 17 In China, a major milestone was achieved with the approval of the first AI-related software for coronary artery fractional flow reserve by the National Medical Products Administration (NMPA) in 2020, 18 highlighting the continuous development and integration of AI technologies in healthcare practices. Following this breakthrough, AI-assisted decision-making software has experienced rising popularity in China. The number of regulatory approvals for AI software expanded from 1 in 2020 to 62 in 2022. 12 Key functions included disease identification, lesion segmentation, and risk prediction, and risk classification, covering therapeutic areas from cardiovascular diseases to various types of cancers. 12 19
Evidence from randomised controlled trials has demonstrated the safety and effectiveness of AI-assisted decision-making software across various therapeutic areas. For disease detection, these tools have facilitated the early identification of patients with low ejection fraction, improved detection rates for actionable lung nodules and increased the identification of easily missed polyps. 20–23 Furthermore, AI software has significantly reduced diagnostic times compared with senior consultants in diagnosing childhood cataracts, improving clinical efficacy. 24 In disease management, AI-assisted decision-making software has decreased treatment delays for cardiovascular diseases and lowered in-hospital mortality for severe sepsis, 25 26 contributing to improved healthcare quality. Additionally, studies have indicated that AI tools are effective across diverse populations, significantly enhancing follow-up rates for diabetic retinopathy in paediatric populations and improving referral adherence in adult patients within low-resource settings. 27 28 However, a priori scoping review found a disparity between substantial research investment and limited real-world clinical implementation. 19 Further, employing stratified cluster sampling from six provinces across China, a study revealed that only 23.75% of the surveyed hospitals had implemented AI-assisted decision-making software. 29 Accordingly, existing literature has emphasised the deficiency in implementation science expertise for understanding AI implementation efforts in clinical settings. 30 To bridge the gap, the current study aimed to explore the barriers and facilitators of implementing existing imaging-based diagnostic AI-assisted decision-making software in China through qualitative interviews, using the updated Consolidated Framework for Implementation Research (CFIR). The key strength of using the updated CFIR lied in its adaptability to capture both the breadth and depth of qualitative data, as well as its applicability to explore the implementation of technology across various healthcare settings, further enhancing the rigour and comprehensiveness. 31 32 In addition, the study provided tailored implementation strategies to address key barriers, exploiting the full potential of imaging-based diagnostic AI-assisted decision-making software for the improved quality of care in China.
Innovation selection
A recent scoping review identified imaging-based diagnostic decision-making software using medical imaging data as the predominant AI-assisted decision-making software in China, especially those designed for the lung nodules detection and diabetic retinopathy screening. 19 To manage the increasing submissions in these areas, the Center for Medical Device Evaluation of NMPA issued two corresponding guidelines in 2022, delineating specific regulatory requirements. 33 34 Therefore, the current study purposively chose to investigate AI-assisted decision-making software for lung nodules and diabetic retinopathy screening, serving as two representative imaging-based diagnostic AI applications. Two lists were obtained from the NMPA database, from which one software for lung nodules and another for diabetic retinopathy were ultimately selected ( online supplemental tables 1 and 2 ). The vendors were selected through convenience sampling and their voluntary consent to participate. Characteristics of the selected AI-assisted decision-making software are shown in table 1 .
Supplemental material
- View inline
Characteristics of the selected AI-assisted decision-making software
Study setting and participants
With the aim of reflecting diversified perspectives from a wide range of stakeholders, participants from both clinical settings and industry were included. Specifically, clinical stakeholders consisted of three different roles, including healthcare practitioners, hospital informatics specialists and hospital administrators. Industry stakeholders were vendors of the selected AI-assisted decision-making software, which was further divided into three subroles, including data scientists, database experts and algorithm engineers. While the perspectives of patients are valuable, the current study aimed to gather in-depth insights regarding practical and systemic challenges from stakeholders who are directly involved in the implementation, deployment and development of the selected AI-assisted decision-making software. The included stakeholders possessed either the operational or technical knowledge necessary to identify specific barriers and facilitators related to the implementation of the selected AI software in clinical settings. Thus, patients were not included as a stakeholder group in this study.
The selection of study participants involved a two-stage process, wherein initial screening occurred at the institutional level, followed by the individual-level selection. For clinical stakeholders, two lists of hospitals that implemented the selected AI-assisted decision-making software were acquired from the corresponding software vendor. Employing stratified purposive sampling, one tertiary hospital and one primary or secondary hospital were selected from each of the lists, respectively. All selected healthcare institutions were located in big cities, including the Cancer Hospital of the Chinese Academy of Medical Sciences, Beijing Hospital, Beijing Shuili Hospital and the community healthcare centre of Qingpu, Shanghai. The snowball sampling technique was subsequently used to select study participants by asking the Scientific Research Division to identify relevant clinical department and related healthcare practitioners, health informatics specialists and hospital administrators. 35 Additionally, healthcare practitioners were stratified by their professional titles, including junior, intermediate and senior. A similar two-stage process was applied to select stakeholders related to AI-assisted software vendors. CY was responsible to contact the hospitals and AI-assisted software vendors for study participation and interview arrangements.
Eligibility criteria for participant selection were as follows:
Inclusion criteria
Participants from clinical settings should either have user experience with the selected AI-assisted decision-making software or have experience in deploying or managing such software at the hospital level.
Participants from the industry should have working experience in the development of AI-assisted CDM software.
Participants should be formal staff at the stakeholder’s institution.
Participants should be at least 18 years old.
Participants should be able to sign the informed consent form voluntarily.
Exclusion criteria
Participants were excluded from the study if:
Participants could not sign the informed consent form.
Participants could not provide at least 15 min for the interview.
No sensitive information was collected during the interviews. To maintain confidentiality, all qualitative data were anonymised, and each participant who signed the informed consent voluntarily was assigned a unique identification number. Deidentified transcriptions and audio recordings were stored securely on a protected research drive with access restricted to the research team. The research data will be destroyed after 5 years of the study’s conclusion.
Theoretical framework: the CFIR
The CFIR, a well-established conceptual framework in implementation science, was originally developed in 2009 to systematically assess complex and multilevel implementation contexts for the identification of determinants impacting the successful implementation of an innovation. 36 The original CFIR is an exhaustive and standardised meta-theoretical framework synthesised from 19 pre-existing implementation theories, models and frameworks, which was modified in 2022 in response to user feedback and critiques. 37 The updated CFIR consists of 48 constructs and 19 subconstructs across 5 domains including innovation, outer setting, inner setting, individual and implementation process. 37 It provided a fundamental structure for the exploratory evaluation of the barriers and facilitators to implementing imaging-based diagnostic AI-assisted decision-making software in China. Studies employing the CFIR in healthcare settings extensively explored various technological areas, including the implementation of electronic health record systems, 38 telemedicine 39 and various innovative tools, such as the frailty identification tool and decision-support systems in emergency care settings. 40 41 The wide adoption of the CFIR across diverse healthcare contexts emphasised its value in capturing the complex dynamics involved in implementing technology innovations. The thorough and flexible application of the updated CFIR in data collection, analysis and reporting within the current study aimed to increase study efficiency, produce generalisable research findings to inform AI implementation practice and build a scientifically sound evidence base for tailoring implementation strategies to address key barriers.
Data collection procedures
Semistructured in-person interviews were conducted. Study-related data were collected subsequent to obtaining informed consent from the participants. Guided by the updated CFIR, different interview guides were developed for four distinct stakeholder roles, including healthcare practitioners, hospital informatics specialists, hospital administrators and vendors of AI-assisted decision-making software ( online supplemental appendix 1 ). The interview guides were designed specifically to elicit participants’ perspectives, experiences and insights in implementing or delivering AI-assisted decision-making software (CY, XL and FJ). Prior to initiating data collection, the interview guides were pilot tested with four non-study participants to ensure clarity and reliability. Necessary modifications were made based on the feedback. The interviews were conducted by two interviewers with extensive training and experience in qualitative interviews (XL and FJ). Interview time, location, stakeholder role and basic demographic information were collected. Interviews continued until constructs of the updated CFIR were adequately represented in the data, indicating data saturation. 42
Data analysis
The interviews were audio recorded, transcribed verbatim in Chinese and coded independently by two coders (XL and FJ). Deductive content analysis was primarily used for data analysis. As a systematic and objective qualitative approach, content analysis is used to describe and quantify phenomena by deriving replicable and reliable inferences from qualitative data within relevant context. 43 For deductive content analysis, data were coded based on existing theories or framework defined a priori. However, the current study allowed new themes that did not fit into any of the pre-existing CFIR constructs to emerge through inductive analysis of the data.
Steps of deductive data analysis were as follows 44 :
Selecting the unit of analysis
Each interview was selected as unit of analysis, wherein conversational turns that contributed to the understanding of research questions were identified as meaning units. A turn consisted of an uninterrupted segment, which could be a single word or a few sentences. Following independent transcription of the audio recordings by two coders (XL and FJ), CY reviewed and compared the transcriptions, finalising the transcript to be analysed. To be immersed in the data, two coders (XL and FJ) engaged in a thorough reading of the transcripts and made relevant annotations.
Developing structured codebook
Before coding, a standardised, publicly available codebook template based on the original CFIR was employed and adapted to the study context collectively (XL, FJ and CY) ( online supplemental appendix 2 ). 45 Adaptations were multifaceted, which included aligning the original CFIR domains and constructs with those of the updated CFIR, tailoring language specific to the implementation of imaging-based diagnostic AI-assisted decision-making software in China, refining operational definitions and developing eligibility criteria for each construct.
Data coding
Two coders (XL and FJ), who were trained rigorously in using the codebook, performed coding independently. To ensure reliability, 10% of the transcripts were randomly selected for pilot testing. Two coders independently applied the codebook to generate preliminary codes for each meaning unit and subsequently categorised them within the updated CFIR framework. On completion, a group discussion with CY or JZ was warranted, which involved a comprehensive review and comparison of coding discrepancies to ensure consistency in the interpretation and categorisation of units. Disagreements were resolved through consensus, and any necessary adjustments to the operational definitions and eligibility criteria were promptly and appropriately made.
The main coding process was then structured into several iterative rounds to ensure coding consistency. In each round, individual coders were responsible to code four distinct transcripts individually and a fifth transcript collaboratively, addressing any inconsistencies through comprehensive discussion until a consensus was reached. ATLAS.ti (V.23.1.1) was used to identify, label and categorise themes and patterns within the qualitative data. 46 Additionally, it facilitated data management, ensuring the storage, systematic organisation and retrieval of interview transcripts.
Reporting the data by category
Identified categories across the five domains of the updated CFIR were reported descriptively with direct quotes from participants.
Trustworthiness
The study employed several methodological strategies to ensure rigour and reliability. Multiple data sources and perspectives were incorporated to achieve triangulation, including distinct stakeholders directly involved in the implementation, deployment and development of the selected AI-assisted decision-making software. Throughout the data coding process, peer debriefing was employed. Two coders independently analysed the transcripts and collaboratively discussed the interpretations and coding decisions to reach a consensus. Moreover, an audit trail was conducted to ensure transparency, with thorough documentation of study processes such as the prespecified research protocol, deidentified transcriptions, informed consent forms, interview codebooks, typed notes, audio recordings and analyses of qualitative data. External audits were further performed to validate credibility. Experts independent of the study reviewed the study protocol, interview guides and findings, providing objective suggestions.
Patient and public involvement
There was no patient or public involvement in this study. Participants were only invited to participate in qualitative interviews.
Characteristics of study setting and participants
Interviews were conducted between May and August 2023. Table 2 provides an overview of the characteristics of the selected healthcare institutions. A total of 43 participants were invited for study enrolment, and 40 (93.0%) agreed to participate, including 23 healthcare practitioners, 6 hospital informatics specialists, 4 hospital administrators and 7 vendors of the selected AI-assisted decision-making software ( table 3 ). Non-participants included two senior healthcare practitioners and one vendor of AI-assisted decision-making software. Most participants held at least a master’s degree, and 57.5% of them were male.
Characteristics of the selected healthcare institutions
Demographic characteristics of study participants
Barriers and facilitators to implementing imaging-based diagnostic AI-assisted decision-making software
Among the 48 CFIR constructs and 19 subconstructs, 21 of them across 5 domains were found to be relevant in the context of implementing imaging-based diagnostic AI-assisted decision-making software in China ( figure 1 ). Specifically, 10 were identified as barriers, 8 as facilitators and 3 as both barriers and facilitators ( tables 4 and 5 ).
- Download figure
- Open in new tab
- Download powerpoint
Identified CFIR constructs and their impact on the implementation of imaging-based diagnostic AI-assisted decision-making software in China. ‘−’ indicated barriers; ‘+’ indicated facilitators. AI, artificial intelligence; CFIR, Consolidated Framework for Implementation Research.
Barriers to implementing imaging-based diagnostic AI-assisted decision-making software using the updated CFIR
Facilitators to implementing imaging-based diagnostic AI-assisted decision-making software using the updated CFIR
Innovation evidence base (+): strong empirical evidence of effectiveness
The innovation evidence base was suggested to be a key determinant facilitating implementation. Participants in this study reported evidence supporting that the clinical performance of AI software was comparable to or even surpassed that of human beings, further leading to decreased diagnostic time, reduced risk of medical errors and enhanced patient outcomes. As the AI software supported healthcare practitioners in making critical judgements regarding patient care, the robust clinical findings of efficacy and accuracy were instrumental in fostering trust and acceptance among participants towards implementation.
Before we started using the AI software in our department, I checked out articles published in some highly respected peer-reviewed journals. They reported that clinical performance of AI was comparable to human performance. This encourages me to start using the software.—intermediate clinician
Innovation relative advantage (±)
Improved clinical efficiency (+).
One of the crucial benefits gained by using AI-assisted decision-making software was the improved clinical efficacy. Some key functions of AI-assisted software included the detection of anomalies and lesions at risk, automated volumetric measurements and classification of disease severity. Study participants noted that the average interpretation time for a human reader was markedly longer than that of AI-assisted software alone or concurrent reading with the software, regardless of the level of clinical experience and complexity of diseases.
The AI software makes decisions very quickly, as compared to my decision-making time. It greatly supports my clinical judgement and improves routine clinical efficacy.—junior clinician
Unsatisfactory clinical performance (−)
However, the real-world clinical performance of the AI-assisted decision-making software remained suboptimal. Despite a strong evidence base, compromised accuracies, high false-positive rates, overestimation of lesion size and misclassification of lesion types were commonly reported by study participants. The participating healthcare practitioners highlighted the need to improve clinical performance of the AI-assisted software, particularly under complex real-world clinical conditions.
In my daily practice, I consider my clinical judgment as the gold standard. The AI software’s performance, especially in distinguishing between part-solid and solid lung nodules, doesn’t meet my expectations. The performance of the software should be improved for better usability.—intermediate clinician
Innovation adaptability (–): lack of adaptability for generated report
The generation of a diagnostic report was recognised as a pivotal function of AI-assisted decision-making software, providing diagnostic recommendations based on the analysis of patient data. The direct and automatic integration of findings into the diagnostic report was a time-saving aspect for healthcare practitioners in terms of medical documentation. However, study participants perceived that diagnostic reports generated by the AI software lacked customisation options necessary to align with the standard documentation practices of healthcare practitioners. This limitation, along with the software’s insufficient flexibility to fully comply with the hospital’s documentation standards, hindered its seamless incorporation into clinical workflow.
Personally, I don’t use the reports generated by the software. The automatically generated repots don’t align with my documentation style or the hospital’s requirement, and it doesn’t allow me to change any elements within the report. I prefer to write the reports by myself.—senior clinician
Innovation trialability (+): AI software trialability
The ability to test or experiment with AI-assisted decision-making software before full implementation was determined as a pivotal factor facilitating successful implementation. Trialability allowed participating healthcare practitioners to assess the AI software on a smaller scale, supporting their familiarity with the new innovation. More importantly, a trial period enabled evaluation of the software’s compatibility with existing workflows, identification of potential implementation barriers, and assessment of the clinical performance and reliability in real-world settings.
As we prepared for the official implementation, our department pilot tested the AI software for several weeks. This allowed me to personally experience the software, making comparisons with our standard clinical practices.—intermediate clinician
Innovation complexity (+): easiness of use
The perceived easiness of use promoted successful integration of AI-assisted decision-making software into healthcare institutions. According to the participants, the AI software featured user-friendly interfaces designed in a straightforward manner for easy navigation. In addition, the AI software generated automated, clear and comprehensible output to support medical decisions, contributing to a smooth learning curve that was conducive to quick adoption and acceptance of study participants.
The good thing is that AI software is straightforward and easy to use. I learned how to use it with minimal hassle because it provided clear and understandable output with just a few mouse clicks—intermediate clinician
Innovation cost (−): financial burden of AI software
Currently, the cost of AI-assisted decision-making software is not covered by any insurance plans. Healthcare institutions sometimes face financial constraints when acquiring the software and managing ongoing maintenance costs. Participated hospital administrators, especially those from primary and secondary hospitals, expressed the need to reallocate budgetary resources from other areas, such as staff resources and infrastructure, to accommodate the high cost associated with AI software. The perceived lack of cost-effectiveness discouraged further investment.
The insurance plans don’t cover the cost of AI software now, and patients are not paying for it either. Cost-effectiveness is one of our top priorities, and we won’t spend a lot money on the software.—hospital administrator
Outer setting
Partnerships and connections (−): lack of a collaborative network between primary/secondary and tertiary hospitals.
AI-assisted decision-making software was valuable in early disease detection and intervention. However, study participants from primary care reported that the absence of partnerships and communication channels with tertiary hospitals created challenges for patients diagnosed with diseases. These challenges included delays in receiving informed referrals to tertiary hospitals, potentially resulting in late medical intervention and discontinuity in care. Further, the lack of established connections impeded the sharing and exchange of patient data between hospitals. Tertiary hospitals received incomplete or insufficient patient profiles from primary hospitals, contributing to an inadequate understanding of the patient’s condition and history. In such scenarios, patients might undergo redundant diagnostic tests at different facilities, leading to both patient inconvenience and increased healthcare costs.
For the efficient and effective utilisation of AI-assisted decision-making software in healthcare settings and optimal patient care, participants highlighted the importance of establishing a mechanism to refer and follow up with patients who have positive or indeterminate disease findings from primary hospitals to tertiary hospitals.
Patients diagnosed at our hospital with positive or indeterminate results usually need to be referred to a tertiary or specialized hospital for further treatment. However, ensuring patient compliance is a challenge. Partnering with those hospitals and establishing some referral and follow-up mechanisms will be beneficial.—intermediate clinician
Policies and laws (±)
National guidelines related to ai (+).
With the rapid advancements in AI technology, China released a series of national policies and guidelines to rigorously promote the interdisciplinary integration of AI into healthcare sector. 47–49 In response, clinical institutions took necessary steps forward, proactively incorporating AI-assisted decision-making software into conventional healthcare practices. To date, well-established regulatory frameworks clearly outlined and regulated the development, approval and classification of AI-assisted decision-making software as a medical device. Compliance with these regulations increased the confidence of study participants in the implementation of AI-assisted software.
We decided to bring this software in our hospital because our country is promoting the widespread adoption of AI, and it’s also the trend across different economic sectors nationwide. There are several national guidelines supporting its development and use in healthcare system, which increased our confidence in implementing the software.—hospital administrator
Lack of information security measures and certification (−)
Conversely, to ensure data security and protect patient privacy, legislation such as the cybersecurity law mandated a multilevel protection scheme. In accordance, the ‘Information Security Technology—Baseline for Classified Protection of Cybersecurity’ defined four levels of security requirements, which provided baseline guidance for securing platforms and systems handling personal information. Information systems in healthcare institutions must comply with level 3 standards, the highest for non-banking institutions, given the sensitivity of patient electronic data. Consequently, participating vendors of AI software seeking collaboration with hospitals were required to have robust information security measures and level 3 security certification as prerequisites to fulfil safety obligations. The absence of such measures and certification, not uncommon among innovative technology companies, posed barriers to successful implementation.
In order to ensure the confidentiality of patient electronic data and comply with cybersecurity protection requirements, our hospital can’t implement AI decision-making software without robust data security measures or Level 3 security certification.—hospital informatics specialist
External pressure–market pressure (+): deployment of AI software in peer hospitals
Study participants noted that the implementation of AI-assisted decision-making software in peer hospitals, such as those within the same academic affiliation, fostered a competitive atmosphere and exerted a form of peer pressure, facilitating its widespread implementation. This was especially evident in the context of China’s medical informatisation development, where hospitals without AI software implementation felt compelled to stay competitive with their peers to gain a strategic advantage.
We’ve learned that some peer hospitals have already been using such software for quite some time. We are late adopters.—hospital informatics specialist
Inner setting
Relational connections (−): lack of collaboration between specialised and non-specialised clinical departments.
When patient care involved multiple clinical departments, ambiguity arose regarding the authorisation of reports generated by AI-assisted decision-making software. These reports were intended to complement the clinical decisions made by human clinicians who ultimately held the responsibility. However, non-specialists faced challenges in endorsing automated reports due to differences in clinical expertise, varying criteria for report validation, and concerns regarding liability. On the other hand, specialists often regarded AI-generated reports as less reliable than their own specialised assessments, potentially leading to reluctance in signing the reports.
Study participants emphasised the importance of establishing an interdepartmental collaborative network between specialty and non-specialty clinical departments and providing clear definitions of the roles and responsibilities within these departments to address this barrier.
As doctors without specialized expertise in ophthalmology, my colleague and I may not be authorized to sign the clinical report produced by the AI software. A collaborative network or mechanism with the department of ophthalmology will be helpful.—intermediate clinician
Communications (+): regular communication channels within department
Participants suggested that establishing regular communication channels, like weekly meetings, ensured that all members of the clinical department stayed informed about AI-assisted decision-making software. It also provided a platform for educational opportunities, such as workshops, to keep healthcare practitioners well informed and up to date. Open communication effectively addressed concerns and questions related to the implementation of AI software, fostering confidence and competence among healthcare practitioners.
I’m glad that I have the opportunity to discuss personal experience with my colleague during our weekly meetings, where case studies are shared and insights are exchanged. The open dialogue enhances our knowledge and improves my proficiency and confidence.—junior clinician
Compatibility (±)
Suboptimal data quality (−).
The effective performance of AI-assisted decision-making software relied on the availability of high-quality and accurate source data. In the process of software development, machine learning and deep learning algorithms used to analyse and interpret imaging data were trained with dataset that underwent meticulous cleaning and curation, ensuring the removal of poor-quality data containing imaging noise and artefacts before analysis. However, in real-world clinical settings, various factors, like equipment limitations, patient motion and varying proficiency of technicians, potentially introduced imperfections in imaging data. As a result, participants pointed out that AI-assisted software was not highly compatible with and adequately trained on data collected during routine clinical practice.
The real-world imaging quality is often less than optimal, which can lead to inaccuracy or failure of AI diagnosis. We can’t always ask the patient to redo the examination for better quality data, in consideration of their time and healthcare cost.—senior clinician
Integration of AI software into existing hospital systems (+)
In contrast, the integration of AI-assisted software into established hospital systems, such as the picture archiving and communication system (PACS), streamlined clinical workflows and facilitated the effective implementation. The compatibility with PACS enabled interoperability between AI-assisted software and healthcare information systems, providing healthcare practitioners with a familiar working environment and mitigating interruptions in workflow.
Our AI software integrates with the PACS. Clinicians don’t have to learn a new standalone system; instead, they can access AI-generated insights directly within their existing PACS environment, minimizing any disruptions to their workflow.—vendor of AI-assisted software
Mission alignment (−): misalignment between software functions and goals of healthcare institution
A misalignment between functions of AI-assisted decision-making software and the core hospital missions, especially for comprehensive tertiary hospitals, was revealed by study participants. Currently, diagnostic AI-assisted software predominantly supports the diagnosis of general and non-complicated diseases, which divergess from the main strategic objectives of tertiary hospitals dedicated to managing complex medical conditions and delivering high-level care through specialised expertise. Alternatively, AI software appeared more suitable for primary hospitals, where it could be used for general disease diagnosis and population-level screening. Tertiary hospitals prioritised other initiatives perceived to be more critical to their mission. This prioritisation further contributed to the reluctance among healthcare practitioners to embrace AI-assisted software, as they identified the introduction of AI software as a distraction from the hospital’s core mission.
At times, it’s difficult for us to establish collaborations with high-level tertiary hospitals. These hospitals often have highly experienced clinicians, focusing on the improvement of care quality for complex diseases and rare conditions. They have the perception that our AI software may not perform well in their setting. Instead, they suggest that our software may be better suited for primary hospitals where initial diagnoses take place.—vendor of AI-assisted decision-making software
Available resources–materials and equipment (–): lack of necessary medical supplies
The availability of essential medical supplies was integral to the successful implementation of AI-assisted decision-making software that relied on medical imaging data as the primary data source for accurate assessment. In primary and secondary hospitals, where resources were relatively scarce, the limited access to equipment, like CT scanners, hindered the implementation of AI-assisted software.
The implementation of AI decision-making software is not possible at hospitals without necessary medical supplies like CT scanners. —hospital informatics specialist
Access to knowledge and information (–): lack of adequate training
For advanced technologies like AI-assisted CDM software, participated healthcare practitioners sometimes lacked the necessary knowledge and information required for effective use. Inadequate training possibly contributed to a reluctance to adopt the technology, due to unfamiliarity with the software’s complete functionalities and challenges in its practical application.
I believe that I haven’t received thorough training on using the AI software. In fact, I’ve explored it on my own, and I’m not completely aware of all its functions.—intermediate clinician
Individuals
High-level and mid-level leaders (+), engagement of hospital administrator (+).
Participants in the study indicated that effective implementation of AI-assisted decision-making software was facilitated by the hospital’s active leadership engagement and promotional initiatives from the hospital to the department level. Hospital administrators took proactive steps to align the AI-assisted software with the institution’s long-term strategic goals through the initiation and oversight of pilot programmes. The endorsement and active support at the hospital level greatly fostered a collaborative environment among the clinical department, information technology department and vendor of AI-assisted decision-support software, positioning the AI-assisted software as an integral component of the hospital.
Strong support from the top level, especially from our hospital administrators, really makes a difference in introducing AI software and running it smoothly. They ensure its fit with the hospital through a pilot program and rigorously and effectively promote multi-stakeholder communication and collaboration.—hospital informatics specialist
Engagement of department head (+)
At the departmental level, leaders, such as department heads, who supported AI-assisted software, actively championed its implementation. They cultivated an atmosphere of support and knowledge-sharing within the department through the organisation of workshops and seminars, stressing the prospective clinical benefits of implementation. Beyond intradepartmental communication, they facilitated efficient interdepartmental communication with the information technology department to ensure seamless integration of AI-assisted decision-making software into the real-world clinical setting.
Our department head actively supports the implementation of AI software by integrating discussions about relevant knowledge and experiences into our weekly meetings, shedding light on the potential clinical benefits. In fact, they play a very important role in facilitating the integration of AI software into the existing PACS, making the entire implementation process much more efficient and effective.—junior clinician
Need (–): unmet clinical needs
Study participants revealed that AI-assisted decision-making software failed to meet the diverse clinical needs of healthcare practitioners. Currently, the underlying AI algorithm was predominantly designed and trained to address general and non-personalised clinical needs. Clinicians perceived AI-assisted software as insufficient in cases that were complex and multifaceted, requiring a comprehensive approach and an in-depth understanding of the patient’s medical history. Incorporating customisation options, enhancing adaptability in AI algorithms and demonstrating a commitment to ongoing improvement were essential to ensure that AI-assisted decision-making software aligned with the disparate needs of healthcare practitioners across various specialties and clinical settings.
The AI software we have is good for the basics, but we definitely expect more. Currently, its functions are too simplified, and it struggles in tricky and complex situations where you need a deep dive into the patient’s history.—senior clinician
Capability (–): incompetence in understanding AI reasoning mechanism
Participated healthcare practitioners faced challenges in implementing AI-assisted decision-making software in clinical practice due to a limited capability in understanding AI algorithms. The deficiency in necessary knowledge and expertise led to difficulties in comprehending the rationale behind the AI’s recommendations and decisions. This lack of clarity contributed to a lack of trust and reluctance towards the implementation of AI-assisted software. Participants suggested that addressing case-specific reasoning and providing global transparency, such as the algorithm’s functionality, strengths and limitations, would be helpful in opening the ‘black box’ of AI technology.
I sometimes find it hard to trust and embrace the software’s recommendations. I struggle with the complexity of the underlying rationale, since the software provides recommendations based on these algorithms. It’s not clear to me what’s inside the black box, like how it works, what its weakness and strengths are, etc. Clarifications on those factors would be helpful.—senior clinician
Implementation process
Engaging–innovation recipient (+): involvement of clinicians.
It was reported that active engagement substantially facilitated the implementation of AI-assisted decision-making software, particularly through the active involvement of key stakeholders during the process of implementation. In specific, the pilot testing phase was conducted to collect valuable insights and suggestions provided by users, determining limitations and identifying areas for improvement that closely aligned with their clinical needs and workflow. The healthcare practitioners, on the other hand, were empowered by actively shaping the software’s functionality and streamlining the process of implementation.
During the pilot testing phase, we collaborated with the entire department to answer any questions and gather suggestions. This active engagement of the clinicians was helpful not only for us to continuously improve the software, but also for the clinicians to feel involved and make an impact; it’s a win-win situation.—vendor of AI-assisted decision-making software
Reflecting and evaluating (–): lack of feedback incorporation
Reflecting and evaluating were central components of the continuous feedback-driven improvements that promoted the seamless integration of AI-assisted decision-making software into clinical settings. However, study participants noted that their suggestions and qualitative feedback, shared during the pilot testing phase, were not adequately reflected and implemented for process enhancement. Furthermore, there was a notable absence of quantitative assessment of the clinical performance of the AI-assisted software following its implementation. The absence of informative reflection on provided feedback and a structured evaluation process contributed to unaddressed challenges and the frustration of participating healthcare practitioners who felt that their inputs were not sufficiently valued.
My colleague and I provided feedback and suggestions about this AI software during the pilot testing phase, but we see no corresponding actions taken by the vendors, which is disappointing.—intermediate clinician I don’t think there is any systematic evaluation mechanism related to the clinical performance of AI software at our hospital. It is, however, important to periodically and systematically evaluate the performance of the software to make it more accurate and usable.—hospital informatics specialist
Comparison of stakeholder perspectives
It should be noted that the perceptions regarding the selected AI-assisted decision-making software varied considerably among different stakeholder roles. Recognising these unique perspectives is essential to the development of effective implementation strategies that address the varied concerns and priorities of each stakeholder group.
Clinicians, as the primary users, juxtaposed the potential benefits and limitations of the software. Junior clinicians, who have limited clinical experiences, generally held positive attitudes towards the implementation, highlighting the software’s ability to support clinical judgement and enhance routine clinical efficacy. While recognising the value of AI implementation, intermediate clinicians, who used the software more insightfully, gained practical perspectives and emphasised the need for strong interdepartmental collaboration, adequate training and referral mechanisms to tertiary or specialised hospitals for patients with positive or indeterminate disease findings. Senior clinicians provided the most critical feedback, expecting higher standard and improved performance in clinical effectiveness, reliability and transparency, particularly in complex clinical scenarios. On the other hand, hospital administrators focused on financial implications, like cost-effectiveness and budgetary constraints, and informatics specialists highlighted the importance of robust information security measures. Moreover, the selected vendors underscored the necessity of aligning the AI functions with the mission of the healthcare institution to ensure successful implementation.
To the best of our knowledge, this study was the first qualitative assessment that leveraged a well-established implementation framework to systematically guide the identification of barriers and facilitators of AI-assisted decision-making software in China’s healthcare system. The implementation of AI-assisted decision-making software in clinical practice is characterised by the inherent complexity and dynamic nature of both AI technology and healthcare environment. Previous literature attempted to synthesise and understand relevant determinants, with minimal application of theories or frameworks in implementation science, particularly in developing countries. 30 50 The use of the updated CFIR played a fundamental role in understanding the context of implementation and establishing a strategic roadmap, consistently and efficiently producing collective and generalisable knowledge for the development of context-specific implementation strategies tailored to China’s healthcare system. 51 The dynamic and continuous interaction among the five domains of the updated CFIR collectively shaped the outcome and effectiveness of imaging-based diagnostic AI-assisted software implementation. 36 52 The current study validated several barriers identified in prior research across diverse clinical settings, including suboptimal clinical performance, 53–55 compromised RWD quality, 56–58 insufficient training, 54 59 60 deficit in transparency and trust, 60–62 financial constraint, 54 57 insufficiency of necessary equipment, 59 60 and limited interdepartmental communication. 54 More importantly, study findings contributed novel insights to the continuous exploration of the implementation of imaging-based diagnostic AI-assisted decision-making software from the unique perspective of China’s healthcare system, establishing a theoretical foundation to guide the development of practical recommendations and implementation strategies for future improvement efforts.
Given the different perspectives of various stakeholder roles, the prioritisation of barriers, as well as the feasibility and cost-effectiveness of recommendations, the following three barriers and their corresponding suggestions were discussed in further detail ( figure 2 ).
Barriers and suggestions for implementing imaging-based diagnostic AI-assisted decision-making software in China. AI, artificial intelligence.
Barrier: misalignment between software functions and goals of healthcare institutions.
Suggestion: shift the focus of imaging-based diagnostic AI-assisted decision-making software implementation towards primary and secondary healthcare settings, where the AI software’s strengths in diagnosing generalised and non-complex conditions can be leveraged effectively.
The AI-assisted decision-making software has been disproportionately implemented in tertiary hospitals in China. 29 However, a notable misalignment between the functionality of imaging-based diagnostic AI-assisted decision-making software and the strategic goals of tertiary hospitals was found in the current study. Specifically, the implementation of AI-assisted decision-making software demonstrated its effectiveness in diagnosing generalised and non-complex medical conditions. Tertiary hospitals, in contrast, mainly served as hubs that provide specialised and advanced healthcare services, particularly for complex medical conditions. Despite the great potential of AI technologies to revolutionise healthcare, it has become evident that the complexity of conditions, frequently encountered by high-level healthcare institutions, has not been adequately addressed by existing AI competency. 55 60 Given the pivotal role that tertiary hospitals play in China’s healthcare system, it is necessary for imaging-based diagnostic AI-assisted decision-making software to further advance to meet the multifaceted clinical needs of tertiary hospitals in the near future. On the other hand, to effectively promote the implementation of existing imaging-based diagnostic AI-assisted software, a shift in the focus of implementation towards the primary or secondary level of healthcare, such as primary hospitals, physical examination centres or secondary hospitals, would offer a more cohesive fit. This shift would create a more suitable context to effectively implement imaging-based diagnostic AI-assisted decision-making software, leveraging its strengths while accommodating the unique challenges faced by healthcare institutes at the primary or secondary level. Primary healthcare in China typically addresses a broader spectrum of clinical needs and medical cases. However, it is often not the initial point of medical contact due to the suboptimal quality of care. 63 With substantial disparities between primary and tertiary care, residents in China perceived primary healthcare as of poor quality, as reflected in a low doctor-to-patient ratio in tertiary care. 64 65 Various contributing factors were reported, including insufficient knowledge among healthcare professionals, a gap between knowledge and practice, disproportionate distribution of health workforce and inadequate continuity of care across the entire healthcare system. 63 66 Implementing imaging-based diagnostic AI-assisted decision-making software at the primary level, aligning its functionality with the overarching goals of primary care, holds promise in addressing these challenges and bridging gaps, thereby potentially diverting patients with common medical needs towards primary healthcare facilities. AI technologies have the potential to facilitate a diagnosis at least equivalent to that of an intermediate-level clinician, complement clinical expertise and optimise medical resource allocation, enhancing early disease detection and ultimately promoting the quality of patient care across the healthcare hierarchy in China. 67–69
Barrier: lack of a collaborative network between primary/secondary and tertiary hospitals.
Suggestion: establish an integrated healthcare ecosystem driven by a hub-and-spoke model to promote the sharing of clinical data and improve patient referrals, ensuring seamless coordination between primary/secondary and tertiary healthcare institutions.
As mentioned above, one of the key challenges in China’s healthcare system lied in the fragmentation of healthcare delivery. The integration of imaging-based diagnostic AI-assisted decision-making software into primary care stressed the absence of a comprehensive collaborative network connecting primary and tertiary healthcare institutions. This deficiency exacerbated inefficiencies in patient referrals, with positive or indeterminate AI-assisted diagnoses at primary hospitals not being effectively referred to tertiary hospitals. As a result, patients could experience delays in receiving specialised treatment. Furthermore, the scattered and isolated electronic medical systems in China posed substantial challenges to joint healthcare initiatives. 63 Sharing and transfer of clinical data related to disease diagnosis were hindered due to the heterogeneity of systems, potentially leading to unnecessary and duplicated medical examinations. To address this issue, establishing an integrated healthcare ecosystem driven by the hub-and-spoke model would be a promising solution to promote the sharing of clinical data and medical knowledge, as well as facilitate best medical practices. This model, when applied in healthcare system, enhances peripheral services by connecting them with resource-replete centres. 70 In this context, basic medical needs are met through spokes, like primary healthcare institutions while medical resources and investments are centralised at hubs, such as tertiary healthcare institutions. The utilisation of imaging-based diagnostic AI-assisted decision-making software in a hub-and-spoke network of stroke care showed improved clinical efficiency, including decreased time to notification and transfer time between spokes to hubs, leading to a shorter length of stay. 71 72 Currently, a similar network tailored to the healthcare system in China has yet to be implemented, and its potential clinical benefits remain unclear. To fully leverage the capabilities of imaging-based diagnostic AI-assisted decision-making software, it is essential to seamlessly refer patients with positive or indeterminate diagnoses at spoke sites to the hub sites for specialised care, minimising delays in early treatment.
Barrier: lack of information security measures and certification.
Suggestion: establish an independent information platform with robust data security measures to ensure the protection of clinical data privacy and facilitate the integration and data exchange across primary/secondary and tertiary healthcare institutions.
The cybersecurity and data protection regulations in China are undergoing rapid changes, positioning it as one of the most stringent globally. The ‘Information security technology—baseline for classified protection of cybersecurity (GB/T 22239-2019)’, jointly issued by the State Market Regulatory Administration and Standardization Administration of China, came into effect in December 2019. 73 The standard defined level 1 to level 4 security requirements and specified the baseline guidance for information security technology to protect information platforms and systems responsible for collecting, storing, transmitting and processing personal information. 73 As China’s healthcare informatisation continues to advance, the security of hospital information infrastructures is becoming increasingly critical, given that any disruptions could have substantial consequences for individuals and society as a whole. To address this concern, the National Health Commission released the ‘Guidelines for Information Security Level Protection in the Healthcare Industry’, stipulating that core systems in healthcare institutions should adhere to level 3 information security protection standards, the highest level for non-banking institutions. 74 Level 3 security protection mainly covers 5 aspects of security technical requirements and 5 aspects of security management requirements. The multidimensional assessment involves 73 categories, with nearly 300 specific requirements, covering aspects such as information protection, security auditing, communication confidentiality, etc. 73 While AI technology software may not be explicitly covered by this specific requirement, it is often a mandatory administrative step for healthcare institutions, particularly tertiary hospitals, to request a level 3 security certification to ensure the protection of clinical data privacy during the integration and data exchange of AI software. As an integrated approach addressing the unique challenges faced by China’s healthcare system, an independent information platform with robust data security measures or level 3 security qualification to facilitate the implementation of imaging-based diagnostic AI-assisted decision-making software should be established. This platform acts as a vital link connecting the AI software, primary and tertiary healthcare institutions. It is designed to collect basic demographic information and medical history while transmitting deidentified imaging data to AI-assisted software for an initial diagnosis in primary care settings. In cases where patients receive positive or indeterminate reports, referral to the collaborated tertiary hospital within the hub-and-spoke network is warranted. Relevant clinical information, including collected demographic data, medical history, clinical reports and referral forms, is seamlessly transferred to enhance overall efficiency. The successful establishment of this platform requires multistakeholder engagement for proficient and collective design and management, addressing interinstitutional data sharing, security and governance challenges stemming from legal, technical and operational requirements. 70 75
Implications for policy-makers and healthcare practitioners
Globally, there are marked differences in the implementation of AI in healthcare systems. These variations are associated with factors including the type of AI software, healthcare infrastructure, existing policies and technological advancements. Despite ongoing criticism and multiple implementation challenges, AI-assisted decision-making software and health information technologies have demonstrated substantial potential for enhancing diagnostic procedures in primary care, especially with strong regulatory support, in both resource-rich and under-resourced settings. 76–79 Primary care is an ideal setting for AI tools to improve clinical efficiency and reduce medical errors due to its role in managing a large number of patients and making decisions under uncertainty. 76
In Germany, the Federal Ministry of Health has been proactive in supporting AI integration in healthcare. The ‘Smart Physician Portal for Patients with Unclear Disease’ project provided ongoing support to general practitioners (GPs) using an AI-based tool to diagnose uncertain cases. 80 This user-centred decision support tool was design specifically to address GP’s essential clinical needs through interviews and workshops, ensuring a seamless fit into the routine workflow while allowing for more efficient patient diagnosis. Similarly, the National Health Service in the UK has actively incorporated AI technologies into primary care through the use of Babylon’s Triage and Diagnostic system streamlining the diagnostic process. 81 Furthermore, the European Union’s 2021 Proposal sought to establish a global standard for safe, reliable and ethical AI by creating a comprehensive legal framework designed to enhance trust and encourage broad implementation, ensuring that the AI systems are both technically and ethically sound. 82 83 Therefore, regulatory support to increase trust, robust technical infrastructure, strong ethical standards and user-centred design are crucial for the extensive integration of AI in healthcare, ultimately improving patient outcomes and clinical efficiency.
In contrast, China faces particular difficulties due to its large and heterogeneous healthcare system, regional disparities in healthcare infrastructure and rapidly evolving regulatory environment. The successful integration of AI is hindered by the disparity in healthcare resources and the critical need for interoperability among various hospital information systems, especially in primary care settings. In particular, primary healthcare facilities in rural areas, constrained by financial and resource limitations, often lack access to the advanced AI technologies that are more steadily available in tertiary hospitals in big cities. 84 More importantly, while electronic medical record (EMR) systems are widely adopted in primary hospitals, their average level and functionality are typically lower as than those in tertiary hospitals. 85 According to the National Health Commission of the People’s Republic of China, as of 2022, the average level of EMR systems in the tertiary public hospitals was 4.0, indicating a medium stage of EMR development that enabled basic clinical decision support. 86 However, to fully facilitate intelligent clinical decision support, EMR systems need to reach at least level 5, posing an even greater challenge to the systematic integration of AI. 87
Financial incentives and policy support for EMR infrastructure facilitating the use of AI in primary healthcare settings could drive broader implementation and improve care quality. Guidelines for the thorough assessment of AI-assisted decision-making software for cost-effectiveness, efficacy and safety are also urgently needed. 88 To improve care coordination across different levels of healthcare institutions, policies should also support collaborative networks and data-sharing platforms. To increase healthcare practitioners’ familiarity with and confidence in AI-assisted decision-making software, major implementation barriers must be addressed and overall trust in AI technologies must be increased through thorough training and continued regulatory support.
Strengths and limitations
The current study has several strengths and limitations. To ensure scientific rigour and validity, the updated CFIR was thoroughly employed to guide the design of interview guides through the reporting of results. Although primarily descriptive, the study extended beyond identifying barriers and facilitators by providing practical suggestions tailored to China’s healthcare system. In order to capture a broad spectrum of perspectives, a wide range of key stakeholders, ranging from healthcare practitioners to industry vendors, were involved, allowing for a qualitative exploration of various roles and the provision of comprehensive insights. However, the inclusion of perspectives from patients should be warranted in future research, particularly through doctor–patient shared decision-making. Given the extensive impact of AI technology on the professional autonomy of healthcare practitioners, existing literature suggested a negative perception among patients towards physicians using AI-assisted software. 89 90 While the current study specifically focused on two representative AI applications, namely imaging-based diagnostic AI-assisted decision-making software for lung nodules and diabetic retinopathy, study findings were further generalised to the general diagnostic AI-assisted decision-making software using medical imaging as source data. Despite employing the updated CFIR as a systematic approach to understanding barriers and facilitators in the implementation process for enhanced generalisability, it is important to acknowledge potential variations across different types of diagnostic AI software. The current study might not fully capture certain software-specific differences and contextual factors associated with implementation. Moreover, purposive sampling was adopted, and all selected healthcare institutions were located in well-resourced areas, potentially leading to limited generalisability of findings beyond the selected healthcare institutions and software. The results should be interpreted considering this context, emphasising the strong need for cross-comparisons of the findings and the validation of recommendations in other settings, particularly in rural areas.
The rapid advancement of AI techniques is fuelling a global shift in the conventional medical decision-making paradigm. By using the updated CFIR, the current study contributed to a comprehensive understanding of the barriers and facilitators in implementing imaging-based diagnostic AI-assisted decision-making software in China’s evolving healthcare landscape. The findings served as a solid theoretical foundation, providing a possible roadmap for future efforts aimed at optimising the effective implementation of imaging-based diagnostic AI-assisted decision-making software. The tangible suggestions could further inform healthcare practitioners, industry stakeholders and policy-makers in both China and other developing countries, facilitating the unleashing of the full potential of imaging-based diagnostic AI-assisted decision-making software for optimal patient care.
Ethics statements
Patient consent for publication.
Not applicable.
Ethics approval
This study involves human participants and was approved by the Institutional Review Board of Peking University (IRB00001052-22138). Participants gave informed consent to participate in the study before taking part.
Acknowledgments
The authors thank all individuals who took the time to participate in the interviews and those who provided constructive suggestions on the manuscript.
- Karimi S , et al
- Greenes RA , et al
- Winters-Miner LA ,
- Bolding PS ,
- Hilbe JM , et al
- Delaney B ,
- Kostopoulou O
- Meunier PY ,
- Raynaud C ,
- Guimaraes E , et al
- Sutton RT ,
- Pincock D ,
- Baumgart DC , et al
- Administration USFaD
- Hogan W , et al
- Center for Medical Device Evaluation N
- Technology CAoIaC
- Administration NMP
- Zhang J , et al
- Zhou J , et al
- Kim J , et al
- Berzin TM , et al
- Rushlow DR ,
- Inselman JW , et al
- Liu Z , et al
- Shimabukuro DW ,
- Barton CW ,
- Feldman MD , et al
- Chang C-H , et al
- Liu TYA , et al
- Mathenge W ,
- Whitestone N ,
- Nkurikiye J , et al
- Genchev GZ , et al
- Chomutare T ,
- Tejedor M ,
- Svenning TO , et al
- Stevenson F ,
- Lau R , et al
- Richardson JE ,
- Abramson EL ,
- Pfoh ER , et al
- Damschroder LJ ,
- Keith RE , et al
- Reardon CM ,
- Widerquist MAO , et al
- Acharya S ,
- Van Citters AD ,
- Scalia P , et al
- Fujimori R ,
- Soeno S , et al
- Sampalli T , et al
- Saunders B ,
- Kingstone T , et al
- Krippendorff K
- Research TCFfI
- Council GOotS
- Wang D , et al
- Hagedorn HJ
- Yankey N , et al
- Schwartz JM ,
- Rossetti SC , et al
- Hehakaya C ,
- Ranschaert ER , et al
- Romero-Brufau S ,
- Boyum P , et al
- Abramoff MD , et al
- de Bruin JS ,
- Hersch F , et al
- Borges do Nascimento IJ ,
- Abdulazeem H ,
- Vasanthan LT , et al
- Zhang Z , et al
- Petitgand C ,
- Motulsky A ,
- Denis JL , et al
- Tanguay-Sela M ,
- Benrimoh D ,
- Popescu C , et al
- Huang J , et al
- Li X , et al
- Hao Y , et al
- Krumholz HM ,
- Yip W , et al
- Jiang Y , et al
- Zhang XL , et al
- Fortenberry JL
- Hassan AE ,
- Ringheanu VM ,
- Rabah RR , et al
- Elijovich L ,
- Dornbos Iii D ,
- Nickele C , et al
- Center NHCSI
- Middendorf M ,
- Heintzman J , et al
- Miyagami T ,
- Kunitomo K , et al
- Cossy-Gantner A ,
- Germann S , et al
- Koçyiğit Burunkaya D , et al
- Gomez-Cabello CA ,
- Pressman S , et al
- Schütze D ,
- Neff MC , et al
- Middleton K , et al
- Thornton J ,
- Wu S , et al
- Wei X , et al
- China NHCotPsRo
- Lorenzini G ,
- Arbelaez Ossa L ,
- Shaw DM , et al
- Shaffer VA ,
- Probst CA ,
- Merkle EC , et al
Contributors CY, JZ and LL designed the study and developed the eligibility criteria. CY contacted the respondents. CY, XL and FJ designed the interview guides. JZ and LL reviewed and made critical comments. XL and FJ conducted interviews and analysed the data. JZ and CY contributed to the review of qualitative analysis through discussion with XL and FJ. XL completed the first draft of the manuscript. CY, JZ and LL reviewed and revised the manuscript. All authors read and approved the final manuscript. CY is the guarantor, has full access to all data in the study and has final responsibility for the decision to submit for publication.
Funding This work was supported by Merck Sharp & Dohme, a subsidiary of Merck & Co., Rahway, New Jersey, USA. The sponsor participated in the design and development of the study, as well as the revision and editing of this manuscript.
Competing interests JZ is an employee of MSD R&D (China). LL is affiliated with Merck Sharp & Dohme, a subsidiary of Merck & Co., Rahway, New Jersey, USA, which funded the study and monitored the conduct of the study.
Patient and public involvement Patients and/or the public were not involved in the design, or conduct, or reporting, or dissemination plans of this research.
Provenance and peer review Not commissioned; externally peer reviewed.
Supplemental material This content has been supplied by the author(s). It has not been vetted by BMJ Publishing Group Limited (BMJ) and may not have been peer-reviewed. Any opinions or recommendations discussed are solely those of the author(s) and are not endorsed by BMJ. BMJ disclaims all liability and responsibility arising from any reliance placed on the content. Where the content includes any translated material, BMJ does not warrant the accuracy and reliability of the translations (including but not limited to local regulations, clinical guidelines, terminology, drug names and drug dosages), and is not responsible for any error and/or omissions arising from translation and adaptation or otherwise.
Read the full text or download the PDF:
- Open access
- Published: 06 September 2024
The state of decentralization of the healthcare system and nutrition programs in the Lao People’s Democratic Republic: an organizational study
- Phonevilay Viphonephom 1 ,
- Sengchanh Kounnavong 2 &
- Daniel Reinharz 1
BMC Health Services Research volume 24 , Article number: 1037 ( 2024 ) Cite this article
Metrics details
The Lao People’s Democratic Republic (Lao PDR), a lower-middle income country, has a higher malnutrition rate than other Southeast Asian countries. The decentralization of healthcare is a determinant of the effectiveness of programs to reduce malnutrition, but no study has focused on this factor in this country. This organizational study explores the state of decentralization of the healthcare system in Lao PDR that underlies the nutrition programs in the country.
A qualitative study, which is based on a neo-institutional theory conceptual framework, explored factors related to dominant structure (laws, regulations, resources) and interpretative schemes (dominant ideas and beliefs) that characterize the nutrition services provided in the Lao healthcare system. Twenty-four semistructured interviews were performed with representatives of healthcare institutions involved in nutrition programs at different government levels, external donors and civil society organizations. The interviews were completed with relevant documents. The analysis focused on the convergence of interpretative schemes of the organizations concerned and the coherence between the structure underpinning the nutrition programs and the interpretative schemes.
Services deployed to reduce malnutrition in the Lao PDR remain largely centralized, despite factors specific to the country that led it to promote decentralization of its services. The convergence of interpretive schemes and the coherence between the observed structure and the interpretative schemes of actors at all governance levels ensure the stability of this state of decentralization, which has persisted for almost 50 years.
Nutrition programs in the Laos PDR are largely under the responsibility of the central government. The transformations in the healthcare system, notably with the use of new information technologies and the fact that the provinces are populated by a growing number of professionals trained in nutrition in addition to factors that push the system to be decentralized, such as ethnic diversity, the increasing availability of human resources in provinces, and the use of communication technologies, are not strong enough to change the balance of power between governance levels. The deconcentration that characterizes decentralization is therefore likely to continue for the foreseeable future.
Peer Review reports
The World Health Organization (WHO) defines malnutrition as deficiencies, excesses, or imbalances in a person’s intake of energy and/or nutrients, encompassing three broad groups of conditions: undernutrition (including wasting, stunting, and underweight); micronutrient-related malnutrition (including deficiencies or excesses of essential vitamins and minerals); and overweight, obesity, and diet-related noncommunicable diseases [ 1 ]. Undernutrition and micronutrient-related malnutrition are the main issues generally addressed by nutrition interventions aimed at five-year-old children in developing countries [ 2 , 3 ]. Children under 5 in lower-middle-income countries are particularly vulnerable to undernutrition and its lifelong effects [ 4 , 5 ], which manifest as wasting (acute undernutrition or severe weight loss due to insufficient food intake and/or infectious disease), stunting (chronic undernutrition leading to low height-for-age and irreversible long-term physical and cognitive damage), and underweight (low weight-for-age, potentially indicating stunting, wasting, or both) [ 5 ]. The WHO highlights that nearly half of deaths among children under 5 years of age are linked to undernutrition. These deaths predominantly occur in low-and middle-income countries [ 1 ].
Undernutrition among children under five years of age is a public health priority in the Lao PDR [ 6 ]. The prevalence of stunting, an indicator often used to estimate the prevalence of chronic malnutrition, is among the highest in East Asia [ 7 ], with a national rate estimated at 31.5% in 2022 [ 8 ]. This rate surpasses those in neighboring lower-middle-income countries, such as Vietnam (20%), Cambodia (22%), and Myanmar (27%) [ 9 ]. According to the WHO standards, stunting is considered critical if it exceeds the threshold of 30% [ 5 ]. Acute malnutrition, estimated by the prevalence of wasting, is still a public health problem among children under five years of age in Lao PDR. The prevalence of wasting is 10%, which is the same as that in Cambodia but higher than that in Myanmar (7%) and Vietnam (5%) [ 9 ]. Furthermore, the problem of malnutrition is unevenly distributed across the territory of Lao PDR. Rural and ethnic minority children are at greater risk of stunting than are urban and majority ethnic groups [ 10 ]. There was a 15% lower risk of stunting for urban children than for their rural counterparts (24% vs. 39%). In Phongsaly Province, the stunting prevalence is as high as 54%, in contrast to the 14% reported in the Vientiane Capital. Additionally, the prevalence of wasting was the lowest in the province of Luangnamtha (3%) and highest in the province of Xayabury (19%) [ 11 ].
The state of healthcare system decentralization has been shown to be a determinant of nutritional status in developing countries [ 12 , 13 ]. Decentralization involves transferring power and responsibility from the central government to lower levels of governance, impacting healthcare service accessibility, quality, and equity [ 14 , 15 , 16 , 17 ]. Decentralization is commonly categorized into three major forms: 1) Deconcentration involves the central government handing over some authority to local administrative offices within the health ministry, allowing local management to handle health-related activities with some discretion; 2) Delegation entails the central government transferring defined managerial and administrative functions to institutions outside the central government's hierarchical structure, which are indirectly controlled by the health ministry; and 3) Devolution refers to the central government legally transferring power to locally elected political organs that operate independently of the central government in specific functions [ 15 ].
Lao PDR has a deconcentration form of decentralization [ 18 , 19 ]. While local levels can provide input for program adaptation to local needs, ultimate control remains with the central government, which can veto decisions made by regional or local health offices [ 20 , 21 ]. In Lao PDR, the dynamics and forces influencing the current state of decentralization are shaped by the country's unique historical-political context and distinctive population characteristics. These factors contribute to the complex nature of power sharing across different government levels. The first factor is related to the historical concept of Lao PDR, which is rooted in the notion of " meuang "[ 22 ]. A meuang is a walled city led by a local ruler overseeing surrounding settlements and villages [ 22 , 23 ]. Each meuang maintained its governance, with oversight and protection from the kingdom but without interference in internal affairs. While the nineteenth century shift toward a centralized state model introduced Western administrative practices, the meuang concept remains deeply ingrained in Lao society, lending historical legitimacy to decentralization forces [ 22 , 24 ]. The second factor is the country's ethnic diversity. The country has 49 official minority groups constituting nearly half the population. Minorities often reside in remote areas [ 25 ]. Decentralizing power and assigning responsibilities to local levels is expected to empower authorities to more effectively customize health programs to meet the diverse needs of the population.
These decentralizing forces are counterbalanced by centralizing forces due in great part to the sociocultural context influenced by Confucianist ideology, which emphasizes social harmony and respect for authority and promotes political centralization in East Asia, including Lao PDR [ 26 , 27 , 28 ]. Moreover, the limited human and financial resources of the central government, which still rely heavily on donor funding, push toward the centralization of the healthcare system and program functioning [ 29 , 30 , 31 ]. From an organizational perspective, these factors create a complex balance between decentralization and centralization in the Lao healthcare system.
Little work has been done in Lao PDR on how the state of decentralization affects the functioning of public health programs, despite being an organizational determinant of health. This organizational study examines the status of decentralization of the healthcare system in Lao PDR and its influence on the National Nutrition Policy.
Conceptual framework
The conceptual framework used in this study was based on neo-institutional theory (NIT) [ 32 , 33 ]. NITs view organizations as social structures that must conform to the expectations and pressures of their institutional environment, which includes normative and regulatory forces [ 34 , 35 ]. This approach was considered relevant for this study because decentralization is an institutionalized concept. The form of decentralization that underlies the nutrition policy in the country arises from sociohistorical and legal factors that institutionalize the distribution of powers that govern the functioning of the policy.
Different NIT conceptual frameworks have been proposed. The one used in this study is the one that was proposed by Hinings and Greenwood in 1988 [ 36 ]. It considers the dialectic between the structure, i.e., the laws, rules, and resources that constrain the work performed in an organization, and the interpretive schemes and their meaning-making processes that shape individual and collective behavior that are dominant in a society, i.e., the dominant ideas and beliefs [ 36 , 37 ]. This dialectic approach has been widely used in studies on public policies concerning the nature of institutional structures [ 38 , 39 , 40 , 41 , 42 ]. From these two dimensions, the structural dimension emphasizes the importance of formalizing corporate values and standards in the organization’s standardized policies and procedures, formalizing decision-making processes, and creating hierarchical structures to ensure compliance with institutionalized environmental factors. On the other hand, the interpretive schemes dimension focuses on the importance of shared meanings and beliefs in shaping organizational behaviors [ 32 , 43 ]. This dimension emphasizes that organizations are influenced by both individual and shared beliefs, values, and norms within their institutional environment. These elements shape the organizational actors' understanding and interpretation of what constitutes legitimate and appropriate behaviors [ 35 , 44 , 45 ]. By doing so, organizations can gain legitimacy and ensure their survival in complex and dynamic institutional environments [ 34 , 35 , 45 , 46 ].
Study design
A case study based on an ethnographic design was conducted to examine patterns of social organization and ideational systems that underlie formal/informal structures, as well as the dominant values, behaviors and beliefs of the groups involved in nutrition interventions in Lao PDR [ 47 , 48 , 49 ]. The data were collected from documents and semistructured interviews with representatives of governmental organizations, nongovernmental organizations (NGOs), and civil society organizations (CSOs) involved in nutrition programs in four provinces of Lao PDR. The nutrition programs in this study are those that align with the Lao National Nutrition Plans and Strategies; they encompass interventions and programs from the health and other sectors that are expected to positively influence nutritional indicators. The data were collected between January and June 2022.
Data collection
Interview Guide
An interview guide (see Fig. 1 ) was developed for this study based on the dimensions of the conceptual framework. This interview guide included a pretest with three members of the Lao Tropical and Public Health Institute (Lao TPHI), who has experience in nutrition research in Lao PDR. These individuals were not part of the study's participants. The participants were asked about their current state of malnutrition in Lao PDR and changes over time, as well as insights into power sharing among government levels and external actors. The questions also covered the organization’s experiences and responsibilities in the field of nutrition. Discussions have further explored perspectives on structural elements (policies, regulations, rules) and common beliefs affecting the healthcare system's ability to address nutrition issues.
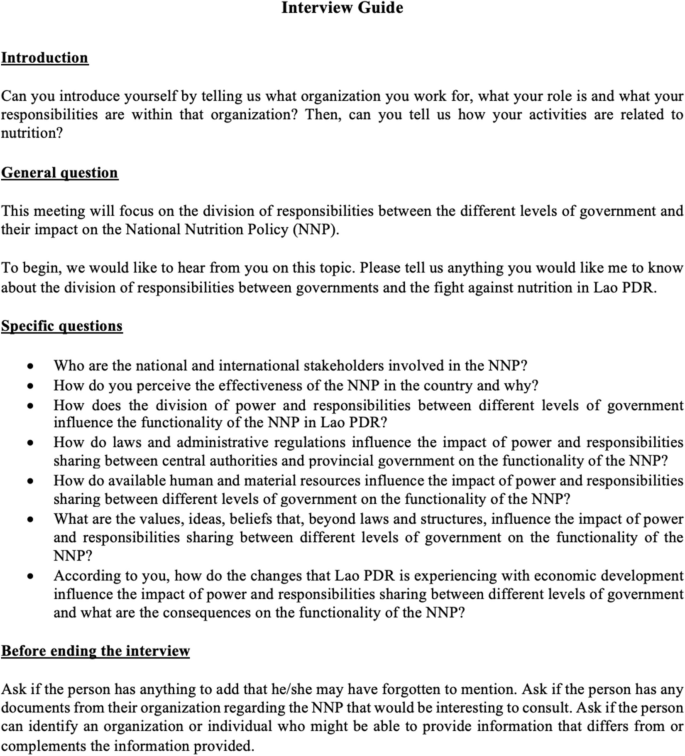
Study sites
To capture the diversity of the country, the latest available national-level data on nutritional status were presented for three provinces with contrasting nutrition situations: Luangnamtha, Xayabury, and Saravan (Table 1 ). Vientiane capital was chosen because of its status as the location of the Ministry of Health (MoH) and national child nutrition-focused institutions (Maternal and Child Health Center and Nutrition Center), as well as the headquarters of development donors and civil society organizations. Luangnamtha, a northern province and one of the most economically developed in the country, has seen remarkable improvements in nutrition indicators. Xayabury, which is also in the north and adjacent to the capital, has experienced little improvement in terms of stunting and underweight rates, with an increase in wasting in recent years. Saravan, in the southern region and one of the poorest provinces of the country, has among the highest prevalence rates of all types of malnutrition, with no significant changes in recent years [ 50 , 51 ].
Source of information
Two sources of information were used for the analysis in this study: interviews with representatives of key institutions and relevant documents [ 52 ].
Representatives of key institutions
The following criteria were used to recruit participants for individual interviews. The participants had to 1) occupy a formal position in relation to nutrition within their organization; 2) be in their current position for at least one year; 3) be authorized to speak on behalf of their organization regarding projects related to nutrition in children under five years of age; and 4) be able to speak one of the languages spoken by the interviewer: Lao (official language), English, or French.
A purposeful sampling approach was used to constitute the sample [ 53 , 54 ]. A preliminary list of participants was created by one of the researchers (SK). At the end of each interview, the participants were asked to suggest additional organizations or individuals who could offer different perspectives. This snowball sampling method aimed to diversify opinions until data saturation was achieved [ 47 , 55 ].
Following administrative protocols, a project summary and discussion topics were initially sent to organization directors for approval. The latter identified individuals within their organization who might have participated in the study. These persons were contacted and asked for their permission to receive project details via fax, WhatsApp, or email. Any queries were addressed through phone calls. If the solicited person agreed, they signed a consent form. The interviews were subsequently scheduled at a mutually convenient time. The participants could choose between face-to-face or online videoconference interviews (Zoom or Google Meet). The interviews, which lasted 45 to 90 min, were audio-recorded with consent, and handwritten notes were taken for documentation and impressions.
To supplement the information provided during the interviews, all documents written in English, Lao, or French on nutrition policies and power sharing between different government levels in Lao PDR were also analyzed. These documents included published academic papers, documents provided by individuals who were interviewed, and documents found on the website of their organization.
Data analysis
All interviews conducted in Lao or French were translated into English by the interviewer and sent to the coauthor who has the least mastery of the Lao language. The realism of the translation was verified by sending a first version of the analyses to three people interviewed, who had to confirm that they found their opinions there. The interviews and document content were analyzed using NVivo 11 software. The interviews and document contents were subsequently analyzed through four main steps: 1) data coding (where data were segmented into meaningful units such as phrases, sentences, or paragraphs); 2) categorization (coded data were then grouped into themes or concepts); 3) coding of themes in connection with the conceptual framework; and 4) comparison of emerging information with published studies on the topic [ 56 ].
The analysis was performed using an inductive-deductive approach [ 57 , 58 ]. The validity of the results was ensured by four elements: 1) credibility based on the triangulation of information provided by multiple sources; 2) transferability through a detailed description of the participants, research process and study context to help the reader judge the relevance of the results for another context [ 59 , 60 ]; 3) reliability based on analyses performed independently by the researcher and one of her supervisors and the search for a consensus between them in case of discrepancy; and 4) confirmability through a detailed and transparent record of the research process, including the data collection, analysis, interpretation and discussion notes from the meetings with coresearchers [ 61 ].
Twenty-four semistructured interviews were conducted with representatives of key organizations: government institutions, United Nations (UN) agencies, international nongovernmental organizations (NGOs), and civil society organizations (CSOs). The participants were primarily women (16 out of 24). The average age was 47 years, with a range from 32 to 61 years. Three participants worked in the healthcare system at the central level, five at the provincial level, five at the district level, and three in health centers (formally known as small hospitals). Three participants worked for UN agencies, and two worked for NGOs. One was a consultant, and two were active members of civil society associations. Twenty-two interviews were conducted in the Lao language (Table 2 ).
Eleven documents were analyzed: 1) Fiscal Decentralization in the People’s Democratic Republic of Lao; 2) Decentralization in Lao PDR: A case study of the Effectiveness of Three Build Directive Policy on Local Authority; 3) the role of Marxism in the Lao political system in the contemporary era; 4) the health system review of Lao PDR in 2014; 5) the Prime Minister’s Decree No. 570 on the organizations and operations of the Ministry of Health in 2021; 6) the National Plan of Action on Nutrition (NPAN) 2021–2025; 7) the National Immunization Programme. Updated Comprehensive Multi-Year Plan Lao PDR 2019–2023; 8) Government spending on health in Lao PDR in 2012; 9) National Nutrition Policy in 2008; 10) National Nutrition strategies to 2025 and Plan of Action 2016–2020; and 11) Operational guideline for the implementation of the National Plan of Action on Nutrition (NPAN) 2021 to 2025 [ 6 , 18 , 19 , 62 , 63 , 64 , 65 , 66 , 67 , 68 , 69 ].
State of decentralization of the Lao healthcare system
Formally, the decentralization of the Lao health system is of the deconcentration type (Fig. 2 ). The Ministry of Health (MoH) oversees the entire healthcare system, which operates across three levels of governance: central, provincial, and district. Provincial and district health authorities’ main responsibility is to ensure that health facilities comply with centrally defined rules [ 6 , 65 ]. Private providers must adhere to centrally defined regulations [ 6 ].
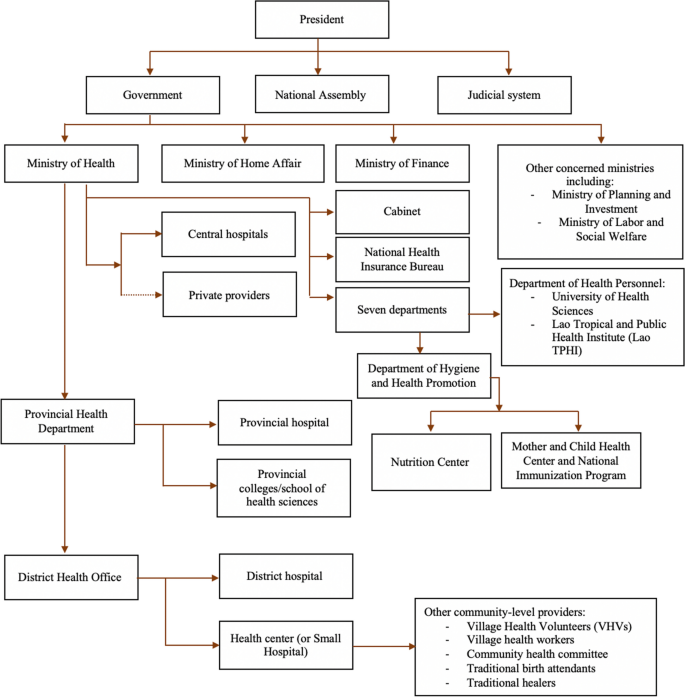
Government-level power sharing in Lao PDR. Sources: Health System Review in Lao 2014; National Plan of Action on Nutrition (NPAN) 2021–2025; National Immunization Programme: Updated Comprehensive Multi-Year Plan Lao PDR 2019–2023; and the Prime Minister’s Decree No. 570 on the organizations and operations of the Ministry of Health in 2021 [ 6 , 63 , 64 , 68 ]
All decisions concerning human and financial resources in the health care sector must be routed through the central level, represented by the MoH and other ministries, notably the Ministry of Finance (MoF), which is responsible for overall fiscal policy and sectoral allocations of the annual recurrent budget. Other ministries are also involved. The Ministry of Planning and Investment (MPI) oversees capital budget allocation, whereas the Ministry of Home Affairs (MHA) manages the total number of civil servants and sectoral allocations of human resource quotas, including the annual recruitment of health personnel at all administrative levels [ 6 , 65 ].
Most respondents (19/24) highlighted the fact that in practice, the power dynamics between levels of governance are complex because constitutional power-sharing arrangements are also under political influence. Official documents support this assertion when they emphasize that in the Lao PDR, the Lao People's Revolutionary Party (LPRP) is the body that has the constitutional mandate to exercise leadership across all levels of government [ 18 , 62 ].
Power sharing between government levels and nutrition programs in Lao PDR
The Lao MoH has the ultimate responsibility for uni- and multisectoral nutrition programs. While the Provincial Health Department (PHD) and District Health Office (DHO) are responsible for implementing and ensuring the functionality of services, the central government retains control over which interventions to offer and over the allocation of human, financial, and material resources [ 66 , 69 ] (Table 3 ).
At the central level, operational leadership in the field of nutrition is formally led by the Multisectoral National Nutrition Committee (MSNNC), which comprises the Ministry of Health, Ministry of Planning and Investment, Ministry of Agriculture and Forestry, Ministry of Education and Sports, and other ministries and mass organizations. The MSNNC defines priorities, assesses local needs, and oversees the implementation of centrally sanctioned interventions at local levels (Table 3 ). Although the responsibility for the MSNNC falls under the MoH, the MSNNC is chaired by the Deputy Prime Minister and deputy chaired by the Deputy Minister of Health. Its members consist of deputy ministers of eight ministries and the vice-director or the secretary of the LPRP’s mass organizations. The MSNNC serves as the central committee overseeing the hierarchical structure of other nutrition committees at the provincial, district, and village levels. The MSNNC structure has been conceived with the idea of having leadership able to promote intersectionality of nutrition interventions [ 6 , 63 , 64 , 66 ].
Yet, under the authority of the Politburo of the LPRP, the Lao national government holds supreme authority, whereas provincial governments possess financial responsibilities and managerial duties. Provincial administration is directly supervised by the central government under the principle of democratic centralism [ 18 , 19 ].
Structure and interpretive schemes underlying the decentralization state of nutrition programs in the Lao PDR
Seven factors related to the structure and interpretative schemes that influence the effectiveness of nutrition programs emerged from the analysis of interviews and documents (Fig. 3 ).
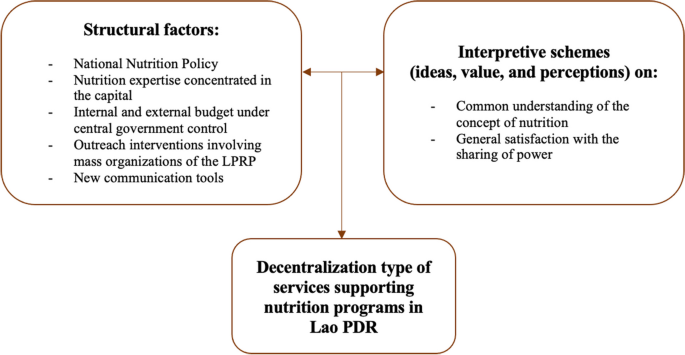
Factors associated with the decentralization type of nutrition programs in Lao PDR
Structural factors
Five main structural factors emerge from the data underlying the decentralization state of nutrition programs in Lao PDR: 1) the existence of a public policy; 2) the availability of human resources; 3) the source of funding; 4) the key role of mass organizations; and 5) communication tools.
A national nutrition public policy
Interventions on malnutrition in Lao PDR are primarily based on a public policy, the National Nutrition Policy (NNP), which was enacted in 2008. This policy provides the legal framework for mobilizing and coordinating actors that can contribute to improving nutrition indicators, regardless of their sector. The policy is operationalized through formal strategic plans developed by the MoH. These official documents define the responsibilities of agencies addressing malnutrition among the different levels of government in the country [ 63 , 66 , 69 ]. All participants agreed that a public policy on nutrition is essential for ensuring intersectoral collaboration at various levels of governance. The policy is seen as a structural foundation that, while centralizing, guarantees the ability of the concerned players to act.
Human resources
The second structuring element that emerges from the data is the fact emphasized by almost all respondents (21/24) and all documents dealing with nutrition [ 6 , 63 , 66 ] that the shortage of expertise outside the capital limits local governments from taking charge of nutrition programs more independently. The respondents also highlighted the fact that a shortage of human resources is compounded by a high turnover rate at all local levels. Turnover weakens the capacity to coordinate the numerous actors involved. Moreover, it drains a considerable part of the local budget, as it leads to continual staff training for nutrition interventions. In brief, all the participants recognized that the state of human resources made it difficult to imagine a program to combat malnutrition run by local authorities.
The health system, from the central to the village levels, is funded from three main sources: a budget allocated by the Ministry of Finance, direct payments at health facilities from users of health services, and funding from external donors, such as NGOs. Funds allocated by the central government are sent to provincial authorities, who then allocate these funds to districts according to planned budgets. These funds are designed for civil servants’ salaries as defined by the central government, as well as for the equipment needs of health establishments. Most of the money paid by service users can be kept by the hospital and used for recurrent costs, including staff wages, allowances, maintenance, utilities, and supplies. The rest (approximately 20%) should be handed over to the provincial or district finance department. Money provided by external donors for projects deployed in provinces and districts must be approved at the central level. One consequence of having multiple sources of financing nutrition activities is the variability in available funds for health services among districts and provinces [ 6 , 18 , 65 ].
The annual budget planning for nutrition interventions involves consultations and reports sent from the district to the provincial levels. The MoH then finalizes and submits it for approval by the National Assembly. In other words, nutrition programs are mainly under central government management. The majority of local-level participants (9/13) feel that this system is not optimal, the main reason being the uncertainty regarding the availability of financial resources over time. These respondents also deplore frequent delays in budget transfers, particularly transfers to remote areas. Above all, nearly all participants at the district and health center levels (6/8) mentioned that nutrition-targeted budgets are often merged with funds intended for non-nutrition public health interventions, as indicated in the following quotation.
“ There’s no other source of money. For the education or agriculture sector, the money would go to each sector separately. For these sectors, they have their own money for nutrition, but we don’t have. So, we need to depend on the integrated work. … Mainly, when we request to the central level, they said they don’t have money for our province anymore; there is only our provincial health office and their help that includes the budget, like they would include the nutrition on the integrated work, and then they manage the staff for it. The structure that divides clearly, it’s not always suitable in real situations ” said by the participant from the provincial level (P11)
The irregular flow of funds is partially offset by the availability of external funding, such as from NGOs [ 63 , 66 ]. However, activities funded by external entities require preapproval from the central government.
The fact that no respondent was able to propose solutions to the problem of funding uncertainty suggests that current funding mechanisms contribute significantly to the solidity of the structure underpinning the decentralization of nutrition programs and hence to the stability of the decentralization type that underlies nutrition programs.
LPRP’s mass organizations
In Lao PDR, the LPRP’s mass organizations, particularly Lao Women’s Union (LWU), are unavoidable collaborators in nearly all programs implemented at the population and community levels that aim to reduce the prevalence of malnutrition. Even if these organizations do not necessarily have expertise in health education which is one of their main contributions, there is a consensus among participants that they have proven to be a valuable substitute for the shortage of professionals in the field. This is expressed in the following quotation:
“…there are the LPRP grassroot members in the meeting (nutrition committee meeting at the district level), who we invited to join us, as well as the standing and district LPRP committee who divides the responsibility for guiding each population group, whoever is responsible for our target group, we would take them to the field, as well as the mass organization, especially the Lao Front for National Development as a main actor to discuss this, second is the Women Union that we can’t forget them, we would take them going to the field with us.” said by the participant from the district level (P17)
This general feeling is also recorded in several official documents [ 6 , 62 , 66 ].
In short, the constant presence of mass organizations whose primary function, in addition to contributing to health education programs, is to increase LPRP values in the population reinforces the power of the central level in nutrition programs deployed in communities.
Communication tools
All the participants underscore the pivotal role of social networks, such as Facebook and WhatsApp, in expediting decision-making processes that previously relied on formal communication channels and informal social gatherings. According to the participants, social media has increased the capacity of nutrition programs to reach their objectives by enabling professionals involved in nutrition programs everywhere to be easily reached and to ask questions without navigating through time consuming and poorly effective bureaucratic procedures. The participants also highlighted that these new technologies inject a sense of dynamism through the competition they foster among teams. This sentiment is captured in the following quotation:
“In the groups, there are staff (DHO staff) and department level (PHD staff) together... If the department (PHD staff) is not in the group, they will send the responsible staff to join the group. So, we always exchange (information) with each other. Whenever the central level (staff) sends messages in the group, everyone always responds. They couldn’t act like they haven’t seen it (messages) because we have the responsibilities for our work, and they also have their responsibilities. If they don’t answer, it means that they haven’t followed up (on the work)” said by the participant from the central level (P01)
There is a consensus that new communication tools have reshaped the power dynamics between central and local levels of governance in Lao PDR. They allow overpassing the traditional hierarchical structure of the healthcare system that is supported by communication channels such as those based on the exchange of information by paper (including faxes). This trend empowers lower levels to directly engage with higher-level actors at the central level, fostering a possible emerging capacity for a more decentralized dynamic inside the field of nutrition programs.
Interpretive schemes
Two main interpretive factors emerged from the data underlying the decentralization state of nutrition programs in Lao PDR: 1) the understanding of the causes and consequences of malnutrition; and 2) the perception of the roles of different levels of government in implementing malnutrition interventions.
Understanding the causes and consequences of malnutrition
The participants across different levels of governance in Lao PDR share a common understanding of the causes and challenges of malnutrition. All the participants recognized that malnutrition is a multifaceted issue requiring attention on both the demand and supply sides. They emphasized the fact that in a resource-limited country such as Lao PDR, factors such as a lack of human and financial resources, combined with contextual challenges in vulnerable communities such as poverty, cultural barriers, and food insecurity must all be considered. Additionally, there is a consensus among participants that addressing malnutrition necessitates multisectoral programs, as programs limited to calories and micronutrients may have a limited impact on improving the condition of malnourished children. Numerous other issues, such as water, sanitation, and hygiene (WASH), gender, and the educational levels of parents, have been found to be key determinants of nutrition. They require significant collaboration from different sectors and stakeholders. In short, there is a broad consensus among stakeholders on interpretive schemes.
Perception of the capacity of the different levels of governance to assume nutrition responsibilities
The majority of participants across various levels of government (10/16) spontaneously declared that interventions to address malnutrition should be led by the central government, citing the fact that the government has expertise and control over the human, financial and material resources necessary for the programs. It is also recognized that district-level health workers, particularly in remote regions, may lack sufficient training and resources. The remaining six participants did not express an opinion on this matter. Although half of the participants at the local level mentioned that regions are increasingly populated by well-trained healthcare professionals, they believe that this trend cannot have a significant impact on the power sharing arrangements for nutrition programs due to the shortage of human resources, especially in remote regions and because local health volunteers and mass organizations largely compensate for human resource needs.
Therefore, there is no question among participants regarding the state of decentralization of services that underpin nutrition programs in the country.
Dialectic between structure and interpretive schemes
In brief, there is coherence between the structure and interpretive schemes that underlie the sharing of responsibilities across governance levels in nutrition programs and interventions in Lao PDR. This coherence is reinforced by the respondents' belief that the desired effects of socioeconomic changes, such as an increase in the number of well-trained nutrition professionals and the use of communication tools empowering regional health workers, cannot sufficiently alleviate human resource needs in the provinces. The system of division of responsibilities between governance levels that has lasted for approximately fifty years is therefore likely to be stable. It is likely to remain so for the foreseeable future.
In Lao PDR, powerful forces are pushing the Lao healthcare system toward more centralization than decentralization, despite factors that support greater autonomy for local authorities.
Every organization concerned with nutrition programs seems to be satisfied with this situation and perceives it as a determinant of the effectiveness of nutrition programs. None of the respondents suggested an alternative approach, despite acknowledging that socioeconomic changes are endowing regions with the capacity to manage public health programs that were previously unimaginable. Therefore, there is convergence of interpretive schemes among concerned actors, as well as coherence between structure and interpretive schemes. This convergence signifies a shared vision of nutrition challenges in the country and the capacity to address them, regardless of organizational concerns or hierarchical levels of governance. The coherence between structure and interpretive schemes reveals a general feeling that the current distribution power among governance levels is the most appropriate way to meet the nutritional needs of the population.
From an organizational perspective seen through a neoinstitutional lens [ 70 ], the main consequence is that the distribution of responsibilities in nutrition services among different government levels has remained stable since the event of the Lao PDR in 1975.
The findings of this study highlight how a country's sociopolitical context influences the state of decentralization and the functioning of its public health programs. In the case of immunization programs in Lao PDR, three notable factors impact their implementation: the Lao sociopolitical background, the availability of resources, and the use of new technologies such as WhatsApp by health professionals. These elements have reshaped the dynamics of the deconcentrated healthcare system and its program operation [ 31 ].
Theoretically, a centralized healthcare system should lead to more standardized public health programs and health services across the country if it ensures consistent application, quality of care, and equitable distribution of resources [ 71 , 72 , 73 ]. However, given the diverse contexts among provinces, a one-size-fits-all approach may not be optimal, especially for complex and multisectoral interventions like nutrition programs. These programs often require adaptation to the specific needs and capacities of local contexts and populations in different communities to be truly effective [ 74 , 75 ].
In a diverse country like Lao PDR, public health interventions require adaptation and more flexible interventions and activities to meet the varied needs of different communities. Empowering local authorities, who are more familiar with local contexts and issues, could improve the management of activities and resources, leading to better interventions that address the specific needs of their populations. In other single-party countries, decentralization has been shown to effectively strengthen public health programs and enhance their effectiveness [ 76 , 77 ].
The documents and interview findings from this study indicate that the formal structure of the healthcare system in Lao PDR is well defined. The organizational structures of the NNP identify the roles and responsibilities of stakeholders from the central level of the MoH to the implementation level of the village. However, in practice, the implemented programs aimed at reducing the prevalence of malnutrition in children under age 5 in the country are far more challenging than the formal structure suggests [ 78 ]. Addressing nutrition outcomes requires a long-term and multifaceted approach, particularly when contributions among actors and power-sharing roles involve local governments working with multiple stakeholders at all levels.
Indeed, Lao PDR faces challenges in reversing the conditions that would allow local governments to put more of their own initiatives into nutrition programs. Unlike in neighboring countries like Vietnam or Thailand, in Lao PDR, the only training on nutrition is a course integrated into a public health master’s program. Additionally, Lao PDR, a country as large as the United Kingdom with a population of 7.7 million inhabitants, is a sparsely populated country. Fifteen of its eighteen provinces have fewer than 500,000 inhabitants. Only one has more than a million [ 79 ]. A small population reduces the likelihood of building a critical mass of people from a given province with expertise in nutrition. Although constraints on qualified human resources are common in many low-resource countries [ 80 , 81 ], these constraints, coupled with the population context in Lao PDR, obviously significantly contribute to the centralization of the Lao healthcare system. In fact, this need for expertise is not limited to nutrition. Optimizing interventions to reduce the prevalence of malnutrition in children under 5 years of age requires tailored approaches that consider the diverse cultural and regional contexts of the population and address multisectoral programs and interventions including food security, water, sanitation, hygiene, maternal education, and social protection. The complexity of these interventions requires multidisciplinary teams [ 63 , 67 , 82 ]. Poorly populated provinces might not be able to gather all this desired expertise. Finally, Lao PDR receives funding for its nutrition program from several external donors and NGOs. This necessitates central-level coordination that might be even more crucial once the country has left, supposedly in 2026, the group of the least developed countries [ 83 ]. Leaving this group will have consequences for the support provided by external actors. Expectations are that the central government will assume more responsibilities for its nutrition programs in the near future.
Finally, advancements in communication technologies and the arrival of better-trained human resources offer hope for addressing the shortage of quality human resources that hinder local authorities' leadership in nutrition programs. An increasing number of graduated individuals living in the provinces are proficient in the use of new technologies [ 84 ]. This increased utilization of technology is anticipated to further transform traditional barriers that govern interactions among stakeholders. Additionally, interpretive patterns are likely to evolve as professionals in provinces become better informed and more willing to customize nutrition programs to meet the specific needs of local populations. However, owing resource shortages and uneven population distribution in Lao PDR, there are unequal opportunities for local levels to assume more responsibility for nutrition interventions, with little to no chance for decision-making at the local level. This is expected to limit the capacity of sub-national levels from contributing more substantially to nutrition programs than they currently do.
None of the respondents evoked a future where nutrition programs would be more decentralized. Nevertheless, they acknowledged that the current state of decentralization is suboptimal. They recognized that one of the undesirable effects of centralization is the difficulty in adapting services to the specific needs of diverse populations.
Limitations
This study has several limitations. First, respondents were selected by their organization directors following administrative procedures mandated by research projects in Lao PDR. While this method has likely allowed to capture a diverse range of perspectives on malnutrition, it may have excluded individuals who disagreed with the official discourse. Although the two coders felt that additional interviews would not yield new information, it is possible that additional insights might have been generated by more interviews if soliciting directly potential participants directly could have been done.
Second, as is common in qualitative studies, unconscious subjectivity and bias among researchers may have influenced some interpretations. However, the risk is mitigated by ensuring the validity of four elements of the results, particularly through the triangulation of information from interviews and document reviews, as well as through independent analyses conducted by two researchers.
Finally, the generalizability of the results may be limited. The Lao context is unique, and nutrition programs have specificities that may differ from those of other national health programs functioning in the country. Consequently, the findings of this study may not be directly applicable to other programs or countries, including those with single-party governance structures.
Decentralization of the Lao healthcare system is of the deconcentrated type, i.e. a system largely under the responsibility of the central government. The transformations taking place in the healthcare system, notably with the use of new information technologies, are changing relationships between individuals across different levels of government and the fact that the provinces are populated by a growing number of professionals trained in nutrition. These factors could lead people to believe that the system would give more responsibility to provincial governments to improve their malnutrition status at local levels. However, the data show that these changes are probably not yet strong enough to change a structure that has endured for approximately fifty years. The deconcentration that characterizes decentralization is therefore likely to continue for the foreseeable future.
Availability of data and materials
The datasets during and/or analyzed during the current study are available from the corresponding author upon reasonable request.
Abbreviations
Civil society organizations
District health offices
Lao People’s Democratic Republic
Lao People's Revolutionary Party
Lao Women's Union
Ministry of Home Affairs
Multi-sectorial National Nutrition Committee
Ministry of finance
Ministry of health
Ministry of planning and investment
Nongovernmental organizations
National Nutrition Policy
National Nutrition Strategy and Plan of Action
National Plan of Action on Nutrition
- Neo-institutional Theory
Provincial health departments
United Nations
Village health volunteers
World Health Organization
World Health Organization. Malnutrition 2024. Available from: https://www.who.int/news-room/fact-sheets/detail/malnutrition .
Caulfield LE, Richard SA, Rivera JA, Musgrove P, Black RE. Stunting, wasting, and micronutrient deficiency disorders. In: Disease Control Priorities in Developing Countries 2nd edition. The International Bank for Reconstruction and Development / The World Bank. New York: Oxford University Press; 2006.
Google Scholar
Muller O, Krawinkel M. Malnutrition and health in developing countries. CMAJ. 2005;173(3):279–86.
Article PubMed PubMed Central Google Scholar
UNICEF. Child Malnutrition 2023. Available from: https://data.unicef.org/topic/nutrition/malnutrition/ .
World Health Organization, United Nations Children's Fund (UNICEF) & World Bank. Levels and trends in child malnutrition: UNICEF / WHO / The World Bank Group joint child malnutrition estimates: key findings of the 2021 edition. World Health Organization. 2021. https://iris.who.int/handle/10665/341135 .
World Health Organization. The Lao People’s Democratic Republic: Health System Review. Asia Pacific Observatory on Health Systems and Policies; 2014. Contract No.: 1.
World Bank. Lao PDR: New Financing to Improve Access to Health and Nutrition Services. 2020.
Save the Children, CARE, EU, CCL, ChildFund. Partnership for Improved Nutrition in Lao PDR Pillar 3: Sustainable Change Achieved through Linking Improved Nutrition and Governance. Vientiane: Lao Social Research; 2022.
UNICEF/WHO/World Bank Joint Child Malnutrition Estimates Expanded Database. Malnutrition data 2023. Available from: https://data.unicef.org/resources/dataset/malnutrition-data/ .
Ministry of Health, World Health Organization. Overview of Lao health system development 2009–2017. Manila, Philippines: World Health Organization Regional Office for the Western Pacific; 2018. Report No.: Licence: CC BY-NC-SA 3.0 IGO.
UNICEF/WHO/World Bank Joint Child Malnutrition Estimates Expanded Database. Malnutrition data 2022. Available from: https://data.unicef.org/resources/dataset/malnutrition-data/ .
Constantinides SV. Determinants of Nutrition Agenda Setting in the Context of the Double Burden of Malnutrition in Tamil Nadu, India. South Carolina: University of South Carolina; 2020.
Acosta AM, Fanzo J. Sussex: Institute of Development Studies; 2012.
Boko SH. Decentralization_Definitions, theories and debates. Decentralization and Reform in Africa. Boston: Sringer; 2002. p. 1–10.
Book Google Scholar
Frumence G, Nyamhanga T, Mwangu M, Hurtig AK. Challenges to the implementation of health sector decentralization in Tanzania: experiences from Kongwa district council. Glob Health Action. 2013;6:20983.
Article PubMed Google Scholar
Mosca I. Decentralization as a determinant of health care expenditure: empirical analysis for OECD countries. Appl Econ Lett. 2007;14(7):511–5.
Article Google Scholar
Saltman RB, Bankauskaite V, Vrangbaek K. Decentralization in Health Care. Maidenhead: Mcgraw-Hill House; 2007.
Martinez-Vazquez J, Gomez JL, Yao G. Fiscal Decentralization in the People’s Democratic Republic of Lao. Georgia, United States: Georgia State University; 2006. Contract No.: Working Paper 06–22.
Luangrath S. Decentralization in Lao PDR: A Case Study of the Effectiveness of Three Build Directive Policy on Local Authority. Seoul, Korea: Seoul National University; 2019.
Litvack J, Seddon J. Decentralization briefly notes. Washington, DC: World Bank Institute; 1999.
Hutchinson PL, LaFond AK. Monitoring and Evaluation of Decentralization Reforms in Developing Country Health Sectors. Maryland, United States: Abt Associates Inc.; 2004.
Stuart-Fox M. Politics and Reform in the Lao People’s Democratic Republic. Working Paper No. 126, Asia Research Center, Murdoch, University. 2005.
Pholsena V, Banomyong R. Laos: From buffer state to crossroads? Chiang Mai, Thailand: Mekong Press; 2006.
Stuart-Fox M. Political Culture and Power in the Lao People’s Democratic Republic. J Asiatic Stud. 2009;138:222–54.
United Nations in Lao PDR. Country Analysis Report: Lao PDR: Analysis to inform the Lao People’s Democratic Republic-United Nations Partnership Framework (2017–2021). Vientiane: United Nations; 2015.
Nawrot KA. Does Confucianism promote cooperation and integration in East Asia? Int Commun Chin Culture. 2020;7(1):1–30.
Jiang Y-H. Confucian political theory in contemporary China. Annu Rev Polit Sci. 2018;21:155–73.
Kim Y, Lee H-K, Park S. The Confucian Tradition and Politics. Oxford Research Encyclopedia of Politics. 2019.
Dickovick JT. Foreign aid and decentralization: Policies for autonomy and programming for responsiveness. Helsinki: The United Nations University World Institute for Development Economics Research (UNU-WIDER); 2013.
Dickovick JT. Foreign aid and decentralization: limitations on impact in autonomy and responsiveness. Public Admin Dev. 2014;34(3):194–206.
Viphonephom P, Kounnavong S, Reinharz D. Decentralization and immunization program in a single-party state: the case of the Lao People’s Democratic Republic. Trop Med Health. 2024;52(1):35.
Meyer JW, Rowan B. Institutionalized organizations: formal structure as myth and ceremony. Am J Sociol. 1977;83(2):340–63.
DiMaggio PJ, Powell WW. The iron cage revisited: institutional isomorphism and collective rationality in organizational fields. Am Sociol Rev. 1983;48(2):147–60.
Marschlich S. Conceptual Framework: Sociological Neo-Institutionalism, Legitimacy, and Public Relations. Corporate Diplomacy: How Multinational Corporations Gain Organizational Legitimacy: A Neo-Institutional Public Relations Perspective. Amsterdam: University of Amsterdam; 2022.
Swaminathan A, Wade JB. Institutional Environment. The Palgrave Encyclopedia of Strategic Management. London: Palgrave Macmillan; 2016. p. 1–7.
Hinings CR, Royston G. The Dynamics of Strategic Change. Oxford: Basil Blackwell Ltd.; 1988.
Hinings CR, Greenwood R, Reay T, Suddaby R. Dynamics of change in Organizational field. Handbook of Organizational Change and Innovation: Oxford University Press; 2003.
Harimanana A, Barennes H, Reinharz D. Organizational analysis of maternal mortality reduction programs in Madagascar. Int J Health Plann Manage. 2011;26(3):e186–96.
Peyrot M. Institutional and organizational dynamics in community-based drug abuse treatment. Soc Probl. 1991;38(1):20–33.
Morrill C, Yalda C, Adelman M, Musheno M, Bejarano C. Telling tales in school- youth culture and conflict narratives. Law Soc Rev. 2000;34(3):521–65.
Addicott R, Furlie E. Sedimented archetype change in public sector organisations: the example of managed clinical networks for cancer. CQUniversity. Conference contribution. 2006. https://hdl.handle.net/10018/8418 .
Breunig KJ, Skjølsvik T. The virtual future of professional service firms: Identifying emerging interpretive schemes caused by technology enactment. Acad Manag Proc. 2016. https://hdl.handle.net/10642/4407 .
Greenwood R, Hinings CR. Understanding radical organizational change: Bringing together the old and the new institutionalism. Acad Manag Rev. 1996;21(4):1022–54.
Senge K. The ‘New Institutionalism’ in organization theory: Bringing society and culture back in. Am Sociol. 2013;44(1):76–95.
Scott WR. Institutions and organizations : ideas, interests and identities. 4th ed. Thousand Oaks: SAGE Publications, Inc.; 2013.
Rouleau L. Théories des organisations : Approches calssiques, contemporaines et de l’avant-garde. Quebec, Canada: Presse de Université du Québec; 2007.
Creswell JW. Research Design: Qualitative, Quantitative, and Mixed Methods Approaches. London, United Kingdom: SAGE Publications Inc; 2013.
Huby G, Hart E, McKevitt C, Sobo E. Addressing the complexity of health care: The practical potential of ethnography. J Health Serv Res Policy. 2007;12(4):193–4.
Kielmann K. The ethnographic lens. In: Gilson L, editor. Health policy and systems research: A methodology reader. Geneva, Switzerland: World Health Organization; 2012. p. 235–52.
Lao Statistic Bureau. Lao PDR: Lao Social Indicator Survey 2011–2012 (Multiple Indicator Cluster Survey/Demographic and Health Survey). Vientiane: Lao Statistic Bureau and UNICEF; 2012.
Lao Statistic Bureau. Lao Social Indicator Survey II 2017. Vientiane: Lao Statistic Bureau and UNICEF; 2018.
Pope C. Qualitative Research in Health Care. Hoboken: Wiley-Blackwell; 2020. p. 2020.
Creswell JW, Plano Clark VL. Designing and Conducting Mixed Methods Research. 2nd ed. Los Angeles: Sage Publications; 2011.
Palinkas AP, Horwitz SM, Green CA, Wisdom JP, Duan N, Hoagwood K. Purposeful sampling for qualitative data collection and analysis in mixed method implementation research. Adm Policy Ment Health. 2015;42(5):533–44.
Naderifar M, Goli H, Ghaljaie F. Snowball sampling: a purposeful method of sampling in qualitative research. Strides Dev Med Educ. 2017;14(3):1–4.
Fortin M-F, Gagnon J. Fondements et étapes du processus de recherche: Méthodes quantitatives et qualitatives. 3rd ed. Montreal: Chenelière éducation; 2016.
Park D, Bahrudin FI, Han J. Circular reasoning for the evolution of research through a strategic construction of research methodologies. Int J Quant Qual Res Methods. 2020;8(3):1–23.
CAS Google Scholar
Proudfoot K. Inductive/Deductive Hybrid Thematic Analysis in Mixed Methods Research. J Mixed Methods Res. 2022;0(0):1–19.
Ponterotto JG. Brief note on the origins, evolution, and meaning of the qualitative research concept “Thick Description.” Qual Rep. 2006;11(3):538–49.
Kharel D. VIsual enthnography, thick description and cultural representation. Dhaulagiri J Sociol Anthropol. 2015;9:147–60.
Korstjens I, Moser A. Series: Practical guidance to qualitative research. Part 4: Trustworthiness and publishing. Eur J Gen Pract. 2018;24(1):120–4.
Dahuai Y, Phoutthaphone S, Jing W. The role of Marxism in the Lao political system in the contemporary era. Int J Soc Sci Human Invent. 2017;4(12):4216–26.
Ministry of Health. National Plan of Action on Nutrition (NPAN) 2021–2025. Vientiane: Ministry of Health; 2021.
Lao PDR National Immunization Programme. Updated Comprehensive Multi-Year Plan Lao PDR 2019–2023. Vientiane: Ministry of Health, GAVI, UNICEF/WHO; 2018.
World Bank. Government spending on health in Lao PDR: Evidence and issues. Vientiane: World Bank; 2012.
Ministry of Health. National Nutrition strategies to 2025 and Plan of Action 2016–2020. Vientiane: Ministry of Health; 2015.
Secretariat National Nutrition Committee. Operational guideline for the implementation of the National Plan of Action on Nutrition (NPAN) 2021 to 2025. Vientiane: National Nutrition Committee Secretariat; 2023.
Bureau PM. Prime Minister’s Decree No. 570 on the organization and activities of the Ministry of Health. Vientiane; 2021.
Ministry of Health. National Nutrition Policy. Vientiane: Ministry of Health; 2008.
Greenwood R, Hinings CRB. Radical organizational change. SAGE Publications Ltd. 2006.
Ostermann M, Vincent J-L. How much centralization of critical care services in the era of telemedicine? Crit Care. 2019;23(1):423.
Kobayashi D, Otsubo T, Imanaka Y. The effect of centralization of health care services on travel time and its equality. Health Policy. 2015;119(3):298–306.
Elson S. Regionalization of health care from a political and structural perspective. Healthc Manage Forum. 2009;22(1):6–11. https://doi.org/10.1016/S0840-4704(10)60279-7 .
Sanders D, Chopra M. Implementing comprehensive and decentralised health systems: the quest for integrated care in post-apartheid South Africa. Int J Integr Care. 2001;1:e33.
Kesale AM, Mahonge C, Muhanga M. Effects of decentralization on the functionality of health facility governing committees in lower and middle-income countries: a systematic literature review. Glob Health Action. 2022;15(1):2074662.
Zhu J, Ge Z, Xia J, Liu Q, Ran Q, Yang Y. Status quo and problem analysis of cervical cancer screening program in China: Based on RE-AIM framework. Front Public Health. 2022;10:987787. https://doi.org/10.3389/fpubh.2022.987787 .
Huang X, Yang B, Liu Q, Zhang R, Tang S, Story M. Improving maternal and child nutrition in China: an analysis of nutrition policies and programs initiated during the 2000–2015 Millennium Development Goals era and implications for achieving the Sustainable Development Goals. J Health Popul Nutr. 2020;39(1):12.
Sengchaleun V, Samri MA, Kounnavong S, Reinharz D. Advocacy Coalition by External Actors and Strategies Used to Influence the Emergence of the National Nutrition Policy in Lao PDR. Health. 2021;13(08):812–29.
World Health Organization. World Health Organization Data 2020. Available from: https://data.who.int/countries .
Delisle H, Shrimpton R, Blaney S, Du Plessis L, Atwood S, Sanders D, et al. Capacity-building for a strong public health nutrition workforce in low-resource countries. Bull World Health Organanization. 2017;95(5):385–8.
Diasso D, Doudou MH, Levrak MC, Sedutto HD, Savadogo A. Municipalities’ organisational capacity to support the implementation of the Multi-Sector Nutrition Plan in Burkina Faso. Glob Health Action. 2021;14(1):1979279.
European Union, UNICEF. Review of costing of the National Plan of Action for Nutrition 2016–2020. Vientiane: European Union and UNICEF; 2020.
Ministry of Foreign Affairs. Lao PDR is recommended to graduate in 2026 from the Least Developed Country Category with an extended preparatory period. Vientiane, Lao PDR: Ministry of Foreign Affairs 2021. Available from: http://www.mofa.gov.la/index.php/activities/state-leaders/3999-lao-pdr-is-recommended-to-graduate-in-2026-from-the-least-developed-country-category-with-an-extended-preparatory-period .
World Bank. Positioning The Lao PDR for a Digital Future. Vientiane: World Bank; 2021.
Download references
Acknowledgements
We would like to express our sincere thanks to all the participants who generously shared their valuable experiences with us. We would also like to express our thanks to the organizations that assisted us in participant recruitment. The first author P.V. extends her special thanks to the Canadian Francophonie Scholarship Program (CFSP) for their support during her doctoral studies.
This research was supported by the Canadian Francophonie Scholarship Program, under the Canadian Bureau for International Education, Government of Canada.
Author information
Authors and affiliations.
Department of Social and Preventive Medicine, Laval University, Quebec City, QC, Canada
Phonevilay Viphonephom & Daniel Reinharz
Lao Tropical and Public Health Institute (Lao TPHI, Ministry of Health, Vientiane, Lao PDR
Sengchanh Kounnavong
You can also search for this author in PubMed Google Scholar
Contributions
PV: conceptualization and design; acquisition of data; analysis and interpretation of data; writing original draft; and writing review and editing of the manuscript. SK: recruitment of participants; writing review and editing the manuscript; and supervision. DR: conceptualization and design; analysis and interpretation of data; writing review and editing of the manuscript and supervision. All authors have read and approved the final manuscript.
Corresponding author
Correspondence to Phonevilay Viphonephom .
Ethics declarations
Ethics approval and consent to participate.
All methods were carried out in accordance with relevant guidelines and regulations. Written consent was obtained from all participants before data collection. Ethical approval for the study was obtained from the Research Ethics Board of Laval University, Quebec, Canada (number “2021–259/23–09-2021”) and the National Ethics Committee for Health Research, Ministry of Health, Vientiane, Lao PDR (number “08/NECHR”, 14/01/2022).
Consent for publication
Not applicable.
Competing interests
The authors declare no competing interests.
Additional information
Publisher’s note.
Springer Nature remains neutral with regard to jurisdictional claims in published maps and institutional affiliations.
Rights and permissions
Open Access This article is licensed under a Creative Commons Attribution 4.0 International License, which permits use, sharing, adaptation, distribution and reproduction in any medium or format, as long as you give appropriate credit to the original author(s) and the source, provide a link to the Creative Commons licence, and indicate if changes were made. The images or other third party material in this article are included in the article's Creative Commons licence, unless indicated otherwise in a credit line to the material. If material is not included in the article's Creative Commons licence and your intended use is not permitted by statutory regulation or exceeds the permitted use, you will need to obtain permission directly from the copyright holder. To view a copy of this licence, visit http://creativecommons.org/licenses/by/4.0/ .
Reprints and permissions
About this article
Cite this article.
Viphonephom, P., Kounnavong, S. & Reinharz, D. The state of decentralization of the healthcare system and nutrition programs in the Lao People’s Democratic Republic: an organizational study. BMC Health Serv Res 24 , 1037 (2024). https://doi.org/10.1186/s12913-024-11513-y
Download citation
Received : 04 October 2023
Accepted : 29 August 2024
Published : 06 September 2024
DOI : https://doi.org/10.1186/s12913-024-11513-y
Share this article
Anyone you share the following link with will be able to read this content:
Sorry, a shareable link is not currently available for this article.
Provided by the Springer Nature SharedIt content-sharing initiative
- Decentralization
- Single-party state
- Lower-middle income country
BMC Health Services Research
ISSN: 1472-6963
- General enquiries: [email protected]

IMAGES
VIDEO
COMMENTS
Frameworks for Qualitative Research ... titative researchers who use a critical or interpretive framework.) 6. Report your workin an objective manner. Typically the form of this report is a journal article, but larger studies may also be published as a monograph or book. Zigler and Muenchow's (1992) book on the impact of
Situating Philosophy and Interpretive Frameworks Within the Research Process. An understanding of the philosophical assumptions behind qualitative research begins with assessing where it fits within the overall process of research, noting its importance . as an element of research, and considering how to actively write it into a study. To help ...
Developing the Organizing Conceptual Framework for the Research ("Domains") 31 Piloting the Data Collection Protocol 35 Sampling, Selecting, and Recruiting Participants 35 ... interpretive qualitative research is particularly rich in analyzing data at both the descriptive (surface) and interpretive (deeper) levels and telling a ...
Hence, the main objective of this article is to highlight philosophical and methodological considerations of leading an interpretive phenomenological study with respect to the qualitative research paradigm, researcher's stance, objectives and research questions, sampling and recruitment, data collection, and data analysis.
Foundations of Qualitative Research: Interpretive and Critical Approaches. Provides a step-by-step guide for doing a real evaluation. It focuses on the main kinds of "big picture" questions that evaluators usually need to answer, and how the nature of such questions is linked to evaluation methodology choices.
The framework includes attention to the key qualitative research concepts transparency, reflexivity, analysis, validity, evidence, and literature. Four questions frame the chapter: What is interpretation, and why are interpretive strategies important in qualitative research?
One of these objectives is "a basic understanding of the philosophical assumptions that form the foundation of interpretive inquiry offers the interpretive researcher a conceptual basis for selecting research methods, thus promoting methodologically sound research design." ... has been used as a framework for qualitative research (Donmoyer ...
The source, size, and power of those explanations vary, but they all link back to an attempt to understand some phenomena. As Anfara and Mertz (2015) have described, scholars have varied perspectives about the use of theoretical frameworks in qualitative research. The following article endeavors to summarize and present variations in usage and ...
Qualitative research projects are informed by a wide range of methodologies and theoretical frameworks. The SAGE Encyclopedia of Qualitative Research Methods presents current and complete information as well as ready-to-use techniques, facts, and examples from the field of qualitative research in a very accessible style. In taking an ...
Qualitative Research: Interpretive Paradigms. All qualitative researchers follow highly abstract principles which bring together beliefs about ontology, epistemology, and methodology. ... to assess the rationale of a study, its conceptual framework and research question(s), quality criteria must take account of the following: lucid context for ...
While many books and articles guide various qualitative research methods and analyses, there is currently no concise resource that explains and differentiates among the most common qualitative approaches. We believe novice qualitative researchers, students planning the design of a qualitative study or taking an introductory qualitative research course, and faculty teaching such courses can ...
Interpretive Frameworks T he research design process in qualitative research begins with philo-sophical assumptions that the inquirers make in deciding to undertake a qualitative study. In addition, researchers bring their own worldviews, par-adigms, or sets of beliefs to the research project, and these inform the con-
Within this paradigm, interpretive researchers recognize the importance of integrating their subjective beliefs into the inquiry process to unravel and share the meanings inherent in qualitative ...
be used and written into qualitative research, and then link them to different interpretive frameworks that operate at a more specific level in the process of research (see Table 2.2). The ontological issue relates to the nature of reality and its characteristics. When researchers conduct qualitative research, they are embracing the idea of ...
Introduction. Research in Political Science is increasingly based on qualitative and interpretative methods. Document analyses, discourse analyses or ethnographic studies have become more and more common (Halperin and Heath Citation 2020; Silverman Citation 2021).However, the application of these methods confronts researchers with a number of principled questions and challenges that concern ...
Phase 1: The Researcher as a Multifaceted Subject. Phase 2: Philosophical Assumptions and Interpretive Frameworks. Phase 3: Research Strategies and Approaches. Phase 4: Methods of Data Collection and Analysis. Phase 5: The Art, Practice, and Politics of Interpretation and Evaluation.
The brief, practical texts in the Essentials of Qualitative Methods series introduce social science and psychology researchers to key approaches to capturing phenomena not easily measured quantitatively, offering exciting, nimble opportunities to gather in-depth qualitative data. This book offers a no-nonsense, step-by-step approach to ...
Robert Elliott and Ladislav Timulak. Qualitative research methods today are a diverse set, encompassing approaches such as empirical phenomenology, grounded theory, ethnography, protocol analysis and dis-course analysis. By one common definition (Polkinghorne, 1983), all these methods rely on linguistic rather than numerical data, and employ ...
The framework includes attention to the key qualitative research concepts transparency, reflexivity, analysis, validity, evidence, and literature. Four questions frame the article: What is interpretation, and why are interpretive strategies important in qualitative research?
Covers the conceptual foundations of interpretive, critical, and post-positivist paradigms: A thorough background of theory and social inquiry is given by looking at the development of each paradigm throughout history. Provides real-world examples: Cases illustrate different approaches to the same research problem so that students can better understand the contrasting features of these paradigms.
Qualitative research questions often ask about lived experience, personal experience, understanding, meaning, and stories. Qualitative research questions may be more general and less specific. Qualitative research questions may also contain only one variable, rather than asking about relationships between multiple variables.
The term 'interpretive research' is often used loosely and synonymously with 'qualitative research', although the two concepts are quite different. Interpretive research is a research paradigm (see Chapter 3) that is based on the assumption that social reality is not singular or objective. Rather, it is shaped by human experiences and ...
2. Philosophical Assumptions and distribute Interpretive Frameworks or. hether we are aware of it or not, we always bring certain beliefs and philosophical assumptions to our research. Sometimes these are deeply Wingrained views about the types ofpost, problems that we need to study, what research questions to ask, or how we go about gathering ...
The objective of this study was to utilise the existential framework as conceptualised by Irvin Yalom to generate a deeper understanding of the underlying factors contributing to the struggles that veterans experience in their return to society. A qualitative interpretive meta-synthesis (QIMS) approach was used to hear the combined voices of a ...
The Epistemic Competence of the Researcher is a critical success factor for ethical, rigorous, and creative research performance, but it requires a deep epistemological and methodological mastery, however, the current scientific literature has not yet achieved a conceptual arrangement, that allows researchers and educators to have a comprehensive theoretical framework for a holistic ...
Co-design with consumers and healthcare professionals is widely used in applied health research. While this approach appears to be ethically the right thing to do, a rigorous evaluation of its process and impact is frequently missing. Evaluation of research co-design is important to identify areas of improvement in the methods and processes, as well as to determine whether research co-design ...
Objectives To identify the barriers and facilitators to the successful implementation of imaging-based diagnostic artificial intelligence (AI)-assisted decision-making software in China, using the updated Consolidated Framework for Implementation Research (CFIR) as a theoretical basis to develop strategies that promote effective implementation. Design This qualitative study involved ...
On the one hand, a framework may provide a sense of the story as it emerges out of the analyses, leading down paths of inquiry that may otherwise be missed (Sandelowski, 1993).In this manner, a question regarding the phenomenon becomes a gateway to the investigation, the theoretical framework suggests an orientation, and rigorous data analysis yields findings (Corbin & Strauss, 2012; Miles et ...
Conceptual framework. The conceptual framework used in this study was based on neo-institutional theory (NIT) [32, 33].NITs view organizations as social structures that must conform to the expectations and pressures of their institutional environment, which includes normative and regulatory forces [34, 35].This approach was considered relevant for this study because decentralization is an ...