Have a language expert improve your writing
Run a free plagiarism check in 10 minutes, generate accurate citations for free.
- Knowledge Base
Methodology
- The 4 Types of Validity in Research | Definitions & Examples

The 4 Types of Validity in Research | Definitions & Examples
Published on September 6, 2019 by Fiona Middleton . Revised on June 22, 2023.
Validity tells you how accurately a method measures something. If a method measures what it claims to measure, and the results closely correspond to real-world values, then it can be considered valid. There are four main types of validity:
- Construct validity : Does the test measure the concept that it’s intended to measure?
- Content validity : Is the test fully representative of what it aims to measure?
- Face validity : Does the content of the test appear to be suitable to its aims?
- Criterion validity : Do the results accurately measure the concrete outcome they are designed to measure?
In quantitative research , you have to consider the reliability and validity of your methods and measurements.
Note that this article deals with types of test validity, which determine the accuracy of the actual components of a measure. If you are doing experimental research, you also need to consider internal and external validity , which deal with the experimental design and the generalizability of results.
Table of contents
Construct validity, content validity, face validity, criterion validity, other interesting articles, frequently asked questions about types of validity.
Construct validity evaluates whether a measurement tool really represents the thing we are interested in measuring. It’s central to establishing the overall validity of a method.
What is a construct?
A construct refers to a concept or characteristic that can’t be directly observed, but can be measured by observing other indicators that are associated with it.
Constructs can be characteristics of individuals, such as intelligence, obesity, job satisfaction, or depression; they can also be broader concepts applied to organizations or social groups, such as gender equality, corporate social responsibility, or freedom of speech.
There is no objective, observable entity called “depression” that we can measure directly. But based on existing psychological research and theory, we can measure depression based on a collection of symptoms and indicators, such as low self-confidence and low energy levels.
What is construct validity?
Construct validity is about ensuring that the method of measurement matches the construct you want to measure. If you develop a questionnaire to diagnose depression, you need to know: does the questionnaire really measure the construct of depression? Or is it actually measuring the respondent’s mood, self-esteem, or some other construct?
To achieve construct validity, you have to ensure that your indicators and measurements are carefully developed based on relevant existing knowledge. The questionnaire must include only relevant questions that measure known indicators of depression.
The other types of validity described below can all be considered as forms of evidence for construct validity.
Receive feedback on language, structure, and formatting
Professional editors proofread and edit your paper by focusing on:
- Academic style
- Vague sentences
- Style consistency
See an example
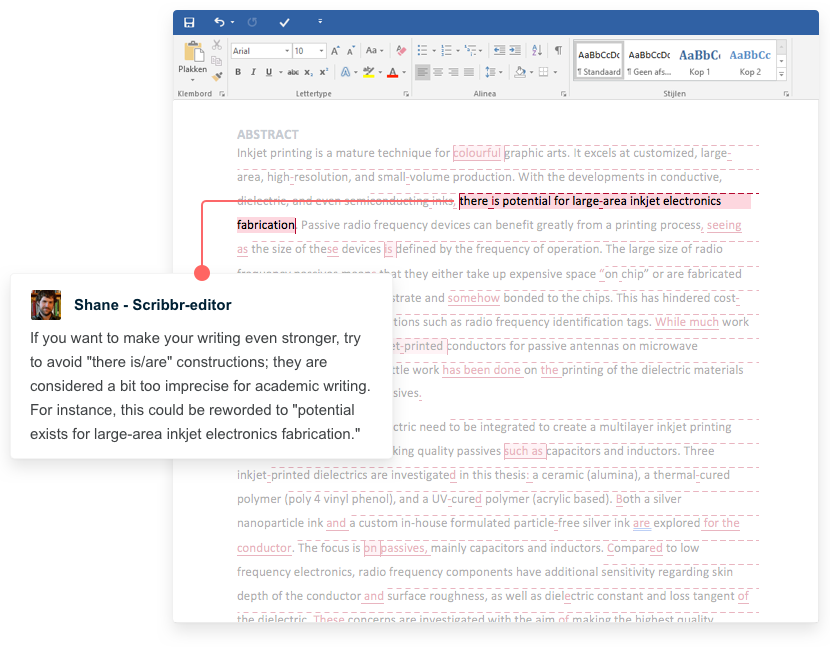
Content validity assesses whether a test is representative of all aspects of the construct.
To produce valid results, the content of a test, survey or measurement method must cover all relevant parts of the subject it aims to measure. If some aspects are missing from the measurement (or if irrelevant aspects are included), the validity is threatened and the research is likely suffering from omitted variable bias .
A mathematics teacher develops an end-of-semester algebra test for her class. The test should cover every form of algebra that was taught in the class. If some types of algebra are left out, then the results may not be an accurate indication of students’ understanding of the subject. Similarly, if she includes questions that are not related to algebra, the results are no longer a valid measure of algebra knowledge.
Face validity considers how suitable the content of a test seems to be on the surface. It’s similar to content validity, but face validity is a more informal and subjective assessment.
You create a survey to measure the regularity of people’s dietary habits. You review the survey items, which ask questions about every meal of the day and snacks eaten in between for every day of the week. On its surface, the survey seems like a good representation of what you want to test, so you consider it to have high face validity.
As face validity is a subjective measure, it’s often considered the weakest form of validity. However, it can be useful in the initial stages of developing a method.
Criterion validity evaluates how well a test can predict a concrete outcome, or how well the results of your test approximate the results of another test.
What is a criterion variable?
A criterion variable is an established and effective measurement that is widely considered valid, sometimes referred to as a “gold standard” measurement. Criterion variables can be very difficult to find.
What is criterion validity?
To evaluate criterion validity, you calculate the correlation between the results of your measurement and the results of the criterion measurement. If there is a high correlation, this gives a good indication that your test is measuring what it intends to measure.
A university professor creates a new test to measure applicants’ English writing ability. To assess how well the test really does measure students’ writing ability, she finds an existing test that is considered a valid measurement of English writing ability, and compares the results when the same group of students take both tests. If the outcomes are very similar, the new test has high criterion validity.
If you want to know more about statistics , methodology , or research bias , make sure to check out some of our other articles with explanations and examples.
- Normal distribution
- Degrees of freedom
- Null hypothesis
- Discourse analysis
- Control groups
- Mixed methods research
- Non-probability sampling
- Quantitative research
- Ecological validity
Research bias
- Rosenthal effect
- Implicit bias
- Cognitive bias
- Selection bias
- Negativity bias
- Status quo bias
Face validity and content validity are similar in that they both evaluate how suitable the content of a test is. The difference is that face validity is subjective, and assesses content at surface level.
When a test has strong face validity, anyone would agree that the test’s questions appear to measure what they are intended to measure.
For example, looking at a 4th grade math test consisting of problems in which students have to add and multiply, most people would agree that it has strong face validity (i.e., it looks like a math test).
On the other hand, content validity evaluates how well a test represents all the aspects of a topic. Assessing content validity is more systematic and relies on expert evaluation. of each question, analyzing whether each one covers the aspects that the test was designed to cover.
A 4th grade math test would have high content validity if it covered all the skills taught in that grade. Experts(in this case, math teachers), would have to evaluate the content validity by comparing the test to the learning objectives.
Criterion validity evaluates how well a test measures the outcome it was designed to measure. An outcome can be, for example, the onset of a disease.
Criterion validity consists of two subtypes depending on the time at which the two measures (the criterion and your test) are obtained:
- Concurrent validity is a validation strategy where the the scores of a test and the criterion are obtained at the same time .
- Predictive validity is a validation strategy where the criterion variables are measured after the scores of the test.
Convergent validity and discriminant validity are both subtypes of construct validity . Together, they help you evaluate whether a test measures the concept it was designed to measure.
- Convergent validity indicates whether a test that is designed to measure a particular construct correlates with other tests that assess the same or similar construct.
- Discriminant validity indicates whether two tests that should not be highly related to each other are indeed not related. This type of validity is also called divergent validity .
You need to assess both in order to demonstrate construct validity. Neither one alone is sufficient for establishing construct validity.
The purpose of theory-testing mode is to find evidence in order to disprove, refine, or support a theory. As such, generalizability is not the aim of theory-testing mode.
Due to this, the priority of researchers in theory-testing mode is to eliminate alternative causes for relationships between variables . In other words, they prioritize internal validity over external validity , including ecological validity .
It’s often best to ask a variety of people to review your measurements. You can ask experts, such as other researchers, or laypeople, such as potential participants, to judge the face validity of tests.
While experts have a deep understanding of research methods , the people you’re studying can provide you with valuable insights you may have missed otherwise.
Cite this Scribbr article
If you want to cite this source, you can copy and paste the citation or click the “Cite this Scribbr article” button to automatically add the citation to our free Citation Generator.
Middleton, F. (2023, June 22). The 4 Types of Validity in Research | Definitions & Examples. Scribbr. Retrieved April 8, 2024, from https://www.scribbr.com/methodology/types-of-validity/
Is this article helpful?
Fiona Middleton
Other students also liked, reliability vs. validity in research | difference, types and examples, construct validity | definition, types, & examples, external validity | definition, types, threats & examples, what is your plagiarism score.
- Privacy Policy
Buy Me a Coffee

Home » Validity – Types, Examples and Guide
Validity – Types, Examples and Guide
Table of Contents

Definition:
Validity refers to the extent to which a concept, measure, or study accurately represents the intended meaning or reality it is intended to capture. It is a fundamental concept in research and assessment that assesses the soundness and appropriateness of the conclusions, inferences, or interpretations made based on the data or evidence collected.
Research Validity
Research validity refers to the degree to which a study accurately measures or reflects what it claims to measure. In other words, research validity concerns whether the conclusions drawn from a study are based on accurate, reliable and relevant data.
Validity is a concept used in logic and research methodology to assess the strength of an argument or the quality of a research study. It refers to the extent to which a conclusion or result is supported by evidence and reasoning.
How to Ensure Validity in Research
Ensuring validity in research involves several steps and considerations throughout the research process. Here are some key strategies to help maintain research validity:
Clearly Define Research Objectives and Questions
Start by clearly defining your research objectives and formulating specific research questions. This helps focus your study and ensures that you are addressing relevant and meaningful research topics.
Use appropriate research design
Select a research design that aligns with your research objectives and questions. Different types of studies, such as experimental, observational, qualitative, or quantitative, have specific strengths and limitations. Choose the design that best suits your research goals.
Use reliable and valid measurement instruments
If you are measuring variables or constructs, ensure that the measurement instruments you use are reliable and valid. This involves using established and well-tested tools or developing your own instruments through rigorous validation processes.
Ensure a representative sample
When selecting participants or subjects for your study, aim for a sample that is representative of the population you want to generalize to. Consider factors such as age, gender, socioeconomic status, and other relevant demographics to ensure your findings can be generalized appropriately.
Address potential confounding factors
Identify potential confounding variables or biases that could impact your results. Implement strategies such as randomization, matching, or statistical control to minimize the influence of confounding factors and increase internal validity.
Minimize measurement and response biases
Be aware of measurement biases and response biases that can occur during data collection. Use standardized protocols, clear instructions, and trained data collectors to minimize these biases. Employ techniques like blinding or double-blinding in experimental studies to reduce bias.
Conduct appropriate statistical analyses
Ensure that the statistical analyses you employ are appropriate for your research design and data type. Select statistical tests that are relevant to your research questions and use robust analytical techniques to draw accurate conclusions from your data.
Consider external validity
While it may not always be possible to achieve high external validity, be mindful of the generalizability of your findings. Clearly describe your sample and study context to help readers understand the scope and limitations of your research.
Peer review and replication
Submit your research for peer review by experts in your field. Peer review helps identify potential flaws, biases, or methodological issues that can impact validity. Additionally, encourage replication studies by other researchers to validate your findings and enhance the overall reliability of the research.
Transparent reporting
Clearly and transparently report your research methods, procedures, data collection, and analysis techniques. Provide sufficient details for others to evaluate the validity of your study and replicate your work if needed.
Types of Validity
There are several types of validity that researchers consider when designing and evaluating studies. Here are some common types of validity:
Internal Validity
Internal validity relates to the degree to which a study accurately identifies causal relationships between variables. It addresses whether the observed effects can be attributed to the manipulated independent variable rather than confounding factors. Threats to internal validity include selection bias, history effects, maturation of participants, and instrumentation issues.
External Validity
External validity concerns the generalizability of research findings to the broader population or real-world settings. It assesses the extent to which the results can be applied to other individuals, contexts, or timeframes. Factors that can limit external validity include sample characteristics, research settings, and the specific conditions under which the study was conducted.
Construct Validity
Construct validity examines whether a study adequately measures the intended theoretical constructs or concepts. It focuses on the alignment between the operational definitions used in the study and the underlying theoretical constructs. Construct validity can be threatened by issues such as poor measurement tools, inadequate operational definitions, or a lack of clarity in the conceptual framework.
Content Validity
Content validity refers to the degree to which a measurement instrument or test adequately covers the entire range of the construct being measured. It assesses whether the items or questions included in the measurement tool represent the full scope of the construct. Content validity is often evaluated through expert judgment, reviewing the relevance and representativeness of the items.
Criterion Validity
Criterion validity determines the extent to which a measure or test is related to an external criterion or standard. It assesses whether the results obtained from a measurement instrument align with other established measures or outcomes. Criterion validity can be divided into two subtypes: concurrent validity, which examines the relationship between the measure and the criterion at the same time, and predictive validity, which investigates the measure’s ability to predict future outcomes.
Face Validity
Face validity refers to the degree to which a measurement or test appears, on the surface, to measure what it intends to measure. It is a subjective assessment based on whether the items seem relevant and appropriate to the construct being measured. Face validity is often used as an initial evaluation before conducting more rigorous validity assessments.
Importance of Validity
Validity is crucial in research for several reasons:
- Accurate Measurement: Validity ensures that the measurements or observations in a study accurately represent the intended constructs or variables. Without validity, researchers cannot be confident that their results truly reflect the phenomena they are studying. Validity allows researchers to draw accurate conclusions and make meaningful inferences based on their findings.
- Credibility and Trustworthiness: Validity enhances the credibility and trustworthiness of research. When a study demonstrates high validity, it indicates that the researchers have taken appropriate measures to ensure the accuracy and integrity of their work. This strengthens the confidence of other researchers, peers, and the wider scientific community in the study’s results and conclusions.
- Generalizability: Validity helps determine the extent to which research findings can be generalized beyond the specific sample and context of the study. By addressing external validity, researchers can assess whether their results can be applied to other populations, settings, or situations. This information is valuable for making informed decisions, implementing interventions, or developing policies based on research findings.
- Sound Decision-Making: Validity supports informed decision-making in various fields, such as medicine, psychology, education, and social sciences. When validity is established, policymakers, practitioners, and professionals can rely on research findings to guide their actions and interventions. Validity ensures that decisions are based on accurate and trustworthy information, which can lead to better outcomes and more effective practices.
- Avoiding Errors and Bias: Validity helps researchers identify and mitigate potential errors and biases in their studies. By addressing internal validity, researchers can minimize confounding factors and alternative explanations, ensuring that the observed effects are genuinely attributable to the manipulated variables. Validity assessments also highlight measurement errors or shortcomings, enabling researchers to improve their measurement tools and procedures.
- Progress of Scientific Knowledge: Validity is essential for the advancement of scientific knowledge. Valid research contributes to the accumulation of reliable and valid evidence, which forms the foundation for building theories, developing models, and refining existing knowledge. Validity allows researchers to build upon previous findings, replicate studies, and establish a cumulative body of knowledge in various disciplines. Without validity, the scientific community would struggle to make meaningful progress and establish a solid understanding of the phenomena under investigation.
- Ethical Considerations: Validity is closely linked to ethical considerations in research. Conducting valid research ensures that participants’ time, effort, and data are not wasted on flawed or invalid studies. It upholds the principle of respect for participants’ autonomy and promotes responsible research practices. Validity is also important when making claims or drawing conclusions that may have real-world implications, as misleading or invalid findings can have adverse effects on individuals, organizations, or society as a whole.
Examples of Validity
Here are some examples of validity in different contexts:
- Example 1: All men are mortal. John is a man. Therefore, John is mortal. This argument is logically valid because the conclusion follows logically from the premises.
- Example 2: If it is raining, then the ground is wet. The ground is wet. Therefore, it is raining. This argument is not logically valid because there could be other reasons for the ground being wet, such as watering the plants.
- Example 1: In a study examining the relationship between caffeine consumption and alertness, the researchers use established measures of both variables, ensuring that they are accurately capturing the concepts they intend to measure. This demonstrates construct validity.
- Example 2: A researcher develops a new questionnaire to measure anxiety levels. They administer the questionnaire to a group of participants and find that it correlates highly with other established anxiety measures. This indicates good construct validity for the new questionnaire.
- Example 1: A study on the effects of a particular teaching method is conducted in a controlled laboratory setting. The findings of the study may lack external validity because the conditions in the lab may not accurately reflect real-world classroom settings.
- Example 2: A research study on the effects of a new medication includes participants from diverse backgrounds and age groups, increasing the external validity of the findings to a broader population.
- Example 1: In an experiment, a researcher manipulates the independent variable (e.g., a new drug) and controls for other variables to ensure that any observed effects on the dependent variable (e.g., symptom reduction) are indeed due to the manipulation. This establishes internal validity.
- Example 2: A researcher conducts a study examining the relationship between exercise and mood by administering questionnaires to participants. However, the study lacks internal validity because it does not control for other potential factors that could influence mood, such as diet or stress levels.
- Example 1: A teacher develops a new test to assess students’ knowledge of a particular subject. The items on the test appear to be relevant to the topic at hand and align with what one would expect to find on such a test. This suggests face validity, as the test appears to measure what it intends to measure.
- Example 2: A company develops a new customer satisfaction survey. The questions included in the survey seem to address key aspects of the customer experience and capture the relevant information. This indicates face validity, as the survey seems appropriate for assessing customer satisfaction.
- Example 1: A team of experts reviews a comprehensive curriculum for a high school biology course. They evaluate the curriculum to ensure that it covers all the essential topics and concepts necessary for students to gain a thorough understanding of biology. This demonstrates content validity, as the curriculum is representative of the domain it intends to cover.
- Example 2: A researcher develops a questionnaire to assess career satisfaction. The questions in the questionnaire encompass various dimensions of job satisfaction, such as salary, work-life balance, and career growth. This indicates content validity, as the questionnaire adequately represents the different aspects of career satisfaction.
- Example 1: A company wants to evaluate the effectiveness of a new employee selection test. They administer the test to a group of job applicants and later assess the job performance of those who were hired. If there is a strong correlation between the test scores and subsequent job performance, it suggests criterion validity, indicating that the test is predictive of job success.
- Example 2: A researcher wants to determine if a new medical diagnostic tool accurately identifies a specific disease. They compare the results of the diagnostic tool with the gold standard diagnostic method and find a high level of agreement. This demonstrates criterion validity, indicating that the new tool is valid in accurately diagnosing the disease.
Where to Write About Validity in A Thesis
In a thesis, discussions related to validity are typically included in the methodology and results sections. Here are some specific places where you can address validity within your thesis:
Research Design and Methodology
In the methodology section, provide a clear and detailed description of the measures, instruments, or data collection methods used in your study. Discuss the steps taken to establish or assess the validity of these measures. Explain the rationale behind the selection of specific validity types relevant to your study, such as content validity, criterion validity, or construct validity. Discuss any modifications or adaptations made to existing measures and their potential impact on validity.
Measurement Procedures
In the methodology section, elaborate on the procedures implemented to ensure the validity of measurements. Describe how potential biases or confounding factors were addressed, controlled, or accounted for to enhance internal validity. Provide details on how you ensured that the measurement process accurately captures the intended constructs or variables of interest.
Data Collection
In the methodology section, discuss the steps taken to collect data and ensure data validity. Explain any measures implemented to minimize errors or biases during data collection, such as training of data collectors, standardized protocols, or quality control procedures. Address any potential limitations or threats to validity related to the data collection process.
Data Analysis and Results
In the results section, present the analysis and findings related to validity. Report any statistical tests, correlations, or other measures used to assess validity. Provide interpretations and explanations of the results obtained. Discuss the implications of the validity findings for the overall reliability and credibility of your study.
Limitations and Future Directions
In the discussion or conclusion section, reflect on the limitations of your study, including limitations related to validity. Acknowledge any potential threats or weaknesses to validity that you encountered during your research. Discuss how these limitations may have influenced the interpretation of your findings and suggest avenues for future research that could address these validity concerns.
Applications of Validity
Validity is applicable in various areas and contexts where research and measurement play a role. Here are some common applications of validity:
Psychological and Behavioral Research
Validity is crucial in psychology and behavioral research to ensure that measurement instruments accurately capture constructs such as personality traits, intelligence, attitudes, emotions, or psychological disorders. Validity assessments help researchers determine if their measures are truly measuring the intended psychological constructs and if the results can be generalized to broader populations or real-world settings.
Educational Assessment
Validity is essential in educational assessment to determine if tests, exams, or assessments accurately measure students’ knowledge, skills, or abilities. It ensures that the assessment aligns with the educational objectives and provides reliable information about student performance. Validity assessments help identify if the assessment is valid for all students, regardless of their demographic characteristics, language proficiency, or cultural background.
Program Evaluation
Validity plays a crucial role in program evaluation, where researchers assess the effectiveness and impact of interventions, policies, or programs. By establishing validity, evaluators can determine if the observed outcomes are genuinely attributable to the program being evaluated rather than extraneous factors. Validity assessments also help ensure that the evaluation findings are applicable to different populations, contexts, or timeframes.
Medical and Health Research
Validity is essential in medical and health research to ensure the accuracy and reliability of diagnostic tools, measurement instruments, and clinical assessments. Validity assessments help determine if a measurement accurately identifies the presence or absence of a medical condition, measures the effectiveness of a treatment, or predicts patient outcomes. Validity is crucial for establishing evidence-based medicine and informing medical decision-making.
Social Science Research
Validity is relevant in various social science disciplines, including sociology, anthropology, economics, and political science. Researchers use validity to ensure that their measures and methods accurately capture social phenomena, such as social attitudes, behaviors, social structures, or economic indicators. Validity assessments support the reliability and credibility of social science research findings.
Market Research and Surveys
Validity is important in market research and survey studies to ensure that the survey questions effectively measure consumer preferences, buying behaviors, or attitudes towards products or services. Validity assessments help researchers determine if the survey instrument is accurately capturing the desired information and if the results can be generalized to the target population.
Limitations of Validity
Here are some limitations of validity:
- Construct Validity: Limitations of construct validity include the potential for measurement error, inadequate operational definitions of constructs, or the failure to capture all aspects of a complex construct.
- Internal Validity: Limitations of internal validity may arise from confounding variables, selection bias, or the presence of extraneous factors that could influence the study outcomes, making it difficult to attribute causality accurately.
- External Validity: Limitations of external validity can occur when the study sample does not represent the broader population, when the research setting differs significantly from real-world conditions, or when the study lacks ecological validity, i.e., the findings do not reflect real-world complexities.
- Measurement Validity: Limitations of measurement validity can arise from measurement error, inadequately designed or flawed measurement scales, or limitations inherent in self-report measures, such as social desirability bias or recall bias.
- Statistical Conclusion Validity: Limitations in statistical conclusion validity can occur due to sampling errors, inadequate sample sizes, or improper statistical analysis techniques, leading to incorrect conclusions or generalizations.
- Temporal Validity: Limitations of temporal validity arise when the study results become outdated due to changes in the studied phenomena, interventions, or contextual factors.
- Researcher Bias: Researcher bias can affect the validity of a study. Biases can emerge through the researcher’s subjective interpretation, influence of personal beliefs, or preconceived notions, leading to unintentional distortion of findings or failure to consider alternative explanations.
- Ethical Validity: Limitations can arise if the study design or methods involve ethical concerns, such as the use of deceptive practices, inadequate informed consent, or potential harm to participants.
Also see Reliability Vs Validity
About the author
Muhammad Hassan
Researcher, Academic Writer, Web developer
You may also like

Alternate Forms Reliability – Methods, Examples...

Construct Validity – Types, Threats and Examples

Internal Validity – Threats, Examples and Guide

Reliability Vs Validity

Internal Consistency Reliability – Methods...

Split-Half Reliability – Methods, Examples and...

Validity & Reliability In Research
A Plain-Language Explanation (With Examples)
By: Derek Jansen (MBA) | Expert Reviewer: Kerryn Warren (PhD) | September 2023
Validity and reliability are two related but distinctly different concepts within research. Understanding what they are and how to achieve them is critically important to any research project. In this post, we’ll unpack these two concepts as simply as possible.
This post is based on our popular online course, Research Methodology Bootcamp . In the course, we unpack the basics of methodology using straightfoward language and loads of examples. If you’re new to academic research, you definitely want to use this link to get 50% off the course (limited-time offer).
Overview: Validity & Reliability
- The big picture
- Validity 101
- Reliability 101
- Key takeaways
First, The Basics…
First, let’s start with a big-picture view and then we can zoom in to the finer details.
Validity and reliability are two incredibly important concepts in research, especially within the social sciences. Both validity and reliability have to do with the measurement of variables and/or constructs – for example, job satisfaction, intelligence, productivity, etc. When undertaking research, you’ll often want to measure these types of constructs and variables and, at the simplest level, validity and reliability are about ensuring the quality and accuracy of those measurements .
As you can probably imagine, if your measurements aren’t accurate or there are quality issues at play when you’re collecting your data, your entire study will be at risk. Therefore, validity and reliability are very important concepts to understand (and to get right). So, let’s unpack each of them.
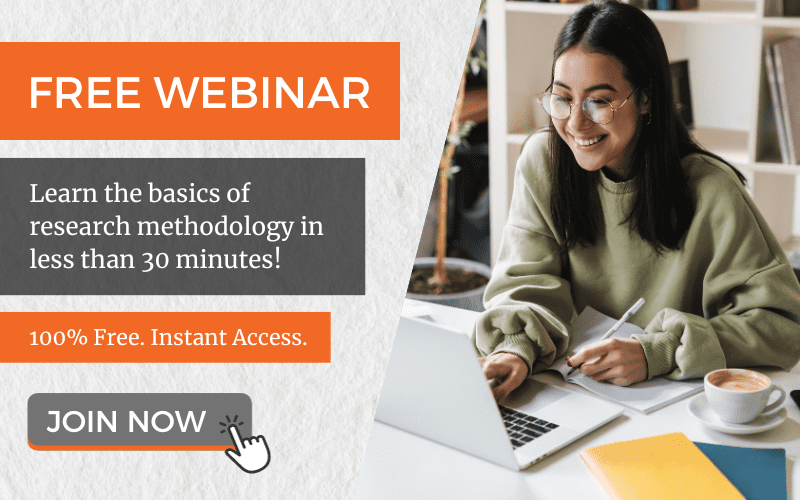
What Is Validity?
In simple terms, validity (also called “construct validity”) is all about whether a research instrument accurately measures what it’s supposed to measure .
For example, let’s say you have a set of Likert scales that are supposed to quantify someone’s level of overall job satisfaction. If this set of scales focused purely on only one dimension of job satisfaction, say pay satisfaction, this would not be a valid measurement, as it only captures one aspect of the multidimensional construct. In other words, pay satisfaction alone is only one contributing factor toward overall job satisfaction, and therefore it’s not a valid way to measure someone’s job satisfaction.
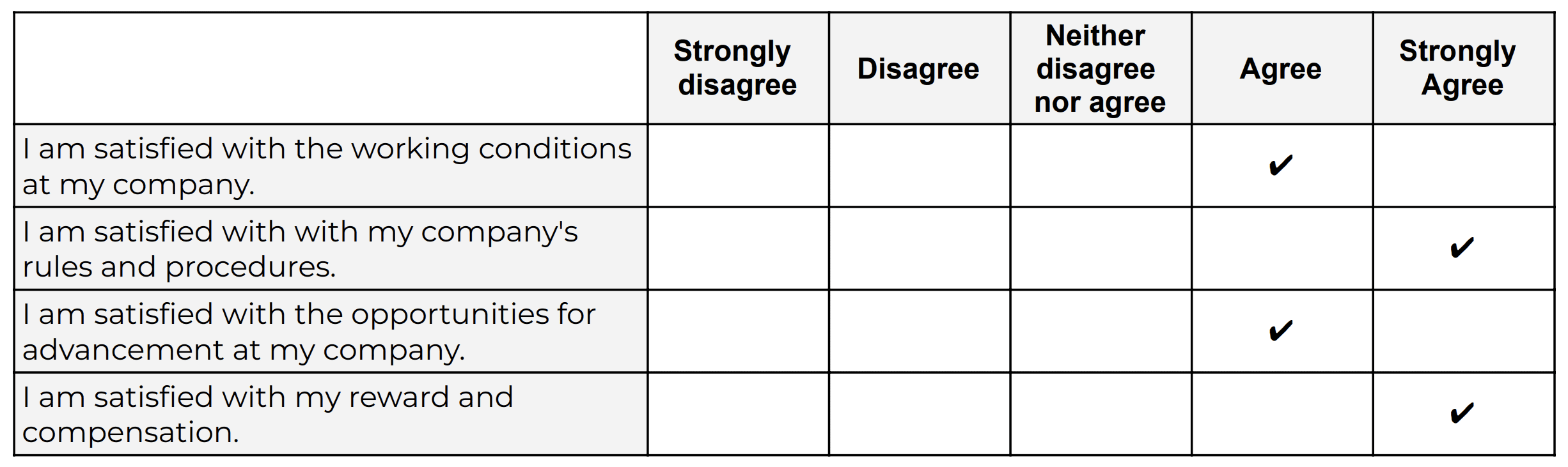
Oftentimes in quantitative studies, the way in which the researcher or survey designer interprets a question or statement can differ from how the study participants interpret it . Given that respondents don’t have the opportunity to ask clarifying questions when taking a survey, it’s easy for these sorts of misunderstandings to crop up. Naturally, if the respondents are interpreting the question in the wrong way, the data they provide will be pretty useless . Therefore, ensuring that a study’s measurement instruments are valid – in other words, that they are measuring what they intend to measure – is incredibly important.
There are various types of validity and we’re not going to go down that rabbit hole in this post, but it’s worth quickly highlighting the importance of making sure that your research instrument is tightly aligned with the theoretical construct you’re trying to measure . In other words, you need to pay careful attention to how the key theories within your study define the thing you’re trying to measure – and then make sure that your survey presents it in the same way.
For example, sticking with the “job satisfaction” construct we looked at earlier, you’d need to clearly define what you mean by job satisfaction within your study (and this definition would of course need to be underpinned by the relevant theory). You’d then need to make sure that your chosen definition is reflected in the types of questions or scales you’re using in your survey . Simply put, you need to make sure that your survey respondents are perceiving your key constructs in the same way you are. Or, even if they’re not, that your measurement instrument is capturing the necessary information that reflects your definition of the construct at hand.
If all of this talk about constructs sounds a bit fluffy, be sure to check out Research Methodology Bootcamp , which will provide you with a rock-solid foundational understanding of all things methodology-related. Remember, you can take advantage of our 60% discount offer using this link.
Need a helping hand?
What Is Reliability?
As with validity, reliability is an attribute of a measurement instrument – for example, a survey, a weight scale or even a blood pressure monitor. But while validity is concerned with whether the instrument is measuring the “thing” it’s supposed to be measuring, reliability is concerned with consistency and stability . In other words, reliability reflects the degree to which a measurement instrument produces consistent results when applied repeatedly to the same phenomenon , under the same conditions .
As you can probably imagine, a measurement instrument that achieves a high level of consistency is naturally more dependable (or reliable) than one that doesn’t – in other words, it can be trusted to provide consistent measurements . And that, of course, is what you want when undertaking empirical research. If you think about it within a more domestic context, just imagine if you found that your bathroom scale gave you a different number every time you hopped on and off of it – you wouldn’t feel too confident in its ability to measure the variable that is your body weight 🙂
It’s worth mentioning that reliability also extends to the person using the measurement instrument . For example, if two researchers use the same instrument (let’s say a measuring tape) and they get different measurements, there’s likely an issue in terms of how one (or both) of them are using the measuring tape. So, when you think about reliability, consider both the instrument and the researcher as part of the equation.
As with validity, there are various types of reliability and various tests that can be used to assess the reliability of an instrument. A popular one that you’ll likely come across for survey instruments is Cronbach’s alpha , which is a statistical measure that quantifies the degree to which items within an instrument (for example, a set of Likert scales) measure the same underlying construct . In other words, Cronbach’s alpha indicates how closely related the items are and whether they consistently capture the same concept .
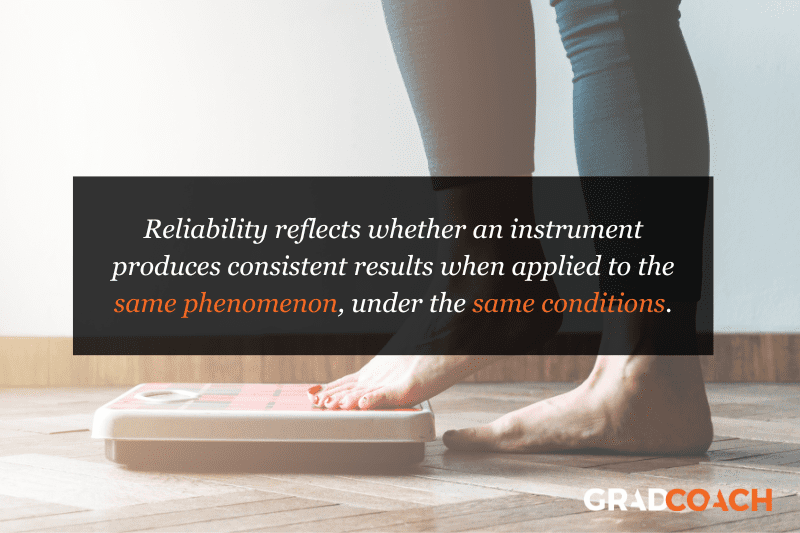
Recap: Key Takeaways
Alright, let’s quickly recap to cement your understanding of validity and reliability:
- Validity is concerned with whether an instrument (e.g., a set of Likert scales) is measuring what it’s supposed to measure
- Reliability is concerned with whether that measurement is consistent and stable when measuring the same phenomenon under the same conditions.
In short, validity and reliability are both essential to ensuring that your data collection efforts deliver high-quality, accurate data that help you answer your research questions . So, be sure to always pay careful attention to the validity and reliability of your measurement instruments when collecting and analysing data. As the adage goes, “rubbish in, rubbish out” – make sure that your data inputs are rock-solid.
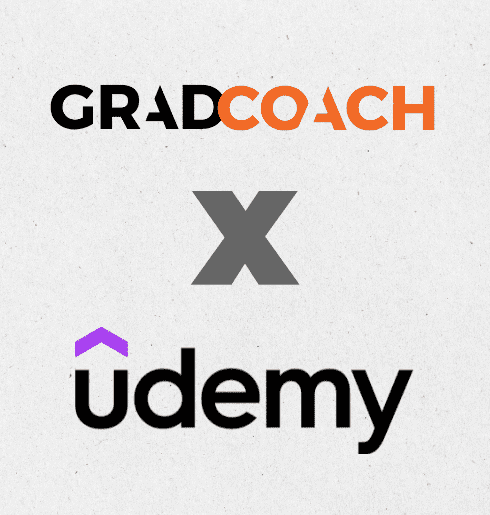
Psst… there’s more!
This post is an extract from our bestselling Udemy Course, Methodology Bootcamp . If you want to work smart, you don't want to miss this .
You Might Also Like:
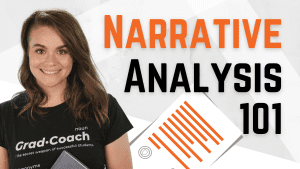
THE MATERIAL IS WONDERFUL AND BENEFICIAL TO ALL STUDENTS.
THE MATERIAL IS WONDERFUL AND BENEFICIAL TO ALL STUDENTS AND I HAVE GREATLY BENEFITED FROM THE CONTENT.
Submit a Comment Cancel reply
Your email address will not be published. Required fields are marked *
Save my name, email, and website in this browser for the next time I comment.
- Print Friendly
Have a language expert improve your writing
Run a free plagiarism check in 10 minutes, automatically generate references for free.
- Knowledge Base
- Methodology
- Reliability vs Validity in Research | Differences, Types & Examples
Reliability vs Validity in Research | Differences, Types & Examples
Published on 3 May 2022 by Fiona Middleton . Revised on 10 October 2022.
Reliability and validity are concepts used to evaluate the quality of research. They indicate how well a method , technique, or test measures something. Reliability is about the consistency of a measure, and validity is about the accuracy of a measure.
It’s important to consider reliability and validity when you are creating your research design , planning your methods, and writing up your results, especially in quantitative research .
Table of contents
Understanding reliability vs validity, how are reliability and validity assessed, how to ensure validity and reliability in your research, where to write about reliability and validity in a thesis.
Reliability and validity are closely related, but they mean different things. A measurement can be reliable without being valid. However, if a measurement is valid, it is usually also reliable.
What is reliability?
Reliability refers to how consistently a method measures something. If the same result can be consistently achieved by using the same methods under the same circumstances, the measurement is considered reliable.
What is validity?
Validity refers to how accurately a method measures what it is intended to measure. If research has high validity, that means it produces results that correspond to real properties, characteristics, and variations in the physical or social world.
High reliability is one indicator that a measurement is valid. If a method is not reliable, it probably isn’t valid.
However, reliability on its own is not enough to ensure validity. Even if a test is reliable, it may not accurately reflect the real situation.
Validity is harder to assess than reliability, but it is even more important. To obtain useful results, the methods you use to collect your data must be valid: the research must be measuring what it claims to measure. This ensures that your discussion of the data and the conclusions you draw are also valid.
Prevent plagiarism, run a free check.
Reliability can be estimated by comparing different versions of the same measurement. Validity is harder to assess, but it can be estimated by comparing the results to other relevant data or theory. Methods of estimating reliability and validity are usually split up into different types.
Types of reliability
Different types of reliability can be estimated through various statistical methods.
Types of validity
The validity of a measurement can be estimated based on three main types of evidence. Each type can be evaluated through expert judgement or statistical methods.
To assess the validity of a cause-and-effect relationship, you also need to consider internal validity (the design of the experiment ) and external validity (the generalisability of the results).
The reliability and validity of your results depends on creating a strong research design , choosing appropriate methods and samples, and conducting the research carefully and consistently.
Ensuring validity
If you use scores or ratings to measure variations in something (such as psychological traits, levels of ability, or physical properties), it’s important that your results reflect the real variations as accurately as possible. Validity should be considered in the very earliest stages of your research, when you decide how you will collect your data .
- Choose appropriate methods of measurement
Ensure that your method and measurement technique are of high quality and targeted to measure exactly what you want to know. They should be thoroughly researched and based on existing knowledge.
For example, to collect data on a personality trait, you could use a standardised questionnaire that is considered reliable and valid. If you develop your own questionnaire, it should be based on established theory or the findings of previous studies, and the questions should be carefully and precisely worded.
- Use appropriate sampling methods to select your subjects
To produce valid generalisable results, clearly define the population you are researching (e.g., people from a specific age range, geographical location, or profession). Ensure that you have enough participants and that they are representative of the population.
Ensuring reliability
Reliability should be considered throughout the data collection process. When you use a tool or technique to collect data, it’s important that the results are precise, stable, and reproducible.
- Apply your methods consistently
Plan your method carefully to make sure you carry out the same steps in the same way for each measurement. This is especially important if multiple researchers are involved.
For example, if you are conducting interviews or observations, clearly define how specific behaviours or responses will be counted, and make sure questions are phrased the same way each time.
- Standardise the conditions of your research
When you collect your data, keep the circumstances as consistent as possible to reduce the influence of external factors that might create variation in the results.
For example, in an experimental setup, make sure all participants are given the same information and tested under the same conditions.
It’s appropriate to discuss reliability and validity in various sections of your thesis or dissertation or research paper. Showing that you have taken them into account in planning your research and interpreting the results makes your work more credible and trustworthy.
Cite this Scribbr article
If you want to cite this source, you can copy and paste the citation or click the ‘Cite this Scribbr article’ button to automatically add the citation to our free Reference Generator.
Middleton, F. (2022, October 10). Reliability vs Validity in Research | Differences, Types & Examples. Scribbr. Retrieved 8 April 2024, from https://www.scribbr.co.uk/research-methods/reliability-or-validity/
Is this article helpful?
Fiona Middleton
Other students also liked, the 4 types of validity | types, definitions & examples, a quick guide to experimental design | 5 steps & examples, sampling methods | types, techniques, & examples.
- Bipolar Disorder
- Therapy Center
- When To See a Therapist
- Types of Therapy
- Best Online Therapy
- Best Couples Therapy
- Best Family Therapy
- Managing Stress
- Sleep and Dreaming
- Understanding Emotions
- Self-Improvement
- Healthy Relationships
- Student Resources
- Personality Types
- Guided Meditations
- Verywell Mind Insights
- 2023 Verywell Mind 25
- Mental Health in the Classroom
- Editorial Process
- Meet Our Review Board
- Crisis Support
Internal Validity vs. External Validity in Research
Both help determine how meaningful the results of the study are
Arlin Cuncic, MA, is the author of The Anxiety Workbook and founder of the website About Social Anxiety. She has a Master's degree in clinical psychology.
:max_bytes(150000):strip_icc():format(webp)/ArlinCuncic_1000-21af8749d2144aa0b0491c29319591c4.jpg)
Rachel Goldman, PhD FTOS, is a licensed psychologist, clinical assistant professor, speaker, wellness expert specializing in eating behaviors, stress management, and health behavior change.
:max_bytes(150000):strip_icc():format(webp)/Rachel-Goldman-1000-a42451caacb6423abecbe6b74e628042.jpg)
Verywell / Bailey Mariner
- Internal Validity
- External Validity
Internal validity is a measure of how well a study is conducted (its structure) and how accurately its results reflect the studied group.
External validity relates to how applicable the findings are in the real world. These two concepts help researchers gauge if the results of a research study are trustworthy and meaningful.
Conclusions are warranted
Controls extraneous variables
Eliminates alternative explanations
Focus on accuracy and strong research methods
Findings can be generalized
Outcomes apply to practical situations
Results apply to the world at large
Results can be translated into another context
What Is Internal Validity in Research?
Internal validity is the extent to which a research study establishes a trustworthy cause-and-effect relationship. This type of validity depends largely on the study's procedures and how rigorously it is performed.
Internal validity is important because once established, it makes it possible to eliminate alternative explanations for a finding. If you implement a smoking cessation program, for instance, internal validity ensures that any improvement in the subjects is due to the treatment administered and not something else.
Internal validity is not a "yes or no" concept. Instead, we consider how confident we can be with study findings based on whether the research avoids traps that may make those findings questionable. The less chance there is for "confounding," the higher the internal validity and the more confident we can be.
Confounding refers to uncontrollable variables that come into play and can confuse the outcome of a study, making us unsure of whether we can trust that we have identified the cause-and-effect relationship.
In short, you can only be confident that a study is internally valid if you can rule out alternative explanations for the findings. Three criteria are required to assume cause and effect in a research study:
- The cause preceded the effect in terms of time.
- The cause and effect vary together.
- There are no other likely explanations for the relationship observed.
Factors That Improve Internal Validity
To ensure the internal validity of a study, you want to consider aspects of the research design that will increase the likelihood that you can reject alternative hypotheses. Many factors can improve internal validity in research, including:
- Blinding : Participants—and sometimes researchers—are unaware of what intervention they are receiving (such as using a placebo on some subjects in a medication study) to avoid having this knowledge bias their perceptions and behaviors, thus impacting the study's outcome
- Experimental manipulation : Manipulating an independent variable in a study (for instance, giving smokers a cessation program) instead of just observing an association without conducting any intervention (examining the relationship between exercise and smoking behavior)
- Random selection : Choosing participants at random or in a manner in which they are representative of the population that you wish to study
- Randomization or random assignment : Randomly assigning participants to treatment and control groups, ensuring that there is no systematic bias between the research groups
- Strict study protocol : Following specific procedures during the study so as not to introduce any unintended effects; for example, doing things differently with one group of study participants than you do with another group
Internal Validity Threats
Just as there are many ways to ensure internal validity, there is also a list of potential threats that should be considered when planning a study.
- Attrition : Participants dropping out or leaving a study, which means that the results are based on a biased sample of only the people who did not choose to leave (and possibly who all have something in common, such as higher motivation)
- Confounding : A situation in which changes in an outcome variable can be thought to have resulted from some type of outside variable not measured or manipulated in the study
- Diffusion : This refers to the results of one group transferring to another through the groups interacting and talking with or observing one another; this can also lead to another issue called resentful demoralization, in which a control group tries less hard because they feel resentful over the group that they are in
- Experimenter bias : An experimenter behaving in a different way with different groups in a study, which can impact the results (and is eliminated through blinding)
- Historical events : May influence the outcome of studies that occur over a period of time, such as a change in the political leader or a natural disaster that occurs, influencing how study participants feel and act
- Instrumentation : This involves "priming" participants in a study in certain ways with the measures used, causing them to react in a way that is different than they would have otherwise reacted
- Maturation : The impact of time as a variable in a study; for example, if a study takes place over a period of time in which it is possible that participants naturally change in some way (i.e., they grew older or became tired), it may be impossible to rule out whether effects seen in the study were simply due to the impact of time
- Statistical regression : The natural effect of participants at extreme ends of a measure falling in a certain direction due to the passage of time rather than being a direct effect of an intervention
- Testing : Repeatedly testing participants using the same measures influences outcomes; for example, if you give someone the same test three times, it is likely that they will do better as they learn the test or become used to the testing process, causing them to answer differently
What Is External Validity in Research?
External validity refers to how well the outcome of a research study can be expected to apply to other settings. This is important because, if external validity is established, it means that the findings can be generalizable to similar individuals or populations.
External validity affirmatively answers the question: Do the findings apply to similar people, settings, situations, and time periods?
Population validity and ecological validity are two types of external validity. Population validity refers to whether you can generalize the research outcomes to other populations or groups. Ecological validity refers to whether a study's findings can be generalized to additional situations or settings.
Another term called transferability refers to whether results transfer to situations with similar characteristics. Transferability relates to external validity and refers to a qualitative research design.
Factors That Improve External Validity
If you want to improve the external validity of your study, there are many ways to achieve this goal. Factors that can enhance external validity include:
- Field experiments : Conducting a study outside the laboratory, in a natural setting
- Inclusion and exclusion criteria : Setting criteria as to who can be involved in the research, ensuring that the population being studied is clearly defined
- Psychological realism : Making sure participants experience the events of the study as being real by telling them a "cover story," or a different story about the aim of the study so they don't behave differently than they would in real life based on knowing what to expect or knowing the study's goal
- Replication : Conducting the study again with different samples or in different settings to see if you get the same results; when many studies have been conducted on the same topic, a meta-analysis can also be used to determine if the effect of an independent variable can be replicated, therefore making it more reliable
- Reprocessing or calibration : Using statistical methods to adjust for external validity issues, such as reweighting groups if a study had uneven groups for a particular characteristic (such as age)
External Validity Threats
External validity is threatened when a study does not take into account the interaction of variables in the real world. Threats to external validity include:
- Pre- and post-test effects : When the pre- or post-test is in some way related to the effect seen in the study, such that the cause-and-effect relationship disappears without these added tests
- Sample features : When some feature of the sample used was responsible for the effect (or partially responsible), leading to limited generalizability of the findings
- Selection bias : Also considered a threat to internal validity, selection bias describes differences between groups in a study that may relate to the independent variable—like motivation or willingness to take part in the study, or specific demographics of individuals being more likely to take part in an online survey
- Situational factors : Factors such as the time of day of the study, its location, noise, researcher characteristics, and the number of measures used may affect the generalizability of findings
While rigorous research methods can ensure internal validity, external validity may be limited by these methods.

Internal Validity vs. External Validity
Internal validity and external validity are two research concepts that share a few similarities while also having several differences.
Similarities
One of the similarities between internal validity and external validity is that both factors should be considered when designing a study. This is because both have implications in terms of whether the results of a study have meaning.
Both internal validity and external validity are not "either/or" concepts. Therefore, you always need to decide to what degree a study performs in terms of each type of validity.
Each of these concepts is also typically reported in research articles published in scholarly journals . This is so that other researchers can evaluate the study and make decisions about whether the results are useful and valid.
Differences
The essential difference between internal validity and external validity is that internal validity refers to the structure of a study (and its variables) while external validity refers to the universality of the results. But there are further differences between the two as well.
For instance, internal validity focuses on showing a difference that is due to the independent variable alone. Conversely, external validity results can be translated to the world at large.
Internal validity and external validity aren't mutually exclusive. You can have a study with good internal validity but be overall irrelevant to the real world. You could also conduct a field study that is highly relevant to the real world but doesn't have trustworthy results in terms of knowing what variables caused the outcomes.
Examples of Validity
Perhaps the best way to understand internal validity and external validity is with examples.
Internal Validity Example
An example of a study with good internal validity would be if a researcher hypothesizes that using a particular mindfulness app will reduce negative mood. To test this hypothesis, the researcher randomly assigns a sample of participants to one of two groups: those who will use the app over a defined period and those who engage in a control task.
The researcher ensures that there is no systematic bias in how participants are assigned to the groups. They do this by blinding the research assistants so they don't know which groups the subjects are in during the experiment.
A strict study protocol is also used to outline the procedures of the study. Potential confounding variables are measured along with mood , such as the participants' socioeconomic status, gender, age, and other factors. If participants drop out of the study, their characteristics are examined to make sure there is no systematic bias in terms of who stays in.
External Validity Example
An example of a study with good external validity would be if, in the above example, the participants used the mindfulness app at home rather than in the laboratory. This shows that results appear in a real-world setting.
To further ensure external validity, the researcher clearly defines the population of interest and chooses a representative sample . They might also replicate the study's results using different technological devices.
A Word From Verywell
Setting up an experiment so that it has both sound internal validity and external validity involves being mindful from the start about factors that can influence each aspect of your research.
It's best to spend extra time designing a structurally sound study that has far-reaching implications rather than to quickly rush through the design phase only to discover problems later on. Only when both internal validity and external validity are high can strong conclusions be made about your results.
San Jose State University. Internal and external validity .
Michael RS. Threats to internal & external validity: Y520 strategies for educational inquiry .
Pahus L, Burgel PR, Roche N, Paillasseur JL, Chanez P. Randomized controlled trials of pharmacological treatments to prevent COPD exacerbations: applicability to real-life patients . BMC Pulm Med . 2019;19(1):127. doi:10.1186/s12890-019-0882-y
By Arlin Cuncic, MA Arlin Cuncic, MA, is the author of The Anxiety Workbook and founder of the website About Social Anxiety. She has a Master's degree in clinical psychology.
Validity in research: a guide to measuring the right things
Last updated
27 February 2023
Reviewed by
Cathy Heath
Validity is necessary for all types of studies ranging from market validation of a business or product idea to the effectiveness of medical trials and procedures. So, how can you determine whether your research is valid? This guide can help you understand what validity is, the types of validity in research, and the factors that affect research validity.
Make research less tedious
Dovetail streamlines research to help you uncover and share actionable insights
- What is validity?
In the most basic sense, validity is the quality of being based on truth or reason. Valid research strives to eliminate the effects of unrelated information and the circumstances under which evidence is collected.
Validity in research is the ability to conduct an accurate study with the right tools and conditions to yield acceptable and reliable data that can be reproduced. Researchers rely on carefully calibrated tools for precise measurements. However, collecting accurate information can be more of a challenge.
Studies must be conducted in environments that don't sway the results to achieve and maintain validity. They can be compromised by asking the wrong questions or relying on limited data.
Why is validity important in research?
Research is used to improve life for humans. Every product and discovery, from innovative medical breakthroughs to advanced new products, depends on accurate research to be dependable. Without it, the results couldn't be trusted, and products would likely fail. Businesses would lose money, and patients couldn't rely on medical treatments.
While wasting money on a lousy product is a concern, lack of validity paints a much grimmer picture in the medical field or producing automobiles and airplanes, for example. Whether you're launching an exciting new product or conducting scientific research, validity can determine success and failure.
- What is reliability?
Reliability is the ability of a method to yield consistency. If the same result can be consistently achieved by using the same method to measure something, the measurement method is said to be reliable. For example, a thermometer that shows the same temperatures each time in a controlled environment is reliable.
While high reliability is a part of measuring validity, it's only part of the puzzle. If the reliable thermometer hasn't been properly calibrated and reliably measures temperatures two degrees too high, it doesn't provide a valid (accurate) measure of temperature.
Similarly, if a researcher uses a thermometer to measure weight, the results won't be accurate because it's the wrong tool for the job.
- How are reliability and validity assessed?
While measuring reliability is a part of measuring validity, there are distinct ways to assess both measurements for accuracy.
How is reliability measured?
These measures of consistency and stability help assess reliability, including:
Consistency and stability of the same measure when repeated multiple times and conditions
Consistency and stability of the measure across different test subjects
Consistency and stability of results from different parts of a test designed to measure the same thing
How is validity measured?
Since validity refers to how accurately a method measures what it is intended to measure, it can be difficult to assess the accuracy. Validity can be estimated by comparing research results to other relevant data or theories.
The adherence of a measure to existing knowledge of how the concept is measured
The ability to cover all aspects of the concept being measured
The relation of the result in comparison with other valid measures of the same concept
- What are the types of validity in a research design?
Research validity is broadly gathered into two groups: internal and external. Yet, this grouping doesn't clearly define the different types of validity. Research validity can be divided into seven distinct groups.
Face validity : A test that appears valid simply because of the appropriateness or relativity of the testing method, included information, or tools used.
Content validity : The determination that the measure used in research covers the full domain of the content.
Construct validity : The assessment of the suitability of the measurement tool to measure the activity being studied.
Internal validity : The assessment of how your research environment affects measurement results. This is where other factors can’t explain the extent of an observed cause-and-effect response.
External validity : The extent to which the study will be accurate beyond the sample and the level to which it can be generalized in other settings, populations, and measures.
Statistical conclusion validity: The determination of whether a relationship exists between procedures and outcomes (appropriate sampling and measuring procedures along with appropriate statistical tests).
Criterion-related validity : A measurement of the quality of your testing methods against a criterion measure (like a “gold standard” test) that is measured at the same time.
- Examples of validity
Like different types of research and the various ways to measure validity, examples of validity can vary widely. These include:
A questionnaire may be considered valid because each question addresses specific and relevant aspects of the study subject.
In a brand assessment study, researchers can use comparison testing to verify the results of an initial study. For example, the results from a focus group response about brand perception are considered more valid when the results match that of a questionnaire answered by current and potential customers.
A test to measure a class of students' understanding of the English language contains reading, writing, listening, and speaking components to cover the full scope of how language is used.
- Factors that affect research validity
Certain factors can affect research validity in both positive and negative ways. By understanding the factors that improve validity and those that threaten it, you can enhance the validity of your study. These include:
Random selection of participants vs. the selection of participants that are representative of your study criteria
Blinding with interventions the participants are unaware of (like the use of placebos)
Manipulating the experiment by inserting a variable that will change the results
Randomly assigning participants to treatment and control groups to avoid bias
Following specific procedures during the study to avoid unintended effects
Conducting a study in the field instead of a laboratory for more accurate results
Replicating the study with different factors or settings to compare results
Using statistical methods to adjust for inconclusive data
What are the common validity threats in research, and how can their effects be minimized or nullified?
Research validity can be difficult to achieve because of internal and external threats that produce inaccurate results. These factors can jeopardize validity.
History: Events that occur between an early and later measurement
Maturation: The passage of time in a study can include data on actions that would have naturally occurred outside of the settings of the study
Repeated testing: The outcome of repeated tests can change the outcome of followed tests
Selection of subjects: Unconscious bias which can result in the selection of uniform comparison groups
Statistical regression: Choosing subjects based on extremes doesn't yield an accurate outcome for the majority of individuals
Attrition: When the sample group is diminished significantly during the course of the study
Maturation: When subjects mature during the study, and natural maturation is awarded to the effects of the study
While some validity threats can be minimized or wholly nullified, removing all threats from a study is impossible. For example, random selection can remove unconscious bias and statistical regression.
Researchers can even hope to avoid attrition by using smaller study groups. Yet, smaller study groups could potentially affect the research in other ways. The best practice for researchers to prevent validity threats is through careful environmental planning and t reliable data-gathering methods.
- How to ensure validity in your research
Researchers should be mindful of the importance of validity in the early planning stages of any study to avoid inaccurate results. Researchers must take the time to consider tools and methods as well as how the testing environment matches closely with the natural environment in which results will be used.
The following steps can be used to ensure validity in research:
Choose appropriate methods of measurement
Use appropriate sampling to choose test subjects
Create an accurate testing environment
How do you maintain validity in research?
Accurate research is usually conducted over a period of time with different test subjects. To maintain validity across an entire study, you must take specific steps to ensure that gathered data has the same levels of accuracy.
Consistency is crucial for maintaining validity in research. When researchers apply methods consistently and standardize the circumstances under which data is collected, validity can be maintained across the entire study.
Is there a need for validation of the research instrument before its implementation?
An essential part of validity is choosing the right research instrument or method for accurate results. Consider the thermometer that is reliable but still produces inaccurate results. You're unlikely to achieve research validity without activities like calibration, content, and construct validity.
- Understanding research validity for more accurate results
Without validity, research can't provide the accuracy necessary to deliver a useful study. By getting a clear understanding of validity in research, you can take steps to improve your research skills and achieve more accurate results.
Get started today
Go from raw data to valuable insights with a flexible research platform
Editor’s picks
Last updated: 21 December 2023
Last updated: 16 December 2023
Last updated: 6 October 2023
Last updated: 5 March 2024
Last updated: 25 November 2023
Last updated: 15 February 2024
Last updated: 11 March 2024
Last updated: 12 December 2023
Last updated: 6 March 2024
Last updated: 10 April 2023
Last updated: 20 December 2023
Latest articles
Related topics, log in or sign up.
Get started for free
Log in using your username and password
- Search More Search for this keyword Advanced search
- Latest content
- Current issue
- Write for Us
- BMJ Journals More You are viewing from: Google Indexer
You are here
- Volume 18, Issue 2
- Issues of validity and reliability in qualitative research
- Article Text
- Article info
- Citation Tools
- Rapid Responses
- Article metrics

- Helen Noble 1 ,
- Joanna Smith 2
- 1 School of Nursing and Midwifery, Queens's University Belfast , Belfast , UK
- 2 School of Human and Health Sciences, University of Huddersfield , Huddersfield , UK
- Correspondence to Dr Helen Noble School of Nursing and Midwifery, Queens's University Belfast, Medical Biology Centre, 97 Lisburn Rd, Belfast BT9 7BL, UK; helen.noble{at}qub.ac.uk
https://doi.org/10.1136/eb-2015-102054
Statistics from Altmetric.com
Request permissions.
If you wish to reuse any or all of this article please use the link below which will take you to the Copyright Clearance Center’s RightsLink service. You will be able to get a quick price and instant permission to reuse the content in many different ways.
Evaluating the quality of research is essential if findings are to be utilised in practice and incorporated into care delivery. In a previous article we explored ‘bias’ across research designs and outlined strategies to minimise bias. 1 The aim of this article is to further outline rigour, or the integrity in which a study is conducted, and ensure the credibility of findings in relation to qualitative research. Concepts such as reliability, validity and generalisability typically associated with quantitative research and alternative terminology will be compared in relation to their application to qualitative research. In addition, some of the strategies adopted by qualitative researchers to enhance the credibility of their research are outlined.
Are the terms reliability and validity relevant to ensuring credibility in qualitative research?
Although the tests and measures used to establish the validity and reliability of quantitative research cannot be applied to qualitative research, there are ongoing debates about whether terms such as validity, reliability and generalisability are appropriate to evaluate qualitative research. 2–4 In the broadest context these terms are applicable, with validity referring to the integrity and application of the methods undertaken and the precision in which the findings accurately reflect the data, while reliability describes consistency within the employed analytical procedures. 4 However, if qualitative methods are inherently different from quantitative methods in terms of philosophical positions and purpose, then alterative frameworks for establishing rigour are appropriate. 3 Lincoln and Guba 5 offer alternative criteria for demonstrating rigour within qualitative research namely truth value, consistency and neutrality and applicability. Table 1 outlines the differences in terminology and criteria used to evaluate qualitative research.
- View inline
Terminology and criteria used to evaluate the credibility of research findings
What strategies can qualitative researchers adopt to ensure the credibility of the study findings?
Unlike quantitative researchers, who apply statistical methods for establishing validity and reliability of research findings, qualitative researchers aim to design and incorporate methodological strategies to ensure the ‘trustworthiness’ of the findings. Such strategies include:
Accounting for personal biases which may have influenced findings; 6
Acknowledging biases in sampling and ongoing critical reflection of methods to ensure sufficient depth and relevance of data collection and analysis; 3
Meticulous record keeping, demonstrating a clear decision trail and ensuring interpretations of data are consistent and transparent; 3 , 4
Establishing a comparison case/seeking out similarities and differences across accounts to ensure different perspectives are represented; 6 , 7
Including rich and thick verbatim descriptions of participants’ accounts to support findings; 7
Demonstrating clarity in terms of thought processes during data analysis and subsequent interpretations 3 ;
Engaging with other researchers to reduce research bias; 3
Respondent validation: includes inviting participants to comment on the interview transcript and whether the final themes and concepts created adequately reflect the phenomena being investigated; 4
Data triangulation, 3 , 4 whereby different methods and perspectives help produce a more comprehensive set of findings. 8 , 9
Table 2 provides some specific examples of how some of these strategies were utilised to ensure rigour in a study that explored the impact of being a family carer to patients with stage 5 chronic kidney disease managed without dialysis. 10
Strategies for enhancing the credibility of qualitative research
In summary, it is imperative that all qualitative researchers incorporate strategies to enhance the credibility of a study during research design and implementation. Although there is no universally accepted terminology and criteria used to evaluate qualitative research, we have briefly outlined some of the strategies that can enhance the credibility of study findings.
- Sandelowski M
- Lincoln YS ,
- Barrett M ,
- Mayan M , et al
- Greenhalgh T
- Lingard L ,
Twitter Follow Joanna Smith at @josmith175 and Helen Noble at @helnoble
Competing interests None.
Read the full text or download the PDF:
- How it works
Reliability and Validity – Definitions, Types & Examples
Published by Alvin Nicolas at August 16th, 2021 , Revised On October 26, 2023
A researcher must test the collected data before making any conclusion. Every research design needs to be concerned with reliability and validity to measure the quality of the research.
What is Reliability?
Reliability refers to the consistency of the measurement. Reliability shows how trustworthy is the score of the test. If the collected data shows the same results after being tested using various methods and sample groups, the information is reliable. If your method has reliability, the results will be valid.
Example: If you weigh yourself on a weighing scale throughout the day, you’ll get the same results. These are considered reliable results obtained through repeated measures.
Example: If a teacher conducts the same math test of students and repeats it next week with the same questions. If she gets the same score, then the reliability of the test is high.
What is the Validity?
Validity refers to the accuracy of the measurement. Validity shows how a specific test is suitable for a particular situation. If the results are accurate according to the researcher’s situation, explanation, and prediction, then the research is valid.
If the method of measuring is accurate, then it’ll produce accurate results. If a method is reliable, then it’s valid. In contrast, if a method is not reliable, it’s not valid.
Example: Your weighing scale shows different results each time you weigh yourself within a day even after handling it carefully, and weighing before and after meals. Your weighing machine might be malfunctioning. It means your method had low reliability. Hence you are getting inaccurate or inconsistent results that are not valid.
Example: Suppose a questionnaire is distributed among a group of people to check the quality of a skincare product and repeated the same questionnaire with many groups. If you get the same response from various participants, it means the validity of the questionnaire and product is high as it has high reliability.
Most of the time, validity is difficult to measure even though the process of measurement is reliable. It isn’t easy to interpret the real situation.
Example: If the weighing scale shows the same result, let’s say 70 kg each time, even if your actual weight is 55 kg, then it means the weighing scale is malfunctioning. However, it was showing consistent results, but it cannot be considered as reliable. It means the method has low reliability.
Internal Vs. External Validity
One of the key features of randomised designs is that they have significantly high internal and external validity.
Internal validity is the ability to draw a causal link between your treatment and the dependent variable of interest. It means the observed changes should be due to the experiment conducted, and any external factor should not influence the variables .
Example: age, level, height, and grade.
External validity is the ability to identify and generalise your study outcomes to the population at large. The relationship between the study’s situation and the situations outside the study is considered external validity.
Also, read about Inductive vs Deductive reasoning in this article.
Looking for reliable dissertation support?
We hear you.
- Whether you want a full dissertation written or need help forming a dissertation proposal, we can help you with both.
- Get different dissertation services at ResearchProspect and score amazing grades!
Threats to Interval Validity
Threats of external validity, how to assess reliability and validity.
Reliability can be measured by comparing the consistency of the procedure and its results. There are various methods to measure validity and reliability. Reliability can be measured through various statistical methods depending on the types of validity, as explained below:
Types of Reliability
Types of validity.
As we discussed above, the reliability of the measurement alone cannot determine its validity. Validity is difficult to be measured even if the method is reliable. The following type of tests is conducted for measuring validity.
Does your Research Methodology Have the Following?
- Great Research/Sources
- Perfect Language
- Accurate Sources
If not, we can help. Our panel of experts makes sure to keep the 3 pillars of Research Methodology strong.
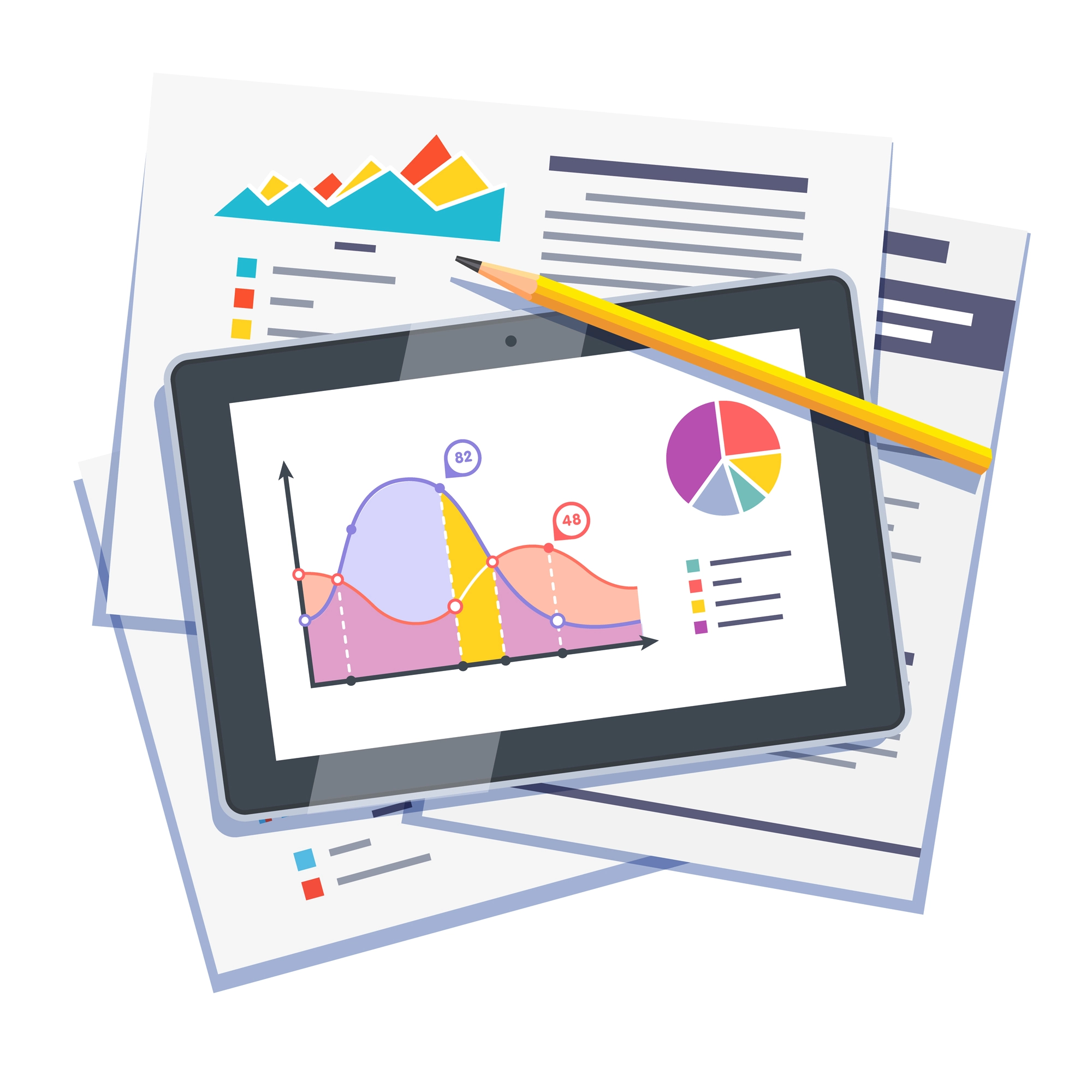
How to Increase Reliability?
- Use an appropriate questionnaire to measure the competency level.
- Ensure a consistent environment for participants
- Make the participants familiar with the criteria of assessment.
- Train the participants appropriately.
- Analyse the research items regularly to avoid poor performance.
How to Increase Validity?
Ensuring Validity is also not an easy job. A proper functioning method to ensure validity is given below:
- The reactivity should be minimised at the first concern.
- The Hawthorne effect should be reduced.
- The respondents should be motivated.
- The intervals between the pre-test and post-test should not be lengthy.
- Dropout rates should be avoided.
- The inter-rater reliability should be ensured.
- Control and experimental groups should be matched with each other.
How to Implement Reliability and Validity in your Thesis?
According to the experts, it is helpful if to implement the concept of reliability and Validity. Especially, in the thesis and the dissertation, these concepts are adopted much. The method for implementation given below:
Frequently Asked Questions
What is reliability and validity in research.
Reliability in research refers to the consistency and stability of measurements or findings. Validity relates to the accuracy and truthfulness of results, measuring what the study intends to. Both are crucial for trustworthy and credible research outcomes.
What is validity?
Validity in research refers to the extent to which a study accurately measures what it intends to measure. It ensures that the results are truly representative of the phenomena under investigation. Without validity, research findings may be irrelevant, misleading, or incorrect, limiting their applicability and credibility.
What is reliability?
Reliability in research refers to the consistency and stability of measurements over time. If a study is reliable, repeating the experiment or test under the same conditions should produce similar results. Without reliability, findings become unpredictable and lack dependability, potentially undermining the study’s credibility and generalisability.
What is reliability in psychology?
In psychology, reliability refers to the consistency of a measurement tool or test. A reliable psychological assessment produces stable and consistent results across different times, situations, or raters. It ensures that an instrument’s scores are not due to random error, making the findings dependable and reproducible in similar conditions.
What is test retest reliability?
Test-retest reliability assesses the consistency of measurements taken by a test over time. It involves administering the same test to the same participants at two different points in time and comparing the results. A high correlation between the scores indicates that the test produces stable and consistent results over time.
How to improve reliability of an experiment?
- Standardise procedures and instructions.
- Use consistent and precise measurement tools.
- Train observers or raters to reduce subjective judgments.
- Increase sample size to reduce random errors.
- Conduct pilot studies to refine methods.
- Repeat measurements or use multiple methods.
- Address potential sources of variability.
What is the difference between reliability and validity?
Reliability refers to the consistency and repeatability of measurements, ensuring results are stable over time. Validity indicates how well an instrument measures what it’s intended to measure, ensuring accuracy and relevance. While a test can be reliable without being valid, a valid test must inherently be reliable. Both are essential for credible research.
Are interviews reliable and valid?
Interviews can be both reliable and valid, but they are susceptible to biases. The reliability and validity depend on the design, structure, and execution of the interview. Structured interviews with standardised questions improve reliability. Validity is enhanced when questions accurately capture the intended construct and when interviewer biases are minimised.
Are IQ tests valid and reliable?
IQ tests are generally considered reliable, producing consistent scores over time. Their validity, however, is a subject of debate. While they effectively measure certain cognitive skills, whether they capture the entirety of “intelligence” or predict success in all life areas is contested. Cultural bias and over-reliance on tests are also concerns.
Are questionnaires reliable and valid?
Questionnaires can be both reliable and valid if well-designed. Reliability is achieved when they produce consistent results over time or across similar populations. Validity is ensured when questions accurately measure the intended construct. However, factors like poorly phrased questions, respondent bias, and lack of standardisation can compromise their reliability and validity.
You May Also Like
A hypothesis is a research question that has to be proved correct or incorrect through hypothesis testing – a scientific approach to test a hypothesis.
A case study is a detailed analysis of a situation concerning organizations, industries, and markets. The case study generally aims at identifying the weak areas.
Discourse analysis is an essential aspect of studying a language. It is used in various disciplines of social science and humanities such as linguistic, sociolinguistics, and psycholinguistic.
USEFUL LINKS
LEARNING RESOURCES
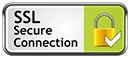
COMPANY DETAILS
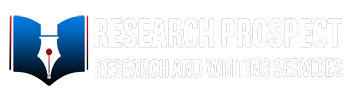
- How It Works
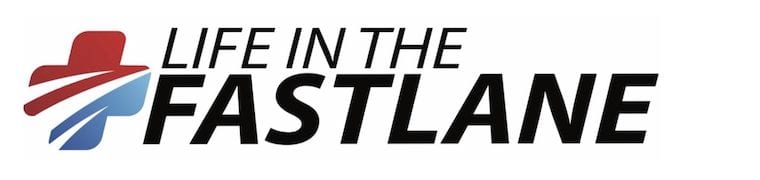
Validity of Research and Measurements
Chris nickson.
- Nov 3, 2020
In general terms, validity is “the quality of being true or correct”, it refers to the strength of results and how accurately they reflect the real world. Thus ‘validity’ can have quite different meanings depending on the context!
- Reliability is distinct from validity, in that it refers to the consistency or repeatability of results
- internal validity
- external validity
- Validity applies to an outcome or measurement, not the instrument used to obtain it and is based on ‘validity evidence’
INTERNAL VALIDITY
- The extent to which the design and conduct of the trial eliminate the possibility of bias, such that observed effects can be attributed to the independent variable
- refers to the accuracy of a trial
- a study that lacks internal validity should not applied to any clinical setting
- power calculation
- details of study context and intervention
- avoid loss of follow up
- standardised treatment conditions
- control groups
- objectivity from blinding and data handling
- Clinical research can be internally valid despite poor external validity
EXTERNAL VALIDITY
- The extent to which the results of a trial provide a correct basis for generalizations to other circumstances
- Also called “generalizability or “applicability”
- Studies can only be applied to clinical settings the same, or similar, to those used in the study
- population validity – how well the study sample can be extrapolated to the population as a whole (based on randomized sampling)
- ecological validity – the extent to which the study environment influences results (can the study be replicated in other contexts?)
- internal/ construct validity – verified relationships between dependent and independent variables
- Research findings cannot have external validity without being internally valid
FACTORS THAT AFFECT EXTERNAL VALIDITY OF CLINICAL RESEARCH (Rothwell, 2006)
Setting of the trial
- healthcare system
- recruitment from primary, secondary or tertiary care
- selection of participating centers
- selection of participating clinicians
Selection of patients
- methods of pre-randomisation diagnosis and investigation
- eligibility criteria
- exclusion criteria
- placebo run-in period
- treatment run-in period
- “enrichment” strategies
- ratio of randomised patients to eligible non-randomised patients in participating centers
- proportion of patients who decline randomisation
Characteristics of randomised patients
- baseline clinical characteristics
- racial group
- uniformity of underlying pathology
- stage in the natural history of disease
- severity of disease
- comorbidity
- absolute risk of a poor outcome in the control group
Differences between trial protocol and routine practice
- trial intervention
- timing of treatment
- appropriateness/ relevance of control intervention
- adequacy of nontrial treatment – both intended and actual
- prohibition of certain non-trial treatments
- Therapeutic or diagnostic advances since trial was performed
Outcome measures and follow up
- clinical relevance of surrogate outcomes
- clinical relevance, validity, and reproducibility of complex scales
- effect of intervention on most relevant components of composite outcomes
- identification of who measured outcome
- use of patient outcomes
- frequency of follow up
- adequacy of length of follow-up
Adverse effects of treatment
- completeness of reporting of relevant adverse effects
- rate of discontinuation of treatment
- selection of trial centers on the basis of skill or experience
- exclusion of patients at risk of complications
- exclusion of patients who experienced adverse events during a run in period
- intensity of trial safety procedures
MEASUREMENT VALIDITY (Downing & Yudkowsky, 2009)
Validity refers to the evidence presented to support or to refute the meaning or interpretation assigned to assessment data or results. It relates to whether a test, tool, instrument or device actually measures what it intends to measure.
Traditionally validity was viewed as a trinatarian concept based on:
- degree to which the the test measures what it is meant to be measuring
- e.g. the ideal depression score would include different variants of depression and be able to distinguish depression from stress and anxiety
- Concurrent validity – compares measurements with an outcome at the same time (e.g. a concurrent “gold standard” test result)
- Predictive validity – compares measurements with an outcome at the same time (e.g. do high exam marks predict subsequent incomes?)
- the degree to which the content of an instrument is an adequate reflection of all the components of the construct
- e.g. a schizophrenia score would need to include both positive and negative symptoms
According to current validity theory in psychometrics, validity is a unitary concept and thus construct validity is the only form of validity. For instance in health professions education, validity evidence for assessments comes from (:
- relationship between test content and the construct of interest
- theory; hypothesis about content
- independent assessment of match between content sampled and domain of interest
- solid, scientific, quantitative evidence
- analysis of individual responses to stimuli
- debriefing of examinees
- process studies aimed at understanding what is measured and the soundness of intended score interpretations
- quality assurance and quality control of assessment data
- data internal to assessments such as: reliability or reproducibility of scores; inter-item correlations; statistical characteristics of items; statistical analysis of item option function; factor studies of dimensionality; Differential Item Functioning (DIF) studies
- a. Convergent and discriminant evidence: relationships between similar and different measures
- b. Test-criterion evidence: relationships between test and criterion measure(s)
- c. Validity generalization: can the validity evidence be generalized? Evidence that the validity studies may generalize to other settings.
- intended and unintended consequences of test use
- differential consequences of test use
- impact of assessment on students, instructors, schools, society
- impact of assessments on curriculum; cost/benefit analysis with respect to tradeoff between instructional time and assessment time.
- Note that strictly speaking we cannot comment on the validity of a test, tool, instrument, or device, only on the measurement that is obtained. This is because the the same test used in a different context (different operator, different subjects, different circumstances, at a different time) may not be valid. In other words, validity evidence applies to the data generated by an instrument, not the instrument itself.
- Validity can be equated with accuracy, and reliability with precision
- Face validity is a term commonly used as an indicator of validity – it is essential worthless! It means at ‘face value’, in other words, the degree to which the measure subjectively looks like what it is intended to measure.
- The higher the stakes of measurement (e.g. test result), the higher the need for validity evidence.
- You can never have too much validity evidence, but the minimum required varies with purpose (e.g. high stakes fellowship exam versus one of many progress tests)
References and Links
Journal articles and Textbooks
- Downing SM, Yudkowsky R. (2009) Assessment in health professions education, Routledge, New York.
- Rothwell PM. Factors that can affect the external validity of randomised controlled trials. PLoS Clin Trials. 2006 May;1(1):e9. [ pubmed ] [ article ]
- Shankar-Hari M, Bertolini G, Brunkhorst FM, et al. Judging quality of current septic shock definitions and criteria. Critical care. 19(1):445. 2015. [ pubmed ] [ article ]
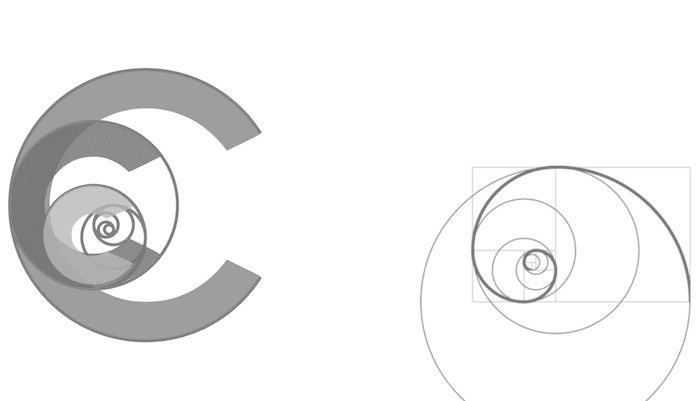
Critical Care
Chris is an Intensivist and ECMO specialist at the Alfred ICU in Melbourne. He is also a Clinical Adjunct Associate Professor at Monash University . He is a co-founder of the Australia and New Zealand Clinician Educator Network (ANZCEN) and is the Lead for the ANZCEN Clinician Educator Incubator programme. He is on the Board of Directors for the Intensive Care Foundation and is a First Part Examiner for the College of Intensive Care Medicine . He is an internationally recognised Clinician Educator with a passion for helping clinicians learn and for improving the clinical performance of individuals and collectives.
After finishing his medical degree at the University of Auckland, he continued post-graduate training in New Zealand as well as Australia’s Northern Territory, Perth and Melbourne. He has completed fellowship training in both intensive care medicine and emergency medicine, as well as post-graduate training in biochemistry, clinical toxicology, clinical epidemiology, and health professional education.
He is actively involved in in using translational simulation to improve patient care and the design of processes and systems at Alfred Health. He coordinates the Alfred ICU’s education and simulation programmes and runs the unit’s education website, INTENSIVE . He created the ‘Critically Ill Airway’ course and teaches on numerous courses around the world. He is one of the founders of the FOAM movement (Free Open-Access Medical education) and is co-creator of litfl.com , the RAGE podcast , the Resuscitology course, and the SMACC conference.
His one great achievement is being the father of three amazing children.
On Twitter, he is @precordialthump .
| INTENSIVE | RAGE | Resuscitology | SMACC
Leave a Reply Cancel reply
This site uses Akismet to reduce spam. Learn how your comment data is processed .
Privacy Overview
- Introduction
- Conclusions
- Article Information
Screenshots of the smartphone cognitive tasks developed by Datacubed Health and included in the ALLFTD Mobile App. Details about the task design and instructions are included in the eMethods in Supplement 1. A, Flanker (Ducks in a Pond) is a task of cognitive control requiring participants to select the direction of the center duck. B, Go/no-go (Go Sushi Go!) requires participants to quickly tap on pieces of sushi (go) but not to tap when they see a fish skeleton (no-go). C, Card sort (Card Shuffle) is a task of cognitive flexibility requiring participants to learn rules that change during the task. D, The adaptative, associative memory task (Humi’s Bistro) requires participants to learn the food orders of several restaurant tables. E, Stroop (Color Clash) is a cognitive inhibition paradigm requiring participants to inhibit their tendency to read words and instead respond based on the color of the word. F, The 2-back task (Animal Parade) requires participants to determine whether animals on a parade float match the animals they saw 2 stimuli previously. G, Participants are asked to complete 3 testing sessions over 2 weeks. Shown in dark blue, they have 3 days to complete each testing session with a washout day between sessions on which no tests are available. Session 2 always begins on day 5 and session 3 on day 9. Screenshots are provided with permission from Datacubed Health.
Forest plots present internal consistency and test-retest reliability results in the discovery and validation cohorts, as well as an estimate in a combined sample of discovery and validation participants. ICC indicates interclass correlation coefficient.
A and B, Correlation matrices display associations of in-clinic criterion standard measures and ALLFTD mobile App (mApp) test scores in discovery and validation cohorts. Below the horizontal dashed lines, the associations among app tests and between app tests and demographic characteristics convergent clinical measures, divergent cognitive tests, and neuroimaging regions of interest can be viewed. Most app tests show strong correlations with each other and with age, convergent clinical measures, and brain volume. The measures show weaker correlations with divergent measures of visuospatial (Benson Figure Copy) and language (Multilingual Naming Test [MINT]) abilities. The strength of convergent correlations between app measures and outcomes is similar to the correlations between criterion standard neuropsychological scores and these outcomes, which can be viewed by looking across the rows above the horizontal black line. C and D, In the discovery and validation cohorts, receiver operating characteristics curves were calculated to determine how well a composite of app tests, the Uniform Data Set, version 3.0, Executive Functioning Composite (UDS3-EF), and the Montreal Cognitive Assessment (MoCA) discriminate individuals without symptoms (Clinical Dementia Rating Scale plus National Alzheimer’s Coordinating Center FTLD module sum of boxes [CDR plus NACC-FTLD-SB] score = 0) from individuals with the mildest symptoms of FTLD (CDR plus NACC-FTLD-SB score = 0.5). AUC indicates area under the curve; CVLT, California Verbal Learning Test.
eMethods. Instruments and Statistical Analysis
eResults. Participants
eTable 1. Participant Characteristics and Test Scores in Original and Validation Cohorts
eTable 2. Comparison of Diagnostic Accuracy for ALLFTD Mobile App Composite Score Across Cohorts
eTable 3. Number of Distractions Reported During the Remote Smartphone Testing Sessions
eTable 4. Qualitative Description of the Distractions Reported During Remote Testing Sessions
eFigure 1. Scatterplots of Test-Retest Reliability in a Mixed Sample of Adults Without Functional Impairment and Participants With FTLD
eFigure 2. Comparison of Test-Retest Reliability Estimates by Endorsement of Distractions
eFigure 3. Comparison of Test-Retest Reliability Estimates by Operating System
eFigure 4. Correlation Matrix in the Combined Cohort
eFigure 5. Neural Correlates of Smartphone Cognitive Test Performance
eReferences
Nonauthor Collaborators
Data Sharing Statement
See More About
Sign up for emails based on your interests, select your interests.
Customize your JAMA Network experience by selecting one or more topics from the list below.
- Academic Medicine
- Acid Base, Electrolytes, Fluids
- Allergy and Clinical Immunology
- American Indian or Alaska Natives
- Anesthesiology
- Anticoagulation
- Art and Images in Psychiatry
- Artificial Intelligence
- Assisted Reproduction
- Bleeding and Transfusion
- Caring for the Critically Ill Patient
- Challenges in Clinical Electrocardiography
- Climate and Health
- Climate Change
- Clinical Challenge
- Clinical Decision Support
- Clinical Implications of Basic Neuroscience
- Clinical Pharmacy and Pharmacology
- Complementary and Alternative Medicine
- Consensus Statements
- Coronavirus (COVID-19)
- Critical Care Medicine
- Cultural Competency
- Dental Medicine
- Dermatology
- Diabetes and Endocrinology
- Diagnostic Test Interpretation
- Drug Development
- Electronic Health Records
- Emergency Medicine
- End of Life, Hospice, Palliative Care
- Environmental Health
- Equity, Diversity, and Inclusion
- Facial Plastic Surgery
- Gastroenterology and Hepatology
- Genetics and Genomics
- Genomics and Precision Health
- Global Health
- Guide to Statistics and Methods
- Hair Disorders
- Health Care Delivery Models
- Health Care Economics, Insurance, Payment
- Health Care Quality
- Health Care Reform
- Health Care Safety
- Health Care Workforce
- Health Disparities
- Health Inequities
- Health Policy
- Health Systems Science
- History of Medicine
- Hypertension
- Images in Neurology
- Implementation Science
- Infectious Diseases
- Innovations in Health Care Delivery
- JAMA Infographic
- Law and Medicine
- Leading Change
- Less is More
- LGBTQIA Medicine
- Lifestyle Behaviors
- Medical Coding
- Medical Devices and Equipment
- Medical Education
- Medical Education and Training
- Medical Journals and Publishing
- Mobile Health and Telemedicine
- Narrative Medicine
- Neuroscience and Psychiatry
- Notable Notes
- Nutrition, Obesity, Exercise
- Obstetrics and Gynecology
- Occupational Health
- Ophthalmology
- Orthopedics
- Otolaryngology
- Pain Medicine
- Palliative Care
- Pathology and Laboratory Medicine
- Patient Care
- Patient Information
- Performance Improvement
- Performance Measures
- Perioperative Care and Consultation
- Pharmacoeconomics
- Pharmacoepidemiology
- Pharmacogenetics
- Pharmacy and Clinical Pharmacology
- Physical Medicine and Rehabilitation
- Physical Therapy
- Physician Leadership
- Population Health
- Primary Care
- Professional Well-being
- Professionalism
- Psychiatry and Behavioral Health
- Public Health
- Pulmonary Medicine
- Regulatory Agencies
- Reproductive Health
- Research, Methods, Statistics
- Resuscitation
- Rheumatology
- Risk Management
- Scientific Discovery and the Future of Medicine
- Shared Decision Making and Communication
- Sleep Medicine
- Sports Medicine
- Stem Cell Transplantation
- Substance Use and Addiction Medicine
- Surgical Innovation
- Surgical Pearls
- Teachable Moment
- Technology and Finance
- The Art of JAMA
- The Arts and Medicine
- The Rational Clinical Examination
- Tobacco and e-Cigarettes
- Translational Medicine
- Trauma and Injury
- Treatment Adherence
- Ultrasonography
- Users' Guide to the Medical Literature
- Vaccination
- Venous Thromboembolism
- Veterans Health
- Women's Health
- Workflow and Process
- Wound Care, Infection, Healing
Get the latest research based on your areas of interest.
Others also liked.
- Download PDF
- X Facebook More LinkedIn
Staffaroni AM , Clark AL , Taylor JC, et al. Reliability and Validity of Smartphone Cognitive Testing for Frontotemporal Lobar Degeneration. JAMA Netw Open. 2024;7(4):e244266. doi:10.1001/jamanetworkopen.2024.4266
Manage citations:
© 2024
- Permissions
Reliability and Validity of Smartphone Cognitive Testing for Frontotemporal Lobar Degeneration
- 1 Department of Neurology, Memory and Aging Center, Weill Institute for Neurosciences, University of California, San Francisco
- 2 Department of Neurology, Columbia University, New York, New York
- 3 Department of Neurology, Mayo Clinic, Rochester, Minnesota
- 4 Department of Quantitative Health Sciences, Division of Biomedical Statistics and Informatics, Mayo Clinic, Rochester, Minnesota
- 5 Department of Neurology, Case Western Reserve University, Cleveland, Ohio
- 6 Department of Neurosciences, University of California, San Diego, La Jolla
- 7 Department of Radiology, University of North Carolina, Chapel Hill
- 8 Department of Neurology, Indiana University, Indianapolis
- 9 Department of Neurology, Vanderbilt University, Nashville, Tennessee
- 10 Department of Neurology, University of Washington, Seattle
- 11 Department of Psychiatry and Psychology, Mayo Clinic, Rochester, Minnesota
- 12 Department of Neurology, Institute for Precision Health, University of California, Los Angeles
- 13 Department of Neurology, Knight Alzheimer Disease Research Center, Washington University, Saint Louis, Missouri
- 14 Department of Psychiatry, Knight Alzheimer Disease Research Center, Washington University, Saint Louis, Missouri
- 15 Department of Neuroscience, Mayo Clinic, Jacksonville, Florida
- 16 Department of Neurology, University of Pennsylvania Perelman School of Medicine, Philadelphia
- 17 Division of Neurology, University of British Columbia, Musqueam, Squamish & Tsleil-Waututh Traditional Territory, Vancouver, Canada
- 18 Department of Neurosciences, University of California, San Diego, La Jolla
- 19 Department of Neurology, Nantz National Alzheimer Center, Houston Methodist and Weill Cornell Medicine, Houston Methodist, Houston, Texas
- 20 Department of Neurology, UCLA (University of California, Los Angeles)
- 21 Department of Neurology, University of Colorado, Aurora
- 22 Department of Neurology, David Geffen School of Medicine, UCLA
- 23 Department of Neurology, University of Alabama, Birmingham
- 24 Tanz Centre for Research in Neurodegenerative Diseases, Division of Neurology, University of Toronto, Toronto, Ontario, Canada
- 25 Department of Neurology, Massachusetts General Hospital and Harvard Medical School, Boston
- 26 Department of Epidemiology and Biostatistics, University of California, San Francisco
- 27 Department of Psychological & Brain Sciences, Washington University, Saint Louis, Missouri
Question Can remote cognitive testing via smartphones yield reliable and valid data for frontotemporal lobar degeneration (FTLD)?
Findings In this cohort study of 360 patients, remotely deployed smartphone cognitive tests showed moderate to excellent reliability comparedwith criterion standard measures (in-person disease severity assessments and neuropsychological tests) and brain volumes. Smartphone tests accurately detected dementia and were more sensitive to the earliest stages of familial FTLD than standard neuropsychological tests.
Meaning These findings suggest that remotely deployed smartphone-based assessments may be reliable and valid tools for evaluating FTLD and may enhance early detection, supporting the inclusion of digital assessments in clinical trials for neurodegeneration.
Importance Frontotemporal lobar degeneration (FTLD) is relatively rare, behavioral and motor symptoms increase travel burden, and standard neuropsychological tests are not sensitive to early-stage disease. Remote smartphone-based cognitive assessments could mitigate these barriers to trial recruitment and success, but no such tools are validated for FTLD.
Objective To evaluate the reliability and validity of smartphone-based cognitive measures for remote FTLD evaluations.
Design, Setting, and Participants In this cohort study conducted from January 10, 2019, to July 31, 2023, controls and participants with FTLD performed smartphone application (app)–based executive functioning tasks and an associative memory task 3 times over 2 weeks. Observational research participants were enrolled through 18 centers of a North American FTLD research consortium (ALLFTD) and were asked to complete the tests remotely using their own smartphones. Of 1163 eligible individuals (enrolled in parent studies), 360 were enrolled in the present study; 364 refused and 439 were excluded. Participants were divided into discovery (n = 258) and validation (n = 102) cohorts. Among 329 participants with data available on disease stage, 195 were asymptomatic or had preclinical FTLD (59.3%), 66 had prodromal FTLD (20.1%), and 68 had symptomatic FTLD (20.7%) with a range of clinical syndromes.
Exposure Participants completed standard in-clinic measures and remotely administered ALLFTD mobile app (app) smartphone tests.
Main Outcomes and Measures Internal consistency, test-retest reliability, association of smartphone tests with criterion standard clinical measures, and diagnostic accuracy.
Results In the 360 participants (mean [SD] age, 54.0 [15.4] years; 209 [58.1%] women), smartphone tests showed moderate-to-excellent reliability (intraclass correlation coefficients, 0.77-0.95). Validity was supported by association of smartphones tests with disease severity ( r range, 0.38-0.59), criterion-standard neuropsychological tests ( r range, 0.40-0.66), and brain volume (standardized β range, 0.34-0.50). Smartphone tests accurately differentiated individuals with dementia from controls (area under the curve [AUC], 0.93 [95% CI, 0.90-0.96]) and were more sensitive to early symptoms (AUC, 0.82 [95% CI, 0.76-0.88]) than the Montreal Cognitive Assessment (AUC, 0.68 [95% CI, 0.59-0.78]) ( z of comparison, −2.49 [95% CI, −0.19 to −0.02]; P = .01). Reliability and validity findings were highly similar in the discovery and validation cohorts. Preclinical participants who carried pathogenic variants performed significantly worse than noncarrier family controls on 3 app tasks (eg, 2-back β = −0.49 [95% CI, −0.72 to −0.25]; P < .001) but not a composite of traditional neuropsychological measures (β = −0.14 [95% CI, −0.42 to 0.14]; P = .32).
Conclusions and Relevance The findings of this cohort study suggest that smartphones could offer a feasible, reliable, valid, and scalable solution for remote evaluations of FTLD and may improve early detection. Smartphone assessments should be considered as a complementary approach to traditional in-person trial designs. Future research should validate these results in diverse populations and evaluate the utility of these tests for longitudinal monitoring.
Frontotemporal lobar degeneration (FTLD) is a neurodegenerative pathology causing early-onset dementia syndromes with impaired behavior, cognition, language, and/or motor functioning. 1 Although over 30 FTLD trials are planned or in progress, there are several barriers to conducting FTLD trials. Clinical trials for neurodegenerative disease are expensive, 2 and frequent in-person trial visits are burdensome for patients, caregivers, and clinicians, 3 a concern magnified in FTLD by behavioral and motor impairments. Given the rarity and geographical dispersion of eligible participants, FTLD trials require global recruitment, 4 particularly for those that are far from expert FTLD clinical trial centers. Furthermore, criterion standard neuropsychological tests are not adequately sensitive until symptoms are already noticeable to families, limiting their usefulness as outcomes in early-stage FTLD treatment trials. 4
Reliable, valid, and scalable remote data collection methods may help surmount these barriers to FTLD clinical trials. Smartphones are garnering interest across neurological conditions as a method for administering remote cognitive and motor evaluations. Preliminary evidence supports the feasibility, reliability, and/or validity of unsupervised smartphone cognitive and motor testing in older adults at risk for Alzheimer disease, 5 - 8 Parkinson disease, 9 and Huntington disease. 10 The clinical heterogeneity of FTLD necessitates a uniquely comprehensive smartphone battery. In the ALLFTD Consortium (Advancing Research and Treatment in Frontotemporal Lobar Degeneration [ARTFLD] and Longitudinal Evaluation of Familial Frontotemporal Dementia Subjects [LEFFTDS]), the ALLFTD mobile Application (ALLFTD-mApp) was designed to remotely monitor cognitive, behavioral, language, and motor functioning in FTLD research. Taylor et al 11 recently reported that unsupervised ALLFTD-mApp data collection through a multicenter North American FTLD research network was feasible and acceptable to participants. Herein, we extend that work by investigating the reliability and validity of unsupervised remote smartphone tests of executive functioning and memory in a cohort with FTLD that has undergone extensive phenotyping.
Participants were enrolled from ongoing FTLD studies requiring in-person assessment, including participants from 18 centers from the ALLFTD study study 12 and University of California, San Francisco (UCSF) FTLD studies. To study the app in older individuals, a small group of older adults without functional impairment was recruited from the UCSF Brain Aging Network for Cognitive Health. All study procedures were approved by the UCSF or Johns Hopkins Central Institutional Review Board. All participants or legally authorized representatives provided written informed consent. The study followed the Strengthening the Reporting of Observational Studies in Epidemiology ( STROBE ) reporting guideline.
Inclusion criteria were age 18 years or older, having access to a smartphone, and reporting English as the primary language. Race and ethnicity were self reported by participants using options consistent with the National Alzheimer’s Coordinating Center (NACC) Uniform Data Set (UDS) and were collected to contextualize the generalizability of these results. Participants were asked to complete tests on their own smartphones. Informants were encouraged for all participants and required for those with symptomatic FTLD (Clinical Dementia Rating Scale plus NACC FTLD module [CDR plus NACC-FTLD] global score ≥1). Recruitment targeted individuals with CDR plus NACC-FTLD global scores less than 2, but sites had discretion to enroll more severely impaired participants. Exclusion criteria were consistent with the parent ALLFTD study. 12
Participants were enrolled in the ALLFTD-mApp study within 90 days of annual ALLFTD study visits (including neuropsychological and neuroimaging data collection). Site research coordinators (including J.C.T., A.B.W., S.D., and M.M.) assisted participants with app download, setup, and orientation and observed participants completing the first questionnaire. All cognitive tasks were self-administered without supervision (except pilot participants, discussed below) in a predefined order with minor adjustments throughout the study. Study partners of participants with symptomatic FTLD were asked to remain nearby during participation to help navigate the ALLFTD-mApp but were asked not to assist with testing.
The baseline participation window was divided into three 25- to 35-minute assessment sessions occurring over 11 days. All cognitive tests were repeated in every session to enhance task reliability 6 , 13 and enable assessment of test-retest reliability, except for card sort, which was administered once every 6 months due to expected practice effects. Adherence was defined as the percentage of all available tasks that were completed. Participants were asked to complete the triplicate of sessions every 6 months for the duration of the app study. Only the baseline triplicate was analyzed in this study.
Replicability was tested by dividing the sample into a discovery cohort (n = 258) comprising all participants enrolled until the initial data freeze (October 1, 2022) and a validation cohort (n = 102) comprising participants enrolled after October 1, 2022, and 18 pilot participants 11 who completed the first session in person with an examiner present during cognitive pretesting. Sensitivity analyses excluded this small pilot cohort.
ALLFTD investigators partnered with Datacubed Health 14 to develop the ALLFTD-mApp on Datacubed Health’s Linkt platform. The app includes cognitive, motor, and speech tasks. This study focuses on 6 cognitive tests developed by Datacubed Health 11 comprising an adaptive associative memory task (Humi’s Bistro) and gamified versions of classic executive functioning paradigms: flanker (Ducks in a Pond), Stroop (Color Clash), 2-back (Animal Parade), go/no-go (Go Sushi Go!), and card sort (Card Shuffle) ( Figure 1 and eMethods in Supplement 1 ). Most participants with symptomatic FTLD (49 [72.1%]) were not administered Stroop or 2-back, as pilot studies identified these as too difficult. 11 The app test results were summarized as a composite score (eMethods in Supplement 1 ). Participants completed surveys to assess technological familiarity (daily or less than daily use of a smartphone) and distractions (present or absent).
Criterion standard clinical data were collected during parent project visits. Syndromic diagnoses were made according to published criteria 15 - 19 based on multidisciplinary conferences that considered neurological history, neurological examination results, and collateral interview. 20
The CDR plus NACC-FTLD module is an 8-domain rating scale based on informant and participant report. 21 A global score was calculated to categorize disease severity as asymptomatic or preclinical if a pathogenic variant carrier (0), prodromal (0.5), or symptomatic (1.0-3.0). 22 A sum of the 8 domain box scores (CDR plus NACC-FTLD sum of boxes) was also calculated. 22
Participants completed the UDS Neuropsychological Battery, version 3.0 23 (eMethods in Supplement 1 ), which includes traditional neuropsychological measures and the Montreal Cognitive Assessment (MoCA), a global cognitive screen. Executive functioning and processing speed measures were summarized into a composite score (UDS3-EF). 24 Participants also completed a 9-item list-learning memory test (California Verbal Learning Test, 2nd edition, Short Form). 25 Most (339 [94.2%]) neuropsychological evaluations were conducted in person. In a subsample (n = 270), motor speed and dexterity were assessed using the Movement Disorder Society Uniform Parkinson Disease Rating Scale 26 Finger Tapping subscale (0 indicates no deficits [n = 240]).
We acquired T1-weighted brain magnetic resonance imaging for 199 participants. Details of image acquisition, harmonization, preprocessing, and processing are provided in eMethods in Supplement 1 and prior publications. 27 Briefly, SPM12 (Statistical Parametric Mapping) was used for segmentation 28 and Large Deformation Diffeomorphic Metric Mapping for generating group templates. 29 Gray matter volumes were calculated in template space by integrating voxels and dividing by total intracranial volume in 2 regions of interest (ROIs) 30 : a frontoparietal and subcortical ROI and a hippocampal ROI. Voxel-based morphometry was used to test unbiased voxel-wise associations of volume with smartphone tests (eMethods in Supplement 1 ). 31 , 32
Participants in the ALLFTD study underwent genetic testing 33 at the University of California, Los Angeles. DNA samples were screened using targeted sequencing of a custom panel of genes previously implicated in neurodegenerative diseases, including GRN ( 138945 ) and MAPT ( 157140 ). Hexanucleotide repeat expansions in C9orf72 ( 614260 ) were detected using both fluorescent and repeat-primed polymerase chain reaction analysis. 34
Statistical analyses were conducted using Stata, version 17.0 (StataCorp LLC), and R, version 4.4.2 (R Project for Statistical Computing). All tests were 2 sided, with a statistical significance threshold of P < .05.
Psychometric properties of the smartphone tests were explored using descriptive statistics. Comparisons between CDR plus NACC-FTLD groups (ie, asymptomatic or preclinical, prodromal, and symptomatic) for continuous variables, including demographic characteristics and cognitive task scores (first exposure to each measure), were analyzed by fitting linear regressions. We used χ 2 difference tests for frequency data (eg, sex and race and ethnicity).
Internal consistency, which measures reliability within a task, was estimated for participants’ first exposure to each test using Cronbach α (details in eMethods in Supplement 1 ). Test-retest reliability was estimated using intraclass correlation coefficients for participants who completed a task at least twice; all exposures were included. Reliability estimates are described as poor (<0.500), moderate (0.500-0.749), good (0.750-0.890), and excellent (≥0.900) 35 ; these are reporting rules of thumb, and clinical interpretation should consider raw estimates. We calculated 95% CIs via bootstrapping with 1000 samples.
Validity analyses used participants’ first exposure to each test. Linear regressions were fitted in participants without symptoms with age, sex, and educational level as independent variables to understand the unique contribution of each demographic factor to cognitive test scores. Correlations and linear regression between the app-based tasks and disease severity (CDR plus NACC-FTLD sum of boxes score), neuropsychological test scores, and gray matter ROIs were used to investigate construct validity in the full sample. Demographic characteristics were not entered as covariates because the primary goal was to assess associations between app-based measures and criterion standards, rather than understand the incremental predictive value of app measures. To address potential motor confounds, associations with disease severity were evaluated in a subsample without finger dexterity deficits on motor examination (using the Movement Disorder Society Uniform Parkinson Disease Rating Scale Finger Tapping subscale). To complement ROI-based neuroimaging analysis based on a priori hypotheses, we conducted voxel-based morphometry (eMethods in Supplement 1 ) to uncover other potential neural correlates of test performance. 31 , 32 Finally, we evaluated the association of the number of distractions and operating system with reliability and validity, controlling for age and disease severity, which are predictive factors associated with test performance in correlation analyses.
To evaluate the app’s ability to select participants with prodromal or symptomatic FTLD for trial enrollment, we tested discrimination of participants without symptoms from those with prodromal and symptomatic FTLD. To understand the app’s utility for screening early cognitive impairment, we fit receiver operating characteristics curves testing the predictive value of the app composite, UDS3-EF, and MoCA for differentiating participants without symptoms and those with preclinical FTLD from those with prodromal FTLD; areas under the curves (AUC) for the app and MoCA were compared using the DeLong test in participants with results for both predictive factors.
We compared app performance in preclinical participants who carried pathogenic variants with that in noncarrier controls using linear regression adjusted for age (a predictive factor in earlier models). For this analysis, we excluded those younger than 45 years to remove participants likely to be years from symptom onset based on natural history studies. 4 We analyzed memory performance in participants who carried MAPT pathogenic variants, as early executive deficits may be less prominent. 34 , 36
Of 1163 eligible participants, 360 were enrolled, 439 were excluded, and 364 refused to participate (additional details are provided in the eResults in Supplement 1 ). Participant characteristics are reported in Table 1 for the full sample. The discovery and validation cohorts did not significantly differ in terms of demographic characteristics, disease severity, or cognition (eTable 1 in Supplement 1 ). In the full sample, there were 209 women (58.1%) and 151 men (41.9%), and the mean (SD) age was 54.0 (15.4) years (range, 18-89 years). The mean (SD) educational level was 16.5 (2.3) years (range, 12-20 years). Among the 358 participants with racial and ethnic data available, 340 (95.0%) identified as White. For the 18 participants self-identifying as being of other race or ethnicity, the specific group was not provided to protect participant anonymity. Among the 329 participants with available CDR plus NACC-FTLD scores ( Table 1 ), 195 (59.3%) were asymptomatic or preclinical (Global Score, 0), 66 (20.1%) were prodromal (Global score, 0.5), and 68 (20.7%) were symptomatic (global score, 1.0 or 2.0). Of those with available genetic testing results (n = 222), 100 (45.0%) carried a pathogenic familial FTLD pathogenic variant, including 63 of 120 participants without symptoms and with available results. On average, participants completed 78% of available smartphone measures over a mean (SD) of 2.6 (0.6) sessions.
Descriptive statistics for each task are presented in Table 2 . Ceiling effects were not observed for any tests. A small percentage of participants were at the floor for flanker (19 [5.3%]), go/no-go (13 [4.0%]), and card sort (9 [3.3%]) scores. Floor effects were only observed in participants with prodromal or symptomatic FTLD.
Except for go/no-go, internal consistency estimates ranged from good to excellent (Cronbach α range, 0.84 [95% CI, 0.81-0.87] to 0.99 [95% CI, 0.99-0.99]), and test-retest reliabilities were moderate to excellent (interclass correlation coefficient [ICC] range, 0.77 [95% CI, 0.69-0.83] to 0.95 [95% CI, 0.93-0.96]), with slightly higher estimates in participants with prodromal or symptomatic FTLD ( Table 2 , Figure 2 , and eFigure 1 in Supplement 1 ). Go/no-go reliability was particularly poor in participants without symptoms (ICC, 0.10 [95% CI, −0.37 to 0.48]) and was removed from subsequent validation analyses except the correlation matrix ( Figure 3 A and B). The 95% CIs for reliability estimates overlapped in the discovery and validation cohorts ( Figure 2 ). Reliability estimates showed overlapping 95% CIs regardless of distractions (eFigure 2 in Supplement 1 ) or operating systems (eFigure 3 in Supplement 1 ), with a pattern of slightly lower reliability estimates when distractions were endorsed for all comparisons except Stroop (Cronbach α).
In 57 participants without symptoms who did not carry pathogenic variants, older age was associated with worse performance on all measures (β range, − 0.40 [95 CI, −0.68 to −0.13] to −0.78 [95 CI, −0.89 to −0.52]; P ≤ .03), except card sort (β = −0.22 [95% CI, −0.54 to 0.09]; P = .16) and go-no/go (β = −0.15 [95% CI, −0.44 to 0.14]; P = .31), though associations were in the expected direction. Associations with sex and educational level were not statistically significant.
Cognitive tests administered using the app showed evidence of convergent and divergent validity (eFigure 4 in Supplement 1 ), with very similar findings in discovery ( Figure 3 A) and validation cohorts ( Figure 3 B). App–based measures of executive functioning were generally correlated with criterion standard in-person measures of these domains and less with measures of other cognitive domains ( r range, 0.40-0.66). For example, the flanker task was associated with the UDS3-EF composite (β = 0.58 [95% CI, 0.48-0.68]; P < .001) and measures of visuoconstruction (β for Benson Figure Copy, 0.43 [95% CI, 0.32-0.54]; P = .01) and naming (β for Multilingual Naming Test, 0.25 [95% CI, 0.14-0.37]; P < .001). The app memory test was associated with criterion standard memory and executive functioning tests.
Worse performance on all app measures was associated with greater disease severity on CDR plus NACC-FTLD ( r range, 0.38-0.59) ( Table 1 , Figure 3 , and eFigure 4 in Supplement 1 ). The same pattern of results was observed after excluding those with finger dexterity issues. Except for go/no-go, performance of participants with prodromal FTLD was statistically significantly worse than that of participants without symptoms on all measures ( P < .001).
The AUC for the app composite to distinguish participants without symptoms from those with dementia was 0.93 (95% CI, 0.90-0.96). The app also accurately differentiated participants without symptoms from those with prodromal or symptomatic FTLD (AUC, 0.87 [95% CI, 0.84-0.92]). Compared with the MoCA (AUC, 0.68 [95% CI, 0.59-0.78), app composite performance (AUC, 0.82 [95% CI, 0.76-0.88]) more accurately differentiated participants without symptoms and with prodromal FTLD ( z of comparison, −2.49 [95% CI, −0.19 to −0.02]; P = .01), with similar accuracy to the UDS3-EF (AUC, 0.81 [95% CI, 0.73-0.88]); highly similar results (eTable 2 in Supplement 1 ) were observed in the discovery ( Figure 3 C) and validation ( Figure 3 D) cohorts.
In 56 participants without symptoms who were older than 45 years, those carrying GRN , C9orf72 , or another rare pathogenic variants performed significantly worse on 3 of 4 executive tests compared with noncarrier controls, including flanker (β = −0.26 [95% CI, −0.46 to −0.05]; P = .02), card sort (β = −0.28 [95% CI, −0.54 to −0.30]; P = .03), and 2-back (β = −0.49 [95% CI, −0.72 to −0.25]; P < .001). The estimated scores of participants who carried pathogenic variants were on average lower than those of carriers on a composite of criterion standard in-person tests, but the difference was not statistically significant (UDS3-EF β = −0.14 [95% CI, −0.42 to 0.14]; P = .32). Participants who carried preclinical MAPT pathogenic variants scored higher than noncarriers on the app Memory test, though the difference was not statistically significant (β = 0.21 [95% CI, −0.50 to 0.58]; P = .19).
In prespecified ROI analyses, worse app executive functioning scores were associated with lower frontoparietal and/or subcortical volume ( Figures 3 A and B) (β range, 0.34 [95% CI, 0.22-0.46] to 0.50 [95 CI, 0.40-0.60]; P < .001 for all) and worse memory scores with smaller hippocampal volume (β = 0.45 [95% CI, 0.34-0.56]; P < .001). Voxel-based morphometry (eFigure 5 in Supplement 1 ) suggested worse app performance was associated with widespread atrophy, particularly in frontotemporal cortices.
Only for card sort were distractions (eTables 3 and 4 in Supplement 1 ) associated with task performance; those experiencing distractions unexpectedly performed better (β = 0.16 [95% CI, 0.05-0.28]; P = .005). The iPhone operating system was associated with better performance on 2 speeded tasks: flanker (β = 0.16 [95% CI, 0.07-0.24]; P < .001) and go/no-go (β = 0.16 [95% CI, 0.06-0.26]; P = .002). In a sensitivity analysis, associations of all app tests with disease severity, UDS3-EF, and regional brain volumes remained after covarying for distractions and operating system, as did the models differentiating participants who carried preclinical pathogenic variants and noncarrier controls.
There is an urgent need to identify reliable and valid digital tools for remote neurobehavioral measurement in neurodegenerative diseases, including FTLD. Prior studies provided preliminary evidence that smartphones collect reliable and valid cognitive data in a variety of age-related and neurodegenerative illnesses. This is the first study, to our knowledge, to provide analogous support for the reliability and validity of remote cognitive testing via smartphones in FTLD and preliminary evidence that this approach improves early detection relative to traditional in-person measures.
Reliability, a prerequisite for a valid clinical trial end point, indicates measurements are consistent. In 2 cohorts, we found smartphone cognitive tests were reliable within a single administration (ie, internally consistent) and across repeated assessments (ie, test-retest reliability) with no apparent differences by operating system. For all measures except go/no-go, reliability estimates were moderate to excellent and on par with other remote digital assessments 5 , 6 , 10 , 37 , 38 and in-clinic criterion standards. 39 - 41 Go/no-go showed similar within- and between-person variability in participants without symptoms (ie, poor reliability), and participant feedback suggested instructions were confusing and the stimuli disappeared too quickly. Those endorsing distractions tended to have lower reliability, though 95% CIs largely overlapped; future research detailing the effect of the home environment on test performance is warranted.
Construct validity was supported by strong associations of smartphone tests with demographics, disease severity, neuroimaging, and criterion standard neuropsychological measures that replicated in a validation sample. These associations were similar to those observed among the criterion standard measures and similar to associations reported in other validation studies of smartphone cognitive tests. 5 , 6 , 10 Associations with disease severity were not explained by motor impairments. The iPhone operating system was associated with better performance on 2 time-based measures, consistent with prior findings. 6
A composite of brief smartphone tests was accurate in distinguishing dementia from cognitively unimpaired participants, screening out participants without symptoms, and detecting prodromal FTLD with greater sensitivity than the MoCA. Moreover, carriers of preclinical C9orf72 and GRN pathogenic variants performed significantly worse than noncarrier controls on 3 tests, whereas they did not significantly differ on criterion standard measures. These findings are consistent with previous studies showing digital executive functioning paradigms may be more sensitive to early FTLD than traditional measures. 42 , 43
This study has some limitations. Validation analyses focused on participants’ initial task exposure. Future studies will explore whether repeated measurements and more sophisticated approaches to composite building (current composite assumes equal weighting of tests) improve reliability and sensitivity, and a normative sample is being collected to better adjust for demographic effects on testing. 24 Longitudinal analyses will explore whether the floor effects in participants with symptomatic FTLD will affect the utility for monitoring. The generalizability of the findings is limited by the study cohort, which comprised participants who were college educated on average, mostly White, and primarily English speakers who owned smartphones and participated in the referring in-person research study. Equity in access to research is a priority in FTLD research 44 , 45 ; translations of the ALLFTD-mApp are in progress, cultural adaptations are being considered, and devices have been purchased for provisioning to improve the diversity of our sample.
The findings of this cohort study, coupled with prior reports indicating that smartphone testing is feasible and acceptable to patients with FTLD, 11 suggest that smartphones may complement traditional in-person research paradigms. More broadly, the scalability, ease of use, reliability, and validity of the ALLFTD-mApp suggest the feasibility and utility of remote digital assessments in dementia clinical trials. Future research should validate these results in diverse populations and evaluate the utility of these tests for longitudinal monitoring.
Accepted for Publication: February 2, 2024.
Published: April 1, 2024. doi:10.1001/jamanetworkopen.2024.4266
Open Access: This is an open access article distributed under the terms of the CC-BY License . © 2024 Staffaroni AM et al. JAMA Network Open .
Corresponding Author: Adam M. Staffaroni, PhD, Weill Institute for Neurosciences, Department of Neurology, Memory and Aging Center, University of California, San Francisco, 675 Nelson Rising Ln, Ste 190, San Francisco, CA 94158 ( [email protected] ).
Author Contributions: Dr Staffaroni had full access to all of the data in the study and takes responsibility for the integrity of the data and the accuracy of the data analysis.
Concept and design: Staffaroni, A. Clark, Taylor, Heuer, Wise, Forsberg, Miller, Hassenstab, Rosen, Boxer.
Acquisition, analysis, or interpretation of data: Staffaroni, A. Clark, Taylor, Heuer, Sanderson-Cimino, Wise, Dhanam, Cobigo, Wolf, Manoochehri, Mester, Rankin, Appleby, Bayram, Bozoki, D. Clark, Darby, Domoto-Reilly, Fields, Galasko, Geschwind, Ghoshal, Graff-Radford, Hsiung, Huey, Jones, Lapid, Litvan, Masdeu, Massimo, Mendez, Miyagawa, Pascual, Pressman, Ramanan, Ramos, Rascovsky, Roberson, Tartaglia, Wong, Kornak, Kremers, Kramer, Boeve, Boxer.
Drafting of the manuscript: Staffaroni, A. Clark, Taylor, Heuer, Wolf, Lapid.
Critical review of the manuscript for important intellectual content: Staffaroni, Taylor, Heuer, Sanderson-Cimino, Wise, Dhanam, Cobigo, Manoochehri, Forsberg, Mester, Rankin, Appleby, Bayram, Bozoki, D. Clark, Darby, Domoto-Reilly, Fields, Galasko, Geschwind, Ghoshal, Graff-Radford, Hsiung, Huey, Jones, Lapid, Litvan, Masdeu, Massimo, Mendez, Miyagawa, Pascual, Pressman, Ramanan, Ramos, Rascovsky, Roberson, Tartaglia, Wong, Miller, Kornak, Kremers, Hassenstab, Kramer, Boeve, Rosen, Boxer.
Statistical analysis: Staffaroni, A. Clark, Taylor, Heuer, Sanderson-Cimino, Cobigo, Kornak, Kremers.
Obtained funding: Staffaroni, Rosen, Boxer.
Administrative, technical, or material support: A. Clark, Taylor, Heuer, Wise, Dhanam, Wolf, Manoochehri, Forsberg, Darby, Domoto-Reilly, Ghoshal, Hsiung, Huey, Jones, Litvan, Massimo, Mendez, Miyagawa, Pascual, Pressman, Ramanan, Kramer, Boeve, Boxer.
Supervision: Geschwind, Miyagawa, Roberson, Kramer, Boxer.
Conflict of Interest Disclosures: Dr Staffaroni reported being a coinventor of 4 ALLFTD mobile application tasks (not analyzed in the present study) and receiving licensing fees from Datacubed Health; receiving research support from the National Institute on Aging (NIA) of the National Institutes of Health (NIH), Bluefield Project to Cure FTD, the Alzheimer’s Association, the Larry L. Hillblom Foundation, and the Rainwater Charitable Foundation; and consulting for Alector Inc, Eli Lilly and Company/Prevail Therapeutics, Passage Bio Inc, and Takeda Pharmaceutical Company. Dr Forsberg reported receiving research support from the NIH. Dr Rankin reported receiving research support from the NIH and the National Science Foundation and serving on the medical advisory board for Eli Lilly and Company. Dr Appleby reported receiving research support from the Centers for Disease Control and Prevention (CDC), the NIH, Ionis Pharmaceuticals Inc, Alector Inc, and the CJD Foundation and consulting for Acadia Pharmaceuticals Inc, Ionis Pharmaceuticals Inc, and Sangamo Therapeutics Inc. Dr Bayram reported receiving research support from the NIH. Dr Domoto-Reilly reported receiving research support from NIH and serving as an investigator for a clinical trial sponsored by Lawson Health Research Institute. Dr Bozoki reported receiving research funding from the NIH, Alector Inc, Cognition Therapeutics Inc, EIP Pharma, and Transposon Therapeutics Inc; consulting for Eisai and Creative Bio-Peptides Inc; and serving on the data safety monitoring board for AviadoBio. Dr Fields reported receiving research support from the NIH. Dr Galasko reported receiving research funding from the NIH; clinical trial funding from Alector Inc and Esai; consulting for Esai, General Electric Health Care, and Fujirebio; and serving on the data safety monitoring board of Cyclo Therapeutics Inc. Dr Geschwind reported consulting for Biogen Inc and receiving research support from Roche and Takeda Pharmaceutical Company for work in dementia. Dr Ghoshal reported participating in clinical trials of antidementia drugs sponsored by Bristol Myers Squibb, Eli Lilly and Company/Avid Radiopharmaceuticals, Janssen Immunotherapy, Novartis AG, Pfizer Inc, Wyeth Pharmaceuticals, SNIFF (The Study of Nasal Insulin to Fight Forgetfulness) study, and A4 (The Anti-Amyloid Treatment in Asymptomatic Alzheimer’s Disease) trial; receiving research support from Tau Consortium and the Association for Frontotemporal Dementia; and receiving funding from the NIH. Dr Graff-Radford reported receiving royalties from UpToDate; reported participating in multicenter therapy studies by sponsored by Biogen Inc, TauRx Therapeutics Ltd, AbbVie Inc, Novartis AG, and Eli Lilly and Company; and receiving research support from the NIH. Dr Grossman reported receiving grant support from the NIH, Avid Radiopharmaceuticals, and Piramal Pharma Ltd; participating in clinical trials sponsored by Biogen Inc, TauRx Therapeutics Ltd, and Alector Inc; consulting for Bracco and UCB; and serving on the editorial board of Neurology . Dr Hsiung reported receiving grant support from the Canadian Institutes of Health Research, the NIH, and the Alzheimer Society of British Columbia; participating in clinical trials sponsored by Anavax Life Sciences Corp, Biogen Inc, Cassava Sciences, Eli Lilly and Company, and Roche; and consulting for Biogen Inc, Novo Nordisk A/S, and Roche. Dr Huey reported receiving research support from the NIH. Dr Jones reported receiving research support from the NIH. Dr Litvan reported receiving research support from the NIH, the Michael J Fox Foundation, the Parkinson Foundation, the Lewy Body Association, CurePSP, Roche, AbbVie Inc, H Lundbeck A/S, Novartis AG, Transposon Therapeutics Inc, and UCB; serving as a member of the scientific advisory board for the Rossy PSP Program at the University of Toronto and for Amydis; and serving as chief editor of Frontiers in Neurology . Dr Masdeu reported consulting for and receiving research funding from Eli Lilly and Company; receiving personal fees from GE Healthcare; receiving grant funding and personal fees from Eli Lilly and Company; and receiving grant funding from Acadia Pharmaceutical Inc, Avanir Pharmaceuticals Inc, Biogen Inc, Eisai, Janssen Global Services LLC, the NIH, and Novartis AG outside the submitted work. Dr Mendez reported receiving research support from the NIH. Dr Miyagawa reported receiving research support from the Zander Family Foundation. Dr Pascual reported receiving research support from the NIH. Dr Pressman reported receiving research support from the NIH. Dr Ramos reported receiving research support from the NIH. Dr Roberson reported receiving research support from the NIA of the NIH, the Bluefield Project, and the Alzheimer’s Drug Discovery Foundation; serving on a data monitoring committee for Eli Lilly and Company; receiving licensing fees from Genentech Inc; and consulting for Applied Genetic Technologies Corp. Dr Tartaglia reported serving as an investigator for clinical trials sponsored by Biogen Inc, Avanex Corp, Green Valley, Roche/Genentech Inc, Bristol Myers Squibb, Eli Lilly and Company/Avid Radiopharmaceuticals, and Janssen Global Services LLC and receiving research support from the Canadian Institutes of Health Research (CIHR). Dr Wong reported receiving research support from the NIH. Dr Kornak reported providing expert witness testimony for Teva Pharmaceuticals Industries Ltd, Apotex Inc, and Puma Biotechnology and receiving research support from the NIH. Dr Kremers reported receiving research funding from NIH. Dr Kramer reported receiving research support from the NIH and royalties from Pearson Inc. Dr Boeve reported serving as an investigator for clinical trials sponsored by Alector Inc, Biogen Inc, and Transposon Therapeutics Inc; receiving royalties from Cambridge Medicine; serving on the Scientific Advisory Board of the Tau Consortium; and receiving research support from NIH, the Mayo Clinic Dorothy and Harry T. Mangurian Jr. Lewy Body Dementia Program, and the Little Family Foundation. Dr Rosen reported receiving research support from Biogen Inc, consulting for Wave Neuroscience and Ionis Pharmaceuticals, and receiving research support from the NIH. Dr Boxer reported being a coinventor of 4 of the ALLFTD mobile application tasks (not the focus of the present study) and previously receiving licensing fees; receiving research support from the NIH, the Tau Research Consortium, the Association for Frontotemporal Degeneration, Bluefield Project to Cure Frontotemporal Dementia, Corticobasal Degeneration Solutions, the Alzheimer’s Drug Discovery Foundation, and the Alzheimer’s Association; consulting for Aeovian Pharmaceuticals Inc, Applied Genetic Technologies Corp, Alector Inc, Arkuda Therapeutics, Arvinas Inc, AviadoBio, Boehringer Ingelheim, Denali Therapeutics Inc, GSK, Life Edit Therapeutics Inc, Humana Inc, Oligomerix, Oscotec Inc, Roche, Transposon Therapeutics Inc, TrueBinding Inc, and Wave Life Sciences; and receiving research support from Biogen Inc, Eisai, and Regeneron Pharmaceuticals Inc. No other disclosures were reported.
Funding/Support: This work was supported by grants AG063911, AG077557, AG62677, AG045390, NS092089, AG032306, AG016976, AG058233, AG038791, AG02350, AG019724, AG062422, NS050915, AG032289-11, AG077557, K23AG061253, and K24AG045333 from the NIH; the Association for Frontotemporal Degeneration; the Bluefield Project to Cure FTD; the Rainwater Charitable Foundation; and grant 2014-A-004-NET from the Larry L. Hillblom Foundation. Samples from the National Centralized Repository for Alzheimer’s Disease and Related Dementias, which receives government support under cooperative agreement grant U24 AG21886 from the NIA, were used in this study.
Role of the Funder/Sponsor: The funders had no role in the design and conduct of the study; collection, management, analysis, and interpretation of the data; preparation, review, or approval of the manuscript; and decision to submit the manuscript for publication.
Group Information: A complete list of the members of the ALLFTD Consortium appears in Supplement 2 .
Data Sharing Statement: See Supplement 3 .
Additional Contributions: We thank the participants and study partners for dedicating their time and effort, and for providing invaluable feedback as we learn how to incorporate digital technologies into FTLD research.
Additional Information: Dr Grossman passed away on April 4, 2023. We want to acknowledge his many contributions to this study, including data acquisition, and design and conduct of the study. He was an ALLFTD site principal investigator and contributed during the development of the ALLFTD mobile app.
- Register for email alerts with links to free full-text articles
- Access PDFs of free articles
- Manage your interests
- Save searches and receive search alerts
Validity of nutrition screening tools for risk of malnutrition among hospitalized adult patients: A systematic review and meta-analysis
Affiliations.
- 1 Son Espases University Hospital, 07120 Palma, Spain. Electronic address: [email protected].
- 2 Primary Care Research Unit of Mallorca, Balearic Islands Health Service, 07002 Palma, Spain. Electronic address: [email protected].
- 3 Research Group on Global Health and Lifestyles, Health Research Institute of the Balearic Islands (IdISBa), 07120 Palma, Spain; Nursing and Physiotherapy Department, University of the Balearic Islands, 07122 Palma, Spain. Electronic address: [email protected].
- 4 Research Group on Global Health and Lifestyles, Health Research Institute of the Balearic Islands (IdISBa), 07120 Palma, Spain; Nursing and Physiotherapy Department, University of the Balearic Islands, 07122 Palma, Spain. Electronic address: [email protected].
- 5 Research Group on Global Health and Lifestyles, Health Research Institute of the Balearic Islands (IdISBa), 07120 Palma, Spain; Nursing and Physiotherapy Department, University of the Balearic Islands, 07122 Palma, Spain. Electronic address: [email protected].
- 6 Research Group on Global Health and Lifestyles, Health Research Institute of the Balearic Islands (IdISBa), 07120 Palma, Spain; Nursing and Physiotherapy Department, University of the Balearic Islands, 07122 Palma, Spain; Centro de Investigación Biomédica en Red (CIBER) de Epidemiología y Salud Pública (CIBERESP), 28029 Madrid, Spain. Electronic address: [email protected].
- PMID: 38582013
- DOI: 10.1016/j.clnu.2024.03.008
Backgrounds & aims: Malnutrition is prevalent among hospitalized patients in developed countries, contributing to negative health outcomes and increased healthcare costs. Timely identification and management of malnutrition are crucial. The lack of a universally accepted definition and standardized diagnostic criteria for malnutrition has led to the development of various screening tools, each with varying validity. This complicates early identification of malnutrition, hindering effective intervention strategies. This systematic review and meta-analysis aimed to identify the most valid and reliable nutritional screening tool for assessing the risk of malnutrition in hospitalized adults.
Methods: A systematic literature search was conducted to identify validation studies published from inception to November 2023, in the Pubmed/MEDLINE, Embase, and CINAHL databases. This systematic review was registered in INPLASY (INPLASY202090028). The risk of bias and quality of included studies were assessed using the Quality Assessment of Diagnostic Accuracy Studies version 2 (QUADAS-2). Meta-analyses were performed for screening tools accuracy using the symmetric hierarchical summary receiver operative characteristics models.
Results: Of the 1646 articles retrieved, 60 met the inclusion criteria and were included in the systematic review, and 21 were included in the meta-analysis. A total of 51 malnutrition risk screening tools and 9 reference standards were identified. The meta-analyses assessed four common malnutrition risk screening tools against two reference standards (Subjective Global Assessment [SGA] and European Society for Clinical Nutrition and Metabolism [ESPEN] criteria). The Malnutrition Universal Screening Tool (MUST) vs SGA had a sensitivity (95% Confidence Interval) of 0.84 (0.73-0.91), and specificity of 0.85 (0.75-0.91). The MUST vs ESPEN had a sensitivity of 0.97 (0.53-0.99) and specificity of 0.80 (0.50-0.94). The Malnutrition Screening Tool (MST) vs SGA had a sensitivity of 0.81 (0.67-0.90) and specificity of 0.79 (0.72-0.74). The Mini Nutritional Assessment-Short Form (MNA-SF) vs ESPEN had a sensitivity of 0.99 (0.41-0.99) and specificity of 0.60 (0.45-0.73). The Nutrition Universal Screening Tool-2002 (NRS-2002) vs SGA had a sensitivity of 0.76 (0.58-0.87) and specificity of 0.86 (0.76-0.93).
Conclusions: The MUST demonstrated high accuracy in detecting malnutrition risk in hospitalized adults. However, the quality of the studies included varied greatly, possibly introducing bias in the results. Future research should compare tools within a specific patient population using a valid and universal gold standard to ensure improved patient care and outcomes.
Keywords: Hospitalized adults; Malnutrition; Malnutrition screening tools; Meta-analysis; Nutritional screening; Systematic review.
Copyright © 2024 The Authors. Published by Elsevier Ltd.. All rights reserved.
- Study Guides
- Homework Questions
Exploring Reliabilty and Validity

An official website of the United States government
The .gov means it’s official. Federal government websites often end in .gov or .mil. Before sharing sensitive information, make sure you’re on a federal government site.
The site is secure. The https:// ensures that you are connecting to the official website and that any information you provide is encrypted and transmitted securely.
- Publications
- Account settings
Preview improvements coming to the PMC website in October 2024. Learn More or Try it out now .
- Advanced Search
- Journal List
- J Grad Med Educ
- v.3(2); 2011 Jun
A Primer on the Validity of Assessment Instruments
1. what is reliability 1.
Reliability refers to whether an assessment instrument gives the same results each time it is used in the same setting with the same type of subjects. Reliability essentially means consistent or dependable results. Reliability is a part of the assessment of validity.
2. What is validity? 1
Validity in research refers to how accurately a study answers the study question or the strength of the study conclusions. For outcome measures such as surveys or tests, validity refers to the accuracy of measurement. Here validity refers to how well the assessment tool actually measures the underlying outcome of interest. Validity is not a property of the tool itself, but rather of the interpretation or specific purpose of the assessment tool with particular settings and learners.
Assessment instruments must be both reliable and valid for study results to be credible. Thus, reliability and validity must be examined and reported, or references cited, for each assessment instrument used to measure study outcomes. Examples of assessments include resident feedback survey, course evaluation, written test, clinical simulation observer ratings, needs assessment survey, and teacher evaluation. Using an instrument with high reliability is not sufficient; other measures of validity are needed to establish the credibility of your study.
3. How is reliability measured? 2 – 4
Reliability can be estimated in several ways; the method will depend upon the type of assessment instrument. Sometimes reliability is referred to as internal validity or internal structure of the assessment tool.
For internal consistency 2 to 3 questions or items are created that measure the same concept, and the difference among the answers is calculated. That is, the correlation among the answers is measured.
Cronbach alpha is a test of internal consistency and frequently used to calculate the correlation values among the answers on your assessment tool. 5 Cronbach alpha calculates correlation among all the variables, in every combination; a high reliability estimate should be as close to 1 as possible.
For test/retest the test should give the same results each time, assuming there are no interval changes in what you are measuring, and they are often measured as correlation, with Pearson r.
Test/retest is a more conservative estimate of reliability than Cronbach alpha, but it takes at least 2 administrations of the tool, whereas Cronbach alpha can be calculated after a single administration. To perform a test/retest, you must be able to minimize or eliminate any change (ie, learning) in the condition you are measuring, between the 2 measurement times. Administer the assessment instrument at 2 separate times for each subject and calculate the correlation between the 2 different measurements.
Interrater reliability is used to study the effect of different raters or observers using the same tool and is generally estimated by percent agreement, kappa (for binary outcomes), or Kendall tau.
Another method uses analysis of variance (ANOVA) to generate a generalizability coefficient, to quantify how much measurement error can be attributed to each potential factor, such as different test items, subjects, raters, dates of administration, and so forth. This model looks at the overall reliability of the results. 6
5. How is the validity of an assessment instrument determined? 4 – 7 , 8
Validity of assessment instruments requires several sources of evidence to build the case that the instrument measures what it is supposed to measure. , 9,10 Determining validity can be viewed as constructing an evidence-based argument regarding how well a tool measures what it is supposed to do. Evidence can be assembled to support, or not support, a specific use of the assessment tool. Evidence can be found in content, response process, relationships to other variables, and consequences.
Content includes a description of the steps used to develop the instrument. Provide information such as who created the instrument (national experts would confer greater validity than local experts, who in turn would have more validity than nonexperts) and other steps that support the instrument has the appropriate content.
Response process includes information about whether the actions or thoughts of the subjects actually match the test and also information regarding training for the raters/observers, instructions for the test-takers, instructions for scoring, and clarity of these materials.
Relationship to other variables includes correlation of the new assessment instrument results with other performance outcomes that would likely be the same. If there is a previously accepted “gold standard” of measurement, correlate the instrument results to the subject's performance on the “gold standard.” In many cases, no “gold standard” exists and comparison is made to other assessments that appear reasonable (eg, in-training examinations, objective structured clinical examinations, rotation “grades,” similar surveys).
Consequences means that if there are pass/fail or cut-off performance scores, those grouped in each category tend to perform the same in other settings. Also, if lower performers receive additional training and their scores improve, this would add to the validity of the instrument.
Different types of instruments need an emphasis on different sources of validity evidence. 7 For example, for observer ratings of resident performance, interrater agreement may be key, whereas for a survey measuring resident stress, relationship to other variables may be more important. For a multiple choice examination, content and consequences may be essential sources of validity evidence. For high-stakes assessments (eg, board examinations), substantial evidence to support the case for validity will be required. 9
There are also other types of validity evidence, which are not discussed here.
6. How can researchers enhance the validity of their assessment instruments?
First, do a literature search and use previously developed outcome measures. If the instrument must be modified for use with your subjects or setting, modify and describe how, in a transparent way. Include sufficient detail to allow readers to understand the potential limitations of this approach.
If no assessment instruments are available, use content experts to create your own and pilot the instrument prior to using it in your study. Test reliability and include as many sources of validity evidence as are possible in your paper. Discuss the limitations of this approach openly.
7. What are the expectations of JGME editors regarding assessment instruments used in graduate medical education research?
JGME editors expect that discussions of the validity of your assessment tools will be explicitly mentioned in your manuscript, in the methods section. If you are using a previously studied tool in the same setting, with the same subjects, and for the same purpose, citing the reference(s) is sufficient. Additional discussion about your adaptation is needed if you (1) have modified previously studied instruments; (2) are using the instrument for different settings, subjects, or purposes; or (3) are using different interpretation or cut-off points. Discuss whether the changes are likely to affect the reliability or validity of the instrument.
Researchers who create novel assessment instruments need to state the development process, reliability measures, pilot results, and any other information that may lend credibility to the use of homegrown instruments. Transparency enhances credibility.
In general, little information can be gleaned from single-site studies using untested assessment instruments; these studies are unlikely to be accepted for publication.
8. What are useful resources for reliability and validity of assessment instruments?
The references for this editorial are a good starting point.
Gail M. Sullivan, MD, MPH, is Editor-in-Chief, Journal of Graduate Medical Education .

COMMENTS
The 4 Types of Validity in Research | Definitions & Examples. Published on September 6, 2019 by Fiona Middleton.Revised on June 22, 2023. Validity tells you how accurately a method measures something. If a method measures what it claims to measure, and the results closely correspond to real-world values, then it can be considered valid.
Validity is important in market research and survey studies to ensure that the survey questions effectively measure consumer preferences, buying behaviors, or attitudes towards products or services. Validity assessments help researchers determine if the survey instrument is accurately capturing the desired information and if the results can be ...
In assessing validity of qualitative research, the challenge can start from the ontology and epistemology of the issue being studied, ... Most qualitative research studies, if not all, are meant to study a specific issue or phenomenon in a certain population or ethnic group, of a focused locality in a particular context, hence generalizability ...
The validity of a research study refers to how well the results among the study participants represent true findings among similar individuals outside the study. This concept of validity applies to all types of clinical studies, including those about prevalence, associations, interventions, and diagnosis. The validity of a research study ...
Validity. Validity is defined as the extent to which a concept is accurately measured in a quantitative study. For example, a survey designed to explore depression but which actually measures anxiety would not be considered valid. The second measure of quality in a quantitative study is reliability, or the accuracy of an instrument.In other words, the extent to which a research instrument ...
In psychology research, validity refers to the extent to which a test or measurement tool accurately measures what it's intended to measure. It ensures that the research findings are genuine and not due to extraneous factors. Validity can be categorized into different types, including construct validity (measuring the intended abstract trait), internal validity (ensuring causal conclusions ...
In simple terms, validity (also called "construct validity") is all about whether a research instrument accurately measures what it's supposed to measure. ... Oftentimes in quantitative studies, the way in which the researcher or survey designer interprets a question or statement can differ from how the study participants interpret it ...
Revised on 10 October 2022. Reliability and validity are concepts used to evaluate the quality of research. They indicate how well a method, technique, or test measures something. Reliability is about the consistency of a measure, and validity is about the accuracy of a measure. It's important to consider reliability and validity when you are ...
The essential difference between internal validity and external validity is that internal validity refers to the structure of a study (and its variables) while external validity refers to the universality of the results. But there are further differences between the two as well. For instance, internal validity focuses on showing a difference ...
Validity in research is the ability to conduct an accurate study with the right tools and conditions to yield acceptable and reliable data that can be reproduced. Researchers rely on carefully calibrated tools for precise measurements. However, collecting accurate information can be more of a challenge.
Evaluating the quality of research is essential if findings are to be utilised in practice and incorporated into care delivery. In a previous article we explored 'bias' across research designs and outlined strategies to minimise bias.1 The aim of this article is to further outline rigour, or the integrity in which a study is conducted, and ensure the credibility of findings in relation to ...
However, the increased importance given to qualitative information in the evidence-based paradigm in health care and social policy requires a more precise conceptualization of validity criteria that goes beyond just academic reflection. After all, one can argue that policy verdicts that are based on qualitative information must be legitimized by valid research, just as quantitative effect ...
The concept of validity is also applied to research studies and their findings. Internal validity examines whether the study design, conduct, and analysis answer the research questions without bias. External validity examines whether the study findings can be generalized to other contexts. Ecological validity examines, specifically, whether the ...
Reliability in research refers to the consistency and stability of measurements or findings. Validity relates to the accuracy and truthfulness of results, measuring what the study intends to. Both are crucial for trustworthy and credible research outcomes.
The goal of this article is to define, examine, and discuss the validity of research problems in primary psychological research. The psychological-research process starts with (0) an idea about the phenomenon of interest, followed by (1) a research-problem statement that includes a literature review of past research on the phenomenon and the research question the studies seek to answer (ideas ...
Thus 'validity' can have quite different meanings depending on the context! Reliability is distinct from validity, in that it refers to the consistency or repeatability of results; Two types of validity are considered when critically appraising clinical research studies: internal validity; external validity
Reliability and validity are among the most important and fundamental domains in the assessment of any measuring methodology for data-collection in a good research. Validity is about what an instrument measures and how well it does so, whereas reliability concerns the truthfulness in the data obtain …
The validity and reliability of the scales used in research are important factors that enable the research to yield healthy results. For this reason, it is useful to understand how the reliability ...
Validity was supported by association of smartphones tests with disease severity (r range, 0.38-0.59), criterion-standard neuropsychological tests (r range, 0.40-0.66), and brain volume (standardized β range, 0.34-0.50). ... (UCSF) FTLD studies. To study the app in older individuals, a small group of older adults without functional impairment ...
The internal validity is the steps taken or standards followed by the researchers in the study environment to obtain the truthful results. The external validity is the generalization followed for wider acceptance of global population. [ 2] Although these validation procedures are essential for the clinical studies, greater care is necessary for ...
The MUST demonstrated high accuracy in detecting malnutrition risk in hospitalized adults. However, the quality of the studies included varied greatly, possibly introducing bias in the results. Future research should compare tools within a specific patient population using a valid and universal gold …
Reliability and Validity 2 Exploring Reliability and Validity Assignment Both reliability and validity are highly considered when determining an instrument or tests success, i.e., it's whether it was a good test with the chosen materials and desired outcomes (Hirschi et al., 2017). Reliability is often the first indicator to examine when determining a test's success rate as it pertains to ...
Study Design, Precision, and Validity in Observational Studies. T he use of observational research methods in the field of palliative care is vital to building the evidence base, identifying best practices, and understanding disparities in access to and delivery of palliative care services. As discussed in the introduction to this series ...
There are few studies examining the face validity of the 40-item version of the Defense Style Questionnaire (DSQ-40). Moreover, the existing studies have provided conflicting results. The present study provides an in-depth examination of the face validity of the DSQ-40. Eight clinicians independently attributed each item of the DSQ-40 to a defense mechanism. The defense mechanisms listed in ...
What is validity? 1. Validity in research refers to how accurately a study answers the study question or the strength of the study conclusions. For outcome measures such as surveys or tests, validity refers to the accuracy of measurement. Here validity refers to how well the assessment tool actually measures the underlying outcome of interest.