S371 Social Work Research - Jill Chonody: What is Quantitative Research?
- Choosing a Topic
- Choosing Search Terms
- What is Quantitative Research?
- Requesting Materials

Quantitative Research in the Social Sciences
This page is courtesy of University of Southern California: http://libguides.usc.edu/content.php?pid=83009&sid=615867
Quantitative methods emphasize objective measurements and the statistical, mathematical, or numerical analysis of data collected through polls, questionnaires, and surveys, or by manipulating pre-existing statistical data using computational techniques . Quantitative research focuses on gathering numerical data and generalizing it across groups of people or to explain a particular phenomenon.
Babbie, Earl R. The Practice of Social Research . 12th ed. Belmont, CA: Wadsworth Cengage, 2010; Muijs, Daniel. Doing Quantitative Research in Education with SPSS . 2nd edition. London: SAGE Publications, 2010.
Characteristics of Quantitative Research
Your goal in conducting quantitative research study is to determine the relationship between one thing [an independent variable] and another [a dependent or outcome variable] within a population. Quantitative research designs are either descriptive [subjects usually measured once] or experimental [subjects measured before and after a treatment]. A descriptive study establishes only associations between variables; an experimental study establishes causality.
Quantitative research deals in numbers, logic, and an objective stance. Quantitative research focuses on numberic and unchanging data and detailed, convergent reasoning rather than divergent reasoning [i.e., the generation of a variety of ideas about a research problem in a spontaneous, free-flowing manner].
Its main characteristics are :
- The data is usually gathered using structured research instruments.
- The results are based on larger sample sizes that are representative of the population.
- The research study can usually be replicated or repeated, given its high reliability.
- Researcher has a clearly defined research question to which objective answers are sought.
- All aspects of the study are carefully designed before data is collected.
- Data are in the form of numbers and statistics, often arranged in tables, charts, figures, or other non-textual forms.
- Project can be used to generalize concepts more widely, predict future results, or investigate causal relationships.
- Researcher uses tools, such as questionnaires or computer software, to collect numerical data.
The overarching aim of a quantitative research study is to classify features, count them, and construct statistical models in an attempt to explain what is observed.
Things to keep in mind when reporting the results of a study using quantiative methods :
- Explain the data collected and their statistical treatment as well as all relevant results in relation to the research problem you are investigating. Interpretation of results is not appropriate in this section.
- Report unanticipated events that occurred during your data collection. Explain how the actual analysis differs from the planned analysis. Explain your handling of missing data and why any missing data does not undermine the validity of your analysis.
- Explain the techniques you used to "clean" your data set.
- Choose a minimally sufficient statistical procedure ; provide a rationale for its use and a reference for it. Specify any computer programs used.
- Describe the assumptions for each procedure and the steps you took to ensure that they were not violated.
- When using inferential statistics , provide the descriptive statistics, confidence intervals, and sample sizes for each variable as well as the value of the test statistic, its direction, the degrees of freedom, and the significance level [report the actual p value].
- Avoid inferring causality , particularly in nonrandomized designs or without further experimentation.
- Use tables to provide exact values ; use figures to convey global effects. Keep figures small in size; include graphic representations of confidence intervals whenever possible.
- Always tell the reader what to look for in tables and figures .
NOTE: When using pre-existing statistical data gathered and made available by anyone other than yourself [e.g., government agency], you still must report on the methods that were used to gather the data and describe any missing data that exists and, if there is any, provide a clear explanation why the missing datat does not undermine the validity of your final analysis.
Babbie, Earl R. The Practice of Social Research . 12th ed. Belmont, CA: Wadsworth Cengage, 2010; Brians, Craig Leonard et al. Empirical Political Analysis: Quantitative and Qualitative Research Methods . 8th ed. Boston, MA: Longman, 2011; McNabb, David E. Research Methods in Public Administration and Nonprofit Management: Quantitative and Qualitative Approaches . 2nd ed. Armonk, NY: M.E. Sharpe, 2008; Quantitative Research Methods . Writing@CSU. Colorado State University; Singh, Kultar. Quantitative Social Research Methods . Los Angeles, CA: Sage, 2007.
Basic Research Designs for Quantitative Studies
Before designing a quantitative research study, you must decide whether it will be descriptive or experimental because this will dictate how you gather, analyze, and interpret the results. A descriptive study is governed by the following rules: subjects are generally measured once; the intention is to only establish associations between variables; and, the study may include a sample population of hundreds or thousands of subjects to ensure that a valid estimate of a generalized relationship between variables has been obtained. An experimental design includes subjects measured before and after a particular treatment, the sample population may be very small and purposefully chosen, and it is intended to establish causality between variables. Introduction The introduction to a quantitative study is usually written in the present tense and from the third person point of view. It covers the following information:
- Identifies the research problem -- as with any academic study, you must state clearly and concisely the research problem being investigated.
- Reviews the literature -- review scholarship on the topic, synthesizing key themes and, if necessary, noting studies that have used similar methods of inquiry and analysis. Note where key gaps exist and how your study helps to fill these gaps or clarifies existing knowledge.
- Describes the theoretical framework -- provide an outline of the theory or hypothesis underpinning your study. If necessary, define unfamiliar or complex terms, concepts, or ideas and provide the appropriate background information to place the research problem in proper context [e.g., historical, cultural, economic, etc.].
Methodology The methods section of a quantitative study should describe how each objective of your study will be achieved. Be sure to provide enough detail to enable the reader can make an informed assessment of the methods being used to obtain results associated with the research problem. The methods section should be presented in the past tense.
- Study population and sampling -- where did the data come from; how robust is it; note where gaps exist or what was excluded. Note the procedures used for their selection;
- Data collection – describe the tools and methods used to collect information and identify the variables being measured; describe the methods used to obtain the data; and, note if the data was pre-existing [i.e., government data] or you gathered it yourself. If you gathered it yourself, describe what type of instrument you used and why. Note that no data set is perfect--describe any limitations in methods of gathering data.
- Data analysis -- describe the procedures for processing and analyzing the data. If appropriate, describe the specific instruments of analysis used to study each research objective, including mathematical techniques and the type of computer software used to manipulate the data.
Results The finding of your study should be written objectively and in a succinct and precise format. In quantitative studies, it is common to use graphs, tables, charts, and other non-textual elements to help the reader understand the data. Make sure that non-textual elements do not stand in isolation from the text but are being used to supplement the overall description of the results and to help clarify key points being made. Further information about how to effectively present data using charts and graphs can be found here .
- Statistical analysis -- how did you analyze the data? What were the key findings from the data? The findings should be present in a logical, sequential order. Describe but do not interpret these trends or negative results; save that for the discussion section. The results should be presented in the past tense.
Discussion Discussions should be analytic, logical, and comprehensive. The discussion should meld together your findings in relation to those identified in the literature review, and placed within the context of the theoretical framework underpinning the study. The discussion should be presented in the present tense.
- Interpretation of results -- reiterate the research problem being investigated and compare and contrast the findings with the research questions underlying the study. Did they affirm predicted outcomes or did the data refute it?
- Description of trends, comparison of groups, or relationships among variables -- describe any trends that emerged from your analysis and explain all unanticipated and statistical insignificant findings.
- Discussion of implications – what is the meaning of your results? Highlight key findings based on the overall results and note findings that you believe are important. How have the results helped fill gaps in understanding the research problem?
- Limitations -- describe any limitations or unavoidable bias in your study and, if necessary, note why these limitations did not inhibit effective interpretation of the results.
Conclusion End your study by to summarizing the topic and provide a final comment and assessment of the study.
- Summary of findings – synthesize the answers to your research questions. Do not report any statistical data here; just provide a narrative summary of the key findings and describe what was learned that you did not know before conducting the study.
- Recommendations – if appropriate to the aim of the assignment, tie key findings with policy recommendations or actions to be taken in practice.
- Future research – note the need for future research linked to your study’s limitations or to any remaining gaps in the literature that were not addressed in your study.
Black, Thomas R. Doing Quantitative Research in the Social Sciences: An Integrated Approach to Research Design, Measurement and Statistics . London: Sage, 1999; Gay,L. R. and Peter Airasain. Educational Research: Competencies for Analysis and Applications . 7th edition. Upper Saddle River, NJ: Merril Prentice Hall, 2003; Hector, Anestine. An Overview of Quantitative Research in Compostion and TESOL . Department of English, Indiana University of Pennsylvania; Hopkins, Will G. “Quantitative Research Design.” Sportscience 4, 1 (2000); A Strategy for Writing Up Research Results . The Structure, Format, Content, and Style of a Journal-Style Scientific Paper. Department of Biology. Bates College; Nenty, H. Johnson. "Writing a Quantitative Research Thesis." International Journal of Educational Science 1 (2009): 19-32; Ouyang, Ronghua (John). Basic Inquiry of Quantitative Research . Kennesaw State University.
- << Previous: Finding Quantitative Research
- Next: Databases >>
- Last Updated: Jul 11, 2023 1:03 PM
- URL: https://libguides.iun.edu/S371socialworkresearch
Social Work Research Methods That Drive the Practice
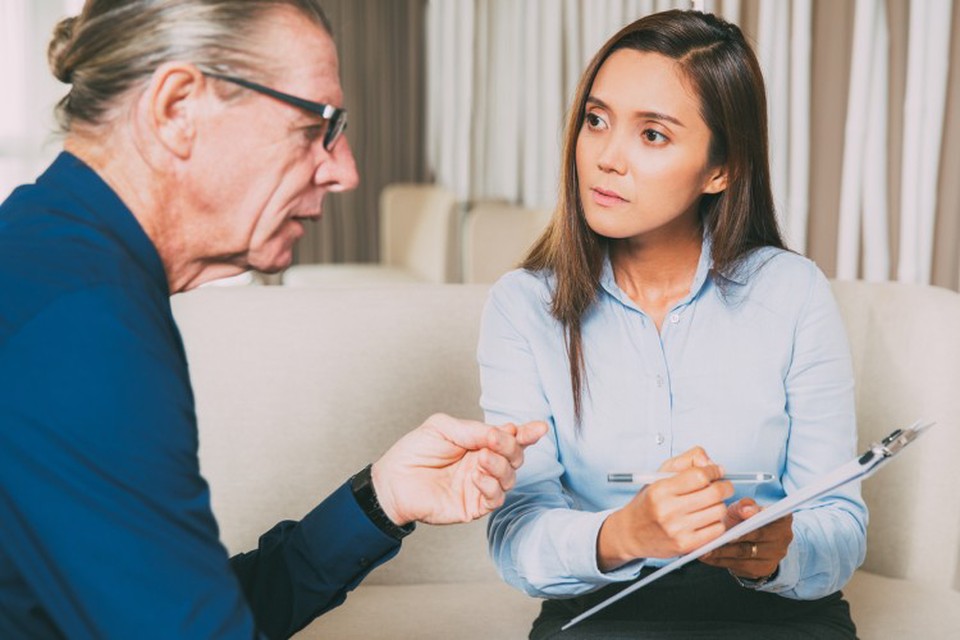
Social workers advocate for the well-being of individuals, families and communities. But how do social workers know what interventions are needed to help an individual? How do they assess whether a treatment plan is working? What do social workers use to write evidence-based policy?
Social work involves research-informed practice and practice-informed research. At every level, social workers need to know objective facts about the populations they serve, the efficacy of their interventions and the likelihood that their policies will improve lives. A variety of social work research methods make that possible.
Data-Driven Work
Data is a collection of facts used for reference and analysis. In a field as broad as social work, data comes in many forms.
Quantitative vs. Qualitative
As with any research, social work research involves both quantitative and qualitative studies.
Quantitative Research
Answers to questions like these can help social workers know about the populations they serve — or hope to serve in the future.
- How many students currently receive reduced-price school lunches in the local school district?
- How many hours per week does a specific individual consume digital media?
- How frequently did community members access a specific medical service last year?
Quantitative data — facts that can be measured and expressed numerically — are crucial for social work.
Quantitative research has advantages for social scientists. Such research can be more generalizable to large populations, as it uses specific sampling methods and lends itself to large datasets. It can provide important descriptive statistics about a specific population. Furthermore, by operationalizing variables, it can help social workers easily compare similar datasets with one another.
Qualitative Research
Qualitative data — facts that cannot be measured or expressed in terms of mere numbers or counts — offer rich insights into individuals, groups and societies. It can be collected via interviews and observations.
- What attitudes do students have toward the reduced-price school lunch program?
- What strategies do individuals use to moderate their weekly digital media consumption?
- What factors made community members more or less likely to access a specific medical service last year?
Qualitative research can thereby provide a textured view of social contexts and systems that may not have been possible with quantitative methods. Plus, it may even suggest new lines of inquiry for social work research.
Mixed Methods Research
Combining quantitative and qualitative methods into a single study is known as mixed methods research. This form of research has gained popularity in the study of social sciences, according to a 2019 report in the academic journal Theory and Society. Since quantitative and qualitative methods answer different questions, merging them into a single study can balance the limitations of each and potentially produce more in-depth findings.
However, mixed methods research is not without its drawbacks. Combining research methods increases the complexity of a study and generally requires a higher level of expertise to collect, analyze and interpret the data. It also requires a greater level of effort, time and often money.
The Importance of Research Design
Data-driven practice plays an essential role in social work. Unlike philanthropists and altruistic volunteers, social workers are obligated to operate from a scientific knowledge base.
To know whether their programs are effective, social workers must conduct research to determine results, aggregate those results into comprehensible data, analyze and interpret their findings, and use evidence to justify next steps.
Employing the proper design ensures that any evidence obtained during research enables social workers to reliably answer their research questions.
Research Methods in Social Work
The various social work research methods have specific benefits and limitations determined by context. Common research methods include surveys, program evaluations, needs assessments, randomized controlled trials, descriptive studies and single-system designs.
Surveys involve a hypothesis and a series of questions in order to test that hypothesis. Social work researchers will send out a survey, receive responses, aggregate the results, analyze the data, and form conclusions based on trends.
Surveys are one of the most common research methods social workers use — and for good reason. They tend to be relatively simple and are usually affordable. However, surveys generally require large participant groups, and self-reports from survey respondents are not always reliable.
Program Evaluations
Social workers ally with all sorts of programs: after-school programs, government initiatives, nonprofit projects and private programs, for example.
Crucially, social workers must evaluate a program’s effectiveness in order to determine whether the program is meeting its goals and what improvements can be made to better serve the program’s target population.
Evidence-based programming helps everyone save money and time, and comparing programs with one another can help social workers make decisions about how to structure new initiatives. Evaluating programs becomes complicated, however, when programs have multiple goal metrics, some of which may be vague or difficult to assess (e.g., “we aim to promote the well-being of our community”).
Needs Assessments
Social workers use needs assessments to identify services and necessities that a population lacks access to.
Common social work populations that researchers may perform needs assessments on include:
- People in a specific income group
- Everyone in a specific geographic region
- A specific ethnic group
- People in a specific age group
In the field, a social worker may use a combination of methods (e.g., surveys and descriptive studies) to learn more about a specific population or program. Social workers look for gaps between the actual context and a population’s or individual’s “wants” or desires.
For example, a social worker could conduct a needs assessment with an individual with cancer trying to navigate the complex medical-industrial system. The social worker may ask the client questions about the number of hours they spend scheduling doctor’s appointments, commuting and managing their many medications. After learning more about the specific client needs, the social worker can identify opportunities for improvements in an updated care plan.
In policy and program development, social workers conduct needs assessments to determine where and how to effect change on a much larger scale. Integral to social work at all levels, needs assessments reveal crucial information about a population’s needs to researchers, policymakers and other stakeholders. Needs assessments may fall short, however, in revealing the root causes of those needs (e.g., structural racism).
Randomized Controlled Trials
Randomized controlled trials are studies in which a randomly selected group is subjected to a variable (e.g., a specific stimulus or treatment) and a control group is not. Social workers then measure and compare the results of the randomized group with the control group in order to glean insights about the effectiveness of a particular intervention or treatment.
Randomized controlled trials are easily reproducible and highly measurable. They’re useful when results are easily quantifiable. However, this method is less helpful when results are not easily quantifiable (i.e., when rich data such as narratives and on-the-ground observations are needed).
Descriptive Studies
Descriptive studies immerse the researcher in another context or culture to study specific participant practices or ways of living. Descriptive studies, including descriptive ethnographic studies, may overlap with and include other research methods:
- Informant interviews
- Census data
- Observation
By using descriptive studies, researchers may glean a richer, deeper understanding of a nuanced culture or group on-site. The main limitations of this research method are that it tends to be time-consuming and expensive.
Single-System Designs
Unlike most medical studies, which involve testing a drug or treatment on two groups — an experimental group that receives the drug/treatment and a control group that does not — single-system designs allow researchers to study just one group (e.g., an individual or family).
Single-system designs typically entail studying a single group over a long period of time and may involve assessing the group’s response to multiple variables.
For example, consider a study on how media consumption affects a person’s mood. One way to test a hypothesis that consuming media correlates with low mood would be to observe two groups: a control group (no media) and an experimental group (two hours of media per day). When employing a single-system design, however, researchers would observe a single participant as they watch two hours of media per day for one week and then four hours per day of media the next week.
These designs allow researchers to test multiple variables over a longer period of time. However, similar to descriptive studies, single-system designs can be fairly time-consuming and costly.
Learn More About Social Work Research Methods
Social workers have the opportunity to improve the social environment by advocating for the vulnerable — including children, older adults and people with disabilities — and facilitating and developing resources and programs.
Learn more about how you can earn your Master of Social Work online at Virginia Commonwealth University . The highest-ranking school of social work in Virginia, VCU has a wide range of courses online. That means students can earn their degrees with the flexibility of learning at home. Learn more about how you can take your career in social work further with VCU.
From M.S.W. to LCSW: Understanding Your Career Path as a Social Worker
How Palliative Care Social Workers Support Patients With Terminal Illnesses
How to Become a Social Worker in Health Care
Gov.uk, Mixed Methods Study
MVS Open Press, Foundations of Social Work Research
Open Social Work Education, Scientific Inquiry in Social Work
Open Social Work, Graduate Research Methods in Social Work: A Project-Based Approach
Routledge, Research for Social Workers: An Introduction to Methods
SAGE Publications, Research Methods for Social Work: A Problem-Based Approach
Theory and Society, Mixed Methods Research: What It Is and What It Could Be
READY TO GET STARTED WITH OUR ONLINE M.S.W. PROGRAM FORMAT?
Bachelor’s degree is required.

Want to create or adapt books like this? Learn more about how Pressbooks supports open publishing practices.
19 11. Quantitative measurement
Chapter outline.
- Overview of measurement (11 minute read)
- Operationalization and levels of measurement (20 minute read)
- Scales and indices (15 minute read)
- Reliability and validity (20 minute read)
- Ethical and social justice considerations for measurement (6 minute read)
Content warning: Discussions of immigration issues, parents and gender identity, anxiety, and substance use.
11.1 Overview of measurement
Learning Objectives
Learners will be able to…
- Provide an overview of the measurement process in social work research
- Describe why accurate measurement is important for research
This chapter begins with an interesting question: Is my apple the same as your apple? Let’s pretend you want to study apples. Perhaps you have read that chemicals in apples may impact neurotransmitters and you want to test if apple consumption improves mood among college students. So, in order to conduct this study, you need to make sure that you provide apples to a treatment group, right? In order to increase the rigor of your study, you may also want to have a group of students, ones who do not get to eat apples, to serve as a comparison group. Don’t worry if this seems new to you. We will discuss this type of design in Chapter 13 . For now, just concentrate on apples.
In order to test your hypothesis about apples, you need to define exactly what is meant by the term “apple” so you ensure everyone is consuming the same thing. You also need to know what you consider a “dose” of this thing that we call “apple” and make sure everyone is consuming the same kind of apples and you need a way to ensure that you give the same amount of apples to everyone in your treatment group. So, let’s start by making sure we understand what the term “apple” means. Say you have an object that you identify as an apple and I have an object that I identify as an apple. Perhaps my “apple” is a chocolate apple, one that looks similar to an apple but made of chocolate and red dye, and yours is a honeycrisp. Perhaps yours is papier-mache and mine is a Macbook Pro. All of these are defined as apples, right?
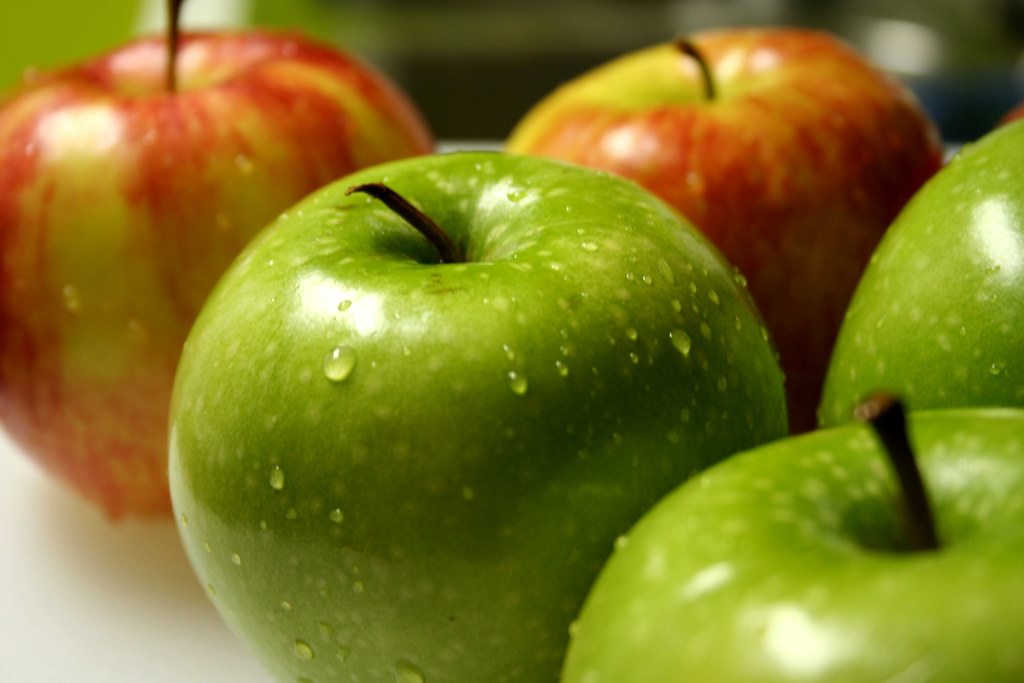
You can see the multitude of ways we could conceptualize “apple,” and how that could create a problem for our research. If I get a Red Delicious (ick) apple and you get a Granny Smith (yum) apple and we observe a change in neurotransmitters, it’s going to be even harder than usual to say the apple influenced the neurotransmitters because we didn’t define “apple” well enough. Measurement in this case is essential to treatment fidelity , which is when you ensure that everyone receives the same, or close to the same, treatment as possible. In other words, you need to make sure everyone is consuming the same kind of apples and you need a way to ensure that you give the same amount of apples to everyone in your treatment group.
In social science, when we use the term measurement , we mean the process by which we describe and ascribe meaning to the key facts, concepts, or other phenomena that we are investigating. At its core, measurement is about defining one’s terms in as clear and precise a way as possible. Of course, measurement in social science isn’t quite as simple as using a measuring cup or spoon, but there are some basic tenets on which most social scientists agree when it comes to measurement. We’ll explore those, as well as some of the ways that measurement might vary depending on your unique approach to the study of your topic.
An important point here is that measurement does not require any particular instruments or procedures. What it does require is some systematic procedure for assigning scores, meanings, and descriptions to individuals or objects so that those scores represent the characteristic of interest. You can measure phenomena in many different ways, but you must be sure that how you choose to measure gives you information and data that lets you answer your research question. If you’re looking for information about a person’s income, but your main points of measurement have to do with the money they have in the bank, you’re not really going to find the information you’re looking for!
What do social scientists measure?
The question of what social scientists measure can be answered by asking yourself what social scientists study. Think about the topics you’ve learned about in other social work classes you’ve taken or the topics you’ve considered investigating yourself. Let’s consider Melissa Milkie and Catharine Warner’s study (2011) [1] of first graders’ mental health. In order to conduct that study, Milkie and Warner needed to have some idea about how they were going to measure mental health. What does mental health mean, exactly? And how do we know when we’re observing someone whose mental health is good and when we see someone whose mental health is compromised? Understanding how measurement works in research methods helps us answer these sorts of questions.
As you might have guessed, social scientists will measure just about anything that they have an interest in investigating. For example, those who are interested in learning something about the correlation between social class and levels of happiness must develop some way to measure both social class and happiness. Those who wish to understand how well immigrants cope in their new locations must measure immigrant status and coping. Those who wish to understand how a person’s gender shapes their workplace experiences must measure gender and workplace experiences. You get the idea. Social scientists can and do measure just about anything you can imagine observing or wanting to study. Of course, some things are easier to observe or measure than others.
In 1964, philosopher Abraham Kaplan (1964) [2] wrote The Conduct of Inquiry, which has since become a classic work in research methodology (Babbie, 2010). [3] In his text, Kaplan describes different categories of things that behavioral scientists observe. One of those categories, which Kaplan called “observational terms,” is probably the simplest to measure in social science. Observational terms are the sorts of things that we can see with the naked eye simply by looking at them. Kaplan roughly defines them as conditions that are easy to identify and verify through direct observation. If, for example, we wanted to know how the conditions of playgrounds differ across different neighborhoods, we could directly observe the variety, amount, and condition of equipment at various playgrounds.
Indirect observables , on the other hand, are less straightforward to assess. In Kaplan’s framework, they are conditions that are subtle and complex that we must use existing knowledge and intuition to define.If we conducted a study for which we wished to know a person’s income, we’d probably have to ask them their income, perhaps in an interview or a survey. Thus, we have observed income, even if it has only been observed indirectly. Birthplace might be another indirect observable. We can ask study participants where they were born, but chances are good we won’t have directly observed any of those people being born in the locations they report.
How do social scientists measure?
Measurement in social science is a process. It occurs at multiple stages of a research project: in the planning stages, in the data collection stage, and sometimes even in the analysis stage. Recall that previously we defined measurement as the process by which we describe and ascribe meaning to the key facts, concepts, or other phenomena that we are investigating. Once we’ve identified a research question, we begin to think about what some of the key ideas are that we hope to learn from our project. In describing those key ideas, we begin the measurement process.
Let’s say that our research question is the following: How do new college students cope with the adjustment to college? In order to answer this question, we’ll need some idea about what coping means. We may come up with an idea about what coping means early in the research process, as we begin to think about what to look for (or observe) in our data-collection phase. Once we’ve collected data on coping, we also have to decide how to report on the topic. Perhaps, for example, there are different types or dimensions of coping, some of which lead to more successful adjustment than others. However we decide to proceed, and whatever we decide to report, the point is that measurement is important at each of these phases.
As the preceding example demonstrates, measurement is a process in part because it occurs at multiple stages of conducting research. We could also think of measurement as a process because it involves multiple stages. From identifying your key terms to defining them to figuring out how to observe them and how to know if your observations are any good, there are multiple steps involved in the measurement process. An additional step in the measurement process involves deciding what elements your measures contain. A measure’s elements might be very straightforward and clear, particularly if they are directly observable. Other measures are more complex and might require the researcher to account for different themes or types. These sorts of complexities require paying careful attention to a concept’s level of measurement and its dimensions. We’ll explore these complexities in greater depth at the end of this chapter, but first let’s look more closely at the early steps involved in the measurement process, starting with conceptualization.
The idea of coming up with your own measurement tool might sound pretty intimidating at this point. The good news is that if you find something in the literature that works for you, you can use it with proper attribution. If there are only pieces of it that you like, you can just use those pieces, again with proper attribution. You don’t always have to start from scratch!
Key Takeaways
- Measurement (i.e. the measurement process) gives us the language to define/describe what we are studying.
- In research, when we develop measurement tools, we move beyond concepts that may be subjective and abstract to a definition that is clear and concise.
- Good social work researchers are intentional with the measurement process.
- Engaging in the measurement process requires us to think critically about what we want to study. This process may be challenging and potentially time-consuming.
- How easy or difficult do you believe it will be to study these topics?
- Think about the chapter on literature reviews. Is there a significant body of literature on the topics you are interested in studying?
- Are there existing measurement tools that may be appropriate to use for the topics you are interested in studying?
11.2 Operationalization and levels of measurement
- Define constructs and operationalization and describe their relationship
- Be able to start operationalizing variables in your research project
- Identify the level of measurement for each type of variable
- Demonstrate knowledge of how each type of variable can be used
Now we have some ideas about what and how social scientists need to measure, so let’s get into the details. In this section, we are going to talk about how to make your variables measurable (operationalization) and how you ultimately characterize your variables in order to analyze them (levels of measurement).
Operationalizing your variables
“Operationalizing” is not a word I’d ever heard before I became a researcher, and actually, my browser’s spell check doesn’t even recognize it. I promise it’s a real thing, though. In the most basic sense, when we operationalize a variable, we break it down into measurable parts. Operationalization is the process of determining how to measure a construct that cannot be directly observed. And a constructs are conditions that are not directly observable and represent states of being, experiences, and ideas. But why construct ? We call them constructs because they are built using different ideas and parameters.
As we know from Section 11.1, sometimes the measures that we are interested in are more complex and more abstract than observational terms or indirect observables . Think about some of the things you’ve learned about in other social work classes—for example, ethnocentrism. What is ethnocentrism? Well, from completing an introduction to social work class you might know that it’s a construct that has something to do with the way a person judges another’s culture. But how would you measure it? Here’s another construct: bureaucracy. We know this term has something to do with organizations and how they operate, but measuring such a construct is trickier than measuring, say, a person’s income. In both cases, ethnocentrism and bureaucracy, these theoretical notions represent ideas whose meaning we have come to agree on. Though we may not be able to observe these abstractions directly, we can observe the things that they are made up of.
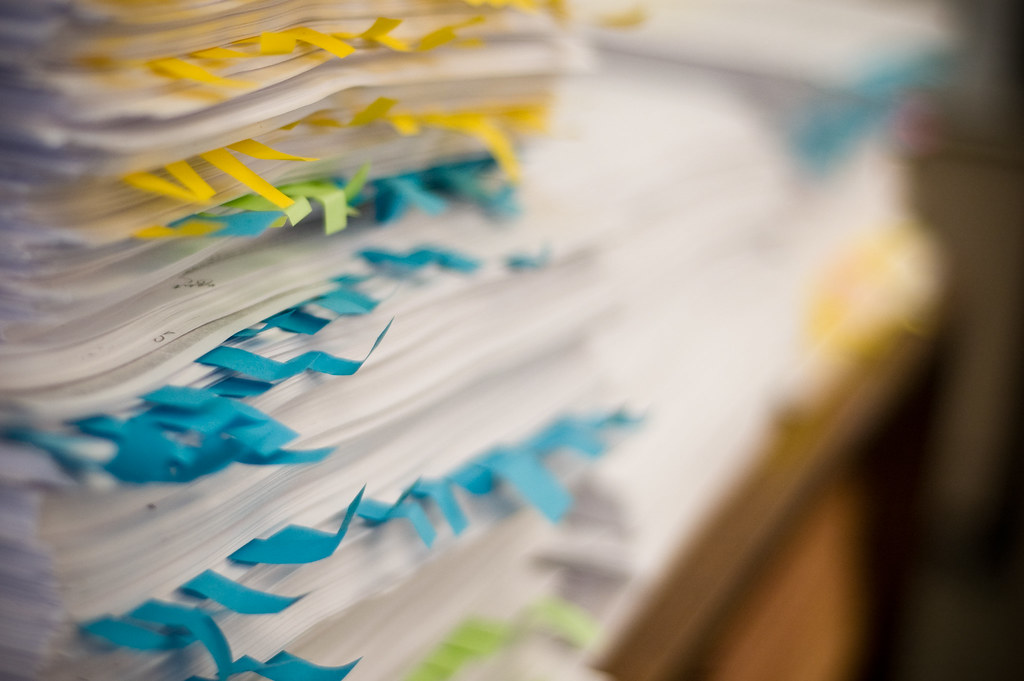
Now, let’s operationalize bureaucracy and ethnocentrism. The construct of bureaucracy could be measured by counting the number of supervisors that need to approve routine spending by public administrators. The greater the number of administrators that must sign off on routine matters, the greater the degree of bureaucracy. Similarly, we might be able to ask a person the degree to which they trust people from different cultures around the world and then assess the ethnocentrism inherent in their answers. We can measure constructs like bureaucracy and ethnocentrism by defining them in terms of what we can observe.
How we operationalize our constructs (and ultimately measure our variables) can affect the conclusions we can draw from our research. Let’s say you’re reviewing a state program to make it more efficient in connecting people to public services. What might be different if we decide to measure bureaucracy by the number of forms someone has to fill out to get a public service instead of the number of people who have to review the forms, like we talked about above? Maybe you find that there is an unnecessary amount of paperwork based on comparisons to other state programs, so you recommend that some of it be eliminated. This is probably a good thing, but will it actually make the program more efficient like eliminating some of the reviews that paperwork has to go through would? I’m not really making a judgment on which way is better to measure bureaucracy, but I encourage you to think about the costs and benefits of each way we operationalized the construct of bureaucracy, and extend this to the way you operationalize your own concepts in your research project.
Levels of Measurement
Now, we’re going to move into some more concrete characterizations of variables. You now hopefully understand how to operationalize your concepts so that you can turn them into variables. Imagine a process kind of like what you see in Figure 11.1 below.
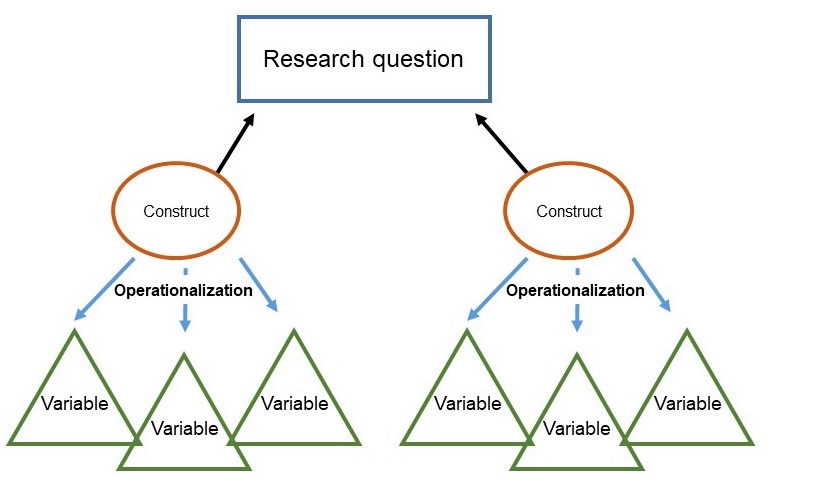
Notice that the arrows from the construct point toward the research question, because ultimately, measuring them will help answer your question!
The level of measuremen t of a variable tells us how the values of the variable relate to each other and what mathematical operations we can perform with the variable. (That second part will become important once we move into quantitative analysis in Chapter 14 and Chapter 15 ). Many students find this definition a bit confusing. What does it mean when we say that the level of measurement tells us about mathematical operations? So before we move on, let’s clarify this a bit.
Let’s say you work for your a community nonprofit that wants to develop programs relevant to community members’ ages (i.e., tutoring for kids in school, job search and resume help for adults, and home visiting for elderly community members). However, you do not have a good understanding of the ages of the people who visit your community center. Below is a part of a questionnaire that you developed to.
- How old are you? – Under 18 years old – 18-30 years old – 31-50 years old – 51-60 years old – Over 60 years old
- How old are you? _____ years
Look at the two items on this questionnaire. They both ask about age, but t he first item asks about age but asks the participant to identify the age range. The second item asks you to identify the actual age in years. These two questions give us data that represent the same information measured at a different level.
It would help your agency if you knew the average age of clients, right? So, which item on the questionnaire will provide this information? Item one’s choices are grouped into categories. Can you compute an average age from these choices? No. Conversely, participants completing item two are asked to provide an actual number, one that you could use to determine an average age. In summary, the two items both ask the participants to report their age. However, the type of data collected from both items is different and must be analyzed differently.
We can think about the four levels of measurement as going from less to more specific, or as it’s more commonly called, lower to higher: nominal, ordinal , interval , and ratio . Each of these levels differ and help the researcher understand something about their data. Think about levels of measurement as a hierarchy.
In order to determine the level of measurement, please examine your data and then ask these four questions (in order).
- Do I have mutually exclusive categories? If the answer is yes, continue to question #2.
- Do my item choices have a hierarchy or order? In other words, can you put your item choices in order? If no, stop–you have nominal level data. If the answer is yes, continue to question #3.
- Can I add, subtract, divide, and multiply my answer choices? If no, stop–you have ordinal level data. If the answer is yes, continue to question #4.
- Is it possible that the answer to this item can be zero? If the answer is no—you have interval level data. If the answer is yes, you are at the ratio level of measurement.
Nominal level . The nominal level of measurement is the lowest level of measurement. It contains categories are mutually exclusive, which means means that anyone who falls into one category cannot not fall into another category. The data can be represented with words (like yes/no) or numbers that correspond to words or a category (like 1 equaling yes and 0 equaling no). Even when the categories are represented as numbers in our data, the number itself does not have an actual numerical value. It is merely a number we have assigned so that we can use the variable in mathematical operations (which we will start talking about in Chapter 14.1 ). We say this level of measurement is lowest or least specific because someone who falls into a category we’ve designated could differ from someone else in the same category. Let’s say on our questionnaire above, we also asked folks whether they own a car. They can answer yes or no, and they fall into mutually exclusive categories. In this case, we would know whether they own a car, but not whether owning a car really affects their life significantly. Maybe they have chosen not to own one and are happy to take the bus, bike, or walk. Maybe they do not own one but would like to own one. We cannot get this information from a nominal variable, which is ok when we have meaningful categories. Nominal variables are especially useful when we just need the frequency of a particular characteristic in our sample.
The nominal level of measurement usually includes many demographic characteristics like race, gender, or marital status.
Ordinal leve l . The ordinal level of measurement is the next level of measurement and contains slightly more specific information than the nominal level. This level has mutually exclusive categories and a hierarchy or order. Let’s go back to the first item on the questionnaire we talked about above.
Do we have mutually exclusive categories? Yes. Someone who selects item A cannot also select item B. So, we know that we have at least nominal level data. However, the next question that we need to ask is “Do my answer choices have order?” or “Can I put my answer choices in order?” The answer is yes, someone who selects A is younger than someone who selects B or C. So, you have at least ordinal level data.
From a data analysis and statistical perspective, ordinal variables get treated exactly like nominal variables because they are both categorical variables , or variables whose values are organized into mutually exclusive groups but whose numerical values cannot be used in mathematical operations. You’ll see this term used again when we get into bivariate analysis in Chapter 15.
Interval level The interval level of measurement is a higher level of measurement. This level marks the point where we are able . This level contains all of the characteristics of the previous levels (mutually exclusive categories and order). What distinguishes it from the ordinal level is that the interval level can be used to conduct mathematical computations with data (like an average, for instance).
Let’s think back to our questionnaire about age again and take a look at the second question where we asked for a person’s exact age in years. Age in years is mutually exclusive – someone can’t be 14 and 15 at the same time – and the order of ages is meaningful, since being 18 means something different than being 32. Now, we can also take the answers to this question and do math with them, like addition, subtraction, multiplication, and division.
Ratio level . Ratio level data is the highest level of measurement. It has mutually exclusive categories, order, and you can perform mathematical operations on it. The main difference between the interval and ratio levels is that the ratio level has an absolute zero, meaning that a value of zero is both possible and meaningful. You might be thinking, “Well, age has an absolute zero,” but someone who is not yet born does not have an age, and the minute they’re born, they are not zero years old anymore.
Data at the ratio level of measurement are usually amounts or numbers of things, and can be negative (if that makes conceptual sense, of course). For example, you could ask someone to report how many A’s they have on their transcript or how many semesters they have earned a 4.0. They could have zero A’s and that would be a valid answer.
From a data analysis and statistical perspective, interval and ratio variables are treated exactly the same because they are both continuous variables , or variables whose values are mutually exclusive and can be used in mathematical operations. Technically, a continuous variable could have an infinite number of values.
What does the level of measurement tell us?
We have spent time learning how to determine our data’s level of measurement. Now what? How could we use this information to help us as we measure concepts and develop measurement tools? First, the types of statistical tests that we are able to use are dependent on our data’s level of measurement. (We will discuss this soon in Chapter 15.) The higher the level of measurement, the more complex statistical tests we are able to conduct. This knowledge may help us decide what kind of data we need to gather, and how. That said, we have to balance this knowledge with the understanding that sometimes, collecting data at a higher level of measurement could negatively impact our studies. For instance, sometimes providing answers in ranges may make prospective participants feel more comfortable responding to sensitive items. Imagine that you were interested in collecting information on topics such as income, number of sexual partners, number of times used illicit drugs, etc. You would have to think about the sensitivity of these items and determine if it would make more sense to collect some data at a lower level of measurement.
Finally, sometimes when analyzing data, researchers find a need to change a data’s level of measurement. For example, a few years ago, a student was interested in studying the relationship between mental health and life satisfaction. This student collected a variety of data. One item asked about the number of mental health diagnoses, reported as the actual number. When analyzing data, my student examined the mental health diagnosis variable and noticed that she had two groups, those with none or one diagnosis and those with many diagnoses. Instead of using the ratio level data (actual number of mental health diagnoses), she collapsed her cases into two categories, few and many. She decided to use this variable in her analyses. It is important to note that you can move a higher level of data to a lower level of data; however, you are unable to move a lower level to a higher level.
- Operationalization involves figuring out how to measure a construct you cannot directly observe.
- Nominal variables have mutually exclusive categories with no natural order. They cannot be used for mathematical operations like addition or subtraction. Race or gender would be one example.
- Ordinal variables have mutually exclusive categories and a natural order. They also cannot be used for mathematical operations like addition or subtraction. Age when measured in categories (i.e., 18-25 years old) would be an example.
- Interval variables have mutually exclusive categories, a natural order, and can be used for mathematical operations. Age as a raw number would be an example.
- Ratio variables have mutually exclusive categories, a natural order, can be used for mathematical operations, and have an absolute zero value. The number of times someone calls a legislator to advocate for a policy would be an example.
- Nominal and ordinal variables are categorical variables, meaning they have mutually exclusive categories and cannot be used for mathematical operations, even when assigned a number.
- Interval and ratio variables are continuous variables, meaning their values are mutually exclusive and can be used in mathematical operations.
- Researchers should consider the costs and benefits of how they operationalize their variables, including what level of measurement they choose, since the level of measurement can affect how you must gather your data.
- What are the primary constructs being explored in the research?
- Could you (or the study authors) have chosen another way to operationalize this construct?
- What are these variables’ levels of measurement?
- Are they categorical or continuous?
11.3 Scales and indices
- Identify different types of scales and compare them to each other
- Understand how to begin the process of constructing scales or indices
Quantitative data analysis requires the construction of two types of measures of variables: indices and scales. These measures are frequently used and are important since social scientists often study variables that possess no clear and unambiguous indicators–for instance, age or gender. Researchers often centralize much of work in regards to the attitudes and orientations of a group of people, which require several items to provide indication of the variables. Secondly, researchers seek to establish ordinal categories from very low to very high (vice-versa), which single data items can not ensure, while an index or scale can.
Although they exhibit differences (which will later be discussed) the two have in common various factors.
- Both are ordinal measures of variables.
- Both can order the units of analysis in terms of specific variables.
- Both are composite measures of variables ( measurements based on more than one one data item ).
In general, indices are a sum of series of individual yes/no questions, that are then combined in a single numeric score. They are usually a measure of the quantity of some social phenomenon and are constructed at a ratio level of measurement. More sophisticated indices weigh individual items according to their importance in the concept being measured (i.e. in a multiple choice test where different questions are worth different numbers of points). Some interval-level indices are not weight counted, but contain other indexes or scales within them (i.e. college admissions that score an applicant based on GPA, SAT scores, essays, and place a different point from each source).
This section discusses two formats used for measurement in research: scales and indices (sometimes called indexes). These two formats are helpful in research because they use multiple indicators to develop a composite (or total) score. Co mposite scores provide a much greater understanding of concepts than a single item could. Although we won’t delve too deeply into the process of scale development, we will cover some important topics for you to understand how scales and indices can be used.
Types of scales
As a student, you are very familiar with end of the semester course evaluations. These evaluations usually include statements such as, “My instructor created an environment of respect” and ask students to use a scale to indicate how much they agree or disagree with the statements. These scales, if developed and administered appropriately, provide a wealth of information to instructors that may be used to refine and update courses. If you examine the end of semester evaluations, you will notice that they are organized, use language that is specific to your course, and have very intentional methods of implementation. In essence, these tools are developed to encourage completion.
As you read about these scales, think about the information that you want to gather from participants. What type or types of scales would be the best for you to use and why? Are there existing scales or do you have to create your own?
The Likert scale
Most people have seen some version of a Likert scale. Designed by Rensis Likert (Likert, 1932) [4] , a Likert scale is a very popular rating scale for measuring ordinal data in social work research. This scale includes Likert items that are simply-worded statements to which participants can indicate their extent of agreement or disagreement on a five- or seven-point scale ranging from “strongly disagree” to “strongly agree.” You will also see Likert scales used for importance, quality, frequency, and likelihood, among lots of other concepts. Below is an example of how we might use a Likert scale to assess your attitudes about research as you work your way through this textbook.
Likert scales are excellent ways to collect information. They are popular; thus, your prospective participants may already be familiar with them. However, they do pose some challenges. You have to be very clear about your question prompts. What does strongly agree mean and how is this differentiated from agree ? In order to clarify this for participants, some researchers will place definitions of these items at the beginning of the tool.
There are a few other, less commonly used, scales discussed next.
Semantic differential scale
This is a composite (multi-item) scale where respondents are asked to indicate their opinions or feelings toward a single statement using different pairs of adjectives framed as polar opposites. For instance, in the above Likert scale, the participant is asked how much they agree or disagree with a statement. In a semantic differential scale, the participant is asked to indicate how they feel about a specific item. This makes the s emantic differential scale an excellent technique for measuring people’s attitudes or feelings toward objects, events, or behaviors. The following is an example of a semantic differential scale that was created to assess participants’ feelings about the content taught in their research class.
Feelings About My Research Class
Directions: Please review the pair of words and then select the one that most accurately reflects your feelings about the content of your research class.
Boring……………………………………….Exciting
Waste of Time…………………………..Worthwhile
Dry…………………………………………….Engaging
Irrelevant…………………………………..Relevant
Guttman scale
This composite scale was designed by Louis Guttman and uses a series of items arranged in increasing order of intensity (least intense to most intense) of the concept. This type of scale allows us to understand the intensity of beliefs or feelings. Each item in the above Guttman scale has a weight (this is not indicated on the tool) which varies with the intensity of that item, and the weighted combination of each response is used as an aggregate measure of an observation. Let’s pretend that you are working with a group of parents whose children have identified as part of the transgender community. You want to know how comfortable they feel with their children. You could develop the following items.
Example Guttman Scale Items
- I would allow my child to use a name that was not gender-specific (e.g., Ryan, Taylor) Yes/No
- I would allow my child to wear clothing of the opposite gender (e.g., dresses for boys) Yes/No
- I would allow my child to use the pronoun of the opposite sex Yes/No
- I would allow my child to live as the opposite gender Yes/No
Notice how the items move from lower intensity to higher intensity. A researcher reviews the yes answers and creates a score for each participant.
Indices (Indexes)
An index is a composite score derived from aggregating measures of multiple concepts (called components) using a set of rules and formulas. It is different from a scale. Scales also aggregate measures; however, these measures examine different dimensions or the same dimension of a single construct. A well-known example of an index is the consumer price index (CPI), which is computed every month by the Bureau of Labor Statistics of the U.S. Department of Labor. The CPI is a measure of how much consumers have to pay for goods and services (in general) and is divided into eight major categories (food and beverages, housing, apparel, transportation, healthcare, recreation, education and communication, and “other goods and services”), which are further subdivided into more than 200 smaller items. Each month, government employees call all over the country to get the current prices of more than 80,000 items. Using a complicated weighting scheme that takes into account the location and probability of purchase for each item, analysts then combine these prices into an overall index score using a series of formulas and rules.
Another example of an index is the Duncan Socioeconomic Index (SEI). This index is used to quantify a person’s socioeconomic status (SES) and is a combination of three concepts: income, education, and occupation. Income is measured in dollars, education in years or degrees achieved, and occupation is classified into categories or levels by status. These very different measures are combined to create an overall SES index score. However, SES index measurement has generated a lot of controversy and disagreement among researchers.
The process of creating an index is similar to that of a scale. First, conceptualize (define) the index and its constituent components. Though this appears simple, there may be a lot of disagreement on what components (concepts/constructs) should be included or excluded from an index. For instance, in the SES index, isn’t income correlated with education and occupation? And if so, should we include one component only or all three components? Reviewing the literature, using theories, and/or interviewing experts or key stakeholders may help resolve this issue. Second, operationalize and measure each component. For instance, how will you categorize occupations, particularly since some occupations may have changed with time (e.g., there were no Web developers before the Internet)? Third, create a rule or formula for calculating the index score. Again, this process may involve a lot of subjectivity. Lastly, validate the index score using existing or new data.
Differences Between Scales and Indices
Though indices and scales yield a single numerical score or value representing a concept of interest, they are different in many ways. First, indices often comprise components that are very different from each other (e.g., income, education, and occupation in the SES index) and are measured in different ways. Conversely, scales typically involve a set of similar items that use the same rating scale (such as a five-point Likert scale about customer satisfaction).
Second, indices often combine objectively measurable values such as prices or income, while scales are designed to assess subjective or judgmental constructs such as attitude, prejudice, or self-esteem. Some argue that the sophistication of the scaling methodology makes scales different from indexes, while others suggest that indexing methodology can be equally sophisticated. Nevertheless, indexes and scales are both essential tools in social science research.
A note on scales and indices
Scales and indices seem like clean, convenient ways to measure different phenomena in social science, but just like with a lot of research, we have to be mindful of the assumptions and biases underneath. What if a scale or an index was developed using only White women as research participants? Is it going to be useful for other groups? It very well might be, but when using a scale or index on a group for whom it hasn’t been tested, it will be very important to evaluate the validity and reliability of the instrument, which we address in the next section.
It’s important to note that while scales and indices are often made up of nominal or ordinal variables, when we analyze them into composite scores, we will treat them as interval/ratio variables.
- Scales and indices are common ways to collect information and involve using multiple indicators in measurement.
- A key difference between a scale and an index is that a scale contains multiple indicators for one concept, whereas an indicator examines multiple concepts (components).
- In order to create scales or indices, researchers must have a clear understanding of the indicators for what they are studying.
- What is the level of measurement for each item on each tool? Take a second and think about why the tool’s creator decided to include these levels of measurement. Identify any levels of measurement you would change and why.
- If these tools don’t exist for what you are interested in studying, why do you think that is?
11.4 Reliability and validity in measurement
- Discuss measurement error, the different types, and how to minimize the probability of them
- Differentiate between reliability and validity and understand how these are related to each other and relevant to understanding the value of a measurement tool
- Compare and contrast the types of reliability and demonstrate how to evaluate each type
- Compare and contrast the types of validity and demonstrate how to evaluate each type
The previous chapter provided insight into measuring concepts in social work research. We discussed the importance of identifying concepts and their corresponding indicators as a way to help us operationalize them. In essence, we now understand that when we think about our measurement process, we must be intentional and thoughtful in the choices that we make. Before we talk about how to evaluate our measurement process, let’s discuss why we want to evaluate our process. We evaluate our process so that we minimize our chances of error . But what is measurement error?
Types of Errors
We need to be concerned with two types of errors in measurement: systematic and random errors. Systematic errors are errors that are generally predictable. These are errors that, “are due to the process that biases the results.” [5] For instance, my cat stepping on the scale with me each morning is a systematic error in measuring my weight. I could predict that each measurement would be off by 13 pounds. (He’s a bit of a chonk.)
There are multiple categories of systematic errors.
- Social desirability , occurs when you ask participants a question and they answer in the way that they feel is the most socially desired . For instance, let's imagine that you want to understand the level of prejudice that participants feel regarding immigrants and decide to conduct face-to-face interviews with participants. Some participants may feel compelled to answer in a way that indicates that they are less prejudiced than they really are.
- [pb_glossary id="2096"]Acquiescence bias occurs when participants answer items in some type of pattern, usually skewed to more favorable responses. For example, imagine that you took a research class and loved it. The professor was great and you learned so much. When asked to complete the end of course questionnaire, you immediately mark "strongly agree" to all items without really reading all of the items. After all, you really loved the class. However, instead of reading and reflecting on each item, you "acquiesced" and used your overall impression of the experience to answer all of the items.
- Leading questions are those questions that are worded in a way so that the participant is "lead" to a specific answer. For instance, think about the question, "Have you ever hurt a sweet, innocent child?" Most people, regardless of their true response, may answer "no" simply because the wording of the question leads the participant to believe that "no" is the correct answer.
In order to minimize these types of errors, you should think about what you are studying and examine potential public perceptions of this issue. Next, think about how your questions are worded and how you will administer your tool (we will discuss these in greater detail in the next chapter). This will help you determine if your methods inadvertently increase the probability of these types of errors.
These errors differ from random errors , whic are "due to chance and are not systematic in any way." [6] Sometimes it is difficult to "tease out" random errors. When you take your statistics class, you will learn more about random errors and what to do about them. They're hard to observe until you start diving deeper into statistical analysis, so put a pin in them for now.
Now that we have a good understanding of the two types of errors, let's discuss what we can do to evaluate our measurement process and minimize the chances of these occurring. Remember, quality projects are clear on what is measured , how it is measured, and why it is measured . In addition, quality projects are attentive to the appropriateness of measurement tools and evaluate whether tools are used correctly and consistently. But how do we do that? Good researchers do not simply assume that their measures work. Instead, they collect data to demonstrate that they work. If their research does not demonstrate that a measure works, they stop using it. There are two key factors to consider in deciding whether your measurements are good: reliability and validity.
Reliability
Reliability refers to the consistency of a measure. Psychologists consider three types of reliability: over time (test-retest reliability), across items (internal consistency), and across different researchers (inter-rater reliability).
Test-retest reliability
When researchers measure a construct that they assume to be consistent across time, then the scores they obtain should also be consistent across time. Test-retest reliability is the extent to which this is actually the case. For example, intelligence is generally thought to be consistent across time. A person who is highly intelligent today will be highly intelligent next week. This means that any good measure of intelligence should produce roughly the same scores for this individual next week as it does today. Clearly, a measure that produces highly inconsistent scores over time cannot be a very good measure of a construct that is supposed to be consistent.
Assessing test-retest reliability requires using the measure on a group of people at one time, using it again on the same group of people at a later time. At neither point has the research participant received any sort of intervention. Once you have these two measurements, you then look at the correlation between the two sets of scores. This is typically done by graphing the data in a scatterplot and computing the correlation coefficient. Figure 11.2 shows the correlation between two sets of scores of several university students on the Rosenberg Self-Esteem Scale, administered two times, a week apart. The correlation coefficient for these data is +.95. In general, a test-retest correlation of +.80 or greater is considered to indicate good reliability.
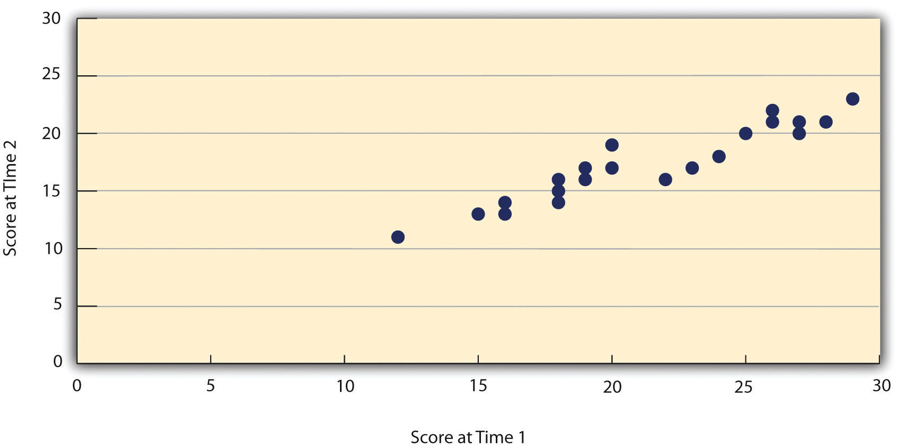
Again, high test-retest correlations make sense when the construct being measured is assumed to be consistent over time, which is the case for intelligence, self-esteem, and the Big Five personality dimensions. But other constructs are not assumed to be stable over time. The very nature of mood, for example, is that it changes. So a measure of mood that produced a low test-retest correlation over a period of a month would not be a cause for concern.
Internal consistency
Another kind of reliability is internal consistency , which is the consistency of people’s responses across the items on a multiple-item measure. In general, all the items on such measures are supposed to reflect the same underlying construct, so people’s scores on those items should be correlated with each other. On the Rosenberg Self-Esteem Scale, people who agree that they are a person of worth should tend to agree that they have a number of good qualities. If people’s responses to the different items are not correlated with each other, then it would no longer make sense to claim that they are all measuring the same underlying construct. This is as true for behavioral and physiological measures as for self-report measures. For example, people might make a series of bets in a simulated game of roulette as a measure of their level of risk seeking. This measure would be internally consistent to the extent that individual participants’ bets were consistently high or low across trials.
Interrater Reliability
Many behavioral measures involve significant judgment on the part of an observer or a rater. Interrater reliability is the extent to which different observers are consistent in their judgments. For example, if you were interested in measuring university students’ social skills, you could make video recordings of them as they interacted with another student whom they are meeting for the first time. Then you could have two or more observers watch the videos and rate each student’s level of social skills. To the extent that each participant does, in fact, have some level of social skills that can be detected by an attentive observer, different observers’ ratings should be highly correlated with each other.
Validity , another key element of assessing measurement quality, is the extent to which the scores from a measure represent the variable they are intended to. But how do researchers make this judgment? We have already considered one factor that they take into account—reliability. When a measure has good test-retest reliability and internal consistency, researchers should be more confident that the scores represent what they are supposed to. There has to be more to it, however, because a measure can be extremely reliable but have no validity whatsoever. As an absurd example, imagine someone who believes that people’s index finger length reflects their self-esteem and therefore tries to measure self-esteem by holding a ruler up to people’s index fingers. Although this measure would have extremely good test-retest reliability, it would have absolutely no validity. The fact that one person’s index finger is a centimeter longer than another’s would indicate nothing about which one had higher self-esteem.
Discussions of validity usually divide it into several distinct “types.” But a good way to interpret these types is that they are other kinds of evidence—in addition to reliability—that should be taken into account when judging the validity of a measure.
Face validity
Face validity is the extent to which a measurement method appears “on its face” to measure the construct of interest. Most people would expect a self-esteem questionnaire to include items about whether they see themselves as a person of worth and whether they think they have good qualities. So a questionnaire that included these kinds of items would have good face validity. The finger-length method of measuring self-esteem, on the other hand, seems to have nothing to do with self-esteem and therefore has poor face validity. Although face validity can be assessed quantitatively—for example, by having a large sample of people rate a measure in terms of whether it appears to measure what it is intended to—it is usually assessed informally.
Face validity is at best a very weak kind of evidence that a measurement method is measuring what it is supposed to. One reason is that it is based on people’s intuitions about human behavior, which are frequently wrong. It is also the case that many established measures in psychology work quite well despite lacking face validity. The Minnesota Multiphasic Personality Inventory-2 (MMPI-2) measures many personality characteristics and disorders by having people decide whether each of over 567 different statements applies to them—where many of the statements do not have any obvious relationship to the construct that they measure. For example, the items “I enjoy detective or mystery stories” and “The sight of blood doesn’t frighten me or make me sick” both measure the suppression of aggression. In this case, it is not the participants’ literal answers to these questions that are of interest, but rather whether the pattern of the participants’ responses to a series of questions matches those of individuals who tend to suppress their aggression.
Content validity
Content validity is the extent to which a measure “covers” the construct of interest. For example, if a researcher conceptually defines test anxiety as involving both sympathetic nervous system activation (leading to nervous feelings) and negative thoughts, then his measure of test anxiety should include items about both nervous feelings and negative thoughts. Or consider that attitudes are usually defined as involving thoughts, feelings, and actions toward something. By this conceptual definition, a person has a positive attitude toward exercise to the extent that they think positive thoughts about exercising, feels good about exercising, and actually exercises. So to have good content validity, a measure of people’s attitudes toward exercise would have to reflect all three of these aspects. Like face validity, content validity is not usually assessed quantitatively. Instead, it is assessed by carefully checking the measurement method against the conceptual definition of the construct.
Criterion validity
Criterion validity is the extent to which people’s scores on a measure are correlated with other variables (known as criteria) that one would expect them to be correlated with. For example, people’s scores on a new measure of test anxiety should be negatively correlated with their performance on an important school exam. If it were found that people’s scores were in fact negatively correlated with their exam performance, then this would be a piece of evidence that these scores really represent people’s test anxiety. But if it were found that people scored equally well on the exam regardless of their test anxiety scores, then this would cast doubt on the validity of the measure.
A criterion can be any variable that one has reason to think should be correlated with the construct being measured, and there will usually be many of them. For example, one would expect test anxiety scores to be negatively correlated with exam performance and course grades and positively correlated with general anxiety and with blood pressure during an exam. Or imagine that a researcher develops a new measure of physical risk taking. People’s scores on this measure should be correlated with their participation in “extreme” activities such as snowboarding and rock climbing, the number of speeding tickets they have received, and even the number of broken bones they have had over the years. When the criterion is measured at the same time as the construct, criterion validity is referred to as concurrent validity ; however, when the criterion is measured at some point in the future (after the construct has been measured), it is referred to as predictive validity (because scores on the measure have “predicted” a future outcome).
Discriminant validity
Discriminant validity , on the other hand, is the extent to which scores on a measure are not correlated with measures of variables that are conceptually distinct. For example, self-esteem is a general attitude toward the self that is fairly stable over time. It is not the same as mood, which is how good or bad one happens to be feeling right now. So people’s scores on a new measure of self-esteem should not be very highly correlated with their moods. If the new measure of self-esteem were highly correlated with a measure of mood, it could be argued that the new measure is not really measuring self-esteem; it is measuring mood instead.
Increasing the reliability and validity of measures
We have reviewed the types of errors and how to evaluate our measures based on reliability and validity considerations. However, what can we do while selecting or creating our tool so that we minimize the potential of errors? Many of our options were covered in our discussion about reliability and validity. Nevertheless, the following table provides a quick summary of things that you should do when creating or selecting a measurement tool.
- In measurement, two types of errors can occur: systematic, which we might be able to predict, and random, which are difficult to predict but can sometimes be addressed during statistical analysis.
- There are two distinct criteria by which researchers evaluate their measures: reliability and validity. Reliability is consistency across time (test-retest reliability), across items (internal consistency), and across researchers (interrater reliability). Validity is the extent to which the scores actually represent the variable they are intended to.
- Validity is a judgment based on various types of evidence. The relevant evidence includes the measure’s reliability, whether it covers the construct of interest, and whether the scores it produces are correlated with other variables they are expected to be correlated with and not correlated with variables that are conceptually distinct.
- Once you have used a measure, you should reevaluate its reliability and validity based on your new data. Remember that the assessment of reliability and validity is an ongoing process.
- Provide a clear statement regarding the reliability and validity of these tools. What strengths did you notice? What were the limitations?
- Think about your target population . Are there changes that need to be made in order for one of these tools to be appropriate for your population?
- If you decide to create your own tool, how will you assess its validity and reliability?
11.5 Ethical and social justice considerations for measurement
- Identify potential cultural, ethical, and social justice issues in measurement.
Just like with other parts of the research process, how we decide to measure what we are researching is influenced by our backgrounds, including our culture, implicit biases, and individual experiences. For me as a middle-class, cisgender white woman, the decisions I make about measurement will probably default to ones that make the most sense to me and others like me, and thus measure characteristics about us most accurately if I don't think carefully about it. There are major implications for research here because this could affect the validity of my measurements for other populations.
This doesn't mean that standardized scales or indices, for instance, won't work for diverse groups of people. What it means is that researchers must not ignore difference in deciding how to measure a variable in their research. Doing so may serve to push already marginalized people further into the margins of academic research and, consequently, social work intervention. Social work researchers, with our strong orientation toward celebrating difference and working for social justice, are obligated to keep this in mind for ourselves and encourage others to think about it in their research, too.
This involves reflecting on what we are measuring, how we are measuring, and why we are measuring. Do we have biases that impacted how we operationalized our concepts? Did we include st a keholders and gatekeepers in the development of our concepts? This can be a way to gain access to vulnerable populations. What feedback did we receive on our measurement process and how was it incorporated into our work? These are all questions we should ask as we are thinking about measurement. Further, engaging in this intentionally reflective process will help us maximize the chances that our measurement will be accurate and as free from bias as possible.
The NASW Code of Ethics discusses social work research and the importance of engaging in practices that do not harm participants. [14] This is especially important considering that many of the topics studied by social workers are those that are disproportionately experienced by marginalized and oppressed populations. Some of these populations have had negative experiences with the research process: historically, their stories have been viewed through lenses that reinforced the dominant culture's standpoint. Thus, when thinking about measurement in research projects, we must remember that the way in which concepts or constructs are measured will impact how marginalized or oppressed persons are viewed. It is important that social work researchers examine current tools to ensure appropriateness for their population(s). Sometimes this may require researchers to use or adapt existing tools. Other times, this may require researchers to develop completely new measures. In summary, the measurement protocols selected should be tailored and attentive to the experiences of the communities to be studied.
But it's not just about reflecting and identifying problems and biases in our measurement, operationalization, and conceptualization - what are we going to do about it? Consider this as you move through this book and become a more critical consumer of research. Sometimes there isn't something you can do in the immediate sense - the literature base at this moment just is what it is. But how does that inform what you will do later?
- Social work researchers must be attentive to personal and institutional biases in the measurement process that affect marginalized groups.
- What are the potential social justice considerations surrounding your methods?
- What are some strategies you could employ to ensure that you engage in ethical research?
- Milkie, M. A., & Warner, C. H. (2011). Classroom learning environments and the mental health of first grade children. Journal of Health and Social Behavior, 52 , 4–22 ↵
- Kaplan, A. (1964). The conduct of inquiry: Methodology for behavioral science . San Francisco, CA: Chandler Publishing Company. ↵
- Earl Babbie offers a more detailed discussion of Kaplan’s work in his text. You can read it in: Babbie, E. (2010). The practice of social research (12th ed.). Belmont, CA: Wadsworth. ↵
- Likert, R. (1932). A technique for the measurement of attitudes. Archives of Psychology, 140, 1–55. ↵
- Engel, R. & Schutt, R. (2013). The practice of research in social work (3rd. ed.) . Thousand Oaks, CA: SAGE. ↵
- Engel, R. & Shutt, R. (2013). The practice of research in social work (3rd. ed.). Thousand Oaks, CA: SAGE. ↵
- Sullivan G. M. (2011). A primer on the validity of assessment instruments. Journal of graduate medical education, 3 (2), 119–120. doi:10.4300/JGME-D-11-00075.1 ↵
- https://www.socialworkers.org/about/ethics/code-of-ethics/code-of-ethics-english ↵
The process by which we describe and ascribe meaning to the key facts, concepts, or other phenomena that we are investigating.
In measurement, conditions that are easy to identify and verify through direct observation.
In measurement, conditions that are subtle and complex that we must use existing knowledge and intuition to define.
The process of determining how to measure a construct that cannot be directly observed
Conditions that are not directly observable and represent states of being, experiences, and ideas.
“a logical grouping of attributes that can be observed and measured and is expected to vary from person to person in a population” (Gillespie & Wagner, 2018, p. 9)
The level that describes the type of operations can be conducted with your data. There are four nominal, ordinal, interval, and ratio.
Level of measurement that follows nominal level. Has mutually exclusive categories and a hierarchy (order).
A higher level of measurement. Denoted by having mutually exclusive categories, a hierarchy (order), and equal spacing between values. This last item means that values may be added, subtracted, divided, and multiplied.
The highest level of measurement. Denoted by mutually exclusive categories, a hierarchy (order), values can be added, subtracted, multiplied, and divided, and the presence of an absolute zero.
variables whose values are organized into mutually exclusive groups but whose numerical values cannot be used in mathematical operations.
variables whose values are mutually exclusive and can be used in mathematical operations
The differerence between that value that we get when we measure something and the true value
Errors that are generally predictable.
Errors lack any perceptable pattern.
The ability of a measurement tool to measure a phenomenon the same way, time after time. Note: Reliability does not imply validity.
The extent to which scores obtained on a scale or other measure are consistent across time
The extent to which different observers are consistent in their assessment or rating of a particular characteristic or item.
The extent to which the scores from a measure represent the variable they are intended to.
The extent to which a measurement method appears “on its face” to measure the construct of interest
The extent to which a measure “covers” the construct of interest, i.e., it's comprehensiveness to measure the construct.
The extent to which people’s scores on a measure are correlated with other variables (known as criteria) that one would expect them to be correlated with.
A type of Criterion validity. Examines how well a tool provides the same scores as an already existing tool.
A type of criterion validity that examines how well your tool predicts a future criterion.
the group of people whose needs your study addresses
individuals or groups who have an interest in the outcome of the study you conduct
the people or organizations who control access to the population you want to study
Graduate research methods in social work Copyright © 2020 by Matthew DeCarlo, Cory Cummings, Kate Agnelli is licensed under a Creative Commons Attribution-NonCommercial-ShareAlike 4.0 International License , except where otherwise noted.
Share This Book
- Search Menu
- Sign in through your institution
- Advance articles
- Editor's Choice
- Author Guidelines
- Submission Site
- Open Access
- About The British Journal of Social Work
- About the British Association of Social Workers
- Editorial Board
- Advertising and Corporate Services
- Journals Career Network
- Self-Archiving Policy
- Dispatch Dates
- Journals on Oxford Academic
- Books on Oxford Academic

Article Contents
- Introduction
- Quantitative research in social work
- Limitations
- Acknowledgements
- < Previous
Nature and Extent of Quantitative Research in Social Work Journals: A Systematic Review from 2016 to 2020
- Article contents
- Figures & tables
- Supplementary Data
Sebastian Kurten, Nausikaä Brimmel, Kathrin Klein, Katharina Hutter, Nature and Extent of Quantitative Research in Social Work Journals: A Systematic Review from 2016 to 2020, The British Journal of Social Work , Volume 52, Issue 4, June 2022, Pages 2008–2023, https://doi.org/10.1093/bjsw/bcab171
- Permissions Icon Permissions
This study reviews 1,406 research articles published between 2016 and 2020 in the European Journal of Social Work (EJSW), the British Journal of Social Work (BJSW) and Research on Social Work Practice (RSWP). It assesses the proportion and complexity of quantitative research designs amongst published articles and investigates differences between the journals. Furthermore, the review investigates the complexity of the statistical methods employed and identifies the most frequently addressed topics. From the 1,406 articles, 504 (35.8 percent) used a qualitative methodology, 389 (27.7 percent) used a quantitative methodology, 85 (6 percent) used the mixed methods (6 percent), 253 (18 percent) articles were theoretical in nature, 148 (10.5 percent) conducted reviews and 27 (1.9 percent) gave project overviews. The proportion of quantitative research articles was higher in RSWP (55.4 percent) than in the EJSW (14.1 percent) and the BJSW (20.5 percent). The topic analysis could identify at least forty different topics addressed by the articles. Although the proportion of quantitative research is rather small in social work research, the review could not find evidence that it is of low sophistication. Finally, this study concludes that future research would benefit from making explicit why a certain methodology was chosen.
Email alerts
Citing articles via.
- X (formerly Twitter)
- Recommend to your Library
Affiliations
- Online ISSN 1468-263X
- Print ISSN 0045-3102
- Copyright © 2024 British Association of Social Workers
- About Oxford Academic
- Publish journals with us
- University press partners
- What we publish
- New features
- Open access
- Institutional account management
- Rights and permissions
- Get help with access
- Accessibility
- Advertising
- Media enquiries
- Oxford University Press
- Oxford Languages
- University of Oxford
Oxford University Press is a department of the University of Oxford. It furthers the University's objective of excellence in research, scholarship, and education by publishing worldwide
- Copyright © 2024 Oxford University Press
- Cookie settings
- Cookie policy
- Privacy policy
- Legal notice
This Feature Is Available To Subscribers Only
Sign In or Create an Account
This PDF is available to Subscribers Only
For full access to this pdf, sign in to an existing account, or purchase an annual subscription.
No internet connection.
All search filters on the page have been cleared., your search has been saved..
- Sign in to my profile My Profile
The Handbook of Social Work Research Methods
- Edited by: Bruce A. Thyer
- Publisher: SAGE Publications, Inc.
- Publication year: 2001
- Online pub date: January 01, 2011
- Discipline: Social Work
- Methods: Measurement , Case study research , Theory
- DOI: https:// doi. org/10.4135/9781412986182
- Keywords: clients , handbooks , knowledge , population , social problems , social welfare , social work practice Show all Show less
- Print ISBN: 9780761919063
- Online ISBN: 9781412986182
- Buy the book icon link
Subject index
"`Not so much a handbook, but an excellent source of reference' - British Journal of Social Work This volume is the definitive resource for anyone doing research in social work. It details both quantitative and qualitative methods and data collection, as well as suggesting the methods appropriate to particular types of studies. It also covers issues such as ethics, gender and ethnicity, and offers advice on how to write up and present your research."
Front Matter
- Acknowledgments
- Overview of Quantitative Research Methods
- Probability and Sampling
- Reliability and Validity in Quantitative Measurement
- Locating Instruments
- Statistics for Social Workers
- Types of Studies
- Descriptive Studies
- Needs Assessments
- Randomized Controlled Trials
- Program Evaluation
- Using Cost → Procedure → Process → Outcome Analysis
- Single-System Designs
- Overview of Qualitative Research Methods
- Reliability and Validity in Qualitative Research
- Narrative Case Studies
- In-Depth Interviews
- Ethnographic Research Methods
- Participant Observation
- Grounded Theory and Other Inductive Research Methods
- Theory Development
- Historical Research
- Literature Reviews
- Critical Analyses
- Ethical Issues
- Gender, Ethnicity, and Race Matters
- Comparative International Research
- Integrating Qualitative and Quantitative Research Methods
- Applying for Research Grants
- Disseminating Research Findings
Back Matter
- About the Editor
- About the Contributors
Sign in to access this content
Get a 30 day free trial, more like this, sage recommends.
We found other relevant content for you on other Sage platforms.
Have you created a personal profile? Login or create a profile so that you can save clips, playlists and searches
- Sign in/register
Navigating away from this page will delete your results
Please save your results to "My Self-Assessments" in your profile before navigating away from this page.
Sign in to my profile
Please sign into your institution before accessing your profile
Sign up for a free trial and experience all Sage Learning Resources have to offer.
You must have a valid academic email address to sign up.
Get off-campus access
- View or download all content my institution has access to.
Sign up for a free trial and experience all Sage Learning Resources has to offer.
- view my profile
- view my lists
- Help & Terms of Use
Quantitative Research Methods for Social Work: Making Social Work Count
- School for Policy Studies
Research output : Book/Report › Authored book
- Quantitative Research Methods
- Social Work
- Persistent link
Fingerprint
- Social Scientists Social Sciences 100%
- UK Social Sciences 100%
- Quantitative Research Method Social Sciences 100%
- Administrative Structure Social Sciences 50%
- Development Project Social Sciences 50%
- Research Councils Social Sciences 50%
- Social Research Social Sciences 50%
- Economic Research Social Sciences 50%
T1 - Quantitative Research Methods for Social Work
T2 - Making Social Work Count
AU - Teater, Barbra
AU - Devaney, John
AU - Forrester, Donald
AU - Scourfield, Jonathan
AU - Carpenter, John
PY - 2017/1/1
Y1 - 2017/1/1
N2 - Social work knowledge and understanding draws heavily on research, and the ability to critically analyse research findings is a core skill for social workers. However, while many social work students are confident in reading qualitative data, a lack of understanding in basic statistical concepts means that this same confidence does not always apply to quantitative data.The book arose from a curriculum development project funded by the Economic and Social Research Council (ESRC), in conjunction with the Higher Education Funding Council for England, the British Academy and the Nuffield Foundation. This was part of a wider initiative to increase the numbers of quantitative social scientists in the UK in order to address an identified skills gap. This gap related to both the conduct of quantitative research and the literacy of social scientists in being able to read and interpret statistical information. The book is a comprehensive resource for students and educators. It is packed with activities and examples from social work covering the basic concepts of quantitative research methods – including reliability, validity, probability, variables and hypothesis testing – and explores key areas of data collection, analysis and evaluation, providing a detailed examination of their application to social work practice.
AB - Social work knowledge and understanding draws heavily on research, and the ability to critically analyse research findings is a core skill for social workers. However, while many social work students are confident in reading qualitative data, a lack of understanding in basic statistical concepts means that this same confidence does not always apply to quantitative data.The book arose from a curriculum development project funded by the Economic and Social Research Council (ESRC), in conjunction with the Higher Education Funding Council for England, the British Academy and the Nuffield Foundation. This was part of a wider initiative to increase the numbers of quantitative social scientists in the UK in order to address an identified skills gap. This gap related to both the conduct of quantitative research and the literacy of social scientists in being able to read and interpret statistical information. The book is a comprehensive resource for students and educators. It is packed with activities and examples from social work covering the basic concepts of quantitative research methods – including reliability, validity, probability, variables and hypothesis testing – and explores key areas of data collection, analysis and evaluation, providing a detailed examination of their application to social work practice.
KW - Quantitative Research Methods
KW - Social Work
M3 - Authored book
SN - 978-1-137-40026-0
BT - Quantitative Research Methods for Social Work
PB - Palgrave Macmillan
CY - London
- Tools and Resources
- Customer Services
- Addictions and Substance Use
- Administration and Management
- Aging and Older Adults
- Biographies
- Children and Adolescents
- Clinical and Direct Practice
- Couples and Families
- Criminal Justice
- Disabilities
- Ethics and Values
- Gender and Sexuality
- Health Care and Illness
- Human Behavior
- International and Global Issues
- Macro Practice
- Mental and Behavioral Health
- Policy and Advocacy
- Populations and Practice Settings
- Race, Ethnicity, and Culture
- Religion and Spirituality
- Research and Evidence-Based Practice
- Social Justice and Human Rights
- Social Work Profession
- Share Facebook LinkedIn Twitter
Article contents
Quantitative research.
- Shenyang Guo Shenyang Guo Wallace H. Kuralt Distinguished Professor, School of Social Work, University of North Carolina at Chapel Hill
- https://doi.org/10.1093/acrefore/9780199975839.013.333
- Published online: 11 June 2013
This entry describes the definition, history, theories, and applications of quantitative methods in social work research. Unlike qualitative research, quantitative research emphasizes precise, objective, and generalizable findings. Quantitative methods are based on numerous probability and statistical theories, with rigorous proofs and support from both simulated and empirical data. Regression analysis plays a paramountly important role in contemporary statistical methods, which include event history analysis, generalized linear modeling, hierarchical linear modeling, propensity score matching, and structural equation modeling. Quantitative methods can be employed in all stages of a scientific inquiry ranging from sample selection to final data analysis.
- event history analysis
- generalized linear modeling
- hierarchical linear modeling
- propensity score matching
- structural equation modeling
You do not currently have access to this article
Please login to access the full content.
Access to the full content requires a subscription
Printed from Encyclopedia of Social Work. Under the terms of the licence agreement, an individual user may print out a single article for personal use (for details see Privacy Policy and Legal Notice).
date: 07 November 2024
- Cookie Policy
- Privacy Policy
- Legal Notice
- Accessibility
- [66.249.64.20|81.177.182.174]
- 81.177.182.174
Character limit 500 /500
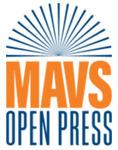
Want to create or adapt books like this? Learn more about how Pressbooks supports open publishing practices.
4.3 Quantitative research questions
Learning objectives.
- Describe how research questions for exploratory, descriptive, and explanatory quantitative questions differ and how to phrase them
- Identify the differences between and provide examples of strong and weak explanatory research questions
Quantitative descriptive questions
The type of research you are conducting will impact the research question that you ask. Probably the easiest questions to think of are quantitative descriptive questions. For example, “What is the average student debt load of MSW students?” is a descriptive question—and an important one. We aren’t trying to build a causal relationship here. We’re simply trying to describe how much debt MSW students carry. Quantitative descriptive questions like this one are helpful in social work practice as part of community scans, in which human service agencies survey the various needs of the community they serve. If the scan reveals that the community requires more services related to housing, child care, or day treatment for people with disabilities, a nonprofit office can use the community scan to create new programs that meet a defined community need.
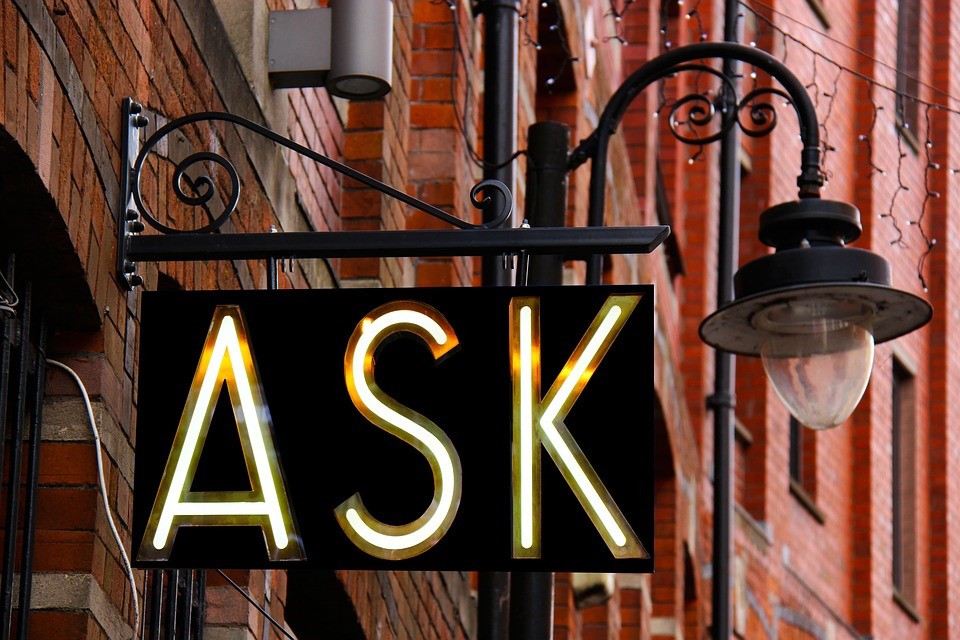
Quantitative descriptive questions will often ask for percentage, count the number of instances of a phenomenon, or determine an average. Descriptive questions may only include one variable, such as ours about debt load, or they may include multiple variables. Because these are descriptive questions, we cannot investigate causal relationships between variables. To do that, we need to use a quantitative explanatory question.
Quantitative explanatory questions
Most studies you read in the academic literature will be quantitative and explanatory. Why is that? Explanatory research tries to build something called nomothetic causal explanations.Matthew DeCarlo says “com[ing]up with a broad, sweeping explanation that is universally true for all people” is the hallmark of nomothetic causal relationships (DeCarlo, 2018, chapter 7.2, para 5 ). They are generalizable across space and time, so they are applicable to a wide audience. The editorial board of a journal wants to make sure their content will be useful to as many people as possible, so it’s not surprising that quantitative research dominates the academic literature.
Structurally, quantitative explanatory questions must contain an independent variable and dependent variable. Questions should ask about the relation between these variables. A standard format for an explanatory quantitative research question is: “What is the relation between [independent variable] and [dependent variable] for [target population]?” You should play with the wording for your research question, revising it as you see fit. The goal is to make the research question reflect what you really want to know in your study.
Let’s take a look at a few more examples of possible research questions and consider the relative strengths and weaknesses of each. Table 4.1 does just that. While reading the table, keep in mind that it only includes some of the most relevant strengths and weaknesses of each question. Certainly each question may have additional strengths and weaknesses not noted in the table.
Making it more specific
A good research question should also be specific and clear about the concepts it addresses. A group of students investigating gender and household tasks knows what they mean by “household tasks.” You likely also have an impression of what “household tasks” means. But are your definition and the students’ definition the same? A participant in their study may think that managing finances and performing home maintenance are household tasks, but the researcher may be interested in other tasks like childcare or cleaning. The only way to ensure your study stays focused and clear is to be specific about what you mean by a concept. The student in our example could pick a specific household task that was interesting to them or that the literature indicated was important—for example, childcare. Or, the student could have a broader view of household tasks, one that encompasses childcare, food preparation, financial management, home repair, and care for relatives. Any option is probably okay, as long as the researchers are clear on what they mean by “household tasks.”
Table 4.2 contains some “watch words” that indicate you may need to be more specific about the concepts in your research question.
It can be challenging in social work research to be this specific, particularly when you are just starting out your investigation of the topic. If you’ve only read one or two articles on the topic, it can be hard to know what you are interested in studying. Broad questions like “What are the causes of chronic homelessness, and what can be done to prevent it?” are common at the beginning stages of a research project. However, social work research demands that you examine the literature on the topic and refine your question over time to be more specific and clear before you begin your study. Perhaps you want to study the effect of a specific anti-homelessness program that you found in the literature. Maybe there is a particular model to fighting homelessness, like Housing First or transitional housing that you want to investigate further. You may want to focus on a potential cause of homelessness such as LGBTQ discrimination that you find interesting or relevant to your practice. As you can see, the possibilities for making your question more specific are almost infinite.
Quantitative exploratory questions
In exploratory research, the researcher doesn’t quite know the lay of the land yet. If someone is proposing to conduct an exploratory quantitative project, the watch words highlighted in Table 4.2 are not problematic at all. In fact, questions such as “What factors influence the removal of children in child welfare cases?” are good because they will explore a variety of factors or causes. In this question, the independent variable is less clearly written, but the dependent variable, family preservation outcomes, is quite clearly written. The inverse can also be true. If we were to ask, “What outcomes are associated with family preservation services in child welfare?”, we would have a clear independent variable, family preservation services, but an unclear dependent variable, outcomes. Because we are only conducting exploratory research on a topic, we may not have an idea of what concepts may comprise our “outcomes” or “factors.” Only after interacting with our participants will we be able to understand which concepts are important.
Key Takeaways
- Quantitative descriptive questions are helpful for community scans but cannot investigate causal relationships between variables.
- Quantitative explanatory questions must include an independent and dependent variable.
Image attributions
Ask by terimakasih0 cc-0.
Guidebook for Social Work Literature Reviews and Research Questions Copyright © 2020 by Rebecca Mauldin and Matthew DeCarlo is licensed under a Creative Commons Attribution-NonCommercial-ShareAlike 4.0 International License , except where otherwise noted.
Share This Book
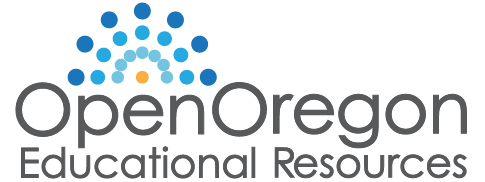
19 11. Quantitative measurement
Chapter outline.
- Conceptual definitions (17 minute read)
- Operational definitions (36 minute read)
- Measurement quality (21 minute read)
- Ethical and social justice considerations (15 minute read)
Content warning: examples in this chapter contain references to ethnocentrism, toxic masculinity, racism in science, drug use, mental health and depression, psychiatric inpatient care, poverty and basic needs insecurity, pregnancy, and racism and sexism in the workplace and higher education.
11.1 Conceptual definitions
Learning objectives.
Learners will be able to…
- Define measurement and conceptualization
- Apply Kaplan’s three categories to determine the complexity of measuring a given variable
- Identify the role previous research and theory play in defining concepts
- Distinguish between unidimensional and multidimensional concepts
- Critically apply reification to how you conceptualize the key variables in your research project
In social science, when we use the term measurement , we mean the process by which we describe and ascribe meaning to the key facts, concepts, or other phenomena that we are investigating. At its core, measurement is about defining one’s terms in as clear and precise a way as possible. Of course, measurement in social science isn’t quite as simple as using a measuring cup or spoon, but there are some basic tenets on which most social scientists agree when it comes to measurement. We’ll explore those, as well as some of the ways that measurement might vary depending on your unique approach to the study of your topic.
An important point here is that measurement does not require any particular instruments or procedures. What it does require is a systematic procedure for assigning scores, meanings, and descriptions to individuals or objects so that those scores represent the characteristic of interest. You can measure phenomena in many different ways, but you must be sure that how you choose to measure gives you information and data that lets you answer your research question. If you’re looking for information about a person’s income, but your main points of measurement have to do with the money they have in the bank, you’re not really going to find the information you’re looking for!
The question of what social scientists measure can be answered by asking yourself what social scientists study. Think about the topics you’ve learned about in other social work classes you’ve taken or the topics you’ve considered investigating yourself. Let’s consider Melissa Milkie and Catharine Warner’s study (2011) [1] of first graders’ mental health. In order to conduct that study, Milkie and Warner needed to have some idea about how they were going to measure mental health. What does mental health mean, exactly? And how do we know when we’re observing someone whose mental health is good and when we see someone whose mental health is compromised? Understanding how measurement works in research methods helps us answer these sorts of questions.
As you might have guessed, social scientists will measure just about anything that they have an interest in investigating. For example, those who are interested in learning something about the correlation between social class and levels of happiness must develop some way to measure both social class and happiness. Those who wish to understand how well immigrants cope in their new locations must measure immigrant status and coping. Those who wish to understand how a person’s gender shapes their workplace experiences must measure gender and workplace experiences (and get more specific about which experiences are under examination). You get the idea. Social scientists can and do measure just about anything you can imagine observing or wanting to study. Of course, some things are easier to observe or measure than others.
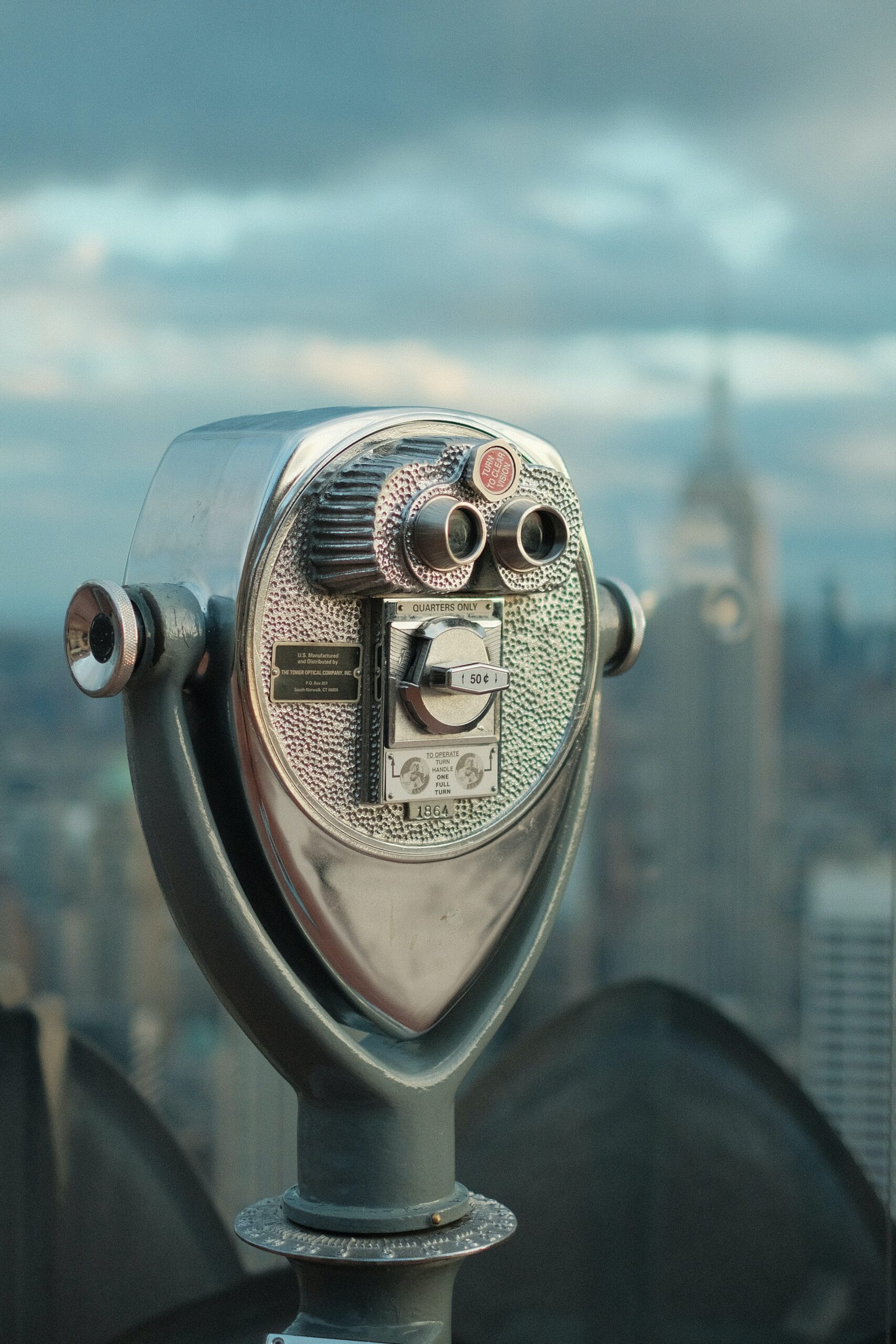
Observing your variables
In 1964, philosopher Abraham Kaplan (1964) [2] wrote The Conduct of Inquiry, which has since become a classic work in research methodology (Babbie, 2010). [3] In his text, Kaplan describes different categories of things that behavioral scientists observe. One of those categories, which Kaplan called “observational terms,” is probably the simplest to measure in social science. Observational terms are the sorts of things that we can see with the naked eye simply by looking at them. Kaplan roughly defines them as conditions that are easy to identify and verify through direct observation. If, for example, we wanted to know how the conditions of playgrounds differ across different neighborhoods, we could directly observe the variety, amount, and condition of equipment at various playgrounds.
Indirect observables , on the other hand, are less straightforward to assess. In Kaplan’s framework, they are conditions that are subtle and complex that we must use existing knowledge and intuition to define. If we conducted a study for which we wished to know a person’s income, we’d probably have to ask them their income, perhaps in an interview or a survey. Thus, we have observed income, even if it has only been observed indirectly. Birthplace might be another indirect observable. We can ask study participants where they were born, but chances are good we won’t have directly observed any of those people being born in the locations they report.
Sometimes the measures that we are interested in are more complex and more abstract than observational terms or indirect observables. Think about some of the concepts you’ve learned about in other social work classes—for example, ethnocentrism. What is ethnocentrism? Well, from completing an introduction to social work class you might know that it has something to do with the way a person judges another’s culture. But how would you measure it? Here’s another construct: bureaucracy. We know this term has something to do with organizations and how they operate but measuring such a construct is trickier than measuring something like a person’s income. The theoretical concepts of ethnocentrism and bureaucracy represent ideas whose meanings we have come to agree on. Though we may not be able to observe these abstractions directly, we can observe their components.
Kaplan referred to these more abstract things that behavioral scientists measure as constructs. Constructs are “not observational either directly or indirectly” (Kaplan, 1964, p. 55), [4] but they can be defined based on observables. For example, the construct of bureaucracy could be measured by counting the number of supervisors that need to approve routine spending by public administrators. The greater the number of administrators that must sign off on routine matters, the greater the degree of bureaucracy. Similarly, we might be able to ask a person the degree to which they trust people from different cultures around the world and then assess the ethnocentrism inherent in their answers. We can measure constructs like bureaucracy and ethnocentrism by defining them in terms of what we can observe. [5]
The idea of coming up with your own measurement tool might sound pretty intimidating at this point. The good news is that if you find something in the literature that works for you, you can use it (with proper attribution, of course). If there are only pieces of it that you like, you can reuse those pieces (with proper attribution and describing/justifying any changes). You don’t always have to start from scratch!
Look at the variables in your research question.
- Classify them as direct observables, indirect observables, or constructs.
- Do you think measuring them will be easy or hard?
- What are your first thoughts about how to measure each variable? No wrong answers here, just write down a thought about each variable.
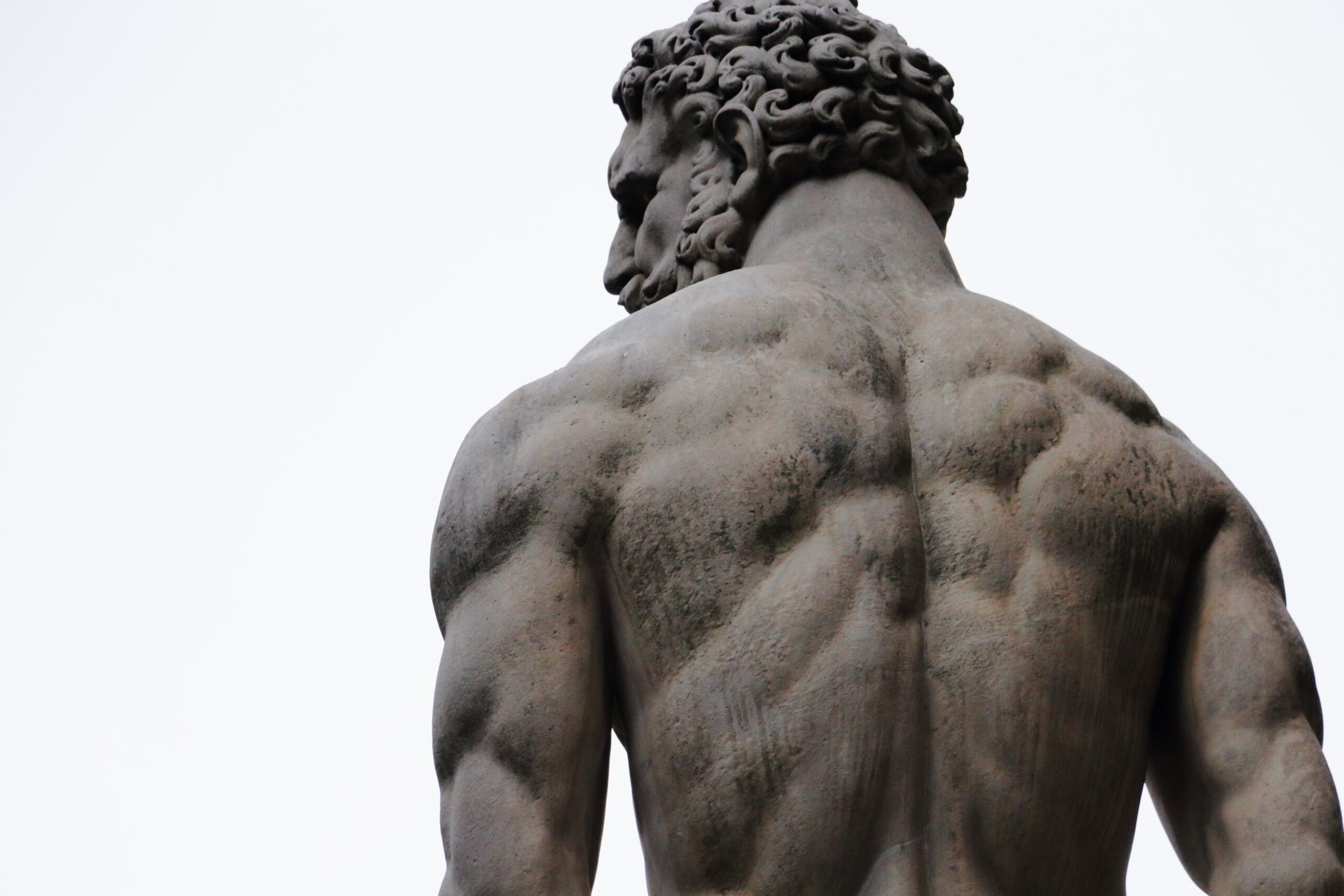
Measurement starts with conceptualization
In order to measure the concepts in your research question, we first have to understand what we think about them. As an aside, the word concept has come up quite a bit, and it is important to be sure we have a shared understanding of that term. A concept is the notion or image that we conjure up when we think of some cluster of related observations or ideas. For example, masculinity is a concept. What do you think of when you hear that word? Presumably, you imagine some set of behaviors and perhaps even a particular style of self-presentation. Of course, we can’t necessarily assume that everyone conjures up the same set of ideas or images when they hear the word masculinity . While there are many possible ways to define the term and some may be more common or have more support than others, there is no universal definition of masculinity. What counts as masculine may shift over time, from culture to culture, and even from individual to individual (Kimmel, 2008). This is why defining our concepts is so important.\
Not all researchers clearly explain their theoretical or conceptual framework for their study, but they should! Without understanding how a researcher has defined their key concepts, it would be nearly impossible to understand the meaning of that researcher’s findings and conclusions. Back in Chapter 7 , you developed a theoretical framework for your study based on a survey of the theoretical literature in your topic area. If you haven’t done that yet, consider flipping back to that section to familiarize yourself with some of the techniques for finding and using theories relevant to your research question. Continuing with our example on masculinity, we would need to survey the literature on theories of masculinity. After a few queries on masculinity, I found a wonderful article by Wong (2010) [6] that analyzed eight years of the journal Psychology of Men & Masculinity and analyzed how often different theories of masculinity were used . Not only can I get a sense of which theories are more accepted and which are more marginal in the social science on masculinity, I am able to identify a range of options from which I can find the theory or theories that will inform my project.
Identify a specific theory (or more than one theory) and how it helps you understand…
- Your independent variable(s).
- Your dependent variable(s).
- The relationship between your independent and dependent variables.
Rather than completing this exercise from scratch, build from your theoretical or conceptual framework developed in previous chapters.
In quantitative methods, conceptualization involves writing out clear, concise definitions for our key concepts. These are the kind of definitions you are used to, like the ones in a dictionary. A conceptual definition involves defining a concept in terms of other concepts, usually by making reference to how other social scientists and theorists have defined those concepts in the past. Of course, new conceptual definitions are created all the time because our conceptual understanding of the world is always evolving.
Conceptualization is deceptively challenging—spelling out exactly what the concepts in your research question mean to you. Following along with our example, think about what comes to mind when you read the term masculinity. How do you know masculinity when you see it? Does it have something to do with men or with social norms? If so, perhaps we could define masculinity as the social norms that men are expected to follow. That seems like a reasonable start, and at this early stage of conceptualization, brainstorming about the images conjured up by concepts and playing around with possible definitions is appropriate. However, this is just the first step. At this point, you should be beyond brainstorming for your key variables because you have read a good amount of research about them
In addition, we should consult previous research and theory to understand the definitions that other scholars have already given for the concepts we are interested in. This doesn’t mean we must use their definitions, but understanding how concepts have been defined in the past will help us to compare our conceptualizations with how other scholars define and relate concepts. Understanding prior definitions of our key concepts will also help us decide whether we plan to challenge those conceptualizations or rely on them for our own work. Finally, working on conceptualization is likely to help in the process of refining your research question to one that is specific and clear in what it asks. Conceptualization and operationalization (next section) are where “the rubber meets the road,” so to speak, and you have to specify what you mean by the question you are asking. As your conceptualization deepens, you will often find that your research question becomes more specific and clear.
If we turn to the literature on masculinity, we will surely come across work by Michael Kimmel , one of the preeminent masculinity scholars in the United States. After consulting Kimmel’s prior work (2000; 2008), [7] we might tweak our initial definition of masculinity. Rather than defining masculinity as “the social norms that men are expected to follow,” perhaps instead we’ll define it as “the social roles, behaviors, and meanings prescribed for men in any given society at any one time” (Kimmel & Aronson, 2004, p. 503). [8] Our revised definition is more precise and complex because it goes beyond addressing one aspect of men’s lives (norms), and addresses three aspects: roles, behaviors, and meanings. It also implies that roles, behaviors, and meanings may vary across societies and over time. Using definitions developed by theorists and scholars is a good idea, though you may find that you want to define things your own way.
As you can see, conceptualization isn’t as simple as applying any random definition that we come up with to a term. Defining our terms may involve some brainstorming at the very beginning. But conceptualization must go beyond that, to engage with or critique existing definitions and conceptualizations in the literature. Once we’ve brainstormed about the images associated with a particular word, we should also consult prior work to understand how others define the term in question. After we’ve identified a clear definition that we’re happy with, we should make sure that every term used in our definition will make sense to others. Are there terms used within our definition that also need to be defined? If so, our conceptualization is not yet complete. Our definition includes the concept of “social roles,” so we should have a definition for what those mean and become familiar with role theory to help us with our conceptualization. If we don’t know what roles are, how can we study them?
Let’s say we do all of that. We have a clear definition of the term masculinity with reference to previous literature and we also have a good understanding of the terms in our conceptual definition…then we’re done, right? Not so fast. You’ve likely met more than one man in your life, and you’ve probably noticed that they are not the same, even if they live in the same society during the same historical time period. This could mean there are dimensions of masculinity. In terms of social scientific measurement, concepts can be said to have multiple dimensions when there are multiple elements that make up a single concept. With respect to the term masculinity , dimensions could based on gender identity, gender performance, sexual orientation, etc.. In any of these cases, the concept of masculinity would be considered to have multiple dimensions.
While you do not need to spell out every possible dimension of the concepts you wish to measure, it is important to identify whether your concepts are unidimensional (and therefore relatively easy to define and measure) or multidimensional (and therefore require multi-part definitions and measures). In this way, how you conceptualize your variables determines how you will measure them in your study. Unidimensional concepts are those that are expected to have a single underlying dimension. These concepts can be measured using a single measure or test. Examples include simple concepts such as a person’s weight, time spent sleeping, and so forth.
One frustrating this is that there is no clear demarcation between concepts that are inherently unidimensional or multidimensional. Even something as simple as age could be broken down into multiple dimensions including mental age and chronological age, so where does conceptualization stop? How far down the dimensional rabbit hole do we have to go? Researchers should consider two things. First, how important is this variable in your study? If age is not important in your study (maybe it is a control variable), it seems like a waste of time to do a lot of work drawing from developmental theory to conceptualize this variable. A unidimensional measure from zero to dead is all the detail we need. On the other hand, if we were measuring the impact of age on masculinity, conceptualizing our independent variable (age) as multidimensional may provide a richer understanding of its impact on masculinity. Finally, your conceptualization will lead directly to your operationalization of the variable, and once your operationalization is complete, make sure someone reading your study could follow how your conceptual definitions informed the measures you chose for your variables.
Write a conceptual definition for your independent and dependent variables.
- Cite and attribute definitions to other scholars, if you use their words.
- Describe how your definitions are informed by your theoretical framework.
- Place your definition in conversation with other theories and conceptual definitions commonly used in the literature.
- Are there multiple dimensions of your variables?
- Are any of these dimensions important for you to measure?
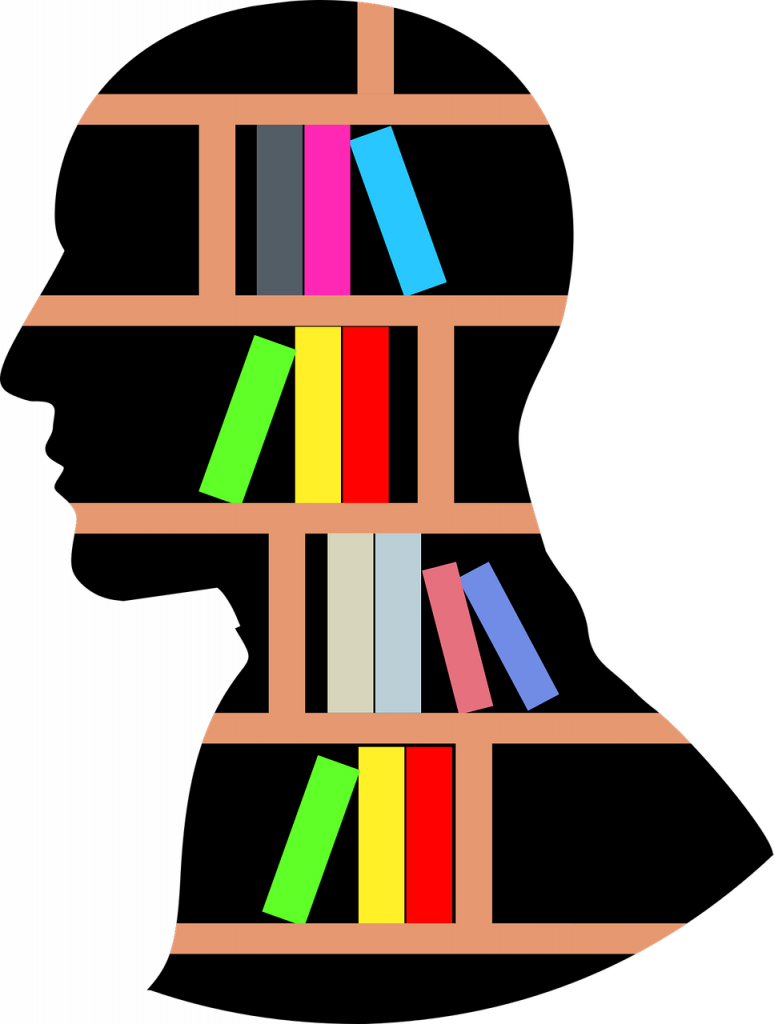
Do researchers actually know what we’re talking about?
Conceptualization proceeds differently in qualitative research compared to quantitative research. Since qualitative researchers are interested in the understandings and experiences of their participants, it is less important for them to find one fixed definition for a concept before starting to interview or interact with participants. The researcher’s job is to accurately and completely represent how their participants understand a concept, not to test their own definition of that concept.
If you were conducting qualitative research on masculinity, you would likely consult previous literature like Kimmel’s work mentioned above. From your literature review, you may come up with a working definition for the terms you plan to use in your study, which can change over the course of the investigation. However, the definition that matters is the definition that your participants share during data collection. A working definition is merely a place to start, and researchers should take care not to think it is the only or best definition out there.
In qualitative inquiry, your participants are the experts (sound familiar, social workers?) on the concepts that arise during the research study. Your job as the researcher is to accurately and reliably collect and interpret their understanding of the concepts they describe while answering your questions. Conceptualization of concepts is likely to change over the course of qualitative inquiry, as you learn more information from your participants. Indeed, getting participants to comment on, extend, or challenge the definitions and understandings of other participants is a hallmark of qualitative research. This is the opposite of quantitative research, in which definitions must be completely set in stone before the inquiry can begin.
The contrast between qualitative and quantitative conceptualization is instructive for understanding how quantitative methods (and positivist research in general) privilege the knowledge of the researcher over the knowledge of study participants and community members. Positivism holds that the researcher is the “expert,” and can define concepts based on their expert knowledge of the scientific literature. This knowledge is in contrast to the lived experience that participants possess from experiencing the topic under examination day-in, day-out. For this reason, it would be wise to remind ourselves not to take our definitions too seriously and be critical about the limitations of our knowledge.
Conceptualization must be open to revisions, even radical revisions, as scientific knowledge progresses. While I’ve suggested consulting prior scholarly definitions of our concepts, you should not assume that prior, scholarly definitions are more real than the definitions we create. Likewise, we should not think that our own made-up definitions are any more real than any other definition. It would also be wrong to assume that just because definitions exist for some concept that the concept itself exists beyond some abstract idea in our heads. Building on the paradigmatic ideas behind interpretivism and the critical paradigm, researchers call the assumption that our abstract concepts exist in some concrete, tangible way is known as reification . It explores the power dynamics behind how we can create reality by how we define it.
Returning again to our example of masculinity. Think about our how our notions of masculinity have developed over the past few decades, and how different and yet so similar they are to patriarchal definitions throughout history. Conceptual definitions become more or less popular based on the power arrangements inside of social science the broader world. Western knowledge systems are privileged, while others are viewed as unscientific and marginal. The historical domination of social science by white men from WEIRD countries meant that definitions of masculinity were imbued their cultural biases and were designed explicitly and implicitly to preserve their power. This has inspired movements for cognitive justice as we seek to use social science to achieve global development.
Key Takeaways
- Measurement is the process by which we describe and ascribe meaning to the key facts, concepts, or other phenomena that we are investigating.
- Kaplan identified three categories of things that social scientists measure including observational terms, indirect observables, and constructs.
- Some concepts have multiple elements or dimensions.
- Researchers often use measures previously developed and studied by other researchers.
- Conceptualization is a process that involves coming up with clear, concise definitions.
- Conceptual definitions are based on the theoretical framework you are using for your study (and the paradigmatic assumptions underlying those theories).
- Whether your conceptual definitions come from your own ideas or the literature, you should be able to situate them in terms of other commonly used conceptual definitions.
- Researchers should acknowledge the limited explanatory power of their definitions for concepts and how oppression can shape what explanations are considered true or scientific.
Think historically about the variables in your research question.
- How has our conceptual definition of your topic changed over time?
- What scholars or social forces were responsible for this change?
Take a critical look at your conceptual definitions.
- How participants might define terms for themselves differently, in terms of their daily experience?
- On what cultural assumptions are your conceptual definitions based?
- Are your conceptual definitions applicable across all cultures that will be represented in your sample?
11.2 Operational definitions
- Define and give an example of indicators and attributes for a variable
- Apply the three components of an operational definition to a variable
- Distinguish between levels of measurement for a variable and how those differences relate to measurement
- Describe the purpose of composite measures like scales and indices
Conceptual definitions are like dictionary definitions. They tell you what a concept means by defining it using other concepts. In this section we will move from the abstract realm (theory) to the real world (measurement). Operationalization is the process by which researchers spell out precisely how a concept will be measured in their study. It involves identifying the specific research procedures we will use to gather data about our concepts. If conceptually defining your terms means looking at theory, how do you operationally define your terms? By looking for indicators of when your variable is present or not, more or less intense, and so forth. Operationalization is probably the most challenging part of quantitative research, but once it’s done, the design and implementation of your study will be straightforward.
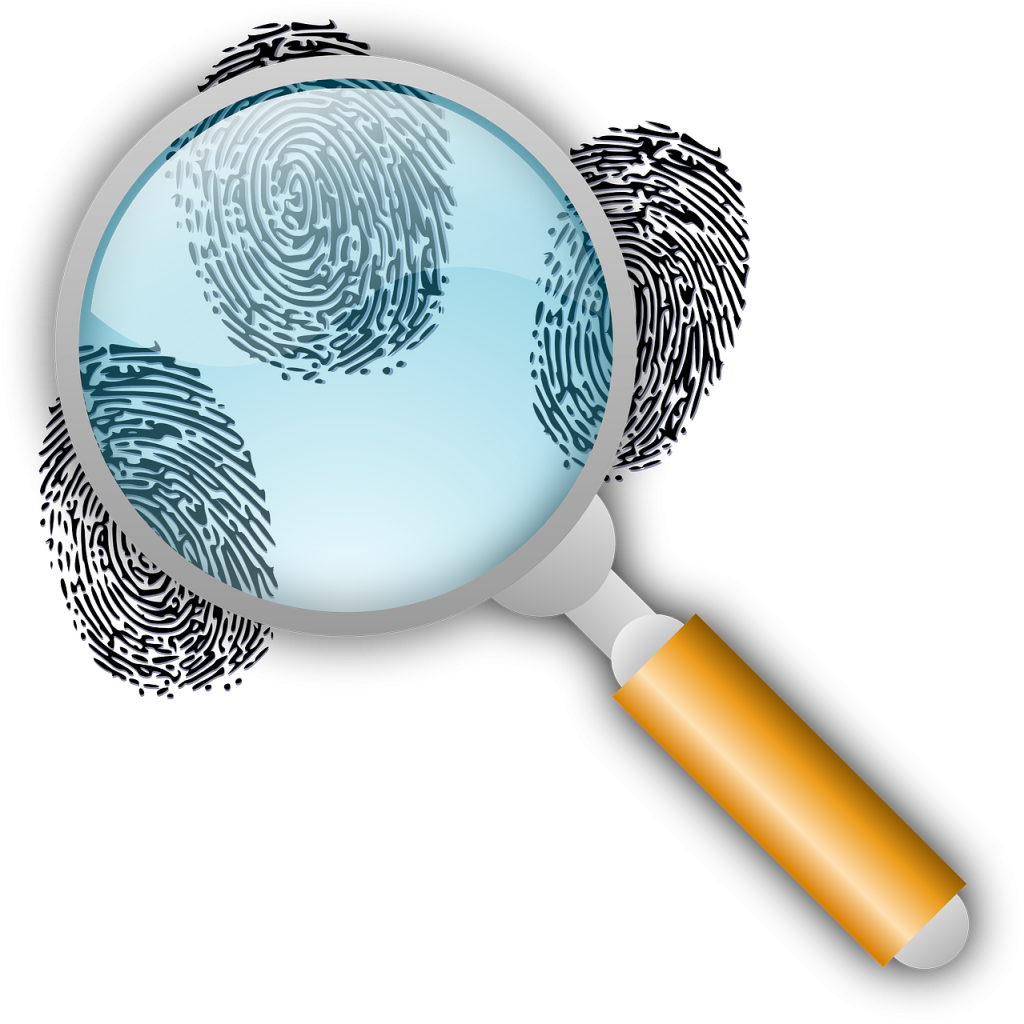
Operationalization works by identifying specific indicators that will be taken to represent the ideas we are interested in studying. If we are interested in studying masculinity, then the indicators for that concept might include some of the social roles prescribed to men in society such as breadwinning or fatherhood. Being a breadwinner or a father might therefore be considered indicators of a person’s masculinity. The extent to which a man fulfills either, or both, of these roles might be understood as clues (or indicators) about the extent to which he is viewed as masculine.
Let’s look at another example of indicators. Each day, Gallup researchers poll 1,000 randomly selected Americans to ask them about their well-being. To measure well-being, Gallup asks these people to respond to questions covering six broad areas: physical health, emotional health, work environment, life evaluation, healthy behaviors, and access to basic necessities. Gallup uses these six factors as indicators of the concept that they are really interested in, which is well-being .
Identifying indicators can be even simpler than the examples described thus far. Political party affiliation is another relatively easy concept for which to identify indicators. If you asked a person what party they voted for in the last national election (or gained access to their voting records), you would get a good indication of their party affiliation. Of course, some voters split tickets between multiple parties when they vote and others swing from party to party each election, so our indicator is not perfect. Indeed, if our study were about political identity as a key concept, operationalizing it solely in terms of who they voted for in the previous election leaves out a lot of information about identity that is relevant to that concept. Nevertheless, it’s a pretty good indicator of political party affiliation.
Choosing indicators is not an arbitrary process. As described earlier, utilizing prior theoretical and empirical work in your area of interest is a great way to identify indicators in a scholarly manner. And you conceptual definitions will point you in the direction of relevant indicators. Empirical work will give you some very specific examples of how the important concepts in an area have been measured in the past and what sorts of indicators have been used. Often, it makes sense to use the same indicators as previous researchers; however, you may find that some previous measures have potential weaknesses that your own study will improve upon.
All of the examples in this chapter have dealt with questions you might ask a research participant on a survey or in a quantitative interview. If you plan to collect data from other sources, such as through direct observation or the analysis of available records, think practically about what the design of your study might look like and how you can collect data on various indicators feasibly. If your study asks about whether the participant regularly changes the oil in their car, you will likely not observe them directly doing so. Instead, you will likely need to rely on a survey question that asks them the frequency with which they change their oil or ask to see their car maintenance records.
- What indicators are commonly used to measure the variables in your research question?
- How can you feasibly collect data on these indicators?
- Are you planning to collect your own data using a questionnaire or interview? Or are you planning to analyze available data like client files or raw data shared from another researcher’s project?
Remember, you need raw data . You research project cannot rely solely on the results reported by other researchers or the arguments you read in the literature. A literature review is only the first part of a research project, and your review of the literature should inform the indicators you end up choosing when you measure the variables in your research question.
Unlike conceptual definitions which contain other concepts, operational definition consists of the following components: (1) the variable being measured and its attributes, (2) the measure you will use, (3) how you plan to interpret the data collected from that measure to draw conclusions about the variable you are measuring.
Step 1: Specifying variables and attributes
The first component, the variable, should be the easiest part. At this point in quantitative research, you should have a research question that has at least one independent and at least one dependent variable. Remember that variables must be able to vary. For example, the United States is not a variable. Country of residence is a variable, as is patriotism. Similarly, if your sample only includes men, gender is a constant in your study, not a variable. A constant is a characteristic that does not change in your study.
When social scientists measure concepts, they sometimes use the language of variables and attributes. A variable refers to a quality or quantity that varies across people or situations. Attributes are the characteristics that make up a variable. For example, the variable hair color would contain attributes like blonde, brown, black, red, gray, etc. A variable’s attributes determine its level of measurement. There are four possible levels of measurement: nominal, ordinal, interval, and ratio. The first two levels of measurement are categorical , meaning their attributes are categories rather than numbers. The latter two levels of measurement are continuous , meaning their attributes are numbers.
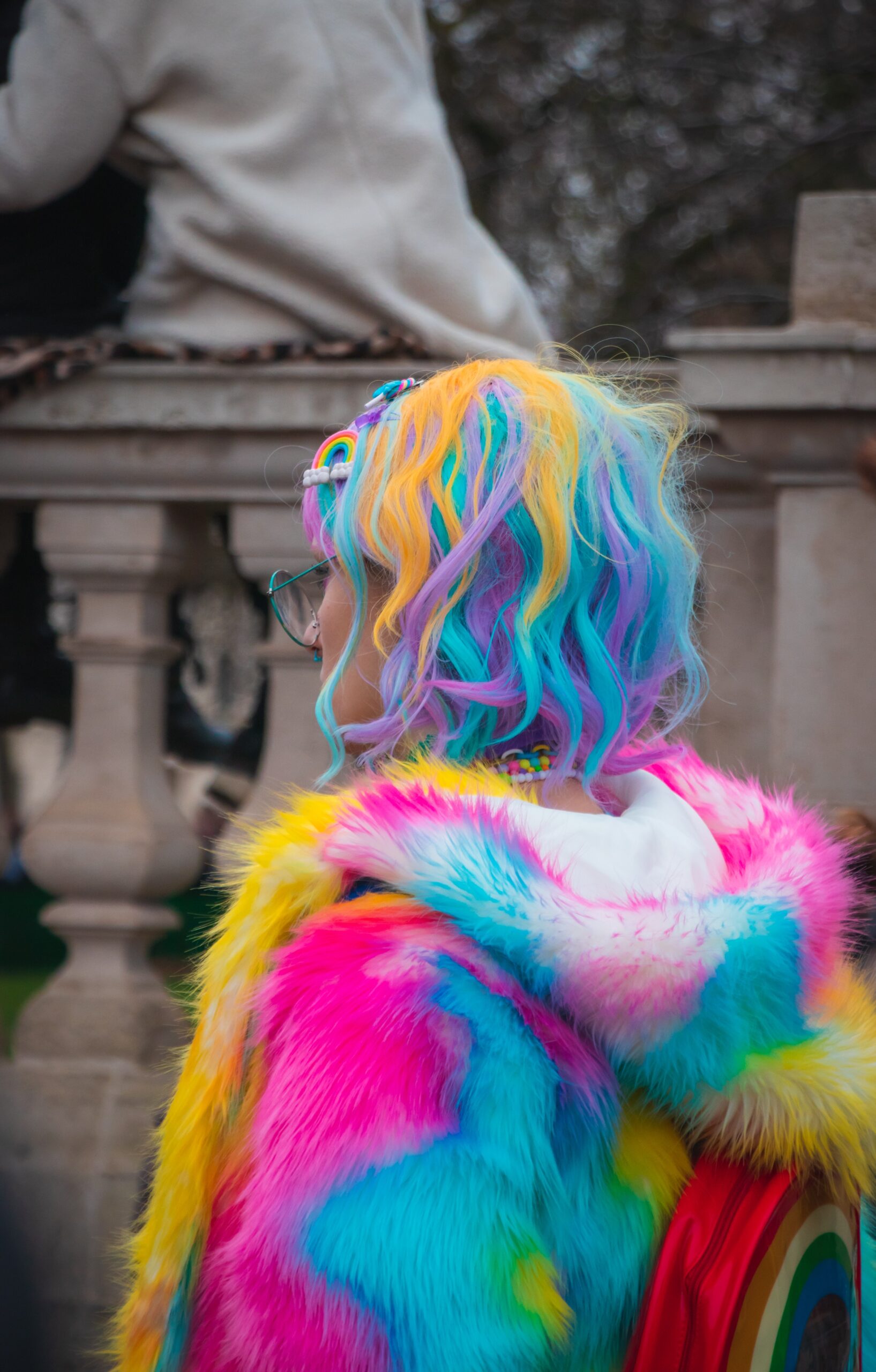
Levels of measurement
Hair color is an example of a nominal level of measurement. Nominal measures are categorical, and those categories cannot be mathematically ranked. As a brown-haired person (with some gray), I can’t say for sure that brown-haired people are better than blonde-haired people. As with all nominal levels of measurement, there is no ranking order between hair colors; they are simply different. That is what constitutes a nominal level of gender and race are also measured at the nominal level.
What attributes are contained in the variable hair color ? While blonde, brown, black, and red are common colors, some people may not fit into these categories if we only list these attributes. My wife, who currently has purple hair, wouldn’t fit anywhere. This means that our attributes were not exhaustive. Exhaustiveness means that all possible attributes are listed. We may have to list a lot of colors before we can meet the criteria of exhaustiveness. Clearly, there is a point at which exhaustiveness has been reasonably met. If a person insists that their hair color is light burnt sienna , it is not your responsibility to list that as an option. Rather, that person would reasonably be described as brown-haired. Perhaps listing a category for other color would suffice to make our list of colors exhaustive.
What about a person who has multiple hair colors at the same time, such as red and black? They would fall into multiple attributes. This violates the rule of mutual exclusivity , in which a person cannot fall into two different attributes. Instead of listing all of the possible combinations of colors, perhaps you might include a multi-color attribute to describe people with more than one hair color.
Making sure researchers provide mutually exclusive and exhaustive is about making sure all people are represented in the data record. For many years, the attributes for gender were only male or female. Now, our understanding of gender has evolved to encompass more attributes that better reflect the diversity in the world. Children of parents from different races were often classified as one race or another, even if they identified with both cultures. The option for bi-racial or multi-racial on a survey not only more accurately reflects the racial diversity in the real world but validates and acknowledges people who identify in that manner. If we did not measure race in this way, we would leave empty the data record for people who identify as biracial or multiracial, impairing our search for truth.
Unlike nominal-level measures, attributes at the ordinal level can be rank ordered. For example, someone’s degree of satisfaction in their romantic relationship can be ordered by rank. That is, you could say you are not at all satisfied, a little satisfied, moderately satisfied, or highly satisfied. Note that even though these have a rank order to them (not at all satisfied is certainly worse than highly satisfied), we cannot calculate a mathematical distance between those attributes. We can simply say that one attribute of an ordinal-level variable is more or less than another attribute.
This can get a little confusing when using rating scales . If you have ever taken a customer satisfaction survey or completed a course evaluation for school, you are familiar with rating scales. “On a scale of 1-5, with 1 being the lowest and 5 being the highest, how likely are you to recommend our company to other people?” That surely sounds familiar. Rating scales use numbers, but only as a shorthand, to indicate what attribute (highly likely, somewhat likely, etc.) the person feels describes them best. You wouldn’t say you are “2” likely to recommend the company, but you would say you are not very likely to recommend the company. Ordinal-level attributes must also be exhaustive and mutually exclusive, as with nominal-level variables.
At the interval level, attributes must also be exhaustive and mutually exclusive and there is equal distance between attributes. Interval measures are also continuous, meaning their attributes are numbers, rather than categories. IQ scores are interval level, as are temperatures in Fahrenheit and Celsius. Their defining characteristic is that we can say how much more or less one attribute differs from another. We cannot, however, say with certainty what the ratio of one attribute is in comparison to another. For example, it would not make sense to say that a person with an IQ score of 140 has twice the IQ of a person with a score of 70. However, the difference between IQ scores of 80 and 100 is the same as the difference between IQ scores of 120 and 140.
While we cannot say that someone with an IQ of 140 is twice as intelligent as someone with an IQ of 70 because IQ is measured at the interval level, we can say that someone with six siblings has twice as many as someone with three because number of siblings is measured at the ratio level. Finally, at the ratio level, attributes are mutually exclusive and exhaustive, attributes can be rank ordered, the distance between attributes is equal, and attributes have a true zero point. Thus, with these variables, we can say what the ratio of one attribute is in comparison to another. Examples of ratio-level variables include age and years of education. We know that a person who is 12 years old is twice as old as someone who is 6 years old. Height measured in meters and weight measured in kilograms are good examples. So are counts of discrete objects or events such as the number of siblings one has or the number of questions a student answers correctly on an exam. The differences between each level of measurement are visualized in Table 11.1.
Levels of measurement=levels of specificity
We have spent time learning how to determine our data’s level of measurement. Now what? How could we use this information to help us as we measure concepts and develop measurement tools? First, the types of statistical tests that we are able to use are dependent on our data’s level of measurement. With nominal-level measurement, for example, the only available measure of central tendency is the mode. With ordinal-level measurement, the median or mode can be used as indicators of central tendency. Interval and ratio-level measurement are typically considered the most desirable because they permit for any indicators of central tendency to be computed (i.e., mean, median, or mode). Also, ratio-level measurement is the only level that allows meaningful statements about ratios of scores. The higher the level of measurement, the more complex statistical tests we are able to conduct. This knowledge may help us decide what kind of data we need to gather, and how.
That said, we have to balance this knowledge with the understanding that sometimes, collecting data at a higher level of measurement could negatively impact our studies. For instance, sometimes providing answers in ranges may make prospective participants feel more comfortable responding to sensitive items. Imagine that you were interested in collecting information on topics such as income, number of sexual partners, number of times someone used illicit drugs, etc. You would have to think about the sensitivity of these items and determine if it would make more sense to collect some data at a lower level of measurement (e.g., asking if they are sexually active or not (nominal) versus their total number of sexual partners (ratio).
Finally, sometimes when analyzing data, researchers find a need to change a data’s level of measurement. For example, a few years ago, a student was interested in studying the relationship between mental health and life satisfaction. This student used a variety of measures. One item asked about the number of mental health symptoms, reported as the actual number. When analyzing data, my student examined the mental health symptom variable and noticed that she had two groups, those with none or one symptoms and those with many symptoms. Instead of using the ratio level data (actual number of mental health symptoms), she collapsed her cases into two categories, few and many. She decided to use this variable in her analyses. It is important to note that you can move a higher level of data to a lower level of data; however, you are unable to move a lower level to a higher level.
- Check that the variables in your research question can vary…and that they are not constants or one of many potential attributes of a variable.
- Think about the attributes your variables have. Are they categorical or continuous? What level of measurement seems most appropriate?
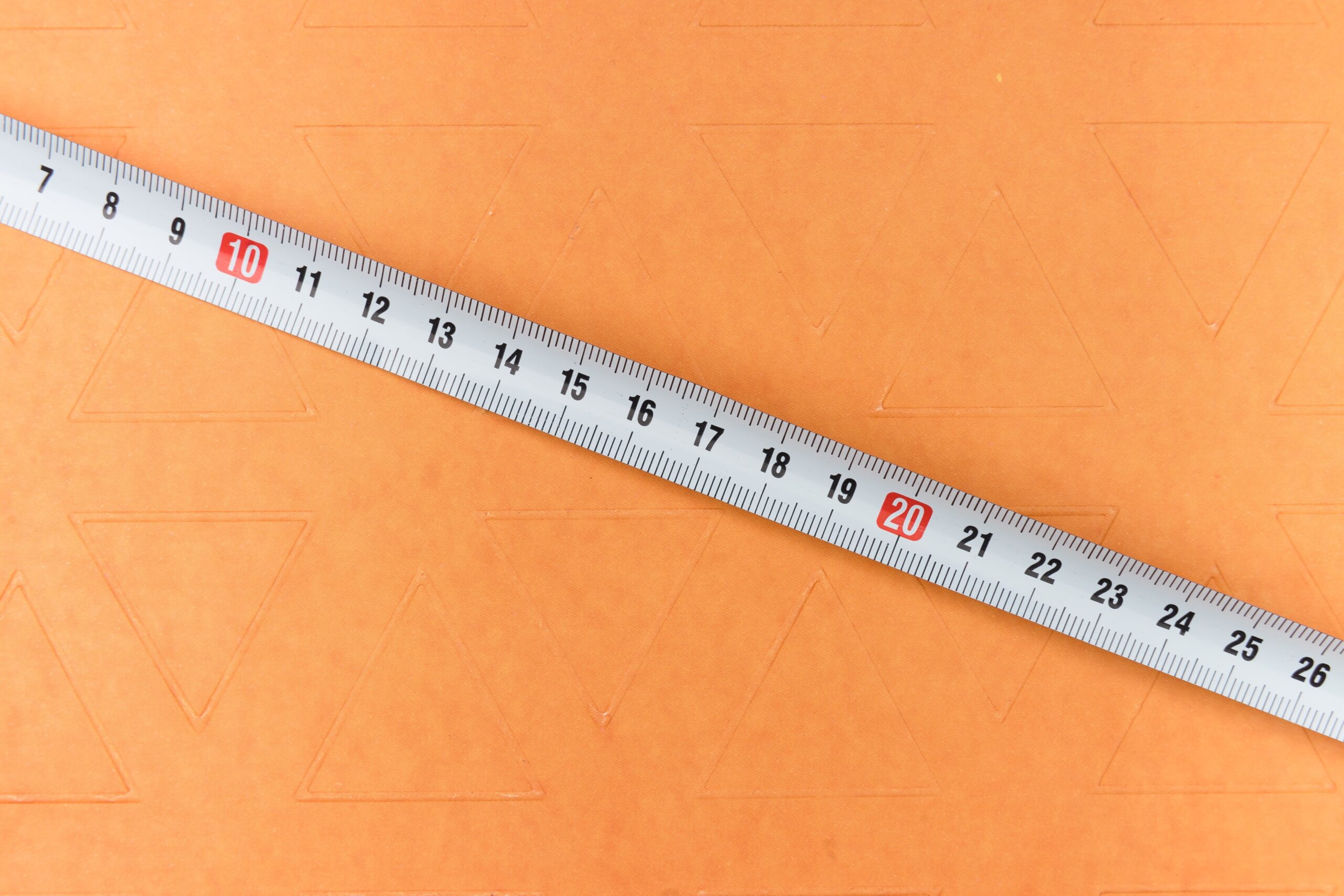
Step 2: Specifying measures for each variable
Let’s pick a social work research question and walk through the process of operationalizing variables to see how specific we need to get. I’m going to hypothesize that residents of a psychiatric unit who are more depressed are less likely to be satisfied with care. Remember, this would be a inverse relationship—as depression increases, satisfaction decreases. In this question, depression is my independent variable (the cause) and satisfaction with care is my dependent variable (the effect). Now we have identified our variables, their attributes, and levels of measurement, we move onto the second component: the measure itself.
So, how would you measure my key variables: depression and satisfaction? What indicators would you look for? Some students might say that depression could be measured by observing a participant’s body language. They may also say that a depressed person will often express feelings of sadness or hopelessness. In addition, a satisfied person might be happy around service providers and often express gratitude. While these factors may indicate that the variables are present, they lack coherence. Unfortunately, what this “measure” is actually saying is that “I know depression and satisfaction when I see them.” While you are likely a decent judge of depression and satisfaction, you need to provide more information in a research study for how you plan to measure your variables. Your judgment is subjective, based on your own idiosyncratic experiences with depression and satisfaction. They couldn’t be replicated by another researcher. They also can’t be done consistently for a large group of people. Operationalization requires that you come up with a specific and rigorous measure for seeing who is depressed or satisfied.
Finding a good measure for your variable depends on the kind of variable it is. Variables that are directly observable don’t come up very often in my students’ classroom projects, but they might include things like taking someone’s blood pressure, marking attendance or participation in a group, and so forth. To measure an indirectly observable variable like age, you would probably put a question on a survey that asked, “How old are you?” Measuring a variable like income might require some more thought, though. Are you interested in this person’s individual income or the income of their family unit? This might matter if your participant does not work or is dependent on other family members for income. Do you count income from social welfare programs? Are you interested in their income per month or per year? Even though indirect observables are relatively easy to measure, the measures you use must be clear in what they are asking, and operationalization is all about figuring out the specifics of what you want to know. For more complicated constructs, you will need compound measures (that use multiple indicators to measure a single variable).
How you plan to collect your data also influences how you will measure your variables. For social work researchers using secondary data like client records as a data source, you are limited by what information is in the data sources you can access. If your organization uses a given measurement for a mental health outcome, that is the one you will use in your study. Similarly, if you plan to study how long a client was housed after an intervention using client visit records, you are limited by how their caseworker recorded their housing status in the chart. One of the benefits of collecting your own data is being able to select the measures you feel best exemplify your understanding of the topic.
Measuring unidimensional concepts
The previous section mentioned two important considerations: how complicated the variable is and how you plan to collect your data. With these in hand, we can use the level of measurement to further specify how you will measure your variables and consider specialized rating scales developed by social science researchers.
Measurement at each level
Nominal measures assess categorical variables. These measures are used for variables or indicators that have mutually exclusive attributes, but that cannot be rank-ordered. Nominal measures ask about the variable and provide names or labels for different attribute values like social work, counseling, and nursing for the variable profession. Nominal measures are relatively straightforward.
Ordinal measures often use a rating scale. It is an ordered set of responses that participants must choose from. Figure 11.1 shows several examples. The number of response options on a typical rating scale is usualy five or seven, though it can range from three to 11. Five-point scales are best for unipolar scales where only one construct is tested, such as frequency (Never, Rarely, Sometimes, Often, Always). Seven-point scales are best for bipolar scales where there is a dichotomous spectrum, such as liking (Like very much, Like somewhat, Like slightly, Neither like nor dislike, Dislike slightly, Dislike somewhat, Dislike very much). For bipolar questions, it is useful to offer an earlier question that branches them into an area of the scale; if asking about liking ice cream, first ask “Do you generally like or dislike ice cream?” Once the respondent chooses like or dislike, refine it by offering them relevant choices from the seven-point scale. Branching improves both reliability and validity (Krosnick & Berent, 1993). [9] Although you often see scales with numerical labels, it is best to only present verbal labels to the respondents but convert them to numerical values in the analyses. Avoid partial labels or length or overly specific labels. In some cases, the verbal labels can be supplemented with (or even replaced by) meaningful graphics. The last rating scale shown in Figure 11.1 is a visual-analog scale, on which participants make a mark somewhere along the horizontal line to indicate the magnitude of their response.
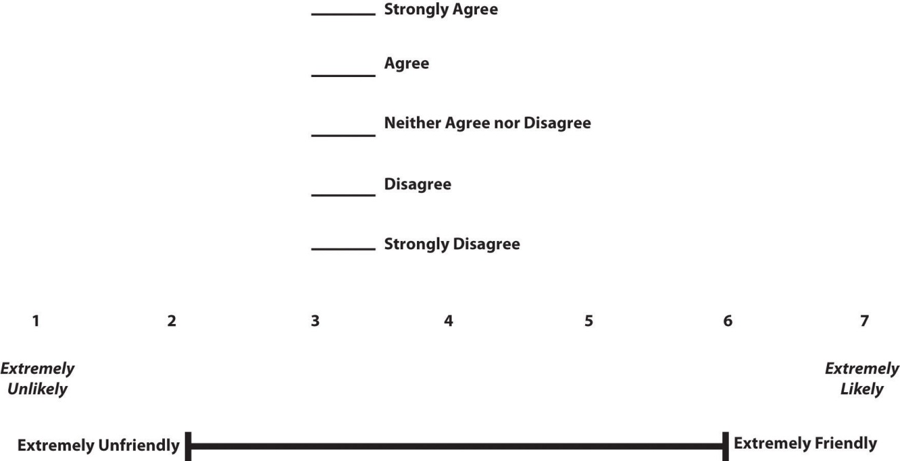
Interval measures are those where the values measured are not only rank-ordered, but are also equidistant from adjacent attributes. For example, the temperature scale (in Fahrenheit or Celsius), where the difference between 30 and 40 degree Fahrenheit is the same as that between 80 and 90 degree Fahrenheit. Likewise, if you have a scale that asks respondents’ annual income using the following attributes (ranges): $0 to 10,000, $10,000 to 20,000, $20,000 to 30,000, and so forth, this is also an interval measure, because the mid-point of each range (i.e., $5,000, $15,000, $25,000, etc.) are equidistant from each other. The intelligence quotient (IQ) scale is also an interval measure, because the measure is designed such that the difference between IQ scores 100 and 110 is supposed to be the same as between 110 and 120 (although we do not really know whether that is truly the case). Interval measures allow us to examine “how much more” is one attribute when compared to another, which is not possible with nominal or ordinal measures. You may find researchers who “pretend” (incorrectly) that ordinal rating scales are actually interval measures so that we can use different statistical techniques for analyzing them. As we will discuss in the latter part of the chapter, this is a mistake because there is no way to know whether the difference between a 3 and a 4 on a rating scale is the same as the difference between a 2 and a 3. Those numbers are just placeholders for categories.
Ratio measures are those that have all the qualities of nominal, ordinal, and interval scales, and in addition, also have a “true zero” point (where the value zero implies lack or non-availability of the underlying construct). Think about how to measure the number of people working in human resources at a social work agency. It could be one, several, or none (if the company contracts out for those services). Measuring interval and ratio data is relatively easy, as people either select or input a number for their answer. If you ask a person how many eggs they purchased last week, they can simply tell you they purchased `a dozen eggs at the store, two at breakfast on Wednesday, or none at all.
Commonly used rating scales in questionnaires
The level of measurement will give you the basic information you need, but social scientists have developed specialized instruments for use in questionnaires, a common tool used in quantitative research. As we mentioned before, if you plan to source your data from client files or previously published results
Although Likert scale is a term colloquially used to refer to almost any rating scale (e.g., a 0-to-10 life satisfaction scale), it has a much more precise meaning. In the 1930s, researcher Rensis Likert (pronounced LICK-ert) created a new approach for measuring people’s attitudes (Likert, 1932) . [10] It involves presenting people with several statements—including both favorable and unfavorable statements—about some person, group, or idea. Respondents then express their agreement or disagreement with each statement on a 5-point scale: Strongly Agree , Agree , Neither Agree nor Disagree , Disagree , Strongly Disagree . Numbers are assigned to each response a nd then summed across all items to produce a score representing the attitude toward the person, group, or idea. For items that are phrased in an opposite direction (e.g., negatively worded statements instead of positively worded statements), reverse coding is used so that the numerical scoring of statements also runs in the opposite direction. The entire set of items came to be called a Likert scale, as indicated in Table 11.2 below.
Unless you are measuring people’s attitude toward something by assessing their level of agreement with several statements about it, it is best to avoid calling it a Likert scale. You are probably just using a rating scale. Likert scales allow for more granularity (more finely tuned response) than yes/no items, including whether respondents are neutral to the statement. Below is an example of how we might use a Likert scale to assess your attitudes about research as you work your way through this textbook.
Semantic differential scales are composite (multi-item) scales in which respondents are asked to indicate their opinions or feelings toward a single statement using different pairs of adjectives framed as polar opposites. Whereas in the above Likert scale, the participant is asked how much they agree or disagree with a statement, in a semantic differential scale the participant is asked to indicate how they feel about a specific item. This makes the s emantic differential scale an excellent technique for measuring people’s attitudes or feelings toward objects, events, or behaviors. Table 11.3 is an example of a semantic differential scale that was created to assess participants’ feelings about this textbook.
This composite scale was designed by Louis Guttman and uses a series of items arranged in increasing order of intensity (least intense to most intense) of the concept. This type of scale allows us to understand the intensity of beliefs or feelings. Each item in the above Guttman scale has a weight (this is not indicated on the tool) which varies with the intensity of that item, and the weighted combination of each response is used as an aggregate measure of an observation.
Example Guttman Scale Items
- I often felt the material was not engaging Yes/No
- I was often thinking about other things in class Yes/No
- I was often working on other tasks during class Yes/No
- I will work to abolish research from the curriculum Yes/No
Notice how the items move from lower intensity to higher intensity. A researcher reviews the yes answers and creates a score for each participant.
Composite measures: Scales and indices
Depending on your research design, your measure may be something you put on a survey or pre/post-test that you give to your participants. For a variable like age or income, one well-worded question may suffice. Unfortunately, most variables in the social world are not so simple. Depression and satisfaction are multidimensional concepts. Relying on a single indicator like a question that asks “Yes or no, are you depressed?” does not encompass the complexity of depression, including issues with mood, sleeping, eating, relationships, and happiness. There is no easy way to delineate between multidimensional and unidimensional concepts, as its all in how you think about your variable. Satisfaction could be validly measured using a unidimensional ordinal rating scale. However, if satisfaction were a key variable in our study, we would need a theoretical framework and conceptual definition for it. That means we’d probably have more indicators to ask about like timeliness, respect, sensitivity, and many others, and we would want our study to say something about what satisfaction truly means in terms of our other key variables. However, if satisfaction is not a key variable in your conceptual framework, it makes sense to operationalize it as a unidimensional concept.
For more complicated measures, researchers use scales and indices (sometimes called indexes) to measure their variables because they assess multiple indicators to develop a composite (or total) score. Co mposite scores provide a much greater understanding of concepts than a single item could. Although we won’t delve too deeply into the process of scale development, we will cover some important topics for you to understand how scales and indices developed by other researchers can be used in your project.
Although they exhibit differences (which will later be discussed) the two have in common various factors.
- Both are ordinal measures of variables.
- Both can order the units of analysis in terms of specific variables.
- Both are composite measures .
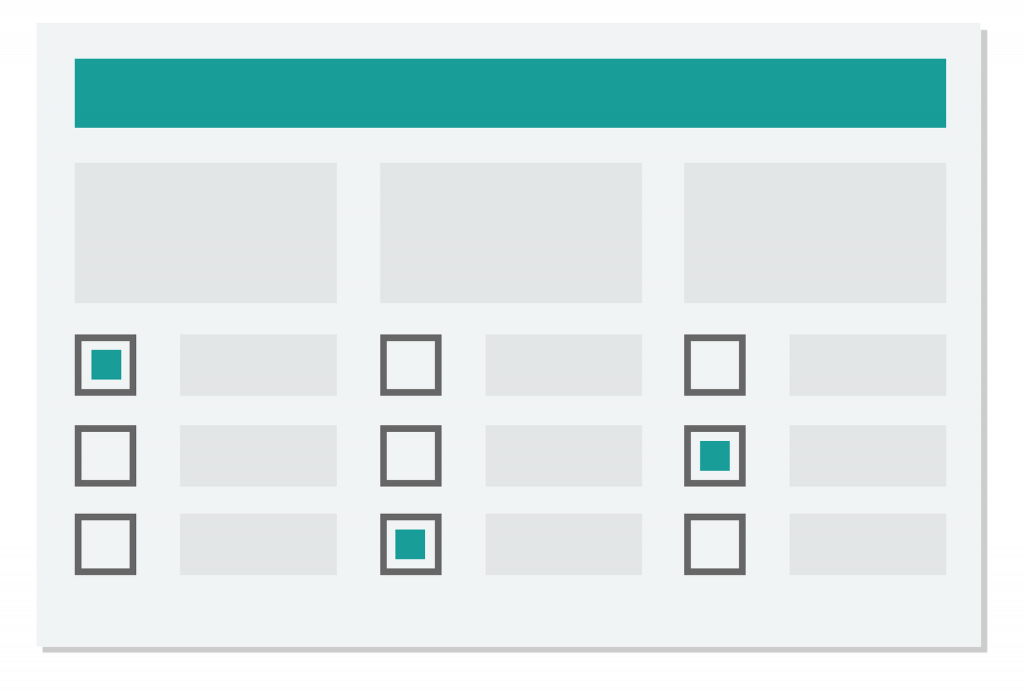
The previous section discussed how to measure respondents’ responses to predesigned items or indicators belonging to an underlying construct. But how do we create the indicators themselves? The process of creating the indicators is called scaling. More formally, scaling is a branch of measurement that involves the construction of measures by associating qualitative judgments about unobservable constructs with quantitative, measurable metric units. Stevens (1946) [11] said, “Scaling is the assignment of objects to numbers according to a rule.” This process of measuring abstract concepts in concrete terms remains one of the most difficult tasks in empirical social science research.
The outcome of a scaling process is a scale , which is an empirical structure for measuring items or indicators of a given construct. Understand that multidimensional “scales”, as discussed in this section, are a little different from “rating scales” discussed in the previous section. A rating scale is used to capture the respondents’ reactions to a given item on a questionnaire. For example, an ordinally scaled item captures a value between “strongly disagree” to “strongly agree.” Attaching a rating scale to a statement or instrument is not scaling. Rather, scaling is the formal process of developing scale items, before rating scales can be attached to those items.
If creating your own scale sounds painful, don’t worry! For most multidimensional variables, you would likely be duplicating work that has already been done by other researchers. Specifically, this is a branch of science called psychometrics. You do not need to create a scale for depression because scales such as the Patient Health Questionnaire (PHQ-9), the Center for Epidemiologic Studies Depression Scale (CES-D), and Beck’s Depression Inventory (BDI) have been developed and refined over dozens of years to measure variables like depression. Similarly, scales such as the Patient Satisfaction Questionnaire (PSQ-18) have been developed to measure satisfaction with medical care. As we will discuss in the next section, these scales have been shown to be reliable and valid. While you could create a new scale to measure depression or satisfaction, a study with rigor would pilot test and refine that new scale over time to make sure it measures the concept accurately and consistently. This high level of rigor is often unachievable in student research projects because of the cost and time involved in pilot testing and validating, so using existing scales is recommended.
Unfortunately, there is no good one-stop=shop for psychometric scales. The Mental Measurements Yearbook provides a searchable database of measures for social science variables, though it woefully incomplete and often does not contain the full documentation for scales in its database. You can access it from a university library’s list of databases. If you can’t find anything in there, your next stop should be the methods section of the articles in your literature review. The methods section of each article will detail how the researchers measured their variables, and often the results section is instructive for understanding more about measures. In a quantitative study, researchers may have used a scale to measure key variables and will provide a brief description of that scale, its names, and maybe a few example questions. If you need more information, look at the results section and tables discussing the scale to get a better idea of how the measure works. Looking beyond the articles in your literature review, searching Google Scholar using queries like “depression scale” or “satisfaction scale” should also provide some relevant results. For example, searching for documentation for the Rosenberg Self-Esteem Scale (which we will discuss in the next section), I found this report from researchers investigating acceptance and commitment therapy which details this scale and many others used to assess mental health outcomes. If you find the name of the scale somewhere but cannot find the documentation (all questions and answers plus how to interpret the scale), a general web search with the name of the scale and “.pdf” may bring you to what you need. Or, to get professional help with finding information, always ask a librarian!
Unfortunately, these approaches do not guarantee that you will be able to view the scale itself or get information on how it is interpreted. Many scales cost money to use and may require training to properly administer. You may also find scales that are related to your variable but would need to be slightly modified to match your study’s needs. You could adapt a scale to fit your study, however changing even small parts of a scale can influence its accuracy and consistency. While it is perfectly acceptable in student projects to adapt a scale without testing it first (time may not allow you to do so), pilot testing is always recommended for adapted scales, and researchers seeking to draw valid conclusions and publish their results must take this additional step.
An index is a composite score derived from aggregating measures of multiple concepts (called components) using a set of rules and formulas. It is different from a scale. Scales also aggregate measures; however, these measures examine different dimensions or the same dimension of a single construct. A well-known example of an index is the consumer price index (CPI), which is computed every month by the Bureau of Labor Statistics of the U.S. Department of Labor. The CPI is a measure of how much consumers have to pay for goods and services (in general) and is divided into eight major categories (food and beverages, housing, apparel, transportation, healthcare, recreation, education and communication, and “other goods and services”), which are further subdivided into more than 200 smaller items. Each month, government employees call all over the country to get the current prices of more than 80,000 items. Using a complicated weighting scheme that takes into account the location and probability of purchase for each item, analysts then combine these prices into an overall index score using a series of formulas and rules.
Another example of an index is the Duncan Socioeconomic Index (SEI). This index is used to quantify a person’s socioeconomic status (SES) and is a combination of three concepts: income, education, and occupation. Income is measured in dollars, education in years or degrees achieved, and occupation is classified into categories or levels by status. These very different measures are combined to create an overall SES index score. However, SES index measurement has generated a lot of controversy and disagreement among researchers.
The process of creating an index is similar to that of a scale. First, conceptualize (define) the index and its constituent components. Though this appears simple, there may be a lot of disagreement on what components (concepts/constructs) should be included or excluded from an index. For instance, in the SES index, isn’t income correlated with education and occupation? And if so, should we include one component only or all three components? Reviewing the literature, using theories, and/or interviewing experts or key stakeholders may help resolve this issue. Second, operationalize and measure each component. For instance, how will you categorize occupations, particularly since some occupations may have changed with time (e.g., there were no Web developers before the Internet)? As we will see in step three below, researchers must create a rule or formula for calculating the index score. Again, this process may involve a lot of subjectivity, so validating the index score using existing or new data is important.
Scale and index development at often taught in their own course in doctoral education, so it is unreasonable for you to expect to develop a consistently accurate measure within the span of a week or two. Using available indices and scales is recommended for this reason.
Differences between scales and indices
Though indices and scales yield a single numerical score or value representing a concept of interest, they are different in many ways. First, indices often comprise components that are very different from each other (e.g., income, education, and occupation in the SES index) and are measured in different ways. Conversely, scales typically involve a set of similar items that use the same rating scale (such as a five-point Likert scale about customer satisfaction).
Second, indices often combine objectively measurable values such as prices or income, while scales are designed to assess subjective or judgmental constructs such as attitude, prejudice, or self-esteem. Some argue that the sophistication of the scaling methodology makes scales different from indexes, while others suggest that indexing methodology can be equally sophisticated. Nevertheless, indexes and scales are both essential tools in social science research.
Scales and indices seem like clean, convenient ways to measure different phenomena in social science, but just like with a lot of research, we have to be mindful of the assumptions and biases underneath. What if a scale or an index was developed using only White women as research participants? Is it going to be useful for other groups? It very well might be, but when using a scale or index on a group for whom it hasn’t been tested, it will be very important to evaluate the validity and reliability of the instrument, which we address in the rest of the chapter.
Finally, it’s important to note that while scales and indices are often made up of nominal or ordinal variables, when we analyze them into composite scores, we will treat them as interval/ratio variables.
- Look back to your work from the previous section, are your variables unidimensional or multidimensional?
- Describe the specific measures you will use (actual questions and response options you will use with participants) for each variable in your research question.
- If you are using a measure developed by another researcher but do not have all of the questions, response options, and instructions needed to implement it, put it on your to-do list to get them.
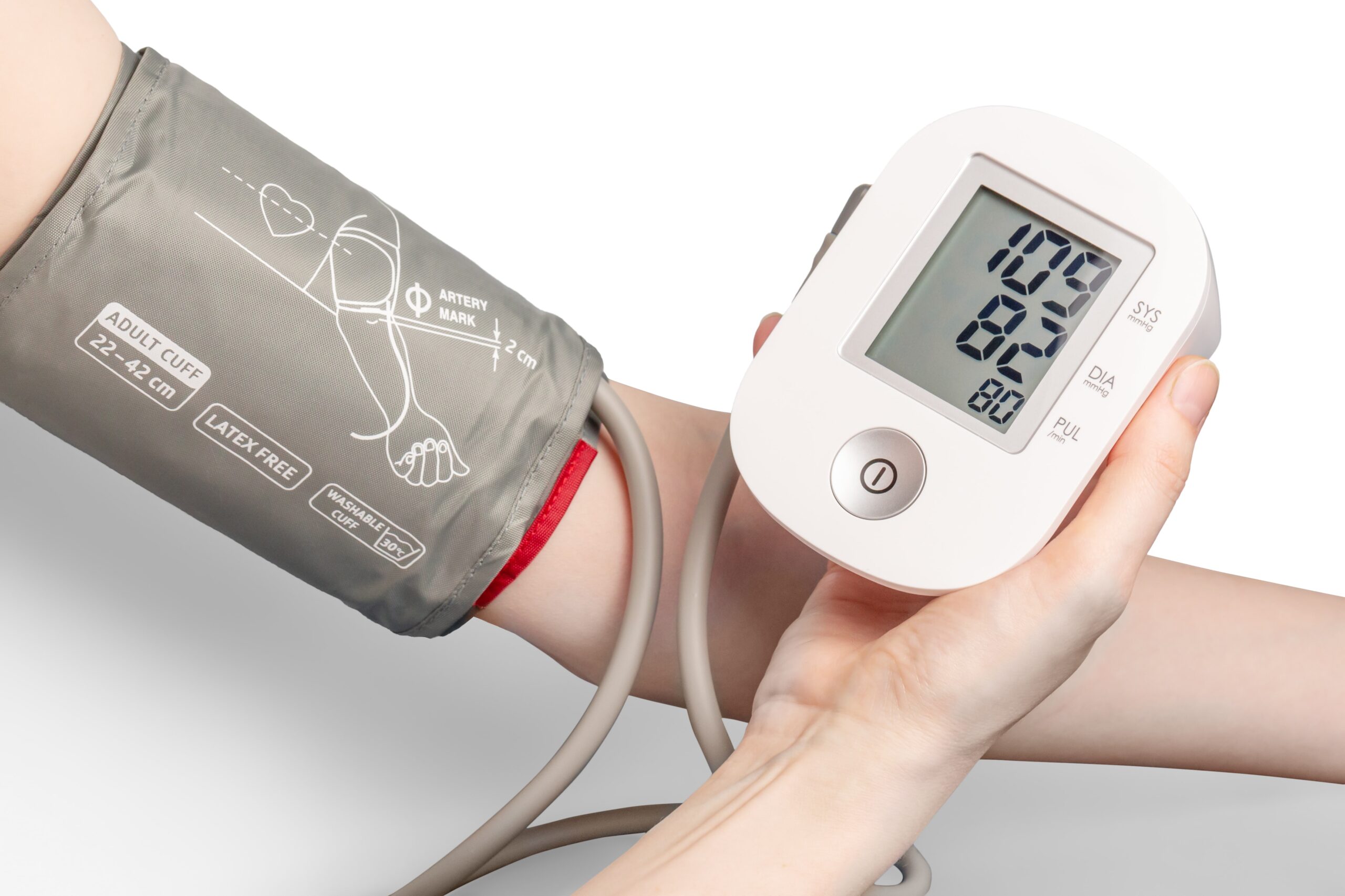
Step 3: How you will interpret your measures
The final stage of operationalization involves setting the rules for how the measure works and how the researcher should interpret the results. Sometimes, interpreting a measure can be incredibly easy. If you ask someone their age, you’ll probably interpret the results by noting the raw number (e.g., 22) someone provides and that it is lower or higher than other people’s ages. However, you could also recode that person into age categories (e.g., under 25, 20-29-years-old, generation Z, etc.). Even scales may be simple to interpret. If there is a scale of problem behaviors, one might simply add up the number of behaviors checked off–with a range from 1-5 indicating low risk of delinquent behavior, 6-10 indicating the student is moderate risk, etc. How you choose to interpret your measures should be guided by how they were designed, how you conceptualize your variables, the data sources you used, and your plan for analyzing your data statistically. Whatever measure you use, you need a set of rules for how to take any valid answer a respondent provides to your measure and interpret it in terms of the variable being measured.
For more complicated measures like scales, refer to the information provided by the author for how to interpret the scale. If you can’t find enough information from the scale’s creator, look at how the results of that scale are reported in the results section of research articles. For example, Beck’s Depression Inventory (BDI-II) uses 21 statements to measure depression and respondents rate their level of agreement on a scale of 0-3. The results for each question are added up, and the respondent is put into one of three categories: low levels of depression (1-16), moderate levels of depression (17-30), or severe levels of depression (31 and over).
One common mistake I see often is that students will introduce another variable into their operational definition. This is incorrect. Your operational definition should mention only one variable—the variable being defined. While your study will certainly draw conclusions about the relationships between variables, that’s not what operationalization is. Operationalization specifies what instrument you will use to measure your variable and how you plan to interpret the data collected using that measure.
Operationalization is probably the trickiest component of basic research methods, so please don’t get frustrated if it takes a few drafts and a lot of feedback to get to a workable definition. At the time of this writing, I am in the process of operationalizing the concept of “attitudes towards research methods.” Originally, I thought that I could gauge students’ attitudes toward research methods by looking at their end-of-semester course evaluations. As I became aware of the potential methodological issues with student course evaluations, I opted to use focus groups of students to measure their common beliefs about research. You may recall some of these opinions from Chapter 1 , such as the common beliefs that research is boring, useless, and too difficult. After the focus group, I created a scale based on the opinions I gathered, and I plan to pilot test it with another group of students. After the pilot test, I expect that I will have to revise the scale again before I can implement the measure in a real social work research project. At the time I’m writing this, I’m still not completely done operationalizing this concept.
- Operationalization involves spelling out precisely how a concept will be measured.
- Operational definitions must include the variable, the measure, and how you plan to interpret the measure.
- There are four different levels of measurement: nominal, ordinal, interval, and ratio (in increasing order of specificity).
- Scales and indices are common ways to collect information and involve using multiple indicators in measurement.
- A key difference between a scale and an index is that a scale contains multiple indicators for one concept, whereas an indicator examines multiple concepts (components).
- Using scales developed and refined by other researchers can improve the rigor of a quantitative study.
Use the research question that you developed in the previous chapters and find a related scale or index that researchers have used. If you have trouble finding the exact phenomenon you want to study, get as close as you can.
- What is the level of measurement for each item on each tool? Take a second and think about why the tool’s creator decided to include these levels of measurement. Identify any levels of measurement you would change and why.
- If these tools don’t exist for what you are interested in studying, why do you think that is?
11.3 Measurement quality
- Define and describe the types of validity and reliability
- Assess for systematic error
The previous chapter provided insight into measuring concepts in social work research. We discussed the importance of identifying concepts and their corresponding indicators as a way to help us operationalize them. In essence, we now understand that when we think about our measurement process, we must be intentional and thoughtful in the choices that we make. This section is all about how to judge the quality of the measures you’ve chosen for the key variables in your research question.
Reliability
First, let’s say we’ve decided to measure alcoholism by asking people to respond to the following question: Have you ever had a problem with alcohol? If we measure alcoholism this way, then it is likely that anyone who identifies as an alcoholic would respond “yes.” This may seem like a good way to identify our group of interest, but think about how you and your peer group may respond to this question. Would participants respond differently after a wild night out, compared to any other night? Could an infrequent drinker’s current headache from last night’s glass of wine influence how they answer the question this morning? How would that same person respond to the question before consuming the wine? In each cases, the same person might respond differently to the same question at different points, so it is possible that our measure of alcoholism has a reliability problem. Reliability in measurement is about consistency.
One common problem of reliability with social scientific measures is memory. If we ask research participants to recall some aspect of their own past behavior, we should try to make the recollection process as simple and straightforward for them as possible. Sticking with the topic of alcohol intake, if we ask respondents how much wine, beer, and liquor they’ve consumed each day over the course of the past 3 months, how likely are we to get accurate responses? Unless a person keeps a journal documenting their intake, there will very likely be some inaccuracies in their responses. On the other hand, we might get more accurate responses if we ask a participant how many drinks of any kind they have consumed in the past week.
Reliability can be an issue even when we’re not reliant on others to accurately report their behaviors. Perhaps a researcher is interested in observing how alcohol intake influences interactions in public locations. They may decide to conduct observations at a local pub by noting how many drinks patrons consume and how their behavior changes as their intake changes. What if the researcher has to use the restroom, and the patron next to them takes three shots of tequila during the brief period the researcher is away from their seat? The reliability of this researcher’s measure of alcohol intake depends on their ability to physically observe every instance of patrons consuming drinks. If they are unlikely to be able to observe every such instance, then perhaps their mechanism for measuring this concept is not reliable.
The following subsections describe the types of reliability that are important for you to know about, but keep in mind that you may see other approaches to judging reliability mentioned in the empirical literature.
Test-retest reliability
When researchers measure a construct that they assume to be consistent across time, then the scores they obtain should also be consistent across time. Test-retest reliability is the extent to which this is actually the case. For example, intelligence is generally thought to be consistent across time. A person who is highly intelligent today will be highly intelligent next week. This means that any good measure of intelligence should produce roughly the same scores for this individual next week as it does today. Clearly, a measure that produces highly inconsistent scores over time cannot be a very good measure of a construct that is supposed to be consistent.
Assessing test-retest reliability requires using the measure on a group of people at one time, using it again on the same group of people at a later time. Unlike an experiment, you aren’t giving participants an intervention but trying to establish a reliable baseline of the variable you are measuring. Once you have these two measurements, you then look at the correlation between the two sets of scores. This is typically done by graphing the data in a scatterplot and computing the correlation coefficient. Figure 11.2 shows the correlation between two sets of scores of several university students on the Rosenberg Self-Esteem Scale, administered two times, a week apart. The correlation coefficient for these data is +.95. In general, a test-retest correlation of +.80 or greater is considered to indicate good reliability.
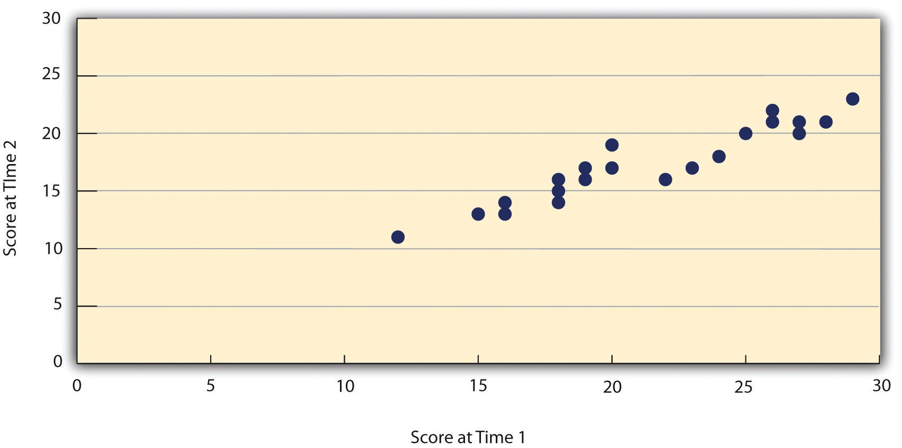
Again, high test-retest correlations make sense when the construct being measured is assumed to be consistent over time, which is the case for intelligence, self-esteem, and the Big Five personality dimensions. But other constructs are not assumed to be stable over time. The very nature of mood, for example, is that it changes. So a measure of mood that produced a low test-retest correlation over a period of a month would not be a cause for concern.
Internal consistency
Another kind of reliability is internal consistency , which is the consistency of people’s responses across the items on a multiple-item measure. In general, all the items on such measures are supposed to reflect the same underlying construct, so people’s scores on those items should be correlated with each other. On the Rosenberg Self-Esteem Scale, people who agree that they are a person of worth should tend to agree that they have a number of good qualities. If people’s responses to the different items are not correlated with each other, then it would no longer make sense to claim that they are all measuring the same underlying construct. This is as true for behavioral and physiological measures as for self-report measures. For example, people might make a series of bets in a simulated game of roulette as a measure of their level of risk seeking. This measure would be internally consistent to the extent that individual participants’ bets were consistently high or low across trials. A specific statistical test known as Cronbach’s Alpha provides a way to measure how well each question of a scale is related to the others.
Interrater reliability
Many behavioral measures involve significant judgment on the part of an observer or a rater. Interrater reliability is the extent to which different observers are consistent in their judgments. For example, if you were interested in measuring university students’ social skills, you could make video recordings of them as they interacted with another student whom they are meeting for the first time. Then you could have two or more observers watch the videos and rate each student’s level of social skills. To the extent that each participant does, in fact, have some level of social skills that can be detected by an attentive observer, different observers’ ratings should be highly correlated with each other.
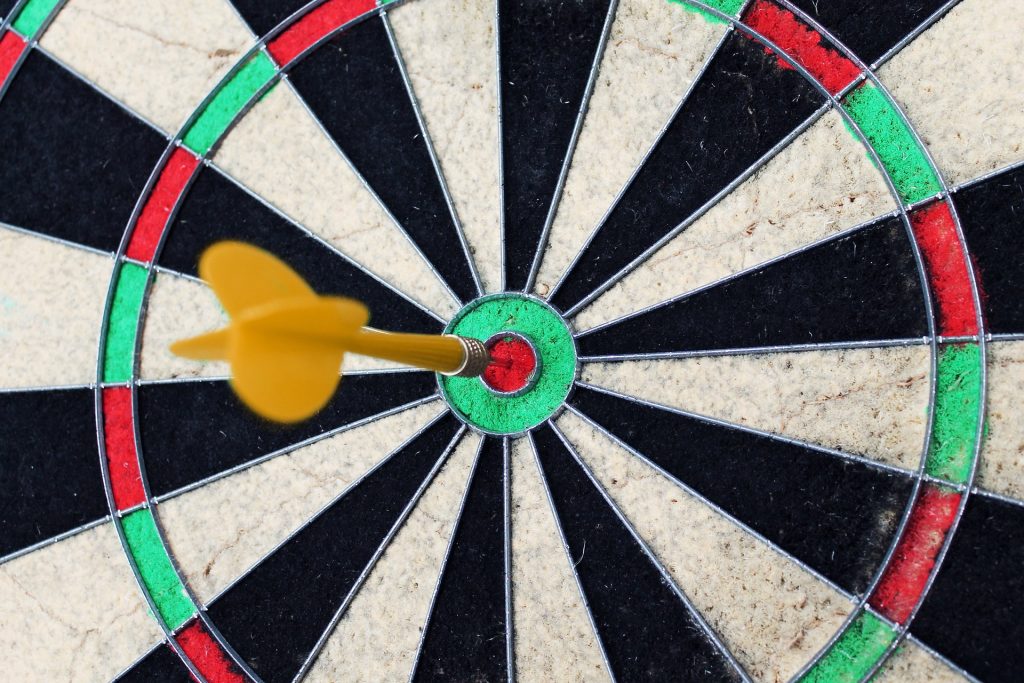
Validity , another key element of assessing measurement quality, is the extent to which the scores from a measure represent the variable they are intended to. But how do researchers make this judgment? We have already considered one factor that they take into account—reliability. When a measure has good test-retest reliability and internal consistency, researchers should be more confident that the scores represent what they are supposed to. There has to be more to it, however, because a measure can be extremely reliable but have no validity whatsoever. As an absurd example, imagine someone who believes that people’s index finger length reflects their self-esteem and therefore tries to measure self-esteem by holding a ruler up to people’s index fingers. Although this measure would have extremely good test-retest reliability, it would have absolutely no validity. The fact that one person’s index finger is a centimeter longer than another’s would indicate nothing about which one had higher self-esteem.
Discussions of validity usually divide it into several distinct “types.” But a good way to interpret these types is that they are other kinds of evidence—in addition to reliability—that should be taken into account when judging the validity of a measure.
Face validity
Face validity is the extent to which a measurement method appears “on its face” to measure the construct of interest. Most people would expect a self-esteem questionnaire to include items about whether they see themselves as a person of worth and whether they think they have good qualities. So a questionnaire that included these kinds of items would have good face validity. The finger-length method of measuring self-esteem, on the other hand, seems to have nothing to do with self-esteem and therefore has poor face validity. Although face validity can be assessed quantitatively—for example, by having a large sample of people rate a measure in terms of whether it appears to measure what it is intended to—it is usually assessed informally.
Face validity is at best a very weak kind of evidence that a measurement method is measuring what it is supposed to. One reason is that it is based on people’s intuitions about human behavior, which are frequently wrong. It is also the case that many established measures in psychology work quite well despite lacking face validity. The Minnesota Multiphasic Personality Inventory-2 (MMPI-2) measures many personality characteristics and disorders by having people decide whether each of over 567 different statements applies to them—where many of the statements do not have any obvious relationship to the construct that they measure. For example, the items “I enjoy detective or mystery stories” and “The sight of blood doesn’t frighten me or make me sick” both measure the suppression of aggression. In this case, it is not the participants’ literal answers to these questions that are of interest, but rather whether the pattern of the participants’ responses to a series of questions matches those of individuals who tend to suppress their aggression.
Content validity
Content validity is the extent to which a measure “covers” the construct of interest. For example, if a researcher conceptually defines test anxiety as involving both sympathetic nervous system activation (leading to nervous feelings) and negative thoughts, then his measure of test anxiety should include items about both nervous feelings and negative thoughts. Or consider that attitudes are usually defined as involving thoughts, feelings, and actions toward something. By this conceptual definition, a person has a positive attitude toward exercise to the extent that they think positive thoughts about exercising, feels good about exercising, and actually exercises. So to have good content validity, a measure of people’s attitudes toward exercise would have to reflect all three of these aspects. Like face validity, content validity is not usually assessed quantitatively. Instead, it is assessed by carefully checking the measurement method against the conceptual definition of the construct.
Criterion validity
Criterion validity is the extent to which people’s scores on a measure are correlated with other variables (known as criteria) that one would expect them to be correlated with. For example, people’s scores on a new measure of test anxiety should be negatively correlated with their performance on an important school exam. If it were found that people’s scores were in fact negatively correlated with their exam performance, then this would be a piece of evidence that these scores really represent people’s test anxiety. But if it were found that people scored equally well on the exam regardless of their test anxiety scores, then this would cast doubt on the validity of the measure.
A criterion can be any variable that one has reason to think should be correlated with the construct being measured, and there will usually be many of them. For example, one would expect test anxiety scores to be negatively correlated with exam performance and course grades and positively correlated with general anxiety and with blood pressure during an exam. Or imagine that a researcher develops a new measure of physical risk taking. People’s scores on this measure should be correlated with their participation in “extreme” activities such as snowboarding and rock climbing, the number of speeding tickets they have received, and even the number of broken bones they have had over the years. When the criterion is measured at the same time as the construct, criterion validity is referred to as concurrent validity ; however, when the criterion is measured at some point in the future (after the construct has been measured), it is referred to as predictive validity (because scores on the measure have “predicted” a future outcome).
Discriminant validity
Discriminant validity , on the other hand, is the extent to which scores on a measure are not correlated with measures of variables that are conceptually distinct. For example, self-esteem is a general attitude toward the self that is fairly stable over time. It is not the same as mood, which is how good or bad one happens to be feeling right now. So people’s scores on a new measure of self-esteem should not be very highly correlated with their moods. If the new measure of self-esteem were highly correlated with a measure of mood, it could be argued that the new measure is not really measuring self-esteem; it is measuring mood instead.
Increasing the reliability and validity of measures
We have reviewed the types of errors and how to evaluate our measures based on reliability and validity considerations. However, what can we do while selecting or creating our tool so that we minimize the potential of errors? Many of our options were covered in our discussion about reliability and validity. Nevertheless, the following table provides a quick summary of things that you should do when creating or selecting a measurement tool. While not all of these will be feasible in your project, it is important to include easy-to-implement measures in your research context.
Make sure that you engage in a rigorous literature review so that you understand the concept that you are studying. This means understanding the different ways that your concept may manifest itself. This review should include a search for existing instruments. [12]
- Do you understand all the dimensions of your concept? Do you have a good understanding of the content dimensions of your concept(s)?
- What instruments exist? How many items are on the existing instruments? Are these instruments appropriate for your population?
- Are these instruments standardized? Note: If an instrument is standardized, that means it has been rigorously studied and tested.
Consult content experts to review your instrument. This is a good way to check the face validity of your items. Additionally, content experts can also help you understand the content validity. [13]
- Do you have access to a reasonable number of content experts? If not, how can you locate them?
- Did you provide a list of critical questions for your content reviewers to use in the reviewing process?
Pilot test your instrument on a sufficient number of people and get detailed feedback. [14] Ask your group to provide feedback on the wording and clarity of items. Keep detailed notes and make adjustments BEFORE you administer your final tool.
- How many people will you use in your pilot testing?
- How will you set up your pilot testing so that it mimics the actual process of administering your tool?
- How will you receive feedback from your pilot testing group? Have you provided a list of questions for your group to think about?
Provide training for anyone collecting data for your project. [15] You should provide those helping you with a written research protocol that explains all of the steps of the project. You should also problem solve and answer any questions that those helping you may have. This will increase the chances that your tool will be administered in a consistent manner.
- How will you conduct your orientation/training? How long will it be? What modality?
- How will you select those who will administer your tool? What qualifications do they need?
When thinking of items, use a higher level of measurement, if possible. [16] This will provide more information and you can always downgrade to a lower level of measurement later.
- Have you examined your items and the levels of measurement?
- Have you thought about whether you need to modify the type of data you are collecting? Specifically, are you asking for information that is too specific (at a higher level of measurement) which may reduce participants’ willingness to participate?
Use multiple indicators for a variable. [17] Think about the number of items that you will include in your tool.
- Do you have enough items? Enough indicators? The correct indicators?
Conduct an item-by-item assessment of multiple-item measures. [18] When you do this assessment, think about each word and how it changes the meaning of your item.
- Are there items that are redundant? Do you need to modify, delete, or add items?
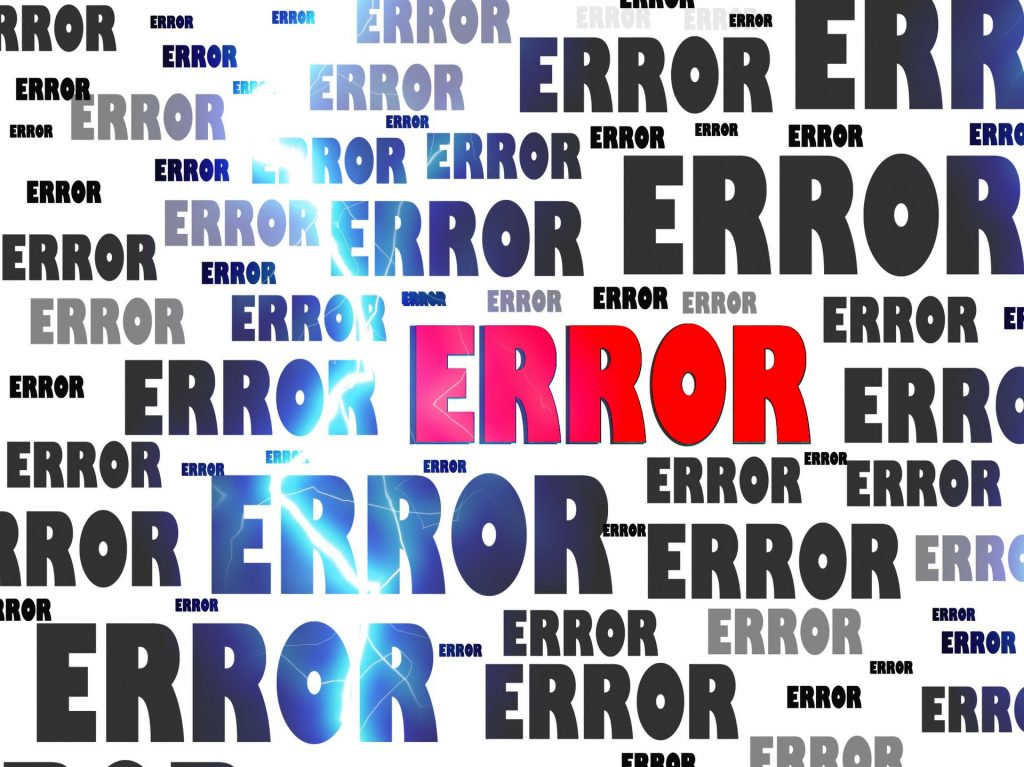
Types of error
As you can see, measures never perfectly describe what exists in the real world. Good measures demonstrate validity and reliability but will always have some degree of error. Systematic error (also called bias) causes our measures to consistently output incorrect data in one direction or another on a measure, usually due to an identifiable process. Imagine you created a measure of height, but you didn’t put an option for anyone over six feet tall. If you gave that measure to your local college or university, some of the taller students might not be measured accurately. In fact, you would be under the mistaken impression that the tallest person at your school was six feet tall, when in actuality there are likely people taller than six feet at your school. This error seems innocent, but if you were using that measure to help you build a new building, those people might hit their heads!
A less innocent form of error arises when researchers word questions in a way that might cause participants to think one answer choice is preferable to another. For example, if I were to ask you “Do you think global warming is caused by human activity?” you would probably feel comfortable answering honestly. But what if I asked you “Do you agree with 99% of scientists that global warming is caused by human activity?” Would you feel comfortable saying no, if that’s what you honestly felt? I doubt it. That is an example of a leading question , a question with wording that influences how a participant responds. We’ll discuss leading questions and other problems in question wording in greater detail in Chapter 12 .
In addition to error created by the researcher, your participants can cause error in measurement. Some people will respond without fully understanding a question, particularly if the question is worded in a confusing way. Let’s consider another potential source or error. If we asked people if they always washed their hands after using the bathroom, would we expect people to be perfectly honest? Polling people about whether they wash their hands after using the bathroom might only elicit what people would like others to think they do, rather than what they actually do. This is an example of social desirability bias , in which participants in a research study want to present themselves in a positive, socially desirable way to the researcher. People in your study will want to seem tolerant, open-minded, and intelligent, but their true feelings may be closed-minded, simple, and biased. Participants may lie in this situation. This occurs often in political polling, which may show greater support for a candidate from a minority race, gender, or political party than actually exists in the electorate.
A related form of bias is called acquiescence bias , also known as “yea-saying.” It occurs when people say yes to whatever the researcher asks, even when doing so contradicts previous answers. For example, a person might say yes to both “I am a confident leader in group discussions” and “I feel anxious interacting in group discussions.” Those two responses are unlikely to both be true for the same person. Why would someone do this? Similar to social desirability, people want to be agreeable and nice to the researcher asking them questions or they might ignore contradictory feelings when responding to each question. You could interpret this as someone saying “yeah, I guess.” Respondents may also act on cultural reasons, trying to “save face” for themselves or the person asking the questions. Regardless of the reason, the results of your measure don’t match what the person truly feels.
So far, we have discussed sources of error that come from choices made by respondents or researchers. Systematic errors will result in responses that are incorrect in one direction or another. For example, social desirability bias usually means that the number of people who say they will vote for a third party in an election is greater than the number of people who actually vote for that candidate. Systematic errors such as these can be reduced, but random error can never be eliminated. Unlike systematic error, which biases responses consistently in one direction or another, random error is unpredictable and does not consistently result in scores that are consistently higher or lower on a given measure. Instead, random error is more like statistical noise, which will likely average out across participants.
Random error is present in any measurement. If you’ve ever stepped on a bathroom scale twice and gotten two slightly different results, maybe a difference of a tenth of a pound, then you’ve experienced random error. Maybe you were standing slightly differently or had a fraction of your foot off of the scale the first time. If you were to take enough measures of your weight on the same scale, you’d be able to figure out your true weight. In social science, if you gave someone a scale measuring depression on a day after they lost their job, they would likely score differently than if they had just gotten a promotion and a raise. Even if the person were clinically depressed, our measure is subject to influence by the random occurrences of life. Thus, social scientists speak with humility about our measures. We are reasonably confident that what we found is true, but we must always acknowledge that our measures are only an approximation of reality.
Humility is important in scientific measurement, as errors can have real consequences. At the time I’m writing this, my wife and I are expecting our first child. Like most people, we used a pregnancy test from the pharmacy. If the test said my wife was pregnant when she was not pregnant, that would be a false positive . On the other hand, if the test indicated that she was not pregnant when she was in fact pregnant, that would be a false negative . Even if the test is 99% accurate, that means that one in a hundred women will get an erroneous result when they use a home pregnancy test. For us, a false positive would have been initially exciting, then devastating when we found out we were not having a child. A false negative would have been disappointing at first and then quite shocking when we found out we were indeed having a child. While both false positives and false negatives are not very likely for home pregnancy tests (when taken correctly), measurement error can have consequences for the people being measured.
- Reliability is a matter of consistency.
- Validity is a matter of accuracy.
- There are many types of validity and reliability.
- Systematic error may arise from the researcher, participant, or measurement instrument.
- Systematic error biases results in a particular direction, whereas random error can be in any direction.
- All measures are prone to error and should interpreted with humility.
Use the measurement tools you located in the previous exercise. Evaluate the reliability and validity of these tools. Hint: You will need to go into the literature to “research” these tools.
- Provide a clear statement regarding the reliability and validity of these tools. What strengths did you notice? What were the limitations?
- Think about your target population . Are there changes that need to be made in order for one of these tools to be appropriate for your population?
- If you decide to create your own tool, how will you assess its validity and reliability?
11.4 Ethical and social justice considerations
- Identify potential cultural, ethical, and social justice issues in measurement.
With your variables operationalized, it’s time to take a step back and look at how measurement in social science impact our daily lives. As we will see, how we measure things is both shaped by power arrangements inside our society, and more insidiously, by establishing what is scientifically true, measures have their own power to influence the world. Just like reification in the conceptual world, how we operationally define concepts can reinforce or fight against oppressive forces.
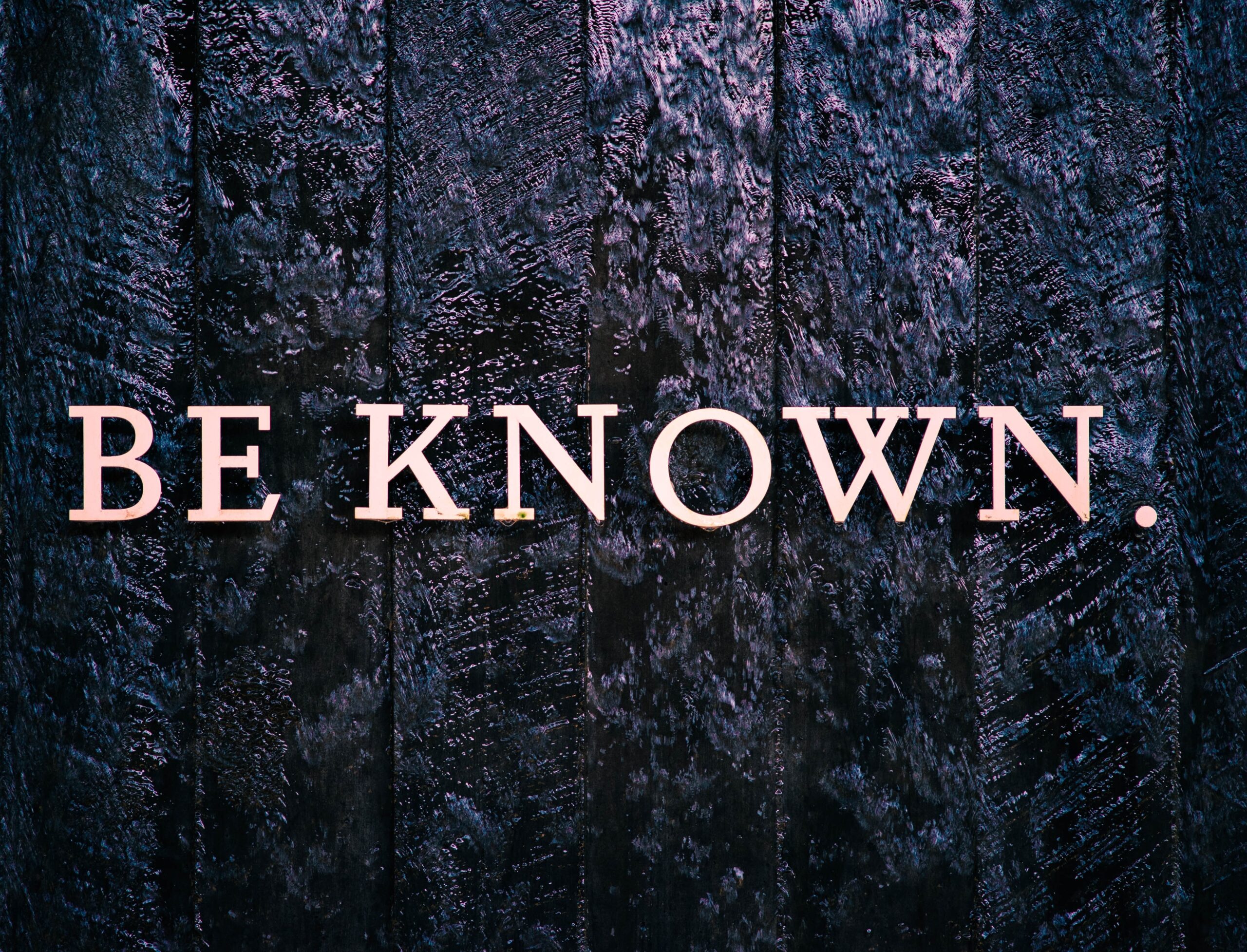
Data equity
How we decide to measure our variables determines what kind of data we end up with in our research project. Because scientific processes are a part of our sociocultural context, the same biases and oppressions we see in the real world can be manifested or even magnified in research data. Jagadish and colleagues (2021) [19] presents four dimensions of data equity that are relevant to consider: in representation of non-dominant groups within data sets; in how data is collected, analyzed, and combined across datasets; in equitable and participatory access to data, and finally in the outcomes associated with the data collection. Historically, we have mostly focused on the outcomes of measures producing outcomes that are biased in one way or another, and this section reviews many such examples. However, it is important to note that equity must also come from designing measures that respond to questions like:
- Are groups historically suppressed from the data record represented in the sample?
- Are equity data gathered by researchers and used to uncover and quantify inequity?
- Are the data accessible across domains and levels of expertise, and can community members participate in the design, collection, and analysis of the public data record?
- Are the data collected used to monitor and mitigate inequitable impacts?
So, it’s not just about whether measures work for one population for another. Data equity is about the context in which data are created from how we measure people and things. We agree with these authors that data equity should be considered within the context of automated decision-making systems and recognizing a broader literature around the role of administrative systems in creating and reinforcing discrimination. To combat the inequitable processes and outcomes we describe below, researchers must foreground equity as a core component of measurement.
Flawed measures & missing measures
At the end of every semester, students in just about every university classroom in the United States complete similar student evaluations of teaching (SETs). Since every student is likely familiar with these, we can recognize many of the concepts we discussed in the previous sections. There are number of rating scale questions that ask you to rate the professor, class, and teaching effectiveness on a scale of 1-5. Scores are averaged across students and used to determine the quality of teaching delivered by the faculty member. SETs scores are often a principle component of how faculty are reappointed to teaching positions. Would it surprise you to learn that student evaluations of teaching are of questionable quality? If your instructors are assessed with a biased or incomplete measure, how might that impact your education?
Most often, student scores are averaged across questions and reported as a final average. This average is used as one factor, often the most important factor, in a faculty member’s reappointment to teaching roles. We learned in this chapter that rating scales are ordinal, not interval or ratio, and the data are categories not numbers. Although rating scales use a familiar 1-5 scale, the numbers 1, 2, 3, 4, & 5 are really just helpful labels for categories like “excellent” or “strongly agree.” If we relabeled these categories as letters (A-E) rather than as numbers (1-5), how would you average them?
Averaging ordinal data is methodologically dubious, as the numbers are merely a useful convention. As you will learn in Chapter 14 , taking the median value is what makes the most sense with ordinal data. Median values are also less sensitive to outliers. So, a single student who has strong negative or positive feelings towards the professor could bias the class’s SETs scores higher or lower than what the “average” student in the class would say, particularly for classes with few students or in which fewer students completed evaluations of their teachers.
We care about teaching quality because more effective teachers will produce more knowledgeable and capable students. However, student evaluations of teaching are not particularly good indicators of teaching quality and are not associated with the independently measured learning gains of students (i.e., test scores, final grades) (Uttl et al., 2017). [20] This speaks to the lack of criterion validity. Higher teaching quality should be associated with better learning outcomes for students, but across multiple studies stretching back years, there is no association that cannot be better explained by other factors. To be fair, there are scholars who find that SETs are valid and reliable. For a thorough defense of SETs as well as a historical summary of the literature see Benton & Cashin (2012). [21]
Even though student evaluations of teaching often contain dozens of questions, researchers often find that the questions are so highly interrelated that one concept (or factor, as it is called in a factor analysis ) explains a large portion of the variance in teachers’ scores on student evaluations (Clayson, 2018). [22] Personally, I believe based on completing SETs myself that factor is probably best conceptualized as student satisfaction, which is obviously worthwhile to measure, but is conceptually quite different from teaching effectiveness or whether a course achieved its intended outcomes. The lack of a clear operational and conceptual definition for the variable or variables being measured in student evaluations of teaching also speaks to a lack of content validity. Researchers check content validity by comparing the measurement method with the conceptual definition, but without a clear conceptual definition of the concept measured by student evaluations of teaching, it’s not clear how we can know our measure is valid. Indeed, the lack of clarity around what is being measured in teaching evaluations impairs students’ ability to provide reliable and valid evaluations. So, while many researchers argue that the class average SETs scores are reliable in that they are consistent over time and across classes, it is unclear what exactly is being measured even if it is consistent (Clayson, 2018). [23]
As a faculty member, there are a number of things I can do to influence my evaluations and disrupt validity and reliability. Since SETs scores are associated with the grades students perceive they will receive (e.g., Boring et al., 2016), [24] guaranteeing everyone a final grade of A in my class will likely increase my SETs scores and my chances at tenure and promotion. I could time an email reminder to complete SETs with releasing high grades for a major assignment to boost my evaluation scores. On the other hand, student evaluations might be coincidentally timed with poor grades or difficult assignments that will bias student evaluations downward. Students may also infer I am manipulating them and give me lower SET scores as a result. To maximize my SET scores and chances and promotion, I also need to select which courses I teach carefully. Classes that are more quantitatively oriented generally receive lower ratings than more qualitative and humanities-driven classes, which makes my decision to teach social work research a poor strategy (Uttl & Smibert, 2017). [25] The only manipulative strategy I will admit to using is bringing food (usually cookies or donuts) to class during the period in which students are completing evaluations. Measurement is impacted by context.
As a white cis-gender male educator, I am adversely impacted by SETs because of their sketchy validity, reliability, and methodology. The other flaws with student evaluations actually help me while disadvantaging teachers from oppressed groups. Heffernan (2021) [26] provides a comprehensive overview of the sexism, racism, ableism, and prejudice baked into student evaluations:
“In all studies relating to gender, the analyses indicate that the highest scores are awarded in subjects filled with young, white, male students being taught by white English first language speaking, able-bodied, male academics who are neither too young nor too old (approx. 35–50 years of age), and who the students believe are heterosexual. Most deviations from this scenario in terms of student and academic demographics equates to lower SET scores. These studies thus highlight that white, able-bodied, heterosexual, men of a certain age are not only the least affected, they benefit from the practice. When every demographic group who does not fit this image is significantly disadvantaged by SETs, these processes serve to further enhance the position of the already privileged” (p. 5).
The staggering consistency of studies examining prejudice in SETs has led to some rather superficial reforms like reminding students to not submit racist or sexist responses in the written instructions given before SETs. Yet, even though we know that SETs are systematically biased against women, people of color, and people with disabilities, the overwhelming majority of universities in the United States continue to use them to evaluate faculty for promotion or reappointment. From a critical perspective, it is worth considering why university administrators continue to use such a biased and flawed instrument. SETs produce data that make it easy to compare faculty to one another and track faculty members over time. Furthermore, they offer students a direct opportunity to voice their concerns and highlight what went well.
As the people with the greatest knowledge about what happened in the classroom as whether it met their expectations, providing students with open-ended questions is the most productive part of SETs. Personally, I have found focus groups written, facilitated, and analyzed by student researchers to be more insightful than SETs. MSW student activists and leaders may look for ways to evaluate faculty that are more methodologically sound and less systematically biased, creating institutional change by replacing or augmenting traditional SETs in their department. There is very rarely student input on the criteria and methodology for teaching evaluations, yet students are the most impacted by helpful or harmful teaching practices.
Students should fight for better assessment in the classroom because well-designed assessments provide documentation to support more effective teaching practices and discourage unhelpful or discriminatory practices. Flawed assessments like SETs, can lead to a lack of information about problems with courses, instructors, or other aspects of the program. Think critically about what data your program uses to gauge its effectiveness. How might you introduce areas of student concern into how your program evaluates itself? Are there issues with food or housing insecurity, mentorship of nontraditional and first generation students, or other issues that faculty should consider when they evaluate their program? Finally, as you transition into practice, think about how your agency measures its impact and how it privileges or excludes client and community voices in the assessment process.
Let’s consider an example from social work practice. Let’s say you work for a mental health organization that serves youth impacted by community violence. How should you measure the impact of your services on your clients and their community? Schools may be interested in reducing truancy, self-injury, or other behavioral concerns. However, by centering delinquent behaviors in how we measure our impact, we may be inattentive to the role of trauma, family dynamics, and other cognitive and social processes beyond “delinquent behavior.” Indeed, we may bias our interventions by focusing on things that are not as important to clients’ needs. Social workers want to make sure their programs are improving over time, and we rely on our measures to indicate what to change and what to keep. If our measures present a partial or flawed view, we lose our ability to establish and act on scientific truths.
While writing this section, one of the authors wrote this commentary article addressing potential racial bias in social work licensing exams. If you are interested in an example of missing or flawed measures that relates to systems your social work practice is governed by (rather than SETs which govern our practice in higher education) check it out!
You may also be interested in similar arguments against the standard grading scale (A-F), and why grades (numerical, letter, etc.) do not do a good job of measuring learning. Think critically about the role that grades play in your life as a student, your self-concept, and your relationships with teachers. Your test and grade anxiety is due in part to how your learning is measured. Those measurements end up becoming an official record of your scholarship and allow employers or funders to compare you to other scholars. The stakes for measurement are the same for participants in your research study.
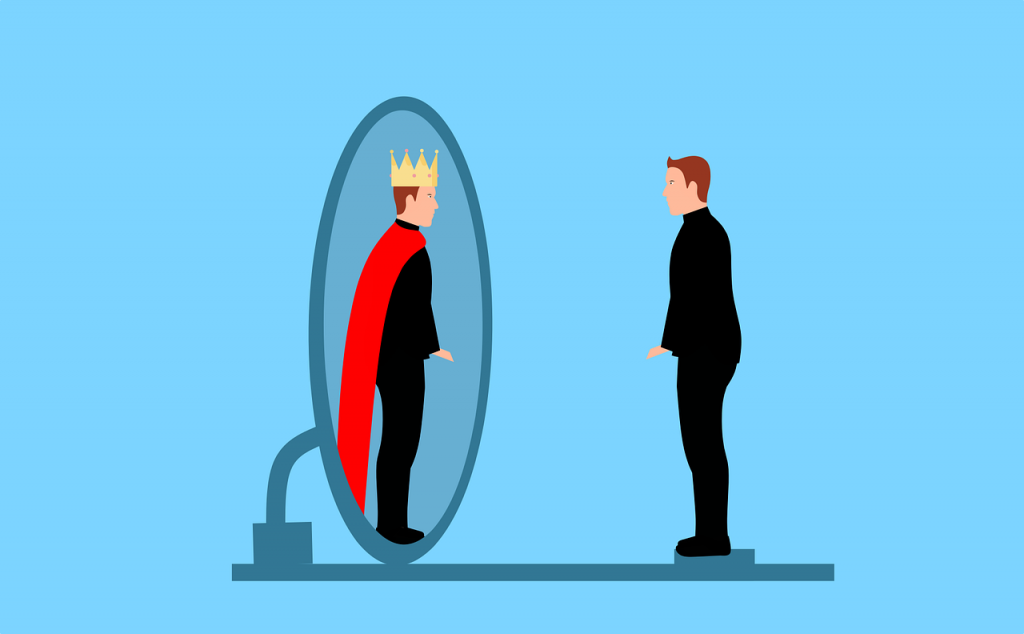
Self-reflection and measurement
Student evaluations of teaching are just like any other measure. How we decide to measure what we are researching is influenced by our backgrounds, including our culture, implicit biases, and individual experiences. For me as a middle-class, cisgender white woman, the decisions I make about measurement will probably default to ones that make the most sense to me and others like me, and thus measure characteristics about us most accurately if I don’t think carefully about it. There are major implications for research here because this could affect the validity of my measurements for other populations.
This doesn’t mean that standardized scales or indices, for instance, won’t work for diverse groups of people. What it means is that researchers must not ignore difference in deciding how to measure a variable in their research. Doing so may serve to push already marginalized people further into the margins of academic research and, consequently, social work intervention. Social work researchers, with our strong orientation toward celebrating difference and working for social justice, are obligated to keep this in mind for ourselves and encourage others to think about it in their research, too.
This involves reflecting on what we are measuring, how we are measuring, and why we are measuring. Do we have biases that impacted how we operationalized our concepts? Did we include stakeholders and gatekeepers in the development of our concepts? This can be a way to gain access to vulnerable populations. What feedback did we receive on our measurement process and how was it incorporated into our work? These are all questions we should ask as we are thinking about measurement. Further, engaging in this intentionally reflective process will help us maximize the chances that our measurement will be accurate and as free from bias as possible.
The NASW Code of Ethics discusses social work research and the importance of engaging in practices that do not harm participants. This is especially important considering that many of the topics studied by social workers are those that are disproportionately experienced by marginalized and oppressed populations. Some of these populations have had negative experiences with the research process: historically, their stories have been viewed through lenses that reinforced the dominant culture’s standpoint. Thus, when thinking about measurement in research projects, we must remember that the way in which concepts or constructs are measured will impact how marginalized or oppressed persons are viewed. It is important that social work researchers examine current tools to ensure appropriateness for their population(s). Sometimes this may require researchers to use existing tools. Other times, this may require researchers to adapt existing measures or develop completely new measures in collaboration with community stakeholders. In summary, the measurement protocols selected should be tailored and attentive to the experiences of the communities to be studied.
Unfortunately, social science researchers do not do a great job of sharing their measures in a way that allows social work practitioners and administrators to use them to evaluate the impact of interventions and programs on clients. Few scales are published under an open copyright license that allows other people to view it for free and share it with others. Instead, the best way to find a scale mentioned in an article is often to simply search for it in Google with “.pdf” or “.docx” in the query to see if someone posted a copy online (usually in violation of copyright law). As we discussed in Chapter 4 , this is an issue of information privilege, or the structuring impact of oppression and discrimination on groups’ access to and use of scholarly information. As a student at a university with a research library, you can access the Mental Measurement Yearbook to look up scales and indexes that measure client or program outcomes while researchers unaffiliated with university libraries cannot do so. Similarly, the vast majority of scholarship in social work and allied disciplines does not share measures, data, or other research materials openly, a best practice in open and collaborative science. It is important to underscore these structural barriers to using valid and reliable scales in social work practice. An invalid or unreliable outcome test may cause ineffective or harmful programs to persist or may worsen existing prejudices and oppressions experienced by clients, communities, and practitioners.
But it’s not just about reflecting and identifying problems and biases in our measurement, operationalization, and conceptualization—what are we going to do about it? Consider this as you move through this book and become a more critical consumer of research. Sometimes there isn’t something you can do in the immediate sense—the literature base at this moment just is what it is. But how does that inform what you will do later?
A place to start: Stop oversimplifying race
We will address many more of the critical issues related to measurement in the next chapter. One way to get started in bringing cultural awareness to scientific measurement is through a critical examination of how we analyze race quantitatively. There are many important methodological objections to how we measure the impact of race. We encourage you to watch Dr. Abigail Sewell’s three-part workshop series called “Nested Models for Critical Studies of Race & Racism” for the Inter-university Consortium for Political and Social Research (ICPSR). She discusses how to operationalize and measure inequality, racism, and intersectionality and critiques researchers’ attempts to oversimplify or overlook racism when we measure concepts in social science. If you are interested in developing your social work research skills further, consider applying for financial support from your university to attend an ICPSR summer seminar like Dr. Sewell’s where you can receive more advanced and specialized training in using research for social change.
- Part 1: Creating Measures of Supraindividual Racism (2-hour video)
- Part 2: Evaluating Population Risks of Supraindividual Racism (2-hour video)
- Part 3: Quantifying Intersectionality (2-hour video)
- Social work researchers must be attentive to personal and institutional biases in the measurement process that affect marginalized groups.
- What is measured and how it is measured is shaped by power, and social workers must be critical and self-reflective in their research projects.
Think about your current research question and the tool(s) that you will use to gather data. Even if you haven’t chosen your tools yet, think of some that you have encountered in the literature so far.
- How does your positionality and experience shape what variables you are choosing to measure and how you measure them?
- Evaluate the measures in your study for potential biases.
- If you are using measures developed by another researcher, investigate whether it is valid and reliable in other studies across cultures.
- Milkie, M. A., & Warner, C. H. (2011). Classroom learning environments and the mental health of first grade children. Journal of Health and Social Behavior, 52 , 4–22 ↵
- Kaplan, A. (1964). The conduct of inquiry: Methodology for behavioral science . San Francisco, CA: Chandler Publishing Company. ↵
- Earl Babbie offers a more detailed discussion of Kaplan’s work in his text. You can read it in: Babbie, E. (2010). The practice of social research (12th ed.). Belmont, CA: Wadsworth. ↵
- In this chapter, we will use the terms concept and construct interchangeably. While each term has a distinct meaning in research conceptualization, we do not believe this distinction is important enough to warrant discussion in this chapter. ↵
- Wong, Y. J., Steinfeldt, J. A., Speight, Q. L., & Hickman, S. J. (2010). Content analysis of Psychology of men & masculinity (2000–2008). Psychology of Men & Masculinity , 11 (3), 170. ↵
- Kimmel, M. (2000). The gendered society . New York, NY: Oxford University Press; Kimmel, M. (2008). Masculinity. In W. A. Darity Jr. (Ed.), International encyclopedia of the social sciences (2nd ed., Vol. 5, p. 1–5). Detroit, MI: Macmillan Reference USA ↵
- Kimmel, M. & Aronson, A. B. (2004). Men and masculinities: A-J . Denver, CO: ABL-CLIO. ↵
- Krosnick, J.A. & Berent, M.K. (1993). Comparisons of party identification and policy preferences: The impact of survey question format. American Journal of Political Science, 27 (3), 941-964. ↵
- Likert, R. (1932). A technique for the measurement of attitudes. Archives of Psychology,140 , 1–55. ↵
- Stevens, S. S. (1946). On the Theory of Scales of Measurement. Science , 103 (2684), 677-680. ↵
- Sullivan G. M. (2011). A primer on the validity of assessment instruments. Journal of graduate medical education, 3 (2), 119–120. doi:10.4300/JGME-D-11-00075.1 ↵
- Engel, R. & Schutt, R. (2013). The practice of research in social work (3rd. ed.) . Thousand Oaks, CA: SAGE. ↵
- Engel, R. & Schutt, R. (2013). The practice of research in social work (3rd. ed.). Thousand Oaks, CA: SAGE. ↵
- Jagadish, H. V., Stoyanovich, J., & Howe, B. (2021). COVID-19 Brings Data Equity Challenges to the Fore. Digital Government: Research and Practice , 2 (2), 1-7. ↵
- Uttl, B., White, C. A., & Gonzalez, D. W. (2017). Meta-analysis of faculty's teaching effectiveness: Student evaluation of teaching ratings and student learning are not related. Studies in Educational Evaluation , 54 , 22-42. ↵
- Benton, S. L., & Cashin, W. E. (2014). Student ratings of instruction in college and university courses. In Higher education: Handbook of theory and research (pp. 279-326). Springer, Dordrecht. ↵
- Clayson, D. E. (2018). Student evaluation of teaching and matters of reliability. Assessment & Evaluation in Higher Education , 43 (4), 666-681. ↵
- Clayson, D. E. (2018). Student evaluation of teaching and matters of reliability. Assessment & Evaluation in Higher Education , 43 (4), 666-681. ↵
- Boring, A., Ottoboni, K., & Stark, P. (2016). Student evaluations of teaching (mostly) do not measure teaching effectiveness. ScienceOpen Research . ↵
- Uttl, B., & Smibert, D. (2017). Student evaluations of teaching: teaching quantitative courses can be hazardous to one’s career. Peer Journal , 5 , e3299. ↵
- Heffernan, T. (2021). Sexism, racism, prejudice, and bias: a literature review and synthesis of research surrounding student evaluations of courses and teaching. Assessment & Evaluation in Higher Education , 1-11. ↵
The process by which we describe and ascribe meaning to the key facts, concepts, or other phenomena under investigation in a research study.
In measurement, conditions that are easy to identify and verify through direct observation.
In measurement, conditions that are subtle and complex that we must use existing knowledge and intuition to define.
Conditions that are not directly observable and represent states of being, experiences, and ideas.
A mental image that summarizes a set of similar observations, feelings, or ideas
developing clear, concise definitions for the key concepts in a research question
concepts that are comprised of multiple elements
concepts that are expected to have a single underlying dimension
assuming that abstract concepts exist in some concrete, tangible way
process by which researchers spell out precisely how a concept will be measured in their study
Clues that demonstrate the presence, intensity, or other aspects of a concept in the real world
unprocessed data that researchers can analyze using quantitative and qualitative methods (e.g., responses to a survey or interview transcripts)
a characteristic that does not change in a study
The characteristics that make up a variable
variables whose values are organized into mutually exclusive groups but whose numerical values cannot be used in mathematical operations.
variables whose values are mutually exclusive and can be used in mathematical operations
The lowest level of measurement; categories cannot be mathematically ranked, though they are exhaustive and mutually exclusive
Exhaustive categories are options for closed ended questions that allow for every possible response (no one should feel like they can't find the answer for them).
Mutually exclusive categories are options for closed ended questions that do not overlap, so people only fit into one category or another, not both.
Level of measurement that follows nominal level. Has mutually exclusive categories and a hierarchy (rank order), but we cannot calculate a mathematical distance between attributes.
An ordered set of responses that participants must choose from.
A level of measurement that is continuous, can be rank ordered, is exhaustive and mutually exclusive, and for which the distance between attributes is known to be equal. But for which there is no zero point.
The highest level of measurement. Denoted by mutually exclusive categories, a hierarchy (order), values can be added, subtracted, multiplied, and divided, and the presence of an absolute zero.
measuring people’s attitude toward something by assessing their level of agreement with several statements about it
Composite (multi-item) scales in which respondents are asked to indicate their opinions or feelings toward a single statement using different pairs of adjectives framed as polar opposites.
A composite scale using a series of items arranged in increasing order of intensity of the construct of interest, from least intense to most intense.
measurements of variables based on more than one one indicator
An empirical structure for measuring items or indicators of the multiple dimensions of a concept.
a composite score derived from aggregating measures of multiple concepts (called components) using a set of rules and formulas
The ability of a measurement tool to measure a phenomenon the same way, time after time. Note: Reliability does not imply validity.
The extent to which scores obtained on a scale or other measure are consistent across time
The consistency of people’s responses across the items on a multiple-item measure. Responses about the same underlying construct should be correlated, though not perfectly.
The extent to which different observers are consistent in their assessment or rating of a particular characteristic or item.
The extent to which the scores from a measure represent the variable they are intended to.
The extent to which a measurement method appears “on its face” to measure the construct of interest
The extent to which a measure “covers” the construct of interest, i.e., it's comprehensiveness to measure the construct.
The extent to which people’s scores on a measure are correlated with other variables (known as criteria) that one would expect them to be correlated with.
A type of criterion validity. Examines how well a tool provides the same scores as an already existing tool administered at the same point in time.
A type of criterion validity that examines how well your tool predicts a future criterion.
The extent to which scores on a measure are not correlated with measures of variables that are conceptually distinct.
(also known as bias) refers to when a measure consistently outputs incorrect data, usually in one direction and due to an identifiable process
When a participant's answer to a question is altered due to the way in which a question is written. In essence, the question leads the participant to answer in a specific way.
Social desirability bias occurs when we create questions that lead respondents to answer in ways that don't reflect their genuine thoughts or feelings to avoid being perceived negatively.
In a measure, when people say yes to whatever the researcher asks, even when doing so contradicts previous answers.
Unpredictable error that does not result in scores that are consistently higher or lower on a given measure but are nevertheless inaccurate.
when a measure indicates the presence of a phenomenon, when in reality it is not present
when a measure does not indicate the presence of a phenomenon, when in reality it is present
the group of people whose needs your study addresses
The value in the middle when all our values are placed in numerical order. Also called the 50th percentile.
individuals or groups who have an interest in the outcome of the study you conduct
the people or organizations who control access to the population you want to study
Graduate research methods in social work Copyright © 2021 by Matthew DeCarlo, Cory Cummings, Kate Agnelli is licensed under a Creative Commons Attribution-NonCommercial-ShareAlike 4.0 International License , except where otherwise noted.
Share This Book

Want to create or adapt books like this? Learn more about how Pressbooks supports open publishing practices.
Matthew DeCarlo
Chapter Outline
- Empirical vs. ethical questions (4 minute read)
- Characteristics of a good research question (4 minute read)
- Quantitative research questions (7 minute read)
- Qualitative research questions (3 minute read)
- Evaluating and updating your research questions (4 minute read)
Content warning: examples in this chapter include references to sexual violence, sexism, substance use disorders, homelessness, domestic violence, the child welfare system, cissexism and heterosexism, and truancy and school discipline.
9.1 Empirical vs. ethical questions
Learning objectives.
Learners will be able to…
- Define empirical questions and provide an example
- Define ethical questions and provide an example
Writing a good research question is an art and a science. It is a science because you have to make sure it is clear, concise, and well-developed. It is an art because often your language needs “wordsmithing” to perfect and clarify the meaning. This is an exciting part of the research process; however, it can also be one of the most stressful.
Creating a good research question begins by identifying a topic you are interested in studying. At this point, you already have a working question. You’ve been applying it to the exercises in each chapter, and after reading more about your topic in the scholarly literature, you’ve probably gone back and revised your working question a few times. We’re going to continue that process in more detail in this chapter. Keep in mind that writing research questions is an iterative process, with revisions happening week after week until you are ready to start your project.
Empirical vs. ethical questions
When it comes to research questions, social science is best equipped to answer empirical questions —those that can be answered by real experience in the real world—as opposed to ethical questions —questions about which people have moral opinions and that may not be answerable in reference to the real world. While social workers have explicit ethical obligations (e.g., service, social justice), research projects ask empirical questions to help actualize and support the work of upholding those ethical principles.
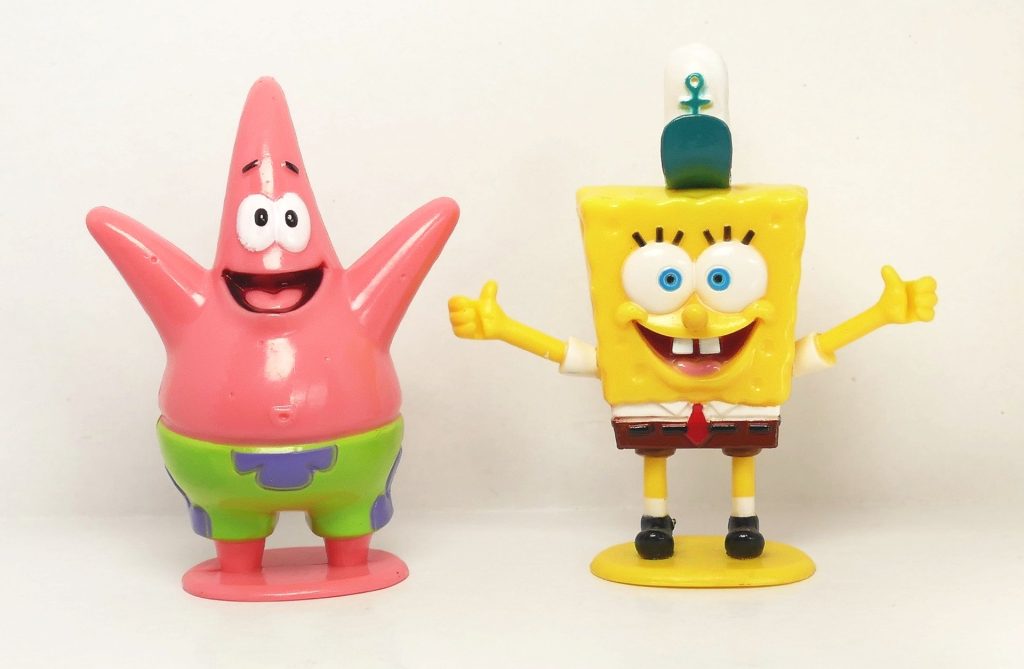
In order to help you better understand the difference between ethical and empirical questions, let’s consider a topic about which people have moral opinions. How about SpongeBob SquarePants? [1] In early 2005, members of the conservative Christian group Focus on the Family (2005) [2] denounced this seemingly innocuous cartoon character as “morally offensive” because they perceived his character to be one that promotes a “pro-gay agenda.” Focus on the Family supported their claim that SpongeBob is immoral by citing his appearance in a children’s video designed to promote tolerance of all family forms (BBC News, 2005). [3] They also cited SpongeBob’s regular hand-holding with his male sidekick Patrick as further evidence of his immorality.
So, can we now conclude that SpongeBob SquarePants is immoral? Not so fast. While your mother or a newspaper or television reporter may provide an answer, a social science researcher cannot. Questions of morality are ethical, not empirical. Of course, this doesn’t mean that social science researchers cannot study opinions about or social meanings surrounding SpongeBob SquarePants (Carter, 2010). [4] We study humans after all, and as you will discover in the following chapters of this textbook, we are trained to utilize a variety of scientific data-collection techniques to understand patterns of human beliefs and behaviors. Using these techniques, we could find out how many people in the United States find SpongeBob morally reprehensible, but we could never learn, empirically, whether SpongeBob is in fact morally reprehensible.
Let’s consider an example from a recent MSW research class I taught. A student group wanted to research the penalties for sexual assault. Their original research question was: “How can prison sentences for sexual assault be so much lower than the penalty for drug possession?” Outside of the research context, that is a darn good question! It speaks to how the War on Drugs and the patriarchy have distorted the criminal justice system towards policing of drug crimes over gender-based violence.
Unfortunately, it is an ethical question, not an empirical one. To answer that question, you would have to draw on philosophy and morality, answering what it is about human nature and society that allows such unjust outcomes. However, you could not answer that question by gathering data about people in the real world. If I asked people that question, they would likely give me their opinions about drugs, gender-based violence, and the criminal justice system. But I wouldn’t get the real answer about why our society tolerates such an imbalance in punishment.
As the students worked on the project through the semester, they continued to focus on the topic of sexual assault in the criminal justice system. Their research question became more empirical because they read more empirical articles about their topic. One option that they considered was to evaluate intervention programs for perpetrators of sexual assault to see if they reduced the likelihood of committing sexual assault again. Another option they considered was seeing if counties or states with higher than average jail sentences for sexual assault perpetrators had lower rates of re-offense for sexual assault. These projects addressed the ethical question of punishing perpetrators of sexual violence but did so in a way that gathered and analyzed empirical real-world data. Our job as social work researchers is to gather social facts about social work issues, not to judge or determine morality.
Key Takeaways
- Empirical questions are distinct from ethical questions.
- There are usually a number of ethical questions and a number of empirical questions that could be asked about any single topic.
- While social workers may research topics about which people have moral opinions, a researcher’s job is to gather and analyze empirical data.
- Take a look at your working question. Make sure you have an empirical question, not an ethical one. To perform this check, describe how you could find an answer to your question by conducting a study, like a survey or focus group, with real people.

9.2 Characteristics of a good research question
- Identify and explain the key features of a good research question
- Explain why it is important for social workers to be focused and clear with the language they use in their research questions
Now that you’ve made sure your working question is empirical, you need to revise that working question into a formal research question. So, what makes a good research question? First, it is generally written in the form of a question. To say that your research question is “the opioid epidemic” or “animal assisted therapy” or “oppression” would not be correct. You need to frame your topic as a question, not a statement. A good research question is also one that is well-focused. A well-focused question helps you tune out irrelevant information and not try to answer everything about the world all at once. You could be the most eloquent writer in your class, or even in the world, but if the research question about which you are writing is unclear, your work will ultimately lack direction.
In addition to being written in the form of a question and being well-focused, a good research question is one that cannot be answered with a simple yes or no. For example, if your interest is in gender norms, you could ask, “Does gender affect a person’s performance of household tasks?” but you will have nothing left to say once you discover your yes or no answer. Instead, why not ask, about the relationship between gender and household tasks. Alternatively, maybe we are interested in how or to what extent gender affects a person’s contributions to housework in a marriage? By tweaking your question in this small way, you suddenly have a much more fascinating question and more to say as you attempt to answer it.
A good research question should also have more than one plausible answer. In the example above, the student who studied the relationship between gender and household tasks had a specific interest in the impact of gender, but she also knew that preferences might be impacted by other factors. For example, she knew from her own experience that her more traditional and socially conservative friends were more likely to see household tasks as part of the female domain, and were less likely to expect their male partners to contribute to those tasks. Thinking through the possible relationships between gender, culture, and household tasks led that student to realize that there were many plausible answers to her questions about how gender affects a person’s contribution to household tasks. Because gender doesn’t exist in a vacuum, she wisely felt that she needed to consider other characteristics that work together with gender to shape people’s behaviors, likes, and dislikes. By doing this, the student considered the third feature of a good research question–she thought about relationships between several concepts. While she began with an interest in a single concept—household tasks—by asking herself what other concepts (such as gender or political orientation) might be related to her original interest, she was able to form a question that considered the relationships among those concepts.
This student had one final component to consider. Social work research questions must contain a target population. Her study would be very different if she were to conduct it on older adults or immigrants who just arrived in a new country. The target population is the group of people whose needs your study addresses. Maybe the student noticed issues with household tasks as part of her social work practice with first-generation immigrants, and so she made it her target population. Maybe she wants to address the needs of another community. Whatever the case, the target population should be chosen while keeping in mind social work’s responsibility to work on behalf of marginalized and oppressed groups.
In sum, a good research question generally has the following features:
- It is written in the form of a question
- It is clearly written
- It cannot be answered with “yes” or “no”
- It has more than one plausible answer
- It considers relationships among multiple variables
- It is specific and clear about the concepts it addresses
- It includes a target population
- A poorly focused research question can lead to the demise of an otherwise well-executed study.
- Research questions should be clearly worded, consider relationships between multiple variables, have more than one plausible answer, and address the needs of a target population.
Okay, it’s time to write out your first draft of a research question.
- Once you’ve done so, take a look at the checklist in this chapter and see if your research question meets the criteria to be a good one.
Brainstorm whether your research question might be better suited to quantitative or qualitative methods.
- Describe why your question fits better with quantitative or qualitative methods.
- Provide an alternative research question that fits with the other type of research method.
9.3 Quantitative research questions
- Describe how research questions for exploratory, descriptive, and explanatory quantitative questions differ and how to phrase them
- Identify the differences between and provide examples of strong and weak explanatory research questions
Quantitative descriptive questions
The type of research you are conducting will impact the research question that you ask. Probably the easiest questions to think of are quantitative descriptive questions. For example, “What is the average student debt load of MSW students?” is a descriptive question—and an important one. We aren’t trying to build a causal relationship here. We’re simply trying to describe how much debt MSW students carry. Quantitative descriptive questions like this one are helpful in social work practice as part of community scans, in which human service agencies survey the various needs of the community they serve. If the scan reveals that the community requires more services related to housing, child care, or day treatment for people with disabilities, a nonprofit office can use the community scan to create new programs that meet a defined community need.
Quantitative descriptive questions will often ask for percentage, count the number of instances of a phenomenon, or determine an average. Descriptive questions may only include one variable, such as ours about student debt load, or they may include multiple variables. Because these are descriptive questions, our purpose is not to investigate causal relationships between variables. To do that, we need to use a quantitative explanatory question.
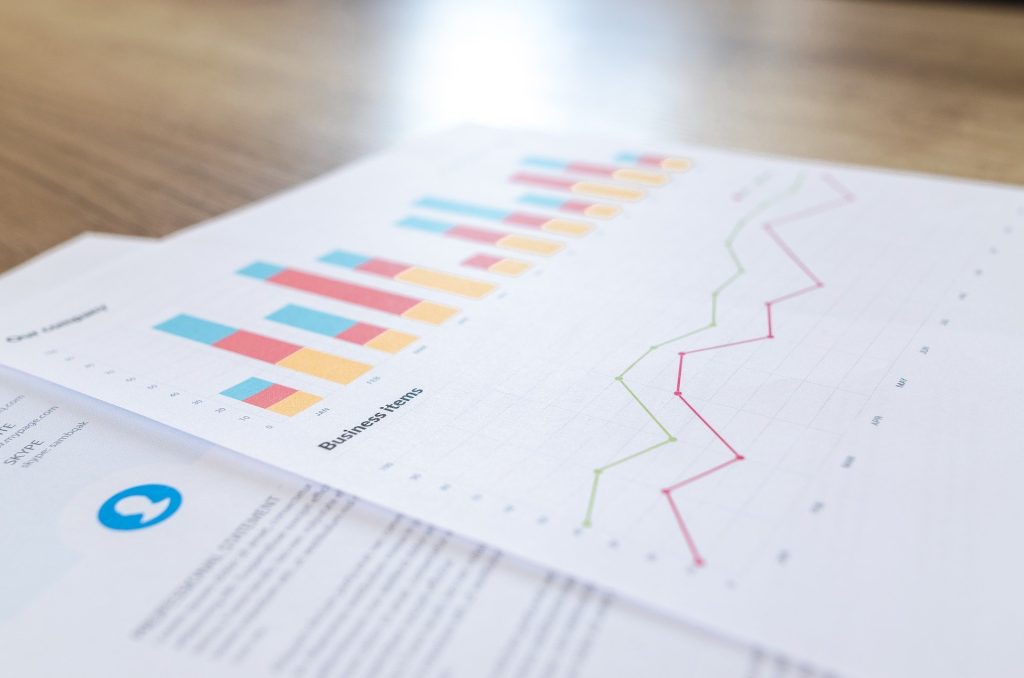
Quantitative explanatory questions
Most studies you read in the academic literature will be quantitative and explanatory. Why is that? If you recall from Chapter 2 , explanatory research tries to build nomothetic causal relationships. They are generalizable across space and time, so they are applicable to a wide audience. The editorial board of a journal wants to make sure their content will be useful to as many people as possible, so it’s not surprising that quantitative research dominates the academic literature.
Structurally, quantitative explanatory questions must contain an independent variable and dependent variable. Questions should ask about the relationship between these variables. The standard format I was taught in graduate school for an explanatory quantitative research question is: “What is the relationship between [independent variable] and [dependent variable] for [target population]?” You should play with the wording for your research question, revising that standard format to match what you really want to know about your topic.
Let’s take a look at a few more examples of possible research questions and consider the relative strengths and weaknesses of each. Table 9.1 does just that. While reading the table, keep in mind that I have only noted what I view to be the most relevant strengths and weaknesses of each question. Certainly each question may have additional strengths and weaknesses not noted in the table. Each of these questions is drawn from student projects in my research methods classes and reflects the work of many students on their research question over many weeks.
Making it more specific
A good research question should also be specific and clear about the concepts it addresses. A student investigating gender and household tasks knows what they mean by “household tasks.” You likely also have an impression of what “household tasks” means. But are your definition and the student’s definition the same? A participant in their study may think that managing finances and performing home maintenance are household tasks, but the researcher may be interested in other tasks like childcare or cleaning. The only way to ensure your study stays focused and clear is to be specific about what you mean by a concept. The student in our example could pick a specific household task that was interesting to them or that the literature indicated was important—for example, childcare. Or, the student could have a broader view of household tasks, one that encompasses childcare, food preparation, financial management, home repair, and care for relatives. Any option is probably okay, as long as the researcher is clear on what they mean by “household tasks.” Clarifying these distinctions is important as we look ahead to specifying how your variables will be measured in Chapter 11 .
Table 9.2 contains some “watch words” that indicate you may need to be more specific about the concepts in your research question.
It can be challenging to be this specific in social work research, particularly when you are just starting out your project and still reading the literature. If you’ve only read one or two articles on your topic, it can be hard to know what you are interested in studying. Broad questions like “What are the causes of chronic homelessness, and what can be done to prevent it?” are common at the beginning stages of a research project as working questions. However, moving from working questions to research questions in your research proposal requires that you examine the literature on the topic and refine your question over time to be more specific and clear. Perhaps you want to study the effect of a specific anti-homelessness program that you found in the literature. Maybe there is a particular model to fighting homelessness, like Housing First or transitional housing, that you want to investigate further. You may want to focus on a potential cause of homelessness such as LGBTQ+ discrimination that you find interesting or relevant to your practice. As you can see, the possibilities for making your question more specific are almost infinite.
Quantitative exploratory questions
In exploratory research, the researcher doesn’t quite know the lay of the land yet. If someone is proposing to conduct an exploratory quantitative project, the watch words highlighted in Table 9.2 are not problematic at all. In fact, questions such as “What factors influence the removal of children in child welfare cases?” are good because they will explore a variety of factors or causes. In this question, the independent variable is less clearly written, but the dependent variable, family preservation outcomes, is quite clearly written. The inverse can also be true. If we were to ask, “What outcomes are associated with family preservation services in child welfare?”, we would have a clear independent variable, family preservation services, but an unclear dependent variable, outcomes. Because we are only conducting exploratory research on a topic, we may not have an idea of what concepts may comprise our “outcomes” or “factors.” Only after interacting with our participants will we be able to understand which concepts are important.
Remember that exploratory research is appropriate only when the researcher does not know much about topic because there is very little scholarly research. In our examples above, there is extensive literature on the outcomes in family reunification programs and risk factors for child removal in child welfare. Make sure you’ve done a thorough literature review to ensure there is little relevant research to guide you towards a more explanatory question.
- Descriptive quantitative research questions are helpful for community scans but cannot investigate causal relationships between variables.
- Explanatory quantitative research questions must include an independent and dependent variable.
- Exploratory quantitative research questions should only be considered when there is very little previous research on your topic.
- Identify the type of research you are engaged in (descriptive, explanatory, or exploratory).
- Create a quantitative research question for your project that matches with the type of research you are engaged in.
Preferably, you should be creating an explanatory research question for quantitative research.
9.4 Qualitative research questions
- List the key terms associated with qualitative research questions
- Distinguish between qualitative and quantitative research questions
Qualitative research questions differ from quantitative research questions. Because qualitative research questions seek to explore or describe phenomena, not provide a neat nomothetic explanation, they are often more general and openly worded. They may include only one concept, though many include more than one. Instead of asking how one variable causes changes in another, we are instead trying to understand the experiences , understandings , and meanings that people have about the concepts in our research question. These keywords often make an appearance in qualitative research questions.
Let’s work through an example from our last section. In Table 9.1, a student asked, “What is the relationship between sexual orientation or gender identity and homelessness for late adolescents in foster care?” In this question, it is pretty clear that the student believes that adolescents in foster care who identify as LGBTQ+ may be at greater risk for homelessness. This is a nomothetic causal relationship—LGBTQ+ status causes changes in homelessness.
However, what if the student were less interested in predicting homelessness based on LGBTQ+ status and more interested in understanding the stories of foster care youth who identify as LGBTQ+ and may be at risk for homelessness? In that case, the researcher would be building an idiographic causal explanation . The youths whom the researcher interviews may share stories of how their foster families, caseworkers, and others treated them. They may share stories about how they thought of their own sexuality or gender identity and how it changed over time. They may have different ideas about what it means to transition out of foster care.
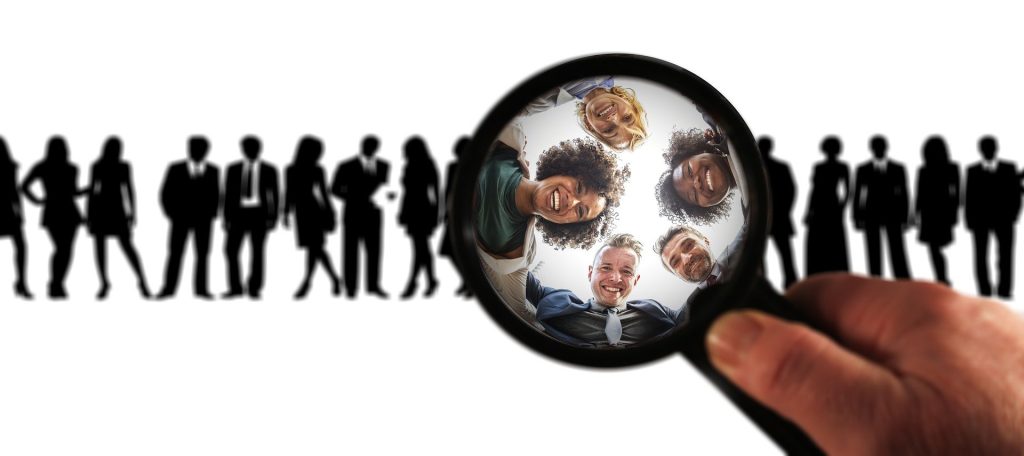
Because qualitative questions usually center on idiographic causal relationships, they look different than quantitative questions. Table 9.3 below takes the final research questions from Table 9.1 and adapts them for qualitative research. The guidelines for research questions previously described in this chapter still apply, but there are some new elements to qualitative research questions that are not present in quantitative questions.
- Qualitative research questions often ask about lived experience, personal experience, understanding, meaning, and stories.
- Qualitative research questions may be more general and less specific.
- Qualitative research questions may also contain only one variable, rather than asking about relationships between multiple variables.
Qualitative research questions have one final feature that distinguishes them from quantitative research questions: they can change over the course of a study. Qualitative research is a reflexive process, one in which the researcher adapts their approach based on what participants say and do. The researcher must constantly evaluate whether their question is important and relevant to the participants. As the researcher gains information from participants, it is normal for the focus of the inquiry to shift.
For example, a qualitative researcher may want to study how a new truancy rule impacts youth at risk of expulsion. However, after interviewing some of the youth in their community, a researcher might find that the rule is actually irrelevant to their behavior and thoughts. Instead, their participants will direct the discussion to their frustration with the school administrators or the lack of job opportunities in the area. This is a natural part of qualitative research, and it is normal for research questions and hypothesis to evolve based on information gleaned from participants.
However, this reflexivity and openness unacceptable in quantitative research for good reasons. Researchers using quantitative methods are testing a hypothesis, and if they could revise that hypothesis to match what they found, they could never be wrong! Indeed, an important component of open science and reproducability is the preregistration of a researcher’s hypotheses and data analysis plan in a central repository that can be verified and replicated by reviewers and other researchers. This interactive graphic from 538 shows how an unscrupulous research could come up with a hypothesis and theoretical explanation after collecting data by hunting for a combination of factors that results in a statistically significant relationship. This is an excellent example of how the positivist assumptions behind quantitative research and intepretivist assumptions behind qualitative research result in different approaches to social science.
- Qualitative research questions often contain words or phrases like “lived experience,” “personal experience,” “understanding,” “meaning,” and “stories.”
- Qualitative research questions can change and evolve over the course of the study.
- Using the guidance in this chapter, write a qualitative research question. You may want to use some of the keywords mentioned above.
9.5 Evaluating and updating your research questions
- Evaluate the feasibility and importance of your research questions
- Begin to match your research questions to specific designs that determine what the participants in your study will do
Feasibility and importance
As you are getting ready to finalize your research question and move into designing your research study, it is important to check whether your research question is feasible for you to answer and what importance your results will have in the community, among your participants, and in the scientific literature
Key questions to consider when evaluating your question’s feasibility include:
- Do you have access to the data you need?
- Will you be able to get consent from stakeholders, gatekeepers, and others?
- Does your project pose risk to individuals through direct harm, dual relationships, or breaches in confidentiality? (see Chapter 6 for more ethical considerations)
- Are you competent enough to complete the study?
- Do you have the resources and time needed to carry out the project?
Key questions to consider when evaluating the importance of your question include:
- Can we answer your research question simply by looking at the literature on your topic?
- How does your question add something new to the scholarly literature? (raises a new issue, addresses a controversy, studies a new population, etc.)
- How will your target population benefit, once you answer your research question?
- How will the community, social work practice, and the broader social world benefit, once you answer your research question?
- Using the questions above, check whether you think your project is feasible for you to complete, given the constrains that student projects face.
- Realistically, explore the potential impact of your project on the community and in the scientific literature. Make sure your question cannot be answered by simply reading more about your topic.
Matching your research question and study design
This chapter described how to create a good quantitative and qualitative research question. In Parts 3 and 4 of this textbook, we will detail some of the basic designs like surveys and interviews that social scientists use to answer their research questions. But which design should you choose?
As with most things, it all depends on your research question. If your research question involves, for example, testing a new intervention, you will likely want to use an experimental design. On the other hand, if you want to know the lived experience of people in a public housing building, you probably want to use an interview or focus group design.
We will learn more about each one of these designs in the remainder of this textbook. We will also learn about using data that already exists, studying an individual client inside clinical practice, and evaluating programs, which are other examples of designs. Below is a list of designs we will cover in this textbook:
- Surveys: online, phone, mail, in-person
- Experiments: classic, pre-experiments, quasi-experiments
- Interviews: in-person or via phone or videoconference
- Focus groups: in-person or via videoconference
- Content analysis of existing data
- Secondary data analysis of another researcher’s data
- Program evaluation
The design of your research study determines what you and your participants will do. In an experiment, for example, the researcher will introduce a stimulus or treatment to participants and measure their responses. In contrast, a content analysis may not have participants at all, and the researcher may simply read the marketing materials for a corporation or look at a politician’s speeches to conduct the data analysis for the study.
I imagine that a content analysis probably seems easier to accomplish than an experiment. However, as a researcher, you have to choose a research design that makes sense for your question and that is feasible to complete with the resources you have. All research projects require some resources to accomplish. Make sure your design is one you can carry out with the resources (time, money, staff, etc.) that you have.
There are so many different designs that exist in the social science literature that it would be impossible to include them all in this textbook. The purpose of the subsequent chapters is to help you understand the basic designs upon which these more advanced designs are built. As you learn more about research design, you will likely find yourself revising your research question to make sure it fits with the design. At the same time, your research question as it exists now should influence the design you end up choosing. There is no set order in which these should happen. Instead, your research project should be guided by whether you can feasibly carry it out and contribute new and important knowledge to the world.
- Research questions must be feasible and important.
- Research questions must match study design.
- Based on what you know about designs like surveys, experiments, and interviews, describe how you might use one of them to answer your research question.
- You may want to refer back to Chapter 2 which discusses how to get raw data about your topic and the common designs used in student research projects.
- Not familiar with SpongeBob SquarePants? You can learn more about him on Nickelodeon’s site dedicated to all things SpongeBob: http://www.nick.com/spongebob-squarepants/ ↵
- Focus on the Family. (2005, January 26). Focus on SpongeBob. Christianity Today . Retrieved from http://www.christianitytoday.com/ct/2005/januaryweb-only/34.0c.html ↵
- BBC News. (2005, January 20). US right attacks SpongeBob video. Retrieved from: http://news.bbc.co.uk/2/hi/americas/4190699.stm ↵
- In fact, an MA thesis examines representations of gender and relationships in the cartoon: Carter, A. C. (2010). Constructing gender and relationships in “SpongeBob SquarePants”: Who lives in a pineapple under the sea . MA thesis, Department of Communication, University of South Alabama, Mobile, AL. ↵
- Writing from an outline (10 minute read plus an 8 minute video, and then a 15 minute video)
- Writing your literature review (30 minute read)
Content warning: TBA
6.1: Writing from an outline
Learners will be able to...
- Integrate facts from the literature into scholarly writing
- Experiment with different approaches to integrating information that do not involve direct quotations from other authors
Congratulations! By now, you should have discovered, retrieved, evaluated, synthesized, and organized the information you need for your literature review. It’s now time to turn that stack of articles, papers, and notes into a literature review–it’s time to start writing!
The first step in research writing is outlining. In Chapter 4, we reviewed how to build a topical outline using quotations and facts from other authors. Use that outline (or one you write now) as a way to organize your thoughts.
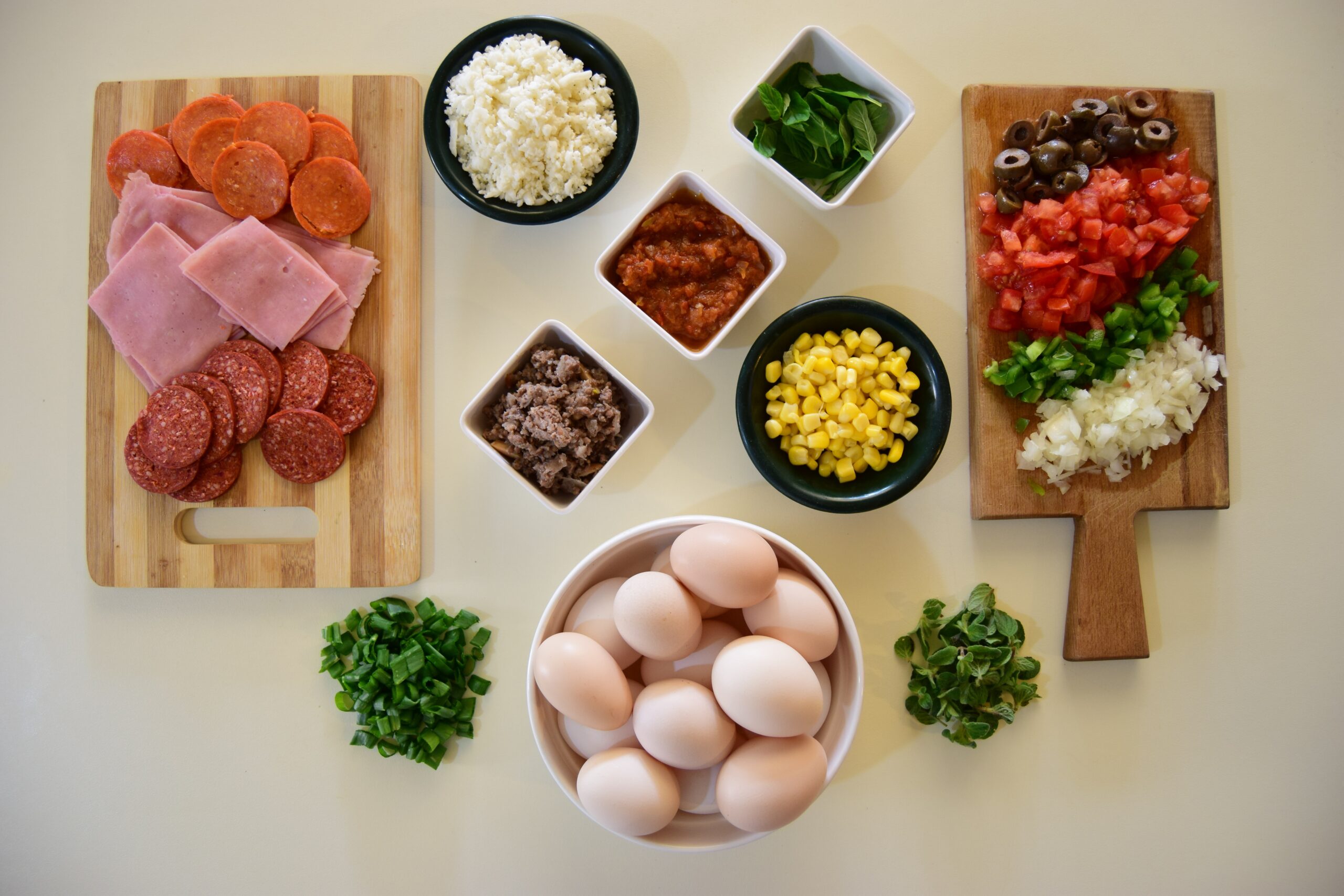
Watch this video from Nicholas Cifuentes-Goodbody on Outlining . As he highlights, outlining is like building a mise en place before a meal--arranging your ingredients in an orderly way so you can create your masterpiece.
From quotations to original writing
Much like combining ingredients on a kitchen countertop, you will need to mix your ingredients together. That means you will not be relying extensively on quotations from other authors in your literature review. In moving from an outline to a literature review, the key intellectual move is relying on your own ideas about the literature, rather than quoting extensively from other sources.
Integrating ideas from other authors
Watch this video from Nicholas Cifuentes-Goodbody on using quotations in academic writing . In the video, he reviews a few different techniques to integrate quotations or ideas from other authors into your writing. All literature reviews use the ideas from other authors, but it's important not to overuse others' words. Your literature review is evaluated by your professor based on how well it shows you are able to make connections between different facts in the scientific literature. The examples in this section should highlight how to get other people's words out of the way of your own. Use these strategies to diversify your writing and show your readers how your sources contributed to your work.
1. Make a claim without a quote
Claim ( Citation )
Some view cities as the storehouse of culture and creativity, and propose that urbanization is a consequence of the attractiveness of these social benefits ( Mumford, 1961 ).
More information
Oftentimes you do not need to directly quote a source to convey its conclusions or arguments – and some disciplines discourage quoting directly! Rather you can paraphrase the main point of a paper in your own words and provide an in-text citation. A benefit of using this strategy is that you can offer support for a claim without using a whole paragraph to introduce and frame a quote. You should make sure that you fully understand the paper's argument and that you are following university citation guidelines before attempting to paraphrase something from a paper.
2. Make a claim that is supported by two or more sources:
Claim ( Citation 1 ; Citation 2 ).
Reviews of this literature concede difficulty in making direct comparisons of emission levels across different sets of analysis ( Bader and Bleischwitz, 2009 ; Kennedy et al., 2009 ; Ramaswami et al., 2012 ).
Sometimes multiple sources support your claim, or there are two major publications that deserve credit for providing evidence on a topic. This is a perfect time to use multiple citations. You can cite two, three, or more sources in a single sentence!
Make a claim that has been supported in multiple contexts:
Context 1 ( Citation ), Context 2 ( Citation ), Context 3 ( Citation ).
These results are supported by more recent research on transportation energy consumption ( Liddle, 2014 ), electricity consumption in buildings ( Lariviere and Lafrance, 1999 ), and overall urban GHG emissions ( Marcotullio et al., 2013b ).
More information:
Use this citation strategy when you want to show that a body of research has found support for some claim across several different contexts. This can show the robustness of an effect or phenomenon and can give your claim some added validity
3. Quote important or unique terms
Claim " Term " ( Citation ).
The spatial implications of this thinking are manifest in the " concentric ring model " of urban expansion and its variants ( Harris and Ullman, 1945 ).
While block or even whole-sentence citations are rare in most research papers in the science and social science disciplines, there is often a need to quote specific terms or phrases that were first coined by a certain source or that were well-explained in a specific paper.
4. Quoting definitions
Contextualize quote , " important word or phrase ."
Role conflict is defined as "A situation in which contradictory, competing, or incompatible expectations are placed on an individual by two or more roles held at the same time" (Open Sociology Dictionary, 2023); whereas, role strain is defined as "a situation caused by higher-than-expected demands placed on an individual performing a specific role that leads to difficulty or stress" (Open Sociology Dictionary, 2023). In our study, we hypothesize that caregivers who reenter higher education experience role conflict between school work, paid work, and care work. Further, we hypothesize that this conflict is greater in individuals who had experienced role strain in employment or caregiving prior to entering college.
A direct quotation can bring attention to specific language in your source. When someone puts something perfectly, you can use a quotation to convey the identical meaning in your work. Definitions are an excellent example of when to use a quotation. In other cases, there may be quotations from important thinkers, clients or community members, and others whose specific wording is important.
I encourage you to use few, if any, direct quotations in your literature review. Personally, I think most students are scared of looking stupid and would rather use a good quotation than risk not getting it right. If you are a student who considers themselves a strong writer, this may not sound relevant to you. However, I'm willing to bet that there are many of your peers for whom this describes a particular bit of research anxiety.
When using quotations, make sure to only include the parts of the quotation that are necessary. You do not need to use quotation marks for statistics you use. And I encourage you to find ways to put others' statistics in your sentences.
Why share information from other sources?
Now that you know some different sentence structures using APA citations, let's examine the purpose behind why you are sharing information from another source. Cited evidence can serve a wide range of purposes in academic papers. These examples will give you an idea of the different ways that you can use citations in your paper.
1. Summarize your source
The studies of Newman and Kenworthy ( 1989, 1999 ) demonstrate a negative relationship between population density and transportation fuel use .
You will help your reader understand your points better if you summarize the key points of a study. Describe the strengths or weaknesses a specific source that has been pivotal in your field. Describe the source's specific methodology, theory, or approach. Be sure to still include a citation. If you mention the name of the author in your text, you still need to provide the date of the study in a parenthetical citation.
2. Cite a method
Despite the popularity of the WUP indicators , they have been routinely criticized because the methodology relies on local- and country-specific definitions of bounding urban areas, resulting in of ten incomparable and widely divergent definitions of the population, density thresholds, or administrative/political units designated ( Satterthwaite, 2007 ).
This is an easy way to give credit to a source that has provided some evidence for the validity of a method or questionnaire. Readers can reference your citation if they are interested in knowing more about the method and its standing in the current literature.
3. Compare sources
Some evidence for this scaling relationship suggests that urban areas with larger population sizes have proportionally smaller energy infrastructures than smaller cities ( Bettencourt et al., 2007 ; Fragkias et al., 2013 ). Other evidence suggests that GHG emissions may increase more than proportionally to population size, such that larger cities exhibit proportionally higher energy demand as they grow than do smaller cities ( Marcotullio et al., 2013 ).
This is one of the most important techniques for creating an effective literature review. This allows you and your readers to consider controversies and discrepancies among the current literature, revealing gaps in the literature or points of contention for further study.
The examples in this guide come from:
Marcotullio, P. J., Hughes, S., Sarzynski, A., Pincetl, S., Sanchez Peña, L., Romero-Lankao, P., Runfola, D. and Seto, K. C. (2014), Urbanization and the carbon cycle: Contributions from social science. Earth's Future, 2: 496–514. doi:10.1002/2014EF000257
Avoiding plagiarism
The most difficult thing about avoiding plagiarism is that reading so much of other people's ideas can make them seem like your own after a while. We recommend you work through this interactive activity on determining how and when to cite other authors.
- Research writing requires outlining, which helps you arrange your facts neatly before writing. It's similar to arranging all of your ingredients before you start cooking.
- Eliminate quotations from your writing as much as possible. Your literature review needs to be your analysis of the literature, not just a summary of other people's good ideas.
- Experiment with the prompts in this chapter as you begin to write your research question.
6.2 Writing your literature review
- Describe the components of a literature review
- Begin to write your literature review
- Identify the purpose of a problem statement
- Apply the components of a formal argument to your topic
- Use elements of formal writing style, including signposting and transitions
- Recognize commons errors in literature reviews
Writing about research is different than other types of writing. Research writing is not like a journal entry or opinion paper. The goal here is not to apply your research question to your life or growth as a practitioner. Research writing is about the provision and interpretation of facts. The tone should be objective and unbiased, and personal experiences and opinions are excluded. Particularly for students who are used to writing case notes, research writing can be a challenge. That's why its important to normalize getting help! If your professor has not built in peer review, consider setting up a peer review group among your peers. You should also reach out to your academic advisor to see if there are writing services on your campus available to graduate students. No one should feel bad for needing help with something they haven't done before, haven't done in a while, or were never taught how to do.
If you’ve followed the steps in this chapter, you likely have an outline, summary table, and concept map from which you can begin the writing process. But what do you need to include in your literature review? We’ve mentioned it before, but to summarize, a literature review should:
- Introduce the topic and define its key terms.
- Establish the importance of the topic.
- Provide an overview of the important literature related to the concepts found in the research question.
- Identify gaps or controversies in the literature.
- Point out consistent findings across studies.
- Synthesize that which is known about a topic, rather than just provide a summary of the articles you read.
- Discuss possible implications and directions for future research.
Do you have enough facts and sources to accomplish these tasks? It’s a good time to consult your outlines and notes on each article you plan to include in your literature review. You may also want to consult with your professor on what is expected of you. If there is something you are missing, you may want to jump back to section 2.3 where we discussed how to search for literature. While you can always fill in material, there is the danger that you will start writing without really knowing what you are talking about or what you want to say. For example, if you don’t have a solid definition of your key concepts or a sense of how the literature has developed over time, it will be difficult to make coherent scholarly claims about your topic.
There is no magical point at which one is ready to write. As you consider whether you are ready, it may be useful to ask yourself these questions:
- How will my literature review be organized?
- What section headings will I be using?
- How do the various studies relate to each other?
- What contributions do they make to the field?
- Where are the gaps or limitations in existing research?
- And finally, but most importantly, how does my own research fit into what has already been done?
The problem statement
Scholarly works often begin with a problem statement, which serves two functions. First, it establishes why your topic is a social problem worth studying. Second, it pulls your reader into the literature review. Who would want to read about something unimportant?
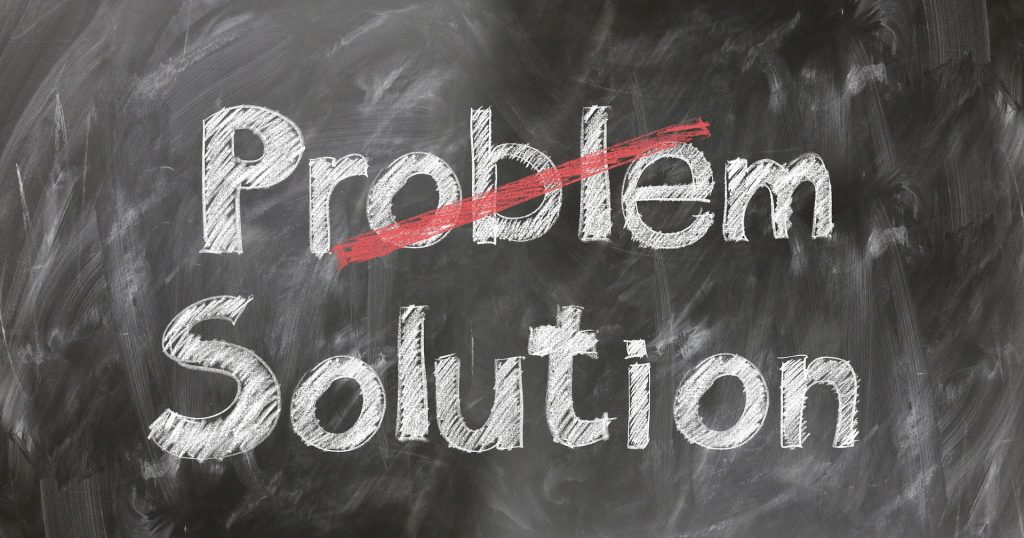
A problem statement generally answers the following questions, though these are far from exhaustive:
- Why is this an important problem to study?
- How many people are affected by this problem?
- How does this problem impact other social issues relevant to social work?
- Why is your target population an important one to study?
A strong problem statement, like the rest of your literature review, should be filled with empirical results, theory, and arguments based on the extant literature. A research proposal differs significantly from other more reflective essays you’ve likely completed during your social work studies. If your topic were domestic violence in rural Appalachia, I’m sure you could come up with answers to the above questions without looking at a single source. However, the purpose of the literature review is not to test your intuition, personal experience, or empathy. Instead, research methods are about gaining specific and articulable knowledge to inform action. With a problem statement, you can take a “boring” topic like the color of rooms used in an inpatient psychiatric facility, transportation patterns in major cities, or the materials used to manufacture baby bottles, and help others see the topic as you see it—an important part of the social world that impacts social work practice.
The structure of a literature review
In general, the problem statement belongs at the beginning of the literature review. We usually advise students to spend no more than a paragraph or two for a problem statement. For the rest of your literature review, there is no set formula by which it needs to be organized. However, a literature review generally follows the format of any other essay—Introduction, Body, and Conclusion.
The introduction to the literature review contains a statement or statements about the overall topic. At a minimum, the introduction should define or identify the general topic, issue, or area of concern. You might consider presenting historical background, mentioning the results of a seminal study, and providing definitions of important terms. The introduction may also point to overall trends in what has been previously published on the topic or on conflicts in theory, methodology, evidence, conclusions, or gaps in research and scholarship. We also suggest putting in a few sentences that walk the reader through the rest of the literature review. Highlight your main arguments from the body of the literature review and preview your conclusion. An introduction should let the reader know what to expect from the rest of your review.
The body of your literature review is where you demonstrate your synthesis and analysis of the literature. Again, do not just summarize the literature. We would also caution against organizing your literature review by source—that is, one paragraph for source A, one paragraph for source B, etc. That structure will likely provide an adequate summary of the literature you’ve found, but it would give you almost no synthesis of the literature. That approach doesn’t tell your reader how to put those facts together, it doesn't highlight points of agreement or contention, or how each study builds on the work of others. In short, it does not demonstrate critical thinking.
Organize your review by argument
Instead, use your outlines and notes as a guide what you have to say about the important topics you need to cover. Literature reviews are written from the perspective of an expert in that field. After an exhaustive literature review, you should feel as though you are able to make strong claims about what is true—so make them! There is no need to hide behind “I believe” or “I think.” Put your voice out in front, loud and proud! But make sure you have facts and sources that back up your claims.
I’ve used the term “ argument ” here in a specific way. An argument in writing means more than simply disagreeing with what someone else said, as this classic Monty Python sketch demonstrates. Toulman, Rieke, and Janik (1984) identify six elements of an argument:
- Claim: the thesis statement—what you are trying to prove
- Grounds: theoretical or empirical evidence that supports your claim
- Warrant: your reasoning (rule or principle) connecting the claim and its grounds
- Backing: further facts used to support or legitimize the warrant
- Qualifier: acknowledging that the argument may not be true for all cases
- Rebuttal: considering both sides (as cited in Burnette, 2012) [1]
Let’s walk through an example. If I were writing a literature review on a negative income tax, a policy in which people in poverty receive an unconditional cash stipend from the government each month equal to the federal poverty level, I would want to lay out the following:
- Claim: the negative income tax is superior to other forms of anti-poverty assistance.
- Grounds: data comparing negative income tax recipients to people receiving anti-poverty assistance in existing programs, theory supporting a negative income tax, data from evaluations of existing anti-poverty programs, etc.
- Warrant: cash-based programs like the negative income tax are superior to existing anti-poverty programs because they allow the recipient greater self-determination over how to spend their money.
- Backing: data demonstrating the beneficial effects of self-determination on people in poverty.
- Qualifier: the negative income tax does not provide taxpayers and voters with enough control to make sure people in poverty are not wasting financial assistance on frivolous items.
- Rebuttal: policy should be about empowering the oppressed, not protecting the taxpayer, and there are ways of addressing taxpayer spending concerns through policy design.
Like any effective argument, your literature review must have some kind of structure. For example, it might begin by describing a phenomenon in a general way along with several studies that provide some detail, then describing two or more competing theories of the phenomenon, and finally presenting a hypothesis to test one or more of the theories. Or, it might describe one phenomenon, then describe another that seems inconsistent with the first, then propose a theory that resolves the inconsistency, and finally present a hypothesis to test that theory. In applied research, it might describe a phenomenon or theory, then describe how that phenomenon or theory applies to some important real-world situation, and finally, may suggest a way to test whether it does, in fact, apply to that situation.
Use signposts
Another important issue is signposting . It may not be a term you are familiar with, but you are likely familiar with the concept. Signposting refers to the words used to identify the organization and structure of your literature review to your reader. The most basic form of signposting is using a topic sentence at the beginning of each paragraph. A topic sentence introduces the argument you plan to make in that paragraph. For example, you might start a paragraph stating, “There is strong disagreement in the literature as to whether psychedelic drugs cause people to develop psychotic disorders, or whether psychotic disorders cause people to use psychedelic drugs.” Within that paragraph, your reader would likely assume you will present evidence for both arguments. The concluding sentence of your paragraph should address the topic sentence, discussing how the facts and arguments from the paragraph you've written support a specific conclusion. To continue with our example, I might say, “There is likely a reciprocal effect in which both the use of psychedelic drugs worsens pre-psychotic symptoms and worsening psychosis increases the desire to use psychedelic drugs.”
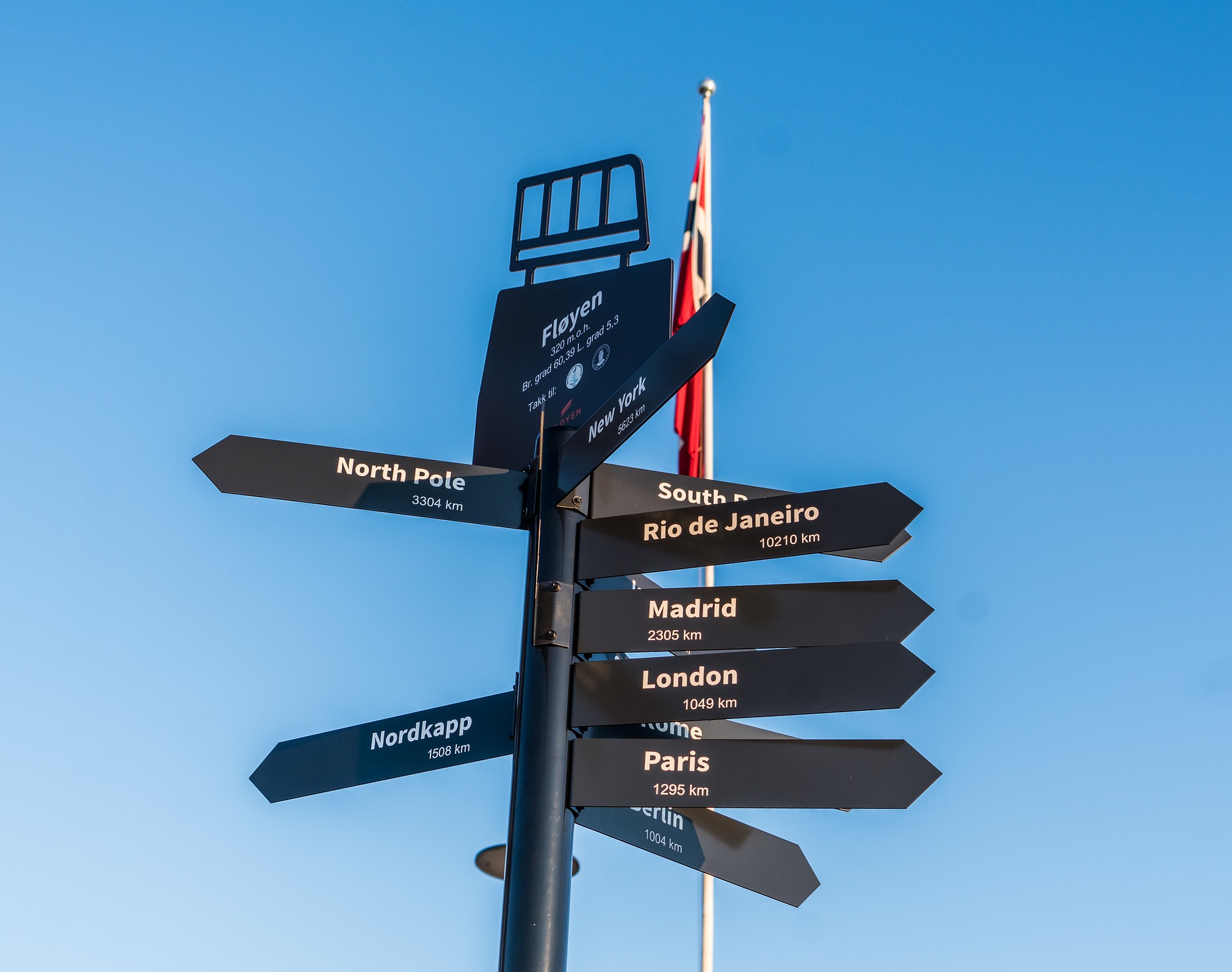
Signposting also involves using headings and subheadings. Your literature review will use APA formatting, which means you need to follow their rules for bolding, capitalization, italicization, and indentation of headings. Headings help your reader understand the structure of your literature review. They can also help if the reader gets lost and needs to re-orient themselves within the document. We often tell our students to assume we know nothing (they don’t mind) and need to be shown exactly where they are addressing each part of the literature review. It’s like walking a small child around, telling them “First we’ll do this, then we’ll do that, and when we’re done, we’ll know this!”
Another way to use signposting is to open each paragraph with a sentence that links the topic of the paragraph with the one before it. Alternatively, one could end each paragraph with a sentence that links it with the next paragraph. For example, imagine we wanted to link a paragraph about barriers to accessing healthcare with one about the relationship between the patient and physician. We could use a transition sentence like this: “Even if patients overcome these barriers to accessing care, the physician-patient relationship can create new barriers to positive health outcomes.” A transition sentence like this builds a connection between two distinct topics. Transition sentences are also useful within paragraphs. They tell the reader how to consider one piece of information in light of previous information. Even simple transitional words like 'however' and 'similarly' can help demonstrate critical thinking and link each building block of your argument together.
Many beginning researchers have difficulty incorporating transitions into their writing. Let’s look at an example. Instead of beginning a sentence or paragraph by launching into a description of a study, such as “Williams (2004) found that…,” it is better to start by indicating something about why you are describing this particular study. Here are some simple examples:
- Another example of this phenomenon comes from the work of Williams (2004)...
- Williams (2004) offers one explanation of this phenomenon...
- An alternative perspective has been provided by Williams (2004)...
Now that we know to use signposts, the natural question is “What goes on the signposts?” First, it is important to start with an outline of the main points that you want to make, organized in the order you want to make them. The basic structure of your argument should then be apparent from the outline itself. Unfortunately, there is no formula we can give you that will work for everyone, but we can provide some general pointers on structuring your literature review.
The literature review tends to move from general to more specific ideas. You can build a review by identifying areas of consensus and areas of disagreement. You may choose to present historical studies—preferably seminal studies that are of significant importance—and close with the most recent research. Another approach is to start with the most distantly related facts and literature and then report on those most closely related to your research question. You could also compare and contrast valid approaches, features, characteristics, theories – that is, one approach, then a second approach, followed by a third approach.
Here are some additional tips for writing the body of your literature review:
- Start broad and then narrow down to more specific information.
- When appropriate, cite two or more sources for a single point, but avoid long strings of references for a single idea.
- Use quotes sparingly. Quotations for definitions are okay, but reserve quotes for when something is said so well you couldn’t possible phrase it differently. Never use quotes for statistics.
- Paraphrase when you need to relay the specific details within an article
- Include only the aspects of the study that are relevant to your literature review. Don’t insert extra facts about a study just to take up space.
- Avoid reflective, personal writing. It is traditional to avoid using first-person language (I, we, us, etc.).
- Avoid informal language like contractions, idioms, and rhetorical questions.
- Note any sections of your review that lack citations from the literature. Your arguments need to be based in empirical or theoretical facts. Do not approach this like a reflective journal entry.
- Point out consistent findings and emphasize stronger studies over weaker ones.
- Point out important strengths and weaknesses of research studies, as well as contradictions and inconsistent findings.
- Implications and suggestions for further research (where there are gaps in the current literature) should be specific.
The conclusion should summarize your literature review, discuss implications, and create a space for further research needed in this area. Your conclusion, like the rest of your literature review, should make a point. What are the important implications of your literature review? How do they inform the question you are trying to answer?
You should consult with your professor and the course syllabus about the final structure your literature review should take. Here is an example of one possible structure:
- Establish the importance of the topic
- Number and type of people affected
- Seriousness of the impact
- Physical, psychological, economic, social, or spiritual consequences of the problem
- Definitions of key terms
- Supporting evidence
- Common findings across studies, gaps in the literature
- Research question(s) and hypothesis(es)
Editing your literature review
Literature reviews are more than a summary of the publications you find on a topic. As you have seen in this brief introduction, literature reviews represent a very specific type of research, analysis, and writing. We will explore these topics further in upcoming chapters. As you begin your literature review, here are some common errors to avoid:
- Accepting a researcher’s finding as valid without evaluating methodology and data
- Ignoring contrary findings and alternative interpretations
- Using findings that are not clearly related to your own study or using findings that are too general
- Dedicating insufficient time to literature searching
- Reporting statistical results from a single study, rather than synthesizing the results of multiple studies to provide a comprehensive view of the literature on a topic
- Relying too heavily on secondary sources
- Overusing quotations
- Not justifying arguments using specific facts or theories from the literature
For your literature review, remember that your goal is to construct an argument for the importance of your research question. As you start editing your literature review, make sure it is balanced. Accurately report common findings, areas where studies contradict each other, new theories or perspectives, and how studies cause us to reaffirm or challenge our understanding of your topic.
It is acceptable to argue that the balance of the research supports the existence of a phenomenon or is consistent with a theory (and that is usually the best that researchers in social work can hope for), but it is not acceptable to ignore contradictory evidence. A large part of what makes a research question interesting is uncertainty about its answer (University of Minnesota, 2016). [2]
In addition to subjectivity and bias, writer's block can obstruct the completion of your literature review. Often times, writer’s block can stem from confusing the creating and editing parts of the writing process. Many writers often start by simply trying to type out what they want to say, regardless of how good it is. Author Anne Lamott (1995) [3] terms these “shitty first drafts,” and we all write them. They are a natural and important part of the writing process.
Even if you have a detailed outline from which to work, the words are not going to fall into place perfectly the first time you start writing. You should consider turning off the editing and critiquing part of your brain for a while and allow your thoughts to flow. Don’t worry about putting a correctly formatted internal citation (as long as you know which source you used there) when you first write. Just get the information out. Only after you’ve reached a natural stopping point might you go back and edit your draft for grammar, APA style, organization, flow, and more. Divorcing the writing and editing process can go a long way to addressing writer’s block—as can picking a topic about which you have something to say!
As you are editing, keep in mind these questions adapted from Green (2012): [4]
- Content: Have I clearly stated the main idea or purpose of the paper? Is the thesis or focus clearly presented and appropriate for the reader?
- Organization: How well is it structured? Is the organization spelled out and easy to follow for the reader ?
- Flow: Is there a logical flow from section to section, paragraph to paragraph, sentence to sentence? Are there transitions between and within paragraphs that link ideas together?
- Development: Have I validated the main idea with supporting material? Are supporting data sufficient? Does the conclusion match the introduction?
- Form: Are there any APA style issues, redundancy, problematic wording and terminology (always know the definition of any word you use!), flawed sentence constructions and selection, spelling, and punctuation?
Social workers use the APA style guide to format and structure their literature reviews. Most students know APA style only as it relates to internal and external citations. If you are confused about them, consult this amazing APA style guide from the University of Texas-Arlington library. Your university's library likely has resources they created to help you with APA style, and you can meet with a librarian or your professor to talk about formatting questions you have. Make sure you budget in a few hours at the end of each project to build a correctly formatted references page and check your internal citations. The highest quality online source of information on APA style is the APA style blog, where you can search questions and answers from the
Of course, APA style is about much more than knowing there is a period after "et al." or citing the location a book was published. APA style is also about what the profession considers to be good writing. If you haven't picked up an APA publication manual because you use citation generators, know that I did the same thing when I was in school. Purchasing the APA manual can help you with a common problem we hear about from students. Every professor (and every website about APA style) seems to have their own peculiar idea of "correct" APA style that you can, if needed, demonstrate is not accurate.
- A literature review is not a book report. Do not organize it by article, with one paragraph for each source in your references. Instead, organize it based on the key ideas and arguments.
- The problem statement draws the reader into your topic by highlighting the importance of the topic to social work and to society overall.
- Signposting is an important component of academic writing that helps your reader follow the structure of your argument and of your literature review.
- Transitions demonstrate critical thinking and help guide your reader through your arguments.
- Editing and writing are separate processes.
- Consult with an APA style guide or a librarian to help you format your paper.
Look at your professor's prompt for the literature review component of your research proposal (or if you don't have one, use the example question provided in this section).
- Write 2-3 facts you would use to address each question or component in the prompt.
- Reflect on which questions you have a lot of information about and which you need to gather more information about in order to answer adequately.
Outline the structure of your literature review using your concept map from Section 5.2 as a guide.
- Identify the key arguments you will make and how they are related to each other.
- Reflect on topic sentences and concluding sentences you would use for each argument.
- Human subjects research (19 minute read)
- Specific ethical issues to consider (12 minute read)
- Benefits and harms of research across the ecosystem (7 minute read)
- Being an ethical researcher (8 minute read)
Content warning: examples in this chapter contain references to numerous incidents of unethical medical experimentation (e.g. intentionally injecting diseases into unknowing participants, withholding proven treatments), social experimentation under extreme conditions (e.g. being directed to deliver electric shocks to test obedience), violations of privacy, gender and racial inequality, research with people who are incarcerated or on parole, experimentation on animals, abuse of people with Autism, community interactions with law enforcement, WWII, the Holocaust, and Nazi activities (especially related to research on humans).
With your literature review underway, you are ready to begin thinking in more concrete terms about your research topic. Recall our discussion in Chapter 2 on practical and ethical considerations that emerge as part of the research process. In this chapter, we will expand on the ethical boundaries that social scientists must abide by when conducting human subjects research. As a result of reading this chapter, you should have a better sense of what is possible and ethical for the research project you create.
6.1 Human subjects research
- Understand what we mean by ethical research and why it is important
- Understand some of the egregious ethical violations that have occurred throughout history
While all research comes with its own set of ethical concerns, those associated with research conducted on human subjects vary dramatically from those of research conducted on nonliving entities. The US Department of Health and Human Services (USDHHS) defines a human subject as “a living individual about whom an investigator (whether professional or student) conducting research obtains (1) data through intervention or interaction with the individual, or (2) identifiable private information” (USDHHS, 1993, para. 1). [5] Some researchers prefer the term "participants" to "subjects'" as it acknowledges the agency of people who participate in the study. For our purposes, we will use the two terms interchangeably.
In some states, human subjects also include deceased individuals and human fetal materials. Nonhuman research subjects, on the other hand, are objects or entities that investigators manipulate or analyze in the process of conducting research. Nonhuman research subjects typically include sources such as newspapers, historical documents, pieces of clothing, television shows, buildings, and even garbage (to name just a few), that are analyzed for unobtrusive research projects. Unsurprisingly, research on human subjects is regulated much more heavily than research on nonhuman subjects. This is why many student research projects use data that is publicly available, rather than recruiting their own study participants. However, there are ethical considerations that all researchers must take into account, regardless of their research subject. We’ll discuss those considerations in addition to concerns that are unique to human subject research.
Why do research participants need protection?
First and foremost, we are professionally bound to engage in the ethical practice of research. This chapter discusses ethical research and will show you how to engage in research that is consistent with the NASW Code of Ethics as well as national and international ethical standards all researchers are accountable to. Before we begin, we need to understand the historical occurrences that were the catalyst for the formation of the current ethical standards . This chapter will enable you to view ethics from a micro, mezzo, and macro perspective.
The research process has led to many life-changing discoveries; these have improved life expectancy, improved living conditions, and helped us understand what contributes to certain social problems. That said, not all research has been conducted in respectful, responsible, or humane ways. Unfortunately, some research projects have dramatically marginalized, oppressed, and harmed participants and whole communities.
Would you believe that the following actions have been carried out in the name of research? I realize there was a content warning at the beginning of the chapter, but it is worth mentioning that the list below of research atrocities may be particularly upsetting or triggering.
- intentionally froze healthy body parts of prisoners to see if they could develop a treatment for freezing [6]
- scaled the body parts of prisoners to how best to treat soldiers who had injuries from being exposed to high temperatures [7]
- intentionally infected healthy individuals to see if they could design effective methods of treatment for infections [8]
- gave healthy people TB to see if they could treat it [9]
- attempted to transplant limbs, bones, and muscles to another person to see if this was possible [10]
- castrated and irradiated genitals to see if they could develop a faster method of sterilization [11]
- starved people and only allowed them to drink seawater to see if they could make saline water drinkable [12]
- artificially inseminated women with animal sperm to see what would happen [13]
- gassed living people to document how they would die [14]
- conducted cruel experiments on people and if they did not die, would kill them so they could undergo an autopsy [15]
- refused to treat syphilis in African American men (when treatment was available) because they wanted to track the progression of the illness [16]
- vivisected humans without anesthesia to see how illnesses that researches gave prisoners impacted their bodies [17]
- intentionally tried to infect prisoners with the Bubonic Plague [18]
- intentionally infected prisoners, prostitutes, soldiers, and children with syphilis to study the disease's progression [19]
- performed gynecological experiments on female slaves without anesthesia to investigate new surgical methods [20]
The sad fact is that not only did all of these occur, in many instances, these travesties continued for years until exposed and halted. Additionally, these examples have contributed to the formation of a legacy of distrust toward research. Specifically, many underrepresented groups have a deep distrust of agencies that implement research and are often skeptical of research findings. This has made it difficult for groups to support and have confidence in medical treatments, advances in social service programs, and evidence-informed policy changes. While the aforementioned unethical examples may have ended, this deep and painful wound on the public's trust remains. Consequently, we must be vigilant in our commitment to ethical research.
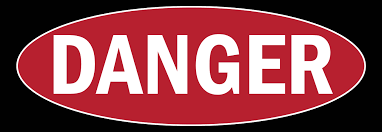
Many of the situations described may seem like extreme historical cases of misuse of power as researchers. However, ethical problems in research don't just happen in these extreme occurrences. None of us are immune to making unethical choices and the ethical practice of research requires conscientious mindful attention to what we are asking of our research participants. A few examples of less noticeable ethical issues might include: failing to fully explain to someone in advance what their participation might involve because you are in a rush to recruit a large enough sample; or only presenting findings that support your ideas to help secure a grant that is relevant to your research area. Remember, any time research is conducted with human beings, there is the chance that ethical violations may occur that pose social, emotional, and even physical risks for groups, and this is especially true when vulnerable or oppressed groups are involved.
A brief history of unethical social science research
Research on humans hasn’t always been regulated in the way it is today. The earliest documented cases of research using human subjects are of medical vaccination trials (Rothman, 1987). [21] One such case took place in the late 1700s, when scientist Edward Jenner exposed an 8-year-old boy to smallpox in order to identify a vaccine for the devastating disease. Medical research on human subjects continued without much law or policy intervention until the mid-1900s when, at the end of World War II, a number of Nazi doctors and scientists were put on trial for conducting human experimentation during the course of which they tortured and murdered many concentration camp inmates (Faden & Beauchamp, 1986). [22] The trials, conducted in Nuremberg, Germany, resulted in the creation of the Nuremberg Code , a 10-point set of research principles designed to guide doctors and scientists who conduct research on human subjects. Today, the Nuremberg Code guides medical and other research conducted on human subjects, including social scientific research.
Medical scientists are not the only researchers who have conducted questionable research on humans. In the 1960s, psychologist Stanley Milgram (1974) [23] conducted a series of experiments designed to understand obedience to authority in which he tricked subjects into believing they were administering an electric shock to other subjects. In fact, the shocks weren’t real at all, but some, though not many, of Milgram’s research participants experienced extreme emotional distress after the experiment (Ogden, 2008). [24] A reaction of emotional distress is understandable. The realization that one is willing to administer painful shocks to another human being just because someone who looks authoritative has told you to do so might indeed be traumatizing—even if you later learn that the shocks weren’t real.
Around the same time that Milgram conducted his experiments, sociology graduate student Laud Humphreys (1970) [25] was collecting data for his dissertation on the tearoom trade, which was the practice of men engaging in anonymous sexual encounters in public restrooms. Humphreys wished to understand who these men were and why they participated in the trade. To conduct his research, Humphreys offered to serve as a “watch queen,” in a local park restroom where the tearoom trade was known to occur. His role would be to keep an eye out for police while also getting the benefit of being able to watch the sexual encounters. What Humphreys did not do was identify himself as a researcher to his research subjects. Instead, he watched his subjects for several months, getting to know several of them, learning more about the tearoom trade practice and, without the knowledge of his research subjects, jotting down their license plate numbers as they pulled into or out of the parking lot near the restroom.
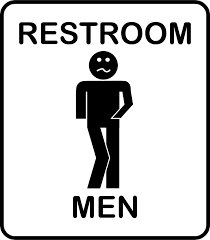
Sometime after participating as a watch queen, with the help of several insiders who had access to motor vehicle registration information, Humphreys used those license plate numbers to obtain the names and home addresses of his research subjects. Then, disguised as a public health researcher, Humphreys visited his subjects in their homes and interviewed them about their lives and their health. Humphreys’ research dispelled a good number of myths and stereotypes about the tearoom trade and its participants. He learned, for example, that over half of his subjects were married to women and many of them did not identify as gay or bisexual. [26]
Once Humphreys’ work became public, there was some major controversy at his home university (e.g., the chancellor tried to have his degree revoked), among scientists in general, and among members of the public, as it raised public concerns about the purpose and conduct of social science research. In addition, the Washington Post journalist Nicholas von Hoffman wrote the following warning about “sociological snoopers”:
We’re so preoccupied with defending our privacy against insurance investigators, dope sleuths, counterespionage men, divorce detectives and credit checkers, that we overlook the social scientists behind the hunting blinds who’re also peeping into what we thought were our most private and secret lives. But they are there, studying us, taking notes, getting to know us, as indifferent as everybody else to the feeling that to be a complete human involves having an aspect of ourselves that’s unknown (von Hoffman, 1970). [27]
In the original version of his report, Humphreys defended the ethics of his actions. In 2008 [28] , years after Humphreys’ death, his book was reprinted with the addition of a retrospect on the ethical implications of his work. In his written reflections on his research and the fallout from it, Humphreys maintained that his tearoom observations constituted ethical research on the grounds that those interactions occurred in public places. But Humphreys added that he would conduct the second part of his research differently. Rather than trace license numbers and interview unwitting tearoom participants in their homes under the guise of public health research, Humphreys instead would spend more time in the field and work to cultivate a pool of informants. Those informants would know that he was a researcher and would be able to fully consent to being interviewed. In the end, Humphreys concluded “there is no reason to believe that any research subjects have suffered because of my efforts, or that the resultant demystification of impersonal sex has harmed society” (Humphreys, 2008, p. 231). [29]
Today, given increasing regulation of social scientific research, chances are slim that a researcher would be allowed to conduct a project similar to Humphreys’. Some argue that Humphreys’ research was deceptive, put his subjects at risk of losing their families and their positions in society, and was therefore unethical (Warwick, 1973; Warwick, 1982). [30] Others suggest that Humphreys’ research “did not violate any premise of either beneficence or the sociological interest in social justice” and that the benefits of Humphreys’ research, namely the dissolution of myths about the tearoom trade specifically and human sexual practice more generally, outweigh the potential risks associated with the work (Lenza, 2004, p. 23). [31] What do you think, and why?
These and other studies (Reverby, 2009) [32] led to increasing public awareness of and concern about research on human subjects. In 1974, the US Congress enacted the National Research Act , which created the National Commission for the Protection of Human Subjects in Biomedical and Behavioral Research. The commission produced The Belmont Report , a document outlining basic ethical principles for research on human subjects (National Commission for the Protection of Human Subjects in Biomedical and Behavioral Research, 1979). [33] The National Research Act (1974) [34] also required that all institutions receiving federal support establish institutional review boards (IRBs) to protect the rights of human research subjects. Since that time, many organizations that do not receive federal support but where research is conducted have also established review boards to evaluate the ethics of the research that they conduct. IRBs are overseen by the federal Office of Human Research Protections .
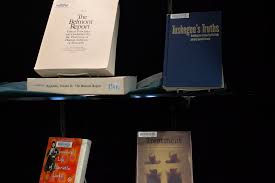
The Belmont Report
As mentioned above, The Belmont Report is a federal document that outlines the foundational principles that guide the ethical practice of research in the United States. These ethical principles include: respect for persons, beneficence, and justice. Each of these terms has specific implications as they are applied to the practice of research. These three principles arose as a response to many of the mistreatment and abuses that have been previously discussed and provide important guidance as researchers consider how they will construct and conduct their research studies. As you are crafting your research proposal, makes sure you are mindful of these important ethical guidelines.
Respect for Persons
As social workers, our professional code of ethics requires that we recognize and respect the "inherent dignity and worth of the person." [35] This is very similar to the ethical research principle of r espect for persons . According to this principle, as researchers, we need to treat all research participants with respect, dignity and inherent autonomy. This is reflected by ensuring that participants have self-determination to make informed decisions about their participation in research, that they have a clear understanding of what they will be asked to do and any risks involved, and that their participation is voluntary and can be stopped at any time. Furthermore, for those persons who may have diminished autonomy (e.g. children, people who are incarcerated), extra protections must be built in to these research studies to ensure that respect for persons continues to be demonstrated towards these groups, as they may be especially vulnerable to exploitation and coercion through the research process. A critical tool in establishing respect for persons in your research is the informed consent process, which will be discussed in more detail below.
Beneficence
You may not be familiar with this word yet, but the concept is pretty straightforward. The main idea with beneficence is that the intent of research is to do good. As researchers, to accomplish this, we seek to maximize benefits and minimize risks. Benefits may be something good or advantageous directly received by the research participant, or they may represent a broader good to a wider group of people or the scientific community at large (such as increasing knowledge about the topic or social problem that you are studying). Risks are potential physical, social, or emotional harm that may come about as a response to participation in a study. These risks may be more immediate (e.g. risk of identifying information about a participant being shared, or a participant being upset or triggered by a particular question), or long-term (e.g. some aspect about the person could be shared that could lead to long-term stigmatization). As researchers, we need to think about risk that might be experienced by the individual, but also risks that might be directed towards the community or population(s) the individual may represent. For instance, if our study is specifically focused on surveying single parents, we need to consider how the sharing of our findings might impact this group and how they are perceived. It is a very rare study in which there is no risk to participants. However, a well-designed and ethically sound study will seek to minimize these risks, provide resources to anticipate and address them, and maximize the benefits that are gained through the study.
The final ethical principle we need to cover is justice. While you likely have some idea what justice is, for the purposes of research, justice is the idea that the benefits and the burdens of research are distributed fairly across populations and groups. To help illustrate the concept of justice in research, research in the area of mental health and psychology has historically been critiqued as failing to adequately represent women and people of diverse racial and ethnic groups in their samples (Cundiff, 2012). [36] This has created a body of knowledge that is overly representative of the white male experience, further reinforcing systems of power and privilege. In addition, consider the influence of language as it relates to research justice. If we create studies that only recruit participants fluent in English, which many studies do, we are often failing to satisfy the ethical principle of justice as it applies to people who don't speak English. It is unrealistic to think that we can represent all people in all studies. However, we do need to thoughtfully acknowledge voices that might not be reflected in our samples and attempt to recruit diverse and representative samples whenever possible.
These three principles provide the foundation for the oversight work that is carried out by Institutional Review Boards, our next topic.
Institutional review boards
Institutional review boards, or IRBs, are tasked with ensuring that the rights and welfare of human research subjects will be protected at all institutions, including universities, hospitals, nonprofit research institutions, and other organizations, that receive federal support for research. IRBs typically consist of members from a variety of disciplines, such as sociology, economics, education, social work, and communications (to name a few). Most IRBs also include representatives from the community in which they reside. For example, representatives from nearby prisons, hospitals, or treatment centers might sit on the IRBs of university campuses near them. The diversity of membership helps to ensure that the many and complex ethical issues that may arise from human subjects research will be considered fully and by a knowledgeable and experienced panel. Investigators conducting research on human subjects are required to submit proposals outlining their research plans to IRBs for review and approval prior to beginning their research. Even students who conduct research on human subjects must have their proposed work reviewed and approved by the IRB before beginning any research (though, on some campuses, exceptions are made for student projects that will not be shared outside of the classroom).
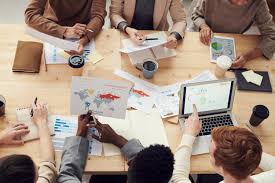
The IRB has three levels of review, defined in statute by the USDHHS.
Exempt review is the lowest level of review. Studies that are considered exempt expose participants to the least potential for harm and often involve little participation by human subjects. In social work, exempt studies often examine data that is publicly available or secondary data from another researcher that has been de-identified by the person who collected it.
Expedited review is the middle level of review. Studies considered under expedited review do not have to go before the full IRB board because they expose participants to minimal risk. However, the studies must be thoroughly reviewed by a member of the IRB committee. While there are many types of studies that qualify for expedited review, the most relevant to social workers include the use of existing medical records, recordings (such as interviews) gathered for research purposes, and research on individual group characteristics or behavior.
Finally, the highest level of review is called a full board review . A full board review will involve multiple members of the IRB evaluating your proposal. When researchers submit a proposal under full board review, the full IRB board will meet, discuss any questions or concerns with the study, invite the researcher to answer questions and defend their proposal, and vote to approve the study or send it back for revision. Full board proposals pose greater than minimal risk to participants. They may also involve the participation of vulnerable populations , or people who need additional protection from the IRB. Vulnerable populations include prisoners, children, people with cognitive impairments, people with physical disabilities, employees, and students. While some of these populations can fall under expedited review in some cases, they will often require the full IRB to approve their study.
It may surprise you to hear that IRBs are not always popular or appreciated by researchers. Who wouldn’t want to conduct ethical research, you ask? In some cases, the concern is that IRBs are most well-versed in reviewing biomedical and experimental research, neither of which is particularly common within social work. Much social work research, especially qualitative research, is open-ended in nature, a fact that can be problematic for IRBs. The members of IRBs often want to know in advance exactly who will be observed, where, when, and for how long, whether and how they will be approached, exactly what questions they will be asked, and what predictions the researcher has for their findings. Providing this level of detail for a year-long participant observation within an activist group of 200-plus members, for example, would be extraordinarily frustrating for the researcher in the best case and most likely would prove to be impossible. Of course, IRBs do not intend to have researchers avoid studying controversial topics or avoid using certain methodologically sound data collection techniques, but unfortunately, that is sometimes the result. The solution is not to eradicate review boards, which serve a necessary and important function, but instead to help educate IRB members about the variety of social scientific research methods and topics covered by social workers and other social scientists.
What we have provided here is only a short summary of federal regulations and international agreements that provide the boundaries between ethical and unethical research.
Here are a few more detailed guides for continued learning about research ethics and human research protections.
- University of California, San Francisco: Levels of IRB Review
- United States Department of Health and Human Services: The Belmont Report
- NIH, National Institute of Environmental Health Sciences: What is Ethics in Research & Why is it important
- NIH: Guiding Principles for Ethical Research
- Council on Social Work Education: National Statement on Research Integrity in Social Work
- Butler, I. (2002). A code of ethics for social work and social care research. British Journal of Social Work , 32 (2), 239-248
- Research on human subjects presents a unique set of challenges and opportunities when it comes to conducting ethical research.
- Research on human subjects has not always been regulated to the extent that it is today.
- All institutions receiving federal support for research must have an IRB. Organizations that do not receive federal support but where research is conducted also often include IRBs as part of their organizational structure.
- Researchers submit studies for IRB review at one of three different levels, depending on the level of harm the study may cause.
- Recall whether your project will gather data from human subjects and sketch out what the data collection process might look like.
- Identify which level of IRB review is most appropriate for your project.
- For many students, your professors may have existing agreements with your university's IRB that allow students to conduct research projects outside the supervision of the IRB. Make sure that your project falls squarely within those parameters. If you feel you may be outside of such an agreement, consult with your professor to see if you will need to submit your study for IRB review before starting your project.
6.2 Specific ethical issues to consider
- Define informed consent, and describe how it works
- Identify the unique concerns related to the study of vulnerable populations
- Differentiate between anonymity and confidentiality
- Explain the ethical responsibilities of social workers conducting research
As should be clear by now, conducting research on humans presents a number of unique ethical considerations. Human research subjects must be given the opportunity to consent to their participation in research, and be fully informed of the study’s risks, benefits, and purpose. Further, subjects’ identities and the information they share should be protected by researchers. Of course, how consent and identity protection are defined may vary by individual researcher, institution, or academic discipline. In this section, we’ll take a look at a few specific topics that individual researchers must consider before embarking on research with human subjects.
Informed consent
An expectation of voluntary participation is presumed in all social work research projects. In other words, we cannot force anyone to participate in our research without that person’s knowledge or consent. Researchers must therefore design procedures to obtain subjects’ informed consent to participate in their research. This specifically relates back to the ethical principle of respect for persons outlined in The Belmont Report . Informed consent is defined as a subject’s voluntary agreement to participate in research based on a full understanding of the research and of the possible risks and benefits involved. Although it sounds simple, ensuring that one has actually obtained informed consent is a much more complex process than you might initially presume.
The first requirement is that, in giving their informed consent, subjects may neither waive nor even appear to waive any of their legal rights. Subjects also cannot release a researcher, her sponsor, or institution from any legal liability should something go wrong during the course of their participation in the research (USDHHS,2009). [37] Because social work research does not typically involve asking subjects to place themselves at risk of physical harm by, for example, taking untested drugs or consenting to new medical procedures, social work researchers do not often worry about potential liability associated with their research projects. However, their research may involve other types of risks.
For example, what if a social work researcher fails to sufficiently conceal the identity of a subject who admits to participating in a local swinger’s club? In this case, a violation of confidentiality may negatively affect the participant’s social standing, marriage, custody rights, or employment. Social work research may also involve asking about intimately personal topics that may be difficult for participants to discuss, such as trauma or suicide. Participants may re-experience traumatic events and symptoms when they participate in your study. Even if you are careful to fully inform your participants of all risks before they consent to the research process, I’m sure you can empathize with thinking you could bear talking about a difficult topic and then finding it too overwhelming once you start. In cases like these, it is important for a social work researcher to have a plan to provide supports. This may mean providing referrals to counseling supports in the community or even calling the police if the participant is an imminent danger to himself or others.
It is vital that social work researchers explain their mandatory reporting duties in the consent form and ensure participants understand them before they participate. Researchers should also emphasize to participants that they can stop the research process at any time or decide to withdraw from the research study for any reason. Importantly, it is not the job of the social work researcher to act as a clinician to the participant. While a supportive role is certainly appropriate for someone experiencing a mental health crisis, social workers must ethically avoid dual roles. Referring a participant in crisis to other mental health professionals who may be better able to help them is the expectation.
Beyond the legal issues, most IRBs require researchers to share some details about the purpose of the research, possible benefits of participation, and, most importantly, possible risks associated with participating in that research with their subjects. In addition, researchers must describe how they will protect subjects’ identities, how, where, and for how long any data collected will be stored, how findings may be shared, and whom to contact for additional information about the study or about subjects’ rights. All this information is typically shared in an informed consent form that researchers provide to subjects. In some cases, subjects are asked to sign the consent form indicating that they have read it and fully understand its contents. In other cases, subjects are simply provided a copy of the consent form and researchers are responsible for making sure that subjects have read and understand the form before proceeding with any kind of data collection. Your IRB will often provide guidance or even templates for what they expect to see included in an informed consent form. This is a document that they will inspect very closely. Table 6.1 outlines elements to include in your informed consent. While these offer a guideline for you, you should always visit your schools, IRB website to see what guidance they offer. They often provide a template that they prefer researchers to use. Using these templates ensures that you are using the language that the IRB reviewers expect to see and this can also save you time.
One last point to consider when preparing to obtain informed consent is that not all potential research subjects are considered equally competent or legally allowed to consent to participate in research. Subjects from vulnerable populations may be at risk of experiencing undue influence or coercion (USDHHS, 2009). [38] The rules for consent are more stringent for vulnerable populations. For example, minors must have the consent of a legal guardian in order to participate in research. In some cases, the minors themselves are also asked to participate in the consent process by signing special, age-appropriate assent forms designed specifically for them. Prisoners and parolees also qualify as vulnerable populations. Concern about the vulnerability of these subjects comes from the very real possibility that prisoners and parolees could perceive that they will receive some highly desired reward, such as early release, if they participate in research or that there could be punitive consequences if they choose not to participate. When a participant faces undue or excess pressure to participate by either favorable or unfavorable means, this is known as coercion and must be avoided by researchers.
Another potential concern regarding vulnerable populations is that they may be underrepresented or left out of research opportunities, specifically because of concerns about their ability to consent. So, on the one hand, researchers must take extra care to ensure that their procedures for obtaining consent from vulnerable populations are not coercive. The procedures for receiving approval to conduct research with these groups may be more rigorous than that for non-vulnerable populations. On the other hand, researchers must work to avoid excluding members of vulnerable populations from participation simply on the grounds that they are vulnerable or that obtaining their consent may be more complex. While there is no easy solution to this ethical research dilemma, an awareness of the potential concerns associated with research on vulnerable populations is important for identifying whatever solution is most appropriate for a specific case.
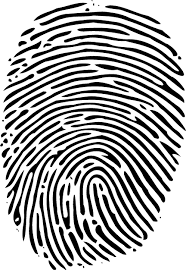
Protection of identities
As mentioned earlier, the informed consent process includes the requirement that researchers outline how they will protect the identities of subjects. This aspect of the research process, however, is one of the most commonly misunderstood. Furthermore, failing to protect identities is one of the greatest risks to participants in social work research studies.
In protecting subjects’ identities, researchers typically promise to maintain either the anonymity or confidentiality of their research subjects. These are two distinctly different terms and they are NOT interchangeable. Anonymity is the more stringent of the two and is very hard to guarantee in most research studies. When a researcher promises anonymity to participants, not even the researcher is able to link participants’ data with their identities. Anonymity may be impossible for some social work researchers to promise due to the modes of data collection many social workers employ. Face-to-face interviewing means that subjects will be visible to researchers and will hold a conversation, making anonymity impossible. In other cases, the researcher may have a signed consent form or obtain personal information on a survey and will therefore know the identities of their research participants. In these cases, a researcher should be able to at least promise confidentiality to participants.
Offering confidentiality means that some identifying information is known at some time by the research team, but only the research team has access to this identifying information and this information will not be linked with their data in any publicly accessible way. Confidentiality in research is quite similar to confidentiality in clinical practice. You know who your clients are, but others do not. You agree to keep their information and identity private. As you can see under the “Risks” section of the consent form in Figure 5.1, sometimes it is not even possible to promise that a subject’s confidentiality will be maintained. This is the case if data are collected in public or in the presence of other research participants in the course of a focus group, for example. Participants who social work researchers deem to be of imminent danger to self or others or those that disclose abuse of children and other vulnerable populations fall under a social worker’s duty to report. Researchers must then violate confidentiality to fulfill their legal obligations.
There are a number of steps that researchers can take to protect the identities of research participants. These include, but are not limited to:
- Collecting data in private spaces
- Not requesting information that will uniquely identify or "out" that person as a participant
- Assigning study identification codes or pseudonyms
- Keeping signed informed consent forms separate from other data provided by the participant
- Making sure that physical data is kept in a locked and secured location, and the virtual data is encrypted or password-protected
- Reporting data in aggregate (only discussing the data collectively, not by individual responses)
Protecting research participants’ identities is not always a simple prospect, especially for those conducting research on stigmatized groups or illegal behaviors. Sociologist Scott DeMuth learned that all too well when conducting his dissertation research on a group of animal rights activists. As a participant observer, DeMuth knew the identities of his research subjects. So when some of his research subjects vandalized facilities and removed animals from several research labs at the University of Iowa, a grand jury called on Mr. DeMuth to reveal the identities of the participants in the raid. When DeMuth refused to do so, he was jailed briefly and then charged with conspiracy to commit animal enterprise terrorism and cause damage to the animal enterprise (Jaschik, 2009). [39]
Publicly, DeMuth’s case raised many of the same questions as Laud Humphreys’ work 40 years earlier. What do social scientists owe the public? Is DeMuth, by protecting his research subjects, harming those whose labs were vandalized? Is he harming the taxpayers who funded those labs? Or is it more important that DeMuth emphasize what he owes his research subjects, who were told their identities would be protected? DeMuth’s case also sparked controversy among academics, some of whom thought that as an academic himself, DeMuth should have been more sympathetic to the plight of the faculty and students who lost years of research as a result of the attack on their labs. Many others stood by DeMuth, arguing that the personal and academic freedom of scholars must be protected whether we support their research topics and subjects or not. DeMuth’s academic adviser even created a new group, Scholars for Academic Justice , to support DeMuth and other academics who face persecution or prosecution as a result of the research they conduct. What do you think? Should DeMuth have revealed the identities of his research subjects? Why or why not?
Discipline-specific considerations
Often times, specific disciplines will provide their own set of guidelines for protecting research subjects and, more generally, for conducting ethical research. For social workers, the National Association of Social Workers (NASW) Code of Ethics section 5.02 describes the responsibilities of social workers in conducting research. Summarized below, these responsibilities are framed as part of a social worker’s responsibility to the profession. As representative of the social work profession, it is your responsibility to conduct and use research in an ethical manner.
A social worker should:
- Monitor and evaluate policies, programs, and practice interventions
- Contribute to the development of knowledge through research
- Keep current with the best available research evidence to inform practice
- Ensure voluntary and fully informed consent of all participants
- Not engage in any deception in the research process
- Allow participants to withdraw from the study at any time
- Provide access to appropriate supportive services for participants
- Protect research participants from harm
- Maintain confidentiality
- Report findings accurately
- Disclose any conflicts of interest
- Researchers must obtain the informed consent of research participants.
- Social workers must take steps to minimize the harms that could arise during the research process.
- If anonymity is promised, individual participants cannot be linked with their data.
- If confidentiality is promised, the identities of research participants cannot be revealed, even if individual participants can be linked with their data.
- The NASW Code of Ethics includes specific responsibilities for social work researchers.
- Talk with your professor to see if an informed consent form is required for your research project. If documentation is required, customize the template provided by your professor or the IRB at your school, using the details of your study. If documentation on consent is not required, for example if consent is given verbally, use the templates as guides to create a guide for what you will say to participants regarding informed consent.
- Identify whether your data will be confidential or anonymous. Describe the measures you will take to protect the identities of individuals in your study. How will you store the data? How will you ensure that no one can identify participants based on what you report in papers and presentations? Be sure to think carefully. People can be identified by characteristics such as age, gender, disability status, location, etc.
6.3 Benefits and harms of research across the ecosystem
- Identify and distinguish between micro-, mezzo-, and macro-level considerations with respect to the ethical conduct of social scientific research
This chapter began with a long list of harmful acts that researchers engaged in while conducting studies on human subjects. Indeed, even the last section on informed consent and protection of confidential information can be seen in light of minimizing harm and maximizing benefits. The benefits of your study should be greater than the harms. But who benefits from your research study, and who might be harmed? The first person who benefits is, most clearly, you as the researcher. You need a project to complete, be it for a grade, a grant, an academic responsibility, etc. However you need to make sure that your benefit does not come at the expense of harming others. Furthermore, research requires resources, including resources from the communities we work with. Part of being good stewards of these resources as social work researchers means that we need to engage in research that benefits the people we serve in meaningful and relevant ways. We need to consider how others are impacted by our research.
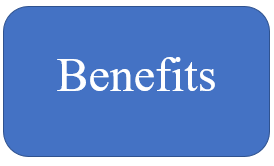
Micro-, mezzo-, and macro-level concerns
One useful way to think about the breadth of ethical questions that might arise out of any research project is to think about potential issues from the perspective of different analytical levels that are familiar to us as social workers. In Chapter 1 , you learned about the micro-, mezzo-, and macro-levels of inquiry and how a researcher’s specific point of focus might vary depending on her level of inquiry. Here we’ll apply this ecological framework to a discussion of research ethics. Within most research projects, there are specific questions that arise for researchers at each of these three levels.
At the micro-level, researchers must consider their own conduct and the impact on individual research participants. For example, did Stanley Milgram behave ethically when he allowed research participants to think that they were administering electric shocks to fellow participants? Did Laud Humphreys behave ethically when he deceived his research subjects about his own identity? Were the rights of individuals in these studies protected? How did these participants benefit themselves from the research that was conducted? While not social workers by trade, would the actions of these two researchers hold up against our professional NASW Code of Ethics? The questions posed here are the sort that you will want to ask yourself as a researcher when considering ethics at the micro-level.
At the mezzo-level, researchers should think about their duty to the community. How will the results of your study impact your target population? Ideally, your results will benefit your target population by identifying important areas for social workers to intervene and to better understand the experiences of the communities they serve. However, it is possible that your study may perpetuate negative stereotypes about your target population or damage its reputation. Indigenous people in particular have highlighted how historically social science has furthered marginalization of indigenous peoples (Smith, 2013). [40] Mezzo-level concerns should also address other groups or organizations that are connected to your target population. This may include the human service agencies with whom you've partnered for your study as well as the communities and peoples they serve. If your study reflected negatively on a particular housing project in your area, for example, will community members seek to remove it from their community? Or might it draw increased law enforcement presence that is unwanted by participants or community members? Research is a powerful tool and can be used for many purposes, not all of them altruistic. In addition, research findings can have many implications, intended and unintended. As responsible researchers, we need to do our best to thoughtfully anticipate these consequences.
Finally, at the macro-level, a researcher should consider duty to, and the expectations of, society. Perhaps the most high-profile case involving macro-level questions of research ethics comes from debates over whether to use data gathered by, or cite published studies based on data gathered from, the Nazis in the course of their unethical and horrendous experiments on humans during World War II (Moe, 1984). [41] Some argue that because the data were gathered in such an unquestionably unethical manner, they should never be used. The data, say these people, are neither valid nor reliable and should therefore not be used in any current scientific investigation (Berger, 1990). [42]
On the other hand, some people argue that data themselves are neutral; that “information gathered is independent of the ethics of the methods and that the two are not linked together” (Pozos, 1992, p. 104). [43] Others point out that not using the data could inadvertently strengthen the claims of those who deny that the Holocaust ever happened. In his striking statement in support of publishing the data, medical ethics professor Velvl Greene (1992) says,
Instead of banning the Nazi data or assigning it to some archivist or custodial committee, I maintain that it be exhumed, printed, and disseminated to every medical school in the world along with the details of methodology and the names of the doctors who did it, whether or not they were indicted, acquitted, or hanged.…Let the students and the residents and the young doctors know that this was not ancient history or an episode from a horror movie where the actors get up after filming and prepare for another role. It was real. It happened yesterday (p. 169–170). [44]
While debates about the use of data collected by the Nazis are typically centered on medical scientists’ use of them, there are conceivable circumstances under which these data might be used by social scientists. Perhaps, for example, a social scientist might wish to examine contemporary reactions to the experiments. Or perhaps the data could be used in a study of the sociology of science. What do you think? Should data gathered by the Nazis be used or cited today? What arguments can you make in support of your position, and how would you respond to those who disagree?
Additionally at the macro-level, you must also consider your responsibilities to the profession of social work. When you engage in social work research, you stand on the reputation the profession has built for over a century. Since research is public-facing, meaning that research findings are intended to be shared publicly, you are an ambassador for the profession. How you conduct yourself as a social work researcher has potential implications for how the public perceives both social work and research. As a social worker, you have a responsibility to work towards greater social, environmental, and economic justice and human rights. Your research should reflect this responsibility. Attending to research ethics helps to fulfill your responsibilities to the profession, in addition to your target population.
Table 6.2 summarizes the key questions that researchers might ask themselves about the ethics of their research at each level of inquiry.
- At the micro-level, researchers should consider their own conduct and the rights of individual research participants.
- At the mezzo-level, researchers should consider the expectations of their profession, any organizations that may have funded their research, and the communities affected by their research.
- At the macro-level, researchers should consider their duty to and the expectations of society with respect to social science research.
- Summarize the benefits and harms at the micro-, mezzo-, and macro-level of inquiry. At which level of inquiry is your research project?
- In a few sentences, identify whether the benefits of your study outweigh the potential harms.
6.4 Being an ethical researcher
- Identify why researchers must provide a detailed description of methodology
- Describe what it means to use science in an ethical way
Research ethics has to do with both how research is conducted and how findings from that research are used. In this section, we’ll consider research ethics from both angles.
Doing science the ethical way
As you should now be aware, researchers must consider their own personal ethical principles in addition to following those of their institution, their discipline, and their community. We’ve already considered many of the ways that social workers strive to ensure the ethical practice of research, such as informing and protecting subjects. But the practice of ethical research doesn’t end once subjects have been identified and data have been collected. Social workers must also fully disclose their research procedures and findings. This means being honest about how research subjects were identified and recruited, how exactly data were collected and analyzed, and ultimately, what findings were reached.
If researchers fully disclose how they conducted their research, then those who use their work to build research projects, create social policies, or make treatment decisions can have greater confidence in the work. By sharing how research was conducted, a researcher helps assure readers they have conducted legitimate research and didn’t simply come to whatever conclusions they wanted to find. A description or presentation of research findings that is not accompanied by information about research methodology is missing relevant information. Sometimes methodological details are left out because there isn’t time or space to share them. This is often the case with news reports of research findings. Other times, there may be a more insidious reason that important information is missing. This may be the case if sharing methodological details would call the legitimacy of a study into question. As researchers, it is our ethical responsibility to fully disclose our research procedures. As consumers of research, it is our ethical responsibility to pay attention to such details. We’ll discuss this more in the next section.
There’s a New Yorker cartoon that depicts a set of filing cabinets that aptly demonstrates what we don’t want to see happen with research. Each filing cabinet drawer in the cartoon is labeled differently. The labels include such headings as, “Our Facts,” “Their Facts,” “Neutral Facts,” “Disputable Facts,” “Absolute Facts,” “Bare Facts,” “Unsubstantiated Facts,” and “Indisputable Facts.” The implication of this cartoon is that one might just choose to open the file drawer of her choice and pick whichever facts one likes best. While this may occur if we use some of the unscientific ways of knowing described in Chapter 1 , it is fortunately not how the discovery of knowledge in social work, or in any other science for that matter, takes place. There actually is a method to this madness we call research. At its best, research reflects a systematic, transparent, informative process.
Honesty in research is facilitated by the scientific principle of replication . Ideally, this means that one scientist could repeat another’s study with relative ease. By replicating a study, we may become more (or less) confident in the original study’s findings. Replication is far more difficult (perhaps impossible) to achieve in the case of many qualitative studies, as our purpose is often a deep understanding of very specific circumstances, rather than the broad, generalizable knowledge we traditionally seek in quantitative studies. Nevertheless, transparency in the research process is an important standard for all social scientific researchers—that we provide as much detail as possible about the processes by which we reach our conclusions. This allows the quality of our research to be evaluated. Along with replication, peer review is another important principle of the scientific process. Peer review involves other knowledgeable researchers in our field of study to evaluate our research and to determine if it is of sufficient quality to share with the public. There are valid critiques of the peer review process: that it is biased towards studies with positive findings, that it may reinforce systemic barriers to oppressed groups accessing and leveraging knowledge, that it is far more subjective and/or unreliable than it claims to be. Despite these critiques, peer review remains a foundational concept for how scientific knowledge is generated.
Full disclosure also includes the need to be honest about a study’s strengths and weaknesses, both with oneself and with others. Being aware of the strengths and weaknesses of your own work can help a researcher make reasonable recommendations about the next steps other researchers might consider taking in their inquiries. Awareness and disclosure of a study’s strengths and weaknesses can also help highlight the theoretical or policy implications of one’s work. In addition, openness about strengths and weaknesses helps those reading the research better evaluate the work and decide for themselves how or whether to rely on its findings. Finally, openness about a study’s sponsors is crucial. How can we effectively evaluate research without knowing who paid the bills? This allows us to assess for potential conflicts of interest that may compromise the integrity of the research.
The standard of replicability, the peer-review process, and openness about a study’s strengths, weaknesses, and funding sources enables those who read the research to evaluate it fairly and completely. Knowledge of funding sources is often raised as an issue in medical research. Understandably, independent studies of new drugs may be more compelling to the Food and Drug Administration (FDA) than studies touting the virtues of a new drug that happen to have been funded by the company who created that drug. But medical researchers aren’t the only ones who need to be honest about their funding. If we know, for example, that a political think tank with ties to a particular party has funded some research, we can take that knowledge into consideration when reviewing the study’s findings and stated policy implications. Lastly, and related to this point, we must consider how, by whom, and for what purpose research may be used.
Using science the ethical way
Science has many uses. By “use” I mean the ways that science is understood and applied (as opposed to the way it is conducted). Some use science to create laws and social policies; others use it to understand themselves and those around them. Some people rely on science to improve their life conditions or those of other people, while still others use it to improve their businesses or other undertakings. In each case, the most ethical way for us to use science is to educate ourselves about the design and purpose of any studies we may wish to use. This helps us to more adequately critique the value of this research, to recognize its strengths and limitations.
As part of my research course, students are asked to critique a research article. I often find in this assignment that students often have very lofty expectations for everything that 'should' be included in the journal article they are reviewing. While I appreciate the high standards, I often give them feedback that it is perhaps unrealistic (even unattainable) for a research study to be perfectly designed and described for public consumption. All research has limitations; this may be a consequence of limited resources, issues related to feasibility, and unanticipated roadblocks or problems as we are carrying out our research. Furthermore, the ways we disseminate or share our research often has restrictions on what and how we can share our findings. This doesn't mean that a study with limitations has no value—every study has limitations! However, as we are reviewing research, we should look for an open discussion about methodology , strengths, and weaknesses of the study that helps us to interpret what took place and in what ways it may be important.
For instance, this can be especially important to think about in terms of a study's sample. It can be challenging to recruit a diverse and representative sample for your study (however, that doesn't mean we shouldn't try!). The next time you are reading research studies that were used to help establish an evidence based practice (EBP), make sure to look at the description of the sample. We cannot assume that what works for one group of people will uniformly work with all groups of people with very different life experiences; however, historically much of our intervention repertoire has been both created by and evaluated on white men. If research studies don't obtain a diverse sample, for whatever reason, we would expect that the authors would identify this as a limitation and an area requiring further study. We need to challenge our profession to provide practices, strategies, models, interventions, and policies that have been evaluated and tested for their efficacy with the diverse range of people that we work with as social workers.
Social scientists who conduct research on behalf of organizations and agencies may face additional ethical questions about the use of their research, particularly when the organization for which a study is conducted controls the final report and the publicity it receives. There is a potential conflict of interest for evaluation researchers who are employees of the agency being evaluated. A similar conflict of interest might exist between independent researchers whose work is being funded by some government agency or private foundation.
So who decides what constitutes ethical conduct or use of research? Perhaps we all do. What qualifies as ethical research may shift over time and across cultures as individual researchers, disciplinary organizations, members of society, and regulatory entities, such as institutional review boards, courts, and lawmakers, all work to define the boundaries between ethical and unethical research.
- Conducting research ethically requires that researchers be ethical not only in their data collection procedures but also in reporting their methods and findings.
- The ethical use of research requires an effort to understand research, an awareness of your own limitations in terms of knowledge and understanding, and the honest application of research findings.
- Think about your research hypothesis at this point. What would happen if your results revealed information that could harm the population you are studying? What are your ethical responsibilities as far as reporting about your research?
- Ultimately, we cannot control how others will use the results of our research. What are the implications of this for how you report on your research?
- Reading the results of empirical studies (16 minute read)
- Annotating empirical journal articles (15 minute read)
- Generalizability and transferability of empirical results (15 minute read)
Content warning: examples in this chapter contain references to domestic violence and details on types of abuse, drug use, poverty, mental health, sexual harassment and details on harassing behaviors, children’s mental health, LGBTQ+ oppression and suicide, obesity, anti-poverty stigma, and psychotic disorders.
5.1 Reading the results of empirical studies
- Describe how statistical significance and confidence intervals demonstrate which results are most important
- Differentiate between qualitative and quantitative results in an empirical journal article
If you recall from section 3.1 , empirical journal articles are those that report the results of quantitative or qualitative data analyzed by the author. They follow a set structure—introduction, methods, results, discussion/conclusions. This section is about reading the most challenging section: results.
I want to normalize not understanding statistics terms and symbols. However, a basic understanding of a results section goes a very long way to understanding the key results in an article. This will take you beyond the two or three sentences in the abstract that summarize the study's results and into the nitty-gritty of what they found for each concept they studied.
Read beyond the abstract
At this point, I have read hundreds of literature reviews written by students. One of the challenges I have noted is that students will report the results as summarized in the abstract, rather than the detailed findings laid out in the results section of the article. This poses a problem when you are writing a literature review because you need to provide specific and clear facts that support your reading of the literature. The abstract may say something like: “we found that poverty is associated with mental health status.” For your literature review, you want the details, not the summary. In the results section of the article, you may find a sentence that states: “children living in households experiencing poverty are three times more likely to have a mental health diagnosis.” This more specific statistical information provides a stronger basis on which to build the arguments in your literature review.
Using the summarized results in an abstract is an understandable mistake to make. The results section often contains figures and tables that may be challenging to understand. Often, without having completed more advanced coursework on statistical or qualitative analysis, some of the terminology, symbols, or diagrams may be difficult to comprehend. This section is all about how to read and interpret the results of an empirical (quantitative or qualitative) journal article. Our discussion here will be basic, and in parts three and four of the textbook, you will learn more about how to interpret results from statistical tests and qualitative data analysis.
Remember, this section only addresses empirical articles. Non-empirical articles (e.g., theoretical articles, literature reviews) don't have results. They cite the analysis of raw data completed by other authors, not the person writing the journal article who is merely summarizing others' work.
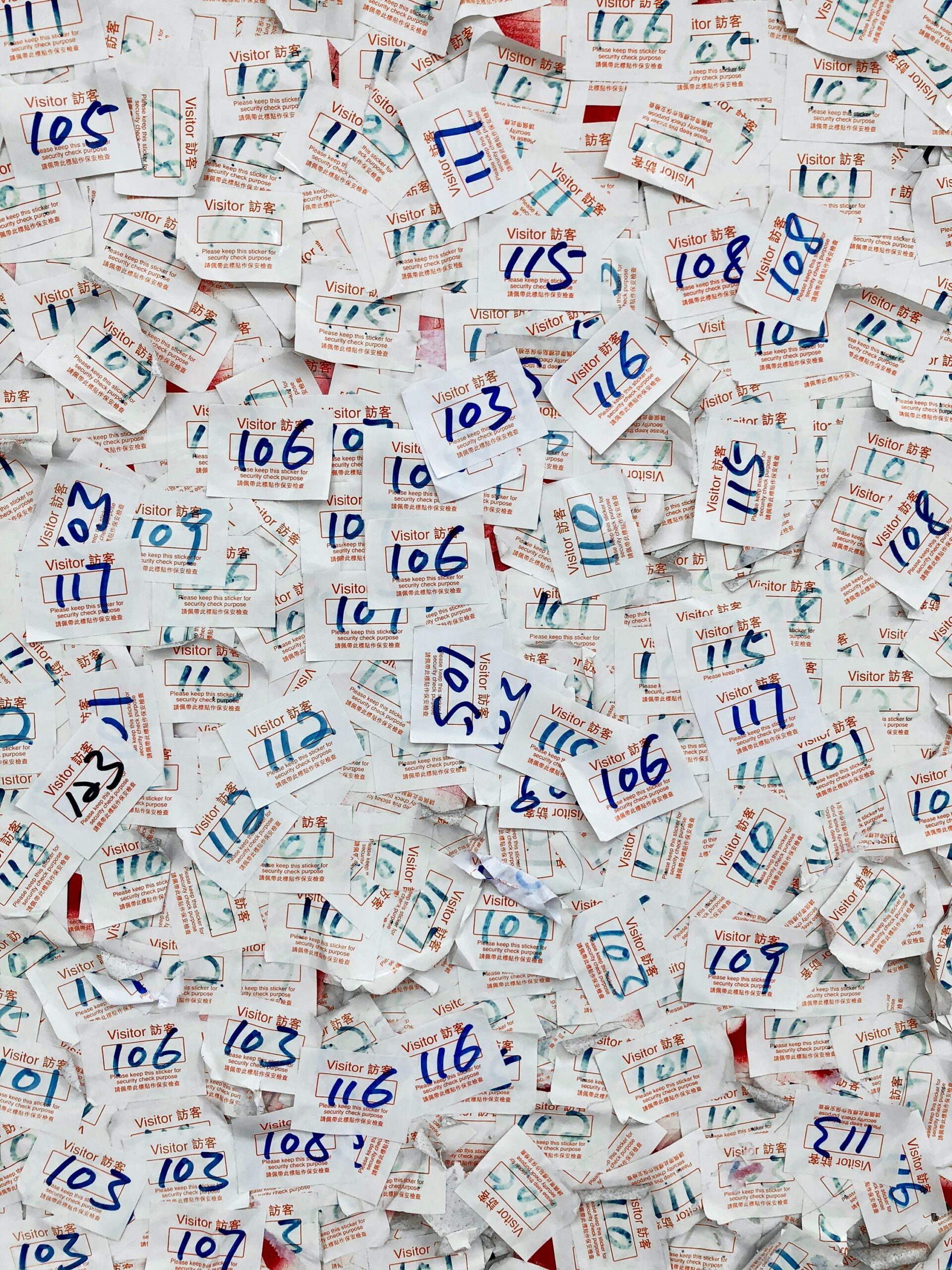
Quantitative results
Quantitative articles often contain tables, and scanning them is a good way to begin reading the results. A table usually provides a quick, condensed summary of the report’s key findings. Tables are a concise way to report large amounts of data. Some tables present descriptive information about a researcher’s sample (often the first table in a results section). These tables will likely contain frequencies (N) and percentages (%). For example, if gender happened to be an important variable for the researcher’s analysis, a descriptive table would show how many and what percent of all study participants are of a particular gender. Frequencies or “how many” will probably be listed as N, while the percent symbol (%) might be used to indicate percentages.
In a table presenting a causal relationship, two sets of variables are represented. The independent variable , or cause, and the dependent variable , the effect. We will discuss these further when we review quantitative conceptualization and measurement. Independent variable attributes are typically presented in the table’s columns, while dependent variable attributes are presented in rows. This allows the reader to scan a table’s rows to see how values on the dependent variable change as the independent variable values change (i.e., changes in the dependent variable depend on changes in the independent variable). Tables displaying results of quantitative analysis will also likely include some information about which relationships are significant or not. We will discuss the details of significance and p-values later in this section.
Let’s look at a specific example: Table 5.1. It presents the causal relationship between gender and experiencing harassing behaviors at work. In this example, gender is the independent variable (the cause) and the harassing behaviors listed are the dependent variables (the effects). [46] Therefore, we place gender in the table’s columns and harassing behaviors in the table’s rows.
Reading across the table’s top row, we see that 2.9% of women in the sample reported experiencing subtle or obvious threats to their safety at work, while 4.7% of men in the sample reported the same. We can read across each of the rows of the table in this way. Reading across the bottom row, we see that 9.4% of women in the sample reported experiencing staring or invasion of their personal space at work while just 2.3% of men in the sample reported having the same experience. We’ll discuss p values later in this section.
While you can certainly scan tables for key results, they are often difficult to understand without reading the text of the article. The article and table were meant to complement each other, and the text should provide information on how the authors interpret their findings. The table is not redundant with the text of the results section. Additionally, the first table in most results sections is a summary of the study's sample, which provides more background information on the study than information about hypotheses and findings. It is also a good idea to look back at the methods section of the article as the data analysis plan the authors outline should walk you through the steps they took to analyze their data which will inform how they report them in the results section.
Statistical significance
The statistics reported in Table 5.1 represent what the researchers found in their sample. The purpose of statistical analysis is usually to generalize from a the small number of people in a study's sample to a larger population of people. Thus, the researchers intend to make causal arguments about harassing behaviors at workplaces beyond those covered in the sample.
Generalizing is key to understanding statistical significance . According to Cassidy and colleagues, (2019) [47] 89% of research methods textbooks in psychology define statistical significance incorrectly. This includes an early draft of this textbook which defined statistical significance as "the likelihood that the relationships we observe could be caused by something other than chance." If you have previously had a research methods class, this might sound familiar to you. It certainly did to me!
But statistical significance is less about "random chance" than more about the null hypothesis . Basically, at the beginning of a study a researcher develops a hypothesis about what they expect to find, usually that there is a statistical relationship between two or more variables . The null hypothesis is the opposite. It is the hypothesis that there is no relationship between the variables in a research study. Researchers then can hopefully reject the null hypothesis because they find a relationship between the variables.
For example, in Table 5.1 researchers were examining whether gender impacts harassment. Of course, researchers assumed that women were more likely to experience harassment than men. The null hypothesis, then, would be that gender has no impact on harassment. Once we conduct the study, our results will hopefully lead us to reject the null hypothesis because we find that gender impacts harassment. We would then generalize from our study's sample to the larger population of people in the workplace.
Statistical significance is calculated using a p-value which is obtained by comparing the statistical results with a hypothetical set of results if the researchers re-ran their study a large number of times. Keeping with our example, imagine we re-ran our study with different men and women from different workplaces hundreds and hundred of times and we assume that the null hypothesis is true that gender has no impact on harassment. If results like ours come up pretty often when the null hypothesis is true, our results probably don't mean much. "The smaller the p-value, the greater the statistical incompatibility with the null hypothesis" (Wasserstein & Lazar, 2016, p. 131). [48] Generally, researchers in the social sciences have used 0.05 as the value at which a result is significant (p is less than 0.05) or not significant (p is greater than 0.05). The p-value 0.05 refers to if 5% of those hypothetical results from re-running our study show the same or more extreme relationships when the null hypothesis is true. Researchers, however, may choose a stricter standard such as 0.01 in which only 1% of those hypothetical results are more extreme or a more lenient standard like 0.1 in which 10% of those hypothetical results are more extreme than what was found in the study.
Let's look back at Table 5.1. Which one of the relationships between gender and harassing behaviors is statistically significant? It's the last one in the table, "staring or invasion of personal space," whose p-value is 0.039 (under the p<0.05 standard to establish statistical significance). Again, this indicates that if we re-ran our study over and over again and gender did not impact staring/invasion of space (i.e., the null hypothesis was true), only 3.9% of the time would we find similar or more extreme differences between men and women than what we observed in our study. Thus, we conclude that for staring or invasion of space only , there is a statistically significant relationship.
For contrast, let's look at "being pushed, hit, or grabbed" and run through the same analysis to see if it is statistically significant. If we re-ran our study over and over again and the null hypothesis was true, 48% of the time (p=.48) we would find similar or more extreme differences between men and women. That means these results are not statistically significant.
This discussion should also highlight a point we discussed previously: that it is important to read the full results section, rather than simply relying on the summary in the abstract. If the abstract stated that most tests revealed no statistically significant relationships between gender and harassment, you would have missed the detail on which behaviors were and were not associated with gender. Read the full results section! And don't be afraid to ask for help from a professor in understanding what you are reading, as results sections are often not written to be easily understood.
Statistical significance and p-values have been critiqued recently for a number of reasons, including that they are misused and misinterpreted (Wasserstein & Lazar, 2016) [49] , that researchers deliberately manipulate their analyses to have significant results (Head et al., 2015) [50] , and factor into the difficulty scientists have today in reproducing many of the results of previous social science studies (Peng, 2015). [51] For this reason, we share these principles, adapted from those put forth by the American Statistical Association, [52] for understanding and using p-values in social science:
- P-values provide evidence against a null hypothesis.
- P-values do not indicate whether the results were produced by random chance alone or if the researcher's hypothesis is true, though both are common misconceptions.
- Statistical significance can be detected in minuscule differences that have very little effect on the real world.
- Nuance is needed to interpret scientific findings, as a conclusion does not become true or false when the p-value passes from p=0.051 to p=0.049.
- Real-world decision-making must use more than reported p-values. It's easy to run analyses of large datasets and only report the significant findings.
- Greater confidence can be placed in studies that pre-register their hypotheses and share their data and methods openly with the public.
- "By itself, a p-value does not provide a good measure of evidence regarding a model or hypothesis. For example, a p-value near 0.05 taken by itself offers only weak evidence against the null hypothesis. Likewise, a relatively large p-value does not imply evidence in favor of the null hypothesis; many other hypotheses may be equally or more consistent with the observed data" (Wasserstein & Lazar, 2016, p. 132).
Confidence intervals
Because of the limitations of p-values, scientists can use other methods to determine whether their models of the world are true. One common approach is to use a confidence interval , or a range of values in which the true value is likely to be found. Confidence intervals are helpful because, as principal #5 above points out, p-values do not measure the size of an effect (Greenland et al., 2016). [53] Remember, something that has very little impact on the world can be statistically significant, and the values in a confidence interval would be helpful. In our example from Table 5.1, imagine our analysis produced a confidence interval that women are 1.2-3.4x more likely to experience "staring or invasion of personal space" than men. As with p-values, calculation for a confidence interval compares what was found in one study with a hypothetical set of results if we repeated the study over and over again. If we calculated 95% confidence intervals for all of the hypothetical set of hundreds and hundreds of studies, that would be our confidence interval.
Confidence intervals are pretty intuitive. As of this writing, my wife and are expecting our second child. The doctor told us our due date was December 11th. But the doctor also told us that December 11th was only their best estimate. They were actually 95% sure our baby might be born any time in the 30-day period between November 27th and December 25th. Confidence intervals are often listed with a percentage, like 90% or 95%, and a range of values, such as between November 27th and December 25th. You can read that as: "we are 95% sure your baby will be born between November 27th and December 25th because we've studied hundreds of thousands of fetuses and mothers, and we're 95% sure your baby will be within these two dates."
Notice that we're hedging our bets here by using words like "best estimate." When testing hypotheses, social scientists generally phrase their findings in a tentative way, talking about what results "indicate" or "support," rather than making bold statements about what their results "prove." Social scientists have humility because they understand the limitations of their knowledge. In a literature review, using a single study or fact to "prove" an argument right or wrong is often a signal to the person reading your literature review (usually your professor) that you may not have appreciated the limitations of that study or its place in the broader literature on the topic. Strong arguments in a literature review include multiple facts and ideas that span across multiple studies.
You can learn more about creating tables, reading tables, and tests of statistical significance in a class focused exclusively on statistical analysis. We provide links to many free and openly licensed resources on statistics in Chapter 16 . For now, we hope this brief introduction to reading tables will improve your confidence in reading and understanding the results sections in quantitative empirical articles.
Qualitative results
Quantitative articles will contain a lot of numbers and the results of statistical tests demonstrating associations between those numbers. Qualitative articles, on the other hand, will consist mostly of quotations from participants. For most qualitative articles, the authors want to put their results in the words of their participants, as they are the experts. Articles that lack quotations make it difficult to assess whether the researcher interpreted the data in a trustworthy, unbiased manner. These types of articles may also indicate how often particular themes or ideas came up in the data, potentially reflective of how important they were to participants.
Authors often organize qualitative results by themes and subthemes. For example, see this snippet from the results section in Bonanno and Veselak (2019) [54] discussion parents' attitudes towards child mental health information sources.
Data analysis revealed four themes related to participants’ abilities to access mental health help and information for their children, and parents’ levels of trust in these sources. These themes are: others’ firsthand experiences family and friends with professional experience, protecting privacy, and uncertainty about schools as information sources. Trust emerged as an overarching and unifying concept for all of these themes. Others’ firsthand experiences. Several participants reported seeking information from other parents who had experienced mental health struggles similar to their own children. They often referenced friends or family members who had been or would be good sources of information due to their own personal experiences. The following quote from Adrienne demonstrates the importance of firsthand experience: [I would only feel comfortable sharing concerns or asking for advice] if I knew that they had been in the same situation. (Adrienne) Similarly, Michelle said: And I talked to a friend of mine who has kids who have IEPs in the district to see, kind of, how did she go about it. (Michelle) ... Friends/family with professional experience . Several respondents referred to friends or family members who had professional experience with or knowledge of child mental health and suggested that these individuals would be good sources of information. For example, Hannah said: Well, what happened with me was I have an uncle who’s a psychiatrist. Sometimes if he’s up in (a city to the north), he’s retired, I can call him sometimes and get information. (Hannah) Michelle, who was in nursing school, echoed this sentiment: At this point, [if my child’s behavioral difficulties continued], I would probably call one of my [nursing] professors. That’s what I’ve done in the past when I’ve needed help with certain things...I have a professor who I would probably consider a friend who I would probably talk to first. She has a big adolescent practice. (Michelle) (p. 402-403)
The terms in bold above refer to the key themes (i.e., qualitative results) that were present in the data. Researchers will state the process by which they interpret each theme, providing a definition and usually some quotations from research participants. Researchers will also draw connections between themes, note consensus or conflict over themes, and situate the themes within the study context.
Qualitative results are specific to the time, place, and culture in which they arise, so you will have to use your best judgment to determine whether these results are relevant to your study. For example, students in my class at Radford University in Southwest Virginia may be studying rural populations. Would a study on group homes in a large urban city transfer well to group homes in a rural area?
Maybe. But even if you were using data from a qualitative study in another rural area, are all rural areas the same? How is the client population and sociocultural context in the article similar or different to the one in your study? Qualitative studies have tremendous depth, but researchers must be intentional about drawing conclusions about one context based on a study in another context. To make conclusions about how a study applies in another context, researchers need to examine each component of an empirical journal article--they need to annotate!
- The results section of empirical articles are often the most difficult to understand.
- To understand a quantitative results section, look for results that were statistically significant and examine the confidence interval, if provided.
- To understand a qualitative results section, look for definitions of themes or codes and use the quotations provided to understand the participants’ perspective.
Select a quantitative empirical article related to your topic.
- Write down the results the authors identify as statistically significant in the results section.
- How do the authors interpret their results in the discussion section?
- Do the authors provide enough information in the introduction for you to understand their results?
Select a qualitative empirical article relevant to your topic.
- Write down the key themes the authors identify and how they were defined by the participants.
5.2 Annotating empirical journal articles
- Define annotation and describe how to use it to identify, extract, and reflect on the information you need from an article
Annotation refers to the process of writing notes on an article. There are many ways to do this. The most basic technique is to print out the article and build a binder related to your topic. Raul Pacheco-Vega's excellent blog has a post on his approach to taking physical notes. Honestly, while you are there, browse around that website. It is full of amazing tips for students conducting a literature review and graduate research projects. I see a lot of benefits to the paper, pen, and highlighter approach to annotating articles. Personally though, I prefer to use a computer to write notes on an article because my handwriting is terrible and typing notes allows me search for keywords. For other students, electronic notes work best because they cannot afford to print every article that they will use in their paper. No matter what you use, the point is that you need to write notes when you're reading. Reading is research!
There are a number of free software tools you can use to help you annotate a journal article. Most PDF readers like Adobe Acrobat have a commenting and highlighting feature, though the PDF readers included with internet browsers like Google Chrome, Microsoft Edge, and Safari do not have this feature. The best approach may be to use a citation manager like Zotero. Using a citation manager, you can build a library of articles, save your annotations, and link annotations across PDFs using keywords. They also provide integration with word processing programs to help with citations in a reference list
Of course, I don't follow this advice because I have a system that works well for me. I have a PDF open in one computer window and a Word document open in a window next to it. I type notes and copy quotes, listing the page number for each note I take. It's a bit low-tech, but it does make my notes searchable. This way, when I am looking for a concept or quote, I can simply search my notes using the Find feature in Word and get to the information I need.
Annotation and reviewing literature does not have to be a solo project. If are working in a group, you can use the Hypothes.is web browser extension to annotate articles collaboratively. You can also use Google Docs to collaboratively annotate a shared PDF using the commenting feature and write collaborative notes in a shared document. By sharing your highlights and comments, you can split the work of getting the most out of each article you read and build off one another's ideas.
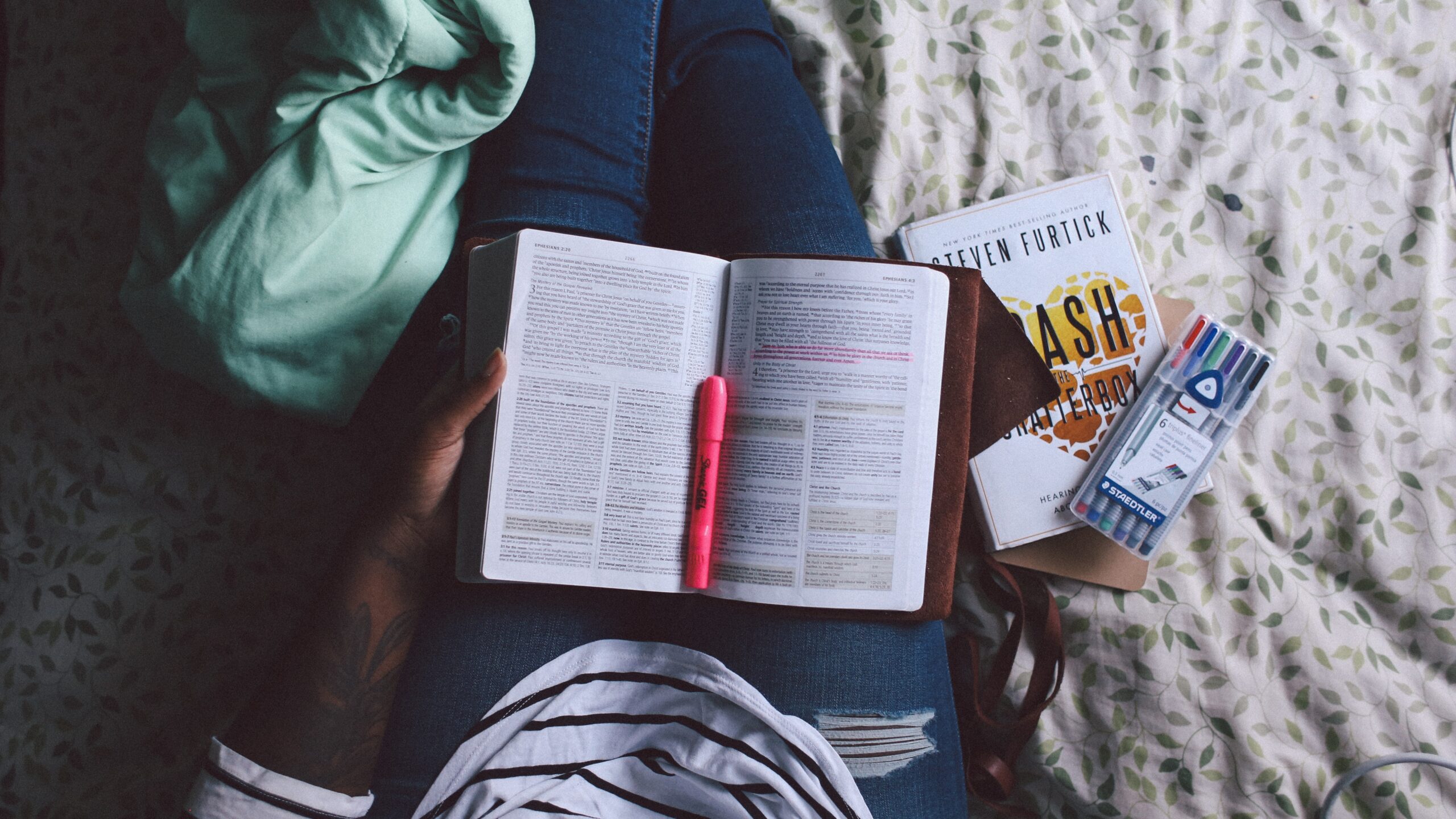
Common annotations
In this section, we present common annotations people make when reading journal articles. These annotations are adapted from Craig Whippo and Raul Pacheco-Vega . If you are annotating on paper, I suggest using different color highlighters for each type of annotation listed below. If you are annotating electronically, you can use the names below as tags to easily find information later. For example, if you are searching for definitions of key concepts, you can either click on the tag for [definitions] in your PDF reader or thumb through a printed copy of article for whatever color or tag you used to indicate definitions of key terms. Most of all, you want to avoid reading through all of your sources again just to find that one thing you know you read somewhere . Time is a graduate student's most valuable resource, so our goal here is to help you spend your time reading the literature wisely.
Personal reflections
Personal reflections are all about you. What do you think? Are there any areas you are confused about? Any new ideas or reflections come to mind while you're reading? Treat these annotations as a means of capturing your first reflections about an article. Write down any questions or thoughts that come to mind as you read. If you think the author says something inaccurate or unsubstantiated, write that down. If you don't understand something, make a note about it and ask your professor. Don't feel bad! Journal articles are hard to understand sometimes, even for professors. Your goal is to critically read the literature, so write down what you think while reading! Table 4.2 contains some questions that might stimulate your thoughts.
Definitions
Note definitions of key terms for your topic. At minimum, you should include a scholarly definition for the concepts represented in your working question. If your working question asks about the process of leaving a relationship with domestic violence, your research proposal will have to explain how you define domestic violence, as well as how you define "leaving" an abusive relationship. While you may already know what you mean by domestic violence, the person reading your research proposal does not.
Annotating definitions also helps you engage with the scholarly debate around your topic. Definitions are often contested among scholars. Some definitions of domestic violence will be more comprehensive, including things such economic abuse or forcing the victim to problematically use substances. Other definitions will be less comprehensive, covering only physical, verbal, and sexual abuse. Often, how someone defines something conceptually is highly related to how they measure it in their study. Since you will have to do both of these things, find a definition that feels right to you or create your own, noting the ways in which it is similar or different from those in the literature.
Definitions are also an important way of dealing with jargon. Becoming familiar with a new content area involves learning the jargon experts use. For example, in the last paragraph I used the term economic abuse, but that's probably not a term you've heard before. If you were conducting a literature review on domestic violence, you would want to search for keywords like economic abuse if they are relevant to your working question. You will also want to know what they mean so you can use them appropriately in designing your study and writing your literature review.
Theoretical perspective
Noting the theoretical perspective of the article can help you interpret the data in the same manner as the author. For example, articles on supervised injection facilities for people who use intravenous drugs most likely come from a harm reduction perspective, and understanding the theory behind harm reduction is important to make sense of empirical results. Articles should be grounded in a theoretical perspective that helps the author conceptualize and understand the data. As we discussed in Chapter 3 , some journal articles are entirely theoretical and help you understand the theories or conceptual models related to your topic. We will help you determine a theoretical perspective for your project in Chapter 7 . For now, it's a good idea to note what theories authors mention when talking about your topic area. Some articles are better about this than others, and many authors make it a bit challenging to find theory (if mentioned at all). In other articles, it may help to note which social work theories are missing from the literature. For example, a study's findings might address issues of oppression and discrimination, but the authors may not use critical theory to make sense of what happened.
Background knowledge
It's a good idea to note any relevant information the author relies on for background. When an author cites facts or opinions from others, you are subsequently able to get information from multiple articles simultaneously. For example, if we were looking at this meta-analysis about domestic violence , in the introduction section, the authors provide facts from many other sources. These facts will likely be relevant to your inquiry on domestic violence, as well.
As you are looking at background information, you should also note any subtopics or concepts about which there is controversy or consensus. The author may present one viewpoint and then an opposing viewpoint, something you may do in your literature review as well. Similarly, they may present facts that scholars in the field have come to consensus on and describe the ways in which different sources support these conclusions.
Sources of interest
Note any relevant sources the author cites. If there is any background information you plan to use, note the original source of that information. When you write your literature review, cite the original source of a piece of information you are using, which may not be where you initially read it . Remember that you should read and refer to the primary source . If you are reading Article A and the author cites a fact from Article B, you should note Article B in your annotations and use Article B when you cite the fact in your paper. You should also make sure Article A interpreted Article B correctly and scan Article B for any other useful facts.
Research question/Purpose
Authors should be clear about the purpose of their article. Charitable authors will give you a sentence that starts with something like this:
- "The purpose of this research project was..."
- "Our research question was..."
- "The research project was designed to test the following hypothesis..."
Unfortunately, not all authors are so clear, and you may to hunt around for the research question or hypothesis. Generally, in an empirical article, the research question or hypothesis is at the end of the introduction. In non-empirical articles, the author will likely discuss the purpose of the article in the abstract or introduction.
We will discuss in greater detail how to read the results of empirical articles in Chapter 5 . For now, just know that you should highlight any of the key findings of an article. They will be described very briefly in the abstract, and in much more detail in the article itself. In an empirical article, you should look at both the 'Results' and 'Discussion' sections. For a non-empirical article, the key findings will likely be in the conclusion. You can also find them in the topic or concluding sentences in a paragraph within the body of the article.
How do researchers know something when they see it? Found in the 'Methods' section of empirical articles, the measures section is where researchers spell out the tools, or measures, they used to gather data. For quantitative studies, you will want to get familiar with the questions researchers typically use to measure key variables. For example, to measure domestic violence, researchers often use the Conflict Tactics Scale . The more frequently used and cited a measure is, the more we know about how well it works (or not). Qualitative studies will often provide at least some of the interview or focus group questions they used with research participants. They will also include information about how their inquiry and hypotheses may have evolved over time. Keep in mind however, sometimes important information is cut out of an article during editing. If you need more information, consider reaching out to the author directly. Before you do so, check if the author provided an appendix with the information you need or if the article links to a their data and measures as part open data sharing practices.
Who exactly were the study participants and how were they recruited? In quantitative studies, you will want to pay attention to the sample size. Generally, the larger the sample, the greater the study's explanatory power. Additionally, randomly drawn samples are desirable because they leave any variation up to chance. Samples that are conducted out of convenience can be biased and non-representative of the larger population. In qualitative studies, non-random sampling is appropriate but consider this: how well does what we find for this group of people transfer to the people who will be in your study? For qualitative studies and quantitative studies, look for how well the sample is described and whether there are important characteristics missing from the article that you would need to determine the quality of the sample.
Limitations
Honest authors will include these at the end of each article. But you should also note any additional limitations you find with their work as well.
Your annotations
These are just a few suggested annotations, but you can come up with your own. For example, maybe there are annotations you would use for different assignments or for the problem statement in your research proposal. If you have an argument or idea that keeps coming to mind when you read, consider creating an annotation for it so you can remember which part of each article supports your ideas. Whatever works for you. The goal with annotation is to extract as much information from each article while reading, so you don't have to go back through everything again. It's useless to read an article and forget most of what you read. Annotate!
- Begin your search by reading thorough and cohesive literature reviews. Review articles are great sources of information to get a broad perspective of your topic.
- Don’t read an article just to say you’ve read it. Annotate and take notes so you don’t have to re-read it later.
- Use software or paper-and-pencil approaches to write notes on articles.
- Annotation is best used when closely reading an empirical study highly similar to your research project.
- Select an empirical article highly related to the study you would like to conduct.
- Annotate the article using the aforementioned annotations and create some of your own.
- Create the first draft of a summary table with key information from this empirical study that you would like to compare to other empirical studies you closely read.
5.3 Generalizability and transferability of empirical results
- Define generalizability and transferability.
- Assess the generalizability and transferability to how researchers use the results from empirical research studies to make arguments about what is objectively true.
- Relate both concepts to the hierarchy of evidence and the types of articles in the scholarly literature
Now that you have read an empirical article in detail, it's important to put its results in conversation with the broader literature on your topic. In this chapter we discuss two important concepts-- generalizability and transferability --and the interrelationship between the two. We also explain how these two properties of empirical data impact your literature review and evidence-based practice.
Generalizability
The figure below provides a common approach to assessing empirical evidence. As you move up the pyramid below, you can be more sure that the data contained in those studies generalizes to all people who experience the issue.
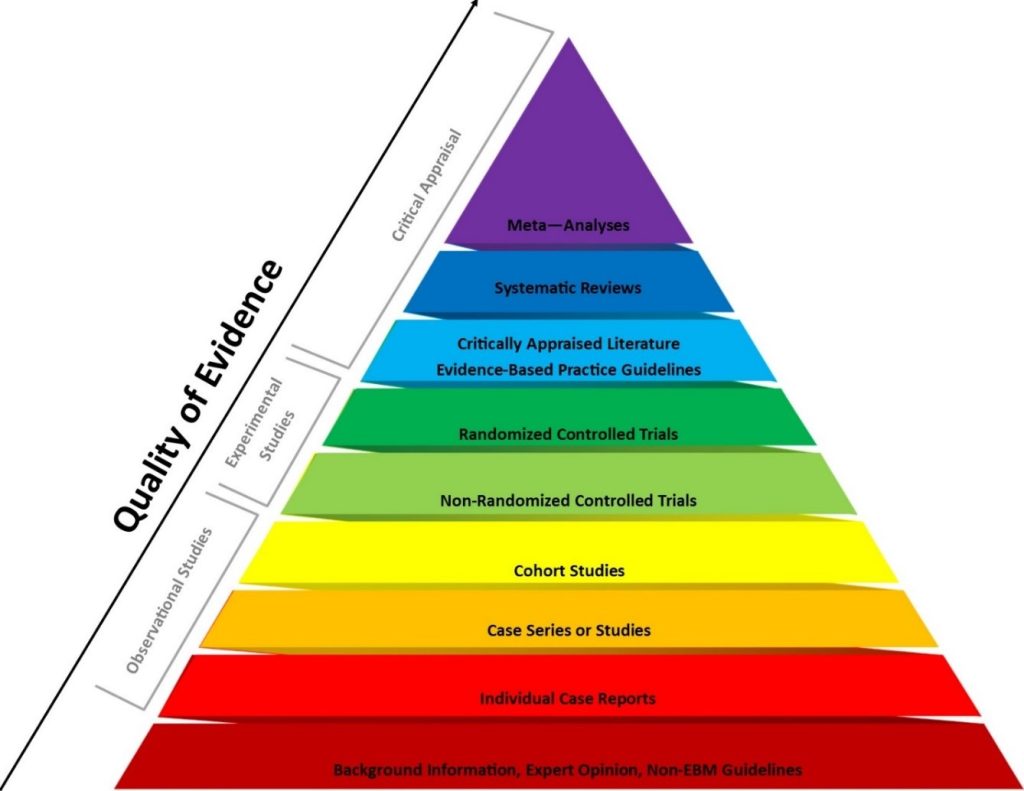
As we reviewed in Chapter 1, objective truth is true for everyone, regardless of context. In other words, objective truths generalize beyond the sample of people from whom data were collected to the larger population of people who experience the issue under examination. You can be much more sure that information from a systematic review or meta-analysis will generalize than something from a case study of a single person, pilot projects, and other studies that do not seek to establish generalizability.
The type of article listed here is also related to the types of research methods the authors used. While we cover many of these approaches in this textbook, some of them (like cohort studies) are somewhat less common in social work. Additionally, there is one important research method, survey design, that does not appear in this diagram. Finally, social work research uses many different types of qualitative research--some of which generates more generalizable data than others.
For a refresher on the different types of evidence available in each type of article, refer back to section 4.1. You'll recall the hierarchy of evidence as described by McNeese & Thyer (2004) [55]
- Systematic reviews and meta-analyses
- Randomized controlled trials
- Quasi-experimental studies
- Case-control and cohort studies
- Pre-experimental (or non-experimental) group studies
- Qualitative studies
Because there is further variation in the types of studies used by social work researchers, I expanded the hierarchy of evidence to cover a greater breadth of research methods in Figure 5.3.
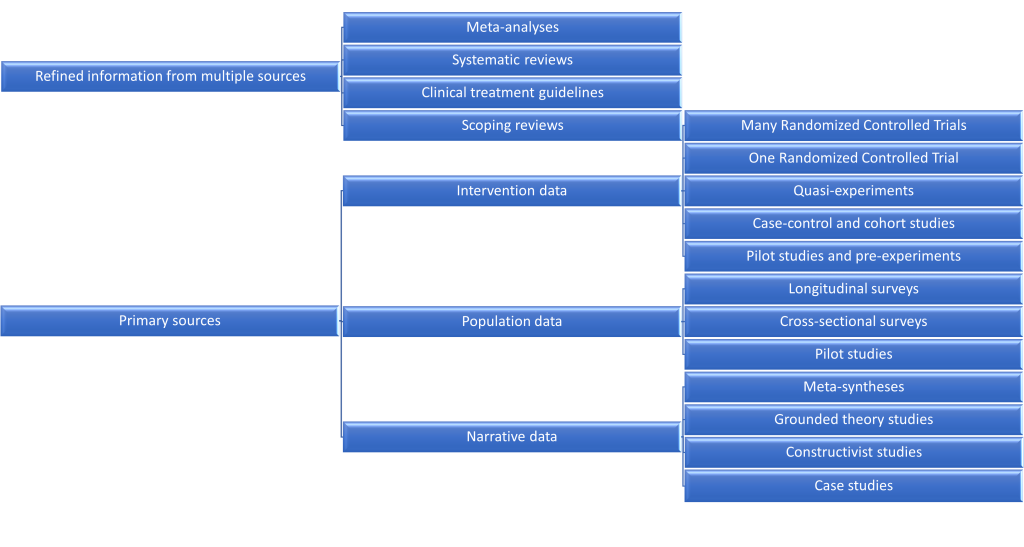
Refined information from multiple sources
The top of the hierarchy represents refined scientific information or meta-research . Meta-research uses the scientific method to analyze and improve the scientific production of knowledge. For example, meta-analyses pull together samples of people from all high-quality studies on a given topic area creating a super-study with far more people than any single researcher could feasibly collect data from. Because scientists (and clinical experts) refine data across multiple studies, these represent the most generalizable research findings.
Of course, not all meta-analyses or systematic reviews are of good quality. As a peer reviewer for a scholarly journal, I have seen poor quality systematic reviews that make methodological mistakes—like not including relevant keywords—that lead to incorrect conclusions. Unfortunately, not all errors are caught in the peer review process, and not all limitations are acknowledged by the authors. Just because you are looking at a systematic review does not mean you are looking at THE OBJECTIVE TRUTH. Nevertheless, you can be pretty sure that results from these studies are generalizable to the population in the study’s research question.
A good way to visualize the process of sampling is by examining the procedure used for systematic reviews and meta-analyses to scientifically search for articles. In Figure 5.4 below, you can see how researchers conducting a systematic review identified a large pool of potentially relevant articles, downloaded and analyzed them for relevance, and in the end, analyzed only 71 articles in their systematic review out of a total of 1,589 potentially relevant articles. Because systematic reviews or meta-analyses are intended to make strong, generalizable conclusions, they often exclude studies that still contain good information.
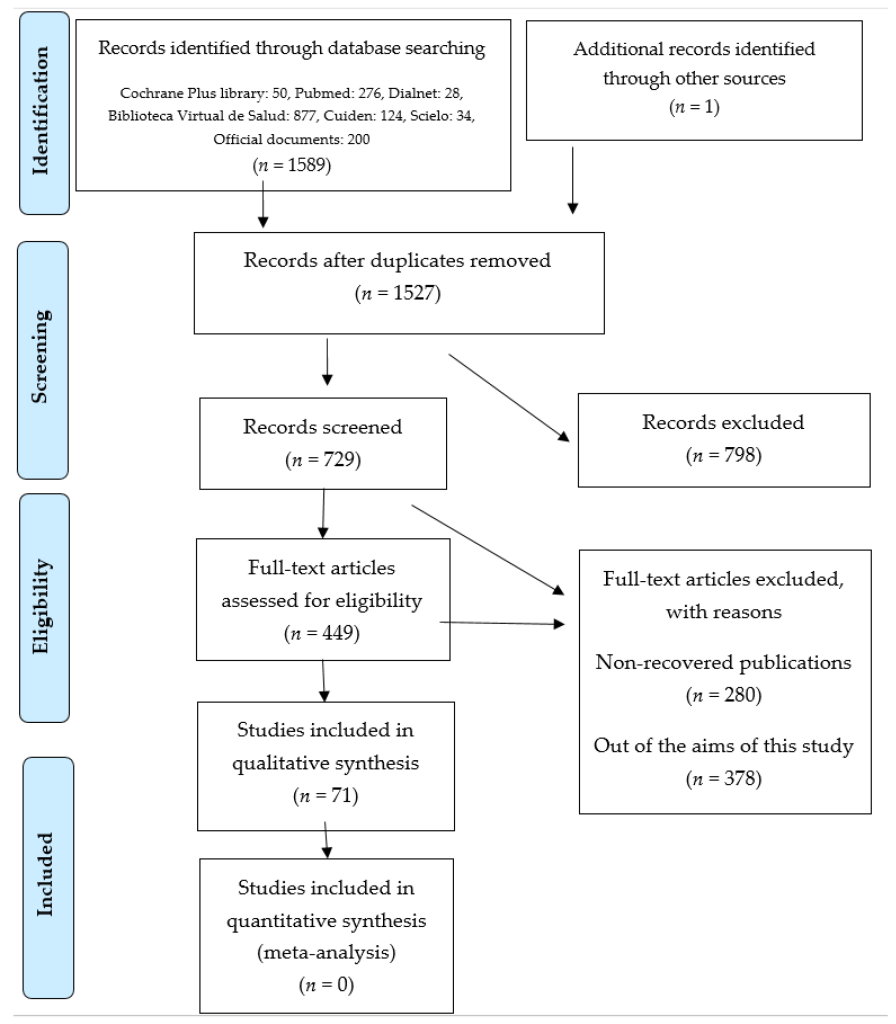
In the process of selecting articles for a meta-analysis and systematic review, researchers may exclude articles with important information for a number of good reasons. No study is perfect, and all research methods decisions come with limitations--including meta-research. Authors conducting a meta-analysis cannot include a study unless researchers provide data for the authors to include in their meta-analysis, and many empirical journal articles do not make their data available. Additionally, a study’s intervention or measures may be a bit different than what researchers want to make conclusions about. This is a key truth applicable across all articles you read—who or what gets selected for analysis in a research project determines how well the project’s results generalize to everyone.
We will talk about this in future chapters as sampling, and in those chapters, we will learn which sampling approaches are intended to support generalizability and which are used for other purposes. For example, availability or convenience sampling is often used to get quick information while random sampling approaches are intended to support generalizability. It is impossible to know everything about your article right now, but by the end of this course, you will have the information you need to critically examine the generalizability of a sample.
Primary sources (empirical studies)
Because refined sources like systematic reviews exclude good studies, they are only a first step in getting to know a topic area. You will need to examine primary sources--the reports of researchers who conducted empirical studies--to make evidence-based conclusions about your topic. Figure 5.3 describes three different types of data and ranks them vertically based on how well you can be sure the information generalizes.
As we will discuss further in our chapter on causal explanations, a key factor in scientifically assessing what happened first. Researchers conducting intervention studies are causing change by providing therapy, housing, or whatever the intervention is and measuring the outcomes of that intervention after they happen. This is unlike survey researchers, who do not introduce an intervention but ask people to self-report information on a questionnaire. Longitudinal surveys are particularly helpful because they can provide a clearer picture of whether the cause came before the effect in a causal relationship, but because they are expensive and time-consuming to conduct, longitudinal studies are relatively rare in the literature and most surveys measure people at only one point in time. Thus, because researchers cannot tightly control the causal variable (an intervention, an experience of abuse, etc.) we can be somewhat less certain of the conclusions of surveys than experiments. At the same time, because surveys measure people in their naturalistic environment rather than in a laboratory or artificial setting, they may do a better job at reducing the potential for the researcher to influence the data a participant provides. Surveys also provide descriptive information--like the number of people with a diagnosis or risk factor--that experiments cannot provide.
Surveys and experiments are commonly used in social work, and we will describe the methods they use in future chapters. When assessing the generalizability of a given survey or experiment, you are looking at whether the methods used by the researchers improve generalizability (or, at least that those methods are intended to improve generalizability). Specifically, there are sampling, measurement, and design decisions that researchers make that can improve generalizability. And once the study is conducted, whether those methods worked as intended also impact generalizability.
We address sampling, measurement, and design in the coming chapters, and you will need more in-depth knowledge of research methods to assess the generalizability of the results you are reading. In the meantime, Figure 5.3 is organized by design, and this is a good starting point for your inquiry since it only requires you to identify the design in each empirical article--which should be included in the abstract and described in detail in the methods section. For more information on how to conduct sampling, measurement, and design in a way that maximizes generalizability, read Part 2 of this textbook.
When searching for design of a study, look for specific keywords that indicate the researcher used methods that do not generalize well like pilot study, pre-experiment, non-experiment, convenience sample, availability sample, and exploratory study. When researchers are seeking to perform a pilot study, they are optimizing for time, not generalizability. Their results may still be useful to you! But, you should not generalize from their study to all people with the issue under analysis without a lot of caution and additional supporting evidence. Instead, you should see whether the lessons from this study might transfer to the context in which you are researching--our next topic.
Qualitative studies use sampling, measures, and designs that do not try to optimize generalizability. Thus, if the results of a qualitative study indicate 10 out of 50 students who participated in the focus group found the mandatory training on harassment to be unhelpful, does that mean 20% of all college students at this university find it unhelpful? Because focus groups and interviews (and other qualitative methods we will discuss) use qualitative methods, they are not concerned with generalizability. It would not make sense to generalize from focus groups to all people in a population. Instead, focus groups methods optimize for trustworthy and authentic research projects that make sure, for example, all themes and quotes in the researcher's report are traceable to quotes from focus group participants. Instead of providing what is generally true, qualitative research provides a thick description of people's experiences so you can understand them. S ubjective inquiry is less generalizable but provides greater depth in understanding people's feelings, beliefs, and decision-making processes within their context.
In Figure 5.3, you will note that some qualitative studies are ranked higher than others in terms of generalizability. Meta-syntheses are ranked highest because they are meta-research, pooling together the themes and raw data from multiple qualitative studies into a super-study. A meta-synthesis is the qualitative equivalent of a meta-analysis, which analyzes quantitative data. Because the researchers conducting the meta-syntheses aim to make more broad generalizations across research studies, even though generalizability is not strictly the goal. In a similar way, grounded theory studies (a type of qualitative design) aim to produce a testable hypothesis that could generalize. At the bottom of the hierarchy are individual case studies, which report what happens with a single person, organization, or event. It's best not to think too long about the generalizability of qualitative results. When examining qualitative articles, you should be examining their transferability, our topic for the next subsection.
Transferability
Generalizability asks one question: How well does the sample of people in this study represent everyone with this issue? If you read in a study that 50% of people in the sample experienced depression, does that mean 50% of everyone experiences depression? We previewed future discussions in this textbook that will discuss the specific quantitative research methods used to optimize the generalizability of results. By adhering strictly to best practices in sampling, measurement, and design, researchers can provide you with good evidence for the generalizability of their study's results.
Of course, generalizability is not the only question worth asking. Just because a study's sample represents a broader population does not mean it is helpful for making conclusions about your working question. In assessing a study's transferability, you are making a weaker but compelling argument that the conclusions of one study can be applied to understanding the people in your working question and research project. Generalizable results may be applicable because they are broadly transferable across situations, and you can be confident in that when they follow the best practices in this textbook for improving generalizability. However, there may be aspects of a study that make its results difficult to transfer to your topic area.
When evaluating the transferability of a research result to your working question, consider the sample, measures, and design. That is, how data was collected from individuals, who those individuals are, and what researchers did with them. You may find that the samples in generalizable studies do not talk about the specific ethnic, cultural, or geographic group that is in your working question. Similarly, studies that measure the outcomes of substance use treatment by measuring sobriety may not match your working question on moderation, medication adherence, or substitution as an outcome in substance use treatment. Evaluating the transferability of designs may help you identify whether the methods the authors used would be similar to those you might use if you were to conduct a study gathering and collecting your own raw data.
Assessing transferability is more subjective. You are using your knowledge of your topic area and research methods (which are always improving!) to make a reasonable argument about why a given piece of evidence from a primary source helps you understand something. Look back at Table 5.2, your annotations, and the researchers' sampling, data analysis, results, and design. Using your critical thinking (and the knowledge you can in Part 2 and Part 3 of this textbook) you will need to make a reasonable argument that these results transfer to the people, places, and culture that you are talking about in your working question.
In the final chapter of Part 1, we will discuss how to assemble the facts you have taken from journal articles into a literature review that represents what you think about the topic.
- Developing your theoretical framework
- Conceptual definitions
- Inductive & deductive reasoning
Nomothetic causal explanations
Content warning: examples in this chapter include references to sexual harassment, domestic violence, gender-based violence, the child welfare system, substance use disorders, neonatal abstinence syndrome, child abuse, racism, and sexism.
11.1 Developing your theoretical framework
- Differentiate between theories that explain specific parts of the social world versus those that are more broad and sweeping in their conclusions
- Identify the theoretical perspectives that are relevant to your project and inform your thinking about it
- Define key concepts in your working question and develop a theoretical framework for how you understand your topic.
Theories provide a way of looking at the world and of understanding human interaction. Paradigms are grounded in big assumptions about the world—what is real, how do we create knowledge—whereas theories describe more specific phenomena. Well, we are still oversimplifying a bit. Some theories try to explain the whole world, while others only try to explain a small part. Some theories can be grouped together based on common ideas but retain their own individual and unique features. Our goal is to help you find a theoretical framework that helps you understand your topic more deeply and answer your working question.
Theories: Big and small
In your human behavior and the social environment (HBSE) class, you were introduced to the major theoretical perspectives that are commonly used in social work. These are what we like to call big-T 'T'heories. When you read about systems theory, you are actually reading a synthesis of decades of distinct, overlapping, and conflicting theories that can be broadly classified within systems theory. For example, within systems theory, some approaches focus more on family systems while others focus on environmental systems, though the core concepts remain similar.
Different theorists define concepts in their own way, and as a result, their theories may explore different relationships with those concepts. For example, Deci and Ryan's (1985) [56] self-determination theory discusses motivation and establishes that it is contingent on meeting one's needs for autonomy, competency, and relatedness. By contrast, ecological self-determination theory, as written by Abery & Stancliffe (1996), [57] argues that self-determination is the amount of control exercised by an individual over aspects of their lives they deem important across the micro, meso, and macro levels. If self-determination were an important concept in your study, you would need to figure out which of the many theories related to self-determination helps you address your working question.
Theories can provide a broad perspective on the key concepts and relationships in the world or more specific and applied concepts and perspectives. Table 7.2 summarizes two commonly used lists of big-T Theoretical perspectives in social work. See if you can locate some of the theories that might inform your project.
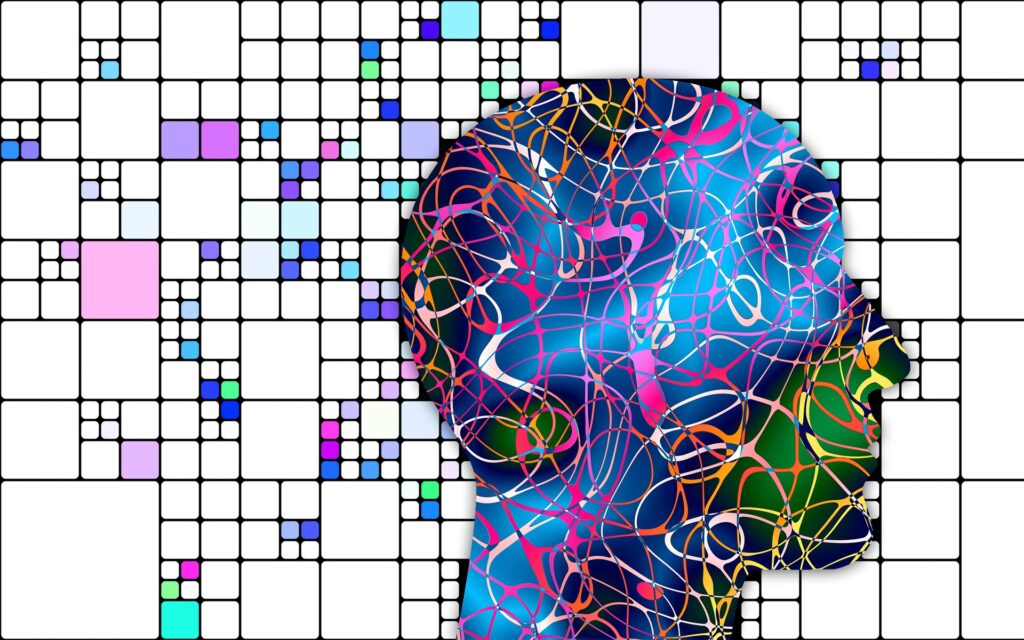
Competing theoretical explanations
Within each area of specialization in social work, there are many other theories that aim to explain more specific types of interactions. For example, within the study of sexual harassment, different theories posit different explanations for why harassment occurs.
One theory, first developed by criminologists, is called routine activities theory. It posits that sexual harassment is most likely to occur when a workplace lacks unified groups and when potentially vulnerable targets and motivated offenders are both present (DeCoster, Estes, & Mueller, 1999). [60]
Other theories of sexual harassment, called relational theories, suggest that one's existing relationships are the key to understanding why and how workplace sexual harassment occurs and how people will respond when it does occur (Morgan, 1999). [61] Relational theories focus on the power that different social relationships provide (e.g., married people who have supportive partners at home might be more likely than those who lack support at home to report sexual harassment when it occurs).
Finally, feminist theories of sexual harassment take a different stance. These theories posit that the organization of our current gender system, wherein those who are the most masculine have the most power, best explains the occurrence of workplace sexual harassment (MacKinnon, 1979). [62] As you might imagine, which theory a researcher uses to examine the topic of sexual harassment will shape the questions asked about harassment. It will also shape the explanations the researcher provides for why harassment occurs.
For a graduate student beginning their study of a new topic, it may be intimidating to learn that there are so many theories beyond what you’ve learned in your theory classes. What’s worse is that there is no central database of theories on your topic. However, as you review the literature in your area, you will learn more about the theories scientists have created to explain how your topic works in the real world. There are other good sources for theories, in addition to journal articles. Books often contain works of theoretical and philosophical importance that are beyond the scope of an academic journal. Do a search in your university library for books on your topic, and you are likely to find theorists talking about how to make sense of your topic. You don't necessarily have to agree with the prevailing theories about your topic, but you do need to be aware of them so you can apply theoretical ideas to your project.
Applying big-T theories to your topic
The key to applying theories to your topic is learning the key concepts associated with that theory and the relationships between those concepts, or propositions . Again, your HBSE class should have prepared you with some of the most important concepts from the theoretical perspectives listed in Table 7.2. For example, the conflict perspective sees the world as divided into dominant and oppressed groups who engage in conflict over resources. If you were applying these theoretical ideas to your project, you would need to identify which groups in your project are considered dominant or oppressed groups, and which resources they were struggling over. This is a very general example. Challenge yourself to find small-t theories about your topic that will help you understand it in much greater detail and specificity. If you have chosen a topic that is relevant to your life and future practice, you will be doing valuable work shaping your ideas towards social work practice.
Integrating theory into your project can be easy, or it can take a bit more effort. Some people have a strong and explicit theoretical perspective that they carry with them at all times. For me, you'll probably see my work drawing from exchange and choice, social constructionist, and critical theory. Maybe you have theoretical perspectives you naturally employ, like Afrocentric theory or person-centered practice. If so, that's a great place to start since you might already be using that theory (even subconsciously) to inform your understanding of your topic. But if you aren't aware of whether you are using a theoretical perspective when you think about your topic, try writing a paragraph off the top of your head or talking with a friend explaining what you think about that topic. Try matching it with some of the ideas from the broad theoretical perspectives from Table 7.2. This can ground you as you search for more specific theories. Some studies are designed to test whether theories apply the real world while others are designed to create new theories or variations on existing theories. Consider which feels more appropriate for your project and what you want to know.
Another way to easily identify the theories associated with your topic is to look at the concepts in your working question. Are these concepts commonly found in any of the theoretical perspectives in Table 7.2? Take a look at the Payne and Hutchison texts and see if any of those look like the concepts and relationships in your working question or if any of them match with how you think about your topic. Even if they don't possess the exact same wording, similar theories can help serve as a starting point to finding other theories that can inform your project. Remember, HBSE textbooks will give you not only the broad statements of theories but also sources from specific theorists and sub-theories that might be more applicable to your topic. Skim the references and suggestions for further reading once you find something that applies well.
Choose a theoretical perspective from Hutchison, Payne, or another theory textbook that is relevant to your project. Using their textbooks or other reputable sources, identify :
- At least five important concepts from the theory
- What relationships the theory establishes between these important concepts (e.g., as x increases, the y decreases)
- How you can use this theory to better understand the concepts and variables in your project?
Developing your own theoretical framework
Hutchison's and Payne's frameworks are helpful for surveying the whole body of literature relevant to social work, which is why they are so widely used. They are one framework, or way of thinking, about all of the theories social workers will encounter that are relevant to practice. Social work researchers should delve further and develop a theoretical or conceptual framework of their own based on their reading of the literature. In Chapter 8 , we will develop your theoretical framework further, identifying the cause-and-effect relationships that answer your working question. Developing a theoretical framework is also instructive for revising and clarifying your working question and identifying concepts that serve as keywords for additional literature searching. The greater clarity you have with your theoretical perspective, the easier each subsequent step in the research process will be.
Getting acquainted with the important theoretical concepts in a new area can be challenging. While social work education provides a broad overview of social theory, you will find much greater fulfillment out of reading about the theories related to your topic area. We discussed some strategies for finding theoretical information in Chapter 3 as part of literature searching. To extend that conversation a bit, some strategies for searching for theories in the literature include:
- Consider searching for these keywords in the title or abstract, specifically
- Looking at the references and cited by links within theoretical articles and textbooks
- Looking at books, edited volumes, and textbooks that discuss theory
- Talking with a scholar on your topic, or asking a professor if they can help connect you to someone
- Nice authors are clear about how they use theory to inform their research project, usually in the introduction and discussion section.
- For example, from the broad umbrella of systems theory, you might pick out family systems theory if you want to understand the effectiveness of a family counseling program.
It's important to remember that knowledge arises within disciplines, and that disciplines have different theoretical frameworks for explaining the same topic. While it is certainly important for the social work perspective to be a part of your analysis, social workers benefit from searching across disciplines to come to a more comprehensive understanding of the topic. Reaching across disciplines can provide uncommon insights during conceptualization, and once the study is completed, a multidisciplinary researcher will be able to share results in a way that speaks to a variety of audiences. A study by An and colleagues (2015) [63] uses game theory from the discipline of economics to understand problems in the Temporary Assistance for Needy Families (TANF) program. In order to receive TANF benefits, mothers must cooperate with paternity and child support requirements unless they have "good cause," as in cases of domestic violence, in which providing that information would put the mother at greater risk of violence. Game theory can help us understand how TANF recipients and caseworkers respond to the incentives in their environment, and highlight why the design of the "good cause" waiver program may not achieve its intended outcome of increasing access to benefits for survivors of family abuse.
Of course, there are natural limits on the depth with which student researchers can and should engage in a search for theory about their topic. At minimum, you should be able to draw connections across studies and be able to assess the relative importance of each theory within the literature. Just because you found one article applying your theory (like game theory, in our example above) does not mean it is important or often used in the domestic violence literature. Indeed, it would be much more common in the family violence literature to find psychological theories of trauma, feminist theories of power and control, and similar theoretical perspectives used to inform research projects rather than game theory, which is equally applicable to survivors of family violence as workers and bosses at a corporation. Consider using the Cited By feature to identify articles, books, and other sources of theoretical information that are seminal or well-cited in the literature. Similarly, by using the name of a theory in the keywords of a search query (along with keywords related to your topic), you can get a sense of how often the theory is used in your topic area. You should have a sense of what theories are commonly used to analyze your topic, even if you end up choosing a different one to inform your project.
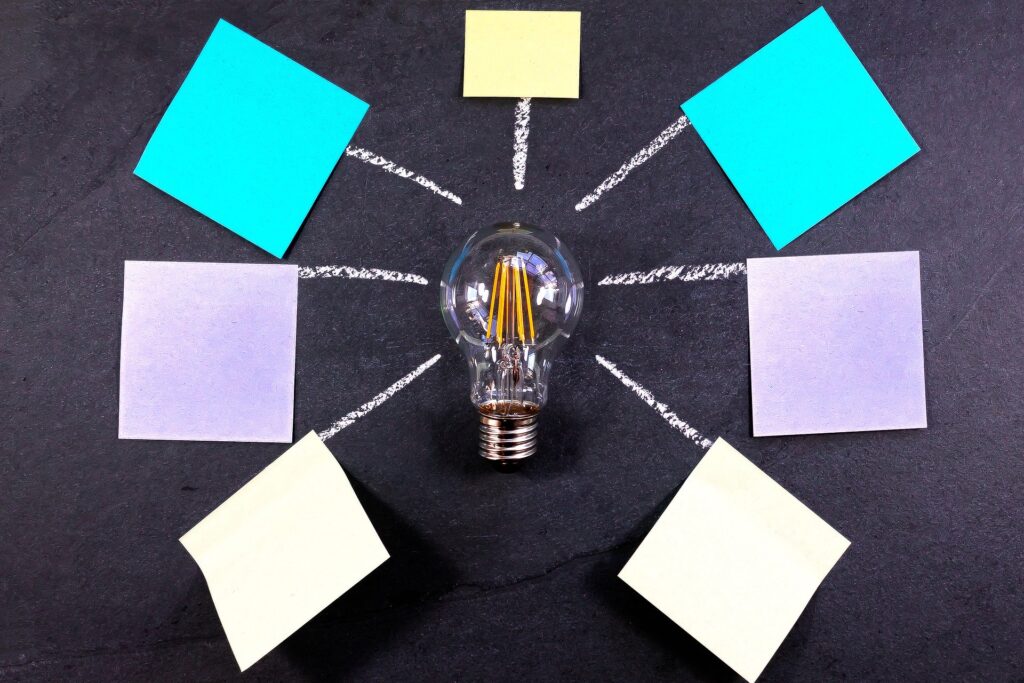
Theories that are not cited or used as often are still immensely valuable. As we saw before with TANF and "good cause" waivers, using theories from other disciplines can produce uncommon insights and help you make a new contribution to the social work literature. Given the privileged position that the social work curriculum places on theories developed by white men, students may want to explore Afrocentricity as a social work practice theory (Pellebon, 2007) [64] or abolitionist social work (Jacobs et al., 2021) [65] when deciding on a theoretical framework for their research project that addresses concepts of racial justice. Start with your working question, and explain how each theory helps you answer your question. Some explanations are going to feel right, and some concepts will feel more salient to you than others. Keep in mind that this is an iterative process. Your theoretical framework will likely change as you continue to conceptualize your research project, revise your research question, and design your study.
By trying on many different theoretical explanations for your topic area, you can better clarify your own theoretical framework. Some of you may be fortunate enough to find theories that match perfectly with how you think about your topic, are used often in the literature, and are therefore relatively straightforward to apply. However, many of you may find that a combination of theoretical perspectives is most helpful for you to investigate your project. For example, maybe the group counseling program for which you are evaluating client outcomes draws from both motivational interviewing and cognitive behavioral therapy. In order to understand the change happening in the client population, you would need to know each theory separately as well as how they work in tandem with one another. Because theoretical explanations and even the definitions of concepts are debated by scientists, it may be helpful to find a specific social scientist or group of scientists whose perspective on the topic you find matches with your understanding of the topic. Of course, it is also perfectly acceptable to develop your own theoretical framework, though you should be able to articulate how your framework fills a gap within the literature.
If you are adapting theoretical perspectives in your study, it is important to clarify the original authors' definitions of each concept. Jabareen (2009) [66] offers that conceptual frameworks are not merely collections of concepts but, rather, constructs in which each concept plays an integral role. [67] A conceptual framework is a network of linked concepts that together provide a comprehensive understanding of a phenomenon. Each concept in a conceptual framework plays an ontological or epistemological role in the framework, and it is important to assess whether the concepts and relationships in your framework make sense together. As your framework takes shape, you will find yourself integrating and grouping together concepts, thinking about the most important or least important concepts, and how each concept is causally related to others.
Much like paradigm, theory plays a supporting role for the conceptualization of your research project. Recall the ice float from Figure 7.1. Theoretical explanations support the design and methods you use to answer your research question. In student projects that lack a theoretical framework, I often see the biases and errors in reasoning that we discussed in Chapter 1 that get in the way of good social science. That's because theories mark which concepts are important, provide a framework for understanding them, and measure their interrelationships. If you are missing this foundation, you will operate on informal observation, messages from authority, and other forms of unsystematic and unscientific thinking we reviewed in Chapter 1 .
Theory-informed inquiry is incredibly helpful for identifying key concepts and how to measure them in your research project, but there is a risk in aligning research too closely with theory. The theory-ladenness of facts and observations produced by social science research means that we may be making our ideas real through research. This is a potential source of confirmation bias in social science. Moreover, as Tan (2016) [68] demonstrates, social science often proceeds by adopting as true the perspective of Western and Global North countries, and cross-cultural research is often when ethnocentric and biased ideas are most visible . In her example, a researcher from the West studying teacher-centric classrooms in China that rely partially on rote memorization may view them as less advanced than student-centered classrooms developed in a Western country simply because of Western philosophical assumptions about the importance of individualism and self-determination. Developing a clear theoretical framework is a way to guard against biased research, and it will establish a firm foundation on which you will develop the design and methods for your study.
- Just as empirical evidence is important for conceptualizing a research project, so too are the key concepts and relationships identified by social work theory.
- Using theory your theory textbook will provide you with a sense of the broad theoretical perspectives in social work that might be relevant to your project.
- Try to find small-t theories that are more specific to your topic area and relevant to your working question.
- In Chapter 2 , you developed a concept map for your proposal. Take a moment to revisit your concept map now as your theoretical framework is taking shape. Make any updates to the key concepts and relationships in your concept map. . If you need a refresher, we have embedded a short how-to video from the University of Guelph Library (CC-BY-NC-SA 4.0) that we also used in Chapter 2 .
11.2 Conceptual definitions
- Define measurement and conceptualization
- Apply Kaplan’s three categories to determine the complexity of measuring a given variable
- Identify the role previous research and theory play in defining concepts
- Distinguish between unidimensional and multidimensional concepts
- Critically apply reification to how you conceptualize the key variables in your research project
In social science, when we use the term measurement , we mean the process by which we describe and ascribe meaning to the key facts, concepts, or other phenomena that we are investigating. At its core, measurement is about defining one’s terms in as clear and precise a way as possible. Of course, measurement in social science isn’t quite as simple as using a measuring cup or spoon, but there are some basic tenets on which most social scientists agree when it comes to measurement. We’ll explore those, as well as some of the ways that measurement might vary depending on your unique approach to the study of your topic.
An important point here is that measurement does not require any particular instruments or procedures. What it does require is a systematic procedure for assigning scores, meanings, and descriptions to individuals or objects so that those scores represent the characteristic of interest. You can measure phenomena in many different ways, but you must be sure that how you choose to measure gives you information and data that lets you answer your research question. If you're looking for information about a person's income, but your main points of measurement have to do with the money they have in the bank, you're not really going to find the information you're looking for!
The question of what social scientists measure can be answered by asking yourself what social scientists study. Think about the topics you’ve learned about in other social work classes you’ve taken or the topics you’ve considered investigating yourself. Let’s consider Melissa Milkie and Catharine Warner’s study (2011) [69] of first graders’ mental health. In order to conduct that study, Milkie and Warner needed to have some idea about how they were going to measure mental health. What does mental health mean, exactly? And how do we know when we’re observing someone whose mental health is good and when we see someone whose mental health is compromised? Understanding how measurement works in research methods helps us answer these sorts of questions.
As you might have guessed, social scientists will measure just about anything that they have an interest in investigating. For example, those who are interested in learning something about the correlation between social class and levels of happiness must develop some way to measure both social class and happiness. Those who wish to understand how well immigrants cope in their new locations must measure immigrant status and coping. Those who wish to understand how a person’s gender shapes their workplace experiences must measure gender and workplace experiences (and get more specific about which experiences are under examination). You get the idea. Social scientists can and do measure just about anything you can imagine observing or wanting to study. Of course, some things are easier to observe or measure than others.
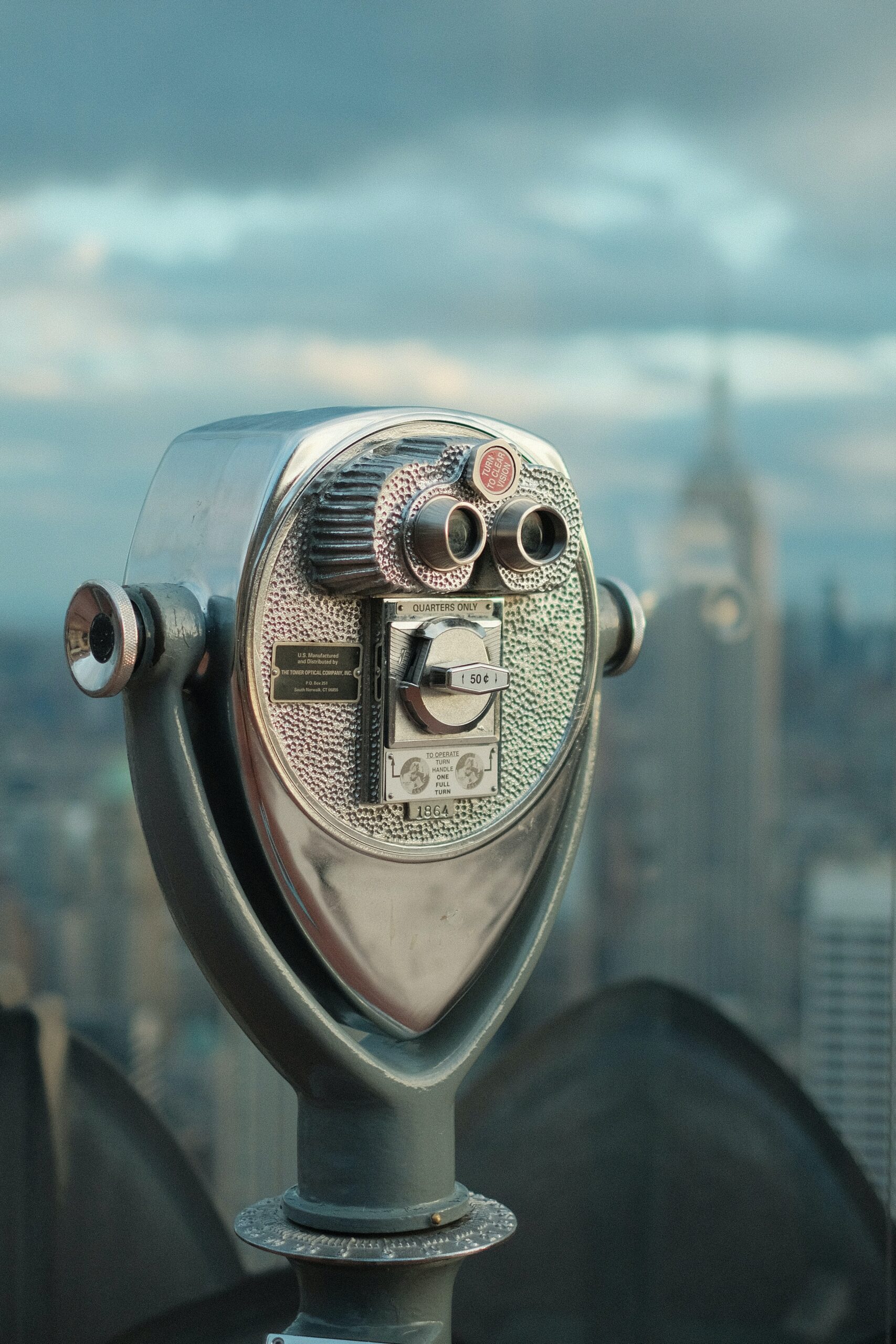
Observing your variables
In 1964, philosopher Abraham Kaplan (1964) [70] wrote The Conduct of Inquiry, which has since become a classic work in research methodology (Babbie, 2010). [71] In his text, Kaplan describes different categories of things that behavioral scientists observe. One of those categories, which Kaplan called “observational terms,” is probably the simplest to measure in social science. Observational terms are the sorts of things that we can see with the naked eye simply by looking at them. Kaplan roughly defines them as conditions that are easy to identify and verify through direct observation. If, for example, we wanted to know how the conditions of playgrounds differ across different neighborhoods, we could directly observe the variety, amount, and condition of equipment at various playgrounds.
Indirect observables , on the other hand, are less straightforward to assess. In Kaplan's framework, they are conditions that are subtle and complex that we must use existing knowledge and intuition to define. If we conducted a study for which we wished to know a person’s income, we’d probably have to ask them their income, perhaps in an interview or a survey. Thus, we have observed income, even if it has only been observed indirectly. Birthplace might be another indirect observable. We can ask study participants where they were born, but chances are good we won’t have directly observed any of those people being born in the locations they report.
Sometimes the measures that we are interested in are more complex and more abstract than observational terms or indirect observables. Think about some of the concepts you’ve learned about in other social work classes—for example, ethnocentrism. What is ethnocentrism? Well, from completing an introduction to social work class you might know that it has something to do with the way a person judges another’s culture. But how would you measure it? Here’s another construct: bureaucracy. We know this term has something to do with organizations and how they operate but measuring such a construct is trickier than measuring something like a person’s income. The theoretical concepts of ethnocentrism and bureaucracy represent ideas whose meanings we have come to agree on. Though we may not be able to observe these abstractions directly, we can observe their components.
Kaplan referred to these more abstract things that behavioral scientists measure as constructs. Constructs are “not observational either directly or indirectly” (Kaplan, 1964, p. 55), [72] but they can be defined based on observables. For example, the construct of bureaucracy could be measured by counting the number of supervisors that need to approve routine spending by public administrators. The greater the number of administrators that must sign off on routine matters, the greater the degree of bureaucracy. Similarly, we might be able to ask a person the degree to which they trust people from different cultures around the world and then assess the ethnocentrism inherent in their answers. We can measure constructs like bureaucracy and ethnocentrism by defining them in terms of what we can observe. [73]
The idea of coming up with your own measurement tool might sound pretty intimidating at this point. The good news is that if you find something in the literature that works for you, you can use it (with proper attribution, of course). If there are only pieces of it that you like, you can reuse those pieces (with proper attribution and describing/justifying any changes). You don't always have to start from scratch!
Look at the variables in your research question.
- Classify them as direct observables, indirect observables, or constructs.
- Do you think measuring them will be easy or hard?
- What are your first thoughts about how to measure each variable? No wrong answers here, just write down a thought about each variable.
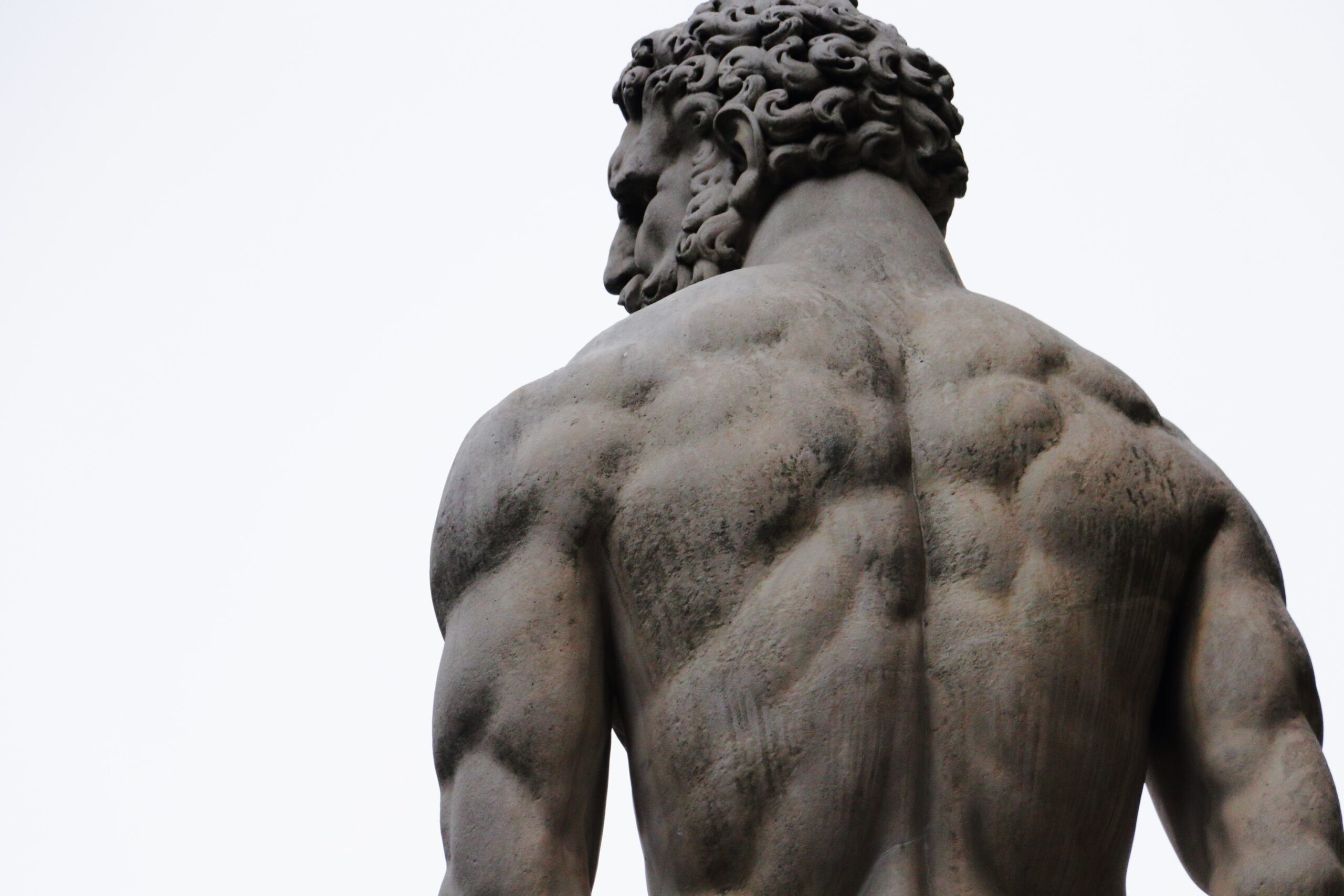
Measurement starts with conceptualization
In order to measure the concepts in your research question, we first have to understand what we think about them. As an aside, the word concept has come up quite a bit, and it is important to be sure we have a shared understanding of that term. A concept is the notion or image that we conjure up when we think of some cluster of related observations or ideas. For example, masculinity is a concept. What do you think of when you hear that word? Presumably, you imagine some set of behaviors and perhaps even a particular style of self-presentation. Of course, we can’t necessarily assume that everyone conjures up the same set of ideas or images when they hear the word masculinity . While there are many possible ways to define the term and some may be more common or have more support than others, there is no universal definition of masculinity. What counts as masculine may shift over time, from culture to culture, and even from individual to individual (Kimmel, 2008). This is why defining our concepts is so important.\
Not all researchers clearly explain their theoretical or conceptual framework for their study, but they should! Without understanding how a researcher has defined their key concepts, it would be nearly impossible to understand the meaning of that researcher’s findings and conclusions. Back in Chapter 7 , you developed a theoretical framework for your study based on a survey of the theoretical literature in your topic area. If you haven't done that yet, consider flipping back to that section to familiarize yourself with some of the techniques for finding and using theories relevant to your research question. Continuing with our example on masculinity, we would need to survey the literature on theories of masculinity. After a few queries on masculinity, I found a wonderful article by Wong (2010) [74] that analyzed eight years of the journal Psychology of Men & Masculinity and analyzed how often different theories of masculinity were used . Not only can I get a sense of which theories are more accepted and which are more marginal in the social science on masculinity, I am able to identify a range of options from which I can find the theory or theories that will inform my project.
Identify a specific theory (or more than one theory) and how it helps you understand...
- Your independent variable(s).
- Your dependent variable(s).
- The relationship between your independent and dependent variables.
Rather than completing this exercise from scratch, build from your theoretical or conceptual framework developed in previous chapters.
In quantitative methods, conceptualization involves writing out clear, concise definitions for our key concepts. These are the kind of definitions you are used to, like the ones in a dictionary. A conceptual definition involves defining a concept in terms of other concepts, usually by making reference to how other social scientists and theorists have defined those concepts in the past. Of course, new conceptual definitions are created all the time because our conceptual understanding of the world is always evolving.
Conceptualization is deceptively challenging—spelling out exactly what the concepts in your research question mean to you. Following along with our example, think about what comes to mind when you read the term masculinity. How do you know masculinity when you see it? Does it have something to do with men or with social norms? If so, perhaps we could define masculinity as the social norms that men are expected to follow. That seems like a reasonable start, and at this early stage of conceptualization, brainstorming about the images conjured up by concepts and playing around with possible definitions is appropriate. However, this is just the first step. At this point, you should be beyond brainstorming for your key variables because you have read a good amount of research about them
In addition, we should consult previous research and theory to understand the definitions that other scholars have already given for the concepts we are interested in. This doesn’t mean we must use their definitions, but understanding how concepts have been defined in the past will help us to compare our conceptualizations with how other scholars define and relate concepts. Understanding prior definitions of our key concepts will also help us decide whether we plan to challenge those conceptualizations or rely on them for our own work. Finally, working on conceptualization is likely to help in the process of refining your research question to one that is specific and clear in what it asks. Conceptualization and operationalization (next section) are where "the rubber meets the road," so to speak, and you have to specify what you mean by the question you are asking. As your conceptualization deepens, you will often find that your research question becomes more specific and clear.
If we turn to the literature on masculinity, we will surely come across work by Michael Kimmel , one of the preeminent masculinity scholars in the United States. After consulting Kimmel’s prior work (2000; 2008), [75] we might tweak our initial definition of masculinity. Rather than defining masculinity as “the social norms that men are expected to follow,” perhaps instead we’ll define it as “the social roles, behaviors, and meanings prescribed for men in any given society at any one time” (Kimmel & Aronson, 2004, p. 503). [76] Our revised definition is more precise and complex because it goes beyond addressing one aspect of men’s lives (norms), and addresses three aspects: roles, behaviors, and meanings. It also implies that roles, behaviors, and meanings may vary across societies and over time. Using definitions developed by theorists and scholars is a good idea, though you may find that you want to define things your own way.
As you can see, conceptualization isn’t as simple as applying any random definition that we come up with to a term. Defining our terms may involve some brainstorming at the very beginning. But conceptualization must go beyond that, to engage with or critique existing definitions and conceptualizations in the literature. Once we’ve brainstormed about the images associated with a particular word, we should also consult prior work to understand how others define the term in question. After we’ve identified a clear definition that we’re happy with, we should make sure that every term used in our definition will make sense to others. Are there terms used within our definition that also need to be defined? If so, our conceptualization is not yet complete. Our definition includes the concept of "social roles," so we should have a definition for what those mean and become familiar with role theory to help us with our conceptualization. If we don't know what roles are, how can we study them?
Let's say we do all of that. We have a clear definition of the term masculinity with reference to previous literature and we also have a good understanding of the terms in our conceptual definition...then we're done, right? Not so fast. You’ve likely met more than one man in your life, and you’ve probably noticed that they are not the same, even if they live in the same society during the same historical time period. This could mean there are dimensions of masculinity. In terms of social scientific measurement, concepts can be said to have multiple dimensions when there are multiple elements that make up a single concept. With respect to the term masculinity , dimensions could based on gender identity, gender performance, sexual orientation, etc.. In any of these cases, the concept of masculinity would be considered to have multiple dimensions.
While you do not need to spell out every possible dimension of the concepts you wish to measure, it is important to identify whether your concepts are unidimensional (and therefore relatively easy to define and measure) or multidimensional (and therefore require multi-part definitions and measures). In this way, how you conceptualize your variables determines how you will measure them in your study. Unidimensional concepts are those that are expected to have a single underlying dimension. These concepts can be measured using a single measure or test. Examples include simple concepts such as a person’s weight, time spent sleeping, and so forth.
One frustrating this is that there is no clear demarcation between concepts that are inherently unidimensional or multidimensional. Even something as simple as age could be broken down into multiple dimensions including mental age and chronological age, so where does conceptualization stop? How far down the dimensional rabbit hole do we have to go? Researchers should consider two things. First, how important is this variable in your study? If age is not important in your study (maybe it is a control variable), it seems like a waste of time to do a lot of work drawing from developmental theory to conceptualize this variable. A unidimensional measure from zero to dead is all the detail we need. On the other hand, if we were measuring the impact of age on masculinity, conceptualizing our independent variable (age) as multidimensional may provide a richer understanding of its impact on masculinity. Finally, your conceptualization will lead directly to your operationalization of the variable, and once your operationalization is complete, make sure someone reading your study could follow how your conceptual definitions informed the measures you chose for your variables.
Write a conceptual definition for your independent and dependent variables.
- Cite and attribute definitions to other scholars, if you use their words.
- Describe how your definitions are informed by your theoretical framework.
- Place your definition in conversation with other theories and conceptual definitions commonly used in the literature.
- Are there multiple dimensions of your variables?
- Are any of these dimensions important for you to measure?
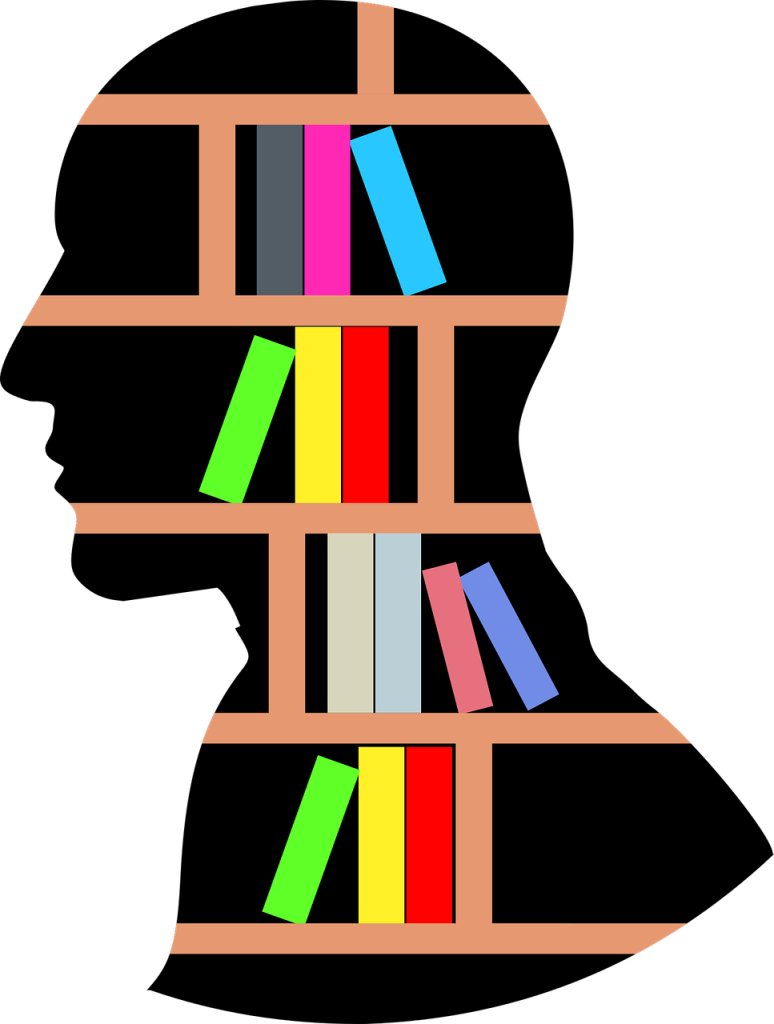
Do researchers actually know what we're talking about?
Conceptualization proceeds differently in qualitative research compared to quantitative research. Since qualitative researchers are interested in the understandings and experiences of their participants, it is less important for them to find one fixed definition for a concept before starting to interview or interact with participants. The researcher’s job is to accurately and completely represent how their participants understand a concept, not to test their own definition of that concept.
If you were conducting qualitative research on masculinity, you would likely consult previous literature like Kimmel’s work mentioned above. From your literature review, you may come up with a working definition for the terms you plan to use in your study, which can change over the course of the investigation. However, the definition that matters is the definition that your participants share during data collection. A working definition is merely a place to start, and researchers should take care not to think it is the only or best definition out there.
In qualitative inquiry, your participants are the experts (sound familiar, social workers?) on the concepts that arise during the research study. Your job as the researcher is to accurately and reliably collect and interpret their understanding of the concepts they describe while answering your questions. Conceptualization of concepts is likely to change over the course of qualitative inquiry, as you learn more information from your participants. Indeed, getting participants to comment on, extend, or challenge the definitions and understandings of other participants is a hallmark of qualitative research. This is the opposite of quantitative research, in which definitions must be completely set in stone before the inquiry can begin.
The contrast between qualitative and quantitative conceptualization is instructive for understanding how quantitative methods (and positivist research in general) privilege the knowledge of the researcher over the knowledge of study participants and community members. Positivism holds that the researcher is the "expert," and can define concepts based on their expert knowledge of the scientific literature. This knowledge is in contrast to the lived experience that participants possess from experiencing the topic under examination day-in, day-out. For this reason, it would be wise to remind ourselves not to take our definitions too seriously and be critical about the limitations of our knowledge.
Conceptualization must be open to revisions, even radical revisions, as scientific knowledge progresses. While I’ve suggested consulting prior scholarly definitions of our concepts, you should not assume that prior, scholarly definitions are more real than the definitions we create. Likewise, we should not think that our own made-up definitions are any more real than any other definition. It would also be wrong to assume that just because definitions exist for some concept that the concept itself exists beyond some abstract idea in our heads. Building on the paradigmatic ideas behind interpretivism and the critical paradigm, researchers call the assumption that our abstract concepts exist in some concrete, tangible way is known as reification . It explores the power dynamics behind how we can create reality by how we define it.
Returning again to our example of masculinity. Think about our how our notions of masculinity have developed over the past few decades, and how different and yet so similar they are to patriarchal definitions throughout history. Conceptual definitions become more or less popular based on the power arrangements inside of social science the broader world. Western knowledge systems are privileged, while others are viewed as unscientific and marginal. The historical domination of social science by white men from WEIRD countries meant that definitions of masculinity were imbued their cultural biases and were designed explicitly and implicitly to preserve their power. This has inspired movements for cognitive justice as we seek to use social science to achieve global development.
- Measurement is the process by which we describe and ascribe meaning to the key facts, concepts, or other phenomena that we are investigating.
- Kaplan identified three categories of things that social scientists measure including observational terms, indirect observables, and constructs.
- Some concepts have multiple elements or dimensions.
- Researchers often use measures previously developed and studied by other researchers.
- Conceptualization is a process that involves coming up with clear, concise definitions.
- Conceptual definitions are based on the theoretical framework you are using for your study (and the paradigmatic assumptions underlying those theories).
- Whether your conceptual definitions come from your own ideas or the literature, you should be able to situate them in terms of other commonly used conceptual definitions.
- Researchers should acknowledge the limited explanatory power of their definitions for concepts and how oppression can shape what explanations are considered true or scientific.
Think historically about the variables in your research question.
- How has our conceptual definition of your topic changed over time?
- What scholars or social forces were responsible for this change?
Take a critical look at your conceptual definitions.
- How participants might define terms for themselves differently, in terms of their daily experience?
- On what cultural assumptions are your conceptual definitions based?
- Are your conceptual definitions applicable across all cultures that will be represented in your sample?
11.3 Inductive and deductive reasoning
- Describe inductive and deductive reasoning and provide examples of each
- Identify how inductive and deductive reasoning are complementary
Congratulations! You survived the chapter on theories and paradigms. My experience has been that many students have a difficult time thinking about theories and paradigms because they perceive them as "intangible" and thereby hard to connect to social work research. I even had one student who said she got frustrated just reading the word "philosophy."
Rest assured, you do not need to become a theorist or philosopher to be an effective social worker or researcher. However, you should have a good sense of what theory or theories will be relevant to your project, as well as how this theory, along with your working question, fit within the three broad research paradigms we reviewed. If you don't have a good idea about those at this point, it may be a good opportunity to pause and read more about the theories related to your topic area.
Theories structure and inform social work research. The converse is also true: research can structure and inform theory. The reciprocal relationship between theory and research often becomes evident to students when they consider the relationships between theory and research in inductive and deductive approaches to research. In both cases, theory is crucial. But the relationship between theory and research differs for each approach.
While inductive and deductive approaches to research are quite different, they can also be complementary. Let’s start by looking at each one and how they differ from one another. Then we’ll move on to thinking about how they complement one another.
Inductive reasoning
A researcher using inductive reasoning begins by collecting data that is relevant to their topic of interest. Once a substantial amount of data have been collected, the researcher will then step back from data collection to get a bird’s eye view of their data. At this stage, the researcher looks for patterns in the data, working to develop a theory that could explain those patterns. Thus, when researchers take an inductive approach, they start with a particular set of observations and move to a more general set of propositions about those experiences. In other words, they move from data to theory, or from the specific to the general. Figure 8.1 outlines the steps involved with an inductive approach to research.
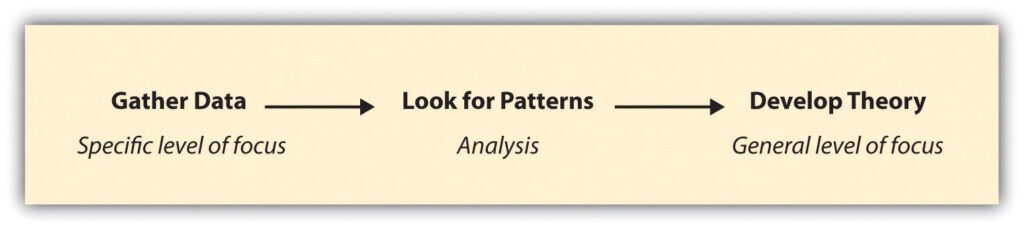
There are many good examples of inductive research, but we’ll look at just a few here. One fascinating study in which the researchers took an inductive approach is Katherine Allen, Christine Kaestle, and Abbie Goldberg’s (2011) [77] study of how boys and young men learn about menstruation. To understand this process, Allen and her colleagues analyzed the written narratives of 23 young cisgender men in which the men described how they learned about menstruation, what they thought of it when they first learned about it, and what they think of it now. By looking for patterns across all 23 cisgender men’s narratives, the researchers were able to develop a general theory of how boys and young men learn about this aspect of girls’ and women’s biology. They conclude that sisters play an important role in boys’ early understanding of menstruation, that menstruation makes boys feel somewhat separated from girls, and that as they enter young adulthood and form romantic relationships, young men develop more mature attitudes about menstruation. Note how this study began with the data—men’s narratives of learning about menstruation—and worked to develop a theory.
In another inductive study, Kristin Ferguson and colleagues (Ferguson, Kim, & McCoy, 2011) [78] analyzed empirical data to better understand how to meet the needs of young people who are homeless. The authors analyzed focus group data from 20 youth at a homeless shelter. From these data they developed a set of recommendations for those interested in applied interventions that serve homeless youth. The researchers also developed hypotheses for others who might wish to conduct further investigation of the topic. Though Ferguson and her colleagues did not test their hypotheses, their study ends where most deductive investigations begin: with a theory and a hypothesis derived from that theory. Section 8.4 discusses the use of mixed methods research as a way for researchers to test hypotheses created in a previous component of the same research project.
You will notice from both of these examples that inductive reasoning is most commonly found in studies using qualitative methods, such as focus groups and interviews. Because inductive reasoning involves the creation of a new theory, researchers need very nuanced data on how the key concepts in their working question operate in the real world. Qualitative data is often drawn from lengthy interactions and observations with the individuals and phenomena under examination. For this reason, inductive reasoning is most often associated with qualitative methods, though it is used in both quantitative and qualitative research.
Deductive reasoning
If inductive reasoning is about creating theories from raw data, deductive reasoning is about testing theories using data. Researchers using deductive reasoning take the steps described earlier for inductive research and reverse their order. They start with a compelling social theory, create a hypothesis about how the world should work, collect raw data, and analyze whether their hypothesis was confirmed or not. That is, deductive approaches move from a more general level (theory) to a more specific (data); whereas inductive approaches move from the specific (data) to general (theory).
A deductive approach to research is the one that people typically associate with scientific investigation. Students in English-dominant countries that may be confused by inductive vs. deductive research can rest part of the blame on Sir Arthur Conan Doyle, creator of the Sherlock Holmes character. As Craig Vasey points out in his breezy introduction to logic book chapter , Sherlock Holmes more often used inductive rather than deductive reasoning (despite claiming to use the powers of deduction to solve crimes). By noticing subtle details in how people act, behave, and dress, Holmes finds patterns that others miss. Using those patterns, he creates a theory of how the crime occurred, dramatically revealed to the authorities just in time to arrest the suspect. Indeed, it is these flashes of insight into the patterns of data that make Holmes such a keen inductive reasoner. In social work practice, rather than detective work, inductive reasoning is supported by the intuitions and practice wisdom of social workers, just as Holmes' reasoning is sharpened by his experience as a detective.
So, if deductive reasoning isn't Sherlock Holmes' observation and pattern-finding, how does it work? It starts with what you have already done in Chapters 3 and 4, reading and evaluating what others have done to study your topic. It continued with Chapter 5, discovering what theories already try to explain how the concepts in your working question operate in the real world. Tapping into this foundation of knowledge on their topic, the researcher studies what others have done, reads existing theories of whatever phenomenon they are studying, and then tests hypotheses that emerge from those theories. Figure 8.2 outlines the steps involved with a deductive approach to research.
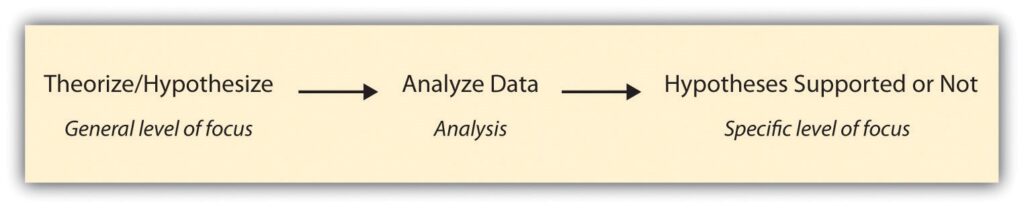
While not all researchers follow a deductive approach, many do. We’ll now take a look at a couple excellent recent examples of deductive research.
In a study of US law enforcement responses to hate crimes, Ryan King and colleagues (King, Messner, & Baller, 2009) [79] hypothesized that law enforcement’s response would be less vigorous in areas of the country that had a stronger history of racial violence. The authors developed their hypothesis from prior research and theories on the topic. They tested the hypothesis by analyzing data on states’ lynching histories and hate crime responses. Overall, the authors found support for their hypothesis and illustrated an important application of critical race theory.
In another recent deductive study, Melissa Milkie and Catharine Warner (2011) [80] studied the effects of different classroom environments on first graders’ mental health. Based on prior research and theory, Milkie and Warner hypothesized that negative classroom features, such as a lack of basic supplies and heat, would be associated with emotional and behavioral problems in children. One might associate this research with Maslow's hierarchy of needs or systems theory. The researchers found support for their hypothesis, demonstrating that policymakers should be paying more attention to the mental health outcomes of children’s school experiences, just as they track academic outcomes (American Sociological Association, 2011). [81]
Complementary approaches
While inductive and deductive approaches to research seem quite different, they can actually be rather complementary. In some cases, researchers will plan for their study to include multiple components, one inductive and the other deductive. In other cases, a researcher might begin a study with the plan to conduct either inductive or deductive research, but then discovers along the way that the other approach is needed to help illuminate findings. Here is an example of each such case.
Dr. Amy Blackstone (n.d.), author of Principles of sociological inquiry: Qualitative and quantitative methods , relates a story about her mixed methods research on sexual harassment.
We began the study knowing that we would like to take both a deductive and an inductive approach in our work. We therefore administered a quantitative survey, the responses to which we could analyze in order to test hypotheses, and also conducted qualitative interviews with a number of the survey participants. The survey data were well suited to a deductive approach; we could analyze those data to test hypotheses that were generated based on theories of harassment. The interview data were well suited to an inductive approach; we looked for patterns across the interviews and then tried to make sense of those patterns by theorizing about them. For one paper (Uggen & Blackstone, 2004) [82] , we began with a prominent feminist theory of the sexual harassment of adult women and developed a set of hypotheses outlining how we expected the theory to apply in the case of younger women’s and men’s harassment experiences. We then tested our hypotheses by analyzing the survey data. In general, we found support for the theory that posited that the current gender system, in which heteronormative men wield the most power in the workplace, explained workplace sexual harassment—not just of adult women but of younger women and men as well. In a more recent paper (Blackstone, Houle, & Uggen, 2006), [83] we did not hypothesize about what we might find but instead inductively analyzed interview data, looking for patterns that might tell us something about how or whether workers’ perceptions of harassment change as they age and gain workplace experience. From this analysis, we determined that workers’ perceptions of harassment did indeed shift as they gained experience and that their later definitions of harassment were more stringent than those they held during adolescence. Overall, our desire to understand young workers’ harassment experiences fully—in terms of their objective workplace experiences, their perceptions of those experiences, and their stories of their experiences—led us to adopt both deductive and inductive approaches in the work. (Blackstone, n.d., p. 21) [84]
Researchers may not always set out to employ both approaches in their work but sometimes find that their use of one approach leads them to the other. One such example is described eloquently in Russell Schutt’s Investigating the Social World (2006). [85] As Schutt describes, researchers Sherman and Berk (1984) [86] conducted an experiment to test two competing theories of the effects of punishment on deterring deviance (in this case, domestic violence).Specifically, Sherman and Berk hypothesized that deterrence theory (see Williams, 2005 [87] for more information on that theory) would provide a better explanation of the effects of arresting accused batterers than labeling theory . Deterrence theory predicts that arresting an accused spouse batterer will reduce future incidents of violence. Conversely, labeling theory predicts that arresting accused spouse batterers will increase future incidents (see Policastro & Payne, 2013 [88] for more information on that theory). Figure 8.3 summarizes the two competing theories and the hypotheses Sherman and Berk set out to test.
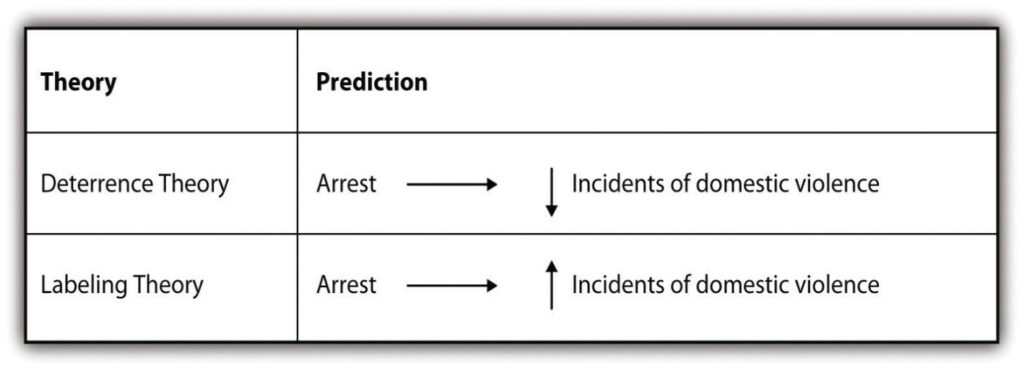
Research from these follow-up studies were mixed. In some cases, arrest deterred future incidents of violence. In other cases, it did not. This left the researchers with new data that they needed to explain. The researchers therefore took an inductive approach in an effort to make sense of their latest empirical observations. The new studies revealed that arrest seemed to have a deterrent effect for those who were married and employed, but that it led to increased offenses for those who were unmarried and unemployed. Researchers thus turned to control theory, which posits that having some stake in conformity through the social ties provided by marriage and employment, as the better explanation (see Davis et al., 2000 [90] for more information on this theory).
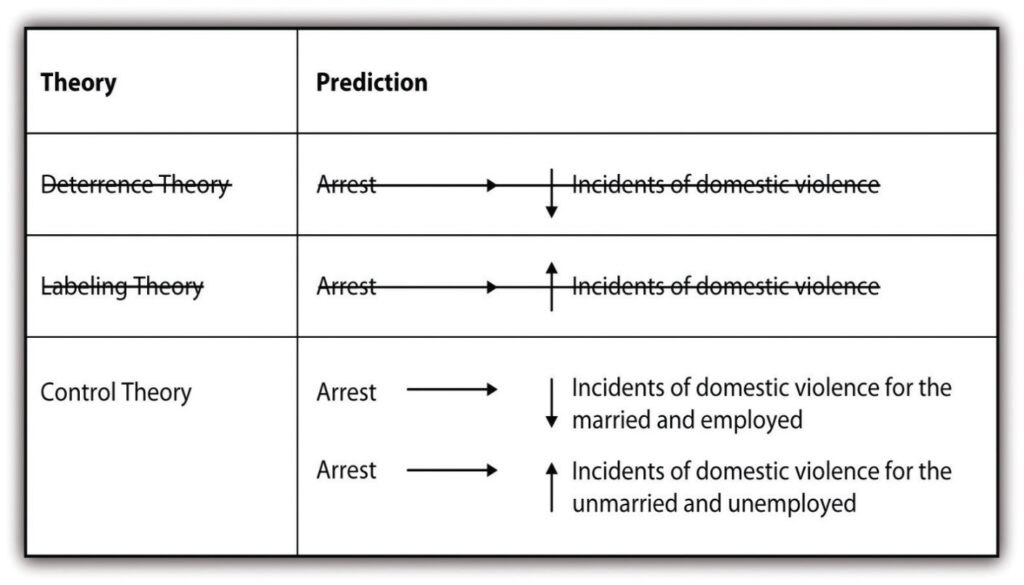
What the original Sherman and Berk study, along with the follow-up studies, show us is that we might start with a deductive approach to research, but then, if confronted by new data we must make sense of, we may move to an inductive approach. We will expand on these possibilities in section 8.4 when we discuss mixed methods research.
Ethical and critical considerations
Deductive and inductive reasoning, just like other components of the research process comes with ethical and cultural considerations for researchers. Specifically, deductive research is limited by existing theory. Because scientific inquiry has been shaped by oppressive forces such as sexism, racism, and colonialism, what is considered theory is largely based in Western, white-male-dominant culture. Thus, researchers doing deductive research may artificially limit themselves to ideas that were derived from this context. Non-Western researchers, international social workers, and practitioners working with non-dominant groups may find deductive reasoning of limited help if theories do not adequately describe other cultures.
While these flaws in deductive research may make inductive reasoning seem more appealing, on closer inspection you'll find similar issues apply. A researcher using inductive reasoning applies their intuition and lived experience when analyzing participant data. They will take note of particular themes, conceptualize their definition, and frame the project using their unique psychology. Since everyone's internal world is shaped by their cultural and environmental context, inductive reasoning conducted by Western researchers may unintentionally reinforcing lines of inquiry that derive from cultural oppression.
Inductive reasoning is also shaped by those invited to provide the data to be analyzed. For example, I recently worked with a student who wanted to understand the impact of child welfare supervision on children born dependent on opiates and methamphetamine. Due to the potential harm that could come from interviewing families and children who are in foster care or under child welfare supervision, the researcher decided to use inductive reasoning and to only interview child welfare workers.
Talking to practitioners is a good idea for feasibility, as they are less vulnerable than clients. However, any theory that emerges out of these observations will be substantially limited, as it would be devoid of the perspectives of parents, children, and other community members who could provide a more comprehensive picture of the impact of child welfare involvement on children. Notice that each of these groups has less power than child welfare workers in the service relationship. Attending to which groups were used to inform the creation of a theory and the power of those groups is an important critical consideration for social work researchers.
As you can see, when researchers apply theory to research they must wrestle with the history and hierarchy around knowledge creation in that area. In deductive studies, the researcher is positioned as the expert, similar to the positivist paradigm presented in Chapter 5. We've discussed a few of the limitations on the knowledge of researchers in this subsection, but the position of the "researcher as expert" is inherently problematic. However, it should also not be taken to an extreme. A researcher who approaches inductive inquiry as a naïve learner is also inherently problematic. Just as competence in social work practice requires a baseline of knowledge prior to entering practice, so does competence in social work research. Because a truly naïve intellectual position is impossible—we all have preexisting ways we view the world and are not fully aware of how they may impact our thoughts—researchers should be well-read in the topic area of their research study but humble enough to know that there is always much more to learn.
- Inductive reasoning begins with a set of empirical observations, seeking patterns in those observations, and then theorizing about those patterns.
- Deductive reasoning begins with a theory, developing hypotheses from that theory, and then collecting and analyzing data to test the truth of those hypotheses.
- Inductive and deductive reasoning can be employed together for a more complete understanding of the research topic.
- Though researchers don’t always set out to use both inductive and deductive reasoning in their work, they sometimes find that new questions arise in the course of an investigation that can best be answered by employing both approaches.
- Identify one theory and how it helps you understand your topic and working question.
I encourage you to find a specific theory from your topic area, rather than relying only on the broad theoretical perspectives like systems theory or the strengths perspective. Those broad theoretical perspectives are okay...but I promise that searching for theories about your topic will help you conceptualize and design your research project.
- Using the theory you identified, describe what you expect the answer to be to your working question.
- Define and provide an example of idiographic causal relationships
- Describe the role of causality in quantitative research as compared to qualitative research
- Identify, define, and describe each of the main criteria for nomothetic causal relationships
- Describe the difference between and provide examples of independent, dependent, and control variables
- Define hypothesis, state a clear hypothesis, and discuss the respective roles of quantitative and qualitative research when it comes to hypotheses
Causality refers to the idea that one event, behavior, or belief will result in the occurrence of another, subsequent event, behavior, or belief. In other words, it is about cause and effect. It seems simple, but you may be surprised to learn there is more than one way to explain how one thing causes another. How can that be? How could there be many ways to understand causality?
Think back to our discussion in Section 5.3 on paradigms [insert chapter link plus link to section 1.2]. You’ll remember the positivist paradigm as the one that believes in objectivity. Positivists look for causal explanations that are universally true for everyone, everywhere because they seek objective truth. Interpretivists, on the other hand, look for causal explanations that are true for individuals or groups in a specific time and place because they seek subjective truths. Remember that for interpretivists, there is not one singular truth that is true for everyone, but many truths created and shared by others.
"Are you trying to generalize or nah?"
One of my favorite classroom moments occurred in the early days of my teaching career. Students were providing peer feedback on their working questions. I overheard one group who was helping someone rephrase their research question. A student asked, “Are you trying to generalize or nah?” Teaching is full of fun moments like that one. Answering that one question can help you understand how to conceptualize and design your research project.
Nomothetic causal explanations are incredibly powerful. They allow scientists to make predictions about what will happen in the future, with a certain margin of error. Moreover, they allow scientists to generalize —that is, make claims about a large population based on a smaller sample of people or items. Generalizing is important. We clearly do not have time to ask everyone their opinion on a topic or test a new intervention on every person. We need a type of causal explanation that helps us predict and estimate truth in all situations.
Generally, nomothetic causal relationships work best for explanatory research projects [INSERT SECTION LINK]. They also tend to use quantitative research: by boiling things down to numbers, one can use the universal language of mathematics to use statistics to explore those relationships. On the other hand, descriptive and exploratory projects often fit better with idiographic causality. These projects do not usually try to generalize, but instead investigate what is true for individuals, small groups, or communities at a specific point in time. You will learn about this type of causality in the next section. Here, we will assume you have an explanatory working question. For example, you may want to know about the risk and protective factors for a specific diagnosis or how a specific therapy impacts client outcomes.
What do nomothetic causal explanations look like?
Nomothetic causal explanations express relationships between variables . The term variable has a scientific definition. This one from Gillespie & Wagner (2018) "a logical grouping of attributes that can be observed and measured and is expected to vary from person to person in a population" (p. 9). [91] More practically, variables are the key concepts in your working question. You know, the things you plan to observe when you actually do your research project, conduct your surveys, complete your interviews, etc. These things have two key properties. First, they vary , as in they do not remain constant. "Age" varies by number. "Gender" varies by category. But they both vary. Second, they have attributes . So the variable "health professions" has attributes or categories, such as social worker, nurse, counselor, etc.
It's also worth reviewing what is not a variable. Well, things that don't change (or vary) aren't variables. If you planned to do a study on how gender impacts earnings but your study only contained women, that concept would not vary . Instead, it would be a constant . Another common mistake I see in students' explanatory questions is mistaking an attribute for a variable. "Men" is not a variable. "Gender" is a variable. "Virginia" is not a variable. The variable is the "state or territory" in which someone or something is physically located.
When one variable causes another, we have what researchers call independent and dependent variables. For example, in a study investigating the impact of spanking on aggressive behavior, spanking would be the independent variable and aggressive behavior would be the dependent variable. An independent variable is the cause, and a dependent variable is the effect. Why are they called that? Dependent variables depend on independent variables. If all of that gets confusing, just remember the graphical relationship in Figure 8.5.
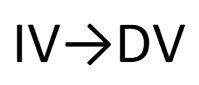
Write out your working question, as it exists now. As we said previously in the subsection, we assume you have an explanatory research question for learning this section.
- Write out a diagram similar to Figure 8.5.
- Put your independent variable on the left and the dependent variable on the right.
- Can your variables vary?
- Do they have different attributes or categories that vary from person to person?
- How does the theory you identified in section 8.1 help you understand this causal relationship?
If the theory you've identified isn't much help to you or seems unrelated, it's a good indication that you need to read more literature about the theories related to your topic.
For some students, your working question may not be specific enough to list an independent or dependent variable clearly. You may have "risk factors" in place of an independent variable, for example. Or "effects" as a dependent variable. If that applies to your research question, get specific for a minute even if you have to revise this later. Think about which specific risk factors or effects you are interested in. Consider a few options for your independent and dependent variable and create diagrams similar to Figure 8.5.
Finally, you are likely to revisit your working question so you may have to come back to this exercise to clarify the causal relationship you want to investigate.
For a ten-cent word like "nomothetic," these causal relationships should look pretty basic to you. They should look like "x causes y." Indeed, you may be looking at your causal explanation and thinking, "wow, there are so many other things I'm missing in here." In fact, maybe my dependent variable sometimes causes changes in my independent variable! For example, a working question asking about poverty and education might ask how poverty makes it more difficult to graduate college or how high college debt impacts income inequality after graduation. Nomothetic causal relationships are slices of reality. They boil things down to two (or often more) key variables and assert a one-way causal explanation between them. This is by design, as they are trying to generalize across all people to all situations. The more complicated, circular, and often contradictory causal explanations are idiographic, which we will cover in the next section of this chapter.
Developing a hypothesis
A hypothesis is a statement describing a researcher’s expectation regarding what they anticipate finding. Hypotheses in quantitative research are a nomothetic causal relationship that the researcher expects to determine is true or false. A hypothesis is written to describe the expected relationship between the independent and dependent variables. In other words, write the answer to your working question using your variables. That's your hypothesis! Make sure you haven't introduced new variables into your hypothesis that are not in your research question. If you have, write out your hypothesis as in Figure 8.5.
A good hypothesis should be testable using social science research methods. That is, you can use a social science research project (like a survey or experiment) to test whether it is true or not. A good hypothesis is also specific about the relationship it explores. For example, a student project that hypothesizes, "families involved with child welfare agencies will benefit from Early Intervention programs," is not specific about what benefits it plans to investigate. For this student, I advised her to take a look at the empirical literature and theory about Early Intervention and see what outcomes are associated with these programs. This way, she could more clearly state the dependent variable in her hypothesis, perhaps looking at reunification, attachment, or developmental milestone achievement in children and families under child welfare supervision.
Your hypothesis should be an informed prediction based on a theory or model of the social world. For example, you may hypothesize that treating mental health clients with warmth and positive regard is likely to help them achieve their therapeutic goals. That hypothesis would be based on the humanistic practice models of Carl Rogers. Using previous theories to generate hypotheses is an example of deductive research. If Rogers’ theory of unconditional positive regard is accurate, a study comparing clinicians who used it versus those who did not would show more favorable treatment outcomes for clients receiving unconditional positive regard.
Let’s consider a couple of examples. In research on sexual harassment (Uggen & Blackstone, 2004), [92] one might hypothesize, based on feminist theories of sexual harassment, that more females than males will experience specific sexually harassing behaviors. What is the causal relationship being predicted here? Which is the independent and which is the dependent variable? In this case, researchers hypothesized that a person’s sex (independent variable) would predict their likelihood to experience sexual harassment (dependent variable).
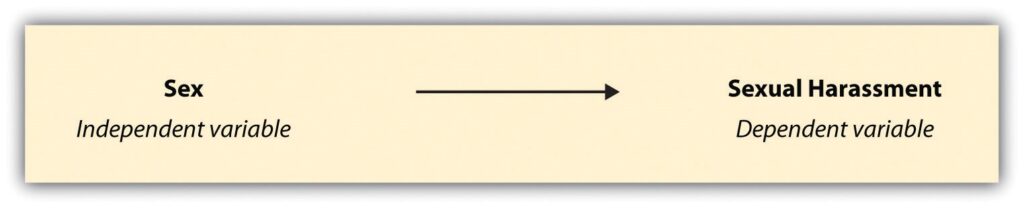
Sometimes researchers will hypothesize that a relationship will take a specific direction. As a result, an increase or decrease in one area might be said to cause an increase or decrease in another. For example, you might choose to study the relationship between age and support for legalization of marijuana. Perhaps you’ve taken a sociology class and, based on the theories you’ve read, you hypothesize that age is negatively related to support for marijuana legalization. [93] What have you just hypothesized?
You have hypothesized that as people get older, the likelihood of their supporting marijuana legalization decreases. Thus, as age (your independent variable) moves in one direction (up), support for marijuana legalization (your dependent variable) moves in another direction (down). So, a direct relationship (or positive correlation) involve two variables going in the same direction and an inverse relationship (or negative correlation) involve two variables going in opposite directions. If writing hypotheses feels tricky, it is sometimes helpful to draw them out and depict each of the two hypotheses we have just discussed.
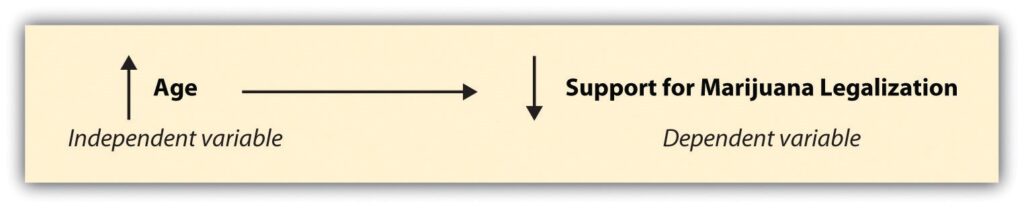
It’s important to note that once a study starts, it is unethical to change your hypothesis to match the data you find. For example, what happens if you conduct a study to test the hypothesis from Figure 8.7 on support for marijuana legalization, but you find no relationship between age and support for legalization? It means that your hypothesis was incorrect, but that’s still valuable information. It would challenge what the existing literature says on your topic, demonstrating that more research needs to be done to figure out the factors that impact support for marijuana legalization. Don’t be embarrassed by negative results, and definitely don’t change your hypothesis to make it appear correct all along!
Criteria for establishing a nomothetic causal relationship
Let’s say you conduct your study and you find evidence that supports your hypothesis, as age increases, support for marijuana legalization decreases. Success! Causal explanation complete, right? Not quite.
You’ve only established one of the criteria for causality. The criteria for causality must include all of the following: covariation, plausibility, temporality, and nonspuriousness. In our example from Figure 8.7, we have established only one criteria—covariation. When variables covary , they vary together. Both age and support for marijuana legalization vary in our study. Our sample contains people of varying ages and varying levels of support for marijuana legalization. If, for example, we only included 16-year-olds in our study, age would be a constant , not a variable.
Just because there might be some correlation between two variables does not mean that a causal relationship between the two is really plausible. Plausibility means that in order to make the claim that one event, behavior, or belief causes another, the claim has to make sense. It makes sense that people from previous generations would have different attitudes towards marijuana than younger generations. People who grew up in the time of Reefer Madness or the hippies may hold different views than those raised in an era of legalized medicinal and recreational use of marijuana. Plausibility is of course helped by basing your causal explanation in existing theoretical and empirical findings.
Once we’ve established that there is a plausible relationship between the two variables, we also need to establish whether the cause occurred before the effect, the criterion of temporality . A person’s age is a quality that appears long before any opinions on drug policy, so temporally the cause comes before the effect. It wouldn’t make any sense to say that support for marijuana legalization makes a person’s age increase. Even if you could predict someone’s age based on their support for marijuana legalization, you couldn’t say someone’s age was caused by their support for legalization of marijuana.
Finally, scientists must establish nonspuriousness. A spurious relationship is one in which an association between two variables appears to be causal but can in fact be explained by some third variable. This third variable is often called a confound or confounding variable because it clouds and confuses the relationship between your independent and dependent variable, making it difficult to discern the true causal relationship is.
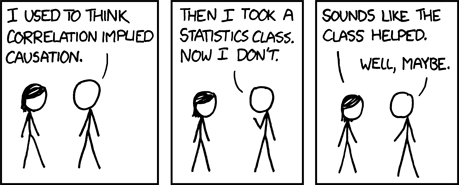
Continuing with our example, we could point to the fact that older adults are less likely to have used marijuana recreationally. Maybe it is actually recreational use of marijuana that leads people to be more open to legalization, not their age. In this case, our confounding variable would be recreational marijuana use. Perhaps the relationship between age and attitudes towards legalization is a spurious relationship that is accounted for by previous use. This is also referred to as the third variable problem , where a seemingly true causal relationship is actually caused by a third variable not in the hypothesis. In this example, the relationship between age and support for legalization could be more about having tried marijuana than the age of the person.
Quantitative researchers are sensitive to the effects of potentially spurious relationships. As a result, they will often measure these third variables in their study, so they can control for their effects in their statistical analysis. These are called control variables , and they refer to potentially confounding variables whose effects are controlled for mathematically in the data analysis process. Control variables can be a bit confusing, and we will discuss them more in Chapter 10, but think about it as an argument between you, the researcher, and a critic.
Researcher: “The older a person is, the less likely they are to support marijuana legalization.” Critic: “Actually, it’s more about whether a person has used marijuana before. That is what truly determines whether someone supports marijuana legalization.” Researcher: “Well, I measured previous marijuana use in my study and mathematically controlled for its effects in my analysis. Age explains most of the variation in attitudes towards marijuana legalization.”
Let’s consider a few additional, real-world examples of spuriousness. Did you know, for example, that high rates of ice cream sales have been shown to cause drowning? Of course, that’s not really true, but there is a positive relationship between the two. In this case, the third variable that causes both high ice cream sales and increased deaths by drowning is time of year, as the summer season sees increases in both (Babbie, 2010). [94]
Here’s another good one: it is true that as the salaries of Presbyterian ministers in Massachusetts rise, so too does the price of rum in Havana, Cuba. Well, duh, you might be saying to yourself. Everyone knows how much ministers in Massachusetts love their rum, right? Not so fast. Both salaries and rum prices have increased, true, but so has the price of just about everything else (Huff & Geis, 1993). [95]
Finally, research shows that the more firefighters present at a fire, the more damage is done at the scene. What this statement leaves out, of course, is that as the size of a fire increases so too does the amount of damage caused as does the number of firefighters called on to help (Frankfort-Nachmias & Leon-Guerrero, 2011). [96] In each of these examples, it is the presence of a confounding variable that explains the apparent relationship between the two original variables.
In sum, the following criteria must be met for a nomothetic causal relationship:
- The two variables must vary together.
- The relationship must be plausible.
- The cause must precede the effect in time.
- The relationship must be nonspurious (not due to a confounding variable).
The hypothetico-dedutive method
The primary way that researchers in the positivist paradigm use theories is sometimes called the hypothetico-deductive method (although this term is much more likely to be used by philosophers of science than by scientists themselves). Researchers choose an existing theory. Then, they make a prediction about some new phenomenon that should be observed if the theory is correct. Again, this prediction is called a hypothesis. The researchers then conduct an empirical study to test the hypothesis. Finally, they reevaluate the theory in light of the new results and revise it if necessary.
This process is usually conceptualized as a cycle because the researchers can then derive a new hypothesis from the revised theory, conduct a new empirical study to test the hypothesis, and so on. As Figure 8.8 shows, this approach meshes nicely with the process of conducting a research project—creating a more detailed model of “theoretically motivated” or “theory-driven” research. Together, they form a model of theoretically motivated research.
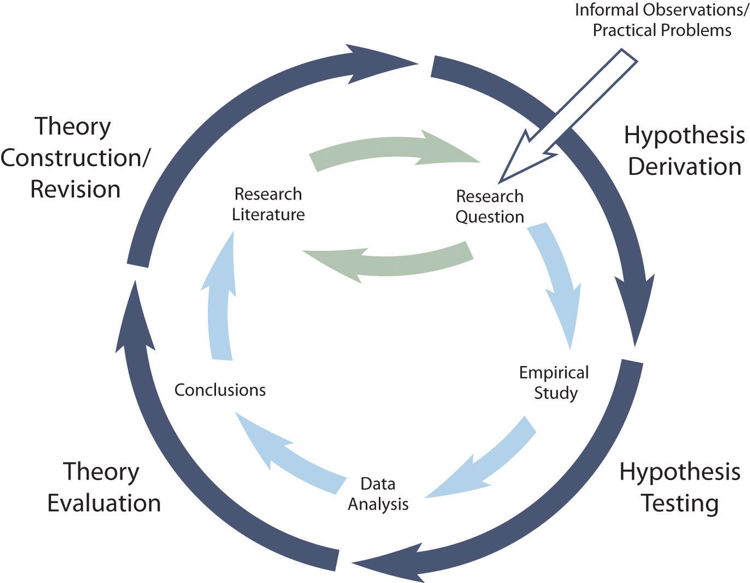
Keep in mind the hypothetico-deductive method is only one way of using social theory to inform social science research. It starts with describing one or more existing theories, deriving a hypothesis from one of those theories, testing your hypothesis in a new study, and finally reevaluating the theory based on the results data analyses. This format works well when there is an existing theory that addresses the research question—especially if the resulting hypothesis is surprising or conflicts with a hypothesis derived from a different theory.
But what if your research question is more interpretive? What if it is less about theory-testing and more about theory-building? This is what our next chapters will cover: the process of inductively deriving theory from people's stories and experiences. This process looks different than that depicted in Figure 8.8. It still starts with your research question and answering that question by conducting a research study. But instead of testing a hypothesis you created based on a theory, you will create a theory of your own that explain the data you collected. This format works well for qualitative research questions and for research questions that existing theories do not address.
- In positivist and quantitative studies, the goal is often to understand the more general causes of some phenomenon rather than the idiosyncrasies of one particular instance, as in an idiographic causal relationship.
- Nomothetic causal explanations focus on objectivity, prediction, and generalization.
- Criteria for nomothetic causal relationships require the relationship be plausible and nonspurious; and that the cause must precede the effect in time.
- In a nomothetic causal relationship, the independent variable causes changes in the dependent variable.
- Hypotheses are statements, drawn from theory, which describe a researcher’s expectation about a relationship between two or more variables.
- Write out your working question and hypothesis.
- Defend your hypothesis in a short paragraph, using arguments based on the theory you identified in section 8.1.
- Review the criteria for a nomothetic causal relationship. Critique your short paragraph about your hypothesis using these criteria.
- Are there potentially confounding variables, issues with time order, or other problems you can identify in your reasoning?
Inductive & deductive (deductive focus)
9. Writing your research question Copyright © 2020 by Matthew DeCarlo is licensed under a Creative Commons Attribution-NonCommercial-ShareAlike 4.0 International License , except where otherwise noted.
Share This Book
Want to create or adapt books like this? Learn more about how Pressbooks supports open publishing practices.
Module 3 Chapter 4: Overview of Quantitative Study Variables
The first thing we need to understand is the nature of variables and how variables are used in a study’s design to answer the study questions. In this chapter you will learn:
- different types of variables in quantitative studies,
- issues surrounding the unit of analysis question.
Understanding Quantitative Variables
The root of the word variable is related to the word “vary,” which should help us understand what variables might be. Variables are elements, entities, or factors that can change (vary); for example, the outdoor temperature, the cost of gasoline per gallon, a person’s weight, and the mood of persons in your extended family are all variables. In other words, they can have different values under different conditions or for different people.
We use variables to describe features or factors of interest. Examples might include the number of members in different households, the distance to healthful food sources in different neighborhoods, the ratio of social work faculty to students in a BSW or MSW program, the proportion of persons from different racial/ethnic groups incarcerated, the cost of transportation to receive services from a social work program, or the rate of infant mortality in different counties. In social work intervention research, variables might include characteristics of the intervention (intensity, frequency, duration) and outcomes associated with the intervention.
Demographic Variables . Social workers are often interested in what we call demographic variables . Demographic variables are used to describe characteristics of a population, group, or sample of the population. Examples of frequently applied demographic variables are
- national origin,
- religious affiliation,
- sexual orientation,
- marital/relationship status,
- employment status,
- political affiliation,
- geographical location,
- education level, and
At a more macro level, the demographics of a community or organization often includes its size; organizations are often measured in terms of their overall budget.
Independent and Dependent Variables . A way that investigators think about study variables has important implications for a study design. Investigators make decisions about having them serve as either independent variables or as dependent variables . This distinction is not something inherent to a variable, it is based on how the investigator chooses to define each variable. Independent variables are the ones you might think of as the manipulated “input” variables, while the dependent variables are the ones where the impact or “output” of that input variation would be observed.
Intentional manipulation of the “input” (independent) variable is not always involved. Consider the example of a study conducted in Sweden examining the relationship between having been the victim of child maltreatment and later absenteeism from high school: no one intentionally manipulated whether the children would be victims of child maltreatment (Hagborg, Berglund, & Fahlke, 2017). The investigators hypothesized that naturally occurring differences in the input variable (child maltreatment history) would be associated with systematic variation in a specific outcome variable (school absenteeism). In this case, the independent variable was a history of being the victim of child maltreatment, and the dependent variable was the school absenteeism outcome. In other words, the independent variable is hypothesized by the investigator to cause variation or change in the dependent variable. This is what it might look like in a diagram where “ x ” is the independent variable and “ y ” is the dependent variable (note: you saw this designation earlier, in Chapter 3, when we discussed cause and effect logic):
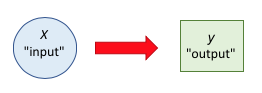
For another example, consider research indicating that being the victim of child maltreatment is associated with a higher risk of substance use during adolescence (Yoon, Kobulsky, Yoon, & Kim, 2017). The independent variable in this model would be having a history of child maltreatment. The dependent variable would be risk of substance use during adolescence. This example is even more elaborate because it specifies the pathway by which the independent variable (child maltreatment) might impose its effects on the dependent variable (adolescent substance use). The authors of the study demonstrated that post-traumatic stress (PTS) was a link between childhood abuse (physical and sexual) and substance use during adolescence.
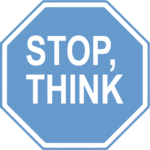
Take a moment to complete the following activity.
Types of Quantitative Variables
There are other meaningful ways to think about variables of interest, as well. Let’s consider different features of variables used in quantitative research studies. Here we explore quantitative variables as being categorical, ordinal, or interval in nature. These features have implications for both measurement and data analysis.
Categorical Variables. Some variables can take on values that vary, but not in a meaningful numerical way. Instead, they might be defined in terms of the categories which are possible. Logically, these are called categorical variables . Statistical software and textbooks sometimes refer to variables with categories as nominal variables . Nominal can be thought of in terms of the Latin root “nom” which means “name,” and should not be confused with number. Nominal means the same thing as categorical in describing variables. In other words, categorical or nominal variables are identified by the names or labels of the represented categories. For example, the color of the last car you rode in would be a categorical variable: blue, black, silver, white, red, green, yellow, or other are categories of the variable we might call car color.
What is important with categorical variables is that these categories have no relevant numeric sequence or order. There is no numeric difference between the different car colors, or difference between “yes” or “no” as the categories in answering if you rode in a blue car. There is no implied order or hierarchy to the categories “Hispanic or Latino” and “Not Hispanic or Latino” in an ethnicity variable; nor is there any relevant order to categories of variables like gender, the state or geographical region where a person resides, or whether a person’s residence is owned or rented.
If a researcher decided to use numbers as symbols related to categories in such a variable, the numbers are arbitrary—each number is essentially just a different, shorter name for each category. For example, the variable gender could be coded in the following ways, and it would make no difference, as long as the code was consistently applied.
Race and ethnicity. One of the most commonly explored categorical variables in social work and social science research is the demographic referring to a person’s racial and/or ethnic background. Many studies utilize the categories specified in past U.S. Census Bureau reports. Here is what the U.S. Census Bureau has to say about the two distinct demographic variables, race and ethnicity ( https://www.census.gov/mso/www/training/pdf/race-ethnicity-onepager.pdf ):
What is race? The Census Bureau defines race as a person’s self-identification with one or more social groups. An individual can report as White, Black or African American, Asian, American Indian and Alaska Native, Native Hawaiian and Other Pacific Islander, or some other race. Survey respondents may report multiple races. What is ethnicity? Ethnicity determines whether a person is of Hispanic origin or not. For this reason, ethnicity is broken out into two categories, Hispanic or Latino and Not Hispanic or Latino. Hispanics may report as any race.
In other words, the Census Bureau defines two categories for the variable called ethnicity (Hispanic or Latino and Not Hispanic or Latino), and seven categories for the variable called race. While these variables and categories are often applied in social science and social work research, they are not without criticism.
Based on these categories, here is what is estimated to be true of the U.S. population in 2016:
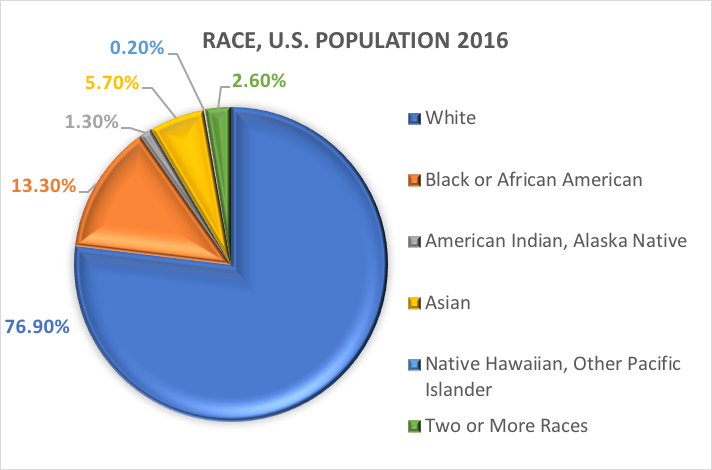
Dichotomous variables. There exists a special category of categorical variable with implications for certain statistical analyses. Categorical variables comprised of exactly two options, no more and no fewer are called dichotomous variables . One example was the U.S. Census Bureau dichotomy of Hispanic/Latino and Non-Hispanic/Non-Latino ethnicity. For another example, investigators might wish to compare people who complete treatment with those who drop out before completing treatment. With the two categories, completed or not completed, this treatment completion variable is not only categorical, it is dichotomous. Variables where individuals respond “yes” or “no” are also dichotomous in nature.
The past tradition of treating gender as either male or female is another example of a dichotomous variable. However, very strong arguments exist for no longer treating gender in this dichotomous manner: a greater variety of gender identities are demonstrably relevant in social work for persons whose identity does not align with the dichotomous (also called binary) categories of man/woman or male/female. These include categories such as agender, androgynous, bigender, cisgender, gender expansive, gender fluid, gender questioning, queer, transgender, and others.
Ordinal Variables. Unlike these categorical variables, sometimes a variable’s categories do have a logical numerical sequence or order. Ordinal, by definition, refers to a position in a series. Variables with numerically relevant categories are called ordinal variables. For example, there is an implied order of categories from least-to-most with the variable called educational attainment. The U.S. Census data categories for this ordinal variable are:
- 1st-4thgrade
- 5th-6thgrade
- 7th-8thgrade
- high school graduate
- some college, no degree
- associate’s degree, occupational
- associate’s degree academic
- bachelor’s degree
- master’s degree
- professional degree
- doctoral degree
In looking at the 2016 Census Bureau estimate data for this variable, we can see that females outnumbered males in the category of having attained a bachelor’s degree: of the 47,718,000 persons in this category, 22,485,000 were male and 25,234,000 were female. While this gendered pattern held for those receiving master’s degrees, the pattern was reversed for receiving doctoral degrees: more males than females obtained this highest level of education. It is also interesting to note that females outnumbered males at the low end of the spectrum: 441,000 females reported no education compared to 374,000 males.
Here is another example of using ordinal variables in social work research: when individuals seek treatment for a problem with alcohol misuse, social workers may wish to know if this is their first, second, third, or whatever numbered serious attempt to change their drinking behavior. Participants enrolled in a study comparing treatment approaches for alcohol use disorders reported that the intervention study was anywhere from their first to eleventh significant change attempt (Begun, Berger, Salm-Ward, 2011). This change attempt variable has implications for how social workers might interpret data evaluating an intervention that was not the first try for everyone involved.
Rating scales. Consider a different but commonly used type of ordinal variable: rating scales. Social, behavioral, and social work investigators often ask study participants to apply a rating scale to describe their knowledge, attitudes, beliefs, opinions, skills, or behavior. Because the categories on such a scale are sequenced (most to least or least to most), we call these ordinal variables.
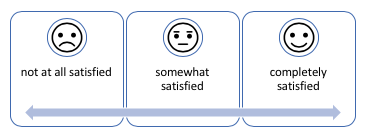
Examples include having participants rate:
- how much they agree or disagree with certain statements (not at all to extremely much);
- how often they engage in certain behaviors (never to always);
- how often they engage in certain behaviors (hourly, daily, weekly, monthly, annually, or less often);
- the quality of someone’s performance (poor to excellent);
- how satisfied they were with their treatment (very dissatisfied to very satisfied)
- their level of confidence (very low to very high).
Interval Variables. Still other variables take on values that vary in a meaningful numerical fashion. From our list of demographic variables, age is a common example. The numeric value assigned to an individual person indicates the number of years since a person was born (in the case of infants, the numeric value may indicate days, weeks, or months since birth). Here the possible values for the variable are ordered, like the ordinal variables, but a big difference is introduced: the nature of the intervals between possible values. With interval variables the “distance” between adjacent possible values are equal. Some statistical software packages and textbooks use the term scale variable : this is exactly the same thing as what we call an interval variable.
For example, in the graph below, the 1 ounce difference between this person consuming 1 ounce or 2 ounces of alcohol (Monday, Tuesday) is exactly the same as the 1 ounce difference between consuming 4 ounces or 5 ounces (Friday, Saturday). If we were to diagram the possible points on the scale, they would all be equidistant; the interval between any two points is measured in standard units (ounces, in this example).
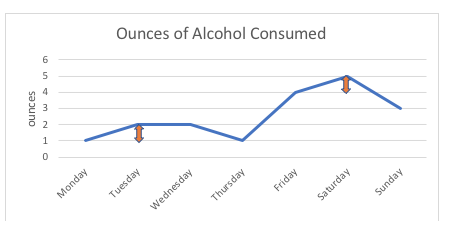
With ordinal variables, such as a rating scales, no one can say for certain that the “distance” between the response options of “never” and “sometimes” is the same as the “distance” between “sometimes” and “often,” even if we used numbers to sequence these response options. Thus, the rating scale remains ordinal, not interval.
What might become a tad confusing is that certain statistical software programs, like SPSS, refer to an interval variable as a “scale” variable. Many variables used in social work research are both ordered and have equal distances between points. Consider for example, the variable of birth order. This variable is interval because:
- the possible values are ordered (e.g., the third-born child came after the first- and second-born and before the fourth-born), and
- the “distances” or intervals are measured in equivalent one-person units.
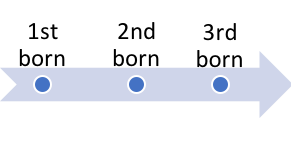
Continuous variables. There exists a special type of numeric interval variable that we call continuous variables. A variable like age might be treated as a continuous variable. Age is ordinal in nature, since higher numbers mean something in relation to smaller numbers. Age also meets our criteria for being an interval variable if we measure it in years (or months or weeks or days) because it is ordinal and there is the same “distance” between being 15 and 30 years old as there is between being 40 and 55 years old (15 calendar years). What makes this a continuous variable is that there are also possible, meaningful “fraction” points between any two intervals. For example, a person can be 20½ (20.5) or 20¼ (20.25) or 20¾ (20.75) years old; we are not limited to just the whole numbers for age. By contrast, when we looked at birth order, we cannot have a meaningful fraction of a person between two positions on the scale.
The Special Case of Income . One of the most abused variables in social science and social work research is the variable related to income. Consider an example about household income (regardless of how many people are in the household). This variable could be categorical (nominal), ordinal, or interval (scale) depending on how it is handled.
Categorical Example: Depending on the nature of the research questions, an investigator might simply choose to use the dichotomous categories of “sufficiently resourced” and “insufficiently resourced” for classifying households, based on some standard calculation method. These might be called “poor” and “not poor” if a poverty line threshold is used to categorize households. These distinct income variable categories are not meaningfully sequenced in a numerical fashion, so it is a categorical variable.
Ordinal Example: Categories for classifying households might be ordered from low to high. For example, these categories for annual income are common in market research:
- Less than $25,000.
- $25,000 to $34,999.
- $35,000 to $49,999.
- $50,000 to $74,999.
- $75,000 to $99,999.
- $100,000 to $149,999.
- $150,000 to $199,999.
- $200,000 or more.
Notice that the categories are not equally sized—the “distance” between pairs of categories are not always the same. They start out in about $10,000 increments, move to $25,000 increments, and end up in about $50,000 increments.
Interval Example . If an investigator asked study participants to report an actual dollar amount for household income, we would see an interval variable. The possible values are ordered and the interval between any possible adjacent units is $1 (as long as dollar fractions or cents are not used). Thus, an income of $10,452 is the same distance on a continuum from $9,452 and $11,452—$1,000 either way.
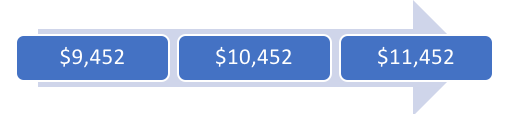
The Special Case of Age . Like income, “age” can mean different things in different studies. Age is usually an indicator of “time since birth.” We can calculate a person’s age by subtracting a date of birth variable from the date of measurement (today’s date minus date of birth). For adults, ages are typically measured in years where adjacent possible values are distanced in 1-year units: 18, 19, 20, 21, 22, and so forth. Thus, the age variable could be a continuous type of interval variable.
However, an investigator might wish to collapse age data into ordered categories or age groups. These still would be ordinal, but might no longer be interval if the increments between possible values are not equivalent units. For example, if we are more interested in age representing specific human development periods, the age intervals might not be equal in span between age criteria. Possibly they might be:
- Infancy (birth to 18 months)
- Toddlerhood (18 months to 2 ½ years)
- Preschool (2 ½ to 5 years)
- School age (6 to 11 years)
- Adolescence (12 to 17 years)
- Emerging Adulthood (18 to 25 years)
- Adulthood (26 to 45 years)
- Middle Adulthood (46 to 60 years)
- Young-Old Adulthood (60 to 74 years)
- Middle-Old Adulthood (75 to 84 years)
- Old-Old Adulthood (85 or more years)
Age might even be treated as a strictly categorical (non-ordinal) variable. For example, if the variable of interest is whether someone is of legal drinking age (21 years or older), or not. We have two categories—meets or does not meet legal drinking age criteria in the United States—and either one could be coded with a “1” and the other as either a “0” or “2” with no difference in meaning.
What is the “right” answer as to how to measure age (or income)? The answer is “it depends.” What it depends on is the nature of the research question: which conceptualization of age (or income) is most relevant for the study being designed.
Alphanumeric Variables. Finally, there are data which do not fit into any of these classifications. Sometimes the information we know is in the form of an address or telephone number, a first or last name, zipcode, or other phrases. These kinds of information are sometimes called alphanumeric variables . Consider the variable “address” for example: a person’s address might be made up of numeric characters (the house number) and letter characters (spelling out the street, city, and state names), such as 1600 Pennsylvania Ave. NW, Washington, DC, 20500.
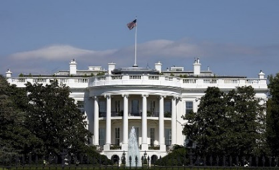
Actually, we have several variables present in this address example:
- the street address: 1600 Pennsylvania Ave.
- the city (and “state”): Washington, DC
- the zipcode: 20500.
This type of information does not represent specific quantitative categories or values with systematic meaning in the data. These are also sometimes called “string” variables in certain software packages because they are made up of a string of symbols. To be useful for an investigator, such a variable would have to be converted or recoded into meaningful values.
A Note about Unit of Analysis
An important thing to keep in mind in thinking about variables is that data may be collected at many different levels of observation. The elements studied might be individual cells, organ systems, or persons. Or, the level of observation might be pairs of individuals, such as couples, brothers and sisters, or parent-child dyads. In this case, the investigator may collect information about the pair from each individual, but is looking at each pair’s data. Thus, we would say that the unit of analysis is the pair or dyad, not each individual person. The unit of analysis could be a larger group, too: for example, data could be collected from each of the students in entire classrooms where the unit of analysis is classrooms in a school or school system. Or, the unit of analysis might be at the level of neighborhoods, programs, organizations, counties, states, or even nations. For example, many of the variables used as indicators of food security at the level of communities, such as affordability and accessibility, are based on data collected from individual households (Kaiser, 2017). The unit of analysis in studies using these indicators would be the communities being compared. This distinction has important measurement and data analysis implications.
A Reminder about Variables versus Variable Levels
A study might be described in terms of the number of variable categories, or levels, that are being compared. For example, you might see a study described as a 2 X 2 design—pronounced as a two by two design. This means that there are 2 possible categories for the first variable and 2 possible categories for the other variable—they are both dichotomous variables. A study comparing 2 categories of the variable “alcohol use disorder” (categories for meets criteria, yes or no) with 2 categories of the variable “illicit substance use disorder” (categories for meets criteria, yes or no) would have 4 possible outcomes (mathematically, 2 x 2=4) and might be diagrammed like this (data based on proportions from the 2016 NSDUH survey, presented in SAMHSA, 2017):
Reading the 4 cells in this 2 X 2 table tells us that in this (hypothetical) survey of 540 individuals, 500 did not meet criteria for either an alcohol or illicit substance use disorder (No, No); 26 met criteria for an alcohol use disorder only (Yes, No); 10 met criteria for an illicit substance use disorder only (No, Yes), and 4 met criteria for both an alcohol and illicit substance use disorder (Yes, Yes). In addition, with a little math applied, we can see that a total of 30 had an alcohol use disorder (26 + 4) and 14 had an illicit substance use disorder (10 + 4). And, we can see that 40 had some sort of substance use disorder (26 + 10 + 4).
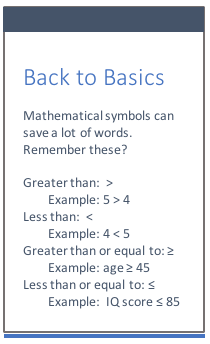
Thus, when you see a study design description that looks like two numbers being multiplied, that is essentially telling you how many categories or levels of each variable there are and leads you to understand how many cells or possible outcomes exist. A 3 X 3 design has 9 cells, a 3 X 4 design has 12 cells, and so forth. This issue becomes important once again when we discuss sample size in Chapter 6.
Interactive Excel Workbook Activities
Complete the following Workbook Activity:
- SWK 3401.3-4.1 Beginning Data Entry
Chapter Summary
In summary, investigators design many of their quantitative studies to test hypotheses about the relationships between variables. Understanding the nature of the variables involved helps in understanding and evaluating the research conducted. Understanding the distinctions between different types of variables, as well as between variables and categories, has important implications for study design, measurement, and samples. Among other topics, the next chapter explores the intersection between the nature of variables studied in quantitative research and how investigators set about measuring those variables.
Social Work 3401 Coursebook Copyright © by Dr. Audrey Begun is licensed under a Creative Commons Attribution-NonCommercial-ShareAlike 4.0 International License , except where otherwise noted.
Share This Book
An official website of the United States government
Official websites use .gov A .gov website belongs to an official government organization in the United States.
Secure .gov websites use HTTPS A lock ( Lock Locked padlock icon ) or https:// means you've safely connected to the .gov website. Share sensitive information only on official, secure websites.
- Publications
- Account settings
- Advanced Search
- Journal List

Family Relationships and Well-Being
Patricia a thomas , phd, hui liu , phd, debra umberson , phd.
- Author information
- Article notes
- Copyright and License information
Address correspondence to: Patricia A. Thomas, PhD, Department of Sociology, Purdue University, 700 W. State Street, West Lafayette, IN 47907. E-mail: [email protected]
Collection date 2017 Nov.
This is an Open Access article distributed under the terms of the Creative Commons Attribution-NonCommercial-NoDerivs licence ( http://creativecommons.org/licenses/by-nc-nd/4.0/ ), which permits non-commercial reproduction and distribution of the work, in any medium, provided the original work is not altered or transformed in any way, and that the work is properly cited. For commercial re-use, please contact [email protected]
Family relationships are enduring and consequential for well-being across the life course. We discuss several types of family relationships—marital, intergenerational, and sibling ties—that have an important influence on well-being. We highlight the quality of family relationships as well as diversity of family relationships in explaining their impact on well-being across the adult life course. We discuss directions for future research, such as better understanding the complexities of these relationships with greater attention to diverse family structures, unexpected benefits of relationship strain, and unique intersections of social statuses.
Keywords: Caregiver stress, Gender issues, Intergenerational, Social support, Well-being
Translational Significance
It is important for future research and health promotion policies to take into account complexities in family relationships, paying attention to family context, diversity of family structures, relationship quality, and intersections of social statuses in an aging society to provide resources to families to reduce caregiving burdens and benefit health and well-being.
For better and for worse, family relationships play a central role in shaping an individual’s well-being across the life course ( Merz, Consedine, Schulze, & Schuengel, 2009 ). An aging population and concomitant age-related disease underlies an emergent need to better understand factors that contribute to health and well-being among the increasing numbers of older adults in the United States. Family relationships may become even more important to well-being as individuals age, needs for caregiving increase, and social ties in other domains such as the workplace become less central in their lives ( Milkie, Bierman, & Schieman, 2008 ). In this review, we consider key family relationships in adulthood—marital, parent–child, grandparent, and sibling relationships—and their impact on well-being across the adult life course.
We begin with an overview of theoretical explanations that point to the primary pathways and mechanisms through which family relationships influence well-being, and then we describe how each type of family relationship is associated with well-being, and how these patterns unfold over the adult life course. In this article, we use a broad definition of well-being, including multiple dimensions such as general happiness, life satisfaction, and good mental and physical health, to reflect the breadth of this concept’s use in the literature. We explore important directions for future research, emphasizing the need for research that takes into account the complexity of relationships, diverse family structures, and intersections of structural locations.
Pathways Linking Family Relationships to Well-Being
A life course perspective draws attention to the importance of linked lives, or interdependence within relationships, across the life course ( Elder, Johnson, & Crosnoe, 2003 ). Family members are linked in important ways through each stage of life, and these relationships are an important source of social connection and social influence for individuals throughout their lives ( Umberson, Crosnoe, & Reczek, 2010 ). Substantial evidence consistently shows that social relationships can profoundly influence well-being across the life course ( Umberson & Montez, 2010 ). Family connections can provide a greater sense of meaning and purpose as well as social and tangible resources that benefit well-being ( Hartwell & Benson, 2007 ; Kawachi & Berkman, 2001 ).
The quality of family relationships, including social support (e.g., providing love, advice, and care) and strain (e.g., arguments, being critical, making too many demands), can influence well-being through psychosocial, behavioral, and physiological pathways. Stressors and social support are core components of stress process theory ( Pearlin, 1999 ), which argues that stress can undermine mental health while social support may serve as a protective resource. Prior studies clearly show that stress undermines health and well-being ( Thoits, 2010 ), and strains in relationships with family members are an especially salient type of stress. Social support may provide a resource for coping that dulls the detrimental impact of stressors on well-being ( Thoits, 2010 ), and support may also promote well-being through increased self-esteem, which involves more positive views of oneself ( Fukukawa et al., 2000 ). Those receiving support from their family members may feel a greater sense of self-worth, and this enhanced self-esteem may be a psychological resource, encouraging optimism, positive affect, and better mental health ( Symister & Friend, 2003 ). Family members may also regulate each other’s behaviors (i.e., social control) and provide information and encouragement to behave in healthier ways and to more effectively utilize health care services ( Cohen, 2004 ; Reczek, Thomeer, Lodge, Umberson, & Underhill, 2014 ), but stress in relationships may also lead to health-compromising behaviors as coping mechanisms to deal with stress ( Ng & Jeffery, 2003 ). The stress of relationship strain can result in physiological processes that impair immune function, affect the cardiovascular system, and increase risk for depression ( Graham, Christian, & Kiecolt-Glaser, 2006 ; Kiecolt-Glaser & Newton, 2001 ), whereas positive relationships are associated with lower allostatic load (i.e., “wear and tear” on the body accumulating from stress) ( Seeman, Singer, Ryff, Love, & Levy-Storms, 2002 ). Clearly, the quality of family relationships can have considerable consequences for well-being.
Marital Relationships
A life course perspective has posited marital relationships as one of the most important relationships that define life context and in turn affect individuals’ well-being throughout adulthood ( Umberson & Montez, 2010 ). Being married, especially happily married, is associated with better mental and physical health ( Carr & Springer, 2010 ; Umberson, Williams, & Thomeer, 2013 ), and the strength of the marital effect on health is comparable to that of other traditional risk factors such as smoking and obesity ( Sbarra, 2009 ). Although some studies emphasize the possibility of selection effects, suggesting that individuals in better health are more likely to be married ( Lipowicz, 2014 ), most researchers emphasize two theoretical models to explain why marital relationships shape well-being: the marital resource model and the stress model ( Waite & Gallager, 2000 ; Williams & Umberson, 2004 ). The marital resource model suggests that marriage promotes well-being through increased access to economic, social, and health-promoting resources ( Rendall, Weden, Favreault, & Waldron, 2011 ; Umberson et al., 2013 ). The stress model suggests that negative aspects of marital relationships such as marital strain and marital dissolutions create stress and undermine well-being ( Williams & Umberson, 2004 ), whereas positive aspects of marital relationships may prompt social support, enhance self-esteem, and promote healthier behaviors in general and in coping with stress ( Reczek, Thomeer, et al., 2014 ; Symister & Friend, 2003 ; Waite & Gallager, 2000 ). Marital relationships also tend to become more salient with advancing age, as other social relationships such as those with family members, friends, and neighbors are often lost due to geographic relocation and death in the later part of the life course ( Liu & Waite, 2014 ).
Married people, on average, enjoy better mental health, physical health, and longer life expectancy than divorced/separated, widowed, and never-married people ( Hughes & Waite, 2009 ; Simon, 2002 ), although the health gap between the married and never married has decreased in the past few decades ( Liu & Umberson, 2008 ). Moreover, marital links to well-being depend on the quality of the relationship; those in distressed marriages are more likely to report depressive symptoms and poorer health than those in happy marriages ( Donoho, Crimmins, & Seeman, 2013 ; Liu & Waite, 2014 ; Umberson, Williams, Powers, Liu, & Needham, 2006 ), whereas a happy marriage may buffer the effects of stress via greater access to emotional support ( Williams, 2003 ). A number of studies suggest that the negative aspects of close relationships have a stronger impact on well-being than the positive aspects of relationships (e.g., Rook, 2014 ), and past research shows that the impact of marital strain on health increases with advancing age ( Liu & Waite, 2014 ; Umberson et al., 2006 ).
Prior studies suggest that marital transitions, either into or out of marriage, shape life context and affect well-being ( Williams & Umberson, 2004 ). National longitudinal studies provide evidence that past experiences of divorce and widowhood are associated with increased risk of heart disease in later life especially among women, irrespective of current marital status ( Zhang & Hayward, 2006 ), and longer duration of divorce or widowhood is associated with a greater number of chronic conditions and mobility limitations ( Hughes & Waite, 2009 ; Lorenz, Wickrama, Conger, & Elder, 2006 ) but only short-term declines in mental health ( Lee & Demaris, 2007 ). On the other hand, entry into marriages, especially first marriages, improves psychological well-being and decreases depression ( Frech & Williams, 2007 ; Musick & Bumpass, 2012 ), although the benefits of remarriage may not be as large as those that accompany a first marriage ( Hughes & Waite, 2009 ). Taken together, these studies show the importance of understanding the lifelong cumulative impact of marital status and marital transitions.
Gender Differences
Gender is a central focus of research on marital relationships and well-being and an important determinant of life course experiences ( Bernard, 1972 ; Liu & Waite, 2014 ; Zhang & Hayward, 2006 ). A long-observed pattern is that men receive more physical health benefits from marriage than women, and women are more psychologically and physiologically vulnerable to marital stress than men ( Kiecolt-Glaser & Newton, 2001 ; Revenson et al., 2016 ; Simon, 2002 ; Williams, 2004 ). Women tend to receive more financial benefits from their typically higher-earning male spouse than do men, but men generally receive more health promotion benefits such as emotional support and regulation of health behaviors from marriage than do women ( Liu & Umberson, 2008 ; Liu & Waite, 2014 ). This is because within a traditional marriage, women tend to take more responsibility for maintaining social connections to family and friends, and are more likely to provide emotional support to their husband, whereas men are more likely to receive emotional support and enjoy the benefit of expanded social networks—all factors that may promote husbands’ health and well-being ( Revenson et al., 2016 ).
However, there is mixed evidence regarding whether men’s or women’s well-being is more affected by marriage. On the one hand, a number of studies have documented that marital status differences in both mental and physical health are greater for men than women ( Liu & Umberson, 2008 ; Sbarra, 2009 ). For example, Williams and Umberson (2004) found that men’s health improves more than women’s from entering marriage. On the other hand, a number of studies reveal stronger effects of marital strain on women’s health than men’s including more depressive symptoms, increases in cardiovascular health risk, and changes in hormones ( Kiecolt-Glaser & Newton, 2001 ; Liu & Waite, 2014 ; Liu, Waite, & Shen, 2016 ). Yet, other studies found no gender differences in marriage and health links (e.g., Umberson et al., 2006 ). The mixed evidence regarding gender differences in the impact of marital relationships on well-being may be attributed to different study samples (e.g., with different age groups) and variations in measurements and methodologies. More research based on representative longitudinal samples is clearly warranted to contribute to this line of investigation.
Race-Ethnicity and SES Heterogeneity
Family scholars argue that marriage has different meanings and dynamics across socioeconomic status (SES) and racial-ethnic groups due to varying social, economic, historical, and cultural contexts. Therefore, marriage may be associated with well-being in different ways across these groups. For example, women who are black or lower SES may be less likely than their white, higher SES counterparts to increase their financial capital from relationship unions because eligible men in their social networks are more socioeconomically challenged ( Edin & Kefalas, 2005 ). Some studies also find that marital quality is lower among low SES and black couples than white couples with higher SES ( Broman, 2005 ). This may occur because the former groups face more stress in their daily lives throughout the life course and these higher levels of stress undermine marital quality ( Umberson, Williams, Thomas, Liu, & Thomeer, 2014 ). Other studies, however, suggest stronger effects of marriage on the well-being of black adults than white adults. For example, black older adults seem to benefit more from marriage than older whites in terms of chronic conditions and disability ( Pienta, Hayward, & Jenkins, 2000 ).
Directions for Future Research
The rapid aging of the U.S. population along with significant changes in marriage and families indicate that a growing number of older adults enter late life with both complex marital histories and great heterogeneity in their relationships. While most research to date focuses on different-sex marriages, a growing body of research has started to examine whether the marital advantage in health and well-being is extended to same-sex couples, which represents a growing segment of relationship types among older couples ( Denney, Gorman, & Barrera, 2013 ; Goldsen et al., 2017 ; Liu, Reczek, & Brown, 2013 ; Reczek, Liu, & Spiker, 2014 ). Evidence shows that same-sex cohabiting couples report worse health than different-sex married couples ( Denney et al., 2013 ; Liu et al., 2013 ), but same-sex married couples are often not significantly different from or are even better off than different-sex married couples in other outcomes such as alcohol use ( Reczek, Liu, et al., 2014 ) and care from their partner during periods of illness ( Umberson, Thomeer, Reczek, & Donnelly, 2016 ). These results suggest that marriage may promote the well-being of same-sex couples, perhaps even more so than for different-sex couples ( Umberson et al., 2016 ). Including same-sex couples in future work on marriage and well-being will garner unique insights into gender differences in marital dynamics that have long been taken for granted based on studies of different-sex couples ( Umberson, Thomeer, Kroeger, Lodge, & Xu, 2015 ). Moreover, future work on same-sex and different-sex couples should take into account the intersection of other statuses such as race-ethnicity and SES to better understand the impact of marital relationships on well-being.
Another avenue for future research involves investigating complexities of marital strain effects on well-being. Some recent studies among older adults suggest that relationship strain may actually benefit certain dimensions of well-being. These studies suggest that strain with a spouse may be protective for certain health outcomes including cognitive decline ( Xu, Thomas, & Umberson, 2016 ) and diabetes control ( Liu et al., 2016 ), while support may not be, especially for men ( Carr, Cornman, & Freedman, 2016 ). Explanations for these unexpected findings among older adults are not fully understood. Family and health scholars suggest that spouses may prod their significant others to engage in more health-promoting behaviors ( Umberson, Crosnoe, et al., 2010 ). These attempts may be a source of friction, creating strain in the relationship; however, this dynamic may still contribute to better health outcomes for older adults. Future research should explore the processes by which strain may have a positive influence on health and well-being, perhaps differently by gender.
Intergenerational Relationships
Children and parents tend to remain closely connected to each other across the life course, and it is well-established that the quality of intergenerational relationships is central to the well-being of both generations ( Merz, Schuengel, & Schulze, 2009 ; Polenick, DePasquale, Eggebeen, Zarit, & Fingerman, 2016 ). Recent research also points to the importance of relationships with grandchildren for aging adults ( Mahne & Huxhold, 2015 ). We focus here on the well-being of parents, adult children, and grandparents. Parents, grandparents, and children often provide care for each other at different points in the life course, which can contribute to social support, stress, and social control mechanisms that influence the health and well-being of each in important ways over the life course ( Nomaguchi & Milkie, 2003 ; Pinquart & Soerensen, 2007 ; Reczek, Thomeer, et al., 2014 ).
Family scholarship highlights the complexities of parent–child relationships, finding that parenthood generates both rewards and stressors, with important implications for well-being ( Nomaguchi & Milkie, 2003 ; Umberson, Pudrovska, & Reczek, 2010 ). Parenthood increases time constraints, producing stress and diminishing well-being, especially when children are younger ( Nomaguchi, Milkie, & Bianchi, 2005 ), but parenthood can also increase social integration, leading to greater emotional support and a sense of belonging and meaning ( Berkman, Glass, Brissette, & Seeman, 2000 ), with positive consequences for well-being. Studies show that adult children play a pivotal role in the social networks of their parents across the life course ( Umberson, Pudrovska, et al., 2010 ), and the effects of parenthood on health and well-being become increasingly important at older ages as adult children provide one of the major sources of care for aging adults ( Seltzer & Bianchi, 2013 ). Norms of filial obligation of adult children to care for parents may be a form of social capital to be accessed by parents when their needs arise ( Silverstein, Gans, & Yang, 2006 ).
Although the general pattern is that receiving support from adult children is beneficial for parents’ well-being ( Merz, Schulze, & Schuengel, 2010 ), there is also evidence showing that receiving social support from adult children is related to lower well-being among older adults, suggesting that challenges to an identity of independence and usefulness may offset some of the benefits of receiving support ( Merz et al., 2010 ; Thomas, 2010 ). Contrary to popular thought, older parents are also very likely to provide instrumental/financial support to their adult children, typically contributing more than they receive ( Grundy, 2005 ), and providing emotional support to their adult children is related to higher well-being for older adults ( Thomas, 2010 ). In addition, consistent with the tenets of stress process theory, most evidence points to poor quality relationships with adult children as detrimental to parents’ well-being ( Koropeckyj-Cox, 2002 ; Polenick et al., 2016 ); however, a recent study found that strain with adult children is related to better cognitive health among older parents, especially fathers ( Thomas & Umberson, 2017 ).
Adult Children
As children and parents age, the nature of the parent–child relationship often changes such that adult children may take on a caregiving role for their older parents ( Pinquart & Soerensen, 2007 ). Adult children often experience competing pressures of employment, taking care of their own children, and providing care for older parents ( Evans et al., 2016 ). Support and strain from intergenerational ties during this stressful time of balancing family roles and work obligations may be particularly important for the mental health of adults in midlife ( Thomas, 2016 ). Most evidence suggests that caregiving for parents is related to lower well-being for adult children, including more negative affect and greater stress response in terms of overall output of daily cortisol ( Bangerter et al., 2017 ); however, some studies suggest that caregiving may be beneficial or neutral for well-being ( Merz et al., 2010 ). Family scholars suggest that this discrepancy may be due to varying types of caregiving and relationship quality. For example, providing emotional support to parents can increase well-being, but providing instrumental support does not unless the caregiver is emotionally engaged ( Morelli, Lee, Arnn, & Zaki, 2015 ). Moreover, the quality of the adult child-parent relationship may matter more for the well-being of adult children than does the caregiving they provide ( Merz, Schuengel, et al., 2009 ).
Although caregiving is a critical issue, adult children generally experience many years with parents in good health ( Settersten, 2007 ), and relationship quality and support exchanges have important implications for well-being beyond caregiving roles. The preponderance of research suggests that most adults feel emotionally close to their parents, and emotional support such as encouragement, companionship, and serving as a confidant is commonly exchanged in both directions ( Swartz, 2009 ). Intergenerational support exchanges often flow across generations or towards adult children rather than towards parents. For example, adult children are more likely to receive financial support from parents than vice versa until parents are very old ( Grundy, 2005 ). Intergenerational support exchanges are integral to the lives of both parents and adult children, both in times of need and in daily life.
Grandparents
Over 65 million Americans are grandparents ( Ellis & Simmons, 2014 ), 10% of children lived with at least one grandparent in 2012 ( Dunifon, Ziol-Guest, & Kopko, 2014 ), and a growing number of American families rely on grandparents as a source of support ( Settersten, 2007 ), suggesting the importance of studying grandparenting. Grandparents’ relationships with their grandchildren are generally related to higher well-being for both grandparents and grandchildren, with some important exceptions such as when they involve more extensive childcare responsibilities ( Kim, Kang, & Johnson-Motoyama, 2017 ; Lee, Clarkson-Hendrix, & Lee, 2016 ). Most grandparents engage in activities with their grandchildren that they find meaningful, feel close to their grandchildren, consider the grandparent role important ( Swartz, 2009 ), and experience lower well-being if they lose contact with their grandchildren ( Drew & Silverstein, 2007 ). However, a growing proportion of children live in households maintained by grandparents ( Settersten, 2007 ), and grandparents who care for their grandchildren without the support of the children’s parents usually experience greater stress ( Lee et al., 2016 ) and more depressive symptoms ( Blustein, Chan, & Guanais, 2004 ), sometimes juggling grandparenting responsibilities with their own employment ( Harrington Meyer, 2014 ). Using professional help and community services reduced the detrimental effects of grandparent caregiving on well-being ( Gerard, Landry-Meyer, & Roe, 2006 ), suggesting that future policy could help mitigate the stress of grandparent parenting and enhance the rewarding aspects of grandparenting instead.
Substantial evidence suggests that the experience of intergenerational relationships varies for men and women. Women tend to be more involved with and affected by intergenerational relationships, with adult children feeling closer to mothers than fathers ( Swartz, 2009 ). Moreover, relationship quality with children is more strongly associated with mothers’ well-being than with fathers’ well-being ( Milkie et al., 2008 ). Motherhood may be particularly salient to women ( McQuillan, Greil, Shreffler, & Tichenor, 2008 ), and women carry a disproportionate share of the burden of parenting, including greater caregiving for young children and aging parents as well as time deficits from these obligations that lead to lower well-being ( Nomaguchi et al., 2005 ; Pinquart & Sorensen, 2006 ). Mothers often report greater parental pressures than fathers, such as more obligation to be there for their children ( Reczek, Thomeer, et al., 2014 ; Stone, 2007 ), and to actively work on family relationships ( Erickson, 2005 ). Mothers are also more likely to blame themselves for poor parent–child relationship quality ( Elliott, Powell, & Brenton, 2015 ), contributing to greater distress for women. It is important to take into account the different pressures and meanings surrounding intergenerational relationships for men and for women in future research.
Family scholars have noted important variations in family dynamics and constraints by race-ethnicity and socioeconomic status. Lower SES can produce and exacerbate family strains ( Conger, Conger, & Martin, 2010 ). Socioeconomically disadvantaged adult children may need more assistance from parents and grandparents who in turn have fewer resources to provide ( Seltzer & Bianchi, 2013 ). Higher SES and white families tend to provide more financial and emotional support, whereas lower SES, black, and Latino families are more likely to coreside and provide practical help, and these differences in support exchanges contribute to the intergenerational transmission of inequality through families ( Swartz, 2009 ). Moreover, scholars have found that a happiness penalty exists such that parents of young children have lower levels of well-being than nonparents; however, policies such as childcare subsidies and paid time off that help parents negotiate work and family responsibilities explain this disparity ( Glass, Simon, & Andersson, 2016 ). Fewer resources can also place strain on grandparent–grandchild relationships. For example, well-being derived from these relationships may be unequally distributed across grandparents’ education level such that those with less education bear the brunt of more stressful grandparenting experiences and lower well-being ( Mahne & Huxhold, 2015 ). Both the burden of parenting grandchildren and its effects on depressive symptoms disproportionately fall upon single grandmothers of color ( Blustein et al., 2004 ). These studies demonstrate the importance of understanding structural constraints that produce greater stress for less advantaged groups and their impact on family relationships and well-being.
Research on intergenerational relationships suggests the importance of understanding greater complexity in these relationships in future work. For example, future research should pay greater attention to diverse family structures and perspectives of multiple family members. There is an increasing trend of individuals delaying childbearing or choosing not to bear children ( Umberson, Pudrovska, et al., 2010 ). How might this influence marital quality and general well-being over the life course and across different social groups? Greater attention to the quality and context of intergenerational relationships from each family member’s perspective over time may prove fruitful by gaining both parents’ and each child’s perceptions. This work has already yielded important insights, such as the ways in which intergenerational ambivalence (simultaneous positive and negative feelings about intergenerational relationships) from the perspectives of parents and adult children may be detrimental to well-being for both parties ( Fingerman, Pitzer, Lefkowitz, Birditt, & Mroczek, 2008 ; Gilligan, Suitor, Feld, & Pillemer, 2015 ). Future work understanding the perspectives of each family member could also provide leverage in understanding the mixed findings regarding whether living in blended families with stepchildren influences well-being ( Gennetian, 2005 ; Harcourt, Adler-Baeder, Erath, & Pettit, 2013 ) and the long-term implications of these family structures when older adults need care ( Seltzer & Bianchi, 2013 ). Longitudinal data linking generations, paying greater attention to the context of these relationships, and collected from multiple family members can help untangle the ways in which family members influence each other across the life course and how multiple family members’ well-being may be intertwined in important ways.
Future studies should also consider the impact of intersecting structural locations that place unique constraints on family relationships, producing greater stress at some intersections while providing greater resources at other intersections. For example, same-sex couples are less likely to have children ( Carpenter & Gates, 2008 ) and are more likely to provide parental caregiving regardless of gender ( Reczek & Umberson, 2016 ), suggesting important implications for stress and burden in intergenerational caregiving for this group. Much of the work on gender, sexuality, race, and socioeconomic status differences in intergenerational relationships and well-being examine one or two of these statuses, but there may be unique effects at the intersection of these and other statuses such as disability, age, and nativity. Moreover, these effects may vary at different stages of the life course.
Sibling Relationships
Sibling relationships are understudied, and the research on adult siblings is more limited than for other family relationships. Yet, sibling relationships are often the longest lasting family relationship in an individual’s life due to concurrent life spans, and indeed, around 75% of 70-year olds have a living sibling ( Settersten, 2007 ). Some suggest that sibling relationships play a more meaningful role in well-being than is often recognized ( Cicirelli, 2004 ). The available evidence suggests that high quality relationships characterized by closeness with siblings are related to higher levels of well-being ( Bedford & Avioli, 2001 ), whereas sibling relationships characterized by conflict and lack of closeness have been linked to lower well-being in terms of major depression and greater drug use in adulthood ( Waldinger, Vaillant, & Orav, 2007 ). Parental favoritism and disfavoritism of children affects the closeness of siblings ( Gilligan, Suitor, & Nam, 2015 ) and depression ( Jensen, Whiteman, Fingerman, & Birditt, 2013 ). Similar to other family relationships, sibling relationships can be characterized by both positive and negative aspects that may affect elements of the stress process, providing both resources and stressors that influence well-being.
Siblings play important roles in support exchanges and caregiving, especially if their sibling experiences physical impairment and other close ties, such as a spouse or adult children, are not available ( Degeneffe & Burcham, 2008 ; Namkung, Greenberg, & Mailick, 2017 ). Although sibling caregivers report lower well-being than noncaregivers, sibling caregivers experience this lower well-being to a lesser extent than spousal caregivers ( Namkung et al., 2017 ). Most people believe that their siblings would be available to help them in a crisis ( Connidis, 1994 ; Van Volkom, 2006 ), and in general support exchanges, receiving emotional support from a sibling is related to higher levels of well-being among older adults ( Thomas, 2010 ). Relationship quality affects the experience of caregiving, with higher quality sibling relationships linked to greater provision of care ( Eriksen & Gerstel, 2002 ) and a lower likelihood of emotional strain from caregiving ( Mui & Morrow-Howell, 1993 ; Quinn, Clare, & Woods, 2009 ). Taken together, these studies suggest the importance of sibling relationships for well-being across the adult life course.
The gender of the sibling dyad may play a role in the relationship’s effect on well-being, with relationships with sisters perceived as higher quality and linked to higher well-being ( Van Volkom, 2006 ), though some argue that brothers do not show their affection in the same way but nevertheless have similar sentiments towards their siblings ( Bedford & Avioli, 2001 ). General social support exchanges with siblings may be influenced by gender and larger family context; sisters exchanged more support with their siblings when they had higher quality relationships with their parents, but brothers exhibited a more compensatory role, exchanging more emotional support with siblings when they had lower quality relationships with their parents ( Voorpostel & Blieszner, 2008 ). Caregiving for aging parents is also distributed differently by gender, falling disproportionately on female siblings ( Pinquart & Sorensen, 2006 ), and sons provide less care to their parents if they have a sister ( Grigoryeva, 2017 ). However, men in same-sex marriages were more likely than men in different-sex marriages to provide caregiving to parents and parents-in-law ( Reczek & Umberson, 2016 ), which may ease the stress and burden on their female siblings.
Although there is less research in this area, family scholars have noted variations in sibling relationships and their effects by race-ethnicity and socioeconomic status. Lower socioeconomic status has been associated with reports of feeling less attached to siblings and this influences several outcomes such as obesity, depression, and substance use ( Van Gundy et al., 2015 ). Fewer socioeconomic resources can also limit the amount of care siblings provide ( Eriksen & Gerstel, 2002 ). These studies suggest sibling relationship quality as an axis of further disadvantage for already disadvantaged individuals. Sibling relationships may influence caregiving experiences by race as well, with black caregivers more likely to have siblings who also provide care to their parents than white caregivers ( White-Means & Rubin, 2008 ) and sibling caregiving leading to lower well-being among white caregivers than minority caregivers ( Namkung et al., 2017 ).
Research on within-family differences has made great strides in our understanding of family relationships and remains a fruitful area of growth for future research (e.g., Suitor et al., 2017 ). Data gathered on multiple members within the same family can help researchers better investigate how families influence well-being in complex ways, including reciprocal influences between siblings. Siblings may have different perceptions of their relationships with each other, and this may vary by gender and other social statuses. This type of data might be especially useful in understanding family effects in diverse family structures, such as differences in treatment and outcomes of biological versus stepchildren, how characteristics of their relationships such as age differences may play a role, and the implications for caregiving for aging parents and for each other. Moreover, it is important to use longitudinal data to understand the consequences of these within-family differences over time as the life course unfolds. In addition, a greater focus on heterogeneity in sibling relationships and their consequences at the intersection of gender, race-ethnicity, SES, and other social statuses merit further investigation.
Relationships with family members are significant for well-being across the life course ( Merz, Consedine, et al., 2009 ; Umberson, Pudrovska, et al., 2010 ). As individuals age, family relationships often become more complex, with sometimes complicated marital histories, varying relationships with children, competing time pressures, and obligations for care. At the same time, family relationships become more important for well-being as individuals age and social networks diminish even as family caregiving needs increase. Stress process theory suggests that the positive and negative aspects of relationships can have a large impact on the well-being of individuals. Family relationships provide resources that can help an individual cope with stress, engage in healthier behaviors, and enhance self-esteem, leading to higher well-being. However, poor relationship quality, intense caregiving for family members, and marital dissolution are all stressors that can take a toll on an individual’s well-being. Moreover, family relationships also change over the life course, with the potential to share different levels of emotional support and closeness, to take care of us when needed, to add varying levels of stress to our lives, and to need caregiving at different points in the life course. The potential risks and rewards of these relationships have a cumulative impact on health and well-being over the life course. Additionally, structural constraints and disadvantage place greater pressures on some families than others based on structural location such as gender, race, and SES, producing further disadvantage and intergenerational transmission of inequality.
Future research should take into account greater complexity in family relationships, diverse family structures, and intersections of social statuses. The rapid aging of the U.S. population along with significant changes in marriage and families suggest more complex marital and family histories as adults enter late life, which will have a large impact on family dynamics and caregiving. Growing segments of family relationships among older adults include same-sex couples, those without children, and those experiencing marital transitions leading to diverse family structures, which all merit greater attention in future research. Moreover, there is some evidence that strain in relationships can be beneficial for certain health outcomes, and the processes by which this occurs merit further investigation. A greater use of longitudinal data that link generations and obtain information from multiple family members will help researchers better understand the ways in which these complex family relationships unfold across the life course and shape well-being. We also highlighted gender, race-ethnicity, and socioeconomic status differences in each of these family relationships and their impact on well-being; however, many studies only consider one status at a time. Future research should consider the impact of intersecting structural locations that place unique constraints on family relationships, producing greater stress or providing greater resources at the intersections of different statuses.
The changing landscape of families combined with population aging present unique challenges and pressures for families and health care systems. With more experiences of age-related disease in a growing population of older adults as well as more complex family histories as these adults enter late life, such as a growing proportion of diverse family structures without children or with stepchildren, caregiving obligations and availability may be less clear. It is important to address ways to ease caregiving or shift the burden away from families through a variety of policies, such as greater resources for in-home aid, creation of older adult residential communities that facilitate social interactions and social support structures, and patient advocates to help older adults navigate health care systems. Adults in midlife may experience competing family pressures from their young children and aging parents, and policies such as childcare subsidies and paid leave to care for family members could reduce burden during this often stressful time ( Glass et al., 2016 ). Professional help and community services can also reduce the burden for grandparents involved in childcare, enabling grandparents to focus on the more positive aspects of grandparent–grandchild relationships. It is important for future research and health promotion policies to take into account the contexts and complexities of family relationships as part of a multipronged approach to benefit health and well-being, especially as a growing proportion of older adults reach late life.
This work was supported in part by grant, 5 R24 HD042849, Population Research Center, awarded to the Population Research Center at The University of Texas at Austin by the Eunice Kennedy Shriver National Institute of Child Health and Human Development.
Conflict of Interest
None reported.
- Bangerter L. R., Liu Y., Kim K., Zarit S. H., Birditt K. S., & Fingerman K. L (2017). Everyday support to aging parents: Links to middle-aged children’s diurnal cortisol and daily mood. The Gerontologist, gnw207. doi:10.1093/geront/gnw207 [ DOI ] [ PMC free article ] [ PubMed ] [ Google Scholar ]
- Bedford V. H., & Avioli P. S (2001). Variations on sibling intimacy in old age. Generations, 25, 34–40. [ Google Scholar ]
- Berkman L. F., Glass T., Brissette I., & Seeman T. E (2000). From social integration to health: Durkheim in the new millennium. Social Science & Medicine, 51, 843–857. doi:10.1016/S0277-9536(00)00065-4 [ DOI ] [ PubMed ] [ Google Scholar ]
- Bernard J. (1972). The future of marriage. New Haven, CT: Yale University Press. [ Google Scholar ]
- Blustein J., Chan S., & Guanais F. C (2004). Elevated depressive symptoms among caregiving grandparents. Health Services Research, 39, 1671–1689. doi:10.1111/j.1475-6773.2004.00312.x [ DOI ] [ PMC free article ] [ PubMed ] [ Google Scholar ]
- Broman C. L. (2005). Marital quality in black and white marriages. Journal of Family Issues, 26, 431–441. doi:10.1177/0192513X04272439 [ Google Scholar ]
- Carpenter C., & Gates G. J (2008). Gay and lesbian partnership: Evidence from California. Demography, 45, 573–590. doi:10.1353/dem.0.0014 [ DOI ] [ PMC free article ] [ PubMed ] [ Google Scholar ]
- Carr D., Cornman J. C., & Freedman V. A (2016). Marital quality and negative experienced well-being: An assessment of actor and partner effects among older married persons. Journals of Gerontology, Series B: Psychological Sciences and Social Sciences, 71, 177–187. doi:10.1093/geronb/gbv073 [ DOI ] [ PMC free article ] [ PubMed ] [ Google Scholar ]
- Carr D., & Springer K. W (2010). Advances in families and health research in the 21st century. Journal of Marriage and Family, 72, 743–761. doi:10.1111/j.1741-3737.2010.00728.x [ Google Scholar ]
- Cicirelli V. G. (2004). Midlife sibling relationships in the context of the family. The Gerontologist, 44, 541. [ Google Scholar ]
- Cohen S. (2004). Social relationships and health. American Psychologist, 59, 676–684. doi:10.1037/0003-066X.59.8.676 [ DOI ] [ PubMed ] [ Google Scholar ]
- Conger R. D., Conger K. J., & Martin M. J (2010). Socioeconomic status, family processes, and individual development. Journal of Marriage and the Family, 72, 685–704. doi:10.1111/j.1741-3737.2010.00725.x [ DOI ] [ PMC free article ] [ PubMed ] [ Google Scholar ]
- Connidis I. A. (1994). Sibling support in older age. Journal of Gerontology, 49, S309–S318. doi:10.1093/geronj/49.6.S309 [ DOI ] [ PubMed ] [ Google Scholar ]
- Degeneffe C. E., & Burcham C. M (2008). Adult sibling caregiving for persons with traumatic brain injury: Predictors of affective and instrumental support. Journal of Rehabilitation, 74, 10–20. [ Google Scholar ]
- Denney J. T., Gorman B. K., & Barrera C. B (2013). Families, resources, and adult health: Where do sexual minorities fit?Journal of Health and Social Behavior, 54, 46. doi:10.1177/0022146512469629 [ DOI ] [ PubMed ] [ Google Scholar ]
- Donoho C. J., Crimmins E. M., & Seeman T. E (2013). Marital quality, gender, and markers of inflammation in the MIDUS cohort. Journal of Marriage and Family, 75, 127–141. doi:10.1111/j.1741-3737.2012.01023.x [ DOI ] [ PMC free article ] [ PubMed ] [ Google Scholar ]
- Drew L. M., & Silverstein M (2007). Grandparents’ psychological well-being after loss of contact with their grandchildren. Journal of Family Psychology, 21, 372–379. doi:10.1037/0893-3200.21.3.372 [ DOI ] [ PubMed ] [ Google Scholar ]
- Dunifon R. E., Ziol-Guest K. M., & Kopko K (2014). Grandparent coresidence and family well-being. The ANNALS of the American Academy of Political and Social Science, 654, 110–126. doi:10.1177/0002716214526530 [ Google Scholar ]
- Edin K., & Kefalas M (2005). Promises I can keep: Why poor women put motherhood before marriage. Berkeley, CA: University of California Press. [ Google Scholar ]
- Elder G. H., Johnson M. K., & Crosnoe R (2003). The emergence and development of life course theory. In Mortimer J. T. & Shanahan M. J. (Eds.), Handbook of the life course (pp. 3–19). New York: Kluwer Academic/Plenum Publishers. doi:10.1007/978-0-306-48247-2_1 [ Google Scholar ]
- Elliott S., Powell R., & Brenton J (2015). Being a good mom: Low-income, black single mothers negotiate intensive mothering. Journal of Family Issues, 36, 351–370. doi:10.1177/0192513X13490279 [ Google Scholar ]
- Ellis R. R., & Simmons T (2014). Coresident grandparents and their grandchildren: 2012. Washington, DC: U.S. Census Bureau. [ Google Scholar ]
- Erickson R. J. (2005). Why emotion work matters: Sex, gender, and the division of household labor. Journal of Marriage and Family, 67, 337–351. doi:10.1111/j.0022-2445.2005.00120.x [ Google Scholar ]
- Eriksen S., & Gerstel N (2002). A labor of love or labor itself. Journal of Family Issues, 23, 836–856. doi:10.1177/019251302236597 [ Google Scholar ]
- Evans K. L., Millsteed J., Richmond J. E., Falkmer M., Falkmer T., & Girdler S. J (2016). Working sandwich generation women utilize strategies within and between roles to achieve role balance. PLOS ONE, 11, e0157469. doi:10.1371/journal.pone.0157469 [ DOI ] [ PMC free article ] [ PubMed ] [ Google Scholar ]
- Fingerman K. L., Pitzer L., Lefkowitz E. S., Birditt K. S., & Mroczek D (2008). Ambivalent relationship qualities between adults and their parents: Implications for the well-being of both parties. The Journals of Gerontology, Series B: Psychological Sciences and Social Sciences, 63, P362–P371. doi:10.1093/geronb/63.6.P362 [ DOI ] [ PMC free article ] [ PubMed ] [ Google Scholar ]
- Frech A., & Williams K (2007). Depression and the psychological benefits of entering marriage. Journal of Health and Social Behavior, 48, 149. doi:10.1177/002214650704800204 [ DOI ] [ PubMed ] [ Google Scholar ]
- Fukukawa Y., Tsuboi S., Niino N., Ando F., Kosugi S., & Shimokata H (2000). Effects of social support and self-esteem on depressive symptoms in Japanese middle-aged and elderly people. Journal of Epidemiology, 10, 63–69. doi:10.2188/jea.10.1sup_63 [ DOI ] [ PubMed ] [ Google Scholar ]
- Gennetian L. A. (2005). One or two parents? Half or step siblings? The effect of family structure on young children’s achievement. Journal of Population Economics, 18, 415–436. doi:10.1007/s00148-004-0215-0 [ Google Scholar ]
- Gerard J. M., Landry-Meyer L., & Roe J. G (2006). Grandparents raising grandchildren: The role of social support in coping with caregiving challenges. The International Journal of Aging and Human Development, 62, 359–383. doi:10.2190/3796-DMB2-546Q-Y4AQ [ DOI ] [ PubMed ] [ Google Scholar ]
- Gilligan M., Suitor J. J., Feld S., & Pillemer K (2015). Do positive feelings hurt? Disaggregating positive and negative components of intergenerational ambivalence. Journal of Marriage and Family, 77, 261–276. doi:10.1111/jomf.12146 [ DOI ] [ PMC free article ] [ PubMed ] [ Google Scholar ]
- Gilligan M., Suitor J. J., & Nam S (2015). Maternal differential treatment in later life families and within-family variations in adult sibling closeness. The Journals of Gerontology, Series B: Psychological Sciences and Social Sciences, 70, 167–177. doi:10.1093/geronb/gbu148 [ DOI ] [ PMC free article ] [ PubMed ] [ Google Scholar ]
- Glass J., Simon R. W., & Andersson M. A (2016). Parenthood and happiness: Effects of work-family reconciliation policies in 22 OECD countries. AJS; American Journal of Sociology, 122, 886–929. doi:10.1086/688892 [ DOI ] [ PMC free article ] [ PubMed ] [ Google Scholar ]
- Goldsen J., Bryan A., Kim H.-J., Muraco A., Jen S., & Fredriksen-Goldsen K (2017). Who says I do: The changing context of marriage and health and quality of life for LGBT older adults. The Gerontologist, 57, S50. doi:10.1093/geront/gnw174 [ DOI ] [ PMC free article ] [ PubMed ] [ Google Scholar ]
- Graham J. E., Christian L. M., & Kiecolt-Glaser J. K (2006). Marriage, health, and immune function: A review of key findings and the role of depression. In Beach S. & Wamboldt M. (Eds.), Relational processes in mental health, Vol. 11. Arlington, VA: American Psychiatric Publishing, Inc. [ Google Scholar ]
- Grigoryeva A. (2017). Own gender, sibling’s gender, parent’s gender: The division of elderly parent care among adult children. American Sociological Review, 82, 116–146. doi:10.1177/0003122416686521 [ Google Scholar ]
- Grundy E. (2005). Reciprocity in relationships: Socio-economic and health influences on intergenerational exchanges between third age parents and their adult children in Great Britain. The British Journal of Sociology, 56, 233–255. doi:10.1111/j.1468-4446.2005.00057.x [ DOI ] [ PubMed ] [ Google Scholar ]
- Harcourt K. T., Adler-Baeder F., Erath S., & Pettit G. S (2013). Examining family structure and half-sibling influence on adolescent well-being. Journal of Family Issues, 36, 250–272. doi:10.1177/0192513X13497350 [ Google Scholar ]
- Harrington Meyer M. (2014). Grandmothers at work - juggling families and jobs. New York, NY: NYU Press. doi:10.18574/nyu/9780814729236.001.0001 [ DOI ] [ PubMed ] [ Google Scholar ]
- Hartwell S. W., & Benson P. R (2007). Social integration: A conceptual overview and two case studies. In Avison W. R., McLeod J. D., & Pescosolido B. (Eds.), Mental health, social mirror (pp. 329–353). New York: Springer. doi:10.1007/978-0-387-36320-2_14 [ Google Scholar ]
- Hughes M. E., & Waite L. J (2009). Marital biography and health at mid-life. Journal of Health and Social Behavior, 50, 344. doi:10.1177/002214650905000307 [ DOI ] [ PMC free article ] [ PubMed ] [ Google Scholar ]
- Jensen A. C., Whiteman S. D., Fingerman K. L., & Birditt K. S (2013). “Life still isn’t fair”: Parental differential treatment of young adult siblings. Journal of Marriage and the Family, 75, 438–452. doi:10.1111/jomf.12002 [ DOI ] [ PMC free article ] [ PubMed ] [ Google Scholar ]
- Kawachi I., & Berkman L. F (2001). Social ties and mental health. Journal of Urban Health-Bulletin of the New York Academy of Medicine, 78, 458–467. doi:10.1093/jurban/78.3.458 [ DOI ] [ PMC free article ] [ PubMed ] [ Google Scholar ]
- Kiecolt-Glaser J. K., & Newton T. L (2001). Marriage and health: His and hers. Psychological Bulletin, 127, 472–503. doi:10.1037//0033-2909.127.4.472 [ DOI ] [ PubMed ] [ Google Scholar ]
- Kim H.-J., Kang H., & Johnson-Motoyama M (2017). The psychological well-being of grandparents who provide supplementary grandchild care: A systematic review. Journal of Family Studies, 23, 118–141. doi:10.1080/13229400.2016.1194306 [ Google Scholar ]
- Koropeckyj-Cox T. (2002). Beyond parental status: Psychological well-being in middle and old age. Journal of Marriage and Family, 64, 957–971. doi:10.1111/j.1741-3737.2002.00957.x [ Google Scholar ]
- Lee E., Clarkson-Hendrix M., & Lee Y (2016). Parenting stress of grandparents and other kin as informal kinship caregivers: A mixed methods study. Children and Youth Services Review, 69, 29–38. doi:10.1016/j.childyouth.2016.07.013 [ Google Scholar ]
- Lee G. R., & Demaris A (2007). Widowhood, gender, and depression: A longitudinal analysis. Research on Aging, 29, 56–72. doi:10.1177/0164027506294098 [ Google Scholar ]
- Lipowicz A. (2014). Some evidence for health-related marriage selection. American Journal of Human Biology, 26, 747–752. doi:10.1002/ajhb.22588 [ DOI ] [ PubMed ] [ Google Scholar ]
- Liu H., Reczek C., & Brown D (2013). Same-sex cohabitors and health: The role of race-ethnicity, gender, and socioeconomic status. Journal of Health and Social Behavior, 54, 25. doi:10.1177/0022146512468280 [ DOI ] [ PubMed ] [ Google Scholar ]
- Liu H., & Umberson D. J (2008). The times they are a changin’: Marital status and health differentials from 1972 to 2003. Journal of Health and Social Behavior, 49, 239–253. doi:10.1177/002214650804900301 [ DOI ] [ PMC free article ] [ PubMed ] [ Google Scholar ]
- Liu H., & Waite L (2014). Bad marriage, broken heart? Age and gender differences in the link between marital quality and cardiovascular risks among older adults. Journal of Health and Social Behavior, 55, 403–423 doi:10.1177/0022146514556893 [ DOI ] [ PMC free article ] [ PubMed ] [ Google Scholar ]
- Liu H., Waite L., & Shen S (2016). Diabetes risk and disease management in later life: A national longitudinal study of the role of marital quality. Journals of Gerontology, Series B: Psychological Sciences and Social Sciences, 71, 1070–1080. doi:10.1093/geronb/gbw061 [ DOI ] [ PMC free article ] [ PubMed ] [ Google Scholar ]
- Lorenz F. O., Wickrama K. A. S., Conger R. D., & Elder G. H (2006). The short-term and decade-long effects of divorce on women’s midlife health. Journal of Health and Social Behavior, 47, 111–125. doi:10.1177/002214650604700202 [ DOI ] [ PubMed ] [ Google Scholar ]
- Mahne K., & Huxhold O (2015). Grandparenthood and subjective well-being: Moderating effects of educational level. The Journals of Gerontology, Series B: Psychological Sciences and Social Sciences, 70, 782–792. doi:10.1093/geronb/gbu147 [ DOI ] [ PubMed ] [ Google Scholar ]
- McQuillan J., Greil A. L., Shreffler K. M., & Tichenor V (2008). The importance of motherhood among women in the contemporary United States. Gender & Society, 22, 477–496. doi:10.1177/0891243208319359 [ DOI ] [ PMC free article ] [ PubMed ] [ Google Scholar ]
- Merz E.-M., Consedine N. S., Schulze H.-J., & Schuengel C (2009). Well-being of adult children and ageing parents: Associations with intergenerational support and relationship quality. Ageing & Society, 29, 783–802. doi:10.1017/s0144686x09008514 [ Google Scholar ]
- Merz E.-M., Schuengel C., & Schulze H.-J (2009). Intergenerational relations across 4 years: Well-being is affected by quality, not by support exchange. Gerontologist, 49, 536–548. doi:10.1093/geront/gnp043 [ DOI ] [ PubMed ] [ Google Scholar ]
- Merz E.-M., Schulze H.-J., & Schuengel C (2010). Consequences of filial support for two generations: A narrative and quantitative review. Journal of Family Issues, 31, 1530–1554. doi:10.1177/0192513x10365116 [ Google Scholar ]
- Milkie M. A., Bierman A., & Schieman S (2008). How adult children influence older parents’ mental health: Integrating stress-process and life-course perspectives. Social Psychology Quarterly, 71, 86. doi:10.1177/019027250807100109 [ Google Scholar ]
- Morelli S. A., Lee I. A., Arnn M. E., & Zaki J (2015). Emotional and instrumental support provision interact to predict well-being. Emotion, 15, 484–493. doi:10.1037/emo0000084 [ DOI ] [ PMC free article ] [ PubMed ] [ Google Scholar ]
- Mui A. C., & Morrow-Howell N (1993). Sources of emotional strain among the oldest caregivers. Research on Aging, 15, 50–69. doi:10.1177/0164027593151003 [ Google Scholar ]
- Musick K., & Bumpass L (2012). Reexamining the case for marriage: Union formation and changes in well-being. Journal of Marriage and Family, 74, 1–18. doi:10.1111/j.1741-3737.2011.00873.x [ DOI ] [ PMC free article ] [ PubMed ] [ Google Scholar ]
- Namkung E. H., Greenberg J. S., & Mailick M. R (2017). Well-being of sibling caregivers: Effects of kinship relationship and race. The Gerontologist, 57, 626–636. doi:10.1093/geront/gnw008 [ DOI ] [ PMC free article ] [ PubMed ] [ Google Scholar ]
- Ng D. M., & Jeffery R. W (2003). Relationships between perceived stress and health behaviors in a sample of working adults. Health Psychology, 22, 638–642. doi:10.1037/0278-6133.22.6.638 [ DOI ] [ PubMed ] [ Google Scholar ]
- Nomaguchi K. M., & Milkie M. A (2003). Costs and rewards of children: The effects of becoming a parent on adults’ lives. Journal of Marriage and Family, 65, 356–374. doi:10.1111/j.1741-3737.2003.00356.x 0022-2445 [ Google Scholar ]
- Nomaguchi K. M., Milkie M. A., & Bianchi S. B (2005). Time strains and psychological well-being: Do dual-earner mothers and fathers differ?Journal of Family Issues, 26, 756–792. doi:10.1177/0192513X05277524 [ Google Scholar ]
- Pearlin L. I. (1999). Stress and mental health: A conceptual overview. In Horwitz A. V. & Scheid T. (Eds.), A Handbook for the study of mental health: Social contexts, theories, and systems (pp. 161–175). Cambridge: Cambridge University Press. [ Google Scholar ]
- Pienta A. M., Hayward M. D., & Jenkins K. R (2000). Health consequences of marriage for the retirement years. Journal of Family Issues, 21, 559–586. doi:10.1177/019251300021005003 [ Google Scholar ]
- Pinquart M., & Soerensen S (2007). Correlates of physical health of informal caregivers: A meta-analysis. Journals of Gerontology, Series B: Psychological Sciences and Social Sciences, 62, P126–P137. doi:10.1093/geronb/62.2.P126 [ DOI ] [ PubMed ] [ Google Scholar ]
- Pinquart M., & Sorensen S (2006). Gender differences in caregiver stressors, social resources, and health: An updated meta-analysis. Journals of Gerontology, Series B: Psychological Sciences and Social Sciences, 61, P33–P45. doi:10.1093/geronb/61.1.P33 [ DOI ] [ PubMed ] [ Google Scholar ]
- Polenick C. A., DePasquale N., Eggebeen D. J., Zarit S. H., & Fingerman K. L (2016). Relationship quality between older fathers and middle-aged children: Associations with both parties’ subjective well-being. The Journals of Gerontology, Series B: Psychological Sciences and Social Sciences, gbw094. doi:10.1093/geronb/gbw094 [ DOI ] [ PMC free article ] [ PubMed ] [ Google Scholar ]
- Quinn C., Clare L., & Woods B (2009). The impact of the quality of relationship on the experiences and wellbeing of caregivers of people with dementia: A systematic review. Aging & Mental Health, 13, 143–154. doi:10.1080/13607860802459799 [ DOI ] [ PubMed ] [ Google Scholar ]
- Reczek C., Liu H., & Spiker R (2014). A population-based study of alcohol use in same-sex and different-sex unions. Journal of Marriage and Family, 76, 557–572. doi:10.1111/jomf.12113 [ DOI ] [ PMC free article ] [ PubMed ] [ Google Scholar ]
- Reczek C., Thomeer M. B., Lodge A. C., Umberson D., & Underhill M (2014). Diet and exercise in parenthood: A social control perspective. Journal of Marriage and Family, 76, 1047–1062. doi:10.1111/jomf.12135 [ DOI ] [ PMC free article ] [ PubMed ] [ Google Scholar ]
- Reczek C., & Umberson D (2016). Greedy spouse, needy parent: The marital dynamics of gay, lesbian, and heterosexual intergenerational caregivers. Journal of Marriage and Family, 78, 957–974. doi:10.1111/jomf.12318 [ DOI ] [ PMC free article ] [ PubMed ] [ Google Scholar ]
- Rendall M. S., Weden M. M., Favreault M. M., & Waldron H (2011). The protective effect of marriage for survival: A review and update. Demography, 48, 481. doi:10.1007/s13524-011-0032-5 [ DOI ] [ PubMed ] [ Google Scholar ]
- Revenson T. A., Griva K., Luszczynska A., Morrison V., Panagopoulou E., Vilchinsky N., & Hagedoorn M (2016). Gender and caregiving: The costs of caregiving for women. In Caregiving in the Illness Context (pp. 48–63). London: Palgrave Macmillan UK. doi:10.1057/9781137558985.0008 [ Google Scholar ]
- Rook K. S. (2014). The health effects of negative social exchanges in later life. Generations, 38, 15–23. [ Google Scholar ]
- Sbarra D. A. (2009). Marriage protects men from clinically meaningful elevations in C-reactive protein: Results from the National Social Life, Health, and Aging Project (NSHAP). Psychosomatic Medicine, 71, 828. doi:10.1097/PSY.0b013e3181b4c4f2 [ DOI ] [ PMC free article ] [ PubMed ] [ Google Scholar ]
- Seeman T. E., Singer B. H., Ryff C. D., Love G. D., & Levy-Storms L (2002). Social relationships, gender, and allostatic load across two age cohorts. Psychosomatic Medicine, 64, 395–406. doi:10.1097/00006842-200205000-00004 [ DOI ] [ PubMed ] [ Google Scholar ]
- Seltzer J. A., & Bianchi S. M (2013). Demographic change and parent-child relationships in adulthood. Annual Review of Sociology, 39, 275–290. doi:10.1146/annurev-soc-071312-145602 [ DOI ] [ PMC free article ] [ PubMed ] [ Google Scholar ]
- Settersten R. A. (2007). Social relationships in the new demographic regime: Potentials and risks, reconsidered. Advances in Life Course Research, 12, 3–28. doi:10.1016/S1040-2608(07)12001–3 [ Google Scholar ]
- Silverstein M., Gans D., & Yang F. M (2006). Intergenerational support to aging parents: The role of norms and needs. Journal of Family Issues, 27, 1068–1084. doi:10.1177/0192513X06288120 [ Google Scholar ]
- Simon R. W. (2002). Revisiting the relationships among gender, marital status, and mental health. The American Journal of Sociology, 107, 1065–1096. doi:10.1086/339225 [ DOI ] [ PubMed ] [ Google Scholar ]
- Stone P. (2007). Opting out? Why women really quit careers and head home. Berkeley, CA: University of California Press. [ Google Scholar ]
- Suitor J. J., Gilligan M., Pillemer K., Fingerman K. L., Kim K., Silverstein M., & Bengtson V. L (2017). Applying within-family differences approaches to enhance understanding of the complexity of intergenerational relations. Journals of Gerontology, Series B: Psychological Sciences and Social Sciences, gbx037. doi:10.1093/geronb/gbx037 [ DOI ] [ PMC free article ] [ PubMed ] [ Google Scholar ]
- Swartz T. (2009). Intergenerational family relations in adulthood: Patterns, variations, and implications in the contemporary United States. Annual Review of Sociology, 35, 191–212. doi:10.1146/annurev.soc.34.040507.134615 [ Google Scholar ]
- Symister P., & Friend R (2003). The influence of social support and problematic support on optimism and depression in chronic illness: A prospective study evaluating self-esteem as a mediator. Health Psychology, 22, 123–129. doi:10.1037/0278-6133.22.2.123 [ DOI ] [ PubMed ] [ Google Scholar ]
- Thoits P. A. (2010). Stress and health: Major findings and policy implications. Journal of Health and Social Behavior, 51, S41–S53. doi:10.1177/0022146510383499 [ DOI ] [ PubMed ] [ Google Scholar ]
- Thomas P. A. (2010). Is it better to give or to receive? Social support and the well-being of older adults. Journal of Gerontology, Series B: Psychological Sciences and Social Sciences, 65, 351–357. doi:10.1093/geronb/gbp113 [ DOI ] [ PubMed ] [ Google Scholar ]
- Thomas P. A. (2016). The impact of relationship-specific support and strain on depressive symptoms across the life course. Journal of Aging and Health, 28, 363–382. doi:10.1177/0898264315591004 [ DOI ] [ PMC free article ] [ PubMed ] [ Google Scholar ]
- Thomas P. A., & Umberson D (2017). Do older parents’ relationships with their adult children affect cognitive limitations, and does this differ for mothers and fathers?Journal of Gerontology, Series B: Psychological Sciences and Social Sciences, gbx009. doi:10.1093/geronb/gbx009 [ DOI ] [ PMC free article ] [ PubMed ] [ Google Scholar ]
- Umberson D., Crosnoe R., & Reczek C (2010). Social relationships and health behavior across the life course. Annual Review of Sociology, Vol 36, 36, 139–157. doi:10.1146/annurev-soc-070308-120011 [ DOI ] [ PMC free article ] [ PubMed ] [ Google Scholar ]
- Umberson D., & Montez J. K (2010). Social relationships and health: A flashpoint for health policy. Journal of Health and Social Behavior, 51, S54–S66. doi:10.1177/0022146510383501 [ DOI ] [ PMC free article ] [ PubMed ] [ Google Scholar ]
- Umberson D., Pudrovska T., & Reczek C (2010). Parenthood, childlessness, and well-being: A life course perspective. Journal of Marriage and Family, 72, 612–629. doi:10.1111/j.1741- 3737.2010.00721.x [ DOI ] [ PMC free article ] [ PubMed ] [ Google Scholar ]
- Umberson D., Thomeer M. B., Kroeger R. A., Lodge A. C., & Xu M (2015). Challenges and opportunities for research on same-sex relationships. Journal of Marriage and Family, 77, 96–111. doi:10.1111/jomf.12155 [ DOI ] [ PMC free article ] [ PubMed ] [ Google Scholar ]
- Umberson D., Thomeer M. B., Reczek C., & Donnelly R (2016). Physical illness in gay, lesbian, and heterosexual marriages: Gendered dyadic experiences. Journal of Health and Social Behavior, 57, 517. doi:10.1177/0022146516671570 [ DOI ] [ PMC free article ] [ PubMed ] [ Google Scholar ]
- Umberson D., Williams K., Powers D. A., Liu H., & Needham B (2006). You make me sick: Marital quality and health over the life course. Journal of Health and Social Behavior, 47, 1–16. doi:10.1177/002214650604700101 [ DOI ] [ PMC free article ] [ PubMed ] [ Google Scholar ]
- Umberson D., Williams K., Thomas P. A., Liu H., & Thomeer M. B (2014). Race, gender, and chains of disadvantage: Childhood adversity, social relationships, and health. Journal of Health and Social Behavior, 55, 20–38. doi:10.1177/ 0022146514521426 [ DOI ] [ PMC free article ] [ PubMed ] [ Google Scholar ]
- Umberson D., Williams K., & Thomeer M. B (2013). Family status and mental health: Recent advances and future directions. In Aneshensel C. S. & Phelan J. C. (Eds.), Handbook of the sociology of mental health (2nd edn, pp. 405–431). Dordrecht: Springer Publishing. doi:10.1007/978-94-007-4276-5_20 [ Google Scholar ]
- Van Gundy K. T., Mills M. L., Tucker C. J., Rebellon C. J., Sharp E. H., & Stracuzzi N. F (2015). Socioeconomic strain, family ties, and adolescent health in a rural northeastern county. Rural Sociology, 80, 60–85. doi:10.1111/ruso.12055 [ Google Scholar ]
- Van Volkom M. (2006). Sibling relationships in middle and older adulthood. Marriage & Family Review, 40, 151–170. doi:10.1300/J002v40n02_08 [ Google Scholar ]
- Voorpostel M., & Blieszner R (2008). Intergenerational solidarity and support between adult siblings. Journal of Marriage and Family, 70, 157–167. doi:10.1111/j.1741-3737.2007.00468.x [ Google Scholar ]
- Waite L. J., & Gallager M (2000). The case for marriage: Why married people are happier, healthier, and better off financially. New York: Doubleday. [ Google Scholar ]
- Waldinger R. J., Vaillant G. E., & Orav E. J (2007). Childhood sibling relationships as a predictor of major depression in adulthood: A 30-year prospective study. American Journal of Psychiatry, 164, 949–954. doi:10.1176/ajp.2007.164.6.949 [ DOI ] [ PubMed ] [ Google Scholar ]
- White-Means S. I., & Rubin R. M (2008). Parent caregiving choices of middle-generation blacks and whites in the United States. Journal of Aging and Health, 20, 560–582. doi:10.1177/0898264308317576 [ DOI ] [ PubMed ] [ Google Scholar ]
- Williams K. (2003). Has the future of marriage arrived? A contemporary examination of gender, marriage, and psychological well-being. Journal of Health and Social Behavior, 44, 470. doi:10.2307/1519794 [ PMC free article ] [ PubMed ] [ Google Scholar ]
- Williams K. (2004). The transition to widowhood and the social regulation of health: Consequences for health and health risk behavior. Journals of Gerontology, Series B: Psychological Sciences and Social Sciences, 59, S343–S349. doi:10.1093/ geronb/59.6.S343 [ DOI ] [ PubMed ] [ Google Scholar ]
- Williams K., & Umberson D (2004). Marital status, marital transitions, and health: A gendered life course perspective. Journal of Health and Social Behavior, 45, 81–98. doi:10.1177/002214650404500106 [ DOI ] [ PMC free article ] [ PubMed ] [ Google Scholar ]
- Xu M., Thomas P. A., & Umberson D (2016). Marital quality and cognitive limitations in late life. The Journals of Gerontology, Series B: Psychological Sciences and Social Sciences, 71, 165–176. doi:10.1093/geronb/gbv014 [ DOI ] [ PMC free article ] [ PubMed ] [ Google Scholar ]
- Zhang Z., & Hayward M. D (2006). Gender, the marital life course, and cardiovascular disease in late midlife. Journal of Marriage and Family, 68, 639–657. doi:10.1111/j.1741-3737.2006.00280.x [ Google Scholar ]
- View on publisher site
- PDF (205.5 KB)
- Collections
Similar articles
Cited by other articles, links to ncbi databases.
- Download .nbib .nbib
- Format: AMA APA MLA NLM
Add to Collections

IMAGES
VIDEO
COMMENTS
Social work is acknowledged to be a high-stress profession that involves working with people in distressing circumstances and complex life situations such as those experiencing abuse, domestic violence, substance misuse, and crime (Stanley & Mettilda, 2016).It has been observed that important sources of occupational stress for social workers include excessive workload, working overtime ...
Quantitative research deals in numbers, logic, and an objective stance. Quantitative research focuses on numberic and unchanging data and detailed, convergent reasoning rather than divergent reasoning [i.e., the generation of a variety of ideas about a research problem in a spontaneous, free-flowing manner]. Its main characteristics are:
This book arose from funding from the Economic and Social Research Council to address the quantitative skills gap in the social sciences. The grants were applied for under the auspices of the Joint University Council Social Work Education Committee to upskill social work academics and develop a curriculum resource with teaching aids.
Social work researchers will send out a survey, receive responses, aggregate the results, analyze the data, and form conclusions based on trends. Surveys are one of the most common research methods social workers use — and for good reason. They tend to be relatively simple and are usually affordable.
The NASW Code of Ethics discusses social work research and the importance of engaging in practices that do not harm participants. [14] This is especially important considering that many of the topics studied by social workers are those that are disproportionately experienced by marginalized and oppressed populations.
Quantitative work seems to present many people in social work with particular problems. Sharland's (2009) authoritative review notes the difficulty 'with people doing qualitative research not by choice but because it's the only thing they feel safe in' (p. 31). Sharland's review is replete with concerns expressed within the social work academic community about quantitative competence.
Nature and Extent of Quantitative Research in Social Work 1521 Introduction Quantitative work seems to present many people in social work with particu lar problems. Sharland's (2009) authoritative review notes the difficulty 'with people doing qualitative research not by choice but because it's the only thing they feel safe in' (p. 31).
Although the proportion of quantitative research is rather small in social work research, the review could not find evidence that it is of low sophistication. Finally, this study concludes that future research would benefit from making explicit why a certain methodology was chosen.
"`Not so much a handbook, but an excellent source of reference' - British Journal of Social Work This volume is the definitive resource for anyone doing research in social work. It details both quantitative and qualitative methods and data collection, as well as suggesting the methods appropriate to particular types of studies. It also covers ...
The book is a comprehensive resource for students and educators. It is packed with activities and examples from social work covering the basic concepts of quantitative research methods - including reliability, validity, probability, variables and hypothesis testing - and explores key areas of data collection, analysis and evaluation ...
This entry describes the definition, history, theories, and applications of quantitative methods in social work research. Unlike qualitative research, quantitative research emphasizes precise, objective, and generalizable findings. Quantitative methods are based on numerous probability and statistical theories, with rigorous proofs and support ...
Quantitative descriptive questions will often ask for figures such as percentages, sums, or averages. Descriptive questions may only include one variable, such as ours included the variable of student debt, or they may include multiple variables. When asking a descriptive question, we cannot investigate causal relationships between variables.
We aren't trying to build a causal relationship here. We're simply trying to describe how much debt MSW students carry. Quantitative descriptive questions like this one are helpful in social work practice as part of community scans, in which human service agencies survey the various needs of the community they serve.
Step 1: Specifying variables and attributes. The first component, the variable, should be the easiest part. At this point in quantitative research, you should have a research question that has at least one independent and at least one dependent variable. Remember that variables must be able to vary.
Define ethical questions and provide an example. Writing a good research question is an art and a science. It is a science because you have to make sure it is clear, concise, and well-developed. It is an art because often your language needs "wordsmithing" to perfect and clarify the meaning. This is an exciting part of the research process ...
Examples might include the number of members in different households, the distance to healthful food sources in different neighborhoods, the ratio of social work faculty to students in a BSW or MSW program, the proportion of persons from different racial/ethnic groups incarcerated, the cost of transportation to receive services from a social ...
The importance of quantitative research in the social sciences generally and social work specifically has been highlighted in recent years, in both an international and a British context. Consensus opinion in the UK is that quantitative work is the 'poor relation' in social work research, leading to a number of initiatives.
BookPDF Available. Quantitative research methods for social work: Making social work count. January 2017. Edition: 1st. Publisher: Palgrave. ISBN: 978-1-137-40026-. Authors: Barbra Teater. City ...
Quantitative research is the opposite of qualitative research, which involves collecting and analyzing non-numerical data (e.g., text, video, or audio). Quantitative research is widely used in the natural and social sciences: biology, chemistry, psychology, economics, sociology, marketing, etc. Quantitative research question examples
Translational Significance. It is important for future research and health promotion policies to take into account complexities in family relationships, paying attention to family context, diversity of family structures, relationship quality, and intersections of social statuses in an aging society to provide resources to families to reduce caregiving burdens and benefit health and well-being.