Have a language expert improve your writing
Run a free plagiarism check in 10 minutes, automatically generate references for free.
- Knowledge Base
- Methodology

Research Design | Step-by-Step Guide with Examples
Published on 5 May 2022 by Shona McCombes . Revised on 20 March 2023.
A research design is a strategy for answering your research question using empirical data. Creating a research design means making decisions about:
- Your overall aims and approach
- The type of research design you’ll use
- Your sampling methods or criteria for selecting subjects
- Your data collection methods
- The procedures you’ll follow to collect data
- Your data analysis methods
A well-planned research design helps ensure that your methods match your research aims and that you use the right kind of analysis for your data.
Table of contents
Step 1: consider your aims and approach, step 2: choose a type of research design, step 3: identify your population and sampling method, step 4: choose your data collection methods, step 5: plan your data collection procedures, step 6: decide on your data analysis strategies, frequently asked questions.
- Introduction
Before you can start designing your research, you should already have a clear idea of the research question you want to investigate.
There are many different ways you could go about answering this question. Your research design choices should be driven by your aims and priorities – start by thinking carefully about what you want to achieve.
The first choice you need to make is whether you’ll take a qualitative or quantitative approach.
Qualitative research designs tend to be more flexible and inductive , allowing you to adjust your approach based on what you find throughout the research process.
Quantitative research designs tend to be more fixed and deductive , with variables and hypotheses clearly defined in advance of data collection.
It’s also possible to use a mixed methods design that integrates aspects of both approaches. By combining qualitative and quantitative insights, you can gain a more complete picture of the problem you’re studying and strengthen the credibility of your conclusions.
Practical and ethical considerations when designing research
As well as scientific considerations, you need to think practically when designing your research. If your research involves people or animals, you also need to consider research ethics .
- How much time do you have to collect data and write up the research?
- Will you be able to gain access to the data you need (e.g., by travelling to a specific location or contacting specific people)?
- Do you have the necessary research skills (e.g., statistical analysis or interview techniques)?
- Will you need ethical approval ?
At each stage of the research design process, make sure that your choices are practically feasible.
Prevent plagiarism, run a free check.
Within both qualitative and quantitative approaches, there are several types of research design to choose from. Each type provides a framework for the overall shape of your research.
Types of quantitative research designs
Quantitative designs can be split into four main types. Experimental and quasi-experimental designs allow you to test cause-and-effect relationships, while descriptive and correlational designs allow you to measure variables and describe relationships between them.
With descriptive and correlational designs, you can get a clear picture of characteristics, trends, and relationships as they exist in the real world. However, you can’t draw conclusions about cause and effect (because correlation doesn’t imply causation ).
Experiments are the strongest way to test cause-and-effect relationships without the risk of other variables influencing the results. However, their controlled conditions may not always reflect how things work in the real world. They’re often also more difficult and expensive to implement.
Types of qualitative research designs
Qualitative designs are less strictly defined. This approach is about gaining a rich, detailed understanding of a specific context or phenomenon, and you can often be more creative and flexible in designing your research.
The table below shows some common types of qualitative design. They often have similar approaches in terms of data collection, but focus on different aspects when analysing the data.
Your research design should clearly define who or what your research will focus on, and how you’ll go about choosing your participants or subjects.
In research, a population is the entire group that you want to draw conclusions about, while a sample is the smaller group of individuals you’ll actually collect data from.
Defining the population
A population can be made up of anything you want to study – plants, animals, organisations, texts, countries, etc. In the social sciences, it most often refers to a group of people.
For example, will you focus on people from a specific demographic, region, or background? Are you interested in people with a certain job or medical condition, or users of a particular product?
The more precisely you define your population, the easier it will be to gather a representative sample.
Sampling methods
Even with a narrowly defined population, it’s rarely possible to collect data from every individual. Instead, you’ll collect data from a sample.
To select a sample, there are two main approaches: probability sampling and non-probability sampling . The sampling method you use affects how confidently you can generalise your results to the population as a whole.
Probability sampling is the most statistically valid option, but it’s often difficult to achieve unless you’re dealing with a very small and accessible population.
For practical reasons, many studies use non-probability sampling, but it’s important to be aware of the limitations and carefully consider potential biases. You should always make an effort to gather a sample that’s as representative as possible of the population.
Case selection in qualitative research
In some types of qualitative designs, sampling may not be relevant.
For example, in an ethnography or a case study, your aim is to deeply understand a specific context, not to generalise to a population. Instead of sampling, you may simply aim to collect as much data as possible about the context you are studying.
In these types of design, you still have to carefully consider your choice of case or community. You should have a clear rationale for why this particular case is suitable for answering your research question.
For example, you might choose a case study that reveals an unusual or neglected aspect of your research problem, or you might choose several very similar or very different cases in order to compare them.
Data collection methods are ways of directly measuring variables and gathering information. They allow you to gain first-hand knowledge and original insights into your research problem.
You can choose just one data collection method, or use several methods in the same study.
Survey methods
Surveys allow you to collect data about opinions, behaviours, experiences, and characteristics by asking people directly. There are two main survey methods to choose from: questionnaires and interviews.
Observation methods
Observations allow you to collect data unobtrusively, observing characteristics, behaviours, or social interactions without relying on self-reporting.
Observations may be conducted in real time, taking notes as you observe, or you might make audiovisual recordings for later analysis. They can be qualitative or quantitative.
Other methods of data collection
There are many other ways you might collect data depending on your field and topic.
If you’re not sure which methods will work best for your research design, try reading some papers in your field to see what data collection methods they used.
Secondary data
If you don’t have the time or resources to collect data from the population you’re interested in, you can also choose to use secondary data that other researchers already collected – for example, datasets from government surveys or previous studies on your topic.
With this raw data, you can do your own analysis to answer new research questions that weren’t addressed by the original study.
Using secondary data can expand the scope of your research, as you may be able to access much larger and more varied samples than you could collect yourself.
However, it also means you don’t have any control over which variables to measure or how to measure them, so the conclusions you can draw may be limited.
As well as deciding on your methods, you need to plan exactly how you’ll use these methods to collect data that’s consistent, accurate, and unbiased.
Planning systematic procedures is especially important in quantitative research, where you need to precisely define your variables and ensure your measurements are reliable and valid.
Operationalisation
Some variables, like height or age, are easily measured. But often you’ll be dealing with more abstract concepts, like satisfaction, anxiety, or competence. Operationalisation means turning these fuzzy ideas into measurable indicators.
If you’re using observations , which events or actions will you count?
If you’re using surveys , which questions will you ask and what range of responses will be offered?
You may also choose to use or adapt existing materials designed to measure the concept you’re interested in – for example, questionnaires or inventories whose reliability and validity has already been established.
Reliability and validity
Reliability means your results can be consistently reproduced , while validity means that you’re actually measuring the concept you’re interested in.
For valid and reliable results, your measurement materials should be thoroughly researched and carefully designed. Plan your procedures to make sure you carry out the same steps in the same way for each participant.
If you’re developing a new questionnaire or other instrument to measure a specific concept, running a pilot study allows you to check its validity and reliability in advance.
Sampling procedures
As well as choosing an appropriate sampling method, you need a concrete plan for how you’ll actually contact and recruit your selected sample.
That means making decisions about things like:
- How many participants do you need for an adequate sample size?
- What inclusion and exclusion criteria will you use to identify eligible participants?
- How will you contact your sample – by mail, online, by phone, or in person?
If you’re using a probability sampling method, it’s important that everyone who is randomly selected actually participates in the study. How will you ensure a high response rate?
If you’re using a non-probability method, how will you avoid bias and ensure a representative sample?
Data management
It’s also important to create a data management plan for organising and storing your data.
Will you need to transcribe interviews or perform data entry for observations? You should anonymise and safeguard any sensitive data, and make sure it’s backed up regularly.
Keeping your data well organised will save time when it comes to analysing them. It can also help other researchers validate and add to your findings.
On their own, raw data can’t answer your research question. The last step of designing your research is planning how you’ll analyse the data.
Quantitative data analysis
In quantitative research, you’ll most likely use some form of statistical analysis . With statistics, you can summarise your sample data, make estimates, and test hypotheses.
Using descriptive statistics , you can summarise your sample data in terms of:
- The distribution of the data (e.g., the frequency of each score on a test)
- The central tendency of the data (e.g., the mean to describe the average score)
- The variability of the data (e.g., the standard deviation to describe how spread out the scores are)
The specific calculations you can do depend on the level of measurement of your variables.
Using inferential statistics , you can:
- Make estimates about the population based on your sample data.
- Test hypotheses about a relationship between variables.
Regression and correlation tests look for associations between two or more variables, while comparison tests (such as t tests and ANOVAs ) look for differences in the outcomes of different groups.
Your choice of statistical test depends on various aspects of your research design, including the types of variables you’re dealing with and the distribution of your data.
Qualitative data analysis
In qualitative research, your data will usually be very dense with information and ideas. Instead of summing it up in numbers, you’ll need to comb through the data in detail, interpret its meanings, identify patterns, and extract the parts that are most relevant to your research question.
Two of the most common approaches to doing this are thematic analysis and discourse analysis .
There are many other ways of analysing qualitative data depending on the aims of your research. To get a sense of potential approaches, try reading some qualitative research papers in your field.
A sample is a subset of individuals from a larger population. Sampling means selecting the group that you will actually collect data from in your research.
For example, if you are researching the opinions of students in your university, you could survey a sample of 100 students.
Statistical sampling allows you to test a hypothesis about the characteristics of a population. There are various sampling methods you can use to ensure that your sample is representative of the population as a whole.
Operationalisation means turning abstract conceptual ideas into measurable observations.
For example, the concept of social anxiety isn’t directly observable, but it can be operationally defined in terms of self-rating scores, behavioural avoidance of crowded places, or physical anxiety symptoms in social situations.
Before collecting data , it’s important to consider how you will operationalise the variables that you want to measure.
The research methods you use depend on the type of data you need to answer your research question .
- If you want to measure something or test a hypothesis , use quantitative methods . If you want to explore ideas, thoughts, and meanings, use qualitative methods .
- If you want to analyse a large amount of readily available data, use secondary data. If you want data specific to your purposes with control over how they are generated, collect primary data.
- If you want to establish cause-and-effect relationships between variables , use experimental methods. If you want to understand the characteristics of a research subject, use descriptive methods.
Cite this Scribbr article
If you want to cite this source, you can copy and paste the citation or click the ‘Cite this Scribbr article’ button to automatically add the citation to our free Reference Generator.
McCombes, S. (2023, March 20). Research Design | Step-by-Step Guide with Examples. Scribbr. Retrieved 21 May 2024, from https://www.scribbr.co.uk/research-methods/research-design/
Is this article helpful?
Shona McCombes
- How it works
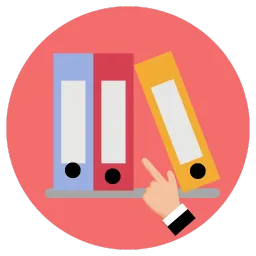
How to Write a Research Design – Guide with Examples
Published by Alaxendra Bets at August 14th, 2021 , Revised On October 3, 2023
A research design is a structure that combines different components of research. It involves the use of different data collection and data analysis techniques logically to answer the research questions .
It would be best to make some decisions about addressing the research questions adequately before starting the research process, which is achieved with the help of the research design.
Below are the key aspects of the decision-making process:
- Data type required for research
- Research resources
- Participants required for research
- Hypothesis based upon research question(s)
- Data analysis methodologies
- Variables (Independent, dependent, and confounding)
- The location and timescale for conducting the data
- The time period required for research
The research design provides the strategy of investigation for your project. Furthermore, it defines the parameters and criteria to compile the data to evaluate results and conclude.
Your project’s validity depends on the data collection and interpretation techniques. A strong research design reflects a strong dissertation , scientific paper, or research proposal .
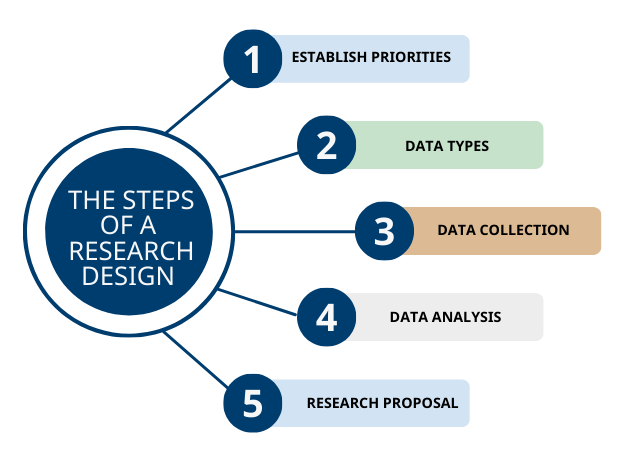
Step 1: Establish Priorities for Research Design
Before conducting any research study, you must address an important question: “how to create a research design.”
The research design depends on the researcher’s priorities and choices because every research has different priorities. For a complex research study involving multiple methods, you may choose to have more than one research design.
Multimethodology or multimethod research includes using more than one data collection method or research in a research study or set of related studies.
If one research design is weak in one area, then another research design can cover that weakness. For instance, a dissertation analyzing different situations or cases will have more than one research design.
For example:
- Experimental research involves experimental investigation and laboratory experience, but it does not accurately investigate the real world.
- Quantitative research is good for the statistical part of the project, but it may not provide an in-depth understanding of the topic .
- Also, correlational research will not provide experimental results because it is a technique that assesses the statistical relationship between two variables.
While scientific considerations are a fundamental aspect of the research design, It is equally important that the researcher think practically before deciding on its structure. Here are some questions that you should think of;
- Do you have enough time to gather data and complete the write-up?
- Will you be able to collect the necessary data by interviewing a specific person or visiting a specific location?
- Do you have in-depth knowledge about the different statistical analysis and data collection techniques to address the research questions or test the hypothesis ?
If you think that the chosen research design cannot answer the research questions properly, you can refine your research questions to gain better insight.
Step 2: Data Type you Need for Research
Decide on the type of data you need for your research. The type of data you need to collect depends on your research questions or research hypothesis. Two types of research data can be used to answer the research questions:
Primary Data Vs. Secondary Data
Qualitative vs. quantitative data.
Also, see; Research methods, design, and analysis .
Need help with a thesis chapter?
- Hire an expert from ResearchProspect today!
- Statistical analysis, research methodology, discussion of the results or conclusion – our experts can help you no matter how complex the requirements are.
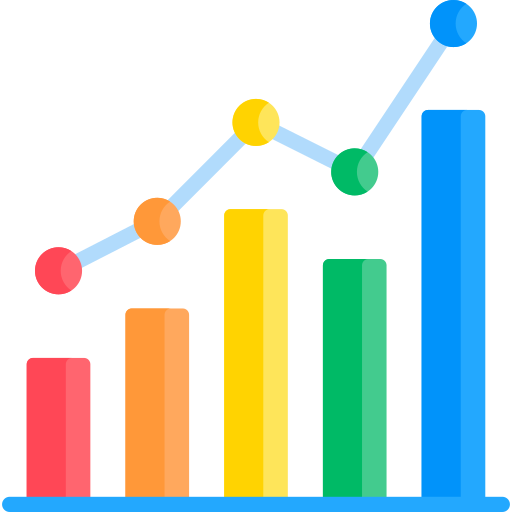
Step 3: Data Collection Techniques
Once you have selected the type of research to answer your research question, you need to decide where and how to collect the data.
It is time to determine your research method to address the research problem . Research methods involve procedures, techniques, materials, and tools used for the study.
For instance, a dissertation research design includes the different resources and data collection techniques and helps establish your dissertation’s structure .
The following table shows the characteristics of the most popularly employed research methods.
Research Methods
Step 4: Procedure of Data Analysis
Use of the correct data and statistical analysis technique is necessary for the validity of your research. Therefore, you need to be certain about the data type that would best address the research problem. Choosing an appropriate analysis method is the final step for the research design. It can be split into two main categories;
Quantitative Data Analysis
The quantitative data analysis technique involves analyzing the numerical data with the help of different applications such as; SPSS, STATA, Excel, origin lab, etc.
This data analysis strategy tests different variables such as spectrum, frequencies, averages, and more. The research question and the hypothesis must be established to identify the variables for testing.
Qualitative Data Analysis
Qualitative data analysis of figures, themes, and words allows for flexibility and the researcher’s subjective opinions. This means that the researcher’s primary focus will be interpreting patterns, tendencies, and accounts and understanding the implications and social framework.
You should be clear about your research objectives before starting to analyze the data. For example, you should ask yourself whether you need to explain respondents’ experiences and insights or do you also need to evaluate their responses with reference to a certain social framework.
Step 5: Write your Research Proposal
The research design is an important component of a research proposal because it plans the project’s execution. You can share it with the supervisor, who would evaluate the feasibility and capacity of the results and conclusion .
Read our guidelines to write a research proposal if you have already formulated your research design. The research proposal is written in the future tense because you are writing your proposal before conducting research.
The research methodology or research design, on the other hand, is generally written in the past tense.
How to Write a Research Design – Conclusion
A research design is the plan, structure, strategy of investigation conceived to answer the research question and test the hypothesis. The dissertation research design can be classified based on the type of data and the type of analysis.
Above mentioned five steps are the answer to how to write a research design. So, follow these steps to formulate the perfect research design for your dissertation .
ResearchProspect writers have years of experience creating research designs that align with the dissertation’s aim and objectives. If you are struggling with your dissertation methodology chapter, you might want to look at our dissertation part-writing service.
Our dissertation writers can also help you with the full dissertation paper . No matter how urgent or complex your need may be, ResearchProspect can help. We also offer PhD level research paper writing services.
Frequently Asked Questions
What is research design.
Research design is a systematic plan that guides the research process, outlining the methodology and procedures for collecting and analysing data. It determines the structure of the study, ensuring the research question is answered effectively, reliably, and validly. It serves as the blueprint for the entire research project.
How to write a research design?
To write a research design, define your research question, identify the research method (qualitative, quantitative, or mixed), choose data collection techniques (e.g., surveys, interviews), determine the sample size and sampling method, outline data analysis procedures, and highlight potential limitations and ethical considerations for the study.
How to write the design section of a research paper?
In the design section of a research paper, describe the research methodology chosen and justify its selection. Outline the data collection methods, participants or samples, instruments used, and procedures followed. Detail any experimental controls, if applicable. Ensure clarity and precision to enable replication of the study by other researchers.
How to write a research design in methodology?
To write a research design in methodology, clearly outline the research strategy (e.g., experimental, survey, case study). Describe the sampling technique, participants, and data collection methods. Detail the procedures for data collection and analysis. Justify choices by linking them to research objectives, addressing reliability and validity.
You May Also Like
Repository of ten perfect research question examples will provide you a better perspective about how to create research questions.
Make sure that your selected topic is intriguing, manageable, and relevant. Here are some guidelines to help understand how to find a good dissertation topic.
How to write a hypothesis for dissertation,? A hypothesis is a statement that can be tested with the help of experimental or theoretical research.
USEFUL LINKS
LEARNING RESOURCES
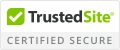
COMPANY DETAILS
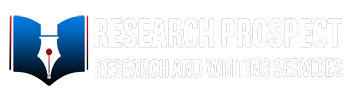
- How It Works
- USC Libraries
- Research Guides
Organizing Your Social Sciences Research Paper
- Types of Research Designs
- Purpose of Guide
- Design Flaws to Avoid
- Independent and Dependent Variables
- Glossary of Research Terms
- Reading Research Effectively
- Narrowing a Topic Idea
- Broadening a Topic Idea
- Extending the Timeliness of a Topic Idea
- Academic Writing Style
- Applying Critical Thinking
- Choosing a Title
- Making an Outline
- Paragraph Development
- Research Process Video Series
- Executive Summary
- The C.A.R.S. Model
- Background Information
- The Research Problem/Question
- Theoretical Framework
- Citation Tracking
- Content Alert Services
- Evaluating Sources
- Primary Sources
- Secondary Sources
- Tiertiary Sources
- Scholarly vs. Popular Publications
- Qualitative Methods
- Quantitative Methods
- Insiderness
- Using Non-Textual Elements
- Limitations of the Study
- Common Grammar Mistakes
- Writing Concisely
- Avoiding Plagiarism
- Footnotes or Endnotes?
- Further Readings
- Generative AI and Writing
- USC Libraries Tutorials and Other Guides
- Bibliography
Introduction
Before beginning your paper, you need to decide how you plan to design the study .
The research design refers to the overall strategy and analytical approach that you have chosen in order to integrate, in a coherent and logical way, the different components of the study, thus ensuring that the research problem will be thoroughly investigated. It constitutes the blueprint for the collection, measurement, and interpretation of information and data. Note that the research problem determines the type of design you choose, not the other way around!
De Vaus, D. A. Research Design in Social Research . London: SAGE, 2001; Trochim, William M.K. Research Methods Knowledge Base. 2006.
General Structure and Writing Style
The function of a research design is to ensure that the evidence obtained enables you to effectively address the research problem logically and as unambiguously as possible . In social sciences research, obtaining information relevant to the research problem generally entails specifying the type of evidence needed to test the underlying assumptions of a theory, to evaluate a program, or to accurately describe and assess meaning related to an observable phenomenon.
With this in mind, a common mistake made by researchers is that they begin their investigations before they have thought critically about what information is required to address the research problem. Without attending to these design issues beforehand, the overall research problem will not be adequately addressed and any conclusions drawn will run the risk of being weak and unconvincing. As a consequence, the overall validity of the study will be undermined.
The length and complexity of describing the research design in your paper can vary considerably, but any well-developed description will achieve the following :
- Identify the research problem clearly and justify its selection, particularly in relation to any valid alternative designs that could have been used,
- Review and synthesize previously published literature associated with the research problem,
- Clearly and explicitly specify hypotheses [i.e., research questions] central to the problem,
- Effectively describe the information and/or data which will be necessary for an adequate testing of the hypotheses and explain how such information and/or data will be obtained, and
- Describe the methods of analysis to be applied to the data in determining whether or not the hypotheses are true or false.
The research design is usually incorporated into the introduction of your paper . You can obtain an overall sense of what to do by reviewing studies that have utilized the same research design [e.g., using a case study approach]. This can help you develop an outline to follow for your own paper.
NOTE : Use the SAGE Research Methods Online and Cases and the SAGE Research Methods Videos databases to search for scholarly resources on how to apply specific research designs and methods . The Research Methods Online database contains links to more than 175,000 pages of SAGE publisher's book, journal, and reference content on quantitative, qualitative, and mixed research methodologies. Also included is a collection of case studies of social research projects that can be used to help you better understand abstract or complex methodological concepts. The Research Methods Videos database contains hours of tutorials, interviews, video case studies, and mini-documentaries covering the entire research process.
Creswell, John W. and J. David Creswell. Research Design: Qualitative, Quantitative, and Mixed Methods Approaches . 5th edition. Thousand Oaks, CA: Sage, 2018; De Vaus, D. A. Research Design in Social Research . London: SAGE, 2001; Gorard, Stephen. Research Design: Creating Robust Approaches for the Social Sciences . Thousand Oaks, CA: Sage, 2013; Leedy, Paul D. and Jeanne Ellis Ormrod. Practical Research: Planning and Design . Tenth edition. Boston, MA: Pearson, 2013; Vogt, W. Paul, Dianna C. Gardner, and Lynne M. Haeffele. When to Use What Research Design . New York: Guilford, 2012.
Action Research Design
Definition and Purpose
The essentials of action research design follow a characteristic cycle whereby initially an exploratory stance is adopted, where an understanding of a problem is developed and plans are made for some form of interventionary strategy. Then the intervention is carried out [the "action" in action research] during which time, pertinent observations are collected in various forms. The new interventional strategies are carried out, and this cyclic process repeats, continuing until a sufficient understanding of [or a valid implementation solution for] the problem is achieved. The protocol is iterative or cyclical in nature and is intended to foster deeper understanding of a given situation, starting with conceptualizing and particularizing the problem and moving through several interventions and evaluations.
What do these studies tell you ?
- This is a collaborative and adaptive research design that lends itself to use in work or community situations.
- Design focuses on pragmatic and solution-driven research outcomes rather than testing theories.
- When practitioners use action research, it has the potential to increase the amount they learn consciously from their experience; the action research cycle can be regarded as a learning cycle.
- Action research studies often have direct and obvious relevance to improving practice and advocating for change.
- There are no hidden controls or preemption of direction by the researcher.
What these studies don't tell you ?
- It is harder to do than conducting conventional research because the researcher takes on responsibilities of advocating for change as well as for researching the topic.
- Action research is much harder to write up because it is less likely that you can use a standard format to report your findings effectively [i.e., data is often in the form of stories or observation].
- Personal over-involvement of the researcher may bias research results.
- The cyclic nature of action research to achieve its twin outcomes of action [e.g. change] and research [e.g. understanding] is time-consuming and complex to conduct.
- Advocating for change usually requires buy-in from study participants.
Coghlan, David and Mary Brydon-Miller. The Sage Encyclopedia of Action Research . Thousand Oaks, CA: Sage, 2014; Efron, Sara Efrat and Ruth Ravid. Action Research in Education: A Practical Guide . New York: Guilford, 2013; Gall, Meredith. Educational Research: An Introduction . Chapter 18, Action Research. 8th ed. Boston, MA: Pearson/Allyn and Bacon, 2007; Gorard, Stephen. Research Design: Creating Robust Approaches for the Social Sciences . Thousand Oaks, CA: Sage, 2013; Kemmis, Stephen and Robin McTaggart. “Participatory Action Research.” In Handbook of Qualitative Research . Norman Denzin and Yvonna S. Lincoln, eds. 2nd ed. (Thousand Oaks, CA: SAGE, 2000), pp. 567-605; McNiff, Jean. Writing and Doing Action Research . London: Sage, 2014; Reason, Peter and Hilary Bradbury. Handbook of Action Research: Participative Inquiry and Practice . Thousand Oaks, CA: SAGE, 2001.
Case Study Design
A case study is an in-depth study of a particular research problem rather than a sweeping statistical survey or comprehensive comparative inquiry. It is often used to narrow down a very broad field of research into one or a few easily researchable examples. The case study research design is also useful for testing whether a specific theory and model actually applies to phenomena in the real world. It is a useful design when not much is known about an issue or phenomenon.
- Approach excels at bringing us to an understanding of a complex issue through detailed contextual analysis of a limited number of events or conditions and their relationships.
- A researcher using a case study design can apply a variety of methodologies and rely on a variety of sources to investigate a research problem.
- Design can extend experience or add strength to what is already known through previous research.
- Social scientists, in particular, make wide use of this research design to examine contemporary real-life situations and provide the basis for the application of concepts and theories and the extension of methodologies.
- The design can provide detailed descriptions of specific and rare cases.
- A single or small number of cases offers little basis for establishing reliability or to generalize the findings to a wider population of people, places, or things.
- Intense exposure to the study of a case may bias a researcher's interpretation of the findings.
- Design does not facilitate assessment of cause and effect relationships.
- Vital information may be missing, making the case hard to interpret.
- The case may not be representative or typical of the larger problem being investigated.
- If the criteria for selecting a case is because it represents a very unusual or unique phenomenon or problem for study, then your interpretation of the findings can only apply to that particular case.
Case Studies. Writing@CSU. Colorado State University; Anastas, Jeane W. Research Design for Social Work and the Human Services . Chapter 4, Flexible Methods: Case Study Design. 2nd ed. New York: Columbia University Press, 1999; Gerring, John. “What Is a Case Study and What Is It Good for?” American Political Science Review 98 (May 2004): 341-354; Greenhalgh, Trisha, editor. Case Study Evaluation: Past, Present and Future Challenges . Bingley, UK: Emerald Group Publishing, 2015; Mills, Albert J. , Gabrielle Durepos, and Eiden Wiebe, editors. Encyclopedia of Case Study Research . Thousand Oaks, CA: SAGE Publications, 2010; Stake, Robert E. The Art of Case Study Research . Thousand Oaks, CA: SAGE, 1995; Yin, Robert K. Case Study Research: Design and Theory . Applied Social Research Methods Series, no. 5. 3rd ed. Thousand Oaks, CA: SAGE, 2003.
Causal Design
Causality studies may be thought of as understanding a phenomenon in terms of conditional statements in the form, “If X, then Y.” This type of research is used to measure what impact a specific change will have on existing norms and assumptions. Most social scientists seek causal explanations that reflect tests of hypotheses. Causal effect (nomothetic perspective) occurs when variation in one phenomenon, an independent variable, leads to or results, on average, in variation in another phenomenon, the dependent variable.
Conditions necessary for determining causality:
- Empirical association -- a valid conclusion is based on finding an association between the independent variable and the dependent variable.
- Appropriate time order -- to conclude that causation was involved, one must see that cases were exposed to variation in the independent variable before variation in the dependent variable.
- Nonspuriousness -- a relationship between two variables that is not due to variation in a third variable.
- Causality research designs assist researchers in understanding why the world works the way it does through the process of proving a causal link between variables and by the process of eliminating other possibilities.
- Replication is possible.
- There is greater confidence the study has internal validity due to the systematic subject selection and equity of groups being compared.
- Not all relationships are causal! The possibility always exists that, by sheer coincidence, two unrelated events appear to be related [e.g., Punxatawney Phil could accurately predict the duration of Winter for five consecutive years but, the fact remains, he's just a big, furry rodent].
- Conclusions about causal relationships are difficult to determine due to a variety of extraneous and confounding variables that exist in a social environment. This means causality can only be inferred, never proven.
- If two variables are correlated, the cause must come before the effect. However, even though two variables might be causally related, it can sometimes be difficult to determine which variable comes first and, therefore, to establish which variable is the actual cause and which is the actual effect.
Beach, Derek and Rasmus Brun Pedersen. Causal Case Study Methods: Foundations and Guidelines for Comparing, Matching, and Tracing . Ann Arbor, MI: University of Michigan Press, 2016; Bachman, Ronet. The Practice of Research in Criminology and Criminal Justice . Chapter 5, Causation and Research Designs. 3rd ed. Thousand Oaks, CA: Pine Forge Press, 2007; Brewer, Ernest W. and Jennifer Kubn. “Causal-Comparative Design.” In Encyclopedia of Research Design . Neil J. Salkind, editor. (Thousand Oaks, CA: Sage, 2010), pp. 125-132; Causal Research Design: Experimentation. Anonymous SlideShare Presentation; Gall, Meredith. Educational Research: An Introduction . Chapter 11, Nonexperimental Research: Correlational Designs. 8th ed. Boston, MA: Pearson/Allyn and Bacon, 2007; Trochim, William M.K. Research Methods Knowledge Base. 2006.
Cohort Design
Often used in the medical sciences, but also found in the applied social sciences, a cohort study generally refers to a study conducted over a period of time involving members of a population which the subject or representative member comes from, and who are united by some commonality or similarity. Using a quantitative framework, a cohort study makes note of statistical occurrence within a specialized subgroup, united by same or similar characteristics that are relevant to the research problem being investigated, rather than studying statistical occurrence within the general population. Using a qualitative framework, cohort studies generally gather data using methods of observation. Cohorts can be either "open" or "closed."
- Open Cohort Studies [dynamic populations, such as the population of Los Angeles] involve a population that is defined just by the state of being a part of the study in question (and being monitored for the outcome). Date of entry and exit from the study is individually defined, therefore, the size of the study population is not constant. In open cohort studies, researchers can only calculate rate based data, such as, incidence rates and variants thereof.
- Closed Cohort Studies [static populations, such as patients entered into a clinical trial] involve participants who enter into the study at one defining point in time and where it is presumed that no new participants can enter the cohort. Given this, the number of study participants remains constant (or can only decrease).
- The use of cohorts is often mandatory because a randomized control study may be unethical. For example, you cannot deliberately expose people to asbestos, you can only study its effects on those who have already been exposed. Research that measures risk factors often relies upon cohort designs.
- Because cohort studies measure potential causes before the outcome has occurred, they can demonstrate that these “causes” preceded the outcome, thereby avoiding the debate as to which is the cause and which is the effect.
- Cohort analysis is highly flexible and can provide insight into effects over time and related to a variety of different types of changes [e.g., social, cultural, political, economic, etc.].
- Either original data or secondary data can be used in this design.
- In cases where a comparative analysis of two cohorts is made [e.g., studying the effects of one group exposed to asbestos and one that has not], a researcher cannot control for all other factors that might differ between the two groups. These factors are known as confounding variables.
- Cohort studies can end up taking a long time to complete if the researcher must wait for the conditions of interest to develop within the group. This also increases the chance that key variables change during the course of the study, potentially impacting the validity of the findings.
- Due to the lack of randominization in the cohort design, its external validity is lower than that of study designs where the researcher randomly assigns participants.
Healy P, Devane D. “Methodological Considerations in Cohort Study Designs.” Nurse Researcher 18 (2011): 32-36; Glenn, Norval D, editor. Cohort Analysis . 2nd edition. Thousand Oaks, CA: Sage, 2005; Levin, Kate Ann. Study Design IV: Cohort Studies. Evidence-Based Dentistry 7 (2003): 51–52; Payne, Geoff. “Cohort Study.” In The SAGE Dictionary of Social Research Methods . Victor Jupp, editor. (Thousand Oaks, CA: Sage, 2006), pp. 31-33; Study Design 101. Himmelfarb Health Sciences Library. George Washington University, November 2011; Cohort Study. Wikipedia.
Cross-Sectional Design
Cross-sectional research designs have three distinctive features: no time dimension; a reliance on existing differences rather than change following intervention; and, groups are selected based on existing differences rather than random allocation. The cross-sectional design can only measure differences between or from among a variety of people, subjects, or phenomena rather than a process of change. As such, researchers using this design can only employ a relatively passive approach to making causal inferences based on findings.
- Cross-sectional studies provide a clear 'snapshot' of the outcome and the characteristics associated with it, at a specific point in time.
- Unlike an experimental design, where there is an active intervention by the researcher to produce and measure change or to create differences, cross-sectional designs focus on studying and drawing inferences from existing differences between people, subjects, or phenomena.
- Entails collecting data at and concerning one point in time. While longitudinal studies involve taking multiple measures over an extended period of time, cross-sectional research is focused on finding relationships between variables at one moment in time.
- Groups identified for study are purposely selected based upon existing differences in the sample rather than seeking random sampling.
- Cross-section studies are capable of using data from a large number of subjects and, unlike observational studies, is not geographically bound.
- Can estimate prevalence of an outcome of interest because the sample is usually taken from the whole population.
- Because cross-sectional designs generally use survey techniques to gather data, they are relatively inexpensive and take up little time to conduct.
- Finding people, subjects, or phenomena to study that are very similar except in one specific variable can be difficult.
- Results are static and time bound and, therefore, give no indication of a sequence of events or reveal historical or temporal contexts.
- Studies cannot be utilized to establish cause and effect relationships.
- This design only provides a snapshot of analysis so there is always the possibility that a study could have differing results if another time-frame had been chosen.
- There is no follow up to the findings.
Bethlehem, Jelke. "7: Cross-sectional Research." In Research Methodology in the Social, Behavioural and Life Sciences . Herman J Adèr and Gideon J Mellenbergh, editors. (London, England: Sage, 1999), pp. 110-43; Bourque, Linda B. “Cross-Sectional Design.” In The SAGE Encyclopedia of Social Science Research Methods . Michael S. Lewis-Beck, Alan Bryman, and Tim Futing Liao. (Thousand Oaks, CA: 2004), pp. 230-231; Hall, John. “Cross-Sectional Survey Design.” In Encyclopedia of Survey Research Methods . Paul J. Lavrakas, ed. (Thousand Oaks, CA: Sage, 2008), pp. 173-174; Helen Barratt, Maria Kirwan. Cross-Sectional Studies: Design Application, Strengths and Weaknesses of Cross-Sectional Studies. Healthknowledge, 2009. Cross-Sectional Study. Wikipedia.
Descriptive Design
Descriptive research designs help provide answers to the questions of who, what, when, where, and how associated with a particular research problem; a descriptive study cannot conclusively ascertain answers to why. Descriptive research is used to obtain information concerning the current status of the phenomena and to describe "what exists" with respect to variables or conditions in a situation.
- The subject is being observed in a completely natural and unchanged natural environment. True experiments, whilst giving analyzable data, often adversely influence the normal behavior of the subject [a.k.a., the Heisenberg effect whereby measurements of certain systems cannot be made without affecting the systems].
- Descriptive research is often used as a pre-cursor to more quantitative research designs with the general overview giving some valuable pointers as to what variables are worth testing quantitatively.
- If the limitations are understood, they can be a useful tool in developing a more focused study.
- Descriptive studies can yield rich data that lead to important recommendations in practice.
- Appoach collects a large amount of data for detailed analysis.
- The results from a descriptive research cannot be used to discover a definitive answer or to disprove a hypothesis.
- Because descriptive designs often utilize observational methods [as opposed to quantitative methods], the results cannot be replicated.
- The descriptive function of research is heavily dependent on instrumentation for measurement and observation.
Anastas, Jeane W. Research Design for Social Work and the Human Services . Chapter 5, Flexible Methods: Descriptive Research. 2nd ed. New York: Columbia University Press, 1999; Given, Lisa M. "Descriptive Research." In Encyclopedia of Measurement and Statistics . Neil J. Salkind and Kristin Rasmussen, editors. (Thousand Oaks, CA: Sage, 2007), pp. 251-254; McNabb, Connie. Descriptive Research Methodologies. Powerpoint Presentation; Shuttleworth, Martyn. Descriptive Research Design, September 26, 2008; Erickson, G. Scott. "Descriptive Research Design." In New Methods of Market Research and Analysis . (Northampton, MA: Edward Elgar Publishing, 2017), pp. 51-77; Sahin, Sagufta, and Jayanta Mete. "A Brief Study on Descriptive Research: Its Nature and Application in Social Science." International Journal of Research and Analysis in Humanities 1 (2021): 11; K. Swatzell and P. Jennings. “Descriptive Research: The Nuts and Bolts.” Journal of the American Academy of Physician Assistants 20 (2007), pp. 55-56; Kane, E. Doing Your Own Research: Basic Descriptive Research in the Social Sciences and Humanities . London: Marion Boyars, 1985.
Experimental Design
A blueprint of the procedure that enables the researcher to maintain control over all factors that may affect the result of an experiment. In doing this, the researcher attempts to determine or predict what may occur. Experimental research is often used where there is time priority in a causal relationship (cause precedes effect), there is consistency in a causal relationship (a cause will always lead to the same effect), and the magnitude of the correlation is great. The classic experimental design specifies an experimental group and a control group. The independent variable is administered to the experimental group and not to the control group, and both groups are measured on the same dependent variable. Subsequent experimental designs have used more groups and more measurements over longer periods. True experiments must have control, randomization, and manipulation.
- Experimental research allows the researcher to control the situation. In so doing, it allows researchers to answer the question, “What causes something to occur?”
- Permits the researcher to identify cause and effect relationships between variables and to distinguish placebo effects from treatment effects.
- Experimental research designs support the ability to limit alternative explanations and to infer direct causal relationships in the study.
- Approach provides the highest level of evidence for single studies.
- The design is artificial, and results may not generalize well to the real world.
- The artificial settings of experiments may alter the behaviors or responses of participants.
- Experimental designs can be costly if special equipment or facilities are needed.
- Some research problems cannot be studied using an experiment because of ethical or technical reasons.
- Difficult to apply ethnographic and other qualitative methods to experimentally designed studies.
Anastas, Jeane W. Research Design for Social Work and the Human Services . Chapter 7, Flexible Methods: Experimental Research. 2nd ed. New York: Columbia University Press, 1999; Chapter 2: Research Design, Experimental Designs. School of Psychology, University of New England, 2000; Chow, Siu L. "Experimental Design." In Encyclopedia of Research Design . Neil J. Salkind, editor. (Thousand Oaks, CA: Sage, 2010), pp. 448-453; "Experimental Design." In Social Research Methods . Nicholas Walliman, editor. (London, England: Sage, 2006), pp, 101-110; Experimental Research. Research Methods by Dummies. Department of Psychology. California State University, Fresno, 2006; Kirk, Roger E. Experimental Design: Procedures for the Behavioral Sciences . 4th edition. Thousand Oaks, CA: Sage, 2013; Trochim, William M.K. Experimental Design. Research Methods Knowledge Base. 2006; Rasool, Shafqat. Experimental Research. Slideshare presentation.
Exploratory Design
An exploratory design is conducted about a research problem when there are few or no earlier studies to refer to or rely upon to predict an outcome . The focus is on gaining insights and familiarity for later investigation or undertaken when research problems are in a preliminary stage of investigation. Exploratory designs are often used to establish an understanding of how best to proceed in studying an issue or what methodology would effectively apply to gathering information about the issue.
The goals of exploratory research are intended to produce the following possible insights:
- Familiarity with basic details, settings, and concerns.
- Well grounded picture of the situation being developed.
- Generation of new ideas and assumptions.
- Development of tentative theories or hypotheses.
- Determination about whether a study is feasible in the future.
- Issues get refined for more systematic investigation and formulation of new research questions.
- Direction for future research and techniques get developed.
- Design is a useful approach for gaining background information on a particular topic.
- Exploratory research is flexible and can address research questions of all types (what, why, how).
- Provides an opportunity to define new terms and clarify existing concepts.
- Exploratory research is often used to generate formal hypotheses and develop more precise research problems.
- In the policy arena or applied to practice, exploratory studies help establish research priorities and where resources should be allocated.
- Exploratory research generally utilizes small sample sizes and, thus, findings are typically not generalizable to the population at large.
- The exploratory nature of the research inhibits an ability to make definitive conclusions about the findings. They provide insight but not definitive conclusions.
- The research process underpinning exploratory studies is flexible but often unstructured, leading to only tentative results that have limited value to decision-makers.
- Design lacks rigorous standards applied to methods of data gathering and analysis because one of the areas for exploration could be to determine what method or methodologies could best fit the research problem.
Cuthill, Michael. “Exploratory Research: Citizen Participation, Local Government, and Sustainable Development in Australia.” Sustainable Development 10 (2002): 79-89; Streb, Christoph K. "Exploratory Case Study." In Encyclopedia of Case Study Research . Albert J. Mills, Gabrielle Durepos and Eiden Wiebe, editors. (Thousand Oaks, CA: Sage, 2010), pp. 372-374; Taylor, P. J., G. Catalano, and D.R.F. Walker. “Exploratory Analysis of the World City Network.” Urban Studies 39 (December 2002): 2377-2394; Exploratory Research. Wikipedia.
Field Research Design
Sometimes referred to as ethnography or participant observation, designs around field research encompass a variety of interpretative procedures [e.g., observation and interviews] rooted in qualitative approaches to studying people individually or in groups while inhabiting their natural environment as opposed to using survey instruments or other forms of impersonal methods of data gathering. Information acquired from observational research takes the form of “ field notes ” that involves documenting what the researcher actually sees and hears while in the field. Findings do not consist of conclusive statements derived from numbers and statistics because field research involves analysis of words and observations of behavior. Conclusions, therefore, are developed from an interpretation of findings that reveal overriding themes, concepts, and ideas. More information can be found HERE .
- Field research is often necessary to fill gaps in understanding the research problem applied to local conditions or to specific groups of people that cannot be ascertained from existing data.
- The research helps contextualize already known information about a research problem, thereby facilitating ways to assess the origins, scope, and scale of a problem and to gage the causes, consequences, and means to resolve an issue based on deliberate interaction with people in their natural inhabited spaces.
- Enables the researcher to corroborate or confirm data by gathering additional information that supports or refutes findings reported in prior studies of the topic.
- Because the researcher in embedded in the field, they are better able to make observations or ask questions that reflect the specific cultural context of the setting being investigated.
- Observing the local reality offers the opportunity to gain new perspectives or obtain unique data that challenges existing theoretical propositions or long-standing assumptions found in the literature.
What these studies don't tell you
- A field research study requires extensive time and resources to carry out the multiple steps involved with preparing for the gathering of information, including for example, examining background information about the study site, obtaining permission to access the study site, and building trust and rapport with subjects.
- Requires a commitment to staying engaged in the field to ensure that you can adequately document events and behaviors as they unfold.
- The unpredictable nature of fieldwork means that researchers can never fully control the process of data gathering. They must maintain a flexible approach to studying the setting because events and circumstances can change quickly or unexpectedly.
- Findings can be difficult to interpret and verify without access to documents and other source materials that help to enhance the credibility of information obtained from the field [i.e., the act of triangulating the data].
- Linking the research problem to the selection of study participants inhabiting their natural environment is critical. However, this specificity limits the ability to generalize findings to different situations or in other contexts or to infer courses of action applied to other settings or groups of people.
- The reporting of findings must take into account how the researcher themselves may have inadvertently affected respondents and their behaviors.
Historical Design
The purpose of a historical research design is to collect, verify, and synthesize evidence from the past to establish facts that defend or refute a hypothesis. It uses secondary sources and a variety of primary documentary evidence, such as, diaries, official records, reports, archives, and non-textual information [maps, pictures, audio and visual recordings]. The limitation is that the sources must be both authentic and valid.
- The historical research design is unobtrusive; the act of research does not affect the results of the study.
- The historical approach is well suited for trend analysis.
- Historical records can add important contextual background required to more fully understand and interpret a research problem.
- There is often no possibility of researcher-subject interaction that could affect the findings.
- Historical sources can be used over and over to study different research problems or to replicate a previous study.
- The ability to fulfill the aims of your research are directly related to the amount and quality of documentation available to understand the research problem.
- Since historical research relies on data from the past, there is no way to manipulate it to control for contemporary contexts.
- Interpreting historical sources can be very time consuming.
- The sources of historical materials must be archived consistently to ensure access. This may especially challenging for digital or online-only sources.
- Original authors bring their own perspectives and biases to the interpretation of past events and these biases are more difficult to ascertain in historical resources.
- Due to the lack of control over external variables, historical research is very weak with regard to the demands of internal validity.
- It is rare that the entirety of historical documentation needed to fully address a research problem is available for interpretation, therefore, gaps need to be acknowledged.
Howell, Martha C. and Walter Prevenier. From Reliable Sources: An Introduction to Historical Methods . Ithaca, NY: Cornell University Press, 2001; Lundy, Karen Saucier. "Historical Research." In The Sage Encyclopedia of Qualitative Research Methods . Lisa M. Given, editor. (Thousand Oaks, CA: Sage, 2008), pp. 396-400; Marius, Richard. and Melvin E. Page. A Short Guide to Writing about History . 9th edition. Boston, MA: Pearson, 2015; Savitt, Ronald. “Historical Research in Marketing.” Journal of Marketing 44 (Autumn, 1980): 52-58; Gall, Meredith. Educational Research: An Introduction . Chapter 16, Historical Research. 8th ed. Boston, MA: Pearson/Allyn and Bacon, 2007.
Longitudinal Design
A longitudinal study follows the same sample over time and makes repeated observations. For example, with longitudinal surveys, the same group of people is interviewed at regular intervals, enabling researchers to track changes over time and to relate them to variables that might explain why the changes occur. Longitudinal research designs describe patterns of change and help establish the direction and magnitude of causal relationships. Measurements are taken on each variable over two or more distinct time periods. This allows the researcher to measure change in variables over time. It is a type of observational study sometimes referred to as a panel study.
- Longitudinal data facilitate the analysis of the duration of a particular phenomenon.
- Enables survey researchers to get close to the kinds of causal explanations usually attainable only with experiments.
- The design permits the measurement of differences or change in a variable from one period to another [i.e., the description of patterns of change over time].
- Longitudinal studies facilitate the prediction of future outcomes based upon earlier factors.
- The data collection method may change over time.
- Maintaining the integrity of the original sample can be difficult over an extended period of time.
- It can be difficult to show more than one variable at a time.
- This design often needs qualitative research data to explain fluctuations in the results.
- A longitudinal research design assumes present trends will continue unchanged.
- It can take a long period of time to gather results.
- There is a need to have a large sample size and accurate sampling to reach representativness.
Anastas, Jeane W. Research Design for Social Work and the Human Services . Chapter 6, Flexible Methods: Relational and Longitudinal Research. 2nd ed. New York: Columbia University Press, 1999; Forgues, Bernard, and Isabelle Vandangeon-Derumez. "Longitudinal Analyses." In Doing Management Research . Raymond-Alain Thiétart and Samantha Wauchope, editors. (London, England: Sage, 2001), pp. 332-351; Kalaian, Sema A. and Rafa M. Kasim. "Longitudinal Studies." In Encyclopedia of Survey Research Methods . Paul J. Lavrakas, ed. (Thousand Oaks, CA: Sage, 2008), pp. 440-441; Menard, Scott, editor. Longitudinal Research . Thousand Oaks, CA: Sage, 2002; Ployhart, Robert E. and Robert J. Vandenberg. "Longitudinal Research: The Theory, Design, and Analysis of Change.” Journal of Management 36 (January 2010): 94-120; Longitudinal Study. Wikipedia.
Meta-Analysis Design
Meta-analysis is an analytical methodology designed to systematically evaluate and summarize the results from a number of individual studies, thereby, increasing the overall sample size and the ability of the researcher to study effects of interest. The purpose is to not simply summarize existing knowledge, but to develop a new understanding of a research problem using synoptic reasoning. The main objectives of meta-analysis include analyzing differences in the results among studies and increasing the precision by which effects are estimated. A well-designed meta-analysis depends upon strict adherence to the criteria used for selecting studies and the availability of information in each study to properly analyze their findings. Lack of information can severely limit the type of analyzes and conclusions that can be reached. In addition, the more dissimilarity there is in the results among individual studies [heterogeneity], the more difficult it is to justify interpretations that govern a valid synopsis of results. A meta-analysis needs to fulfill the following requirements to ensure the validity of your findings:
- Clearly defined description of objectives, including precise definitions of the variables and outcomes that are being evaluated;
- A well-reasoned and well-documented justification for identification and selection of the studies;
- Assessment and explicit acknowledgment of any researcher bias in the identification and selection of those studies;
- Description and evaluation of the degree of heterogeneity among the sample size of studies reviewed; and,
- Justification of the techniques used to evaluate the studies.
- Can be an effective strategy for determining gaps in the literature.
- Provides a means of reviewing research published about a particular topic over an extended period of time and from a variety of sources.
- Is useful in clarifying what policy or programmatic actions can be justified on the basis of analyzing research results from multiple studies.
- Provides a method for overcoming small sample sizes in individual studies that previously may have had little relationship to each other.
- Can be used to generate new hypotheses or highlight research problems for future studies.
- Small violations in defining the criteria used for content analysis can lead to difficult to interpret and/or meaningless findings.
- A large sample size can yield reliable, but not necessarily valid, results.
- A lack of uniformity regarding, for example, the type of literature reviewed, how methods are applied, and how findings are measured within the sample of studies you are analyzing, can make the process of synthesis difficult to perform.
- Depending on the sample size, the process of reviewing and synthesizing multiple studies can be very time consuming.
Beck, Lewis W. "The Synoptic Method." The Journal of Philosophy 36 (1939): 337-345; Cooper, Harris, Larry V. Hedges, and Jeffrey C. Valentine, eds. The Handbook of Research Synthesis and Meta-Analysis . 2nd edition. New York: Russell Sage Foundation, 2009; Guzzo, Richard A., Susan E. Jackson and Raymond A. Katzell. “Meta-Analysis Analysis.” In Research in Organizational Behavior , Volume 9. (Greenwich, CT: JAI Press, 1987), pp 407-442; Lipsey, Mark W. and David B. Wilson. Practical Meta-Analysis . Thousand Oaks, CA: Sage Publications, 2001; Study Design 101. Meta-Analysis. The Himmelfarb Health Sciences Library, George Washington University; Timulak, Ladislav. “Qualitative Meta-Analysis.” In The SAGE Handbook of Qualitative Data Analysis . Uwe Flick, editor. (Los Angeles, CA: Sage, 2013), pp. 481-495; Walker, Esteban, Adrian V. Hernandez, and Micheal W. Kattan. "Meta-Analysis: It's Strengths and Limitations." Cleveland Clinic Journal of Medicine 75 (June 2008): 431-439.
Mixed-Method Design
- Narrative and non-textual information can add meaning to numeric data, while numeric data can add precision to narrative and non-textual information.
- Can utilize existing data while at the same time generating and testing a grounded theory approach to describe and explain the phenomenon under study.
- A broader, more complex research problem can be investigated because the researcher is not constrained by using only one method.
- The strengths of one method can be used to overcome the inherent weaknesses of another method.
- Can provide stronger, more robust evidence to support a conclusion or set of recommendations.
- May generate new knowledge new insights or uncover hidden insights, patterns, or relationships that a single methodological approach might not reveal.
- Produces more complete knowledge and understanding of the research problem that can be used to increase the generalizability of findings applied to theory or practice.
- A researcher must be proficient in understanding how to apply multiple methods to investigating a research problem as well as be proficient in optimizing how to design a study that coherently melds them together.
- Can increase the likelihood of conflicting results or ambiguous findings that inhibit drawing a valid conclusion or setting forth a recommended course of action [e.g., sample interview responses do not support existing statistical data].
- Because the research design can be very complex, reporting the findings requires a well-organized narrative, clear writing style, and precise word choice.
- Design invites collaboration among experts. However, merging different investigative approaches and writing styles requires more attention to the overall research process than studies conducted using only one methodological paradigm.
- Concurrent merging of quantitative and qualitative research requires greater attention to having adequate sample sizes, using comparable samples, and applying a consistent unit of analysis. For sequential designs where one phase of qualitative research builds on the quantitative phase or vice versa, decisions about what results from the first phase to use in the next phase, the choice of samples and estimating reasonable sample sizes for both phases, and the interpretation of results from both phases can be difficult.
- Due to multiple forms of data being collected and analyzed, this design requires extensive time and resources to carry out the multiple steps involved in data gathering and interpretation.
Burch, Patricia and Carolyn J. Heinrich. Mixed Methods for Policy Research and Program Evaluation . Thousand Oaks, CA: Sage, 2016; Creswell, John w. et al. Best Practices for Mixed Methods Research in the Health Sciences . Bethesda, MD: Office of Behavioral and Social Sciences Research, National Institutes of Health, 2010Creswell, John W. Research Design: Qualitative, Quantitative, and Mixed Methods Approaches . 4th edition. Thousand Oaks, CA: Sage Publications, 2014; Domínguez, Silvia, editor. Mixed Methods Social Networks Research . Cambridge, UK: Cambridge University Press, 2014; Hesse-Biber, Sharlene Nagy. Mixed Methods Research: Merging Theory with Practice . New York: Guilford Press, 2010; Niglas, Katrin. “How the Novice Researcher Can Make Sense of Mixed Methods Designs.” International Journal of Multiple Research Approaches 3 (2009): 34-46; Onwuegbuzie, Anthony J. and Nancy L. Leech. “Linking Research Questions to Mixed Methods Data Analysis Procedures.” The Qualitative Report 11 (September 2006): 474-498; Tashakorri, Abbas and John W. Creswell. “The New Era of Mixed Methods.” Journal of Mixed Methods Research 1 (January 2007): 3-7; Zhanga, Wanqing. “Mixed Methods Application in Health Intervention Research: A Multiple Case Study.” International Journal of Multiple Research Approaches 8 (2014): 24-35 .
Observational Design
This type of research design draws a conclusion by comparing subjects against a control group, in cases where the researcher has no control over the experiment. There are two general types of observational designs. In direct observations, people know that you are watching them. Unobtrusive measures involve any method for studying behavior where individuals do not know they are being observed. An observational study allows a useful insight into a phenomenon and avoids the ethical and practical difficulties of setting up a large and cumbersome research project.
- Observational studies are usually flexible and do not necessarily need to be structured around a hypothesis about what you expect to observe [data is emergent rather than pre-existing].
- The researcher is able to collect in-depth information about a particular behavior.
- Can reveal interrelationships among multifaceted dimensions of group interactions.
- You can generalize your results to real life situations.
- Observational research is useful for discovering what variables may be important before applying other methods like experiments.
- Observation research designs account for the complexity of group behaviors.
- Reliability of data is low because seeing behaviors occur over and over again may be a time consuming task and are difficult to replicate.
- In observational research, findings may only reflect a unique sample population and, thus, cannot be generalized to other groups.
- There can be problems with bias as the researcher may only "see what they want to see."
- There is no possibility to determine "cause and effect" relationships since nothing is manipulated.
- Sources or subjects may not all be equally credible.
- Any group that is knowingly studied is altered to some degree by the presence of the researcher, therefore, potentially skewing any data collected.
Atkinson, Paul and Martyn Hammersley. “Ethnography and Participant Observation.” In Handbook of Qualitative Research . Norman K. Denzin and Yvonna S. Lincoln, eds. (Thousand Oaks, CA: Sage, 1994), pp. 248-261; Observational Research. Research Methods by Dummies. Department of Psychology. California State University, Fresno, 2006; Patton Michael Quinn. Qualitiative Research and Evaluation Methods . Chapter 6, Fieldwork Strategies and Observational Methods. 3rd ed. Thousand Oaks, CA: Sage, 2002; Payne, Geoff and Judy Payne. "Observation." In Key Concepts in Social Research . The SAGE Key Concepts series. (London, England: Sage, 2004), pp. 158-162; Rosenbaum, Paul R. Design of Observational Studies . New York: Springer, 2010;Williams, J. Patrick. "Nonparticipant Observation." In The Sage Encyclopedia of Qualitative Research Methods . Lisa M. Given, editor.(Thousand Oaks, CA: Sage, 2008), pp. 562-563.
Philosophical Design
Understood more as an broad approach to examining a research problem than a methodological design, philosophical analysis and argumentation is intended to challenge deeply embedded, often intractable, assumptions underpinning an area of study. This approach uses the tools of argumentation derived from philosophical traditions, concepts, models, and theories to critically explore and challenge, for example, the relevance of logic and evidence in academic debates, to analyze arguments about fundamental issues, or to discuss the root of existing discourse about a research problem. These overarching tools of analysis can be framed in three ways:
- Ontology -- the study that describes the nature of reality; for example, what is real and what is not, what is fundamental and what is derivative?
- Epistemology -- the study that explores the nature of knowledge; for example, by what means does knowledge and understanding depend upon and how can we be certain of what we know?
- Axiology -- the study of values; for example, what values does an individual or group hold and why? How are values related to interest, desire, will, experience, and means-to-end? And, what is the difference between a matter of fact and a matter of value?
- Can provide a basis for applying ethical decision-making to practice.
- Functions as a means of gaining greater self-understanding and self-knowledge about the purposes of research.
- Brings clarity to general guiding practices and principles of an individual or group.
- Philosophy informs methodology.
- Refine concepts and theories that are invoked in relatively unreflective modes of thought and discourse.
- Beyond methodology, philosophy also informs critical thinking about epistemology and the structure of reality (metaphysics).
- Offers clarity and definition to the practical and theoretical uses of terms, concepts, and ideas.
- Limited application to specific research problems [answering the "So What?" question in social science research].
- Analysis can be abstract, argumentative, and limited in its practical application to real-life issues.
- While a philosophical analysis may render problematic that which was once simple or taken-for-granted, the writing can be dense and subject to unnecessary jargon, overstatement, and/or excessive quotation and documentation.
- There are limitations in the use of metaphor as a vehicle of philosophical analysis.
- There can be analytical difficulties in moving from philosophy to advocacy and between abstract thought and application to the phenomenal world.
Burton, Dawn. "Part I, Philosophy of the Social Sciences." In Research Training for Social Scientists . (London, England: Sage, 2000), pp. 1-5; Chapter 4, Research Methodology and Design. Unisa Institutional Repository (UnisaIR), University of South Africa; Jarvie, Ian C., and Jesús Zamora-Bonilla, editors. The SAGE Handbook of the Philosophy of Social Sciences . London: Sage, 2011; Labaree, Robert V. and Ross Scimeca. “The Philosophical Problem of Truth in Librarianship.” The Library Quarterly 78 (January 2008): 43-70; Maykut, Pamela S. Beginning Qualitative Research: A Philosophic and Practical Guide . Washington, DC: Falmer Press, 1994; McLaughlin, Hugh. "The Philosophy of Social Research." In Understanding Social Work Research . 2nd edition. (London: SAGE Publications Ltd., 2012), pp. 24-47; Stanford Encyclopedia of Philosophy . Metaphysics Research Lab, CSLI, Stanford University, 2013.
Sequential Design
- The researcher has a limitless option when it comes to sample size and the sampling schedule.
- Due to the repetitive nature of this research design, minor changes and adjustments can be done during the initial parts of the study to correct and hone the research method.
- This is a useful design for exploratory studies.
- There is very little effort on the part of the researcher when performing this technique. It is generally not expensive, time consuming, or workforce intensive.
- Because the study is conducted serially, the results of one sample are known before the next sample is taken and analyzed. This provides opportunities for continuous improvement of sampling and methods of analysis.
- The sampling method is not representative of the entire population. The only possibility of approaching representativeness is when the researcher chooses to use a very large sample size significant enough to represent a significant portion of the entire population. In this case, moving on to study a second or more specific sample can be difficult.
- The design cannot be used to create conclusions and interpretations that pertain to an entire population because the sampling technique is not randomized. Generalizability from findings is, therefore, limited.
- Difficult to account for and interpret variation from one sample to another over time, particularly when using qualitative methods of data collection.
Betensky, Rebecca. Harvard University, Course Lecture Note slides; Bovaird, James A. and Kevin A. Kupzyk. "Sequential Design." In Encyclopedia of Research Design . Neil J. Salkind, editor. (Thousand Oaks, CA: Sage, 2010), pp. 1347-1352; Cresswell, John W. Et al. “Advanced Mixed-Methods Research Designs.” In Handbook of Mixed Methods in Social and Behavioral Research . Abbas Tashakkori and Charles Teddle, eds. (Thousand Oaks, CA: Sage, 2003), pp. 209-240; Henry, Gary T. "Sequential Sampling." In The SAGE Encyclopedia of Social Science Research Methods . Michael S. Lewis-Beck, Alan Bryman and Tim Futing Liao, editors. (Thousand Oaks, CA: Sage, 2004), pp. 1027-1028; Nataliya V. Ivankova. “Using Mixed-Methods Sequential Explanatory Design: From Theory to Practice.” Field Methods 18 (February 2006): 3-20; Bovaird, James A. and Kevin A. Kupzyk. “Sequential Design.” In Encyclopedia of Research Design . Neil J. Salkind, ed. Thousand Oaks, CA: Sage, 2010; Sequential Analysis. Wikipedia.
Systematic Review
- A systematic review synthesizes the findings of multiple studies related to each other by incorporating strategies of analysis and interpretation intended to reduce biases and random errors.
- The application of critical exploration, evaluation, and synthesis methods separates insignificant, unsound, or redundant research from the most salient and relevant studies worthy of reflection.
- They can be use to identify, justify, and refine hypotheses, recognize and avoid hidden problems in prior studies, and explain data inconsistencies and conflicts in data.
- Systematic reviews can be used to help policy makers formulate evidence-based guidelines and regulations.
- The use of strict, explicit, and pre-determined methods of synthesis, when applied appropriately, provide reliable estimates about the effects of interventions, evaluations, and effects related to the overarching research problem investigated by each study under review.
- Systematic reviews illuminate where knowledge or thorough understanding of a research problem is lacking and, therefore, can then be used to guide future research.
- The accepted inclusion of unpublished studies [i.e., grey literature] ensures the broadest possible way to analyze and interpret research on a topic.
- Results of the synthesis can be generalized and the findings extrapolated into the general population with more validity than most other types of studies .
- Systematic reviews do not create new knowledge per se; they are a method for synthesizing existing studies about a research problem in order to gain new insights and determine gaps in the literature.
- The way researchers have carried out their investigations [e.g., the period of time covered, number of participants, sources of data analyzed, etc.] can make it difficult to effectively synthesize studies.
- The inclusion of unpublished studies can introduce bias into the review because they may not have undergone a rigorous peer-review process prior to publication. Examples may include conference presentations or proceedings, publications from government agencies, white papers, working papers, and internal documents from organizations, and doctoral dissertations and Master's theses.
Denyer, David and David Tranfield. "Producing a Systematic Review." In The Sage Handbook of Organizational Research Methods . David A. Buchanan and Alan Bryman, editors. ( Thousand Oaks, CA: Sage Publications, 2009), pp. 671-689; Foster, Margaret J. and Sarah T. Jewell, editors. Assembling the Pieces of a Systematic Review: A Guide for Librarians . Lanham, MD: Rowman and Littlefield, 2017; Gough, David, Sandy Oliver, James Thomas, editors. Introduction to Systematic Reviews . 2nd edition. Los Angeles, CA: Sage Publications, 2017; Gopalakrishnan, S. and P. Ganeshkumar. “Systematic Reviews and Meta-analysis: Understanding the Best Evidence in Primary Healthcare.” Journal of Family Medicine and Primary Care 2 (2013): 9-14; Gough, David, James Thomas, and Sandy Oliver. "Clarifying Differences between Review Designs and Methods." Systematic Reviews 1 (2012): 1-9; Khan, Khalid S., Regina Kunz, Jos Kleijnen, and Gerd Antes. “Five Steps to Conducting a Systematic Review.” Journal of the Royal Society of Medicine 96 (2003): 118-121; Mulrow, C. D. “Systematic Reviews: Rationale for Systematic Reviews.” BMJ 309:597 (September 1994); O'Dwyer, Linda C., and Q. Eileen Wafford. "Addressing Challenges with Systematic Review Teams through Effective Communication: A Case Report." Journal of the Medical Library Association 109 (October 2021): 643-647; Okoli, Chitu, and Kira Schabram. "A Guide to Conducting a Systematic Literature Review of Information Systems Research." Sprouts: Working Papers on Information Systems 10 (2010); Siddaway, Andy P., Alex M. Wood, and Larry V. Hedges. "How to Do a Systematic Review: A Best Practice Guide for Conducting and Reporting Narrative Reviews, Meta-analyses, and Meta-syntheses." Annual Review of Psychology 70 (2019): 747-770; Torgerson, Carole J. “Publication Bias: The Achilles’ Heel of Systematic Reviews?” British Journal of Educational Studies 54 (March 2006): 89-102; Torgerson, Carole. Systematic Reviews . New York: Continuum, 2003.
- << Previous: Purpose of Guide
- Next: Design Flaws to Avoid >>
- Last Updated: May 21, 2024 11:14 AM
- URL: https://libguides.usc.edu/writingguide

17 Research Proposal Examples
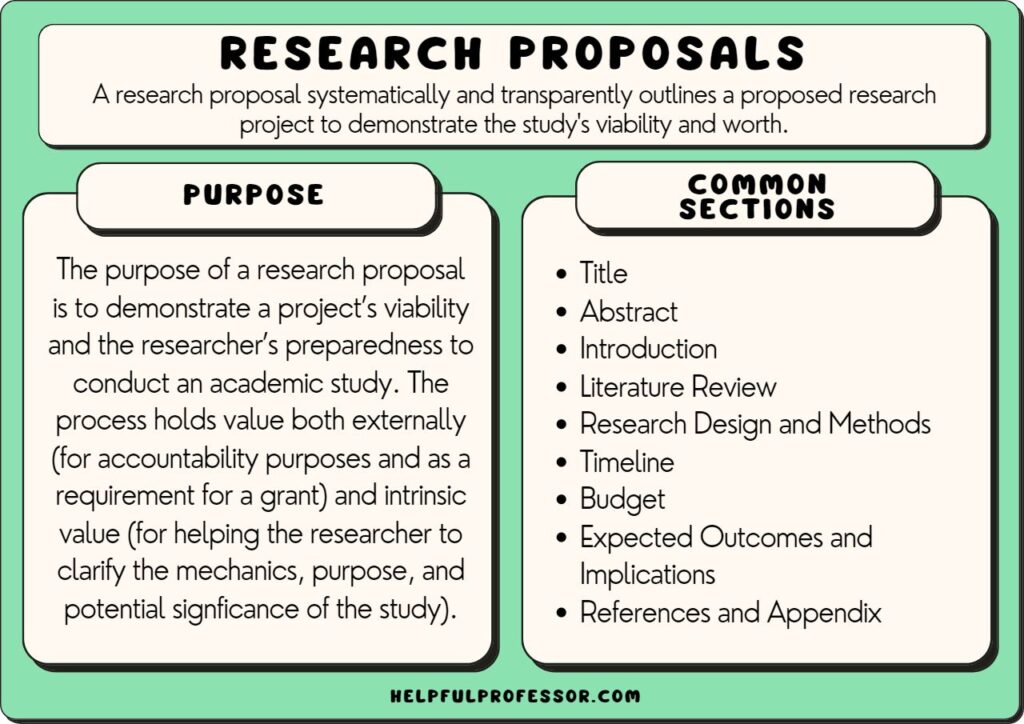
A research proposal systematically and transparently outlines a proposed research project.
The purpose of a research proposal is to demonstrate a project’s viability and the researcher’s preparedness to conduct an academic study. It serves as a roadmap for the researcher.
The process holds value both externally (for accountability purposes and often as a requirement for a grant application) and intrinsic value (for helping the researcher to clarify the mechanics, purpose, and potential signficance of the study).
Key sections of a research proposal include: the title, abstract, introduction, literature review, research design and methods, timeline, budget, outcomes and implications, references, and appendix. Each is briefly explained below.
Watch my Guide: How to Write a Research Proposal
Get your Template for Writing your Research Proposal Here (With AI Prompts!)
Research Proposal Sample Structure
Title: The title should present a concise and descriptive statement that clearly conveys the core idea of the research projects. Make it as specific as possible. The reader should immediately be able to grasp the core idea of the intended research project. Often, the title is left too vague and does not help give an understanding of what exactly the study looks at.
Abstract: Abstracts are usually around 250-300 words and provide an overview of what is to follow – including the research problem , objectives, methods, expected outcomes, and significance of the study. Use it as a roadmap and ensure that, if the abstract is the only thing someone reads, they’ll get a good fly-by of what will be discussed in the peice.
Introduction: Introductions are all about contextualization. They often set the background information with a statement of the problem. At the end of the introduction, the reader should understand what the rationale for the study truly is. I like to see the research questions or hypotheses included in the introduction and I like to get a good understanding of what the significance of the research will be. It’s often easiest to write the introduction last
Literature Review: The literature review dives deep into the existing literature on the topic, demosntrating your thorough understanding of the existing literature including themes, strengths, weaknesses, and gaps in the literature. It serves both to demonstrate your knowledge of the field and, to demonstrate how the proposed study will fit alongside the literature on the topic. A good literature review concludes by clearly demonstrating how your research will contribute something new and innovative to the conversation in the literature.
Research Design and Methods: This section needs to clearly demonstrate how the data will be gathered and analyzed in a systematic and academically sound manner. Here, you need to demonstrate that the conclusions of your research will be both valid and reliable. Common points discussed in the research design and methods section include highlighting the research paradigm, methodologies, intended population or sample to be studied, data collection techniques, and data analysis procedures . Toward the end of this section, you are encouraged to also address ethical considerations and limitations of the research process , but also to explain why you chose your research design and how you are mitigating the identified risks and limitations.
Timeline: Provide an outline of the anticipated timeline for the study. Break it down into its various stages (including data collection, data analysis, and report writing). The goal of this section is firstly to establish a reasonable breakdown of steps for you to follow and secondly to demonstrate to the assessors that your project is practicable and feasible.
Budget: Estimate the costs associated with the research project and include evidence for your estimations. Typical costs include staffing costs, equipment, travel, and data collection tools. When applying for a scholarship, the budget should demonstrate that you are being responsible with your expensive and that your funding application is reasonable.
Expected Outcomes and Implications: A discussion of the anticipated findings or results of the research, as well as the potential contributions to the existing knowledge, theory, or practice in the field. This section should also address the potential impact of the research on relevant stakeholders and any broader implications for policy or practice.
References: A complete list of all the sources cited in the research proposal, formatted according to the required citation style. This demonstrates the researcher’s familiarity with the relevant literature and ensures proper attribution of ideas and information.
Appendices (if applicable): Any additional materials, such as questionnaires, interview guides, or consent forms, that provide further information or support for the research proposal. These materials should be included as appendices at the end of the document.
Research Proposal Examples
Research proposals often extend anywhere between 2,000 and 15,000 words in length. The following snippets are samples designed to briefly demonstrate what might be discussed in each section.
1. Education Studies Research Proposals
See some real sample pieces:
- Assessment of the perceptions of teachers towards a new grading system
- Does ICT use in secondary classrooms help or hinder student learning?
- Digital technologies in focus project
- Urban Middle School Teachers’ Experiences of the Implementation of
- Restorative Justice Practices
- Experiences of students of color in service learning
Consider this hypothetical education research proposal:
The Impact of Game-Based Learning on Student Engagement and Academic Performance in Middle School Mathematics
Abstract: The proposed study will explore multiplayer game-based learning techniques in middle school mathematics curricula and their effects on student engagement. The study aims to contribute to the current literature on game-based learning by examining the effects of multiplayer gaming in learning.
Introduction: Digital game-based learning has long been shunned within mathematics education for fears that it may distract students or lower the academic integrity of the classrooms. However, there is emerging evidence that digital games in math have emerging benefits not only for engagement but also academic skill development. Contributing to this discourse, this study seeks to explore the potential benefits of multiplayer digital game-based learning by examining its impact on middle school students’ engagement and academic performance in a mathematics class.
Literature Review: The literature review has identified gaps in the current knowledge, namely, while game-based learning has been extensively explored, the role of multiplayer games in supporting learning has not been studied.
Research Design and Methods: This study will employ a mixed-methods research design based upon action research in the classroom. A quasi-experimental pre-test/post-test control group design will first be used to compare the academic performance and engagement of middle school students exposed to game-based learning techniques with those in a control group receiving instruction without the aid of technology. Students will also be observed and interviewed in regard to the effect of communication and collaboration during gameplay on their learning.
Timeline: The study will take place across the second term of the school year with a pre-test taking place on the first day of the term and the post-test taking place on Wednesday in Week 10.
Budget: The key budgetary requirements will be the technologies required, including the subscription cost for the identified games and computers.
Expected Outcomes and Implications: It is expected that the findings will contribute to the current literature on game-based learning and inform educational practices, providing educators and policymakers with insights into how to better support student achievement in mathematics.
2. Psychology Research Proposals
See some real examples:
- A situational analysis of shared leadership in a self-managing team
- The effect of musical preference on running performance
- Relationship between self-esteem and disordered eating amongst adolescent females
Consider this hypothetical psychology research proposal:
The Effects of Mindfulness-Based Interventions on Stress Reduction in College Students
Abstract: This research proposal examines the impact of mindfulness-based interventions on stress reduction among college students, using a pre-test/post-test experimental design with both quantitative and qualitative data collection methods .
Introduction: College students face heightened stress levels during exam weeks. This can affect both mental health and test performance. This study explores the potential benefits of mindfulness-based interventions such as meditation as a way to mediate stress levels in the weeks leading up to exam time.
Literature Review: Existing research on mindfulness-based meditation has shown the ability for mindfulness to increase metacognition, decrease anxiety levels, and decrease stress. Existing literature has looked at workplace, high school and general college-level applications. This study will contribute to the corpus of literature by exploring the effects of mindfulness directly in the context of exam weeks.
Research Design and Methods: Participants ( n= 234 ) will be randomly assigned to either an experimental group, receiving 5 days per week of 10-minute mindfulness-based interventions, or a control group, receiving no intervention. Data will be collected through self-report questionnaires, measuring stress levels, semi-structured interviews exploring participants’ experiences, and students’ test scores.
Timeline: The study will begin three weeks before the students’ exam week and conclude after each student’s final exam. Data collection will occur at the beginning (pre-test of self-reported stress levels) and end (post-test) of the three weeks.
Expected Outcomes and Implications: The study aims to provide evidence supporting the effectiveness of mindfulness-based interventions in reducing stress among college students in the lead up to exams, with potential implications for mental health support and stress management programs on college campuses.
3. Sociology Research Proposals
- Understanding emerging social movements: A case study of ‘Jersey in Transition’
- The interaction of health, education and employment in Western China
- Can we preserve lower-income affordable neighbourhoods in the face of rising costs?
Consider this hypothetical sociology research proposal:
The Impact of Social Media Usage on Interpersonal Relationships among Young Adults
Abstract: This research proposal investigates the effects of social media usage on interpersonal relationships among young adults, using a longitudinal mixed-methods approach with ongoing semi-structured interviews to collect qualitative data.
Introduction: Social media platforms have become a key medium for the development of interpersonal relationships, particularly for young adults. This study examines the potential positive and negative effects of social media usage on young adults’ relationships and development over time.
Literature Review: A preliminary review of relevant literature has demonstrated that social media usage is central to development of a personal identity and relationships with others with similar subcultural interests. However, it has also been accompanied by data on mental health deline and deteriorating off-screen relationships. The literature is to-date lacking important longitudinal data on these topics.
Research Design and Methods: Participants ( n = 454 ) will be young adults aged 18-24. Ongoing self-report surveys will assess participants’ social media usage, relationship satisfaction, and communication patterns. A subset of participants will be selected for longitudinal in-depth interviews starting at age 18 and continuing for 5 years.
Timeline: The study will be conducted over a period of five years, including recruitment, data collection, analysis, and report writing.
Expected Outcomes and Implications: This study aims to provide insights into the complex relationship between social media usage and interpersonal relationships among young adults, potentially informing social policies and mental health support related to social media use.
4. Nursing Research Proposals
- Does Orthopaedic Pre-assessment clinic prepare the patient for admission to hospital?
- Nurses’ perceptions and experiences of providing psychological care to burns patients
- Registered psychiatric nurse’s practice with mentally ill parents and their children
Consider this hypothetical nursing research proposal:
The Influence of Nurse-Patient Communication on Patient Satisfaction and Health Outcomes following Emergency Cesarians
Abstract: This research will examines the impact of effective nurse-patient communication on patient satisfaction and health outcomes for women following c-sections, utilizing a mixed-methods approach with patient surveys and semi-structured interviews.
Introduction: It has long been known that effective communication between nurses and patients is crucial for quality care. However, additional complications arise following emergency c-sections due to the interaction between new mother’s changing roles and recovery from surgery.
Literature Review: A review of the literature demonstrates the importance of nurse-patient communication, its impact on patient satisfaction, and potential links to health outcomes. However, communication between nurses and new mothers is less examined, and the specific experiences of those who have given birth via emergency c-section are to date unexamined.
Research Design and Methods: Participants will be patients in a hospital setting who have recently had an emergency c-section. A self-report survey will assess their satisfaction with nurse-patient communication and perceived health outcomes. A subset of participants will be selected for in-depth interviews to explore their experiences and perceptions of the communication with their nurses.
Timeline: The study will be conducted over a period of six months, including rolling recruitment, data collection, analysis, and report writing within the hospital.
Expected Outcomes and Implications: This study aims to provide evidence for the significance of nurse-patient communication in supporting new mothers who have had an emergency c-section. Recommendations will be presented for supporting nurses and midwives in improving outcomes for new mothers who had complications during birth.
5. Social Work Research Proposals
- Experiences of negotiating employment and caring responsibilities of fathers post-divorce
- Exploring kinship care in the north region of British Columbia
Consider this hypothetical social work research proposal:
The Role of a Family-Centered Intervention in Preventing Homelessness Among At-Risk Youthin a working-class town in Northern England
Abstract: This research proposal investigates the effectiveness of a family-centered intervention provided by a local council area in preventing homelessness among at-risk youth. This case study will use a mixed-methods approach with program evaluation data and semi-structured interviews to collect quantitative and qualitative data .
Introduction: Homelessness among youth remains a significant social issue. This study aims to assess the effectiveness of family-centered interventions in addressing this problem and identify factors that contribute to successful prevention strategies.
Literature Review: A review of the literature has demonstrated several key factors contributing to youth homelessness including lack of parental support, lack of social support, and low levels of family involvement. It also demonstrates the important role of family-centered interventions in addressing this issue. Drawing on current evidence, this study explores the effectiveness of one such intervention in preventing homelessness among at-risk youth in a working-class town in Northern England.
Research Design and Methods: The study will evaluate a new family-centered intervention program targeting at-risk youth and their families. Quantitative data on program outcomes, including housing stability and family functioning, will be collected through program records and evaluation reports. Semi-structured interviews with program staff, participants, and relevant stakeholders will provide qualitative insights into the factors contributing to program success or failure.
Timeline: The study will be conducted over a period of six months, including recruitment, data collection, analysis, and report writing.
Budget: Expenses include access to program evaluation data, interview materials, data analysis software, and any related travel costs for in-person interviews.
Expected Outcomes and Implications: This study aims to provide evidence for the effectiveness of family-centered interventions in preventing youth homelessness, potentially informing the expansion of or necessary changes to social work practices in Northern England.
Research Proposal Template
Get your Detailed Template for Writing your Research Proposal Here (With AI Prompts!)
This is a template for a 2500-word research proposal. You may find it difficult to squeeze everything into this wordcount, but it’s a common wordcount for Honors and MA-level dissertations.
Your research proposal is where you really get going with your study. I’d strongly recommend working closely with your teacher in developing a research proposal that’s consistent with the requirements and culture of your institution, as in my experience it varies considerably. The above template is from my own courses that walk students through research proposals in a British School of Education.
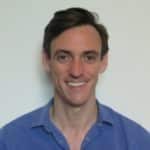
Chris Drew (PhD)
Dr. Chris Drew is the founder of the Helpful Professor. He holds a PhD in education and has published over 20 articles in scholarly journals. He is the former editor of the Journal of Learning Development in Higher Education. [Image Descriptor: Photo of Chris]
- Chris Drew (PhD) https://helpfulprofessor.com/author/chris-drew-phd/ 15 Animism Examples
- Chris Drew (PhD) https://helpfulprofessor.com/author/chris-drew-phd/ 10 Magical Thinking Examples
- Chris Drew (PhD) https://helpfulprofessor.com/author/chris-drew-phd/ Social-Emotional Learning (Definition, Examples, Pros & Cons)
- Chris Drew (PhD) https://helpfulprofessor.com/author/chris-drew-phd/ What is Educational Psychology?
8 thoughts on “17 Research Proposal Examples”
Very excellent research proposals
very helpful
Very helpful
Dear Sir, I need some help to write an educational research proposal. Thank you.
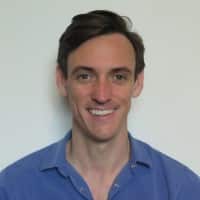
Hi Levi, use the site search bar to ask a question and I’ll likely have a guide already written for your specific question. Thanks for reading!
very good research proposal
Thank you so much sir! ❤️
Very helpful 👌
Leave a Comment Cancel Reply
Your email address will not be published. Required fields are marked *

How To Write A Research Proposal
A Straightforward How-To Guide (With Examples)
By: Derek Jansen (MBA) | Reviewed By: Dr. Eunice Rautenbach | August 2019 (Updated April 2023)
Writing up a strong research proposal for a dissertation or thesis is much like a marriage proposal. It’s a task that calls on you to win somebody over and persuade them that what you’re planning is a great idea. An idea they’re happy to say ‘yes’ to. This means that your dissertation proposal needs to be persuasive , attractive and well-planned. In this post, I’ll show you how to write a winning dissertation proposal, from scratch.
Before you start:
– Understand exactly what a research proposal is – Ask yourself these 4 questions
The 5 essential ingredients:
- The title/topic
- The introduction chapter
- The scope/delimitations
- Preliminary literature review
- Design/ methodology
- Practical considerations and risks
What Is A Research Proposal?
The research proposal is literally that: a written document that communicates what you propose to research, in a concise format. It’s where you put all that stuff that’s spinning around in your head down on to paper, in a logical, convincing fashion.
Convincing is the keyword here, as your research proposal needs to convince the assessor that your research is clearly articulated (i.e., a clear research question) , worth doing (i.e., is unique and valuable enough to justify the effort), and doable within the restrictions you’ll face (time limits, budget, skill limits, etc.). If your proposal does not address these three criteria, your research won’t be approved, no matter how “exciting” the research idea might be.
PS – if you’re completely new to proposal writing, we’ve got a detailed walkthrough video covering two successful research proposals here .
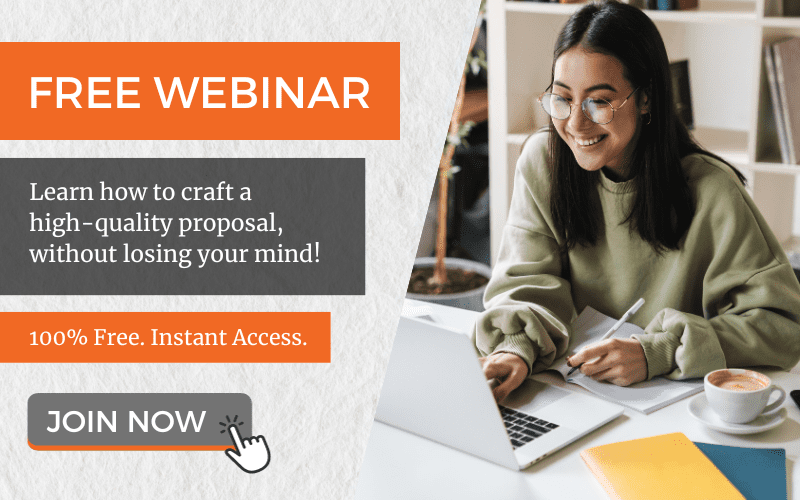
How do I know I’m ready?
Before starting the writing process, you need to ask yourself 4 important questions . If you can’t answer them succinctly and confidently, you’re not ready – you need to go back and think more deeply about your dissertation topic .
You should be able to answer the following 4 questions before starting your dissertation or thesis research proposal:
- WHAT is my main research question? (the topic)
- WHO cares and why is this important? (the justification)
- WHAT data would I need to answer this question, and how will I analyse it? (the research design)
- HOW will I manage the completion of this research, within the given timelines? (project and risk management)
If you can’t answer these questions clearly and concisely, you’re not yet ready to write your research proposal – revisit our post on choosing a topic .
If you can, that’s great – it’s time to start writing up your dissertation proposal. Next, I’ll discuss what needs to go into your research proposal, and how to structure it all into an intuitive, convincing document with a linear narrative.
The 5 Essential Ingredients
Research proposals can vary in style between institutions and disciplines, but here I’ll share with you a handy 5-section structure you can use. These 5 sections directly address the core questions we spoke about earlier, ensuring that you present a convincing proposal. If your institution already provides a proposal template, there will likely be substantial overlap with this, so you’ll still get value from reading on.
For each section discussed below, make sure you use headers and sub-headers (ideally, numbered headers) to help the reader navigate through your document, and to support them when they need to revisit a previous section. Don’t just present an endless wall of text, paragraph after paragraph after paragraph…
Top Tip: Use MS Word Styles to format headings. This will allow you to be clear about whether a sub-heading is level 2, 3, or 4. Additionally, you can view your document in ‘outline view’ which will show you only your headings. This makes it much easier to check your structure, shift things around and make decisions about where a section needs to sit. You can also generate a 100% accurate table of contents using Word’s automatic functionality.

Ingredient #1 – Topic/Title Header
Your research proposal’s title should be your main research question in its simplest form, possibly with a sub-heading providing basic details on the specifics of the study. For example:
“Compliance with equality legislation in the charity sector: a study of the ‘reasonable adjustments’ made in three London care homes”
As you can see, this title provides a clear indication of what the research is about, in broad terms. It paints a high-level picture for the first-time reader, which gives them a taste of what to expect. Always aim for a clear, concise title . Don’t feel the need to capture every detail of your research in your title – your proposal will fill in the gaps.
Need a helping hand?
Ingredient #2 – Introduction
In this section of your research proposal, you’ll expand on what you’ve communicated in the title, by providing a few paragraphs which offer more detail about your research topic. Importantly, the focus here is the topic – what will you research and why is that worth researching? This is not the place to discuss methodology, practicalities, etc. – you’ll do that later.
You should cover the following:
- An overview of the broad area you’ll be researching – introduce the reader to key concepts and language
- An explanation of the specific (narrower) area you’ll be focusing, and why you’ll be focusing there
- Your research aims and objectives
- Your research question (s) and sub-questions (if applicable)
Importantly, you should aim to use short sentences and plain language – don’t babble on with extensive jargon, acronyms and complex language. Assume that the reader is an intelligent layman – not a subject area specialist (even if they are). Remember that the best writing is writing that can be easily understood and digested. Keep it simple.
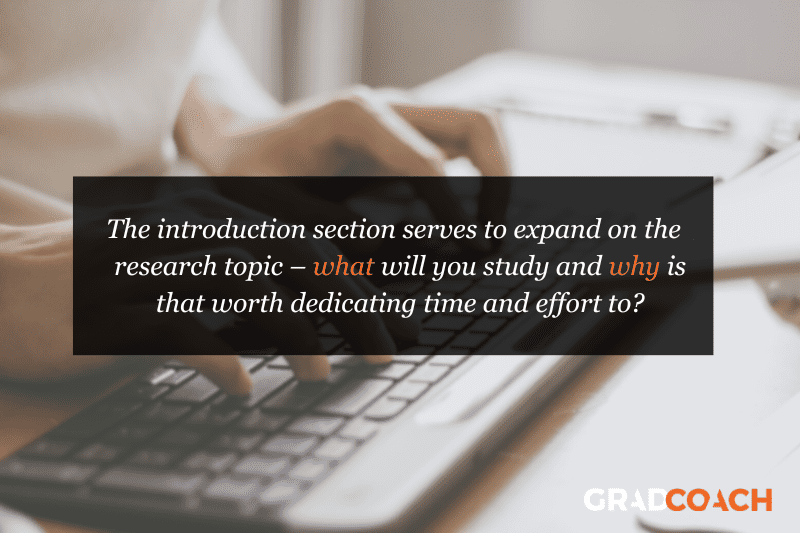
Note that some universities may want some extra bits and pieces in your introduction section. For example, personal development objectives, a structural outline, etc. Check your brief to see if there are any other details they expect in your proposal, and make sure you find a place for these.
Ingredient #3 – Scope
Next, you’ll need to specify what the scope of your research will be – this is also known as the delimitations . In other words, you need to make it clear what you will be covering and, more importantly, what you won’t be covering in your research. Simply put, this is about ring fencing your research topic so that you have a laser-sharp focus.
All too often, students feel the need to go broad and try to address as many issues as possible, in the interest of producing comprehensive research. Whilst this is admirable, it’s a mistake. By tightly refining your scope, you’ll enable yourself to go deep with your research, which is what you need to earn good marks. If your scope is too broad, you’re likely going to land up with superficial research (which won’t earn marks), so don’t be afraid to narrow things down.
Ingredient #4 – Literature Review
In this section of your research proposal, you need to provide a (relatively) brief discussion of the existing literature. Naturally, this will not be as comprehensive as the literature review in your actual dissertation, but it will lay the foundation for that. In fact, if you put in the effort at this stage, you’ll make your life a lot easier when it’s time to write your actual literature review chapter.
There are a few things you need to achieve in this section:
- Demonstrate that you’ve done your reading and are familiar with the current state of the research in your topic area.
- Show that there’s a clear gap for your specific research – i.e., show that your topic is sufficiently unique and will add value to the existing research.
- Show how the existing research has shaped your thinking regarding research design . For example, you might use scales or questionnaires from previous studies.
When you write up your literature review, keep these three objectives front of mind, especially number two (revealing the gap in the literature), so that your literature review has a clear purpose and direction . Everything you write should be contributing towards one (or more) of these objectives in some way. If it doesn’t, you need to ask yourself whether it’s truly needed.
Top Tip: Don’t fall into the trap of just describing the main pieces of literature, for example, “A says this, B says that, C also says that…” and so on. Merely describing the literature provides no value. Instead, you need to synthesise it, and use it to address the three objectives above.
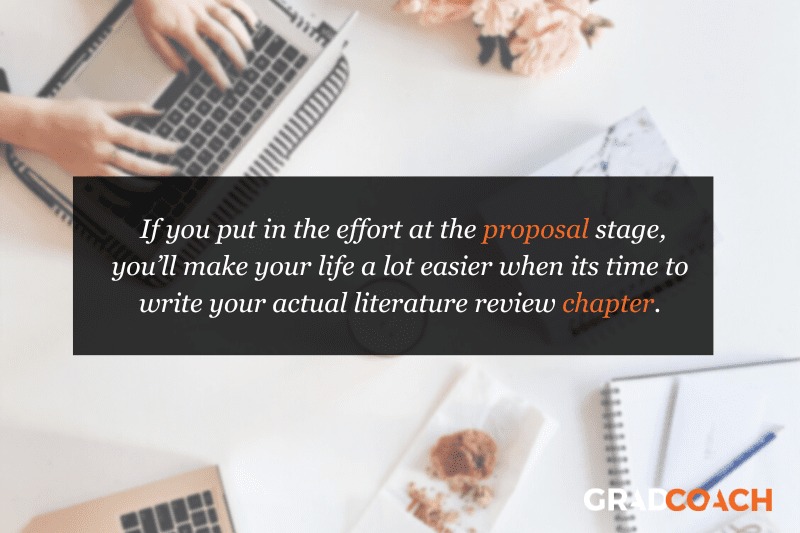
Ingredient #5 – Research Methodology
Now that you’ve clearly explained both your intended research topic (in the introduction) and the existing research it will draw on (in the literature review section), it’s time to get practical and explain exactly how you’ll be carrying out your own research. In other words, your research methodology.
In this section, you’ll need to answer two critical questions :
- How will you design your research? I.e., what research methodology will you adopt, what will your sample be, how will you collect data, etc.
- Why have you chosen this design? I.e., why does this approach suit your specific research aims, objectives and questions?
In other words, this is not just about explaining WHAT you’ll be doing, it’s also about explaining WHY. In fact, the justification is the most important part , because that justification is how you demonstrate a good understanding of research design (which is what assessors want to see).
Some essential design choices you need to cover in your research proposal include:
- Your intended research philosophy (e.g., positivism, interpretivism or pragmatism )
- What methodological approach you’ll be taking (e.g., qualitative , quantitative or mixed )
- The details of your sample (e.g., sample size, who they are, who they represent, etc.)
- What data you plan to collect (i.e. data about what, in what form?)
- How you plan to collect it (e.g., surveys , interviews , focus groups, etc.)
- How you plan to analyse it (e.g., regression analysis, thematic analysis , etc.)
- Ethical adherence (i.e., does this research satisfy all ethical requirements of your institution, or does it need further approval?)
This list is not exhaustive – these are just some core attributes of research design. Check with your institution what level of detail they expect. The “ research onion ” by Saunders et al (2009) provides a good summary of the various design choices you ultimately need to make – you can read more about that here .
Don’t forget the practicalities…
In addition to the technical aspects, you will need to address the practical side of the project. In other words, you need to explain what resources you’ll need (e.g., time, money, access to equipment or software, etc.) and how you intend to secure these resources. You need to show that your project is feasible, so any “make or break” type resources need to already be secured. The success or failure of your project cannot depend on some resource which you’re not yet sure you have access to.
Another part of the practicalities discussion is project and risk management . In other words, you need to show that you have a clear project plan to tackle your research with. Some key questions to address:
- What are the timelines for each phase of your project?
- Are the time allocations reasonable?
- What happens if something takes longer than anticipated (risk management)?
- What happens if you don’t get the response rate you expect?
A good way to demonstrate that you’ve thought this through is to include a Gantt chart and a risk register (in the appendix if word count is a problem). With these two tools, you can show that you’ve got a clear, feasible plan, and you’ve thought about and accounted for the potential risks.
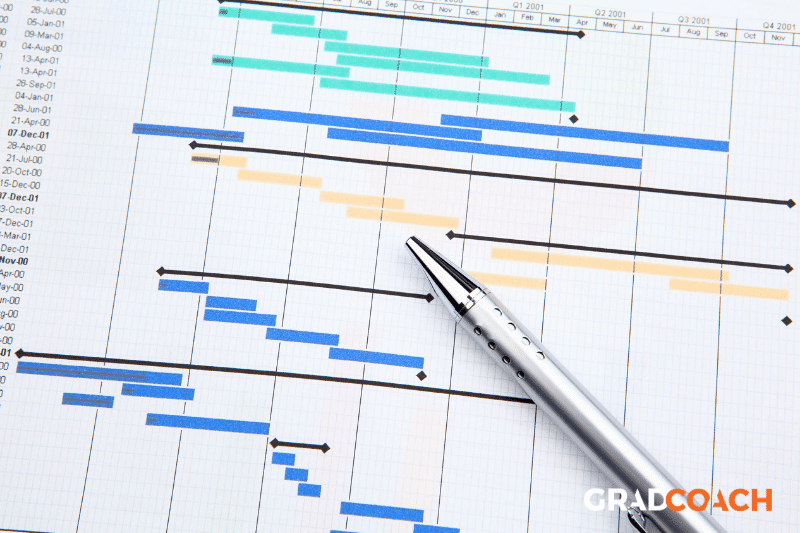
Tip – Be honest about the potential difficulties – but show that you are anticipating solutions and workarounds. This is much more impressive to an assessor than an unrealistically optimistic proposal which does not anticipate any challenges whatsoever.
Final Touches: Read And Simplify
The final step is to edit and proofread your proposal – very carefully. It sounds obvious, but all too often poor editing and proofreading ruin a good proposal. Nothing is more off-putting for an assessor than a poorly edited, typo-strewn document. It sends the message that you either do not pay attention to detail, or just don’t care. Neither of these are good messages. Put the effort into editing and proofreading your proposal (or pay someone to do it for you) – it will pay dividends.
When you’re editing, watch out for ‘academese’. Many students can speak simply, passionately and clearly about their dissertation topic – but become incomprehensible the moment they turn the laptop on. You are not required to write in any kind of special, formal, complex language when you write academic work. Sure, there may be technical terms, jargon specific to your discipline, shorthand terms and so on. But, apart from those, keep your written language very close to natural spoken language – just as you would speak in the classroom. Imagine that you are explaining your project plans to your classmates or a family member. Remember, write for the intelligent layman, not the subject matter experts. Plain-language, concise writing is what wins hearts and minds – and marks!
Let’s Recap: Research Proposal 101
And there you have it – how to write your dissertation or thesis research proposal, from the title page to the final proof. Here’s a quick recap of the key takeaways:
- The purpose of the research proposal is to convince – therefore, you need to make a clear, concise argument of why your research is both worth doing and doable.
- Make sure you can ask the critical what, who, and how questions of your research before you put pen to paper.
- Title – provides the first taste of your research, in broad terms
- Introduction – explains what you’ll be researching in more detail
- Scope – explains the boundaries of your research
- Literature review – explains how your research fits into the existing research and why it’s unique and valuable
- Research methodology – explains and justifies how you will carry out your own research
Hopefully, this post has helped you better understand how to write up a winning research proposal. If you enjoyed it, be sure to check out the rest of the Grad Coach Blog . If your university doesn’t provide any template for your proposal, you might want to try out our free research proposal template .
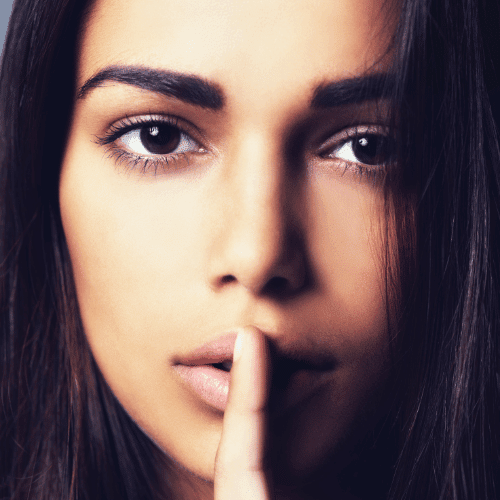
Psst… there’s more!
This post is an extract from our bestselling short course, Research Proposal Bootcamp . If you want to work smart, you don't want to miss this .
You Might Also Like:
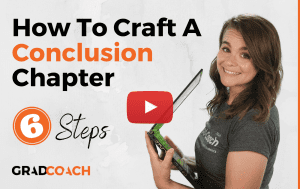
30 Comments
Thank you so much for the valuable insight that you have given, especially on the research proposal. That is what I have managed to cover. I still need to go back to the other parts as I got disturbed while still listening to Derek’s audio on you-tube. I am inspired. I will definitely continue with Grad-coach guidance on You-tube.
Thanks for the kind words :). All the best with your proposal.
First of all, thanks a lot for making such a wonderful presentation. The video was really useful and gave me a very clear insight of how a research proposal has to be written. I shall try implementing these ideas in my RP.
Once again, I thank you for this content.
I found reading your outline on writing research proposal very beneficial. I wish there was a way of submitting my draft proposal to you guys for critiquing before I submit to the institution.
Hi Bonginkosi
Thank you for the kind words. Yes, we do provide a review service. The best starting point is to have a chat with one of our coaches here: https://gradcoach.com/book/new/ .
Hello team GRADCOACH, may God bless you so much. I was totally green in research. Am so happy for your free superb tutorials and resources. Once again thank you so much Derek and his team.
You’re welcome, Erick. Good luck with your research proposal 🙂
thank you for the information. its precise and on point.
Really a remarkable piece of writing and great source of guidance for the researchers. GOD BLESS YOU for your guidance. Regards
Thanks so much for your guidance. It is easy and comprehensive the way you explain the steps for a winning research proposal.
Thank you guys so much for the rich post. I enjoyed and learn from every word in it. My problem now is how to get into your platform wherein I can always seek help on things related to my research work ? Secondly, I wish to find out if there is a way I can send my tentative proposal to you guys for examination before I take to my supervisor Once again thanks very much for the insights
Thanks for your kind words, Desire.
If you are based in a country where Grad Coach’s paid services are available, you can book a consultation by clicking the “Book” button in the top right.
Best of luck with your studies.
May God bless you team for the wonderful work you are doing,
If I have a topic, Can I submit it to you so that you can draft a proposal for me?? As I am expecting to go for masters degree in the near future.
Thanks for your comment. We definitely cannot draft a proposal for you, as that would constitute academic misconduct. The proposal needs to be your own work. We can coach you through the process, but it needs to be your own work and your own writing.
Best of luck with your research!
I found a lot of many essential concepts from your material. it is real a road map to write a research proposal. so thanks a lot. If there is any update material on your hand on MBA please forward to me.
GradCoach is a professional website that presents support and helps for MBA student like me through the useful online information on the page and with my 1-on-1 online coaching with the amazing and professional PhD Kerryen.
Thank you Kerryen so much for the support and help 🙂
I really recommend dealing with such a reliable services provider like Gradcoah and a coach like Kerryen.
Hi, Am happy for your service and effort to help students and researchers, Please, i have been given an assignment on research for strategic development, the task one is to formulate a research proposal to support the strategic development of a business area, my issue here is how to go about it, especially the topic or title and introduction. Please, i would like to know if you could help me and how much is the charge.
This content is practical, valuable, and just great!
Thank you very much!
Hi Derek, Thank you for the valuable presentation. It is very helpful especially for beginners like me. I am just starting my PhD.
This is quite instructive and research proposal made simple. Can I have a research proposal template?
Great! Thanks for rescuing me, because I had no former knowledge in this topic. But with this piece of information, I am now secured. Thank you once more.
I enjoyed listening to your video on how to write a proposal. I think I will be able to write a winning proposal with your advice. I wish you were to be my supervisor.
Dear Derek Jansen,
Thank you for your great content. I couldn’t learn these topics in MBA, but now I learned from GradCoach. Really appreciate your efforts….
From Afghanistan!
I have got very essential inputs for startup of my dissertation proposal. Well organized properly communicated with video presentation. Thank you for the presentation.
Wow, this is absolutely amazing guys. Thank you so much for the fruitful presentation, you’ve made my research much easier.
this helps me a lot. thank you all so much for impacting in us. may god richly bless you all
How I wish I’d learn about Grad Coach earlier. I’ve been stumbling around writing and rewriting! Now I have concise clear directions on how to put this thing together. Thank you!
Fantastic!! Thank You for this very concise yet comprehensive guidance.
Even if I am poor in English I would like to thank you very much.
Thank you very much, this is very insightful.
Submit a Comment Cancel reply
Your email address will not be published. Required fields are marked *
Save my name, email, and website in this browser for the next time I comment.
- Print Friendly

An official website of the United States government
The .gov means it’s official. Federal government websites often end in .gov or .mil. Before sharing sensitive information, make sure you’re on a federal government site.
The site is secure. The https:// ensures that you are connecting to the official website and that any information you provide is encrypted and transmitted securely.
- Publications
- Account settings
Preview improvements coming to the PMC website in October 2024. Learn More or Try it out now .
- Advanced Search
- Journal List
- Indian J Anaesth
- v.60(9); 2016 Sep
How to write a research proposal?
Department of Anaesthesiology, Bangalore Medical College and Research Institute, Bengaluru, Karnataka, India
Devika Rani Duggappa
Writing the proposal of a research work in the present era is a challenging task due to the constantly evolving trends in the qualitative research design and the need to incorporate medical advances into the methodology. The proposal is a detailed plan or ‘blueprint’ for the intended study, and once it is completed, the research project should flow smoothly. Even today, many of the proposals at post-graduate evaluation committees and application proposals for funding are substandard. A search was conducted with keywords such as research proposal, writing proposal and qualitative using search engines, namely, PubMed and Google Scholar, and an attempt has been made to provide broad guidelines for writing a scientifically appropriate research proposal.
INTRODUCTION
A clean, well-thought-out proposal forms the backbone for the research itself and hence becomes the most important step in the process of conduct of research.[ 1 ] The objective of preparing a research proposal would be to obtain approvals from various committees including ethics committee [details under ‘Research methodology II’ section [ Table 1 ] in this issue of IJA) and to request for grants. However, there are very few universally accepted guidelines for preparation of a good quality research proposal. A search was performed with keywords such as research proposal, funding, qualitative and writing proposals using search engines, namely, PubMed, Google Scholar and Scopus.
Five ‘C’s while writing a literature review

BASIC REQUIREMENTS OF A RESEARCH PROPOSAL
A proposal needs to show how your work fits into what is already known about the topic and what new paradigm will it add to the literature, while specifying the question that the research will answer, establishing its significance, and the implications of the answer.[ 2 ] The proposal must be capable of convincing the evaluation committee about the credibility, achievability, practicality and reproducibility (repeatability) of the research design.[ 3 ] Four categories of audience with different expectations may be present in the evaluation committees, namely academic colleagues, policy-makers, practitioners and lay audiences who evaluate the research proposal. Tips for preparation of a good research proposal include; ‘be practical, be persuasive, make broader links, aim for crystal clarity and plan before you write’. A researcher must be balanced, with a realistic understanding of what can be achieved. Being persuasive implies that researcher must be able to convince other researchers, research funding agencies, educational institutions and supervisors that the research is worth getting approval. The aim of the researcher should be clearly stated in simple language that describes the research in a way that non-specialists can comprehend, without use of jargons. The proposal must not only demonstrate that it is based on an intelligent understanding of the existing literature but also show that the writer has thought about the time needed to conduct each stage of the research.[ 4 , 5 ]
CONTENTS OF A RESEARCH PROPOSAL
The contents or formats of a research proposal vary depending on the requirements of evaluation committee and are generally provided by the evaluation committee or the institution.
In general, a cover page should contain the (i) title of the proposal, (ii) name and affiliation of the researcher (principal investigator) and co-investigators, (iii) institutional affiliation (degree of the investigator and the name of institution where the study will be performed), details of contact such as phone numbers, E-mail id's and lines for signatures of investigators.
The main contents of the proposal may be presented under the following headings: (i) introduction, (ii) review of literature, (iii) aims and objectives, (iv) research design and methods, (v) ethical considerations, (vi) budget, (vii) appendices and (viii) citations.[ 4 ]
Introduction
It is also sometimes termed as ‘need for study’ or ‘abstract’. Introduction is an initial pitch of an idea; it sets the scene and puts the research in context.[ 6 ] The introduction should be designed to create interest in the reader about the topic and proposal. It should convey to the reader, what you want to do, what necessitates the study and your passion for the topic.[ 7 ] Some questions that can be used to assess the significance of the study are: (i) Who has an interest in the domain of inquiry? (ii) What do we already know about the topic? (iii) What has not been answered adequately in previous research and practice? (iv) How will this research add to knowledge, practice and policy in this area? Some of the evaluation committees, expect the last two questions, elaborated under a separate heading of ‘background and significance’.[ 8 ] Introduction should also contain the hypothesis behind the research design. If hypothesis cannot be constructed, the line of inquiry to be used in the research must be indicated.

Review of literature
It refers to all sources of scientific evidence pertaining to the topic in interest. In the present era of digitalisation and easy accessibility, there is an enormous amount of relevant data available, making it a challenge for the researcher to include all of it in his/her review.[ 9 ] It is crucial to structure this section intelligently so that the reader can grasp the argument related to your study in relation to that of other researchers, while still demonstrating to your readers that your work is original and innovative. It is preferable to summarise each article in a paragraph, highlighting the details pertinent to the topic of interest. The progression of review can move from the more general to the more focused studies, or a historical progression can be used to develop the story, without making it exhaustive.[ 1 ] Literature should include supporting data, disagreements and controversies. Five ‘C's may be kept in mind while writing a literature review[ 10 ] [ Table 1 ].
Aims and objectives
The research purpose (or goal or aim) gives a broad indication of what the researcher wishes to achieve in the research. The hypothesis to be tested can be the aim of the study. The objectives related to parameters or tools used to achieve the aim are generally categorised as primary and secondary objectives.
Research design and method
The objective here is to convince the reader that the overall research design and methods of analysis will correctly address the research problem and to impress upon the reader that the methodology/sources chosen are appropriate for the specific topic. It should be unmistakably tied to the specific aims of your study.
In this section, the methods and sources used to conduct the research must be discussed, including specific references to sites, databases, key texts or authors that will be indispensable to the project. There should be specific mention about the methodological approaches to be undertaken to gather information, about the techniques to be used to analyse it and about the tests of external validity to which researcher is committed.[ 10 , 11 ]
The components of this section include the following:[ 4 ]
Population and sample
Population refers to all the elements (individuals, objects or substances) that meet certain criteria for inclusion in a given universe,[ 12 ] and sample refers to subset of population which meets the inclusion criteria for enrolment into the study. The inclusion and exclusion criteria should be clearly defined. The details pertaining to sample size are discussed in the article “Sample size calculation: Basic priniciples” published in this issue of IJA.
Data collection
The researcher is expected to give a detailed account of the methodology adopted for collection of data, which include the time frame required for the research. The methodology should be tested for its validity and ensure that, in pursuit of achieving the results, the participant's life is not jeopardised. The author should anticipate and acknowledge any potential barrier and pitfall in carrying out the research design and explain plans to address them, thereby avoiding lacunae due to incomplete data collection. If the researcher is planning to acquire data through interviews or questionnaires, copy of the questions used for the same should be attached as an annexure with the proposal.
Rigor (soundness of the research)
This addresses the strength of the research with respect to its neutrality, consistency and applicability. Rigor must be reflected throughout the proposal.
It refers to the robustness of a research method against bias. The author should convey the measures taken to avoid bias, viz. blinding and randomisation, in an elaborate way, thus ensuring that the result obtained from the adopted method is purely as chance and not influenced by other confounding variables.
Consistency
Consistency considers whether the findings will be consistent if the inquiry was replicated with the same participants and in a similar context. This can be achieved by adopting standard and universally accepted methods and scales.
Applicability
Applicability refers to the degree to which the findings can be applied to different contexts and groups.[ 13 ]
Data analysis
This section deals with the reduction and reconstruction of data and its analysis including sample size calculation. The researcher is expected to explain the steps adopted for coding and sorting the data obtained. Various tests to be used to analyse the data for its robustness, significance should be clearly stated. Author should also mention the names of statistician and suitable software which will be used in due course of data analysis and their contribution to data analysis and sample calculation.[ 9 ]
Ethical considerations
Medical research introduces special moral and ethical problems that are not usually encountered by other researchers during data collection, and hence, the researcher should take special care in ensuring that ethical standards are met. Ethical considerations refer to the protection of the participants' rights (right to self-determination, right to privacy, right to autonomy and confidentiality, right to fair treatment and right to protection from discomfort and harm), obtaining informed consent and the institutional review process (ethical approval). The researcher needs to provide adequate information on each of these aspects.
Informed consent needs to be obtained from the participants (details discussed in further chapters), as well as the research site and the relevant authorities.
When the researcher prepares a research budget, he/she should predict and cost all aspects of the research and then add an additional allowance for unpredictable disasters, delays and rising costs. All items in the budget should be justified.
Appendices are documents that support the proposal and application. The appendices will be specific for each proposal but documents that are usually required include informed consent form, supporting documents, questionnaires, measurement tools and patient information of the study in layman's language.
As with any scholarly research paper, you must cite the sources you used in composing your proposal. Although the words ‘references and bibliography’ are different, they are used interchangeably. It refers to all references cited in the research proposal.
Successful, qualitative research proposals should communicate the researcher's knowledge of the field and method and convey the emergent nature of the qualitative design. The proposal should follow a discernible logic from the introduction to presentation of the appendices.
Financial support and sponsorship
Conflicts of interest.
There are no conflicts of interest.
- Open access
- Published: 15 May 2024
Alzheimer blood biomarkers: practical guidelines for study design, sample collection, processing, biobanking, measurement and result reporting
- Xuemei Zeng 1 ,
- Yijun Chen 2 ,
- Anuradha Sehrawat 1 ,
- Jihui Lee 1 ,
- Tara K. Lafferty 1 ,
- Julia Kofler 3 ,
- Sarah B. Berman 4 ,
- Robert A. Sweet 1 , 4 ,
- Dana L. Tudorascu 1 ,
- William E. Klunk 1 ,
- Milos D. Ikonomovic 1 , 4 , 5 ,
- Anna Pfister 6 , 7 ,
- Henrik Zetterberg 6 , 7 , 8 , 9 , 10 , 11 ,
- Beth E. Snitz 4 ,
- Anne D. Cohen 1 ,
- Victor L. Villemagne 1 ,
- Tharick A. Pascoal 1 , 4 ,
- M. llyas Kamboh 12 ,
- Oscar I. Lopez 4 ,
- Kaj Blennow 6 , 7 &
- Thomas K. Karikari ORCID: orcid.org/0000-0003-1422-4358 1 , 6
Molecular Neurodegeneration volume 19 , Article number: 40 ( 2024 ) Cite this article
829 Accesses
33 Altmetric
Metrics details
Alzheimer’s disease (AD), the most common form of dementia, remains challenging to understand and treat despite decades of research and clinical investigation. This might be partly due to a lack of widely available and cost-effective modalities for diagnosis and prognosis. Recently, the blood-based AD biomarker field has seen significant progress driven by technological advances, mainly improved analytical sensitivity and precision of the assays and measurement platforms. Several blood-based biomarkers have shown high potential for accurately detecting AD pathophysiology. As a result, there has been considerable interest in applying these biomarkers for diagnosis and prognosis, as surrogate metrics to investigate the impact of various covariates on AD pathophysiology and to accelerate AD therapeutic trials and monitor treatment effects. However, the lack of standardization of how blood samples and collected, processed, stored analyzed and reported can affect the reproducibility of these biomarker measurements, potentially hindering progress toward their widespread use in clinical and research settings. To help address these issues, we provide fundamental guidelines developed according to recent research findings on the impact of sample handling on blood biomarker measurements. These guidelines cover important considerations including study design, blood collection, blood processing, biobanking, biomarker measurement, and result reporting. Furthermore, the proposed guidelines include best practices for appropriate blood handling procedures for genetic and ribonucleic acid analyses. While we focus on the key blood-based AD biomarkers for the AT(N) criteria (e.g., amyloid-beta [Aβ]40, Aβ42, Aβ42/40 ratio, total-tau, phosphorylated-tau, neurofilament light chain, brain-derived tau and glial fibrillary acidic protein), we anticipate that these guidelines will generally be applicable to other types of blood biomarkers. We also anticipate that these guidelines will assist investigators in planning and executing biomarker research, enabling harmonization of sample handling to improve comparability across studies.
Alzheimer’s disease (AD), the most common form of dementia, poses significant economic and social burden on affected individuals, as well as their families, caregivers, communities, and healthcare systems worldwide [ 1 ]. An estimated excess of 50 million people are living with AD globally, including 6.7 million in the United States. By 2050, these numbers are expected to rise to 152 million and 13.8 million, respectively [ 2 , 3 ]. Neuropathologically, AD is characterized by two hallmark lesions in the brain; amyloid-β (Aβ) plaques and tau neurofibrillary tangles [ 4 , 5 ]. Most individuals with brain pathophysiological evidence of AD clinically show progressive cognitive impairment [ 6 ]. Unfortunately, despite decades of research and numerous clinical trials, AD remains difficult to treat, with only a few FDA-approved drugs available for treatment [ 1 ]. Among them, lecanemab, donanemab and aducanumab, humanized antibodies designed to reduce the amyloid plaque burden, are the only ones expected to provide disease-modifying therapy [ 7 , 8 , 9 ]. The other drugs are palliative treatments that reduce the symptoms temporarily but are not directed toward preventing or slowing disease progression.
The slow pace of AD drug development is partly due to a lack of accessible and cost-effective biomarkers for participant enrollment and stratification in clinical trials. The National Institute on Aging and the Alzheimer’s Association (NIA-AA) research framework recommends the use of biomarker criteria for amyloid pathology, tau pathology, and neurodegeneration [AT(N)] for a biological definition of AD [ 10 ]. However, these assessments are currently performed using expensive, time-consuming, and sometimes invasive procedures with limited global accessibility such as magnetic resonance imaging (MRI), positron emission tomography (PET) scans, and/or cerebrospinal fluid (CSF) biomarkers [ 11 , 12 , 13 ], which are unsuitable for large-scale clinical applications and population screenings. It has been estimated that screening a single participant for AD clinical trials with PET and MRI could cost at least US $8,000 [ 14 ]. Given the typically high screen-failure rate (percentage of screened participants not meeting the enrollment criteria), it is not surprising that participant screening may cost 50–70% of total per-participant costs [ 14 ]. These costs could be prohibitive for large-scale clinical trials. In terms of clinical applications, imaging all patients with suspected cognitive impairment due to AD using PET and MRI would be difficult to achieve due to the low throughput and the limited availability of the specialized facilities and expertise needed to administer and interpret these tests [ 13 ].
To address this issue, there is a growing need to develop less invasive, more cost-effective, and scalable biomarkers that can reliably identify AD pathology. Blood-based AD biomarkers are a desirable choice due to availability of blood specimens through routine clinical practice and research programs. In clinical trials, blood biomarkers have already shown utility as pre-screening measures to streamline the identification and inclusion of individuals who fit pre-defined criteria for biological evidence of disease [ 15 , 16 ]. Importantly, clinical prescriptions of the recent FDA-approved anti-amyloid drugs require prior confirmation of brain amyloidosis. However, since amyloid PET and CSF Aβ42/Aβ 40 assessments are not feasible in many hospital settings, blood biomarkers would be very useful proxies.
The development of such biomarkers has been hindered by the extreme complexity of the blood proteome, low biomarker abundance, and signal dilution from peripheral tissues. However, significant advances have been achieved in the past decade, benefiting partly from the development of ultra-sensitive immunoassays and high-performance mass spectrometry technology platforms [ 17 , 18 , 19 , 20 ]. Blood-based biomarkers with high potential of providing accurate assessment of the AT(N) criteria include the Aβ42/40 ratio for amyloid pathology, phosphorylated tau (p-tau) for tau pathology, and neurofilament light-chain (NfL) and brain-derived tau for neurodegeneration/axonal injury [ 11 , 21 ]. In addition, plasma glial fibrillary acidic protein (GFAP), an indicator of reactive astrogliosis often associated with brain Aβ plaques, has also been proposed as an early marker for amyloid pathology [ 22 , 23 , 24 , 25 ].
The anticipated next stage in the development of highly sensitive and specific blood biomarkers for AD is to employ them in real-world settings for clinical diagnosis, population studies, and eligibility screening for therapeutic trials. However, a major challenge facing the field is the need for increased standardization of collection, processing, and storage procedures. Another important challenge is the need for agreed-upon procedures to monitor and maintain long-term stability in the biomarker measurements, especially since none of the blood-based AD biomarkers in use currently has certified reference measurement procedures. These obstacles must be overcome before blood-based biomarkers can be effectively adopted in clinical and research-based settings, and these measurements can be appropriately harmonized. A survey of studies across fifteen centers revealed variations in sample processing, such as the time of day for collection, fasting status, time from collection to centrifugation, the temperature at various steps, and centrifugation parameters [ 26 ]. The lack of standardization can introduce measurement variations, reducing clinical reliability and making it challenging to compare results across laboratories and establish clinical thresholds. These variations can be introduced at three phases: preanalytical, analytical, and post-analytical. The advancement of analytical technologies, such as automation in sample preparation, has dramatically reduced errors in the analytical phase. It is now thought that the preanalytical stage is the most error-prone phase (over 60%), followed by the post-analytical phase (over 20%) [ 27 ].
Decades of research have led to the development of a consensus protocol for standardized collection and biobanking of CSF-based AD biomarkers [ 28 ], which has been key to the much improved inter-laboratory reproducibility of the core CSF biomarkers in recent years [ 29 ]. Blood-based AD biomarkers often have smaller effect sizes than the corresponding CSF biomarkers (with the exception of GFAP) [ 25 ], possibly due to signal attenuation caused by counterpart proteins secreted or biomarkers sequestered by peripheral tissues and the increased biological complexity of blood [ 13 , 30 ] (further discussed below). Therefore, minimizing preanalytical variations is even more critical for blood-based AD biomarkers. O’Bryant et al., in 2015, proposed a set of guidelines to standardize blood sample collection [ 31 ]. Yet, the evidence evaluating defined preanalytical factors was limited at that time. Since then, many research studies have been published, and an updated evidence-based plasma handling standardized operating procedure (SOP) was proposed in 2022 by Verberk et al. [ 26 ]. However, this SOP was limited to preanalytical factors and was directed at experienced blood biomarker laboratories and scientists. As blood biomarkers become more widely available and simplified commercial technologies get increasingly accessible, fundamental guidelines that: (1) take into account the preanalytical, analytical, and post-analytical pipeline; (2) accommodate the needs of investigators new to the blood biomarker space; and (3) are applicable to both traditional (blood collection and assessments in a clinical setting) and non-traditional (home collections, population-based evaluations, resource-limited settings) environments, are needed.
Standardization of blood AD biomarker research across sites, studies and investigators must consider several steps, including study design, blood collection, blood processing, biobanking, biomarker measurement, and result reporting (Fig. 1 ). This review aims to expand on earlier guidelines by employing the evidence base from recent research findings and our own experiences to provide a detailed description of the general considerations associated with each of these steps. Furthermore, we present an easy-to-follow SOP to aid in the design and implementation of high-quality blood-based AD biomarker research projects, covering the preanalytical, analytical, and post-analytical phases. It is worth noting that although we focus on the key blood-based AD biomarkers for the AT(N) criteria (e.g., Aβ40, Aβ42, Aβ42/40 ratio, total-tau (t-tau), p-tau, NfL, brain-derived tau [BD-tau] and GFAP), we anticipate that these guidelines will generally be applicable to other types of blood biomarkers as well as discovery proteomic investigations.
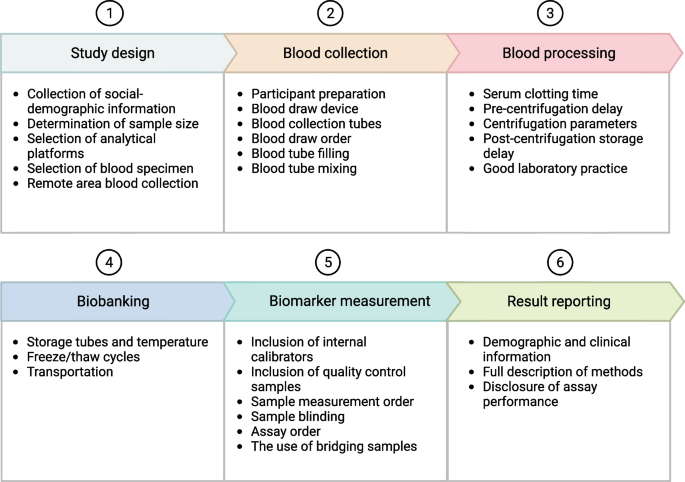
Proposed general workflow for conducting high-quality blood AD biomarker research and the important considerations associated with each step. The major steps are divided into six, namely study design, blood collection, blood processing, biobanking, biomarker measurement and results reporting. The important considerations under each step are listed in the Figure. Detailed discussions are provided in the text
Considerations during study design
Item 1: collection of sociodemographic, lifestyle, and health information.
Numerous sociodemographic, lifestyle, health, and environmental factors have been associated with an increased risk of AD [ 32 , 33 , 34 ]. For example, age, education, sex, race, creatinine levels, concomitant medication (including anti-amyloid therapies), medical history, and comorbidities such as diabetes, hypertension, impaired kidney function, liver diseases, and cardiovascular diseases have been found to be significantly associated with blood AD biomarkers levels in some research cohorts compared with unaffected controls [ 7 , 35 , 36 , 37 , 38 , 39 , 40 , 41 , 42 , 43 ]. Additionally, conditions such as pregnancy, menopausal symptoms and hormone therapy use are critical as biomarker changes have been reported [ 44 , 45 ] but require further investigation. While it may not be feasible to include all demographic and clinical information, we recommend recording as much information as possible during subject recruitment and through the medical record extraction to prevent over-/under-interpretation of results and to enable adjustment of reference ranges according to population characteristics if needed. Importantly, the collection, processing, storage, and application of personal data must conform with the prevailing ethical guidelines and legal frameworks in the countries concerned. For example, there is the General Data Protection Regulation (GDPR) in Europe and Health Insurance Portability and Accountability Act of 1996 (HIPAA) in the United States.
Item 2: sample size consideration
Sample size planning is critical in studies evaluating the diagnostic and prognostic capabilities of blood AD biomarkers and the impact of covariates. Insufficient sample size reduces the power to detect true significance. In contrast, excess sample size may magnify the importance of clinically non-meaningful differences, increase costs, prolong the study, and potentially expose more participants to needless evaluations [ 46 ]. Blood AD biomarkers often have smaller effect sizes than CSF biomarkers due to the expected dilution effect from peripheral tissues. Aβ42/40 ratio, for example, has reduced difference between Aβ+ vs. Aβ- individuals from ~ 40% in CSF to ~ 10% in blood [ 13 , 25 , 30 ]. Similarly, p-tau fold change is comparatively decreased from 166% in CSF to 85.6% in the blood [ 13 ], despite plasma p-tau having larger effect sizes than plasma Aβ42/40 ratio when compared directly [ 25 , 47 ]. Therefore, blood AD biomarker studies will require larger sample sizes than CSF biomarker studies. To determine the optimal sample size, one must consider factors such as expected effect size, population variance, desirable type I and type II error rates, participant dropout rate for longitudinal studies, and adjustment of covariates [ 46 ]. It is important to note that sample size calculation can become challenging for complex studies and will require statisticians’ advice during study design.
Item 3: selection of analytical platforms
Blood AD biomarkers tend to be near or below the detection limit of traditional enzyme-linked immunosorbent assays (ELISAs). However, in the past decade, many technologies have emerged that have significantly accelerated research in this area [ 18 , 19 , 48 , 49 , 50 , 51 , 52 , 53 , 54 , 55 , 56 , 57 , 58 , 59 , 60 , 61 , 62 ]. Among them, Single molecule array (Simoa), mass spectrometry, as well as immunoassay technologies available on platforms such as the Elecsys and Cobas systems from Roche Diagnostics, Meso Scale Discovery (MSD), and immunomagnetic reduction (MagQu) are the most used. Recently, the Lumipulse G system, widely used for running electrochemiluminescence (ECL) immunoassays in CSF samples for research and in vitro diagnostic purposes, has also moved into the blood biomarker space, with assays now available for plasma Aβ peptides, NfL, p-tau181 and p-tau217 [ 63 ]. In addition, Ella, a novel platform for running multi-analyte automated microfluidic immunoassays (Simple Plex™) has now entered the AD biomarker field, with assays available for plasma NfL [ 64 , 65 ]. Emerging platforms include Nano Mosaic and NULISA. We have summarized in Table 1 the commonly used platforms for the key AD blood biomarkers, but the list is not meant to be exhaustive. For more detailed descriptions, readers should refer to recent review articles [ 20 , 66 , 67 , 68 ] and references therein.
Extensive studies have been conducted to examine associations of blood biomarkers with AD pathology and compare the performance of different platforms. While a comprehensive review of the literature is beyond the scope of this work, a brief summary of the key findings is provided below:
Amyloid plaques, one of the primary pathological features of AD, consist mainly of amyloid beta peptides [ 77 , 78 ]. While CSF Aβ42/40 has been used in clinical settings to assess brain Aβ plaques, the association of blood Aβ42/40 with AD pathologies has been controversial [ 79 , 80 ]. Several immunoassays and MS assays are available to measure blood Aβ peptides [ 20 ], but overall, there is low inter-platform reproducibility [ 75 , 81 ]. MS assays generally exhibit superior predictive power for brain Aβ compared to immunoassays, possibly due to higher specificity obtained through MS assays [ 81 ].
CSF t-tau is a biomarker for neurodegeneration or neuronal injury [ 82 ]. However, plasma t-tau shows low correlation with CSF t-tau due to potential contamination with tau from peripheral sources [ 83 , 84 ]. Improved plasma t-tau assays have been reported recently [ 85 , 86 ]. In addition, recently developed Simoa assay targeting brain-derived tau showed a better correlation with CSF t-tau and improved biomarker performance [ 21 ].
CSF p-tau is a biomarker for neurofibrillary tangles [ 15 , 87 , 88 ]. Despite their low abundance in the blood, several assays are available to measure p-tau species in the blood [ 13 , 89 , 90 , 91 , 92 ]. Unlike plasma Aβ assays, p-tau assays exhibited overall strong inter-platform concordance [ 20 , 93 , 94 , 95 , 96 ]. P-tau181, p-tau217, and p-tau231 are the most widely studied p-tau species. P-tau212 is a new marker recently reported [ 97 ]. Different p-tau species might increase at different stages of the AD continuum [ 91 , 98 ]. Unlike their CSF counterparts, blood p-tau exhibits better association with Aβ plaques rather than neurofibrillary tangles.
GFAP is a biomarker for reactive astrogliosis [ 99 ], a cellular response often associated with brain Aβ plaque pathology in AD [ 100 ]. Plasma GFAP positively correlated with Aβ burden and tau pathology in AD [ 101 , 102 ]. Plasma GFAP level may be impacted by non-AD brain injuries and is an FDA-approved biomarker for detecting intracranial lesions after brain injury [ 103 ].
Neuronal damage/injury leads to elevated secretion of NfL into the extracellular space [ 104 ]. Although non-AD specific, NfL is an excellent biomarker for neurodegeneration to monitor the disease progression of AD patients [ 105 , 106 ]. Head-to-head comparison of Simoa and Ella assays in a multiple sclerosis cohort demonstrated a strong correlation between the platforms [ 64 , 65 ]. Plasma/serum brain-derived tau showed stronger specificity to AD pathophysiology versus related non-AD disorders.
Item 4: selection of blood specimen
Both plasma and serum have been utilized for measuring AD biomarkers. Studies comparing AD biomarker levels in serum and plasma have shown that some analytes, including Aβ peptides, t-tau, BD-tau and multiple p-tau species, are present at lower levels in serum, possibly due to a loss from clot trapping [ 26 , 107 , 108 , 109 , 110 , 111 ]. This makes it more challenging to measure such biomarkers in serum, especially for individuals whose biomarker levels are close to the lower detection limit. Nonetheless, biomarkers such as p-tau231, p-tau181 and BD-tau have been shown to have equivalent diagnostic accuracies in plasma and serum [ 21 , 89 , 107 , 109 , 111 ]. P-tau217 [ 112 ] and p-tau212 [ 97 ] are currently measurable in plasma but not serum. It is important to note that the choice of the blood specimen depends on the overall research objectives and sample availability. For example, serum may be a better choice for studies that evaluate the integrity of the blood-brain barrier since the CSF/serum albumin ratio is a well-established indicator of blood-CSF barrier function [ 113 ]. Additionally, serum is more widely used in hospital systems, with more clinical tests using serum instead of plasma as the specimen, according to the Mayo Clinic 2023 Test Catalog [ 114 ].
On the other hand, many research cohorts collect plasma instead of serum. Ethylenediaminetetraacetic acid (EDTA), heparin, and citrate are the most commonly used anticoagulants in clinics for plasma collection [ 115 ]. EDTA is the most universal in AD biomarker research [ 26 ]. Several studies have suggested that citrate plasma has lower levels of several biomarkers, including Aβ peptides, NfL, GFAP, and t-tau, compared with EDTA and heparin plasma [ 116 , 117 ]. However, studies comparing heparin vs. EDTA have generated mixed results. For example, one study found most biomarkers to be more abundant in heparin plasma than EDTA plasma [ 107 ], while another found higher levels of t-tau and p-tau181 but similar levels of Aβ40 and Aβ42 in heparin compared with EDTA plasma samples [ 118 ]. Rózga et al., on the other hand, reported that the levels of t-tau were significantly lower in heparin plasma [ 116 ].
Regardless of the type of blood specimen chosen, it is important to use the same type of specimen throughout the study. Although biomarkers may show a similar trend in different specimens, they are not necessarily linearly correlated in samples from all individuals. For example, despite strong correlations and similar diagnostic accuracy between paired serum and plasma p-tau levels, Kac et al. observed larger disagreements in samples with lower p-tau concentrations [ 109 ].
Item 5: blood collection from remote areas, under-resourced settings, or home care
Advanced laboratory equipment, such as ultra-low temperature freezers and centrifuges, are typically required for the processing of traditional venipuncture-based blood specimen. This can create significant obstacles for community-based studies utilizing home sampling, as well as for studies in remote areas, where access to such equipment may be limited. In addition, venipuncture may be difficult and painful for individuals with small or fragile veins [ 119 ]. To overcome these challenges, some research initiatives have explored alternative blood collection methods. For instance, Walter et al. compared Aβ40 and Aβ42 levels in conventional venous blood vs. capillary blood collected by finger insertion using microvettes. They found a good correlation between the two specimen types, despite slightly lower levels in capillary blood [ 120 ]. Similarly, Lombardi et al. and Simrén et al. investigated the use of dried blood spots (DBS) and dried plasma spots (DPS) for NfL measurement and found that NfL levels in both DBS and DPS samples correlated strongly with those in EDTA plasma samples with a stronger correlation observed for DPS samples [ 121 , 122 ].
Considerations during blood collection
Item 1: preparation of participants for the blood draw.
Studies examining the impact of pre-blood draw activities of participants, such as fasting, physical exercise, medication use, and the time of day for blood collection, are limited. However, preliminary evidence shows that some of these factors can impact blood biomarker levels. For example, Rózga et al. found that the levels of Aβ40 and Aβ42 were 5–9% higher in blood samples collected in the afternoon compared with those collected in the morning, with the opposite trend noted for t-tau [ 116 ]. Meyer et al. found significantly higher Aβ40 and Aβ42 levels in non-fasting blood when measured with Simoa assays [ 123 ]. Signal variation from plasma samples obtained on consecutive days from the same individual or within a cohort, which allows for the evaluation of short term fluctuation, have also been reported [ 124 ].
To minimize potential bias arising from these effects, we recommend pre-defining the participant preparation protocol upfront and following it throughout the study. Ideally, blood should be drawn at the same time of the day for all participants with the same fasting status throughout the study. If practical in the population under study, fasting blood samples may be more reliable in general. It is also important to record information such as blood draw time, fasting status, date and time of last meal, hours of sleep the previous night, pre-blood draw exercise activity, and medication use, to facilitate downstream interpretation of results.
Item 2: blood draw devices
Blood can be collected from participants in various ways, including venipuncture using an evacuated system (Vacutainer®), a syringe, butterfly needles, intravenous (IV) catheters, fingersticks, or heelsticks. The choice of blood draw methods depends on factors such as patient characteristics, the type of tests to be performed, and the preference and experience of clinical staff. Venipuncture using a needle and vacutainer tubes are the most used for routine blood draw. It is important to note that the devices used for the blood draw may influence the blood sample quality. For example, IV catheters and smaller-bore needles are sometimes used for patients with hard-to-access veins or when multiple blood draws over an extended period are needed. However, both have been found to have a higher hemolysis rate [ 125 , 126 ]. Additionally, lubricant coating and needle material, if released into the blood, can potentially contaminate the specimens, which have been shown to affect antigen-antibody binding in some immunoassays [ 127 ]. There is a lack of published research on the impact of blood draw devices on blood AD biomarker levels, aside from the type of blood collection tubes used (described below). To harmonize the blood collection procedures across different labs, O’Bryant et al. recommended using 21-gauge needles for blood draw in adults [ 31 ]. When possible, new straight needle venipuncture is preferred over the IV start. Any variation from standard procedures should be carefully documented.
Item 3: blood collection tubes
Most biomarker studies use evacuated tube systems for blood collection. There are many different brands of evacuated tube systems available, including BD’s Vacutainer®, Sarstedt’s Monovette®, and Greiner Bio-One’s VACUETTE®. Different blood collection tubes vary in materials, shape, size, additives used, and safety features. The color of the tube closure typically indicates the additives. We have listed some commonly used blood collection tubes and their intended clinical applications in Fig. 2 . Various components of the evacuated tubes, such as their surface coating, stopper materials, stopper lubricants, gel separators, and additives, can interfere with clinical laboratory assays and are potential sources for preanalytical variation [ 115 , 127 , 128 ]. For instance, gel separators – inert gels used as barriers for better separation of serum or plasma from cells/clots after centrifugation – have been shown to absorb blood constituents and interfere with various clinical tests for therapeutic drug monitoring [ 129 ]. It is worth noting that several studies indicated that AD biomarkers, especially Aβ peptides in the CSF matrix, may exhibit varying absorption rates to tube walls based on the materials they are made of. Specifically, there is a higher overall loss when polystyrene tubes are utilized [ 130 , 131 , 132 ]. Pre-treating the tubes with detergent Tween-20 might mitigate the absorption [ 133 , 134 ]. However, currently, there is no evidence to suggest that the primary materials used in blood tubes have any effect on AD biomarker levels [ 116 , 135 ]. To reduce the risk of variability caused by using different types of tubes, we suggest using the same brand of tubes consistently throughout the studies and limiting the number of lots to as few as possible.
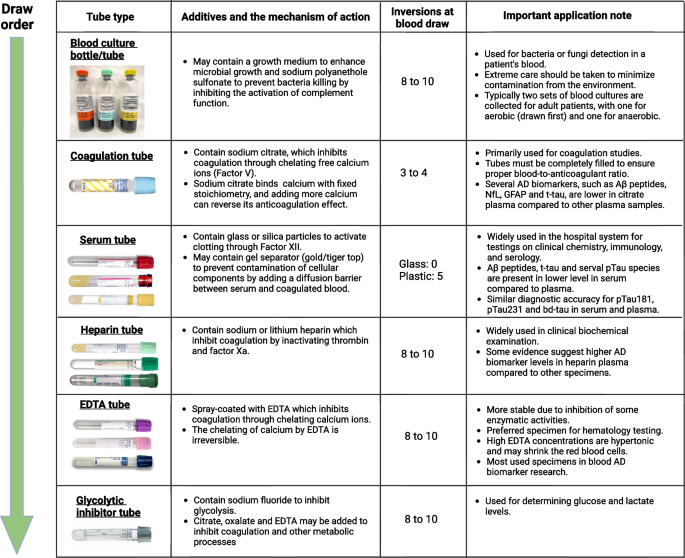
Commonly used human blood collection tubes, their draw order, additives, and important application notes. The numbers of inversions are based on BD’s recommendations for Vacutainer® tubes
Item 4: blood draw order
Different blood collection tubes contain distinctive additives. To minimize the impact of additive cross-contamination when collecting samples from a single blood draw into different types of tubes, the Clinical and Laboratory Standards Institute [ 136 ] CLSI; https://clsi.org/ ) recommended a specific blood draw order (as shown in Fig. 2 ): (1) blood culture tube or bottle; (2) sodium citrate tube; (3) serum tube; (4) heparin tube; (5) EDTA tube; (6) sodium fluoride/potassium oxalate glycolytic inhibitor tube. Following the recommended blood draw order is crucial to avoid carry-over additives that may result in inaccurate results. For example, if an EDTA tube is drawn before a serum tube, some of the EDTA may carry over into the serum tube and interfere with the coagulation.
Item 5: blood collection tube filling height
Some collection tubes, such as those for plasma collection, contain spray-coated or liquid additives. Therefore, different tube filling heights may cause variations in the blood-to-additive ratio, potentially influencing the protein composition. In support of this, Rózga et al. found a lower level of plasma t-tau when K 2 -EDTA tubes were filled only to 50% compared to 100% [ 116 ]. Therefore, to minimize variation, we recommend adhering to manufacturers’ recommendations for blood volume filling to maintain a consistent additive-to-blood ratio among all samples.
Item 6: proper mixing of blood samples
For blood collection tubes containing additives, it is crucial to gently invert the tubes immediately after the blood draw to ensure proper mixing of the additive with the blood. Failure to do so may result in non-homogenous samples and the formation of microclots or residual fibrins that can obstruct the sample probe of analytical instruments. The number of required inversions varies by tube type. We suggest following the manufacturer’s guidelines for the mixing. The recommended number of inversions for BD’s Vacutainer® tubes is listed in Fig. 2 .
Considerations during blood processing
Both serum and plasma are liquid components derived from blood after separating the blood cells, typically through centrifugation. The main difference is that serum is collected from clotted blood, while plasma is collected without clotting through anti-coagulants, thus retaining the clotting factors. Apart from the variations mentioned above for blood collection, several factors during blood processing, including pre-centrifugation delay time, centrifugation conditions, post-centrifugation storage delay, and temperature at various processing steps, may also contribute to the pre-analytical variation of the resulting blood specimens. In the following sections, we summarize current research findings on the impact of these variables and provide general guidelines for blood processing. Additionally, we have included a detailed step-by-step SOP for collecting plasma from EDTA tubes in Additional file 1 .
Item 1: serum clotting time
Harmonizing SOPs for serum collection can be challenging, partly because of the difficulty in setting the optimal clotting time. Insufficient clotting may lead to the formation of residual fibrin, which may clog the biomarker-measuring instruments [ 137 ]. In contrast, prolonged clotting may lead to cell lysis, resulting in serum contamination with cellular components [ 138 ]. The ideal clotting time varies not only by tube type but also by patient characteristics. Plain red top tubes (glass with no additive or plastic coated with silica as clot activator) and serum separator tubes (SST; gold top or tiger top) with clot activators and gel separators are commonly used in the clinics for serum collection. BD Diagnostic recommends a 30-min clotting time for SST and a 60-min clotting time for the red top tubes. Patients with certain diseases, such as liver diseases and multiple myeloma, or those on anticoagulant therapy, may require a longer clotting time. It has therefore been recommended that blood samples should be left to sit upright at room temperature for at least 30 min but no more than 60 min to allow clots to form and minimize the interference of blood cell lysis [ 31 ].
Item 2: pre-centrifugation delay time
Several studies have investigated the impact of pre-centrifugation delay on AD blood biomarker measurements. A long delay has been associated with a more significant decrease in biomarker levels. This impact can be mitigated by storing blood at 4 °C rather than room temperature prior to centrifugation [ 26 , 116 , 120 ]. Among the AD blood biomarkers, Aβ peptides are particularly susceptible to loss from pre-centrifugation delay. Their levels drop in a time-dependent manner when stored at room temperature [ 139 ]. To minimize interference of blood cell lysis and protein degradation, it is recommended completing the whole process within 2 h [ 31 ] and, if not feasible, keeping the blood refrigerated for no more than 24 h [ 26 ]. However, the shorter the pre-centrifugation delay, the better for all the blood biomarkers.
Item 3: centrifugation settings, including speed, time, and temperature
Optimal centrifugation settings are crucial for obtaining high-quality serum/plasma samples. Prolonged or excessive-speed centrifugation may cause blood cell lysis, while centrifugation that is too short and/or at an insufficient speed may result in incomplete separation of serum/plasma from blood cells [ 140 ]. Centrifugation settings may vary by blood collection tube type. For example, coagulated tubes require longer centrifugation than plasma tubes to ensure complete serum separation from the clot (CLSI H21). According to a recent survey [ 26 ] the common practice in the blood AD biomarker field is to centrifuge for 5–15 min at 1500–3000 xg. However, there are still very limited studies evaluating the impact of centrifugation parameters on AD blood biomarker measurements. A preliminary investigation found no significant difference between room temperature and 4 °C centrifugation for most AD biomarkers except t-tau, whose abundance was lower with 4 °C centrifugation [ 26 ].
Item 4: post-centrifugation storage delay
Post-centrifugation delay may also contribute to a decrease in biomarker abundance, although the rate of decline appears slower than during pre-centrifugation delay [ 26 , 120 ]. Similarly, keeping samples on wet ice while waiting for storage has been found to greatly mitigate the impact of storage delay.
Item 5: good laboratory practice (GLP)
Adhering to GLP during blood processing is crucial for ensuring the safety, quality, and integrity of research studies. Below are some key practices that should be followed:
All blood samples and associated collection devices should be considered potentially infectious, and proper personal protective equipment (PPE) should always be used to minimize exposure risk.
To protect the confidentiality of research participants, personal information should not be included on specimen labels. To avoid sample mix-up, all tubes should be clearly labeled, preferably using printed labels or barcodes rather than handwritten ones. This labeling should be done in advance of the participants’ visits for blood collection.
Good pipetting skills are essential for ensuring high sample quality. When pipetting plasma/serum from the blood collection tubes, gently draw the liquid from the top and gradually move the pipette down with the liquid. It is important to avoid disturbing the buffy coat and the cell layers in the plasma tubes and clots in the serum tubes. If allowed, leave the bottom ~ 10% of plasma/serum behind to prevent cross-layer contamination.
If plasma/serum samples are to be aliquoted into more than one tube, it is important to transfer them from the blood collection tubes to a second, intermediary tube (such as low protein binding conical tubes) after centrifugation. Before aliquoting, the samples should be mixed by inverting the conical intermediary tube or pipetting up and down multiple times to ensure homogeneity. Direct aliquoting from the blood collection tubes right after centrifugation may lead to heterogeneity among aliquots due to the impact of centrifugation forces.
Hemolysis significantly deteriorates sample quality and is the primary cause of unusable specimens for clinical assays [ 140 ]. Therefore, samples should be inspected for signs of hemolysis which may impact the assay results. We recommend using a quick reference chart (such as the CDC Hemolysis Reference Palette) to record the hemolysis scale during specimen collection and checking the influence of hemolysis during data analysis.
Item 6: general procedures for serum collection
Figure 3 A illustrates the general procedures for collecting serum. We recommend collecting at least 5 ml of blood, yielding approximately 2.5 ml of serum to ensure sufficient specimens for multianalyte measurement. To minimize the impact of freeze/thaw, samples should be aliquoted. Both plain red-top tubes and serum separator tubes are commonly used for serum collection. Other than glass plain red top tubes, all other tube types require five inversions immediately after the blood draw to mix the blood with clot activators. Below are the general procedures for serum collection.
CRITICAL: If not using the glass red top tubes, phlebotomists should gently invert the blood tubes 5 times immediately after blood draw.
CRITICAL: Place the filled blood collection tubes upright at room temperature for 30 to 60 min to allow the clot to form.
CRITICAL: If the blood is not centrifuged immediately after the clotting time (30 to 60 min at room temperature), the tubes should be refrigerated (4 °C) for no longer than 2 h.
Centrifuge clotted tubes balanced by weight for 10 min at 1500 to 2000 × g at 4 °C.
Use the disposable transfer pipette to transfer the serum (top layer) to a 15 mL conical tube (or 50 mL conical tube if collecting 30 to 100 mL of blood). Be careful not to disturb the clot containing red blood cells, white blood cells, platelets, etc.
If more than one tube is collected, combine the serum samples from all tubes into the same conical tube.
Gently invert the conical tube 8–10 times to mix. Aliquot 250 μl to 1 ml into labeled microtubes or cryovials with O-ring-sealed screw leads. Residual aliquots can be saved and pooled as QC samples for repeated analysis.
Store all aliquots upright in a specimen box in an -80 °C or colder freezer.
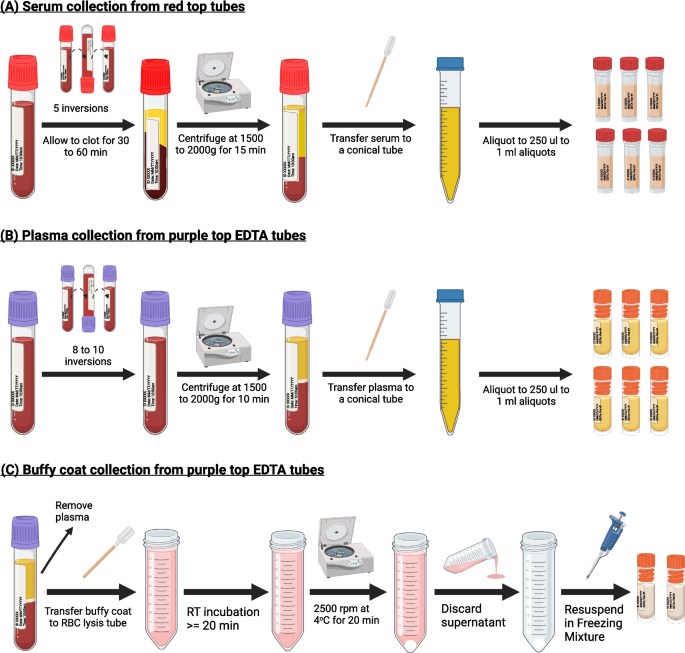
General procedures for serum ( A ), plasma ( B ), and buffy coat ( C ) collection from whole blood
Item 7: general procedures for plasma collection
Figure 3 B depicts the general procedures for collecting plasma. We recommend using EDTA or heparin tubes for blood collection, filling the tubes until the vacuum is exhausted, and following the procedures below.
CRITICAL: Immediately after blood collection, gently invert/mix (180-degree turns) the EDTA tubes 8–10 times. Place the tubes upright on a rack until centrifugation.
CRITICAL: It is advisable to store collected blood at 4 °C instead of at room temperature before centrifugation. Blood samples should be centrifuged within 2 h of blood collection to minimize degradation of AD biomarkers.
CRITICAL: In case of unavoidable prolonged centrifugation delay, place blood samples in the refrigerator for no more than 24 h. Avoid direct contact of blood tubes with ice to minimize cell lysis.
Centrifuge balanced blood collection tubes for 10 min at 1500 to 2000 × g at 4 °C.
Use the disposable transfer pipette to transfer the plasma (top layer) to a 15 mL conical tube (or 50 mL conical tube if collecting 30 to 100 mL of blood). Be careful not to disturb the buffy coat layer (the whitish layer in the middle) and the red blood cell layer (the red layer at the bottom).
If more than one tube is collected, combine the plasma samples from all blood collection tubes into the same conical tube.
Item 8: general procedures for buffy coat collection
Buffy coat contains most of the white blood cells and platelets of the anti-coagulated blood and is useful for a variety of clinical applications including genomic/genetic analysis. The remaining blood fractions after plasma collection can be used to collect buffy coat. To ensure high quality DNA, we recommend further enriching the buffy coats using a hypotonic solution to lyse the residual red blood cells. Figure 3 C illustrates the general procedures for buffy coat collection.
Note: Prepare the following reagents ahead of time and store them at 4 °C.
Ammonium chloride solution: 7.72 g/L
Ammonium bicarbonate solution: 0.79 g/L
Freezing mixture: TriPotassium Citrate: 17.8 g, Sodium Phosphate, monobasic: 2.4 g, Sodium Phosphate, dibasic: 2.8 g, Glycerin (Glycerol): 400 ml; bring volume to 1 L with distilled water.
Freshly prepare RBC lysis buffer by combining 45 ml ammonium chloride solution and 5 ml ammonium bicarbonate solution.
After removing the plasma (top layer) from the EDTA or heparin tubes, use another transfer pipette to draw the buffy coat (the whitish layer on top of the RBC layer) and place into the RBC lysis buffer tube (50 ml).
Mix by pipetting up and down to separate any leftover cells from within transfer pipette.
Cap the 50 ml tubes with lysis buffer + buffy coat and gently invert several times to mix.
Incubate at room temp for at least 20 min.
Add 10% bleach or Cavicide to the used blood tubes (lower layer with RBC) with leftover blood in them; discard in an appropriate biohazard bag.
After 20 min incubation, centrifuge 50 ml tubes at 4 °C for 20 min at 2500 rpm
After centrifuging, a white pellet will be visible at the bottom of the tube.
If no pellet is visible, centrifuge for an additional 20 min.
If pellet is visible, pour the red supernatant into a beaker filled with 10% bleach or Cavicide.
Let pellet dry (approximately 10–20 min).
Add 1 ml of freezing mixture to pellet. [ Freezing Mixture: TriPotassium Citrate: 17.8 g, Sodium Phosphate, monobasic: 2.4 g, Sodium Phosphate, dibasic: 2.8 g, Glycerin (Glycerol): 400 ml; bring volume to 1 L with distilled water].
Gently mix to break the pellet into single cell suspension.
Transfer whole (cells + freezing mix) into cryotubes.
Store at -80 °C for subsequent DNA isolation for genetic studies.
Item 9: detailed step-by-step SOP for blood collection and processing
We have combined the points discussed above and our own experiences to develop an SOP for the handling of blood for biomarker measurements (see Additional file 1 ). This SOP, which was primarily developed to streamline handling procedures for neurodegenerative disease cohort studies at the University of Pittsburgh Alzheimer’s Disease Research Center and ancillary centers and studies, can also be used by other investigators including those who are new to blood biomarker studies. Importantly, the SOP is adapted to allow for the concurrent processing of whole blood into plasma/serum for protein biomarker evaluation, and buffy coat for genetic/genomic studies. Moreover, the design of the SOP enables multiple tube aliquots to be collected and stored, and residual volumes pooled for quality control (QC) purposes.
Considerations for biobanking
Item 1: storage tubes and temperature.
To minimize analyte loss caused by adherence to the tube surfaces, it is recommended to use low protein binding microcentrifuge tubes or cryovials to store serum or plasma samples. For long-term storage, tubes with O-ring-sealed screws should be used to prevent evaporation. To prevent degradation, samples should be stored at ultra-low temperature freezers (-80 °C) or liquid N 2 tanks instead of -20 °C freezers. If feasible, aliquots of the same sample should be stored in separate freezers to prevent complete loss in the case of a freezer failure.
Item 2: freeze/thaw cycles
Several studies have investigated the impact of freeze/thaw cycles on blood biomarkers [ 107 , 116 , 141 , 142 , 143 ]. The overall results suggest that plasma AD biomarkers are stable for at least three freeze/thaw cycles. However, serum AD biomarkers may be more sensitive to freeze/thaw cycles. For example, serum Aβ40 level significantly decreased after any freeze/thaw cycle, while serum Aβ42 level showed a significant decrease at the third freeze-thaw cycle [ 107 ]. Therefore, to minimize the impact of freeze/thaw cycles, we recommend aliquoting samples in adequate volume, limiting the total number of freeze/thaw cycles to as few as possible, but never more than three.
Item 3: transportation
To transport samples to different facilities, it is recommended to use abundant dry ice sufficient to last at least 24 h post the expected delivery time to avoid sample thawing due to delivery delays. If possible, use a courier that replenishes dry ice mid-way, and avoid weekend delivery. It is worth noting that dry ice inside storage tubes may change the pH of the specimen during thawing and potentially influence the assays [ 144 ]. Therefore, samples may be stored free of dry ice in -80 °C freezers for at least 24 h before thawing for measurement. To provide guidance to readers, we have compiled a list of recommended practices during blood transportation, created based on issues regularly encountered at a major biomarker laboratory.
Include an accurate sample list (including accurate volumes). Ideally, provide a Microsoft Excel sheet (or similar) with columns for sample IDs, their corresponding volumes, and their location in the box. Adding a pictorial illustration of sample arrangements in the boxes is also helpful.
Inform the receiving laboratory ahead of time so that they will keep a lookout and be able to receive and store them in good time. The receiving lab may need to find freezer space before your samples arrive. Therefore, it is important that they are informed ahead of time.
Ensure that the package contains an adequate amount of dry ice. For long-distance transportation that spans multiple days, choose a reliable company that can refill the dry ice midway.
Print labels using a computer, rather than handwriting them, to ensure better legibility.
Use tubes with caps that do not become loose accidentally.
Sort the samples in the order you want them analyzed. If you are unsure of the order, there are two main rules:
If your samples are in groups: randomize them, so that all groups are represented in all analytical runs.
If you have longitudinal samples, keep all samples from the same participant together and in the order in which they were collected. Ensure your sample coding reflects this ordering.
Do the final sorting of the samples BEFORE you send them. It might take longer and be too time-consuming for the receiving lab to do it.
Considerations during biomarker measurements
The quality of laboratory and clinical assays has greatly improved due to advances in instrument technology, particularly the use of automated equipment, which has led to higher reproducibility through standardized procedures. However, analytical errors/variations from various sources may still occur and render results unusable or confound study findings. Common sources of analytical errors/variations include instrument malfunction, operators’ failure to follow procedures, inherent batch-to-batch variation of the assay, and matrix interference. To minimize errors/variations, it is important to use good process controls and follow good laboratory practices. Below, we outline several considerations that are critical for ensuring high-quality results and monitoring/maintaining the long-term stability of the AD blood biomarker measurements.
Item 1: preparation of samples for measurement
All blood specimens should be considered potential biohazards and handled with appropriate PPE. To ensure accurate measurement, it is crucial to homogenize samples before measurement. Samples should be completely thawed and mixed thoroughly. To minimize the impact of particulates that could clot cartridges or sample probes in the instrumentation, samples should be centrifuged before dispensing into a measurement container. It is also important to minimize the bubbles during pipetting. Any remaining samples should be promptly returned to the -80 °C freezer to minimize protein degradation. Place a dot on the tube lid with a permanent marker after each freeze-thaw cycle and keep track of the total number of cycles in updated inventory of samples. It is also recommended to keep samples with the same number of freeze-thaw cycles together and separate from the original, un-thawed samples.
Item 2: inclusion of calibrators
Despite significant technological advances, batch-to-batch variation remains an inherent issue in clinical chemistry. Contributing factors may include variations in instrument performance, changes in reagents and consumables, operator variability, etc. To address this issue, calibrators should be included in every batch to help correct for batch effects. Some platforms, such as Lumipulse, have been built to circumvent this issue such that there is an internal, manufacturer-provided calibration curve against which all sample results are plotted. However, this approach only works if the manufacturer can adjust all reagent batches to perform equivalently to the initial batch used to generate the built-in curve. The acceptance criteria for the calibration curve must be predefined to ensure accuracy and consistency. Although these criteria may vary depending on the platform used, certain parameters such as the regression coefficient, the variance between predicted and actual concentrations, and the repeatability for each calibrator should be considered.
Item 3: inclusion of QC samples
QC samples play a critical role in process control and should be included in every analytical run to evaluate assay performance and address potential errors. At a minimum, QC samples should be run in duplicates at the beginning and end of each analytical run. These samples should be selected in a way that they cover a range of concentrations across the standard curve or the typical range of concentrations for the measured sample values. It is typically advisable to use use three QC samples – low, average, and high concentrations relative to the assay standard curve. For optimal results, the same lot of QC samples should be used over time to help detect system or operator performance changes. Whenever possible, QC samples should be the same specimen types as the test samples. When switching between batches of QC samples, bridging is recommended – the relative comparison of QC samples to adjust the biomarker distribution on one plate to the other. These factors can then be applied to all samples measured on each plate/run to normalize the entire dataset. In Fig. 4 , we present a hypothetical example of a cohort consisting of 280 samples that were analyzed across four plates for Biomarker X. As shown in Fig. 4 A, the mean and overall data distribution of the uncorrected results from plates 1 and 2 appear similar, while plates 3 and 4 show a noticeable shift (simulation datasets in Additional file 2 ). However, after normalization using the plate-specific scaling factors based on QC readings, as shown in Fig. 4 B, the results from all four plates become more comparable.
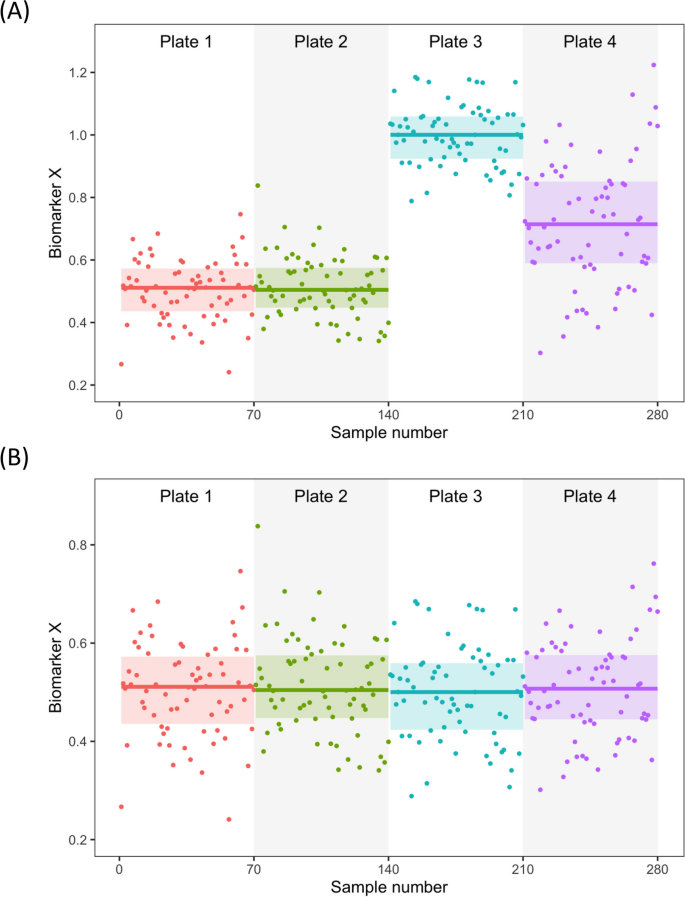
Visualization of data generated from measuring a hypothetical biomarker on 280 samples spread across four plates. The figure shows the results before ( A ) and after ( B ) adjustment based on the signals generated for identical aliquots of the same quality control samples measured on each plate, assuming all other key variables remain the same
Similar to calibrators, a predefined set of criteria should be applied to determine whether a run passes or fails. Key parameters to consider include the deviation from target values established through prior repeated analyses and the reproducibility of the QC measurements. An article by Schindler et al. [ 145 ] which tracked CSF assay performances over approximately a decade sheds light on this issue and potential corrective factors.
Item 4: sample measurement order
Batch-to-batch variation and within-batch run order effects can bias results, leading to erroneous conclusions. One way to address these issues is to randomize the order of sample analysis. There are various methods of randomization, including simple randomization, block randomization, stratified randomization, and covariate adaptive randomization [ 146 ]. Simple randomization involves using a single random sequence and is easy to implement, for example, by using the random number generator in Excel. However, simple randomization may result in an unequal distribution of groups and may not be suitable for studies with a small sample size ( n < 200). Block randomization addresses this issue by randomly assigning an equal number of samples from each group to each block and is more appropriate for small-size studies. For studies with multiple covariates, stratified randomization or covariate adaptive randomization may be necessary to control the potential uneven distribution of covariates. Additionally, for longitudinal measures, we recommend measuring all time points for the same participant simultaneously to minimize the impact of batch variation.
Item 5: sample blinding
Knowledge of grouping information may bias the analysis results and taint the data. Whenever possible, scientists performing the measurements and the analysts/statisticians who work with the ensuing data should be blinded from grouping allocation until measurement results have been finalized. A third party should perform the randomization to generate sample analysis orders.
Item 6: assay order
If multiple biomarkers are to be measured from the same aliquots, it is best practice to start with the biomarker most sensitive to degradation/denaturation from repeated freeze/thaw cycles. For example, among the core AD blood biomarkers, Aβ40 and Aβ42 tend to be most prone to degradation and should therefore be measured first, if possible, followed by t-tau, p-tau, BD-tau and finally GFAP and NfL. In addition, using multiplexed assays, if available, can help minimize the number of freeze/thaw cycles required.
Item 7: the use of bridging samples
For discovery research, it is recommended to process all samples at the same time with the same batch of reagents. However, in cases where it is unavoidable to change the reagent lots or run the analysis at different times or in various laboratories, harmonization can be achieved by repeating the measurement for bridging samples. Ideally, bridging samples should be from the same sample cohort and cover the full range of values of the study samples. The bridging samples are analyzed using the same reagents and consumables for each batch, allowing the determination of batch-specific normalization factors. The same approach described in Fig. 4 for normalizing run data from different plates is applicable here to between-batch normalization.
Item 8: longitudinal samples
Longitudinal studies, which involve continuous tracking of the same individuals over time, are commonly employed to assess the influence of various interventions or risk factors on disease outcomes. In contrast to cross-sectional studies, which capture snapshots of participants at a single point in time, longitudinal studies typically require an extended duration to collect specimens for the entire study. In some cases, researchers may be able to wait until all samples are collected to run them simultaneously. However, there are instances where running batches of samples along the way becomes necessary to gain preliminary insights into the data. To minimize the impact of temporal variation, proper control measures must be implemented to monitor and maintain the long-term stability of measurements. Several practical guidelines, proposed by Palmqvist et al. for AD CSF biomarkers, can be adopted for blood AD biomarkers [ 78 ]. These include controlling preanalytical variables, following good laboratory practices, utilizing calibrators and QC samples, and assessing the batch effect through batch-bridging. Ideally, one would like to use the same QCs throughout a longitudinal study. However, if this is not possible, it is important to perform batch-bridging and re-run samples from the previous analysis round to normalize runs. Therefore, it is crucial to properly plan for the longitudinal studies, including stocking up reagents (including QC and bridging samples) and consumables for the entire study if shelf life is allowed. While most blood AD biomarker research has been conducted in research settings, there is an increasing number of studies being initiated to evaluate the use of these biomarkers in real clinical practice, where specimens are continuously analyzed. Maintaining measurement stability becomes even more important in these real-world settings.
Considerations for result reporting
Item 1: demographic and clinical information.
To minimize potential bias caused by demographic and clinical factors, it is important to include the distribution of available demographic and clinical characteristics among groups in the report, along with their associated statistical findings. These information help identify potential moderating variables and adjust for their effect in the analysis. Additionally, including this information in the report allows better comparison with results from different studies.
Item 2: full description of methods
To facilitate the coherent pooling of data in future meta-analyses, it is important to include detailed descriptions of blood collection/handling protocols and laboratory assay protocols in the Method section of peer-reviewed publications. In addition, as preanalytical variables, such as blood draw devices and parameters during blood processing, may interfere with AD biomarker measurements, it is important to describe these variables and to indicated if they were changed during the study.
Item 3: disclosure of assay performance
It is important to include assay performance in the publication to enable other researchers to evaluate the quality of the reported results. Multiple attributes can be useful for assessing assay performance, such as calibration curves’ R -value, linearity range, the limit of detection, and limit of quantification, as well as QC performance measures such as repeatability and intermediate precision. Moreover, the reports should also disclose if plate adjustments, such as with the use of bridging samples or QC samples, have been performed to account for batch variation.
Unresolved issues
Certified reference materials.
A major source of confusion in the field is that blood biomarker assays for the same analyte (e.g., plasma p-tau181 or p-tau217) from various sources return very different numerical values in terms of concentrations. We have sought to explain the analytical reasons behind this phenomenon – that it is often due to the use of different calibrators as well as variability of signals from the technical platforms [ 13 ]. However, the issue limits the comparability of assay results across platforms, which might become even more acute once cutpoints are generated. The use of certified reference materials could provide a solution, by providing a focal point for comparing and calibrating the standards used in the different assays. An example of the use of certified reference materials in mitigating between-assay bias was demonstrated by the application by Boulo S et al. in CSF samples [ 147 ].
Real world applications
Despite excellent performances of blood biomarkers demonstrated in many publications, we should be mindful that the results have mostly come from highly selected research cohorts that additionally have prior confirmation of pathology using CSF or neuroimaging biomarkers or even sometimes both. As we venture into applying blood biomarkers as potential first line screeners in clinical practice and therapeutic trials, it is important to note that this might be the real “test drive” for the biomarkers. As such, their performances may not be as stellar as observed for multiple reasons. This includes the much lower prevalence of AD pathophysiology in the community compared with the research cohorts, and the high heterogeneity of diseases presented in the clinics contrary to those included in defined cohorts. Moreover, such heterogenous cases would not benefit from prior categorization using CSF and neuroimaging biomarkers as often done for the existing cohorts that have recorded high accuracies.
Clinical use of blood biomarkers is much anticipated. However, it is often forgotten that one of the fundamental factors that need addressing prior to this happening is the generation and validation of assay thresholds. Such threshold points would not only be based on the detection of amyloid and/or tau pathology but would additionally investigate potential clinical scenarios such as if and how the set cutpoints respond to given demographic and clinical variables.
The blood AD biomarker research field has experienced rapid growth in recent years, with numerous studies highlighting the potential of blood-based AD biomarkers in supporting clinical decision-making and accelerating therapeutic development. We have outlined in this review general guidelines for the various steps involved in blood AD biomarker measurement, to promote good laboratory practices to minimize analytical errors and facilitate the development of standardized protocols that can improve reproducibility and enable cross-validation across different research centers. Table 2 provides a summary of the important preanalytical, analytical and post-analytical factors to consider in AD blood biomarker research. However, further research is needed to fully understand the impact of preanalytical and analytical variables, and these guidelines should be updated as new research findings become available. Finally, we provide an adaptable SOP that can be applied to blood collection, processing, and downstream handling in biomarker evaluations.
Availability of data and materials
All the data are included in the manuscript and the supporting information.
Abbreviations
Amyloid-beta
- Alzheimer’s disease
Brain-derived tau
Cerebrospinal fluid
Dried blood spot
Dried plasma spot
Electrochemiluminescence
Ethylenediaminetetraacetic acid
Enzyme-linked immunosorbent assay
General Data Protection Regulation
Glial fibrillary acidic protein
Good laboratory practice
Health Insurance Portability and Accountability Act of 1996
Interdigitated microelectrode sensor
Immunomagnetic reduction
Immunoprecipitation coupled with mass spectrometry
Intravenous
Magnetic resonance imaging
Mass spectrometry
Meso Scale Discovery
Neurofilament light-chain
Phosphorylated tau
Positron emission tomography
Personal protective equipment
Quality control
Single-molecule array
Standardized operating procedure
Serum separator tubes
Alzheimer’s Association. 2023. Alzheimer’s disease facts and figures. Alzheimers Dement. 2023;19:1598–695.
Hebert LE, Weuve J, Scherr PA, Evans DA. Alzheimer disease in the United States (2010–2050) estimated using the 2010 census. Neurology. 2013;80:1778–83.
Article PubMed PubMed Central Google Scholar
Patterson C. World Alzheimer Report 2018: The state of the art of dementia research; New frontiers. London: Alzheimer’s Disease International; 2018.
Alzheimer A, Stelzmann RA, Schnitzlein HN, Murtagh FR. An English translation of Alzheimer’s 1907 paper, “Uber eine eigenartige Erkankung der Hirnrinde.” Clin Anat. 1995;8:429–31.
Article CAS PubMed Google Scholar
DeTure MA, Dickson DW. The neuropathological diagnosis of Alzheimer’s disease. Mol Neurodegener. 2019;14:32.
Tarawneh R, Holtzman DM. The clinical problem of symptomatic Alzheimer disease and mild cognitive impairment. Cold Spring Harb Perspect Med. 2012;2:a006148.
van Dyck CH, Swanson CJ, Aisen P, Bateman RJ, Chen C, Gee M, Kanekiyo M, Li D, Reyderman L, Cohen S, et al. Lecanemab in early Alzheimer’s disease. N Engl J Med. 2022;388:9–21.
Article PubMed Google Scholar
Budd Haeberlein S, Aisen PS, Barkhof F, Chalkias S, Chen T, Cohen S, Dent G, Hansson O, Harrison K, von Hehn C, et al. Two randomized phase 3 studies of aducanumab in early Alzheimer’s disease. J Prev Alzheimers Dis. 2022;9:197–210.
CAS PubMed Google Scholar
Reardon S. FDA approves Alzheimer’s drug lecanemab amid safety concerns. Nature. 2023;613:227–8.
Jack CR Jr, Bennett DA, Blennow K, Carrillo MC, Dunn B, Haeberlein SB, Holtzman DM, Jagust W, Jessen F, Karlawish J, et al. NIA-AA research framework: toward a biological definition of Alzheimer’s disease. Alzheimers Dement. 2018;14:535–62.
Alawode DOT, Heslegrave AJ, Ashton NJ, Karikari TK, Simrén J, Montoliu-Gaya L, Pannee J, O’Connor A, Weston PSJ, Lantero-Rodriguez J, et al. Transitioning from cerebrospinal fluid to blood tests to facilitate diagnosis and disease monitoring in Alzheimer’s disease. J Intern Med. 2021;290:583–601.
Article CAS PubMed PubMed Central Google Scholar
Balogun WG, Zetterberg H, Blennow K, Karikari TK. Plasma biomarkers for neurodegenerative disorders: ready for prime time? Curr Opin Psychiatry. 2023;36(2):112.
Karikari TK, Ashton NJ, Brinkmalm G, Brum WS, Benedet AL, Montoliu-Gaya L, Lantero-Rodriguez J, Pascoal TA, Suárez-Calvet M, Rosa-Neto P, et al. Blood phospho-tau in Alzheimer disease: analysis, interpretation, and clinical utility. Nat Rev Neurol. 2022;18:400–18.
Malzbender K, Lavin-Mena L, Hughes L, Bose N, Goldman D, Patel D. Key Barriers to Clinical Trials for Alzheimer’s Disease. Leonard D., editor. Los Angeles, CA, USA: Schaeffer Center for Health Policy and Economics; 2020.
Moscoso A, Karikari TK, Grothe MJ, Ashton NJ, Lantero-Rodriguez J, Snellman A, Zetterberg H, Blennow K, Schöll M. CSF biomarkers and plasma p-tau181 as predictors of longitudinal tau accumulation: Implications for clinical trial design. Alzheimers Dement. 2022;18:2614–26.
Cullen NC, Leuzy A, Janelidze S, Palmqvist S, Svenningsson AL, Stomrud E, Dage JL, Mattsson-Carlgren N, Hansson O. Plasma biomarkers of Alzheimer’s disease improve prediction of cognitive decline in cognitively unimpaired elderly populations. Nat Commun. 2021;12:3555.
Rissin DM, Kan CW, Campbell TG, Howes SC, Fournier DR, Song L, Piech T, Patel PP, Chang L, Rivnak AJ, et al. Single-molecule enzyme-linked immunosorbent assay detects serum proteins at subfemtomolar concentrations. Nat Biotechnol. 2010;28:595–9.
Nakamura A, Kaneko N, Villemagne VL, Kato T, Doecke J, Doré V, Fowler C, Li Q-X, Martins R, Rowe C, et al. High performance plasma amyloid-β biomarkers for Alzheimer’s disease. Nature. 2018;554:249–54.
Ovod V, Ramsey KN, Mawuenyega KG, Bollinger JG, Hicks T, Schneider T, Sullivan M, Paumier K, Holtzman DM, Morris JC, et al. Amyloid β concentrations and stable isotope labeling kinetics of human plasma specific to central nervous system amyloidosis. Alzheimers Dement. 2017;13:841–9.
Chong JR, Ashton NJ, Karikari TK, Tanaka T, Schöll M, Zetterberg H, Blennow K, Chen CP, Lai MKP. Blood-based high sensitivity measurements of beta-amyloid and phosphorylated tau as biomarkers of Alzheimer’s disease: a focused review on recent advances. J Neurol Neurosurg Psychiatry. 2021;92:1231–41.
Gonzalez-Ortiz F, Turton M, Kac PR, Smirnov D, Premi E, Ghidoni R, Benussi L, Cantoni V, Saraceno C, Rivolta J, et al. Brain-derived tau: a novel blood-based biomarker for Alzheimer’s disease-type neurodegeneration. Brain. 2023;146:1152–65.
Pereira JB, Janelidze S, Smith R, Mattsson-Carlgren N, Palmqvist S, Zetterberg H, Stomrud E, Ashton NJ, Blennow K, Hansson O. Plasma glial fibrillary acidic protein is an early marker of Aβ pathology in Alzheimer’s disease. medRxiv. 2021:2021.2004.2011.21255152.
Osborn LM, Kamphuis W, Wadman WJ, Hol EM. Astrogliosis: an integral player in the pathogenesis of Alzheimer’s disease. Prog Neurobiol. 2016;144:121–41.
Bellaver B, Povala G, Ferreira PCL, Ferrari-Souza JP, Leffa DT, Lussier FZ, Benedet AL, Ashton NJ, Triana-Baltzer G, Kolb HC, et al. Astrocyte reactivity influences amyloid-β effects on tau pathology in preclinical Alzheimer’s disease. Nat Med. 2023;29(7):1775–81.
Benedet AL, Brum WS, Hansson O, Karikari TK, Zimmer ER, Zetterberg H, Blennow K, Ashton NJ. The accuracy and robustness of plasma biomarker models for amyloid PET positivity. Alzheimers Res Ther. 2022;14:26.
Verberk IMW, Misdorp EO, Koelewijn J, Ball AJ, Blennow K, Dage JL, Fandos N, Hansson O, Hirtz C, Janelidze S, et al. Characterization of pre-analytical sample handling effects on a panel of Alzheimer’s disease–related blood-based biomarkers: Results from the Standardization of Alzheimer’s Blood Biomarkers (SABB) working group. Alzheimers Dement. 2022;18:1484–97.
Plebani M. Exploring the iceberg of errors in laboratory medicine. Clin Chim Acta. 2009;404:16–23.
Teunissen CE, Petzold A, Bennett JL, Berven FS, Brundin L, Comabella M, Franciotta D, Frederiksen JL, Fleming JO, Furlan R, et al. A consensus protocol for the standardization of cerebrospinal fluid collection and biobanking. Neurology. 2009;73:1914–22.
Gobom J, Parnetti L, Rosa-Neto P, Vyhnalek M, Gauthier S, Cataldi S, Lerch O, Laczo J, Cechova K, Clarin M, et al. Validation of the LUMIPULSE automated immunoassay for the measurement of core AD biomarkers in cerebrospinal fluid. Clin Chem Lab Med. 2022;60:207–19.
Schindler SE, Bollinger JG, Ovod V, Mawuenyega KG, Li Y, Gordon BA, Holtzman DM, Morris JC, Benzinger TLS, Xiong C, et al. High-precision plasma β-amyloid 42/40 predicts current and future brain amyloidosis. Neurology. 2019;93:e1647–59.
O’Bryant SE, Gupta V, Henriksen K, Edwards M, Jeromin A, Lista S, Bazenet C, Soares H, Lovestone S, Hampel H, et al. Guidelines for the standardization of preanalytic variables for blood-based biomarker studies in Alzheimer’s disease research. Alzheimers Dement. 2015;11:549–60.
Armstrong RA. Risk factors for Alzheimer’s disease. Folia Neuropathol. 2019;57:87–105.
Article Google Scholar
Henderson AS. The risk factors for Alzheimer’s disease: a review and a hypothesis. Acta Psychiatr Scand. 1988;78:257–75.
Killin LOJ, Starr JM, Shiue IJ, Russ TC. Environmental risk factors for dementia: a systematic review. BMC Geriatr. 2016;16:175.
Mielke MM, Dage JL, Frank RD, Algeciras-Schimnich A, Knopman DS, Lowe VJ, Bu G, Vemuri P, Graff-Radford J, Jack CR Jr, Petersen RC. Performance of plasma phosphorylated tau 181 and 217 in the community. Nat Med. 2022;28:1398–405.
Toledo JB, Vanderstichele H, Figurski M, Aisen PS, Petersen RC, Weiner MW, Jack CR, Jagust W, Decarli C, Toga AW, et al. Factors affecting Aβ plasma levels and their utility as biomarkers in ADNI. Acta Neuropathol. 2011;122:401.
Schindler SE, Karikari TK, Ashton NJ, Henson RL, Yarasheski KE, West T, Meyer MR, Kirmess KM, Li Y, Saef B, et al. Effect of race on prediction of brain amyloidosis by plasma Aβ42/Aβ40, phosphorylated tau, and neurofilament light. Neurology. 2022;99:e245–57.
Syrjanen JA, Campbell MR, Algeciras-Schimnich A, Vemuri P, Graff-Radford J, Machulda MM, Bu G, Knopman DS, Jack CR Jr, Petersen RC, Mielke MM. Associations of amyloid and neurodegeneration plasma biomarkers with comorbidities. Alzheimers Dement. 2022;18:1128–40.
Arvanitakis Z, Lucas JA, Younkin LH, Younkin SG, Graff-Radford NR. Serum creatinine levels correlate with plasma amyloid β protein. Alzheimer Dis Assoc Disord. 2002;16:187–90.
Blasko I, Kemmler G, Krampla W, Jungwirth S, Wichart I, Jellinger K, Tragl KH, Fischer P. Plasma amyloid beta protein 42 in non-demented persons aged 75 years: effects of concomitant medication and medial temporal lobe atrophy. Neurobiol Aging. 2005;26:1135–43.
Chatterjee P, Pedrini S, Ashton NJ, Tegg M, Goozee K, Singh AK, Karikari TK, Simrén J, Vanmechelen E, Armstrong NJ, et al. Diagnostic and prognostic plasma biomarkers for preclinical Alzheimer’s disease. Alzheimers Dement. 2022;18:1141–54.
Lopez OL, Chang Y, Ives DG, Snitz BE, Fitzpatrick AL, Carlson MC, Rapp SR, Williamson JD, Tracy RP, DeKosky ST, Kuller LH. Blood amyloid levels and risk of dementia in the Ginkgo Evaluation of Memory Study (GEMS): a longitudinal analysis. Alzheimers Dement. 2019;15:1029–38.
Pontecorvo MJ, Lu M, Burnham SC, Schade AE, Dage JL, Shcherbinin S, Collins EC, Sims JR, Mintun MA. Association of donanemab treatment with exploratory plasma biomarkers in early symptomatic Alzheimer disease: a secondary analysis of the TRAILBLAZER-ALZ randomized clinical trial. JAMA Neurol. 2022;79:1250–9.
Depypere H, Vergallo A, Lemercier P, Lista S, Benedet A, Ashton N, Cavedo E, Zetterberg H, Blennow K, Vanmechelen E, et al. Menopause hormone therapy significantly alters pathophysiological biomarkers of Alzheimer’s disease. Alzheimers Dement. 2023;19:1320–30.
Thurston RC, Maki P, Chang Y, Wu M, Aizenstein HJ, Derby CA, Karikari TK. Menopausal vasomotor symptoms and plasma Alzheimer disease biomarkers. Am J Obstet Gynecol. 2023. https://doi.org/10.1016/j.ajog.2023.11.002 .
Sakpal TV. Sample size estimation in clinical trial. Perspect Clin Res. 2010;1:67–9.
PubMed PubMed Central Google Scholar
Milà-Alomà M, Ashton NJ, Shekari M, Salvadó G, Ortiz-Romero P, Montoliu-Gaya L, Benedet AL, Karikari TK, Lantero-Rodriguez J, Vanmechelen E, et al. Plasma p-tau231 and p-tau217 as state markers of amyloid-β pathology in preclinical Alzheimer’s disease. Nat Med. 2022;28:1797–801.
Fandos N, Pérez-Grijalba V, Pesini P, Olmos S, Bossa M, Villemagne VL, Doecke J, Fowler C, Masters CL, Sarasa M. Plasma amyloid β 42/40 ratios as biomarkers for amyloid β cerebral deposition in cognitively normal individuals. Alzheimers Dement (Amst). 2017;8:179–87.
De Meyer S, Schaeverbeke JM, Verberk IMW, Gille B, De Schaepdryver M, Luckett ES, Gabel S, Bruffaerts R, Mauroo K, Thijssen EH, et al. Comparison of ELISA- and SIMOA-based quantification of plasma Aβ ratios for early detection of cerebral amyloidosis. Alzheimers Res Ther. 2020;12:162.
Doecke JD, Pérez-Grijalba V, Fandos N, Fowler C, Villemagne VL, Masters CL, Pesini P, Sarasa M. Total Aβ(42)/Aβ(40) ratio in plasma predicts amyloid-PET status, independent of clinical AD diagnosis. Neurology. 2020;94:e1580–91.
Vogelgsang J, Shahpasand-Kroner H, Vogelgsang R, Streit F, Vukovich R, Wiltfang J. Multiplex immunoassay measurement of amyloid-β42 to amyloid-β40 ratio in plasma discriminates between dementia due to Alzheimer’s disease and dementia not due to Alzheimer’s disease. Exp Brain Res. 2018;236:1241–50.
Palmqvist S, Janelidze S, Stomrud E, Zetterberg H, Karl J, Zink K, Bittner T, Mattsson N, Eichenlaub U, Blennow K, Hansson O. Performance of fully automated plasma assays as screening tests for Alzheimer disease-related β-amyloid status. JAMA Neurol. 2019;76:1060–9.
Wilson DH, Rissin DM, Kan CW, Fournier DR, Piech T, Campbell TG, Meyer RE, Fishburn MW, Cabrera C, Patel PP, et al. The Simoa HD-1 analyzer: a novel fully automated digital immunoassay analyzer with single-molecule sensitivity and multiplexing. J Lab Autom. 2016;21:533–47.
Keshavan A, Pannee J, Karikari TK, Rodriguez JL, Ashton NJ, Nicholas JM, Cash DM, Coath W, Lane CA, Parker TD, et al. Population-based blood screening for preclinical Alzheimer’s disease in a British birth cohort at age 70. Brain. 2021;144:434–49.
Aldo P, Marusov G, Svancara D, David J, Mor G. Simple Plex(™): a novel multi-analyte, automated microfluidic immunoassay platform for the detection of human and mouse cytokines and chemokines. Am J Reprod Immunol. 2016;75:678–93.
Kaneko N, Nakamura A, Washimi Y, Kato T, Sakurai T, Arahata Y, Bundo M, Takeda A, Niida S, Ito K, et al. Novel plasma biomarker surrogating cerebral amyloid deposition. Proc Jpn Acad Ser B Phys Biol Sci. 2014;90:353–64.
Kirmess KM, Meyer MR, Holubasch MS, Knapik SS, Hu Y, Jackson EN, Harpstrite SE, Verghese PB, West T, Fogelman I, et al. The PrecivityAD™ test: accurate and reliable LC-MS/MS assays for quantifying plasma amyloid beta 40 and 42 and apolipoprotein E proteotype for the assessment of brain amyloidosis. Clin Chim Acta. 2021;519:267–75.
Hong C-Y, Wu CC, Chiu YC, Yang SY, Horng HE, Yang HC. Magnetic susceptibility reduction method for magnetically labeled immunoassay. Appl Phys Lett. 2006;88:212512.
Chieh JJ, Yang SY, Jian ZF, Wang WC, Horng HE, Yang HC, Hong C-Y. Hyper-high-sensitivity wash-free magnetoreduction assay on biomolecules using high-Tc superconducting quantum interference devices. J Appl Phys. 2008;103:014703.
Yang Y-H, Situmeang RFV, Ong PA. Can blood amyloid levels be used as a biomarker for Alzheimer’s disease? Brain Sci Adv. 2021;7:17–25.
Lue L-F, Kuo Y-M, Sabbagh M. Advance in plasma AD core biomarker development: current findings from immunomagnetic reduction-based SQUID technology. Neurol Ther. 2019;8:95–111.
Kim Y, Yoo YK, Kim HY, Roh JH, Kim J, Baek S, Lee JC, Kim HJ, Chae M-S, Jeong D, et al. Comparative analyses of plasma amyloid-β levels in heterogeneous and monomerized states by interdigitated microelectrode sensor system. Sci Adv. 2019;5:eaav1388.
Wilson EN, Young CB, Ramos Benitez J, Swarovski MS, Feinstein I, Vandijck M, Le Guen Y, Kasireddy NM, Shahid M, Corso NK, et al. Performance of a fully-automated Lumipulse plasma phospho-tau181 assay for Alzheimer’s disease. Alzheimers Res Ther. 2022;14:172.
Gauthier A, Viel S, Perret M, Brocard G, Casey R, Lombard C, Laurent-Chabalier S, Debouverie M, Edan G, Vukusic S, et al. Comparison of SimoaTM and EllaTM to assess serum neurofilament-light chain in multiple sclerosis. Ann Clin Transl Neurol. 2021;8:1141–50.
Truffi M, Garofalo M, Ricciardi A, Cotta Ramusino M, Perini G, Scaranzin S, Gastaldi M, Albasini S, Costa A, Chiavetta V, et al. Neurofilament-light chain quantification by Simoa and Ella in plasma from patients with dementia: a comparative study. Sci Rep. 2023;13:4041.
Ding XL, Tuo QZ, Lei P. An introduction to ultrasensitive assays for plasma tau detection. J Alzheimers Dis. 2021;80:1353–62.
Lue LF, Guerra A, Walker DG. Amyloid beta and tau as Alzheimer’s disease blood biomarkers: promise from new technologies. Neurol Ther. 2017;6:25–36.
Li D, Mielke MM. An update on blood-based markers of Alzheimer’s disease using the SiMoA platform. Neurol Ther. 2019;8:73–82.
Barthélemy NR, Horie K, Sato C, Bateman RJ. Blood plasma phosphorylated-tau isoforms track CNS change in Alzheimer’s disease. J Exp Med. 2020;217(11):e20200861.
Jang H, Kim JS, Lee HJ, Kim C-H, Na DL, Kim HJ, Allué JA, Sarasa L, Castillo S, Pesini P, et al. Performance of the plasma Aβ42/Aβ40 ratio, measured with a novel HPLC-MS/MS method, as a biomarker of amyloid PET status in a DPUK-KOREAN cohort. Alzheimers Res Ther. 2021;13:179.
Montoliu-Gaya L, Benedet AL, Tissot C, Vrillon A, Ashton NJ, Brum WS, Lantero-Rodriguez J, Stevenson J, Nilsson J, Sauer M, et al. Mass spectrometric simultaneous quantification of tau species in plasma shows differential associations with amyloid and tau pathologies. Nat Aging. 2023;3:661–9.
Palmqvist S, Stomrud E, Cullen N, Janelidze S, Manuilova E, Jethwa A, Bittner T, Eichenlaub U, Suridjan I, Kollmorgen G, et al. An accurate fully automated panel of plasma biomarkers for Alzheimer’s disease. Alzheimers Dement. 2023;19:1204–15.
Feng W, Beer JC, Hao Q, Ariyapala IS, Sahajan A, Komarov A, Cha K, Moua M, Qiu X, Xu X, et al. NULISA: a proteomic liquid biopsy platform with attomolar sensitivity and high multiplexing. Nat Commun. 2023;14:7238.
Yang S-Y, Chiu M-J, Chen T-F, Horng H-E. Detection of plasma biomarkers using immunomagnetic reduction: a promising method for the early diagnosis of Alzheimer’s disease. Neurol Ther. 2017;6:37–56.
Pannee J, Shaw LM, Korecka M, Waligorska T, Teunissen CE, Stoops E, Vanderstichele HMJ, Mauroo K, Verberk IMW, Keshavan A, et al. The global Alzheimer’s Association round robin study on plasma amyloid β methods. Alzheimers Dement (Amst). 2021;13:e12242.
Korecka M, Shaw LM. Mass spectrometry-based methods for robust measurement of Alzheimer’s disease biomarkers in biological fluids. J Neurochem. 2021;159:211–33.
Palmqvist S, Zetterberg H, Mattsson N, Johansson P, Minthon L, Blennow K, Olsson M, Hansson O. Detailed comparison of amyloid PET and CSF biomarkers for identifying early Alzheimer disease. Neurology. 2015;85:1240–9.
Palmqvist S, Zetterberg H, Blennow K, Vestberg S, Andreasson U, Brooks DJ, Owenius R, Hägerström D, Wollmer P, Minthon L, Hansson O. Accuracy of brain amyloid detection in clinical practice using cerebrospinal fluid β-amyloid 42: a cross-validation study against amyloid positron emission tomography. JAMA Neurol. 2014;71:1282–9.
Qu Y, Ma Y-H, Huang Y-Y, Ou Y-N, Shen X-N, Chen S-D, Dong Q, Tan L, Yu J-T. Blood biomarkers for the diagnosis of amnestic mild cognitive impairment and Alzheimer’s disease: a systematic review and meta-analysis. Neurosci Biobehav Rev. 2021;128:479–86.
Koychev I, Jansen K, Dette A, Shi L, Holling H. Blood-based ATN biomarkers of Alzheimer’s disease: a meta-analysis. J Alzheimers Dis. 2021;79:177–95.
Janelidze S, Teunissen CE, Zetterberg H, Allué JA, Sarasa L, Eichenlaub U, Bittner T, Ovod V, Verberk IMW, Toba K, et al. Head-to-head comparison of 8 plasma amyloid-β 42/40 assays in Alzheimer disease. JAMA Neurol. 2021;78:1375–82.
Mattsson N, Insel PS, Palmqvist S, Portelius E, Zetterberg H, Weiner M, Blennow K, Hansson O. Initiative tAsDN: cerebrospinal fluid tau, neurogranin, and neurofilament light in Alzheimer’s disease. EMBO Mol Med. 2016;8:1184–96.
Zetterberg H, Wilson D, Andreasson U, Minthon L, Blennow K, Randall J, Hansson O. Plasma tau levels in Alzheimer’s disease. Alzheimers Res Ther. 2013;5:9.
Mattsson N, Zetterberg H, Janelidze S, Insel PS, Andreasson U, Stomrud E, Palmqvist S, Baker D, Tan Hehir CA, Jeromin A, et al. Plasma tau in Alzheimer disease. Neurology. 2016;87:1827–35.
Chen Z, Mengel D, Keshavan A, Rissman RA, Billinton A, Perkinton M, Percival-Alwyn J, Schultz A, Properzi M, Johnson K, et al. Learnings about the complexity of extracellular tau aid development of a blood-based screen for Alzheimer’s disease. Alzheimers Dement. 2019;15:487–96.
Snellman A, Lantero-Rodriguez J, Emeršič A, Vrillon A, Karikari TK, Ashton NJ, Gregorič Kramberger M, Čučnik S, Paquet C, Rot U, et al. N-terminal and mid-region tau fragments as fluid biomarkers in neurological diseases. Brain. 2022;145:2834–48.
Ashton NJ, Benedet AL, Pascoal TA, Karikari TK, Lantero-Rodriguez J, Brum WS, Mathotaarachchi S, Therriault J, Savard M, Chamoun M, et al. Cerebrospinal fluid p-tau231 as an early indicator of emerging pathology in Alzheimer’s disease. eBioMedicine. 2022;76:103836.
Karikari TK, Emeršič A, Vrillon A, Lantero-Rodriguez J, Ashton NJ, Kramberger MG, Dumurgier J, Hourregue C, Čučnik S, Brinkmalm G, et al. Head-to-head comparison of clinical performance of CSF phospho-tau T181 and T217 biomarkers for Alzheimer’s disease diagnosis. Alzheimers Dement. 2021;17:755–67.
Karikari TK, Pascoal TA, Ashton NJ, Janelidze S, Benedet AL, Rodriguez JL, Chamoun M, Savard M, Kang MS, Therriault J, et al. Blood phosphorylated tau 181 as a biomarker for Alzheimer’s disease: a diagnostic performance and prediction modelling study using data from four prospective cohorts. Lancet Neurol. 2020;19:422–33.
Karikari TK, Benedet AL, Ashton NJ, Lantero Rodriguez J, Snellman A, Suárez-Calvet M, Saha-Chaudhuri P, Lussier F, Kvartsberg H, Rial AM, et al. Diagnostic performance and prediction of clinical progression of plasma phospho-tau181 in the Alzheimer’s Disease Neuroimaging Initiative. Mol Psychiatry. 2021;26:429–42.
Ashton NJ, Pascoal TA, Karikari TK, Benedet AL, Lantero-Rodriguez J, Brinkmalm G, Snellman A, Schöll M, Troakes C, Hye A, et al. Plasma p-tau231: a new biomarker for incipient Alzheimer’s disease pathology. Acta Neuropathol. 2021;141:709–24.
Palmqvist S, Janelidze S, Quiroz YT, Zetterberg H, Lopera F, Stomrud E, Su Y, Chen Y, Serrano GE, Leuzy A, et al. Discriminative accuracy of plasma phospho-tau217 for Alzheimer disease vs other neurodegenerative disorders. JAMA. 2020;324:772–81.
Mielke MM, Frank RD, Dage JL, Jeromin A, Ashton NJ, Blennow K, Karikari TK, Vanmechelen E, Zetterberg H, Algeciras-Schimnich A, et al. Comparison of plasma phosphorylated tau species with amyloid and tau positron emission tomography, neurodegeneration, vascular pathology, and cognitive outcomes. JAMA Neurol. 2021;78:1108–17.
Ashton NJ, Puig-Pijoan A, Milà-Alomà M, Fernández-Lebrero A, García-Escobar G, González-Ortiz F, Kac PR, Brum WS, Benedet AL, Lantero-Rodriguez J, et al. Plasma and CSF biomarkers in a memory clinic: Head-to-head comparison of phosphorylated tau immunoassays. Alzheimers Dement. 2023;19:1913–24.
Bayoumy S, Verberk IMW, den Dulk B, Hussainali Z, Zwan M, van der Flier WM, Ashton NJ, Zetterberg H, Blennow K, Vanbrabant J, et al. Clinical and analytical comparison of six Simoa assays for plasma P-tau isoforms P-tau181, P-tau217, and P-tau231. Alzheimers Res Ther. 2021;13:198.
Janelidze S, Bali D, Ashton NJ, Barthélemy NR, Vanbrabant J, Stoops E, Vanmechelen E, He Y, Dolado AO, Triana-Baltzer G, et al. Head-to-head comparison of 10 plasma phospho-tau assays in prodromal Alzheimer’s disease. Brain. 2023;146:1592–601.
Kac PR, González-Ortiz F, Emeršič A, Dulewicz M, Koutarapu S, Turton M, An Y, Smirnov D, Kulczyńska-Przybik A, Varma V, et al. Plasma p-tau212: antemortem diagnostic performance and prediction of autopsy verification of Alzheimer’s disease neuropathology. medRxiv. 2023:2023.2012.2011.23299806.
Ashton NJ, Janelidze S, Mattsson-Carlgren N, Binette AP, Strandberg O, Brum WS, Karikari TK, González-Ortiz F, Di Molfetta G, Meda FJ, et al. Differential roles of Aβ42/40, p-tau231 and p-tau217 for Alzheimer’s trial selection and disease monitoring. Nat Med. 2022;28:2555–62.
Yang Z, Wang KK. Glial fibrillary acidic protein: from intermediate filament assembly and gliosis to neurobiomarker. Trends Neurosci. 2015;38:364–74.
Monterey MD, Wei H, Wu X, Wu JQ. The many faces of astrocytes in Alzheimer’s disease. Front Neurol. 2021;12:619626.
Chatterjee P, Pedrini S, Stoops E, Goozee K, Villemagne VL, Asih PR, Verberk IMW, Dave P, Taddei K, Sohrabi HR, et al. Plasma glial fibrillary acidic protein is elevated in cognitively normal older adults at risk of Alzheimer’s disease. Transl Psychiatry. 2021;11:27.
Benedet AL, Milà-Alomà M, Vrillon A, Ashton NJ, Pascoal TA, Lussier F, Karikari TK, Hourregue C, Cognat E, Dumurgier J, et al. Differences between plasma and cerebrospinal fluid glial fibrillary acidic protein levels across the Alzheimer disease continuum. JAMA Neurol. 2021;78:1471–83.
Papa L, Ladde JG, O’Brien JF, Thundiyil JG, Tesar J, Leech S, Cassidy DD, Roa J, Hunter C, Miller S, et al. Evaluation of glial and neuronal blood biomarkers compared with clinical decision rules in assessing the need for computed tomography in patients with mild traumatic brain injury. JAMA Netw Open. 2022;5:e221302.
Sjögren M, Blomberg M, Jonsson M, Wahlund LO, Edman A, Lind K, Rosengren L, Blennow K, Wallin A. Neurofilament protein in cerebrospinal fluid: a marker of white matter changes. J Neurosci Res. 2001;66:510–6.
Gaiottino J, Norgren N, Dobson R, Topping J, Nissim A, Malaspina A, Bestwick JP, Monsch AU, Regeniter A, Lindberg RL, et al. Increased neurofilament light chain blood levels in neurodegenerative neurological diseases. PLoS One. 2013;8:e75091.
Ashton NJ, Janelidze S, Al Khleifat A, Leuzy A, van der Ende EL, Karikari TK, Benedet AL, Pascoal TA, Lleó A, Parnetti L, et al. A multicentre validation study of the diagnostic value of plasma neurofilament light. Nat Commun. 2021;12:3400.
Ashton NJ, Suárez-Calvet M, Karikari TK, Lantero-Rodriguez J, Snellman A, Sauer M, Simrén J, Minguillon C, Fauria K, Blennow K, Zetterberg H. Effects of pre-analytical procedures on blood biomarkers for Alzheimer’s pathophysiology, glial activation, and neurodegeneration. Alzheimers Dement (Amst). 2021;13:e12168.
O’Connell GC, Alder ML, Webel AR, Moore SM. Neuro biomarker levels measured with high-sensitivity digital ELISA differ between serum and plasma. Bioanalysis. 2019;11:2087–94.
Kac PR, Gonzalez-Ortiz F, Simrén J, Dewit N, Vanmechelen E, Zetterberg H, Blennow K, Ashton NJ, Karikari TK. Diagnostic value of serum versus plasma phospho-tau for Alzheimer’s disease. Alzheimers Res Ther. 2022;14:65.
Jonaitis EM, Zetterberg H, Koscik RL, Betthauser TJ, Van Hulle CA, Hogan K, Hegge L, Kollmorgen G, Suridjan I, Gleason CE, et al. Crosswalk study on blood collection-tube types for Alzheimer’s disease biomarkers. Alzheimers Dement. 2022;14:e12266.
Google Scholar
Gonzalez-Ortiz F, Dias A, Turton M, Magalhães R, Kac PR, Correia M, Harrison P, Zetterberg H, Maia LF, Blennow K, Karikari TK. Preanalytical stability of plasma/serum brain-derived tau. Alzheimers Dement. 2023;19(10):4764–70.
Gonzalez-Ortiz F, Ferreira PCL, González-Escalante A, Montoliu-Gaya L, Ortiz-Romero P, Kac PR, Turton M, Kvartsberg H, Ashton NJ, Zetterberg H, et al. A novel ultrasensitive assay for plasma p-tau217: performance in individuals with subjective cognitive decline and early Alzheimer’s disease. Alzheimers Dement. 2023. https://doi.org/10.1002/alz.13525 .
Tumani H, Hegen H. CSF albumin: albumin CSF/serum ratio (marker for blood-CSF barrier function). 2015. pp. 111–114.
Mayo Clinic Test Catalog. https://www.mayocliniclabs.com/en/test-catalog .
Bowen RA, Remaley AT. Interferences from blood collection tube components on clinical chemistry assays. Biochem Med (Zagreb). 2014;24:31–44.
Rózga M, Bittner T, Batrla R, Karl J. Preanalytical sample handling recommendations for Alzheimer’s disease plasma biomarkers. Alzheimers Dement (Amst). 2019;11:291–300.
van Lierop Z, Verberk IMW, van Uffelen KWJ, Koel-Simmelink MJA, In ‘t Veld L, Killestein J, Teunissen CE. Pre-analytical stability of serum biomarkers for neurological disease: neurofilament-light, glial fibrillary acidic protein and contactin-1. Clin Chem Lab Med. 2022;60:842–50.
Jiang L, Ding X, Wang W, Yang X, Li T, Lei P. Head-to-head comparison of different blood collecting tubes for quantification of Alzheimer’s disease biomarkers in plasma. Biomolecules. 2022;12:1194.
Walsh G. Difficult peripheral venous access: recognizing and managing the patient at risk. J Assoc Vasc Access. 2008;13:198–203.
Walter M, Wiltfang J, Vogelgsang J. Pre-analytical sampling and storage conditions of amyloid-β peptides in venous and capillary blood. J Alzheimers Dis. 2020;78:529–35.
Lombardi V, Carassiti D, Giovannoni G, Lu CH, Adiutori R, Malaspina A. The potential of neurofilaments analysis using dry-blood and plasma spots. Sci Rep. 2020;10:97.
Simrén J, Ashton NJ, Blennow K, Zetterberg H. Blood neurofilament light in remote settings: alternative protocols to support sample collection in challenging pre-analytical conditions. Alzheimers Dement. 2021;13:e12145.
Meyer P-F, Ashton NJ, Karikari TK, Strikwerda-Brown C, Köbe T, Gonneaud J, Pichet Binette A, Ozlen H, Yakoub Y, Simrén J, et al. Plasma p-tau231, p-tau181, PET biomarkers, and cognitive change in older adults. Ann Neurol. 2022;91:548–60.
Brum WS, Ashton NJ, Simrén J, di Molfetta G, Karikari TK, Benedet AL, Zimmer ER, Lantero-Rodriguez J, Montoliu-Gaya L, Jeromin A, et al. Biological variation estimates of Alzheimer’s disease plasma biomarkers in healthy individuals. Alzheimers Dement. 2024;20:1284–97.
Heyer NJ, Derzon JH, Winges L, Shaw C, Mass D, Snyder SR, Epner P, Nichols JH, Gayken JA, Ernst D, Liebow EB. Effectiveness of practices to reduce blood sample hemolysis in EDs: a laboratory medicine best practices systematic review and meta-analysis. Clin Biochem. 2012;45:1012–32.
Dugan L, Leech L, Speroni KG, Corriher J. Factors affecting hemolysis rates in blood samples drawn from newly placed IV sites in the emergency department. J Emerg Nurs. 2005;31:338–45.
Bowen RA, Hortin GL, Csako G, Otañez OH, Remaley AT. Impact of blood collection devices on clinical chemistry assays. Clin Biochem. 2010;43:4–25.
Bowen RA, Sattayapiwat A, Gounden V, Remaley AT. Blood collection tube-related alterations in analyte concentrations in quality control material and serum specimens. Clin Biochem. 2014;47:150–7.
Schrapp A, Mory C, Duflot T, Pereira T, Imbert L, Lamoureux F. The right blood collection tube for therapeutic drug monitoring and toxicology screening procedures: standard tubes, gel or mechanical separator? Clin Chim Acta. 2019;488:196–201.
Lewczuk P, Beck G, Esselmann H, Bruckmoser R, Zimmermann RD, Fiszer M, Bibl M, Maler JM, Kornhuber J, Wiltfang J. Effect of sample collection tubes on cerebrospinal fluid concentrations of tau proteins and amyloid β peptides. Clin Chem. 2006;52:332–4.
Perret-Liaudet A, Pelpel M, Tholance Y, Dumont B, Vanderstichele H, Zorzi W, ElMoualij B, Schraen S, Moreaud O, Gabelle A, et al. Cerebrospinal fluid collection tubes: a critical issue for Alzheimer disease diagnosis. Clin Chem. 2012;58:787–9.
Bjerke M, Portelius E, Minthon L, Wallin A, Anckarsäter H, Anckarsäter R, Andreasen N, Zetterberg H, Andreasson U, Blennow K. Confounding factors influencing amyloid Beta concentration in cerebrospinal fluid. Int J Alzheimers Dis. 2010;2010:986310.
Pica-Mendez AM, Tanen M, Dallob A, Tanaka W, Laterza OF. Nonspecific binding of Aβ42 to polypropylene tubes and the effect of Tween-20. Clin Chim Acta. 1833;2010:411.
Toombs J, Paterson RW, Schott JM, Zetterberg H. Amyloid-beta 42 adsorption following serial tube transfer. Alzheimers Res Ther. 2014;6:5.
Lachno DR, Vanderstichele H, De Groote G, Kostanjevecki V, De Meyer G, Siemers ER, Willey MB, Bourdage JS, Konrad RJ, Dean RA. The influence of matrix type, diurnal rhythm and sample collection and processing on the measurement of plasma β-amyloid isoforms using the INNO-BIA plasma Aβ forms multiplex assay. J Nutr Health Aging. 2009;13:220–5.
(CLSI). CaLSI: collection, transport, and processing of blood specimens for testing plasma-based coagulation assays and molecular hemostasis assays; approved guideline—fourth edition. CLSI document H21–A4. Wayne: Clinical and Laboratory Standards Institute; 2017.
Reichstein E. The importance of preanalytical factors in immunodiagnostic testing. EJIFCC. 2003;14:124–7.
Timms JF, Arslan-Low E, Gentry-Maharaj A, Luo Z, T’Jampens D, Podust VN, Ford J, Fung ET, Gammerman A, Jacobs I, Menon U. Preanalytic influence of sample handling on SELDI-TOF serum protein profiles. Clin Chem. 2007;53:645–56.
Sunde AL, Alsnes IV, Aarsland D, Ashton NJ, Tovar-Rios DA, De Santis G, Blennow K, Zetterberg H, Kjosavik SR. Preanalytical stability of plasma biomarkers for Alzheimer’s disease pathology. Alzheimers Dement. 2023;15:e12439.
Lippi G, Blanckaert N, Bonini P, Green S, Kitchen S, Palicka V, Vassault AJ, Plebani M. Haemolysis: an overview of the leading cause of unsuitable specimens in clinical laboratories. Clin Chem Lab Med. 2008;46:764–72.
Keshavan A, Heslegrave A, Zetterberg H, Schott JM. Stability of blood-based biomarkers of Alzheimer’s disease over multiple freeze-thaw cycles. Alzheimers Dement. 2018;10:448–51.
Lierop ZYGJv, Verberk IMW, Uffelen KWJv, Koel-Simmelink MJA, Veld Lit, Killestein J, Teunissen CE. Pre-analytical stability of serum biomarkers for neurological disease: neurofilament-light, glial fibrillary acidic protein and contactin-1. Clin Chem Lab Med. 2022;60:842–850.
Liu HC, Chiu MJ, Lin CH, Yang SY. Stability of plasma amyloid-β 1–40, amyloid-β 1–42, and total tau protein over repeated freeze/thaw cycles. Dement Geriatr Cogn Dis Extra. 2020;10:46–55.
Murphy BM, Swarts S, Mueller BM, van der Geer P, Manning MC, Fitchmun MI. Protein instability following transport or storage on dry ice. Nat Methods. 2013;10:278–9.
Schindler SE, Sutphen CL, Teunissen C, McCue LM, Morris JC, Holtzman DM, Mulder SD, Scheltens P, Xiong C, Fagan AM. Upward drift in cerebrospinal fluid amyloid β 42 assay values for more than 10 years. Alzheimers Dement. 2018;14:62–70.
Kang M, Ragan BG, Park JH. Issues in outcomes research: an overview of randomization techniques for clinical trials. J Athl Train. 2008;43:215–21.
Boulo S, Kuhlmann J, Andreasson U, Brix B, Venkataraman I, Herbst V, Rutz S, Manuilova E, Vandijck M, Dekeyser F, et al. First amyloid β1-42 certified reference material for re-calibrating commercial immunoassays. Alzheimers Dement. 2020;16:1493–503.
Download references
Acknowledgements
R01 AG064877 (MIK), P30 AG066468 (University of Pittsburgh ADRC, OIL as PI), R01 MH116046 (RAS, JK), P01 AG14449 and P01 AG025204 (MDI). HZ is a Wallenberg Scholar supported by grants from the Swedish Research Council (#2022-01018 and #2019-02397), the European Union’s Horizon Europe research and innovation programme under grant agreement No 101053962, Swedish State Support for Clinical Research (#ALFGBG-71320), the Alzheimer Drug Discovery Foundation (ADDF), USA (#201809-2016862), the AD Strategic Fund and the Alzheimer’s Association (#ADSF-21-831376-C, #ADSF-21-831381-C, and #ADSF-21-831377-C), the Bluefield Project, the Olav Thon Foundation, the Erling-Persson Family Foundation, Stiftelsen för Gamla Tjänarinnor, Hjärnfonden, Sweden (#FO2022-0270), the European Union’s Horizon 2020 research and innovation programme under the Marie Skłodowska-Curie grant agreement No 860197 (MIRIADE), the European Union Joint Programme – Neurodegenerative Disease Research (JPND2021-00694), the National Institute for Health and Care Research University College London Hospitals Biomedical Research Centre, and the UK Dementia Research Institute at UCL (UKDRI-1003). KB is supported by the Swedish Research Council (#2017-00915 and #2022-00732), Hjärnfonden, Sweden (#FO2017-0243 and #ALZ2022-0006), and the Swedish state under the agreement between the Swedish government and the County Councils, the ALF-agreement (#ALFGBG-715986 and #ALFGBG-965240). TKK was supported by the NIH (R01 AG083874, U24 AG082930, P30 AG066468, RF1 AG052525-01A1, R01 AG053952-05, R37 AG023651-17, RF1 AG025516-12A1, R01 AG073267-02, R01 AG075336-01, R01 AG072641-02, P01 AG025204-16), the Swedish Research Council (Vetenskåpradet; #2021-03244), the Alzheimer’s Association (#AARF-21-850325), the Swedish Alzheimer Foundation (Alzheimerfonden), the Aina (Ann) Wallströms and Mary-Ann Sjöbloms stiftelsen, and the Emil och Wera Cornells stiftelsen.
Author information
Authors and affiliations.
Department of Psychiatry, School of Medicine, University of Pittsburgh, 3811 O’Hara Street, Pittsburgh, PA, 15213, USA
Xuemei Zeng, Anuradha Sehrawat, Jihui Lee, Tara K. Lafferty, Robert A. Sweet, Dana L. Tudorascu, William E. Klunk, Milos D. Ikonomovic, Anne D. Cohen, Victor L. Villemagne, Tharick A. Pascoal & Thomas K. Karikari
Department of Chemistry, University of Pittsburgh, Pittsburgh, PA, 15213, USA
Department of Pathology, School of Medicine, University of Pittsburgh, Pittsburgh, PA, 15213, USA
Julia Kofler
Department of Neurology, School of Medicine, University of Pittsburgh, Pittsburgh, PA, 15213, USA
Sarah B. Berman, Robert A. Sweet, Milos D. Ikonomovic, Beth E. Snitz, Tharick A. Pascoal & Oscar I. Lopez
Geriatric Research Education and Clinical Center, VA Pittsburgh HS, Pittsburgh, PA, USA
Milos D. Ikonomovic
Department of Psychiatry and Neurochemistry, Institute of Neuroscience and Physiology, The Sahlgrenska Academy, University of Gothenburg, Mölndal, Sweden
Anna Pfister, Henrik Zetterberg, Kaj Blennow & Thomas K. Karikari
Clinical Neurochemistry Laboratory, Sahlgrenska University Hospital, Mölndal, Sweden
Anna Pfister, Henrik Zetterberg & Kaj Blennow
Department of Neurodegenerative Disease, UCL Queen Square Institute of Neurology, London, UK
Henrik Zetterberg
UK Dementia Research Institute at UCL, London, UK
Hong Kong Center for Neurodegenerative Diseases, Hong Kong, China
Department of Medicine, School of Medicine and Public Health, University of Wisconsin-Madison, Madison, WI, USA
Department of Human Genetics, School of Public Health, University of Pittsburgh, Pittsburgh, PA, 15213, USA
M. llyas Kamboh
You can also search for this author in PubMed Google Scholar
Contributions
XZ and TKK contributed to the conception and design of the review. XZ, YC, AS and TKL prepared the first draft of the manuscript, which was critically revised by all authors. All authors contributed to and approved the final version of the manuscript.
Authors’ information
Corresponding author.
Correspondence to Thomas K. Karikari .
Ethics declarations
Ethics approval and consent to participate.
Not applicable.
Consent for publication
Competing interests.
MDI has received research funding from GE Healthcare and Avid Radiopharmaceuticals. HZ has served at scientific advisory boards and/or as a consultant for Abbvie, Acumen, Alector, Alzinova, ALZPath, Annexon, Apellis, Artery Therapeutics, AZTherapies, CogRx, Denali, Eisai, Nervgen, Novo Nordisk, Optoceutics, Passage Bio, Pinteon Therapeutics, Prothena, Red Abbey Labs, reMYND, Roche, Samumed, Siemens Healthineers, Triplet Therapeutics, and Wave, has given lectures in symposia sponsored by Cellectricon, Fujirebio, Alzecure, Biogen, and Roche, and is a co-founder of Brain Biomarker Solutions in Gothenburg AB (BBS), which is a part of the GU Ventures Incubator Program (outside submitted work). KB has served as a consultant and at advisory boards for Acumen, ALZPath, BioArctic, Biogen, Eisai, Lilly, Moleac Pte. Ltd, Novartis, Ono Pharma, Prothena, Roche Diagnostics, and Siemens Healthineers; has served at data monitoring committees for Julius Clinical and Novartis; has given lectures, produced educational materials and participated in educational programs for AC Immune, Biogen, Celdara Medical, Eisai and Roche Diagnostics; and is a co-founder of Brain Biomarker Solutions in Gothenburg AB (BBS), which is a part of the GU Ventures Incubator Program, outside the work presented in this paper. The other authors declare no competing interests.
Additional information
Publisher’s note.
Springer Nature remains neutral with regard to jurisdictional claims in published maps and institutional affiliations.
Supplementary Information
Additional file 1..
Step-by-step blood specimen collection procedures.
Additional file 2.
Simulation datasets used to generate the plots in Fig. 4 .
Rights and permissions
Open Access This article is licensed under a Creative Commons Attribution 4.0 International License, which permits use, sharing, adaptation, distribution and reproduction in any medium or format, as long as you give appropriate credit to the original author(s) and the source, provide a link to the Creative Commons licence, and indicate if changes were made. The images or other third party material in this article are included in the article's Creative Commons licence, unless indicated otherwise in a credit line to the material. If material is not included in the article's Creative Commons licence and your intended use is not permitted by statutory regulation or exceeds the permitted use, you will need to obtain permission directly from the copyright holder. To view a copy of this licence, visit http://creativecommons.org/licenses/by/4.0/ . The Creative Commons Public Domain Dedication waiver ( http://creativecommons.org/publicdomain/zero/1.0/ ) applies to the data made available in this article, unless otherwise stated in a credit line to the data.
Reprints and permissions
About this article
Cite this article.
Zeng, X., Chen, Y., Sehrawat, A. et al. Alzheimer blood biomarkers: practical guidelines for study design, sample collection, processing, biobanking, measurement and result reporting. Mol Neurodegeneration 19 , 40 (2024). https://doi.org/10.1186/s13024-024-00711-1
Download citation
Received : 25 July 2023
Accepted : 13 February 2024
Published : 15 May 2024
DOI : https://doi.org/10.1186/s13024-024-00711-1
Share this article
Anyone you share the following link with will be able to read this content:
Sorry, a shareable link is not currently available for this article.
Provided by the Springer Nature SharedIt content-sharing initiative
- Blood biomarker
- Standardization
- Preanalytical factors
- Neurodegeneration
Molecular Neurodegeneration
ISSN: 1750-1326
- Submission enquiries: Access here and click Contact Us
- General enquiries: [email protected]
- Privacy Policy

Home » Research Methodology – Types, Examples and writing Guide
Research Methodology – Types, Examples and writing Guide
Table of Contents

Research Methodology
Definition:
Research Methodology refers to the systematic and scientific approach used to conduct research, investigate problems, and gather data and information for a specific purpose. It involves the techniques and procedures used to identify, collect , analyze , and interpret data to answer research questions or solve research problems . Moreover, They are philosophical and theoretical frameworks that guide the research process.
Structure of Research Methodology
Research methodology formats can vary depending on the specific requirements of the research project, but the following is a basic example of a structure for a research methodology section:
I. Introduction
- Provide an overview of the research problem and the need for a research methodology section
- Outline the main research questions and objectives
II. Research Design
- Explain the research design chosen and why it is appropriate for the research question(s) and objectives
- Discuss any alternative research designs considered and why they were not chosen
- Describe the research setting and participants (if applicable)
III. Data Collection Methods
- Describe the methods used to collect data (e.g., surveys, interviews, observations)
- Explain how the data collection methods were chosen and why they are appropriate for the research question(s) and objectives
- Detail any procedures or instruments used for data collection
IV. Data Analysis Methods
- Describe the methods used to analyze the data (e.g., statistical analysis, content analysis )
- Explain how the data analysis methods were chosen and why they are appropriate for the research question(s) and objectives
- Detail any procedures or software used for data analysis
V. Ethical Considerations
- Discuss any ethical issues that may arise from the research and how they were addressed
- Explain how informed consent was obtained (if applicable)
- Detail any measures taken to ensure confidentiality and anonymity
VI. Limitations
- Identify any potential limitations of the research methodology and how they may impact the results and conclusions
VII. Conclusion
- Summarize the key aspects of the research methodology section
- Explain how the research methodology addresses the research question(s) and objectives
Research Methodology Types
Types of Research Methodology are as follows:
Quantitative Research Methodology
This is a research methodology that involves the collection and analysis of numerical data using statistical methods. This type of research is often used to study cause-and-effect relationships and to make predictions.
Qualitative Research Methodology
This is a research methodology that involves the collection and analysis of non-numerical data such as words, images, and observations. This type of research is often used to explore complex phenomena, to gain an in-depth understanding of a particular topic, and to generate hypotheses.
Mixed-Methods Research Methodology
This is a research methodology that combines elements of both quantitative and qualitative research. This approach can be particularly useful for studies that aim to explore complex phenomena and to provide a more comprehensive understanding of a particular topic.
Case Study Research Methodology
This is a research methodology that involves in-depth examination of a single case or a small number of cases. Case studies are often used in psychology, sociology, and anthropology to gain a detailed understanding of a particular individual or group.
Action Research Methodology
This is a research methodology that involves a collaborative process between researchers and practitioners to identify and solve real-world problems. Action research is often used in education, healthcare, and social work.
Experimental Research Methodology
This is a research methodology that involves the manipulation of one or more independent variables to observe their effects on a dependent variable. Experimental research is often used to study cause-and-effect relationships and to make predictions.
Survey Research Methodology
This is a research methodology that involves the collection of data from a sample of individuals using questionnaires or interviews. Survey research is often used to study attitudes, opinions, and behaviors.
Grounded Theory Research Methodology
This is a research methodology that involves the development of theories based on the data collected during the research process. Grounded theory is often used in sociology and anthropology to generate theories about social phenomena.
Research Methodology Example
An Example of Research Methodology could be the following:
Research Methodology for Investigating the Effectiveness of Cognitive Behavioral Therapy in Reducing Symptoms of Depression in Adults
Introduction:
The aim of this research is to investigate the effectiveness of cognitive-behavioral therapy (CBT) in reducing symptoms of depression in adults. To achieve this objective, a randomized controlled trial (RCT) will be conducted using a mixed-methods approach.
Research Design:
The study will follow a pre-test and post-test design with two groups: an experimental group receiving CBT and a control group receiving no intervention. The study will also include a qualitative component, in which semi-structured interviews will be conducted with a subset of participants to explore their experiences of receiving CBT.
Participants:
Participants will be recruited from community mental health clinics in the local area. The sample will consist of 100 adults aged 18-65 years old who meet the diagnostic criteria for major depressive disorder. Participants will be randomly assigned to either the experimental group or the control group.
Intervention :
The experimental group will receive 12 weekly sessions of CBT, each lasting 60 minutes. The intervention will be delivered by licensed mental health professionals who have been trained in CBT. The control group will receive no intervention during the study period.
Data Collection:
Quantitative data will be collected through the use of standardized measures such as the Beck Depression Inventory-II (BDI-II) and the Generalized Anxiety Disorder-7 (GAD-7). Data will be collected at baseline, immediately after the intervention, and at a 3-month follow-up. Qualitative data will be collected through semi-structured interviews with a subset of participants from the experimental group. The interviews will be conducted at the end of the intervention period, and will explore participants’ experiences of receiving CBT.
Data Analysis:
Quantitative data will be analyzed using descriptive statistics, t-tests, and mixed-model analyses of variance (ANOVA) to assess the effectiveness of the intervention. Qualitative data will be analyzed using thematic analysis to identify common themes and patterns in participants’ experiences of receiving CBT.
Ethical Considerations:
This study will comply with ethical guidelines for research involving human subjects. Participants will provide informed consent before participating in the study, and their privacy and confidentiality will be protected throughout the study. Any adverse events or reactions will be reported and managed appropriately.
Data Management:
All data collected will be kept confidential and stored securely using password-protected databases. Identifying information will be removed from qualitative data transcripts to ensure participants’ anonymity.
Limitations:
One potential limitation of this study is that it only focuses on one type of psychotherapy, CBT, and may not generalize to other types of therapy or interventions. Another limitation is that the study will only include participants from community mental health clinics, which may not be representative of the general population.
Conclusion:
This research aims to investigate the effectiveness of CBT in reducing symptoms of depression in adults. By using a randomized controlled trial and a mixed-methods approach, the study will provide valuable insights into the mechanisms underlying the relationship between CBT and depression. The results of this study will have important implications for the development of effective treatments for depression in clinical settings.
How to Write Research Methodology
Writing a research methodology involves explaining the methods and techniques you used to conduct research, collect data, and analyze results. It’s an essential section of any research paper or thesis, as it helps readers understand the validity and reliability of your findings. Here are the steps to write a research methodology:
- Start by explaining your research question: Begin the methodology section by restating your research question and explaining why it’s important. This helps readers understand the purpose of your research and the rationale behind your methods.
- Describe your research design: Explain the overall approach you used to conduct research. This could be a qualitative or quantitative research design, experimental or non-experimental, case study or survey, etc. Discuss the advantages and limitations of the chosen design.
- Discuss your sample: Describe the participants or subjects you included in your study. Include details such as their demographics, sampling method, sample size, and any exclusion criteria used.
- Describe your data collection methods : Explain how you collected data from your participants. This could include surveys, interviews, observations, questionnaires, or experiments. Include details on how you obtained informed consent, how you administered the tools, and how you minimized the risk of bias.
- Explain your data analysis techniques: Describe the methods you used to analyze the data you collected. This could include statistical analysis, content analysis, thematic analysis, or discourse analysis. Explain how you dealt with missing data, outliers, and any other issues that arose during the analysis.
- Discuss the validity and reliability of your research : Explain how you ensured the validity and reliability of your study. This could include measures such as triangulation, member checking, peer review, or inter-coder reliability.
- Acknowledge any limitations of your research: Discuss any limitations of your study, including any potential threats to validity or generalizability. This helps readers understand the scope of your findings and how they might apply to other contexts.
- Provide a summary: End the methodology section by summarizing the methods and techniques you used to conduct your research. This provides a clear overview of your research methodology and helps readers understand the process you followed to arrive at your findings.
When to Write Research Methodology
Research methodology is typically written after the research proposal has been approved and before the actual research is conducted. It should be written prior to data collection and analysis, as it provides a clear roadmap for the research project.
The research methodology is an important section of any research paper or thesis, as it describes the methods and procedures that will be used to conduct the research. It should include details about the research design, data collection methods, data analysis techniques, and any ethical considerations.
The methodology should be written in a clear and concise manner, and it should be based on established research practices and standards. It is important to provide enough detail so that the reader can understand how the research was conducted and evaluate the validity of the results.
Applications of Research Methodology
Here are some of the applications of research methodology:
- To identify the research problem: Research methodology is used to identify the research problem, which is the first step in conducting any research.
- To design the research: Research methodology helps in designing the research by selecting the appropriate research method, research design, and sampling technique.
- To collect data: Research methodology provides a systematic approach to collect data from primary and secondary sources.
- To analyze data: Research methodology helps in analyzing the collected data using various statistical and non-statistical techniques.
- To test hypotheses: Research methodology provides a framework for testing hypotheses and drawing conclusions based on the analysis of data.
- To generalize findings: Research methodology helps in generalizing the findings of the research to the target population.
- To develop theories : Research methodology is used to develop new theories and modify existing theories based on the findings of the research.
- To evaluate programs and policies : Research methodology is used to evaluate the effectiveness of programs and policies by collecting data and analyzing it.
- To improve decision-making: Research methodology helps in making informed decisions by providing reliable and valid data.
Purpose of Research Methodology
Research methodology serves several important purposes, including:
- To guide the research process: Research methodology provides a systematic framework for conducting research. It helps researchers to plan their research, define their research questions, and select appropriate methods and techniques for collecting and analyzing data.
- To ensure research quality: Research methodology helps researchers to ensure that their research is rigorous, reliable, and valid. It provides guidelines for minimizing bias and error in data collection and analysis, and for ensuring that research findings are accurate and trustworthy.
- To replicate research: Research methodology provides a clear and detailed account of the research process, making it possible for other researchers to replicate the study and verify its findings.
- To advance knowledge: Research methodology enables researchers to generate new knowledge and to contribute to the body of knowledge in their field. It provides a means for testing hypotheses, exploring new ideas, and discovering new insights.
- To inform decision-making: Research methodology provides evidence-based information that can inform policy and decision-making in a variety of fields, including medicine, public health, education, and business.
Advantages of Research Methodology
Research methodology has several advantages that make it a valuable tool for conducting research in various fields. Here are some of the key advantages of research methodology:
- Systematic and structured approach : Research methodology provides a systematic and structured approach to conducting research, which ensures that the research is conducted in a rigorous and comprehensive manner.
- Objectivity : Research methodology aims to ensure objectivity in the research process, which means that the research findings are based on evidence and not influenced by personal bias or subjective opinions.
- Replicability : Research methodology ensures that research can be replicated by other researchers, which is essential for validating research findings and ensuring their accuracy.
- Reliability : Research methodology aims to ensure that the research findings are reliable, which means that they are consistent and can be depended upon.
- Validity : Research methodology ensures that the research findings are valid, which means that they accurately reflect the research question or hypothesis being tested.
- Efficiency : Research methodology provides a structured and efficient way of conducting research, which helps to save time and resources.
- Flexibility : Research methodology allows researchers to choose the most appropriate research methods and techniques based on the research question, data availability, and other relevant factors.
- Scope for innovation: Research methodology provides scope for innovation and creativity in designing research studies and developing new research techniques.
Research Methodology Vs Research Methods
About the author.
Muhammad Hassan
Researcher, Academic Writer, Web developer
You may also like

How to Cite Research Paper – All Formats and...

Data Collection – Methods Types and Examples

Delimitations in Research – Types, Examples and...

Research Paper Format – Types, Examples and...

Research Process – Steps, Examples and Tips

Research Design – Types, Methods and Examples
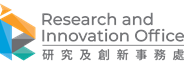
Quick Access
- PolyU 85th Anniversary
- Faculties, Schools and Departments
- Facts and Figures
- Research Grants and Awards
- Online Systems
- Technology Showcase
- Technology & Collaborative Platform
- External Partnership Opportunities
- Intellectual Property
- Research Facilities
- Event Calendar

Research Highlight
- Researchers
- Research Outputs
Funding & Awards
- Funding and Award Opportunities
- Major External Funding/Awards Obtained
Guidelines, Forms & Systems
- Handbooks and Guidelines
Research Resources
- Detailed Arrangements
- List of Eligible Funding / Award Schemes
- InnoHK R&D Centres
- Research Labs Institutes and Centres
- Research Equipment
- Mainland Translational Research Institutes
- Research Committee (RC)
- Areas of Excellence Committee (AoEC)
- DRC Secretaries
- FRC Secretaries
- Research Salons / Briefing Sessions
- IP Governance @ PolyU
- Patent Alert Service
- Patent Search
- Research and Development Cash Rebate Scheme
News and Events
- Media Coverage
- Promotional Materials
- e-Newsletters
- RAE 2026 Overview
- PolyU Impact Cases
- RAE Systems
- RAE Taskforce
- Useful Resources
- Service Contact
- General Enquiries
PolyU study reveals effectiveness of GBGI infrastructure in mitigating urban heat, proposing nine-stage framework for development of a sustainable city
17 May 2024
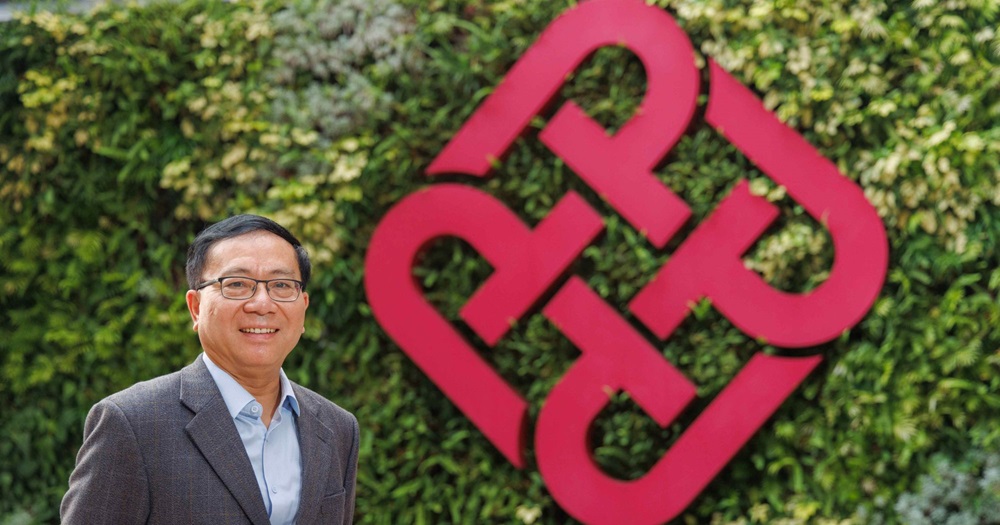
A study by Prof. Hai Guo, Professor of the Department of Civil and Environmental Engineering at PolyU and global researchers shows the effectiveness of green interventions in cooling temperatures across various regions.
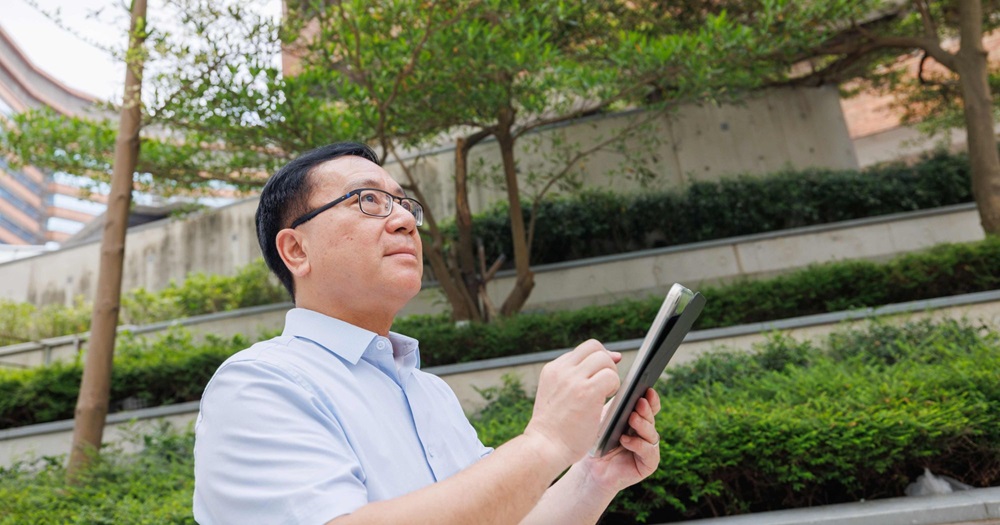
Prof. Hai Guo’s team found that constructed grey infrastructures in Asian cities, especially roof gardens and pergolas, are the most beneficial for urban cooling, with a temperature reduction of up to 17.7°C.
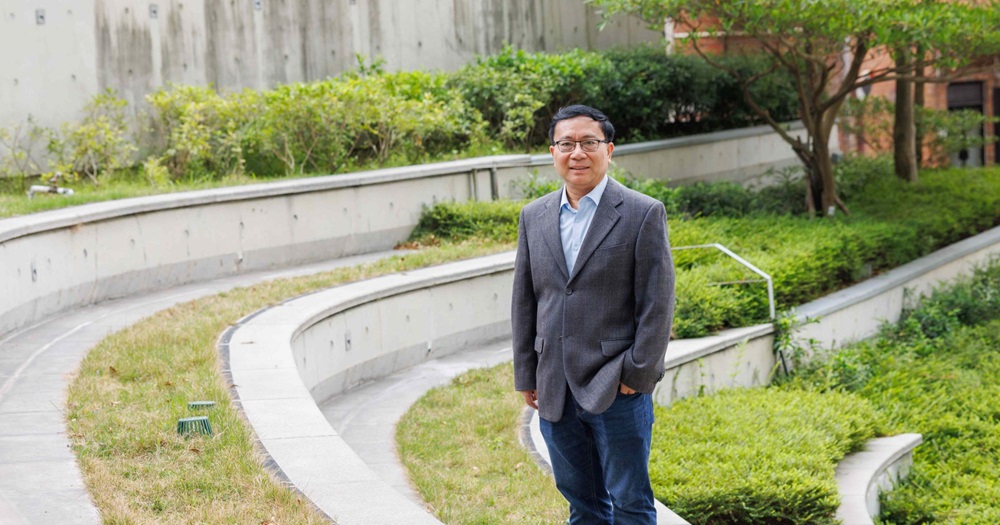
Linearly planted hedges and street trees have contributed to lowering temperatures by up to 10.8°C. Street trees are also highly recommended for their substantial potential to create other positive environmental impacts.
Primarily due to the impact of urbanisation and global warming, urban heatwaves have become a challenging issue worldwide, with Hong Kong persistently experiencing record-breaking high-temperature days. Mitigating urban heat through green and blue infrastructures is essential for creating a sustainable environment. Prof. Hai GUO, Professor of the Department of Civil and Environmental Engineering at The Hong Kong Polytechnic University (PolyU) and global researchers have conducted a first-of-its-kind study on the effectiveness of green interventions in cooling urban heat across various regions that can assist policymakers in prioritising effective interventions to develop sustainable cities. The study findings have been published in the international interdisciplinary journal The Innovation .
Globally, the most efficient air cooling was observed in botanical gardens, wetlands, green walls, street trees and vegetated balconies. In light of this, the research team conducted a global review of the effectiveness of green-blue-grey infrastructure (GBGI) in air cooling. GBGI refers to green infrastructures that encompass naturally vegetation-based elements like trees, grass and hedges; blue infrastructures are related to water-based features like pools, lakes and rivers; and grey infrastructures comprise engineered structures including green walls, green facades and roofs.
The Study revealed regional and city-specific variations in the effectiveness of GBGI for mitigating urban heat. In Europe, Asia, North America and Australia, the overall cooling effect of GBGI is up to 18.9°C, 17.7°C, 12°C and 9.63°C respectively. In addition, the implementation of green and blue infrastructures has proven to be highly effective in lowering air temperatures globally. While green infrastructures can regulate urban heat through evaporation, transpiration, shading and thermal insulation, blue infrastructures absorb heat and cool the surrounding area through evaporation.
In Asian cities, constructed grey infrastructures, especially roof gardens and pergolas, are found to be the most effective for urban cooling. Roof gardens in Singapore achieved the most significant temperature reduction of 17.7°C. Pergolas and green roofs in Japan and South Korea also had substantial impact, resulting in cooling temperatures by 16.2°C and 10.8°C, respectively. Linearly planted hedges and street trees contributed to lowering temperatures by up to 10.8°C. Authorities are advised to plant more street trees, not only for their impressive cooling efficiency but also for their substantial potential to create other positive environmental impacts.
The Study also showed notable effects of various GBGI features in mitigating urban heat in Mainland China cities. The most effective means include botanical gardens, wetlands, green walls and attenuation ponds which exhibited temperature reductions of up to 10°C, 9.27°C, 8°C and 7°C respectively. Although the cooling effect ranges are generally similar in the north and south of China, there is variability within the same region. For example, in Beijing, botanical garden could result in up to 10°C temperature decrease while that in Shaanxi province only contributed to 2.7°C. In Hong Kong, parks, green roofs and golf courses were found to play substantial roles in cooling urban heat, resulting in temperature reductions of 4.9°C, 4.9°C and 4.2°C respectively.
A “Shining City Project” was proposed by the Hong Kong government in last year’s Policy Address to enhance urban green space. This initiative includes the greening of riverbanks to turn them into flower viewing points and the extensive planting of trees in government venues and at roundabouts on major roads.
Prof. Guo said, “With their distinctive location and natural environment, the types of GBGI in Hong Kong are unique. The city features a network of oceans, rivers, wetlands and reservoirs, with remarkable vegetation cover, encompassing approximately 70% of its land area of which country parks occupy around 40%, and possesses a precious natural asset in the Victoria Harbour. Meanwhile, the Government actively promotes GBGI in new development areas and the adoption of green building design in new government projects. These forward-looking initiatives highlight Hong Kong’s dedication to sustainable and resilient urban development.”
Globally, the types of GBGI vary significantly across continents due to diverse regional contexts, climate conditions and urban planning priorities. The Study’s GBGI heat mitigation inventory can assist policymakers and urban planners in prioritising effective interventions to reduce the risk of urban overheating and promote community resilience. At this point, the research team has introduced a nine-stage framework to facilitate the implementation of GBGI that outlines stages of stakeholder engagement, feasibility studies, design, policy development, implementation, monitoring, evaluation, and eventual upscaling and replication.
Prof. Guo emphasised, “This framework serves as a strategic roadmap, optimising GBGI implementation to maximise benefits. Policymakers should conduct thorough investigation and planning tailored to the specific context and needs of their cities. In Asia, the extensive development of GBGI is a response to challenges posed by rapid urbanisation and cultural preferences that prioritise green areas for community activities, together with environmental goals focused on biodiversity conservation, improved air quality and mitigation of the urban heat island effect. It is crucial for future GBGI implementation to adopt a holistic approach, optimising their multifunctional benefits to effectively address sustainability goals.”
Sign up to receive our e-Newsletter
We use Cookies to give you a better experience on our website. By continuing to browse the site without changing your privacy settings, you are consenting to our use of Cookies. For more information, please see our Privacy Policy Statement .
Your browser is not the latest version. If you continue to browse our website, Some pages may not function properly. You are recommended to upgrade to a newer version or switch to a different browser. A list of the web browsers that we support can be found here
What are you looking for?

Popular Search
- Partnership
- Human Subjects Ethics
- Research Collaboration

COMMENTS
A research design is a strategy for answering your research question using empirical data. Creating a research design means making decisions about: Your overall research objectives and approach. Whether you'll rely on primary research or secondary research. Your sampling methods or criteria for selecting subjects. Your data collection methods.
Step 1: Consider your aims and approach. Step 2: Choose a type of research design. Step 3: Identify your population and sampling method. Step 4: Choose your data collection methods. Step 5: Plan your data collection procedures. Step 6: Decide on your data analysis strategies. Frequently asked questions.
Step 2: Data Type you Need for Research. Decide on the type of data you need for your research. The type of data you need to collect depends on your research questions or research hypothesis. Two types of research data can be used to answer the research questions: Primary Data Vs. Secondary Data.
Research design refers to the overall plan, structure or strategy that guides a research project, from its conception to the final analysis of data. Research designs for quantitative studies include descriptive, correlational, experimental and quasi-experimenta l designs. Research designs for qualitative studies include phenomenological ...
A well-structured research proposal includes a title page, abstract and table of contents, introduction, literature review, research design and methodology, contribution to knowledge, research schedule, timeline and budget. Visme's research proposal examples and templates offer a great starting point for creating engaging and well-structured ...
Research Proposal Example/Sample. Detailed Walkthrough + Free Proposal Template. If you're getting started crafting your research proposal and are looking for a few examples of research proposals, you've come to the right place. In this video, we walk you through two successful (approved) research proposals, one for a Master's-level ...
The purpose of the research proposal (its job, so to speak) is to convince your research supervisor, committee or university that your research is suitable (for the requirements of the degree program) and manageable (given the time and resource constraints you will face). The most important word here is "convince" - in other words, your ...
Failure to develop a coherent and persuasive argument for the proposed research. This is critical. In many workplace settings, the research proposal is a formal document intended to argue for why a study should be funded. ... Describe the overall research design by building upon and drawing examples from your review of the literature. Consider ...
Before beginning your paper, you need to decide how you plan to design the study.. The research design refers to the overall strategy and analytical approach that you have chosen in order to integrate, in a coherent and logical way, the different components of the study, thus ensuring that the research problem will be thoroughly investigated. It constitutes the blueprint for the collection ...
Here is an explanation of each step: 1. Title and Abstract. Choose a concise and descriptive title that reflects the essence of your research. Write an abstract summarizing your research question, objectives, methodology, and expected outcomes. It should provide a brief overview of your proposal. 2.
Research Proposal Format Example. Following is a general outline of the material that should be included in your project proposal. I. Title Page II. Introduction and Literature Review (Chapters 2 and 3) A. Identification of specific problem area (e.g., what is it, why it is important). B. Prevalence, scope of problem.
A strong research design is crucial to a successful research proposal, scientific paper, or dissertation. In this video, you'll get an idea of the series of ...
Here's an example of a research proposal to give you an idea of how it can be structured: [Your Name] [Your Affiliation or Institution] [Date] ... 3.1 Research Design: This study will adopt a mixed-methods approach, combining quantitative surveys and qualitative interviews. The quantitative phase will involve administering standardized ...
17 Research Proposal Examples. By Chris Drew (PhD) / January 12, 2024. A research proposal systematically and transparently outlines a proposed research project. The purpose of a research proposal is to demonstrate a project's viability and the researcher's preparedness to conduct an academic study. It serves as a roadmap for the researcher.
Make sure you can ask the critical what, who, and how questions of your research before you put pen to paper. Your research proposal should include (at least) 5 essential components : Title - provides the first taste of your research, in broad terms. Introduction - explains what you'll be researching in more detail.
Step 1: Define your variables. You should begin with a specific research question. We will work with two research question examples, one from health sciences and one from ecology: Example question 1: Phone use and sleep. You want to know how phone use before bedtime affects sleep patterns.
A proposal needs to show how your work fits into what is already known about the topic and what new paradigm will it add to the literature, while specifying the question that the research will answer, establishing its significance, and the implications of the answer. [ 2] The proposal must be capable of convincing the evaluation committee about ...
This will guide your research design and help you select appropriate methods. Select a research design: There are many different research designs to choose from, including experimental, survey, case study, and qualitative designs. Choose a design that best fits your research question and objectives.
Depending on the length of your research proposal, you may wish to include a contents page for the proposal itself (not for your main research project: suggested contents for this are included in your Proposed Chapter Outline, section 9), as follows (add page numbers/subsections when you know them, depending on your research).As you introduce sub-sections into your different sections, number ...
While many books and articles guide various qualitative research methods and analyses, there is currently no concise resource that explains and differentiates among the most common qualitative approaches. We believe novice qualitative researchers, students planning the design of a qualitative study or taking an introductory qualitative research course, and faculty teaching such courses can ...
Example #9: Corporate Proposal Template. This template is professionally to grab the attention of potential business partners and give your corporate image a boost. You can customize the proposal for a new product development project or corporate initiative. Visme's brand design tool can help ensure your proposal stays on brand.
Alzheimer's disease (AD), the most common form of dementia, remains challenging to understand and treat despite decades of research and clinical investigation. This might be partly due to a lack of widely available and cost-effective modalities for diagnosis and prognosis. Recently, the blood-based AD biomarker field has seen significant progress driven by technological advances, mainly ...
Qualitative Research Methodology. This is a research methodology that involves the collection and analysis of non-numerical data such as words, images, and observations. This type of research is often used to explore complex phenomena, to gain an in-depth understanding of a particular topic, and to generate hypotheses.
A "Shining City Project" was proposed by the Hong Kong government in last year's Policy Address to enhance urban green space. ... the research team has introduced a nine-stage framework to facilitate the implementation of GBGI that outlines stages of stakeholder engagement, feasibility studies, design, policy development, implementation ...