- Our Mission
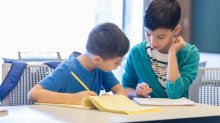

Using Mathematical Modeling to Get Real With Students
Unlike canned word problems, mathematical modeling plunges students into the messy complexities of real-world problem solving.
How do you bring math to life for kids? Illustrating the boundless possibilities of mathematics can be difficult if students are only asked to examine hypothetical situations like divvying up a dessert equally or determining how many apples are left after sharing with friends, writes third- and fourth- grade teacher Matthew Kandel for Mathematics Teacher: Learning and Teaching PK-12 .
In the early years of instruction, it’s not uncommon for students to think they’re learning math for the sole purpose of being able to solve word problems or help fictional characters troubleshoot issues in their imaginary lives, Kandel says. “A word problem is a one-dimensional world,” he writes. “Everything is distilled down to the quantities of interest. To solve a word problem, students can pick out the numbers and decide on an operation.”
But through the use of mathematical modeling, students are plucked out of the hypothetical realm and plunged into the complexities of reality—presented with opportunities to help solve real-world problems with many variables by generating questions, making assumptions, learning and applying new skills, and ultimately arriving at an answer.
In Kandel’s classroom, this work begins with breaking students into small groups, providing them with an unsharpened pencil and a simple, guiding question: “How many times can a pencil be sharpened before it is too small to use?”
Setting the Stage for Inquiry
The process of tackling the pencil question is not unlike the scientific method. After defining a question to investigate, students begin to wonder and hypothesize—what information do we need to know?—in order to identify a course of action. This step is unique to mathematical modeling: Whereas a word problem is formulaic, leading students down a pre-existing path toward a solution, a modeling task is “free-range,” empowering students to use their individual perspectives to guide them as they progress through their investigation, Kandel says.
Modeling problems also have a number of variables, and students themselves have the agency to determine what to ignore and what to focus their attention on.
After inter-group discussions, students in Kandel’s classroom came to the conclusion that they’d need answers to a host of other questions to proceed with answering their initial inquiry:
- How much does the pencil sharpener remove?
- What is the length of a brand new, unsharpened pencil?
- Does the pencil sharpener remove the same amount of pencil each time it is used?
Introducing New Skills in Context
Once students have determined the first mathematical question they’d like to tackle (does the pencil sharpener remove the same amount of pencil each time it is used?), they are met with a roadblock. How were they to measure the pencil if the length did not fall conveniently on an inch or half inch? Kandel took the opportunity to introduce a new target skill which the class could begin using immediately: measuring to the nearest quarter inch.
“One group of students was not satisfied with the precision of measuring to the nearest quarter inch and asked to learn how to measure to the nearest eighth of an inch,” Kandel explains. “The attention and motivation exhibited by students is unrivaled by the traditional class in which the skill comes first, the problem second.”
Students reached a consensus and settled on taking six measurements total: the initial length of the new, unsharpened pencil as well as the lengths of the pencil after each of five sharpenings. To ensure all students can practice their newly acquired skill, Kandel tells the class that “all group members must share responsibility, taking turns measuring and checking the measurements of others.”
Next, each group created a simple chart to record their measurements, then plotted their data as a line graph—though exploring other data visualization techniques or engaging students in alternative followup activities would work as well.
“We paused for a quick lesson on the number line and the introduction of a new term—mixed numbers,” Kandel explains. “Armed with this new information, students had no trouble marking their y-axis in half- or quarter-inch increments.”
Sparking Mathematical Discussions
Mathematical modeling presents a multitude of opportunities for class-wide or small-group discussions, some which evolve into debates in which students state their hypotheses, then subsequently continue working to confirm or refute them.
Kandel’s students, for example, had a wide range of opinions when it came to answering the question of how small of a pencil would be deemed unusable. Eventually, the class agreed that once a pencil reached 1 ¼ inch, it could no longer be sharpened—though some students said they would be able to still write with it.
“This discussion helped us better understand what it means to make an assumption and how our assumptions affected our mathematical outcomes,” Kandel writes. Students then indicated the minimum size with a horizontal line across their respective graphs.
Many students independently recognized the final step of extending their line while looking at their graphs. With each of the six points representing their measurements, the points descended downward toward the newly added horizontal “line of inoperability.”
With mathematical modeling, Kandel says, there are no right answers, only models that are “more or less closely aligned with real-world observations.” Each group of students may come to a different conclusion, which can lead to a larger class discussion about accuracy. To prove their group had the most accurate conclusion, students needed to compare and contrast their methods as well as defend their final result.
Developing Your Own Mathematical Models
The pencil problem is a great starting point for introducing mathematical modeling and free-range problem solving to your students, but you can customize based on what you have available and the particular needs of each group of students.
Depending on the type of pencil sharpener you have, for example, students can determine what constitutes a “fair test” and set the terms of their own inquiry.
Additionally, Kandel suggests putting scaffolds in place to allow students who are struggling with certain elements to participate: Simplified rulers can be provided for students who need accommodations; charts can be provided for students who struggle with data collection; graphs with prelabeled x- and y-axes can be prepared in advance.
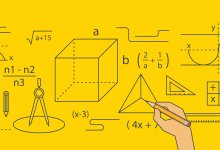
.css-1sk4066:hover{background:#d1ecfa;} 7 Real-World Math Strategies
Students can also explore completely different free-range problem solving and real world applications for math . At North Agincourt Jr. Public School in Scarborough, Canada, kids in grades 1-6 learn to conduct water audits. By adding, subtracting, finding averages, and measuring liquids—like the flow rate of all the water foundations, toilets, and urinals—students measure the amount of water used in their school or home in a single day.
Or you can ask older students to bring in common household items—anything from a measuring cup to a recipe card—and identify three ways the item relates to math. At Woodrow Petty Elementary School in Taft, Texas, fifth-grade students display their chosen objects on the class’s “real-world math wall.” Even acting out restaurant scenarios can provide students with an opportunity to reinforce critical mathematical skills like addition and subtraction, while bolstering an understanding of decimals and percentages. At Suzhou Singapore International School in China, third- to fifth- graders role play with menus, ordering fictional meals and learning how to split the check when the bill arrives.

- school Campus Bookshelves
- menu_book Bookshelves
- perm_media Learning Objects
- login Login
- how_to_reg Request Instructor Account
- hub Instructor Commons
- Download Page (PDF)
- Download Full Book (PDF)
- Periodic Table
- Physics Constants
- Scientific Calculator
- Reference & Cite
- Tools expand_more
- Readability
selected template will load here
This action is not available.
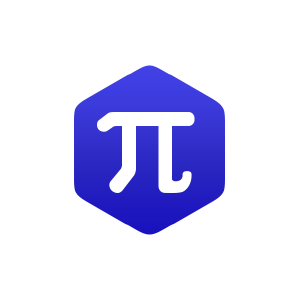
12: Modeling
- Last updated
- Save as PDF
- Page ID 57725
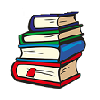
- Peter L. Moore
- Iowa State University
12.1 What is a model?
A model is a representation of reality that allows us to understand something better. There are many types of models, including conceptual , mathematical , and physical models. A physical model is a physical object or set of objects intended to represent something else that is too large, small, complex or otherwise inaccessible for direct investigation. A conceptual model is a collection of hypothesized relationships between different objects or variables, and is usually described in narrative. From an early age, we learn how to construct both physical and conceptual models. Children create conceptual models to help them understand cause and effect relationships that lead to either desirable or unwanted outcomes (‘if I jump down one or two steps, it’s fun, but if I jump down three or more steps it hurts my legs: jumping farther hurts more’). When my gradeschool son builds a spaceship from Legos, he is creating a physical model of a spaceship he has seen in a movie or book. These are not particularly sophisticated models, but they are nevertheless ways of representing some aspect of reality (or imagined reality).
As with Legos, mathematical models can serve mostly a desire for creative play. Like Lego models, it is perfectly possible to create a mathematical model that represents reality poorly, and is therefore not very useful. Perhaps we claim to have created a model of a car, but if we’ve only stacked rectangular bricks together and failed to add wheels, it is not a particularly good or useful model of a car. Thus, model construction and use should be done with the broader problem context in mind. The means should justify the desired ends.
Mathematical models are only as useful as the conceptual models on which they are based.
In this book, we are interested in mathematical and conceptual models and the connections between them. Ultimately, our goal isn’t necessarily to become mathematical modelers, but rather to be able to construct, use, and understand models that can assist with problem-solving. Indeed, many mathematical models originate from a desire to quantify the relationships in a conceptual model devised to address a problem. Several possible approaches to quantification lead to a handful of varieties of mathematical models. We’ll focus our discussion on three distinct but related types of mathematical models that differ in their origins and implementation. The first two are grounded in theory, while the third often arises from statistical data analysis.
- Analytical models are usually developed from theory based on fundamental physical, chemical or biological principles. A hypothesis that a tree’s height should scale with it’s trunk diameter raised to the 2/3 power in order to retain structural integrity is such a model. These models are often the most general and abstract, and can sometimes be solved with paper and pencil. However, they can become hopelessly complex and un-solvable when one tries to incorporate realistic details and context. The idealizations necessary to make an analytical model solvable can also sometimes limit its utility.
- Numerical models may be created and motivated in the same manner as analytical models, but employ techniques for mathematical approximation that permit relaxation of analytical idealizations and introduction of detail without making the equations too difficult to solve. Numerical models can be solved by hand for very small systems, but are more appropriately implemented in computer programs.
- Empirical models may have analytical or numerical components, but contain parameters that must be quantified by experiment or systematic observation. Data must be incorporated and usually analyzed statistically in order to define parameter values. In some cases, regression is used to constrain the functional relationships between variables or to identify the value of coefficients. Thus, a fully empirical model is data-driven or data-calibrated.
We have already seen or worked with a few examples of models. The Logistic population growth model that we discussed briefly in Section 10.1.3 is a theoretical model that can be implemented either in numerical or analytical form. Even that model, however, has empirical components, since it’s use in practical problem-solving requires some observational constraints on r and K . When we solved for total brook trout population in Section 11.2.1, we employed an empirical model known as the Leslie method, which is based on a conceptual model of the change in catch probability under declining population.
12.1.1 Example: The Universal Soil Loss Equation (USLE)
The widely-used Universal Soil Loss Equation (USLE) is an example of an empirical model. The master equation for USLE is:
\[A = RKLSCP \label{12.1} \]
where A is the soil loss (usually in tons/acre/year), R is a rainfall erosivity factor, K is a soil erodibility factor, L and S are the slope length and angle factors, C is a ground-cover factor and P is a parameter that accounts for soil conservation practices or structures.
The factors in USLE are quantities whose values cannot be measured directly. Instead, the numerical values are each derived from a combination of carefully-designed field experiments where all but one factor is held constant. The factor values are then derived from measured differences in soil loss.
The great value of the USLE and it’s kin is that it is sufficiently easy to use that farmers with little formal training in math or computing can easily get satisfactory results. Most factor values can either be looked up in tables or measured on the ground or from maps.
The ease of use comes at a cost, however. Because factor values are derived from experiments, they are strictly valid only within the range of conditions considered within the experiments. In other words, if applied in settings where – for example – rainfall intensity is twice as large as the largest observed in experiments, the reliability of results is uncertain. Fully empirical models can therefore sometimes be unreliable in conditions outside the range of the conditions under which factor values were determined.
12.1.2 Example: probability of deer-automobile encounters (Problem 3.3)
As we have already seen, simple theoretical models can sometimes be sufficient to explore a range of system behaviors, even when functional relationships are uncertain. These models will inevitable by limited in power by the simplifying assumptions or idealizations used, but when the science or management problem permit a solution with substantial uncertainty, this approach is still warranted.
Let’s assume that deer in our county are randomly distributed in space, and that they have no particular reason to either avoid or seek out roads. Call the total area of the county A \(_{c}\) and the proportion of the area occupied by roads f , so that the area of roads A \(_{r}\) = f A \(_{c}\). Let’s assume that there are N \(_{0}\) deer in the county. It follows that – if the deer are randomly distributed – there will be approximately f N \(_{0}\) deer on the road at any moment. What is that number according to the numbers we produced earlier for Story County, IA? The value of f was estimated to be approximately 0.0076, so if there are say 1000 deer in the county, we should expect either 7 or 8 of them on the road at any given time. That seems reasonable, but that isn’t what we’re after. We’d like to know about how likely collisions are between deer and automobiles. So we need to work in something about the number and distance of car trips through the road system, right? This is left as an exercise for the student, as there are many possible ways to approach this.
12.2 Dealing with higher mathematics
Many powerful mathematical models have been devised to explore and describe phenomena in nature. Some of the most powerful are those that allow predictions of unobserved or future events or patterns. These can directly inform management decisions provided that managers trust and understand their results. Unfortunately, many of these powerful models employ mathematical concepts and methods that are beyond the typical undergraduate training in math. Does that mean that most people are doomed to never understand or use these models? Absolutely not! There isn’t any inherent reason that students need to take calculus, linear algebra, or differential equations courses before they can comprehend the gist of a model constructed with those skills. It certainly helps to have at least a conceptual grasp of some key concepts in calculus, but that doesn’t translate to a pre-requisite.
12.2.1 Example: prairie dog plague (Problem 3.4)
Since this problem deals with hypothetical future events, it may not be possible to glean the answer directly from past work or from observation. Instead, we can construct a simple model of the prairie dog community with random, probabilistic interactions among wellmixed individuals.
A common way to model disease transmission is with a compartment model often called SIR. We consider individuals in a population to be in one of three (or four) states: Susceptible (S), Infected (I), and Removed (R) or Recovered. Individuals move from compartment S to compartment I by disease transmission. Infected individuals in compartment I then either recover and move to compartment R, or are removed from the population by death or isolation. These transfers between compartments are often described with a system of differential equations :
\(\frac{dS}{dt}\) = − βSI (12.2)
\(\frac{dI}{dt}\) = β SI − γI (12.3)
\(\frac{dR}{dt}\) = γI (12.4)
These differential equations are not easily solved in most cases, but we can use them as a basis for a numerical simulation of disease dynamics if we are able to estimate the parameters β and γ . A numerical representation of the first equation might look something like this, for example:
S \(_{t+1}\) = S \(_{t}\) − βS \(_{t}\) I \(_{t}\) (12.5)
I \(_{t+1}\) = I \(_{t}\) + βS \(_{t}\) I \(_{t}\) − γI \(_{t}\) (12.6)
R \(_{t+1}\) = R \(_{t}\) + γI \(_{t}\) (12.7)
This says that in a given time increment, susceptible individuals are moved from the S compartment to the I (infected) compartment at a rate that is proportional to the product of the numbers of individuals in each compartment and the transmission rate constant β . You can see in the first and second equations above that when a number of idividuals infected according to the β SI term in lost from the S compartment (because it is negative), it is gained (positive) in the I compartment. All individuals are accounted for in moving into or out of the I compartment. Similarly, individuals move from the I compartment to the R compartment at a rate governed by the rate constant γ . Selection of these rate constants to a large extent governs the behavior of the model, and thus the predicted fate of the prairie dog colony. But implementing management options informed by positive model outputs is where the biggest challenge arises.
12.3 Power-Law Scaling
Consider this seemingly innocuous question: are larger animals heavier than smaller animals?
You: Hmmm, well, yeah I think so?! An adult bear weights more than a snowshoe hare, for instance.
OK, great, but how would we know if this is true more generally? And what exactly do we mean by larger? Does that mean taller? Larger volume? This brings up a few issues that become important when we’re talking about real quantities rather than abstract variables. Unambiguously defining quantities can be an important first step in communicating quantitative information. In the next section we’ll be specific about what information is required to fully define a quantity. For now let’s agree that we’re satisfied with relating the mass of an animal to its volume. Do animals that take up more space (i.e., have greater volume) also weigh more? Maybe we can say it another way: is the weight or mass of an animal proportional to its body size? We could write this in symbols:
M ∝ V ? (12.8)
The symbol ‘∝’ between M (body mass) and V (volume) means “proportional to”. So this isn’t an equation yet because we’re not sure anything is equal. And of course it’s nonsense that an animal’s weight is equal to its volume. There must be some other parameter that transforms an animal’s volume into a mass. Let’s call it c , and try it out in an equation:
M = cV (12.9)
But what is c ? As we said above, we’d prefer to have some meaning for the symbols we throw around in equations. Let’s use one of our old algebraic tools for manipulating equations and “solve the equation for c ”. By that we mean get c onto one side of the equation all by itself. To get there, we just need to divide both sides of the equation by V , yielding:
\(\frac{M}{V}\) = c (12.10)
Now recall that the definition of density is mass per unit volume. That’s exactly what we have on the left-hand side of the equation! So our equation now says that c , the parameter we used to transform volume into mass, is the same as density! So for an individual animal, the parameter that relates mass to volume is density. As we have done previously, let’s assume that most animals have a density close to that of water so this proportionality parameter c doesn’t vary significantly among species. So to the extent that it is correct to say that most animal’s body density is close to that of water, we can argue that larger animals do indeed weigh more, in general.
This is probably not a very profound revelation to you . But with only a few more small leaps in logic, we can get somewhere considerably more interesting. For more than a century, biologists have been intrigued by a remarkable relationship between the basal metabolic rate and body mass for animals of a wide range of sizes and shapes. Amazingly, if one assembles a large set of data and plots it on a graph with a logarithmic scale, mice, humans and elephants and most of the rest fall along a straight line! An equation that describes this relationship and the line on the graph looks like this:
B = B \(_{0}\)M\(^{b}\) (12.11)
where B is the basal metabolic rate, M is body mass as before, and B \(_{0}\) and b are constants (we’ll see what they mean later!). This equation is yet another power law, and equations with this form pop up surprisingly often in ecology once you start looking. We’ll get more into functions and power laws later on. But for now, some important points should be made:
- The argument that there should be a proportionality between body mass and metabolic rate was originally conceived theoretically on the basis that energy given off by an animal to its surroundings might depend mostly on the animal’s surface area, while its mass scales with volume.
- Measurements by many researchers over more than a century have been compared against this theoretical prediction, with varying degrees of success. In most cases however, the power-law relationship holds.
We’ll look into this in more detail a bit later.
Mathematical modeling and problem solving: from fundamentals to applications
- Open access
- Published: 15 March 2024
Cite this article
You have full access to this open access article
- Masahito Ohue 1 ,
- Kotoyu Sasayama 2 &
- Masami Takata 3
580 Accesses
Explore all metrics
The rapidly advancing fields of machine learning and mathematical modeling, greatly enhanced by the recent growth in artificial intelligence, are the focus of this special issue. This issue compiles extensively revised and improved versions of the top papers from the workshop on Mathematical Modeling and Problem Solving at PDPTA'23, the 29th International Conference on Parallel and Distributed Processing Techniques and Applications. Covering fundamental research in matrix operations and heuristic searches to real-world applications in computer vision and drug discovery, the issue underscores the crucial role of supercomputing and parallel and distributed computing infrastructure in research. Featuring nine key studies, this issue pushes forward computational technologies in mathematical modeling, refines techniques for analyzing images and time-series data, and introduces new methods in pharmaceutical and materials science, making significant contributions to these areas.
Similar content being viewed by others
Large-Scale and Distributed Optimization: An Introduction
Optimization Theory
WORHP: Development and Applications of the ESA NLP SOLVER
Avoid common mistakes on your manuscript.
The field of machine learning and mathematical modeling is rapidly evolving, significantly impacting diverse research areas. The recent surge in artificial intelligence technologies has further accelerated this trend, highlighting the growing importance of “mathematical modeling and problem solving” in scientific endeavors [ 1 ]. Modeling natural phenomena and engineering systems not only deepens our understanding of fundamental principles but also drives the development of innovative technologies for effective control. These advancements have considerable implications for both industry and academia.
This special issue showcases the latest advancements in mathematical modeling and problem solving across various disciplines. The scope of topics is wide, encompassing everything from foundational research in new matrix operation methods, heuristic search, and constrained optimization techniques to practical research in computer vision, drug discovery, materials science, financial engineering, and mechanical processes.
A key aspect of contemporary mathematical modeling research is its integration with supercomputing, which involves extensive parallel and distributed computing. The sheer volume and augmented data often require rapid computational strategies. The infrastructure, including hardware and software, supporting parallel and distributed computing is thus vital for applied research. This issue includes a selection of research presented at the “Mathematical Modeling and Problem Solving” workshop during the 29th International Conference on Parallel and Distributed Processing Techniques and Applications (PDPTA’23). After a thorough selection process, nine significant studies were chosen as articles on this issue.
Four papers focus on computational technologies foundational to mathematical modeling. Chiyonobu and colleagues enhance the two-sided Jacobi method for singular value decomposition for complex matrices, previously effective only for real matrices [ 2 ]. They incorporate QR decomposition for complex matrix scenarios, offering two distinct implementations for both complex and real matrices. Zhong et al. introduce a novel hyper-heuristic algorithm, the evolutionary multi-mode slime mold optimization (EMSMO), inspired by slime mold behaviors [ 3 ]. This algorithm demonstrates superior performance in benchmarks and engineering problems, outperforming traditional evolutionary and hyper-heuristic algorithms. Zhang et al. unveil the meta-generative data augmentation optimization (MGDAO), a method that advances data augmentation in foundational machine learning for image and natural language processing [ 4 ]. This technique surpasses standard auto-augmentation methods in few-shot image and text classification benchmarks. Matsuzaki and colleagues propose a mixed-integer programming (MIP)-based method for scheduling machining operations in automated manufacturing, considering worker conditions [ 5 ]. They validate this method through computer experiments modeled on real-world machining tasks.
Two papers address applications involving image and time-series data, traditional targets of mathematical modeling. Ishikawa et al. enhance concrete crack detection by using strongly blurred images in training data, improving recognizer accuracy [ 6 ]. Takata et al. develop a method for recommending stock combinations by analyzing price change waveforms, showing potential for diversifying portfolios and minimizing risks [ 7 ].
Last but not least, three papers focus on pharmaceutical and materials science applications. Ueki and Ohue assess AlphaFold2 and binder hallucination techniques for improving antibody binding affinity, indicating a more efficient method than traditional experimental approaches [ 8 ]. Morikawa et al. introduce a machine learning method using graph kernels for predicting metal–organic frameworks (MOFs) combinations, demonstrating accurate MOF structure prediction without physical synthesis [ 9 ]. Furui and Ohue present an enhanced version of the lead optimization mapper (Lomap) algorithm for drug discovery [ 10 ]. This improved algorithm offers a faster approach to create free energy perturbation (FEP) graphs for numerous compounds, while maintaining the quality of the output.
In summary, this special issue represents a significant contribution to the fields of mathematical modeling and application, providing innovative methods to the community. As editors, we extend our gratitude to all researchers who contributed to this collection, paving the way for the next era of mathematical modeling and problem solving.
Yüksel N, Börklü HR, Sezer HK, Canyurt OE (2023) Review of artificial intelligence applications in engineering design perspective. Eng Appl Artif Intell 118:105697
Article Google Scholar
Chiyonobu M, Miyamae T, Takata M, Harayama J, Kimura K, Nakamura Y (2024) Singular value decomposition for complex matrices using two-sided Jacobi method. J Supercomput. https://doi.org/10.1007/s11227-024-05903-6
Zhong R, Zhang E, Munetomo M (2024) Evolutionary multi-mode slime mold optimization: a hyper-heuristic algorithm inspired by slime mold foraging behaviors. J Supercomput. https://doi.org/10.1007/s11227-024-05909-0
Zhang E, Dong B, Wahib M, Zhong R, Munetomo M (2024) Meta generative image and text data augmentation optimization. J Supercomput. https://doi.org/10.1007/s11227-024-05912-5
Matsuzaki J, Sakakibara K, Nakamura M, Watanabe S (2024) Large neighborhood local search method with MIP techniques for large-scale machining scheduling with many constraints. J Supercomput. https://doi.org/10.1007/s11227-024-05912-5
Ishikawa S, Chiyonobu M, Iida S, Takata M (2024) Improvement of recognition rate using data augmentation with blurred images. J Supercomput. https://doi.org/10.1007/s11227-024-05901-8
Takata M, Kidoguchi N, Chiyonobu M (2024) Stock recommendation methods for stability. J Supercomput. https://doi.org/10.1007/s11227-024-05902-7
Ueki T, Ohue M (2024) Antibody complementarity-determining region design using AlphaFold2 and DDG Predictor. J Supercomput. https://doi.org/10.1007/s11227-023-05887-9
Morikawa Y, Shin K, Ohshima H, Kubouchi M (2024) Prediction of specific surface area of metal–organic frameworks by graph kernels. J Supercomput. https://doi.org/10.1007/s11227-024-05914-3
Furui K, Ohue M (2024) FastLomap: faster lead optimization mapper algorithm for large-scale relative free energy perturbation. J Supercomput
Download references
This study was partially supported by JSPS KAKENHI (23H04887) (M.O.).
Author information
Authors and affiliations.
Department of Computer Science, School of Computing, Tokyo Institute of Technology, Kanagawa, 226-8501, Japan
Masahito Ohue
Elevator and Escalator Development Department, Mitsubishi Electric Building Solutions Corporation, Aichi, 492-8682, Japan
Kotoyu Sasayama
Research Group of Information and Communication Technology for Life, Nara Women’s University, Nara, 630-8506, Japan
Masami Takata
You can also search for this author in PubMed Google Scholar
Contributions
All authors listed have made a substantial, direct, and intellectual contribution to the work and approved it for publication.
Corresponding author
Correspondence to Masahito Ohue .
Ethics declarations
Conflict of interest.
All authors declare that the research was conducted without any commercial or financial relationships that could be construed as a potential conflict of interest.
Additional information
Publisher's note.
Springer Nature remains neutral with regard to jurisdictional claims in published maps and institutional affiliations.
Rights and permissions
Open Access This article is licensed under a Creative Commons Attribution 4.0 International License, which permits use, sharing, adaptation, distribution and reproduction in any medium or format, as long as you give appropriate credit to the original author(s) and the source, provide a link to the Creative Commons licence, and indicate if changes were made. The images or other third party material in this article are included in the article's Creative Commons licence, unless indicated otherwise in a credit line to the material. If material is not included in the article's Creative Commons licence and your intended use is not permitted by statutory regulation or exceeds the permitted use, you will need to obtain permission directly from the copyright holder. To view a copy of this licence, visit http://creativecommons.org/licenses/by/4.0/ .
Reprints and permissions

About this article
Ohue, M., Sasayama, K. & Takata, M. Mathematical modeling and problem solving: from fundamentals to applications. J Supercomput (2024). https://doi.org/10.1007/s11227-024-06007-x
Download citation
Accepted : 17 February 2024
Published : 15 March 2024
DOI : https://doi.org/10.1007/s11227-024-06007-x
Share this article
Anyone you share the following link with will be able to read this content:
Sorry, a shareable link is not currently available for this article.
Provided by the Springer Nature SharedIt content-sharing initiative
- Mathematical modeling
- Machine learning
- Parallel and distributed computing
- Drug discovery and materials science
- Find a journal
- Publish with us
- Track your research
- STEM Ambassadors
- School trusts
- ITE and governors
- Invest in schools
- STEM careers inspiration
- Benefits and impact
- Our supporters
- Become a STEM Ambassador
- Request a STEM Ambassador
- Employer information
- Training and support
- STEM Ambassadors Partners
- Working with community groups
- Search icon
- Join the STEM Community
Mathematics: Problem Solving and Investigations
A list of resources containing activities which could be integrated into your scheme of work with the purpose of integrating problem solving and investigational maths work into the natural process of learning mathematics and not just as a bolt-on added extra.
The first few resources are aimed at primary mathematics with the remainder aimed at Key Stages 3 & 4.
Making Molecules
Quality Assured Category: Mathematics Publisher: cre8ate maths
This activity asks students to investigate the structure of hydrocarbons. A printed sheet representing carbon and hydrogen atoms is cut up and used by students to build as many isomers as they can for two chemical formulae.
Students are then challenged to discover a 3-D shape with 60 vertices, made from regular pentagons and hexagons. Teachers may choose to give 12 pentagons and 20 hexagons to groups of students for them to build the model or make up the shape from its net.
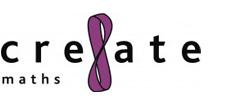
Problem Cards
Quality Assured Collection Category: Mathematics Publisher: Nuffield Foundation
This collection contains two packs of problem cards that have been designed for use by students in conjunction with the main work described in the Teachers' Guides. It is intended that the majority of students should at least be able to 'have a go' at most of the questions, but should also be encouraged, to the full extent of their individual abilities, to think around a problem and to devise alternatives and generalisations.
There are two packs of problem cards, purple and red, each accompanied by a teachers' guide.
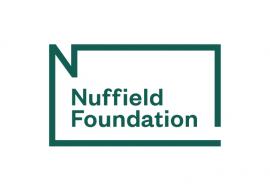
Starting Investigations
Quality Assured Category: Mathematics Publisher: Collins Educational
A book of mathematical investigations aimed at students working at National Curriculum levels 1 to 3.
Contains forty simple investigations covering topics such as Odds and Evens, Place Value, Number patterns and many more.
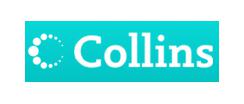
Badger Maths problem solving
Quality Assured Category: Mathematics Publisher: Badger
A series of resources aimed at years one through to six aimed at developing problem solving skills. Each resource gives an example of a four step problem solving approach and task cards split into levels.
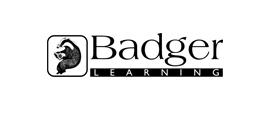
Problem solving with EYFS, Key Stage One and Key Stage Two children
Quality Assured Category: Computing Publisher: Department for Education
A set of resources from the National Strategies aimed at years one to six designed to help students become proficient problem solvers in mathematics.
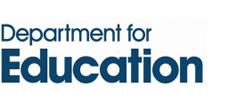
Problem Solving Tasks
Quality Assured Collection Category: Mathematics Publisher:
The Spode Group have produced a number of resources to support problem solving in mathematics through real life problems.
The resources contain a wide range of open-ended tasks, practical tasks, investigations and real life problems still useful today to place the mathematics learnt in the classroom into a real world context.
Bowland Maths: Assessment Tasks
Quality Assured Collection Category: Mathematics Publisher: Bowland Charitable Trust
Bowland Maths includes over thirty tasks designed to help assess students’ achievements and progression against key processes. To help with this assessment, each task contains sample work, and a 'progression table' showing how students’ work on the task can provide evidence of their progress with the four key processes: representing, analysing, communicating and reflecting.
These materials are also ideal for formative assessment that concentrates on providing the types of rich feedback that have been proven to help students improve their reasoning.
Graded Assessment in Mathematics (GAIM)
Quality Assured Collection Category: Mathematics Publisher: Nelson Thornes
GAIM is a teacher assessment scheme for Key Stages Three and Four. The scheme is designed to encourage teaching and learning through practical problem solving and investigations, involve students in all assessment and record keeping and introduce continuous assessment into normal classroom practice.
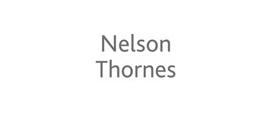
Investigative and Problem-Solving Approaches to Mathematics and Their Assessment
Quality Assured Category: Mathematics Publisher: Institute of Physics
This resource was written in response to the Cockroft Report to address the recommendation that all mathematics teaching should include opportunities for exposition by the teacher, discussion between teachers and students and between students themselves, practical work, consolidation and practice, problem solving and investigational work. The purpose of the pack was to address these issues and support their introduction and assessment.
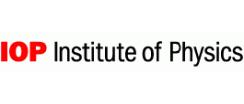
Design a Board Game
Quality Assured Category: Mathematics Publisher: Shell Centre for Mathematical Education
In this Shell Centre module, groups carefully design and produce their own board games. These games are then played and evaluated by other class members.
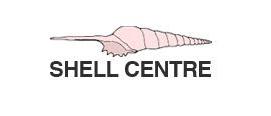
Plan a Trip
In this Shell Centre module students plan and undertake a class trip using costings, scheduling, surveys and everyday arithmetic.
Produce a Quiz Show
In this Shell Centre modlue, Produce a Quiz Show, students devise, schedule, run and evaluate their own classroom quizzes.
Pure Investigations
These two books from the Shell centre focus on the pure investigations.

- All courses
- For Teachers
- For Students
Problem Solving, Mathematical Investigation, and Modeling (6081)
This course intends to enhance the students’ knowledge and skills in dealing with real-life and/or non-routine applications of mathematics. Students will have the opportunity to explore the use of problem-solving strategies or heuristics as they engage in mathematical investigations, formulate and justify conjectures, make generalizations, and communicate mathematical ideas.
- Teacher: MELANIE GURAT
- Enrolled students: 2
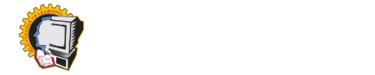
Let's Connect
Contact details.
Brgy. Atabay, Hilongos, Leyte, Philippines 6524
+639 17-306-5169
Get in Touch
Your Phone Number
Your E-mail
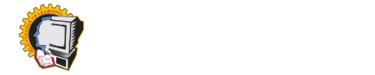
- Glenn’s Memoir
- Online Admission
Want to receive push notifications for all major on-site activities?
This Area is Widget-Ready
You can place here any widget you want!
You can also display any layout saved in Divi Library.
Let’s try with contact form:
Insert/edit link
Enter the destination URL
Or link to existing content
- Share full article
For more audio journalism and storytelling, download New York Times Audio , a new iOS app available for news subscribers.

- May 10, 2024 • 27:42 Stormy Daniels Takes the Stand
- May 9, 2024 • 34:42 One Strongman, One Billion Voters, and the Future of India
- May 8, 2024 • 28:28 A Plan to Remake the Middle East
- May 7, 2024 • 27:43 How Changing Ocean Temperatures Could Upend Life on Earth
- May 6, 2024 • 29:23 R.F.K. Jr.’s Battle to Get on the Ballot
- May 3, 2024 • 25:33 The Protesters and the President
- May 2, 2024 • 29:13 Biden Loosens Up on Weed
- May 1, 2024 • 35:16 The New Abortion Fight Before the Supreme Court
- April 30, 2024 • 27:40 The Secret Push That Could Ban TikTok
- April 29, 2024 • 47:53 Trump 2.0: What a Second Trump Presidency Would Bring
- April 26, 2024 • 21:50 Harvey Weinstein Conviction Thrown Out
- April 25, 2024 • 40:33 The Crackdown on Student Protesters
Stormy Daniels Takes the Stand
The porn star testified for eight hours at donald trump’s hush-money trial. this is how it went..
Hosted by Michael Barbaro
Featuring Jonah E. Bromwich
Produced by Olivia Natt and Michael Simon Johnson
Edited by Lexie Diao
With Paige Cowett
Original music by Will Reid and Marion Lozano
Engineered by Alyssa Moxley
Listen and follow The Daily Apple Podcasts | Spotify | Amazon Music | YouTube
This episode contains descriptions of an alleged sexual liaison.
What happened when Stormy Daniels took the stand for eight hours in the first criminal trial of former President Donald J. Trump?
Jonah Bromwich, one of the lead reporters covering the trial for The Times, was in the room.
On today’s episode

Jonah E. Bromwich , who covers criminal justice in New York for The New York Times.

Background reading
In a second day of cross-examination, Stormy Daniels resisted the implication she had tried to shake down Donald J. Trump by selling her story of a sexual liaison.
Here are six takeaways from Ms. Daniels’s earlier testimony.
There are a lot of ways to listen to The Daily. Here’s how.
We aim to make transcripts available the next workday after an episode’s publication. You can find them at the top of the page.
The Daily is made by Rachel Quester, Lynsea Garrison, Clare Toeniskoetter, Paige Cowett, Michael Simon Johnson, Brad Fisher, Chris Wood, Jessica Cheung, Stella Tan, Alexandra Leigh Young, Lisa Chow, Eric Krupke, Marc Georges, Luke Vander Ploeg, M.J. Davis Lin, Dan Powell, Sydney Harper, Mike Benoist, Liz O. Baylen, Asthaa Chaturvedi, Rachelle Bonja, Diana Nguyen, Marion Lozano, Corey Schreppel, Rob Szypko, Elisheba Ittoop, Mooj Zadie, Patricia Willens, Rowan Niemisto, Jody Becker, Rikki Novetsky, John Ketchum, Nina Feldman, Will Reid, Carlos Prieto, Ben Calhoun, Susan Lee, Lexie Diao, Mary Wilson, Alex Stern, Dan Farrell, Sophia Lanman, Shannon Lin, Diane Wong, Devon Taylor, Alyssa Moxley, Summer Thomad, Olivia Natt, Daniel Ramirez and Brendan Klinkenberg.
Our theme music is by Jim Brunberg and Ben Landsverk of Wonderly. Special thanks to Sam Dolnick, Paula Szuchman, Lisa Tobin, Larissa Anderson, Julia Simon, Sofia Milan, Mahima Chablani, Elizabeth Davis-Moorer, Jeffrey Miranda, Renan Borelli, Maddy Masiello, Isabella Anderson and Nina Lassam.
Jonah E. Bromwich covers criminal justice in New York, with a focus on the Manhattan district attorney’s office and state criminal courts in Manhattan. More about Jonah E. Bromwich
Advertisement

COMMENTS
Problem Solving, Mathematical Investigation and Modeling: Learning Guide | PDF | Triangle | Area. LG-M114-10-13 - Free download as PDF File (.pdf), Text File (.txt) or read online for free.
This module is designed to provide you with a background of knowledge in Problem Solving, Mathematical Investigation, and Modeling. It focuses on the following: (1) Problem Solving and Mathematics Education ( a) Problem Solving: Definition. and Process ( b) Problem Solving and Mathematics Education ( c) Problem Solving and the Conceptual
To solve a word problem, students can pick out the numbers and decide on an operation.". But through the use of mathematical modeling, students are plucked out of the hypothetical realm and plunged into the complexities of reality—presented with opportunities to help solve real-world problems with many variables by generating questions ...
Optimization and Mathematical Programming. • Optimization models (also called mathematical programs) represent problem choices as decision variables and seek values that maximize or minimize objective functions of the decision variables subject to constraints on variable values expressing the limits on possible decision choices. [1.3]
The Mathematical Investigation and Modeling is a 3-unit course that introduces mathematical modeling, based on the use of elementary functions, to describe and explore real-world data and phenomena. Graphical, numerical, symbolic, and verbal methods are used in the investigation of data, functions, ... develop a personal framework of problem ...
Mathematics investigation Overview. At its core, mathematics is about problem-solving and modelling the world around us. By giving students meaningful problems to solve they are engaged and can apply their learning, thereby deepening their understanding. ... Using a guided investigation model ensures that students stay focused on the ...
Based on these models but with some modifications, an investigation model (see Fig. 1) was developed for this study to describe the interaction of these processes. An important difference between a mathematical investigation model and a problem-solving model is the additional phase of problem posing after understanding the task in investigation.
Summary. Investigation can play a vital part in the learning of mathematical concepts and in problem-solving. At all stages the teacher has an essential part to play. He sets the scene, providing real materials or a challenging problem when necessary. He observes what his pupils do with these and asks questions which will help their learning.
Mathematics provides a rich source of tools for problem solving and it would be advisable to use such tried and tested methods. However, mathematics can only be applied to mathematical problems and mathematical modeling is a way of formulating a given problem in a mathematical form. These problem-solving phases are depicted in Fig. 2.2.
12.1 What is a model? A model is a representation of reality that allows us to understand something better. There are many types of models, including conceptual, mathematical, and physical models.A physical model is a physical object or set of objects intended to represent something else that is too large, small, complex or otherwise inaccessible for direct investigation.
The rapidly advancing fields of machine learning and mathematical modeling, greatly enhanced by the recent growth in artificial intelligence, are the focus of this special issue. This issue compiles extensively revised and improved versions of the top papers from the workshop on Mathematical Modeling and Problem Solving at PDPTA'23, the 29th International Conference on Parallel and Distributed ...
George Polya (1887-1985) was known as the father of mathematical problem solving in. mathematics education. Polya defines that [2] t here are 4 stages in the problem solving process such. as: (1 ...
The mathematical modeling competence and the problem solving competence are closely related, because the use of different types of models that represent real objects and situations is the premise ...
Graded Assessment in Mathematics (GAIM) GAIM is a teacher assessment scheme for Key Stages Three and Four. The scheme is designed to encourage teaching and learning through practical problem solving and investigations, involve students in all assessment and record keeping and introduce continuous assessment into normal classroom practice.
Problem Solving, Mathematical Investigation, and Modeling (6081) This course intends to enhance the students' knowledge and skills in dealing with real-life and/or non-routine applications of mathematics. Students will have the opportunity to explore the use of problem-solving strategies or heuristics as they engage in mathematical ...
This course will introduce you to mathematical approach in solving routine and non-routine problem, as well as investigating and modeling elementary functions to describe and explore real-world data and phenomena. Problem Solving involves the application of mathematical skills and reasoning to problems encountered in everyday life. Real world problems are not presented in a neat and orderly ...
Solving Mathematical Problems by Investigation. Joseph B. W. Yeo, Yeap Ban Har. Published 1 May 2009. Mathematics, Education. TLDR. This chapter discusses the relationship between problem solving and investigation by differentiating investigation as a task, as a process and as an activity, and shows how the process of investigation can occur in ...
workplace MM problems into school mathematics. Mathematical Problem-Solving The investigation of mathematical PS was pioneered by Pólya [25], who defined it as a heuristic with a four-step process: understanding the problem, devising a plan for solving the problem, carrying out the plan, and looking back to examine the solution.
Taking into consideration the aforementioned different theories of leaning embedded on mathematical modeling, this study attempted to determine the effects of integrating mathematical modeling to the problem solving perform ance and math anxiety of Grade 9 students. In this study, much is given importance to the process rather than the product.
Topic 11.3 Mathematical Investigation: Process versus ActivityTopic 11.4 Mathematical Modelling and the Real-World
Problems and Problem Solving. Problem, defined. Before we engage into mathematical problem solving, let us first explore some definition of the term "problem" as defined by great mathematicians. In general, a problem is a statement or a situation where there is an obstacle between what we have and what we want.
The study explores the capabilities of large language models (LLMs), particularly GPT-4, in understanding and solving geotechnical problems, a specialised area that has not been extensively examined in previous research. Employing a question bank obtained from a commonly used textbook in geotechnical engineering, the research assesses GPT-4's performance across various topics and cognitive ...
On today's episode. Jonah E. Bromwich, who covers criminal justice in New York for The New York Times. Stormy Daniels leaving court on Thursday, after a second day of cross-examination in the ...