
An official website of the United States government
The .gov means it’s official. Federal government websites often end in .gov or .mil. Before sharing sensitive information, make sure you’re on a federal government site.
The site is secure. The https:// ensures that you are connecting to the official website and that any information you provide is encrypted and transmitted securely.
- Publications
- Account settings
Preview improvements coming to the PMC website in October 2024. Learn More or Try it out now .
- Advanced Search
- Journal List
- Springer Nature - PMC COVID-19 Collection


A systematic literature review of how and whether social media data can complement traditional survey data to study public opinion
Maud reveilhac.
1 Lausanne University (Switzerland), Faculty of Social and Political Sciences, Institute of Social Sciences, Life Course and Social Inequality Research Centre, Lausanne, Switzerland
Stephanie Steinmetz
Davide morselli.
2 Swiss Centre of Expertise in Life Course Research LIVES, Lausanne, Switzerland
Associated Data
Please refer to the appendix.
In this article, we review existing research on the complementarity of social media data and survey data for the study of public opinion. We start by situating our review in the extensive literature (N = 187) about the uses, challenges, and frameworks related to the use of social media for studying public opinion. Based on 187 relevant articles (141 empirical and 46 theoretical) - we identify within the 141 empircal ones six main research approaches concerning the complementarity of both data sources. Results show that the biggest share of the research has focused on how social media can be used to confirm survey findings, especially for election predictions. The main contribution of our review is to detail and classify other growing complementarity approaches, such as comparing both data sources on a given phenomenon, using survey measures as a proxy in social media research, enriching surveys with SMD, recruiting individuals on social media to conduct a second survey phase, and generating new insight on “old” or “under-investigated” topics or theories using SMD. We discuss the advantages and disadvantages associated with each of these approaches in relation to four main research purposes, namely the improvement of validity, sustainability, reliability, and interpretability. We conclude by discussing some limitations of our study and highlighting future paths for research.
Introduction
This paper provides a systematic literature review of how social media data (SMD) and traditional survey data have been used complementarily to study public opinion (PO) over the last decade. As social media users represent more than half of the world’s population (see [ 26 ]) and provide continuous reactions to daily socio-political events, it is not surprising that traditional survey research has been concerned about whether such data would make surveys obsolete or whether they could be used complementarily. Addressing these questions is particularly relevant in the area of PO. Social media plays a growing role in the formation of PO as user-generated content on these platforms is increasingly deployed as representations of PO (e.g. [ 27 , 56 ]). In addition, politicians increasingly consider social media, especially Twitter, to be a “barometer” of PO [ 44 ].
Despite the extensive literature about the benefits and challenges of using SMD to answer social and political questions, as well as about SMD as a possible replacement for traditional surveys, a comprehensive overview of the complementarity of both data sources remains limited. The aim of this paper is to fill this gap by providing a systematic literature review focusing on how SMD and survey data can complement each other to study PO. Inspired by the influential study of Japec et al. [ 45 ] which elaborated on the complementarity of survey data and “big data” (rather broadly defined), we want to concentrate, however, on one type of “big data”, namely SMD. There are two main reasons for this choice. First, SMD are a specific type of “non-survey” data which possess specific arrangements (or conventions) and paradata that are different from other types of administrative or “big data”, especially when it come to the assessment of PO. Second, whereas there is substantial research on augmenting survey data with administrative (e.g. electricity or water consumption) or other type of “web data” (e.g. Google searches or citation metrics) to improve estimates of PO or official statistics, we still lack an overarching picture of the (new) developments and approaches of complementing SMD and surveys with each other.
Our analysis is based on an extensive survey of the literature capturing a representative sample of the best published theoretical and empirical scientific papers on the topic (N = 187). We have restricted the analytical period to the last decade (2010–2020) as the discussion on complementarity is still a young field of study (e.g. [ 58 ]). On this basis, we have been able to identify six complementarity approaches which can be synthesised to four major purposes, namely predicting, substituting, comparing, and linking SMD and survey data.
In the next section, we situate our review within the existing literature by demonstrating how the scientific discussion surrounding the opportunities and challenges offered by SMD within survey research has evolved, especially by highlighting the complementary understanding of PO offered by both data sources. Then, we discuss more specifically which research approaches have emerged, and we classify them according to four main research purposes using both data sources complementarily. The analysis of the empirical studies aims to act as a guide for other researchers by identifying research gaps and highlighting the pros and cons of each approach. Furthermore, we underline areas for future improvements and point to technical and ethical considerations. We conclude by mentioning the main contributions and limitations of our review.
Background – The complementary understandings of PO
Surveys have long been the most predictive and accurate tools for collecting and measuring opinion. However, over the last decade, decreasing response rates have called into question the potential of using a random sample of individuals to represent an entire population (e.g. [ 37 , 49 ]), thus posing important concerns about the sustainability of survey research. Even by adapting to new modes, such as push-to-web, to increase response rates, it remains unclear whether surveys will maintain this dominant role as communication habits continue to change (e.g. [ 68 ]). Given the recent “survey crisis” (e.g. [ 13 , 22 ]), an increasingly rich source of PO data is commonly referred to as “big data”. These “new” data take the form of extraordinarily large and complex datasets. There are three attributes that are generally agreed upon to describe this type of data (e.g. [ 19 ]), namely volume, velocity, and variety. Social media are a sub-type of big data where people express their thoughts and opinions with the purpose of sharing them with others [ 18 ]. Due to their inherent properties, SMD have been seen as a promising complementary, and even alternative, source of data for exploring PO. However, researchers acknowledged early on that, almost universally, SMD are non-random, and thus discouraged using them as a means of making generalisable claims. This challenge is well highlighted by Schober et al. [ 68 ], who claim that, while the social media researcher seeks to achieve topic coverage, the survey researcher emphasises population coverage as a central endeavour.
An entire strand of research thus focussed on how surveys and social media differ in several aspects. Table Table1 1 attempts to classify the most prominent differences along which SMD and survey data are typically compared. We have identified several dimensions based on recurring criteria mentioned in the literature concerning the nature of and the relationship between both data sources. Often-cited criteria include the type of population and data signal, the unit of observation and analysis, and the available meta-data (for a thorough discussion of the differences see [ 18 , 68 ], and [ 77 ]).
Differences between SMD and survey data to study PO
SM | Survey | |
---|---|---|
Type of population | Selective | Representative |
Data signals | Platform users and their signals (e.g. posts or tweets, #hashtags, @mentions, retweets, replies) | Opinion survey of individuals with a defined sampling frame |
Types of data | Unstructured texts containing opinions and opinion strength or merely information (e.g. links) | Structured opinions measured by answers to pre-defined and pre-tested survey questions (scales) |
Unit of observation or the level at which data are collected | Can be any of the following: users, search queries or keywords, #hashtags or @mentions, retweets, or replies, likes or emotional reactions, location | Individuals from a sampling frame representing a target population |
Unit of analysis or level at which the data are analysed | Can be any of the following: users, location, texts from a specific topic or sentiment, overall texts, links, or other metadata | Individuals’ responses to survey items or aggregated responses at the country, region or household level |
Meta-data | Set of users’ behavioural information (e.g. network, frequency of use, interactions) and contextual information (e.g. time and location) | Precise and quasi-complete socio-demographic information on individuals and auxiliary data (e.g. number of contact attempts, number of persons in the household) |
To understand how to best use both data sources complementarily, it is also essential to reflect on how they construct PO differently. This is increasingly important, as what constitutes “the public” tends to be forged by the methods and data from which it is derived [ 56 ]. In survey research, PO is equivalent to the private opinion of a representative public, operationalised as a set of positions on a given topic. PO can thus be conceptualised as a reflection of a shared position among citizens on specific issues that are then amplified and reviewed by news media and political actors [ 42 ]. Survey measures of PO are constrained by the scope of the questionnaires, which usually provide little room for spontaneous expressions of opinion (except in open-ended survey questions). The diversity of opinions is thereby reduced into a set of discrete and aggregate data (e.g. [ 75 ]). Conversely, the reliance on social media for measuring PO expands the societal and collective components of opinions [ 59 ] by conceptualising it in Habermas’ [ 39 ] terms as a complex system of representations. In this respect, SMD are better suited to capturing the conversational and relational nature of PO formation [ 3 ]. Hence, where survey data weigh precision and standardisation, SMD excel in multidimensionality and polyphony. In addition to their focus on solicited private opinions, surveys are also less reactive to opinion changes than SMD. In theory, opinion changes could be assessed by frequent short opinion surveys (e.g. every two months). However, the advantage of SMD is that they can cover opinion change more rapidly (and on an ad hoc basis), thus reacting faster to events, which is almost impossible for surveys (e.g. it takes more time to set up probability-based surveys for the study of COVID compared to what can be done with SMD).
Despite the advantages offered by social media for measuring more social and timelier opinions, the reliance on SMD raises important questions for empirical research on (automated) measurements of opinions and on the choice of the indicators employed to model opinions. Indeed, constructing measures of PO based on SMD can be very time consuming and can involve a lot of pre-processing effort before the data can be translated into meaningful measures of expressed opinions. Furthermore, it sometimes remains quite difficult to know what is driving the evolution of ideas and concerns found in online conversations. Consequently, a current strand of research seeks to better understand the issues of representativeness of social media communities and the validity of measured opinion, especially opinions stemming from sentiment analysis. While there is a rising interest in applying SMD to understand opinion, and even to replace traditional surveys (e.g. [ 3 , 32 ]), SMD alone are of limited use for social scientific research as they usually provide incomplete and imprecise information. However, the issues associated with SMD are not necessarily fatal to the proposition that they can be used to generate social insights, especially in complementing survey data. An efficient strategy to enhance research lies, therefore, in the analysis of how both data sources can complement each other in ways that maximise their strengths.
In the next sections, we aim to show that there is a plethora of research practices in which both data sources complement each other for the study of PO. To date, however, there is still no consensus about the best way to use SMD for studying PO [ 58 ]. We are now at a point where we should reflect on what has been done so far, what lessons we can learn from it, and then specify suitable trends for social research. In this paper, we seek to fill this gap by reviewing research that uses both data sources complementarily for the purposes of measuring PO and by providing a critical evaluation of the identified research paths.
Method of analysis: Building a corpus of relevant articles
To build our corpus of scientific articles, we carried out several searches in bibliographic databases (focusing on Scopus and Google Scholar ) using the software PublishOrPerish [ 40 ]. We obtained an initial corpus of 3596 unique papers, which we reduced to papers that were relevant for the scope of our review. The initial corpus was deliberately based on a search-query that was broad enough to collect the relevant literature, while not missing important papers. We used the query “(social media OR twitter OR facebook OR instagram OR reddit) AND (survey OR surveys OR polls)” and specified that it should appear in the body of the text (using the keyword field) instead of appearing only in the title or abstract, which were found to be too restrictive to capture the literature of interest. The query was designed to restrict the focus of our review to SMD, thus ignoring other types of “big data” or “digital trace” data.
A first filter was applied to reduce the number of papers to journal articles, book chapters, and scientific reports (thus excluding books, theses, and conference papers) as we wanted to concentrate on high-valued scientific sources which have already been approved by the scientific community. In this respect, including conference papers would have drastically inflated the number of (duplicated) papers concerned with predictions and with replicating previous studies using alternative methods of analysis and algorithms. Among the remaining papers, we applied two eligibility criteria to disregard those that were not pertinent to the analysis as i) their focus was not on PO, ii) they were oriented towards a specific aspect of data treatment (e.g. estimating socio-demographics from texts or profile pictures) or an analytical strategy (e.g. elaborating algorithms). We also excluded articles mentioning survey findings without an explicit aim of supplementing, comparing, or combining those with SMD.
Results of the literature review on the uses of social media as a complement to surveys
Overall, the collection protocol left us with 187 papers - 141 of an empirical and 46 of a theoretical nature (these papers can be found in the Appendix). Most of these papers stem from political communication and computational social sciences journals. Although the sample of 187 papers may not cover the whole corpus of research on the subject, it is nonetheless sufficient to highlight the main research directions that have been endorsed on the topic of complementarity. Figure Figure1 1 provides an overview of the yearly repartition of the retrieved papers differentiating between those with a theoretical (N = 46) and an empirical (N = 141) focus. While the number of theoretical papers remains stable over the years, we can see a steady increase in empirical papers over time.

Number of empirical and theoretical articles according to our meta-review of the existing literature using surveys and SMD
Theoretical insights
Starting with the theoretical papers in our review (N = 46, see Table Table2 2 in the Appendix), survey and social media researchers have explored ways in which social media and survey data can yield congruent conclusions (e.g. [ 68 ]). One part of these articles (n = 14) tries to establish a framework regarding the predictive power of SMD as a potential substitute for surveys. This line of research stems principally from the fields of election and economy forecasting (for recent reviews see [ 15 , 66 ]).
List of theoretical papers focusing on the combination of survey and social media data (n = 46, continues next pages)
Author(s) | Date | Title | Source | Focus |
---|---|---|---|---|
Blumenthal | 2005 | The Public Opinion Quarterly | General | |
Gayo-Avello | 2011 | Communications of the ACM | Prediction | |
Sobkowicz et al. | 2012 | Government Information Quarterly | Prediction | |
Boyd & Crawford | 2012 | Information, Communication & Society | General | |
Metaxas & Mustafaraj | 2012 | Science | Prediction | |
Gayo-Avello | 2012 | arXiv Preprint | Prediction | |
Gayo-Avello | 2012 | IEEE Internet Computing | Prediction | |
Smith | 2013 | International Journal of Public Opinion Research | Ontology | |
Couper | 2013 | Survey Research Methods | General | |
Baker et al. | 2013 | Journal of Survey Statistics and Methodology | General | |
Schoen et al. | 2013 | Internet Research | Prediction | |
Gayo-Avello | 2013 | Social Science Computer Review | Prediction | |
Stieglitz & Dang-Xuan | 2013 | Social Network Analysis and Mining volume | General | |
Gayo-Avello et al. | 2013 | Internet Research | Prediction | |
Ruths & Pfeffer | 2014 | Science | Behaviour | |
Tufekci | 2014 | arXiv Preprint | Error sources | |
Murphy et al. | 2014 | Book chapter (1): Social Media, Sociality, and Survey Research | Ontology | |
Murphy et al. | 2014 | Public Opinion Quarterly | General | |
Hill & Dever | 2014 | Book chapter (12): Social Media, Sociality, and Survey Research | General | |
Tang et al. | 2014 | ACM SIGKDD Explorations Newsletter | Ontology | |
Zagheni & Weber | 2015 | International Journal of Manpower | Demographic | |
Resnick et al. | 2015 | The American Academy of Political and Social Science | General | |
Ampofo et ak. | 2015 | Book chapter (8): Innovations in Digital Research Methods | Ontology | |
Hargittai | 2015 | The American Academy of Political and Social Science | General | |
Schober et al. | 2016 | Public Opinion Quarterly | General | |
Olteanu et al. | 2016 | Frontiers in Big Data | Error sources | |
Junngherr | 2016 | Journal of Information Technology & Politics | Prediction | |
Spiro | 2016 | Current Opinion in Psychology | Linking | |
RJ Dalton | 2016 | International Journal of Sociology | Behaviour | |
Johnson & Smith | 2017 | Book chapter: Seeing Cities Through Big Data | Linking | |
Hsieh & Murphy | 2017 | Book chapter (2): Total Survey Error in Practice | Error sources | |
Salleh | 2017 | Science | General | |
Pal | 2017 | Current Opinion in Behavioral Sciences | Small data | |
Salunkhe et al. | 2017 | International Journal of Advanced Research in Science, Communication and Technology | Prediction | |
Klašnja et al. | 2018 | Book chapter: The Oxford Handbook of Polling and Survey Methods | General | |
Jungherr | 2018 | Book chapter: Digital Discussions | General | |
Kwak & Cho | 2018 | Asian Journal for Public Opinion Research | Prediction | |
Szreder | 2018 | Archives of Data Science | General | |
Freelon | 2019 | Book chapter: Digital Discussions | Demographic | |
Trottier | 2019 | Fast Capitalism | General | |
Sen et al. | 2019 | arXiv Preprint | Error sources | |
Salvatore et al. | 2020 | Social Indicators Research | Error sources | |
Stier et al. | 2020 | Social Science Computer Review | Linking | |
Romele et al. | 2020 | AI & SOCIETY | Ontology | |
Skoric et al. | 2020 | Information | Prediction | |
Rousidis et al. | 2020 | Multimedia Tools and Applications | Prediction | |
Chauhan et al. | 2020 | Journal of Ambient Intelligence and Humanized Computing | Prediction |
Another strand of theoretical articles (n = 14) focuses instead on the compliance of social media research with established reporting standards so as to guarantee transparency and replicability (e.g. [ 51 ]). Finding ways of integrating data obtained from different sources (n = 3) also constitutes a fertile path of research [ 46 ]. In this respect, Stier et al. [ 72 ] provide the most advanced guide on how to systematically link survey data with information from external data sources, including SMD, at different level of analysis. The authors demonstrate that integrating traditional survey data and digital trace data is of growing interest, notably because of the limited reliability of self-reported behavioural measures and declining response rates. Additionally, enriching survey data with SMD could also help to reduce unit non-response and to control for the unrepresentativeness of SMD, as they are limited to those respondents having social media profiles and consenting to the linkage. Finally, a smaller share of research (n = 5) focuses on developing a quality assessment framework for SMD which is similar to the Total Survey Error (TSE) [ 11 , 38 ]. The TSE framework has been extended to encompass SMD and their inherent quality challenges (see the studies by Sen et al. [ 70 ] on Twitter-based studies and Jungherr [ 47 ] for a measurement theory to account for the pitfalls of digital traces). In a similar vein, Hsieh and Murphy [ 43 ] analysed the potential benefits of evaluating estimates from surveys and SMD in common terms and arrived at a general error framework for Twitter opinion research. Olteanu et al. [ 61 ] went a step further by pointing to the errors and biases that could potentially affect studies based on digital behavioural data, outlining them in an idealised study framework. The paper by Sen et al. [ 70 ] provides the most advanced framework to date. It involves potential measurement and representation errors in a digital trace-based study lifecycle where they are classified according to their sources.
Other research (n = 5) tackles the ontology of SMD as compared to survey data. In these papers, prevalent discussions revolve around the conception of opinion as measured by both data sources, as well as debates related to the evolution of “new” research “paradigms” or “digital hermeneutics”. The remaining papers concentrate on behavioural research (n = 2), demographic research (n = 2), and small data analysis in political communication (n = 1).
Overall, the considered theoretical articles stress the importance of developing a framework that accounts for possible biases of SMD while remaining in, or mirroring, the TSE. Moreover, they also emphasize the need, in this debate, to focus on the complementarity rather than the replacing aspect, notably by developing clear and reliable linking strategies. These articles also encourage researchers to go beyond the dominant model for understanding PO from probability sample surveys to encompass other (“new”) expressions of opinions (e.g. Murphy et al. 2014) that can possibly supplement or even replace survey-based approaches.
Empirical insights
The empirical literature (N = 141) focuses on a rather narrow set of topics, such as elections, political issues, and approval ratings for the presidency (64%). Another important area of PO research using SMD complementarily with survey data is related to health (e.g. vaccination, drugs, etc.), equality issues, and climate or environment-related concerns. Most empirical studies in our review are based on Twitter data (73%), followed by Facebook (18%) and other social media (9%). This is related to the fact that not all social media platforms provide the same degree of data accessibility [ 8 ]. For instance, Facebook imposes severe limitations on the scope of retrievable data, whereas Twitter has less strong privacy settings, allowing researchers to get access to Twitter’s historical data.
Overall, we derived six major approaches on how survey data and SMD can complement each other namely i) predicting social and political outcomes using SMD (n = 48), ii) comparing both data sources on a given phenomenon (n = 26), iii) using survey measures as a proxy in social media research (n = 18), iv) enriching surveys with SMD (n = 9), v) recruiting individuals on social media to conduct a second survey phase (n = 8), and vi) generating new insight on “old” or “under-investigated” topics or theories using SMD (n = 32). These approaches can be synthesised in four, partly overlapping, ‘data complementing’ research purposes: i) validating survey findings with SMD, ii) improving the sustainability of the research by diversifying the views on a phenomenon, iii) improving the reliability of survey measures by specifying measurements, and iv) improving the interpretability of social or political issues. Figure Figure2 2 summarises the relationship between the six approaches and the four research purposes. Furthermore, it shows that each purpose leads to a typical way of using both data sources complementarily. For instance, improving reliability by specifying a research question involves data linkage strategies, while generating new insights involves a sequential use of social media and survey stages.

Complementary approaches using SMD and survey data for the study of PO
The analysis of our corpus suggests that the biggest part of research concentrates on whether SMD can potentially substitute survey data (n = 48, see Table Table3 3 in the Appendix). This has mostly been done by trying to replicate survey findings by using SMD for forecasting (see recent review by [ 66 ]). The aim to predict real-world outcomes with SMD in the realm of PO has essentially been applied to elections. Most of these papers directly refer to the much-cited study of O’Connor et al. [ 60 ] which purpose is to validate SMD against survey findings. While research in this area has tested a range of different methodologies, the results remain inconclusive, and only in some cases could elections be accurately predicted (e.g. [ 31 , 47 ]). Recent literature reviews on the use of SMD for running electoral predictions (e.g. [ 15 ]) classify studies according to the employed methods of prediction, such as volume, sentiment, or network approaches. These reviews show considerable variance in the accuracy of predictions, which, on average, lag behind the established survey measurements. A common problem of the aforementioned studies lies in the decision about which approach can most accurately yield predictions (but also which social media platforms are better suited, and how that varies in different geographical or temporal contexts). This inference problem is quite complex as various elements are involved in skewing the samples in social media debates. To date, the inconclusive state of the research has led to a research agenda aiming to respond to the plea from Gayo-Avello et al. [ 33 ] for a “model explaining the predictive power of social media” (p. 490). In this realm, for instance, the study of Pasek et al. (2019) assesses how patterns of approval among population subgroups compare to tweets about the president, while disentangling effects at the individual and group levels of analysis. On a more theoretical level, the study by Schober et al. [ 69 ] seeks to elaborate when and under what conditions SMD can be used to make valid inferences. However, the inconclusive state of the research may also be linked to the fact that predictions are often done based on the content created by users and overlook the characteristics of the creating users. For instance, SMD can be biased towards a particular group (see [ 5 , 24 ]). Moreover, interactions on social media platforms are not always the product of individuals, but also bots, organisations, political parties, etc. [ 80 ]. Based on the evaluation of the body of articles falling under the ‘substitution paradigm’, a path for future research could be to better account for the characteristics of social media users, insofar as these characteristics can be useful for assessing how individual tweets can be converted into meaningful measures of expressed opinion. To do so, future studies could survey social media users identified using relevant key terms (e.g. hashtags or mentions) to gauge the relationship between social media measures of their sentiment and survey measures of their attitudes.
List of publications combining social media and survey data for prediction purposes (n = 48, continues next pages)
Author(s) | Date | Title | Source | SM | Topic | Level of analysis |
---|---|---|---|---|---|---|
Tumasjan et al. | 2011 | Social Science Computer Review | election | national | ||
Aparaschivei | 2011 | Journal of Media Research-Revista de Studii Media | Twitter & Facebook & Youtube | election | national | |
Jungherr et al. | 2012 | Social science computer review | election | national | ||
Borondo et al. | 2012 | Chaos An Interdisciplinary Journal of Nonlinear Science | election | national | ||
Jungherr et al. | 2012 | Social science computer review | election | national | ||
Choy et al. | 2012 | arXiv Preprint | election | national | ||
Borondo et al. | 2012 | Chaos: An Interdisciplinary Journal of Nonlinear Science | election | national | ||
González-Bailón et al. | 2012 | Human Communication Research | other social media (Usenet) | presidential approval | national | |
Franch | 2013 | ocial Media | Journal of Information Technology & Politics | Facebook, Twitter, Google, & YouTube | election | national |
Kermanidis & Maragoudakis | 2013 | Int. J. Social Network Mining | election | national | ||
Fu & Chan | 2013 | Cyberpsychology, Behavior, and Social Networking | online discussion forums, personal blogs, and microblogs | election | local | |
DiGrazia et al. | 2013 | PloS one | politics | district | ||
Paul & Dredze | 2014 | PloS one | health | national | ||
Cheng & Chen | 2014 | social media | Aslib Journal of Information Management | politics | regional | |
Ceron et al. | 2014 | New media & society | politics | national | ||
Ceron et al. | 2015 | Social Science Computer Review | election | national | ||
Murthy | 2015 | Information, Communication & Society | election | State | ||
Eom et al. | 2015 | PloS one | election | national | ||
Wong et al. | 2015 | Journal of medical internet research | health | national | ||
Durahim & Coşkun | 2015 | Technological Forecasting and Social Change | well-being | national & regional | ||
Huberty | 2015 | ocial Media | International Journal of Forecasting | election | national | |
Jungherr et al. | 2015 | Social Science Computer Review | election | national | ||
Burnap et al. | 2016 | Electoral Studies | election | national | ||
Cody et al. | 2016 | arXiv Preprint | presidential approval | national | ||
Beauchamp | 2017 | American Journal of Political Science | election | State | ||
Yaqub et al. | 2017 | Government Information Quarterly | election | national | ||
Lopez et al. | 2017 | Statistics, Politics and Policy | Brexit | national | ||
Vepsäläinen et al. | 2017 | Government Information Quarterly | election | national | ||
Feng et al. | 2017 | Tobacco Control | health | national | ||
Kristensen et al. | 2017 | PloS one | politics | national | ||
Beauchamp | 2017 | American Journal of Political Science | election | national | ||
Oliveira et al. | 2017 | Journal of Information Technology & Politics | election | national | ||
Masoomali et al. | 2018 | World Development | equality (gender, immigration, LGB, etc.) | international | ||
Bastos & Mercea | 2018 | Information, Communication & Society | Brexit | constituencies | ||
Chmielewska-Szlajfer | 2018 | versus | Media, Culture & Society | election | national | |
Pasek et al. | 2018 | Public Opinion Quarterly | economic satisfaction | national | ||
Heredia et al. | 2018 | ocial media | Social Network Analysis and Mining | election | national | |
Zhang | 2018 | ocial media | PloS one | election | national | |
Bansal & Srivastava | 2018 | Procedia Computer Science | election | State | ||
Grimaldi | 2019 | Social Network Analysis and Mining | election | national | ||
Awais et al. | 2019 | Journal of Ambient Intelligence and Humanized Computing | election | national | ||
Pasek et al. | 2019 | Social Science Computer Review | presidential approval | national | ||
Jaidka et al. | 2019 | Asian Journal of Communication | election | international | ||
Pasek et al. | 2020 | Social Science Computer Review | presidential approval | national | ||
Chin & Wang | 2020 | Journal of Forecasting | election | County & city | ||
Stieglitz et al. | 2020 | Information Systems Frontiers | election | international | ||
Gong et al. | 2020 | social media | Plos one | election | State | |
Sepúlveda & Norambuena | 2020 | Intelligent Data Analysis | election | national |
The second dominant approach in our review is related to how surveys can be enriched with SMD (n = 9, see Table Table4 4 in the Appendix). Here, SMD are collected with the intention of improving the reliability of survey measures at the individual or aggregate level. Replication of survey-based opinions can be difficult, either because of improper interpretation of the findings or because insufficient information has been provided. Such issues undermine the credibility of survey research and make it difficult to evaluate the contributions of a given study. Research aiming to enrich surveys with SMD most often implies the adoption of a data-linking strategy. This can be done, for instance, either at the user level, public actor level, geographic level, or temporal level (see [ 72 ]). Enriching surveys with SMD can serve several goals. First, it can help to augment the explanatory potential of survey measures. For instance, De Sio & Weber [ 23 ] adopted an innovative research design to explain election outcomes based on party strategy on social media with respect to policy issue salience. They did this by linking representative mass surveys from six European countries with Twitter analysis of campaign activity. Second, enrichment of survey data with SMD can also help to test research hypotheses by relying on “true” behavioural measures (instead of self-reported survey measures). For instance, Karlsen and Enjolras [ 48 ] linked candidate survey data with Twitter data to study styles of social media campaigning. These differences in campaigning styles were then related to the extent to which candidates were successful on Twitter. Third, SMD also offer an opportunity to address issues of item non-response and calibration of novel measures. For instance, Shin [ 71 ] studied the extent to which social media users selectively consumed like-minded news stories by linking survey responses from Twitter users with their media following and exposure to news via their friends. The study further showed some differences between self-reports and digital measures, such as more pronounced patterns of selective exposure in the SMD. Finally, linking social survey and SMD further provides an opportunity to explore the relationship between attitudes and beliefs reported through surveys and content (and behaviours) generated online. For instance, Cardenal et al. [ 14 ] combined survey and Web-tracking data to analyse how Facebook-referred news consumption influenced social media users’ agendas. They found that selective exposure increased with amplified news consumption. The core problem in these studies lies in gaining consent to carry out the data linkage. This constitutes a complex procedure in which issues of anonymity, security, and disclosure all come to the fore. An additional problem is that social media measurements provide only one partial view of opinions. For instance, while researchers can measure how many times a given message has been liked, shared, or retweeted, it is much harder to account for (or measure) how often a given message has been seen or has attracted attention. Moreover, our corpus shows that research relying on linking strategies tends to remain at the individual and public actor levels of analysis, which requires requesting consent to use the linked data. This may, in turn, introduce consent or selection bias. To mitigate such difficulties, future studies should also explore the potentials of linking both data sources at higher levels of analysis, such as country or according to topicality level.
List of publications combining social media and survey data for enrichment purposes (n = 9)
Author(s) | Date | Title | Source | SM | Topic | Level of analysis |
---|---|---|---|---|---|---|
Vaccari et al. | 2015 | social media | Journal of Computer-Mediated Communication | politics | national | |
Karlsen & Enjolras | 2016 | The International Journal of Press/Politics | election | national | ||
Hofstra et al. | 2017 | American Sociological Review | equality (gender, immigration, LGB, etc.) | national | ||
Quinlan et al. | 2018 | Information, Communication & Society | Twitter & Facebook | political communication | national | |
Stier et al. | 2018 | social media | Political communication | Twitter & Facebook | politics | national |
Cardenal et al. | 2019 | International Journal of Public Opinion Research | politics | national | ||
Jacbs & Spierings | 2019 | Information, Communication & Society | politics | national | ||
De Sio & Weber | 2020 | West European Politics | politics | international | ||
Shin | 2020 | ocial Media | Social Media + Society | politics | national |
A third purpose is to use surveys as a proxy in social media research. This approach therefore reverses the logic that SMD are always used as a complementary (side) element of the main survey-based analyses. In this kind of “survey proxy approach” (n = 18, see Table Table5 5 in the Appendix), SMD are used as the main source of analysis, while the survey data are used for contextualising or calibrating SMD. A first strand of research relies on SMD to complement traditional research approaches in political communication and citizens’ political engagement. For instance, the assessment of the importance of given public concerns in PO has been measured extensively with the “most important problem” survey item. Social media provide another way to measure this concern in an unintrusive way by (semi-)automatically classifying the content of social media texts, while also accounting for the extent to which different actors are responsive to these concerns. Following this logic, the study conducted by Eberl et al. [ 28 ] investigated the effects of sentiment and issue salience on emotionally labelled responses to posts written by political actors on Facebook. Another study, by Plescia et al. [ 64 ], analysed the responsiveness of populist parties to the issue salience amongst the public. They did this by relying on survey data to measure public salience and tweets to assess salience issue for parties. A second strand of studies aims at facilitating cross-national comparisons. For instance, a possible application consists in using survey data for classifying parties and voters along important dimensions (e.g. see [ 30 ]). Here, parties were placed on a left and right spectrum using the Chapel Hill Expert Survey [ 4 ]. Party score on the overall ideological stance was then used as an explanatory variable in subsequent analysis. Another example is the study by Park et al. [ 62 ] which investigated the consumption of popular YouTube videos in countries that differ in cultural values, language, gross domestic product, and Internet penetration rate. A possible issue encountered by these studies is linked to spurious effects between survey and social media measurements (e.g. misleading or unexplained correlations). Furthermore, these studies tend to remain poorly equipped to explain actual motives behind social media users’ expression of opinions or reactions. The “survey as proxy” approach requires a considerable dose of ingenuity and methodological innovation to mine social media for producing opinion estimates that can be merged with survey estimates. For instance, SMD corpora often deviate from a predefined (survey) coding scheme. Substantively, a future path of research should take advantage of the fact that a growing number of societal issues have become transnational, such as immigration, terrorism, women’s rights, and climate change. Such research could involve the combination of word embeddings and survey opinion measures at the country level.
List of publications using survey as proxy with social media data (n = 18, continues next page)
Author(s) | Date | Title | Source | SM | Topic | Level of analysis |
---|---|---|---|---|---|---|
Vaccari & Nielsen | 2013 | Journal of Information Technology & Politics | Facebook, Twitter, and YouTube | election | national | |
LaMarre & Suzuki-Lambrecht | 2013 | Public relations review | election | users | ||
Jensen & Anstead | 2013 | Policy & Internet | election | State | ||
Larsson | 2015 | Journal of Information Technology & Politics | political communication | international | ||
Theocharis et al. | 2016 | Journal of Communication | politics | national | ||
Ceron & d’Adda | 2016 | New media & society | politics | national | ||
Park et al. | 2017 | PLoS one | Youtube | other | international | |
Ernst et al. | 2017 | Information, Communication & Society | Twitter & Facebook | politics | national | |
Stier et al. | 2018 | social media | Political communication | Twitter & Facebook | political communication | national |
Barberá & Zeitzoff | 2018 | social media | International Studies Quarterly | Twitter & Facebook | political communication | international |
Rossini et al. | 2018 | Journal of Information Technology & Politics | Twitter & Facebook | political communication | national | |
Rossini et al. | 2018 | Social Media | Social Media + Society | Twitter & Facebook | political communication | national |
Rossini et al. | 2018 | ocial media | Social Media + Society | Twitter & Facebook | political communication | national |
Plescia et al. | 2019 | Representation | politics | international | ||
Wells et al. | 2020 | New Media & Society | politics | national | ||
Lazarus & Thornton | 2020 | Social Science Computer Review | politics | national | ||
Daniel & Obholzer | 2020 | Research & Politics | political communication | international | ||
Eberl et al. | 2020 | Journal of Information Technology & Politics | politics | national |
A fourth approach aims to compare SMD with survey responses that directly measure PO. Studies comparing SMD with survey data (n = 26, see Table Table6 6 in Appendix) essentially aim at improving sustainability of the research, which consists in the ability to gauge PO consistently over time. Sustainability thus implies that we should develop designs that include opportunities for “holistic merging” of the data that will generate more inclusive and fine-grained research insights. There are several reasons that comparing both data sources is meaningful for social research. Firstly, comparing SMD and survey data can be very useful in times of protests and collective actions, notably due to the difficulty of generating survey data to properly assess these disruptive changes (see critique of survey data by Lee [ 53 ]). The timing of an event might indeed not coincide with the timing of a survey, which is often done ex-post. For instance, Davis et al. [ 21 ] examined the extent to which tweets about the affordable care act (“Obamacare”) could be used to measure PO over time. Secondly, social media can be compared to surveys for research questions that require chronicity, on a weekly or daily basis, thus going beyond the few ongoing surveys that collect data monthly or yearly. For instance, Diaz et al. [ 25 ] demonstrated how social media activity functions like an “opt-in panel” where users repeatedly discuss the same topics. This allows us to study, longitudinally, quite rapid shifts in individual opinions and behaviours, thus complementing survey panels which are prohibitively expensive. Another example is the study by Loureiro & Alló [ 54 ], which aimed to complement surveys by providing up-to-date measurements about social concerns when debating mitigation and energy transition paths. Thirdly, survey questions are often designed to capture internal attitudes toward a specific object. However, the relevance of certain survey questions might vary over time and, in some cases, might no longer correspond to the issues discussed spontaneously online. For instance, at a geographical level, the study by Scarborough [ 67 ] compared gender equality attitudes found in survey data to sentiments emanating from tweets. Fourth, SMD can produce quicker and less expensive statistics for enabling informed policy and program decisions. However, this requires gaining knowledge of where any possible disparities in attitude distributions between SMD and survey data may lie. In this respect, the study by Amaya et al. [ 2 ] presented recent advancements. The authors compared attitude distributions between Reddit users and survey measures of political leaning, political interest, and policy issues. They showed that Reddit users tend to have more centrist and normally distributed scores than the survey data, skewing estimates toward the conservative end of the spectrum on all attitude measures. Another study, from Pasek et al. (2020), explained that SMD might be better conceived as providing insights about public attention rather than (“survey like”) attitudes or opinions. To do so, the authors compared tweets mentioning the presidential candidates and open-ended survey questions about the candidates to assess whether spikes surrounding political events correlate between both data sources. Results display some support for the correlation between social media attention and survey data, but they also show systematic differences that need to be better understood to assess when SMD can best generate insights about select topics. The research comparing both data sources tends to remain focused on volume analysis and tonality assessment. This type of research also tends to pay little attention to the domain-specificity of the SMD collected as well as to ways of mitigating replicability and consistency issues (e.g. [ 34 ]). For instance, the evolution of search queries around a given theme might lack precision and consistency over time. The connotation of hashtags can change or whole hashtags can even disappear. Better combining both data sources also requires elaborating more sophisticated measures of opinion and attitudes. One could think about pushing forward “stance detection” in complement to “sentiment detection”, but also about advancing “narrative analysis” in complement to “topic or frame detection”. These are avenues where computational social research would benefit from the expertise of applied computational linguistics.
List of publications combining social media and survey data for comparison purposes (n = 26, continues next pages)
Author(s) | Date | Title | Source | SM | Topic | Level of analysis |
---|---|---|---|---|---|---|
King et al. | 2013 | Health policy | health | national | ||
Tsou et al. | 2013 | Cartography and Geographic Information Science | Twitter (+ web pages) | election | national | |
Kim et al. | 2013 | Book chapter (3): Social Media, Sociality, and Survey Research | politics | national | ||
Ceron et al. | 2014 | New media & society | election | national | ||
Barry | 2014 | Environmental management | FlickrTM (pictures+comments) | climate & environment & energy | national | |
Van Dalen et al. | 2015 | Journal of Information Technology & Politics | politics | national | ||
Jungherr et al. | 2016 | Journal of Computer-Mediated Communication | election | national | ||
Bhattacharya et al. | 2016 | Journal of the Association for Information Science and Technology | politics | national | ||
Diaz et al. | 2017 | PloS one | politics | international | ||
Grčar et al. | 2017 | Computational social networks | Brexit | national | ||
Davis et al. | 2017 | Journal of medical Internet research | health | national | ||
Heikinheimo et al. | 2017 | User-generated geographic information for visitor monitoring in a national park: A comparison of social media data and visitor survey | International Journal of Fgeo-Information | other | regional | |
Bajaj | 2017 | Asian Survey | political communication | national | ||
Wang et al. | 2018 | Sustainability | other social media | other | local | |
Farhadloo et al. | 2018 | JMIR Public Health Surveillance | health | national | ||
Howell et al. | 2018 | Politics and the Life Sciences | health | national | ||
Wainger et al. | 2018 | Ecological Economics | climate & environment & energy | national | ||
Nawa et al. | 2018 | American Journal of Transplantation | health | national | ||
Scarborough | 2018 | Socius | equality (gender, immigration, LGB, etc.) | region & State & national | ||
Mancosu & Bobba | 2019 | PloS one | Facebbok | politics | national | |
Merkley et al. | 2020 | Canadian Journal of Political Science | Twitter (+GoogleTrend) | health | national | |
Loureiro & Alló | 2020 | Energy Policy | climate & environment & energy | international | ||
Amaya et al. | 2020 | book chapter (5): Big Data Meets Survey Science: A Collection of Innovative Methods | health | national | ||
Klingeren et al. | 2020 | Public opinion on Twitter? How vote choice and arguments on Twitter comply with patterns in survey data, evidence from the 2016 Ukraine referendum in the Netherlands | Acta Politica | politics | national | |
Pasek et al. | 2020 | book chapter (6): Big Data Meets Survey Science: A Collection of Innovative Methods | campaign events | national | ||
Nowak et al. | 2020 | Comparing covariation among vaccine hesitancy and broader beliefs within Twitter and survey data | PloS one | health | national |
A fifth approach implicates using SMD to generate new insights. This is especially useful when survey data are not available or when survey data are not recent enough (n = 32, see Table Table7 7 in Appendix). Here, the main purpose is to improve the interpretability of the research by adopting an “ethnographic” methodology. By avoiding rigid research design plans, SMD can remain responsive to, and pursue, new paths of discovery as they emerge. Based on the papers collected, we found typical reasons for relying on SMD to generate new insights, such as capturing emergent opinions, expanding the scope of survey measures, validating survey measures, proposing novel approaches to get a more nuanced or dynamic perspective on PO, and making causal analyses (see column “Reason to complement” in Table Table6 6 in Appendix). When used for capturing emergent opinions, SMD allow us to study the topical and normative climate around specific issues for which we have no theoretically grounded ideas yet. In this exploratory design, social media can provide survey researchers with a snapshot of important societal and political concerns worth surveying in future research. This is especially useful for emerging topics, such as nuclear power (e.g. [ 50 ]) or health-related policies [ 65 , 74 ]. On these emerging issues, SMD can be used in an exploratory or ethnographic perspective to generate initial and qualitative insights into under-studied research objects in order to develop quantitative survey measurements. SMD can also be useful for expanding the scope of survey measures on topics that are difficult to survey. For instance, Hatipoğlu et al. [ 41 ] used SMD to study international relationships with a case study on Turkish sentiments towards Syrian refugees using Twitter. Another study by Guan et al. (2020) relied on the social media platform Weibo to study Chinese views of the United States. SMD can also be useful for validating survey measures. For instance, the study by Dahlberg et al. [ 20 ] investigated the meanings of democracy in a cross-country perspective to better understand differences in the usage of the term “democracy” across languages and countries. The authors’ findings aimed to inform survey measurements about the different conceptualisations of democracy, notably by highlighting translations and language equivalence issues in survey items. Another reason is to propose novel approaches for achieving a more nuanced or dynamic perspective on PO. For instance, researchers can add new components and improve “old findings”, which are difficult to measure with survey data. In this view, the study by Barberá et al. [ 7 ] modelled policy issue responsiveness using Twitter data, thus going beyond the more static perspective on issue congruence offered by surveys. In another study, Clark et al. [ 16 ] investigated organisational legitimacy in a case study about public reactions on social media to the Supreme Court’s same-sex marriage cases. The authors argued that SMD can lessen some of the limitations of survey research in the field, notably by accessing not just policy positioning among individuals but also a variety of features of political discourse, such as opinion intensity and emotions like anger or happiness. SMD can also be used to make causal inferences in order to understand changes in opinion before and after an event, such as measuring the effect of a promulgated law on PO [ 1 ]. Here, SMD allow researchers to rely on spontaneous opinions expressed online rather than on retrospective survey questions, and this can help develop policy initiatives. For instance, Tavoschi et al. [ 73 ] used Twitter as a “sentinel system” to assess the orientation of PO in relation to vaccination. Despite the advantages of SMD in providing new research insights, these studies tend to lack a rigorous contextualisation of the findings derived from SMD. In this respect, a reliance on SMD would benefit from implementing sequential designs, where social media help to identify specific populations or sub-topics, which could then lead to a second quantitative survey phase. Whenever possible, SMD would further benefit from a comparison with longitudinal surveys to assess the extent to which both data sources reveal similar dynamics of change. Future studies could further exploit SMD’s ability to generate new insights for research in sensitive fields, such as war, racism, sexual orientation, and religious beliefs. These are often topics on which it remains difficult to collect survey data, notably because of the social desirability bias (e.g. [ 52 ]) and the like (e.g. extreme response style, moderacy bias, and acquiescence), but also because of the fear of being denounced or because the topic is controversial.
List of publications combining social media and survey data for generating new insights (n = 32, continues next pages)
Author(s) | Date | Title | Source | SM | Topic | Reason to complement | Level of analysis |
---|---|---|---|---|---|---|---|
Ampofo et al. | 2011 | Information, Communication & Society | election | what citizens think about surveys | national | ||
Robillard et al. | 2013 | Journal of Medical Internet Research | Yahoo! Answers | health | capture emergent opinions | international | |
Cavazos-Rehg et al. | 2014 | Journal of Medical Internet Research | health | capture emergent opinions | international | ||
Russell Neuman et al. | 2014 | Journal of Communication | Twitter, blogs, forum commentaries, and traditional media news stories | politics | alternative to self-reported measures | national | |
Kim & Kim | 2014 | International Journal of Multimedia and Ubiquitous Engineering | climate & environment & energy | capture emergent opinions | national | ||
Trilling | 2015 | Social science computer review | Twitter (+ transcript of TV debate) | election | more nuanced approach of PO | national | |
Williams et al. | 2015 | Global Environmental Change | climate & environment & energy | more dynamic perspective of PO | international | ||
Kirilenko et al. | 2015 | Global Environmental Change | climate & environment & energy | “passive survey” of PO | national & regional | ||
Thompson et al. | 2015 | Cyberpsychology, Behavior, and Social Networking | health | capture emergent opinions | national | ||
Sajuria & Fábrega | 2016 | Digital Methods for Social Science | politics | alternative to self-reported measures | national | ||
Settle et al. | 2016 | Political Science Research and Methods | politics | alternative to self-reported measures | State | ||
Marchetti & Ceccobelli | 2016 | Journalism Practice | election | more nuanced approach of PO | national | ||
Krauss et al. | 2017 | American Journal of Health Promotion | health | capture emergent opinions | national | ||
Barisione & Ceron | 2017 | ocial Media | Social Media and European Politics | economic satisfaction | national | ||
Chan & Fu | 2017 | Journal of Computer-Mediated Communication | politics | more dynamic perspective of PO | city (Hong Kong) | ||
Flores | 2017 | American Journal of Sociology | equality (gender, immigration, LGB, etc.) | causal inference | State | ||
Stautz et al. | 2017 | BMJ open | health | more nuanced approach of PO | national | ||
Chadwick & Dennis | 2017 | Social media | Political Studies | Twitter (+ campaign emails & online news articles) | politics | more nuanced approach of PO | national |
Etter at al. | 2018 | social media | Business & Society | economic satisfaction | expand the scope of survey focus | national | |
Karami et al. | 2018 | International Journal of Strategic Decision Sciences | election | more nuanced approach of PO | national | ||
Clark et al. | 2018 | Journal of Law and Courts | equality (gender, immigration, LGB, etc.) | expand the scope of survey focus | national | ||
Couper et al. | 2019 | Biological Conservation | Twitter (+ GoogleSearches & media) | climate & environment & energy | expand the scope of survey focus | International | |
Aydogan et al. | 2019 | Journal of Representative Democracy | politics | novel approach | national | ||
Hatipoğlu et al. | 2019 | All Azimuth: A Journal of Foreign Policy and Peace | politics | expand the scope of survey focus | national | ||
Vidal-Alaball et al. | 2019 | JMIR Formative Research | health | validate survey measurements | international | ||
Barberá et al. | 2019 | American Political Science Review | politics | expand the scope of survey focus | national | ||
Dahlberg et al. | 2020 | Zeitschrift für Vergleichende Politikwissenschaft | Different social media (+ online news) | politics | validate survey measurements | international | |
Lovari et al. | 2020 | American Behavioral Scientist | health | national | |||
Adams-Cohen | 2020 | American Politics Research | equality (gender, immigration, LGB, etc.) | causal inference | national & State | ||
Tavoschi et al. | 2020 | Human Vaccines & Immunotherapeutics | health | capture emergent opinions | national | ||
Guan et al. | 2020 | International Relations of the Asia-Pacific | politics | capture emergent opinions | national | ||
Kinra et al. | 2020 | Transport Policy | other | expand the scope of survey focus | national |
The last approach using SMD and survey data complementarily focuses on using social media to recruit survey respondents. However, in comparison with the previous approach, the studies collected here usually analyse SMD and survey data in sequential phases. As we only consider papers that are in some way also related to PO and are not solely about recruitment of survey respondents and their socio-demographic characteristics, the number of studies we were able to analyse is much smaller (n = 8, see Table Table8 8 in the Appendix). Our review demonstrates that the papers essentially tackle the problem surveys have in recruiting specific politically involved sub-groups of the population. In particular, the research relies on social media to access representative samples of social media users, for instance, those who commented on their countries’ elections (see [ 9 , 12 ]) or who posted at least one election-related tweet [ 79 ]. Furthermore, in these studies, ethical concerns (e.g. privacy, tracking, etc.), but also the technical affordability of the social media platform used, are discussed. The latter issue is important, as each social media platform has particular arrangements which are likely to influence the group of individuals that can be reached. Overall, future studies could think about extending the recruitment approach to enhance our knowledge of reactions to systematic events, topics, or other repetitive features (such as supporting an issue or taking part in actions), while eliminating recall errors. Furthermore, relying on SMD can help researchers pre-test their hypotheses for future surveys by uncovering relevant underlying discursive patterns or by making smaller-scale qualitative observations.
List of publications using social media as a recruitment tool (n = 8)
Author(s) | Date | Title | Source | SM | Topic | Level of analysis |
---|---|---|---|---|---|---|
Bekafigo & McBride | 2013 | Social Science Computer Review | election | State | ||
Bode & Dalrymple | 2014 | Journal of Political Marketing | politics | national | ||
Vaccari et al. | 2014 | Rivista Italiana di Scienza Politica | politics | national | ||
Vaccari et al. | 2015 | social media | Journal of Communication | politics | national | |
Vaccari et al. | 2015 | social media | Journal of Computer-Mediated Communication | politics | national | |
Kobayashi & Ichifuji | 2015 | Tweets that matter: Evidence from a randomized field experiment in Japan | Political Communication | politics | national | |
Burke & Kraut | 2016 | The relationship between Facebook use and well-being depends on communication type and tie strength | Journal of computer-mediated communication | well-being | international | |
Vaccari et al. | 2016 | Social Media + Society | politics | national |
Summary and concluding remarks
The aim of this article was to provide a review of published papers on the complementarity of SMD and survey data for PO research. We started this review by situating our work within theoretical advances concerning the complementarity of both data source. There has been extensive work underlying the opportunities and (quality) challenges of SMD for answering social research questions. However, research attention has only recently turned to SMD as a source of expression of PO and of its measurement. Consequently, there is a need for more research to uncover the ways in which SMD can be best used for fostering the understanding of PO.
The main contribution of our review is to provide a complete picture of the empirical research on the topic while calling attention to the pros and cons of each approach and possible future paths of advancements. Though this review might not be exhaustive, it has enabled us to show six major complementarity approaches which were identified as responding to four different research purposes. Below we highlight the main research paths for each approach. Using both data sources complementarily for prediction purposes was by far the most prominent approach and it remains a research area which raises many questions about the potential generalisability of the findings, namely in terms of the representativeness and validity of social media measurements of PO. We believe that the most important difficulty lies perhaps in the manner in which these studies deduce political opinions or attitudes from SMD. Survey researchers readily admit that opinions are more difficult to measure than behaviour because they involve what people think and not just how they act. Thereby, the choice to rely on sentiment analysis or merely on volume metrics (such as the number of retweets or mentions) seems unclear, at least for the near future.
Approaches concerned with improving sustainability have a significant potential for advancing social research, as they allow researchers to combine the richness of SMD content with established survey measures. When SMD are used in similar contexts to survey data, we believe that a critical view should prevail, informed by current social science best practices and expertise. For instance, whereas surveys draw a sample of carefully worded and standardised questions, social media can cover many topics as well as different facets of the same topic, which are not necessarily defined a priori on a theoretical basis. This research avenue is most likely to be fruitful for studies aiming to augment surveys by mapping discussions that are topical on social media, while allowing variations at country or regional levels of analysis to be discerned (e.g. Bennett et al. [ 10 ] on climate change opinions). Studies aiming to compare both data sources are certainly the most suitable to help improve our understanding about when and how both data sources can be validly combined. Survey methodologists can play a decisive role, notably by paying attention to the type of (open-ended) questions that can be more directly comparable with SMD. This direction can also inform the lack of consistent evidence for the first prediction approach.
Alternatively, studies aiming to improve reliability see research as mostly requiring control for the still severe limitations of using SMD appropriately in a PO context. In this respect, studies enriching survey data with SMD offer a solution to the fact that social media often lack relevant individual information, such as respondent’s attributes (e.g. sociodemographic characteristics or personality traits) or key outcome variables (e.g. voting, social, or political attitudes). Additionally, the “survey as proxy” approach enables researchers to calibrate SMD according to standardized survey measures at the actor (e.g. political candidates or parties) or context levels by reversing the data linking strategy. Future paths for both approaches implicate opening up the analysis to non-individual levels.
Studies aiming to improve the interpretability of survey research by generating new insights or by recruiting respondents on social media for a second survey phase, and that use both data sources complementarily, offer additional fertile ways to consider for new analyses that would not be possible using survey data alone. In this view, SMD do not aim to replace opinion surveys, but aim to provide a broader context for interpreting opinion, which will then serve to improve the quality of survey questions. This research avenue is most likely to be useful for knowing more about hard-to-reach populations (e.g. the LGBTQI* or disabled persons communities) or topics that are difficult to survey (e.g. violence and racism), especially when conducting iterative phases of analysis. It is also useful to get “opinion climates” about topics which have long been under survey scrutiny (e.g. emerging concerns related to feminism or social inclusion) in order to develop “updated” survey measurements.
Bringing together the opportunities offered by these different approaches shows that samples of social media users do not necessarily have to be representative of the general public to be used meaningfully as a complement to surveys. Most importantly, we believe that SMD should supplement, but not replace, traditional methods and data sources in the study of PO. By keeping up with current developments, we believe that remaining in the framework of survey research when using both data sources complementarily is paramount for identifying potential non-survey data sources, accessing them, and assessing their quality and usefulness for the study of PO. Like mixed-method approaches combining qualitative and quantitative data (e.g. [ 36 ]), the primary motive for complementing survey and SMD with one another is to allow researchers to mix datasets in a meaningful way for developing an overall interpretation.
Technical and ethical note
Regarding sustainability, it is important to consider that the patterns of social media consumption are influenced not only by user preferences, but also by technological changes and the availability of the platforms. For instance, social media companies may not survive and whole platforms could disappear, thus impeding data access. With changes in consumption patterns, PO may be difficult to measure consistently over time. From a more technical perspective, it is also important to assess the extent to which databases composed of social media texts collected by different means (e.g. different search queries or different platform algorithms) might raise consistency and replicability issues (e.g. [ 34 ]). As for reliability, several issues are worth considering. Even though SMD can provide complementary information to survey estimates though linkage, there are sometimes concerns about the veracity or honesty of the information collected. For instance, SMD may increase the potential for social stigmatisation, causing users to be more reluctant to share their true opinions [ 63 ]. However, the opposite may also be true: users could express more radical opinions to gain social approval (e.g. disinhibition effect). The identity of those who post can also raise veracity concerns [ 55 ], and it may be difficult to distinguish sarcastic content from texts that are straight-forwardly positive or negative (e.g. [ 35 ]). Another important issue is that we usually know how many people have liked a post, clicked on a link, or retweeted a message, but we rarely know how many people have seen the item and chosen not to take any action [ 77 ]. Furthermore, due to algorithms that favour selective exposure and homophily of opinion [ 6 , 17 ], it is important to assess the extent to which findings derived from online opinion generate more polarised opinions than the ones that would be obtained through the private setting of surveys.
When researchers aim to generate new insights, they should consider that each social media platform has particular arrangements. For instance, the orientation of the content (e.g. political, family-oriented, business-oriented) as well as the scope of the content (e.g. possible bias toward more visible events) can play a decisive role on what content is available and which user profiles are most likely to be active on the social media platform. Furthermore, the nature of the platform allows for different levels of engagement in debates (e.g. Twitter is mostly used for short text content, while YouTube and Instagram allow sharing and commenting on videos and pictures). Functional capabilities can not only influence the ways of recruiting respondents for a second survey phase (e.g. direct messages), but also the identifying of sub-groups of interest (e.g. differences between friend and follower networks, and the reciprocity of follower networks). In addition, social media platforms may give users control over the availability of the information (e.g. to suppress or filter unwanted comments), which will again impact what is available from whom and on what.
For each research purpose, we should also consider that there are important ethical factors that are likely to influence the possible paths of research relying on SMD. Each platform has its own rules which are subject to change at any time. For instance, anonymity settings also affect the content of SMD, with growing concerns about surveillance and the resulting loss of privacy [ 29 , 76 , 78 ], thus influencing what people are willing to post. There are also evolving rules about the banning of particular words and behaviours, as well as users, which may influence research findings (especially when conducting longitudinal research). SMD are private property of tech companies and can be arbitrarily erased or made inaccessible, compromising the replicability of research.
Our review has several limitations. First, it focuses on social media but do not include other data sources that are frequently compared to survey data to model PO (e.g. Google trends, mainstream media, or administrative data). We thus encourage future research to extend the proposed complementary framework to additional data sources. This would allow the building of knowledge about the most suitable ways of combining these data for answering specific research purposes. Furthermore, our review entails a conceptual aim with less focus on the variety of methods used to either collect, clean, analyse, and aggregate the data to generate statistics. Discussing the pros and cons of methodologies employed by these papers could constitute the object of another review.
Notwithstanding these limitations, our study is not only of interest for social and political scientists concerned by the declining response rates and restrictive budgeting for survey research [ 57 ]. As social media have been established as multifunctional tools, and many companies and researchers implement strategies based on social media to collect opinions, make predictions, study behaviours, conduct experiments, or recruit hard-to-reach populations, this review is also of interest for practitioners.
Extracting PO from social media text can foster social sciences by moving it forward as an applied field, thus bridging gaps between computational models and interpretative research. We see this collaboration as particularly important for developing more advanced and reliable measures of opinion from social media texts. This also constitutes an opportunity to challenge the opposition of the so-called data-driven and theory-driven approaches , a simplistic dichotomy which further consolidates the misconception that social research can be conducted by relying solely on text-based data. We encourage researchers to acknowledge the different conceptualisation of opinion when measured by SMD and surveys, and we advise them to adopt a mixed-method strategy where the complementarity of both data is paramount.
Code availability
Not applicable;
Authors contribution
All authors contributed to the design of the study. Maud Reveilhac planned the study, conducted the data analysis, and wrote the manuscript. All authors contributed to the review of the manuscript and approved the final version.
Open access funding provided by University of Lausanne.
Data availability
Declarations.
We have no conflict of interests to disclose;
Publisher’s note
Springer Nature remains neutral with regard to jurisdictional claims in published maps and institutional affiliations.
Contributor Information
Maud Reveilhac, Email: [email protected] .
Stephanie Steinmetz, Email: [email protected] .
Davide Morselli, Email: [email protected] .
Click through the PLOS taxonomy to find articles in your field.
For more information about PLOS Subject Areas, click here .
Loading metrics
Open Access
Peer-reviewed
Research Article
Social impact in social media: A new method to evaluate the social impact of research
Roles Investigation, Writing – original draft
* E-mail: [email protected]
Affiliation Department of Journalism and Communication Studies, Universitat Autonoma de Barcelona, Barcelona, Spain

Affiliation Department of Psychology and Sociology, Universidad de Zaragoza, Zaragoza, Spain
Roles Conceptualization, Investigation, Methodology, Supervision, Writing – review & editing
Affiliation Department of Sociology, Universitat Autonoma de Barcelona, Barcelona, Spain
Affiliation Department of Sociology, Universitat de Barcelona (UB), Barcelona, Spain
- Cristina M. Pulido,
- Gisela Redondo-Sama,
- Teresa Sordé-Martí,
- Ramon Flecha
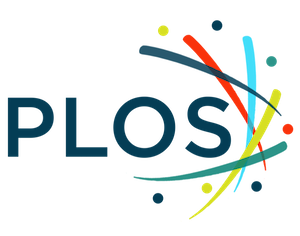
- Published: August 29, 2018
- https://doi.org/10.1371/journal.pone.0203117
- Reader Comments
The social impact of research has usually been analysed through the scientific outcomes produced under the auspices of the research. The growth of scholarly content in social media and the use of altmetrics by researchers to track their work facilitate the advancement in evaluating the impact of research. However, there is a gap in the identification of evidence of the social impact in terms of what citizens are sharing on their social media platforms. This article applies a social impact in social media methodology (SISM) to identify quantitative and qualitative evidence of the potential or real social impact of research shared on social media, specifically on Twitter and Facebook. We define the social impact coverage ratio (SICOR) to identify the percentage of tweets and Facebook posts providing information about potential or actual social impact in relation to the total amount of social media data found related to specific research projects. We selected 10 projects in different fields of knowledge to calculate the SICOR, and the results indicate that 0.43% of the tweets and Facebook posts collected provide linkages with information about social impact. However, our analysis indicates that some projects have a high percentage (4.98%) and others have no evidence of social impact shared in social media. Examples of quantitative and qualitative evidence of social impact are provided to illustrate these results. A general finding is that novel evidences of social impact of research can be found in social media, becoming relevant platforms for scientists to spread quantitative and qualitative evidence of social impact in social media to capture the interest of citizens. Thus, social media users are showed to be intermediaries making visible and assessing evidence of social impact.
Citation: Pulido CM, Redondo-Sama G, Sordé-Martí T, Flecha R (2018) Social impact in social media: A new method to evaluate the social impact of research. PLoS ONE 13(8): e0203117. https://doi.org/10.1371/journal.pone.0203117
Editor: Sergi Lozano, Institut Català de Paleoecologia Humana i Evolució Social (IPHES), SPAIN
Received: November 8, 2017; Accepted: August 15, 2018; Published: August 29, 2018
Copyright: © 2018 Pulido et al. This is an open access article distributed under the terms of the Creative Commons Attribution License , which permits unrestricted use, distribution, and reproduction in any medium, provided the original author and source are credited.
Data Availability: All relevant data are within the paper and its Supporting Information files.
Funding: The research leading to these results has received funding from the 7th Framework Programme of the European Commission under the Grant Agreement n° 613202 P.I. Ramon Flecha, https://ec.europa.eu/research/fp7/index_en.cfm . The funders had no role in study design, data collection and analysis, decision to publish, or preparation of the manuscript.
Competing interests: The authors have declared that no competing interests exist.
Introduction
The social impact of research is at the core of some of the debates influencing how scientists develop their studies and how useful results for citizens and societies may be obtained. Concrete strategies to achieve social impact in particular research projects are related to a broader understanding of the role of science in contemporary society. There is a need to explore dialogues between science and society not only to communicate and disseminate science but also to achieve social improvements generated by science. Thus, the social impact of research emerges as an increasing concern within the scientific community [ 1 ]. As Bornmann [ 2 ] said, the assessment of this type of impact is badly needed and is more difficult than the measurement of scientific impact; for this reason, it is urgent to advance in the methodologies and approaches to measuring the social impact of research.
Several authors have approached the conceptualization of social impact, observing a lack of generally accepted conceptual and instrumental frameworks [ 3 ]. It is common to find a wide range of topics included in the contributions about social impact. In their analysis of the policies affecting land use, Hemling et al. [ 4 ] considered various domains in social impact, for instance, agricultural employment or health risk. Moving to the field of flora and fauna, Wilder and Walpole [ 5 ] studied the social impact of conservation projects, focusing on qualitative stories that provided information about changes in attitudes, behaviour, wellbeing and livelihoods. In an extensive study by Godin and Dore [ 6 ], the authors provided an overview and framework for the assessment of the contribution of science to society. They identified indicators of the impact of science, mentioning some of the most relevant weaknesses and developing a typology of impact that includes eleven dimensions, with one of them being the impact on society. The subdimensions of the impact of science on society focus on individuals (wellbeing and quality of life, social implication and practices) and organizations (speeches, interventions and actions). For the authors, social impact “refers to the impact knowledge has on welfare, and on the behaviours, practices and activities of people and groups” (p. 7).
In addition, the terms “social impact” and “societal impact” are sometimes used interchangeably. For instance, Bornmann [ 2 ] said that due to the difficulty of distinguishing social benefits from the superior term of societal benefits, “in much literature the term ‘social impact’ is used instead of ‘societal impact’”(p. 218). However, in other cases, the distinction is made [ 3 ], as in the present research. Similar to the definition used by the European Commission [ 7 ], social impact is used to refer to economic impact, societal impact, environmental impact and, additionally, human rights impact. Therefore, we use the term social impact as the broader concept that includes social improvements in all the above mentioned areas obtained from the transference of research results and representing positive steps towards the fulfilment of those officially defined social goals, including the UN Sustainable Development Goals, the EU 2020 Agenda, or similar official targets. For instance, the Europe 2020 strategy defines five priority targets with concrete indicators (employment, research and development, climate change and energy, education and poverty and social exclusion) [ 8 ], and we consider the targets addressed by objectives defined in the specific call that funds the research project.
This understanding of the social impact of research is connected to the creation of the Social Impact Open Repository (SIOR), which constitutes the first open repository worldwide that displays, cites and stores the social impact of research results [ 9 ]. The SIOR has linked to ORCID and Wikipedia to allow the synergies of spreading information about the social impact of research through diverse channels and audiences. It is relevant to mention that currently, SIOR includes evidence of real social impact, which implies that the research results have led to actual improvements in society. However, it is common to find evidence of potential social impact in research projects. The potential social impact implies that in the development of the research, there has been some evidence of the effectiveness of the research results in terms of social impact, but the results have not yet been transferred.
Additionally, a common confusion is found among the uses of dissemination, transference (policy impact) and social impact. While dissemination means to disseminate the knowledge created by research to citizens, companies and institutions, transference refers to the use of this knowledge by these different actors (or others), and finally, as already mentioned, social impact refers to the actual improvements resulting from the use of this knowledge in relation to the goals motivating the research project (such as the United Nations Sustainable Development Goals). In the present research [ 3 ], it is argued that “social impact can be understood as the culmination of the prior three stages of the research” (p.3). Therefore, this study builds on previous contributions measuring the dissemination and transference of research and goes beyond to propose a novel methodological approach to track social impact evidences.
In fact, the contribution that we develop in this article is based on the creation of a new method to evaluate the evidence of social impact shared in social media. The evaluation proposed is to measure the social impact coverage ratio (SICOR), focusing on the presence of evidence of social impact shared in social media. Then, the article first presents some of the contributions from the literature review focused on the research on social media as a source for obtaining key data for monitoring or evaluating different research purposes. Second, the SISM (social impact through social media) methodology[ 10 ] developed is introduced in detail. This methodology identifies quantitative and qualitative evidence of the social impact of the research shared on social media, specifically on Twitter and Facebook, and defines the SICOR, the social impact coverage ratio. Next, the results are discussed, and lastly, the main conclusions and further steps are presented.
Literature review
Social media research includes the analysis of citizens’ voices on a wide range of topics [ 11 ]. According to quantitative data from April 2017 published by Statista [ 12 ], Twitter and Facebook are included in the top ten leading social networks worldwide, as ranked by the number of active users. Facebook is at the top of the list, with 1,968 million active users, and Twitter ranks 10 th , with 319 million active users. Between them are the following social networks: WhatsApp, YouTube, Facebook Messenger, WeChat, QQ, Instagram,Qzone and Tumblr. If we look at altmetrics, the tracking of social networks for mentions of research outputs includes Facebook, Twitter, Google+,LinkedIn, Sina Weibo and Pinterest. The social networks common to both sources are Facebook and Twitter. These are also popular platforms that have a relevant coverage of scientific content and easy access to data, and therefore, the research projects selected here for application of the SISM methodology were chosen on these platforms.
Chew and Eysenbach [ 13 ] studied the presence of selected keywords in Twitter related to public health issues, particularly during the 2009 H1N1 pandemic, identifying the potential for health authorities to use social media to respond to the concerns and needs of society. Crooks et al.[ 14 ] investigated Twitter activity in the context of a 5.8 magnitude earthquake in 2011 on the East Coast of the United States, concluding that social media content can be useful for event monitoring and can complement other sources of data to improve the understanding of people’s responses to such events. Conversations among young Canadians posted on Facebook and analysed by Martinello and Donelle [ 15 ] revealed housing and transportation as main environmental concerns, and the project FoodRisc examined the role of social media to illustrate consumers’ quick responses during food crisis situations [ 16 ]. These types of contributions illustrate that social media research implies the understanding of citizens’ concerns in different fields, including in relation to science.
Research on the synergies between science and citizens has increased over the years, according to Fresco [ 17 ], and there is a growing interest among researchers and funding agencies in how to facilitate communication channels to spread scientific results. For instance, in 1998, Lubchenco [ 18 ] advocated for a social contract that “represents a commitment on the part of all scientists to devote their energies and talents to the most pressing problems of the day, in proportion to their importance, in exchange for public funding”(p.491).
In this framework, the recent debates on how to increase the impact of research have acquired relevance in all fields of knowledge, and major developments address the methods for measuring it. As highlighted by Feng Xia et al. [ 19 ], social media constitute an emerging approach to evaluating the impact of scholarly publications, and it is relevant to consider the influence of the journal, discipline, publication year and user type. The authors revealed that people’s concerns differ by discipline and observed more interest in papers related to everyday life, biology, and earth and environmental sciences. In the field of biomedical sciences, Haustein et al. [ 20 ] analysed the dissemination of journal articles on Twitter to explore the correlations between tweets and citations and proposed a framework to evaluate social media-based metrics. In fact, different studies address the relationship between the presence of articles on social networks and citations [ 21 ]. Bornmann [ 22 ] conducted a case study using a sample of 1,082 PLOS journal articles recommended in F1000 to explore the usefulness of altmetrics for measuring the broader impact of research. The author presents evidence about Facebook and Twitter as social networks that may indicate which papers in the biomedical sciences can be of interest to broader audiences, not just to specialists in the area. One aspect of particular interest resulting from this contribution is the potential to use altmetrics to measure the broader impacts of research, including the societal impact. However, most of the studies investigating social or societal impact lack a conceptualization underlying its measurement.
To the best of our knowledge, the assessment of social impact in social media (SISM) has developed according to this gap. At the core of this study, we present and discuss the results obtained through the application of the SICOR (social impact coverage ratio) with examples of evidence of social impact shared in social media, particularly on Twitter and Facebook, and the implications for further research.
Following these previous contributions, our research questions were as follows: Is there evidence of social impact of research shared by citizens in social media? If so, is there quantitative or qualitative evidence? How can social media contribute to identifying the social impact of research?
Methods and data presentation
A group of new methodologies related to the analysis of online data has recently emerged. One of these emerging methodologies is social media analytics [ 23 ], which was initially used most in the marketing research field but also came to be used in other domains due to the multiple possibilities opened up by the availability and richness of the data for different research purposes. Likewise, the concern of how to evaluate the social impact of research as well as the development of methodologies for addressing this concern has occupied central attention. The development of SISM (Social Impact in Social Media) and the application of the SICOR (Social Impact Coverage Ratio) is a contribution to advancement in the evaluation of the social impact of research through the analysis of the social media selected (in this case, Twitter and Facebook). Thus, SISM is novel in both social media analytics and among the methodologies used to evaluate the social impact of research. This development has been made under IMPACT-EV, a research project funded under the Framework Program FP7 of the Directorate-General for Research and Innovation of the European Commission. The main difference from other methodologies for measuring the social impact of research is the disentanglement between dissemination and social impact. While altmetrics is aimed at measuring research results disseminated beyond academic and specialized spheres, SISM contribute to advancing this measurement by shedding light on to what extent evidence of the social impact of research is found in social media data. This involves the need to differentiate between tweets or Facebook posts (Fb/posts) used to disseminate research findings from those used to share the social impact of research. We focus on the latter, investigating whether there is evidence of social impact, including both potential and real social impact. In fact, the question is whether research contributes and/or has the potential to contribute to improve the society or living conditions considering one of these goals defined. What is the evidence? Next, we detail the application of the methodology.
Data collection
To develop this study, the first step was to select research projects with social media data to be analysed. The selection of research projects for application of the SISM methodology was performed according to three criteria.
Criteria 1. Selection of success projects in FP7. The projects were success stories of the 7 th Framework Programme (FP7) highlighted by the European Commission [ 24 ] in the fields of knowledge of medicine, public health, biology and genomics. The FP7 published calls for project proposals from 2007 to 2013. This implies that most of the projects funded in the last period of the FP7 (2012 and 2013) are finalized or in the last phase of implementation.
Criteria 2. Period of implementation. We selected projects in the 2012–2013 period because they combine recent research results with higher possibilities of having Twitter and Facebook accounts compared with projects of previous years, as the presence of social accounts in research increased over this period.
Criteria 3. Twitter and Facebook accounts. It was crucial that the selected projects had active Twitter and Facebook accounts.
Table 1 summarizes the criteria and the final number of projects identified. As shown, 10 projects met the defined criteria. Projects in medical research and public health had higher presence.
- PPT PowerPoint slide
- PNG larger image
- TIFF original image
https://doi.org/10.1371/journal.pone.0203117.t001
After the selection of projects, we defined the timeframe of social media data extraction on Twitter and Facebook from the starting date of the project until the day of the search, as presented in Table 2 .
https://doi.org/10.1371/journal.pone.0203117.t002
The second step was to define the search strategies for extracting social media data related to the research projects selected. In this line, we defined three search strategies.
Strategy 1. To extract messages published on the Twitter account and the Facebook page of the selected projects. We listed the Twitter accounts and Facebook pages related to each project in order to look at the available information. In this case, it is important to clarify that the tweets published under the corresponding Twitter project account are original tweets or retweets made from this account. It is relevant to mention that in one case, the Twitter account and Facebook page were linked to the website of the research group leading the project. In this case, we selected tweets and Facebook posts related to the project. For instance, in the case of the Twitter account, the research group created a specific hashtag to publish messages related to the project; therefore, we selected only the tweets published under this hashtag. In the analysis, we prioritized the analysis of the tweets and Facebook posts that received some type of interaction (likes, retweets or shares) because such interaction is a proxy for citizens’ interest. In doing so, we used the R program and NVivoto extract the data and proceed with the analysis. Once we obtained the data from Twitter and Facebook, we were able to have an overview of the information to be further analysed, as shown in Table 3 .
https://doi.org/10.1371/journal.pone.0203117.t003
We focused the second and third strategies on Twitter data. In both strategies, we extracted Twitter data directly from the Twitter Advanced Search tool, as the API connected to NVivo and the R program covers only a specific period of time limited to 7/9 days. Therefore, the use of the Twitter Advanced Search tool made it possible to obtain historic data without a period limitation. We downloaded the results in PDF and then uploaded them to NVivo.
Strategy 2. To use the project acronym combined with other keywords, such as FP7 or EU. This strategy made it possible to obtain tweets mentioning the project. Table 4 presents the number of tweets obtained with this strategy.
https://doi.org/10.1371/journal.pone.0203117.t004
Strategy 3. To use searchable research results of projects to obtain Twitter data. We defined a list of research results, one for each project, and converted them into keywords. We selected one searchable keyword for each project from its website or other relevant sources, for instance, the brief presentations prepared by the European Commission and published in CORDIS. Once we had the searchable research results, we used the Twitter Advanced Search tool to obtain tweets, as presented in Table 5 .
https://doi.org/10.1371/journal.pone.0203117.t005
The sum of the data obtained from these three strategies allowed us to obtain a total of 3,425 tweets and 1,925 posts on public Facebook pages. Table 6 presents a summary of the results.
https://doi.org/10.1371/journal.pone.0203117.t006
We imported the data obtained from the three search strategies into NVivo to analyse. Next, we select tweets and Facebook posts providing linkages with quantitative or qualitative evidence of social impact, and we complied with the terms of service for the social media from which the data were collected. By quantitative and qualitative evidence, we mean data or information that shows how the implementation of research results has led to improvements towards the fulfilment of the objectives defined in the EU2020 strategy of the European Commission or other official targets. For instance, in the case of quantitative evidence, we searched tweets and Facebook posts providing linkages with quantitative information about improvements obtained through the implementation of the research results of the project. In relation to qualitative evidence, for example, we searched for testimonies that show a positive evaluation of the improvement due to the implementation of research results. In relation to this step, it is important to highlight that social media users are intermediaries making visible evidence of social impact. Users often share evidence, sometimes sharing a link to an external resource (e.g., a video, an official report, a scientific article, news published on media). We identified evidence of social impact in these sources.
Data analysis
γ i is the total number of messages obtained about project i with evidence of social impact on social media platforms (Twitter, Facebook, Instagram, etc.);
T i is the total number of messages from project i on social media platforms (Twitter, Facebook, Instagram, etc.); and
n is the number of projects selected.
Analytical categories and codebook
The researchers who carried out the analysis of the social media data collected are specialists in the social impact of research and research on social media. Before conducting the full analysis, two aspects were guaranteed. First, how to identify evidence of social impact relating to the targets defined by the EU2020 strategy or to specific goals defined by the call addressed was clarified. Second, we held a pilot to test the methodology with one research project that we know has led to considerable social impact, which allowed us to clarify whether or not it was possible to detect evidence of social impact shared in social media. Once the pilot showed positive results, the next step was to extend the analysis to another set of projects and finally to the whole sample. The construction of the analytical categories was defined a priori, revised accordingly and lastly applied to the full sample.
Different observations should be made. First, in this previous analysis, we found that the tweets and Facebook users play a key role as “intermediaries,” serving as bridges between the larger public and the evidence of social impact. Social media users usually share a quote or paragraph introducing evidence of social impact and/or link to an external resource, for instance, a video, official report, scientific article, news story published on media, etc., where evidence of the social impact is available. This fact has implications for our study, as our unit of analysis is all the information included in the tweets or Facebook posts. This means that our analysis reaches the external resources linked to find evidence of social impact, and for this reason, we defined tweets or Facebook posts providing linkages with information about social impact.
Second, the other important aspect is the analysis of the users’ profile descriptions, which requires much more development in future research given the existing limitations. For instance, some profiles are users’ restricted due to privacy reasons, so the information is not available; other accounts have only the name of the user with no description of their profile available. Therefore, we gave priority to the identification of evidence of social impact including whether a post obtained interaction (retweets, likes or shares) or was published on accounts other than that of the research project itself. In the case of the profile analysis, we added only an exploratory preliminary result because this requires further development. Considering all these previous details, the codebook (see Table 7 ) that we present as follows is a result of this previous research.
https://doi.org/10.1371/journal.pone.0203117.t007
How to analyse Twitter and Facebook data
To illustrate how we analysed data from Twitter and Facebook, we provide one example of each type of evidence of social impact defined, considering both real and potential social impact, with the type of interaction obtained and the profiles of those who have interacted.
QUANESISM. Tweet by ZeroHunger Challenge @ZeroHunger published on 3 May 2016. Text: How re-using food waste for animal feed cuts carbon emissions.-NOSHAN project hubs.ly/H02SmrP0. 7 retweets and 5 likes.
The unit of analysis is all the content of the tweet, including the external link. If we limited our analysis to the tweet itself, it would not be evidence. Examining the external link is necessary to find whether there is evidence of social impact. The aim of this project was to investigate the process and technologies needed to use food waste for feed production at low cost, with low energy consumption and with a maximal evaluation of the starting wastes. This tweet provides a link to news published in the PHYS.org portal [ 25 ], which specializes in science news. The news story includes an interview with the main researcher that provides the following quotation with quantitative evidence:
'Our results demonstrated that with a NOSHAN 10 percent mix diet, for every kilogram of broiler chicken feed, carbon dioxide emissions were reduced by 0.3 kg compared to a non-food waste diet,' explains Montse Jorba, NOSHAN project coordinator. 'If 1 percent of total chicken broiler feed in Europe was switched to the 10 percent NOSHAN mix diet, the total amount of CO2 emissions avoided would be 0.62 million tons each year.'[ 25 ]
This quantitative evidence “a NOSHAN 10 percent mix diet, for every kilogram of broiler chicken feed, carbon dioxide emissions carbon dioxide emissions were reduced by 0.3 kg to a non-food waste diet” is linked directly with the Europe 2020 target of Climate Change & Energy, specifically with the target of reducing greenhouse gas emissions by 20% compared to the levels in 1990 [ 8 ]. The illustrative extrapolation the coordinator mentioned in the news is also an example of quantitative evidence, although is an extrapolation based on the specific research result.
This tweet was captured by the Acronym search strategy. It is a message tweeted by an account that is not related to the research project. The twitter account is that of the Zero Hunger Challenge movement, which supports the goals of the UN. The interaction obtained is 7 retweets and 5 likes. Regarding the profiles of those who retweeted and clicked “like”, there were activists, a journalist, an eco-friendly citizen, a global news service, restricted profiles (no information is available on those who have retweeted) and one account with no information in its profile.
The following example illustrates the analysis of QUALESISM: Tweet by @eurofitFP7 published on4 October 2016. Text: See our great new EuroFIT video on youtube! https://t.co/TocQwMiW3c 9 retweets and 5 likes.
The aim of this project is to improve health through the implementation of two novel technologies to achieve a healthier lifestyle. The tweet provides a link to a video on YouTube on the project’s results. In this video, we found qualitative evidence from people who tested the EuroFit programme; there are quotes from men who said that they have experienced improved health results using this method and that they are more aware of how to manage their health:
One end-user said: I have really amazing results from the start, because I managed to change a lot of things in my life. And other one: I was more conscious of what I ate, I was more conscious of taking more steps throughout the day and also standing up a little more. [ 26 ]
The research applies the well researched scientific evidence to the management of health issues in daily life. The video presents the research but also includes a section where end-users talk about the health improvements they experienced. The quotes extracted are some examples of the testimonies collected. All agree that they have improved their health and learned healthy habits for their daily lives. These are examples of qualitative evidence linked with the target of the call HEALTH.2013.3.3–1—Social innovation for health promotion [ 27 ] that has the objectives of reducing sedentary habits in the population and promoting healthy habits. This research contributes to this target, as we see in the video testimonies. Regarding the interaction obtained, this tweet achieved 9 retweets and 5 likes. In this case, the profiles of the interacting citizens show involvement in sport issues, including sport trainers, sport enthusiasts and some researchers.
To summarize the analysis, in Table 8 below, we provide a summary with examples illustrating the evidence found.
https://doi.org/10.1371/journal.pone.0203117.t008
Quantitative evidence of social impact in social media
There is a greater presence of tweets/Fb posts with quantitative evidence (14) than with qualitative evidence (9) in the total number of tweets/Fb posts identified with evidence of social impact. Most of the tweets/Fb posts with quantitative evidence of social impact are from scientific articles published in peer-reviewed international journals and show potential social impact. In Table 8 , we introduce 3 examples of this type of tweets/Fb posts with quantitative evidence:
The first tweet with quantitative social impact selected is from project 7. The aim of this project was to provide high-quality scientific evidence for preventing vitamin D deficiency in European citizens. The tweet highlighted the main contribution of the published study, that is, “Weekly consumption of 7 vitamin D-enhanced eggs has an important impact on winter vitamin D status in adults” [ 28 ]. The quantitative evidence shared in social media was extracted from a news publication in a blog on health news. This blog collects scientific articles of research results. In this case, the blog disseminated the research result focused on how vitamin D-enhanced eggs improve vitamin D deficiency in wintertime, with the published results obtained by the research team of the project selected. The quantitative evidence illustrates that the group of adults who consumed vitamin D-enhanced eggs did not suffer from vitamin D deficiency, as opposed to the control group, which showed a significant decrease in vitamin D over the winter. The specific evidence is the following extracted from the article [ 28 ]:
With the use of a within-group analysis, it was shown that, although serum 25(OH) D in the control group significantly decreased over winter (mean ± SD: -6.4 ± 6.7 nmol/L; P = 0.001), there was no change in the 2 groups who consumed vitamin D-enhanced eggs (P>0.1 for both. (p. 629)
This evidence contributes to achievement of the target defined in the call addressed that is KBBE.2013.2.2–03—Food-based solutions for the eradication of vitamin D deficiency and health promotion throughout the life cycle [ 29 ]. The quantitative evidence shows how the consumption of vitamin D-enhanced eggs reduces vitamin D deficiency.
The second example of this table corresponds to the example of quantitative evidence of social impact provided in the previous section.
The third example is a Facebook post from project 3 that is also tweeted. Therefore, this evidence was published in both social media sources analysed. The aim of this project was to measure a range of chemical and physical environmental hazards in food, consumer products, water, air, noise, and the built environment in the pre- and postnatal early-life periods. This Facebook post and tweet links directly to a scientific article [ 30 ] that shows the precision of the spectroscopic platform:
Using 1H NMR spectroscopy we characterized short-term variability in urinary metabolites measured from 20 children aged 8–9 years old. Daily spot morning, night-time and pooled (50:50 morning and night-time) urine samples across six days (18 samples per child) were analysed, and 44 metabolites quantified. Intraclass correlation coefficients (ICC) and mixed effect models were applied to assess the reproducibility and biological variance of metabolic phenotypes. Excellent analytical reproducibility and precision was demonstrated for the 1H NMR spectroscopic platform (median CV 7.2%) . (p.1)
This evidence is linked to the target defined in the call “ENV.2012.6.4–3—Integrating environmental and health data to advance knowledge of the role of environment in human health and well-being in support of a European exposome initiative” [ 31 ]. The evidence provided shows how the project’s results have contributed to building technology for improving the data collection to advance in the knowledge of the role of the environment in human health, especially in early life. The interaction obtained is one retweet from a citizen from Nigeria interested in health issues, according to the information available in his profile.
Qualitative evidence of social impact in social media
We found qualitative evidence of the social impact of different projects, as shown in Table 9 . Similarly to the quantitative evidence, the qualitative cases also demonstrate potential social impact. The three examples provided have in common that they are tweets or Facebook posts that link to videos where the end users of the research project explain their improvements once they have implemented the research results.
https://doi.org/10.1371/journal.pone.0203117.t009
The first tweet with qualitative evidence selected is from project 4. The aim of this project is to produce a system that helps in the prevention of obesity and eating disorders, targeting young people and adults [ 32 ]. The twitter account that published this tweet is that of the Future and Emerging Technologies Programme of the European Commission, and a link to a Euronews video is provided. This video shows how the patients using the technology developed in the research achieved control of their eating disorders, through the testimonies of patients commenting on the positive results they have obtained. These testimonies are included in the news article that complements the video. An example of these testimonies is as follows:
Pierre Vial has lost 43 kilos over the past nine and a half months. He and other patients at the eating disorder clinic explain the effects obesity and anorexia have had on their lives. Another patient, Karin Borell, still has some months to go at the clinic but, after decades of battling anorexia, is beginning to be able to visualise life without the illness: “On a good day I see myself living a normal life without an eating disorder, without problems with food. That’s really all I wish right now”.[ 32 ]
This qualitative evidence shows how the research results contribute to the achievement of the target goals of the call addressed:“ICT-2013.5.1—Personalised health, active ageing, and independent living”. [ 33 ] In this case, the results are robust, particularly for people suffering chronic diseases and desiring to improve their health; people who have applied the research findings are improving their eating disorders and better managing their health. The value of this evidence is the inclusion of the patients’ voices stating the impact of the research results on their health.
The second example is a Facebook post from project 9, which provides a link to a Euronews video. The aim of this project is to bring some tools from the lab to the farm in order to guarantee a better management of the farm and animal welfare. In this video [ 34 ], there are quotes from farmers using the new system developed through the research results of the project. These quotes show how use of the new system is improving the management of the farm and the health of the animals; some examples are provided:
Cameras and microphones help me detect in real time when the animals are stressed for whatever reason,” explained farmer Twan Colberts. “So I can find solutions faster and in more efficient ways, without me being constantly here, checking each animal.”
This evidence shows how the research results contribute to addressing the objectives specified in the call “KBBE.2012.1.1–02—Animal and farm-centric approach to precision livestock farming in Europe” [ 29 ], particularly, to improve the precision of livestock farming in Europe. The interaction obtained is composed of6 likes and 1 share. The profiles are diverse, but some of them do not disclose personal information; others have not added a profile description, and only their name and photo are available.
Interrater reliability (kappa)
The analysis of tweets and Facebook posts providing linkages with information about social impact was conducted following a content analysis method in which reliability was based on a peer review process. This sample is composed of 3,425 tweets and 1,925 Fb/posts. Each tweet and Facebook post was analysed to identify whether or not it contains evidence of social impact. Each researcher has the codebook a priori. We used interrater reliability in examining the agreement between the two raters on the assignment of the categories defined through Cohen’s kappa. We used SPSS to calculate this coefficient. We exported an excel sheet with the sample coded by the two researchers being 1 (is evidence of social impact, either potential or real) and 0 (is not evidence of social impact) to SPSS. The cases where agreement was not achieved were not considered as containing evidence of social impact. The result obtained is 0.979; considering the interpretation of this number according to Landis & Koch [ 35 ], our level of agreement is almost perfect, and thus, our analysis is reliable. To sum up the data analysis, the description of the steps followed is explained:
Step 1. Data analysis I. We included all data collected in an excel sheet to proceed with the analysis. Prior to the analysis, researchers read the codebook to keep in mind the information that should be identified.
Step 2. Each researcher involved reviewed case by case the tweets and Facebook posts to identify whether they provide links with evidence of social impact or not. If the researcher considers there to be evidence of social impact, he or she introduces the value of 1into the column, and if not, the value of 0.
Step 3. Once all the researchers have finished this step, the next step is to export the excel sheet to SPSS to extract the kappa coefficient.
Step 4. Data Analysis II. The following step was to analyse case by case the tweets and Facebook posts identified as providing linkages with information of social impact and classify them as quantitative or qualitative evidence of social impact.
Step 5. The interaction received was analysed because this determines to which extent this evidence of social impact has captured the attention of citizens (in the form of how many likes, shares, or retweets the post has).
Step 6. Finally, if available, the profile descriptions of the citizens interacting through retweeting or sharing the Facebook post were considered.
Step 7. SICOR was calculated. It could be applied to the complete sample (all data projects) or to each project, as we will see in the next section.
The total number of tweets and Fb/posts collected from the 10 projects is 5,350. After the content analysis, we identified 23 tweets and Facebook posts providing linkages to information about social impact. To respond to the research question, which considered whether there is evidence of social impact shared by citizens in social media, the answer was affirmative, although the coverage ratio is low. Both Twitter and Facebook users retweeted or shared evidence of social impact, and therefore, these two social media networks are valid sources for expanding knowledge on the assessment of social impact. Table 10 shows the social impact coverage ratio in relation to the total number of messages analysed.
https://doi.org/10.1371/journal.pone.0203117.t010
The analysis of each of the projects selected revealed some results to consider. Of the 10 projects, 7 had evidence, but those projects did not necessarily have more Tweets and Facebook posts. In fact, some projects with fewer than 70 tweets and 50 Facebook posts have more evidence of social impact than other projects with more than 400 tweets and 400 Facebook posts. This result indicates that the number of tweets and Facebook posts does not determine the existence of evidence of social impact in social media. For example, project 2 has 403 tweets and 423 Facebooks posts, but it has no evidence of social impact on social media. In contrast, project 9 has 62 tweets, 43 Facebook posts, and 2 pieces of evidence of social impact in social media, as shown in Table 11 .
https://doi.org/10.1371/journal.pone.0203117.t011
The ratio of tweets/Fb posts to evidence is 0.43%, and it differs depending on the project, as shown below in Table 12 . There is one project (P7) with a ratio of 4.98%, which is a social impact coverage ratio higher than that of the other projects. Next, a group of projects (P3, P9, P10) has a social impact coverage ratio between 1.41% and 2,99%.The next slot has three projects (P1, P4, P5), with a ratio between 0.13% and 0.46%. Finally, there are three projects (P2, P6, P8) without any tweets/Fb posts evidence of social impact.
https://doi.org/10.1371/journal.pone.0203117.t012
Considering the three strategies for obtaining data, each is related differently to the evidence of social impact. In terms of the social impact coverage ratio, as shown in Table 13 , the most successful strategy is number 3 (searchable research results), as it has a relation of 17.86%, which is much higher than the ratios for the other 2 strategies. The second strategy (acronym search) is more effective than the first (profile accounts),with 1.77% for the former as opposed to 0.27% for the latter.
https://doi.org/10.1371/journal.pone.0203117.t013
Once tweets and Facebook posts providing linkages with information about social impact(ESISM)were identified, we classified them in terms of quantitative (QUANESISM) or qualitative evidence (QUALESISM)to determine which type of evidence was shared in social media. Table 14 indicates the amount of quantitative and qualitative evidence identified for each search strategy.
https://doi.org/10.1371/journal.pone.0203117.t014
First, the results obtained indicated that the SISM methodology aids in calculating the social impact coverage ratio of the research projects selected and evaluating whether the social impact of the corresponding research is shared by citizens in social media. The social impact coverage ratio applied to the sample selected is low, but when we analyse the SICOR of each project separately, we can observe that some projects have a higher social impact coverage ratio than others. Complementary to altmetrics measuring the extent to which research results reach out society, the SICOR considers the question whether this process includes evidence of potential or real social impact. In this sense, the overall methodology of SISM contributes to advancement in the evaluation of the social impact of research by providing a more precise approach to what we are evaluating.
This contribution complements current evaluation methodologies of social impact that consider which improvements are shared by citizens in social media. Exploring the results in more depth, it is relevant to highlight that of the ten projects selected, there is one research project with a social impact coverage ratio higher than those of the others, which include projects without any tweets or Facebook posts with evidence of social impact. This project has a higher ratio of evidence than the others because evidence of its social impact is shared more than is that of other projects. This also means that the researchers produced evidence of social impact and shared it during the project. Another relevant result is that the quantity of tweets and Fb/posts collected did not determine the number of tweets and Fb/posts found with evidence of social impact. Moreover, the analysis of the research projects selected showed that there are projects with less social media interaction but with more tweets and Fb/posts containing evidence of social media impact. Thus, the number of tweets and Fb/posts with evidence of social impact is not determined by the number of publication messages collected; it is determined by the type of messages published and shared, that is, whether they contain evidence of social impact or not.
The second main finding is related to the effectiveness of the search strategies defined. Related to the strategies carried out under this methodology, one of the results found is that the most effective search strategy is the searchable research results, which reveals a higher percentage of evidence of social impact than the own account and acronym search strategies. However, the use of these three search strategies is highly recommended because the combination of all of them makes it possible to identify more tweets and Facebook posts with evidence of social impact.
Another result is related to the type of evidence of social impact found. There is both quantitative and qualitative evidence. Both types are useful for understanding the type of social impact achieved by the corresponding research project. In this sense, quantitative evidence allows us to understand the improvements obtained by the implementation of the research results and capture their impact. In contrast, qualitative evidence allows us to deeply understand how the resultant improvements obtained from the implementation of the research results are evaluated by the end users by capturing their corresponding direct quotes. The social impact includes the identification of both real and potential social impact.
Conclusions
After discussing the main results obtained, we conclude with the following points. Our study indicates that there is incipient evidence of social impact, both potential and real, in social media. This demonstrates that researchers from different fields, in the present case involved in medical research, public health, animal welfare and genomics, are sharing the improvements generated by their research and opening up new venues for citizens to interact with their work. This would imply that scientists are promoting not only the dissemination of their research results but also the evidence on how their results may lead to the improvement of societies. Considering the increasing relevance and presence of the dissemination of research, the results indicate that scientists still need to include in their dissemination and communication strategies the aim of sharing the social impact of their results. This implies the publication of concrete qualitative or quantitative evidence of the social impact obtained. Because of the inclusion of this strategy, citizens will pay more attention to the content published in social media because they are interested in knowing how science can contribute to improving their living conditions and in accessing crucial information. Sharing social impact in social media facilitates access to citizens of different ages, genders, cultural backgrounds and education levels. However, what is most relevant for our argument here is how citizens should also be able to participate in the evaluation of the social impact of research, with social media a great source to reinforce this democratization process. This contributes not only to greatly improving the social impact assessment, as in addition to experts, policy makers and scientific publications, citizens through social media contribute to making this assessment much more accurate. Thus, citizens’ contribution to the dissemination of evidence of the social impact of research yields access to more diverse sectors of society and information that might be unknown by the research or political community. Two future steps are opened here. On the one hand, it is necessary to further examine the profiles of users who interact with this evidence of social impact considering the limitations of the privacy and availability of profile information. A second future task is to advance in the articulation of the role played by citizens’ participation in social impact assessment, as citizens can contribute to current worldwide efforts by shedding new light on this process of social impact assessment and contributing to making science more relevant and useful for the most urgent and poignant social needs.
Supporting information
S1 file. interrater reliability (kappa) result..
This file contains the SPSS file with the result of the calculation of Cohen’s Kappa regards the interrater reliability. The word document exported with the obtained result is also included.
https://doi.org/10.1371/journal.pone.0203117.s001
S2 File. Data collected and SICOR calculation.
This excel contains four sheets, the first one titled “data collected” contains the number of tweets and Facebook posts collected through the three defined search strategies; the second sheet titled “sample” contains the sample classified by project indicating the ID of the message or code assigned, the type of message (tweet or Facebook post) and the codification done by researchers being 1 (is evidence of social impact, either potential or real) and 0 (is not evidence of social impact); the third sheet titled “evidence found” contains the number of type of evidences of social impact founded by project (ESISM-QUANESIM or ESISM-QUALESIM), search strategy and type of message (tweet or Facebook posts); and the last sheet titled “SICOR” contains the Social Impact Coverage Ratio calculation by projects in one table and type of search strategy done in another one.
https://doi.org/10.1371/journal.pone.0203117.s002
Acknowledgments
The research leading to these results received funding from the 7 th Framework Programme of the European Commission under Grant Agreement n° 613202. The extraction of available data using the list of searchable keywords on Twitter and Facebook followed the ethical guidelines for social media research supported by the Economic and Social Research Council (UK) [ 36 ] and the University of Aberdeen [ 37 ]. Furthermore, the research results have already been published and made public, and hence, there are no ethical issues.
- View Article
- PubMed/NCBI
- Google Scholar
- 6. Godin B, Dore C. Measuring the impacts of science; beyond the economic dimension. INRS Urbanisation, Culture et Sociult, HSIT Lecture. 2005. Helsinki, Finland: Helsinki Institute for Science and Technology Studies.
- 7. European Commission. Better regulation Toolbox. 2017: 129–130. Available from https://ec.europa.eu/info/sites/info/files/better-regulation-toolbox_0.pdf
- 8. European 2020 Strategy. 2010. Available from https://ec.europa.eu/info/business-economy-euro/economic-and-fiscal-policy-coordination/eu-economic-governance-monitoring-prevention-correction/european-semester/framework/europe-2020-strategy_en
- 10. Flecha R, Sordé-Martí T. SISM Methodology (Social Impact through Social Media).2016. Available from https://archive.org/details/SISMMethodology
- 12. Statista. Statistics and facts about social media usage. 2017. Available from https://www.statista.com/topics/1164/social-networks/
- 16. FoodRisc Consortium, European Commission, Main researcher: Patrick Wall. FoodRisc—Result in Brief (Seventh Framework Programme). 2017. Available from http://cordis.europa.eu/result/rcn/90678_en.html
- 23. Murphy, L. Grit Report. Greenbook research industry trends report. Grit Q3-Q4 2016; (Vol. 3–4). New York. Retrieved from https://www.greenbook.org/grit
- 24. EC Research & Innovation. Success Stories [Internet]. 2017 [cited 2017 Apr 25]. Available from: http://ec.europa.eu/research/infocentre/success_stories_en.cfm
- 25. PhysOrg. How re-using food waste for animal feed cuts carbon emissions. 2016. Available from: https://phys.org/news/2016-04-re-using-food-animal-carbon-emissions.html
- 26. EuroFIT. EuroFIT International PP Youtube. 2016; minute 2.05–2.20. Available from: https://www.youtube.com/watch?time_continue=155&v=CHkbnD8IgZw
- 27. European Commission<. Work Programme 2013. Cooperation Theme 1 Health. (2013). Available from: https://ec.europa.eu/research/participants/portal/doc/call/fp7/common/1567645-1._health_upd_2013_wp_27_june_2013_en.pdf
- 29. European Commission. Work Programme 2013. Cooperation Theme 2. Food, agriculture, and fisheries, and biotechnology. (2013). Available from: http://ec.europa.eu/research/participants/data/ref/fp7/192042/b-wp-201302_en.pdf
- 31. European Commission. Work Programme 2012. Cooperation Theme 6 Environment (including climate change). (2011). Available from: http://ec.europa.eu/research/participants/data/ref/fp7/89467/f-wp-201201_en.pdf
- 32. Research Information Center. Technology trialled in fight against ticking timebomb of obesity. 2016. Available from: http://www.euronews.com/2016/08/10/technology-trialled-in-fight-against-ticking-timebomb-of-obesity
- 33. European Commission. Work Programme 2013. Cooperation Theme 3. ICT (Information and Communication Technologies) (2012). Available from: http://ec.europa.eu/research/participants/data/ref/fp7/132099/c-wp-201301_en.pdf
- 34. Euronews. Big farmer is watching! Surveillance technology monitors animal wellbeing. 2016. Available from http://www.euronews.com/2016/05/09/big-farmer-is-watching-surveillance-technology-monitors-animal-wellbeing
- 36. Economic and Social Research Council. Social Media Best Practice and Guidance. Using Social Media. 2017. Available from: http://www.esrc.ac.uk/research/impact-toolkit/social-media/using-social-media/
- 37. Townsend, L. & Wallace, C. Social Media Research: A Guide to Ethics. 2016. Available from http://www.gla.ac.uk/media/media_487729_en.pdf
Social Media and Higher Education: A Literature Review
- Conference paper
- Cite this conference paper
- Yuanqiong Wang 14 &
- Gabriele Meiselwitz 14
Part of the book series: Lecture Notes in Computer Science ((LNISA,volume 9182))
Included in the following conference series:
- International Conference on Social Computing and Social Media
53k Accesses
4 Citations
1 Altmetric
This paper presents a literature review of empirical research related to the use and effects of social media in higher education settings. The adoption of social media has been steadily increasing. However, a majority of the research reported focuses on students’ perception on the effects of social media in learning. The research on the effects of social media on student learning and faculty perspectives are still limited. This literature review focused on the empirical studies that involved the use of social media in higher education in the computing field. Recommendations for future research directions were presented as the result of this literature review.
You have full access to this open access chapter, Download conference paper PDF
Similar content being viewed by others
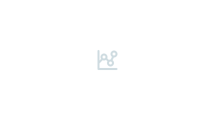
Social media usage by higher education academics: A scoping review of the literature
The opportunities and challenges of social media in higher education: a literature review.
Social Media in Higher Education: A Review of Their Uses, Benefits and Limitations
- Social media
- Higher education
- Student learning
1 Introduction
The popularity of social media sites has been steadily increasing over the last few years, and over 70 % of online adults are now using a social networking site of some kind. Many users of social networking sites have more than one account, and check these accounts several times daily [ 6 ]. But even as social media has been widely adopted by many users, its use for higher education has also been questioned by educators. Although faculty in higher education often utilizes social networking sites in a professional context, many are reluctant to use social networking sites for teaching and learning. Moreover, even though computing faculty members may have more experience with the technology, their adoption of social media for teaching purpose has been at a lower rate comparing to faculty in other fields such as Humanities and Arts, Professions and Applied Sciences, and Social Sciences [ 6 , 20 ].
Web 2.0 (often referred to as the “social web”), with its many benefits such as social networking and user-generated content, has drawn much attention for teaching and learning [ 2 ]. Learning paradigms have shifted over the last decades from a traditional classroom setting to include online learning, e-learning, collaborative learning, and many hybrid forms. This shift indicates a move from instructor-led and instructor-centered learning environments to learner-centered environments, which focus on knowledge creation and building rather than knowledge transmission [ 3 , 5 ]. At first glance, Web 2.0 applications such as social networks, wikis, blogging, and micro blogging seem to be well suited for learner-centered environments, but a closer look reveals that the adoption of Web 2.0 technologies and applications in higher education learning is lagging behind the adoption of Web 2.0 technologies overall. Although roughly 90 % of young adults (18-29 years old) use some social network site, many faculty members also see limitations and potential problems with the use of online and interactive technologies in higher education [ 6 , 20 ]. In a survey, 56 % of faculty members stated that they see online and mobile technologies as more distracting than helpful to students for academic work [ 20 ].
Several studies have investigated the use of social media in higher education, many concentrating on the use of Facebook in their courses. Facebook still dominates the social media landscape, and is popular across a diverse mix of demographic profiles, but other sites have gained popularity and many users now participate in multiple networks [ 20 ]. However, the popularity of Facebook has prompted many educators to integrate some elements into their learning environments.
Some studies point out that it is an obligation to prepare students for what they will encounter once they graduate from college and enter the workplace [ 1 , 5 ]. Other studies examine the connection between social networking and informal and formal learning. Learning in a constructivist environment focuses on the individual learner and the situational context in which learning occurs, and the variety of options and tools that are available through social networking could support this type of situational learning. Students with different backgrounds, learning styles, and preferences can choose which tools they prefer for their individual learning process [ 19 ]. In addition, these technologies may create a higher level of student engagement that will build and support a community of scholars [ 9 , 12 , 23 ].
The majority of studies are experimental studies investigating specific social networking tools (e.g. MySpace, Facebook, Twitter) in specific settings (Business education, communication, medical school), and several studies focusing on pedagogy, learning outcomes, or teaching styles are emerging [ 19 ]. There is little discussion to date about some practical concerns for educators when integrating this technology into the higher education learning process. The fast pace in which technology changes, privacy and security concerns, intellectual property, accessibility for students with disabilities, or the increased workload for instructors have not received much attention [ 19 , 20 ]. Many educators are concerned about the short lifespan of certain applications. MySpace, for example, once the top site for young adults, is practically non-existent in the list of social networks used by this age group [ 6 ]. Moreover, it recently resorted to mass-mailing its former users to convince them to reactivate their still existing accounts [ 25 ]. Many young adults also have moved on from Facebook to other social networking sites, are participating in several sites, and check only their preferred site frequently [ 6 ].
The purpose of this paper is to review existing literature related to the use of social media in computing education at higher education level, the effects of social media on learning, and the concerns of adopting social media in learning. Empirical studies that focused on the use of social media for computer education in colleges, the effects of social media on student learning, and potential barriers of the social media adoption are presented in this paper. This literature review attempts to answer the following research questions:
Does social media lead to any improvement in higher education for learning computing related subjects?
What are the general objective benefits associated to the use of social media in higher education for learning computing related subjects?
What are the perceived benefits associated to the use of social media in higher education for learning computing related subjects?
What are the barriers or concerns that computer faculties have toward the use of social media in higher education for learning computing related subjects?
Literature focusing on the use of social media in higher education for computing subjects was collected and reviewed. The following online databases were utilized for the literature search: EBSCO, IEEE, ACM digital library.
The focus of the search was to gather full-text articles presenting empirical studies which involve the use of social media in higher education setting, especially the ones used for computing related subjects. To manage the scope and the comprehensiveness of the study, the following criteria were used to determine the inclusion of the paper for the review:
The study involved social media tools.
The study investigated the effects of the social media to students’ learning performance and behavior, the effects of the social media to students’ perception of learning process, the perception of the faculty members related to the use of social media.
The study focused on higher education preferably in computing related field. Therefore, studies conducted at K-12 level were excluded from this review.
The study must include a clear discussion on the research method utilized.
The study must be published between 2010 and 2014.
The paper must be written in English.
This section discusses the findings of this literature research. The findings are organized based on the key perspectives of the study.
3.1 Student Perspectives
Majority of the studies reviewed are focused on students’ perspectives of the social media use for instructional purpose, using various social media tools, such as Facebook, Blog, Wiki, and in-house social network tools, etc. Facebook has been the most frequently used site for the studies. This is consistent with the findings reported by Pearson’s social media for teaching and learning survey [ 20 ]. Based on a survey to 191 students in the use of Facebook for a closed group discussion, Gonzalez-Ramirez, Gasco, and Taverner [ 7 ] reported that students’ perceived weaknesses of Facebook in teaching included privacy issues, time required, and technological deficit; while the potential strengths that students predict include performance, communication, participation, and motivation. Students’ perceived usefulness of the tool and students’ learning achievements were the most frequently studied factors.
Student Perceived Learning Experience. In order to study the impact of social media in higher education setting, many researchers conducted explorative studies to investigate the students’ perceived learning experience [ 4 , 7 , 12 , 17 , 21 – 23 ]. Veletsianos and Navarrete [ 24 ] conducted a case study utilizing Elgg as the online social network in an online course, and investigated students’ perceived learning experience. The students reported to have overall enjoyed the experience. When being asked to compare the experience of using social network site (SNS) for class purpose to their previous experience of using traditional learning management systems (LMS), most students preferred SNS over LMS. However, when investigating further in terms of how students were using the tool, they noticed that there was limited participation to course related and graded activities, and little use for social networking and sharing purpose. In addition, students requested more support in managing the amount of information offered in SNS showing the potential of information overload by using SNS. While SNS provide more ways of communication and have the potential of accessing more resources, some students reported to have lacked the ability to effectively find and categorize content for future retrieval. However, all the findings from their study was based on students’ self-reported usage and perception. No investigation in terms of students’ actual usage of the site was done.
Li, Ganeshan and Xu [ 12 ] conducted an online survey to 300 students and a follow-up interview with nine of the respondents to investigate students’ preference of the communication tools and social networking sites. They reported that the students preferred Facebook in general. However, when it is time to discuss course related topics, Facebook is much less preferred than email. Some of the factors that affected the use of SNS for learning purpose included network speed, security and privacy.
Ozmen and Atici [ 17 ] conducted semi-structured interviews with 15 students in the use of LMS supported by SNS. They’ve incorporated Ning to support a Blackboard site. When being asked the overall perception of their learning experience, students responded that although Ning may have the potential to enhance the communication by using the chat tool, the overuse of chat actually lead to more distraction than to help them learn. Therefore, it is suggested that more pedagogical considerations need to be taken when incorporating SNS to the class environment. Finding the appropriate level of integration with the existing LMS and identify the appropriate activities may be the key to improve perceived learning experience.
In addition to general learning experience and preferences, several studies focused on the specific elements that may have the potential of affecting students learning by incorporating SNS to their classes. One of the common studied effect was the social support provided by SNS. For example, DeAndrea, Ellison, LaRose, Steinfield, and Fiore [ 4 ] presented an experiment they conducted that involved first year college students utilizing SpartanConnect, a social media site they designed, to study the effect of SNS in enhancing students’ perceptions of social support. Students were asked to create an account on the site before the semester started. The researchers then distributed pre-test survey after the first two weeks of classes to all first year students, and a post-test survey after using the site for the semester. Out of 1616 first year students who completed post-test survey, 265 students filled out both surveys. Higher level of perceived social support was reported after the use of the site.
Thoms, Eryilmaz and Gerbino [ 22 ] conducted a quasi-experiment in which students were asked to use an in-house online social network (OSN) to receive peer support recommendation. They also found that the use of OSN has improved students’ perceived level of course interaction and peer support, which in turn may lead to better learning.
Taylor [ 21 ] conducted a case study that investigated the possible effect of SNS on students’ retention rate in lower level computing class. The author reported to have seen an increased retention rate after the use of Facebook in CS1 class.
Unfortunately, although much previous research [ 21 , 22 ] reported improvements in students’ perceived learning experience and social support, there are also negative impacts reported. For example, Junco [ 9 ] conducted a survey to investigate the relationship between the use of Facebook and students’ engagement in learning. A negative relationship was reported between the self-reported frequency of Facebook use and students’ engagement. Based on the self-reported data, it shows a negative relationship between the frequency of engaging in Facebook chat and time spent preparing for class as well. This finding seems to be consistent with the study reported by Ozmen and Atici [ 17 ] that overuse of chat can become a distraction for learning since chat takes away the time that initially should have been allocated for study.
Student Learning Achievements. Unlike the studies of the impact on students’ perceived learning experience, the actual learning achievements were not investigated as heavily. Laru, Naykki, Jarvela [ 11 ] conducted a case study that involved 21 students work in groups of four to five for 12 weeks to complete a wiki project. A number of social media tools were introduced to the students, such as ShoZu, Flickr, Google Reader Mobile, Wordpress.com, Wikispaces, FeedBlendr, and FeedBurner RSS. Data was captured by using video recordings, social software usage activity and pre-and post-tests of students’ conceptual understanding of the materials. The comparison between the pre- and post- conceptual knowledge test showed an improvement in test scores received. Looking into more detail in terms of the relationship between the actual activities and the learning outcome, the researchers reported that the higher level of wiki-related activities was an indicator for determining the students improved scores.
Hernandez et al. [ 8 ] conducted an experiment to investigate the impact of different tools supporting students’ learning and perception of interaction. The students were assigned into groups that used Facebook with wiki-style document creation and wall/comment feature, Google Docs, or LMS discussion forum. After comparing the level of activities in each group setting and their final product, it was reported that the number of messages posted was higher in SNS compared to those using traditional LMS forum, time between messages posted was shorter in Facebook compared to other groups, and groups using Facebook also reported higher level of perceived interaction. However, the final result was the same among all groups. No effect in the learning outcome was reported when comparing groups using different tools for group communication.
Instead of an objective measure of the students’ learning achievement while SNS was used, many studies either overlooked the impact of SNS for learning outcomes or used only students’ self-reported data for this purpose [ 9 , 11 ]. More research is needed to look into the impact of SNS on student learning in addition to the impact on student learning experience.
Student SNS Usage Pattern. In addition to the potential impact of SNS for learning, the patterns of the posts in different tools were also investigated. Maleko et al. [ 13 ] reported their findings based on a case study conducted that compares Facebook and Blackboard usage by the students. They reported that posts in Facebook were unique in that they were concentrated in the expression of dissatisfaction, course admin, encouragement, discussions outside programming, and general advice; while the posts in Blackboard tend to be more for the purpose of community building and question to the lecturer. In addition, students reported to have preferred the use of Facebook for learning support where no authoritative figure was present.
Kear et al. [ 10 ] conducted a survey after students were asked to use an in-house wiki to co-edit a document after completing an online tutorial of how the wiki can be used. They collected data about students’ use of wiki over time. The authors reported decreased use overtime although students learned how to use wiki. After further investigation through the survey instrument, they found that the students were unhappy about editing other students’ work. When being asked to compare wiki and traditional discussion forum for this kind of collaborative activities, students preferred forum over wiki.
3.2 Faculty Perspectives
Unlike the studies focused on students’ perspectives, only a handful of studies that we investigated looked into the SNS use from faculty members’ perspectives.
Faculty Perception. Faculty perception of the SNS use for learning purpose has been mixed comparing to students perception. The survey conducted by Pearson [ 20 ] indicated that one of the reasons for faculty members not incorporating SNS in their teaching was because they consider the use of SNS as a distraction. Roblyer et al. [ 18 ] reported different perceptions of the faculty members compared to students. They found that students are more open to use Facebook when comparing to email for the communication purpose. Faculty members were more prone to traditional technologies, such as email. Brown also did a survey and a follow-up more in depth interview with the faculty members regarding the use of Web 2.0 technologies for learning purposes. The responses received from the faculty members indicated that promoting active student participation, enhancing distribution of and access to tutor-selected or generated learning content are the potential benefits indicated.
Faculty Concerns. The hesitance of faculty members regarding the use of SNS in classrooms may be explained by the concerns identified by previous studies. For example, Brown [ 2 ] reported that misalignments between the increasing amount of collaborative group work expected and continuing individual assessment, no “added value” to teaching, and too many constraints (because of the university policy) are the major concerns from faculty members. Kear et al. [ 10 ] reported that, in addition to the above mentioned concerns, performance of the SNS used, the difficulties in marking and monitoring students’ work, workload issues are also among the concerns raised by the teaching staff members.
4 Discussion
The literature review has shown some empirical findings that this paper attempted to investigate. In terms of the question regarding whether social media lead to any improvement in higher education for learning computing related subjects, the literature shows some evidence of improvement. Although the empirical study is extremely limited in gathering objective performance data to showcase the improvement of learning, the student self-reported data shows promising potential of the effectiveness of SNS use in higher education. In addition, literature indicated that careful pedagogical consideration needs to be made in order to ensure the effective use of SNS. However, more investigation is definitely needed.
The general objective benefits associated to the use of social media in higher education for learning computing related subjects has been identified by case studies and survey data. The identified benefits included improved social support, improved retention rate through peer support, and improved perceived interaction. However, the empirical study also showed that there can be negative impact of the use of SNS to the students’ engagement in learning.
When answering the perceived benefits associated with the use of social media in higher education for learning computing related subjects, the answers from students and faculty members are similar. Students tend to enjoy the activities using SNS considering it to help improve the interaction, and motivation to learning. In addition to the benefits identified by students, faculty members value the possibility of enhancing distribution of and access to tutor-selected or generated learning content through the use of SNS.
The specific concerns from computer faculty members were not identified from the literature review. However, the investigation of the literature shows that there is a list of potential concerns that are common for most of the faculty members. In general, faculty members share similar concerns as the students which include security, privacy and performance of the site/tool being used. In addition, faculty members are also concerned about the work load issue, the difficulty of performance evaluation and monitoring, and need for careful pedagogical design when it comes to the use of SNS for learning. Faculty members in computing field may be more concerned about the potential distraction of SNS and its security issues because of their familiarity of the technology. However, this was not identified in the literature and further investigation is definitely needed.
5 Recommendations and Future Research
Even though the literature review shows potential of social media usage for learning purpose, the use of the technology is still limited and not many controlled evaluations and in-depth studies in higher education settings have been conducted. First, more empirical study is needed to investigate the actual “added” benefits of SNS comparing to the use of traditional LMS. One of the major limitations of current literature is that most of the studies focused on self-report data to study the effect of the technology. Therefore, the actual usage and learning outcome should be addressed and investigated in more depth.
Although computing faculty members may know the technology better than faculty members in other field, their adoption of SNS is lagging behind. Is there any specific reason? Is it because of the nature of the topic that is sometimes hard to describe in texts? Is it because of the higher security concern from the faculty members? More investigation is needed to address this issue.
Alexander, B.: Web 2.0: A new wave of innovation for teaching and learning? Educause review 41.2 (2006)
Google Scholar
Brown, S.: Seeing Web 2.0 in context: A study of academic perceptions. Internet High. Educ. 15 (1), 50–57 (2012)
Article Google Scholar
Brownson, S.: Embedding social media tools in online learning courses. J. Res. Innovative Teach. 7 (1), 112–118 (2014)
DeAndrea, D.: Serious social media: on the use of social media for improving students adjustment to college. Internet High. Educ. 15 (1), 15–23 (2012)
Duffy, P., Bruns A.: The use of blogs, wikis and RSS in education: In: A conversation of possibilities, pp. 31–38 (2006)
Duggan, M., Smith, A.: Social media update 2013. Pew Internet and American Life Project (2013)
González-Ramírez, R., Gascó, J., Taverner, J.: Facebook in teaching: strengths and weaknesses. Int. J. Inf. Learn. Technol. 32 (1), 65–78 (2015)
Hernández, R. et al.: Facebook for CSCL, Latin-American experience for professors. In: Proceedings of the IEEE 12th International Conference on Advanced Learning Technologies (ICALT), IEEE (2012)
Junco, R.: The relationship between frequency of Facebook use, participation in Facebook activities, and student engagement. Comput. Educ. 58 (1), 162–171 (2012)
Kear, K., et al.: From forums to wikis: perspectives on tools for collaboration. Internet High. Educ. 13 (4), 218–225 (2010)
Laru, J., Näykki, P., Järvelä, S.: Supporting small-group learning using multiple Web 2.0 tools: a case study in the higher education context. Internet High. Educ. 15 (1), 29–38 (2012)
Li, X., Ganeshan, K., Xu, G.: The role of social networking sites in e-learning, In: Proceedings of the FIE 2012 Conference on 42nd ASEE/IEEE Frontiers in Education (2012)
Maleko, M., et al.: Facebook versus Blackboard for supporting the learning of programming in a fully online course: the changing face of computing education. In: Proceedings of the Learning and Teaching in Computing and Engineering (LaTiCE), IEEE (2013)
Mamaqi, X.: The efficiency of different ways of informal learning on firm performance: A comparison between, classroom, web 2 and workplace training. Computers in Human Behavior (2015)
Moran, M., Seaman, J., Tinti-Kane, H.: Teaching, Learning, and Sharing: How Today’s Higher Education Faculty Use Social Media. Babson Survey Research Group, UK (2011)
O’Keeffe, G., Clarke-Pearson, K.: The impact of social media on children, adolescents, and families. Pediatrics 127 (4), 800–804 (2011)
Özmen, B., Atıcı, B.: Learners views regarding the use of social networking sites in distance learning. Int. Rev. Res. Open Distrib. Learn. 15 (4), 161–177 (2014)
Roblyer, M.D., et al.: Findings on Facebook in higher education: A comparison of college faculty and student uses and perceptions of social networking sites. Internet High. Educ. 13 (3), 134–140 (2010)
Rodriguez, J.: Social media use in higher education: key areas to consider for educators. MERLOT J. Online Learn. Teach. 7 (4), 539–550 (2011)
MathSciNet Google Scholar
Seaman, J., Tinti-Kane, H.: Social Media for Teaching and Learning. Pearson Learning Systems, UK (2013)
Taylor, K.: Can utilizing social media and visual programming increase retention of minorities in programming classes? In: Frontiers in Education Conference 2013, vol. 54 pp. 1046-1048. IEEE (2013)
Thoms, B., Eryilmaz, E., Gerbino, S.: Designing a peer support system for computer programming courses using online social networking software(HICSS), In: 47th Hawaii International Conference on System Sciences, IEEE (2014)
Veletsianos, G.: Higher education scholars participation and practices on Twitter. J. Comput. Assist. Learn. 28 (4), 336–349 (2012)
Veletsianos, G., Navarrete, C.: Online social networks as formal learning environments: Learner experiences and activities. Int. Rev. Res. Open Distrib. Learn. 13 (1), 144–166 (2012)
Washington Post. http://www.washingtonpost.com/news/the-intersect/wp/2014/06/02/myspace-still-exists-and-its-desperate-enough-to-blackmail-you-into-logging-in/
Download references
Author information
Authors and affiliations.
Towson University, Annapolis, MD, USA
Yuanqiong Wang & Gabriele Meiselwitz
You can also search for this author in PubMed Google Scholar
Corresponding author
Correspondence to Yuanqiong Wang .
Editor information
Editors and affiliations.
Towson University, Towson, MD, USA
Gabriele Meiselwitz
Rights and permissions
Reprints and permissions
Copyright information
© 2015 Springer International Publishing Switzerland
About this paper
Cite this paper.
Wang, Y., Meiselwitz, G. (2015). Social Media and Higher Education: A Literature Review. In: Meiselwitz, G. (eds) Social Computing and Social Media. SCSM 2015. Lecture Notes in Computer Science(), vol 9182. Springer, Cham. https://doi.org/10.1007/978-3-319-20367-6_11
Download citation
DOI : https://doi.org/10.1007/978-3-319-20367-6_11
Publisher Name : Springer, Cham
Print ISBN : 978-3-319-20366-9
Online ISBN : 978-3-319-20367-6
eBook Packages : Computer Science Computer Science (R0)
Share this paper
Anyone you share the following link with will be able to read this content:
Sorry, a shareable link is not currently available for this article.
Provided by the Springer Nature SharedIt content-sharing initiative
- Publish with us
Policies and ethics
- Find a journal
- Track your research
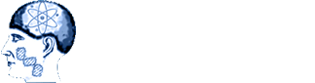
Asian Journal of Education and Social Studies
Published: 2024-03-16
DOI: 10.9734/ajess/2024/v50i41334
Page: 320-328
Issue: 2024 - Volume 50 [Issue 4]
Review Article

Article Metrics
Impact of social media on society: a literature review.
Zahid Ahmad Wani
Shantha College of Allied Health Science, Affiliated to RGUHS, Bangalore, India.
Ayesha Bhat
Shantha College of Physiotherapy, Affiliated to RGUHS, Bangalore, India.
Vartika vishnoi
Vivek University Bijnor, UP, India.
Hina Praveen
Gautham College, Affiliated to RGUHS, Bangalore, India.
Naveen H Simon *
Shantha College of Nursing, Affiliated to RGUHS, Bangalore, India.
Diana Hephzibah
*Author to whom correspondence should be addressed.
This manuscript explores the profound impact of social media on society, with a focus on social behavior, politics, and cultural norms. Employing a systematic literature review, including diverse sources, case studies, and real-world examples, the study provides a nuanced and comprehensive analysis. The brevity enforced by character limits on platforms like Twitter has shaped concise and direct communication, with emojis, memes, and hashtags becoming integral to online discourse. The rapid spread of information on social media has, however, led to the dissemination of misinformation, influencing public opinion and potentially affecting political outcomes. Collaboration among individuals, policymakers, and technology developers is crucial to shape the future of social media responsibly. This necessitates cultivating a culture of responsible use, promoting digital literacy, and advocating for policies that safeguard user rights and well-being. Striking a balance between innovation and ethical considerations is paramount to ensure the continued positive contribution of social media to society.
Keywords: Cultural landscapes, interpersonal relationships, paradigm shift, social media
How to Cite
- Download Citation
- Endnote/Zotero/Mendeley (RIS)
Shahjahan ATM, Chisty K, Social media research and its effect on our society. International Journal of Information 7 Communication Engineering. 2014;8(6).
Aalbers G, McNally RJ, Heeren A, de Wit S, Fried EI. Social media and depression symptoms: A network perspective. J. Exp. Psychol. Gen. 2018;148:1454–1462 DOI: 10.1037/xge0000528
Abbas R, Mesch G. Do rich teens get richer? Facebook use and the link between offline and online social capital among Palestinian youth in Israel. Inf. Commun. Soc. 2018;21:63–79.
DOI: 10.1080/1369118X.2016.1261168
Alahmar AT. The impact of social media on the academic performance of second year medical students at College of Medicine, University of Babylon, Iraq. J. Med. Allied Sci. 2016;6:77–83 DOI: 10.5455/jmas.236927
Barbosa B, Chkoniya V, Simoes D, Filipe S, Santos CA. Always connected: Generation Y smartphone use and social capital. Rev. Ibérica Sist.Tecnol. Inf. 2020; E35:152–166.
Bin Zhao et al. Online detection of unusual events in multimedia via dynamic sparse coding; 2011.
Chang Y. The relationship between maladaptive perfectionism with burnout: Testing mediating effect of emotion-focused coping. Pers. Individ. Differ. 2012; 53:635–639.
DOI: 10.1016/j.paid.2012.05.002
Chen M, Xiao X. The effect of social media on the development of students’ affective variables. Front. Psychol. 2022;13: 1010766 DOI: 10.3389/fpsyg.2022.1010766
Drouin M, Reining L, Flanagan M, Carpenter M, Toscos T. College students in distress: Can social media be a source of social support? Coll. Stud. J. 2018;52: 494–504.
Festinger L. A theory of social comparison processes. Hum. Relat. 1954; 7:117–140 DOI: 10.1177/001872675400700202
Frison E, Eggermont S. Exploring the relationships between different types of Facebook use, perceived online social support, and adolescents’ depressed mood. Soc. Sci. Compu. Rev. 2016;34: 153–171.
DOI: 10.1177/0894439314567449
Hair JF, Sarstedt M, Pieper TM, Ringle CM. The use of partial least squares structural equation modeling in strategic management research: A review of past practices and recommendations for future applications. Long Range Plann. 2012;45: 320–340 DOI: 10.1016/j.lrp.2012.09.008
Keles B, McCrae N, Grealish A. A systematic review: The influence of social media on depression, anxiety and psychological distress in adolescents. Int. J. Adolesc. Youth. 2020;25: 79–93.
DOI: 10.1080/02673843.2019.1590851
Mahmoudi Sidi Ahmed et al., Detection of abnormal motions in multimedia, Chania ICMI-MIAUCE’08 workshop, Crete, Greece; 2008.
Mathewson M. The impact of social media usage on students’ mental health. J. Stud. Affairs. 2020;29:146–160.
Nagar, Himanshu, Chetna Dabas, Gupta JP. Navie bayes and K-means hybrid analysis for extracting extremist tweets, ACM Conference. 2020;27-32.
Ostic D, Qalati SA, Barbosa B, Shah SMM, Galvan Vela E, Herzallah AM and Liu F. Effects of social media use on psychological well-being: A mediated model. Front. Psychol. 2021;12:678766 DOI: 10.3389/fpsyg.2021.67876
Willium S. Network security and communication, IEEE Transaction. 2012; 31(4):123-141.
Shabnoor S, Tajinder S, Social media its impact with positive and Negative Aspects IJCATR. 2016;5(2):71 - 75.
Sampasa-Kanyinga H, Lewis RF. Frequent use of social networking sites is associated with poor psychological functioning among children and adolescents. Cyberpsychol. Behav. Soc. Network. 2015;18:380–385 DOI: 10.1089/cyber.2015.0055
Stapel DA, Koomen W. Distinctiveness of others, mutability of selves: Their impact on self-evaluations. J. Pers. Soc. Psychol. 2000;79:1068–1087 DOI: 10.1037//0022-3514.79.6.1068
Vernon L, Modecki KL, Barber BL. Tracking effects of problematic social networking on adolescent psychopathology: The mediating role of sleep disruptions. J. Clin. Child Adolesc. Psychol. 2017;46:269–28 DOI: 10.1080/15374416.2016.1188702
Tariq W, Mehboob M, Khan MA, Ullah F. The impact of social media and social networking on education and Students of Pakistan. International Journal of Computer sciences issues. July 2012;9(3).
Weng L, Menczer F. Topicality and impact in social media: Diverse messages, focused messengers. PLoS One. 2015; 10:e0118410 DOI: 10.1371/journal.pone.0118410
Zareen N, Karim N, Khan UA. Psycho-emotional impact of social media emojis. ISRA Med. J. 2016;8:257–262.
© Copyright 2010-Till Date, Asian Journal of Education and Social Studies. All rights reserved.
- DOI: 10.62754/joe.v3i3.3386
- Corpus ID: 271712167
Silenced Voices on the Net: A Systematic Literature Review on Gender-Based in Social Media Networks
- A. Duche-Pérez , Ricardo Enrique Grundy-López , +2 authors Ángela Valeria Mya Pazo Romero
- Published in Journal of Ecohumanism 25 June 2024
126 References
Social media and the public sphere.
- Highly Influential
Politicizing Gender in Discourse: Feminist Critical Discourse Analysis as Political Perspective and Praxis
Impact of social media influencers on consumers' well-being and purchase intention: a tiktok perspective, impact of green hrm practices on environmental performance: the mediating role of green innovation, impulsive and compulsive buying tendencies and consumer resistance to digital innovations: the moderating role of perceived threat of covid-19, the difference between emergency remote teaching and e-learning, investigating the role of social media abuse in gender-based violence: the experiences of women police officers, content analysis of responses to the line, an australian primary prevention of violence against women campaign on facebook, rethinking male peer support theory: social network responses to young men’s violence against women, declaración prisma 2020: una guía actualizada para la publicación de revisiones sistemáticas, related papers.
Showing 1 through 3 of 0 Related Papers
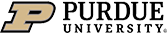
ORIGINAL RESEARCH article
“left on read” examining social media users’ lurking behavior: an integration of anxiety and social media fatigue.
- 1 School of Culture and Media, Shanxi College of Applied Science and Technology, Taiyuan, China
- 2 School of Humanities and Social Sciences, City University of Macau, Macao, China
- 3 School of Broadcast Announcing Arts, Communication University of Zhejiang, Hangzhou, China
Introduction: With the widespread use of social media, the behavior and mindset of users have been transformed, leading to a gradual increase in lurking users, which can impede the sustainable development of social media platforms. In this study, we aim to investigate the impact of intrinsic and extrinsic motivational factors on social media users’ anxiety, social media fatigue, and lurking behavior.
Methodology: For the confirmation of these phenomena and to validate the theories, a structural equation model was constructed based on the SSO (Stressor-Strain-Outcome) theoretical framework. The model was then tested and validated with data from 836 valid online surveys. These data were analyzed using SPSS 27.0 and AMOS 24.0 software.
Results: The results indicate that intrinsic motivations (such as social comparison and privacy concerns) and extrinsic motivations (including information overload, functional overload, and social overload) are positively associated with users’ lurking behavior through the mediating effects of social media fatigue and anxiety. Additionally, for the mediator variables, social media fatigue was found to be positively associated with anxiety.
Discussion: These findings underscore the importance of social media platforms considering both intrinsic and extrinsic motivational factors to mitigate user anxiety and social media fatigue. By addressing these factors, platforms can foster user satisfaction and increase engagement, ultimately contributing to the sustainable development of social media platforms.
1 Introduction
Advances in Internet technology have fueled the rapid growth of social media platforms. WeChat, as one of the most popular social media platforms among Chinese users, seamlessly integrates various functions such as communication, payment, official accounts, Moments, Mini apps, and life services, fundamentally reshaping people’s lifestyles. Nevertheless, as social media becomes deeply intertwined with people’s professional, educational, and personal lives, users are increasingly fatigued by the constant demands for engagement such as liking, retweeting, commenting, bargaining, price cutting, voting, and other overwhelming social requests ( Guo et al., 2020 ). Faced with the ever-expanding WeChat Moments and countless group chats, users are increasingly choosing to “leave on read” (a term commonly used in messaging and social media contexts to indicate that a person has read a message but has not responded to it), gradually transforming into a “silent presence” within the social media platform ( Barnidge et al., 2023 ). These users receive information without actively responding, view information without providing feedback, read content without sharing it, and are often referred to as “lurkers” ( Takahashi et al., 2003 ). Their behavior of exclusively searching or reading information that meets their needs on social networks, while seldom or never creating content, sharing information, or posting insights, is defined as lurking behavior ( Edelmann, 2013 ). In the realm of social media, consistent user engagement and active content creation are essential to platform activity, and the presence of lurkers significantly hampers the platform’s sustainable development ( Kang and Shin, 2008 ; Ortiz et al., 2018 ). Hence, identifying the underlying reasons for lurking is imperative for the advancement of social media platforms.
Existing literature attributes lurking behavior to factors such as computer anxiety ( Osatuyi, 2015 ), technology overload ( Karr-Wisniewski and Lu, 2010 ), information security awareness ( Ortiz et al., 2018 ), and knowledge-collecting behavior ( Nguyen et al., 2022 ). However, the majority of these studies concentrate on investigating individual factors, thereby neglecting the broader psychological motivations driving lurkers and the comprehensive impact of the external environment on their behavior. Thus, this study draws upon self-determination theory ( Deci et al., 1991 ; Hung et al., 2011 ) to propose that user lurking behavior is influenced by a combination of intrinsic and extrinsic motivations.
This study aims to investigate the factors influencing WeChat users’ lurking behavior by addressing the following questions: First, what intrinsic and extrinsic motivations contribute to social media users’ lurking behavior amidst the prevalence of social media? Second, how do these motivating factors impact users’ lurking behavior and what tailored preventive measures can be implemented? To delve deeper into these inquiries, the study adopts the SSO model to uncover the underlying motivations driving social media users’ lurking behavior and to shed light on the essence of “left on read” behavior. By doing so, the research seeks to fill the gaps in existing literature concerning the complexities of social media users’ lurking behavior. Additionally, it strives to unveil users’ interaction patterns and communication needs within the virtual realm of social media, with the ultimate goal of optimizing the functionality of social media platforms and offering enhanced solutions to mitigate issues related to users’ lurking behavior. Through this investigation, it also aims to provide concrete recommendations for refining the functions of social media platforms and enhancing overall user experience in the virtual space.
2 Literature review
2.1 sso model.
The SSO model consists of stressor, strain, and outcome ( Koeske and Koeske, 1993 ), with the strain mediating between the stressor and the behavioral outcome. This model delves into the micro-psychological processes within the stimulus-organism-response paradigm and investigates the impacts of various stressors on an individual’s psychology and behavior. Stressors in this context refer to environmental stimuli that induce stress in individuals, while strain manifests as negative disruptions in attention, cognition, and emotion that individuals experience when under the influence of a stressor. Outcomes signify the enduring feelings of stress or tension that individuals undergo when exposed to a stressor, resulting in sustained negative behavioral or psychological reactions ( Hu et al., 2024 ).
The SSO model, serving as a comprehensive framework for explaining an individual’s intrinsic psychological perceptions and behavioral responses to environmental stimuli, is extensively utilized in the realms of psychosocial wellbeing ( Dhir et al., 2018 ), emotional dissonance ( Takahashi et al., 2003 ), social media fatigue ( Dhir et al., 2019 ), and other health communication fields. In this study, intrinsic user stress and extrinsic social media stress collectively form a stressor for social media users, triggering a negative focal strain response that ultimately manifests as lurking behavior. Consequently, the utilization of the SSO model to explore the lurking behavior of social media users in this study is considered fitting.
2.2 The self-determination theory
The Self-Determination Theory (SDT) is a comprehensive theory of individual motivation, emotions, and personality qualities that focuses on the underlying reasons for individual behavior ( Deci and Ryan, 2000 ). According to the theory, motivation serves as the driving force behind human behavior, determining the specific actions individuals take in response to external circumstances and internal needs. Previous research has demonstrated the significant impact of motivation on technology acceptance behavior ( Teo et al., 1999 ; Venkatesh et al., 2002 ), job stress and employees’ well-being ( Luo, 1999 ), as well as physical activity ( Teo et al., 1999 ; Vallerand, 2001 ).
Individual motivation can be classified into three main types based on the perceived level of autonomy: intrinsic motivation, extrinsic motivation, and amotivation ( Deci and Ryan, 2000 ). Intrinsic motivation stems from internal factors that bring satisfaction to an individual during a specific activity, reflecting an internal drive. On the other hand, extrinsic motivation is driven by external factors such as rewards, promotions, punishments, and coercion. Both intrinsic and extrinsic motivation play a crucial role in shaping an individual’s intentions and actions toward an activity ( Olatokun and Nwafor, 2012 ). Therefore, this study examines both intrinsic and extrinsic motivations and their impact on lurking behavior in a holistic approach.
2.3 Social media lurking
As users become more knowledgeable and experienced in using social media, their engagement and contribution to social media platforms evolve over time ( Hong et al., 2023 ). Online knowledge communities categorize participants into two groups: lurkers and posters. Lurkers are individuals who read posts without actively contributing, while posters are those who share knowledge within online communities ( Nguyen, 2020 , 2021 ). Some previous studies have portrayed lurking behavior as a negative behavior among social media users, characterized by a typical “free-riding” phenomenon ( Sun et al., 2014 ), where lurkers consume information without actively sharing it, resulting in minimal contribution to the online community ( Van Mierlo, 2014 ).
However, some scholars argue that lurking behavior serves as a social media participation strategy for users aiming to safeguard the privacy of their personal information within social media networks ( Osatuyi, 2015 ). Permission to lurk is seen as an essential requirement for users to integrate into unfamiliar online communities, allowing them to understand and adapt to the social environment through passive observation upon initial entry ( Neelen and Fetter, 2010 ). Building on scholars’ definitions of lurking behavior, this study defines lurking behavior as the observation-only behavior of social media users without active participation on social media platforms ( Ortiz et al., 2018 ).
Various triggers prompt lurking behavior, some users perceive lurking as a method to protect their private information ( Osatuyi, 2015 ; Ortiz et al., 2018 ), while others, concerned about their reputation within the community, opt to lurk to avoid comparisons with other users regarding information dissemination and knowledge contribution ( Nisar et al., 2019 ). Moreover, when users experience information and social overload on social media, they may develop negative emotions like anxiety and fatigue, leading to a deliberate reduction in content contribution and engagement in information avoidance behaviors ( Guo et al., 2020 ; Nguyen, 2020 ). Social media fatigue is also considered a trigger for lurking behaviors ( Hong et al., 2023 ). Social media fatigue refers to the feelings of weariness, monotony, and ennui experienced by users during social media usage, influenced by personal, platform, and social factors ( Maier et al., 2015 ).
However, the majority of existing studies have examined the reasons behind social media users’ lurking behavior from a singular standpoint, either negative or positive, thereby hindering a comprehensive understanding of the underlying factors contributing to such behavior. In an effort to address this gap, this study leverages the Self-Determination Theory (SDT) to investigate the impact of anxiety and social media fatigue as mediating variables on users’ lurking behaviors, taking into account two primary factors: intrinsic motivation and extrinsic motivation.
3 Research hypothesis and model
Motivation plays a pivotal role in influencing user behavior ( Deci and Ryan, 2000 ). In this study, intrinsic and extrinsic motivations are closely examined. Intrinsic motivation, which stems from within an individual, is scrutinized through the lenses of social comparison and privacy concerns; whereas extrinsic motivation, originating from external sources, is analyzed in terms of information overload, functional overload, and social overload. These motivations are chosen for their ability to capture the essence of social media characteristics and user behaviors.
Social comparison unveils the negative emotional responses users experience when comparing themselves to more specialized individuals, potentially diminishing their positive engagement and resulting in lurking behavior ( Nisar et al., 2019 ; Verduyn et al., 2020 ; Okano and Nomura, 2023 ). Privacy concerns signify users’ apprehensions regarding data protection. If users feel their personal data is being mishandled, they perceive privacy risks and are hesitant to engage in information sharing activities ( Osatuyi, 2015 ; Ortiz et al., 2018 ).
Regarding extrinsic motivations, information overload manifests as users’ unease with the abundance of information on social media, potentially diminishing the perceived value of knowledge. Functional overload refers to the disruption in user behavior due to platform over functionality. Social overload amplifies the online burdens on users through excessive social demands, consuming time and attention, consequently resulting in lurking behavior ( Guo et al., 2020 ; Liu et al., 2020 ; Hong et al., 2023 ).
Moreover, the inclusion of anxiety and social media fatigue as psychological strain responses more accurately captures the negative emotions contributing to users’ lurking behavior ( Dhir et al., 2018 ; Hong et al., 2023 ; Li et al., 2024 ). Users experiencing anxiety due to factors like lack of expertise and self-confidence may refrain from engaging in sharing activities ( Okano and Nomura, 2023 ). Social media fatigue symbolizes users’ weariness and burnout from prolonged social media use and the inability to find gratification in sharing activities, thus resulting in lurking behavior ( Hong et al., 2023 ).
3.1 Intrinsic motivation
3.1.1 social comparison.
Social comparison involves using others as a reference point to gage our own behavior in relation to theirs (ability comparisons) or to determine how we should think, feel, and act (opinion comparisons). These comparisons offer insights into our own abilities, social standing, and performance, as well as those of others ( Festinger, 1954 ). In online social settings, users frequently engage in comparisons with other community members, evoking a range of emotions. Individuals typically focus on self-evaluation as the central aspect of social comparison ( Lim and Yang, 2019 ). As users of social media platforms, individuals tend to concentrate on their impact within the community and may consciously or subconsciously measure themselves against those who have achieved greater success, leading to feelings of inadequacy and negative sentiment ( Nisar et al., 2019 ). This behavior of social comparison diminishes self-confidence in one’s knowledge, abilities, and other facets of life, heightens anxiety, and diminishes motivation to participate in activities, potentially resulting in escalating fatigue and burnout associated with social media usage.
Moreover, upward social comparison (where individuals compare themselves to those in more favorable circumstances) represents a common social phenomenon ( Okano and Nomura, 2023 ). On social media platforms, individuals engage in self-comparisons by observing the content (e.g., posts, photos, etc.) presented by others online, with the number of likes and comments serving as indications of individual social competence and popularity. This uptick in social comparison behavior notably intensifies the frequency of comparisons made by social media users, perpetuating feelings of social fatigue stemming from continual self-evaluation against others. Consequently, users’ interest in social interactions may dwindle, making lurking behavior more prevalent. Therefore, the following hypotheses are proposed:
H 1a: Social comparison is positively associated with anxiety.
H 1b: Social comparison is positively associated with social media fatigue.
3.1.2 Privacy concerns
In today’s digital landscape, an increasing number of users are persistently mindful of the significance of online privacy issues, indicating their apprehensions about potential data breaches associated with social media use ( Zhang et al., 2022 ). Despite this heightened awareness, numerous personal data points are susceptible to unauthorized exposure on social media platforms without users’ explicit consent, leading to heightened levels of privacy-related anxiety. Individuals troubled by privacy concerns often harbor suspicions that the online community and its members could potentially exploit their personal information, fostering a substantial sense of privacy risk that dissuades them from freely sharing information within the community ( Kim et al., 2021 ). In a notable instance, Winder (2021) reported a data breach in which the personal data of 419 million Facebook users was compromised and exposed to unsecured databases in 2019. Additionally, many interface interactions necessitate users to click the “consent” button to proceed, inadvertently granting platforms permission to gather information about their browsing habits, potentially leading to unauthorized dissemination of data without the user’s explicit consent. This heightened awareness regarding privacy issues can contribute to psychological fatigue ( Fan et al., 2020 ). Furthermore, privacy concerns may cultivate user distrust in social media usage. A study by Ortiz et al. (2018) explored the connection between personal privacy concerns and users’ behavioral intentions, noting that such concerns are likely to induce anxiety, subsequently influencing users to adopt negative usage patterns that ultimately culminate in reduced social media engagement. Therefore, the following hypotheses are proposed:
H 2a: Privacy concerns are positively associated with anxiety.
H 2b: Privacy concerns are positively associated with social media fatigue.
3.2 Extrinsic motivation
Building upon the technology overload framework proposed by Karr-Wisniewski and Lu (2010) , Zhang et al. (2016) introduced three types of perceptual overload within social network service environments: system functional overload, information overload, and social overload. Perceptual overload has been highlighted as a significant precursor to various negative outcomes such as anxiety, exhaustion, regret, and fatigue ( Guo et al., 2020 ; Huang et al., 2022 ; Li et al., 2022 ). This overload can instigate feelings of fatigue, subsequently influencing users’ intentions or behaviors toward discontinuing usage. Nevertheless, the impact of perceived overload on users’ lurking behavior, whether indirect or direct, remains ambiguous. Therefore, this study examines it as an extrinsic environmental stimulus that motivates lurking behavior.
3.2.1 Functional overload
While the current features of social media platforms generally cater to the basic requirements of users, platforms frequently introduce new features via system updates to address issues, improve the interface, or enhance overall user experience. As the functionality of these platforms continues to expand, there is a risk of surpassing the genuine needs of users, leading to functional overload ( Thompson et al., 2005 ; Zhou and Xie, 2023 ). Platform operators often introduce these new features with the intention of enhancing the user experience; however, some features may not align with users’ actual needs, potentially adding to users’ burdens and anxieties ( Fu et al., 2020 ). Given that many users engage with multiple social media platforms simultaneously, they must invest considerable time and effort in familiarizing themselves with these new features that may exceed their requirements. If the benefits derived from learning and utilizing these additional features do not outweigh the associated costs, users may experience feelings of monotony and fatigue ( Lee et al., 2016 ). Furthermore, certain added features could heighten the complexity of social media platforms, diminishing usability and, consequently, fostering user dissatisfaction. Therefore, the following hypotheses are proposed:
H 3a: Functional overload is positively associated with anxiety.
H 3b: Functional overload is positively associated with social media fatigue.
3.2.2 Information overload
In the era of rapid technological advancement, users are inundated with vast quantities of intricate and often indistinguishable information across social media platforms. While information serves to alleviate uncertainty, an excessive influx of data can exacerbate uncertainty and impose cognitive burdens ( Hogan and Brashers, 2015 ; Zhang et al., 2016 ). Information overload ensues when users are inundated with information that surpasses their limited processing capabilities, eliciting negative emotional responses ( Lee et al., 2016 ). This overload stands as a primary driver of user fatigue; heightened perceptions of information overload correspond to increased stress levels and subsequent fatigue ( Kim and Park, 2015 ). Users may experience discontent stemming from a perceived lack of effective information filtering mechanisms on the platform. Moreover, when information surpasses users’ cognitive thresholds, an excess of irrelevant and nebulous data compounds user fatigue, potentially leading to mental distress, stress, and anxiety ( Fu et al., 2020 ). Therefore, the following hypotheses are proposed:
H 4a: Information overload is positively associated with anxiety.
H 4b: Information overload is positively associated with social media fatigue.

3.2.3 Social overload
The advent of mobile technology has facilitated pervasive social interaction and communication through social networks. Research by Dunbar (1992) suggests that individuals can maintain stable relationships with up to 150 friends. However, the prevalence of social media in everyday life has led many users to accumulate online connections far surpassing Dunbar (1992) proposed limit. Within the realm of social media, users are often inundated with various social demands from their network, and social overload manifests when individuals are confronted with more requests than they can manage ( Zhang et al., 2016 ). Social overload can disrupt users’ regular activities, divert their attention, induce feelings of stress and anxiety, impede their engagement, and foster unsustainable patterns of social media use ( Cao and Sun, 2018 ). Consequently, users may find themselves struggling to cope with the demands placed upon them, leading to heightened levels of anxiety and fatigue. Therefore, the following hypotheses are proposed:
H 5a: Social overload is positively associated with anxiety.
H 5b: Social overload is positively associated with social media fatigue.
3.3 Anxiety
Anxiety is a prevalent emotional state that arises when individuals find themselves in uncertain circumstances, experiencing internal tension and distress, while the autonomic nervous system initiates coping mechanisms in response to perceived threats ( Bekker et al., 2003 ). In the realm of social media, anxiety stands out as a common negative psychological repercussion often stemming from social media overload ( Dhir et al., 2018 ). This state of anxiety can prompt various perceptual and evaluative hindrances, such as distortions in perception, hyper-vigilance toward potential dangers and threats, as well as impairments in rational judgment and information processing abilities. Within the sphere of social media, anxiety may deter users from actively engaging in information sharing and interactive pursuits, resulting in adverse usage behaviors ( Huang et al., 2022 ). Moreover, individuals may harbor concerns about privacy breaches or subpar content quality when posting on social media, impacting the construction of their personal image. Consequently, anxious users may gravitate toward an observer role or ‘lurking’ mode ( Okano and Nomura, 2023 ) to mitigate the risk of errors and bolster their self-efficacy on social media platforms ( Li et al., 2024 ). Consequently, users grappling with anxiety on social media are prone to abstain from active participation in discussions or knowledge dissemination, opting instead to maintain a low profile. Therefore, the following hypothesis is proposed:
H 6: Anxiety is positively associated with lurking behavior.
3.4 Social media fatigue
Social media fatigue manifests when users grow disenchanted with the myriad features and overwhelming content present on social media platforms, prompting them to seek respite ( Guo et al., 2020 ). This psychological weariness, known as social media fatigue, is intrinsically linked to the emergence of anxiety. Previous studies have highlighted that individuals may experience cognitive impairment when grappling with burnout, hindering their ability to effectively regulate their emotions ( Lee et al., 2016 ). The adverse consequences of social media fatigue may drive users toward a passive role on social media platforms, exacerbating both psychological and physical exhaustion, potentially prompting them to temporarily or permanently retreat from online interactions to alleviate distress ( Fan et al., 2020 ; Hsu et al., 2023 ). Scholars also posit that fatigue could elicit users’ discontent with social media, serving as a key catalyst for negative behaviors like discontinuing usage ( Zhang et al., 2016 , 2022 ). Therefore, the following hypotheses are proposed:
H 7a: Social media fatigue is positively associated with anxiety.
H 7b: Social media fatigue is positively associated with lurking behavior.
In summary, utilizing the SSO model and the preceding discussion, this study puts forth a theoretical model for examining the lurking behavior of social media users. The conceptual framework is visually depicted in Figure 1 .
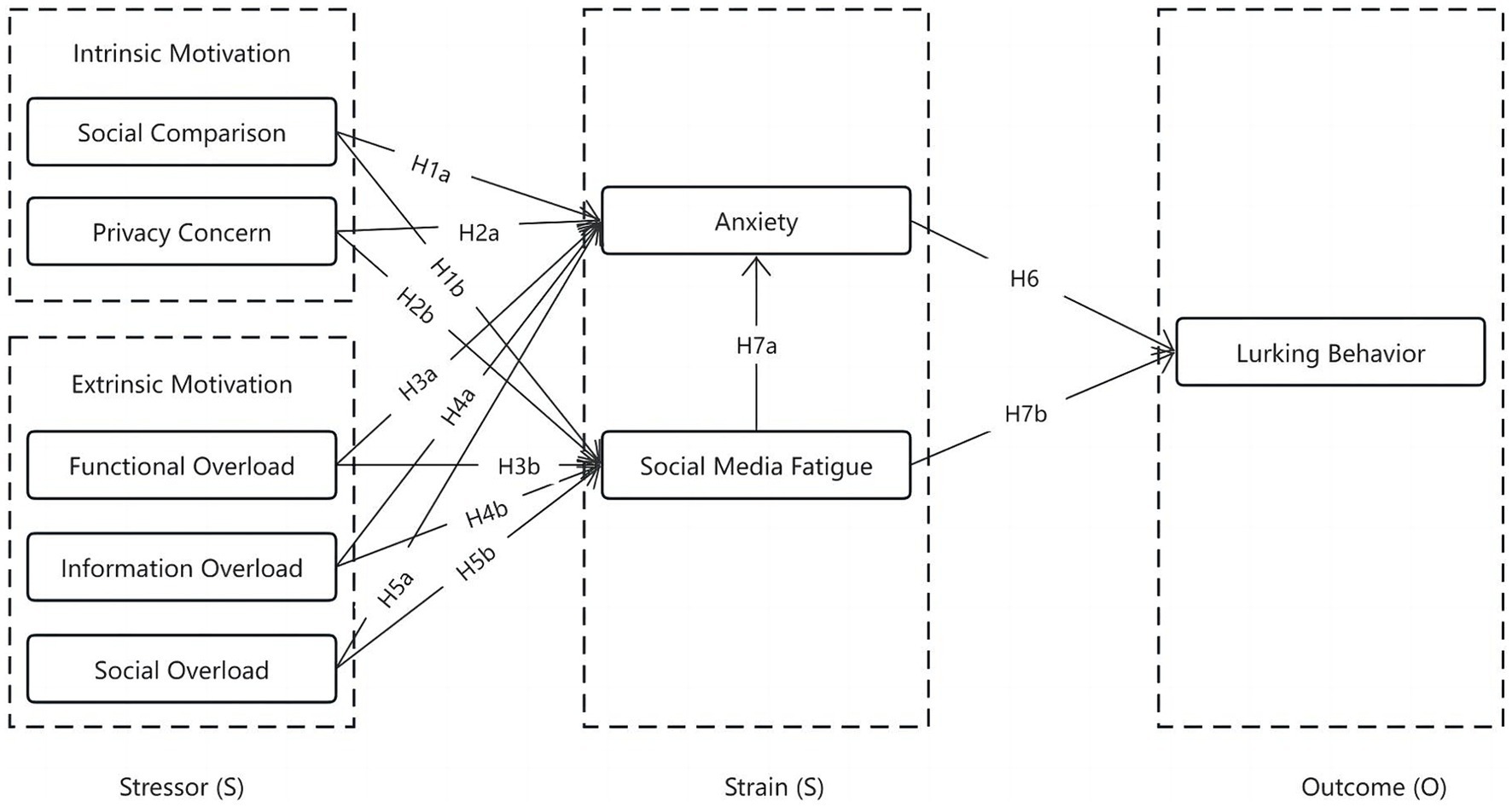
Figure 1 . Conceptual framework and research hypotheses.
4 Methodology
4.1 measurement development.
A two-part questionnaire was meticulously crafted to gather data from the participants. The first segment aimed to capture demographic information including gender, age, education level, daily frequency and duration of WeChat usage, WeChat experience, and the number of WeChat contacts. The subsequent part encompassed all the constructs outlined in the research model. The research inquiries in this study were adapted from established scales, with slight modifications made to align them with the research context and the practical experiences of WeChat users.
The questionnaire underwent a rigorous validation process, inclusive of back-translation and pre-testing procedures. Initially, the English questionnaire was translated into Chinese and subsequently retranslated back into English by an independent individual to refine and ensure the content’s accuracy. A panel of experts carefully scrutinized the data collection tool to evaluate the appropriateness of the chosen indicators for representing the constructs. Following this, the questionnaire underwent a pre-test involving 60 WeChat users to validate the efficacy of the tool. Based on the feedback from the pre-survey, suggestions from respondents and professional scholars were incorporated and ambiguous statements in the questions were corrected to ensure the accuracy and clarity of the questionnaire. The final measurement items are shown in Supplementary Table 1 .
4.2 Sample and data collection
We selected WeChat users in China for our study due to the platform’s widespread popularity ( Chen et al., 2019 ). The survey took place in January and February of 2024. Random samples were obtained through the online survey platform, Credamo, which is known for its reliability and wide usage in China. Respondents who completed valid questionnaires were offered material or monetary incentives. A total of 862 responses were collected. First, questionnaires completed in under 3 min were eliminated to ensure thoughtful responses. Second, all responses were carefully scrutinized, and those indicating “I do not use WeChat” in the first question were excluded. Third, 14 responses were disqualified due to missing data. Ultimately, 836 completed surveys were obtained, resulting in a recovery rate of 96.98%.
4.3 Measurement of variables
The question items in this study were sourced from established scales, with minor adjustments made to tailor the inquiries to the specific research context. All items were assessed using a 5-point Likert scale ranging from strongly disagree (1) to strongly agree (5). Among these, social comparison, privacy concern, information overload, functional overload, and social overload were considered independent variables, while social media fatigue and anxiety served as mediating variables, and lurking behavior was the dependent variable. The detailed measurements are outlined below:
The measurement scales for social comparison were adapted from studies by Nisar et al. (2019) and Jabeen et al. (2023) .The measurement scale for privacy concerns was sourced from the work of Cain and Imre (2022) .
Measurement scales for functional overload were adopted from Guo et al. (2020) an Lee et al. (2016) ; information overload measurements were derived from Zhang et al. (2016) and Guo et al. (2020) ; social overload scales were based on studies by Guo et al. (2020) and Fu et al. (2020) .
The measure of anxiety was taken from the research of Liu et al. (2020) . Social media fatigue measurements were sourced from studies by Jabeen et al. (2023) and Kaur et al. (2021) .
The scale for lurking behavior was adapted from studies conducted by Zhang et al. (2021) and Hong et al. (2023) . The final measurement items can be found in Supplementary Table 1 .
Moreover, individual-level differences, particularly sociodemographic variables such as age ( Reer et al., 2019 ), may influence behaviors and outcomes related to social media use. Hence, in line with prior research ( Dhir et al., 2021 ), we included age, gender, and education level as control variables.
4.4 Demographic data
The demographics of the sample are outlined in Table 1 below. The data reveals that there were 388 males (46.4%) and 448 females (53.6%). Regarding age distribution, individuals aged 21 to 30 accounted for 36.1%, closely followed by the 31 to 40 age group at 34.4%. This distribution aligns with the typical age demographics of social media users, predominantly consisting of middle-aged and young individuals. Furthermore, a significant portion of the sample indicated high frequency and duration of WeChat usage, with 60.2% using WeChat more than 10 times a day, 63% using the platform for over 2 h daily, 60.2% having over 5 years of WeChat experience, and 82.1% having more than 100 WeChat friends.
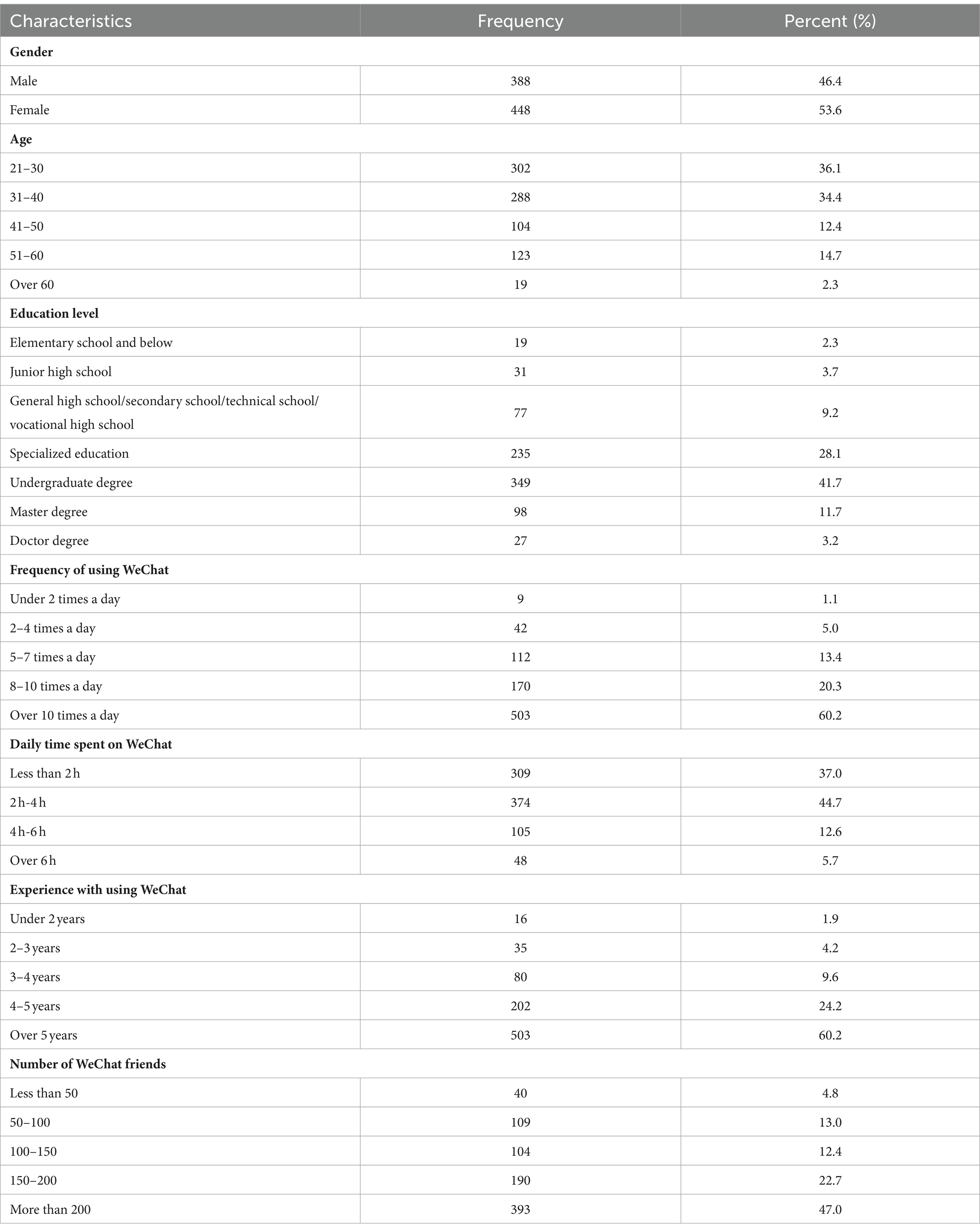
Table 1 . Demographic characteristics of participants ( N = 836).
For this study, data analysis was conducted using SPSS 27.0 and AMOS 24.0 software. Initially, the acquired data underwent descriptive statistical analysis, reliability and validity tests. Subsequently, structural equation modeling was utilized for validation factor analysis, fit analysis, and path coefficient analysis of the model. Finally, the study examined the mediating role of social media fatigue and anxiety in a chain mediation analysis.
5.1 Validation factor analysis
Before proceeding with the structural equation modeling, aside from evaluating the questionnaire’s reliability, assessing the scale’s validity is essential, encompassing content validity, convergent validity, and discriminant validity. Firstly, considering that the scales used to measure latent variables in this study are sourced from established and well-tested scales, the content validity is deemed satisfactory. Secondly, examination of Table 2 reveals that all factor loadings exceed 0.6, with a KMO coefficient of 0.940, and an overall Bartlett’s ball test chi-square value of 20278.000 (df = 861, p < 0.001), indicating a cumulative variance contribution ratio of 66.121%. Moreover, the combined reliability (CR) of all latent variables surpassed 0.8, while the average variance extracted value (AVE) was consistently above 0.5, signifying strong internal consistency and aggregation within the questionnaire. Additionally, as illustrated in Table 3 , to assess the differentiation between latent variables, following the approach suggested by Fornell and Larcker (1981) , comparing correlation coefficients between the square root of AVE and the corresponding latent variables is necessary to test discriminant validity. The analysis reveals that the square root of AVE for each latent variable exceeds the respective correlation coefficient, affirming the scale’s clear differentiation validity.
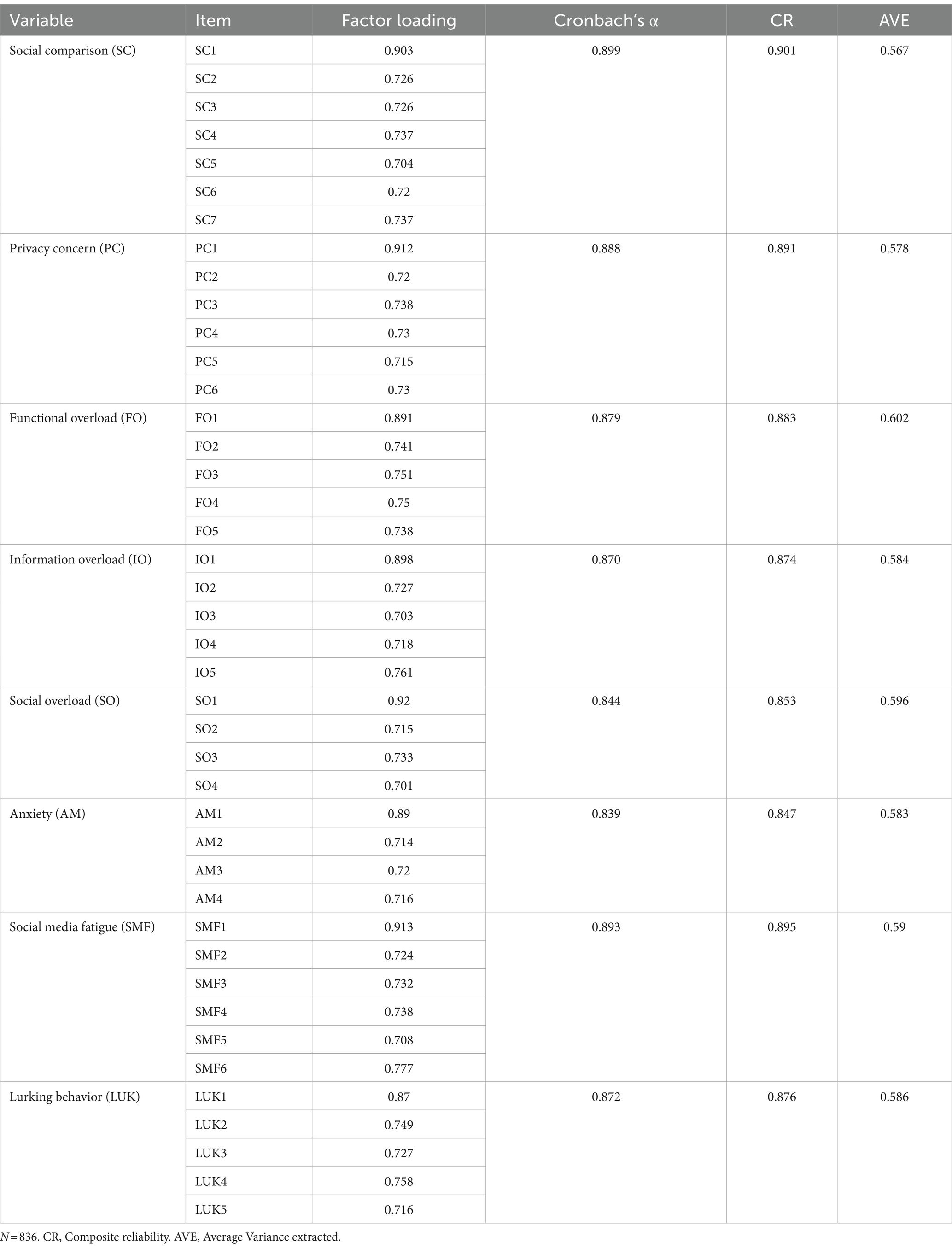
Table 2 . Reliability and convergent validity.
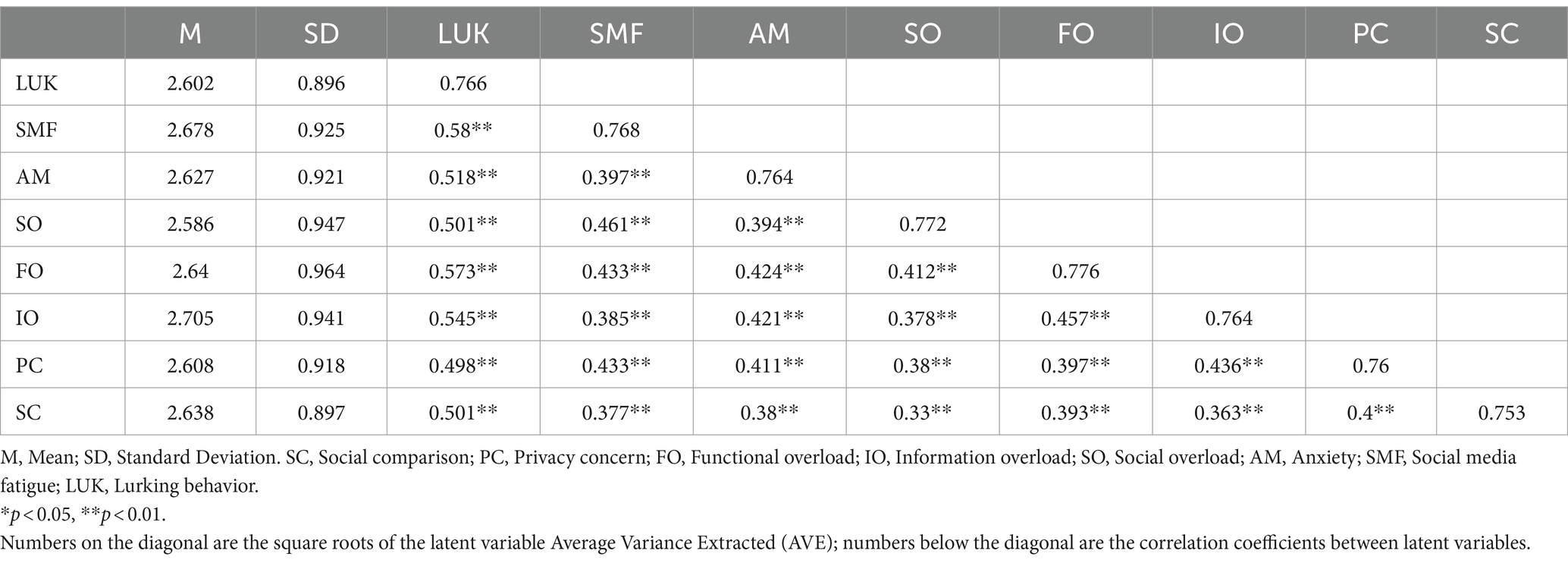
Table 3 . Discriminant validity of constructs.
The discriminant validity test results are presented in Table 3 below. The diagonal line represents the mean variance extraction (AVE) values, which are higher than the correlation coefficients on the non-diagonal line. This suggests that the scale demonstrates good discriminant validity, and the data provides support for further testing and analysis.
Table 3 also demonstrates a significant correlation ( p < 0.01) between social comparison, privacy concerns, information overload, functional overload, social overload, anxiety, social media fatigue, and lurking behaviors. This indicates that the latent variables exhibit both discriminative and correlative relationships with each other, thereby suggesting that the data’s discriminative validity of the scale is optimal.
5.2 Common method bias
In this study, common method bias was mitigated through procedural measures, such as ensuring anonymity in measurements. Data obtained were evaluated for common method bias using the Harman single-factor test. The unrotated exploratory factor analysis results revealed a total of eight factors, each with eigenvalues >1. The highest proportion of variance explained by a factor was 32.87%, which falls below the 40% threshold. Thus, it can be inferred that the results of this study were not significantly influenced by common method bias.
5.3 Model fitting and hypothesis testing
The purpose of this study is to discuss the mechanisms of intrinsic motivation based on social media users (WeChat) and extrinsic motivation for social media perceptual overload on users’ lurking behavior, and to test the mediating role of anxiety and social media fatigue from a social psychology perspective. To achieve this, AMOS 24.0 was utilized to construct a structural equation model (SEM) in order to analyze the relationships between latent variables. Initial scrutiny involved assessing and refining the model fit based on the Modification Index (MI) values provided by the structural equations. As indicated in Table 4 , the chi-square degrees of freedom ratio falls below the critical value of 3, and key fit indices such as GFI, CFI, NFI, IFI, and TLI surpass the threshold of 0.9. Additionally, the RMSEA value is below 0.08, collectively suggesting a strong fit for the proposed model.

Table 4 . Model fit indices ( N = 836).
Figure 2 illustrates the results of hypothesis testing for structural equation modeling (SEM). In the SSO theoretical framework, stressors stem from both intrinsic and extrinsic motivation dimensions. The results of the data on the intrinsic motivation dimension reveal that social comparison is positively associated with social media fatigue ( β = 0.137, p < 0.01) and anxiety ( β = 0.190, p < 0.01), while privacy concern is positively associated with social media fatigue ( β = 0.207, p < 0.01) and anxiety ( β = 0.209, p < 0.01), supporting hypotheses H1a, H1b, H2a, and H2b.
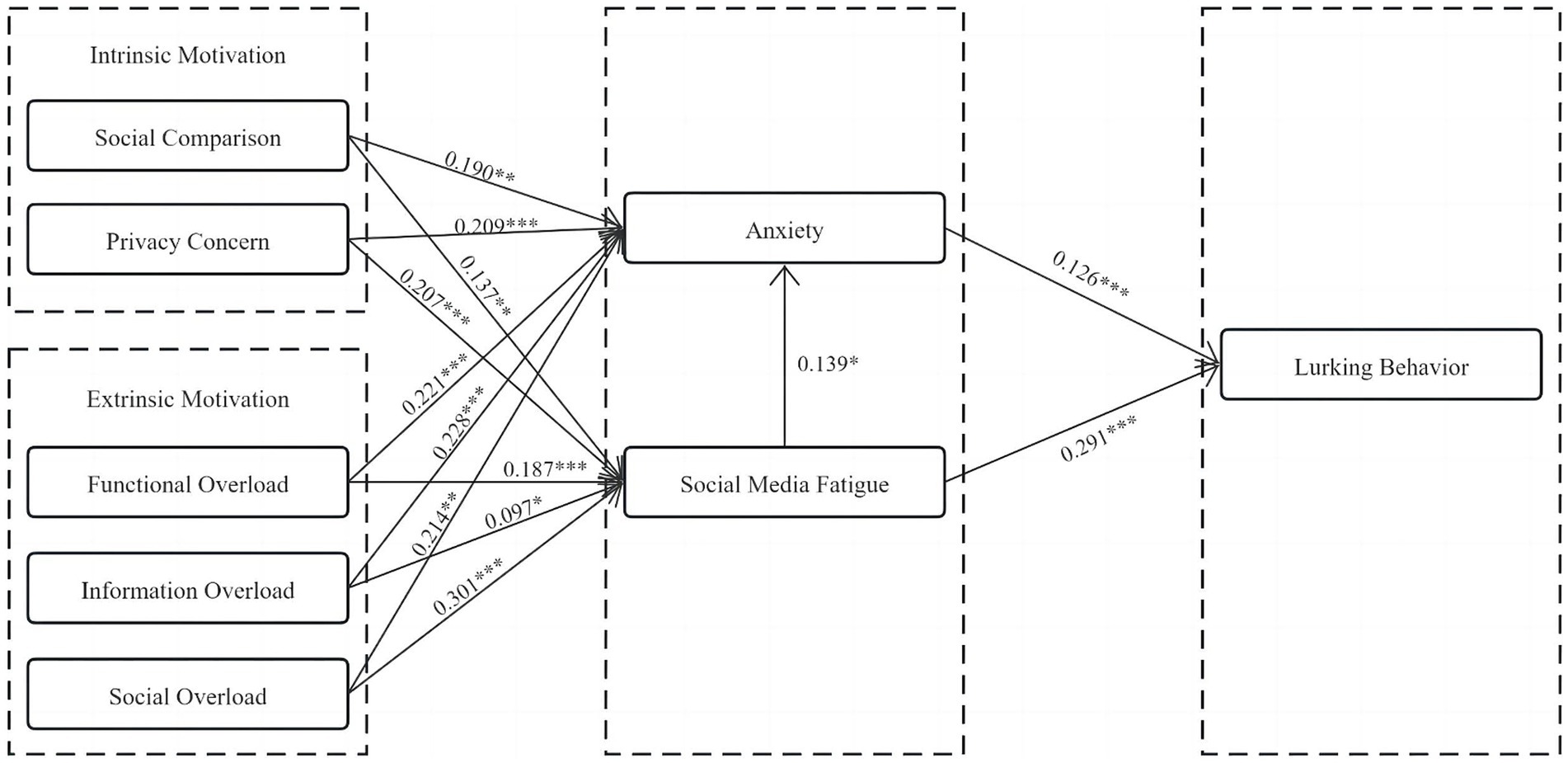
Figure 2 . Results of model testing.
The findings related to extrinsic motivation show that information overload is positively associated with social media fatigue ( β = 0.097, p < 0.01) and anxiety ( β = 0.228, p < 0.01); functional overload is positively associated with social media fatigue ( β = 0.187, p < 0.01) and anxiety ( β = 0.221, p < 0.01); and social overload is positively associated with social media fatigue ( β = 0.301, p < 0.01) and anxiety ( β = 0.214, p < 0.01), supporting hypotheses H3a, H3b, H4a, H4b, H5a, and H5b.
Regarding the impact of mediating variables on lurking behavior, the data demonstrates that anxiety is positively associated with lurking behavior ( β = 0.126, p < 0.01), supporting hypothesis H6; social media fatigue is positively associated with lurking behavior ( β = 0.291, p < 0.01), supporting hypothesis H7a; additionally, social media fatigue is positively associated with anxiety ( β = 0.139, p < 0.01), supporting hypothesis H7b.
5.4 Mediating effects test
To examine the mediation mechanism in our study, we employed the percentile Bootstrap method of bias correction introduced by Hayes (2017) for conducting the mediation effect analysis. With a confidence interval set at 95% and 5,000 iterations of resampling, the results of the analysis indicate that the confidence intervals for all path effects do not contain 0, confirming the presence of mediating effects ( Table 5 ).
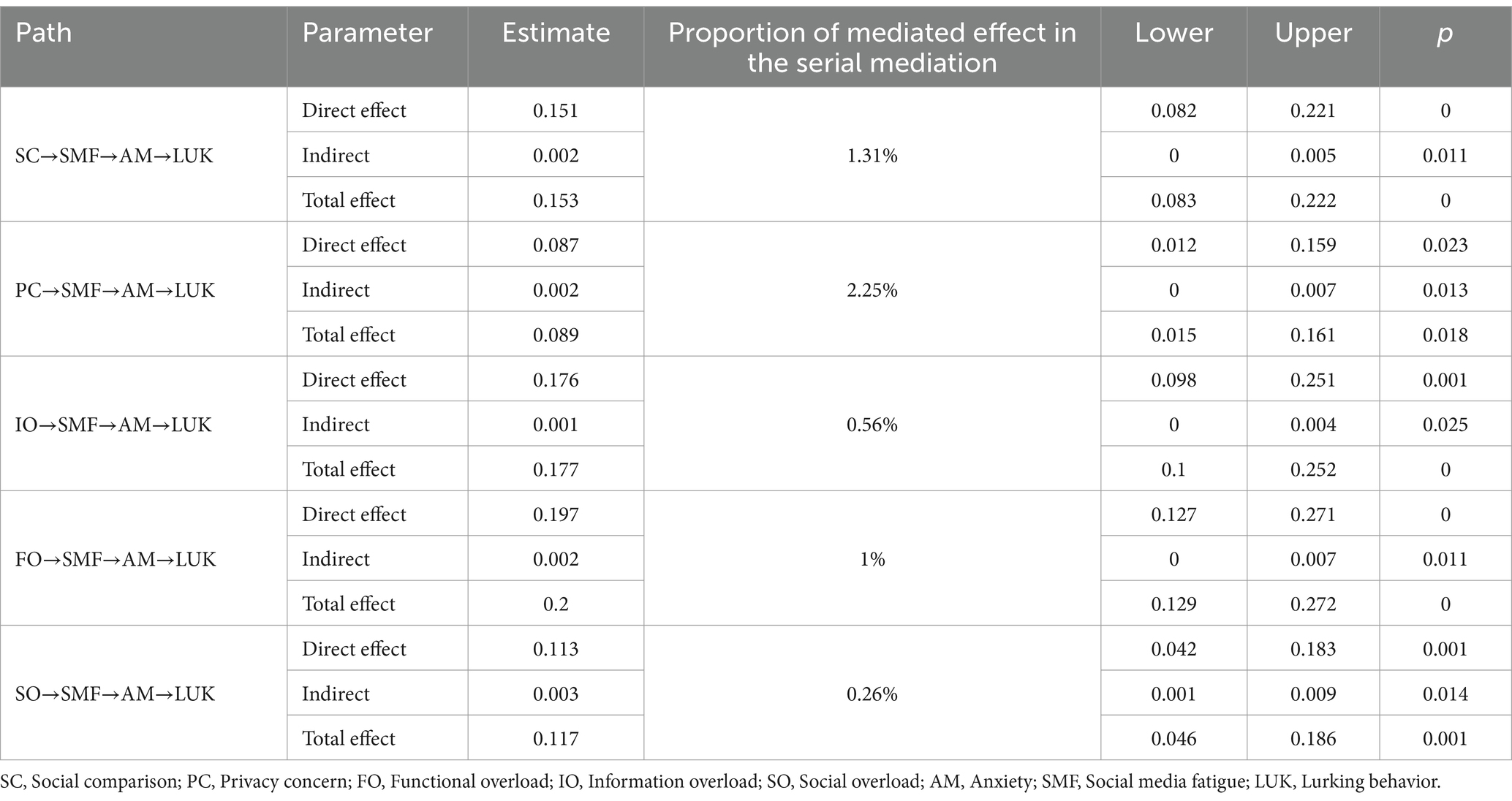
Table 5 . Mediation effect test.
In the first pathway, social comparison exhibited a significant impact on lurking behavior with a direct effect size of 0.151. Further analysis revealed that social media fatigue and anxiety acted as mediators between social comparison and lurking behavior. Together, social media fatigue and anxiety influenced lurking behavior with an indirect effect size of 0.002, accounting for 1.31%. This suggests that individuals may opt for lurking behavior under the influence of social comparison through the pathways of social media fatigue and anxiety.
Moving on to the second pathway, privacy concern also showed a significant influence on lurking behavior, with a direct effect size of 0.087. Social media fatigue and anxiety played intermediary roles between privacy concern and lurking behavior, collectively affecting lurking behavior with an indirect effect size of 0.002, accounting for 2.25%. This indicates that privacy concerns can trigger social media fatigue and anxiety, subsequently impacting an individual’s lurking behavior.
In the third pathway, information overload displayed a notable impact on lurking behavior with a direct effect size of 0.176. Social media fatigue and anxiety jointly mediated the relationship between information overload and lurking behavior, resulting in an indirect effect size of 0.001, representing 0.56%. This suggests that information overload may lead to social media fatigue and anxiety, thereby influencing users’ decisions to engage in lurking behavior.
Likewise, in the fourth pathway, functional overload significantly influenced lurking behavior with a direct effect size of 0.197. Social media fatigue and anxiety jointly served as mediators between functional overload and lurking behavior, presenting an indirect effect size of 0.002, accounting for 1%. This implies that functional overload could escalate social media fatigue and anxiety, prompting users to seek lurking behavior as a coping mechanism.
The fifth pathway illustrated that social overload had a comparatively minor impact on lurking behavior, with a direct effect size of 0.113. Social media fatigue and anxiety jointly mediated the association between social overload and lurking behavior, resulting in an indirect effect size of 0.003, accounting for 0.26%. This indicates that social overload could instigate social media fatigue and anxiety, subsequently influencing users’ decisions to engage in lurking behavior.
6 Discussion
Utilizing the SSO theoretical model as a foundational framework, this study delved into how users’ intrinsic motivational factors and social media’s extrinsic motivational factors of perceptual overload contribute to social media fatigue and anxiety, subsequently prompting lurking behavior. Diverging from prior research that primarily examined lurking behavior through a singular lens ( Sun et al., 2014 ), this study integrates individual internal factors and environmental external factors, incorporating social media fatigue and anxiety as mediating variables to examine their impact on users’ lurking behavior. The findings consistently supported the hypotheses. Intrinsic motives such as social comparison and privacy concerns were found to be positively associated with social media fatigue and anxiety, while extrinsic motives including functional overload, information overload, and social overload were also positively associated with social media fatigue and anxiety. Furthermore, social media fatigue and anxiety were identified as key determinants of users’ lurking behavior. Additionally, by considering the psychological aspects of users, the study confirmed that social media fatigue is positively associated with anxiety.
First, both social comparison and privacy concern, as intrinsic motivational aspects, were positively associated with anxiety and social media fatigue. This aligns with the findings of previous researchers ( Ortiz et al., 2018 ; Nisar et al., 2019 ; Liu et al., 2020 ), highlighting the critical role of social comparison and privacy concerns in users’ social media experiences. Social comparison metrics suggest that when users compare themselves to individuals on WeChat they view as more skilled or knowledgeable, it can negatively impact their own self-perceptions. This struggle to manage their self-image within WeChat Moments can heighten anxiety levels and contribute to social media fatigue. The primary reason users refrain from sharing information is the apprehension of personal data breaches and misuse by the mobile app. Users often need to disclose sensitive details like location, phone number, identity information, and home address to access new features or services. Neglecting to address privacy breach risks results in users avoiding specific platform features and services. Hence, the importance of privacy concerns cannot be overstressed, and social media platforms ought to enhance privacy protection technologies to mitigate the exposure of users’ personal data, safeguard information confidentiality, and alleviate privacy anxieties.
Secondly, extrinsic factors such as functional overload, information overload, and social overload have been positively associated with social media fatigue and anxiety. Users are inundated with various stimuli such as group messages, public accounts notifications, new feature introductions, short video recommendations, likes, friends’ comments, advertisements, and other interface elements. When the quantity of these services surpasses the user’s capacity, the user feels the urge to escape from social media. Functional overload, information overload, and social overload collectively play a significant role in contributing to social media fatigue, in accordance with findings by Liu et al. (2020) , Lee et al. (2016) , and Guo et al. (2020) . The saturated social media environment blurs the boundaries between users’ personal and professional lives, causing users to experience pressures related to information processing, technical functions, and social interactions. This results in work-related boundaries encroaching into personal life boundaries, leading to private leisure time being dominated by social media content. The negative user experience stemming from these processes acts as a direct stressor, triggering negative emotions such as disinterest, boredom, and anxiety. In response, users may modify their behaviors by reducing their social media usage, lurking, dropping out, ignoring content, quitting platforms, fleeing from interactions, or transitioning to alternative platforms. This gradual disengagement from social media, as seen in users retiring from WeChat Moments, serves as a defense mechanism to preserve the boundaries between work, social, and personal life domains. This aligns with the findings of Liu et al. (2020) that suggest social media saturation can lead to user anxiety. Active user participation plays a pivotal role in sustaining social media platforms by generating new knowledge and value for business, economic, and public administration purposes. Therefore, social media platforms should prioritize improving their technical infrastructure and services through extensive user research. By refining product design and establishing a secure, streamlined, and user-centric service system, platforms can address user apprehensions, minimize societal repercussions, and address operational hurdles. This strategic approach can enhance users’ emotional satisfaction and foster increased engagement in information dissemination.
Third, the study findings revealed a positive association between social media fatigue and anxiety, with both factors correlating positively with lurking behavior. Prolonged negative emotional states often drive users toward lurking, a passive observation mode devoid of active engagement, as a coping mechanism to alleviate social media-induced stress. Therefore, social media platforms should prioritize addressing users’ negative emotional states by emphasizing the generalization of Moments, optimizing platform management, reducing commercial intrusions in social interactions like WeChat Moments, and offering sophisticated, empathetic tools for managing contextual relationships. These personalized contextual relationship management tools can aid users in navigating intricate social networks, thereby enriching the social ambiance of WeChat Moments interactions and fostering users’ motivation to share information. Additionally, it is recommended that social media platforms introduce features like “short-term lurking” or “short-term disconnection” to empower technology firms and service providers to leverage algorithms for monitoring users’ information consumption, browsing patterns, engagement levels, and screen time. By integrating this data with a meticulous analysis of users’ social media behaviors, these algorithms could automatically suggest users engage in “short-term lurking” or “short-term disconnection” within specified timeframes. This feature should allow user customization and control, enabling individuals to decide whether to enable social media access during these intervals. In the contemporary era of information overload post-connectionism, the notion of “digital escape” is as vital as “digital connectivity” and merits significant consideration.
7 Conclusion
7.1 theoretical contributions.
Based on the SSO model, our study investigated the lurking behaviors of users on social media platforms and analyzed the effects of social comparison, privacy concerns, information overload, functional overload, and social overload on social media fatigue, anxiety, and lurking behaviors. The contributions of this study will be discussed in the subsequent sections.
With the pervasive influence of social media in the era of big data, the prevalence of negative emotions and detrimental usage patterns among users has emerged as a significant trend. In contrast to prior research primarily concentrating on addictive or persistent usage tendencies, our study investigates the less-explored aspects of prolonged usage, unraveling the mechanisms underlying users’ anxiety and social media fatigue across cognitive, affective, and behavioral dimensions within the social media sphere. Furthermore, it scrutinizes the interplay between users’ lurking behaviors and negative emotions like fatigue and anxiety, bridging a scholarly void that traditionally focused solely on active or continuous usage behaviors, failing to elucidate the intrinsic impact of negative emotions on adverse usage patterns. This unique perspective not only introduces a fresh theoretical framework but also provides pragmatic insights for understanding and addressing these complex dynamics.
Additionally, this study establishes an integrated explanatory model that incorporates intrinsic and extrinsic influencing factors, shifting the discussion from static factor analysis to dynamic mechanism explanation. It provides a comprehensive account of the change mechanism in users’ information behavior from active engagement to lurking. Many previous studies have focused on either extrinsic social factors or intrinsic individual factors when discussing user lurking behaviors ( Sun et al., 2014 ; Ortiz et al., 2018 ; Nguyen, 2020 ), neglecting the interplay between these factors and failing to systematically elucidate the mechanism of information behavior change among users. The results suggest that exploring lurking behaviors on social media from both intrinsic motivation and extrinsic motivation perspectives is both reasonable and effective. Perceived high risks associated with privacy maintenance and image management, coupled with the pressure of an overloaded social media environment, influence users’ perceptions of information sharing and online interactions, diminishing the value and necessity of information behavior within their social media platforms. This leads to the emergence of fatigue and anxiety, leading users to transition from active engagement to lurking behaviors.
7.2 Practical implications
The study of the factors influencing lurking behavior is essential in the advancement of social media and holds practical significance.
First, social media functions as a dynamic platform enabling content creation and exchange grounded in user relationships. The active engagement of users remains pivotal in driving information dissemination and enriching the vibrancy of social media ecosystems. Yet, lurking behaviors have the potential to obstruct the evolution of social media platforms, impeding operators from efficiently aggregating data, understanding user preferences, and elevating service quality via user-generated content. Our study underscores the significance of users’ inherent psychological stimuli, such as privacy concerns and social comparisons, in diminishing their involvement in online social interactions. Consequently, social media providers must prioritize enhancing and innovating the features and functionalities of their applications to fortify users’ confidence. For instance, empowering users to configure privacy settings for their communications can amplify trust and alleviate concerns about the platform. Furthermore, continual improvements in privacy policy adherence and simplification of application terms and conditions are essential to assure users that their privacy is shielded, thereby bolstering their sense of security.
Second, it is imperative for social media operators to recognize that the benefits derived from different user behaviors vary significantly. Merely increasing the number of registered users without focusing on user activity and engagement is counterproductive. Operators need to carefully address factors that can induce anxiety in users and pay heed to users’ psychological behaviors. Operators can alleviate users’ psychosocial burden by creating a relaxed social environment through technical interventions, as well as implementing measures to automatically detect and block spam to mitigate the stress of perceived social media overload.
Finally, operators should diligently analyze users’ habits and preferences to enhance personalized information recommendations and implement effective information blocking mechanisms. By alleviating users’ tendency to engage in social upward comparison, satisfaction levels and user activity can be significantly improved. By studying the factors that trigger user anxiety and lurking behavior, operators can offer targeted psychological support and interventions to users, thereby encouraging user retention and fostering sustainable development for all stakeholders within the social media business ecosystem.
7.3 Limitations and future directions
Our research has several limitations that need to be noted. Firstly, the collected questionnaire samples primarily represent middle-aged and young individuals, with less representation from the elderly group. Additionally, the research area is limited to China, thereby restricting the generalizability of the findings. Future studies should aim to utilize more comprehensive and representative data samples. Secondly, this study focused on lurking phenomenon in WeChat, a popular social media platform in China. However, it is important to note that lurking behavior may vary across different social media platforms such as Tiktok, Facebook, and Twitter. Therefore, it is recommended to extend the study of lurking to other social media platforms to gain a more comprehensive understanding. Lastly, while this study primarily explores the negative outcomes of lurking behavior and provides suggestions for operators to enhance user engagement, it is crucial to acknowledge that there may be rationalities and positive aspects associated with the emergence of lurking. Hence, future research should delve deeper into exploring the positive aspects of social media lurking.
Data availability statement
The raw data supporting the conclusions of this article will be made available by the authors, without undue reservation.
Ethics statement
Ethical review and approval was not required for the study on human participants in accordance with the local legislation and institutional requirements. Written informed consent from the patients/ participants or patients/participants legal guardian/next of kin was not required to participate in this study in accordance with the national legislation and the institutional requirements.
Author contributions
XL: Conceptualization, Data curation, Formal analysis, Investigation, Methodology, Software, Supervision, Writing – original draft, Writing – review & editing. RF: Resources, Supervision, Validation, Writing – review & editing. XC: Methodology, Supervision, Writing – review & editing. YY: Writing – review & editing, Software, Formal analysis, Data curation.
The author(s) declare that no financial support was received for the research, authorship, and/or publication of this article.
Acknowledgments
The authors gratefully acknowledge all the participants of this study, who were duly informed about the purpose and content of the research and willingly agreed to take part. Participants were assured that they could withdraw from the study at any point without facing any consequences.
Conflict of interest
The authors declare that the research was conducted in the absence of any commercial or financial relationships that could be construed as a potential conflict of interest.
Publisher’s note
All claims expressed in this article are solely those of the authors and do not necessarily represent those of their affiliated organizations, or those of the publisher, the editors and the reviewers. Any product that may be evaluated in this article, or claim that may be made by its manufacturer, is not guaranteed or endorsed by the publisher.
Supplementary material
The Supplementary material for this article can be found online at: https://www.frontiersin.org/articles/10.3389/fpsyg.2024.1406895/full#supplementary-material
Barnidge, M., Peacock, C., Kim, B., Kim, Y., and Xenos, M. A. (2023). Networks and selective avoidance: how social media networks influence unfriending and other avoidance behaviors. Soc. Sci. Comput. Rev. 41, 1017–1038. doi: 10.1177/08944393211069628
Crossref Full Text | Google Scholar
Bekker, H. L., Legare, F., Stacey, D., O'Connor, A., and Lemyre, L. (2003). Is anxiety a suitable measure of decision aid effectiveness: a systematic review? Patient Educ. Couns. 50, 255–262. doi: 10.1016/S0738-3991(03)00045-4
PubMed Abstract | Crossref Full Text | Google Scholar
Cain, J. A., and Imre, I. (2022). Everybody wants some: collection and control of personal information, privacy concerns, and social media use. New Media Soc. 24, 2705–2724. doi: 10.1177/14614448211000327
Cao, X., and Sun, J. (2018). Exploring the effect of overload on the discontinuous intention of social media users: an S-O-R perspective. Comput. Hum. Behav. 81, 10–18. doi: 10.1016/J.CHB.2017.11.035
Chen, X., Sun, M. X., Wu, D., and Song, X. Y. (2019). Information-sharing behavior on WeChat moments: the role of anonymity, familiarity, and intrinsic motivation. Front. Psychol. 10:2540. doi: 10.3389/FPSYG.2019.02540
Deci, E. L., and Ryan, R. M. (2000). The "what" and "why" of goal pursuits: human needs and the self-determination of behavior. Psychol. Inq. 11, 227–268. doi: 10.1207/S15327965PLI1104_01
Deci, E. L., Ryan, R. M., Vallerand, R. J., and Pelletier, L. G. (1991). Motivation and education: the self-determination perspective. Educ. Psychol. 26, 325–346. doi: 10.1080/00461520.1991.9653137
Dhir, A., Kaur, P., Chen, S., and Pallesen, S. (2019). Antecedents and consequences of social media fatigue. Int. J. Inf. Manag. 48, 193–202. doi: 10.1016/J.IJINFOMGT.2019.05.021
Dhir, A., Talwar, S., Kaur, P., Budhiraja, S., and Islam, N. (2021). The dark side of social media: stalking, online self-disclosure and problematic sleep. Int. J. Consum. Stud. 45, 1373–1391. doi: 10.1111/IJCS.12659
Dhir, A., Yossatorn, Y., Kaur, P., and Chen, S. (2018). Online social media fatigue and psychological wellbeing: a study of compulsive use, fear of missing out, fatigue, anxiety and depression. Int. J. Inf. Manag. 40, 141–152. doi: 10.1016/J.IJINFOMGT.2018.01.012
Dunbar, R. I. M. (1992). Neocortex size as a constraint on group size in primates. J. Hum. Evol. 22, 469–493. doi: 10.1016/0047-2484(92)90081-J
Edelmann, N. (2013). Reviewing the definitions of "lurkers" and some implications for online research. Cyberpsychol. Behav. Soc. Netw. 16, 645–649. doi: 10.1089/CYBER.2012.0362
Fan, X., Jiang, X., Deng, N., Dong, X., and Lin, Y. (2020). Does role conflict influence discontinuous usage intentions? Privacy concerns, social media fatigue and self-esteem. Inf. Technol. People 34, 1152–1174. doi: 10.1108/ITP-08-2019-0416
Festinger, L. (1954). A theory of social comparison processes. Hum. Relat. 7, 117–140. doi: 10.1177/001872675400700202
Fornell, C., and Larcker, D. F. (1981). Evaluating structural equation models with unobservable variables and measurement error. J. Mark. Res. 18, 39–50. doi: 10.1177/002224378101800104
Fu, S., Li, H., Liu, Y., Pirkkalainen, H., and Salo, M. (2020). Social media overload, exhaustion, and use discontinuance: examining the effects of information overload, system feature overload, and social overload. Inf. Process. Manag. 57:102307. doi: 10.1016/J.IPM.2020.102307
Guo, Y., Lu, Z., Kuang, H., and Wang, C. (2020). Information avoidance behavior on social network sites: information irrelevance, overload, and the moderating role of time pressure. Int. J. Inf. Manag. 52:102067. doi: 10.1016/J.IJINFOMGT.2020.102067
Hayes, A. F. (2017). Introduction to mediation, moderation, and conditional process analysis: A regression-based approach . New York: Guilford Publications.
Google Scholar
Hogan, T. P., and Brashers, D. E. (2015). “The theory of communication and uncertainty management: implications from the wider realm of information behavior” in Uncertainty, information management, and disclosure decisions . eds. T. Afifi and W. Afifi (London: Routledge), 45–66.
Hong, Y., Hu, J., and Zhao, Y. (2023). Would you go invisible on social media? An empirical study on the antecedents of users' lurking behavior. Technol. Forecast. Soc. Change 187:122237. doi: 10.1016/J.TECHFORE.2022.122237
Hsu, J. S. C., Chiu, C. M., Chang-Chien, Y. T., and Tang, K. (2023). How social media fatigue feigning and altering emotion discourage the use of social media. Internet Res. in press 34, 1488–1518. doi: 10.1108/INTR-06-2022-0390
Hu, B., Zhu, Y., Liu, C., Zheng, S., Zhao, Z., and Bao, R. (2024). Collectivism, face concern and Chinese-style lurking among university students: the moderating role of trait mindfulness. Front. Psychol. 15:1298357. doi: 10.3389/FPSYG.2024.1298357
Huang, Q., Lei, S., and Ni, B. (2022). Perceived information overload and unverified information sharing on WeChat amid the COVID-19 pandemic: a moderated mediation model of anxiety and perceived herd. Front. Psychol. 13:837820. doi: 10.3389/FPSYG.2022.837820
Hung, S. Y., Durcikova, A., Lai, H. M., and Lin, W. M. (2011). The influence of intrinsic and extrinsic motivation on individuals knowledge sharing behavior. Int. J. Hum.-Comput. Stud. 69, 415–427. doi: 10.1016/J.IJHCS.2011.02.004
Jabeen, F., Tandon, A., Sithipolvanichgul, J., Srivastava, S., and Dhir, A. (2023). Social media-induced fear of missing out (FoMO) and social media fatigue: the role of narcissism, comparison and disclosure. J. Bus. Res. 159:113693. doi: 10.1016/J.JBUSRES.2023.113693
Kang, K., and Shin, S. K. (2008). "A model of virtual community knowledge exchange intentions: Perceived network structure, self-efficacy and individual motivations," in Proceedings of the 39th decision sciences institute (DSI) annual meeting (Fresno, TX: Decision Sciences Institute).
Karr-Wisniewski, P., and Lu, Y. (2010). When more is too much: operationalizing technology overload and exploring its impact on knowledge worker productivity. Comput. Hum. Behav. 26, 1061–1072. doi: 10.1016/J.CHB.2010.03.008
Kaur, P., Islam, N., Tandon, A., and Dhir, A. (2021). Social media users’ online subjective well-being and fatigue: a network heterogeneity perspective. Technol. Forecast. Soc. Change 172:121039. doi: 10.1016/J.TECHFORE.2021.121039
Kim, J., Kim, H. M., and Kim, M. (2021). The impact of a sense of virtual community on online community: does online privacy concern matter? Internet Res. 31, 519–539. doi: 10.1108/INTR-01-2020-0015
Kim, S., and Park, H. (2015). Empirical study on antecedents and consequences of users' fatigue on SNS and the moderating effect of habit. J. Korea Soc. IT Serv. 14, 137–157. doi: 10.9716/KITS.2015.14.4.137
Koeske, G. F., and Koeske, R. D. (1993). A preliminary test of a stress-strain-outcome model for reconceptualizing the burnout phenomenon. J. Soc. Serv. Res. 17, 107–135. doi: 10.1300/J079V17N03_06
Lee, A. R., Son, S. M., and Kim, K. K. (2016). Information and communication technology overload and social networking service fatigue: a stress perspective. Comput. Hum. Behav. 55, 51–61. doi: 10.1016/J.CHB.2015.08.011
Li, J., Guo, F., Qu, Q. X., and Hao, D. (2022). How does perceived overload in mobile social media influence users’ passive usage intentions? Considering the mediating roles of privacy concerns and social media fatigue. Int. J. Hum. Comput. Interact. 38, 983–992. doi: 10.1080/10447318.2021.1986318
Li, K., Jiang, S., Yan, X., and Li, J. (2024). Mechanism study of social media overload on health self-efficacy and anxiety. Heliyon 10:E23326. doi: 10.1016/J.HELIYON.2023.E23326
Lim, M., and Yang, Y. (2019). Upward social comparison and Facebook users’ grandiosity: examining the effect of envy on loneliness and subjective well-being. Online Inf. Rev. 43, 635–652. doi: 10.1108/OIR-04-2017-0137
Liu, X., Min, Q., Wu, D., and Liu, Z. (2020). How does social network diversity affect users’ lurking intention toward social network services? A role perspective. Inf. Manag. 57:103258. doi: 10.1016/J.IM.2019.103258
Luo, L. (1999). Work motivation, job stress and employees' well-being. J. Appl. Manag. Stud. 8, 61–72.
Maier, C., Laumer, S., Eckhardt, A., and Weitzel, T. (2015). Giving too much social support: social overload on social networking sites. Eur. J. Inf. Syst. 24, 447–464. doi: 10.1057/EJIS.2014.3
Neelen, M., and Fetter, S. (2010). Lurking: a challenge or a fruitful strategy? A comparison between lurkers and active participants in an online corporate community of practice. Int. J. Knowl. Learn. 6, 269–284. doi: 10.1504/IJKL.2010.038649
Nguyen, T. M. (2020). Four-dimensional model: a literature review on reasons behind lurking behavior. VINE J. Inf. Knowl. Manag. Syst. 51, 302–317. doi: 10.1108/VJIKMS-10-2019-0168
Nguyen, T. M. (2021). Four-dimensional model: a literature review in online organisational knowledge sharing. VINE J. Inf. Knowl. Manag. Syst. 51, 109–138. doi: 10.1108/VJIKMS-05-2019-0077
Nguyen, T. M., Viet Ngo, L., and Paramita, W. (2022). Turning lurkers into innovation agents: an interactionist perspective of self-determinant theory. J. Bus. Res. 141, 822–835. doi: 10.1016/J.JBUSRES.2021.11.087
Nisar, T. M., Prabhakar, G., Ilavarasan, P. V., and Baabdullah, A. M. (2019). Facebook usage and mental health: an empirical study of role of non-directional social comparisons in the UK. Int. J. Inf. Manag. 48, 53–62. doi: 10.1016/J.IJINFOMGT.2019.01.017
Okano, H., and Nomura, M. (2023). Examining social anxiety and dual aspects of social comparison orientation: the moderating role of self-evaluation of social skills. Front. Psychol. 14:1270143. doi: 10.3389/FPSYG.2023.1270143
Olatokun, W., and Nwafor, C. I. (2012). The effect of extrinsic and intrinsic motivation on knowledge sharing intentions of civil servants in Ebonyi state, Nigeria. Inf. Dev. 28, 216–234. doi: 10.1177/0266666912438567
Ortiz, J., Chih, W. H., and Tsai, F. S. (2018). Information privacy, consumer alienation, and lurking behavior in social networking sites. Comput. Hum. Behav. 80, 143–157. doi: 10.1016/J.CHB.2017.11.005
Osatuyi, B. (2015). Is lurking an anxiety-masking strategy on social media sites? The effects of lurking and computer anxiety on explaining information privacy concern on social media platforms. Comput. Hum. Behav. 49, 324–332. doi: 10.1016/J.CHB.2015.02.062
Reer, F., Tang, W. Y., and Quandt, T. (2019). Psychosocial well-being and social media engagement: the mediating roles of social comparison orientation and fear of missing out. New Media Soc. 21, 1486–1505. doi: 10.1177/1461444818823719
Sun, N., Rau, P. P. L., and Ma, L. (2014). Understanding lurkers in online communities: a literature review. Comput. Hum. Behav. 38, 110–117. doi: 10.1016/J.CHB.2014.05.022
Takahashi, M., Fujimoto, M., and Yamasaki, N. (2003). "The active lurker: influence of an in-house online community on its outside environment," in Proceedings of the 2003 ACM international conference on supporting group work (New York: Association for Computing Machinery), 1–10.
Teo, T. S. H., Lim, V. K. G., and Lai, R. Y. C. (1999). Intrinsic and extrinsic motivation in internet usage. Omega 27, 25–37. doi: 10.1016/S0305-0483(98)00028-0
Thompson, D. V., Hamilton, R. W., and Rust, R. T. (2005). Feature fatigue: when product capabilities become too much of a good thing. J. Mark. Res. 42, 431–442. doi: 10.1509/JMKR.2005.42.4.431
Vallerand, R. (2001). “JA hierarchical model of intrinsic and extrinsic motivation in sport and exercise” in Advances in motivation in sport and exercise . eds. G. C. Roberts and D. Treasure (Champaign, IL: Human Kinetics), 263–319.
Van Mierlo, T. (2014). The 1% rule in four digital health social networks: an observational study. J. Med. Internet Res. 16:e2966. doi: 10.2196/JMIR.2966
Venkatesh, V., Speier, C., and Morris, M. G. (2002). User acceptance enablers in individual decision making about technology: towards an integrated model. Decis. Sci. 33, 297–316. doi: 10.1111/J.1540-5915.2002.TB01646.X
Verduyn, P., Gugushvili, N., Massar, K., Täht, K., and Kross, E. (2020). Social comparison on social networking sites. Curr. Opin. Psychol. 36, 32–37. doi: 10.1016/J.COPSYC.2020.04.002
Winder, D. (2021). Unsecured Facebook databases leak data of 419 million users. Available at: https://www.forbes.com/sites/daveywinder/2019/09/05/facebook-security-snafu-exposes-419-million-user-phone-numbers/?sh526d8da141ab7 [Accessed May 1, 2024].
Zhang, Y., He, W., and Peng, L. (2022). How perceived pressure affects users’ social media fatigue behavior: a case on WeChat. J. Comput. Inf. Syst. 62, 337–348. doi: 10.1080/08874417.2020.1824596
Zhang, Y., Shi, S., Guo, S., Chen, X., and Piao, Z. (2021). Audience management, online turbulence and lurking in social networking services: a transactional process of stress perspective. Int. J. Inf. Manag. 56:102233. doi: 10.1016/J.IJINFOMGT.2020.102233
Zhang, S., Zhao, L., Lu, Y., and Yang, J. (2016). Do you get tired of socializing? An empirical explanation of discontinuous usage behaviour in social network services. Inf. Manag. 53, 904–914. doi: 10.1016/J.IM.2016.03.006
Zhou, T., and Xie, Y. (2023). Understanding social media users' information avoidance intention: a C-A-C perspective. Aslib J. Inf. Manag. 76, 570–584. doi: 10.1108/AJIM-10-2022-0471
Keywords: perceived overload, social comparison, privacy concern, social media fatigue, anxiety, lurking behavior
Citation: Liu X, Feng R, Chen X and Yuan Y (2024) “Left on read” examining social media users’ lurking behavior: an integration of anxiety and social media fatigue. Front. Psychol . 15:1406895. doi: 10.3389/fpsyg.2024.1406895
Received: 25 March 2024; Accepted: 24 July 2024; Published: 02 August 2024.
Reviewed by:
Copyright © 2024 Liu, Feng, Chen and Yuan. This is an open-access article distributed under the terms of the Creative Commons Attribution License (CC BY) . The use, distribution or reproduction in other forums is permitted, provided the original author(s) and the copyright owner(s) are credited and that the original publication in this journal is cited, in accordance with accepted academic practice. No use, distribution or reproduction is permitted which does not comply with these terms.
*Correspondence: Xiaoyu Liu, [email protected]
Disclaimer: All claims expressed in this article are solely those of the authors and do not necessarily represent those of their affiliated organizations, or those of the publisher, the editors and the reviewers. Any product that may be evaluated in this article or claim that may be made by its manufacturer is not guaranteed or endorsed by the publisher.

An official website of the United States government
The .gov means it’s official. Federal government websites often end in .gov or .mil. Before sharing sensitive information, make sure you’re on a federal government site.
The site is secure. The https:// ensures that you are connecting to the official website and that any information you provide is encrypted and transmitted securely.
- Publications
- Account settings
- My Bibliography
- Collections
- Citation manager
Save citation to file
Email citation, add to collections.
- Create a new collection
- Add to an existing collection
Add to My Bibliography
Your saved search, create a file for external citation management software, your rss feed.
- Search in PubMed
- Search in NLM Catalog
- Add to Search
Automated data analysis of unstructured grey literature in health research: A mapping review
Affiliations.
- 1 National Institute for Health and Care Research Innovation Observatory, Population Health Sciences Institute, Newcastle University, Newcastle upon Tyne, UK.
- 2 Interdisciplinary Computing and Complex BioSystems (ICOS) Research Group, School of Computing, Newcastle University, Newcastle upon Tyne, UK.
- PMID: 38115736
- DOI: 10.1002/jrsm.1692
The amount of grey literature and 'softer' intelligence from social media or websites is vast. Given the long lead-times of producing high-quality peer-reviewed health information, this is causing a demand for new ways to provide prompt input for secondary research. To our knowledge, this is the first review of automated data extraction methods or tools for health-related grey literature and soft data, with a focus on (semi)automating horizon scans, health technology assessments (HTA), evidence maps, or other literature reviews. We searched six databases to cover both health- and computer-science literature. After deduplication, 10% of the search results were screened by two reviewers, the remainder was single-screened up to an estimated 95% sensitivity; screening was stopped early after screening an additional 1000 results with no new includes. All full texts were retrieved, screened, and extracted by a single reviewer and 10% were checked in duplicate. We included 84 papers covering automation for health-related social media, internet fora, news, patents, government agencies and charities, or trial registers. From each paper, we extracted data about important functionalities for users of the tool or method; information about the level of support and reliability; and about practical challenges and research gaps. Poor availability of code, data, and usable tools leads to low transparency regarding performance and duplication of work. Financial implications, scalability, integration into downstream workflows, and meaningful evaluations should be carefully planned before starting to develop a tool, given the vast amounts of data and opportunities those tools offer to expedite research.
Keywords: artificial intelligence; automation; grey literature; literature review; natural language processing.
© 2023 The Authors. Research Synthesis Methods published by John Wiley & Sons Ltd.
PubMed Disclaimer
Similar articles
- Folic acid supplementation and malaria susceptibility and severity among people taking antifolate antimalarial drugs in endemic areas. Crider K, Williams J, Qi YP, Gutman J, Yeung L, Mai C, Finkelstain J, Mehta S, Pons-Duran C, Menéndez C, Moraleda C, Rogers L, Daniels K, Green P. Crider K, et al. Cochrane Database Syst Rev. 2022 Feb 1;2(2022):CD014217. doi: 10.1002/14651858.CD014217. Cochrane Database Syst Rev. 2022. PMID: 36321557 Free PMC article.
- Beyond the black stump: rapid reviews of health research issues affecting regional, rural and remote Australia. Osborne SR, Alston LV, Bolton KA, Whelan J, Reeve E, Wong Shee A, Browne J, Walker T, Versace VL, Allender S, Nichols M, Backholer K, Goodwin N, Lewis S, Dalton H, Prael G, Curtin M, Brooks R, Verdon S, Crockett J, Hodgins G, Walsh S, Lyle DM, Thompson SC, Browne LJ, Knight S, Pit SW, Jones M, Gillam MH, Leach MJ, Gonzalez-Chica DA, Muyambi K, Eshetie T, Tran K, May E, Lieschke G, Parker V, Smith A, Hayes C, Dunlop AJ, Rajappa H, White R, Oakley P, Holliday S. Osborne SR, et al. Med J Aust. 2020 Dec;213 Suppl 11:S3-S32.e1. doi: 10.5694/mja2.50881. Med J Aust. 2020. PMID: 33314144
- FreeStyle Libre Flash Glucose Self-Monitoring System: A Single-Technology Assessment [Internet]. Bidonde J, Fagerlund BC, Frønsdal KB, Lund UH, Robberstad B. Bidonde J, et al. Oslo, Norway: Knowledge Centre for the Health Services at The Norwegian Institute of Public Health (NIPH); 2017 Aug 21. Report from the Norwegian Institute of Public Health No. 2017-07. Oslo, Norway: Knowledge Centre for the Health Services at The Norwegian Institute of Public Health (NIPH); 2017 Aug 21. Report from the Norwegian Institute of Public Health No. 2017-07. PMID: 29553668 Free Books & Documents. Review.
- Recovery schools for improving behavioral and academic outcomes among students in recovery from substance use disorders: a systematic review. Hennessy EA, Tanner-Smith EE, Finch AJ, Sathe N, Kugley S. Hennessy EA, et al. Campbell Syst Rev. 2018 Oct 4;14(1):1-86. doi: 10.4073/csr.2018.9. eCollection 2018. Campbell Syst Rev. 2018. PMID: 37131375 Free PMC article.
- The use of artificial intelligence for automating or semi-automating biomedical literature analyses: A scoping review. Santos ÁOD, da Silva ES, Couto LM, Reis GVL, Belo VS. Santos ÁOD, et al. J Biomed Inform. 2023 Jun;142:104389. doi: 10.1016/j.jbi.2023.104389. Epub 2023 May 13. J Biomed Inform. 2023. PMID: 37187321 Review.
- Blumenfeld P, Pfeffer RM, Symon Z, et al. The lag time in initiating clinical testing of new drugs in combination with radiation therapy, a significant barrier to progress? Br J Cancer. 2014;111(7):1305-1309. doi:10.1038/bjc.2014.448
- Morris ZS, Wooding S, Grant J. The answer is 17 years, what is the question: understanding time lags in translational research. J R Soc Med. 2011;104(12):510-520. doi:10.1258/jrsm.2011.110180
- Van Norman GA. Drugs, devices, and the FDA: part 2: an overview of approval processes: FDA approval of medical devices. JACC: Basic Transl Sci. 2016;1(4):277-287. doi:10.1016/j.jacbts.2016.03.009
- DeYoung J, Beltagy I, van Zuylen M, Kuehl B, Wang LL. MS2: a dataset for multi-document summarization of medical studies. ArXiv. 2021. doi:10.48550/arXiv.2104.06486
- Goodman CS, Church F. HTA 101 Introduction to health technology assessment. 2004.
Publication types
- Search in MeSH
Related information
Grants and funding.
- HSRIC-2016-10009/National Institute for Health and Care Research
LinkOut - more resources
Full text sources.
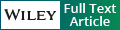
- Citation Manager
NCBI Literature Resources
MeSH PMC Bookshelf Disclaimer
The PubMed wordmark and PubMed logo are registered trademarks of the U.S. Department of Health and Human Services (HHS). Unauthorized use of these marks is strictly prohibited.
Unraveling Social Media’s Issues on Public Opinion and Activism: A Thematic Review
- August 2024
- In book: Board Diversity and Corporate Governance (pp.155-163)

- This person is not on ResearchGate, or hasn't claimed this research yet.

Discover the world's research
- 25+ million members
- 160+ million publication pages
- 2.3+ billion citations
No full-text available

To read the full-text of this research, you can request a copy directly from the authors.
- Kukuh Buntoro
- Suswanta Suswanta

- Chaoyuan She

- Valeria Bondarenko

- Fabio da Silva Gomes

- Saba Rasheed
- Beenish Zahoor

- BMC PUBLIC HEALTH
- Anne Rogers

- Qual Res Psychol

- Estella Chee Li Lim
- Chu-Ting Chang

- Jerome Turner

- Austin J. Hill

- TECHNOL FORECAST SOC

- Mohammad Dalvi-Esfahani
- Lam Wai Leong

- Mehrbakhsh Nilashi

- FOOD POLICY

- C.J.A.M. Termeer

- Rasha Abdulla

- Bernhard Rieder
- Liesbeth Zack
- AUST J PSYCHOL
- Fiona H. McKay

- J K Chaddah
- S M I R Naqvi
- N K Khouzani
- Recruit researchers
- Join for free
- Login Email Tip: Most researchers use their institutional email address as their ResearchGate login Password Forgot password? Keep me logged in Log in or Continue with Google Welcome back! Please log in. Email · Hint Tip: Most researchers use their institutional email address as their ResearchGate login Password Forgot password? Keep me logged in Log in or Continue with Google No account? Sign up
Thank you for visiting nature.com. You are using a browser version with limited support for CSS. To obtain the best experience, we recommend you use a more up to date browser (or turn off compatibility mode in Internet Explorer). In the meantime, to ensure continued support, we are displaying the site without styles and JavaScript.
- View all journals
- Explore content
- About the journal
- Publish with us
- Sign up for alerts
- Open access
- Published: 08 August 2024
Investor attention and environmental performance of Chinese high-tech companies: the moderating effects of media attention and coverage sentiment
- Yanpeng Chen ORCID: orcid.org/0009-0003-2706-416X 1 &
- Wenjun Mai ORCID: orcid.org/0009-0002-4205-5731 2
Humanities and Social Sciences Communications volume 11 , Article number: 1020 ( 2024 ) Cite this article
78 Accesses
Metrics details
- Business and management
- Environmental studies
In the context of China’s rapid economic development, corporate pollutant emissions are increasing, underscoring the imperative for further enhancement of corporate environmental performance. Given the significance of high-tech enterprises in the environmental domain, this study examines the impact of investor attention on the corporate environmental performance of Chinese high-tech enterprises. This study utilized data from 463 high-tech enterprises in mainland China from 2011 to 2022 and employed the dynamic panel generalized method of moments (GMM) technique. The findings indicate that investor attention has a negative impact on the corporate environmental performance of high-tech enterprises. This research also finds that both media attention and coverage sentiment mitigate the negative relationship between investor attention and environmental performance of high-tech firms. Heterogeneity analysis reveals that in the sample of IT service industry, heightened media attention exacerbates the negative impact of investor attention on corporate environmental performance, and media coverage sentiment exhibits no significant moderating effect. Within samples of non-polluting and state-owned enterprises, increased media coverage sentiment amplifies the negative impact of investor attention on corporate environmental performance. This paper suggests that policymakers should foster a media environment that supports accurate and thorough environmental reporting while businesses should proactively engage with media to align investor perceptions with sustainable environmental practices.
Similar content being viewed by others
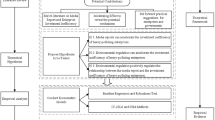
Assessing the moderating effect of environmental regulation on the process of media reports affecting enterprise investment inefficiency in China
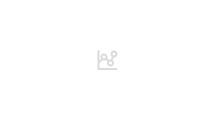
ESG performance on the value of family firms: international evidence during Covid-19
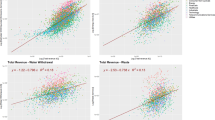
Assessments of the environmental performance of global companies need to account for company size
Introduction.
China’s rapid industrialization and economic expansion have brought about significant environmental challenges. Environmental severe issues such as air and water pollution, hazardous waste, and high carbon emissions are prevalent, particularly in areas with concentrated industrial activities. These environmental problems not only pose health risks but also threaten sustainable development (Guo et al. 2020 ). The high-tech industry, while a driver of economic growth, has been notably impacted by these issues, leading to increased scrutiny and regulatory pressures. Figure 1 displays the total carbon emissions of high-tech companies from 2011 to 2021. According to the figure, there is a trend of annual increase in the carbon emission levels of high-tech firms. The environmental issue is primarily attributed to prioritizing economic goals over environmental stewardship, often exacerbated by lax enforcement of environmental regulations and insufficient transparency in environmental reporting (Chen et al. 2021 ). Several studies have highlighted the environmental repercussions of China’s industrial policies, which have emphasized GDP growth over environmental health. Yu and Wang ( 2021 ) discuss how regional disparities in enforcing environmental regulations contribute to uneven environmental degradation across provinces. They suggest that while some regions have begun to adopt more stringent environmental policies, others continue to experience deteriorating environmental conditions due to weaker regulatory frameworks. Similarly, environmental investments, while increasing, often fail to offset the damage caused by industrial expansion, as noted by Jiang et al. ( 2022 ). Their findings indicate that although investments in environmental protection are mandated, they do not lead to reduced pollution levels or enhanced corporate innovation in environmental practices.
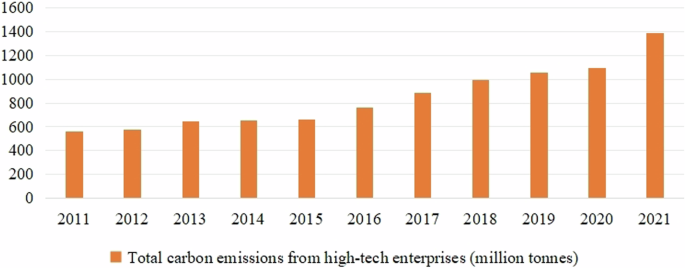
Total carbon emissions of high-tech enterprises from 2011–2021. Source: China National Bureau of Statistics.
Investor attention, as an increasingly significant driver for corporations, is becoming increasingly prominent (Chen et al. 2024 ). Investors are no longer solely pursuing financial returns but also demonstrating a keen interest in corporate environmental performance (Faller and zu Knyphausen-Aufseß 2018 ). This is because investors recognize the long-term risks and opportunities associated with environmental issues such as resource scarcity, climate change, and pollution (Dam and Scholtens 2012 ). Furthermore, investor attention enhances corporate green innovation and environmental performance by increasing information transparency, alleviating financing constraints, and mitigating institutional costs (He et al. 2022 ). While investor attention may have the potential to monitor corporate behavior and enhance corporate environmental performance, it can also negatively impact corporate environmental performance, particularly in emerging economies where inexperienced and irrational individual investors dominate the capital markets (Chen et al. 2023 ).
With the rapid advancement of information technology and the widespread penetration of the internet, social media has emerged as a principal conduit for individuals to access information, exchange perspectives, and share experiences (Murphy et al. 2014 ). Social media comprises various user-driven platforms that facilitate the dissemination of captivating content, dialogues, and communication with a broader audience (Kapoor et al. 2018 ). It furnishes an environment conducive to interaction and networking between individuals and businesses, offering a platform for engagement (Anstead and O’Loughlin 2015 ). In this era of digitization, social media platforms have evolved into vital bridges facilitating interaction between enterprises and stakeholders such as consumers, investors, and other relevant parties (Chatterjee and Kumar Kar, 2020 ).
As an external factor, media attention can enhance corporate motivation to improve their environmental performance (Aerts and Cormier 2009 ; Brammer and Pavelin 2006 ). Reverte ( 2009 ) argues that media attention significantly influences voluntary corporate social responsibility information disclosure, wherein a higher degree of media attention garnered by companies on environmental matters leads to a greater willingness on the part of these firms to disclose their environmental performance information. Brammer and Pavelin ( 2006 ) noted that media attention could oversee companies in enhancing the quality of environmental disclosure and regulating inappropriate environmental management practices within corporations. The sustained media attention and the pressure from public have mitigated managers’ self-serving behaviors, propelling companies to enhance their environmental awareness. Faced with media pressure, companies are inclined to allocate more resources to environmental expenditures to ameliorate their environmental performance (Aerts and Cormier 2009 ). Regarding media coverage sentiment, Liu and McConnell ( 2013 ) argue that media coverage sentiment influences corporate behavior, subsequently affecting corporate environmental performance. Lei and Zhang ( 2020 ) examined the impact of media content and sentiment in news reports on the performance of environmental responsibility investments. Their findings revealed a correlation between coverage sentiment and corporate environmental responsibility, emphasizing the significance of media coverage on environmental responsibility-related issues and its potential influence on financial indices.
Based on the above discussion, this paper aims to address the problem by employing data from 463 high-tech enterprises from 2011 to 2022. By utilizing the dynamic panel generalized method of moments (GMM) technique, this study seeks to investigate the influence of investor attention on the environmental performance of high-tech enterprises, while examining the moderating effects of media attention and media coverage sentiment within this context. The reasons for selecting high-tech enterprises as the research sample in this paper are as follows: Firstly, the high-tech sector has revolutionized corporate production methodologies and significantly influenced human lifestyles (Chen et al. 2023 ). Moreover, it has become increasingly crucial in mitigating climate change impacts. Secondly, the activities of logistics, supply chains, and global manufacturing units within high-tech enterprises profoundly affect carbon emissions (Christmann and Taylor 2002 ). Additionally, high-tech firms display a solid commitment to environmental issues, as they are uniquely positioned to implement innovative measures for environmental protection compared to other industries (Al-Najjar and Salama, 2022 ).
This paper presents several primary contributions. Firstly, previous research has predominantly concentrated on internal factors (Zhang and Fu 2023 ), with limited attention given to the impact of external factors, such as investor attention, on corporate environmental performance. This paper extends the literature by substantiating the role of investor attention in the environmental performance of high-tech enterprises. Secondly, given the limited research on media factors in the relationship between investor attention and corporate environmental performance, there needs to be more studies that employ media attention and coverage sentiment as a moderating variable to delve into the underlying relationship between investor attention and corporate environmental performance. Thirdly, scant research has centered on high-tech enterprises as a sample to delve into the intricate relationship between investor attention and corporate environmental performance. Within the existing research, the focal point has often gravitated toward other industries or conglomerates, thereby leaving the examination of this specific domain of high-tech enterprises relatively underexplored. Therefore, by focusing on high-tech enterprises, this study takes a more comprehensive perspective to examine the relationship between investor attention and corporate environmental performance while investigating the moderating role of media attention and coverage sentiment.
The organization of the paper is as follows: Section 2 provides a comprehensive review of the relevant literature. Section 3 outlines the methodology used in this study. Section 4 presents the findings, while Section 5 discusses these results. Finally, Section 6 concludes the paper.
Literature review
Corporate environmental performance is a metric used to assess a company’s performance in terms of environmental impacts, resource utilization, and relevant financial factors. It also measures a company’s efforts to mitigate these impacts and implement preventative measures (Dragomir 2018 ). The measurement of corporate environmental performance mainly involves the pollutant emissions (Braam et al. 2016 ), the ASSET4 ESG database (Gonenc and Scholtens 2017 ), and the Sino-Securities Index (Jiang et al. 2023 ). Corporations should bear a certain degree of responsibility towards society and the environment (Ibrahim and Varn 2021 ). While the positive impact of corporate environmental responsibility on environmental performance is marginal, corporate environmental performance exerts a significant and positive influence on environmental responsibility (Peng et al. 2021 ). Previous studies have also confirmed that factors like digital technologies (Li et al. 2020 ), corporate governance (Abedin et al. 2023 ), and R&D investment (Alam et al. 2019 ) impact corporate environmental performance. However, far from being peripheral, informal regulation such as media scrutiny and community activism plays a pivotal role in enhancing corporate environmental performance, especially by shaping corporate environmental strategies and outcomes under the external scrutiny of investor attention and media coverage. The discourse surrounding informal external regulation pivots on its unique capacity to influence corporate behavior beyond formal legislative frameworks, leveraging public opinion and stakeholder expectations as powerful motivators for environmental stewardship within the corporate sector (Chen et al. 2022 ). For example, Chen et al. ( 2022 ) seminal work elucidates how informal environmental regulation (IER), mainly via environmental media coverage, exerts considerable pressure on firms to comply with existing environmental standards and innovate and elevate their environmental performance. The synthesis offered by Miroshnychenko et al. ( 2019 ) further delineates the significant impact of external corporate governance mechanisms, including informal regulatory pressures, on driving corporate environmental performance improvements. Their research provides compelling evidence that the external governance environment is a critical determinant of environmental performance, highlighting the multifaceted nature of corporate environmental governance. Lastly, Jacoby et al. ( 2019 ) illuminate the pivotal role of external monitoring, including media coverage, in enhancing firms’ environmental transparency and, by extension, their overall environmental performance. This insight affirms the critical function of informal external regulation in promoting greater accountability and transparency within the corporate sector, fostering a culture of environmental responsibility.
Investor attention and corporate environmental performance
In recent years, investors have demonstrated an elevated environmental consciousness (Chiou et al. 2011 ), exhibiting a propensity to monitor corporate environmental conduct. Investor attention refers to the interest and significance that investors attribute to a particular company or a series of activities within a market (Chen et al. 2022 ). This attention may manifest as investors closely monitor and focus on relevant information, news, financial reports, market trends, and the performance and prospects of specific companies. The measurement of investor attention mainly involve the Google Search Volume Index (Vlastakis and Markellos 2012 ), the Baidu Search Volume Index (Chu et al. 2022 ), and the number of searches for stock names (Clarke et al. 2021 ). Investor attention can significantly impact a company’s environmental performance (Deng et al. 2022 ). This effect arises from the proactive efforts of investors to collect detailed information about the company, which in turn enhances transparency and can drive improvements in the firm’s environmental performance (Hao and Xiong 2021 ). Although existing literature identifies a relationship between investor attention and corporate environmental performance, the nature of this impact, whether positive or negative, remains uncertain. That is to say, investor attention may have both positive (Huang et al. 2019 ) and negative effects (Chen et al. 2023 ) on corporate environmental performance.
On the one hand, investor attention may exert a positive influence on corporate environmental performance. Under the heightened scrutiny of investors, companies are inclined to enhance their environmental performance to avert reputational losses stemming from environmental incidents (Ming et al. 2023 ). Wang et al. ( 2023 ) elucidate the direct positive association between investor attention and corporate environmental performance in China, emphasizing the mediating roles of corporate governance and information disclosure quality. Their study underscores the significance of incorporating investor attention as a strategic avenue for enhancing environmental performance in firms. Moreover, Dilla et al. ( 2019 ) expand on how investor perceptions regarding the significance of environmental responsibility modulate the influence of environmental performance information on investment decisions. This modulation suggests a psychological mechanism where investor values and beliefs about environmental stewardship dictate their responsiveness to corporate environmental disclosures. Consequently, this underlines the critical role of aligning corporate environmental narratives with investor environmental values to foster positive investment behaviors. Furthermore, the study by Setyowati et al. ( 2021 ) explores the relationship between corporate environmental responsibility and financial performance in the energy sector, revealing that positive environmental actions elicit favorable responses from investors. This finding elucidates a mechanism where corporate environmental responsibility signals investors about the firm’s commitment to sustainability, potentially influencing investors future goals and expectations. In addition, Harimauwan and Lukman ( 2023 ) assess the impact of environmental performance and corporate social responsibility (CSR) on stock returns, finding that while environmental performance does not directly affect stock returns, CSR has a negative impact. This highlights the complex relationship between environmental strategies and investor reactions, with implications for how firms should present their environmental performance to attract investor attention. Lastly, Pham and Luu Duc Huynh ( 2020 ) delve into the relationship between investor attention and the green bond market performance, demonstrating that investor attention significantly influences green bond returns and volatility. However, this relationship varies over time, suggesting the evolving nature of investor influence on sustainable investment avenues. These studies reinforce the notion that investor attention plays a crucial role in promoting corporate environmental performance.
On the other hand, investor attention may exert a negative influence on corporate environmental performance. These effects stem from a range of mechanisms, including market pressures, short-termism, and the potentially distracting influence of intense public attention. Firstly, studies conducted by Chen et al. ( 2023 ) have pointed out that environmental information disclosure is a reference for companies in fulfilling their environmental responsibilities. However, long-term online investor attention is negatively correlated with the quality of environmental performance by companies. Luo et al. ( 2019 ) argue that while environmental information disclosure quality negatively impacts debt financing costs, the mediating effect of public attention can exacerbate this by highlighting negative environmental practices to the public, thereby increasing the cost of debt due to perceived higher risks. Furthermore, research by Wei et al. ( 2020 ) delves into the trading strategies of institutional investors in response to negative environmental event disclosures. The findings suggest that institutional investors’ preemptive reactions to anticipated negative disclosures can mitigate the immediate stock price effects but may encourage short-termism, potentially undermining long-term environmental commitments and innovation due to market volatility. Guo and Lu ( 2021 ) highlight how public attention, while generally positive, can lead to more significant governmental scrutiny and pressure, which could lead firms to adopt superficial or compliance-focused environmental strategies rather than substantive, long-term improvements in environmental performance. The work by Shen ( 2019 ) brings an interesting perspective on institutional investor heterogeneity, indicating that the overall shareholding by institutional investors negatively correlated with the level of corporate environmental responsibility. This suggests that investor pressures could disincentivize firms from undertaking more robust environmental actions due to concerns over short-term financial impacts. Additionally, Budhijana ( 2022 ) explores the relationship between dividend policy, financial performance, and environmental performance on investor reactions. The findings indicate that while environmental performance initiatives are crucial, they might not always translate to positive investor reactions if perceived to be at the expense of financial returns, highlighting a potential misalignment between investor expectations and corporate environmental strategies. Collectively, these studies underscore a critical tension between the push for improved corporate environmental performance and the mechanisms through which investor attention might inadvertently foster an environment of short-termism or superficial compliance.
Based on the above review, we formulate the opposite hypotheses:
H1: Investor attention affects corporate environmental performance.
H1a: Investor attention has a positive impact on corporate environmental performance.
H1b: Investor attention has a negative impact on corporate environmental performance.
Role of media attention
Media attention refers to the sustained focus and coverage by the media on specific topics, events, or entities, encompassing the allocation of media resources over time, the frequency and depth of reporting, and the persistence of interest in particular subjects (Erlich et al. 2021 ). This concept is paramount as media attention and coverage can significantly influence public perspectives and behaviors. The measurement of media attention mainly involves the tweets from Sina Weibo (Sun et al. 2020 ), the CEO Media Exposure Index (Bai et al. 2019 ), and the news article (Erlich et al. 2021 ). The application of legitimacy theory in the context of media attention regulating the relationship between investor attention and corporate environmental performance highlights the interplay of societal expectations and corporate conduct. Legitimacy theory posits that businesses must operate within the bounds of societal norms and values to be deemed legitimate by their stakeholders, which includes investors, consumers, and the broader public affected by their environmental practices (Suchman 1995 ). Media plays a critical role in shaping perceptions of corporate legitimacy, especially concerning environmental performance. When media highlights a company’s environmental actions or inactions, it influences public perception and, by extension, investor decisions. Recent work underscores the moderating role of media attention in the relationship between investor attention and corporate environmental performance, suggesting a complex interaction that can significantly enhance a firm’s environmental outcomes. Wang and Zhang ( 2023 ) delve into the effects of media coverage on corporate governance and environmental protection investment. They find that media attention positively moderates the relationship between corporate governance mechanisms and environmental investment, suggesting that media visibility can enhance the influence of investor attention on firms’ environmental actions, leading to increased investment in environmental protection. Lee and Cho ( 2020 ) contribute to the discussion by highlighting the role of media attention in shaping corporate environmental strategies. They found that consumers’ perceived media credibility enhanced the relationship between media exposure and environmental protection behavior intentions, suggesting that credible media coverage could significantly influence corporate environmental initiatives by shaping public perceptions and investor attention. This finding underscores the critical role of media in bridging the gap between corporate environmental actions and investor awareness, thus potentially guiding investor attention toward firms with commendable environmental practices. Tavakolifar et al. ( 2021 ) examine the effect of media attention on firms’ commitment to climate change actions, finding that heightened media visibility significantly increases the likelihood of firms undertaking more robust climate change initiatives. This study illustrates how media coverage can be a critical moderating variable, strengthening the relationship between investor attention and corporate environmental commitments. Li et al. ( 2020 ) assess the impact of individual investor attention on tourism firms’ restructured performance, emphasizing the moderating effect of media attention. Their results indicate that media attention, varying by time and media heterogeneity, can significantly influence the relationship between investor attention and corporate environmental performance, highlighting the immediate effect of investor attention and the time-dependent nature of media attention’s moderating role.
Based on the above analyses, we propose the hypotheses:
H2: There is a moderating effect of media attention in the relationship between investor attention and corporate environmental performance.
Role of media coverage sentiment
Media coverage sentiment refers to the emotional stance and inclination presented in media coverage towards specific topics, events, or entities. This encompasses the emotional tone, biased attitudes, and sentimental implications conveyed within the reports (Gervais and Fessler 2017 ). The exploration of media coverage sentiment and its moderating effects on the relationship between investor attention and corporate environmental performance has garnered academic interest. These research unveils the nuanced ways in which coverage sentiment not only reflects but also shapes corporate behaviors and investor perceptions, particularly in the context of environmental stewardship within high-tech enterprises in China. The study by Lei and Zhang ( 2020 ) highlights how coverage sentiment regarding corporate social responsibility (CSR) influences socially responsible investment performance. Their findings suggest that positive coverage sentiment can amplify the effects of investor attention on corporate environmental performance, showcasing the power of positive media portrayal in enhancing corporate reputation. Wang et al. ( 2021 ) investigate the effects of environmental news on investor sentiment and green industry stock returns. Their findings reveal that positive media coverage regarding environmental issues can sway investor sentiment favorably towards companies within the green industry, subsequently improving their environmental performance through increased investments in sustainable practices. Qing et al. ( 2023 ) examine the relationship between green technology innovations and corporate financial performance, with a particular focus on the moderating effect of media coverage sentiment. Their study underscores how positive coverage sentiment towards green innovations can significantly bolster investor support for such initiatives, thereby enhancing corporate environmental performance.
In conclusion, we propose the hypotheses:
H3: There is a moderating effect of coverage sentiment in the relationship between investor attention and corporate environmental performance.
Research gap
Although scholars have conducted extensive research in related fields, some research gaps still need to be addressed. Firstly, the relationship between investor attention and corporate environmental performance remains to be determined. Secondly, limited research incorporates media attention and coverage sentiment as moderating variables in the relationship between investor attention and corporate environmental performance. Thirdly, prior research has predominantly focused on manufacturing and polluting enterprises, with scarce investigation into high-tech enterprises as subjects to explore the relationship between investor attention and corporate environmental performance. In summary, this paper aims to address the research gap, complement existing literature, delve deeper into the mechanisms by which investor attention influences the environmental performance of high-tech enterprises, and incorporate media factors into the research framework. The research framework of this paper is shown in Fig. 2 .
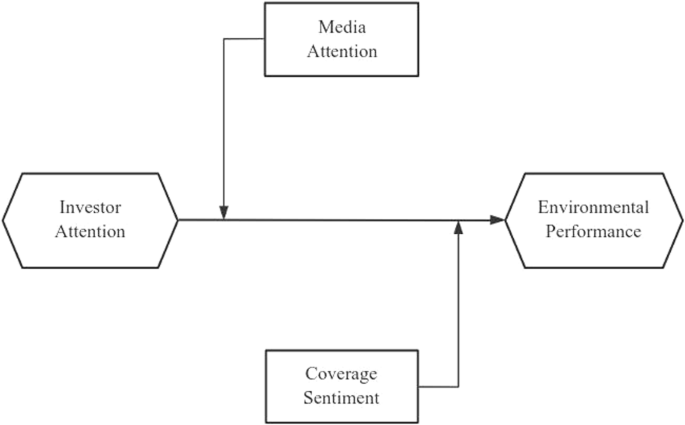
The theoretical framework.
Methodology
Research model.
This study constructs a model grounded in an extensive review of existing literature and prior investigations, represented as follows:
where EP, IA, SIZE, EMP, TOBINQ, LISTAGE, and ROE are environmental performance, investor attention, enterprise size, number of employees, Tobin’s Q ratio, listing age, and return on equity, respectively. The \({\rm{\varepsilon }}\) denotes the error terms, with i indicating the cross-sectional unit and t denoting the time period.
This paper employed panel estimation methods to evaluate the specified equation, as these methods offer greater informational content and reduce collinearity among variables (Baltagi 2008 ). The dynamic panel model is distinguished by incorporating lagged dependent variables on the right-hand side, represented as follows:
where γ is the coefficient of the lagged dependent variable.
This study also examines the moderating effects of media attention and the sentiment of media coverage on the relationship between investor attention and corporate environmental performance. Following the methodology outlined by Zhuo and Chen ( 2023 ), this research incorporates interaction terms into the baseline regression model to formulate an empirical testing framework, which is presented as follows:
where MA is media attention, and MCS is media coverage sentiment.
As Brambor et al. ( 2006 ) suggest, it is essential to focus on the interaction term rather than the individual components (IA or MA) within the interaction model. This focus is necessary because, in the absence of investor attention (or media attention), the coefficients reflect solely the influence of media attention (or investor attention) on corporate environmental performance. The derivative of the equation concerning IA is taken to calculate the marginal effects. The formula is provided below:
To determine the significance of the marginal effects, new standard errors must be calculated. Therefore, mean, minimum and maximum values are employed to compute t-statistics, which are then used to evaluate the significance of these marginal effects.
This research utilizes a standard panel data model for estimation, explicitly employing the generalized method of moments (GMM) due to the nature of the data. GMM optimizes an objective function and estimates parameters based on observed data within the model’s framework (Hansen, 1982 ). The difference GMM approach addresses endogeneity bias by establishing moment conditions through observations at two different time points (Holtz-Eakin et al. 1988 ). System GMM, which extends the difference GMM method, leverages both first-order and second-order moment conditions for endogenous variables, thereby enhancing the robustness and utilization of panel data against issues such as endogeneity and serial correlation (Blundell and Bond 1998 ).
Dependent variable
To measure the dependent variable of this paper, namely corporate environmental performance (EP), we rely on the ESG rating score constructed by Sino-Securities Index Information Service (Jiang et al. 2023 ). The Sino-Securities Index (SNSI) encompasses the most recent ESG reporting guidelines issued by the Hong Kong Exchange and aligns with international standards. Furthermore, it integrates China’s specific national context into the SNSI ESG Ratings Methodology. This study’s corporate environmental performance data is derived from the “environmental” score within the SNSI ESG Ratings. The environmental evaluation indicators of SNSI include climate change, resource utilization, environmental pollution, environmentally friendly, and environmental management.
Independent variable
We obtained data from the Guba Database, part of the Chinese Research Data Services (CNRDS), to evaluate investor attention. This database is a specialized financial text resource that analyzes statistical data from comments about listed companies on Chinese online stock forums. It primarily focuses on the textual analysis and quantitative statistics of comments made by Chinese stock investors on various stock forums and social media platforms. This extensive and diverse data source provides us with detailed research information. In this study, we measure investor attention by using the logarithm of the number of stock investors’ comments, as suggested by Clarke et al. ( 2021 ).
Moderating variable
This study obtained media attention (MA) and media coverage sentiment (MCS) data from the Chinese Financial News Database of Chinese Research Data Services (CNRDS). The data sources cover more than 400 online media and more than 600 newspapers and publications, covering not only mainstream financial websites, national websites, local websites, and industry websites on the internet but also mainstream newspapers, national newspapers, and other important newspapers and magazines, with tens of millions of news data, which can fully satisfy the research needs. The logarithm of the frequency of mentions of listed companies in news reports is selected as a measure of media attention (Byun and Oh 2018 ), while the Janis-Fadner coefficient is employed to assess the media coverage sentiment generated by news reports (Deephouse 1996 ). MCS is calculated based on positive and negative news coverage. If news coverage is more optimistic, the value of MCS is closer to 1. If news coverage is more harmful, the value of MCS is closer to −1 (Huang et al. 2021 ). The formula for MCS is shown as follows:
where \({\rm{pos}}\) represents the number of positive news reports; \({\rm{neg}}\) represents the number of adverse news reports.
Control variables
Building upon prior research on corporate environmental performance (Nguyen et al. 2021 ), this paper has selected a series of variables representing corporate-level characteristics that could potentially impact EP as control variables. The control variables in this study include enterprise size (Chen et al. 2023 ), number of employees (López-Gamero et al. 2009 ), Tobin’s Q (He et al. 2022 ), listing age (Zhang et al. 2022a , b ), and return on equity (Chen et al. 2023 ). The control variable data are sourced from the China Stock Market and Accounting Research Database (CSMAR).
Research sample
This study’s sample comprises high-tech companies listed on the Shanghai and Shenzhen Stock Exchanges from 2011 to 2022. Adhering to the selection criteria outlined in the “Administrative Measures for the Recognition of High-tech Enterprises” as utilized by Qu et al. ( 2023 ), we meticulously selected the high-tech firms. The selection methodology included several critical steps: excluding ST and PT firms due to their unique financial conditions, excluding financial and insurance firms to maintain industry relevance, eliminating firms with incomplete financial data for precision, and adjusting for outliers to ensure the data’s integrity. This thorough process resulted in a comprehensive final dataset of 5556 firm-year observations, forming a robust basis for empirical analysis. The definitions and sources for all variables are presented in Table 1 .
Descriptive statistics and correlation coefficient tests
To begin our analysis, we conduct descriptive and correlation analyses before delving into the panel data investigation. Table 2 presents the descriptive statistics and correlation analysis for independent and dependent variables. The estimated results reveal a positive correlation between investor attention and the environmental performance of high-tech firms, aligning with prior literature (Zhang et al. 2022a , b ). In conclusion, our examination indicates no substantial issues with multicollinearity within this study.
Benchmark regression
The benchmark regression results for the models are presented in Table 3 . Before discussing the results, we conducted various diagnostic tests to ensure the model’s validity. The Hansen test did not reject the null hypothesis of no over-identifying restrictions. The serial correlation test rejected the null hypothesis for first-order autocorrelation (AR(1)) but did not reject it for second-order autocorrelation (AR(2)). These outcomes confirm the validity of the model. Based on our findings, both difference GMM and system GMM consistently support the hypothesis of a statistically significant negative impact of investor attention on the environmental performance of high-tech listed companies. This finding aligns with Chen et al. ( 2023 ).
Robustness check
To ensure our findings were not biased by the potential impact of the COVID-19 pandemic and to further enhance the robustness of our research, this paper re-conducted GMM regression analysis using data from 2011–2019, a period that excludes the potential effects of the COVID-19 outbreak, with results presented in Table 4 . This approach allowed us to test the timeliness and universality of the impact of investor attention on corporate environmental performance. According to the results in Table 4 , the coefficient for investor attention is −1.89 and is significant at the 1% level, confirming that investor attention has a significant negative effect on the environmental performance of Chinese high-tech companies. This outcome, consistent with our benchmark regression analysis, bolsters the credibility and robustness of our findings, indicating that investor attention negatively impacts corporate environmental performance even when excluding the effects of the COVID-19 pandemic.
To further validate the findings on the impact of investor attention on the environmental performance of Chinese high-tech companies, this study uses environmental information disclosure (EID) as an alternative measure of corporate environmental performance. EID involves the transparent provision of detailed information regarding a company’s environmental performance, impacts, and initiatives, encompassing data, reports, and other pertinent details related to environmental aspects of its operations, products, and services (Li et al. 2022 ). The premise for this substitution is that the disclosure of environmental information serves as a proxy for corporate environmental performance, reflecting a firm’s commitment to transparency and environmental responsibility (Zeng et al. 2020 ). The results in Table 5 indicate that the coefficient for investor attention on environmental information disclosure is −0.22, significant at the 1% level. This is consistent with the benchmark regression results, further substantiating the negative impact of investor attention on corporate environmental behavior.
Moderating effect
Based on the results in Table 4 and Table 5 , the coefficients of investor attention on environmental performance are negative, indicating a significant negative impact of IA on environmental performance among high-tech enterprises. This outcome further substantiates the accuracy of the findings in Table 3 . However, companies garner increased investor attention with the widespread use of social media platforms (Kapoor et al. 2018 ). Without adequate regulation, this heightened attention could lead to a decline in corporate environmental performance. Hence, this study introduces moderate variables, namely media attention and coverage sentiment, to address this issue. These variables are used to explore whether the media factor moderates the relationship between investor attention and environmental performance in high-tech enterprises, as presented in Table 6 .
Based on the findings presented in Table 6 , the interaction term between investor attention and media attention (IA*MA) shows significance across all models. The positive and statistically significant interaction coefficient between investor and media attention indicates that the relationship between investor intention and corporate environmental performance differs depending on media attention. The interaction term between investor attention and media coverage sentiment (IA*MCS) reflects a similar conclusion. In other words, as media coverage sentiment increases, the negative impact generated by investor attention might diminish.
Marginal effect
After confirming the moderation effects, the next phase calculates the marginal effects (Cole et al. 2006 ). We re-estimate standard errors to determine the significance of the marginal influence on corporate environmental performance due to shifts in investor attention. Figure 3 showcases the marginal effects of media attention and sentiment as moderating factors. The results reveal a notably positive trend in the marginal effect with increased media attention and sentiment, partly driven by investor attention. Table 6 further illustrates that investor and media attention exhibit positive and statistically significant relationships at mean and maximum levels. However, this association diminishes with a negative marginal effect at the minimum level. For example, a 1% rise in IA corresponds to a 2.01% increase in the annual growth of corporate environmental performance at the mean level. The impact is even more significant at the maximum level, where a 1% increase in IA results in a 5.95% rise in corporate environmental performance, exceeding the effect observed when media attention is at the mean level. This suggests that higher media attention mitigates the adverse effects of investor attention on the environmental performance of high-tech enterprises. Moreover, media coverage sentiment also alleviates the negative relationship between investor attention and corporate environmental performance, with a more robust moderating effect than media attention.
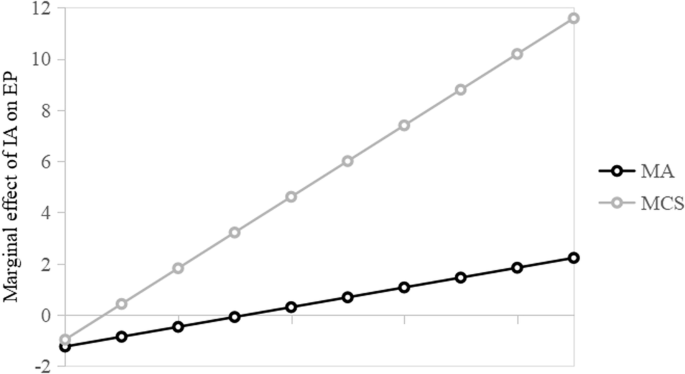
The marginal effect of IA on EP, conditional upon MA and MCS.
Heterogeneity analysis
To further substantiate the research findings, this study conducts heterogeneity analysis to investigate how media attention and sentiment in media coverage influence the relationship between investor attention and the environmental performance of high-tech firms across different sub-samples. Utilizing Qu et al. ( 2023 ) to classify high-tech enterprises, the samples were categorized into high-tech manufacturing firms and high-tech information technology service firms. The results of this heterogeneity analysis are detailed in Table 7 .
Based on the results from Table 7 , we observed that heightened investor attention in high-tech enterprises within both the manufacturing and IT service industries leads to a reduction in the environmental performance of these enterprises. In high-tech manufacturing enterprises, media attention and media coverage sentiment mitigate the negative relationship between investor attention and corporate environmental performance. However, in the sample of IT service enterprises, the interaction term coefficient between media attention and investor attention is −4.54, indicating that media attention intensifies the negative relationship between investor attention and environmental performance. Additionally, we found no significant moderating effect of media coverage sentiment within the sampled IT service enterprises.
Following the methodology outlined by Zhou et al. ( 2022 ), we categorized the sample into polluted and non-polluted high-tech enterprises for heterogeneity analysis. The findings, detailed in Table 8 , indicate that investor attention negatively impacts the environmental performance of both types of enterprises. Moreover, media attention mitigates this negative impact across both sample groups. Specifically, media coverage sentiment inversely moderates the relationship between investor attention and corporate environmental performance in the polluted high-tech enterprises' sample. However, within the non-polluted enterprises' sample, the coefficient of IA*MCS is −11.91, indicating that media coverage sentiment exacerbates the negative impact of investor attention on corporate environmental performance.
In accordance with the methodology employed by Wang et al. ( 2022 ), we categorized the sample into state-owned and private enterprises to examine the underlying causal mechanisms within different ownership frameworks. The findings are illustrated in Table 9 . Based on the results, we found that both state-owned and private high-tech enterprises experience a negative impact on their environmental performance due to investor attention. Additionally, in both samples, media attention can mitigate the negative impact of investor attention on corporate environmental performance. However, in the case of state-owned enterprises, the coefficient of IA*MCS is −5.22, indicating that an increase in media coverage sentiment exacerbates the negative relationship between investor attention and corporate environmental performance. Conversely, within private enterprises, increased media coverage sentiment mitigates the negative impact of investor attention on corporate environmental performance.
This study constructed a model delineating the role of investor attention on environmental performance within high-tech firms while validating the moderating effects of media attention and media coverage sentiment. This study draws several conclusions based on the empirical analysis conducted on data from 463 Chinese high-tech enterprises.
First, investor attention can significantly negatively impact the environmental performance of high-tech enterprises. This counterintuitive result suggests that increased investor attention may pressure firms to prioritize short-term financial returns over long-term environmental strategies. This phenomenon is supported by literature indicating that high levels of investor attention may lead firms to engage in earnings management and other short-term behaviors that detract from genuine environmental improvements (Bushee 1998 ; El Ouadghiri et al. 2022 ). Furthermore, profit-focused investors may demand cost reductions from the companies, including environmental expenditures, which could result in firms cutting their environmental protection spending and adopting cost-effective yet environmentally less beneficial practices, consequently diminishing their environmental performance (Radu and Smaili 2021 ). Comparatively, this finding diverges from studies like Wang et al. ( 2023 ), who identified a positive association between investor attention and environmental performance in different contexts. This discrepancy highlights the unique challenges faced by high-tech firms in China, where rapid innovation cycles and intense competition might amplify the pressure to generate immediate financial results at the expense of environmental integrity (Guo and Lu 2021 ).
Second, the interaction term between investor attention and media attention is positively significant, suggesting that media attention mitigates the negative impact of investor attention on the environmental performance of Chinese high-tech companies. This finding illuminates how media attention can alleviate some adverse effects associated with investor pressures. Media attention influences corporate behavior by increasing transparency and accountability in environmental practices. Theoretical insights from the legitimacy theory in media studies suggest that media attention can heighten the demand for corporate legitimacy in environmental performance. Media attention can compel corporations to enhance their environmental strategies to maintain their legitimacy in the eyes of the public, thereby mitigating the negative pressures associated with investor attention (Suchman 1995 ). This theory is supported by findings from (Ge 2024 ), who demonstrate that media attention leads to enhanced corporate ESG performance by imposing financial constraints that encourage better governance practices. Additionally, media attention often shapes public expectations and evaluations of corporations. Sustained media attention on corporate environmental performance constructs public expectations regarding corporate environmental responsibility (Geng et al. 2023 ). This might lead investors to perceive environmental performance more positively, aligning with societal expectations and potentially exerting a positive influence on the environmental performance of high-tech companies.
Third, the interaction term between investor attention and coverage sentiment is positive, indicating that favorable coverage can mitigate the negative impacts of investor attention on the environmental performance of high-tech companies in China. This result highlights the complexity of the relationship between media influence and corporate environmental strategies. The moderation effect of coverage sentiment can also be explained through legitimacy theory (Suchman 1995 ). Favorable media coverage likely enhances public perceptions of a company’s legitimacy, particularly regarding its environmental efforts, thus encouraging more substantial and sincere environmental practices despite investor pressures that might otherwise prioritize short-term gains (Deephouse 1996 ). This alignment is supported by findings that suggest media coverage informs the public and reshapes corporate priorities to align with prevailing social and environmental norms (Brammer and Pavelin 2006 ). In addition, coverage sentiment can influence corporate brand image and social identification (Kissel and Büttgen 2015 ). When media emotions portray a company’s positive stance or concern regarding environmental issues, this could bolster investors’ sense of identification with the company and elevate attention toward its environmental performance.
Fourth, heterogeneity analysis reveals that while media attention and coverage sentiment moderate the relationship between investor attention and environmental performance in the high-tech manufacturing sector, these factors exert a positive and non-significant moderating influence, respectively, in the high-tech IT sector. This divergence in effects underscores the sector-specific dynamics of media impact on corporate environmental strategies. The moderation observed in the manufacturing sector may be attributable to the more tangible and direct environmental impacts associated with manufacturing activities, which garnered significant media attention. This scrutiny motivates companies to enhance their environmental practices under investor attention. In contrast, the IT sector’s less tangible environmental impacts might not translate media attention effectively into environmental performance improvements, possibly due to the abstract nature of IT services and lesser direct environmental effects. This sector-specific response aligns with the studies suggesting that the role of media in shaping corporate behavior can vary greatly depending on industry characteristics and the type of environmental impact involved (Bansal and Clelland 2004 ; Christensen et al. 2007 ).
Fifth, media attention and sentiment mitigate this negative relationship within pollution-intensive sectors, whereas in non-polluted high-tech industries, media attention retains a negative moderation, but coverage sentiment exerts a positive moderate effect. This distinction is due to these sectors’ differing inherent environmental impacts and public visibility. Polluted industries are subject to more stringent regulatory scrutiny and public pressure, making positive coverage sentiment a critical enhancer of corporate reputation and environmental compliance. In contrast, the less tangible environmental impacts of non-polluted firms may lead to a disconnect where positive coverage sentiment does not align as effectively with actual environmental performance, possibly due to overemphasis on symbolic rather than substantive environmental actions (Christensen et al. 2007 ). The findings align with existing literature that has endorsed the beneficial impacts of media coverage on corporate environmental strategies (Dhaliwal et al. 2011 ). However, the unique moderation by coverage sentiment in non-polluting sectors introduces a novel perspective, indicating that the effectiveness of media influences is highly contingent on industry-specific characteristics and the nature of environmental impacts.
Sixth, the heterogeneity analysis differentiates the moderating effects of media attention and coverage sentiment on investor attention related to environmental performance, with contrasting impacts observed in state-owned versus private high-tech companies in China. In private enterprises, media attention and sentiment mitigate the negative relationship between investor attention and environmental performance. Conversely, while media attention exhibits a negative moderation in state-owned enterprises, coverage sentiment demonstrates a positive moderating effect. This differentiation likely arises from the distinct operational and regulatory environments that characterize state-owned and private enterprises. State-owned enterprises are often under stricter governmental scrutiny, which can align coverage sentiment more closely with governmental agendas rather than genuine environmental performance, potentially leading to negative publicity effects even when investor interest is high. In contrast, private companies, with more operational flexibility and less political interference, might leverage positive coverage sentiment more effectively to enhance their environmental performance, reflecting a more straightforward relationship between media coverage and corporate behavior, as suggested by legitimacy theory (Suchman 1995 ). This suggests that while media can bolster corporate environmental efforts, its impact is contingent on the nature of ownership and inherent motivations of the firms involved.
Given the significance of high-tech enterprises in the environment, this paper is intrigued by the underlying mechanisms through which investor attention and media coverage influence the corporate environmental performance of high-tech firms. Empirically, we employed the dynamic panel generalized method of moments (GMM) technique to examine how investor attention influences corporate environmental performance among 463 high-tech enterprises from 2011 to 2022. The findings indicate that investor attention exerts a statistically significant negative impact on corporate environmental performance within high-tech enterprises. Further, we tested the moderating effects of media attention and coverage sentiment. The results revealed that both media attention and media coverage sentiment mitigate the negative relationship between investor attention and environmental performance. Finally, through heterogeneity analysis, we observed that within the sample of high-tech enterprises in the IT service industry, heightened media attention exacerbates the negative impact of investor attention on corporate environmental performance. Simultaneously, media coverage sentiment exhibits no significant moderating effect. Within samples of non-polluting and state-owned enterprises, increased media coverage sentiment exacerbates the negative impact of investor attention on corporate environmental performance.
The empirical findings that investor attention negatively impacts the environmental performance of Chinese high-tech enterprises, it is crucial to develop policy recommendations that can mitigate these adverse effects while promoting sustainable business practices. Therefore, an integrated approach involving regulatory interventions and strategic business initiatives is essential. First, regulatory bodies need to introduce and enforce comprehensive ESG reporting standards. By mandating detailed disclosure of environmental risks and the measures taken to mitigate them, regulators would increase transparency and enhance investor awareness regarding the environmental implications of their investment decisions. Such policies should encourage investors to consider environmental as a critical factor in their investment strategy, aligning financial returns with environmental sustainability. Second, the development of ‘green’ investment indices and benchmarks could guide investors towards more environmentally friendly enterprises. These indices should be designed to reward companies that demonstrate robust environmental management systems and penalize those that perform poorly in this regard. Inclusion in or exclusion from these indices could be based on verified environmental performance metrics, thus motivating companies to improve their practices to attract conscious investment. Last, to directly counter the negative impacts of investor attention, policymakers should consider implementing incentives for investments in green technologies and sustainable practices. These could take the form of tax breaks, subsidies, or even public recognition programs for investors and companies that prioritize environmental performance.
The empirical findings elucidate the moderating effects of media attention and coverage sentiment on the negative relationship between investor attention and environmental performance. These results underscore the pivotal role of media in shaping corporate environmental strategies and investor perceptions, which policymakers can leverage to enhance the environmental accountability of high-tech enterprises. To effectively transform these insights into action, it is imperative for policymakers to facilitate a media landscape that supports comprehensive reporting on corporate environmental practices. This could involve the introduction of policies that encourage journalistic thoroughness and accuracy in environmental reporting, such as subsidizing professional training for journalists in environmental science and corporate environmental responsibility. Furthermore, regulatory frameworks could be designed to ensure that media coverage highlights environmental shortcomings and celebrates exemplary practices, thereby fostering a balanced perspective that enhances investor understanding and engagement. Simultaneously, the moderate effect of coverage sentiment suggests that enhancing the emotional resonance of media narratives around corporate environmental initiatives could be beneficial. This could be achieved by supporting media formats and platforms that effectively convey the successes and challenges of corporate environmental management, potentially incorporating multimedia and interactive content to engage a broader audience. Additionally, encouraging corporations to openly communicate their environmental strategies and progress in accessible and relatable terms can help align media coverage sentiment with corporate environmental realities.
Businesses, on the other hand, should strategically engage with the media to mold investor perceptions and behaviors positively. This involves proactive public relations campaigns and transparency initiatives that provide deep insights into the firms’ environmental management practices and innovations. By doing so, companies not only capitalize on the modulation provided by media attention but also cultivate a robust corporate image against negative investor reactions based on environmental risks. Moreover, integrating media intelligence into corporate strategy formulation could enable businesses to address areas of potential environmental concern highlighted by media, thereby aligning investor attention with strategic environmental improvements. This strategic alignment helps in not only mitigating risks but also in harnessing investor interest to propel environmental innovations. The relationship between media coverage, investor attention, and corporate environmental performance forms a complex feedback loop that can be optimized through coordinated efforts between the state, media, and the corporate sector. By constructing a regulatory and operational ecosystem that promotes transparency, encourages informed media engagement, and facilitates comprehensive environmental disclosures, policymakers can significantly advance the environmental performance within high-tech companies.
In addition, this study has limitations, notably excluding certain enterprise data. Our findings suggest that future research should identify other factors that mitigate the adverse effects of investor attention on corporate environmental performance. This approach could provide a more comprehensive understanding and uncover new strategies to improve corporate transparency and sustainability practices.
Data availability
The data that support the findings of this study are available from the corresponding author upon reasonable request.
Abedin SH, Subha S, Anwar M, Kabir MN, Tahat YA, Hossain M (2023) Environmental performance and corporate governance: evidence from Japan. Sustainability 15(4):4. https://doi.org/10.3390/su15043273
Article Google Scholar
Aerts W, Cormier D (2009) Media legitimacy and corporate environmental communication. Account Organ Soc 34(1):1–27. https://doi.org/10.1016/j.aos.2008.02.005
Alam MdS, Atif M, Chien-Chi C, Soytaş U (2019) Does corporate R&D investment affect firm environmental performance? Evidence from G-6 countries. Energy Econ 78:401–411. https://doi.org/10.1016/j.eneco.2018.11.031
Al-Najjar B, Salama A (2022) Mind the gap: are female directors and executives more sensitive to the environment in high-tech us firms? Technol Forecast Soc Change 184:122024. https://doi.org/10.1016/j.techfore.2022.122024
Anstead N, O’Loughlin B (2015) Social media analysis and public opinion: the 2010 UK general election. J Comput Mediat Commun 20(2):204–220. https://doi.org/10.1111/jcc4.12102
Bai L, Yan X, Yu G (2019) Impact of CEO media appearance on corporate performance in social media. North Am J Econ Financ 50:100996. https://doi.org/10.1016/j.najef.2019.100996
Baltagi BH (2008) Forecasting with panel data. J Forecast 27(2):153–173. https://doi.org/10.1002/for.1047
Article MathSciNet Google Scholar
Bansal P, Clelland I (2004) Talking trash: legitimacy, impression management, and unsystematic risk in the context of the natural environment. Acad Manag J 47(1):93–103. https://doi.org/10.5465/20159562
Blundell R, Bond S (1998). Initial conditions and moment restrictions in dynamic panel data models. J Econom
Braam GJM, Uit de Weerd L, Hauck M, Huijbregts MAJ (2016) Determinants of corporate environmental reporting: the importance of environmental performance and assurance. J Clean Prod 129:724–734. https://doi.org/10.1016/j.jclepro.2016.03.039
Brambor T, Clark WR, Golder M (2006) Understanding interaction models: improving empirical analyses. Political Anal 14(1):63–82. https://doi.org/10.1093/pan/mpi014
Brammer S, Pavelin S (2006) Voluntary environmental disclosures by large UK companies. J Bus Financ Account 33(7–8):1168–1188. https://doi.org/10.1111/j.1468-5957.2006.00598.x
Budhijana RB (2022) The effect of dividend policy, financial performance and environmental performance on investor reactions. J Soc Sci 3(5):5. https://doi.org/10.46799/jss.v3i5.420
Bushee BJ (1998) The influence of institutional investors on myopic R&D investment behavior. Account Rev 73(3):305–333
Google Scholar
Byun SK, Oh J-M (2018) Local corporate social responsibility, media coverage, and shareholder value. J Bank Financ 87:68–86. https://doi.org/10.1016/j.jbankfin.2017.09.010
Chatterjee S, Kumar Kar A (2020) Why do small and medium enterprises use social media marketing and what is the impact: empirical insights from India. Int J Inf Manag 53:102103. https://doi.org/10.1016/j.ijinfomgt.2020.102103
Chen CX, Lin RF, Zheng X, Li Y (2021) Environmental regulations and corporate green investment: evidence from heavy polluting companies in China. E3S Web Conf 275:02051. https://doi.org/10.1051/e3sconf/202127502051
Chen H, Fang X, Xiang E, Ji X, An M (2023) Do online media and investor attention affect corporate environmental information disclosure? Evidence from Chinese listed companies. Int Rev Econ Financ 86:1022–1040. https://doi.org/10.1016/j.iref.2023.01.022
Chen J, Tang G, Yao J, Zhou G (2022) Investor attention and stock returns. J Financ Quant Anal 57(2):455–484. https://doi.org/10.1017/S0022109021000090
Chen L, Khurram MU, Gao Y, Abedin MZ, Lucey B (2023) ESG disclosure and technological innovation capabilities of the Chinese listed companies. Res Int Bus Financ 65:101974. https://doi.org/10.1016/j.ribaf.2023.101974
Chen L, Li W, Yuan K, Zhang X (2022) Can informal environmental regulation promote industrial structure upgrading? Evidence from China. Appl Econ 54(19):2161–2180. https://doi.org/10.1080/00036846.2021.1985073
Chen Y, Masron TA, Mai W (2024) Role of investor attention and executive green awareness on environmental information disclosure of Chinese high-tech listed companies. J Environ Manag 365:121552. https://doi.org/10.1016/j.jenvman.2024.121552
Chiou T-Y, Chan HK, Lettice F, Chung SH (2011) The influence of greening the suppliers and green innovation on environmental performance and competitive advantage in Taiwan. Transport Res Part E Logist Transport Rev 47(6):822–836. https://doi.org/10.1016/j.tre.2011.05.016
Christensen LJ, Peirce E, Hartman LP, Hoffman WM, Carrier J (2007) Ethics, CSR, and sustainability education in the financial times top 50 global business schools: baseline data and future research directions. J Bus Ethics 73(4):347–368. https://doi.org/10.1007/s10551-006-9211-5
Christmann P, Taylor G (2002) Globalization and the environment: strategies for international voluntary environmental initiatives. Acad Manag Perspect 16(3):121–135. https://doi.org/10.5465/ame.2002.8540373
Chu G, Li X, Zhang Y (2022) Information demand and net selling around earnings announcement. Res Int Bus Financ 59:101522. https://doi.org/10.1016/j.ribaf.2021.101522
Clarke J, Chen H, Du D, Hu YJ (2021) Fake news, investor attention, and market reaction. Inf Syst Res 32(1):35–52. https://doi.org/10.1287/isre.2019.0910
Cole MA, Elliott RJR, Fredriksson PG (2006) Endogenous pollution havens: does FDI influence environmental regulations? Scand J Econ 108(1):157–178. https://doi.org/10.1111/j.1467-9442.2006.00439.x
Dam L, Scholtens B (2012) Does ownership type matter for corporate social responsibility? Corp Gov: Int Rev 20(3):233–252. https://doi.org/10.1111/j.1467-8683.2011.00907.x
Deephouse DL (1996) Does isomorphism legitimate? Acad Manag J 39(4):1024–1039. https://doi.org/10.5465/256722
Deng C, Zhou X, Peng C, Zhu H (2022) Going green: insight from asymmetric risk spillover between investor attention and pro-environmental investment. Financ Res Lett 47:102565. https://doi.org/10.1016/j.frl.2021.102565
Dhaliwal DS, Li OZ, Tsang A, Yang YG (2011) Voluntary nonfinancial disclosure and the cost of equity capital: the initiation of corporate social responsibility reporting. Account Rev 86(1):59–100
Dilla W, Janvrin D, Perkins J, Raschke R (2019) Do environmental responsibility views influence investors’ use of environmental performance and assurance information? Sustainability Account, Manag Policy J 10(3):476–497. https://doi.org/10.1108/SAMPJ-12-2018-0357
Dragomir VD (2018) How do we measure corporate environmental performance? A critical review. J Clean Prod 196:1124–1157. https://doi.org/10.1016/j.jclepro.2018.06.014
El Ouadghiri I, Erragragui E, Jaballah J, Peillex J (2022) Institutional investor attention and stock market volatility and liquidity: international evidence. Appl Econ 54(42):4839–4854. https://doi.org/10.1080/00036846.2022.2036689
Erlich A, Berliner D, Palmer-Rubin B, Bagozzi BE (2021) Media attention and bureaucratic responsiveness. J Public Adm Res Theory 31(4):687–703. https://doi.org/10.1093/jopart/muab001
Faller CM, zu Knyphausen-Aufseß D (2018) Does equity ownership matter for corporate social responsibility? A literature review of theories and recent empirical findings. J Bus Ethics 150(1):15–40. https://doi.org/10.1007/s10551-016-3122-x
Ge C (2024) Negative media coverage and corporate ESG performance: evidence from China. Businesses 4(1):1. https://doi.org/10.3390/businesses4010007
Geng Y, Chen J, Liu T, Tao D (2023) Public environmental attention, media coverage, and corporate green innovation: evidence from heavily polluting industries in China. Environ Sci Pollut Res 30(37):86911–86926. https://doi.org/10.1007/s11356-023-28369-0
Gervais MM, Fessler DMT (2017) On the deep structure of social affect: attitudes, emotions, sentiments, and the case of “contempt. Behav Brain Sci 40:e225. https://doi.org/10.1017/S0140525X16000352
Article PubMed Google Scholar
Gonenc H, Scholtens B (2017) Environmental and financial performance of fossil fuel firms: a closer inspection of their interaction. Ecol Econ 132:307–328. https://doi.org/10.1016/j.ecolecon.2016.10.004
Guo M, Kuai Y, Liu X (2020) Stock market response to environmental policies: evidence from heavily polluting firms in China. Econ Model 86:306–316. https://doi.org/10.1016/j.econmod.2019.09.028
Guo Z, Lu C (2021) Corporate environmental performance in China: the moderating effects of the media versus the approach of local governments. Int J Environ Res Public Health 18(1):1. https://doi.org/10.3390/ijerph18010150
Hansen LP (1982) Large sample properties of generalized method of moments estimators. Econometrica 50(4):1029. https://doi.org/10.2307/1912775
Hao J, Xiong X (2021) Retail investor attention and firms’ idiosyncratic risk: evidence from China. Int Rev Financ Anal 74:101675. https://doi.org/10.1016/j.irfa.2021.101675
Harimauwan J, Lukman H (2023) The effect of environmental performance, corporate social responsibility, earnings per share, and return on assets on stock returns on manufacturing companies. Int J Application Econ Bus 1(1):61–69
He F, Yan Y, Hao J, Wu JG(2022) Retail investor attention and corporate green innovation: evidence from China Energy Econ 115:106308. https://doi.org/10.1016/j.eneco.2022.106308
Holtz-Eakin D, Newey W, Rosen HS (1988) Estimating vector autoregressions with panel data. Econometrica 56(6):1371. https://doi.org/10.2307/1913103
Huang M, Li M, Liao Z (2021) Do politically connected CEOs promote Chinese listed industrial firms’ green innovation? The mediating role of external governance environments. J Clean Prod 278:123634. https://doi.org/10.1016/j.jclepro.2020.123634
Huang Z, Liao G, Li Z (2019) Loaning scale and government subsidy for promoting green innovation. Technol Forecast Soc Change 144:148–156. https://doi.org/10.1016/j.techfore.2019.04.023
Ibrahim H, Varn M (2021) A literature review of the history and evolution of corporate social responsibility. Research 6(1):1. https://doi.org/10.1234/re.v6.i1.1
Jacoby G, Liu M, Wang Y, Wu Z, Zhang Y (2019) Corporate governance, external control, and environmental information transparency: evidence from emerging markets. J Int Financ Mark, Inst Money 58:269–283. https://doi.org/10.1016/j.intfin.2018.11.015
Jiang X, Akbar A, Hysa E, Akbar M (2022) Environmental protection investment and enterprise innovation: evidence from Chinese listed companies. Kybernetes 52(2):708–727. https://doi.org/10.1108/K-12-2021-1292
Jiang Z, Xu Y, Fang M, Tang Z, Tao C (2023) How does the bond market price corporate ESG engagement? Evidence from China. Econ Anal Policy 78:1406–1423. https://doi.org/10.1016/j.eap.2023.05.019
Kapoor KK, Tamilmani K, Rana NP, Patil P, Dwivedi YK, Nerur S (2018) Advances in social media research: past, present and future. Inf Syst Front 20(3):531–558. https://doi.org/10.1007/s10796-017-9810-y
Kissel P, Büttgen M (2015) Using social media to communicate employer brand identity: the impact on corporate image and employer attractiveness. J Brand Manag 22(9):755–777. https://doi.org/10.1057/bm.2015.42
Lee J, Cho M (2020) The effects of consumers’ media exposure, attention, and credibility on pro-environmental behaviors. J Promot Manag 26(3):434–455. https://doi.org/10.1080/10496491.2019.1699629
Lei S, Zhang Y (2020) The role of the media in socially responsible investing. Int J Bank Mark 38(4):823–841. https://doi.org/10.1108/IJBM-09-2019-0332
Li G, Xue Q, Qin J (2022) Environmental information disclosure and green technology innovation: empirical evidence from China. Technol Forecast Soc Change 176:121453. https://doi.org/10.1016/j.techfore.2021.121453
Li H, Liu Y-F, Liang S, Zhou Q (2020) Tourism firm restructuring: does the attention of individual investor matter? Tour Manag 80:104126. https://doi.org/10.1016/j.tourman.2020.104126
Li Y, Dai J, Cui L (2020) The impact of digital technologies on economic and environmental performance in the context of industry 4.0: a moderated mediation model. Int J Prod Econ 229:107777. https://doi.org/10.1016/j.ijpe.2020.107777
Liu B, McConnell JJ (2013) The role of the media in corporate governance: do the media influence managers’ capital allocation decisions? J Financ Econ 110(1):1–17. https://doi.org/10.1016/j.jfineco.2013.06.003
López-Gamero MD, Molina-Azorín JF, Claver-Cortés E (2009) The whole relationship between environmental variables and firm performance: competitive advantage and firm resources as mediator variables. J Environ Manag 90(10):3110–3121. https://doi.org/10.1016/j.jenvman.2009.05.007
Luo W, Guo X, Zhong S, Wang J (2019) Environmental information disclosure quality, media attention and debt financing costs: evidence from Chinese heavy polluting listed companies. J Clean Prod 231:268–277. https://doi.org/10.1016/j.jclepro.2019.05.237
Ming Y, Li Y, Liu N, Li J (2023) Retail investor attention and corporate environmental performance: evidence from China. Financ Res Lett 56:104143. https://doi.org/10.1016/j.frl.2023.104143
Miroshnychenko I, Barontini R, Testa F (2019). Corporate governance and environmental performance: a systematic overview. Corporate social responsibility, ethics and sustainable prosperity. World Sci: 127–150. https://doi.org/10.1142/9789811206887_0005
Murphy J, Link MW, Childs JH, Tesfaye CL, Dean E, Stern M, Pasek J, Cohen J, Callegaro M, Harwood P (2014) Social media in public opinion research: executive summary of the Aapor task force on emerging technologies in public opinion research. Public Opin Q 78(4):788–794. https://doi.org/10.1093/poq/nfu053
Nguyen THH, Elmagrhi MH, Ntim CG, Wu Y (2021) Environmental performance, sustainability, governance and financial performance: evidence from heavily polluting industries in China. Bus Strategy Environ 30(5):2313–2331. https://doi.org/10.1002/bse.2748
Peng B, Chen S, Elahi E, Wan A (2021) Can corporate environmental responsibility improve environmental performance? An inter-temporal analysis of Chinese chemical companies. Environ Sci Pollut Res 28(10):12190–12201. https://doi.org/10.1007/s11356-020-11636-9
Article CAS Google Scholar
Pham L, Luu Duc Huynh T (2020) How does investor attention influence the green bond market? Financ Res Lett 35:101533. https://doi.org/10.1016/j.frl.2020.101533
Qing L, Alnafrah I, Dagestani AA (2023). Does green technology innovation benefit corporate financial performance? Investigating the moderating effect of media coverage. Corp Social Responsib Environ Manag, csr.2659. https://doi.org/10.1002/csr.2659
Qu S, Wang J, Li Y, Wang K (2023) How does risk-taking affect the green technology innovation of high-tech enterprises in China: the moderating role of financial mismatch. Environ Sci Pollut Res 30(9):23747–23763. https://doi.org/10.1007/s11356-022-23820-0
Radu C, Smaili N (2021) Corporate performance patterns of Canadian listed firms: balancing financial and corporate social responsibility outcomes. Bus Strategy Environ 30(7):3344–3359. https://doi.org/10.1002/bse.2806
Reverte C (2009) Determinants of corporate social responsibility disclosure ratings by Spanish listed firms. J Bus Ethics 88(2):351–366. https://doi.org/10.1007/s10551-008-9968-9
Setyowati A, Purnomowati NH, Sari DN, Ramadhan ES (2021) Does corporate environmental responsibility affect investor future goal in the energy sector firms? IOP Conf Ser Earth Environ Sci 905(1):012140. https://doi.org/10.1088/1755-1315/905/1/012140
Shen C (2019) Research on the relationship between institutional investor heterogeneity and corporate environmental responsibility. IOP Conf Ser Mater Sci Eng 688(5):055007. https://doi.org/10.1088/1757-899X/688/5/055007
Suchman MC (1995) Managing legitimacy: strategic and institutional approaches. Acad Manag Rev 20(3):571–610. https://doi.org/10.2307/258788
Sun Y, Liu X, Chen G, Hao Y, Zhang ZJ(2020) How mood affects the stock market: empirical evidence from microblogs Inf Manag 57(5):103181. https://doi.org/10.1016/j.im.2019.103181
Tavakolifar M, Omar A, Lemma TT, Samkin G (2021) Media attention and its impact on corporate commitment to climate change action. J Clean Prod 313:127833. https://doi.org/10.1016/j.jclepro.2021.127833
Vlastakis N, Markellos RN (2012) Information demand and stock market volatility. J Bank Financ 36(6):1808–1821. https://doi.org/10.1016/j.jbankfin.2012.02.007
Wang G, Yu G, Shen X (2021) The effect of online environmental news on green industry stocks: the mediating role of investor sentiment. Phys A Stat Mech Appl 573:125979. https://doi.org/10.1016/j.physa.2021.125979
Wang Q, Liu M, Zhang B (2022) Do state-owned enterprises really have better environmental performance in China? Environmental regulation and corporate environmental strategies. Resour Conserv Recycling 185:106500. https://doi.org/10.1016/j.resconrec.2022.106500
Wang Y, Zhang J (2023) Corporate governance, media coverage, and corporate environmental protection investment: empirical evidence from listed companies in China’s high-pollution industries. Sustainability 15(11):11. https://doi.org/10.3390/su15118643
Wang Z, Zhang J, Ullah H (2023) Exploring the multidimensional perspective of retail investors’ attention: the mediating influence of corporate governance and information disclosure on corporate environmental performance in China. Sustainability 15(15):15. https://doi.org/10.3390/su151511818
Wei P, Mao X, Chen X (2020) Institutional investors’ attention to environmental information, trading strategies, and market impacts: evidence from China. Bus Strategy Environ 29(2):566–591. https://doi.org/10.1002/bse.2387
Yu X, Wang P (2021) Economic effects analysis of environmental regulation policy in the process of industrial structure upgrading: evidence from Chinese provincial panel data. Sci Total Environ 753:142004. https://doi.org/10.1016/j.scitotenv.2020.142004
Article CAS PubMed Google Scholar
Zeng Y, Gulzar MA, Wang Z, Zhao X (2020) The effect of expected financial performance on corporate environmental responsibility disclosure: evidence from China. Environ Sci Pollut Res 27(30):37946–37962. https://doi.org/10.1007/s11356-020-09719-8
Zhang R, Fu W (2023) Multiple large shareholders and corporate environmental performance. Financ Res Lett 51:103487. https://doi.org/10.1016/j.frl.2022.103487
Zhang Y, Zhang R, Zhang C (2022a) Insight into the driving force of environmental performance improvement: environmental regulation or media coverage. J Clean Prod 358:132024. https://doi.org/10.1016/j.jclepro.2022.132024
Zhang Y, Zhang R, Zhang C (2022b) Insight into the driving force of environmental performance improvement: environmental regulation or media coverage. J Clean Prod 358:132024. https://doi.org/10.1016/j.jclepro.2022.132024
Zhou X, Jia M, Wang L, Sharma GD, Zhao X, Ma X (2022) Modelling and simulation of a four-group evolutionary game model for green innovation stakeholders: contextual evidence in lens of sustainable development. Renew Energy 197:500–517. https://doi.org/10.1016/j.renene.2022.07.068
Zhuo C, Chen J (2023) Can digital transformation overcome the enterprise innovation dilemma: effect, mechanism and effective boundary. Technol Forecast Soc Change 190:122378. https://doi.org/10.1016/j.techfore.2023.122378
Download references
Author information
Authors and affiliations.
School of Management, Universiti Sains Malaysia, Minden, Penang, Malaysia
Yanpeng Chen
National Higher Education Research Institute, Universiti Sains Malaysia, Gelugor, Malaysia
You can also search for this author in PubMed Google Scholar
Contributions
Yanpeng Chen, as the first author of this paper, took the lead in the primary research work, including experimental design, data collection, and initial analysis. Yanpeng Chen also took responsibility for drafting and revising the initial manuscript, ensuring the clear communication of scientific information within the text. Wenjun Mai provided valuable professional advice, ensuring the scientific rigor of the methodology and analysis. She also contributed to the data and the logic of the full paper.
Corresponding authors
Correspondence to Yanpeng Chen or Wenjun Mai .
Ethics declarations
Competing interests.
The authors declare no competing interests.
Ethics approval
This article does not contain any studies with human participants performed by any of the authors.
Informed consent
Additional information.
Publisher’s note Springer Nature remains neutral with regard to jurisdictional claims in published maps and institutional affiliations.
Supplementary information
Rights and permissions.
Open Access This article is licensed under a Creative Commons Attribution-NonCommercial-NoDerivatives 4.0 International License, which permits any non-commercial use, sharing, distribution and reproduction in any medium or format, as long as you give appropriate credit to the original author(s) and the source, provide a link to the Creative Commons licence, and indicate if you modified the licensed material. You do not have permission under this licence to share adapted material derived from this article or parts of it. The images or other third party material in this article are included in the article’s Creative Commons licence, unless indicated otherwise in a credit line to the material. If material is not included in the article’s Creative Commons licence and your intended use is not permitted by statutory regulation or exceeds the permitted use, you will need to obtain permission directly from the copyright holder. To view a copy of this licence, visit http://creativecommons.org/licenses/by-nc-nd/4.0/ .
Reprints and permissions
About this article
Cite this article.
Chen, Y., Mai, W. Investor attention and environmental performance of Chinese high-tech companies: the moderating effects of media attention and coverage sentiment. Humanit Soc Sci Commun 11 , 1020 (2024). https://doi.org/10.1057/s41599-024-03489-1
Download citation
Received : 23 December 2023
Accepted : 18 July 2024
Published : 08 August 2024
DOI : https://doi.org/10.1057/s41599-024-03489-1
Share this article
Anyone you share the following link with will be able to read this content:
Sorry, a shareable link is not currently available for this article.
Provided by the Springer Nature SharedIt content-sharing initiative
Quick links
- Explore articles by subject
- Guide to authors
- Editorial policies


IMAGES
COMMENTS
Kaplan and Haenlein (2010) de ne social media as "a group of Internet-based. applications that build on the ideological and technological foundations of Web. 2.0, and that allow the creation and ...
In business world social media became popular after 2012 and academic literature also indicates social media evolved after 2000 ( Boyd & Ellison, 2007 ). Therefore, the document published in 2000 and after had been considered for the review only. Firstly the keyword "social media" was searched in Scopus database.
Keywords: social media, social networks, social media definition, social media applications, literature review. Introduction. The term "social media" (SM) was first used in 1994 on a Tokyo online media environment, called Matisse. 1 It was in these early days of the commercial Internet that the first SM platforms were developed and launched ...
Abstract. This manuscript explores the profound impact of social media on society, with a focus on social behavior, politics, and cultural norms. Employing a systematic literature review ...
Social media has become an integral part of contemporary society, profoundly transforming communication, social behavior, political engagement, and cultural norms. This paper presents a ...
The literature on social media in innovation typically refers to product innovation (Ghezzi et al., 2016), process innovation ... The purpose of this paper is not to review the social media typologies extensively. Nevertheless, the systematic review of the literature identified four main possible types of social media used by organisations for ...
This systematic literature review has revealed that the second-most used theory in social media for knowledge sharing research, is the Technology Acceptance Model (TAM). This model explains the perceptions of using new technologies, with a focus on ease of use and usefulness, in turn effecting the intention of adopting social media as a tool ...
In the past, there have been several insightful attempts to review the social media marketing literature. For example, Lamberton and Stephen reviewed and synthesized 160 articles on digital, social media, and mobile marketing published during the period from 2000 to 2015, while ...
A matrix summary of 20 social media literature reviews published from 2011 through early 2014 is provided in Table 1. ... (2013) reported continents of origin for corresponding authors when discussing the results of a literature review regarding social media use by health-care professionals and trainees. Although limited by the fact that the ...
Systematic Literature Review Social media provides a space to disclose minority experiences and share ways to cope and get support; constant surveillance of one's social media profile can become a stressor, potentially leading to depression. O'Reilly et al. Potential of Social Media in Promoting Mental Health in Adolescents
The role of social media in the global society and economy has increased substantially over the years across different populations, age groups, and contexts. ... (CSML) through a systematic literature review to determine whether and how its components—users' goals, use context, inquiry, reflection, and action—have been addressed in the ...
In this article, we review existing research on the complementarity of social media data and survey data for the study of public opinion. We start by situating our review in the extensive literature (N = 187) about the uses, challenges, and frameworks related to the use of social media for studying public opinion. Based on 187 relevant articles (141 empirical and 46 theoretical) - we identify ...
Alalwan AA, Rana NP, Dwivedi Yet al. Social media in marketing: a review and analysis of the existing literature. Telem Inform 2017; 34: 1177-1190. Crossref. Google Scholar. 20. ... Alves H, Fernandes C, Raposo M. Social media marketing: A literature review and implications. Psychology and Marketing 2016; 33: 1029-1038. Crossref. Google ...
In the age of widespread connectivity, social media has emerged as a dynamic platform shaping public opinion and activism globally. This chapter aims to systematically review literature spanning 2013-2022, aiming to uncover prevalent social media issues influencing public opinion and activism.
Results of the literature review on the uses of social media as a complement to surveys. Overall, the collection protocol left us with 187 papers - 141 of an empirical and 46 of a theoretical nature (these papers can be found in the Appendix). Most of these papers stem from political communication and computational social sciences journals.
The relevant literature on social media is reviewed to yield a better understanding of how it has transformed the way people communicate, acquire and use information. The development and expansion of social media have rapidly changed the interaction and communication of people, thereby attract- ing attention in an unprecedented scale. This paper reviews the relevant literature on social media ...
Literature review. Social media research includes the analysis of citizens' voices on a wide range of topics [].According to quantitative data from April 2017 published by Statista [], Twitter and Facebook are included in the top ten leading social networks worldwide, as ranked by the number of active users.Facebook is at the top of the list, with 1,968 million active users, and Twitter ...
In this article, the authors present the results from a structured review of the literature, identifying and analyzing the most quoted and dominant definitions of social media (SM) and alternative terms that were used between 1994 and 2019 to identify their major applications. Similarities and differences in the definitions are highlighted to provide guidelines for researchers and managers who ...
This section discusses the findings of this literature research. The findings are organized based on the key perspectives of the study. 3.1 Student Perspectives. Majority of the studies reviewed are focused on students' perspectives of the social media use for instructional purpose, using various social media tools, such as Facebook, Blog, Wiki, and in-house social network tools, etc ...
This manuscript explores the profound impact of social media on society, with a focus on social behavior, politics, and cultural norms. Employing a systematic literature review, including diverse sources, case studies, and real-world examples, the study provides a nuanced and comprehensive analysis. The brevity enforced by character limits on ...
In their literature review of 43 articles examining literacy practices and social media, Stornaiuolo et al. (2013) found that many scholars studying social media in schools looked at identity development and expression, security issues, relationships, and friending behaviors.
Gender-based violence against women on social media networks is a significant problem due to its pervasive and harmful impact on individuals and society as a whole. This issue manifests in various forms, including harassment, threats, and abuse, which can have severe psychological, emotional, and physical consequences for the victims. This systematic review identifies and analyzes the dynamics ...
Literature reviews on how social media use affects adolescent mental health have accumulated at an unprecedented rate of late. Yet, a higher-level integration of the evidence is still lacking. We fill this gap with an up-to-date umbrella review, a review of reviews published between 2019 and mid-2021. Our search yielded 25 reviews: seven meta ...
In study 2 the purpose was to identify the instructional strategies and technology affordances used while integrating technology that facilitated the development of student creativity by completing a systematic literature review about how technology (i.e., social media) is being used by educators to foster creativity.
This paper reviews the relevant literature on social media to yield a better understanding of how it has transformed the way people communicate, acquire and use information. To elucidate on the ...
1 School of Culture and Media, Shanxi College of Applied Science and Technology, Taiyuan, China; 2 School of Humanities and Social Sciences, City University of Macau, Macao, China; 3 School of Broadcast Announcing Arts, Communication University of Zhejiang, Hangzhou, China; Introduction: With the widespread use of social media, the behavior and mindset of users have been transformed, leading ...
The amount of grey literature and 'softer' intelligence from social media or websites is vast. Given the long lead-times of producing high-quality peer-reviewed health information, this is causing a demand for new ways to provide prompt input for secondary research. To our knowledge, this is the fir …
Through a systematic literature review, the characteristics of affordances research in social media are uncovered: the most prominent areas of application, research approaches, and dominant typologies and conceptualisations. Significant blurriness of the term 'affordance' is identified as well as an inconsistent use in research.
In the age of widespread connectivity, social media has emerged as a dynamic platform shaping public opinion and activism globally. This chapter aims to systematically review literature spanning ...
Social media comprises various user-driven platforms that facilitate the ... Ibrahim H, Varn M (2021) A literature review of the history and evolution of corporate social responsibility. ...