Have a language expert improve your writing
Run a free plagiarism check in 10 minutes, generate accurate citations for free.
- Knowledge Base
Methodology
- How to Write a Strong Hypothesis | Steps & Examples

How to Write a Strong Hypothesis | Steps & Examples
Published on May 6, 2022 by Shona McCombes . Revised on November 20, 2023.
A hypothesis is a statement that can be tested by scientific research. If you want to test a relationship between two or more variables, you need to write hypotheses before you start your experiment or data collection .
Example: Hypothesis
Daily apple consumption leads to fewer doctor’s visits.
Table of contents
What is a hypothesis, developing a hypothesis (with example), hypothesis examples, other interesting articles, frequently asked questions about writing hypotheses.
A hypothesis states your predictions about what your research will find. It is a tentative answer to your research question that has not yet been tested. For some research projects, you might have to write several hypotheses that address different aspects of your research question.
A hypothesis is not just a guess – it should be based on existing theories and knowledge. It also has to be testable, which means you can support or refute it through scientific research methods (such as experiments, observations and statistical analysis of data).
Variables in hypotheses
Hypotheses propose a relationship between two or more types of variables .
- An independent variable is something the researcher changes or controls.
- A dependent variable is something the researcher observes and measures.
If there are any control variables , extraneous variables , or confounding variables , be sure to jot those down as you go to minimize the chances that research bias will affect your results.
In this example, the independent variable is exposure to the sun – the assumed cause . The dependent variable is the level of happiness – the assumed effect .
Receive feedback on language, structure, and formatting
Professional editors proofread and edit your paper by focusing on:
- Academic style
- Vague sentences
- Style consistency
See an example
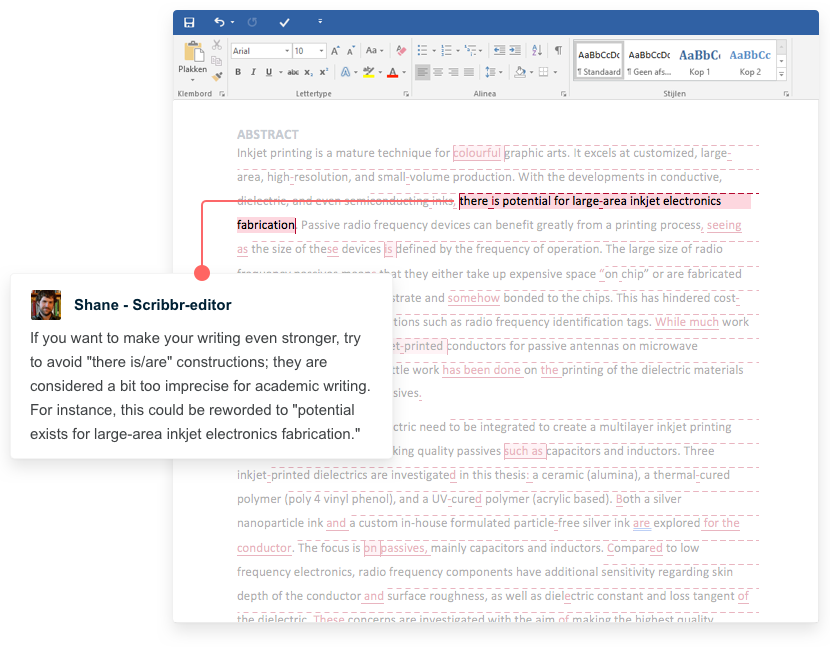
Step 1. Ask a question
Writing a hypothesis begins with a research question that you want to answer. The question should be focused, specific, and researchable within the constraints of your project.
Step 2. Do some preliminary research
Your initial answer to the question should be based on what is already known about the topic. Look for theories and previous studies to help you form educated assumptions about what your research will find.
At this stage, you might construct a conceptual framework to ensure that you’re embarking on a relevant topic . This can also help you identify which variables you will study and what you think the relationships are between them. Sometimes, you’ll have to operationalize more complex constructs.
Step 3. Formulate your hypothesis
Now you should have some idea of what you expect to find. Write your initial answer to the question in a clear, concise sentence.
4. Refine your hypothesis
You need to make sure your hypothesis is specific and testable. There are various ways of phrasing a hypothesis, but all the terms you use should have clear definitions, and the hypothesis should contain:
- The relevant variables
- The specific group being studied
- The predicted outcome of the experiment or analysis
5. Phrase your hypothesis in three ways
To identify the variables, you can write a simple prediction in if…then form. The first part of the sentence states the independent variable and the second part states the dependent variable.
In academic research, hypotheses are more commonly phrased in terms of correlations or effects, where you directly state the predicted relationship between variables.
If you are comparing two groups, the hypothesis can state what difference you expect to find between them.
6. Write a null hypothesis
If your research involves statistical hypothesis testing , you will also have to write a null hypothesis . The null hypothesis is the default position that there is no association between the variables. The null hypothesis is written as H 0 , while the alternative hypothesis is H 1 or H a .
- H 0 : The number of lectures attended by first-year students has no effect on their final exam scores.
- H 1 : The number of lectures attended by first-year students has a positive effect on their final exam scores.
If you want to know more about the research process , methodology , research bias , or statistics , make sure to check out some of our other articles with explanations and examples.
- Sampling methods
- Simple random sampling
- Stratified sampling
- Cluster sampling
- Likert scales
- Reproducibility
Statistics
- Null hypothesis
- Statistical power
- Probability distribution
- Effect size
- Poisson distribution
Research bias
- Optimism bias
- Cognitive bias
- Implicit bias
- Hawthorne effect
- Anchoring bias
- Explicit bias
Here's why students love Scribbr's proofreading services
Discover proofreading & editing
A hypothesis is not just a guess — it should be based on existing theories and knowledge. It also has to be testable, which means you can support or refute it through scientific research methods (such as experiments, observations and statistical analysis of data).
Null and alternative hypotheses are used in statistical hypothesis testing . The null hypothesis of a test always predicts no effect or no relationship between variables, while the alternative hypothesis states your research prediction of an effect or relationship.
Hypothesis testing is a formal procedure for investigating our ideas about the world using statistics. It is used by scientists to test specific predictions, called hypotheses , by calculating how likely it is that a pattern or relationship between variables could have arisen by chance.
Cite this Scribbr article
If you want to cite this source, you can copy and paste the citation or click the “Cite this Scribbr article” button to automatically add the citation to our free Citation Generator.
McCombes, S. (2023, November 20). How to Write a Strong Hypothesis | Steps & Examples. Scribbr. Retrieved April 17, 2024, from https://www.scribbr.com/methodology/hypothesis/
Is this article helpful?
Shona McCombes
Other students also liked, construct validity | definition, types, & examples, what is a conceptual framework | tips & examples, operationalization | a guide with examples, pros & cons, unlimited academic ai-proofreading.
✔ Document error-free in 5minutes ✔ Unlimited document corrections ✔ Specialized in correcting academic texts
Have a language expert improve your writing
Run a free plagiarism check in 10 minutes, automatically generate references for free.
- Knowledge Base
- Methodology
- How to Write a Strong Hypothesis | Guide & Examples
How to Write a Strong Hypothesis | Guide & Examples
Published on 6 May 2022 by Shona McCombes .
A hypothesis is a statement that can be tested by scientific research. If you want to test a relationship between two or more variables, you need to write hypotheses before you start your experiment or data collection.
Table of contents
What is a hypothesis, developing a hypothesis (with example), hypothesis examples, frequently asked questions about writing hypotheses.
A hypothesis states your predictions about what your research will find. It is a tentative answer to your research question that has not yet been tested. For some research projects, you might have to write several hypotheses that address different aspects of your research question.
A hypothesis is not just a guess – it should be based on existing theories and knowledge. It also has to be testable, which means you can support or refute it through scientific research methods (such as experiments, observations, and statistical analysis of data).
Variables in hypotheses
Hypotheses propose a relationship between two or more variables . An independent variable is something the researcher changes or controls. A dependent variable is something the researcher observes and measures.
In this example, the independent variable is exposure to the sun – the assumed cause . The dependent variable is the level of happiness – the assumed effect .
Prevent plagiarism, run a free check.
Step 1: ask a question.
Writing a hypothesis begins with a research question that you want to answer. The question should be focused, specific, and researchable within the constraints of your project.
Step 2: Do some preliminary research
Your initial answer to the question should be based on what is already known about the topic. Look for theories and previous studies to help you form educated assumptions about what your research will find.
At this stage, you might construct a conceptual framework to identify which variables you will study and what you think the relationships are between them. Sometimes, you’ll have to operationalise more complex constructs.
Step 3: Formulate your hypothesis
Now you should have some idea of what you expect to find. Write your initial answer to the question in a clear, concise sentence.
Step 4: Refine your hypothesis
You need to make sure your hypothesis is specific and testable. There are various ways of phrasing a hypothesis, but all the terms you use should have clear definitions, and the hypothesis should contain:
- The relevant variables
- The specific group being studied
- The predicted outcome of the experiment or analysis
Step 5: Phrase your hypothesis in three ways
To identify the variables, you can write a simple prediction in if … then form. The first part of the sentence states the independent variable and the second part states the dependent variable.
In academic research, hypotheses are more commonly phrased in terms of correlations or effects, where you directly state the predicted relationship between variables.
If you are comparing two groups, the hypothesis can state what difference you expect to find between them.
Step 6. Write a null hypothesis
If your research involves statistical hypothesis testing , you will also have to write a null hypothesis. The null hypothesis is the default position that there is no association between the variables. The null hypothesis is written as H 0 , while the alternative hypothesis is H 1 or H a .
Hypothesis testing is a formal procedure for investigating our ideas about the world using statistics. It is used by scientists to test specific predictions, called hypotheses , by calculating how likely it is that a pattern or relationship between variables could have arisen by chance.
A hypothesis is not just a guess. It should be based on existing theories and knowledge. It also has to be testable, which means you can support or refute it through scientific research methods (such as experiments, observations, and statistical analysis of data).
A research hypothesis is your proposed answer to your research question. The research hypothesis usually includes an explanation (‘ x affects y because …’).
A statistical hypothesis, on the other hand, is a mathematical statement about a population parameter. Statistical hypotheses always come in pairs: the null and alternative hypotheses. In a well-designed study , the statistical hypotheses correspond logically to the research hypothesis.
Cite this Scribbr article
If you want to cite this source, you can copy and paste the citation or click the ‘Cite this Scribbr article’ button to automatically add the citation to our free Reference Generator.
McCombes, S. (2022, May 06). How to Write a Strong Hypothesis | Guide & Examples. Scribbr. Retrieved 15 April 2024, from https://www.scribbr.co.uk/research-methods/hypothesis-writing/
Is this article helpful?
Shona McCombes
Other students also liked, operationalisation | a guide with examples, pros & cons, what is a conceptual framework | tips & examples, a quick guide to experimental design | 5 steps & examples.

What Is A Research (Scientific) Hypothesis? A plain-language explainer + examples
By: Derek Jansen (MBA) | Reviewed By: Dr Eunice Rautenbach | June 2020
If you’re new to the world of research, or it’s your first time writing a dissertation or thesis, you’re probably noticing that the words “research hypothesis” and “scientific hypothesis” are used quite a bit, and you’re wondering what they mean in a research context .
“Hypothesis” is one of those words that people use loosely, thinking they understand what it means. However, it has a very specific meaning within academic research. So, it’s important to understand the exact meaning before you start hypothesizing.
Research Hypothesis 101
- What is a hypothesis ?
- What is a research hypothesis (scientific hypothesis)?
- Requirements for a research hypothesis
- Definition of a research hypothesis
- The null hypothesis
What is a hypothesis?
Let’s start with the general definition of a hypothesis (not a research hypothesis or scientific hypothesis), according to the Cambridge Dictionary:
Hypothesis: an idea or explanation for something that is based on known facts but has not yet been proved.
In other words, it’s a statement that provides an explanation for why or how something works, based on facts (or some reasonable assumptions), but that has not yet been specifically tested . For example, a hypothesis might look something like this:
Hypothesis: sleep impacts academic performance.
This statement predicts that academic performance will be influenced by the amount and/or quality of sleep a student engages in – sounds reasonable, right? It’s based on reasonable assumptions , underpinned by what we currently know about sleep and health (from the existing literature). So, loosely speaking, we could call it a hypothesis, at least by the dictionary definition.
But that’s not good enough…
Unfortunately, that’s not quite sophisticated enough to describe a research hypothesis (also sometimes called a scientific hypothesis), and it wouldn’t be acceptable in a dissertation, thesis or research paper . In the world of academic research, a statement needs a few more criteria to constitute a true research hypothesis .
What is a research hypothesis?
A research hypothesis (also called a scientific hypothesis) is a statement about the expected outcome of a study (for example, a dissertation or thesis). To constitute a quality hypothesis, the statement needs to have three attributes – specificity , clarity and testability .
Let’s take a look at these more closely.
Need a helping hand?
Hypothesis Essential #1: Specificity & Clarity
A good research hypothesis needs to be extremely clear and articulate about both what’ s being assessed (who or what variables are involved ) and the expected outcome (for example, a difference between groups, a relationship between variables, etc.).
Let’s stick with our sleepy students example and look at how this statement could be more specific and clear.
Hypothesis: Students who sleep at least 8 hours per night will, on average, achieve higher grades in standardised tests than students who sleep less than 8 hours a night.
As you can see, the statement is very specific as it identifies the variables involved (sleep hours and test grades), the parties involved (two groups of students), as well as the predicted relationship type (a positive relationship). There’s no ambiguity or uncertainty about who or what is involved in the statement, and the expected outcome is clear.
Contrast that to the original hypothesis we looked at – “Sleep impacts academic performance” – and you can see the difference. “Sleep” and “academic performance” are both comparatively vague , and there’s no indication of what the expected relationship direction is (more sleep or less sleep). As you can see, specificity and clarity are key.
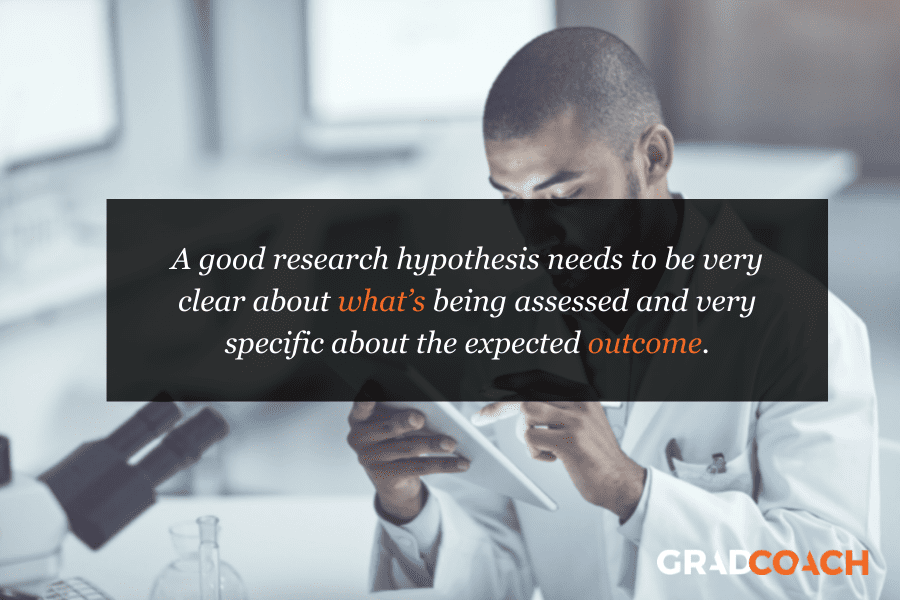
Hypothesis Essential #2: Testability (Provability)
A statement must be testable to qualify as a research hypothesis. In other words, there needs to be a way to prove (or disprove) the statement. If it’s not testable, it’s not a hypothesis – simple as that.
For example, consider the hypothesis we mentioned earlier:
Hypothesis: Students who sleep at least 8 hours per night will, on average, achieve higher grades in standardised tests than students who sleep less than 8 hours a night.
We could test this statement by undertaking a quantitative study involving two groups of students, one that gets 8 or more hours of sleep per night for a fixed period, and one that gets less. We could then compare the standardised test results for both groups to see if there’s a statistically significant difference.
Again, if you compare this to the original hypothesis we looked at – “Sleep impacts academic performance” – you can see that it would be quite difficult to test that statement, primarily because it isn’t specific enough. How much sleep? By who? What type of academic performance?
So, remember the mantra – if you can’t test it, it’s not a hypothesis 🙂
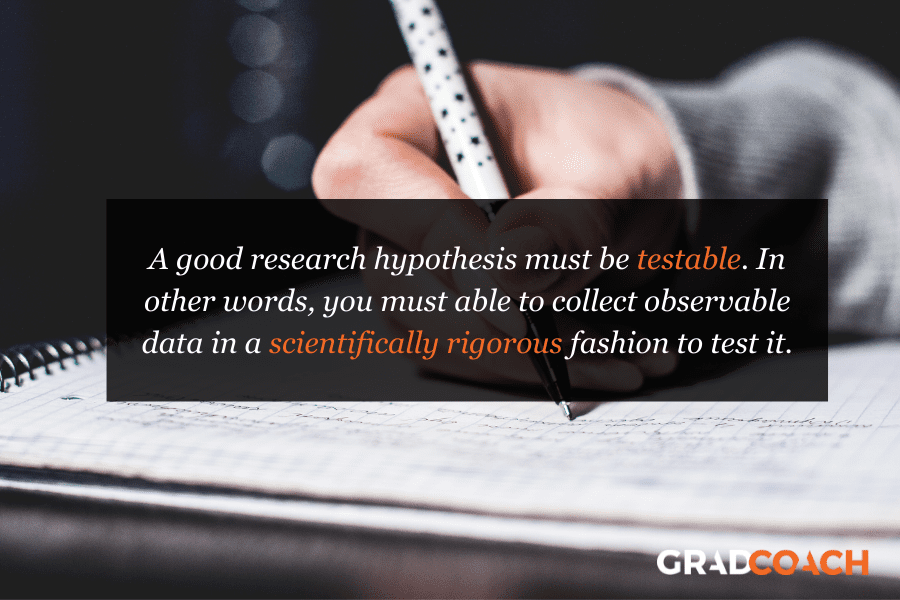
Defining A Research Hypothesis
You’re still with us? Great! Let’s recap and pin down a clear definition of a hypothesis.
A research hypothesis (or scientific hypothesis) is a statement about an expected relationship between variables, or explanation of an occurrence, that is clear, specific and testable.
So, when you write up hypotheses for your dissertation or thesis, make sure that they meet all these criteria. If you do, you’ll not only have rock-solid hypotheses but you’ll also ensure a clear focus for your entire research project.
What about the null hypothesis?
You may have also heard the terms null hypothesis , alternative hypothesis, or H-zero thrown around. At a simple level, the null hypothesis is the counter-proposal to the original hypothesis.
For example, if the hypothesis predicts that there is a relationship between two variables (for example, sleep and academic performance), the null hypothesis would predict that there is no relationship between those variables.
At a more technical level, the null hypothesis proposes that no statistical significance exists in a set of given observations and that any differences are due to chance alone.
And there you have it – hypotheses in a nutshell.
If you have any questions, be sure to leave a comment below and we’ll do our best to help you. If you need hands-on help developing and testing your hypotheses, consider our private coaching service , where we hold your hand through the research journey.
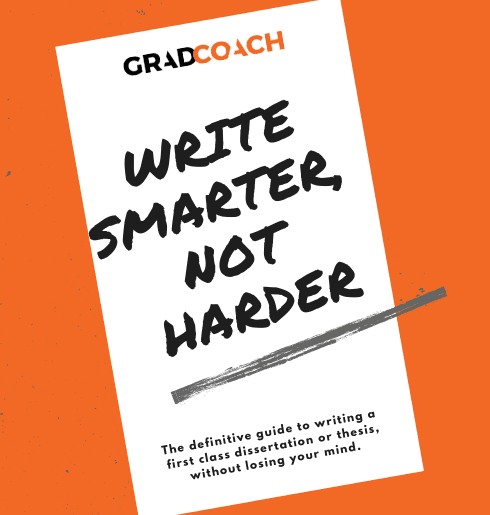
Psst… there’s more (for free)
This post is part of our dissertation mini-course, which covers everything you need to get started with your dissertation, thesis or research project.
You Might Also Like:
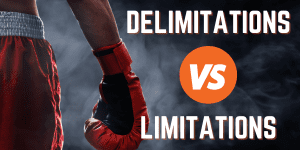
16 Comments
Very useful information. I benefit more from getting more information in this regard.
Very great insight,educative and informative. Please give meet deep critics on many research data of public international Law like human rights, environment, natural resources, law of the sea etc
In a book I read a distinction is made between null, research, and alternative hypothesis. As far as I understand, alternative and research hypotheses are the same. Can you please elaborate? Best Afshin
This is a self explanatory, easy going site. I will recommend this to my friends and colleagues.
Very good definition. How can I cite your definition in my thesis? Thank you. Is nul hypothesis compulsory in a research?
It’s a counter-proposal to be proven as a rejection
Please what is the difference between alternate hypothesis and research hypothesis?
It is a very good explanation. However, it limits hypotheses to statistically tasteable ideas. What about for qualitative researches or other researches that involve quantitative data that don’t need statistical tests?
In qualitative research, one typically uses propositions, not hypotheses.
could you please elaborate it more
I’ve benefited greatly from these notes, thank you.
This is very helpful
well articulated ideas are presented here, thank you for being reliable sources of information
Excellent. Thanks for being clear and sound about the research methodology and hypothesis (quantitative research)
I have only a simple question regarding the null hypothesis. – Is the null hypothesis (Ho) known as the reversible hypothesis of the alternative hypothesis (H1? – How to test it in academic research?
this is very important note help me much more
Trackbacks/Pingbacks
- What Is Research Methodology? Simple Definition (With Examples) - Grad Coach - […] Contrasted to this, a quantitative methodology is typically used when the research aims and objectives are confirmatory in nature. For example,…
Submit a Comment Cancel reply
Your email address will not be published. Required fields are marked *
Save my name, email, and website in this browser for the next time I comment.
- Print Friendly
- Bipolar Disorder
- Therapy Center
- When To See a Therapist
- Types of Therapy
- Best Online Therapy
- Best Couples Therapy
- Best Family Therapy
- Managing Stress
- Sleep and Dreaming
- Understanding Emotions
- Self-Improvement
- Healthy Relationships
- Student Resources
- Personality Types
- Guided Meditations
- Verywell Mind Insights
- 2023 Verywell Mind 25
- Mental Health in the Classroom
- Editorial Process
- Meet Our Review Board
- Crisis Support
How to Write a Great Hypothesis
Hypothesis Definition, Format, Examples, and Tips
Kendra Cherry, MS, is a psychosocial rehabilitation specialist, psychology educator, and author of the "Everything Psychology Book."
:max_bytes(150000):strip_icc():format(webp)/IMG_9791-89504ab694d54b66bbd72cb84ffb860e.jpg)
Amy Morin, LCSW, is a psychotherapist and international bestselling author. Her books, including "13 Things Mentally Strong People Don't Do," have been translated into more than 40 languages. Her TEDx talk, "The Secret of Becoming Mentally Strong," is one of the most viewed talks of all time.
:max_bytes(150000):strip_icc():format(webp)/VW-MIND-Amy-2b338105f1ee493f94d7e333e410fa76.jpg)
Verywell / Alex Dos Diaz
- The Scientific Method
Hypothesis Format
Falsifiability of a hypothesis.
- Operationalization
Hypothesis Types
Hypotheses examples.
- Collecting Data
Frequently Asked Questions
A hypothesis is a tentative statement about the relationship between two or more variables. It is a specific, testable prediction about what you expect to happen in a study. It is a preliminary answer to your question that helps guide the research process.
Consider a study designed to examine the relationship between sleep deprivation and test performance. The hypothesis might be: "This study is designed to assess the hypothesis that sleep-deprived people will perform worse on a test than individuals who are not sleep-deprived."
At a Glance
A hypothesis is crucial to scientific research because it offers a clear direction for what the researchers are looking to find. This allows them to design experiments to test their predictions and add to our scientific knowledge about the world. This article explores how a hypothesis is used in psychology research, how to write a good hypothesis, and the different types of hypotheses you might use.
The Hypothesis in the Scientific Method
In the scientific method , whether it involves research in psychology, biology, or some other area, a hypothesis represents what the researchers think will happen in an experiment. The scientific method involves the following steps:
- Forming a question
- Performing background research
- Creating a hypothesis
- Designing an experiment
- Collecting data
- Analyzing the results
- Drawing conclusions
- Communicating the results
The hypothesis is a prediction, but it involves more than a guess. Most of the time, the hypothesis begins with a question which is then explored through background research. At this point, researchers then begin to develop a testable hypothesis.
Unless you are creating an exploratory study, your hypothesis should always explain what you expect to happen.
In a study exploring the effects of a particular drug, the hypothesis might be that researchers expect the drug to have some type of effect on the symptoms of a specific illness. In psychology, the hypothesis might focus on how a certain aspect of the environment might influence a particular behavior.
Remember, a hypothesis does not have to be correct. While the hypothesis predicts what the researchers expect to see, the goal of the research is to determine whether this guess is right or wrong. When conducting an experiment, researchers might explore numerous factors to determine which ones might contribute to the ultimate outcome.
In many cases, researchers may find that the results of an experiment do not support the original hypothesis. When writing up these results, the researchers might suggest other options that should be explored in future studies.
In many cases, researchers might draw a hypothesis from a specific theory or build on previous research. For example, prior research has shown that stress can impact the immune system. So a researcher might hypothesize: "People with high-stress levels will be more likely to contract a common cold after being exposed to the virus than people who have low-stress levels."
In other instances, researchers might look at commonly held beliefs or folk wisdom. "Birds of a feather flock together" is one example of folk adage that a psychologist might try to investigate. The researcher might pose a specific hypothesis that "People tend to select romantic partners who are similar to them in interests and educational level."
Elements of a Good Hypothesis
So how do you write a good hypothesis? When trying to come up with a hypothesis for your research or experiments, ask yourself the following questions:
- Is your hypothesis based on your research on a topic?
- Can your hypothesis be tested?
- Does your hypothesis include independent and dependent variables?
Before you come up with a specific hypothesis, spend some time doing background research. Once you have completed a literature review, start thinking about potential questions you still have. Pay attention to the discussion section in the journal articles you read . Many authors will suggest questions that still need to be explored.
How to Formulate a Good Hypothesis
To form a hypothesis, you should take these steps:
- Collect as many observations about a topic or problem as you can.
- Evaluate these observations and look for possible causes of the problem.
- Create a list of possible explanations that you might want to explore.
- After you have developed some possible hypotheses, think of ways that you could confirm or disprove each hypothesis through experimentation. This is known as falsifiability.
In the scientific method , falsifiability is an important part of any valid hypothesis. In order to test a claim scientifically, it must be possible that the claim could be proven false.
Students sometimes confuse the idea of falsifiability with the idea that it means that something is false, which is not the case. What falsifiability means is that if something was false, then it is possible to demonstrate that it is false.
One of the hallmarks of pseudoscience is that it makes claims that cannot be refuted or proven false.
The Importance of Operational Definitions
A variable is a factor or element that can be changed and manipulated in ways that are observable and measurable. However, the researcher must also define how the variable will be manipulated and measured in the study.
Operational definitions are specific definitions for all relevant factors in a study. This process helps make vague or ambiguous concepts detailed and measurable.
For example, a researcher might operationally define the variable " test anxiety " as the results of a self-report measure of anxiety experienced during an exam. A "study habits" variable might be defined by the amount of studying that actually occurs as measured by time.
These precise descriptions are important because many things can be measured in various ways. Clearly defining these variables and how they are measured helps ensure that other researchers can replicate your results.
Replicability
One of the basic principles of any type of scientific research is that the results must be replicable.
Replication means repeating an experiment in the same way to produce the same results. By clearly detailing the specifics of how the variables were measured and manipulated, other researchers can better understand the results and repeat the study if needed.
Some variables are more difficult than others to define. For example, how would you operationally define a variable such as aggression ? For obvious ethical reasons, researchers cannot create a situation in which a person behaves aggressively toward others.
To measure this variable, the researcher must devise a measurement that assesses aggressive behavior without harming others. The researcher might utilize a simulated task to measure aggressiveness in this situation.
Hypothesis Checklist
- Does your hypothesis focus on something that you can actually test?
- Does your hypothesis include both an independent and dependent variable?
- Can you manipulate the variables?
- Can your hypothesis be tested without violating ethical standards?
The hypothesis you use will depend on what you are investigating and hoping to find. Some of the main types of hypotheses that you might use include:
- Simple hypothesis : This type of hypothesis suggests there is a relationship between one independent variable and one dependent variable.
- Complex hypothesis : This type suggests a relationship between three or more variables, such as two independent and dependent variables.
- Null hypothesis : This hypothesis suggests no relationship exists between two or more variables.
- Alternative hypothesis : This hypothesis states the opposite of the null hypothesis.
- Statistical hypothesis : This hypothesis uses statistical analysis to evaluate a representative population sample and then generalizes the findings to the larger group.
- Logical hypothesis : This hypothesis assumes a relationship between variables without collecting data or evidence.
A hypothesis often follows a basic format of "If {this happens} then {this will happen}." One way to structure your hypothesis is to describe what will happen to the dependent variable if you change the independent variable .
The basic format might be: "If {these changes are made to a certain independent variable}, then we will observe {a change in a specific dependent variable}."
A few examples of simple hypotheses:
- "Students who eat breakfast will perform better on a math exam than students who do not eat breakfast."
- "Students who experience test anxiety before an English exam will get lower scores than students who do not experience test anxiety."
- "Motorists who talk on the phone while driving will be more likely to make errors on a driving course than those who do not talk on the phone."
- "Children who receive a new reading intervention will have higher reading scores than students who do not receive the intervention."
Examples of a complex hypothesis include:
- "People with high-sugar diets and sedentary activity levels are more likely to develop depression."
- "Younger people who are regularly exposed to green, outdoor areas have better subjective well-being than older adults who have limited exposure to green spaces."
Examples of a null hypothesis include:
- "There is no difference in anxiety levels between people who take St. John's wort supplements and those who do not."
- "There is no difference in scores on a memory recall task between children and adults."
- "There is no difference in aggression levels between children who play first-person shooter games and those who do not."
Examples of an alternative hypothesis:
- "People who take St. John's wort supplements will have less anxiety than those who do not."
- "Adults will perform better on a memory task than children."
- "Children who play first-person shooter games will show higher levels of aggression than children who do not."
Collecting Data on Your Hypothesis
Once a researcher has formed a testable hypothesis, the next step is to select a research design and start collecting data. The research method depends largely on exactly what they are studying. There are two basic types of research methods: descriptive research and experimental research.
Descriptive Research Methods
Descriptive research such as case studies , naturalistic observations , and surveys are often used when conducting an experiment is difficult or impossible. These methods are best used to describe different aspects of a behavior or psychological phenomenon.
Once a researcher has collected data using descriptive methods, a correlational study can examine how the variables are related. This research method might be used to investigate a hypothesis that is difficult to test experimentally.
Experimental Research Methods
Experimental methods are used to demonstrate causal relationships between variables. In an experiment, the researcher systematically manipulates a variable of interest (known as the independent variable) and measures the effect on another variable (known as the dependent variable).
Unlike correlational studies, which can only be used to determine if there is a relationship between two variables, experimental methods can be used to determine the actual nature of the relationship—whether changes in one variable actually cause another to change.
The hypothesis is a critical part of any scientific exploration. It represents what researchers expect to find in a study or experiment. In situations where the hypothesis is unsupported by the research, the research still has value. Such research helps us better understand how different aspects of the natural world relate to one another. It also helps us develop new hypotheses that can then be tested in the future.
Some examples of how to write a hypothesis include:
- "Staying up late will lead to worse test performance the next day."
- "People who consume one apple each day will visit the doctor fewer times each year."
- "Breaking study sessions up into three 20-minute sessions will lead to better test results than a single 60-minute study session."
The four parts of a hypothesis are:
(1) The research question
(2) The independent variable (IV)
(3) The dependent variable (DV)
(4) The proposed relationship between the IV and DV
No, a hypothesis and a theory are not the same thing. A hypothesis is a testable prediction about a specific research question. A theory, on the other hand, is an explanation supported by an existing body of scientific research.
Thompson WH, Skau S. On the scope of scientific hypotheses . R Soc Open Sci . 2023;10(8):230607. doi:10.1098/rsos.230607
Taran S, Adhikari NKJ, Fan E. Falsifiability in medicine: what clinicians can learn from Karl Popper [published correction appears in Intensive Care Med. 2021 Jun 17;:]. Intensive Care Med . 2021;47(9):1054-1056. doi:10.1007/s00134-021-06432-z
Eyler AA. Research Methods for Public Health . 1st ed. Springer Publishing Company; 2020. doi:10.1891/9780826182067.0004
Nosek BA, Errington TM. What is replication ? PLoS Biol . 2020;18(3):e3000691. doi:10.1371/journal.pbio.3000691
Aggarwal R, Ranganathan P. Study designs: Part 2 - Descriptive studies . Perspect Clin Res . 2019;10(1):34-36. doi:10.4103/picr.PICR_154_18
Nevid J. Psychology: Concepts and Applications. Wadworth, 2013.
By Kendra Cherry, MSEd Kendra Cherry, MS, is a psychosocial rehabilitation specialist, psychology educator, and author of the "Everything Psychology Book."
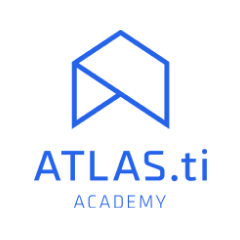
How to Write a Hypothesis: A Step-by-Step Guide
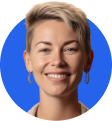
Introduction
An overview of the research hypothesis, different types of hypotheses, variables in a hypothesis, how to formulate an effective research hypothesis, designing a study around your hypothesis.
The scientific method can derive and test predictions as hypotheses. Empirical research can then provide support (or lack thereof) for the hypotheses. Even failure to find support for a hypothesis still represents a valuable contribution to scientific knowledge. Let's look more closely at the idea of the hypothesis and the role it plays in research.
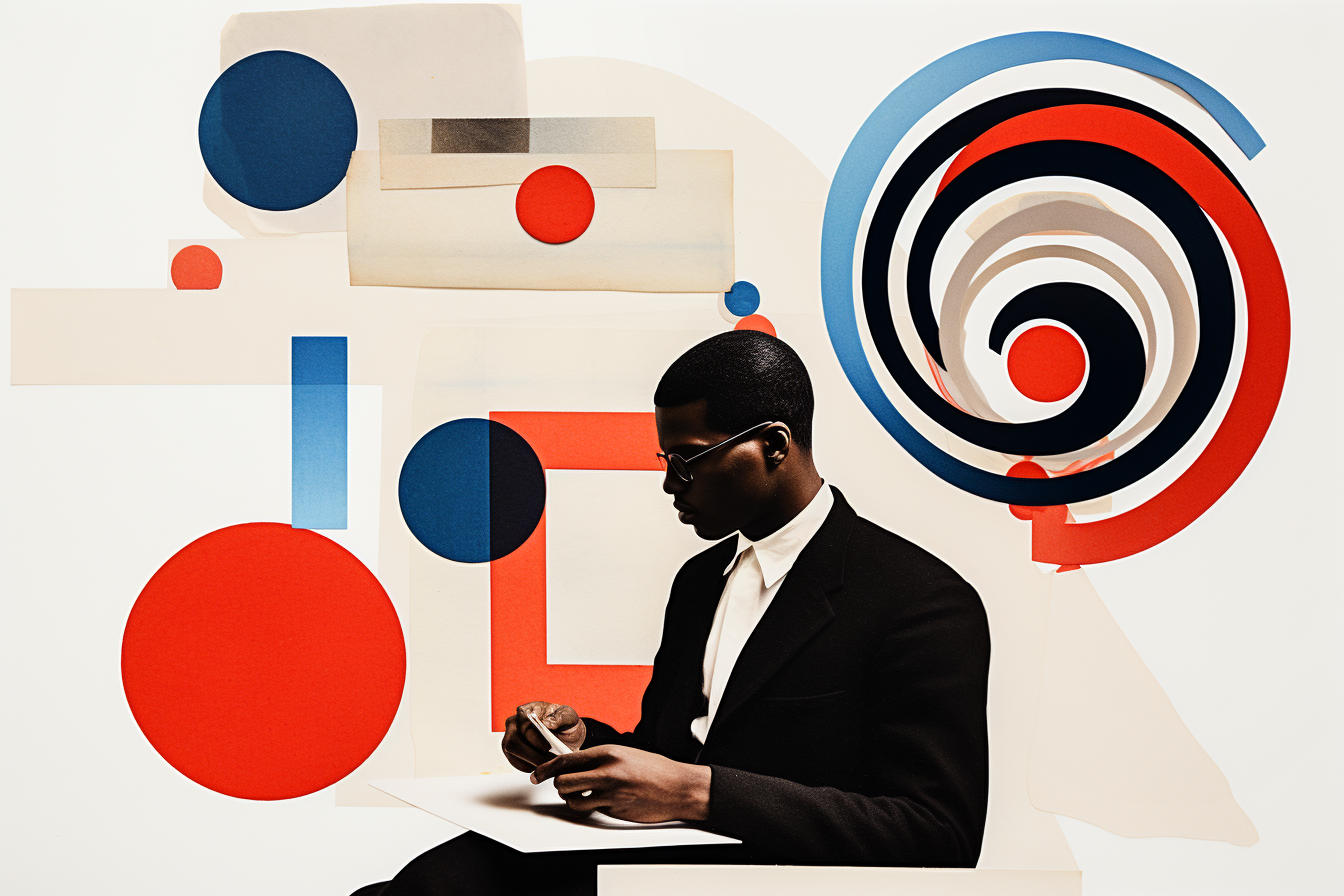
As much as the term exists in everyday language, there is a detailed development that informs the word "hypothesis" when applied to research. A good research hypothesis is informed by prior research and guides research design and data analysis , so it is important to understand how a hypothesis is defined and understood by researchers.
What is the simple definition of a hypothesis?
A hypothesis is a testable prediction about an outcome between two or more variables . It functions as a navigational tool in the research process, directing what you aim to predict and how.
What is the hypothesis for in research?
In research, a hypothesis serves as the cornerstone for your empirical study. It not only lays out what you aim to investigate but also provides a structured approach for your data collection and analysis.
Essentially, it bridges the gap between the theoretical and the empirical, guiding your investigation throughout its course.
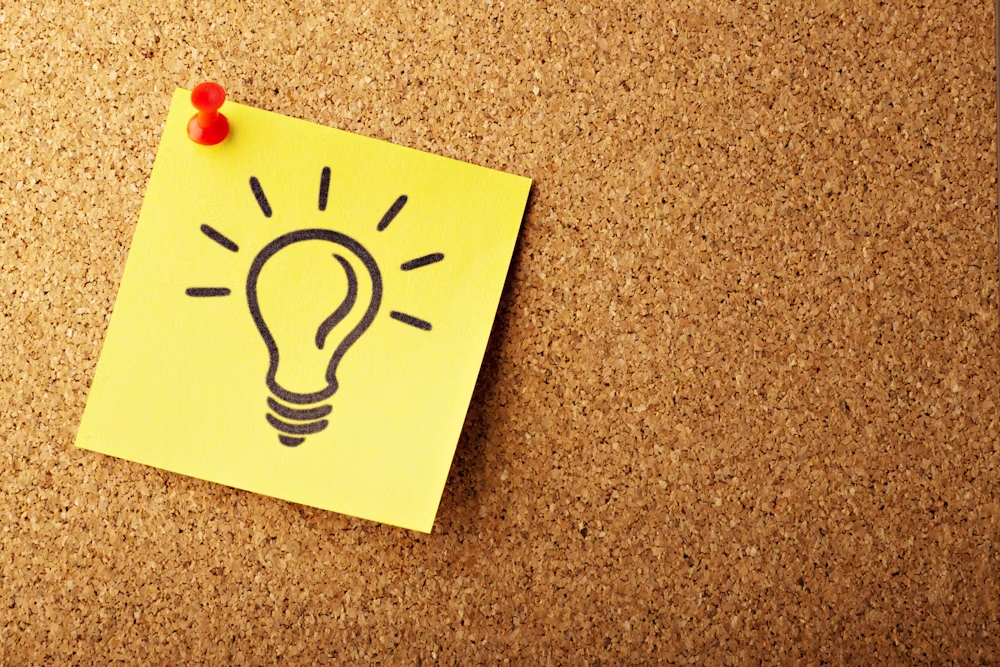
What is an example of a hypothesis?
If you are studying the relationship between physical exercise and mental health, a suitable hypothesis could be: "Regular physical exercise leads to improved mental well-being among adults."
This statement constitutes a specific and testable hypothesis that directly relates to the variables you are investigating.
What makes a good hypothesis?
A good hypothesis possesses several key characteristics. Firstly, it must be testable, allowing you to analyze data through empirical means, such as observation or experimentation, to assess if there is significant support for the hypothesis. Secondly, a hypothesis should be specific and unambiguous, giving a clear understanding of the expected relationship between variables. Lastly, it should be grounded in existing research or theoretical frameworks , ensuring its relevance and applicability.
Understanding the types of hypotheses can greatly enhance how you construct and work with hypotheses. While all hypotheses serve the essential function of guiding your study, there are varying purposes among the types of hypotheses. In addition, all hypotheses stand in contrast to the null hypothesis, or the assumption that there is no significant relationship between the variables .
Here, we explore various kinds of hypotheses to provide you with the tools needed to craft effective hypotheses for your specific research needs. Bear in mind that many of these hypothesis types may overlap with one another, and the specific type that is typically used will likely depend on the area of research and methodology you are following.
Null hypothesis
The null hypothesis is a statement that there is no effect or relationship between the variables being studied. In statistical terms, it serves as the default assumption that any observed differences are due to random chance.
For example, if you're studying the effect of a drug on blood pressure, the null hypothesis might state that the drug has no effect.
Alternative hypothesis
Contrary to the null hypothesis, the alternative hypothesis suggests that there is a significant relationship or effect between variables.
Using the drug example, the alternative hypothesis would posit that the drug does indeed affect blood pressure. This is what researchers aim to prove.
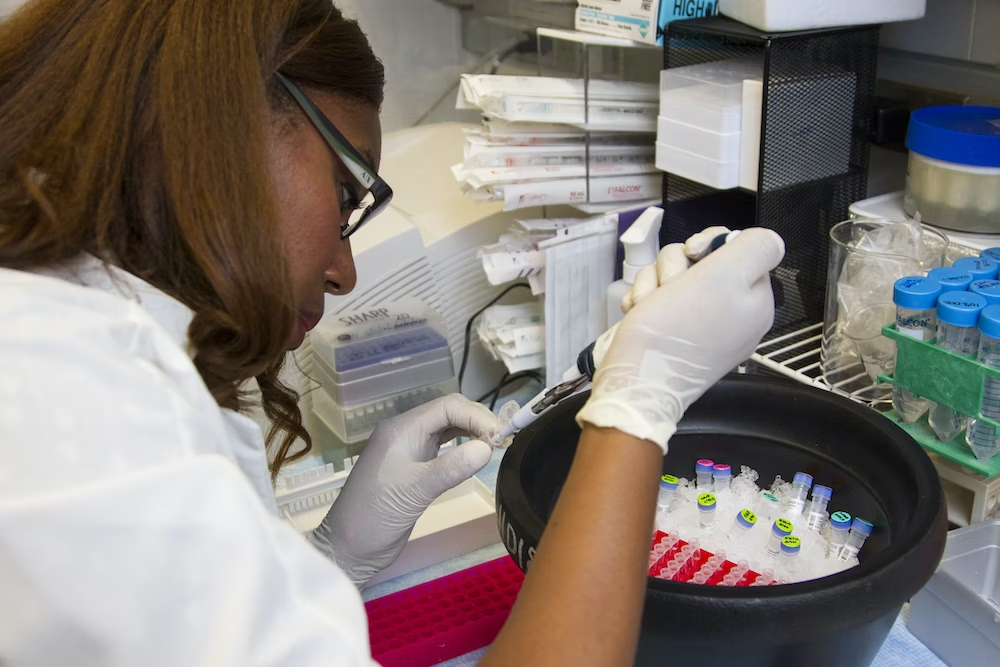
Simple hypothesis
A simple hypothesis makes a prediction about the relationship between two variables, and only two variables.
For example, "Increased study time results in better exam scores." Here, "study time" and "exam scores" are the only variables involved.
Complex hypothesis
A complex hypothesis, as the name suggests, involves more than two variables. For instance, "Increased study time and access to resources result in better exam scores." Here, "study time," "access to resources," and "exam scores" are all variables.
This hypothesis refers to multiple potential mediating variables. Other hypotheses could also include predictions about variables that moderate the relationship between the independent variable and dependent variable .
Directional hypothesis
A directional hypothesis specifies the direction of the expected relationship between variables. For example, "Eating more fruits and vegetables leads to a decrease in heart disease."
Here, the direction of heart disease is explicitly predicted to decrease, due to effects from eating more fruits and vegetables. All hypotheses typically specify the expected direction of the relationship between the independent and dependent variable, such that researchers can test if this prediction holds in their data analysis .
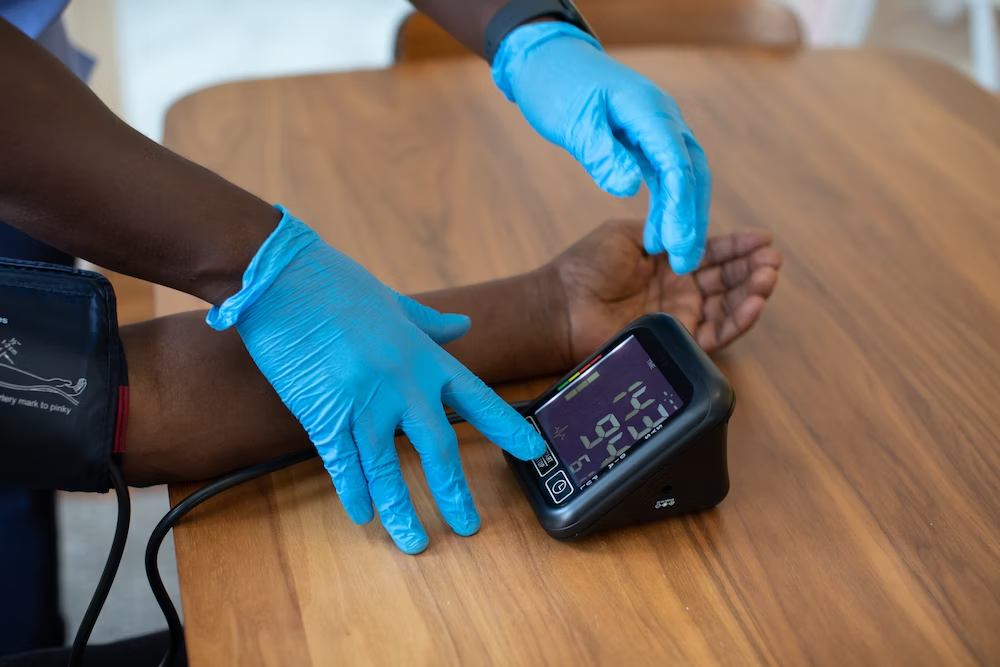
Statistical hypothesis
A statistical hypothesis is one that is testable through statistical methods, providing a numerical value that can be analyzed. This is commonly seen in quantitative research .
For example, "There is a statistically significant difference in test scores between students who study for one hour and those who study for two."
Empirical hypothesis
An empirical hypothesis is derived from observations and is tested through empirical methods, often through experimentation or survey data . Empirical hypotheses may also be assessed with statistical analyses.
For example, "Regular exercise is correlated with a lower incidence of depression," could be tested through surveys that measure exercise frequency and depression levels.
Causal hypothesis
A causal hypothesis proposes that one variable causes a change in another. This type of hypothesis is often tested through controlled experiments.
For example, "Smoking causes lung cancer," assumes a direct causal relationship.
Associative hypothesis
Unlike causal hypotheses, associative hypotheses suggest a relationship between variables but do not imply causation.
For instance, "People who smoke are more likely to get lung cancer," notes an association but doesn't claim that smoking causes lung cancer directly.
Relational hypothesis
A relational hypothesis explores the relationship between two or more variables but doesn't specify the nature of the relationship.
For example, "There is a relationship between diet and heart health," leaves the nature of the relationship (causal, associative, etc.) open to interpretation.
Logical hypothesis
A logical hypothesis is based on sound reasoning and logical principles. It's often used in theoretical research to explore abstract concepts, rather than being based on empirical data.
For example, "If all men are mortal and Socrates is a man, then Socrates is mortal," employs logical reasoning to make its point.
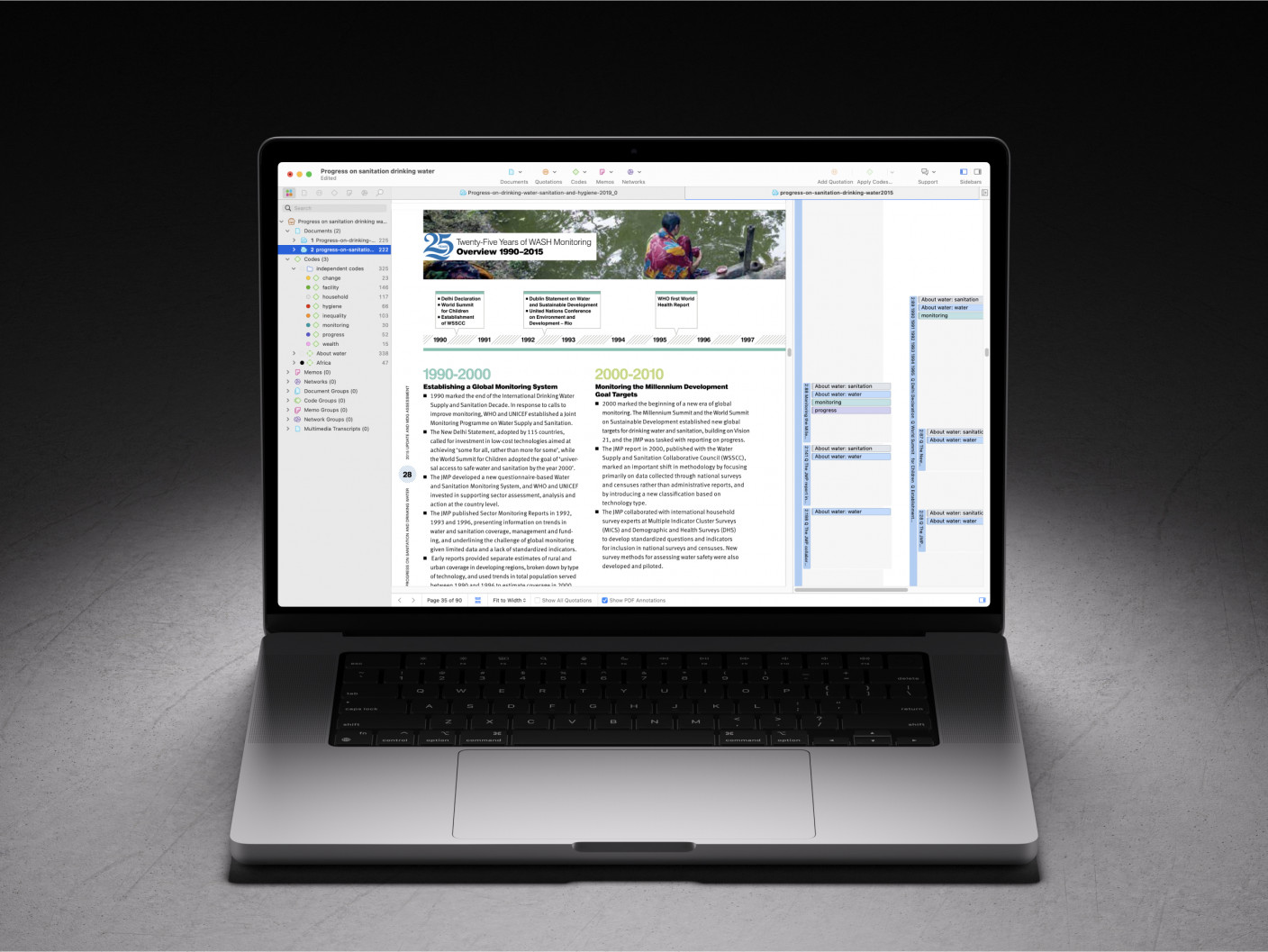
Let ATLAS.ti take you from research question to key insights
Get started with a free trial and see how ATLAS.ti can make the most of your data.
In any research hypothesis, variables play a critical role. These are the elements or factors that the researcher manipulates, controls, or measures. Understanding variables is essential for crafting a clear, testable hypothesis and for the stages of research that follow, such as data collection and analysis.
In the realm of hypotheses, there are generally two types of variables to consider: independent and dependent. Independent variables are what you, as the researcher, manipulate or change in your study. It's considered the cause in the relationship you're investigating. For instance, in a study examining the impact of sleep duration on academic performance, the independent variable would be the amount of sleep participants get.
Conversely, the dependent variable is the outcome you measure to gauge the effect of your manipulation. It's the effect in the cause-and-effect relationship. The dependent variable thus refers to the main outcome of interest in your study. In the same sleep study example, the academic performance, perhaps measured by exam scores or GPA, would be the dependent variable.
Beyond these two primary types, you might also encounter control variables. These are variables that could potentially influence the outcome and are therefore kept constant to isolate the relationship between the independent and dependent variables . For example, in the sleep and academic performance study, control variables could include age, diet, or even the subject of study.
By clearly identifying and understanding the roles of these variables in your hypothesis, you set the stage for a methodologically sound research project. It helps you develop focused research questions, design appropriate experiments or observations, and carry out meaningful data analysis . It's a step that lays the groundwork for the success of your entire study.
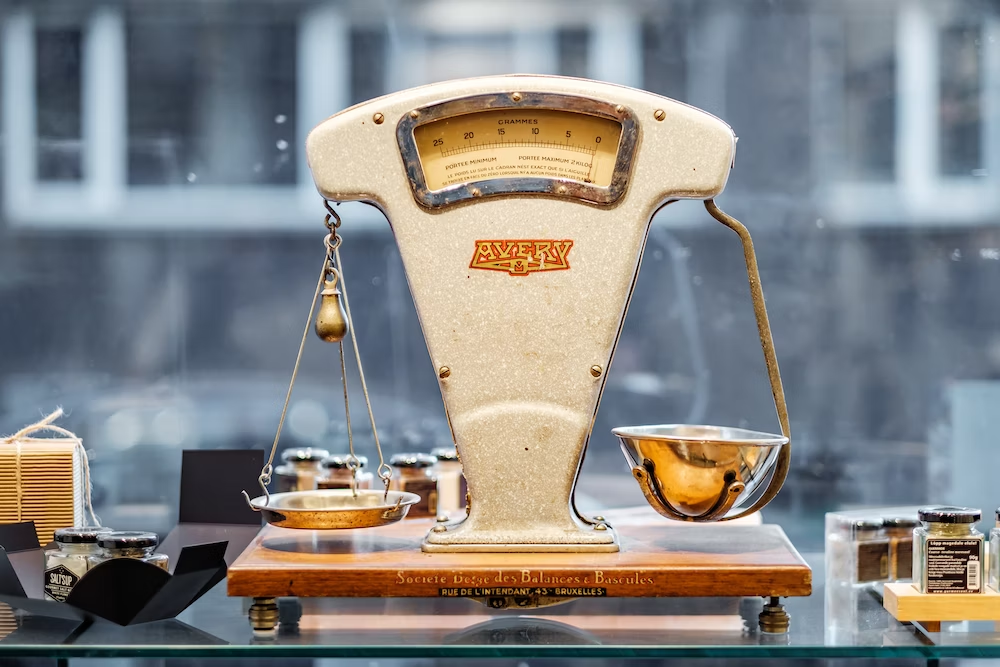
Crafting a strong, testable hypothesis is crucial for the success of any research project. It sets the stage for everything from your study design to data collection and analysis . Below are some key considerations to keep in mind when formulating your hypothesis:
- Be specific : A vague hypothesis can lead to ambiguous results and interpretations . Clearly define your variables and the expected relationship between them.
- Ensure testability : A good hypothesis should be testable through empirical means, whether by observation , experimentation, or other forms of data analysis.
- Ground in literature : Before creating your hypothesis, consult existing research and theories. This not only helps you identify gaps in current knowledge but also gives you valuable context and credibility for crafting your hypothesis.
- Use simple language : While your hypothesis should be conceptually sound, it doesn't have to be complicated. Aim for clarity and simplicity in your wording.
- State direction, if applicable : If your hypothesis involves a directional outcome (e.g., "increase" or "decrease"), make sure to specify this. You also need to think about how you will measure whether or not the outcome moved in the direction you predicted.
- Keep it focused : One of the common pitfalls in hypothesis formulation is trying to answer too many questions at once. Keep your hypothesis focused on a specific issue or relationship.
- Account for control variables : Identify any variables that could potentially impact the outcome and consider how you will control for them in your study.
- Be ethical : Make sure your hypothesis and the methods for testing it comply with ethical standards , particularly if your research involves human or animal subjects.
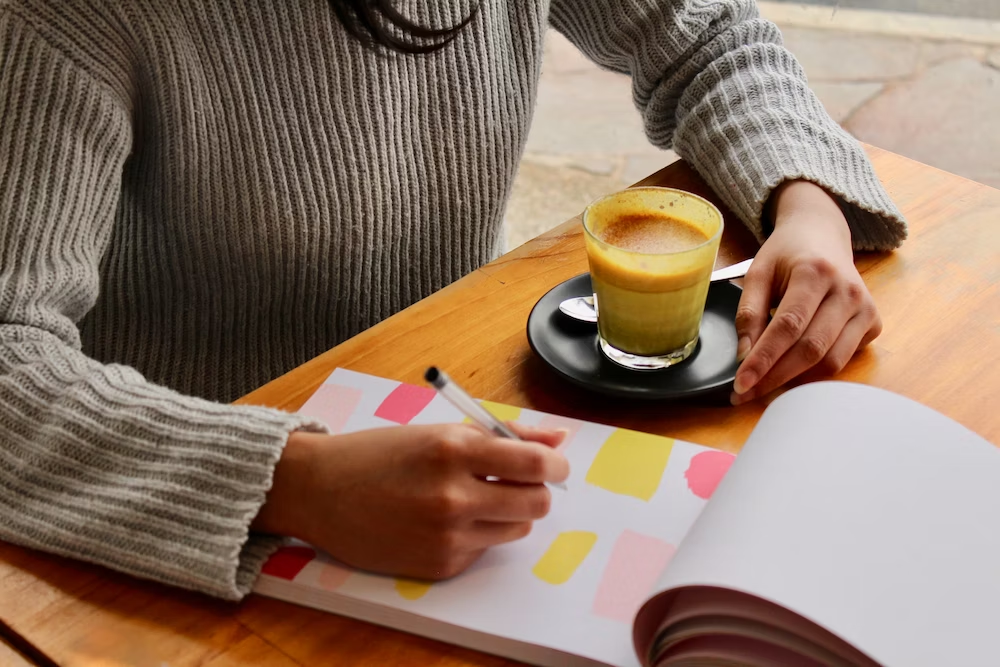
Designing your study involves multiple key phases that help ensure the rigor and validity of your research. Here we discuss these crucial components in more detail.
Literature review
Starting with a comprehensive literature review is essential. This step allows you to understand the existing body of knowledge related to your hypothesis and helps you identify gaps that your research could fill. Your research should aim to contribute some novel understanding to existing literature, and your hypotheses can reflect this. A literature review also provides valuable insights into how similar research projects were executed, thereby helping you fine-tune your own approach.
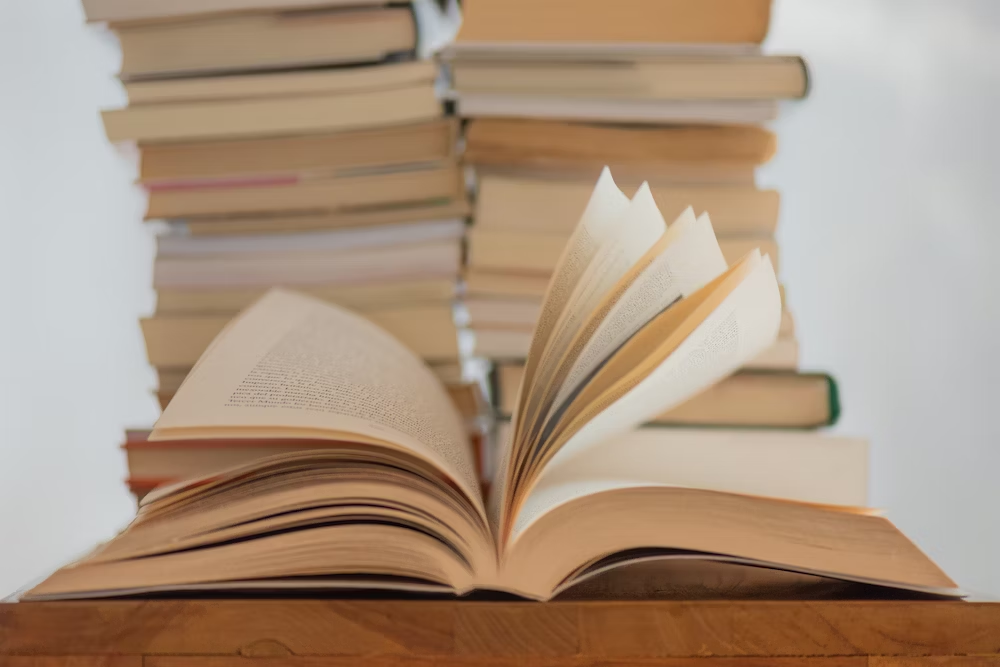
Research methods
Choosing the right research methods is critical. Whether it's a survey, an experiment, or observational study, the methodology should be the most appropriate for testing your hypothesis. Your choice of methods will also depend on whether your research is quantitative, qualitative, or mixed-methods. Make sure the chosen methods align well with the variables you are studying and the type of data you need.
Preliminary research
Before diving into a full-scale study, it’s often beneficial to conduct preliminary research or a pilot study . This allows you to test your research methods on a smaller scale, refine your tools, and identify any potential issues. For instance, a pilot survey can help you determine if your questions are clear and if the survey effectively captures the data you need. This step can save you both time and resources in the long run.
Data analysis
Finally, planning your data analysis in advance is crucial for a successful study. Decide which statistical or analytical tools are most suited for your data type and research questions . For quantitative research, you might opt for t-tests, ANOVA, or regression analyses. For qualitative research , thematic analysis or grounded theory may be more appropriate. This phase is integral for interpreting your results and drawing meaningful conclusions in relation to your research question.
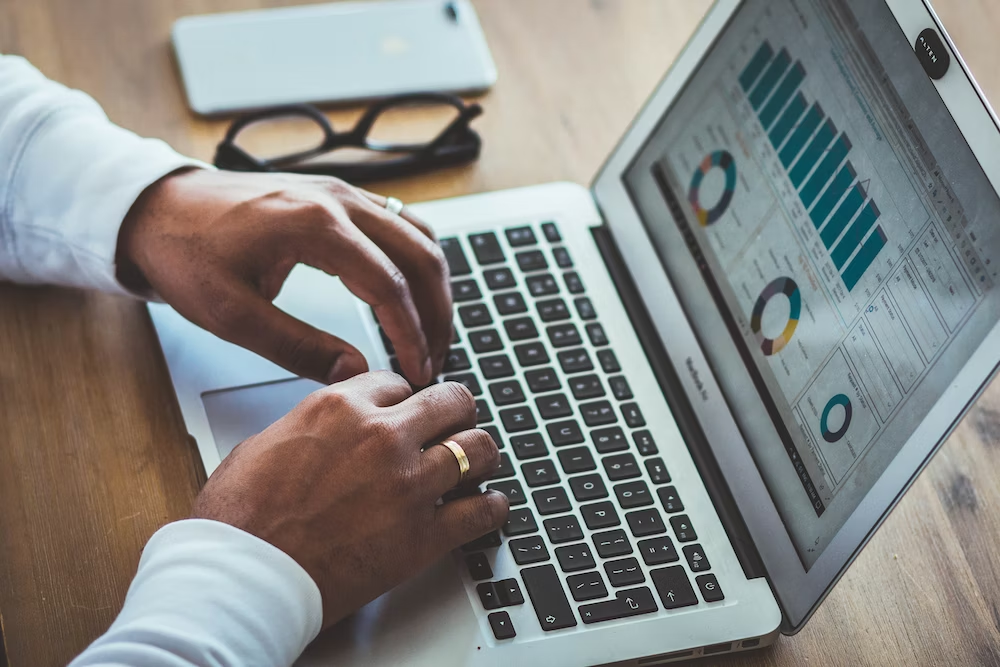
Turn data into evidence for insights with ATLAS.ti
Powerful analysis for your research paper or presentation is at your fingertips starting with a free trial.
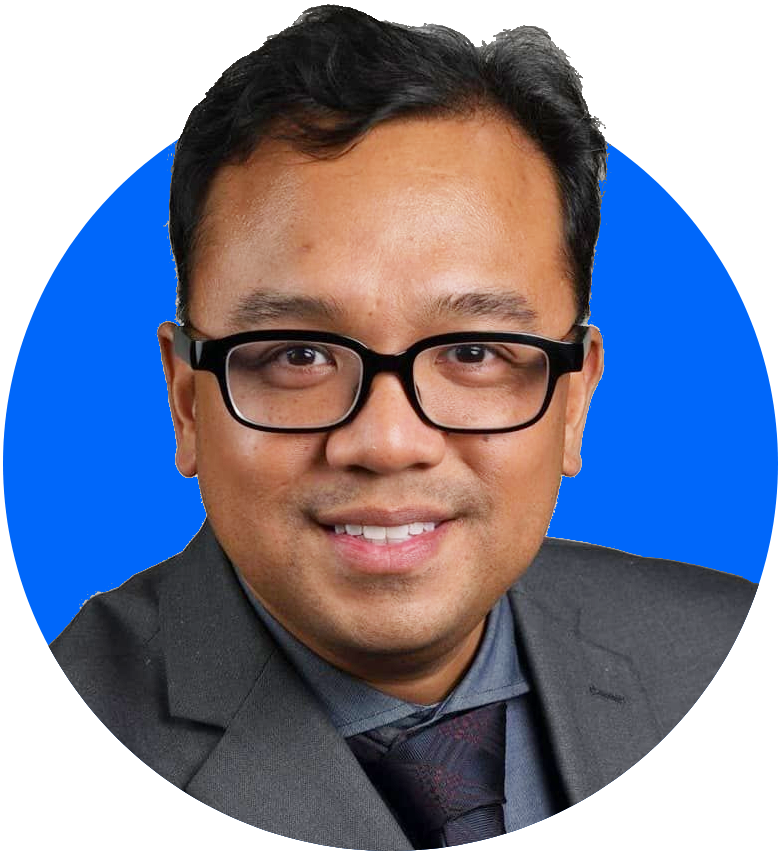
- Resources Home 🏠
- Try SciSpace Copilot
- Search research papers
- Add Copilot Extension
- Try AI Detector
- Try Paraphraser
- Try Citation Generator
- April Papers
- June Papers
- July Papers

The Craft of Writing a Strong Hypothesis

Table of Contents
Writing a hypothesis is one of the essential elements of a scientific research paper. It needs to be to the point, clearly communicating what your research is trying to accomplish. A blurry, drawn-out, or complexly-structured hypothesis can confuse your readers. Or worse, the editor and peer reviewers.
A captivating hypothesis is not too intricate. This blog will take you through the process so that, by the end of it, you have a better idea of how to convey your research paper's intent in just one sentence.
What is a Hypothesis?
The first step in your scientific endeavor, a hypothesis, is a strong, concise statement that forms the basis of your research. It is not the same as a thesis statement , which is a brief summary of your research paper .
The sole purpose of a hypothesis is to predict your paper's findings, data, and conclusion. It comes from a place of curiosity and intuition . When you write a hypothesis, you're essentially making an educated guess based on scientific prejudices and evidence, which is further proven or disproven through the scientific method.
The reason for undertaking research is to observe a specific phenomenon. A hypothesis, therefore, lays out what the said phenomenon is. And it does so through two variables, an independent and dependent variable.
The independent variable is the cause behind the observation, while the dependent variable is the effect of the cause. A good example of this is “mixing red and blue forms purple.” In this hypothesis, mixing red and blue is the independent variable as you're combining the two colors at your own will. The formation of purple is the dependent variable as, in this case, it is conditional to the independent variable.
Different Types of Hypotheses

Types of hypotheses
Some would stand by the notion that there are only two types of hypotheses: a Null hypothesis and an Alternative hypothesis. While that may have some truth to it, it would be better to fully distinguish the most common forms as these terms come up so often, which might leave you out of context.
Apart from Null and Alternative, there are Complex, Simple, Directional, Non-Directional, Statistical, and Associative and casual hypotheses. They don't necessarily have to be exclusive, as one hypothesis can tick many boxes, but knowing the distinctions between them will make it easier for you to construct your own.
1. Null hypothesis
A null hypothesis proposes no relationship between two variables. Denoted by H 0 , it is a negative statement like “Attending physiotherapy sessions does not affect athletes' on-field performance.” Here, the author claims physiotherapy sessions have no effect on on-field performances. Even if there is, it's only a coincidence.
2. Alternative hypothesis
Considered to be the opposite of a null hypothesis, an alternative hypothesis is donated as H1 or Ha. It explicitly states that the dependent variable affects the independent variable. A good alternative hypothesis example is “Attending physiotherapy sessions improves athletes' on-field performance.” or “Water evaporates at 100 °C. ” The alternative hypothesis further branches into directional and non-directional.
- Directional hypothesis: A hypothesis that states the result would be either positive or negative is called directional hypothesis. It accompanies H1 with either the ‘<' or ‘>' sign.
- Non-directional hypothesis: A non-directional hypothesis only claims an effect on the dependent variable. It does not clarify whether the result would be positive or negative. The sign for a non-directional hypothesis is ‘≠.'
3. Simple hypothesis
A simple hypothesis is a statement made to reflect the relation between exactly two variables. One independent and one dependent. Consider the example, “Smoking is a prominent cause of lung cancer." The dependent variable, lung cancer, is dependent on the independent variable, smoking.
4. Complex hypothesis
In contrast to a simple hypothesis, a complex hypothesis implies the relationship between multiple independent and dependent variables. For instance, “Individuals who eat more fruits tend to have higher immunity, lesser cholesterol, and high metabolism.” The independent variable is eating more fruits, while the dependent variables are higher immunity, lesser cholesterol, and high metabolism.
5. Associative and casual hypothesis
Associative and casual hypotheses don't exhibit how many variables there will be. They define the relationship between the variables. In an associative hypothesis, changing any one variable, dependent or independent, affects others. In a casual hypothesis, the independent variable directly affects the dependent.
6. Empirical hypothesis
Also referred to as the working hypothesis, an empirical hypothesis claims a theory's validation via experiments and observation. This way, the statement appears justifiable and different from a wild guess.
Say, the hypothesis is “Women who take iron tablets face a lesser risk of anemia than those who take vitamin B12.” This is an example of an empirical hypothesis where the researcher the statement after assessing a group of women who take iron tablets and charting the findings.
7. Statistical hypothesis
The point of a statistical hypothesis is to test an already existing hypothesis by studying a population sample. Hypothesis like “44% of the Indian population belong in the age group of 22-27.” leverage evidence to prove or disprove a particular statement.
Characteristics of a Good Hypothesis
Writing a hypothesis is essential as it can make or break your research for you. That includes your chances of getting published in a journal. So when you're designing one, keep an eye out for these pointers:
- A research hypothesis has to be simple yet clear to look justifiable enough.
- It has to be testable — your research would be rendered pointless if too far-fetched into reality or limited by technology.
- It has to be precise about the results —what you are trying to do and achieve through it should come out in your hypothesis.
- A research hypothesis should be self-explanatory, leaving no doubt in the reader's mind.
- If you are developing a relational hypothesis, you need to include the variables and establish an appropriate relationship among them.
- A hypothesis must keep and reflect the scope for further investigations and experiments.
Separating a Hypothesis from a Prediction
Outside of academia, hypothesis and prediction are often used interchangeably. In research writing, this is not only confusing but also incorrect. And although a hypothesis and prediction are guesses at their core, there are many differences between them.
A hypothesis is an educated guess or even a testable prediction validated through research. It aims to analyze the gathered evidence and facts to define a relationship between variables and put forth a logical explanation behind the nature of events.
Predictions are assumptions or expected outcomes made without any backing evidence. They are more fictionally inclined regardless of where they originate from.
For this reason, a hypothesis holds much more weight than a prediction. It sticks to the scientific method rather than pure guesswork. "Planets revolve around the Sun." is an example of a hypothesis as it is previous knowledge and observed trends. Additionally, we can test it through the scientific method.
Whereas "COVID-19 will be eradicated by 2030." is a prediction. Even though it results from past trends, we can't prove or disprove it. So, the only way this gets validated is to wait and watch if COVID-19 cases end by 2030.
Finally, How to Write a Hypothesis
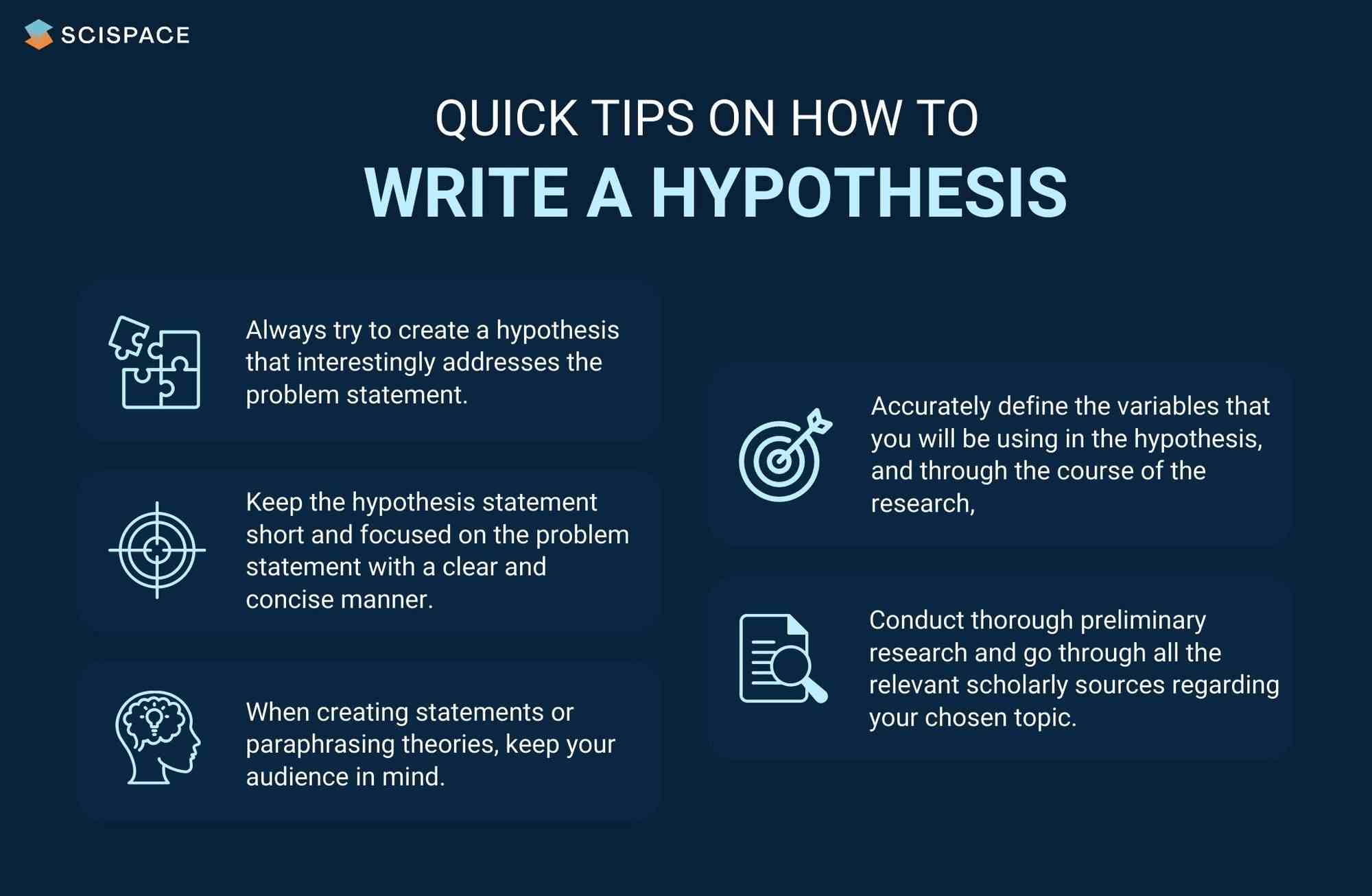
Quick tips on writing a hypothesis
1. Be clear about your research question
A hypothesis should instantly address the research question or the problem statement. To do so, you need to ask a question. Understand the constraints of your undertaken research topic and then formulate a simple and topic-centric problem. Only after that can you develop a hypothesis and further test for evidence.
2. Carry out a recce
Once you have your research's foundation laid out, it would be best to conduct preliminary research. Go through previous theories, academic papers, data, and experiments before you start curating your research hypothesis. It will give you an idea of your hypothesis's viability or originality.
Making use of references from relevant research papers helps draft a good research hypothesis. SciSpace Discover offers a repository of over 270 million research papers to browse through and gain a deeper understanding of related studies on a particular topic. Additionally, you can use SciSpace Copilot , your AI research assistant, for reading any lengthy research paper and getting a more summarized context of it. A hypothesis can be formed after evaluating many such summarized research papers. Copilot also offers explanations for theories and equations, explains paper in simplified version, allows you to highlight any text in the paper or clip math equations and tables and provides a deeper, clear understanding of what is being said. This can improve the hypothesis by helping you identify potential research gaps.
3. Create a 3-dimensional hypothesis
Variables are an essential part of any reasonable hypothesis. So, identify your independent and dependent variable(s) and form a correlation between them. The ideal way to do this is to write the hypothetical assumption in the ‘if-then' form. If you use this form, make sure that you state the predefined relationship between the variables.
In another way, you can choose to present your hypothesis as a comparison between two variables. Here, you must specify the difference you expect to observe in the results.
4. Write the first draft
Now that everything is in place, it's time to write your hypothesis. For starters, create the first draft. In this version, write what you expect to find from your research.
Clearly separate your independent and dependent variables and the link between them. Don't fixate on syntax at this stage. The goal is to ensure your hypothesis addresses the issue.
5. Proof your hypothesis
After preparing the first draft of your hypothesis, you need to inspect it thoroughly. It should tick all the boxes, like being concise, straightforward, relevant, and accurate. Your final hypothesis has to be well-structured as well.
Research projects are an exciting and crucial part of being a scholar. And once you have your research question, you need a great hypothesis to begin conducting research. Thus, knowing how to write a hypothesis is very important.
Now that you have a firmer grasp on what a good hypothesis constitutes, the different kinds there are, and what process to follow, you will find it much easier to write your hypothesis, which ultimately helps your research.
Now it's easier than ever to streamline your research workflow with SciSpace Discover . Its integrated, comprehensive end-to-end platform for research allows scholars to easily discover, write and publish their research and fosters collaboration.
It includes everything you need, including a repository of over 270 million research papers across disciplines, SEO-optimized summaries and public profiles to show your expertise and experience.
If you found these tips on writing a research hypothesis useful, head over to our blog on Statistical Hypothesis Testing to learn about the top researchers, papers, and institutions in this domain.
Frequently Asked Questions (FAQs)
1. what is the definition of hypothesis.
According to the Oxford dictionary, a hypothesis is defined as “An idea or explanation of something that is based on a few known facts, but that has not yet been proved to be true or correct”.
2. What is an example of hypothesis?
The hypothesis is a statement that proposes a relationship between two or more variables. An example: "If we increase the number of new users who join our platform by 25%, then we will see an increase in revenue."
3. What is an example of null hypothesis?
A null hypothesis is a statement that there is no relationship between two variables. The null hypothesis is written as H0. The null hypothesis states that there is no effect. For example, if you're studying whether or not a particular type of exercise increases strength, your null hypothesis will be "there is no difference in strength between people who exercise and people who don't."
4. What are the types of research?
• Fundamental research
• Applied research
• Qualitative research
• Quantitative research
• Mixed research
• Exploratory research
• Longitudinal research
• Cross-sectional research
• Field research
• Laboratory research
• Fixed research
• Flexible research
• Action research
• Policy research
• Classification research
• Comparative research
• Causal research
• Inductive research
• Deductive research
5. How to write a hypothesis?
• Your hypothesis should be able to predict the relationship and outcome.
• Avoid wordiness by keeping it simple and brief.
• Your hypothesis should contain observable and testable outcomes.
• Your hypothesis should be relevant to the research question.
6. What are the 2 types of hypothesis?
• Null hypotheses are used to test the claim that "there is no difference between two groups of data".
• Alternative hypotheses test the claim that "there is a difference between two data groups".
7. Difference between research question and research hypothesis?
A research question is a broad, open-ended question you will try to answer through your research. A hypothesis is a statement based on prior research or theory that you expect to be true due to your study. Example - Research question: What are the factors that influence the adoption of the new technology? Research hypothesis: There is a positive relationship between age, education and income level with the adoption of the new technology.
8. What is plural for hypothesis?
The plural of hypothesis is hypotheses. Here's an example of how it would be used in a statement, "Numerous well-considered hypotheses are presented in this part, and they are supported by tables and figures that are well-illustrated."
9. What is the red queen hypothesis?
The red queen hypothesis in evolutionary biology states that species must constantly evolve to avoid extinction because if they don't, they will be outcompeted by other species that are evolving. Leigh Van Valen first proposed it in 1973; since then, it has been tested and substantiated many times.
10. Who is known as the father of null hypothesis?
The father of the null hypothesis is Sir Ronald Fisher. He published a paper in 1925 that introduced the concept of null hypothesis testing, and he was also the first to use the term itself.
11. When to reject null hypothesis?
You need to find a significant difference between your two populations to reject the null hypothesis. You can determine that by running statistical tests such as an independent sample t-test or a dependent sample t-test. You should reject the null hypothesis if the p-value is less than 0.05.

You might also like

Consensus GPT vs. SciSpace GPT: Choose the Best GPT for Research
Literature Review and Theoretical Framework: Understanding the Differences

Types of Essays in Academic Writing - Quick Guide (2024)
Research Hypothesis In Psychology: Types, & Examples
Saul Mcleod, PhD
Editor-in-Chief for Simply Psychology
BSc (Hons) Psychology, MRes, PhD, University of Manchester
Saul Mcleod, PhD., is a qualified psychology teacher with over 18 years of experience in further and higher education. He has been published in peer-reviewed journals, including the Journal of Clinical Psychology.
Learn about our Editorial Process
Olivia Guy-Evans, MSc
Associate Editor for Simply Psychology
BSc (Hons) Psychology, MSc Psychology of Education
Olivia Guy-Evans is a writer and associate editor for Simply Psychology. She has previously worked in healthcare and educational sectors.
On This Page:
A research hypothesis, in its plural form “hypotheses,” is a specific, testable prediction about the anticipated results of a study, established at its outset. It is a key component of the scientific method .
Hypotheses connect theory to data and guide the research process towards expanding scientific understanding
Some key points about hypotheses:
- A hypothesis expresses an expected pattern or relationship. It connects the variables under investigation.
- It is stated in clear, precise terms before any data collection or analysis occurs. This makes the hypothesis testable.
- A hypothesis must be falsifiable. It should be possible, even if unlikely in practice, to collect data that disconfirms rather than supports the hypothesis.
- Hypotheses guide research. Scientists design studies to explicitly evaluate hypotheses about how nature works.
- For a hypothesis to be valid, it must be testable against empirical evidence. The evidence can then confirm or disprove the testable predictions.
- Hypotheses are informed by background knowledge and observation, but go beyond what is already known to propose an explanation of how or why something occurs.
Predictions typically arise from a thorough knowledge of the research literature, curiosity about real-world problems or implications, and integrating this to advance theory. They build on existing literature while providing new insight.
Types of Research Hypotheses
Alternative hypothesis.
The research hypothesis is often called the alternative or experimental hypothesis in experimental research.
It typically suggests a potential relationship between two key variables: the independent variable, which the researcher manipulates, and the dependent variable, which is measured based on those changes.
The alternative hypothesis states a relationship exists between the two variables being studied (one variable affects the other).
A hypothesis is a testable statement or prediction about the relationship between two or more variables. It is a key component of the scientific method. Some key points about hypotheses:
- Important hypotheses lead to predictions that can be tested empirically. The evidence can then confirm or disprove the testable predictions.
In summary, a hypothesis is a precise, testable statement of what researchers expect to happen in a study and why. Hypotheses connect theory to data and guide the research process towards expanding scientific understanding.
An experimental hypothesis predicts what change(s) will occur in the dependent variable when the independent variable is manipulated.
It states that the results are not due to chance and are significant in supporting the theory being investigated.
The alternative hypothesis can be directional, indicating a specific direction of the effect, or non-directional, suggesting a difference without specifying its nature. It’s what researchers aim to support or demonstrate through their study.
Null Hypothesis
The null hypothesis states no relationship exists between the two variables being studied (one variable does not affect the other). There will be no changes in the dependent variable due to manipulating the independent variable.
It states results are due to chance and are not significant in supporting the idea being investigated.
The null hypothesis, positing no effect or relationship, is a foundational contrast to the research hypothesis in scientific inquiry. It establishes a baseline for statistical testing, promoting objectivity by initiating research from a neutral stance.
Many statistical methods are tailored to test the null hypothesis, determining the likelihood of observed results if no true effect exists.
This dual-hypothesis approach provides clarity, ensuring that research intentions are explicit, and fosters consistency across scientific studies, enhancing the standardization and interpretability of research outcomes.
Nondirectional Hypothesis
A non-directional hypothesis, also known as a two-tailed hypothesis, predicts that there is a difference or relationship between two variables but does not specify the direction of this relationship.
It merely indicates that a change or effect will occur without predicting which group will have higher or lower values.
For example, “There is a difference in performance between Group A and Group B” is a non-directional hypothesis.
Directional Hypothesis
A directional (one-tailed) hypothesis predicts the nature of the effect of the independent variable on the dependent variable. It predicts in which direction the change will take place. (i.e., greater, smaller, less, more)
It specifies whether one variable is greater, lesser, or different from another, rather than just indicating that there’s a difference without specifying its nature.
For example, “Exercise increases weight loss” is a directional hypothesis.
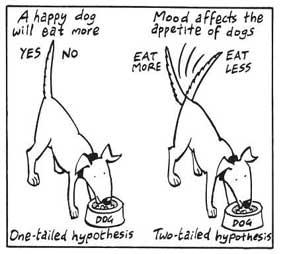
Falsifiability
The Falsification Principle, proposed by Karl Popper , is a way of demarcating science from non-science. It suggests that for a theory or hypothesis to be considered scientific, it must be testable and irrefutable.
Falsifiability emphasizes that scientific claims shouldn’t just be confirmable but should also have the potential to be proven wrong.
It means that there should exist some potential evidence or experiment that could prove the proposition false.
However many confirming instances exist for a theory, it only takes one counter observation to falsify it. For example, the hypothesis that “all swans are white,” can be falsified by observing a black swan.
For Popper, science should attempt to disprove a theory rather than attempt to continually provide evidence to support a research hypothesis.
Can a Hypothesis be Proven?
Hypotheses make probabilistic predictions. They state the expected outcome if a particular relationship exists. However, a study result supporting a hypothesis does not definitively prove it is true.
All studies have limitations. There may be unknown confounding factors or issues that limit the certainty of conclusions. Additional studies may yield different results.
In science, hypotheses can realistically only be supported with some degree of confidence, not proven. The process of science is to incrementally accumulate evidence for and against hypothesized relationships in an ongoing pursuit of better models and explanations that best fit the empirical data. But hypotheses remain open to revision and rejection if that is where the evidence leads.
- Disproving a hypothesis is definitive. Solid disconfirmatory evidence will falsify a hypothesis and require altering or discarding it based on the evidence.
- However, confirming evidence is always open to revision. Other explanations may account for the same results, and additional or contradictory evidence may emerge over time.
We can never 100% prove the alternative hypothesis. Instead, we see if we can disprove, or reject the null hypothesis.
If we reject the null hypothesis, this doesn’t mean that our alternative hypothesis is correct but does support the alternative/experimental hypothesis.
Upon analysis of the results, an alternative hypothesis can be rejected or supported, but it can never be proven to be correct. We must avoid any reference to results proving a theory as this implies 100% certainty, and there is always a chance that evidence may exist which could refute a theory.
How to Write a Hypothesis
- Identify variables . The researcher manipulates the independent variable and the dependent variable is the measured outcome.
- Operationalized the variables being investigated . Operationalization of a hypothesis refers to the process of making the variables physically measurable or testable, e.g. if you are about to study aggression, you might count the number of punches given by participants.
- Decide on a direction for your prediction . If there is evidence in the literature to support a specific effect of the independent variable on the dependent variable, write a directional (one-tailed) hypothesis. If there are limited or ambiguous findings in the literature regarding the effect of the independent variable on the dependent variable, write a non-directional (two-tailed) hypothesis.
- Make it Testable : Ensure your hypothesis can be tested through experimentation or observation. It should be possible to prove it false (principle of falsifiability).
- Clear & concise language . A strong hypothesis is concise (typically one to two sentences long), and formulated using clear and straightforward language, ensuring it’s easily understood and testable.
Consider a hypothesis many teachers might subscribe to: students work better on Monday morning than on Friday afternoon (IV=Day, DV= Standard of work).
Now, if we decide to study this by giving the same group of students a lesson on a Monday morning and a Friday afternoon and then measuring their immediate recall of the material covered in each session, we would end up with the following:
- The alternative hypothesis states that students will recall significantly more information on a Monday morning than on a Friday afternoon.
- The null hypothesis states that there will be no significant difference in the amount recalled on a Monday morning compared to a Friday afternoon. Any difference will be due to chance or confounding factors.
More Examples
- Memory : Participants exposed to classical music during study sessions will recall more items from a list than those who studied in silence.
- Social Psychology : Individuals who frequently engage in social media use will report higher levels of perceived social isolation compared to those who use it infrequently.
- Developmental Psychology : Children who engage in regular imaginative play have better problem-solving skills than those who don’t.
- Clinical Psychology : Cognitive-behavioral therapy will be more effective in reducing symptoms of anxiety over a 6-month period compared to traditional talk therapy.
- Cognitive Psychology : Individuals who multitask between various electronic devices will have shorter attention spans on focused tasks than those who single-task.
- Health Psychology : Patients who practice mindfulness meditation will experience lower levels of chronic pain compared to those who don’t meditate.
- Organizational Psychology : Employees in open-plan offices will report higher levels of stress than those in private offices.
- Behavioral Psychology : Rats rewarded with food after pressing a lever will press it more frequently than rats who receive no reward.

How to write a research hypothesis
Last updated
19 January 2023
Reviewed by
Miroslav Damyanov
Start with a broad subject matter that excites you, so your curiosity will motivate your work. Conduct a literature search to determine the range of questions already addressed and spot any holes in the existing research.
Narrow the topics that interest you and determine your research question. Rather than focusing on a hole in the research, you might choose to challenge an existing assumption, a process called problematization. You may also find yourself with a short list of questions or related topics.
Use the FINER method to determine the single problem you'll address with your research. FINER stands for:
I nteresting
You need a feasible research question, meaning that there is a way to address the question. You should find it interesting, but so should a larger audience. Rather than repeating research that others have already conducted, your research hypothesis should test something novel or unique.
The research must fall into accepted ethical parameters as defined by the government of your country and your university or college if you're an academic. You'll also need to come up with a relevant question since your research should provide a contribution to the existing research area.
This process typically narrows your shortlist down to a single problem you'd like to study and the variable you want to test. You're ready to write your hypothesis statements.
Make research less tedious
Dovetail streamlines research to help you uncover and share actionable insights
- Types of research hypotheses
It is important to narrow your topic down to one idea before trying to write your research hypothesis. You'll only test one problem at a time. To do this, you'll write two hypotheses – a null hypothesis (H0) and an alternative hypothesis (Ha).
You'll come across many terms related to developing a research hypothesis or referring to a specific type of hypothesis. Let's take a quick look at these terms.
Null hypothesis
The term null hypothesis refers to a research hypothesis type that assumes no statistically significant relationship exists within a set of observations or data. It represents a claim that assumes that any observed relationship is due to chance. Represented as H0, the null represents the conjecture of the research.
Alternative hypothesis
The alternative hypothesis accompanies the null hypothesis. It states that the situation presented in the null hypothesis is false or untrue, and claims an observed effect in your test. This is typically denoted by Ha or H(n), where “n” stands for the number of alternative hypotheses. You can have more than one alternative hypothesis.
Simple hypothesis
The term simple hypothesis refers to a hypothesis or theory that predicts the relationship between two variables - the independent (predictor) and the dependent (predicted).
Complex hypothesis
The term complex hypothesis refers to a model – either quantitative (mathematical) or qualitative . A complex hypothesis states the surmised relationship between two or more potentially related variables.

Directional hypothesis
When creating a statistical hypothesis, the directional hypothesis (the null hypothesis) states an assumption regarding one parameter of a population. Some academics call this the “one-sided” hypothesis. The alternative hypothesis indicates whether the researcher tests for a positive or negative effect by including either the greater than (">") or less than ("<") sign.
Non-directional hypothesis
We refer to the alternative hypothesis in a statistical research question as a non-directional hypothesis. It includes the not equal ("≠") sign to show that the research tests whether or not an effect exists without specifying the effect's direction (positive or negative).
Associative hypothesis
The term associative hypothesis assumes a link between two variables but stops short of stating that one variable impacts the other. Academic statistical literature asserts in this sense that correlation does not imply causation. So, although the hypothesis notes the correlation between two variables – the independent and dependent - it does not predict how the two interact.
Logical hypothesis
Typically used in philosophy rather than science, researchers can't test a logical hypothesis because the technology or data set doesn't yet exist. A logical hypothesis uses logic as the basis of its assumptions.
In some cases, a logical hypothesis can become an empirical hypothesis once technology provides an opportunity for testing. Until that time, the question remains too expensive or complex to address. Note that a logical hypothesis is not a statistical hypothesis.
Empirical hypothesis
When we consider the opposite of a logical hypothesis, we call this an empirical or working hypothesis. This type of hypothesis considers a scientifically measurable question. A researcher can consider and test an empirical hypothesis through replicable tests, observations, and measurements.
Statistical hypothesis
The term statistical hypothesis refers to a test of a theory that uses representative statistical models to test relationships between variables to draw conclusions regarding a large population. This requires an existing large data set, commonly referred to as big data, or implementing a survey to obtain original statistical information to form a data set for the study.
Testing this type of hypothesis requires the use of random samples. Note that the null and alternative hypotheses are used in statistical hypothesis testing.
Causal hypothesis
The term causal hypothesis refers to a research hypothesis that tests a cause-and-effect relationship. A causal hypothesis is utilized when conducting experimental or quasi-experimental research.
Descriptive hypothesis
The term descriptive hypothesis refers to a research hypothesis used in non-experimental research, specifying an influence in the relationship between two variables.
- What makes an effective research hypothesis?
An effective research hypothesis offers a clearly defined, specific statement, using simple wording that contains no assumptions or generalizations, and that you can test. A well-written hypothesis should predict the tested relationship and its outcome. It contains zero ambiguity and offers results you can observe and test.
The research hypothesis should address a question relevant to a research area. Overall, your research hypothesis needs the following essentials:
Hypothesis Essential #1: Specificity & Clarity
Hypothesis Essential #2: Testability (Provability)
- How to develop a good research hypothesis
In developing your hypothesis statements, you must pre-plan some of your statistical analysis. Once you decide on your problem to examine, determine three aspects:
the parameter you'll test
the test's direction (left-tailed, right-tailed, or non-directional)
the hypothesized parameter value
Any quantitative research includes a hypothesized parameter value of a mean, a proportion, or the difference between two proportions. Here's how to note each parameter:
Single mean (μ)
Paired means (μd)
Single proportion (p)
Difference between two independent means (μ1−μ2)
Difference between two proportions (p1−p2)
Simple linear regression slope (β)
Correlation (ρ)
Defining these parameters and determining whether you want to test the mean, proportion, or differences helps you determine the statistical tests you'll conduct to analyze your data. When writing your hypothesis, you only need to decide which parameter to test and in what overarching way.
The null research hypothesis must include everyday language, in a single sentence, stating the problem you want to solve. Write it as an if-then statement with defined variables. Write an alternative research hypothesis that states the opposite.
- What is the correct format for writing a hypothesis?
The following example shows the proper format and textual content of a hypothesis. It follows commonly accepted academic standards.
Null hypothesis (H0): High school students who participate in varsity sports as opposed to those who do not, fail to score higher on leadership tests than students who do not participate.
Alternative hypothesis (H1): High school students who play a varsity sport as opposed to those who do not participate in team athletics will score higher on leadership tests than students who do not participate in athletics.
The research question tests the correlation between varsity sports participation and leadership qualities expressed as a score on leadership tests. It compares the population of athletes to non-athletes.
- What are the five steps of a hypothesis?
Once you decide on the specific problem or question you want to address, you can write your research hypothesis. Use this five-step system to hone your null hypothesis and generate your alternative hypothesis.
Step 1 : Create your research question. This topic should interest and excite you; answering it provides relevant information to an industry or academic area.
Step 2 : Conduct a literature review to gather essential existing research.
Step 3 : Write a clear, strong, simply worded sentence that explains your test parameter, test direction, and hypothesized parameter.
Step 4 : Read it a few times. Have others read it and ask them what they think it means. Refine your statement accordingly until it becomes understandable to everyone. While not everyone can or will comprehend every research study conducted, any person from the general population should be able to read your hypothesis and alternative hypothesis and understand the essential question you want to answer.
Step 5 : Re-write your null hypothesis until it reads simply and understandably. Write your alternative hypothesis.
What is the Red Queen hypothesis?
Some hypotheses are well-known, such as the Red Queen hypothesis. Choose your wording carefully, since you could become like the famed scientist Dr. Leigh Van Valen. In 1973, Dr. Van Valen proposed the Red Queen hypothesis to describe coevolutionary activity, specifically reciprocal evolutionary effects between species to explain extinction rates in the fossil record.
Essentially, Van Valen theorized that to survive, each species remains in a constant state of adaptation, evolution, and proliferation, and constantly competes for survival alongside other species doing the same. Only by doing this can a species avoid extinction. Van Valen took the hypothesis title from the Lewis Carroll book, "Through the Looking Glass," which contains a key character named the Red Queen who explains to Alice that for all of her running, she's merely running in place.
- Getting started with your research
In conclusion, once you write your null hypothesis (H0) and an alternative hypothesis (Ha), you’ve essentially authored the elevator pitch of your research. These two one-sentence statements describe your topic in simple, understandable terms that both professionals and laymen can understand. They provide the starting point of your research project.
Get started today
Go from raw data to valuable insights with a flexible research platform
Editor’s picks
Last updated: 21 December 2023
Last updated: 16 December 2023
Last updated: 6 October 2023
Last updated: 5 March 2024
Last updated: 25 November 2023
Last updated: 15 February 2024
Last updated: 11 March 2024
Last updated: 12 December 2023
Last updated: 6 March 2024
Last updated: 10 April 2023
Last updated: 20 December 2023
Latest articles
Related topics, log in or sign up.
Get started for free

How to Develop a Good Research Hypothesis
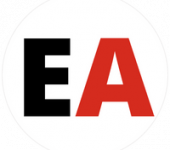
The story of a research study begins by asking a question. Researchers all around the globe are asking curious questions and formulating research hypothesis. However, whether the research study provides an effective conclusion depends on how well one develops a good research hypothesis. Research hypothesis examples could help researchers get an idea as to how to write a good research hypothesis.
This blog will help you understand what is a research hypothesis, its characteristics and, how to formulate a research hypothesis
Table of Contents
What is Hypothesis?
Hypothesis is an assumption or an idea proposed for the sake of argument so that it can be tested. It is a precise, testable statement of what the researchers predict will be outcome of the study. Hypothesis usually involves proposing a relationship between two variables: the independent variable (what the researchers change) and the dependent variable (what the research measures).
What is a Research Hypothesis?
Research hypothesis is a statement that introduces a research question and proposes an expected result. It is an integral part of the scientific method that forms the basis of scientific experiments. Therefore, you need to be careful and thorough when building your research hypothesis. A minor flaw in the construction of your hypothesis could have an adverse effect on your experiment. In research, there is a convention that the hypothesis is written in two forms, the null hypothesis, and the alternative hypothesis (called the experimental hypothesis when the method of investigation is an experiment).
Characteristics of a Good Research Hypothesis
As the hypothesis is specific, there is a testable prediction about what you expect to happen in a study. You may consider drawing hypothesis from previously published research based on the theory.
A good research hypothesis involves more effort than just a guess. In particular, your hypothesis may begin with a question that could be further explored through background research.
To help you formulate a promising research hypothesis, you should ask yourself the following questions:
- Is the language clear and focused?
- What is the relationship between your hypothesis and your research topic?
- Is your hypothesis testable? If yes, then how?
- What are the possible explanations that you might want to explore?
- Does your hypothesis include both an independent and dependent variable?
- Can you manipulate your variables without hampering the ethical standards?
- Does your research predict the relationship and outcome?
- Is your research simple and concise (avoids wordiness)?
- Is it clear with no ambiguity or assumptions about the readers’ knowledge
- Is your research observable and testable results?
- Is it relevant and specific to the research question or problem?
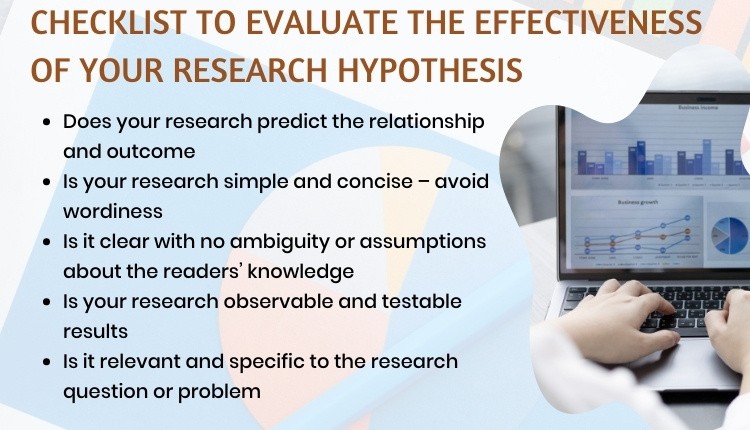
The questions listed above can be used as a checklist to make sure your hypothesis is based on a solid foundation. Furthermore, it can help you identify weaknesses in your hypothesis and revise it if necessary.
Source: Educational Hub
How to formulate a research hypothesis.
A testable hypothesis is not a simple statement. It is rather an intricate statement that needs to offer a clear introduction to a scientific experiment, its intentions, and the possible outcomes. However, there are some important things to consider when building a compelling hypothesis.
1. State the problem that you are trying to solve.
Make sure that the hypothesis clearly defines the topic and the focus of the experiment.
2. Try to write the hypothesis as an if-then statement.
Follow this template: If a specific action is taken, then a certain outcome is expected.
3. Define the variables
Independent variables are the ones that are manipulated, controlled, or changed. Independent variables are isolated from other factors of the study.
Dependent variables , as the name suggests are dependent on other factors of the study. They are influenced by the change in independent variable.
4. Scrutinize the hypothesis
Evaluate assumptions, predictions, and evidence rigorously to refine your understanding.
Types of Research Hypothesis
The types of research hypothesis are stated below:
1. Simple Hypothesis
It predicts the relationship between a single dependent variable and a single independent variable.
2. Complex Hypothesis
It predicts the relationship between two or more independent and dependent variables.
3. Directional Hypothesis
It specifies the expected direction to be followed to determine the relationship between variables and is derived from theory. Furthermore, it implies the researcher’s intellectual commitment to a particular outcome.
4. Non-directional Hypothesis
It does not predict the exact direction or nature of the relationship between the two variables. The non-directional hypothesis is used when there is no theory involved or when findings contradict previous research.
5. Associative and Causal Hypothesis
The associative hypothesis defines interdependency between variables. A change in one variable results in the change of the other variable. On the other hand, the causal hypothesis proposes an effect on the dependent due to manipulation of the independent variable.
6. Null Hypothesis
Null hypothesis states a negative statement to support the researcher’s findings that there is no relationship between two variables. There will be no changes in the dependent variable due the manipulation of the independent variable. Furthermore, it states results are due to chance and are not significant in terms of supporting the idea being investigated.
7. Alternative Hypothesis
It states that there is a relationship between the two variables of the study and that the results are significant to the research topic. An experimental hypothesis predicts what changes will take place in the dependent variable when the independent variable is manipulated. Also, it states that the results are not due to chance and that they are significant in terms of supporting the theory being investigated.
Research Hypothesis Examples of Independent and Dependent Variables
Research Hypothesis Example 1 The greater number of coal plants in a region (independent variable) increases water pollution (dependent variable). If you change the independent variable (building more coal factories), it will change the dependent variable (amount of water pollution).
Research Hypothesis Example 2 What is the effect of diet or regular soda (independent variable) on blood sugar levels (dependent variable)? If you change the independent variable (the type of soda you consume), it will change the dependent variable (blood sugar levels)
You should not ignore the importance of the above steps. The validity of your experiment and its results rely on a robust testable hypothesis. Developing a strong testable hypothesis has few advantages, it compels us to think intensely and specifically about the outcomes of a study. Consequently, it enables us to understand the implication of the question and the different variables involved in the study. Furthermore, it helps us to make precise predictions based on prior research. Hence, forming a hypothesis would be of great value to the research. Here are some good examples of testable hypotheses.
More importantly, you need to build a robust testable research hypothesis for your scientific experiments. A testable hypothesis is a hypothesis that can be proved or disproved as a result of experimentation.
Importance of a Testable Hypothesis
To devise and perform an experiment using scientific method, you need to make sure that your hypothesis is testable. To be considered testable, some essential criteria must be met:
- There must be a possibility to prove that the hypothesis is true.
- There must be a possibility to prove that the hypothesis is false.
- The results of the hypothesis must be reproducible.
Without these criteria, the hypothesis and the results will be vague. As a result, the experiment will not prove or disprove anything significant.
What are your experiences with building hypotheses for scientific experiments? What challenges did you face? How did you overcome these challenges? Please share your thoughts with us in the comments section.
Frequently Asked Questions
The steps to write a research hypothesis are: 1. Stating the problem: Ensure that the hypothesis defines the research problem 2. Writing a hypothesis as an 'if-then' statement: Include the action and the expected outcome of your study by following a ‘if-then’ structure. 3. Defining the variables: Define the variables as Dependent or Independent based on their dependency to other factors. 4. Scrutinizing the hypothesis: Identify the type of your hypothesis
Hypothesis testing is a statistical tool which is used to make inferences about a population data to draw conclusions for a particular hypothesis.
Hypothesis in statistics is a formal statement about the nature of a population within a structured framework of a statistical model. It is used to test an existing hypothesis by studying a population.
Research hypothesis is a statement that introduces a research question and proposes an expected result. It forms the basis of scientific experiments.
The different types of hypothesis in research are: • Null hypothesis: Null hypothesis is a negative statement to support the researcher’s findings that there is no relationship between two variables. • Alternate hypothesis: Alternate hypothesis predicts the relationship between the two variables of the study. • Directional hypothesis: Directional hypothesis specifies the expected direction to be followed to determine the relationship between variables. • Non-directional hypothesis: Non-directional hypothesis does not predict the exact direction or nature of the relationship between the two variables. • Simple hypothesis: Simple hypothesis predicts the relationship between a single dependent variable and a single independent variable. • Complex hypothesis: Complex hypothesis predicts the relationship between two or more independent and dependent variables. • Associative and casual hypothesis: Associative and casual hypothesis predicts the relationship between two or more independent and dependent variables. • Empirical hypothesis: Empirical hypothesis can be tested via experiments and observation. • Statistical hypothesis: A statistical hypothesis utilizes statistical models to draw conclusions about broader populations.

Wow! You really simplified your explanation that even dummies would find it easy to comprehend. Thank you so much.
Thanks a lot for your valuable guidance.
I enjoy reading the post. Hypotheses are actually an intrinsic part in a study. It bridges the research question and the methodology of the study.
Useful piece!
This is awesome.Wow.
It very interesting to read the topic, can you guide me any specific example of hypothesis process establish throw the Demand and supply of the specific product in market
Nicely explained
It is really a useful for me Kindly give some examples of hypothesis
It was a well explained content ,can you please give me an example with the null and alternative hypothesis illustrated
clear and concise. thanks.
So Good so Amazing
Good to learn
Thanks a lot for explaining to my level of understanding
Explained well and in simple terms. Quick read! Thank you
It awesome. It has really positioned me in my research project
Rate this article Cancel Reply
Your email address will not be published.
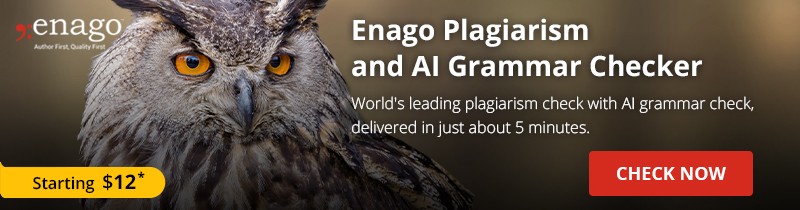
Enago Academy's Most Popular Articles
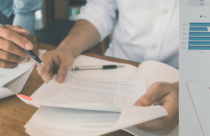
- Reporting Research
Choosing the Right Analytical Approach: Thematic analysis vs. content analysis for data interpretation
In research, choosing the right approach to understand data is crucial for deriving meaningful insights.…
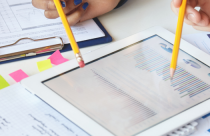
Comparing Cross Sectional and Longitudinal Studies: 5 steps for choosing the right approach
The process of choosing the right research design can put ourselves at the crossroads of…
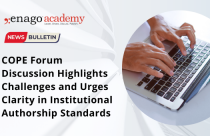
- Industry News
COPE Forum Discussion Highlights Challenges and Urges Clarity in Institutional Authorship Standards
The COPE forum discussion held in December 2023 initiated with a fundamental question — is…
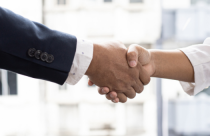
- Career Corner
Unlocking the Power of Networking in Academic Conferences
Embarking on your first academic conference experience? Fear not, we got you covered! Academic conferences…
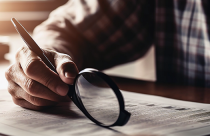
Research Recommendations – Guiding policy-makers for evidence-based decision making
Research recommendations play a crucial role in guiding scholars and researchers toward fruitful avenues of…
Choosing the Right Analytical Approach: Thematic analysis vs. content analysis for…
Comparing Cross Sectional and Longitudinal Studies: 5 steps for choosing the right…
How to Design Effective Research Questionnaires for Robust Findings

Sign-up to read more
Subscribe for free to get unrestricted access to all our resources on research writing and academic publishing including:
- 2000+ blog articles
- 50+ Webinars
- 10+ Expert podcasts
- 50+ Infographics
- 10+ Checklists
- Research Guides
We hate spam too. We promise to protect your privacy and never spam you.
I am looking for Editing/ Proofreading services for my manuscript Tentative date of next journal submission:
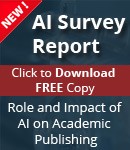
What should universities' stance be on AI tools in research and academic writing?
- Skip to main content
- Skip to primary sidebar
- Skip to footer
- QuestionPro

- Solutions Industries Gaming Automotive Sports and events Education Government Travel & Hospitality Financial Services Healthcare Cannabis Technology Use Case NPS+ Communities Audience Contactless surveys Mobile LivePolls Member Experience GDPR Positive People Science 360 Feedback Surveys
- Resources Blog eBooks Survey Templates Case Studies Training Help center

Home Market Research
Data Analysis in Research: Types & Methods
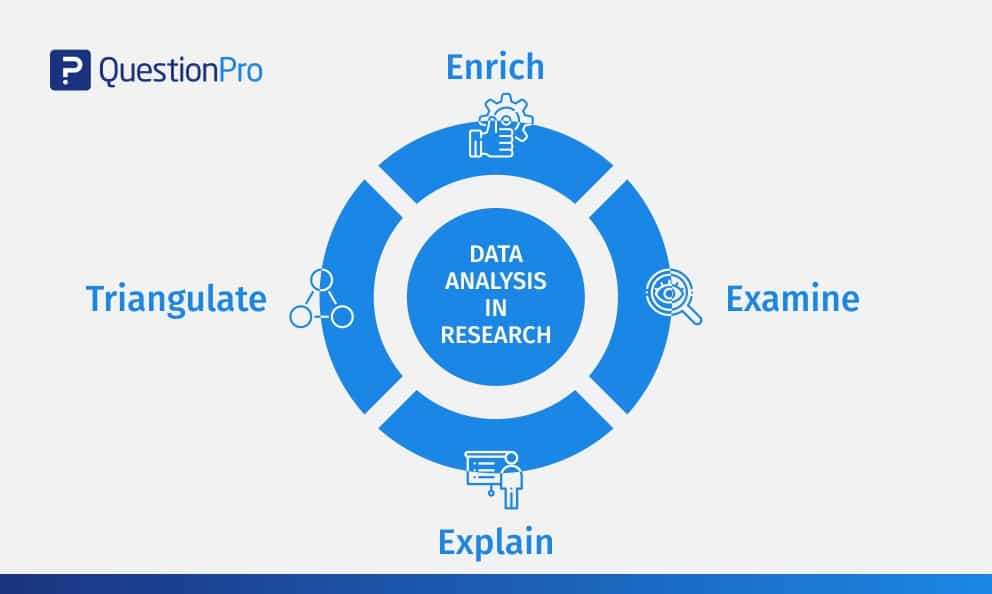
Content Index
Why analyze data in research?
Types of data in research, finding patterns in the qualitative data, methods used for data analysis in qualitative research, preparing data for analysis, methods used for data analysis in quantitative research, considerations in research data analysis, what is data analysis in research.
Definition of research in data analysis: According to LeCompte and Schensul, research data analysis is a process used by researchers to reduce data to a story and interpret it to derive insights. The data analysis process helps reduce a large chunk of data into smaller fragments, which makes sense.
Three essential things occur during the data analysis process — the first is data organization . Summarization and categorization together contribute to becoming the second known method used for data reduction. It helps find patterns and themes in the data for easy identification and linking. The third and last way is data analysis – researchers do it in both top-down and bottom-up fashion.
LEARN ABOUT: Research Process Steps
On the other hand, Marshall and Rossman describe data analysis as a messy, ambiguous, and time-consuming but creative and fascinating process through which a mass of collected data is brought to order, structure and meaning.
We can say that “the data analysis and data interpretation is a process representing the application of deductive and inductive logic to the research and data analysis.”
Researchers rely heavily on data as they have a story to tell or research problems to solve. It starts with a question, and data is nothing but an answer to that question. But, what if there is no question to ask? Well! It is possible to explore data even without a problem – we call it ‘Data Mining’, which often reveals some interesting patterns within the data that are worth exploring.
Irrelevant to the type of data researchers explore, their mission and audiences’ vision guide them to find the patterns to shape the story they want to tell. One of the essential things expected from researchers while analyzing data is to stay open and remain unbiased toward unexpected patterns, expressions, and results. Remember, sometimes, data analysis tells the most unforeseen yet exciting stories that were not expected when initiating data analysis. Therefore, rely on the data you have at hand and enjoy the journey of exploratory research.
Create a Free Account
Every kind of data has a rare quality of describing things after assigning a specific value to it. For analysis, you need to organize these values, processed and presented in a given context, to make it useful. Data can be in different forms; here are the primary data types.
- Qualitative data: When the data presented has words and descriptions, then we call it qualitative data . Although you can observe this data, it is subjective and harder to analyze data in research, especially for comparison. Example: Quality data represents everything describing taste, experience, texture, or an opinion that is considered quality data. This type of data is usually collected through focus groups, personal qualitative interviews , qualitative observation or using open-ended questions in surveys.
- Quantitative data: Any data expressed in numbers of numerical figures are called quantitative data . This type of data can be distinguished into categories, grouped, measured, calculated, or ranked. Example: questions such as age, rank, cost, length, weight, scores, etc. everything comes under this type of data. You can present such data in graphical format, charts, or apply statistical analysis methods to this data. The (Outcomes Measurement Systems) OMS questionnaires in surveys are a significant source of collecting numeric data.
- Categorical data: It is data presented in groups. However, an item included in the categorical data cannot belong to more than one group. Example: A person responding to a survey by telling his living style, marital status, smoking habit, or drinking habit comes under the categorical data. A chi-square test is a standard method used to analyze this data.
Learn More : Examples of Qualitative Data in Education
Data analysis in qualitative research
Data analysis and qualitative data research work a little differently from the numerical data as the quality data is made up of words, descriptions, images, objects, and sometimes symbols. Getting insight from such complicated information is a complicated process. Hence it is typically used for exploratory research and data analysis .
Although there are several ways to find patterns in the textual information, a word-based method is the most relied and widely used global technique for research and data analysis. Notably, the data analysis process in qualitative research is manual. Here the researchers usually read the available data and find repetitive or commonly used words.
For example, while studying data collected from African countries to understand the most pressing issues people face, researchers might find “food” and “hunger” are the most commonly used words and will highlight them for further analysis.
LEARN ABOUT: Level of Analysis
The keyword context is another widely used word-based technique. In this method, the researcher tries to understand the concept by analyzing the context in which the participants use a particular keyword.
For example , researchers conducting research and data analysis for studying the concept of ‘diabetes’ amongst respondents might analyze the context of when and how the respondent has used or referred to the word ‘diabetes.’
The scrutiny-based technique is also one of the highly recommended text analysis methods used to identify a quality data pattern. Compare and contrast is the widely used method under this technique to differentiate how a specific text is similar or different from each other.
For example: To find out the “importance of resident doctor in a company,” the collected data is divided into people who think it is necessary to hire a resident doctor and those who think it is unnecessary. Compare and contrast is the best method that can be used to analyze the polls having single-answer questions types .
Metaphors can be used to reduce the data pile and find patterns in it so that it becomes easier to connect data with theory.
Variable Partitioning is another technique used to split variables so that researchers can find more coherent descriptions and explanations from the enormous data.
LEARN ABOUT: Qualitative Research Questions and Questionnaires
There are several techniques to analyze the data in qualitative research, but here are some commonly used methods,
- Content Analysis: It is widely accepted and the most frequently employed technique for data analysis in research methodology. It can be used to analyze the documented information from text, images, and sometimes from the physical items. It depends on the research questions to predict when and where to use this method.
- Narrative Analysis: This method is used to analyze content gathered from various sources such as personal interviews, field observation, and surveys . The majority of times, stories, or opinions shared by people are focused on finding answers to the research questions.
- Discourse Analysis: Similar to narrative analysis, discourse analysis is used to analyze the interactions with people. Nevertheless, this particular method considers the social context under which or within which the communication between the researcher and respondent takes place. In addition to that, discourse analysis also focuses on the lifestyle and day-to-day environment while deriving any conclusion.
- Grounded Theory: When you want to explain why a particular phenomenon happened, then using grounded theory for analyzing quality data is the best resort. Grounded theory is applied to study data about the host of similar cases occurring in different settings. When researchers are using this method, they might alter explanations or produce new ones until they arrive at some conclusion.
LEARN ABOUT: 12 Best Tools for Researchers
Data analysis in quantitative research
The first stage in research and data analysis is to make it for the analysis so that the nominal data can be converted into something meaningful. Data preparation consists of the below phases.
Phase I: Data Validation
Data validation is done to understand if the collected data sample is per the pre-set standards, or it is a biased data sample again divided into four different stages
- Fraud: To ensure an actual human being records each response to the survey or the questionnaire
- Screening: To make sure each participant or respondent is selected or chosen in compliance with the research criteria
- Procedure: To ensure ethical standards were maintained while collecting the data sample
- Completeness: To ensure that the respondent has answered all the questions in an online survey. Else, the interviewer had asked all the questions devised in the questionnaire.
Phase II: Data Editing
More often, an extensive research data sample comes loaded with errors. Respondents sometimes fill in some fields incorrectly or sometimes skip them accidentally. Data editing is a process wherein the researchers have to confirm that the provided data is free of such errors. They need to conduct necessary checks and outlier checks to edit the raw edit and make it ready for analysis.
Phase III: Data Coding
Out of all three, this is the most critical phase of data preparation associated with grouping and assigning values to the survey responses . If a survey is completed with a 1000 sample size, the researcher will create an age bracket to distinguish the respondents based on their age. Thus, it becomes easier to analyze small data buckets rather than deal with the massive data pile.
LEARN ABOUT: Steps in Qualitative Research
After the data is prepared for analysis, researchers are open to using different research and data analysis methods to derive meaningful insights. For sure, statistical analysis plans are the most favored to analyze numerical data. In statistical analysis, distinguishing between categorical data and numerical data is essential, as categorical data involves distinct categories or labels, while numerical data consists of measurable quantities. The method is again classified into two groups. First, ‘Descriptive Statistics’ used to describe data. Second, ‘Inferential statistics’ that helps in comparing the data .
Descriptive statistics
This method is used to describe the basic features of versatile types of data in research. It presents the data in such a meaningful way that pattern in the data starts making sense. Nevertheless, the descriptive analysis does not go beyond making conclusions. The conclusions are again based on the hypothesis researchers have formulated so far. Here are a few major types of descriptive analysis methods.
Measures of Frequency
- Count, Percent, Frequency
- It is used to denote home often a particular event occurs.
- Researchers use it when they want to showcase how often a response is given.
Measures of Central Tendency
- Mean, Median, Mode
- The method is widely used to demonstrate distribution by various points.
- Researchers use this method when they want to showcase the most commonly or averagely indicated response.
Measures of Dispersion or Variation
- Range, Variance, Standard deviation
- Here the field equals high/low points.
- Variance standard deviation = difference between the observed score and mean
- It is used to identify the spread of scores by stating intervals.
- Researchers use this method to showcase data spread out. It helps them identify the depth until which the data is spread out that it directly affects the mean.
Measures of Position
- Percentile ranks, Quartile ranks
- It relies on standardized scores helping researchers to identify the relationship between different scores.
- It is often used when researchers want to compare scores with the average count.
For quantitative research use of descriptive analysis often give absolute numbers, but the in-depth analysis is never sufficient to demonstrate the rationale behind those numbers. Nevertheless, it is necessary to think of the best method for research and data analysis suiting your survey questionnaire and what story researchers want to tell. For example, the mean is the best way to demonstrate the students’ average scores in schools. It is better to rely on the descriptive statistics when the researchers intend to keep the research or outcome limited to the provided sample without generalizing it. For example, when you want to compare average voting done in two different cities, differential statistics are enough.
Descriptive analysis is also called a ‘univariate analysis’ since it is commonly used to analyze a single variable.
Inferential statistics
Inferential statistics are used to make predictions about a larger population after research and data analysis of the representing population’s collected sample. For example, you can ask some odd 100 audiences at a movie theater if they like the movie they are watching. Researchers then use inferential statistics on the collected sample to reason that about 80-90% of people like the movie.
Here are two significant areas of inferential statistics.
- Estimating parameters: It takes statistics from the sample research data and demonstrates something about the population parameter.
- Hypothesis test: I t’s about sampling research data to answer the survey research questions. For example, researchers might be interested to understand if the new shade of lipstick recently launched is good or not, or if the multivitamin capsules help children to perform better at games.
These are sophisticated analysis methods used to showcase the relationship between different variables instead of describing a single variable. It is often used when researchers want something beyond absolute numbers to understand the relationship between variables.
Here are some of the commonly used methods for data analysis in research.
- Correlation: When researchers are not conducting experimental research or quasi-experimental research wherein the researchers are interested to understand the relationship between two or more variables, they opt for correlational research methods.
- Cross-tabulation: Also called contingency tables, cross-tabulation is used to analyze the relationship between multiple variables. Suppose provided data has age and gender categories presented in rows and columns. A two-dimensional cross-tabulation helps for seamless data analysis and research by showing the number of males and females in each age category.
- Regression analysis: For understanding the strong relationship between two variables, researchers do not look beyond the primary and commonly used regression analysis method, which is also a type of predictive analysis used. In this method, you have an essential factor called the dependent variable. You also have multiple independent variables in regression analysis. You undertake efforts to find out the impact of independent variables on the dependent variable. The values of both independent and dependent variables are assumed as being ascertained in an error-free random manner.
- Frequency tables: The statistical procedure is used for testing the degree to which two or more vary or differ in an experiment. A considerable degree of variation means research findings were significant. In many contexts, ANOVA testing and variance analysis are similar.
- Analysis of variance: The statistical procedure is used for testing the degree to which two or more vary or differ in an experiment. A considerable degree of variation means research findings were significant. In many contexts, ANOVA testing and variance analysis are similar.
- Researchers must have the necessary research skills to analyze and manipulation the data , Getting trained to demonstrate a high standard of research practice. Ideally, researchers must possess more than a basic understanding of the rationale of selecting one statistical method over the other to obtain better data insights.
- Usually, research and data analytics projects differ by scientific discipline; therefore, getting statistical advice at the beginning of analysis helps design a survey questionnaire, select data collection methods , and choose samples.
LEARN ABOUT: Best Data Collection Tools
- The primary aim of data research and analysis is to derive ultimate insights that are unbiased. Any mistake in or keeping a biased mind to collect data, selecting an analysis method, or choosing audience sample il to draw a biased inference.
- Irrelevant to the sophistication used in research data and analysis is enough to rectify the poorly defined objective outcome measurements. It does not matter if the design is at fault or intentions are not clear, but lack of clarity might mislead readers, so avoid the practice.
- The motive behind data analysis in research is to present accurate and reliable data. As far as possible, avoid statistical errors, and find a way to deal with everyday challenges like outliers, missing data, data altering, data mining , or developing graphical representation.
LEARN MORE: Descriptive Research vs Correlational Research The sheer amount of data generated daily is frightening. Especially when data analysis has taken center stage. in 2018. In last year, the total data supply amounted to 2.8 trillion gigabytes. Hence, it is clear that the enterprises willing to survive in the hypercompetitive world must possess an excellent capability to analyze complex research data, derive actionable insights, and adapt to the new market needs.
LEARN ABOUT: Average Order Value
QuestionPro is an online survey platform that empowers organizations in data analysis and research and provides them a medium to collect data by creating appealing surveys.
MORE LIKE THIS
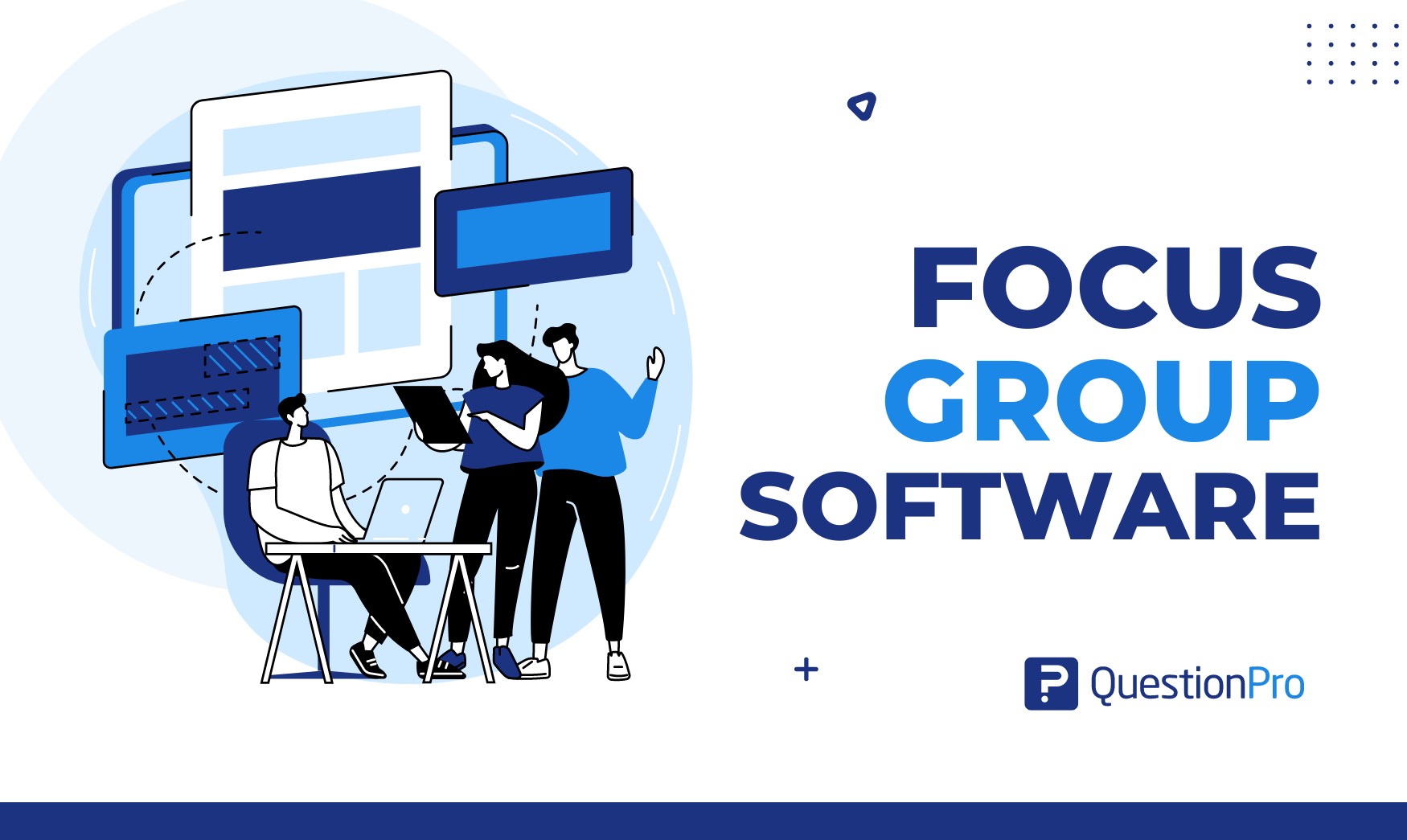
Top 7 Focus Group Software for Comprehensive Research
Apr 17, 2024
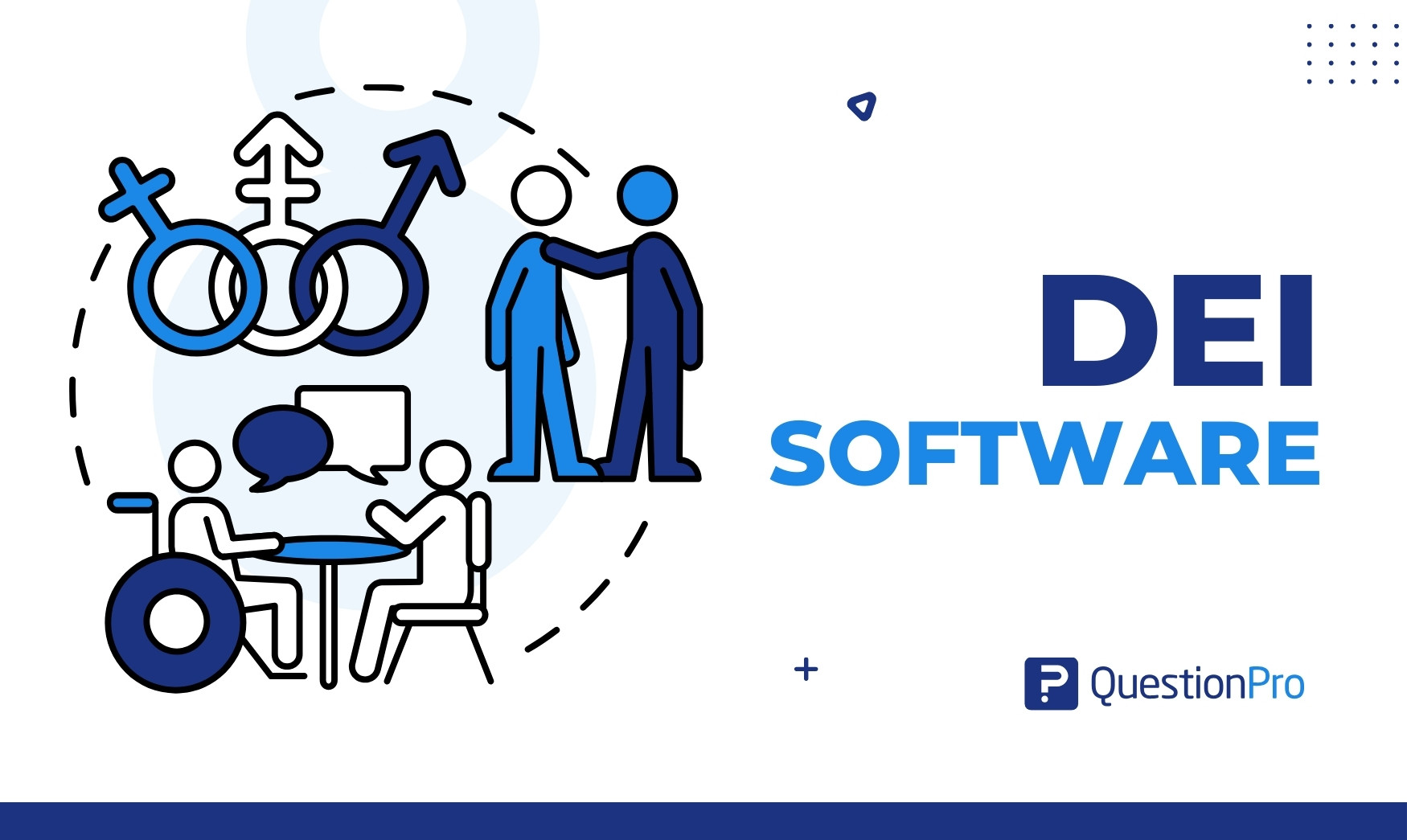
Top 7 DEI Software Solutions to Empower Your Workplace
Apr 16, 2024
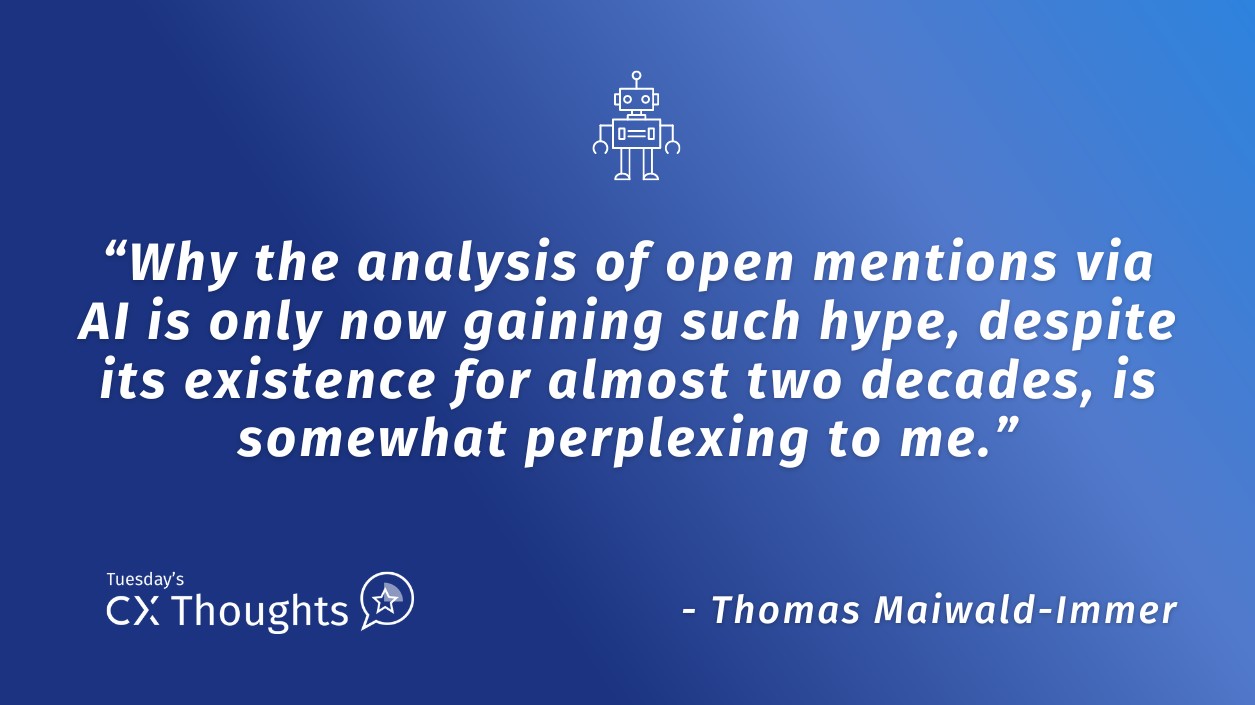
The Power of AI in Customer Experience — Tuesday CX Thoughts
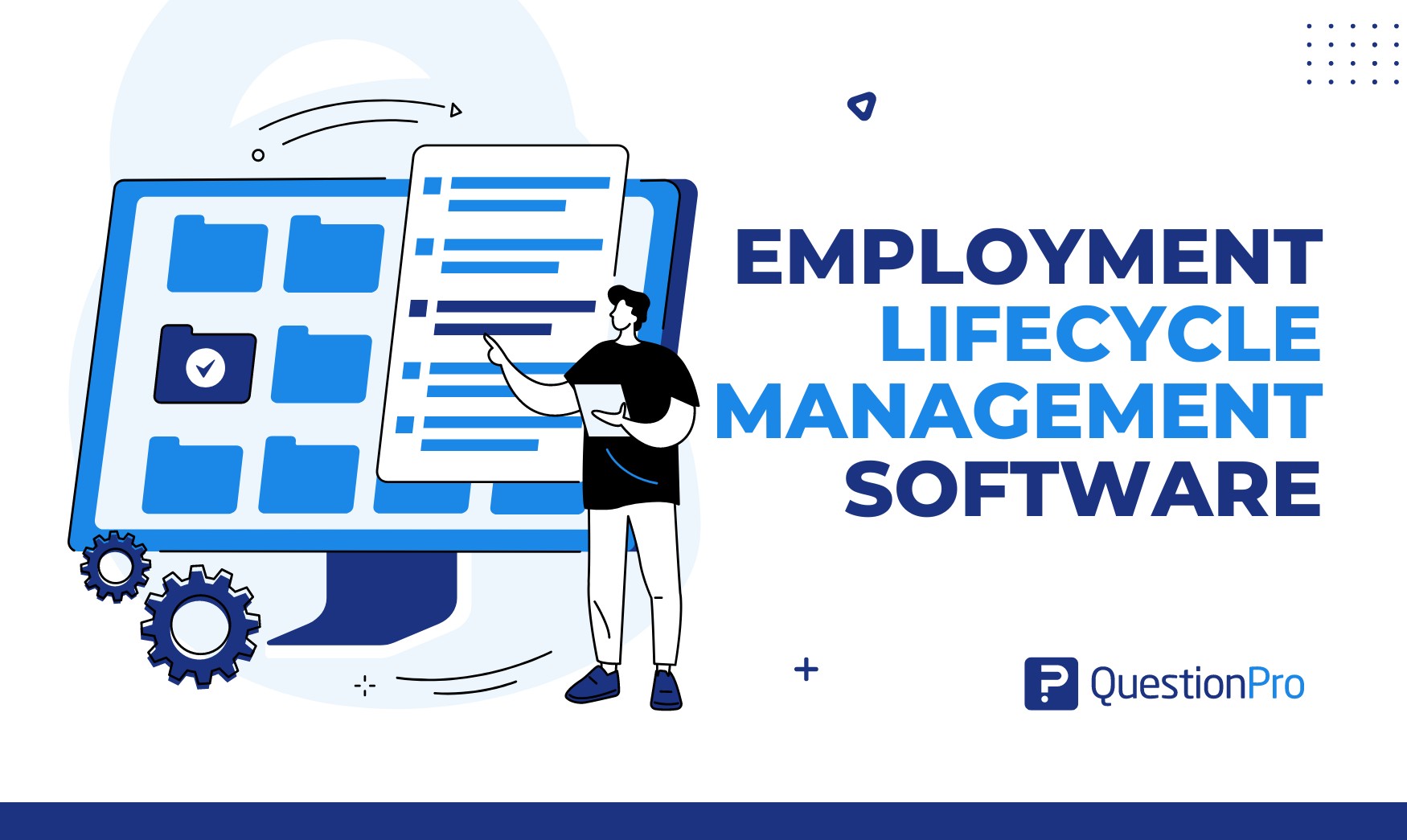
Employee Lifecycle Management Software: Top of 2024
Apr 15, 2024
Other categories
- Academic Research
- Artificial Intelligence
- Assessments
- Brand Awareness
- Case Studies
- Communities
- Consumer Insights
- Customer effort score
- Customer Engagement
- Customer Experience
- Customer Loyalty
- Customer Research
- Customer Satisfaction
- Employee Benefits
- Employee Engagement
- Employee Retention
- Friday Five
- General Data Protection Regulation
- Insights Hub
- Life@QuestionPro
- Market Research
- Mobile diaries
- Mobile Surveys
- New Features
- Online Communities
- Question Types
- Questionnaire
- QuestionPro Products
- Release Notes
- Research Tools and Apps
- Revenue at Risk
- Survey Templates
- Training Tips
- Uncategorized
- Video Learning Series
- What’s Coming Up
- Workforce Intelligence

Evaluating the mismatch hypothesis: An analysis of the evolutionary history of Type II Diabetes
Evolutionary mismatch is a mechanism whereby disease-associated genetic variants that experienced positive natural selection in past environments because they were beneficial to fitness, may now contribute to negative health consequences in the context of present, modern environments. This project investigates the plausibility of the mismatch hypothesis for Type II Diabetes (T2D) by integrating genome-wide association data for individuals with South Asian ancestry (n=40,850) and evolutionary genomics.
HYPOTHESIS AND THEORY article
This article is part of the research topic.
The Evolution and Sustainability of Societal Systems
Redefining those Subtle Borders: Transhumanism, Frontiers, Retroprogression and Hermeneutics at the crossroads of Teaching (Article Type: Conceptual Analysis ) Provisionally Accepted
- 1 University of Valladolid, Spain
The final, formatted version of the article will be published soon.
The world of education is undergoing many changes, this implies that worthwhile views coming from the past can be totally lost in the acceleration we are experiencing. Transhumanism, as a tendency, seems to imply a moving forward at a increasing speed. Therefore, it would be interesting to reflect on Transhumanism but in relation to Retro-progression and Phronesis, to be able to understand how limits work in the realm of education. Interpretation, then, is one of the basic tools for the educators in this very complex environment, their role as meta-interpreters can be founded in a return to hermeneutics as an art and a science. In so doing, past and future are reconciled in the present and the transhumanist principles do keep in mind the human part of the term. We conclude that Education is always an act of human understanding that implies the full attention and presence of the educator who is therefore able to offer meaningful actions by understanding properly the hereand-now and uniqueness of every teaching situation.
Keywords: Transhumanism, Frontiers, Education, retro-progression, Teaching, hermeneutics
Received: 20 Feb 2024; Accepted: 17 Apr 2024.
Copyright: © 2024 Francisco Carrera. This is an open-access article distributed under the terms of the Creative Commons Attribution License (CC BY) . The use, distribution or reproduction in other forums is permitted, provided the original author(s) or licensor are credited and that the original publication in this journal is cited, in accordance with accepted academic practice. No use, distribution or reproduction is permitted which does not comply with these terms.
* Correspondence: Dr. Francisco José Francisco Carrera, University of Valladolid, Valladolid, Spain
People also looked at
- Open access
- Published: 12 April 2024
Subclinical epileptiform discharges in Alzheimer’s disease are associated with increased hippocampal blood flow
- Christian Sandøe Musaeus 1 ,
- Troels Wesenberg Kjaer 1 ,
- Ulrich Lindberg 3 ,
- Mark B. Vestergaard 3 ,
- Henrik Bo 1 ,
- Wiberg Larsson 3 ,
- Daniel Zvi Press 6 ,
- Birgitte Bo Andersen 1 ,
- Peter Høgh 2 , 4 ,
- Preben Kidmose 5 ,
- Martin Christian Hemmsen 7 ,
- Mike Lind Rank 7 ,
- Steen Gregers Hasselbalch 1 , 4 ,
- Gunhild Waldemar 1 , 4 &
- Kristian Steen Frederiksen 1 , 4
Alzheimer's Research & Therapy volume 16 , Article number: 80 ( 2024 ) Cite this article
155 Accesses
Metrics details
In epilepsy, the ictal phase leads to cerebral hyperperfusion while hypoperfusion is present in the interictal phases. Patients with Alzheimer’s disease (AD) have an increased prevalence of epileptiform discharges and a study using intracranial electrodes have shown that these are very frequent in the hippocampus. However, it is not known whether there is an association between hippocampal hyperexcitability and regional cerebral blood flow (rCBF). The objective of the study was to investigate the association between rCBF in hippocampus and epileptiform discharges as measured with ear-EEG in patients with Alzheimer’s disease. Our hypothesis was that increased spike frequency may be associated with increased rCBF in hippocampus.
A total of 24 patients with AD, and 15 HC were included in the analysis. Using linear regression, we investigated the association between rCBF as measured with arterial spin-labelling MRI (ASL-MRI) in the hippocampus and the number of spikes/sharp waves per 24 h as assessed by ear-EEG.
No significant difference in hippocampal rCBF was found between AD and HC ( p -value = 0.367). A significant linear association between spike frequency and normalized rCBF in the hippocampus was found for patients with AD (estimate: 0.109, t-value = 4.03, p -value < 0.001). Changes in areas that typically show group differences (temporal-parietal cortex) were found in patients with AD, compared to HC.
Conclusions
Increased spike frequency was accompanied by a hemodynamic response of increased blood flow in the hippocampus in patients with AD. This phenomenon has also been shown in patients with epilepsy and supports the hypothesis of hyperexcitability in patients with AD. The lack of a significant difference in hippocampal rCBF may be due to an increased frequency of epileptiform discharges in patients with AD.
Trial registration
The study is registered at clinicaltrials.gov (NCT04436341).
Patients with Alzheimer’s disease (AD) and dementia with Lewy bodies (DLB) are at an increased risk of developing epileptic seizures [ 1 , 2 , 3 , 4 , 5 , 6 , 7 ]. A common indicator of seizures are epileptiform discharges identified with electroencephalography (EEG). Recently, studies using long-term EEG monitoring have found an increased frequency of epileptiform discharges in patients with AD as compared to healthy controls (HC) [ 8 , 9 , 10 ], and those with discharges showed accelerated disease progression [ 8 , 10 ].
In patients with temporal lobe epilepsy, ictal activity leads to increased regional cerebral blood flow [ 11 , 12 , 13 ], while interictal periods are associated with a decreased rCBF [ 14 , 15 , 16 ]. Studies in AD have found that epileptiform discharges are more frequent than in HC using long-term EEG monitoring [ 8 , 9 , 10 ]. Although epileptiform discharges are not the same as ictal activity, the former are often associated with epilepsy and may also be due to underlying hyperexcitability. As hyperexcitability can be defined as an increased probability of neuronal activation by a certain stimulus [ 17 ], it may be less transient compared to ictal activity and may underly the increased number of epileptiform discharges. In general, most studies that measured the rCBF changes in patients with AD have shown a decreased rCBF in the regions affected by neurodegeneration, which includes the hippocampus [ 18 , 19 , 20 , 21 ]. However, a few studies found increased rCBF in the temporal lobes including the hippocampus [ 22 , 23 ]. It is unclear whether the conflicting results can be attributed to a biological difference as opposed to methodological differences but if so, it may be due to varying levels of hyperexcitability. Although the epileptiform discharges seen in AD using long-term in-patient EEG monitoring only involve spikes/sharp waves and not ictal activity [ 8 , 9 , 10 ], it is possible that a similar hemodynamic response may underly epileptiform discharges if the hyperexcitability is constantly present. This is supported by a study showing hippocampal hyperexcitability [ 24 ] but this association has not been investigated.
Ear-EEG is a new method for long-term EEG recording in the out-patient setting. Ear-EEG is a method where EEG signals are recorded from electrodes placed within a customized earpiece inserted into each ear allowing the individual to engage in their daily routine [ 25 , 26 , 27 ]. The signals recorded using ear-EEG has been shown to correspond to the temporal electrodes on scalp EEG [ 25 ] and a study in patients with temporal lobe epilepsy has shown that epileptiform discharges can be detected with ear-EEG [ 28 ]. Recently, the ear-EEG has been shown to be safe and feasible in patients with AD [ 29 ] and a recent study using the same cohort as presented here have found a significantly increased spike frequency in AD [ 30 ]. Arterial spin labeling MRI (ASL-MRI) is a non-invasive imaging technique that allows for quantitative measurements of rCBF [ 19 , 20 , 31 , 32 , 33 , 34 , 35 ]. This makes it a useful technique for measuring rCBF in specific brain regions in AD [ 19 , 20 , 31 , 32 , 33 , 34 , 35 ]. While most studies have shown a decrease in rCBF in temporal lobe structures, a few studies have found an increased rCBF in AD [ 22 , 35 ]. Although the heterogeneous findings of both increased and decreased rCBF could be due to methodological challenges, it could also be due to a response to an underlying hyperexcitability, which should be explored. By combining these techniques, it is possible to understand the relationship between epileptiform discharges and rCBF in the hippocampus.
The aim of this study was to investigate the association between rCBF in hippocampus and the frequency of epileptiform discharges in patients with AD as measured with ear-EEG. The reason for hippocampus being investigated was due to previous finding of hippocampal hyperexcitability in patients with AD [ 24 ]. Our hypothesis was that increased spike frequency as a measure of hyperexcitability was associated with increased rCBF in hippocampus as seen in patients with epilepsy.
Participants
In the current study, patients with mild to moderate AD, and healthy controls (HC) were recruited from the Memory Clinic at Copenhagen University Hospital – Rigshospitalet and the Memory Clinic at Zealand University Hospital, Roskilde. Patients with AD met the NIA-AA criteria for probable AD with amnestic presentation [ 36 ] with the diagnosis being determined based on a consensus conference. The consensus conference included information from structural imaging and, in most instances, [ 18 F]FDG positron emission tomography (PET). Some patients underwent lumbar puncture (n AD =18) with evaluation of amyloid-β42, phosphorylated tau, and total tau while some underwent a [ 11 C] Pittsburgh compound-B-PET scan (n AD =2) or both (n AD =3) as part of the clinical work-up.
In patients with AD, the inclusion criteria included (1) a mini-mental state examination (MMSE) [ 37 ] score of 16–28, (2) age between 50 and 90 years, (3) native Danish speaker, (4) at least 7 years of education, (5) hearing and vision sufficient for neuropsychological examination, (6) no alcohol or drug abuse within the last two years, (7) no contraindications for MRI, (8) an MRI or CT scan that supported the diagnosis of AD, (9) the general health conditions of the patient allowed participation in the study (as judged by the principal investigator), and (10) living with a caregiver who was able to assist the patient with the home EEG recordings.
The following exclusion criteria were applied: (1) epilepsy prior to the diagnosis of AD, (2) focal pathology (except AD related atrophy) in the hippocampus, i.e. hippocampal sclerosis, (3) living with a relative with serious illness or impaired activities of daily living since the participant may need help to participate in the study, (4) living in a nursing home, (5) psychiatric (except mild depression) or neurological conditions that affects the brain except AD, (6) currently treated with anti-epileptic medication, tricyclic antidepressants or antipsychotics, (7) daily or almost daily administration of medication with known anticholinergic or adrenergic effect, which may affect cognitive abilities or EEG, (8) large cerebral infarctions or more than four lacunar infarctions on MRI, (9) suffering from facial tics/facial hyperkinetic disorders or (10) daily use of hearing aids.
The HC were recruited from a pool of participants in other studies who had expressed interest in participating in new studies. The inclusion criteria were (1) normal cognition (as judged by the principal investigator), (2) a general health compatible with participation in the study as well as criteria 2–7 as applied in patients with AD. The following exclusion criteria were applied: (1) diagnosed with epilepsy, (2) focal pathology in the brain (except mild hippocampal atrophy) as well as exclusion criteria 5–10 as applied in patients with AD but without AD.
The study was approved by the Capital Region Ethics Committee (H-17,035,751), and by the Danish Medicines Agency (2,017,112,288), and registered at the Data Protection Agency (P-2021-866). All participants gave written and oral informed consent before participating in the study. The study is registered at clinicaltrials.gov (NCT04436341).
Study design
In this cross-sectional study, a total of four visits were planned for patients with AD, and HC, see Fig. 1 .
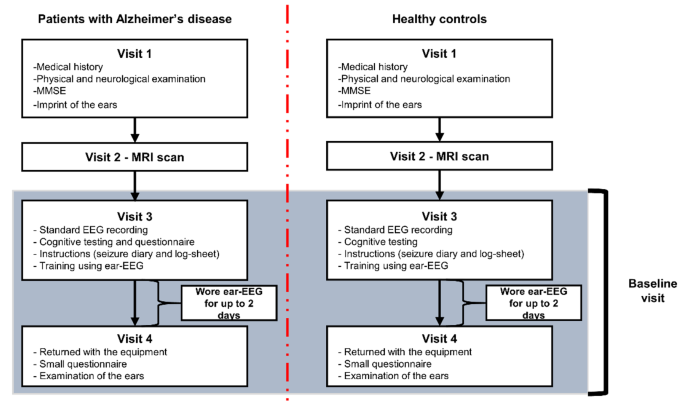
Study design. The patients with AD underwent the same procedures as HC except that no questionnaires were administered for the HC in visit 3
At visit 1, informed consent was obtained followed by assessment of medical history, a physical and neurological examination, the Mini-Mental State Examination (MMSE) (for assessment of global cognitive function) and an imprint of the ears using Otoform A Soft X (Dreve, Germany), a soft ear impression silicone. Subsequently, the patient underwent the following: visit 2) MRI scan (either before or after the ear-EEG recording), visit 3) standard EEG recording together with ear-EEG, Functional Assessment Questionnaire IADL (FAQ IADL) [ 38 ] (to assess everyday function), and the neuropsychiatric inventory (NPI) [ 39 ] (to assess behavioral and psychological symptoms).
Ear-EEG recording and review
The participants underwent up to two days of out-patient ear-EEG recording. A full description of the ear-EEG equipment and pre-processing can be found in the supplementary material.
A sharp asymmetric negative potential of 20–200 ms duration was considered an epileptiform discharge (spike/sharp wave) if it was clearly distinct from ongoing background activity and unlikely to be artifactual [ 40 ]. Due to the nature of ear-EEG, we could not investigate the spatial distribution of the spikes/sharp wave. All annotations performed by CSM underwent review by a board-certified clinical neurophysiologist (TWK), who made the final ruling. The term epileptiform discharges cover both spikes and sharp waves and assumes an underlying irritative process as seen in epilepsy, even if this cannot be stated with absolute certainty. Both CSM and TWK were blinded to the diagnosis when reviewing the EEGs.
The spike frequency was calculated by dividing the number of spikes by the amount of time (in days) when data from at least one electrode in each ear was being recorded.
The results from the ear-EEG recordings from the patients with AD and HC have been presented elsewhere [ 30 ].
MRI acquisition
All scans were recorded on a 3T Achieva dStream (Philips, Best, The Netherlands) with a 32-channel head receive coil.
Structural image acquisition and analysis
A sagittal 3D T1-weighted magnetization prepared rapid acquisition gradient echo (T1-MPRAGE) was recorded with the following acquisition parameters: repetition time (TR) 6.9 ms, echo time (TE) 2.82 ms, flip angle 9, matrix size 256 × 255, 155 slices, voxel size 1.1 × 1.1 × 1.1 mm 3 , The T1-weighted images were segmented using Freesurfer (version 7.2.0, https://surfer.nmr.mgh.harvard.edu/ ) and the volumes for each hippocampus and the inferior lateral ventricles were obtained. Both were normalized to the intracranial volume (ICV). The estimated total intracranial volume (eTIV) generated by FreeSurfer was used as an estimate for ICV in this study as has previously been shown [ 41 ].
Phase contrast mapping
The mean global cerebral blood flow (CBF) was obtained using velocity sensitive phase contrast mapping (PCM) MRI [ 42 , 43 ]. Blood velocity contrast maps were acquired by a turbo field echo sequence. Measurements were acquired from an imaging plane perpendicular to the carotid arteries and one perpendicular to the basilar artery.
The blood flow in both internal carotids and the basilar artery was calculated by multiplying the mean blood velocity by the cross-sectional area from regions of interest defining each vessel. The global mean CBF was calculated by normalizing the total blood flow from each artery to the total brain weight, which was estimated from the segmentation of the structural MRI images with an assumed brain density of 1.05 g/mL. Calculations were performed using a custom-built script in Python ( https://github.com/MarkVestergaard/PCMCalculator ).
Arterial spin labeling MRI analysis
A pseudo continuous ASL (PCASL) sequence with Look-Locker Echo Planar Imaging was chosen. The labeling plane was placed across the neck 9 cm beneath the center of the imaging slab and the labeling duration was 1650 ms. The acquisition parameters were: 13 slices, TE 10.8 ms, voxel size 3.44 × 3.44 × 6.6 mm 3 , FoV 220 × 220 × 85 mm 3 , TR was 300 ms, Look-Locker Flip-Angle 40 o , slice acquisition duration 22 ms, SENSE factor 2.3, The post-labeling delays were set at [100, 400, 700, 1000, 1300, 1600, 1900 ms]. Each ASL pair (label and control) took 8 s. In the current study, the region of interest was the hippocampus, which resulted in the acquisition plane being placed parallel to the inferior lateral ventricles. After each ASL scan, a single equilibrium magnetization scan (M0) was acquired with the same parameters as the previously described ASL images except for a 10,000 ms TR.
ASL images were quantified using BASIL in FSL (FMRIB software library, version 6.0.5.1, www.fmrib.ox.ac.uk ) with quantification [ 44 ] and fitting of the macrovascular compartment [ 45 ]. The initial prior of bolus arrival time was adjusted to account for the delayed arrival as seen in our sample, which resulted in a selected arrival time prior of 1.6 s. Lastly, the ASL data were registered to the T1wscan. The ASL quantification was not corrected for differences in hematocrit values.
To quantify rCBF in the hippocampus, we first extracted all the values within the hippocampus ROI segmented with FreeSurfer and then removed the voxels with zero values as these were assumed to be contaminated by CSF. Afterwards, any values more than two standard deviations above the mean were removed since they were assumed most likely to represent arteries. Finally, the median value of CBF was extracted for each hippocampus and normalized to the global mean CBF as measured with PCM and the mean of the two values were computed. Due to the both the structural and functional connectivity between the hippocampus and the precuneus [ 46 ], we wanted to investigate if a similar association between spike frequency and rCBF in precuneus was present. Here, the same approach for computing the normalized rCBF in the precuneus was used. No other regions were investigated.
The statistical analyses were performed in RStudio (v1.2.1335). When comparing age, education, MMSE, and the time difference between the ear-EEG recording and MRI scan, we performed t-test between AD and HC. Chi-squared tests was performed for testing for sexual distribution.
When comparing the hippocampus volume, global cerebral blood flow, and rCBF in the hippocampus or precuneus, we performed a t-test between AD and HC. Here, the distribution of the data as well as variance between groups were investigated before performing t-tests.
When comparing the number of spikes or sharp waves/24 hours (spike frequency) between HC, and AD, we calculated the rate ratio using the function rateratio from the epitools toolbox.
Simple linear regression was used to test if spike frequency significantly predicted the normalized mean rCBF in the hippocampus in patients with AD. Since it has been hypothesized that the number of epileptiform discharges increases with AD severity, we performed the same analysis with MMSE as a covariate. In the exploratory analysis, we tested if the spike frequency was associated with the normalized rCBF in the precuneus and whether a similar association between rCBF and spike frequency could be seen in HC.
The R code and output from the subsequent analyses can be found in the supplementary material.
When conducting the voxel-to-voxel analysis to compare normalized rCBF between HC and AD, we performed two-sample unpaired t-tests in randomize from FSL with cluster correction.
Patient characteristics
A total of 25 patients with AD, and 15 HC were included. One patient with AD had less than one hour of ear-EEG data after pre-processing and was not included in the linear regression analyses. The average time from MRI scan to ear-EEG recording was 19.18 days and was not significantly different between groups ( p -value = 0.332). Both left and right hippocampus were significantly smaller in patients with AD as compared to HC ( p -value < 0.05). See Table 1 for baseline demographics.
Spike frequency measured with ear-EEG
The spike frequency was significantly higher in patients with AD (range: 0–13.04 (mean: 3.03 spikes/24 hours) as compared to HC (range: 0–6.66 (mean: 1.04 spikes/24 hours) with a risk ratio of 2.9 (CI: 1.77–5.01, p -value = < 0.001).
Global and regional CBF
Global cerebral blood flow was not significantly ( p -value = 0.872, t-value = 0.16, df = 38) different between AD (mean (SD): 43.23 ml/100 g/min (12.28)) and HC (mean (SD): 42.60 ml/100 g/min (11.01)).
No significant differences were found for the normalized rCBF value in the hippocampus between the AD, and HC for left hippocampus ( p -value = 0.367, t-value = -0.91, df = 38), right hippocampus ( p -value = 0.092, t-value = -1.73, df = 38), or mean hippocampus ( p -value = 0.118, t-value = -1.60, df = 38). See Fig. 2 . A significant difference was found between AD and HC for the normalized rCBF in the precuneus ( p -value = 0.010, t-value = -2.73, df = 38) with a higher normalized rCBF in the HC, see Supplementary Fig. 1 .
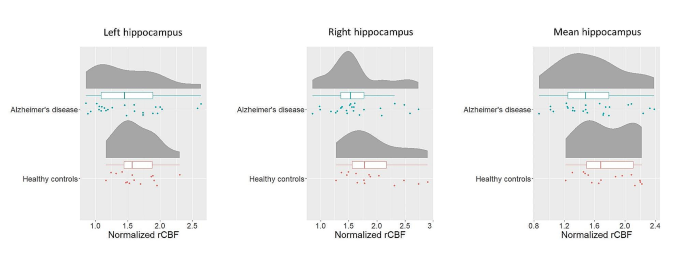
Raincloud plots showing mean normalized rCBF. No significant differences were found between AD, and HC for left hippocampus, right hippocampus, or mean hippocampus
In an exploratory manner, we conducted a voxel-to-voxel comparison and found significant bilateral decreased rCBF in the temporal and parietal lobe in patients with AD as compared to HC, see Fig. 3 .
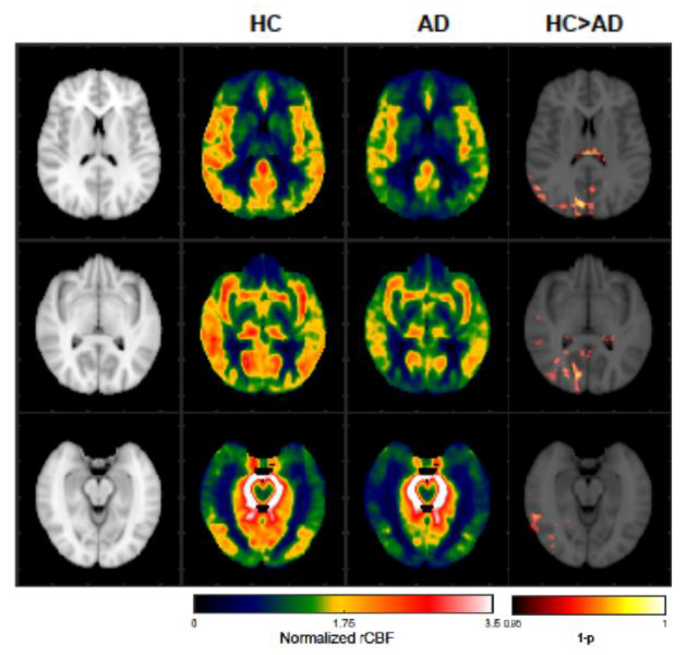
Exploratory analysis of rCBF. A significantly decreased normalized rCBF in the temporal and parietal lobes (including precuneus) in AD as compared to HC
Association between ASL and spike frequency
A significant positive linear association (estimate: 0.109, t-value = 4.03, p -value < 0.001) between spike frequency and normalized rCBF in the hippocampus was found for AD, see Fig. 4 . This effect was also present when the outlier was kept in the model ( p -value = 0.028). See Supplementary Fig. 2 and page 4–7 in the supplementary material for detection of outliers. In the following analysis with MMSE as a covariate the association between spike frequency and rCBF was significant ( p -value < 0.001) but MMSE was not significant (estimate = 0.006, p -value = 0.756), indicating that its inclusion did not significantly alter the association between spike frequency and rCBF. No significant association between rCBF and spike frequency was found for the HC (estimate: 0.021, t-value = 0.491, p -value = 0.632), see Supplementary Fig. 5 .
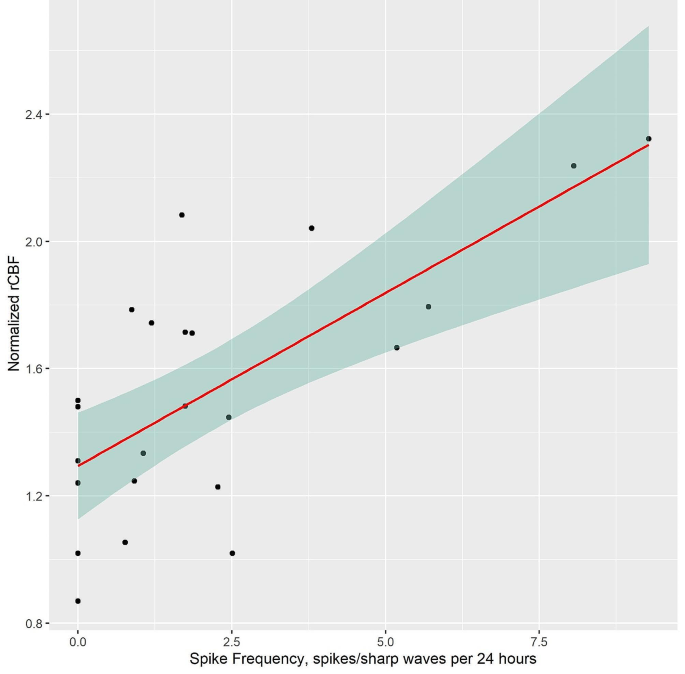
Association between normalized rCBF in hippocampus and spike frequency as measured with ear-EEG. Simple linear regression was applied to test if spike frequency was associated with the normalized mean rCBF in the hippocampus in patients with AD ( p < 0.001)
As part of the exploratory analysis, we also found a positive association between normalized rCBF in precuneus and spike frequency in patients with AD (estimate: 0.069, p -value = 0.037), see Supplementary Fig. 6 . This may be explained by the positive association between normalized rCBF in precuneus and hippocampus (estimate: 0.504, p -value = 0.013), see Supplementary Fig. 7 .
In the present study, we investigated the association between rCBF in the hippocampus and epileptiform discharges (in the form of spike/wave activity) in patients with AD. As hypothesized, a significant linear association between spike frequency and the normalized rCBF in the hippocampus was found in patients with AD. This association was not affected by adjusting for disease severity as measured with the MMSE. In an exploratory analysis a significant positive association between spike frequency and the normalized rCBF in precuneus was found in patients with AD. Spike frequency was significantly higher in patients with AD compared to HC. No difference in the mean hippocampal rCBF was found between AD and HC. The exploratory analysis revealed a significantly lower normalized rCBF in the parietal lobes including precuneus in AD as compared to HC.
The findings of an association between spike frequency and normalized rCBF in the hippocampus suggests that these two biological phenomena may be coupled in AD. Similarly, in patients with epilepsy, rCBF increases at the epileptic focus during the ictal phase [ 11 , 12 , 13 ]. Even though we only measured spikes/sharp waves and not ictal activity, the results indicate a common hemodynamic response to epileptiform discharges in both conditions. Due to this resemblance with epilepsy, it is likely that the increasing rCBF in hippocampus with more epileptiform discharges is linked to an increased metabolic demand [ 47 ], which may again be linked to accelerated neurodegeneration although this remains speculative. The epileptiform discharges measured in patients with AD in this study may represent a hyperexcitable state of neurons in the hippocampus that does not directly resemble the pathophysiological mechanisms in epilepsy. Using a more sensitive measuring technique, which enables recordings of much smaller amplitudes of spikes, it was found that these discharges are much more common in the hippocampus as compared to what is detected using scalp EEG and possibly indicating a hyperexcitable state [ 24 ]. Such a hyperexcitable state may also represent a less transient state than seen during an epileptic seizure. This would also explain the positive association despite the difference in time between ASL acquisition and ear-EEG recording. Furthermore, the association between hippocampal rCBF and spike frequency in HC was not significant, which suggests that this coupling is specific to AD, but more studies are needed. To test the effect of anti-seizure medication on this hyperexcitability, a recent study investigated the changes in rCBF in patients with AD after administration of levetiracetam using ASL-MRI but without long-term EEG monitoring and found an increased hippocampal rCBF [ 48 ], which is in contrast to the current findings. Based on the current findings of an association between spike frequency and hippocampal rCBF, we would expect a decrease in rCBF after administration of levetiracetam due to a decrease in the hippocampal excitability. Due to the limited studies in this field, further investigations of the rCBF response to anti-seizure medications while performing long-term EEG monitoring are needed to better understand the relationship between epileptiform discharges and rCBF in AD. Specifically, it remains to be determined how chronic administration of anti-seizure medication in patients with AD will alter hippocampal perfusion and if increased perfusion due to epileptiform discharges will be decreased (i.e., normalization) after administration of anti-seizure medication in patients with AD.
In the current study, no significant difference in rCBF was observed between AD and HC. In the existing literature investigating changes in rCBF in AD using ASL [ 19 , 20 , 22 , 31 , 32 , 33 , 34 , 35 ] for the most part found decreased rCBF have been reported while a few studies found increased rCBF in temporal lobe structures as well as hippocampus [ 22 , 35 ]. Hippocampal rCBF is affected by both technical (e.g., partial volume effect due to atrophy) and physiological factors that may lead to the heterogeneous findings in the literature. As for the physiological role, the rCBF in AD could be driven by epileptiform discharges leading to a pathophysiological increased rCBF and a lack of difference between the two groups.
In the exploratory analysis, we found significantly decreased normalized rCBF in the precuneus in patients with AD as compared to HC. This could be due to the neuropathological abnormalities being present in the precuneus in the early stages of the disease [ 49 ]. Using resting-state functional MRI, the precuneus is considered a main hub of the default mode network and is both structurally and functionally connected to the hippocampus [ 46 , 50 ] and lower default mode network connectivity has been linked to faster cortical thinning in older adults with amyloid depositions [ 51 ]. Due to the interconnectivity between the precuneus and the hippocampus, we investigated the role of the precuneus in epileptiform discharges and found a positive association between spike frequency and rCBF in the precuneus in patients with AD. Another explanation for the association is that the precuneus has reduced rCBF from the deposition of amyloid, as the precuneus is one of the earliest regions to show amyloid deposition in AD, but that the epileptiform discharges lead to a relative increase from a lower baseline. It is possible that the epileptiform discharges measured with ear-EEG in the study originate from the precuneus, but as previous studies have found that most epileptiform discharges originate from the temporal lobes and due to low spatial resolution of ear-EEG, it is most obvious to conclude that the epileptiform discharges originate from the temporal lobes. However, more studies are needed to understand the role of the intrinsic network between hippocampus and precuneus in relation to epileptiform discharges.
The study has several limitations. Due to the focus on the hippocampus, we did not acquire ASL images from the whole brain, which limits further exploration into the possible association between epileptiform discharges and other brain regions. In addition, the MRI acquisition was not performed on the same day as the beginning of the ear-EEG recording, which may lead to the association being weaker due to variability in the measured epileptiform discharges. Although we do not have any knowledge on the day-to-day variations of hippocampal rCBF in patients with AD, it is possible that it may have affected the results. To overcome this, future studies should arrange the study visits to limit any time lag when comparing the two modalities to better understand the association. Furthermore, due to the low spatial resolution of the ear-EEG, it will be of importance to systematically compare epileptiform discharges between ear-EEG and scalp EEG, which was not possible in the current study since only 30 min of scalp EEG was recorded. Although scalp EEG is the gold standard for detection of epileptiform discharges, a study has shown that scalp EEG is unable to detect a large proportion of the epileptiform discharges [ 24 ], which may be an advantage of ear-EEG but more studies are needed. Although ear-EEG has been able to detect epileptiform discharges in patients with epilepsy [ 28 ], no studies have so far directly compared ear-EEG to scalp EEG in detection of epileptiform discharges. Therefore, it is possible that accuracy is lower with ear-EEG than conventional scalp EEG. Lastly, we did not perform any measurement of AD biomarkers or more extensive neuropsychological examination of the HC and therefore subtle cognitive decline, or the presence of AD pathology cannot be ruled out. Although it is speculative, the presence of pathology or covert disease could potentially have explained the few HC with epileptiform discharges. Overall, we were able to demonstrate in a relatively small sample that an association between spike frequency and normalized rCBF is present in AD, which underlines the neuropathological link between epileptiform discharges and hippocampal rCBF.
As hypothesized, increasing spike frequency was associated with increasing rCBF in the hippocampus in patients with AD while this could not be shown in HC. Surprisingly, no significant difference in the hippocampal rCBF was found between AD and HC, which may be due to an increased frequency of epileptiform discharges in patients with AD increasing rCBF. The hemodynamic coupling between epileptiform discharges and blood flow has previously been found in patients with epilepsy and may be a shared mechanism across pathologies. Our findings indicate that hyperperfusion may be an accompanying phenomenon to hyperexcitability in patients with AD, which is in support of the theory of a hyperexcitable hippocampus. However, the current study has some limitations and more studies investigating the association between rCBF and epileptiform discharges in AD are needed.
Data availability
The datasets generated and/or analyzed during the current study are not publicly available due Danish data protection regulations but are available from the corresponding author on reasonable request.
Beagle AJ, Darwish SM, Ranasinghe KG, La AL, Karageorgiou E, Vossel KA. Relative incidence of seizures and myoclonus in Alzheimer’s Disease, Dementia with Lewy Bodies, and Frontotemporal Dementia. J Alzheimers Dis. 2017;60(1):211–23.
Article PubMed PubMed Central Google Scholar
Scarmeas N, Honig LS, Choi H, Cantero J, Brandt J, Blacker D et al. Seizures in Alzheimer Disease. Arch Neurol [Internet]. 2009;66(8). https://doi.org/10.1001/archneurol.2009.130.
Amatniek JC, Hauser WA, DelCastillo-Castaneda C, Jacobs DM, Marder K, Bell K et al. Incidence and Predictors of Seizures in Patients with Alzheimer’s Disease. Epilepsia [Internet]. 2006;47(5):867–72. https://doi.org/10.1111/j.1528-1167.2006.00554.x.
Lozsadi DA, Larner AJ. Prevalence and Causes of Seizures at the Time of Diagnosis of Probable Alzheimer’s Disease. Dement Geriatr Cogn Disord [Internet]. 2006;22(2):121–4. https://doi.org/10.1159/000093664.
Bernardi S, Scaldaferri N, Vanacore N, Trebbastoni A, Francia A, D’Amico A et al. Seizures in Alzheimer’s disease: a retrospective study of a cohort of outpatients. Epileptic Disord [Internet]. 2010;12(1):16–21. https://doi.org/10.1684/epd.2010.0290.
Rao SC, Dove G, Cascino GD, Petersen RC. Recurrent seizures in patients with dementia: Frequency, seizure types, and treatment outcome. Epilepsy Behav [Internet]. 2009;14(1):118–20. https://doi.org/10.1016/j.yebeh.2008.08.012.
Risse SC, Lampe TH, Bird TD, Nochlin D, Sumi SM, Keenan T et al. Myoclonus, Seizures, and Paratonia in Alzheimer Disease. Alzheimer Dis Assoc Disord [Internet]. 1990;4(4):217–25. https://doi.org/10.1097/00002093-199040400-00003.
Horvath AA, Papp A, Zsuffa J, Szucs A, Luckl J, Radai F, et al. Subclinical epileptiform activity accelerates the progression of Alzheimer’s disease: a long-term EEG study. Clin Neurophysiol off J Int Fed Clin Neurophysiol. 2021;132(8):1982–9.
Article Google Scholar
Lam AD, Sarkis RA, Pellerin KR, Jing J, Dworetzky BA, Hoch DB, et al. Association of epileptiform abnormalities and seizures in Alzheimer disease. Neurology. 2020;95(16):e2259–70.
Vossel KA, Ranasinghe KG, Beagle AJ, Mizuiri D, Honma SM, Dowling AF et al. Incidence and impact of subclinical epileptiform activity in Alzheimer’s disease. Ann Neurol [Internet]. 2016;80(6):858–70. https://doi.org/10.1002/ana.24794
Duncan R. Epilepsy, cerebral blood flow, and cerebral metabolic rate. Cerebrovasc Brain Metab Rev. 1992;4(2):105–21.
CAS PubMed Google Scholar
Dupont P, Zaknun JJ, Maes A, Tepmongkol S, Vasquez S, Bal CS, et al. Dynamic perfusion patterns in temporal lobe epilepsy. Eur J Nucl Med Mol Imaging. 2009;36(5):823–30.
Article PubMed Google Scholar
Nguyen D, Kapina V, Seeck M, Viallon M, Fedespiel A, Lovblad KO. Ictal hyperperfusion demonstrated by arterial spin-labeling MRI in status epilepticus. Journal of neuroradiology = Journal de neuroradiologie. Volume 37. France; 2010. pp. 250–1.
Pendse N, Wissmeyer M, Altrichter S, Vargas M, Delavelle J, Viallon M, et al. Interictal arterial spin-labeling MRI perfusion in intractable epilepsy. J Neuroradiol = J Neuroradiol. 2010;37(1):60–3.
Article CAS PubMed Google Scholar
Lim YM, Cho YW, Shamim S, Solomon J, Birn R, Luh WM, et al. Usefulness of pulsed arterial spin labeling MR imaging in mesial temporal lobe epilepsy. Epilepsy Res. 2008;82(2–3):183–9.
Haller S, Zaharchuk G, Thomas DL, Lovblad KO, Barkhof F, Golay X. Arterial Spin Labeling Perfusion of the Brain: Emerging Clinical Applications. Radiology [Internet]. 2016;281(2):337–56. https://doi.org/10.1148/radiol.2016150789
Targa Dias Anastacio H, Matosin N, Ooi L. Neuronal hyperexcitability in Alzheimer’s disease: what are the drivers behind this aberrant phenotype? Transl Psychiatry [Internet]. 2022;12(1):257. https://doi.org/10.1038/s41398-022-02024-7
Roquet D, Sourty M, Botzung A, Armspach JP, Blanc F. Brain perfusion in dementia with Lewy bodies and Alzheimer’s disease: an arterial spin labeling MRI study on prodromal and mild dementia stages. Alzheimers Res Ther [Internet]. 2016;8(1). https://doi.org/10.1186/s13195-016-0196-8
Johnson NA, Jahng GH, Weiner MW, Miller BL, Chui HC, Jagust WJ et al. Pattern of Cerebral Hypoperfusion in Alzheimer Disease and Mild Cognitive Impairment Measured with Arterial Spin-labeling MR Imaging: Initial Experience. Radiology [Internet]. 2005;234(3):851–9. https://doi.org/10.1148/radiol.2343040197
Yoshiura T, Hiwatashi A, Yamashita K, Ohyagi Y, Monji A, Takayama Y et al. Simultaneous Measurement of Arterial Transit Time, Arterial Blood Volume, and Cerebral Blood Flow Using Arterial Spin-Labeling in Patients with Alzheimer Disease. Am J Neuroradiol [Internet]. 2009;30(7):1388–93. https://doi.org/10.3174/ajnr.a1562
Wolk DA, Detre JA. Arterial spin labeling MRI: an emerging biomarker for Alzheimer’s disease and other neurodegenerative conditions. Curr Opin Neurol. 2012;25(4):421–8.
Article CAS PubMed PubMed Central Google Scholar
Alsop DC, Casement M, de Bazelaire C, Fong T, Press DZ. Hippocampal hyperperfusion in Alzheimer’s disease. Neuroimage [Internet]. 2008;42(4):1267–74. https://doi.org/10.1016/j.neuroimage.2008.06.006
Fleisher AS, Podraza KM, Bangen KJ, Taylor C, Sherzai A, Sidhar K et al. Cerebral perfusion and oxygenation differences in Alzheimer’s disease risk. Neurobiol Aging [Internet]. 2009;30(11):1737–48. https://doi.org/10.1016/j.neurobiolaging.2008.01.012
Lam AD, Deck G, Goldman A, Eskandar EN, Noebels J, Cole AJ. Silent hippocampal seizures and spikes identified by foramen ovale electrodes in Alzheimer’s disease. Nat Med. 2017;23(6):678–80.
Kappel SL, Rank ML, Toft HO, Andersen M, Kidmose P. Dry-Contact Electrode Ear-EEG. IEEE Trans Biomed Eng [Internet]. 2019;66(1):150–8. https://doi.org/10.1109/tbme.2018.2835778.
Looney D, Kidmose P, Park C, Ungstrup M, Rank M, Rosenkranz K et al. The In-the-Ear Recording Concept: User-Centered and Wearable Brain Monitoring. IEEE Pulse [Internet]. 2012;3(6):32–42. https://doi.org/10.1109/mpul.2012.2216717.
Looney D, Park C, Kidmose P, Rank ML, Ungstrup M, Rosenkranz K et al. An in-the-ear platform for recording electroencephalogram. In: 2011 Annual International Conference of the IEEE Engineering in Medicine and Biology Society [Internet]. IEEE; 2011. https://doi.org/10.1109/iembs.2011.6091733.
Zibrandtsen IC, Kidmose P, Christensen CB, Kjaer TW. Ear-EEG detects ictal and interictal abnormalities in focal and generalized epilepsy - A comparison with scalp EEG monitoring. Clin Neurophysiol [Internet]. 2017;128(12):2454–61. https://doi.org/10.1016/j.clinph.2017.09.115.
Musaeus CS, Waldemar G, Andersen BB, Høgh P, Kidmose P, Hemmsen MC et al. Long-Term EEG Monitoring in Patients with Alzheimer’s Disease Using Ear-EEG: A Feasibility Study. J Alzheimer’s Dis [Internet]. 2022;90(4):1713–23. Available from: https://www.medra.org/servlet/aliasResolver?alias=iospress&doi=10.3233/JAD-220491
Musaeus CS, Frederiksen KS, Andersen BB, Høgh P, Kidmose P, Fabricius M et al. Detection of subclinical epileptiform discharges in Alzheimer’s disease using long-term outpatient EEG monitoring. Neurobiol Dis [Internet]. 2023;183:106149. Available from: https://linkinghub.elsevier.com/retrieve/pii/S0969996123001638
Alsop DC, Detre JA, Grossman M. Assessment of cerebral blood flow in Alzheimer’s disease by spin-labeled magnetic resonance imaging. Ann Neurol [Internet]. 2000;47(1):93–100. https://doi.org/10.1002/1531-8249(200001)47:1%3C93 ::aid-ana15%3E3.0.co;2-8.
Asllani I, Habeck C, Scarmeas N, Borogovac A, Brown TR, Stern Y. Multivariate and Univariate Analysis of Continuous Arterial Spin Labeling Perfusion MRI in Alzheimer’s Disease. J Cereb Blood Flow Metab [Internet]. 2007;28(4):725–36. https://doi.org/10.1038/sj.jcbfm.9600570
Dai W, Lopez OL, Carmichael OT, Becker JT, Kuller LH, Gach HM. Mild Cognitive Impairment and Alzheimer Disease: Patterns of Altered Cerebral Blood Flow at MR Imaging. Radiology [Internet]. 2009;250(3):856–66. https://doi.org/10.1148/radiol.2503080751
Binnewijzend MAA, Kuijer JPA, Benedictus MR, van der Flier WM, Wink AM, Wattjes MP et al. Cerebral Blood Flow Measured with 3D Pseudocontinuous Arterial Spin-labeling MR Imaging in Alzheimer Disease and Mild Cognitive Impairment: A Marker for Disease Severity. Radiology [Internet]. 2013;267(1):221–30. https://doi.org/10.1148/radiol.12120928
Ding B, Ling HW, Huang J, Zhang H, Wang T, Yan FH, et al. Pattern of cerebral hyperperfusion in Alzheimer's disease and amnestic mild cognitive impairment using voxel-based analysis of 3D arterial spin-labeling imaging: initial experience. Clin Interv Aging [Internet]. 2014;493. Available from: http://dx.doi.org/10.2147/cia.s58879
McKhann GM, Knopman DS, Chertkow H, Hyman BT, Jack CR, Kawas CH, et al. The diagnosis of dementia due to Alzheimer’s disease: recommendations from the National Institute on Aging-Alzheimer’s Association workgroups on diagnostic guidelines for Alzheimer’s disease. Alzheimers Dement [Internet]. 2011;7(3):263–9. https://doi.org/10.1016/j.jalz.2011.03.005. Available from:.
Folstein MF, Folstein SE, McHugh PR. Mini-mental state. A practical method for grading the cognitive state of patients for the clinician. J Psychiatr Res. 1975/11/01. 1975;12(3):189–98.
Pfeffer RI, Kurosaki TT, Harrah CH, Chance JM, Filos S. Measurement of Functional Activities in Older Adults in the Community. J Gerontol [Internet]. 1982;37(3):323–9. https://doi.org/10.1093/geronj/37.3.323.
Cummings JL, Mega M, Gray K, Rosenberg-Thompson S, Carusi DA, Gornbein J. The Neuropsychiatric Inventory: Comprehensive assessment of psychopathology in dementia. Neurology [Internet]. 1994;44(12):2308. https://doi.org/10.1212/wnl.44.12.2308.
Kural MA, Duez L, Sejer Hansen V, Larsson PG, Rampp S, Schulz R et al. Criteria for defining interictal epileptiform discharges in EEG. Neurology [Internet]. 2020;94(20):e2139 LP-e2147. Available from: http://n.neurology.org/content/94/20/e2139.abstract
Voevodskaya O, Simmons A, Nordenskjöld R, Kullberg J, Ahlström H, Lind L et al. The effects of intracranial volume adjustment approaches on multiple regional MRI volumes in healthy aging and Alzheimer’s disease [Internet]. Vol. 6, Frontiers in Aging Neuroscience. 2014. Available from: https://www.frontiersin.org/articles/ https://doi.org/10.3389/fnagi.2014.00264
Bakker CJ, Hartkamp MJ, Mali WP. Measuring blood flow by nontriggered 2D phase-contrast MR Angiography. Magn Reson Imaging. 1996;14(6):609–14.
Vestergaard MB, Lindberg U, Aachmann-Andersen NJ, Lisbjerg K, Christensen SJ, Rasmussen P, et al. Comparison of global cerebral blood flow measured by phase-contrast mapping MRI with (15) O-H(2) o positron emission tomography. J Magn Reson Imaging. 2017;45(3):692–9.
Chappell MA, Groves AR, Whitcher B, Woolrich MW. Variational Bayesian Inference for a Nonlinear Forward Model. Trans Sig Proc [Internet]. 2009;57(1):223–236. https://doi.org/10.1109/TSP.2008.2005752
Chappell MA, MacIntosh BJ, Donahue MJ, Günther M, Jezzard P, Woolrich MW. Separation of macrovascular signal in multi-inversion time arterial spin labelling MRI. Magn Reson Med. 2010;63(5):1357–65.
Cunningham SI, Tomasi D, Volkow ND. Structural and functional connectivity of the precuneus and thalamus to the default mode network. Hum Brain Mapp. 2017;38(2):938–56.
Alavi A, Yakir S, Newberg AB. Positron emission tomography in seizure disorders. Ann N Y Acad Sci. 2011;1228:E1–12.
Press DZ, Musaeus CS, Zhao L, Breton JM, Shafi MM, Dai W, et al. Levetiracetam increases hippocampal blood Flow in Alzheimer’s Disease as measured by arterial spin labelling MRI. J Alzheimers Dis. 2023;93(3):939–48.
Yokoi T, Watanabe H, Yamaguchi H, Bagarinao E, Masuda M, Imai K et al. Involvement of the Precuneus/Posterior Cingulate Cortex Is Significant for the Development of Alzheimer’s Disease: A PET (THK5351, PiB) and Resting fMRI Study [Internet]. Vol. 10, Frontiers in Aging Neuroscience. 2018. Available from: https://www.frontiersin.org/articles/ https://doi.org/10.3389/fnagi.2018.00304
Wang L, Laviolette P, O’Keefe K, Putcha D, Bakkour A, Van Dijk KRA, et al. Intrinsic connectivity between the hippocampus and posteromedial cortex predicts memory performance in cognitively intact older individuals. NeuroImage. 2010;51(2):910–7.
Hampton OL, Buckley RF, Manning LK, Scott MR, Properzi MJ, Peña-Gómez C et al. Resting-state functional connectivity and amyloid burden influence longitudinal cortical thinning in the default mode network in preclinical Alzheimer’s disease. NeuroImage Clin [Internet]. 2020;28:102407. Available from: https://www.sciencedirect.com/science/article/pii/S2213158220302448
Download references
Acknowledgements
We would like to thank specialist nurses Susanne Kristiansen and Oda Jakobsen for their assistance in recruiting participants and helping during study visits.
The study was funded by the Alzheimer Research Foundation (grant number: 181003), Toyota Foundation (KJ/BG-9171 F), Axel Muusfeldts Foundation (2016 − 527), Ellen Mørchs foundation (J.nr. 32491419), Rigshospitalet Research Foundation and T&W Engineering. None of the funding parties had a role in the collection, analysis, and interpretation of data or in the writing of the manuscript or whether to publish the results of the study. CSM received funding from the Lundbeck Foundation (R358-2020-2344).
Open access funding provided by Copenhagen University
Author information
Authors and affiliations.
Danish Dementia Research Centre (DDRC), Department of Neurology, Copenhagen University Hospital – Rigshospitalet, Inge Lehmanns vej 8, Copenhagen, 2100, Denmark
Christian Sandøe Musaeus, Troels Wesenberg Kjaer, Henrik Bo, Birgitte Bo Andersen, Steen Gregers Hasselbalch, Gunhild Waldemar & Kristian Steen Frederiksen
Regional Dementia Research Centre, Department of Neurology, Zealand University Hospital, Vestermarksvej 11, Roskilde, 4000, Denmark
Functional Imaging Unit, Department of Clinical Physiology and Nuclear Medicine, University of Copenhagen, Valdemar Hansens Vej 13, Glostrup, 2600, Denmark
Ulrich Lindberg, Mark B. Vestergaard & Wiberg Larsson
Department of Clinical Medicine, University of Copenhagen, Blegdamsvej 3B, Copenhagen, 2200, Denmark
Peter Høgh, Steen Gregers Hasselbalch, Gunhild Waldemar & Kristian Steen Frederiksen
Department of Electrical and Computer Engineering, Aarhus University, Finlandsgade 22, Aarhus N, 8200, Denmark
Preben Kidmose
Berenson-Allen Center for Non-invasive Brain Stimulation, Beth Israel Deaconess Medical Center, Harvard Medical School, 330 Brookline Ave, Boston, MA, 02215, USA
Daniel Zvi Press
T&W Engineering, Borupvang 2, Lillerød, 3450, Denmark
Martin Christian Hemmsen & Mike Lind Rank
You can also search for this author in PubMed Google Scholar
Contributions
CSM: Conceptualization, Data Curation, Formal Analysis, Funding Acquisition, Investigation, Methodology, Project Administration, Software, Visualization, Writing – Original Draft PreparationTWK: Methodology, Formal analysis, Resources, Writing - Review & Editing, Visualization, Supervision, Project administration UL: Conceptualization, Data Curation, Formal Analysis, Resources, Methodology, Software, Visualization, Writing – Review & EditingMBV: Conceptualization, Formal Analysis, Resources, Methodology, Software, Writing – Review & EditingHBWL: Conceptualization, Resources, Methodology, Writing – Review & EditingDZP: Conceptualization, Methodology, Writing – Review & EditingBBA: Conceptualization, Investigation, Resources, Writing – Review & EditingPH: Conceptualization, Investigation, Resources, Writing – Review & EditingPK: Conceptualization, Methodology, Software, Visualization, Writing – Review & EditingMCH: Conceptualization, Methodology, Resources, Writing – Review & EditingMLR: Conceptualization, Methodology, Resources, Writing – Review & EditingSGH: Conceptualization, Writing – Review & EditingGW: Conceptualization, Methodology, Resources, Investigation, Writing - Review & Editing, Supervision, Project administration, Funding acquisitionKSF: Conceptualization, Methodology, Investigation, Writing - Review & Editing, Supervision, Project administration.
Corresponding author
Correspondence to Christian Sandøe Musaeus .
Ethics declarations
Ethics approval and consent to participate.
The study was approved by the Capital Region Ethics Committee (H-17035751), and by the Danish Medicines Agency (2017112288). All participants gave written and oral informed consent before participating in the study.
Consent for publication
Not applicable.
Competing interests
CSM received an unrestricted grant from T&W engineering. Two authors (Mike Lind Rank, and Martin Christian Hemmsen) who are employees of T&W Engineering contributed to the interpretation and writing of the report. TWK consults for T&W engineering. KSF serves as an associate editor for Alzheimer’s Research & Therapy.
Additional information
Publisher’s note.
Springer Nature remains neutral with regard to jurisdictional claims in published maps and institutional affiliations.
Electronic supplementary material
Below is the link to the electronic supplementary material.
Supplementary Material 1
Rights and permissions.
Open Access This article is licensed under a Creative Commons Attribution 4.0 International License, which permits use, sharing, adaptation, distribution and reproduction in any medium or format, as long as you give appropriate credit to the original author(s) and the source, provide a link to the Creative Commons licence, and indicate if changes were made. The images or other third party material in this article are included in the article’s Creative Commons licence, unless indicated otherwise in a credit line to the material. If material is not included in the article’s Creative Commons licence and your intended use is not permitted by statutory regulation or exceeds the permitted use, you will need to obtain permission directly from the copyright holder. To view a copy of this licence, visit http://creativecommons.org/licenses/by/4.0/ . The Creative Commons Public Domain Dedication waiver ( http://creativecommons.org/publicdomain/zero/1.0/ ) applies to the data made available in this article, unless otherwise stated in a credit line to the data.
Reprints and permissions
About this article
Cite this article.
Musaeus, C.S., Kjaer, T.W., Lindberg, U. et al. Subclinical epileptiform discharges in Alzheimer’s disease are associated with increased hippocampal blood flow. Alz Res Therapy 16 , 80 (2024). https://doi.org/10.1186/s13195-024-01432-9
Download citation
Received : 26 October 2023
Accepted : 19 March 2024
Published : 12 April 2024
DOI : https://doi.org/10.1186/s13195-024-01432-9
Share this article
Anyone you share the following link with will be able to read this content:
Sorry, a shareable link is not currently available for this article.
Provided by the Springer Nature SharedIt content-sharing initiative
- Alzheimer’s disease
- Hyperperfusion
- Epileptiform discharges
- Spike frequency
Alzheimer's Research & Therapy
ISSN: 1758-9193
- General enquiries: [email protected]

An official website of the United States government
The .gov means it’s official. Federal government websites often end in .gov or .mil. Before sharing sensitive information, make sure you’re on a federal government site.
The site is secure. The https:// ensures that you are connecting to the official website and that any information you provide is encrypted and transmitted securely.
- Publications
- Account settings
Preview improvements coming to the PMC website in October 2024. Learn More or Try it out now .
- Advanced Search
- Journal List
- v.53(4); 2010 Aug

Research questions, hypotheses and objectives
Patricia farrugia.
* Michael G. DeGroote School of Medicine, the
Bradley A. Petrisor
† Division of Orthopaedic Surgery and the
Forough Farrokhyar
‡ Departments of Surgery and
§ Clinical Epidemiology and Biostatistics, McMaster University, Hamilton, Ont
Mohit Bhandari
There is an increasing familiarity with the principles of evidence-based medicine in the surgical community. As surgeons become more aware of the hierarchy of evidence, grades of recommendations and the principles of critical appraisal, they develop an increasing familiarity with research design. Surgeons and clinicians are looking more and more to the literature and clinical trials to guide their practice; as such, it is becoming a responsibility of the clinical research community to attempt to answer questions that are not only well thought out but also clinically relevant. The development of the research question, including a supportive hypothesis and objectives, is a necessary key step in producing clinically relevant results to be used in evidence-based practice. A well-defined and specific research question is more likely to help guide us in making decisions about study design and population and subsequently what data will be collected and analyzed. 1
Objectives of this article
In this article, we discuss important considerations in the development of a research question and hypothesis and in defining objectives for research. By the end of this article, the reader will be able to appreciate the significance of constructing a good research question and developing hypotheses and research objectives for the successful design of a research study. The following article is divided into 3 sections: research question, research hypothesis and research objectives.
Research question
Interest in a particular topic usually begins the research process, but it is the familiarity with the subject that helps define an appropriate research question for a study. 1 Questions then arise out of a perceived knowledge deficit within a subject area or field of study. 2 Indeed, Haynes suggests that it is important to know “where the boundary between current knowledge and ignorance lies.” 1 The challenge in developing an appropriate research question is in determining which clinical uncertainties could or should be studied and also rationalizing the need for their investigation.
Increasing one’s knowledge about the subject of interest can be accomplished in many ways. Appropriate methods include systematically searching the literature, in-depth interviews and focus groups with patients (and proxies) and interviews with experts in the field. In addition, awareness of current trends and technological advances can assist with the development of research questions. 2 It is imperative to understand what has been studied about a topic to date in order to further the knowledge that has been previously gathered on a topic. Indeed, some granting institutions (e.g., Canadian Institute for Health Research) encourage applicants to conduct a systematic review of the available evidence if a recent review does not already exist and preferably a pilot or feasibility study before applying for a grant for a full trial.
In-depth knowledge about a subject may generate a number of questions. It then becomes necessary to ask whether these questions can be answered through one study or if more than one study needed. 1 Additional research questions can be developed, but several basic principles should be taken into consideration. 1 All questions, primary and secondary, should be developed at the beginning and planning stages of a study. Any additional questions should never compromise the primary question because it is the primary research question that forms the basis of the hypothesis and study objectives. It must be kept in mind that within the scope of one study, the presence of a number of research questions will affect and potentially increase the complexity of both the study design and subsequent statistical analyses, not to mention the actual feasibility of answering every question. 1 A sensible strategy is to establish a single primary research question around which to focus the study plan. 3 In a study, the primary research question should be clearly stated at the end of the introduction of the grant proposal, and it usually specifies the population to be studied, the intervention to be implemented and other circumstantial factors. 4
Hulley and colleagues 2 have suggested the use of the FINER criteria in the development of a good research question ( Box 1 ). The FINER criteria highlight useful points that may increase the chances of developing a successful research project. A good research question should specify the population of interest, be of interest to the scientific community and potentially to the public, have clinical relevance and further current knowledge in the field (and of course be compliant with the standards of ethical boards and national research standards).
FINER criteria for a good research question
Adapted with permission from Wolters Kluwer Health. 2
Whereas the FINER criteria outline the important aspects of the question in general, a useful format to use in the development of a specific research question is the PICO format — consider the population (P) of interest, the intervention (I) being studied, the comparison (C) group (or to what is the intervention being compared) and the outcome of interest (O). 3 , 5 , 6 Often timing (T) is added to PICO ( Box 2 ) — that is, “Over what time frame will the study take place?” 1 The PICOT approach helps generate a question that aids in constructing the framework of the study and subsequently in protocol development by alluding to the inclusion and exclusion criteria and identifying the groups of patients to be included. Knowing the specific population of interest, intervention (and comparator) and outcome of interest may also help the researcher identify an appropriate outcome measurement tool. 7 The more defined the population of interest, and thus the more stringent the inclusion and exclusion criteria, the greater the effect on the interpretation and subsequent applicability and generalizability of the research findings. 1 , 2 A restricted study population (and exclusion criteria) may limit bias and increase the internal validity of the study; however, this approach will limit external validity of the study and, thus, the generalizability of the findings to the practical clinical setting. Conversely, a broadly defined study population and inclusion criteria may be representative of practical clinical practice but may increase bias and reduce the internal validity of the study.
PICOT criteria 1
A poorly devised research question may affect the choice of study design, potentially lead to futile situations and, thus, hamper the chance of determining anything of clinical significance, which will then affect the potential for publication. Without devoting appropriate resources to developing the research question, the quality of the study and subsequent results may be compromised. During the initial stages of any research study, it is therefore imperative to formulate a research question that is both clinically relevant and answerable.
Research hypothesis
The primary research question should be driven by the hypothesis rather than the data. 1 , 2 That is, the research question and hypothesis should be developed before the start of the study. This sounds intuitive; however, if we take, for example, a database of information, it is potentially possible to perform multiple statistical comparisons of groups within the database to find a statistically significant association. This could then lead one to work backward from the data and develop the “question.” This is counterintuitive to the process because the question is asked specifically to then find the answer, thus collecting data along the way (i.e., in a prospective manner). Multiple statistical testing of associations from data previously collected could potentially lead to spuriously positive findings of association through chance alone. 2 Therefore, a good hypothesis must be based on a good research question at the start of a trial and, indeed, drive data collection for the study.
The research or clinical hypothesis is developed from the research question and then the main elements of the study — sampling strategy, intervention (if applicable), comparison and outcome variables — are summarized in a form that establishes the basis for testing, statistical and ultimately clinical significance. 3 For example, in a research study comparing computer-assisted acetabular component insertion versus freehand acetabular component placement in patients in need of total hip arthroplasty, the experimental group would be computer-assisted insertion and the control/conventional group would be free-hand placement. The investigative team would first state a research hypothesis. This could be expressed as a single outcome (e.g., computer-assisted acetabular component placement leads to improved functional outcome) or potentially as a complex/composite outcome; that is, more than one outcome (e.g., computer-assisted acetabular component placement leads to both improved radiographic cup placement and improved functional outcome).
However, when formally testing statistical significance, the hypothesis should be stated as a “null” hypothesis. 2 The purpose of hypothesis testing is to make an inference about the population of interest on the basis of a random sample taken from that population. The null hypothesis for the preceding research hypothesis then would be that there is no difference in mean functional outcome between the computer-assisted insertion and free-hand placement techniques. After forming the null hypothesis, the researchers would form an alternate hypothesis stating the nature of the difference, if it should appear. The alternate hypothesis would be that there is a difference in mean functional outcome between these techniques. At the end of the study, the null hypothesis is then tested statistically. If the findings of the study are not statistically significant (i.e., there is no difference in functional outcome between the groups in a statistical sense), we cannot reject the null hypothesis, whereas if the findings were significant, we can reject the null hypothesis and accept the alternate hypothesis (i.e., there is a difference in mean functional outcome between the study groups), errors in testing notwithstanding. In other words, hypothesis testing confirms or refutes the statement that the observed findings did not occur by chance alone but rather occurred because there was a true difference in outcomes between these surgical procedures. The concept of statistical hypothesis testing is complex, and the details are beyond the scope of this article.
Another important concept inherent in hypothesis testing is whether the hypotheses will be 1-sided or 2-sided. A 2-sided hypothesis states that there is a difference between the experimental group and the control group, but it does not specify in advance the expected direction of the difference. For example, we asked whether there is there an improvement in outcomes with computer-assisted surgery or whether the outcomes worse with computer-assisted surgery. We presented a 2-sided test in the above example because we did not specify the direction of the difference. A 1-sided hypothesis states a specific direction (e.g., there is an improvement in outcomes with computer-assisted surgery). A 2-sided hypothesis should be used unless there is a good justification for using a 1-sided hypothesis. As Bland and Atlman 8 stated, “One-sided hypothesis testing should never be used as a device to make a conventionally nonsignificant difference significant.”
The research hypothesis should be stated at the beginning of the study to guide the objectives for research. Whereas the investigators may state the hypothesis as being 1-sided (there is an improvement with treatment), the study and investigators must adhere to the concept of clinical equipoise. According to this principle, a clinical (or surgical) trial is ethical only if the expert community is uncertain about the relative therapeutic merits of the experimental and control groups being evaluated. 9 It means there must exist an honest and professional disagreement among expert clinicians about the preferred treatment. 9
Designing a research hypothesis is supported by a good research question and will influence the type of research design for the study. Acting on the principles of appropriate hypothesis development, the study can then confidently proceed to the development of the research objective.
Research objective
The primary objective should be coupled with the hypothesis of the study. Study objectives define the specific aims of the study and should be clearly stated in the introduction of the research protocol. 7 From our previous example and using the investigative hypothesis that there is a difference in functional outcomes between computer-assisted acetabular component placement and free-hand placement, the primary objective can be stated as follows: this study will compare the functional outcomes of computer-assisted acetabular component insertion versus free-hand placement in patients undergoing total hip arthroplasty. Note that the study objective is an active statement about how the study is going to answer the specific research question. Objectives can (and often do) state exactly which outcome measures are going to be used within their statements. They are important because they not only help guide the development of the protocol and design of study but also play a role in sample size calculations and determining the power of the study. 7 These concepts will be discussed in other articles in this series.
From the surgeon’s point of view, it is important for the study objectives to be focused on outcomes that are important to patients and clinically relevant. For example, the most methodologically sound randomized controlled trial comparing 2 techniques of distal radial fixation would have little or no clinical impact if the primary objective was to determine the effect of treatment A as compared to treatment B on intraoperative fluoroscopy time. However, if the objective was to determine the effect of treatment A as compared to treatment B on patient functional outcome at 1 year, this would have a much more significant impact on clinical decision-making. Second, more meaningful surgeon–patient discussions could ensue, incorporating patient values and preferences with the results from this study. 6 , 7 It is the precise objective and what the investigator is trying to measure that is of clinical relevance in the practical setting.
The following is an example from the literature about the relation between the research question, hypothesis and study objectives:
Study: Warden SJ, Metcalf BR, Kiss ZS, et al. Low-intensity pulsed ultrasound for chronic patellar tendinopathy: a randomized, double-blind, placebo-controlled trial. Rheumatology 2008;47:467–71.
Research question: How does low-intensity pulsed ultrasound (LIPUS) compare with a placebo device in managing the symptoms of skeletally mature patients with patellar tendinopathy?
Research hypothesis: Pain levels are reduced in patients who receive daily active-LIPUS (treatment) for 12 weeks compared with individuals who receive inactive-LIPUS (placebo).
Objective: To investigate the clinical efficacy of LIPUS in the management of patellar tendinopathy symptoms.
The development of the research question is the most important aspect of a research project. A research project can fail if the objectives and hypothesis are poorly focused and underdeveloped. Useful tips for surgical researchers are provided in Box 3 . Designing and developing an appropriate and relevant research question, hypothesis and objectives can be a difficult task. The critical appraisal of the research question used in a study is vital to the application of the findings to clinical practice. Focusing resources, time and dedication to these 3 very important tasks will help to guide a successful research project, influence interpretation of the results and affect future publication efforts.
Tips for developing research questions, hypotheses and objectives for research studies
- Perform a systematic literature review (if one has not been done) to increase knowledge and familiarity with the topic and to assist with research development.
- Learn about current trends and technological advances on the topic.
- Seek careful input from experts, mentors, colleagues and collaborators to refine your research question as this will aid in developing the research question and guide the research study.
- Use the FINER criteria in the development of the research question.
- Ensure that the research question follows PICOT format.
- Develop a research hypothesis from the research question.
- Develop clear and well-defined primary and secondary (if needed) objectives.
- Ensure that the research question and objectives are answerable, feasible and clinically relevant.
FINER = feasible, interesting, novel, ethical, relevant; PICOT = population (patients), intervention (for intervention studies only), comparison group, outcome of interest, time.
Competing interests: No funding was received in preparation of this paper. Dr. Bhandari was funded, in part, by a Canada Research Chair, McMaster University.
- Open access
- Published: 11 April 2024
The Fremantle Back Awareness Questionnaire: cross-cultural adaptation, reliability, and validity of the Italian version in people with chronic low back pain
- Marco Monticone 1 ,
- Carolina Maurandi 2 ,
- Elisa Porcu 3 ,
- Federico Arippa 4 ,
- Benedict M. Wand 5 &
- Giorgio Corona 6
BMC Musculoskeletal Disorders volume 25 , Article number: 279 ( 2024 ) Cite this article
88 Accesses
Metrics details
Background and aim
There is evidence to suggest that assessing back-specific altered self-perception may be useful when seeking to understand and manage low back pain (LBP). The Fremantle Back Awareness Questionnaire (FreBAQ) is a patient-reported measure of back-specific body perception that has never been adapted and psychometrically analysed in Italian. Hence, the objectives of this research were to cross-culturally adapt and validate the Italian version of this outcome measure (namely, the FreBAQ-I), to make it available for use with Italians suffering from chronic LBP.
The FreBAQ-I was developed by forward and backward translation, review by a committee skilled in patient-reported measures and test of the pre-final version to assess its clarity, acceptability, and relevance. The statistical analyses examined: structural validity based on Rasch analysis; hypotheses testing by investigating correlations of the FreBAQ-I with the Roland Morris Disability Questionnaire (RMDQ), a pain intensity numerical rating scale (PI-NRS), the Pain Catastrophising Scale (PCS), and the Tampa Scale of Kinesiophobia (TSK) (Pearson’s correlations); reliability by internal consistency (Cronbach’s alpha) and test–retest repeatability (intraclass correlation coefficient, ICC (2,1)); and measurement error by determining the minimum detectable change (MDC). After the development of a consensus-based translation of the FreBAQ-I, the new outcome measure was delivered to 100 people with chronic LBP.
Rasch analysis confirmed the substantial unidimensionality and the structural validity of the FreBAQ-I. Hypothesis testing was considered good as at least 75% of the hypotheses were confirmed; correlations: RMDQ ( r = 0.35), PI-NRS ( r = 0.25), PCS ( r = 0.41) and TSK ( r = 0.38). Internal consistency was acceptable (alpha = 0.82) and test–retest repeatability was excellent (ICC (2,1) = 0.88, 95% CI: 0.83, 0.92). The MDC 95 corresponded to 6.7 scale points.
The FreBAQ-I was found to be a unidimensional, valid, and reliable outcome measure in Italians with chronic LBP. Its application is advised for clinical and research use within the Italian speaking community.
Peer Review reports
Low back pain (LBP) is a very common condition with a highly variable course[ 1 ]. Most episodes improve considerably within 6 weeks [ 2 ]; however, about two-thirds of persons still report some pain at 3 and 12 months, leading to chronic ill health[ 3 , 4 ]. Chronic LBP has a wide range of deleterious effects on the individual, limiting functional capacity, work participation, and social engagement, as well as negatively impacting personal relationships, and mental and physical health [ 2 ].
An extensive research effort over many years has suggested multiple factors that might impact the chronic LBP experience, including changes in the way the back is perceived or experienced by the individual. Previous studies have reported that people with chronic LBP represent the back differently when asked to draw how it feels to them [ 5 ], have reduced tactile acuity [ 6 ], deficits in proprioception [ 7 ], reduced motor-imagery ability [ 8 ], and changes in tactile processing similar to the spatial neglect seen following cerebrovascular accidents [ 9 ].
Based on these premises, the Fremantle Back Awareness Questionnaire (FreBAQ) –a self-report questionnaire designed to assess back-specific body perception– was specifically developed for persons with chronic LBP [ 10 ]. The questionnaire was shown to be feasible, reliable, and valid, by means of associations with measures of pain duration, pain intensity, disability, and pain catastrophising [ 10 ]. Further, a later study on chronic LBP demonstrated the FreBAQ’s unidimensionality and acceptable internal consistency, as well as offering further support for the relationship between FreBAQ scores and clinical status, including measures of fear-avoidance and psychological distress [ 11 ].
The FreBAQ represents a helpful tool for assessing warning signs of compromised self-perception of the lower back in people with chronic LBP [ 10 , 11 ] and in women with lumbopelvic pain during pregnancy and postpartum [ 12 ]. This questionnaire seems a promising instrument for identifying additional factors involved in the persistence of back problems, and could serve to guide targeted treatment strategies [ 10 , 11 , 13 ]. Indeed, preliminary studies suggest that treatment programs aimed at improving disturbed body perception (through sensorimotor retraining) may have positive effects on pain and function in individuals with non-specific LBP [ 14 , 15 ].
However, the quality of a patient-reported outcome measure (PROM) may differ noticeably when cross-culturally adapted and used in a country different to where it was initially developed [ 16 ]. Well-established methodological criteria are recommended when validation studies are performed [ 17 ]. Application of these criteria are designed to ensure the quality of the new measurement tool and permit more confident comparison of findings across populations.
The FreBAQ has previously been adapted and psychometrically examined in Japanese, Dutch, German, Turkish, Chinese, Indian, Spanish and Persian populations [ 13 , 18 , 19 , 20 , 21 , 22 , 23 , 24 ], but an Italian version (FreBAQ-I) has not yet been cross-culturally adapted and psychometrically analysed in a similar population. Therefore, the purpose of this research was to develop a FreBAQ-I and examine its psychometric properties in Italians suffering from chronic LBP.
The COSMIN (COnsensus-based Standards for the selection of health status Measurement Instruments) guidelines were adopted [ 16 ].
The Institutional Review Board endorsed this cross-sectional study (no. 7/16, April 5th 2016). The research was carried out in accordance with the ethical and humane principles of research specified in the Declaration of Helsinki.
Participants
This research engaged people attending an outpatient Hospital Rehabilitation Unit, meeting the following inclusion criteria: diagnosis of chronic non-specific LBP (i.e. a pain localised below the costal margin and above the inferior gluteal folds lasting for more than three months, without a distinguishable, specific, patho-anatomical cause or disease [ 25 ]); people speaking Italian as their first language (as well as those who had an adequate knowledge of Italian) and aged over 18. Exclusion criteria: acute (lasting up to one month) and subacute non-specific LBP (lasting up to three months); specific LBP (i.e. fracture, spinal deformity, disc herniation, canal stenosis, spondylolisthesis, or infections); peripheral or central neurological disorders assessed by means of imaging (e.g. radiographs, CT scans, or MRI) and/or anamnesis; systemic illness (including rheumatologic diseases); cognitive disorders (Mini Mental State Examination of < 24); recent myocardial infarctions; any past cerebrovascular accidents; and not capable or reluctant to give informed consent.
Participants were assessed by two physical and rehabilitation medicine physicians, who were under the supervision of the principal investigator (MM). Both physicians had at least a fifteen years’ experience, were involved in the assessments of the participants during the research process but not in the treatment procedure. Those who satisfied the criteria for inclusion were provided with information about the research aims and procedures and invited to sign a written informed consent form. After that, demographic and clinical characteristics were collected, and all participants completed the outcome measures listed below. Participants were invited to fill in the FreBAQ-I a second time, 7–10 days after their initial assessment to side-step variations in symptoms associated with possible memory effects [ 26 ].
Cross-cultural adaptation
The Italian translation and adaptation of the FreBAQ was performed following the American Association of Orthopaedic Surgeon Outcomes Committee’s recommended protocol and according to the standards for good practice used in the translation and cultural adaptation procedure for PROM [ 27 , 28 ].
Step 1: Italian translation
The original FreBAQ [ 10 ] was independently translated into Italian by two bilingual professionals with distinct backgrounds and some experience in the PROM field. They strove to select terms capturing the connotative meanings of the source text and –at the same time– reflecting everyday-spoken language. Conflicts between translations were examined by the principal investigator and then settled by consensus, in order to consolidate a preliminary Italian version.
Step 2: Back-translation Into English
Two native English-speaking bilingual professional translators, separately back-translated the preliminary adaptation. Then, the principal investigator and the translators checked and clarified potential inconsistencies between the different versions and agreed an advanced Italian version of the questionnaire.
Step 3: Expert Committee
This advanced version was submitted to a team of six bilingual clinicians, methodologists, and translators. They investigated the idiomatic, semantic, and theoretical similarity of items and response categories. This phase finished when a consensus was reached on a prefinal version.
Step 4: Test of the prefinal version
A pilot test was then performed to explore intelligibility, appropriateness, cultural relevance, and potential ambiguity of the prefinal version. Cognitive interviews were performed by a qualified psychologist after administering the tool to 10 persons with chronic LBP, representatives of the target population. The team of experts examined the findings of this test in order to detect any useful refinement and then agreed the final version of FreBAQ-I. These deliberations are available from the corresponding author on request.
Acceptability and feasibility
Participants were interviewed about the comprehensibility of each part of the questionnaire, and the data were verified for missing or multiple answers. The time to compile each questionnaire was gathered (Fig. 1 ).
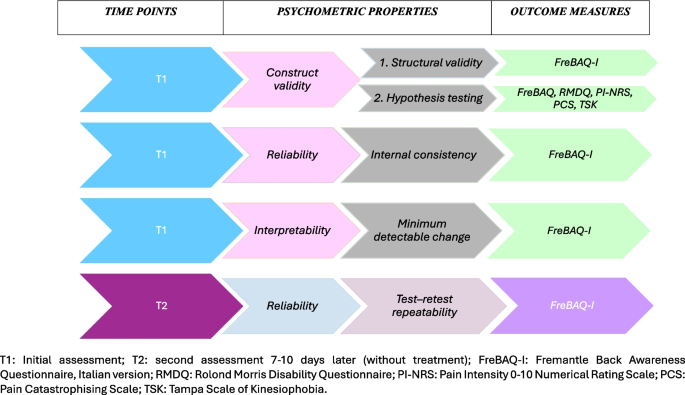
The study procedure
Psychometric properties
Construct validity.
It represents the degree to which the scores of a measurement instrument are consistent with hypotheses, with regard to internal relationships, or relationships with scores of other instruments or differences between relevant groups [ 29 ] and was assessed by structural validity and hypothesis testing.
1. Structural validity (i.e. the degree to which the scores of a measurement instrument are an adequate reflection of the dimensionality of the construct to be measured [ 29 ]) . Rasch analysis (Winsteps software v. 4.8.0) examined the FreBAQ-I using the rating scale model (because all items shared the same rating scale structure). Our detailed iterative procedure has been reported in previous studies [ 30 , 31 ]. In short, the following psychometric issues were investigated:
a) diagnostic assessment of the rating categories, by investigating whether each response category was being used consistently and effectively; for that, the transition thresholds between categories (i.e. the points where two adjacent categories have an equal probability to be endorsed) and average category measures should be ordered from less to more on the underlying latent continuum [ 32 ];
b) internal construct validity, assessed checking how well the observed responses to the items align with the responses predicted by the Rasch model, using chi-square fit statistics (infit and outfit mean-square statistics, MnSq). Based on the sample size, values from 0.75 to 1.30 [ 33 ] were considered as indicating an acceptable fit;
c) reliability, in terms of both person reliability index and item reliability index [ 32 ], providing an estimate of the degree of replicability (across different samples) of person and item placements along the trait continuum (range 0–1; coefficients > 0.80 are considered as good, > 0.90 as excellent). High reliability levels (of persons or items) mean that there is a high probability that persons (or items) estimated with high Rasch measures actually do have higher measures than persons (or items) estimated with low measures [ 33 ].
d) unidimensionality of the scale, examining the unexplained variance after the Rasch dimension is extracted, as obtained by a Principal Component Analysis of the residuals (PCAr). Additional factors are not likely to be present in the residuals if the eigenvalue of the first residual component is < 2 [ 33 ];
e) local item dependence. For any pair of items, no residual correlation > 0.20 (above the average observed residual correlation) should be detected once the variable under measurement (Rasch factor) has been filtered out [ 34 ].
2. Hypothesis testing , which takes place when hypotheses are formulated a priori on the relationships of scores on the instrument under investigation with scores deriving from other measures evaluating related or dissimilar constructs, by also describing the expected direction (i.e. positive or negative) and magnitude (i.e. low, moderate, large) [ 29 ] . Based on what previously assumed in a previous study on the same matter [ 11 ], it was hypothesized a priori the FreBAQ-I would achieve positive moderate correlations (from 0.30 to 0.60) with measures of disability, and catastrophizing, and low correlations (< 0.30) with measures of pain intensity and kinesiophobia. Pearson’s correlation coefficients were calculated, and construct validity was considered as satisfactory if at least 75% of the hypotheses was reached [ 26 ].
- Reliability
It represents the degree to which the measurement is free from measurement error [ 29 ] and was calculated as detailed below.
1. Internal consistency is the degree of interrelatedness among the items [ 29 ]) and was evaluated by calculating Cronbach’s alpha (values of > 0.70 being considered acceptable).
2. Test–retest repeatability is the degree to which the measurement is free from measurement error over time [ 29 ]) and was examined 7–10 days later without treatment using the intraclass correlation coefficient, ICC (2,1) (values of 0.70–0.85 were considered good and > 0.85 excellent) [ 26 ].
3. The standard error of measurement (SEM) is the difference between an amount that can be measured and its true value [ 29 ]) and was assessed using the formula:
where SD represents the standard deviation of the measurements at baseline.
Interpretability
It represents the degree to which one can assign qualitative meaning to an instrument’s quantitative scores or change in scores [ 29 ] and was calculated by the minimum detectable change (MDC) (i.e. the change beyond measurement error [ 29 ]) by using the following equation:
A z value of 1.96 was used to derive a 95% confidence level MDC (MDC 95 ).
Descriptive statistics were further calculated to identify floor/ceiling effects, which were present if > 15% of the scores achieve their lowest or highest potential value, respectively [ 26 ].
Questionnaires
This questionnaire quantifies distorted perception of the back. It is self-administered and includes 9 items. Each item is scored by a five-point response scale (range: 0 = ‘never’ up to 4 = ‘always’); the final score is obtained by summing the responses from each of the items and ranges from 0 to 36, with higher scores corresponding to greater levels of back-perception distortion [ 10 ].
Roland Morris Disability Questionnaire
This questionnaire assesses LBP related disability. It comprises 24 items, with a total score ranging from 0 (no disability) to 24 (highest level of disability) [ 35 ].
Pain Numerical Rating Scale (PI-NRS)
An 11-point pain numerical rating scale ranging from 0 (no pain at all) to 10 (the worst imaginable pain) was used [ 36 ], asking participants to rate their current pain intensity.
Pain Catastrophising Scale (PCS)
This is a 13-item self-administered questionnaire. People are asked to classify the frequency with which they experience the thoughts listed in the tool, based on a five-point scale, which ranges from 0 (never) to 4 (always). The total score is obtained summing up the scores of the individual items and can vary from 0 to 52 [ 37 ]. Higher scores denote greater levels of pain catastrophising.
Tampa Scale of Kinesiophobia (TSK)
This questionnaire is self-administered and composed of 13 items [ 38 ]. Each item is scored using a four-point Likert scale ranging from 1 (strongly disagree) to 4 (strongly agree), and the total score is calculated by adding the scores of the individual items (range 13–52). Higher values correspond to greater fear of movement [ 38 ].
All outcome measures were administered in their validated Italian versions [ 35 , 37 , 38 , 39 , 40 , 41 ]. The FreBAQ-I was systematically distributed first, then the RMDQ, the NRS, the TSK and the PCS during the first assessment, respectively; only the FreBAQ-I was delivered during the second assessment. Statistical analyses were performed with STATA 13.1 software (StataCorp LP, College Station, TX, USA).
The sample size of 100 was determined to provide adequate statistical power for test–retest reliability, expecting to obtain, with 90% probability, an ICC of about 0.85, with the lower limit of the 95% CI not less than 0.75 [ 42 ]. Moreover, a sample size of 100 participants is able to ensure stability in Rasch item calibrations within ± 0.5 logits with 95% confidence [ 43 ].
One hundred and thirty-five persons with chronic non-specific LBP were consecutively assessed, of whom 25 were excluded. The reasons for exclusion were: cognitive impairment ( n = 5); systemic illness ( n = 4); recent cerebrovascular event ( n = 2); recent myocardial infarction ( n = 7); and reluctance to take part ( n = 7). Of the remaining people, 10 dropped out before starting the study because of: logistic issues ( n = 4); economic constraints ( n = 3); or personal problems ( n = 3). Hence, our final sample was comprised of 100 subjects. All participants returned to the hospital for a second assessment within a period of 7 to 10 days, facilitated by a telephonic follow-up conducted by a research assistant. Average pain duration was 49 months (SD 80). Socio-demographic and clinical characteristics of the participants are described in Tables 1 and 2 (Fig. 2 ).
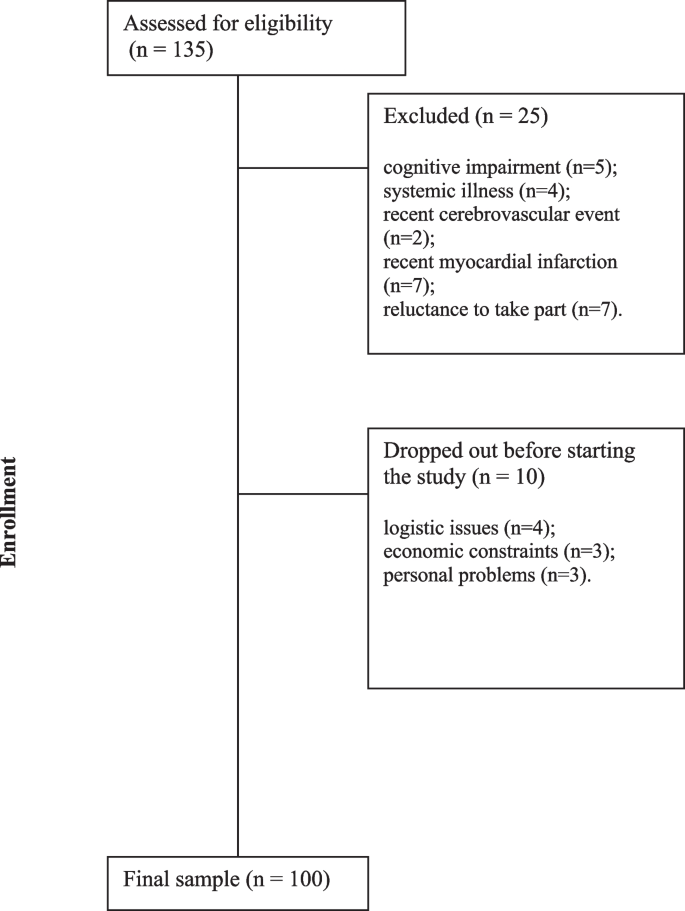
Flow chart of participants inclusion
Translation and cross-cultural adaptation
The adaptation process took four weeks to settle upon a culturally appropriate version. All the terms were easily forward and back translated and there was no need of any major local adjustment. The term “occasionally” of one rating category was translated in Italian with “qualche volta” because it was judged as more suitable for a middle response category, while the translation “occasionalmente” would have been hardly discernible in Italian from the adjacent category “rarely/raramente”.
The appropriateness of the whole procedure and related results was endorsed by the team of experts, who also reviewed the findings from the cognitive interviews and made only minor changes based on concerns raised by some participants, to enhance the questionnaire’s comprehensibility. After that, the principal investigator and experts confirmed the final version of the FreBAQ-I, in agreement with the developer (BMW).
Acceptability
The questionnaire took 1.97 ± 1.13 min to complete. The questions were well received. No missing responses or multiple responses were observed, nor were any comprehension difficulties raised during the instrument completion.
Scale psychometric properties
Structural validity.
The 5-level rating scale of the FreBAQ-I showed a monotonical advance of both the transition thresholds between categories and the average category measure. According to the mean-square goodness-of-fit statistics, all items fitted the Rasch model (Table 3 ).
The mean person ability was -0.97 logits (ability range from -3.06 to 3.34). The item reliability was 0.96, while person reliability was 0.75. The PCAr showed that FreBAQ was essentially unidimensional: the measured variable explained 50.3% of the variance in the data, while the secondary component explained only 9.9% of the variance (corresponding to an eigenvalue of 1.8). No local item dependence was detected, (i.e., no strong > 0.20 residual correlation between items was found).
Hypothesis testing
It was considered good as 3 out of 4 a priori hypotheses were confirmed (i.e., ≥ 75%). Related results are shown in Table 4 .
Cronbach’s α was 0.82. Test–retest reliability was found to be high: ICC (2,1) = 0.88 (95% CI: 0.83—0.92). The SEM was 2.44.
The MDC 95 was 6.7 points.
The mean score for the FreBAQ-I was 9.68 points (SD 6.98). No ceiling or floor effects were detected in any of the used scales, including the FreBAQ-I.
This research describes the cross-cultural adaptation of the FreBAQ and the assessment of its validity, reliability, and measurement error, in Italian-speaking people with chronic non-specific LBP. The FreBAQ-I demonstrated unidimensionality, good validity, and adequate reliability. International recommendations were followed in the current study and all the steps suggested that the process of translation and cross-cultural adaptation was accurate and efficient. Our methodological approach comprised forward and backward translation, minor amendments by a team of experts, cognitive debriefing, and discussion and resolution through consensus among the committee members. The procedure established the initial conceptual, semantic, and content equivalence between source and target language. The final version was well accepted, and easily understood and self-administered. The respondent burden was minimal as the questionnaire needs only a few minutes to complete. Overall, the FreBAQ-I appears to be appropriate for everyday clinical practice.
Our results corroborated the findings of the previous structural validations of this outcome measure [ 11 , 13 , 44 ]. The tool proved to be unidimensional (a key measurement requirement), with items acceptably fitting the mathematical model, and a rating scale functioning as expected. No local item dependence was found. In our sample the average difficulty of the items (endorsability) was about 1 logit lower than the mean sample ability (agreeability): in that condition the scale better assesses persons with moderate to high levels of disturbed body perception. These findings indicate that the item selection was appropriate and able to correctly measure the variable of interest. A minor deviation from unidimensionality was found in a sample of Indian people with chronic LBP but no issue was expected as for the clinical application of the FreBAQ in this population [ 22 ].
The correlation with disability (RMDQ) was as expected (Table 3 ), indicating that higher levels of back pain related disability are associated with enhanced levels of body perception disruption relating specifically to the back. This is in line with the findings of the developers, where a moderate correlation with disability was observed (0.32)[ 11 ]. As predicted, we also noted a positive correlation between pain intensity and disrupted perception of the back, though this relationship was weaker than that noted for disability, also consistent with previous research [ 11 ]. Taken together, these results suggest that disrupted body perception is more strongly related to disability than pain in people with chronic LBP [ 11 ]. This issue was also seen in most other adapted studies of the FreBAQ (i.e. Japanese, Dutch, German, Turkish and Persian) [ 13 , 18 , 19 , 20 , 24 ], probably indicating disrupted body perception impacts more on the functional consequences of pain, rather than the experience of pain itself. A higher correlation with disability than pain was otherwise found in the Spanish sample (0.48 vs 0.38) [ 23 ]. Very low correlations with disability and pain were found in the Indian study [ 22 ].
With respect to catastrophizing, we noted a similar correlation to what was reported in the original English version (0.36) [ 11 ]. Our results are also consistent with results obtained from the Japanese (0.38) [ 13 ], Turkish (0.41) [ 20 ], Spanish (0.46) [ 23 ] and Persian (0.60) [ 24 ] cross-cultural adaptation studies. These findings support the idea of a relationship between high levels of pain catastrophizing and disrupted body perception. For kinesiophobia, our estimates are slightly higher than those reported by the original developers (0.26) [ 11 ], however this study utilized a different measure of kinesiophobia (i.e. the Fear Avoidance Beliefs Questionnaire, physical activity subscale), which makes direct comparison difficult. Our results are somewhat in line with the Turkish and Spanish estimates (0.60 and 0.37) [ 20 , 23 ], while divergent from what was found in Japanese, Dutch and Persian samples (0.22, 0.10, and 0.17 respectively) [ 13 , 18 , 24 ], and, therefore, more analyses are recommended before firm conclusions can be drawn about the relationship between kinesiophobia and altered body perception.
The internal consistency of the FreBAQ-I was good (0.82) and quite similar to that reported by the developers (0.80) [ 11 ]. Similar results were found in other versions of the questionnaires: Japanese 0.80 [ 13 ]; Dutch 0.82 [ 18 ]; German 0.91 [ 19 ]; Turkish 0.87 [ 20 ]; Chinese 0.83 [ 21 ], Indian 0.91 [ 22 ], Spanish 0.82 [ 23 ] and Persian 0.74 [ 24 ].
Test–retest repeatability demonstrated an excellent level of agreement between the results on days 1 and 8 (ICC (2,1) = 0.88), a value higher than those reported in the original study (0.65) [ 10 ], while in the other validations, values were of 0.69 [ 18 ], 0.78 [ 23 ] and 0.90 [ 21 ]. The better reliability noted in this investigation may reflect the fact that no treatment was provided to participants between the two testing occasions, a control not enacted in the original study [ 10 ]. The measurement error of the FreBAQ-I was acceptable. Due to the high repeatability of the test–retest the SEM and MDC were rather low.
The MDC 95 demonstrated that a change of more than 7 points after a given intervention (~ 19% of the FreBAQ score range of 36 points) would not be the result of an error in measurement. Slightly different findings were achieved by the Dutch study (where the MDC was estimated at 10.8 points) [ 18 ] and the Persian study (2.52 points) [ 24 ], while the validation of the Chinese and the Spanish version of the FreBAQ reported an MDC 95 of 5.99 and 5.12 points, respectively [ 21 ].
This study should acknowledge some limitations. First, the study design is cross-sectional and thus responsiveness and minimal important change could not be assessed. Second, no external anchor such as a global rating of change was used to assess clinical stability during the assessment of reliability and participants may have improved or worsened between the first and second assessment of the FreBAQ-I. Third, the association between back-related perceptual dysfunction and physical performance measures was not investigated as only questionnaires were employed. Fourth, relationships with other psychological characteristics (e.g. Pain Self-Efficacy Questionnaire or the Coping Strategies Questionnaire-27 revised) [ 45 , 46 , 47 ], or quality of life (e.g. the Short-Form Health Survey 36-items) [ 48 ], as well as with clinical tests that might have the ability to detect alterations in the sensorimotor system [ 49 ], were not examined. Fifth, our research was limited to people with chronic non-specific LBP and it is doubtful if these results can be expanded to individuals with other causes of lumbar pain (e.g. canal stenosis, fracture, or disk herniation) or pain of another duration. Therefore, studies in these populations are advised.
Conclusions
The FreBAQ-I displays a one-factor structure, it is valid and reliable, and has an adequate measurement error. This Italian version can be recommended for use in clinical and research settings for the assessment of Italian-speaking people with chronic LBP.
Availability of data and materials
The datasets used and/or analysed during the current study are available from the first author under a reasonable request.
Abbreviations
Confidence Interval
Computed Tomography
Fremantle Back Awareness Questionnaire
Fremantle Back Awareness Questionnaire-Italian
Intraclass correlation coefficient
International Society for Pharmacoeconomics and Outcomes Research
- Low back pain
Minimum detectable change
Mean-square statistics
Magnetic Resonance Imaging
Principal Component Analysis of the residuals
Pain Catastrophising Scale
Pain intensity numerical rating scale
Patient-reported outcomes measures
Standard error
Standard error of measurement
Tampa Scale of Kinesiophobia
Dunn KM, Hestbaek L, Cassidy JD. Low back pain across the life course. Best Prac Res Clin Rheumatol. 2013;27:591–600.
Article Google Scholar
Hartvigsen J, Hancock MJ, Kongsted A, Louw Q, Ferreira ML, Genevay S, et al. What low back pain is and why we need to pay attention. The Lancet. 2018;391:2356–67.
Menezes Costa LDC, Maher CG, Hancock MJ, McAuley JH, Herbert RD, Costa LOP. The prognosis of acute and persistent low-back pain: a meta-analysis. CMAJ. 2012;184:E613–24.
Article PubMed Central Google Scholar
Itz CJ, Geurts JW, Van Kleef M, Nelemans P. Clinical course of non-specific low back pain: A systematic review of prospective cohort studies set in primary care: Clinical course of non-specific low back pain. EJP. 2013;17:5–15.
Article CAS PubMed Google Scholar
Moseley LG. I can’t find it! Distorted body image and tactile dysfunction in patients with chronic back pain. Pain. 2008;140:239–43.
Article PubMed Google Scholar
Wand BM, Di Pietro F, George P, O’Connell NE. Tactile thresholds are preserved yet complex sensory function is impaired over the lumbar spine of chronic non-specific low back pain patients: a preliminary investigation. Physiotherapy. 2010;96:317–23.
Sheeran L, Sparkes V, Caterson B, Busse-Morris M, Van Deursen R. Spinal Position Sense and Trunk Muscle Activity During Sitting and Standing in Nonspecific Chronic Low Back Pain: Classification Analysis. Spine. 2012;37:E486–95.
Bray H, Moseley GL. Disrupted working body schema of the trunk in people with back pain. Br J Sports Med. 2011;45:168–73.
Moseley GL, Gallagher L, Gallace A. Neglect-like tactile dysfunction in chronic back pain. Neurology. 2012;79:327–32.
Wand BM, James M, Abbaszadeh S, George PJ, Formby PM, Smith AJ, et al. Assessing self-perception in patients with chronic low back pain: Development of a back-specific body-perception questionnaire. BMR. 2014;27:463–73.
Wand BM, Catley MJ, Rabey MI, O’Sullivan PB, O’Connell NE, Smith AJ. Disrupted Self-Perception in People With Chronic Low Back Pain. Further Evaluation of the Fremantle Back Awareness Questionnaire. J Pain. 2016;17:1001–12.
Goossens N, Geraerts I, Vandenplas L, Van Veldhoven Z, Asnong A, Janssens L. Body perception disturbances in women with pregnancy-related lumbopelvic pain and their role in the persistence of pain postpartum. BMC Pregnancy Childbirth. 2021;21:219.
Article PubMed PubMed Central Google Scholar
Nishigami T, Mibu A, Tanaka K, Yamashita Y, Shimizu ME, Wand BM, et al. Validation of the Japanese Version of the Fremantle Back Awareness Questionnaire in Patients with Low Back Pain. Pain Pract. 2018;18:170–9.
Wälti P, Kool J, Luomajoki H. Short-term effect on pain and function of neurophysiological education and sensorimotor retraining compared to usual physiotherapy in patients with chronic or recurrent non-specific low back pain, a pilot randomized controlled trial. BMC Musculoskelet Disord. 2015;16:83.
Wand BM, O’Connell NE, Di Pietro F, Bulsara M. Managing Chronic Nonspecific Low Back Pain With a Sensorimotor Retraining Approach: Exploratory Multiple-Baseline Study of 3 Participants. Phys Ther. 2011;91:535–46.
Prinsen CAC, Mokkink LB, Bouter LM, Alonso J, Patrick DL, De Vet HCW, et al. COSMIN guideline for systematic reviews of patient-reported outcome measures. Qual Life Res. 2018;27:1147–57.
Article CAS PubMed PubMed Central Google Scholar
Mokkink LB, De Vet HCW, Prinsen CAC, Patrick DL, Alonso J, Bouter LM, et al. COSMIN Risk of Bias checklist for systematic reviews of Patient-Reported Outcome Measures. Qual Life Res. 2018;27:1171–9.
Janssens L, Goossens N, Wand BM, Pijnenburg M, Thys T, Brumagne S. The development of the Dutch version of the Fremantle Back Awareness Questionnaire. Musculoskeletal Science and Practice. 2017;32:84–91.
Ehrenbrusthoff K, Ryan CG, Grüneberg C, Wand BM, Martin DJ. The translation, validity and reliability of the German version of the Fremantle Back Awareness Questionnaire. PLoS ONE. 2018;13:e0205244.
Erol E, Yildiz A, Yildiz R, Apaydin U, Gokmen D, Elbasan B. Reliability and Validity of the Turkish Version of the Fremantle Back Awareness Questionnaire. Spine. 2019;44:E549–54.
Hu F, Liu C, Cao S, Wang X, Liu W, Li T, et al. Cross-cultural adaptation and validation of the simplified chinese version of the fremantle back awareness questionnaire in patients with low back Pain. Eur Spine J. 2022;31:935–42.
Rao P, Jain M, Barman A, Bansal S, Sahu R, Singh N. Fremantle Back awareness questionnaire in chronic low Back pain (Frebaq-I): translation and validation in the Indian population. Asian J Neurosurg. 2021;16:113–8.
García-Dopico N, La Torre-Luque D, Wand BM, Velasco-Roldán O, Sitges C. The cross-cultural adaptation, validity, and reliability of the Spanish version of the Fremantle Back Awareness Questionnaire. Front Psychol. 2023;14:1070411.
Mahmoudzadeh A, Abbaszadeh S, Baharlouei H, Karimi A. Translation and Cross-cultural Adaptation of the Fremantle Back Awareness Questionnaire into Persian language and the assessment of reliability and validity in patients with chronic low back pain. J Res Med Sci. 2020;25:74.
Negrini S, Bonaiuti D, Monticone M, Trevisan C. Medical causes of low back pain. In: Interventional Spine. Elsevier; 2008. p. 803–11.
Chapter Google Scholar
Monticone M, Galeoto G, Berardi A, Tofani M. Psychometric properties of assessment tools. Measuring Spinal Cord Injury: A Practical Guide of Outcome Measures; 2021. p. 7–15.
Google Scholar
Beaton DE, Bombardier C, Guillemin F, Ferraz MB. Guidelines for the Process of Cross-Cultural Adaptation of Self-Report Measures: Spine. 2000;25:3186–91.
CAS PubMed Google Scholar
Wild D, Grove A, Martin M, Eremenco S, McElroy S, Verjee-Lorenz A, et al. Principles of Good Practice for the Translation and Cultural Adaptation Process for Patient-Reported Outcomes (PRO) Measures: Report of the ISPOR Task Force for Translation and Cultural Adaptation. Value in Health. 2005;8:94–104.
De Vet HC, Terwee CB, Mokkink LB, Knol DL. Measurement in medicine: a practical guide. Cambridge university press; 2011.
Book Google Scholar
Franchignoni F, Monticone M, Giordano A, Rocca B. Rasch validation of the Prosthetic Mobility Questionnaire: A new outcome measure for assessing mobility in people with lower limb amputation. J Rehabil Med. 2015;47:460–5.
Franchignoni F, Giordano A, Vercelli S, Bravini E, Stissi V, Ferriero G. Rasch Analysis of the Patient and Observer Scar Assessment Scale in Linear Scars: Suggestions for a Patient and Observer Scar Assessment Scale v21 Plastic Recons Surg. 2019;144:1073E-9E.
CAS Google Scholar
Bond T, Yan Z, Heene M. Applying the Rasch model: Fundamental measurement in the human sciences. Routledge; 2020.
Linacre JM, Wright BD. Winsteps. https://www.winsteps.com/index.htm . 2000.Accessed 27–06–2013
Christensen KB, Makransky G, Horton M. Critical values for Yen’s Q 3: Identification of local dependence in the Rasch model using residual correlations. Appl Psychol Meas. 2017;41:178–94.
Padua R, Padua L, Ceccarelli E, Romanini E, Zanoli G, Bondì R, et al. Italian version of the Roland Disability Questionnaire, specific for low back pain: cross-cultural adaptation and validation. Eur Spine J. 2002;11:126–9.
Farrar JT, Young JP Jr, LaMoreaux L, Werth JL, Poole RM. Clinical importance of changes in chronic pain intensity measured on an 11-point numerical pain rating scale. Pain. 2001;94:149–58.
Monticone M, Baiardi P, Ferrari S, Foti C, Mugnai R, Pillastrini P, et al. Development of the Italian version of the Pain Catastrophising Scale (PCS-I): cross-cultural adaptation, factor analysis, reliability, validity and sensitivity to change. Qual Life Res. 2012;21:1045–50.
Monticone M, Giorgi I, Baiardi P, Barbieri M, Rocca B, Bonezzi C. Development of the Italian Version of the Tampa Scale of Kinesiophobia (TSK-I): Cross-Cultural Adaptation. Factor Analysis, Reliability, and Validity: Spine. 2010;35:1241–6.
PubMed Google Scholar
Monticone M, Baiardi P, Vanti C, Ferrari S, Pillastrini P, Mugnai R, et al. Responsiveness of the Oswestry Disability Index and the Roland Morris Disability Questionnaire in Italian subjects with sub-acute and chronic low back pain. Eur Spine J. 2012;21:122–9.
Monticone M, Ambrosini E, Rocca B, Foti C, Ferrante S. Responsiveness of the Tampa Scale of Kinesiophobia in Italian subjects with chronic low back pain undergoing motor and cognitive rehabilitation. European Spine J. 2016;25:2882–8.
Monticone M, Portoghese I, Rocca B, Giordano A, Campagna M, Franchignoni F. Responsiveness and minimal important change of the Pain Catastrophizing Scale in people with chronic low back pain undergoing multidisciplinary rehabilitation. Eur J Phys Rehab Med. 2022;58:68.
Zou GY. Sample size formulas for estimating intraclass correlation coefficients with precision and assurance. Stat Med. 2012;31:3972–81.
Linacre JM. Sample size and item calibration stability. Rasch measurement transactions. 1994;7:328.
Schäfer A, Wand BM, Lüdtke K, Ehrenbrusthoff K, Schöttker-Königer T. Validation and investigation of cross cultural equivalence of the Fremantle back awareness questionnaire-German version (FreBAQ-G). BMC Musculoskelet Disord. 2021;22:1–14.
Chiarotto A, Vanti C, Ostelo RW, Ferrari S, Tedesco G, Rocca B, et al. The pain self-efficacy questionnaire: cross-cultural adaptation into Italian and assessment of its measurement properties. Pain Pract. 2015;15:738–47.
Monticone M, Giordano A, Franchignoni F. Scale shortening and decrease in measurement precision: Analysis of the Pain Self-Efficacy Questionnaire and its short forms in an Italian-speaking population with neck pain disorders. Physical Therapy. 2021;101:pzab039.
Monticone M, Ferrante S, Giorgi I, Galandra C, Rocca B, Foti C. The 27-item coping strategies questionnaire—revised: confirmatory factor analysis, reliability and validity in italian-speaking subjects with chronic pain. Pain Res Manage. 2014;19:153–8.
Apolone G, Mosconi P. The Italian SF-36 Health Survey: translation, validation and norming. J Clin Epidemiol. 1998;51:1025–36.
Meier R, Emch C, Gross-Wolf C, Pfeiffer F, Meichtry A, Schmid A, et al. Sensorimotor and body perception assessments of nonspecific chronic low back pain: a cross-sectional study. BMC Musculoskelet Disord. 2021;22:1–10.
Download references
Acknowledgements
The authors would like to thank all the people who got involved into the study, along with Dr Franco Franchignoni and Dr Andrea Giordano, which gave important suggestions and support for the data analysis.
This research did not receive any specific grant from funding agencies in the public, commercial, or not-for-profit sectors.
Author information
Authors and affiliations.
Department of Surgical Sciences, University of Cagliari, Cagliari, Italy
Marco Monticone
Ambulatorio di quartiere, Cagliari, Italy
Carolina Maurandi
Rehabilitation Medicine and Neurorehabilitation, P.O. San Martino, Oristano, Italy
Elisa Porcu
Department of Mechanical, Chemical, and Materials Engineering, University of Cagliari, Cagliari, Italy
Federico Arippa
The Faculty of Medicine, Nursing & Midwifery and Health Sciences, The University of Notre Dame Australia, Fremantle, WA, Australia
Benedict M. Wand
Studio Fisioterapico Corona, Cagliari, Italy
Giorgio Corona
You can also search for this author in PubMed Google Scholar
Contributions
MM initiated study conception and design. CM, GC and EP performed the data collection and acquisition of data. FA performed the data analysis. MM interpreted the data. MM wrote the manuscript. MM, EP and FA edited the manuscript. BMW had a role in critical revision of different parts of the work. All authors have read and approved the final manuscript.
Corresponding author
Correspondence to Federico Arippa .
Ethics declarations
Ethics approval and consent to participate.
This cross-sectional study was approved by our Local Ethical Committee (Salvatore Maugeri Foundation, Scientific Institute of Lissone, Monza Brianza, Italy) on the 05/04/2016 (committee’s reference number: no. 7/16), and conducted in accordance with ethical and humane principles of research of the Declaration of Helsinki. Informed consent, written and verbal, was obtained from all participants. Before inclusion, all participants signed a written informed consent that detailed the study’s aims, attendance modalities in the trial, and that asked for permission to use their clinical and socio-demographic data for the purpose of the present research.
Consent for publication
Not applicable.
Competing interests
The authors declare that they have no competing interests.
Additional information
Publisher’s note.
Springer Nature remains neutral with regard to jurisdictional claims in published maps and institutional affiliations.
Rights and permissions
Open Access This article is licensed under a Creative Commons Attribution 4.0 International License, which permits use, sharing, adaptation, distribution and reproduction in any medium or format, as long as you give appropriate credit to the original author(s) and the source, provide a link to the Creative Commons licence, and indicate if changes were made. The images or other third party material in this article are included in the article's Creative Commons licence, unless indicated otherwise in a credit line to the material. If material is not included in the article's Creative Commons licence and your intended use is not permitted by statutory regulation or exceeds the permitted use, you will need to obtain permission directly from the copyright holder. To view a copy of this licence, visit http://creativecommons.org/licenses/by/4.0/ . The Creative Commons Public Domain Dedication waiver ( http://creativecommons.org/publicdomain/zero/1.0/ ) applies to the data made available in this article, unless otherwise stated in a credit line to the data.
Reprints and permissions
About this article
Cite this article.
Monticone, M., Maurandi, C., Porcu, E. et al. The Fremantle Back Awareness Questionnaire: cross-cultural adaptation, reliability, and validity of the Italian version in people with chronic low back pain. BMC Musculoskelet Disord 25 , 279 (2024). https://doi.org/10.1186/s12891-024-07420-2
Download citation
Received : 07 November 2023
Accepted : 05 April 2024
Published : 11 April 2024
DOI : https://doi.org/10.1186/s12891-024-07420-2
Share this article
Anyone you share the following link with will be able to read this content:
Sorry, a shareable link is not currently available for this article.
Provided by the Springer Nature SharedIt content-sharing initiative
- Rehabilitation
- Rasch analysis
- Measurement error
BMC Musculoskeletal Disorders
ISSN: 1471-2474
- Submission enquiries: [email protected]
- General enquiries: [email protected]

IMAGES
VIDEO
COMMENTS
The predicted outcome of the experiment or analysis; 5. Phrase your hypothesis in three ways. To identify the variables, you can write a simple prediction in ... Write a null hypothesis. If your research involves statistical hypothesis testing, you will also have to write a null hypothesis. The null hypothesis is the default position that there ...
It seeks to explore and understand a particular aspect of the research subject. In contrast, a research hypothesis is a specific statement or prediction that suggests an expected relationship between variables. It is formulated based on existing knowledge or theories and guides the research design and data analysis. 7.
A research hypothesis is your proposed answer to your research question. The research hypothesis usually includes an explanation ('x affects y because …'). A statistical hypothesis, on the other hand, is a mathematical statement about a population parameter. Statistical hypotheses always come in pairs: the null and alternative hypotheses.
A research hypothesis (also called a scientific hypothesis) is a statement about the expected outcome of a study (for example, a dissertation or thesis). To constitute a quality hypothesis, the statement needs to have three attributes - specificity, clarity and testability. Let's take a look at these more closely.
A specific hypothesis helps to define the research question, which is important in the research process as it guides the selection of an appropriate research design and methodology. Testability of the hypothesis means that it can be proven or disproven through empirical data collection and analysis.
Statistical hypothesis: This hypothesis uses statistical analysis to evaluate a representative sample of the population and then generalizes the findings to the larger group. ... In situations where the hypothesis is unsupported by the research, the research still has value. Such research helps us better understand how different aspects of the ...
Variables in a hypothesis. In any research hypothesis, variables play a critical role. These are the elements or factors that the researcher manipulates, controls, or measures. Understanding variables is essential for crafting a clear, testable hypothesis and for the stages of research that follow, such as data collection and analysis.
3. Simple hypothesis. A simple hypothesis is a statement made to reflect the relation between exactly two variables. One independent and one dependent. Consider the example, "Smoking is a prominent cause of lung cancer." The dependent variable, lung cancer, is dependent on the independent variable, smoking. 4.
A research hypothesis helps test theories. A hypothesis plays a pivotal role in the scientific method by providing a basis for testing existing theories. For example, a hypothesis might test the predictive power of a psychological theory on human behavior. It serves as a great platform for investigation activities.
A research hypothesis, in its plural form "hypotheses," is a specific, testable prediction about the anticipated results of a study, established at its outset. It is a key component of the scientific method. Hypotheses connect theory to data and guide the research process towards expanding scientific understanding.
A research hypothesis defines the theory or problem your research intends to test. It is the "educated guess" of what the final results of your research or experiment will be. ... In developing your hypothesis statements, you must pre-plan some of your statistical analysis. Once you decide on your problem to examine, determine three aspects ...
A snapshot analysis of citation activity of hypothesis articles may reveal interest of the global scientific community towards their implications across various disciplines and countries. As a prime example, Strachan's hygiene hypothesis, published in 1989,10 is still attracting numerous citations on Scopus, the largest bibliographic database ...
A research question is what a study aims to answer after data analysis and interpretation. ... This statement is based on background research and current knowledge.8,9 The research hypothesis makes a specific prediction about a new phenomenon10 or a formal statement on the expected relationship between an independent variable and a dependent ...
It's essentially an educated guess—based on observations—of what the results of your experiment or research will be. Some hypothesis examples include: If I water plants daily they will grow faster. Adults can more accurately guess the temperature than children can. Butterflies prefer white flowers to orange ones.
The steps to write a research hypothesis are: 1. Stating the problem: Ensure that the hypothesis defines the research problem. 2. Writing a hypothesis as an 'if-then' statement: Include the action and the expected outcome of your study by following a 'if-then' structure. 3.
Introduction. Statistical analysis is necessary for any research project seeking to make quantitative conclusions. The following is a primer for research-based statistical analysis. It is intended to be a high-level overview of appropriate statistical testing, while not diving too deep into any specific methodology.
Definition of research in data analysis: According to LeCompte and Schensul, research data analysis is a process used by researchers to reduce data to a story and interpret it to derive insights. The data analysis process helps reduce a large chunk of data into smaller fragments, which makes sense. Three essential things occur during the data ...
Medical providers often rely on evidence-based medicine to guide decision-making in practice. Often a research hypothesis is tested with results provided, typically with p values, confidence intervals, or both. Additionally, statistical or research significance is estimated or determined by the investigators. Unfortunately, healthcare providers may have different comfort levels in interpreting ...
Evolutionary mismatch is a mechanism whereby disease-associated genetic variants that experienced positive natural selection in past environments because they were beneficial to fitness, may now contribute to negative health consequences in the context of present, modern environments. This project investigates the plausibility of the mismatch hypothesis for Type II Diabetes
The world of education is undergoing many changes, this implies that worthwhile views coming from the past can be totally lost in the acceleration we are experiencing. Transhumanism, as a tendency, seems to imply a moving forward at a increasing speed. Therefore, it would be interesting to reflect on Transhumanism but in relation to Retro-progression and Phronesis, to be able to understand how ...
AI tools can perform tasks like data analysis and hypothesis generation quickly and efficiently. This reduction in cognitive load for scientists can lead them to accept conclusions drawn by AI without sufficient scrutiny. ... The dual edges of AI in scientific research 2024-04-18 - ADITYA SINHA Aditya Sinha (X: @adityasinha004) is Officer ...
Research Article. Contingent claim analysis and Minsky's financial instability hypothesis ... the same idea is used in the growing literature on Contingent Claim Analysis as a novel tool that can be used by regulatory authorities in their evaluation of risk assumed by financial institutions. This paper combines these approaches with Minsky ...
Formulating Hypotheses for Different Study Designs. Generating a testable working hypothesis is the first step towards conducting original research. Such research may prove or disprove the proposed hypothesis. Case reports, case series, online surveys and other observational studies, clinical trials, and narrative reviews help to generate ...
Our hypothesis was that increased spike frequency may be associated with increased rCBF in hippocampus. A total of 24 patients with AD, and 15 HC were included in the analysis. Using linear regression, we investigated the association between rCBF as measured with arterial spin-labelling MRI (ASL-MRI) in the hippocampus and the number of spikes ...
The development of the research question, including a supportive hypothesis and objectives, is a necessary key step in producing clinically relevant results to be used in evidence-based practice. A well-defined and specific research question is more likely to help guide us in making decisions about study design and population and subsequently ...
There is evidence to suggest that assessing back-specific altered self-perception may be useful when seeking to understand and manage low back pain (LBP). The Fremantle Back Awareness Questionnaire (FreBAQ) is a patient-reported measure of back-specific body perception that has never been adapted and psychometrically analysed in Italian. Hence, the objectives of this research were to cross ...