On the Use of Language in Science-Fiction Literature
Published: Jan 4, 2020 at 04:07pm
Categories: language • science-fiction • literature
Many science-fiction stories utilize language to some extent. Some use it just to add depth to the setting, where others may make it a quintessential element of the plot. I really like language (like… a lot ), so I wanted to compare some of the different uses of language in science fiction. One of the best ways to compare things is to attempt to arbitrarily classify them and post that classification online for people to debate and tell you how wrong you are, so I’ve developed a Scale of Linguistic Integration that I will explain here.

The Scale of Linguistic Integration
The scale is as follows:
- A Type-1 Work , also called a glossarial work , is a work which introduces or repurposes individual specific terms or phrases.
- A Type-2 Work , also called a culturally linguistic work , is a work which intends to give a sense of the existence of a culture by using custom language.
- A Type-3 Work , also called a linguistic work , is a work which makes extensive use of language to affect either the reader or the characters deeply.
Works should be classified by their highest attributable type . That is to say that a particular work might well have elements that could be classified as any of Types 1, 2, or 3, in which case it should be labeled as a Type-3 work.
Type 1: Glossarial Works
Type-1 works contain words invented by or specially defined by the author to name specific phenomena within the work.
At the Type-1 level, there may be only a few invented words and they may not be directly connected to one another, either within the work or without. Often, though, the terms will be related and used in a more orchestrated manner.
Some Type-1 examples:
- “Liar!” (Isaac Asimov, 1941) sees the first written use of the word robotics , which is defined (by context) as the study of robots. Asimov then went on to provide the terms roboticist , roboticide , psychoroboticist , and a few other related terms in other robot-related works. However, in my opinion these terms alone do not constitute the sense of culture that is required for the Robot novels to be considered Type-2.
- A Wrinkle in Time (Madeleine L’Engle, 1962) uses its own definition of the term tesseract quite extensively, but this is (as I recall) the only specially-defined word in the book.
Type 2: Culturally Linguistic Works
A work that is Type-2 utilizes multiple terms or phrases which coexist to build the sense of a unique culture within the work. They may also (or alternatively) introduce new idioms or other expressions that cause the reader or some character(s) to feel that there is an entirely separate culture from their own due to the use of these words.
There are many examples of Type-2 works in science fiction:
- Dune (Frank Herbert, 1965) famously includes a large glossary at the end of the book to help the reader through the many invented words. These are all meant to give the reader the impression of centuries-old secret societies and governments.
- The Naked Sun (Isaac Asimov, 1957) features a society where a dichotomy is drawn between seeing and viewing , which poses problems for the Earth-born protagonist when he is first exposed to this.
- The Red Rising series (Pierce Brown, 2014) invents many words and phrases which are then used by characters according to their different social castes. At some points, this is even a direct plot device.
- Nineteen Eighty-Four (George Orwell, 1949) is particularly noteworthy for its portrayal of language as a weapon of oppression. The Eurasian government’s official langue, Newspeak , is the result of taking the Sapir-Whorf hypothesis too far, insisting that (for example) the lack of negative modifiers in the language will prevent citizens from even thinking about disagreeing with the government. Although Newspeak is labeled as a language within the book, I think its use is not quite extensive enough to warrant classification as a Type-3 work.
The Left Hand of Darkness (Ursula K. Le Guin, 1969) introduces the concept of shifgrethor , which plays an important role in the development of some of the protagonist’s relationships with members of an alien (but human) society. I don’t consider Left Hand to be merely Type-1 because the (lack of) comprehension of this term is a crucial element of the plot at some points, causing much distress to the protagonist as he attempts to understand the culture. The book also introduces a few other terms or confusions of terms (e.g., island ), but these are not as relevant to the protagonist other than in passing comments.
Additionally, the use of gendered pronouns is a point of much contention among readers of Left Hand , since the non-protagonist characters are all androgynous most of the time. (Approximately 26 days of their 28-day months are spent without having a biological sex or any related organs, but for those two days each month each person will sort-of-randomly acquire a sex.) The protagonist (a xeno-anthropologist) reflects on this in his writings, and notes that he chooses to exclusively use the masculine pronouns “he” and “him” in referring to the locals, unless they are currently female.
Type 3: Deeply Linguistic Works
Some works go above and beyond the classification as Type 2 and instead use language as a whole to help tell the story. These works might use language for the sake of the reader, or they might use language for the characters to interact with. Sometimes the work is written in a specific way with the intent of causing the reader to think about things in a different light.
I can think of fewer examples of Type-3 works, but these are among my favorites:
- A Fire Upon the Deep (Vernor Vinge, 1992) opens with two characters who refer to themselves using first-person plural pronouns and grammatical constructions while simultaneously referring to themselves as individuals. Within the first two or three chapters it becomes apparent that these creatures are actually small packs of lower-minded beings who, when in close proximity to one another, telepathically link to form a collective individual. This is one of my favorite uses of language in all of science fiction.
- The Expanse series (James S.A. Corey, 2011) is set a few hundred years in our future when humans have settled Mars and parts of the asteroid belt. The Belters (those who have been living in the asteroid belt for a few generations now) have experienced significant dialectal drift and evolution over those centuries. The culture of the Belters is an amalgamation of the cultures of people from many different backgrounds on Earth, and the books show its use regularly. There are invented words whose roots to old Earth languages can be deduced by the keen-eyed, as well as entirely new words to serve as complement.
Additional Types
Of course, we can enumerate further types if we so choose!
Type 0: Alinguistic Works
A work is Type-0 if it makes no special use of language. It invents no new terms. All people speak as though they are real people today, so there is no interesting juxtaposition of linguistic constructs.
It seems that most science-fiction authors invent (or repurpose) at least a few words in their works. This is perhaps unsurprising: science fiction is described as a genre all about speculating on the future and how humans will interact with it, so giving names to the different phenomena of the fictional setting seems quintessential.
Even going back to the earliest days of science fiction, we can see that works were using their own terms. The War of the Worlds (H.G. Wells, 1898) introduces the concept of the heat-ray — a terrifying device that can instantly incinerate humans with ease. But we have to ask: does this really constitute Type-1 classification? After all, the device is really just a ray that inflicts massive amounts of heat in whatever direction it is pointed. What else could it be called? Further, Wells tells us of the black smoke (sinister smoke which is black) and tripods (devices with three legs). While these terms have a particular meaning within the work, they do not seem incredibly inventive.
I think arguments could definitely be made for classifying The War of the Worlds as either Type 0 or Type 1, and I imagine many early science-fiction works could be similarly subjected to debate.
Type 4: Fundamentally Linguistic Works
These are all works said to be above Type 3. An example of a qualification for being Type-4 might be the construction of a full language which is used within the work.
I do not know of a science-fiction work of literature that could be categorized as Type 4. However, J.R.R. Tolkien’s The Lord of the Rings (1954) absolutely qualifies. What do all those songs and poems translate to? Hell if I know — it’s not all spelled out for the reader! There’s a (comparatively) complete language back there, most of which was never explicitly published in any sort of book meant for learning the languages. It’s more than just a culture or a deep presence of language; it’s an entire history, embedded within the pages of the book.
I think The Expanse comes closest within science fiction, but it falls short because the Belter language is not as complete. It’s really more of a creole resulting from the amalgamation of various Earth language whose meaning can be determined by the observant (and appropriately-educated) reader. However, many fans of the series seem to have taken up development of the language, and I can’t decide whether this should impact the series’s classification here. You tell me!
A Dichotomy Further
I realized after developing this scale that there is actually an additional dimension to the analysis: whether the given use of language is relevant in-universe or not. We might say that a work’s language has in-universe relevance (IUR) if the presence of this new language-stuff has a direct impact on the story.
For example: the new words in Asimov’s early robot-focused short stories ( robotics , roboticist , etc) do not have IUR. They merely exist and are used by characters as though they have always existed, more or less. But the see / view distinction in Asimov’s The Naked Sun has IUR because the presence of this distinction causes conflict for the protagonist.
Nineteen Eighty-Four ’s Newspeak language definitely has IUR, as it was specifically designed in-universe for the oppression of people. But the unique grammatical constructions in A Fire Upon the Deep do not have IUR, because no character is really impacted by them.
Finishing Thoughts
When reading, I really love to pay attention to how the author uses language to augment the story-telling. Sometimes they just introduce new words, and other times they use language in a fundamental way that has a deeper impact on the reader or characters in the work without which the work would not be complete. As far as I know, there is no existing classification for these different levels of linguistic integration — so I have given you one!
Of course, these are just thoughts regurgitated out of my head. I’m sure many people will find ways to disagree about either the scale itself or my classification of works within this post. I’d love to hear your thoughts!
Lastly, if you think there’s a work that should rank highly on the scale but which I did not mention, I would very much appreciate a recommendation. I’m always looking for new books to read!
Computer Speech and Language
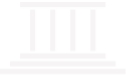
Subject Area and Category
- Human-Computer Interaction
- Theoretical Computer Science
Academic Press Inc.
Publication type
08852308, 10958363
1986-1987, 1989-2023
Information
How to publish in this journal
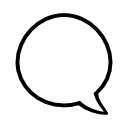
The set of journals have been ranked according to their SJR and divided into four equal groups, four quartiles. Q1 (green) comprises the quarter of the journals with the highest values, Q2 (yellow) the second highest values, Q3 (orange) the third highest values and Q4 (red) the lowest values.
The SJR is a size-independent prestige indicator that ranks journals by their 'average prestige per article'. It is based on the idea that 'all citations are not created equal'. SJR is a measure of scientific influence of journals that accounts for both the number of citations received by a journal and the importance or prestige of the journals where such citations come from It measures the scientific influence of the average article in a journal, it expresses how central to the global scientific discussion an average article of the journal is.
Evolution of the number of published documents. All types of documents are considered, including citable and non citable documents.
This indicator counts the number of citations received by documents from a journal and divides them by the total number of documents published in that journal. The chart shows the evolution of the average number of times documents published in a journal in the past two, three and four years have been cited in the current year. The two years line is equivalent to journal impact factor ™ (Thomson Reuters) metric.
Evolution of the total number of citations and journal's self-citations received by a journal's published documents during the three previous years. Journal Self-citation is defined as the number of citation from a journal citing article to articles published by the same journal.
Evolution of the number of total citation per document and external citation per document (i.e. journal self-citations removed) received by a journal's published documents during the three previous years. External citations are calculated by subtracting the number of self-citations from the total number of citations received by the journal’s documents.
International Collaboration accounts for the articles that have been produced by researchers from several countries. The chart shows the ratio of a journal's documents signed by researchers from more than one country; that is including more than one country address.
Not every article in a journal is considered primary research and therefore "citable", this chart shows the ratio of a journal's articles including substantial research (research articles, conference papers and reviews) in three year windows vs. those documents other than research articles, reviews and conference papers.
Ratio of a journal's items, grouped in three years windows, that have been cited at least once vs. those not cited during the following year.
Leave a comment
Name * Required
Email (will not be published) * Required
* Required Cancel
The users of Scimago Journal & Country Rank have the possibility to dialogue through comments linked to a specific journal. The purpose is to have a forum in which general doubts about the processes of publication in the journal, experiences and other issues derived from the publication of papers are resolved. For topics on particular articles, maintain the dialogue through the usual channels with your editor.
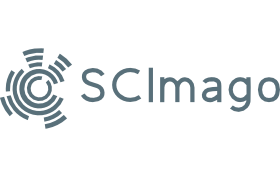
Follow us on @ScimagoJR Scimago Lab , Copyright 2007-2022. Data Source: Scopus®

Cookie settings
Cookie Policy
Legal Notice
Privacy Policy
Help | Advanced Search
Computer Science > Computation and Language
Title: wavllm: towards robust and adaptive speech large language model.
Abstract: The recent advancements in large language models (LLMs) have revolutionized the field of natural language processing, progressively broadening their scope to multimodal perception and generation. However, effectively integrating listening capabilities into LLMs poses significant challenges, particularly with respect to generalizing across varied contexts and executing complex auditory tasks. In this work, we introduce WavLLM, a robust and adaptive speech large language model with dual encoders, and a prompt-aware LoRA weight adapter, optimized by a two-stage curriculum learning approach. Leveraging dual encoders, we decouple different types of speech information, utilizing a Whisper encoder to process the semantic content of speech, and a WavLM encoder to capture the unique characteristics of the speaker's identity. Within the curriculum learning framework, WavLLM first builds its foundational capabilities by optimizing on mixed elementary single tasks, followed by advanced multi-task training on more complex tasks such as combinations of the elementary tasks. To enhance the flexibility and adherence to different tasks and instructions, a prompt-aware LoRA weight adapter is introduced in the second advanced multi-task training stage. We validate the proposed model on universal speech benchmarks including tasks such as ASR, ST, SV, ER, and also apply it to specialized datasets like Gaokao English listening comprehension set for SQA, and speech Chain-of-Thought (CoT) evaluation set. Experiments demonstrate that the proposed model achieves state-of-the-art performance across a range of speech tasks on the same model size, exhibiting robust generalization capabilities in executing complex tasks using CoT approach. Furthermore, our model successfully completes Gaokao tasks without specialized training. The codes, models, audio, and Gaokao evaluation set can be accessed at \url{ this http URL }.
Submission history
Access paper:.
- HTML (experimental)
- Other Formats
References & Citations
- Google Scholar
- Semantic Scholar
BibTeX formatted citation

Bibliographic and Citation Tools
Code, data and media associated with this article, recommenders and search tools.
- Institution
arXivLabs: experimental projects with community collaborators
arXivLabs is a framework that allows collaborators to develop and share new arXiv features directly on our website.
Both individuals and organizations that work with arXivLabs have embraced and accepted our values of openness, community, excellence, and user data privacy. arXiv is committed to these values and only works with partners that adhere to them.
Have an idea for a project that will add value for arXiv's community? Learn more about arXivLabs .
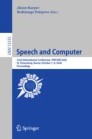
- Conference proceedings
- © 2020
Speech and Computer
22nd International Conference, SPECOM 2020, St. Petersburg, Russia, October 7–9, 2020, Proceedings
- Alexey Karpov ORCID: https://orcid.org/0000-0003-3424-652X 0 ,
- Rodmonga Potapova ORCID: https://orcid.org/0000-0002-7532-9156 1
St. Petersburg Institute for Informatics and Automation, Russian Academy of Sciences, St. Petersburg, Russia
You can also search for this editor in PubMed Google Scholar
Institute for Applied and Mathematical Linguistics, Moscow State Linguistic University, Moscow, Russia
Part of the book series: Lecture Notes in Computer Science (LNCS, volume 12335)
Part of the book sub series: Lecture Notes in Artificial Intelligence (LNAI)
Conference series link(s): SPECOM: International Conference on Speech and Computer
105k Accesses
178 Citations
2 Altmetric
Conference proceedings info: SPECOM 2020.
- Table of contents
- Other volumes
About this book
Editors and affiliations, bibliographic information.
- Publish with us
Buying options
- Available as EPUB and PDF
- Read on any device
- Instant download
- Own it forever
- Compact, lightweight edition
- Dispatched in 3 to 5 business days
- Free shipping worldwide - see info
Tax calculation will be finalised at checkout
Other ways to access
This is a preview of subscription content, log in via an institution to check for access.
Table of contents (65 papers)
Front matter, lightweight cnn for robust voice activity detection.
- Tanvirul Alam, Akib Khan
Hate Speech Detection Using Transformer Ensembles on the HASOC Dataset
- Pedro Alonso, Rajkumar Saini, György Kovács
MP3 Compression to Diminish Adversarial Noise in End-to-End Speech Recognition
- Iustina Andronic, Ludwig Kürzinger, Edgar Ricardo Chavez Rosas, Gerhard Rigoll, Bernhard U. Seeber
Exploration of End-to-End ASR for OpenSTT – Russian Open Speech-to-Text Dataset
- Andrei Andrusenko, Aleksandr Laptev, Ivan Medennikov
Directional Clustering with Polyharmonic Phase Estimation for Enhanced Speaker Localization
- Sergei Astapov, Dmitriy Popov, Vladimir Kabarov
Speech Emotion Recognition Using Spectrogram Patterns as Features
Pragmatic markers in dialogue and monologue: difficulties of identification and typical formation models.
- Natalia Bogdanova-Beglarian, Olga Blinova, Tatiana Sherstinova, Daria Gorbunova, Kristina Zaides, Tatiana Popova
Data Augmentation and Loss Normalization for Deep Noise Suppression
- Sebastian Braun, Ivan Tashev
Automatic Information Extraction from Scanned Documents
- Lukáš Bureš, Petr Neduchal, Luděk Müller
Dealing with Newly Emerging OOVs in Broadcast Programs by Daily Updates of the Lexicon and Language Model
- Petr Cerva, Veronika Volna, Lenka Weingartova
A Rumor Detection in Russian Tweets
- Aleksandr Chernyaev, Alexey Spryiskov, Alexander Ivashko, Yuliya Bidulya
Automatic Prediction of Word Form Reduction in Russian Spontaneous Speech
- Maria Dayter, Elena Riekhakaynen
Formant Frequency Analysis of MSA Vowels in Six Algerian Regions
- Ghania Droua-Hamdani
Emotion Recognition and Sentiment Analysis of Extemporaneous Speech Transcriptions in Russian
- Anastasia Dvoynikova, Oxana Verkholyak, Alexey Karpov
Predicting a Cold from Speech Using Fisher Vectors; SVM and XGBoost as Classifiers
- José Vicente Egas-López, Gábor Gosztolya
Toxicity in Texts and Images on the Internet
- Denis Gordeev, Vsevolod Potapov
An Automated Pipeline for Robust Image Processing and Optical Character Recognition of Historical Documents
- Ivan Gruber, Pavel Ircing, Petr Neduchal, Marek Hrúz, Miroslav Hlaváč, Zbyněk Zajíc et al.
Lipreading with LipsID
- Miroslav Hlaváč, Ivan Gruber, Miloš Železný, Alexey Karpov
Automated Destructive Behavior State Detection on the 1D CNN-Based Voice Analysis
- Anastasia Iskhakova, Daniyar Wolf, Roman Meshcheryakov
Other Volumes
This book constitutes the proceedings of the 22nd International Conference on Speech and Computer, SPECOM 2020, held in St. Petersburg, Russia, in October 2020. The 65 papers presented were carefully reviewed and selected from 160 submissions. The papers present current research in the area of computer speech processing including speech science, speech technology, natural language processing, human-computer interaction, language identification, multimedia processing, human-machine interaction, deep learning for audio processing, computational paralinguistics, affective computing, speech and language resources, speech translation systems, text mining and sentiment analysis, voice assistants, etc.
Due to the Corona pandemic SPECOM 2020 was held as a virtual event.
- artificial intelligence
- computational linguistics
- computer science
- computer systems
- correlation analysis
- engineering
- linguistics
- machine learning
- mathematics
- Natural Language Processing (NLP)
- natural languages
- signal processing
- speech analysis
- speech communication
- speech processing
- speech recognition
- speech signals
Alexey Karpov
Rodmonga Potapova
Book Title : Speech and Computer
Book Subtitle : 22nd International Conference, SPECOM 2020, St. Petersburg, Russia, October 7–9, 2020, Proceedings
Editors : Alexey Karpov, Rodmonga Potapova
Series Title : Lecture Notes in Computer Science
DOI : https://doi.org/10.1007/978-3-030-60276-5
Publisher : Springer Cham
eBook Packages : Computer Science , Computer Science (R0)
Copyright Information : Springer Nature Switzerland AG 2020
Softcover ISBN : 978-3-030-60275-8 Published: 05 October 2020
eBook ISBN : 978-3-030-60276-5 Published: 04 October 2020
Series ISSN : 0302-9743
Series E-ISSN : 1611-3349
Edition Number : 1
Number of Pages : XIV, 689
Number of Illustrations : 67 b/w illustrations, 155 illustrations in colour
Topics : Artificial Intelligence , Computer Appl. in Social and Behavioral Sciences , Computers and Education , Data Mining and Knowledge Discovery , Information Systems Applications (incl. Internet) , Computer Imaging, Vision, Pattern Recognition and Graphics
Policies and ethics
- Find a journal
- Track your research
Thank you for visiting nature.com. You are using a browser version with limited support for CSS. To obtain the best experience, we recommend you use a more up to date browser (or turn off compatibility mode in Internet Explorer). In the meantime, to ensure continued support, we are displaying the site without styles and JavaScript.
- View all journals
- My Account Login
- Explore content
- About the journal
- Publish with us
- Sign up for alerts
- Open access
- Published: 08 April 2024
A neural speech decoding framework leveraging deep learning and speech synthesis
- Xupeng Chen 1 na1 ,
- Ran Wang 1 na1 ,
- Amirhossein Khalilian-Gourtani ORCID: orcid.org/0000-0003-1376-9583 2 ,
- Leyao Yu 2 , 3 ,
- Patricia Dugan 2 ,
- Daniel Friedman 2 ,
- Werner Doyle 4 ,
- Orrin Devinsky 2 ,
- Yao Wang ORCID: orcid.org/0000-0003-3199-3802 1 , 3 na2 &
- Adeen Flinker ORCID: orcid.org/0000-0003-1247-1283 2 , 3 na2
Nature Machine Intelligence ( 2024 ) Cite this article
17 Altmetric
Metrics details
- Neural decoding
A preprint version of the article is available at bioRxiv.
Decoding human speech from neural signals is essential for brain–computer interface (BCI) technologies that aim to restore speech in populations with neurological deficits. However, it remains a highly challenging task, compounded by the scarce availability of neural signals with corresponding speech, data complexity and high dimensionality. Here we present a novel deep learning-based neural speech decoding framework that includes an ECoG decoder that translates electrocorticographic (ECoG) signals from the cortex into interpretable speech parameters and a novel differentiable speech synthesizer that maps speech parameters to spectrograms. We have developed a companion speech-to-speech auto-encoder consisting of a speech encoder and the same speech synthesizer to generate reference speech parameters to facilitate the ECoG decoder training. This framework generates natural-sounding speech and is highly reproducible across a cohort of 48 participants. Our experimental results show that our models can decode speech with high correlation, even when limited to only causal operations, which is necessary for adoption by real-time neural prostheses. Finally, we successfully decode speech in participants with either left or right hemisphere coverage, which could lead to speech prostheses in patients with deficits resulting from left hemisphere damage.
Similar content being viewed by others
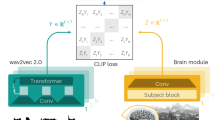
Decoding speech perception from non-invasive brain recordings
Alexandre Défossez, Charlotte Caucheteux, … Jean-Rémi King
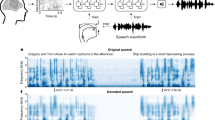
Speech synthesis from neural decoding of spoken sentences
Gopala K. Anumanchipalli, Josh Chartier & Edward F. Chang
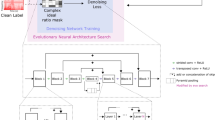
Restoring speech intelligibility for hearing aid users with deep learning
Peter Udo Diehl, Yosef Singer, … Veit M. Hofmann
Speech loss due to neurological deficits is a severe disability that limits both work life and social life. Advances in machine learning and brain–computer interface (BCI) systems have pushed the envelope in the development of neural speech prostheses to enable people with speech loss to communicate 1 , 2 , 3 , 4 , 5 . An effective modality for acquiring data to develop such decoders involves electrocorticographic (ECoG) recordings obtained in patients undergoing epilepsy surgery 4 , 5 , 6 , 7 , 8 , 9 , 10 . Implanted electrodes in patients with epilepsy provide a rare opportunity to collect cortical data during speech with high spatial and temporal resolution, and such approaches have produced promising results in speech decoding 4 , 5 , 8 , 9 , 10 , 11 .
Two challenges are inherent to successfully carrying out speech decoding from neural signals. First, the data to train personalized neural-to-speech decoding models are limited in duration, and deep learning models require extensive training data. Second, speech production varies in rate, intonation, pitch and so on, even within a single speaker producing the same word, complicating the underlying model representation 12 , 13 . These challenges have led to diverse speech decoding approaches with a range of model architectures. Currently, public code to test and replicate findings across research groups is limited in availability.
Earlier approaches to decoding and synthesizing speech spectrograms from neural signals focused on linear models. These approaches achieved a Pearson correlation coefficient (PCC) of ~0.6 or lower, but with simple model architectures that are easy to interpret and do not require large training datasets 14 , 15 , 16 . Recent research has focused on deep neural networks leveraging convolutional 8 , 9 and recurrent 5 , 10 , 17 network architectures. These approaches vary across two major dimensions: the intermediate latent representation used to model speech and the speech quality produced after synthesis. For example, cortical activity has been decoded into an articulatory movement space, which is then transformed into speech, providing robust decoding performance but with a non-natural synthetic voice reconstruction 17 . Conversely, some approaches have produced naturalistic reconstruction leveraging wavenet vocoders 8 , generative adversarial networks (GAN) 11 and unit selection 18 , but achieve limited accuracy. A recent study in one implanted patient 19 provided both robust accuracies and a naturalistic speech waveform by leveraging quantized HuBERT features 20 as an intermediate representation space and a pretrained speech synthesizer that converts the HuBERT features into speech. However, HuBERT features do not carry speaker-dependent acoustic information and can only be used to generate a generic speaker’s voice, so they require a separate model to translate the generic voice to a specific patient’s voice. Furthermore, this study and most previous approaches have employed non-causal architectures, which may limit real-time applications, which typically require causal operations.
To address these issues, in this Article we present a novel ECoG-to-speech framework with a low-dimensional intermediate representation guided by subject-specific pre-training using speech signal only (Fig. 1 ). Our framework consists of an ECoG decoder that maps the ECoG signals to interpretable acoustic speech parameters (for example, pitch, voicing and formant frequencies), as well as a speech synthesizer that translates the speech parameters to a spectrogram. The speech synthesizer is differentiable, enabling us to minimize the spectrogram reconstruction error during training of the ECoG decoder. The low-dimensional latent space, together with guidance on the latent representation generated by a pre-trained speech encoder, overcomes data scarcity issues. Our publicly available framework produces naturalistic speech that highly resembles the speaker’s own voice, and the ECoG decoder can be realized with different deep learning model architectures and using different causality directions. We report this framework with multiple deep architectures (convolutional, recurrent and transformer) as the ECoG decoder, and apply it to 48 neurosurgical patients. Our framework performs with high accuracy across the models, with the best performance obtained by the convolutional (ResNet) architecture (PCC of 0.806 between the original and decoded spectrograms). Our framework can achieve high accuracy using only causal processing and relatively low spatial sampling on the cortex. We also show comparable speech decoding from grid implants on the left and right hemispheres, providing a proof of concept for neural prosthetics in patients suffering from expressive aphasia (with damage limited to the left hemisphere), although such an approach must be tested in patients with damage to the left hemisphere. Finally, we provide a publicly available neural decoding pipeline ( https://github.com/flinkerlab/neural_speech_decoding ) that offers flexibility in ECoG decoding architectures to push forward research across the speech science and prostheses communities.
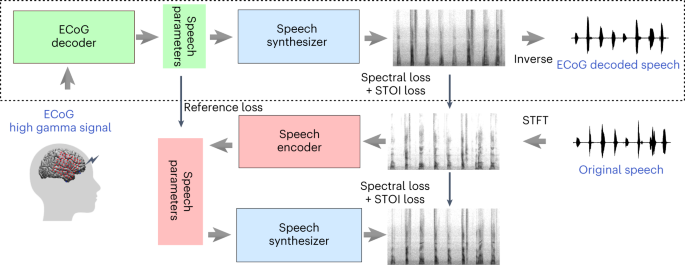
The upper part shows the ECoG-to-speech decoding pipeline. The ECoG decoder generates time-varying speech parameters from ECoG signals. The speech synthesizer generates spectrograms from the speech parameters. A separate spectrogram inversion algorithm converts the spectrograms to speech waveforms. The lower part shows the speech-to-speech auto-encoder, which generates the guidance for the speech parameters to be produced by the ECoG decoder during its training. The speech encoder maps an input spectrogram to the speech parameters, which are then fed to the same speech synthesizer to reproduce the spectrogram. The speech encoder and a few learnable subject-specific parameters in the speech synthesizer are pre-trained using speech signals only. Only the upper part is needed to decode the speech from ECoG signals once the pipeline is trained.
ECoG-to-speech decoding framework
Our ECoG-to-speech framework consists of an ECoG decoder and a speech synthesizer (shown in the upper part of Fig. 1 ). The neural signals are fed into an ECoG decoder, which generates speech parameters, followed by a speech synthesizer, which translates the parameters into spectrograms (which are then converted to a waveform by the Griffin–Lim algorithm 21 ). The training of our framework comprises two steps. We first use semi-supervised learning on the speech signals alone. An auto-encoder, shown in the lower part of Fig. 1 , is trained so that the speech encoder derives speech parameters from a given spectrogram, while the speech synthesizer (used here as the decoder) reproduces the spectrogram from the speech parameters. Our speech synthesizer is fully differentiable and generates speech through a weighted combination of voiced and unvoiced speech components generated from input time series of speech parameters, including pitch, formant frequencies, loudness and so on. The speech synthesizer has only a few subject-specific parameters, which are learned as part of the auto-encoder training (more details are provided in the Methods Speech synthesizer section). Currently, our speech encoder and speech synthesizer are subject-specific and can be trained using any speech signal of a participant, not just those with corresponding ECoG signals.
In the next step, we train the ECoG decoder in a supervised manner based on ground-truth spectrograms (using measures of spectrogram difference and short-time objective intelligibility, STOI 8 , 22 ), as well as guidance for the speech parameters generated by the pre-trained speech encoder (that is, reference loss between speech parameters). By limiting the number of speech parameters (18 at each time step; Methods section Summary of speech parameters ) and using the reference loss, the ECoG decoder can be trained with limited corresponding ECoG and speech data. Furthermore, because our speech synthesizer is differentiable, we can back-propagate the spectral loss (differences between the original and decoded spectrograms) to update the ECoG decoder. We provide multiple ECoG decoder architectures to choose from, including 3D ResNet 23 , 3D Swin Transformer 24 and LSTM 25 . Importantly, unlike many methods in the literature, we employ ECoG decoders that can operate in a causal manner, which is necessary for real-time speech generation from neural signals. Note that, once the ECoG decoder and speech synthesizer are trained, they can be used for ECoG-to-speech decoding without using the speech encoder.
Data collection
We employed our speech decoding framework across N = 48 participants who consented to complete a series of speech tasks (Methods section Experiments design). These participants, as part of their clinical care, were undergoing treatment for refractory epilepsy with implanted electrodes. During the hospital stay, we acquired synchronized neural and acoustic speech data. ECoG data were obtained from five participants with hybrid-density (HB) sampling (clinical-research grid) and 43 participants with low-density (LD) sampling (standard clinical grid), who took part in five speech tasks: auditory repetition (AR), auditory naming (AN), sentence completion (SC), word reading (WR) and picture naming (PN). These tasks were designed to elicit the same set of spoken words across tasks while varying the stimulus modality. We provided 50 repeated unique words (400 total trials per participant), all of which were analysed locked to the onset of speech production. We trained a model for each participant using 80% of available data for that participant and evaluated the model on the remaining 20% of data (with the exception of the more stringent word-level cross-validation).
Speech decoding performance and causality
We first aimed to directly compare the decoding performance across different architectures, including those that have been employed in the neural speech decoding literature (recurrent and convolutional) and transformer-based models. Although any decoder architecture could be used for the ECoG decoder in our framework, employing the same speech encoder guidance and speech synthesizer, we focused on three representative models for convolution (ResNet), recurrent (LSTM) and transformer (Swin) architectures. Note that any of these models can be configured to use temporally non-causal or causal operations. Our results show that ResNet outperformed the other models, providing the highest PCC across N = 48 participants (mean PCC = 0.806 and 0.797 for non-causal and causal, respectively), closely followed by Swin (mean PCC = 0.792 and 0.798 for non-causal and causal, respectively) (Fig. 2a ). We found the same when evaluating the three models using STOI+ (ref. 26 ), as shown in Supplementary Fig. 1a . The causality of machine learning models for speech production has important implications for BCI applications. A causal model only uses past and current neural signals to generate speech, whereas non-causal models use past, present and future neural signals. Previous reports have typically employed non-causal models 5 , 8 , 10 , 17 , which can use neural signals related to the auditory and speech feedback that is unavailable in real-time applications. Optimally, only the causal direction should be employed. We thus compared the performance of the same models with non-causal and causal temporal operations. Figure 2a compares the decoding results of causal and non-causal versions of our models. The causal ResNet model (PCC = 0.797) achieved a performance comparable to that of the non-causal model (PCC = 0.806), with no significant differences between the two (Wilcoxon two-sided signed-rank test P = 0.093). The same was true for the causal Swin model (PCC = 0.798) and its non-causal (PCC = 0.792) counterpart (Wilcoxon two-sided signed-rank test P = 0.196). In contrast, the performance of the causal LSTM model (PCC = 0.712) was significantly inferior to that of its non-causal (PCC = 0.745) version (Wilcoxon two-sided signed-rank test P = 0.009). Furthermore, the LSTM model showed consistently lower performance than ResNet and Swin. However, we did not find significant differences between the causal ResNet and causal Swin performances (Wilcoxon two-sided signed-rank test P = 0.587). Because the ResNet and Swin models had the highest performance and were on par with each other and their causal counterparts, we chose to focus further analyses on these causal models, which we believe are best suited for prosthetic applications.
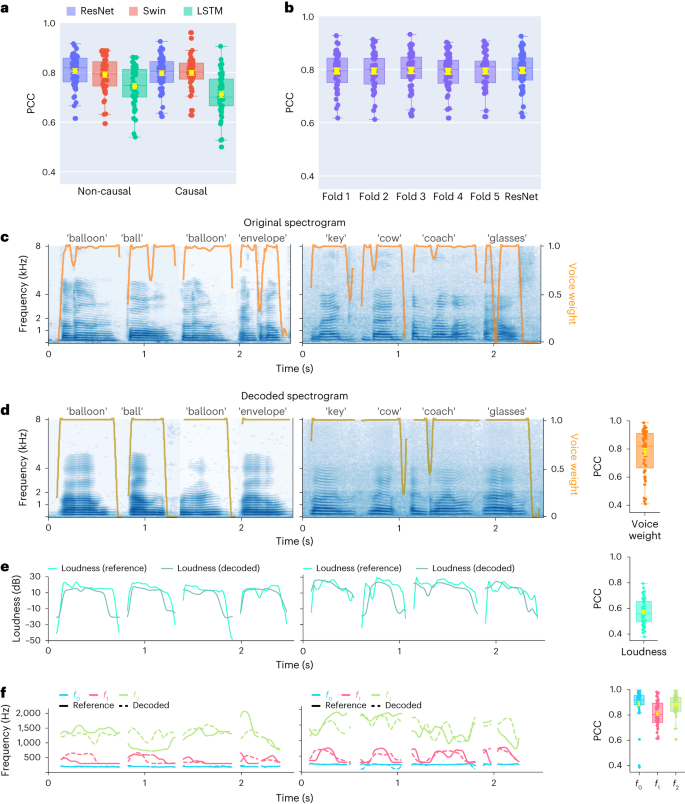
a , Performances of ResNet, Swin and LSTM models with non-causal and causal operations. The PCC between the original and decoded spectrograms is evaluated on the held-out testing set and shown for each participant. Each data point corresponds to a participant’s average PCC across testing trials. b , A stringent cross-validation showing the performance of the causal ResNet model on unseen words during training from five folds; we ensured that the training and validation sets in each fold did not overlap in unique words. The performance across all five validation folds was comparable to our trial-based validation, denoted for comparison as ResNet (identical to the ResNet causal model in a ). c – f , Examples of decoded spectrograms and speech parameters from the causal ResNet model for eight words (from two participants) and the PCC values for the decoded and reference speech parameters across all participants. Spectrograms of the original ( c ) and decoded ( d ) speech are shown, with orange curves overlaid representing the reference voice weight learned by the speech encoder ( c ) and the decoded voice weight from the ECoG decoder ( d ). The PCC between the decoded and reference voice weights is shown on the right across all participants. e , Decoded and reference loudness parameters for the eight words, and the PCC values of the decoded loudness parameters across participants (boxplot on the right). f , Decoded (dashed) and reference (solid) parameters for pitch ( f 0 ) and the first two formants ( f 1 and f 2 ) are shown for the eight words, as well as the PCC values across participants (box plots to the right). All box plots depict the median (horizontal line inside the box), 25th and 75th percentiles (box) and 25th or 75th percentiles ± 1.5 × interquartile range (whiskers) across all participants ( N = 48). Yellow error bars denote the mean ± s.e.m. across participants.
Source data
To ensure our framework can generalize well to unseen words, we added a more stringent word-level cross-validation in which random (ten unique) words were entirely held out during training (including both pre-training of the speech encoder and speech synthesizer and training of the ECoG decoder). This ensured that different trials from the same word could not appear in both the training and testing sets. The results shown in Fig. 2b demonstrate that performance on the held-out words is comparable to our standard trial-based held-out approach (Fig. 2a , ‘ResNet’). It is encouraging that the model can decode unseen validation words well, regardless of which words were held out during training.
Next, we show the performance of the ResNet causal decoder on the level of single words across two representative participants (LD grids). The decoded spectrograms accurately preserve the spectro-temporal structure of the original speech (Fig. 2c,d ). We also compare the decoded speech parameters with the reference parameters. For each parameter, we calculated the PCC between the decoded time series and the reference sequence, showing average PCC values of 0.781 (voice weight, Fig. 2d ), 0.571 (loudness, Fig. 2e ), 0.889 (pitch f 0 , Fig. 2f ), 0.812 (first formant f 1 , Fig. 2f ) and 0.883 (second formant f 2 , Fig. 2f ). Accurate reconstruction of the speech parameters, especially the pitch, voice weight and first two formants, is essential for accurate speech decoding and naturalistic reconstruction that mimics a participant’s voice. We also provide a non-causal version of Fig. 2 in Supplementary Fig. 2 . The fact that both non-causal and causal models can yield reasonable decoding results is encouraging.
Left-hemisphere versus right-hemisphere decoding
Most speech decoding studies have focused on the language- and speech-dominant left hemisphere 27 . However, little is known about decoding speech representations from the right hemisphere. To this end, we compared left- versus right-hemisphere decoding performance across our participants to establish the feasibility of a right-hemisphere speech prosthetic. For both our ResNet and Swin decoders, we found robust speech decoding from the right hemisphere (ResNet PCC = 0.790, Swin PCC = 0.798) that was not significantly different from that of the left (Fig. 3a , ResNet independent t -test, P = 0.623; Swin independent t -test, P = 0.968). A similar conclusion held when evaluating STOI+ (Supplementary Fig. 1b , ResNet independent t -test, P = 0.166; Swin independent t -test, P = 0.114). Although these results suggest that it may be feasible to use neural signals in the right hemisphere to decode speech for patients who suffer damage to the left hemisphere and are unable to speak 28 , it remains unknown whether intact left-hemisphere cortex is necessary to allow for speech decoding from the right hemisphere until tested in such patients.
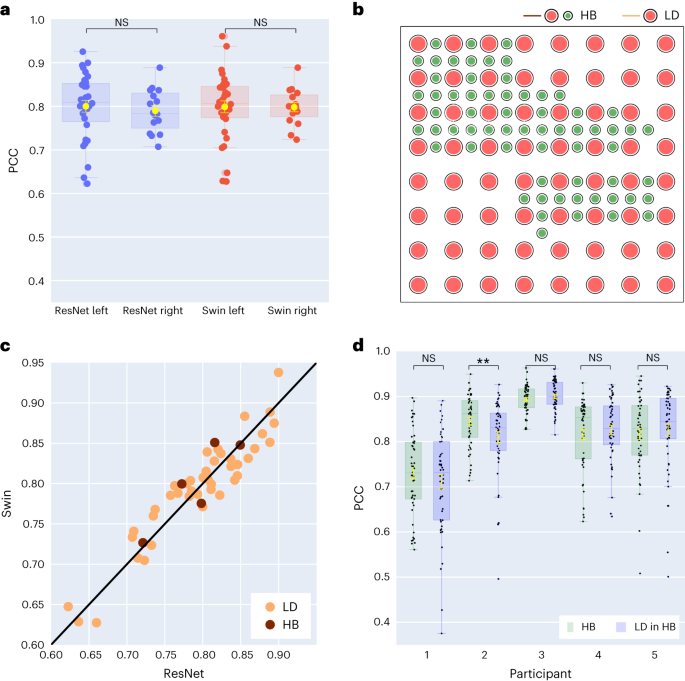
a , Comparison between left- and right-hemisphere participants using causal models. No statistically significant differences (ResNet independent t -test, P = 0.623; Swin Wilcoxon independent t -test, P = 0.968) in PCC values exist between left- ( N = 32) and right- ( N = 16) hemisphere participants. b , An example hybrid-density ECoG array with a total of 128 electrodes. The 64 electrodes marked in red correspond to a LD placement. The remaining 64 green electrodes, combined with red electrodes, reflect HB placement. c , Comparison between causal ResNet and causal Swin models for the same participant across participants with HB ( N = 5) or LD ( N = 43) ECoG grids. The two models show similar decoding performances from the HB and LD grids. d , Decoding PCC values across 50 test trials by the ResNet model for HB ( N = 5) participants when all electrodes are used versus when only LD-in-HB electrodes ( N = 5) are considered. There are no statistically significant differences for four out of five participants (Wilcoxon two-sided signed-rank test, P = 0.114, 0.003, 0.0773, 0.472 and 0.605, respectively). All box plots depict the median (horizontal line inside box), 25th and 75th percentiles (box) and 25th or 75th percentiles ± 1.5 × interquartile range (whiskers). Yellow error bars denote mean ± s.e.m. Distributions were compared with each other as indicated, using the Wilcoxon two-sided signed-rank test and independent t -test. ** P < 0.01; NS, not significant.
Effect of electrode density
Next, we assessed the impact of electrode sampling density on speech decoding, as many previous reports use higher-density grids (0.4 mm) with more closely spaced contacts than typical clinical grids (1 cm). Five participants consented to hybrid grids (Fig. 3b , HB), which typically had LD electrode sampling but with additional electrodes interleaved. The HB grids provided a decoding performance similar to clinical LD grids in terms of PCC values (Fig. 3c ), with a slight advantage in STOI+, as shown in Supplementary Fig. 3b . To ascertain whether the additional spatial sampling indeed provides improved speech decoding, we compared models that decode speech based on all the hybrid electrodes versus only the LD electrodes in participants with HB grids (comparable to our other LD participants). Our findings (Fig. 3d ) suggest that the decoding results were not significantly different from each other (with the exception of participant 2) in terms of PCC and STOI+ (Supplementary Fig. 3c ). Together, these results suggest that our models can learn speech representations well from both high and low spatial sampling of the cortex, with the exciting finding of robust speech decoding from the right hemisphere.
Contribution analysis
Finally, we investigated which cortical regions contribute to decoding to provide insight for the targeted implantation of future prosthetics, especially on the right hemisphere, which has not yet been investigated. We used an occlusion approach to quantify the contributions of different cortical sites to speech decoding. If a region is involved in decoding, occluding the neural signal in the corresponding electrode (that is, setting the signal to zero) will reduce the accuracy (PCC) of the speech reconstructed on testing data (Methods section Contribution analysis ). We thus measured each region’s contribution by decoding the reduction in the PCC when the corresponding electrode was occluded. We analysed all electrodes and participants with causal and non-causal versions of the ResNet and Swin decoders. The results in Fig. 4 show similar contributions for the ResNet and Swin models (Supplementary Figs. 8 and 9 describe the noise-level contribution). The non-causal models show enhanced auditory cortex contributions compared with the causal models, implicating auditory feedback in decoding, and underlying the importance of employing only causal models during speech decoding because neural feedback signals are not available for real-time decoding applications. Furthermore, across the causal models, both the right and left hemispheres show similar contributions across the sensorimotor cortex, especially on the ventral portion, suggesting the potential feasibility of right-hemisphere neural prosthetics.
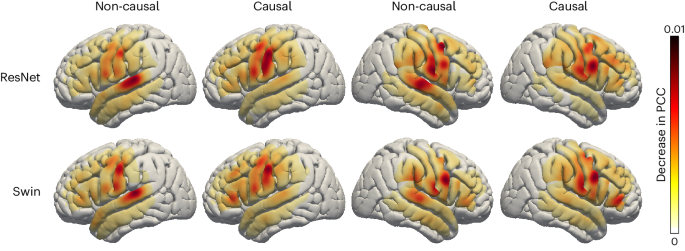
Visualization of the contribution of each cortical location to the decoding result achieved by both causal and non-causal decoding models through an occlusion analysis. The contribution of each electrode region in each participant is projected onto the standardized Montreal Neurological Institute (MNI) brain anatomical map and then averaged over all participants. Each subplot shows the causal or non-causal contribution of different cortical locations (red indicates a higher contribution; yellow indicates a lower contribution). For visualization purposes, we normalized the contribution of each electrode location by the local grid density, because there were multiple participants with non-uniform density.
Our novel pipeline can decode speech from neural signals by leveraging interchangeable architectures for the ECoG decoder and a novel differentiable speech synthesizer (Fig. 5 ). Our training process relies on estimating guidance speech parameters from the participants’ speech using a pre-trained speech encoder (Fig. 6a ). This strategy enabled us to train ECoG decoders with limited corresponding speech and neural data, which can produce natural-sounding speech when paired with our speech synthesizer. Our approach was highly reproducible across participants ( N = 48), providing evidence for successful causal decoding with convolutional (ResNet; Fig. 6c ) and transformer (Swin; Fig. 6d ) architectures, both of which outperformed the recurrent architecture (LSTM; Fig. 6e ). Our framework can successfully decode from both high and low spatial sampling with high levels of decoding performance. Finally, we provide potential evidence for robust speech decoding from the right hemisphere as well as the spatial contribution of cortical structures to decoding across the hemispheres.
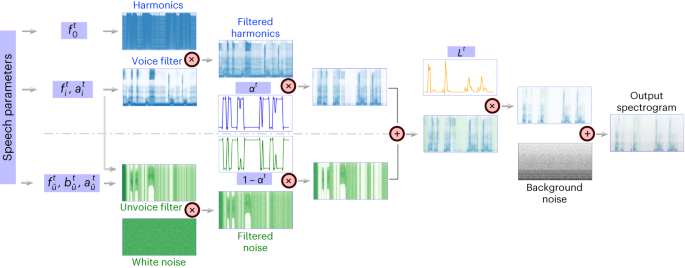
Our speech synthesizer generates the spectrogram at time t by combining a voiced component and an unvoiced component based on a set of speech parameters at t . The upper part represents the voice pathway, which generates the voiced component by passing a harmonic excitation with fundamental frequency \({f}_{0}^{\;t}\) through a voice filter (which is the sum of six formant filters, each specified by formant frequency \({f}_{i}^{\;t}\) and amplitude \({a}_{i}^{t}\) ). The lower part describes the noise pathway, which synthesizes the unvoiced sound by passing white noise through an unvoice filter (consisting of a broadband filter defined by centre frequency \({f}_{\hat{u}}^{\;t}\) , bandwidth \({b}_{\hat{u}}^{t}\) and amplitude \({a}_{\hat{u}}^{t}\) , and the same six formant filters used for the voice filter). The two components are next mixed with voice weight α t and unvoice weight 1 − α t , respectively, and then amplified by loudness L t . A background noise (defined by a stationary spectrogram B ( f )) is finally added to generate the output spectrogram. There are a total of 18 speech parameters at any time t , indicated in purple boxes.
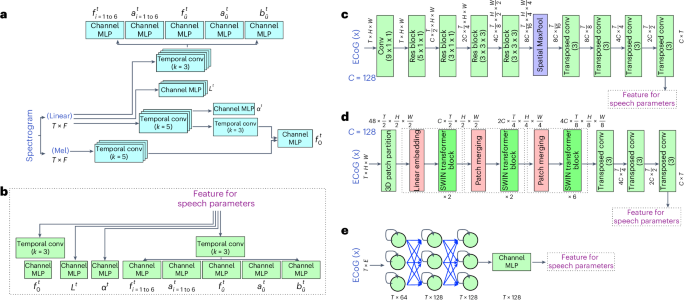
a , The speech encoder architecture. We input a spectrogram into a network of temporal convolution layers and channel MLPs that produce speech parameters. b , c , The ECoG decoder ( c ) using the 3D ResNet architecture. We first use several temporal and spatial convolutional layers with residual connections and spatiotemporal pooling to generate downsampled latent features, and then use corresponding transposed temporal convolutional layers to upsample the features to the original temporal dimension. We then apply temporal convolution layers and channel MLPs to map the features to speech parameters, as shown in b . The non-causal version uses non-causal temporal convolution in each layer, whereas the causal version uses causal convolution. d , The ECoG decoder using the 3D Swin architecture. We use three or four stages of 3D Swin blocks with spatial-temporal attention (three blocks for LD and four blocks for HB) to extract the features from the ECoG signal. We then use the transposed versions of temporal convolution layers as in c to upsample the features. The resulting features are mapped to the speech parameters using the same structure as shown in b . Non-causal versions apply temporal attention to past, present and future tokens, whereas the causal version applies temporal attention only to past and present tokens. e , The ECoG decoder using LSTM layers. We use three LSTM layers and one layer of channel MLP to generate features. We then reuse the prediction layers in b to generate the corresponding speech parameters. The non-causal version employs bidirectional LSTM in each layer, whereas the causal version uses unidirectional LSTM.
Our decoding pipeline showed robust speech decoding across participants, leading to PCC values within the range 0.62–0.92 (Fig. 2a ; causal ResNet mean 0.797, median 0.805) between the decoded and ground-truth speech across several architectures. We attribute our stable training and accurate decoding to the carefully designed components of our pipeline (for example, the speech synthesizer and speech parameter guidance) and the multiple improvements ( Methods sections Speech synthesizer , ECoG decoder and Model training ) over our previous approach on the subset of participants with hybrid-density grids 29 . Previous reports have investigated speech- or text-decoding using linear models 14 , 15 , 30 , transitional probability 4 , 31 , recurrent neural networks 5 , 10 , 17 , 19 , convolutional neural networks 8 , 29 and other hybrid or selection approaches 9 , 16 , 18 , 32 , 33 . Overall, our results are similar to (or better than) many previous reports (54% of our participants showed higher than 0.8 for the decoding PCC; Fig. 3c ). However, a direct comparison is complicated by multiple factors. Previous reports vary in terms of the reported performance metrics, as well as the stimuli decoded (for example, continuous speech versus single words) and the cortical sampling (that is, high versus low density, depth electrodes compared with surface grids). Our publicly available pipeline, which can be used across multiple neural network architectures and tested on various performance metrics, can facilitate the research community to conduct more direct comparisons while still adhering to a high accuracy of speech decoding.
The temporal causality of decoding operations, critical for real-time BCI applications, has not been considered by most previous studies. Many of these non-causal models relied on auditory (and somatosensory) feedback signals. Our analyses show that non-causal models rely on a robust contribution from the superior temporal gyrus (STG), which is mostly eliminated using a causal model (Fig. 4 ). We believe that non-causal models would show limited generalizability to real-time BCI applications due to their over-reliance on feedback signals, which may be absent (if no delay is allowed) or incorrect (if a short latency is allowed during real-time decoding). Some approaches used imagined speech, which avoids feedback during training 16 , or showed generalizability to mimed production lacking auditory feedback 17 , 19 . However, most reports still employ non-causal models, which cannot rule out feedback during training and inference. Indeed, our contribution maps show robust auditory cortex recruitment for the non-causal ResNet and Swin models (Fig. 4 , in contrast to their causal counterparts, which decode based on more frontal regions. Furthermore, the recurrent neural networks that are widely used in the literature 5 , 19 are typically bidirectional, producing non-causal behaviours and longer latencies for prediction during real-time applications. Unidirectional causal results are typically not reported. The recurrent network we tested performed the worst when trained with one direction (Fig. 2a , causal LSTM). Although our current focus was not real-time decoding, we were able to synthesize speech from neural signals with a delay of under 50 ms (Supplementary Table 1 ), which provides minimal auditory delay interference and allows for normal speech production 34 , 35 . Our data suggest that causal convolutional and transformer models can perform on par with their non-causal counterparts and recruit more relevant cortical structures for real-time decoding.
In our study we have leveraged an intermediate speech parameter space together with a novel differentiable speech synthesizer to decode subject-specific naturalistic speech (Fig. 1 . Previous reports used varying approaches to model speech, including an intermediate kinematic space 17 , an acoustically relevant intermediate space using HuBERT features 19 derived from a self-supervised speech masked prediction task 20 , an intermediate random vector (that is, GAN) 11 or direct spectrogram representations 8 , 17 , 36 , 37 . Our choice of speech parameters as the intermediate representation allowed us to decode subject-specific acoustics. Our intermediate acoustic representation led to significantly more accurate speech decoding than directly mapping ECoG to the speech spectrogram 38 , and than mapping ECoG to a random vector, which is then fed to a GAN-based speech synthesizer 11 (Supplementary Fig. 10 ). Unlike the kinematic representation, our acoustic intermediate representation using speech parameters and the associated speech synthesizer enables our decoding pipeline to produce natural-sounding speech that preserves subject-specific characteristics, which would be lost with the kinematic representation.
Our speech synthesizer is motivated by classical vocoder models for speech production (generating speech by passing an excitation source, harmonic or noise, through a filter 39 , 40 and is fully differentiable, facilitating the training of the ECoG decoder using spectral losses through backpropagation. Furthermore, the guidance speech parameters needed for training the ECoG decoder can be obtained using a speech encoder that can be pre-trained without requiring neural data. Thus, it could be trained using older speech recordings or a proxy speaker chosen by the patient in the case of patients without the ability to speak. Training the ECoG decoder using such guidance, however, would require us to revise our current training strategy to overcome the challenge of misalignment between neural signals and speech signals, which is a scope of our future work. Additionally, the low-dimensional acoustic space and pre-trained speech encoder (for generating the guidance) using speech signals only alleviate the limited data challenge in training the ECoG-to-speech decoder and provide a highly interpretable latent space. Finally, our decoding pipeline is generalizable to unseen words (Fig. 2b ). This provides an advantage compared to the pattern-matching approaches 18 that produce subject-specific utterances but with limited generalizability.
Many earlier studies employed high-density electrode coverage over the cortex, providing many distinct neural signals 5 , 10 , 17 , 30 , 37 . One question we directly addressed was whether higher-density coverage improves decoding. Surprisingly, we found a high decoding performance in terms of spectrogram PCC with both low-density and higher (hybrid) density grid coverages (Fig. 3c ). Furthermore, comparing the decoding performance obtained using all electrodes in our hybrid-density participants versus using only the low-density electrodes in the same participants revealed that the decoding did not differ significantly (albeit for one participant; Fig. 3d ). We attribute these results to the ability of our ECoG decoder to extract speech parameters from neural signals as long as there is sufficient perisylvian coverage, even in low-density participants.
A striking result was the robust decoding from right hemisphere cortical structures as well as the clear contribution of the right perisylvian cortex. Our results are consistent with the idea that syllable-level speech information is represented bilaterally 41 . However, our findings suggest that speech information is well-represented in the right hemisphere. Our decoding results could directly lead to speech prostheses for patients who suffer from expressive aphasia or apraxia of speech. Some previous studies have shown limited right-hemisphere decoding of vowels 42 and sentences 43 . However, the results were mostly mixed with left-hemisphere signals. Although our decoding results provide evidence for a robust representation of speech in the right hemisphere, it is important to note that these regions are likely not critical for speech, as evidenced by the few studies that have probed both hemispheres using electrical stimulation mapping 44 , 45 . Furthermore, it is unclear whether the right hemisphere would contain sufficient information for speech decoding if the left hemisphere were damaged. It would be necessary to collect right-hemisphere neural data from left-hemisphere-damaged patients to verify we can still achieve acceptable speech decoding. However, we believe that right-hemisphere decoding is still an exciting avenue as a clinical target for patients who are unable to speak due to left-hemisphere cortical damage.
There are several limitations in our study. First, our decoding pipeline requires speech training data paired with ECoG recordings, which may not exist for paralysed patients. This could be mitigated by using neural recordings during imagined or mimed speech and the corresponding older speech recordings of the patient or speech by a proxy speaker chosen by the patient. As discussed earlier, we would need to revise our training strategy to overcome the temporal misalignment between the neural signal and the speech signal. Second, our ECoG decoder models (3D ResNet and 3D Swin) assume a grid-based electrode sampling, which may not be the case. Future work should develop model architectures that are capable of handling non-grid data, such as strips and depth electrodes (stereo intracranial electroencephalogram (sEEG)). Importantly, such decoders could replace our current grid-based ECoG decoders while still being trained using our overall pipeline. Finally, our focus in this study was on word-level decoding limited to a vocabulary of 50 words, which may not be directly comparable to sentence-level decoding. Specifically, two recent studies have provided robust speech decoding in a few chronic patients implanted with intracranial ECoG 19 or a Utah array 46 that leveraged a large amount of data available in one patient in each study. It is noteworthy that these studies use a range of approaches in constraining their neural predictions. Metzger and colleagues employed a pre-trained large transformer model leveraging directional attention to provide the guidance HuBERT features for their ECoG decoder. In contrast, Willet and colleagues decoded at the level of phonemes and used transition probability models at both phoneme and word levels to constrain decoding. Our study is much more limited in terms of data. However, we were able to achieve good decoding results across a large cohort of patients through the use of a compact acoustic representation (rather than learnt contextual information). We expect that our approach can help improve generalizability for chronically implanted patients.
To summarize, our neural decoding approach, capable of decoding natural-sounding speech from 48 participants, provides the following major contributions. First, our proposed intermediate representation uses explicit speech parameters and a novel differentiable speech synthesizer, which enables interpretable and acoustically accurate speech decoding. Second, we directly consider the causality of the ECoG decoder, providing strong support for causal decoding, which is essential for real-time BCI applications. Third, our promising decoding results using low sampling density and right-hemisphere electrodes shed light on future neural prosthetic devices using low-density grids and in patients with damage to the left hemisphere. Last, but not least, we have made our decoding framework open to the community with documentation ( https://github.com/flinkerlab/neural_speech_decoding ), and we trust that this open platform will help propel the field forward, supporting reproducible science.
Experiments design
We collected neural data from 48 native English-speaking participants (26 female, 22 male) with refractory epilepsy who had ECoG subdural electrode grids implanted at NYU Langone Hospital. Five participants underwent HB sampling, and 43 LD sampling. The ECoG array was implanted on the left hemisphere for 32 participants and on the right for 16. The Institutional Review Board of NYU Grossman School of Medicine approved all experimental procedures. After consulting with the clinical-care provider, a research team member obtained written and oral consent from each participant. Each participant performed five tasks 47 to produce target words in response to auditory or visual stimuli. The tasks were auditory repetition (AR, repeating auditory words), auditory naming (AN, naming a word based on an auditory definition), sentence completion (SC, completing the last word of an auditory sentence), visual reading (VR, reading aloud written words) and picture naming (PN, naming a word based on a colour drawing).
For each task, we used the exact 50 target words with different stimulus modalities (auditory, visual and so on). Each word appeared once in the AN and SC tasks and twice in the others. The five tasks involved 400 trials, with corresponding word production and ECoG recording for each participant. The average duration of the produced speech in each trial was 500 ms.
Data collection and preprocessing
The study recorded ECoG signals from the perisylvian cortex (including STG, inferior frontal gyrus (IFG), pre-central and postcentral gyri) of 48 participants while they performed five speech tasks. A microphone recorded the subjects’ speech and was synchronized to the clinical Neuroworks Quantum Amplifier (Natus Biomedical), which captured ECoG signals. The ECoG array consisted of 64 standard 8 × 8 macro contacts (10-mm spacing) for 43 participants with low-density sampling. For five participants with hybrid-density sampling, the ECoG array also included 64 additional interspersed smaller electrodes (1 mm) between the macro contacts (providing 10-mm centre-to-centre spacing between macro contacts and 5-mm centre-to-centre spacing between micro/macro contacts; PMT Corporation) (Fig. 3b ). This Food and Drug Administration (FDA)-approved array was manufactured for this study. A research team member informed participants that the additional contacts were for research purposes during consent. Clinical care solely determined the placement location across participants (32 left hemispheres; 16 right hemispheres). The decoding models were trained separately for each participant using all trials except ten randomly selected ones from each task, leading to 350 trials for training and 50 for testing. The reported results are for testing data only.
We sampled ECoG signals from each electrode at 2,048 Hz and downsampled them to 512 Hz before processing. Electrodes with artefacts (for example, line noise, poor contact with the cortex, high-amplitude shifts) were rejected. The electrodes with interictal and epileptiform activity were also excluded from the analysis. The mean of a common average reference (across all remaining valid electrodes and time) was subtracted from each individual electrode. After the subtraction, a Hilbert transform extracted the envelope of the high gamma (70–150 Hz) component from the raw signal, which was then downsampled to 125 Hz. A reference signal was obtained by extracting a silent period of 250 ms before each trial’s stimulus period within the training set and averaging the signals over these silent periods. Each electrode’s signal was normalized to the reference mean and variance (that is, z -score). The data-preprocessing pipeline was coded in MATLAB and Python. For participants with noisy speech recordings, we applied spectral gating to remove stationary noise from the speech using an open-source tool 48 . We ruled out the possibility that our neural data suffer from a recently reported acoustic contamination (Supplementary Fig. 5 ) by following published approaches 49 .
To pre-train the auto-encoder, including the speech encoder and speech synthesizer, unlike our previous work in ref. 29 , which completely relied on unsupervised training, we provided supervision for some speech parameters to improve their estimation accuracy further. Specifically, we used the Praat method 50 to estimate the pitch and four formant frequencies ( \({f}_{ {{{\rm{i}}}} = {1}\,{{{\rm{to}}}}\,4}^{t}\) , in hertz) from the speech waveform. The estimated pitch and formant frequency were resampled to 125 Hz, the same as the ECoG signal and spectrogram sampling frequency. The mean square error between these speech parameters generated by the speech encoder and those estimated by the Praat method was used as a supervised reference loss, in addition to the unsupervised spectrogram reconstruction and STOI losses, making the training of the auto-encoder semi-supervised.
Speech synthesizer
Our speech synthesizer was inspired by the traditional speech vocoder, which generates speech by switching between voiced and unvoiced content, each generated by filtering a specific excitation signal. Instead of switching between the two components, we use a soft mix of the two components, making the speech synthesizer differentiable. This enables us to train the ECoG decoder and the speech encoder end-to-end by minimizing the spectrogram reconstruction loss with backpropagation. Our speech synthesizer can generate a spectrogram from a compact set of speech parameters, enabling training of the ECoG decoder with limited data. As shown in Fig. 5 , the synthesizer takes dynamic speech parameters as input and contains two pathways. The voice pathway applies a set of formant filters (each specified by the centre frequency \({f}_{i}^{\;t}\) , bandwidth \({b}_{i}^{t}\) that is dependent on \({f}_{i}^{\;t}\) , and amplitude \({a}_{i}^{t}\) ) to the harmonic excitation (with pitch frequency f 0 ) and generates the voiced component, V t ( f ), for each time step t and frequency f . The noise pathway filters the input white noise with an unvoice filter (consisting of a broadband filter defined by centre frequency \({f}_{\hat{u}}^{\;t}\) , bandwidth \({b}_{\hat{u}}^{t}\) and amplitude \({a}_{\hat{u}}^{t}\) and the same six formant filters used for the voice filter) and produces the unvoiced content, U t ( f ). The synthesizer combines the two components with a voice weight α t ∈ [0, 1] to obtain the combined spectrogram \({\widetilde{S}}^{t}{(\;f\;)}\) as
Factor α t acts as a soft switch for the gradient to flow back through the synthesizer. The final speech spectrogram is given by
where L t is the loudness modulation and B ( f ) the background noise. We describe the various components in more detail in the following.
Formant filters in the voice pathway
We use multiple formant filters in the voice pathway to model formants that represent vowels and nasal information. The formant filters capture the resonance in the vocal tract, which can help recover a speaker’s timbre characteristics and generate natural-sounding speech. We assume the filter for each formant is time-varying and can be derived from a prototype filter G i ( f ), which achieves maximum at a centre frequency \({f}_{i}^{{{\;{\rm{proto}}}}}\) and has a half-power bandwidth \({b}_{i}^{{{{\rm{proto}}}}}\) . The prototype filters have learnable parameters and will be discussed later. The actual formant filter at any time is written as a shifted and scaled version of G i ( f ). Specifically, at time t , given an amplitude \({\left({a}_{i}^{t}\right)}\) , centre frequency \({\left(\;{f}_{i}^{\;t}\right)}\) and bandwidth \({\left({b}_{i}^{t}\right)}\) , the frequency-domain representation of the i th formant filter is
where f max is half of the speech sampling frequency, which in our case is 8,000 Hz.
Rather than letting the bandwidth parameters \({b}_{i}^{t}\) be independent variables, based on the empirically observed relationships between \({b}_{i}^{t}\) and the centre frequencies \({f}_{i}^{\;t}\) , we set
The threshold frequency f θ , slope a and baseline bandwidth b 0 are three parameters that are learned during the auto-encoder training, shared among all six formant filters. This parameterization helps to reduce the number of speech parameters to be estimated at every time sample, making the representation space more compact.
Finally the filter for the voice pathway with N formant filters is given by \({F}_{{{{\rm{v}}}}}^{\;t}{(\;f\;)}={\mathop{\sum }\nolimits_{i = 1}^{N}{F}_{i}^{\;t}(\;f\;)}\) . Previous studies have shown that two formants ( N = 2) are enough for intelligible reconstruction 51 , but we use N = 6 for more accurate synthesis in our experiments.
Unvoice filters
We construct the unvoice filter by adding a single broadband filter \({F}_{\hat{u}}^{\;t}{(\;f\;)}\) to the formant filters for each time step t . The broadband filter \({F}_{\hat{u}}^{\;t}{(\;f\;)}\) has the same form as equation ( 1 ) but has its own learned prototype filter \({G}_{\hat{u}}{(f)}\) . The speech parameters corresponding to the broadband filter include \({\left({\alpha }_{\hat{u}}^{t},\,{f}_{\hat{u}}^{\;t},\,{b}_{\hat{u}}^{t}\right)}\) . We do not impose a relationship between the centre frequency \({f}_{\hat{u}}^{\;t}\) and the bandwidth \({b}_{\hat{u}}^{t}\) . This allows more flexibility in shaping the broadband unvoice filter. However, we constrain \({b}_{\hat{u}}^{t}\) to be larger than 2,000 Hz to capture the wide spectral range of obstruent phonemes. Instead of using only the broadband filter, we also retain the N formant filters in the voice pathway \({F}_{i}^{\;t}\) for the noise pathway. This is based on the observation that humans perceive consonants such as /p/ and /d/ not only by their initial bursts but also by their subsequent formant transitions until the next vowel 52 . We use identical formant filter parameters to encode these transitions. The overall unvoice filter is \({F}_{{{{\rm{u}}}}}^{\;t}{(\;f\;)}={F}_{\hat{u}}^{\;t}(\;f\;)+\mathop{\sum }\nolimits_{i = 1}^{N}{F}_{i}^{\;t}{(\;f\;)}\) .
Voice excitation
We use the voice filter in the voice pathway to modulate the harmonic excitation. Following ref. 53 , we define the harmonic excitation as \({h}^{t}={\mathop{\sum }\nolimits_{k = 1}^{K}{h}_{k}^{t}}\) , where K = 80 is the number of harmonics.
The value of the k th resonance at time step t is \({h}_{k}^{t}={\sin (2\uppi k{\phi }^{t})}\) with \({\phi }^{t}={\mathop{\sum }\nolimits_{\tau = 0}^{t}{f}_{0}^{\;\tau }}\) , where \({f}_{0}^{\;\tau }\) is the fundamental frequency at time τ . The spectrogram of h t forms the harmonic excitation in the frequency domain H t ( f ), and the voice excitation is \({V}^{\;t}{(\;f\;)}={F}_{{{{\rm{v}}}}}^{t}{(\;f\;)}{H}^{\;t}{(\;f\;)}\) .
Noise excitation
The noise pathway models consonant sounds (plosives and fricatives). It is generated by passing a stationary Gaussian white noise excitation through the unvoice filter. We first generate the noise signal n ( t ) in the time domain by sampling from the Gaussian process \({{{\mathcal{N}}}}{(0,\,1)}\) and then obtain its spectrogram N t ( f ). The spectrogram of the unvoiced component is \({U}^{\;t}{(\;f\;)}={F}_{u}^{\;t}{(\;f\;)}{N}^{\;t}{(\;f\;)}\) .
Summary of speech parameters
The synthesizer generates the voiced component at time t by driving a harmonic excitation with pitch frequency \({f}_{0}^{\;t}\) through N formant filters in the voice pathway, each described by two parameters ( \({f}_{ i}^{\;t},\,{a}_{ i}^{t}\) ). The unvoiced component is generated by filtering a white noise through the unvoice filter consisting of an additional broadband filter with three parameters ( \({f}_{\hat{u}}^{\;t},\,{b}_{\hat{u}}^{t},\,{a}_{\hat{u}}^{t}\) ). The two components are mixed based on the voice weight α t and further amplified by the loudness value L t . In total, the synthesizer input includes 18 speech parameters at each time step.
Unlike the differentiable digital signal processing (DDSP) in ref. 53 , we do not directly assign amplitudes to the K harmonics. Instead, the amplitude in our model depends on the formant filters, which has two benefits:
The representation space is more compact. DDSP requires 80 amplitude parameters \({a}_{k}^{t}\) for each of the 80 harmonic components \({f}_{k}^{\;t}\) ( k = 1, 2, …, 80) at each time step. In contrast, our synthesizer only needs a total of 18 parameters.
The representation is more disentangled. For human speech, the vocal tract shape (affecting the formant filters) is largely independent of the vocal cord tension (which determines the pitch). Modelling these two separately leads to a disentangled representation.
In contrast, DDSP specifies the amplitude for each harmonic component directly resulting in entanglement and redundancy between these amplitudes. Furthermore, it remains uncertain whether the amplitudes \({a}_{k}^{t}\) could be effectively controlled and encoded by the brain. In our approach, we explicitly model the formant filters and fundamental frequency, which possess clear physical interpretations and are likely to be directly controlled by the brain. Our representation also enables a more robust and direct estimation of the pitch.
Speaker-specific synthesizer parameters
Prototype filters.
Instead of using a predetermined prototype formant filter shape, for example, a standard Gaussian function, we learn a speaker-dependent prototype filter for each formant to allow more expressive and flexible formant filter shapes. We define the prototype filter G i ( f ) of the i th formant as a piecewise linear function, linearly interpolated from g i [ m ], m = 1, …, M , with the amplitudes of the filter at M being uniformly sampled frequencies in the range [0, f max ]. We constrain g i [ m ] to increase and then decrease monotonically so that G i ( f ) is unimodal and has a single peak value of 1. Given g i [ m ], m = 1, …, M , we can determine the peak frequency \({f}_{i}^{\;{{{\rm{proto}}}}}\) and the half-power bandwidth \({b}_{i}^{{{{\rm{proto}}}}}\) of G i ( f ).
The prototype parameters g i [ m ], m = 1, …, M of each formant filter are time-invariant and are determined during the auto-encoder training. Compared with ref. 29 , we increase M from 20 to 80 to enable more expressive formant filters, essential for synthesizing male speakers’ voices.
We similarly learn a prototype filter for the broadband filter G û ( f ) for the unvoiced component, which is specified by M parameters g û ( m ).
Background noise
The recorded sound typically contains background noise. We assume that the background noise is stationary and has a specific frequency distribution, depending on the speech recording environment. This frequency distribution B ( f ) is described by K parameters, where K is the number of frequency bins ( K = 256 for females and 512 for males). The K parameters are also learned during auto-encoder training. The background noise is added to the mixed speech components to generate the final speech spectrogram.
To summarize, our speech synthesizer has the following learnable parameters: the M = 80 prototype filter parameters for each of the N = 6 formant filters and the broadband filters (totalling M ( N + 1) = 560), the three parameters f θ , a and b 0 relating the centre frequency and bandwidth for the formant filters (totalling 18), and K parameters for the background noise (256 for female and 512 for male). The total number of parameters for female speakers is 834, and that for male speakers is 1,090. Note that these parameters are speaker-dependent but time-independent, and they can be learned together with the speech encoder during the training of the speech-to-speech auto-encoder, using the speaker’s speech only.
Speech encoder
The speech encoder extracts a set of (18) speech parameters at each time point from a given spectrogram, which are then fed to the speech synthesizer to reproduce the spectrogram.
We use a simple network architecture for the speech encoder, with temporal convolutional layers and multilayer perceptron (MLP) across channels at the same time point, as shown in Fig. 6a . We encode pitch \({f}_{0}^{\;t}\) by combining features generated from linear and Mel-scale spectrograms. The other 17 speech parameters are derived by applying temporal convolutional layers and channel MLP to the linear-scale spectrogram. To generate formant filter centre frequencies \({f}_{i = 1\,{{{\rm{to}}}}\,6}^{\;t}\) , broadband unvoice filter frequency \({f}_{\hat{u}}^{\;t}\) and pitch \({f}_{0}^{\;t}\) , we use sigmoid activation at the end of the corresponding channel MLP to map the output to [0, 1], and then de-normalize it to real values by scaling [0, 1] to predefined [ f min , f max ]. The [ f min , f max ] values for each frequency parameter are chosen based on previous studies 54 , 55 , 56 , 57 . Our compact speech parameter space facilitates stable and easy training of our speech encoder. Models were coded using PyTorch version 1.21.1 in Python.
ECoG decoder
In this section we present the design details of three ECoG decoders: the 3D ResNet ECoG decoder, the 3D Swin transformer ECoG decoder and the LSTM ECoG decoder. The models were coded using PyTorch version 1.21.1 in Python.
3D ResNet ECoG decoder
This decoder adopts the ResNet architecture 23 for the feature extraction backbone of the decoder. Figure 6c illustrates the feature extraction part. The model views the ECoG input as 3D tensors with spatiotemporal dimensions. In the first layer, we apply only temporal convolution to the signal from each electrode, because the ECoG signal exhibits more temporal than spatial correlations. In the subsequent parts of the decoder, we have four residual blocks that extract spatiotemporal features using 3D convolution. After downsampling the electrode dimension to 1 × 1 and the temporal dimension to T /16, we use several transposed Conv layers to upsample the features to the original temporal size T . Figure 6b shows how to generate the different speech parameters from the resulting features using different temporal convolution and channel MLP layers. The temporal convolution operation can be causal (that is, using only past and current samples as input) or non-causal (that is, using past, current and future samples), leading to causal and non-causal models.
3D Swin Transformer ECoG decoder
Swin Transformer 24 employs the window and shift window methods to enable self-attention of small patches within each window. This reduces the computational complexity and introduces the inductive bias of locality. Because our ECoG input data have three dimensions, we extend Swin Transformer to three dimensions to enable local self-attention in both temporal and spatial dimensions among 3D patches. The local attention within each window gradually becomes global attention as the model merges neighbouring patches in deeper transformer stages.
Figure 6d illustrates the overall architecture of the proposed 3D Swin Transformer. The input ECoG signal has a size of T × H × W , where T is the number of frames and H × W is the number of electrodes at each frame. We treat each 3D patch of size 2 × 2 × 2 as a token in the 3D Swin Transformer. The 3D patch partitioning layer produces \({\frac{T}{2}\times \frac{H}{2}\times \frac{W}{2}}\) 3D tokens, each with a 48-dimensional feature. A linear embedding layer then projects the features of each token to a higher dimension C (=128).
The 3D Swin Transformer comprises three stages with two, two and six layers, respectively, for LD participants and four stages with two, two, six and two layers for HB participants. It performs 2 × 2 × 2 spatial and temporal downsampling in the patch-merging layer of each stage. The patch-merging layer concatenates the features of each group of 2 × 2 × 2 temporally and spatially adjacent tokens. It applies a linear layer to project the concatenated features to one-quarter of their original dimension after merging. In the 3D Swin Transformer block, we replace the multi-head self-attention (MSA) module in the original Swin Transformer with the 3D shifted window multi-head self-attention module. It adapts the other components to 3D operations as well. A Swin Transformer block consists of a 3D shifted window-based MSA module followed by a feedforward network (FFN), a two-layer MLP. Layer normalization is applied before each MSA module and FFN, and a residual connection is applied after each module.
Consider a stage with T × H × W input tokens. If the 3D window size is P × M × M , we partition the input into \({\lceil \frac{T}{P}\rceil \times \lceil \frac{H}{M}\rceil \times \lceil \frac{W}{M}\rceil}\) non-overlapping 3D windows evenly. We choose P = 16, M = 2. We perform the multi-head self-attention within each 3D window. However, this design lacks connection across adjacent windows, which may limit the representation power of the architecture. Therefore, we extend the shifted 2D window mechanism of the Swin Transformer to shifted 3D windows. In the second layer of the stage, we shift the window by \(\left({\frac{P}{2},\,\frac{M}{2},\,\frac{M}{2}}\right)\) tokens along the temporal, height and width axes from the previous layer. This creates cross-window connections for the self-attention module. This shifted 3D window design enables the interaction of electrodes with longer spatial and temporal distances by connecting neighbouring tokens in non-overlapping 3D windows in the previous layer.
The temporal attention in the self-attention operation can be constrained to be causal (that is, each token only attends to tokens temporally before it) or non-causal (that is, each token can attend to tokens temporally before or after it), leading to the causal and non-causal models, respectively.
LSTM decoder
The decoder uses the LSTM architecture 25 for the feature extraction in Fig. 6e . Each LSTM cell is composed of a set of gates that control the flow of information: the input gate, the forget gate and the output gate. The input gate regulates the entry of new data into the cell state, the forget gate decides what information is discarded from the cell state, and the output gate determines what information is transferred to the next hidden state and can be output from the cell.
In the LSTM architecture, the ECoG input would be processed through these cells sequentially. For each time step T , the LSTM would take the current input x t and the previous hidden state h t − 1 and would produce a new hidden state h t and output y t . This process allows the LSTM to maintain information over time and is particularly useful for tasks such as speech and neural signal processing, where temporal dependencies are critical. Here we use three layers of LSTM and one linear layer to generate features to map to speech parameters. Unlike 3D ResNet and 3D Swin, we keep the temporal dimension unchanged across all layers.
Model training
Training of the speech encoder and speech synthesizer.
As described earlier, we pre-train the speech encoder and the learnable parameters in the speech synthesizer to perform a speech-to-speech auto-encoding task. We use multiple loss terms for the training. The modified multi-scale spectral (MSS) loss is inspired by ref. 53 and is defined as
Here, S t ( f ) denotes the ground-truth spectrogram and \({\widehat{S}}^{t}{(\;f\;)}\) the reconstructed spectrogram in the linear scale, \({S}_{{{{\rm{mel}}}}}^{t}{(\;f\;)}\) and \({\widehat{S}}_{{{{\rm{mel}}}}}^{t}{(\;f\;)}\) are the corresponding spectrograms in the Mel-frequency scale. We sample the frequency range [0, 8,000 Hz] with K = 256 bins for female participants. For male participants, we set K = 512 because they have lower f 0 , and it is better to have a higher resolution in frequency.
To improve the intelligibility of the reconstructed speech, we also introduce the STOI loss by implementing the STOI+ metric 26 , which is a variation of the original STOI metric 8 , 22 . STOI+ 26 discards the normalization and clipping step in STOI and has been shown to perform best among intelligibility evaluation metrics. First, a one-third octave band analysis 22 is performed by grouping Discrete Fourier transform (DFT) bins into 15 one-third octave bands with the lowest centre frequency set equal to 150 Hz and the highest centre frequency equal to ~4.3 kHz. Let \({\hat{x}(k,\,m)}\) denote the k th DFT bin of the m th frame of the ground-truth speech. The norm of the j th one-third octave band, referred to as a time-frequency (TF) unit, is then defined as
where k 1 ( j ) and k 2 ( j ) denote the one-third octave band edges rounded to the nearest DFT bin. The TF representation of the processed speech \({\hat{y}}\) is obtained similarly and denoted by Y j ( m ). We then extract the short-time temporal envelopes in each band and frame, denoted X j , m and Y j , m , where \({X}_{j,\,m}={\left[{X}_{j}{(m-N+1)},\,{X}_{j}{(m-N+2)},\,\ldots ,\,{X}_{j}{(m)}\right]}^{\rm{T}}\) , with N = 30. The STOI+ metric is the average of the PCC d j , m between X j , m and Y j , m , overall j and m (ref. 26 ):
We use the negative of the STOI+ metric as the STOI loss:
where J and M are the total numbers of frequency bins ( J = 15) and frames, respectively. Note that L STOI is differentiable with respect to \({\widehat{S}}^{t}{(\;f\;)}\) , and thus can be used to update the model parameters generating the predicted spectrogram \({\widehat{S}}^{t}{(\;f\;)}\) .
To further improve the accuracy for estimating the pitch \({\widetilde{f}}_{0}^{\;t}\) and formant frequencies \({\widetilde{f}}_{{{{\rm{i}}}} = {1}\,{{{\rm{to}}}}\,4}^{\;t}\) , we add supervisions to them using the formant frequencies extracted by the Praat method 50 . The supervision loss is defined as
where the weights β i are chosen to be β 1 = 0.1, β 2 = 0.06, β 3 = 0.03 and β 4 = 0.02, based on empirical trials. The overall training loss is defined as
where the weighting parameters λ i are empirically optimized to be λ 1 = 1.2 and λ 2 = 0.1 through testing the performances on three hybrid-density participants with different parameter choices.
Training of the ECoG decoder
With the reference speech parameters generated by the speech encoder and the target speech spectrograms as ground truth, the ECoG decoder is trained to match these targets. Let us denote the decoded speech parameters as \({\widetilde{C}}_{j}^{\;t}\) , and their references as \({C}_{j}^{\;t}\) , where j enumerates all speech parameters fed to the speech synthesizer. We define the reference loss as
where weighting parameters λ j are chosen as follows: voice weight λ α = 1.8, loudness λ L = 1.5, pitch \({\lambda }_{{f}_{0}}={0.4}\) , formant frequencies \({\lambda }_{{f}_{1}}={3},\,{\lambda }_{{f}_{2}}={1.8},\,{\lambda }_{{f}_{3}}={1.2},\,{\lambda }_{{f}_{4}}={0.9},\,{\lambda }_{{f}_{5}}={0.6},\,{\lambda }_{{f}_{6}}={0.3}\) , formant amplitudes \({\lambda }_{{a}_{1}}={4},\,{\lambda }_{{a}_{2}}={2.4},\,{\lambda }_{{a}_{3}}={1.2},\,{\lambda }_{{a}_{4}}={0.9},\,{\lambda }_{{a}_{5}}={0.6},\,{\lambda }_{{a}_{6}}={0.3}\) , broadband filter frequency \({\lambda }_{{f}_{\hat{u}}}={10}\) , amplitude \({\lambda }_{{a}_{\hat{u}}}={4}\) , bandwidth \({\lambda }_{{b}_{\hat{u}}}={4}\) . Similar to speech-to-speech auto-encoding, we add supervision loss for pitch and formant frequencies derived by the Praat method and use the MSS and STOI loss to measure the difference between the reconstructed spectrograms and the ground-truth spectrogram. The overall training loss for the ECoG decoder is
where weighting parameters λ i are empirically optimized to be λ 1 = 1.2, λ 2 = 0.1 and λ 3 = 1, through the same parameter search process as described for training the speech encoder.
We use the Adam optimizer 58 with hyper-parameters lr = 10 −3 , β 1 = 0.9 and β 2 = 0.999 to train both the auto-encoder (including the speech encoder and speech synthesizer) and the ECoG decoder. We train a separate set of models for each participant. As mentioned earlier, we randomly selected 50 out of 400 trials per participant as the test data and used the rest for training.
Evaluation metrics
In this Article, we use the PCC between the decoded spectrogram and the actual speech spectrogram to evaluate the objective quality of the decoded speech, similar to refs. 8 , 18 , 59 .
We also use STOI+ 26 , as described in Methods section Training of the ECoG decoder to measure the intelligibility of the decoded speech. The STOI+ value ranges from −1 to 1 and has been reported to have a monotonic relationship with speech intelligibility.
Contribution analysis with the occlusion method
To measure the contribution of the cortex region under each electrode to the decoding performance, we adopted an occlusion-based method that calculates the change in the PCC between the decoded and the ground-truth spectrograms when an electrode signal is occluded (that is, set to zeros), as in ref. 29 . This method enables us to reveal the critical brain regions for speech production. We used the following notations: S t ( f ), the ground-truth spectrogram; \({\hat{{{{{S}}}}}}^{t}{(\;f\;)}\) , the decoded spectrogram with ‘intact’ input (that is, all ECoG signals are used); \({\hat{{{{{S}}}}}}_{i}^{t}{(\;f\;)}\) , the decoded spectrogram with the i th ECoG electrode signal occluded; r ( ⋅ , ⋅ ), correlation coefficient between two signals. The contribution of i th electrode for a particular participant is defined as
where Mean{ ⋅ } denotes averaging across all testing trials of the participant.
We generate the contribution map on the standardized Montreal Neurological Institute (MNI) brain anatomical map by diffusing the contribution of each electrode of each participant (with a corresponding location in the MNI coordinate) into the adjacent area within the same anatomical region using a Gaussian kernel and then averaging the resulting map from all participants. To account for the non-uniform density of the electrodes in different regions and across the participants, we normalize the sum of the diffused contribution from all the electrodes at each brain location by the total number of electrodes in the region across all participants.
We estimate the noise level for the contribution map to assess the significance of our contribution analysis. To derive the noise level, we train a shuffled model for each participant by randomly pairing the mismatched speech segment and ECoG segment in the training set. We derive the average contribution map from the shuffled models for all participants using the same occlusion analysis as described earlier. The resulting contribution map is used as the noise level. Contribution levels below the noise levels at corresponding cortex locations are assigned a value of 0 (white) in Fig. 4 .
Reporting summary
Further information on research design is available in the Nature Portfolio Reporting Summary linked to this Article.
Data availability
The data of one participant who consented to the release of the neural and audio data are publicly available through Mendeley Data at https://data.mendeley.com/datasets/fp4bv9gtwk/2 (ref. 60 ). Although all participants consented to share their data for research purposes, not all participants agreed to share their audio publicly. Given the sensitive nature of audio speech data we will share data with researchers that directly contact the corresponding author and provide documentation that the data will be strictly used for research purposes and will comply with the terms of our study IRB. Source data are provided with this paper.
Code availability
The code is available at https://github.com/flinkerlab/neural_speech_decoding ( https://doi.org/10.5281/zenodo.10719428 ) 61 .
Schultz, T. et al. Biosignal-based spoken communication: a survey. IEEE / ACM Trans. Audio Speech Lang. Process. 25 , 2257–2271 (2017).
Google Scholar
Miller, K. J., Hermes, D. & Staff, N. P. The current state of electrocorticography-based brain-computer interfaces. Neurosurg. Focus 49 , E2 (2020).
Article Google Scholar
Luo, S., Rabbani, Q. & Crone, N. E. Brain-computer interface: applications to speech decoding and synthesis to augment communication. Neurotherapeutics 19 , 263–273 (2022).
Moses, D. A., Leonard, M. K., Makin, J. G. & Chang, E. F. Real-time decoding of question-and-answer speech dialogue using human cortical activity. Nat. Commun. 10 , 3096 (2019).
Moses, D. A. et al. Neuroprosthesis for decoding speech in a paralyzed person with anarthria. N. Engl. J. Med. 385 , 217–227 (2021).
Herff, C. & Schultz, T. Automatic speech recognition from neural signals: a focused review. Front. Neurosci. 10 , 429 (2016).
Rabbani, Q., Milsap, G. & Crone, N. E. The potential for a speech brain-computer interface using chronic electrocorticography. Neurotherapeutics 16 , 144–165 (2019).
Angrick, M. et al. Speech synthesis from ECoG using densely connected 3D convolutional neural networks. J. Neural Eng. 16 , 036019 (2019).
Sun, P., Anumanchipalli, G. K. & Chang, E. F. Brain2Char: a deep architecture for decoding text from brain recordings. J. Neural Eng. 17 , 066015 (2020).
Makin, J. G., Moses, D. A. & Chang, E. F. Machine translation of cortical activity to text with an encoder–decoder framework. Nat. Neurosci. 23 , 575–582 (2020).
Wang, R. et al. Stimulus speech decoding from human cortex with generative adversarial network transfer learning. In Proc. 2020 IEEE 17th International Symposium on Biomedical Imaging ( ISBI ) (ed. Amini, A.) 390–394 (IEEE, 2020).
Zelinka, P., Sigmund, M. & Schimmel, J. Impact of vocal effort variability on automatic speech recognition. Speech Commun. 54 , 732–742 (2012).
Benzeghiba, M. et al. Automatic speech recognition and speech variability: a review. Speech Commun. 49 , 763–786 (2007).
Martin, S. et al. Decoding spectrotemporal features of overt and covert speech from the human cortex. Front. Neuroeng. 7 , 14 (2014).
Herff, C. et al. Towards direct speech synthesis from ECoG: a pilot study. In Proc. 2016 38th Annual International Conference of the IEEE Engineering in Medicine and Biology Society ( EMBC ) (ed. Patton, J.) 1540–1543 (IEEE, 2016).
Angrick, M. et al. Real-time synthesis of imagined speech processes from minimally invasive recordings of neural activity. Commun. Biol 4 , 1055 (2021).
Anumanchipalli, G. K., Chartier, J. & Chang, E. F. Speech synthesis from neural decoding of spoken sentences. Nature 568 , 493–498 (2019).
Herff, C. et al. Generating natural, intelligible speech from brain activity in motor, premotor and inferior frontal cortices. Front. Neurosci. 13 , 1267 (2019).
Metzger, S. L. et al. A high-performance neuroprosthesis for speech decoding and avatar control. Nature 620 , 1037–1046 (2023).
Hsu, W.-N. et al. Hubert: self-supervised speech representation learning by masked prediction of hidden units. IEEE/ACM Trans. Audio Speech Lang. Process. 29 , 3451–3460 (2021).
Griffin, D. & Lim, J. Signal estimation from modified short-time fourier transform. IEEE Trans. Acoustics Speech Signal Process. 32 , 236–243 (1984).
Taal, C. H., Hendriks, R. C., Heusdens, R. & Jensen, J. A short-time objective intelligibility measure for time-frequency weighted noisy speech. In Proc. 2010 IEEE International Conference on Acoustics, Speech and Signal Processing (ed. Douglas, S.) 4214–4217 (IEEE, 2010).
He, K., Zhang, X., Ren, S. & Sun, J. Deep residual learning for image recognition. In Proc. 2016 IEEE Conference on Computer Vision and Pattern Recognition ( CVPR ) (ed. Bajcsy, R.) 770–778 (IEEE, 2016).
Liu, Z. et al. Swin Transformer: hierarchical vision transformer using shifted windows. In Proc. 2021 IEEE / CVF International Conference on Computer Vision ( ICCV ) (ed. Dickinson, S.) 9992–10002 (IEEE, 2021).
Hochreiter, S. & Schmidhuber, J. Long short-term memory. Neural Comput. 9 , 1735–1780 (1997).
Graetzer, S. & Hopkins, C. Intelligibility prediction for speech mixed with white Gaussian noise at low signal-to-noise ratios. J. Acoust. Soc. Am. 149 , 1346–1362 (2021).
Hickok, G. & Poeppel, D. The cortical organization of speech processing. Nat. Rev. Neurosci. 8 , 393–402 (2007).
Trupe, L. A. et al. Chronic apraxia of speech and Broca’s area. Stroke 44 , 740–744 (2013).
Wang, R. et al. Distributed feedforward and feedback cortical processing supports human speech production. Proc. Natl Acad. Sci. USA 120 , e2300255120 (2023).
Mugler, E. M. et al. Differential representation ofÿ articulatory gestures and phonemes in precentral and inferior frontal gyri. J. Neurosci. 38 , 9803–9813 (2018).
Herff, C. et al. Brain-to-text: decoding spoken phrases from phone representations in the brain. Front. Neurosci. 9 , 217 (2015).
Kohler, J. et al. Synthesizing speech from intracranial depth electrodes using an encoder-decoder framework. Neurons Behav. Data Anal. Theory https://doi.org/10.51628/001c.57524 (2022).
Angrick, M. et al. Towards closed-loop speech synthesis from stereotactic EEG: a unit selection approach. In Proc. 2022 IEEE International Conference on Acoustics , Speech and Signal Processing ( ICASSP ) (ed. Li, H.) 1296–1300 (IEEE, 2022).
Ozker, M., Doyle, W., Devinsky, O. & Flinker, A. A cortical network processes auditory error signals during human speech production to maintain fluency. PLoS Biol. 20 , e3001493 (2022).
Stuart, A., Kalinowski, J., Rastatter, M. P. & Lynch, K. Effect of delayed auditory feedback on normal speakers at two speech rates. J. Acoust. Soc. Am. 111 , 2237–2241 (2002).
Verwoert, M. et al. Dataset of speech production in intracranial electroencephalography. Sci. Data 9 , 434 (2022).
Berezutskaya, J. et al. Direct speech reconstruction from sensorimotor brain activity with optimized deep learning models. J. Neural Eng. 20 , 056010 (2023).
Wang, R., Wang, Y. & Flinker, A. Reconstructing speech stimuli from human auditory cortex activity using a WaveNet approach. In Proc. 2018 IEEE Signal Processing in Medicine and Biology Symposium ( SPMB ) (ed. Picone, J.) 1–6 (IEEE, 2018).
Flanagan, J. L. Speech Analysis Synthesis and Perception Vol. 3 (Springer, 2013).
Serra, X. & Smith, J. Spectral modeling synthesis: a sound analysis/synthesis system based on a deterministic plus stochastic decomposition. Comput. Music J. 14 , 12–24 (1990).
Cogan, G. B. et al. Sensory–motor transformations for speech occur bilaterally. Nature 507 , 94–98 (2014).
Ibayashi, K. et al. Decoding speech with integrated hybrid signals recorded from the human ventral motor cortex. Front. Neurosci. 12 , 221 (2018).
Soroush, P. Z. et al. The nested hierarchy of overt, mouthed and imagined speech activity evident in intracranial recordings. NeuroImage 269 , 119913 (2023).
Tate, M. C., Herbet, G., Moritz-Gasser, S., Tate, J. E. & Duffau, H. Probabilistic map of critical functional regions of the human cerebral cortex: Broca’s area revisited. Brain 137 , 2773–2782 (2014).
Long, M. A. et al. Functional segregation of cortical regions underlying speech timing and articulation. Neuron 89 , 1187–1193 (2016).
Willett, F. R. et al. A high-performance speech neuroprosthesis. Nature 620 , 1031–1036 (2023).
Shum, J. et al. Neural correlates of sign language production revealed by electrocorticography. Neurology 95 , e2880–e2889 (2020).
Sainburg, T., Thielk, M. & Gentner, T. Q. Finding, visualizing and quantifying latent structure across diverse animal vocal repertoires. PLoS Comput. Biol. 16 , e1008228 (2020).
Roussel, P. et al. Observation and assessment of acoustic contamination of electrophysiological brain signals during speech production and sound perception. J. Neural Eng. 17 , 056028 (2020).
Boersma, P. & Van Heuven, V. Speak and unSpeak with PRAAT. Glot Int. 5 , 341–347 (2001).
Chang, E. F., Raygor, K. P. & Berger, M. S. Contemporary model of language organization: an overview for neurosurgeons. J. Neurosurgery 122 , 250–261 (2015).
Jiang, J., Chen, M. & Alwan, A. On the perception of voicing in syllable-initial plosives in noise. J. Acoust. Soc. Am. 119 , 1092–1105 (2006).
Engel, J., Hantrakul, L., Gu, C. & Roberts, A. DDSP: differentiable digital signal processing. In Proc. 8th International Conference on Learning Representations https://openreview.net/forum?id=B1x1ma4tDr (Open.Review.net, 2020).
Flanagan, J. L. A difference limen for vowel formant frequency. J. Acoust. Soc. Am. 27 , 613–617 (1955).
Schafer, R. W. & Rabiner, L. R. System for automatic formant analysis of voiced speech. J. Acoust. Soc. Am. 47 , 634–648 (1970).
Fitch, J. L. & Holbrook, A. Modal vocal fundamental frequency of young adults. Arch. Otolaryngol. 92 , 379–382 (1970).
Stevens, S. S. & Volkmann, J. The relation of pitch to frequency: a revised scale. Am. J. Psychol. 53 , 329–353 (1940).
Kingma, D. P. & Ba, J. Adam: a method for stochastic optimization. In Proc. 3rd International Conference on Learning Representations (eds Bengio, Y. & LeCun, Y.) http://arxiv.org/abs/1412.6980 (arXiv, 2015).
Angrick, M. et al. Interpretation of convolutional neural networks for speech spectrogram regression from intracranial recordings. Neurocomputing 342 , 145–151 (2019).
Chen, X. ECoG_HB_02. Mendeley data, V2 (Mendeley, 2024); https://doi.org/10.17632/fp4bv9gtwk.2
Chen, X. & Wang, R. Neural speech decoding 1.0 (Zenodo, 2024); https://doi.org/10.5281/zenodo.10719428
Download references
Acknowledgements
This Work was supported by the National Science Foundation under grants IIS-1912286 and 2309057 (Y.W. and A.F.) and National Institute of Health grants R01NS109367, R01NS115929 and R01DC018805 (A.F.).

Author information
These authors contributed equally: Xupeng Chen, Ran Wang.
These authors jointly supervised this work: Yao Wang, Adeen Flinker.
Authors and Affiliations
Electrical and Computer Engineering Department, New York University, Brooklyn, NY, USA
Xupeng Chen, Ran Wang & Yao Wang
Neurology Department, New York University, Manhattan, NY, USA
Amirhossein Khalilian-Gourtani, Leyao Yu, Patricia Dugan, Daniel Friedman, Orrin Devinsky & Adeen Flinker
Biomedical Engineering Department, New York University, Brooklyn, NY, USA
Leyao Yu, Yao Wang & Adeen Flinker
Neurosurgery Department, New York University, Manhattan, NY, USA
Werner Doyle
You can also search for this author in PubMed Google Scholar
Contributions
Y.W. and A.F. supervised the research. X.C., R.W., Y.W. and A.F. conceived research. X.C., R.W., A.K.-G., L.Y., P.D., D.F., W.D., O.D. and A.F. performed research. X.C., R.W., Y.W. and A.F. contributed new reagents/analytic tools. X.C., R.W., A.K.-G., L.Y. and A.F. analysed data. P.D. and D.F. provided clinical care. W.D. provided neurosurgical clinical care. O.D. assisted with patient care and consent. X.C., Y.W. and A.F. wrote the paper.
Corresponding author
Correspondence to Adeen Flinker .
Ethics declarations
Competing interests.
The authors declare no competing interests.
Peer review
Peer review information.
Nature Machine Intelligence thanks the anonymous reviewer(s) for their contribution to the peer review of this work.
Additional information
Publisher’s note Springer Nature remains neutral with regard to jurisdictional claims in published maps and institutional affiliations.
Supplementary information
Supplementary information.
Supplementary Figs. 1–10, Table 1 and audio files list.
Reporting Summary
Supplementary audio 1.
Example original and decoded audios for eight words.
Supplementary Audio 2
Example original and decoded words from low density participants.
Supplementary Audio 3
Example original and decoded words from hybrid density participants.
Supplementary Audio 4
Example original and decoded words from left hemisphere low density participants.
Supplementary Audio 5
Example original and decoded words from right hemisphere low density participants.
Source Data Fig. 2
Data for Fig, 2a,b,d,e,f.
Source Data Fig. 3
Data for Fig, 3a,c,d.
Rights and permissions
Open Access This article is licensed under a Creative Commons Attribution 4.0 International License, which permits use, sharing, adaptation, distribution and reproduction in any medium or format, as long as you give appropriate credit to the original author(s) and the source, provide a link to the Creative Commons licence, and indicate if changes were made. The images or other third party material in this article are included in the article’s Creative Commons licence, unless indicated otherwise in a credit line to the material. If material is not included in the article’s Creative Commons licence and your intended use is not permitted by statutory regulation or exceeds the permitted use, you will need to obtain permission directly from the copyright holder. To view a copy of this licence, visit http://creativecommons.org/licenses/by/4.0/ .
Reprints and permissions
About this article
Cite this article.
Chen, X., Wang, R., Khalilian-Gourtani, A. et al. A neural speech decoding framework leveraging deep learning and speech synthesis. Nat Mach Intell (2024). https://doi.org/10.1038/s42256-024-00824-8
Download citation
Received : 29 July 2023
Accepted : 08 March 2024
Published : 08 April 2024
DOI : https://doi.org/10.1038/s42256-024-00824-8
Share this article
Anyone you share the following link with will be able to read this content:
Sorry, a shareable link is not currently available for this article.
Provided by the Springer Nature SharedIt content-sharing initiative
Quick links
- Explore articles by subject
- Guide to authors
- Editorial policies
Sign up for the Nature Briefing newsletter — what matters in science, free to your inbox daily.

Which Language Do You Want to Learn?
- Inside Babbel
- Babbel Bytes
ARTICLES ABOUT
4 sci-fi universal translators (and 1 possibly real one).

Photo: LucasFilm
Science fiction is often applauded for its ability to predict future technologies. These tools might be for the better (the smart watches shown on The Jetsons) or for the worse (the obscene surveillance state shown in Nineteen Eighty-Four ). But sci-fi doesn’t just predict the future; it can create it, too. The imaginations of Isaac Asimov, Ursula K. Le Guin and countless other writers have inspired scientists to create the tech-centric world we live in. It should come as no surprise, then, that the most sought-after linguistic technology was first thought of in First Contact , a 1945 novella by Murray Leinster. In the story, creatures from around the universe are able to communicate using what’s called a universal translator.
The concept of the universal translator is simple enough: it’s a device that can translate between any two languages, ideally as fast as possible. In execution, though, it’s not simple at all. The problem of human language is that it’s so complex that even our best artificial intelligence hasn’t mastered it. But as you can imagine, almost every tech company in the world is attempting to crack it , and each year brings incredible developments.
If you go from the real world to sci-fi, though, there are universal translators everywhere. Why? Well, frankly, it solves a lot of story problems. Sure, the characters could spend decades mastering alien languages, but why not just have a machine that does that all for them? As we wait for a device that will break down all language barriers forever, we rounded up our favorite fictional translation devices. We also threw in one of the most recent attempts at a real universal translator.
Tardis Translation Circuit — Doctor Who
In the long-running British TV show Doctor Who , the Tardis is a machine able to go anywhere throughout time and space. So sure, why not throw a translation device in there? The titular Doctor, being a 2,000-year-old alien, already speaks millions of languages, of course, but he does need translation services to offer his traveling companions. The Tardis employs a telepathic field that automatically translates both text and speech for people. The Tardis Translation Circuit is particularly complicated because it’s connected to the Doctor himself (or, thanks to Jodie Whittaker, herself ). This means that the Doctor’s language abilities control who gets access to it, and also apparently allows him to briefly speak in other languages, like with the 10th Doctor’s near-constant yelling of the French Allons-y! (“Let’s go!”). That’s also why in the scene above, the Tardis’ translation doesn’t work until the Doctor comes out of what was essentially a coma.
Confused yet? Doctor Who doesn’t mind being very vague about how its technology works (it describes the space-time continuum as a “big ball of wibbly-wobbly timey-wimey stuff”), so asking for a realistic explanation is futile. The show takes the easier route by just ignoring any inconsistencies in the way the translation circuit works.
Additional fun fact: In the Doctor Who book Only Human , it’s revealed that the Tardis has a censorship feature — no swearing in space, apparently — which helps explain why the show can keep its PG rating.
Babel Fish — The Hitchhiker’s Guide to the Galaxy
The Hitchhiker’s Guide to the Galaxy is “a trilogy in five parts,” which might give you a hint that the whole thing is meant to be slightly ridiculous. So it only makes sense that their universal translator is the silliest of them all. It’s called a Babel fish, and it’s a creature that naturally evolved so that when you stick it in your ear, it eats your brain waves and excretes thought, translating any language in the universe for you. To help distract from the extraordinary implausibility of such a fish, the book launches into an explanation as to why the Babel fish disproves God’s existence.
The argument goes something like this: “I refuse to prove that I exist,” says God, “for proof denies faith, and without faith, I am nothing.”
“But,” says Man, “the Babel fish is a dead giveaway, isn’t it? It could not have evolved by chance. It proves you exist, and, by your own arguments, you don’t. QED.”
“Oh dear,” says God, “I hadn’t thought of that,” and vanishes in a puff of logic.
Universal Translator — Star Trek
Star Trek ‘s universal translator, or UT, is the closest to a “real” gadget so far on this list. There’s no telepathy or fish involved. The UT is the sci-fi standard for translation technology, and any article about the concept of a universal translator is bound to reference it. In execution, it’s still more of a plot device than a technological one, but the creators of Star Trek have put at least a little effort into explaining how translation works. It’s essentially a really advanced Google Translate that takes in language information and figures out the best way to translate it. There are some inconsistencies in how it works, but that’s bound to happen with a franchise that’s been rebooted so many times.
In the above clip, Hoshi Sato explains how the UT operates, and says it was invented in the 22nd century (so the clock is ticking for us). In addition to the UT’s ability to translate any Earth language, Hoshi used the UT to invent the linguacode, which can be used to communicate with any species in existence. The linguacode ventures a bit more into the realm of impossibility, because it’s unlikely it’ll ever be that easy to crack alien languages.
In the Star Trek universe, the invention of the UT broke down all of Earth’s language barriers and created planet-wide world peace and cooperation. It will probably take more than translation to do that in real life.
We would be remiss not to mention Star Trek’s biggest contribution to language: Klingon . The Klingons are skeptical of the UT and prefer speaking in their own language, allowing many opportunities to hear Klingon throughout the various shows and movies. The language itself was developed into a full language, which is pretty much a sci-fi nerd prerequisite at this point. One of the most recent iterations of Star Trek — the TV show Star Trek: Discovery — hired official Klingon translator Robyn Stewart to create dialogue for the alien species, which is then translated on-screen. Even in the future, there’s no escape from subtitles.
C-3PO — Star Wars
In Star Wars , despite the existence of laser swords, spaceships and massive, planet-shaped weapons (OK, fine, moon-shaped ), everyone is still forced to use language interpreters. Granted, C-3PO is a super-advanced robot that speaks six million languages. But even so, it seems pretty inconvenient to have to drag him with you every time you need a mediator. C-3PO tends to show a lot of flaws in droid design. For example, why would you build a two-legged robot? Sure, humans pull it off, but when C-3PO is walking around, he looks like a baby taking its first steps. Plus, C-3PO is scared of everything and is in fact kind of irritating (which is why R2-D2 has always been the more popular of the duo). There’s a reason this golden boy is always falling over and getting his limbs pulled off.
My overall point here is that when technology advances, I have to imagine we’ll be able to make more efficient translation devices. Nothing against C-3PO, but there’s just gotta be a better way to talk to Ewoks.
Pixel Buds — Google
Compared to the sci-fi we’ve covered so far, reality is lagging. There isn’t any tool that will immediately and seamlessly translate from one language to another. But the idea of a universal translator is looking more and more possible with every passing year.
While many companies are vying for linguistic supremacy, Google takes the blue ribbon for now (Microsoft isn’t too far behind with its Skype Translator) . The release of Google Pixel Buds , which, as seen in the clip above, have the ability to automatically translate speech, got the tech world very excited in October 2017, and it is now a feature in many Google products.
There are two main flaws to the Pixel Buds translations: they’re not quite quick enough to count as “instant” translation, and they only work for a limited number of languages. With each new update, though, they’re improving. They’ve gone from 12 to 40 supported languages in the past three years, and each iteration is faster than the last. Competing earbuds are even working on allowing “interruptions,” meaning two people can talk over each other but the device will still be able to translate both people at the same time.
Google Translate isn’t taking the place of real human multilingualism — yet. There are a lot of flaws that come up. But instead of just pointing out all of those, we can look at the positives. A few decades ago, instant translation seemed nearly impossible. In 2020, you can communicate in some capacity with almost anyone on the internet. No, it’s not perfect. No, not every language is represented. But still, it’s a technology that for years ago was purely the stuff of sci-fi. Basically, we’re living in the future.

An official website of the United States government
The .gov means it’s official. Federal government websites often end in .gov or .mil. Before sharing sensitive information, make sure you’re on a federal government site.
The site is secure. The https:// ensures that you are connecting to the official website and that any information you provide is encrypted and transmitted securely.
- Publications
- Account settings
Preview improvements coming to the PMC website in October 2024. Learn More or Try it out now .
- Advanced Search
- Journal List
- Springer Nature - PMC COVID-19 Collection

Visions of Artificial Intelligence and Robots in Science Fiction: a computational analysis
Hirotaka osawa.
University of Tsukuba, Tsukuba, Japan
Dohjin Miyamoto
Satoshi hase, reina saijo, kentaro fukuchi, yoichiro miyake, associated data.
The datasets generated during and/or analyzed during the current study are available from the corresponding author upon reasonable request.
Driven by the rapid development of artificial intelligence (AI) and anthropomorphic robotic systems, the various possibilities and risks of such technologies have become a topic of urgent discussion. Although science fiction (SF) works are often cited as references for visions of future developments, this framework of discourse may not be appropriate for serious discussions owing to technical inaccuracies resulting from its reliance on entertainment media. However, these science fiction works could help researchers understand how people might react to new AI and robotic systems. Hence, classifying depictions of artificial intelligence in science fiction may be expected to help researchers to communicate more clearly by identifying science fiction elements to which their works may be similar or dissimilar. In this study, we analyzed depictions of artificial intelligence in SF together with expert critics and writers. First, 115 AI systems described in SF were selected based on three criteria, including diversity of intelligence, social aspects, and extension of human intelligence. Nine elements representing their characteristics were analyzed using clustering and principal component analysis. The results suggest the prevalence of four distinctive categories, including human-like characters, intelligent machines, helpers such as vehicles and equipment, and infrastructure, which may be mapped to a two-dimensional space with axes representing intelligence and humanity. This research contributes to the public relations of AI and robotic technologies by analyzing shared imaginative visions of AI in society based on SF works.
Introduction
Science fiction (SF) as a literary genre draws on the interaction between technology and society. SF describes the influence of technology on society in terms of human drama based on compelling storytelling. The SF genre is an important component of our contemporary society owing to its popularization of science and technology along with an understanding of their transformative potential. The impact of SF is significant in both academic and industrial fields. For example, iRobot, manufacturer of the Roomba brand of cleaning robots, named their company based on Isaac Asimov’s novel “I, Robot.” Palmer Luckey, who founded the VR headset company Oculus, expressed influence of Neal Stephenson’s “Snow Crash”, Ernes Cline’s “Ready Player One”, and Reki Kawahara’s “Sword Art Online” on his work. Researchers in academic fields including information science, mechanics, robotics, and artificial intelligence (AI) are also typically broadly familiar with SF. For example, Nature Publishing Group has organized a series of short SF stories in “Nature” magazine since 2009. These stories help researchers in many different disciplines and the general public understand visions of future technologies more easily. Along these lines, Microsoft commissioned short stories from several SF writers based on their laboratory technology. The short stories were organized as a free anthology titled “Future Visions”. In China, international SF conferences are often actively supported by government organs. In Japan, the Society of Artificial Intelligence, the Japanese Society for Robotics, the Society for Automatic Measurement and Control, the Society of Human Interface, and other academic societies related to information, machinery, and electricity have continuously created special articles on SF. An increasing number of academic organizations also specialize in SF, such as the SF Film Institute on HCD-Net. Many researchers have attested as to the influence of SF on their work, and many technical terms are derived from SF, including “robot,” “robotics,” “technical singularity,” and “cyberspace.”
Science fiction is widely understood to have motivated a broad variety of research and development. Kurosu 2014 ; Marcus et al. 1999 ; Mubin et al. 2016 ; Nagy et al. 2018 ; Schmitz et al. 2008 ; Tanenbaum, Tanenbaum, and Wakkary 2012 ; Troiano, Tiab, and Lim 2016 ). From a more positive perspective, there are several notable examples in which SF writers have participated in various projects as technical advisors. Science fiction writers such as Bruce Sterling and Cory Doctorow are frequently involved in conferences and policy decisions on information technology (Sterling 2009 ). Satoshi Hase and Taiyo Fujii have participated in the ethics committee of the Japanese Society for Artificial Intelligence and are involved in the creation of ethical standards, and the Japanese Writer’s Community also cooperated with a survey (Ema et al. 2016 ). Liu Cixin, the author of “The Three-Body Problem”, joined a Chinese company (Cixin, Nahm, and Ascher 2013 ). The acceptance of AI and robotic anthropomorphic systems in society has been a major theme in SF for many years. Various concepts have been generated in the interaction of both fields, from Isaac Asimov’s Three Laws of Robotics (Asimov 1950 ) (McCauley 2007 ) to Verner Vinge’s technological singularity (Singularity) (Vinge 1993 ). For example, Isaac Asimov’s SF stories exploring robotics as a theme have been discussed as a future vision of humans and AI. His Three Laws of Robotics (Asimov 1950 ; McCauley 2007 ) are referred to in the Chiba University Robot Charter (Matsuo 2017 ) and Korea’s Robot Ethics Charter (Shaw-Garlock 2009 ). Moreover, SF has always exerted a significant influence on the development of AI technologies. Shedroff et al. defined four ways in which SF influences designers and researchers, including through (1) inspiration, (2) by establishing expectations, (3) by creating a social context, and (4) by describing new paradigms (Shedroff and Noessel 2012 ). SF has also been used as a teaching method for AI ethics (Burton et al. 2018 ).
While SF stories and images have helped to envision the future, fictional depictions to involve some important constraints. First, SF stories are generally produced for the primary purpose of entertainment rather than as a scientific investigation of the possibilities of future societies. There are also concerns as to the dark visions depicted by some SF media, which sometimes involve themes such as those of robotic systems replacing humans or going catastrophically out of control. Although SF writers are professional storytellers that rely on themes involving science and technology in their work, they are not typically science and technology professionals. Hence, there is some risk that the narrative logic inherent in SF may neglect the context of real social situations. For example, the AI referred to as Skynet appearing in the Terminator franchise is occasionally referenced as a negative vision of AI in the technical literature (Mubin et al. 2016 ). In addition, there are works that do substantially involve social problems that may exist in the background of future societies. Owing to recent rapid development of AI, the ethical problems posed by the use of such technology in society have been discussed as a practical matter in various contexts. As a result, caution should be exercised in applying ideas from classic SF, including visions of intelligent anthropomorphic robotic systems to real-world problems directly. Out-of-context applications of science fiction ideas have also been criticized. For example, Jean-Gabriel Ganascia says that various technologies have been overhyped as a result of an abuse of the term technical singularity by Ray Kurzweil (Ganascia 2010 , 2017 ). A humanities scholar, Jennifer Robertson, described the vision of the future depicted by the Japanese government as problematic, stating that it tended to confirm sexist representations inherited from the classic SF works (Robertson 2011 ). Given that some scientists and technicians have used ideas from SF unscrupulously in scientific communications, researchers must attend to the possibility that the deliverables of such advanced technologies envisioned in fiction may not be compatible with society, or that some implementations may be impractical, unethical, or ill-advisedi.e., Skynet, etc. While the scenarios presented in SF have the advantage of helping depict the future, they are limited by their fictional nature. In addition, in some works, it is important to consider the social problems and conditions that contributed to the background of the art, rather than the specifics of fictional techniques. The application of SF thus requires careful attention, as such works were necessarily created within the context of a specific time period. Hence, fictional ideas should not be uncritically adopted as a basis for future developments without an appropriate consideration of the context of such works.
These examples suggest that science fiction can help us understand how the public imagines future AI and robots, as opposed to directly predicting the future. Science fiction stories and the new technologies they describe provide good indicators of how the general public perceives technology. Therefore, by analyzing AI and robots depicted in existing science fiction works, the general reception of new technologies developed by researchers and engineers may be more effectively predicted. In this study, we analyzed popular preconceptions of AI and robotic systems by investigating depictions of such technologies in existing science fiction.
This study surveyed depictions of AI and robotic systems in SF with the help of SF experts to analyze types of stereotypes applied to anthropomorphic systems and visions of their development and adoption in SF. The remainder of this study is organized as follows. Section 2 explains the background of the relationship between science fiction and science and technology, including artificial intelligence. Section 3 explains how we determined the SF criteria to avoid arbitrary selection. Section 4 explains the statistical methods used to perform the data analysis. Section 5 discusses the stereotypes and visions exhibited by these works in the SF genre. The contribution and limitations of the present work are described in Sect. 6, and Sect. 7 presents our final conclusions along with some possible avenues for future research.
The impact of Science Fiction: speculative inferences of Social Development from Scientific reasoning
SF is a literary genre centered on stories developed based on themes relating to various types of science, technology, or scientific methods. The definition of modern SF is significantly broader than that of the content that was originally called SF. The conventional definition of the genre is imprecise, varying by author, critic, and reader and is often controversial (Tatsumi 2000 ). The concept of SF, in terms of stories based on scientific thinking, has a long history. However, such genres attracted increasing attention owing to the development of science in the context of the Industrial Revolution. For example, in Bram Stoker’s ”Dracula”, characters try to save a person who was attacked by a vampire via a blood transfusion. The work itself is a horror novel, but such literary techniques have been widely used in horror, action, and drama genres. Therefore, such works can be regarded as having some overlap with SF. Overall, SF is heavily influenced by science and technology in the fields of physics, chemistry, biology, space engineering, mechanical engineering, electrical engineering, and information technology.
There are several reasons for the widespread popularity of SF. For example, owing to the development of science and technology, there are many well-known cases in which conventional scientific knowledge was overturned by groundbreaking research. Typically classic SF works have rarely addressed science and technology in a rigorous or truly scientific manner. However, ideas of sci-fi technologies envisioned by these works, such as robots and space travel, have been maintained over several generations and widely explored in the genre. Often, the scientific framework of such stories may be improved over time to reflect changing contemporary ideas, so such radical possibilities are often explored usefully in SF despite its typical lack of true scientific rigor and process. For example, SF stories based on time machines or faster-than-light navigation can be considered as such works. SF has explored a wide variety.
of conceptually conceivable worlds, such as planets or universes with different physical laws. The plausibility of such descriptions cannot be easily assessed, even if though such speculative descriptions may be based on patterns derived from scientific inference. Many sci-fi works are based settings that diverge dramatically from the real world, such as fictional worlds in which the speed of light is extremely slow, stories that unfold under high gravity (Robert Forward ‘dragon’s egg’), or works that explore the concept of planetary intelligences (Stanislaw Lem’s Solaris’). Furthermore, there are examples in which the reactions of society to new technologies are realistic, though the presented technologies or scientific advancements themselves may be fictional. For example, Sakyo Komatsu’s “Virus” described a pandemic that decreased the population of society through a depiction of characters onboard a train. His explanation is referred to as having predicted the COVID-19 pandemic in Japan, even though the virus in the story is fictional (Omori 2020 ). Hence, we can consider that SF works may sometimes accurately predict future events or scenarios. For example, although the specific technologies of the Internet itself were not directly predicted, novels which foresee a world connected by the communication networks have a long history. For example, Shinichi Hoshi wrote ‘Voice Net’, which featured an AI-based intra-net service based on telephone networks. Some works focus on portraying human beings and society through fictional technology.
In this paper, we define SF as genre of stories that depict the imaginative settings and the reactions of people in fictional societies, with themes involving scientific techniques and reasoning. It includes stories based on technologies that may not necessarily be accurate according to current scientific knowledge or have not yet been achieved.
How AI and robotic Systems are portrayed in Science Fiction: Social Agents and Human Extension
Artificial intelligence and robots are often portrayed in SF as social agents or technologies that extend human capabilities. Several depictions of artificial slave appear in classic stories. For example, golems in Jewish folklore might be considered as a representative example of an animated construct. Golems are anthropomorphic beings that can be controlled by a human, in a manner somewhat analogous to that of a computational agent. Similar to stories involving robots, these stories often involve a theme of golems breaking free of human control or escaping. Mary Shelley’s “Frankenstein” is widely known as a classic work that may be considered as a predecessor of later SF, which tells the story of a monster created by Dr. Frankenstein by stitching together dead and dismembered bodies, which then escapes and kills Frankenstein’s family and friends in revenge for his unfortunate creation. Karel Capek’s ”R.U.R.” is a story about artificial agents that revolt against their creators. The robots depicted in this work are not mechanical artifacts, but rather biological workers created via technology. Similarly, his work “War with the Newts” does not deal with artificial intelligence itself, but it does detail the consequences of human training of intelligent salamanders on which society depends, and a revolt is foreseen. There are several works on the controllability of artifacts, which consider new technologies and their social impact. Stories about robots have often centered on the theme of fear of artificial creations going out of control. Several reasons for this revolt have been explored, but one common criteria of such story is that events cannot be foreseen beforehand.
These fears are called the Frankenstein Complex, after Frankenstein’s monster (Mccauley and Hall 2007 ). Concerned about the tendency to equate artifacts with monsters, Isaac Asimov, a prominent classic SF writer, introduced the Three Laws of Robotics in his work ‘I, robot.‘ (Asimov 1978 ). In many of Asmiov’s works, robots function as autonomous artifacts programmed to adhere to the following principles in order of priority. 1. A robot may not injure a human being or, through inaction, allow a human being to come to harm. 2. A robot must obey the orders given it by human beings except where such orders would conflict with the First Law. 3. A robot must protect its own existence as long as such protection does not conflict with the First or Second Laws. The Three Principles of Robotics were used repeatedly and extensively in Asimov’s own later works, and are known to have greatly influenced many other authors. There have also been proposals, such as Chiba University’s ‘Chiba University Robot Charter’ and Korea’s Robot Ethics Charter, to establish actual control codes based on Asimov’s three laws (Matsuo 2017 ; Shaw-Garlock 2009 ). However, it should be noted that these three principles are merely a narrative device. In fact most of the short stories comprising ”I, Robot.” are centered on interactions between humans and constructs that cannot be predicted solely based on the Three Laws. There are many more examples of similar literary themes in which an artifact seeks or gains a human soul. For example, “The Adventures of Pinocchio”, a children’s story by Carlo Collodi in 1883, also describes examples of intentional behavior by artifacts. Many stories focus on the of nonhumans entities obtaining intelligence or souls similar to those of humans. For example, the Greek myth of Pygmalion involves a sculpture of a woman that behaves like a human being and marries the sculptor who created her(Kaplan 2004 ). In many of these narrative forms, artificial intelligence questions the nature of intelligence itself. Barrington Bayley’s “Soul of the Robot” tells the story by a robot in the first person, and incorporates some material on artificial intelligence material, including the frame problem. However, in essence the work describes how the robot protagonist’s autonomy is a result of human intelligence. The theme of women’s souls or autonomy being limited or controlled by men has been portrayed in Western literature alongside critical investigations of sex and gender divergence. Amy Thomson’s ”Virtual Girl” presents a critical exploration of these themes from the perspective of a female robot.
Human augmentation through information technology is another common theme in SF works on artificial intelligence and robotics. For example, the impact of VR and HCI has been frequently explored in cyberpunk SF. Cyberpunk emerged as a trend in SF in the 1980s. The genre often presuppose a futures in which the bodies or minds of humans are augmented with technological systems. Alice Bradley Sheldon, better known by her pen name James Tiptree, Jr., was an early writer of cyberpunk SF who explored the idea of a woman who remotely controls a mindless but living artificially constructed separate body as an advertisement for a corporation in “ The Girl Who Was Plugged In”. The idea that technology can compensate for basic disparities such as gender was later inherited by Donna Haraway’s “Cyborg Manifesto” and the associated movement (Haraway 2000 ). Similarly, the artist Sputniko!, who creates art to overcome gender differences with technology, stated that her work “Crowbot Jenny” was influenced by Donna Haraway. Many SF works discuss the theme of transforming a person into a superhuman by expanding their intelligence or changing their values. Writers such as William Gibson and Bruce Sterling have contributed to this trend. Jun Rekimoto was influenced by this idea as an HCI researcher at SONY CSL/Tokyo University. For example, JackIn, a remote presence technology that seamlessly superimposes a users’ body on remote viewpoints, was named after a phrase from William Gibson’s Neuromancer (Kasahara et al. 2017 ). Augmented Human, as he put it, was based on the expansive ideas of human nature proposed by cyberpunks (Rekimoto 2014 ). Masahiko Inami, a VR researcher, likewise pointed out the influence of cyberpunk SF on research, stating that the use of retroreflective materials for transparency (Inami, Kawakami, and Tachi 2003 ) was influenced by optical camouflage depicted in the cyberpunk SF “Ghost in the Shell”. In Superhuman Sports, of which he is an advocate, this extension of humanity has been tested in other ways (Orikasa et al. 2017 ). Post-cyberpunk SF is often seen as a positive indicator of this orientation. For example, Verner Vinge, an advocate of the idea of a technological singularity, proposed in his work that the intelligence tends to extend itself, and defined the singularity in terms of such extension, without making a fundamental distinction between human and machine. This concept is sometimes called intelligence amplification (IA) in comparison with AI (Leinweber 2009 ). Greg Egan used his knowledge of physics and cognitive science to actively describe changes in humanity as an author (Nichols, Smith, and Miller 2007 ). In addition, stories focused on one Internet technology and social media networks, which are relatively novel developments in society that augment human capabilities, are considered to be included in these works. Dave Eggers’ “The Circle,” for example, depicts the consequences of a world built on corporate social network approval, with each technology being presented as a realistic manifestation of future concern.
Designing an analysis of AI in Science Fiction
Making criteria for review.
Following previous studies (Mubin et al. 2016 ; Reeves 2012 ), we first established a set of review criteria to avoid an arbitrary survey. Previous research on the use of robots in SF suggests the importance of selecting works based on unified criteria (Mubin et al. 2019 ). Hence, we used the Science Fiction Hall of Fame as a specific organization to limit the scope of the SF literature review. However, representations of AI in SF are more diverse than those of robots, and thus simply following existing standards was difficult. It was also difficult to conduct cleanly separated surveys of robots and artificial intelligence. Intelligent information processing technologies that emerged before the name artificial intelligence existed were often referred to as robots. Both robots without physical bodies and artificial intelligence with physical bodies have appeared, so narrowing the range of the two terms was not useful. Importantly, AI in earlier SF works are generally not labeled as “AI” as they were written before the definition of AI was established; hence, it was not possible to collect such works simply by searching for the word.
To establish the review criteria, we conducted online discussions with 15 experts from the organization Science Fiction and Fantasy Writers of Japan and selected seven experts, including six critics and one writer, with different specialties in foreign and domestic SF works, including comics, young adult novels, visual works, and drama. This writer’s association is founded on 57 years ago, and includes authors, critics, translators, and researchers. It is widely considered most authoritative association for the study of Sci-Fi. Therefore, we selected the organization as the partners in this study. Based on a half-day face-to-face discussion between them and ourselves (a scientist, two engineers, and a philosopher), we established the following criteria to select AI systems portrayed in SF stories.
In the prior discussion, including the above review of the literature, we identified three different roles for AI technology described in SF stories.
- - Stories considering the possibilities of alien intelligence. These depict different forms of intelligence such as programs, robots, and extraterrestrial intelligence. Stevelts, the group intelligence of nanomachines in Greg Egan’s “Steve Fever,” was mentioned in the discussion.
- - Stories considering aspect of social intelligence. Even if a detailed implementation of intelligence is not described in the story, this category included works focused on social interactions with AI. In the discussion, Bokko-chan from Shinichi Hoshi’s “Bokko-chan,” a parrot toss response robot, was mentioned.
- - Stories considering the possibility of artificial extension of human intelligence. The theme of this category was the expansion of human cognitive ability through advanced interfaces between humans and robots or machines, augmented humans, the internet, and social networks. In the discussion, Chohei Kanbayashi’s “Yukikaze” was cited as an AI for a combat aircraft designed to extends the operator’s ability.
We collected stores on artificial intelligence and robotic technologies from science fiction on as broad a basis as possible with the cooperation of experts. Therefore, it was necessary to collect AI and robots that appeared in the works by setting a broad standard including as wide a range of intelligence technologies as possible to cover the diversity of the subject. This is reflected in the first policy on the diversity of intelligence. Artificial intelligence and robotics in science fiction are applicable to social agents or extensions of human intelligence. This background is explained in Sect. 2.2 , and the criteria from this aspect are reflected in policies 2 and 3.
The information used to classify AI in the selected SF using the above criteria was examined, as shown in Table 1 . Considering the characteristics of AI that are important in literature and those that are important in terms of AI technology, the following 20 factors and work summaries were collected. In addition, we obtained an overview of each story to verify the correctness of the factor.
Collected AI factors (11 factors shown in gray were quantified and normalized after data collection in Sect. 2.2 )
First, we designed the following review priorities for the survey requested of SF experts. These three characteristics have been cited as the impact of AI in science fiction on readers.
- - Diversity. The age of the publication and the media in which the work was published must not be biased toward a specific field.
- - Impact. Works with a significant social impact should be included. Those with less social impact but unique characteristics were also appropriate for collection.
- - Uniqueness. In the case of AI with similar characteristics, the original work was included inserted. When multiple AI systems appear in a single work, the system with the most unique features was collected.
Collecting Data
We collected 115 portrayals of AI from experts after they performed mutual quality check. The average year of publication of the works collected was 1981 (with a standard deviation (SD) of 26.8 years). The oldest character was the human cyborg described in “Rakouské celní úřady” written by Jaroslav Hašek in 1912, and the newest was Girl M, a bionic AI controlled by slime mold, in “Long Dreaming Day,” written by Katsuie Shibata in 2019. Among the works collected, 55 were published in Japan, 52 in the US, three in the UK, two in Poland, and one in the Czech Republic, with two works being published worldwide simultaneously. 93 were first released as novels, 12 as comics, seven as movies (two of which were animations), and three as plays. Fourteen works were published before 1945, 64 works were published after World War II until 1995, before the widespread adoption of the Internet, and 37 works were published after 1995. We confirmed that the distribution had sufficient diversity in each decade, as shown in Table 2 .
Each factor in four clusters (* An asterisk indicates that a significant difference of p < .05 was obtained on Tukey’s test.)
Here, we discuss 20 factors. We first estimated that 11 factors were quantifiable, including maker and independence factors. However, deeper discussion revealed that the latter two factors were not appropriate to scalar value (darker gray shading). We then selected nine factors, as displayed in the light gray cells in Table 1 , for normalization. The participants were divided into five steps using two experts. For example, in the case of an animal-type AI, the human-type value was 0.25, and in the case of an AI with a part such as a neck or a hand, the human-type value was 0.75. The Cohen’s Kappa value of two experts results was 0.86 (> 0.8) and we estimated that the collected data were of sufficient accuracy.
We used principal component analysis (PCA) to extract the main factors from multiple factors. As a result, of 43.3% the information in the second main component and 64.4% in the fourth main component was extracted. We decided that up to the fourth main component represented our data well. The first axis (24.4%) with a high contribution ratio was labeled intelligence , whereas the second axis (19.0%) was labeled humanity , which suggests familiarity with human society. The third axis (12.0%) with a high contribution ratio of independence was labeled as independence , and the fourth axis (9.1%) with a high maker factor contribution ratio was labeled maker . Hierarchical cluster analysis was also performed for the 11 factors. The distance between each element was measured in terms of the Euclidean distance and classified using Ward’s method. The cluster tree was divided into four clusters based on the distance between 10 distinctive points. These four clusters were characteristically separated on a PCA map constructed with the first (intelligence) and second (humanity) factors as primary axes, as Fig. 1 is mainly constructed by nine factors. There was no distinctive clustering in the PCA map constructed by the third (independence) and fourth (maker) factors.

AI in SF on a PCA Map (each AI name has a label in front of the name)
Based on the characteristics of the four clusters, we labeled them as Buddy, Machine, Infrastructure, and Human. As shown in Fig. 1 (arrows), several factors exhibited mutual relationships. For example, both the pairing of human shape with friendliness and consciousness with language ability contributed to increased intelligence and humanity, as shown in Fig. 1 . Increasing generality, learning, and higher network connectivity contributed to increased intelligence, but also decreased humanity. The crowd factor did not contribute to intelligence, but did contribute to decreased humanity. Physical factors contributed to increased humanity but also decreased intelligence. Independence and maker did not contribute to either axis Electricity was the most common energy source, and other sources were diverse, with no significant trends.
The average values of the axes for each cluster are given in Table 3 . The combinations that showed significant differences on Tukey’s multiple comparison test are listed in the first table cell. The distribution by year of publication is shown in Table 2 . These averages exhibit a weak tendency in which Machine and Human type AIs are typically slightly older archetypes than Buddy and Infrastructure. In addition, it is remarkable that all works with Infrastrcuture-type AIs were written after 1970 (The year of publication of “Voice Net,” written by Shin’ichi Hoshi). This suggests that most of this AI type was generated after computer and telecommunication technologies were developed.
Four clusters with years
Machine-type AI
The cluster was populated by AI that are shown as being less intelligent than humans (as regarded by human beings). In contrast to the human type, this type is unique in that it exhibited low generality (0.04 (SD 0.13)), low consciousness (0.08 (SD 0.26)), low language ability (0.08 (SD 0.19)), and low learning ability (0.15 (SD. 35)), and low human-shape (0.17 (SD 0.27)). Representative examples of this type include the Robot Mother in “The Mechanical Mice” by Maurice Hugi, “Hog-Belly Honey” by R.A Lafferty, and KEIGI-1 in “Inter Ice Age 4” The tasks performed by AI range from babysitting to weapons, but many of them do not learn from the environment. These appear in stories as unintelligent automated machines for solving specific problems. Alternatively, these machines are provided in an environment in which the protagonists cannot interfere. Their inflexibility often damages human society.
Human type AI
Human-type AI was the most common of the four types (40%). From Table 2 , it may be observed that this type of AI has been depicted in SF from the beginning to the present day in every decade. Specificities in this type included moderate generality (0.41 (SD 0.32)), high consciousness (0.85 (SD 0.31)), high language skills (0.97 (SD 0.12)), moderate learning skills (0.56 (SD 0.43)), high physical appearance (0.97 (SD 0.11)), and high human-shape (0.89 (SD 0.22)). Representative examples of this type include Atom in Osamu Tezuka’s “Astro Boy” and several robots in Isaac Asimov’s “I, Robot”. This type is thought to include the traditional SF theme of artificial humans taking the place of humans. Regarding the tasks performed by the AI, housework was the most common task (11 cases), followed by outdoor physical labor (5 cases) follows. Human-type AI act independently as members of society, learn from their environment, and perform general tasks, just as humans do. In general, most of these characters are treated as a metaphor for humans. This type of AI was concentrated in a relatively small cluster, as illustrated in Fig. 1 . This seems to be because the human image was the norm for these characters.
Buddy-type AI
The buddy-type AI were identified as human-dependent, conscious, and collaborative agents that typically helped with work. They are similar to the human type, but distinct in terms of their low generality (0.24 (SD. 45)), slightly lower language skills (0.74 (SD 0.32)), and low human-shape (0.06 (SD 0.22)). Representative examples of this type include HAL 9000 (a spaceship AI) in “2001: A Space Odyssey” by Arthur C. Clark, Yukikaze (a combat aircraft) in “Yukikaze” by Chohei Kanbayashi, and Asurada (a semiautomatic car) in “Future GPX Cyber Formula” by Mitsuo Fukuda. The buddy-type AI typically exhibits a tool-type shape such as that of a vehicle, performs specific tasks for each tool, and sometimes accepts commands via non-verbal input. Among the tasks performed by the AI, the most common task was military (eight cases), followed by automatic operation (four cases). The buddy type of AI works with humans to extend their cognitive abilities. These AI’s unique consciousness (autonomy) interferes with and effects human consciousness. There are also cases in which they run out of control owing to dilemmas involving human orders.
Infrastructure-type AI
Infrastructure-type AI are often less physically active, include substantial network connectivity and language capabilities, and are often used as social infrastructure. In contrast to the human type, this type is characterized by high network connectivity (0.98 (SD.07)), slightly lower physical appearance (0.61 (SD 0.40)), and moderate human-shape (0.43 (SD 0.44)). Representative examples of this type include Skynet in “Terminator” by James Cameron, Wintermute in “Neuromancer” by William Gibson, and Lacia in “Beatless” by Satoshi Hase. The most common task performed by the AI was facility management (15 cases). The image of infrastructure-type AI is thought to have been created mainly after World War II with the development of computer and communication technologies. The average year of publication was 1994 (SD 18.0), as shown in Table 2 , which was more recent than the other categories. The implementation of computer networks has varied over time, and some works have been represented as AI over telephone networks (“Voice Net” by Shin’ichi Hoshi). In “Voice Net,“ a recommendation system and evaluation economy similar to that of the Internet society is achieved by an AI system composed of telephone networks installed in buildings.
Discussion of SF stereotypes and possibilities
Ai factors that imply contributions to intelligence and humanity.
In order to explain the developed framework for AI and robot systems, we identified some factors suggestive of intelligence and humanity in fictional AI. Our analysis of fiction, shown in the PCA map ( Fig. 1 ), contributed to revealing these hidden relationships.
Embodiment is an important factor in the field of AI (Brooks 1991 ). From an SF viewpoint, it has been considered as a factor contributing to humanity in terms of familiarity with human society. However, our analysis suggests that embodiment exhibits a two-sided influence on how intelligence influences people. If a humanlike shape is attributes AI, portrays typically involved increased intelligence. However, general physical attribution contributed to decreased intelligence. This tendency was the same as that suggested in the robotics design and human-computer interaction (HCI) design principle called the adaptation gap (Komatsu, Kurosawa, and Yamada 2012 ), in which humanlike attributes contribute to more intelligence.
Language ability, consciousness, learning ability, generality, and network connection exhibited similar tendencies in contributing to an increase in portrayed intelligence. However, their contributions to humanity were slightly different. The language ability of AI and their consciousness showed the same tendency to weakly increase humanity. In contrast, learning ability and generality contributed weakly to decreased humanity, and network connection marginally contributed to decreased humanity. It may be useful to explain language capabilities rather than the versatility of AI to convey the advantage of AI to non-experts.
It is also remarkable that the crowd factor simply contributes to decreased humanity. It is difficult for people to imagine an intelligence comprised of many less complex intelligences. Hence, care should be taken to communicate the harmlessness of this kind of artificial crowd intelligence.
Avoiding stereotypes: human and machine
Human-type AI in SF seems to have been used as a motif for humans from different cultures, and machine-type AI in SF seems to have been used as a motif for an uncontrollable machines, with each being a stereotypical aspect. Human-type agents, like Karel Capek’s R.U.R. which coined the term “robot,” are reflective of racial discrimination and slave labor engaged in housework and labor. This is thought to have functioned in the narratives to describe aspects of the coexistence of different human beings in society such as fear.
Machine-type AI are another stereotype in SF, representing the theme of machines that work independently and may cause problems by going out of control. The fear of this type of uncontrollable machine goes back to the stories of golems. The main features of this type of AI are its apparent lack of intelligence and inflexibility.
Although we acknowledge that these AI images work well in the literature, we are concerned that they may not present a technically realistic image. We are also concerned that these fictional works may induce stereotypes of AI. Although creating human-like intelligence is a primary goal of AI, today’s AI are not as intelligent as humans, but they are also not necessarily unsophisticated machines regulated by simple rules easily understood by humans. Human-like images are often used, especially in the design and promotion of commercialized AI; however, we think researchers and technologists should take care to avoid the overuse of this image, and should explain their technologies to avoid these stereotypes.
Beyond Anthropomorphism: non-human buddies and social infrastructure
We believe that buddy-type and infrastructure-type AI will be more important in communicating the vision of future AI designs. Buddy-type AIs are not like a human, but they performs tasks in cooperation with humans. A Buddy-type AI’s work deals with the problem of how to compromise between AI and human decision-making as a unified working system, including issues such as the division of roles between humans and AI in automated driving. These examples can shed light on how the coupling of humans and AI my function under extreme conditions. Yukikaze is a typical example. It depicts the process in which a human pilot interacts with a heterogeneous helper intelligence, and in the process, accepts decisions and makes heterogeneous decisions. This is a challenge that needs to be addressed when dealing with autonomous weapons, autonomous vehicles, and other similar issues.
The infrastructure type is a new image that appeared alongside the development of the computer. These systems were imagined as information technology progressively developed. For example, “Beatless” by Satoshi Hase depicts a world of AI after a singularity, and Lacia is depicted as a humanoid interface. In this story, human activities are monitored and predicted by AI as operating as infrastructure, and human agents “hack” the human mind socially through several human factors, including gender. “Beatless” is considered a key SF story for referenced in future AI design, with addressed the ethical problems associated with the introduction of AI into society, the ethical problems of persuasive engineering, as discussed by Fogg et al. (Fogg 1999 ), and gender difference problems in social factors, as explored by Nass et al. (Nass and Moon 2000 ) and corresponding cases were described experimentally. This provides a realistic example of the risks that interactive agent affective computing technology may present to decision-making (Picard 1997 ). These works will help the public to understand the pressing problems of information technology.
6 Contribution and Limitations .
This research has contributed guidelines for AI researchers on how to explain their work in the society. For instance, it is not appropriate to use SF related to human-like AI as an example of a system that operates as infrastructure for a connected society. In “Beatless,” for example, the question of who bears responsibility for decision-making in the decisions of infrastructuralized artificial intelligence is debated as an essential issue. When explaining similar social infrastructure AI, “Beatless” can be used to discuss the decision-making of infrastructure AI, showing that concerns based on human AI are not appropriate metaphors.
Our categorization also allows researchers to identify works that provide inspiring and simulative visions of AI. Important works are selected according to the criteria, and the results produce knowledge about stereotypes. By evaluating the artificial intelligence developed by engineers with the same parameters as these fictional portrayals, and using the results to classify their similarity to fictional AI, it is possible to address the problems described in the fictional as a possible virtual problem in advance. Based on the results of this survey, we believe it is appropriate to collect more extensive surveys from the general public using crowdsourcing and other methods.
The contribution of this research is to derive the range of contemporary popular imaginations of artificial intelligence and robots from sci-fi works based on the analysis of experts. Therefore, it is difficult at present to directly derive a future vision using SF alone. In the future, to understand through science fiction how AI fits into the various imaginary futures presented in literature and media, attention needs to be paid to the philosophical and empirical aspects of each work, as well as to the computation of narrative components. For example, what would it be like for people in the imaginary world to interact with AI or robotics and to live under the socio-technical conditions created thereby? Do patterns of human life continue to exist, what changes are likely, and what conditions exist? Is the story fundamentally optimistic about human nature and the ability to self-govern, or does it suggest that people need monitoring and guidance, and how does that human concept relate to the types of AI in the story, and how does it work? As a next step in this research, we believe that additional verification should be conducted from a multifaceted perspective, including literary scholars. Popular concerns about artificial intelligence technology can be addressed by separating the concerns that come from the literary visions of humans and tools from the real concerns that are extrapolated from real technology.
In a future work, we plan to use crowdsourcing to collect more data. The present work also involves a bias towards Japanese and American fiction. Science fiction from the United States has a strong influence on every country, including Japan. However, there is a risk that several results of this research may reflect a Japanese cultural background. It is possible that different trends may be observed in other countries. In the future, we plan to translate the questionnaire items into other languages and conduct international surveys. It should be noted that these analyses were correlative, not causal.
7 Conclusion .
We have surveyed and analyzed depictions of AI and robotic systems in the SF. As a result, stereotypes that AI researchers need to know in reference to science fiction have been identified, and areas that are important in communicating about future AI and robotics technologies to the public have been discovered. We also analyzed the contribution of several factors to the various vision of AI.
In this study, we hired critics and an author living in Japan with the help of a writer’s organization. Therefore, many of the selected works were limited to Japan or the United States, and most were novels. Many Japanese films are based on novels, which typically consider science from a multidisciplinary perspective. However, related research includes many studies on the influence of visual work, and we hope to extend future work to include more movies. The next step in this research is to develop a more detailed methods of communication. For example, the best fiction for conveying actual AI and robots to people can be selected by classifying actual AI according to the parameters of this study and searching for similar stories.
This study was supported by JST RISTEX Grant Number JPMJRX18H6, Japan.
Data Availability
Declarations.
The authors declare that they have no conflict of interest.
Publisher’s note
Springer Nature remains neutral with regard to jurisdictional claims in published maps and institutional affiliations.
- Asimov I. I, Robot. New York: Doubleday; 1950. [ Google Scholar ]
- Asimov I (1978) “The Machine and The Robot.” in Science Fiction: Contemporary Mythology , edited by P. S. Warrick, M. H. Greenberg, and J. D. Olander. Harper and Row
- Brooks RA (1991) “Intelligence without Representation.” Artificial Intelligence
- Burton E, Goldsmith J, Mattei N. How to Teach Computer Ethics through Science Fiction. Commun ACM. 2018; 61 (8):54–64. doi: 10.1145/3154485. [ CrossRef ] [ Google Scholar ]
- Cixin L, Translated by Gabriel Ascher (2013) Translated by Holger Nahm, and. “Beyond Narcissism: What Science Fiction Can Offer Literature.” Science Fiction Studies 40(1):22–32
- Ema A, Akiya N, Osawa H, Hattori H, Oie S, Ichise R, Kanzaki N, Kukita M, Saijo R, Otani T, Miyano N, Yoshimi Yashiro Future Relations between Humans and Artificial Intelligence: A Stakeholder Opinion Survey in Japan. IEEE Technol Soc Mag. 2016; 35 (4):68–75. doi: 10.1109/MTS.2016.2618719. [ CrossRef ] [ Google Scholar ]
- Fogg BJ. Persuasive Technologies. Commun ACM. 1999; 42 (5):26–29. doi: 10.1145/301353.301396. [ CrossRef ] [ Google Scholar ]
- Ganascia J-G. Epistemology of AI Revisited in the Light of the Philosophy of Information. Knowl Technol Policy. 2010; 23 (1):57–73. doi: 10.1007/s12130-010-9101-0. [ CrossRef ] [ Google Scholar ]
- Ganascia J-G (2017) Intelligence Artificielle: Vers Une Domination Programmée ?
- Haraway D (2000) “A Cyborg Manifest: Science, Technology, and Socialist-Feminism in the Late Twentieth Century. The cybercultures reader. Psychology Press, pp 291–324
- Inami M, Kawakami N, and Susumu Tachi (2003) Optical Camouflage Using Retro-Reflective Projection Technology. IEEE Computer Society
- Kaplan F. Who Is Afraid of the Humanoid? Investigating Cultural Differences in the Acceptation of Robots. Int J Humanoid Rob. 2004; 01 (03):465–480. doi: 10.1142/S0219843604000289. [ CrossRef ] [ Google Scholar ]
- Kasahara S, Nagai S, Rekimoto J. JackIn Head: Immersive Visual Telepresence System with Omnidirectional Wearable Camera. IEEE Trans Vis Comput Graph. 2017; 23 (3):1222–1234. doi: 10.1109/TVCG.2016.2642947. [ PubMed ] [ CrossRef ] [ Google Scholar ]
- Komatsu T, Kurosawa R. How Does the Difference Between Users’ Expectations and Perceptions About a Robotic Agent Affect Their Behavior? Int J Social Robot. 2012; 4 (2):109–116. doi: 10.1007/s12369-011-0122-y. [ CrossRef ] [ Google Scholar ]
- Kurosu M (2014) “User Interfaces That Appeared in SciFi Movies and Their Reality.” Pp. 580–88 in Design, User Experience, and Usability. Theories, Methods, and Tools for Designing the User Experience , edited by A. Marcus. Cham: Springer International Publishing
- Leinweber DJ. Nerds on Wall Street. Hoboken, NJ, USA: John Wiley & Sons, Inc.; 2009. “Artificial Intelligence and Intelligence Amplification; pp. 149–158. [ Google Scholar ]
- Marcus A, Sterling B, Swanwick M, Soloway E, and Vernor Vinge (1999) Opening Pleanary: Sci-Fi @ CHI-99: Science-Fiction Authors Predict Future User Interfaces. In Extended Abstracts on Human Factors in Computing Systems, 95–96. 10.1145/632716.63277
- Matsuo T (2017) “The Current Status of Japanese Robotics Law: Focusing on Automated Vehicles.” Pp. 151–70 in Robotics, Autonomics and the Law
- McCauley L. AI Armageddon and the Three Laws of Robotics. Ethics Inf Technol. 2007; 9 (2):153–164. doi: 10.1007/s10676-007-9138-2. [ CrossRef ] [ Google Scholar ]
- Mccauley L (2007) and Dunn Hall. “The Frankenstein Complex and Asimov’s Three Laws.” 9–14
- Mubin O, Billinghurst M, Obaid M, Jordan P, Alves-Oliveria P, Eriksson T, Barendregt W, Sjolle D, Fjeld M (2016) and Simeon Simoff. “Towards an Agenda for Sci-Fi Inspired HCI Research.” Pp. 1–6 in Proceedings of the 13th International Conference on Advances in Computer Entertainment Technology . New York, New York, USA: ACM Press
- Mubin O, Wadibhasme K, Jordan P. Reflecting on the Presence of Science Fiction Robots in Computing Literature. ACM Trans Human-Robot Interact. 2019; 8 (1):1–25. doi: 10.1145/3303706. [ CrossRef ] [ Google Scholar ]
- Nagy P, Wylie R, Eschrich J, Finn Ed. Why Frankenstein Is a Stigma Among Scientists. Sci Eng Ethics. 2018; 24 (4):1143–1159. doi: 10.1007/s11948-017-9936-9. [ PubMed ] [ CrossRef ] [ Google Scholar ]
- Nass C. Machines and Mindlessness: Social Responses to Computers. J Soc Issues. 2000; 56 (1):81–103. doi: 10.1111/0022-4537.00153. [ CrossRef ] [ Google Scholar ]
- Nichols R, Smith ND (2007) and Fred Miller. “Philosophy Through Science Fiction: A Coursebook with Readings.” 448
- Omori N (2020) “Komatsu Sakyō: Japan’s Apocalyptic Sci-Fi Author in the Spotlight in 2020.” Nippon.Com . Retrieved ( https://www.nippon.com/en/japan-topics/g00943/)
- Orikasa M, Inukai H, Eto K, Minamizawa K (2017) and Masahiko Inami. “Design of Sports Creation Workshop for Superhuman Sports.” Pp. 1–4 in Proceedings of the Virtual Reality International Conference . New York, New York, USA: ACM Press
- Picard RW (1997) Affective Computing. MIT Press
- Reeves S (2012) “Envisioning Ubiquitous Computing.” Pp. 1573–1582 in Proceedings of the SIGCHI Conference on Human Factors in Computing Systems , CHI ’12 . New York, NY, USA: Association for Computing Machinery
- Rekimoto J (2014) “A New You: From Augmented Reality to Augmented Human.” Pp. 1–1 in International Conference on Interactive Tabletops and Surfaces . New York, New York, USA: ACM Press
- Robertson J (2011) “Gendering Robots:Posthuman Traditionalism in Japan.” Pp. 277–303 in Recreating Japanese Men
- Schmitz M, Endres C, Butz A (2008) A Survey of Human-Computer Interaction Design in Science Fiction Movies. Institute for Computer Sciences, Social-Informatics and Telecommunications Engineering
- Shaw-Garlock G. Looking Forward to Sociable Robots. Int J Social Robot. 2009; 1 (3):249–260. doi: 10.1007/s12369-009-0021-7. [ CrossRef ] [ Google Scholar ]
- Shedroff N (2012) and Chris Noessel. “Make It so: Learning from Sci-Fi Interfaces.” Pp. 7–8 in International Working Conference on Advanced Visual Interfaces . New York, New York, USA: ACM Press
- Sterling B. “Design Fiction ” Interactions. 2009; 16 (3):20–24. doi: 10.1145/1516016.1516021. [ CrossRef ] [ Google Scholar ]
- Tanenbaum J, Tanenbaum K (2012) and Ron Wakkary. “Steampunk as Design Fiction.” Pp. 1583–1592 in Conference on Human Factors in Computing System , CHI ’12 . New York, NY, USA: Association for Computing Machinery
- Tatsumi T. Generations and Controversies: An Overview of Japanese Science Fiction, 1957–1997. Sci Fiction Stud. 2000; 27 (1):105–114. [ Google Scholar ]
- Troiano G, Tiab J, Youn-kyung Lim (2016) “SCI-FI: Shape-Changing Interfaces, Future Interactions.” Pp. 1–10 in the 9th Nordic Conference
- Vinge V (1993) “The Coming Technological Singularity: How to Survive in the Post-Human Era.”
Student Guide
Master's Programme in Computer, Communication and Information Sciences
Programme main page.
Master’s Programme in Computer, Communication and Information Sciences (CCIS) merges all the ICT related education in Aalto University under 10 majors. Programme is jointly organized by the School of Science (SCI) and the School of Electrical Engineering (ELEC), and coordinated by the School of Science.
The programme majors are the following:
- Acoustics and Audio Technology (ELEC, SCI)
- Communications Engineering (ELEC)
- Computer Science (SCI)
- Game Design and Development (SCI, ARTS)
- Human-Computer Interaction (ELEC, SCI)
- Machine Learning, Data Science and Artificial Intelligence (SCI)
- Security and Cloud Computing (SCI)
- Signal Processing and Data Science (ELEC)
- Software and Service Engineering (SCI)
- Speech and Language Technology (ELEC)
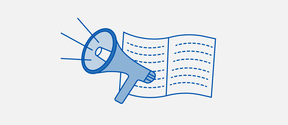
Join Funilab and have a say in how Sisu develops!
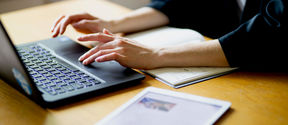
Master’s thesis management functionality in MyStudies to be launched in autumn 2024
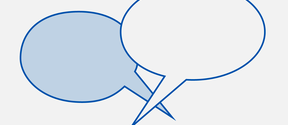
UniPID Virtual Studies Course Catalogue
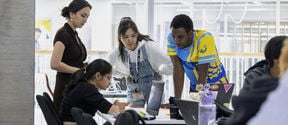
See your programme's Student Guide pages for 2024–2025, 2025–2026 degree requirements

Aalto Readers' Club
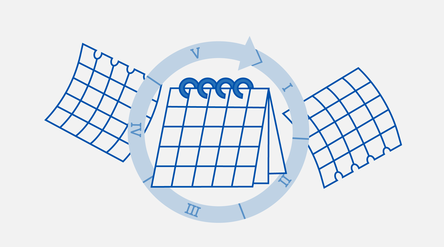
Beat the Blues: I'm enough - the power of self compassion
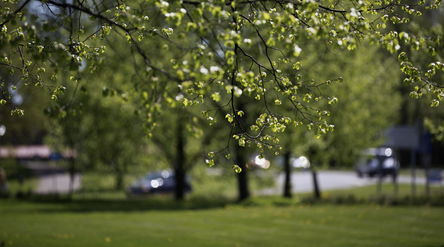
Alpacas, AYY, Starting Point of Wellbeing, and refreshments in Alvar Aalto Park
Start your studies with pomodoro, continuing studies in ccis progamme: instructions for bachelor students at aalto university.
Here you can find information how to continue your studies in this Master's programme.
Changing your master’s programme in the School of Science
If you are a student of School of Science and want to change your master’s programme, first check whether you meet the criteria for starting studies in the programme. The criteria for transferring to the programme must be met in bachelor's degree.
About changing programmes
- You may change your master’s programme once during your master’s studies. After the change, you cannot return to your original programme.
- You may change your master’s programme within the School of Science.
- You may not change into a master’s programme that requires a separate admission process (e.g. Master’s Programme in ICT Innovation, Master’s Programme in Security and Cloud Computing, the major Game Design and Development of the Master’s Programme in Computer, Communication and Information Sciences).
- You may change your master’s programme only if you were originally granted a study right for both bachelor’s and master’s degree at Aalto.
- If you were granted a study right for a master’s degree only, you cannot change your master’s programme. However, you can apply for the desired programme in the separate Aalto admission round for master’s studies .
- You may not change your master’s programme if, after you have you completed your bachelor’s degree at Aalto, you accept an admission offer for a master’s programme for which you have applied for in a separate admission round.
- If you were granted a scholarship for your master’s studies, the scholarship will continue in your new programme. Changing your master’s programme will not affect the duration of your scholarship.
How to apply
- Create a new version of your personal study plan (HOPS) in Sisu for your desired master’s programme.
- Schedule your studies. You must be able to complete all studies of the new programme within the study time that you have left.
- Apply for the change by contacting the planning officer of your desired master’s programme. You can find their contact information on the programme’s Contact page.
- The planning officer checks that you meet the criteria and notifies you.
- A decision of the change will be made by the dean of the School of Science.
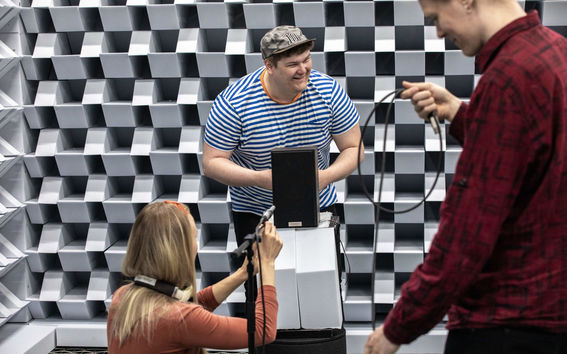
Acoustics and Audio Technology - Computer, Communication and Information Sciences, Master of Science (Technology)
The major in Acoustics and Audio Technology equips students with a fundamental understanding of human hearing, audio perception, and physics of sound. The skills they acquire enable, for example, reducing noise pollution, planning harmonic environments and designing coherent sound experiences.

Communications Engineering - Computer, Communication and Information Sciences, Master of Science (Technology)
In our digitally revolutionised world, the ability to develop, build, and maintain networks is in greater demand than ever before.
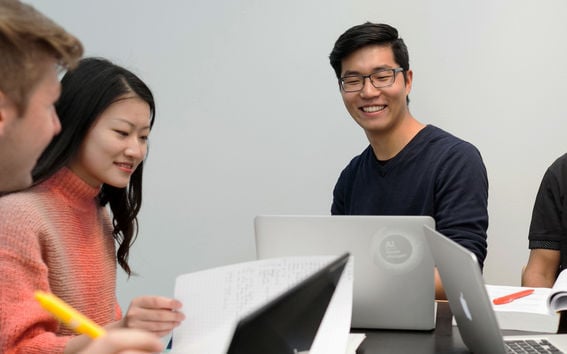
Computer Science - Computer, Communication and Information Sciences, Master of Science (Technology)
The Master of Science in Computer Science is grounded in leading-edge computing research at Aalto University, which is routinely ranked among the top 10 Computer Science departments in Europe. The programme offers a deep understanding on the design and analysis of algorithms, software, and computing technologies.

Game Design and Development - Computer, Communication and Information Sciences, Master of Science (Technology)
Game Design and Development is a multidisciplinary major that tightly integrates students from different backgrounds. Students create and analyze games, experiment with new technologies and design approaches, and meet like-minded talent with whom to build the future of games and interactive experiences.
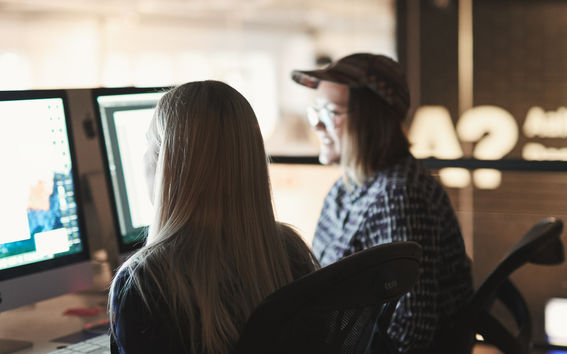
Human-Computer Interaction - Computer, Communication and Information Sciences, Master of Science (Technology)
By creating user-friendly next-generation computing products, HCI experts help society make the most out of revolutionary technologies.

Machine Learning, Data Science and Artificial Intelligence - Computer, Communication and Information Sciences, Master of Science (Technology)
The data-intensive major in Machine Learning, Data Science and Artificial Intelligence deals with some of the most challenging problems of the 21st century. Be it finding new solutions to tackle climate change or better understanding the causes of an epidemic, this field has an integral part to play.
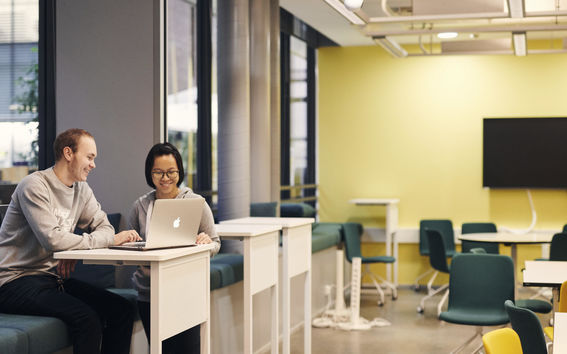
Signal Processing and Data Science - Computer, Communication and Information Sciences, Master of Science (Technology)
In the Signal Processing and Data Science major, you will learn to extract useful information, discover patterns, and make predictions from large amounts of signal or data. You get to apply your knowledge to the physical world – making devices, systems and entities smarter and more environmentally friendly.
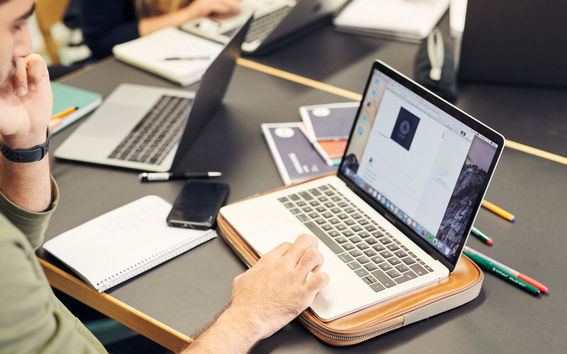
Software and Service Engineering - Computer, Communication and Information Sciences, Master of Science (Technology)
Software and Service Engineering is the backbone of modern society and economy. The Master’s programme in Computer, Communication and Information Sciences – Software and Service Engineering equips students with some of the most sought after skills in today’s job market, across a wide range of industries.

Speech and Language Technology - Computer, Communication and Information Sciences, Master of Science (Technology)
Aalto University’s Speech and Language Technology major benefits from leading research in machine learning and speech technology, with researchers actively involved in teaching. Graduates of the programme are well-equipped to launch their careers in the fast-growing field.
Feedback about the page
- Published: 10.1.2023
- Updated: 11.3.2024
Language and Linguistics in Sci-Fi
The joys of reading fiction with undergraduate linguists, format + mechanics.
I’m currently teaching Language & Linguistics in Sci-Fi every spring as a 1-credit Linguistics elective. It’s a Directed Reading, which means (among other things) that I teach it on top of my regular courses. The enrollment cap is 12. Students need to have taken one linguistics class or get my permission to enroll.
Here’s a sample syllabus . We meet once a week for 75 minutes. Each week we discuss an assigned short story or novella. Students take turn leading the discussions, which tend to follow a similar format:
- We start off talking informally about our affective responses: did we enjoy the story; how hard was it to read; did we find the characters likeable or relatable; what other stories or real-world events did this story remind us of?
- We make sure everyone shares a basic understanding of the story. Some stories start in medias res and then have a point where the narration pauses and there’s some background explanation. We re-read that passage together, as well as other passages that provide clues, to make sure everyone has the key information. Other stories leave large aspects of the plot and setting ambiguous (e.g. ‘The Third Tower’). We discuss our shared confusion, re-read passages that offer hints, and compare our interpretations.
- By themselves, students usually come around to the question ‘Why did Dr. Pak have us read this story? What does it have to do with language and linguistics?’ If all goes well, we linger here for a while and work to appreciate how the story makes us see language differently.
For the end of the semester, each student independently reads a sci-fi novel from this list (they can borrow the books from me or our library):
- Mind of My Mind by Octavia Butler
- The Girl with All the Gifts by Mike Carey
- Klara and the Sun by Kazuo Ishiguro
- The Buried Giant by Kazuo Ishiguro
- Metamorphosis by Franz Kafka
- The Left Hand of Darkness by Ursula K. Le Guin
- Embassytown (in its entirety) by China Miéville
- The Color of Distance by Amy Thomson
- Strange Bodies by Marcel Theroux
- Project Hail Mary by Andy Weir
Students can read a different novel if they want, as long as I approve it ahead of time.
During the final exam period, each student schedules a time to have an informal interview/conversation with me about their selected book. I record each conversation and share it with the student as a memento. If a student strongly prefers to write an essay rather than having an interview, I allow them to, but I’ve found the interviews to be more enjoyable and more suitable for this kind of class: the student and I can give each other immediate feedback, ask for clarification, reread passages together, and chat informally about tangents, with less pressure to impress or evaluate.
Alternative formats
Scaled-back . If you’d like to teach some of these stories but can’t commit to a weekly class, here are some other ideas I’ve tried:
- Incorporate one or two sci-fi readings into a regular linguistics class. I’ve included ‘The Truth of Fact, the Truth of Feeling’ in a formal semantics class, as a way to get students thinking more deeply about what truth is. I also once spent the last day of my Foundations of Linguistics class on a discussion of ‘Story of Your Life.’
- Host a stand-alone reading discussion as an extra-credit event. I did this once with ‘Story of Your Life’: I made the reading available to my two classes (Languages of the World and Morphology & Syntax) and invited students to an evening discussion. They got a point of extra credit if they participated in the discussion and submitted a short write-up.
- Or you can try a non-credit book-club format. Put out an interest email, decide on a regular time and place, and decide on each reading at the end of the previous discussion. This is how I first taught my course, and I think it worked because it was the summer of 2020, when people had lots of extra time and were desperate for connection. I’m afraid my students have now reverted to their pre-pandemic levels of busyness, so as much as they might like to, they probably wouldn’t attend a noncredit book club regularly.
Scaled-up. In Spring 2021 I scaled taught Language & Linguistics in Sci-Fi as a full 3-credit class. It was a first-year seminar, which at Emory is a context where instructors often experiment with more eclectic content. Here’s the syllabus . You’ll see that I scaled up the class by doing a few different things:
- I added three novels to the core required readings: Mind of My Mind by Octavia Butler, Strange Bodies by Marcel Theroux and Embassytown by China Miéville .
- I added some traditional intro-linguistics content. We talked about the linguistic science behind the stories, e.g. aphasia in ‘Speech Sounds,’ speech acts in ‘The Easthound,’ literacy and writing systems in ‘The Truth of Fact, the Truth of Feeling.’ We also spent a couple days on modalities and formal features of various sci-fi languages. (See other approaches for more info on this.)
- Students did more extensive end-of-semester final projects and longer in-class presentations.
One thing I’d do differently if I taught it again: simplify the Evidence Exercises and Reflections (syllabus p. 3). We were advised that semester to offer lots of low-stakes assignments, but I had too many of these, and they ended up being burdensome for both the students and me.
Department of Computer Science
Library item label woz ere --> undergraduate undergraduate home courses course structure entry requirements scholarships accreditation careers student profiles postgraduate postgraduate home why sheffield postgraduate taught taught courses student profiles accreditation scholarships phd study apply now fees and funding studentships and phd opportunities phd profiles research research home innovation and impact collaborate with us current grant portfolio previous grant portfolio centres and institutes research groups ref2021 fellowship programme research themes speech and language healthcare technologies bioinspired machine intelligence dependable and secure systems people people home academic staff admin and professional staff academic visitors technical staff research staff department about contact us facilities alumni meet our staff news blog events jobs genesys industrial advisory board prizes equality, diversity and inclusion information for current staff and students speech and language.
The cluster is unique in its ability to conduct research across a broad spectrum, from computational models of language and human hearing, to commercially deployed automatic speech recognition (ASR) and text engineering systems.
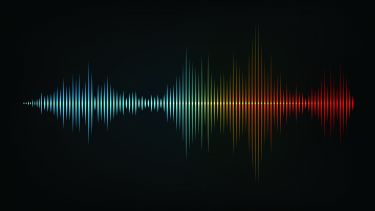
We address Speech and Language challenges through one of the strongest concentrations of researchers worldwide, comprising academics from our Natural Language Processing and Speech and Hearing groups
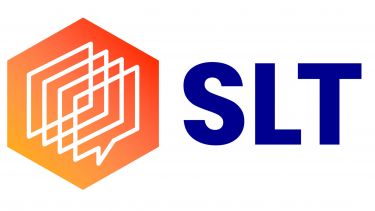
Our UKRI (UK Research and Innovation) Centre for Doctoral Training in Speech and Language Technologies and their applications will equip computer scientists with the skills, knowledge and confidence to tackle today’s evolving issues, and future challenges.
The Centre for Speech & Language Technology brings together expertise from researchers in the Department of Computer Science and Silicon Valley based company, VoiceBase , the leading provider of AI-powered speech analytics, to pioneer the future of speech recognition and speech analytics
Research groups
Natural language processing, speech and hearing, news and highlights.
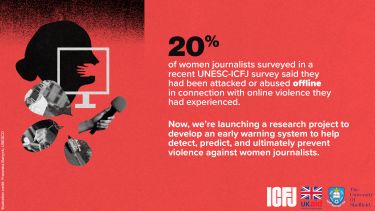
Towards an Early Warning System for Violence Against Women Journalists
Researchers from the Department are part of a major UK-government funded research project to work towards an early warning system to help detect, predict, and ultimately prevent violence against women journalists
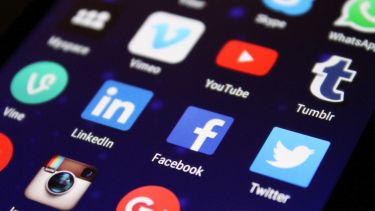
Global disinformation report calls for internet companies to fact-check all political content
Internet companies should apply fact-checking to all political content published by politicians, political parties and their affiliates to help tackle the spread of disinformation, according to a new report.
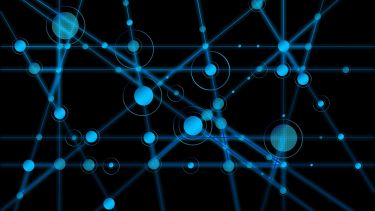
AI can predict Twitter users likely to spread disinformation before they do it
A new artificial intelligence-based algorithm that can accurately predict which Twitter users will spread disinformation before they actually do it has been developed by researchers from the University of Sheffield.
Combating disinformation and abuse in social media
Sheffield’s big data analytics has probed the veracity, sentiment, and sharing patterns of social media posts and exposed the ways social media can be used and abused to shape opinions about significant political events, such as elections or the Brexit referendum. The methods and findings have been used to promote truth in public discourse, underpinning UK and international policy responses to misinformation and the misuse of social media in relation to various issues.
Discover more
Finding our voice: the rise and impact of voice recognition technology
Automatic Speech Recognition (ASR) for conversational speech is a challenging research problem, particularly in the context of adverse acoustic conditions such as over the telephone or in multi-party meetings. Researchers in the department have developed, with industry, state-of-the-art ASR tools.
Machine translation
Machine translation (MT) is inexpensive, fast, and accessible, but it lacks the reliability of human translators. Sheffield research on quality estimation (QE) in MT has enabled the identification of the likelihood of error, allowing MT to be used with greater confidence and underpinning impacts for multiple organisations.
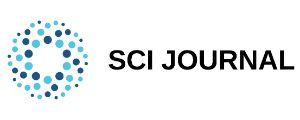
Computer Speech and Language Impact Factor & Key Scientometrics
Computer speech and language overview, impact factor.
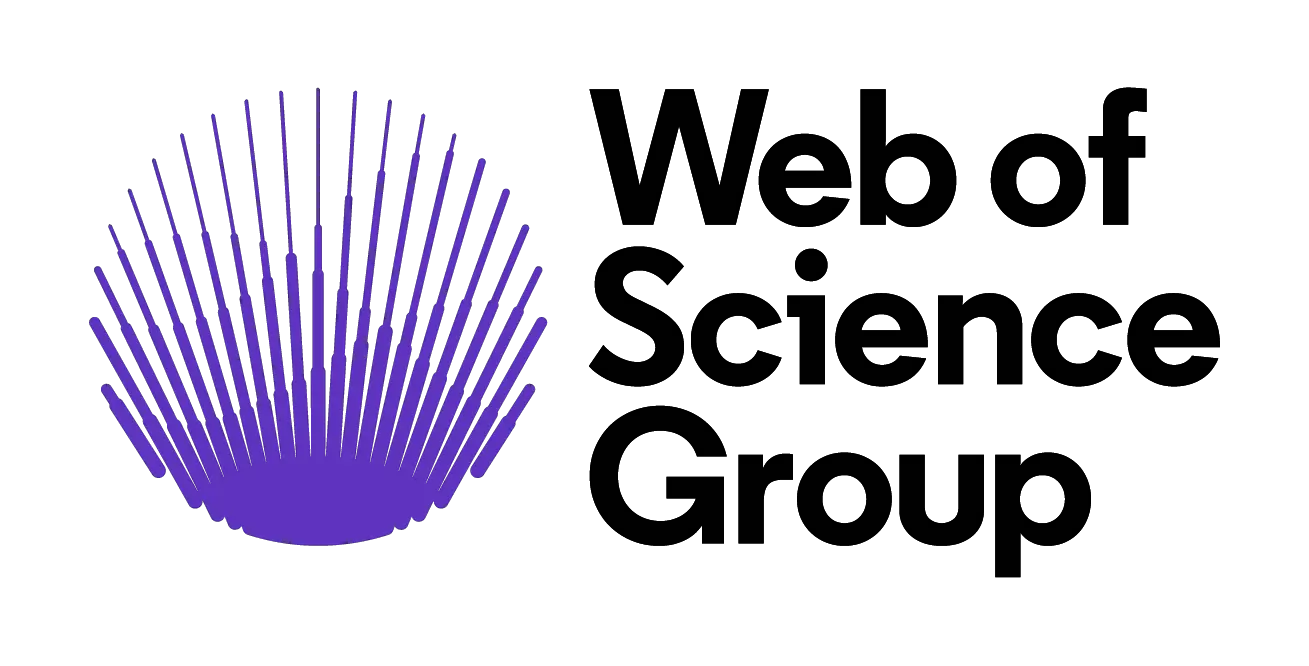
I. Basic Journal Info

Journal ISSN: 08852308, 10958363
Publisher: elsevier inc., history: 1986-1987, 1989-ongoing, journal hompage: link, how to get published:, research categories, scope/description:.
--------------------------------
Best Academic Tools
- Academic Writing Tools
- Proofreading Tools
- Academic Search Engines
- Project Management Tools
- Survey Tools for Research
- Transcription Tools
- Reference Management Software
- AI-Based Summary Generators
- Academic Social Network Sites
- Plagiarism Checkers
- Science Communication Tools
- Jasper AI Review
II. Science Citation Report (SCR)
Computer speech and language scr impact factor, computer speech and language scr journal ranking, computer speech and language scimago sjr rank.
SCImago Journal Rank (SJR indicator) is a measure of scientific influence of scholarly journals that accounts for both the number of citations received by a journal and the importance or prestige of the journals where such citations come from.
Computer Speech and Language Scopus 2-Year Impact Factor Trend
Computer speech and language scopus 3-year impact factor trend, computer speech and language scopus 4-year impact factor trend, computer speech and language impact factor history.
- 2022 Impact Factor 5.385 4.703 4.315
- 2021 Impact Factor 4.479 3.95 4.044
- 2020 Impact Factor 2.73 3.262 2.922
- 2019 Impact Factor 3.901 3.382 3.415
- 2018 Impact Factor 2.862 3.019 3.121
- 2017 Impact Factor 2.975 3.217 3.041
- 2016 Impact Factor 3.13 3.068 2.956
- 2015 Impact Factor 3.253 3.154 3.192
- 2014 Impact Factor 2.615 NA NA
- 2013 Impact Factor 3.134 NA NA
- 2012 Impact Factor 3.86 NA NA
- 2011 Impact Factor 3.729 NA NA
- 2010 Impact Factor 3.02 NA NA
- 2009 Impact Factor 2.672 NA NA
- 2008 Impact Factor 3.569 NA NA
- 2007 Impact Factor 3 NA NA
- 2006 Impact Factor 1.766 NA NA
- 2005 Impact Factor 1.22 NA NA
- 2004 Impact Factor 2.488 NA NA
- 2003 Impact Factor 2.146 NA NA
- 2002 Impact Factor 1.167 NA NA
- 2001 Impact Factor 1.541 NA NA
- 2000 Impact Factor 1.659 NA NA
See what other people are reading
HIGHEST PAID JOBS
- Highest Paying Nursing Jobs
- Highest Paying Non-Physician Jobs
- Highest Paying Immunology Jobs
- Highest Paying Microbiology Jobs
LATEX TUTORIALS
- LaTeX Installation Guide – Easy to Follow Steps to Install LaTeX
- 6 Easy Steps to Create Your First LaTeX Document
- How to Use LaTeX Paragraphs and Sections
- How to Use LaTeX Packages with Examples
MUST-READ BOOKS
- Multidisciplinary
- Health Science
Impact factor (IF) is a scientometric factor based on the yearly average number of citations on articles published by a particular journal in the last two years. A journal impact factor is frequently used as a proxy for the relative importance of a journal within its field. Find out more: What is a good impact factor?
III. Other Science Influence Indicators
Any impact factor or scientometric indicator alone will not give you the full picture of a science journal. There are also other factors such as H-Index, Self-Citation Ratio, SJR, SNIP, etc. Researchers may also consider the practical aspect of a journal such as publication fees, acceptance rate, review speed. ( Learn More )
Computer Speech and Language H-Index
The h-index is an author-level metric that attempts to measure both the productivity and citation impact of the publications of a scientist or scholar. The index is based on the set of the scientist's most cited papers and the number of citations that they have received in other publications
Computer Speech and Language H-Index History
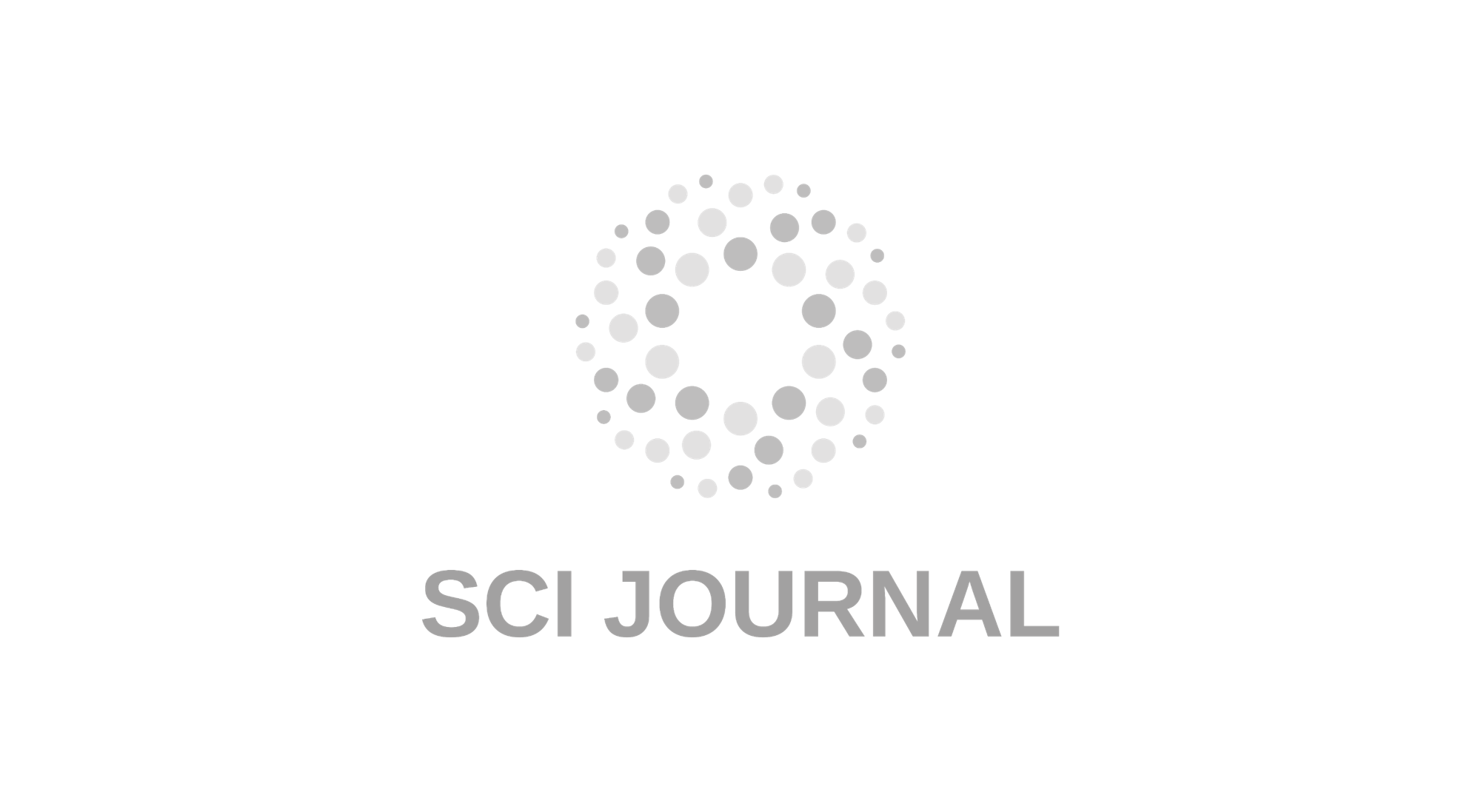
scijournal.org is a platform dedicated to making the search and use of impact factors of science journals easier.

IMAGES
VIDEO
COMMENTS
An official publication of the International Speech Communication Association (ISCA) Computer Speech & Language publishes reports of original research related to the recognition, understanding, production, coding and mining of speech and language. The speech and language sciences have a long history, but it is only relatively recently that large-scale implementation of and experimentation with ...
Pierce Darragh. Many science-fiction stories utilize language to some extent. Some use it just to add depth to the setting, where others may make it a quintessential element of the plot. I really like language (like… a lot), so I wanted to compare some of the different uses of language in science fiction. One of the best ways to compare ...
Teaching a computer to process and generate human language is a fundamental aspect of enabling a computer to process thought, problem solve, and communicate in a way that humans can understand.
Corrigendum to 'Unsupervised sign language validation process based on hand-motion parameter clustering' <Computer Speech & Language Volume 71, January 2022, 101256> Mehrez Boulares, Ahmed Barnawi. Article 101319 View PDF; Special Issue on State-of-the-art Handcrafted Feature Extraction for Speech and Voice Analysis.
A physical exertion inspired multi-task learning framework for detecting out-of-breath speech. Physical exertion is a stress condition that affects how we normally produce speech. It alters both the temporal and spectral pattern of speech characteristics. Therefore, speech utterances can be used as a cost-effective telehealth solution to ...
Scope. Computer Speech & Language publishes reports of original research related to the recognition, understanding, production, coding and mining of speech and language. The speech and language sciences have a long history, but it is only relatively recently that large-scale implementation of and experimentation with complex models of speech ...
In this work, we introduce WavLLM, a robust and adaptive speech large language model with dual encoders, and a prompt-aware LoRA weight adapter, optimized by a two-stage curriculum learning approach. Leveraging dual encoders, we decouple different types of speech information, utilizing a Whisper encoder to process the semantic content of speech ...
Neuromancer came out in 1984, a fantastic year to release a book about brain hacking, and described a whole host of new concepts and inventions that are the cornerstone for what sci-fi, and ...
Aalto University's Speech and Language Technology major benefits from leading research in machine learning and speech technology, with researchers actively involved in teaching. ... Applicants are expected to have a high-quality Bachelor's degree in computer science, software engineering, communications engineering, or electrical ...
The papers present current research in the area of computer speech processing including speech science, speech technology, natural language processing, human-computer interaction, language identification, multimedia processing, human-machine interaction, deep learning for audio processing, computational paralinguistics, affective computing ...
Linguistics has an intrinsic connection to science fiction stories given the nature of the genre and its frequent use of alien settings and cultures. As mentioned in Aliens and Linguists: Language Study and Science Fiction by Walter E. Meyers, science fiction is almost always concerned with the idea of communication, such as communication with aliens and machines, or communication using dead ...
But three research teams recently made progress in turning data from electrodes surgically placed on the brain into computer-generated speech. Using computational models known as neural networks, they reconstructed words and sentences that were, in some cases, intelligible to human listeners. None of the efforts, described in papers in recent ...
This would exclude where a computer interprets human speech, and where we 'assume' a human is programming as they can be seen making input to a computer, but we don't see that input. ... (royalty-free) in his new Sci-Fi novels as an esoteric language by some galactic empire (I've only read a fantasy book from him "Kajjám, a Tévedés", so I ...
An analysis of observation length requirements for machine understanding of human behaviors from spoken language. Sandeep Nallan Chakravarthula, Brian R.W. Baucom, Shrikanth Narayanan, Panayiotis Georgiou. Article 101162.
Decoding human speech from neural signals is essential for brain-computer interface (BCI) technologies that aim to restore speech in populations with neurological deficits. However, it remains a ...
The language itself was developed into a full language, which is pretty much a sci-fi nerd prerequisite at this point. One of the most recent iterations of Star Trek — the TV show Star Trek: Discovery — hired official Klingon translator Robyn Stewart to create dialogue for the alien species, which is then translated on-screen.
Abstract. Driven by the rapid development of artificial intelligence (AI) and anthropomorphic robotic systems, the various possibilities and risks of such technologies have become a topic of urgent discussion. Although science fiction (SF) works are often cited as references for visions of future developments, this framework of discourse may ...
Speech and Language Technology - Computer, Communication and Information Sciences, Master of Science (Technology) Aalto University's Speech and Language Technology major benefits from leading research in machine learning and speech technology, with researchers actively involved in teaching.
The illness in 'Speech Sounds' attacks the brain, not the vocal tract; it's a kind of aphasia. Left-handed people 'tend to be less impaired' (p. 5), suggesting that the illness targets the left hemisphere (where language is lateralized for almost all right-handed people but far fewer left-handed people). Sometimes the illness affects ...
We talked about the linguistic science behind the stories, e.g. aphasia in 'Speech Sounds,' speech acts in 'The Easthound,' literacy and writing systems in 'The Truth of Fact, the Truth of Feeling.' We also spent a couple days on modalities and formal features of various sci-fi languages. (See other approaches for more info on this.)
Department of Computer Science. Speech and Language. The cluster is unique in its ability to conduct research across a broad spectrum, from computational models of language and human hearing, to commercially deployed automatic speech recognition (ASR) and text engineering systems. We address Speech and Language challenges through one of the ...
Scope/Description: Computer Speech & Language publishes reports of original research related to the recognition, understanding, production, coding and mining of speech and language. The speech and language sciences have a long history, but it is only relatively recently that large-scale implementation of and experimentation with complex models ...