- Privacy Policy

Home » Inductive Reasoning – Definition, Types and Guide

Inductive Reasoning – Definition, Types and Guide
Table of Contents

Inductive Reasoning
Definition :
Inductive reasoning is a type of reasoning that involves deriving generalizations or conclusions from specific observations or instances. In other words, inductive reasoning involves making inferences about the properties or characteristics of a group or category based on observations or data collected from a smaller subset of that group or category.
Steps in Inductive Reasoning
The steps in inductive reasoning can be summarized as follows:
- Observation : The first step in inductive reasoning is to make observations of a particular phenomenon or group of instances. These observations can be made through various methods, such as experiments, surveys, or data analysis.
- Pattern Recognition : Once observations are made, the next step is to identify patterns or regularities in the data. This involves looking for similarities or commonalities among the instances or data points.
- Hypothesis Formulation: Based on the patterns or regularities identified, a hypothesis or tentative explanation is formulated. This hypothesis should account for the observations made and provide a possible explanation for the patterns identified.
- Testing : The next step is to test the hypothesis through further observations or experiments. This involves collecting additional data and comparing it to the hypothesis to determine if it holds up or if it needs to be modified.
- Evaluation: Once testing is completed, the results are evaluated to determine the strength of the hypothesis. If the results support the hypothesis, it may be considered a valid explanation for the observed phenomenon. However, if the results do not support the hypothesis, it may need to be revised or discarded altogether.
- Conclusion : The final step is to draw a conclusion based on the evaluation of the hypothesis. If the hypothesis is supported by the data, it may be used to make predictions or generalizations about the larger population or phenomenon. However, if the hypothesis is not supported, further research may be needed to better understand the phenomenon.
Types of Inductive Reasoning
Types of Inductive Reasoning are as follows:
Generalization
Generalization is the most common type of inductive reasoning. It involves making conclusions about a larger population based on observations made on a smaller sample of that population. For example, a researcher may study a sample of college students to draw general conclusions about the attitudes and behaviors of college students as a whole. Generalization is useful when it is not feasible to study an entire population, and when the sample is representative of the population being studied. However, generalizations may not always hold true for the entire population and may need to be revised if new evidence is found.
Analogy is another type of inductive reasoning that involves drawing conclusions about a particular phenomenon based on similarities to other phenomena that have been observed. For example, a doctor may diagnose a patient’s illness based on similarities between the patient’s symptoms and those of other patients with a similar illness. Analogy can be a useful tool for making predictions or drawing conclusions when direct observations are not possible or when limited information is available. However, it is important to note that analogies may not always be accurate or applicable to every situation.
Causal Inference
Causal inference involves making predictions or drawing conclusions about the causes of a particular phenomenon based on observed correlations or relationships between different variables. For example, a researcher may study the relationship between smoking and lung cancer to determine if smoking is a causal factor in the development of lung cancer. Causal inference can be a powerful tool for identifying causes and predicting outcomes. However, it is important to recognize that correlation does not always imply causation, and other factors may be at play.
Statistical Reasoning
Statistical reasoning involves making predictions or drawing conclusions about a particular population based on statistical analysis of data collected from a sample of that population. For example, a marketer may use statistical analysis to predict consumer behavior based on data collected from a sample of consumers. Statistical reasoning can be a powerful tool for making predictions and identifying trends. However, it is important to use appropriate statistical methods and ensure that the sample is representative of the population being studied.
Predictive Reasoning
Predictive reasoning involves making predictions about future events or outcomes based on patterns or trends observed in past events or outcomes. For example, a stock analyst may use predictive reasoning to make predictions about the future performance of a particular stock based on past trends in the stock market. Predictive reasoning can be a useful tool for making informed decisions and planning for the future. However, it is important to recognize that past performance does not always predict future outcomes, and other factors may come into play.
Applications of Inductive Reasoning
Here are some of the most common applications of inductive reasoning:
inductive reasoning is widely used in research fields to develop theories and models based on observed data. Researchers use inductive reasoning to analyze data, identify patterns and trends, and generate hypotheses about the relationships between variables. This process is used in many fields of research, including social sciences, education, and environmental science.
Mathematics
In mathematics, inductive reasoning is used to make conjectures and prove mathematical statements. Mathematicians use inductive reasoning to identify patterns in numbers and formulas, and then use those patterns to make predictions about the behavior of mathematical systems. For example, in geometry, mathematicians might observe that the sum of the angles of a triangle always adds up to 180 degrees, and then use inductive reasoning to make a general statement about all triangles.
In psychology, inductive reasoning is used to develop theories about human cognition and behavior. Psychologists use observational and experimental methods to gather data about human behavior, and then use inductive reasoning to develop hypotheses about the underlying psychological processes that explain that behavior. For example, a psychologist might observe that children tend to imitate the behavior of their parents, and then use inductive reasoning to develop a theory about the role of social learning in child development.
Inductive reasoning is a key part of the scientific method. Scientists use inductive reasoning to make generalizations about the natural world based on observed data. This process is used to develop hypotheses, test them through experimentation, and refine theories about how the world works. Inductive reasoning is used in many fields of science, including physics, biology, and psychology.
Inductive reasoning is an important tool in medical research. It is used to generate hypotheses about the causes of diseases, test potential treatments through clinical trials, and analyze data to identify patterns and trends. Inductive reasoning is also used in the diagnosis of diseases, where doctors use observed symptoms and test results to make a diagnosis.
Inductive reasoning is used in marketing to analyze consumer behavior and predict trends in the market. Marketers use data on consumer preferences, buying habits, and demographics to make predictions about future sales and develop marketing strategies to reach their target audience. Inductive reasoning is also used in market research to generate hypotheses about the factors that influence consumer behavior.
Inductive reasoning is used in finance to analyze market trends and make predictions about future stock prices and other financial indicators. Financial analysts use data on past market performance and economic indicators to make predictions about future market performance. Inductive reasoning is also used in risk management, where analysts use data on past losses and other risk factors to predict the likelihood of future losses.
Inductive reasoning is used in law to make decisions based on past legal cases. Lawyers and judges use past legal cases as analogies to make decisions about current cases. This process is known as legal reasoning by analogy and is a key part of common law legal systems.
Inductive Reasoning Examples
Here are some real-time examples of inductive reasoning:
- Research studies: Psychologists use inductive reasoning to make generalizations about human behavior based on observations of study participants. By analyzing patterns in behavior, thoughts, and emotions, researchers can make predictions about how people will respond to different stimuli or situations.
- Weather forecasting: Weather forecasting uses inductive reasoning to make predictions about the weather based on past observations. By analyzing patterns in temperature, wind direction, and atmospheric pressure, meteorologists can predict future weather conditions with a high degree of accuracy.
- Medical diagnosis: Doctors use inductive reasoning to diagnose medical conditions by observing a patient’s symptoms and using those observations to make a generalization about the underlying cause of the symptoms. For example, a doctor might observe a patient’s fever, cough, and fatigue and make a diagnosis of the flu based on those observations.
- Market research: Market researchers use inductive reasoning to make predictions about consumer behavior based on past observations. By analyzing patterns in consumer spending, purchasing habits, and demographic data, market researchers can make predictions about future trends and help businesses make informed decisions.
- Criminal investigations: Law enforcement officers use inductive reasoning to solve crimes by observing evidence and using those observations to make inferences about what happened. For example, a detective might observe a broken window and a missing laptop and infer that a burglary occurred.
- Scientific research: Scientists use inductive reasoning to make hypotheses based on observations and data. By analyzing patterns in experimental data, scientists can make predictions about how natural phenomena work and test those predictions through further experimentation. For example, a biologist might observe a particular behavior in an animal and make a hypothesis about the underlying biological mechanism that drives that behavior.
How to conduct Inductive Reasoning
Here are the steps to conduct inductive reasoning:
- Make specific observations: The first step in conducting inductive reasoning is to make specific observations about a particular phenomenon or event. This might involve collecting data, conducting experiments, or simply observing what is happening around you.
- Identify patterns: Once you have made your observations, the next step is to identify patterns or trends in the data. Look for similarities or commonalities among the observations you have made.
- Formulate a hypothesis: Based on the patterns you have identified, formulate a hypothesis or tentative generalization about the phenomenon you are studying. This hypothesis should be based on the specific observations you have made and should be testable through further observation or experimentation.
- Test the hypothesis: The final step in conducting inductive reasoning is to test your hypothesis. This might involve collecting additional data, conducting experiments, or making further observations. The goal is to determine whether your hypothesis is supported by the data you have collected.
- Revise the hypothesis: If your hypothesis is not supported by the data, revise it and try again. If your hypothesis is supported by the data, you can use it to make predictions about future observations or events.
Purpose of Inductive Reasoning
The purpose of inductive reasoning is to make generalizations based on specific observations. It allows us to make predictions about what we might expect to observe in the future, based on what we have observed in the past. Inductive reasoning is a key tool in scientific research, as it allows researchers to develop hypotheses and theories based on empirical observations.
Inductive reasoning is also important in everyday life. For example, when we encounter a new situation, we may use inductive reasoning to make predictions about how people are likely to behave based on our past experiences. Similarly, we may use inductive reasoning to make predictions about future events based on our past observations of similar events.
Inductive reasoning allows us to make predictions and draw conclusions even when we do not have all the information we might like. However, it is important to note that inductive reasoning is not foolproof and can be subject to biases and errors. Therefore, it’s important to approach inductive reasoning with a critical eye and to continually evaluate and revise our hypotheses based on new data and observations.
When to use Inductive Reasoning
Inductive reasoning is typically used when we want to make generalizations or predictions based on specific observations. Here are some situations where inductive reasoning may be particularly useful:
- Exploratory research: Inductive reasoning is often used in exploratory research, where the goal is to gather data and identify patterns or trends that may inform future research or hypotheses.
- Observational studies: In fields such as sociology, psychology, and anthropology, researchers often use inductive reasoning to develop theories based on their observations of human behavior and social interactions.
- Problem-solving: When faced with a new problem or situation, we may use inductive reasoning to make predictions or generate potential solutions based on our past experiences and observations.
- Decision-making: Inductive reasoning can be useful when making decisions based on limited information, as it allows us to make predictions and draw conclusions even when we don’t have all the facts.
- Scientific research: In scientific research, inductive reasoning is often used to develop hypotheses and theories based on empirical observations. Researchers may use inductive reasoning to identify patterns or trends in their data, which can then be used to develop testable hypotheses.
Advantages of Inductive Reasoning
There are several advantages to using inductive reasoning:
- Flexibility : Inductive reasoning allows us to make generalizations and predictions based on specific observations, even when we do not have complete information or a clear understanding of the underlying mechanisms at work.
- Creativity : Inductive reasoning can be a useful tool for generating new ideas and hypotheses. By observing patterns and trends in our data, we can develop new theories or identify areas for further exploration.
- Accessibility : Inductive reasoning is accessible to people with varying levels of expertise or knowledge. By relying on empirical observations, we can develop insights and draw conclusions without requiring specialized training or expertise.
- Real-world applicability: Inductive reasoning is used in a variety of fields, from scientific research to business and decision-making. By making predictions based on past observations, we can make informed decisions and develop effective strategies for achieving our goals.
- Development of theories: Inductive reasoning can be used to develop theories that can be tested through further observation or experimentation. This allows us to refine our understanding of complex phenomena and develop a more nuanced understanding of how things work.
Limitations of Inductive Reasoning
While inductive reasoning has several advantages, it also has some limitations that should be considered:
- Limited scope : Inductive reasoning relies on specific observations to make generalizations, which means that the conclusions we draw are limited to the scope of our observations. This means that our conclusions may not be applicable to broader contexts or situations.
- Potential for bias: Inductive reasoning can be influenced by our own biases and preconceptions, as well as by the specific observations we choose to focus on. This can lead to inaccurate or incomplete conclusions.
- Lack of certainty: Inductive reasoning is probabilistic in nature, meaning that the conclusions we draw are based on probability rather than certainty. This means that our predictions and generalizations may not always be accurate.
- Limited explanatory power: Inductive reasoning can tell us what is likely to happen based on past observations, but it may not tell us why something is happening or how it works. This can limit our ability to develop deeper understanding of complex phenomena.
- Lack of predictive power: Inductive reasoning is limited to predicting what is likely to happen based on past observations. It may not be able to predict new or novel phenomena that have not been observed before.
About the author
Muhammad Hassan
Researcher, Academic Writer, Web developer
You may also like

Deductive Reasoning – Definition, Types and...

Abductive Reasoning – Definition, Types and...

Research Approach – Types Methods and Examples
What's the difference between deductive reasoning and inductive reasoning?
Deductive reasoning and inductive reasoning are easy to mix up. Learn what the difference is and see examples of each type of scientific reasoning.
Sherlock Holmes, the fictional sleuth who famously resides on Baker Street, is known for his impressive powers of logical reasoning. With a quick visual sweep of a crime scene, he generates hypotheses, gathers observations and draws inferences that ultimately reveal the responsible criminal's methods and identity.
Holmes is often said to be a master of deductive reasoning, but he also leans heavily on inductive reasoning. Because of their similar names, however, these concepts are easy to mix up.
So what's the difference between deductive and inductive reasoning? Read on to learn the key distinctions between these two modes of logic used by literary detectives and real-life scientists alike.
Related: Sherlock Holmes' famous memory trick really works
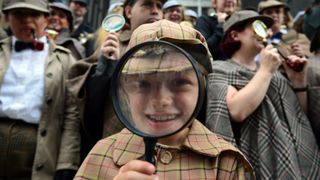
What is deductive reasoning?
Deductive reasoning, also known as deduction, is a basic form of reasoning that uses a general principle or premise as grounds to draw specific conclusions.
This type of reasoning leads to valid conclusions when the premise is known to be true — for example, "all spiders have eight legs" is known to be a true statement. Based on that premise, one can reasonably conclude that, because tarantulas are spiders, they, too, must have eight legs.
The scientific method uses deduction to test scientific hypotheses and theories , which predict certain outcomes if they are correct, said Sylvia Wassertheil-Smoller , a researcher and professor emerita at Albert Einstein College of Medicine.
"We go from the general — the theory — to the specific — the observations," Wassertheil-Smoller told Live Science. In other words, theories and hypotheses can be built on past knowledge and accepted rules, and then tests are conducted to see whether those known principles apply to a specific case.
Deductive reasoning begins with a first premise, which is followed by a second premise and an inference, or a conclusion based on reasoning and evidence. A common form of deductive reasoning is the "syllogism," in which two statements — a major premise and a minor premise — together reach a logical conclusion.
For example, the major premise "Every A is B" could be followed by the minor premise "This C is A." Those statements would lead to the conclusion that "This C is B." Syllogisms are considered a good way to test deductive reasoning to make sure the argument is valid.
In deductive reasoning, if something is true of a class of things in general, it is also true for all members of that class.
Deductive conclusions are reliable provided that the premises they're based on are true, but you run into trouble if they're false, according to Norman Herr , a professor of secondary education at California State University, Northridge. For instance the argument "All bald men are grandfathers. Harold is bald. Therefore, Harold is a grandfather," is logically valid, but it is untrue because the original premise is false.
Related: Crows outthink monkeys, can grasp recursive patterns
Deductive reasoning examples
Here are some examples of deductive reasoning:
Major premise: All mammals have backbones. Minor premise: Humans are mammals. Conclusion: Humans have backbones.
Major premise: All birds lay eggs. Minor premise: Pigeons are birds. Conclusion: Pigeons lay eggs.
Major premise: All plants perform photosynthesis. Minor premise: A cactus is a plant. Conclusion: A cactus performs photosynthesis.
What is inductive reasoning?
Inductive reasoning uses specific and limited observations to draw general conclusions that can be applied more widely. So while deductive reasoning is more of a top-down approach — moving from a general premise to a specific case — inductive reasoning is the opposite. It uses a bottom-up approach to generate new premises, or hypotheses, based on observed patterns, according to the University of Illinois .
Inductive reasoning is also called inductive logic or inference. "In inductive inference, we go from the specific to the general," Wassertheil-Smoller told Live Science. "We make many observations, discern a pattern, make a generalization, and infer an explanation or a theory."
In science, she added, there is a constant interplay between inductive and deductive reasoning that leads researchers steadily closer to a truth that can be verified with certainty,
The reliability of a conclusion made with inductive logic depends on the completeness of the observations. For instance, let's say you have a bag of coins; you pull three coins from the bag, and each coin is a penny. Using inductive logic, you might then propose that all of the coins in the bag are pennies.
Even though all of the initial observations — that each coin taken from the bag was a penny — are correct, inductive reasoning does not guarantee that the conclusion will be true. The next coin you pull could be a quarter.
Here's another example: " Penguins are birds. Penguins can't fly. Therefore, no birds can fly." The conclusion does not follow logically from the statements, because the only birds included in the sample were penguins.
Despite this inherent limitation, inductive reasoning has its place in the scientific method, and scientists use it to form hypotheses and theories. Researchers then use deductive reasoning to apply the theories to specific situations.
Related: Does everyone have an inner monologue?
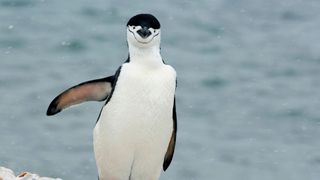
Inductive reasoning examples
Here are some examples of inductive reasoning:
Data: I see fireflies in my backyard every summer. Hypothesis: This summer, I will probably see fireflies in my backyard.
Data: I tend to catch colds when people around me are sick. Hypothesis: Colds are infectious.
Data: Every dog I meet is friendly. Hypothesis: Most dogs are usually friendly.
What is abductive reasoning?
Another form of scientific reasoning that diverges from inductive and deductive reasoning is called abductive. Abductive reasoning is a form of logic that starts with an incomplete set of observations and proceeds to the likeliest possible explanation for that data, according to Butte College in Oroville, California.
It is based on making and testing hypotheses using the best information available. It often entails making an educated guess after observing a phenomenon for which there is no clear explanation.
For example, a person walks into their living room and finds torn-up papers all over the floor. The person's dog has been alone in the apartment all day. The person concludes that the dog tore up the papers because it is the most likely scenario. It's possible that a family member with a key to the apartment swung by and destroyed the papers, or it may have been done by the landlord. But the dog theory is the most likely conclusion based on the data at hand.
Abductive reasoning is useful for forming hypotheses to be tested. For instance, abductive reasoning is used by doctors when they're assessing which ailment a patient likely has based on their symptoms. They then check which potential diagnosis is correct using medical tests. Jurors also use abductive reasoning to make decisions based on the select evidence presented to them by lawyers and witnesses.
Related: What is Occam's razor?
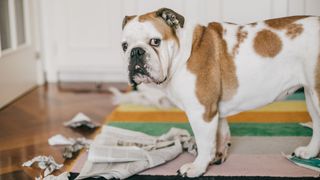
Abductive reasoning examples
Here are some examples of abductive reasoning:
— How many calories can the brain burn by thinking?
— What is the Dunning-Kruger effect?
— Can we ever stop thinking?
Observation: The grass is wet outside when you get up in the morning, but you haven't recently watered the lawn. Best-guess explanation: It likely rained last night.
Observation: At a restaurant, you see a bag and a half-eaten sandwich at an empty table. Best-guess explanation: The table's occupant is probably in the restroom.
Observation: You enter a basketball court and see a group of people in red shirts celebrating while another group in blue shirts sulks. Best-guess explanation: The red team probably just beat the blue team in a game.
Editor's note: This article was updated on March 7, 2024.
Sign up for the Live Science daily newsletter now
Get the world’s most fascinating discoveries delivered straight to your inbox.
Live Science x HowTheLightGetsIn — Get discounted tickets to the world’s largest ideas and music festival
Do opposites really attract in relationships?
Possible 'mega' fort found in Wales hints at tension between Romans and Celtics
Most Popular
- 2 Silver is being buried beneath the sea, and it's all because of climate change, study finds
- 3 Kamikaze termites blow themselves up with 'explosive' backpacks — and scientists just figured out how
- 4 Sunspots surge to 23-year high as solar maximum continues to intensify far beyond initial expectations
- 5 Aviron Strong Series Rower review
Educational resources and simple solutions for your research journey
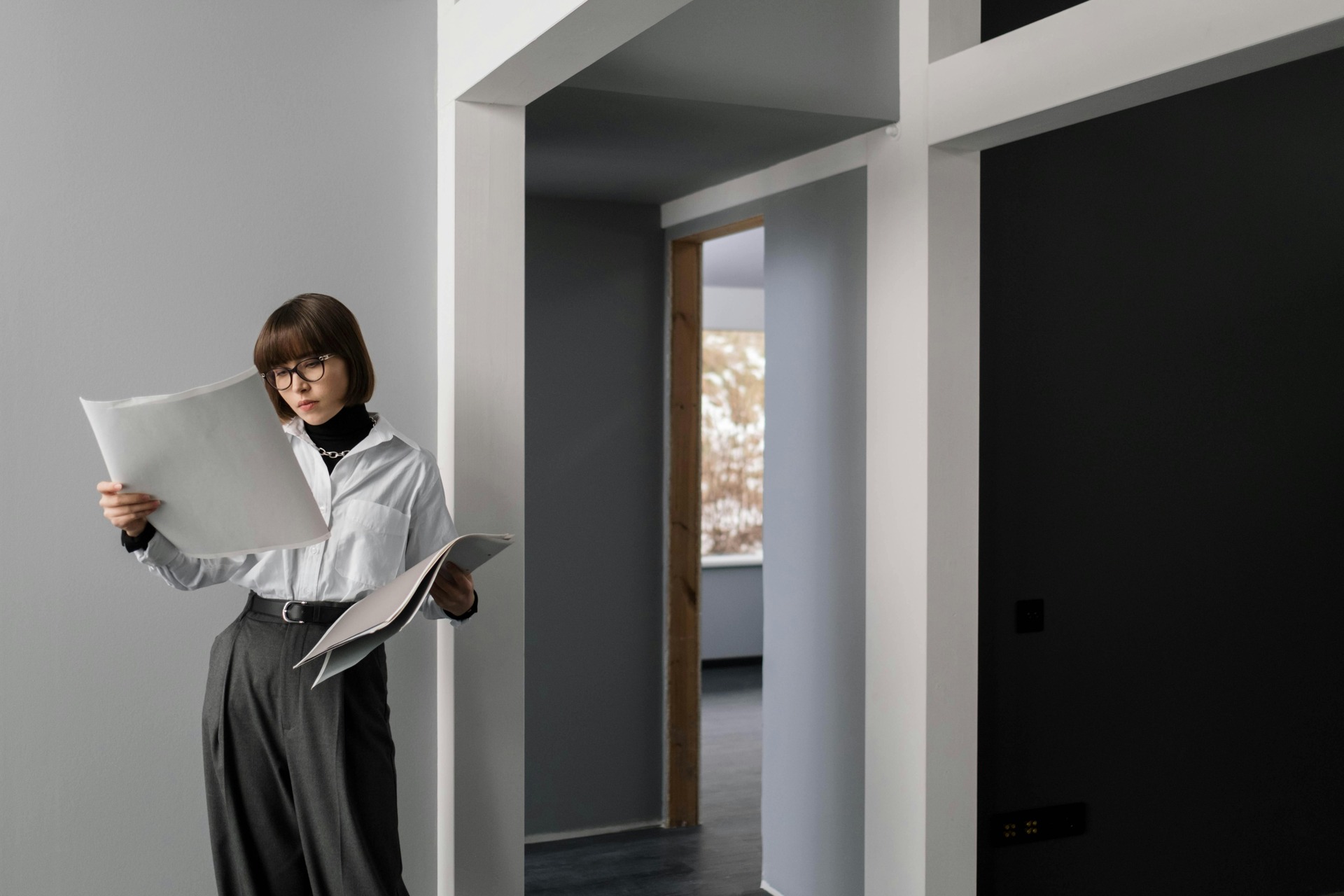
What is Inductive Reasoning? Definition, Types and Examples
Inductive reasoning is a type of reasoning method that analyzes specific evidence or observations to form general inferences. In inductive reasoning, a bottom-up approach is used, in which specific instances are considered to reach a broader conclusion—often to predict future outcomes or infer general rules or principles. Many often confuse inductive reasoning with deductive reasoning, as both are logical reasoning methods but with different approaches and outcomes. I n deductive reasoning, conclusions are drawn from a set of premises or assumptions by moving from general assumptions to more specific conclusions. Hence, deductive reasoning is a top-down approach in which one moves from more general assumptions to specific conclusions, usually to prove a fact or hypothesis. In this article, we will discuss inductive and deductive reasoning, evaluate the benefits and limitations of inductive and deductive approaches, and provide examples of the two logical reasoning methods.
Table of Contents
- What is inductive reasoning?
Inductive reasoning is a logical reasoning method with widespread usage in day-to-day decision-making, statistics, research, and probability analysis. It makes use of more specific observations or instances to derive general principles or conclusions. Because of these features, inductive reasoning is a valuable tool to form hypotheses for research and experimental studies. It is interesting to note that, without really thinking about it , each of us uses inductive reasoning in our lives to reach specific conclusions. Before, we discuss how we use the inductive reasoning approach, it is wise to first understand the basic elements of an inductive reasoning approach. The inductive reasoning approach can be broken down into a series of three progressive statements, leading from a more specific observation to a general assumption.
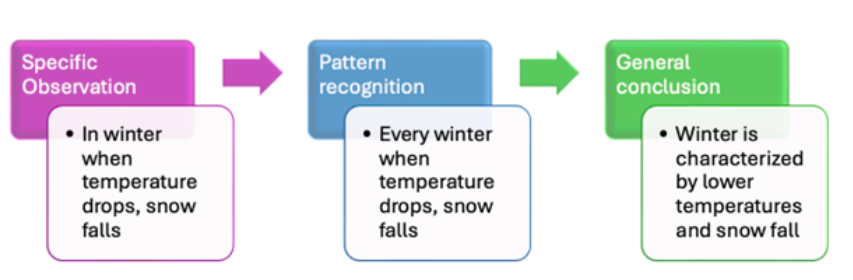
Here are some inductive reasoning examples :
Stages | Specific observation | Pattern recognition | General conclusion |
Example 1 | The sun rises in the east each morning | The sun continues to rise in the east each morning | The sun will always rise in the east each morning |
Example 2 | Eating spicy food makes you sweat with a hot sensation in your mouth | Every time you eat spicy food it makes you sweat and causes a hot sensation in your mouth | Spicy food triggers sweating and a hot sensation in your mouth |
Example 3 | Watering plants makes them grow and become taller | A positive correlation is found between watering plants and their growth patterns | It can be concluded that consistent watering leads to taller plants and improved flower production |
Inductive reasoning in research
Now, we have seen how inductive reasoning is used in day-to-day life to make assumptions and reach conclusions. Next, we will explore how to use inductive reasoning in research. Inductive reasoning is widely used in exploratory (qualitative) research where the goal is to understand a phenomenon and form a hypothesis for further studies. However, inductive reasoning can also be used in quantitative research in the early stages of hypothesis formation. The inductive reasoning in research is usually done in three parts: data (observations) collection, pattern recognition, and general conclusions based on the patterns.
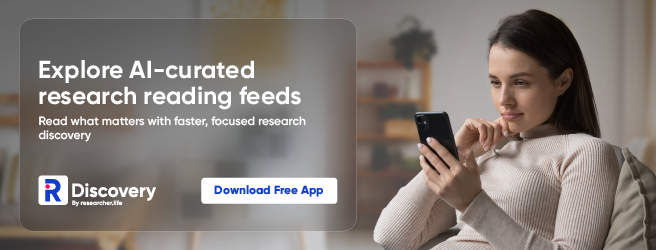
Let’s have a look at an example of inductive reasoning in research:
Research Question: Does regular exercise improve mental health?
Observation and data collection: To address the question, you would give surveys to people who workout to collect their responses on whether regular exercise helps them improve their mental health.
Pattern recognition: Next, you will try to identify any patterns and correlations between exercising and mental health by analyzing the data. For example, you identify a consistent pattern showing a positive correlation between regular exercise and mental health indicators such as reduced stress levels, improved mood and sleep, and enhanced cognitive function.
General conclusion: Based on the research findings and observed pattern s , you form a general conclusion that regular exercise is associated with improved mental health outcomes. However, the conclusion does not imply a causal relationship; it simply suggests a correlation between exercise and mental health.
Tip: It is important to be open-minded while applying inductive reasoning in research, as preconceived notions or biases can influence the interpretation of data and introduce research biases such as confirmation bias. You should allow the patterns to emerge naturally from the data to avoid any biases while also being open to alternate explanations as new data become available in iterative stages.
Inductive vs deductive reasoning
Inductive reasoning and deductive reasoning are both logical forms of reasoning approaches that are used to make conclusions—the difference is that they operate differently. The question of inductive vs deductive reasoning has puzzled many as they are often confused with one another. Here is a rule of thumb to differentiate between the two: for inductive reasoning, the reasoning follows a bottoms-up approach, meaning the logic flows from a specific to a more general conclusion. In the case of deductive reasoning, the reasoning uses the top-down approach in which the logic flows from general to more specific observation. See the diagram below to easily remember the difference between deductive and inductive research methods .
Tip: Inductive reasoning is used to make an educated guess about the outcome by using experiences and proven observations. Deductive reasoning uses theories and general observations to reach a specific conclusion. The idea is to prove a fact.
Let’s look at inductive and deductive reasoning in detail for better understanding:
Inductive reasoning
Definition: Inductive reasoning involves drawing general conclusions from specific observations or examples. It moves from particular to general.
Process: The process of inductive reasoning starts with specific instances or observations and then the stage of pattern/trend identification among them. From these specific observations, a general hypothesis or conclusion is then formed.
Example: I get sad when the weather is gloomy. Many people feel sad when there is no sun and the weather is gloomy. People usually feel depressed when the weather is gloomy and no sunshine.
Strengths: Inductive reasoning is useful for generating hypotheses, exploring new phenomena, and identifying patterns in data.
Weaknesses: Inductive reasoning does not guarantee certainty; conclusions drawn from inductive reasoning are probabilistic rather than definite.
Deductive reasoning
Definition: Deductive reasoning involves deriving specific conclusions from general principles or premises. It moves from the general to the particular (you would have noticed deductive reasoning skills portrayed by Sherlock Holmes to solve a mystery).
Process: In deductive reasoning, the reasoning makes use of general principles or known facts to apply logical rules and derive specific conclusions that necessarily follow from those premises.
Example: All calico cats are female. My (fostered) kitten Luna is a calico. Hence, Luna must be a female.
Strengths: Deductive reasoning ensures certainty; if the premises are true and the reasoning is valid, then the conclusion must also be true. It is often used in mathematics, logic, and formal systems.
Weaknesses: Deductive reasoning relies strongly on the notion that the premise is true; if the premises are incorrect, the conclusion will also be incorrect. It may not be suitable for exploring new phenomena or generating hypotheses.

Inductive vs abductive reasoning
Inductive vs abductive reasoning are other similar terms people often confus e. L ike inductive reasoning, abductive reasoning is also a form of analyzing premises or observations to predict outcomes. One of the major differences between inductive and abductive reasoning is that the latter uses incomplete information to reach the conclusion. Abductive reasoning allows one to conclude in lieu of complete information, providing freedom . H owever, this feature makes it unreliable as it might lead to several wrong conclusions before reaching the true answer. Abductive reasoning is commonly used in the medical field especially for diagnosis purposes in the absence of more concrete information such as lab tests. For e xample: Doctors often use abductive reasoning to diagnose their patients based on their knowledge and logical guesswork considering the patient’s symptoms. In such scenarios, patients often present some symptoms , which might or might not reflect the exact problem.
Benefits of inductive reasoning
Inductive reasoning is a valuable reasoning tool to form hypotheses for exploratory and other kinds of research. The major benefits of inductive reasoning are listed below:
- Flexibility: Inductive reasoning workflow begins with a specific observation leading to a more general conclusion , t hus, providing ranges of probabilities and possibilities to work with.
- Generate new hypothesis: As inductive reasoning involves observing specific instances and patterns it can be a valuable tool to generate new hypotheses, sometimes an entirely novel hypothesis that has not been explored earlier. This allows researchers to innovate solutions to problems that advance the field further.
- Applicability to diverse fields: Inductive reasoning has applications in a wide range of disciplines such as science, social sciences, humanities, and everyday decision-making. It allows researchers and practitioners in various fields to derive insights and make predictions based on observed patterns.
- Encourages exploration: Inductive reasoning encourages exploration, as analyzing patterns and existing information provides new hypotheses , which open up avenues for future research and opportunities for newer discoveries.
Limitations of inductive reasoning
Now, that we have learned about the benefits of inductive reasoning, let’s also talk about the limitations of inductive reasoning which you should take into consideration before applying inductive reasoning in your research.
- Inaccurate hypothesis generation: If limited information is available to form a hypothesis, it may lead to the generation of a wrong theory , which further results in incorrect interpretation as you generalize the findings.
- Findings are subject to change: Inductive reasoning-based hypothesis generation runs a risk of becoming obsolete if contra di cting new data becomes available. Care must be taken while forming a hypothesis with limited information or instances.
- Uncertainty: Inductive reasoning cannot guarantee the truth of its conclusions. The conclusions can be wrong even if all the premises are true. As you move from specific to general principles, there is always a possibility that new observations could contradict those principles .
- Overgeneralizations: This approach can lead to overgeneralizations where broad conclusions are drawn from limited observations , leading to stereotypes and incorrect assumptions about groups of people, objects, or phenomena.
Key takeaways
The key takeaways of inductive reasoning are listed below for your easy reference:
- Inductive reasoning is a logical bottom-up reasoning method that uses specific observations to make generalized assumptions.
For example:
- Specific observation: Noticing that the supermarket near my house in Milan closes at 7 pm every day.
- Pattern recognition: All the supermarkets in my locality close at 7 pm.
- Generalized assumption: All the supermarkets in my city close at 7 pm.
- Inductive and deductive reasoning are both logical reasoning methods, but vice – versa approaches to addressing the phenomenon. Deductive reasoning is a top-down approach in which reasoning follows from general observations to more specific inferences.
- General observation: If it rains, the streets will be wet.
- Pattern recognition: It is raining now.
- Specific observation: The streets were wet as it rained.
- Since inductive reasoning is exploratory while deductive reasoning is confirmatory in nature, the former is usually used for hypothesis generation while the latter is used for validating facts. However, both can be used in combination within a single research study.
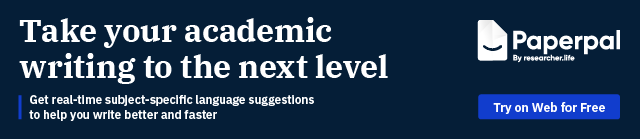
Frequently asked questions
Inductive reasoning is a method of reasoning where conclusions are drawn from specific observations or patterns to form general principles or hypotheses.
- What are types of inductive reasoning?
There are different types of inductive reasoning, including:
- Inductive generalization: Generalizing from specific cases or observations to form assumptions about the population.
- Statistical generalization: Making predictions/generalization about the population by applying statistical analysis on the observed data. It is more specific than inductive generalization as it deals with numerical data.
- Causal relationships: Inferring causal relationships based on correlations observed in data. It is used to make a cause-and-effect relationship between two different observations to reach a general conclusion
- Analogical reasoning: This type of inductive reasoning is applied to draw conclusions about a certain element based on its similarities with another. If a particular conclusion holds true for one element, it should be true for the other.
- What is the process of inductive reasoning in research?
The process of inductive reasoning in research typically involves:
- Observing specific instances or phenomena.
- Identifying patterns or regularities in the observed data.
- Formulating a general hypothesis or principle based on these patterns.
- Testing the hypothesis through further observation, experimentation, or data collection.
- Refining or revising the hypothesis based on new evidence or findings.
- Iterating the process to continually refine and develop a deeper understanding of the phenomenon under study.
- Can inductive reasoning be combined with deductive reasoning in research?
Yes, inductive reasoning and deductive reasoning can be combined in research to form a comprehensive approach when conducting a study. Researchers often use inductive reasoning to generate hypotheses or theories based on observed patterns or data. Then, they use deductive reasoning to test these hypotheses by making specific predictions and designing experiments or studies to gather evidence. After collecting data through deductive methods, researchers may analyze it inductively to identify further patterns or refine their hypotheses. This iterative process allows for the integration of both inductive and deductive reasoning in the research endeavor, leading to a more robust understanding of the phenomena under investigation.
- What are some common misconceptions about inductive reasoning in research?
Some misconceptions about inductive reasoning in research are mentioned below.
- Inductive reasoning guarantees conclusions: Despite generating hypotheses from observed patterns, inductive reasoning doesn’t always lead to definite conclusions; hypotheses are subject to revision.
- Inductive reasoning lacks scientific validity: Despite its association with deductive reasoning, inductive reasoning is a fundamental part of the scientific method, generating testable hypotheses.
- Inductive reasoning is entirely subjective: Inductive reasoning can exhibit subjectivity due to the interpretation involved, potentially leading to confirmation bias.The usage of systematic methods can reduce subjectivity in drawing conclusions from data.
- Inductive conclusions are always valid: The reliability of inductive conclusions can be influenced by factors like sample size and data representativeness.
- Inductive and deductive reasoning are opposites: Although different, inductive and deductive reasoning often complement each other in research, forming a comprehensive approach.
- How is inductive reasoning used in qualitative research?
Inductive reasoning in qualitative research involves:
- Formulating research questions based on specific observations
- Collecting descriptive data
- Analyzing data to recognize patterns or themes
- Developing theories grounded in the data
- Iteratively refining understanding through reflexivity and iterations
We hope this article has provided you with the information you need about inductive and deductive reasoning to allow you to apply these effectively in your research.
R Discovery is a literature search and research reading platform that accelerates your research discovery journey by keeping you updated on the latest, most relevant scholarly content. With 250M+ research articles sourced from trusted aggregators like CrossRef, Unpaywall, PubMed, PubMed Central, Open Alex and top publishing houses like Springer Nature, JAMA, IOP, Taylor & Francis, NEJM, BMJ, Karger, SAGE, Emerald Publishing and more, R Discovery puts a world of research at your fingertips.
Try R Discovery Prime FREE for 1 week or upgrade at just US$72 a year to access premium features that let you listen to research on the go, read in your language, collaborate with peers, auto sync with reference managers, and much more. Choose a simpler, smarter way to find and read research – Download the app and start your free 7-day trial today !
Related Posts

Research in Shorts: R Discovery’s New Feature Helps Academics Assess Relevant Papers in 2mins

Research Paper Appendix: Format and Examples
2.4 Developing a Hypothesis
Learning objectives.
- Distinguish between a theory and a hypothesis.
- Discover how theories are used to generate hypotheses and how the results of studies can be used to further inform theories.
- Understand the characteristics of a good hypothesis.
Theories and Hypotheses
Before describing how to develop a hypothesis it is imporant to distinguish betwee a theory and a hypothesis. A theory is a coherent explanation or interpretation of one or more phenomena. Although theories can take a variety of forms, one thing they have in common is that they go beyond the phenomena they explain by including variables, structures, processes, functions, or organizing principles that have not been observed directly. Consider, for example, Zajonc’s theory of social facilitation and social inhibition. He proposed that being watched by others while performing a task creates a general state of physiological arousal, which increases the likelihood of the dominant (most likely) response. So for highly practiced tasks, being watched increases the tendency to make correct responses, but for relatively unpracticed tasks, being watched increases the tendency to make incorrect responses. Notice that this theory—which has come to be called drive theory—provides an explanation of both social facilitation and social inhibition that goes beyond the phenomena themselves by including concepts such as “arousal” and “dominant response,” along with processes such as the effect of arousal on the dominant response.
Outside of science, referring to an idea as a theory often implies that it is untested—perhaps no more than a wild guess. In science, however, the term theory has no such implication. A theory is simply an explanation or interpretation of a set of phenomena. It can be untested, but it can also be extensively tested, well supported, and accepted as an accurate description of the world by the scientific community. The theory of evolution by natural selection, for example, is a theory because it is an explanation of the diversity of life on earth—not because it is untested or unsupported by scientific research. On the contrary, the evidence for this theory is overwhelmingly positive and nearly all scientists accept its basic assumptions as accurate. Similarly, the “germ theory” of disease is a theory because it is an explanation of the origin of various diseases, not because there is any doubt that many diseases are caused by microorganisms that infect the body.
A hypothesis , on the other hand, is a specific prediction about a new phenomenon that should be observed if a particular theory is accurate. It is an explanation that relies on just a few key concepts. Hypotheses are often specific predictions about what will happen in a particular study. They are developed by considering existing evidence and using reasoning to infer what will happen in the specific context of interest. Hypotheses are often but not always derived from theories. So a hypothesis is often a prediction based on a theory but some hypotheses are a-theoretical and only after a set of observations have been made, is a theory developed. This is because theories are broad in nature and they explain larger bodies of data. So if our research question is really original then we may need to collect some data and make some observation before we can develop a broader theory.
Theories and hypotheses always have this if-then relationship. “ If drive theory is correct, then cockroaches should run through a straight runway faster, and a branching runway more slowly, when other cockroaches are present.” Although hypotheses are usually expressed as statements, they can always be rephrased as questions. “Do cockroaches run through a straight runway faster when other cockroaches are present?” Thus deriving hypotheses from theories is an excellent way of generating interesting research questions.
But how do researchers derive hypotheses from theories? One way is to generate a research question using the techniques discussed in this chapter and then ask whether any theory implies an answer to that question. For example, you might wonder whether expressive writing about positive experiences improves health as much as expressive writing about traumatic experiences. Although this question is an interesting one on its own, you might then ask whether the habituation theory—the idea that expressive writing causes people to habituate to negative thoughts and feelings—implies an answer. In this case, it seems clear that if the habituation theory is correct, then expressive writing about positive experiences should not be effective because it would not cause people to habituate to negative thoughts and feelings. A second way to derive hypotheses from theories is to focus on some component of the theory that has not yet been directly observed. For example, a researcher could focus on the process of habituation—perhaps hypothesizing that people should show fewer signs of emotional distress with each new writing session.
Among the very best hypotheses are those that distinguish between competing theories. For example, Norbert Schwarz and his colleagues considered two theories of how people make judgments about themselves, such as how assertive they are (Schwarz et al., 1991) [1] . Both theories held that such judgments are based on relevant examples that people bring to mind. However, one theory was that people base their judgments on the number of examples they bring to mind and the other was that people base their judgments on how easily they bring those examples to mind. To test these theories, the researchers asked people to recall either six times when they were assertive (which is easy for most people) or 12 times (which is difficult for most people). Then they asked them to judge their own assertiveness. Note that the number-of-examples theory implies that people who recalled 12 examples should judge themselves to be more assertive because they recalled more examples, but the ease-of-examples theory implies that participants who recalled six examples should judge themselves as more assertive because recalling the examples was easier. Thus the two theories made opposite predictions so that only one of the predictions could be confirmed. The surprising result was that participants who recalled fewer examples judged themselves to be more assertive—providing particularly convincing evidence in favor of the ease-of-retrieval theory over the number-of-examples theory.
Theory Testing
The primary way that scientific researchers use theories is sometimes called the hypothetico-deductive method (although this term is much more likely to be used by philosophers of science than by scientists themselves). A researcher begins with a set of phenomena and either constructs a theory to explain or interpret them or chooses an existing theory to work with. He or she then makes a prediction about some new phenomenon that should be observed if the theory is correct. Again, this prediction is called a hypothesis. The researcher then conducts an empirical study to test the hypothesis. Finally, he or she reevaluates the theory in light of the new results and revises it if necessary. This process is usually conceptualized as a cycle because the researcher can then derive a new hypothesis from the revised theory, conduct a new empirical study to test the hypothesis, and so on. As Figure 2.2 shows, this approach meshes nicely with the model of scientific research in psychology presented earlier in the textbook—creating a more detailed model of “theoretically motivated” or “theory-driven” research.

Figure 2.2 Hypothetico-Deductive Method Combined With the General Model of Scientific Research in Psychology Together they form a model of theoretically motivated research.
As an example, let us consider Zajonc’s research on social facilitation and inhibition. He started with a somewhat contradictory pattern of results from the research literature. He then constructed his drive theory, according to which being watched by others while performing a task causes physiological arousal, which increases an organism’s tendency to make the dominant response. This theory predicts social facilitation for well-learned tasks and social inhibition for poorly learned tasks. He now had a theory that organized previous results in a meaningful way—but he still needed to test it. He hypothesized that if his theory was correct, he should observe that the presence of others improves performance in a simple laboratory task but inhibits performance in a difficult version of the very same laboratory task. To test this hypothesis, one of the studies he conducted used cockroaches as subjects (Zajonc, Heingartner, & Herman, 1969) [2] . The cockroaches ran either down a straight runway (an easy task for a cockroach) or through a cross-shaped maze (a difficult task for a cockroach) to escape into a dark chamber when a light was shined on them. They did this either while alone or in the presence of other cockroaches in clear plastic “audience boxes.” Zajonc found that cockroaches in the straight runway reached their goal more quickly in the presence of other cockroaches, but cockroaches in the cross-shaped maze reached their goal more slowly when they were in the presence of other cockroaches. Thus he confirmed his hypothesis and provided support for his drive theory. (Zajonc also showed that drive theory existed in humans (Zajonc & Sales, 1966) [3] in many other studies afterward).
Incorporating Theory into Your Research
When you write your research report or plan your presentation, be aware that there are two basic ways that researchers usually include theory. The first is to raise a research question, answer that question by conducting a new study, and then offer one or more theories (usually more) to explain or interpret the results. This format works well for applied research questions and for research questions that existing theories do not address. The second way is to describe one or more existing theories, derive a hypothesis from one of those theories, test the hypothesis in a new study, and finally reevaluate the theory. This format works well when there is an existing theory that addresses the research question—especially if the resulting hypothesis is surprising or conflicts with a hypothesis derived from a different theory.
To use theories in your research will not only give you guidance in coming up with experiment ideas and possible projects, but it lends legitimacy to your work. Psychologists have been interested in a variety of human behaviors and have developed many theories along the way. Using established theories will help you break new ground as a researcher, not limit you from developing your own ideas.
Characteristics of a Good Hypothesis
There are three general characteristics of a good hypothesis. First, a good hypothesis must be testable and falsifiable . We must be able to test the hypothesis using the methods of science and if you’ll recall Popper’s falsifiability criterion, it must be possible to gather evidence that will disconfirm the hypothesis if it is indeed false. Second, a good hypothesis must be logical. As described above, hypotheses are more than just a random guess. Hypotheses should be informed by previous theories or observations and logical reasoning. Typically, we begin with a broad and general theory and use deductive reasoning to generate a more specific hypothesis to test based on that theory. Occasionally, however, when there is no theory to inform our hypothesis, we use inductive reasoning which involves using specific observations or research findings to form a more general hypothesis. Finally, the hypothesis should be positive. That is, the hypothesis should make a positive statement about the existence of a relationship or effect, rather than a statement that a relationship or effect does not exist. As scientists, we don’t set out to show that relationships do not exist or that effects do not occur so our hypotheses should not be worded in a way to suggest that an effect or relationship does not exist. The nature of science is to assume that something does not exist and then seek to find evidence to prove this wrong, to show that really it does exist. That may seem backward to you but that is the nature of the scientific method. The underlying reason for this is beyond the scope of this chapter but it has to do with statistical theory.
Key Takeaways
- A theory is broad in nature and explains larger bodies of data. A hypothesis is more specific and makes a prediction about the outcome of a particular study.
- Working with theories is not “icing on the cake.” It is a basic ingredient of psychological research.
- Like other scientists, psychologists use the hypothetico-deductive method. They construct theories to explain or interpret phenomena (or work with existing theories), derive hypotheses from their theories, test the hypotheses, and then reevaluate the theories in light of the new results.
- Practice: Find a recent empirical research report in a professional journal. Read the introduction and highlight in different colors descriptions of theories and hypotheses.
- Schwarz, N., Bless, H., Strack, F., Klumpp, G., Rittenauer-Schatka, H., & Simons, A. (1991). Ease of retrieval as information: Another look at the availability heuristic. Journal of Personality and Social Psychology, 61 , 195–202. ↵
- Zajonc, R. B., Heingartner, A., & Herman, E. M. (1969). Social enhancement and impairment of performance in the cockroach. Journal of Personality and Social Psychology, 13 , 83–92. ↵
- Zajonc, R.B. & Sales, S.M. (1966). Social facilitation of dominant and subordinate responses. Journal of Experimental Social Psychology, 2 , 160-168. ↵

Share This Book
- Increase Font Size

15 Hypothesis Examples
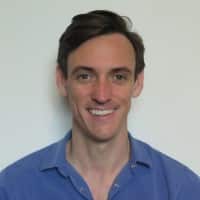
Chris Drew (PhD)
Dr. Chris Drew is the founder of the Helpful Professor. He holds a PhD in education and has published over 20 articles in scholarly journals. He is the former editor of the Journal of Learning Development in Higher Education. [Image Descriptor: Photo of Chris]
Learn about our Editorial Process
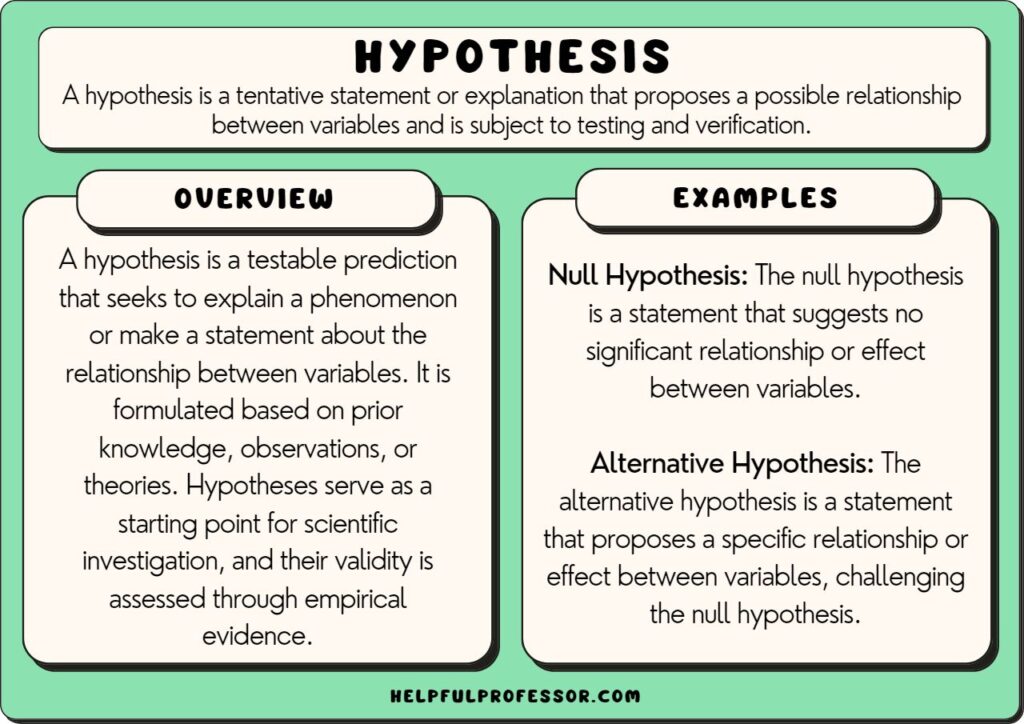
A hypothesis is defined as a testable prediction , and is used primarily in scientific experiments as a potential or predicted outcome that scientists attempt to prove or disprove (Atkinson et al., 2021; Tan, 2022).
In my types of hypothesis article, I outlined 13 different hypotheses, including the directional hypothesis (which makes a prediction about an effect of a treatment will be positive or negative) and the associative hypothesis (which makes a prediction about the association between two variables).
This article will dive into some interesting examples of hypotheses and examine potential ways you might test each one.
Hypothesis Examples
1. “inadequate sleep decreases memory retention”.
Field: Psychology
Type: Causal Hypothesis A causal hypothesis explores the effect of one variable on another. This example posits that a lack of adequate sleep causes decreased memory retention. In other words, if you are not getting enough sleep, your ability to remember and recall information may suffer.
How to Test:
To test this hypothesis, you might devise an experiment whereby your participants are divided into two groups: one receives an average of 8 hours of sleep per night for a week, while the other gets less than the recommended sleep amount.
During this time, all participants would daily study and recall new, specific information. You’d then measure memory retention of this information for both groups using standard memory tests and compare the results.
Should the group with less sleep have statistically significant poorer memory scores, the hypothesis would be supported.
Ensuring the integrity of the experiment requires taking into account factors such as individual health differences, stress levels, and daily nutrition.
Relevant Study: Sleep loss, learning capacity and academic performance (Curcio, Ferrara & De Gennaro, 2006)
2. “Increase in Temperature Leads to Increase in Kinetic Energy”
Field: Physics
Type: Deductive Hypothesis The deductive hypothesis applies the logic of deductive reasoning – it moves from a general premise to a more specific conclusion. This specific hypothesis assumes that as temperature increases, the kinetic energy of particles also increases – that is, when you heat something up, its particles move around more rapidly.
This hypothesis could be examined by heating a gas in a controlled environment and capturing the movement of its particles as a function of temperature.
You’d gradually increase the temperature and measure the kinetic energy of the gas particles with each increment. If the kinetic energy consistently rises with the temperature, your hypothesis gets supporting evidence.
Variables such as pressure and volume of the gas would need to be held constant to ensure validity of results.
3. “Children Raised in Bilingual Homes Develop Better Cognitive Skills”
Field: Psychology/Linguistics
Type: Comparative Hypothesis The comparative hypothesis posits a difference between two or more groups based on certain variables. In this context, you might propose that children raised in bilingual homes have superior cognitive skills compared to those raised in monolingual homes.
Testing this hypothesis could involve identifying two groups of children: those raised in bilingual homes, and those raised in monolingual homes.
Cognitive skills in both groups would be evaluated using a standard cognitive ability test at different stages of development. The examination would be repeated over a significant time period for consistency.
If the group raised in bilingual homes persistently scores higher than the other, the hypothesis would thereby be supported.
The challenge for the researcher would be controlling for other variables that could impact cognitive development, such as socio-economic status, education level of parents, and parenting styles.
Relevant Study: The cognitive benefits of being bilingual (Marian & Shook, 2012)
4. “High-Fiber Diet Leads to Lower Incidences of Cardiovascular Diseases”
Field: Medicine/Nutrition
Type: Alternative Hypothesis The alternative hypothesis suggests an alternative to a null hypothesis. In this context, the implied null hypothesis could be that diet has no effect on cardiovascular health, which the alternative hypothesis contradicts by suggesting that a high-fiber diet leads to fewer instances of cardiovascular diseases.
To test this hypothesis, a longitudinal study could be conducted on two groups of participants; one adheres to a high-fiber diet, while the other follows a diet low in fiber.
After a fixed period, the cardiovascular health of participants in both groups could be analyzed and compared. If the group following a high-fiber diet has a lower number of recorded cases of cardiovascular diseases, it would provide evidence supporting the hypothesis.
Control measures should be implemented to exclude the influence of other lifestyle and genetic factors that contribute to cardiovascular health.
Relevant Study: Dietary fiber, inflammation, and cardiovascular disease (King, 2005)
5. “Gravity Influences the Directional Growth of Plants”
Field: Agronomy / Botany
Type: Explanatory Hypothesis An explanatory hypothesis attempts to explain a phenomenon. In this case, the hypothesis proposes that gravity affects how plants direct their growth – both above-ground (toward sunlight) and below-ground (towards water and other resources).
The testing could be conducted by growing plants in a rotating cylinder to create artificial gravity.
Observations on the direction of growth, over a specified period, can provide insights into the influencing factors. If plants consistently direct their growth in a manner that indicates the influence of gravitational pull, the hypothesis is substantiated.
It is crucial to ensure that other growth-influencing factors, such as light and water, are uniformly distributed so that only gravity influences the directional growth.
6. “The Implementation of Gamified Learning Improves Students’ Motivation”
Field: Education
Type: Relational Hypothesis The relational hypothesis describes the relation between two variables. Here, the hypothesis is that the implementation of gamified learning has a positive effect on the motivation of students.
To validate this proposition, two sets of classes could be compared: one that implements a learning approach with game-based elements, and another that follows a traditional learning approach.
The students’ motivation levels could be gauged by monitoring their engagement, performance, and feedback over a considerable timeframe.
If the students engaged in the gamified learning context present higher levels of motivation and achievement, the hypothesis would be supported.
Control measures ought to be put into place to account for individual differences, including prior knowledge and attitudes towards learning.
Relevant Study: Does educational gamification improve students’ motivation? (Chapman & Rich, 2018)
7. “Mathematics Anxiety Negatively Affects Performance”
Field: Educational Psychology
Type: Research Hypothesis The research hypothesis involves making a prediction that will be tested. In this case, the hypothesis proposes that a student’s anxiety about math can negatively influence their performance in math-related tasks.
To assess this hypothesis, researchers must first measure the mathematics anxiety levels of a sample of students using a validated instrument, such as the Mathematics Anxiety Rating Scale.
Then, the students’ performance in mathematics would be evaluated through standard testing. If there’s a negative correlation between the levels of math anxiety and math performance (meaning as anxiety increases, performance decreases), the hypothesis would be supported.
It would be crucial to control for relevant factors such as overall academic performance and previous mathematical achievement.
8. “Disruption of Natural Sleep Cycle Impairs Worker Productivity”
Field: Organizational Psychology
Type: Operational Hypothesis The operational hypothesis involves defining the variables in measurable terms. In this example, the hypothesis posits that disrupting the natural sleep cycle, for instance through shift work or irregular working hours, can lessen productivity among workers.
To test this hypothesis, you could collect data from workers who maintain regular working hours and those with irregular schedules.
Measuring productivity could involve examining the worker’s ability to complete tasks, the quality of their work, and their efficiency.
If workers with interrupted sleep cycles demonstrate lower productivity compared to those with regular sleep patterns, it would lend support to the hypothesis.
Consideration should be given to potential confounding variables such as job type, worker age, and overall health.
9. “Regular Physical Activity Reduces the Risk of Depression”
Field: Health Psychology
Type: Predictive Hypothesis A predictive hypothesis involves making a prediction about the outcome of a study based on the observed relationship between variables. In this case, it is hypothesized that individuals who engage in regular physical activity are less likely to suffer from depression.
Longitudinal studies would suit to test this hypothesis, tracking participants’ levels of physical activity and their mental health status over time.
The level of physical activity could be self-reported or monitored, while mental health status could be assessed using standard diagnostic tools or surveys.
If data analysis shows that participants maintaining regular physical activity have a lower incidence of depression, this would endorse the hypothesis.
However, care should be taken to control other lifestyle and behavioral factors that could intervene with the results.
Relevant Study: Regular physical exercise and its association with depression (Kim, 2022)
10. “Regular Meditation Enhances Emotional Stability”
Type: Empirical Hypothesis In the empirical hypothesis, predictions are based on amassed empirical evidence . This particular hypothesis theorizes that frequent meditation leads to improved emotional stability, resonating with numerous studies linking meditation to a variety of psychological benefits.
Earlier studies reported some correlations, but to test this hypothesis directly, you’d organize an experiment where one group meditates regularly over a set period while a control group doesn’t.
Both groups’ emotional stability levels would be measured at the start and end of the experiment using a validated emotional stability assessment.
If regular meditators display noticeable improvements in emotional stability compared to the control group, the hypothesis gains credit.
You’d have to ensure a similar emotional baseline for all participants at the start to avoid skewed results.
11. “Children Exposed to Reading at an Early Age Show Superior Academic Progress”
Type: Directional Hypothesis The directional hypothesis predicts the direction of an expected relationship between variables. Here, the hypothesis anticipates that early exposure to reading positively affects a child’s academic advancement.
A longitudinal study tracking children’s reading habits from an early age and their consequent academic performance could validate this hypothesis.
Parents could report their children’s exposure to reading at home, while standardized school exam results would provide a measure of academic achievement.
If the children exposed to early reading consistently perform better acadically, it gives weight to the hypothesis.
However, it would be important to control for variables that might impact academic performance, such as socioeconomic background, parental education level, and school quality.

12. “Adopting Energy-efficient Technologies Reduces Carbon Footprint of Industries”
Field: Environmental Science
Type: Descriptive Hypothesis A descriptive hypothesis predicts the existence of an association or pattern related to variables. In this scenario, the hypothesis suggests that industries adopting energy-efficient technologies will resultantly show a reduced carbon footprint.
Global industries making use of energy-efficient technologies could track their carbon emissions over time. At the same time, others not implementing such technologies continue their regular tracking.
After a defined time, the carbon emission data of both groups could be compared. If industries that adopted energy-efficient technologies demonstrate a notable reduction in their carbon footprints, the hypothesis would hold strong.
In the experiment, you would exclude variations brought by factors such as industry type, size, and location.
13. “Reduced Screen Time Improves Sleep Quality”
Type: Simple Hypothesis The simple hypothesis is a prediction about the relationship between two variables, excluding any other variables from consideration. This example posits that by reducing time spent on devices like smartphones and computers, an individual should experience improved sleep quality.
A sample group would need to reduce their daily screen time for a pre-determined period. Sleep quality before and after the reduction could be measured using self-report sleep diaries and objective measures like actigraphy, monitoring movement and wakefulness during sleep.
If the data shows that sleep quality improved post the screen time reduction, the hypothesis would be validated.
Other aspects affecting sleep quality, like caffeine intake, should be controlled during the experiment.
Relevant Study: Screen time use impacts low‐income preschool children’s sleep quality, tiredness, and ability to fall asleep (Waller et al., 2021)
14. Engaging in Brain-Training Games Improves Cognitive Functioning in Elderly
Field: Gerontology
Type: Inductive Hypothesis Inductive hypotheses are based on observations leading to broader generalizations and theories. In this context, the hypothesis deduces from observed instances that engaging in brain-training games can help improve cognitive functioning in the elderly.
A longitudinal study could be conducted where an experimental group of elderly people partakes in regular brain-training games.
Their cognitive functioning could be assessed at the start of the study and at regular intervals using standard neuropsychological tests.
If the group engaging in brain-training games shows better cognitive functioning scores over time compared to a control group not playing these games, the hypothesis would be supported.
15. Farming Practices Influence Soil Erosion Rates
Type: Null Hypothesis A null hypothesis is a negative statement assuming no relationship or difference between variables. The hypothesis in this context asserts there’s no effect of different farming practices on the rates of soil erosion.
Comparing soil erosion rates in areas with different farming practices over a considerable timeframe could help test this hypothesis.
If, statistically, the farming practices do not lead to differences in soil erosion rates, the null hypothesis is accepted.
However, if marked variation appears, the null hypothesis is rejected, meaning farming practices do influence soil erosion rates. It would be crucial to control for external factors like weather, soil type, and natural vegetation.
The variety of hypotheses mentioned above underscores the diversity of research constructs inherent in different fields, each with its unique purpose and way of testing.
While researchers may develop hypotheses primarily as tools to define and narrow the focus of the study, these hypotheses also serve as valuable guiding forces for the data collection and analysis procedures, making the research process more efficient and direction-focused.
Hypotheses serve as a compass for any form of academic research. The diverse examples provided, from Psychology to Educational Studies, Environmental Science to Gerontology, clearly demonstrate how certain hypotheses suit specific fields more aptly than others.
It is important to underline that although these varied hypotheses differ in their structure and methods of testing, each endorses the fundamental value of empiricism in research. Evidence-based decision making remains at the heart of scholarly inquiry, regardless of the research field, thus aligning all hypotheses to the core purpose of scientific investigation.
Testing hypotheses is an essential part of the scientific method . By doing so, researchers can either confirm their predictions, giving further validity to an existing theory, or they might uncover new insights that could potentially shift the field’s understanding of a particular phenomenon. In either case, hypotheses serve as the stepping stones for scientific exploration and discovery.
Atkinson, P., Delamont, S., Cernat, A., Sakshaug, J. W., & Williams, R. A. (2021). SAGE research methods foundations . SAGE Publications Ltd.
Curcio, G., Ferrara, M., & De Gennaro, L. (2006). Sleep loss, learning capacity and academic performance. Sleep medicine reviews , 10 (5), 323-337.
Kim, J. H. (2022). Regular physical exercise and its association with depression: A population-based study short title: Exercise and depression. Psychiatry Research , 309 , 114406.
King, D. E. (2005). Dietary fiber, inflammation, and cardiovascular disease. Molecular nutrition & food research , 49 (6), 594-600.
Marian, V., & Shook, A. (2012, September). The cognitive benefits of being bilingual. In Cerebrum: the Dana forum on brain science (Vol. 2012). Dana Foundation.
Tan, W. C. K. (2022). Research Methods: A Practical Guide For Students And Researchers (Second Edition) . World Scientific Publishing Company.
Waller, N. A., Zhang, N., Cocci, A. H., D’Agostino, C., Wesolek‐Greenson, S., Wheelock, K., … & Resnicow, K. (2021). Screen time use impacts low‐income preschool children’s sleep quality, tiredness, and ability to fall asleep. Child: care, health and development, 47 (5), 618-626.
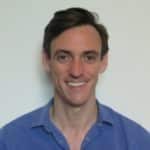
- Chris Drew (PhD) https://helpfulprofessor.com/author/chris-drew-phd-2/ 10 Reasons you’re Perpetually Single
- Chris Drew (PhD) https://helpfulprofessor.com/author/chris-drew-phd-2/ 20 Montessori Toddler Bedrooms (Design Inspiration)
- Chris Drew (PhD) https://helpfulprofessor.com/author/chris-drew-phd-2/ 21 Montessori Homeschool Setups
- Chris Drew (PhD) https://helpfulprofessor.com/author/chris-drew-phd-2/ 101 Hidden Talents Examples
Leave a Comment Cancel Reply
Your email address will not be published. Required fields are marked *
- Bipolar Disorder
- Therapy Center
- When To See a Therapist
- Types of Therapy
- Best Online Therapy
- Best Couples Therapy
- Managing Stress
- Sleep and Dreaming
- Understanding Emotions
- Self-Improvement
- Healthy Relationships
- Student Resources
- Personality Types
- Sweepstakes
- Guided Meditations
- Verywell Mind Insights
- 2024 Verywell Mind 25
- Mental Health in the Classroom
- Editorial Process
- Meet Our Review Board
- Crisis Support
How to Write a Great Hypothesis
Hypothesis Definition, Format, Examples, and Tips
Verywell / Alex Dos Diaz
- The Scientific Method
Hypothesis Format
Falsifiability of a hypothesis.
- Operationalization
Hypothesis Types
Hypotheses examples.
- Collecting Data
A hypothesis is a tentative statement about the relationship between two or more variables. It is a specific, testable prediction about what you expect to happen in a study. It is a preliminary answer to your question that helps guide the research process.
Consider a study designed to examine the relationship between sleep deprivation and test performance. The hypothesis might be: "This study is designed to assess the hypothesis that sleep-deprived people will perform worse on a test than individuals who are not sleep-deprived."
At a Glance
A hypothesis is crucial to scientific research because it offers a clear direction for what the researchers are looking to find. This allows them to design experiments to test their predictions and add to our scientific knowledge about the world. This article explores how a hypothesis is used in psychology research, how to write a good hypothesis, and the different types of hypotheses you might use.
The Hypothesis in the Scientific Method
In the scientific method , whether it involves research in psychology, biology, or some other area, a hypothesis represents what the researchers think will happen in an experiment. The scientific method involves the following steps:
- Forming a question
- Performing background research
- Creating a hypothesis
- Designing an experiment
- Collecting data
- Analyzing the results
- Drawing conclusions
- Communicating the results
The hypothesis is a prediction, but it involves more than a guess. Most of the time, the hypothesis begins with a question which is then explored through background research. At this point, researchers then begin to develop a testable hypothesis.
Unless you are creating an exploratory study, your hypothesis should always explain what you expect to happen.
In a study exploring the effects of a particular drug, the hypothesis might be that researchers expect the drug to have some type of effect on the symptoms of a specific illness. In psychology, the hypothesis might focus on how a certain aspect of the environment might influence a particular behavior.
Remember, a hypothesis does not have to be correct. While the hypothesis predicts what the researchers expect to see, the goal of the research is to determine whether this guess is right or wrong. When conducting an experiment, researchers might explore numerous factors to determine which ones might contribute to the ultimate outcome.
In many cases, researchers may find that the results of an experiment do not support the original hypothesis. When writing up these results, the researchers might suggest other options that should be explored in future studies.
In many cases, researchers might draw a hypothesis from a specific theory or build on previous research. For example, prior research has shown that stress can impact the immune system. So a researcher might hypothesize: "People with high-stress levels will be more likely to contract a common cold after being exposed to the virus than people who have low-stress levels."
In other instances, researchers might look at commonly held beliefs or folk wisdom. "Birds of a feather flock together" is one example of folk adage that a psychologist might try to investigate. The researcher might pose a specific hypothesis that "People tend to select romantic partners who are similar to them in interests and educational level."
Elements of a Good Hypothesis
So how do you write a good hypothesis? When trying to come up with a hypothesis for your research or experiments, ask yourself the following questions:
- Is your hypothesis based on your research on a topic?
- Can your hypothesis be tested?
- Does your hypothesis include independent and dependent variables?
Before you come up with a specific hypothesis, spend some time doing background research. Once you have completed a literature review, start thinking about potential questions you still have. Pay attention to the discussion section in the journal articles you read . Many authors will suggest questions that still need to be explored.
How to Formulate a Good Hypothesis
To form a hypothesis, you should take these steps:
- Collect as many observations about a topic or problem as you can.
- Evaluate these observations and look for possible causes of the problem.
- Create a list of possible explanations that you might want to explore.
- After you have developed some possible hypotheses, think of ways that you could confirm or disprove each hypothesis through experimentation. This is known as falsifiability.
In the scientific method , falsifiability is an important part of any valid hypothesis. In order to test a claim scientifically, it must be possible that the claim could be proven false.
Students sometimes confuse the idea of falsifiability with the idea that it means that something is false, which is not the case. What falsifiability means is that if something was false, then it is possible to demonstrate that it is false.
One of the hallmarks of pseudoscience is that it makes claims that cannot be refuted or proven false.
The Importance of Operational Definitions
A variable is a factor or element that can be changed and manipulated in ways that are observable and measurable. However, the researcher must also define how the variable will be manipulated and measured in the study.
Operational definitions are specific definitions for all relevant factors in a study. This process helps make vague or ambiguous concepts detailed and measurable.
For example, a researcher might operationally define the variable " test anxiety " as the results of a self-report measure of anxiety experienced during an exam. A "study habits" variable might be defined by the amount of studying that actually occurs as measured by time.
These precise descriptions are important because many things can be measured in various ways. Clearly defining these variables and how they are measured helps ensure that other researchers can replicate your results.
Replicability
One of the basic principles of any type of scientific research is that the results must be replicable.
Replication means repeating an experiment in the same way to produce the same results. By clearly detailing the specifics of how the variables were measured and manipulated, other researchers can better understand the results and repeat the study if needed.
Some variables are more difficult than others to define. For example, how would you operationally define a variable such as aggression ? For obvious ethical reasons, researchers cannot create a situation in which a person behaves aggressively toward others.
To measure this variable, the researcher must devise a measurement that assesses aggressive behavior without harming others. The researcher might utilize a simulated task to measure aggressiveness in this situation.
Hypothesis Checklist
- Does your hypothesis focus on something that you can actually test?
- Does your hypothesis include both an independent and dependent variable?
- Can you manipulate the variables?
- Can your hypothesis be tested without violating ethical standards?
The hypothesis you use will depend on what you are investigating and hoping to find. Some of the main types of hypotheses that you might use include:
- Simple hypothesis : This type of hypothesis suggests there is a relationship between one independent variable and one dependent variable.
- Complex hypothesis : This type suggests a relationship between three or more variables, such as two independent and dependent variables.
- Null hypothesis : This hypothesis suggests no relationship exists between two or more variables.
- Alternative hypothesis : This hypothesis states the opposite of the null hypothesis.
- Statistical hypothesis : This hypothesis uses statistical analysis to evaluate a representative population sample and then generalizes the findings to the larger group.
- Logical hypothesis : This hypothesis assumes a relationship between variables without collecting data or evidence.
A hypothesis often follows a basic format of "If {this happens} then {this will happen}." One way to structure your hypothesis is to describe what will happen to the dependent variable if you change the independent variable .
The basic format might be: "If {these changes are made to a certain independent variable}, then we will observe {a change in a specific dependent variable}."
A few examples of simple hypotheses:
- "Students who eat breakfast will perform better on a math exam than students who do not eat breakfast."
- "Students who experience test anxiety before an English exam will get lower scores than students who do not experience test anxiety."
- "Motorists who talk on the phone while driving will be more likely to make errors on a driving course than those who do not talk on the phone."
- "Children who receive a new reading intervention will have higher reading scores than students who do not receive the intervention."
Examples of a complex hypothesis include:
- "People with high-sugar diets and sedentary activity levels are more likely to develop depression."
- "Younger people who are regularly exposed to green, outdoor areas have better subjective well-being than older adults who have limited exposure to green spaces."
Examples of a null hypothesis include:
- "There is no difference in anxiety levels between people who take St. John's wort supplements and those who do not."
- "There is no difference in scores on a memory recall task between children and adults."
- "There is no difference in aggression levels between children who play first-person shooter games and those who do not."
Examples of an alternative hypothesis:
- "People who take St. John's wort supplements will have less anxiety than those who do not."
- "Adults will perform better on a memory task than children."
- "Children who play first-person shooter games will show higher levels of aggression than children who do not."
Collecting Data on Your Hypothesis
Once a researcher has formed a testable hypothesis, the next step is to select a research design and start collecting data. The research method depends largely on exactly what they are studying. There are two basic types of research methods: descriptive research and experimental research.
Descriptive Research Methods
Descriptive research such as case studies , naturalistic observations , and surveys are often used when conducting an experiment is difficult or impossible. These methods are best used to describe different aspects of a behavior or psychological phenomenon.
Once a researcher has collected data using descriptive methods, a correlational study can examine how the variables are related. This research method might be used to investigate a hypothesis that is difficult to test experimentally.
Experimental Research Methods
Experimental methods are used to demonstrate causal relationships between variables. In an experiment, the researcher systematically manipulates a variable of interest (known as the independent variable) and measures the effect on another variable (known as the dependent variable).
Unlike correlational studies, which can only be used to determine if there is a relationship between two variables, experimental methods can be used to determine the actual nature of the relationship—whether changes in one variable actually cause another to change.
The hypothesis is a critical part of any scientific exploration. It represents what researchers expect to find in a study or experiment. In situations where the hypothesis is unsupported by the research, the research still has value. Such research helps us better understand how different aspects of the natural world relate to one another. It also helps us develop new hypotheses that can then be tested in the future.
Thompson WH, Skau S. On the scope of scientific hypotheses . R Soc Open Sci . 2023;10(8):230607. doi:10.1098/rsos.230607
Taran S, Adhikari NKJ, Fan E. Falsifiability in medicine: what clinicians can learn from Karl Popper [published correction appears in Intensive Care Med. 2021 Jun 17;:]. Intensive Care Med . 2021;47(9):1054-1056. doi:10.1007/s00134-021-06432-z
Eyler AA. Research Methods for Public Health . 1st ed. Springer Publishing Company; 2020. doi:10.1891/9780826182067.0004
Nosek BA, Errington TM. What is replication ? PLoS Biol . 2020;18(3):e3000691. doi:10.1371/journal.pbio.3000691
Aggarwal R, Ranganathan P. Study designs: Part 2 - Descriptive studies . Perspect Clin Res . 2019;10(1):34-36. doi:10.4103/picr.PICR_154_18
Nevid J. Psychology: Concepts and Applications. Wadworth, 2013.
By Kendra Cherry, MSEd Kendra Cherry, MS, is a psychosocial rehabilitation specialist, psychology educator, and author of the "Everything Psychology Book."

Want to create or adapt books like this? Learn more about how Pressbooks supports open publishing practices.
5.5 Introduction to Hypothesis Tests
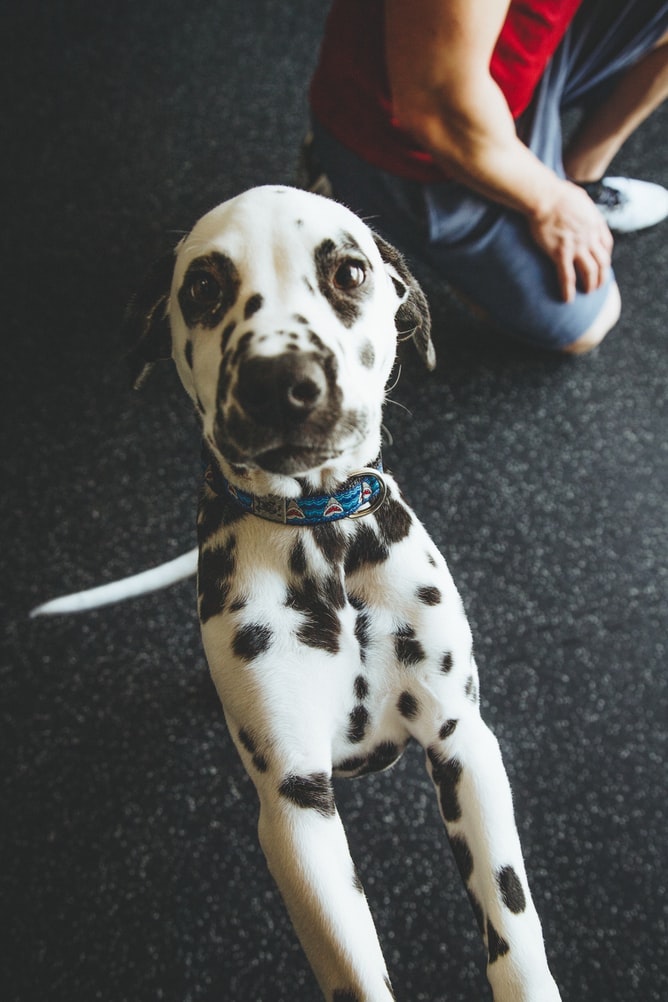
One job of a statistician is to make statistical inferences about populations based on samples taken from the population. Confidence intervals are one way to estimate a population parameter.
Another way to make a statistical inference is to make a decision about a parameter. For instance, a car dealership advertises that its new small truck gets 35 miles per gallon on average. A tutoring service claims that its method of tutoring helps 90% of its students get an A or a B. A company says that female managers in their company earn an average of $60,000 per year. A statistician may want to make a decision about or evaluate these claims. A hypothesis test can be used to do this.
A hypothesis test involves collecting data from a sample and evaluating the data. Then the statistician makes a decision as to whether or not there is sufficient evidence to reject the null hypothesis based upon analyses of the data.
In this section, you will conduct hypothesis tests on single means when the population standard deviation is known.
Hypothesis testing consists of two contradictory hypotheses or statements, a decision based on the data, and a conclusion. To perform a hypothesis test, a statistician will perform some variation of these steps:
- Define hypotheses.
- Collect and/or use the sample data to determine the correct distribution to use.
- Calculate test statistic.
- Make a decision.
- Write a conclusion.
Defining your hypotheses
The actual test begins by considering two hypotheses: the null hypothesis and the alternative hypothesis. These hypotheses contain opposing viewpoints.
The null hypothesis ( H 0 ) is often a statement of the accepted historical value or norm. This is your starting point that you must assume from the beginning in order to show an effect exists.
The alternative hypothesis ( H a ) is a claim about the population that is contradictory to H 0 and what we conclude when we reject H 0 .
Since the null and alternative hypotheses are contradictory, you must examine evidence to decide if you have enough evidence to reject the null hypothesis or not. The evidence is in the form of sample data.
After you have determined which hypothesis the sample supports, you make a decision . There are two options for a decision. They are “reject H 0 ” if the sample information favors the alternative hypothesis or “do not reject H 0 ” or “decline to reject H 0 ” if the sample information is insufficient to reject the null hypothesis.
The following table shows mathematical symbols used in H 0 and H a :
equal (=) | not equal (≠) greater than (>) less than (<) |
equal (=) | less than (<) |
equal (=) | more than (>) |
NOTE: H 0 always has a symbol with an equal in it. H a never has a symbol with an equal in it. The choice of symbol in the alternative hypothesis depends on the wording of the hypothesis test. Despite this, many researchers may use =, ≤, or ≥ in the null hypothesis. This practice is acceptable because our only decision is to reject or not reject the null hypothesis.
We want to test whether the mean GPA of students in American colleges is 2.0 (out of 4.0). The null hypothesis is: H 0 : μ = 2.0. What is the alternative hypothesis?
A medical trial is conducted to test whether or not a new medicine reduces cholesterol by 25%. State the null and alternative hypotheses.
Using the Sample to Test the Null Hypothesis
Once you have defined your hypotheses, the next step in the process is to collect sample data. In a classroom context, the data or summary statistics will usually be given to you.
Then you will have to determine the correct distribution to perform the hypothesis test, given the assumptions you are able to make about the situation. Right now, we are demonstrating these ideas in a test for a mean when the population standard deviation is known using the z distribution. We will see other scenarios in the future.
Calculating a Test Statistic
Next you will start evaluating the data. This begins with calculating your test statistic , which is a measure of the distance between what you observed and what you are assuming to be true. In this context, your test statistic, z ο , quantifies the number of standard deviations between the sample mean, x, and the population mean, µ . Calculating the test statistic is analogous to the previously discussed process of standardizing observations with z -scores:

where µ o is the value assumed to be true in the null hypothesis.
Making a Decision
Once you have your test statistic, there are two methods to use it to make your decision:
- Critical value method (discussed further in later chapters)
- p -value method (our current focus)
p -Value Method
To find a p -value , we use the test statistic to calculate the actual probability of getting the test result. Formally, the p -value is the probability that, if the null hypothesis is true, the results from another randomly selected sample will be as extreme or more extreme as the results obtained from the given sample.
A large p -value calculated from the data indicates that we should not reject the null hypothesis. The smaller the p -value, the more unlikely the outcome and the stronger the evidence is against the null hypothesis. We would reject the null hypothesis if the evidence is strongly against it.
Draw a graph that shows the p -value. The hypothesis test is easier to perform if you use a graph because you see the problem more clearly.
Suppose a baker claims that his bread height is more than 15 cm on average. Several of his customers do not believe him. To persuade his customers that he is right, the baker decides to do a hypothesis test. He bakes ten loaves of bread. The mean height of the sample loaves is 17 cm. The baker knows from baking hundreds of loaves of bread that the standard deviation for the height is 0.5 cm and the distribution of heights is normal.
The null hypothesis could be H 0 : μ ≤ 15.
The alternate hypothesis is H a : μ > 15.
The words “is more than” calls for the use of the > symbol, so “ μ > 15″ goes into the alternate hypothesis. The null hypothesis must contradict the alternate hypothesis.

Suppose the null hypothesis is true (the mean height of the loaves is no more than 15 cm). Then, is the mean height (17 cm) calculated from the sample unexpectedly large? The hypothesis test works by asking how unlikely the sample mean would be if the null hypothesis were true. The graph shows how far out the sample mean is on the normal curve. The p -value is the probability that, if we were to take other samples, any other sample mean would fall at least as far out as 17 cm.
This means that the p -value is the probability that a sample mean is the same or greater than 17 cm when the population mean is, in fact, 15 cm. We can calculate this probability using the normal distribution for means.
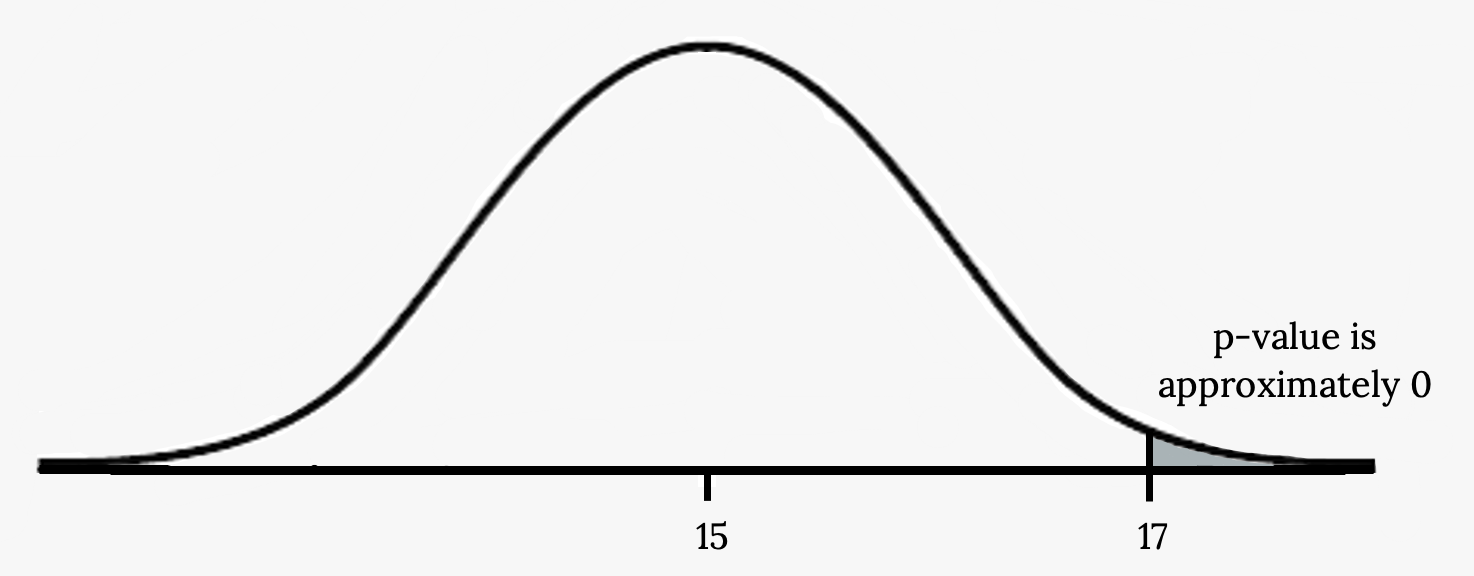
A p -value of approximately zero tells us that it is highly unlikely that a loaf of bread rises no more than 15 cm on average. That is, almost 0% of all loaves of bread would be at least as high as 17 cm purely by CHANCE had the population mean height really been 15 cm. Because the outcome of 17 cm is so unlikely (meaning it is happening NOT by chance alone), we conclude that the evidence is strongly against the null hypothesis that the mean height would be at most 15 cm. There is sufficient evidence that the true mean height for the population of the baker’s loaves of bread is greater than 15 cm.
A normal distribution has a standard deviation of one. We want to verify a claim that the mean is greater than 12. A sample of 36 is taken with a sample mean of 12.5.
Find the p -value.
Decision and Conclusion
A systematic way to decide whether to reject or not reject the null hypothesis is to compare the p -value and a preset or preconceived α (also called a significance level ). A preset α is the probability of a type I error (rejecting the null hypothesis when the null hypothesis is true). It may or may not be given to you at the beginning of the problem. If there is no given preconceived α , then use α = 0.05.
When you make a decision to reject or not reject H 0 , do as follows:
- If α > p -value, reject H 0 . The results of the sample data are statistically significant . You can say there is sufficient evidence to conclude that H 0 is an incorrect belief and that the alternative hypothesis, H a , may be correct.
- If α ≤ p -value, fail to reject H 0 . The results of the sample data are not significant. There is not sufficient evidence to conclude that the alternative hypothesis, H a , may be correct.
After you make your decision, write a thoughtful conclusion in the context of the scenario incorporating the hypotheses.
NOTE: When you “do not reject H 0 ,” it does not mean that you should believe that H 0 is true. It simply means that the sample data have failed to provide sufficient evidence to cast serious doubt about the truthfulness of H o .
When using the p -value to evaluate a hypothesis test, the following rhymes can come in handy:
If the p -value is low, the null must go.
If the p -value is high, the null must fly.
This memory aid relates a p -value less than the established alpha (“the p -value is low”) as rejecting the null hypothesis and, likewise, relates a p -value higher than the established alpha (“the p -value is high”) as not rejecting the null hypothesis.
Fill in the blanks:
- Reject the null hypothesis when .
- The results of the sample data .
- Do not reject the null when hypothesis when .
It’s a Boy Genetics Labs claim their procedures improve the chances of a boy being born. The results for a test of a single population proportion are as follows:
- H 0 : p = 0.50, H a : p > 0.50
- p -value = 0.025
Interpret the results and state a conclusion in simple, non-technical terms.
Click here for more multimedia resources, including podcasts, videos, lecture notes, and worked examples.
Figure References
Figure 5.11: Alora Griffiths (2019). dalmatian puppy near man in blue shorts kneeling. Unsplash license. https://unsplash.com/photos/7aRQZtLsvqw
Figure 5.13: Kindred Grey (2020). Bread height probability. CC BY-SA 4.0.
A decision-making procedure for determining whether sample evidence supports a hypothesis
The claim that is assumed to be true and is tested in a hypothesis test
A working hypothesis that is contradictory to the null hypothesis
A measure of the difference between observations and the hypothesized (or claimed) value
The probability that an event will occur, assuming the null hypothesis is true
Probability that a true null hypothesis will be rejected, also known as type I error and denoted by α
Finding sufficient evidence that the observed effect is not just due to variability, often from rejecting the null hypothesis
Significant Statistics Copyright © 2024 by John Morgan Russell, OpenStaxCollege, OpenIntro is licensed under a Creative Commons Attribution-ShareAlike 4.0 International License , except where otherwise noted.
Share This Book
Have a language expert improve your writing
Run a free plagiarism check in 10 minutes, generate accurate citations for free.
- Knowledge Base
Hypothesis Testing | A Step-by-Step Guide with Easy Examples
Published on November 8, 2019 by Rebecca Bevans . Revised on June 22, 2023.
Hypothesis testing is a formal procedure for investigating our ideas about the world using statistics . It is most often used by scientists to test specific predictions, called hypotheses, that arise from theories.
There are 5 main steps in hypothesis testing:
- State your research hypothesis as a null hypothesis and alternate hypothesis (H o ) and (H a or H 1 ).
- Collect data in a way designed to test the hypothesis.
- Perform an appropriate statistical test .
- Decide whether to reject or fail to reject your null hypothesis.
- Present the findings in your results and discussion section.
Though the specific details might vary, the procedure you will use when testing a hypothesis will always follow some version of these steps.
Table of contents
Step 1: state your null and alternate hypothesis, step 2: collect data, step 3: perform a statistical test, step 4: decide whether to reject or fail to reject your null hypothesis, step 5: present your findings, other interesting articles, frequently asked questions about hypothesis testing.
After developing your initial research hypothesis (the prediction that you want to investigate), it is important to restate it as a null (H o ) and alternate (H a ) hypothesis so that you can test it mathematically.
The alternate hypothesis is usually your initial hypothesis that predicts a relationship between variables. The null hypothesis is a prediction of no relationship between the variables you are interested in.
- H 0 : Men are, on average, not taller than women. H a : Men are, on average, taller than women.
Here's why students love Scribbr's proofreading services
Discover proofreading & editing
For a statistical test to be valid , it is important to perform sampling and collect data in a way that is designed to test your hypothesis. If your data are not representative, then you cannot make statistical inferences about the population you are interested in.
There are a variety of statistical tests available, but they are all based on the comparison of within-group variance (how spread out the data is within a category) versus between-group variance (how different the categories are from one another).
If the between-group variance is large enough that there is little or no overlap between groups, then your statistical test will reflect that by showing a low p -value . This means it is unlikely that the differences between these groups came about by chance.
Alternatively, if there is high within-group variance and low between-group variance, then your statistical test will reflect that with a high p -value. This means it is likely that any difference you measure between groups is due to chance.
Your choice of statistical test will be based on the type of variables and the level of measurement of your collected data .
- an estimate of the difference in average height between the two groups.
- a p -value showing how likely you are to see this difference if the null hypothesis of no difference is true.
Based on the outcome of your statistical test, you will have to decide whether to reject or fail to reject your null hypothesis.
In most cases you will use the p -value generated by your statistical test to guide your decision. And in most cases, your predetermined level of significance for rejecting the null hypothesis will be 0.05 – that is, when there is a less than 5% chance that you would see these results if the null hypothesis were true.
In some cases, researchers choose a more conservative level of significance, such as 0.01 (1%). This minimizes the risk of incorrectly rejecting the null hypothesis ( Type I error ).
The results of hypothesis testing will be presented in the results and discussion sections of your research paper , dissertation or thesis .
In the results section you should give a brief summary of the data and a summary of the results of your statistical test (for example, the estimated difference between group means and associated p -value). In the discussion , you can discuss whether your initial hypothesis was supported by your results or not.
In the formal language of hypothesis testing, we talk about rejecting or failing to reject the null hypothesis. You will probably be asked to do this in your statistics assignments.
However, when presenting research results in academic papers we rarely talk this way. Instead, we go back to our alternate hypothesis (in this case, the hypothesis that men are on average taller than women) and state whether the result of our test did or did not support the alternate hypothesis.
If your null hypothesis was rejected, this result is interpreted as “supported the alternate hypothesis.”
These are superficial differences; you can see that they mean the same thing.
You might notice that we don’t say that we reject or fail to reject the alternate hypothesis . This is because hypothesis testing is not designed to prove or disprove anything. It is only designed to test whether a pattern we measure could have arisen spuriously, or by chance.
If we reject the null hypothesis based on our research (i.e., we find that it is unlikely that the pattern arose by chance), then we can say our test lends support to our hypothesis . But if the pattern does not pass our decision rule, meaning that it could have arisen by chance, then we say the test is inconsistent with our hypothesis .
If you want to know more about statistics , methodology , or research bias , make sure to check out some of our other articles with explanations and examples.
- Normal distribution
- Descriptive statistics
- Measures of central tendency
- Correlation coefficient
Methodology
- Cluster sampling
- Stratified sampling
- Types of interviews
- Cohort study
- Thematic analysis
Research bias
- Implicit bias
- Cognitive bias
- Survivorship bias
- Availability heuristic
- Nonresponse bias
- Regression to the mean
Hypothesis testing is a formal procedure for investigating our ideas about the world using statistics. It is used by scientists to test specific predictions, called hypotheses , by calculating how likely it is that a pattern or relationship between variables could have arisen by chance.
A hypothesis states your predictions about what your research will find. It is a tentative answer to your research question that has not yet been tested. For some research projects, you might have to write several hypotheses that address different aspects of your research question.
A hypothesis is not just a guess — it should be based on existing theories and knowledge. It also has to be testable, which means you can support or refute it through scientific research methods (such as experiments, observations and statistical analysis of data).
Null and alternative hypotheses are used in statistical hypothesis testing . The null hypothesis of a test always predicts no effect or no relationship between variables, while the alternative hypothesis states your research prediction of an effect or relationship.
Cite this Scribbr article
If you want to cite this source, you can copy and paste the citation or click the “Cite this Scribbr article” button to automatically add the citation to our free Citation Generator.
Bevans, R. (2023, June 22). Hypothesis Testing | A Step-by-Step Guide with Easy Examples. Scribbr. Retrieved September 3, 2024, from https://www.scribbr.com/statistics/hypothesis-testing/
Is this article helpful?
Rebecca Bevans
Other students also liked, choosing the right statistical test | types & examples, understanding p values | definition and examples, what is your plagiarism score.
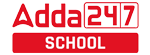
- Mathematical Reasoning and Statements: Meaning, Types, Examples
A mathematical statement is a form of a sentence that is either true or false, but not both. Mathematical reasoning involves determining the truth values of given statements within the field of mathematics. Check the meaning, types and solved examples of Mathematical Reasoning and Statements here
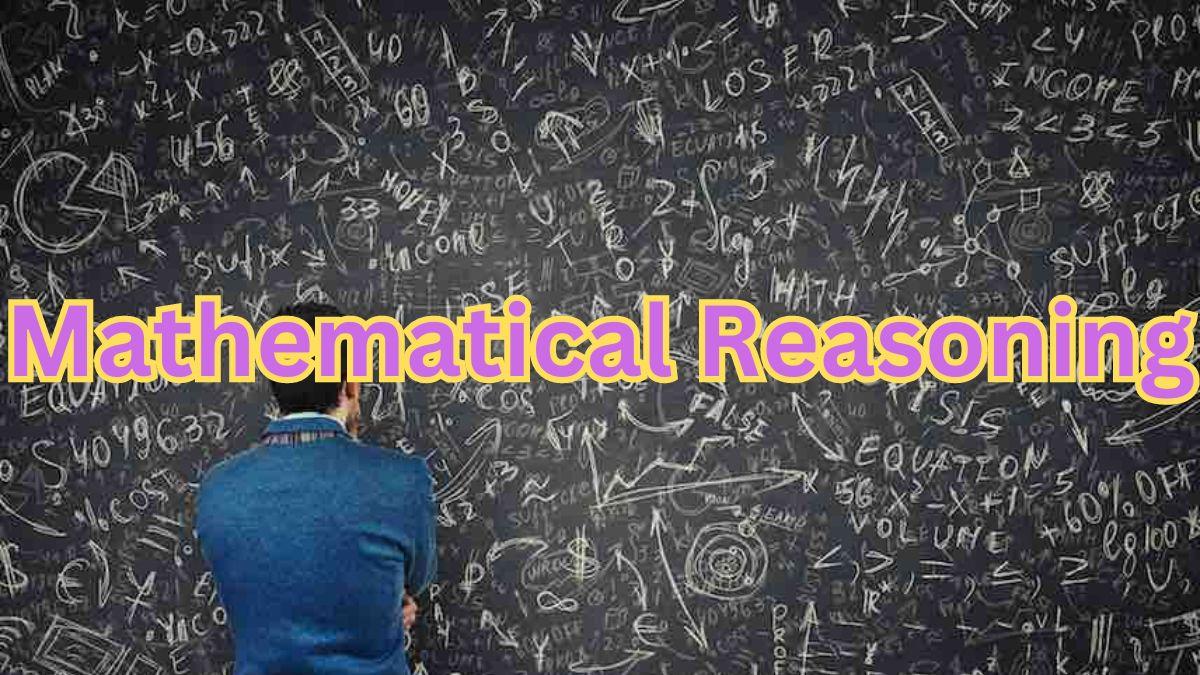
Table of Contents
Long before the beginning of the modern era of science and mathematics, the concept or mathematical reasoning existed. The mathematical reasoning can therefore be termed as one of the earliest concepts of Mathematics. Reasoning can be inferred or applied only if we have been provided with the statements. The statements used in the case of reasoning in mathematics is also known as mathematical statements or statements in mathematical reasoning.
Mathematical Reasoning
Mathematical reasoning, also known as the principle of mathematical reasoning, involves determining the truth values of provided statements within the field of mathematics. These types of logical statements are frequently seen in competitive exams such as JEE and the problems are quite simple and enjoyable to solve. In this article, we will explore the concept of mathematical reasoning and learn effective problem-solving techniques.
Mathematical Reasoning and Statements
The core or prelude of the reasoning in mathematics is the mathematical statements. The statements and mathematical reasoning completes each other. Without the presence of mathematical statements, we cannot derive any reasoning. In other words, we can say that statement is the basic unit of reasoning in mathematics. To learn the concept of reasoning and statements used in applied mathematics, we will have to explore its meaning and examples.
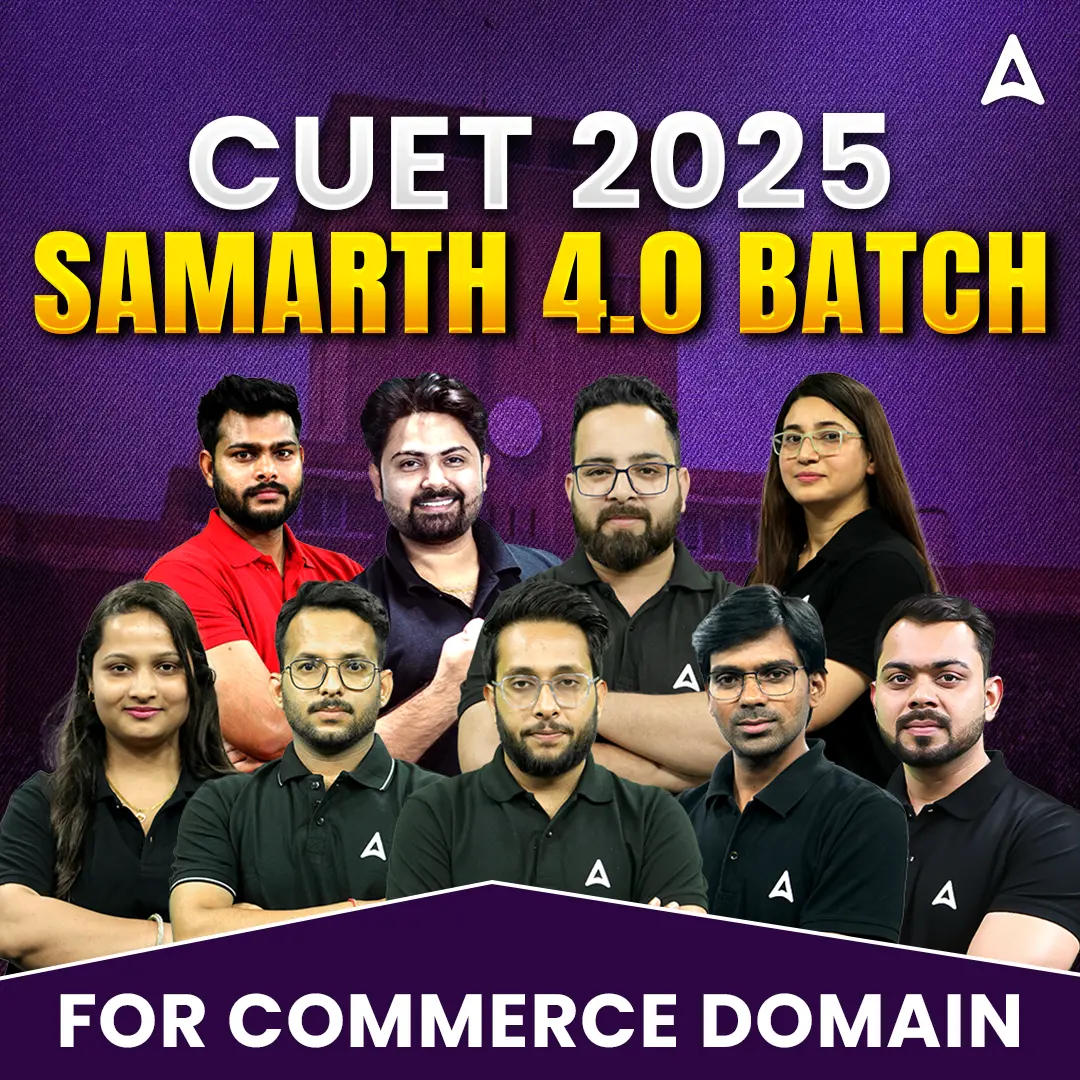
What is Meant by Mathematical Reasoning?
Mathematical reasoning is an important ability that allows students to assess a given hypothesis without considering any specific context or significance. Simply put, when it comes to analyzing a scientific investigation or claim, the logic used is not influenced by personal viewpoints.
Derivations and proofs necessitate a factual and scientific foundation. The skills of mathematical critical thinking and logical reasoning are essential for solving mathematical reasoning problems. It is a part of mathematics where we learn to obtain the true values of the statements presented before us.
What is a Mathematical Statement?
A sentence is considered a statement if it can be classified as correct or incorrect, true or false. It cannot be both, as a sentence that is both true and false is not a statement. Additionally, if a sentence is neither true nor false, it is also not considered a statement. For example,
Sentence 1: Independence Day is on 15 August
Sentence 2: The weight of ant is greater than the weight of the lion.
Upon reading these statements, we can quickly determine that sentence 1 is accurate while sentence 2 is incorrect. Therefore, these sentences are considered to be statements because they can be proven as either true or false, and do not contain any ambiguity.
Mathematically Acceptable Statements
To know whether a sentence is mathematical statement or not, we have to look at its certain aspects.
A statement in mathematics is any sentence that abides by certain rules given below.
- For a statement to be mathematically valid, it must be either true or false, but not both simultaneously.
- A sentence is not considered a mathematically validated statement if it is imperative, interrogative, or exclamatory.
- An open statement is defined as a sentence that contains one or more variables. An open statement can transform into a closed statement by substituting specific values for the variables in the sentence.
It is important to understand that a sentence cannot be considered a statement if:
- The given statement includes pronouns such as ‘she’, ‘he’, ‘they’ etc.
- The given statement is a question.
- The statement is an order or request.
- It is in exclamation format.
- The given statement involves variable places such as ‘here’, ‘there’, ‘everywhere’ etc.
- The given statement includes variable time such as ‘today’, ‘tomorrow’, ‘yesterday’ etc.
Mathematical Reasoning Types
There are seven types of mathematical reasoning: intuition, counterfactual thinking, critical thinking, backward induction, inductive reasoning, deductive reasoning, and abductive induction. Among these 7 types, the following two are considered the primary types.
Inductive Reasoning
Inductive reasoning involves checking the validity of a statement using a set of rules and then making a generalization based on the given statement. In simpler terms, inductive reasoning is a form of reasoning that is not strict, where statements are generalized.
In the Inductive method of mathematical reasoning, a particular set of rules is used to verify the validity of a statement before generalizing it. The concept of inductive reasoning is utilized in the principle of mathematical induction. Inductive reasoning is not included in geometrical proofs due to its generalization. Here is an illustration that will aid in the comprehension of inductive reasoning in mathematics.
The example of inductive reasoning is given hereunder:
Statement: The cost of labor to create the item is Rs. 5, while the cost of goods is Rs. 10. The item has a sales price of Rs. 50.
Reasoning: Based on the statement made, it can be concluded that the product will generate a satisfactory amount of profit for the retailers that sell it.
Deductive Reasoning
Deductive reasoning is a rigorous form of reasoning where statements are treated as true if the assumptions being made are true. In the field of mathematics, deductive reasoning holds greater significance than inductive reasoning.
Deductive reasoning is the antithesis of inductive reasoning. In contrast to inductive reasoning, deductive reasoning involves using the principles of a broad scenario to validate specific statements. The concept of mathematical induction relies on deductive reasoning (despite its misleading name). The example provided below will aid in gaining a better understanding of deductive reasoning in mathematics. The example is given below:
Statement: The Theorem of Pythagoras holds true for any right-angled triangle.
Reasoning: If triangle ABC is a right triangle, it will follow the Pythagoras Theorem.
Types of Reasoning Statements
There are 3 major types of reasoning statements in Mathematics. These are:
- Simple Statements
- Compound Statements
- If-Then Statements
Let us learn these mathematical statements one by one:
Simple Mathematical Statements
A statement is considered simple if its truth value is not reliant on another statement. Put differently, a basic statement should not be made up of basic statements.
So, a basic statement cannot be further simplified into simpler statements. Working with straightforward statements is the most simple, and we can easily use a direct reasoning method. Some instances have been given to elucidate the idea of straightforward declarations.
Example: Square is a parallelogram.
Reasoning: Modifiers are absent in the provided statement. Hence, it can be concluded that the statement provided is simple.
Compound Mathematical Statements
Put simply, when simple statements are combined together, they form a compound statement. Hence, these statements consist of a combination of at least two simple statements connected by words such as ‘and’ or ‘or’.
Various alternative connectives can be used in place of the two connectives listed. These statements are essential for using Deduction reasoning in Mathematics. Take a closer look at the detailed example provided below to gain a clearer insight. Let us understand this statements through an example.
Example: We have selected two basic statements that can be linked with a connector.
Statement 1: Transversal lines make equal alternate angles with parallel lines
Statement 2: Parallel lines do not intersect.
Compound Statement: Transversal lines make equal alternate angles with parallel lines and Parallel lines do not intersect.
If-Then Mathematical Statements
If-Then statements are also known as conditional statements. Conditional statements that consist of an if-then proposition, with a hypothesis leading to a conclusion, are referred to as If-then statements. If the assumption is correct and the result is incorrect, the if-then statement is considered incorrect. Similarly, if the assumption is incorrect, the entire assertion is incorrect.
If a condition leads to a result, proving the condition true will also prove the result true, and proving the result false will also prove the condition false. If we come across a statement that says ‘a if and only if b’, we can justify it by demonstrating that if a is true, then b is also true and if b is true, then a is also true. Go through the example given below to understand this concept.
Example 1: If 60% population is female then 40% population is male.
Reasoning: Here the 60% female is the hypothesis and if that condition is met then the conclusion is satisfying.
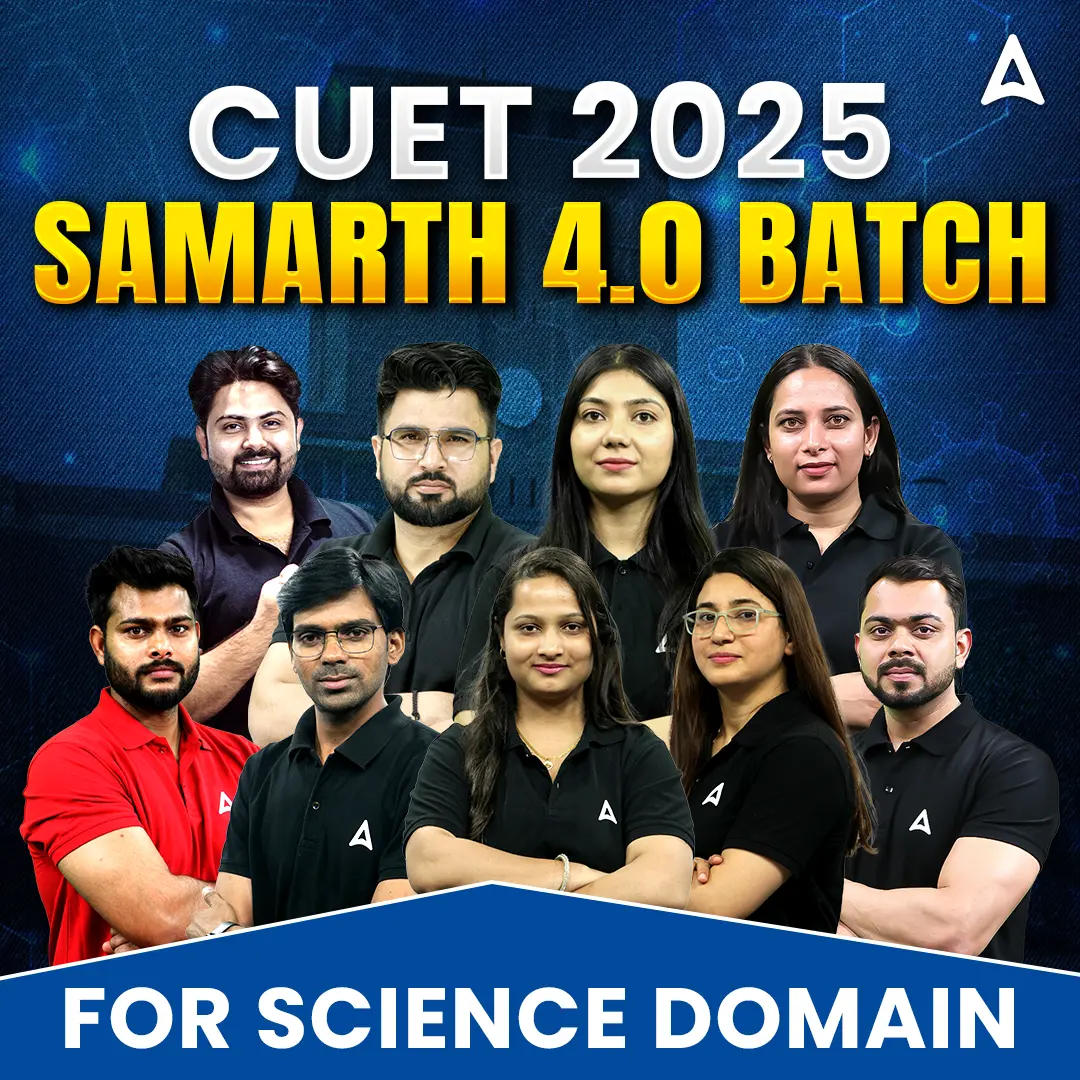
Mathematical Reasoning and Statements Examples
Go through the solved examples provided below to make your concept of mathematical reasoning and mathematical statements strong.
Example 1: SQUARE:PERIMETER::CIRCLE 😕
A.RADIUS B. CHORD C. SECTOR D. CIRCUMFERENCE
Ans: D. CIRCUMFERENCE
Reasoning: The perimeter of a square represents its boundary, while the circumference is the boundary of a circle.
Example 2: Review the following set of statements and indicate which ones are considered valid mathematically.
i) The Sun rises in the east.
ii) New Delhi is a country.
iii) Red rose is more beautiful than a yellow rose.
Solution: Upon reading the initial statement, it is clear that the first statement is true while the second statement is false without a doubt. Different individuals’ perceptions may influence the interpretation of the third statement. Therefore, it may be accurate for certain individuals and simultaneously incorrect for others. However, ambiguous statements are not permissible for logical reasoning in mathematics.
Therefore, a statement is considered valid in mathematics only if it is either true or false, but not both simultaneously. Therefore, statements 1 and 2 are deemed mathematically valid, whereas statement 3 is not mathematically acceptable.
Example 3: The total of x, y, and z, three natural numbers, is always negative.
Solution: This statement is deemed acceptable. It will always be false because every positive number is greater than zero, so the total of positive numbers cannot be negative.
Sharing is caring!
What is reasoning in mathematics?
In simple terms, the study of logic through mathematical symbols is called mathematical reasoning.
Is mathematical reasoning hard?
It requires more time and exploration than what is usually provided in the classroom to learn and apply mathematical reasoning to problems. It also demands that children deeply ponder and struggle with ideas, which may initially make them uncomfortable, particularly if they are accustomed to quickly solving problems.
What are the 4 types of reasoning in math?
Four types of reasoning will be our focus here: deductive reasoning, inductive reasoning, abductive reasoning and reasoning by analogy.
What is the another name for mathematical reasoning?
The mathematical reasoning is also known as the principle of mathematical reasoning.
Hi there, I am Ashish and have completed my education from Science Domain. I have 2 years of experience in content creation, catering to the demands of young students. I provide written content related to NEET, JEE, Board Exams, CLAT, CUET (UG & PG) and management exams in a simple manner. My content provides important insights on several topics in depth.
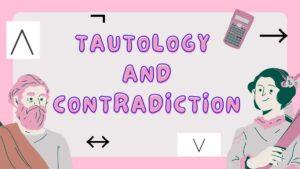
Leave a comment
Your email address will not be published. Required fields are marked *
Save my name, email, and website in this browser for the next time I comment.
Trending Articles
- Most Trending Teachers Day Speech
- NEET Syllabus 2025
- NEET Cut Off 2024
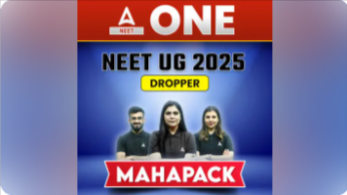
CBSE Board Exam 2024
- CBSE Previous Year Papers
- CUET Syllabus
- CUET Previous Year paper
- CUET Participating College & Universities
- JEE Main Syllabus 2025
- NEET State wise Cut off
- NEET Rank Predictor
- NEET OMR Sheet
- NEET College Predictor
Recent Posts
- Tautology and Contradiction – Definition, Examples, Truth Table
- Divisibility Rule of 3- Definition, Proof, Examples
- Divisibility Rules- Check Divisibility Tests from 1 to 13
- Polynomials- Definition, Formula, Types, Example
- Zero of a Polynomial for Class 9 and 10 Students, Learn Zero Polynomial with Examples
- Divisibility Rule of 4, How to Know a Number is Divisible by 4
- Divisibility Rule of 9, Check the Test of Divisibility of 9
- Divisibility Rule of 13, Check Divisibility Rules for 13 with Examples
- Divisibility Rule of 6, What is the Divisibility Rule for 6
- Divisibility Rule of 11- Check Divisibility Test of 11 With Examples
- Types of Angles for Class 5 to 9 in Maths- Check Definitions
- Divisibility Rule of 7, 7 Divisibility Rule with Examples
- Algebra Formulas PDF, Get All Algebraic Identities Formula Chart for Class 9
- Integers: Definition, Number, Rules, Formula and Examples
- Composite Numbers Definition, Examples and List from 1 to 100
- Greater Than and More Than Symbols Meaning in Maths
- Table of 16 | Download 16 Times Table in Maths
- Differentiation Formulas, Definition, Chart, PDF, Solved Examples
- Trigonometry Formulas for Class 11 and 12 PDF Download
- Prime Numbers 1 to 100: Definition, List, Chart, Properties, How to Identify
- Perfect Squares- Definition, Formula, Examples, Tips and Tricks, Chart
- Fraction to Percent- Conversion Formula, Steps, Table, Examples
- Discount Formula- Check Loss Discount Percentage Formula
- Profit and Loss Formula, Get Tricks PDF Download
- Sphere Formula For Diameter, Surface Area and Volume
- a2+b2 Formula- Check a2-b2 Formula Proof, Questions & Answers
- Maths Formulas for Class 6 to 10, 12 PDF Download
- 0 Factorial is Equal to, Check Value
- GP Formula- Sum of n Terms, Sum of Infinite GP
IMPORTANT EXAMS
Ncert solutions.
- NCERT Class 12
- NCERT Class 11
- NCERT Class 10
- NCERT Class 9
NCERT Books
School syllabus.
- CBSE Class 12
- CBSE Class 11
- CBSE Class 10
- CBSE Class 9
- JEE Mains 2024
Our Other Websites
- Teachers Adda
- Bankers Adda
- Current Affairs
- Adda Bengali
- Engineers Adda
- Adda Marathi
- Adda School
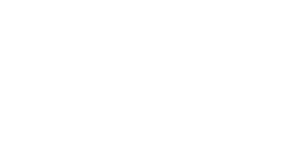
Get all your queries solved in one single place. We at Adda247 school strive each day to provide you the best material across the online education industry. We consider your struggle as our motivation to work each day.
Download Adda247 App
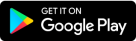
Follow us on

- Responsible Disclosure Program
- Cancellation & Refunds
- Terms & Conditions
- Privacy Policy

IMAGES
VIDEO
COMMENTS
So in hypothetical reasoning what we're doing is making a leap from the evidence we have available to the rule or principle or theory which explains that evidence. The hypothesis is the link between the two. We have some finite evidence available to us, and we hypothesize an explanation.
Examples: Inductive reasoning. Nala is an orange cat and she purrs loudly. Baby Jack said his first word at the age of 12 months. Every orange cat I've met purrs loudly. All observed babies say their first word at the age of 12 months. All orange cats purr loudly. All babies say their first word at the age of 12 months.
A hypothesis is a statement that explains the predictions and reasoning of your research—an "educated guess" about how your scientific experiments will end. As a fundamental part of the scientific method, a good hypothesis is carefully written, but even the simplest ones can be difficult to put into words. ... Some hypothesis examples ...
5. Phrase your hypothesis in three ways. To identify the variables, you can write a simple prediction in if…then form. The first part of the sentence states the independent variable and the second part states the dependent variable. If a first-year student starts attending more lectures, then their exam scores will improve.
10 out of 20 dogs didn't have fleas = reject hypothesis; All land mammal species depend on water = support hypothesis; Limitations of a deductive approach. The conclusions of deductive reasoning can only be true if all the premises set in the inductive study are true and the terms are clear. Example. All dogs have fleas (premise) Benno is a ...
Here are some real-time examples of inductive reasoning: ... Test the hypothesis: The final step in conducting inductive reasoning is to test your hypothesis. This might involve collecting additional data, conducting experiments, or making further observations. The goal is to determine whether your hypothesis is supported by the data you have ...
Inductive reasoning in math involves making generalizations based on observed patterns. It's a process where you notice a pattern from specific cases, form a hypothesis about a general rule, and then test and verify this hypothesis with additional examples. Again, however, the conclusion isn't guaranteed to be true for all cases.
This chapter will cover a series of types of induction. First, we'll cover Hypothetical Reasoning, which is the kind of reasoning that people call the "scientific method.". After that, we'll cover the basics of Causal Reasoning, Statistical Generalization, and Arguing from Analogy. We'll also explore the logic of each a bit and look ...
For example, if there are 20 balls—either black or white—in an urn, to estimate their respective numbers, a sample of four balls is drawn, three are black and one is white. ... Inductive reasoning is also known as hypothesis construction because any conclusions made are based on current knowledge and predictions. [citation needed] ...
Here are some examples of inductive reasoning: Data: I see fireflies in my backyard every summer. Hypothesis: This summer, I will probably see fireflies in my backyard.
Well-Formulated Inductive Reasoning Examples. 1. Polling and Surveys. "We surveyed 1,000 people across the county and 520 of them said they will vote to re-elect the mayor. We estimate that 52% of the county will vote for the mayor and he will be re-elected.". Many statisticians make a living from conducting tried-and-true inductive ...
Simple Hypothesis Examples. Increasing the amount of natural light in a classroom will improve students' test scores. Drinking at least eight glasses of water a day reduces the frequency of headaches in adults. Plant growth is faster when the plant is exposed to music for at least one hour per day.
Inductive reasoning is a logical reasoning method with widespread usage in day-to-day decision-making, statistics, research, and probability analysis. It makes use of more specific observations or instances to derive general principles or conclusions. Because of these features, inductive reasoning is a valuable tool to form hypotheses for ...
First, a good hypothesis must be testable and falsifiable. We must be able to test the hypothesis using the methods of science and if you'll recall Popper's falsifiability criterion, it must be possible to gather evidence that will disconfirm the hypothesis if it is indeed false. Second, a good hypothesis must be logical.
15 Hypothesis Examples. A hypothesis is defined as a testable prediction, and is used primarily in scientific experiments as a potential or predicted outcome that scientists attempt to prove or disprove (Atkinson et al., 2021; Tan, 2022). In my types of hypothesis article, I outlined 13 different hypotheses, including the directional hypothesis ...
Here are seven types of reasoning and examples of situations when they're best used: 1. Deductive reasoning Deductive reasoning is a type of reasoning that uses formal logic and observations to prove a theory or hypothesis. In deductive reasoning, you start with an assumption and then make observations or rational thoughts to validate or refute ...
A hypothesis is a tentative statement about the relationship between two or more variables. It is a specific, testable prediction about what you expect to happen in a study. It is a preliminary answer to your question that helps guide the research process. Consider a study designed to examine the relationship between sleep deprivation and test ...
Deductive reasoning is a top-down approach, while inductive reasoning is a bottom-up approach. In deductive reasoning, you start with general ideas and work toward specific conclusions through inferences. Based on theories, you form a hypothesis. Using empirical observations, you test that hypothesis using inferential statistics and form a ...
When using the p-value to evaluate a hypothesis test, the following rhymes can come in handy:. If the p-value is low, the null must go.. If the p-value is high, the null must fly.. This memory aid relates a p-value less than the established alpha ("the p-value is low") as rejecting the null hypothesis and, likewise, relates a p-value higher than the established alpha ("the p-value is ...
4. Formulate your hypothesis. After collecting background information and making a prediction based on your question, plan a statement that lays out your variables, subjects and predicted outcome. Whether you write it as an "if/then" or declarative statement, your hypothesis should include the prediction to be tested.
Introduction. Understanding the relationship between sampling distributions, probability distributions, and hypothesis testing is the crucial concept in the NHST — Null Hypothesis Significance Testing — approach to inferential statistics. is crucial, and many introductory text books are excellent here. I will add some here to their discussion, perhaps with a different approach, but the ...
Present the findings in your results and discussion section. Though the specific details might vary, the procedure you will use when testing a hypothesis will always follow some version of these steps. Table of contents. Step 1: State your null and alternate hypothesis. Step 2: Collect data. Step 3: Perform a statistical test.
Reasoning: Here the 60% female is the hypothesis and if that condition is met then the conclusion is satisfying. Mathematical Reasoning and Statements Examples. Go through the solved examples provided below to make your concept of mathematical reasoning and mathematical statements strong. Example 1: SQUARE:PERIMETER::CIRCLE 😕. A.RADIUS B. CHORD