
MARKET INTEL
INITIATIVES
LEADERSHIP DEVELOPMENT
TRENDING TOPICS

Why is Precision Agriculture Important?
Bernt nelson.
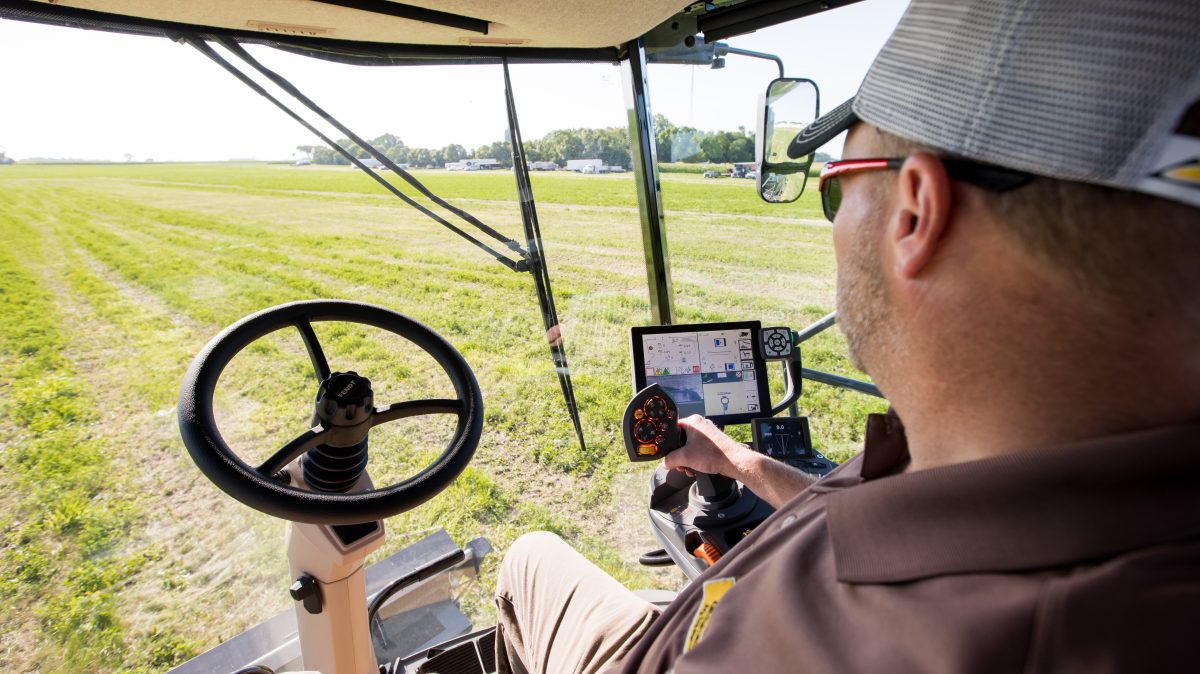
photo credit: AFBF
When agriculture began at the end of the Stone Age, the world had approximately 5 million people to feed, and few, if any, farmers were feeding people beyond their extended family. Farmers today use technology to plant and harvest mile-long fields with equipment guided by satellites for sub-inch accuracy, allowing them to feed nearly 8 billion people across the world with fewer resources than ever before.
The term “precision agriculture” means managing, tracking or enhancing crop or livestock production inputs, including seed, feed, fertilizer, chemicals, water and time, at a heightened level of accuracy to improve efficiencies and commodity quality and yield, while positively impacting environmental stewardship.
To understand advancements in precision agriculture technology, we have to start at the beginning when precision agriculture wasn’t yet guided by GPS. Dr. Pierre Robert, sometimes referred to as “the father of precision agriculture,” conducted some of the earliest research on soil variability. In 1983, Dr. Robert was the first to research variable rate fertilizer spreading, which acknowledges that different areas of a field have different crop yields and thus have different nutrient requirements to obtain optimal yields. This understanding eventually led to the variable rate field management systems that farmers use today. According to a publication by USDA’s Economic Research Service, variable rate technology is used to plant between 5-25% of total U.S. planted acreage for winter wheat, cotton, sorghum and rice.
Through much of the 1990s, data was gearing up to become the new crop of the 21st century. Now farmers just needed a method to gather information more efficiently. The first yield monitor, created in 1992, did just that. Yield monitors allowed farmers to record observable changes in crop yields throughout an entire field. This data could be paired with grid sampling, taking soil samples from grid points mapped out on a field, to create a map of input adjustments needed to improve yields.
Precision agriculture allows farmers to deliver exactly what a plant needs, exactly when and where it needs it and in the exact amount.
Advancement
The remaining piece of the puzzle was how to make applying variable rate technology more efficient. GPS auto-guidance systems revolutionized the way farmers operate machinery. The first GPS auto-guidance system was used on a salt harvester in 1996. By the early 2000s precision farming began to pick up speed. According to the previously referenced ERS report, today, the majority of corn, cotton, rice, sorghum, soybeans and winter wheat acres are planted using auto-guidance. These guidance systems are the foundation of precision agriculture across all brands of equipment and electronics that are used to produce food, fiber and renewable fuel for the world.
GPS systems are environmentally friendly and allow for more efficient use of inputs. These technologies deliver exactly what a plant needs, exactly where it’s needed, reducing waste and runoff that would be the result of excessive use. Thanks to auto-guidance systems that allowed a GPS signal to steer tractors with pinpoint accuracy, this can all be done while simultaneously gathering and recording field data.
Most precision agriculture systems operate today using real-time kinematic positioning signals. These satellite signals use measurements from the signal’s carrier wave in addition to information sent from a base station to correct errors in positioning for sub-inch or even sub-centimeter accuracy. These systems are also capable of using a wide-area augmentation system signal with 6- to 8-inch accuracy as a backup if RTK is having problems.
RTK can be used for field operations such as tillage, planting, harvesting, spraying and a wide range of other field activities. This technology is used to collect data relating to soil temperature, soil moisture, seeding depth, seeds per acre, yield and more while a farmer carries out normal operations. This data is accumulated and can be used to provide production performance over time. Topography data can even be gathered and used to design tile drainage systems that help more efficiently manage water.
Precision agriculture allows farmers to deliver exactly what a plant needs, exactly when and where it needs it and in the exact amount. This increases efficiency for chemical and fertilizer use and helps avoid excess application, making it more environmentally friendly. Chemicals aren’t wasted and fertilizer is utilized by the plant rather than empty space where roots are not able to reach.
Bernt Nelson is an economist at the American Farm Bureau Federation. This column is a condensed version of a previously published Market Intel piece .
Trending Topics
Front Desk: (202) 406-3600 General Inquiries: [email protected] Media Inquiries: [email protected]
600 Maryland Avenue SW Suite 1000 Washington DC 20024
© 2024 American Farm Bureau Federation ® | Privacy Policy | Terms & Conditions
Thank you for visiting nature.com. You are using a browser version with limited support for CSS. To obtain the best experience, we recommend you use a more up to date browser (or turn off compatibility mode in Internet Explorer). In the meantime, to ensure continued support, we are displaying the site without styles and JavaScript.
- View all journals
- Explore content
- About the journal
- Publish with us
- Sign up for alerts
- Published: 27 April 2017
Technology: The Future of Agriculture
- Anthony King
Nature volume 544 , pages S21–S23 ( 2017 ) Cite this article
199k Accesses
219 Citations
225 Altmetric
Metrics details
- Agriculture
A technological revolution in farming led by advances in robotics and sensing technologies looks set to disrupt modern practice.
Over the centuries, as farmers have adopted more technology in their pursuit of greater yields, the belief that 'bigger is better' has come to dominate farming, rendering small-scale operations impractical. But advances in robotics and sensing technologies are threatening to disrupt today's agribusiness model. “There is the potential for intelligent robots to change the economic model of farming so that it becomes feasible to be a small producer again,” says robotics engineer George Kantor at Carnegie Mellon University in Pittsburgh, Pennsylvania.
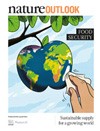
Twenty-first century robotics and sensing technologies have the potential to solve problems as old as farming itself. “I believe, by moving to a robotic agricultural system, we can make crop production significantly more efficient and more sustainable,” says Simon Blackmore, an engineer at Harper Adams University in Newport, UK. In greenhouses devoted to fruit and vegetable production, engineers are exploring automation as a way to reduce costs and boost quality (see ‘ Ripe for the picking ’). Devices to monitor vegetable growth, as well as robotic pickers, are currently being tested. For livestock farmers, sensing technologies can help to manage the health and welfare of their animals (‘ Animal trackers ’). And work is underway to improve monitoring and maintenance of soil quality (‘ Silicon soil saviours ’), and to eliminate pests and disease without resorting to indiscriminate use of agrichemicals (‘ Eliminating enemies ’).
Although some of these technologies are already available, most are at the research stage in labs and spin-off companies. “Big-machinery manufacturers are not putting their money into manufacturing agricultural robots because it goes against their current business models,” says Blackmore. Researchers such as Blackmore and Kantor are part of a growing body of scientists with plans to revolutionize agricultural practice. If they succeed, they'll change how we produce food forever. “We can use technology to double food production,” says Richard Green, agricultural engineer at Harper Adams.
Ripe for the picking
The Netherlands is famed for the efficiency of its fruit- and vegetable-growing greenhouses, but these operations rely on people to pick the produce. “Humans are still better than robots, but there is a lot of effort going into automatic harvesting,” says Eldert van Henten, an agricultural engineer at Wageningen University in the Netherlands, who is working on a sweet-pepper harvester. The challenge is to quickly and precisely identify the pepper and avoid cutting the main stem of the plant. The key lies in fast, precise software. “We are performing deep learning with the machine so it can interpret all the data from a colour camera fast,” says van Henten. “We even feed data from regular street scenes into the neural network to better train it.”
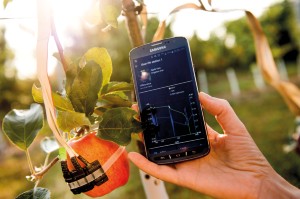
In the United Kingdom, Green has developed a strawberry harvester that he says can pick the fruit faster than humans. It relies on stereoscopic vision with RGB cameras to capture depth, but it is its powerful algorithms that allow it to pick a strawberry every two seconds. People can pick 15 to 20 a minute, Green estimates. “Our partners at the National Physical Laboratory worked on the problem for two years, but had a brainstorm one day and finally cracked it,” says Green, adding that the solution is too commercially sensitive to share. He thinks that supervised groups of robots can step into the shoes of strawberry pickers in around five years. Harper Adams University is considering setting up a spin-off company to commercialize the technology. The big hurdle to commercialization, however, is that food producers demand robots that can pick all kinds of vegetables, says van Henten. The variety of shapes, sizes and colours of tomatoes, for instance, makes picking them a tough challenge, although there is already a robot available to remove unwanted leaves from the plants.
Another key place to look for efficiencies is timing. Picking too early is wasteful because you miss out on growth, but picking too late slashes weeks off the storage time. Precision-farming engineer Manuela Zude-Sasse at the Leibniz Institute for Agricultural Engineering and Bioeconomy in Potsdam, Germany, is attaching sensors to apples to detect their size, and levels of the pigments chlorophyll and anthocyanin. The data are fed into an algorithm to calculate developmental stage, and, when the time is ripe for picking, growers are alerted by smartphone.
So far, Zude-Sasse has put sensors on pears, citrus fruits, peaches, bananas and apples ( pictured ). She is set to start field trials later this year in a commercial tomato greenhouse and an apple orchard. She is also developing a smartphone app for cherry growers. The app will use photographs of cherries taken by growers to calculate growth rate and a quality score.
Growing fresh fruit and vegetables is all about keeping the quality high while minimizing costs. “If you can schedule harvest to optimum fruit development, then you can reap an economic benefit and a quality one,” says Zude-Sasse.
Eliminating enemies
The Food and Agriculture Organization of the United Nations estimates that 20–40% of global crop yields are lost each year to pests and diseases, despite the application of around two-million tonnes of pesticide. Intelligent devices, such as robots and drones, could allow farmers to slash agrichemical use by spotting crop enemies earlier to allow precise chemical application or pest removal, for example. “The market is demanding foods with less herbicide and pesticide, and with greater quality,” says Red Whittaker, a robotics engineer at Carnegie Mellon who designed and patented an automated guidance system for tractors in 1997. “That challenge can be met by robots.”
“We predict drones, mounted with RGB or multispectral cameras, will take off every morning before the farmer gets up, and identify where within the field there is a pest or a problem,” says Green. As well as visible light, these cameras would be able to collect data from the invisible parts of the electromagnetic spectrum that could allow farmers to pinpoint a fungal disease, for example, before it becomes established. Scientists from Carnegie Mellon have begun to test the theory in sorghum ( Sorghum bicolor ), a staple in many parts of Africa and a potential biofuel crop in the United States.
Agribotix, an agriculture data-analysis company in Boulder, Colorado, supplies drones and software that use near-infrared images to map patches of unhealthy vegetation in large fields. Images can also reveal potential causes, such as pests or problems with irrigation. The company processes drone data from crop fields in more than 50 countries. It is now using machine learning to train its systems to differentiate between crops and weeds, and hopes to have this capability ready for the 2017 growing season. “We will be able to ping growers with an alert saying you have weeds growing in your field, here and here,” says crop scientist Jason Barton, an executive at Agribotix.
Modern technology that can autonomously eliminate pests and target agrichemicals better will reduce collateral damage to wildlife, lower resistance and cut costs. “We are working with a pesticide company keen to apply from the air using a drone,” says Green. Rather than spraying a whole field, the pesticide could be delivered to the right spot in the quantity needed, he says. The potential reductions in pesticide use are impressive. According to researchers at the University of Sydney's Australian Centre for Field Robotics, targeted spraying of vegetables used 0.1% of the volume of herbicide used in conventional blanket spraying. Their prototype robot is called RIPPA (Robot for Intelligent Perception and Precision Application) and shoots weeds with a directed micro-dose of liquid. Scientists at Harper Adams are going even further, testing a robot that does away with chemicals altogether by blasting weeds close to crops with a laser. “Cameras identify the growing point of the weed and our laser, which is no more than a concentrated heat source, heats it up to 95 °C, so the weed either dies or goes dormant,” says Blackmore.
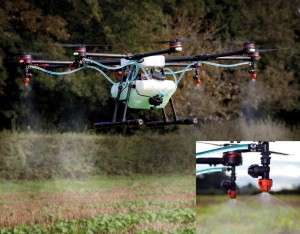
Animal trackers
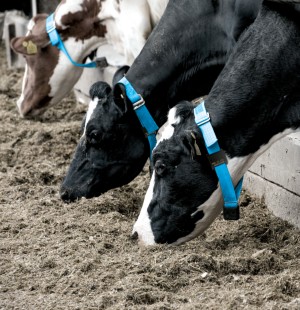
Smart collars — a bit like the wearable devices designed to track human health and fitness — have been used to monitor cows in Scotland since 2010. Developed by Glasgow start-up Silent Herdsman, the collar monitors fertility by tracking activity — cows move around more when they are fertile — and uses this to alert farmers to when a cow is ready to mate, sending a message to his or her laptop or smartphone. The collars ( pictured ), which are now being developed by Israeli dairy-farm-technology company Afimilk after they acquired Silent Herdsman last year, also detect early signs of illness by monitoring the average time each cow spends eating and ruminating, and warning the farmer via a smartphone if either declines.
“We are now looking at more subtle behavioural changes and how they might be related to animal health, such as lameness or acidosis,” says Richard Dewhurst, an animal nutritionist at Scotland's Rural College (SRUC) in Edinburgh, who is involved in research to expand the capabilities of the collar. Scientists are developing algorithms to interrogate data collected by the collars.
In a separate project, Dewhurst is analysing levels of exhaled ketones and sulfides in cow breath to reveal underfeeding and tissue breakdown or excess protein in their diet. “We have used selected-ionflow-tube mass spectrometry, but there are commercial sensors available,” says Dewhurst.
Cameras are also improving the detection of threats to cow health. The inflammatory condition mastitis — often the result of a bacterial infection — is one of the biggest costs to the dairy industry, causing declines in milk production or even death. Thermal-imaging cameras installed in cow sheds can spot hot, inflamed udders, allowing animals to be treated early.
Carol-Anne Duthie, an animal scientist at SRUC, is using 3D cameras to film cattle at water troughs to estimate the carcass grade (an assessment of the quality of a culled cow) and animal weight. These criteria determine the price producers are paid. Knowing the optimum time to sell would maximize profit and provide abattoirs with more-consistent animals. “This has knock on effects in terms of overall efficiency of the entire supply chain, reducing the animals which are out of specification reaching the abattoir,” Duthie explains.
And researchers in Belgium have developed a camera system to monitor broiler chickens in sheds. Three cameras continually track the movements of thousands of individual birds to spot problems quickly. “Analysing the behaviour of broilers can give an early warning for over 90% of problems,” says bioengineer Daniel Berckmans at the University of Leuven. The behaviour-monitoring system is being sold by Fancom, a livestock-husbandry firm in Panningen, the Netherlands. The Leuven researchers have also launched a cough monitor to flag respiratory problems in pigs, through a spin-off company called SoundTalks. This can give a warning 12 days earlier than farmers or vets would normally be able to detect a problem, says Berckmans. The microphone, which is positioned above animals in their pen, identifies sick individuals so that treatment can be targeted. “The idea was to reduce the use of antibiotics,” says Berckmans.
Berckmans is now working on downsizing a stress monitor designed for people so that it will attach to a cow's ear tag. “The more you stress an animal, the less energy is available from food for growth,” he says. The monitor takes 200 physiological measurements a second, alerting farmers through a smartphone when there is a problem.
Silicon soil saviours
The richest resource for arable farmers is soil. But large harvesters damage and compact soil, and overuse of agrichemicals such as nitrogen fertilizer are bad for both the environment and a farmer's bottom line. Robotics and autonomous machines could help.
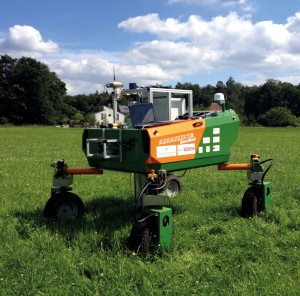
Data from drones are being used for smarter application of nitrogen fertilizer. “Healthy vegetation reflects more near-infrared light than unhealthy vegetation,” explains Barton. The ratio of red to near-infrared bands on a multispectral image can be used to estimate chlorophyll concentration and, therefore, to map biomass and see where interventions such as fertilization are needed after weather or pest damage, for example. When French agricultural technology company Airinov, which offers this type of drone survey, partnered with a French farming cooperative, they found that over a period of 3 years, in 627 fields of oilseed rape ( Brassica napus ), farmers used on average 34 kilograms less nitrogen fertilizer per hectare than they would without the survey data. This saved on average €107 (US$115) per hectare per year.
Bonirob ( pictured ) — a car-sized robot originally developed by a team of scientists including those at Osnabrück University of Applied Sciences in Germany — can measure other indicators of soil quality using various sensors and modules, including a moisture sensor and a penetrometer, which is used to assess soil compaction. According to Arno Ruckelshausen, an agricultural technologist at Osnabrück, Bonirob can take a sample of soil, liquidize it and analyse it to precisely map in real time characteristics such as pH and phosphorous levels. The University of Sydney's smaller RIPPA robot can also detect soil characteristics that affect crop production, by measuring soil conductivity.
Soil mapping opens the door to sowing different crop varieties in one field to better match shifting soil properties such as water availability. “You could differentially seed a field, for example, planting deep-rooting barley or wheat varieties in more sandy parts,” says Maurice Moloney, chief executive of the Global Institute for Food Security in Saskatoon, Canada. Growing multiple crops together could also lead to smarter use of agrichemicals. “Nature is strongly against monoculture, which is one reason we have to use massive amounts of herbicide and pesticides,” says van Henten. “It is about making the best use of resources.”
Mixed sowing would challenge an accepted pillar of agricultural wisdom: that economies of scale and the bulkiness of farm machinery mean vast fields of a single crop is the most-efficient way to farm, and the bigger the machine, the more-efficient the process. Some of the heaviest harvesters weigh 60 tonnes, cost more than a top-end sports car and leave a trail of soil compaction in their wake that can last for years.
But if there is no need for the farmer to drive the machine, then one large vehicle that covers as much area as possible is no longer needed. “As soon as you remove the human component, size is irrelevant,” says van Henten. Small, autonomous robots make mixed planting feasible and would not crush the soil.
In April, researchers at Harpers Adams began a proof-of-concept experiment with a hectare of barley. “We plan to grow and harvest the entire crop from start to finish with no humans entering the field,” says Green. The experiment will use existing machinery, such as tractors, that have been made autonomous, rather than new robots, but their goal is to use the software developed during this trial as the brains of purpose-built robots in the future. “Robots can facilitate a new way of doing agriculture,” says van Henten. Many of these disruptive technologies may not be ready for the prime time just yet, but the revolution is coming.
You can also search for this author in PubMed Google Scholar
Related links
Related links in nature research.
Bioengineering: Solar upgrade
Agrobiodiversity: The living library
Outlook on agriculture and drought
Related external links
Hands free hectare 2
Rights and permissions
Reprints and permissions
About this article
Cite this article.
King, A. Technology: The Future of Agriculture. Nature 544 , S21–S23 (2017). https://doi.org/10.1038/544S21a
Download citation
Published : 27 April 2017
Issue Date : 27 April 2017
DOI : https://doi.org/10.1038/544S21a
Share this article
Anyone you share the following link with will be able to read this content:
Sorry, a shareable link is not currently available for this article.
Provided by the Springer Nature SharedIt content-sharing initiative
This article is cited by
Positive public attitudes towards agricultural robots.
- Hendrik Hilmar Zeddies
Scientific Reports (2024)
Enhancing Hill Farming Efficiency Using Unmanned Agricultural Vehicles: A Comprehensive Review
- Mrutyunjay Padhiary
- Laxmi Narayan Sethi
- Avinash Kumar
Transactions of the Indian National Academy of Engineering (2024)
How to make sense of 3D representations for plant phenotyping: a compendium of processing and analysis techniques
- Negin Harandi
- Breght Vandenberghe
- Arnout Van Messem
Plant Methods (2023)
Precise in-field molecular diagnostics of crop diseases by smartphone-based mutation-resolved pathogenic RNA analysis
- Qingdong Zeng
- Ruijie Deng
Nature Communications (2023)
Quick links
- Explore articles by subject
- Guide to authors
- Editorial policies
Sign up for the Nature Briefing: Anthropocene newsletter — what matters in anthropocene research, free to your inbox weekly.

Information
- Author Services
Initiatives
You are accessing a machine-readable page. In order to be human-readable, please install an RSS reader.
All articles published by MDPI are made immediately available worldwide under an open access license. No special permission is required to reuse all or part of the article published by MDPI, including figures and tables. For articles published under an open access Creative Common CC BY license, any part of the article may be reused without permission provided that the original article is clearly cited. For more information, please refer to https://www.mdpi.com/openaccess .
Feature papers represent the most advanced research with significant potential for high impact in the field. A Feature Paper should be a substantial original Article that involves several techniques or approaches, provides an outlook for future research directions and describes possible research applications.
Feature papers are submitted upon individual invitation or recommendation by the scientific editors and must receive positive feedback from the reviewers.
Editor’s Choice articles are based on recommendations by the scientific editors of MDPI journals from around the world. Editors select a small number of articles recently published in the journal that they believe will be particularly interesting to readers, or important in the respective research area. The aim is to provide a snapshot of some of the most exciting work published in the various research areas of the journal.
Original Submission Date Received: .
- Active Journals
- Find a Journal
- Proceedings Series
- For Authors
- For Reviewers
- For Editors
- For Librarians
- For Publishers
- For Societies
- For Conference Organizers
- Open Access Policy
- Institutional Open Access Program
- Special Issues Guidelines
- Editorial Process
- Research and Publication Ethics
- Article Processing Charges
- Testimonials
- Preprints.org
- SciProfiles
- Encyclopedia
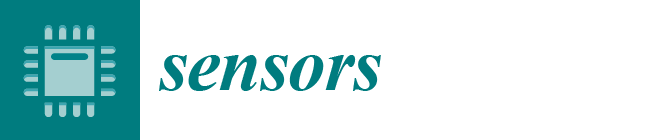
Article Menu

- Subscribe SciFeed
- Recommended Articles
- PubMed/Medline
- Google Scholar
- on Google Scholar
- Table of Contents
Find support for a specific problem in the support section of our website.
Please let us know what you think of our products and services.
Visit our dedicated information section to learn more about MDPI.
JSmol Viewer
Smart sensors and smart data for precision agriculture: a review.
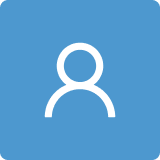

1. Introduction
2. types of smart sensors in precision agriculture, 3. data collection and management techniques, 4. applications and case studies, 5. challenges and future directions, 6. conclusions, author contributions, conflicts of interest, abbreviations.
ADC | Analog-to-Digital Converter | MLR | Multiple Regression Analysis |
AI | Artificial Intelligence | N | Nitrogen |
ANFIS | Adaptive Periodic Threshold-sensitive Energy Efficient sensor Network protocol | NC | Network Coordinator |
APTEEN | Adaptive Periodic Threshold-sensitive Energy Efficient sensor Network protocol | NCS | Neural Compute Stick |
AWS | Amazon Web Services | NDVI | Normalized Difference Vegetation Index |
BOP | Beacon Only Period | OASNDFA | Optimized Algorithm of Sensor Node Deployment for intelligent Agricultural monitoring |
CDC | Complex Dielectric Constant | OSRS | Open-Source Remote Sensing |
CNN | Convolutional Neural Network | PA | Precision Agriculture |
CNSVMC | Convolutional Neural Support Vector Machine Classifier | PAF | Precision Agriculture and Farming |
CRF | Controlled-Release Fertilizer | PAR | Photosynthetically Active Radiation |
D-ATR | Diamond Attenuated Total Internal Reflectance | PCR | Principal Component Regression |
DCTA | Dynamic Converge cast Tree Algorithm | PIS | Passive Infrared Sensor |
DSS | Decision Support Systems | PLC | Programmable Logic Controller |
EC | Electrical Conductivity | PLSR | Partial Least Squares Regression |
ET | Evapotranspiration | PWM | Pulse-Width Modulation |
FLFL | Five-Layer, Fifteen-Level | reNDVI | red-edge NDVI |
FTIR | Fourier Transform Infrared Spectroscopy | RFEH | Radio Frequency Energy Harvesting |
GCPs | Ground Control Points | RMSE | Root Mean Square Error |
GD | Gradient Descent | RNN | Recurrent Neural Network |
GHS | Greenhouse Gas | RTU | Remote Terminal Unit |
G-IoT | Green IoT | SDGs | Sustainable Development Goals |
GIS | Geographic Information Systems | SIS-PAF | Smart Irrigation System for Precision Agriculture and Farming |
GNDVI | Green Normalized Difference Vegetation Index | SNR | Signal-To-Noise Ratio |
GPR | Gaussian Process Regression | SPAD | Soil Plant Analysis Development |
GPS | Global Positioning Systems | SSIM | Structural Similarity Index |
GPU | Graphics Processing Unit | SVM | Support Vector Machines |
IAS | Irrigation Advisory Services | TAK | Title, Abstract, and Keywords |
IoE | Internet of Everything | TVWS | TV Whitespace |
IoT | Internet of Things | UAVs | Unmanned Aerial Vehicles |
IWUE | Irrigation Water Use Efficiency | UWB | Ultra-Wide Band |
Lasso | Least absolute shrinkage and selection operator regression | VLRGD | Variable Learning Rate Gradient Descent |
LCIS | Low-cost System for agricultural Irrigation Support | VWC | Volumetric Water Content |
LDR | Light-Dependent Resistor | Wi-Fi | Wireless Fidelity |
LIBS | Laser-Induced Breakdown Spectroscopy | W-Mod | Water balance simulation Modeling |
LoRa | Long-Range | WS | Web Server |
LPWA | Low-Power Wide-Area | WSAN | Wireless Sensor and Actuator Network |
LTMN | Long Short-Term Memory | WSNs | Wireless Sensor Networks |
MAPE | Mean Absolute Percentage Error | WUSN | Wireless Underground Sensor Networks |
MC | Moisture Content | ZigBee | Zonal Intercommunication Global standard |
ML | Machine Learning |
- Bhakta, I.; Phadikar, S.; Majumder, K. State-of-the-art Technologies in Precision Agriculture: A Systematic Review. J. Sci. Food Agric. 2019 , 99 , 4878–4888. [ Google Scholar ] [ CrossRef ] [ PubMed ]
- Kendall, H.; Clark, B.; Li, W.; Jin, S.; Jones, G.D.; Chen, J.; Taylor, J.; Li, Z.; Frewer, L.J. Precision Agriculture Technology Adoption: A Qualitative Study of Small-Scale Commercial “Family Farms” Located in the North China Plain. Precis. Agric. 2022 , 23 , 319–351. [ Google Scholar ] [ CrossRef ]
- Liu, J.; Xiang, J.; Jin, Y.; Liu, R.; Yan, J.; Wang, L. Boost Precision Agriculture with Unmanned Aerial Vehicle Remote Sensing and Edge Intelligence: A Survey. Remote Sens. 2021 , 13 , 4387. [ Google Scholar ] [ CrossRef ]
- Segarra, J.; Buchaillot, M.L.; Araus, J.L.; Kefauver, S.C. Remote Sensing for Precision Agriculture: Sentinel-2 Improved Features and Applications. Agronomy 2020 , 10 , 641. [ Google Scholar ] [ CrossRef ]
- Radoglou-Grammatikis, P.; Sarigiannidis, P.; Lagkas, T.; Moscholios, I. A Compilation of UAV Applications for Precision Agriculture. Comput. Netw. 2020 , 172 , 107148. [ Google Scholar ] [ CrossRef ]
- Zhang, F.; Cao, N. Application and Research Progress of Geographic Information System (GIS) in Agriculture. In Proceedings of the 2019 8th International Conference on Agro-Geoinformatics (Agro-Geoinformatics), Istanbul, Turkey, 16–19 July 2019; pp. 1–5. [ Google Scholar ] [ CrossRef ]
- Leroux, C.; Jones, H.; Pichon, L.; Guillaume, S.; Lamour, J.; Taylor, J.; Naud, O.; Crestey, T.; Lablee, J.-L.; Tisseyre, B. GeoFIS: An Open Source, Decision-Support Tool for Precision Agriculture Data. Agriculture 2018 , 8 , 73. [ Google Scholar ] [ CrossRef ]
- Mavridou, E.; Vrochidou, E.; Papakostas, G.A.; Pachidis, T.; Kaburlasos, V.G. Machine Vision Systems in Precision Agriculture for Crop Farming. J. Imaging 2019 , 5 , 89. [ Google Scholar ] [ CrossRef ] [ PubMed ]
- Tamirat, T.W.; Pedersen, S.M.; Lind, K.M. Farm and Operator Characteristics Affecting Adoption of Precision Agriculture in Denmark and Germany. Acta Agric. Scand. B Soil Plant Sci. 2018 , 68 , 349–357. [ Google Scholar ] [ CrossRef ]
- Guo, J.; Li, X.; Li, Z.; Hu, L.; Yang, G.; Zhao, C.; Fairbairn, D.; Watson, D.; Ge, M. Multi-GNSS Precise Point Positioning for Precision Agriculture. Precis. Agric. 2018 , 19 , 895–911. [ Google Scholar ] [ CrossRef ]
- Coble, K.H.; Mishra, A.K.; Ferrell, S.; Griffin, T. Big Data in Agriculture: A Challenge for the Future. Appl. Econ. Perspect. Policy 2018 , 40 , 79–96. [ Google Scholar ] [ CrossRef ]
- Wolfert, S.; Ge, L.; Verdouw, C.; Bogaardt, M.-J. Big Data in Smart Farming—A Review. Agric. Syst. 2017 , 153 , 69–80. [ Google Scholar ] [ CrossRef ]
- Bersani, C.; Ruggiero, C.; Sacile, R.; Soussi, A.; Zero, E. Internet of Things Approaches for Monitoring and Control of Smart Greenhouses in Industry 4.0. Energies 2022 , 15 , 3834. [ Google Scholar ] [ CrossRef ]
- Ahmed, N.; De, D.; Hussain, I. Internet of Things (IoT) for Smart Precision Agriculture and Farming in Rural Areas. IEEE Internet Things J. 2018 , 5 , 4890–4899. [ Google Scholar ] [ CrossRef ]
- Bhat, S.A.; Huang, N.-F. Big Data and AI Revolution in Precision Agriculture: Survey and Challenges. IEEE Access 2021 , 9 , 110209–110222. [ Google Scholar ] [ CrossRef ]
- Shadrin, D.; Menshchikov, A.; Ermilov, D.; Somov, A. Designing Future Precision Agriculture: Detection of Seeds Germination Using Artificial Intelligence on a Low-Power Embedded System. IEEE Sens. J. 2019 , 19 , 11573–11582. [ Google Scholar ] [ CrossRef ]
- Patrício, D.I.; Rieder, R. Computer Vision and Artificial Intelligence in Precision Agriculture for Grain Crops: A Systematic Review. Comput. Electron. Agric. 2018 , 153 , 69–81. [ Google Scholar ] [ CrossRef ]
- Abioye, E.A.; Abidin, M.S.Z.; Mahmud, M.S.A.; Buyamin, S.; Ishak, M.H.I.; Rahman, M.K.I.A.; Otuoze, A.O.; Onotu, P.; Ramli, M.S.A. A Review on Monitoring and Advanced Control Strategies for Precision Irrigation. Comput. Electron. Agric. 2020 , 173 , 105441. [ Google Scholar ] [ CrossRef ]
- Köksal, Ö.; Tekinerdogan, B. Architecture Design Approach for IoT-Based Farm Management Information Systems. Precis. Agric. 2019 , 20 , 926–958. [ Google Scholar ] [ CrossRef ]
- Ruan, J.; Jiang, H.; Zhu, C.; Hu, X.; Shi, Y.; Liu, T.; Rao, W.; Chan, F.T.S. Agriculture IoT: Emerging Trends, Cooperation Networks, and Outlook. IEEE Wirel. Commun. 2019 , 26 , 56–63. [ Google Scholar ] [ CrossRef ]
- Dholu, M.; Ghodinde, K.A. Internet of Things (IoT) for Precision Agriculture Application. In Proceedings of the 2018 2nd International Conference on Trends in Electronics and Informatics (ICOEI), Tirunelveli, India, 11–12 May 2018; pp. 339–342. [ Google Scholar ] [ CrossRef ]
- Sharma, A.; Jain, A.; Gupta, P.; Chowdary, V. Machine Learning Applications for Precision Agriculture: A Comprehensive Review. IEEE Access 2021 , 9 , 4843–4873. [ Google Scholar ] [ CrossRef ]
- Kok, Z.H.; Mohamed Shariff, A.R.; Alfatni, M.S.M.; Khairunniza-Bejo, S. Support Vector Machine in Precision Agriculture: A Review. Comput. Electron. Agric. 2021 , 191 , 106546. [ Google Scholar ] [ CrossRef ]
- Kour, V.P.; Arora, S. Recent Developments of the Internet of Things in Agriculture: A Survey. IEEE Access 2020 , 8 , 129924–129957. [ Google Scholar ] [ CrossRef ]
- Ayoub Shaikh, T.; Rasool, T.; Rasheed Lone, F. Towards Leveraging the Role of Machine Learning and Artificial Intelligence in Precision Agriculture and Smart Farming. Comput. Electron. Agric. 2022 , 198 , 107119. [ Google Scholar ] [ CrossRef ]
- Kerry, R.; Escolà, A. (Eds.) Sensing Approaches for Precision Agriculture ; Springer International Publishing: Cham, Switzerland, 2021; ISBN 978-3-030-78430-0. [ Google Scholar ]
- Chlingaryan, A.; Sukkarieh, S.; Whelan, B. Machine Learning Approaches for Crop Yield Prediction and Nitrogen Status Estimation in Precision Agriculture: A Review. Comput. Electron. Agric. 2018 , 151 , 61–69. [ Google Scholar ] [ CrossRef ]
- Karunathilake, E.M.B.M.; Le, A.T.; Heo, S.; Chung, Y.S.; Mansoor, S. The Path to Smart Farming: Innovations and Opportunities in Precision Agriculture. Agriculture 2023 , 13 , 1593. [ Google Scholar ] [ CrossRef ]
- Sadowski, S.; Spachos, P. Wireless Technologies for Smart Agricultural Monitoring Using Internet of Things Devices with Energy Harvesting Capabilities. Comput. Electron. Agric. 2020 , 172 , 105338. [ Google Scholar ] [ CrossRef ]
- Sharma, H.; Haque, A.; Jaffery, Z.A. Maximization of Wireless Sensor Network Lifetime Using Solar Energy Harvesting for Smart Agriculture Monitoring. Ad Hoc Netw. 2019 , 94 , 101966. [ Google Scholar ] [ CrossRef ]
- Rathinam, D.D.K.; Surendran, D.; Shilpa, A.; Grace, A.S.; Sherin, J. Modern Agriculture Using Wireless Sensor Network (WSN). In Proceedings of the 2019 5th International Conference on Advanced Computing & Communication Systems (ICACCS), Coimbatore, India, 15–16 March 2019; pp. 515–519. [ Google Scholar ] [ CrossRef ]
- Bwambale, E.; Abagale, F.K.; Anornu, G.K. Smart Irrigation Monitoring and Control Strategies for Improving Water Use Efficiency in Precision Agriculture: A Review. Agric. Water Manag. 2022 , 260 , 107324. [ Google Scholar ] [ CrossRef ]
- Huong, T.T.; Huu Thanh, N.; Van, N.T.; Tien Dat, N.; Van Long, N.; Marshall, A. Water and Energy-Efficient Irrigation Based on Markov Decision Model for Precision Agriculture. In Proceedings of the 2018 IEEE Seventh International Conference on Communications and Electronics (ICCE), Hue, Vietnam, 18–20 July 2018; pp. 51–56. [ Google Scholar ] [ CrossRef ]
- Torky, M.; Hassanein, A.E. Integrating Blockchain and the Internet of Things in Precision Agriculture: Analysis, Opportunities, and Challenges. Comput. Electron. Agric. 2020 , 178 , 105476. [ Google Scholar ] [ CrossRef ]
- Thakur, D.; Kumar, Y.; Kumar, A.; Singh, P.K. Applicability of Wireless Sensor Networks in Precision Agriculture: A Review. Wirel. Pers. Commun. 2019 , 107 , 471–512. [ Google Scholar ] [ CrossRef ]
- Narayanavaram, B.; Reddy, E.M.K.; Rashmi, M.R. Arduino Based Automation of Agriculture A Step towards Modernization of Agriculture. In Proceedings of the 2020 4th International Conference on Electronics, Communication and Aerospace Technology (ICECA), Coimbatore, India, 5–7 November 2020; pp. 1184–1189. [ Google Scholar ] [ CrossRef ]
- Cicioğlu, M.; Çalhan, A. Smart Agriculture with Internet of Things in Cornfields. Comput. Electr. Eng. 2021 , 90 , 106982. [ Google Scholar ] [ CrossRef ]
- Shaikh, T.A.; Mir, W.A.; Rasool, T.; Sofi, S. Machine Learning for Smart Agriculture and Precision Farming: Towards Making the Fields Talk. Arch. Comput. Methods Eng. 2022 , 29 , 4557–4597. [ Google Scholar ] [ CrossRef ]
- Anisi, M.H.; Abdul-Salaam, G.; Abdullah, A.H. A Survey of Wireless Sensor Network Approaches and Their Energy Consumption for Monitoring Farm Fields in Precision Agriculture. Precis. Agric. 2015 , 16 , 216–238. [ Google Scholar ] [ CrossRef ]
- Ferrández-Pastor, F.; García-Chamizo, J.; Nieto-Hidalgo, M.; Mora-Martínez, J. Precision Agriculture Design Method Using a Distributed Computing Architecture on Internet of Things Context. Sensors 2018 , 18 , 1731. [ Google Scholar ] [ CrossRef ] [ PubMed ]
- Santilli, M.; Carpio, R.F.; Gasparri, A. A Framework for Tasks Allocation and Scheduling in Precision Agriculture Settings. In Proceedings of the 2021 20th International Conference on Advanced Robotics (ICAR), Ljubljana, Slovenia, 6–10 December 2021; pp. 996–1002. [ Google Scholar ] [ CrossRef ]
- Sorbelli, F.B.; Pinotti, C.M.; Silvestri, S.; Das, S.K. Measurement Errors in Range-Based Localization Algorithms for UAVs: Analysis and Experimentation. IEEE Trans. Mob. Comput. 2022 , 21 , 1291–1304. [ Google Scholar ] [ CrossRef ]
- Rudolph, S.; Marchant, B.P.; Weihermüller, L.; Vereecken, H. Assessment of the Position Accuracy of a Single-Frequency GPS Receiver Designed for Electromagnetic Induction Surveys. Precis. Agric. 2019 , 20 , 19–39. [ Google Scholar ] [ CrossRef ]
- Filgueiras, R.; Almeida, T.S.; Mantovani, E.C.; Dias, S.H.B.; Fernandes-Filho, E.I.; da Cunha, F.F.; Venancio, L.P. Soil Water Content and Actual Evapotranspiration Predictions Using Regression Algorithms and Remote Sensing Data. Agric. Water Manag. 2020 , 241 , 106346. [ Google Scholar ] [ CrossRef ]
- Jo, H.-S.; Park, C.; Lee, E.; Choi, H.K.; Park, J. Path Loss Prediction Based on Machine Learning Techniques: Principal Component Analysis, Artificial Neural Network, and Gaussian Process. Sensors 2020 , 20 , 1927. [ Google Scholar ] [ CrossRef ] [ PubMed ]
- Mishra, P.; Nikzad-Langerodi, R. Partial Least Square Regression versus Domain Invariant Partial Least Square Regression with Application to Near-Infrared Spectroscopy of Fresh Fruit. Infrared Phys. Technol. 2020 , 111 , 103547. [ Google Scholar ] [ CrossRef ]
- Waluyo; Widura, A.; Hadiatna, F.; Anugerah, D. Fuzzy-Based Smart Farming and Consumed Energy Comparison Using the Internet of Things. IEEE Access 2023 , 11 , 69241–69251. [ Google Scholar ] [ CrossRef ]
- Rajput, A.; Kumaravelu, V.B. Fuzzy Logic–Based Distributed Clustering Protocol to Improve Energy Efficiency and Stability of Wireless Smart Sensor Networks for Farmland Monitoring Systems. Int. J. Commun. Syst. 2020 , 33 , e4239. [ Google Scholar ] [ CrossRef ]
- Zhang, B.; Wang, S.; Wang, M. Area Double Cluster Head APTEEN Routing Protocol-Based Particle Swarm Optimization for Wireless Sensor Networks. EURASIP J. Wirel. Commun. Netw. 2020 , 2020 , 155. [ Google Scholar ] [ CrossRef ]
- Triantafyllou, A.; Tsouros, D.C.; Sarigiannidis, P.; Bibi, S. An Architecture Model for Smart Farming. In Proceedings of the 2019 15th International Conference on Distributed Computing in Sensor Systems (DCOSS), Santorini, Greece, 29–31 May 2019; pp. 385–392. [ Google Scholar ] [ CrossRef ]
- Bauer, J.; Aschenbruck, N. Design and Implementation of an Agricultural Monitoring System for Smart Farming. In Proceedings of the 2018 IoT Vertical and Topical Summit on Agriculture—Tuscany (IOT Tuscany), Tuscany, Italy, 8–9 May 2018; pp. 1–6. [ Google Scholar ] [ CrossRef ]
- Maroli, A.; Narwane, V.S.; Gardas, B.B. Applications of IoT for Achieving Sustainability in Agricultural Sector: A Comprehensive Review. J. Environ. Manag. 2021 , 298 , 113488. [ Google Scholar ] [ CrossRef ] [ PubMed ]
- Paul, K.; Chatterjee, S.S.; Pai, P.; Varshney, A.; Juikar, S.; Prasad, V.; Bhadra, B.; Dasgupta, S. Viable Smart Sensors and Their Application in Data Driven Agriculture. Comput. Electron. Agric. 2022 , 198 , 107096. [ Google Scholar ] [ CrossRef ]
- Bhoyar, P.; Sahare, P.; Dhok, S.B.; Deshmukh, R.B. Communication Technologies and Security Challenges for Internet of Things: A Comprehensive Review. AEU—Int. J. Electron. Commun. 2019 , 99 , 81–99. [ Google Scholar ] [ CrossRef ]
- Singh, D.K.; Sobti, R. Wireless Communication Technologies for Internet of Things and Precision Agriculture: A Review. In Proceedings of the 2021 6th International Conference on Signal Processing, Computing and Control (ISPCC), Solan, India, 7–9 October 2021; pp. 765–769. [ Google Scholar ] [ CrossRef ]
- Dai, J.; Sugano, M. Low-Cost Sensor Network for Collecting Real-Time Data for Agriculture by Combining Energy Harvesting and LPWA Technology. In Proceedings of the 2019 IEEE Global Humanitarian Technology Conference (GHTC), Seattle, WA, USA, 17–20 October 2019; pp. 1–4. [ Google Scholar ] [ CrossRef ]
- Tao, W.; Zhao, L.; Wang, G.; Liang, R. Review of the Internet of Things Communication Technologies in Smart Agriculture and Challenges. Comput. Electron. Agric. 2021 , 189 , 106352. [ Google Scholar ] [ CrossRef ]
- Elijah, O.; Rahman, T.A.; Orikumhi, I.; Leow, C.Y.; Hindia, M.H.D.N. An Overview of Internet of Things (IoT) and Data Analytics in Agriculture: Benefits and Challenges. IEEE Internet Things J. 2018 , 5 , 3758–3773. [ Google Scholar ] [ CrossRef ]
- Afaqui, M.S.; Garcia-Villegas, E.; Lopez-Aguilera, E. IEEE 802.11ax: Challenges and Requirements for Future High Efficiency WiFi. IEEE Wirel. Commun. 2017 , 24 , 130–137. [ Google Scholar ] [ CrossRef ]
- Deep, V.; Elarabi, T. Efficient IEEE 802.15.4 ZigBee Standard Hardware Design for IoT Applications. In Proceedings of the 2017 International Conference on Signals and Systems (ICSigSys), Bali, Indonesia, 16–18 May 2017; pp. 261–265. [ Google Scholar ]
- Fraile, L.P.; Tsampas, S.; Mylonas, G.; Amaxilatis, D. A Comparative Study of LoRa and IEEE 802.15.4-Based IoT Deployments Inside School Buildings. IEEE Access 2020 , 8 , 160957–160981. [ Google Scholar ] [ CrossRef ]
- Bacco, M.; Berton, A.; Gotta, A.; Caviglione, L. IEEE 802.15.4 Air-Ground UAV Communications in Smart Farming Scenarios. IEEE Commun. Lett. 2018 , 22 , 1910–1913. [ Google Scholar ] [ CrossRef ]
- Hu, J.-G.; Mei, W.-Z.; Wu, J.; Li, J.-W.; Wang, D.-M. A Fully Integrated RFID Reader SoC. Micromachines 2023 , 14 , 1691. [ Google Scholar ] [ CrossRef ] [ PubMed ]
- Pesovic, U.; Djurasevic, S.; Lukovic, V.; Planinsic, P. Interference Classification for IEEE 802.15.4 Networks. In Proceedings of the 2020 International Conference on Broadband Communications for Next Generation Networks and Multimedia Applications (CoBCom), Graz, Austria, 7–9 July 2020; pp. 1–4. [ Google Scholar ]
- Wang, Y.-P.E.; Lin, X.; Adhikary, A.; Grovlen, A.; Sui, Y.; Blankenship, Y.; Bergman, J.; Razaghi, H.S. A Primer on 3GPP Narrowband Internet of Things. IEEE Commun. Mag. 2017 , 55 , 117–123. [ Google Scholar ] [ CrossRef ]
- Savitha, A.C.; Aravind, H.S.; Jayaram, M.N.; Harshith, K.; Nagaraj, V. Monitoring Plant Growth in a Greenhouse Using IoT with the Energy-Efficient Wireless Sensor Network. In Recent Innovations in Artificial Intelligence and Smart Applications ; Studies in Computational Intelligence; Al-Emran, M., Shaalan, K., Eds.; Springer: Cham, Switzerland, 2022; Volume 1061, pp. 85–104. [ Google Scholar ] [ CrossRef ]
- Soheli, S.J.; Jahan, N.; Hossain, M.B.; Adhikary, A.; Khan, A.R.; Wahiduzzaman, M. Smart Greenhouse Monitoring System Using Internet of Things and Artificial Intelligence. Wirel. Pers. Commun. 2022 , 124 , 3603–3634. [ Google Scholar ] [ CrossRef ]
- Touhami, A.; Benahmed, K.; Bounaama, F. Monitoring of Greenhouse Based on Internet of Things and Wireless Sensor Network. In Proceedings of the 8th International Conference on Sciences of Electronics, Technologies of Information and Telecommunications (SETIT’18), SETIT 2018, Genoa, Italy and Hammamet, Tunisia, 18–20 December 2018 ; Smart Innovation, Systems and Technologies; Bouhlel, M., Rovetta, S., Eds.; Springer: Cham, Switzerland, 2020; Volume 147, pp. 281–289. [ Google Scholar ] [ CrossRef ]
- Qian, W.; Zhen, J.; Shen, T. ONENET-Based Greenhouse Remote Monitoring and Control System for Greenhouse Environment. In Communications, Signal Processing, and Systems. CSPS 2019. Lecture Notes in Electrical Engineering ; Liang, Q., Wang, W., Liu, X., Na, Z., Jia, M., Zhang, B., Eds.; Springer: Singapore, 2020; Volume 571, pp. 1703–1708. [ Google Scholar ] [ CrossRef ]
- Ali, T.A.A.; Choksi, V.; Potdar, M.B. Precision Agriculture Monitoring System Using Green Internet of Things (G-IoT). In Proceedings of the 2018 2nd International Conference on Trends in Electronics and Informatics (ICOEI), Tirunelveli, India, 11–12 May 2018; pp. 481–487. [ Google Scholar ] [ CrossRef ]
- Vuran, M.C.; Salam, A.; Wong, R.; Irmak, S. Internet of Underground Things in Precision Agriculture: Architecture and Technology Aspects. Ad Hoc Netw. 2018 , 81 , 160–173. [ Google Scholar ] [ CrossRef ]
- Reka, S.S.; Chezian, B.K.; Chandra, S.S. A Novel Approach of IoT-Based Smart Greenhouse Farming System. In Green Buildings and Sustainable Engineering ; Springer Transactions in Civil and Environmental Engineering; Drück, H., Pillai, R., Tharian, M., Majeed, A., Eds.; Springer: Singapore, 2019; pp. 227–235. [ Google Scholar ] [ CrossRef ]
- Keswani, B.; Mohapatra, A.G.; Mohanty, A.; Khanna, A.; Rodrigues, J.J.P.C.; Gupta, D.; de Albuquerque, V.H.C. Adapting Weather Conditions Based IoT Enabled Smart Irrigation Technique in Precision Agriculture Mechanisms. Neural Comput. Appl. 2019 , 31 , 277–292. [ Google Scholar ] [ CrossRef ]
- Erler, A.; Riebe, D.; Beitz, T.; Löhmannsröben, H.-G.; Gebbers, R. Soil Nutrient Detection for Precision Agriculture Using Handheld Laser-Induced Breakdown Spectroscopy (LIBS) and Multivariate Regression Methods (PLSR, Lasso and GPR). Sensors 2020 , 20 , 418. [ Google Scholar ] [ CrossRef ] [ PubMed ]
- Chen, W.-L.; Lin, Y.-B.; Lin, Y.-W.; Chen, R.; Liao, J.-K.; Ng, F.-L.; Chan, Y.-Y.; Liu, Y.-C.; Wang, C.-C.; Chiu, C.-H.; et al. AgriTalk: IoT for Precision Soil Farming of Turmeric Cultivation. IEEE Internet Things J. 2019 , 6 , 5209–5223. [ Google Scholar ] [ CrossRef ]
- Wohwe Sambo, D.; Forster, A.; Yenke, B.O.; Sarr, I.; Gueye, B.; Dayang, P. Wireless Underground Sensor Networks Path Loss Model for Precision Agriculture (WUSN-PLM). IEEE Sens. J. 2020 , 20 , 5298–5313. [ Google Scholar ] [ CrossRef ]
- Tripodi, P.; Massa, D.; Venezia, A.; Cardi, T. Sensing Technologies for Precision Phenotyping in Vegetable Crops: Current Status and Future Challenges. Agronomy 2018 , 8 , 57. [ Google Scholar ] [ CrossRef ]
- Sun, H.; Li, M.; Zhang, Q. Crop Sensing in Precision Agriculture. In Soil and Crop Sensing for Precision Crop Production. Agriculture Automation and Control ; Li, M., Yang, C., Zhang, Q., Eds.; Springer: Cham, Switzerland, 2022; pp. 251–293. [ Google Scholar ] [ CrossRef ]
- Brewer, K.; Clulow, A.; Sibanda, M.; Gokool, S.; Naiken, V.; Mabhaudhi, T. Predicting the Chlorophyll Content of Maize over Phenotyping as a Proxy for Crop Health in Smallholder Farming Systems. Remote Sens. 2022 , 14 , 518. [ Google Scholar ] [ CrossRef ]
- Lozoya, C.; Eyzaguirre, E.; Espinoza, J.; Montes-Fonseca, S.L.; Rosas-Perez, G. Spectral Vegetation Index Sensor Evaluation for Greenhouse Precision Agriculture. In Proceedings of the 2019 IEEE SENSORS, Montreal, QC, Canada, 27–30 October 2019; pp. 1–4. [ Google Scholar ] [ CrossRef ]
- Wahab, I.; Hall, O.; Jirström, M. Remote Sensing of Yields: Application of UAV Imagery-Derived NDVI for Estimating Maize Vigor and Yields in Complex Farming Systems in Sub-Saharan Africa. Drones 2018 , 2 , 28. [ Google Scholar ] [ CrossRef ]
- Alam, M.; Alam, M.S.; Roman, M.; Tufail, M.; Khan, M.U.; Khan, M.T. Real-Time Machine-Learning Based Crop/Weed Detection and Classification for Variable-Rate Spraying in Precision Agriculture. In Proceedings of the 2020 7th International Conference on Electrical and Electronics Engineering (ICEEE), Antalya, Turkey, 14–16 April 2020; pp. 273–280. [ Google Scholar ] [ CrossRef ]
- Monteiro, A.; Santos, S.; Gonçalves, P. Precision Agriculture for Crop and Livestock Farming—Brief Review. Animals 2021 , 11 , 2345. [ Google Scholar ] [ CrossRef ] [ PubMed ]
- Chandra Pandey, P.; Tripathi, A.K.; Sharma, J.K. An Evaluation of GPS Opportunity in Market for Precision Agriculture. In GPS and GNSS Technology in Geosciences ; Elsevier: Amsterdam, The Netherlands, 2021; pp. 337–349. [ Google Scholar ] [ CrossRef ]
- Mazzia, V.; Comba, L.; Khaliq, A.; Chiaberge, M.; Gay, P. UAV and Machine Learning Based Refinement of a Satellite-Driven Vegetation Index for Precision Agriculture. Sensors 2020 , 20 , 2530. [ Google Scholar ] [ CrossRef ] [ PubMed ]
- Brook, A.; De Micco, V.; Battipaglia, G.; Erbaggio, A.; Ludeno, G.; Catapano, I.; Bonfante, A. A Smart Multiple Spatial and Temporal Resolution System to Support Precision Agriculture from Satellite Images: Proof of Concept on Aglianico Vineyard. Remote Sens. Environ. 2020 , 240 , 111679. [ Google Scholar ] [ CrossRef ]
- Mukherjee, A.; Misra, S.; Raghuwanshi, N.S. A Survey of Unmanned Aerial Sensing Solutions in Precision Agriculture. J. Netw. Comput. Appl. 2019 , 148 , 102461. [ Google Scholar ] [ CrossRef ]
- Mancini, A.; Frontoni, E.; Zingaretti, P. Satellite and UAV Data for Precision Agriculture Applications. In Proceedings of the 2019 International Conference on Unmanned Aircraft Systems (ICUAS), Atlanta, GA, USA, 11–14 June 2019; pp. 491–497. [ Google Scholar ] [ CrossRef ]
- Fisher, J.B.; Lee, B.; Purdy, A.J.; Halverson, G.H.; Dohlen, M.B.; Cawse-Nicholson, K.; Wang, A.; Anderson, R.G.; Aragon, B.; Arain, M.A.; et al. ECOSTRESS: NASA’s Next Generation Mission to Measure Evapotranspiration From the International Space Station. Water Resour. Res. 2020 , 56 , e2019WR026058. [ Google Scholar ] [ CrossRef ]
- Vecchiotti, F.; Tilch, N.; Kociu, A. The Use of TERRA-ASTER Satellite for Landslide Detection. Geosciences 2021 , 11 , 258. [ Google Scholar ] [ CrossRef ]
- Han, Y.; Bai, X.; Shao, W.; Wang, J. Retrieval of Soil Moisture by Integrating Sentinel-1A and MODIS Data over Agricultural Fields. Water 2020 , 12 , 1726. [ Google Scholar ] [ CrossRef ]
- Bukowiecki, J.; Rose, T.; Kage, H. Sentinel-2 Data for Precision Agriculture?—A UAV-Based Assessment. Sensors 2021 , 21 , 2861. [ Google Scholar ] [ CrossRef ] [ PubMed ]
- Onojeghuo, A.O.; Blackburn, G.A.; Huang, J.; Kindred, D.; Huang, W. Applications of Satellite ‘Hyper-Sensing’ in Chinese Agriculture: Challenges and Opportunities. Int. J. Appl. Earth Obs. Geoinf. 2018 , 64 , 62–86. [ Google Scholar ] [ CrossRef ]
- Dong, T.; Shang, J.; Liu, J.; Qian, B.; Jing, Q.; Ma, B.; Huffman, T.; Geng, X.; Sow, A.; Shi, Y.; et al. Using RapidEye Imagery to Identify Within-Field Variability of Crop Growth and Yield in Ontario, Canada. Precis. Agric. 2019 , 20 , 1231–1250. [ Google Scholar ] [ CrossRef ]
- de Lara, A.; Longchamps, L.; Khosla, R. Soil Water Content and High-Resolution Imagery for Precision Irrigation: Maize Yield. Agronomy 2019 , 9 , 174. [ Google Scholar ] [ CrossRef ]
- Mulla, D.J. Satellite Remote Sensing for Precision Agriculture. In Sensing Approaches for Precision Agriculture ; Kerry, R., Escolà, A., Eds.; Progress in Precision Agriculture; Springer: Cham, Switzerland, 2021. [ Google Scholar ] [ CrossRef ]
- García, L.; Parra, L.; Jimenez, J.M.; Lloret, J.; Mauri, P.V.; Lorenz, P. DronAway: A Proposal on the Use of Remote Sensing Drones as Mobile Gateway for WSN in Precision Agriculture. Appl. Sci. 2020 , 10 , 6668. [ Google Scholar ] [ CrossRef ]
- Kpienbaareh, D.; Kansanga, M.; Luginaah, I. Examining the Potential of Open Source Remote Sensing for Building Effective Decision Support Systems for Precision Agriculture in Resource-Poor Settings. GeoJournal 2019 , 84 , 1481–1497. [ Google Scholar ] [ CrossRef ]
- Toscano, P.; Castrignanò, A.; Di Gennaro, S.F.; Vonella, A.V.; Ventrella, D.; Matese, A. A Precision Agriculture Approach for Durum Wheat Yield Assessment Using Remote Sensing Data and Yield Mapping. Agronomy 2019 , 9 , 437. [ Google Scholar ] [ CrossRef ]
- Han, X.; Thomasson, J.A.; Wang, T.; Swaminathan, V. Autonomous Mobile Ground Control Point Improves Accuracy of Agricultural Remote Sensing through Collaboration with UAV. Inventions 2020 , 5 , 12. [ Google Scholar ] [ CrossRef ]
- González-García, J.; Swenson, R.L.; Gómez-Espinosa, A. Real-Time Kinematics Applied at Unmanned Aerial Vehicles Positioning for Orthophotography in Precision Agriculture. Comput. Electron. Agric. 2020 , 177 , 105695. [ Google Scholar ] [ CrossRef ]
- García, L.; Parra, L.; Jimenez, J.M.; Lloret, J.; Lorenz, P. IoT-Based Smart Irrigation Systems: An Overview on the Recent Trends on Sensors and IoT Systems for Irrigation in Precision Agriculture. Sensors 2020 , 20 , 1042. [ Google Scholar ] [ CrossRef ] [ PubMed ]
- Meeradevi; Supreetha, M.A.; Mundada, M.R.; Pooja, J.N. Design of a Smart Water-Saving Irrigation System for Agriculture Based on a Wireless Sensor Network for Better Crop Yield. In Proceedings of the ICCCE 2018, Hyderabad, India, 24–25 January 2018; Lecture Notes in Electrical Engineering. Kumar, A., Mozar, S., Eds.; Springer: Singapore, 2018; Volume 500, pp. 93–104. [ Google Scholar ] [ CrossRef ]
- Bonfante, A.; Monaco, E.; Manna, P.; De Mascellis, R.; Basile, A.; Buonanno, M.; Cantilena, G.; Esposito, A.; Tedeschi, A.; De Michele, C.; et al. LCIS DSS—An Irrigation Supporting System for Water Use Efficiency Improvement in Precision Agriculture: A Maize Case Study. Agric. Syst. 2019 , 176 , 102646. [ Google Scholar ] [ CrossRef ]
- Sanjeevi, P.; Prasanna, S.; Siva Kumar, B.; Gunasekaran, G.; Alagiri, I.; Vijay Anand, R. Precision Agriculture and Farming Using Internet of Things Based on Wireless Sensor Network. Trans. Emerg. Telecommun. Technol. 2020 , 31 , e3978. [ Google Scholar ] [ CrossRef ]
- Placidi, P.; Morbidelli, R.; Fortunati, D.; Papini, N.; Gobbi, F.; Scorzoni, A. Monitoring Soil and Ambient Parameters in the IoT Precision Agriculture Scenario: An Original Modeling Approach Dedicated to Low-Cost Soil Water Content Sensors. Sensors 2021 , 21 , 5110. [ Google Scholar ] [ CrossRef ] [ PubMed ]
- Kashyap, P.K.; Kumar, S.; Jaiswal, A.; Prasad, M.; Gandomi, A.H. Towards Precision Agriculture: IoT-Enabled Intelligent Irrigation Systems Using Deep Learning Neural Network. IEEE Sens. J. 2021 , 21 , 17479–17491. [ Google Scholar ] [ CrossRef ]
- Yang, C. Remote Sensing and Precision Agriculture Technologies for Crop Disease Detection and Management with a Practical Application Example. Engineering 2020 , 6 , 528–532. [ Google Scholar ] [ CrossRef ]
- Che’Ya, N.N.; Mohidem, N.A.; Roslin, N.A.; Saberioon, M.; Tarmidi, M.Z.; Arif Shah, J.; Fazlil Ilahi, W.F.; Man, N. Mobile Computing for Pest and Disease Management Using Spectral Signature Analysis: A Review. Agronomy 2022 , 12 , 967. [ Google Scholar ] [ CrossRef ]
- Albanese, A.; Nardello, M.; Brunelli, D. Automated Pest Detection With DNN on the Edge for Precision Agriculture. IEEE J. Emerg. Sel. Top. Circuits Syst. 2021 , 11 , 458–467. [ Google Scholar ] [ CrossRef ]
- Lloret, J.; Sendra, S.; Garcia, L.; Jimenez, J.M. A Wireless Sensor Network Deployment for Soil Moisture Monitoring in Precision Agriculture. Sensors 2021 , 21 , 7243. [ Google Scholar ] [ CrossRef ] [ PubMed ]
- Brunelli, D.; Albanese, A.; d’Acunto, D.; Nardello, M. Energy Neutral Machine Learning Based IoT Device for Pest Detection in Precision Agriculture. IEEE Internet Things Mag. 2019 , 2 , 10–13. [ Google Scholar ] [ CrossRef ]
- Morais, R.; Silva, N.; Mendes, J.; Adão, T.; Pádua, L.; López-Riquelme, J.A.; Pavón-Pulido, N.; Sousa, J.J.; Peres, E. MySense: A Comprehensive Data Management Environment to Improve Precision Agriculture Practices. Comput. Electron. Agric. 2019 , 162 , 882–894. [ Google Scholar ] [ CrossRef ]
- Deng, L.; Mao, Z.; Li, X.; Hu, Z.; Duan, F.; Yan, Y. UAV-Based Multispectral Remote Sensing for Precision Agriculture: A Comparison between Different Cameras. ISPRS J. Photogramm. Remote Sens. 2018 , 146 , 124–136. [ Google Scholar ] [ CrossRef ]
- Karimi, N.; Arabhosseini, A.; Karimi, M.; Kianmehr, M.H. Web-Based Monitoring System Using Wireless Sensor Networks for Traditional Vineyards and Grape Drying Buildings. Comput. Electron. Agric. 2018 , 144 , 269–283. [ Google Scholar ] [ CrossRef ]
- Akhter, R.; Sofi, S.A. Precision Agriculture Using IoT Data Analytics and Machine Learning. J. King Saud Univ.—Comput. Inf. Sci. 2022 , 34 , 5602–5618. [ Google Scholar ] [ CrossRef ]
- Feng, X.; Yan, F.; Liu, X. Study of Wireless Communication Technologies on Internet of Things for Precision Agriculture. Wirel. Pers. Commun. 2019 , 108 , 1785–1802. [ Google Scholar ] [ CrossRef ]
- Shadrin, D.; Menshchikov, A.; Somov, A.; Bornemann, G.; Hauslage, J.; Fedorov, M. Enabling Precision Agriculture Through Embedded Sensing With Artificial Intelligence. IEEE Trans. Instrum. Meas. 2020 , 69 , 4103–4113. [ Google Scholar ] [ CrossRef ]
- Tiglao, N.M.; Alipio, M.; Balanay, J.V.; Saldivar, E.; Tiston, J.L. Agrinex: A Low-Cost Wireless Mesh-Based Smart Irrigation System. Measurement 2020 , 161 , 107874. [ Google Scholar ] [ CrossRef ]
- Singh, R.; Aernouts, M.; De Meyer, M.; Weyn, M.; Berkvens, R. Leveraging LoRaWAN Technology for Precision Agriculture in Greenhouses. Sensors 2020 , 20 , 1827. [ Google Scholar ] [ CrossRef ] [ PubMed ]
- Shrishti, R.; Dubey, A.K.; Upadhyay, D. Precision Agriculture Using Cloud-Based Mobile Application for Sensing and Monitoring of Farms. In Emerging Technologies in Data Mining and Information Security ; Hassanien, A.E., Bhattacharyya, S., Chakrabati, S., Bhattacharya, A., Dutta, S., Eds.; Advances in Intelligent Systems and Computing; Springer: Singapore, 2021; Volume 1300, pp. 417–425. [ Google Scholar ] [ CrossRef ]
- Ampatzidis, Y.; Partel, V.; Costa, L. Agroview: Cloud-Based Application to Process, Analyze and Visualize UAV-Collected Data for Precision Agriculture Applications Utilizing Artificial Intelligence. Comput. Electron. Agric. 2020 , 174 , 105457. [ Google Scholar ] [ CrossRef ]
- Suresh Kumar, K.; Balakrishnan, S.; Janet, J. A Cloud-Based Prototype for the Monitoring and Predicting of Data in Precision Agriculture Based on Internet of Everything. J. Ambient. Intell. Humaniz. Comput. 2021 , 12 , 8719–8730. [ Google Scholar ] [ CrossRef ]
- Huang, Y.; Chen, Z.; Yu, T.; Huang, X.; Gu, X. Agricultural Remote Sensing Big Data: Management and Applications. J. Integr. Agric. 2018 , 17 , 1915–1931. [ Google Scholar ] [ CrossRef ]
- Filippi, P.; Jones, E.J.; Wimalathunge, N.S.; Somarathna, P.D.S.N.; Pozza, L.E.; Ugbaje, S.U.; Jephcott, T.G.; Paterson, S.E.; Whelan, B.M.; Bishop, T.F.A. An Approach to Forecast Grain Crop Yield Using Multi-Layered, Multi-Farm Data Sets and Machine Learning. Precis. Agric. 2019 , 20 , 1015–1029. [ Google Scholar ] [ CrossRef ]
- Shafi, U.; Mumtaz, R.; García-Nieto, J.; Hassan, S.A.; Zaidi, S.A.R.; Iqbal, N. Precision Agriculture Techniques and Practices: From Considerations to Applications. Sensors 2019 , 19 , 3796. [ Google Scholar ] [ CrossRef ]
- González-Teruel, J.; Torres-Sánchez, R.; Blaya-Ros, P.; Toledo-Moreo, A.; Jiménez-Buendía, M.; Soto-Valles, F. Design and Calibration of a Low-Cost SDI-12 Soil Moisture Sensor. Sensors 2019 , 19 , 491. [ Google Scholar ] [ CrossRef ] [ PubMed ]
- Vohra, A.; Pandey, N.; Khatri, S.K. Prevention of Agricultural Commodities Using Artificial Intelligence. In Proceedings of the 2019 2nd International Conference on Power Energy, Environment and Intelligent Control (PEEIC), Greater Noida, India, 18–19 October 2019; pp. 52–54. [ Google Scholar ] [ CrossRef ]
- Louki, I.I.; Al-Omran, A.M. Calibration of Soil Moisture Sensors (ECH2O-5TE) in Hot and Saline Soils with New Empirical Equation. Agronomy 2022 , 13 , 51. [ Google Scholar ] [ CrossRef ]
- Wu, B.; Han, H.; He, J.; Zhang, J.; Cui, L.; Jia, Z.; Yang, W. Field-Specific Calibration and Evaluation of ECH 2 O EC-5 Sensor for Sandy Soils. Soil Sci. Soc. Am. J. 2014 , 78 , 70–78. [ Google Scholar ] [ CrossRef ]
- López Riquelme, J.A.; Soto, F.; Suardíaz, J.; Sánchez, P.; Iborra, A.; Vera, J.A. Wireless Sensor Networks for Precision Horticulture in Southern Spain. Comput. Electron. Agric. 2009 , 68 , 25–35. [ Google Scholar ] [ CrossRef ]
- Radi; Murtiningrum; Ngadisih; Muzdrikah, F.S.; Nuha, M.S.; Rizqi, F.A. Calibration of Capacitive Soil Moisture Sensor (SKU:SEN0193). In Proceedings of the 2018 4th International Conference on Science and Technology (ICST), Yogyakarta, Indonesia, 7–8 August 2018; pp. 1–6. [ Google Scholar ] [ CrossRef ]
- Goap, A.; Sharma, D.; Shukla, A.K.; Rama Krishna, C. An IoT Based Smart Irrigation Management System Using Machine Learning and Open Source Technologies. Comput. Electron. Agric. 2018 , 155 , 41–49. [ Google Scholar ] [ CrossRef ]
- Ahmad, Y.A.; Surya Gunawan, T.; Mansor, H.; Hamida, B.A.; Fikri Hishamudin, A.; Arifin, F. On the Evaluation of DHT22 Temperature Sensor for IoT Application. In Proceedings of the 2021 8th International Conference on Computer and Communication Engineering (ICCCE), Kuala Lumpur, Malaysia, 22–23 June 2021; pp. 131–134. [ Google Scholar ] [ CrossRef ]
- Margret Sharmila, F.; Suryaganesh, P.; Abishek, M.; Benny, U. Iot Based Smart Window Using Sensor Dht11. In Proceedings of the 2019 5th International Conference on Advanced Computing & Communication Systems (ICACCS), Coimbatore, India, 15–16 March 2019; pp. 782–784. [ Google Scholar ] [ CrossRef ]
- McCauley, D.M.; Nackley, L.L.; Kelley, J. Demonstration of a Low-Cost and Open-Source Platform for on-Farm Monitoring and Decision Support. Comput. Electron. Agric. 2021 , 187 , 106284. [ Google Scholar ] [ CrossRef ]
- Gupta, G.S.; Quan, V.M. Multi-Sensor Integrated System for Wireless Monitoring of Greenhouse Environment. In Proceedings of the 2018 IEEE Sensors Applications Symposium (SAS), Seoul, Republic of Korea, 12–14 March 2018; pp. 1–6. [ Google Scholar ] [ CrossRef ]
- Lata, S.; Verma, H.K.; Roy, N.R.; Sagar, K. Development of Greenhouse-Application-Specific Wireless Sensor Node and Graphical User Interface. Int. J. Inf. Technol. 2023 , 15 , 211–218. [ Google Scholar ] [ CrossRef ]
- Imam, M.Y.; Jannat, N.; Bibi, F.; Khan, G.S. Effective Study of Home Plants in Purity of Territory by Utilizing Wireless Sensor System. In Proceedings of the 2019 16th International Bhurban Conference on Applied Sciences and Technology (IBCAST), Islamabad, Pakistan, 8–12 January 2019; pp. 322–327. [ Google Scholar ] [ CrossRef ]
- Ocheltree, T.W.; Mueller, K.M.; Chesus, K.; LeCain, D.R.; Kray, J.A.; Blumenthal, D.M. Identification of Suites of Traits That Explains Drought Resistance and Phenological Patterns of Plants in a Semi-Arid Grassland Community. Oecologia 2020 , 192 , 55–66. [ Google Scholar ] [ CrossRef ]
- Kramlikh, A.V.; Lomaka, I.A. Nanosatellite’s Rotational Motion Parameters Determination Using Light Sensor and Angular Velocity Sensor Measurements. In Proceedings of the 2018 25th Saint Petersburg International Conference on Integrated Navigation Systems (ICINS), Saint Petersburg, Russia, 28–30 May 2018; pp. 1–3. [ Google Scholar ] [ CrossRef ]
- Schefer, T.A.; Kostylev, M. Effect of Hydrogen Gas on the FMR Absorption Amplitude of Pd/Co Layered Films. IEEE Trans. Magn. 2018 , 54 , 4001305. [ Google Scholar ] [ CrossRef ]
- Chandrappa, V.Y.; Chandrappa, V.Y.; Ray, B.; Ray, B.; Ashwath, N.; Ashwath, N.; Shrestha, P.; Shrestha, P. Application of Internet of Things (IoT) to Develop a Smart Watering System for Cairns Parklands—A Case Study. In Proceedings of the 2020 IEEE Region 10 Symposium (TENSYMP), Dhaka, Bangladesh, 5–7 June 2020; pp. 1118–1122. [ Google Scholar ] [ CrossRef ]
- Ma, M.; Liu, Y.; Zhang, Y.; Qin, W.; Wang, Z.; Zhang, Y.; Lu, C.; Lu, Q. In Situ Measurements of Winter Wheat Diurnal Changes in Photosynthesis and Environmental Factors Reveal New Insight into Photosynthesis Improvement by Super-High-Yield Cultivation. J. Integr. Agric. 2021 , 20 , 527–539. [ Google Scholar ] [ CrossRef ]
- Chegoonian, A.M.; Zolfaghari, K.; Leavitt, P.R.; Baulch, H.M.; Duguay, C.R. Improvement of Field Fluorometry Estimates of Chlorophyll a Concentration in a Cyanobacteria-rich Eutrophic Lake. Limnol. Oceanogr. Methods 2022 , 20 , 193–209. [ Google Scholar ] [ CrossRef ]
- Costa-Filho, E.; Chávez, J.L.; Zhang, H.; Andales, A.A. An Optimized Surface Aerodynamic Temperature Approach to Estimate Maize Sensible Heat Flux and Evapotranspiration. Agric. For. Meteorol. 2021 , 311 , 108683. [ Google Scholar ] [ CrossRef ]
- Awawda, J.; Ishaq, I. IoT Smart Irrigation System for Precision Agriculture. In Intelligent Sustainable Systems ; Nagar, A.K., Singh Jat, D., Mishra, D.K., Joshi, A., Eds.; Lecture Notes in Networks and Systems; Springer: Singapore, 2023; Volume 579, pp. 335–346. [ Google Scholar ] [ CrossRef ]
- Suciu, G.; Marcu, I.; Balaceanu, C.; Dobrea, M.; Botezat, E. Efficient IoT System for Precision Agriculture. In Proceedings of the 2019 15th International Conference on Engineering of Modern Electric Systems (EMES), Oradea, Romania, 13–14 June 2019; pp. 173–176. [ Google Scholar ] [ CrossRef ]
- Kamienski, C.; Soininen, J.-P.; Taumberger, M.; Dantas, R.; Toscano, A.; Salmon Cinotti, T.; Filev Maia, R.; Torre Neto, A. Smart Water Management Platform: IoT-Based Precision Irrigation for Agriculture. Sensors 2019 , 19 , 276. [ Google Scholar ] [ CrossRef ] [ PubMed ]
- Boursianis, A.D.; Papadopoulou, M.S.; Gotsis, A.; Wan, S.; Sarigiannidis, P.; Nikolaidis, S.; Goudos, S.K. Smart Irrigation System for Precision Agriculture—The AREThOU5A IoT Platform. IEEE Sens. J. 2021 , 21 , 17539–17547. [ Google Scholar ] [ CrossRef ]
- Anguraj, D.K.; Mandhala, V.N.; Bhattacharyya, D.; Kim, T. Hybrid Neural Network Classification for Irrigation Control in WSN Based Precision Agriculture. J. Ambient. Intell. Humaniz. Comput. 2021 , 1–12. [ Google Scholar ] [ CrossRef ]
- Schneps-Schneppe, M.; Lacis, G. On Smart Greenhouse Issues. In Internet of Things, Smart Spaces, and Next Generation Networks and Systems. In Proceedings of the NEW2AN ruSMART 2021, St. Petersburg, Russia, 26–27 August 2021; Koucheryavy, Y., Balandin, S., Andreev, S., Eds.; Lecture Notes in Computer Science. Springer: Cham, Switzerland, 2022; Volume 13158, pp. 9–21. [ Google Scholar ] [ CrossRef ]
- Du, C.; Zhang, L.; Ma, X.; Lou, X.; Shan, Y.; Li, H.; Zhou, R. A Cotton High-Efficiency Water-Fertilizer Control System Using Wireless Sensor Network for Precision Agriculture. Processes 2021 , 9 , 1693. [ Google Scholar ] [ CrossRef ]
- Kollu, P.K.; Bangare, M.L.; Hari Prasad, P.V.; Bangare, P.M.; Rane, K.P.; Arias-Gonzáles, J.L.; Lalar, S.; Shabaz, M. Internet of Things Driven Multilinear Regression Technique for Fertilizer Recommendation for Precision Agriculture. SN Appl. Sci. 2023 , 5 , 264. [ Google Scholar ] [ CrossRef ]
- Bristow, N.; Rengaraj, S.; Chadwick, D.R.; Kettle, J.; Jones, D.L. Development of a LoRaWAN IoT Node with Ion-Selective Electrode Soil Nitrate Sensors for Precision Agriculture. Sensors 2022 , 22 , 9100. [ Google Scholar ] [ CrossRef ] [ PubMed ]
- Rogovska, N.; Laird, D.A.; Chiou, C.-P.; Bond, L.J. Development of Field Mobile Soil Nitrate Sensor Technology to Facilitate Precision Fertilizer Management. Precis. Agric. 2019 , 20 , 40–55. [ Google Scholar ] [ CrossRef ]
- Freidenreich, A.; Barraza, G.; Jayachandran, K.; Khoddamzadeh, A.A. Precision Agriculture Application for Sustainable Nitrogen Management of Justicia Brandegeana Using Optical Sensor Technology. Agriculture 2019 , 9 , 98. [ Google Scholar ] [ CrossRef ]
- Colaço, A.F.; Bramley, R.G.V. Do Crop Sensors Promote Improved Nitrogen Management in Grain Crops? Field Crops Res. 2018 , 218 , 126–140. [ Google Scholar ] [ CrossRef ]
- Higgins, S.; Schellberg, J.; Bailey, J.S. Improving Productivity and Increasing the Efficiency of Soil Nutrient Management on Grassland Farms in the UK and Ireland Using Precision Agriculture Technology. Eur. J. Agron. 2019 , 106 , 67–74. [ Google Scholar ] [ CrossRef ]
- Kalambukattu, J.G.; Kumar, S.; Arya Raj, R. Digital Soil Mapping in a Himalayan Watershed Using Remote Sensing and Terrain Parameters Employing Artificial Neural Network Model. Environ. Earth Sci. 2018 , 77 , 203. [ Google Scholar ] [ CrossRef ]
- Lee, G.; Wei, Q.; Zhu, Y. Emerging Wearable Sensors for Plant Health Monitoring. Adv. Funct. Mater. 2021 , 31 , 2106475. [ Google Scholar ] [ CrossRef ]
- Huang, Y.; Huang, Y.; Gao, M.; Tian, S.; Xie, S. Wearable Plant Sensors Based on Nanometer-Thick Ag Films on Polyethylene Glycol Terephthalate Substrates for Real-Time Monitoring of Plant Growth. ACS Appl. Nano Mater. 2023 , 6 , 19010–19017. [ Google Scholar ] [ CrossRef ]
- Sharma, R.P.; Dharavath, R.; Edla, D.R. IoFT-FIS: Internet of Farm Things Based Prediction for Crop Pest Infestation Using Optimized Fuzzy Inference System. Internet Things 2023 , 21 , 100658. [ Google Scholar ] [ CrossRef ]
- Koubaa, A.; Aldawood, A.; Saeed, B.; Hadid, A.; Ahmed, M.; Saad, A.; Alkhouja, H.; Ammar, A.; Alkanhal, M. Smart Palm: An IoT Framework for Red Palm Weevil Early Detection. Agronomy 2020 , 10 , 987. [ Google Scholar ] [ CrossRef ]
Click here to enlarge figure
IoT Platform | Application | Reference |
---|---|---|
Phytoprove (2019) | Develop tools for early, rapid, and non-destructive determination of the nitrogen and water supply status of plants. | (accessed on 1 March 2024) |
Telemetry2u | Provides an integrated hardware and software system for measuring integrated temperature and irrigation control. | (accessed on 1 March 2024) |
Sensoterra | Provides an integrated hardware and software system for measuring soil moisture. | (accessed on 1 March 2024) |
Cropx (2013) | Offers an integrated hardware and software system designed for the precise measurement of electrical conductivity, soil moisture, and temperature. | (accessed on 1 March 2024) |
ONFARM | Easily accessible critical information can be viewed in the office or on the go through a user-friendly dashboard. | (accessed on 1 March 2024) |
Phytech | Optimizing irrigation through data-driven insights for enhanced plant health and resource management. | (accessed on 1 March 2024) |
Semios (2020) | Tool for enhancing yields by providing real-time assessments and responses to insect activity, disease, and overall plant health conditions. | (accessed on 1 March 2024) |
Parameters | Standard | Frequency | Data Rate | Transmission Range | Energy Consumption | Cost | Limitations |
---|---|---|---|---|---|---|---|
Wi-Fi | IEEE 802.11 [ ] | 2.4 GHz and 5 GHz bands | 11–50 and 150 Mbps | 20–100 m | High | High | High power consumption, limited range compared to other long-range technologies, and susceptibility to interference. |
ZigBee | Zigbee Alliance IEEE 802.15.4 [ ] | 2.4 GHz and 868/915 MHz bands | 20, 40, and 250 kbps | 10–100 m | Low | Low | Line-of-sight connectivity should exist between the sensor node and the coordinator node. |
Long-Range (LoRa) | Lora Alliance IEEE 802.15.4 [ ] | Unlicensed ISM bands 868/915 MHz | 50 kbps | <30 km | Very low | High | Low data rates, not suitable for high-bandwidth applications, scalability of the network, and capacity for messages. |
SigFox | IEEE 802.15.4 [ ] | Unlicensed ISM bands 868/915/433 MHz | 100 bps | 10–40 km | Low | Medium | Very low data rates, limited uplink capabilities, and a low payload limit for transmitted messages. |
RFID | ISO/IEC 14443, ISO/IEC 15693. [ ] | 25 kHz, 13.56 MHz, and 860–960 MHz | 40 to 160 kbp/s | 1–5 m | Low | Low | Short-range, limited data storage on passive RFID tags, and potential for signal reflection and interference. |
Mobile communication | N/A | Licensed bands 900–1800 MHz | Up to 170 kbps | 1–10 km | Medium | Medium | Relatively high power consumption, especially for mobile devices, and may not be cost-effective for certain IoT applications. |
Bluetooth | IEEE 802.15.1 [ ] | 2.4 GHz ISM band | 1 to 3 Mps | 1 to 10 m | Low | Low | Moderate range, higher power consumption compared to low-power technologies, and potential interference in crowded areas. |
NB-IoT | 3GPP release 13 [ ] | LTE frequency bands | 200 kbps | 11–0 Km | Medium | High | Limited data rates, not suitable for applications requiring high bandwidth, and potential latency in communication. |
Satellite Name | Application in PA |
---|---|
ECOSTRESS | Evapotranspiration (ET) [ ] |
TERRA-ASTER | Water management [ ] |
Sentinel-1A | Soil Moisture [ ] |
Sentinel-2 | Crop management [ ] |
Terra/Aqua MODIS | Crop yield [ ] |
KOMPSAT-2 | Crop yield [ ] |
RapidEye | Crop yield [ ] Soil water [ ] |
GeoEye-1 | Nutrient management [ ] |
Sensor Name | Parameters | Reference |
---|---|---|
SDI-12 Sensor | Soil Moisture | [ ] |
Hydra probe II soil sensor | Soil Moisture, Soil Temperature, Conductivity and Salinity level | [ ] |
ECH2O-5TE Sensor | Soil Moisture content levels, Soil Electric Conductivity, Soil Temperature, Soil Organic Content, Soil Texture, and Soil Bulk Density | [ ] |
ECH2O EC-5 Sensor | Soil Moisture, Soil Temperature, Soil Water | [ ] |
EC250 sensor | Soil Temperature, Soil Moisture, Salinity level, and Conductivity | [ ] |
SKU: SEN0193 sensor | Soil Moisture, Soil Temperature | [ ] |
VH-400 sensor | Soil Moisture sensor, Soil Temperature | [ ] |
DHT11/DHT22 sensor | Temperature and Humidity sensor | [ , ] |
SHT31 Sensor | Temperature and Humidity sensor | [ ] |
SHT71, SHT75 | Temperature and Humidity sensor | [ ] |
TAOS TSL262R | Luminosity sensor | [ ] |
TGS4161 | CO sensor | [ ] |
S-THB-M002 sensor | Temperature and Humidity sensor | [ ] |
TCS3472 RGB sensor | Light sensor | [ ] |
Sense H2 sensor | Hydrogen, Plant Wetness, Plant Temperature, and CO | [ ] |
MP406 sensor | Soil Moisture, Soil Temperature, Soil Dielectric Permittivity | [ ] |
Cl-340 photosynthesis | Plant Moisture, Photosynthesis, Plant Wetness, Hydrogen level, Plant Temperature and CO | [ ] |
PTM-48A photosynthesis monitor | Plant Moisture, Photosynthesis, Plant Wetness, Plant Temperature and CO | [ ] |
YSI 6025 and YSI 6131 chlorophyll sensors | Photosynthesis | [ ] |
HMP45C sensor | Air Humidity, Air Temperature, and Air Pressure | [ ] |
The statements, opinions and data contained in all publications are solely those of the individual author(s) and contributor(s) and not of MDPI and/or the editor(s). MDPI and/or the editor(s) disclaim responsibility for any injury to people or property resulting from any ideas, methods, instructions or products referred to in the content. |
Share and Cite
Soussi, A.; Zero, E.; Sacile, R.; Trinchero, D.; Fossa, M. Smart Sensors and Smart Data for Precision Agriculture: A Review. Sensors 2024 , 24 , 2647. https://doi.org/10.3390/s24082647
Soussi A, Zero E, Sacile R, Trinchero D, Fossa M. Smart Sensors and Smart Data for Precision Agriculture: A Review. Sensors . 2024; 24(8):2647. https://doi.org/10.3390/s24082647
Soussi, Abdellatif, Enrico Zero, Roberto Sacile, Daniele Trinchero, and Marco Fossa. 2024. "Smart Sensors and Smart Data for Precision Agriculture: A Review" Sensors 24, no. 8: 2647. https://doi.org/10.3390/s24082647
Article Metrics
Article access statistics, further information, mdpi initiatives, follow mdpi.
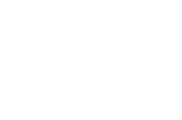
Subscribe to receive issue release notifications and newsletters from MDPI journals
Academia.edu no longer supports Internet Explorer.
To browse Academia.edu and the wider internet faster and more securely, please take a few seconds to upgrade your browser .
Enter the email address you signed up with and we'll email you a reset link.
- We're Hiring!
- Help Center
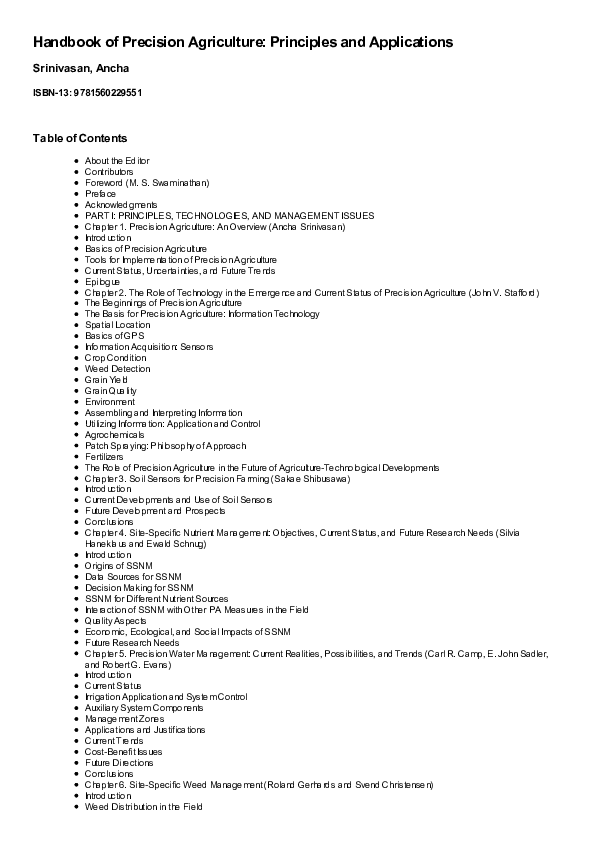
Handbook of precision agriculture: principles and applications

Related Papers
The University of …
Michele Marra
Roland K. Roberts and Burton C. English are Professors, and James A. Larson is an Associate Professor, Rebecca L. Cochran is a Research Associate at the University of Tennessee. Bob Goodman is an Associate Professor at Auburn University, Sherry Larkin is an Assistant ...
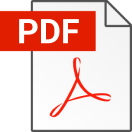
Eduardo Segarra
… of the Beltwide Cotton Conference …
Traditionally, optimal fertilizer input use in agriculture has assumed spatial and temporal field homogeneity with respect to soil fertility, soil moisture, pest populations, and crop characteristics. That is, optimal fertilizer input decision rules do not account for these ...
Marcelo Stabile
Wiley eBooks
Francis Pierce
Spatial optimal nitrogen fertilizer application levels and associated net revenues in irrigated cotton production were derived. Results indicate that precision farming can improve the profitability and increase the efficiency associated with nitrogen fertilizer use in irrigated cotton production.
Computers and Electronics in Agriculture
Johnie Jenkins
Spatial optimal nitrogen fertilizer application levels and net revenues in irrigated cotton production were derived. Results indicate that precision farming can improve the profitability, and potentially reduce the environmental damages associated with nitrogen fertilizer use in irrigated cotton production.
Peter Ellsworth
An Integrated Cotton Management (ICM) Demonstration project was conducted on the Demonstration Farm at the Maricopa Agricultural Center in 1999 for the second year. In this project, all current guidelines and recommendations disseminated by the University of Arizona were integrated in a systems approach for cotton production. The Extension Specialists in agronomy, entomology, irrigation management, weed sciences, and plant pathology following the University recommendations made the management decisions. On a 52.7 acre field, 78% Bt and 22% non-Bt cotton was planted into moisture on April 9, 1999. Because of problems with cool temperatures and deep seeding, a stand of only 25,000 plants/acre was established. Weed control was achieved with one preplant application and two cultivations. The field was sprayed three times for lygus and two times for whitefly control. Approximately 38.6 acre-inches of irrigation water was applied. An average of 3005 lb/acre of seed cotton were harvested. ...
Loading Preview
Sorry, preview is currently unavailable. You can download the paper by clicking the button above.
RELATED PAPERS
Journal of Agribusiness
Agricultural Systems
Debbie Boykin , Johnie Jenkins
Joe Ellington
Gretchen Sassenrath
Agronomy Journal
Global Science Books
California Agriculture
Jeffrey Mitchell
H. Booltink , jan verhagen
Journal of nematology
Charles Overstreet
Paul Mask , Randy Raper
T.A. Gemtos
Mahesh Pandit
H. Melakeberhan
allah bakhsh
Renewable Agriculture and Food Systems
diego alejandro castillon nieto
Field Crops Research
Debbie Boykin
Harry Schomberg
RELATED TOPICS
- We're Hiring!
- Help Center
- Find new research papers in:
- Health Sciences
- Earth Sciences
- Cognitive Science
- Mathematics
- Computer Science
- Academia ©2024
Precision Agriculture
An International Journal on Advances in Precision Agriculture
- Addresses within-field natural resources variability, including soil and crop variability and characteristics.
- Explores engineering technology, focusing on sensor systems, computational techniques, positioning systems and control systems.
- Present content on Managing variability, including sampling techniques and methods, nutrient and crop protection chemicals recommendation and crop quality
- Covers topics on engineering technology, focusing on sensor systems, computational techniques, positioning systems and control systems for site-specific application
- Encourages submissions on measurement, management, technology and impact of spatial variability at the within-field scale.
- Prioritizes environmental coverage including sediments, leaching, runoff and drainage related to within-field spatial variability.
- Davide Cammarano
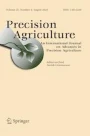
Latest issue
Volume 25, Issue 4
Latest articles
Mangodetnet: a novel label-efficient weakly supervised fruit detection framework.
- Alessandro Rocco Denarda
- Francesco Crocetti
- Mario Luca Fravolini
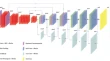
Integrating NDVI and agronomic data to optimize the variable-rate nitrogen fertilization
- Nicola Silvestri
- Leonardo Ercolini
- Massimiliano Ruggeri
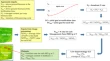
Evaluation of the PROMET model for yield estimation and N fertilization in on-farm research
- B. Brandenburg
- Y. Reckleben
- H. W. Griepentrog
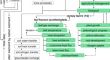
A holistic simulation model of solid-set sprinkler irrigation systems for precision irrigation
- M. Morcillo
- J. F. Ortega
- M. A. Moreno
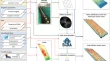
Combining 2D image and point cloud deep learning to predict wheat above ground biomass
- Shaolong Zhu
- Weijun Zhang
- Chengming Sun
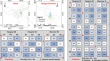
Journal updates
Special issues in plant sciences, precision ag definition.
The International Society of Precision Agriculture ( https://www.ispag.org/ ) adopted the following definition of precision agriculture in 2019:
‘Precision Agriculture is a management strategy that gathers, processes and analyzes temporal, spatial and individual data and combines it with other information to support management decisions according to estimated variability for improved resource use efficiency, productivity, quality, profitability and sustainability of agricultural production.’
Journal information
- Astrophysics Data System (ADS)
- Biological Abstracts
- CAB Abstracts
- Chemical Abstracts Service (CAS)
- Current Contents/Agriculture, Biology & Environmental Sciences
- Engineering Village – GEOBASE
- Google Scholar
- Japanese Science and Technology Agency (JST)
- Norwegian Register for Scientific Journals and Series
- OCLC WorldCat Discovery Service
- Science Citation Index Expanded (SCIE)
- TD Net Discovery Service
- UGC-CARE List (India)
Rights and permissions
Editorial policies
© Springer Science+Business Media, LLC, part of Springer Nature
- Find a journal
- Publish with us
- Track your research
- Advanced Searches
- Legislation
- Congressional Record
- Search Tools
- Browse the Help Center
Examples: "Trade Relations", "Export Controls"
Examples: hr5, h.r.5, sjres8, sa2, pl116-21, 86Stat1326
Examples: trade sanctions reform, small modular reactor
Examples: hr5, h.r.5, sjres8, s2, 90stat2495
Examples: baseball, "standing rules"
Examples: 5, 20, 37
Examples: hr5021, H.Res.866, sconres15, S.51, 117pl2, 117-2
Examples: "enrolled bill signed", "leak detection dog"
Examples: "diplomatic service", retired
Examples: PN4, pn12, pn1633-2, 118PN345
Examples: Morris, Beck
Examples: general, "deputy under secretary"
Examples: judiciary, "Coast Guard"
Examples: marine mammals, "5 U.S.C. 801", "Presidential Address"
Example: peace corps
Examples: EC6228, r12313, PM45, PT83, ML160
Example: 5 U.S.C. 801
Examples: "trade relations", "Export Control Act"
Examples: EC2, PM32, POM43
Examples: UNESCO, "sea turtles"
Examples: 115-3, 114-13(A)
Example: 106-1
H.R.1339 - Precision Agriculture Satellite Connectivity Act 118th Congress (2023-2024) | $(document).ready(function () { $('#alert-BILL-437258-191').congress_Alert({ type: 'BILL', id: '437258', buttonDivId: 'alert-BILL-437258-191', buttonText: 'Get alerts', buttonTextIfLoggedIn: 'Get alerts', buttonTextIfHasAlert: 'Cancel Alerts', buttonTextIfHasDialog: 'Edit Alerts', dialogDivId: 'alert-dialog-BILL-437258-191', titleText: 'To get email alerts ', alertSourceType: ' legislation', alertMessageText: "You will receive an alert for any updates to actions, bill text, cosponsors, or summaries.", titleTextIfLoggedIn: 'Get email alerts ', titleTextAddendum: 'for this', titleTextIfHasAlert: 'Cancel this alert?', showEditDialogue: 'true', editAlertDialogTitle: 'Track Changes - Choose one or more (Optional) Help ', hideEditLink: 'false', dataSet: '', countLimitReached: 'false', cannotAddNewAlertDialogTitle: 'Cannot add new alert', cannotAddNewAlertDialogMessage: '' }); });
Sponsor: | (Introduced 03/03/2023) |
---|---|
Committees: | House - Energy and Commerce | Senate - Commerce, Science, and Transportation |
Committee Meetings: | |
Committee Reports: | |
Latest Action: | Senate - 05/01/2023 Received in the Senate and Read twice and referred to the Committee on Commerce, Science, and Transportation. ( ) |
Roll Call Votes: | There has been |
Tracker: Tip | This bill has the status Passed House Here are the steps for Status of Legislation: |
Subject — policy area:.
- Science, Technology, Communications
- View subjects
Give Feedback on This Bill
- Contact Your Member
Summary: H.R.1339 — 118th Congress (2023-2024) All Information (Except Text)
Bill summaries are authored by CRS .
Shown Here: Passed House (04/26/2023)
Precision Agriculture Satellite Connectivity Act
This bill requires the Federal Communications Commission (FCC) to review, and recommend changes to, its rules for fixed, mobile, and earth exploration satellites to promote precision agriculture (an information- and technology-based management system used to identify, analyze, and manage variability in agricultural production for optimum profitability, sustainability, and environmental protection).
In conducting its review, the FCC must consult with a task force that advises the FCC on ways to assess and advance broadband internet on unserved agricultural land and promote precision agriculture.

IMAGES
COMMENTS
Precision agriculture employs cutting-edge technologies to increase agricultural productivity while reducing adverse impacts on the environment. Precision agriculture is a farming approach that uses advanced technology and data analysis to maximize crop yields, cut waste, and increase productivity. It is a potential strategy for tackling some of the major issues confronting contemporary ...
Precision agriculture is a management concept, which relies on intensive data collection and data processing for guiding targeted actions that improve the efficiency, productivity, and sustainability of agricultural operations. Several studies have assessed the adoption rate of precision agriculture technologies at regional or national scale, but the literature lacks global evaluations of the ...
Beginnings. The term "precision agriculture" means managing, tracking or enhancing crop or livestock production inputs, including seed, feed, fertilizer, chemicals, water and time, at a heightened level of accuracy to improve efficiencies and commodity quality and yield, while positively impacting environmental stewardship.
Precision agriculture is the collection of hardware and software technologies that allow a farmer to make informed, differentiated decisions regarding agricultural operations such as planting ...
Examining 952 papers with precision, our research not only provides fundamental insights but also practical perspectives, casting a spotlight on the intricate dimensions of precision agriculture. Delving into critical concerns and pivotal issues, our findings present valuable insights tailored for practitioners, policymakers, and researchers alike.
This book chapter discusses the need for precision agriculture, sustainable agriculture, and the environment, methods for achieving it, methods for using it as a step towards improving rural ...
Precision-farming engineer Manuela Zude-Sasse at the Leibniz Institute for Agricultural Engineering and Bioeconomy in Potsdam, Germany, is attaching sensors to apples to detect their size, and ...
The agri-food industry faces a great challenge due to the growing global population. When considering land scarcity, this can be solved only by a higher production efficiency. Precision agriculture (PA) provides a potential answer. Most farms, especially in developing countries, are small-scale units that have difficulties in applying precision agriculture technologies. On the basis of the ...
Precision agriculture or satellite farming or site-specific crop management is a farming based on observing, measuring, and responding to inter- and intra-field variability in crops. Or. PA is an information- and technology-based farm management system to identity, analyze, and manage variability within fields for optimum profitability ...
Precision agriculture (PA) has become an increasingly popular approach to sustainable intensification in agriculture, with the intent of a balance between agricultural productivity and environmental stewardship. Sustainable intensification aims to increase agricultural productivity while minimizing negative environmental impacts.
This study elucidates the multi-faceted determinants that influence the adoption of precision agriculture within the small-scale farming sector. The study adopts a systematic literature review approach, meticulously selecting and analysing 29 relevant papers.
Precision Farming or Precision Agr iculture is generally defined as informat ion and technology based farm. management system to identify, analyse an d manage spatial and temporal variability ...
Precision agriculture, driven by the convergence of smart sensors and advanced technologies, has emerged as a transformative force in modern farming practices. The present review synthesizes insights from a multitude of research papers, exploring the dynamic landscape of precision agriculture. The main focus is on the integration of smart sensors, coupled with technologies such as the Internet ...
PLOS' precision agriculture research explores and assesses the very latest agricultural technologies. Whether in controlled environments or directly in the field, our research highlights new methods and technologies for agricultural surveillance and intervention, such as sensors and chemical testing, or high-tech farm machinery and machine learning that measures, analyses, and improves crop ...
The combined use of geostatistics and GIS for precision farming was detailed in a series of papers by Mulla (1989, 1991, 1993). The use of geostatistics in precision agriculture is extensively documented by Oliver (2010). 1.4 FARMING BY SOIL Pierre Robert is often regarded as the father of precision farming because of his active promotion
The central premise of precision farming lies in the collection, analysis, and application of vast amounts of data related to agricultural fields. Through sophisticated technologies such as GPS ...
The Role of Technology in the Emergence and Current Status of Precision Agriculture (John V. Stafford) The Beginnings of Precision Agriculture The Basis for Precision Agriculture: Information Technology Spatial Location Basics of GPS Information Acquisition: Sensors Crop Condition Weed Detection Grain Yield Grain Quality Environment Assembling ...
Precision agriculture is a management strategy that utilizes information technologies to collect useful data from distinct sources, with the aim of supporting the decisions associated to the production of crops. ... CCTA 2009, Beijing, China, October 14-17, 2009, Revised Selected Papers. Vol. 317. Springer Science & Business Media. Google ...
precision agriculture technologies because of their capacity to invest in relatively expensive tools (compared to farms in developing countries), and because of their large field ... The 17 papers provided numbers for the adoption rate of one or more precision agriculture technologies for 73 observations, each observation being defined by two ...
Precision agriculture (PA) is one such "data-driven strategy" to improve soil and resource management plans, and manage crops and livestock (Botta et al., 2022, p. 831). PA integrates information technology into farm machinery and farm management using innovations such as satellites, drones, sensors, and AI systems to help farmers make site ...
Precision livestock farming (PLF) is defined as the application of precision agriculture to the management of livestock production. Processes of precision livestock farming approach focus on animal growth, egg and milk production, detection and monitoring of diseases and aspects related to animal behavior. Systems include monitoring of milk to ...
Precision Ag definition. 'Precision Agriculture is a management strategy that gathers, processes and analyzes temporal, spatial and individual data and combines it with other information to support management decisions according to estimated variability for improved resource use efficiency, productivity, quality, profitability and ...
The term "precision. farming" describes the integration of GIS and GPS tools to provide extensive detailed information. on crop growth, crop health, crop y ield, water absorption, nutrient levels ...
Shown Here: Passed House (04/26/2023) Precision Agriculture Satellite Connectivity Act. This bill requires the Federal Communications Commission (FCC) to review, and recommend changes to, its rules for fixed, mobile, and earth exploration satellites to promote precision agriculture (an information- and technology-based management system used to identify, analyze, and manage variability in ...