Dask “Column assignment doesn’t support type numpy.ndarray”
I’m trying to use Dask instead of pandas since the data size I’m analyzing is quite large. I wanted to add a flag column based on several conditions.
But, then I got the following error message. The above code works perfectly when using np.where with pandas dataframe, but didn’t work with dask.array.where .


Advertisement
If numpy works and the operation is row-wise, then one solution is to use .map_partitions :
Type Support in Pandas API on Spark ¶
In this chapter, we will briefly show you how data types change when converting pandas-on-Spark DataFrame from/to PySpark DataFrame or pandas DataFrame.
Type casting between PySpark and pandas API on Spark ¶
When converting a pandas-on-Spark DataFrame from/to PySpark DataFrame, the data types are automatically casted to the appropriate type.
The example below shows how data types are casted from PySpark DataFrame to pandas-on-Spark DataFrame.
The example below shows how data types are casted from pandas-on-Spark DataFrame to PySpark DataFrame.
Type casting between pandas and pandas API on Spark ¶
When converting pandas-on-Spark DataFrame to pandas DataFrame, the data types are basically the same as pandas.
However, there are several data types only provided by pandas.
These kinds of pandas specific data types below are not currently supported in the pandas API on Spark but planned to be supported.
pd.Timedelta
pd.Categorical
pd.CategoricalDtype
The pandas specific data types below are not planned to be supported in the pandas API on Spark yet.
pd.SparseDtype
pd.DatetimeTZDtype
pd.UInt*Dtype
pd.BooleanDtype
pd.StringDtype
Internal type mapping ¶
The table below shows which NumPy data types are matched to which PySpark data types internally in the pandas API on Spark.
The table below shows which Python data types are matched to which PySpark data types internally in pandas API on Spark.
For decimal type, pandas API on Spark uses Spark’s system default precision and scale.
You can check this mapping by using the as_spark_type function.
You can also check the underlying PySpark data type of Series or schema of DataFrame by using Spark accessor.
Pandas API on Spark currently does not support multiple types of data in a single column.
Transform and apply a function
Type Hints in Pandas API on Spark
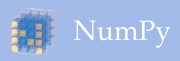
- NumPy v1.18 Manual
- NumPy Reference
Array objects
Table of Contents
- Constructing arrays
- Indexing arrays
- Internal memory layout of an ndarray
- Memory layout
- Other attributes
- Array interface
- ctypes foreign function interface
- Array conversion
- Shape manipulation
- Item selection and manipulation
- Calculation
- Arithmetic, matrix multiplication, and comparison operations
- Special methods
Previous topic
numpy.ndarray
Quick search
The n-dimensional array ( ndarray ) ¶.
An ndarray is a (usually fixed-size) multidimensional container of items of the same type and size. The number of dimensions and items in an array is defined by its shape , which is a tuple of N non-negative integers that specify the sizes of each dimension. The type of items in the array is specified by a separate data-type object (dtype) , one of which is associated with each ndarray.
As with other container objects in Python, the contents of an ndarray can be accessed and modified by indexing or slicing the array (using, for example, N integers), and via the methods and attributes of the ndarray .
Different ndarrays can share the same data, so that changes made in one ndarray may be visible in another. That is, an ndarray can be a “view” to another ndarray, and the data it is referring to is taken care of by the “base” ndarray. ndarrays can also be views to memory owned by Python strings or objects implementing the buffer or array interfaces.
A 2-dimensional array of size 2 x 3, composed of 4-byte integer elements:
The array can be indexed using Python container-like syntax:
For example slicing can produce views of the array:
Constructing arrays ¶
New arrays can be constructed using the routines detailed in Array creation routines , and also by using the low-level ndarray constructor:
Indexing arrays ¶
Arrays can be indexed using an extended Python slicing syntax, array[selection] . Similar syntax is also used for accessing fields in a structured data type .
Array Indexing .
Internal memory layout of an ndarray ¶
An instance of class ndarray consists of a contiguous one-dimensional segment of computer memory (owned by the array, or by some other object), combined with an indexing scheme that maps N integers into the location of an item in the block. The ranges in which the indices can vary is specified by the shape of the array. How many bytes each item takes and how the bytes are interpreted is defined by the data-type object associated with the array.
Both the C and Fortran orders are contiguous , i.e., single-segment, memory layouts, in which every part of the memory block can be accessed by some combination of the indices.
While a C-style and Fortran-style contiguous array, which has the corresponding flags set, can be addressed with the above strides, the actual strides may be different. This can happen in two cases:
If self.shape[k] == 1 then for any legal index index[k] == 0 . This means that in the formula for the offset and thus and the value of = self.strides[k] is arbitrary. If an array has no elements ( self.size == 0 ) there is no legal index and the strides are never used. Any array with no elements may be considered C-style and Fortran-style contiguous.
Point 1. means that self and self.squeeze() always have the same contiguity and aligned flags value. This also means that even a high dimensional array could be C-style and Fortran-style contiguous at the same time.
An array is considered aligned if the memory offsets for all elements and the base offset itself is a multiple of self.itemsize . Understanding memory-alignment leads to better performance on most hardware.
Points (1) and (2) are not yet applied by default. Beginning with NumPy 1.8.0, they are applied consistently only if the environment variable NPY_RELAXED_STRIDES_CHECKING=1 was defined when NumPy was built. Eventually this will become the default.
You can check whether this option was enabled when your NumPy was built by looking at the value of np.ones((10,1), order='C').flags.f_contiguous . If this is True , then your NumPy has relaxed strides checking enabled.
It does not generally hold that self.strides[-1] == self.itemsize for C-style contiguous arrays or self.strides[0] == self.itemsize for Fortran-style contiguous arrays is true.
Data in new ndarrays is in the row-major (C) order, unless otherwise specified, but, for example, basic array slicing often produces views in a different scheme.
Several algorithms in NumPy work on arbitrarily strided arrays. However, some algorithms require single-segment arrays. When an irregularly strided array is passed in to such algorithms, a copy is automatically made.
Array attributes ¶
Array attributes reflect information that is intrinsic to the array itself. Generally, accessing an array through its attributes allows you to get and sometimes set intrinsic properties of the array without creating a new array. The exposed attributes are the core parts of an array and only some of them can be reset meaningfully without creating a new array. Information on each attribute is given below.
Memory layout ¶
The following attributes contain information about the memory layout of the array:
Data type ¶
Data type objects
The data type object associated with the array can be found in the dtype attribute:
Other attributes ¶
Array interface ¶.
The Array Interface .
ctypes foreign function interface ¶
Array methods ¶.
An ndarray object has many methods which operate on or with the array in some fashion, typically returning an array result. These methods are briefly explained below. (Each method’s docstring has a more complete description.)
For the following methods there are also corresponding functions in numpy : all , any , argmax , argmin , argpartition , argsort , choose , clip , compress , copy , cumprod , cumsum , diagonal , imag , max , mean , min , nonzero , partition , prod , ptp , put , ravel , real , repeat , reshape , round , searchsorted , sort , squeeze , std , sum , swapaxes , take , trace , transpose , var .

Array conversion ¶
Shape manipulation ¶.
For reshape, resize, and transpose, the single tuple argument may be replaced with n integers which will be interpreted as an n-tuple.
Item selection and manipulation ¶
For array methods that take an axis keyword, it defaults to None . If axis is None , then the array is treated as a 1-D array. Any other value for axis represents the dimension along which the operation should proceed.
Calculation ¶
Many of these methods take an argument named axis . In such cases,
If axis is None (the default), the array is treated as a 1-D array and the operation is performed over the entire array. This behavior is also the default if self is a 0-dimensional array or array scalar. (An array scalar is an instance of the types/classes float32, float64, etc., whereas a 0-dimensional array is an ndarray instance containing precisely one array scalar.)
If axis is an integer, then the operation is done over the given axis (for each 1-D subarray that can be created along the given axis).
Example of the axis argument
A 3-dimensional array of size 3 x 3 x 3, summed over each of its three axes
The parameter dtype specifies the data type over which a reduction operation (like summing) should take place. The default reduce data type is the same as the data type of self . To avoid overflow, it can be useful to perform the reduction using a larger data type.
For several methods, an optional out argument can also be provided and the result will be placed into the output array given. The out argument must be an ndarray and have the same number of elements. It can have a different data type in which case casting will be performed.
Arithmetic, matrix multiplication, and comparison operations ¶
Arithmetic and comparison operations on ndarrays are defined as element-wise operations, and generally yield ndarray objects as results.
Each of the arithmetic operations ( + , - , * , / , // , % , divmod() , ** or pow() , << , >> , & , ^ , | , ~ ) and the comparisons ( == , < , > , <= , >= , != ) is equivalent to the corresponding universal function (or ufunc for short) in NumPy. For more information, see the section on Universal Functions .
Comparison operators:
Truth value of an array ( bool ):
Truth-value testing of an array invokes ndarray.__bool__ , which raises an error if the number of elements in the array is larger than 1, because the truth value of such arrays is ambiguous. Use .any() and .all() instead to be clear about what is meant in such cases. (If the number of elements is 0, the array evaluates to False .)
Unary operations:
Arithmetic:
Any third argument to pow is silently ignored, as the underlying ufunc takes only two arguments.
The three division operators are all defined; div is active by default, truediv is active when __future__ division is in effect.
Because ndarray is a built-in type (written in C), the __r{op}__ special methods are not directly defined.
The functions called to implement many arithmetic special methods for arrays can be modified using __array_ufunc__ .
Arithmetic, in-place:
In place operations will perform the calculation using the precision decided by the data type of the two operands, but will silently downcast the result (if necessary) so it can fit back into the array. Therefore, for mixed precision calculations, A {op}= B can be different than A = A {op} B . For example, suppose a = ones((3,3)) . Then, a += 3j is different than a = a + 3j : while they both perform the same computation, a += 3 casts the result to fit back in a , whereas a = a + 3j re-binds the name a to the result.
Matrix Multiplication:
Matrix operators @ and @= were introduced in Python 3.5 following PEP465. NumPy 1.10.0 has a preliminary implementation of @ for testing purposes. Further documentation can be found in the matmul documentation.
Special methods ¶
For standard library functions:
Basic customization:
Container customization: (see Indexing )
Conversion; the operations int , float and complex . . They work only on arrays that have one element in them and return the appropriate scalar.
String representations:
- © Copyright 2008-2019, The SciPy community.
- Last updated on May 24, 2020.
- Created using Sphinx 2.4.4.

Statistics Made Easy
How to Fix: ‘numpy.float64’ object does not support item assignment
One common error you may encounter when using Python is:
This error usually occurs when you attempt to use brackets to assign a new value to a NumPy variable that has a type of float64 .
The following example shows how to resolve this error in practice.
How to Reproduce the Error
Suppose we create some NumPy variable that has a value of 15.22 and we attempt to use brackets to assign it a new value of 13.7 :
We receive the error that ‘numpy.float64’ object does not support item assignment .
We received this error because one_float is a scalar but we attempted to treat it like an array where we could use brackets to change the value in index position 0.
Since one_float is not an array, we can’t use brackets when attempting to change its value.
How to Fix the Error
The way to resolve this error is to simply not use brackets when assigning a new value to the float:
We’re able to successfully change the value from 15.22 to 13.7 because we didn’t use brackets.
Note that it’s fine to use brackets to change values in specific index positions as long as you’re working with an array.
For example, the following code shows how to change the first element in a NumPy array from 15.22 to 13.7 by using bracket notation:
This time we don’t receive an error either because we’re working with a NumPy array so it makes sense to use brackets.
Additional Resources
The following tutorials explain how to fix other common errors in Python:
How to Fix in Python: ‘numpy.ndarray’ object is not callable How to Fix: TypeError: ‘numpy.float64’ object is not callable How to Fix: Typeerror: expected string or bytes-like object
Featured Posts

Hey there. My name is Zach Bobbitt. I have a Master of Science degree in Applied Statistics and I’ve worked on machine learning algorithms for professional businesses in both healthcare and retail. I’m passionate about statistics, machine learning, and data visualization and I created Statology to be a resource for both students and teachers alike. My goal with this site is to help you learn statistics through using simple terms, plenty of real-world examples, and helpful illustrations.
Leave a Reply Cancel reply
Your email address will not be published. Required fields are marked *
Navigation Menu
Search code, repositories, users, issues, pull requests..., provide feedback.
We read every piece of feedback, and take your input very seriously.
Saved searches
Use saved searches to filter your results more quickly.
To see all available qualifiers, see our documentation .
- Notifications
Have a question about this project? Sign up for a free GitHub account to open an issue and contact its maintainers and the community.
By clicking “Sign up for GitHub”, you agree to our terms of service and privacy statement . We’ll occasionally send you account related emails.
Already on GitHub? Sign in to your account
False positive: numpy ndarray .T does not support item assignment #3932
anuppari commented Nov 1, 2020
hippo91 commented Nov 17, 2020
Sorry, something went wrong.
No branches or pull requests

IMAGES
VIDEO
COMMENTS
This answer isn't elegant but is functional. I found the select function was about 20 seconds quicker on an 11m row dataset in pandas. I also found that even if I performed the same function in dask that the result would return a numpy (pandas) array.
Dask "Column assignment doesn't support type numpy.ndarray" ... I wanted to add a flag column based on several conditions. ... 283 Questions keras 211 Questions list 709 Questions loops 176 Questions machine-learning 204 Questions matplotlib 561 Questions numpy 879 Questions opencv 223 Questions pandas 2949 Questions pyspark 157 Questions ...
Hi, from looking into the available resources irt to adding a new column to dask dataframe from an array I figured sth like this should work import dask.dataframe as dd import dask.array as da w = dd.from_dask_array(da.from_npy_stack('/h...
Basically I create a column group in order to make the groupby on consecutive elements. Using a dask data frame instead directly does not work: TypeError: Column assignment doesn't support type ndarray which I can understand. I have tried to create a dask array instead but as my divisions are not representative of the length I don't know how to determine the chunks.
numpy.ndarray# class numpy. ndarray (shape, dtype = float, buffer = None, offset = 0, strides = None, order = None) [source] #. An array object represents a multidimensional, homogeneous array of fixed-size items. An associated data-type object describes the format of each element in the array (its byte-order, how many bytes it occupies in memory, whether it is an integer, a floating point ...
The problem is that train_test_split(X, y, ...) returns numpy arrays and not pandas dataframes. Numpy arrays have no attribute named columns. If you want to see what features SelectFromModel kept, you need to substitute X_train (which is a numpy.array) with X which is a pandas.DataFrame.. selected_feat= X.columns[(sel.get_support())] This will return a list of the columns kept by the feature ...
You are trying to assign an object of type dask.....DataFrame to a column. A column needs a 2d data structure like a series/list etc. This may be a quirk of how dask does things so you could try explicitly converting your assigned value to a series before assigning it.
method. ndarray.astype(dtype, order='K', casting='unsafe', subok=True, copy=True) #. Copy of the array, cast to a specified type. Parameters: dtypestr or dtype. Typecode or data-type to which the array is cast. order{'C', 'F', 'A', 'K'}, optional. Controls the memory layout order of the result. 'C' means C order, 'F ...
2. Unfortunately, even df.assign did not solve the problem and I was getting the same error: I had to do this: ks.reset_option('compute.ops_on_diff_frames') # convert target to a koalas series so that it can be assigned to the dataframe as a column. ks_series = ks.Series(iris.target) df["target"] = ks_series.
If it is known in advance that an operation _will_ perform a 0D-array -> scalar cast, then one can consider manually remedying the situation with either typing.cast or a # type: ignore comment. Record array dtypes# The dtype of numpy.recarray, and the numpy.rec functions in general, can be specified in one of two ways: Directly via the dtype ...
Dask "Column assignment doesn't support type numpy.ndarray" I'm trying to use Dask instead of pandas since the data size I'm analyzing is quite large. I wanted to add a flag column based on several conditions. import dask.array as da data['Flag'] = da.where((data['col1']>0) & (data['col2']>data['col4'] | data['col3']>data['col4']), 1, 0 ...
In the first case pure client is created without settings={'use_numpy': True} and data for insertion must be provided in lists or tuples. In the second case numpy client is created with settings={'use_numpy': True} and data for insertion must be provided in numpy arrays of pandas dataframe.
The table below shows which Python data types are matched to which PySpark data types internally in pandas API on Spark. For decimal type, pandas API on Spark uses Spark's system default precision and scale. You can check this mapping by using the as_spark_type function. You can also check the underlying PySpark data type of Series or schema ...
OK, my mistake, unlike Pytorch, numpy.array () ONLY creates 1D arrays. The correct behaviour would be to do something like total_array = np.zeros () or np.empty () np.array can create 2d arrsys - if you give a nested list. total_array[0,0]=1 is the more idiomatic way of indexing a 2d array.
An ndarray is a (usually fixed-size) multidimensional container of items of the same type and size. The number of dimensions and items in an array is defined by its shape , which is a tuple of N non-negative integers that specify the sizes of each dimension. The type of items in the array is specified by a separate data-type object (dtype), one ...
Suppose we create some NumPy variable that has a value of 15.22 and we attempt to use brackets to assign it a new value of 13.7: import numpy as np #define some float value one_float = np. float64 (15.22) #attempt to modify float value to be 13.7 one_float[0] = 13.7 TypeError: 'numpy.float64' object does not support item assignment
Warning: numpy.int64 data type is not supported. Data is converted to float64. Warning: numpy.int64 data type is not supported. Data is converted to float64. SqlSatelliteCall function failed. Please see the console output for more information.
I've solved this problem by setting client = Client('localhost', settings={"use_numpy":True}) thank you for your try to help me. Would you like to improve the docs about insert_dataframe() with client settings? This would help others to solve the problem.
Not an answer to your question, but a numpy suggestion: you can get a 2D numpy array of random numbers by doing np.random.rand(5,10) (using the legacy method). Although, you seem to have each row being the same value, which I am not sure was intended or not.
Callum027 mentioned this issue on May 17, 2020. List type not supported for annotating functions for apply #1506. Closed. ueshin mentioned this issue on Jul 9, 2020. Enable to assign list. #1644. Merged. HyukjinKwon closed this as completed in #1644 on Jul 9, 2020. HyukjinKwon pushed a commit that referenced this issue on Jul 9, 2020.
Code to reproduce: import numpy as np a = np.array([[1,2,3],[4,5,6]]) print(a) a.T[1,1] = 10 print(a) Current behavior Pylint gives E1137: 'a.T' does not support item assignment (unsupported-assignment-operation) but the program runs fin...