
An official website of the United States government
The .gov means it’s official. Federal government websites often end in .gov or .mil. Before sharing sensitive information, make sure you’re on a federal government site.
The site is secure. The https:// ensures that you are connecting to the official website and that any information you provide is encrypted and transmitted securely.
- Publications
- Account settings
Preview improvements coming to the PMC website in October 2024. Learn More or Try it out now .
- Advanced Search
- Journal List
- Indian Dermatol Online J
- v.10(1); Jan-Feb 2019

Types of Variables, Descriptive Statistics, and Sample Size
Feroze kaliyadan.
Department of Dermatology, King Faisal University, Al Hofuf, Saudi Arabia
Vinay Kulkarni
1 Department of Dermatology, Prayas Amrita Clinic, Pune, Maharashtra, India
This short “snippet” covers three important aspects related to statistics – the concept of variables , the importance, and practical aspects related to descriptive statistics and issues related to sampling – types of sampling and sample size estimation.
What is a variable?[ 1 , 2 ] To put it in very simple terms, a variable is an entity whose value varies. A variable is an essential component of any statistical data. It is a feature of a member of a given sample or population, which is unique, and can differ in quantity or quantity from another member of the same sample or population. Variables either are the primary quantities of interest or act as practical substitutes for the same. The importance of variables is that they help in operationalization of concepts for data collection. For example, if you want to do an experiment based on the severity of urticaria, one option would be to measure the severity using a scale to grade severity of itching. This becomes an operational variable. For a variable to be “good,” it needs to have some properties such as good reliability and validity, low bias, feasibility/practicality, low cost, objectivity, clarity, and acceptance. Variables can be classified into various ways as discussed below.
Quantitative vs qualitative
A variable can collect either qualitative or quantitative data. A variable differing in quantity is called a quantitative variable (e.g., weight of a group of patients), whereas a variable differing in quality is called a qualitative variable (e.g., the Fitzpatrick skin type)
A simple test which can be used to differentiate between qualitative and quantitative variables is the subtraction test. If you can subtract the value of one variable from the other to get a meaningful result, then you are dealing with a quantitative variable (this of course will not apply to rating scales/ranks).
Quantitative variables can be either discrete or continuous
Discrete variables are variables in which no values may be assumed between the two given values (e.g., number of lesions in each patient in a sample of patients with urticaria).
Continuous variables, on the other hand, can take any value in between the two given values (e.g., duration for which the weals last in the same sample of patients with urticaria). One way of differentiating between continuous and discrete variables is to use the “mid-way” test. If, for every pair of values of a variable, a value exactly mid-way between them is meaningful, the variable is continuous. For example, two values for the time taken for a weal to subside can be 10 and 13 min. The mid-way value would be 11.5 min which makes sense. However, for a number of weals, suppose you have a pair of values – 5 and 8 – the midway value would be 6.5 weals, which does not make sense.
Under the umbrella of qualitative variables, you can have nominal/categorical variables and ordinal variables
Nominal/categorical variables are, as the name suggests, variables which can be slotted into different categories (e.g., gender or type of psoriasis).
Ordinal variables or ranked variables are similar to categorical, but can be put into an order (e.g., a scale for severity of itching).
Dependent and independent variables
In the context of an experimental study, the dependent variable (also called outcome variable) is directly linked to the primary outcome of the study. For example, in a clinical trial on psoriasis, the PASI (psoriasis area severity index) would possibly be one dependent variable. The independent variable (sometime also called explanatory variable) is something which is not affected by the experiment itself but which can be manipulated to affect the dependent variable. Other terms sometimes used synonymously include blocking variable, covariate, or predictor variable. Confounding variables are extra variables, which can have an effect on the experiment. They are linked with dependent and independent variables and can cause spurious association. For example, in a clinical trial for a topical treatment in psoriasis, the concomitant use of moisturizers might be a confounding variable. A control variable is a variable that must be kept constant during the course of an experiment.
Descriptive Statistics
Statistics can be broadly divided into descriptive statistics and inferential statistics.[ 3 , 4 ] Descriptive statistics give a summary about the sample being studied without drawing any inferences based on probability theory. Even if the primary aim of a study involves inferential statistics, descriptive statistics are still used to give a general summary. When we describe the population using tools such as frequency distribution tables, percentages, and other measures of central tendency like the mean, for example, we are talking about descriptive statistics. When we use a specific statistical test (e.g., Mann–Whitney U-test) to compare the mean scores and express it in terms of statistical significance, we are talking about inferential statistics. Descriptive statistics can help in summarizing data in the form of simple quantitative measures such as percentages or means or in the form of visual summaries such as histograms and box plots.
Descriptive statistics can be used to describe a single variable (univariate analysis) or more than one variable (bivariate/multivariate analysis). In the case of more than one variable, descriptive statistics can help summarize relationships between variables using tools such as scatter plots.
Descriptive statistics can be broadly put under two categories:
- Sorting/grouping and illustration/visual displays
- Summary statistics.
Sorting and grouping
Sorting and grouping is most commonly done using frequency distribution tables. For continuous variables, it is generally better to use groups in the frequency table. Ideally, group sizes should be equal (except in extreme ends where open groups are used; e.g., age “greater than” or “less than”).
Another form of presenting frequency distributions is the “stem and leaf” diagram, which is considered to be a more accurate form of description.
Suppose the weight in kilograms of a group of 10 patients is as follows:
56, 34, 48, 43, 87, 78, 54, 62, 61, 59
The “stem” records the value of the “ten's” place (or higher) and the “leaf” records the value in the “one's” place [ Table 1 ].
Stem and leaf plot
0 | - |
1 | - |
2 | - |
3 | 4 |
4 | 3 8 |
5 | 4 6 9 |
6 | 1 2 |
7 | 8 |
8 | 7 |
9 | - |
Illustration/visual display of data
The most common tools used for visual display include frequency diagrams, bar charts (for noncontinuous variables) and histograms (for continuous variables). Composite bar charts can be used to compare variables. For example, the frequency distribution in a sample population of males and females can be illustrated as given in Figure 1 .

Composite bar chart
A pie chart helps show how a total quantity is divided among its constituent variables. Scatter diagrams can be used to illustrate the relationship between two variables. For example, global scores given for improvement in a condition like acne by the patient and the doctor [ Figure 2 ].

Scatter diagram
Summary statistics
The main tools used for summary statistics are broadly grouped into measures of central tendency (such as mean, median, and mode) and measures of dispersion or variation (such as range, standard deviation, and variance).
Imagine that the data below represent the weights of a sample of 15 pediatric patients arranged in ascending order:
30, 35, 37, 38, 38, 38, 42, 42, 44, 46, 47, 48, 51, 53, 86
Just having the raw data does not mean much to us, so we try to express it in terms of some values, which give a summary of the data.
The mean is basically the sum of all the values divided by the total number. In this case, we get a value of 45.
The problem is that some extreme values (outliers), like “'86,” in this case can skew the value of the mean. In this case, we consider other values like the median, which is the point that divides the distribution into two equal halves. It is also referred to as the 50 th percentile (50% of the values are above it and 50% are below it). In our previous example, since we have already arranged the values in ascending order we find that the point which divides it into two equal halves is the 8 th value – 42. In case of a total number of values being even, we choose the two middle points and take an average to reach the median.
The mode is the most common data point. In our example, this would be 38. The mode as in our case may not necessarily be in the center of the distribution.
The median is the best measure of central tendency from among the mean, median, and mode. In a “symmetric” distribution, all three are the same, whereas in skewed data the median and mean are not the same; lie more toward the skew, with the mean lying further to the skew compared with the median. For example, in Figure 3 , a right skewed distribution is seen (direction of skew is based on the tail); data values' distribution is longer on the right-hand (positive) side than on the left-hand side. The mean is typically greater than the median in such cases.

Location of mode, median, and mean
Measures of dispersion
The range gives the spread between the lowest and highest values. In our previous example, this will be 86-30 = 56.
A more valuable measure is the interquartile range. A quartile is one of the values which break the distribution into four equal parts. The 25 th percentile is the data point which divides the group between the first one-fourth and the last three-fourth of the data. The first one-fourth will form the first quartile. The 75 th percentile is the data point which divides the distribution into a first three-fourth and last one-fourth (the last one-fourth being the fourth quartile). The range between the 25 th percentile and 75 th percentile is called the interquartile range.
Variance is also a measure of dispersion. The larger the variance, the further the individual units are from the mean. Let us consider the same example we used for calculating the mean. The mean was 45.
For the first value (30), the deviation from the mean will be 15; for the last value (86), the deviation will be 41. Similarly we can calculate the deviations for all values in a sample. Adding these deviations and averaging will give a clue to the total dispersion, but the problem is that since the deviations are a mix of negative and positive values, the final total becomes zero. To calculate the variance, this problem is overcome by adding squares of the deviations. So variance would be the sum of squares of the variation divided by the total number in the population (for a sample we use “n − 1”). To get a more realistic value of the average dispersion, we take the square root of the variance, which is called the “standard deviation.”
The box plot
The box plot is a composite representation that portrays the mean, median, range, and the outliers [ Figure 4 ].

The concept of skewness and kurtosis
Skewness is a measure of the symmetry of distribution. Basically if the distribution curve is symmetric, it looks the same on either side of the central point. When this is not the case, it is said to be skewed. Kurtosis is a representation of outliers. Distributions with high kurtosis tend to have “heavy tails” indicating a larger number of outliers, whereas distributions with low kurtosis have light tails, indicating lesser outliers. There are formulas to calculate both skewness and kurtosis [Figures [Figures5 5 – 8 ].

Positive skew

High kurtosis (positive kurtosis – also called leptokurtic)

Negative skew

Low kurtosis (negative kurtosis – also called “Platykurtic”)
Sample Size
In an ideal study, we should be able to include all units of a particular population under study, something that is referred to as a census.[ 5 , 6 ] This would remove the chances of sampling error (difference between the outcome characteristics in a random sample when compared with the true population values – something that is virtually unavoidable when you take a random sample). However, it is obvious that this would not be feasible in most situations. Hence, we have to study a subset of the population to reach to our conclusions. This representative subset is a sample and we need to have sufficient numbers in this sample to make meaningful and accurate conclusions and reduce the effect of sampling error.
We also need to know that broadly sampling can be divided into two types – probability sampling and nonprobability sampling. Examples of probability sampling include methods such as simple random sampling (each member in a population has an equal chance of being selected), stratified random sampling (in nonhomogeneous populations, the population is divided into subgroups – followed be random sampling in each subgroup), systematic (sampling is based on a systematic technique – e.g., every third person is selected for a survey), and cluster sampling (similar to stratified sampling except that the clusters here are preexisting clusters unlike stratified sampling where the researcher decides on the stratification criteria), whereas nonprobability sampling, where every unit in the population does not have an equal chance of inclusion into the sample, includes methods such as convenience sampling (e.g., sample selected based on ease of access) and purposive sampling (where only people who meet specific criteria are included in the sample).
An accurate calculation of sample size is an essential aspect of good study design. It is important to calculate the sample size much in advance, rather than have to go for post hoc analysis. A sample size that is too less may make the study underpowered, whereas a sample size which is more than necessary might lead to a wastage of resources.
We will first go through the sample size calculation for a hypothesis-based design (like a randomized control trial).
The important factors to consider for sample size calculation include study design, type of statistical test, level of significance, power and effect size, variance (standard deviation for quantitative data), and expected proportions in the case of qualitative data. This is based on previous data, either based on previous studies or based on the clinicians' experience. In case the study is something being conducted for the first time, a pilot study might be conducted which helps generate these data for further studies based on a larger sample size). It is also important to know whether the data follow a normal distribution or not.
Two essential aspects we must understand are the concept of Type I and Type II errors. In a study that compares two groups, a null hypothesis assumes that there is no significant difference between the two groups, and any observed difference being due to sampling or experimental error. When we reject a null hypothesis, when it is true, we label it as a Type I error (also denoted as “alpha,” correlating with significance levels). In a Type II error (also denoted as “beta”), we fail to reject a null hypothesis, when the alternate hypothesis is actually true. Type II errors are usually expressed as “1- β,” correlating with the power of the test. While there are no absolute rules, the minimal levels accepted are 0.05 for α (corresponding to a significance level of 5%) and 0.20 for β (corresponding to a minimum recommended power of “1 − 0.20,” or 80%).
Effect size and minimal clinically relevant difference
For a clinical trial, the investigator will have to decide in advance what clinically detectable change is significant (for numerical data, this is could be the anticipated outcome means in the two groups, whereas for categorical data, it could correlate with the proportions of successful outcomes in two groups.). While we will not go into details of the formula for sample size calculation, some important points are as follows:
In the context where effect size is involved, the sample size is inversely proportional to the square of the effect size. What this means in effect is that reducing the effect size will lead to an increase in the required sample size.
Reducing the level of significance (alpha) or increasing power (1-β) will lead to an increase in the calculated sample size.
An increase in variance of the outcome leads to an increase in the calculated sample size.
A note is that for estimation type of studies/surveys, sample size calculation needs to consider some other factors too. This includes an idea about total population size (this generally does not make a major difference when population size is above 20,000, so in situations where population size is not known we can assume a population of 20,000 or more). The other factor is the “margin of error” – the amount of deviation which the investigators find acceptable in terms of percentages. Regarding confidence levels, ideally, a 95% confidence level is the minimum recommended for surveys too. Finally, we need an idea of the expected/crude prevalence – either based on previous studies or based on estimates.
Sample size calculation also needs to add corrections for patient drop-outs/lost-to-follow-up patients and missing records. An important point is that in some studies dealing with rare diseases, it may be difficult to achieve desired sample size. In these cases, the investigators might have to rework outcomes or maybe pool data from multiple centers. Although post hoc power can be analyzed, a better approach suggested is to calculate 95% confidence intervals for the outcome and interpret the study results based on this.
Financial support and sponsorship
Conflicts of interest.
There are no conflicts of interest.
Educational resources and simple solutions for your research journey
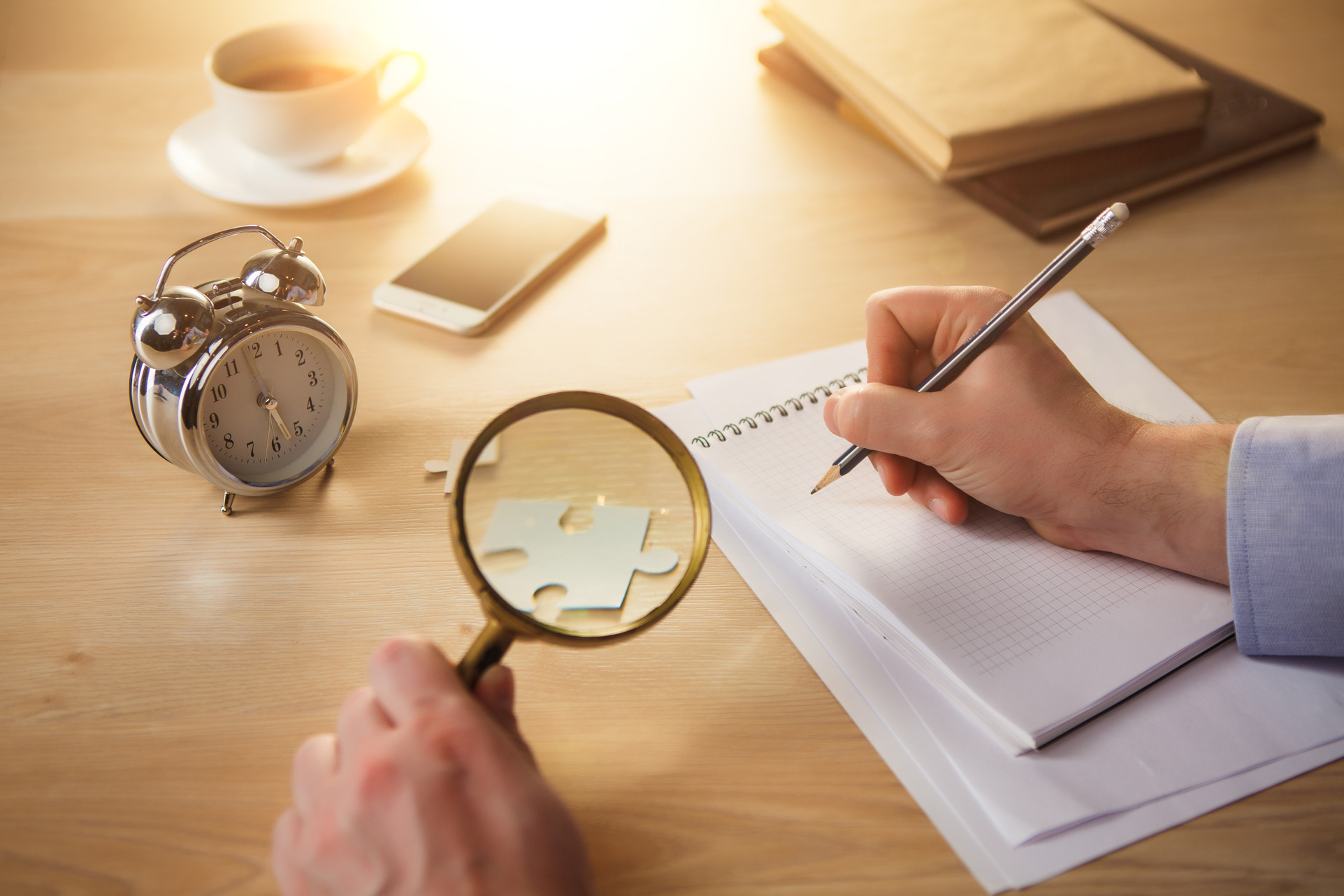
What is Descriptive Research? Definition, Methods, Types and Examples
Descriptive research is a methodological approach that seeks to depict the characteristics of a phenomenon or subject under investigation. In scientific inquiry, it serves as a foundational tool for researchers aiming to observe, record, and analyze the intricate details of a particular topic. This method provides a rich and detailed account that aids in understanding, categorizing, and interpreting the subject matter.
Descriptive research design is widely employed across diverse fields, and its primary objective is to systematically observe and document all variables and conditions influencing the phenomenon.
After this descriptive research definition, let’s look at this example. Consider a researcher working on climate change adaptation, who wants to understand water management trends in an arid village in a specific study area. She must conduct a demographic survey of the region, gather population data, and then conduct descriptive research on this demographic segment. The study will then uncover details on “what are the water management practices and trends in village X.” Note, however, that it will not cover any investigative information about “why” the patterns exist.
Table of Contents
What is descriptive research?
If you’ve been wondering “What is descriptive research,” we’ve got you covered in this post! In a nutshell, descriptive research is an exploratory research method that helps a researcher describe a population, circumstance, or phenomenon. It can help answer what , where , when and how questions, but not why questions. In other words, it does not involve changing the study variables and does not seek to establish cause-and-effect relationships.

Importance of descriptive research
Now, let’s delve into the importance of descriptive research. This research method acts as the cornerstone for various academic and applied disciplines. Its primary significance lies in its ability to provide a comprehensive overview of a phenomenon, enabling researchers to gain a nuanced understanding of the variables at play. This method aids in forming hypotheses, generating insights, and laying the groundwork for further in-depth investigations. The following points further illustrate its importance:
Provides insights into a population or phenomenon: Descriptive research furnishes a comprehensive overview of the characteristics and behaviors of a specific population or phenomenon, thereby guiding and shaping the research project.
Offers baseline data: The data acquired through this type of research acts as a reference for subsequent investigations, laying the groundwork for further studies.
Allows validation of sampling methods: Descriptive research validates sampling methods, aiding in the selection of the most effective approach for the study.
Helps reduce time and costs: It is cost-effective and time-efficient, making this an economical means of gathering information about a specific population or phenomenon.
Ensures replicability: Descriptive research is easily replicable, ensuring a reliable way to collect and compare information from various sources.
When to use descriptive research design?
Determining when to use descriptive research depends on the nature of the research question. Before diving into the reasons behind an occurrence, understanding the how, when, and where aspects is essential. Descriptive research design is a suitable option when the research objective is to discern characteristics, frequencies, trends, and categories without manipulating variables. It is therefore often employed in the initial stages of a study before progressing to more complex research designs. To put it in another way, descriptive research precedes the hypotheses of explanatory research. It is particularly valuable when there is limited existing knowledge about the subject.
Some examples are as follows, highlighting that these questions would arise before a clear outline of the research plan is established:
- In the last two decades, what changes have occurred in patterns of urban gardening in Mumbai?
- What are the differences in climate change perceptions of farmers in coastal versus inland villages in the Philippines?
Characteristics of descriptive research
Coming to the characteristics of descriptive research, this approach is characterized by its focus on observing and documenting the features of a subject. Specific characteristics are as below.
- Quantitative nature: Some descriptive research types involve quantitative research methods to gather quantifiable information for statistical analysis of the population sample.
- Qualitative nature: Some descriptive research examples include those using the qualitative research method to describe or explain the research problem.
- Observational nature: This approach is non-invasive and observational because the study variables remain untouched. Researchers merely observe and report, without introducing interventions that could impact the subject(s).
- Cross-sectional nature: In descriptive research, different sections belonging to the same group are studied, providing a “snapshot” of sorts.
- Springboard for further research: The data collected are further studied and analyzed using different research techniques. This approach helps guide the suitable research methods to be employed.
Types of descriptive research
There are various descriptive research types, each suited to different research objectives. Take a look at the different types below.
- Surveys: This involves collecting data through questionnaires or interviews to gather qualitative and quantitative data.
- Observational studies: This involves observing and collecting data on a particular population or phenomenon without influencing the study variables or manipulating the conditions. These may be further divided into cohort studies, case studies, and cross-sectional studies:
- Cohort studies: Also known as longitudinal studies, these studies involve the collection of data over an extended period, allowing researchers to track changes and trends.
- Case studies: These deal with a single individual, group, or event, which might be rare or unusual.
- Cross-sectional studies : A researcher collects data at a single point in time, in order to obtain a snapshot of a specific moment.
- Focus groups: In this approach, a small group of people are brought together to discuss a topic. The researcher moderates and records the group discussion. This can also be considered a “participatory” observational method.
- Descriptive classification: Relevant to the biological sciences, this type of approach may be used to classify living organisms.
Descriptive research methods
Several descriptive research methods can be employed, and these are more or less similar to the types of approaches mentioned above.
- Surveys: This method involves the collection of data through questionnaires or interviews. Surveys may be done online or offline, and the target subjects might be hyper-local, regional, or global.
- Observational studies: These entail the direct observation of subjects in their natural environment. These include case studies, dealing with a single case or individual, as well as cross-sectional and longitudinal studies, for a glimpse into a population or changes in trends over time, respectively. Participatory observational studies such as focus group discussions may also fall under this method.
Researchers must carefully consider descriptive research methods, types, and examples to harness their full potential in contributing to scientific knowledge.
Examples of descriptive research
Now, let’s consider some descriptive research examples.
- In social sciences, an example could be a study analyzing the demographics of a specific community to understand its socio-economic characteristics.
- In business, a market research survey aiming to describe consumer preferences would be a descriptive study.
- In ecology, a researcher might undertake a survey of all the types of monocots naturally occurring in a region and classify them up to species level.
These examples showcase the versatility of descriptive research across diverse fields.
Advantages of descriptive research
There are several advantages to this approach, which every researcher must be aware of. These are as follows:
- Owing to the numerous descriptive research methods and types, primary data can be obtained in diverse ways and be used for developing a research hypothesis .
- It is a versatile research method and allows flexibility.
- Detailed and comprehensive information can be obtained because the data collected can be qualitative or quantitative.
- It is carried out in the natural environment, which greatly minimizes certain types of bias and ethical concerns.
- It is an inexpensive and efficient approach, even with large sample sizes
Disadvantages of descriptive research
On the other hand, this design has some drawbacks as well:
- It is limited in its scope as it does not determine cause-and-effect relationships.
- The approach does not generate new information and simply depends on existing data.
- Study variables are not manipulated or controlled, and this limits the conclusions to be drawn.
- Descriptive research findings may not be generalizable to other populations.
- Finally, it offers a preliminary understanding rather than an in-depth understanding.
To reiterate, the advantages of descriptive research lie in its ability to provide a comprehensive overview, aid hypothesis generation, and serve as a preliminary step in the research process. However, its limitations include a potential lack of depth, inability to establish cause-and-effect relationships, and susceptibility to bias.
Frequently asked questions
When should researchers conduct descriptive research.
Descriptive research is most appropriate when researchers aim to portray and understand the characteristics of a phenomenon without manipulating variables. It is particularly valuable in the early stages of a study.
What is the difference between descriptive and exploratory research?
Descriptive research focuses on providing a detailed depiction of a phenomenon, while exploratory research aims to explore and generate insights into an issue where little is known.
What is the difference between descriptive and experimental research?
Descriptive research observes and documents without manipulating variables, whereas experimental research involves intentional interventions to establish cause-and-effect relationships.
Is descriptive research only for social sciences?
No, various descriptive research types may be applicable to all fields of study, including social science, humanities, physical science, and biological science.
How important is descriptive research?
The importance of descriptive research lies in its ability to provide a glimpse of the current state of a phenomenon, offering valuable insights and establishing a basic understanding. Further, the advantages of descriptive research include its capacity to offer a straightforward depiction of a situation or phenomenon, facilitate the identification of patterns or trends, and serve as a useful starting point for more in-depth investigations. Additionally, descriptive research can contribute to the development of hypotheses and guide the formulation of research questions for subsequent studies.
Editage All Access is a subscription-based platform that unifies the best AI tools and services designed to speed up, simplify, and streamline every step of a researcher’s journey. The Editage All Access Pack is a one-of-a-kind subscription that unlocks full access to an AI writing assistant, literature recommender, journal finder, scientific illustration tool, and exclusive discounts on professional publication services from Editage.
Based on 22+ years of experience in academia, Editage All Access empowers researchers to put their best research forward and move closer to success. Explore our top AI Tools pack, AI Tools + Publication Services pack, or Build Your Own Plan. Find everything a researcher needs to succeed, all in one place – Get All Access now starting at just $14 a month !
Related Posts

Back to School – Lock-in All Access Pack for a Year at the Best Price

Journal Turnaround Time: Researcher.Life and Scholarly Intelligence Join Hands to Empower Researchers with Publication Time Insights
- Privacy Policy

Home » Descriptive Research Design – Types, Methods and Examples
Descriptive Research Design – Types, Methods and Examples
Table of Contents

Descriptive Research Design
Definition:
Descriptive research design is a type of research methodology that aims to describe or document the characteristics, behaviors, attitudes, opinions, or perceptions of a group or population being studied.
Descriptive research design does not attempt to establish cause-and-effect relationships between variables or make predictions about future outcomes. Instead, it focuses on providing a detailed and accurate representation of the data collected, which can be useful for generating hypotheses, exploring trends, and identifying patterns in the data.
Types of Descriptive Research Design
Types of Descriptive Research Design are as follows:
Cross-sectional Study
This involves collecting data at a single point in time from a sample or population to describe their characteristics or behaviors. For example, a researcher may conduct a cross-sectional study to investigate the prevalence of certain health conditions among a population, or to describe the attitudes and beliefs of a particular group.
Longitudinal Study
This involves collecting data over an extended period of time, often through repeated observations or surveys of the same group or population. Longitudinal studies can be used to track changes in attitudes, behaviors, or outcomes over time, or to investigate the effects of interventions or treatments.
This involves an in-depth examination of a single individual, group, or situation to gain a detailed understanding of its characteristics or dynamics. Case studies are often used in psychology, sociology, and business to explore complex phenomena or to generate hypotheses for further research.
Survey Research
This involves collecting data from a sample or population through standardized questionnaires or interviews. Surveys can be used to describe attitudes, opinions, behaviors, or demographic characteristics of a group, and can be conducted in person, by phone, or online.
Observational Research
This involves observing and documenting the behavior or interactions of individuals or groups in a natural or controlled setting. Observational studies can be used to describe social, cultural, or environmental phenomena, or to investigate the effects of interventions or treatments.
Correlational Research
This involves examining the relationships between two or more variables to describe their patterns or associations. Correlational studies can be used to identify potential causal relationships or to explore the strength and direction of relationships between variables.
Data Analysis Methods
Descriptive research design data analysis methods depend on the type of data collected and the research question being addressed. Here are some common methods of data analysis for descriptive research:
Descriptive Statistics
This method involves analyzing data to summarize and describe the key features of a sample or population. Descriptive statistics can include measures of central tendency (e.g., mean, median, mode) and measures of variability (e.g., range, standard deviation).
Cross-tabulation
This method involves analyzing data by creating a table that shows the frequency of two or more variables together. Cross-tabulation can help identify patterns or relationships between variables.
Content Analysis
This method involves analyzing qualitative data (e.g., text, images, audio) to identify themes, patterns, or trends. Content analysis can be used to describe the characteristics of a sample or population, or to identify factors that influence attitudes or behaviors.
Qualitative Coding
This method involves analyzing qualitative data by assigning codes to segments of data based on their meaning or content. Qualitative coding can be used to identify common themes, patterns, or categories within the data.
Visualization
This method involves creating graphs or charts to represent data visually. Visualization can help identify patterns or relationships between variables and make it easier to communicate findings to others.
Comparative Analysis
This method involves comparing data across different groups or time periods to identify similarities and differences. Comparative analysis can help describe changes in attitudes or behaviors over time or differences between subgroups within a population.
Applications of Descriptive Research Design
Descriptive research design has numerous applications in various fields. Some of the common applications of descriptive research design are:
- Market research: Descriptive research design is widely used in market research to understand consumer preferences, behavior, and attitudes. This helps companies to develop new products and services, improve marketing strategies, and increase customer satisfaction.
- Health research: Descriptive research design is used in health research to describe the prevalence and distribution of a disease or health condition in a population. This helps healthcare providers to develop prevention and treatment strategies.
- Educational research: Descriptive research design is used in educational research to describe the performance of students, schools, or educational programs. This helps educators to improve teaching methods and develop effective educational programs.
- Social science research: Descriptive research design is used in social science research to describe social phenomena such as cultural norms, values, and beliefs. This helps researchers to understand social behavior and develop effective policies.
- Public opinion research: Descriptive research design is used in public opinion research to understand the opinions and attitudes of the general public on various issues. This helps policymakers to develop effective policies that are aligned with public opinion.
- Environmental research: Descriptive research design is used in environmental research to describe the environmental conditions of a particular region or ecosystem. This helps policymakers and environmentalists to develop effective conservation and preservation strategies.
Descriptive Research Design Examples
Here are some real-time examples of descriptive research designs:
- A restaurant chain wants to understand the demographics and attitudes of its customers. They conduct a survey asking customers about their age, gender, income, frequency of visits, favorite menu items, and overall satisfaction. The survey data is analyzed using descriptive statistics and cross-tabulation to describe the characteristics of their customer base.
- A medical researcher wants to describe the prevalence and risk factors of a particular disease in a population. They conduct a cross-sectional study in which they collect data from a sample of individuals using a standardized questionnaire. The data is analyzed using descriptive statistics and cross-tabulation to identify patterns in the prevalence and risk factors of the disease.
- An education researcher wants to describe the learning outcomes of students in a particular school district. They collect test scores from a representative sample of students in the district and use descriptive statistics to calculate the mean, median, and standard deviation of the scores. They also create visualizations such as histograms and box plots to show the distribution of scores.
- A marketing team wants to understand the attitudes and behaviors of consumers towards a new product. They conduct a series of focus groups and use qualitative coding to identify common themes and patterns in the data. They also create visualizations such as word clouds to show the most frequently mentioned topics.
- An environmental scientist wants to describe the biodiversity of a particular ecosystem. They conduct an observational study in which they collect data on the species and abundance of plants and animals in the ecosystem. The data is analyzed using descriptive statistics to describe the diversity and richness of the ecosystem.
How to Conduct Descriptive Research Design
To conduct a descriptive research design, you can follow these general steps:
- Define your research question: Clearly define the research question or problem that you want to address. Your research question should be specific and focused to guide your data collection and analysis.
- Choose your research method: Select the most appropriate research method for your research question. As discussed earlier, common research methods for descriptive research include surveys, case studies, observational studies, cross-sectional studies, and longitudinal studies.
- Design your study: Plan the details of your study, including the sampling strategy, data collection methods, and data analysis plan. Determine the sample size and sampling method, decide on the data collection tools (such as questionnaires, interviews, or observations), and outline your data analysis plan.
- Collect data: Collect data from your sample or population using the data collection tools you have chosen. Ensure that you follow ethical guidelines for research and obtain informed consent from participants.
- Analyze data: Use appropriate statistical or qualitative analysis methods to analyze your data. As discussed earlier, common data analysis methods for descriptive research include descriptive statistics, cross-tabulation, content analysis, qualitative coding, visualization, and comparative analysis.
- I nterpret results: Interpret your findings in light of your research question and objectives. Identify patterns, trends, and relationships in the data, and describe the characteristics of your sample or population.
- Draw conclusions and report results: Draw conclusions based on your analysis and interpretation of the data. Report your results in a clear and concise manner, using appropriate tables, graphs, or figures to present your findings. Ensure that your report follows accepted research standards and guidelines.
When to Use Descriptive Research Design
Descriptive research design is used in situations where the researcher wants to describe a population or phenomenon in detail. It is used to gather information about the current status or condition of a group or phenomenon without making any causal inferences. Descriptive research design is useful in the following situations:
- Exploratory research: Descriptive research design is often used in exploratory research to gain an initial understanding of a phenomenon or population.
- Identifying trends: Descriptive research design can be used to identify trends or patterns in a population, such as changes in consumer behavior or attitudes over time.
- Market research: Descriptive research design is commonly used in market research to understand consumer preferences, behavior, and attitudes.
- Health research: Descriptive research design is useful in health research to describe the prevalence and distribution of a disease or health condition in a population.
- Social science research: Descriptive research design is used in social science research to describe social phenomena such as cultural norms, values, and beliefs.
- Educational research: Descriptive research design is used in educational research to describe the performance of students, schools, or educational programs.
Purpose of Descriptive Research Design
The main purpose of descriptive research design is to describe and measure the characteristics of a population or phenomenon in a systematic and objective manner. It involves collecting data that describe the current status or condition of the population or phenomenon of interest, without manipulating or altering any variables.
The purpose of descriptive research design can be summarized as follows:
- To provide an accurate description of a population or phenomenon: Descriptive research design aims to provide a comprehensive and accurate description of a population or phenomenon of interest. This can help researchers to develop a better understanding of the characteristics of the population or phenomenon.
- To identify trends and patterns: Descriptive research design can help researchers to identify trends and patterns in the data, such as changes in behavior or attitudes over time. This can be useful for making predictions and developing strategies.
- To generate hypotheses: Descriptive research design can be used to generate hypotheses or research questions that can be tested in future studies. For example, if a descriptive study finds a correlation between two variables, this could lead to the development of a hypothesis about the causal relationship between the variables.
- To establish a baseline: Descriptive research design can establish a baseline or starting point for future research. This can be useful for comparing data from different time periods or populations.
Characteristics of Descriptive Research Design
Descriptive research design has several key characteristics that distinguish it from other research designs. Some of the main characteristics of descriptive research design are:
- Objective : Descriptive research design is objective in nature, which means that it focuses on collecting factual and accurate data without any personal bias. The researcher aims to report the data objectively without any personal interpretation.
- Non-experimental: Descriptive research design is non-experimental, which means that the researcher does not manipulate any variables. The researcher simply observes and records the behavior or characteristics of the population or phenomenon of interest.
- Quantitative : Descriptive research design is quantitative in nature, which means that it involves collecting numerical data that can be analyzed using statistical techniques. This helps to provide a more precise and accurate description of the population or phenomenon.
- Cross-sectional: Descriptive research design is often cross-sectional, which means that the data is collected at a single point in time. This can be useful for understanding the current state of the population or phenomenon, but it may not provide information about changes over time.
- Large sample size: Descriptive research design typically involves a large sample size, which helps to ensure that the data is representative of the population of interest. A large sample size also helps to increase the reliability and validity of the data.
- Systematic and structured: Descriptive research design involves a systematic and structured approach to data collection, which helps to ensure that the data is accurate and reliable. This involves using standardized procedures for data collection, such as surveys, questionnaires, or observation checklists.
Advantages of Descriptive Research Design
Descriptive research design has several advantages that make it a popular choice for researchers. Some of the main advantages of descriptive research design are:
- Provides an accurate description: Descriptive research design is focused on accurately describing the characteristics of a population or phenomenon. This can help researchers to develop a better understanding of the subject of interest.
- Easy to conduct: Descriptive research design is relatively easy to conduct and requires minimal resources compared to other research designs. It can be conducted quickly and efficiently, and data can be collected through surveys, questionnaires, or observations.
- Useful for generating hypotheses: Descriptive research design can be used to generate hypotheses or research questions that can be tested in future studies. For example, if a descriptive study finds a correlation between two variables, this could lead to the development of a hypothesis about the causal relationship between the variables.
- Large sample size : Descriptive research design typically involves a large sample size, which helps to ensure that the data is representative of the population of interest. A large sample size also helps to increase the reliability and validity of the data.
- Can be used to monitor changes : Descriptive research design can be used to monitor changes over time in a population or phenomenon. This can be useful for identifying trends and patterns, and for making predictions about future behavior or attitudes.
- Can be used in a variety of fields : Descriptive research design can be used in a variety of fields, including social sciences, healthcare, business, and education.
Limitation of Descriptive Research Design
Descriptive research design also has some limitations that researchers should consider before using this design. Some of the main limitations of descriptive research design are:
- Cannot establish cause and effect: Descriptive research design cannot establish cause and effect relationships between variables. It only provides a description of the characteristics of the population or phenomenon of interest.
- Limited generalizability: The results of a descriptive study may not be generalizable to other populations or situations. This is because descriptive research design often involves a specific sample or situation, which may not be representative of the broader population.
- Potential for bias: Descriptive research design can be subject to bias, particularly if the researcher is not objective in their data collection or interpretation. This can lead to inaccurate or incomplete descriptions of the population or phenomenon of interest.
- Limited depth: Descriptive research design may provide a superficial description of the population or phenomenon of interest. It does not delve into the underlying causes or mechanisms behind the observed behavior or characteristics.
- Limited utility for theory development: Descriptive research design may not be useful for developing theories about the relationship between variables. It only provides a description of the variables themselves.
- Relies on self-report data: Descriptive research design often relies on self-report data, such as surveys or questionnaires. This type of data may be subject to biases, such as social desirability bias or recall bias.
About the author
Muhammad Hassan
Researcher, Academic Writer, Web developer
You may also like

Basic Research – Types, Methods and Examples

Survey Research – Types, Methods, Examples

Qualitative Research Methods

One-to-One Interview – Methods and Guide

Triangulation in Research – Types, Methods and...

Focus Groups – Steps, Examples and Guide
- What is descriptive research?
Last updated
5 February 2023
Reviewed by
Cathy Heath
Short on time? Get an AI generated summary of this article instead
Descriptive research is a common investigatory model used by researchers in various fields, including social sciences, linguistics, and academia.
Read on to understand the characteristics of descriptive research and explore its underlying techniques, processes, and procedures.
Analyze your descriptive research
Dovetail streamlines analysis to help you uncover and share actionable insights
Descriptive research is an exploratory research method. It enables researchers to precisely and methodically describe a population, circumstance, or phenomenon.
As the name suggests, descriptive research describes the characteristics of the group, situation, or phenomenon being studied without manipulating variables or testing hypotheses . This can be reported using surveys , observational studies, and case studies. You can use both quantitative and qualitative methods to compile the data.
Besides making observations and then comparing and analyzing them, descriptive studies often develop knowledge concepts and provide solutions to critical issues. It always aims to answer how the event occurred, when it occurred, where it occurred, and what the problem or phenomenon is.
- Characteristics of descriptive research
The following are some of the characteristics of descriptive research:
Quantitativeness
Descriptive research can be quantitative as it gathers quantifiable data to statistically analyze a population sample. These numbers can show patterns, connections, and trends over time and can be discovered using surveys, polls, and experiments.
Qualitativeness
Descriptive research can also be qualitative. It gives meaning and context to the numbers supplied by quantitative descriptive research .
Researchers can use tools like interviews, focus groups, and ethnographic studies to illustrate why things are what they are and help characterize the research problem. This is because it’s more explanatory than exploratory or experimental research.
Uncontrolled variables
Descriptive research differs from experimental research in that researchers cannot manipulate the variables. They are recognized, scrutinized, and quantified instead. This is one of its most prominent features.
Cross-sectional studies
Descriptive research is a cross-sectional study because it examines several areas of the same group. It involves obtaining data on multiple variables at the personal level during a certain period. It’s helpful when trying to understand a larger community’s habits or preferences.
Carried out in a natural environment
Descriptive studies are usually carried out in the participants’ everyday environment, which allows researchers to avoid influencing responders by collecting data in a natural setting. You can use online surveys or survey questions to collect data or observe.
Basis for further research
You can further dissect descriptive research’s outcomes and use them for different types of investigation. The outcomes also serve as a foundation for subsequent investigations and can guide future studies. For example, you can use the data obtained in descriptive research to help determine future research designs.
- Descriptive research methods
There are three basic approaches for gathering data in descriptive research: observational, case study, and survey.
You can use surveys to gather data in descriptive research. This involves gathering information from many people using a questionnaire and interview .
Surveys remain the dominant research tool for descriptive research design. Researchers can conduct various investigations and collect multiple types of data (quantitative and qualitative) using surveys with diverse designs.
You can conduct surveys over the phone, online, or in person. Your survey might be a brief interview or conversation with a set of prepared questions intended to obtain quick information from the primary source.
Observation
This descriptive research method involves observing and gathering data on a population or phenomena without manipulating variables. It is employed in psychology, market research , and other social science studies to track and understand human behavior.
Observation is an essential component of descriptive research. It entails gathering data and analyzing it to see whether there is a relationship between the two variables in the study. This strategy usually allows for both qualitative and quantitative data analysis.
Case studies
A case study can outline a specific topic’s traits. The topic might be a person, group, event, or organization.
It involves using a subset of a larger group as a sample to characterize the features of that larger group.
You can generalize knowledge gained from studying a case study to benefit a broader audience.
This approach entails carefully examining a particular group, person, or event over time. You can learn something new about the study topic by using a small group to better understand the dynamics of the entire group.
- Types of descriptive research
There are several types of descriptive study. The most well-known include cross-sectional studies, census surveys, sample surveys, case reports, and comparison studies.
Case reports and case series
In the healthcare and medical fields, a case report is used to explain a patient’s circumstances when suffering from an uncommon illness or displaying certain symptoms. Case reports and case series are both collections of related cases. They have aided the advancement of medical knowledge on countless occasions.
The normative component is an addition to the descriptive survey. In the descriptive–normative survey, you compare the study’s results to the norm.
Descriptive survey
This descriptive type of research employs surveys to collect information on various topics. This data aims to determine the degree to which certain conditions may be attained.
You can extrapolate or generalize the information you obtain from sample surveys to the larger group being researched.
Correlative survey
Correlative surveys help establish if there is a positive, negative, or neutral connection between two variables.
Performing census surveys involves gathering relevant data on several aspects of a given population. These units include individuals, families, organizations, objects, characteristics, and properties.
During descriptive research, you gather different degrees of interest over time from a specific population. Cross-sectional studies provide a glimpse of a phenomenon’s prevalence and features in a population. There are no ethical challenges with them and they are quite simple and inexpensive to carry out.
Comparative studies
These surveys compare the two subjects’ conditions or characteristics. The subjects may include research variables, organizations, plans, and people.
Comparison points, assumption of similarities, and criteria of comparison are three important variables that affect how well and accurately comparative studies are conducted.
For instance, descriptive research can help determine how many CEOs hold a bachelor’s degree and what proportion of low-income households receive government help.
- Pros and cons
The primary advantage of descriptive research designs is that researchers can create a reliable and beneficial database for additional study. To conduct any inquiry, you need access to reliable information sources that can give you a firm understanding of a situation.
Quantitative studies are time- and resource-intensive, so knowing the hypotheses viable for testing is crucial. The basic overview of descriptive research provides helpful hints as to which variables are worth quantitatively examining. This is why it’s employed as a precursor to quantitative research designs.
Some experts view this research as untrustworthy and unscientific. However, there is no way to assess the findings because you don’t manipulate any variables statistically.
Cause-and-effect correlations also can’t be established through descriptive investigations. Additionally, observational study findings cannot be replicated, which prevents a review of the findings and their replication.
The absence of statistical and in-depth analysis and the rather superficial character of the investigative procedure are drawbacks of this research approach.
- Descriptive research examples and applications
Several descriptive research examples are emphasized based on their types, purposes, and applications. Research questions often begin with “What is …” These studies help find solutions to practical issues in social science, physical science, and education.
Here are some examples and applications of descriptive research:
Determining consumer perception and behavior
Organizations use descriptive research designs to determine how various demographic groups react to a certain product or service.
For example, a business looking to sell to its target market should research the market’s behavior first. When researching human behavior in response to a cause or event, the researcher pays attention to the traits, actions, and responses before drawing a conclusion.
Scientific classification
Scientific descriptive research enables the classification of organisms and their traits and constituents.
Measuring data trends
A descriptive study design’s statistical capabilities allow researchers to track data trends over time. It’s frequently used to determine the study target’s current circumstances and underlying patterns.
Conduct comparison
Organizations can use a descriptive research approach to learn how various demographics react to a certain product or service. For example, you can study how the target market responds to a competitor’s product and use that information to infer their behavior.
- Bottom line
A descriptive research design is suitable for exploring certain topics and serving as a prelude to larger quantitative investigations. It provides a comprehensive understanding of the “what” of the group or thing you’re investigating.
This research type acts as the cornerstone of other research methodologies . It is distinctive because it can use quantitative and qualitative research approaches at the same time.
What is descriptive research design?
Descriptive research design aims to systematically obtain information to describe a phenomenon, situation, or population. More specifically, it helps answer the what, when, where, and how questions regarding the research problem rather than the why.
How does descriptive research compare to qualitative research?
Despite certain parallels, descriptive research concentrates on describing phenomena, while qualitative research aims to understand people better.
How do you analyze descriptive research data?
Data analysis involves using various methodologies, enabling the researcher to evaluate and provide results regarding validity and reliability.
Should you be using a customer insights hub?
Do you want to discover previous research faster?
Do you share your research findings with others?
Do you analyze research data?
Start for free today, add your research, and get to key insights faster
Editor’s picks
Last updated: 18 April 2023
Last updated: 27 February 2023
Last updated: 22 August 2024
Last updated: 5 February 2023
Last updated: 16 August 2024
Last updated: 9 March 2023
Last updated: 30 April 2024
Last updated: 12 December 2023
Last updated: 11 March 2024
Last updated: 4 July 2024
Last updated: 6 March 2024
Last updated: 5 March 2024
Last updated: 13 May 2024
Latest articles
Related topics, .css-je19u9{-webkit-align-items:flex-end;-webkit-box-align:flex-end;-ms-flex-align:flex-end;align-items:flex-end;display:-webkit-box;display:-webkit-flex;display:-ms-flexbox;display:flex;-webkit-flex-direction:row;-ms-flex-direction:row;flex-direction:row;-webkit-box-flex-wrap:wrap;-webkit-flex-wrap:wrap;-ms-flex-wrap:wrap;flex-wrap:wrap;-webkit-box-pack:center;-ms-flex-pack:center;-webkit-justify-content:center;justify-content:center;row-gap:0;text-align:center;max-width:671px;}@media (max-width: 1079px){.css-je19u9{max-width:400px;}.css-je19u9>span{white-space:pre;}}@media (max-width: 799px){.css-je19u9{max-width:400px;}.css-je19u9>span{white-space:pre;}} decide what to .css-1kiodld{max-height:56px;display:-webkit-box;display:-webkit-flex;display:-ms-flexbox;display:flex;-webkit-align-items:center;-webkit-box-align:center;-ms-flex-align:center;align-items:center;}@media (max-width: 1079px){.css-1kiodld{display:none;}} build next, decide what to build next, log in or sign up.
Get started for free
- Skip to main content
- Skip to primary sidebar
- Skip to footer
- QuestionPro

- Solutions Industries Gaming Automotive Sports and events Education Government Travel & Hospitality Financial Services Healthcare Cannabis Technology Use Case AskWhy Communities Audience Contactless surveys Mobile LivePolls Member Experience GDPR Positive People Science 360 Feedback Surveys
- Resources Blog eBooks Survey Templates Case Studies Training Help center

Home Market Research
Descriptive Research: Definition, Characteristics, Methods + Examples
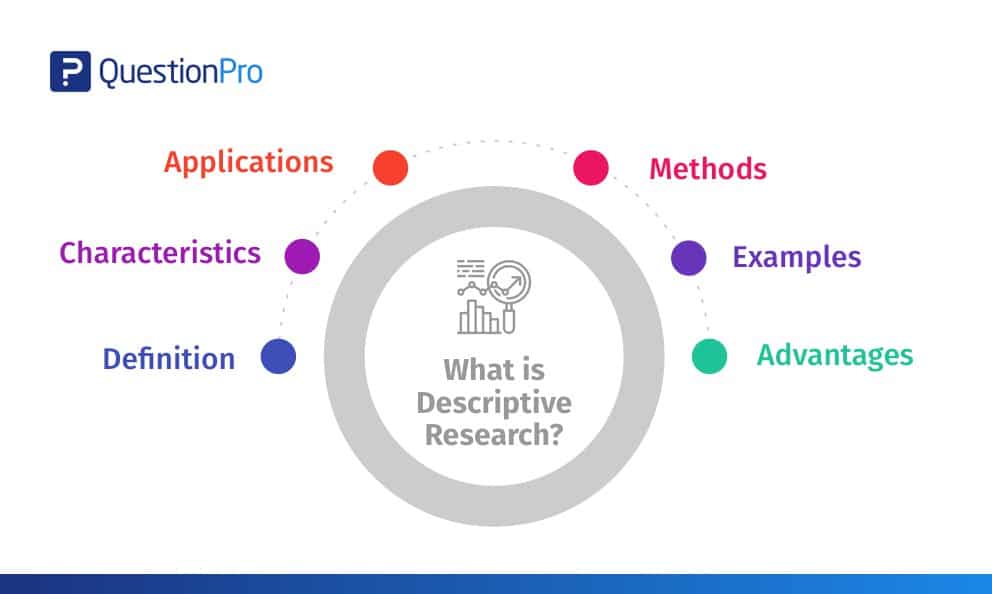
Suppose an apparel brand wants to understand the fashion purchasing trends among New York’s buyers, then it must conduct a demographic survey of the specific region, gather population data, and then conduct descriptive research on this demographic segment.
The study will then uncover details on “what is the purchasing pattern of New York buyers,” but will not cover any investigative information about “ why ” the patterns exist. Because for the apparel brand trying to break into this market, understanding the nature of their market is the study’s main goal. Let’s talk about it.
What is descriptive research?
Descriptive research is a research method describing the characteristics of the population or phenomenon studied. This descriptive methodology focuses more on the “what” of the research subject than the “why” of the research subject.
The method primarily focuses on describing the nature of a demographic segment without focusing on “why” a particular phenomenon occurs. In other words, it “describes” the research subject without covering “why” it happens.
Characteristics of descriptive research
The term descriptive research then refers to research questions, the design of the study, and data analysis conducted on that topic. We call it an observational research method because none of the research study variables are influenced in any capacity.
Some distinctive characteristics of descriptive research are:
- Quantitative research: It is a quantitative research method that attempts to collect quantifiable information for statistical analysis of the population sample. It is a popular market research tool that allows us to collect and describe the demographic segment’s nature.
- Uncontrolled variables: In it, none of the variables are influenced in any way. This uses observational methods to conduct the research. Hence, the nature of the variables or their behavior is not in the hands of the researcher.
- Cross-sectional studies: It is generally a cross-sectional study where different sections belonging to the same group are studied.
- The basis for further research: Researchers further research the data collected and analyzed from descriptive research using different research techniques. The data can also help point towards the types of research methods used for the subsequent research.
Applications of descriptive research with examples
A descriptive research method can be used in multiple ways and for various reasons. Before getting into any survey , though, the survey goals and survey design are crucial. Despite following these steps, there is no way to know if one will meet the research outcome. How to use descriptive research? To understand the end objective of research goals, below are some ways organizations currently use descriptive research today:
- Define respondent characteristics: The aim of using close-ended questions is to draw concrete conclusions about the respondents. This could be the need to derive patterns, traits, and behaviors of the respondents. It could also be to understand from a respondent their attitude, or opinion about the phenomenon. For example, understand millennials and the hours per week they spend browsing the internet. All this information helps the organization researching to make informed business decisions.
- Measure data trends: Researchers measure data trends over time with a descriptive research design’s statistical capabilities. Consider if an apparel company researches different demographics like age groups from 24-35 and 36-45 on a new range launch of autumn wear. If one of those groups doesn’t take too well to the new launch, it provides insight into what clothes are like and what is not. The brand drops the clothes and apparel that customers don’t like.
- Conduct comparisons: Organizations also use a descriptive research design to understand how different groups respond to a specific product or service. For example, an apparel brand creates a survey asking general questions that measure the brand’s image. The same study also asks demographic questions like age, income, gender, geographical location, geographic segmentation , etc. This consumer research helps the organization understand what aspects of the brand appeal to the population and what aspects do not. It also helps make product or marketing fixes or even create a new product line to cater to high-growth potential groups.
- Validate existing conditions: Researchers widely use descriptive research to help ascertain the research object’s prevailing conditions and underlying patterns. Due to the non-invasive research method and the use of quantitative observation and some aspects of qualitative observation , researchers observe each variable and conduct an in-depth analysis . Researchers also use it to validate any existing conditions that may be prevalent in a population.
- Conduct research at different times: The analysis can be conducted at different periods to ascertain any similarities or differences. This also allows any number of variables to be evaluated. For verification, studies on prevailing conditions can also be repeated to draw trends.
Advantages of descriptive research
Some of the significant advantages of descriptive research are:
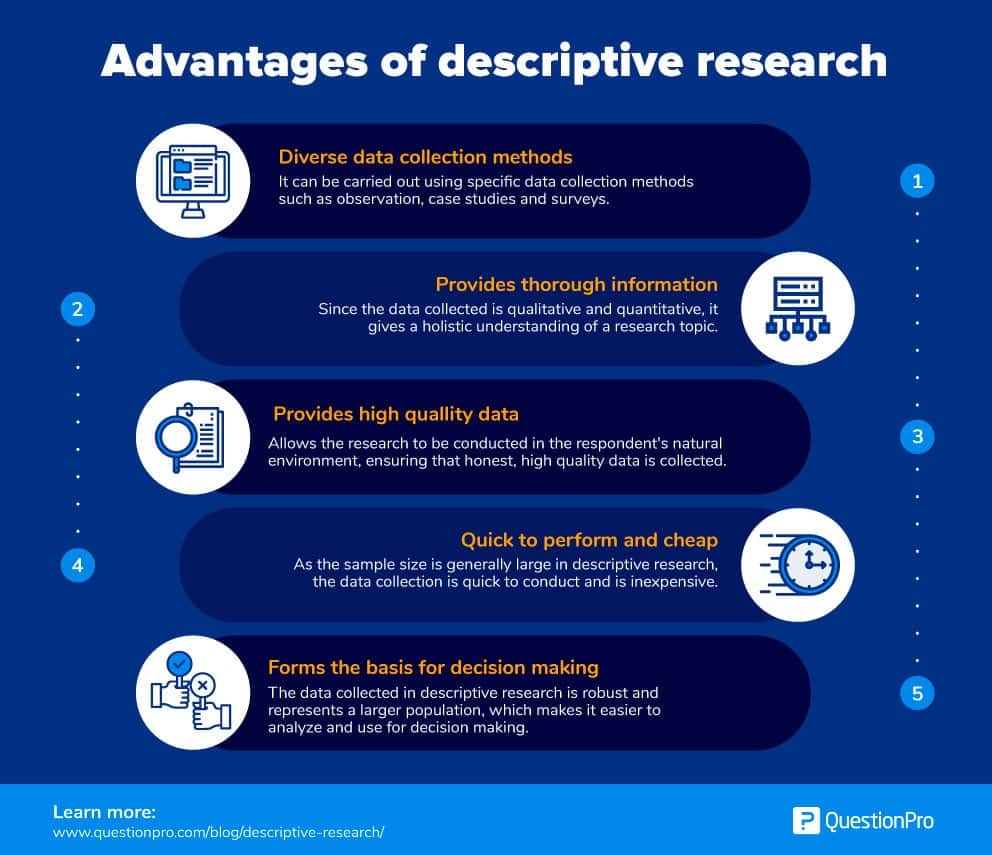
- Data collection: A researcher can conduct descriptive research using specific methods like observational method, case study method, and survey method. Between these three, all primary data collection methods are covered, which provides a lot of information. This can be used for future research or even for developing a hypothesis for your research object.
- Varied: Since the data collected is qualitative and quantitative, it gives a holistic understanding of a research topic. The information is varied, diverse, and thorough.
- Natural environment: Descriptive research allows for the research to be conducted in the respondent’s natural environment, which ensures that high-quality and honest data is collected.
- Quick to perform and cheap: As the sample size is generally large in descriptive research, the data collection is quick to conduct and is inexpensive.
Descriptive research methods
There are three distinctive methods to conduct descriptive research. They are:
Observational method
The observational method is the most effective method to conduct this research, and researchers make use of both quantitative and qualitative observations.
A quantitative observation is the objective collection of data primarily focused on numbers and values. It suggests “associated with, of or depicted in terms of a quantity.” Results of quantitative observation are derived using statistical and numerical analysis methods. It implies observation of any entity associated with a numeric value such as age, shape, weight, volume, scale, etc. For example, the researcher can track if current customers will refer the brand using a simple Net Promoter Score question .
Qualitative observation doesn’t involve measurements or numbers but instead just monitoring characteristics. In this case, the researcher observes the respondents from a distance. Since the respondents are in a comfortable environment, the characteristics observed are natural and effective. In a descriptive research design, the researcher can choose to be either a complete observer, an observer as a participant, a participant as an observer, or a full participant. For example, in a supermarket, a researcher can from afar monitor and track the customers’ selection and purchasing trends. This offers a more in-depth insight into the purchasing experience of the customer.
Case study method
Case studies involve in-depth research and study of individuals or groups. Case studies lead to a hypothesis and widen a further scope of studying a phenomenon. However, case studies should not be used to determine cause and effect as they can’t make accurate predictions because there could be a bias on the researcher’s part. The other reason why case studies are not a reliable way of conducting descriptive research is that there could be an atypical respondent in the survey. Describing them leads to weak generalizations and moving away from external validity.
Survey research
In survey research, respondents answer through surveys or questionnaires or polls . They are a popular market research tool to collect feedback from respondents. A study to gather useful data should have the right survey questions. It should be a balanced mix of open-ended questions and close ended-questions . The survey method can be conducted online or offline, making it the go-to option for descriptive research where the sample size is enormous.
Examples of descriptive research
Some examples of descriptive research are:
- A specialty food group launching a new range of barbecue rubs would like to understand what flavors of rubs are favored by different people. To understand the preferred flavor palette, they conduct this type of research study using various methods like observational methods in supermarkets. By also surveying while collecting in-depth demographic information, offers insights about the preference of different markets. This can also help tailor make the rubs and spreads to various preferred meats in that demographic. Conducting this type of research helps the organization tweak their business model and amplify marketing in core markets.
- Another example of where this research can be used is if a school district wishes to evaluate teachers’ attitudes about using technology in the classroom. By conducting surveys and observing their comfortableness using technology through observational methods, the researcher can gauge what they can help understand if a full-fledged implementation can face an issue. This also helps in understanding if the students are impacted in any way with this change.
Some other research problems and research questions that can lead to descriptive research are:
- Market researchers want to observe the habits of consumers.
- A company wants to evaluate the morale of its staff.
- A school district wants to understand if students will access online lessons rather than textbooks.
- To understand if its wellness questionnaire programs enhance the overall health of the employees.
LEARN MORE FREE TRIAL
MORE LIKE THIS
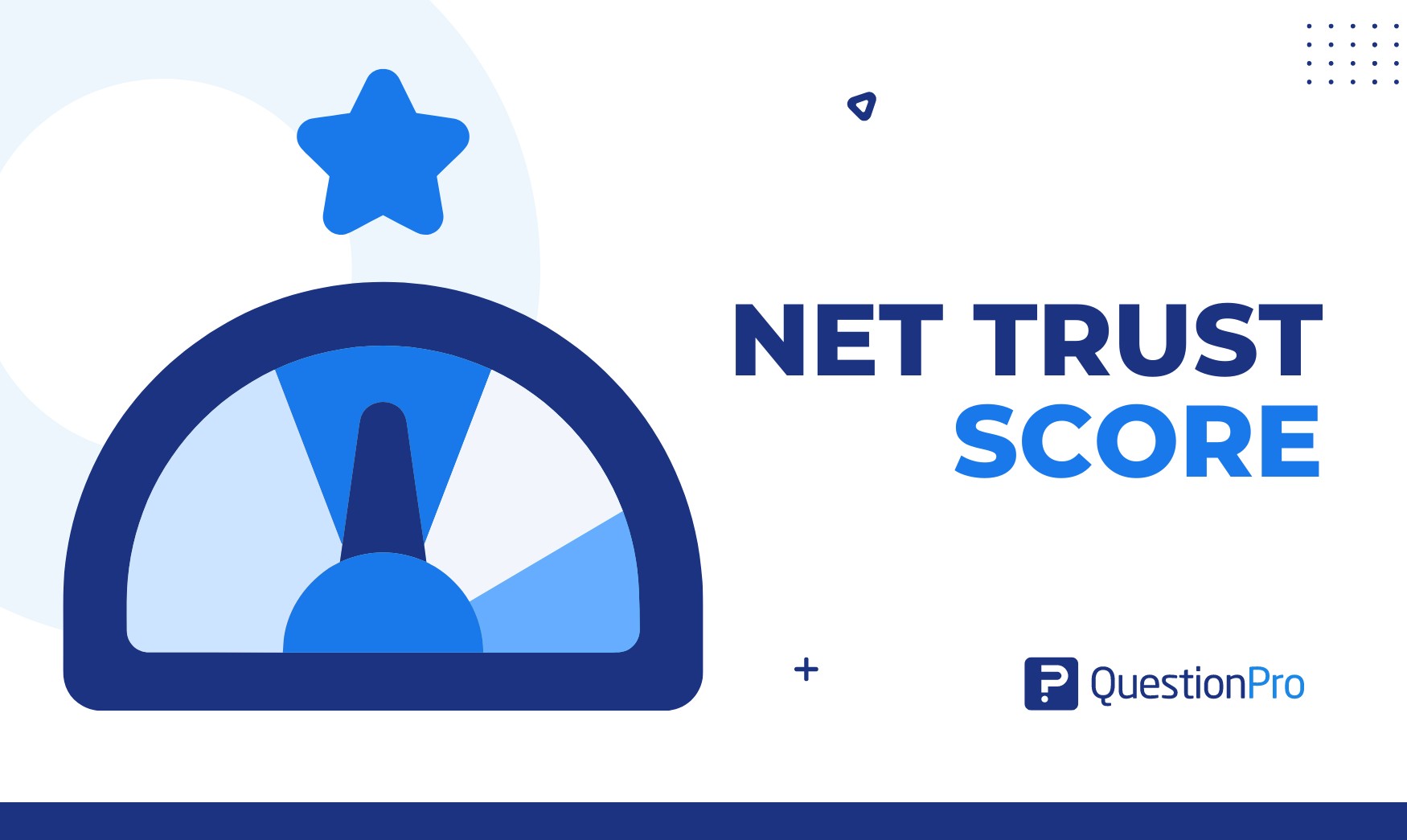
Net Trust Score: Tool for Measuring Trust in Organization
Sep 2, 2024
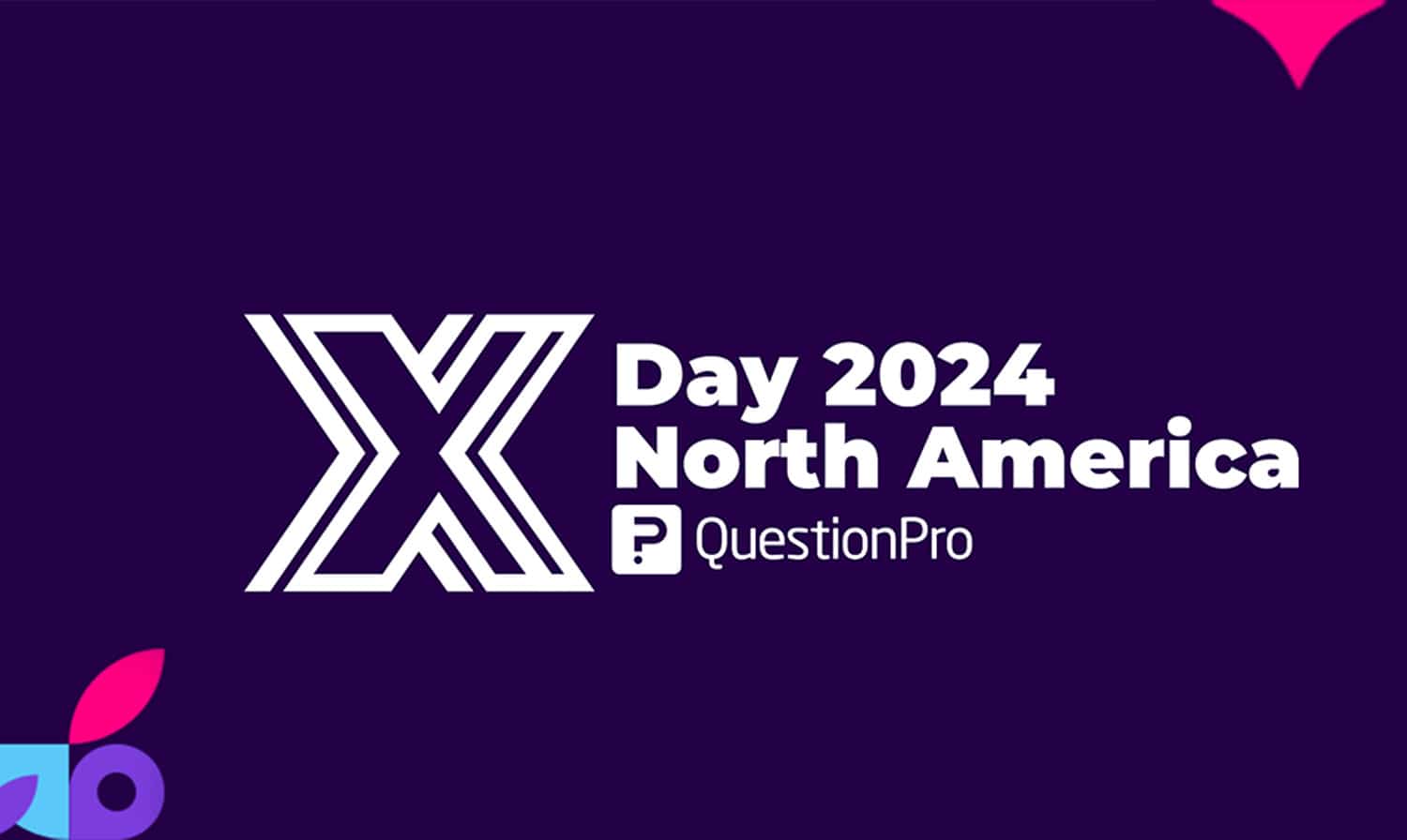
Why You Should Attend XDAY 2024
Aug 30, 2024
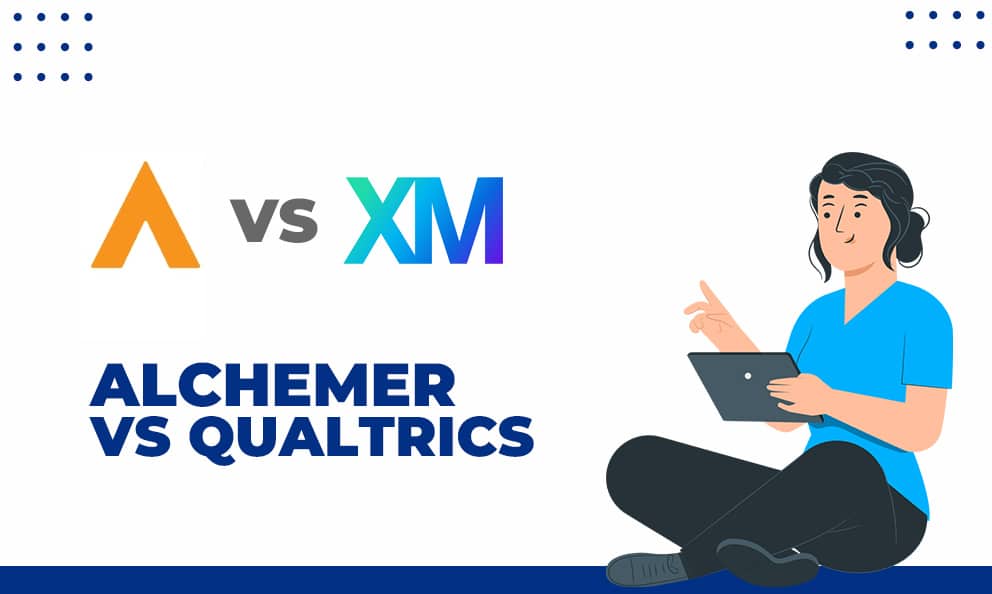
Alchemer vs Qualtrics: Find out which one you should choose
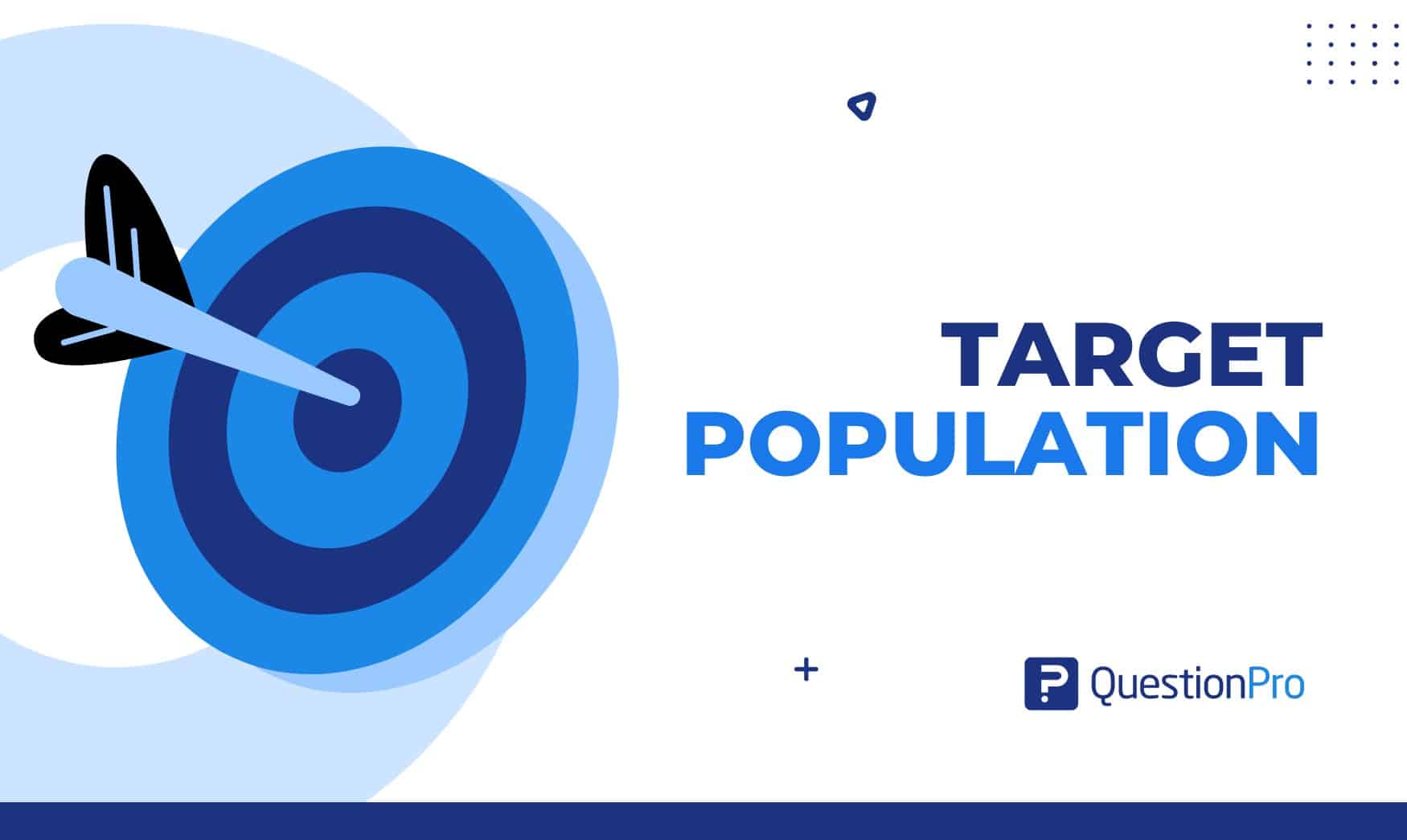
Target Population: What It Is + Strategies for Targeting
Aug 29, 2024
Other categories
- Academic Research
- Artificial Intelligence
- Assessments
- Brand Awareness
- Case Studies
- Communities
- Consumer Insights
- Customer effort score
- Customer Engagement
- Customer Experience
- Customer Loyalty
- Customer Research
- Customer Satisfaction
- Employee Benefits
- Employee Engagement
- Employee Retention
- Friday Five
- General Data Protection Regulation
- Insights Hub
- Life@QuestionPro
- Market Research
- Mobile diaries
- Mobile Surveys
- New Features
- Online Communities
- Question Types
- Questionnaire
- QuestionPro Products
- Release Notes
- Research Tools and Apps
- Revenue at Risk
- Survey Templates
- Training Tips
- Tuesday CX Thoughts (TCXT)
- Uncategorized
- What’s Coming Up
- Workforce Intelligence
Copyright © SurveySparrow Inc. 2024 Privacy Policy Terms of Service SurveySparrow Inc.
Descriptive Research 101: Definition, Methods and Examples

Parvathi Vijayamohan
Last Updated: 16 July 2024
10 min read
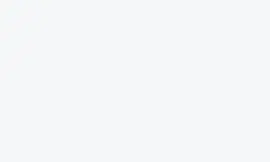
Table Of Contents
- Descriptive Research 101: The Definitive Guide
What is Descriptive Research?
- Key Characteristics
- Observation
- Case Studies
- Types of Descriptive Research
- Question Examples
- Real-World Examples
Tips to Excel at Descriptive Research
- More Interesting Reads
Imagine you are a detective called to a crime scene. Your job is to study the scene and report whatever you find: whether that’s the half-smoked cigarette on the table or the large “RACHE” written in blood on the wall. That, in a nutshell, is descriptive research .
Researchers often need to do descriptive research on a problem before they attempt to solve it. So in this guide, we’ll take you through:
- What is descriptive research + its characteristics
- Descriptive research methods
- Types of descriptive research
- Descriptive research examples
- Tips to excel at the descriptive method
Click to jump to the section that interests you.
Let’s begin by going through what descriptive studies can and cannot do.
Definition: As its name says, descriptive research describes the characteristics of the problem, phenomenon, situation, or group under study.
So the goal of all descriptive studies is to explore the background, details, and existing patterns in the problem to fully understand it. In other words, preliminary research.
However, descriptive research can be both preliminary and conclusive . You can use the data from a descriptive study to make reports and get insights for further planning.
What descriptive research isn’t: Descriptive research finds the what/when/where of a problem, not the why/how .
Because of this, we can’t use the descriptive method to explore cause-and-effect relationships where one variable (like a person’s job role) affects another variable (like their monthly income).
Key Characteristics of Descriptive Research
- Answers the “what,” “when,” and “where” of a research problem. For this reason, it is popularly used in market research , awareness surveys , and opinion polls .
- Sets the stage for a research problem. As an early part of the research process, descriptive studies help you dive deeper into the topic.
- Opens the door for further research. You can use descriptive data as the basis for more profound research, analysis and studies.
- Qualitative and quantitative research . It is possible to get a balanced mix of numerical responses and open-ended answers from the descriptive method.
- No control or interference with the variables . The researcher simply observes and reports on them. However, specific research software has filters that allow her to zoom in on one variable.
- Done in natural settings . You can get the best results from descriptive research by talking to people, surveying them, or observing them in a suitable environment. For example, suppose you are a website beta testing an app feature. In that case, descriptive research invites users to try the feature, tracking their behavior and then asking their opinions .
- Can be applied to many research methods and areas. Examples include healthcare, SaaS, psychology, political studies, education, and pop culture.
Descriptive Research Methods: The Top Three You Need to Know!
In short, survey research is a brief interview or conversation with a set of prepared questions about a topic. So you create a questionnaire, share it, and analyze the data you collect for further action.
Read more : The difference between surveys vs questionnaires
- Surveys can be hyper-local, regional, or global, depending on your objectives.
- Share surveys in-person, offline, via SMS, email, or QR codes – so many options!
- Easy to automate if you want to conduct many surveys over a period.
FYI: If you’re looking for the perfect tool to conduct descriptive research, SurveySparrow’s got you covered. Our AI-powered text and sentiment analysis help you instantly capture detailed insights for your studies.
With 1,000+ customizable (and free) survey templates , 20+ question types, and 1500+ integrations , SurveySparrow makes research super-easy.
Want to try out our platform? Click on the template below to start using it.👇
Product Market Research Survey Template
Preview Template
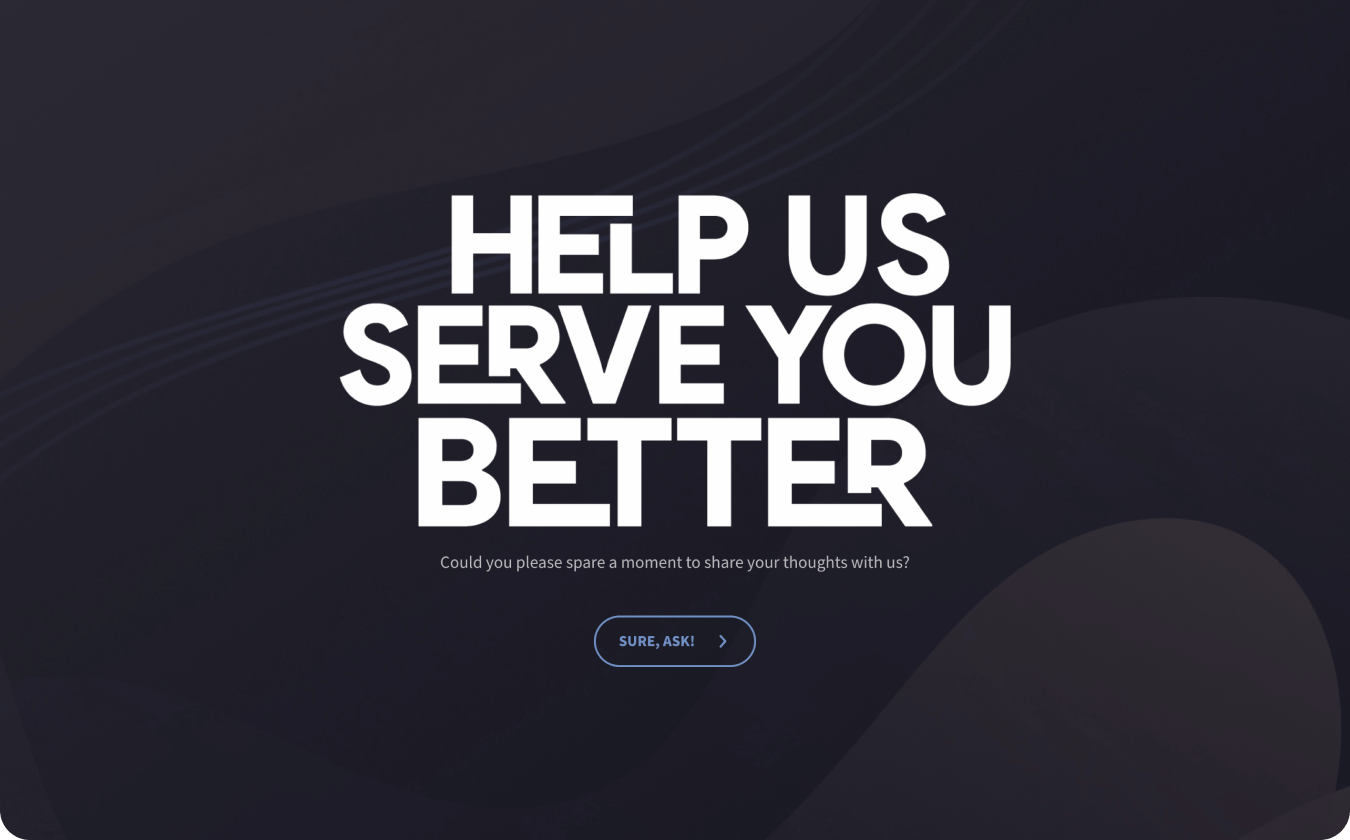
2. Observation
The observational method is a type of descriptive research in which you, the researcher, observe ongoing behavior.
Now, there are several (non-creepy) ways you can observe someone. In fact, observational research has three main approaches:
- Covert observation: In true spy fashion, the researcher mixes in with the group undetected or observes from a distance.
- Overt observation : The researcher identifies himself as a researcher – “The name’s Bond. J. Bond.” – and explains the purpose of the study.
- Participatory observation : The researcher participates in what he is observing to understand his topic better.
- Observation is one of the most accurate ways to get data on a subject’s behavior in a natural setting.
- You don’t need to rely on people’s willingness to share information.
- Observation is a universal method that can be applied to any area of research.
3. Case Studies
In the case study method, you do a detailed study of a specific group, person, or event over a period.
This brings us to a frequently asked question: “What’s the difference between case studies and longitudinal studies?”
A case study will go very in-depth into the subject with one-on-one interviews, observations, and archival research. They are also qualitative, though sometimes they will use numbers and stats.
An example of longitudinal research would be a study of the health of night shift employees vs. general shift employees over a decade. An example of a case study would involve in-depth interviews with Casey, an assistant director of nursing who’s handled the night shift at the hospital for ten years now.
- Due to the focus on a few people, case studies can give you a tremendous amount of information.
- Because of the time and effort involved, a case study engages both researchers and participants.
- Case studies are helpful for ethically investigating unusual, complex, or challenging subjects. An example would be a study of the habits of long-term cocaine users.
7 Types of Descriptive Research
Cross-sectional research | Studies a particular group of people or their sections at a given point in time. Example: current social attitudes of Gen Z in the US |
Longitudinal research | Studies a group of people over a long period of time. Example: tracking changes in social attitudes among Gen-Zers from 2022 – 2032. |
Normative research | Compares the results of a study against the existing norms. Example: comparing a verdict in a legal case against similar cases. |
Correlational/relational research | Investigates the type of relationship and patterns between 2 variables. Example: music genres and mental states. |
Comparative research | Compares 2 or more similar people, groups or conditions based on specific traits. Example: job roles of employees in similar positions from two different companies. |
Classification research | Arranges the data into classes according to certain criteria for better analysis. Example: the classification of newly discovered insects into species. |
Archival research | Searching for and extracting information from past records. Example: Tracking US Census data over the decades. |
Descriptive Research Question Examples
- How have teen social media habits changed in 10 years?
- What causes high employee turnover in tech?
- How do urban and rural diets differ in India?
- What are consumer preferences for electric vs. gasoline cars in Germany?
- How common is smartphone addiction among UK college students?
- What drives customer satisfaction in banking?
- How have adolescent mental health issues changed in 15 years?
- What leisure activities are popular among retirees in Japan?
- How do commute times vary in US metro areas?
- What makes e-commerce websites successful?
Descriptive Research: Real-World Examples To Build Your Next Study
1. case study: airbnb’s growth strategy.
In an excellent case study, Tam Al Saad, Principal Consultant, Strategy + Growth at Webprofits, deep dives into how Airbnb attracted and retained 150 million users .
“What Airbnb offers isn’t a cheap place to sleep when you’re on holiday; it’s the opportunity to experience your destination as a local would. It’s the chance to meet the locals, experience the markets, and find non-touristy places.
Sure, you can visit the Louvre, see Buckingham Palace, and climb the Empire State Building, but you can do it as if it were your hometown while staying in a place that has character and feels like a home.” – Tam al Saad, Principal Consultant, Strategy + Growth at Webprofits
2. Observation – Better Tech Experiences for the Elderly
We often think that our elders are so hopeless with technology. But we’re not getting any younger either, and tech is changing at a hair trigger! This article by Annemieke Hendricks shares a wonderful example where researchers compare the levels of technological familiarity between age groups and how that influences usage.
“It is generally assumed that older adults have difficulty using modern electronic devices, such as mobile telephones or computers. Because this age group is growing in most countries, changing products and processes to adapt to their needs is increasingly more important. “ – Annemieke Hendricks, Marketing Communication Specialist, Noldus
3. Surveys – Decoding Sleep with SurveySparrow
SRI International (formerly Stanford Research Institute) – an independent, non-profit research center – wanted to investigate the impact of stress on an adolescent’s sleep. To get those insights, two actions were essential: tracking sleep patterns through wearable devices and sending surveys at a pre-set time – the pre-sleep period.
“With SurveySparrow’s recurring surveys feature, SRI was able to share engaging surveys with their participants exactly at the time they wanted and at the frequency they preferred.”
Read more about this project : How SRI International decoded sleep patterns with SurveySparrow
1: Answer the six Ws –
- Who should we consider?
- What information do we need?
- When should we collect the information?
- Where should we collect the information?
- Why are we obtaining the information?
- Way to collect the information
#2: Introduce and explain your methodological approach
#3: Describe your methods of data collection and/or selection.
#4: Describe your methods of analysis.
#5: Explain the reasoning behind your choices.
#6: Collect data.
#7: Analyze the data. Use software to speed up the process and reduce overthinking and human error.
#8: Report your conclusions and how you drew the results.
Wrapping Up
Whether it’s social media habits, consumer preferences, or mental health trends, descriptive research provides a clear snapshot into what people actually think.
If you want to know more about feedback methodology, or research, check out some of our other articles below.
👉 Desk Research 101: Definition, Methods, and Examples
👉 Exploratory Research: Your Guide to Unraveling Insights
👉 Design Research: Types, Methods, and Importance

Content marketer at SurveySparrow.
Parvathi is a sociologist turned marketer. After 6 years as a copywriter, she pivoted to B2B, diving into growth marketing for SaaS. Now she uses content and conversion optimization to fuel growth - focusing on CX, reputation management and feedback methodology for businesses.
You Might Also Like
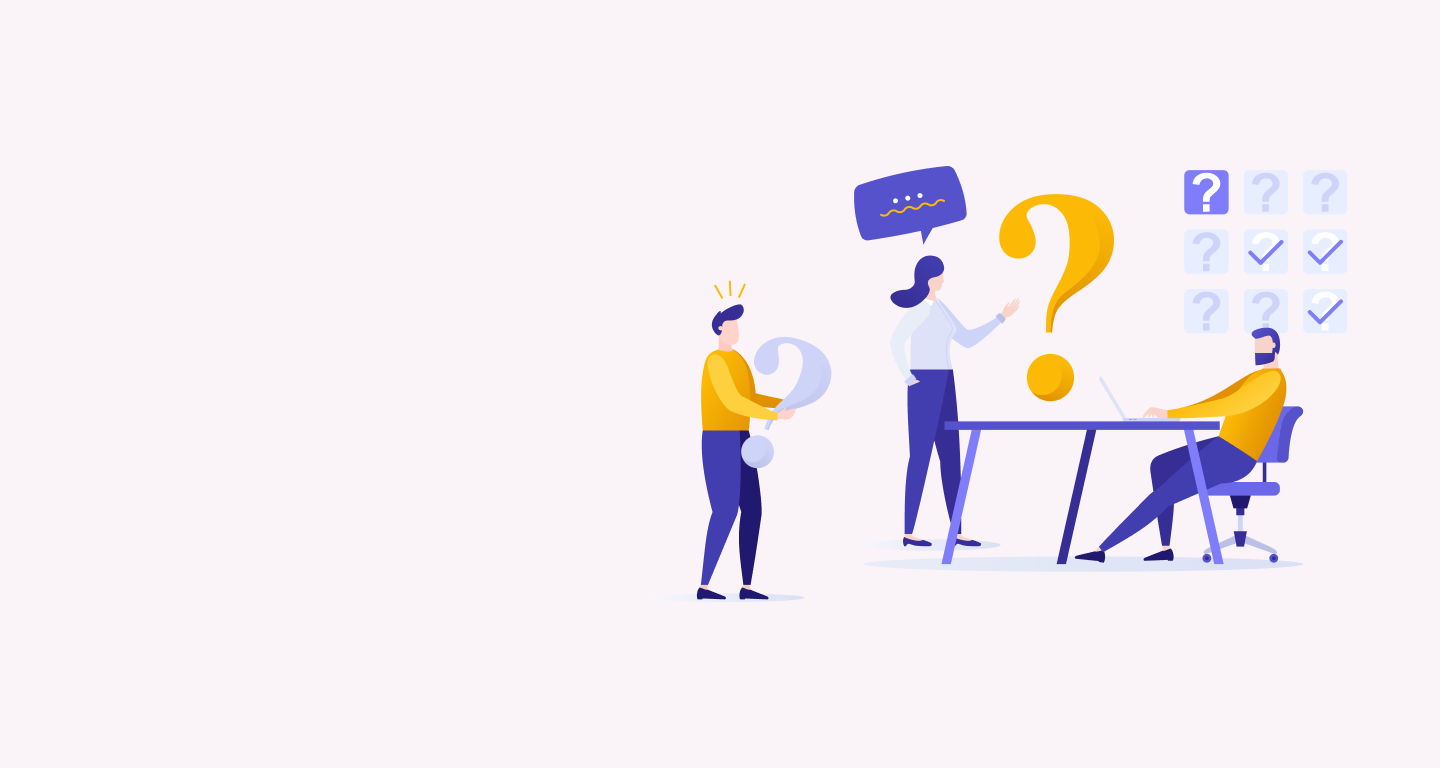
How to Craft the Perfect Coaching Effectiveness Survey Questions
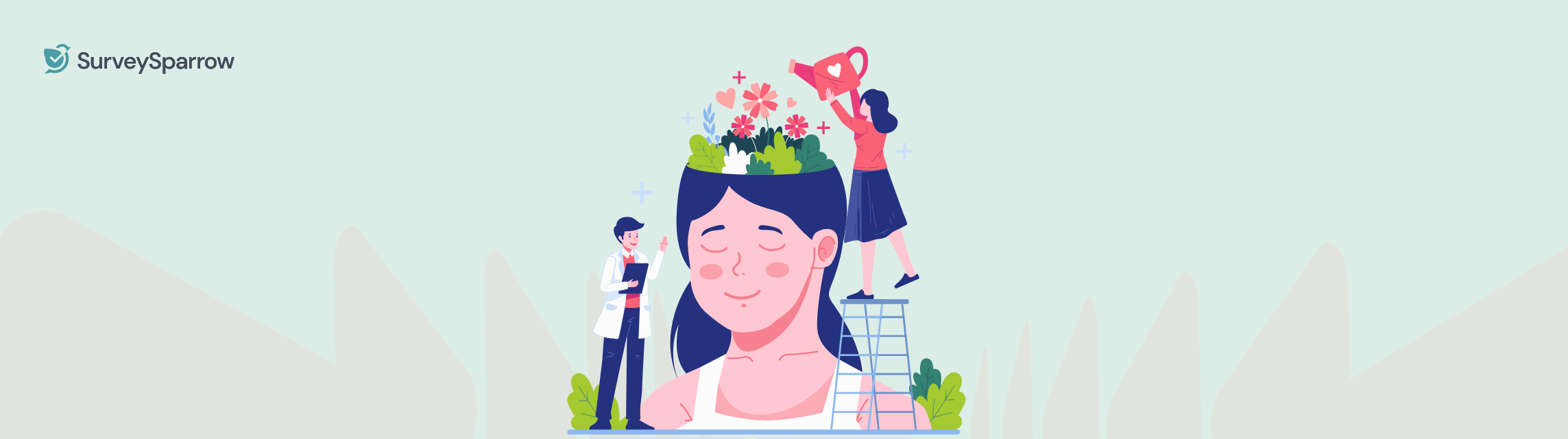
70+ Mental Health Survey Questions for Students (Types & Free Survey Questionnaire)
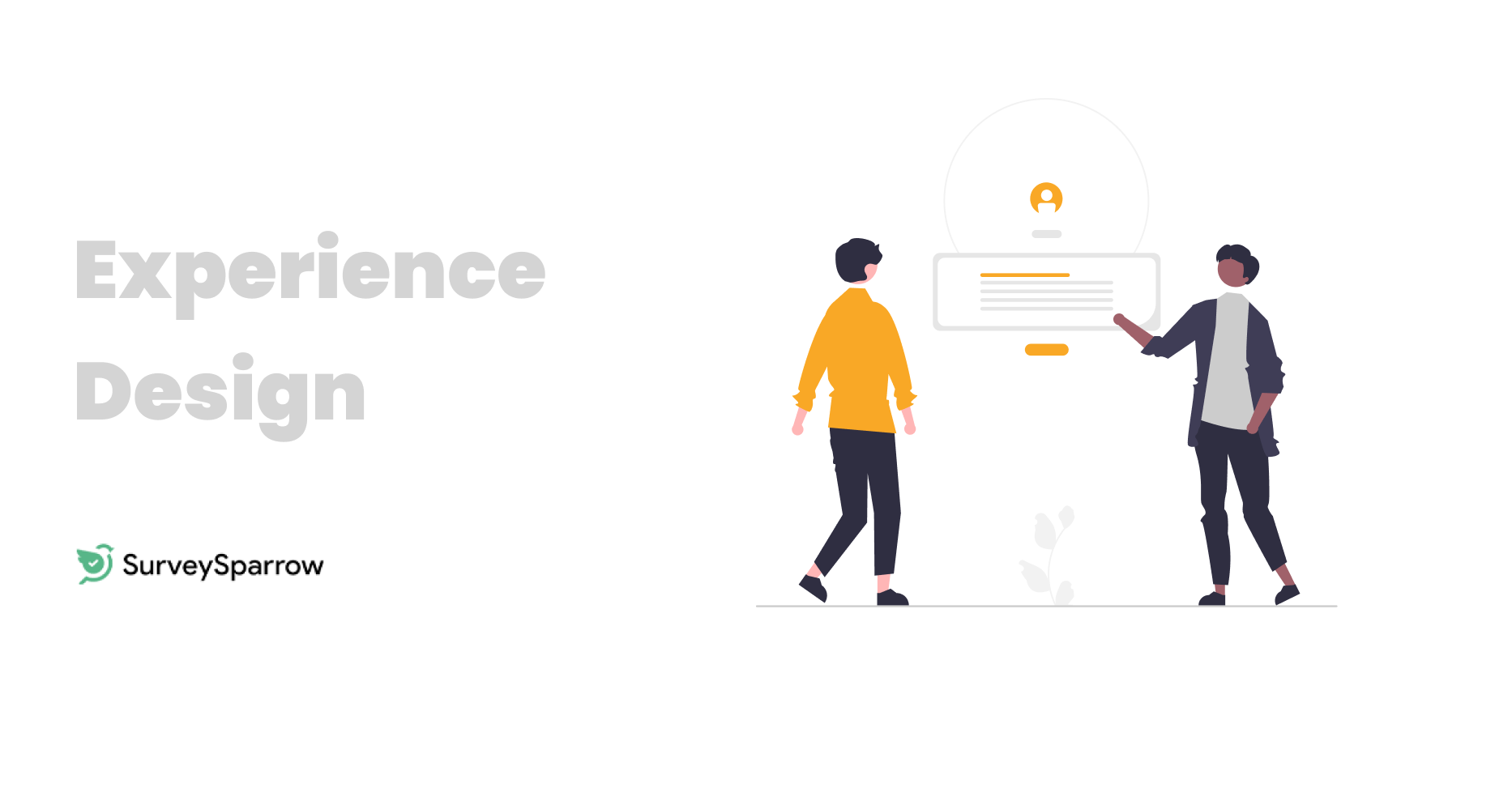
Customer Experience
Experience Design 101: A Guide to Enhancing User Experience in 2024
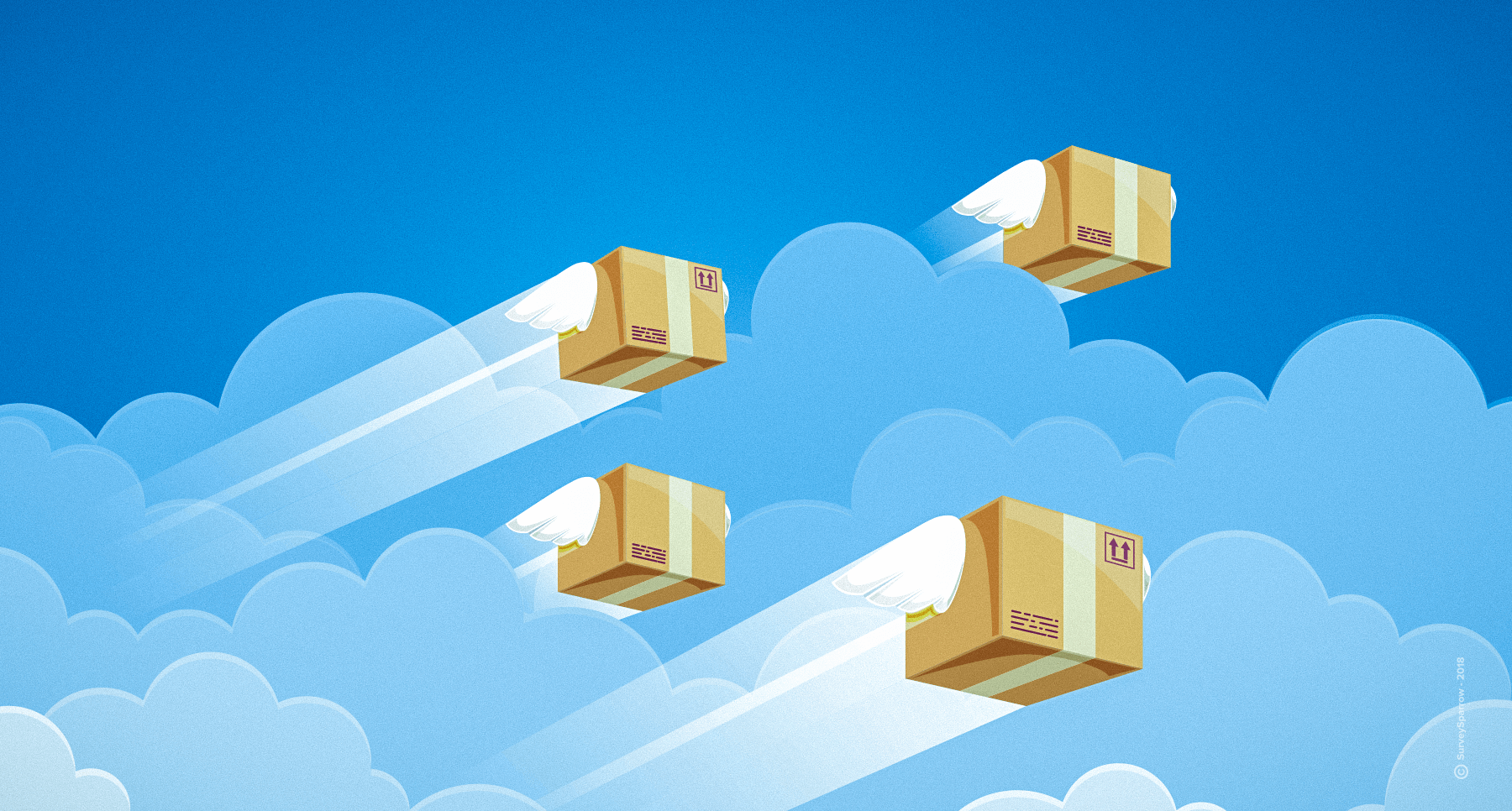
Startup Journey
Why This June is Brighter Than The 4th of July!
Turn every feedback into a growth opportunity.
14-day free trial • Cancel Anytime • No Credit Card Required • Need a Demo?

What is Descriptive Research and How is it Used?

Introduction
What does descriptive research mean, why would you use a descriptive research design, what are the characteristics of descriptive research, examples of descriptive research, what are the data collection methods in descriptive research, how do you analyze descriptive research data, ensuring validity and reliability in the findings.
Conducting descriptive research offers researchers a way to present phenomena as they naturally occur. Rooted in an open-ended and non-experimental nature, this type of research focuses on portraying the details of specific phenomena or contexts, helping readers gain a clearer understanding of topics of interest.
From businesses gauging customer satisfaction to educators assessing classroom dynamics, the data collected from descriptive research provides invaluable insights across various fields.
This article aims to illuminate the essence, utility, characteristics, and methods associated with descriptive research, guiding those who wish to harness its potential in their respective domains.

At its core, descriptive research refers to a systematic approach used by researchers to collect, analyze, and present data about real-life phenomena to describe it in its natural context. It primarily aims to describe what exists, based on empirical observations .
Unlike experimental research, where variables are manipulated to observe outcomes, descriptive research deals with the "as-is" scenario to facilitate further research by providing a framework or new insights on which continuing studies can build.
Definition of descriptive research
Descriptive research is defined as a research method that observes and describes the characteristics of a particular group, situation, or phenomenon.
The goal is not to establish cause and effect relationships but rather to provide a detailed account of the situation.
The difference between descriptive and exploratory research
While both descriptive and exploratory research seek to provide insights into a topic or phenomenon, they differ in their focus. Exploratory research is more about investigating a topic to develop preliminary insights or to identify potential areas of interest.
In contrast, descriptive research offers detailed accounts and descriptions of the observed phenomenon, seeking to paint a full picture of what's happening.
The evolution of descriptive research in academia
Historically, descriptive research has played a foundational role in numerous academic disciplines. Anthropologists, for instance, used this approach to document cultures and societies. Psychologists have employed it to capture behaviors, emotions, and reactions.
Over time, the method has evolved, incorporating technological advancements and adapting to contemporary needs, yet its essence remains rooted in describing a phenomenon or setting as it is.

Descriptive research serves as a cornerstone in the research landscape for its ability to provide a detailed snapshot of life. Its unique qualities and methods make it an invaluable method for various research purposes. Here's why:
Benefits of obtaining a clear picture
Descriptive research captures the present state of phenomena, offering researchers a detailed reflection of situations. This unaltered representation is crucial for sectors like marketing, where understanding current consumer behavior can shape future strategies.
Facilitating data interpretation
Given its straightforward nature, descriptive research can provide data that's easier to interpret, both for researchers and their audiences. Rather than analyzing complex statistical relationships among variables, researchers present detailed descriptions of their qualitative observations . Researchers can engage in in depth analysis relating to their research question , but audiences can also draw insights from their own interpretations or reflections on potential underlying patterns.
Enhancing the clarity of the research problem
By presenting things as they are, descriptive research can help elucidate ambiguous research questions. A well-executed descriptive study can shine light on overlooked aspects of a problem, paving the way for further investigative research.
Addressing practical problems
In real-world scenarios, it's not always feasible to manipulate variables or set up controlled experiments. For instance, in social sciences, understanding cultural norms without interference is paramount. Descriptive research allows for such non-intrusive insights, ensuring genuine understanding.
Building a foundation for future research
Often, descriptive studies act as stepping stones for more complex research endeavors. By establishing baseline data and highlighting patterns, they create a platform upon which more intricate hypotheses can be built and tested in subsequent studies.
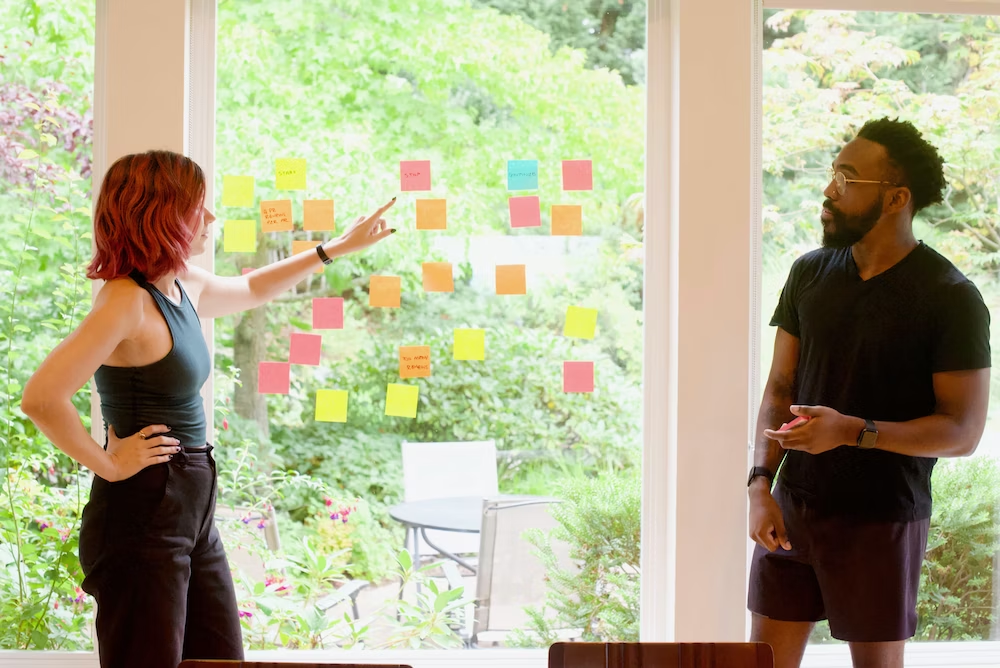
Descriptive research is distinguished by a set of hallmark characteristics that set it apart from other research methodologies . Recognizing these features can help researchers effectively design, implement , and interpret descriptive studies.
Specificity in the research question
As with all research, descriptive research starts with a well-defined research question aiming to detail a particular phenomenon. The specificity ensures that the study remains focused on gathering relevant data without unnecessary deviations.
Focus on the present situation
While some research methods aim to predict future trends or uncover historical truths, descriptive research is predominantly concerned with the present. It seeks to capture the current state of affairs, such as understanding today's consumer habits or documenting a newly observed phenomenon.
Standardized and structured methodology
To ensure credibility and consistency in results, descriptive research often employs standardized methods. Whether it's using a fixed set of survey questions or adhering to specific observation protocols, this structured approach ensures that data is collected uniformly, making it easier to compare and analyze.
Non-manipulative approach in observation
One of the standout features of descriptive research is its non-invasive nature. Researchers observe and document without influencing the research subject or the environment. This passive stance ensures that the data gathered is a genuine reflection of the phenomenon under study.
Replicability and consistency in results
Due to its structured methodology, findings from descriptive research can often be replicated in different settings or with different samples. This consistency adds to the credibility of the results, reinforcing the validity of the insights drawn from the study.

Analyze data quickly and efficiently with ATLAS.ti
Download a free trial to see how you can make sense of complex qualitative data.
Numerous fields and sectors conduct descriptive research for its versatile and detailed nature. Through its focus on presenting things as they naturally occur, it provides insights into a myriad of scenarios. Here are some tangible examples from diverse domains:
Conducting market research
Businesses often turn to data analysis through descriptive research to understand the demographics of their target market. For instance, a company launching a new product might survey potential customers to understand their age, gender, income level, and purchasing habits, offering valuable data for targeted marketing strategies.
Evaluating employee behaviors
Organizations rely on descriptive research designs to assess the behavior and attitudes of their employees. By conducting observations or surveys , companies can gather data on workplace satisfaction, collaboration patterns, or the impact of a new office layout on productivity.
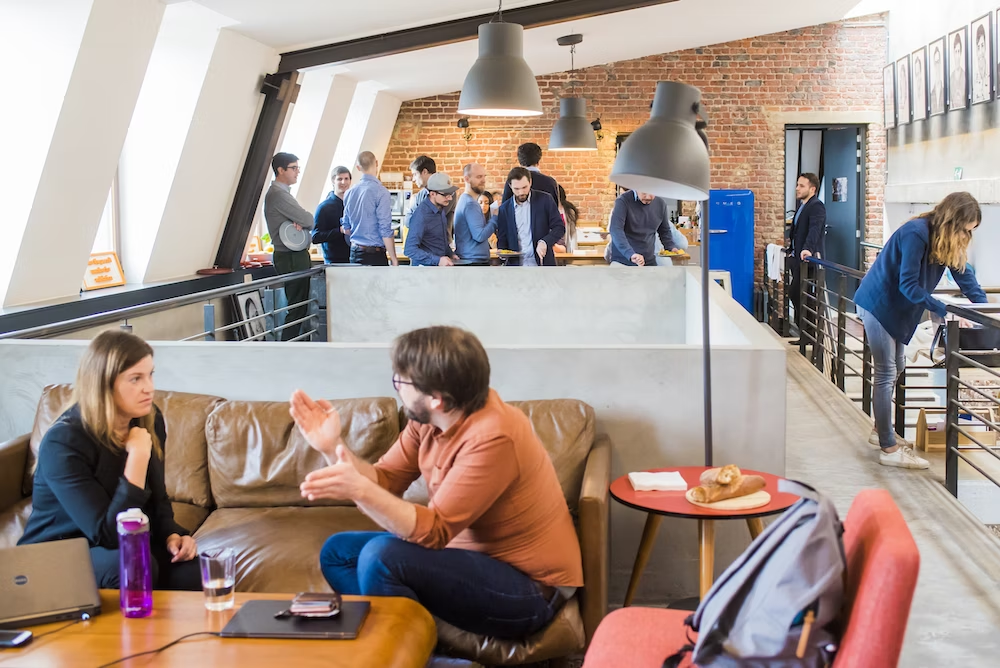
Understanding consumer preferences
Brands aiming to understand their consumers' likes and dislikes often use descriptive research. By observing shopping behaviors or conducting product feedback surveys , they can gauge preferences and adjust their offerings accordingly.
Documenting historical patterns
Historians and anthropologists employ descriptive research to identify patterns through analysis of events or cultural practices. For instance, a historian might detail the daily life in a particular era, while an anthropologist might document rituals and ceremonies of a specific tribe.
Assessing student performance
Educational researchers can utilize descriptive studies to understand the effectiveness of teaching methodologies. By observing classrooms or surveying students, they can measure data trends and gauge the impact of a new teaching technique or curriculum on student engagement and performance.

Descriptive research methods aim to authentically represent situations and phenomena. These techniques ensure the collection of comprehensive and reliable data about the subject of interest.
The most appropriate descriptive research method depends on the research question and resources available for your research study.
Surveys and questionnaires
One of the most familiar tools in the researcher's arsenal, surveys and questionnaires offer a structured means of collecting data from a vast audience. Through carefully designed questions, researchers can obtain standardized responses that lend themselves to straightforward comparison and analysis in quantitative and qualitative research .
Survey research can manifest in various formats, from face-to-face interactions and telephone conversations to digital platforms. While surveys can reach a broad audience and generate quantitative data ripe for statistical analysis, they also come with the challenge of potential biases in design and rely heavily on respondent honesty.
Observations and case studies
Direct or participant observation is a method wherein researchers actively watch and document behaviors or events. A researcher might, for instance, observe the dynamics within a classroom or the behaviors of shoppers in a market setting.
Case studies provide an even deeper dive, focusing on a thorough analysis of a specific individual, group, or event. These methods present the advantage of capturing real-time, detailed data, but they might also be time-intensive and can sometimes introduce observer bias .
Interviews and focus groups
Interviews , whether they follow a structured script or flow more organically, are a powerful means to extract detailed insights directly from participants. On the other hand, focus groups gather multiple participants for discussions, aiming to gather diverse and collective opinions on a particular topic or product.
These methods offer the benefit of deep insights and adaptability in data collection . However, they necessitate skilled interviewers, and focus group settings might see individual opinions being influenced by group dynamics.
Document and content analysis
Here, instead of generating new data, researchers examine existing documents or content . This can range from studying historical records and newspapers to analyzing media content or literature.
Analyzing existing content offers the advantage of accessibility and can provide insights over longer time frames. However, the reliability and relevance of the content are paramount, and researchers must approach this method with a discerning eye.

Descriptive research data, rich in details and insights, necessitates meticulous analysis to derive meaningful conclusions. The analysis process transforms raw data into structured findings that can be communicated and acted upon.
Qualitative content analysis
For data collected through interviews , focus groups , observations , or open-ended survey questions , qualitative content analysis is a popular choice. This involves examining non-numerical data to identify patterns, themes, or categories.
By coding responses or observations , researchers can identify recurring elements, making it easier to comprehend larger data sets and draw insights.
Using descriptive statistics
When dealing with quantitative data from surveys or experiments, descriptive statistics are invaluable. Measures such as mean, median, mode, standard deviation, and frequency distributions help summarize data sets, providing a snapshot of the overall patterns.
Graphical representations like histograms, pie charts, or bar graphs can further help in visualizing these statistics.
Coding and categorizing the data
Both qualitative and quantitative data often require coding. Coding involves assigning labels to specific responses or behaviors to group similar segments of data. This categorization aids in identifying patterns, especially in vast data sets.
For instance, responses to open-ended questions in a survey can be coded based on keywords or sentiments, allowing for a more structured analysis.
Visual representation through graphs and charts
Visual aids like graphs, charts, and plots can simplify complex data, making it more accessible and understandable. Whether it's showcasing frequency distributions through histograms or mapping out relationships with networks, visual representations can elucidate trends and patterns effectively.
In the realm of research , the credibility of findings is paramount. Without trustworthiness in the results, even the most meticulously gathered data can lose its value. Two cornerstones that bolster the credibility of research outcomes are validity and reliability .
Validity: Measuring the right thing
Validity addresses the accuracy of the research. It seeks to answer the question: Is the research genuinely measuring what it aims to measure? In descriptive research, where the objective is to paint an authentic picture of the current state of affairs, ensuring validity is crucial.
For instance, if a study aims to understand consumer preferences for a product category, the questions posed should genuinely reflect those preferences and not veer into unrelated territories. Multiple forms of validity, including content, criterion, and construct validity, can be examined to ensure that the research instruments and processes are aligned with the research goals.
Reliability: Consistency in findings
Reliability, on the other hand, pertains to the consistency of the research findings. When a study demonstrates reliability, this suggests that others could repeat the study and the outcomes would remain consistent across repetitions.
In descriptive research, factors like the clarity of survey questions , the training of observers , and the standardization of interview protocols play a role in enhancing reliability. Techniques such as test-retest and internal consistency measurements can be employed to assess and improve reliability.

Make your research happen with ATLAS.ti
Analyze descriptive research with our powerful data analysis interface. Download a free trial of ATLAS.ti.

Have a language expert improve your writing
Run a free plagiarism check in 10 minutes, automatically generate references for free.
- Knowledge Base
- Methodology
- Descriptive Research Design | Definition, Methods & Examples

Descriptive Research Design | Definition, Methods & Examples
Published on 5 May 2022 by Shona McCombes . Revised on 10 October 2022.
Descriptive research aims to accurately and systematically describe a population, situation or phenomenon. It can answer what , where , when , and how questions , but not why questions.
A descriptive research design can use a wide variety of research methods to investigate one or more variables . Unlike in experimental research , the researcher does not control or manipulate any of the variables, but only observes and measures them.
Table of contents
When to use a descriptive research design, descriptive research methods.
Descriptive research is an appropriate choice when the research aim is to identify characteristics, frequencies, trends, and categories.
It is useful when not much is known yet about the topic or problem. Before you can research why something happens, you need to understand how, when, and where it happens.
- How has the London housing market changed over the past 20 years?
- Do customers of company X prefer product Y or product Z?
- What are the main genetic, behavioural, and morphological differences between European wildcats and domestic cats?
- What are the most popular online news sources among under-18s?
- How prevalent is disease A in population B?
Prevent plagiarism, run a free check.
Descriptive research is usually defined as a type of quantitative research , though qualitative research can also be used for descriptive purposes. The research design should be carefully developed to ensure that the results are valid and reliable .
Survey research allows you to gather large volumes of data that can be analysed for frequencies, averages, and patterns. Common uses of surveys include:
- Describing the demographics of a country or region
- Gauging public opinion on political and social topics
- Evaluating satisfaction with a company’s products or an organisation’s services
Observations
Observations allow you to gather data on behaviours and phenomena without having to rely on the honesty and accuracy of respondents. This method is often used by psychological, social, and market researchers to understand how people act in real-life situations.
Observation of physical entities and phenomena is also an important part of research in the natural sciences. Before you can develop testable hypotheses , models, or theories, it’s necessary to observe and systematically describe the subject under investigation.
Case studies
A case study can be used to describe the characteristics of a specific subject (such as a person, group, event, or organisation). Instead of gathering a large volume of data to identify patterns across time or location, case studies gather detailed data to identify the characteristics of a narrowly defined subject.
Rather than aiming to describe generalisable facts, case studies often focus on unusual or interesting cases that challenge assumptions, add complexity, or reveal something new about a research problem .
Cite this Scribbr article
If you want to cite this source, you can copy and paste the citation or click the ‘Cite this Scribbr article’ button to automatically add the citation to our free Reference Generator.
McCombes, S. (2022, October 10). Descriptive Research Design | Definition, Methods & Examples. Scribbr. Retrieved 3 September 2024, from https://www.scribbr.co.uk/research-methods/descriptive-research-design/
Is this article helpful?
Shona McCombes
Other students also liked, a quick guide to experimental design | 5 steps & examples, correlational research | guide, design & examples, qualitative vs quantitative research | examples & methods.
- Descriptive Research Designs: Types, Examples & Methods

One of the components of research is getting enough information about the research problem—the what, how, when and where answers, which is why descriptive research is an important type of research. It is very useful when conducting research whose aim is to identify characteristics, frequencies, trends, correlations, and categories.
This research method takes a problem with little to no relevant information and gives it a befitting description using qualitative and quantitative research method s. Descriptive research aims to accurately describe a research problem.
In the subsequent sections, we will be explaining what descriptive research means, its types, examples, and data collection methods.
What is Descriptive Research?
Descriptive research is a type of research that describes a population, situation, or phenomenon that is being studied. It focuses on answering the how, what, when, and where questions If a research problem, rather than the why.
This is mainly because it is important to have a proper understanding of what a research problem is about before investigating why it exists in the first place.
For example, an investor considering an investment in the ever-changing Amsterdam housing market needs to understand what the current state of the market is, how it changes (increasing or decreasing), and when it changes (time of the year) before asking for the why. This is where descriptive research comes in.
What Are The Types of Descriptive Research?
Descriptive research is classified into different types according to the kind of approach that is used in conducting descriptive research. The different types of descriptive research are highlighted below:
- Descriptive-survey
Descriptive survey research uses surveys to gather data about varying subjects. This data aims to know the extent to which different conditions can be obtained among these subjects.
For example, a researcher wants to determine the qualification of employed professionals in Maryland. He uses a survey as his research instrument , and each item on the survey related to qualifications is subjected to a Yes/No answer.
This way, the researcher can describe the qualifications possessed by the employed demographics of this community.
- Descriptive-normative survey
This is an extension of the descriptive survey, with the addition being the normative element. In the descriptive-normative survey, the results of the study should be compared with the norm.
For example, an organization that wishes to test the skills of its employees by a team may have them take a skills test. The skills tests are the evaluation tool in this case, and the result of this test is compared with the norm of each role.
If the score of the team is one standard deviation above the mean, it is very satisfactory, if within the mean, satisfactory, and one standard deviation below the mean is unsatisfactory.
- Descriptive-status
This is a quantitative description technique that seeks to answer questions about real-life situations. For example, a researcher researching the income of the employees in a company, and the relationship with their performance.
A survey will be carried out to gather enough data about the income of the employees, then their performance will be evaluated and compared to their income. This will help determine whether a higher income means better performance and low income means lower performance or vice versa.
- Descriptive-analysis
The descriptive-analysis method of research describes a subject by further analyzing it, which in this case involves dividing it into 2 parts. For example, the HR personnel of a company that wishes to analyze the job role of each employee of the company may divide the employees into the people that work at the Headquarters in the US and those that work from Oslo, Norway office.
A questionnaire is devised to analyze the job role of employees with similar salaries and who work in similar positions.
- Descriptive classification
This method is employed in biological sciences for the classification of plants and animals. A researcher who wishes to classify the sea animals into different species will collect samples from various search stations, then classify them accordingly.
- Descriptive-comparative
In descriptive-comparative research, the researcher considers 2 variables that are not manipulated, and establish a formal procedure to conclude that one is better than the other. For example, an examination body wants to determine the better method of conducting tests between paper-based and computer-based tests.
A random sample of potential participants of the test may be asked to use the 2 different methods, and factors like failure rates, time factors, and others will be evaluated to arrive at the best method.
- Correlative Survey
Correlative surveys are used to determine whether the relationship between 2 variables is positive, negative, or neutral. That is, if 2 variables say X and Y are directly proportional, inversely proportional or are not related to each other.
Examples of Descriptive Research
There are different examples of descriptive research, that may be highlighted from its types, uses, and applications. However, we will be restricting ourselves to only 3 distinct examples in this article.
- Comparing Student Performance:
An academic institution may wish 2 compare the performance of its junior high school students in English language and Mathematics. This may be used to classify students based on 2 major groups, with one group going ahead to study while courses, while the other study courses in the Arts & Humanities field.
Students who are more proficient in mathematics will be encouraged to go into STEM and vice versa. Institutions may also use this data to identify students’ weak points and work on ways to assist them.
- Scientific Classification
During the major scientific classification of plants, animals, and periodic table elements, the characteristics and components of each subject are evaluated and used to determine how they are classified.
For example, living things may be classified into kingdom Plantae or kingdom animal is depending on their nature. Further classification may group animals into mammals, pieces, vertebrae, invertebrae, etc.
All these classifications are made a result of descriptive research which describes what they are.
- Human Behavior
When studying human behaviour based on a factor or event, the researcher observes the characteristics, behaviour, and reaction, then use it to conclude. A company willing to sell to its target market needs to first study the behaviour of the market.
This may be done by observing how its target reacts to a competitor’s product, then use it to determine their behaviour.
What are the Characteristics of Descriptive Research?
The characteristics of descriptive research can be highlighted from its definition, applications, data collection methods, and examples. Some characteristics of descriptive research are:
- Quantitativeness
Descriptive research uses a quantitative research method by collecting quantifiable information to be used for statistical analysis of the population sample. This is very common when dealing with research in the physical sciences.
- Qualitativeness
It can also be carried out using the qualitative research method, to properly describe the research problem. This is because descriptive research is more explanatory than exploratory or experimental.
- Uncontrolled variables
In descriptive research, researchers cannot control the variables like they do in experimental research.
- The basis for further research
The results of descriptive research can be further analyzed and used in other research methods. It can also inform the next line of research, including the research method that should be used.
This is because it provides basic information about the research problem, which may give birth to other questions like why a particular thing is the way it is.
Why Use Descriptive Research Design?
Descriptive research can be used to investigate the background of a research problem and get the required information needed to carry out further research. It is used in multiple ways by different organizations, and especially when getting the required information about their target audience.
- Define subject characteristics :
It is used to determine the characteristics of the subjects, including their traits, behaviour, opinion, etc. This information may be gathered with the use of surveys, which are shared with the respondents who in this case, are the research subjects.
For example, a survey evaluating the number of hours millennials in a community spends on the internet weekly, will help a service provider make informed business decisions regarding the market potential of the community.
- Measure Data Trends
It helps to measure the changes in data over some time through statistical methods. Consider the case of individuals who want to invest in stock markets, so they evaluate the changes in prices of the available stocks to make a decision investment decision.
Brokerage companies are however the ones who carry out the descriptive research process, while individuals can view the data trends and make decisions.
Descriptive research is also used to compare how different demographics respond to certain variables. For example, an organization may study how people with different income levels react to the launch of a new Apple phone.
This kind of research may take a survey that will help determine which group of individuals are purchasing the new Apple phone. Do the low-income earners also purchase the phone, or only the high-income earners do?
Further research using another technique will explain why low-income earners are purchasing the phone even though they can barely afford it. This will help inform strategies that will lure other low-income earners and increase company sales.
- Validate existing conditions
When you are not sure about the validity of an existing condition, you can use descriptive research to ascertain the underlying patterns of the research object. This is because descriptive research methods make an in-depth analysis of each variable before making conclusions.
- Conducted Overtime
Descriptive research is conducted over some time to ascertain the changes observed at each point in time. The higher the number of times it is conducted, the more authentic the conclusion will be.
What are the Disadvantages of Descriptive Research?
- Response and Non-response Bias
Respondents may either decide not to respond to questions or give incorrect responses if they feel the questions are too confidential. When researchers use observational methods, respondents may also decide to behave in a particular manner because they feel they are being watched.
- The researcher may decide to influence the result of the research due to personal opinion or bias towards a particular subject. For example, a stockbroker who also has a business of his own may try to lure investors into investing in his own company by manipulating results.
- A case-study or sample taken from a large population is not representative of the whole population.
- Limited scope:The scope of descriptive research is limited to the what of research, with no information on why thereby limiting the scope of the research.
What are the Data Collection Methods in Descriptive Research?
There are 3 main data collection methods in descriptive research, namely; observational method, case study method, and survey research.
1. Observational Method
The observational method allows researchers to collect data based on their view of the behaviour and characteristics of the respondent, with the respondents themselves not directly having an input. It is often used in market research, psychology, and some other social science research to understand human behaviour.
It is also an important aspect of physical scientific research, with it being one of the most effective methods of conducting descriptive research . This process can be said to be either quantitative or qualitative.
Quantitative observation involved the objective collection of numerical data , whose results can be analyzed using numerical and statistical methods.
Qualitative observation, on the other hand, involves the monitoring of characteristics and not the measurement of numbers. The researcher makes his observation from a distance, records it, and is used to inform conclusions.
2. Case Study Method
A case study is a sample group (an individual, a group of people, organizations, events, etc.) whose characteristics are used to describe the characteristics of a larger group in which the case study is a subgroup. The information gathered from investigating a case study may be generalized to serve the larger group.
This generalization, may, however, be risky because case studies are not sufficient to make accurate predictions about larger groups. Case studies are a poor case of generalization.
3. Survey Research
This is a very popular data collection method in research designs. In survey research, researchers create a survey or questionnaire and distribute it to respondents who give answers.
Generally, it is used to obtain quick information directly from the primary source and also conducting rigorous quantitative and qualitative research. In some cases, survey research uses a blend of both qualitative and quantitative strategies.
Survey research can be carried out both online and offline using the following methods
- Online Surveys: This is a cheap method of carrying out surveys and getting enough responses. It can be carried out using Formplus, an online survey builder. Formplus has amazing tools and features that will help increase response rates.
- Offline Surveys: This includes paper forms, mobile offline forms , and SMS-based forms.
What Are The Differences Between Descriptive and Correlational Research?
Before going into the differences between descriptive and correlation research, we need to have a proper understanding of what correlation research is about. Therefore, we will be giving a summary of the correlation research below.
Correlational research is a type of descriptive research, which is used to measure the relationship between 2 variables, with the researcher having no control over them. It aims to find whether there is; positive correlation (both variables change in the same direction), negative correlation (the variables change in the opposite direction), or zero correlation (there is no relationship between the variables).
Correlational research may be used in 2 situations;
(i) when trying to find out if there is a relationship between two variables, and
(ii) when a causal relationship is suspected between two variables, but it is impractical or unethical to conduct experimental research that manipulates one of the variables.
Below are some of the differences between correlational and descriptive research:
- Definitions :
Descriptive research aims is a type of research that provides an in-depth understanding of the study population, while correlational research is the type of research that measures the relationship between 2 variables.
- Characteristics :
Descriptive research provides descriptive data explaining what the research subject is about, while correlation research explores the relationship between data and not their description.
- Predictions :
Predictions cannot be made in descriptive research while correlation research accommodates the possibility of making predictions.
Descriptive Research vs. Causal Research
Descriptive research and causal research are both research methodologies, however, one focuses on a subject’s behaviors while the latter focuses on a relationship’s cause-and-effect. To buttress the above point, descriptive research aims to describe and document the characteristics, behaviors, or phenomena of a particular or specific population or situation.
It focuses on providing an accurate and detailed account of an already existing state of affairs between variables. Descriptive research answers the questions of “what,” “where,” “when,” and “how” without attempting to establish any causal relationships or explain any underlying factors that might have caused the behavior.
Causal research, on the other hand, seeks to determine cause-and-effect relationships between variables. It aims to point out the factors that influence or cause a particular result or behavior. Causal research involves manipulating variables, controlling conditions or a subgroup, and observing the resulting effects. The primary objective of causal research is to establish a cause-effect relationship and provide insights into why certain phenomena happen the way they do.
Descriptive Research vs. Analytical Research
Descriptive research provides a detailed and comprehensive account of a specific situation or phenomenon. It focuses on describing and summarizing data without making inferences or attempting to explain underlying factors or the cause of the factor.
It is primarily concerned with providing an accurate and objective representation of the subject of research. While analytical research goes beyond the description of the phenomena and seeks to analyze and interpret data to discover if there are patterns, relationships, or any underlying factors.
It examines the data critically, applies statistical techniques or other analytical methods, and draws conclusions based on the discovery. Analytical research also aims to explore the relationships between variables and understand the underlying mechanisms or processes involved.
Descriptive Research vs. Exploratory Research
Descriptive research is a research method that focuses on providing a detailed and accurate account of a specific situation, group, or phenomenon. This type of research describes the characteristics, behaviors, or relationships within the given context without looking for an underlying cause.
Descriptive research typically involves collecting and analyzing quantitative or qualitative data to generate descriptive statistics or narratives. Exploratory research differs from descriptive research because it aims to explore and gain firsthand insights or knowledge into a relatively unexplored or poorly understood topic.
It focuses on generating ideas, hypotheses, or theories rather than providing definitive answers. Exploratory research is often conducted at the early stages of a research project to gather preliminary information and identify key variables or factors for further investigation. It involves open-ended interviews, observations, or small-scale surveys to gather qualitative data.
Read More – Exploratory Research: What are its Method & Examples?
Descriptive Research vs. Experimental Research
Descriptive research aims to describe and document the characteristics, behaviors, or phenomena of a particular population or situation. It focuses on providing an accurate and detailed account of the existing state of affairs.
Descriptive research typically involves collecting data through surveys, observations, or existing records and analyzing the data to generate descriptive statistics or narratives. It does not involve manipulating variables or establishing cause-and-effect relationships.
Experimental research, on the other hand, involves manipulating variables and controlling conditions to investigate cause-and-effect relationships. It aims to establish causal relationships by introducing an intervention or treatment and observing the resulting effects.
Experimental research typically involves randomly assigning participants to different groups, such as control and experimental groups, and measuring the outcomes. It allows researchers to control for confounding variables and draw causal conclusions.
Related – Experimental vs Non-Experimental Research: 15 Key Differences
Descriptive Research vs. Explanatory Research
Descriptive research focuses on providing a detailed and accurate account of a specific situation, group, or phenomenon. It aims to describe the characteristics, behaviors, or relationships within the given context.
Descriptive research is primarily concerned with providing an objective representation of the subject of study without explaining underlying causes or mechanisms. Explanatory research seeks to explain the relationships between variables and uncover the underlying causes or mechanisms.
It goes beyond description and aims to understand the reasons or factors that influence a particular outcome or behavior. Explanatory research involves analyzing data, conducting statistical analyses, and developing theories or models to explain the observed relationships.
Descriptive Research vs. Inferential Research
Descriptive research focuses on describing and summarizing data without making inferences or generalizations beyond the specific sample or population being studied. It aims to provide an accurate and objective representation of the subject of study.
Descriptive research typically involves analyzing data to generate descriptive statistics, such as means, frequencies, or percentages, to describe the characteristics or behaviors observed.
Inferential research, however, involves making inferences or generalizations about a larger population based on a smaller sample.
It aims to draw conclusions about the population characteristics or relationships by analyzing the sample data. Inferential research uses statistical techniques to estimate population parameters, test hypotheses, and determine the level of confidence or significance in the findings.
Related – Inferential Statistics: Definition, Types + Examples
Conclusion
The uniqueness of descriptive research partly lies in its ability to explore both quantitative and qualitative research methods. Therefore, when conducting descriptive research, researchers have the opportunity to use a wide variety of techniques that aids the research process.
Descriptive research explores research problems in-depth, beyond the surface level thereby giving a detailed description of the research subject. That way, it can aid further research in the field, including other research methods .
It is also very useful in solving real-life problems in various fields of social science, physical science, and education.
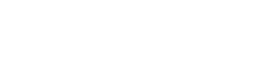
Connect to Formplus, Get Started Now - It's Free!
- descriptive research
- descriptive research method
- example of descriptive research
- types of descriptive research
- busayo.longe
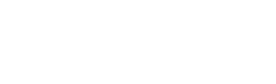
You may also like:
Cross-Sectional Studies: Types, Pros, Cons & Uses
In this article, we’ll look at what cross-sectional studies are, how it applies to your research and how to use Formplus to collect...

Type I vs Type II Errors: Causes, Examples & Prevention
This article will discuss the two different types of errors in hypothesis testing and how you can prevent them from occurring in your research
Acceptance Sampling: Meaning, Examples, When to Use
In this post, we will discuss extensively what acceptance sampling is and when it is applied.
Extrapolation in Statistical Research: Definition, Examples, Types, Applications
In this article we’ll look at the different types and characteristics of extrapolation, plus how it contrasts to interpolation.
Formplus - For Seamless Data Collection
Collect data the right way with a versatile data collection tool. try formplus and transform your work productivity today..

Bridging the Gap: Overcome these 7 flaws in descriptive research design
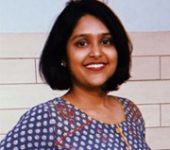
Descriptive research design is a powerful tool used by scientists and researchers to gather information about a particular group or phenomenon. This type of research provides a detailed and accurate picture of the characteristics and behaviors of a particular population or subject. By observing and collecting data on a given topic, descriptive research helps researchers gain a deeper understanding of a specific issue and provides valuable insights that can inform future studies.
In this blog, we will explore the definition, characteristics, and common flaws in descriptive research design, and provide tips on how to avoid these pitfalls to produce high-quality results. Whether you are a seasoned researcher or a student just starting, understanding the fundamentals of descriptive research design is essential to conducting successful scientific studies.
Table of Contents
What Is Descriptive Research Design?
The descriptive research design involves observing and collecting data on a given topic without attempting to infer cause-and-effect relationships. The goal of descriptive research is to provide a comprehensive and accurate picture of the population or phenomenon being studied and to describe the relationships, patterns, and trends that exist within the data.
Descriptive research methods can include surveys, observational studies , and case studies, and the data collected can be qualitative or quantitative . The findings from descriptive research provide valuable insights and inform future research, but do not establish cause-and-effect relationships.
Importance of Descriptive Research in Scientific Studies
1. understanding of a population or phenomenon.
Descriptive research provides a comprehensive picture of the characteristics and behaviors of a particular population or phenomenon, allowing researchers to gain a deeper understanding of the topic.
2. Baseline Information
The information gathered through descriptive research can serve as a baseline for future research and provide a foundation for further studies.
3. Informative Data
Descriptive research can provide valuable information and insights into a particular topic, which can inform future research, policy decisions, and programs.
4. Sampling Validation
Descriptive research can be used to validate sampling methods and to help researchers determine the best approach for their study.
5. Cost Effective
Descriptive research is often less expensive and less time-consuming than other research methods , making it a cost-effective way to gather information about a particular population or phenomenon.
6. Easy to Replicate
Descriptive research is straightforward to replicate, making it a reliable way to gather and compare information from multiple sources.
Key Characteristics of Descriptive Research Design
The primary purpose of descriptive research is to describe the characteristics, behaviors, and attributes of a particular population or phenomenon.
2. Participants and Sampling
Descriptive research studies a particular population or sample that is representative of the larger population being studied. Furthermore, sampling methods can include convenience, stratified, or random sampling.
3. Data Collection Techniques
Descriptive research typically involves the collection of both qualitative and quantitative data through methods such as surveys, observational studies, case studies, or focus groups.
4. Data Analysis
Descriptive research data is analyzed to identify patterns, relationships, and trends within the data. Statistical techniques , such as frequency distributions and descriptive statistics, are commonly used to summarize and describe the data.
5. Focus on Description
Descriptive research is focused on describing and summarizing the characteristics of a particular population or phenomenon. It does not make causal inferences.
6. Non-Experimental
Descriptive research is non-experimental, meaning that the researcher does not manipulate variables or control conditions. The researcher simply observes and collects data on the population or phenomenon being studied.
When Can a Researcher Conduct Descriptive Research?
A researcher can conduct descriptive research in the following situations:
- To better understand a particular population or phenomenon
- To describe the relationships between variables
- To describe patterns and trends
- To validate sampling methods and determine the best approach for a study
- To compare data from multiple sources.
Types of Descriptive Research Design
1. survey research.
Surveys are a type of descriptive research that involves collecting data through self-administered or interviewer-administered questionnaires. Additionally, they can be administered in-person, by mail, or online, and can collect both qualitative and quantitative data.
2. Observational Research
Observational research involves observing and collecting data on a particular population or phenomenon without manipulating variables or controlling conditions. It can be conducted in naturalistic settings or controlled laboratory settings.
3. Case Study Research
Case study research is a type of descriptive research that focuses on a single individual, group, or event. It involves collecting detailed information on the subject through a variety of methods, including interviews, observations, and examination of documents.
4. Focus Group Research
Focus group research involves bringing together a small group of people to discuss a particular topic or product. Furthermore, the group is usually moderated by a researcher and the discussion is recorded for later analysis.
5. Ethnographic Research
Ethnographic research involves conducting detailed observations of a particular culture or community. It is often used to gain a deep understanding of the beliefs, behaviors, and practices of a particular group.
Advantages of Descriptive Research Design
1. provides a comprehensive understanding.
Descriptive research provides a comprehensive picture of the characteristics, behaviors, and attributes of a particular population or phenomenon, which can be useful in informing future research and policy decisions.
2. Non-invasive
Descriptive research is non-invasive and does not manipulate variables or control conditions, making it a suitable method for sensitive or ethical concerns.
3. Flexibility
Descriptive research allows for a wide range of data collection methods , including surveys, observational studies, case studies, and focus groups, making it a flexible and versatile research method.
4. Cost-effective
Descriptive research is often less expensive and less time-consuming than other research methods. Moreover, it gives a cost-effective option to many researchers.
5. Easy to Replicate
Descriptive research is easy to replicate, making it a reliable way to gather and compare information from multiple sources.
6. Informs Future Research
The insights gained from a descriptive research can inform future research and inform policy decisions and programs.
Disadvantages of Descriptive Research Design
1. limited scope.
Descriptive research only provides a snapshot of the current situation and cannot establish cause-and-effect relationships.
2. Dependence on Existing Data
Descriptive research relies on existing data, which may not always be comprehensive or accurate.
3. Lack of Control
Researchers have no control over the variables in descriptive research, which can limit the conclusions that can be drawn.
The researcher’s own biases and preconceptions can influence the interpretation of the data.
5. Lack of Generalizability
Descriptive research findings may not be applicable to other populations or situations.
6. Lack of Depth
Descriptive research provides a surface-level understanding of a phenomenon, rather than a deep understanding.
7. Time-consuming
Descriptive research often requires a large amount of data collection and analysis, which can be time-consuming and resource-intensive.
7 Ways to Avoid Common Flaws While Designing Descriptive Research
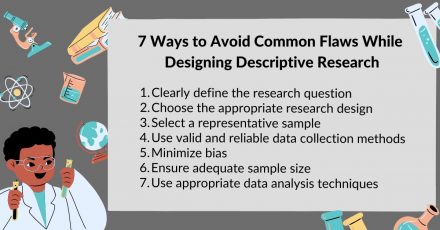
1. Clearly define the research question
A clearly defined research question is the foundation of any research study, and it is important to ensure that the question is both specific and relevant to the topic being studied.
2. Choose the appropriate research design
Choosing the appropriate research design for a study is crucial to the success of the study. Moreover, researchers should choose a design that best fits the research question and the type of data needed to answer it.
3. Select a representative sample
Selecting a representative sample is important to ensure that the findings of the study are generalizable to the population being studied. Researchers should use a sampling method that provides a random and representative sample of the population.
4. Use valid and reliable data collection methods
Using valid and reliable data collection methods is important to ensure that the data collected is accurate and can be used to answer the research question. Researchers should choose methods that are appropriate for the study and that can be administered consistently and systematically.
5. Minimize bias
Bias can significantly impact the validity and reliability of research findings. Furthermore, it is important to minimize bias in all aspects of the study, from the selection of participants to the analysis of data.
6. Ensure adequate sample size
An adequate sample size is important to ensure that the results of the study are statistically significant and can be generalized to the population being studied.
7. Use appropriate data analysis techniques
The appropriate data analysis technique depends on the type of data collected and the research question being asked. Researchers should choose techniques that are appropriate for the data and the question being asked.
Have you worked on descriptive research designs? How was your experience creating a descriptive design? What challenges did you face? Do write to us or leave a comment below and share your insights on descriptive research designs!

extremely very educative
Indeed very educative and useful. Well explained. Thank you
Simple,easy to understand
Excellent and easy to understand queries and questions get answered easily. Its rather clear than any confusion. Thanks a million Shritika Sirisilla.
Easy to understand. Well written , educative and informative
Rate this article Cancel Reply
Your email address will not be published.
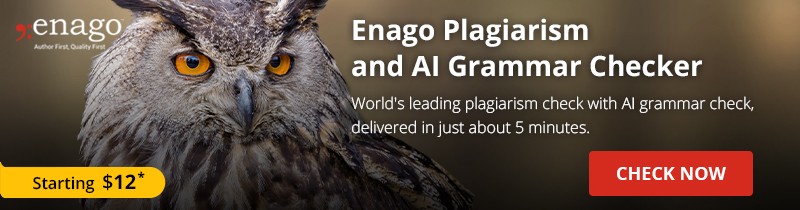
Enago Academy's Most Popular Articles
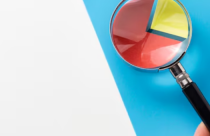
- Promoting Research
Graphical Abstracts Vs. Infographics: Best practices for using visual illustrations for increased research impact
Dr. Sarah Chen stared at her computer screen, her eyes staring at her recently published…
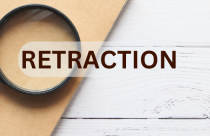
- Publishing Research
10 Tips to Prevent Research Papers From Being Retracted
Research paper retractions represent a critical event in the scientific community. When a published article…
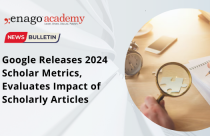
- Industry News
Google Releases 2024 Scholar Metrics, Evaluates Impact of Scholarly Articles
Google has released its 2024 Scholar Metrics, assessing scholarly articles from 2019 to 2023. This…
![variables of descriptive research What is Academic Integrity and How to Uphold it [FREE CHECKLIST]](https://www.enago.com/academy/wp-content/uploads/2024/05/FeatureImages-59-210x136.png)
Ensuring Academic Integrity and Transparency in Academic Research: A comprehensive checklist for researchers
Academic integrity is the foundation upon which the credibility and value of scientific findings are…
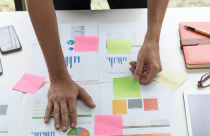
- Reporting Research
How to Optimize Your Research Process: A step-by-step guide
For researchers across disciplines, the path to uncovering novel findings and insights is often filled…
Choosing the Right Analytical Approach: Thematic analysis vs. content analysis for…
Comparing Cross Sectional and Longitudinal Studies: 5 steps for choosing the right…
Research Recommendations – Guiding policy-makers for evidence-based decision making

Sign-up to read more
Subscribe for free to get unrestricted access to all our resources on research writing and academic publishing including:
- 2000+ blog articles
- 50+ Webinars
- 10+ Expert podcasts
- 50+ Infographics
- 10+ Checklists
- Research Guides
We hate spam too. We promise to protect your privacy and never spam you.
- AI in Academia
- Career Corner
- Diversity and Inclusion
- Infographics
- Expert Video Library
- Other Resources
- Enago Learn
- Upcoming & On-Demand Webinars
- Peer Review Week 2024
- Open Access Week 2023
- Conference Videos
- Enago Report
- Journal Finder
- Enago Plagiarism & AI Grammar Check
- Editing Services
- Publication Support Services
- Research Impact
- Translation Services
- Publication solutions
- AI-Based Solutions
- Thought Leadership
- Call for Articles
- Call for Speakers
- Author Training
- Edit Profile
I am looking for Editing/ Proofreading services for my manuscript Tentative date of next journal submission:
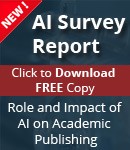
In your opinion, what is the most effective way to improve integrity in the peer review process?
Root out friction in every digital experience, super-charge conversion rates, and optimize digital self-service
Uncover insights from any interaction, deliver AI-powered agent coaching, and reduce cost to serve
Increase revenue and loyalty with real-time insights and recommendations delivered to teams on the ground
Know how your people feel and empower managers to improve employee engagement, productivity, and retention
Take action in the moments that matter most along the employee journey and drive bottom line growth
Whatever they’re are saying, wherever they’re saying it, know exactly what’s going on with your people
Get faster, richer insights with qual and quant tools that make powerful market research available to everyone
Run concept tests, pricing studies, prototyping + more with fast, powerful studies designed by UX research experts
Track your brand performance 24/7 and act quickly to respond to opportunities and challenges in your market
Explore the platform powering Experience Management
- Free Account
- Product Demos
- For Digital
- For Customer Care
- For Human Resources
- For Researchers
- Financial Services
- All Industries
Popular Use Cases
- Customer Experience
- Employee Experience
- Net Promoter Score
- Voice of Customer
- Customer Success Hub
- Product Documentation
- Training & Certification
- XM Institute
- Popular Resources
- Customer Stories
- Artificial Intelligence
Market Research
- Partnerships
- Marketplace
The annual gathering of the experience leaders at the world’s iconic brands building breakthrough business results, live in Salt Lake City.
- English/AU & NZ
- Español/Europa
- Español/América Latina
- Português Brasileiro
- REQUEST DEMO
- Experience Management
- Descriptive Research
Try Qualtrics for free
Descriptive research: what it is and how to use it.
8 min read Understanding the who, what and where of a situation or target group is an essential part of effective research and making informed business decisions.
For example you might want to understand what percentage of CEOs have a bachelor’s degree or higher. Or you might want to understand what percentage of low income families receive government support – or what kind of support they receive.
Descriptive research is what will be used in these types of studies.
In this guide we’ll look through the main issues relating to descriptive research to give you a better understanding of what it is, and how and why you can use it.
Free eBook: 2024 global market research trends report
What is descriptive research?
Descriptive research is a research method used to try and determine the characteristics of a population or particular phenomenon.
Using descriptive research you can identify patterns in the characteristics of a group to essentially establish everything you need to understand apart from why something has happened.
Market researchers use descriptive research for a range of commercial purposes to guide key decisions.
For example you could use descriptive research to understand fashion trends in a given city when planning your clothing collection for the year. Using descriptive research you can conduct in depth analysis on the demographic makeup of your target area and use the data analysis to establish buying patterns.
Conducting descriptive research wouldn’t, however, tell you why shoppers are buying a particular type of fashion item.
Descriptive research design
Descriptive research design uses a range of both qualitative research and quantitative data (although quantitative research is the primary research method) to gather information to make accurate predictions about a particular problem or hypothesis.
As a survey method, descriptive research designs will help researchers identify characteristics in their target market or particular population.
These characteristics in the population sample can be identified, observed and measured to guide decisions.
Descriptive research characteristics
While there are a number of descriptive research methods you can deploy for data collection, descriptive research does have a number of predictable characteristics.
Here are a few of the things to consider:
Measure data trends with statistical outcomes
Descriptive research is often popular for survey research because it generates answers in a statistical form, which makes it easy for researchers to carry out a simple statistical analysis to interpret what the data is saying.
Descriptive research design is ideal for further research
Because the data collection for descriptive research produces statistical outcomes, it can also be used as secondary data for another research study.
Plus, the data collected from descriptive research can be subjected to other types of data analysis .
Uncontrolled variables
A key component of the descriptive research method is that it uses random variables that are not controlled by the researchers. This is because descriptive research aims to understand the natural behavior of the research subject.
It’s carried out in a natural environment
Descriptive research is often carried out in a natural environment. This is because researchers aim to gather data in a natural setting to avoid swaying respondents.
Data can be gathered using survey questions or online surveys.
For example, if you want to understand the fashion trends we mentioned earlier, you would set up a study in which a researcher observes people in the respondent’s natural environment to understand their habits and preferences.
Descriptive research allows for cross sectional study
Because of the nature of descriptive research design and the randomness of the sample group being observed, descriptive research is ideal for cross sectional studies – essentially the demographics of the group can vary widely and your aim is to gain insights from within the group.
This can be highly beneficial when you’re looking to understand the behaviors or preferences of a wider population.
Descriptive research advantages
There are many advantages to using descriptive research, some of them include:
Cost effectiveness
Because the elements needed for descriptive research design are not specific or highly targeted (and occur within the respondent’s natural environment) this type of study is relatively cheap to carry out.
Multiple types of data can be collected
A big advantage of this research type, is that you can use it to collect both quantitative and qualitative data. This means you can use the stats gathered to easily identify underlying patterns in your respondents’ behavior.
Descriptive research disadvantages
Potential reliability issues.
When conducting descriptive research it’s important that the initial survey questions are properly formulated.
If not, it could make the answers unreliable and risk the credibility of your study.
Potential limitations
As we’ve mentioned, descriptive research design is ideal for understanding the what, who or where of a situation or phenomenon.
However, it can’t help you understand the cause or effect of the behavior. This means you’ll need to conduct further research to get a more complete picture of a situation.
Descriptive research methods
Because descriptive research methods include a range of quantitative and qualitative research, there are several research methods you can use.
Use case studies
Case studies in descriptive research involve conducting in-depth and detailed studies in which researchers get a specific person or case to answer questions.
Case studies shouldn’t be used to generate results, rather it should be used to build or establish hypothesis that you can expand into further market research .
For example you could gather detailed data about a specific business phenomenon, and then use this deeper understanding of that specific case.
Use observational methods
This type of study uses qualitative observations to understand human behavior within a particular group.
By understanding how the different demographics respond within your sample you can identify patterns and trends.
As an observational method, descriptive research will not tell you the cause of any particular behaviors, but that could be established with further research.
Use survey research
Surveys are one of the most cost effective ways to gather descriptive data.
An online survey or questionnaire can be used in descriptive studies to gather quantitative information about a particular problem.
Survey research is ideal if you’re using descriptive research as your primary research.
Descriptive research examples
Descriptive research is used for a number of commercial purposes or when organizations need to understand the behaviors or opinions of a population.
One of the biggest examples of descriptive research that is used in every democratic country, is during elections.
Using descriptive research, researchers will use surveys to understand who voters are more likely to choose out of the parties or candidates available.
Using the data provided, researchers can analyze the data to understand what the election result will be.
In a commercial setting, retailers often use descriptive research to figure out trends in shopping and buying decisions.
By gathering information on the habits of shoppers, retailers can get a better understanding of the purchases being made.
Another example that is widely used around the world, is the national census that takes place to understand the population.
The research will provide a more accurate picture of a population’s demographic makeup and help to understand changes over time in areas like population age, health and education level.
Where Qualtrics helps with descriptive research
Whatever type of research you want to carry out, there’s a survey type that will work.
Qualtrics can help you determine the appropriate method and ensure you design a study that will deliver the insights you need.
Our experts can help you with your market research needs , ensuring you get the most out of Qualtrics market research software to design, launch and analyze your data to guide better, more accurate decisions for your organization.
Related resources
Market intelligence 10 min read, marketing insights 11 min read, ethnographic research 11 min read, qualitative vs quantitative research 13 min read, qualitative research questions 11 min read, qualitative research design 12 min read, primary vs secondary research 14 min read, request demo.
Ready to learn more about Qualtrics?
Child Care and Early Education Research Connections
Descriptive research studies.
Descriptive research is a type of research that is used to describe the characteristics of a population. It collects data that are used to answer a wide range of what, when, and how questions pertaining to a particular population or group. For example, descriptive studies might be used to answer questions such as: What percentage of Head Start teachers have a bachelor's degree or higher? What is the average reading ability of 5-year-olds when they first enter kindergarten? What kinds of math activities are used in early childhood programs? When do children first receive regular child care from someone other than their parents? When are children with developmental disabilities first diagnosed and when do they first receive services? What factors do programs consider when making decisions about the type of assessments that will be used to assess the skills of the children in their programs? How do the types of services children receive from their early childhood program change as children age?
Descriptive research does not answer questions about why a certain phenomenon occurs or what the causes are. Answers to such questions are best obtained from randomized and quasi-experimental studies . However, data from descriptive studies can be used to examine the relationships (correlations) among variables. While the findings from correlational analyses are not evidence of causality, they can help to distinguish variables that may be important in explaining a phenomenon from those that are not. Thus, descriptive research is often used to generate hypotheses that should be tested using more rigorous designs.
A variety of data collection methods may be used alone or in combination to answer the types of questions guiding descriptive research. Some of the more common methods include surveys, interviews, observations, case studies, and portfolios. The data collected through these methods can be either quantitative or qualitative. Quantitative data are typically analyzed and presenting using descriptive statistics . Using quantitative data, researchers may describe the characteristics of a sample or population in terms of percentages (e.g., percentage of population that belong to different racial/ethnic groups, percentage of low-income families that receive different government services) or averages (e.g., average household income, average scores of reading, mathematics and language assessments). Quantitative data, such as narrative data collected as part of a case study, may be used to organize, classify, and used to identify patterns of behaviors, attitudes, and other characteristics of groups.
Descriptive studies have an important role in early care and education research. Studies such as the National Survey of Early Care and Education and the National Household Education Surveys Program have greatly increased our knowledge of the supply of and demand for child care in the U.S. The Head Start Family and Child Experiences Survey and the Early Childhood Longitudinal Study Program have provided researchers, policy makers and practitioners with rich information about school readiness skills of children in the U.S.
Each of the methods used to collect descriptive data have their own strengths and limitations. The following are some of the strengths and limitations of descriptive research studies in general.
Study participants are questioned or observed in a natural setting (e.g., their homes, child care or educational settings).
Study data can be used to identify the prevalence of particular problems and the need for new or additional services to address these problems.
Descriptive research may identify areas in need of additional research and relationships between variables that require future study. Descriptive research is often referred to as "hypothesis generating research."
Depending on the data collection method used, descriptive studies can generate rich datasets on large and diverse samples.
Limitations:
Descriptive studies cannot be used to establish cause and effect relationships.
Respondents may not be truthful when answering survey questions or may give socially desirable responses.
The choice and wording of questions on a questionnaire may influence the descriptive findings.
Depending on the type and size of sample, the findings may not be generalizable or produce an accurate description of the population of interest.
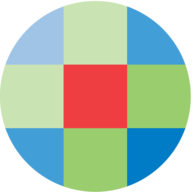
- Get new issue alerts Get alerts
- Submit a Manuscript
Secondary Logo
Journal logo.
Colleague's E-mail is Invalid
Your message has been successfully sent to your colleague.
Save my selection
Types of Variables, Descriptive Statistics, and Sample Size
Kaliyadan, Feroze; Kulkarni, Vinay 1
Department of Dermatology, King Faisal University, Al Hofuf, Saudi Arabia
1 Department of Dermatology, Prayas Amrita Clinic, Pune, Maharashtra, India
Address for correspondence: Dr. Feroze Kaliyadan, Department of Dermatology, King Faisal University, Saudi Arabia. E-mail:
Received December , 2018
Accepted December , 2018
This short “snippet” covers three important aspects related to statistics – the concept of variables , the importance, and practical aspects related to descriptive statistics and issues related to sampling – types of sampling and sample size estimation.
What is a variable?[ 1 2 ] To put it in very simple terms, a variable is an entity whose value varies. A variable is an essential component of any statistical data. It is a feature of a member of a given sample or population, which is unique, and can differ in quantity or quantity from another member of the same sample or population. Variables either are the primary quantities of interest or act as practical substitutes for the same. The importance of variables is that they help in operationalization of concepts for data collection. For example, if you want to do an experiment based on the severity of urticaria, one option would be to measure the severity using a scale to grade severity of itching. This becomes an operational variable. For a variable to be “good,” it needs to have some properties such as good reliability and validity, low bias, feasibility/practicality, low cost, objectivity, clarity, and acceptance. Variables can be classified into various ways as discussed below.
Quantitative vs qualitative
A variable can collect either qualitative or quantitative data. A variable differing in quantity is called a quantitative variable (e.g., weight of a group of patients), whereas a variable differing in quality is called a qualitative variable (e.g., the Fitzpatrick skin type)
A simple test which can be used to differentiate between qualitative and quantitative variables is the subtraction test. If you can subtract the value of one variable from the other to get a meaningful result, then you are dealing with a quantitative variable (this of course will not apply to rating scales/ranks).
Quantitative variables can be either discrete or continuous
Discrete variables are variables in which no values may be assumed between the two given values (e.g., number of lesions in each patient in a sample of patients with urticaria).
Continuous variables, on the other hand, can take any value in between the two given values (e.g., duration for which the weals last in the same sample of patients with urticaria). One way of differentiating between continuous and discrete variables is to use the “mid-way” test. If, for every pair of values of a variable, a value exactly mid-way between them is meaningful, the variable is continuous. For example, two values for the time taken for a weal to subside can be 10 and 13 min. The mid-way value would be 11.5 min which makes sense. However, for a number of weals, suppose you have a pair of values – 5 and 8 – the midway value would be 6.5 weals, which does not make sense.
Under the umbrella of qualitative variables, you can have nominal/categorical variables and ordinal variables
Nominal/categorical variables are, as the name suggests, variables which can be slotted into different categories (e.g., gender or type of psoriasis).
Ordinal variables or ranked variables are similar to categorical, but can be put into an order (e.g., a scale for severity of itching).
Dependent and independent variables
In the context of an experimental study, the dependent variable (also called outcome variable) is directly linked to the primary outcome of the study. For example, in a clinical trial on psoriasis, the PASI (psoriasis area severity index) would possibly be one dependent variable. The independent variable (sometime also called explanatory variable) is something which is not affected by the experiment itself but which can be manipulated to affect the dependent variable. Other terms sometimes used synonymously include blocking variable, covariate, or predictor variable. Confounding variables are extra variables, which can have an effect on the experiment. They are linked with dependent and independent variables and can cause spurious association. For example, in a clinical trial for a topical treatment in psoriasis, the concomitant use of moisturizers might be a confounding variable. A control variable is a variable that must be kept constant during the course of an experiment.
Descriptive Statistics
Statistics can be broadly divided into descriptive statistics and inferential statistics.[ 3 4 ] Descriptive statistics give a summary about the sample being studied without drawing any inferences based on probability theory. Even if the primary aim of a study involves inferential statistics, descriptive statistics are still used to give a general summary. When we describe the population using tools such as frequency distribution tables, percentages, and other measures of central tendency like the mean, for example, we are talking about descriptive statistics. When we use a specific statistical test (e.g., Mann–Whitney U-test) to compare the mean scores and express it in terms of statistical significance, we are talking about inferential statistics. Descriptive statistics can help in summarizing data in the form of simple quantitative measures such as percentages or means or in the form of visual summaries such as histograms and box plots.
Descriptive statistics can be used to describe a single variable (univariate analysis) or more than one variable (bivariate/multivariate analysis). In the case of more than one variable, descriptive statistics can help summarize relationships between variables using tools such as scatter plots.
Descriptive statistics can be broadly put under two categories:
- Sorting/grouping and illustration/visual displays
- Summary statistics.
Sorting and grouping
Sorting and grouping is most commonly done using frequency distribution tables. For continuous variables, it is generally better to use groups in the frequency table. Ideally, group sizes should be equal (except in extreme ends where open groups are used; e.g., age “greater than” or “less than”).
Another form of presenting frequency distributions is the “stem and leaf” diagram, which is considered to be a more accurate form of description.
Suppose the weight in kilograms of a group of 10 patients is as follows:
56, 34, 48, 43, 87, 78, 54, 62, 61, 59
The “stem” records the value of the “ten's” place (or higher) and the “leaf” records the value in the “one's” place [ Table 1 ].

Illustration/visual display of data
The most common tools used for visual display include frequency diagrams, bar charts (for noncontinuous variables) and histograms (for continuous variables). Composite bar charts can be used to compare variables. For example, the frequency distribution in a sample population of males and females can be illustrated as given in Figure 1 .

A pie chart helps show how a total quantity is divided among its constituent variables. Scatter diagrams can be used to illustrate the relationship between two variables. For example, global scores given for improvement in a condition like acne by the patient and the doctor [ Figure 2 ].

Summary statistics
The main tools used for summary statistics are broadly grouped into measures of central tendency (such as mean, median, and mode) and measures of dispersion or variation (such as range, standard deviation, and variance).
Imagine that the data below represent the weights of a sample of 15 pediatric patients arranged in ascending order:
30, 35, 37, 38, 38, 38, 42, 42, 44, 46, 47, 48, 51, 53, 86
Just having the raw data does not mean much to us, so we try to express it in terms of some values, which give a summary of the data.
The mean is basically the sum of all the values divided by the total number. In this case, we get a value of 45.
The problem is that some extreme values (outliers), like “'86,” in this case can skew the value of the mean. In this case, we consider other values like the median, which is the point that divides the distribution into two equal halves. It is also referred to as the 50 th percentile (50% of the values are above it and 50% are below it). In our previous example, since we have already arranged the values in ascending order we find that the point which divides it into two equal halves is the 8 th value – 42. In case of a total number of values being even, we choose the two middle points and take an average to reach the median.
The mode is the most common data point. In our example, this would be 38. The mode as in our case may not necessarily be in the center of the distribution.
The median is the best measure of central tendency from among the mean, median, and mode. In a “symmetric” distribution, all three are the same, whereas in skewed data the median and mean are not the same; lie more toward the skew, with the mean lying further to the skew compared with the median. For example, in Figure 3 , a right skewed distribution is seen (direction of skew is based on the tail); data values' distribution is longer on the right-hand (positive) side than on the left-hand side. The mean is typically greater than the median in such cases.

Measures of dispersion
The range gives the spread between the lowest and highest values. In our previous example, this will be 86-30 = 56.
A more valuable measure is the interquartile range. A quartile is one of the values which break the distribution into four equal parts. The 25 th percentile is the data point which divides the group between the first one-fourth and the last three-fourth of the data. The first one-fourth will form the first quartile. The 75 th percentile is the data point which divides the distribution into a first three-fourth and last one-fourth (the last one-fourth being the fourth quartile). The range between the 25 th percentile and 75 th percentile is called the interquartile range.
Variance is also a measure of dispersion. The larger the variance, the further the individual units are from the mean. Let us consider the same example we used for calculating the mean. The mean was 45.
For the first value (30), the deviation from the mean will be 15; for the last value (86), the deviation will be 41. Similarly we can calculate the deviations for all values in a sample. Adding these deviations and averaging will give a clue to the total dispersion, but the problem is that since the deviations are a mix of negative and positive values, the final total becomes zero. To calculate the variance, this problem is overcome by adding squares of the deviations. So variance would be the sum of squares of the variation divided by the total number in the population (for a sample we use “n − 1”). To get a more realistic value of the average dispersion, we take the square root of the variance, which is called the “standard deviation.”
The box plot
The box plot is a composite representation that portrays the mean, median, range, and the outliers [ Figure 4 ].

The concept of skewness and kurtosis
Skewness is a measure of the symmetry of distribution. Basically if the distribution curve is symmetric, it looks the same on either side of the central point. When this is not the case, it is said to be skewed. Kurtosis is a representation of outliers. Distributions with high kurtosis tend to have “heavy tails” indicating a larger number of outliers, whereas distributions with low kurtosis have light tails, indicating lesser outliers. There are formulas to calculate both skewness and kurtosis [Figures 5 – 8 ].

Sample Size
In an ideal study, we should be able to include all units of a particular population under study, something that is referred to as a census.[ 5 6 ] This would remove the chances of sampling error (difference between the outcome characteristics in a random sample when compared with the true population values – something that is virtually unavoidable when you take a random sample). However, it is obvious that this would not be feasible in most situations. Hence, we have to study a subset of the population to reach to our conclusions. This representative subset is a sample and we need to have sufficient numbers in this sample to make meaningful and accurate conclusions and reduce the effect of sampling error.
We also need to know that broadly sampling can be divided into two types – probability sampling and nonprobability sampling. Examples of probability sampling include methods such as simple random sampling (each member in a population has an equal chance of being selected), stratified random sampling (in nonhomogeneous populations, the population is divided into subgroups – followed be random sampling in each subgroup), systematic (sampling is based on a systematic technique – e.g., every third person is selected for a survey), and cluster sampling (similar to stratified sampling except that the clusters here are preexisting clusters unlike stratified sampling where the researcher decides on the stratification criteria), whereas nonprobability sampling, where every unit in the population does not have an equal chance of inclusion into the sample, includes methods such as convenience sampling (e.g., sample selected based on ease of access) and purposive sampling (where only people who meet specific criteria are included in the sample).
An accurate calculation of sample size is an essential aspect of good study design. It is important to calculate the sample size much in advance, rather than have to go for post hoc analysis. A sample size that is too less may make the study underpowered, whereas a sample size which is more than necessary might lead to a wastage of resources.
We will first go through the sample size calculation for a hypothesis-based design (like a randomized control trial).
The important factors to consider for sample size calculation include study design, type of statistical test, level of significance, power and effect size, variance (standard deviation for quantitative data), and expected proportions in the case of qualitative data. This is based on previous data, either based on previous studies or based on the clinicians' experience. In case the study is something being conducted for the first time, a pilot study might be conducted which helps generate these data for further studies based on a larger sample size). It is also important to know whether the data follow a normal distribution or not.
Two essential aspects we must understand are the concept of Type I and Type II errors. In a study that compares two groups, a null hypothesis assumes that there is no significant difference between the two groups, and any observed difference being due to sampling or experimental error. When we reject a null hypothesis, when it is true, we label it as a Type I error (also denoted as “alpha,” correlating with significance levels). In a Type II error (also denoted as “beta”), we fail to reject a null hypothesis, when the alternate hypothesis is actually true. Type II errors are usually expressed as “1- β,” correlating with the power of the test. While there are no absolute rules, the minimal levels accepted are 0.05 for α (corresponding to a significance level of 5%) and 0.20 for β (corresponding to a minimum recommended power of “1 − 0.20,” or 80%).
Effect size and minimal clinically relevant difference
For a clinical trial, the investigator will have to decide in advance what clinically detectable change is significant (for numerical data, this is could be the anticipated outcome means in the two groups, whereas for categorical data, it could correlate with the proportions of successful outcomes in two groups.). While we will not go into details of the formula for sample size calculation, some important points are as follows:
In the context where effect size is involved, the sample size is inversely proportional to the square of the effect size. What this means in effect is that reducing the effect size will lead to an increase in the required sample size.
Reducing the level of significance (alpha) or increasing power (1-β) will lead to an increase in the calculated sample size.
An increase in variance of the outcome leads to an increase in the calculated sample size.
A note is that for estimation type of studies/surveys, sample size calculation needs to consider some other factors too. This includes an idea about total population size (this generally does not make a major difference when population size is above 20,000, so in situations where population size is not known we can assume a population of 20,000 or more). The other factor is the “margin of error” – the amount of deviation which the investigators find acceptable in terms of percentages. Regarding confidence levels, ideally, a 95% confidence level is the minimum recommended for surveys too. Finally, we need an idea of the expected/crude prevalence – either based on previous studies or based on estimates.
Sample size calculation also needs to add corrections for patient drop-outs/lost-to-follow-up patients and missing records. An important point is that in some studies dealing with rare diseases, it may be difficult to achieve desired sample size. In these cases, the investigators might have to rework outcomes or maybe pool data from multiple centers. Although post hoc power can be analyzed, a better approach suggested is to calculate 95% confidence intervals for the outcome and interpret the study results based on this.
Financial support and sponsorship
Conflicts of interest.
There are no conflicts of interest.
- Cited Here |
- PubMed | CrossRef
Biostatistics ; descriptive statistics ; sample size ; variables
- + Favorites
- View in Gallery
Readers Of this Article Also Read
A study of the prevalence and precipitating factors of pruritus in pityriasis....
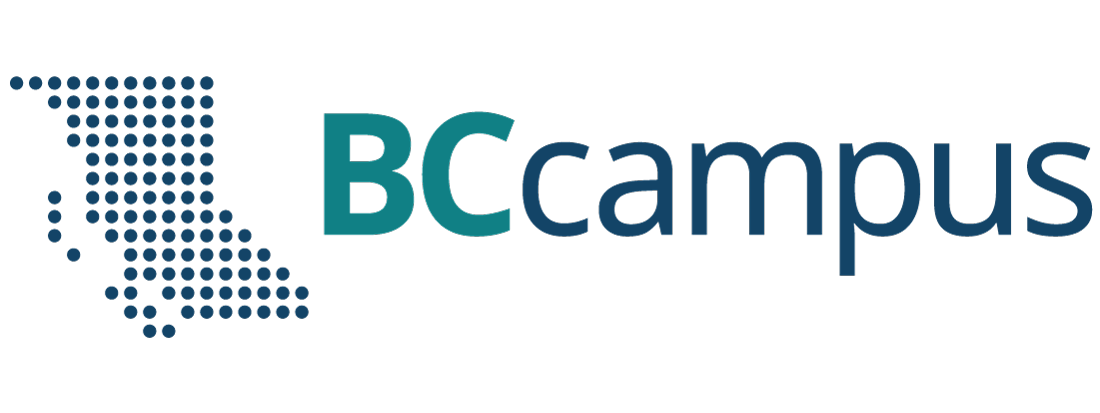
Want to create or adapt books like this? Learn more about how Pressbooks supports open publishing practices.
Chapter 3. Psychological Science
3.2 Psychologists Use Descriptive, Correlational, and Experimental Research Designs to Understand Behaviour
Learning objectives.
- Differentiate the goals of descriptive, correlational, and experimental research designs and explain the advantages and disadvantages of each.
- Explain the goals of descriptive research and the statistical techniques used to interpret it.
- Summarize the uses of correlational research and describe why correlational research cannot be used to infer causality.
- Review the procedures of experimental research and explain how it can be used to draw causal inferences.
Psychologists agree that if their ideas and theories about human behaviour are to be taken seriously, they must be backed up by data. However, the research of different psychologists is designed with different goals in mind, and the different goals require different approaches. These varying approaches, summarized in Table 3.2, are known as research designs . A research design is the specific method a researcher uses to collect, analyze, and interpret data . Psychologists use three major types of research designs in their research, and each provides an essential avenue for scientific investigation. Descriptive research is research designed to provide a snapshot of the current state of affairs . Correlational research is research designed to discover relationships among variables and to allow the prediction of future events from present knowledge . Experimental research is research in which initial equivalence among research participants in more than one group is created, followed by a manipulation of a given experience for these groups and a measurement of the influence of the manipulation . Each of the three research designs varies according to its strengths and limitations, and it is important to understand how each differs.
Research design | Goal | Advantages | Disadvantages |
---|---|---|---|
Descriptive | To create a snapshot of the current state of affairs | Provides a relatively complete picture of what is occurring at a given time. Allows the development of questions for further study. | Does not assess relationships among variables. May be unethical if participants do not know they are being observed. |
Correlational | To assess the relationships between and among two or more variables | Allows testing of expected relationships between and among variables and the making of predictions. Can assess these relationships in everyday life events. | Cannot be used to draw inferences about the causal relationships between and among the variables. |
Experimental | To assess the causal impact of one or more experimental manipulations on a dependent variable | Allows drawing of conclusions about the causal relationships among variables. | Cannot experimentally manipulate many important variables. May be expensive and time consuming. |
Source: Stangor, 2011. |
Descriptive Research: Assessing the Current State of Affairs
Descriptive research is designed to create a snapshot of the current thoughts, feelings, or behaviour of individuals. This section reviews three types of descriptive research : case studies , surveys , and naturalistic observation (Figure 3.4).
Sometimes the data in a descriptive research project are based on only a small set of individuals, often only one person or a single small group. These research designs are known as case studies — descriptive records of one or more individual’s experiences and behaviour . Sometimes case studies involve ordinary individuals, as when developmental psychologist Jean Piaget used his observation of his own children to develop his stage theory of cognitive development. More frequently, case studies are conducted on individuals who have unusual or abnormal experiences or characteristics or who find themselves in particularly difficult or stressful situations. The assumption is that by carefully studying individuals who are socially marginal, who are experiencing unusual situations, or who are going through a difficult phase in their lives, we can learn something about human nature.
Sigmund Freud was a master of using the psychological difficulties of individuals to draw conclusions about basic psychological processes. Freud wrote case studies of some of his most interesting patients and used these careful examinations to develop his important theories of personality. One classic example is Freud’s description of “Little Hans,” a child whose fear of horses the psychoanalyst interpreted in terms of repressed sexual impulses and the Oedipus complex (Freud, 1909/1964).
Another well-known case study is Phineas Gage, a man whose thoughts and emotions were extensively studied by cognitive psychologists after a railroad spike was blasted through his skull in an accident. Although there are questions about the interpretation of this case study (Kotowicz, 2007), it did provide early evidence that the brain’s frontal lobe is involved in emotion and morality (Damasio et al., 2005). An interesting example of a case study in clinical psychology is described by Rokeach (1964), who investigated in detail the beliefs of and interactions among three patients with schizophrenia, all of whom were convinced they were Jesus Christ.
In other cases the data from descriptive research projects come in the form of a survey — a measure administered through either an interview or a written questionnaire to get a picture of the beliefs or behaviours of a sample of people of interest . The people chosen to participate in the research (known as the sample) are selected to be representative of all the people that the researcher wishes to know about (the population). In election polls, for instance, a sample is taken from the population of all “likely voters” in the upcoming elections.
The results of surveys may sometimes be rather mundane, such as “Nine out of 10 doctors prefer Tymenocin” or “The median income in the city of Hamilton is $46,712.” Yet other times (particularly in discussions of social behaviour), the results can be shocking: “More than 40,000 people are killed by gunfire in the United States every year” or “More than 60% of women between the ages of 50 and 60 suffer from depression.” Descriptive research is frequently used by psychologists to get an estimate of the prevalence (or incidence ) of psychological disorders.
A final type of descriptive research — known as naturalistic observation — is research based on the observation of everyday events . For instance, a developmental psychologist who watches children on a playground and describes what they say to each other while they play is conducting descriptive research, as is a biopsychologist who observes animals in their natural habitats. One example of observational research involves a systematic procedure known as the strange situation , used to get a picture of how adults and young children interact. The data that are collected in the strange situation are systematically coded in a coding sheet such as that shown in Table 3.3.
Coder name: | ||||
This table represents a sample coding sheet from an episode of the “strange situation,” in which an infant (usually about one year old) is observed playing in a room with two adults — the child’s mother and a stranger. Each of the four coding categories is scored by the coder from 1 (the baby makes no effort to engage in the behaviour) to 7 (the baby makes a significant effort to engage in the behaviour). More information about the meaning of the coding can be found in Ainsworth, Blehar, Waters, and Wall (1978). | ||||
Coding categories explained | ||||
Proximity | The baby moves toward, grasps, or climbs on the adult. | |||
Maintaining contact | The baby resists being put down by the adult by crying or trying to climb back up. | |||
Resistance | The baby pushes, hits, or squirms to be put down from the adult’s arms. | |||
Avoidance | The baby turns away or moves away from the adult. | |||
Episode | Coding categories | |||
---|---|---|---|---|
Proximity | Contact | Resistance | Avoidance | |
Mother and baby play alone | 1 | 1 | 1 | 1 |
Mother puts baby down | 4 | 1 | 1 | 1 |
Stranger enters room | 1 | 2 | 3 | 1 |
Mother leaves room; stranger plays with baby | 1 | 3 | 1 | 1 |
Mother re-enters, greets and may comfort baby, then leaves again | 4 | 2 | 1 | 2 |
Stranger tries to play with baby | 1 | 3 | 1 | 1 |
Mother re-enters and picks up baby | 6 | 6 | 1 | 2 |
Source: Stang0r, 2011. |
The results of descriptive research projects are analyzed using descriptive statistics — numbers that summarize the distribution of scores on a measured variable . Most variables have distributions similar to that shown in Figure 3.5 where most of the scores are located near the centre of the distribution, and the distribution is symmetrical and bell-shaped. A data distribution that is shaped like a bell is known as a normal distribution .
A distribution can be described in terms of its central tendency — that is, the point in the distribution around which the data are centred — and its dispersion, or spread . The arithmetic average, or arithmetic mean , symbolized by the letter M , is the most commonly used measure of central tendency . It is computed by calculating the sum of all the scores of the variable and dividing this sum by the number of participants in the distribution (denoted by the letter N ). In the data presented in Figure 3.5 the mean height of the students is 67.12 inches (170.5 cm). The sample mean is usually indicated by the letter M .
In some cases, however, the data distribution is not symmetrical. This occurs when there are one or more extreme scores (known as outliers ) at one end of the distribution. Consider, for instance, the variable of family income (see Figure 3.6), which includes an outlier (a value of $3,800,000). In this case the mean is not a good measure of central tendency. Although it appears from Figure 3.6 that the central tendency of the family income variable should be around $70,000, the mean family income is actually $223,960. The single very extreme income has a disproportionate impact on the mean, resulting in a value that does not well represent the central tendency.
The median is used as an alternative measure of central tendency when distributions are not symmetrical. The median is the score in the center of the distribution, meaning that 50% of the scores are greater than the median and 50% of the scores are less than the median . In our case, the median household income ($73,000) is a much better indication of central tendency than is the mean household income ($223,960).
A final measure of central tendency, known as the mode , represents the value that occurs most frequently in the distribution . You can see from Figure 3.6 that the mode for the family income variable is $93,000 (it occurs four times).
In addition to summarizing the central tendency of a distribution, descriptive statistics convey information about how the scores of the variable are spread around the central tendency. Dispersion refers to the extent to which the scores are all tightly clustered around the central tendency , as seen in Figure 3.7.
Or they may be more spread out away from it, as seen in Figure 3.8.
One simple measure of dispersion is to find the largest (the maximum ) and the smallest (the minimum ) observed values of the variable and to compute the range of the variable as the maximum observed score minus the minimum observed score. You can check that the range of the height variable in Figure 3.5 is 72 – 62 = 10. The standard deviation , symbolized as s , is the most commonly used measure of dispersion . Distributions with a larger standard deviation have more spread. The standard deviation of the height variable is s = 2.74, and the standard deviation of the family income variable is s = $745,337.
An advantage of descriptive research is that it attempts to capture the complexity of everyday behaviour. Case studies provide detailed information about a single person or a small group of people, surveys capture the thoughts or reported behaviours of a large population of people, and naturalistic observation objectively records the behaviour of people or animals as it occurs naturally. Thus descriptive research is used to provide a relatively complete understanding of what is currently happening.
Despite these advantages, descriptive research has a distinct disadvantage in that, although it allows us to get an idea of what is currently happening, it is usually limited to static pictures. Although descriptions of particular experiences may be interesting, they are not always transferable to other individuals in other situations, nor do they tell us exactly why specific behaviours or events occurred. For instance, descriptions of individuals who have suffered a stressful event, such as a war or an earthquake, can be used to understand the individuals’ reactions to the event but cannot tell us anything about the long-term effects of the stress. And because there is no comparison group that did not experience the stressful situation, we cannot know what these individuals would be like if they hadn’t had the stressful experience.
Correlational Research: Seeking Relationships among Variables
In contrast to descriptive research, which is designed primarily to provide static pictures, correlational research involves the measurement of two or more relevant variables and an assessment of the relationship between or among those variables. For instance, the variables of height and weight are systematically related (correlated) because taller people generally weigh more than shorter people. In the same way, study time and memory errors are also related, because the more time a person is given to study a list of words, the fewer errors he or she will make. When there are two variables in the research design, one of them is called the predictor variable and the other the outcome variable . The research design can be visualized as shown in Figure 3.9, where the curved arrow represents the expected correlation between these two variables.
One way of organizing the data from a correlational study with two variables is to graph the values of each of the measured variables using a scatter plot . As you can see in Figure 3.10 a scatter plot is a visual image of the relationship between two variables . A point is plotted for each individual at the intersection of his or her scores for the two variables. When the association between the variables on the scatter plot can be easily approximated with a straight line , as in parts (a) and (b) of Figure 3.10 the variables are said to have a linear relationship .
When the straight line indicates that individuals who have above-average values for one variable also tend to have above-average values for the other variable , as in part (a), the relationship is said to be positive linear . Examples of positive linear relationships include those between height and weight, between education and income, and between age and mathematical abilities in children. In each case, people who score higher on one of the variables also tend to score higher on the other variable. Negative linear relationships , in contrast, as shown in part (b), occur when above-average values for one variable tend to be associated with below-average values for the other variable. Examples of negative linear relationships include those between the age of a child and the number of diapers the child uses, and between practice on and errors made on a learning task. In these cases, people who score higher on one of the variables tend to score lower on the other variable.
Relationships between variables that cannot be described with a straight line are known as nonlinear relationships . Part (c) of Figure 3.10 shows a common pattern in which the distribution of the points is essentially random. In this case there is no relationship at all between the two variables, and they are said to be independent . Parts (d) and (e) of Figure 3.10 show patterns of association in which, although there is an association, the points are not well described by a single straight line. For instance, part (d) shows the type of relationship that frequently occurs between anxiety and performance. Increases in anxiety from low to moderate levels are associated with performance increases, whereas increases in anxiety from moderate to high levels are associated with decreases in performance. Relationships that change in direction and thus are not described by a single straight line are called curvilinear relationships .
The most common statistical measure of the strength of linear relationships among variables is the Pearson correlation coefficient , which is symbolized by the letter r . The value of the correlation coefficient ranges from r = –1.00 to r = +1.00. The direction of the linear relationship is indicated by the sign of the correlation coefficient. Positive values of r (such as r = .54 or r = .67) indicate that the relationship is positive linear (i.e., the pattern of the dots on the scatter plot runs from the lower left to the upper right), whereas negative values of r (such as r = –.30 or r = –.72) indicate negative linear relationships (i.e., the dots run from the upper left to the lower right). The strength of the linear relationship is indexed by the distance of the correlation coefficient from zero (its absolute value). For instance, r = –.54 is a stronger relationship than r = .30, and r = .72 is a stronger relationship than r = –.57. Because the Pearson correlation coefficient only measures linear relationships, variables that have curvilinear relationships are not well described by r , and the observed correlation will be close to zero.
It is also possible to study relationships among more than two measures at the same time. A research design in which more than one predictor variable is used to predict a single outcome variable is analyzed through multiple regression (Aiken & West, 1991). Multiple regression is a statistical technique, based on correlation coefficients among variables, that allows predicting a single outcome variable from more than one predictor variable . For instance, Figure 3.11 shows a multiple regression analysis in which three predictor variables (Salary, job satisfaction, and years employed) are used to predict a single outcome (job performance). The use of multiple regression analysis shows an important advantage of correlational research designs — they can be used to make predictions about a person’s likely score on an outcome variable (e.g., job performance) based on knowledge of other variables.
An important limitation of correlational research designs is that they cannot be used to draw conclusions about the causal relationships among the measured variables. Consider, for instance, a researcher who has hypothesized that viewing violent behaviour will cause increased aggressive play in children. He has collected, from a sample of Grade 4 children, a measure of how many violent television shows each child views during the week, as well as a measure of how aggressively each child plays on the school playground. From his collected data, the researcher discovers a positive correlation between the two measured variables.
Although this positive correlation appears to support the researcher’s hypothesis, it cannot be taken to indicate that viewing violent television causes aggressive behaviour. Although the researcher is tempted to assume that viewing violent television causes aggressive play, there are other possibilities. One alternative possibility is that the causal direction is exactly opposite from what has been hypothesized. Perhaps children who have behaved aggressively at school develop residual excitement that leads them to want to watch violent television shows at home (Figure 3.13):
Although this possibility may seem less likely, there is no way to rule out the possibility of such reverse causation on the basis of this observed correlation. It is also possible that both causal directions are operating and that the two variables cause each other (Figure 3.14).
Still another possible explanation for the observed correlation is that it has been produced by the presence of a common-causal variable (also known as a third variable ). A common-causal variable is a variable that is not part of the research hypothesis but that causes both the predictor and the outcome variable and thus produces the observed correlation between them . In our example, a potential common-causal variable is the discipline style of the children’s parents. Parents who use a harsh and punitive discipline style may produce children who like to watch violent television and who also behave aggressively in comparison to children whose parents use less harsh discipline (Figure 3.15)
In this case, television viewing and aggressive play would be positively correlated (as indicated by the curved arrow between them), even though neither one caused the other but they were both caused by the discipline style of the parents (the straight arrows). When the predictor and outcome variables are both caused by a common-causal variable, the observed relationship between them is said to be spurious . A spurious relationship is a relationship between two variables in which a common-causal variable produces and “explains away” the relationship . If effects of the common-causal variable were taken away, or controlled for, the relationship between the predictor and outcome variables would disappear. In the example, the relationship between aggression and television viewing might be spurious because by controlling for the effect of the parents’ disciplining style, the relationship between television viewing and aggressive behaviour might go away.
Common-causal variables in correlational research designs can be thought of as mystery variables because, as they have not been measured, their presence and identity are usually unknown to the researcher. Since it is not possible to measure every variable that could cause both the predictor and outcome variables, the existence of an unknown common-causal variable is always a possibility. For this reason, we are left with the basic limitation of correlational research: correlation does not demonstrate causation. It is important that when you read about correlational research projects, you keep in mind the possibility of spurious relationships, and be sure to interpret the findings appropriately. Although correlational research is sometimes reported as demonstrating causality without any mention being made of the possibility of reverse causation or common-causal variables, informed consumers of research, like you, are aware of these interpretational problems.
In sum, correlational research designs have both strengths and limitations. One strength is that they can be used when experimental research is not possible because the predictor variables cannot be manipulated. Correlational designs also have the advantage of allowing the researcher to study behaviour as it occurs in everyday life. And we can also use correlational designs to make predictions — for instance, to predict from the scores on their battery of tests the success of job trainees during a training session. But we cannot use such correlational information to determine whether the training caused better job performance. For that, researchers rely on experiments.
Experimental Research: Understanding the Causes of Behaviour
The goal of experimental research design is to provide more definitive conclusions about the causal relationships among the variables in the research hypothesis than is available from correlational designs. In an experimental research design, the variables of interest are called the independent variable (or variables ) and the dependent variable . The independent variable in an experiment is the causing variable that is created (manipulated) by the experimenter . The dependent variable in an experiment is a measured variable that is expected to be influenced by the experimental manipulation . The research hypothesis suggests that the manipulated independent variable or variables will cause changes in the measured dependent variables. We can diagram the research hypothesis by using an arrow that points in one direction. This demonstrates the expected direction of causality (Figure 3.16):
Research Focus: Video Games and Aggression
Consider an experiment conducted by Anderson and Dill (2000). The study was designed to test the hypothesis that viewing violent video games would increase aggressive behaviour. In this research, male and female undergraduates from Iowa State University were given a chance to play with either a violent video game (Wolfenstein 3D) or a nonviolent video game (Myst). During the experimental session, the participants played their assigned video games for 15 minutes. Then, after the play, each participant played a competitive game with an opponent in which the participant could deliver blasts of white noise through the earphones of the opponent. The operational definition of the dependent variable (aggressive behaviour) was the level and duration of noise delivered to the opponent. The design of the experiment is shown in Figure 3.17
Two advantages of the experimental research design are (a) the assurance that the independent variable (also known as the experimental manipulation ) occurs prior to the measured dependent variable, and (b) the creation of initial equivalence between the conditions of the experiment (in this case by using random assignment to conditions).
Experimental designs have two very nice features. For one, they guarantee that the independent variable occurs prior to the measurement of the dependent variable. This eliminates the possibility of reverse causation. Second, the influence of common-causal variables is controlled, and thus eliminated, by creating initial equivalence among the participants in each of the experimental conditions before the manipulation occurs.
The most common method of creating equivalence among the experimental conditions is through random assignment to conditions, a procedure in which the condition that each participant is assigned to is determined through a random process, such as drawing numbers out of an envelope or using a random number table . Anderson and Dill first randomly assigned about 100 participants to each of their two groups (Group A and Group B). Because they used random assignment to conditions, they could be confident that, before the experimental manipulation occurred, the students in Group A were, on average, equivalent to the students in Group B on every possible variable, including variables that are likely to be related to aggression, such as parental discipline style, peer relationships, hormone levels, diet — and in fact everything else.
Then, after they had created initial equivalence, Anderson and Dill created the experimental manipulation — they had the participants in Group A play the violent game and the participants in Group B play the nonviolent game. Then they compared the dependent variable (the white noise blasts) between the two groups, finding that the students who had viewed the violent video game gave significantly longer noise blasts than did the students who had played the nonviolent game.
Anderson and Dill had from the outset created initial equivalence between the groups. This initial equivalence allowed them to observe differences in the white noise levels between the two groups after the experimental manipulation, leading to the conclusion that it was the independent variable (and not some other variable) that caused these differences. The idea is that the only thing that was different between the students in the two groups was the video game they had played.
Despite the advantage of determining causation, experiments do have limitations. One is that they are often conducted in laboratory situations rather than in the everyday lives of people. Therefore, we do not know whether results that we find in a laboratory setting will necessarily hold up in everyday life. Second, and more important, is that some of the most interesting and key social variables cannot be experimentally manipulated. If we want to study the influence of the size of a mob on the destructiveness of its behaviour, or to compare the personality characteristics of people who join suicide cults with those of people who do not join such cults, these relationships must be assessed using correlational designs, because it is simply not possible to experimentally manipulate these variables.
Key Takeaways
- Descriptive, correlational, and experimental research designs are used to collect and analyze data.
- Descriptive designs include case studies, surveys, and naturalistic observation. The goal of these designs is to get a picture of the current thoughts, feelings, or behaviours in a given group of people. Descriptive research is summarized using descriptive statistics.
- Correlational research designs measure two or more relevant variables and assess a relationship between or among them. The variables may be presented on a scatter plot to visually show the relationships. The Pearson Correlation Coefficient ( r ) is a measure of the strength of linear relationship between two variables.
- Common-causal variables may cause both the predictor and outcome variable in a correlational design, producing a spurious relationship. The possibility of common-causal variables makes it impossible to draw causal conclusions from correlational research designs.
- Experimental research involves the manipulation of an independent variable and the measurement of a dependent variable. Random assignment to conditions is normally used to create initial equivalence between the groups, allowing researchers to draw causal conclusions.
Exercises and Critical Thinking
- There is a negative correlation between the row that a student sits in in a large class (when the rows are numbered from front to back) and his or her final grade in the class. Do you think this represents a causal relationship or a spurious relationship, and why?
- Think of two variables (other than those mentioned in this book) that are likely to be correlated, but in which the correlation is probably spurious. What is the likely common-causal variable that is producing the relationship?
- Imagine a researcher wants to test the hypothesis that participating in psychotherapy will cause a decrease in reported anxiety. Describe the type of research design the investigator might use to draw this conclusion. What would be the independent and dependent variables in the research?
Image Attributions
Figure 3.4: “ Reading newspaper ” by Alaskan Dude (http://commons.wikimedia.org/wiki/File:Reading_newspaper.jpg) is licensed under CC BY 2.0
Aiken, L., & West, S. (1991). Multiple regression: Testing and interpreting interactions . Newbury Park, CA: Sage.
Ainsworth, M. S., Blehar, M. C., Waters, E., & Wall, S. (1978). Patterns of attachment: A psychological study of the strange situation . Hillsdale, NJ: Lawrence Erlbaum Associates.
Anderson, C. A., & Dill, K. E. (2000). Video games and aggressive thoughts, feelings, and behavior in the laboratory and in life. Journal of Personality and Social Psychology, 78 (4), 772–790.
Damasio, H., Grabowski, T., Frank, R., Galaburda, A. M., Damasio, A. R., Cacioppo, J. T., & Berntson, G. G. (2005). The return of Phineas Gage: Clues about the brain from the skull of a famous patient. In Social neuroscience: Key readings. (pp. 21–28). New York, NY: Psychology Press.
Freud, S. (1909/1964). Analysis of phobia in a five-year-old boy. In E. A. Southwell & M. Merbaum (Eds.), Personality: Readings in theory and research (pp. 3–32). Belmont, CA: Wadsworth. (Original work published 1909).
Kotowicz, Z. (2007). The strange case of Phineas Gage. History of the Human Sciences, 20 (1), 115–131.
Rokeach, M. (1964). The three Christs of Ypsilanti: A psychological study . New York, NY: Knopf.
Stangor, C. (2011). Research methods for the behavioural sciences (4th ed.). Mountain View, CA: Cengage.
Long Descriptions
Figure 3.6 long description: There are 25 families. 24 families have an income between $44,000 and $111,000 and one family has an income of $3,800,000. The mean income is $223,960 while the median income is $73,000. [Return to Figure 3.6]
Figure 3.10 long description: Types of scatter plots.
- Positive linear, r=positive .82. The plots on the graph form a rough line that runs from lower left to upper right.
- Negative linear, r=negative .70. The plots on the graph form a rough line that runs from upper left to lower right.
- Independent, r=0.00. The plots on the graph are spread out around the centre.
- Curvilinear, r=0.00. The plots of the graph form a rough line that goes up and then down like a hill.
- Curvilinear, r=0.00. The plots on the graph for a rough line that goes down and then up like a ditch.
[Return to Figure 3.10]
Introduction to Psychology - 1st Canadian Edition Copyright © 2014 by Jennifer Walinga and Charles Stangor is licensed under a Creative Commons Attribution-NonCommercial-ShareAlike 4.0 International License , except where otherwise noted.
Share This Book
Have a language expert improve your writing
Run a free plagiarism check in 10 minutes, generate accurate citations for free.
- Knowledge Base
Methodology
- Types of Research Designs Compared | Guide & Examples
Types of Research Designs Compared | Guide & Examples
Published on June 20, 2019 by Shona McCombes . Revised on June 22, 2023.
When you start planning a research project, developing research questions and creating a research design , you will have to make various decisions about the type of research you want to do.
There are many ways to categorize different types of research. The words you use to describe your research depend on your discipline and field. In general, though, the form your research design takes will be shaped by:
- The type of knowledge you aim to produce
- The type of data you will collect and analyze
- The sampling methods , timescale and location of the research
This article takes a look at some common distinctions made between different types of research and outlines the key differences between them.
Table of contents
Types of research aims, types of research data, types of sampling, timescale, and location, other interesting articles.
The first thing to consider is what kind of knowledge your research aims to contribute.
Type of research | What’s the difference? | What to consider |
---|---|---|
Basic vs. applied | Basic research aims to , while applied research aims to . | Do you want to expand scientific understanding or solve a practical problem? |
vs. | Exploratory research aims to , while explanatory research aims to . | How much is already known about your research problem? Are you conducting initial research on a newly-identified issue, or seeking precise conclusions about an established issue? |
aims to , while aims to . | Is there already some theory on your research problem that you can use to develop , or do you want to propose new theories based on your findings? |
Prevent plagiarism. Run a free check.
The next thing to consider is what type of data you will collect. Each kind of data is associated with a range of specific research methods and procedures.
Type of research | What’s the difference? | What to consider |
---|---|---|
Primary research vs secondary research | Primary data is (e.g., through or ), while secondary data (e.g., in government or scientific publications). | How much data is already available on your topic? Do you want to collect original data or analyze existing data (e.g., through a )? |
, while . | Is your research more concerned with measuring something or interpreting something? You can also create a research design that has elements of both. | |
vs | Descriptive research gathers data , while experimental research . | Do you want to identify characteristics, patterns and or test causal relationships between ? |
Finally, you have to consider three closely related questions: how will you select the subjects or participants of the research? When and how often will you collect data from your subjects? And where will the research take place?
Keep in mind that the methods that you choose bring with them different risk factors and types of research bias . Biases aren’t completely avoidable, but can heavily impact the validity and reliability of your findings if left unchecked.
Type of research | What’s the difference? | What to consider |
---|---|---|
allows you to , while allows you to draw conclusions . | Do you want to produce knowledge that applies to many contexts or detailed knowledge about a specific context (e.g. in a )? | |
vs | Cross-sectional studies , while longitudinal studies . | Is your research question focused on understanding the current situation or tracking changes over time? |
Field research vs laboratory research | Field research takes place in , while laboratory research takes place in . | Do you want to find out how something occurs in the real world or draw firm conclusions about cause and effect? Laboratory experiments have higher but lower . |
Fixed design vs flexible design | In a fixed research design the subjects, timescale and location are begins, while in a flexible design these aspects may . | Do you want to test hypotheses and establish generalizable facts, or explore concepts and develop understanding? For measuring, testing and making generalizations, a fixed research design has higher . |
Choosing between all these different research types is part of the process of creating your research design , which determines exactly how your research will be conducted. But the type of research is only the first step: next, you have to make more concrete decisions about your research methods and the details of the study.
Read more about creating a research design
If you want to know more about statistics , methodology , or research bias , make sure to check out some of our other articles with explanations and examples.
- Normal distribution
- Degrees of freedom
- Null hypothesis
- Discourse analysis
- Control groups
- Mixed methods research
- Non-probability sampling
- Quantitative research
- Ecological validity
Research bias
- Rosenthal effect
- Implicit bias
- Cognitive bias
- Selection bias
- Negativity bias
- Status quo bias
Cite this Scribbr article
If you want to cite this source, you can copy and paste the citation or click the “Cite this Scribbr article” button to automatically add the citation to our free Citation Generator.
McCombes, S. (2023, June 22). Types of Research Designs Compared | Guide & Examples. Scribbr. Retrieved September 3, 2024, from https://www.scribbr.com/methodology/types-of-research/
Is this article helpful?
Shona McCombes
Other students also liked, what is a research design | types, guide & examples, qualitative vs. quantitative research | differences, examples & methods, what is a research methodology | steps & tips, "i thought ai proofreading was useless but..".
I've been using Scribbr for years now and I know it's a service that won't disappoint. It does a good job spotting mistakes”
Pardon Our Interruption
As you were browsing something about your browser made us think you were a bot. There are a few reasons this might happen:
- You've disabled JavaScript in your web browser.
- You're a power user moving through this website with super-human speed.
- You've disabled cookies in your web browser.
- A third-party browser plugin, such as Ghostery or NoScript, is preventing JavaScript from running. Additional information is available in this support article .
To regain access, please make sure that cookies and JavaScript are enabled before reloading the page.
People-environment relations following COVID-19 pandemic lifestyle restrictions: a multinational, explorative analysis of intended biophilic design changes
- Open access
- Published: 02 September 2024
- Volume 5 , article number 229 , ( 2024 )
Cite this article
You have full access to this open access article
- Kalterina Shulla 1 ,
- Bernd-Friedrich Voigt 2 ,
- Salim Lardjane 3 ,
- Kerstin Fischer 4 ,
- Piotr Kędzierski 5 ,
- Giuseppe Scandone 6 &
- Thomas Süße 7
The study analyzes the consequences of the COVID-19 pandemic restrictions for the human–environment relations through the lenses of biophilic design. The mixed-method quantitative and qualitative explanatory research combines contextual and personal variables, such as, among others, country, age group, gender, overcrowding, time spent outside, access to nature/food and the exposure to biophilic elements, during and after the lockdown. The results indicate that psychological pressure on individuals caused by pandemic restrictions imposed early 2020, triggered changes in human-environmental relation. More precisely, our comparative analysis of six European countries (Italy, Germany, Poland, Spain, Denmark and Sweden) indicates that people-environment relations do not depend on the objective severity of country-wise restrictions, but rather on the individual perceptions of these restrictions. The results complement the lack of the research for the role of biophilic design in understanding and enhancing human–environment relations during the COVID-19 pandemic restrictions and thereafter.
Avoid common mistakes on your manuscript.
1 Introduction
The power of nature for physical and mental well-being and the healing role in stressful conditions is undeniable, as human aesthetic, intellectual, cognitive and spiritual cravings are fulfilled [ 1 ]. Exposure to real or simulated natural views can quickly trigger restorative activity in the brain and reduce stress levels [ 2 ]. Contemplating a nature-integrated urban environment can enhance positive emotions [ 3 , 4 ] as deprivation from it can worsen negative states [ 5 ]. In early 2020, the COVID-19 pandemic imposed worldwide restrictions that were unique because of their variety and differing severity which ultimately resulted in graduated states of psychological pressure on individuals [ 6 , 7 ]. However, when people are forced to cope with crises in unusual circumstances, changes are triggered in their patterns of living and working toward a more sustainable and resilient lifestyle [ 8 ]. “Worry” can divert life priorities, but a strong self-concept of nature can serve as a buffer for a moderate impact on environmental values [ 9 ], enabling “salutogenic” experiences out of stress and psychological states even in extreme environments [ 10 ]. The restrictions of the COVID-19 global pandemic highlighted an attention towards Biophilic Design, as it embraces elements of direct and indirect experience of nature.
As personal growth is attributed to societal dynamics and changes in norms [ 11 ], the way people use and perceive the environment can be encouraged or limited by such norms and cultural contexts [ 12 ]. Ironically, despite its far-reaching negative effects, the pandemic also created a new environment for self-reflection and changes in personal perceptions and actions toward its natural surroundings [ 13 ] and the individuals’ lifestyle choices, such as choice of food, housing, mobility, etc.; factors which are mainly not beyond individual control [ 14 ]. While the ecological footprint and environmental impact of the crisis have been widely considered in research of sustainable design practices [ 15 ], biophilic design (BD) encompasses the mutual benefits of connecting with nature to both humans (physiological and psychological benefits) and the environment [ 16 ]. Therefore, this study aims to understand the effects of the COVID-19 pandemic on changes of people-environment relations considering objective as well as perceived severity of lifestyle restrictions. Beyond this, the research aims to depict a pattern of predictive variables regarding the likelihood of integrating BD in future life. We do so in combining a country-comparative research approach, explorative quantitative and qualitative analysis of intended BD changes.
2 Conceptual background
The term ‘biophilia’ (love of life), composed of the ancient Greek words for “life” (bio) and “love” (philia), describes harmonious relationships between humans and the biosphere [ 17 ]. The term was first used by Erich Fromm in “The Heart of Man” (1964) and later by Edward Wilson in “Biophilia” [ 18 ]. The individual’s physiological and psychological response to nature enables the effects of BD elements such as the direct experience of nature in the built environment (natural light, air, plants, animals, water, landscapes), the indirect experience of nature (contact with the representation or image of nature, natural materials, etc.) and the experience of space and place (spatial features of prospect refuge, etc.) [ 19 , 20 ].
The human response to design stimuli allows BD elements to improve quality and sustainability by enhancing health and well-being, productivity, biodiversity, circularity, and resilience [ 21 ]. The green building movement in the early 1990s enforced the link between improved environmental quality and worker productivity [ 22 ] through the use of BD to connect with the indoor environment. Additional benefits include addressing workplace stress, student performance, patient recovery, and community cohesiveness [ 23 ] and improving well-being in prisons [ 24 ]. The BD elements in the landscape (even those not perceived as such) enable the incorporation of diverse strategies into the built environment [ 25 ]. In urban settings, these elements, the new “Hanging Gardens of Babylon”, are indicators of sustainability and resilience. When there is freedom to choose for home or workplace environment relations, the choice is often dominated by a viewpoint with a generous prospect, elevated position, open, savanna-like terrain, proximity to a body of water, etc. [ 26 ].
BD research is mainly related to two theoretical concepts from environmental psychology: The first is Stress Reduction Theory (SRT) [ 27 , 28 ] which explains the extent to which contemplating nature can trigger restorative activity in the brain, which in turn is responsible for reduced stress levels and positive emotions. The second explanatory concept comes from Attention Restoration Theory (ART) [ 29 ], which states that a lack of concentration as well as mental fatigue, which can be attributed to a prolonged direct attention span, can be positively influenced by a visual or physical stay in nature and the increase in concentration can be achieved through restorative processes with less energy-draining attention [ 30 ]. When a person is facing an unpleasant and stressful change in its person-environment relation because of a perceived external behavioral control, patterns of BD (see Annex 2) and biophilia values [ 31 ] can trigger individual restorative responses [ 10 ]. These responses might ultimately result in an adjustment of the environmental surroundings, or at least enhance a person`s motivation to do so by effecting the likelihood of using BD. Mindsponge Theory [ 32 ] conceptualizes this relation of perceived behavioral control in nature and models it with intentions towards behavior change [ 33 ]. A crucial part of this systemic conceptualization of the person-environment relation is the element of “perception of external information by the sensory systems, such as visual or auditory information” [ 34 ].
Literature shows that pandemic restrictions have divergent but socioeconomically moderate psychological effects (either positively or negatively related to states of stress) and that enforced restrictions can be perceived differently (i.e., at the individual level). In addition, deprivation from one of the domains can have such great importance that it can dominate the totality of the measures and, as a consequence, can result in a perceived stronger severity of the measures despite the moderate or weak objective status of the country [ 35 ]. The severe restrictions imposed in Europe and all over the world (although differing across countries), especially during the first wave of the pandemic (March–June 2020), limited life choices [ 36 , 37 ]. These restrictions were accompanied by psychological distress and a decrease in psychological well-being in the general public [ 38 ], among others, due to limited access to physical activity, lack of blue/green landscapes, views of nature from home [ 39 ] and remote interactions, which caused loneliness, especially for women and younger adults [ 40 ]. During this period in Italy, the lack of adequate space, terraces and gardens resulted in increased stress and aggressiveness [ 41 ], where the correlation with the “home satisfaction” factor in those conditions was related to spatial features of adequacy, flexibility, and crowding [ 42 ]. As human risk perception can lead to immediate action, in France, hours and days before the lockdown, people moved from their homes to other places, closer to family, or with better living conditions in terms of size, crowding, landscape, etc. [ 43 ]. Additional challenges in the living environment were also due to the necessity of adapting to working from home [ 44 , 45 ]. During the first wave, for instance, more than 60% of the workers in Germany were obligated to work from home, confronting the lack of a separable home-office working space and triggering a large-scale invasion of work into the private sphere [ 46 ].
The related post pandemic research has analyzed the role of biophilic features for recovery from COVID-19. Afacan (2021) explores the role of biophilic design in enhancing psychological resilience during the pandemic, related to recovery tension mood, depression and anger [ 47 ]. Furthermore, integrating natural elements into both residential and public spaces, especially in times of crisis, can significantly improve mental and physical health and foster a sense of community and connection [ 48 ]. BD principles are vital for enhancing post-pandemic living spaces, through maximizing natural light and ventilation, incorporating plants and green spaces, using natural materials, and designing flexible, multi-functional spaces. These approaches not only create aesthetically pleasing environments but also support well-being and sustainability, making living spaces more adaptable and resilient to future crises [ 49 ]. Incorporating natural elements into architectural design not only create aesthetically pleasing environments but also encourage deeper connections with nature, leading to healthier, more resilient living spaces, and better mental and physical health [ 50 ]. Furthermore, investigations on the relevance of various influential factors for the efficiency and effectiveness of working from home, for physical and mental well-being have been conducted [ 51 , 52 ]. There is a need for coordinated cross-disciplinary research to address COVID-19's mental health impacts and understanding the pandemic's psychological effects during and after the pandemic [ 53 ]. However, the role of BD as an indicator of enhancing connection between nature and humans triggered by lived experiences during the pandemic is under-researched. This study aims to fill this gap, by analyzing people-environment relations following COVID-19 pandemic lifestyle restrictions, through a multinational, explorative analysis of intended BD changes.
3 Systematization of restrictive measures during the pandemic in Italy, Germany, Poland, Spain, Denmark and Sweden
The restrictive measures taken during the COVID-19 pandemic for most of the countries, consists on establishing lockdowns, declaring state of emergency, ban on outside activities, border and travel/international flights, and events. The Oxford COVID-19 Government Response Tracker (OxCGRT) defines the stringency of the measures in eight domains: school and workplace closings, canceling public events, restrictions on gathering size, close public transport, stay-at-home requirements, restrictions on internal movement and international travel [ 54 , 55 ]. These restrictions were considered as basis for defining the comparative groups, contrasting case/country selections: (1) countries that experienced strong/moderate restrictions (Italy, Spain, Germany and Poland) and ‘(2) countries with relatively weak restrictions (Denmark and Sweden). Countries are used as proxies, not considering internal differences (i.e., Italy, “in November 2020, was divided into three zones (red, orange, and yellow)) depending on the severity of the outbreak, with different restrictions applied in each zone.
The six selected countries were affected differently by the pandemic, as reflected by the varying severity of the measures taken. During the first wave of the pandemic, the state of emergency was declared in Italy, Spain, and Denmark. The lockdown was implemented in Italy, Spain and Poland, partially in Germany, while Denmark and Sweden had no national lockdowns (see Table 1 , below) for an overview of the restrictions considering the above domains, plus the lockdown status and the state of the emergency in the six countries). Sections 3.1 . and 3.2 . display detailed illustrations of the restrictive measures in the groups.
3.1 Countries with strong/moderate restrictions, Italy and Spain
Italy, one of the first countries in Europe to be heavily impacted by the COVID-19 pandemic, took a series of strict measures to curb the spread of the virus, with a significant impact on citizens, among the positioned in the group of countries with more self-protective measures [ 56 ]. The Italian government declared a state of emergency on January 31st, 2020 (which lasted until 1st of April 2022), and a nationwide lockdown on March 9th, 2020, closing all nonessential businesses and allowing people to leave their homes for essential reasons, such as buying groceries or going to the doctor [ 57 ]. Face masks were mandatory in all public spaces, and social distancing was enforced. In the summer of 2020, restrictions were gradually lifted, and people were allowed to travel within the country for tourism purposes. However, new restrictions were imposed in the fall due to a rise in cases; nevertheless, these restrictions were less severe, although social distancing was still enforced. Vaccination campaigns were underway, and people who were fully vaccinated had more freedom, such as attending events and travelling abroad. The pandemic resulted in an increase in remote work, with many companies allowing their employees to work from home [ 58 ]. This consequently led people to move out of cities toward smaller towns and villages with more affordable housing and space. The pandemic has accelerated the trend of suburbanization in Italy, with more people looking for larger homes with outdoor spaces [ 59 ]. According to the Digital Innovation Observatories of the School of Management of the Politecnico di Milano, in 2022, there were approximately 3.6 million remote workers, almost 500 thousand fewer than in 2021, with a decrease in particular in the Public Administration (PA) and Small Medium Enterprises (SMEs); however, there is slight but constant growth in large companies, which, with 1.84 million workers, accounted for approximately half of the total smart workers. Despite this, there is increased awareness and action in organizations to create workspace environments that motivate and give meaning to work in the office. Approximately 52% of large companies, 30% of SMEs and 25% of PAs have already carried out interventions to modify the environment or are doing so in recent months. In the future, these initiatives are planned or under evaluation for 26% of large companies, 21% of public administrations and 14% of SMEs [ 60 ]. Furthermore, during the pandemic parks and public gardens have become more important as places for people to exercise and relax while maintaining social distance. A study conducted in Italy during the first COVID-19 pandemic wave (April–May 2020) highlighted the fact that restrictions influenced citizens’ perceptions of urban green spaces, with a consequent increase in general interest in parks and public garden [ 61 ].
Spain was one of the European countries with the highest incidence during the first wave [ 62 ], and in the global context, Spain experienced one of the worst situations [ 63 ]. The government imposed a nationwide lockdown by mid-March 2020, which included the prohibition of nonessential transit and blanket recommendations for WFH. Easing measures started later in May through several phases. Having high heterogeneity across the territory, the lifting of limitations would progress through the phases as rearranged during the process, making the progressive lifting of the restrictions challenging due to the highly decentralized system [ 64 ]. First, outside exercise was allowed, but borders remained closed, and no travel between different territorial units was permitted. Face masks were highly recommended both on public transport and outside. Afterward, shops, food markets and restaurants reopened with social distancing and reduced capacity, while public transit reopened with full service but reduced passenger numbers. The country entered a ‘new normality’ in late June, where travel between provinces was also allowed again. These restrictions were difficult to implement because of the conflicted political environment, which resulted in some territorial administrations taking preliminary measures at the subnational level in an uncoordinated manner [ 65 ]. Apart from the economic impact, the lockdown measures also had a significant social impact in Spain. Official data indicate an increase in gender-based violence (a 48% increase in calls for gender violence helplines during the first weeks of April 2020 compared to before the lockdown) [ 66 ]. Regarding WFH in Spain, nearly 83% of professionals were not granted the opportunity to WFH in 2020, whereas only 9.8% were telecommuting. In 2021, however, there was an increase in home office use to 25.2%. During the first month of the pandemic, there was a 38% reduction in physical activity [ 67 ].
Germany has taken a medium amount of protective measures during the pandemic [ 56 ]. The first contact restrictions were already announced in March 2020, followed by restrictions on travel and the closing of small shops and schools. In April, the obligation to remain in quarantine for 2 weeks when returning from another country and the recommendation to wear a face mask were lifted. Soon after, face masks were required for public transportation. In May 2020, schools and small shops opened slowly again. Contact restrictions depended on the number of cases in the district. In October, there were increasing lockdowns and contact restrictions introduced across the country, which lasted until January 2021. In August 2021, shops, restaurants, etc., are increasingly being opened for people who have gone through an infection, have been vaccinated twice or have a recent negative test. In December, these opportunities held wide only for people who were fully vaccinated or had recovered. The pandemic resulted in intensified suburbanization in Germany during 2020, although this process has steadily increased due to internal migration because of the lowest rates since the mid-1990s [ 68 ]. The residential green spaces attached to residential buildings, mostly designed with “semi-public access”, were appreciated by residents as creating refugia in challenging times and were more actively used than they were in prepandemic times, especially when sitting in parks was not allowed [ 69 ]. During the pandemic in Hamburg, a larger number of visitors were recorded in protected nature areas and local nature reserves, to an extent causing considerable problems for the wildlife there. For instance, in the nature reserve Duvenstedter Brook, many people nature parks more as urban recreational areas, chasing deer or playing badminton on protected stretches of heath.
Poland experienced a relatively mild first phase of the pandemic compared to other European countries, where the first case was identified a month later than in Germany and France. The government declared lockdown and enforced self-isolation measures (24 March 2020) and even applied measures that were not yet recommended by international institutions; for example, the first EU country to shut down its external borders, including those with other EU Member States. Factors slowing the progression of the initial phases of the pandemic include the relatively younger population compared to the most affected European countries, the larger population living in rural areas and the low rate of mobility domestically and internationally among the Polish people [ 70 ]. The impact of COVID-19 also resulted in changes in real estate and suburbanization in Poland, driving a wave of people to buy property (houses with land plots) to escape the dread of living in apartment buildings, either occasionally or permanently [ 71 ]. In addition, the pandemic fostered many measures by the Polish government related to the economy, taxes, employment and extraordinary changes in court proceedings and the system of justice.
3.2 Countries with weak restrictions, Denmark and Sweden
In Denmark, the anti-COVID-19 measures taken were comparatively brief [ 72 ]. Denmark was indeed in the group of countries in which there were fewer self-protective measures [ 56 ]. Starting in the middle of March 2020, schools, public institutions, hairdressers, restaurants, shopping malls, etc., were closed down, but on April 20th, 2020, these were opened again, with fitness centers and swimming pools being the last ones to open again on June 1st. Over the course of the summer, face masks were first recommended and then needed, first for public transport and later for restaurants, shops and public institutions. In northern Jutland, seven municipalities were completely isolated from their environment due to an outbreak of a new variant on some mink farms. Apart from those restrictions, no mobility restrictions were imposed within the country. This changed shortly before Christmas 2020, when schools, restaurants, public institutions, theatres, etc., were closed for almost two months; starting on February 28th, the country started opening everything again. On May 21st, almost all restrictions were lifted. Furthermore, many Danes have their own houses, gardens and/or summer houses. In 2020, 2.7 million Danes lived in detached houses, whereas 1.6 million lived in multiunit houses where they did not own themselves; this relationship has not changed between 2021 and 2022 [ 73 ]. Given the short period in which public life was restricted and given access to nature for a large proportion of the population, it can be expected that the impact of COVID-19 measures on the Danish population’s attitude towards the environment is not very pronounced.
Sweden chose a different strategy during the pandemic, mainly based on voluntary measures and citizen behaviors and recommendations rather than restrictions, and a complete lockdown was never implemented (Sweden country snapshot: public health agencies and services in the response to COVID-19, according to World Health Organization WHO. No state of emergency was declared because the Swedish Constitution does not provide for a state of emergency during a public health crisis. This less rigid approach focused more on mitigation measures for slowing, but not stopping, the pandemic and relied on existing high levels of institutional and interpersonal trust. The affected geographical regions or households were not under enforced quarantine, and facemasks were not recommended outside health care [ 74 ]. Recommendations consisted mainly of “staying at home even with the slightest symptom of an infection, physical distancing, enhanced hygiene measures, avoiding public transportation, and working from home if possible” [ 75 ]. Physical distancing was recommended in public spaces but mandatory in bars, restaurants and events. A maximum of 50 people was allowed to gather. In some opinions, this was considered to have caused less serious consequences than did the severe policies used in most countries [ 76 ]. WFH, which accounts for approximately 40% of the total workforce in Sweden and is independent of previous work experience, influences the establishment of new habits [ 77 ]. Studies suggest that workload, performance and well-being decreased during the pandemic [ 78 ].
Assuming positive effects on well-being through a stronger connection between individuals and the natural environment while also considering the unusual circumstances of the world pandemic, this study addresses individuals’ likelihood of using BD after the pandemic. More precisely, the research interest stretches out to identify contextual variables (country, overcrowding, time spent outside, and access to nature/food,) and personal variables (age group and sex) influencing the likelihood of using BD by focusing on the following:
Individuals’ exposure (during and after the lockdown) to several BD elements intentionally or unintentionally, including indoors (color, water, air, sunlight, plants, animals, natural materials, views and vistas, façade greening, geology and landscape, habitats and ecosystems) and outdoors (location, green neighborhood, wide prospect, proximity to natural resorts, etc.), as reported through a questionnaire to test whether the severity of the lockdown restriction of the COVID-19 pandemic fostered stronger people-environment relations, as valued by the likelihood of using BD elements.
The role of the context of (strong/weak) restrictions in several European countries (Italy, Germany, Poland, Spain, Denmark and Sweden) to test whether people-environment relations differ according to the objective and/or perceived severity of the measures in a country context.
The study design was exploratory, mixed-method, cross-sectoral and comparative. The data were collected through a survey directed to European countries via an online Google form conducted from 30 January to 28 February 2023 (see Annex 1). Following a random, uncontrolled sampling strategy, the survey was shared with learning networks such as the Bosch Alumni Network (an international network across 140 countries currently hosting more than 8000 members), the network of European RCEs (Regional Centers of Expertise on Education for Sustainable Development), and the COST (European Cooperation in Science and Technology) action networks of Indoor Air Pollution and Circular city, as well as with practitioners of several universities in Europe. The questions were closed and open (with the purpose of revealing the unexpected elements of change) and organized into four sections: (1) background questions containing variables such as age (different generations have different attitudes and approaches to restrictions), gender and countries (which are used as proxies); (2) questions about exposure to BD elements (color, water, air, sunlight, plants, animals, views and vistas, geology and landscape, habitats and ecosystems) indoors and outdoors, before and after the lockdown; based on the indoor and outdoor elements of the BD [ 80 , 81 , 82 ], from the framework of 14 Biophilic Patterns [ 31 ]; (3) questions about flexibility and adaptation of the living environment after the lockdown concerning the elements of BD; and (4) additional information on any major changes incentivized by the lockdown restrictions concerning lifestyle and wellbeing.
The data were processed through mixed methods, namely, descriptive statistics, statistical model building and testing and a thematic analysis [ 83 ] of the qualitative data using affinity diagramming (Lucero) [ 84 ]. Table 2 provides an overview of the methodological approach.
To facilitate quantitative correspondence analyses [ 88 , 89 ], numerical variables were recorded in three modalities (less, same, more) or (low, intermediate, high). Data visualizations were derived as two-dimensional planes using the FactoMiner package [ 85 ] of R statistical software [ 90 ]. Finally, a logistic model of the included variables was estimated to explain the likelihood of including BD using R statistical software.
The qualitative data were analyzed using affinity diagrams [ 84 ] to identify the categories that emerged. Affinity diagramming is a variant of thematic analysis [ 83 ]. In this process, all the comments were printed in different colors depending on the country and were clustered and labeled in several iterative steps so that the categories emerged bottom up in several different steps: (a) initial familiarization with the data, (b) creating initial codes, (c) collating codes with supporting quotes, (d) grouping codes into themes, (e) reviewing and revising themes, and (f) writing up the narrative based on the categories emerging. This thematic analysis process was carried out using the affinity mapping technique by creating large visual representations of the data points (chart making and ‘walking the wall’). Affinity diagrams allow identifying patterns in participants’ answers, illustrating what consequences of the restrictions on their lifestyles they were foregrounding themselves. For the quantification of the comments, the instances in each category per country were counted and divided by the total number of comments for each country, in line with the recommendations provided by Lucero [ 84 ]. For instance, seven Swedish participants made a comment that reported a change toward a healthier lifestyle, which corresponds to 16.7% of the total number of comments (42) made by the Swedish participants.
5.1 General descriptive analyses
The 403 participants in the survey were mainly from European Union countries and the United Kingdom (89%), such as Italy (17%), Germany (16%), Sweden (14%), Poland (11%), Denmark (9%), Spain (9%), the UK (3%), France (2%), and other EU countries (8% Czech Republic, Belgium, Greece, Romania, Bulgaria, The Netherlands, Portugal and Lithuania). The rest were from EU neighboring countries (9%, Albania, Serbia, Bosnia-Herzegovina, Belarus, Moldova and Turkey) and from other countries in the world (2% United States of America, Canada, Cameroon, Jordan, Kenya and Saudi Arabia). Ninety percent of the respondents had a level of education as a graduate/postgraduate from different fields. The majority of respondents belong to the 90-ties and 80-ties (37% and 27%, respectively). The rest were born on 70-ties (16.5%), 60-ties (9.5%), and 2000s (6%) in the 50-ties (2%). A total of 58% of the respondents were female, 41% were male, and 1% other.
The perceived severity of the restrictions from the respondents corresponds with the objective severity of restriction in Italy and Spain (strong) and in Germany and Poland (moderate) for Sweden (weak); for the Danish participants, the restrictive measures are perceived as moderate and strong by the majority of the respondents in contrast with the countries’ weak objective status. Table 3 displays objective restrictions (based on the criteria followed by the Oxford Covid-19 government response tracker; as also displayed in Table 1 ) and subjective restrictions as perceived by the respondents of the six countries. In total, 403 participants in this survey perceived the restrictive measures taken in their countries as strong and moderate.
The descriptive statistics revealed that the most influential variables were (1) overcrowding/limited space discomfort (58%), (2) difficulties to work (50%), and 3) difficulties accessing green spaces (33%). Although no significant limits were reported for the choice of food (only 10%), the respondents reported changes in their nutritional status after the pandemic related to: the use of regional products (52.7%), switching to organic products (48.7%) and growing their own vegetation through urban gardening or farming (43.3%). Approximately 80% of the respondents considered visual and nonvisual connections with nature after the pandemic to be very important.
A comparison of the “time spent outside in nature”, “during” and “after” the pandemic with that “before” the pandemic revealed that “during”, for 43% of the respondents is “less”, and “after” for, 60% of the participants is “more”. One-quarter of the participants had a steady attitude “same” for “before” and “after” the pandemic.
Figure 1 shows the “Likelihood of including Biophilic design” in relation to the “Time spent outside”, indicating that this is more likely for respondents who have spent “more” or “less” time outside. The graph was generated using the data from the six selected countries: Denmark, Germany, Italy, Poland, Spain, and Sweden.
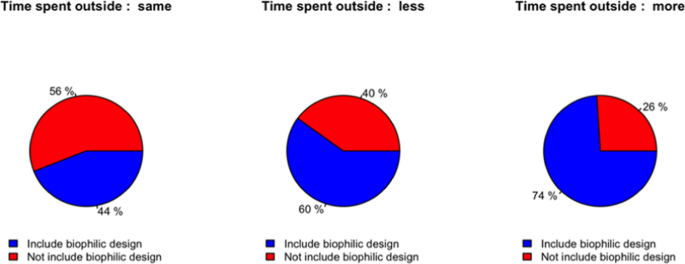
Likelihood of including biophilic design in relation to “time spent outside” during the pandemic
Figure 2 shows the exposure to BD in the living environment before and after the pandemic, specifically to the following elements: balcony/terrace; private garden/common garden; green roof/façade; views and vistas from home, green or blue; plants/vegetation growing in home gardens/roofs/vases; glass surfaces, sunlight illumination (dynamic & diffuse light); orientation, ventilation, thermal and airflow variability; natural materials (natural wood grains; leather; stone, fossil textures; bamboo, rattan, dried grasses, cork, organic palette. There were no major changes in the specific elements of BD in indoor living environments despite slight increases in the amount of vegetation growing in home gardens/roofs/vases and in vistas from home and glass surfaces, sunlight and illumination. Nevertheless, the majority of respondents reported that they would like to include these BD elements in the future.
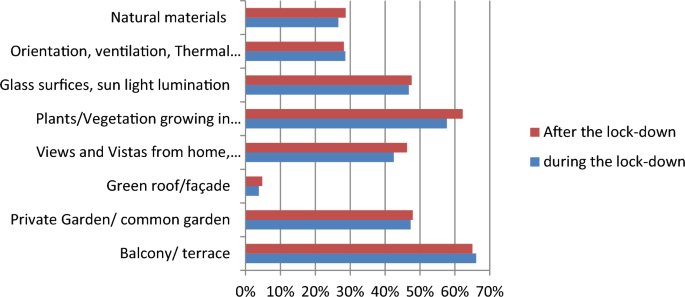
Exposure to BD elements before and after the pandemic: balcony/terrace; private garden/common garden; green roof/façade; views and vistas from home, green or blue; plants/vegetation growing in home gardens/roofs/vases; glass surfaces, sunlight illumination (dynamic & diffuse light); orientation, ventilation, thermal and airflow variability; natural materials (natural wood grains; leather; stone, fossil textures; bamboo, rattan, dried grasses, cork, organic palette. Axes x- represents the BD elements. Axes y-represent the % of survey participants
Furthermore, the likelihood of BD outdoors when changing habitation is considered important, especially linked to proximity to urban gardens/green areas (53.1%), proximity to a water body (sea, lake, river, etc.) 45.1%, proximity to rural areas/suburbs (natural terrain with trees and vegetation) 42.7%, proximity to city centers and services (39.1%), proximity to relatives or family (36.7%), and elevated position (i.e., looking downhill or a viewpoint with a wide prospect) by 22.7% of respondents. Other changes are related to working habits, where 65% of respondents preferred flexible virtual/office presence time, 44% fewer working hours, 21% preferred to switch to a full-time home office and only 8% preferred full-time presence. One-quarter of the respondents had changed jobs/occupations after the lockdown. As a result, 63% of participants reported having adopted their home to create space for home office and 39% for recreational activities.
Only a small percentage (11%) of respondents reported having created an indoor individual space for Prospect (an unimpeded view over a distance for surveillance and planning), Refuge (withdrawal from environmental conditions or the main flow of activity, in which the individual is protected from behind and overhead), Mystery (partially obscured views or other sensory devices that entice the individual to travel deeper into the environment) and Risk (an identifiable threat coupled with a reliable safeguard). Furthermore, these elements are considered very likely to be included in the future by the majority of the respondents. Fifty-three percent of the respondents reported other changes, especially related to activities in nature, meditation and self-reflection, work, nutrition towards a more vegetal diet and taking a pet.
5.2 Statistical analysis
A first logistic model was estimated using all the available data (all countries) (see Table 3 ) to evaluate the effect of variables on the high vs. low/moderate Likelihood of including BD elements in the environment”. Variables that have significant descriptive power can be described by three cluster types: age and sex; variables pertaining to the severity of the experience lived during lockdown (overcrowding, limited choice of food, and time spent outside during lockdown); and variables reflecting the need for interaction (pet). The most significant variable is undoubtedly the degree of “overcrowding” experienced during lockdown, which may, as the time spent outside during lockdown, act as a proxy for the experienced severity of the restrictions (as distinguished from the perceived severity and from the objective severity of the restrictions-) see Table 4 ).
Based on these overall results, we conducted narrower correspondence analyses to focus the dependence of the “likelihood” on the above identified variables with respect to the subsample of the six selected cluster countries. Figures 3 , 4 and 5 show the results for the variables “Age”, “Time spent outside during the pandemic” and “Overcrowding”.
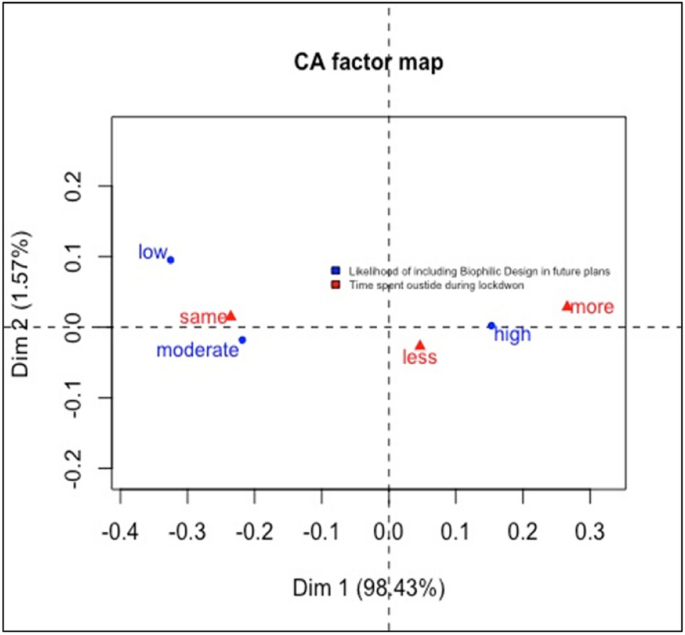
Correspondence Analyses factors maps for the likelihood of using biophilic design related to the variable: age
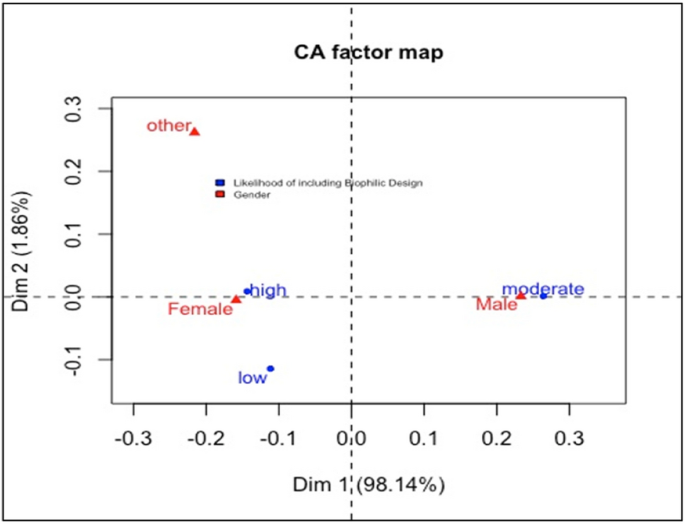
Correspondence Analyses factors maps for the likelihood of using biophilic design related to the variable: time spent outside during the pandemic
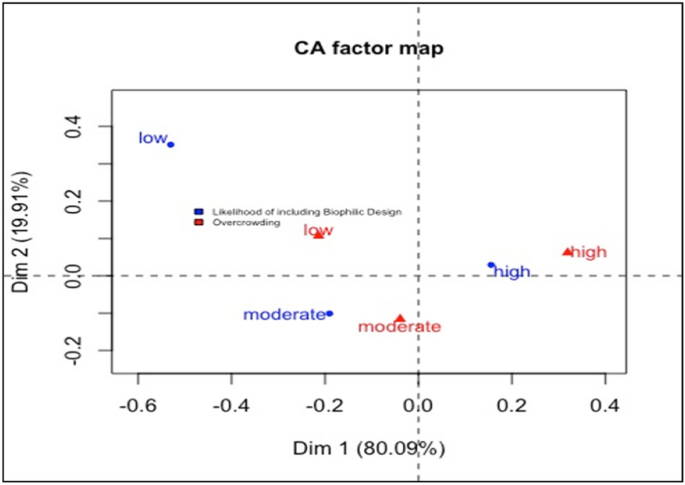
Correspondence Analyses factors maps for the likelihood of using biophilic design related to the variable: overcrowding
Figure 3 indicates a moderate “Likelihood of including BD in the environment” for males, while females and individuals who chose the “other” option have more extreme points of view (either low or high likelihood). Figure 4 indicates that the “Likelihood of including BD elements in the environment” is high for respondents who went outside “less” during the pandemic but also for those who went outside “more”. The other dependencies are as follows: the more severe the effect of the lockdown is, the higher the “Likelihood of including BD elements in the environment” as shown in Fig. 5 , for the dependence on overcrowding. An analysis of the variable “Choice of food” did not reveal relevant additional information.
Based on these insights, we ran further analyses on the subsample of 6 countries, aggregated by country. We included objective variables, such as the severity of the restrictions and country. However, neither variable showed a significant influence. After recursively discarding the nonsignificant factors, the final logistic model obtained is displayed in Table 5 .
Two factors appear to correlate the most with the” Likelihood of including BD in the environment” (which was also found by the descriptive analysis):”Time spent outside during lockdown” and the “Will to change the environment”. In particular, it was found that (1) having spent “less” time outside or “more” time outside during lockdown was positively correlated with the likelihood of including BD in the environment, and (2) the higher the willingness to change the environment after experiencing COVID-19 lockdown restrictions was, the higher the likelihood of including BD in the environment. Those who spent “less” time outside during lockdown had approximately two times greater chances of including BD in their environment in the future than those who spent the “same” amount of time outside. Those who spent “more” time outside during lockdown had approximately four times greater chances of including BD in their environment in the future (than those who spent the “same” amount of time outside). Those for whom the “Will to change the environment” is “high” have approximately seven times more chances to include BD in their environment in the future (than those for which the “Will to change the environment is “low”). Those for whom the “Will to change the environment” is “moderate” have approximately the same chances of including BD in their environment in the future (as those for whom the “Will to change the environment” is “low”) (Figs. 6 , 7 and 8 ).
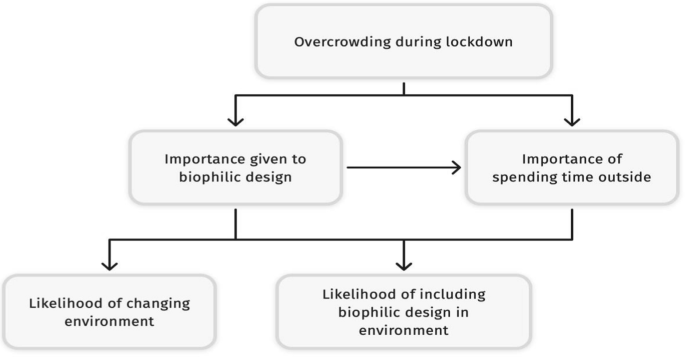
Causal structure diagram explaining the likelihood of including biophilic design in person-environment relation
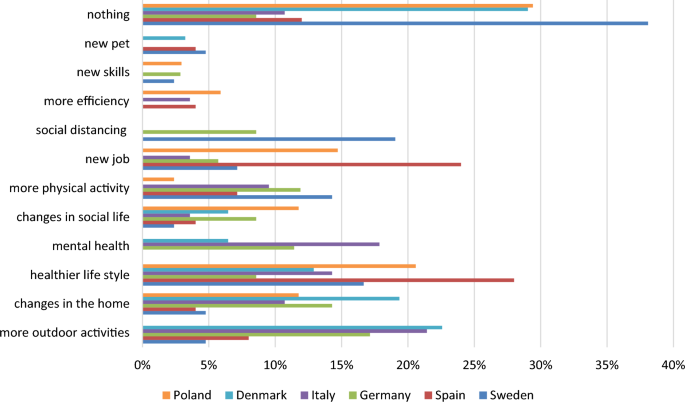
Lifestyle changes as reported by the survey participants by country. Axes y represent the % of survey participants and Axes x represent the reporting lifestyle changes
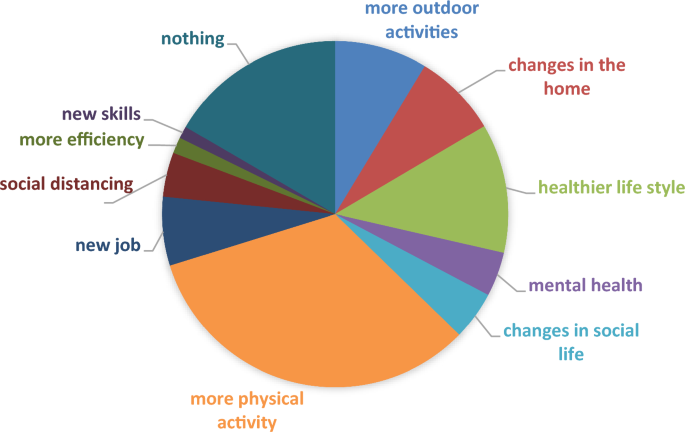
The relative role of lifestyle changes as reported by the participants
The strongest combination of factors appears to be: “Will to change the environment”- “high” and “Time spent outside during lockdown”- “more”. For this combination, the probability of including BD in the environment in the future is estimated to be 91%. The weakest combination of factors appears to be: “Will to change the environment”- “low”, and”Time spent outside during lockdown”- “same”. For this combination, the probability of including BD in the environment in the future is estimated to be 24%. Finally, these two variables may act as proxies for the severity of the restrictions experienced (as a subjective indicator of severity rather than objective severity by lockdown measures, as indicated through indices of the Oxford COVID-19 government response tracker).
We conducted further analysis to explain the motivation to include BD elements in the future environment by running a Bayesian causal analysis [ 88 , 89 ] using the R package bnlearn [ 90 ]. The dataset included the six cluster countries. A first Bayesian network representation of the joint distribution of all variables coded at two levels (high vs. low or moderate, more vs. less or same) was obtained and gradually modified to account for causal dependencies while not degrading the fitness measure retained (BIC criterion). For the final model, two main causal factors explain the” Likelihood of including BD elements in the future environment”: “Importance given to BD” and” “Importance of spending time outside”. Moreover, following the causal path, the lockdown had a causal impact on these two factors, mainly through the experience of “Overcrowding during lockdown”, which may be considered a good proxy of the perceived severity of restrictions. The obtained causal structure can be summarized by the following diagram:
As indicated, individuals who experienced strong overcrowding during lockdown are more likely to attach high importance to BD elements in their environment and hence are more likely to change their environment through BD elements and to value spending time outside. Interestingly, people who are less willing to change their environment, give less importance to BD in general and are less likely to have experienced overcrowding during lockdown. Furthermore, people who experienced more overcrowding during lockdown may have had fewer opportunities to go outside during lockdown and may have had problems accessing food, and being “stuck at home”, which explains the relevance of these variables in our logistic regressions. We confirmed this insight by running the following contingency Tables 6 and 7 :
5.3 Qualitative analyses
Altogether and across countries, 32 comments concerned a healthier lifestyle, which amounted to 16.4% of the total number of comments (195). The qualitative analysis of a total of 210 qualitative comments from the Danish, German, Italian, Polish, Spanish and Swedish participants yielded 12 different thematic categories, while participants were free to write as much as they wanted; most participants made exactly one point; only three comments were assigned to two different categories. First, there is one group of 20 comments that seem to refer to the time during the pandemic, not afterwards. In the following, we disregard these comments, leaving us with 193 valid data points. In addition, 44 comments (mostly by the Swedish participants) suggested that there were no changes in their lifestyle due to the pandemic.
The next largest category comprises comments in which participants describe how they perform more outdoor activities. For instance, participants reported on longer walks, spending time in the garden and enjoying fresh air; for example, participants wrote “ I spend more time in my city’s park ”, that they “ try to integrate exercise and fresh air ” or that they “ take time to enjoy nature .” Relatedly, eighteen participants reported having resumed a healthier lifestyle, eating less meat, performing more physical activity and cooking more at home. One participant writes, “ The COVID-19 pandemic and lockdown were starting points for changing what I don’t like in my life. The years after the lockdown were full of news in my life and radically changed myself. ” An additional ten participants reported doing more sports, for instance, “ starting fitness at home ”, “ doing more sports ” or doing “ more physical activities outdoors ”. In addition, eleven participants reported more attention given to their mental health. Many have taken up regular meditation exercises, while others report more awareness and appreciation of the small things of life; for instance, some simply take “ more time for meditation ”, another wrote, “ The overwhelming anxiety forced me to become more meditative ” and again, others state that they prioritize time for themselves now and value time alone, outside or time for recreational purposes more.
Like those with greater appreciation of life and nature, ten participants also reported greater appreciation of social connections; one participant reported moving into the city because the countryside was lonely. More generally, participants described spending more time with friends and family; many stated that they go out more often and “ spend more time with family ” organize “ weekly potlucks with my friends to create a sense of community ” or “ think about friendship in a new way ”.
Eighteen participants also reported changes in their homes; several had obtained a pet or more plants, i.e., “ took a pet and changed home ”, but some also described creating a separate space for a home office; for instance, “ I created a home office with a good amount of daylight and a view outside ”. Some even bought a house or moved into the countryside—one even changed country. Eight participants reported on improvements to their homes: “ cleaning my room more often and having less things there, making it a productive space ” or “ general home and garden improvements ”. Furthermore, participants reported that they improved the efficiency of their work or achieved a better work-life balance, i.e., by means of “ smart working ” or the use of “ new technologies that allow me to rest better ”. Eight participants had found a new job or changed careers. Three reported new skills that they acquired during the pandemic. Finally, three participants state that they are still careful and attend to social distancing; for instance, they say that they are still “ careful ”, “ keep distance from people ” and “ pay more attention to hygiene in public spaces ”. A family in Poland sold their apartment to buy a house in the suburbs, which they had never considered before the pandemic, to obtain more living space and better air quality, with a sizable plot of land, a vegetable garden, and a small orchard.
Participants’ qualitative data thus align with the quantitative survey data, suggesting that the pandemic indeed had an effect on lifestyle choices such that they became more attentive to nature, value nature and outdoor activities in the sun and fresh air. For some, these new preferences have led to changes in habitation. Regarding the differences among the six countries under consideration, the Polish participants reported more career changes than did the participants from other countries and less than the effect of the pandemic on their outdoor activities and attention to mental health and physical activity; however, they seem to have used the pandemic widely to change their eating habits. Among the Swedish, Polish and Danish participants, many reported no changes; especially among the Swedish participants, 38.1% stated that there were no changes at all. These results are in line with the severity of the restrictions imposed in these countries during the pandemic. Furthermore, extended social distancing effects were mentioned only by German and Swedish participants. In all other respects, participants from all six countries reported similar changes in lifestyle; this is interesting in its own right since across countries, people seem to have used the pandemic to rethink their lifestyles, where many of the changes reported are towards a more biophilic and sustainable lifestyle. However, it needs to be stated that these conclusions do only apply for those participants who responded to the qualitative part of the questionnaire.
The qualitative analysis added further insights to the interpretation of our quantitative analysis because it indicated that whether people changed their lifestyles was related to the severity of the country lockdown measures, but how and what changed depended to some extent on the respondents’ personal circumstances. There are also other changes that are not related to BD concepts, such as new jobs, increased efficiency, and new education.
6 Conclusions
The study background reinforces the idea that the COVID-19 pandemic restrictions and lockdowns were accompanied by general discomfort due to alterations in livelihood, work, and activities in nature and in-person social interaction. Based on the findings of this study, it can be argued that the severity of restrictive measures (strong/moderate/weak) imposed by countries during the global COVID-19 pandemic influenced the likelihood of including BD for changes in people-environment relationships. However, this effect occurs mainly when restrictions are individually perceived or experienced as severe due to personal circumstances. Our findings suggest that pandemic restrictions triggered a motivation to include BD and by this to change the person-environment relation. In this regard, individual-level perceived severity of restrictions appears to be a stronger proxy of the intended environmental behavior change than country-level objective indictors. These findings underline the relevance of the element of individual environment perception within the systemic reasoning of mindsponge theory [ 22 ]. It can also be concluded, that, in times of perceived crisis of the person-environment relation, the restorative effects of BD proposed by SRT [] and ART [] may serve as an explanation of an individual`s intention to change the lifestyle towards more natural surroundings. The mixed method analyses conducted at different sample levels revealed that, despite drastic social distancing measures, the experienced discomfort created by “Overcrowding” was identified as the most influential variable in relation to the “Time spent outside nature”, independent of other variables such as country, gender, and age. Likewise, the comparative data evaluation between Italy, Spain, Germany, Poland, Sweden and Denmark does not reinforce the assumption that people-environment relations differ according to the severity of the measures in a country context but rather according to individual responses to crises.
Experienced restrictions (mainly defined by “Overcrowding” and “Time spent outside in nature”) influence the likelihood of including BD elements in the future. Individuals who experienced changes in their individual person-environment relations through pandemic restrictions by having “more” or “less” access to nature were more likely to change their behavior, as indicated by the use of BD elements, both indoors and outdoors, compared to individuals who experienced “same” (unaffected) nature access. However, “Overcrowding” here is also subjective to individual perceptions (unrelated, for example, to the official definition for person/sqm2) and may have created more discomfort for individuals who were less likely to have had the opportunity to go outside during lockdown and may have had problems accessing food, being “stuck at home” or “difficulties to work”, as also explained by the role of these variables in the logistic regressions.
The COVID-19 pandemic appears to have influenced the trend toward relocation in proximity to urban gardens/green areas, water (sea, lake, river, etc.) or to rural areas/suburbs and not so much in specific elements indoors (as supported by the suburbanization statistics in the 6 countries). Often, the desired changes contradict with possibilities, as the results indicate an appreciation of natural elements but not always being possible to change, linked with “whenever is freedom to choose”.
To conclude, there is a post pandemic tendency toward greater connection with nature and healthier habits regarding nutrition and lifestyle within the freedom of individual choice. The likelihood of changing the environment through BD elements is related to experienced and perceived changes during the pandemic. Individuals who were experiencing “Overcrowding” are more likely to place high importance on BD elements in their environment now and hence are more willing to change their person-environment relationships. Given these preconditions, respondents revealed higher likelihood of including BD elements in the future, while a relatively unchanged routine during the pandemic did not result in post pandemic changes or increased attention to BD elements, both indoors and outdoors.
7 Limitations and implications for theory and practice
This is a cross-sectoral and not longitudinal survey-based data collection study due to the impossibility of starting a survey during the pandemic. Thus, we referred to people recalling, considering the survey time in early 2023, when the pandemic had not yet officially ended. For the purpose of this study, the survey questions that relate to the perceived severity of the restrictions are limited to discomfort by overcrowding, difficulties working, access to green spaces, exposure to BD elements and choice of food, excluding other elements, for example, travelling (local and international), and access to social events and contacts.
Regarding the lifestyle changes reported by the participants, it must be considered that all those who did not complete the open question in the survey may not have actually changed anything (the reason for not providing information on lifestyle changes is not known). Furthermore, when reporting on their lifestyle changes, the participants had recently been primed to consider biophilic lifestyle changes since they were asked about those changes in the questions before. The order in which the questions were asked may thus have introduced a certain bias regarding the reporting of biophilic lifestyle changes, meaning that these changes may have occurred, but people may have been less inclined to report on other kinds of changes.
Our study relies on data of a specific demographic group, particularly educational background, which may not be representative of the general population. The survey was distributed among participants from particular organizations or institutions, and 90% of the respondents had a higher level of education which may not mirror a generalization for the broader population. Therefore, our evaluations must be interpreted in light of the preferences of this specific demographic group. Furthermore, our conclusions consider the data of only six countries. While the country selection was specifically motivated by a classification of objective severity of measures, we understand, that a different or extended set of countries may have resulted in different results and interpretations. Future research can extend the range and heterogeneity of the data based on country and further demographic variables to enhance generalizability of conclusions. At the same time, this may serve as an evaluation of our conclusion, that it is the perceived severity (at most over-crowding) rather than the objective severity of restrictions that has the stronger impact on the likelihood of including BD in future life. To this regard, a shift of level of analysis towards regional or local surroundings may provide further insights on the relevance of (perceived) overcrowding for the likelihood of BD in person-environment relations. This will ultimately help extending the scope of this research approach beyond effects related to COVID-19 pandemic.
The pandemic highlighted the value of nature in cities and the living environment for the health and well-being of citizens and it uncovered the unhealthy aspects of current urbanization and living-working styles. Our qualitative data underline the general finding that the pandemic has increased the trend of suburbanization, stressing that accessible urban nature is a key component of creating sustainable urban communities and human health and well-being. However, we need to point out, that the majority of respondents in our study wishes/plans for changes rather than does. As such, our results emphasize that proper societal structures and long-term measures are important for enabling larger-scale changes in people-environment relations. Future longitudinal studies will have to find out if such measures will be effective.
Data availability
The datasets generated during and/or analyses during the current study are available from the corresponding author upon reasonable request.
Kellert SR. Introduction. In: Kellert SR, Wilson EO, editors. The biophilia hypothesis. Washington, DC: Island Press; 1993.
Google Scholar
Ulrich R, Simons R, Losito B, Fiorito E, Miles M, Zelson M. Stress Recovery During Exposure to Natural and Urban Environments. J Environ Psychol. 1991;11:201–30. https://doi.org/10.1016/S0272-4944(05)80184-7 .
Article Google Scholar
Li Q, Otsuka T, Kobayashi M, Wakayama Y, Inagaki H, Katsumata M, Kagawa T. Acute effects of walking in forest environments on cardiovascular and metabolic parameters. Eur J Appl Physiol. 2011;111:2845–53. https://doi.org/10.1007/s00421-011-1918-z .
Article CAS Google Scholar
Berto R. The role of nature in coping with psycho-physiological stress: a literature review on restorativeness. Behav Sci. 2014;4(4):394–409. https://doi.org/10.3390/bs4040394 .
Downton P, Jones D, Zeunert J, Roös P. Biophilic design applications: putting theory and patterns into built environment practice. Knowledge E. 2017. https://doi.org/10.18502/keg.v2i2.596 .
Lee KO, Mai KM, Park S. Green space accessibility helps buffer declined mental health during the COVID-19 pandemic: evidence from big data in the United Kingdom. Nat Mental Health. 2023;1:124–34. https://doi.org/10.1038/s44220-023-00018-y .
Wang J, Fan Y, Palacios J, et al. Global evidence of expressed sentiment alterations during the COVID-19 pandemic. Nat Hum Behav. 2022;6:349–58. https://doi.org/10.1038/s41562-022-01312-y .
European Environmental Agency, EEA. (2022). Strand, R., Kovacic, Z., Funtowicz, S. (European Centre for Governance in Complexity) Benini, L., Jesus, A. (EEA) COVID-19: lessons for sustainability?—European Environment Agency (europa.eu).
Sneddon J, Daniel E, Fischer R, et al. The impact of the COVID-19 pandemic on environmental values. Sustain Sci. 2022;17:2155–63. https://doi.org/10.1007/s11625-022-01151-w .
Nicolas M, Martinent G, Palinkas L, Suedfeld P. Dynamics of stress and recovery and relationships with perceived environmental mastery in extreme environments. J Environ Psychol. 2022;83:101853. https://doi.org/10.1016/j.jenvp.2022.101853 .
Yabe T, Bueno BGB, Dong X, Pentland A, Moro E. Behavioral changes during the COVID-19 pandemic decreased income diversity of urban encounters. Nat Commun. 2023;14(1):2310. https://doi.org/10.1038/s41467-023-37913-y .
Rainisio N, Inghilleri P. Culture, environmental psychology, and well-being: An emergent theoretical framework. In: Rainisio N, editor. Well-being and cultures: Perspectives from positive psychology. Dordrecht: Springer, Netherlands; 2012. p. 103–16. https://doi.org/10.1007/978-94-007-4611-4_7 .
Chapter Google Scholar
Kalantidou E. Not going back to normal: designing psychologies toward environmental and social resilience. Hu Arenas. 2023;6:131–46. https://doi.org/10.1007/s42087-021-00198-y .
Akenji L. Consumer scapegoatism and limits to green consumerism. J Clean Prod. 2014;63:13–23. https://doi.org/10.1016/j.jclepro.2013.05.022 .
Mihelcic JR, Zimmerman JB. Environmental engineering: fundamentals, sustainability, design. Hoboken: John Wiley & Sons; 2014.
Wijesooriya N, Brambilla A. Bridging biophilic design and environmentally sustainable design: a critical review. J Cleaner Prod. 2021;283: 124591. https://doi.org/10.1016/j.jclepro.2020.124591 .
Barbiero G, Berto R. Biophilia as evolutionary adaptation: an onto-and phylogenetic framework for biophilic design. Front Psychol. 2021;12: 700709. https://doi.org/10.3389/fpsyg.2021.700709 .
Wilson EO. Biophilia. Cambridge: Harvard University Press; 1984.
Book Google Scholar
Kellert SR, Wilson EO. The biophilia hypothesis. Washington, DC: Island Press; 1993.
Kellert SR, Calabrese EF. 2015. The practice of biophilic design. http://www.biophilic-design.com
Zhong W, Schröder T, Bekkering J. Biophilic design in architecture and its contributions to health, well-being, and sustainability: a critical review. Front Architect Res. 2022;11(1):114–41. https://doi.org/10.1016/j.foar.2021.07.006 .
Romm J, Browning WD. Greening the building and the bottom line. Terrapin Bright Green; 1994. https://terrapinbrightgreen.com
Patterns of Biophilic Design (terrapinbrightgreen.com)
Söderlund J, Newman P. Improving Mental Health in Prisons Through Biophilic Design. The Prison Journal. 2017;97(6):750–72. https://doi.org/10.1177/0032885517734516 .
Hady SIMA. Activating biophilic design patterns as a sustainable landscape approach. J Eng Appl Sci. 2021;68:46. https://doi.org/10.1186/s44147-021-00031-x .
Roös P, Downton PJ, D. Zeunert, J. (2016). Biophilia in urban design—patterns and principles for smart Australian cities.
Ulrich RS, Simons RF, Losito BD, Fiorito E, Miles MA, Zelson M. Stress recovery during exposure to natural and urban environments. J Environ Psychol. 1991;11(3):201–30. https://doi.org/10.1016/S0272-4944(05)80184-7 .
Gaekwad JS, Moslehian AS, Roös PB. A meta-analysis of physiological stress responses to natural environments: biophilia and stress recovery theory perspectives. J Environ Psychol. 2023. https://doi.org/10.1016/j.jenvp.2023.102085 .
Kaplan S. The restorative benefits of nature: toward an integrative framework. J Environ Psychol. 1995;15:169–82. https://doi.org/10.1016/0272-4944(95)90001-2 .
Stevenson MP, Schilhab T, Bentsen P. Attention restoration theory II: a systematic review to clarify attention processes affected by exposure to natural environments. J Toxicol Environ Health Part B. 2018;21(4):227–68. https://doi.org/10.1080/10937404.2016.1196155 .
Browning WD, Ryan CO, Clancy JO. 14 patterns of biophilic design. New York: Terrapin Bright Green, LLC; 2014. https://terrapinbrightgreen.com
Vuong Q-H. Mindsponge theory. De Gruyter. 2023. https://doi.org/10.2478/9788367405157-002 .
Nguyen M-H, La V-P, Le T-T, Vuong Q-H. Introduction to Bayesian mindsponge framework analytics: an innovative method for social and psychological research. MethodsX. 2022;9: 101808. https://doi.org/10.1016/j.mex.2022.101808 .
Montemayor C, Haladjian HH. Perception and cognition are largely independent, but still affect each other in systematic ways: arguments from evolution and the consciousness-attention dissociation. Front Psychol. 2017;8:40. https://doi.org/10.3389/fpsyg.2017.00040 .
Ren X. Pandemic and lockdown: a territorial approach to COVID-19 in China, Italy and the United States. Eur Geogr Econ. 2020;61(4–5):423–34. https://doi.org/10.1080/15387216.2020.1762103 .
Mækelæ M, Reggev N, Dutra N, Tamayo R, et al. Perceived efficacy of COVID-19 restrictions, reactions and their impact on mental health during the early phase of the outbreak in six countries. Royal Soc Open Sci. 2020;7: 200644. https://doi.org/10.1098/rsos.200644 .
Fors Connolly F, Olofsson J, Malmberg G, Stattin M. SHARE Working Paper Series 62–2021: adjustment of daily activities to restrictions and reported spread of the COVID-19 pandemic across Europe. 2021; https://doi.org/10.17617/2.3292885
Vindegaard N, Benros ME. COVID-19 pandemic and mental health consequences: systematic review of the current evidence. Brain Behav Immun. 2020;89:531–42. https://doi.org/10.1016/j.bbi.2020.05.048 .
Cumbrera MG, Foley R, Correa-Fernández J, González-Marín A, Braçe O, Hewlett D. The importance for wellbeing of having views of nature from and in the home during the COVID-19 pandemic. Results from the GreenCOVID study. J Environ Psychol. 2022;83:101864. https://doi.org/10.1016/j.jenvp.2022.101864 .
Caro JC, Clark AE, D’Ambrosio C, Vögele C. The impact of COVID-19 lockdown stringency on loneliness in five European countries. Soc Sci Med. 2022;314:15492. https://doi.org/10.1016/j.socscimed.2022.115492 .
D’Alessandro D, Gola M, Appolloni L, Dettori M, Fara GM, Rebecchi A, Settimo G, Capolongo S. COVID-19 and living space challenge Well-being and public health recommendations for a healthy, safe, and sustainable housing. Acta Biomed. 2020. https://doi.org/10.23750/abm.v91i9-S.10115 .
Fornara F, Mosca O, Bosco A, et al. Space at home and psychological distress during the Covid-19 lockdown in Italy. J Environ Psychol. 2022;79(2022):101747. https://doi.org/10.1016/j.jenvp.2021.101747 .
Atkinson-Clement C, Pigalle E. What can we learn from Covid-19 pandemic’s impact on human behaviour? The case of France’s lockdown. Hum Soc Sci Commun. 2021;8:81. https://doi.org/10.1057/s41599-021-00749-2 .
Bertoni M, Cavapozzi D, Pasini G, Pavese C. Remote working and mental health during the first wave of the COVID-19 pandemic. SSRN Electron J. 2022. https://doi.org/10.2139/ssrn.4111999 .
Awada M, Lucas G, Becerik-Gerber B, Roll S. Working from home during the COVID-19 pandemic: Impact on office worker productivity and work experience. Work. 2021. https://doi.org/10.3233/WOR-210301 .
Shulla K, Voigt BF, Cibian S, et al. Effects of COVID-19 on the sustainable development goals (SDGs). Dis Sustain. 2021;2:15. https://doi.org/10.1007/s43621-021-00026-x .
Afacan Y. Impacts of biophilic design on the development of gerotranscendence and the profile of mood states during the COVID-19 pandemic. Ageing Soc. 2021;43:1–25. https://doi.org/10.1017/S0144686X21001860 .
Gür M, Kaprol T. The participation of biophilic design in the design of the post-pandemic living space. Hershey: IGI Global; 2022. https://doi.org/10.4018/978-1-7998-6725-8.ch004 .
Biophilic Design: The future of greener living spaces—Econyl
Role of Biophilic Design in Sustainable Architecture (archiplexgroup.com)
Bolisani E, Scarso E, Ipsen C, Kirchner K, Hansen J. Working from home during COVID-19 pandemic: lessons learned and issues. Manag Market. 2020;15(1):458–76. https://doi.org/10.2478/mmcks-2020-0027 .
Deepa V, Baber H, Shukla B, Sujatha R, Khan D. Does lack of social interaction act as a barrier to effectiveness in work from home? COVID-19 and gender. J Organ Effective. 2023;10(1):94–111. https://doi.org/10.1108/JOEPP-11-2021-0311 .
Holmes EA, O’Connor RC, Perry VH, Tracey I, Wessely S, Arseneault L, Bullmore E. Multidisciplinary research priorities for the COVID-19 pandemic: a call for action for mental health science. Lancet Psychiatry. 2020;7(6):547–60. https://doi.org/10.1016/S2215-0366(20)30168-1 .
Hale T, Angrist N, Goldszmidt R, et al. A global panel database of pandemic policies (Oxford COVID-19 government response tracker). Nat Hum Behav. 2021;5:529–38. https://doi.org/10.1038/s41562-021-01079-8 .
Hale T. 2023. Working paper version 15.0.docx (ox.ac.uk)
SHARE-ERIC 2021. Results of the 1st SHARE Corona Survey; Project SHARE-COVID19 (Project Number 101015924, Report No. 1, March 2021). Munich: SHARE-ERIC. https://doi.org/10.17617/2.3356927
Protezionecivile. Coronavirus: state of emergency ends on March 31. https://www.protezionecivile.gov.it/en/notizia/coronavirus-state-emergency-ends-march-31/
Ipsen C, van Veldhoven M, Kirchner K, Hansen JP. Six key advantages and disadvantages of working from home in Europe during COVID-19. Int J Environ Res Public Health. 2021;18(4):1826. https://doi.org/10.3390/ijerph18041826 .
Kercuku A, Curci F, Lanzani A, Zanfi F. Italia di mezzo: The emerging marginality of intermediate territories between metropolises and inner areas. REGION. 2023;10:89–112. https://doi.org/10.18335/region.v10i1.397 .
Osservatori. Smart working in Italia: Numeri e trend. https://www.osservatori.net/it/ricerche/comunicati-stampa/smart-working-italia-numeri-trend
Larcher F, Pomatto E, Battisti L, Gullino P, Devecchi M. Perceptions of urban green areas during the social distancing period for COVID-19 containment in Italy. Horticulturae. 2021. https://doi.org/10.3390/horticulturae7030055 .
López MG, Chiner-Oms Á, García de Viedma D, et al. The first wave of the COVID-19 epidemic in Spain was associated with early introductions and fast spread of a dominating genetic variant. Nat Genet. 2021;53:1405–14. https://doi.org/10.1038/s41588-021-00936-6 .
Guirao A. The Covid-19 outbreak in Spain. A simple dynamics model, some lessons, and a theoretical framework for control response. Infect Dis Model. 2020;5:652–69. https://doi.org/10.1016/j.idm.2020.08.010 .
Monge S, Latasa Zamalloa P, Sierra Moros MJ, et al. Lifting COVID-19 mitigation measures in Spain (may–june 2020). Enferm Infecc Microbiol Clin. 2023;41(1):11–7. https://doi.org/10.1016/j.eimce.2021.05.019 .
Viguria AU, Casamitjana N. Early interventions and impact of COVID-19 in Spain. Int J Environ Res Public Health. 2021. https://doi.org/10.3390/ijerph18084026 .
Instituto de la Mujer. Impacto de género del COVID-19. 2020. https://www.inmujeres.gob.es/
FitBit the impact of coronavirus on global activity. https://blog.fitbit.com/covid-19-global-activity . Accessed 22 Mar 2020.
Stawarz N, Rosenbaum-Feldbrügge M, Sander N, Sulak H, Knobloch V. The impact of the COVID-19 pandemic on internal migration in Germany: a descriptive analysis. Popul Space Place. 2022. https://doi.org/10.1002/psp.2566 .
Säumel I, Sanft SJ. Crisis mediated new discoveries, claims and encounters: changing use and perception of residential greenery in multistory housing in Berlin, Germany. Urban For Urban Green. 2022. https://doi.org/10.1016/j.ufug.2022.127622 .
Gruszczynski L, Zatoński M, Mckee M. Do regulations matter in fighting the COVID-19 pandemic? Lessons from Poland. European Journal of Risk Regulation. 2021;12(4):739–57. https://doi.org/10.1017/err.2021.53 .
The New York Times. House hunting in Poland: why more buyers are looking there. https://www.nytimes.com/2020/08/26/realestate/house-hunting-poland.html
Statens Serum Institut. COVID-19 ramte verden og Danmark: Se tidslinjen her. https://www.ssi.dk/aktuelt/nyheder/2022/da-covid-19-ramte-verden-og-danmark-se-tidslinjen-her
Statistics Denmark. Housing conditions. https://www.dst.dk/en/Statistik/emner/borgere/boligforhold
Ludvigsson JF. The first eight months of Sweden’s COVID-19 strategy and the key actions and actors that were involved. Acta Paediatr. 2020;109(12):2459–71. https://doi.org/10.1111/apa.15582 .
Carlson J, Tegnell A. Swedish response to COVID-19. China CDC Wkly. 2020. https://doi.org/10.46234/ccdcw2020.215 .
Björkman A, Gisslén M, Gullberg M, Ludvigsson J. The Swedish COVID-19 approach: a scientific dialogue on mitigation policies. Front Public Health. 2023. https://doi.org/10.3389/fpubh.2023.1206732 .
Vilhelmson, et al. Sustained work from home post-pandemic? A Swedish case. Findings. 2023. https://doi.org/10.32866/001c.74470 .
Hallman DM, Januario LB, Mathiassen SE, et al. Working from home during the COVID-19 outbreak in Sweden: effects on 24-h time-use in office workers. BMC Public Health. 2021;21:528. https://doi.org/10.1186/s12889-021-10582-6 .
Politico. Europe’s coronavirus lockdown measures compared. https://www.politico.eu/article/europes-coronavirus-lockdown-measures-compared/
Kellert SR. Dimensions, elements, and attributes of biophilic design. Biophilic Design. 2008;2015:3–19.
Beatley T. Biophilic cities: integrating nature into urban design and planning. Washington, DC: Island Press; 2010.
Beatley T. Handbook of biophilic design. Washington, DC: Island Press; 2016.
Braun V, Clarke V. Using thematic analysis in psychology. Qual Res Psychol. 2006;3(2):77–101.
Lucero A. Using affinity diagrams to evaluate interactive prototypes. In: Abascal J, Barbosa S, Fetter M, Gross T, Palanque P, Winckler M, editors. Human-computer interaction—INTERACT 2015. INTERACT 2015. Lecture notes in computer science, vol. 9297. Cham: Springer; 2015. https://doi.org/10.1007/978-3-319-22668-2_19 .
Lê S, Josse J, Husson F. FactoMineR: an r package for multivariate analysis. J Stat Softw. 2008;25(1):1–18. https://doi.org/10.18637/jss.v025.i015 .
Venables WN, Ripley BD. Modern applied statistics with S. New York: Springer; 2002.
Selvin S. Statistical analysis of epidemiologic data (monographs in epidemiology and biostatistics, V. 35). 3rd ed. Oxford: Oxford University Press; 2004.
Greenacre M. Theory and application of correspondence analysis. London: Academic Press; 1983.
Greenacre M. Correspondence Analysis in Practice. 2nd ed. Boca Raton: Chapman and Hall/CRC; 2007.
R Core Team. R: a language and environment for statistical computing. Vienna: R Foundation for Statistical Computing; 2023.
Download references
Acknowledgements
The study is the third part of the project “Publication series: Sustainability in post pandemic society”, funded by the International Alumni Centre Berlin (iac), a center of excellence funded by the Robert Bosch Stiftung for impact-oriented alumni work and networks in philanthropy.
Open Access funding enabled and organized by Projekt DEAL.
Author information
Authors and affiliations.
University of Bonn, Bonn, Germany
Kalterina Shulla
South Westphalia University of Applied Sciences, Meschede, Germany
Bernd-Friedrich Voigt
LMBA UMR CNRS 6205, University of South Brittany, Lorient, France
Salim Lardjane
University of Southern Denmark, Odense, Denmark
Kerstin Fischer
Otwock, Poland
Piotr Kędzierski
QG Enviro, Lecce, Italy
Giuseppe Scandone
University of Applied Sciences and Arts, Bielefeld, Germany
Thomas Süße
You can also search for this author in PubMed Google Scholar
Contributions
KSH and BFV contributed to the conceptualization and design, writing and revision and methodological framework, the SL contributed to the statistical analyses, the KF contributed to the qualitative analyses and country background, and the PL, GS and TS contributed to corresponding countries’ background and introduction. All the authors contributed to the data collection.
Corresponding authors
Correspondence to Kalterina Shulla or Bernd-Friedrich Voigt .
Ethics declarations
Ethics approval and consent to participate.
Ethics approval and consent to participate were obtained from all the participants.
Consent for publication
The research does not involve human research participants. The survey was anonymous.
Competing interests
The authors declare no competing interests.
Additional information
Publisher's note.
Springer Nature remains neutral with regard to jurisdictional claims in published maps and institutional affiliations.
1.1 Survey: changes in people-environment relations in a post-pandemic society
Section : General and background questions 1. Country 2. Decade of birth (1960, 1970, 1980, 1990, 2000) 3. Gender 4. Occupation/level of education 5. In your opinion, the COVID-19 lockdown restrictions in your country/city were relatively (weak, moderate, strong) 6. During the lockdown, did you experience discomfort related to (overcrowding/limited home space, no access to parks or natural areas, recreation, limited choice of food, difficulties to work)? 7. On a scale from 1 (not at all) to 7 (very much), did you have problems accessing parks or green spaces? 8. On a scale from 1 (not at all) to 7 (very much), did you have limited choice of foods? 9. On a scale from 1 (not at all) to 7 (very much), did you experience difficulties to work? 10. Are you a member of the Bosch Alumni Network? (Yes/No) | |
Section : Exposure to biophilic design (design that reconnects us with nature and helps healing, reduces stress, improves creativity and our well-being) elements, indoors and outdoors, before and after the lockdown of the COVID-19 pandemic (color, water, air, sunlight, plants, animals, views and vistas, geology and landscape, habitats and ecosystems) 11. The time you spent outside during the lockdown, compared to before that (walking, sport activities; visual (a view to elements of nature living systems and natural processes)/nonvisual (auditory or gustatory stimuli that engender a deliberate and positive reference to nature, living systems or natural processes) connection with nature; in the presence of water (a condition that enhances the experience of a place through seeing, hearing or touching water); and sounds (nonrhythmic sensory stimuli) is? (Less/the same/more) 12. The time you spend outside now compared to before the lockdown (walking, sport activities, visual/nonvisual connection with nature, presence of water, sounds) is? (Less/the same/more) 13. On a scale from 1 to 7, how important are these elements for you? 14. Which of the elements of biophilic design were present in your indoor living environment before the lockdown? balcony/terrace; private garden/common garden; green roof/façade; views and vistas from home, green or blue; plants/vegetation growing in home gardens/roofs/vases; glass surfaces, sunlight illumination (dynamic & diffuse light and varying intensities of light and shadow that change over time to create conditions that occur in nature); orientation, ventilation, thermal and airflow variability (subtle changes in air temperature, relative humidity, airflow across the skin, and surface temperatures that mimic natural environments); any of these natural materials (natural wood grains; leather; stone, fossil textures; bamboo, rattan, dried grasses, cork, organic palette) 15. Which of the elements of biophilic design are present in your living environment now? balcony/terrace/private garden/common garden/green roof/façade/views and vistas from home, green or blue/plants/vegetation growing in home gardens/roofs/vases/glass surfaces, sunlight illumination/Orientation, ventilation, thermal and airflow variability/any of these natural materials (natural wood grains; leather; stone, fossil textures; bamboo, rattan, dried grasses, cork, organic palette) 16. On a scale from 1 to 7 (1- the lowest- 7 the highest), how likely is it that you will include the biophilic design elements in the future in your living environment (if possible)? | |
Section : Flexibility and adaptation of the living environment after lockdown in relation to the elements of "Biophilic Design" and "Biophilic Urban Design" 17. Did you adapt your home environment after the lockdown to create space for: Home office/Recreational activities/Individual space for (biophilic elements of Prospect (An unimpeded view over a distance, for surveillance and planning) Refuge (A place for withdrawal from environmental conditions or the main flow of activity, in which the individual is protected from behind and overhead)], Mystery (The promise of more information, achieved through partially obscured views or other sensory devices that entice the individual to travel deeper into the environment) and Risk (An identifiable threat coupled with a reliable safeguard) 18. On a scale from 1 to 7 (1- the lowest- 7 the highest), how likely is that you will change your environment (home office, recreational/physical activities, individual space) in the future? 19. If you changed/would change your habitation after the lockdown, did/would you consider any of the elements of Biophilic Urban Design? Proximity to urban gardens, green areas/proximity to water bodies (see, lake, river, etc.), proximity to city centres and services/proximity to rural areas/suburbs (natural terrain with trees and vegetation), and elevated position (i.e., looking downhill or a view point with a wide prospect/proximity to relatives or family/other (please specify) 20. On a scale 1 less to 7 very, how important are for you the elements of Biophilic Urban Design for choosing your habitation? 21. After the lockdown, would you consider any of these changes in your working conditions: flexible virtual/office presence time/switch to full-time home office/total office presence/change in job/occupation/working fewer hours/other? 22. After the lockdown, would you consider any of these changes related to food: Organic/Regional/Growing your own vegetation through urban gardening or farming? | |
Section : Additional information 23. After the lockdown, did you/would you consider taking a pet/animal? 24. Please describe any major changes that you have made incentivized by the lockdown restrictions concerning your lifestyle and wellbeing, based on the above or other factors |
2.1 Three categories of the 14 biophilic patterns according to Browning, Ryan and Clancy [ 67 ]
Nature in the Space Patterns 1. Visual Connection with Nature (A view to elements of nature, living systems and natural processes) 2. Non-Visual Connection with Nature (auditory, haptic, olfactory, or gustatory stimuli that engender a deliberate and positive reference to nature, living systems or natural processes) 3. Non-Rhythmic Sensory Stimuli (Stochastic and ephemeral connections with nature that may be analysed statistically but may not be predicted precisely) 4. Thermal & Airflow Variability (Subtle changes in air temperature, relative humidity, airflow across the skin, and surface temperatures that mimic natural environments) 5. Presence of Water (A condition that enhances the experience of a place through seeing, hearing or touching water) 6. Dynamic & Diffuse Light (averages varying intensities of light and shadow that change over time to create conditions that occur in nature) 7. Connection with Natural Systems (Awareness of natural processes, especially seasonal and temporal changes characteristic of a healthy ecosystem) | |
Natural Analogues Patterns 8. Biomorphic Forms & Patterns (Symbolic references to contoured, patterned, textured or numerical arrangements that persist in nature) 9. Material Connection with Nature (Materials and elements from nature that, through minimal processing, reflect the local ecology or geology and create a distinct sense of place) 10. Complexity & Order (Rich sensory information that adheres to a spatial hierarchy similar to those encountered in nature) | |
Nature of the Space Patterns 11. Prospect (An unimpeded view over a distance for surveillance and planning) 12. Refuge (A place for withdrawal from environmental conditions or the main flow of activity in which the individual is protected from behind and overhead) 13. Mystery (The promise of more information, achieved through partially obscured views or other sensory devices that entice the individual to travel deeper into the environment) 14. Risk/Peril (An identifiable threat coupled with a reliable safeguard) |
Rights and permissions
Open Access This article is licensed under a Creative Commons Attribution 4.0 International License, which permits use, sharing, adaptation, distribution and reproduction in any medium or format, as long as you give appropriate credit to the original author(s) and the source, provide a link to the Creative Commons licence, and indicate if changes were made. The images or other third party material in this article are included in the article's Creative Commons licence, unless indicated otherwise in a credit line to the material. If material is not included in the article's Creative Commons licence and your intended use is not permitted by statutory regulation or exceeds the permitted use, you will need to obtain permission directly from the copyright holder. To view a copy of this licence, visit http://creativecommons.org/licenses/by/4.0/ .
Reprints and permissions
About this article
Shulla, K., Voigt, BF., Lardjane, S. et al. People-environment relations following COVID-19 pandemic lifestyle restrictions: a multinational, explorative analysis of intended biophilic design changes. Discov Sustain 5 , 229 (2024). https://doi.org/10.1007/s43621-024-00423-y
Download citation
Received : 16 May 2024
Accepted : 16 August 2024
Published : 02 September 2024
DOI : https://doi.org/10.1007/s43621-024-00423-y
Share this article
Anyone you share the following link with will be able to read this content:
Sorry, a shareable link is not currently available for this article.
Provided by the Springer Nature SharedIt content-sharing initiative
- Biophilic design
- Environmental psychology
- People-environment relation
- COVID-19 pandemic
Advertisement
- Find a journal
- Publish with us
- Track your research

IMAGES
VIDEO
COMMENTS
Descriptive research aims to accurately and systematically describe a population, situation or phenomenon. It can answer what, where, when and how questions, but not why questions. A descriptive research design can use a wide variety of research methods to investigate one or more variables. Unlike in experimental research, the researcher does ...
Abstract. This short "snippet" covers three important aspects related to statistics - the concept of variables, the importance, and practical aspects related to descriptive statistics and issues related to sampling - types of sampling and sample size estimation. Keywords: Biostatistics, descriptive statistics, sample size, variables.
Descriptive research design is a suitable option when the research objective is to discern characteristics, frequencies, trends, and categories without manipulating variables. It is therefore often employed in the initial stages of a study before progressing to more complex research designs.
Descriptive research design does not attempt to establish cause-and-effect relationships between variables or make predictions about future outcomes. Instead, it focuses on providing a detailed and accurate representation of the data collected, which can be useful for generating hypotheses, exploring trends, and identifying patterns in the data.
Descriptive research is an exploratory research method.It enables researchers to precisely and methodically describe a population, circumstance, or phenomenon.. As the name suggests, descriptive research describes the characteristics of the group, situation, or phenomenon being studied without manipulating variables or testing hypotheses.This can be reported using surveys, observational ...
Characteristics of descriptive research. The term descriptive research then refers to research questions, the design of the study, and data analysis conducted on that topic. We call it an observational research method because none of the research study variables are influenced in any capacity. Some distinctive characteristics of descriptive ...
What descriptive research isn't: Descriptive research finds the what/when/where of a problem, not the why/how. Because of this, we can't use the descriptive method to explore cause-and-effect relationships where one variable (like a person's job role) affects another variable (like their monthly income).
Definition of descriptive research. Descriptive research is defined as a research method that observes and describes the characteristics of a particular group, situation, or phenomenon. The goal is not to establish cause and effect relationships but rather to provide a detailed account of the situation.
Descriptive research aims to accurately and systematically describe a population, situation or phenomenon. It can answer what, where, when, and how questions, but not why questions. A descriptive research design can use a wide variety of research methods to investigate one or more variables. Unlike in experimental research, the researcher does ...
Types of descriptive statistics. There are 3 main types of descriptive statistics: The distribution concerns the frequency of each value. The central tendency concerns the averages of the values. The variability or dispersion concerns how spread out the values are. You can apply these to assess only one variable at a time, in univariate ...
Correlational research is a type of descriptive research, which is used to measure the relationship between 2 variables, with the researcher having no control over them. It aims to find whether there is; positive correlation (both variables change in the same direction), negative correlation (the variables change in the opposite direction), or ...
Hence, descriptive research cannot describe what caused a situation. Thus, descriptive research cannot be used as the basis of a causal relationship, where one variable affects another. In other words, descriptive research can be said to have a low requirement for internal validity. The description is used for frequencies, averages, and other ...
We call this process "describing data". In the process of producing summaries of the sample, we use measures like mean, median, variance, graphs, charts, frequencies, histograms, box and whisker plots, and percentages. For datasets with just one variable, we use univariate descriptive statistics. For datasets with multiple variables, we use ...
Descriptive research relies on existing data, which may not always be comprehensive or accurate. 3. Lack of Control. Researchers have no control over the variables in descriptive research, which can limit the conclusions that can be drawn. 4. Bias. The researcher's own biases and preconceptions can influence the interpretation of the data. 5.
Descriptive research design is ideal for further research. Because the data collection for descriptive research produces statistical outcomes, it can also be used as secondary data for another research study. Plus, the data collected from descriptive research can be subjected to other types of data analysis. Uncontrolled variables
Examples. Discrete variables (aka integer variables) Counts of individual items or values. Number of students in a class. Number of different tree species in a forest. Continuous variables (aka ratio variables) Measurements of continuous or non-finite values. Distance.
Descriptive research may identify areas in need of additional research and relationships between variables that require future study. Descriptive research is often referred to as "hypothesis generating research." Depending on the data collection method used, descriptive studies can generate rich datasets on large and diverse samples. ...
Descriptive research may be an initial step before the other two types of psychological research are conducted: Correlational research: examines two variables at once, and may be used to identify ...
A descriptive research design was used to observe and describe the characteristics of the sample without manipulating any of the variables (Siedlecki, 2020), which allowed for data collection ...
A control variable is a variable that must be kept constant during the course of an experiment. Descriptive Statistics. Statistics can be broadly divided into descriptive statistics and inferential statistics.[3 4] Descriptive statistics give a summary about the sample being studied without drawing any inferences based on probability theory ...
The results of descriptive research projects are analyzed using descriptive statistics — numbers that summarize the distribution of scores on a measured variable. Most variables have distributions similar to that shown in Figure 3.5 where most of the scores are located near the centre of the distribution, and the distribution is symmetrical ...
You can also create a mixed methods research design that has elements of both. Descriptive research vs experimental research. Descriptive research gathers data without controlling any variables, while experimental research manipulates and controls variables to determine cause and effect.
Independent variable is a variable that stands alone and isn't changed by the other variables you are trying to measure while a dependent variable is a variable or something that depends on other factors. ... Descriptive research, requires the researcher to go to the field and write exactly what he/she saw.
The study analyzes the consequences of the COVID-19 pandemic restrictions for the human-environment relations through the lenses of biophilic design. The mixed-method quantitative and qualitative explanatory research combines contextual and personal variables, such as, among others, country, age group, gender, overcrowding, time spent outside, access to nature/food and the exposure to ...