Jump to navigation


Cochrane Training
Chapter 15: interpreting results and drawing conclusions.
Holger J Schünemann, Gunn E Vist, Julian PT Higgins, Nancy Santesso, Jonathan J Deeks, Paul Glasziou, Elie A Akl, Gordon H Guyatt; on behalf of the Cochrane GRADEing Methods Group
Key Points:
- This chapter provides guidance on interpreting the results of synthesis in order to communicate the conclusions of the review effectively.
- Methods are presented for computing, presenting and interpreting relative and absolute effects for dichotomous outcome data, including the number needed to treat (NNT).
- For continuous outcome measures, review authors can present summary results for studies using natural units of measurement or as minimal important differences when all studies use the same scale. When studies measure the same construct but with different scales, review authors will need to find a way to interpret the standardized mean difference, or to use an alternative effect measure for the meta-analysis such as the ratio of means.
- Review authors should not describe results as ‘statistically significant’, ‘not statistically significant’ or ‘non-significant’ or unduly rely on thresholds for P values, but report the confidence interval together with the exact P value.
- Review authors should not make recommendations about healthcare decisions, but they can – after describing the certainty of evidence and the balance of benefits and harms – highlight different actions that might be consistent with particular patterns of values and preferences and other factors that determine a decision such as cost.
Cite this chapter as: Schünemann HJ, Vist GE, Higgins JPT, Santesso N, Deeks JJ, Glasziou P, Akl EA, Guyatt GH. Chapter 15: Interpreting results and drawing conclusions [last updated August 2023]. In: Higgins JPT, Thomas J, Chandler J, Cumpston M, Li T, Page MJ, Welch VA (editors). Cochrane Handbook for Systematic Reviews of Interventions version 6.5. Cochrane, 2024. Available from www.training.cochrane.org/handbook .
15.1 Introduction
The purpose of Cochrane Reviews is to facilitate healthcare decisions by patients and the general public, clinicians, guideline developers, administrators and policy makers. They also inform future research. A clear statement of findings, a considered discussion and a clear presentation of the authors’ conclusions are, therefore, important parts of the review. In particular, the following issues can help people make better informed decisions and increase the usability of Cochrane Reviews:
- information on all important outcomes, including adverse outcomes;
- the certainty of the evidence for each of these outcomes, as it applies to specific populations and specific interventions; and
- clarification of the manner in which particular values and preferences may bear on the desirable and undesirable consequences of the intervention.
A ‘Summary of findings’ table, described in Chapter 14 , Section 14.1 , provides key pieces of information about health benefits and harms in a quick and accessible format. It is highly desirable that review authors include a ‘Summary of findings’ table in Cochrane Reviews alongside a sufficient description of the studies and meta-analyses to support its contents. This description includes the rating of the certainty of evidence, also called the quality of the evidence or confidence in the estimates of the effects, which is expected in all Cochrane Reviews.
‘Summary of findings’ tables are usually supported by full evidence profiles which include the detailed ratings of the evidence (Guyatt et al 2011a, Guyatt et al 2013a, Guyatt et al 2013b, Santesso et al 2016). The Discussion section of the text of the review provides space to reflect and consider the implications of these aspects of the review’s findings. Cochrane Reviews include five standard subheadings to ensure the Discussion section places the review in an appropriate context: ‘Summary of main results (benefits and harms)’; ‘Potential biases in the review process’; ‘Overall completeness and applicability of evidence’; ‘Certainty of the evidence’; and ‘Agreements and disagreements with other studies or reviews’. Following the Discussion, the Authors’ conclusions section is divided into two standard subsections: ‘Implications for practice’ and ‘Implications for research’. The assessment of the certainty of evidence facilitates a structured description of the implications for practice and research.
Because Cochrane Reviews have an international audience, the Discussion and Authors’ conclusions should, so far as possible, assume a broad international perspective and provide guidance for how the results could be applied in different settings, rather than being restricted to specific national or local circumstances. Cultural differences and economic differences may both play an important role in determining the best course of action based on the results of a Cochrane Review. Furthermore, individuals within societies have widely varying values and preferences regarding health states, and use of societal resources to achieve particular health states. For all these reasons, and because information that goes beyond that included in a Cochrane Review is required to make fully informed decisions, different people will often make different decisions based on the same evidence presented in a review.
Thus, review authors should avoid specific recommendations that inevitably depend on assumptions about available resources, values and preferences, and other factors such as equity considerations, feasibility and acceptability of an intervention. The purpose of the review should be to present information and aid interpretation rather than to offer recommendations. The discussion and conclusions should help people understand the implications of the evidence in relation to practical decisions and apply the results to their specific situation. Review authors can aid this understanding of the implications by laying out different scenarios that describe certain value structures.
In this chapter, we address first one of the key aspects of interpreting findings that is also fundamental in completing a ‘Summary of findings’ table: the certainty of evidence related to each of the outcomes. We then provide a more detailed consideration of issues around applicability and around interpretation of numerical results, and provide suggestions for presenting authors’ conclusions.
15.2 Issues of indirectness and applicability
15.2.1 the role of the review author.
“A leap of faith is always required when applying any study findings to the population at large” or to a specific person. “In making that jump, one must always strike a balance between making justifiable broad generalizations and being too conservative in one’s conclusions” (Friedman et al 1985). In addition to issues about risk of bias and other domains determining the certainty of evidence, this leap of faith is related to how well the identified body of evidence matches the posed PICO ( Population, Intervention, Comparator(s) and Outcome ) question. As to the population, no individual can be entirely matched to the population included in research studies. At the time of decision, there will always be differences between the study population and the person or population to whom the evidence is applied; sometimes these differences are slight, sometimes large.
The terms applicability, generalizability, external validity and transferability are related, sometimes used interchangeably and have in common that they lack a clear and consistent definition in the classic epidemiological literature (Schünemann et al 2013). However, all of the terms describe one overarching theme: whether or not available research evidence can be directly used to answer the health and healthcare question at hand, ideally supported by a judgement about the degree of confidence in this use (Schünemann et al 2013). GRADE’s certainty domains include a judgement about ‘indirectness’ to describe all of these aspects including the concept of direct versus indirect comparisons of different interventions (Atkins et al 2004, Guyatt et al 2008, Guyatt et al 2011b).
To address adequately the extent to which a review is relevant for the purpose to which it is being put, there are certain things the review author must do, and certain things the user of the review must do to assess the degree of indirectness. Cochrane and the GRADE Working Group suggest using a very structured framework to address indirectness. We discuss here and in Chapter 14 what the review author can do to help the user. Cochrane Review authors must be extremely clear on the population, intervention and outcomes that they intend to address. Chapter 14, Section 14.1.2 , also emphasizes a crucial step: the specification of all patient-important outcomes relevant to the intervention strategies under comparison.
In considering whether the effect of an intervention applies equally to all participants, and whether different variations on the intervention have similar effects, review authors need to make a priori hypotheses about possible effect modifiers, and then examine those hypotheses (see Chapter 10, Section 10.10 and Section 10.11 ). If they find apparent subgroup effects, they must ultimately decide whether or not these effects are credible (Sun et al 2012). Differences between subgroups, particularly those that correspond to differences between studies, should be interpreted cautiously. Some chance variation between subgroups is inevitable so, unless there is good reason to believe that there is an interaction, review authors should not assume that the subgroup effect exists. If, despite due caution, review authors judge subgroup effects in terms of relative effect estimates as credible (i.e. the effects differ credibly), they should conduct separate meta-analyses for the relevant subgroups, and produce separate ‘Summary of findings’ tables for those subgroups.
The user of the review will be challenged with ‘individualization’ of the findings, whether they seek to apply the findings to an individual patient or a policy decision in a specific context. For example, even if relative effects are similar across subgroups, absolute effects will differ according to baseline risk. Review authors can help provide this information by identifying identifiable groups of people with varying baseline risks in the ‘Summary of findings’ tables, as discussed in Chapter 14, Section 14.1.3 . Users can then identify their specific case or population as belonging to a particular risk group, if relevant, and assess their likely magnitude of benefit or harm accordingly. A description of the identifying prognostic or baseline risk factors in a brief scenario (e.g. age or gender) will help users of a review further.
Another decision users must make is whether their individual case or population of interest is so different from those included in the studies that they cannot use the results of the systematic review and meta-analysis at all. Rather than rigidly applying the inclusion and exclusion criteria of studies, it is better to ask whether or not there are compelling reasons why the evidence should not be applied to a particular patient. Review authors can sometimes help decision makers by identifying important variation where divergence might limit the applicability of results (Rothwell 2005, Schünemann et al 2006, Guyatt et al 2011b, Schünemann et al 2013), including biologic and cultural variation, and variation in adherence to an intervention.
In addressing these issues, review authors cannot be aware of, or address, the myriad of differences in circumstances around the world. They can, however, address differences of known importance to many people and, importantly, they should avoid assuming that other people’s circumstances are the same as their own in discussing the results and drawing conclusions.
15.2.2 Biological variation
Issues of biological variation that may affect the applicability of a result to a reader or population include divergence in pathophysiology (e.g. biological differences between women and men that may affect responsiveness to an intervention) and divergence in a causative agent (e.g. for infectious diseases such as malaria, which may be caused by several different parasites). The discussion of the results in the review should make clear whether the included studies addressed all or only some of these groups, and whether any important subgroup effects were found.
15.2.3 Variation in context
Some interventions, particularly non-pharmacological interventions, may work in some contexts but not in others; the situation has been described as program by context interaction (Hawe et al 2004). Contextual factors might pertain to the host organization in which an intervention is offered, such as the expertise, experience and morale of the staff expected to carry out the intervention, the competing priorities for the clinician’s or staff’s attention, the local resources such as service and facilities made available to the program and the status or importance given to the program by the host organization. Broader context issues might include aspects of the system within which the host organization operates, such as the fee or payment structure for healthcare providers and the local insurance system. Some interventions, in particular complex interventions (see Chapter 17 ), can be only partially implemented in some contexts, and this requires judgements about indirectness of the intervention and its components for readers in that context (Schünemann 2013).
Contextual factors may also pertain to the characteristics of the target group or population, such as cultural and linguistic diversity, socio-economic position, rural/urban setting. These factors may mean that a particular style of care or relationship evolves between service providers and consumers that may or may not match the values and technology of the program.
For many years these aspects have been acknowledged when decision makers have argued that results of evidence reviews from other countries do not apply in their own country or setting. Whilst some programmes/interventions have been successfully transferred from one context to another, others have not (Resnicow et al 1993, Lumley et al 2004, Coleman et al 2015). Review authors should be cautious when making generalizations from one context to another. They should report on the presence (or otherwise) of context-related information in intervention studies, where this information is available.
15.2.4 Variation in adherence
Variation in the adherence of the recipients and providers of care can limit the certainty in the applicability of results. Predictable differences in adherence can be due to divergence in how recipients of care perceive the intervention (e.g. the importance of side effects), economic conditions or attitudes that make some forms of care inaccessible in some settings, such as in low-income countries (Dans et al 2007). It should not be assumed that high levels of adherence in closely monitored randomized trials will translate into similar levels of adherence in normal practice.
15.2.5 Variation in values and preferences
Decisions about healthcare management strategies and options involve trading off health benefits and harms. The right choice may differ for people with different values and preferences (i.e. the importance people place on the outcomes and interventions), and it is important that decision makers ensure that decisions are consistent with a patient or population’s values and preferences. The importance placed on outcomes, together with other factors, will influence whether the recipients of care will or will not accept an option that is offered (Alonso-Coello et al 2016) and, thus, can be one factor influencing adherence. In Section 15.6 , we describe how the review author can help this process and the limits of supporting decision making based on intervention reviews.
15.3 Interpreting results of statistical analyses
15.3.1 confidence intervals.
Results for both individual studies and meta-analyses are reported with a point estimate together with an associated confidence interval. For example, ‘The odds ratio was 0.75 with a 95% confidence interval of 0.70 to 0.80’. The point estimate (0.75) is the best estimate of the magnitude and direction of the experimental intervention’s effect compared with the comparator intervention. The confidence interval describes the uncertainty inherent in any estimate, and describes a range of values within which we can be reasonably sure that the true effect actually lies. If the confidence interval is relatively narrow (e.g. 0.70 to 0.80), the effect size is known precisely. If the interval is wider (e.g. 0.60 to 0.93) the uncertainty is greater, although there may still be enough precision to make decisions about the utility of the intervention. Intervals that are very wide (e.g. 0.50 to 1.10) indicate that we have little knowledge about the effect and this imprecision affects our certainty in the evidence, and that further information would be needed before we could draw a more certain conclusion.
A 95% confidence interval is often interpreted as indicating a range within which we can be 95% certain that the true effect lies. This statement is a loose interpretation, but is useful as a rough guide. The strictly correct interpretation of a confidence interval is based on the hypothetical notion of considering the results that would be obtained if the study were repeated many times. If a study were repeated infinitely often, and on each occasion a 95% confidence interval calculated, then 95% of these intervals would contain the true effect (see Section 15.3.3 for further explanation).
The width of the confidence interval for an individual study depends to a large extent on the sample size. Larger studies tend to give more precise estimates of effects (and hence have narrower confidence intervals) than smaller studies. For continuous outcomes, precision depends also on the variability in the outcome measurements (i.e. how widely individual results vary between people in the study, measured as the standard deviation); for dichotomous outcomes it depends on the risk of the event (more frequent events allow more precision, and narrower confidence intervals), and for time-to-event outcomes it also depends on the number of events observed. All these quantities are used in computation of the standard errors of effect estimates from which the confidence interval is derived.
The width of a confidence interval for a meta-analysis depends on the precision of the individual study estimates and on the number of studies combined. In addition, for random-effects models, precision will decrease with increasing heterogeneity and confidence intervals will widen correspondingly (see Chapter 10, Section 10.10.4 ). As more studies are added to a meta-analysis the width of the confidence interval usually decreases. However, if the additional studies increase the heterogeneity in the meta-analysis and a random-effects model is used, it is possible that the confidence interval width will increase.
Confidence intervals and point estimates have different interpretations in fixed-effect and random-effects models. While the fixed-effect estimate and its confidence interval address the question ‘what is the best (single) estimate of the effect?’, the random-effects estimate assumes there to be a distribution of effects, and the estimate and its confidence interval address the question ‘what is the best estimate of the average effect?’ A confidence interval may be reported for any level of confidence (although they are most commonly reported for 95%, and sometimes 90% or 99%). For example, the odds ratio of 0.80 could be reported with an 80% confidence interval of 0.73 to 0.88; a 90% interval of 0.72 to 0.89; and a 95% interval of 0.70 to 0.92. As the confidence level increases, the confidence interval widens.
There is logical correspondence between the confidence interval and the P value (see Section 15.3.3 ). The 95% confidence interval for an effect will exclude the null value (such as an odds ratio of 1.0 or a risk difference of 0) if and only if the test of significance yields a P value of less than 0.05. If the P value is exactly 0.05, then either the upper or lower limit of the 95% confidence interval will be at the null value. Similarly, the 99% confidence interval will exclude the null if and only if the test of significance yields a P value of less than 0.01.
Together, the point estimate and confidence interval provide information to assess the effects of the intervention on the outcome. For example, suppose that we are evaluating an intervention that reduces the risk of an event and we decide that it would be useful only if it reduced the risk of an event from 30% by at least 5 percentage points to 25% (these values will depend on the specific clinical scenario and outcomes, including the anticipated harms). If the meta-analysis yielded an effect estimate of a reduction of 10 percentage points with a tight 95% confidence interval, say, from 7% to 13%, we would be able to conclude that the intervention was useful since both the point estimate and the entire range of the interval exceed our criterion of a reduction of 5% for net health benefit. However, if the meta-analysis reported the same risk reduction of 10% but with a wider interval, say, from 2% to 18%, although we would still conclude that our best estimate of the intervention effect is that it provides net benefit, we could not be so confident as we still entertain the possibility that the effect could be between 2% and 5%. If the confidence interval was wider still, and included the null value of a difference of 0%, we would still consider the possibility that the intervention has no effect on the outcome whatsoever, and would need to be even more sceptical in our conclusions.
Review authors may use the same general approach to conclude that an intervention is not useful. Continuing with the above example where the criterion for an important difference that should be achieved to provide more benefit than harm is a 5% risk difference, an effect estimate of 2% with a 95% confidence interval of 1% to 4% suggests that the intervention does not provide net health benefit.
15.3.2 P values and statistical significance
A P value is the standard result of a statistical test, and is the probability of obtaining the observed effect (or larger) under a ‘null hypothesis’. In the context of Cochrane Reviews there are two commonly used statistical tests. The first is a test of overall effect (a Z-test), and its null hypothesis is that there is no overall effect of the experimental intervention compared with the comparator on the outcome of interest. The second is the (Chi 2 ) test for heterogeneity, and its null hypothesis is that there are no differences in the intervention effects across studies.
A P value that is very small indicates that the observed effect is very unlikely to have arisen purely by chance, and therefore provides evidence against the null hypothesis. It has been common practice to interpret a P value by examining whether it is smaller than particular threshold values. In particular, P values less than 0.05 are often reported as ‘statistically significant’, and interpreted as being small enough to justify rejection of the null hypothesis. However, the 0.05 threshold is an arbitrary one that became commonly used in medical and psychological research largely because P values were determined by comparing the test statistic against tabulations of specific percentage points of statistical distributions. If review authors decide to present a P value with the results of a meta-analysis, they should report a precise P value (as calculated by most statistical software), together with the 95% confidence interval. Review authors should not describe results as ‘statistically significant’, ‘not statistically significant’ or ‘non-significant’ or unduly rely on thresholds for P values , but report the confidence interval together with the exact P value (see MECIR Box 15.3.a ).
We discuss interpretation of the test for heterogeneity in Chapter 10, Section 10.10.2 ; the remainder of this section refers mainly to tests for an overall effect. For tests of an overall effect, the computation of P involves both the effect estimate and precision of the effect estimate (driven largely by sample size). As precision increases, the range of plausible effects that could occur by chance is reduced. Correspondingly, the statistical significance of an effect of a particular magnitude will usually be greater (the P value will be smaller) in a larger study than in a smaller study.
P values are commonly misinterpreted in two ways. First, a moderate or large P value (e.g. greater than 0.05) may be misinterpreted as evidence that the intervention has no effect on the outcome. There is an important difference between this statement and the correct interpretation that there is a high probability that the observed effect on the outcome is due to chance alone. To avoid such a misinterpretation, review authors should always examine the effect estimate and its 95% confidence interval.
The second misinterpretation is to assume that a result with a small P value for the summary effect estimate implies that an experimental intervention has an important benefit. Such a misinterpretation is more likely to occur in large studies and meta-analyses that accumulate data over dozens of studies and thousands of participants. The P value addresses the question of whether the experimental intervention effect is precisely nil; it does not examine whether the effect is of a magnitude of importance to potential recipients of the intervention. In a large study, a small P value may represent the detection of a trivial effect that may not lead to net health benefit when compared with the potential harms (i.e. harmful effects on other important outcomes). Again, inspection of the point estimate and confidence interval helps correct interpretations (see Section 15.3.1 ).
MECIR Box 15.3.a Relevant expectations for conduct of intervention reviews
Interpreting results ( ) | |
. | Authors commonly mistake a lack of evidence of effect as evidence of a lack of effect. |
15.3.3 Relation between confidence intervals, statistical significance and certainty of evidence
The confidence interval (and imprecision) is only one domain that influences overall uncertainty about effect estimates. Uncertainty resulting from imprecision (i.e. statistical uncertainty) may be no less important than uncertainty from indirectness, or any other GRADE domain, in the context of decision making (Schünemann 2016). Thus, the extent to which interpretations of the confidence interval described in Sections 15.3.1 and 15.3.2 correspond to conclusions about overall certainty of the evidence for the outcome of interest depends on these other domains. If there are no concerns about other domains that determine the certainty of the evidence (i.e. risk of bias, inconsistency, indirectness or publication bias), then the interpretation in Sections 15.3.1 and 15.3.2 . about the relation of the confidence interval to the true effect may be carried forward to the overall certainty. However, if there are concerns about the other domains that affect the certainty of the evidence, the interpretation about the true effect needs to be seen in the context of further uncertainty resulting from those concerns.
For example, nine randomized controlled trials in almost 6000 cancer patients indicated that the administration of heparin reduces the risk of venous thromboembolism (VTE), with a risk ratio of 43% (95% CI 19% to 60%) (Akl et al 2011a). For patients with a plausible baseline risk of approximately 4.6% per year, this relative effect suggests that heparin leads to an absolute risk reduction of 20 fewer VTEs (95% CI 9 fewer to 27 fewer) per 1000 people per year (Akl et al 2011a). Now consider that the review authors or those applying the evidence in a guideline have lowered the certainty in the evidence as a result of indirectness. While the confidence intervals would remain unchanged, the certainty in that confidence interval and in the point estimate as reflecting the truth for the question of interest will be lowered. In fact, the certainty range will have unknown width so there will be unknown likelihood of a result within that range because of this indirectness. The lower the certainty in the evidence, the less we know about the width of the certainty range, although methods for quantifying risk of bias and understanding potential direction of bias may offer insight when lowered certainty is due to risk of bias. Nevertheless, decision makers must consider this uncertainty, and must do so in relation to the effect measure that is being evaluated (e.g. a relative or absolute measure). We will describe the impact on interpretations for dichotomous outcomes in Section 15.4 .
15.4 Interpreting results from dichotomous outcomes (including numbers needed to treat)
15.4.1 relative and absolute risk reductions.
Clinicians may be more inclined to prescribe an intervention that reduces the relative risk of death by 25% than one that reduces the risk of death by 1 percentage point, although both presentations of the evidence may relate to the same benefit (i.e. a reduction in risk from 4% to 3%). The former refers to the relative reduction in risk and the latter to the absolute reduction in risk. As described in Chapter 6, Section 6.4.1 , there are several measures for comparing dichotomous outcomes in two groups. Meta-analyses are usually undertaken using risk ratios (RR), odds ratios (OR) or risk differences (RD), but there are several alternative ways of expressing results.
Relative risk reduction (RRR) is a convenient way of re-expressing a risk ratio as a percentage reduction:

For example, a risk ratio of 0.75 translates to a relative risk reduction of 25%, as in the example above.
The risk difference is often referred to as the absolute risk reduction (ARR) or absolute risk increase (ARI), and may be presented as a percentage (e.g. 1%), as a decimal (e.g. 0.01), or as account (e.g. 10 out of 1000). We consider different choices for presenting absolute effects in Section 15.4.3 . We then describe computations for obtaining these numbers from the results of individual studies and of meta-analyses in Section 15.4.4 .
15.4.2 Number needed to treat (NNT)
The number needed to treat (NNT) is a common alternative way of presenting information on the effect of an intervention. The NNT is defined as the expected number of people who need to receive the experimental rather than the comparator intervention for one additional person to either incur or avoid an event (depending on the direction of the result) in a given time frame. Thus, for example, an NNT of 10 can be interpreted as ‘it is expected that one additional (or less) person will incur an event for every 10 participants receiving the experimental intervention rather than comparator over a given time frame’. It is important to be clear that:
- since the NNT is derived from the risk difference, it is still a comparative measure of effect (experimental versus a specific comparator) and not a general property of a single intervention; and
- the NNT gives an ‘expected value’. For example, NNT = 10 does not imply that one additional event will occur in each and every group of 10 people.
NNTs can be computed for both beneficial and detrimental events, and for interventions that cause both improvements and deteriorations in outcomes. In all instances NNTs are expressed as positive whole numbers. Some authors use the term ‘number needed to harm’ (NNH) when an intervention leads to an adverse outcome, or a decrease in a positive outcome, rather than improvement. However, this phrase can be misleading (most notably, it can easily be read to imply the number of people who will experience a harmful outcome if given the intervention), and it is strongly recommended that ‘number needed to harm’ and ‘NNH’ are avoided. The preferred alternative is to use phrases such as ‘number needed to treat for an additional beneficial outcome’ (NNTB) and ‘number needed to treat for an additional harmful outcome’ (NNTH) to indicate direction of effect.
As NNTs refer to events, their interpretation needs to be worded carefully when the binary outcome is a dichotomization of a scale-based outcome. For example, if the outcome is pain measured on a ‘none, mild, moderate or severe’ scale it may have been dichotomized as ‘none or mild’ versus ‘moderate or severe’. It would be inappropriate for an NNT from these data to be referred to as an ‘NNT for pain’. It is an ‘NNT for moderate or severe pain’.
We consider different choices for presenting absolute effects in Section 15.4.3 . We then describe computations for obtaining these numbers from the results of individual studies and of meta-analyses in Section 15.4.4 .
15.4.3 Expressing risk differences
Users of reviews are liable to be influenced by the choice of statistical presentations of the evidence. Hoffrage and colleagues suggest that physicians’ inferences about statistical outcomes are more appropriate when they deal with ‘natural frequencies’ – whole numbers of people, both treated and untreated (e.g. treatment results in a drop from 20 out of 1000 to 10 out of 1000 women having breast cancer) – than when effects are presented as percentages (e.g. 1% absolute reduction in breast cancer risk) (Hoffrage et al 2000). Probabilities may be more difficult to understand than frequencies, particularly when events are rare. While standardization may be important in improving the presentation of research evidence (and participation in healthcare decisions), current evidence suggests that the presentation of natural frequencies for expressing differences in absolute risk is best understood by consumers of healthcare information (Akl et al 2011b). This evidence provides the rationale for presenting absolute risks in ‘Summary of findings’ tables as numbers of people with events per 1000 people receiving the intervention (see Chapter 14 ).
RRs and RRRs remain crucial because relative effects tend to be substantially more stable across risk groups than absolute effects (see Chapter 10, Section 10.4.3 ). Review authors can use their own data to study this consistency (Cates 1999, Smeeth et al 1999). Risk differences from studies are least likely to be consistent across baseline event rates; thus, they are rarely appropriate for computing numbers needed to treat in systematic reviews. If a relative effect measure (OR or RR) is chosen for meta-analysis, then a comparator group risk needs to be specified as part of the calculation of an RD or NNT. In addition, if there are several different groups of participants with different levels of risk, it is crucial to express absolute benefit for each clinically identifiable risk group, clarifying the time period to which this applies. Studies in patients with differing severity of disease, or studies with different lengths of follow-up will almost certainly have different comparator group risks. In these cases, different comparator group risks lead to different RDs and NNTs (except when the intervention has no effect). A recommended approach is to re-express an odds ratio or a risk ratio as a variety of RD or NNTs across a range of assumed comparator risks (ACRs) (McQuay and Moore 1997, Smeeth et al 1999). Review authors should bear these considerations in mind not only when constructing their ‘Summary of findings’ table, but also in the text of their review.
For example, a review of oral anticoagulants to prevent stroke presented information to users by describing absolute benefits for various baseline risks (Aguilar and Hart 2005, Aguilar et al 2007). They presented their principal findings as “The inherent risk of stroke should be considered in the decision to use oral anticoagulants in atrial fibrillation patients, selecting those who stand to benefit most for this therapy” (Aguilar and Hart 2005). Among high-risk atrial fibrillation patients with prior stroke or transient ischaemic attack who have stroke rates of about 12% (120 per 1000) per year, warfarin prevents about 70 strokes yearly per 1000 patients, whereas for low-risk atrial fibrillation patients (with a stroke rate of about 2% per year or 20 per 1000), warfarin prevents only 12 strokes. This presentation helps users to understand the important impact that typical baseline risks have on the absolute benefit that they can expect.
15.4.4 Computations
Direct computation of risk difference (RD) or a number needed to treat (NNT) depends on the summary statistic (odds ratio, risk ratio or risk differences) available from the study or meta-analysis. When expressing results of meta-analyses, review authors should use, in the computations, whatever statistic they determined to be the most appropriate summary for meta-analysis (see Chapter 10, Section 10.4.3 ). Here we present calculations to obtain RD as a reduction in the number of participants per 1000. For example, a risk difference of –0.133 corresponds to 133 fewer participants with the event per 1000.
RDs and NNTs should not be computed from the aggregated total numbers of participants and events across the trials. This approach ignores the randomization within studies, and may produce seriously misleading results if there is unbalanced randomization in any of the studies. Using the pooled result of a meta-analysis is more appropriate. When computing NNTs, the values obtained are by convention always rounded up to the next whole number.
15.4.4.1 Computing NNT from a risk difference (RD)
A NNT may be computed from a risk difference as

where the vertical bars (‘absolute value of’) in the denominator indicate that any minus sign should be ignored. It is convention to round the NNT up to the nearest whole number. For example, if the risk difference is –0.12 the NNT is 9; if the risk difference is –0.22 the NNT is 5. Cochrane Review authors should qualify the NNT as referring to benefit (improvement) or harm by denoting the NNT as NNTB or NNTH. Note that this approach, although feasible, should be used only for the results of a meta-analysis of risk differences. In most cases meta-analyses will be undertaken using a relative measure of effect (RR or OR), and those statistics should be used to calculate the NNT (see Section 15.4.4.2 and 15.4.4.3 ).
15.4.4.2 Computing risk differences or NNT from a risk ratio
To aid interpretation of the results of a meta-analysis of risk ratios, review authors may compute an absolute risk reduction or NNT. In order to do this, an assumed comparator risk (ACR) (otherwise known as a baseline risk, or risk that the outcome of interest would occur with the comparator intervention) is required. It will usually be appropriate to do this for a range of different ACRs. The computation proceeds as follows:

As an example, suppose the risk ratio is RR = 0.92, and an ACR = 0.3 (300 per 1000) is assumed. Then the effect on risk is 24 fewer per 1000:

The NNT is 42:

15.4.4.3 Computing risk differences or NNT from an odds ratio
Review authors may wish to compute a risk difference or NNT from the results of a meta-analysis of odds ratios. In order to do this, an ACR is required. It will usually be appropriate to do this for a range of different ACRs. The computation proceeds as follows:

As an example, suppose the odds ratio is OR = 0.73, and a comparator risk of ACR = 0.3 is assumed. Then the effect on risk is 62 fewer per 1000:

The NNT is 17:
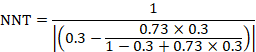
15.4.4.4 Computing risk ratio from an odds ratio
Because risk ratios are easier to interpret than odds ratios, but odds ratios have favourable mathematical properties, a review author may decide to undertake a meta-analysis based on odds ratios, but to express the result as a summary risk ratio (or relative risk reduction). This requires an ACR. Then
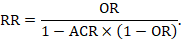
It will often be reasonable to perform this transformation using the median comparator group risk from the studies in the meta-analysis.
15.4.4.5 Computing confidence limits
Confidence limits for RDs and NNTs may be calculated by applying the above formulae to the upper and lower confidence limits for the summary statistic (RD, RR or OR) (Altman 1998). Note that this confidence interval does not incorporate uncertainty around the ACR.
If the 95% confidence interval of OR or RR includes the value 1, one of the confidence limits will indicate benefit and the other harm. Thus, appropriate use of the words ‘fewer’ and ‘more’ is required for each limit when presenting results in terms of events. For NNTs, the two confidence limits should be labelled as NNTB and NNTH to indicate the direction of effect in each case. The confidence interval for the NNT will include a ‘discontinuity’, because increasingly smaller risk differences that approach zero will lead to NNTs approaching infinity. Thus, the confidence interval will include both an infinitely large NNTB and an infinitely large NNTH.
15.5 Interpreting results from continuous outcomes (including standardized mean differences)
15.5.1 meta-analyses with continuous outcomes.
Review authors should describe in the study protocol how they plan to interpret results for continuous outcomes. When outcomes are continuous, review authors have a number of options to present summary results. These options differ if studies report the same measure that is familiar to the target audiences, studies report the same or very similar measures that are less familiar to the target audiences, or studies report different measures.
15.5.2 Meta-analyses with continuous outcomes using the same measure
If all studies have used the same familiar units, for instance, results are expressed as durations of events, such as symptoms for conditions including diarrhoea, sore throat, otitis media, influenza or duration of hospitalization, a meta-analysis may generate a summary estimate in those units, as a difference in mean response (see, for instance, the row summarizing results for duration of diarrhoea in Chapter 14, Figure 14.1.b and the row summarizing oedema in Chapter 14, Figure 14.1.a ). For such outcomes, the ‘Summary of findings’ table should include a difference of means between the two interventions. However, when units of such outcomes may be difficult to interpret, particularly when they relate to rating scales (again, see the oedema row of Chapter 14, Figure 14.1.a ). ‘Summary of findings’ tables should include the minimum and maximum of the scale of measurement, and the direction. Knowledge of the smallest change in instrument score that patients perceive is important – the minimal important difference (MID) – and can greatly facilitate the interpretation of results (Guyatt et al 1998, Schünemann and Guyatt 2005). Knowing the MID allows review authors and users to place results in context. Review authors should state the MID – if known – in the Comments column of their ‘Summary of findings’ table. For example, the chronic respiratory questionnaire has possible scores in health-related quality of life ranging from 1 to 7 and 0.5 represents a well-established MID (Jaeschke et al 1989, Schünemann et al 2005).
15.5.3 Meta-analyses with continuous outcomes using different measures
When studies have used different instruments to measure the same construct, a standardized mean difference (SMD) may be used in meta-analysis for combining continuous data. Without guidance, clinicians and patients may have little idea how to interpret results presented as SMDs. Review authors should therefore consider issues of interpretability when planning their analysis at the protocol stage and should consider whether there will be suitable ways to re-express the SMD or whether alternative effect measures, such as a ratio of means, or possibly as minimal important difference units (Guyatt et al 2013b) should be used. Table 15.5.a and the following sections describe these options.
Table 15.5.a Approaches and their implications to presenting results of continuous variables when primary studies have used different instruments to measure the same construct. Adapted from Guyatt et al (2013b)
|
|
|
| ||
1a. Generic standard deviation (SD) units and guiding rules | It is widely used, but the interpretation is challenging. It can be misleading depending on whether the population is very homogenous or heterogeneous (i.e. how variable the outcome was in the population of each included study, and therefore how applicable a standard SD is likely to be). See Section . | Use together with other approaches below. |
1b. Re-express and present as units of a familiar measure | Presenting data with this approach may be viewed by users as closer to the primary data. However, few instruments are sufficiently used in clinical practice to make many of the presented units easily interpretable. See Section . | When the units and measures are familiar to the decision makers (e.g. healthcare providers and patients), this presentation should be seriously considered. Conversion to natural units is also an option for expressing results using the MID approach below (row 3). |
1c. Re-express as result for a dichotomous outcome | Dichotomous outcomes are very familiar to clinical audiences and may facilitate understanding. However, this approach involves assumptions that may not always be valid (e.g. it assumes that distributions in intervention and comparator group are roughly normally distributed and variances are similar). It allows applying GRADE guidance for large and very large effects. See Section . | Consider this approach if the assumptions appear reasonable. If the minimal important difference for an instrument is known describing the probability of individuals achieving this difference may be more intuitive. Review authors should always seriously consider this option. Re-expressing SMDs is not the only way of expressing results as dichotomous outcomes. For example, the actual outcomes in the studies can be dichotomized, either directly or using assumptions, prior to meta-analysis. |
| ||
2. Ratio of means | This approach may be easily interpretable to clinical audiences and involves fewer assumptions than some other approaches. It allows applying GRADE guidance for large and very large effects. It cannot be applied when measure is a change from baseline and therefore negative values possible and the interpretation requires knowledge and interpretation of comparator group mean. See Section | Consider as complementing other approaches, particularly the presentation of relative and absolute effects. |
3. Minimal important difference units | This approach may be easily interpretable for audiences but is applicable only when minimal important differences are known. See Section . | Consider as complementing other approaches, particularly the presentation of relative and absolute effects. |
15.5.3.1 Presenting and interpreting SMDs using generic effect size estimates
The SMD expresses the intervention effect in standard units rather than the original units of measurement. The SMD is the difference in mean effects between the experimental and comparator groups divided by the pooled standard deviation of participants’ outcomes, or external SDs when studies are very small (see Chapter 6, Section 6.5.1.2 ). The value of a SMD thus depends on both the size of the effect (the difference between means) and the standard deviation of the outcomes (the inherent variability among participants or based on an external SD).
If review authors use the SMD, they might choose to present the results directly as SMDs (row 1a, Table 15.5.a and Table 15.5.b ). However, absolute values of the intervention and comparison groups are typically not useful because studies have used different measurement instruments with different units. Guiding rules for interpreting SMDs (or ‘Cohen’s effect sizes’) exist, and have arisen mainly from researchers in the social sciences (Cohen 1988). One example is as follows: 0.2 represents a small effect, 0.5 a moderate effect and 0.8 a large effect (Cohen 1988). Variations exist (e.g. <0.40=small, 0.40 to 0.70=moderate, >0.70=large). Review authors might consider including such a guiding rule in interpreting the SMD in the text of the review, and in summary versions such as the Comments column of a ‘Summary of findings’ table. However, some methodologists believe that such interpretations are problematic because patient importance of a finding is context-dependent and not amenable to generic statements.
15.5.3.2 Re-expressing SMDs using a familiar instrument
The second possibility for interpreting the SMD is to express it in the units of one or more of the specific measurement instruments used by the included studies (row 1b, Table 15.5.a and Table 15.5.b ). The approach is to calculate an absolute difference in means by multiplying the SMD by an estimate of the SD associated with the most familiar instrument. To obtain this SD, a reasonable option is to calculate a weighted average across all intervention groups of all studies that used the selected instrument (preferably a pre-intervention or post-intervention SD as discussed in Chapter 10, Section 10.5.2 ). To better reflect among-person variation in practice, or to use an instrument not represented in the meta-analysis, it may be preferable to use a standard deviation from a representative observational study. The summary effect is thus re-expressed in the original units of that particular instrument and the clinical relevance and impact of the intervention effect can be interpreted using that familiar instrument.
The same approach of re-expressing the results for a familiar instrument can also be used for other standardized effect measures such as when standardizing by MIDs (Guyatt et al 2013b): see Section 15.5.3.5 .
Table 15.5.b Application of approaches when studies have used different measures: effects of dexamethasone for pain after laparoscopic cholecystectomy (Karanicolas et al 2008). Reproduced with permission of Wolters Kluwer
|
|
|
|
|
| |
|
| |||||
|
| |||||
1a. Post-operative pain, standard deviation units Investigators measured pain using different instruments. Lower scores mean less pain. | The pain score in the dexamethasone groups was on average than in the placebo groups). | – | 539 (5) | OO Low |
As a rule of thumb, 0.2 SD represents a small difference, 0.5 a moderate and 0.8 a large. | |
1b. Post-operative pain Measured on a scale from 0, no pain, to 100, worst pain imaginable. | The mean post-operative pain scores with placebo ranged from 43 to 54. | The mean pain score in the intervention groups was on average
| – | 539 (5) |
OO Low | Scores calculated based on an SMD of 0.79 (95% CI –1.41 to –0.17) and rescaled to a 0 to 100 pain scale. The minimal important difference on the 0 to 100 pain scale is approximately 10. |
1c. Substantial post-operative pain, dichotomized Investigators measured pain using different instruments. | 20 per 100 | 15 more (4 more to 18 more) per 100 patients in dexamethasone group achieved important improvement in the pain score. | RR = 0.25 (95% CI 0.05 to 0.75) | 539 (5) | OO Low | Scores estimated based on an SMD of 0.79 (95% CI –1.41 to –0.17).
|
2. Post-operative pain Investigators measured pain using different instruments. Lower scores mean less pain. | The mean post-operative pain scores with placebo was 28.1. | On average a 3.7 lower pain score (0.6 to 6.1 lower) | Ratio of means 0.87 (0.78 to 0.98) | 539 (5) | OO Low | Weighted average of the mean pain score in dexamethasone group divided by mean pain score in placebo. |
3. Post-operative pain Investigators measured pain using different instruments. | The pain score in the dexamethasone groups was on average less than the control group. | – | 539 (5) | OO Low | An effect less than half the minimal important difference suggests a small or very small effect. |
1 Certainty rated according to GRADE from very low to high certainty. 2 Substantial unexplained heterogeneity in study results. 3 Imprecision due to wide confidence intervals. 4 The 20% comes from the proportion in the control group requiring rescue analgesia. 5 Crude (arithmetic) means of the post-operative pain mean responses across all five trials when transformed to a 100-point scale.
15.5.3.3 Re-expressing SMDs through dichotomization and transformation to relative and absolute measures
A third approach (row 1c, Table 15.5.a and Table 15.5.b ) relies on converting the continuous measure into a dichotomy and thus allows calculation of relative and absolute effects on a binary scale. A transformation of a SMD to a (log) odds ratio is available, based on the assumption that an underlying continuous variable has a logistic distribution with equal standard deviation in the two intervention groups, as discussed in Chapter 10, Section 10.6 (Furukawa 1999, Guyatt et al 2013b). The assumption is unlikely to hold exactly and the results must be regarded as an approximation. The log odds ratio is estimated as
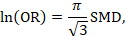
(or approximately 1.81✕SMD). The resulting odds ratio can then be presented as normal, and in a ‘Summary of findings’ table, combined with an assumed comparator group risk to be expressed as an absolute risk difference. The comparator group risk in this case would refer to the proportion of people who have achieved a specific value of the continuous outcome. In randomized trials this can be interpreted as the proportion who have improved by some (specified) amount (responders), for instance by 5 points on a 0 to 100 scale. Table 15.5.c shows some illustrative results from this method. The risk differences can then be converted to NNTs or to people per thousand using methods described in Section 15.4.4 .
Table 15.5.c Risk difference derived for specific SMDs for various given ‘proportions improved’ in the comparator group (Furukawa 1999, Guyatt et al 2013b). Reproduced with permission of Elsevier
|
|
|
|
|
|
|
|
|
| ||||||||
Situations in which the event is undesirable, reduction (or increase if intervention harmful) in adverse events with the intervention | |||||||||||||||||
| −3% | −5% | −7% | −8% | −8% | −8% | −7% | −6% | −4% | ||||||||
| −6% | −11% | −15% | −17% | −19% | −20% | −20% | −17% | −12% | ||||||||
| −8% | −15% | −21% | −25% | −29% | −31% | −31% | −28% | −22% | ||||||||
| −9% | −17% | −24% | −23% | −34% | −37% | −38% | −36% | −29% | ||||||||
Situations in which the event is desirable, increase (or decrease if intervention harmful) in positive responses to the intervention | |||||||||||||||||
| 4% | 6% | 7% | 8% | 8% | 8% | 7% | 5% | 3% | ||||||||
| 12% | 17% | 19% | 20% | 19% | 17% | 15% | 11% | 6% | ||||||||
| 22% | 28% | 31% | 31% | 29% | 25% | 21% | 15% | 8% | ||||||||
| 29% | 36% | 38% | 38% | 34% | 30% | 24% | 17% | 9% | ||||||||
15.5.3.4 Ratio of means
A more frequently used approach is based on calculation of a ratio of means between the intervention and comparator groups (Friedrich et al 2008) as discussed in Chapter 6, Section 6.5.1.3 . Interpretational advantages of this approach include the ability to pool studies with outcomes expressed in different units directly, to avoid the vulnerability of heterogeneous populations that limits approaches that rely on SD units, and for ease of clinical interpretation (row 2, Table 15.5.a and Table 15.5.b ). This method is currently designed for post-intervention scores only. However, it is possible to calculate a ratio of change scores if both intervention and comparator groups change in the same direction in each relevant study, and this ratio may sometimes be informative.
Limitations to this approach include its limited applicability to change scores (since it is unlikely that both intervention and comparator group changes are in the same direction in all studies) and the possibility of misleading results if the comparator group mean is very small, in which case even a modest difference from the intervention group will yield a large and therefore misleading ratio of means. It also requires that separate ratios of means be calculated for each included study, and then entered into a generic inverse variance meta-analysis (see Chapter 10, Section 10.3 ).
The ratio of means approach illustrated in Table 15.5.b suggests a relative reduction in pain of only 13%, meaning that those receiving steroids have a pain severity 87% of those in the comparator group, an effect that might be considered modest.
15.5.3.5 Presenting continuous results as minimally important difference units
To express results in MID units, review authors have two options. First, they can be combined across studies in the same way as the SMD, but instead of dividing the mean difference of each study by its SD, review authors divide by the MID associated with that outcome (Johnston et al 2010, Guyatt et al 2013b). Instead of SD units, the pooled results represent MID units (row 3, Table 15.5.a and Table 15.5.b ), and may be more easily interpretable. This approach avoids the problem of varying SDs across studies that may distort estimates of effect in approaches that rely on the SMD. The approach, however, relies on having well-established MIDs. The approach is also risky in that a difference less than the MID may be interpreted as trivial when a substantial proportion of patients may have achieved an important benefit.
The other approach makes a simple conversion (not shown in Table 15.5.b ), before undertaking the meta-analysis, of the means and SDs from each study to means and SDs on the scale of a particular familiar instrument whose MID is known. For example, one can rescale the mean and SD of other chronic respiratory disease instruments (e.g. rescaling a 0 to 100 score of an instrument) to a the 1 to 7 score in Chronic Respiratory Disease Questionnaire (CRQ) units (by assuming 0 equals 1 and 100 equals 7 on the CRQ). Given the MID of the CRQ of 0.5, a mean difference in change of 0.71 after rescaling of all studies suggests a substantial effect of the intervention (Guyatt et al 2013b). This approach, presenting in units of the most familiar instrument, may be the most desirable when the target audiences have extensive experience with that instrument, particularly if the MID is well established.
15.6 Drawing conclusions
15.6.1 conclusions sections of a cochrane review.
Authors’ conclusions in a Cochrane Review are divided into implications for practice and implications for research. While Cochrane Reviews about interventions can provide meaningful information and guidance for practice, decisions about the desirable and undesirable consequences of healthcare options require evidence and judgements for criteria that most Cochrane Reviews do not provide (Alonso-Coello et al 2016). In describing the implications for practice and the development of recommendations, however, review authors may consider the certainty of the evidence, the balance of benefits and harms, and assumed values and preferences.
15.6.2 Implications for practice
Drawing conclusions about the practical usefulness of an intervention entails making trade-offs, either implicitly or explicitly, between the estimated benefits, harms and the values and preferences. Making such trade-offs, and thus making specific recommendations for an action in a specific context, goes beyond a Cochrane Review and requires additional evidence and informed judgements that most Cochrane Reviews do not provide (Alonso-Coello et al 2016). Such judgements are typically the domain of clinical practice guideline developers for which Cochrane Reviews will provide crucial information (Graham et al 2011, Schünemann et al 2014, Zhang et al 2018a). Thus, authors of Cochrane Reviews should not make recommendations.
If review authors feel compelled to lay out actions that clinicians and patients could take, they should – after describing the certainty of evidence and the balance of benefits and harms – highlight different actions that might be consistent with particular patterns of values and preferences. Other factors that might influence a decision should also be highlighted, including any known factors that would be expected to modify the effects of the intervention, the baseline risk or status of the patient, costs and who bears those costs, and the availability of resources. Review authors should ensure they consider all patient-important outcomes, including those for which limited data may be available. In the context of public health reviews the focus may be on population-important outcomes as the target may be an entire (non-diseased) population and include outcomes that are not measured in the population receiving an intervention (e.g. a reduction of transmission of infections from those receiving an intervention). This process implies a high level of explicitness in judgements about values or preferences attached to different outcomes and the certainty of the related evidence (Zhang et al 2018b, Zhang et al 2018c); this and a full cost-effectiveness analysis is beyond the scope of most Cochrane Reviews (although they might well be used for such analyses; see Chapter 20 ).
A review on the use of anticoagulation in cancer patients to increase survival (Akl et al 2011a) provides an example for laying out clinical implications for situations where there are important trade-offs between desirable and undesirable effects of the intervention: “The decision for a patient with cancer to start heparin therapy for survival benefit should balance the benefits and downsides and integrate the patient’s values and preferences. Patients with a high preference for a potential survival prolongation, limited aversion to potential bleeding, and who do not consider heparin (both UFH or LMWH) therapy a burden may opt to use heparin, while those with aversion to bleeding may not.”
15.6.3 Implications for research
The second category for authors’ conclusions in a Cochrane Review is implications for research. To help people make well-informed decisions about future healthcare research, the ‘Implications for research’ section should comment on the need for further research, and the nature of the further research that would be most desirable. It is helpful to consider the population, intervention, comparison and outcomes that could be addressed, or addressed more effectively in the future, in the context of the certainty of the evidence in the current review (Brown et al 2006):
- P (Population): diagnosis, disease stage, comorbidity, risk factor, sex, age, ethnic group, specific inclusion or exclusion criteria, clinical setting;
- I (Intervention): type, frequency, dose, duration, prognostic factor;
- C (Comparison): placebo, routine care, alternative treatment/management;
- O (Outcome): which clinical or patient-related outcomes will the researcher need to measure, improve, influence or accomplish? Which methods of measurement should be used?
While Cochrane Review authors will find the PICO domains helpful, the domains of the GRADE certainty framework further support understanding and describing what additional research will improve the certainty in the available evidence. Note that as the certainty of the evidence is likely to vary by outcome, these implications will be specific to certain outcomes in the review. Table 15.6.a shows how review authors may be aided in their interpretation of the body of evidence and drawing conclusions about future research and practice.
Table 15.6.a Implications for research and practice suggested by individual GRADE domains
Domain | Implications for research | Examples for research statements | Implications for practice |
Risk of bias | Need for methodologically better designed and executed studies. | All studies suffered from lack of blinding of outcome assessors. Trials of this type are required. | The estimates of effect may be biased because of a lack of blinding of the assessors of the outcome. |
Inconsistency | Unexplained inconsistency: need for individual participant data meta-analysis; need for studies in relevant subgroups. | Studies in patients with small cell lung cancer are needed to understand if the effects differ from those in patients with pancreatic cancer. | Unexplained inconsistency: consider and interpret overall effect estimates as for the overall certainty of a body of evidence. Explained inconsistency (if results are not presented in strata): consider and interpret effects estimates by subgroup. |
Indirectness | Need for studies that better fit the PICO question of interest. | Studies in patients with early cancer are needed because the evidence is from studies in patients with advanced cancer. | It is uncertain if the results directly apply to the patients or the way that the intervention is applied in a particular setting. |
Imprecision | Need for more studies with more participants to reach optimal information size. | Studies with approximately 200 more events in the experimental intervention group and the comparator intervention group are required. | Same uncertainty interpretation as for certainty of a body of evidence: e.g. the true effect may be substantially different. |
Publication bias | Need to investigate and identify unpublished data; large studies might help resolve this issue. | Large studies are required. | Same uncertainty interpretation as for certainty of a body of evidence (e.g. the true effect may be substantially different). |
Large effects | No direct implications. | Not applicable. | The effect is large in the populations that were included in the studies and the true effect is likely going to cross important thresholds. |
Dose effects | No direct implications. | Not applicable. | The greater the reduction in the exposure the larger is the expected harm (or benefit). |
Opposing bias and confounding | Studies controlling for the residual bias and confounding are needed. | Studies controlling for possible confounders such as smoking and degree of education are required. | The effect could be even larger or smaller (depending on the direction of the results) than the one that is observed in the studies presented here. |
The review of compression stockings for prevention of deep vein thrombosis (DVT) in airline passengers described in Chapter 14 provides an example where there is some convincing evidence of a benefit of the intervention: “This review shows that the question of the effects on symptomless DVT of wearing versus not wearing compression stockings in the types of people studied in these trials should now be regarded as answered. Further research may be justified to investigate the relative effects of different strengths of stockings or of stockings compared to other preventative strategies. Further randomised trials to address the remaining uncertainty about the effects of wearing versus not wearing compression stockings on outcomes such as death, pulmonary embolism and symptomatic DVT would need to be large.” (Clarke et al 2016).
A review of therapeutic touch for anxiety disorder provides an example of the implications for research when no eligible studies had been found: “This review highlights the need for randomized controlled trials to evaluate the effectiveness of therapeutic touch in reducing anxiety symptoms in people diagnosed with anxiety disorders. Future trials need to be rigorous in design and delivery, with subsequent reporting to include high quality descriptions of all aspects of methodology to enable appraisal and interpretation of results.” (Robinson et al 2007).
15.6.4 Reaching conclusions
A common mistake is to confuse ‘no evidence of an effect’ with ‘evidence of no effect’. When the confidence intervals are too wide (e.g. including no effect), it is wrong to claim that the experimental intervention has ‘no effect’ or is ‘no different’ from the comparator intervention. Review authors may also incorrectly ‘positively’ frame results for some effects but not others. For example, when the effect estimate is positive for a beneficial outcome but confidence intervals are wide, review authors may describe the effect as promising. However, when the effect estimate is negative for an outcome that is considered harmful but the confidence intervals include no effect, review authors report no effect. Another mistake is to frame the conclusion in wishful terms. For example, review authors might write, “there were too few people in the analysis to detect a reduction in mortality” when the included studies showed a reduction or even increase in mortality that was not ‘statistically significant’. One way of avoiding errors such as these is to consider the results blinded; that is, consider how the results would be presented and framed in the conclusions if the direction of the results was reversed. If the confidence interval for the estimate of the difference in the effects of the interventions overlaps with no effect, the analysis is compatible with both a true beneficial effect and a true harmful effect. If one of the possibilities is mentioned in the conclusion, the other possibility should be mentioned as well. Table 15.6.b suggests narrative statements for drawing conclusions based on the effect estimate from the meta-analysis and the certainty of the evidence.
Table 15.6.b Suggested narrative statements for phrasing conclusions
|
|
High certainty of the evidence | |
Large effect | X results in a large reduction/increase in outcome |
Moderate effect | X reduces/increases outcome X results in a reduction/increase in outcome |
Small important effect | X reduces/increases outcome slightly X results in a slight reduction/increase in outcome |
Trivial, small unimportant effect or no effect | X results in little to no difference in outcome X does not reduce/increase outcome |
Moderate certainty of the evidence | |
Large effect | X likely results in a large reduction/increase in outcome X probably results in a large reduction/increase in outcome |
Moderate effect | X likely reduces/increases outcome X probably reduces/increases outcome X likely results in a reduction/increase in outcome X probably results in a reduction/increase in outcome |
Small important effect | X probably reduces/increases outcome slightly X likely reduces/increases outcome slightly X probably results in a slight reduction/increase in outcome X likely results in a slight reduction/increase in outcome |
Trivial, small unimportant effect or no effect | X likely results in little to no difference in outcome X probably results in little to no difference in outcome X likely does not reduce/increase outcome X probably does not reduce/increase outcome |
Low certainty of the evidence | |
Large effect | X may result in a large reduction/increase in outcome The evidence suggests X results in a large reduction/increase in outcome |
Moderate effect | X may reduce/increase outcome The evidence suggests X reduces/increases outcome X may result in a reduction/increase in outcome The evidence suggests X results in a reduction/increase in outcome |
Small important effect | X may reduce/increase outcome slightly The evidence suggests X reduces/increases outcome slightly X may result in a slight reduction/increase in outcome The evidence suggests X results in a slight reduction/increase in outcome |
Trivial, small unimportant effect or no effect | X may result in little to no difference in outcome The evidence suggests that X results in little to no difference in outcome X may not reduce/increase outcome The evidence suggests that X does not reduce/increase outcome |
Very low certainty of the evidence | |
Any effect | The evidence is very uncertain about the effect of X on outcome X may reduce/increase/have little to no effect on outcome but the evidence is very uncertain |
Another common mistake is to reach conclusions that go beyond the evidence. Often this is done implicitly, without referring to the additional information or judgements that are used in reaching conclusions about the implications of a review for practice. Even when additional information and explicit judgements support conclusions about the implications of a review for practice, review authors rarely conduct systematic reviews of the additional information. Furthermore, implications for practice are often dependent on specific circumstances and values that must be taken into consideration. As we have noted, review authors should always be cautious when drawing conclusions about implications for practice and they should not make recommendations.
15.7 Chapter information
Authors: Holger J Schünemann, Gunn E Vist, Julian PT Higgins, Nancy Santesso, Jonathan J Deeks, Paul Glasziou, Elie Akl, Gordon H Guyatt; on behalf of the Cochrane GRADEing Methods Group
Acknowledgements: Andrew Oxman, Jonathan Sterne, Michael Borenstein and Rob Scholten contributed text to earlier versions of this chapter.
Funding: This work was in part supported by funding from the Michael G DeGroote Cochrane Canada Centre and the Ontario Ministry of Health. JJD receives support from the National Institute for Health Research (NIHR) Birmingham Biomedical Research Centre at the University Hospitals Birmingham NHS Foundation Trust and the University of Birmingham. JPTH receives support from the NIHR Biomedical Research Centre at University Hospitals Bristol NHS Foundation Trust and the University of Bristol. The views expressed are those of the author(s) and not necessarily those of the NHS, the NIHR or the Department of Health.
15.8 References
Aguilar MI, Hart R. Oral anticoagulants for preventing stroke in patients with non-valvular atrial fibrillation and no previous history of stroke or transient ischemic attacks. Cochrane Database of Systematic Reviews 2005; 3 : CD001927.
Aguilar MI, Hart R, Pearce LA. Oral anticoagulants versus antiplatelet therapy for preventing stroke in patients with non-valvular atrial fibrillation and no history of stroke or transient ischemic attacks. Cochrane Database of Systematic Reviews 2007; 3 : CD006186.
Akl EA, Gunukula S, Barba M, Yosuico VE, van Doormaal FF, Kuipers S, Middeldorp S, Dickinson HO, Bryant A, Schünemann H. Parenteral anticoagulation in patients with cancer who have no therapeutic or prophylactic indication for anticoagulation. Cochrane Database of Systematic Reviews 2011a; 1 : CD006652.
Akl EA, Oxman AD, Herrin J, Vist GE, Terrenato I, Sperati F, Costiniuk C, Blank D, Schünemann H. Using alternative statistical formats for presenting risks and risk reductions. Cochrane Database of Systematic Reviews 2011b; 3 : CD006776.
Alonso-Coello P, Schünemann HJ, Moberg J, Brignardello-Petersen R, Akl EA, Davoli M, Treweek S, Mustafa RA, Rada G, Rosenbaum S, Morelli A, Guyatt GH, Oxman AD, Group GW. GRADE Evidence to Decision (EtD) frameworks: a systematic and transparent approach to making well informed healthcare choices. 1: Introduction. BMJ 2016; 353 : i2016.
Altman DG. Confidence intervals for the number needed to treat. BMJ 1998; 317 : 1309-1312.
Atkins D, Best D, Briss PA, Eccles M, Falck-Ytter Y, Flottorp S, Guyatt GH, Harbour RT, Haugh MC, Henry D, Hill S, Jaeschke R, Leng G, Liberati A, Magrini N, Mason J, Middleton P, Mrukowicz J, O'Connell D, Oxman AD, Phillips B, Schünemann HJ, Edejer TT, Varonen H, Vist GE, Williams JW, Jr., Zaza S. Grading quality of evidence and strength of recommendations. BMJ 2004; 328 : 1490.
Brown P, Brunnhuber K, Chalkidou K, Chalmers I, Clarke M, Fenton M, Forbes C, Glanville J, Hicks NJ, Moody J, Twaddle S, Timimi H, Young P. How to formulate research recommendations. BMJ 2006; 333 : 804-806.
Cates C. Confidence intervals for the number needed to treat: Pooling numbers needed to treat may not be reliable. BMJ 1999; 318 : 1764-1765.
Clarke MJ, Broderick C, Hopewell S, Juszczak E, Eisinga A. Compression stockings for preventing deep vein thrombosis in airline passengers. Cochrane Database of Systematic Reviews 2016; 9 : CD004002.
Cohen J. Statistical Power Analysis in the Behavioral Sciences . 2nd edition ed. Hillsdale (NJ): Lawrence Erlbaum Associates, Inc.; 1988.
Coleman T, Chamberlain C, Davey MA, Cooper SE, Leonardi-Bee J. Pharmacological interventions for promoting smoking cessation during pregnancy. Cochrane Database of Systematic Reviews 2015; 12 : CD010078.
Dans AM, Dans L, Oxman AD, Robinson V, Acuin J, Tugwell P, Dennis R, Kang D. Assessing equity in clinical practice guidelines. Journal of Clinical Epidemiology 2007; 60 : 540-546.
Friedman LM, Furberg CD, DeMets DL. Fundamentals of Clinical Trials . 2nd edition ed. Littleton (MA): John Wright PSG, Inc.; 1985.
Friedrich JO, Adhikari NK, Beyene J. The ratio of means method as an alternative to mean differences for analyzing continuous outcome variables in meta-analysis: a simulation study. BMC Medical Research Methodology 2008; 8 : 32.
Furukawa T. From effect size into number needed to treat. Lancet 1999; 353 : 1680.
Graham R, Mancher M, Wolman DM, Greenfield S, Steinberg E. Committee on Standards for Developing Trustworthy Clinical Practice Guidelines, Board on Health Care Services: Clinical Practice Guidelines We Can Trust. Washington, DC: National Academies Press; 2011.
Guyatt G, Oxman AD, Akl EA, Kunz R, Vist G, Brozek J, Norris S, Falck-Ytter Y, Glasziou P, DeBeer H, Jaeschke R, Rind D, Meerpohl J, Dahm P, Schünemann HJ. GRADE guidelines: 1. Introduction-GRADE evidence profiles and summary of findings tables. Journal of Clinical Epidemiology 2011a; 64 : 383-394.
Guyatt GH, Juniper EF, Walter SD, Griffith LE, Goldstein RS. Interpreting treatment effects in randomised trials. BMJ 1998; 316 : 690-693.
Guyatt GH, Oxman AD, Vist GE, Kunz R, Falck-Ytter Y, Alonso-Coello P, Schünemann HJ. GRADE: an emerging consensus on rating quality of evidence and strength of recommendations. BMJ 2008; 336 : 924-926.
Guyatt GH, Oxman AD, Kunz R, Woodcock J, Brozek J, Helfand M, Alonso-Coello P, Falck-Ytter Y, Jaeschke R, Vist G, Akl EA, Post PN, Norris S, Meerpohl J, Shukla VK, Nasser M, Schünemann HJ. GRADE guidelines: 8. Rating the quality of evidence--indirectness. Journal of Clinical Epidemiology 2011b; 64 : 1303-1310.
Guyatt GH, Oxman AD, Santesso N, Helfand M, Vist G, Kunz R, Brozek J, Norris S, Meerpohl J, Djulbegovic B, Alonso-Coello P, Post PN, Busse JW, Glasziou P, Christensen R, Schünemann HJ. GRADE guidelines: 12. Preparing summary of findings tables-binary outcomes. Journal of Clinical Epidemiology 2013a; 66 : 158-172.
Guyatt GH, Thorlund K, Oxman AD, Walter SD, Patrick D, Furukawa TA, Johnston BC, Karanicolas P, Akl EA, Vist G, Kunz R, Brozek J, Kupper LL, Martin SL, Meerpohl JJ, Alonso-Coello P, Christensen R, Schünemann HJ. GRADE guidelines: 13. Preparing summary of findings tables and evidence profiles-continuous outcomes. Journal of Clinical Epidemiology 2013b; 66 : 173-183.
Hawe P, Shiell A, Riley T, Gold L. Methods for exploring implementation variation and local context within a cluster randomised community intervention trial. Journal of Epidemiology and Community Health 2004; 58 : 788-793.
Hoffrage U, Lindsey S, Hertwig R, Gigerenzer G. Medicine. Communicating statistical information. Science 2000; 290 : 2261-2262.
Jaeschke R, Singer J, Guyatt GH. Measurement of health status. Ascertaining the minimal clinically important difference. Controlled Clinical Trials 1989; 10 : 407-415.
Johnston B, Thorlund K, Schünemann H, Xie F, Murad M, Montori V, Guyatt G. Improving the interpretation of health-related quality of life evidence in meta-analysis: The application of minimal important difference units. . Health Outcomes and Qualithy of Life 2010; 11 : 116.
Karanicolas PJ, Smith SE, Kanbur B, Davies E, Guyatt GH. The impact of prophylactic dexamethasone on nausea and vomiting after laparoscopic cholecystectomy: a systematic review and meta-analysis. Annals of Surgery 2008; 248 : 751-762.
Lumley J, Oliver SS, Chamberlain C, Oakley L. Interventions for promoting smoking cessation during pregnancy. Cochrane Database of Systematic Reviews 2004; 4 : CD001055.
McQuay HJ, Moore RA. Using numerical results from systematic reviews in clinical practice. Annals of Internal Medicine 1997; 126 : 712-720.
Resnicow K, Cross D, Wynder E. The Know Your Body program: a review of evaluation studies. Bulletin of the New York Academy of Medicine 1993; 70 : 188-207.
Robinson J, Biley FC, Dolk H. Therapeutic touch for anxiety disorders. Cochrane Database of Systematic Reviews 2007; 3 : CD006240.
Rothwell PM. External validity of randomised controlled trials: "to whom do the results of this trial apply?". Lancet 2005; 365 : 82-93.
Santesso N, Carrasco-Labra A, Langendam M, Brignardello-Petersen R, Mustafa RA, Heus P, Lasserson T, Opiyo N, Kunnamo I, Sinclair D, Garner P, Treweek S, Tovey D, Akl EA, Tugwell P, Brozek JL, Guyatt G, Schünemann HJ. Improving GRADE evidence tables part 3: detailed guidance for explanatory footnotes supports creating and understanding GRADE certainty in the evidence judgments. Journal of Clinical Epidemiology 2016; 74 : 28-39.
Schünemann HJ, Puhan M, Goldstein R, Jaeschke R, Guyatt GH. Measurement properties and interpretability of the Chronic respiratory disease questionnaire (CRQ). COPD: Journal of Chronic Obstructive Pulmonary Disease 2005; 2 : 81-89.
Schünemann HJ, Guyatt GH. Commentary--goodbye M(C)ID! Hello MID, where do you come from? Health Services Research 2005; 40 : 593-597.
Schünemann HJ, Fretheim A, Oxman AD. Improving the use of research evidence in guideline development: 13. Applicability, transferability and adaptation. Health Research Policy and Systems 2006; 4 : 25.
Schünemann HJ. Methodological idiosyncracies, frameworks and challenges of non-pharmaceutical and non-technical treatment interventions. Zeitschrift für Evidenz, Fortbildung und Qualität im Gesundheitswesen 2013; 107 : 214-220.
Schünemann HJ, Tugwell P, Reeves BC, Akl EA, Santesso N, Spencer FA, Shea B, Wells G, Helfand M. Non-randomized studies as a source of complementary, sequential or replacement evidence for randomized controlled trials in systematic reviews on the effects of interventions. Research Synthesis Methods 2013; 4 : 49-62.
Schünemann HJ, Wiercioch W, Etxeandia I, Falavigna M, Santesso N, Mustafa R, Ventresca M, Brignardello-Petersen R, Laisaar KT, Kowalski S, Baldeh T, Zhang Y, Raid U, Neumann I, Norris SL, Thornton J, Harbour R, Treweek S, Guyatt G, Alonso-Coello P, Reinap M, Brozek J, Oxman A, Akl EA. Guidelines 2.0: systematic development of a comprehensive checklist for a successful guideline enterprise. CMAJ: Canadian Medical Association Journal 2014; 186 : E123-142.
Schünemann HJ. Interpreting GRADE's levels of certainty or quality of the evidence: GRADE for statisticians, considering review information size or less emphasis on imprecision? Journal of Clinical Epidemiology 2016; 75 : 6-15.
Smeeth L, Haines A, Ebrahim S. Numbers needed to treat derived from meta-analyses--sometimes informative, usually misleading. BMJ 1999; 318 : 1548-1551.
Sun X, Briel M, Busse JW, You JJ, Akl EA, Mejza F, Bala MM, Bassler D, Mertz D, Diaz-Granados N, Vandvik PO, Malaga G, Srinathan SK, Dahm P, Johnston BC, Alonso-Coello P, Hassouneh B, Walter SD, Heels-Ansdell D, Bhatnagar N, Altman DG, Guyatt GH. Credibility of claims of subgroup effects in randomised controlled trials: systematic review. BMJ 2012; 344 : e1553.
Zhang Y, Akl EA, Schünemann HJ. Using systematic reviews in guideline development: the GRADE approach. Research Synthesis Methods 2018a: doi: 10.1002/jrsm.1313.
Zhang Y, Alonso-Coello P, Guyatt GH, Yepes-Nunez JJ, Akl EA, Hazlewood G, Pardo-Hernandez H, Etxeandia-Ikobaltzeta I, Qaseem A, Williams JW, Jr., Tugwell P, Flottorp S, Chang Y, Zhang Y, Mustafa RA, Rojas MX, Schünemann HJ. GRADE Guidelines: 19. Assessing the certainty of evidence in the importance of outcomes or values and preferences-Risk of bias and indirectness. Journal of Clinical Epidemiology 2018b: doi: 10.1016/j.jclinepi.2018.1001.1013.
Zhang Y, Alonso Coello P, Guyatt G, Yepes-Nunez JJ, Akl EA, Hazlewood G, Pardo-Hernandez H, Etxeandia-Ikobaltzeta I, Qaseem A, Williams JW, Jr., Tugwell P, Flottorp S, Chang Y, Zhang Y, Mustafa RA, Rojas MX, Xie F, Schünemann HJ. GRADE Guidelines: 20. Assessing the certainty of evidence in the importance of outcomes or values and preferences - Inconsistency, Imprecision, and other Domains. Journal of Clinical Epidemiology 2018c: doi: 10.1016/j.jclinepi.2018.1005.1011.
For permission to re-use material from the Handbook (either academic or commercial), please see here for full details.

An official website of the United States government
The .gov means it’s official. Federal government websites often end in .gov or .mil. Before sharing sensitive information, make sure you’re on a federal government site.
The site is secure. The https:// ensures that you are connecting to the official website and that any information you provide is encrypted and transmitted securely.
- Publications
- Account settings
Preview improvements coming to the PMC website in October 2024. Learn More or Try it out now .
- Advanced Search
- Journal List
- J Grad Med Educ
- v.5(4); 2013 Dec
Analyzing and Interpreting Data From Likert-Type Scales
Likert-type scales are frequently used in medical education and medical education research. Common uses include end-of-rotation trainee feedback, faculty evaluations of trainees, and assessment of performance after an educational intervention. A sizable percentage of the educational research manuscripts submitted to the Journal of Graduate Medical Education employ a Likert scale for part or all of the outcome assessments. Thus, understanding the interpretation and analysis of data derived from Likert scales is imperative for those working in medical education and education research. The goal of this article is to provide readers who do not have extensive statistics background with the basics needed to understand these concepts.
Developed in 1932 by Rensis Likert 1 to measure attitudes, the typical Likert scale is a 5- or 7-point ordinal scale used by respondents to rate the degree to which they agree or disagree with a statement ( table ). In an ordinal scale, responses can be rated or ranked, but the distance between responses is not measurable. Thus, the differences between “always,” “often,” and “sometimes” on a frequency response Likert scale are not necessarily equal. In other words, one cannot assume that the difference between responses is equidistant even though the numbers assigned to those responses are. This is in contrast to interval data, in which the difference between responses can be calculated and the numbers do refer to a measureable “something.” An example of interval data would be numbers of procedures done per resident: a score of 3 means the resident has conducted 3 procedures. Interestingly, with computer technology, survey designers can create continuous measure scales that do provide interval responses as an alternative to a Likert scale. The various continuous measures for pain are well-known examples of this ( figure 1 ).

Continuous Measure Example
Please tell us your current pain level by sliding the pointer to the appropriate point along the continuous pain scale above.
Typical Likert Scales

The Controversy
In the medical education literature, there has been a long-standing controversy regarding whether ordinal data, converted to numbers, can be treated as interval data. 2 That is, can means, standard deviations, and parametric statistics, which depend upon data that are normally distributed ( figure 2 ), be used to analyze ordinal data?

A Normal Distribution
When conducting research, we measure data from a sample of the total population of interest, not from all members of the population. Parametric tests make assumptions about the underlying population from which the research data have been obtained—usually that these population data are normally distributed. Nonparametric tests do not make this assumption about the “shape” of the population from which the study data have been drawn. Nonparametric tests are less powerful than parametric tests and usually require a larger sample size (n value) to have the same power as parametric tests to find a difference between groups when a difference actually exists. Descriptive statistics, such as means and standard deviations, have unclear meanings when applied to Likert scale responses. For example, what does the average of “never” and “rarely” really mean? Does “rarely and a half” have a useful meaning? 3 Furthermore, if responses are clustered at the high and low extremes, the mean may appear to be the neutral or middle response, but this may not fairly characterize the data. This clustering of extremes is common, for example, in trainee evaluations of experiences that may be very popular with one group and perceived as unnecessary by others (eg, an epidemiology course in medical school). Other non-normal distributions of response data can similarly result in a mean score that is not a helpful measure of the data's central tendency.
Because of these observations, experts over the years have argued that the median should be used as the measure of central tendency for Likert scale data. 3 Similarly, experts have contended that frequencies (percentages of responses in each category), contingency tables, χ 2 tests, the Spearman rho assessment, or the Mann-Whitney U test should be used for analysis instead of parametric tests, which, strictly speaking, require interval data (eg, t tests, analysis of variance, Pearson correlations, regression). 3 However, other experts assert that if there is an adequate sample size (at least 5–10 observations per group) and if the data are normally distributed (or nearly normal), parametric tests can be used with Likert scale ordinal data. 3
Fortunately, Dr. Geoff Norman, one of world's leaders in medical education research methodology, has comprehensively reviewed this controversy. He provides compelling evidence, with actual examples using real and simulated data, that parametric tests not only can be used with ordinal data, such as data from Likert scales, but also that parametric tests are generally more robust than nonparametric tests. That is, parametric tests tend to give “the right answer” even when statistical assumptions—such as a normal distribution of data—are violated, even to an extreme degree. 4 Thus, parametric tests are sufficiently robust to yield largely unbiased answers that are acceptably close to “the truth” when analyzing Likert scale responses. 4
Educators and researchers also commonly create several Likert-type items, group them into a “survey scale,” and then calculate a total score or mean score for the scale items. Often this practice is recommended, particularly when researchers are attempting to measure less concrete concepts, such as trainee motivation, patient satisfaction, and physician confidence—where a single survey item is unlikely to be capable of fully capturing the concept being assessed. 5 In these cases, experts suggest using the Cronbach alpha or Kappa test or factor analysis technique to provide evidence that the components of the scale are sufficiently intercorrelated and that the grouped items measure the underlying variable.
The Bottom Line
Now that many experts have weighed in on this debate, the conclusions are fairly clear: parametric tests can be used to analyze Likert scale responses. However, to describe the data, means are often of limited value unless the data follow a classic normal distribution and a frequency distribution of responses will likely be more helpful. Furthermore, because the numbers derived from Likert scales represent ordinal responses, presentation of a mean to the 100th decimal place is usually not helpful or enlightening to readers.
In summary, we recommend that authors determine how they will describe and analyze their data as a first step in planning educational or research projects. Then they should discuss, in the Methods section or in a cover letter if the explanation is too lengthy, why they have chosen to portray and analyze their data in a particular way. Reviewers, readers, and especially editors will greatly appreciate this additional effort.
Gail M. Sullivan, MD, MPH, is Editor-in-Chief of the Journal of Graduate Medical Education, and Anthony R. Artino Jr, PhD, is Associate Professor of Medicine and Preventive Medicine and Biometrics, Uniformed Services University of the Health Sciences.
Academia.edu no longer supports Internet Explorer.
To browse Academia.edu and the wider internet faster and more securely, please take a few seconds to upgrade your browser .
Enter the email address you signed up with and we'll email you a reset link.
- We're Hiring!
- Help Center
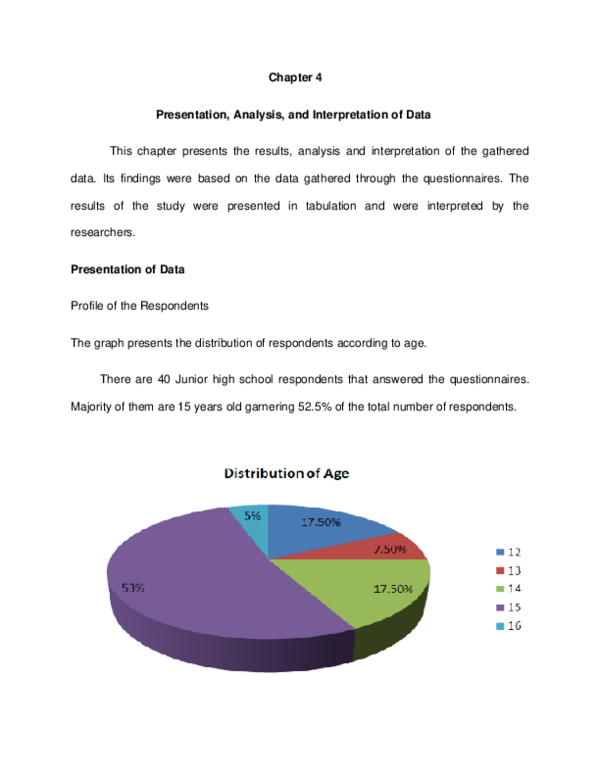
Chapter 4 Presentation, Analysis, and Interpretation of Data

Related Papers
The analysis and interpretation of data about wearing high heels for female students of Ligao community college.
James Joel Delos Reyes
The analysis and interpretation of data about wearing high heels for female students of Ligao community college. To complete this study properly, it is necessary to analyze the data collected in order to answer the research questions. Data is interpreted in a descriptive form. This chapter comprises the analysis, presentation and interpretation of the findings resulting from this study. The analysis and interpretation of data is carried out in two phases. The first part, which is based on the results of the questionnaire, deals with a qualitative analysis of data. The second, which is based on quantitative analysis. The unit of analysis is the major entity that the researcher going to analyze in the study. It is not 'what' or 'who' that is being studied. Researchers were collecting the data or information from the following female student for the completion of the study 100 questionnaires were distributed, only 80 was retrieve, some students did not completely answer the given data, few of them with a lot of missing data, while the remaining students answer well on the given questionnaire. The researchers use table in order to easily identify the data and interpret it according to the response of the following female students. In order to get the percentage of the following data we use the formula P=the value or the frequency/ by total respondent, (100) multiply (100). Table 1.
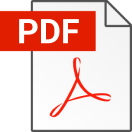
Eğitimde Kuram ve Uygulama
Tamer Kutluca
1.Uluslararası İnsan Çalışmaları Kongresi (ICHUS2018)
ARİF DURĞUN , Burhanettin Uysal
Giriş ve Amaç: Bu çalışma ile hastanelerde çalışan sağlık meslek mensuplarının mesleki ve örgütsel bağlılık düzeyleri arasındaki ilişkiyi incelemek ve daha önce hemşireler üzerinde yapılan araştırmalardan yola çıkarak sağlık meslek mensupları üzerinde uygulayarak literatüre veri kazandırılmak amaçlanmaktadır. Gereç ve Yöntem: Araştırma, Bolu ili İzzet Baysal Üniversitesi İzzet Baysal Eğitim ve Araştırma Hastanesinde sağlık meslek mensupları üzerinde 2018 yılı Ekim-Kasım ayında yapılmıştır. Araştırmanın evrenini tüm sağlık meslek mensupları oluşturmaktadır. Araştırma etik kurul onayı alındıktan sonra hastanede görev yapan 684 sağlık meslek mensubundan tesadüfî örnekleme bağlı kalarak %95 güven aralığında %5 hata payı ile 247 çalışan üzerinde planlandı ancak araştırmanın sınırlılıklarından dolayı 201 çalışan üzerinde uygulandı. Anketlerden elde edilen veriler SPSS (Statistical Package for the Social Sciences) programı ile analiz edildi. Ayrıca sosyo-demografik özelliklere göre sağlık meslek mensupları arasında önemli farklılıklar gösterip göstermediğini analiz etmek için t testi, tek yönlü varyans analizi, ölçeklerin boyutları arasında korelasyon ve regresyon analizleri yapıldı. Her iki ölçeğe de Lisrel programı ile doğrulayıcı faktör analizi uygulandı. Bulgular ve Sonuç: Araştırmadan elde edilen bulgulara göre örgütsel bağlılık ve mesleki bağlılık güvenirlik analizi Cronbach’s alpha değerleri yüksek ve çok yüksek bulundu. Demografik faktörlerle ölçeklerin toplam puanları ile yapılan t-testi ve tek yönlü anova sonuçlarına göre cinsiyet, medeni durum değişkenleri açısından mesleki bağlılık ve örgütsel bağlılık anlamlı farklılaşmamaktadır. Eğitim değişkenine göre mesleki bağlılığın mesleki üyeliği sürdürme alt boyutunda anlamlı farklılaştığı, farklılığın doktora-lisans ve doktora-lise arasından kaynaklandığı bulundu. Unvan değişkenine göre çaba gösterme alt boyutunda anlamlı farklılaştı. Çalışma saatleri değişkeninde ise her iki ölçekte de anlamlı farklılık bulundu. Boyutlar arasında pozitif yönlü anlamlı ilişkiler bulundu (p<0,01;0,05). Regresyon analizine göre mesleki bağlılık, örgütsel bağlılığı etkilemektedir (p<0,01; R²:0,108;B=0,328).
bilal yaman
Bu araştırmanın amacı, gençlik merkezi faaliyetlerine katılan bireylerin bazı değişkenlere göre serbest zaman tatmin düzeylerinin incelenmesidir. Evreni Türkiye İç Anadolu bölgesindeki Gençlik merkezine üye gençler oluşturmaktadır. Araştırma grubunu ise bu bölgede bulunan 11 ildeki Gençlik merkezlerine üye olan yaşları 13-27 arasında değişen 906 birey oluşturmaktadır. Araştırma verileri toplanmasında, serbest zaman tatmin düzeylerini belirlemek amacıyla Beard ve Ragheb' in (1980) geliştirdikleri, Karlı ve arkadaşlarının (2008) yılında geçerlilik güvenilirlik çalışmasını yaparak Türkçe literatüre kazandırdıkları 39 sorudan ve altı alt boyuttan oluşan iç tutarlılık (Chronbach Alfa) katsayısı 92 olarak bulunmuş Serbest Zaman Tatmin Ölçeği (Leisure Satisfaction Scale/LSS) kullanılmıştır. Verilerin analizinde değişkenlerin gruplara göre dağılımları incelenmiş, dağılımların normalliği ve varyansların homojenliği değerlendirilerek dağılımların parametrik özellik sergilemediği sonucuna ...
Chris Isokpenhi
abdurrahman kırtepe
Mehari Tesfai
International Journal of Anatolia Sport Sciences
serap çolak
DergiPark (Istanbul University)
mehmet Atlar
michaelroy yabes
Loading Preview
Sorry, preview is currently unavailable. You can download the paper by clicking the button above.
RELATED PAPERS
Venilyn April Barrientos
Beden Egitimi Ve Spor Bilimleri Dergisi
Talha Murathan
Hacettepe Üniversitesi Hemşirelik Fakültesi dergisi
Emine Geçkil
Emine Önder
Turkiye Klinikleri Journal of Medical Sciences
Ahmet Sunter
Accountability in Human Resource Management
Manmeet Bali Nag
İstanbul Gelişim Üniversitesi Sosyal Bilimler Dergisi
Yağmur Callak
Kastamonu Eğitim Dergisi
özgür Kalafat
Opus uluslararası toplum araştırmaları dergisi
Ilknur Maya
Journal of Turkish Studies
Ana Dili Eğitimi Dergisi
Gıyasettin Aytaş
Gül KALELİ YILMAZ
tutku başöz
Journal of International Social Research
Okan SARIGÖZ
Cevdet Cengiz
Ismail gürler
Yadigar Polat
Düzce Tıp Fakültesi Dergisi
melih Şahin
Sebahattin Devecioglu
The Journal of Academic Social Science Studies
Erhan görmez
Kuramsal Eğitimbilim
kübra erbey
Melike Yoncalık
Cukurova University Faculty of Education Journal
mehmet ali kandemir
International journal of Science Culture and Sport
Mehmet güllü
- We're Hiring!
- Help Center
- Find new research papers in:
- Health Sciences
- Earth Sciences
- Cognitive Science
- Mathematics
- Computer Science
- Academia ©2024

Sometimes, often, and always: Exploring the vague meanings of frequency expressions
- Published: 07 July 2011
- Volume 44 , pages 144–157, ( 2012 )
Cite this article
- Franziska Bocklisch 1 ,
- Steffen F. Bocklisch 2 &
- Josef F. Krems 1
24k Accesses
38 Citations
3 Altmetric
Explore all metrics
The article describes a general two-step procedure for the numerical translation of vague linguistic terms (LTs). The suggested procedure consists of empirical and model components, including (1) participants’ estimates of numerical values corresponding to verbal terms and (2) modeling of the empirical data using fuzzy membership functions (MFs), respectively. The procedure is outlined in two studies for data from N = 89 and N = 109 participants, who were asked to estimate numbers corresponding to 11 verbal frequency expressions (e.g., sometimes ). Positions and shapes of the resulting MFs varied considerably in symmetry, vagueness, and overlap and are indicative of the different meanings of the vague frequency expressions. Words were not distributed equidistantly across the numerical scale. This has important implications for the many questionnaires that use verbal rating scales, which consist of frequency expressions and operate on the premise of equidistance. These results are discussed for an exemplar questionnaire (COPSOQ). Furthermore, the variation of the number of prompted LTs (5 vs. 11) showed no influence on the words’ interpretations.
Similar content being viewed by others
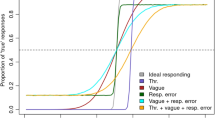
Most quantifiers have many meanings
Fuzzy Rating vs. Fuzzy Conversion Scales: An Empirical Comparison through the MSE
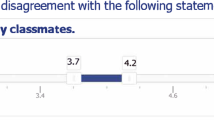
On some properties of Cronbach’s α coefficient for interval-valued data in questionnaires
Avoid common mistakes on your manuscript.
Since the 1960s, researchers in different scientific areas have sustained an interest in studying the relationship between verbal and numerical expressions—particularly, probability words and quantifiers (Bocklisch, Bocklisch, & Krems, 2010 ; Dhami & Wallsten, 2005 ; Lichtenstein & Newman, 1967 ; Teigen & Brun, 2003 ). Moreover, expressions of intensity or frequency of occurrence (e.g., sometimes or often ) are of interest with regard to their wide application in questionnaires. Several studies consistently showed that people prefer to use words instead of numbers to indicate their opinions and uncertainty (e.g., Wallsten, Budescu, Zwick, & Kemp, 1993 ). Even experts such as doctors or lawyers frequently use qualitative rather than quantitative terms to express their beliefs, on the grounds that words are more natural and are easier to understand and communicate. Words are especially useful in most everyday situations when subjective belief or uncertainty cannot be precisely verbalized in quantitative terms. Therefore, while it may be more natural for people to use language to express their beliefs, it is also potentially more advantageous to use numerical estimates: Their standard interpretation renders them easily comparable, and they form the basis of calculations and computational inferences. Accordingly, many researchers have developed translation procedures (e.g., Beyth-Marom, 1982 ; Bocklisch et al., 2010 ; Budescu, Karelitz, & Wallsten, 2003 ) and have established numerical equivalents for common linguistic expressions (for a broader literature review, see Teigen & Brun, 2003 ). One outcome of these efforts is that linguistic terms have often been conceptualized as fuzzy sets and mathematically described using fuzzy membership functions (MFs; Budescu et al., 2003 ; Zadeh, 1965 ; Zimmer, 1984 ).
Figure 1 shows an example of the fuzzy MF for the linguistic term probable reported by Bocklisch et al. ( 2010 ). The function’s shape and position represent the vague meaning of probable on a 0–1.0 probability scale. The numerical probabilities occurring between approximately P = .6 and P = .75 show the highest membership values and, therefore, are most representative and describe the meaning of probable best. Because the vague linguistic term has no sharp boundary, the membership values for the other numerical probabilities decrease continuously from the function’s peak. Hence, they are less representative of the meaning of probable .
Example of a fuzzy membership function for the linguistic term probable (see Bocklisch, Bocklisch, & Krems, 2010 )
The two studies presented herein support the objectives of our article. First, we present a general two-step procedure for the translation of linguistic expressions into numbers and show that this is a methodological innovation. To this end, in study 1, we outline the method exemplarily for verbal frequency expressions. Second, we apply the procedure to the field of verbal rating scales and, thereby, test and construct scales with nearly equidistant response categories. In study 2 we use the verbal response scale of the Copenhagen Psychosocial Questionnaire (COPSOQ; Kristensen, Hannerz, Høgh, & Borg, 2005 ) as an example. In the Conclusions section, we summarize and outline implications of our results, which include recommendations for the construction of verbal rating scales. Additionally, we discuss interesting future prospects using fuzzy methodology.
Translation procedure as a methodological innovation
The translation procedure is composed of (1) a direct empirical estimation method that yields data from participants who assign numbers to presented words and (2) a fuzzy approach for the analysis of data resulting in parametric MFs of potential type (Bocklisch & Bitterlich, 1994 ). Our method differs from existing approaches, and the proposed MF type offers advantages over other MF concepts. First, the direct estimation method is very frugal, efficient, and easy to use for yielding empirical data from decision makers. Moreover, our method conserves resources (e.g., as compared with Budescu et al., 2003 ) because only three numbers per verbal expression are required for estimation. In our opinion, this is an important criterion regarding potential fields of application (such as medicine) where expert knowledge is crucial but difficult to obtain or expensive. In contrast, Budescu and colleagues proposed a multistimuli method where participants viewed one phrase and 11 probability values (0, .1, . . . , .9, 1) and then judged the degree to which the phrase accurately described each probability. Thus, while these judgments were used to create individualized MFs, they were only partly defined according to the 11 numerical probability values reported by participants. Second, our parametric MFs are defined for a sample or specific population so that a generalized model for the vague linguistic expressions that are suitable for a group of people is obtained. It is a well-known fact that the interindividual variability of estimates is large (Teigen & Brun, 2003 ). Therefore, if group MFs are fitted, it is necessary to consider variability and potential contradictions in the estimation behavior of participants. The presented MF approach takes this into account by using parameters (see the Method section). Furthermore, we argue that continuous modeling of group MFs of verbal expressions is useful in that it serves as a flexible basis for further calculations. Additionally, such modeling is easily implemented in a variety of existing models or applications, such as decision support systems (Boegl, Adlassnig, Hayashi, Rothenfluh, & Leitich, 2004 ).
In Bocklisch et al. ( 2010 ), the suggested translation method was outlined for verbal probability expressions (e.g., probable ). The proposed general procedure can be broadly applied to other linguistic terms. In this article, we present the results of two studies. Study 1 included 11 expressions indicative of frequency of occurrence (e.g., occasionally ) with regard to the potential interest of different research areas and applications that apply verbal rating scales with frequency expressions. After presenting the method, results are discussed with respect to the selection of frequency terms considered appropriate for verbal rating scales in questionnaires. Study 2 employed the translation procedure to explore the COPSOQ response scale in more detail.
Application in verbal response scales
In psychology and the social sciences, many research questions are addressed by directly interrogating participants with the help of questionnaires. Often, responses to presented questions are given by choosing a category of a related verbal answering scale. Although such data collection is determined directly by the verbal categories of the scales, little systematic research has been done (Rohrmann, 1978 ), as compared with the construction of questionnaire items. Spector ( 1976 ) summarized the consequences of how response categories are commonly selected: “This selection is often made on no more solid basis than habit, imitation, or subjective judgment. Yet the equal interval properties of the response continuum is assumed even though this assumption may, in fact, be false. . . . When faced with a scale of unequal intervals, subjects sometimes complain of a difficulty in making responses because some adjacent choices are closer together than others. To eliminate this problem, equal interval response categories should be used” (p. 374). Here, we show that our proposed translation procedure can serve as a useful basis for testing and constructing verbal rating scales and determining equidistant verbal response categories.
For the selection of frequency terms, three main criteria are suggested: equidistance, percentage of correct reclassifications, and discriminatory power of the MFs. First, frequency words should be distributed equidistantly along the numerical scale so that data can be interpreted as having interval-scale properties and, therefore, further statistical analyses are feasible. Generally, verbal rating scale categories are assumed to have rank order, but the distance between intervals is not necessarily equal (Jamieson, 2004 ). That is, verbal rating scale responses comprise ordinal- but not interval-level data, and this precludes the application of parametric statistical analyses. It is common practice to apply mathematical operations, such as multiplication or division (necessary for the calculation of means, etc.) to such data, although these operations are not valid for ordinal data. Moreover, employing inappropriate statistical techniques may lead to the misinterpretation of results and to incorrect conclusions.
Second, the percentage of correct reclassifications—that is, how many original data points were reclassified correctly according to the frequency expression to which they originally belonged—gives information about the discriminability and steadiness of the words’ meanings. Third, the criterion of discriminatory power reveals whether MFs differ considerably or not. On the basis of this measure, it is possible to conclude whether the meanings of LTs are interpreted similarly or differently by study participants.
In study 2, fuzzy MFs for the scale of an example questionnaire—namely, the COPSOQ (Kristensen et al., 2005 )—are discussed. The COPSOQ is a free screening instrument for evaluating psychosocial factors at work, including stress and employee well-being, as well as selected personality factors. The questionnaire consists of five frequency words: almost never , infrequently , sometimes , often , and always . We constructed three response scales with alternative frequency expressions and empirically tested an alternative scale consisting of never , sometimes , in half of the cases , often , and always . We hypothesized that the distance between each of the alternative response labels is nearly equal and compared results of both scales (original vs. alternative COPSOQ).
Two-step translation procedure
Here, we present details of the two-step translation procedure for the numerical translation of verbal frequency expressions. First, the estimation technique and method applied in the empirical study are outlined. Thereafter, fuzzy analysis and MFs are specified.
Step One: empirical investigation
Participants.
Eighty-nine undergraduate students (9 males) at Chemnitz University of Technology with an average age of 21.5 years ( SD = 2.7) took part in the study. Four persons stated that they did not understand the task and were therefore excluded from further data analyses.
Materials and procedure
The survey instrument was a paper questionnaire and consisted of two parts. In the first part, participants were asked to consider their workload and related requirements that their course of study imposed on them. Then they were asked to answer the following three questions of the COPSOQ (the original material was presented in German): (1) Is it always necessary to work at a rapid pace? (2) Is your work unevenly distributed such that it piles up? (3) How often do you not have enough time to complete all of your work tasks? An explanation as to how the paper questionnaire should be filled out followed, and participants were then asked to assign three numerical values to each of the 11 exemplars of frequency expressions (see translations from the original German in Table 1 ). Words were chosen according to their frequent usage in questionnaires and in daily communication and on the basis of former research (e.g., Rohrmann, 1978 ). Three numerical values were estimated: (1) the typical value that best represented the given frequency word, (2) the minimal value , and (3) maximal value that corresponded to the given verbal expression. The semantic meaning of the words can be characterized as follows: The first value identifies the most typical numerical equivalent for the word, whereas other values indicate lower and upper boundaries of the verbal frequency expression. Participants were instructed to give their estimates in frequency format (e.g., Is it hardly ever necessary to work at a rapid pace means “in X of 100 work tasks/cases”). We used this format because it is a natural mode of representing information and it turned out that encoding and estimating information in frequency format is easier than in probability or percentage form (Gigerenzer & Hoffrage, 1995 ; Hoffrage, Lindsey, Hertwig, & Gigerenzer, 2000 ).
Step two: Fuzzy analysis
- Fuzzy membership functions
MFs are truth value functions. The membership value ( μ ) represents the value of the truth that an object belongs to a specific class (e.g., the numerical frequency that 70 of 100 cases belong to the class frequency expression often ). For the analysis of empirical data provided by the 85 participants, a parametric MF of the potential type (Bocklisch & Bitterlich, 1994 ; Hempel & Bocklisch, 2009 ) was used (see Fig. 2 ).
Parametric membership function of potential type
This function is based on a set of eight parameters: r marks the position of the mean value of the empirical estimates of the typical value , while a represents the maximum value of the MF. Regarding class structure, a expresses class weight in the given structure (we used a = 1 for all classes in this investigation, such that all frequency terms were weighted equally). The parameters c l and c r characterize left- and right-sided expansions of the class and, therefore, mark the range of the class, in a crisp sense. In addition to the mean of typical estimates ( M typ ), the means of minimum ( M min ) and maximum ( M max ) correspondence values estimated by participants were used for the calculation: c l = M typ − M min and c r = M max − M typ . A special feature of this function type is that there is no intersection with the x -axis ( μ is always >0). This characteristic is founded on the assumption that sample estimates are not representative of the whole population; therefore, no definite end-points are defined. The parameters b l and b r assign left- and right-sided membership values at the boundaries of the function. Therefore, b l and b r represent border membership, whereas d l and d r specify continuous decline of the MF starting from the class center and are denoted as representative of a class. The d parameters determine the shape of the function and, hence, the fuzziness of the class. The b and d parameters were calculated from the distribution of the empirical data using Fuzzy Toolbox software (Bocklisch, 2008 ), which is specialized for fuzzy analyses and modeling of MFs.
In contrast to the nonparametric individualized MF approaches of Wallsten, Budescu, Rapoport, Zwick, and Forsyth ( 1986 ) and Budescu et al. ( 2003 ), we fit group MFs to obtain a generalized model of a sample or certain population of participants. Furthermore, our MFs are defined continuously, such that, in addition to the expansions of the class ( c parameters), the MFs’ shape ( d parameters) carries information about the distribution of the empirical estimates. This is an advantage insofar as potential contradictions between participants’ estimates are considered. In contrast, a triangular MF type describes the graded interval between μ = 0 and μ = 1 with a rather arbitrary linear model and, thus, does not account for the empirical data provided by many individuals. On the level of individual estimates, a triangular MF would model the data appropriately, but on the level of a certain sample or population, this is not the case. Additional parameters are needed to model the expansion ( c ) and the distribution of the estimates ( d ), as well as the membership value at the border of the function ( b ), which is by definition always >0. A continuous variation of MFs, ranging from highly fuzzy to crisp, is available through this parametric function type. It also allows for asymmetry in fuzzy classes by providing individual parameters for the left- and right-hand branches of the function. As the results of former research show (Bocklisch et al., 2010 ; Budescu et al., 2003 ), many verbal expressions are best described by asymmetric MFs. Therefore, we expect this feature to be especially important for the present study.
We first present the descriptive statistics of the data set. Thereafter, the fuzzy MF procedure is specified. In our opinion, it is valuable to present both results for purposes of completeness and comparison, even though we favor the latter approach. It is important that the two approaches be understood independently. Moreover, fuzzy analysis and modeling of the MFs, by definition, do not refer to the background of probability theory and statistics. Although some parameters of our MF type can be interpreted statistically in this case (e.g., r values are equal to the arithmetic mean), an MF is not a probability density function, and conventional requirements (i.e., the integral of the variable’s density is equal to 1) are not valid. A more general comparative discussion of the statistical and fuzzy approaches is provided in Singpurwalla and Booker ( 2004 ).
Descriptive statistics
Table 1 shows the typical values that corresponded to the frequency expressions presented. Minimum and maximum estimates of the semantic meaning of linguistic terms were necessary for modeling the MFs ( c parameters). Hence, they are not presented here.
At first glance, the results show that frequency expressions are distributed almost over the entire numerical frequency scale with varying distances, ranging from never ( M = 1.37) to always ( M = 97.46). Clearly, the 11 expressions are divided into three frequency categories: lower and higher frequency categories, which refer to the middle point of the scale ( M = 50), and a medium frequency category consisting of one LT ( in half of the cases : M = 50.14). The first 5 expressions (ranging from never to sometimes ) are characterized by means less than M = 35 and, therefore, belong to the lower frequency group, whereas the remaining expressions (ranging from frequently to always ) show mean values larger than M = 65 and belong to the higher frequency category. Between the expressions sometimes and in half of the cases and between in half of the cases and frequently , there are intervals measuring approximately 15. These are the largest two intervals among all the intervals between the LTs. Similar findings were reported by Bocklisch et al. ( 2010 ) for verbal probability expressions, which are also split according to three categories (low, medium, and high probability). Standard deviation ( SD ) values show a systematic pattern: Frequency expressions near the borders of the numerical frequency scale have smaller SD s. Starting with the minimum of the verbal scale ( never : SD = 2.23), the SDs increase up to midscale, reaching their highest values with the words occasionally ( SD = 12.23) and sometimes ( SD = 10.96), as well as frequently ( SD = 15.43) and often ( SD = 12.91), and subsequently decrease again ( always : SD = 6.17). Again, the frequency expression that covers the middle of the scale ( in half of the cases : SD = 1.21) is an exception, because its SD is the smallest one. By tendency, skews are higher at the borders of the verbal scale. Expressions belonging to the lower category (e.g., never ) are slightly skewed to the right, and in the higher category (e.g., always ), they tend to be skewed to the left. Kurtosis values are considerably higher for the expressions in half of the cases , almost always , and always , while values for the other frequency expressions are almost normally distributed (i.e., kurtosis = 0 according to the SPSS software’s definition). These findings are consistent with results reported by Bocklisch et al. ( 2010 ) as well as Budescu et al. ( 2003 ) that investigated verbal probability expressions.
Fuzzy analysis
Figure 3 shows the MFs for the 11 verbal frequency expressions. The representative values ( r ) indicating the highest memberships are identical to the reported means in Table 1 . Obviously, the functions differ in shape, symmetry, overlap, and vagueness. The functions for the verbal frequency expressions at the borders of the scale, never and always , are narrower than those in the middle, such as sometimes or often , which is in accordance with reported SD s and kurtosis values. Most of the functions are slightly asymmetric and are clearly not distributed equidistantly along the scale. Some (neighbor) functions overlap to a large extent (e.g., occasionally and sometimes ), while others are quite distinct (e.g., in half of the cases and frequently ).
Membership functions of the 11 verbal frequency expressions
The area of MF overlap A ov (see Fig. 4 , gray area) is informative about the similarity of the words’ meanings. Overlap is defined as the surface imbedded by the MFs and the x -axis. One important characteristic of our parametric potential MF type is that the function has no points of intersection with the x -axis and, therefore, the surface integral is infinite. Additionally, the function type has no general integral solution. Hence, the surface covered by the function (in a certain range) can only be approximated, which is done with the help of Fuzzy Toolbox software (Bocklisch, 2008 ) and operates as follows. The range of the MFs is identified: Here, the minimum is 0 and the maximum is 100 according to the numerical frequency scale. Thereafter, μ min is calculated numerous times using a high sampling rate with equidistant sample points along the numerical scale. Then the area of overlap A ov is determined by adding up the products of the sampling distance and μ min values for the whole number of sampling points. Thereafter, areas covered by MF1 and MF2 ( A MF1 and A MF2 ) are defined using the same procedure. A standardized quotient ( ov ) of the overlapping area of the MFs ( A ov ) is obtained by calculating the arithmetic mean: ov = 0.5 × [( A ov : A MF1 ) + ( A ov : A MF2 )].
Approximation of the discriminatory power of two membership functions
The ov is used to define the discriminatory power ( dp ) between two MFs: dp = 1 – ov (Bocklisch, 2008 ). The dp is standardized taking values from 0 (MFs are identical) to 1 (no overlap at all). Hence, the larger the overlap (e.g., occasionally and sometimes ), the smaller the dp and the more similar the meanings of the verbal expressions are. The ov of the MFs in Fig. 4 is approximately .37 which corresponds to dp = .63. Table 2 shows dp values for the 11 LTs.
If dp values are greater than or equal to .7, then MFs (and LTs) are interpreted as being considerably different, because the area of shared overlap is less than 30%. This is the case for a lot of LTs (see Table 2 ), except for infrequently and occasionally ( dp = .46), occasionally and sometimes ( dp = .25), frequentl y and often ( dp = .19), often and most of the time ( dp = .32), frequently and most of the time ( dp = .38), and most of the time and almost always ( dp = .69). Most of these LT pairs are direct “neighbors.”
The COPSOQ answer scale (Kristensen et al., 2005 ) consists of five frequency expressions: almost never , infrequently , sometimes , often , and always . Figure 5 shows the MFs of the verbal rating scale utilized in the COPSOQ (upper left corner) and three proposed alternative scales that are almost equidistant, consisting of four and five frequency expressions.
Membership functions of the original COPSOQ and alternative COPSOQ (I-III) response scales
In the original COPSOQ scale, the distances between the representative values vary. The LTs almost never and infrequently have approximately the same distance (10.21) as infrequently and sometimes (14.61), but the words sometimes and often (36.53), as well as often and always (27.8), are separated by a greater distance. Therefore, this scale is not equidistant. Furthermore, no verbal term is associated with the middle of the scale, which indicates a frequency of occurrence of approximately 50 out of 100. That is, such a term is unavailable, even to participants who should wish to express this frequency.
The interpretation of verbal frequency scales as interval scales relies on the premise of equidistance (Jamieson, 2004 ). While authors of the COPSOQ may have wanted the frequency words to be distributed as shown in Fig. 5 , such a distribution is rather unlikely, for two reasons: First, if a middle category is not intended, an even number of LTs is usually chosen for a verbal response scale. Second, a scale that combines highly similar words (such as almost never and infrequentl y) with highly discriminatory terms (e.g., often and always ) seems to be inconsistent.
To remedy this problem, we propose three scales that meet the criterion of equidistance quite well (see Fig. 5 ): first, two 5-point scales consisting of the frequency terms never , sometimes , in half of the cases , often , and always (alternative COPSOQ I) and almost never , sometimes , in half of the cases , often , and almost always (alternative COPSOQ II) and, second, a 4-point scale with the expressions almost never , sometimes , often , and almost always (alternative COPSOQ III). The frequency expressions for these scales were chosen according to results presented in Table 2 and Fig. 3 . Both 5-point scales (alternative COPSOQs I and II) are distributed almost equidistantly, do not overlap to a great extent (see dp values in Table 2 ), and are almost symmetric in shape. However, they differ according to their psychological width, which “. . . refers to the extent of the psychological continuum suggested by the rating labels” (Lam & Stevens, 1994 , p.142). Therefore, alternative COPSOQ I is wider, because the LTs at the borders of the scale approximate the numerical endpoints ( never , M = 1.37; always , M = 97.46) and, hence, mark a wider psychological continuum than the LTs of alternative COPSOQ II ( almost never , M = 8.31; almost always , M = 88.11). The 4-point alternative COPSOQ III (see Fig. 5 , lower left) is also nearly equidistant, where MFs are highly distinct and the middle of the scale is not covered.
In addition to the criteria of equidistance, symmetry, and overlap of the MFs’ distribution, the percentage of correct reclassifications of the participants’ original estimates is informative of the quality of the scales. For the reclassification task, the original data were used and reassigned to the MFs. Basically this is done by using a participant’s typical estimate for a certain verbal expression and entering it into the equations of all MFs (see Fig. 2 ) as u . Then the membership values ( μ ) can be calculated. Therefore, 11 membership values (i.e., for the 11 MFs of the 11 frequency expressions) are generated for one data point (i.e., estimate of a respondent). Among these, the highest membership value indicates the frequency word to which the estimate is reclassified. The reclassification is correct if this frequency word is the same as the one for which the estimate was originally given. The reclassification step was done with the help of Fuzzy Toolbox software (Bocklisch, 2008 ). Table 3 shows reclassification results obtained by counting the number of original data points correctly reclassified according to the frequency expression to which they originally belong.
For the original scale consisting of 11 frequency expressions, the correct reclassification percentages lie between 1.18% for occasionally (only 1.18% of the typical estimates for occasionally were reclassified as belonging to occasionally , and the other 98.82% were erroneously reclassified as belonging to other frequency expressions) and 98.82% for in half of the cases (nearly all estimates for in half of the cases were reclassified as belonging to in half of the cases ). The mean percentage of reclassification for this scale ( M = 56.35) is rather low, which is mainly due to the large overlap of the MFs of the frequency expressions (see Fig. 3 ). The original COPSOQ scale ( M = 79.99) and all alternative scales ( M > 85.3) with four to five linguistic terms have higher mean percentages of correct reclassification. Hence, the more terms that are included in a scale, the lower the reclassification percentages will be, due to the similarity of the words’ meanings that can be observed in the overlap of the MFs. In summary, all suggested alternative COPSOQ scales showed better reclassification results and were nearly e quidistant, as compared with the original COPSOQ scale. To optimize all criteria, it would be advisable to choose the alternative COPSOQ I with the five frequency expressions never , sometimes , in half of the cases , often , and always.
In study 1, we outlined a general procedure for the translation of verbal expressions based on empirical estimates and using fuzzy MFs for modeling. The results (see Table 1 and Fig. 3 ) showed that the MFs of frequency expressions at borders of the numerical scale (i.e., never and always ) showed less vagueness than did midscale expressions (i.e., often and sometimes ), suggesting that they more clearly reflected the given expression. This was also found for probability expressions (Bocklisch et al., 2010 ) that differed even more in vagueness when midscale terms and boundary terms are compared. The LT in half of the cases is an exception ( SD = 1.21; see MF in Fig. 3 ): Its meaning is rather crisp with regard to other frequency expressions in the middle of the scale and as compared with the midscale probability LTs thinkable ( SD = 20.24) and possible ( SD = 21.60) in Bocklisch et al. ( 2010 ). This could be due to the relatively “precise” meaning of the word “half.”
The dp values (see Table 2 ) and percentages of correct reclassification (see Table 3 ) were introduced as means for measuring the disparity and steadiness of the MFs. Hence, a differentiated evaluation of the MFs is possible, and conclusions concerning the meaning of the modeled LTs are straightforward. For a few MFs, dp values are rather low, and therefore, the meanings of the corresponding LTs are very similar. However, most of the words are distinct. The percentages of correct reclassification are very high for never (81.18), in half of the cases (98.82), and always (91.57), which supports the idea that these LTs are more precise in their meanings.
The emerging categories, low, middle, and high frequencies, may be due to the actual sample of verbal expressions. It would be interesting to determine whether the estimation of more or fewer LTs would lead to the same categories as those found in this study and in Bocklisch et al. ( 2010 ) or not.
Many questionnaires utilize verbal rating scales consisting of verbal frequency expressions. Thus, we exemplarily tested a well-established questionnaire, the COPSOQ, concerning equidistant distribution of its linguistic expressions and the quality of the scale (i.e., percentages of correct reclassification of the original data). It was found that the scale is in need of improvement because it fails to satisfy the criterion of an equidistant distribution. At present, strictly speaking, the scale cannot be interpreted as having interval level, and hence, further statistical analyses (e.g., the calculation of means for groups of participants) are not appropriate. To solve this problem we proposed three alternative COPSOQ scales with four or five frequency expressions distributed nearly equidistantly (see Fig. 5 ). The suggested 4-point scale (alternative COPSOQ III) should be employed for research questions where no middle category is intended. Alternatives I and II differ concerning LTs at the borders, and alternative I offers a wider psychological continuum for frequency estimation. Both scales produced positive results for mean reclassification percentages, dp s of the MFs, and equidistance and can thus both be applied according to intended utilization. Wyatt and Meyers ( 1987 ) found that scales with less extreme endpoints (e.g., alternative COPSOQ II: almost never and almost always ) lead to greater variability in respondents’ estimates than do scales with more extreme endpoints (e.g., alternative COPSOQ I: never and always ). However, it is not yet clear whether this finding can be generalized to other words and contexts (Lam & Stevens, 1994 ).
In summary, we showed that our translation procedure is a methodological innovation and, therefore, has potential for application in research. In study 2 we use the method again, exploring the COPSOQ scale in greater detail. That is, one could argue that the total number of frequency expressions influences the resulting MFs. If this were the case, it might be inappropriate to draw conclusions from a study that presented 11 LTs to a scale (COPSOQ) that consisted of only 5 LTs. Therefore, in study 2, we presented the 5 LTs and compared the results with those of study 1. Additionally, we manipulated scales of the original COPSOQ and alternative COPSOQ I, which allowed us to test whether our conclusions based on the MFs in study 1 were indeed correct.
One hundred nine undergraduate students (19 males) of Chemnitz University of Technology with an average age of 23.4 years ( SD = 3.3) took part in the study. Fifteen persons did not understand the task and were therefore excluded from further analyses.
The paper questionnaire employed in study 2 was identical to that used in study 1, except that the number of presented frequency expressions differed (study 1, 11 LTs vs. study 2, 5 LTs). Again, participants first answered three questions of the COPSOQ. One group of participants ( N = 51) received the original COPSOQ response scale ( almost never , infrequently , sometimes , often , and always ), while the other group ( n = 42) obtained an alternative COPSOQ answering scale ( never , sometimes , in half of the cases, often , and always ). In the second part, the study 1 translation procedure was also used to translate the five frequency expressions.
Table 4 shows the descriptive results of the typical values that corresponded to the frequency expressions of the original and alternative COPSOQ scales (middle and right columns), as well as the results of study 1 (left column; see also Table 1 ) for purposes of comparison.
For the LTs sometimes , often , and always , a direct comparison between all conditions is possible. In sum, mean values for often and always are very similar. The largest difference is 5.3 between always in the context of 11 LTs and always in the original COPSOQ scale using 5 LTs. For sometimes , the original COPSOQ ( M = 41.08) stands out, as compared with the other conditions (alternative COPSOQ, M = 29.0 and the 11-LT version, M = 33.13). The differences between conditions for never and in half of the cases (11 LTs vs. 5LTs. alternative COPSOQ) as well as for almost always and infrequently (11 LTs vs. 5LTs, original COPSOQ) are also rather small. The SD s are comparable in size between groups for a certain LT, except always (original COPSOQ: SD = 19.04), which has a larger SD than the other conditions.
Figure 6 shows the resulting MFs for the five verbal frequency expressions of the original versus alternative COPSOQ response scales in the context of 5 LTs vs. 11 LTs (see also Fig. 5 ).
Membership functions of the verbal frequency expressions of the original versus alternative COPSOQ I response scales for 5 versus 11 LTs
In the alternative scale version (5 LTs), the verbal terms at the borders ( never and always ) are closer to the borders of the underlying numerical scale, as compared with the original scale (5 LTs). The scales also differ in the extent of the MFs’ overlaps. For instance, in the original COPSOQ, the overlaps occurring at border terms are larger, and in the alternative version, midscale terms overlap more. The distribution of MFs is closer to equidistance for the suggested alternative response scale. The functions’ shapes of the word often are very similar, while the others differ slightly—for instance, in expansion (e.g., the MF for sometimes is broader in the alternative scale version). The frequency expression in half of the cases marks the middle of the scale. The function’s shape is salient; it is asymmetric, and the left-hand branch is very crisp, as compared with the right-hand branch.
A comparison of frequency expressions between the 5- and 11-LT versions of the original COPSOQ (see Fig. 6 , left side) and of the alternative COPSOQ (see Fig. 6 , right side) shows a highly similar appearance of MFs in terms of r -value positions (equal to the means in Table 4 ), shapes, and overlaps. MFs tend to be slightly narrower in the 11-LT versions of the two scales, and the border term always tends to be more extreme, as compared with the 5-LT versions. The frequency expression in half of the cases has equal r values (5 LTs, r = 50.24; 11 LTs, r = 50.14), but the MF’s shape deviates. In the 5-LT version of the alternative COPSOQ, it is rather fuzzy and asymmetric, whereas in the 11-LT version, it is very crisp and symmetric. For the evaluation of the differences between the 5- and 11-LT versions, again, dp values are calculated. Table 5 shows the dp values.
For instance, for sometimes , the difference between the 5- and 11-LT versions of the original COPSOQ scale is slightly larger ( dp = .29) than for the 5- and 11-LT versions of the alternative COPSOQ I scale ( dp = .14). Generally, dp values for never , almost never , infrequently , sometimes , in half of the cases , and often are all rather small ( dp s < .49), which means that the MFs are very similar and overlap in 50% to 90%. However, for always , there is a considerable difference between MFs in the alternative COPSOQ I (5 vs. 11 LTs: dp = .74), but not for the original COPSOQ (5 vs. 11 LTs: dp = .53).
Study 2 aimed to clarify (1) whether the suggested alternative response labels (see Fig. 5 : alternative COPSOQ I) also have equal distances in the context of 5 LTs and (2) whether the total number of prompted LTs (5 vs. 11) influences the interpretation of frequency words. First, we found that alternative COPSOQ I has nearly equal distances between the response categories (see Table 4 and Fig. 6 ). Hence, our presented method is generally suitable for application in choosing LTs for answering scales. Second, the resulting dp values (see Table 5 ) show that the total number of prompted LTs seems to have no systematic influence on the words’ interpretation, since nearly all MFs are identical to a great extent ( dp s < .53). There is only one considerable difference: MFs of always (alternative COPSOQ I) are distinct ( dp = .74). That is, always in the 5-LT version is broader and covers more of the numerical frequency scale than always in the 11-LT version does. Nevertheless, the difference is rather small, because the criterion value of dp > .7 is just met. Accordingly, this tendency is also the case for always in the original version (see Fig. 6 , left side). Our results show that the number of prompted LTs has no considerable influence on the interpretation of the LTs meanings, although there are, at least to some extent, small differences between the MFs depending on the number of LTs presented (see also Table 4 ).
Conclusions
This article presents a general two-step procedure for the numerical translation of linguistic terms that are exemplars of frequency expressions. In two studies, we showed that the presented procedure is a methodological innovation and can serve as basis for choosing LTs for applications such as questionnaires. In study 1, the procedure was presented for 11 frequency expressions. First, three numerical values for each linguistic term (i.e., most typical, minimal, and maximal correspondence values) were estimated. Second, the resulting data were modeled using the parametric MFs of the potential type. While most alternative procedures are more costly (Budescu et al., 2003 ) or are not based on empirical estimates (Boegl et al., 2004 ), our approach is very frugal and efficient in terms of data collection.
Results show that the functions are capable of modeling the data in a very efficient way, yielding averaged MFs that describe the LTs continuously along a numerical frequency scale. They also take into account the asymmetry of the empirical data, resulting due to the parameters that model the left- and right-hand branches of the function (e.g., c l and c r ). MFs with fewer parameters would model the data without considering asymmetry and would, therefore, be less accurate and suitable for the reported data. The b and d parameters reflect features of the distribution of the empirical estimates and carry information about between-subjects differences. Another advantage of the proposed function type is that the semantic content of parameters can be interpreted at a meta-level. Hence, they render the vague meaning of linguistic terms more tangible. In addition to existing methods (e.g., Boegl et al., 2004 ; Budescu et al., 2003 ; Wallsten et al., 1986 ), this parametric MF approach is an interesting alternative that yields group MFs and contributes to the investigation of vague linguistic terms. Future research would benefit from a comparison of different translation procedures and MF concepts (e.g., individualized MFs vs. group MFs).
In study 2, we explored the COPSOQ scale in detail. Questionnaires are widely used in the social sciences and humanities to address empirical research questions. We exemplarily tested the COPSOQ questionnaire (see the Results sections of studies 1 and 2) and found that the scale employed in this tool is in need of improvement because its verbal labels fail to satisfy the criterion of an equidistant distribution. At present, this questionnaire scale is ordinal rather than interval level, and therefore, statistical analyses such as the calculation of arithmetic means for groups of participants are not valid. A counterargument might suggest that missing equidistance is compensated for by the conventional visual arrangement of scales. This might, indeed, have an influence on the interpretation of the words’ meanings. To clarify this issue, our translation approach may be useful for further studies. We suggest three nearly equidistant verbal frequency scales (see Fig. 5 ) with four or five frequency expressions as a starting point for such studies.
In constructing verbal response scales, we recommend adapting the context of the cover story according to the topic (e.g., psychology, medicine, or economy), because context is known to influence a word’s interpretation (Pepper & Prytulak, 1974 ; Teigen & Brun, 2003 ). Additionally, the purpose for which the LTs will be used afterward (e.g., questionnaire or decision support system) should also be considered. Future studies may benefit from choosing estimators from the target population—for example, medical experts or participants in experimental studies. According to the desired psychological width of the response scale, “choosing a scale for a particular application must take into account what needs to be measured” (Wyatt & Meyers, 1987 , p. 34).
Different samples of participants and different languages of investigation should also be considered in future studies. We report data from a student sample using German LTs. Although this might limit the generalizability of our results, the presented methodology (translation procedure) is not restricted to a certain sample or language. Therefore, it would be interesting to study how different samples of people (such as experts vs. novices in medicine) interpret LTs and whether or not the meanings of verbal expressions are understood similarly in different languages.
The reported MFs, especially in study 1, show large overlaps, indicating that contiguous expressions are very similar or almost identical in their meanings. It is noteworthy that despite the vagueness of natural language, MFs are a convenient tool for identifying words that are more distinct (i.e., with small overlap) in their meaning than others. The identification of unambiguous and distinct words that can be used for communication is of tremendous importance in areas such as medicine or the military, where misunderstandings could lead to severe consequences. Currently, we are exploring the availability of such distinct words for communication purposes with the help of our MFs. Karelitz and Budescu ( 2004 ) devised promising criteria for the conversion of phrases from a communicator’s to a recipient’s lexicon—for instance, the peak rank order between MFs. Our MF approach could contribute additional criteria to such an approach, such as the mathematical quantification of MF overlaps.
Beyth-Marom, R. (1982). How probable is probable? A numerical translation of verbal probability expressions. Journal of Forecasting, 1, 257–269.
Article Google Scholar
Bocklisch, S. F. (2008). Handbook Fuzzy Toolbox. GWT-TUD . Chemnitz, Germany: Chemnitz University of Technoloogy, Department of Electrical Engineering.
Google Scholar
Bocklisch, S. F., & Bitterlich, N. (1994). Fuzzy pattern classification—methodology and application. In R. Kruse, J. Gebhardt, & R. Palm (Eds.), Fuzzy systems in computer science (pp. 295–301). Wiesbaden, Germany: Vieweg.
Chapter Google Scholar
Bocklisch, F., Bocklisch, S.F., & Krems, J.F. (2010). How to translate words into numbers? A fuzzy approach for the numerical translation of verbal probabilities. In E. Hüllermeier, R. Kruse, & F. Hoffmann (Eds.), IPMU 2010, Lecture Notes in Artificial Intelligence 6178 (pp. 614–623). Springer.
Boegl, K., Adlassnig, K.-P., Hayashi, Y., Rothenfluh, T. E., & Leitich, H. (2004). Knowledge acquisition in the fuzzy knowledge representation framework of a medical consultation system. Artificial Intelligence in Medicine, 30, 1–26.
Article PubMed Google Scholar
Budescu, D. V., Karelitz, T. M., & Wallsten, T. S. (2003). Predicting the directionality of probability words from their membership functions. Journal of Behavioral Decision Making, 16, 159–180.
Dhami, M. K., & Wallsten, T. S. (2005). Interpersonal comparison of subjective probabilities: Towards translating linguistic probabilities. Memory & Cognition, 33, 1057–1068.
Gigerenzer, G., & Hoffrage, U. (1995). How to improve Bayesian reasoning without instruction: Frequency formats. Psychological Review, 102, 684–704.
Hempel, A.-J., & Bocklisch, S. F. (2009). Parametric fuzzy modelling for complex data-inherent structures. In Proceedings of the Joint 2009 International Fuzzy Systems Association World Congress and 2009 European Society of Fuzzy Logic and Technology Conference (IFSA-EUSFLAT 2009) (pp. 885–890). Lisbon.
Hoffrage, U., Lindsey, S., Hertwig, R., & Gigerenzer, G. (2000). Communicating statistical information. Science, 290, 2261–2262.
Jamieson, S. (2004). Likert scales: How to (ab)use them. Medical Education, 38, 1217–1218.
Karelitz, T. M., & Budescu, D. V. (2004). You Say “probable” and I say “likely”: Improving interpersonal communication with verbal probability phrases. Journal of Experimental Psychology. Applied, 10, 25–41.
Kristensen, T. S., Hannerz, H., Høgh, A., & Borg, V. (2005). The Copenhagen Psychosocial Questionnaire (COPSOQ)—a tool for the assessment and improvement of the psychosocial work environment. Scandinavian Journal of Work and Environmental Health, 31, 438–449.
Lam, T. C. M., & Stevens, J. J. (1994). Effects of content polarization, item wording, and rating scale width on rating responses. Applied Measurement in Education, 7, 141–158.
Lichtenstein, S., & Newman, J. R. (1967). Empirical scaling of common verbal phrases associated with numerical probabilities. Psychonomic Science, 9, 563–564.
Pepper, S., & Prytulak, L. S. (1974). Sometimes frequently means seldom: Context effects in the interpretation of quantitative expressions. Journal of Research in Personality, 8, 95–101.
Rohrmann, B. (1978). Empirische Studien zur Entwicklung von Antwortskalen für die sozialwissenschaftliche Forschung. Zeitschrift für Sozialpsychologie, 9, 222–245.
Singpurwalla, N. D., & Booker, J. M. (2004). Membership functions and probability measures of fuzzy sets. Journal of the American Statistical Association, 467, 867–877.
Spector, P. E. (1976). Choosing response categories for summated rating scales. Journal of Applied Psychology, 61, 374–375.
Teigen, K. H., & Brun, W. (2003). Verbal expressions of uncertainty and probability. In D. Hardman (Ed.), Thinking: Psychological perspectives on reasoning, judgment and decision making (pp. 125–145). New York: Wiley.
Wallsten, T. S., Budescu, D. V., Rapoport, A., Zwick, R., & Forsyth, B. (1986). Measuring the vague meaning of probability terms. Journal of Experimental Psychology. General, 115, 348–365.
Wallsten, T. S., Budescu, D. V., Zwick, R., & Kemp, S. M. (1993). Preferences and reasons for communicating probabilistic information in numerical or verbal terms. Bulletin of the Psychonomic Society, 31, 135–138.
Wyatt, R. C., & Meyers, L. S. (1987). Psychometric properties of four 5-point Likert type response scales. Educational and Psychological Measurement, 47, 27–35.
Zadeh, L. A. (1965). Fuzzy sets. Information and Control, 8, 338–353.
Zimmer, A. (1984). A model for the interpretation of verbal predictions. International Journal of Man–Machine Studies, 20, 121–134.
Download references
Author Note
Thanks to Martin Baumann, Marta Pereira, Diana Rösler, Andreas Neubert, Lydia Obermann, Thomas Schäfer, David V. Budescu, and the students of Chemnitz University of Technology for their contributions and support.
Author information
Authors and affiliations.
Department of Psychology, Chemnitz University of Technology, 09107, Chemnitz, Germany
Franziska Bocklisch & Josef F. Krems
Department of Automation, Chemnitz University of Technology, 09107, Chemnitz, Germany
Steffen F. Bocklisch
You can also search for this author in PubMed Google Scholar
Corresponding author
Correspondence to Franziska Bocklisch .
Rights and permissions
Reprints and permissions
About this article
Bocklisch, F., Bocklisch, S.F. & Krems, J.F. Sometimes, often, and always: Exploring the vague meanings of frequency expressions. Behav Res 44 , 144–157 (2012). https://doi.org/10.3758/s13428-011-0130-8
Download citation
Published : 07 July 2011
Issue Date : March 2012
DOI : https://doi.org/10.3758/s13428-011-0130-8
Share this article
Anyone you share the following link with will be able to read this content:
Sorry, a shareable link is not currently available for this article.
Provided by the Springer Nature SharedIt content-sharing initiative
- Translation procedure
- Linguistic terms
- Frequency expressions
- Verbal rating scale
- Find a journal
- Publish with us
- Track your research
The University of Chicago The Law School
Innovation clinic—significant achievements for 2023-24.
The Innovation Clinic continued its track record of success during the 2023-2024 school year, facing unprecedented demand for our pro bono services as our reputation for providing high caliber transactional and regulatory representation spread. The overwhelming number of assistance requests we received from the University of Chicago, City of Chicago, and even national startup and venture capital communities enabled our students to cherry-pick the most interesting, pedagogically valuable assignments offered to them. Our focus on serving startups, rather than all small- to medium-sized businesses, and our specialization in the needs and considerations that these companies have, which differ substantially from the needs of more traditional small businesses, has proven to be a strong differentiator for the program both in terms of business development and prospective and current student interest, as has our further focus on tackling idiosyncratic, complex regulatory challenges for first-of-their kind startups. We are also beginning to enjoy more long-term relationships with clients who repeatedly engage us for multiple projects over the course of a year or more as their legal needs develop.
This year’s twelve students completed over twenty projects and represented clients in a very broad range of industries: mental health and wellbeing, content creation, medical education, biotech and drug discovery, chemistry, food and beverage, art, personal finance, renewable energy, fintech, consumer products and services, artificial intelligence (“AI”), and others. The matters that the students handled gave them an unparalleled view into the emerging companies and venture capital space, at a level of complexity and agency that most junior lawyers will not experience until several years into their careers.
Representative Engagements
While the Innovation Clinic’s engagements are highly confidential and cannot be described in detail, a high-level description of a representative sample of projects undertaken by the Innovation Clinic this year includes:
Transactional/Commercial Work
- A previous client developing a symptom-tracking wellness app for chronic disease sufferers engaged the Innovation Clinic again, this time to restructure its cap table by moving one founder’s interest in the company to a foreign holding company and subjecting the holding company to appropriate protections in favor of the startup.
- Another client with whom the Innovation Clinic had already worked several times engaged us for several new projects, including (1) restructuring their cap table and issuing equity to an additional, new founder, (2) drafting several different forms of license agreements that the company could use when generating content for the platform, covering situations in which the company would license existing content from other providers, jointly develop new content together with contractors or specialists that would then be jointly owned by all creators, or commission contractors to make content solely owned by the company, (3) drafting simple agreements for future equity (“Safes”) for the company to use in its seed stage fundraising round, and (4) drafting terms of service and a privacy policy for the platform.
- Yet another repeat client, an internet platform that supports independent artists by creating short films featuring the artists to promote their work and facilitates sales of the artists’ art through its platform, retained us this year to draft a form of independent contractor agreement that could be used when the company hires artists to be featured in content that the company’s Fortune 500 brand partners commission from the company, and to create capsule art collections that could be sold by these Fortune 500 brand partners in conjunction with the content promotion.
- We worked with a platform using AI to accelerate the Investigational New Drug (IND) approval and application process to draft a form of license agreement for use with its customers and an NDA for prospective investors.
- A novel personal finance platform for young, high-earning individuals engaged the Innovation Clinic to form an entity for the platform, including helping the founders to negotiate a deal among them with respect to roles and equity, terms that the equity would be subject to, and other post-incorporation matters, as well as to draft terms of service and a privacy policy for the platform.
- Students also formed an entity for a biotech therapeutics company founded by University of Chicago faculty members and an AI-powered legal billing management platform founded by University of Chicago students.
- A founder the Innovation Clinic had represented in connection with one venture engaged us on behalf of his other venture team to draft an equity incentive plan for the company as well as other required implementing documentation. His venture with which we previously worked also engaged us this year to draft Safes to be used with over twenty investors in a seed financing round.
More information regarding other types of transactional projects that we typically take on can be found here .
Regulatory Research and Advice
- A team of Innovation Clinic students invested a substantial portion of our regulatory time this year performing highly detailed and complicated research into public utilities laws of several states to advise a groundbreaking renewable energy technology company as to how its product might be regulated in these states and its clearest path to market. This project involved a review of not only the relevant state statutes but also an analysis of the interplay between state and federal statutes as it relates to public utilities law, the administrative codes of the relevant state executive branch agencies, and binding and non-binding administrative orders, decisions and guidance from such agencies in other contexts that could shed light on how such states would regulate this never-before-seen product that their laws clearly never contemplated could exist. The highly varied approach to utilities regulation in all states examined led to a nuanced set of analysis and recommendations for the client.
- In another significant research project, a separate team of Innovation Clinic students undertook a comprehensive review of all settlement orders and court decisions related to actions brought by the Consumer Financial Protection Bureau for violations of the prohibition on unfair, deceptive, or abusive acts and practices under the Consumer Financial Protection Act, as well as selected relevant settlement orders, court decisions, and other formal and informal guidance documents related to actions brought by the Federal Trade Commission for violations of the prohibition on unfair or deceptive acts or practices under Section 5 of the Federal Trade Commission Act, to assemble a playbook for a fintech company regarding compliance. This playbook, which distilled very complicated, voluminous legal decisions and concepts into a series of bullet points with clear, easy-to-follow rules and best practices, designed to be distributed to non-lawyers in many different facets of this business, covered all aspects of operations that could subject a company like this one to liability under the laws examined, including with respect to asset purchase transactions, marketing and consumer onboarding, usage of certain terms of art in advertising, disclosure requirements, fee structures, communications with customers, legal documentation requirements, customer service and support, debt collection practices, arrangements with third parties who act on the company’s behalf, and more.
Miscellaneous
- Last year’s students built upon the Innovation Clinic’s progress in shaping the rules promulgated by the Financial Crimes Enforcement Network (“FinCEN”) pursuant to the Corporate Transparency Act to create a client alert summarizing the final rule, its impact on startups, and what startups need to know in order to comply. When FinCEN issued additional guidance with respect to that final rule and changed portions of the final rule including timelines for compliance, this year’s students updated the alert, then distributed it to current and former clients to notify them of the need to comply. The final bulletin is available here .
- In furtherance of that work, additional Innovation Clinic students this year analyzed the impact of the final rule not just on the Innovation Clinic’s clients but also its impact on the Innovation Clinic, and how the Innovation Clinic should change its practices to ensure compliance and minimize risk to the Innovation Clinic. This also involved putting together a comprehensive filing guide for companies that are ready to file their certificates of incorporation to show them procedurally how to do so and explain the choices they must make during the filing process, so that the Innovation Clinic would not be involved in directing or controlling the filings and thus would not be considered a “company applicant” on any client’s Corporate Transparency Act filings with FinCEN.
- The Innovation Clinic also began producing thought leadership pieces regarding AI, leveraging our distinct and uniquely University of Chicago expertise in structuring early-stage companies and analyzing complex regulatory issues with a law and economics lens to add our voice to those speaking on this important topic. One student wrote about whether non-profits are really the most desirable form of entity for mitigating risks associated with AI development, and another team of students prepared an analysis of the EU’s AI Act, comparing it to the Executive Order on AI from President Biden, and recommended a path forward for an AI regulatory environment in the United States. Both pieces can be found here , with more to come!
Innovation Trek
Thanks to another generous gift from Douglas Clark, ’89, and managing partner of Wilson, Sonsini, Goodrich & Rosati, we were able to operationalize the second Innovation Trek over Spring Break 2024. The Innovation Trek provides University of Chicago Law School students with a rare opportunity to explore the innovation and venture capital ecosystem in its epicenter, Silicon Valley. The program enables participating students to learn from business and legal experts in a variety of different industries and roles within the ecosystem to see how the law and economics principles that students learn about in the classroom play out in the real world, and facilitates meaningful connections between alumni, students, and other speakers who are leaders in their fields. This year, we took twenty-three students (as opposed to twelve during the first Trek) and expanded the offering to include not just Innovation Clinic students but also interested students from our JD/MBA Program and Doctoroff Business Leadership Program. We also enjoyed four jam-packed days in Silicon Valley, expanding the trip from the two and a half days that we spent in the Bay Area during our 2022 Trek.
The substantive sessions of the Trek were varied and impactful, and enabled in no small part thanks to substantial contributions from numerous alumni of the Law School. Students were fortunate to visit Coinbase’s Mountain View headquarters to learn from legal leaders at the company on all things Coinbase, crypto, and in-house, Plug & Play Tech Center’s Sunnyvale location to learn more about its investment thesis and accelerator programming, and Google’s Moonshot Factory, X, where we heard from lawyers at a number of different Alphabet companies about their lives as in-house counsel and the varied roles that in-house lawyers can have. We were also hosted by Wilson, Sonsini, Goodrich & Rosati and Fenwick & West LLP where we held sessions featuring lawyers from those firms, alumni from within and outside of those firms, and non-lawyer industry experts on topics such as artificial intelligence, climate tech and renewables, intellectual property, biotech, investing in Silicon Valley, and growth stage companies, and general advice on career trajectories and strategies. We further held a young alumni roundtable, where our students got to speak with alumni who graduated in the past five years for intimate, candid discussions about life as junior associates. In total, our students heard from more than forty speakers, including over twenty University of Chicago alumni from various divisions.
The Trek didn’t stop with education, though. Throughout the week students also had the opportunity to network with speakers to learn more from them outside the confines of panel presentations and to grow their networks. We had a networking dinner with Kirkland & Ellis, a closing dinner with all Trek participants, and for the first time hosted an event for admitted students, Trek participants, and alumni to come together to share experiences and recruit the next generation of Law School students. Several speakers and students stayed in touch following the Trek, and this resulted not just in meaningful relationships but also in employment for some students who attended.
More information on the purposes of the Trek is available here , the full itinerary is available here , and one student participant’s story describing her reflections on and descriptions of her experience on the Trek is available here .
The Innovation Clinic is grateful to all of its clients for continuing to provide its students with challenging, high-quality legal work, and to the many alumni who engage with us for providing an irreplaceable client pipeline and for sharing their time and energy with our students. Our clients are breaking the mold and bringing innovations to market that will improve the lives of people around the world in numerous ways. We are glad to aid in their success in any way that we can. We look forward to another productive year in 2024-2025!
Have a language expert improve your writing
Run a free plagiarism check in 10 minutes, generate accurate citations for free.
- Knowledge Base
Methodology
- What Is a Likert Scale? | Guide & Examples
What Is a Likert Scale? | Guide & Examples
Published on July 3, 2020 by Pritha Bhandari and Kassiani Nikolopoulou. Revised on June 22, 2023.
A Likert scale is a rating scale used to measure opinions, attitudes, or behaviors.
It consists of a statement or a question, followed by a series of five or seven answer statements. Respondents choose the option that best corresponds with how they feel about the statement or question.
Because respondents are presented with a range of possible answers, Likert scales are great for capturing the level of agreement or their feelings regarding the topic in a more nuanced way. However, Likert scales are prone to response bias , where respondents either agree or disagree with all the statements due to fatigue or social desirability or have a tendency toward extreme responding or other demand characteristics .
Likert scales are common in survey research , as well as in fields like marketing, psychology, or other social sciences.
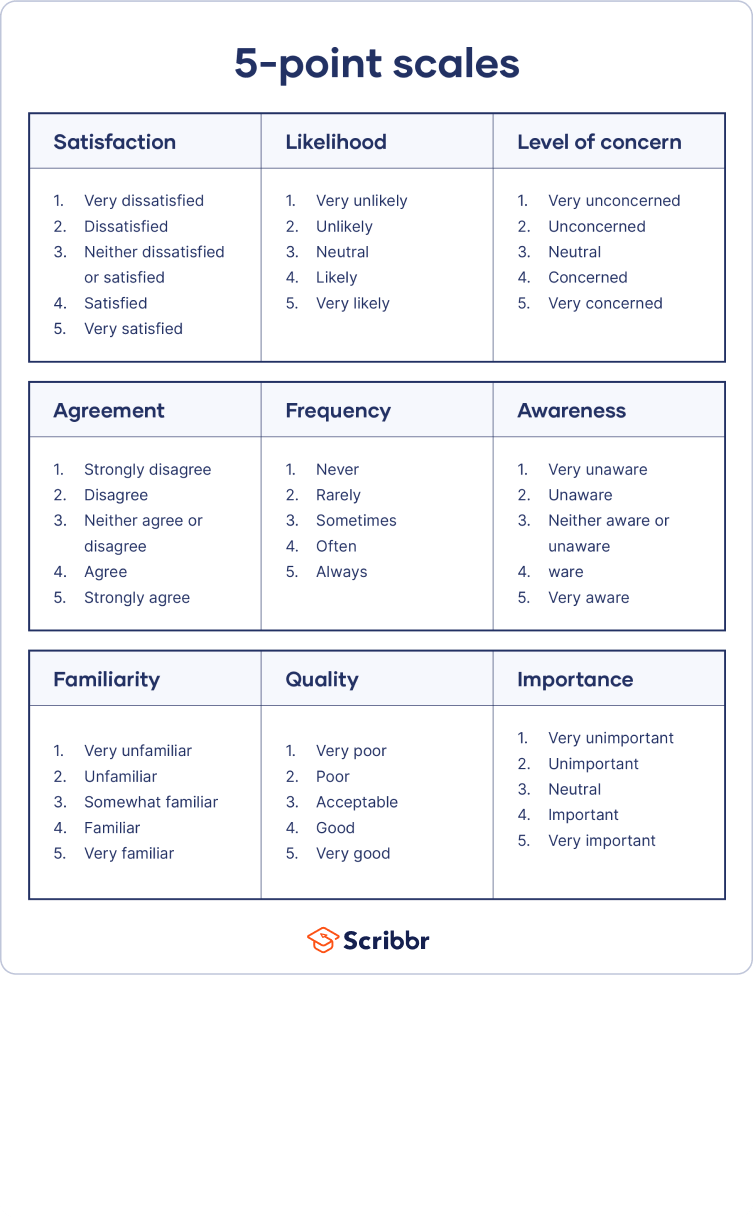
Download Likert scale response options
Table of contents
What are likert scale questions, when to use likert scale questions, how to write strong likert scale questions, how to write likert scale responses, how to analyze data from a likert scale, advantages and disadvantages of likert scales, other interesting articles, frequently asked questions about likert scales.
Likert scales commonly comprise either five or seven options. The options on each end are called response anchors . The midpoint is often a neutral item, with positive options on one side and negative options on the other. Each item is given a score from 1 to 5 or 1 to 7.
The format of a typical five-level Likert question, for example, could be:
- Strongly disagree
- Neither agree nor disagree
- Strongly agree
In addition to measuring the level of agreement or disagreement, Likert scales can also measure other spectrums, such as frequency, satisfaction, or importance.
Receive feedback on language, structure, and formatting
Professional editors proofread and edit your paper by focusing on:
- Academic style
- Vague sentences
- Style consistency
See an example
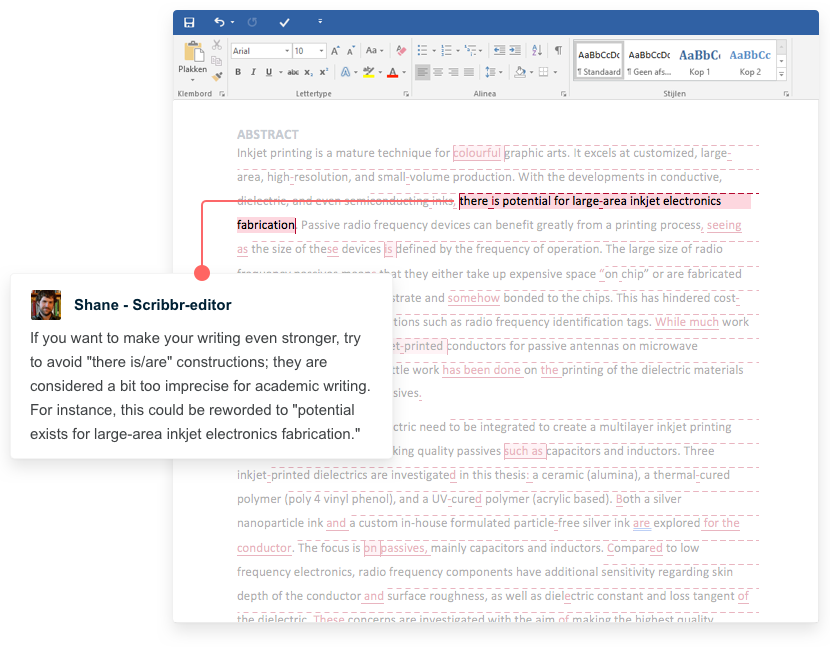
Researchers use Likert scale questions when they are seeking a greater degree of nuance than possible from a simple “yes or no” question.
For example, let’s say you are conducting a survey about customer views on a pair of running shoes. You ask survey respondents “Are you satisfied with the shoes you purchased?”
A dichotomous question like the above gives you very limited information. There is no way you can tell how satisfied or dissatisfied customers really are. You get more specific and interesting information by asking a Likert scale question instead:
“How satisfied are you with the shoes you purchased?”
- 1 – Very dissatisfied
- 2 – Dissatisfied
- 4 – Satisfied
- 5 – Very satisfied
Likert scales are most useful when you are measuring unobservable individual characteristics , or characteristics that have no concrete, objective measurement. These can be elements like attitudes, feelings, or opinions that cause variations in behavior.
Each Likert scale–style question should assess a single attitude or trait. In order to get accurate results, it is important to word your questions precisely. As a rule of thumb, make sure each question only measures one aspect of your topic.
For example, if you want to assess attitudes towards environmentally friendly behaviors, you can design a Likert scale with a variety of questions that measure different aspects of this topic.
Here are a few pointers:
Include both questions and statements
Use both positive and negative framing, avoid double negatives, ask about only one thing at a time, be crystal clear.
A good rule of thumb is to use a mix of both to keep your participants engaged during the survey. When deciding how to phrase questions and statements, it’s important that they are easily understood and do not bias your respondents in one way or another.
If all of your questions only ask about things in socially desirable ways, your participants may be biased towards agreeing with all of them to show themselves in a positive light.
- Positive framing
- Negative framing
Environmental damage caused by single-use water bottles is a serious problem. | ||||
---|---|---|---|---|
Strongly disagree | Disagree | Neither agree nor disagree | Agree | Strongly agree |
Banning single-use water bottles is pointless for reducing environmental damage. | ||||
---|---|---|---|---|
Strongly disagree | Disagree | Neither agree nor disagree | Agree | Strongly agree |
Respondents who agree with the first statement should also disagree with the second. By including both of these statements in a long survey, you can also check whether the participants’ responses are reliable and consistent.
Double negatives can lead to confusion and misinterpretations, as respondents may be unsure of what they are agreeing or disagreeing with.
- Bad example
- Good example
I never buy non-organic products. | ||||
---|---|---|---|---|
Strongly disagree | Disagree | Neither agree nor disagree | Agree | Strongly agree |
I try to buy organic products whenever possible. | ||||
---|---|---|---|---|
Strongly disagree | Disagree | Neither agree nor disagree | Agree | Strongly agree |
Avoid double-barreled questions (asking about two different topics within the same question). When faced with such questions, your respondents may selectively answer about one topic and ignore the other. Questions like this may also confuse respondents, leading them to choose a neutral but inaccurate answer in an attempt to answer both questions simultaneously.
How would you rate your knowledge of climate change and food systems? | ||||
---|---|---|---|---|
Very poor | Poor | Fair | Good | Excellent |
How would you rate your knowledge of climate change? | ||||
---|---|---|---|---|
Very poor | Poor | Fair | Good | Excellent |
How would you rate your knowledge of food systems? | ||||
---|---|---|---|---|
Very poor | Poor | Fair | Good | Excellent |
The accuracy of your data also relies heavily on word choice:
- Pose your questions clearly, leaving no room for misunderstanding.
- Make language and stylistic choices that resonate with your target demographic.
- Stay away from jargon that could discourage or confuse your respondents.
When using Likert scales, how you phrase your response options is just as crucial as how you phrase your questions.
Here are a few tips to keep in mind.
Decide on a number of response options
Choose the type of response option, choose between unipolar and bipolar options, make sure that you use mutually exclusive options.
More options give you deeper insights but can make it harder for participants to decide on one answer. Fewer options mean you capture less detail, but the scale is more user-friendly.
Usually, researchers include five or seven response options. It’s a good idea to include an odd number so that there is a midpoint. However, if you want to force your respondents to choose, an even number of responses removes the neutral option.
How frequently do you buy biodegradable products? | ||||
---|---|---|---|---|
Never | Occasionally | Sometimes | Often | Always |
How frequently do you buy biodegradable products? | ||||||
---|---|---|---|---|---|---|
Never | Rarely | Occasionally | Sometimes | Often | Very often | Always |
You can measure a wide range of perceptions, motivations, and intentions using Likert scales. Response options should strive to cover the full range of opinions you anticipate a participant can have.
Some of the most common types of items include:
- Agreement: Strongly Agree, Agree, Neither Agree nor Disagree, Disagree, Strongly Disagree
- Quality: Very Poor, Poor, Fair, Good, Excellent
- Likelihood: Extremely Unlikely, Somewhat Unlikely, Likely, Somewhat Likely, Extremely Likely
- Experience: Very Negative, Somewhat Negative, Neutral, Somewhat Positive, Very Positive
Some researchers also include a “don’t know” option. This allows them to distinguish between respondents who do not feel sufficiently informed to give an opinion and those who are “neutral” on the topic. However, including a “don’t know” option may trigger unmotivated respondents to select that for every question.
On a unipolar scale, you measure only one attribute (e.g., satisfaction). On a bipolar scale, you can measure two attributes (e.g., satisfaction or dissatisfaction) along a continuum.
How satisfied are you with the range of organic products available? | ||||
---|---|---|---|---|
Not at all satisfied | Somewhat satisfied | Satisfied | Very satisfied | Extremely satisfied |
How satisfied are you with the range of organic products available? | ||||
---|---|---|---|---|
Extremely dissatisfied | Dissatisfied | Neither dissatisfied nor satisfied | Satisfied | Extremely satisfied |
Your choice depends on your research questions and aims. If you want finer-grained details about one attribute, select unipolar items. If you want to allow a broader range of responses, select bipolar items.
Unipolar scales are most accurate when five-point scales are used. Conversely, bipolar scales are most accurate when a seven-point scale is used (with three scale points on each side of a truly neutral midpoint.)
Avoid overlaps in the response items. If two items have similar meanings, it risks making your respondent’s choice random.
Environmental damage caused by single-use water bottles is a serious problem. | |||||
---|---|---|---|---|---|
Strongly agree | Agree | Neither agree nor disagree | Indifferent | Disagree | Strongly disagree |
Environmental damage caused by single-use water bottles is a serious problem. | ||||
---|---|---|---|---|
Strongly agree | Agree | Neither agree nor disagree | Disagree | Strongly disagree |
Before analyzing your data, it’s important to consider what type of data you are dealing with. Likert-derived data can be treated either as ordinal-level or interval-level data . However, most researchers treat Likert-derived data as ordinal: assuming there is not an equal distance between responses.
Furthermore, you need to decide which descriptive statistics and/or inferential statistics may be used to describe and analyze the data obtained from your Likert scale.
You can use descriptive statistics to summarize the data you collected in simple numerical or visual form.
- Ordinal data: To get an overall impression of your sample, you find the mode, or most common score, for each question. You also create a bar chart for each question to visualize the frequency of each item choice.
- Interval data: You add up the scores from each question to get the total score for each participant. You find the mean , or average, score and the standard deviation , or spread, of the scores for your sample.
You can use inferential statistics to test hypotheses , such as correlations between different responses or patterns in the whole dataset.
- Ordinal data: You hypothesize that knowledge of climate change is related to belief that environmental damage is a serious problem. You use a chi-square test of independence to see if these two attributes are correlated.
- Interval data: You investigate whether age is related to attitudes towards environmentally friendly behavior. Using a Pearson correlation test, you assess whether the overall score for your Likert scale is related to age.
Lastly, be sure to clearly state in your analysis whether you treat the data at interval level or at ordinal level.
Analyzing data at the ordinal level
Researchers usually treat Likert-derived data as ordinal . Here, response categories are presented in a ranking order, but the distances between the categories cannot be presumed to be equal.
For example, consider a scale where 1 = strongly agree, 2 = agree, 3 = neutral, 4 = disagree, and 5 = strongly disagree.
In this scale, 4 is more negative than 3, 2, or 1. However, it cannot be inferred that a response of 4 is twice as negative as a response of 2.
Treating Likert-derived data as ordinal, you can use descriptive statistics to summarize the data you collected in simple numerical or visual form. The median or mode generally is used as the measure of central tendency . In addition, you can create a bar chart for each question to visualize the frequency of each item choice.
Appropriate inferential statistics for ordinal data are, for example, Spearman’s correlation or a chi-square test for independence .
Analyzing data at the interval level
However, you can also choose to treat Likert-derived data at the interval level . Here, response categories are presented in a ranking order, and the distance between categories is presumed to be equal.
Appropriate inferential statistics used here are an analysis of variance (ANOVA) or Pearson’s correlation . Such analysis is legitimate, provided that you state the assumption that the data are at interval level.
In terms of descriptive statistics, you add up the scores from each question to get the total score for each participant. You find the mean , or average, score and the standard deviation , or spread, of the scores for your sample.
Likert scales are a practical and accessible method of collecting data.
- Quantitative: Likert scales easily operationalize complex topics by breaking down abstract phenomena into recordable observations. This enables statistical testing of your hypotheses.
- Fine-grained: Because Likert-type questions aren’t binary ( yes/no , true/false , etc.) you can get detailed insights into perceptions, opinions, and behaviors.
- User-friendly: Unlike open-ended questions, Likert scales are closed-ended and don’t ask respondents to generate ideas or justify their opinions. This makes them quick for respondents to fill out and ensures they can easily yield data from large samples.
Problems with Likert scales often come from inappropriate design choices.
- Response bias: Due to social desirability bias , people often avoid selecting the extreme items or disagreeing with statements to seem more “normal” or show themselves in a favorable light.
- Fatigue/inattention: In Likert scales with many questions, respondents can get bored and lose interest. They may absent-mindedly select responses regardless of their true feelings. This results in invalid responses.
- Subjective interpretation: Some items can be vague and interpreted very differently by respondents. Words like “somewhat” or “fair” don’t have precise or narrow definitions.
- Restricted choice: Since Likert-type questions are closed-ended, respondents sometimes have to choose the most relevant answer even if it may not accurately reflect reality.
If you want to know more about statistics , methodology , or research bias , make sure to check out some of our other articles with explanations and examples.
- Student’s t -distribution
- Normal distribution
- Null and Alternative Hypotheses
- Chi square tests
- Confidence interval
- Quartiles & Quantiles
- Cluster sampling
- Stratified sampling
- Data cleansing
- Reproducibility vs Replicability
- Peer review
- Prospective cohort study
Research bias
- Implicit bias
- Cognitive bias
- Placebo effect
- Hawthorne effect
- Hindsight bias
- Affect heuristic
- Social desirability bias
A Likert scale is a rating scale that quantitatively assesses opinions, attitudes, or behaviors. It is made up of 4 or more questions that measure a single attitude or trait when response scores are combined.
To use a Likert scale in a survey , you present participants with Likert-type questions or statements, and a continuum of items, usually with 5 or 7 possible responses, to capture their degree of agreement.
Individual Likert-type questions are generally considered ordinal data , because the items have clear rank order, but don’t have an even distribution.
Overall Likert scale scores are sometimes treated as interval data. These scores are considered to have directionality and even spacing between them.
The type of data determines what statistical tests you should use to analyze your data.
Operationalization means turning abstract conceptual ideas into measurable observations.
For example, the concept of social anxiety isn’t directly observable, but it can be operationally defined in terms of self-rating scores, behavioral avoidance of crowded places, or physical anxiety symptoms in social situations.
Before collecting data , it’s important to consider how you will operationalize the variables that you want to measure.
Cite this Scribbr article
If you want to cite this source, you can copy and paste the citation or click the “Cite this Scribbr article” button to automatically add the citation to our free Citation Generator.
Bhandari, P. & Nikolopoulou, K. (2023, June 22). What Is a Likert Scale? | Guide & Examples. Scribbr. Retrieved September 3, 2024, from https://www.scribbr.com/methodology/likert-scale/
Is this article helpful?
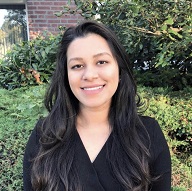
Pritha Bhandari
Other students also liked, survey research | definition, examples & methods, what is quantitative research | definition, uses & methods, levels of measurement | nominal, ordinal, interval and ratio, "i thought ai proofreading was useless but..".
I've been using Scribbr for years now and I know it's a service that won't disappoint. It does a good job spotting mistakes”

- SUGGESTED TOPICS
- The Magazine
- Newsletters
- Managing Yourself
- Managing Teams
- Work-life Balance
- The Big Idea
- Data & Visuals
- Reading Lists
- Case Selections
- HBR Learning
- Topic Feeds
- Account Settings
- Email Preferences
Can AI Assistants Add Value to Your Sales Team?
- Prabhakant Sinha,
- Arun Shastri,
- Sally E. Lorimer

The benefits — and challenges — of bringing these tools onboard.
AI assistants are transforming sales by acting as digital coaches, analysts, and advisors to salespeople. They analyze sales pitches and provide personalized feedback, helping salespeople refine their communication and engagement strategies. By leveraging AI’s analytical and verbal-visual capabilities, companies can drive customer engagement, increase conversion rates, and enhance overall sales performance. They can streamline tasks like content creation and customer interaction analysis, allowing sales teams to focus on higher-value activities. Adoption challenges include upfront costs, data integration, and managing change, but a phased implementation can mitigate risks.
A salesperson is preparing for an important customer meeting. They rehearse a pitch with an AI-powered digital coaching tool which is tailored to the company’s objectives and sales philosophy. The system analyzes the salesperson’s tone, words, and pacing. It points out areas for improvement, for instance, suggesting use of phrases that emphasize collaboration (“let’s explore this together…”) and reminding the salesperson to schedule a next meeting with the prospect. Further, the salesperson gets data-driven insights about the customer’s needs and preferences, including recommendations about sales actions and cross-selling opportunities.
- Prabhakant Sinha is a cofounder of ZS, a global professional-services firm. He is a coauthor of the HBR Sales Management Handbook , forthcoming October 2024.
- Arun Shastri is a leader of the artificial intelligence practice at ZS, a global professional-services firm, and teaches sales executives at Northwestern’s Kellogg School of Management. He is a coauthor of the HBR Sales Management Handbook , forthcoming October 2024.
- Sally E. Lorimer is a principal at ZS, a global professional-services firm. She is a coauthor of the HBR Sales Management Handbook , forthcoming October 2024.
Partner Center

COMMENTS
Then, to identify the verbal interpretation of the range of mean score, the writer used Bringula's interval (Table 2) of 5-point scale (Bringula, 2012). The open-ended questionnaires were analyzed ...
The analysis and interpretation of data is carried out in two phases. The. first part, which is based on the results of the questionnaire, deals with a quantitative. analysis of data. The second, which is based on the results of the interview and focus group. discussions, is a qualitative interpretation.
In a thesis or dissertation, the discussion is an in-depth exploration of the results, going into detail about the meaning of your findings and citing relevant sources to put them in context. The conclusion is more shorter and more general: it concisely answers your main research question and makes recommendations based on your overall findings.
Verbal analysis is also to be differentiated from methods whereby a researcher undertakes qualitative observations in a messy context, but then analyzes only the quantitative data from that messy situation. For example, suppose one observes how the introduction of new technological equipment effects the operation in a trauma unit, but then ...
ABSTRACT. This chapter formulates the issues and choices researchers should be aware of when adopting or adapting various methods of analysing verbal data such as transcripts of classroom discourse and small group dialogues, talk-aloud protocols from reasoning and problem solving tasks, students' written work, textbook passages and test items ...
Interpretation is ultimately a communal endeavor: Initial interpretations may be incomplete, nearsighted, and/or narrow, but eventually, these interpretations become richer, broader, and more inclusive. Feminist revisionist history projects are an exemplary case. Over time, the writing, art, and cultural contributions of countless women ...
Part 4: Chapter 22. An assertion differs from an interpretation by providing perspective on an underlying pattern, a perspective that implies what it means to you and why you think it's significant. Without such a perspective, an interpretation merely becomes a statement with no potential for development. Just as one might utter a statement ...
The Verbal Interpretation of Social Documents. PAUL HANLY FURFEY. V ERBAL interpetation means the systematic discovery of the literal sense of recorded language. The literal sense is the meaning which follows naturally from the words themselves, taken singly and in groups. The term social document is understood broadly in the present paper.
The segmentation of verbal transcription data is a difficult process in qualitative analysis and requires many constructs to be accounted for including syntax and semantics (Lemke, 2012). Using a ...
Textual analysis is a broad term for various research methods used to describe, interpret and understand texts. All kinds of information can be gleaned from a text - from its literal meaning to the subtext, symbolism, assumptions, and values it reveals. The methods used to conduct textual analysis depend on the field and the aims of the ...
Critical discourse analysis (or discourse analysis) is a research method for studying written or spoken language in relation to its social context. It aims to understand how language is used in real life situations. When you conduct discourse analysis, you might focus on: The purposes and effects of different types of language.
Key Points: This chapter provides guidance on interpreting the results of synthesis in order to communicate the conclusions of the review effectively. Methods are presented for computing, presenting and interpreting relative and absolute effects for dichotomous outcome data, including the number needed to treat (NNT).
This chapter addresses a wide range of concepts related to interpretation in qualitative research, examines the meaning and importance of interpretation in qualitative inquiry, and explores the ways methodology, data, and the self/researcher as instrument interact and impact interpretive processes. Additionally, the chapter presents a series of ...
Thus, understanding the interpretation and analysis of data derived from Likert scales is imperative for those working in medical education and education research. The goal of this article is to provide readers who do not have extensive statistics background with the basics needed to understand these concepts.
The analysis and interpretation of data about wearing high heels for female students of Ligao community college. To complete this study properly, it is necessary to analyze the data collected in order to answer the research questions. ... 4.18 0.59 Agree 3.2 Situational problems and essays improve the verbal skills of students 3.85 1.05 Agree 3 ...
Abstract. Questionnaires are the dominant data collection method in psychology and the social sciences in general, and most use rating scales as response mode. Within category scaling, verbal labelling of rating scales has become the primary approach to enhancing useability. The labels are used as "qualifiers", either for endpoints or for each ...
The methods of discourse analysis of verbal data can be used to compare curriculum documents, textbooks, and tests with classroom dialogue, teacher discourse, student writing, etc. They make possible rich descriptions of the lived curriculum, its relation to official curriculum plans, and to the web of intertextuality among all the spoken and ...
non-verbal means between a speaker/writer and a listener/reader which takes place in a certain context (Seidlhofer and Widdowson 1999: 207). This approach assumes the potential of discourse to (re)construct a ... interpretation while using exemplifications from different types of discourse. It opens with Alimuradov et al.'s investigation into ...
The findings indicate that (1) the English Academic Writing Readiness scale dimensions encompass vocabulary, grammar, structure, formatting, and time management. (2) The structural equation model ...
The article describes a general two-step procedure for the numerical translation of vague linguistic terms (LTs). The suggested procedure consists of empirical and model components, including (1) participants' estimates of numerical values corresponding to verbal terms and (2) modeling of the empirical data using fuzzy membership functions (MFs), respectively. The procedure is outlined in ...
Download Table | Data Verbal Interpretation Guide from publication: Students' Perception on Outcome-Based Education (OBE) Implementation: A Preliminary Study in UniKL MSI | This study aimed to ...
General The Innovation Clinic continued its track record of success during the 2023-2024 school year, facing unprecedented demand for our pro bono services as our reputation for providing high caliber transactional and regulatory representation spread. The overwhelming number of assistance requests we received from the University of Chicago, City of Chicago, and even national startup and ...
Revised on June 22, 2023. A Likert scale is a rating scale used to measure opinions, attitudes, or behaviors. It consists of a statement or a question, followed by a series of five or seven answer statements. Respondents choose the option that best corresponds with how they feel about the statement or question.
They can streamline tasks like content creation and customer interaction analysis, allowing sales teams to focus on higher-value activities. ... By leveraging AI's analytical and verbal-visual ...
Meanwhile, using the arbitrary scores, the researchers created corresponding verbal interpretation (VI) adapted from Akcaoğlu (2011) for each range, as presented in Table 1. Initially, there were ...