- Skip to main content
- Skip to primary sidebar
- Skip to footer
- QuestionPro

- Solutions Industries Gaming Automotive Sports and events Education Government Travel & Hospitality Financial Services Healthcare Cannabis Technology Use Case NPS+ Communities Audience Contactless surveys Mobile LivePolls Member Experience GDPR Positive People Science 360 Feedback Surveys
- Resources Blog eBooks Survey Templates Case Studies Training Help center

Home Market Research

Data Analysis in Research: Types & Methods
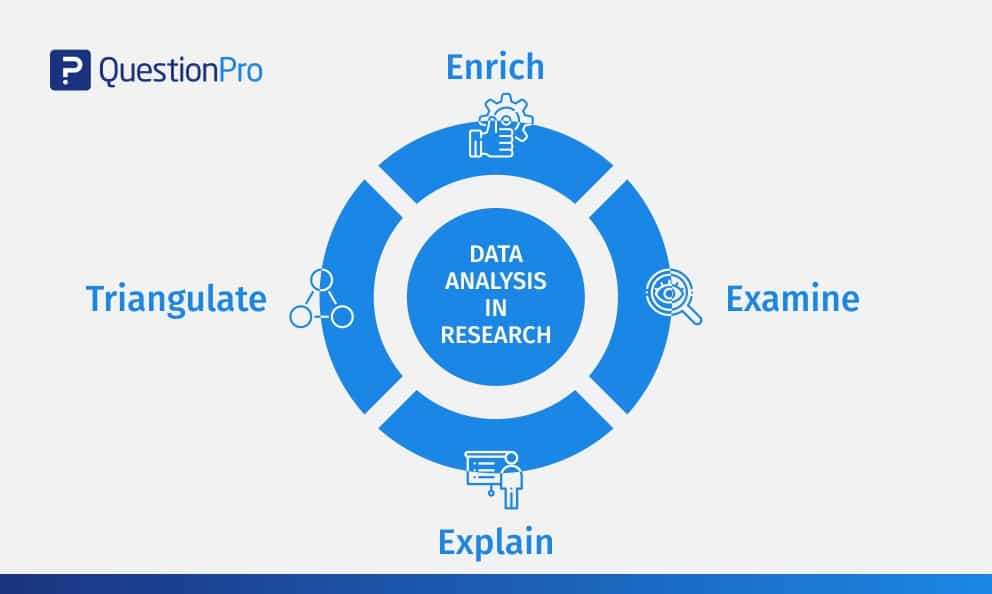
Content Index
Why analyze data in research?
Types of data in research, finding patterns in the qualitative data, methods used for data analysis in qualitative research, preparing data for analysis, methods used for data analysis in quantitative research, considerations in research data analysis, what is data analysis in research.
Definition of research in data analysis: According to LeCompte and Schensul, research data analysis is a process used by researchers to reduce data to a story and interpret it to derive insights. The data analysis process helps reduce a large chunk of data into smaller fragments, which makes sense.
Three essential things occur during the data analysis process — the first is data organization . Summarization and categorization together contribute to becoming the second known method used for data reduction. It helps find patterns and themes in the data for easy identification and linking. The third and last way is data analysis – researchers do it in both top-down and bottom-up fashion.
LEARN ABOUT: Research Process Steps
On the other hand, Marshall and Rossman describe data analysis as a messy, ambiguous, and time-consuming but creative and fascinating process through which a mass of collected data is brought to order, structure and meaning.
We can say that “the data analysis and data interpretation is a process representing the application of deductive and inductive logic to the research and data analysis.”
Researchers rely heavily on data as they have a story to tell or research problems to solve. It starts with a question, and data is nothing but an answer to that question. But, what if there is no question to ask? Well! It is possible to explore data even without a problem – we call it ‘Data Mining’, which often reveals some interesting patterns within the data that are worth exploring.
Irrelevant to the type of data researchers explore, their mission and audiences’ vision guide them to find the patterns to shape the story they want to tell. One of the essential things expected from researchers while analyzing data is to stay open and remain unbiased toward unexpected patterns, expressions, and results. Remember, sometimes, data analysis tells the most unforeseen yet exciting stories that were not expected when initiating data analysis. Therefore, rely on the data you have at hand and enjoy the journey of exploratory research.
Create a Free Account
Every kind of data has a rare quality of describing things after assigning a specific value to it. For analysis, you need to organize these values, processed and presented in a given context, to make it useful. Data can be in different forms; here are the primary data types.
- Qualitative data: When the data presented has words and descriptions, then we call it qualitative data . Although you can observe this data, it is subjective and harder to analyze data in research, especially for comparison. Example: Quality data represents everything describing taste, experience, texture, or an opinion that is considered quality data. This type of data is usually collected through focus groups, personal qualitative interviews , qualitative observation or using open-ended questions in surveys.
- Quantitative data: Any data expressed in numbers of numerical figures are called quantitative data . This type of data can be distinguished into categories, grouped, measured, calculated, or ranked. Example: questions such as age, rank, cost, length, weight, scores, etc. everything comes under this type of data. You can present such data in graphical format, charts, or apply statistical analysis methods to this data. The (Outcomes Measurement Systems) OMS questionnaires in surveys are a significant source of collecting numeric data.
- Categorical data: It is data presented in groups. However, an item included in the categorical data cannot belong to more than one group. Example: A person responding to a survey by telling his living style, marital status, smoking habit, or drinking habit comes under the categorical data. A chi-square test is a standard method used to analyze this data.
Learn More : Examples of Qualitative Data in Education
Data analysis in qualitative research
Data analysis and qualitative data research work a little differently from the numerical data as the quality data is made up of words, descriptions, images, objects, and sometimes symbols. Getting insight from such complicated information is a complicated process. Hence it is typically used for exploratory research and data analysis .
Although there are several ways to find patterns in the textual information, a word-based method is the most relied and widely used global technique for research and data analysis. Notably, the data analysis process in qualitative research is manual. Here the researchers usually read the available data and find repetitive or commonly used words.
For example, while studying data collected from African countries to understand the most pressing issues people face, researchers might find “food” and “hunger” are the most commonly used words and will highlight them for further analysis.
LEARN ABOUT: Level of Analysis
The keyword context is another widely used word-based technique. In this method, the researcher tries to understand the concept by analyzing the context in which the participants use a particular keyword.
For example , researchers conducting research and data analysis for studying the concept of ‘diabetes’ amongst respondents might analyze the context of when and how the respondent has used or referred to the word ‘diabetes.’
The scrutiny-based technique is also one of the highly recommended text analysis methods used to identify a quality data pattern. Compare and contrast is the widely used method under this technique to differentiate how a specific text is similar or different from each other.
For example: To find out the “importance of resident doctor in a company,” the collected data is divided into people who think it is necessary to hire a resident doctor and those who think it is unnecessary. Compare and contrast is the best method that can be used to analyze the polls having single-answer questions types .
Metaphors can be used to reduce the data pile and find patterns in it so that it becomes easier to connect data with theory.
Variable Partitioning is another technique used to split variables so that researchers can find more coherent descriptions and explanations from the enormous data.
LEARN ABOUT: Qualitative Research Questions and Questionnaires
There are several techniques to analyze the data in qualitative research, but here are some commonly used methods,
- Content Analysis: It is widely accepted and the most frequently employed technique for data analysis in research methodology. It can be used to analyze the documented information from text, images, and sometimes from the physical items. It depends on the research questions to predict when and where to use this method.
- Narrative Analysis: This method is used to analyze content gathered from various sources such as personal interviews, field observation, and surveys . The majority of times, stories, or opinions shared by people are focused on finding answers to the research questions.
- Discourse Analysis: Similar to narrative analysis, discourse analysis is used to analyze the interactions with people. Nevertheless, this particular method considers the social context under which or within which the communication between the researcher and respondent takes place. In addition to that, discourse analysis also focuses on the lifestyle and day-to-day environment while deriving any conclusion.
- Grounded Theory: When you want to explain why a particular phenomenon happened, then using grounded theory for analyzing quality data is the best resort. Grounded theory is applied to study data about the host of similar cases occurring in different settings. When researchers are using this method, they might alter explanations or produce new ones until they arrive at some conclusion.
LEARN ABOUT: 12 Best Tools for Researchers
Data analysis in quantitative research
The first stage in research and data analysis is to make it for the analysis so that the nominal data can be converted into something meaningful. Data preparation consists of the below phases.
Phase I: Data Validation
Data validation is done to understand if the collected data sample is per the pre-set standards, or it is a biased data sample again divided into four different stages
- Fraud: To ensure an actual human being records each response to the survey or the questionnaire
- Screening: To make sure each participant or respondent is selected or chosen in compliance with the research criteria
- Procedure: To ensure ethical standards were maintained while collecting the data sample
- Completeness: To ensure that the respondent has answered all the questions in an online survey. Else, the interviewer had asked all the questions devised in the questionnaire.
Phase II: Data Editing
More often, an extensive research data sample comes loaded with errors. Respondents sometimes fill in some fields incorrectly or sometimes skip them accidentally. Data editing is a process wherein the researchers have to confirm that the provided data is free of such errors. They need to conduct necessary checks and outlier checks to edit the raw edit and make it ready for analysis.
Phase III: Data Coding
Out of all three, this is the most critical phase of data preparation associated with grouping and assigning values to the survey responses . If a survey is completed with a 1000 sample size, the researcher will create an age bracket to distinguish the respondents based on their age. Thus, it becomes easier to analyze small data buckets rather than deal with the massive data pile.
LEARN ABOUT: Steps in Qualitative Research
After the data is prepared for analysis, researchers are open to using different research and data analysis methods to derive meaningful insights. For sure, statistical analysis plans are the most favored to analyze numerical data. In statistical analysis, distinguishing between categorical data and numerical data is essential, as categorical data involves distinct categories or labels, while numerical data consists of measurable quantities. The method is again classified into two groups. First, ‘Descriptive Statistics’ used to describe data. Second, ‘Inferential statistics’ that helps in comparing the data .
Descriptive statistics
This method is used to describe the basic features of versatile types of data in research. It presents the data in such a meaningful way that pattern in the data starts making sense. Nevertheless, the descriptive analysis does not go beyond making conclusions. The conclusions are again based on the hypothesis researchers have formulated so far. Here are a few major types of descriptive analysis methods.
Measures of Frequency
- Count, Percent, Frequency
- It is used to denote home often a particular event occurs.
- Researchers use it when they want to showcase how often a response is given.
Measures of Central Tendency
- Mean, Median, Mode
- The method is widely used to demonstrate distribution by various points.
- Researchers use this method when they want to showcase the most commonly or averagely indicated response.
Measures of Dispersion or Variation
- Range, Variance, Standard deviation
- Here the field equals high/low points.
- Variance standard deviation = difference between the observed score and mean
- It is used to identify the spread of scores by stating intervals.
- Researchers use this method to showcase data spread out. It helps them identify the depth until which the data is spread out that it directly affects the mean.
Measures of Position
- Percentile ranks, Quartile ranks
- It relies on standardized scores helping researchers to identify the relationship between different scores.
- It is often used when researchers want to compare scores with the average count.
For quantitative research use of descriptive analysis often give absolute numbers, but the in-depth analysis is never sufficient to demonstrate the rationale behind those numbers. Nevertheless, it is necessary to think of the best method for research and data analysis suiting your survey questionnaire and what story researchers want to tell. For example, the mean is the best way to demonstrate the students’ average scores in schools. It is better to rely on the descriptive statistics when the researchers intend to keep the research or outcome limited to the provided sample without generalizing it. For example, when you want to compare average voting done in two different cities, differential statistics are enough.
Descriptive analysis is also called a ‘univariate analysis’ since it is commonly used to analyze a single variable.
Inferential statistics
Inferential statistics are used to make predictions about a larger population after research and data analysis of the representing population’s collected sample. For example, you can ask some odd 100 audiences at a movie theater if they like the movie they are watching. Researchers then use inferential statistics on the collected sample to reason that about 80-90% of people like the movie.
Here are two significant areas of inferential statistics.
- Estimating parameters: It takes statistics from the sample research data and demonstrates something about the population parameter.
- Hypothesis test: I t’s about sampling research data to answer the survey research questions. For example, researchers might be interested to understand if the new shade of lipstick recently launched is good or not, or if the multivitamin capsules help children to perform better at games.
These are sophisticated analysis methods used to showcase the relationship between different variables instead of describing a single variable. It is often used when researchers want something beyond absolute numbers to understand the relationship between variables.
Here are some of the commonly used methods for data analysis in research.
- Correlation: When researchers are not conducting experimental research or quasi-experimental research wherein the researchers are interested to understand the relationship between two or more variables, they opt for correlational research methods.
- Cross-tabulation: Also called contingency tables, cross-tabulation is used to analyze the relationship between multiple variables. Suppose provided data has age and gender categories presented in rows and columns. A two-dimensional cross-tabulation helps for seamless data analysis and research by showing the number of males and females in each age category.
- Regression analysis: For understanding the strong relationship between two variables, researchers do not look beyond the primary and commonly used regression analysis method, which is also a type of predictive analysis used. In this method, you have an essential factor called the dependent variable. You also have multiple independent variables in regression analysis. You undertake efforts to find out the impact of independent variables on the dependent variable. The values of both independent and dependent variables are assumed as being ascertained in an error-free random manner.
- Frequency tables: The statistical procedure is used for testing the degree to which two or more vary or differ in an experiment. A considerable degree of variation means research findings were significant. In many contexts, ANOVA testing and variance analysis are similar.
- Analysis of variance: The statistical procedure is used for testing the degree to which two or more vary or differ in an experiment. A considerable degree of variation means research findings were significant. In many contexts, ANOVA testing and variance analysis are similar.
- Researchers must have the necessary research skills to analyze and manipulation the data , Getting trained to demonstrate a high standard of research practice. Ideally, researchers must possess more than a basic understanding of the rationale of selecting one statistical method over the other to obtain better data insights.
- Usually, research and data analytics projects differ by scientific discipline; therefore, getting statistical advice at the beginning of analysis helps design a survey questionnaire, select data collection methods, and choose samples.
LEARN ABOUT: Best Data Collection Tools
- The primary aim of data research and analysis is to derive ultimate insights that are unbiased. Any mistake in or keeping a biased mind to collect data, selecting an analysis method, or choosing audience sample il to draw a biased inference.
- Irrelevant to the sophistication used in research data and analysis is enough to rectify the poorly defined objective outcome measurements. It does not matter if the design is at fault or intentions are not clear, but lack of clarity might mislead readers, so avoid the practice.
- The motive behind data analysis in research is to present accurate and reliable data. As far as possible, avoid statistical errors, and find a way to deal with everyday challenges like outliers, missing data, data altering, data mining , or developing graphical representation.
LEARN MORE: Descriptive Research vs Correlational Research The sheer amount of data generated daily is frightening. Especially when data analysis has taken center stage. in 2018. In last year, the total data supply amounted to 2.8 trillion gigabytes. Hence, it is clear that the enterprises willing to survive in the hypercompetitive world must possess an excellent capability to analyze complex research data, derive actionable insights, and adapt to the new market needs.
LEARN ABOUT: Average Order Value
QuestionPro is an online survey platform that empowers organizations in data analysis and research and provides them a medium to collect data by creating appealing surveys.
MORE LIKE THIS
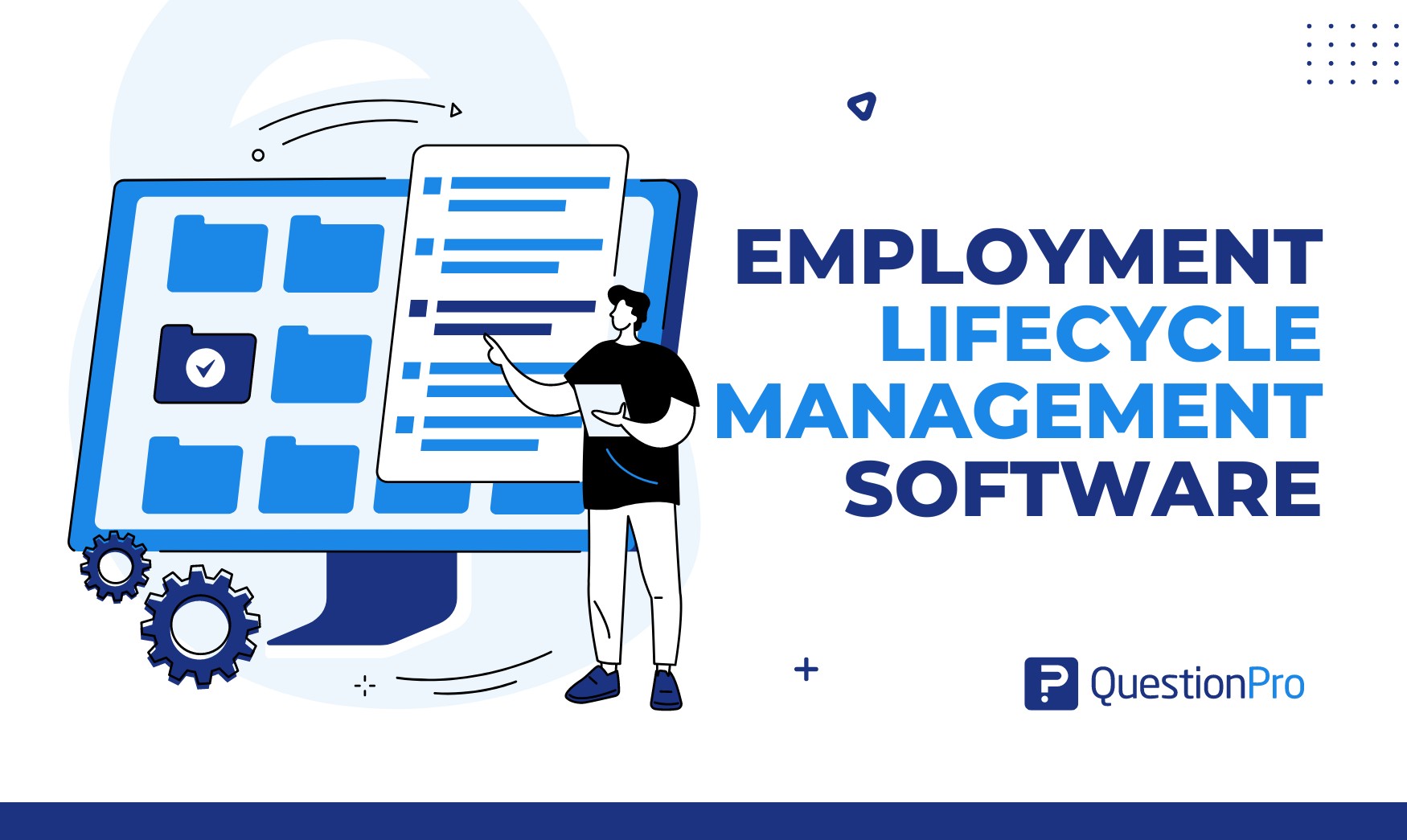
Employee Lifecycle Management Software: Top of 2024
Apr 15, 2024
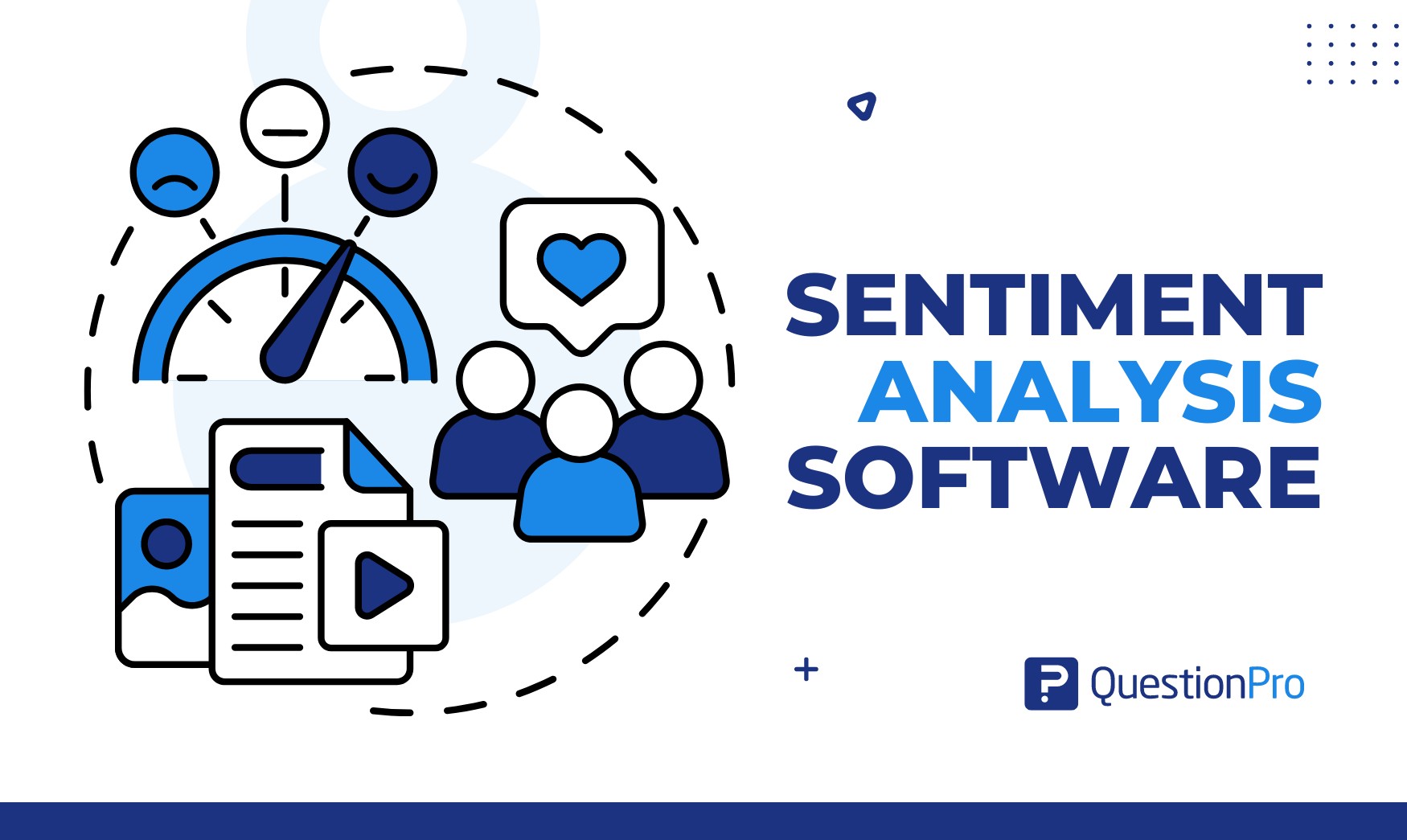
Top 15 Sentiment Analysis Software That Should Be on Your List
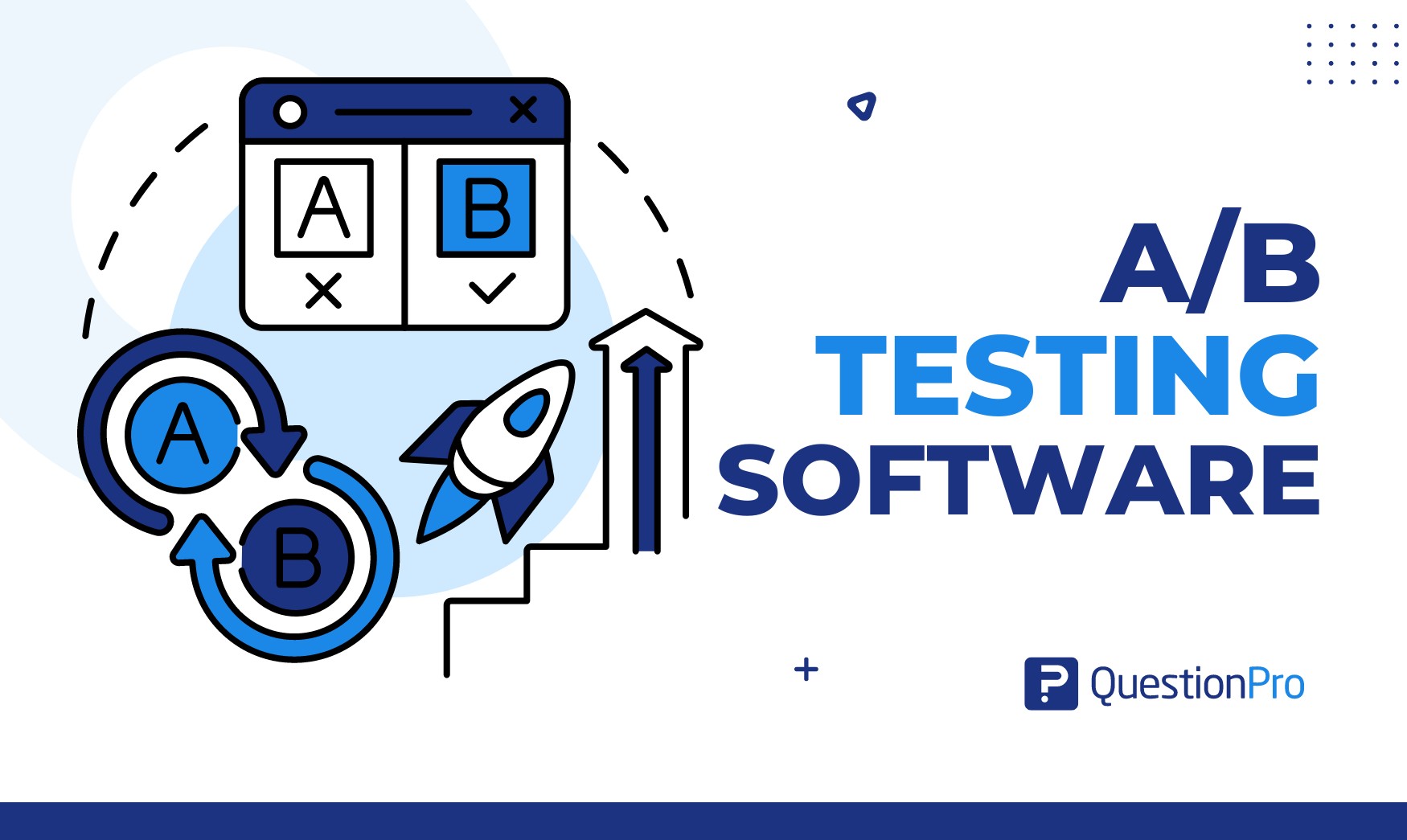
Top 13 A/B Testing Software for Optimizing Your Website
Apr 12, 2024
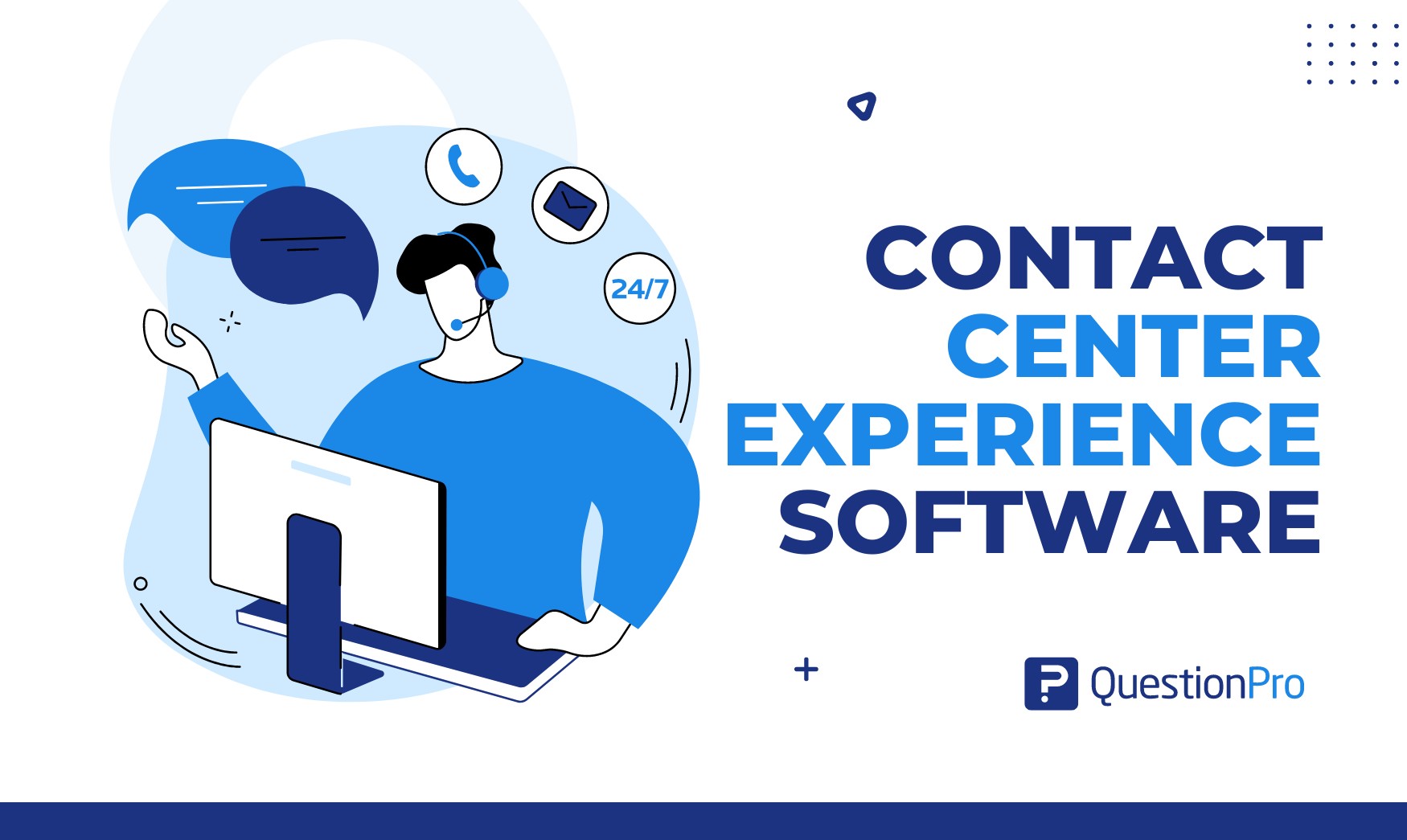
21 Best Contact Center Experience Software in 2024
Other categories.
- Academic Research
- Artificial Intelligence
- Assessments
- Brand Awareness
- Case Studies
- Communities
- Consumer Insights
- Customer effort score
- Customer Engagement
- Customer Experience
- Customer Loyalty
- Customer Research
- Customer Satisfaction
- Employee Benefits
- Employee Engagement
- Employee Retention
- Friday Five
- General Data Protection Regulation
- Insights Hub
- Life@QuestionPro
- Market Research
- Mobile diaries
- Mobile Surveys
- New Features
- Online Communities
- Question Types
- Questionnaire
- QuestionPro Products
- Release Notes
- Research Tools and Apps
- Revenue at Risk
- Survey Templates
- Training Tips
- Uncategorized
- Video Learning Series
- What’s Coming Up
- Workforce Intelligence
Your Modern Business Guide To Data Analysis Methods And Techniques

Table of Contents
1) What Is Data Analysis?
2) Why Is Data Analysis Important?
3) What Is The Data Analysis Process?
4) Types Of Data Analysis Methods
5) Top Data Analysis Techniques To Apply
6) Quality Criteria For Data Analysis
7) Data Analysis Limitations & Barriers
8) Data Analysis Skills
9) Data Analysis In The Big Data Environment
In our data-rich age, understanding how to analyze and extract true meaning from our business’s digital insights is one of the primary drivers of success.
Despite the colossal volume of data we create every day, a mere 0.5% is actually analyzed and used for data discovery , improvement, and intelligence. While that may not seem like much, considering the amount of digital information we have at our fingertips, half a percent still accounts for a vast amount of data.
With so much data and so little time, knowing how to collect, curate, organize, and make sense of all of this potentially business-boosting information can be a minefield – but online data analysis is the solution.
In science, data analysis uses a more complex approach with advanced techniques to explore and experiment with data. On the other hand, in a business context, data is used to make data-driven decisions that will enable the company to improve its overall performance. In this post, we will cover the analysis of data from an organizational point of view while still going through the scientific and statistical foundations that are fundamental to understanding the basics of data analysis.
To put all of that into perspective, we will answer a host of important analytical questions, explore analytical methods and techniques, while demonstrating how to perform analysis in the real world with a 17-step blueprint for success.
What Is Data Analysis?
Data analysis is the process of collecting, modeling, and analyzing data using various statistical and logical methods and techniques. Businesses rely on analytics processes and tools to extract insights that support strategic and operational decision-making.
All these various methods are largely based on two core areas: quantitative and qualitative research.
To explain the key differences between qualitative and quantitative research, here’s a video for your viewing pleasure:
Gaining a better understanding of different techniques and methods in quantitative research as well as qualitative insights will give your analyzing efforts a more clearly defined direction, so it’s worth taking the time to allow this particular knowledge to sink in. Additionally, you will be able to create a comprehensive analytical report that will skyrocket your analysis.
Apart from qualitative and quantitative categories, there are also other types of data that you should be aware of before dividing into complex data analysis processes. These categories include:
- Big data: Refers to massive data sets that need to be analyzed using advanced software to reveal patterns and trends. It is considered to be one of the best analytical assets as it provides larger volumes of data at a faster rate.
- Metadata: Putting it simply, metadata is data that provides insights about other data. It summarizes key information about specific data that makes it easier to find and reuse for later purposes.
- Real time data: As its name suggests, real time data is presented as soon as it is acquired. From an organizational perspective, this is the most valuable data as it can help you make important decisions based on the latest developments. Our guide on real time analytics will tell you more about the topic.
- Machine data: This is more complex data that is generated solely by a machine such as phones, computers, or even websites and embedded systems, without previous human interaction.
Why Is Data Analysis Important?
Before we go into detail about the categories of analysis along with its methods and techniques, you must understand the potential that analyzing data can bring to your organization.
- Informed decision-making : From a management perspective, you can benefit from analyzing your data as it helps you make decisions based on facts and not simple intuition. For instance, you can understand where to invest your capital, detect growth opportunities, predict your income, or tackle uncommon situations before they become problems. Through this, you can extract relevant insights from all areas in your organization, and with the help of dashboard software , present the data in a professional and interactive way to different stakeholders.
- Reduce costs : Another great benefit is to reduce costs. With the help of advanced technologies such as predictive analytics, businesses can spot improvement opportunities, trends, and patterns in their data and plan their strategies accordingly. In time, this will help you save money and resources on implementing the wrong strategies. And not just that, by predicting different scenarios such as sales and demand you can also anticipate production and supply.
- Target customers better : Customers are arguably the most crucial element in any business. By using analytics to get a 360° vision of all aspects related to your customers, you can understand which channels they use to communicate with you, their demographics, interests, habits, purchasing behaviors, and more. In the long run, it will drive success to your marketing strategies, allow you to identify new potential customers, and avoid wasting resources on targeting the wrong people or sending the wrong message. You can also track customer satisfaction by analyzing your client’s reviews or your customer service department’s performance.
What Is The Data Analysis Process?

When we talk about analyzing data there is an order to follow in order to extract the needed conclusions. The analysis process consists of 5 key stages. We will cover each of them more in detail later in the post, but to start providing the needed context to understand what is coming next, here is a rundown of the 5 essential steps of data analysis.
- Identify: Before you get your hands dirty with data, you first need to identify why you need it in the first place. The identification is the stage in which you establish the questions you will need to answer. For example, what is the customer's perception of our brand? Or what type of packaging is more engaging to our potential customers? Once the questions are outlined you are ready for the next step.
- Collect: As its name suggests, this is the stage where you start collecting the needed data. Here, you define which sources of data you will use and how you will use them. The collection of data can come in different forms such as internal or external sources, surveys, interviews, questionnaires, and focus groups, among others. An important note here is that the way you collect the data will be different in a quantitative and qualitative scenario.
- Clean: Once you have the necessary data it is time to clean it and leave it ready for analysis. Not all the data you collect will be useful, when collecting big amounts of data in different formats it is very likely that you will find yourself with duplicate or badly formatted data. To avoid this, before you start working with your data you need to make sure to erase any white spaces, duplicate records, or formatting errors. This way you avoid hurting your analysis with bad-quality data.
- Analyze : With the help of various techniques such as statistical analysis, regressions, neural networks, text analysis, and more, you can start analyzing and manipulating your data to extract relevant conclusions. At this stage, you find trends, correlations, variations, and patterns that can help you answer the questions you first thought of in the identify stage. Various technologies in the market assist researchers and average users with the management of their data. Some of them include business intelligence and visualization software, predictive analytics, and data mining, among others.
- Interpret: Last but not least you have one of the most important steps: it is time to interpret your results. This stage is where the researcher comes up with courses of action based on the findings. For example, here you would understand if your clients prefer packaging that is red or green, plastic or paper, etc. Additionally, at this stage, you can also find some limitations and work on them.
Now that you have a basic understanding of the key data analysis steps, let’s look at the top 17 essential methods.
17 Essential Types Of Data Analysis Methods
Before diving into the 17 essential types of methods, it is important that we go over really fast through the main analysis categories. Starting with the category of descriptive up to prescriptive analysis, the complexity and effort of data evaluation increases, but also the added value for the company.
a) Descriptive analysis - What happened.
The descriptive analysis method is the starting point for any analytic reflection, and it aims to answer the question of what happened? It does this by ordering, manipulating, and interpreting raw data from various sources to turn it into valuable insights for your organization.
Performing descriptive analysis is essential, as it enables us to present our insights in a meaningful way. Although it is relevant to mention that this analysis on its own will not allow you to predict future outcomes or tell you the answer to questions like why something happened, it will leave your data organized and ready to conduct further investigations.
b) Exploratory analysis - How to explore data relationships.
As its name suggests, the main aim of the exploratory analysis is to explore. Prior to it, there is still no notion of the relationship between the data and the variables. Once the data is investigated, exploratory analysis helps you to find connections and generate hypotheses and solutions for specific problems. A typical area of application for it is data mining.
c) Diagnostic analysis - Why it happened.
Diagnostic data analytics empowers analysts and executives by helping them gain a firm contextual understanding of why something happened. If you know why something happened as well as how it happened, you will be able to pinpoint the exact ways of tackling the issue or challenge.
Designed to provide direct and actionable answers to specific questions, this is one of the world’s most important methods in research, among its other key organizational functions such as retail analytics , e.g.
c) Predictive analysis - What will happen.
The predictive method allows you to look into the future to answer the question: what will happen? In order to do this, it uses the results of the previously mentioned descriptive, exploratory, and diagnostic analysis, in addition to machine learning (ML) and artificial intelligence (AI). Through this, you can uncover future trends, potential problems or inefficiencies, connections, and casualties in your data.
With predictive analysis, you can unfold and develop initiatives that will not only enhance your various operational processes but also help you gain an all-important edge over the competition. If you understand why a trend, pattern, or event happened through data, you will be able to develop an informed projection of how things may unfold in particular areas of the business.
e) Prescriptive analysis - How will it happen.
Another of the most effective types of analysis methods in research. Prescriptive data techniques cross over from predictive analysis in the way that it revolves around using patterns or trends to develop responsive, practical business strategies.
By drilling down into prescriptive analysis, you will play an active role in the data consumption process by taking well-arranged sets of visual data and using it as a powerful fix to emerging issues in a number of key areas, including marketing, sales, customer experience, HR, fulfillment, finance, logistics analytics , and others.

As mentioned at the beginning of the post, data analysis methods can be divided into two big categories: quantitative and qualitative. Each of these categories holds a powerful analytical value that changes depending on the scenario and type of data you are working with. Below, we will discuss 17 methods that are divided into qualitative and quantitative approaches.
Without further ado, here are the 17 essential types of data analysis methods with some use cases in the business world:
A. Quantitative Methods
To put it simply, quantitative analysis refers to all methods that use numerical data or data that can be turned into numbers (e.g. category variables like gender, age, etc.) to extract valuable insights. It is used to extract valuable conclusions about relationships, differences, and test hypotheses. Below we discuss some of the key quantitative methods.
1. Cluster analysis
The action of grouping a set of data elements in a way that said elements are more similar (in a particular sense) to each other than to those in other groups – hence the term ‘cluster.’ Since there is no target variable when clustering, the method is often used to find hidden patterns in the data. The approach is also used to provide additional context to a trend or dataset.
Let's look at it from an organizational perspective. In a perfect world, marketers would be able to analyze each customer separately and give them the best-personalized service, but let's face it, with a large customer base, it is timely impossible to do that. That's where clustering comes in. By grouping customers into clusters based on demographics, purchasing behaviors, monetary value, or any other factor that might be relevant for your company, you will be able to immediately optimize your efforts and give your customers the best experience based on their needs.
2. Cohort analysis
This type of data analysis approach uses historical data to examine and compare a determined segment of users' behavior, which can then be grouped with others with similar characteristics. By using this methodology, it's possible to gain a wealth of insight into consumer needs or a firm understanding of a broader target group.
Cohort analysis can be really useful for performing analysis in marketing as it will allow you to understand the impact of your campaigns on specific groups of customers. To exemplify, imagine you send an email campaign encouraging customers to sign up for your site. For this, you create two versions of the campaign with different designs, CTAs, and ad content. Later on, you can use cohort analysis to track the performance of the campaign for a longer period of time and understand which type of content is driving your customers to sign up, repurchase, or engage in other ways.
A useful tool to start performing cohort analysis method is Google Analytics. You can learn more about the benefits and limitations of using cohorts in GA in this useful guide . In the bottom image, you see an example of how you visualize a cohort in this tool. The segments (devices traffic) are divided into date cohorts (usage of devices) and then analyzed week by week to extract insights into performance.
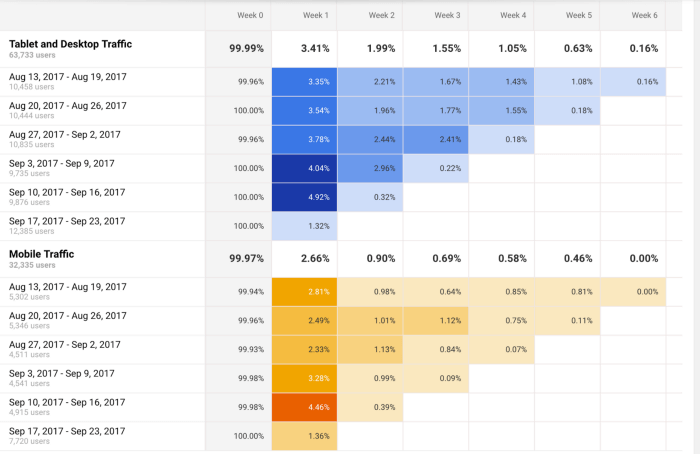
3. Regression analysis
Regression uses historical data to understand how a dependent variable's value is affected when one (linear regression) or more independent variables (multiple regression) change or stay the same. By understanding each variable's relationship and how it developed in the past, you can anticipate possible outcomes and make better decisions in the future.
Let's bring it down with an example. Imagine you did a regression analysis of your sales in 2019 and discovered that variables like product quality, store design, customer service, marketing campaigns, and sales channels affected the overall result. Now you want to use regression to analyze which of these variables changed or if any new ones appeared during 2020. For example, you couldn’t sell as much in your physical store due to COVID lockdowns. Therefore, your sales could’ve either dropped in general or increased in your online channels. Through this, you can understand which independent variables affected the overall performance of your dependent variable, annual sales.
If you want to go deeper into this type of analysis, check out this article and learn more about how you can benefit from regression.
4. Neural networks
The neural network forms the basis for the intelligent algorithms of machine learning. It is a form of analytics that attempts, with minimal intervention, to understand how the human brain would generate insights and predict values. Neural networks learn from each and every data transaction, meaning that they evolve and advance over time.
A typical area of application for neural networks is predictive analytics. There are BI reporting tools that have this feature implemented within them, such as the Predictive Analytics Tool from datapine. This tool enables users to quickly and easily generate all kinds of predictions. All you have to do is select the data to be processed based on your KPIs, and the software automatically calculates forecasts based on historical and current data. Thanks to its user-friendly interface, anyone in your organization can manage it; there’s no need to be an advanced scientist.
Here is an example of how you can use the predictive analysis tool from datapine:

**click to enlarge**
5. Factor analysis
The factor analysis also called “dimension reduction” is a type of data analysis used to describe variability among observed, correlated variables in terms of a potentially lower number of unobserved variables called factors. The aim here is to uncover independent latent variables, an ideal method for streamlining specific segments.
A good way to understand this data analysis method is a customer evaluation of a product. The initial assessment is based on different variables like color, shape, wearability, current trends, materials, comfort, the place where they bought the product, and frequency of usage. Like this, the list can be endless, depending on what you want to track. In this case, factor analysis comes into the picture by summarizing all of these variables into homogenous groups, for example, by grouping the variables color, materials, quality, and trends into a brother latent variable of design.
If you want to start analyzing data using factor analysis we recommend you take a look at this practical guide from UCLA.
6. Data mining
A method of data analysis that is the umbrella term for engineering metrics and insights for additional value, direction, and context. By using exploratory statistical evaluation, data mining aims to identify dependencies, relations, patterns, and trends to generate advanced knowledge. When considering how to analyze data, adopting a data mining mindset is essential to success - as such, it’s an area that is worth exploring in greater detail.
An excellent use case of data mining is datapine intelligent data alerts . With the help of artificial intelligence and machine learning, they provide automated signals based on particular commands or occurrences within a dataset. For example, if you’re monitoring supply chain KPIs , you could set an intelligent alarm to trigger when invalid or low-quality data appears. By doing so, you will be able to drill down deep into the issue and fix it swiftly and effectively.
In the following picture, you can see how the intelligent alarms from datapine work. By setting up ranges on daily orders, sessions, and revenues, the alarms will notify you if the goal was not completed or if it exceeded expectations.
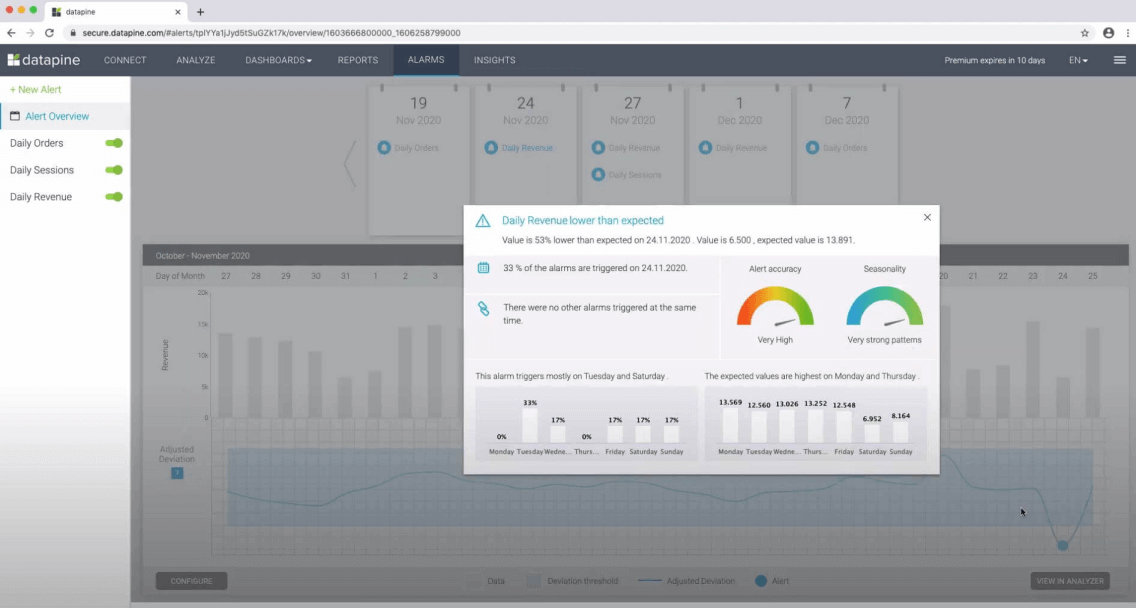
7. Time series analysis
As its name suggests, time series analysis is used to analyze a set of data points collected over a specified period of time. Although analysts use this method to monitor the data points in a specific interval of time rather than just monitoring them intermittently, the time series analysis is not uniquely used for the purpose of collecting data over time. Instead, it allows researchers to understand if variables changed during the duration of the study, how the different variables are dependent, and how did it reach the end result.
In a business context, this method is used to understand the causes of different trends and patterns to extract valuable insights. Another way of using this method is with the help of time series forecasting. Powered by predictive technologies, businesses can analyze various data sets over a period of time and forecast different future events.
A great use case to put time series analysis into perspective is seasonality effects on sales. By using time series forecasting to analyze sales data of a specific product over time, you can understand if sales rise over a specific period of time (e.g. swimwear during summertime, or candy during Halloween). These insights allow you to predict demand and prepare production accordingly.
8. Decision Trees
The decision tree analysis aims to act as a support tool to make smart and strategic decisions. By visually displaying potential outcomes, consequences, and costs in a tree-like model, researchers and company users can easily evaluate all factors involved and choose the best course of action. Decision trees are helpful to analyze quantitative data and they allow for an improved decision-making process by helping you spot improvement opportunities, reduce costs, and enhance operational efficiency and production.
But how does a decision tree actually works? This method works like a flowchart that starts with the main decision that you need to make and branches out based on the different outcomes and consequences of each decision. Each outcome will outline its own consequences, costs, and gains and, at the end of the analysis, you can compare each of them and make the smartest decision.
Businesses can use them to understand which project is more cost-effective and will bring more earnings in the long run. For example, imagine you need to decide if you want to update your software app or build a new app entirely. Here you would compare the total costs, the time needed to be invested, potential revenue, and any other factor that might affect your decision. In the end, you would be able to see which of these two options is more realistic and attainable for your company or research.
9. Conjoint analysis
Last but not least, we have the conjoint analysis. This approach is usually used in surveys to understand how individuals value different attributes of a product or service and it is one of the most effective methods to extract consumer preferences. When it comes to purchasing, some clients might be more price-focused, others more features-focused, and others might have a sustainable focus. Whatever your customer's preferences are, you can find them with conjoint analysis. Through this, companies can define pricing strategies, packaging options, subscription packages, and more.
A great example of conjoint analysis is in marketing and sales. For instance, a cupcake brand might use conjoint analysis and find that its clients prefer gluten-free options and cupcakes with healthier toppings over super sugary ones. Thus, the cupcake brand can turn these insights into advertisements and promotions to increase sales of this particular type of product. And not just that, conjoint analysis can also help businesses segment their customers based on their interests. This allows them to send different messaging that will bring value to each of the segments.
10. Correspondence Analysis
Also known as reciprocal averaging, correspondence analysis is a method used to analyze the relationship between categorical variables presented within a contingency table. A contingency table is a table that displays two (simple correspondence analysis) or more (multiple correspondence analysis) categorical variables across rows and columns that show the distribution of the data, which is usually answers to a survey or questionnaire on a specific topic.
This method starts by calculating an “expected value” which is done by multiplying row and column averages and dividing it by the overall original value of the specific table cell. The “expected value” is then subtracted from the original value resulting in a “residual number” which is what allows you to extract conclusions about relationships and distribution. The results of this analysis are later displayed using a map that represents the relationship between the different values. The closest two values are in the map, the bigger the relationship. Let’s put it into perspective with an example.
Imagine you are carrying out a market research analysis about outdoor clothing brands and how they are perceived by the public. For this analysis, you ask a group of people to match each brand with a certain attribute which can be durability, innovation, quality materials, etc. When calculating the residual numbers, you can see that brand A has a positive residual for innovation but a negative one for durability. This means that brand A is not positioned as a durable brand in the market, something that competitors could take advantage of.
11. Multidimensional Scaling (MDS)
MDS is a method used to observe the similarities or disparities between objects which can be colors, brands, people, geographical coordinates, and more. The objects are plotted using an “MDS map” that positions similar objects together and disparate ones far apart. The (dis) similarities between objects are represented using one or more dimensions that can be observed using a numerical scale. For example, if you want to know how people feel about the COVID-19 vaccine, you can use 1 for “don’t believe in the vaccine at all” and 10 for “firmly believe in the vaccine” and a scale of 2 to 9 for in between responses. When analyzing an MDS map the only thing that matters is the distance between the objects, the orientation of the dimensions is arbitrary and has no meaning at all.
Multidimensional scaling is a valuable technique for market research, especially when it comes to evaluating product or brand positioning. For instance, if a cupcake brand wants to know how they are positioned compared to competitors, it can define 2-3 dimensions such as taste, ingredients, shopping experience, or more, and do a multidimensional scaling analysis to find improvement opportunities as well as areas in which competitors are currently leading.
Another business example is in procurement when deciding on different suppliers. Decision makers can generate an MDS map to see how the different prices, delivery times, technical services, and more of the different suppliers differ and pick the one that suits their needs the best.
A final example proposed by a research paper on "An Improved Study of Multilevel Semantic Network Visualization for Analyzing Sentiment Word of Movie Review Data". Researchers picked a two-dimensional MDS map to display the distances and relationships between different sentiments in movie reviews. They used 36 sentiment words and distributed them based on their emotional distance as we can see in the image below where the words "outraged" and "sweet" are on opposite sides of the map, marking the distance between the two emotions very clearly.

Aside from being a valuable technique to analyze dissimilarities, MDS also serves as a dimension-reduction technique for large dimensional data.
B. Qualitative Methods
Qualitative data analysis methods are defined as the observation of non-numerical data that is gathered and produced using methods of observation such as interviews, focus groups, questionnaires, and more. As opposed to quantitative methods, qualitative data is more subjective and highly valuable in analyzing customer retention and product development.
12. Text analysis
Text analysis, also known in the industry as text mining, works by taking large sets of textual data and arranging them in a way that makes it easier to manage. By working through this cleansing process in stringent detail, you will be able to extract the data that is truly relevant to your organization and use it to develop actionable insights that will propel you forward.
Modern software accelerate the application of text analytics. Thanks to the combination of machine learning and intelligent algorithms, you can perform advanced analytical processes such as sentiment analysis. This technique allows you to understand the intentions and emotions of a text, for example, if it's positive, negative, or neutral, and then give it a score depending on certain factors and categories that are relevant to your brand. Sentiment analysis is often used to monitor brand and product reputation and to understand how successful your customer experience is. To learn more about the topic check out this insightful article .
By analyzing data from various word-based sources, including product reviews, articles, social media communications, and survey responses, you will gain invaluable insights into your audience, as well as their needs, preferences, and pain points. This will allow you to create campaigns, services, and communications that meet your prospects’ needs on a personal level, growing your audience while boosting customer retention. There are various other “sub-methods” that are an extension of text analysis. Each of them serves a more specific purpose and we will look at them in detail next.
13. Content Analysis
This is a straightforward and very popular method that examines the presence and frequency of certain words, concepts, and subjects in different content formats such as text, image, audio, or video. For example, the number of times the name of a celebrity is mentioned on social media or online tabloids. It does this by coding text data that is later categorized and tabulated in a way that can provide valuable insights, making it the perfect mix of quantitative and qualitative analysis.
There are two types of content analysis. The first one is the conceptual analysis which focuses on explicit data, for instance, the number of times a concept or word is mentioned in a piece of content. The second one is relational analysis, which focuses on the relationship between different concepts or words and how they are connected within a specific context.
Content analysis is often used by marketers to measure brand reputation and customer behavior. For example, by analyzing customer reviews. It can also be used to analyze customer interviews and find directions for new product development. It is also important to note, that in order to extract the maximum potential out of this analysis method, it is necessary to have a clearly defined research question.
14. Thematic Analysis
Very similar to content analysis, thematic analysis also helps in identifying and interpreting patterns in qualitative data with the main difference being that the first one can also be applied to quantitative analysis. The thematic method analyzes large pieces of text data such as focus group transcripts or interviews and groups them into themes or categories that come up frequently within the text. It is a great method when trying to figure out peoples view’s and opinions about a certain topic. For example, if you are a brand that cares about sustainability, you can do a survey of your customers to analyze their views and opinions about sustainability and how they apply it to their lives. You can also analyze customer service calls transcripts to find common issues and improve your service.
Thematic analysis is a very subjective technique that relies on the researcher’s judgment. Therefore, to avoid biases, it has 6 steps that include familiarization, coding, generating themes, reviewing themes, defining and naming themes, and writing up. It is also important to note that, because it is a flexible approach, the data can be interpreted in multiple ways and it can be hard to select what data is more important to emphasize.
15. Narrative Analysis
A bit more complex in nature than the two previous ones, narrative analysis is used to explore the meaning behind the stories that people tell and most importantly, how they tell them. By looking into the words that people use to describe a situation you can extract valuable conclusions about their perspective on a specific topic. Common sources for narrative data include autobiographies, family stories, opinion pieces, and testimonials, among others.
From a business perspective, narrative analysis can be useful to analyze customer behaviors and feelings towards a specific product, service, feature, or others. It provides unique and deep insights that can be extremely valuable. However, it has some drawbacks.
The biggest weakness of this method is that the sample sizes are usually very small due to the complexity and time-consuming nature of the collection of narrative data. Plus, the way a subject tells a story will be significantly influenced by his or her specific experiences, making it very hard to replicate in a subsequent study.
16. Discourse Analysis
Discourse analysis is used to understand the meaning behind any type of written, verbal, or symbolic discourse based on its political, social, or cultural context. It mixes the analysis of languages and situations together. This means that the way the content is constructed and the meaning behind it is significantly influenced by the culture and society it takes place in. For example, if you are analyzing political speeches you need to consider different context elements such as the politician's background, the current political context of the country, the audience to which the speech is directed, and so on.
From a business point of view, discourse analysis is a great market research tool. It allows marketers to understand how the norms and ideas of the specific market work and how their customers relate to those ideas. It can be very useful to build a brand mission or develop a unique tone of voice.
17. Grounded Theory Analysis
Traditionally, researchers decide on a method and hypothesis and start to collect the data to prove that hypothesis. The grounded theory is the only method that doesn’t require an initial research question or hypothesis as its value lies in the generation of new theories. With the grounded theory method, you can go into the analysis process with an open mind and explore the data to generate new theories through tests and revisions. In fact, it is not necessary to collect the data and then start to analyze it. Researchers usually start to find valuable insights as they are gathering the data.
All of these elements make grounded theory a very valuable method as theories are fully backed by data instead of initial assumptions. It is a great technique to analyze poorly researched topics or find the causes behind specific company outcomes. For example, product managers and marketers might use the grounded theory to find the causes of high levels of customer churn and look into customer surveys and reviews to develop new theories about the causes.
How To Analyze Data? Top 17 Data Analysis Techniques To Apply

Now that we’ve answered the questions “what is data analysis’”, why is it important, and covered the different data analysis types, it’s time to dig deeper into how to perform your analysis by working through these 17 essential techniques.
1. Collaborate your needs
Before you begin analyzing or drilling down into any techniques, it’s crucial to sit down collaboratively with all key stakeholders within your organization, decide on your primary campaign or strategic goals, and gain a fundamental understanding of the types of insights that will best benefit your progress or provide you with the level of vision you need to evolve your organization.
2. Establish your questions
Once you’ve outlined your core objectives, you should consider which questions will need answering to help you achieve your mission. This is one of the most important techniques as it will shape the very foundations of your success.
To help you ask the right things and ensure your data works for you, you have to ask the right data analysis questions .
3. Data democratization
After giving your data analytics methodology some real direction, and knowing which questions need answering to extract optimum value from the information available to your organization, you should continue with democratization.
Data democratization is an action that aims to connect data from various sources efficiently and quickly so that anyone in your organization can access it at any given moment. You can extract data in text, images, videos, numbers, or any other format. And then perform cross-database analysis to achieve more advanced insights to share with the rest of the company interactively.
Once you have decided on your most valuable sources, you need to take all of this into a structured format to start collecting your insights. For this purpose, datapine offers an easy all-in-one data connectors feature to integrate all your internal and external sources and manage them at your will. Additionally, datapine’s end-to-end solution automatically updates your data, allowing you to save time and focus on performing the right analysis to grow your company.
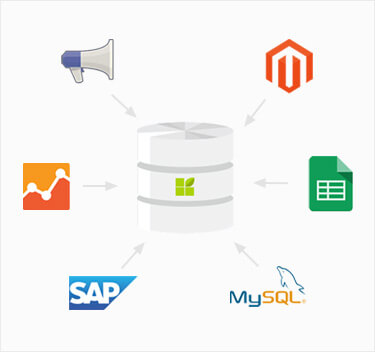
4. Think of governance
When collecting data in a business or research context you always need to think about security and privacy. With data breaches becoming a topic of concern for businesses, the need to protect your client's or subject’s sensitive information becomes critical.
To ensure that all this is taken care of, you need to think of a data governance strategy. According to Gartner , this concept refers to “ the specification of decision rights and an accountability framework to ensure the appropriate behavior in the valuation, creation, consumption, and control of data and analytics .” In simpler words, data governance is a collection of processes, roles, and policies, that ensure the efficient use of data while still achieving the main company goals. It ensures that clear roles are in place for who can access the information and how they can access it. In time, this not only ensures that sensitive information is protected but also allows for an efficient analysis as a whole.
5. Clean your data
After harvesting from so many sources you will be left with a vast amount of information that can be overwhelming to deal with. At the same time, you can be faced with incorrect data that can be misleading to your analysis. The smartest thing you can do to avoid dealing with this in the future is to clean the data. This is fundamental before visualizing it, as it will ensure that the insights you extract from it are correct.
There are many things that you need to look for in the cleaning process. The most important one is to eliminate any duplicate observations; this usually appears when using multiple internal and external sources of information. You can also add any missing codes, fix empty fields, and eliminate incorrectly formatted data.
Another usual form of cleaning is done with text data. As we mentioned earlier, most companies today analyze customer reviews, social media comments, questionnaires, and several other text inputs. In order for algorithms to detect patterns, text data needs to be revised to avoid invalid characters or any syntax or spelling errors.
Most importantly, the aim of cleaning is to prevent you from arriving at false conclusions that can damage your company in the long run. By using clean data, you will also help BI solutions to interact better with your information and create better reports for your organization.
6. Set your KPIs
Once you’ve set your sources, cleaned your data, and established clear-cut questions you want your insights to answer, you need to set a host of key performance indicators (KPIs) that will help you track, measure, and shape your progress in a number of key areas.
KPIs are critical to both qualitative and quantitative analysis research. This is one of the primary methods of data analysis you certainly shouldn’t overlook.
To help you set the best possible KPIs for your initiatives and activities, here is an example of a relevant logistics KPI : transportation-related costs. If you want to see more go explore our collection of key performance indicator examples .

7. Omit useless data
Having bestowed your data analysis tools and techniques with true purpose and defined your mission, you should explore the raw data you’ve collected from all sources and use your KPIs as a reference for chopping out any information you deem to be useless.
Trimming the informational fat is one of the most crucial methods of analysis as it will allow you to focus your analytical efforts and squeeze every drop of value from the remaining ‘lean’ information.
Any stats, facts, figures, or metrics that don’t align with your business goals or fit with your KPI management strategies should be eliminated from the equation.
8. Build a data management roadmap
While, at this point, this particular step is optional (you will have already gained a wealth of insight and formed a fairly sound strategy by now), creating a data governance roadmap will help your data analysis methods and techniques become successful on a more sustainable basis. These roadmaps, if developed properly, are also built so they can be tweaked and scaled over time.
Invest ample time in developing a roadmap that will help you store, manage, and handle your data internally, and you will make your analysis techniques all the more fluid and functional – one of the most powerful types of data analysis methods available today.
9. Integrate technology
There are many ways to analyze data, but one of the most vital aspects of analytical success in a business context is integrating the right decision support software and technology.
Robust analysis platforms will not only allow you to pull critical data from your most valuable sources while working with dynamic KPIs that will offer you actionable insights; it will also present them in a digestible, visual, interactive format from one central, live dashboard . A data methodology you can count on.
By integrating the right technology within your data analysis methodology, you’ll avoid fragmenting your insights, saving you time and effort while allowing you to enjoy the maximum value from your business’s most valuable insights.
For a look at the power of software for the purpose of analysis and to enhance your methods of analyzing, glance over our selection of dashboard examples .
10. Answer your questions
By considering each of the above efforts, working with the right technology, and fostering a cohesive internal culture where everyone buys into the different ways to analyze data as well as the power of digital intelligence, you will swiftly start to answer your most burning business questions. Arguably, the best way to make your data concepts accessible across the organization is through data visualization.
11. Visualize your data
Online data visualization is a powerful tool as it lets you tell a story with your metrics, allowing users across the organization to extract meaningful insights that aid business evolution – and it covers all the different ways to analyze data.
The purpose of analyzing is to make your entire organization more informed and intelligent, and with the right platform or dashboard, this is simpler than you think, as demonstrated by our marketing dashboard .

This visual, dynamic, and interactive online dashboard is a data analysis example designed to give Chief Marketing Officers (CMO) an overview of relevant metrics to help them understand if they achieved their monthly goals.
In detail, this example generated with a modern dashboard creator displays interactive charts for monthly revenues, costs, net income, and net income per customer; all of them are compared with the previous month so that you can understand how the data fluctuated. In addition, it shows a detailed summary of the number of users, customers, SQLs, and MQLs per month to visualize the whole picture and extract relevant insights or trends for your marketing reports .
The CMO dashboard is perfect for c-level management as it can help them monitor the strategic outcome of their marketing efforts and make data-driven decisions that can benefit the company exponentially.
12. Be careful with the interpretation
We already dedicated an entire post to data interpretation as it is a fundamental part of the process of data analysis. It gives meaning to the analytical information and aims to drive a concise conclusion from the analysis results. Since most of the time companies are dealing with data from many different sources, the interpretation stage needs to be done carefully and properly in order to avoid misinterpretations.
To help you through the process, here we list three common practices that you need to avoid at all costs when looking at your data:
- Correlation vs. causation: The human brain is formatted to find patterns. This behavior leads to one of the most common mistakes when performing interpretation: confusing correlation with causation. Although these two aspects can exist simultaneously, it is not correct to assume that because two things happened together, one provoked the other. A piece of advice to avoid falling into this mistake is never to trust just intuition, trust the data. If there is no objective evidence of causation, then always stick to correlation.
- Confirmation bias: This phenomenon describes the tendency to select and interpret only the data necessary to prove one hypothesis, often ignoring the elements that might disprove it. Even if it's not done on purpose, confirmation bias can represent a real problem, as excluding relevant information can lead to false conclusions and, therefore, bad business decisions. To avoid it, always try to disprove your hypothesis instead of proving it, share your analysis with other team members, and avoid drawing any conclusions before the entire analytical project is finalized.
- Statistical significance: To put it in short words, statistical significance helps analysts understand if a result is actually accurate or if it happened because of a sampling error or pure chance. The level of statistical significance needed might depend on the sample size and the industry being analyzed. In any case, ignoring the significance of a result when it might influence decision-making can be a huge mistake.
13. Build a narrative
Now, we’re going to look at how you can bring all of these elements together in a way that will benefit your business - starting with a little something called data storytelling.
The human brain responds incredibly well to strong stories or narratives. Once you’ve cleansed, shaped, and visualized your most invaluable data using various BI dashboard tools , you should strive to tell a story - one with a clear-cut beginning, middle, and end.
By doing so, you will make your analytical efforts more accessible, digestible, and universal, empowering more people within your organization to use your discoveries to their actionable advantage.
14. Consider autonomous technology
Autonomous technologies, such as artificial intelligence (AI) and machine learning (ML), play a significant role in the advancement of understanding how to analyze data more effectively.
Gartner predicts that by the end of this year, 80% of emerging technologies will be developed with AI foundations. This is a testament to the ever-growing power and value of autonomous technologies.
At the moment, these technologies are revolutionizing the analysis industry. Some examples that we mentioned earlier are neural networks, intelligent alarms, and sentiment analysis.
15. Share the load
If you work with the right tools and dashboards, you will be able to present your metrics in a digestible, value-driven format, allowing almost everyone in the organization to connect with and use relevant data to their advantage.
Modern dashboards consolidate data from various sources, providing access to a wealth of insights in one centralized location, no matter if you need to monitor recruitment metrics or generate reports that need to be sent across numerous departments. Moreover, these cutting-edge tools offer access to dashboards from a multitude of devices, meaning that everyone within the business can connect with practical insights remotely - and share the load.
Once everyone is able to work with a data-driven mindset, you will catalyze the success of your business in ways you never thought possible. And when it comes to knowing how to analyze data, this kind of collaborative approach is essential.
16. Data analysis tools
In order to perform high-quality analysis of data, it is fundamental to use tools and software that will ensure the best results. Here we leave you a small summary of four fundamental categories of data analysis tools for your organization.
- Business Intelligence: BI tools allow you to process significant amounts of data from several sources in any format. Through this, you can not only analyze and monitor your data to extract relevant insights but also create interactive reports and dashboards to visualize your KPIs and use them for your company's good. datapine is an amazing online BI software that is focused on delivering powerful online analysis features that are accessible to beginner and advanced users. Like this, it offers a full-service solution that includes cutting-edge analysis of data, KPIs visualization, live dashboards, reporting, and artificial intelligence technologies to predict trends and minimize risk.
- Statistical analysis: These tools are usually designed for scientists, statisticians, market researchers, and mathematicians, as they allow them to perform complex statistical analyses with methods like regression analysis, predictive analysis, and statistical modeling. A good tool to perform this type of analysis is R-Studio as it offers a powerful data modeling and hypothesis testing feature that can cover both academic and general data analysis. This tool is one of the favorite ones in the industry, due to its capability for data cleaning, data reduction, and performing advanced analysis with several statistical methods. Another relevant tool to mention is SPSS from IBM. The software offers advanced statistical analysis for users of all skill levels. Thanks to a vast library of machine learning algorithms, text analysis, and a hypothesis testing approach it can help your company find relevant insights to drive better decisions. SPSS also works as a cloud service that enables you to run it anywhere.
- SQL Consoles: SQL is a programming language often used to handle structured data in relational databases. Tools like these are popular among data scientists as they are extremely effective in unlocking these databases' value. Undoubtedly, one of the most used SQL software in the market is MySQL Workbench . This tool offers several features such as a visual tool for database modeling and monitoring, complete SQL optimization, administration tools, and visual performance dashboards to keep track of KPIs.
- Data Visualization: These tools are used to represent your data through charts, graphs, and maps that allow you to find patterns and trends in the data. datapine's already mentioned BI platform also offers a wealth of powerful online data visualization tools with several benefits. Some of them include: delivering compelling data-driven presentations to share with your entire company, the ability to see your data online with any device wherever you are, an interactive dashboard design feature that enables you to showcase your results in an interactive and understandable way, and to perform online self-service reports that can be used simultaneously with several other people to enhance team productivity.
17. Refine your process constantly
Last is a step that might seem obvious to some people, but it can be easily ignored if you think you are done. Once you have extracted the needed results, you should always take a retrospective look at your project and think about what you can improve. As you saw throughout this long list of techniques, data analysis is a complex process that requires constant refinement. For this reason, you should always go one step further and keep improving.
Quality Criteria For Data Analysis
So far we’ve covered a list of methods and techniques that should help you perform efficient data analysis. But how do you measure the quality and validity of your results? This is done with the help of some science quality criteria. Here we will go into a more theoretical area that is critical to understanding the fundamentals of statistical analysis in science. However, you should also be aware of these steps in a business context, as they will allow you to assess the quality of your results in the correct way. Let’s dig in.
- Internal validity: The results of a survey are internally valid if they measure what they are supposed to measure and thus provide credible results. In other words , internal validity measures the trustworthiness of the results and how they can be affected by factors such as the research design, operational definitions, how the variables are measured, and more. For instance, imagine you are doing an interview to ask people if they brush their teeth two times a day. While most of them will answer yes, you can still notice that their answers correspond to what is socially acceptable, which is to brush your teeth at least twice a day. In this case, you can’t be 100% sure if respondents actually brush their teeth twice a day or if they just say that they do, therefore, the internal validity of this interview is very low.
- External validity: Essentially, external validity refers to the extent to which the results of your research can be applied to a broader context. It basically aims to prove that the findings of a study can be applied in the real world. If the research can be applied to other settings, individuals, and times, then the external validity is high.
- Reliability : If your research is reliable, it means that it can be reproduced. If your measurement were repeated under the same conditions, it would produce similar results. This means that your measuring instrument consistently produces reliable results. For example, imagine a doctor building a symptoms questionnaire to detect a specific disease in a patient. Then, various other doctors use this questionnaire but end up diagnosing the same patient with a different condition. This means the questionnaire is not reliable in detecting the initial disease. Another important note here is that in order for your research to be reliable, it also needs to be objective. If the results of a study are the same, independent of who assesses them or interprets them, the study can be considered reliable. Let’s see the objectivity criteria in more detail now.
- Objectivity: In data science, objectivity means that the researcher needs to stay fully objective when it comes to its analysis. The results of a study need to be affected by objective criteria and not by the beliefs, personality, or values of the researcher. Objectivity needs to be ensured when you are gathering the data, for example, when interviewing individuals, the questions need to be asked in a way that doesn't influence the results. Paired with this, objectivity also needs to be thought of when interpreting the data. If different researchers reach the same conclusions, then the study is objective. For this last point, you can set predefined criteria to interpret the results to ensure all researchers follow the same steps.
The discussed quality criteria cover mostly potential influences in a quantitative context. Analysis in qualitative research has by default additional subjective influences that must be controlled in a different way. Therefore, there are other quality criteria for this kind of research such as credibility, transferability, dependability, and confirmability. You can see each of them more in detail on this resource .
Data Analysis Limitations & Barriers
Analyzing data is not an easy task. As you’ve seen throughout this post, there are many steps and techniques that you need to apply in order to extract useful information from your research. While a well-performed analysis can bring various benefits to your organization it doesn't come without limitations. In this section, we will discuss some of the main barriers you might encounter when conducting an analysis. Let’s see them more in detail.
- Lack of clear goals: No matter how good your data or analysis might be if you don’t have clear goals or a hypothesis the process might be worthless. While we mentioned some methods that don’t require a predefined hypothesis, it is always better to enter the analytical process with some clear guidelines of what you are expecting to get out of it, especially in a business context in which data is utilized to support important strategic decisions.
- Objectivity: Arguably one of the biggest barriers when it comes to data analysis in research is to stay objective. When trying to prove a hypothesis, researchers might find themselves, intentionally or unintentionally, directing the results toward an outcome that they want. To avoid this, always question your assumptions and avoid confusing facts with opinions. You can also show your findings to a research partner or external person to confirm that your results are objective.
- Data representation: A fundamental part of the analytical procedure is the way you represent your data. You can use various graphs and charts to represent your findings, but not all of them will work for all purposes. Choosing the wrong visual can not only damage your analysis but can mislead your audience, therefore, it is important to understand when to use each type of data depending on your analytical goals. Our complete guide on the types of graphs and charts lists 20 different visuals with examples of when to use them.
- Flawed correlation : Misleading statistics can significantly damage your research. We’ve already pointed out a few interpretation issues previously in the post, but it is an important barrier that we can't avoid addressing here as well. Flawed correlations occur when two variables appear related to each other but they are not. Confusing correlations with causation can lead to a wrong interpretation of results which can lead to building wrong strategies and loss of resources, therefore, it is very important to identify the different interpretation mistakes and avoid them.
- Sample size: A very common barrier to a reliable and efficient analysis process is the sample size. In order for the results to be trustworthy, the sample size should be representative of what you are analyzing. For example, imagine you have a company of 1000 employees and you ask the question “do you like working here?” to 50 employees of which 49 say yes, which means 95%. Now, imagine you ask the same question to the 1000 employees and 950 say yes, which also means 95%. Saying that 95% of employees like working in the company when the sample size was only 50 is not a representative or trustworthy conclusion. The significance of the results is way more accurate when surveying a bigger sample size.
- Privacy concerns: In some cases, data collection can be subjected to privacy regulations. Businesses gather all kinds of information from their customers from purchasing behaviors to addresses and phone numbers. If this falls into the wrong hands due to a breach, it can affect the security and confidentiality of your clients. To avoid this issue, you need to collect only the data that is needed for your research and, if you are using sensitive facts, make it anonymous so customers are protected. The misuse of customer data can severely damage a business's reputation, so it is important to keep an eye on privacy.
- Lack of communication between teams : When it comes to performing data analysis on a business level, it is very likely that each department and team will have different goals and strategies. However, they are all working for the same common goal of helping the business run smoothly and keep growing. When teams are not connected and communicating with each other, it can directly affect the way general strategies are built. To avoid these issues, tools such as data dashboards enable teams to stay connected through data in a visually appealing way.
- Innumeracy : Businesses are working with data more and more every day. While there are many BI tools available to perform effective analysis, data literacy is still a constant barrier. Not all employees know how to apply analysis techniques or extract insights from them. To prevent this from happening, you can implement different training opportunities that will prepare every relevant user to deal with data.
Key Data Analysis Skills
As you've learned throughout this lengthy guide, analyzing data is a complex task that requires a lot of knowledge and skills. That said, thanks to the rise of self-service tools the process is way more accessible and agile than it once was. Regardless, there are still some key skills that are valuable to have when working with data, we list the most important ones below.
- Critical and statistical thinking: To successfully analyze data you need to be creative and think out of the box. Yes, that might sound like a weird statement considering that data is often tight to facts. However, a great level of critical thinking is required to uncover connections, come up with a valuable hypothesis, and extract conclusions that go a step further from the surface. This, of course, needs to be complemented by statistical thinking and an understanding of numbers.
- Data cleaning: Anyone who has ever worked with data before will tell you that the cleaning and preparation process accounts for 80% of a data analyst's work, therefore, the skill is fundamental. But not just that, not cleaning the data adequately can also significantly damage the analysis which can lead to poor decision-making in a business scenario. While there are multiple tools that automate the cleaning process and eliminate the possibility of human error, it is still a valuable skill to dominate.
- Data visualization: Visuals make the information easier to understand and analyze, not only for professional users but especially for non-technical ones. Having the necessary skills to not only choose the right chart type but know when to apply it correctly is key. This also means being able to design visually compelling charts that make the data exploration process more efficient.
- SQL: The Structured Query Language or SQL is a programming language used to communicate with databases. It is fundamental knowledge as it enables you to update, manipulate, and organize data from relational databases which are the most common databases used by companies. It is fairly easy to learn and one of the most valuable skills when it comes to data analysis.
- Communication skills: This is a skill that is especially valuable in a business environment. Being able to clearly communicate analytical outcomes to colleagues is incredibly important, especially when the information you are trying to convey is complex for non-technical people. This applies to in-person communication as well as written format, for example, when generating a dashboard or report. While this might be considered a “soft” skill compared to the other ones we mentioned, it should not be ignored as you most likely will need to share analytical findings with others no matter the context.
Data Analysis In The Big Data Environment
Big data is invaluable to today’s businesses, and by using different methods for data analysis, it’s possible to view your data in a way that can help you turn insight into positive action.
To inspire your efforts and put the importance of big data into context, here are some insights that you should know:
- By 2026 the industry of big data is expected to be worth approximately $273.4 billion.
- 94% of enterprises say that analyzing data is important for their growth and digital transformation.
- Companies that exploit the full potential of their data can increase their operating margins by 60% .
- We already told you the benefits of Artificial Intelligence through this article. This industry's financial impact is expected to grow up to $40 billion by 2025.
Data analysis concepts may come in many forms, but fundamentally, any solid methodology will help to make your business more streamlined, cohesive, insightful, and successful than ever before.
Key Takeaways From Data Analysis
As we reach the end of our data analysis journey, we leave a small summary of the main methods and techniques to perform excellent analysis and grow your business.
17 Essential Types of Data Analysis Methods:
- Cluster analysis
- Cohort analysis
- Regression analysis
- Factor analysis
- Neural Networks
- Data Mining
- Text analysis
- Time series analysis
- Decision trees
- Conjoint analysis
- Correspondence Analysis
- Multidimensional Scaling
- Content analysis
- Thematic analysis
- Narrative analysis
- Grounded theory analysis
- Discourse analysis
Top 17 Data Analysis Techniques:
- Collaborate your needs
- Establish your questions
- Data democratization
- Think of data governance
- Clean your data
- Set your KPIs
- Omit useless data
- Build a data management roadmap
- Integrate technology
- Answer your questions
- Visualize your data
- Interpretation of data
- Consider autonomous technology
- Build a narrative
- Share the load
- Data Analysis tools
- Refine your process constantly
We’ve pondered the data analysis definition and drilled down into the practical applications of data-centric analytics, and one thing is clear: by taking measures to arrange your data and making your metrics work for you, it’s possible to transform raw information into action - the kind of that will push your business to the next level.
Yes, good data analytics techniques result in enhanced business intelligence (BI). To help you understand this notion in more detail, read our exploration of business intelligence reporting .
And, if you’re ready to perform your own analysis, drill down into your facts and figures while interacting with your data on astonishing visuals, you can try our software for a free, 14-day trial .
News alert: UC Berkeley has announced its next university librarian
Secondary menu
- Log in to your Library account
- Hours and Maps
- Connect from Off Campus
- UC Berkeley Home
Search form
Research methods--quantitative, qualitative, and more: overview.
- Quantitative Research
- Qualitative Research
- Data Science Methods (Machine Learning, AI, Big Data)
- Text Mining and Computational Text Analysis
- Evidence Synthesis/Systematic Reviews
- Get Data, Get Help!
About Research Methods
This guide provides an overview of research methods, how to choose and use them, and supports and resources at UC Berkeley.
As Patten and Newhart note in the book Understanding Research Methods , "Research methods are the building blocks of the scientific enterprise. They are the "how" for building systematic knowledge. The accumulation of knowledge through research is by its nature a collective endeavor. Each well-designed study provides evidence that may support, amend, refute, or deepen the understanding of existing knowledge...Decisions are important throughout the practice of research and are designed to help researchers collect evidence that includes the full spectrum of the phenomenon under study, to maintain logical rules, and to mitigate or account for possible sources of bias. In many ways, learning research methods is learning how to see and make these decisions."
The choice of methods varies by discipline, by the kind of phenomenon being studied and the data being used to study it, by the technology available, and more. This guide is an introduction, but if you don't see what you need here, always contact your subject librarian, and/or take a look to see if there's a library research guide that will answer your question.
Suggestions for changes and additions to this guide are welcome!
START HERE: SAGE Research Methods
Without question, the most comprehensive resource available from the library is SAGE Research Methods. HERE IS THE ONLINE GUIDE to this one-stop shopping collection, and some helpful links are below:
- SAGE Research Methods
- Little Green Books (Quantitative Methods)
- Little Blue Books (Qualitative Methods)
- Dictionaries and Encyclopedias
- Case studies of real research projects
- Sample datasets for hands-on practice
- Streaming video--see methods come to life
- Methodspace- -a community for researchers
- SAGE Research Methods Course Mapping
Library Data Services at UC Berkeley
Library Data Services Program and Digital Scholarship Services
The LDSP offers a variety of services and tools ! From this link, check out pages for each of the following topics: discovering data, managing data, collecting data, GIS data, text data mining, publishing data, digital scholarship, open science, and the Research Data Management Program.
Be sure also to check out the visual guide to where to seek assistance on campus with any research question you may have!
Library GIS Services
Other Data Services at Berkeley
D-Lab Supports Berkeley faculty, staff, and graduate students with research in data intensive social science, including a wide range of training and workshop offerings Dryad Dryad is a simple self-service tool for researchers to use in publishing their datasets. It provides tools for the effective publication of and access to research data. Geospatial Innovation Facility (GIF) Provides leadership and training across a broad array of integrated mapping technologies on campu Research Data Management A UC Berkeley guide and consulting service for research data management issues
General Research Methods Resources
Here are some general resources for assistance:
- Assistance from ICPSR (must create an account to access): Getting Help with Data , and Resources for Students
- Wiley Stats Ref for background information on statistics topics
- Survey Documentation and Analysis (SDA) . Program for easy web-based analysis of survey data.
Consultants
- D-Lab/Data Science Discovery Consultants Request help with your research project from peer consultants.
- Research data (RDM) consulting Meet with RDM consultants before designing the data security, storage, and sharing aspects of your qualitative project.
- Statistics Department Consulting Services A service in which advanced graduate students, under faculty supervision, are available to consult during specified hours in the Fall and Spring semesters.
Related Resourcex
- IRB / CPHS Qualitative research projects with human subjects often require that you go through an ethics review.
- OURS (Office of Undergraduate Research and Scholarships) OURS supports undergraduates who want to embark on research projects and assistantships. In particular, check out their "Getting Started in Research" workshops
- Sponsored Projects Sponsored projects works with researchers applying for major external grants.
- Next: Quantitative Research >>
- Last Updated: Apr 3, 2023 3:14 PM
- URL: https://guides.lib.berkeley.edu/researchmethods

Qualitative Data Analysis Methods 101:
The “big 6” methods + examples.
By: Kerryn Warren (PhD) | Reviewed By: Eunice Rautenbach (D.Tech) | May 2020 (Updated April 2023)
Qualitative data analysis methods. Wow, that’s a mouthful.
If you’re new to the world of research, qualitative data analysis can look rather intimidating. So much bulky terminology and so many abstract, fluffy concepts. It certainly can be a minefield!
Don’t worry – in this post, we’ll unpack the most popular analysis methods , one at a time, so that you can approach your analysis with confidence and competence – whether that’s for a dissertation, thesis or really any kind of research project.
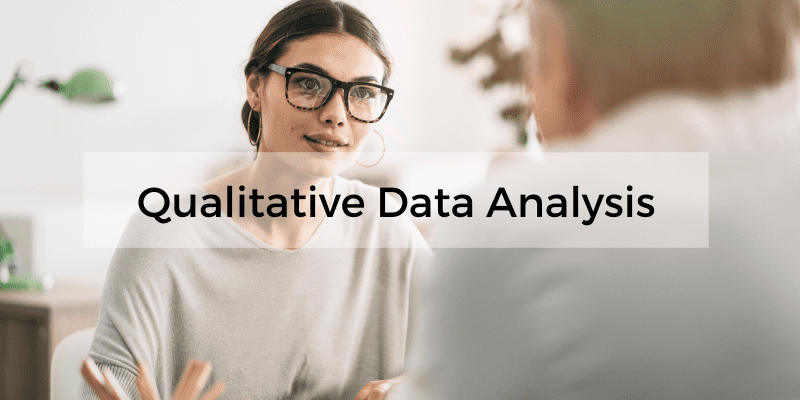
What (exactly) is qualitative data analysis?
To understand qualitative data analysis, we need to first understand qualitative data – so let’s step back and ask the question, “what exactly is qualitative data?”.
Qualitative data refers to pretty much any data that’s “not numbers” . In other words, it’s not the stuff you measure using a fixed scale or complex equipment, nor do you analyse it using complex statistics or mathematics.
So, if it’s not numbers, what is it?
Words, you guessed? Well… sometimes , yes. Qualitative data can, and often does, take the form of interview transcripts, documents and open-ended survey responses – but it can also involve the interpretation of images and videos. In other words, qualitative isn’t just limited to text-based data.
So, how’s that different from quantitative data, you ask?
Simply put, qualitative research focuses on words, descriptions, concepts or ideas – while quantitative research focuses on numbers and statistics . Qualitative research investigates the “softer side” of things to explore and describe , while quantitative research focuses on the “hard numbers”, to measure differences between variables and the relationships between them. If you’re keen to learn more about the differences between qual and quant, we’ve got a detailed post over here .
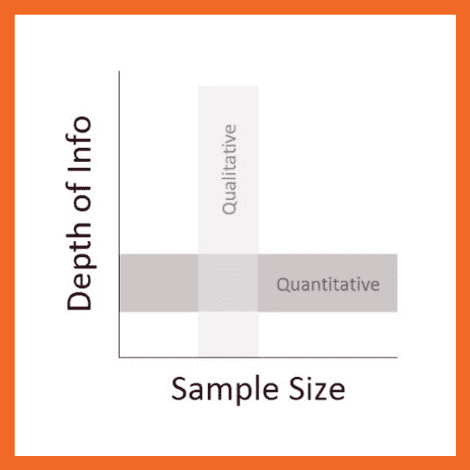
So, qualitative analysis is easier than quantitative, right?
Not quite. In many ways, qualitative data can be challenging and time-consuming to analyse and interpret. At the end of your data collection phase (which itself takes a lot of time), you’ll likely have many pages of text-based data or hours upon hours of audio to work through. You might also have subtle nuances of interactions or discussions that have danced around in your mind, or that you scribbled down in messy field notes. All of this needs to work its way into your analysis.
Making sense of all of this is no small task and you shouldn’t underestimate it. Long story short – qualitative analysis can be a lot of work! Of course, quantitative analysis is no piece of cake either, but it’s important to recognise that qualitative analysis still requires a significant investment in terms of time and effort.
Need a helping hand?
In this post, we’ll explore qualitative data analysis by looking at some of the most common analysis methods we encounter. We’re not going to cover every possible qualitative method and we’re not going to go into heavy detail – we’re just going to give you the big picture. That said, we will of course includes links to loads of extra resources so that you can learn more about whichever analysis method interests you.
Without further delay, let’s get into it.
The “Big 6” Qualitative Analysis Methods
There are many different types of qualitative data analysis, all of which serve different purposes and have unique strengths and weaknesses . We’ll start by outlining the analysis methods and then we’ll dive into the details for each.
The 6 most popular methods (or at least the ones we see at Grad Coach) are:
- Content analysis
- Narrative analysis
- Discourse analysis
- Thematic analysis
- Grounded theory (GT)
- Interpretive phenomenological analysis (IPA)
Let’s take a look at each of them…
QDA Method #1: Qualitative Content Analysis
Content analysis is possibly the most common and straightforward QDA method. At the simplest level, content analysis is used to evaluate patterns within a piece of content (for example, words, phrases or images) or across multiple pieces of content or sources of communication. For example, a collection of newspaper articles or political speeches.
With content analysis, you could, for instance, identify the frequency with which an idea is shared or spoken about – like the number of times a Kardashian is mentioned on Twitter. Or you could identify patterns of deeper underlying interpretations – for instance, by identifying phrases or words in tourist pamphlets that highlight India as an ancient country.
Because content analysis can be used in such a wide variety of ways, it’s important to go into your analysis with a very specific question and goal, or you’ll get lost in the fog. With content analysis, you’ll group large amounts of text into codes , summarise these into categories, and possibly even tabulate the data to calculate the frequency of certain concepts or variables. Because of this, content analysis provides a small splash of quantitative thinking within a qualitative method.
Naturally, while content analysis is widely useful, it’s not without its drawbacks . One of the main issues with content analysis is that it can be very time-consuming , as it requires lots of reading and re-reading of the texts. Also, because of its multidimensional focus on both qualitative and quantitative aspects, it is sometimes accused of losing important nuances in communication.
Content analysis also tends to concentrate on a very specific timeline and doesn’t take into account what happened before or after that timeline. This isn’t necessarily a bad thing though – just something to be aware of. So, keep these factors in mind if you’re considering content analysis. Every analysis method has its limitations , so don’t be put off by these – just be aware of them ! If you’re interested in learning more about content analysis, the video below provides a good starting point.
QDA Method #2: Narrative Analysis
As the name suggests, narrative analysis is all about listening to people telling stories and analysing what that means . Since stories serve a functional purpose of helping us make sense of the world, we can gain insights into the ways that people deal with and make sense of reality by analysing their stories and the ways they’re told.
You could, for example, use narrative analysis to explore whether how something is being said is important. For instance, the narrative of a prisoner trying to justify their crime could provide insight into their view of the world and the justice system. Similarly, analysing the ways entrepreneurs talk about the struggles in their careers or cancer patients telling stories of hope could provide powerful insights into their mindsets and perspectives . Simply put, narrative analysis is about paying attention to the stories that people tell – and more importantly, the way they tell them.
Of course, the narrative approach has its weaknesses , too. Sample sizes are generally quite small due to the time-consuming process of capturing narratives. Because of this, along with the multitude of social and lifestyle factors which can influence a subject, narrative analysis can be quite difficult to reproduce in subsequent research. This means that it’s difficult to test the findings of some of this research.
Similarly, researcher bias can have a strong influence on the results here, so you need to be particularly careful about the potential biases you can bring into your analysis when using this method. Nevertheless, narrative analysis is still a very useful qualitative analysis method – just keep these limitations in mind and be careful not to draw broad conclusions . If you’re keen to learn more about narrative analysis, the video below provides a great introduction to this qualitative analysis method.
QDA Method #3: Discourse Analysis
Discourse is simply a fancy word for written or spoken language or debate . So, discourse analysis is all about analysing language within its social context. In other words, analysing language – such as a conversation, a speech, etc – within the culture and society it takes place. For example, you could analyse how a janitor speaks to a CEO, or how politicians speak about terrorism.
To truly understand these conversations or speeches, the culture and history of those involved in the communication are important factors to consider. For example, a janitor might speak more casually with a CEO in a company that emphasises equality among workers. Similarly, a politician might speak more about terrorism if there was a recent terrorist incident in the country.
So, as you can see, by using discourse analysis, you can identify how culture , history or power dynamics (to name a few) have an effect on the way concepts are spoken about. So, if your research aims and objectives involve understanding culture or power dynamics, discourse analysis can be a powerful method.
Because there are many social influences in terms of how we speak to each other, the potential use of discourse analysis is vast . Of course, this also means it’s important to have a very specific research question (or questions) in mind when analysing your data and looking for patterns and themes, or you might land up going down a winding rabbit hole.
Discourse analysis can also be very time-consuming as you need to sample the data to the point of saturation – in other words, until no new information and insights emerge. But this is, of course, part of what makes discourse analysis such a powerful technique. So, keep these factors in mind when considering this QDA method. Again, if you’re keen to learn more, the video below presents a good starting point.
QDA Method #4: Thematic Analysis
Thematic analysis looks at patterns of meaning in a data set – for example, a set of interviews or focus group transcripts. But what exactly does that… mean? Well, a thematic analysis takes bodies of data (which are often quite large) and groups them according to similarities – in other words, themes . These themes help us make sense of the content and derive meaning from it.
Let’s take a look at an example.
With thematic analysis, you could analyse 100 online reviews of a popular sushi restaurant to find out what patrons think about the place. By reviewing the data, you would then identify the themes that crop up repeatedly within the data – for example, “fresh ingredients” or “friendly wait staff”.
So, as you can see, thematic analysis can be pretty useful for finding out about people’s experiences , views, and opinions . Therefore, if your research aims and objectives involve understanding people’s experience or view of something, thematic analysis can be a great choice.
Since thematic analysis is a bit of an exploratory process, it’s not unusual for your research questions to develop , or even change as you progress through the analysis. While this is somewhat natural in exploratory research, it can also be seen as a disadvantage as it means that data needs to be re-reviewed each time a research question is adjusted. In other words, thematic analysis can be quite time-consuming – but for a good reason. So, keep this in mind if you choose to use thematic analysis for your project and budget extra time for unexpected adjustments.
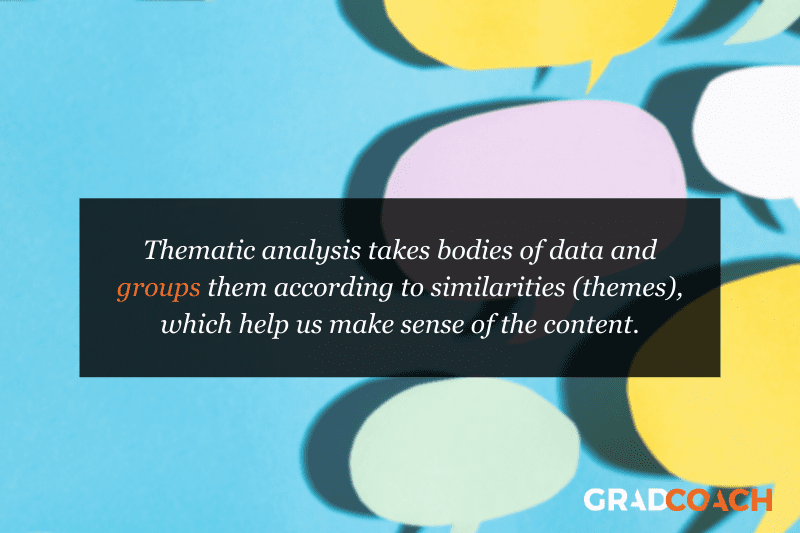
QDA Method #5: Grounded theory (GT)
Grounded theory is a powerful qualitative analysis method where the intention is to create a new theory (or theories) using the data at hand, through a series of “ tests ” and “ revisions ”. Strictly speaking, GT is more a research design type than an analysis method, but we’ve included it here as it’s often referred to as a method.
What’s most important with grounded theory is that you go into the analysis with an open mind and let the data speak for itself – rather than dragging existing hypotheses or theories into your analysis. In other words, your analysis must develop from the ground up (hence the name).
Let’s look at an example of GT in action.
Assume you’re interested in developing a theory about what factors influence students to watch a YouTube video about qualitative analysis. Using Grounded theory , you’d start with this general overarching question about the given population (i.e., graduate students). First, you’d approach a small sample – for example, five graduate students in a department at a university. Ideally, this sample would be reasonably representative of the broader population. You’d interview these students to identify what factors lead them to watch the video.
After analysing the interview data, a general pattern could emerge. For example, you might notice that graduate students are more likely to read a post about qualitative methods if they are just starting on their dissertation journey, or if they have an upcoming test about research methods.
From here, you’ll look for another small sample – for example, five more graduate students in a different department – and see whether this pattern holds true for them. If not, you’ll look for commonalities and adapt your theory accordingly. As this process continues, the theory would develop . As we mentioned earlier, what’s important with grounded theory is that the theory develops from the data – not from some preconceived idea.
So, what are the drawbacks of grounded theory? Well, some argue that there’s a tricky circularity to grounded theory. For it to work, in principle, you should know as little as possible regarding the research question and population, so that you reduce the bias in your interpretation. However, in many circumstances, it’s also thought to be unwise to approach a research question without knowledge of the current literature . In other words, it’s a bit of a “chicken or the egg” situation.
Regardless, grounded theory remains a popular (and powerful) option. Naturally, it’s a very useful method when you’re researching a topic that is completely new or has very little existing research about it, as it allows you to start from scratch and work your way from the ground up .
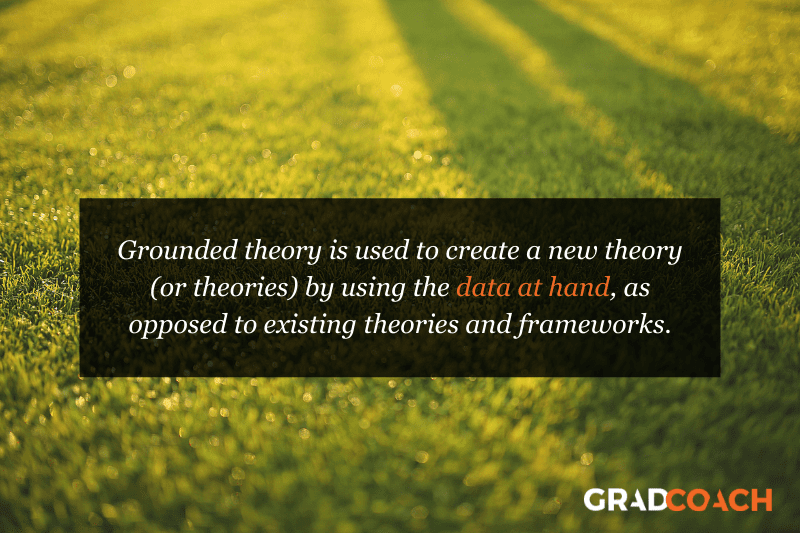
QDA Method #6: Interpretive Phenomenological Analysis (IPA)
Interpretive. Phenomenological. Analysis. IPA . Try saying that three times fast…
Let’s just stick with IPA, okay?
IPA is designed to help you understand the personal experiences of a subject (for example, a person or group of people) concerning a major life event, an experience or a situation . This event or experience is the “phenomenon” that makes up the “P” in IPA. Such phenomena may range from relatively common events – such as motherhood, or being involved in a car accident – to those which are extremely rare – for example, someone’s personal experience in a refugee camp. So, IPA is a great choice if your research involves analysing people’s personal experiences of something that happened to them.
It’s important to remember that IPA is subject – centred . In other words, it’s focused on the experiencer . This means that, while you’ll likely use a coding system to identify commonalities, it’s important not to lose the depth of experience or meaning by trying to reduce everything to codes. Also, keep in mind that since your sample size will generally be very small with IPA, you often won’t be able to draw broad conclusions about the generalisability of your findings. But that’s okay as long as it aligns with your research aims and objectives.
Another thing to be aware of with IPA is personal bias . While researcher bias can creep into all forms of research, self-awareness is critically important with IPA, as it can have a major impact on the results. For example, a researcher who was a victim of a crime himself could insert his own feelings of frustration and anger into the way he interprets the experience of someone who was kidnapped. So, if you’re going to undertake IPA, you need to be very self-aware or you could muddy the analysis.
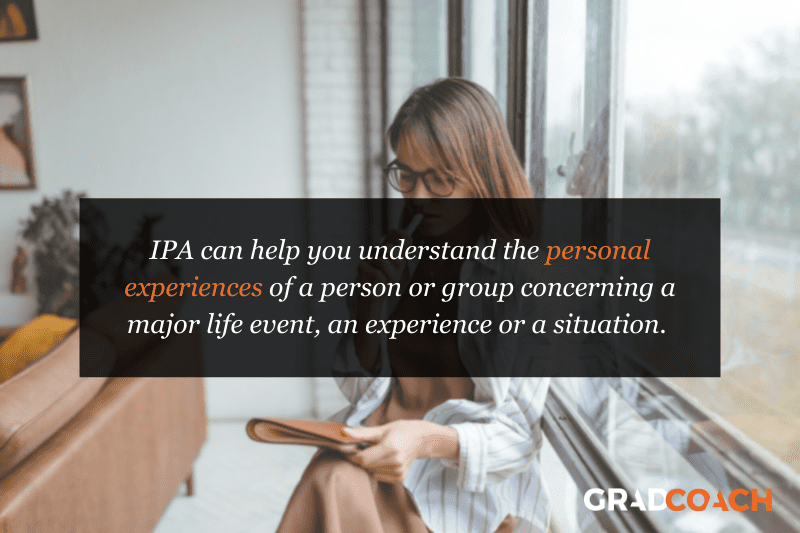
How to choose the right analysis method
In light of all of the qualitative analysis methods we’ve covered so far, you’re probably asking yourself the question, “ How do I choose the right one? ”
Much like all the other methodological decisions you’ll need to make, selecting the right qualitative analysis method largely depends on your research aims, objectives and questions . In other words, the best tool for the job depends on what you’re trying to build. For example:
- Perhaps your research aims to analyse the use of words and what they reveal about the intention of the storyteller and the cultural context of the time.
- Perhaps your research aims to develop an understanding of the unique personal experiences of people that have experienced a certain event, or
- Perhaps your research aims to develop insight regarding the influence of a certain culture on its members.
As you can probably see, each of these research aims are distinctly different , and therefore different analysis methods would be suitable for each one. For example, narrative analysis would likely be a good option for the first aim, while grounded theory wouldn’t be as relevant.
It’s also important to remember that each method has its own set of strengths, weaknesses and general limitations. No single analysis method is perfect . So, depending on the nature of your research, it may make sense to adopt more than one method (this is called triangulation ). Keep in mind though that this will of course be quite time-consuming.
As we’ve seen, all of the qualitative analysis methods we’ve discussed make use of coding and theme-generating techniques, but the intent and approach of each analysis method differ quite substantially. So, it’s very important to come into your research with a clear intention before you decide which analysis method (or methods) to use.
Start by reviewing your research aims , objectives and research questions to assess what exactly you’re trying to find out – then select a qualitative analysis method that fits. Never pick a method just because you like it or have experience using it – your analysis method (or methods) must align with your broader research aims and objectives.
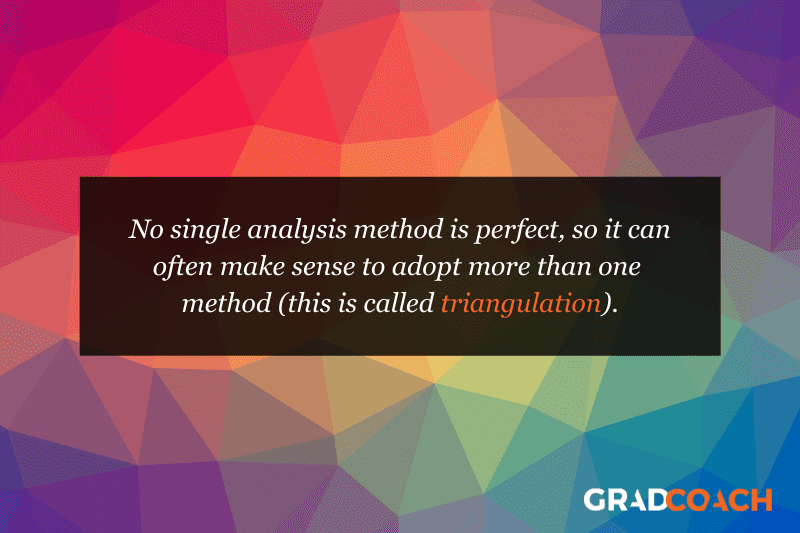
Let’s recap on QDA methods…
In this post, we looked at six popular qualitative data analysis methods:
- First, we looked at content analysis , a straightforward method that blends a little bit of quant into a primarily qualitative analysis.
- Then we looked at narrative analysis , which is about analysing how stories are told.
- Next up was discourse analysis – which is about analysing conversations and interactions.
- Then we moved on to thematic analysis – which is about identifying themes and patterns.
- From there, we went south with grounded theory – which is about starting from scratch with a specific question and using the data alone to build a theory in response to that question.
- And finally, we looked at IPA – which is about understanding people’s unique experiences of a phenomenon.
Of course, these aren’t the only options when it comes to qualitative data analysis, but they’re a great starting point if you’re dipping your toes into qualitative research for the first time.
If you’re still feeling a bit confused, consider our private coaching service , where we hold your hand through the research process to help you develop your best work.
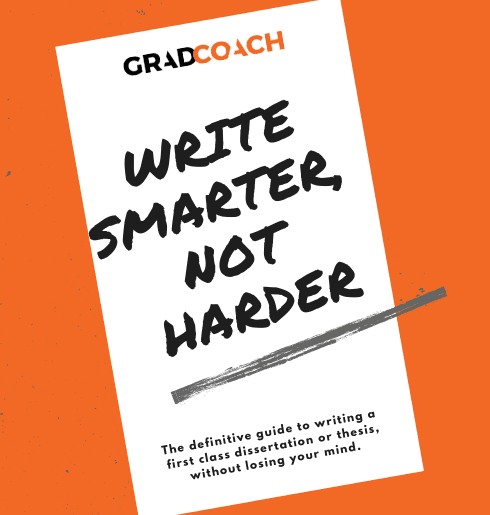
Psst… there’s more (for free)
This post is part of our dissertation mini-course, which covers everything you need to get started with your dissertation, thesis or research project.
You Might Also Like:
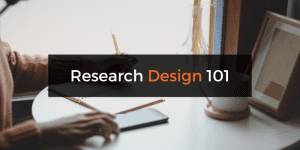
84 Comments
This has been very helpful. Thank you.
Thank you madam,
Thank you so much for this information
I wonder it so clear for understand and good for me. can I ask additional query?
Very insightful and useful
Good work done with clear explanations. Thank you.
Thanks so much for the write-up, it’s really good.
Thanks madam . It is very important .
thank you very good
This has been very well explained in simple language . It is useful even for a new researcher.
Great to hear that. Good luck with your qualitative data analysis, Pramod!
This is very useful information. And it was very a clear language structured presentation. Thanks a lot.
Thank you so much.
very informative sequential presentation
Precise explanation of method.
Hi, may we use 2 data analysis methods in our qualitative research?
Thanks for your comment. Most commonly, one would use one type of analysis method, but it depends on your research aims and objectives.
You explained it in very simple language, everyone can understand it. Thanks so much.
Thank you very much, this is very helpful. It has been explained in a very simple manner that even a layman understands
Thank nicely explained can I ask is Qualitative content analysis the same as thematic analysis?
Thanks for your comment. No, QCA and thematic are two different types of analysis. This article might help clarify – https://onlinelibrary.wiley.com/doi/10.1111/nhs.12048
This is my first time to come across a well explained data analysis. so helpful.
I have thoroughly enjoyed your explanation of the six qualitative analysis methods. This is very helpful. Thank you!
Thank you very much, this is well explained and useful
i need a citation of your book.
Thanks a lot , remarkable indeed, enlighting to the best
Hi Derek, What other theories/methods would you recommend when the data is a whole speech?
Keep writing useful artikel.
It is important concept about QDA and also the way to express is easily understandable, so thanks for all.
Thank you, this is well explained and very useful.
Very helpful .Thanks.
Hi there! Very well explained. Simple but very useful style of writing. Please provide the citation of the text. warm regards
The session was very helpful and insightful. Thank you
This was very helpful and insightful. Easy to read and understand
As a professional academic writer, this has been so informative and educative. Keep up the good work Grad Coach you are unmatched with quality content for sure.
Keep up the good work Grad Coach you are unmatched with quality content for sure.
Its Great and help me the most. A Million Thanks you Dr.
It is a very nice work
Very insightful. Please, which of this approach could be used for a research that one is trying to elicit students’ misconceptions in a particular concept ?
This is Amazing and well explained, thanks
great overview
What do we call a research data analysis method that one use to advise or determining the best accounting tool or techniques that should be adopted in a company.
Informative video, explained in a clear and simple way. Kudos
Waoo! I have chosen method wrong for my data analysis. But I can revise my work according to this guide. Thank you so much for this helpful lecture.
This has been very helpful. It gave me a good view of my research objectives and how to choose the best method. Thematic analysis it is.
Very helpful indeed. Thanku so much for the insight.
This was incredibly helpful.
Very helpful.
very educative
Nicely written especially for novice academic researchers like me! Thank you.
choosing a right method for a paper is always a hard job for a student, this is a useful information, but it would be more useful personally for me, if the author provide me with a little bit more information about the data analysis techniques in type of explanatory research. Can we use qualitative content analysis technique for explanatory research ? or what is the suitable data analysis method for explanatory research in social studies?
that was very helpful for me. because these details are so important to my research. thank you very much
I learnt a lot. Thank you
Relevant and Informative, thanks !
Well-planned and organized, thanks much! 🙂
I have reviewed qualitative data analysis in a simplest way possible. The content will highly be useful for developing my book on qualitative data analysis methods. Cheers!
Clear explanation on qualitative and how about Case study
This was helpful. Thank you
This was really of great assistance, it was just the right information needed. Explanation very clear and follow.
Wow, Thanks for making my life easy
This was helpful thanks .
Very helpful…. clear and written in an easily understandable manner. Thank you.
This was so helpful as it was easy to understand. I’m a new to research thank you so much.
so educative…. but Ijust want to know which method is coding of the qualitative or tallying done?
Thank you for the great content, I have learnt a lot. So helpful
precise and clear presentation with simple language and thank you for that.
very informative content, thank you.
You guys are amazing on YouTube on this platform. Your teachings are great, educative, and informative. kudos!
Brilliant Delivery. You made a complex subject seem so easy. Well done.
Beautifully explained.
Thanks a lot
Is there a video the captures the practical process of coding using automated applications?
Thanks for the comment. We don’t recommend using automated applications for coding, as they are not sufficiently accurate in our experience.
content analysis can be qualitative research?
THANK YOU VERY MUCH.
Thank you very much for such a wonderful content
do you have any material on Data collection
What a powerful explanation of the QDA methods. Thank you.
Great explanation both written and Video. i have been using of it on a day to day working of my thesis project in accounting and finance. Thank you very much for your support.
very helpful, thank you so much
Submit a Comment Cancel reply
Your email address will not be published. Required fields are marked *
Save my name, email, and website in this browser for the next time I comment.
- Print Friendly
- Resources Home 🏠
- Try SciSpace Copilot
- Search research papers
- Add Copilot Extension
- Try AI Detector
- Try Paraphraser
- Try Citation Generator
- April Papers
- June Papers
- July Papers

A Comprehensive Guide to Methodology in Research
Table of Contents
Research methodology plays a crucial role in any study or investigation. It provides the framework for collecting, analyzing, and interpreting data, ensuring that the research is reliable, valid, and credible. Understanding the importance of research methodology is essential for conducting rigorous and meaningful research.
In this article, we'll explore the various aspects of research methodology, from its types to best practices, ensuring you have the knowledge needed to conduct impactful research.
What is Research Methodology?
Research methodology refers to the system of procedures, techniques, and tools used to carry out a research study. It encompasses the overall approach, including the research design, data collection methods, data analysis techniques, and the interpretation of findings.
Research methodology plays a crucial role in the field of research, as it sets the foundation for any study. It provides researchers with a structured framework to ensure that their investigations are conducted in a systematic and organized manner. By following a well-defined methodology, researchers can ensure that their findings are reliable, valid, and meaningful.
When defining research methodology, one of the first steps is to identify the research problem. This involves clearly understanding the issue or topic that the study aims to address. By defining the research problem, researchers can narrow down their focus and determine the specific objectives they want to achieve through their study.
How to Define Research Methodology
Once the research problem is identified, researchers move on to defining the research questions. These questions serve as a guide for the study, helping researchers to gather relevant information and analyze it effectively. The research questions should be clear, concise, and aligned with the overall goals of the study.
After defining the research questions, researchers need to determine how data will be collected and analyzed. This involves selecting appropriate data collection methods, such as surveys, interviews, observations, or experiments. The choice of data collection methods depends on various factors, including the nature of the research problem, the target population, and the available resources.
Once the data is collected, researchers need to analyze it using appropriate data analysis techniques. This may involve statistical analysis, qualitative analysis, or a combination of both, depending on the nature of the data and the research questions. The analysis of data helps researchers to draw meaningful conclusions and make informed decisions based on their findings.
Role of Methodology in Research
Methodology plays a crucial role in research, as it ensures that the study is conducted in a systematic and organized manner. It provides a clear roadmap for researchers to follow, ensuring that the research objectives are met effectively. By following a well-defined methodology, researchers can minimize bias, errors, and inconsistencies in their study, thus enhancing the reliability and validity of their findings.
In addition to providing a structured approach, research methodology also helps in establishing the reliability and validity of the study. Reliability refers to the consistency and stability of the research findings, while validity refers to the accuracy and truthfulness of the findings. By using appropriate research methods and techniques, researchers can ensure that their study produces reliable and valid results, which can be used to make informed decisions and contribute to the existing body of knowledge.
Steps in Choosing the Right Research Methodology
Choosing the appropriate research methodology for your study is a critical step in ensuring the success of your research. Let's explore some steps to help you select the right research methodology:
Identifying the Research Problem
The first step in choosing the right research methodology is to clearly identify and define the research problem. Understanding the research problem will help you determine which methodology will best address your research questions and objectives.
Identifying the research problem involves a thorough examination of the existing literature in your field of study. This step allows you to gain a comprehensive understanding of the current state of knowledge and identify any gaps that your research can fill. By identifying the research problem, you can ensure that your study contributes to the existing body of knowledge and addresses a significant research gap.
Once you have identified the research problem, you need to consider the scope of your study. Are you focusing on a specific population, geographic area, or time frame? Understanding the scope of your research will help you determine the appropriate research methodology to use.
Reviewing Previous Research
Before finalizing the research methodology, it is essential to review previous research conducted in the field. This will allow you to identify gaps, determine the most effective methodologies used in similar studies, and build upon existing knowledge.
Reviewing previous research involves conducting a systematic review of relevant literature. This process includes searching for and analyzing published studies, articles, and reports that are related to your research topic. By reviewing previous research, you can gain insights into the strengths and limitations of different methodologies and make informed decisions about which approach to adopt.
During the review process, it is important to critically evaluate the quality and reliability of the existing research. Consider factors such as the sample size, research design, data collection methods, and statistical analysis techniques used in previous studies. This evaluation will help you determine the most appropriate research methodology for your own study.
Formulating Research Questions
Once the research problem is identified, formulate specific and relevant research questions. These questions will guide your methodology selection process by helping you determine what type of data you need to collect and how to analyze it.
Formulating research questions involves breaking down the research problem into smaller, more manageable components. These questions should be clear, concise, and measurable. They should also align with the objectives of your study and provide a framework for data collection and analysis.
When formulating research questions, consider the different types of data that can be collected, such as qualitative or quantitative data. Depending on the nature of your research questions, you may need to employ different data collection methods, such as interviews, surveys, observations, or experiments. By carefully formulating research questions, you can ensure that your chosen methodology will enable you to collect the necessary data to answer your research questions effectively.
Implementing the Research Methodology
After choosing the appropriate research methodology, it is time to implement it. This stage involves collecting data using various techniques and analyzing the gathered information. Let's explore two crucial aspects of implementing the research methodology:
Data Collection Techniques
Data collection techniques depend on the chosen research methodology. They can include surveys, interviews, observations, experiments, or document analysis. Selecting the most suitable data collection techniques will ensure accurate and relevant data for your study.
Data Analysis Methods
Data analysis is a critical part of the research process. It involves interpreting and making sense of the collected data to draw meaningful conclusions. Depending on the research methodology, data analysis methods can include statistical analysis, content analysis, thematic analysis, or grounded theory.
Ensuring the Validity and Reliability of Your Research
In order to ensure the validity and reliability of your research findings, it is important to address these two key aspects:
Understanding Validity in Research
Validity refers to the accuracy and soundness of a research study. It is crucial to ensure that the research methods used effectively measure what they intend to measure. Researchers can enhance validity by using proper sampling techniques, carefully designing research instruments, and ensuring accurate data collection.
Ensuring Reliability in Your Study
Reliability refers to the consistency and stability of the research results. It is important to ensure that the research methods and instruments used yield consistent and reproducible results. Researchers can enhance reliability by using standardized procedures, ensuring inter-rater reliability, and conducting pilot studies.
A comprehensive understanding of research methodology is essential for conducting high-quality research. By selecting the right research methodology, researchers can ensure that their studies are rigorous, reliable, and valid. It is crucial to follow the steps in choosing the appropriate methodology, implement the chosen methodology effectively, and address validity and reliability concerns throughout the research process. By doing so, researchers can contribute valuable insights and advances in their respective fields.
You might also like

AI for Meta-Analysis — A Comprehensive Guide
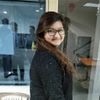
How To Write An Argumentative Essay

Beyond Google Scholar: Why SciSpace is the best alternative

An official website of the United States government
The .gov means it’s official. Federal government websites often end in .gov or .mil. Before sharing sensitive information, make sure you’re on a federal government site.
The site is secure. The https:// ensures that you are connecting to the official website and that any information you provide is encrypted and transmitted securely.
- Publications
- Account settings
Preview improvements coming to the PMC website in October 2024. Learn More or Try it out now .
- Advanced Search
- Journal List
- AIMS Public Health
- v.3(1); 2016

What Synthesis Methodology Should I Use? A Review and Analysis of Approaches to Research Synthesis
Kara schick-makaroff.
1 Faculty of Nursing, University of Alberta, Edmonton, AB, Canada
Marjorie MacDonald
2 School of Nursing, University of Victoria, Victoria, BC, Canada
Marilyn Plummer
3 College of Nursing, Camosun College, Victoria, BC, Canada
Judy Burgess
4 Student Services, University Health Services, Victoria, BC, Canada
Wendy Neander
Associated data, additional file 1.
When we began this process, we were doctoral students and a faculty member in a research methods course. As students, we were facing a review of the literature for our dissertations. We encountered several different ways of conducting a review but were unable to locate any resources that synthesized all of the various synthesis methodologies. Our purpose is to present a comprehensive overview and assessment of the main approaches to research synthesis. We use ‘research synthesis’ as a broad overarching term to describe various approaches to combining, integrating, and synthesizing research findings.
We conducted an integrative review of the literature to explore the historical, contextual, and evolving nature of research synthesis. We searched five databases, reviewed websites of key organizations, hand-searched several journals, and examined relevant texts from the reference lists of the documents we had already obtained.
We identified four broad categories of research synthesis methodology including conventional, quantitative, qualitative, and emerging syntheses. Each of the broad categories was compared to the others on the following: key characteristics, purpose, method, product, context, underlying assumptions, unit of analysis, strengths and limitations, and when to use each approach.
Conclusions
The current state of research synthesis reflects significant advancements in emerging synthesis studies that integrate diverse data types and sources. New approaches to research synthesis provide a much broader range of review alternatives available to health and social science students and researchers.
1. Introduction
Since the turn of the century, public health emergencies have been identified worldwide, particularly related to infectious diseases. For example, the Severe Acute Respiratory Syndrome (SARS) epidemic in Canada in 2002-2003, the recent Ebola epidemic in Africa, and the ongoing HIV/AIDs pandemic are global health concerns. There have also been dramatic increases in the prevalence of chronic diseases around the world [1] – [3] . These epidemiological challenges have raised concerns about the ability of health systems worldwide to address these crises. As a result, public health systems reform has been initiated in a number of countries. In Canada, as in other countries, the role of evidence to support public health reform and improve population health has been given high priority. Yet, there continues to be a significant gap between the production of evidence through research and its application in practice [4] – [5] . One strategy to address this gap has been the development of new research synthesis methodologies to deal with the time-sensitive and wide ranging evidence needs of policy makers and practitioners in all areas of health care, including public health.
As doctoral nursing students facing a review of the literature for our dissertations, and as a faculty member teaching a research methods course, we encountered several ways of conducting a research synthesis but found no comprehensive resources that discussed, compared, and contrasted various synthesis methodologies on their purposes, processes, strengths and limitations. To complicate matters, writers use terms interchangeably or use different terms to mean the same thing, and the literature is often contradictory about various approaches. Some texts [6] , [7] – [9] did provide a preliminary understanding about how research synthesis had been taken up in nursing, but these did not meet our requirements. Thus, in this article we address the need for a comprehensive overview of research synthesis methodologies to guide public health, health care, and social science researchers and practitioners.
Research synthesis is relatively new in public health but has a long history in other fields dating back to the late 1800s. Research synthesis, a research process in its own right [10] , has become more prominent in the wake of the evidence-based movement of the 1990s. Research syntheses have found their advocates and detractors in all disciplines, with challenges to the processes of systematic review and meta-analysis, in particular, being raised by critics of evidence-based healthcare [11] – [13] .
Our purpose was to conduct an integrative review of the literature to explore the historical, contextual, and evolving nature of research synthesis [14] – [15] . We synthesize and critique the main approaches to research synthesis that are relevant for public health, health care, and social scientists. Research synthesis is the overarching term we use to describe approaches to combining, aggregating, integrating, and synthesizing primary research findings. Each synthesis methodology draws on different types of findings depending on the purpose and product of the chosen synthesis (see Additional File 1 ).
3. Method of Review
Based on our current knowledge of the literature, we identified these approaches to include in our review: systematic review, meta-analysis, qualitative meta-synthesis, meta-narrative synthesis, scoping review, rapid review, realist synthesis, concept analysis, literature review, and integrative review. Our first step was to divide the synthesis types among the research team. Each member did a preliminary search to identify key texts. The team then met to develop search terms and a framework to guide the review.
Over the period of 2008 to 2012 we extensively searched the literature, updating our search at several time points, not restricting our search by date. The dates of texts reviewed range from 1967 to 2015. We used the terms above combined with the term “method* (e.g., “realist synthesis” and “method*) in the database Health Source: Academic Edition (includes Medline and CINAHL). This search yielded very few texts on some methodologies and many on others. We realized that many documents on research synthesis had not been picked up in the search. Therefore, we also searched Google Scholar, PubMed, ERIC, and Social Science Index, as well as the websites of key organizations such as the Joanna Briggs Institute, the University of York Centre for Evidence-Based Nursing, and the Cochrane Collaboration database. We hand searched several nursing, social science, public health and health policy journals. Finally, we traced relevant documents from the references in obtained texts.
We included works that met the following inclusion criteria: (1) published in English; (2) discussed the history of research synthesis; (3) explicitly described the approach and specific methods; or (4) identified issues, challenges, strengths and limitations of the particular methodology. We excluded research reports that resulted from the use of particular synthesis methodologies unless they also included criteria 2, 3, or 4 above.
Based on our search, we identified additional types of research synthesis (e.g., meta-interpretation, best evidence synthesis, critical interpretive synthesis, meta-summary, grounded formal theory). Still, we missed some important developments in meta-analysis, for example, identified by the journal's reviewers that have now been discussed briefly in the paper. The final set of 197 texts included in our review comprised theoretical, empirical, and conceptual papers, books, editorials and commentaries, and policy documents.
In our preliminary review of key texts, the team inductively developed a framework of the important elements of each method for comparison. In the next phase, each text was read carefully, and data for these elements were extracted into a table for comparison on the points of: key characteristics, purpose, methods, and product; see Additional File 1 ). Once the data were grouped and extracted, we synthesized across categories based on the following additional points of comparison: complexity of the process, degree of systematization, consideration of context, underlying assumptions, unit of analysis, and when to use each approach. In our results, we discuss our comparison of the various synthesis approaches on the elements above. Drawing only on documents for the review, ethics approval was not required.
We identified four broad categories of research synthesis methodology: Conventional, quantitative, qualitative, and emerging syntheses. From our dataset of 197 texts, we had 14 texts on conventional synthesis, 64 on quantitative synthesis, 78 on qualitative synthesis, and 41 on emerging syntheses. Table 1 provides an overview of the four types of research synthesis, definitions, types of data used, products, and examples of the methodology.
Although we group these types of synthesis into four broad categories on the basis of similarities, each type within a category has unique characteristics, which may differ from the overall group similarities. Each could be explored in greater depth to tease out their unique characteristics, but detailed comparison is beyond the scope of this article.
Additional File 1 presents one or more selected types of synthesis that represent the broad category but is not an exhaustive presentation of all types within each category. It provides more depth for specific examples from each category of synthesis on the characteristics, purpose, methods, and products than is found in Table 1 .
4.1. Key Characteristics
4.1.1. what is it.
Here we draw on two types of categorization. First, we utilize Dixon Woods et al.'s [49] classification of research syntheses as being either integrative or interpretive . (Please note that integrative syntheses are not the same as an integrative review as defined in Additional File 1 .) Second, we use Popay's [80] enhancement and epistemological models .
The defining characteristics of integrative syntheses are that they involve summarizing the data achieved by pooling data [49] . Integrative syntheses include systematic reviews, meta-analyses, as well as scoping and rapid reviews because each of these focus on summarizing data. They also define concepts from the outset (although this may not always be true in scoping or rapid reviews) and deal with a well-specified phenomenon of interest.
Interpretive syntheses are primarily concerned with the development of concepts and theories that integrate concepts [49] . The analysis in interpretive synthesis is conceptual both in process and outcome, and “the product is not aggregations of data, but theory” [49] , [p.12]. Interpretive syntheses involve induction and interpretation, and are primarily conceptual in process and outcome. Examples include integrative reviews, some systematic reviews, all of the qualitative syntheses, meta-narrative, realist and critical interpretive syntheses. Of note, both quantitative and qualitative studies can be either integrative or interpretive
The second categorization, enhancement versus epistemological , applies to those approaches that use multiple data types and sources [80] . Popay's [80] classification reflects the ways that qualitative data are valued in relation to quantitative data.
In the enhancement model , qualitative data adds something to quantitative analysis. The enhancement model is reflected in systematic reviews and meta-analyses that use some qualitative data to enhance interpretation and explanation. It may also be reflected in some rapid reviews that draw on quantitative data but use some qualitative data.
The epistemological model assumes that quantitative and qualitative data are equal and each has something unique to contribute. All of the other review approaches, except pure quantitative or qualitative syntheses, reflect the epistemological model because they value all data types equally but see them as contributing different understandings.
4.1.2. Data type
By and large, the quantitative approaches (quantitative systematic review and meta-analysis) have typically used purely quantitative data (i.e., expressed in numeric form). More recently, both Cochrane [81] and Campbell [82] collaborations are grappling with the need to, and the process of, integrating qualitative research into a systematic review. The qualitative approaches use qualitative data (i.e., expressed in words). All of the emerging synthesis types, as well as the conventional integrative review, incorporate qualitative and quantitative study designs and data.
4.1.3. Research question
Four types of research questions direct inquiry across the different types of syntheses. The first is a well-developed research question that gives direction to the synthesis (e.g., meta-analysis, systematic review, meta-study, concept analysis, rapid review, realist synthesis). The second begins as a broad general question that evolves and becomes more refined over the course of the synthesis (e.g., meta-ethnography, scoping review, meta-narrative, critical interpretive synthesis). In the third type, the synthesis begins with a phenomenon of interest and the question emerges in the analytic process (e.g., grounded formal theory). Lastly, there is no clear question, but rather a general review purpose (e.g., integrative review). Thus, the requirement for a well-defined question cuts across at least three of the synthesis types (e.g., quantitative, qualitative, and emerging).
4.1.4. Quality appraisal
This is a contested issue within and between the four synthesis categories. There are strong proponents of quality appraisal in the quantitative traditions of systematic review and meta-analysis based on the need for strong studies that will not jeopardize validity of the overall findings. Nonetheless, there is no consensus on pre-defined criteria; many scales exist that vary dramatically in composition. This has methodological implications for the credibility of findings [83] .
Specific methodologies from the conventional, qualitative, and emerging categories support quality appraisal but do so with caveats. In conventional integrative reviews appraisal is recommended, but depends on the sampling frame used in the study [18] . In meta-study, appraisal criteria are explicit but quality criteria are used in different ways depending on the specific requirements of the inquiry [54] . Among the emerging syntheses, meta-narrative review developers support appraisal of a study based on criteria from the research tradition of the primary study [67] , [84] – [85] . Realist synthesis similarly supports the use of high quality evidence, but appraisal checklists are viewed with scepticism and evidence is judged based on relevance to the research question and whether a credible inference may be drawn [69] . Like realist, critical interpretive syntheses do not judge quality using standardized appraisal instruments. They will exclude fatally flawed studies, but there is no consensus on what ‘fatally flawed’ means [49] , [71] . Appraisal is based on relevance to the inquiry, not rigor of the study.
There is no agreement on quality appraisal among qualitative meta-ethnographers with some supporting and others refuting the need for appraisal. [60] , [62] . Opponents of quality appraisal are found among authors of qualitative (grounded formal theory and concept analysis) and emerging syntheses (scoping and rapid reviews) because quality is not deemed relevant to the intention of the synthesis; the studies being reviewed are not effectiveness studies where quality is extremely important. These qualitative synthesis are often reviews of theoretical developments where the concept itself is what is important, or reviews that provide quotations from the raw data so readers can make their own judgements about the relevance and utility of the data. For example, in formal grounded theory, the purpose of theory generation and authenticity of data used to generate the theory is not as important as the conceptual category. Inaccuracies may be corrected in other ways, such as using the constant comparative method, which facilitates development of theoretical concepts that are repeatedly found in the data [86] – [87] . For pragmatic reasons, evidence is not assessed in rapid and scoping reviews, in part to produce a timely product. The issue of quality appraisal is unresolved across the terrain of research synthesis and we consider this further in our discussion.
4.2. Purpose
All research syntheses share a common purpose -- to summarize, synthesize, or integrate research findings from diverse studies. This helps readers stay abreast of the burgeoning literature in a field. Our discussion here is at the level of the four categories of synthesis. Beginning with conventional literature syntheses, the overall purpose is to attend to mature topics for the purpose of re-conceptualization or to new topics requiring preliminary conceptualization [14] . Such syntheses may be helpful to consider contradictory evidence, map shifting trends in the study of a phenomenon, and describe the emergence of research in diverse fields [14] . The purpose here is to set the stage for a study by identifying what has been done, gaps in the literature, important research questions, or to develop a conceptual framework to guide data collection and analysis.
The purpose of quantitative systematic reviews is to combine, aggregate, or integrate empirical research to be able to generalize from a group of studies and determine the limits of generalization [27] . The focus of quantitative systematic reviews has been primarily on aggregating the results of studies evaluating the effectiveness of interventions using experimental, quasi-experimental, and more recently, observational designs. Systematic reviews can be done with or without quantitative meta-analysis but a meta-analysis always takes place within the context of a systematic review. Researchers must consider the review's purpose and the nature of their data in undertaking a quantitative synthesis; this will assist in determining the approach.
The purpose of qualitative syntheses is broadly to synthesize complex health experiences, practices, or concepts arising in healthcare environments. There may be various purposes depending on the qualitative methodology. For example, in hermeneutic studies the aim may be holistic explanation or understanding of a phenomenon [42] , which is deepened by integrating the findings from multiple studies. In grounded formal theory, the aim is to produce a conceptual framework or theory expected to be applicable beyond the original study. Although not able to generalize from qualitative research in the statistical sense [88] , qualitative researchers usually do want to say something about the applicability of their synthesis to other settings or phenomena. This notion of ‘theoretical generalization’ has been referred to as ‘transferability’ [89] – [90] and is an important criterion of rigour in qualitative research. It applies equally to the products of a qualitative synthesis in which the synthesis of multiple studies on the same phenomenon strengthens the ability to draw transferable conclusions.
The overarching purpose of emerging syntheses is challenging the more traditional types of syntheses, in part by using data from both quantitative and qualitative studies with diverse designs for analysis. Beyond this, however, each emerging synthesis methodology has a unique purpose. In meta-narrative review, the purpose is to identify different research traditions in the area, synthesize a complex and diverse body of research. Critical interpretive synthesis shares this characteristic. Although a distinctive approach, critical interpretive synthesis utilizes a modification of the analytic strategies of meta-ethnography [61] (e.g., reciprocal translational analysis, refutational synthesis, and lines of argument synthesis) but goes beyond the use of these to bring a critical perspective to bear in challenging the normative or epistemological assumptions in the primary literature [72] – [73] . The unique purpose of a realist synthesis is to amalgamate complex empirical evidence and theoretical understandings within a diverse body of literature to uncover the operative mechanisms and contexts that affect the outcomes of social interventions. In a scoping review, the intention is to find key concepts, examine the range of research in an area, and identify gaps in the literature. The purpose of a rapid review is comparable to that of a scoping review, but done quickly to meet the time-sensitive information needs of policy makers.
4.3. Method
4.3.1. degree of systematization.
There are varying degrees of systematization across the categories of research synthesis. The most systematized are quantitative systematic reviews and meta-analyses. There are clear processes in each with judgments to be made at each step, although there are no agreed upon guidelines for this. The process is inherently subjective despite attempts to develop objective and systematic processes [91] – [92] . Mullen and Ramirez [27] suggest that there is often a false sense of rigour implied by the terms ‘systematic review’ and ‘meta-analysis’ because of their clearly defined procedures.
In comparison with some types of qualitative synthesis, concept analysis is quite procedural. Qualitative meta-synthesis also has defined procedures and is systematic, yet perhaps less so than concept analysis. Qualitative meta-synthesis starts in an unsystematic way but becomes more systematic as it unfolds. Procedures and frameworks exist for some of the emerging types of synthesis [e.g., [50] , [63] , [71] , [93] ] but are not linear, have considerable flexibility, and are often messy with emergent processes [85] . Conventional literature reviews tend not to be as systematic as the other three types. In fact, the lack of systematization in conventional literature synthesis was the reason for the development of more systematic quantitative [17] , [20] and qualitative [45] – [46] , [61] approaches. Some authors in the field [18] have clarified processes for integrative reviews making them more systematic and rigorous, but most conventional syntheses remain relatively unsystematic in comparison with other types.
4.3.2. Complexity of the process
Some synthesis processes are considerably more complex than others. Methodologies with clearly defined steps are arguably less complex than the more flexible and emergent ones. We know that any study encounters challenges and it is rare that a pre-determined research protocol can be followed exactly as intended. Not even the rigorous methods associated with Cochrane [81] systematic reviews and meta-analyses are always implemented exactly as intended. Even when dealing with numbers rather than words, interpretation is always part of the process. Our collective experience suggests that new methodologies (e.g., meta-narrative synthesis and realist synthesis) that integrate different data types and methods are more complex than conventional reviews or the rapid and scoping reviews.
4.4. Product
The products of research syntheses usually take three distinct formats (see Table 1 and Additional File 1 for further details). The first representation is in tables, charts, graphical displays, diagrams and maps as seen in integrative, scoping and rapid reviews, meta-analyses, and critical interpretive syntheses. The second type of synthesis product is the use of mathematical scores. Summary statements of effectiveness are mathematically displayed in meta-analyses (as an effect size), systematic reviews, and rapid reviews (statistical significance).
The third synthesis product may be a theory or theoretical framework. A mid-range theory can be produced from formal grounded theory, meta-study, meta-ethnography, and realist synthesis. Theoretical/conceptual frameworks or conceptual maps may be created in meta-narrative and critical interpretive syntheses, and integrative reviews. Concepts for use within theories are produced in concept analysis. While these three product types span the categories of research synthesis, narrative description and summary is used to present the products resulting from all methodologies.
4.5. Consideration of context
There are diverse ways that context is considered in the four broad categories of synthesis. Context may be considered to the extent that it features within primary studies for the purpose of the review. Context may also be understood as an integral aspect of both the phenomenon under study and the synthesis methodology (e.g., realist synthesis). Quantitative systematic reviews and meta-analyses have typically been conducted on studies using experimental and quasi-experimental designs and more recently observational studies, which control for contextual features to allow for understanding of the ‘true’ effect of the intervention [94] .
More recently, systematic reviews have included covariates or mediating variables (i.e., contextual factors) to help explain variability in the results across studies [27] . Context, however, is usually handled in the narrative discussion of findings rather than in the synthesis itself. This lack of attention to context has been one criticism leveled against systematic reviews and meta-analyses, which restrict the types of research designs that are considered [e.g., [95] ].
When conventional literature reviews incorporate studies that deal with context, there is a place for considering contextual influences on the intervention or phenomenon. Reviews of quantitative experimental studies tend to be devoid of contextual considerations since the original studies are similarly devoid, but context might figure prominently in a literature review that incorporates both quantitative and qualitative studies.
Qualitative syntheses have been conducted on the contextual features of a particular phenomenon [33] . Paterson et al. [54] advise researchers to attend to how context may have influenced the findings of particular primary studies. In qualitative analysis, contextual features may form categories by which the data can be compared and contrasted to facilitate interpretation. Because qualitative research is often conducted to understand a phenomenon as a whole, context may be a focus, although this varies with the qualitative methodology. At the same time, the findings in a qualitative synthesis are abstracted from the original reports and taken to a higher level of conceptualization, thus removing them from the original context.
Meta-narrative synthesis [67] , [84] , because it draws on diverse research traditions and methodologies, may incorporate context into the analysis and findings. There is not, however, an explicit step in the process that directs the analyst to consider context. Generally, the research question guiding the synthesis is an important factor in whether context will be a focus.
More recent iterations of concept analysis [47] , [96] – [97] explicitly consider context reflecting the assumption that a concept's meaning is determined by its context. Morse [47] points out, however, that Wilson's [98] approach to concept analysis, and those based on Wilson [e.g., [45] ], identify attributes that are devoid of context, while Rodgers' [96] , [99] evolutionary method considers context (e.g., antecedents, consequences, and relationships to other concepts) in concept development.
Realist synthesis [69] considers context as integral to the study. It draws on a critical realist logic of inquiry grounded in the work of Bhaskar [100] , who argues that empirical co-occurrence of events is insufficient for inferring causation. One must identify generative mechanisms whose properties are causal and, depending on the situation, may nor may not be activated [94] . Context interacts with program/intervention elements and thus cannot be differentiated from the phenomenon [69] . This approach synthesizes evidence on generative mechanisms and analyzes contextual features that activate them; the result feeds back into the context. The focus is on what works, for whom, under what conditions, why and how [68] .
4.6. Underlying Philosophical and Theoretical Assumptions
When we began our review, we ‘assumed’ that the assumptions underlying synthesis methodologies would be a distinguishing characteristic of synthesis types, and that we could compare the various types on their assumptions, explicit or implicit. We found, however, that many authors did not explicate the underlying assumptions of their methodologies, and it was difficult to infer them. Kirkevold [101] has argued that integrative reviews need to be carried out from an explicit philosophical or theoretical perspective. We argue this should be true for all types of synthesis.
Authors of some emerging synthesis approaches have been very explicit about their assumptions and philosophical underpinnings. An implicit assumption of most emerging synthesis methodologies is that quantitative systematic reviews and meta-analyses have limited utility in some fields [e.g., in public health – [13] , [102] ] and for some kinds of review questions like those about feasibility and appropriateness versus effectiveness [103] – [104] . They also assume that ontologically and epistemologically, both kinds of data can be combined. This is a significant debate in the literature because it is about the commensurability of overarching paradigms [105] but this is beyond the scope of this review.
Realist synthesis is philosophically grounded in critical realism or, as noted above, a realist logic of inquiry [93] , [99] , [106] – [107] . Key assumptions regarding the nature of interventions that inform critical realism have been described above in the section on context. See Pawson et al. [106] for more information on critical realism, the philosophical basis of realist synthesis.
Meta-narrative synthesis is explicitly rooted in a constructivist philosophy of science [108] in which knowledge is socially constructed rather than discovered, and what we take to be ‘truth’ is a matter of perspective. Reality has a pluralistic and plastic character, and there is no pre-existing ‘real world’ independent of human construction and language [109] . See Greenhalgh et al. [67] , [85] and Greenhalgh & Wong [97] for more discussion of the constructivist basis of meta-narrative synthesis.
In the case of purely quantitative or qualitative syntheses, it may be an easier matter to uncover unstated assumptions because they are likely to be shared with those of the primary studies in the genre. For example, grounded formal theory shares the philosophical and theoretical underpinnings of grounded theory, rooted in the theoretical perspective of symbolic interactionism [110] – [111] and the philosophy of pragmatism [87] , [112] – [114] .
As with meta-narrative synthesis, meta-study developers identify constructivism as their interpretive philosophical foundation [54] , [88] . Epistemologically, constructivism focuses on how people construct and re-construct knowledge about a specific phenomenon, and has three main assumptions: (1) reality is seen as multiple, at times even incompatible with the phenomenon under consideration; (2) just as primary researchers construct interpretations from participants' data, meta-study researchers also construct understandings about the primary researchers' original findings. Thus, meta-synthesis is a construction of a construction, or a meta-construction; and (3) all constructions are shaped by the historical, social and ideological context in which they originated [54] . The key message here is that reports of any synthesis would benefit from an explicit identification of the underlying philosophical perspectives to facilitate a better understanding of the results, how they were derived, and how they are being interpreted.
4.7. Unit of Analysis
The unit of analysis for each category of review is generally distinct. For the emerging synthesis approaches, the unit of analysis is specific to the intention. In meta-narrative synthesis it is the storyline in diverse research traditions; in rapid review or scoping review, it depends on the focus but could be a concept; and in realist synthesis, it is the theories rather than programs that are the units of analysis. The elements of theory that are important in the analysis are mechanisms of action, the context, and the outcome [107] .
For qualitative synthesis, the units of analysis are generally themes, concepts or theories, although in meta-study, the units of analysis can be research findings (“meta-data-analysis”), research methods (“meta-method”) or philosophical/theoretical perspectives (“meta-theory”) [54] . In quantitative synthesis, the units of analysis range from specific statistics for systematic reviews to effect size of the intervention for meta-analysis. More recently, some systematic reviews focus on theories [115] – [116] , therefore it depends on the research question. Similarly, within conventional literature synthesis the units of analysis also depend on the research purpose, focus and question as well as on the type of research methods incorporated into the review. What is important in all research syntheses, however, is that the unit of analysis needs to be made explicit. Unfortunately, this is not always the case.
4.8. Strengths and Limitations
In this section, we discuss the overarching strengths and limitations of synthesis methodologies as a whole and then highlight strengths and weaknesses across each of our four categories of synthesis.
4.8.1. Strengths of Research Syntheses in General
With the vast proliferation of research reports and the increased ease of retrieval, research synthesis has become more accessible providing a way of looking broadly at the current state of research. The availability of syntheses helps researchers, practitioners, and policy makers keep up with the burgeoning literature in their fields without which evidence-informed policy or practice would be difficult. Syntheses explain variation and difference in the data helping us identify the relevance for our own situations; they identify gaps in the literature leading to new research questions and study designs. They help us to know when to replicate a study and when to avoid excessively duplicating research. Syntheses can inform policy and practice in a way that well-designed single studies cannot; they provide building blocks for theory that helps us to understand and explain our phenomena of interest.
4.8.2. Limitations of Research Syntheses in General
The process of selecting, combining, integrating, and synthesizing across diverse study designs and data types can be complex and potentially rife with bias, even with those methodologies that have clearly defined steps. Just because a rigorous and standardized approach has been used does not mean that implicit judgements will not influence the interpretations and choices made at different stages.
In all types of synthesis, the quantity of data can be considerable, requiring difficult decisions about scope, which may affect relevance. The quantity of available data also has implications for the size of the research team. Few reviews these days can be done independently, in particular because decisions about inclusion and exclusion may require the involvement of more than one person to ensure reliability.
For all types of synthesis, it is likely that in areas with large, amorphous, and diverse bodies of literature, even the most sophisticated search strategies will not turn up all the relevant and important texts. This may be more important in some synthesis methodologies than in others, but the omission of key documents can influence the results of all syntheses. This issue can be addressed, at least in part, by including a library scientist on the research team as required by some funding agencies. Even then, it is possible to miss key texts. In this review, for example, because none of us are trained in or conduct meta-analyses, we were not even aware that we had missed some new developments in this field such as meta-regression [117] – [118] , network meta-analysis [119] – [121] , and the use of individual patient data in meta-analyses [122] – [123] .
One limitation of systematic reviews and meta-analyses is that they rapidly go out of date. We thought this might be true for all types of synthesis, although we wondered if those that produce theory might not be somewhat more enduring. We have not answered this question but it is open for debate. For all types of synthesis, the analytic skills and the time required are considerable so it is clear that training is important before embarking on a review, and some types of review may not be appropriate for students or busy practitioners.
Finally, the quality of reporting in primary studies of all genres is variable so it is sometimes difficult to identify aspects of the study essential for the synthesis, or to determine whether the study meets quality criteria. There may be flaws in the original study, or journal page limitations may necessitate omitting important details. Reporting standards have been developed for some types of reviews (e.g., systematic review, meta-analysis, meta-narrative synthesis, realist synthesis); but there are no agreed upon standards for qualitative reviews. This is an important area for development in advancing the science of research synthesis.
4.8.3. Strengths and Limitations of the Four Synthesis Types
The conventional literature review and now the increasingly common integrative review remain important and accessible approaches for students, practitioners, and experienced researchers who want to summarize literature in an area but do not have the expertise to use one of the more complex methodologies. Carefully executed, such reviews are very useful for synthesizing literature in preparation for research grants and practice projects. They can determine the state of knowledge in an area and identify important gaps in the literature to provide a clear rationale or theoretical framework for a study [14] , [18] . There is a demand, however, for more rigour, with more attention to developing comprehensive search strategies and more systematic approaches to combining, integrating, and synthesizing the findings.
Generally, conventional reviews include diverse study designs and data types that facilitate comprehensiveness, which may be a strength on the one hand, but can also present challenges on the other. The complexity inherent in combining results from studies with diverse methodologies can result in bias and inaccuracies. The absence of clear guidelines about how to synthesize across diverse study types and data [18] has been a challenge for novice reviewers.
Quantitative systematic reviews and meta-analyses have been important in launching the field of evidence-based healthcare. They provide a systematic, orderly and auditable process for conducting a review and drawing conclusions [25] . They are arguably the most powerful approaches to understanding the effectiveness of healthcare interventions, especially when intervention studies on the same topic show very different results. When areas of research are dogged by controversy [25] or when study results go against strongly held beliefs, such approaches can reduce the uncertainty and bring strong evidence to bear on the controversy.
Despite their strengths, they also have limitations. Systematic reviews and meta-analyses do not provide a way of including complex literature comprising various types of evidence including qualitative studies, theoretical work, and epidemiological studies. Only certain types of design are considered and qualitative data are used in a limited way. This exclusion limits what can be learned in a topic area.
Meta-analyses are often not possible because of wide variability in study design, population, and interventions so they may have a narrow range of utility. New developments in meta-analysis, however, can be used to address some of these limitations. Network meta-analysis is used to explore relative efficacy of multiple interventions, even those that have never been compared in more conventional pairwise meta-analyses [121] , allowing for improved clinical decision making [120] . The limitation is that network meta-analysis has only been used in medical/clinical applications [119] and not in public health. It has not yet been widely accepted and many methodological challenges remain [120] – [121] . Meta-regression is another development that combines meta-analytic and linear regression principles to address the fact that heterogeneity of results may compromise a meta-analysis [117] – [118] . The disadvantage is that many clinicians are unfamiliar with it and may incorrectly interpret results [117] .
Some have accused meta-analysis of combining apples and oranges [124] raising questions in the field about their meaningfulness [25] , [28] . More recently, the use of individual rather than aggregate data has been useful in facilitating greater comparability among studies [122] . In fact, Tomas et al. [123] argue that meta-analysis using individual data is now the gold standard although access to the raw data from other studies may be a challenge to obtain.
The usefulness of systematic reviews in synthesizing complex health and social interventions has also been challenged [102] . It is often difficult to synthesize their findings because such studies are “epistemologically diverse and methodologically complex” [ [69] , p.21]. Rigid inclusion/exclusion criteria may allow only experimental or quasi-experimental designs into consideration resulting in lost information that may well be useful to policy makers for tailoring an intervention to the context or understanding its acceptance by recipients.
Qualitative syntheses may be the type of review most fraught with controversy and challenge, while also bringing distinct strengths to the enterprise. Although these methodologies provide a comprehensive and systematic review approach, they do not generally provide definitive statements about intervention effectiveness. They do, however, address important questions about the development of theoretical concepts, patient experiences, acceptability of interventions, and an understanding about why interventions might work.
Most qualitative syntheses aim to produce a theoretically generalizable mid-range theory that explains variation across studies. This makes them more useful than single primary studies, which may not be applicable beyond the immediate setting or population. All provide a contextual richness that enhances relevance and understanding. Another benefit of some types of qualitative synthesis (e.g., grounded formal theory) is that the concept of saturation provides a sound rationale for limiting the number of texts to be included thus making reviews potentially more manageable. This contrasts with the requirements of systematic reviews and meta-analyses that require an exhaustive search.
Qualitative researchers debate about whether the findings of ontologically and epistemological diverse qualitative studies can actually be combined or synthesized [125] because methodological diversity raises many challenges for synthesizing findings. The products of different types of qualitative syntheses range from theory and conceptual frameworks, to themes and rich descriptive narratives. Can one combine the findings from a phenomenological study with the theory produced in a grounded theory study? Many argue yes, but many also argue no.
Emerging synthesis methodologies were developed to address some limitations inherent in other types of synthesis but also have their own issues. Because each type is so unique, it is difficult to identify overarching strengths of the entire category. An important strength, however, is that these newer forms of synthesis provide a systematic and rigorous approach to synthesizing a diverse literature base in a topic area that includes a range of data types such as: both quantitative and qualitative studies, theoretical work, case studies, evaluations, epidemiological studies, trials, and policy documents. More than conventional literature reviews and systematic reviews, these approaches provide explicit guidance on analytic methods for integrating different types of data. The assumption is that all forms of data have something to contribute to knowledge and theory in a topic area. All have a defined but flexible process in recognition that the methods may need to shift as knowledge develops through the process.
Many emerging synthesis types are helpful to policy makers and practitioners because they are usually involved as team members in the process to define the research questions, and interpret and disseminate the findings. In fact, engagement of stakeholders is built into the procedures of the methods. This is true for rapid reviews, meta-narrative syntheses, and realist syntheses. It is less likely to be the case for critical interpretive syntheses.
Another strength of some approaches (realist and meta-narrative syntheses) is that quality and publication standards have been developed to guide researchers, reviewers, and funders in judging the quality of the products [108] , [126] – [127] . Training materials and online communities of practice have also been developed to guide users of realist and meta-narrative review methods [107] , [128] . A unique strength of critical interpretive synthesis is that it takes a critical perspective on the process that may help reconceptualize the data in a way not considered by the primary researchers [72] .
There are also challenges of these new approaches. The methods are new and there may be few published applications by researchers other than the developers of the methods, so new users often struggle with the application. The newness of the approaches means that there may not be mentors available to guide those unfamiliar with the methods. This is changing, however, and the number of applications in the literature is growing with publications by new users helping to develop the science of synthesis [e.g., [129] ]. However, the evolving nature of the approaches and their developmental stage present challenges for novice researchers.
4.9. When to Use Each Approach
Choosing an appropriate approach to synthesis will depend on the question you are asking, the purpose of the review, and the outcome or product you want to achieve. In Additional File 1 , we discuss each of these to provide guidance to readers on making a choice about review type. If researchers want to know whether a particular type of intervention is effective in achieving its intended outcomes, then they might choose a quantitative systemic review with or without meta-analysis, possibly buttressed with qualitative studies to provide depth and explanation of the results. Alternately, if the concern is about whether an intervention is effective with different populations under diverse conditions in varying contexts, then a realist synthesis might be the most appropriate.
If researchers' concern is to develop theory, they might consider qualitative syntheses or some of the emerging syntheses that produce theory (e.g., critical interpretive synthesis, realist review, grounded formal theory, qualitative meta-synthesis). If the aim is to track the development and evolution of concepts, theories or ideas, or to determine how an issue or question is addressed across diverse research traditions, then meta-narrative synthesis would be most appropriate.
When the purpose is to review the literature in advance of undertaking a new project, particularly by graduate students, then perhaps an integrative review would be appropriate. Such efforts contribute towards the expansion of theory, identify gaps in the research, establish the rationale for studying particular phenomena, and provide a framework for interpreting results in ways that might be useful for influencing policy and practice.
For researchers keen to bring new insights, interpretations, and critical re-conceptualizations to a body of research, then qualitative or critical interpretive syntheses will provide an inductive product that may offer new understandings or challenges to the status quo. These can inform future theory development, or provide guidance for policy and practice.
5. Discussion
What is the current state of science regarding research synthesis? Public health, health care, and social science researchers or clinicians have previously used all four categories of research synthesis, and all offer a suitable array of approaches for inquiries. New developments in systematic reviews and meta-analysis are providing ways of addressing methodological challenges [117] – [123] . There has also been significant advancement in emerging synthesis methodologies and they are quickly gaining popularity. Qualitative meta-synthesis is still evolving, particularly given how new it is within the terrain of research synthesis. In the midst of this evolution, outstanding issues persist such as grappling with: the quantity of data, quality appraisal, and integration with knowledge translation. These topics have not been thoroughly addressed and need further debate.
5.1. Quantity of Data
We raise the question of whether it is possible or desirable to find all available studies for a synthesis that has this requirement (e.g., meta-analysis, systematic review, scoping, meta-narrative synthesis [25] , [27] , [63] , [67] , [84] – [85] ). Is the synthesis of all available studies a realistic goal in light of the burgeoning literature? And how can this be sustained in the future, particularly as the emerging methodologies continue to develop and as the internet facilitates endless access? There has been surprisingly little discussion on this topic and the answers will have far-reaching implications for searching, sampling, and team formation.
Researchers and graduate students can no longer rely on their own independent literature search. They will likely need to ask librarians for assistance as they navigate multiple sources of literature and learn new search strategies. Although teams now collaborate with library scientists, syntheses are limited in that researchers must make decisions on the boundaries of the review, in turn influencing the study's significance. The size of a team may also be pragmatically determined to manage the search, extraction, and synthesis of the burgeoning data. There is no single answer to our question about the possibility or necessity of finding all available articles for a review. Multiple strategies that are situation specific are likely to be needed.
5.2. Quality Appraisal
While the issue of quality appraisal has received much attention in the synthesis literature, scholars are far from resolution. There may be no agreement about appraisal criteria in a given tradition. For example, the debate rages over the appropriateness of quality appraisal in qualitative synthesis where there are over 100 different sets of criteria and many do not overlap [49] . These differences may reflect disciplinary and methodological orientations, but diverse quality appraisal criteria may privilege particular types of research [49] . The decision to appraise is often grounded in ontological and epistemological assumptions. Nonetheless, diversity within and between categories of synthesis is likely to continue unless debate on the topic of quality appraisal continues and evolves toward consensus.
5.3. Integration with Knowledge Translation
If research syntheses are to make a difference to practice and ultimately to improve health outcomes, then we need to do a better job of knowledge translation. In the Canadian Institutes of Health Research (CIHR) definition of knowledge translation (KT), research or knowledge synthesis is an integral component [130] . Yet, with few exceptions [131] – [132] , very little of the research synthesis literature even mentions the relationship of synthesis to KT nor does it discuss strategies to facilitate the integration of synthesis findings into policy and practice. The exception is in the emerging synthesis methodologies, some of which (e.g., realist and meta-narrative syntheses, scoping reviews) explicitly involve stakeholders or knowledge users. The argument is that engaging them in this way increases the likelihood that the knowledge generated will be translated into policy and practice. We suggest that a more explicit engagement with knowledge users in all types of synthesis would benefit the uptake of the research findings.
Research synthesis neither makes research more applicable to practice nor ensures implementation. Focus must now turn seriously towards translation of synthesis findings into knowledge products that are useful for health care practitioners in multiple areas of practice and develop appropriate strategies to facilitate their use. The burgeoning field of knowledge translation has, to some extent, taken up this challenge; however, the research-practice gap continues to plague us [133] – [134] . It is a particular problem for qualitative syntheses [131] . Although such syntheses have an important place in evidence-informed practice, little effort has gone into the challenge of translating the findings into useful products to guide practice [131] .
5.4. Limitations
Our study took longer than would normally be expected for an integrative review. Each of us were primarily involved in our own dissertations or teaching/research positions, and so this study was conducted ‘off the sides of our desks.’ A limitation was that we searched the literature over the course of 4 years (from 2008–2012), necessitating multiple search updates. Further, we did not do a comprehensive search of the literature after 2012, thus the more recent synthesis literature was not systematically explored. We did, however, perform limited database searches from 2012–2015 to keep abreast of the latest methodological developments. Although we missed some new approaches to meta-analysis in our search, we did not find any new features of the synthesis methodologies covered in our review that would change the analysis or findings of this article. Lastly, we struggled with the labels used for the broad categories of research synthesis methodology because of our hesitancy to reinforce the divide between quantitative and qualitative approaches. However, it was very difficult to find alternative language that represented the types of data used in these methodologies. Despite our hesitancy in creating such an obvious divide, we were left with the challenge of trying to find a way of characterizing these broad types of syntheses.
6. Conclusion
Our findings offer methodological clarity for those wishing to learn about the broad terrain of research synthesis. We believe that our review makes transparent the issues and considerations in choosing from among the four broad categories of research synthesis. In summary, research synthesis has taken its place as a form of research in its own right. The methodological terrain has deep historical roots reaching back over the past 200 years, yet research synthesis remains relatively new to public health, health care, and social sciences in general. This is rapidly changing. New developments in systematic reviews and meta-analysis, and the emergence of new synthesis methodologies provide a vast array of options to review the literature for diverse purposes. New approaches to research synthesis and new analytic methods within existing approaches provide a much broader range of review alternatives for public health, health care, and social science students and researchers.
Acknowledgments
KSM is an assistant professor in the Faculty of Nursing at the University of Alberta. Her work on this article was largely conducted as a Postdoctoral Fellow, funded by KRESCENT (Kidney Research Scientist Core Education and National Training Program, reference #KRES110011R1) and the Faculty of Nursing at the University of Alberta.
MM's work on this study over the period of 2008-2014 was supported by a Canadian Institutes of Health Research Applied Public Health Research Chair Award (grant #92365).
We thank Rachel Spanier who provided support with reference formatting.
List of Abbreviations (in Additional File 1 )
Conflict of interest: The authors declare that they have no conflicts of interest in this article.
Authors' contributions: KSM co-designed the study, collected data, analyzed the data, drafted/revised the manuscript, and managed the project.
MP contributed to searching the literature, developing the analytic framework, and extracting data for the Additional File.
JB contributed to searching the literature, developing the analytic framework, and extracting data for the Additional File.
WN contributed to searching the literature, developing the analytic framework, and extracting data for the Additional File.
All authors read and approved the final manuscript.
Additional Files: Additional File 1 – Selected Types of Research Synthesis
This Additional File is our dataset created to organize, analyze and critique the literature that we synthesized in our integrative review. Our results were created based on analysis of this Additional File.
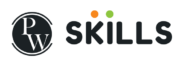
Data Analysis Techniques in Research – Methods, Tools & Examples
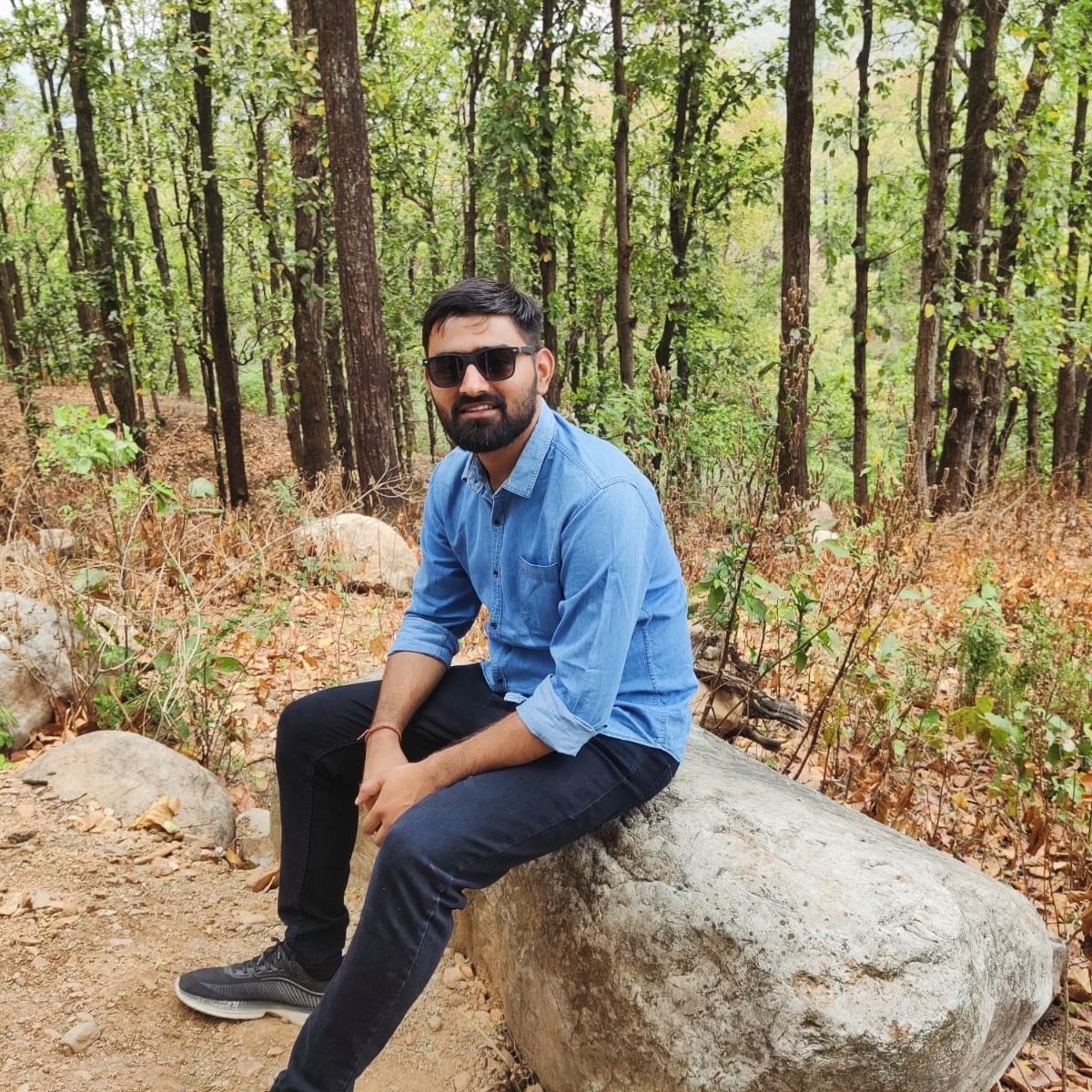
Varun Saharawat is a seasoned professional in the fields of SEO and content writing. With a profound knowledge of the intricate aspects of these disciplines, Varun has established himself as a valuable asset in the world of digital marketing and online content creation.
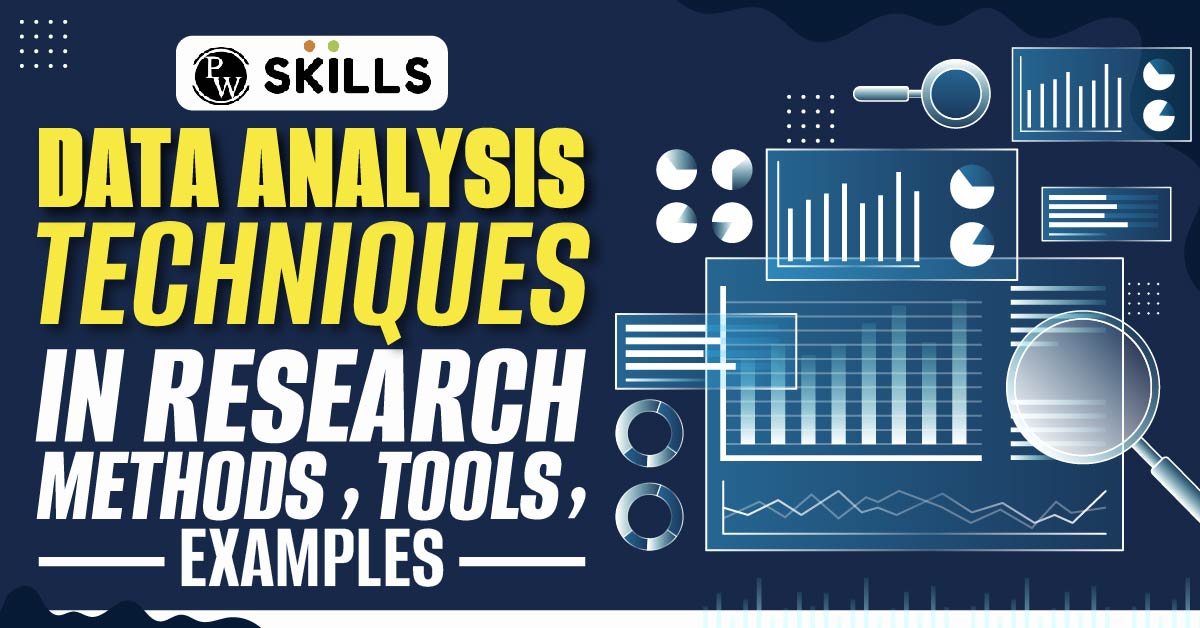
Data analysis techniques in research are essential because they allow researchers to derive meaningful insights from data sets to support their hypotheses or research objectives.
Data Analysis Techniques in Research : While various groups, institutions, and professionals may have diverse approaches to data analysis, a universal definition captures its essence. Data analysis involves refining, transforming, and interpreting raw data to derive actionable insights that guide informed decision-making for businesses.
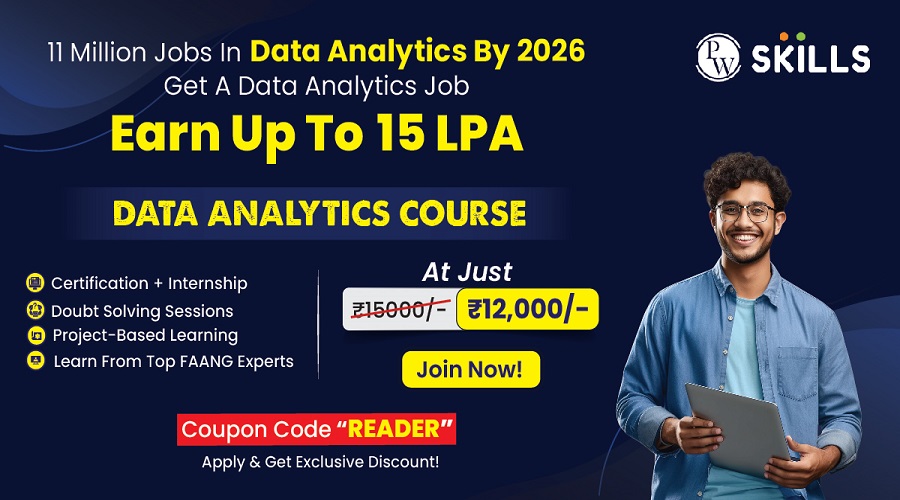
A straightforward illustration of data analysis emerges when we make everyday decisions, basing our choices on past experiences or predictions of potential outcomes.
If you want to learn more about this topic and acquire valuable skills that will set you apart in today’s data-driven world, we highly recommend enrolling in the Data Analytics Course by Physics Wallah . And as a special offer for our readers, use the coupon code “READER” to get a discount on this course.
Table of Contents
What is Data Analysis?
Data analysis is the systematic process of inspecting, cleaning, transforming, and interpreting data with the objective of discovering valuable insights and drawing meaningful conclusions. This process involves several steps:
- Inspecting : Initial examination of data to understand its structure, quality, and completeness.
- Cleaning : Removing errors, inconsistencies, or irrelevant information to ensure accurate analysis.
- Transforming : Converting data into a format suitable for analysis, such as normalization or aggregation.
- Interpreting : Analyzing the transformed data to identify patterns, trends, and relationships.
Types of Data Analysis Techniques in Research
Data analysis techniques in research are categorized into qualitative and quantitative methods, each with its specific approaches and tools. These techniques are instrumental in extracting meaningful insights, patterns, and relationships from data to support informed decision-making, validate hypotheses, and derive actionable recommendations. Below is an in-depth exploration of the various types of data analysis techniques commonly employed in research:
1) Qualitative Analysis:
Definition: Qualitative analysis focuses on understanding non-numerical data, such as opinions, concepts, or experiences, to derive insights into human behavior, attitudes, and perceptions.
- Content Analysis: Examines textual data, such as interview transcripts, articles, or open-ended survey responses, to identify themes, patterns, or trends.
- Narrative Analysis: Analyzes personal stories or narratives to understand individuals’ experiences, emotions, or perspectives.
- Ethnographic Studies: Involves observing and analyzing cultural practices, behaviors, and norms within specific communities or settings.

2) Quantitative Analysis:
Quantitative analysis emphasizes numerical data and employs statistical methods to explore relationships, patterns, and trends. It encompasses several approaches:
Descriptive Analysis:
- Frequency Distribution: Represents the number of occurrences of distinct values within a dataset.
- Central Tendency: Measures such as mean, median, and mode provide insights into the central values of a dataset.
- Dispersion: Techniques like variance and standard deviation indicate the spread or variability of data.
Diagnostic Analysis:
- Regression Analysis: Assesses the relationship between dependent and independent variables, enabling prediction or understanding causality.
- ANOVA (Analysis of Variance): Examines differences between groups to identify significant variations or effects.
Predictive Analysis:
- Time Series Forecasting: Uses historical data points to predict future trends or outcomes.
- Machine Learning Algorithms: Techniques like decision trees, random forests, and neural networks predict outcomes based on patterns in data.
Prescriptive Analysis:
- Optimization Models: Utilizes linear programming, integer programming, or other optimization techniques to identify the best solutions or strategies.
- Simulation: Mimics real-world scenarios to evaluate various strategies or decisions and determine optimal outcomes.
Specific Techniques:
- Monte Carlo Simulation: Models probabilistic outcomes to assess risk and uncertainty.
- Factor Analysis: Reduces the dimensionality of data by identifying underlying factors or components.
- Cohort Analysis: Studies specific groups or cohorts over time to understand trends, behaviors, or patterns within these groups.
- Cluster Analysis: Classifies objects or individuals into homogeneous groups or clusters based on similarities or attributes.
- Sentiment Analysis: Uses natural language processing and machine learning techniques to determine sentiment, emotions, or opinions from textual data.
Also Read: AI and Predictive Analytics: Examples, Tools, Uses, Ai Vs Predictive Analytics
Data Analysis Techniques in Research Examples
To provide a clearer understanding of how data analysis techniques are applied in research, let’s consider a hypothetical research study focused on evaluating the impact of online learning platforms on students’ academic performance.
Research Objective:
Determine if students using online learning platforms achieve higher academic performance compared to those relying solely on traditional classroom instruction.
Data Collection:
- Quantitative Data: Academic scores (grades) of students using online platforms and those using traditional classroom methods.
- Qualitative Data: Feedback from students regarding their learning experiences, challenges faced, and preferences.
Data Analysis Techniques Applied:
1) Descriptive Analysis:
- Calculate the mean, median, and mode of academic scores for both groups.
- Create frequency distributions to represent the distribution of grades in each group.
2) Diagnostic Analysis:
- Conduct an Analysis of Variance (ANOVA) to determine if there’s a statistically significant difference in academic scores between the two groups.
- Perform Regression Analysis to assess the relationship between the time spent on online platforms and academic performance.
3) Predictive Analysis:
- Utilize Time Series Forecasting to predict future academic performance trends based on historical data.
- Implement Machine Learning algorithms to develop a predictive model that identifies factors contributing to academic success on online platforms.
4) Prescriptive Analysis:
- Apply Optimization Models to identify the optimal combination of online learning resources (e.g., video lectures, interactive quizzes) that maximize academic performance.
- Use Simulation Techniques to evaluate different scenarios, such as varying student engagement levels with online resources, to determine the most effective strategies for improving learning outcomes.
5) Specific Techniques:
- Conduct Factor Analysis on qualitative feedback to identify common themes or factors influencing students’ perceptions and experiences with online learning.
- Perform Cluster Analysis to segment students based on their engagement levels, preferences, or academic outcomes, enabling targeted interventions or personalized learning strategies.
- Apply Sentiment Analysis on textual feedback to categorize students’ sentiments as positive, negative, or neutral regarding online learning experiences.
By applying a combination of qualitative and quantitative data analysis techniques, this research example aims to provide comprehensive insights into the effectiveness of online learning platforms.
Also Read: Learning Path to Become a Data Analyst in 2024
Data Analysis Techniques in Quantitative Research
Quantitative research involves collecting numerical data to examine relationships, test hypotheses, and make predictions. Various data analysis techniques are employed to interpret and draw conclusions from quantitative data. Here are some key data analysis techniques commonly used in quantitative research:
1) Descriptive Statistics:
- Description: Descriptive statistics are used to summarize and describe the main aspects of a dataset, such as central tendency (mean, median, mode), variability (range, variance, standard deviation), and distribution (skewness, kurtosis).
- Applications: Summarizing data, identifying patterns, and providing initial insights into the dataset.
2) Inferential Statistics:
- Description: Inferential statistics involve making predictions or inferences about a population based on a sample of data. This technique includes hypothesis testing, confidence intervals, t-tests, chi-square tests, analysis of variance (ANOVA), regression analysis, and correlation analysis.
- Applications: Testing hypotheses, making predictions, and generalizing findings from a sample to a larger population.
3) Regression Analysis:
- Description: Regression analysis is a statistical technique used to model and examine the relationship between a dependent variable and one or more independent variables. Linear regression, multiple regression, logistic regression, and nonlinear regression are common types of regression analysis .
- Applications: Predicting outcomes, identifying relationships between variables, and understanding the impact of independent variables on the dependent variable.
4) Correlation Analysis:
- Description: Correlation analysis is used to measure and assess the strength and direction of the relationship between two or more variables. The Pearson correlation coefficient, Spearman rank correlation coefficient, and Kendall’s tau are commonly used measures of correlation.
- Applications: Identifying associations between variables and assessing the degree and nature of the relationship.
5) Factor Analysis:
- Description: Factor analysis is a multivariate statistical technique used to identify and analyze underlying relationships or factors among a set of observed variables. It helps in reducing the dimensionality of data and identifying latent variables or constructs.
- Applications: Identifying underlying factors or constructs, simplifying data structures, and understanding the underlying relationships among variables.
6) Time Series Analysis:
- Description: Time series analysis involves analyzing data collected or recorded over a specific period at regular intervals to identify patterns, trends, and seasonality. Techniques such as moving averages, exponential smoothing, autoregressive integrated moving average (ARIMA), and Fourier analysis are used.
- Applications: Forecasting future trends, analyzing seasonal patterns, and understanding time-dependent relationships in data.
7) ANOVA (Analysis of Variance):
- Description: Analysis of variance (ANOVA) is a statistical technique used to analyze and compare the means of two or more groups or treatments to determine if they are statistically different from each other. One-way ANOVA, two-way ANOVA, and MANOVA (Multivariate Analysis of Variance) are common types of ANOVA.
- Applications: Comparing group means, testing hypotheses, and determining the effects of categorical independent variables on a continuous dependent variable.
8) Chi-Square Tests:
- Description: Chi-square tests are non-parametric statistical tests used to assess the association between categorical variables in a contingency table. The Chi-square test of independence, goodness-of-fit test, and test of homogeneity are common chi-square tests.
- Applications: Testing relationships between categorical variables, assessing goodness-of-fit, and evaluating independence.
These quantitative data analysis techniques provide researchers with valuable tools and methods to analyze, interpret, and derive meaningful insights from numerical data. The selection of a specific technique often depends on the research objectives, the nature of the data, and the underlying assumptions of the statistical methods being used.
Also Read: Analysis vs. Analytics: How Are They Different?
Data Analysis Methods
Data analysis methods refer to the techniques and procedures used to analyze, interpret, and draw conclusions from data. These methods are essential for transforming raw data into meaningful insights, facilitating decision-making processes, and driving strategies across various fields. Here are some common data analysis methods:
- Description: Descriptive statistics summarize and organize data to provide a clear and concise overview of the dataset. Measures such as mean, median, mode, range, variance, and standard deviation are commonly used.
- Description: Inferential statistics involve making predictions or inferences about a population based on a sample of data. Techniques such as hypothesis testing, confidence intervals, and regression analysis are used.
3) Exploratory Data Analysis (EDA):
- Description: EDA techniques involve visually exploring and analyzing data to discover patterns, relationships, anomalies, and insights. Methods such as scatter plots, histograms, box plots, and correlation matrices are utilized.
- Applications: Identifying trends, patterns, outliers, and relationships within the dataset.
4) Predictive Analytics:
- Description: Predictive analytics use statistical algorithms and machine learning techniques to analyze historical data and make predictions about future events or outcomes. Techniques such as regression analysis, time series forecasting, and machine learning algorithms (e.g., decision trees, random forests, neural networks) are employed.
- Applications: Forecasting future trends, predicting outcomes, and identifying potential risks or opportunities.
5) Prescriptive Analytics:
- Description: Prescriptive analytics involve analyzing data to recommend actions or strategies that optimize specific objectives or outcomes. Optimization techniques, simulation models, and decision-making algorithms are utilized.
- Applications: Recommending optimal strategies, decision-making support, and resource allocation.
6) Qualitative Data Analysis:
- Description: Qualitative data analysis involves analyzing non-numerical data, such as text, images, videos, or audio, to identify themes, patterns, and insights. Methods such as content analysis, thematic analysis, and narrative analysis are used.
- Applications: Understanding human behavior, attitudes, perceptions, and experiences.
7) Big Data Analytics:
- Description: Big data analytics methods are designed to analyze large volumes of structured and unstructured data to extract valuable insights. Technologies such as Hadoop, Spark, and NoSQL databases are used to process and analyze big data.
- Applications: Analyzing large datasets, identifying trends, patterns, and insights from big data sources.
8) Text Analytics:
- Description: Text analytics methods involve analyzing textual data, such as customer reviews, social media posts, emails, and documents, to extract meaningful information and insights. Techniques such as sentiment analysis, text mining, and natural language processing (NLP) are used.
- Applications: Analyzing customer feedback, monitoring brand reputation, and extracting insights from textual data sources.
These data analysis methods are instrumental in transforming data into actionable insights, informing decision-making processes, and driving organizational success across various sectors, including business, healthcare, finance, marketing, and research. The selection of a specific method often depends on the nature of the data, the research objectives, and the analytical requirements of the project or organization.
Also Read: Quantitative Data Analysis: Types, Analysis & Examples
Data Analysis Tools
Data analysis tools are essential instruments that facilitate the process of examining, cleaning, transforming, and modeling data to uncover useful information, make informed decisions, and drive strategies. Here are some prominent data analysis tools widely used across various industries:
1) Microsoft Excel:
- Description: A spreadsheet software that offers basic to advanced data analysis features, including pivot tables, data visualization tools, and statistical functions.
- Applications: Data cleaning, basic statistical analysis, visualization, and reporting.
2) R Programming Language:
- Description: An open-source programming language specifically designed for statistical computing and data visualization.
- Applications: Advanced statistical analysis, data manipulation, visualization, and machine learning.
3) Python (with Libraries like Pandas, NumPy, Matplotlib, and Seaborn):
- Description: A versatile programming language with libraries that support data manipulation, analysis, and visualization.
- Applications: Data cleaning, statistical analysis, machine learning, and data visualization.
4) SPSS (Statistical Package for the Social Sciences):
- Description: A comprehensive statistical software suite used for data analysis, data mining, and predictive analytics.
- Applications: Descriptive statistics, hypothesis testing, regression analysis, and advanced analytics.
5) SAS (Statistical Analysis System):
- Description: A software suite used for advanced analytics, multivariate analysis, and predictive modeling.
- Applications: Data management, statistical analysis, predictive modeling, and business intelligence.
6) Tableau:
- Description: A data visualization tool that allows users to create interactive and shareable dashboards and reports.
- Applications: Data visualization , business intelligence , and interactive dashboard creation.
7) Power BI:
- Description: A business analytics tool developed by Microsoft that provides interactive visualizations and business intelligence capabilities.
- Applications: Data visualization, business intelligence, reporting, and dashboard creation.
8) SQL (Structured Query Language) Databases (e.g., MySQL, PostgreSQL, Microsoft SQL Server):
- Description: Database management systems that support data storage, retrieval, and manipulation using SQL queries.
- Applications: Data retrieval, data cleaning, data transformation, and database management.
9) Apache Spark:
- Description: A fast and general-purpose distributed computing system designed for big data processing and analytics.
- Applications: Big data processing, machine learning, data streaming, and real-time analytics.
10) IBM SPSS Modeler:
- Description: A data mining software application used for building predictive models and conducting advanced analytics.
- Applications: Predictive modeling, data mining, statistical analysis, and decision optimization.
These tools serve various purposes and cater to different data analysis needs, from basic statistical analysis and data visualization to advanced analytics, machine learning, and big data processing. The choice of a specific tool often depends on the nature of the data, the complexity of the analysis, and the specific requirements of the project or organization.
Also Read: How to Analyze Survey Data: Methods & Examples
Importance of Data Analysis in Research
The importance of data analysis in research cannot be overstated; it serves as the backbone of any scientific investigation or study. Here are several key reasons why data analysis is crucial in the research process:
- Data analysis helps ensure that the results obtained are valid and reliable. By systematically examining the data, researchers can identify any inconsistencies or anomalies that may affect the credibility of the findings.
- Effective data analysis provides researchers with the necessary information to make informed decisions. By interpreting the collected data, researchers can draw conclusions, make predictions, or formulate recommendations based on evidence rather than intuition or guesswork.
- Data analysis allows researchers to identify patterns, trends, and relationships within the data. This can lead to a deeper understanding of the research topic, enabling researchers to uncover insights that may not be immediately apparent.
- In empirical research, data analysis plays a critical role in testing hypotheses. Researchers collect data to either support or refute their hypotheses, and data analysis provides the tools and techniques to evaluate these hypotheses rigorously.
- Transparent and well-executed data analysis enhances the credibility of research findings. By clearly documenting the data analysis methods and procedures, researchers allow others to replicate the study, thereby contributing to the reproducibility of research findings.
- In fields such as business or healthcare, data analysis helps organizations allocate resources more efficiently. By analyzing data on consumer behavior, market trends, or patient outcomes, organizations can make strategic decisions about resource allocation, budgeting, and planning.
- In public policy and social sciences, data analysis is instrumental in developing and evaluating policies and interventions. By analyzing data on social, economic, or environmental factors, policymakers can assess the effectiveness of existing policies and inform the development of new ones.
- Data analysis allows for continuous improvement in research methods and practices. By analyzing past research projects, identifying areas for improvement, and implementing changes based on data-driven insights, researchers can refine their approaches and enhance the quality of future research endeavors.
However, it is important to remember that mastering these techniques requires practice and continuous learning. That’s why we highly recommend the Data Analytics Course by Physics Wallah . Not only does it cover all the fundamentals of data analysis, but it also provides hands-on experience with various tools such as Excel, Python, and Tableau. Plus, if you use the “ READER ” coupon code at checkout, you can get a special discount on the course.
For Latest Tech Related Information, Join Our Official Free Telegram Group : PW Skills Telegram Group
Data Analysis Techniques in Research FAQs
What are the 5 techniques for data analysis.
The five techniques for data analysis include: Descriptive Analysis Diagnostic Analysis Predictive Analysis Prescriptive Analysis Qualitative Analysis
What are techniques of data analysis in research?
Techniques of data analysis in research encompass both qualitative and quantitative methods. These techniques involve processes like summarizing raw data, investigating causes of events, forecasting future outcomes, offering recommendations based on predictions, and examining non-numerical data to understand concepts or experiences.
What are the 3 methods of data analysis?
The three primary methods of data analysis are: Qualitative Analysis Quantitative Analysis Mixed-Methods Analysis
What are the four types of data analysis techniques?
The four types of data analysis techniques are: Descriptive Analysis Diagnostic Analysis Predictive Analysis Prescriptive Analysis
- Finance Data Analysis: What is a Financial Data Analysis?
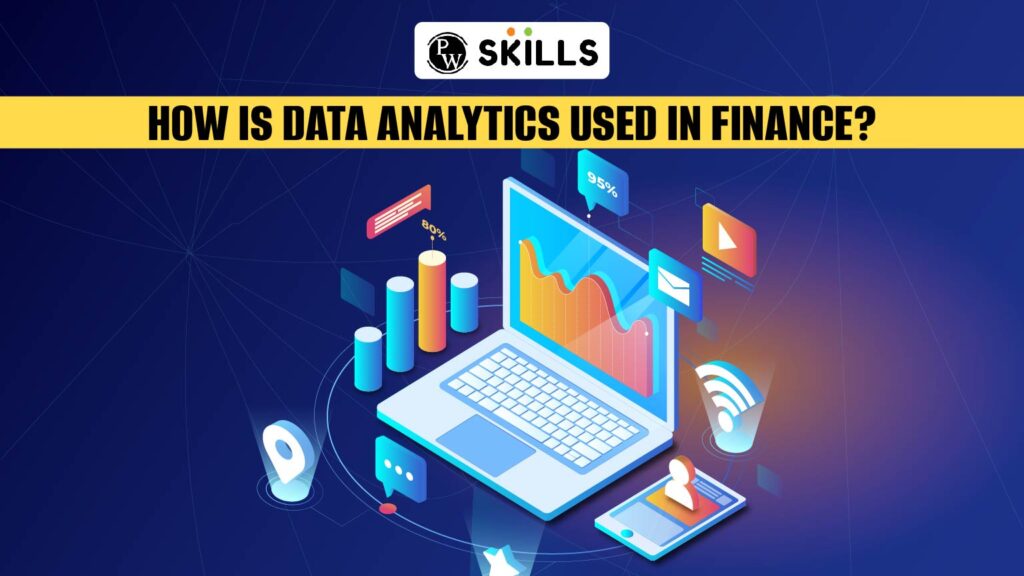
Finance data analysis is used increasingly by many companies worldwide. Data analysis in finance helps to collect various financial-related raw…
- What are Data Analysis Tools?
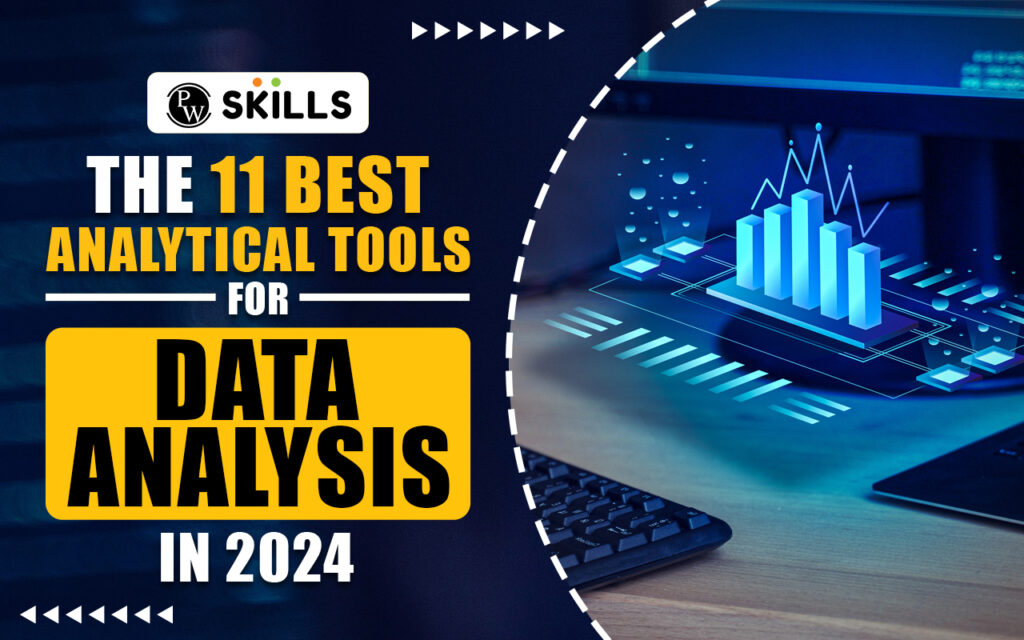
Data Analytical tools help to extract important insights from raw and unstructured data. Read this article to get a list…
- Which Course is Best for Business Analyst? (Business Analysts Online Courses)
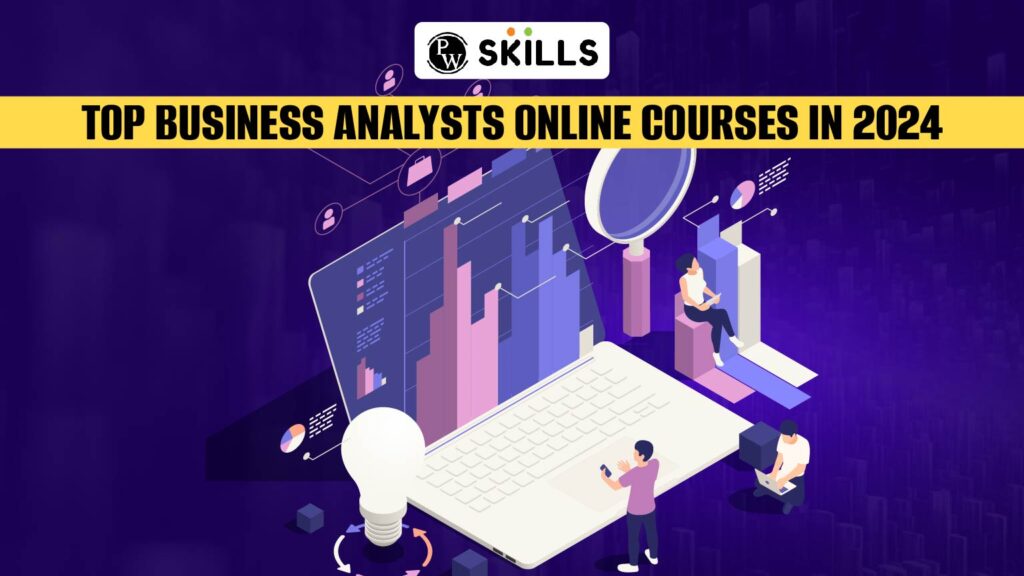
Many reputed platforms and institutions offer online certification courses which can help you land job offers in relevant companies. In…
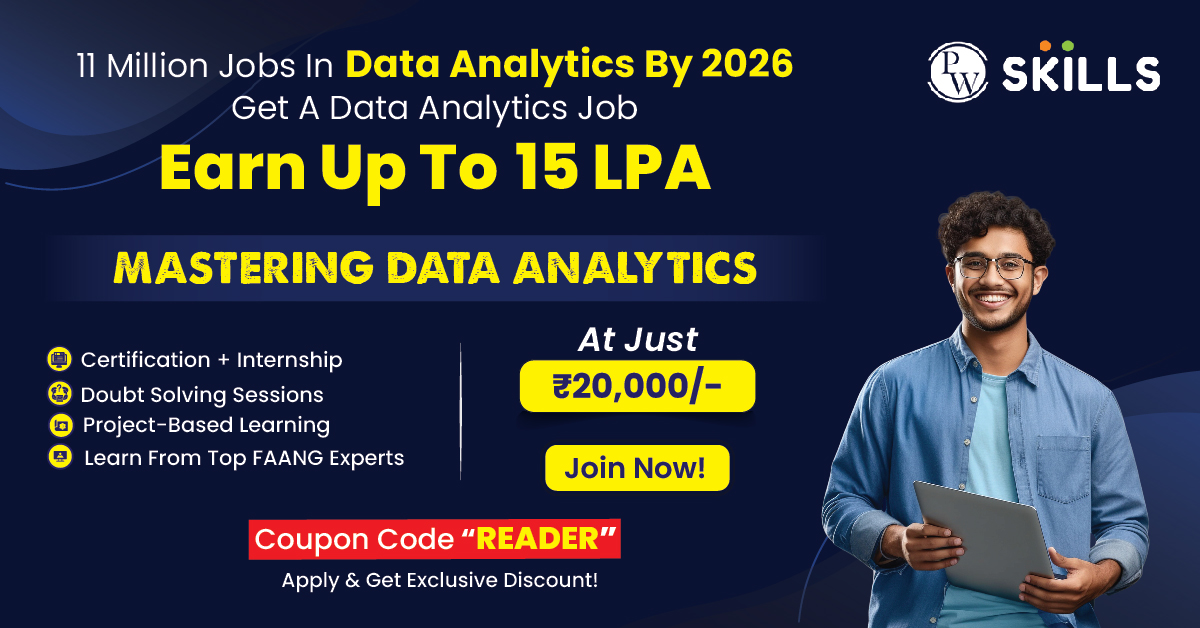
Related Articles
- What is Data Analytics in Database?
- Best Courses For Data Analytics: Top 10 Courses For Your Career in Trend
- Big Data: What Do You Mean By Big Data?
- Top 20 Big Data Tools Used By Professionals
- 10 Most Popular Big Data Analytics Tools
- Top Best Big Data Analytics Classes 2024
- Big Data and Analytics – Definition, Benefits, and More
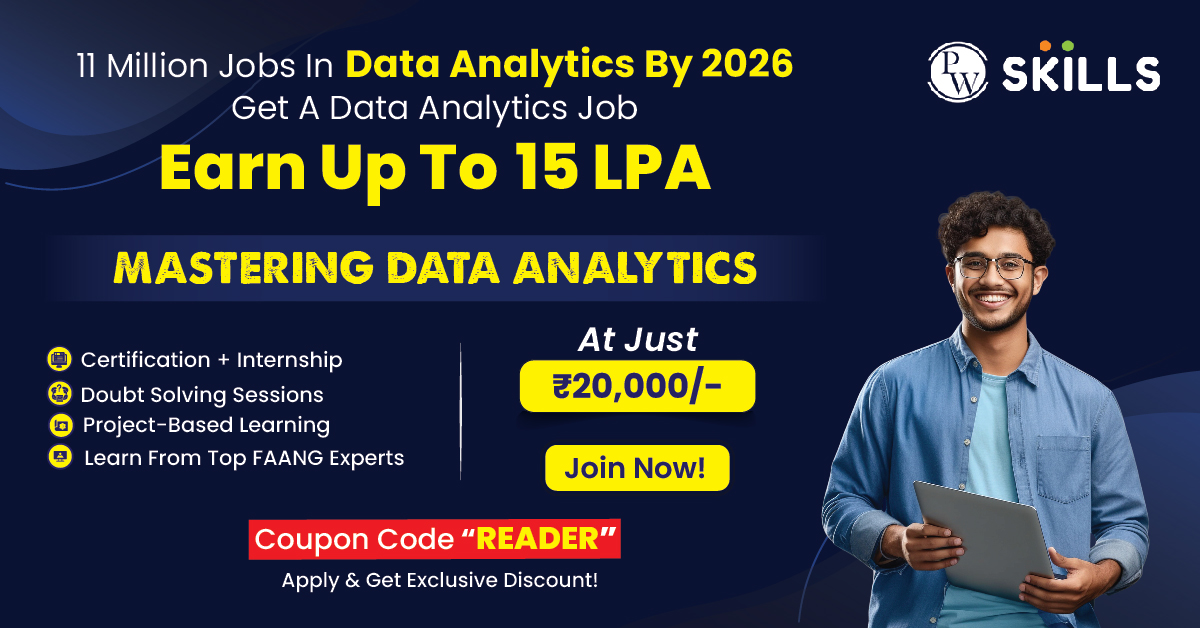
- Technical Support
- Find My Rep
You are here
Job and Work Analysis Methods, Research, and Applications for Human Resource Management
- Frederick P. Morgeson - Michigan State University, USA
- Michael T. Brannick - University of South Florida, USA
- Edward L. Levine - University of South Florida, USA
- Description
See what’s new to this edition by selecting the Features tab on this page. Should you need additional information or have questions regarding the HEOA information provided for this title, including what is new to this edition, please email [email protected] . Please include your name, contact information, and the name of the title for which you would like more information. For information on the HEOA, please go to http://ed.gov/policy/highered/leg/hea08/index.html .
For assistance with your order: Please email us at [email protected] or connect with your SAGE representative.
SAGE 2455 Teller Road Thousand Oaks, CA 91320 www.sagepub.com
“This book is the go-to source of information on job analysis. [A] unique, rich book, and a must-read for future job analysts, I/O psychologists, and HR professionals in training.”
“The book comprehensively covers both techniques for job analysis and uses for job analysis results. It is highly academically informative and easy to read with touches of humor throughout.”
“This is a step by step guide to successful job analysis.”
“This text is an HRM resource that shows the evolution of jobs through job analysis and the progression of job analysis in effective HRM.”
The text contains all the necessary elements to work the analysis and design of positions. Although I expected it to have more examples and tools, it has updated and didactic material for this type of courses.
NEW TO THIS EDITION:
- New references and the latest research findings offer the most current information available.
- Expanded discussion of competency models and teams in organizations recognizes the latest workplace trends.
- Expanded discussion of O*NET offers the latest information on this open-source, web-based database from the Department of Labor.
KEY FEATURES:
- Substantial coverage is offered on O*NET, strategic job analysis, competencies and competency modeling, and inaccuracy in job analysis ratings.
- Numerous samples, models, and templates provide readers with tools they can put into practice.
- Numerous examples illustrate the "how-to" of job analysis in real-life settings.
Sample Materials & Chapters
1. Introduction
8. Staffing and Training
For instructors
Select a purchasing option.

This title is also available on SAGE Knowledge , the ultimate social sciences online library. If your library doesn’t have access, ask your librarian to start a trial .
Popular searches
- How to Get Participants For Your Study
- How to Do Segmentation?
- Conjoint Preference Share Simulator
- MaxDiff Analysis
- Likert Scales
- Reliability & Validity
Request consultation
Do you need support in running a pricing or product study? We can help you with agile consumer research and conjoint analysis.
Looking for an online survey platform?
Conjointly offers a great survey tool with multiple question types, randomisation blocks, and multilingual support. The Basic tier is always free.
Research Methods Knowledge Base
- Navigating the Knowledge Base
- Foundations
- Measurement
- Research Design
- Conclusion Validity
- Data Preparation
- Descriptive Statistics
- Inferential Statistics
- Table of Contents
Fully-functional online survey tool with various question types, logic, randomisation, and reporting for unlimited number of surveys.
Completely free for academics and students .
By the time you get to the analysis of your data, most of the really difficult work has been done. It’s much more difficult to: define the research problem; develop and implement a sampling plan; conceptualize, operationalize and test your measures; and develop a design structure. If you have done this work well, the analysis of the data is usually a fairly straightforward affair.
In most social research the data analysis involves three major steps, done in roughly this order:
- Cleaning and organizing the data for analysis ( Data Preparation )
- Describing the data ( Descriptive Statistics )
- Testing Hypotheses and Models ( Inferential Statistics )
Data Preparation involves checking or logging the data in; checking the data for accuracy; entering the data into the computer; transforming the data; and developing and documenting a database structure that integrates the various measures.
Descriptive Statistics are used to describe the basic features of the data in a study. They provide simple summaries about the sample and the measures. Together with simple graphics analysis, they form the basis of virtually every quantitative analysis of data. With descriptive statistics you are simply describing what is, what the data shows.
Inferential Statistics investigate questions, models and hypotheses. In many cases, the conclusions from inferential statistics extend beyond the immediate data alone. For instance, we use inferential statistics to try to infer from the sample data what the population thinks. Or, we use inferential statistics to make judgments of the probability that an observed difference between groups is a dependable one or one that might have happened by chance in this study. Thus, we use inferential statistics to make inferences from our data to more general conditions; we use descriptive statistics simply to describe what’s going on in our data.
In most research studies, the analysis section follows these three phases of analysis. Descriptions of how the data were prepared tend to be brief and to focus on only the more unique aspects to your study, such as specific data transformations that are performed. The descriptive statistics that you actually look at can be voluminous. In most write-ups, these are carefully selected and organized into summary tables and graphs that only show the most relevant or important information. Usually, the researcher links each of the inferential analyses to specific research questions or hypotheses that were raised in the introduction, or notes any models that were tested that emerged as part of the analysis. In most analysis write-ups it’s especially critical to not “miss the forest for the trees.” If you present too much detail, the reader may not be able to follow the central line of the results. Often extensive analysis details are appropriately relegated to appendices, reserving only the most critical analysis summaries for the body of the report itself.
Cookie Consent
Conjointly uses essential cookies to make our site work. We also use additional cookies in order to understand the usage of the site, gather audience analytics, and for remarketing purposes.
For more information on Conjointly's use of cookies, please read our Cookie Policy .
Which one are you?
I am new to conjointly, i am already using conjointly.
Thank you for visiting nature.com. You are using a browser version with limited support for CSS. To obtain the best experience, we recommend you use a more up to date browser (or turn off compatibility mode in Internet Explorer). In the meantime, to ensure continued support, we are displaying the site without styles and JavaScript.
- View all journals
- Explore content
- About the journal
- Publish with us
- Sign up for alerts
- Published: 12 April 2024
Pretraining a foundation model for generalizable fluorescence microscopy-based image restoration
- Chenxi Ma 1 na1 ,
- Weimin Tan ORCID: orcid.org/0000-0001-7677-4772 1 na1 ,
- Ruian He 1 &
- Bo Yan ORCID: orcid.org/0000-0001-5692-3486 1
Nature Methods ( 2024 ) Cite this article
1434 Accesses
34 Altmetric
Metrics details
- Confocal microscopy
- Image processing
- Super-resolution microscopy
- Wide-field fluorescence microscopy
Fluorescence microscopy-based image restoration has received widespread attention in the life sciences and has led to significant progress, benefiting from deep learning technology. However, most current task-specific methods have limited generalizability to different fluorescence microscopy-based image restoration problems. Here, we seek to improve generalizability and explore the potential of applying a pretrained foundation model to fluorescence microscopy-based image restoration. We provide a universal fluorescence microscopy-based image restoration (UniFMIR) model to address different restoration problems, and show that UniFMIR offers higher image restoration precision, better generalization and increased versatility. Demonstrations on five tasks and 14 datasets covering a wide range of microscopy imaging modalities and biological samples demonstrate that the pretrained UniFMIR can effectively transfer knowledge to a specific situation via fine-tuning, uncover clear nanoscale biomolecular structures and facilitate high-quality imaging. This work has the potential to inspire and trigger new research highlights for fluorescence microscopy-based image restoration.
This is a preview of subscription content, access via your institution
Access options
Access Nature and 54 other Nature Portfolio journals
Get Nature+, our best-value online-access subscription
24,99 € / 30 days
cancel any time
Subscribe to this journal
Receive 12 print issues and online access
251,40 € per year
only 20,95 € per issue
Buy this article
- Purchase on Springer Link
- Instant access to full article PDF
Prices may be subject to local taxes which are calculated during checkout
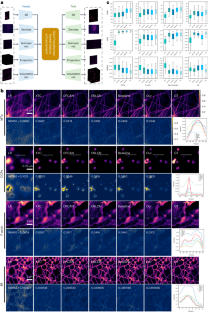
Similar content being viewed by others
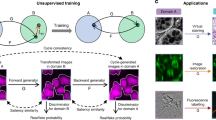
Unsupervised content-preserving transformation for optical microscopy
Xinyang Li, Guoxun Zhang, … Qionghai Dai
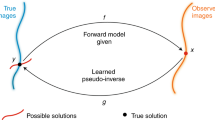
Applications, promises, and pitfalls of deep learning for fluorescence image reconstruction
Chinmay Belthangady & Loic A. Royer
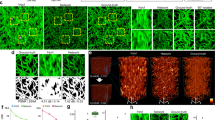
Deep learning enables reference-free isotropic super-resolution for volumetric fluorescence microscopy
Hyoungjun Park, Myeongsu Na, … Jong Chul Ye
Data availability
All training and testing data involved in the experiments come from existing literature and can be downloaded from the corresponding links provided in Supplementary Table 2 or via Zenodo at https://doi.org/10.5281/zenodo.8401470 (ref. 55 ).
Code availability
The PyTorch code of our UniFMIR, together with trained models, as well as some example images for inference are publicly available at https://github.com/cxm12/UNiFMIR ( https://doi.org/10.5281/zenodo.10117581 ) 56 . Furthermore, We also provide a live demo for UniFMIR at http://unifmir.fdudml.cn/ . Users can also access the colab at https://colab.research.google.com/github/cxm12/UNiFMIR/blob/main/UniFMIR.ipynb or use the steps in our GitHub documentation to run the demo locally. This newly built interactive software platform facilitates users to freely and easily use the pretrained foundation model. It also makes it easy for us to continuously train the foundation model with new data and share it with the community. Finally, we shared all models on BioImage.IO at https://bioimage.io/#/ . Data are available via Zenodo at https://doi.org/10.5281/zenodo.10577218 , https://doi.org/10.5281/zenodo.10579778 , https://doi.org/10.5281/zenodo.10579822 , https://doi.org/10.5281/zenodo.10595428 , https://doi.org/10.5281/zenodo.10595460 , https://doi.org/10.5281/zenodo.8420081 and https://doi.org/10.5281/zenodo.8420100 (refs. 57 , 58 , 59 , 60 , 61 , 62 , 63 ). We used the Pycharm software for code development.
Preibisch, S. et al. Efficient bayesian-based multiview deconvolution. Nat. Methods 11 , 645–648 (2014).
Article CAS PubMed PubMed Central Google Scholar
Gustafsson, N. et al. Fast live-cell conventional fluorophore nanoscopy with ImageJ through super-resolution radial fluctuations. Nat. Commun. 7 , 12471 (2016).
Arigovindan, M. et al. High-resolution restoration of 3D structures from widefield images with extreme low signal-to-noise-ratio. Proc. Natl Acad. Sci. USA 110 , 17344–17349 (2013).
Weigert, M. et al. Content-aware image restoration: pushing the limits of fluorescence microscopy. Nat. Methods 15 , 1090–1097 (2018).
Article CAS PubMed Google Scholar
Qiao, C. et al. Evaluation and development of deep neural networks for image super-resolution in optical microscopy. Nat. Methods 18 , 194–202 (2021).
Chen, J. et al. Three-dimensional residual channel attention networks denoise and sharpen fluorescence microscopy image volumes. Nat. Methods 18 , 678–687 (2021).
Wang, Z., Xie, Y. & Ji, S. Global voxel transformer networks for augmented microscopy. Nat. Mach. Intell. 3 , 161–171 (2021).
Article Google Scholar
Wang, Z. et al. Real-time volumetric reconstruction of biological dynamics with light-field microscopy and deep learning. Nat. Methods 18 , 551–556 (2021).
Li, X. et al. Reinforcing neuron extraction and spike inference in calcium imaging using deep self-supervised denoising. Nat. Methods 18 , 1395–1400 (2021).
Qiao, C. et al. Rationalized deep neural network for sustained super-resolution live imaging of rapid subcellular processes. Nat. Biotechol. 41 , 367–377 (2022).
Belthangady, C. & Royer, L. A. Applications, promises, and pitfalls of deep learning for fluorescence image reconstruction. Nat. Methods 16 , 1215–1225 (2019).
Wu, Y. & Shroff, H. Faster, sharper, and deeper: structured illumination microscopy for biological imaging. Nat. Methods 15 , 1011–1019 (2018).
Wu, Y. et al. Multiview confocal super-resolution microscopy. Nature 600 , 279–284 (2021).
Chen, R. et al. Single-frame deep-learning super-resolution microscopy for intracellular dynamics imaging. Nat. Commun. 14 , 2854 (2023).
Xu, Y. K. T. et al. Cross-modality supervised image restoration enables nanoscale tracking of synaptic plasticity in living mice. Nat. Methods 20 , 935–944 (2023).
Bommasani, R. et al. On the opportunities and risks of foundation models. Preprint at https://arxiv.org/abs/2108.07258 (2021).
Fei, N. et al. Towards artificial general intelligence via a multimodal foundation model. Nat. Commun. 13 , 3094 (2022).
Zhang, Y. et al. DialoGPT: large-scale generative pre-training for conversational response generation. In Proceedings of the 58th Annual Meeting of the Association for Computational Linguistics: System Demonstrations. 270–278 (2020).
Yang, Z. et al. Xlnet: generalized autoregressive pretraining for language understanding. In Conference on Neural Information Processing Systems (NeurIPS) (2019).
Dai, Z. et al. Coatnet: marrying convolution and attention for all data sizes. In IEEE/CVF Conference on Computer Vision and Pattern Recognition (CVPR) (2021).
Kirillov, A. et al. Segment anything. In Proceedings of the IEEE/CVF International Conference on Computer Vision , 4015–4026 (2023).
Achiam, J. et al. Gpt-4 technical report. Preprint at https://arxiv.org/abs/2303.08774 (2023).
Bao, F. et al. One transformer fits all distributions in multi-modal diffusion at scale. In International Conference on Machine Learning (ICML) (2023).
Bi, K. et al. Accurate medium-range global weather forecasting with 3D neural networks. Nature 619 , 533–538 (2023).
Singhal, K. et al. Large language models encode clinical knowledge. Nature 620 , 172–180 (2023).
Jiang, L. Y. et al. Health system-scale language models are all-purpose prediction engines. Nature 619 , 357–362 (2023).
Huang, Z. et al. A visual-language foundation model for pathology image analysis using medical twitter. Nat. Methods 29 , 2307–2316 (2023).
CAS Google Scholar
Zhou, Y. et al. A foundation model for generalizable disease detection from retinal images. Nature 622 , 156–163 (2023).
Moor, M. et al. Foundation models for generalist medical artificial intelligence. Nature 616 , 259–265 (2023).
Madani, A. et al. Large language models generate functional protein sequences across diverse families. Nature Biotechnol. 41 , 1099–1106 (2023).
Article CAS Google Scholar
Theodoris, C. V. et al. Transfer learning enables predictions in network biology. Nature 618 , 616–624 (2023).
Henighan, T. et al. Scaling laws for autoregressive generative modeling. Preprint at https://arxiv.org/abs/2010.14701 (2020).
Zamir, A. et al. Taskonomy: disentangling task transfer learning. In Twenty-Eighth International Joint Conference on Artificial Intelligence (IJCAI) , 3712–3722 (2019).
Liu, Z. et al. Swin transformer: hierarchical vision transformer using shifted windows. In IEEE/CVF Conference on Computer Vision and Pattern Recognition (CVPR) (2021).
Xia, B. et al. Efficient non-local contrastive attention for image super-resolution. In Association for the Advancement of Artificial Intelligence (AAAI) (2022).
Descloux, A., Grubmayer, K. S. & Radenovic, A. Parameter-free image resolution estimation based on decorrelation analysis. Nat. Methods 16 , 918–924 (2019).
Nieuwenhuizen, R. et al. Measuring image resolution in optical nanoscopy. Nat. Methods 10 , 557–562 (2013).
Culley, S. et al. Quantitative mapping and minimization of super-resolution optical imaging artifacts. Nat. Methods 15 , 263–266 (2018).
Li, X. et al. Three-dimensional structured illumination microscopy with enhanced axial resolution. Nat. Biotechnol. 41 , 1307–1319 (2023).
Spahn, C. et al. DeepBacs for multi-task bacterial image analysis using open-source deep learning approaches. Commun. Biol. 5 , 688 (2022).
Ouyang, W. et al. ShareLoc—an open platform for sharing localization microscopy data. Nat. Methods 19 , 1331–1333 (2022).
Zhang, X. C. et al. Zoom to learn, learn to zoom. In IEEE/CVF Conference on Computer Vision and Pattern Recognition (CVPR) (2019).
Nehme, E. et al. Deep-storm: super-resolution single-molecule microscopy by deep learning. Optica 5 , 458–464 (2018).
Guo, L. L. et al. EHR foundation models improve robustness in the presence of temporal distribution shift. Sci. Rep. 13 , 3767 (2023).
Liang, J. et al. Swinir: image restoration using swin transformer. In IEEE/CVF International Conference on Computer Vision Workshops (ICCVW) , 1833–1844 (2021).
Simonyan, K. & Zisserman, A. Very deep convolutional networks for large-scale image recognition. In International Conference on Machine Learning (ICLR) (2015).
Kingma, D. & Ba, J. Adam: a method for stochastic optimization. Preprint at https://arxiv.org/abs/1412.6980 (2014).
Wang, Z. et al. Image quality assessment: from error visibility to structural similarity. IEEE Trans. Image Process. 13 , 600–612 (2004).
Article PubMed Google Scholar
Abbe, E. Beiträge zur theorie des mikroskops und der mikroskopischen wahrnehmung. Archiv. f. Mikrosk. Anatomie 9 , 413–418 (1873).
Koho, S. et al. Fourier ring correlation simplifies image restoration in fluorescence microscopy. Nat. Commun. 10 , 3103 (2019).
Article PubMed PubMed Central Google Scholar
Baskin, C. et al. UNIQ: uniform noise injection for non-uniform quantization of neural networks. ACM Transactions on Computer Systems (TOCS) , 37 (1–4), 1–15 (2021).
Arganda, C. et al. Trainable weka segmentation: a machine learning tool for microscopy pixel classification. Bioinformatics 33 , 2424–2426 (2017).
Jacob, B. et al. Quantization and training of neural networks for efficient integer-arithmetic-only inference. In IEEE/CVF Conference on Computer Vision and Pattern Recognition (CVPR) , 2704–2713 (2018).
Ma, C., Tan, W., He, R. & Yan, B. UniFMIR: pre-training a foundation model for universal fluorescence microscopy image restoration (2023.10.03). Zenodo https://doi.org/10.5281/zenodo.8401470 (2023).
Ma, C., Tan, W., He, R., & Yan, B. UniFMIR: pre-training a foundation model for universal fluorescence microscopy image restoration (version 2023.11.13). Zenodo https://doi.org/10.5281/zenodo.10117581 (2023).
Ma, C., Tan, W., He, R. & Yan, B. UniFMIRProjectionOnFlyWing. Zenodo https://doi.org/10.5281/zenodo.10577218 (2024).
Ma, C., Tan, W., He, R. & Yan, B. UniFMIRDenoiseOnPlanaria. Zenodo https://doi.org/10.5281/zenodo.10579778 (2024).
Ma, C., Tan, W., He, R. & Yan, B. UniFMIRDenoiseOnTribolium. Zenodo https://doi.org/10.5281/zenodo.10579822 (2024).
Ma, C., Tan, W., He, R. & Yan, B. UniFMIRVolumetricReconstructionOnVCD. Zenodo https://doi.org/10.5281/zenodo.10595428 (2024).
Ma, C., Tan, W., He, R. & Yan, B. UniFMIRIsotropicReconstructionOnLiver. Zenodo https://doi.org/10.5281/zenodo.10595460 (2024) .
Ma, C., Tan, W., He, R. & Yan, B. UniFMIRSuperResolutionOnMicrotubules. Zenodo https://doi.org/10.5281/zenodo.8420081 (2023).
Ma, C., Tan, W., He, R. & Yan, B. UniFMIRSuperResolutionOnFactin. Zenodo https://doi.org/10.5281/zenodo.8420100 (2023).
Download references
Acknowledgements
We gratefully acknowledge support for this work provided by the National Natural Science Foundation of China (NSFC) (grant nos. U2001209 to B.Y. and 62372117 to W.T.) and the Natural Science Foundation of Shanghai (grant no. 21ZR1406600 to W.T.).
Author information
These authors contributed equally: Chenxi Ma, Weimin Tan.
Authors and Affiliations
School of Computer Science, Shanghai Key Laboratory of Intelligent Information Processing, Fudan University, Shanghai, China
Chenxi Ma, Weimin Tan, Ruian He & Bo Yan
You can also search for this author in PubMed Google Scholar
Contributions
B.Y. and W.T. supervised the research. C.M. and W.T. conceived of the technique. C.M. implemented the algorithm. C.M. and W.T. designed the validation experiments. C.M. trained the network and performed the validation experiments. R.H. implemented the interactive software platform and organized the codes and models. All authors had access to the study and wrote the paper.
Corresponding author
Correspondence to Bo Yan .
Ethics declarations
Competing interests.
The authors declare no competing interests.
Peer review
Peer review information.
Nature Methods thanks Ricardo Henriques and the other, anonymous, reviewer(s) for their contribution to the peer review of this work. Primary Handling Editor: Rita Strack, in collaboration with the Nature Methods team.
Additional information
Publisher’s note Springer Nature remains neutral with regard to jurisdictional claims in published maps and institutional affiliations.
Extended data
Extended data fig. 1 overall architecture of the unifmir..
The proposed UniFMIR approach is composed of three submodules: a multihead module, a Swin transformer-based feature enhancement module, and a multitail module. The numbers of parameters (M) and calculations (GFLOPs) required for the head, feature enhancement and tail modules for different tasks are marked below the structures of the respective modules. The input sizes and output sizes of training batches for different tasks are also marked below the images.
Extended Data Fig. 2 Network architecture of the Swin transformer-based feature enhancement module 46 .
The feature enhancement module consists of convolutional layers and a series of Swin transformer blocks (STB), each of which includes several Swin transformer layers (STL), a convolutional layer and a residual connection. The STL is composed of layer normalization operations, a multihead self-attention (MSA) mechanism and a multilayer perceptron (MLP). In the MSA mechanism, the input features are first divided into multiple small patches with a moving window operation, and then the self-attention in each patch is calculated to output features f out . The MLP is composed of two fully connected layers (FCs) and Gaussian-error linear unit (GELU) activation.
Extended Data Fig. 3 Generalization ability analysis of super-resolution on unseen modality of single-molecule localization microscopy data from the Shareloc platform 52 .
a, SR results obtained by the SOTA model (DeepSTORM 54 ), the pretrained UniFMIR model without fine-tuning, Baseline (same network structure as UniFMIR trained from scratch), and our fine-tuned UniFMIR model. The GT dSTORM images of microtubules stained with Alexa 647 in U2OS cells incubated with nocodazole and the input synthesized LR images are also shown. The PSNR/NRMSE results of the SR outputs obtained on n = 16 synthetic inputs are shown on the right. b, SR results obtained on the real-world wide-field images. The NRMSE values are depicted on the residual images under different SR results and the raw input images. The PSNR/NRMSE results on n = 9 real-world inputs are shown on the right. Box-plot elements are defined as follows: center line (median); box limits (upper and lower quartiles); whiskers (1.5x interquartile range). The line plots show the pixel intensities along the dashed lines in the corresponding images. Scale bar: 6.5 μ m.
Supplementary information
Supplementary information.
Supplementary Notes 1–5, Figs. 1–17 and Tables 1 and 2.
Reporting Summary
Rights and permissions.
Springer Nature or its licensor (e.g. a society or other partner) holds exclusive rights to this article under a publishing agreement with the author(s) or other rightsholder(s); author self-archiving of the accepted manuscript version of this article is solely governed by the terms of such publishing agreement and applicable law.
Reprints and permissions
About this article
Cite this article.
Ma, C., Tan, W., He, R. et al. Pretraining a foundation model for generalizable fluorescence microscopy-based image restoration. Nat Methods (2024). https://doi.org/10.1038/s41592-024-02244-3
Download citation
Received : 27 July 2023
Accepted : 13 March 2024
Published : 12 April 2024
DOI : https://doi.org/10.1038/s41592-024-02244-3
Share this article
Anyone you share the following link with will be able to read this content:
Sorry, a shareable link is not currently available for this article.
Provided by the Springer Nature SharedIt content-sharing initiative
Quick links
- Explore articles by subject
- Guide to authors
- Editorial policies
Sign up for the Nature Briefing newsletter — what matters in science, free to your inbox daily.

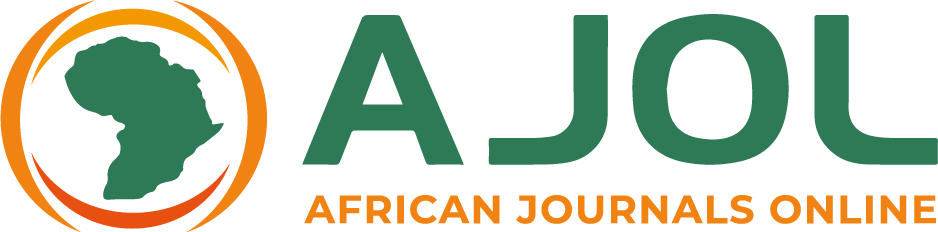
Southern African Linguistics and Applied Language Studies Journal / Southern African Linguistics and Applied Language Studies / Vol. 42 No. 1 (2024) / Articles (function() { function async_load(){ var s = document.createElement('script'); s.type = 'text/javascript'; s.async = true; var theUrl = 'https://www.journalquality.info/journalquality/ratings/2404-www-ajol-info-salas'; s.src = theUrl + ( theUrl.indexOf("?") >= 0 ? "&" : "?") + 'ref=' + encodeURIComponent(window.location.href); var embedder = document.getElementById('jpps-embedder-ajol-salas'); embedder.parentNode.insertBefore(s, embedder); } if (window.attachEvent) window.attachEvent('onload', async_load); else window.addEventListener('load', async_load, false); })();
Article sidebar, article details, main article content, connecting lexical bundles and moves in medical research articles’ methods section.

This study employs a corpus-driven approach to identify the four-word lexical bundles in the Methods section of 1 000 medical research articles (MRAs) from ten leading medical journals representing ten medical sub-fields. The bundles are first structurally and functionally analysed and further connected to rhetorical moves to fill the form-function gap of lexical bundle studies. Results showed that, structurally, the Methods section is dominated by clausal bundles (types and tokens). Functionally, the Methods section is dominated by research-oriented bundles (types and tokens). Our analysis of the bundle-move connection in the Methods section showed that all move-specific bundles (i.e. bundles occurring in only one move) are strongly associated with the rhetorical function of the moves they occurred in, while most cross-move bundles (i.e. bundles occurring in multiple moves) seem to display no clear associations with moves. In addition, the structural and functional analysis of move-specific bundles and cross-move bundles showed apparent structural and functional similarities. Our study may have valuable pedagogical implications for medical academic writing.
AJOL is a Non Profit Organisation that cannot function without donations. AJOL and the millions of African and international researchers who rely on our free services are deeply grateful for your contribution. AJOL is annually audited and was also independently assessed in 2019 by E&Y.
Your donation is guaranteed to directly contribute to Africans sharing their research output with a global readership.
- For annual AJOL Supporter contributions, please view our Supporters page.
Journal Identifiers
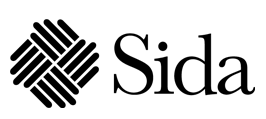
Search Cornell
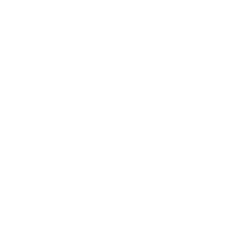
Class Roster
Section menu.
- Toggle Navigation
- Summer 2024
- Spring 2024
- Winter 2024
- Archived Rosters
Last Updated
- Schedule of Classes - April 15, 2024 7:36PM EDT
- Course Catalog - April 15, 2024 7:06PM EDT
NBA 5220 Equity Investment Research and Analysis
Course description.
Course information provided by the Courses of Study 2023-2024 . Courses of Study 2024-2025 is scheduled to publish mid-June.
This course is an introduction to the theory and practice of equity research and is similar to that provided to aspiring analysts, as apprentices, in buy-side investment firms. The course provides a comprehensive framework for analyzing equity securities and developing formal target prices and BUY/SELL/HOLD recommendations. Each student defines an industry to study and prepares an "industry review." Each student analyzes in detail one stock in the industry and prepares a stock report. A live portfolio is invested in late March with student picks. Topics include the research process, analysis strategies, valuation techniques and portfolio construction methods. Templates, examples and detailed feedback on draft reports are provided. Students should be prepared to conduct rigorous, creative research based upon their own work and insights.
When Offered Spring.
Permission Note Enrollment limited to: non-Johnson School students.
Comments Video summarizing course can be viewed here.
View Enrollment Information
Regular Academic Session. Combined with: NBA 4120
Credits and Grading Basis
3 Credits Graded (Letter grades only)
Class Number & Section Details
19905 NBA 5220 LEC 001
Meeting Pattern
- TR 11:40am - 12:55pm
- Aug 27 - Dec 5, 2024
Instructors
To be determined. There are currently no textbooks/materials listed, or no textbooks/materials required, for this section. Additional information may be found on the syllabus provided by your professor.
For the most current information about textbooks, including the timing and options for purchase, see the Cornell Store .
Additional Information
Instruction Mode: In Person
Or send this URL:
Available Syllabi
About the class roster.
The schedule of classes is maintained by the Office of the University Registrar . Current and future academic terms are updated daily . Additional detail on Cornell University's diverse academic programs and resources can be found in the Courses of Study . Visit The Cornell Store for textbook information .
Please contact [email protected] with questions or feedback.
If you have a disability and are having trouble accessing information on this website or need materials in an alternate format, contact [email protected] for assistance.
Cornell University ©2024
Analysis of the impact of terrain factors and data fusion methods on uncertainty in intelligent landslide detection
- Original Paper
- Published: 16 April 2024
Cite this article
- Rui Zhang 1 ,
- Jichao Lv ORCID: orcid.org/0000-0003-2082-945X 1 ,
- Yunjie Yang 1 ,
- Tianyu Wang 1 &
- Guoxiang Liu 1
Current research on deep learning-based intelligent landslide detection modeling has focused primarily on improving and innovating model structures. However, the impact of terrain factors and data fusion methods on the prediction accuracy of models remains underexplored. To clarify the contribution of terrain information to landslide detection modeling, 1022 landslide samples compiled from Planet remote sensing images and DEM data in the Sichuan–Tibet area. We investigate the impact of digital elevation models (DEMs), remote sensing image fusion, and feature fusion techniques on the landslide prediction accuracy of models. First, we analyze the role of DEM data in landslide modeling using models such as Fast_SCNN, the SegFormer, and the Swin Transformer. Next, we use a dual-branch network for feature fusion to assess different data fusion methods. We then conduct both quantitative and qualitative analyses of the modeling uncertainty, including examining the validation set accuracy, test set confusion matrices, prediction probability distributions, segmentation results, and Grad-CAM results. The findings indicate the following: (1) model predictions become more reliable when fusing DEM data with remote sensing images, enhancing the robustness of intelligent landslide detection modeling; (2) the results obtained through dual-branch network data feature fusion lead to slightly greater accuracy than those from data channel fusion; and (3) under consistent data conditions, deep convolutional neural network models and attention mechanism models show comparable capabilities in predicting landslides. These research outcomes provide valuable references and insights for deep learning-based intelligent landslide detection.
This is a preview of subscription content, log in via an institution to check access.
Access this article
Price includes VAT (Russian Federation)
Instant access to the full article PDF.
Rent this article via DeepDyve
Institutional subscriptions
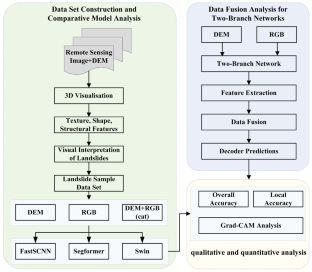
Amankwah SOY, Wang G, Gnyawali K, Hagan DFT, Sarfo I, Zhen D, Nooni IK, Ullah W, Duan Z (2022) Landslide detection from bitemporal satellite imagery using attention-based deep neural networks. Landslides 19:2459–2471. https://doi.org/10.1007/s10346-022-01915-6
Article Google Scholar
Catani F (2021) Landslide detection by deep learning of non-nadiral and crowdsourced optical images. Landslides 18:1025–1044. https://doi.org/10.1007/s10346-020-01513-4
Chen L-C, Zhu Y, Papandreou G, Schroff F, Adam H (2018) Encoder-decoder with atrous separable convolution for semantic image segmentation
Dosovitskiy A, Beyer L, Kolesnikov A, Weissenborn D, Zhai X, Unterthiner T, Dehghani M, Minderer M, Heigold G, Gelly S, Uszkoreit J, Houlsby N (2021) An image is worth 16x16 words: transformers for image recognition at scale
Dou J, Yunus AP, Bui DT, Merghadi A, Sahana M, Zhu Z, Chen C-W, Han Z, Pham BT (2020) Improved landslide assessment using support vector machine with bagging, boosting, and stacking ensemble machine learning framework in a mountainous watershed, Japan. Landslides 17:641–658. https://doi.org/10.1007/s10346-019-01286-5
Đurić D, Mladenović A, Pešić-Georgiadis M, Marjanović M, Abolmasov B (2017) Using multiresolution and multitemporal satellite data for post-disaster landslide inventory in the Republic of Serbia. Landslides 14:1467–1482. https://doi.org/10.1007/s10346-017-0847-2
Fan X, Scaringi G, Xu Q, Zhan W, Dai L, Li Y, Pei X, Yang Q, Huang R (2018) Coseismic landslides triggered by the 8th August 2017 Ms 7.0 Jiuzhaigou earthquake (Sichuan, China): factors controlling their spatial distribution and implications for the seismogenic blind fault identification. Landslides 15:967–983. https://doi.org/10.1007/s10346-018-0960-x
Fang Z, Wang Y, Peng L, Hong H (2020) Integration of convolutional neural network and conventional machine learning classifiers for landslide susceptibility mapping. Comput Geosci 139:104470. https://doi.org/10.1016/j.cageo.2020.104470
Ghorbanzadeh O, Xu Y, Ghamisi P, Kopp M, Kreil D (2022) Landslide4Sense: reference benchmark data and deep learning models for landslide detection. IEEE Trans Geosci Remote Sensing 60:1–17. https://doi.org/10.1109/TGRS.2022.3215209
Haque U, Da Silva PF, Devoli G, Pilz J, Zhao B, Khaloua A, Wilopo W, Andersen P, Lu P, Lee J, Yamamoto T, Keellings D, Wu J-H, Glass GE (2019) The human cost of global warming: deadly landslides and their triggers (1995–2014). Sci Total Environ 682:673–684. https://doi.org/10.1016/j.scitotenv.2019.03.415
Article CAS Google Scholar
He K, Zhang X, Ren S, Sun J (2015) Deep residual learning for image recognition
Ji S, Yu D, Shen C, Li W, Xu Q (2020) Landslide detection from an open satellite imagery and digital elevation model dataset using attention boosted convolutional neural networks. Landslides 17:1337–1352. https://doi.org/10.1007/s10346-020-01353-2
Lei T, Zhang Y, Lv Z, Li S, Liu S, Nandi AK (2019) Landslide inventory mapping from bitemporal images using deep convolutional neural networks. IEEE Geosci Remote Sensing Lett 16:982–986. https://doi.org/10.1109/LGRS.2018.2889307
Li D, Tang X, Tu Z, Fang C, Ju Y (2023a) Automatic detection of forested landslides: a case study in Jiuzhaigou County. China Remote Sensing 15:3850. https://doi.org/10.3390/rs15153850
Li W, Fu Y, Fan S, Xin M, Bai H (2023b) DCI-PGCN: dual-channel interaction portable graph convolutional network for landslide detection. IEEE Trans Geosci Remote Sensing 61:1–16. https://doi.org/10.1109/TGRS.2023.3273623
Liu X, Peng Y, Lu Z, Li W, Yu J, Ge D, Xiang W (2023) Feature-fusion segmentation network for landslide detection using high-resolution remote sensing images and digital elevation model data. IEEE Trans Geosci Remote Sensing 61:1–14. https://doi.org/10.1109/TGRS.2022.3233637
Liu Z, Lin Y, Cao Y, Hu H, Wei Y, Zhang Z, Lin S, Guo B (2021) Swin transformer: hierarchical vision transformer using shifted windows
Lu P, Qin Y, Li Z, Mondini AC, Casagli N (2019) Landslide mapping from multi-sensor data through improved change detection-based Markov random field. Remote Sens Environ 231:111235. https://doi.org/10.1016/j.rse.2019.111235
Lu W, Hu Y, Zhang Z, Cao W (2023) A dual-encoder U-Net for landslide detection using Sentinel-2 and DEM data. Landslides 20(9):1975–1987
Poudel RPK, Liwicki S, Cipolla R (2019) Fast-SCNN: fast semantic segmentation network
Sangelantoni L, Gioia E, Marincioni F (2018) Impact of climate change on landslides frequency: the Esino river basin case study (Central Italy). Nat Hazards 93:849–884. https://doi.org/10.1007/s11069-018-3328-6
Selvaraju RR, Cogswell M, Das A, Vedantam R, Parikh D, Batra D (2017) Grad-CAM: visual explanations from deep networks via gradient-based localization, in: 2017 IEEE International Conference on Computer Vision (ICCV). Presented at the 2017 IEEE International Conference on Computer Vision (ICCV), IEEE, Venice, pp. 618–626. https://doi.org/10.1109/ICCV.2017.74
Soares LP, Dias HC, Grohmann CH (2020) Landslide segmentation with U-Net: evaluating different sampling methods and patch sizes.
Su Z, Chow JK, Tan PS, Wu J, Ho YK, Wang Y-H (2021) Deep convolutional neural network–based pixel-wise landslide inventory mapping. Landslides 18:1421–1443. https://doi.org/10.1007/s10346-020-01557-6
Xie E, Wang W, Yu Z, Anandkumar A, Alvarez JM, Luo P (2021) SegFormer: simple and efficient design for semantic segmentation with transformers
Xu Q, Ouyang C, Jiang T, Yuan X, Fan X, Cheng D (2022) MFFENet and ADANet: a robust deep transfer learning method and its application in high precision and fast cross-scene recognition of earthquake-induced landslides. Landslides 19:1617–1647. https://doi.org/10.1007/s10346-022-01847-1
Yang Z, Xu C, Li L (2022) Landslide detection based on ResU-Net with transformer and CBAM embedded: two examples with geologically different environments. Remote Sensing 14:2885. https://doi.org/10.3390/rs14122885
Yu B, Chen F, Xu C, Wang L, Wang N (2021) Matrix SegNet: a practical deep learning framework for landslide mapping from images of different areas with different spatial resolutions. Remote Sensing 13:3158. https://doi.org/10.3390/rs13163158
Zeng T, Glade T, Xie Y, Yin K, Peduto D (2023a) Deep learning powered long-term warning systems for reservoir landslides. International Journal of Disaster Risk Reduction 94:103820. https://doi.org/10.1016/j.ijdrr.2023.103820
Zeng T, Gong Q, Wu L, Zhu Y, Yin K, Peduto D (2023b) Double-index rainfall warning and probabilistic physically based model for fast-moving landslide hazard analysis in subtropical-typhoon area. Landslides. https://doi.org/10.1007/s10346-023-02187-4
Zeng T, Wu L, Peduto D, Glade T, Hayakawa YS, Yin K (2023c) Ensemble learning framework for landslide susceptibility mapping: different basic classifier and ensemble strategy. Geosci Front 14:101645. https://doi.org/10.1016/j.gsf.2023.101645
Zeng T, Jin B, Glade T, Xie Y, Li Y, Zhu Y, Yin K (2024a) Assessing the imperative of conditioning factor grading in machine learning-based landslide susceptibility modeling: a critical inquiry. CATENA 236:107732. https://doi.org/10.1016/j.catena.2023.107732
Zeng T, Wu L, Hayakawa YS, Yin K, Gui L, Jin B, Guo Z, Peduto D (2024b) Advanced integration of ensemble learning and MT-InSAR for enhanced slow-moving landslide susceptibility zoning. Eng Geol 331:107436. https://doi.org/10.1016/j.enggeo.2024.107436
Zhang X, Yu W, Pun M-O, Shi W (2023) Cross-domain landslide mapping from large-scale remote sensing images using prototype-guided domain-aware progressive representation learning. ISPRS J Photogramm Remote Sens 197:1–17. https://doi.org/10.1016/j.isprsjprs.2023.01.018
Zhou B, Khosla A, Lapedriza A, Oliva A, Torralba A (2015) Learning deep features for discriminative localization
Zhou Y, Xu H, Zhang W, Gao B, Heng PA (2021) C 3 -SemiSeg: contrastive semi-supervised segmentation via cross-set learning and dynamic class-balancing, in: 2021 IEEE/CVF International Conference on Computer Vision (ICCV). Presented at the 2021 IEEE/CVF International Conference on Computer Vision (ICCV), IEEE, Montreal, QC, Canada, pp. 7016–7025. https://doi.org/10.1109/ICCV48922.2021.00695
Download references
Acknowledgements
We wish to express our gratitude to Planet for providing high-resolution remote-sensing imagery.
This research was jointly funded by the National Key Research and Development Program of China (Grant No. 2023YFB2604001) and the National Natural Science Foundation of China (Grant Nos. 42371460, U22A20565, and 42171355).
Author information
Authors and affiliations.
Faculty of Geosciences and Engineering, Southwest Jiaotong University, Chengdu, 611756, Sichuan, China
Rui Zhang, Jichao Lv, Yunjie Yang, Tianyu Wang & Guoxiang Liu
You can also search for this author in PubMed Google Scholar
Corresponding author
Correspondence to Jichao Lv .
Ethics declarations
Ethics approval.
Not applicable to studies not involving humans or animals.
Informed consent
Not applicable to studies not involving humans.
Competing interests
The authors declare no competing interests.
Rights and permissions
Springer Nature or its licensor (e.g. a society or other partner) holds exclusive rights to this article under a publishing agreement with the author(s) or other rightsholder(s); author self-archiving of the accepted manuscript version of this article is solely governed by the terms of such publishing agreement and applicable law.
Reprints and permissions
About this article
Zhang, R., Lv, J., Yang, Y. et al. Analysis of the impact of terrain factors and data fusion methods on uncertainty in intelligent landslide detection. Landslides (2024). https://doi.org/10.1007/s10346-024-02260-6
Download citation
Received : 11 January 2024
Accepted : 05 April 2024
Published : 16 April 2024
DOI : https://doi.org/10.1007/s10346-024-02260-6
Share this article
Anyone you share the following link with will be able to read this content:
Sorry, a shareable link is not currently available for this article.
Provided by the Springer Nature SharedIt content-sharing initiative
- Landslide detection
- Terrain factors
- Data fusion
- Deep learning
- Find a journal
- Publish with us
- Track your research
- Open access
- Published: 14 November 2023
Employment of patients with rheumatoid arthritis - a systematic review and meta-analysis
- Lilli Kirkeskov 1 , 2 &
- Katerina Bray 1 , 3
BMC Rheumatology volume 7 , Article number: 41 ( 2023 ) Cite this article
1682 Accesses
1 Citations
7 Altmetric
Metrics details
Patients with rheumatoid arthritis (RA) have difficulties maintaining employment due to the impact of the disease on their work ability. This review aims to investigate the employment rates at different stages of disease and to identify predictors of employment among individuals with RA.
The study was carried out according to the Preferred Reporting Items for Systematic Reviews and Meta-Analysis (PRISMA) guidelines focusing on studies reporting employment rate in adults with diagnosed RA. The literature review included cross-sectional and cohort studies published in the English language between January 1966 and January 2023 in the PubMed, Embase and Cochrane Library databases. Data encompassing employment rates, study demographics (age, gender, educational level), disease-related parameters (disease activity, disease duration, treatment), occupational factors, and comorbidities were extracted. Quality assessment was performed employing Newcastle–Ottawa Scale. Meta-analysis was conducted to ascertain predictors for employment with odds ratios and confidence intervals, and test for heterogeneity, using chi-square and I 2 -statistics were calculated. This review was registered with PROSPERO (CRD42020189057).
Ninety-one studies, comprising of a total of 101,831 participants, were included in the analyses. The mean age of participants was 51 years and 75.9% were women. Disease duration varied between less than one year to more than 18 years on average. Employment rates were 78.8% (weighted mean, range 45.4–100) at disease onset; 47.0% (range 18.5–100) at study entry, and 40.0% (range 4–88.2) at follow-up. Employment rates showed limited variations across continents and over time. Predictors for sustained employment included younger age, male gender, higher education, low disease activity, shorter disease duration, absence of medical treatment, and the absence of comorbidities.
Notably, only some of the studies in this review met the requirements for high quality studies. Both older and newer studies had methodological deficiencies in the study design, analysis, and results reporting.
Conclusions
The findings in this review highlight the prevalence of low employment rates among patients with RA, which increases with prolonged disease duration and higher disease activity. A comprehensive approach combining clinical and social interventions is imperative, particularly in early stages of the disease, to facilitate sustained employment among this patient cohort.
Peer Review reports
Rheumatoid arthritis (RA) is a chronic, inflammatory joint disease that can lead to joint destruction. RA particularly attacks peripheral joints and joint tissue, gradually resulting in bone erosion, destruction of cartilage, and, ultimately, loss of joint integrity. The prevalence of RA varies globally, ranging from 0.1- 2.0% of the population worldwide [ 1 , 2 ]. RA significantly reduces functional capacity, quality of life, and results in an increase in sick leave, unemployment, and early retirement [ 3 , 4 , 5 ]. The loss of productivity due to RA is substantial [ 2 , 5 , 6 , 7 ]. A 2015 American study estimated the cost of over $250 million annually from RA-related absenteeism in United States alone [ 8 ].
Research has highlighted the importance of maintaining a connection to the labour market [ 3 , 9 ], Even a short cessation from work entails a pronounced risk of enduring work exclusion [ 10 ]. In Denmark merely 55% on sick leave for 13 weeks succeeded in re-joining the workforce within one year. Among those on sick leave for 26 weeks, only 40% returned to work within the same timeframe [ 11 ]. Sustained employment is associated with an improved health-related quality of life [ 12 , 13 ]. Early and aggressive treatment of RA is crucial for importance in achieving remission and a favourable prognosis reducing the impact of the disease [ 2 , 14 , 15 , 16 ]. Therefore, initiating treatment in a timely manner and supporting patients with RA in maintaining their jobs with inclusive and flexible workplaces if needed is critical [ 3 , 17 ].
International studies have indicated, that many patients with RA are not employed [ 18 ]. In 2020, the average employment rate across Organization for Economic Co-operation and Development (OECD) countries was 69% in the general population (15 to 64 years of age), exhibiting variations among countries, ranging from 46–47% in South Africa and India to 85% in Iceland [ 19 ]. Employment rates were lower for individuals with educational levels below upper secondary level compared to those with upper secondary level or higher education [ 19 ]. For individuals suffering with chronic diseases, the employment rates tend to be lower. Prognostic determinants for employment in the context of other chronic diseases encompasses the disease’s severity, employment status prior to getting a chronic disease, and baseline educational level [ 20 , 21 , 22 ]. These somatic and social factors may similarly influence employment status of patients with RA. Several factors, including the type of job (especially physically demanding occupations), support from employers and co-workers, social safety net, and disease factors such as duration and severity, could have an impact on whether patients with RA are employed [ 17 , 23 , 24 ]. Over the years, politicians and social welfare systems have tried to improve the employment rates for patients with chronic diseases. In some countries, rehabilitation clinics have been instrumental in supporting patients to remain in paid work. Healthcare professionals who care for patients with RA occupy a pivotal role in preventing work-related disability and support the patients to remain in work. Consequently, knowledge of the factors that contribute to retention of patients with RA at work is imperative [ 17 , 25 ].
The aim of this study is therefore to conduct a systematic review, with a primary focus on examining employment rates among patients with RA at the onset of the disease, at study entry, and throughout follow-up. Additionally, this study intends to identify predictors of employment. The predefined predictors, informed by the author’s comprehensive understanding of the field and specific to RA, encompass socioeconomic factors such as age, gender, level of education, employment status prior to the disease, disease stage and duration, treatment modalities, and comorbidities, including depression, which are relevant both to RA and other chronic conditions [ 26 ].
This systematic review was carried out according to Preferred Reporting Items for Systematic Reviews and Meta-Analysis (PRISMA) for studies that included employment rate in patients with rheumatoid arthritis [ 27 ]. PROSPERO registration number: CRD42020189057.
Selection criteria and search strategies
A comprehensive literature search was conducted, covering the period from January 1966 to January 2023 across the PubMed, Embase, and Cochrane Library databases using the following search terms: (Rheumatoid arthritis OR RA) AND (employment OR return to work). Only studies featuring a minimum cohort size of thirty patients and articles in the English language were deemed eligible for inclusion.
The initial screening of articles was based on the titles and abstracts. Studies comprising a working-age population, with current or former employment status, and with no limitations to gender, demographics, or ethnicity were included in this review. Articles addressing topics of employment, work ability or disability, return to work or disability pension were encompassed within the scope of this review. Full-time and part-time employment, but not ‘working as housewives’ was included in this review’s definition of employment. Studies involving other inflammatory diseases than RA were excluded. Reference lists in the selected articles were reviewed, and more articles were included if relevant. A review of the reference lists in the initially selected articles was conducted, with additional articles incorporated if they proved relevant to the research objectives. The eligible study designs encompassed cohort studies, case–control studies, and cross-sectional studies. All other study designs, including reviews, case series/case reports, in vitro studies, qualitative studies, and studies based on health economics were systematically excluded from the review.
Data extraction, quality assessment and risk-of-bias
The data extraction from the selected articles included author names, year of publication, study design, date for data collection, employment rate, study population, age, gender, educational level, ethnicity, disease duration, and pharmacological treatment. To ensure comprehensive evaluation of study quality and potential bias, quality assessment was independently assessed by two reviewers (LK and KB) using the Newcastle–Ottawa Scale (NOS) for cross-sectional and cohort studies [ 28 ]. Any disparities in the assessment were resolved by discussion until consensus was reached. For cross-sectional studies the quality assessment included: 1) Selection (maximum 5 points): representativeness of the sample, sample size, non-respondents, ascertainment of the risk factor; 2) Comparability (maximum 2 points); study controls for the most important, and any additional factor; 3) Outcome (maximum 3 points): assessment of outcome, and statistical testing. For cohort studies the assessment included: 1) Selection (maximum 4 points): representativeness of the exposed cohort, selection of the non-exposed cohort, ascertainment of exposure, demonstration that the outcome of interest was not present at start of study; 2) Comparability (maximum 2 points): comparability of cohorts on the basis of the design or analysis; 3) Outcome (maximum 3 points): assessment of outcome, was the follow-up long enough for outcomes to occur, and adequacy of follow up of cohorts. The rating scale was based on 9–10 items dividing the studies into high (7–9/10), moderate (4–6) or low (0–3) quality. A low NOS score (range 0–3) indicated a high risk of bias, and a high NOS score (range 7–9/10) indicated a lower risk of bias.
Analytical approach
For outcomes reported in numerical values or percentages, the odds ratio along with their 95% confidence intervals (CI) were calculated, whenever feasible. Weighted means were calculated, and comparisons between these were conducted using t-test for unpaired data. Furthermore, meta-analysis concerning the pre-determined and potentially pivotal predictors for employment status, both at disease onset, study entry, and follow-up was undertaken. The predictors included age, gender, ethnicity, level of education, duration of disease, treatment, and the presence of comorbities, contingent upon the availability of the adequate data. Additionally, attempts have been made to find information regarding on job categorizations, disease activity (quantified through DAS28; disease activity score for number of swollen joints), and quality of life (SF-36 scores ranging from 0 (worst) to 100 (best)). Age was defined as (< = 50/ > 50 years), gender (male/female), educational level college education or more/no college education), race (Caucasian/not Caucasian), job type (non-manual/manual), comorbidities (not present/present), MTX ever (no/yes), biological treatment ever (no/yes), prednisolone ever (no/yes), disease duration, HAQ score (from 0–3)), joint pain (VAS from 1–10), and DAS28 score. Age, disease duration, HAQ score, VAS score, SF36 and DAS28 were in the studies reported by mean values and standard deviations (SD). Challenges were encountered during attempts to find data which could be used for analysing predictors of employment status before disease onset, and at follow-up, as well as factors related to treatments beyond MTX, prednisolone, and biological as predictors for being employed after disease onset. Test for heterogeneity was done using Chi-squared statistics and I 2 , where I 2 below 40% might not be important; 30–60% may represent moderate heterogeneity; 50–90% substantial heterogeneity; and 75–100% considerable heterogeneity. Meta-analysis for predictors for employment and odds ratio; confidence intervals; and test for heterogeneity were calculated using the software Review Manager (RevMan, version 5.3. Copenhagen: The Nordic Cochrane Centre, The Cochrane Collaboration, 2014).
General description of included studies
The search yielded a total of 2277 references addressing RA its association with employment. Following the initial title screen, 199 studies were considered relevant for further evaluation. Of those, 91 studies ultimately met the inclusion criteria. Figure 1 shows the results of the systematic search strategy.
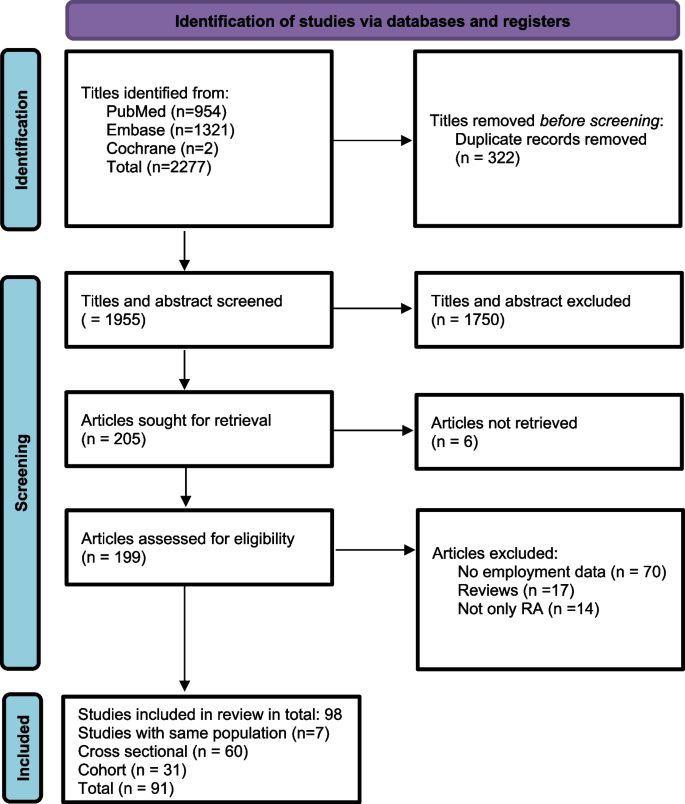
Flow chart illustrating the systematic search for studies examining employment outcome in patients with rheumatoid arthritis
Table 1 summarizes the general characteristics of the included studies. The publication year of the included studies ranged from 1971 to 2022. Among the studies, 60 (66%) adopted a cross-sectional research design [ 13 , 18 , 29 , 30 , 31 , 32 , 33 , 34 , 35 , 36 , 37 , 38 , 39 , 40 , 41 , 42 , 43 , 44 , 45 , 46 , 47 , 48 , 49 , 50 , 51 , 52 , 53 , 54 , 55 , 56 , 57 , 58 , 59 , 60 , 61 , 62 , 63 , 64 , 65 , 66 , 67 , 68 , 69 , 70 , 71 , 72 , 73 , 74 , 75 , 76 , 77 , 78 , 79 , 80 , 81 , 82 , 83 , 84 , 85 , 86 , 87 , 88 , 129 ] with a total of 41,857 participants analysing data at a specific point in time. Concurrently, 31 studies (34%) adopted a cohort design [ 89 , 90 , 91 , 92 , 93 , 94 , 95 , 96 , 97 , 98 , 99 , 100 , 101 , 102 , 103 , 104 , 105 , 106 , 107 , 108 , 109 , 110 , 111 , 112 , 113 , 114 , 115 , 116 , 117 , 118 , 119 , 120 , 121 , 122 , 130 ] with a total of 59,974 participants. Most of these studies exhibited a small to moderate sample size, with a median of 652 participants. Additionally, single centre studies and studies from high-income countries were predominant. Study details are shown in Table 1 .
General description of study participants
On average, patients with RA were 51 years old, with an age range spanning from 42 to 64 years. Furthermore, the female population accounted for 75.9% of the patient cohort, with a range from 41 to 92%. The duration of the disease at study entry exhibited significant variability, ranging from less than one year up to more than 18 years on average.
- Employment rate
At disease onset, the employment rate was 78.8% (weighted mean, range 45.4–100), at study entry 47.0% (range 18.5–100), and during the follow-up period 40.0% (range 4–88.2), as shown in Table 2 . Notably, a comparative analysis of the employment rates between Europe and North America indicated no substantial difference ( p = 0.93). However, the comparison between Europe, North America and ‘other continents’ did yield significant differences (or nearly differences) with p -values of 0.003 and 0.08, respectively.
The employment rate exhibited no change, when comparing studies from the 1980s through to 2022. Specifically, the weighted mean for the years 1981–2000 was 49.2%, aligning closely with the corresponding figures for the years 2001–2010 (49.2%) and 2011–2022 43.6%. These findings were statistically non-significant, with p -values of 0.80 for comparison between year 1981–2000 and 2001–2010; 0.66 for 2001–2010 and 2011–2022, and 0.94 for 1981–2000 and 2011–2022, shown in Figure S 1 , see Additional file.
Among the studies included in the analysis, nineteen studies included data of employment at follow-up, with durations ranging from 1 to 20 years, Table 2 . For instance, Jäntti, 1999 [ 97 ] reported an employment rate 69% one year after disease onset, which gradually declined to 50% after 15 years and further to 20% after 20 years. Similarly, Mäkisara, 1982 [ 63 ] demonstrated that 60% of the patients were employed 5 years after disease onset, 50% after 10 years, and 33% after 15 years. Nikiphorou, 2012 [ 101 ] reported an employment rate of 67% at study entry, which decreased to 43% after 10 years.
In addition, seven studies included data of employment rate among patients comparing different medical treatments [ 18 , 44 , 56 , 91 , 105 , 110 , 119 ]. These studies indicated that, on average, 55.0% (weighted mean) of the patients were employed after receiving treatment with MTX, while 42.8% after undergoing treatment with a combination of MTX + Adalimumab (all patients were employed at disease onset in these specific studies).
Predictors for employment
Information of normative comparison data to use for meta-analysis of predictors for employment at study entry was available for age, gender, educational level, race, job type, comorbidities, MTX at any time, biological treatment at any time, prednisolone at any time, disease duration, HAQ score, joint pain (VAS-score), and disease activity (DAS28 score). Predictors for employment at study entry was being younger /age below 50 years, being a male, higher educational level (college or more), non-manual work, having no comorbidities, no medical treatment, short disease duration, and low HAQ score, VAS-score, or DAS28 score. Heterogeneity was small for age, gender, medical treatment, and moderate for educational level, and job type as indicted by the I 2 values, Table 3 , and shown in detail in Figures S 2 , S 3 , S 4 , S 5 , S 6 , S 7 , S 8 , S 9 , S 10 , S 11 , S 12 , S 13 , S 14 , S 15 and S 16 , see Additional file.
Assessment of quality of included studies
All studies were subject to rigorous quality assessment. These assessments resulted in categorisation of either medium quality ( n = 64; 70%) or high-quality studies ( n = 27; 30%), with no studies falling into the low-quality category. The quality assessment is shown in Tables 4 and 5 .
Notably, many studies were characterised by several common attributes, including cross-sectional study design, single-centre-settings, relatively small sample sizes, and the reliance on self-reported patient data. When including only the high-quality studies in the analyses, the employment rates at study entry changed from 47% (weighted mean, all studies) to 50% (weighted mean, high quality studies).
Key findings
This systematic review has identified a decline in the employment rate among patients with RA, with a notable decrease from disease onset during the study entry to follow-up, where only half of the patients were employed. These findings corroborate earlier research that indicated a substantial decline in employment rates among patients with RA over time. Notably, previous studies have reported that approximately one third of patients with RA stopped working within 2 to 3 years after disease onset, and more than half was unable to work after 10 to 15 years [ 23 , 63 , 93 , 97 , 101 ]. Only few studies have included data from the general population, comparing the employment rates with the rates for patients with RA [ 89 , 90 ]. Comparisons with the general population further underscored the challenges faced by RA patients, as their employment rates were consistently lower.
Despite changes in medical treatment, social security systems, and societal norms over the past decades, there was no significant improvement in the employment for patients with RA. This pattern aligns with data from the Global Burden of Disease studies, highlighting the persistent need for novel approaches and dedicated efforts to support patients with RA in sustaining employment [ 2 , 123 ]. Recent recommendations from EULAR (European Alliance of Associations for Rheumatology) and ACR (American College of Rheumatology) have emphasized the importance of enabling individuals with rheumatic and musculoskeletal diseases to engage in healthy and sustainable work [ 17 , 124 , 125 ].
While different countries possess different social laws and health care systems for supporting patients with chronic diseases, the variations in the weighted mean of employment rates across countries were relatively minor.
In the meta-analysis, one of the strongest predictors for maintaining employment was younger age at disease onset [ 43 , 51 , 101 , 116 ]. Verstappen, 2004 found that older patients with RA had an increased risk of becoming work disabled, potentially caused by the cumulative effects of long-standing RA, joint damage, and diminished coping mechanisms, compared to younger patients [ 23 ].
More women than men develop RA, however this study showed that a higher proportion of men managed to remain employed compared to women [ 18 , 36 , 42 , 43 , 46 , 62 , 71 , 89 , 101 , 116 ]. Previous studies have shown inconsistent results in this regard. Eberhart, 2007 found that a significantly higher number of men with RA worked even though there was no difference in any disease state between the sexes [ 93 ]. De Roos,1999 showed that work-disabled women were less likely to be well-educated and more likely to be in a nonprofessional occupation than working women. Interestingly, there was no association of these variables among men. Type of work and disease activity may influence work capacity more in women than in men [ 46 ]. Sokka, 2010 demonstrated a lower DAS28 and HAQ-score in men compared to women among the still working patients with RA, which indicated that women continued working at higher disability and disease activity levels compared with men [ 18 ].
Disease duration also played a significant role as a predictor of employment outcomes [ 33 , 36 , 45 , 71 , 77 , 86 , 102 , 111 ]. Longer disease duration correlate with decreased employment likelihood, which could be attributed to older age and increased joint damage and disability in patients with longer-standing RA.
Higher educational levels were associated with a greater possibility of employment [ 30 , 43 , 45 , 46 , 51 , 62 , 86 ]. This is probably due to enhanced job opportunities, flexibility, lower physical workload, better insurance coverage, and improved health care for well-educated individuals. This is further supported by the fact that having a manual work was a predictor for not being employed [ 30 , 39 , 43 , 44 , 45 ].
Furthermore, health-related quality of life, as measured by SF 36, lower disease activity (DAS28 scores), reduced joint pain (VAS-score), and lower disability (HAQ score) were additionally predictors for being employed [ 33 , 35 , 36 , 45 , 71 , 86 ]. This support the statement that the fewer symptoms from RA, the greater the possibility of being able to work.
The results showed that the presence of comorbidity was a predictor for not being employed, aligning with findings from previous studies that chronic diseases such as cardiovascular disease, lung disease, diabetes, cancer, and depression reduced the chances of being employed [ 126 ]. Moreover, the risk of exiting paid work increased with multimorbidity [ 127 ].
While limited data were available for assessing the impact of treatment on employment, indications suggested that patients with RA were receiving medical treatments, such as MTX or biological medicine, were more likely to be unemployed. One possible explanation for this phenomenon could be that patients with RA, who were receiving medical treatment, had a more severe and a longer duration of RA compared to those, who had never been on medical treatment. However, the scarcity of relevant studies necessitates caution when drawing definitive conclusions in this regard.
Therefore, the predictors for employment found in this review were being younger, being a male, having higher education, low disease activity, low disease duration, and being without comorbidities. This is supported by previous studies [ 93 , 116 ]
In summary, this review underscores the importance of managing disease activity, offering early support to patients upon diagnosis, and reducing physically demanding work to maintain employment among patients with RA. Achieving success in this endeavour requires close cooperation among healthcare professionals, rehabilitation institutions, companies, and employers. Furthermore, it is important that these efforts are underpinned by robust social policies that ensure favourable working conditions and provide financial support for individuals with physical disabilities, enabling them to remain active in the labour market.
Strengths and limitations
The strength of this review and meta-analysis lies in the inclusion of a large number of articles originating from various countries. Furthermore, the data showed a consistent employment rate in high quality studies compared to all studies. However, there are some limitations to this review. No librarian was used to define search terms and only three databases were searched. Furthermore, the initial search, selection of articles, data extraction, and analysis was undertaken only by one author, potentially leading to the omission of relevant literature and data. The review also extended back to 1966, with some articles from the 1970s and 1980s included. Given the significant changes in medical treatment, social security systems, and society over the past decades, the generalizability of the findings may be limited.
Moreover, the majority of studies did not include a control group from the general population, which limited the ability to compare employment rates with the general population in the respective countries. Many studies were cross-sectional in design, which limits the evidence of causality between employment rate and having RA. However, the employment rate was approximately the same in high quality studies compared to all studies, which supports an association. A substantial number of studies relied on self-reported employment rates, introducing the potential for recall bias. Additionally, many studies did not account for all relevant risk factors for unemployment failing to control for all relevant confounders.
EULAR have made recommendation for point to consider when designing, analysing, and reporting of studies with work participation as an outcome domain in patients with inflammatory arthritis. These recommendations include study design, study duration, and the choice of work participation outcome domains (e.g., job type, social security system) and measurement instruments, the power to detect meaningful effects, interdependence among different work participation outcome domains (e.g., between absenteeism and presentism), the populations included in the analysis of each work participation outcome domain and relevant characteristics should be described. In longitudinal studies work-status should be regularly assessed and changes reported, and both aggregated results and proportions of predefined meaningful categories should be considered [ 128 ]. Only some of the studies in this review met the requirements for high quality studies. In both older and newer studies methodological deficiencies persisted in study design, analysis, and reporting of results, as recommended by EULAR.
Perspectives for future studies
Future research in this area should focus on developing and evaluating new strategies to address the ongoing challenges faced by patients with RA in maintaining employment. Despite many initiatives over the years, there has been no success in increasing employment rates for patients with RA in many countries. Therefore, there is a pressing need for controlled studies that investigated the effectiveness of interventions such as education, social support, and workplace adaptations in improving employment outcomes for these individuals.
This systematic review underscores the low employment rate among patients with RA. Key predictors of sustained employment include being younger, having higher educational level, short disease duration, and lower disease activity, along with fewer comorbidities. Importantly, the review reveals that the employment rate has not changed significantly across different time periods. To support patients with RA in maintaining their employment, a comprehensive approach that combines early clinical treatment with social support is crucial. This approach can play a pivotal role in helping patients with RA stay connected to the labour market.
Availability of data and materials
The datasets used and/or analyzed during the current study are available in the supplementary file.
Abbreviations
- Rheumatoid arthritis
Methotrexate
Newcastle Ottawa Quality Assessment Scale
Standard deviation
Not analyzed
Not relevant
Disease activity
Health Assessment Questionnaire
Visual analog scale for pain
European Alliance of Associations for Rheumatology
American College of Rheumatology
Almutairi K, Nossent J, Preen D, Keen H, Inderjeeth C. The global prevalence of rheumatoid arthritis: a meta-analysis based on a systematic review. Rheumatol Int. 2021;41:863–77.
Article PubMed Google Scholar
Safiri S, Kolahi AA, Hoy D, Smith E, Bettampadi D, Mansournia MA, et al. Global, regional and national burden of rheumatoid arthritis 1990–2017: a systematic analysis of the Global Burden of Disease study 2017. Ann Rheum Dis. 2019;78:1463–71.
Verstappen SMM. Rheumatoid arthritis and work: The impact of rheumatoid arthritis on absenteeism and presenteeism. Best Pract Res Clin Rheumatol. 2015;29:495–511.
Madsen CMT, Bisgaard SK, Primdahl J, Christensen JR, von Bülow C. A systematic review of job loss prevention interventions for persons with inflammatory arthritis. J Occup Rehabil. 2021;4:866–85.
Kessler RC, Maclean JR, Petukhova M, Sarawate CA, Short L, Li TT, et al. The effects of rheumatoid arthritis on labor force participation, work performance, and healthcare costs in two workplace samples. J Occup Environ Med. 2008;50:88–98.
Filipovic I, Walker D, Forster F, Curry AS. Quantifying the economic burden of productivity loss in rheumatoid arthritis. Rheumatology. 2011;50:1083–90.
Burton W, Morrison A, Maclean R, Ruderman E. Systematic review of studies of productivity loss due to rheumatoid arthritis. Occup Med. 2006;56:18–27.
Article Google Scholar
Gunnarsson C, Chen J, Rizzo JA, Ladapo JA, Naim A, Lofland JH. The employee absenteeism costs of reumatoid arthritis. Evidence from US National Survey Data. J Occup Environ Med. 2015;57:635–42.
van der Noordt M, Ijzelenberg H, Droomers M, Proper KI. Health effects of employment: a systematic review of prospective studies. Occup Environ Health. 2014;71:730–6.
Google Scholar
Virtanen M, Kivimäki M, Vahtera J, Elovainio M, Sund R, Virtanen P, et al. Sickness absence as a risk factor for job termination, unemployment, and disability pension among temporary and permanent employees. Occup Environ Med. 2006;63:212–7.
Article CAS PubMed PubMed Central Google Scholar
Vilhelmsen J. Længerevarende sygefravær øger risikoen for udstødning [long-term sick-leave increase the risk of job termination]. 2007. https://www.ae.dk/analyse/2007-10-laengerevarende-sygefravaer-oeger-risikoen-for-udstoedning .
Grønning K, Rødevand E, Steinsbekk A. Paid work is associated with improved health-related quality of life in patients with rheumatoid arthritis. Clin Rheumatol. 2010;29:1317–22.
Article PubMed PubMed Central Google Scholar
Chorus AMJ, Miedema HS, Boonen A, van der Linden S. Quality of life and work in patients with rheumatoid arthritis and ankylosing spondylitis of working age. Ann Rheum Dis. 2003;62:7.
Ma MHY, Kingsley GH, Scott DL. A systematic comparison of combination DMARD therapy and tumour necrosis inhibitor therapy with methotrexate in patients with early rheumatoid arthritis. Rheumatology (Oxford). 2010;49:91–8.
Article CAS PubMed Google Scholar
Vermeer M, Kuper HH, Hoekstra M, Haagsma CJ, Posthumus MD, Brus HL, et al. Implementation of a treat-to-target strategy in very early rheumatoid arthritis. Results of the Dutch arthritis monitoring remission induction cohort study. Arthritis Rheum. 2011;63:2865–72.
Vermeer M, Kuper HH, Bernelot Moens HJ, Drossaers-Bakker KW, van der Bijl AE, van Riel PL, et al. Sustained beneficial effects of a protocolized treat-to-target strategy in very early rheumatoid arthritis: three-year results of the Dutch rheumatoid arthritis monitoring remission induction cohort. Arthritis Care Res. 2013;65:1219–26.
Article CAS Google Scholar
Boonen A, Webers C, Butink M, Barten B, Betteridge N, Black DC, et al. 2021 EULAR points to consider to support people with rheumatic and musculoskeletal diseases to participate in healthy and sustainable paid work. Ann Rheum Dis. 2023;82:57–64.
Sokka T, Kautianen H, Pincus T, Verstappen SMM, Aggarwai A, Alten R, et al. Work disability remains a major problem in rheumatoid arthritis in the 2000s: data from 32 countries in the QUEST-RA study. Arthritis Res Ther. 2010;1(R42):1–10.
OECD. Employment rate (indicator). 2020. https://dataoecd.org/emp/employment-rate.htm . Assessed on 11 May.
Hannerz H, Pedersen BH, Poulsen OM, Humle F, Andersen LL. A nationwide prospective cohort study on return to gainful occupation after stroke in Denmark 1996–2006. BMJ Open. 2011;1:1–5.
Tumin D, Chou H, Hayes D Jr, Tobias JD, Galantowicz M, McConnell PI. Employment after hearth transplantation among adults with congenital heart disease. Congenit Heart Dis. 2017;12:794–9.
Islam T, Dahlui M, Majid HA, Nahar AM, MohdTaib NA, Su TT, MyBCC study group. Factors associated with return to work of breast cancer survivors: a systematic review. BMC Public Health. 2014;14:1–13.
Verstappen SMM, Bijlsma JWJ, Verkleij H, Buskens E, Blaauw AAM, Borg EJ, Jacobs JWG. Overview of work disability in rheumatoid arthritis patients as observed in cross-sectional and longitudinal surveys. Arthritis Rheum. 2004;51:488–97.
Wilkie R, Bjork M, Costa-Black KM, Parker M, Pransky G. Managing work participation for people with rheumatic and musculoskeletal diseases. Best Pract Res. 2020;34:1–16.
Varekamp I, Haafkens JA, Detaille SI, Tak PP, van Dijk FJH. Preventing work disability among employees with rheumatoid arthritis: what medical preofessionals can learn form patients’ perspective. Arthritis Rheum. 2005;53:965–72.
Kirkeskov L, Carlsen RK, Lund T, Buus NH. Emloyment of patients with kidney failure treated with dialysis or kidney transplantation - a systematic review and metaanalysis. BMC Nephrol. 2021;22–348:1–17.
Page MJ, McKenzie JE, Bossuyt PM, Boutron I, Hoffmann TC, Mulrow CD, et al. The PRISMA 2020 statement: an updated guideline for reporting systematic reviews. BMJ. 2020;2021:372. https://doi.org/10.1136/bmj.n71 .
Wells GA, Shea B, O’Connell D, Peterson J, Welch V, Losos M, et al. Newcastle-Ottawa Scale (NOS) for assessing the quality if nonrandomized studies in meta-analyses. 2009. http://www.ohri.ca/programs/clinical_epidemiology/oxford.asp .
Al-Jabi SW, Seleit DI, Badran A, Koni A, Zyoud SH. Impact of socio-demographic and clinical characteristics on functional disability and health-related quality of life in patients with rheumatoid arthritis: a cross-sectional study from Palestine. Health Qual Life Outcomes. 2021;19:241.
Allaire SH, Anderson JJ, Meenan RF. Reducing work disability associated with rheumatoid arthritis: Identifiction of additional risk factors and persons likely to benefit from intervention. Arthritis Care Res. 1996;9(5):9.
Allaire S, Wolfe F, Niu J, Lavalley MP. Comtemporary prevalence and incidence of work disability associated with rheumatoid arthritis in the US. Arthritis Rheum. 2008;59(4):7.
Anno S, Sugioka Y, Inui K, Tada M, Okano T, Mamoto K. Evaluation of work disability in Japanese patients with rheumatoid arthritis: from the TOMORROW study. Clin Rheumatol. 2018;37:9.
Azevedo ABC, Ferraz MB, Ciconelli RM. Indirect costs of rheumatoid arthritis in Brazil. Value Health. 2008;11:869–77.
Backman CL, Kennedy SM, Chalmers A, Singer J. Participation in paid and unpaid work by adults with rheumatoid arthritis. J Rheumatol. 2004;31:47–57.
PubMed Google Scholar
Berner C, Haider S, Grabovac I, Lamprecht T, Fenzl KH, Erlacher L, et al. Work ability and employment in rheumatoid arthritis: a cross-sectional study on the role of muscle strength and lower extremity function. Int J Rheumatol. 2018;2018:11.
Bertin P, Fagnani F, Duburcq A, Woronoff AS, Chauvin P, Cukierman G, et al. Impact of rheumatoid arthritis on career progression, productivity, and employability: the PRET Study. Joint Bone Spine. 2016;83:6.
Bodur H, Borman P, Alper B, Keskin D. Work status and related variables in patients with rheumatoid arthitis and ankylosing spondylitis. Turk J Rheumatol. 2011;26(2):19.
Cadena J, Vinaccia S, Perez A, Rico MI, Hinojosa R, Anaya JM. The impact of disease activity on the quality of life, mental health status, and family dysfunction in Colombian patients with rheumatoid arthritis. Clin Rheumatol. 2003;9:142–50.
Callahan LF, Bloch DA, Pincus T. Identification of work disability in rheumatoid arthritis : physical, radiographic and laboratory variables do not add explanatory power to demographic and functional variables. J Clin Epidemiol. 1992;45(2):12.
Camilleri JP, Jessop AM, Davis S, Jessop JD, Hall M. A survey of factors affecting the capacity to work in patients with rheumatoid arthritis in South Wales. Clin Rehabil. 1995;9:4.
Chen MH, Lee MH, Liao HT, Chen WS, Lai CC, Tsai CY. Health-related quality of life outcomes in patients with rehumatoid arthritis and ankylosing spondylitis after tapering biologic treatment. Clin Rheumatol. 2018;37:429–38.
Chorus AMJ, Miedema HS, Wevers CJ, van der Linden S. Labour force participation among patients with rheumtoid arthritis. Ann Rheum Dis. 2000;59:6.
Chorus AMJ, Miedema HS, Wevers CJ, van der Linden S. Work factors and behavioural coping in relation to withdrawal from the labour force in patients with rheumatoid arthritis. Ann Rheum Dis. 2001;60:8.
Chung CP, Sokka T, Arbogast PG, Pincus T. Work disability in early rheumatoid arthritis: higher rates but better clinical status in Finland compared with the US. Ann Rheum Dis. 2006;65:5.
Dadoniene J, Stropuviene S, Venalis A, Boonen A. High work disability rate among rheumatoid arthritis patients in Lithuania. Arthritis Rheum. 2004;51:433–9.
De Roos AJ, Callahan LF. Differences by sex in correlates of work status in rheumatoid arthritis patients. Arthritis Care Res. 1999;12:381–91.
Dejaco C, Mueller T, Zamani O, Kurtz U, Egger S, Resch-Passini J, et al. A prospective study to evaluate the impact of Golimumab therapy on work productivity and activity, and quality of life in patients with rheumatoid arthritis, psoriatic arthritis and axil spondylarthritis in a real life setting in AUSTRIA. The Go-ACTIVE Study. Front Med. 2022;9:1–9.
Doeglas D, Suurmeijer T, Krol B, Sanderman R, van Leeuwen M, van Rijswijk M. Work disability in early rheumatoid arthritis. Ann Rheum Dis. 1995;54:6.
Fara N, Recchia O, Sequeira G, Sanchez K. Disability due to rheumatic diseases in the city of Junín, Argentina. Rheumatol Int. 2019;39:729–33.
Fifield J, Reisine S, Sheehan TJ, McQuillan J. Gender, paid work, and symptoms of emotional distress in rheumatoid arthritis patients. Arthritis Rheum. 1996;39:427–35.
Gomes RKS, Schreiner LC, Vieira MO, Machado PH, Nobre MRC. Staying in the labor force among patients with rheumatoid arthritis and associated factors in Southern Brazil. Adv Rheumatol. 2018;58(14):1–9.
Hamdeh HA, Al-Jabi SW, Koni A, Zyoud SH. Health-related quality of life and treatment satisfaction in Palestinians with rheumatoid arthritis: a cross-sectional study. BMC Rheumatol. 2022;6(19):1–12.
Hazes JM, Taylor P, Strand V, Purcaru O, Coteur G, Mease P. Physical function improvements and relief from fatigue and pain are associated with incresed productivity at work and at home in rheumatoid arthritis patients treated with certolizumab pegol. Rheumatology. 2010;49:1900–10.
Hulander E, Lindqvist HM, Wadell AT, Gjertsson I, Winkvist A, Bärebring L. Improvements in body composition after a proposed anti-inflammatory diet are modified by employment status in weight-stable patients with rheumatoid arthritis, a randomized controlled crossover trial. Nutrients. 2022;14:1058.
Intriago M, Maldonado G, Guerrero R, Moreno M, Moreno L, Rios C. Functional disability and its determinants in Ecudorian patients with rheumatoid arthritis. Open Access Rheumatol. 2020;12:97–104.
Kavanaugh A, Smolen JS, Emery P, Purcaru O, Keystone E, Richard L, et al. Effect of certolizumab pegol with ethotrexate on home and work place productivity and social activities in patients with active rheumatoid arthritis. Arthritis Rheum. 2009;61:1592–600.
Kwon JM, Rhee J, Ku H, Lee EK. Socioeconomic and employment status of patients with rheumatoid arthritis in Korea. Epidemiol Health. 2003;34:1–7.
Lacaille D, Sheps S, Spinelli JJ, Chalmers A, Esdaile JM. Identification of modifiable work-related factors that influence the risk of work disability in rheumatoid arthritis. Arthritis Rheum. 2004;51:843–52.
Lahiri M, Cheung PPM, Dhanasekaran P, Wong SR, Yap A, Tan DSH, et al. Evaluation of a multidisciplinary care model to improve quality of life in rheumatoid arthritis: a randomised controlled trial. Qual Life Res. 2022;31:1749–59.
Lapcevic M, Vukovic M, Gvozdenovic BS, Mioljevic V, Marjanovic S. Socioeconomic and therapy factor influence on self-reported fatigue, anxiety and depression in rheumatoid arthritis patients. Rev Bras Reumatol. 2017;57(6):12.
Mattila K, Buttgereit F, Tuominen R. Impact of morning stiffness on working behaviour and performance in people with rhematoid arthritis. Rheumatol Int. 2014;34:1751–8.
McQuillan J, Andersen JA, Berdahl TA, Willett J. Associations of rheumatoid arthritis and depressive symptoms over time: Are there differences by education, race/ethnicity, and gender? Arthritis Care Res. 2022;0:1–9.
Mäkisara GL, Mäkisara P. Prognosis of funcrional capacity and work capacity in rheumatoid arthritis. Clin Rheumatol. 1982;1(2):9.
Meenan RF, Yelin EH, Nevitt M, Epstein WV. The impact of chronic disease. A sociomedical profile of rheumatoid arthritis. Arthritis Rheum. 1981;24:544–9.
Morf H, Castelar-Pinheiro GR, Vargas-Santos AB, Baerwald C, Seifert O. Impact of clinical and psychological factors associated with depression in patients with rheumatoid arthritis: comparative study between Germany and Brazil. Clin Rheumatol. 2021;40:1779–87.
Newhall-Perry K, Law NJ, Ramos B, Sterz M, Wong WK, Bulpitt KJ, et al. Direct and indirect costs associated with the onset of seropositive rheumatoid arthritis. J Rheumatol. 2000;27:1156–63.
CAS PubMed Google Scholar
Osterhaus JT, Purcaru O, Richard L. Discriminant validity, responsiveness and reliability of the rheumatoid arthritis-specific Work Productivity Survey (WPS-RA). Arthritis Res Ther. 2009;11(R73):1–12.
Pieringer H, Puchner R, Pohanka E, Danninger K. Power of national economy, disease control and employment status in patients with RA - an analytical multi-site ecological study. Clin Rheumatol. 2016;35:5.
Rosa-Gocalves D, Bernardes M, Costa L. Quality of life and functional capacity in patients with rheumatoid arthritis - Cross-sectional study. Reumatol Clin. 2018;14:360–6.
Sacilotto NC, Giorgi RDN, Vargas-Santos AB, Albuquerque CP, Radominski SC, Pereira IA, et al. Real - rheumatoid arthritis in real life - study cohort: a sociodemographic profile of rheumatoid arthritis in Brazil. Adv Rheumatol. 2020;60:20.
Shanahan EM, Smith M, Roberts-Thomson L, Esterman A, Ahern M. Influence of rheumatoid arthritis on work participation in Australia. Intern Med J. 2008;38:166–73.
Smolen JS, van der Heijde DM, Keystone EC, van Vollenhoven RF, Golding MB, Guérette B, et al. Association of joint space narrowing with impairment of physical function and work ability in patients with early rheumatoid arthritis: protection beyond disease control by adalimumab plus methotrexate. Ann Rheum Dis. 2012;72:1156–62.
Syngle D, Singh A, Verma A. Impact of rheumatoid arthritis on work capacity impairment and its predictors. Clin Rheumatol. 2020;39:1101–9.
Tamborenea MN, Pisoni C, Toloza S, Mysler E, Tate G. Pereira D et al Work instability in rheumatoid arthritis patients from Argentina: prevalence and associated factors. Rheumatol Int. 2015;35:107–14.
Tanaka Y, Kameda H, Saito K, Kanedo Y, Tanaka E, Yasuda S, et al. Response to tocilizumab and work productivity in patients with rheumatoid arthritis: 2-year follow-up of FIRST ACT-SC study. Mod Rheumatol. 2021;21:42–52.
van der Zee-Neuen A, Putrik P, Ramiro S, Keszei AP, Hmamouchi I, Dougados M, Boonen A. Large country differences in work outcomes in patients with RA - an analysis in the multinational study COMORA. Arthritis Res Ther. 2017;19:216.
van Jaarsveld CHM, Jacobs JWG, Schrijvers AJP, van Albada-Kuipers GA, Hofman DM, Bijlsma JWJ. Effects of rheumatoid arthritis on employment and social participation during the first years of disease in the Netherlands. Br J Rheumatol. 1998;37:848–53.
Verstappen SMM, Boonen A, Bijlsma JWJ, Buskens E, Verkleij H, Schenk Y, et al. Working status among Dutch patients with rheumatoid arthritis: work disability and working conditions. Rheumatology. 2005;44:202–6.
Vliet Vlieland TPM, Buitenhuis NA, van Zeben D, Vandenbroucke JP, Breedveld FC, Hazes JMW. Sociodemographic factors and the outcome of rheumatoid arthritis in young women. Ann Rheum Dis. 1994;53:803–6.
Li F, Ai W, Ye J, Wang C, Yuan S, Xie Y, et al. Inflammatory markers and risk factors of RA patients with depression and application of different scales in judging depression. Clin Rheumatol. 2022;41:2309–17.
Wan SW, He HG, Mak A, Lahiri M, Luo N, Cheung PP, et al. Health-related quality of life and its predictors among patients with rheumatoid arthritis. Appl Nurs Res. 2016;30:176–83.
Xavier RM, Zerbini CAF, Pollak DF, Morales-Torres JLA, Chalem P, Restrepo JFM, et al. Burden of rheumatoid arthritis on patients’ work productivity and quality of life. Adv Rheumatol. 2019;59:47.
Yajima N, Kawaguchi T, Takahashi R, Nishiwaki H, Toyoshima Y, Oh K, et al. Adherence to methotrexate and associated factors considering social desirability in patients with rheumatoid arthritis: a multicenter cross-sectional study. BMC Rheumatol. 2022;6(75):1–8.
Yates M, Ledingham JM, Hatcher PA, Adas M, Hewitt S, Bartlett-Pestell S, et al. Disease activity and its predictors in early inflammatory arthritis: findings from a national cohort. Rheumatology. 2021;60:4811–20.
Yelin E, Henke C, Epstein W. The work dynamics of the person with rheumatoid arthritis. Arthritis Rheum. 1987;30:507–12.
Zhang W, Bansback N, Guh D, Li X, Nosyk B, Marra CA, et al. Short-term influence of adalimumab on work productivity outcomes in patients with rheumatoid arthritis. J Rheumatol. 2008;35:1729–36.
Żołnierczyk-Zreda D, Jędryka-Góral A, Bugajska J, Bedyńska S, Brzosko M, Pazdur J. The relationship between work, mental health, physical health, and fatigue in patients with rheumatoid arthritis: a cross-sectional study. J Health Psychol. 2020;25:665–73.
da Rocha Castellar Pinheiro G, Khandker RK, Sato R, Rose A, Piercy J. Impact of rheumatoid arthritis on quality of life, work productivity and resource utilisation: an observational, cross-sectional study in Brazil. Clin Exp Rheumatol. 2013;31:334–40.
Albers JMC, Kuper HH, van Riel PLCM, Prevoo MLL, Van’t Hof MA, van Gestel AM, et al. Socio-economic consequences of rheumatoid arthritis in the first year of the disease. Rheumatology. 1999;38:423–30.
Barrett EM, Scott DGI, Wiles NJ. The impact of rheumatoid arthritis on employment status in the early years of disease: a UK community-based study. Rheumatology. 2000;39:7.
Bejano V, Quinn M, Conaghan PG, Reece R, Keenan AM, Walker D, et al. Effect of the early use of the anti–tumor necrosis factor Adalimumab on the prevention of job loss in patients with early rheumatoid arthritis. Arthritis Care Res. 2008;59:1467–74.
Eberhardt K, Larsson BM, Nived K. Early rheumatoid arthritis – some social, economical, and psychological aspects. Scand J Rheum. 1993;22:119–23.
Eberhardt K, Larsson BM, Nived K, Lindqvist E. Work disability in rheumatoid arthritis- development over 15 years and evaluation of predictive factors over time. J Rheumatol. 2007;34:481–7.
Halpern MT, Cifaldi MA, Kvien TK. Impact of adalimumab on work participation in rheumatoid arthritis: comparison of an open-label extension study and a registry-based control group. Ann Rheum Dis. 2009;68:930–7.
Herenius MMJ, Hoving JI, Sluiter JK, Raterman HG, Lems WF, Dijkmans BAC, et al. Improvement of work ability, quality of life, and fatique in patients with rheumatoid arthritis treated with adalimumab. J Occup Environ Health. 2010;52:618–21.
Hoving JL, Bartelds GM, Sluiter JK, Sadiraj K, Groot I, Lems WF, et al. Perceived work ability, quality of life, and fatigue in patients with rheumatoid arthritis after a 6-month course of TNF inhibitors: prospective intervention study and partial economic evaluation. Scand J Rheumatol. 2009;38:246–50.
Jäntti J, Aho K, Kaarela K, Kautiainen H. Work disability in an inception cohort of patients with seropositive rheumatoid arthritis: a 20 year study. Rheumatology. 1999;38:4.
Kaarela K, Lehtinen K, Luukkainen R. Work capacity of patients with inflammatory joint diseases: an eight-year follow-up study. Scand J Rheumatol. 1987;16:403–6.
McWilliams DF, Varughese S, Young A, Kiely PD, Walsh DA. Work disability and state benefit claims in early rheumatoid arthritis: the ERAN cohort. Rheumatology. 2014;53:9.
Mau W, Bornmann M, Weber H, Weidemann HF, Hecker H, Raspe HH. Prediction of permanent work disability in a follow-up study of early rheumatoid arthritis: results of a tree structured analysis using RECPAM. Br J Rheumatol. 1996;35:652–9.
Nikiphorou E, Guh D, Bansback N, Zhang W, Dixey J, Williams P, et al. Work disability rates in RA. Results from an inception cohort with 24 years follow-up. Rheumatology. 2012;51:8.
Nordmark B, Blomqvist P, Andersson B, Hägerström M, Nordh-Grate K, Rönnqvist R, et al. A two-year follow-up of work capacity in early rheumatoid arthritis: a study of multidisciplinary team care with emphasis on vocational support. Scand J Rheumatol. 2006;35:7–14.
Pincus T, Callahan LF, Sale WG, Brooks AL, Payne LE, Vaughn WK. Severe functional declines, work disability, and increased mortality in seventy-five rheumatoid arthritis patients studied over mine years. Arthritis Rheum. 1984;27:864–72.
Puolakka K, Kautiainen H, Möttönen T, Hannonen P, Hakala M, Korpela M, et al. Predictors of productivity loss in early rheumatoid arthritis: a 5 year follow up study. Ann Rheum Dis. 2005;64:130–3.
Puolakka K, Kautiainen H, Möttönen T, Hannonen P, Korpela M, Julkunen H, et al. Impact of initial aggressive drug treatment with a combination of disease-modifying antirheumatic drugs on the development of work disability in early rheumatoid arthritis. Arthritis Rheum. 2004;50:55–62.
Reisine S, Fifield J, Walsh S, Feinn R. Factors associated with continued employment among patients with rheumatoid arthritis: a survival model. J Rheumatol. 2001;28:2400–8.
Reisine S, Fifield J, Walsh S, Dauser D. Work disability among two cohorts of women with recent onset rheumatoid arthritis: a survival analysis. Arthritis Rheum. 2007;57:372–80.
Robinson HS, Walters K. Return to work after treatment of rheumatoid arthritis. Can Med Assoc J. 1971;105:166–9.
CAS PubMed PubMed Central Google Scholar
Smolen JS, Han C, van der Heijde D, Emery P, Bathon JM, Keystone E, et al. Infliximab treatment maintains employability in patients with early rheumatoid arthritis. Arthritis Rheum. 2006;54:716–22.
van Vollenhoven RF, Cifaldi MA, Ray S, Chen N, Weisman MH. Improvement in work place and household productivity for patients with early rheumatoid arthritis treated with adalimumab plus methotrexate: work outcomes and their correlations with clinical and radiographic measures from a randomized controlled trial companion study. Arthritis Care Res. 2010;62:226–34.
Vazquez-Villegas ML, Gamez-Nava JI, Celis A, Sanchez-Mosco D, de la Cerda-Trujillo LF, Murillo-Vazquez JD, et al. Prognostic factors for permanent work disability in patients with rheumatoid arthritis who received combination therapy of conventional synthetic disease-modifying antirheumatic drugs. A retrospective cohort study. J Clin Rheumatol. 2017;23:376–82.
Verstappen SMM, Jacobs JWG, Kruize AA, Erlich JC, van Albada-Kuipers GA, Verkleij H, et al. Trends in economic consequences of rheumatoid arthritis over two subsequent years. Rheumatology. 2007;46:968–74.
Vlak T, Eldar R. Disability in rheumatoid arthritis after monotherapy with DMARDs. Int J Rehabil Res. 2003;26:207–12.
Yelin E, Trupin L, Katz P, Lubeck D, Rush S, Wanke L. Association between etanercept use and employment outcomes among patients with rheumatoid arthritis. Arthritis & Rheum. 2003;48:3046–54.
Young A, Dixey J, Cox N, Davies P, Devlin J, Emery P, et al. How does functional disability in early rheumatoid arthritis (RA) affect patients and their lifes? Results of 5 years of follow-up in 732 patients from the Early RA Study (ERAS). Rheumatology. 2000;39:603–11.
Young A, Dixey J, Kulinskaya E, Cox N, Davies P, Devlin J, et al. Which patients stop working because of rheumatoid arthritis? Results of five years’ follow up in 732 patients from the Early RA Study (ERAS). Ann Rheum Dis. 2002;61:335–40.
Zirkzee EJM, Sneep AC, de Buck PDM, Allaart CF, Peeters AJ, Ronday HK, et al. Sick leave and work disability in patients with early arthritis. Clin Rheumatol. 2008;27:9.
Reisine S, McQuillan J, Fifield J. Predictors of work disability in rheumatoid arthritis patients. Arthritis Rheum. 2005;38:1630–7.
Verstappen SMM, Watson KD, Lunt M, McGrother K, Symmons PM, Hyrich KL. Working status in patients with rheumatoid arthritis, ankylosing spondylitis and psoriatic arthritis: results from the British Society for Rheumatology Biogics Register. Rheumatology. 2010;49:1570–7.
Nissilä M, Isomäki H, Kaarela K, Kiviniemi P, Martio J, Sarna S. Prognosis of inflammatory joint diseases. A three-year follow-up study. Scand J Rheumatol. 1983;12:33–8.
Han C, Smolen J, Kavanaugh A, St.Clair EW, Baker D, Bala M. Comparison of employability outcomes among patients with early or long-standing rheumatoid arthritis. Arthritis Rheum. 2008;59:510–4.
Gwinnutt JM, Leggett S, Lunt M, Barton A, Hyrich KL, Walker-Bone K, et al. Predictors of presenteeism, absenteeism and job loss in patients commencing methotrexate or biologic therapy for rheumatoid arthritis. Rheumatology. 2020;59:2908–19.
Cieza A, Causey K, Kamenov K, Hanson SW, Chatterji S, Vos T. Global estimates of the need for rehabilitation based on the Global Burden of Disease study 2019: a systematic analysis for the Global Burden of Disease Study 2019. Lancet. 2020. https://doi.org/10.1016/S0140-6736(20)32340-0:1-12 .
Gwinnutt JM, Wieczorek M, Balanescu A, Bischoff-Ferrari HA, Boonen A, Cavalli G, et al. 2021 EULAR recommendations regarding lifestyle behaviours and work participation to prevent progression of rheumatic and musculoskeletal diseases. Ann Rheum Dis. 2023;82:48–56.
England BR, Smith BJ, Baker NA, Barton JL, Oatis CA, Guyatt G, et al. 2022 American College of Rheumatology Guideline for exercise, rehabilitation, diet, and additional integrative interventions for rheumatoid arthritis. Arthritis Care Res. 2023;75:1603–15.
Vu M, Carvalho N, Clarke PM, Buchbinder R, Tran-Duy A. Impact of comorbid conditions on healtcare expenditure and work-related outcomes in patients with rheumatoid arthritis. J Rheumatol. 2021;48:1221–9.
Amaral GSG, Ots P, Brouwer S, van Zon SKR. Multimorbidity and exit from paid employment: the effect of specific combinations of chronic health conditions. Eur J Public Health. 2022;32:392–7.
Boonen A, Putrik P, Marques ML, Alunno A, Abasolo L, Beaton D, et al. EULAR Points to Consider (PtC) for designing, analysing and reporting of studies with work participation as an outcome domain in patients with inflammatory arthritis. Ann Rheum Dis. 2021;80:1116–23.
Lajas C, Abasolo L, Bellajdel B, Hernandez-Garcia C, Carmona L, Vargas E, et al. Costs and predictors of costs in rheumatoid arthritis: A prevalence-based study. Arthritis Care Res. 2003;49:64–70.
Reisine S, McQuillan J, Fifield J. Predictors of work disability in rheumatoid arthritis patients. Arthritis Rheum. 1995;38:1630–7.
Download references
Acknowledgements
Open access funding provided by Royal Library, Copenhagen University Library
Author information
Authors and affiliations.
Department of Social Medicine, University Hospital Bispebjerg-Frederiksberg, Copenhagen, Denmark
Lilli Kirkeskov & Katerina Bray
Department of Social Medicine, University Hospital Bispebjerg-Frederiksberg, Nordre Fasanvej 57, Vej 8, Opgang 2.2., 2000, Frederiksberg, Denmark
Lilli Kirkeskov
Department of Occupational and Social Medicine, Holbaek Hospital, Holbaek, Denmark
Katerina Bray
You can also search for this author in PubMed Google Scholar
Contributions
LK performed the systematic research, including reading articles, performed the blinded quality assessment and the meta-analysis, and drafted and revised the article. KM performed the blinded quality assessment and the discussion afterwards of articles to be included in the research and the scores, and drafted and revised the article.
Corresponding author
Correspondence to Lilli Kirkeskov .
Ethics declarations
Ethics approval and consent to participate.
Not applicable as this is a systematic review. All the studies that are included have obtained ethical approval and consent as appreciated by the journal in which they have been published.
Consent for publication
Not applicable.
Competing interests
The authors declare no competing interests.
Additional information
Publisher’s note.
Springer Nature remains neutral with regard to jurisdictional claims in published maps and institutional affiliations.
Supplementary Information
Additional file 1: figure s1..
Employment; year of investigation.
Additional file 2: Figure S2.
Forest Plot of Comparison: Predictors for employment. Outcome: Younger or older age.
Additional file 3: Figure S3.
Forest Plot of Comparison: Predictors for employment. Outcome: >50 yr or <50 yr of age.
Additional file 4: Figure S4.
Forest Plot of Comparison: Predictors for employment. Outcome: Gender: Male or Female.
Additional file 5: Figure S5.
Forest Plot of Comparison: Predictors for employment. Outcome: Educational level: no college education or college education or higher.
Additional file 6: Figure S6.
Forest Plot of Comparison: Predictors for employment. Outcome: no comorbidities present or one or more comorbidities present.
Additional file 7: Figure S7.
Forest Plot of Comparison: Predictors for employment. Outcome: Ethnicity: Caucasian or other than Caucasian.
Additional file 8: Figure S8.
Forest Plot of Comparison: Predictors for employment. Outcome: Short or long disease duration.
Additional file 9: Figure S9.
Forest Plot of Comparison: Predictors for employment. Outcome: Low or high Health Assessment Questionnaire, HAQ-score.
Additional file 10: Figure S10.
Forest Plot of Comparison: Predictors for employment. Outcome: Low or high VAS-score.
Additional file 11: Figure S11.
Forest Plot of Comparison: Predictors for employment. Outcome: Job type: blue collar workers or other job types.
Additional file 12: Figure S12.
Forest Plot of Comparison: Predictors for employment. Outcome: No MTX or MTX.
Additional file 13: Figure S13.
Forest Plot of Comparison: Predictors for employment. Outcome: No biological or biological.
Additional file 14: Figure S14.
Forest Plot of Comparison: Predictors for employment. Outcome: No prednisolone or prednisolone.
Additional file 15: Figure S15.
Forest Plot of Comparison: Predictors for employment. Outcome: Low or high DAS score.
Additional file 16: Figure S16.
Forest Plot of Comparison: Predictors for employment. Outcome: Low or high SF 36-score.
Rights and permissions
Open Access This article is licensed under a Creative Commons Attribution 4.0 International License, which permits use, sharing, adaptation, distribution and reproduction in any medium or format, as long as you give appropriate credit to the original author(s) and the source, provide a link to the Creative Commons licence, and indicate if changes were made. The images or other third party material in this article are included in the article's Creative Commons licence, unless indicated otherwise in a credit line to the material. If material is not included in the article's Creative Commons licence and your intended use is not permitted by statutory regulation or exceeds the permitted use, you will need to obtain permission directly from the copyright holder. To view a copy of this licence, visit http://creativecommons.org/licenses/by/4.0/ . The Creative Commons Public Domain Dedication waiver ( http://creativecommons.org/publicdomain/zero/1.0/ ) applies to the data made available in this article, unless otherwise stated in a credit line to the data.
Reprints and permissions
About this article
Cite this article.
Kirkeskov, L., Bray, K. Employment of patients with rheumatoid arthritis - a systematic review and meta-analysis. BMC Rheumatol 7 , 41 (2023). https://doi.org/10.1186/s41927-023-00365-4
Download citation
Received : 07 June 2023
Accepted : 20 October 2023
Published : 14 November 2023
DOI : https://doi.org/10.1186/s41927-023-00365-4
Share this article
Anyone you share the following link with will be able to read this content:
Sorry, a shareable link is not currently available for this article.
Provided by the Springer Nature SharedIt content-sharing initiative
- Return to work
- Unemployment
BMC Rheumatology
ISSN: 2520-1026
- Submission enquiries: [email protected]
- General enquiries: [email protected]
Have a language expert improve your writing
Run a free plagiarism check in 10 minutes, generate accurate citations for free.
- Knowledge Base
Methodology
- What Is Qualitative Research? | Methods & Examples
What Is Qualitative Research? | Methods & Examples
Published on June 19, 2020 by Pritha Bhandari . Revised on June 22, 2023.
Qualitative research involves collecting and analyzing non-numerical data (e.g., text, video, or audio) to understand concepts, opinions, or experiences. It can be used to gather in-depth insights into a problem or generate new ideas for research.
Qualitative research is the opposite of quantitative research , which involves collecting and analyzing numerical data for statistical analysis.
Qualitative research is commonly used in the humanities and social sciences, in subjects such as anthropology, sociology, education, health sciences, history, etc.
- How does social media shape body image in teenagers?
- How do children and adults interpret healthy eating in the UK?
- What factors influence employee retention in a large organization?
- How is anxiety experienced around the world?
- How can teachers integrate social issues into science curriculums?
Table of contents
Approaches to qualitative research, qualitative research methods, qualitative data analysis, advantages of qualitative research, disadvantages of qualitative research, other interesting articles, frequently asked questions about qualitative research.
Qualitative research is used to understand how people experience the world. While there are many approaches to qualitative research, they tend to be flexible and focus on retaining rich meaning when interpreting data.
Common approaches include grounded theory, ethnography , action research , phenomenological research, and narrative research. They share some similarities, but emphasize different aims and perspectives.
Note that qualitative research is at risk for certain research biases including the Hawthorne effect , observer bias , recall bias , and social desirability bias . While not always totally avoidable, awareness of potential biases as you collect and analyze your data can prevent them from impacting your work too much.
Here's why students love Scribbr's proofreading services
Discover proofreading & editing
Each of the research approaches involve using one or more data collection methods . These are some of the most common qualitative methods:
- Observations: recording what you have seen, heard, or encountered in detailed field notes.
- Interviews: personally asking people questions in one-on-one conversations.
- Focus groups: asking questions and generating discussion among a group of people.
- Surveys : distributing questionnaires with open-ended questions.
- Secondary research: collecting existing data in the form of texts, images, audio or video recordings, etc.
- You take field notes with observations and reflect on your own experiences of the company culture.
- You distribute open-ended surveys to employees across all the company’s offices by email to find out if the culture varies across locations.
- You conduct in-depth interviews with employees in your office to learn about their experiences and perspectives in greater detail.
Qualitative researchers often consider themselves “instruments” in research because all observations, interpretations and analyses are filtered through their own personal lens.
For this reason, when writing up your methodology for qualitative research, it’s important to reflect on your approach and to thoroughly explain the choices you made in collecting and analyzing the data.
Qualitative data can take the form of texts, photos, videos and audio. For example, you might be working with interview transcripts, survey responses, fieldnotes, or recordings from natural settings.
Most types of qualitative data analysis share the same five steps:
- Prepare and organize your data. This may mean transcribing interviews or typing up fieldnotes.
- Review and explore your data. Examine the data for patterns or repeated ideas that emerge.
- Develop a data coding system. Based on your initial ideas, establish a set of codes that you can apply to categorize your data.
- Assign codes to the data. For example, in qualitative survey analysis, this may mean going through each participant’s responses and tagging them with codes in a spreadsheet. As you go through your data, you can create new codes to add to your system if necessary.
- Identify recurring themes. Link codes together into cohesive, overarching themes.
There are several specific approaches to analyzing qualitative data. Although these methods share similar processes, they emphasize different concepts.
Qualitative research often tries to preserve the voice and perspective of participants and can be adjusted as new research questions arise. Qualitative research is good for:
- Flexibility
The data collection and analysis process can be adapted as new ideas or patterns emerge. They are not rigidly decided beforehand.
- Natural settings
Data collection occurs in real-world contexts or in naturalistic ways.
- Meaningful insights
Detailed descriptions of people’s experiences, feelings and perceptions can be used in designing, testing or improving systems or products.
- Generation of new ideas
Open-ended responses mean that researchers can uncover novel problems or opportunities that they wouldn’t have thought of otherwise.
Prevent plagiarism. Run a free check.
Researchers must consider practical and theoretical limitations in analyzing and interpreting their data. Qualitative research suffers from:
- Unreliability
The real-world setting often makes qualitative research unreliable because of uncontrolled factors that affect the data.
- Subjectivity
Due to the researcher’s primary role in analyzing and interpreting data, qualitative research cannot be replicated . The researcher decides what is important and what is irrelevant in data analysis, so interpretations of the same data can vary greatly.
- Limited generalizability
Small samples are often used to gather detailed data about specific contexts. Despite rigorous analysis procedures, it is difficult to draw generalizable conclusions because the data may be biased and unrepresentative of the wider population .
- Labor-intensive
Although software can be used to manage and record large amounts of text, data analysis often has to be checked or performed manually.
If you want to know more about statistics , methodology , or research bias , make sure to check out some of our other articles with explanations and examples.
- Chi square goodness of fit test
- Degrees of freedom
- Null hypothesis
- Discourse analysis
- Control groups
- Mixed methods research
- Non-probability sampling
- Quantitative research
- Inclusion and exclusion criteria
Research bias
- Rosenthal effect
- Implicit bias
- Cognitive bias
- Selection bias
- Negativity bias
- Status quo bias
Quantitative research deals with numbers and statistics, while qualitative research deals with words and meanings.
Quantitative methods allow you to systematically measure variables and test hypotheses . Qualitative methods allow you to explore concepts and experiences in more detail.
There are five common approaches to qualitative research :
- Grounded theory involves collecting data in order to develop new theories.
- Ethnography involves immersing yourself in a group or organization to understand its culture.
- Narrative research involves interpreting stories to understand how people make sense of their experiences and perceptions.
- Phenomenological research involves investigating phenomena through people’s lived experiences.
- Action research links theory and practice in several cycles to drive innovative changes.
Data collection is the systematic process by which observations or measurements are gathered in research. It is used in many different contexts by academics, governments, businesses, and other organizations.
There are various approaches to qualitative data analysis , but they all share five steps in common:
- Prepare and organize your data.
- Review and explore your data.
- Develop a data coding system.
- Assign codes to the data.
- Identify recurring themes.
The specifics of each step depend on the focus of the analysis. Some common approaches include textual analysis , thematic analysis , and discourse analysis .
Cite this Scribbr article
If you want to cite this source, you can copy and paste the citation or click the “Cite this Scribbr article” button to automatically add the citation to our free Citation Generator.
Bhandari, P. (2023, June 22). What Is Qualitative Research? | Methods & Examples. Scribbr. Retrieved April 15, 2024, from https://www.scribbr.com/methodology/qualitative-research/
Is this article helpful?
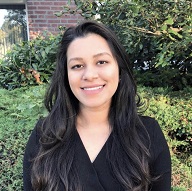
Pritha Bhandari
Other students also liked, qualitative vs. quantitative research | differences, examples & methods, how to do thematic analysis | step-by-step guide & examples, "i thought ai proofreading was useless but..".
I've been using Scribbr for years now and I know it's a service that won't disappoint. It does a good job spotting mistakes”

IMAGES
VIDEO
COMMENTS
To situate your research in an existing body of work, or to evaluate trends within a research topic. Case study: Either: ... so you have to reflect carefully on your choices and assumptions and be careful to avoid research bias. Quantitative analysis methods. Quantitative analysis uses numbers and statistics to understand frequencies, ...
Definition of research in data analysis: According to LeCompte and Schensul, research data analysis is a process used by researchers to reduce data to a story and interpret it to derive insights. The data analysis process helps reduce a large chunk of data into smaller fragments, which makes sense. Three essential things occur during the data ...
For many researchers unfamiliar with qualitative research, determining how to conduct qualitative analyses is often quite challenging. Part of this challenge is due to the seemingly limitless approaches that a qualitative researcher might leverage, as well as simply learning to think like a qualitative researcher when analyzing data. From framework analysis (Ritchie & Spencer, 1994) to content ...
Step 1: Explain your methodological approach. Step 2: Describe your data collection methods. Step 3: Describe your analysis method. Step 4: Evaluate and justify the methodological choices you made. Tips for writing a strong methodology chapter. Other interesting articles.
A method of data analysis that is the umbrella term for engineering metrics and insights for additional value, direction, and context. By using exploratory statistical evaluation, data mining aims to identify dependencies, relations, patterns, and trends to generate advanced knowledge.
About Research Methods. This guide provides an overview of research methods, how to choose and use them, and supports and resources at UC Berkeley. As Patten and Newhart note in the book Understanding Research Methods, "Research methods are the building blocks of the scientific enterprise. They are the "how" for building systematic knowledge.
QDA Method #1: Qualitative Content Analysis. Content analysis is possibly the most common and straightforward QDA method. At the simplest level, content analysis is used to evaluate patterns within a piece of content (for example, words, phrases or images) or across multiple pieces of content or sources of communication. For example, a collection of newspaper articles or political speeches.
Step 1: Consider your aims and approach. Step 2: Choose a type of research design. Step 3: Identify your population and sampling method. Step 4: Choose your data collection methods. Step 5: Plan your data collection procedures. Step 6: Decide on your data analysis strategies. Other interesting articles.
Abstract. This paper aims to provide an overview of the use and assessment of qualitative research methods in the health sciences. Qualitative research can be defined as the study of the nature of phenomena and is especially appropriate for answering questions of why something is (not) observed, assessing complex multi-component interventions ...
Table of contents. Step 1: Write your hypotheses and plan your research design. Step 2: Collect data from a sample. Step 3: Summarize your data with descriptive statistics. Step 4: Test hypotheses or make estimates with inferential statistics.
Research methodology refers to the system of procedures, techniques, and tools used to carry out a research study. It encompasses the overall approach, including the research design, data collection methods, data analysis techniques, and the interpretation of findings. Research methodology plays a crucial role in the field of research, as it ...
A GFT is a mid-range GT that has "fit, work and grab": that is, it fits the data (concepts and categories from primary studies), works to explain the phenomenon under review, and resonates with the readers' experiences and understandings. ... the units of analysis can be research findings ("meta-data-analysis"), research methods ...
Thematic analysis is a research method used to identify and interpret patterns or themes in a data set; it often leads to new insights and understanding (Boyatzis, 1998; Elliott, 2018; Thomas, 2006).However, it is critical that researchers avoid letting their own preconceptions interfere with the identification of key themes (Morse & Mitcham, 2002; Patton, 2015).
Data Analysis. Definition: Data analysis refers to the process of inspecting, cleaning, transforming, and modeling data with the goal of discovering useful information, drawing conclusions, and supporting decision-making. It involves applying various statistical and computational techniques to interpret and derive insights from large datasets.
Data analysis techniques in research are categorized into qualitative and quantitative methods, each with its specific approaches and tools. These techniques are instrumental in extracting meaningful insights, patterns, and relationships from data to support informed decision-making, validate hypotheses, and derive actionable recommendations.
Qualitative Research. Qualitative research is a type of research methodology that focuses on exploring and understanding people's beliefs, attitudes, behaviors, and experiences through the collection and analysis of non-numerical data. It seeks to answer research questions through the examination of subjective data, such as interviews, focus ...
The research methodology is an important section of any research paper or thesis, as it describes the methods and procedures that will be used to conduct the research. It should include details about the research design, data collection methods, data analysis techniques, and any ethical considerations.
Job and Work Analysis: Methods, Research, and Applications for Human Resource Management provides students and professionals alike with an in-depth exploration of job analysis. Job analysis encompasses a wide range of crucial topics that help us understand what people do at work and why. This one-of-a-kind text expertly unpacks the best job ...
In most social research the data analysis involves three major steps, done in roughly this order: Data Preparation involves checking or logging the data in; checking the data for accuracy; entering the data into the computer; transforming the data; and developing and documenting a database structure that integrates the various measures.
Thoroughly updated and revised, the Second Edition of Job and Work Analysis presents the most important and commonly used methods in human resource management in much detail. The authors clearly outline how organizations can create programs to improve hiring and training, make jobs safer, provide a satisfying work environment, and help employees to work smarter. Throughout, they provide ...
ISBN: 9781544329529. TITLE: Job and Work Analysis: Methods, Research, and Applications for Human Resource Management, 3rd Edition. AUTHOR: Michael T. Brannick
Here are some key characteristics of quantitative research: Numerical data: Quantitative research involves collecting numerical data through standardized methods such as surveys, experiments, and observational studies. This data is analyzed using statistical methods to identify patterns and relationships.
This work has the potential to inspire and trigger new research highlights for fluorescence microscopy-based image restoration. ... A visual-language foundation model for pathology image analysis ...
This research addresses the growing need for fast and cost-efficient methods for microplastic (MP) analysis. We present a thermo-analytical method that enables the identification and quantification of different polymer types in sediment and sand composite samples based on their phase transition behavior. Differential scanning calorimetry (DSC) was performed, and the results were evaluated by ...
This study employs a corpus-driven approach to identify the four-word lexical bundles in the Methods section of 1 000 medical research articles (MRAs) from ten leading medical journals representing ten medical sub-fields. The bundles are first structurally and functionally analysed and further connected to rhetorical moves to fill the form-function gap of lexical bundle studies.
Topics include the research process, analysis strategies, valuation techniques and portfolio construction methods. Templates, examples and detailed feedback on draft reports are provided. ... Students should be prepared to conduct rigorous, creative research based upon their own work and insights. Fall 2024 - NBA 5220 - This course is an ...
Current research on deep learning-based intelligent landslide detection modeling has focused primarily on improving and innovating model structures. However, the impact of terrain factors and data fusion methods on the prediction accuracy of models remains underexplored. To clarify the contribution of terrain information to landslide detection modeling, 1022 landslide samples compiled from ...
Background Patients with rheumatoid arthritis (RA) have difficulties maintaining employment due to the impact of the disease on their work ability. This review aims to investigate the employment rates at different stages of disease and to identify predictors of employment among individuals with RA. Methods The study was carried out according to the Preferred Reporting Items for Systematic ...
Qualitative research involves collecting and analyzing non-numerical data (e.g., text, video, or audio) to understand concepts, opinions, or experiences. It can be used to gather in-depth insights into a problem or generate new ideas for research. Qualitative research is the opposite of quantitative research, which involves collecting and ...
The importance of our study is that it is unique, pro-public, and self-funded, to identify potential toxic ingredients. We performed an exhaustive protein-quantitative, product-qualitative, and chemical and toxicology analysis based on industrial standards, on popular brands of protein supplements sold in the Indian market. 2. Methods 2.1.