The short-term mean reversion of stock price and the change in trading volume
Journal of Derivatives and Quantitative Studies: 선물연구
ISSN : 1229-988X
Article publication date: 18 June 2021
Issue publication date: 23 September 2021
This study aims to analyze the effect of change in trading volume on the short-term mean reversion of the stock price in the Korean stock market. Through the variance ratio test, this paper finds that the market shows the mean reversion pattern after 2000, but not before. This study also confirms that the mean reversion property is significantly reduced if the effect of change in trading volume is excluded from the return of a stock with a significant contemporaneous correlation between return and change in trading volume in the post-2000 market. The results appear in both the Korea Composite Stock Price Index and Korea Securities Dealers Automated Quotation. This phenomenon stems from the significance of the return response to change in trading volume per se and not the sign of the response. Additionally, the findings imply that the trading volume has a term structure because of the mean reversion of the trading volume and the return also has a partial term structure because of the contemporaneous correlation between return and change in trading volume. This conclusion suggests that considering the short-term impact of change in trading volume enables a more efficient observation of the market and avoidance of asset misallocation.
- Trading volume
- Granger causality
- Variance ratio
- Korean stock market
- Contemporaneous correlation
- Mean-reversion
Jung, W. and Kang, M. (2021), "The short-term mean reversion of stock price and the change in trading volume", Journal of Derivatives and Quantitative Studies: 선물연구 , Vol. 29 No. 3, pp. 190-214. https://doi.org/10.1108/JDQS-01-2021-0003
Emerald Publishing Limited
Copyright © 2021, Woosung Jung and Mhin Kang.
Published in Journal of Derivatives and Quantitative Studies: 선물연구. Published by Emerald Publishing Limited. This article is published under the Creative Commons Attribution (CC BY 4.0) licence. Anyone may reproduce, distribute, translate and create derivative works of this article (for both commercial and non-commercial purposes), subject to full attribution to the original publication and authors. The full terms of this licence maybe seen at http://creativecommons.org/licences/by/4.0/legalcode

1. Introduction
If the market is efficient, stock prices fully reflect all available information and it is impossible to make economic profits by trading based on the information set ( Fama, 1970 ; Malkiel, 1989 ). Therefore, stock prices follow the martingale process [ 1 ] and stock returns are not predictable. However, various studies have been conducted on the mean reversion of stock returns, which suggests that stock returns can be predictable. These findings are inconsistent with the efficient market hypothesis. Poterba and Summers (1988) observe that the divergence between market and fundamental values will eventually be eliminated by speculative forces, causing the stock price to mean-revert. This correction of “erroneous” market movements leads to the argument that stock returns must be negatively correlated at some frequency. More recently, Nagel (2012) shows that individual stocks have a negative serial correlation at daily and even monthly frequencies. Therefore, a short-term reversal strategy that buys losers and sells winners over the prior days generates profits. The mean-reverting phenomenon is not US-specific. Earlier literature shows that mean-reverting stock index returns exist in the Korean stock market ( Lee, 2002 ; Bae, 2006 ).
However, earlier research mainly focuses on the return-generating process and trading volume level. Recent studies investigate another possible channel for the mean-reversion of stock returns: the change in trading volume. Kang and Chae (2019b) find that in addition to the relationship between the trading volume and stock return, the change in trading volume also has a separate contemporaneous correlation with stock returns (hereinafter, CCRV) in the Korean stock market. They also argue that the illiquidity premium hypothesis offers an appropriate explanation for this phenomenon. Specifically, increased liquidity simultaneously causes larger trading volume and higher prices because of lowered illiquidity premiums, thereby leading to a significant and positive CCRV. Furthermore, Kang and Chae (2019a) confirm the presence of mean reversion of the trading volume in the Korean market. These findings imply that trading volume has a term structure and through the CCRV channel, this term structure affects stock price movements.
This study sheds light on the role of trading volume change in stock price mean reversion. If the trading volume mean-reverts and has a significant contemporaneous correlation with stock returns, the return is predictable, conditional on the current trading volume. In other words, the stock return process reflects the mean reversion of the trading volume and implies that the price term structure is related to the trading volume term structure. Therefore, if we extract the return component orthogonal to the volume change effect, the component becomes more comparable to the martingale process. We use the variance ratio (VR) test to empirically investigate how CCRV-orthogonalized returns become closely comparable to the martingale process than the original returns.
Why is it important to examine how trading volume change affects the mean reversion of stock returns? The study on it has important implications for investors’ risk perceptions and asset allocations. If we do not consider the impact of volume change on stock returns, the return volatility may be misestimated according to the term length. Consequently, investors may misallocate their wealth to assets, leading to market inefficiency.
Our sample includes all stocks in the Korea Composite Stock Price Index (KOSPI) and Korea Securities Dealers Automated Quotation (KOSDAQ) from September 1987 to December 2017. First, we verify whether the volume change affects the return contemporaneously in the Korean stock market. Consistent with Kang and Chae’s (2019b) findings, the distribution of CCRV in our sample shows that most stocks have positive and significant CCRV. In total, 74% of stocks have positive CCRV and 11% of stocks have negative CCRV at the 10% significance level. Only 15% of the stocks had insignificant CCRV. This result does not change in the sub-periods and the different exchanges, indicating that the mean-reversion of trading volume affects the stock price mean reversion through the CCRV channel. Based on this implication, we hypothesize that CCRV-orthogonalized returns are more comparable to random walks.
Next, we analyze our hypothesis using the VR test following Lo and MacKinlay (1988) and Poterba and Summers (1988) . If we remove the CCRV effects on stock returns, the stock returns are closer to the martingale process, leading to the VR of CCRV-orthogonalized returns being closer to 1 than the original stock returns. Our empirical results support this prediction. The differences in the average VRs between CCRV-orthogonalized returns and original stock returns are significantly positive and increase as the test horizon becomes longer. For example, the difference in the average VRs of 10-day to 5-day between CCRV-orthogonalized returns and original returns is 0.0017 ( t -values: 4.12), but the difference in the average VRs of 100-day to 5-day increases to 0.01 ( t -values: 21.91).
For robustness, we conduct a subsample analysis to examine whether the effect changes in the different subsamples. First, we divide the entire sample into two groups as of 2000. As earlier literature notes that the 1997 currency crisis had a severe impact on the Korean economy, stock returns after the crisis behave quite differently from before and the mean reversion phenomenon of stock returns may have changed. Our subsample results also show structural changes in the stock return behavior. In the first half of the sample, all VRs are greater than 1, indicating mean aversion. This result is consistent with Bae (2006) , which finds that the KOSPI shows a mean aversion phenomenon. However, in the second half of the sample, we find that stock returns show mean reversion. Bae (2006) suggests that this change contributes to an increase in foreign and institutional investors who pursue long-term investments. In both subsamples, the VRs of CCRV-orthogonalized returns are always greater than those of the original returns, consistent with the main result.
Second, stocks in the KOSPI and KOSDAQ markets have different characteristics such as investor and industry composition. Therefore, it is necessary to conduct VR tests separately in each market. However, the evidence shows no significant difference.
Finally, we also investigate whether both the significance and sign of the CCRV variable affect the results. We find that VR increases when we eliminate the volume effect of CCRV in stocks with both positive and negative CCRV. However, securities with insignificant CCRV do not show a significant change in the VR value, even after the volume effect of the CCRV is eliminated. The result suggests that regardless of its sign, the significance of CCRV plays a role in increasing the short-term variance in stock returns.
Overall, our empirical evidence supports the hypothesis that stock returns are affected by the volume change through the CCRV channel. Therefore, if we eliminate the volume change effect on stock returns, the stock returns become closely comparable to the martingale process. This evidence suggests that the mean reversion of trading volume affects the variance of short-term and long-term returns differently. Thus, we need to consider the volume effect on short-term stock returns to avoid asset misallocation.
The remainder of this paper is organized as follows. Section 2 summarizes previous studies. Section 3 describes the data and methodology. Section 4 presents our empirical results. Section 5 presents the results of robustness tests. Section 6 concludes.
2. Literature review
Our work builds on long-lasting literature on the mean reversion of stock returns. Tversky and Kahneman (1974) argue that a representativeness heuristic inclines people to overreact to new information. Many studies show that investor sentiment causes prices to swing away from the true value in the financial market ( De Bondt and Thaler , 1985, 1987 ; Shefrin and Statman, 1985 ; De Long et al. , 1990 ; Lehmann, 1990 ). If market values diverge from fundamental values, speculative forces may eliminate the difference, leading to a negative serial correlation in stock returns ( Poterba and Summers, 1988 ). Fama and French (1988) also describe how long-horizon returns lead to predictability because of slowly decaying price components. They note that the long-term mean reversion of stock returns is consistent with two alternate explanations: overreaction by irrational investors or time-varying equilibrium expected returns in an efficient market.
Some research has challenged the evidence of stock return mean reversion based on the test methodology. Richardson and Stock (1989) show that small-sample bias correction provides evidence for the mean reversion in long-horizon returns of the NYSE index and size decile portfolios. Using US data, McQueen (1992) argues that the general least square (GLS) randomization test does not reject the random walk of returns for horizons of 1 to 10 years. Jegadeesh (1991) also provides evidence against mean reversion by noting that the mean reversion phenomenon is concentrated only in January. A similar result is also observed in the market index of the London Stock Exchange. However, other studies support the mean-reverting phenomenon with more powerful panel methods or new data. Balvers et al. (2000) use a more robust test with annual panel data from 18 countries and find the mean reversion of index returns with a reversion speed of 18 to 20% per year. Gropp (2004) uses a panel method and discovers the mean reversion for the NYSE, AMEX and NASDAQ. More recently, Mukherji (2011) shows that mean reversion persists for small company stocks in one-, four- and five-year returns. Cecchetti, Lam and Mark (1990) described how the desire for consumption smoothing leads to negative autocorrelations without overreaction.
The mean reversion evidence varies across investment horizons and whether the focus is on the index or individual stocks. Sims (1980) argues that systematic short-term variations in fundamental values should be negligible in a competitive market. Therefore, price should follow a martingale process over brief time intervals, even if stock returns include a component that varies predictably over the long horizon. Early studies focus on the long-run mean reversion of stock index returns. Using the VR test, Poterba and Summers (1988) show that the S&P composite stock index has a long-term negative correlation but a short-term positive correlation because of the transitory component in stock returns. Fama and French (1988) also show that size and industry portfolio autocorrelations are weak for daily and weekly holding periods. Temporary components account for 40% of the predictable price variation of three- to five-year returns for small firms and 25% for large firms. However, stock index returns have a positive autocorrelation in the short horizon such as weekly or monthly, especially for small stocks. Lo and MacKinlay (1988) suggest that this result is mainly attributed to small firms and is not entirely explained by time-varying risk premiums and infrequent trading. Lo and MacKinlay (1990) describe further how despite negative autocorrelation in individual stock returns, weekly portfolio returns are strongly positively auto-correlated and result from important cross-autocorrelation. Nagel (2012) shows that individual stocks still have small negative autocorrelations daily, weekly and monthly. A positive high-frequency autocorrelation of the index declines and has even been negative in recent years ( Campbell, 2017 , p. 162).
There have been few studies of mean reversion in the Korean market. Lee (2002) finds that the KOSPI’s daily returns revert to the mean by using a fractionally integrated process. Bae (2006) also shows that the KOSPI and KOSDAQ’s monthly returns followed a weak mean-reverting process after the 1997 currency crisis. Our study focuses on short-term reversion in individual stock returns and suggests this phenomenon as a possible factor.
We also extend the literature on the relationship between abnormal trading volumes and stock returns. One pillar of research shows that heterogeneity in investment environments causes abnormal trading volumes. As this heterogeneity is resolved by trading between investors from different backgrounds, the abnormal trading volume decreases and the price becomes stable. Therefore, abnormal trading volume per se does not affect future returns. The differences in investment environments arise because of asymmetric information among investors ( Wang, 1994 ; Llorente et al. , 2002 ; Tetlock, 2010 ), differences in opinion among traders ( Harris and Raviv, 1993 ; Kandel and Pearson, 1995 ; Garfinkel and Sokobin, 2006 ) and irrational behavior by investors ( Campbell et al. , 1993 ; Odean, 1998 ; Scheinkman and Xiong, 2003 ; Baker and Stein, 2004 ; Grinblatt and Han, 2005 ; Statman Thorley and Vorkink, 2006 ; Choi et al. , 2010 ).
Another pillar suggests that abnormal trading volumes and market frictions affect stock returns. According to the investor recognition hypothesis ( Miller, 1977 ; Mayshar, 1983 ; Merton, 1987 ), stocks can be overvalued when a market has short-selling constraints. In particular, Odean and Barber (2009) argue that salient events affecting a stock such as unpredicted news, rapid price changes or unusual trading volumes, increase investors’ buying and selling demand. However, increased selling demand is not activated because of short-selling restrictions. Therefore, these events create net buying demand and higher returns on average. Gervais et al. (2001) suggest the visibility hypothesis that a sharp increase in trading volumes would attract investors’ attention to the stock, resulting in net buying pressure and, thus, in stock price increases. They find evidentiary support for this hypothesis that future returns with high trading volumes are significantly higher than those with low trading volumes. Kaniel et al. (2012) also show consistent results for stock markets across 41 countries.
Earlier research on stock returns and trading volumes in the Korean stock market has mainly focused on the causality test between these two variables. As for the stock index, various studies find that index returns are positively correlated with both trading volumes and changes in trading volumes ( Chung, 1987 ; Kho, 1997 ; Silvapulle and Choi, 1999 ; Kim and Kim, 1996 ; Chang, 1997 ). However, Lim (2016) finds that using the TGARCH model, the change in the KOSPI trading volume is affected by the return but not vice versa.
In a study on the relationship between trading volume and individual stock returns, Lee (2009) shows that using GARCH and regression methods, large and medium-sized stocks in the KOSPI market positively correlate and have a two-way Granger causal relationship with trading volumes. Small stocks, however, do not show a simultaneous relationship but only a Granger causal relationship. Eom (2013) uses the VAR model of the KOSDAQ stock to show that both past individual stock and market returns have a positive relationship with current trading volumes. Using the TGARCH and EGARCH models, Jheon and Park (2014) show a simultaneous correlation between trading volumes and returns in the KOSDAQ market. They also show that the degree of correlation between trading volumes and previous trading volumes depends significantly on the size of the stock. Finally, Lee et al. (2015) use the variance decomposition method to represent a more significant impact of the return on trading volume than the opposite.
In a study of the relationship between the change in trading volume and returns, Jinn et al. (1994) show that changes in the trading volume positively correlate with the return. Kook and Jung (2001) find that while an increase in past trading volume is likely to reverse the current return sign, the decrease continues the return trend. Kang and Chae (2019b) note that the CCRV of most stocks in the Korean market is significantly positive and the CCRV accounts for 4.22% of the total volatility of stocks. Furthermore, they argue that the liquidity premium hypothesis supports positive CCRV in the Korean market. Our study does not focus on the relationship between trading volume and returns but sheds light on how the trading volume change affects the short-term mean reversion of stock returns through the CCRV channel.
Among the studies on the relationship between abnormal trading volumes and stock returns, certain studies investigate how trading volumes positively affect the mean reversion of stock returns. Campbell et al. (1993) argue this relationship theoretically. According to their model, noisy trading causes price movements, but those movements are reverted when absorbed by liquidity providers. Further, the model assumes that such noisy trading is followed by high trading volume, while informed trading is not. The model implicitly assumes a downward-sloping demand curve and not a perfectly elastic demand curve. This assumption allows the price to be affected by trading volume. Empirically, Conrad et al. (1994) , based on a sample of NASDAQ stocks, find that high trading activity increases with reversal profitability. Avramov et al. (2006) show that the weekly or monthly negative autocorrelation of an individual stock, measured by a profit of contrarian strategy, is stronger for firms with high trading volume and high illiquidity. However, our study focuses on the impact of change, instead of the level of trading volume, on the mean-reversion of stock returns through the CCRV channel suggested by Kang and Chae (2019b) . Mean-reversion of trading volume can affect the returns when a stock has significant CCRV. Therefore, if we eliminate volume effects from returns, the short-term mean-reversion phenomenon in stock returns can be partially mitigated. This point is an additional aspect that differentiates this study from previous ones. Furthermore, to the best of our knowledge, this study is the first to investigate the relation between trading volume and mean reversion in the Korean stock market.
3. Data and methodology
3.1 methodology.
This study mainly argues that the mean reversion of stock prices is overestimated by responding to the trading volume change. Kang and Chae (2019b) show that daily volatility caused by trading volume change in the Korean stock market, on average, accounts for about 4% of the total daily volatility. However, as Kang and Chae (2019b) have stated, the volatility caused by the volume change will disappear in the long run because the trading volume mean-reverts and the price changes resulting from the change in trading volume will be restored to the original position. Therefore, Kang and Chae (2019b) also argue that it is desirable to consider the effect of the trading volume change on the return when estimating future prices based on current information. A stock return can be decomposed into the induced return by the change in trading volume and fundamental return. Figure 1 shows the concept for this argument. Figure 1(a) represents the fundamental return path of a stock, Figure 1(b) presents the return movements induced by trading volume change and Figure 1(c) is the original return path, which is the sum of Figures 1(a) and 1(b) .
If we assume that the fundamental return path follows the Wiener process, for t < T, the relation between the short-term return volatility σ f ( t ) 2 and the long-term return volatility σ f ( T ) 2 can be expressed as σ f ( t ) 2 / t = σ f ( T ) 2 / T . In contrast, the relation between long- and short-term volatility observed in a separate CCRV induced return path, which has negative autocorrelation caused by mean-reversion of trading volume and CCRV, can be expressed as σ CCRV ( t ) 2 / t > σ CCRV ( T ) 2 / T . Therefore, the long-term original return volatility σ ( T ) 2 / T = σ f ( T ) 2 / T + σ CCRV ( T ) 2 / T is less than σ ( t ) 2 / t = σ f ( t ) 2 / t + σ CCRV ( t ) 2 / t and σ ( T ) 2 / T < σ ( t ) 2 / t holds. As short-term volatility is more affected by CCRV-induced components having negative autocorrelation than long-term volatility, a short-term structural mean-reversion in price is observed. To confirm this, we conduct the following process.
First, we extract the effect of change in trading volume from the original return path to compare the mean reversion phenomenon in the original return with that in the CCRV-orthogonalized return. We construct CCRV-orthogonalized time-series returns for each stock by using the following regression specification: (1) r i , t = a i + β i Δ V i , t + ε i , t , where Δ V i , t is defined as the change in log volume turnover of stock i at time t. We use ε i,t s as CCRV-orthogonalized stock returns. Significant β i implies that the return of stock i is affected by the trading volume change.
Next, we compare the mean reversion of original returns with that of CCRV-orthogonalized returns using the VR test following Lo and MacKinlay (1988) and Poterba and Summers (1988) [ 2 ]. The test is based on the fact that the stock variance should be proportional to the return horizon if the returns follow a random walk. We examine the variance of returns at different horizons relative to the variance over the base interval. For daily returns, the VR statistics are, therefore: (2) V R l ( k ) = v a r ( r t k ) k / v a r ( r t l ) l , where r t τ = ∑ i = 0 τ − 1 r t − i , t is the test interval and l is the base interval. When conducting the VR test for the CCRV-orthogonalized returns, we substitute r t with ε t .
If daily returns do not have serial autocorrelations through time, the VR l ( k ) statistics should converge to 1. However, if daily returns are autocorrelative, VR l ( k ) varies depending on the sign of autocorrelation and the length of the test interval. If a positive (negative) autocorrelation exists in the daily returns, VR l ( k ) is greater (less) than one and increases (decreases) as k increases.
We set the base interval, l , as five days [ 3 ] and the test interval, k , to 1, 10, 20, 30, 50 and 100 days to calculate the VR statistics for short-, mid- and long-term test intervals. We compute two VR statistics for each test interval using the original and CCRV-orthogonalized returns and compare these statistics. If both VR values are less than 1, but the VR value from CCRV-orthogonalized returns is greater than that from the original stock returns, the mean reversion phenomenon of stock returns is weakened after excluding the volume effects. We suggest that CCRV-orthogonalized returns are more comparable to random walks.
Our sample covers all common stocks listed on the KOSPI and KOSDAQ from September 1, 1978 to December 31, 2015. We exclude ETNs, ETFs, REITs, SPACs, KDRs, preferred stocks and common stocks with less than one year of stock returns. We obtain stock information from FnDataGuide.
4. Empirical analysis
4.1 distribution of contemporaneous correlation between return and change in trading volume.
We eventually show that the stock price’s mean reversion phenomenon in the CCRV-orthogonalized returns becomes weaker than that in the original returns. However, before conducting this discussion, we first need to verify whether the volume change affects the return contemporaneously. Although Kang and Chae (2019b) show the Korean stock market’s result, it is applied to each stock each year. As our analysis is performed without year-by-year classification, it is essential to reaffirm the volume effect on the price for each level. Therefore, we use equation (1) as a regression specification to estimate β i , which refers to the sensitivity of returns to volume changes. Figure 2 shows the histogram of the t -values of the estimated β i and Panel A in Table 1 shows the overall distribution of the estimated β i .
Figure 2 shows that stocks with significantly positive CCRV (located on the right side of t -values 1.645 on the x-axis) are the majority, while stocks with significantly negative CCRV (located on the left side of t -values −1.645 on the x-axis) are the minority. Panel A in Table 1 represents the supporting result that the positive CCRV stocks account for 74% of the total, while the negative CCRV stocks account only for 11%. These findings are consistent with those of Kang and Chae (2019b) . This panel also describes the results for the sub-period before and after 2000 and for the KOSPI and KOSDAQ markets after 2000. These sub-sample results are not different from those in the entire sample, except that the proportion of negative CCRV stocks decreases to approximately 7% before 2000. The fact that more than 80% of stocks in our sample have significant CCRV indicates that the returns of most stocks are affected by trading volume changes.
In addition, we analyze the difference in characteristics of the significant and insignificant CCRV stocks. First, we classify each stock’s size, Be/Me ratio and return volatility into 10 groups each year, assigning one to the smallest group and 10 to the largest group by sequentially increasing them. Additionally, stocks belonging to KOSPI are assigned 0 and stocks belonging to KOSDAQ are assigned 1 to distinguish the exchanges. Panel B in Table 1 shows the statistics for the group numbers calculated through this process. We then apply the daily Carhart model ( Carhart, 1997 ) to calculate the adjusted- R 2 and alpha of each stock. Panel B also reports the statistics for these results. Significant CCRV stocks have a larger size and less volatility than insignificant CCRV stocks. Further, adjusted- R 2 and alpha of Significant CCRV stocks are also significantly higher. However, the difference in size is only 1.24 and considering the dispersion of standard deviation within each group, it may be unreasonable to consider size as a major determinant of the significance of CCRV [ 4 ]. This evidence is also applicable for adjusted- R 2 and alpha.
4.2 Contemporaneous correlation between return and change in trading volume effect on mean reversion of stock return
4.2.1 determination of the base interval for variance ratio test..
As we have seen in Section 4.1, our sample confirms that most shares are affected by a change in trading volume. For stocks with significant CCRV, β i Δ V i , t in equation (1) operates as a determinant factor for the stock return to explain the discrepancy between the original return, r i , t and the CCRV-orthogonalized return, ε i , t . Therefore, in this section, we demonstrate that using the VR test, these stocks have significant mean reversion differences between CCRV-orthogonalized and original returns.
Before we proceed with the VR test, it is necessary to select the base interval in equation (2) . As described in Section 3.1, stock returns and variances are measured at frequencies of 1, 5, 10, 20, 30, 50 and 100 days. In measuring variance, we exclude data with fewer than 20 consecutive observations. The left side of Table 2 shows the statistics of the estimated variances and the right side displays the return variance scaled by the interval for comparison between the results of each interval.
Panel A shows the entire sample result. The average variances scaled by each interval peak at 0.00205 for the test interval of one day and gradually decreases to 0.00158 as the test interval increases to 100 days. Panel B represents the result of significant CCRV stocks. The average return variance/interval is 0.00207 for the one-day interval, similar to that in Panel A, but peaks at 0.00224 for the five-day interval. Then, it gradually declines to 0.00169 for the 100-day return. This difference is caused by approximately 400 stocks with insignificant CCRV, excluded from the sample in Panel B. This result is consistent with the prediction in Figure 1 that greater variance will be observed in stocks significantly affected by trading volume changes. Return variance/interval of stocks with significant CCRV is maximized at the five-day test interval because, as demonstrated by Kang and Chae (2019a) , the abnormal trading volume is halved in two to three days; thus, most of the variance enhanced by the trading volume change disappears in five days. Based on these results, we determined five days as the base interval, l , in equation (2) .
Additionally, Panel C provides the result for CCRV-orthogonalized return. Comparing the results in Panel B and Panel C, we confirm that CCRV-orthogonalization decreases the return variance. This result may be expected because the Panel B and Panel C samples are composed of significant CCRV stocks. However, it contains more information. As shown in the return variance/interval column, the proportion of decrement for each interval is different. The proportion decreases gradually as the interval increases. This evidence implies that the CCRV effect is relatively stronger on short-term prices.
These results have important implications for option pricing. One of the most important requirements for option pricing and hedging is the volatility estimation of the forward price. Options are often traded through the over-the-counter (OTC) market for hedging the existing portfolios, stock grants and product development for customers. For options that are not traded in such a market, implied volatility cannot be used for volatility estimation, and therefore, it relies heavily on historical volatility. As shown in Panel C of Table 2 , if the long-term forward price volatility is estimated based on the short-term historical return, the long-term volatility of significant CCRV stock can be over-estimated. In this case, because of the convexity of the options price, a small error in volatility estimation may cause a large error in the options price [ 5 ]. In addition, volatility misestimation also affects Greeks, causes errors in the hedging ratio, increases book management costs and may also affect risk management. As described above, misestimation of volatility has important implications in dealing with derivatives. Therefore, the forward prices’ volatility from historical returns must be estimated carefully.
4.2.2 Variance ratio test.
In this section, we compare the mean reversion in original stock returns with that in CCRV-orthogonalized returns. Table 3 presents the results of the VR test for each test interval. First, column (a) shows that the average of VR (1) in the whole sample is 1.0669, which is significantly greater than 1. This result suggests that the variance of the five-day return is less than five times that of the unit-day return because of a negative autocorrelation between one-day returns within five days.
On the other hand, VR (10) to VR (100) are significantly smaller than 1 and monotonically decrease as the test interval increases. This evidence indicates a negative autocorrelation between the unit-day returns, even for longer than 10 days. The evidence suggests that, overall, the mean reversion of stock returns exists across all test intervals.
Unlike the results for the whole sample, the original returns of significant CCRV stocks have different results for VR (1). The mean of VR (1) is 0.9548, which is significantly less than 1. This evidence shows that positive autocorrelations exist between one-day returns within a base interval of five days. On the other hand, for the test intervals of more than 10 days, all VR values are significantly less than 1 and monotonically decrease as the test interval increases, consistent with results for the whole sample. The original return of significant CCRV stocks shows momentum for a short period of fewer than five days and reversal for a more extended period.
CCRV-orthogonalized returns show similar results in most cases to the original returns of stocks with significant CCRV. All test values of VR (1) through VR (100) are significantly less than 1. In other words, even CCRV-orthogonalized returns present momentum for shorter periods of less than five days and reversal for longer periods.
Despite similar VR values between the original return and CCRV-orthogonalized returns, we should pay attention to the change in VR values. The last column (d) indicates that the VRs of the CCRV-orthogonalized return in all test intervals except VR (1) is significantly greater than those of the original return. If we consider the average half-lives of abnormal trading volumes in the Korean stock market, five days may be insufficient for the trading volume shock to disappear. As a result, the abnormal volume-induced returns do not disappear in five days. This hypothesis is consistent with the evidence that there is no difference in VR (1) between the original and CCRV-orthogonalized returns. Meanwhile, VR (10) to VR (100) in column (d) indicate that mean reversion of CCRV-orthogonalized returns is weaker for the test intervals of more than 10 days. These findings suggest that the market can be more efficient if we eliminate the short-term price impact of trading volume changes [ 6 ].
In addition to the above analysis, we conduct VR tests for each sub-period by dividing the entire sample period since 2000 into two. Bae (2006) demonstrates that the KOSPI shows momentum before the financial crisis but changes to mean reversion subsequently. The KOSDAQ index also presents mean reversion after the financial crisis. A sub-period analysis is necessary to investigate whether these results persist, even in short-term returns of individual stocks, not the index. Moreover, with a sub-period analysis, we examine how volatility in the original and CCRV-orthogonalized returns of stocks with signature CCRV varies over time.
Panel A of Table 4 shows the sub-period result from 1987 to 1999, which is quite different from Table 3 . All values of VR (10) to VR (100) are significantly greater than 1 for both the entire sample and significant CCRV stocks. This result implies that stock prices do not show a short-term mean-reversion pattern [ 7 ]. Meanwhile, Panel B of Table 4 shows the sub-period result after 2000, similar to Table 3 . Among them, in the results of the whole sample, the values of VR (10) to VR (100) are less than 1, which is consistent with Bae (2006) , who argues that stock prices revert to the mean after the 1997 currency crisis. Likewise, in both the original and CCRV-orthogonalized return of significant CCRV stocks, short-term mean-reversion patterns are observed at intervals greater than 10.
The last columns (d) of Panels A and B in Table 4 show that the difference in VR values is significantly positive for the test interval of greater than 10 days, consistent with the results in Table 3 . However, this should be interpreted carefully. The methodology of this study, which examines the short-term effects of trading volumes on returns, cannot be applied because we do not observe the short-term mean reversion phenomenon of stock returns before 2000. Therefore, the previous argument that the stock market becomes more efficient when we eliminate short-term impact in stock returns because of trading volume changes should apply only to the market after 2000.
Next, we divide the whole sample into KOSPI and KOSDAQ samples and proceed with the above analysis. The KOSPI and KOSDAQ markets may show different market efficiencies because of different investors and industry compositions ( Lee et al. , 2006 ; Park et al. , 2007 ). Such differences in market efficiency may cause differences in the mean reversion patterns between the two markets. We conduct the analysis only on the post-2000 sample where the mean reversion phenomenon of the stock price exists. Table 5 describes the results.
First, results between the KOSPI and KOSDAQ markets do not contrast significantly for the whole sample. In both markets, VR (1) is significantly greater than 1. VR (10) to VR (100) are significantly less than 1 and monotonically decrease as the test interval increases. This evidence shows a short-term mean reversion in the KOSPI and KOSDAQ markets after 2000.
However, we observe discrepant results from the original return in the significant CCRV stock sample. In both markets, VR (10) to VR (100) are smaller than 1 and decrease as the test interval increases. However, while VR (1) in the KOSPI market is not significantly different from 1 (mean: 1.0194, standard error: 0.0143), VR (1) in the KOSDAQ market is significantly smaller than 1 (mean = 0.9745, standard error = 0.0097). The results suggest that while short-term momentum is observed in the KOSDAQ market even for less than five days, this is not the case in the KOSPI market. These results are similar to the results of the CCRV-orthogonalized returns.
In addition, the results in the different columns show that the VR (10) to VR (100) of CCRV-orthogonalized returns are significantly greater than those of original returns in both the KOSPI and KOSDAQ markets. Therefore, our argument that the market can be observed more efficiently when the CCRV effect is eliminated does not depend on the market.
4.3 Sign of contemporaneous correlation between return and change in trading volume and mean reversion of stock return
In the previous sections, we examine our hypothesis that CCRV affects stock mean reversion and, eventually, market efficiency. However, as we have seen in Table 1 , CCRV is categorized into three groups based on its significance and sign: a significantly positive CCRV, a significantly negative CCRV and an insignificant CCRV. Thus, the overall response of the return to trading volume changes will be different for each group, as shown in Figure 3 .
The price moves in the same direction as the volume change in the significantly positive CCRV stocks and moves in the opposite direction in the significantly negative CCRV stocks. In the insignificant CCRV stocks, the price is uncorrelated with the volume change. As the mean reversion of trading volume is related to price movements only in stocks with significant CCRV, the VR of the CCRV-orthogonalized return is significantly larger than that of the original return in both the significantly positive and significantly negative CCRV stock samples but do not show significant differences in the insignificant CCRV stock sample. To demonstrate this argument empirically, we divide the post-2000 sample into three groups based on the sign of CCRV and investigate the mean reversion phenomenon in each subsample [ 8 ].
Table 6 presents the results for each subsample. First, in the positive and negative CCRV groups, the VR difference between the original and CCRV-orthogonalized returns is significantly positive in all test intervals of greater than 10 days. However, the difference in the insignificant CCRV group is small (0.0001) and the t -values are also not significant. This evidence is consistent with our expectation that the impact of CCRV on return mean reversion depends on the significance of CCRV, not on the sign.
On the other hand, in the positive CCRV group, VR (1) is significantly less than 1, at 0.9376, indicating short-term momentum in returns within five days, while in the negative CCRV group, VR (1) is 1.0714, indicating short-term mean reversion. However, this difference may result from each stock’s characteristics rather than the CCRV’s sign because CCRV is the mechanical response of returns to volume changes. According to Kang and Chae (2019b) , the sign of CCRV depends on stock characteristics such as size and liquidity. This evidence suggests that the difference in VR (1) between stocks with positive and negative CCRV is caused by latent stock characteristics rather than the sign of CCRV per se [ 9 ].
Table 7 shows the subsample results of applying the analysis of Table 6 to the KOSPI and KOSDAQ markets. In most cases, the results do not differ between markets and are similar to those in Table 6 . VR (10) to VR (100) in both the positive and negative CCRV groups are significantly larger than before when the CCRV effect is removed from stock returns, but there is no significant change in the insignificant CCRV group. Although the differences of VR (30) and VR (50) in the insignificant CCRV group in the KOSDAQ market are significant ( t = 1.69 for VR(30) and t = 2.65 for VR(50)), the difference is 0.0005, which is not economically meaningful. Based on the empirical results, we can conclude that the significance of CCRV per se , rather than its sign, induces the impact of trading volume change on return and, thus, we can observe the market more efficiently by considering the CCRV.
Moreover, column (a) of Panel A in Table 7 shows that the VR (1) for the KOSPI market is 0.9893 in the positive CCRV group and 1.2105 in the negative CCRV group. As shown in Table 5 , the VR (1) of the KOSPI is 1.0194, which is not significant and is larger than 1. Now, we can confirm that the result is because of differences in the VR (1) between the two different CCRV groups. These results are similar to VR (1) for the KOSDAQ market. In Table 5 , the VR (1) for the KOSDAQ market is 0.9745, but in Table 7 , it is 0.9476 in the positive CCRV group and 1.1336 in the negative CCRV group. In addition, VR (1) increases significantly in the positive CCRV group when the volume effect is removed from stock returns and decreases significantly to close to 1 in the negative CCRV group. This evidence also suggests that removing the volume effect from stock returns enables a more efficient observation of the market [ 10 ].
5. Robustness test
5.1 controlling the effects of the previous trading volume level.
Previous studies focus on the relationship between trading volume level and return and the effect of the current trading volume on the following return. In the context of those studies, Kang and Chae (2019b) argue that the CCRV is a phenomenon that acts in addition to the existing relationship between the trading volume level and returns.
Following Kang and Chae (2019b) , we assume that the effect of CCRV on stock price mean-reversion is an additional phenomenon overlapping the relation between trading volume level and return. Therefore, the results of this study must hold when the effect of trading volume level on stock return is removed. To confirm this, we must control the lead-lag effect observed in the time series of trading volumes and returns. In this regard, Lee (2002) , Lee (2009) and Lee et al. (2015) verify the Granger causality for the Korean stock market, but the results of daily data at the individual stock level are yet to be reported. Therefore, we check whether the Granger causality appears in the daily trading volume and return in the Korean market as a prerequisite for the main analysis. Panel A in Table 8 below shows the results of a Granger causality test for the Korean market from 2001 to 2015 by using the five lagged daily returns and trading volumes from t −1 to t −5.
As shown in Panel A of Table 8 , in the Korean stock market, 84.36% of the stocks’ returns Granger-cause the trading volumes, 48.87% of the stocks’ trading volumes Granger-cause the returns and 44.81% of the stocks shows a bidirectional Granger causality.
Further, a VR test is conducted to verify if VR appears closer to one in the CCRV-orthogonalized return when the influence of previous trading volumes and returns on the current return is removed. The test is conducted on the variance observed in the two-type residuals of the following two-stage regression equations. The following regression equation (3a) controls the five lagged returns and trading volumes applied to the Granger causality test. In addition, in the regression equation (3b) , the residual of the regression equation (3a) , ϵ 1, i , t , is the dependent variable and the change in trading volume is the explanatory variable: (3a) r i , t = α 1 , i + ∑ j = 1 m γ i , j r t − j + ∑ j = 1 m β i , j T V t − j + ϵ 1 , i , t , (3b) ϵ i , t = α 2 , i + β i ′ Δ T V t + ϵ 2 , i , t ,
Panel B in Table 8 shows the VR test results for the two residuals ϵ 1, i , t and ϵ 2, i , t calculated in the above process. As shown in column (a), in ϵ 1, i , t , where the previous return and trading volume level are controlled, VR(1) is 1.0415 and in VR(10)∼VR(100), it is significantly smaller than 1. This evidence shows the mean-reversion pattern that appears. The result of ϵ 2, i , t is similar, wherein VR(1) is 1.0365 and VR(10) ∼ VR(100) shows a mean-reversion pattern that is significantly smaller than 1, between 0.9630 and 0.9756. In addition, the difference between the two residuals in VR(10) to VR(100) is 0.0045 to 0.0119, which increases as the period increases and all t -values appear at statistically significant levels. The difference is similar to the difference observed in the Korean market from 2000 to 2015 in panel B of Table 4 , which is 0.0019 to 0.0097. Therefore, even when the Granger causality between the return and the trading volume level in the Korean market is controlled, the VR of the CCRV-orthogonalized return appears closer to one. Further, this result supports our main argument that the mean reversion of stock prices is overestimated by responding to the trading volume change.
5.2 Testing for efficiency by using the approximate entropy
We use the approximate entropy methodology (hereinafter, ApEn ) proposed by Pincus (1991) to supplement the results from the previous test.
ApEn is a measure that quantifies and represents the complexity, unpredictability and irregularity observed in time series and is similar to the verification contents of the market efficiency hypothesis of Fama (1970) . Pincus and Kalman (2004) , Kim et al. (2005) , Oh et al. (2007) , Bhaduri (2014) and Pele et al. (2017) tests the market efficiency by applying this methodology to the asset market. The method of calculating ApEn is as follows: A p E n ( S N , m , r ) = ∑ i = 1 N − m + 1 ln [ C m i ( r ) ] ( N − m + 1 ) − ∑ i = 1 N − m ln [ C m + 1 i ( r ) ] ( N − m ) C m i ( r ) = B i ( N − m + 1 ) , C m + 1 i ( r ) = B i ( N − m ) B i = θ ( r − d [ x ( u ) , x ( j ) ] (4) d [ x ( u ) , x ( j ) ] = max k = 1 , 2 , … , m ( | S i + k − 1 − S j + k − 1 | ) , where S N is an instantaneous time-series, m is a pattern length based on embedding dimension, r is similarity based on the threshold, d is the maximum distance between x ( i ) elements and x(j) elements and B i is Heaviside function having 1 if d < r and 0 if d ≥ r . We set m as two and r as 0.2 times the standard deviation of returns following the definition and assumption of Kim et al. (2005) .
As shown in the above equation (4) , the value of ApEn decreases as similar patterns appear in the time series and its value increases as predictions become difficult. Thus, higher values imply higher efficiency.
We compare the ApEn value in each stock’s original return with that in the CCRV- orthogonalized return. The results are reported in Table 9 . The average value of ApEn is 1.5802 in the original return and 1.5883 in the CCRV-orthogonalized return. The average difference between the two values measured in each stock appears to be 0.0081 and the corresponding t -values are 12.65. The significant increase in the ApEn value observed in the CCRV-orthogonalized return indicates the increased randomness in the time series of the CCRV-orthogonalized return. Therefore, the results of the ApEn analysis are consistent with the VR test. This evidence shows that the market can be observed more efficiently if the trading volume change is considered.
6. Conclusion
This study analyzed the short-term mean reversion of stock return in the Korean market from 1987 to 2015. Mainly focusing on the effect of the change in trading volume on stock returns, we compare the mean reversion patterns in the CCRV-orthogonalized return with that in the original return using the VR test.
The empirical analysis confirms the existence of short-term mean reversion of stock price in the Korean market after 2000, but not before 2000. In addition, for stocks whose price has been affected by changes in trading volume since 2000, the VR increases from the original and is closer to 1 if the trading volume effect is excluded. These results appear in the significant CCRV stocks in both KOSPI and KOSDAQ, regardless of the sign of CCRV.
Based on the above results, we confirm that the partial term structure of the stock return is related to the term structure of the trading volume and CCRV and the stock return’s term structure also partially affects the short-term mean reversion of price. This term structure of stock prices amplifies the volatility in short-term returns over volatility in long-term returns. However, such a trading volume effect is predictable and extractable by the consideration of the CCRV channel. Consequently, we conclude that the market is observed more efficiently if we remove the effect of trading volume change on the stock price. Moreover, by considering the CCRV effect, we can avoid the misestimation of the forward price volatility that may cause mispricing and hedging errors when dealing with OTC market’s options, which usually depend on the historical data when estimating volatility.
On the subject of mean reversion of a stock price, it would be a good research topic to compare the long-term and short-term mean reversions for each stock and examine the factors that affect each phenomenon. Moreover, revealing the determinants of the mean reversion of stock prices can be a promising future work. Given our finding that the mean reversion pattern appears differently because of the sign of CCRV, other factors may play an important role in the mean reversion of a stock price. If we can reveal the causal relationship between these hidden determinants and the mean reversion, we can have a deeper understanding of market efficiency and we can better understand the characteristics of the market. Finally, the discussion on CCRV, the main variable of this study, is still in progress and more research is needed. Therefore, we hope that this study enriches the existing discussion and serves as a useful ingredient for future research.
Return path decomposition
CCRV distribution (histogram)
Return response to the change in trading volume
CCRV distribution
This table reports the estimated approximate entropy for original return and CCRV-orthogonalized return in the Korean stock market from 2000 to 2015. Values in parentheses are standard errors
More precisely, the stock price is not a martingale in the dividend discount model because
E t [ P t + 1 ] = ( 1 + R ) P t − E t [ D t + 1 ] .
However, we can obtain a martingale process if we substitute P t with V t , which is the dividend-adjusted total value:
V t = N t P t ( 1 + R ) t a n d N t + 1 = N t ( 1 + D t + 1 P t + 1 )
For the testing of mean reversion of the stock price, the methodologies of Fama and French (1988) , Jegadeesh (1991) and Poterba and Summers (1988) are usually applied. We choose the last one because building the pseudo return path is only possible by this methodology.
According to Kang and Chae (2019a) , the half-life, which is the time necessary for half of the deviated amount of trading volume to decay, is about two to three days. Thus, we suppose that five days is enough time for trading volume shock to almost disappear.
Refer to the difference in CCRV distribution according to size and liquidity level in Table 10 of Kang and Chae (2019b) .
This tendency is more extreme in out-of-the-money (OTM). For example, when the volatility is 30% for a one-year 120% OTM call option, a 1% change in volatility has a 6% effect on the option price; in a 150% OTM call option, it has an 18% effect or higher.
If the market is efficient, the VR value will be 1.
For the pre-2000 data, even when we adjust the base interval to 10 or 20 days instead of 5 days, we do not find the short-term mean reversion patterns.
As for CCRV, more research is still needed on its cause and we expect this analysis to be useful for discussion.
Alternately, it is possible that the difference in mean reversion in the one-to-five-day interval will be one of the determinants of the sign of the CCRV.
This result is also observed in Table 6 . In Tables 3 , 4 and 5 , without the CCRV sign classification, the result is not observed.
Avramov , D. , Chordia , T. and Goyal , A. ( 2006 ), “ Liquidity and autocorrelations in individual stock returns ”, The Journal of Finance , Vol. 61 No. 5 , pp. 2365 - 2394 .
Bae , J.H. ( 2006 ), “ A reexamination of mean reversion and aversion in Korean stock prices ”, Journal of Economics Studies , Vol. 24 , pp. 85 - 105 .
Baker , M. and Stein , J.C. ( 2004 ), “ Market liquidity as a sentiment indicator ”, Journal of Financial Markets , Vol. 7 No. 3 , pp. 271 - 299 .
Balvers , R. , Wu , Y. and Gilliland , E. ( 2000 ), “ Mean reversion across national stock markets and parametric contrarian investment strategies ”, The Journal of Finance , Vol. 55 No. 2 , pp. 745 - 772 .
Bhaduri , S.N. ( 2014 ), “ Applying approximate entropy (ApEn) to speculative bubble in the stock market ”, Journal of Emerging Market Finance , Vol. 13 No. 1 , pp. 43 - 68 .
Campbell , J.Y. ( 2017 ), Financial Decisions and Markets: A Course in Asset Pricing , ( Princeton University Press ).
Campbell , J.Y. , Grossman , S.J. and Wang , J. ( 1993 ), “ Trading volume and serial correlation in stock returns ”, The Quarterly Journal of Economics , Vol. 108 No. 4 , pp. 905 - 939 .
Carhart , M.M. ( 1997 ), “ On persistence in mutual fund performance ”, The Journal of Finance , Vol. 52 No. 1 , pp. 57 - 82 .
Cecchetti , S.G. , Lam , P.S. and Mark , N.C. ( 1990 ), “ Mean reversion in equilibrium asset price ”, American Economic Review , Vol. 80 No. 3 , pp. 398 - 418 .
Chang , K.H. ( 1997 ), “ Trading volume, volume volatility, and stock return predictability ”, Asian Review of Financial Research , Vol. 14 , pp. 1 - 27 .
Choi , W. , Hoyem , K. and Kim , J.W. ( 2010 ), “ Capital gains overhang and the earnings announcement volume premium ”, Financial Analysts Journal , Vol. 66 No. 2 , pp. 40 - 53 .
Chung , J.R. ( 1987 ), “ Stock price volatility and trading volume – theory and empirical verification ”, Korean Journal of Financial Studies , Vol. 9 No. 1 , pp. 309 - 336 .
Conrad , J.S. , Hameed , A. and Niden , C. ( 1994 ), “ Volume and autocovariances in short‐horizon individual security returns ”, The Journal of Finance , Vol. 49 No. 4 , pp. 1305 - 1329 .
De Bondt , W.F. and Thaler , R. ( 1985 ), “ Does the stock market overreact? ”, The Journal of Finance , Vol. 40 No. 3 , pp. 793 - 805 .
De Bondt , W.F. and Thaler , R.H. ( 1987 ), “ Further evidence on investor overreaction and stock market seasonality ”, The Journal of Finance , Vol. 42 No. 3 , pp. 557 - 581 .
De Long , J.B. , Shleifer , A. , Summers , L.H. and Waldmann , R.J. ( 1990 ), “ Positive feedback investment strategies and destabilizing rational speculation ”, The Journal of Finance , Vol. 45 No. 2 , pp. 379 - 395 .
Dimson , E. ( 1979 ), “ Risk measurement when shares are subject to infrequent trading ”, Journal of Financial Economics , Vol. 7 No. 2 , pp. 197 - 226 .
Eom , Y.S. ( 2013 ), “ Trading volume, investor overconfidence, and the disposition effect ”, The Korean Journal of Financial Management , Vol. 30 No. 3 , pp. 1 - 33 .
Fama , E.F. ( 1970 ), “ Efficient capital markets: a review of theory and empirical work ”, The Journal of Finance , Vol. 25 No. 2 , pp. 383 - 417 .
Fama , E.F. and French , K.R. ( 1988 ), “ Permanent and temporary components of stock prices ”, Journal of Political Economy , Vol. 96 No. 2 , pp. 246 - 273 .
Garfinkel , J.A. and Sokobin , J. ( 2006 ), “ Volume, opinion divergence, and returns: a study of post-earnings announcement drift ”, Journal of Accounting Research , Vol. 44 No. 1 , pp. 85 - 112 .
Gervais , S. , Kaniel , R. and Mingelgrin , D.H. ( 2001 ), “ The high‐volume return premium ”, The Journal of Finance , Vol. 56 No. 3 , pp. 877 - 919 .
Grinblatt , M. and Han , B. ( 2005 ), “ Prospect theory, mental accounting, and momentum ”, Journal of Financial Economics , Vol. 78 No. 2 , pp. 311 - 339 .
Gropp , J. ( 2004 ), “ Mean reversion of industry stock returns in the US, 1926–1998 ”, Journal of Empirical Finance , Vol. 11 No. 4 , pp. 537 - 551 .
Harris , M. and Raviv , A. ( 1993 ), “ Differences of opinion make a horse race ”, Review of Financial Studies , Vol. 6 No. 3 , pp. 473 - 506 .
Jegadeesh , N. ( 1991 ), “ Seasonality in stock price mean reversion: evidence from the US and the UK ”, The Journal of Finance , Vol. 46 No. 4 , pp. 1427 - 1444 .
Jheon , S.K and Park , K.S. ( 2014 ), “ A study on the dynamic relation between stock return and trading volume in KOSDAQ market ”, Financial Planning Review , Vol. 7 No. 1 , pp. 55 - 85 .
Jinn , T. , Lee , J-h. and Nam , J-h. ( 1994 ), “ Research on the trading volume and stock price change ”, Korean Journal of Financial Studies , Vol. 16 No. 1 , pp. 513 - 526 .
Kandel , E. and Pearson , N.D. ( 1995 ), “ Differential interpretation of public signals and trade in speculative markets ”, Journal of Political Economy , Vol. 103 No. 4 , pp. 831 - 872 .
Kang , M. and Chae , J. ( 2019a ), “ Mean-reversion of the trading volume ”, Asian Review of Financial Research , Vol. 32 No. 2 , pp. 149 - 186 .
Kang , M. and Chae , J. ( 2019b ), “ Contemporaneous correlation between the return and the change in trading volume ”, Journal of Derivatives and Quantitative Studies , Vol. 27 No. 4 , pp. 425 - 473 .
Kaniel , R. , Ozoguz , A. and Starks , L. ( 2012 ), “ The high volume return premium: cross-country evidence ”, Journal of Financial Economics , Vol. 103 No. 2 , pp. 255 - 279 .
Kho , B.C. ( 1997 ), “ Stock prices and volume: a semi – nonparametric approach ”, Asian Review of Financial Research , Vol. 13 No. 1 , pp. 1 - 35 .
Kim , K.Y. and Kim , Y.B. ( 1996 ), “ Linear and nonlinear granger causality in the stock price – volume Relation – an empirical investigation in the korean stock market ”, Asian Review of Financial Research , Vol. 9 No. 2 , pp. 167 - 186 .
Kim , T.H. , Um , C.J. and Oh , G.J. ( 2005 ), “ A comparative test for market efficiency between international market indices: using approximate entropy ”, Asian Review of Financial Research , Vol. 18 No. 2 , pp. 239 - 262 .
Kook , C.P. and Jung , W.H. ( 2001 ), “ An empirical study on information effect of increase or decrease in stock trading volume ”, Korean Journal of Financial Studies , Vol. 29 No. 1 , pp. 87 - 115 .
Lee , C.S. ( 2009 ), “ A study on the trading volume and market volatility ”, Journal of Industrial Economics and Business , Vol. 22 No. 2 , pp. 495 - 511 .
Lee , J.W. ( 2002 ), “ A test on granger causality between trading volume and stock returns in the Korean stock market ”, The Korean Journal of Financial Engineering , Vol. 1 , pp. 1 - 32 .
Lee , C.W. , Guahk , S. and Wi , H.J. ( 2006 ), “ Over-reaction of stock prices in KOSDAQ market ”, Korean Journal of Business Administration , Vol. 19 No. 1 , pp. 181 - 198 .
Lee , Y.D. , Park , H.K. and Kim , S.O. ( 2015 ), “ The dynamic relationship between stock return and trading volume in the Korea stock market ”, Journal of Industrial Economics and Business , Vol. 28 No. 2 , pp. 739 - 758 .
Lehmann , B.N. ( 1990 ), “ Fads, martingales, and market efficiency ”, The Quarterly Journal of Economics , Vol. 105 No. 1 , pp. 1 - 28 .
Lim , S.S. ( 2016 ), “ The effect of the global financial crisis on the return and trading volume in KOSPI ”, Journal of Industrial Economics and Business , Vol. 29 No. 3 , pp. 961 - 981 .
Llorente , G. , Michaely , R. , Saar , G. and Wang , J. ( 2002 ), “ Dynamic volume-return relation of individual stocks ”, Review of Financial Studies , Vol. 15 No. 4 , pp. 1005 - 1047 .
Lo , A.W. and MacKinlay , A.C. ( 1988 ), “ Stock market prices do not follow random walks: evidence from a simple specification test ”, Review of Financial Studies , Vol. 1 No. 1 , pp. 41 - 66 .
Lo , A.W. and MacKinlay , A.C. ( 1990 ), “ When are contrarian profits due to stock market overreaction? ”, “ Review of Financial Studies , Vol. 3 No. 2 , pp. 175 - 205 .
McQueen , G. ( 1992 ), “ Long-horizon mean-reverting stock prices revisited ”, The Journal of Financial and Quantitative Analysis , Vol. 27 No. 1 , pp. 1 - 18 .
Malkiel , B.G. ( 1989 ), “ Efficient market hypothesis ”, Finance , Palgrave Macmillan , London , pp. 127 - 134 .
Mayshar , J. ( 1983 ), “ On divergence of opinion and imperfections in capital markets ”, The American Economic Review , Vol. 73 No. 1 , pp. 114 - 128 .
Merton , R.C. ( 1987 ), “ A simple model of capital market equilibrium with incomplete information ”.
Miller , E.M. ( 1977 ), “ Risk, uncertainty, and divergence of opinion ”, The Journal of Finance , Vol. 32 No. 4 , pp. 1151 - 1168 .
Mukherji , S. ( 2011 ), “ Are stock returns still mean-reverting? ”, Review of Financial Economics , Vol. 20 No. 1 , pp. 22 - 27 .
Nagel , S. ( 2012 ), “ Evaporating liquidity ”, Review of Financial Studies , Vol. 25 No. 7 , pp. 2005 - 2039 .
Odean , T. ( 1998 ), “ Volume, volatility, price, and profit when all traders are above average ”, The Journal of Finance , Vol. 53 No. 6 , pp. 1887 - 1934 .
Odean , T. and Barber , B.M. ( 2009 ), “ Just how much do individual investors lose by trading? ”, Review of Financial Studies , Vol. 22 No. 2 , pp. 609 - 632 .
Oh , G. , Kim , S. and Eom , C. ( 2007 ), “ Market efficiency in foreign exchange markets ”, Physica A: Statistical Mechanics and Its Applications , Vol. 382 No. 1 , pp. 209 - 212 .
Park , J.H. , Nam , S.K. and Eom , K.S. ( 2007 ), “ Market efficiency in KOSDAQ: a volatility comparison between main boards and new markets using a permanent and transitory component model ”, Korean Journal of Financial Studies , Vol. 36 No. 4 , pp. 533 - 566 .
Pele , D.T. , Lazar , E. and Dufour , A. ( 2017 ), “ Information entropy and measures of market risk ”, Entropy , Vol. 19 No. 5 , p. 226 .
Pincus , S.M. ( 1991 ), “ Approximate entropy as a measure of system complexity ”, Proceedings of the National Academy of Sciences , Vol. 88 No. 6 , pp. 2297 - 2301 .
Pincus , S. and Kalman , R.E. ( 2004 ), “ Irregularity, volatility, risk, and financial market time series ”, Proceedings of the National Academy of Sciences , Vol. 101 No. 38 , pp. 13709 - 13714 .
Poterba , J.M. and Summers , L.H. ( 1988 ), “ Mean reversion in stock prices: evidence and implications ”, Journal of Financial Economics , Vol. 22 No. 1 , pp. 27 - 59 .
Richardson , M. and Stock , J.H. ( 1989 ), “ Drawing inferences from statistics based on multiyear asset returns ”, Journal of Financial Economics , Vol. 25 No. 2 , pp. 323 - 348 .
Scheinkman , J.A. and Xiong , W. ( 2003 ), “ Overconfidence and speculative bubbles ”, Journal of Political Economy , Vol. 111 No. 6 , pp. 1183 - 1220 .
Shefrin , H. and Statman , M. ( 1985 ), “ The disposition to sell winners too early and ride losers too long: Theory and evidence ”, The Journal of Finance , Vol. 40 No. 3 , pp. 777 - 790 .
Silvapulle , P. and Choi , J.S. ( 1999 ), “ Testing for linear and nonlinear granger causality in the stock price-volume relation: Korean evidence ”, The Quarterly Review of Economics and Finance , Vol. 39 No. 1 , pp. 59 - 76 .
Sims , C.A. ( 1980 ), “ Martingale-like behavior of prices ”, working paper , National Bureau of Economic Research , June .
Statman , M. , Thorley , S. and Vorkink , K. ( 2006 ), “ Investor overconfidence and trading volume ”, Review of Financial Studies , Vol. 19 No. 4 , pp. 1531 - 1565 .
Tetlock , P.C. ( 2010 ), “ Does public financial news resolve asymmetric information? ”, Review of Financial Studies , Vol. 23 No. 9 , pp. 3520 - 3557 .
Tversky , A. and Kahneman , D. ( 1974 ), “ Judgment under uncertainty: heuristics and biases ”, Science , Vol. 185 No. 4157 , pp. 1124 - 1131 .
Wang , J. ( 1994 ), “ A model of competitive stock trading volume ”, Journal of Political Economy , Vol. 102 No. 1 , pp. 127 - 168 .
Further reading
Rhee , I.K. ( 2002 ), “ Fractionally integrated processes in securities markets ”, The Korean Journal of Financial Management , Vol. 19 No. 2 , pp. 159 - 185 .
Acknowledgements
We are grateful to the anonymous referees for their valuable comments.
Corresponding author
Related articles, we’re listening — tell us what you think, something didn’t work….
Report bugs here
All feedback is valuable
Please share your general feedback
Join us on our journey
Platform update page.
Visit emeraldpublishing.com/platformupdate to discover the latest news and updates
Questions & More Information
Answers to the most commonly asked questions here
Subscribe to the PwC Newsletter
Join the community, edit social preview.

Add a new code entry for this paper
Remove a code repository from this paper, mark the official implementation from paper authors, add a new evaluation result row, remove a task, add a method, remove a method, edit datasets, pamr: passive aggressive mean reversion strategy for portfolio selection.
Machine Learning 2012 · Bin Li , Peilin Zhao , Steven C. H. Hoi · Edit social preview
This article proposes a novel online portfolio selection strategy named “Passive Aggressive Mean Reversion” (PAMR). Unlike traditional trend following approaches, the proposed approach relies upon the mean reversion relation of financial markets. Equipped with online passive aggressive learning technique from machine learning, the proposed portfolio selection strategy can effectively exploit the mean reversion property of markets. By analyzing PAMR’s update scheme, we find that it nicely trades off between portfolio returnand volatility risk and reflects the mean reversion trading principle. We also present several variants of PAMR algorithm, including a mixture algorithm which mixes PAMR and other strategies. We conduct extensive numerical experiments to evaluate the empirical performance of the proposed algorithms on various real datasets. The encouraging results show that in most cases the proposed PAMR strategy outperforms all benchmarks and almost all state-of-the-art portfolio selection strategies under various performance metrics. In addition to its superior performance, the proposed PAMR runs extremely fast and thus is very suitable for real-life online trading applications. The experimental testbed including source codes and data sets is available athttp://www.cais.ntu.edu.sg/~chhoi/PAMR/.
Code Edit Add Remove Mark official
Tasks edit add remove, datasets edit, results from the paper edit add remove, methods edit add remove.
High frequency trading strategies, market fragility and price spikes: an agent based model perspective
- S.I.: Application of O. R. to Financial Markets
- Open access
- Published: 25 August 2018
- Volume 282 , pages 217–244, ( 2019 )
Cite this article
You have full access to this open access article
- Frank McGroarty ORCID: orcid.org/0000-0003-2962-0927 1 ,
- Ash Booth 2 ,
- Enrico Gerding 2 &
- V. L. Raju Chinthalapati 3
20k Accesses
28 Citations
3 Altmetric
Explore all metrics
Given recent requirements for ensuring the robustness of algorithmic trading strategies laid out in the Markets in Financial Instruments Directive II, this paper proposes a novel agent-based simulation for exploring algorithmic trading strategies. Five different types of agents are present in the market. The statistical properties of the simulated market are compared with equity market depth data from the Chi-X exchange and found to be significantly similar. The model is able to reproduce a number of stylised market properties including: clustered volatility, autocorrelation of returns, long memory in order flow, concave price impact and the presence of extreme price events. The results are found to be insensitive to reasonable parameter variations.
Similar content being viewed by others
Rock around the clock: an agent-based model of low- and high-frequency trading.
Agent-Based Modelling of Stock Markets Using Existing Order Book Data
Heterogeneity, spontaneous coordination and extreme events within large-scale and small-scale agent-based financial market models.
Avoid common mistakes on your manuscript.
1 Introduction
Over the last three decades, there has been a significant change in the financial trading ecosystem. Markets have transformed from exclusively human-driven systems to predominantly computer driven. These machine driven markets have laid the foundations for a new breed to trader: the algorithm. According to Angel et al. ( 2010 ), algorithmically generated orders are now thought to account for over 80% of volume traded on US equity markets, with figures continuing to rise.
The rise of algorithmic trading has not been a smooth one. Since its introduction, recurring periods of high volatility and extreme stock price behaviour have plagued the markets. The SEC and CFTC ( 2010 ) report, among others, has linked such periods to trading algorithms, and their frequent occurrence has undermined investors confidence in the current market structure and regulation. Indeed, Johnson et al. ( 2013 ) reports that so called extreme price movement Flash Crashes are becoming ever more frequent with over 18,000 of them occurring between 2006 and 2011 in various stocks.
Thus, in this paper, we describe for the first time an agent-based simulation environment that is realistic and robust enough for the analysis of algorithmic trading strategies. In detail, we describe an agent-based market simulation that centres around a fully functioning limit order book (LOB) and populations of agents that represent common market behaviours and strategies: market makers, fundamental traders, high-frequency momentum traders, high-frequency mean reversion traders and noise traders.
The model described in this paper includes agents that operate on different timescales and whose strategic behaviours depend on other market participants. The decoupling of actions across timescales combined with dynamic behaviour of agents is lacking from previous models and is essential in dictating the more complex patterns seen in high-frequency order-driven markets. Consequently, this paper presents a model that represents a richer set of trading behaviours and is able to replicate more of the empirically observed empirical regularities than any other paper. Such abilities provide a crucial step towards a viable platform for the testing of trading algorithms as outlined in MiFID II.
We compare the output of our model to depth-of-book market data from the Chi-X equity exchange and find that our model accurately reproduces empirically observed values for: autocorrelation of price returns, volatility clustering, kurtosis, the variance of price return and order-sign time series and the price impact function of individual orders. Interestingly, we find that, in certain proportions, the presence of high-frequency trading agents gives rise to the occurrence of extreme price events. We asses the sensitivity of the model to parameter variation and find the proportion of high-frequency strategies in the market to have the largest influence on market dynamics.
This paper is structured as follows: Sect. 2 gives a background on the need for increased regulation and the rise of MiFID II. Section 3 gives an overview of the relevant literature while Sect. 4 provides a description of the model structure and agent behaviours in detail. In Sect. 5 the results are summarised while Sect. 6 gives concluding remarks and discusses potential future work.
2 The need for improved oversight and the scope of MiFID II
One of the more well known incidents of market turbulence is the extreme price spike of the 6th May 2010. At 14:32, began a trillion dollar stock market crash that lasted for a period of only 36 min (Kirilenko et al. 2014 ). Particularly shocking was not the large intra-day loss but the sudden rebound of most securities to near their original values. This breakdown resulted in the second-largest intraday point swing ever witnessed, at 1010.14 points. Only 2 weeks after the crash, the SEC and CFTC released a joint report that did little but quash rumours of terrorist involvement. During the months that followed, there was a great deal of speculation about the events on May 6th with the identification of a cause made particularly difficult by the increased number of exchanges, use of algorithmic trading systems and speed of trading. Finally, the SEC and CFTC released their report on September 30th concluding that the event was initiated by a single algorithmic order that executed a large sale of futures contracts in an extraordinarily short amount of time from fund management firm Waddell and Reed (W&R) (SEC 2010 ).
The report was met with mixed responses and a number of academics have expressed disagreement with the SEC report. Menkveld and Yueshen ( 2013 ) analysed W&Rs orderflow and identified an alternative narrative. They did not conclude that the crash was simply the price W&R were required to take for demanding immediacy in the S&P. Instead, they found that cross-market arbitrage, which provided e-mini sellers with increased liquidity from S&P buyers in other markets, broke down minutes before the crash. As a result of the breakdown, W&R were forced to find buyers only in E-mini and so they decelerated their selling. An extreme response (in terms of price and selling behaviour) then resulted in W&R paying a disproportionately high price for demanding liquidity.
Easley and Prado ( 2011 ) show that major liquidity issues were percolating over the days that preceded the price spike. They note that immediately prior to the large W&R trade, volume was high and liquidity was low. Using a technique developed in previous research (Easley et al. 2010 ), they suggest that, during the period in question, order flow was becoming increasingly toxic. They go on to demonstrate how, in a high-frequency world, such toxicity may cause market makers to exit - sowing the seeds for episodic liquidity. Of particular note, the authors express their concern that an anomaly like this is highly likely to occur, once again, in the future.
Another infamous crash occurred on the 23rd March 2012 during the IPO of a firm called BATS. The stock began trading at 11:14 a.m. with an initial price of $15.25. Within 900 ms of opening, the stock price had fallen to $0.28 and within 1.5 s, the price bottomed at $0.0007. Yet another technological incident was witnessed when, on the 1st August 2012, the new market-making system of Knight Capital was deployed. Knight Capital was a world leader in automated market making and a vocal advocate of automated trading. The error occurred when testing software was released alongside the final market-making software. According to the official statement of Knight Capital Group ( 2012 ):
Knight experienced a technology issue at the open of trading...this issue was related to Knights installation of trading software and resulted in Knight sending numerous erroneous orders in NYSE-listed securities...which has resulted in a realised pre-tax loss of approximately 440 million [dollars].
This 30 min of bogus trading brought an end to Knights 17 year existence, with the firm subsequently merging with a rival.
The all-too-common extreme price spikes are a dramatic consequence of the growing complexity of modern financial markets and have not gone unnoticed by the regulators. In November 2011, the European Union ( 2011 ) made proposals for a revision of the Markets in Financial Instruments Directive (MiFID). Although this directive only governs the European markets, according to the World Bank ( 2012 ) (in terms of market capitalisation), the EU represents a market around two thirds of the size of the US. In the face of declining investor confidence and rapidly changing markets, a draft of MiFID II was produced. After nearly three years of debate, on the 14th January 2014, the European Parliament and the Council reached an agreement on the updated rules for MiFID II, with a clear focus on transparency and the regulation of automated trading systems (European Union 2014 ).
MiFID II came to be as a result of increasing fears that algorithmic trading had the potential to cause market distortion over unprecedented timescales. Particularly, there were concerns over increased volatility, high cancellation rates and the ability of algorithmic systems to withdraw liquidity at any time. Thus, MiFID II introduces tighter regulation over algorithmic trading, imposing specific and detailed requirements over those that operate such strategies. This increased oversight requires clear definitions of the strategies under regulation.
MiFID II defines algorithmic trading as the use of computer algorithms to automatically determine the parameters of orders, including: trade initiation, timing, price and modification/cancellation of orders, with no human intervention. This definition specifically excludes any systems that only deal with order routing, order processing, or post trade processing where no determination of parameters is involved.
The level of automation of algorithmic trading strategies varies greatly. Brokers and large sell side institutions tend to focus on optimal execution, where the aim of the algorithmic trading is to minimise the market impact of orders. These algorithms focus on order slicing and timing. Other institutions, often quantitative buy-side firms, attempt to automate the entire trading process. These algorithms may have full discretion regarding their trading positions and encapsulate: price modelling and prediction to determine trade direction, initiation, closeout and monitoring of portfolio risk. This type of trading tends to occur via direct market access (DMA) or sponsored access.
Under MiFID II, HFT is considered as a subset of algorithmic trading. The European Commission defines HFT as any computerised technique that executes large numbers of transactions in fractions of a second using:
Infrastructure designed for minimising latencies, such as proximity hosting, collocation or DMA.
Systematic determination of trade initiation, closeout or routing with-out any human intervention for individual orders; and
High intra-day message rates due to volumes of orders, quotes or cancellations.
Specifically, MiFID II introduces rules on algorithmic trading in financial instruments. Any firm participating in algorithmic trading is required to ensure it has effective controls in place, such as circuit breakers to halt trading if price volatility becomes too high. Also, any algorithms used must be tested and authorised by regulators. We find the last requirement particularly interesting as MiFID II is not specific about how algorithmic trading strategies are to be tested.
Given the clear need for robust methods for testing these strategies in such a new, relatively ill-explored and data-rich complex system, an agent-oriented approach, with its emphasis on autonomous actions and interactions, is an ideal approach for addressing questions of stability and robustness.
3 Background and related work
This section begins by exploring the literature on the various universal statistical properties (or stylised facts) associated with financial markets. Next, modelling techniques from the market microstructure literature are explored before discussing the current state of the art in agent-based modelling of financial markets.
3.1 The statistical properties of limit order markets
The empirical literature on LOBs is very large and several non-trivial regularities, so-called stylised facts, have been observed across different asset classes, exchanges, levels of liquidity and markets. These stylised facts are particularly useful as indicators of the validity of a model (Buchanan 2012 ). For example, Lo and MacKinlay ( 2001 ) show the persistence of volatility clustering across markets and asset classes, which disappears with a simple random walk model for the evolution of price time series, as clustered volatility suggests that large variation in price are more like to follow other large variations.
3.1.1 Fat-tailed distribution of returns
Across all timescales, distributions of price returns have been found to have positive kurtosis, that is to say they are fat-tailed. An understanding of positively kurtotic distribution is paramount for trading and risk management as large price movements are more likely than in commonly assumed normal distributions.
Fat tails have been observed in the returns distributions of many markets including: the American Stock Exchange, Euronext, the LSE, NASDAQ, and the Shenzhen Stock Exchange (see Cont 2001 ; Plerou and Stanley 2008 ; Chakraborti et al. 2011 ) but the precise form of the distribution varies with the timescale used. Gu et al. ( 2008 ) found that across various markets, the tails of the distribution at very short timescales are well-approximated by a power law with exponent \(\alpha \approx 3\) . Drozdz et al. ( 2007 ) found tails to be less heavy ( \(\alpha > 3\) ) in high-frequency data for various indices from 2004 to 2006, suggesting that the specific form of the stylised facts may have evolved over time with trading behaviours and technology. Both Gopikrishnan et al. ( 1998 ) and Cont ( 2001 ) have found that at longer timescales, returns distributions become increasingly similar to the standard normal distribution.
3.1.2 Volatility clustering
Volatility clustering refers to the long memory of absolute or square mid-price returns and means that large changes in price tend to follow other large price changes. Cont ( 2001 ), and Stanley et al. ( 2008 ) found this long memory phenomenon to exist on timescales of weeks and months while its existence has been documented across a number of markets, including: the NYSE, Paris Bourse, S&P 500 index futures and the USD/JPY currency pair (Cont 2005 ; Gu and Zhou 2009 ; Chakraborti et al. 2011 ). Lillo and Farmer ( 2004 ) formalise the concept as follows. Let \(X = X(t_1),X(t_2),\ldots ,X(t_k)\) denote a real-valued, wide-sense stationary time series. Then, we can characterise long memory using the diffusion properties of the integrated series Y :
A stationary process \(Y_t\) (with finite variance) is then said to have long range dependence if its autocorrelation function, \(C(l) = corr(Y_t,Y_{t+\tau })\) , decays as a power of the lag:
for some \(H \in (0.5,1)\) . The exponent H is known as the Hurst exponent.
In the empirical research studies outlined above, the values of the Hurst exponent varied from \(H \approx 0.58\) on the Shenzhen Stock Exchange to \(H \approx 0.815\) for the USD/JPY currency pair. There are a number of potential explanations for volatility clustering and Bouchaud et al. ( 2009 ) suggest the arrival of news and the splicing of large orders by traders.
3.1.3 Autocorrelation of returns
Stanley et al. ( 2008 ) and Chakraborti et al. ( 2011 ) observed that, across a number of markets, returns series lacked significant autocorrelation, except for weak, negative autocorrelation on very short timescales. This includes: Euronext, FX markets, the NYSE and the S&P500 index (Chakraborti et al. 2011 ; At-Sahalia et al. 2011 ). Cont ( 2001 ) explains the absence of strong autocorrelations by proposing that, if returns were correlated, traders would use simple strategies to exploit the autocorrelation and generate profit. Such actions would, in turn, reduce the autocorrelation such that the autocorrelation would no longer remain. Evidence suggests that the small but significant negative autocorrelation found on short time-scales has disappeared more quickly in recent years, perhaps an artefact of the new financial ecosystem. Bouchaud and Potters ( 2003 ) report that from 1991 to 1995, negative autocorrelation persisted on timescales of up to 20–30 min but no longer for the GBP/USD currency pair. Moreover, Cont et al. ( 2013 ), discovered no significant autocorrelation for timescales of over 20 s in the NYSE during 2010.
3.1.4 Long memory in order flow
The probability of observing a given type of order in the future is positively correlated with its empirical frequency in the past. In fact, analysis of the time series generated by assigning the value \(+\) 1 to incoming buy orders and − 1 to incoming sell orders has been shown to display long memory on the NYSE, the Paris Bourse and the Shenzhen stock Exchange (Gu and Zhou 2009 ). Study of the LSE has been particularly active, with a number of reports finding similar results for limit order arrivals, market order arrivals and order cancellations, while Axioglou and Skouras ( 2011 ) suggest that the long memory reported by Lillo and Farmer ( 2004 ) was simply an artefact caused by market participants changing trading strategies each day.
3.1.5 Long memory in returns
The long memory in order flow discussed above has lead some to expect long memory in return series, yet has not been found to be the case. Studies on the Deutsche Bourse, the LSE and on the Paris Bourse have all reported Hurst exponents of around 0.5, i.e. no long memory (Carbone et al. 2004 ; Lillo and Farmer 2004 ). Bouchaud et al. ( 2004 ) have suggested that this may be due to the long memory of market orders being negatively correlated with the long memory of price changes caused by the long memory of limit order arrival and cancellation.
3.1.6 Price impact
The changes in best quoted prices that occur as a result of a trader’s actions is termed the price impact. The importance of monitoring and minimising price impact precedes the extensive adoption of electronic order driven markets. This paper will specifically focus on the impact of single transactions in limit order markets (as opposed to the impact of a large parent order) with volume v .
A Great deal of research has investigated the impact of individual orders, and has conclusively found that impact follows a concave function of volume. That is, the impact increases more quickly with changes at small volumes and less quickly at larger volumes. However, the detailed functional form has been contested and varies across markets and market protocols (order priority, tick size, etc.).
Some of the earliest literature found strongly concave functions though did not attempt to identify a functional form (Hasbrouck 1991 ; Hausman et al. 1992 ). In a study of the NYSE, Lillo et al. ( 2003 ) analysed the stocks of 1000 companies and divided them into groups according to their market capitalisation. Fitting a price impact curve to each group, they found that the curves could be collapsed into a single function that followed a power law distribution of the following form:
where \(\Delta p\) is the change in the mid-price caused by a traders action, v is the volume of the trade, \(\eta \) takes the value 1 in the event of a sell and \(+\) 1 in the event of a buy and \(\lambda \) allows for adjustment for market capitalisation. They found the exponent \(\beta \) to be approximately 0.5 for small volumes and 0.2 for large volumes. After normalising for daily volumes, \(\lambda \) was found to vary significantly across stocks with a clear dependence on market capitalisation M approximated by \(M \approx \lambda ^\delta \) , with \(\delta \) in the region of 0.4.
Good approximations of the value for the exponent \(\beta \) have also been found by Lillo and Farmer ( 2004 ) on the London Stock Exchange and Hopman ( 2007 ) on the Paris Bourse to fall in the range 0.3–0.4. Consequently, all explorations have identified strongly concave impact functions for individual orders but find slight variations in functional form owing to differences in market protocols.
3.1.7 Extreme price events
Though the fat-tailed distribution of returns and the high probability of large price movements has been observed across financial markets for many years (as documented in Sect. 3.1.1 ), the new technology-driven marketplace has introduced a particularly extreme kind of price event.
Since the introduction of automated and algorithmic trading, recurring periods of high volatility and extreme stock price behaviour have plagued the markets. Johnson et al. ( 2013 ) define these so called price spikes as an occurrence of a stock price ticking down [up] at least ten times before ticking up [down] and with a price change exceeding 0.8% of the initial price. Remarkably, they found 18,520 crashes and spikes with durations less than 1500 ms to have occurred between January 3rd 2006 and February 3rd 2011 in various stocks. One of the many aims of recent regulation such as MiFID II and the DoddFrank Wall Street Reform and Consumer Protection Act is to curtail such extreme price events.
3.2 Modelling limit order books
The financial community has expressed an active interest in developing models of LOB markets that are realistic, practical and tractable (see Predoiu et al. 2011 ; Obizhaeva and Wang 2013 ). The literature on this topic is divided into four main streams: theoretical equilibrium models from financial economics, statistical order book models from econophysics, stochastic models from the mathematical finance community, and agent-based models (ABMs) from complexity science. Each of these methodologies is described below with a detailed discussion of ABMs in Sect. 3.3 .
Financial economics models tend to be built upon the idea of liquidity being consumed during a trade and then replenished as liquidity providers try to benefit. Foucault et al. ( 2005 ) and Goettler et al. ( 2005 ), for example, describe theoretical models of LOB markets with finite levels of resilience in equilibrium that depend mainly on the characteristics of the market participants. In these models, the level of resilience reflects the volume of hidden liquidity. Many models are partial equilibrium in nature. taking the dynamics of the limit order book as given. For example, Predoiu et al. ( 2011 ) provide a framework that allows discrete orders and more general dynamics, while Alfinsi et al. ( 2010 ) implement general but continuous limit order books. In order to operate in a full equilibrium setting, models have to heavily limit the set of possible order-placement strategies. Rosu ( 2009 ), for example, allows only orders of a given size, while Goettler et al. ( 2005 ) only explore single-shot strategies. Though these simplifications enable the models to more precisely describe the tradeoffs presented by market participants, it comes at the cost of unrealistic assumptions and simplified settings. It is rarely possible to estimate the parameters of these models from real data and their practical applicability is limited (Farmer and Foley 2009 ). Descriptive statistical models, on the other hand, tend to fit the data well but often lack economic rigour and typically involve the tuning of a number of free parameters (Cont et al. 2010 ). Consequently, their practicability is questioned.
Stochastic order book models attempt to balance descriptive power and analytical tractability. Such models are distinguished by their representation of aggregate order flows by a random process, commonly a Poisson process (as in Farmer et al. 2005 ; Cont et al. 2010 ). Unfortunately, the high level statistical description of participant behaviour inherent in stochastic order book models ignores important complex interactions between market participants and fails to explain many phenomena that arise (Johnson et al. 2013 ). As such, a richer bottom-up modelling approach is needed to enable the further exploration and understanding of limit order markets.
3.3 Agent-based models
Grimm et al. ( 2006 ) provides a simple yet adept definition of ABMs as models in which a number of heterogeneous agents interact with each other and their environment in a particular way. One of the key advantages of ABMs, compared to the aforementioned modelling methods, is their ability to model heterogeneity of agents. Moreover, ABMs can provide insight into not just the behaviour of individual agents but also the aggregate effects that emerge from the interactions of all agents. This type of modelling lends itself perfectly to capturing the complex phenomena often found in financial systems and, consequently, has led to a number of prominent models that have proven themselves incredibly useful in understanding, e.g. the interactions between trading algorithms and human traders (De Luca and Cliff 2011 ), empirical regularities in the inter-bank foreign exchange market Chakrabarti ( 2000 ), the links between leveraged investment and bubbles/crashes in financial markets (Thurner et al. 2012 ), and the complexities of systemic risk in the wider economy (Geanakoplos et al. 2012 ).
The effectiveness of ABMs has also been demonstrated with LOBs. The first ABMs of LOBs assume the sequential arrival of agents and the emptying of the LOB after each time step (see e.g. Foucault 1999 ). Unfortunately, Smith et al. ( 2003 ) notes that approaches such as this fail to appreciate the function of the LOB to store liquidity for future consumption. More recently, ABMs have begun to closely mimic true order books and successfully reproduce a number of the statistical features described in Sect. 3 .
To this end, Cont and Bouchaud ( 2000 ) demonstrate that in a simplified market where trading agents imitate each other, the resultant returns series fits a fat-tailed distribution and exhibits clustered volatility. Furthermore, Chiarella and Iori ( 2002 ) describe a model in which agents share a common valuation for the asset traded in a LOB. They find that the volatility produced in their model is far lower than is found in the real world and there is no volatility clustering. They thus suggest that significant heterogeneity is required for the properties of volatility to emerge.
Additionally, Challet and Stinchcombe ( 2003 ) note that most LOB mod-els assume that trader parameters remain constant through time and explore how varying such parameters through time affected the price time series. They find that time dependence results in the emergence of autocorrelated mid-price returns, volatility clustering and the fat-tailed distribution of mid-price changes and they suggest that many empirical regularities might be a result of traders modifying their actions through time.
Correspondingly, Preis et al. ( 2006 ) reproduced the main findings of the state-of-the-art stochastic models using an ABM rather than and independent Poisson process, while Preis et al. ( 2007 ) digs deeper and explores the effects of individual agents in the model. They found that the Hurst expo-nent of the mid-price return series depends strongly on the relative numbers of agent types in the model.
In similar vein, Mastromatteo et al. ( 2014 ) use a dynamical-systems / agent-based approach to understand the non-additive, square-root dependence of the impact of meta-orders in financial markets. Their model finds that this function is independent of epoch, microstructure and execution style. Although their study lends strong support to the idea that the square-root impact function is both highly generic and robust, Johnson et al. ( 2013 ) notes that it is somewhat specialised and lacks some of the important agent-agent interactions that give rise to crashes that spikes and crashes in price that have been seen to regularly occur in LOB markets.
Similarly, Oesch ( 2014 ) describes an ABM that highlights the importance of the long memory of order flow and the selective liquidity behaviour of agents in replicating the concave price impact function of order sizes. Although the model is able to replicate the existence of temporary and permanent price impact, its use as an environment for developing and testing trade execution strategies is limited. In its current form, the model lacks agents whose strategic behaviours depend on other market participants.
Though each of the models described above are able to replicate or explain one or two of the stylised facts reported in Sect. 3.1 , no one model exists that demonstrates all empirically observed regularities a clear requirement of a model intended for real-world validation. Also, no paper has yet presented agents that are operate on varying timescales. Against this background, we propose a novel modelling environment that includes a number of agents with strategic behaviours that act on differing timescales as it is these features, we believe, that are essential in dictating the more complex patterns seen in high-frequency order-driven markets.
4 The model
This paper describes a model Footnote 1 that implements a fully functioning limit order book as used in most electronic financial markets. By following the principle of Occam’s razor, “the simplest explanation is more likely the correct one”, we consider a limited set of parameters that would show a possible path of the system dynamics. The main objective of the proposed agent based model is to identify the emerging patterns due to the complex/complicated interactions within the market. We consider five categories of traders (simplest explanation of the market ecology) which enables us to credibly mimic (including extreme price changes) price patterns in the market. The model is stated in pseudo-continuous time. That is, a simulated day is divided into \(T = 300{,}000\) periods (approximately the number of 10ths of a second in an 8.5 h trading day) and during each period there is a possibility for each agent to act a close approximation to reality. The model comprises of 5 agent types: Market makers, liquidity consumers, mean reversion traders, momentum traders and noise traders that are each presented in detail later in this section.
To replicate the mismatch in the timescales upon which market participants can act [as highlighted by Johnson et al. ( 2013 )], during each period every agent is given the opportunity to act based on probability, \(\delta _{\tau }\) , that is determined by their type, \(\tau \) , (market maker, trend follower, etc.). In more detail, to represent a high-frequency trader’s ability to react more quickly to market events than, say, a long term fundamental investor, we assigned a higher delta providing a higher chance of being chosen to act. Importantly, when chosen, agents are not required to act. This facet allows agents to vary their activity through time and in response the market, as with real-world market participants. A more formal treatment of the simulation logic is presented in Algorithm 1 :

The probability of a member of each agent group acting is denoted \(\delta _{mm}\) for market makers, \(\delta _{lc}\) for liquidity consumers, \(\delta _{mr}\) for mean reversion traders, \(\delta _{mt}\) for momentum traders and \(\delta _{nt}\) for noise traders. Upon being chosen to act, if an agent wishes to submit an order, it will communicate an order type, volume and price determined by that agent’s internal logic. The order is then submitted to the LOB where it is matched using price-time priority. If no match occurs then the order is stored in the book until it is later filled or canceled by the originating trader. Such a model conforms to the adaptive market hypothesis proposed by Lo ( 2004 ) as the market dynamics emerge from the interactions of a number of species of agents adapting to a changing environment using simple heuristics. Although the model contains a fair number of free parameters, those parameters are determined through experiment (see Sect. 5.1 ) and found to be relatively insensitive to reasonable variation. Below we define the 5 agent types.
As mentioned above, MiFID II characterises HFT as transactions executing in fractions of a second. Since the model considers that the minimum possible time for execution of transactions is approximately \(\frac{1}{10}\) th of a second, the artificial market with the agents represents HFT environment. The market ecology of traders is described by the participating agents types, with the trading speed of the individual agent being determined by that agent’s action probability \(\delta _{\tau }\) . We set the parametric values for \(\delta _{\tau }\) such that the artificial market summary statistics most closely resembles those of the real market. For example, in Sect. 5 , we identify the required action probabilities in order to calibrate the agent based models are \(\delta _{mm}=0.1, \delta _{lc}=0.1, \delta _{mr}=0.4, \delta _{mt}=0.4, \delta _{nt}=0.75\) . One can see that the chances of participation of the noise traders at each and every tick of the market is high which means that noise traders are very high frequency traders. Similarly, the trading speed of the traders from the other categories can be verified. Lower action probabilities correspond to slower the trading speeds.
4.1 Market makers
Market makers represent market participants who attempt to earn the spread by supplying liquidity on both sides of the LOB. In traditional markets, market makers were appointed but in modern electronic exchanges any agent is able to follow such a strategy. Footnote 2 These agents simultaneously post an order on each side of the book, maintaining an approximately neutral position throughout the day. They make their income from the difference between their bids and oers. If one or both limit orders is executed, it will be replaced by a new one the next time the market maker is chosen to trade. In this paper we implement an intentionally simple market making strategy based on the liquidity provider strategy described by Oesch ( 2014 ). Each round, the market maker generates a prediction for the sign of the next period’s order using a simple w period rolling-mean estimate. When a market maker predicts that a buy order will arrive next, she will set her sell limit order volume to a uniformly distributed random number between \(v_{min}\) and \(v_{max}\) and her buy limit order volume to \(v^{-}\) . An algorithm describing the market makers logic is given in Algorithm 2 .

4.2 Liquidity consumers
Liquidity consumers represent large slower moving funds that make long term trading decisions based on the rebalancing of portfolios. In real world markets, these are likely to be large institutional investors. These agents are either buying or selling a large order of stock over the course of a day for which they hope to minimise price impact and trading costs. Whether these agents are buying or selling is assigned with equal probability. The initial volume \(h_0\) of a large order is drawn from a uniform distribution between \(h_{min}\) and \(h_{max}\) . To execute the large order, a liquidity consumer agent looks at the current volume available at the opposite best price, \(\varPhi _t\) . If the remaining volume of his large order, \(h_t\) , is less than \(\varPhi _t\) the agent sets this periods volume to \(v_t = h_t\) , otherwise he takes all available volume at the best price, \(v_t = \varPhi _t\) . For simplicity liquidity consumers only utilise market orders. An algorithm describing the Liquidity Consumer’s logic is given in Algorithm 3 .
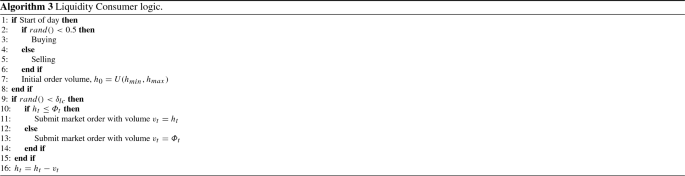

4.3 Momentum traders
This group of agents represents the first of two high frequency traders. This set of agents invest based on the belief that price changes have inertia a strategy known to be widely used (Keim and Madhavan 1995 ). A momentum strategy involves taking a long position when prices have been recently rising, and a short position when they have recently been falling. Specifically, we implement simple momentum trading agents that rely on calculating a rate of change (ROC) to detect momentum, given by:
When \(\text {roc}_t\) is greater than some threshold \(\kappa \) the momentum trader enters buy market orders of a value proportional to the strength of the momentum. That is, the volume of the market order will be:
where \(W_{a,t}\) is the wealth of agent a at time t . A complete description of the momentum trader’s logic is given in Algorithm 4 .

4.4 Mean reversion traders
The second group of high-frequency agents are the mean-reversion traders. Again, this is a well documented strategy (Serban 2010 ) in which traders believe that asset prices tend to revert towards their a historical average (though this may be a very short term average). They attempt to generate profit by taking long positions when the market price is below the historical average price, and short positions when it is above. Specifically, we define agents that, when chosen to trade, compare the current price to an exponential moving average of the asset price, ema \(_t\) , at time t calculated as:
where \(p_t\) is the price at time t and \(\alpha \) is a discount factor that adjust the recency bias. If the current price, \(p_t\) , is k standard deviations above \(ema_t\) the agent enters a sell limit order at a single tick size improvement of the best price offer, and if it is k standard deviations below then he enters a buy. The volume of a mean reversion trader’s order is denoted by \(v_{mr}\) . An algorithm describing the mean reversion traders logic is given in Algorithm 5 .

4.5 Noise traders
These agents are defined so as to capture all other market activity and are modelled very closely to Cui and Brabazon ( 2012 ). There parameters are fitted using empirical order probabilities. The noise traders are randomly assigned whether to submit a buy or sell order in each period with equal probability. Once assigned, they then randomly place either a market or limit order or cancel an existing order according to the probabilities \(\lambda _m\) , \(\lambda _l\) and \(\lambda _c\) respectively.
When submitting an order, the size of that order, \(v_t\) , is drawn from a log-normal distribution described by:
where \(\mu \) and \(\sigma \) represent the mean and standard deviation of the \(v_t\) s natural logarithm and \(u_v\) is a uniformly distributed random variable between 0 and 1. If a limit order is required the noise trader faces four further possibilities:
With probability \(\lambda _{\text {crs}}\) the agent crosses the spread and places a limit order at the opposing best ensuring immediate (but potentially partial) order fulfillment. If the order is not completely filled, it will remain in the order book.
With probability \(\lambda _{\text {inspr}}\) the agent places a limit order at a price within the bid and ask spread, \(p_{\text {inspr}}\) , that is uniformly distributed between the best bid and ask.
With probability \(\lambda _{\text {spr}}\) the agent places a limit order at the best price available on their side of the book.
With probability \(\lambda _{\text {offspr}}\) the agent will place a limit order deeper in the book, at a price, \(p_{\text {offspr}}\) , distributed with the power law:
where \(u_0\) is a uniformly distributed random variable between 0 and 1 while \(\text {xmin}_{\text {offspr}}\) and \(\beta \) are parameters of the power law that are fitted to empirical data.
The sum of these probabilities must equal one \((\lambda _\text {crs}+\lambda _\text {inspr}+\lambda _\text {spr}+\lambda _\text {ospr} = 1)\) . To prevent spurious price processes, noise traders market orders are limited in volume such that they cannot consume more than half of the total opposing side’s available volume. Another restriction is that noise traders will make sure that no side of the order book is empty and place limit orders appropriately. The full noise trader logic is described in Algorithm 6 .
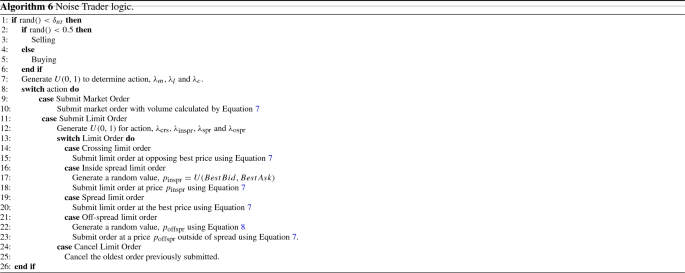
We believe that our range of 5 types of market participant reflects a more realistically diverse market ecology than is normally considered in models of financial markets. Some traders in our model are uninformed and their noise trades only ever contribute random perturbations to the price path. While other trader types are informed, it would be unrealistic to think that that these could monitor the market and exploit anomalies in an unperturbed way. In reality, there are always time lags between observation and consequent action between capturing market data, deducing an opportunity, and implementing a trade to exploit it. These time gaps may persist for only a few milliseconds but in todays most liquid assets, many quotes, cancellations and trades can occur in a few milliseconds. Even in such small time intervals, a sea of different informed and uninformed traders compete with each other. Among the informed traders, some perceived trading opportunities will be based on analysis of long-horizon returns, while others will come into focus only when looking at short-term return horizons. Traders will possess differing amounts of information, and some will make cognitive errors or omissions. The upshot of all this is that some traders perceive a buying opportunity where others will seek to sell. That conclusion should not be controversial. Buyers and sellers must exist in the same time interval for any trading to occur. Real financial markets are maelstroms of competing forces and perspectives, and the only way to model them with any degree of realism is by using some sort of random selection process.
Our analysis shows that the standard models of market microstructure are too Spartan to be used directly as the basis for agent-based simulations. However, by enriching these standard market microstructure model with insights from behavioural finance, we develop a usable agent based model for finance. OHara ( 1995 ) identifies three main market-microstructure agent types: market-makers, uninformed (noise) traders and informed traders. The first two agent-types are clearly identifiable in our framework. Our three remaining types of agent are different types of informed agent. While the market microstructure literature does not distinguish between different types of informed agent, behavioural finance researchers make precisely this distinction e.g. Using a multi-month return horizon, Jegadeesh and Titman ( 1993 ) showed that exploiting observed momentum (i.e. positive serial correlation) effects in empirical data by buying winners and selling losers was a robust profitable trading strategy. De Bondt and Thaler ( 1985 ) found the opposite effect at a different time horizon. They showed how persistent reversal (negative serial correlation) observed in multi-year stock returns can be profitably exploited by a similar, but opposite, buy-losers and sell-winners trading rule strategy. A re-examination of the market microstructure literature bearing these ideas in mind is revealing.
Almost all market microstructure models about informed trading, dating back to Bagehot ( 1971 ), assume that private information is exogenously derived. This is consistent with our liquidity consumer agent type and also with the view of information being based on fundamental information about intrinsic value but it is at odds with our momentum and mean reversion traders. However, an empirical market microstructure paper by Evans and Lyons ( 2002 ) opens the door to the idea that private information could be based on endogenous technical (i.e. price and volume) information, such as drives our momentum and mean-reversion agents. Evans and Lyons ( 2002 ) show that price behaviour in the foreign exchange markets is a function of cumulative order flow. Order flow is the difference between buyer-initiated trading volume and seller-initiated trading volume. It can be thought of as a measure of net buying (selling) pressure. Crucially, order flow does not require any fundamental model to be specified. Endogenous technical price behaviour is sufficient to generate it. The preceding enables us to conclude that while our 5 types of market participant initially seem at odds with the standard market microstructure model, closer scrutiny reveals that all 5 of our agent types have very firm roots in the market microstructure literature.
In this section we begin by performing a global sensitivity analysis to explore the influence of the parameters on market dynamics and ensure the robustness of the model. Subsequently, we explore the existence of the following stylised facts in depth-of-book data from the Chi-X exchange compared with our model: fat tailed distribution of returns, volatility clustering, autocorrelation of returns, long memory in order flow, concave price impact function and the existence of extreme price events.
5.1 Sensitivity analysis
In this section, we asses the sensitivity of the agent-based model described above. To do so, we employ an established approach to global sensitivity analysis known as variance-based global sensitivity (Sobol 2001 ). In variance-based global sensitivity analysis, the inputs to an agent-based model are treated as random variables with probability density functions representing their associated uncertainty. The impact of the set of input variables on a model’s output measures may be independent or cooperative and so the output f ( x ) may be expressed as a finite hierarchical cooperative function expansion using an analysis of variance (ANOVA). Thus, the mapping between input variables \(x_1,\ldots ,x_n\) and output variables \(f(x) = f(x_1,\ldots ,x_n)\) may be expressed in the following functional form:
where \(f_0\) is the zeroth order mean effect, \(f_i(x_i)\) is a first order term that describes the effect of variable \(x_i\) on the output f ( x ), and \(f_{i,j}(x_i,x_j)\) is a second order term that describes the cooperative impact of variables \(x_i\) and \(x_j\) on the output. The final term, \(f_{1,2,\ldots ,n}(x_1,x_2,\ldots ,x_n)\) describe the residual n th order cooperative effect of all of the input variables. Consequently, the total variance is calculated as follows:
where \(\rho (x)\) is the probability distribution over input variables. Partial variances are then defined as:
Now, the total partial variances \(D_{i}^{tot}\) for each parameter \(x_i\) , \(i = \overline{1,n}\) , is computed as
where \(\langle i \rangle \) refers to the summations over all D that contains i . Once the above is computed, the total sensitivity indicies can be calculated as:
It follows that the total partial variance for each parameter \(x_i\) is
In this paper, twenty three input parameters and four output parameters are considered. The input parameters include: The probabilities of each of the five agent groups performing an action ( \(\delta _{mm}, \delta _{lc}, \delta _{mr}, \delta _{mt}, \delta _{nt}\) ), the market makers parameters ( w , the period length of the rolling mean, and \(v_{max}\) , the max order volume for limit order), the upper limit of the distribution from which liquidity consumers order volume is drawn ( \(h_{max}\) ), the momentum traders’ parameters ( \(n_r\) , the lag parameter of the ROC, and \(\kappa \) , the trade entry point threshold), as well as the following noise trader parameters:
Probability of submitting a market order, \(\lambda _m\)
Probability of submitting a limit order, \(\lambda _l\)
Probability of canceling a limit order, \(\lambda _c\)
Probability of a crossing limit order, \(\lambda _{crs}\)
Probability of a inside-spread limit order, \(\lambda _{inspr}\)
Probability of a spread limit order, \(\lambda {spr}\)
Probability of a off-spread limit order, \(\lambda _{offspr}\)
Market order size distribution parameters, \(\mu _{mo}\) and \(\sigma _{mo}\)
Limit order size distribution parameters, \(\mu _{lo}\) and \(\sigma _{lo}\)
Off-spread relative price distribution parameters, \(xmin_{offspr}\) and \(\beta _{offspr}\)
The following output parameters are monitored: the Hurst exponent H of volatility [as calculated using the DFA method described by Peng et al. ( 1994 )], the mean autocorrelation of mid-price returns R ( m ), the mean first lag autocorrelation term of the order-sign series R ( o ), and the best t exponent \(\beta \) of the price impact function as in Eq. 3 .
As our model is stochastic (agents’ actions are defined over probability distributions), there is inherent uncertainty in the range of outputs, even for fixed input parameters. In the following, ten thousand samples from within the parameter space were generated with the input parameters distributed uniformly in the ranges displayed in Table 1 .
For each sample of the parameters space, the model is run for 300, 000 trading periods to approximately simulate a trading day on a high-frequency timescale. The global variance sensitivity, as defined in Eq. 14 is presented in Fig. 1 .
The global variance sensitivities clearly identify the upper limit of the distribution from which liquidity consumers order volume is drawn ( \(h_{max}\) ) and the probabilities of each of the agent groups acting (particularly those of the high-frequency traders, \(\delta _{mr}\) and \(\delta _{mt}\) ) as the most important input parameters for all outputs. The biggest influence of each of these parameters was on the mean first lag autocorrelation term of the order-sign series R ( o ) followed by the exponent of the price impact function \(\beta \) .
To find the set of parameters that produces outputs most similar to those reported in the literature and to further explore the influence of input parameters we perform a large scale grid search of the input space. This yields the optimal set of parameters displayed in Table 2 . With this set of parameters we go on to explore the model’s ability to reproduce the various statistical properties that are outlined in Sect. 3 .
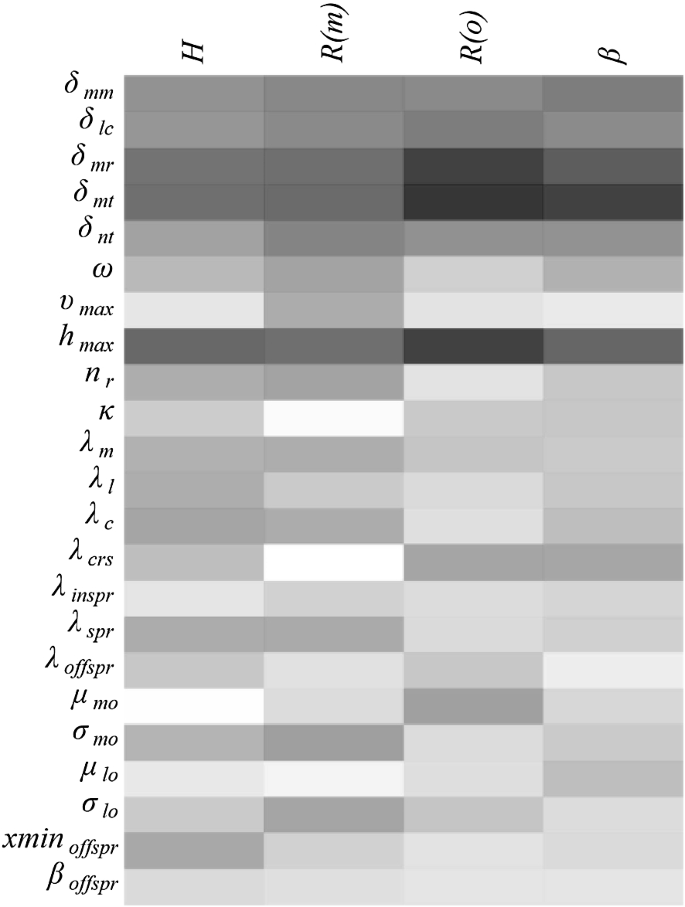
Heatmap of the global variance sensitivity
5.2 Fat tailed distribution of returns
Figure 2 displays a side-by-side comparison of how the kurtosis of the mid-price return series varies with lag length for our model and an average of the top 5 most actively traded stocks on the Chi-X exchange in a period of 100 days of trading from 12th February 2013 to 3rd July 2013. A value of 1000 on the x-axis mean that the return was taken as \(\log (p_{t+1000}) - \log (p_t)\) . In our LOB model, only substantial cancellations, orders that fall inside the spread, and large orders that cross the spread are able to alter the mid price. This generates many periods with returns of 0 which significantly reduces the variance estimate and generates a leptokurtic distribution in the short run, as can be seen in Fig. 2 a.
Kurtosis is found to be relatively high for short timescales but falls to match levels of the normal distribution at longer timescales. This not only closely matches the pattern of decay seen in the empirical data displayed in Fig. 2 b but also agrees with the findings of Cont ( 2001 ) and Gu et al. ( 2008 ).
5.3 Volatility clustering
To test for volatility clustering, we compute the Hurst exponent of volatility using the DFA method described by Peng et al. ( 1994 ). Figure 3 details the percentage of simulations runs with significant volatility clustering defined as \(0.6< H < 1\) . Once again, in the shortest time lags volatility clustering seems to be present at short timescales in all the simulations but rapidly disappears for longer lags in agreement with Lillo and Farmer ( 2004 ).
5.4 Autocorrelation of returns
Table 3 reports descriptive statistics for the first lag autocorrelation of the returns series for our agent based model and for the Chi-X data. In both instances, there is a very weak but significant autocorrelation in both the mid-price and trade price returns. The median autocorrelation of mid-price returns for the agent-based model and the Chi-X data were found to be − 0.0034 and − 0.0044, respectively. Using a non-parametric test, the distributions of the two groups were not found to differ significantly (Mann–Whitney \(U = 300,P > 0.1\) two-tailed).
This has been empirically observed in other studies (see Sect. 3.1.3 ) and is commonly thought to be due to the refilling effect of the order book after a trade that changes the best price. The result is similar for the trade price autocorrelation but as a trade price will always occur at the best bid or ask price a slight oscillation is to be expected and is observed.
5.5 Long memory in order flow
As presented in Table 4 , we find the mean first lag autocorrelation term of the order-sign series for our model to be 0.2079 which is close to that calculated for the empirical data and those reported in the literature. Most studies find the order sign autocorrelation to be between 0.2 and 0.3 (see Lillo and Farmer 2004 for example). In Table 4 , H order signs shows a mean Hurst exponent of the order signs time series for our model of \(\approx \) 0.7 which indicates a long-memory process and corresponds with the findings of previous studies and with our own empirical results [see Lillo and Farmer ( 2004 ) and Mike and Farmer ( 2008 )].
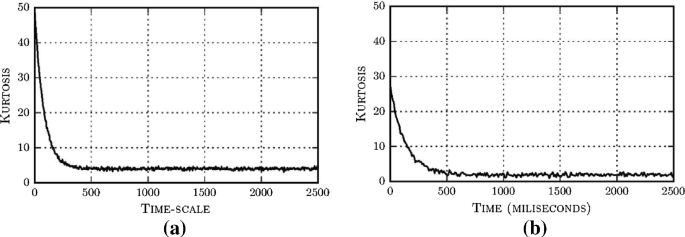
Comparing Kurtosis. a Kurtosis by timescale in our model. b Kurtosis by time (ms) of order book data for Chi-X
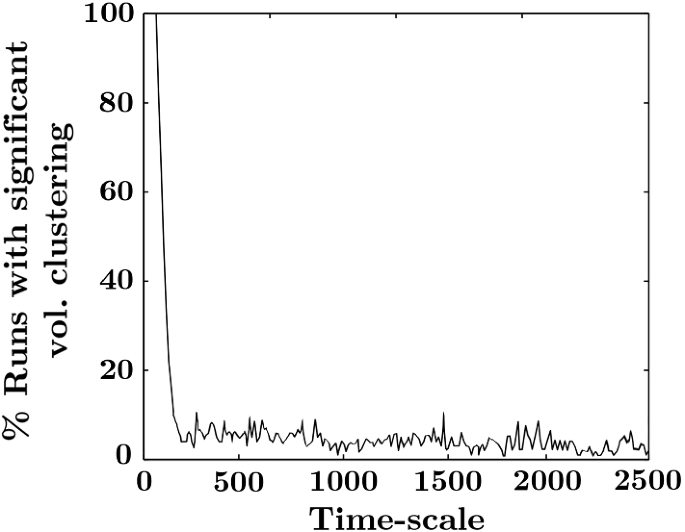
Volatility clustering by timescale
5.6 Concave price impact
Figure 4 a illustrates the price impact in the model as a function of order size on a log-log scale. The concavity of the function is clear. The shape of this curve is very similar t that of the empirical data from Chi-X shown in Fig. 4 b. The price impact is for the model is found to be best fit by the relation \(\Delta p \propto v^{0.28}\) , while the empirically measured impact was best fit by \(\Delta p \propto v^{0.35}\) . Both of these estimates of the exponent of the impact function agree with the findings of Lillo et al. ( 2003 ), Lillo and Farmer ( 2004 ) and Hopman ( 2007 ) but the model is sensitive to the volume provided by the market makers. When the market order volume is reduced, the volume at the opposing best price reduces compared to the rest of the book. This allows smaller trades to eat further into the liquidity stretching the right-most side of the curve.
Figure 5 demonstrates the effects of varying consumers’ volume parameter \(h_{max}\) on the price impact curve. This parameter appears to have very little influence on the shape of the price impact function. However, it does appear to have an effect on the size of the impact. Although \(h_{max}\) is relatively insensitive to minor changes, when the volume traded by the liquidity consumers is reduced dramatically, the relative amount of available liquidity in the market increases to the point where price impact is reduced. Very similar results are seen as the market makers’ order size \((v_{max})\) is increased.
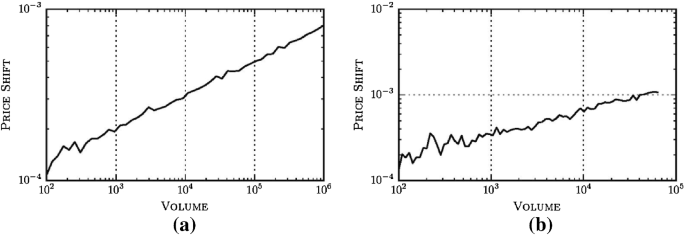
Log–log price impact. a Log–log price impact function for the agent-based model. b Log–log price impact function for the Chi-X data
Figure 6 shows the effects on the price impact function of adjusting the relative probabilities of events from the high frequency traders. It is clear that strong concavity is retained across all parameter combinations but some subtle artefacts can be seen. Firstly, increasing the probability of both types of high frequency traders equally seems to have very little effect on the shape of the impact function. This is likely due to the strategies of the high frequency traders restraining one another. Although the momentum traders are more active—jumping on price movements and consuming liquidity at the top of the book—they are counterbalanced by the increased activity of the mean reversion traders who replenish top-of-book liquidity when substantial price movements occur. In the regime where the probability of momentum traders acting is high but the probability for mean reversion traders is low (the dotted line) we see an increase in price impact across the entire range of order sizes. In this scenario, when large price movements occur, the activity of the liquidity consuming trend followers outweighs that of the liquidity providing mean reverters, leading to less volume being available in the book and thus a greater impact for incoming orders.
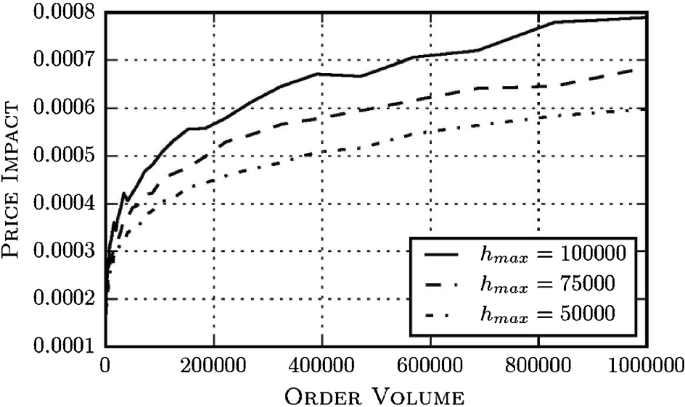
The price impact function with different liquidity consumer parameterisations. Each line represents a different setting for \(h_{max}\)
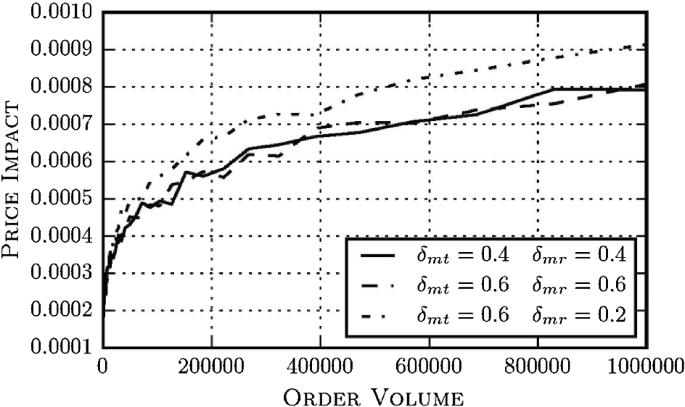
Price impact for various values for the probability of the high frequency traders acting
5.7 Extreme price events
We follow the definition of Johnson et al. ( 2013 ) and define an extreme price event as an occurrence of a stock price ticking down [up] at least ten times before ticking up [down] and with a price change exceeding 0.8% of the initial price. Figure 7 shows a plot the mid-price time-series provides with an illustrative example of a flash occurring in the simulation. During this event, the number of sequential down ticks is 11, the price change is \(1.3\%\) , and the event lasts for 12 simulation steps.
Table 5 shows statistics for the number of events for each day in the Chi-X data and per simulated day in our ABM. On average, in our model, there are 0.8286 events per day very close to the average average number observed in empirical data.
Upon inspection, we can see that such events occur when an agent makes a particularly large order that eats through the best price (and sometimes further price levels). This causes the momentum traders to submit particularly large orders on the same side, setting off a positive feedback chain that pushes the price further in the same direction. The price begins to revert when the momentum traders begin to run out of cash while the mean reversion traders become increasingly active.
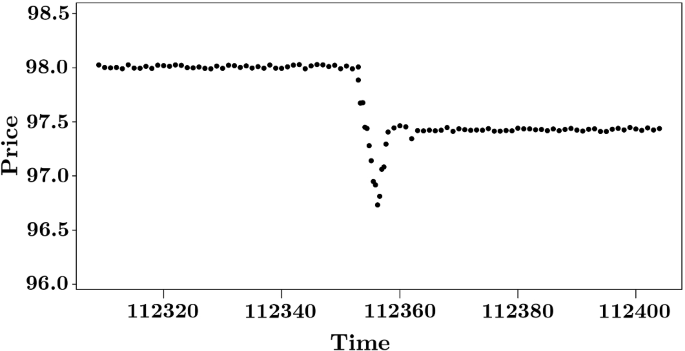
Price spike example
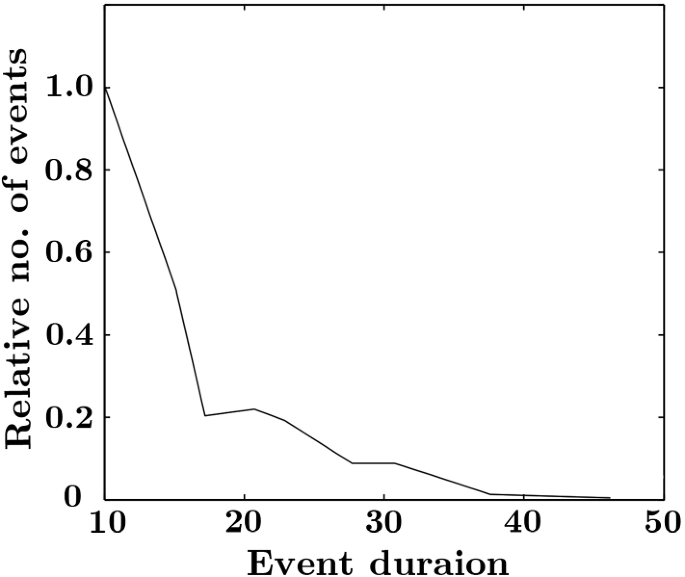
Relative numbers of crash/spike events as a function of their duration
Figure 8 illustrates the relative numbers of extreme price events as a function of their duration. The event duration is the time difference (in simulation time) between the first and last tick in the sequence of jumps in a particular direction. It is clear that these extreme price events are more likely to occur quickly than over a longer timescale. This is due to the higher probability of momentum traders acting during such events. It is very rare to see an event that lasts longer than 35 time steps.
Figure 9 shows the relative number of crash and spike events as a function of their duration for different schemes of high frequency activity. The solid line shows the result with the standard parameter setting from Table 2 . The dashed line shows results from a scheme with an increased probability of both types of high frequency trader acting. Here, we see that there is an increased incidence of short duration flash events. It seems that the increased activity of the trend follows causes price jumps to be more common while the increased activity of the mean reverts ensures that the jump is short lived. In the scenario where the activity of the momentum followers is high but that of the mean reverts is low (the dotted line) we see an increase in the number of events cross all time scales. This follows from our previous analogy.
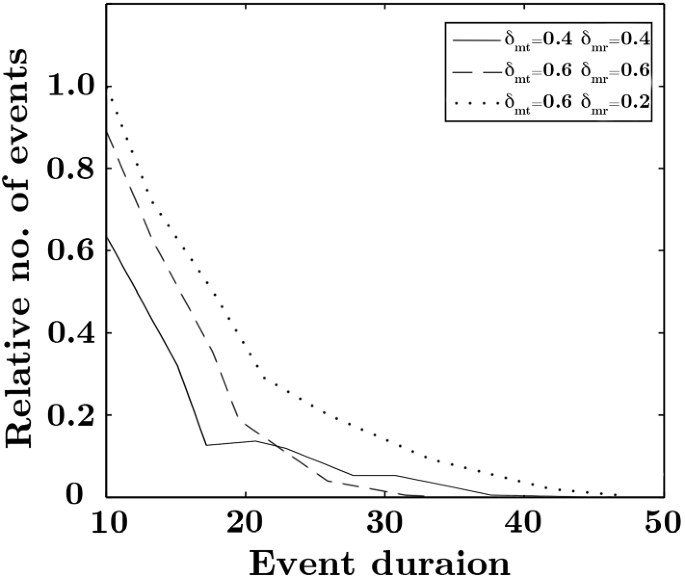
Price spike occurrence with various values for the probability of the high frequency traders acting
6 Conclusion
In light of the requirements of the forthcoming MiFID II laws, an interactive simulation environment for trading algorithms is an important endeavour. Not only would it allow regulators to understand the effects of algorithms on the market dynamics but it would also allow trading firms to optimise proprietary algorithms. The agent-based simulation proposed in this paper is designed for such a task and is able to replicate a number of well-known statistical characteristics of financial markets including: clustered volatility, autocorrelation of returns, long memory in order flow, concave price impact and the presence of extreme price events, with values that closely match those identified in depth-of-book equity data from the Chi-X exchange. This supports prevailing empirical findings from microstructure research.
On top of model validation, a number of interesting facets are explored. Firstly, we find that increasing the total number of high frequency participants has no discernible effect on the shape of the price impact function while increased numbers do lead to an increase in price spike events. We also find that the balance of trading strategies is important in determining the shape of the price impact function. Specifically, excess activity from aggressive liquidity-consuming strategies leads to a market that yields increased price impact.
The strategic interaction of the agents and the differing time-scales on which they act are, at present, unique to this model and crucial in dictating the complexities of high-frequency order-driven markets. As a result, this paper presents the first model capable of replicating all of the aforementioned stylised facts of limit order books, an important step towards an environment for testing automated trading algorithms. Such environment not only fulfills a requirement of MiFID II, more than that, it makes an important step towards increased transparency and improved resilience of the complex socio-technical system that is our brave new marketplace.
Our model offers regulators a lens through which they can scrutinise the risk of extreme prices for any given state of the market ecology. MiFID II requires that all the firms participating in algorithmic trading must get tested and authorised by the regulators for their trading algorithms. Our analysis demonstrates that there is a strong relationship between market ecology and the size/duration of price movements (see Fig. 9 ). Furthermore, our agent based model setting offers a means of testing any individual automated trading strategy or any combination of strategies for the systemic risk posed, which aims specifically to satisfy the MiFID II requirement . “ ..... that algorithms should undergo testing, and thus facilities will be required for such testing.” (p. 19, MiFID 2012 ). Moreover, insights from our model and the continuous monitoring of market ecology would enable regulators and policy makers to assess the evolving likelihood of extreme price swings. The proposed agent based model fulfils one of the main objectives of MiFID II that is testing the automated trading strategies and the associated risk.
While this model has been shown to accurately produce a number of order book dynamics, the intra-day volume profile has not been examined. Future work will involve the exploration of the relative volumes traded throughout a simulated day and extensions made so as to replicate the well known u-shaped volume profiles (see Jain and Joh 1988 ; McInish and Wood 1992 ).
Note that the financial markets evolved from concentrated markets to fragmented Multilateral Trading Facilities (MTFs). Recent studies by Upson and Ness ( 2017 ), Thierry and Albert ( 2014 ) and Félez-Viñas ( 2018 ) verify that the market fragmentation is not the root cause for market instability and moreover, fragmentation is associated with improved market liquidity. As there is no evidence that fragmentation is a likely cause of extreme price spikes and the complexity introduced by including market fragmentation would make it harder to find a stable viable agent based model, we consider only a concentrated single market in our model.
Although, at present, any player in a LOB may follow a market making strategy, MIFiD II is likely to require all participants that wish to operate such a strategy to register as a market maker. This will require them to continually provide liquidity at the best prices no matter what.
Alfinsi, A., Fruth, A., & Schied, A. (2010). Optimal execution strategies in limit order books with general shape functions. Quantitative Finance , 10 , 143–157.
Google Scholar
Angel, J. J., Harris, L. E., Katz, G., Levitt, A., Mathisson, D., Niederauer, D. L., et al. (2010). Current perspectives on modern equity markets: A collection of essays by financial industry experts . New York: Knight Capital Group, Inc.
At-Sahalia, Y., Mykland, P. A., & Zhang, L. (2011). Ultra high frequency volatility estimation with dependent microstructure noise. Journal of Econometrics , 160 (1), 160–175.
Axioglou, C., & Skouras, S. (2011). Markets change every day: Evidence from the memory of trade direction. Journal of Empirical Finance , 18 (3), 423–446.
Bagehot, W. (1971). The only game in town. Financial Analysts Journal , 27 , 12–14.
Bouchaud, J. P., Farmer, J. D., & Lillo, F. (2009). How markets slowly digest changes in supply and demand. In T. Hens & K. R. Schenk-Hoppe (Eds.), Handbook of financial markets: Dynamics and evolution (pp. 57–160). North Holland: Elsevier.
Bouchaud, J. P., Gefen, Y., Potters, M., & Wyart, M. (2004). Fluctuations and response in financial markets: The subtle nature of ‘random’ price changes. Quantitative Finance , 4 (2), 176–190.
Bouchaud, J. P., & Potters, M. (2003). Theory of financial risk and derivative pricing: From statistical physics to risk management . Cambridge: Cambridge University Press.
Buchanan, M. (2012). It’s a (stylized) fact!. Nature Physics , 8 (1), 3.
Carbone, A., Castelli, G., & Stanley, H. E. (2004). Time-dependent Hurst exponent in financial time series. Physica A: Statistical Mechanics and its Applications , 344 (1), 267–271.
Chakrabarti, R. (2000). Just another day in the inter-bank foreign exchange market. Journal of Financial Economics , 56 , 2–32.
Chakraborti, A., Toke, I. M., Patriarca, M., & Abergel, F. (2011). Econophysics review: I. Empirical facts. Quantitative Finance , 11 (7), 991–1012.
Challet, D., & Stinchcombe, R. (2003). Non-constant rates and over-diffusive prices in a simple model of limit order markets. Quantitative finance , 3 (3), 155–162.
Chiarella, C., & Iori, G. (2002). A simulation analysis of the microstructure of double auction markets. Quantitative Finance , 2 (5), 346–353.
Cont, R. (2001). Empirical properties of asset returns: Stylized facts and statistical issues. Quantitative Finance , 1 (2), 223–236.
Cont, R. (2005). Long range dependence in financial markets. In J. Lévy-Véhel & E. Lutton (Eds.), Fractals in engineering (pp. 159–179). London: Springer.
Cont, R., & Bouchaud, J. P. (2000). Herd behavior and aggregate fluctuations in financial markets. Macroeconomic Dynamics , 4 (2), 170–196.
Cont, R., Kukanov, A., & Stoikov, S. (2013). The price impact of order book events. Journal of Financial Econometrics , 12 (1), 47–88.
Cont, R., Stoikov, S., & Talreja, R. (2010). A stochastic model for order book dynamics. Operations Research , 58 (3), 549–563.
Cui, W., & Brabazon, A. (2012). An agent-based modeling approach to study price impact. In 2012 IEEE Conference on Computational Intelligence for Financial Engineering & Economics (CIFEr) (pp. 1–8). IEEE.
De Bondt, W., & Thaler, R. (1985). Does the stock market overreact? Journal of Finance , 40 , 793–807.
De Luca, M., & Cliff, D. (2011). Human-agent auction interactions : Adaptive-aggressive agents dominate. In Twenty-second international joint conference on artificial intelligence (p. 178).
Drozdz, S., Forczek, M., Kwapien, J., Oswiecimka, P., & Rak, R. (2007). Stock market return distributions: From past to present. Physica A: Statistical Mechanics and its Applications , 383 (1), 59–64.
Easley, D., De Prado, M., & O’Hara, M. (2010). The microstructure of the “flash crash”: flow toxicity, liquidity crashes, and the probability of informed trading. Technical Report. Unpublished Cornell University working paper.
Easley, D., & De Prado, M. M. Lopez. (2011). The microstructure of the “flash crash”: Flow toxicity, liquidity crashes, and the probability of informed trading. Journal of Portfolio Management , 37 , 118–128.
European Union. (2011). Proposal for a directive of the European Parliment and of the council on markets in financial instruments repealing Directive 2004/39/EC of the European Parliament and of the Council (Recast). Official Journal of the European Union. http://eur-lex.europa.eu/legal-content/EN/TXT/?qid=1398344410276&uri=CELEX:52011PC0656 .
European Union. (2014). Markets in Financial Instruments (MiFID): Commissioner Michel Barnier welcomes agreement in trilogue on revised European rules. Memo. http://europa.eu/rapid/press-release_MEMO-14-15_en.htm?locale=en .
Evans, M. D. D., & Lyons, R. K. (2002). Order flow and exchange rate dynamics. Journal of Political Economy , 110 , 170–180.
Farmer, J. D., & Foley, D. (2009). The economy needs agent-based modelling. Nature , 460 , 685–686.
Farmer, J. D., Patelli, P., & Zovko, I. I. (2005). The predictive power of zero intelligence in financial markets. Proceedings of the National Academy of Sciences of the United States of America , 102 (6), 2254–9.
Félez-Viñas, E. (2018). Market fragmentation, mini flash crashes and liquidity. Working paper presented at the FMA European Conference, Kristiansand, Norway.
Foucault, T. (1999). Order flow composition and trading costs in a dynamic limit order market. Journal of Financial Markets , 2 (2), 99–134.
Foucault, T., Kandan, O., & Kandel, E. (2005). Limit order book as a market for liquidity. The Review of Financial Studies , 18 , 1171–1217.
Geanakoplos, J., Axtell, R., Farmer, J., Howitt, P., Conlee, B., Goldstein, J., et al. (2012). Getting at systemic risk via an agent-based model of the housing market. The American economic review , 102 (3), 53–58.
Goettler, R. L., Parlour, C. A., & Rajan, U. (2005). Equilibrium in a dynamic limit order market. Journal of Finance , 60 , 1–44.
Gopikrishnan, P., Meyer, M., Amaral, L. A. N., & Stanley, H. E. (1998). Inverse cubic law for the distribution of stock price variations. The European Physical Journal B-Condensed Matter and Complex Systems , 3 (2), 139–140.
Grimm, V., Berger, U., Bastiansen, F., Eliassen, S., Ginot, V., Giske, J., et al. (2006). A standard protocol for describing individual-based and agent-based models. Ecological Modelling , 198 (1–2), 115–126.
Gu, G. F., Chen, W., & Zhou, W. X. (2008). Empirical distributions of Chinese stock returns at different microscopic timescales. Physica A: Statistical Mechanics and its Applications , 387 (2), 495–502.
Gu, G. F., & Zhou, W. X. (2009). Emergence of long memory in stock volatility from a modified Mike-Farmer model. EPL (Europhysics Letters) , 86 (4), 48,002.
Hasbrouck, J. (1991). Measuring the information content of stock trades. The Journal of Finance , 46 , 179–207.
Hausman, J. A., Lo, A. W., & Mackinlay, A. C. (1992). An ordered probit analysis of transaction stock prices. Journal of Financial Economics , 31 , 319–379.
Hopman, C. (2007). Do supply and demand drive stock prices? Quantitative Finance , 7 (1), 37–53.
Jain, P. C., & Joh, G. H. (1988). The dependence between hourly prices and trading volume. The Journal of Financial and Quantitative Analysis , 23 , 269–283.
Jegadeesh, N., & Titman, S. (1993). Returns to buying winners and selling losers: Implications for stock market efficiency. Journal of Finance , 48 , 65–91.
Johnson, N., Zhao, G., Hunsader, E., Qi, H., Johnson, N., Meng, J., et al. (2013). Abrupt rise of new machine ecology beyond human response time. Scientific Reports, Nature Publishing Group , 3 , 2627.
Keim, D. B., & Madhavan, A. (1995). Anatomy of the trading process empirical evidence on the behavior of institutional traders. Journal of Financial Economics , 37 (3), 371–398.
Kirilenko, A., Kyle, A.S., Samadi, M., & Tuzun, T. (2014). The flash crash: The impact of high frequency trading on an electronic market. Available at SSRN 1686004.
Knight Capital Group. (2012). Knight capital group provides update regarding august 1st disruption to routing in NYSE-listed securities. Retrieved from http://www.knight.com/investorRelations/pressReleases.asp?compid=105070&releaseID=1721599 .
Lillo, F., & Farmer, J. D. (2004). The long memory of the efficient market. Studies in Nonlinear Dynamics & Econometrics , 8 (3), 1–33.
Lillo, F., Farmer, J. D., & Mantegna, R. N. (2003). Master curve for price impact function. Nature , 421 (6919), 129–130.
Lo, A., & MacKinlay, A. (2001). A non-random walk down Wall Street . Princeton, NJ: Princeton University Press.
Lo, A. W. (2004). The adaptive markets hypothesis. The Journal of Portfolio Management , 30 (5), 15–29.
Mastromatteo, I., Toth, B., & Bouchaud, J. P. (2014). Agent-based models for latent liquidity and concave price impact. Physical Review E , 89 (4), 042,805.
McInish, T. H., & Wood, R. A. (1992). An analysis of intraday patterns in bid/ask spreads for NYSE stocks. The Journal of Finance , 47 , 753–764.
Menkveld, A.J., & Yueshen, B.Z. (2013). Anatomy of the flash crash. SSRN Electronic Journal. Available at SSRN 2243520.
MiFID II Hand book, Thomson Reuters. (2012). Retrieved from http://online.thomsonreuters.com/edm/assets/BG636126646047157632e/MiFID%20II%20Handbook.pdf .
Mike, S., & Farmer, J. D. (2008). An empirical behavioral model of liquidity and volatility. Journal of Economic Dynamics and Control , 32 (1), 200–234.
Obizhaeva, Aa, & Wang, J. (2013). Optimal trading strategy and supply/demand dynamics. Journal of Financial Markets , 16 (1), 1–32.
Oesch, C. (2014). An agent-based model for market impact. In 2014 IEEE symposium on computational intelligence for financial engineering and economics (CIFEr) .
OHara, M. (1995). Market microstructure . New York: Wiley.
Peng, C. K., Buldyrev, S. V., Havlin, S., Simons, M., Stanley, H. E., & Goldberger, A. L. (1994). Mosaic organization of DNA nucleotides. Physical Review E , 49 , 1685–1689.
Plerou, V., & Stanley, H. E. (2008). Stock return distributions: Tests of scaling and universality from three distinct stock markets. Physical Review E—Statistical, Nonlinear, and Soft Matter Physics , 77 (3), 037,101.
Predoiu, S., Shaikhet, G., & Shreve, S. (2011). Optimal execution in a general one-sided limit-order book. SIAM Journal on Financial Mathematics , 2 (1), 183–212.
Preis, T., Golke, S., Paul, W., & Schneider, J. J. (2006). Multi-agent-based order book model of financial markets. Europhysics Letters (EPL) , 75 (3), 510–516.
Preis, T., Golke, S., Paul, W., & Schneider, J. J. (2007). Statistical analysis of financial returns for a multiagent order book model of asset trading. Physical Review E—Statistical, Nonlinear, and Soft Matter Physics , 76 (1), 016,108.
Rosu, I. (2009). A dynamic model of the limit order book. Review of Financial Studies , 22 , 4601–4641.
SEC, CFTC. (2010). Findings regarding the market events of May 6, 2010. Technical report, Report of the Staffs of the CFTC and SEC to the Joint Advisory Committee on Emerging Regulatory Issues.
Serban, A. F. (2010). Combining mean reversion and momentum trading strategies in foreign exchange markets. Journal of Banking and Finance , 34 , 2720–2727.
Smith, E., Farmer, J., Gillemot, L., & Krishnamurthy, S. (2003). Statistical theory of the continuous double auction. Quantitative Finance , 3 (6), 481–514.
Sobol, I. M. (2001). Global sensitivity indices for nonlinear mathematical models and their Monte Carlo estimates. Mathematics and Computers in Simulation , 55 , 271–280.
Stanley, H. E., Plerou, V., & Gabaix, X. (2008). A statistical physics view of financial fluctuations: Evidence for scaling and universality. Physica A: Statistical Mechanics and its Applications , 387 (15), 3967–3981.
Thierry, F., & Albert, M. (2014). Competition for order flow and smart order routing systems. Journal of Finance , 63 , 119–158.
Thurner, S., Farmer, J. D., & Geanakoplos, J. (2012). Leverage causes fat tails and clustered volatility. Quantitative Finance , 12 (5), 695–707.
Upson, J., & Van Ness, R. A. (2017). Multiple markets, algorithmic trading, and market liquidity. Journal of Financial Markets , 32 , 49–68.
World Bank. (2012). Data retrieved from http://data.worldbank.org/indicator/CM.MKT.LCAP.CD .
Download references
Acknowledgements
This work was supported by an EPSRC Doctoral Training Centre Grant (EP/G03690X/1).
Author information
Authors and affiliations.
Southampton Business School, University of Southampton, Southampton, SO17 1BJ, UK
Frank McGroarty
Electronics and Computer Science, University of Southampton, Southampton, SO17 1BJ, UK
Ash Booth & Enrico Gerding
Business School, University of Greenwich, London, SE10 9LS, UK
V. L. Raju Chinthalapati
You can also search for this author in PubMed Google Scholar
Corresponding author
Correspondence to Frank McGroarty .
Rights and permissions
Open Access This article is distributed under the terms of the Creative Commons Attribution 4.0 International License ( http://creativecommons.org/licenses/by/4.0 /), which permits unrestricted use, distribution, and reproduction in any medium, provided you give appropriate credit to the original author(s) and the source, provide a link to the Creative Commons license, and indicate if changes were made.
Reprints and permissions
About this article
McGroarty, F., Booth, A., Gerding, E. et al. High frequency trading strategies, market fragility and price spikes: an agent based model perspective. Ann Oper Res 282 , 217–244 (2019). https://doi.org/10.1007/s10479-018-3019-4
Download citation
Published : 25 August 2018
Issue Date : November 2019
DOI : https://doi.org/10.1007/s10479-018-3019-4
Share this article
Anyone you share the following link with will be able to read this content:
Sorry, a shareable link is not currently available for this article.
Provided by the Springer Nature SharedIt content-sharing initiative
- Agent-based model
- Limit order book
- Stylised facts
- Algorithmic trading
- Find a journal
- Publish with us
- Track your research
Click through the PLOS taxonomy to find articles in your field.
For more information about PLOS Subject Areas, click here .
Loading metrics
Open Access
Peer-reviewed
Research Article
Multi-strategy modified sparrow search algorithm for hyperparameter optimization in arbitrage prediction models
Roles Conceptualization, Methodology, Software, Validation, Writing – original draft
Affiliation School of Software, Henan Polytechnic University, Jiaozuo, China

Roles Conceptualization, Funding acquisition, Methodology, Resources, Supervision, Writing – review & editing
* E-mail: [email protected]
Affiliations School of Software, Henan Polytechnic University, Jiaozuo, China, Hebi National Optoelectronic Technology Co, Ltd, Hebi, China
Roles Funding acquisition, Project administration, Resources, Writing – review & editing
Roles Funding acquisition, Resources, Supervision, Writing – review & editing
Roles Software, Validation, Visualization
Roles Validation, Visualization
Roles Formal analysis
Roles Investigation
Roles Data curation
- Shenjie Cheng,
- Panke Qin,
- Baoyun Lu,
- Jinxia Yu,
- Yongli Tang,
- Zeliang Zeng,
- Sensen Tu,
- Haoran Qi,
- Bo Ye,
- Zhongqi Cai
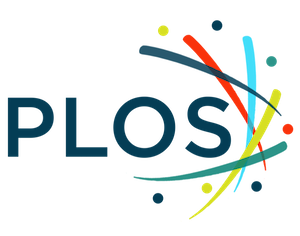
- Published: May 15, 2024
- https://doi.org/10.1371/journal.pone.0303688
- Reader Comments
Deep learning models struggle to effectively capture data features and make accurate predictions because of the strong non-linear characteristics of arbitrage data. Therefore, to fully exploit the model performance, researchers have focused on network structure and hyperparameter selection using various swarm intelligence algorithms for optimization. Sparrow Search Algorithm (SSA), a classic heuristic method that simulates the sparrows’ foraging and anti-predatory behavior, has demonstrated excellent performance in various optimization problems. Hence, in this study, the Multi-Strategy Modified Sparrow Search Algorithm (MSMSSA) is applied to the Long Short-Term Memory (LSTM) network to construct an arbitrage spread prediction model (MSMSSA-LSTM). In the modified algorithm, the good point set theory, the proportion-adaptive strategy, and the improved location update method are introduced to further enhance the spatial exploration capability of the sparrow. The proposed model was evaluated using the real spread data of rebar and hot coil futures in the Chinese futures market. The obtained results showed that the mean absolute percentage error, root mean square error, and mean absolute error of the proposed model had decreased by a maximum of 58.5%, 65.2%, and 67.6% compared to several classical models. The model has high accuracy in predicting arbitrage spreads, which can provide some reference for investors.
Citation: Cheng S, Qin P, Lu B, Yu J, Tang Y, Zeng Z, et al. (2024) Multi-strategy modified sparrow search algorithm for hyperparameter optimization in arbitrage prediction models. PLoS ONE 19(5): e0303688. https://doi.org/10.1371/journal.pone.0303688
Editor: David Alaminos, University of Barcelona: Universitat de Barcelona, SPAIN
Received: January 19, 2024; Accepted: April 29, 2024; Published: May 15, 2024
Copyright: © 2024 Cheng et al. This is an open access article distributed under the terms of the Creative Commons Attribution License , which permits unrestricted use, distribution, and reproduction in any medium, provided the original author and source are credited.
Data Availability: The raw data related to this study can be obtained from the OPEN ICPSR database (accession numbers: openicpsr-199341).
Funding: This work was supported by the Henan University Science and Technology Innovation Team Support Plan (20IRTSTHN013) and the Henan Province Key R&D and Promotion Special Project (212102210166). The funders are responsible for the entire program.
Competing interests: The authors have declared that no competing interests exist.
1 Introduction
The futures market plays a significant contribution to stabilizing commodity prices and promoting capital flow. Among them, cross-variety arbitrage trading in futures has attracted widespread attention from investors due to its low cost, small risk, and relatively stable returns. Its essence is spread arbitrage, obtaining returns based on the regression of spreads between futures contracts with stronger correlation. Therefore, in order to assist investors in formulating more scientific trading strategies to obtain substantial profits, it is extremely important to accurately predict the trend of arbitrage spreads between futures contracts. However, the market is dynamic and volatile, and the pricing of contracts is often based on a variety of complex dynamic conditions [ 1 ]. These factors make the prediction of arbitrage spread trends a significant challenge.
The traditional solution to the problem of arbitrage spread trend prediction is to utilize time series models, such as the autoregressive conditional heteroskedasticity (ARCH) model, the autoregressive moving average (ARMA) model, the autoregressive integrated moving average (ARIMA) model, etc [ 2 – 5 ]. Although these models can fit the current data well, the prediction effect is not ideal when facing out-of-sample data.
In recent years, with the development and perfection of artificial intelligence technology, numerous machine learning models have been widely used in the field of financial time series prediction due to their outstanding fitting ability. Tay and Cao [ 6 ] used support vector machines (SVM) to predict the closing price of the Standard&Poor 500 stock index futures. The experimental results show that this method is more effective than the back propagation (BP) neural network model. Nayak et al. [ 7 ] combined the artificial chemical reaction optimization (ACRO) algorithm with the multilayer perceptron (MLP) to construct an artificial chemical reaction neural network (ACRNN) for predicting stock market indices. Li [ 8 ] achieved the prediction of the settlement price of China’s stock index futures through empirical mode decomposition (EMD) and radial basis function (RBF) neural networks. Compared with the traditional time series model, the aforementioned methods have achieved a significant improvement in prediction accuracy. Meanwhile, with the advent of the big data era, scholars are conducting more and more research on deep neural network (DNN) models [ 9 , 10 ]. Hu [ 11 ] applied a convolutional neural network (CNN) to predict stock prices. However, since CNNs are better at handling image problems, their accuracy is lower when facing time series prediction. Berradi and Lazaar [ 12 ] predicted the stock price of Total Maroc from the Casablanca Stock Exchange through a recurrent neural network (RNN) and used principal component analysis (PCA) for dimensionality reduction, ultimately obtaining superior prediction results. It is noteworthy that despite their effectiveness, there are still some issues with the RNN model. Hochreiter and Schmidhuber [ 13 ] improved its unit structure and proposed the long short-term memory (LSTM) network model, which effectively addressed their shortcomings such as insufficient long-term memory capacity through the design of gate structures. Numerous studies have shown that LSTM networks are able to discover long-term dependencies in sequence information well, and are therefore widely used in the field of financial forecasting [ 14 – 17 ].
To further enhance the predictive performance of the LSTM model, it is necessary to optimize its hyperparameters. However, there is no clear function relationship between model performance and hyperparameters. Therefore, in practical applications, researchers often determine the optimal values of hyperparameters based on their own experience, existing research, and abundant experimental results. This approach not only results in a significant waste of manpower and computational resources, but also introduces subjective factors that make it difficult to ensure the optimality of the model. When the hyperparameter space is more complex, the entire optimization process can be extremely time-consuming and inefficient. Therefore, finding the optimal combination of hyperparameters in neural network models has also become a challenging task.
In addition, in the futures market, arbitrage methods are mainly divided into two categories: mean reversion arbitrage methods and neural network arbitrage methods [ 18 ]. The mean reversion method uses financial time series analysis methods to study the long-term relationships that exist between futures, so as to design arbitrage strategies. For example, Liu and Lan [ 19 ] constructed cointegration regression and vector error correction model to analyze the monthly average closing price of polyvinyl chloride futures contracts. The results found that there is a long-term equilibrium and short-term deviation relationship of the contract spread, confirming the existence of intertemporal arbitrage opportunities in this contract. Liu [ 20 ] applied the cointegration method to empirically analyze the prices of hogs, corn, and soybean meal. It was found that there is a long-term mean relationship between the three, and the trading simulation also confirmed the possibility of profit. The neural network arbitrage method utilizes neural network models to predict spreads and formulate arbitrage strategies. At present, the existing domestic research is mainly based on the mean reversion principle of spreads. There are fewer studies on using neural network for arbitrage, and most of them have the disadvantages of single model and low prediction accuracy [ 21 , 22 ]. Therefore, to develop scientific and efficient arbitrage strategies, it is extremely important to accurately predict the arbitrage spreads between futures.
Given the above reasons, this article proposes an arbitrage spread prediction model based on multi-strategy modified SSA-optimized LSTM (MSMSSA-LSTM) by combining the sparrow search algorithm [ 23 ] (SSA) with the LSTM network. This research have constructed a regression model with high prediction accuracy by using MSMSSA to match the futures data features and LSTM neural network topology structure. On this basis, this paper conducts an empirical analysis using the spread dataset of rebar and hot coil futures in the Chinese futures market.
The main contributions of this article can be summarized as follows:
- This research has constructed the MSMSSA-LSTM model by improving the sparrow search algorithm to achieve the trend prediction of arbitrage spread between futures.
- To verify the effectiveness of the MSMSSA-LSTM model, this research selected the MLP model, RNN model, LSTM model, gated recurrent unit (GRU) model, and LSTM model optimized by traditional sparrow search algorithm (SSA-LSTM) as comparative experiments. The experimental results indicate that the MSMSSA-LSTM model has high prediction accuracy and is more suitable for predicting the trend of arbitrage spread between futures.
The remaining chapters of this paper are organized as follows. Section 2 introduces the hyperparameter optimization problem in the LSTM network model and its solutions. Section 3 analyzes some literature on optimizing LSTM models using metaheuristic algorithms. Section 4 describes the sparrow search algorithm and its improvement strategies, and construct the MSMSSA-LSTM model. Section 5 validates the effectiveness of the MSMSSA-LSTM model in predicting arbitrage spread trends through comparative experiments. Finally, the conclusion is given in Section 6.
2 Problem description
In neural networks, there are many parameters that need to be set manually which are hyperparameters. The selection of suitable hyperparameter values plays a crucial role in the final prediction performance of the model. Therefore, how to choose appropriate hyperparameters based on the characteristics of the data has always been a widely studied topic.
This section first described the hyperparameter optimization problem using mathematical formulas. Secondly, several existing solutions to this problem and their respective drawbacks were given. Finally, a new solution was proposed.
The traditional hyperparameter optimization techniques include grid search, random search, and Bayesian optimization. Among them, grid search determines the optimal solution by exhaustively traversing all combinations of hyperparameters. Random search searches for the global optimal solution by randomly selecting sample points. Bayesian optimization attempts to seek the optimal solution by constructing a posterior probability of the black box function output. Compared to the three, Bayesian optimization fully considers the existing hyperparameter combination information, while grid search and random search ignore this information, which can lead to serious resource waste. Although Bayesian optimization has shown good results in finding the optimal combination of hyperparameters, it still has the disadvantages of slow search speed and easy to fall into local optimal solutions. Therefore, this paper effectively solves the above problems by combining the improved SSA algorithm with the LSTM network model.
3 Related works
In related work, there is a lot of research on using heuristic algorithms to optimize LSTM models. Some of them are listed below.
In 2022, Drewil and Al-Bahadili [ 24 ] applied genetic algorithms to find the optimal values of window size and number of units in the LSTM network model. They selected air pollution prediction for experiments and proved that the model modified by the optimization algorithm outperformed the benchmark model. In 2023, Bacanin et al. [ 25 ] optimized the learning rate, dropout rate, number of epochs, number of layers, and number of neuron cells in each layer in the LSTM network using the improved particle swarm optimization algorithm. The experimental results of cloud load prediction show that the optimized LSTM has superior performance to other performed techniques. In 2020, Kumar and Haider [ 26 ] used the flower pollination algorithm and particle swarm optimization algorithm to optimize the time lag, number of hidden layers, number of hidden neurons, batch size, and epochs in the RNN-LSTM model, respectively. The experimental results demonstrate that the optimized model enhances performance and has higher accuracy. In 2022, Jovanovic et al. [ 27 ] applied the salp swarm algorithm with a disputation operator to optimize the learning rate, dropout rate, number of neurons in the LSTM layer, and the number of training epochs in the LSTM network. They selected the West Texas Intermediate dataset for testing. The obtained results demonstrate that the proposed model outperforms all other competitors and exhibits the best performance. In 2024, Zhang et al. [ 28 ] optimized the Bi-LSTM model by using the whale optimization algorithm with circle mapping and self-adaptive weight adjustment. The accuracy of the proposed method is proved by the plug-load electricity consumption prediction. In 2022, Bacanin et al. [ 29 ] achieved smart air quality prediction and node localization based on the Graph LSTM and the improved dragonfly optimizer algorithm. In 2023, Gülmez [ 30 ] optimized the learning rate, dropout rate, optimizer algorithm, layer existing or not existing, and number of neurons in the LSTM model using the artificial rabbit optimization algorithm. Dow Jones Index stock price data was used for testing. The results indicate that the model has certain universality and good prediction accuracy.
4 Methodology
Section 4.1 introduces the traditional sparrow search algorithm. Section 4.2 presents three improvement strategies and name the improved algorithm as the multi-strategy modified sparrow search algorithm (MSMSSA). Additionally, this section also provides the pseudocode of MSMSSA. Section 4.3 constructs the MSMSSA-LSTM model and introduce its structure and execution process.
4.1 Sparrow search algorithm
The sparrow search algorithm is a swarm intelligence optimization algorithm proposed based on the foraging and anti-predation behavior of sparrow populations. When foraging, sparrows will be divided into two types, discoverers and followers, based on the quality of the food searched. The discoverer is responsible for finding food and providing the foraging area and direction for the followers. The followers follow the discoverer to get better food. If the follower’s position is poor, it will fly to other areas to forage. In addition, when a sparrow individual discovers predators around the population, it will send out an alarm signal and move to a safe area. Sparrows in the middle of the group will randomly approach other sparrows. Once the alarm value is higher than the safety value, the discoverer will immediately lead the followers out of the danger zone and fly to other safe areas to forage.
Assuming there are n sparrows in the sparrow population and the dimension of the search space is d, then the position information of all sparrows can be regarded as an n×d matrix. The position of each sparrow can be represented as x i,j , where i = 1, 2, 3, …, n, j = 1, 2, 3, …, d. x i,j indicates the position information of the i-th sparrow in the j-th dimension. The quality of the food searched by each sparrow is reflected by the fitness function. The fitness value of each sparrow can be expressed as F xi = f([x i,1 , x i,2 , x i,3 , …, x i,d ]).
In this expression, t denotes the current number of iterations and T is a constant representing the total number of iterations. X i denotes the position corresponding to the sparrow whose fitness value is ranked i in the population. α is a random number between (0, 1]. Q is a random number that follows a standard normal distribution. L is a 1×d matrix with all elements being 1. R 2 and ST indicate the alarm value and safety value, respectively, with their ranges being [0, 1] and [0.5, 1], respectively. When R 2 < ST, it represents that no predators are detected at this time, and the discoverer can perform a wide range of searches. When R 2 ≥ ST, it indicates that there are a large number of predators around the foraging environment at this time, and the discoverer needs to immediately lead the followers to forage in other safe areas.
Among them, X p represents the optimal position of the current discoverer. X worst denotes the worst global position currently. A indicates a 1×d matrix, and each of its elements randomly takes the value of 1 or -1. A + satisfies the equation A + = A T (AA T ) -1 . When i > n / 2, it represents that the fitness value of the i-th follower is poor, has not obtained food, is in a state of starvation, and needs to fly to other areas to forage. When i ≤ n / 2, the follower follows the discoverer who is in the optimal position at this time to forage.
Where X best indicates the best global position currently. β and K are both step control parameters. β denotes a random number that follows a standard normal distribution, and K represents a random number in the range of [–1, 1]. f i indicates the fitness value of the current watcher. f g and f ω denote the best and worst fitness values in the present entire sparrow population, respectively. Ɛ is a very small constant to avoid the denominator being 0. When f i > f g , it represents that the current watcher is on the boundary of the population and is easy to become the target of predators. When f i = f g , it indicates that the watcher in the middle of the population has sensed danger and needs to approach other companions to ensure its own safety.
4.2 Improved strategies
4.2.1 good point set theory..
Fig 1(A) is the initial population generated by random initialization. Fig 1(B) is the initial population generated by good point set initialization. By comparison, it can be found that the effect of initializing the population with a good point set is better.
- PPT PowerPoint slide
- PNG larger image
- TIFF original image
(a) Random Initialization. (b) Good point set Initialization.
https://doi.org/10.1371/journal.pone.0303688.g001
4.2.2 Proportion-Adaptive strategy.
Where max_ and min_ represent the maximum and minimum values of the proportion of the population occupied by the discoverer, respectively. t is the current number of iterations. T is a constant, denoting the total number of iterations. When max_ = 0.7, min_ = 0.2, and T = 20, the change in the proportion of the population occupied by the discoverer sparrow is shown in Fig 2 .
https://doi.org/10.1371/journal.pone.0303688.g002
Fig 2 shows the changes in the proportion of discoverers throughout the entire iteration process. It can be observed that in the early stage, the proportion of discoverers is larger, which allows for sufficient global search. In the later stage, the proportion of discoverers gradually decreases, the proportion of followers increases, and the local search capability is enhanced.
4.2.3 Improved location update method.
This paper improved the traditional SSA through the above three strategies and named it the multi-strategy modified sparrow search algorithm (MSMSSA). The implementation procedure of MSMSSA is illustrated in Algorithm 1.
Algorithm 1: The framework of the MSMSSA.
T: the total number of iterations
max_: the maximum proportion of discoverers in the population
min_: the minimum proportion of discoverers in the population
number: the number of sentinels
ST: the safety value
Initializing a population of n sparrows using the good point set method
Establish a fitness function F ( X ), where X = ( x 1 , x 2 ,⋯ x d )
Output: X best , F g
Calculate fitness values and sort them, recording the current best and worst individuals
while (t ≤ T)
Calculate P
R 2 = rand(1)
for i = 1 to P*n do
Update the location of discoverers according to Eq ( 9 )
end for
for i = P*n+1 to n do
Update the location of followers according to Eq ( 3 )
for i = 1 to number do
Update the location of sentinels according to Eq ( 4 )
Calculate the fitness values of the new location and update if it is better
t = t + 1
return X best , F g
4.3 MSMSSA-LSTM model
The real target of cross-variety arbitrage trading is the spread between different futures contracts. When the spread is higher than the equilibrium state, a short strategy is adopted, and when it is lower than the equilibrium state, a long strategy is adopted. Profits can be obtained through the regression of spread. Therefore, in order to seek higher returns, it is particularly important to accurately predict the spread. Since the LSTM model performs excellently in dealing with time series problems, this paper builds a spread prediction model for futures data based on it. Selecting appropriate hyperparameters in LSTM can effectively improve the topology of the network model and enhance its fitting and generalization capabilities. As a consequence, to match the network model structure with the characteristics of futures data, this paper combines the MSMSSA algorithm with the LSTM model to construct a MSMSSA-LSTM prediction model.
4.3.1 Structure of MSMSSA-LSTM.
According to the fitness values of each individual in the sparrow population, they are divided into discoverers and followers, and their positions are updated by Formulas ( 9 ) and ( 3 ). A certain number of vigilant sparrows are randomly selected and updated according to Formula ( 4 ). It is judged whether the termination condition is satisfied. If it is satisfied, the optimal value of the target parameter is output. Otherwise, it is re-divided. Continue to update position information and calculate fitness values until the termination condition is satisfied. Finally, the LSTM model is constructed based on the obtained optimal value of the target parameter to realize arbitrage spread prediction. The structure of the MSMSSA-LSTM model is shown in Fig 3 .
https://doi.org/10.1371/journal.pone.0303688.g003
4.3.2 Algorithm flow of MSMSSA-LSTM.
The specific steps for optimizing LSTM network hyperparameters using the MSMSSA algorithm are as follows:
Step 1 . Process the data. Determine the input features of the model. Check whether the data is missing, abnormal, disordered, etc. If it exists, process the data through corresponding preprocessing operations. Normalize the data. Divide the data into training sets, validation sets, and test sets according to a certain proportion.
Step 2 . Set the parameters. Set the parameters in the MSMSSA algorithm, such as population size, number of iterations, maximum and minimum proportions of the discoverer sparrow in the population, number of watchers, safety values, etc.
Step 3 . Generate the initial population. Based on parameters such as the number of populations, the dimension of the search space, the upper and lower limits of each target parameter value, etc., generate the initial population through the method of initializing the good point set.
Step 4 . Calculate the fitness value. Perform LSTM modeling according to the target parameters corresponding to each sparrow, return the mean square error on the validation set as its fitness value, sort the fitness values, and find out the best and worst sparrow individuals.
Step 5 . Update the location information. Calculate the number of discoverers, followers, and watchers, update their location information according to Formulas ( 9 ), ( 3 )~( 4 ), compare the global optimal solution, and update the optimal fitness value.
Step 6 . Determine the termination condition. When the number of iterations reaches the maximum, return the optimal value of the target parameters. Otherwise, go back to step 4 and continue execution.
Step 7 . Build the model. Build the LSTM model according to the optimal value of the target parameters.
Step 8 . Make a prediction. Train the model with the training set and validation set data, use the trained model to predict the test set, and get the prediction results.
The flowchart of the MSMSSA-LSTM algorithm is shown in Fig 4 .
https://doi.org/10.1371/journal.pone.0303688.g004
5 Experiments
To prove the effectiveness of MSMSSA-LSTM, this paper compared this method with MLP, RNN, LSTM, GRU, and SSA-LSTM using the same training set and test set data under the same operating environment. All the experiments are based on the TensorFlow deep learning framework under the CentOS operating system, configured with NVIDIA CUDA 10.1 and cuDNN 7.6 deep learning libraries to accelerate GPU computing. The Python version is 3.7, and the TensorFlow version is 2.3. According to the influence factors, including the opening price spread, highest price spread, lowest price spread, closing price spread, moving average convergence and divergence (MACD), differential exponential average (DEA), difference (DIF), and price spread fluctuation, the next minute’s closing price spread is predicted.
5.1.1 Data description.
This article selects the main contract data of rebar and hot coil futures from December 4, 2020, to February 16, 2023, as the research object. To ensure the continuity of the data and avoid the impact of contract delisting, another main contract data is used as a replacement when it is two months away from the delivery period. At the same time, to improve the predictive performance of the model, a large amount of data is needed for training. Therefore, this article uses high-frequency trading data of 1-minute prices of rebar and hot coil futures for research, totaling 180000 sets of data. They are divided into training and testing sets in an 8:2 ratio. The training set is mainly used for optimizing target parameters and training the model, while the testing set is mainly used to verify the predictive effect of the model outside of the sample. The data is sourced from Eastern Wealth Choice data. Fig 5 shows the 1-minute trend of the closing prices of two futures in 2021.
https://doi.org/10.1371/journal.pone.0303688.g005
As can be visualized from the chart above, the price trends of rebar and hot coil futures are extremely similar. Table 1 shows the result of a basic statistical analysis of the price data for the two futures.
https://doi.org/10.1371/journal.pone.0303688.t001
5.1.2 Correlation analysis.
https://doi.org/10.1371/journal.pone.0303688.t002
Table 2 shows that the correlation coefficient of rebar and hot coil is 0.9905, indicating that there is a very strong correlation between the two, and arbitrage trading can be constructed. However, the strength of the correlation cannot reflect the stability of the spread between the two futures varieties, so a cointegration test needs to be performed.
5.1.3 Cointegration test.
The cointegration test can only be performed if each data series satisfies the same order of single integrality. Therefore, this research needs to carry out the unit root test on the price series of rebar and hot coil futures first to determine their smoothness and the order of single integrality. This research uses Eviews software to conduct an ADF test to get Table 3 .
https://doi.org/10.1371/journal.pone.0303688.t003
As can be seen from the table, the ADF test values of HC and RB are both greater than the critical values at the 1%, 5%, and 10% significance levels, and the P values are all greater than 0.05. Therefore, the price series of hot coil and rebar futures have a unit root and are non-stationary series. On this basis, the first-order difference is performed to obtain the series ρHC and ρRB. The ADF test values are all less than the critical values at the 1%, 5%, and 10% significance levels, and the P values are all less than 0.05. Therefore, there is no unit root and it is a stationary series. Therefore, the price series of these two futures varieties are both first-order integrated, meet the cointegration conditions, and can undergo cointegration testing.
Among them, HC is the explained variable and RB is the explanatory variable. Ɛ t0 is random error. In this case, the value of R 2 is 0.981099, which indicates that the model has a 98.1% probability of explaining the real situation well, and the fitting effect is good. Next, the smoothness of the residual series is tested by the ADF method and the results are shown in Table 4 .
https://doi.org/10.1371/journal.pone.0303688.t004
Table 4 illustrates that at the 1% significance level, the ADF test value of the residual series is less than the critical value, that is, the null hypothesis is rejected and the series is considered to be stationary. According to EG cointegration theory, the price series of hot coil and rebar futures have a stable long-term equilibrium relationship.
5.2 Evaluation criteria
5.3 Model implementation
5.3.1 initialization of parameters..
In the MSMSSA-LSTM model, this research chooses the mean square error MSE as the loss function, uses the Adam algorithm as the optimizer, and sets Dropout to 0.1 to prevent overfitting. The number of sparrows in the population is 15, in which the maximum value of the proportion of discoverers is 0.7 and the minimum value is 0.2. The safe value is 0.8. The percentage of watchers is set to 20%. The number of iterations of the algorithm is 20. There are five objective parameters to be optimized by the MSMSSA algorithm, which are the learning rate, the number of iterations, the number of neurons in the two hidden layers, and the time step. Before starting the optimization, each objective parameter should be limited to a reasonable search range to avoid the waste of resources. In this paper, combined with relevant references and existing research [ 34 – 36 ], the appropriate search range of target parameters is finally selected, as shown in Table 5 .
https://doi.org/10.1371/journal.pone.0303688.t005
5.3.2 Comparison of algorithms.
To verify the performance of the improved sparrow search algorithm, the SSA-LSTM model was designed for comparison. Meanwhile, in order to avoid deviations in the results of a single run, this study conducted 10 independent experiments on MSMSSA-LSTM and SSA-LSTM, respectively. During the experiments, the same parameter settings were used for both methods. Specific details can be found in 5.3.1. Table 6 shows the optimization results of the objective function.
https://doi.org/10.1371/journal.pone.0303688.t006
It can be found that the best value, the worst value, the average value, and the median value of the optimization results of the MSMSSA algorithm are better than those of the SSA algorithm, which indicates that MSMSSA has stronger spatial search capability and higher convergence accuracy. The standard deviation and variance of the MSMSSA algorithm are also smaller than the SSA algorithm, indicating that MSMSSA has higher stability.
In addition, this research also plots the optimization results of the two algorithms on the objective function into a box plot. As shown in Fig 6 . It is not difficult to see that the median and mean of box-plot produced by the MSMSSA algorithm are smaller than those of the SSA algorithm. Therefore, the box-plot of the MSMSSA algorithm is in a lower position, which indicates that the overall quality of the solution generated by the MSMSSA algorithm is better than that of the SSA algorithm. At the same time, the IQR of the box-plot generated by the MSMSSA algorithm and the SSA algorithm are 8.91e-06 and 1.178e-05, respectively, indicating that the MSMSSA algorithm produces smaller discrete degrees and more stable optimization results.
https://doi.org/10.1371/journal.pone.0303688.g006
Fig 7 shows the convergence curves of the best optimization results of the two algorithms. The MSMSSA algorithm found the minimum value of the objective function 1.6646e-04 at the 6th iteration. The SSA algorithm found the optimal objective function value at the 10th iteration, which is 1.6807e-04. Therefore, the MSMSSA algorithm outperforms the SSA algorithm both in terms of convergence speed and optimization accuracy.
https://doi.org/10.1371/journal.pone.0303688.g007
According to the above analysis, the MSMSSA algorithm has stronger space exploration performance, more accurate optimization precision, better robustness, and faster convergence speed than the standard sparrow search algorithm. This indicates that the MSMSSA algorithm can find a better combination of hyperparameters in the LSTM model, and provide help for constructing high-precision arbitrage spread prediction model.
5.3.3 Optimization of target parameters.
In 10 independent experiments, when the objective function achieves the minimum value, the changes of each parameter during the optimization process are shown in Fig 8 and Table 7 . It is not difficult to find that when the MSMSSA algorithm is executed to the 6th round, the fitness value is 0.00016646. At the same time, this value remains stable in the subsequent iteration process and no longer changes, indicating that the optimal parameter combination in the model has been found, that is, the learning rate is 0.00775587, the number of epochs is 95, the number of neurons in the LSTM layer is 40, the number of neurons in the Dense layer is 2, and the time step is 45. This paper constructs a high-precision LSTM model to achieve arbitrage spread prediction through the optimal value of these parameters.
https://doi.org/10.1371/journal.pone.0303688.g008
https://doi.org/10.1371/journal.pone.0303688.t007
5.4 Experimental results and analysis
In this paper, MLP, RNN, LSTM, and GRU, which are more widely used time series forecasting models in the financial field, are chosen as contrast experiments. At the same time, to demonstrate the effectiveness of our improvement on the SSA, an SSA-LSTM model was designed for validation. In the previous 10 independent experiments, the optimal combination of parameters searched by the SSA is as follows: the learning rate is 0.00827520, the number of model training is 48, the number of neurons in the first and second hidden layers is 70 and 3, and the time step is 24. The same training set data is used to train each model, and the test set data is predicted based on the trained model. Figs 9 – 14 show the prediction results.
https://doi.org/10.1371/journal.pone.0303688.g009
https://doi.org/10.1371/journal.pone.0303688.g010
https://doi.org/10.1371/journal.pone.0303688.g011
https://doi.org/10.1371/journal.pone.0303688.g012
https://doi.org/10.1371/journal.pone.0303688.g013
https://doi.org/10.1371/journal.pone.0303688.g014
In Figs 9 – 14 , among the six forecasting models, the broken line fitting degree of real value and predicted value is MSMSSA-LSTM, SSA-LSTM, GRU, LSTM, RNN, MLP from high to low. Among them, MSMSSA-LSTM has the highest degree of broken line fitting which almost coincides with each other, and MLP has the lowest degree of broken line fitting.
In order to more intuitively reflect the predictive performance of various models on futures spread data and to demonstrate the effectiveness and superiority of the MSMSSA-LSTM model, this paper calculated the evaluation indicators for each model based on their predicted values and real value. The results are shown in Table 8 .
https://doi.org/10.1371/journal.pone.0303688.t008
As shown in Table 8 , the MLP model has the largest MAPE, RMSE, and MAE values of 0.0212, 7.5799, and 5.6275, respectively, and the smallest R 2 value of 0.9918, indicating that the model is hard to fit effectively to futures spread data and has poor predictive performance. Compared with MLP, the predictive performance of RNN has been improved, with MAPE, RMSE, MAE, and R 2 being 0.0148, 4.7028, 3.4576, and 0.9968, respectively. However, due to the defects of gradient vanishing, gradient explosion, and insufficient long-term memory ability in RNN models, there is still plenty of room for improvement in their prediction accuracy. LSTM effectively solves the problems of the RNN model by introducing gate structure and significantly improves the predictive performance. The four evaluation indicators are, in order, 0.0109, 3.3225, 2.4217, and 0.9984. As a variant of the LSTM model, GRU has MAPE, RMSE, MAE, and R 2 values of 0.0115, 3.2126, 2.3824, and 0.9985, respectively. From the evaluation indicators, GRU is slightly better than LSTM. The SSA-LSTM model reduces prediction error by using the traditional sparrow search algorithm to find the optimal hyperparameter combination in the LSTM network, with evaluation indicators of 0.0095, 2.8525, 1.9701, and 0.9988, respectively. This paper constructs a MSMSSA-LSTM model by improving the SSA algorithm. Its MAPE, RMSE, and MAE are the smallest, at 0.0088, 2.6409, and 1.8251 respectively, and its R 2 is the largest, at 0.9990. Compared with the other five models, the MAPE of MSMSSA-LSTM decreased by 58.5%, 40.5%, 19.3%, 23.5%, and 7.4%, respectively. The RMSE decreased by 65.2%, 43.8%, 20.5%, 17.8%, and 7.4%, respectively. The MAE decreased by 67.6%, 47.2%, 24.6%, 23.4%, and 7.4%, respectively. The experimental results show that the MSMSSA-LSTM model proposed in this paper has significantly better prediction accuracy than the other five methods, and the effect is the best.
According to the above analysis, the MSMSSA-LSTM model has a good predictive ability for the trend of arbitrage spread. This can help investors formulate more scientific trading strategies and seek higher returns.
6 Conclusion
This research proposes a novel technique for the problem of arbitrage spread forecasting named MSMSSA-LSTM. The technique utilizes MSMSSA to automatically seek the optimal combination of hyperparameters in the LSTM model. This effectively solves the problem that hyperparameters in LSTM are difficult to determine and cannot be adjusted with training. Based on the standard sparrow search algorithm, MSMSSA introduces the good point set theory, the proportion-adaptive strategy, and the improved location update method to further enhance the spatial search capability of SSA. This paper innovatively applies the MSMSSA algorithm and LSTM model to the field of futures arbitrage and has achieved good results. The newly proposed model is evaluated using real spread data of rebar and hot coil futures in the Chinese futures market, and compared with the SSA-LSTM model and several classical machine learning methods. The key findings are as follows:
- Compared with the SSA algorithm, the MSMSSA algorithm has stronger global optimization ability and better robustness. Faced with hyperparameter optimization problems in LSTM models, the MSMSSA algorithm has shown better applicability.
- Compared with several classical machine learning methods, the mean absolute error of the proposed model is reduced by at least 23.4%. This indicates that using the MSMSSA algorithm to optimize the LSTM network can minimize the influence of human factors and improve the generalization ability and prediction effect of the model.
In summary, the experimental results show that the MSMSSA-LSTM model outperforms all comparative experiments and has the highest accuracy in arbitrage spread trend prediction. The limitation of this model is that the training time is long. During the hyperparameter optimization process, the LSTM network may run hundreds or thousands of times. The time cost is very high. Therefore, future research will continue to accelerate algorithm optimization and improve model performance.
- 1. Zheng Y. Neural Network and Order Flow, Technical Analysis: Predicting short-term direction of futures contract[J]. arXiv preprint arXiv:2203.12457, 2022.
- View Article
- Google Scholar
- 4. Ariyo A A, Adewumi A O, Ayo C K. Stock price prediction using the ARIMA model[C]//2014 UKSim-AMSS 16th international conference on computer modelling and simulation. IEEE, 2014: 106-112.
- 14. Chen K, Zhou Y, Dai F. A LSTM-based method for stock returns prediction: A case study of China stock market[C]//2015 IEEE international conference on big data (big data). IEEE, 2015: 2823-2824.
- 15. Nelson D M Q, Pereira A C M, De Oliveira R A. Stock market’s price movement prediction with LSTM neural networks[C]//2017 International joint conference on neural networks (IJCNN). Ieee, 2017: 1419-1426.

IMAGES
VIDEO
COMMENTS
Abstract. This paper presents a comprehensive analysis of a mean reversion trading strategy, centered around the True Strength Index (TSI), applied to the SPY (S&P 500) and QQQ (Nasdaq Index) ETFs. The study spans historical data from 1996 to 2022, encompassing various market conditions to assess the strategy's robustness.
Mean reversion trading strategies are widely used in industry. However, not all strategies ensure that the ... In the paper, we summarize the statistics results for nine pairs and display the detailed results for two pairs. The rest of the paper is structured as follows. We introduce our methodology in Section 2 followed by
INTRODUCTION. market maker is a firm, individual or trading strategy that always or often quotes both a buy and a sell price for a financial instrument or commodity, hoping to make a profit by exploiting the difference between the two prices, known as the spread. Intuitively, a market maker wishes to buy and sell equal volumes of the instrument ...
Stock network. Mean reversion. Distributed optimization. 1. Introduction. Portfolio selection is a practical engineering task in finance that aims to minimize portfolio risk or maximize its return by allocating wealth in different assets. The research for portfolio strategy can be divided into two major schools.
1. Introduction. Momentum and mean reversion trading strategies are two of the most commonly used algorithmic trading strategies. Momentum strategies identify recent trends in prices; traders generally buy when recent trends point upwards and sell when recent trends point downwards. Mean reversion strategies assess whether prices tend to revert ...
to be reverting towards its long-term mean if the price shows a downward trend when greater than and upward trend when less than . Prices of commodities such as oil [11, 13] and foreign exchange rates [8] have been empirically observed to exhibit mean reversion. Mean-reverting stochas-tic processes are studied as a major class of price models, as
Recent studies have shown that online portfolio selection strategies that exploit the mean reversion property can achieve excess return from equity markets. This paper empirically investigates the performance of state-of-the-art mean reversion strategies on real market data. The aims of the study are twofold. The first is to find out why the mean reversion strategies perform extremely well on ...
Abstract and Figures. In this paper, we review briefly existing approaches to statistical arbitrage and mean reversion and then proceed to present a new approach that combines model-independence ...
Research Papers Optimal mean-reversion strategy in the presence of bid-ask spread and delays in capital allocations Sergey Isaenko The John Molson School of Business, Concordia University, 1455 de Maisonneuve Blvd. W., Montreal, Quebec, H3G 1M8 Canada Correspondence [email protected]
This study analyzed the short-term mean reversion of stock return in the Korean market from 1987 to 2015. Mainly focusing on the effect of the change in trading volume on stock returns, we compare the mean reversion patterns in the CCRV-orthogonalized return with that in the original return using the VR test. The empirical analysis confirms the ...
The PAMR strategy is based on the mean reversion idea as described in Sect. 4.1, and is equipped with Passive Aggressive (PA) online learning technique (Crammer et al. 2006 ). First of all, given a portfolio vector b and a price relative vector x t , we define a ϵ -insensitive loss function for the t th trading day as.
This paper examines the evidence on the extent to which stock prices exhibit mean—reverting behavior. The question of whether stock prices contain transitory components is important for financial practice and theory. For example, consider the question of investment strategy. If stock price movements
Online portfolio selection (OLPS) is a procedure for allocating portfolio assets using only past information to maximize an expected return. There have been successful mean reversion strategies that have achieved large excess returns on the traditional OLPS benchmark datasets. We propose a genetic mean reversion strategy that evolves a population of portfolio vectors using a hybrid genetic ...
Understanding mean reversion provides valuable insights into market dynamics, investor behavior, and the potential for profitable trading strategies. The aim of this study was to empirically ...
Mean-reversion trading strategies, often referred to as 'follow the loser' strategies, assume losers (winners) over some lookback window will be winners (losers) in the ... and sensor data has led to a plethora of research in this field. ... in an attempt to remove linear trend in the mean. Throughout this paper, for brevity, we will refer ...
This article proposes a novel online portfolio selection strategy named "Passive Aggressive Mean Reversion" (PAMR). Unlike traditional trend following approaches, the proposed approach relies upon the mean reversion relation of financial markets. Equipped with online passive aggressive learning technique from machine learning, the proposed ...
4.4 Mean reversion traders. The second group of high-frequency agents are the mean-reversion traders. Again, this is a well documented strategy (Serban 2010) in which traders believe that asset prices tend to revert towards their a historical average (though this may be a very short term average). They attempt to generate profit by taking long ...
Cointegration, Mean reversion, Cryptocurrency market Paper type — Research paper 2. 1 Introduction ... So, the performance of the research's strategy is questionable. Furthermore, they did not compare their method with a naive strategy, i.e., buy-and-hold strategy, and besides, the required investment to deploy the strategy
In addition, in the futures market, arbitrage methods are mainly divided into two categories: mean reversion arbitrage methods and neural network arbitrage methods . The mean reversion method uses financial time series analysis methods to study the long-term relationships that exist between futures, so as to design arbitrage strategies.