What is explanatory research?
Last updated
12 June 2023
Reviewed by
Miroslav Damyanov
The search for knowledge and understanding never stops in the field of research. Researchers are always finding new techniques to help analyze and make sense of the world. Explanatory research is one such technique. It provides a new perspective on various areas of study.
So, what exactly is explanatory research? This article will provide an in-depth overview of everything you need to know about explanatory research and its purpose. You’ll also get to know the different types of explanatory research and how they’re conducted.

Analyze explanatory research
Get a deeper understanding of your explanatory research when you analyze it in Dovetail
- Explanatory research: definition
Explanatory research is a technique used to gain a deeper understanding of the underlying reasons for, causes of, and relationships behind a particular phenomenon that has yet to be extensively studied.
Researchers use this method to understand why and how a particular phenomenon occurs the way it does. Since there is limited information regarding the phenomenon being studied, it’s up to the researcher to develop fresh ideas and collect more data.
The results and conclusions drawn from explanatory research give researchers a deeper understanding and help predict future occurrences.
- Descriptive research vs. explanatory research
Descriptive research aims to define or summarize an event or population without explaining why it exists. It focuses on acquiring and conveying facts.
On the other hand, explanatory research aims to explain why a phenomenon occurs by working to understand the causes and correlations between variables.
Unlike descriptive research, which focuses on providing descriptions and characteristics of a given phenomenon, explanatory research goes a step further to explain different mechanisms and the reasons behind them. Explanatory research is never concerned with producing new knowledge or solving problems. Instead, it aims to explain why and how something happens.
- Exploratory research vs. explanatory research
Explanatory research explains why specific phenomena function as they do. Meanwhile, exploratory research examines and investigates an issue that is not clearly defined. Both methods are crucial for problem analysis.
Researchers use exploratory research at the outset to discover new ideas, concepts, and opportunities. Once exploratory research has identified a potential area of interest or problem, researchers employ explanatory research to delve further into the specific subject matter.
Researchers employ the explanatory research technique when they want to explain why and how something occurs in a certain way. Researchers who employ this approach usually have an outcome in mind, and carrying it out is their top priority.
- When to use explanatory research
Explanatory research may be helpful in the following situations:
When testing a theoretical model: explanatory research can help researchers develop a theory. It can provide sufficient evidence to validate or refine existing theories based on the available data.
When establishing causality: this research method can determine the cause-and-effect relationships between study variables and determine which variable influences the predicted outcome most. Explanatory research explores all the factors that lead to a certain outcome or phenomenon.
When making informed decisions: the results and conclusions drawn from explanatory research can provide a basis for informed decision-making. It can be helpful in different industries and sectors. For example, entrepreneurs in the business sector can use explanatory research to implement informed marketing strategies to increase sales and generate more revenue.
When addressing research gaps: a research gap is an unresolved problem or unanswered question due to inadequate research in that space. Researchers can use explanatory research to gather information about a certain phenomenon and fill research gaps. It also enables researchers to answer previously unanswered questions and explain different mechanisms that haven’t yet been studied.
When conducting program evaluation: researchers can also use the technique to determine the effectiveness of a particular program and identify all the factors that are likely to contribute to its success or failure.
- Types of explanatory research
Here are the different types of explanatory research:
Case study research: this method involves the in-depth analysis of a given individual, company, organization, or event. It allows researchers to study individuals or organizations that have faced the same situation. This way, they can determine what worked for them and what didn’t.
Experimental research: this involves manipulating independent variables and observing how they affect dependent variables. This method allows researchers to establish a cause-and-effect relationship between different variables.
Quasi-experimental research: this type of research is quite similar to experimental research, but it lacks complete control over variables. It’s best suited to situations where manipulating certain variables is difficult or impossible.
Correlational research: this involves identifying underlying relationships between two or more variables without manipulating them. It determines the strength and direction of the relationship between different variables.
Historical research: this method involves studying past events to gain a better understanding of their causes and effects. It’s mostly used in fields like history and sociology.
Survey research: this type of explanatory research involves collecting data using a set of structured questionnaires or interviews given to a representative sample of participants. It helps researchers gather information about individuals’ attitudes, opinions, and behaviors toward certain phenomena.
Observational research: this involves directly observing and recording people in their natural setting, like the home, the office, or a shop. By studying their actions, needs, and challenges, researchers can gain valuable insights into their behavior, preferences, and pain points. This results in explanatory conclusions.
- How to conduct explanatory research
Take the following steps when conducting explanatory research:
Develop the research question
The first step is to familiarize yourself with the topic you’re interested in and clearly articulate your specific goals. This will help you define the research question you want to answer or the problem you want to solve. Doing this will guide your research and ensure you collect the right data.
Formulate a hypothesis
The next step is to formulate a hypothesis that will address your expectations. Some researchers find that literature material has already covered their topic in the past. If this is the case with you, you can use such material as the main foundation of your hypothesis. However, if it doesn’t exist, you must formulate a hypothesis based on your own instincts or literature material on closely related topics.
Select the research type
Choose an appropriate research type based on your research questions, available resources, and timeline. Consider the level of control you need over the variables.
Next, design and develop instruments such as surveys, interview guides, or observation guidelines to gather relevant data.
Collect the data
Collecting data involves implementing the research instruments and gathering information from a representative sample of your target audience. Ensure proper data collection protocol, ethical considerations , and appropriate documentation for the data you collect.
Analyze the data
Once you have collected the data you need for your research, you’ll need to organize, code, and interpret it.
Use appropriate analytical methods, such as statistical analysis or thematic coding , to uncover patterns, relationships, and explanations that address your research goals and questions. You may have to suggest or conduct further research based on the results to elaborate on certain areas.
Communicate the results
Finally, communicate your results to relevant stakeholders , such as team members, clients, or other involved partners. Present your insights clearly and concisely through reports, slides, or visualizations. Provide actionable recommendations and avenues for future research.
- Examples of explanatory research
Here are some real-life examples of explanatory research:
Understanding what causes high crime rates in big cities
Law enforcement organizations use explanatory research to pinpoint what causes high crime rates in particular cities. They gather information about various influencing factors, such as gang involvement, drug misuse, family structures, and firearm availability.
They then use regression analysis to examine the data further to understand the factors contributing to the high crime rates.
Factors that influence students’ academic performance
Educators and stakeholders in the Department of Education use questionnaires and interviews to gather data on factors that affect academic performance. These factors include parental engagement, learning styles, motivation, teaching quality, and peer pressure.
The data is used to ascertain how these variables affect students’ academic performance.
Examining what causes economic disparity in certain areas
Researchers use correlational and experimental research approaches to gather information on variables like education levels, household income, and employment rates. They use the information to examine the causes of economic disparity in certain regions.
- Advantages of explanatory research
Here are some of the benefits you can expect from explanatory research:
Deeper understanding : the technique helps fill research gaps in previous studies by explaining the reasons, causes, and relationships behind particular behaviors or phenomena.
Competitive edge: by understanding the underlying factors that drive customer satisfaction and behavior, companies can create more engaging products and desirable services.
Predictable capabilities: it helps researchers and teams make predictions regarding certain phenomena like user behavior or future iterations of product features.
Informed decision-making: explanatory research generates insights that can help individuals make informed decisions in various sectors.
- Disadvantages of explanatory research
Explanatory research is a great approach for better understanding various phenomena, but it has some limitations.
It’s time-consuming: explanatory research can be a time-consuming process, requiring careful planning, data collection, analysis, and interpretation. The technique might extend your timeline.
It’s resource intensive: explanatory research often requires a significant allocation of resources, including financial, human, and technological. This could pose challenges for organizations with limited budgets or constraints.
You have limited control over real-world factors: this type of research often takes place in controlled environments. Researchers may find this limits their ability to capture real-world complexities and variables that influence a particular behavior or phenomenon.
Depth and breadth are difficult to balance : explanatory research mainly focuses on a narrow hypothesis, which can limit the scope of the research and prevent researchers from understanding a problem more broadly.
Should you be using a customer insights hub?
Do you want to discover previous research faster?
Do you share your research findings with others?
Do you analyze research data?
Start for free today, add your research, and get to key insights faster
Editor’s picks
Last updated: 11 January 2024
Last updated: 15 January 2024
Last updated: 17 January 2024
Last updated: 12 May 2023
Last updated: 30 April 2024
Last updated: 18 May 2023
Last updated: 25 November 2023
Last updated: 13 May 2024
Latest articles
Related topics, .css-je19u9{-webkit-align-items:flex-end;-webkit-box-align:flex-end;-ms-flex-align:flex-end;align-items:flex-end;display:-webkit-box;display:-webkit-flex;display:-ms-flexbox;display:flex;-webkit-flex-direction:row;-ms-flex-direction:row;flex-direction:row;-webkit-box-flex-wrap:wrap;-webkit-flex-wrap:wrap;-ms-flex-wrap:wrap;flex-wrap:wrap;-webkit-box-pack:center;-ms-flex-pack:center;-webkit-justify-content:center;justify-content:center;row-gap:0;text-align:center;max-width:671px;}@media (max-width: 1079px){.css-je19u9{max-width:400px;}.css-je19u9>span{white-space:pre;}}@media (max-width: 799px){.css-je19u9{max-width:400px;}.css-je19u9>span{white-space:pre;}} decide what to .css-1kiodld{max-height:56px;display:-webkit-box;display:-webkit-flex;display:-ms-flexbox;display:flex;-webkit-align-items:center;-webkit-box-align:center;-ms-flex-align:center;align-items:center;}@media (max-width: 1079px){.css-1kiodld{display:none;}} build next, decide what to build next.

Users report unexpectedly high data usage, especially during streaming sessions.

Users find it hard to navigate from the home page to relevant playlists in the app.

It would be great to have a sleep timer feature, especially for bedtime listening.

I need better filters to find the songs or artists I’m looking for.
Log in or sign up
Get started for free
- Explanatory Research: Types, Examples, Pros & Cons

Explanatory research is designed to do exactly what it sounds like: explain, and explore. You ask questions, learn about your target market, and develop hypotheses for testing in your study. This article will take you through some of the types of explanatory research and what they are used for.
What is Explanatory Research?
Explanatory research is defined as a strategy used for collecting data for the purpose of explaining a phenomenon. Because the phenomenon being studied began with a single piece of data, it is up to the researcher to collect more pieces of data.
In other words, explanatory research is a method used to investigate a phenomenon (a situation worth studying) that had not been studied before or had not been well explained previously in a proper way. It is a process in which the purpose is to find out what would be a potential answer to the problem.
This method of research enables you to find out what does not work as well as what does and once you have found this information, you can take measures for developing better alternatives that would improve the process being studied. The goal of explanatory research is to answer the question “How,” and it is most often conducted by people who want to understand why something works the way it does, or why something happens as it does.
Read: How to Write a Problem Statement for your Research
By using this method, researchers are able to explain why something is happening and how it happens. In other words, explanatory research can be used to “explain” something, by providing the right context. This is usually done through the use of surveys and interviews.
Importance of Explanatory Research
Explanatory research helps researchers to better understand a subject, but it does not help them to predict what might happen in the future. Explanatory research is also known by other names, such as ex post facto (Latin for “after the fact”) and causal research.
The most important goal of explanatory research is to help understand a given phenomenon. This can be done through basic or applied research .
Basic explanatory research, also known as pure or fundamental research, is conducted without any specific real-world application in mind. Applied explanatory research attempts to develop new knowledge that can be used to improve humans’ everyday lives.
Read: How to Write a Thesis Statement for Your Research: Tips + Examples
For example, you might want to know why people buy certain products, why companies change their business processes, or what motivates people in the workplace. Explanatory research starts with a theory or hypothesis and then gathers evidence to prove or disprove the theory.
Most explanatory research uses surveys to gather information from a pool of respondents . The results will then provide information about the target population as a whole.
Purpose of Explanatory Research
The purpose of explanatory research is to explore a topic and develop a deeper understanding of it so that it can be described or explained more fully. The researcher sets out with a specific question or hypothesis in mind, which will guide the data collection and analysis process.
Explanatory research can take any number of forms, from experimental studies in which researchers test a hypothesis by manipulating variables, to interviews and surveys that are used to gather insights from participants about their experiences. Explanatory research seeks neither to generate new knowledge nor solve a specific problem; rather it seeks to understand why something happens.
For example, imagine that you would like to know whether one’s age affects his or her ability to use a particular type of computer software. You develop the hypothesis that older people will have more difficulty using the software than younger people.
In order to test your hypothesis and learn more about the relationship between age and software usage, you design and conduct an explanatory study.
Read: How to Write An Abstract For Research Papers: Tips & Examples
Characteristics of Explanatory Research
Explanatory research is used to explain something that has already happened but it doesn’t try to control anything, nor does it seek to predict what will happen. Instead, its aim is to understand what has happened when it comes to a certain phenomenon.
Here are some of the characteristics of explanatory research, they include:
- It is used when the researcher wants to explain the relationship between two variables that the researcher cannot manipulate. This means that the researcher must rely on secondary data instead to understand the variables.
- In explanatory research, the data is collected before the study begins and is usually collected by a different individual/organization than that of the researcher.
- Explanatory research does not involve random sampling or random allocation (the process of assigning subjects and participants to different study groups).
Types of Explanatory Research
Explanatory research generally focuses on the “why” questions. For example, a business might ask why customers aren’t buying their product or how they can improve their sales process. Types of explanatory research include:
1. Case studies: Case studies allow researchers to examine companies that experienced the same situation as them. This helps them understand what worked and what didn’t work for the other company.
Explore: Formplus Customer Success Stories and Case Studies
2. Literature research: Literature research involves examining and reviewing existing academic literature on a topic related to your projects, such as a particular strategy or method. Literature research allows researchers to see how other people have discussed a similar problem and how they arrived at their conclusions.
3. Observations: Observations involve gathering information by observing events without interfering with them. They’re useful for gathering information about social interactions, such as who talks to whom on a subway platform or how people react to certain ads in public spaces, like billboards and bus shelters.
4. Pilot studies: Pilot studies are small versions of larger studies that help researchers prepare for larger studies by testing out methods, procedures, or instruments before using them in the final study design.
Read: Research Report: Definition, Types + [Writing Guide]
5. Focus groups: Focus groups involves gathering a group of people so participants can share opinions, instead of answering questions
Difference between Explanatory and Exploratory Research
Explanatory research is a type of research that answers the question “why.” It explains why something happens and it helps to understand what caused something to happen.
Explanatory research always has a clear objective in mind, and it’s all about the execution of that objective. Its main focus is to answer questions like “why?” and “how?”
Exploratory research on the other hand is a form of observational research, meaning that it involves observing and measuring what already exists. Exploratory research is also used when the researcher doesn’t know what they’re looking for.
Its purpose is to help researchers better understand a subject so that they can develop a theory. It is not about drawing any conclusion but about learning more about the subject.
Examples of Explanatory Research
Explanatory research will make it easier to find explanations for things that are difficult to understand.
For example, if you’re trying to figure out why someone got sick, explanatory research can help you look at all of your options and figure out what happened.
In this way, it is also used in order to determine whether or not something was caused by a person or an event. If a person was involved, you might want to consider looking at other people who may have been involved as well.
It can also be useful for determining whether or not the person who caused the problem has changed over time. This can be especially helpful when you’re dealing with a long-term relationship where there have been many changes.
Read: 21 Chrome Extensions for Academic Researchers in 2022
Let us assume a researcher wants to figure out what happened during an accident and how it happened.
Explanatory research will try to understand if a person was driving while intoxicated, or if the person had been under the influence of alcohol or drugs at the time of their death. If they were not, then they may have had some other medical condition that caused them to pass away unexpectedly.
In the two examples, explanatory research wanted to answer the question of what happened and why did it happen.
Advantages of Explanatory Research
Here are some of the advantages of explanatory research:
- Explanatory research can explain how something happened
- It also helps to understand a cause of a phenomenon
- It is great in predicting what will happen in the future based on observations made today.
- It is also a great way to start your research if you are unfamiliar with the subject.
Disadvantages of Explanatory Research
Explanatory research is beneficial in many ways as listed above, but here are a few of the disadvantages of explanatory research.
1. Clarity on what is not known: The first disadvantage is that this kind of research is not always clear about what is and isn’t known. Which means it doesn’t always make the best use of existing information or knowledge.
You need to be specific about what you know already and how much more there might be left for future studies in order for this kind of research project to be useful at all times. This can help avoid wasting time by focusing on an issue that has already been studied enough without knowing it yet (or vice versa).
2. No clear hypothesis: Another disadvantage is that when designing experiments using this method there often isn’t any clear hypothesis about what will happen next which makes it impossible for scientists to predict
Explanatory research is taking a topic and explaining it thoroughly so that audiences have a better understanding of the topic in question. With explanatory research, having great explanations takes on more importance, so if you are a researcher in the social science field, you might want to put it to use.
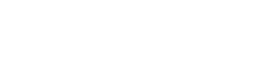
Connect to Formplus, Get Started Now - It's Free!
- analytical thesis statement
- causal research
- explanatory research
- exploratory research
- target population
- busayo.longe
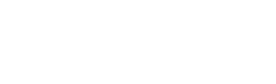
You may also like:
Exploratory Research: What are its Method & Examples?
Overview on exploratory research, examples and methodology. Shows guides on how to conduct exploratory research with online surveys

How to do a Meta Analysis: Methodology, Pros & Cons
In this article, we’ll go through the concept of meta-analysis, what it can be used for, and how you can use it to improve how you...
Descriptive Research Designs: Types, Examples & Methods
Ultimate guide to Descriptive research. Definitions, designs, types, examples and methodology.
How to Write a Thesis Statement for Your Research: Tips + Examples
In this article, we’ll show you how to create different types of thesis statements plus examples you can learn from.
Formplus - For Seamless Data Collection
Collect data the right way with a versatile data collection tool. try formplus and transform your work productivity today..
- Skip to main content
- Skip to primary sidebar
- Skip to footer
- QuestionPro

- Solutions Industries Gaming Automotive Sports and events Education Government Travel & Hospitality Financial Services Healthcare Cannabis Technology Use Case NPS+ Communities Audience Contactless surveys Mobile LivePolls Member Experience GDPR Positive People Science 360 Feedback Surveys
- Resources Blog eBooks Survey Templates Case Studies Training Help center

Home Market Research Research Tools and Apps
Analytical Research: What is it, Importance + Examples
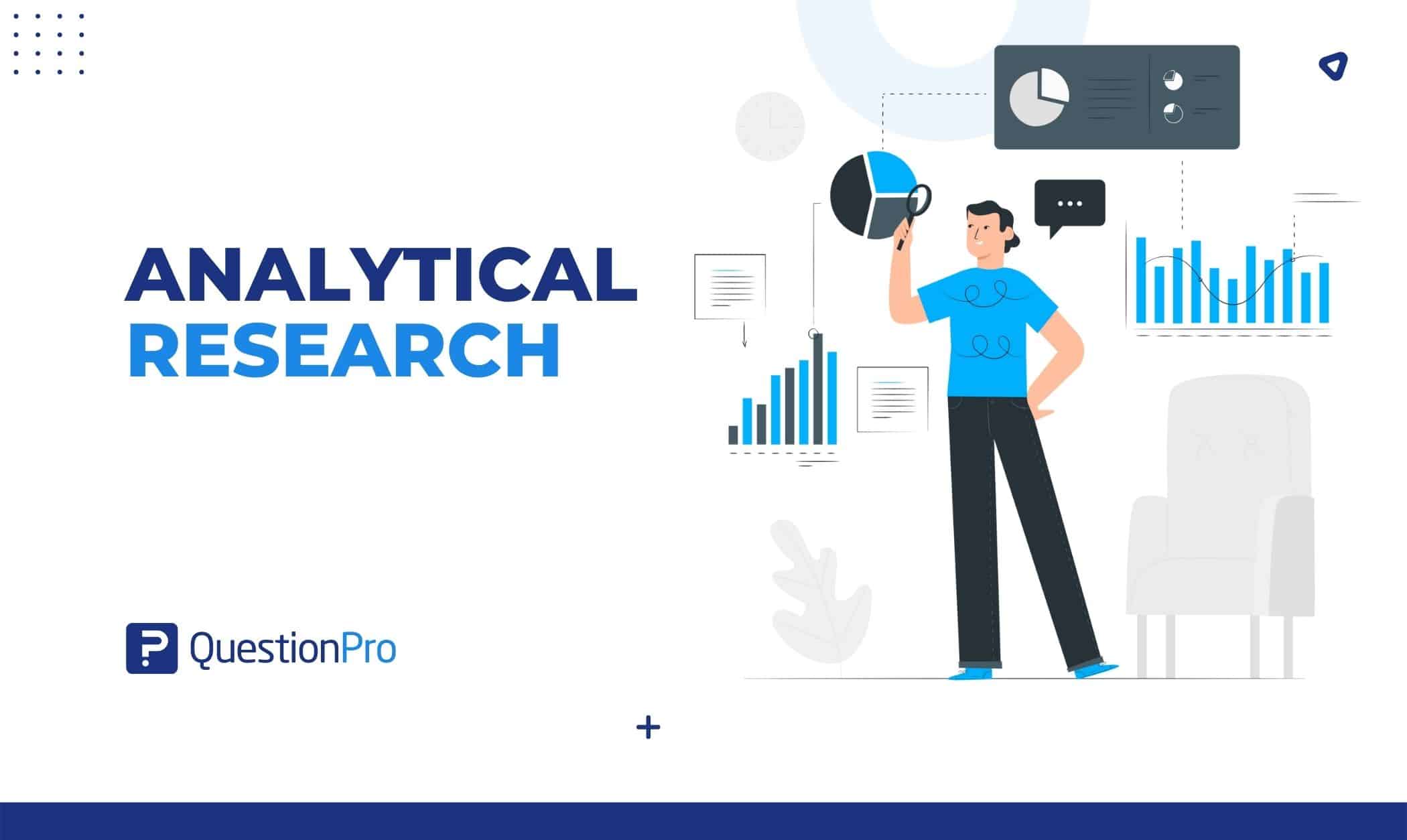
Finding knowledge is a loose translation of the word “research.” It’s a systematic and scientific way of researching a particular subject. As a result, research is a form of scientific investigation that seeks to learn more. Analytical research is one of them.
Any kind of research is a way to learn new things. In this research, data and other pertinent information about a project are assembled; after the information is gathered and assessed, the sources are used to support a notion or prove a hypothesis.
An individual can successfully draw out minor facts to make more significant conclusions about the subject matter by using critical thinking abilities (a technique of thinking that entails identifying a claim or assumption and determining whether it is accurate or untrue).
What is analytical research?
This particular kind of research calls for using critical thinking abilities and assessing data and information pertinent to the project at hand.
Determines the causal connections between two or more variables. The analytical study aims to identify the causes and mechanisms underlying the trade deficit’s movement throughout a given period.
It is used by various professionals, including psychologists, doctors, and students, to identify the most pertinent material during investigations. One learns crucial information from analytical research that helps them contribute fresh concepts to the work they are producing.
Some researchers perform it to uncover information that supports ongoing research to strengthen the validity of their findings. Other scholars engage in analytical research to generate fresh perspectives on the subject.
Various approaches to performing research include literary analysis, Gap analysis , general public surveys, clinical trials, and meta-analysis.
Importance of analytical research
The goal of analytical research is to develop new ideas that are more believable by combining numerous minute details.
The analytical investigation is what explains why a claim should be trusted. Finding out why something occurs is complex. You need to be able to evaluate information critically and think critically.
This kind of information aids in proving the validity of a theory or supporting a hypothesis. It assists in recognizing a claim and determining whether it is true.
Analytical kind of research is valuable to many people, including students, psychologists, marketers, and others. It aids in determining which advertising initiatives within a firm perform best. In the meantime, medical research and research design determine how well a particular treatment does.
Thus, analytical research can help people achieve their goals while saving lives and money.
Methods of Conducting Analytical Research
Analytical research is the process of gathering, analyzing, and interpreting information to make inferences and reach conclusions. Depending on the purpose of the research and the data you have access to, you can conduct analytical research using a variety of methods. Here are a few typical approaches:
Quantitative research
Numerical data are gathered and analyzed using this method. Statistical methods are then used to analyze the information, which is often collected using surveys, experiments, or pre-existing datasets. Results from quantitative research can be measured, compared, and generalized numerically.
Qualitative research
In contrast to quantitative research, qualitative research focuses on collecting non-numerical information. It gathers detailed information using techniques like interviews, focus groups, observations, or content research. Understanding social phenomena, exploring experiences, and revealing underlying meanings and motivations are all goals of qualitative research.
Mixed methods research
This strategy combines quantitative and qualitative methodologies to grasp a research problem thoroughly. Mixed methods research often entails gathering and evaluating both numerical and non-numerical data, integrating the results, and offering a more comprehensive viewpoint on the research issue.
Experimental research
Experimental research is frequently employed in scientific trials and investigations to establish causal links between variables. This approach entails modifying variables in a controlled environment to identify cause-and-effect connections. Researchers randomly divide volunteers into several groups, provide various interventions or treatments, and track the results.
Observational research
With this approach, behaviors or occurrences are observed and methodically recorded without any outside interference or variable data manipulation . Both controlled surroundings and naturalistic settings can be used for observational research . It offers useful insights into behaviors that occur in the actual world and enables researchers to explore events as they naturally occur.
Case study research
This approach entails thorough research of a single case or a small group of related cases. Case-control studies frequently include a variety of information sources, including observations, records, and interviews. They offer rich, in-depth insights and are particularly helpful for researching complex phenomena in practical settings.
Secondary data analysis
Examining secondary information is time and money-efficient, enabling researchers to explore new research issues or confirm prior findings. With this approach, researchers examine previously gathered information for a different reason. Information from earlier cohort studies, accessible databases, or corporate documents may be included in this.
Content analysis
Content research is frequently employed in social sciences, media observational studies, and cross-sectional studies. This approach systematically examines the content of texts, including media, speeches, and written documents. Themes, patterns, or keywords are found and categorized by researchers to make inferences about the content.
Depending on your research objectives, the resources at your disposal, and the type of data you wish to analyze, selecting the most appropriate approach or combination of methodologies is crucial to conducting analytical research.
Examples of analytical research
Analytical research takes a unique measurement. Instead, you would consider the causes and changes to the trade imbalance. Detailed statistics and statistical checks help guarantee that the results are significant.
For example, it can look into why the value of the Japanese Yen has decreased. This is so that an analytical study can consider “how” and “why” questions.
Another example is that someone might conduct analytical research to identify a study’s gap. It presents a fresh perspective on your data. Therefore, it aids in supporting or refuting notions.
Descriptive vs analytical research
Here are the key differences between descriptive research and analytical research:
The study of cause and effect makes extensive use of analytical research. It benefits from numerous academic disciplines, including marketing, health, and psychology, because it offers more conclusive information for addressing research issues.
QuestionPro offers solutions for every issue and industry, making it more than just survey software. For handling data, we also have systems like our InsightsHub research library.
You may make crucial decisions quickly while using QuestionPro to understand your clients and other study subjects better. Make use of the possibilities of the enterprise-grade research suite right away!
LEARN MORE FREE TRIAL
MORE LIKE THIS
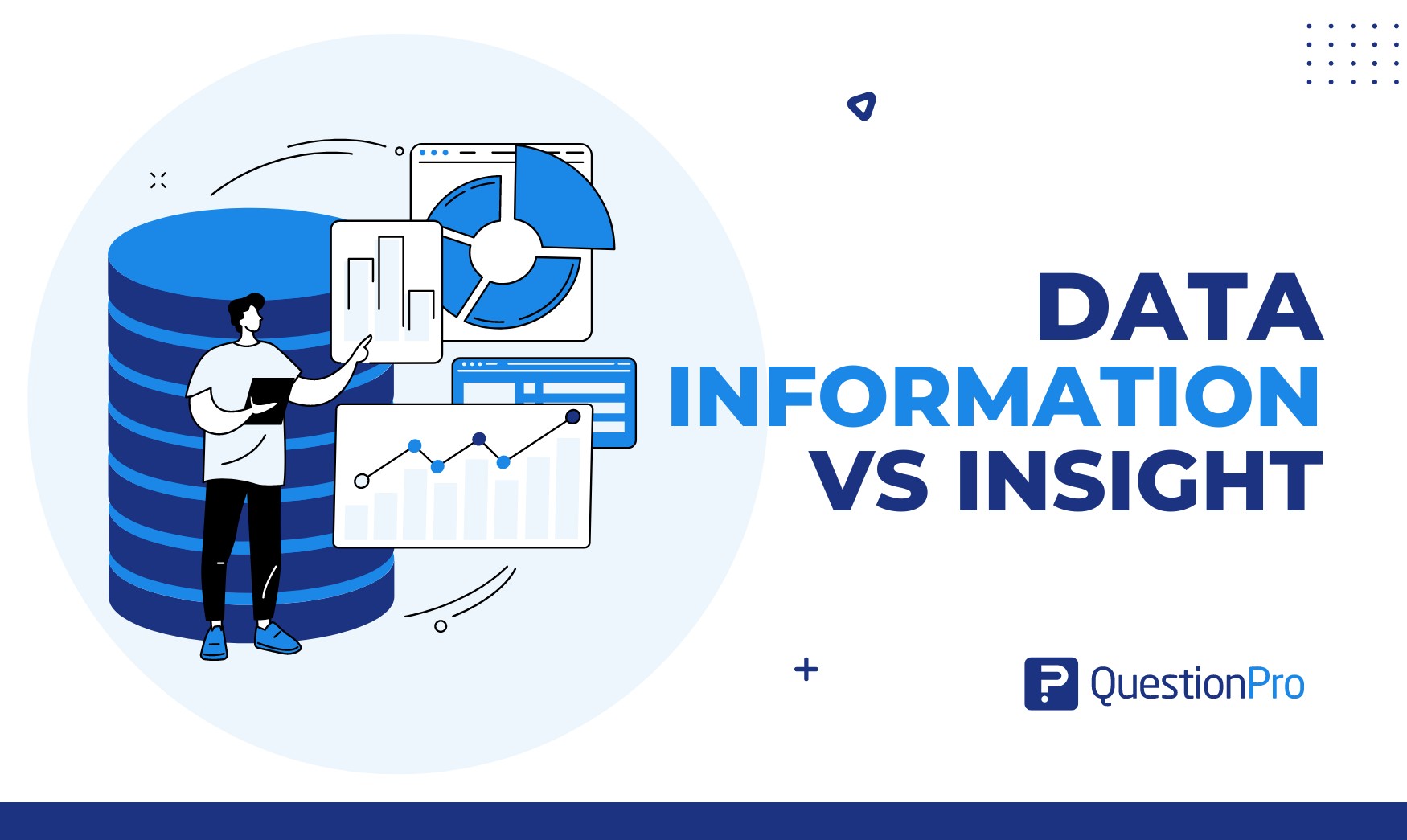
Data Information vs Insight: Essential differences
May 14, 2024
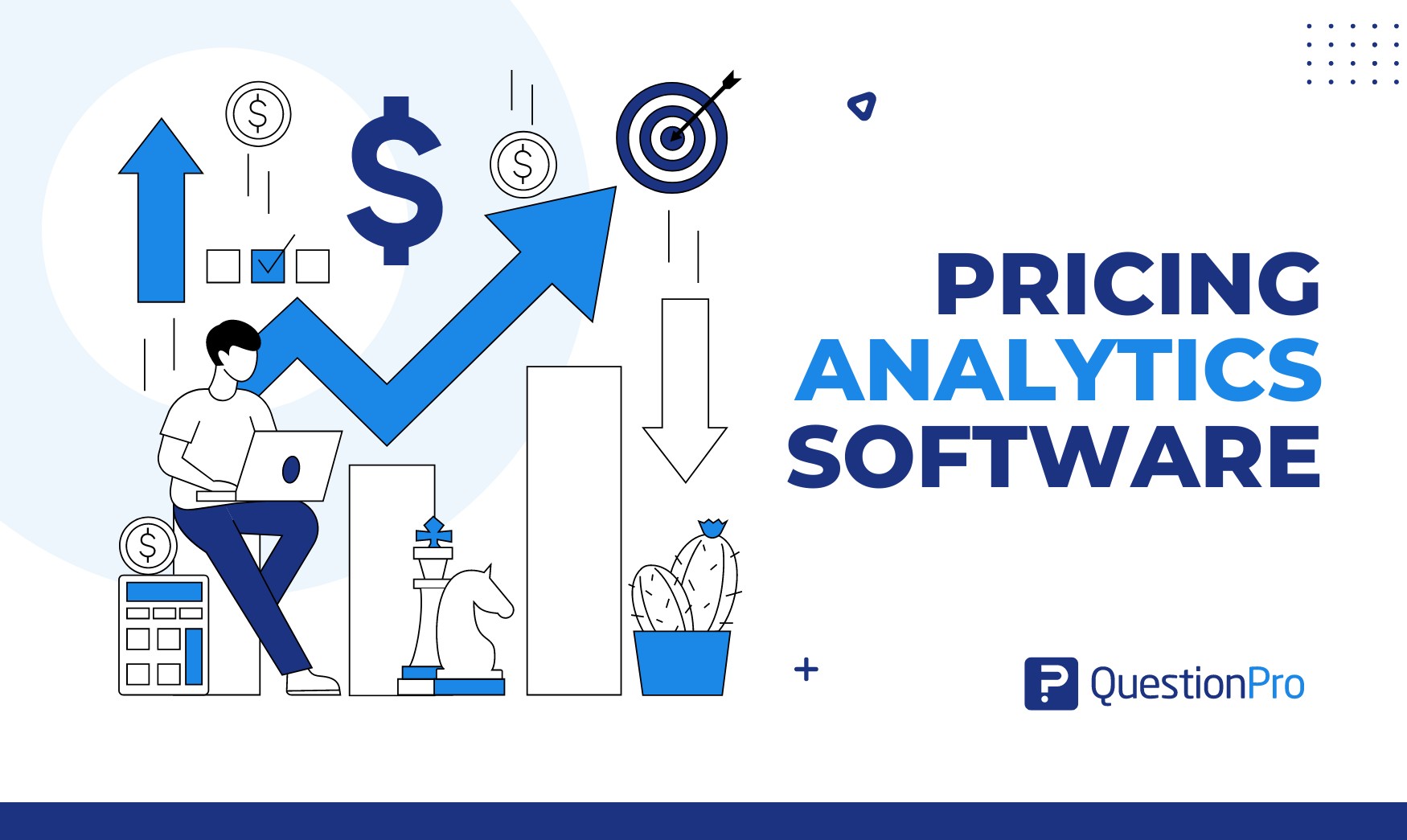
Pricing Analytics Software: Optimize Your Pricing Strategy
May 13, 2024
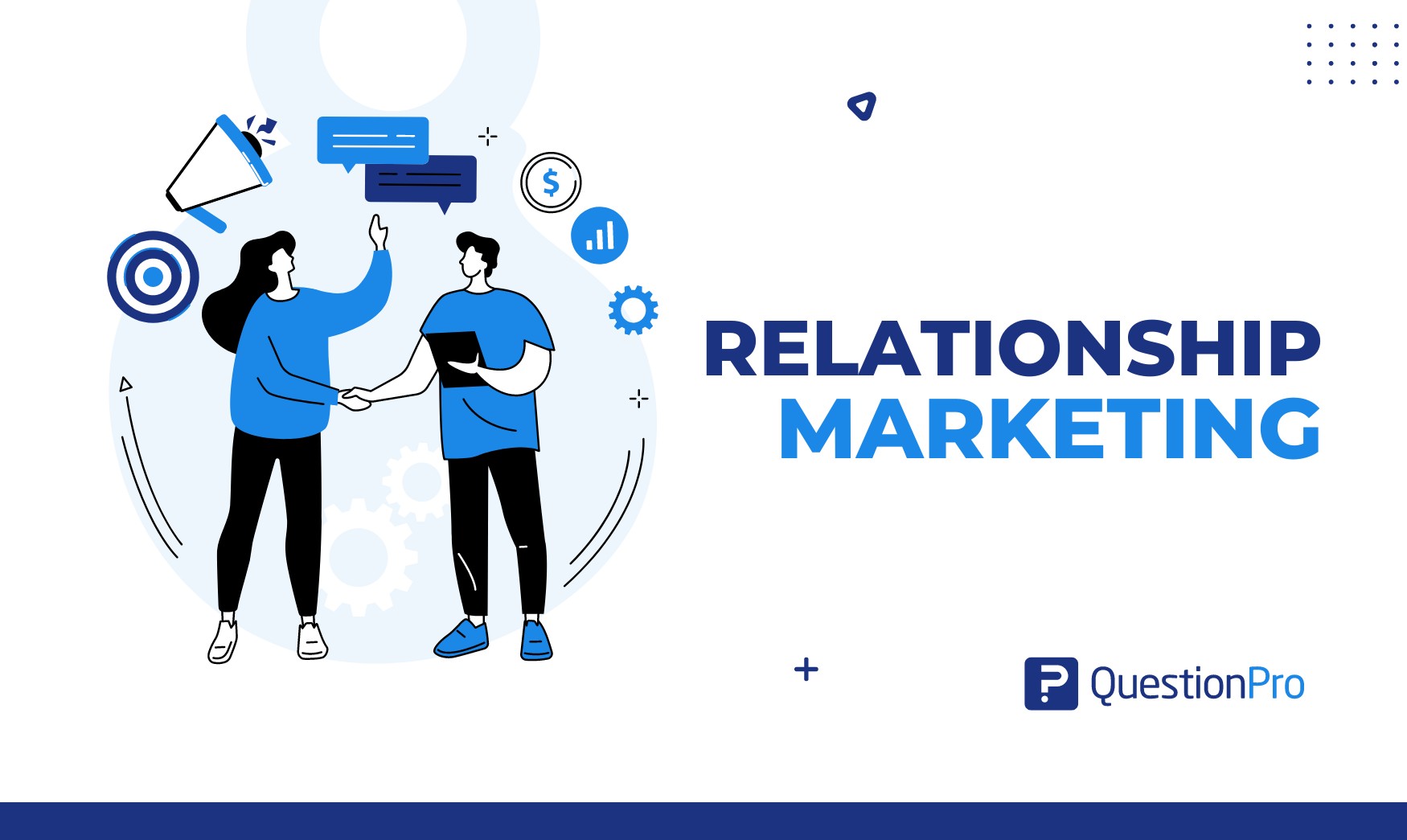
Relationship Marketing: What It Is, Examples & Top 7 Benefits
May 8, 2024
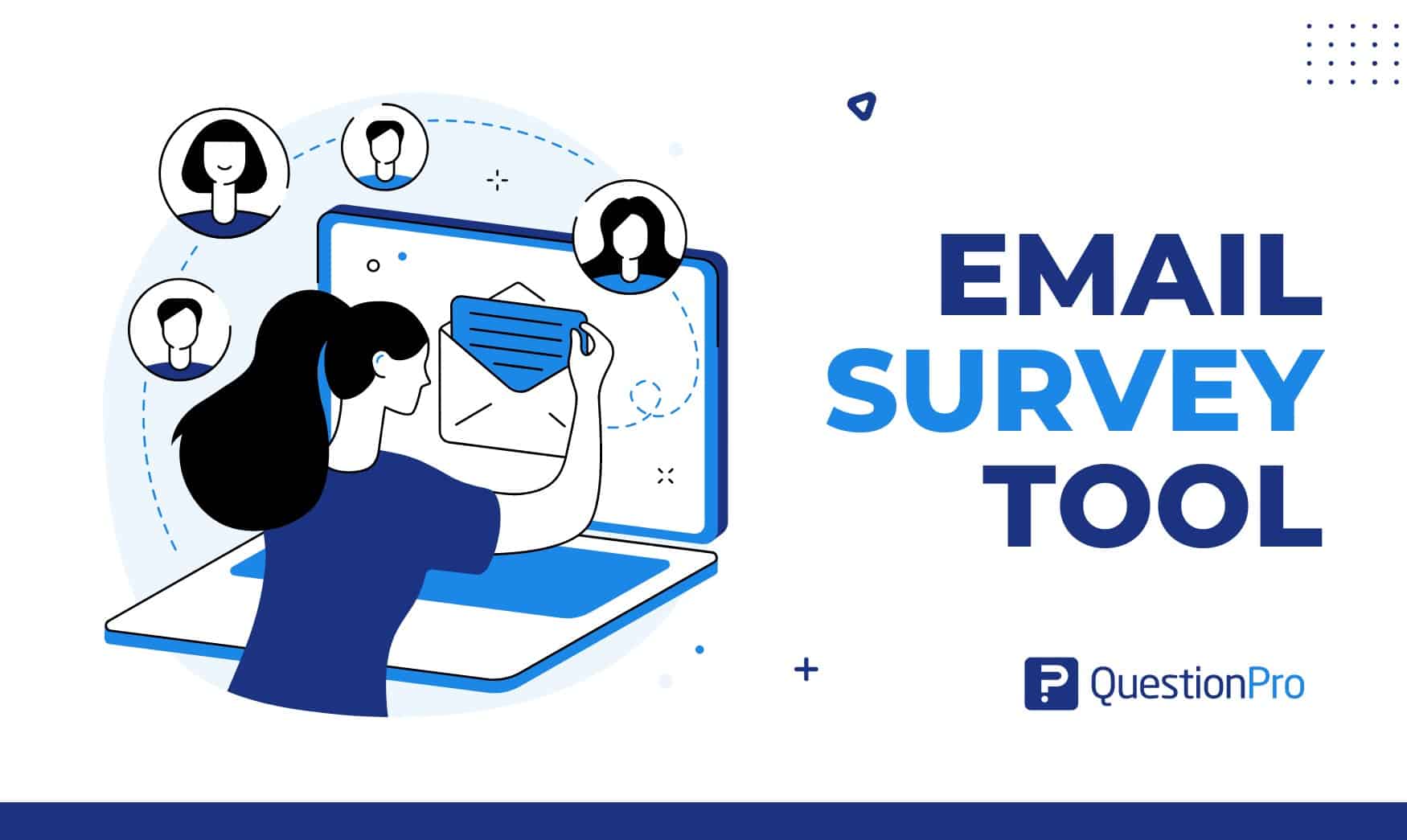
The Best Email Survey Tool to Boost Your Feedback Game
May 7, 2024
Other categories
- Academic Research
- Artificial Intelligence
- Assessments
- Brand Awareness
- Case Studies
- Communities
- Consumer Insights
- Customer effort score
- Customer Engagement
- Customer Experience
- Customer Loyalty
- Customer Research
- Customer Satisfaction
- Employee Benefits
- Employee Engagement
- Employee Retention
- Friday Five
- General Data Protection Regulation
- Insights Hub
- Life@QuestionPro
- Market Research
- Mobile diaries
- Mobile Surveys
- New Features
- Online Communities
- Question Types
- Questionnaire
- QuestionPro Products
- Release Notes
- Research Tools and Apps
- Revenue at Risk
- Survey Templates
- Training Tips
- Uncategorized
- Video Learning Series
- What’s Coming Up
- Workforce Intelligence
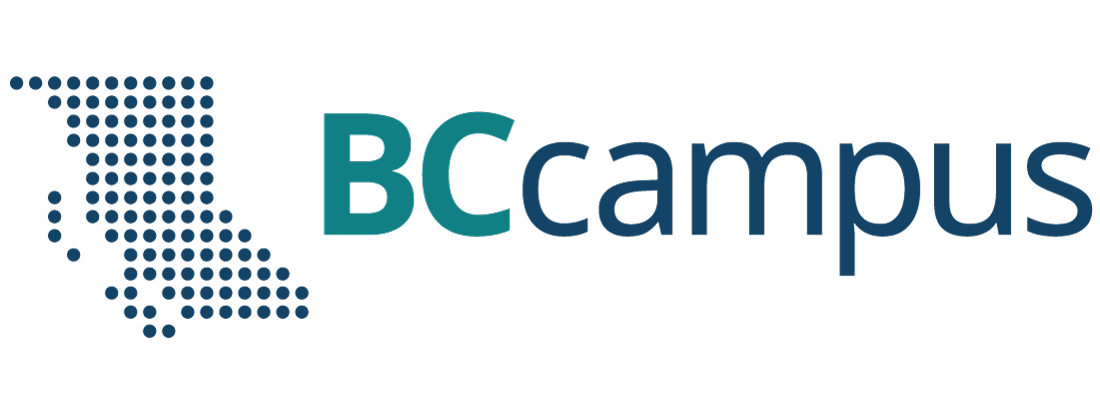
Want to create or adapt books like this? Learn more about how Pressbooks supports open publishing practices.
Chapter 3: Developing a Research Question
3.2 Exploration, Description, Explanation
As you can see, there is much to think about and many decisions to be made as you begin to define your research question and your research project. Something else you will need to consider in the early stages is whether your research will be exploratory, descriptive, or explanatory. Each of these types of research has a different aim or purpose, consequently, how you design your research project will be determined in part by this decision. In the following paragraphs we will look at these three types of research.
Exploratory research
Researchers conducting exploratory research are typically at the early stages of examining their topics. These sorts of projects are usually conducted when a researcher wants to test the feasibility of conducting a more extensive study; he or she wants to figure out the lay of the land with respect to the particular topic. Perhaps very little prior research has been conducted on this subject. If this is the case, a researcher may wish to do some exploratory work to learn what method to use in collecting data, how best to approach research participants, or even what sorts of questions are reasonable to ask. A researcher wanting to simply satisfy his or her own curiosity about a topic could also conduct exploratory research. Conducting exploratory research on a topic is often a necessary first step, both to satisfy researcher curiosity about the subject and to better understand the phenomenon and the research participants in order to design a larger, subsequent study. See Table 2.1 for examples.
Descriptive research
Sometimes the goal of research is to describe or define a particular phenomenon. In this case, descriptive research would be an appropriate strategy. A descriptive may, for example, aim to describe a pattern. For example, researchers often collect information to describe something for the benefit of the general public. Market researchers rely on descriptive research to tell them what consumers think of their products. In fact, descriptive research has many useful applications, and you probably rely on findings from descriptive research without even being aware that that is what you are doing. See Table 3.1 for examples.
Explanatory research
The third type of research, explanatory research, seeks to answer “why” questions. In this case, the researcher is trying to identify the causes and effects of whatever phenomenon is being studied. An explanatory study of college students’ addictions to their electronic gadgets, for example, might aim to understand why students become addicted. Does it have anything to do with their family histories? Does it have anything to do with their other extracurricular hobbies and activities? Does it have anything to do with the people with whom they spend their time? An explanatory study could answer these kinds of questions. See Table 3.1 for examples.
Table 3.1 Exploratory, descriptive and explanatory research differences (Adapted from Adjei, n.d.).
Research Methods for the Social Sciences: An Introduction Copyright © 2020 by Valerie Sheppard is licensed under a Creative Commons Attribution-NonCommercial-ShareAlike 4.0 International License , except where otherwise noted.
Share This Book
- Privacy Policy

Home » Exploratory Vs Explanatory Research
Exploratory Vs Explanatory Research
Table of Contents

Exploratory research and explanatory research are two fundamental types of research studies, and they have different objectives, approaches, and outcomes.
Exploratory Research
Exploratory research is usually conducted when the researcher is trying to gain a deeper understanding of a particular phenomenon, situation, or problem. The primary purpose of exploratory research is to explore and generate ideas, hypotheses, and theories about a topic or issue that is not well understood. The researcher typically uses qualitative research methods, such as in-depth interviews, focus groups, or observational studies, to collect data. The data collected in exploratory research is usually descriptive and helps the researcher to identify patterns and trends, generate hypotheses, and develop a deeper understanding of the research problem. Exploratory research is usually the first step in a larger research project, and its results are used to guide the design of subsequent studies.
Explanatory Research
Explanatory research , on the other hand, is conducted when the researcher is trying to explain the relationship between variables or to test hypotheses that have been generated through exploratory research. The primary purpose of explanatory research is to explain why and how things happen. The researcher typically uses quantitative research methods, such as surveys or experiments, to collect data. The data collected in explanatory research is usually analyzed statistically to test hypotheses and to establish cause-and-effect relationships between variables.
Differences Between Exploratory and Explanatory Research
In summary, exploratory research is used to gain a deeper understanding of a research problem, while explanatory research is used to explain the relationship between variables or to test hypotheses. Both types of research are important and complement each other in the research process. Exploratory research is usually the first step in a larger research project, while explanatory research is conducted after exploratory research to test hypotheses and to establish cause-and-effect relationships between variables.
About the author
Muhammad Hassan
Researcher, Academic Writer, Web developer
You may also like

Inductive Vs Deductive Research

Basic Vs Applied Research

Generative Vs Evaluative Research

Reliability Vs Validity

Longitudinal Vs Cross-Sectional Research

Qualitative Vs Quantitative Research
Have a language expert improve your writing
Run a free plagiarism check in 10 minutes, automatically generate references for free.
- Knowledge Base
- Methodology
- Explanatory Research | Definition, Guide, & Examples
Explanatory Research | Definition, Guide & Examples
Published on 7 May 2022 by Tegan George and Julia Merkus. Revised on 20 January 2023.
Explanatory research is a research method that explores why something occurs when limited information is available. It can help you increase your understanding of a given topic, ascertain how or why a particular phenomenon is occurring, and predict future occurrences.
Explanatory research can also be explained as a ’cause and effect’ model, investigating patterns and trends in existing data that haven’t been previously investigated. For this reason, it is often considered a type of causal research .
Table of contents
When to use explanatory research, explanatory research questions, explanatory research data collection, explanatory research data analysis, step-by-step example of explanatory research, explanatory vs exploratory research, advantages and disadvantages of exploratory research, frequently asked questions about explanatory research.
Explanatory research is used to investigate how or why a phenomenon takes place. Therefore, this type of research is often one of the first stages in the research process, serving as a jumping-off point for future research. While there is often data available about your topic, it’s possible the particular causal relationship you are interested in has not been robustly studied.
Explanatory research helps you analyse these patterns, formulating hypotheses that can guide future endeavors. If you are seeking a more complete understanding of a relationship between variables, explanatory research is a great place to start. However, keep in mind that it will likely not yield conclusive results.
You analysed their final grades and noticed that the students who take your course in the first semester always obtain higher grades than students who take the same course in the second semester.
Prevent plagiarism, run a free check.
Explanatory research answers ‘why’ and ‘what’ questions, leading to an improved understanding of a previously unresolved problem or providing clarity for related future research initiatives.
Here are a few examples:
- Why do undergraduate students obtain higher average grades in the first semester than in the second semester?
- How does marital status affect labour market participation?
- Why do multilingual individuals show more risky behaviour during business negotiations than monolingual individuals?
- How does a child’s ability to delay immediate gratification predict success later in life?
- Why are teenagers more likely to litter in a highly littered area than in a clean area?
After choosing your research question, there is a variety of options for research and data collection methods to choose from.
A few of the most common research methods include:
- Literature reviews
- Interviews and focus groups
- Pilot studies
- Observations
- Experiments
The method you choose depends on several factors, including your timeline, your budget, and the structure of your question.
If there is already a body of research on your topic, a literature review is a great place to start. If you are interested in opinions and behaviour, consider an interview or focus group format. If you have more time or funding available, an experiment or pilot study may be a good fit for you.
In order to ensure you are conducting your explanatory research correctly, be sure your analysis is definitively causal in nature, and not just correlated.
Always remember the phrase ‘correlation doesn’t imply causation’. Correlated variables are merely associated with one another: when one variable changes, so does the other. However, this isn’t necessarily due to a direct or indirect causal link.
Causation means that changes in the independent variable bring about changes in the dependent variable. In other words, there is a direct cause-and-effect relationship between variables.
Causal evidence must meet three criteria:
- Temporal : What you define as the ’cause’ must precede what you define as the ‘effect’.
- Variation : Intervention must be systematic between your independent variable and dependent variable.
- Non-spurious : Be careful that there are no mitigating factors or hidden third variables that confound your results.
Correlation doesn’t imply causation, but causation always implies correlation. In order to get conclusive causal results, you’ll need to conduct a full experimental design .
Your explanatory research design depends on the research method you choose to collect your data . In most cases, you’ll use an experiment to investigate potential causal relationships. We’ll walk you through the steps using an example.
Step 1: Develop the research question
The first step in conducting explanatory research is getting familiar with the topic you’re interested in, so that you can develop a research question .
Let’s say you’re interested in language retention rates in adults.
You are interested in finding out how the duration of exposure to language influences language retention ability later in life.
Step 2: Formulate a hypothesis
The next step is to address your expectations. In some cases, there is literature available on your subject or on a closely related topic that you can use as a foundation for your hypothesis . In other cases, the topic isn’t well studied, and you’ll have to develop your hypothesis based on your instincts or on existing literature on more distant topics.
- H 0 : The duration of exposure to a language in infancy does not influence language retention in adults who were adopted from abroad as children.
- H 1 : The duration of exposure to a language in infancy has a positive effect on language retention in adults who were adopted from abroad as children.
Step 3: Design your methodology and collect your data
Next, decide what data collection and data analysis methods you will use and write them up. After carefully designing your research, you can begin to collect your data.
- Adults who were adopted from Colombia between 0 and 6 months of age
- Adults who were adopted from Colombia between 6 and 12 months of age
- Adults who were adopted from Colombia between 12 and 18 months of age
- Monolingual adults who have not been exposed to a different language
During the study, you test their Spanish language proficiency twice in a research design that has three stages:
- Pretest : You conduct several language proficiency tests to establish any differences between groups pre-intervention.
- Intervention : You provide all groups with 8 hours of Spanish class.
- Posttest : You again conduct several language proficiency tests to establish any differences between groups post-intervention.
You made sure to control for any confounding variables , such as age, gender, and proficiency in other languages.

Step 4: Analyse your data and report results
After data collection is complete, proceed to analyse your data and report the results.
- The pre-exposed adults showed higher language proficiency in Spanish than those who had not been pre-exposed. The difference is even greater for the posttest.
- The adults who were adopted between 12 and 18 months of age had a higher Spanish language proficiency level than those who were adopted between 0 and 6 months or 6 and 12 months of age, but there was no difference found between the latter two groups.
To determine whether these differences are significant, you conduct a mixed ANOVA. The ANOVA shows that all differences are not significant for the pretest, but they are significant for the posttest.
Step 5: Interpret your results and provide suggestions for future research
As you interpret the results, try to come up with explanations for the results that you did not expect. In most cases, you want to provide suggestions for future research.
However, this difference is only significant after the intervention (the Spanish class).
You decide it’s worth it to further research the matter, and propose a few additional research ideas:
- Replicate the study with a larger sample
- Replicate the study for other maternal languages (e.g., Korean, Lingala, Arabic)
- Replicate the study for other language aspects, such as nativeness of the accent
It can be easy to confuse explanatory research with exploratory research. If you’re in doubt about the relationship between exploratory and explanatory research, just remember that exploratory research lays the groundwork for later explanatory research.
Exploratory research questions often begin with ‘what’. They are designed to guide future research and do not usually have conclusive results. Exploratory research is often utilised as a first step in your research process, to help you focus your research question and fine-tune your hypotheses.
Explanatory research questions often start with ‘why’ or ‘how’. They help you study why and how a previously studied phenomenon takes place.
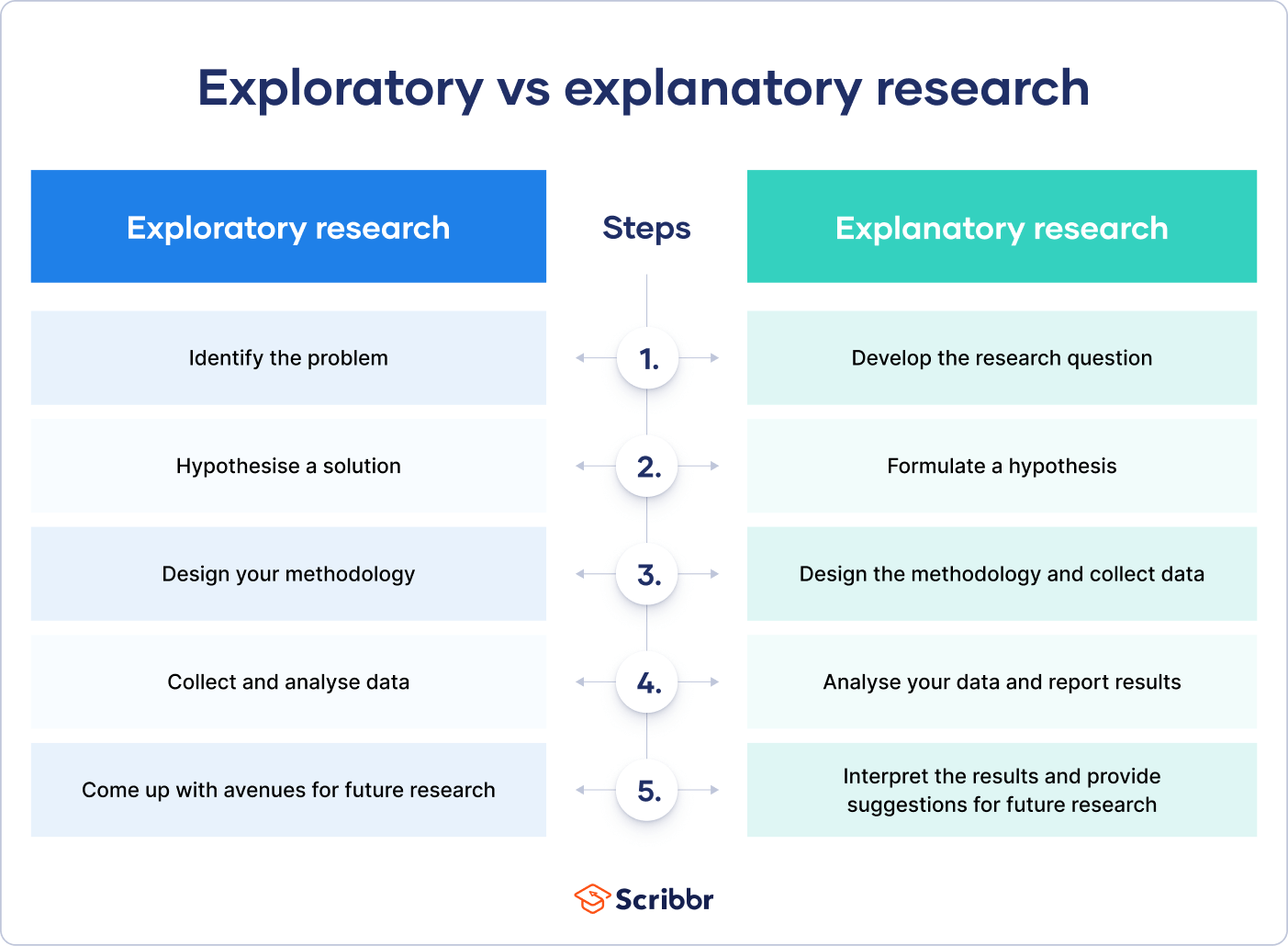
Like any other research design , exploratory research has its trade-offs: while it provides a unique set of benefits, it also has significant downsides:
- It gives more meaning to previous research. It helps fill in the gaps in existing analyses and provides information on the reasons behind phenomena.
- It is very flexible and often replicable, since the internal validity tends to be high when done correctly.
- As you can often use secondary research, explanatory research is often very cost- and time-effective, allowing you to utilise pre-existing resources to guide your research before committing to heavier analyses.
Disadvantages
- While explanatory research does help you solidify your theories and hypotheses, it usually lacks conclusive results.
- Results can be biased or inadmissible to a larger body of work and are not generally externally valid . You will likely have to conduct more robust (often quantitative ) research later to bolster any possible findings gleaned from explanatory research.
- Coincidences can be mistaken for causal relationships , and it can sometimes be challenging to ascertain which is the causal variable and which is the effect.
Explanatory research is a research method used to investigate how or why something occurs when only a small amount of information is available pertaining to that topic. It can help you increase your understanding of a given topic.
Explanatory research is used to investigate how or why a phenomenon occurs. Therefore, this type of research is often one of the first stages in the research process , serving as a jumping-off point for future research.
Exploratory research explores the main aspects of a new or barely researched question.
Explanatory research explains the causes and effects of an already widely researched question.
Quantitative research deals with numbers and statistics, while qualitative research deals with words and meanings.
Quantitative methods allow you to test a hypothesis by systematically collecting and analysing data, while qualitative methods allow you to explore ideas and experiences in depth.
Cite this Scribbr article
If you want to cite this source, you can copy and paste the citation or click the ‘Cite this Scribbr article’ button to automatically add the citation to our free Reference Generator.
George, T. & Merkus, J. (2023, January 20). Explanatory Research | Definition, Guide & Examples. Scribbr. Retrieved 14 May 2024, from https://www.scribbr.co.uk/research-methods/explanatory-research-design/
Is this article helpful?
Tegan George
Other students also liked, exploratory research | definition, guide, & examples, descriptive research design | definition, methods & examples, a quick guide to experimental design | 5 steps & examples.
- Log in / Register

- Getting started
- Criteria for a problem formulation
- Find who and what you are looking for
- Too broad, too narrow, or o.k.?
- Test your knowledge
- Lesson 5: Meeting your supervisor
- Getting started: summary
- Literature search
- Searching for articles
- Searching for Data
- Databases provided by your library
- Other useful search tools
- Free text, truncating and exact phrase
- Combining search terms – Boolean operators
- Keep track of your search strategies
- Problems finding your search terms?
- Different sources, different evaluations
- Extract by relevance
- Lesson 4: Obtaining literature
- Literature search: summary
- Research methods
- Combining qualitative and quantitative methods
- Collecting data
- Analysing data
- Strengths and limitations
Explanatory, analytical and experimental studies
- The Nature of Secondary Data
- How to Conduct a Systematic Review
- Directional Policy Research
- Strategic Policy Research
- Operational Policy Research
- Conducting Research Evaluation
- Research Methods: Summary
- Project management
- Project budgeting
- Data management plan
- Quality Control
- Project control
- Project management: Summary
- Writing process
- Title page, abstract, foreword, abbreviations, table of contents
- Introduction, methods, results
- Discussion, conclusions, recomendations, references, appendices, layout
- Use citations correctly
- Use references correctly
- Bibliographic software
- Writing process – summary
- Research methods /
- Lesson 2: Empirical studies /
- Explanatory, analytical and ex…
Explanatory, analytical and experimental studies…
- Explain Why a phenomenon is going on
- Can be used for hypothesis testing
- Allow for inferences to be drawn about associations and causality
- Examples: Case-control study, Cohort study (follow-up), Intervention trial
A common form of an Explanatory/Analytical study is a case control study. The diagram below displays a classic case control study during which a researcher who wants to test the effect of a particular medicine on an illness will design a study in which a group of patients is divided into two groups – one group will receive the treatment while another group receives a placebo (control). The patients will be followed and their health outcomes will be compared to see if the treatment course resulted in a lessening or elimination of the illness in the treated group as compared to the untreated group.
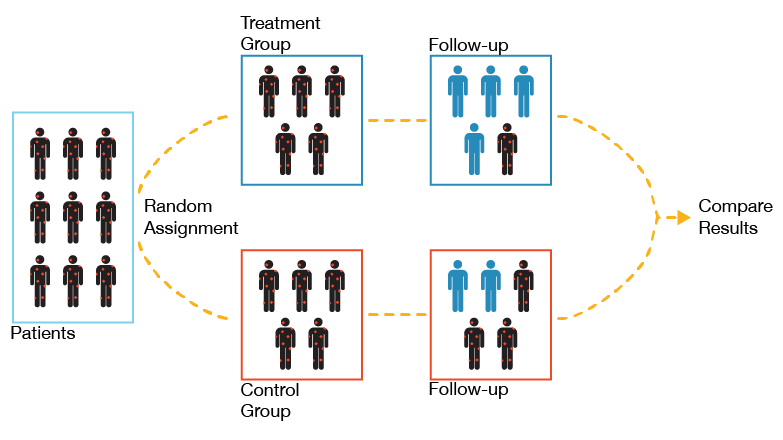
There are also quasi-experimental studies, such as uncontrolled before and after studies. Uncontrolled before and after studies measure the situation before and after the introduction of an intervention in the same study site(s) and any observed differences in performance are assumed to be due to the intervention.
For example, a reseacher may aim to test whether a book reading club in a retirment home reduces feelings of loneliness and isolation among elderly residents. In a quasi-experimental study, the research would use an accepted research tool (i.e. a loneliness survey) to measure feelings of loneliness and isolation among a group of residents and then implement the book reading club for some period of time. After the defined period of time has passed, the researcher would administer the same accepted research tool to the same group of residents a second time and compare the results of the pre-club survey to the post-club survey to measure any change in the levels or loneliness and isolation experienced among the group of elderly residents. Any changes would be ascribed to the implementation of the reading club.
Uncontrolled before and after studies are relatively simple to conduct and for the purpose of attributing causation are considered superior to observational studies; however, they may have intrinsic weaknesses as evaluative designs, as other trends or sudden changes make it difficult to attribute observed changes to the intervention. For example, in the case of the book club described above, a reduction in feelings of loneliness and isolation could be the result of another phenomonon, like weekly arts and crafts sessions, introduced into the elderly resident population. Without a control group, it is difficult to determine if the positive benefit experienced by the elderly residents is as a result of the book club, the arts and crafts sessions, or some other unknown factor.
Furthermore, in such studies the intervention is confounded by the Hawthorne effect – which is an effect sometimes experienced by participants in research projects in which they experience a positive or beneficial outcome simply as a result of participating in a research project. This effect could lead to an overestimate of the effectiveness of an intervention. That being said, because of the ease with which before and after studies can be implemented, they are often a good study type for graduate level research.
Please find an overview of the strengths and limiations of various study types in the following.
Your friend's e-mail
Message (Note: The link to the page is attached automtisk in the message to your friend)

Short and sweet: multiple mini case studies as a form of rigorous case study research
- Original Article
- Open access
- Published: 15 May 2024
Cite this article
You have full access to this open access article
- Sebastian Käss ORCID: orcid.org/0000-0002-0640-3500 1 ,
- Christoph Brosig ORCID: orcid.org/0000-0001-7809-0796 1 ,
- Markus Westner ORCID: orcid.org/0000-0002-6623-880X 2 &
- Susanne Strahringer ORCID: orcid.org/0000-0002-9465-9679 1
Case study research is one of the most widely used research methods in Information Systems (IS). In recent years, an increasing number of publications have used case studies with few sources of evidence, such as single interviews per case. While there is much methodological guidance on rigorously conducting multiple case studies, it remains unclear how researchers can achieve an acceptable level of rigour for this emerging type of multiple case study with few sources of evidence, i.e., multiple mini case studies. In this context, we synthesise methodological guidance for multiple case study research from a cross-disciplinary perspective to develop an analytical framework. Furthermore, we calibrate this analytical framework to multiple mini case studies by reviewing previous IS publications that use multiple mini case studies to provide guidelines to conduct multiple mini case studies rigorously. We also offer a conceptual definition of multiple mini case studies, distinguish them from other research approaches, and position multiple mini case studies as a pragmatic and rigorous approach to research emerging and innovative phenomena in IS.
Avoid common mistakes on your manuscript.
1 Introduction
Case study research has become a widely used research method in Information Systems (IS) research (Palvia et al. 2015 ) that allows for a comprehensive analysis of a contemporary phenomenon in its real-world context (Dubé and Paré, 2003 ). This research method is particularly useful due to its flexibility in covering complex phenomena with multiple contextual variables, different types of evidence, and a wide range of analytical options (Voss et al. 2002 ; Yin 2018 ). Although case study research is particularly useful for studying contemporary phenomena, some researchers feel that it lacks rigour, particularly in terms of the validity of findings (Lee and Hubona 2009 ). In response to these criticisms, Yin ( 2018 ) provides comprehensive methodological steps to conduct case studies rigorously. In addition, many other publications with a partly discipline-specific view on case study research, offer guidelines for achieving rigour in case study research, e.g., Benbasat et al. ( 1987 ), Dubé and Paré ( 2003 ), Pan and Tan ( 2011 ), or Voss et al. ( 2002 ). Most publications on case study methodology converge on four criteria for ensuring rigour in case study research: (1) construct validity, (2) internal validity, (3) external validity, and (4) reliability (Gibbert et al. 2008 ; Voss et al. 2002 ; Yin 2018 ).
A key element of rigour in case study research is to look at the unit of analysis of a case from multiple perspectives in order to draw informed conclusions (Dubois and Gadde 2002 ). Case study researchers refer to this as triangulation, for example, by using multiple sources of evidence per case to support findings (Benbasat et al. 1987 ; Yin 2018 ). However, in our own research experience, we have come across numerous IS publications with a limited number of sources of evidence per case, such as a single interview per case. Some researchers refer to these studies as mini case studies (e.g., McBride 2009 ; Weill and Olson 1989 ), while others refer to them as multiple mini cases (e.g., Eisenhardt 1989 ). We were unable to find a definition or conceptualisation of this type of case study. Therefore, we will refer to this type of case study as a multiple mini case study (MMCS). Interestingly, many researchers use these MMCSs to study emerging and innovative phenomena.
From a methodological perspective, multiple case study publications with limited sources of evidence, also known as MMCSs, may face criticism for their lack of rigour (Dubé and Paré 2003 ). Alternatively, they may be referred to as “marginal case studies” (Piekkari et al. 2009 , p. 575) if they fail to establish a connection between theory and empirical evidence, provide only limited context, or merely offer illustrative aspects (Piekkari et al. 2009 ). IS scholars advocate conducting case study research in a mindful manner by balancing methodological blueprints and justified design choices (Keutel et al. 2014 ). Consequently, we propose MMCSs as a mindful approach with the potential for rigour, distinguishing them from marginal case studies. The following research question guides our study:
RQ: How can researchers rigorously conduct MMCSs in the IS discipline?
As shown in Fig. 1 , we develop an analytical framework by synthesising methodological guidance on how to rigorously conduct multiple case study research. We then address three aspects of our research question: For aspect (1), we analyse published MMCSs in the IS discipline to derive a "Research in Practice" definition of MMCSs and research situations for MMCSs. For aspect (2), we use the analytical framework to analyse how researchers in the IS discipline ensure that existing MMCSs follow a rigorous methodology. For aspect (3), we discuss the methodological findings about rigorous MMCSs in order to derive methodological guidelines for MMCSs that researchers in the IS discipline can follow.
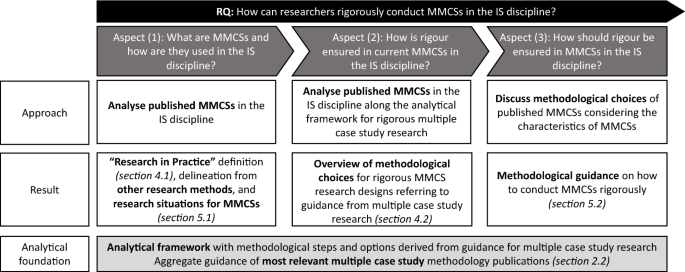
Overview of the research approach
We approach these aspects by introducing the conceptual foundation for case study research in Sect. 2 . We define commonly accepted criteria for ensuring validity in case study research, introduce the concept of MMCSs, and distinguish them from other types of case studies. Furthermore, as a basis for analysis, we present an analytical framework of methodological steps and options for the rigorous conduct of multiple case study research. Section 3 presents our methodological approach to identifying published MMCSs in the IS discipline. In Sect. 4 , we first define MMCSs from a research in practice perspective (Sect. 4.1 ). Second, we present an overview of methodological options for rigorous MMCSs based on our analytical framework (Sect. 4.2 ). In Sect. 5 , we differentiate MMCSs from other research approaches, identify research situations of MMCSs (i.e., to study emerging and innovative phenomena), and provide guidance on how to ensure rigour in MMCSs. In our conclusion, we clarify the limitations of our study and provide an outlook for future research with MMCSs.
2 Conceptual foundation
2.1 case study research.
Case study research is about understanding phenomena by studying one or multiple cases in their context. Creswell and Poth ( 2016 ) define it as an “approach in which the investigator explores a bounded system (a case) or multiple bounded systems (cases) over time, through detailed, in-depth data collection” (p. 73). Therefore, it is suitable for complex topics with little available knowledge, needing an in-depth investigation, or where the research subject is inseparable from its context (Paré 2004 ). Additionally, Yin ( 2018 ) states that case study research is useful if the research focuses on contemporary events where no control of behavioural events is required. Typically, this type of research is most suitable for how and why research questions (Yin 2018 ). Eventually, the inferences from case study research are based on analytic or logical generalisation (Yin 2018 ). Instead of drawing conclusions from a representative statistical sample towards the population, case study research builds on analytical findings from the observed cases (Dubois and Gadde 2002 ; Eisenhardt and Graebner 2007 ). Case studies can be descriptive, exploratory, or explanatory (Dubé and Paré 2003 ).
The contribution of research to theory can be divided into the steps of theory building , development and testing , which is a continuum (Ridder 2017 ; Welch et al. 2011 ), and case studies are useful at all stages (Ridder 2017 ). In theory building, there is no theory to explain a phenomenon, and the researcher identifies new concepts, constructs, and relationships based on the data (Ridder 2017 ). In theory development, a tentative theory already exists that is extended or refined (e.g., by adding new antecedents, moderators, mediators, and outcomes) (Ridder 2017 ). In theory testing, an existing theory is challenged through empirical investigation (Ridder 2017 ).
In case study research, there are different paradigms for obtaining research results, either positivist or interpretivist (Dubé and Paré 2003 ; Orlikowski and Baroudi 1991 ). The positivist paradigm assumes that a set of variables and relationships can be objectively identified by the researcher (Orlikowski and Baroudi 1991 ). In contrast, the interpretivist paradigm assumes that the results are inherently rooted in the researcher’s worldview (Orlikowski and Baroudi 1991 ). Nowadays, researchers find that there are similar numbers of positivist and interpretivist case studies in the IS discipline compared to almost 20 years ago when positivist research was perceived as dominant (Keutel et al. 2014 ; Klein and Myers 1999 ). As we aim to understand how to conduct MMCSs rigorously, we focus on methodological guidance for positivist case study research.
The literature proposes a four-phased approach to conducting a case study: (1) the definition of the research design, (2) the data collection, (3) the data analysis, and (4) the composition (Yin 2018 ). Table 1 provides an overview and explanation of the four phases.
Case studies can be classified based on their depth and breadth, as shown in Fig. 2 . We can distinguish five types of case studies: in-depth single case studies , marginal case studies , multiple case studies , MMCSs , and extensive in-depth multiple case studies . Each type has distinct characteristics, yet the boundaries between the different types of case studies is blurred. Except for the marginal case studies, the italic references in Fig. 2 are well-established publications that define the respective type and provide methodological guidance. The shading is to visualise the different types of case studies. The italic references in Fig. 2 for marginal case studies refer to publications that conceptualise them.
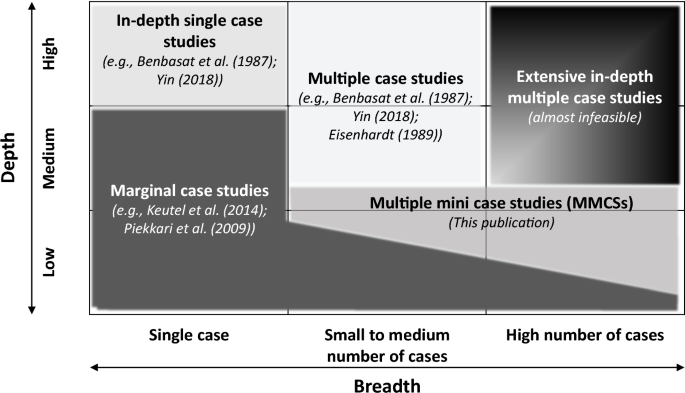
Simplistic conceptualisation of MMCS
In-depth single case studies focus on a single bounded system as a case (Creswell and Poth 2016 ; Paré 2004 ; Yin 2018 ). According to the literature, a single case study should only be used if a case meets one or more of the following five characteristics: it is a critical, unusual, common, revelatory, or longitudinal case (Benbasat et al. 1987 ; Yin 2018 ). Single case studies are more often used for descriptive research (Dubé and Paré 2003 ).
A second type of case studies are marginal case studies , which generally have low depth (Keutel et al. 2014 ; Piekkari et al. 2009 ). Marginal case studies lack a clear link between theory and empirical evidence, a clear contextualisation of the case, and are often used for illustration purposes (Keutel et al. 2014 ; Piekkari et al. 2009 ). Therefore, marginal case studies provide only marginal insights with a lack of generalisability.
In contrast, multiple case studies employ multiple cases to obtain a broader picture of the researched phenomenon from different perspectives (Creswell and Poth 2016 ; Paré 2004 ; Yin 2018 ). These multiple case studies are often considered to provide more robust results due to the multiplicity of their insights (Eisenhardt and Graebner 2007 ). However, often discussed criticisms of multiple case studies are high costs, difficult access to multiple sources of evidence for each case, and long duration (Dubé and Paré 2003 ; Meredith 1998 ; Voss et al. 2002 ). Eisenhardt ( 1989 ) considers four to ten in-depth cases as a suitable number of cases for multiple case study research. With fewer than four cases, the empirical grounding is less convincing, and with more than ten cases, researchers quickly get overwhelmed by the complexity and volume of data (Eisenhardt 1989 ). Therefore, methodological literature views extensive in-depth multiple case studies as almost infeasible due to their high complexity and resource demands, which can easily overwhelm the research team and the readers (Stake 2013 ). Hence, we could not find a methodological publication outlining the approach for this case study type.
To solve the complexity and resource issues for multiple case studies, a new phenomenon has emerged: MMCS . An MMCS is a special type of multiple case study that focuses on an investigation's breadth by using a relatively high number of cases while having a somewhat limited depth per case. We characterise breadth not only by the number of cases but also by the variety of the cases. Even though there is no formal conceptualisation of the term, we understand MMCSs as a type of multiple case study research with few sources of evidence per case. Due to the limited depth per case, one can overcome the resource and complexity issues of classical multiple case studies. However, having only some sources of evidence per case may be considered a threat to rigour. Therefore, in this publication, we provide suggestions on how to address these threats.
2.2 Rigour in case study research
Rigour is essential for case study research (Dubé and Paré 2003 ; Yin 2018 ) and, in the early 2000s, researchers criticised case study research for inadequate rigour (e.g., Dubé and Paré 2003 ; Gibbert et al. 2008 ). Based on this, various methodological publications provide guidance for rigorous case study research (e.g., Dubé and Paré 2003 ; Gibbert et al. 2008 ).
Methodological literature proposes four criteria to ensure rigour in case study research: Construct validity , internal validity , external validity , and reliability (Dubé and Paré 2003 ; Gibbert et al. 2008 ; Yin 2018 ). Table 2 outlines these criteria and states in which research phase they should be addressed (Yin 2018 ). Methodological literature agrees that all four criteria must be met for rigorous case study research (Dubé and Paré 2003 ).
The methodological literature discusses multiple options for achieving rigour in case study research (e.g., Benbasat et al. 1987 ; Dubé and Paré 2003 ; Eisenhardt 1989 ; Yin 2018 ). We aggregated guidance from multiple sources by conducting a cross-disciplinary literature review to build our analytical foundation (cf. Fig. 1 ). This literature review aims to identify the most relevant multiple case study methodology publications from a cross-disciplinary and IS-specific perspective. We focus on the most cited methodology publications, while being aware that this may over-represent disciplines with a higher number of case study publications. However, this approach helps to capture an implicit consensus among case study researchers on how to conduct multiple case studies rigorously. The literature review produced an analytical framework of methodological steps and options for conducting multiple case studies rigorously. Appendix A Footnote 1 provides a detailed documentation of the literature review process. The analytical framework derived from the set of methodological publications is presented in Table 3 . We identified required and optional steps for each research stage. The analytical framework is the basis for the further analysis of MMCS and an explanation of all methodological steps is provided in Appendix B. Footnote 2
3 Research methodology
For our research, we analysed published MMCSs in the IS discipline with the goal of understanding how these publications ensured rigour. This section outlines the methodology of how we identified our MMCS publications.
First, we searched bibliographic databases and citation indexing services (Vom Brocke et al. 2009 ; Vom Brocke et al. 2015 ) to retrieve IS-specific MMCSs (Hanelt et al. 2015 ). As shown in Fig. 3 , we used two sets of keywords, the first set focusing on multiple case studies and the second set explicitly on mini case studies. We decided to follow this approach as many MMCSs are positioned as multiple case studies, avoiding the connotation “mini” or “short”. We restricted our search to completed research publications written in English from litbaskets.io size “S”, a set of 29 highly ranked IS journals (Boell and Wang 2019 ) Footnote 3 and leading IS conference proceedings from AMCIS, ECIS, HICSS, ICIS, and PACIS (published until end of June 2023). We focused on these outlets, as they can be taken as a representative sample of high quality IS research (Gogan et al. 2014 ; Sørensen and Landau 2015 ).
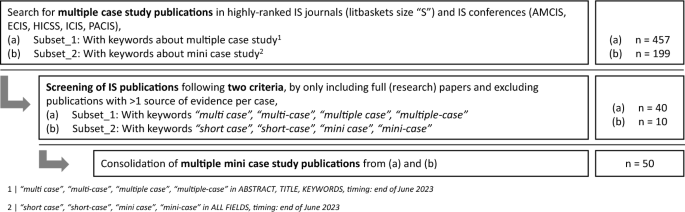
The search process for published MMCSs in the IS discipline
Second, we screened the obtained set of IS publications to identify MMCSs. We only included publications with positivist multiple cases where the majority of cases was captured with only one primary source of evidence. Further, we excluded all publications which were interview studies rather than case studies (i.e., they do not have a clearly defined case). In some cases, it was unclear from the full text whether a publication fulfils this requirement. Therefore, we contacted the authors and clarified the research methodology with them. Eventually, our final set contained 50 publications using MMCSs.
For qualitative data analysis, we employed axial coding (Recker 2012 ) based on the pre-defined analytical framework shown in Table 3 . For the coding, we followed the explanations of the authors in the manuscripts. The coding was conducted and reviewed by two of the authors. We coded the first five publications of the set of IS MMCS publications together and discussed our decisions. After the initial coding was completed, we checked the reliability and validity by re-coding a sample of the other author’s set. In this sample, we achieved inter-coder reliability of 91% as a percent agreement in the decisions made (Nili et al. 2020 ). Hence, we consider our coding as highly consistent.
In the results section, we illustrate the chosen methodological steps for each MMCS type (descriptive, exploratory, and explanatory). For this purpose, we selected three publications based on two criteria: only journal publications, as they have more details about their methodological steps and publications which applied most of the analytical framework’s methodology steps. This led to three exemplary IS MMCS publications: (1) McBride ( 2009 ) for descriptive MMCSs, (2) Baker and Niederman ( 2014 ) for exploratory MMCSs, and (3) van de Weerd et al. ( 2016 ) for explanatory MMCSs.
4.1 MMCS from a “Research in Practice" perspective
In this section, we explain MMCSs from a "Research in Practice" perspective and identify different types based on our sample of 50 MMCS publications. As outlined in Sect. 2.1 , an MMCS is a special type of a multiple case study, which focuses on an investigation’s breadth by using a relatively high number of cases while having a limited depth per case. In the most extreme scenario, an MMCS only has one source of evidence per case. Moreover, breadth is not only characterised by the number of cases, but also by the variety of the cases. MMCSs have been used widely but hardly labelled as such, i.e., only 10 of our analysed 50 MMCS publications explicitly use the terms mini or short case in the manuscript . Multiple case study research distinguishes between descriptive, exploratory, and explanatory case studies (Dubé and Paré 2003 ). The MMCSs in our sample follow the same classification with three descriptive, 40 exploratory, and seven explanatory MMCSs. Descriptive and exploratory MMCSs are used in the early stages of research , and exploratory and explanatory MMCSs are used to corroborate findings .
Descriptive MMCSs provide little information on the methodological steps for the design, data collection, analysis, and presentation of results. They are used to illustrate novel phenomena and create research questions, not solutions, and can be useful for developing research agendas (e.g., McBride 2009 ; Weill and Olson 1989 ). The descriptive MMCS publications analysed contained between four to six cases, with an average of 4.6 cases per publication. Of the descriptive MMCSs analysed, one did not state research questions, one answered a how question and the third answered how and what questions. Descriptive MMCSs are illustrative and have a low depth per case, resulting in the highest risk of being considered a marginal case study.
Exploratory MMCSs are used to explore new phenomena quickly, generate first research results, and corroborate findings. Most of the analysed exploratory MMCSs answer what and how questions or combinations. However, six publications do not explicitly state a research question, and some MMCSs use why, which, or whether research questions. The analysed exploratory MMCSs have three to 27 cases, with an average of 10.2 cases per publication. An example of an exploratory MMCS is the study by Baker and Niederman ( 2014 ), who explore the impacts of strategic alignment during merger and acquisition (M&A) processes. They argue that previous research with multiple case studies (mostly with three cases) shows some commonalities, but much remains unclear due to the low number of cases. Moreover, they justify the limited depth of their research with the “proprietary and sensitive nature of the questions” (Baker and Niederman 2014 , p. 123).
Explanatory MMCSs use an a priori framework with a relatively high number of cases to find groups of cases that share similar characteristics. Most explanatory MMCSs answer how questions, yet some publications answer what, why, or combinations of the three questions. The analysed explanatory MMCSs have three to 18 cases, with an average of 7.2 cases per publication. An example of an explanatory MMCS publication is van de Weerd et al. ( 2016 ), who researched the influence of organisational factors on the adoption of Software as a Service (SaaS) in Indonesia.
4.2 Applied MMCS methodology in IS publications
4.2.1 overarching.
In the following sections, we present the results of our analysis. For this purpose, we mapped our 50 IS MMCS publications to the methodological options (Table 3 ) and present one example per MMCS type. We extended some methodological steps with options from methodology-in-use. A full coding table can be found in Appendix D Footnote 4 . Tables 4 , 5 , 6 and 7 summarise the absolute and percentual occurrences of each methodological option in descriptive, exploratory, and explanatory IS MMCS publications. All tables are structured in the same way and show the number of absolute and, in parentheses, the percentual occurrences of each methodological option. The percentages may not add up to 100% due to rounding. The bold numbers show the most common methodological option for each MMCS type and step. Most publications were classified in previously identified options. Some IS MMCS publications lacked detail on methodological steps, so we classified them as "step not evident". Only 16% (8 out of 50) explained how they addressed validity and reliability threats.
4.2.2 Research design phase
There are six methodological steps in the research design phase, as shown in Table 4 . Descriptive MMCSs usually define the research question (2 out of 3, 67%), clarify the unit of analysis (2 out of 3, 67%), bound the case (2 out of 3, 67%), or specify an a priori theoretical framework (2 out of 3, 67%). The case replication logic is mostly not evident (2 out of 3, 67%). Descriptive MMCS use a criterion-based selection (1 out of 3, 33%), a maximum variation selection (1 out of 3, 33%), or do not specify the selection logic (1 out of 3, 33%). Descriptive MMCSs have a high risk of becoming a marginal case study due to their illustrative nature–our chosen example is not different. McBride ( 2009 ) does not define the research question, does not have a priori theoretical framework, nor does he justify the case replication and the case selection logic. However, he clarifies the unit of analysis and extensively bounds each case with significant context about the case organisation and its setup.
The majority of exploratory MMCSs define the research question (34 out of 40, 85%) clarify the unit of analysis (35 out of 40, 88%), and specify an a priori theoretical framework (33 out of 40, 83%). However, only a minority (6 out of 40, 15%) follow the instructions of bounding the case or justify the case replication logic (13 out of 40, 33%). The most used case selection logic is the criterion-based selection (23 out of 40, 58%), followed by step not evident (5 out of 40, 13%), other selection approaches (3 of 40, 13%), maximum variation selection (3 out of 40, 13%), a combination of approaches (2 out of 40, 5%), snowball selection (2 out of 40, 5%), typical case selection (1 out of 40, 3%), and convenience-based selection (1 out of 40, 3%). Baker and Niederman ( 2014 ) build their exploratory MMCS on previous multiple case studies with three cases that showed ambiguous results. Hence, Baker and Niederman ( 2014 ) formulate three research objectives instead of defining a research question. They clearly define the unit of analysis (i.e., the integration of the IS function after M&A) but lack the bounding of the case. The authors use a rather complex a priori framework, leading to a high number of required cases. This a priori framework is also used for the “theoretical replication logic [to choose] conforming and disconfirming cases” (Baker and Niederman 2014 , p. 116). A combination of maximum variation and snowball selection is used to select the cases (Baker and Niederman 2014 ). The maximum variation is chosen to get evidence for all elements of their rather complex a priori framework (i.e., the breadth), and the snowball sampling is chosen to get more details for each framework element.
All explanatory MMCS s define the research question, clarify the unit of analysis, and specify an a priori theoretical framework. However, only one (14%) bounds the case. The case replication logic is mostly a mixture of theoretical and literal replication (3 out of 7, 43%) and one (14%) MMCS does a literal replication. For 43% (3 out of 7) of the publications, the step is not evident. Most explanatory MMCSs use criterion-based selection (4 out of 7, 57%), followed by maximum variation selection (2 out of 7, 29%) and snowball selection (1 out of 7, 14%). In their publication, van de Weerd et al. ( 2016 ) define the research question and clarify the unit of analysis (i.e., the influence of organisational factors on SaaS adoption in Indonesian SMEs). Further, they specify an a priori framework (i.e., based on organisational size, organisational readiness, and top management support) to target the research (van de Weerd et al. 2016 ). A combination of theoretical (between the groups of cases) and literal (within the groups of cases) replication was used. To strengthen the findings, van de Weerd et al. ( 2016 ) find at least one other literally replicated case for each theoretically replicated case.
To summarize this phase, we see that in all three types of MMCSs, the majority of publications define the research question, clarify the unit of analysis, and specify an a priori theoretical framework. Moreover, descriptive MMCSs are more likely to bound the case than exploratory and explanatory MMCSs. However, only a minority across all MMCSs justify the case replication logic, whereas the majority does not. Most MMCSs justify the case selection logic, with criterion-based case selection being the most often applied methodological option.
4.2.3 Data collection phase
In the data collection phase, there are four methodological steps, as summarised in Table 5 .
One descriptive MMCS applies triangulation via multiple sources, whereas for the majority (2 out of 3, 67%), the step is not evident. One (33%) of the analysed descriptive MMCSs creates a full chain of evidence, none creates a case study database, and one (33%) uses a case study protocol. McBride ( 2009 ) applies triangulation via multiple sources, as he followed “up practitioner talks delivered at several UK annual conferences” (McBride 2009 , p. 237). Therefore, we view the follow-up interviews as the primary source of evidence per case, as dedicated questions to the unit of analysis can be asked per case. Triangulation via multiple sources was then conducted by combining practitioner talks and documents with follow-up interviews. McBride ( 2009 ) does not create a full chain of evidence, a case study database, nor a case study protocol. This design decision might be rooted in the objective of a descriptive MMCS to illustrate and open up new questions rather than find clear solutions (McBride 2009 ).
Most exploratory MMCSs triangulate via multiple sources (20 out of 40, 50%) or via multiple investigators (4 out of 40, 10%). Eight (20%) exploratory MMCSs apply multiple triangulation types and for eight (20%), no triangulation is evident. At first glance, a triangulation via multiple sources may seem contradictory to the definition of MMCSs–yet it is not. MMCSs that triangulate via multiple sources have one source per case as the primary, detailed evidence (e.g., an interview), which is combined with easily available supplementary sources of evidence (e.g., public reports and documents (Baker and Niederman 2014 ), press articles (Hahn et al. 2015 ), or online data (Kunduru and Bandi 2019 )). As this leads to multiple sources of evidence, we understand this as a triangulation via multiple sources; however, on a different level than triangulating via multiple in-depth interviews per case. Only a minority of exploratory MMCSs create a full chain of evidence (14 out of 40, 35%), and a majority (23 out of 40, 58%) use a case study database or a case study protocol (20 out of 40, 50%). Baker and Niederman ( 2014 ) triangulate with multiple sources (i.e., financial reports as supplementary sources) to increase the validity of their research. Further, the authors create a full chain of evidence from their research question through an identical interview protocol to the case study’s results. For every case, an individual case report is created and stored in the case study database (Baker and Niederman 2014 ).
All explanatory MMCSs triangulate during the data collection phase, either via multiple sources (2 out of 7, 29%) or a combination of multiple investigators and sources (5 out of 7, 71%). Interestingly, only three explanatory MMCSs (43%) create a full chain of evidence. All create a case study database (7 out of 7, 100%) and the majority creates a case study protocol (6 out of 7, 86%). In their explanatory MMCS, van de Weerd et al. ( 2016 ) use semi-structured interviews as the primary data collection method. The interview data is complemented “with field notes and (online) documentation” (van de Weerd et al. 2016 , p. 919), e.g., data from corporate websites or annual reports. Moreover, a case study protocol and a case study database in NVivo are created to increase reliability.
To summarise the data collection phase, we see that most (40 out of 50, 80%) of MMCSs apply some type of triangulation. However, only 36% (18 out of 50) of the analysed MMCSs create a full chain of evidence. Moreover, descriptive MMCSs are less likely to create a case study database (0 out of 3, 0%) or a case study protocol (1 out of 3, 33%). In contrast, most exploratory and explanatory MMCS publications create a case study database and case study protocol.
4.2.4 Data analysis phase
There are three methodological steps (cf. Table 6 ) for the data analysis phase, each with multiple methodological options.
One descriptive MMCS (33%) corroborates findings through triangulation, and two do not (67%). Further, one (33%) uses a rich description of findings as other corroboration approaches, whereas for the majority (2 out of 3, 67%), the corroboration with other approaches is not evident. Descriptive MMCSs mostly do not define their within-case analysis strategy (2 out of 3, 67%). However, pre-defined patterns are used to conduct a cross-case analysis (2 out of 3, 67%). In the data analysis, McBride ( 2009 ) triangulates via multiple sources of evidence (i.e., talks at practitioner conferences and resulting follow-up interviews), but does not apply other corroboration approaches or provides methodological explanations for the within or cross-case analysis. This design decision might be rooted in the illustrative nature of his descriptive MMCS and the focus on analysing each case standalone.
Exploratory MMCSs mostly corroborate findings through a combination of triangulation via multiple investigators and sources (15 out of 40, 38%) or triangulation via multiple sources (9 out of 40, 23%). However, for ten (25%) exploratory MMCSs, this step is not evident. For the other corroboration approaches, a combination of approaches is mostly used (15 out of 40, 38%), followed by rich description of findings (11 out of 40, 28%), peer review (6 out of 40, 15%), and prolonged field visits (1 out of 40, 3%). For five (13%) publications, other corroboration approaches are not evident. Pattern matching (17 out of 40, 43%) and explanation building (5 out of 40, 13%) are the most used methodological options for the within-case analysis. To conduct a cross-case analysis, 11 (28%) MMCSs use a comparison of pairs or groups of cases, nine (23%) pre-defined patterns, and six (15%) structure their data along themes. Interestingly, for 14 (35%) exploratory MMCSs, no methodological step to conduct the cross-case analysis is evident. Baker and Niederman ( 2014 ) use a combination of triangulation via multiple investigators (“The interviews were coded by both researchers independently […], with a subsequent discussion to reach complete agreement” (Baker and Niederman 2014 , p. 117)) and sources to increase internal validity. Moreover, the authors use a rich description of the findings. An explanation-building strategy is used for the within-case analysis, and the cross-case analysis is done based on pre-defined patterns (Baker and Niederman 2014 ). This decision for the cross-case analysis is justified by a citation of Dubé and Paré ( 2003 , p. 619), who see it as “a form of pattern-matching in which the analysis of the case study is carried out by building a textual explanation of the case.”
Explanatory MMCSs corroborate findings through a triangulation via multiple sources (4 out of 7, 57%) or a combination of multiple investigators and sources (3 out of 7, 43%). For the other corroboration approaches, a rich description of findings (3 out of 7, 43%), a combination of approaches (3 out of 7, 43%), or peer review (1 out of 7, 14%) are used. To conduct a within-case analysis, pattern matching (5 out of 7, 71%) or explanation building (1 out of 7, 14%) are used. For the cross-case analysis, pre-defined patterns (3 out of 7, 43%) and a comparison of pairs or groups of cases (2 out of 7, 29%) are used; yet, for two (29%) explanatory MMCSs a cross-case analysis step is not evident. van de Weerd et al. ( 2016 ) corroborate their findings through a triangulation via multiple sources, a combination of rich description of findings and solicitation of participants’ views (“summarizing the interview results of each case company for feedback and approval” (van de Weerd et al. 2016 , p. 920)) as other corroboration approaches. Moreover, for the within-case analysis, the authors “followed an explanation-building procedure to strengthen […] [the] internal validity” (van de Weerd et al. 2016 , p. 920). For the cross-case, the researchers compare groups of cases. They refer to this approach as an informal qualitative comparative analysis.
To summarize the results of the data analysis phase, we see that some type of triangulation is used by most of the MMCSs, with source triangulation (alone or in combination with another approach) being the most often used methodological option. For the within-case analysis, pattern matching (22 of 50, 44%) is the most often used methodological option. For the cross-case analysis, pre-defined patterns are most often used (14 out of 50, 28%). However, depending on the type of MMCS, there are differences in the options used and some methodological options are never used (e.g., time-series analysis and solicitation of participants’ views).
4.2.5 Composition phase
We can find two methodological steps for the composition phase, as summarized in Table 7 .
Descriptive MMCSs do not apply triangulation in the composition phase (3 out of 3, 100%), nor do they use the methodological step to let key informants review the draft of the case study report (3 of 3, 100%). Also, the descriptive MMCS by McBride ( 2009 ) does not apply any of the methodological steps.
Exploratory MMCSs mostly use triangulation via multiple sources (25 out of 40, 63%), a combination of multiple sources and theories (2 out of 40, 5%), triangulation via multiple investigators (1 out of 40, 3%), and a combination of multiple sources and methods (1 out of 40, 3%). However, for 11 (28%) exploratory MMCS publications, no triangulation step is evident. Moreover, the majority (24 out of 40, 85%) do not let key informants review a draft of the case study report. Baker and Niederman ( 2014 ) do not use triangulation in the composition phase nor let key informants review the draft of the case study report. An example of an exploratory publication that applies both methodological steps is the publication by Kurnia et al. ( 2015 ). The authors triangulate via multiple sources and let key informants review their interview transcripts and the case study report to increase construct validity.
Explanatory MMCSs mostly use triangulation via multiple sources (5 out of 7, 71%) and for two (29%), the step is not evident. Furthermore, only two MMCS (29%) publications let key informants review the draft of the case study report, whereas the majority (5 out of 7, 71%) do not. In their publication , van de Weerd et al. ( 2016 ) use both methodological steps of the composition phase. The authors triangulate via multiple sources by presenting interview snippets from different cases for each result in the case study manuscript. Moreover, each case and the final case study report were shared with key informants for review and approval to reduce the risk of misinterpretations and increase construct validity.
To summarize, most exploratory and explanatory MMCSs use triangulation in the composition phase, whereas descriptive MMCSs do not. Moreover, only a fraction of all MMCSs let key informants review a draft of the case study report (8 out of 50, 16%).
5 Discussion
5.1 mmcs from a “research in practice" perspective, 5.1.1 delineating mmcs from other research approaches.
In this section, we delineate MMCSs from related research approaches. In the subsequent sections, we outline research situations for which MMCSs can be used and the benefits MMCSs provide.
Closely related research approaches from which we delineate MMCSs are multiple case studies , interviews, and vignettes . As shown in Fig. 2 , MMCSs differ from multiple case studies in that they focus on breadth by using a high number of cases with limited depth per case. In the most extreme situation, an MMCS only has one primary source of evidence per case. Moreover, MMCSs can also consider a greater variety of cases. In contrast, multiple case studies have a high depth per case and multiple sources of evidence per case to allow for a source triangulation (Benbasat et al. 1987 ; Yin 2018 ). Moreover, multiple case studies mainly focus on how and why research questions (Yin 2018 ), whereas MMCSs can additionally answer what, whether, and which research questions. The rationale why MMCSs are used for more types of research questions is their breadth, allowing them to also answer rather explorative research questions.
Distinguishing MMCSs from interviews is more difficult . Yet, we see two differences. First, interview studies do not have a clear unit of analysis. Interview studies may choose interviewees based on expertise (expert interviews), whereas case study researchers select informants based on the ability to inform about the case (key informants) (Yin 2018 ). Most of the 50 analysed MMCS (88%) specify their unit of analysis. Second, MMCSs can use multiple data collection methods (e.g., observations, interviews, documents), while interviews only use one (the interview) (Lamnek and Krell 2010 ). An example showing these delineation difficulties between MMCSs and interviews is the publication of Demlehner and Laumer ( 2020 ). The authors claim to take “a multiple case study approach including 39 expert interviews” (Demlehner and Laumer 2020 , p. 1). However, our criteria classify this as an interview study. Demlehner and Laumer ( 2020 ) contend that the interviewees were chosen using a “purposeful sampling strategy” (p. 5). However, case study research selects cases based on replication logic, not sampling (Yin 2018 ). Moreover, the results are not presented on a per-case basis (as usual for case studies); instead, the findings are presented on an aggregated level, similar to expert interviews. Therefore, we would not classify this publication as an MMCS but find that it is a very good example to discuss this delineation.
MMCSs differ from vignettes, which are used for (1) data collection , (2) data analysis , and (3) research communication (Klotz et al. 2022 ; Urquhart 2001 ). Researchers use vignettes for data collection as stimuli to which participants react (Klotz et al. 2022 ), i.e., a carefully constructed description of a person, object, or situation (Atzmüller and Steiner 2010 ; Hughes and Huby 2002 ). We can delineate MMCS from vignettes for data collection based on this definition. First, MMCSs are not used as a stimulus to which participants can react, as in MMCSs, data is collected without the stimulus requirement. Furthermore, vignettes for data collection are carefully constructed, which contradicts the characteristics of MMCS, that are all based on collected empirical data and not constructed descriptions.
A data analysis vignette is used as a retrospective tool (Klotz et al. 2022 ) and is very short, which makes it difficult to analyse deeper relationships between constructs. MMCSs differ from vignettes for data analysis in two ways. First, MMCSs are a complete research methodology with four steps, whereas vignettes for data analysis cover only one step (the data analysis) (e.g., Zamani and Pouloudi 2020 ). Second, vignettes are too short to conduct a thorough analysis of relationships, whereas MMCSs foster a more comprehensive analysis, allowing for a deeper analysis of relationships.
Finally, a vignette used for research communication “(1) is bounded to a short time span, a location, a special situation, or one or a few key actors, (2) provides vivid, authentic, and evocative accounts of the events with a narrative flow, (3) is rather short, and (4) is rooted in empirical data, sometimes inspired by data or constructed.” (Klotz et al. 2022 , p. 347). Based on the four elements for the vignettes’ definition, we can delineate MMCS from vignettes used for research communication. First, MMCSs are not necessarily bounded to a short time span, location, special situation, or key actors; instead, with MMCSs, a clearly defined case bounded in its context is researched. Second, the focus of MMCSs is not on the narrative flow; instead, the focus is on describing (c.f., McBride ( 2009 )), exploring (c.f., Baker and Niederman ( 2014 )), or explaining (c.f., van de Weerd et al. ( 2016 )) a phenomenon. Third, while MMCSs do not have the depth of multiple case studies, they are much more comprehensive than vignettes (e.g., the majority of analysed publications (42 of 50, 84%) specify an a priori theoretical framework). Fourth, every MMCS must be based on empirical data, i.e., all of our 50 MMCSs collect data for their study and base their results on this data. This is a key difference from vignettes, which can be completely fictitious (Klotz et al. 2022 ).
5.1.2 MMCS research situations
The decision to use an MMCS as a research method depends on the research context. MMCSs can be used in the early stages of research (descriptive and exploratory MMCS) and to corroborate findings (exploratory and explanatory MMCS). Academic literature has yet to agree on a uniform categorisation of research questions. For instance, Marshall and Rossman ( 2016 ) distinguish between descriptive, exploratory, explanatory, and emancipatory research questions. In contrast, Yin ( 2018 ) distinguishes between who , what , where , how , and why questions, where he argues that the latter two are especially suitable for explanatory case study research. MMCSs can answer more types of research questions than Yin ( 2018 ) proposed. The reason for this is rooted in the higher breadth of MMCSs, which allows MMCSs to also answer rather exploratory what , whether , or which questions, besides the how and why questions that are suggested by Yin ( 2018 ).
For descriptive MMCSs , the main goal of the how and what questions is to describe the phenomenon. However, in our sample of analysed MMCSs, the analysis stops after the description of the phenomenon. The main goal of the five types of exploratory MMCS research questions is to investigate little-known aspects of a particular phenomenon. The how and why questions analyse operational links between different constructs (e.g., “How do different types of IS assets account for synergies between business units to create business value?” (Mandrella et al. 2016 , p. 2)). Exploratory what questions can be answered by case study research and other research methods (e.g., surveys or archival analysis) (Yin 2018 ). Nevertheless, all whether and which MMCS research questions can also be re-formulated as exploratory what questions. The reason why many MMCSs answer what , whether , or which research questions lies in the breadth (i.e., higher number and variety of cases) of MMCS, that allow them to answer these rather exploratory research questions to a satisfactory level. Finally, the research questions of the explanatory MMCSs aim to analyse operational links (i.e., how or why something is happening). This is also in line with the findings of Yin ( 2018 ) for multiple case study research. However, for MMCSs, this view must be extended, as explanatory MMCSs are also able to answer what questions. We explain this with the higher breadth of MMCS.
To discuss an MMCS’s contribution to theory, we use the idea of the theory continuum proposed by Ridder ( 2017 ) (cf. Section 2.1 ). Despite being used in the early phase of research (descriptive and exploratory), we do not recommend using MMCSs to build theory . We argue that for theory building, data with “as much depth as […] feasible” (Eisenhardt 1989 , p. 539) is required on a per-case basis. However, a key characteristic of MMCSs is the limited depth per case, which conflicts with the in-depth requirements of theory building. Moreover, a criterion for theory building is that there is no theory available which explains the phenomenon (Ridder 2017 ). Nevertheless, in our analysed MMCSs, 84% (42 out of 50) have an a priori theoretical framework. Furthermore, for theory building, the recommendation is to use between four to ten cases; with more, “it quickly becomes difficult to cope with the complexity and volume of the data” (Eisenhardt 1989 , p. 545). However, a characteristic of MMCSs is to have a relatively high number of cases, i.e., the analysed MMCSs often have more than 20 cases, which is significantly above the recommendation for theory building.
The next phase in the theory continuum is theory development , where a tentative theory is extended or refined (Ridder 2017 ). MMCSs should and are used for theory development, i.e., 84% (42 out of 50) of analysed MMCS publications have an a priori theoretical framework extended and refined using the MMCS. An MMCS example for theory development is the research of Karunagaran et al. ( 2016 ), who use a combination of the diffusion of innovation theory and technology organisation environment framework as tentative theories to research the adoption of cloud computing. As Ridder ( 2017 ) outlined, for theory development, literal replication and pattern matching should be used. Both methodological steps are used by Karunagaran et al. ( 2016 ) to identify the mechanisms of cloud adoption more precisely.
The next step in the theory continuum is theory testing , where existing theory is challenged by finding anomalies that existing theory cannot explain (Ridder 2017 ). The boundaries between theory development and testing are often blurred (Ridder 2017 ). In theory testing, the phenomenon is understood, and the research strategy focuses on testing if the theory also holds under different circumstances, i.e., hypotheses can be formed and tested based on existing theory (Ridder 2017 ). In multiple case study research, theory testing uses theoretical replication with pattern matching or addressing rival explanations (Ridder 2017 ). In our MMCS publications, no publication addresses rival explanations, and only a few apply theoretical replication and pattern matching–yet not for theory testing. A few publications claim to test propositions derived from an a priori theoretical framework (e.g., Schäfferling et al. 2011 ; Spiegel and Lazic 2010 ; Wagner and Ettrich-Schmitt 2009 ). However, these publications either do not state their replication logic (e.g., Spiegel and Lazic 2010 ; Wagner and Ettrich-Schmitt 2009 ) or use a literal replication (e.g., Schäfferling et al. 2011 ), both of which weaken the value of their theory testing.
5.1.3 MMCS research benefits
MMCSs are beneficial in multiple research situations and can be an avenue to address the frequent criticism of multiple case study research of being time-consuming and costly (Voss et al. 2002 ; Yin 2018 ).
Firstly, MMCSs can be used for time-critical topics where it is beneficial to publish results quicker and discuss them instead of conducting in-depth multiple case studies (e.g., COVID-19 (e.g., dos Santos Tavares et al. 2021 ) or emergent technology adoption (e.g., Bremser 2017 )). Especially with COVID-19, research publishing saw a significantly higher speed due to special issues of journals and faster review processes. Further, due to the fast technological advancements, there is a higher risk that the results are obsolete and of less practical use when researched with time-consuming multiple in-depth case studies.
Secondly, MMCSs can be used in research situations when it is challenging to gather in-depth data from multiple sources of evidence for each case due to the limited availability of sources of evidence or limited accessibility of sources of evidence. When researching novel phenomena (e.g., the adoption of new technologies in organisations), managers and decision-makers are usually interviewed as sources of evidence. However, in most organisations, only one (or very few) decision-makers have the ability to inform and should be interviewed, limiting the potential sources of evidence per case. These decision-makers often have limited availability for multiple in-depth interviews. Furthermore, the sources of evidence are often difficult to access, as professional organisations have regulations that prevent sharing documents with researchers.
Thirdly, MMCSs can be beneficial when the research framework is complex and requires many cases for validation (e.g., Baker and Niederman ( 2014 ) validate their rather complex a priori framework with 22 cases) or when previous research has led to contradictory results . Therefore, in both situations, a higher breadth of cases is required to also research combinatorial effects (e.g., van de Weerd et al. 2016 ). However, conducting an in-depth multiple case study would take time and effort. Therefore, MMCSs can be a mindful way to collect many cases, but in the same vein, being time and cost-efficient.
5.2 MMCS research rigour
Table 8 outlines two types of methodological steps for MMCSs. The first are methodological steps, where MMCSs should follow multiple case study methodological guidance (e.g., clarify the unit of analysis ), while the second is unique to MMCSs due to its characteristics. This section focuses on the latter, exploring MMCS characteristics, problems, validity threats, and proposed solutions.
The characteristics of MMCSs of having only one primary source of evidence per case prevents MMCSs from using source triangulation, which is often used in multiple case study research (Stake 2013 ; Voss et al. 2002 ; Yin 2018 ). By only having one source of evidence, researchers can fail to develop a sufficient set of operational measures and instead rely on subjective judgements, which threatens construct validity (Yin 2018 ). The threats to construct validity must be addressed throughout the MMCS research process. To do so, we propose to use easily accessible supplementary data or other triangulation approaches to increase construct validity in a MMCS. For the other triangulation approaches, we see that the majority of publications use supplementary data (e.g., publicly available documents) as further sources of evidence, multiple investigators, multiple methods (e.g., quantitative and qualitative), multiple theories, or combinations of these (cf. Tables 5 , 6 and 7 ). Having one or, in the best case, all of them reduces the risk of reporting spurious relationships and subjective judgements of the researchers, as a phenomenon is analysed from multiple perspectives. Besides the above-mentioned types of triangulation, we propose to apply a new type of triangulation, which is specific to MMCSs and triangulates findings across similar cases combined to groups instead of multiple sources per case. We propose that all reported findings have to be found in more than one case in a group of cases. This is also in line with previous methodological guidelines, which suggest that findings should only be reported if they have at least three confirmations (Stake 2013 ). To triangulate across multiple cases in one group, researchers have to identify multiple similar cases by applying a literal case replication logic to reinforce similar results. One should also apply a theoretical replication to compare different groups of literally replicated cases (i.e., searching for contrary results). Therefore, researchers have to justify their case replication logic . However, in our sample of MMCS, the majority (32 of 50, 64%) does not justify their replication logic, whereas the remaining publications use either literal replication (8 of 50, 16%), theoretical replication (6 of 50, 12%), or a combination (4 of 50, 8%). We encourage researchers to use a combination of literal and theoretical replication because it allows triangulation across different groups of cases. An exemplary MMCS that uses this approach is the publication of van de Weerd et al. ( 2016 ), who use theoretical replication to find cases with different outcomes (e.g., adoption and non-adoption) and use literal replication to find cases with similar characteristics and form groups of them.
Two further methodological steps, which are not exclusive to MMCS but recommended for increasing the construct validity, are creating a chain of evidence and letting key informants review a draft of the case study report . Only 36% (18 out of 50) of the analysed MMCS publications establish a chain of evidence. One reason for this lower usage may be that the majority (35 out of 50, 70%) of the publications analysed are conference proceedings. While we understand that these publications face space limitations, we note that no publication offers a supplementary appendix with in-depth insights. However, we encourage researchers to create a full chain of evidence with as much transparency as possible. Therefore, online directories for supplementary appendices could be a valuable addition. As opposed to a few years ago, these repositories today are widely available and using them for such purposes could become a good research practice for qualitative research. Interestingly, only 16% (8 of 50) analysed MMCS publications let key informants review the draft of the case study report . As MMCSs only have one source of evidence per case, misinterpretations and subjective judgement by the researcher have a significantly higher impact on the results compared to multiple case study research. Therefore, MMCS researchers should let key informants review the case study report before publishing.
MMCSs only have few (one) sources of evidence per case, so the risk of focusing on spurious relationships is higher, threatening internal validity (Dubé and Paré 2003 ). This threat to internal validity must be addressed in the data analysis phase. In the context of MMCSs, researchers may aggregate fewer data points to obtain a within-case overview. Therefore, having a clear perspective of the existing data points and rigorously applying the within-case analysis methodological steps (e.g., pattern matching) is even more critical. However, due to the limited depth of data at MMCSs, the within-case analysis must be combined with an analysis across groups of cases (to allow triangulation via multiple groups of cases). For MMCSs, we propose not doing the cross-case analysis on a per-case basis. Instead, we propose to build groups of similar cases across which researchers could conduct an analysis across groups of cases. This solidifies internal validity in case study research (Eisenhardt 1989 ) by viewing and synthesising insights from multiple perspectives (Paré 2004 ; Yin 2018 ).
Another risk of MMCSs is the relatively high number of cases (i.e., we found up to 27 for exploratory MMCSs) that is higher than Eisenhardt’s ( 1989 ) recommendation of maximal ten cases in multiple case study research. With more than ten in-depth cases, researchers struggled to manage the complexity and data volume, resulting in models with low generalisability and reduced external validity (Eisenhardt 1989 ). We propose to use two methodological steps to address the threat to external validity.
First, like Yin’s ( 2018 ) recommendation to use theory for single case studies, we suggest an a priori theoretical framework for MMCSs. 84% (42 out of 50) of the analysed MMCS publications use such a framework. An a priori theoretical framework has two advantages: it simplifies research by pre-defining constructs and relationships, and it enables analytical techniques like pattern matching. Second, instead of doing the within and then cross-case analysis on a per-case basis, for MMCSs, we propose first doing the within-case analysis and then forming groups of similar cases. Then, the cross-case analysis is performed on the formed groups of cases. To form case groups, replication logic (literal and theoretical) must be chosen carefully. Cross-group analysis (with at least two cases per group) can increase the generalisability of results.
To increase MMCS reliability, a case study database and protocol should be created, similar to multiple case studies. To ensure higher reliability, researchers should document MMCS design decisions in more detail. As outlined in the results section, the documentation on why design decisions were taken is often relatively short and should be more detailed. This call for better documentation is not exclusive to MMCSs, as Benbasat et al. ( 1987 ) and Dubé and Paré ( 2003 ) also criticised this for multiple case study research.To ensure rigour in MMCS, we suggest following the steps for multiple case study research. However, MMCSs have unique characteristics, such as an inability to source triangulate on a per-case level, a higher risk of marginal cases, and difficulty in managing a high number of cases. Therefore, for some methodological steps (cf. Table 8 ), we propose MMCS-specific methodological options. First, MMCS should include supplementary data per case (to increase construct validity). Second, instead of doing a cross-case analysis, we propose to form groups of similar cases and focus on the cross-group analysis (i.e., in each group, there must be at least two cases). Third, researchers should justify their case replication logic , i.e., a combination of theoretical replication (to form different groups) and literal replication (to find the same cases within groups) should be conducted to allow for this cross-group analysis.
6 Conclusion
Our publication contributes to case study research in the IS discipline and beyond by making four methodological contributions. First, we provide a conceptual definition of MMCSs and distinguish them from other research approaches. Second, we provide a contemporary collection of exemplary MMCS publications and their methodological choices. Third, we outline methodological guidelines for rigorous MMCS research and provide examples of good practice. Fourth, we identify research situations for which MMCSs can be used as a pragmatic and rigorous approach.
Our findings have three implications for research practice: First, we found that MMCSs can be descriptive, exploratory, or explanatory and can be considered as a type of multiple case study. Our set of IS MMCS publications shows that this pragmatic approach is advantageous in three situations. First, for time-sensitive topics, where rapid discussion of results, especially in the early stages of research, is beneficial. Second, when it is difficult to collect comprehensive data from multiple sources for each case, either because of limited availability or limited accessibility to the data source. Third, in situations where the research setting is complex, many cases are needed to validate effects (e.g., combinatorial effects) or previous research has produced conflicting results. It is important, however, that the pragmatism of the MMCS should not be misunderstood as a lack of methodological rigour.
Second, we have provided guidelines that researchers can follow to conduct MMCSs rigorously. As we observe an increasing number of MMCSs being published, we encourage their authors to clarify their methodological approach by referring to our analytical MMCS framework. Our analytical framework helps researchers to justify their approach and to distinguish it from approaches that lack methodological rigour.
Third, throughout our collection of MMCS publications, we contacted several authors to clarify their case study research methodology. In many cases, these publications lacked critical details that would be important to classify them as MMCS or marginal cases. Many researchers responded that some details were not mentioned due to space limitations. While we understand these constraints, we suggest that researchers still present these details, for example, by considering online appendices in research repositories.
Our paper has five limitations that could be addressed by future research. First, we focus exclusively on methodological guidelines for positivist multiple case study research. Therefore, we have not explicitly covered methodological approaches from other research paradigms.
Second, we aggregated methodological guidance on multiple case study research from the most relevant publications by citation count only. As a result, we did not capture evidence from publications with far fewer citations or that are relevant in specific niches. However, our design choice is still justified as the aim was to identify established and widely accepted methodological strategies to ensure rigour in case study research.
Third, the literature reviews were keyword-based. Therefore, concepts that fall within our understanding of MMCS but do not include the keywords used for the literature search could not be identified. However, due to the different search terms and versatile search approaches, our search should have captured the most relevant contributions.
Fourth, we selected publications from highly ranked IS MMCS publications and proceedings of leading IS conferences to analyse how rigour is ensured in MMCSs in the IS discipline. We therefore excluded all other research outlets. As with the limitations arising from the keyword-based search, we may have omitted IS MMCS publications that refer to short or mini case studies. However, the limitation of our search is justified as it helps us to ensure that all selected publications have undergone a substantial peer review process and qualify as a reference base in IS.
Fifth, we coded our variables based on the characteristics explicitly stated in the manuscript (i.e., if authors position their MMCS as exploratory, we coded it as exploratory). However, for some variables, researchers do not have a consistent understanding (e.g., the discussion of what constitutes exploratory research by cf., Sarker et al. ( 2018 )). Therefore, we took the risk that MMCS may have different understandings of the coded variables.
For the future, our manuscript on positivist MMCSs provides researchers with guidance for an emerging type of case study research. Based on our study, we can identify promising areas for future research. By limiting ourselves to the most established strategies for ensuring rigour, we also invite authors to enrich our methodological guidelines with other, less commonly used steps. In addition, future research could compare the use of MMCSs in IS with other disciplines in order to solidify our findings.
Data availability
Provided at https://doi.org/10.6084/m9.figshare.24916458
The information can be found in the online Appendix: https://doi.org/10.6084/m9.figshare.24916458 .
litbaskets.io is a web interface that allows searching for literature across the top 847 IS journals. It offers ranging from 2XS (Basket of Eight) to 3XL (847) essential IS journals and a full list of 29 journals which are the basis for this study can be found in Appendix C ( https://doi.org/10.6084/m9.figshare.24916458 ).
Atzmüller C, Steiner PM (2010) Experimental vignette studies in survey research. Method Eur J Res Methods Behav Soc Sci. https://doi.org/10.1027/1614-2241/a000014
Article Google Scholar
Baker EW, Niederman F (2014) Integrating the IS functions after mergers and acquisitions: analyzing business-IT alignment. J Strateg Inf Syst 23(2):112–127. https://doi.org/10.1016/j.jsis.2013.08.002
Benbasat I, Goldstein DK, Mead M (1987) The case research strategy in studies of information systems. MIS Q 11(3):369–386. https://doi.org/10.2307/248684
Boell S, Wang B (2019) www.litbaskets.io , an IT artifact supporting exploratory literature searches for information systems research. In: Proceedings ACIS 2019
Bremser CP, Gunther Rothlauf F (2017) Strategies and influencing factors for big data exploration. In: proceedings AMCIS 2017
Vom Brocke J, Simons A, Niehaves B, Riemer K, Plattfaut R, Cleven A (2009) Reconstructing the giant: on the importance of rigour in documenting the literature search process. In: Proceedings ECIS 2009
Creswell JW, Poth CN (2016) Qualitative inquiry and research design: choosing among five approaches, 4th edn. Sage Publications, California
Google Scholar
Demlehner Q, Laumer S (2020) Shall we use it or not? Explaining the adoption of artificial intelligence for car manufacturing purposes. In: Proceedings ECIS 2020
Dubé L, Paré G (2003) Rigor in information systems positivist case research: current practices, trends, and recommendations. MIS Q 27(4):597–636. https://doi.org/10.2307/30036550
Dubois A, Gadde L-E (2002) Systematic combining: an abductive approach to case research. J Bus Res 55(7):553–560. https://doi.org/10.1016/S0148-2963(00)00195-8
Eisenhardt KM (1989) Building theories from case study research. Acad Manag Rev 14(4):532–550. https://doi.org/10.2307/258557
Eisenhardt KM, Graebner ME (2007) Theory building from cases: opportunities and challenges. Acad Manag J 50(1):25–32. https://doi.org/10.5465/amj.2007.24160888
Gibbert M, Ruigrok W, Wicki B (2008) What passes as a rigorous case study? Strateg Manag J 29(13):1465–1474. https://doi.org/10.1002/smj.722
Gogan JL, McLaughlin MD, Thomas D (2014) Critical incident technique in the basket. In: Proceedings ICIS 2014
Hahn C, Röher D, Zarnekow R (2015) A value proposition oriented typology of electronic marketplaces for B2B SaaS applications. In: Proceedings AMCIS 2015
Hanelt A, Hildebrandt B, Polier J (2015) Uncovering the role of IS in business model innovation: a taxonomy-driven approach to structure the field. In: Proceedings ECIS 2015
Hughes R, Huby M (2002) The application of vignettes in social and nursing research. J Adv Nurs 37(4):382–386. https://doi.org/10.1046/j.1365-2648.2002.02100.x
Karunagaran S, Mathew S, Lehner F (2016) Differential adoption of cloud technology: a multiple case study of large firms and SMEs. In: Proceedings ICIS 2016
Keutel M, Michalik B, Richter J (2014) Towards mindful case study research in IS: a critical analysis of the past ten years. Eur J Inf Syst 23(3):256–272. https://doi.org/10.1057/ejis.2013.26
Klein HK, Myers MD (1999) A set of principles for conducting and evaluating interpretive field studies in information systems. MIS Q 23(1):67–93. https://doi.org/10.2307/249410
Klotz S, Kratzer S, Westner M, Strahringer S (2022) Literary sketches in information systems research: conceptualization and guidance for using vignettes as a narrative form. Inf Syst Manag. https://doi.org/10.1080/10580530.2021.1996661
Kunduru SR, Bandi RK (2019) Fluidity of power structures underpinning public discourse on social media: a multi-case study on twitter discourse in India. In: Proceedings AMCIS 2019
Kurnia S, Karnali RJ, Rahim MM (2015) A qualitative study of business-to-business electronic commerce adoption within the indonesian grocery industry: a multi-theory perspective. Inf Manag 52(4):518–536. https://doi.org/10.1016/j.im.2015.03.003
Lamnek S, Krell C (2010) Qualitative sozialforschung: mit online-materialien, 6th edn. Beltz Verlangsgruppe, Germany
Lee AS, Hubona GS (2009) A scientific basis for rigor in information systems research. MIS Q 33(2):237–262. https://doi.org/10.2307/20650291
Mandrella M, Zander S, Trang S (2016) How different types of IS assets account for synergy-enabled value in multi-unit firms: mapping of critical success factors and key performance indicators. In: Proceedings AMCIS 2016
Marshall C, Rossman GB (2016) Designing qualitative research, 6th edn. SAGE Publications, Inc., California
McBride N (2009) Exploring service issues within the IT organisation: four mini-case studies. Int J Inf Manag 29(3):237–242. https://doi.org/10.1016/j.ijinfomgt.2008.11.010
Meredith J (1998) Building operations management theory through case and field research. J Oper Manag 16:441–454. https://doi.org/10.1016/S0272-6963(98)00023-0
Nili A, Tate M, Barros A, Johnstone D (2020) An approach for selecting and using a method of inter-coder reliability in information management research. Int J Inf Manage 54:102154. https://doi.org/10.1016/j.ijinfomgt.2020.102154
Orlikowski WJ, Baroudi JJ (1991) Studying information technology in organizations: research approaches and assumptions. Inf Syst Res 2(1):1–28
Palvia P, Daneshvar Kakhki M, Ghoshal T, Uppala V, Wang W (2015) Methodological and topic trends in information systems research: a meta-analysis of IS journals. Commun Assoc Inf Syst 37(1):30. https://doi.org/10.17705/1CAIS.03730
Pan SL, Tan B (2011) Demystifying case research: a structured–pragmatic–situational (SPS) approach to conducting case studies. Inf Organ 21(3):161–176. https://doi.org/10.1016/j.infoandorg.2011.07.001
Paré G (2004) Investigating information systems with positivist case research. Commun Assoc Inf Syst 13(1):18. https://doi.org/10.17705/1CAIS.01318
Piekkari R, Welch C, Paavilainen E (2009) The case study as disciplinary convention: evidence from international business journals. Organ Res Methods 12(3):567–589. https://doi.org/10.1177/109442810831990
Recker J (2012) Scientific research in information systems: a beginner’s guide. Springer, Berlin
Ridder H-G (2017) The theory contribution of case study research. Bus Res 10(2):281–305. https://doi.org/10.1007/s40685-017-0045-z
dos Santos Tavares AP, Fornazin M, Joia LA (2021) The good, the bad, and the ugly: digital transformation and the Covid-19 pandemic. In: Proceedings AMCIS 2021
Sarker S, Xiao X, Beaulieu T, Lee AS (2018) Learning from first-generation qualitative approaches in the IS discipline: an evolutionary view and some implications for authors and evaluators (PART 1/2). J Assoc Inf Syst 19(8):752–774. https://doi.org/10.17705/1jais.00508
Schäfferling A, Wagner H-T, Schulz M, Dum T (2011) The effect of knowledge management systems on absorptive capacity: findings from international law firms. In: Proceedings PACIS 2011
Sørensen C, Landau JS (2015) Academic agility in digital innovation research: the case of mobile ICT publications within information systems 2000–2014. J Strateg Inf Syst 24(3):158–170. https://doi.org/10.1016/j.jsis.2015.07.001
Spiegel F, Lazic M (2010) Incentive and control mechanisms for mitigating relational risk in IT outsourcing relationships. In: Proceedings AMCIS 2010
Stake RE (2013) Multiple case study analysis. The Guilford Press
Urquhart C (2001) Bridging information requirements and information needs assessment: Do scenarios and vignettes provide a link? Inf Res 6(2):6–2
van de Weerd I, Mangula IS, Brinkkemper S (2016) Adoption of software as a service in indonesia: examining the influence of organizational factors. Inf Manag 53(7):915–928. https://doi.org/10.1016/j.im.2016.05.008
Vom Brocke J, Simons A, Riemer K, Niehaves B, Plattfaut R, Cleven A (2015) Standing on the shoulders of giants: challenges and recommendations of literature search in information systems research. Commun Assoc Inf Syst 37(1):9. https://doi.org/10.17705/1CAIS.03709
Voss C, Tsikriktsis N, Frohlich M (2002) Case research in operations management. Int J Oper Prod Manag 22(2):195–219
Wagner H-T, Ettrich-Schmitt K (2009) Integrating value-adding mobile services into an emergency management system for tourist destinations. In: Proceedings ECIS 2009
Welch C, Piekkari R, Plakoyiannaki E. et al (2011) Theorising from case studies: Towards a pluralist future for international business research. J Int Bus Stud 42, 740–762. https://doi.org/10.1057/jibs.2010.55
Weill P, Olson MH (1989) Managing investment in information technology: mini case examples and implications. MIS Q 13(1):3–17. https://doi.org/10.2307/248694
Yin RK (2018) Case study research and applications: design and methods, 5th edn. Sage Publications, California
Zamani E, Pouloudi N (2020) Generative mechanisms of workarounds, discontinuance and reframing: a study of negative disconfirmation with consumerised IT. Inf Syst J 31(3):284–428. https://doi.org/10.1111/isj.12315
Download references
Open Access funding enabled and organized by Projekt DEAL. No funding was received for conducting this study.
Author information
Authors and affiliations.
Technische Universität Dresden, Dresden, Germany
Sebastian Käss, Christoph Brosig & Susanne Strahringer
OTH Regensburg, Seybothstr 2, 93053, Regensburg, Germany
Markus Westner
You can also search for this author in PubMed Google Scholar
Contributions
All authors contributed to the study conception and design. Literature search and analyses were performed by the first two authors, and reviewed by the other two. All authors contributed to the interpretation and the discussion of the results. The first draft of the manuscript was written by the first two authors and all authors commented on the previous versions of the manuscript and critically revised the work. All authors read and approved the final manuscript.
Corresponding author
Correspondence to Susanne Strahringer .
Ethics declarations
Conflict of interest.
The authors have no competing interests to declare that are relevant to the content of this study.
Ethical approval
Not Applicable, no human participants.
Additional information
Publisher's note.
Springer Nature remains neutral with regard to jurisdictional claims in published maps and institutional affiliations.
Rights and permissions
Open Access This article is licensed under a Creative Commons Attribution 4.0 International License, which permits use, sharing, adaptation, distribution and reproduction in any medium or format, as long as you give appropriate credit to the original author(s) and the source, provide a link to the Creative Commons licence, and indicate if changes were made. The images or other third party material in this article are included in the article's Creative Commons licence, unless indicated otherwise in a credit line to the material. If material is not included in the article's Creative Commons licence and your intended use is not permitted by statutory regulation or exceeds the permitted use, you will need to obtain permission directly from the copyright holder. To view a copy of this licence, visit http://creativecommons.org/licenses/by/4.0/ .
Reprints and permissions
About this article
Käss, S., Brosig, C., Westner, M. et al. Short and sweet: multiple mini case studies as a form of rigorous case study research. Inf Syst E-Bus Manage (2024). https://doi.org/10.1007/s10257-024-00674-2
Download citation
Received : 24 January 2024
Accepted : 23 February 2024
Published : 15 May 2024
DOI : https://doi.org/10.1007/s10257-024-00674-2
Share this article
Anyone you share the following link with will be able to read this content:
Sorry, a shareable link is not currently available for this article.
Provided by the Springer Nature SharedIt content-sharing initiative
- Case study research
- Multiple mini case study
- Short case study
- Methodological guidance
- Find a journal
- Publish with us
- Track your research

An official website of the United States government
The .gov means it’s official. Federal government websites often end in .gov or .mil. Before sharing sensitive information, make sure you’re on a federal government site.
The site is secure. The https:// ensures that you are connecting to the official website and that any information you provide is encrypted and transmitted securely.
- Publications
- Account settings
Preview improvements coming to the PMC website in October 2024. Learn More or Try it out now .
- Advanced Search
- Journal List
- J Korean Med Sci
- v.37(16); 2022 Apr 25

A Practical Guide to Writing Quantitative and Qualitative Research Questions and Hypotheses in Scholarly Articles
Edward barroga.
1 Department of General Education, Graduate School of Nursing Science, St. Luke’s International University, Tokyo, Japan.
Glafera Janet Matanguihan
2 Department of Biological Sciences, Messiah University, Mechanicsburg, PA, USA.
The development of research questions and the subsequent hypotheses are prerequisites to defining the main research purpose and specific objectives of a study. Consequently, these objectives determine the study design and research outcome. The development of research questions is a process based on knowledge of current trends, cutting-edge studies, and technological advances in the research field. Excellent research questions are focused and require a comprehensive literature search and in-depth understanding of the problem being investigated. Initially, research questions may be written as descriptive questions which could be developed into inferential questions. These questions must be specific and concise to provide a clear foundation for developing hypotheses. Hypotheses are more formal predictions about the research outcomes. These specify the possible results that may or may not be expected regarding the relationship between groups. Thus, research questions and hypotheses clarify the main purpose and specific objectives of the study, which in turn dictate the design of the study, its direction, and outcome. Studies developed from good research questions and hypotheses will have trustworthy outcomes with wide-ranging social and health implications.
INTRODUCTION
Scientific research is usually initiated by posing evidenced-based research questions which are then explicitly restated as hypotheses. 1 , 2 The hypotheses provide directions to guide the study, solutions, explanations, and expected results. 3 , 4 Both research questions and hypotheses are essentially formulated based on conventional theories and real-world processes, which allow the inception of novel studies and the ethical testing of ideas. 5 , 6
It is crucial to have knowledge of both quantitative and qualitative research 2 as both types of research involve writing research questions and hypotheses. 7 However, these crucial elements of research are sometimes overlooked; if not overlooked, then framed without the forethought and meticulous attention it needs. Planning and careful consideration are needed when developing quantitative or qualitative research, particularly when conceptualizing research questions and hypotheses. 4
There is a continuing need to support researchers in the creation of innovative research questions and hypotheses, as well as for journal articles that carefully review these elements. 1 When research questions and hypotheses are not carefully thought of, unethical studies and poor outcomes usually ensue. Carefully formulated research questions and hypotheses define well-founded objectives, which in turn determine the appropriate design, course, and outcome of the study. This article then aims to discuss in detail the various aspects of crafting research questions and hypotheses, with the goal of guiding researchers as they develop their own. Examples from the authors and peer-reviewed scientific articles in the healthcare field are provided to illustrate key points.
DEFINITIONS AND RELATIONSHIP OF RESEARCH QUESTIONS AND HYPOTHESES
A research question is what a study aims to answer after data analysis and interpretation. The answer is written in length in the discussion section of the paper. Thus, the research question gives a preview of the different parts and variables of the study meant to address the problem posed in the research question. 1 An excellent research question clarifies the research writing while facilitating understanding of the research topic, objective, scope, and limitations of the study. 5
On the other hand, a research hypothesis is an educated statement of an expected outcome. This statement is based on background research and current knowledge. 8 , 9 The research hypothesis makes a specific prediction about a new phenomenon 10 or a formal statement on the expected relationship between an independent variable and a dependent variable. 3 , 11 It provides a tentative answer to the research question to be tested or explored. 4
Hypotheses employ reasoning to predict a theory-based outcome. 10 These can also be developed from theories by focusing on components of theories that have not yet been observed. 10 The validity of hypotheses is often based on the testability of the prediction made in a reproducible experiment. 8
Conversely, hypotheses can also be rephrased as research questions. Several hypotheses based on existing theories and knowledge may be needed to answer a research question. Developing ethical research questions and hypotheses creates a research design that has logical relationships among variables. These relationships serve as a solid foundation for the conduct of the study. 4 , 11 Haphazardly constructed research questions can result in poorly formulated hypotheses and improper study designs, leading to unreliable results. Thus, the formulations of relevant research questions and verifiable hypotheses are crucial when beginning research. 12
CHARACTERISTICS OF GOOD RESEARCH QUESTIONS AND HYPOTHESES
Excellent research questions are specific and focused. These integrate collective data and observations to confirm or refute the subsequent hypotheses. Well-constructed hypotheses are based on previous reports and verify the research context. These are realistic, in-depth, sufficiently complex, and reproducible. More importantly, these hypotheses can be addressed and tested. 13
There are several characteristics of well-developed hypotheses. Good hypotheses are 1) empirically testable 7 , 10 , 11 , 13 ; 2) backed by preliminary evidence 9 ; 3) testable by ethical research 7 , 9 ; 4) based on original ideas 9 ; 5) have evidenced-based logical reasoning 10 ; and 6) can be predicted. 11 Good hypotheses can infer ethical and positive implications, indicating the presence of a relationship or effect relevant to the research theme. 7 , 11 These are initially developed from a general theory and branch into specific hypotheses by deductive reasoning. In the absence of a theory to base the hypotheses, inductive reasoning based on specific observations or findings form more general hypotheses. 10
TYPES OF RESEARCH QUESTIONS AND HYPOTHESES
Research questions and hypotheses are developed according to the type of research, which can be broadly classified into quantitative and qualitative research. We provide a summary of the types of research questions and hypotheses under quantitative and qualitative research categories in Table 1 .
Research questions in quantitative research
In quantitative research, research questions inquire about the relationships among variables being investigated and are usually framed at the start of the study. These are precise and typically linked to the subject population, dependent and independent variables, and research design. 1 Research questions may also attempt to describe the behavior of a population in relation to one or more variables, or describe the characteristics of variables to be measured ( descriptive research questions ). 1 , 5 , 14 These questions may also aim to discover differences between groups within the context of an outcome variable ( comparative research questions ), 1 , 5 , 14 or elucidate trends and interactions among variables ( relationship research questions ). 1 , 5 We provide examples of descriptive, comparative, and relationship research questions in quantitative research in Table 2 .
Hypotheses in quantitative research
In quantitative research, hypotheses predict the expected relationships among variables. 15 Relationships among variables that can be predicted include 1) between a single dependent variable and a single independent variable ( simple hypothesis ) or 2) between two or more independent and dependent variables ( complex hypothesis ). 4 , 11 Hypotheses may also specify the expected direction to be followed and imply an intellectual commitment to a particular outcome ( directional hypothesis ) 4 . On the other hand, hypotheses may not predict the exact direction and are used in the absence of a theory, or when findings contradict previous studies ( non-directional hypothesis ). 4 In addition, hypotheses can 1) define interdependency between variables ( associative hypothesis ), 4 2) propose an effect on the dependent variable from manipulation of the independent variable ( causal hypothesis ), 4 3) state a negative relationship between two variables ( null hypothesis ), 4 , 11 , 15 4) replace the working hypothesis if rejected ( alternative hypothesis ), 15 explain the relationship of phenomena to possibly generate a theory ( working hypothesis ), 11 5) involve quantifiable variables that can be tested statistically ( statistical hypothesis ), 11 6) or express a relationship whose interlinks can be verified logically ( logical hypothesis ). 11 We provide examples of simple, complex, directional, non-directional, associative, causal, null, alternative, working, statistical, and logical hypotheses in quantitative research, as well as the definition of quantitative hypothesis-testing research in Table 3 .
Research questions in qualitative research
Unlike research questions in quantitative research, research questions in qualitative research are usually continuously reviewed and reformulated. The central question and associated subquestions are stated more than the hypotheses. 15 The central question broadly explores a complex set of factors surrounding the central phenomenon, aiming to present the varied perspectives of participants. 15
There are varied goals for which qualitative research questions are developed. These questions can function in several ways, such as to 1) identify and describe existing conditions ( contextual research question s); 2) describe a phenomenon ( descriptive research questions ); 3) assess the effectiveness of existing methods, protocols, theories, or procedures ( evaluation research questions ); 4) examine a phenomenon or analyze the reasons or relationships between subjects or phenomena ( explanatory research questions ); or 5) focus on unknown aspects of a particular topic ( exploratory research questions ). 5 In addition, some qualitative research questions provide new ideas for the development of theories and actions ( generative research questions ) or advance specific ideologies of a position ( ideological research questions ). 1 Other qualitative research questions may build on a body of existing literature and become working guidelines ( ethnographic research questions ). Research questions may also be broadly stated without specific reference to the existing literature or a typology of questions ( phenomenological research questions ), may be directed towards generating a theory of some process ( grounded theory questions ), or may address a description of the case and the emerging themes ( qualitative case study questions ). 15 We provide examples of contextual, descriptive, evaluation, explanatory, exploratory, generative, ideological, ethnographic, phenomenological, grounded theory, and qualitative case study research questions in qualitative research in Table 4 , and the definition of qualitative hypothesis-generating research in Table 5 .
Qualitative studies usually pose at least one central research question and several subquestions starting with How or What . These research questions use exploratory verbs such as explore or describe . These also focus on one central phenomenon of interest, and may mention the participants and research site. 15
Hypotheses in qualitative research
Hypotheses in qualitative research are stated in the form of a clear statement concerning the problem to be investigated. Unlike in quantitative research where hypotheses are usually developed to be tested, qualitative research can lead to both hypothesis-testing and hypothesis-generating outcomes. 2 When studies require both quantitative and qualitative research questions, this suggests an integrative process between both research methods wherein a single mixed-methods research question can be developed. 1
FRAMEWORKS FOR DEVELOPING RESEARCH QUESTIONS AND HYPOTHESES
Research questions followed by hypotheses should be developed before the start of the study. 1 , 12 , 14 It is crucial to develop feasible research questions on a topic that is interesting to both the researcher and the scientific community. This can be achieved by a meticulous review of previous and current studies to establish a novel topic. Specific areas are subsequently focused on to generate ethical research questions. The relevance of the research questions is evaluated in terms of clarity of the resulting data, specificity of the methodology, objectivity of the outcome, depth of the research, and impact of the study. 1 , 5 These aspects constitute the FINER criteria (i.e., Feasible, Interesting, Novel, Ethical, and Relevant). 1 Clarity and effectiveness are achieved if research questions meet the FINER criteria. In addition to the FINER criteria, Ratan et al. described focus, complexity, novelty, feasibility, and measurability for evaluating the effectiveness of research questions. 14
The PICOT and PEO frameworks are also used when developing research questions. 1 The following elements are addressed in these frameworks, PICOT: P-population/patients/problem, I-intervention or indicator being studied, C-comparison group, O-outcome of interest, and T-timeframe of the study; PEO: P-population being studied, E-exposure to preexisting conditions, and O-outcome of interest. 1 Research questions are also considered good if these meet the “FINERMAPS” framework: Feasible, Interesting, Novel, Ethical, Relevant, Manageable, Appropriate, Potential value/publishable, and Systematic. 14
As we indicated earlier, research questions and hypotheses that are not carefully formulated result in unethical studies or poor outcomes. To illustrate this, we provide some examples of ambiguous research question and hypotheses that result in unclear and weak research objectives in quantitative research ( Table 6 ) 16 and qualitative research ( Table 7 ) 17 , and how to transform these ambiguous research question(s) and hypothesis(es) into clear and good statements.
a These statements were composed for comparison and illustrative purposes only.
b These statements are direct quotes from Higashihara and Horiuchi. 16
a This statement is a direct quote from Shimoda et al. 17
The other statements were composed for comparison and illustrative purposes only.
CONSTRUCTING RESEARCH QUESTIONS AND HYPOTHESES
To construct effective research questions and hypotheses, it is very important to 1) clarify the background and 2) identify the research problem at the outset of the research, within a specific timeframe. 9 Then, 3) review or conduct preliminary research to collect all available knowledge about the possible research questions by studying theories and previous studies. 18 Afterwards, 4) construct research questions to investigate the research problem. Identify variables to be accessed from the research questions 4 and make operational definitions of constructs from the research problem and questions. Thereafter, 5) construct specific deductive or inductive predictions in the form of hypotheses. 4 Finally, 6) state the study aims . This general flow for constructing effective research questions and hypotheses prior to conducting research is shown in Fig. 1 .

Research questions are used more frequently in qualitative research than objectives or hypotheses. 3 These questions seek to discover, understand, explore or describe experiences by asking “What” or “How.” The questions are open-ended to elicit a description rather than to relate variables or compare groups. The questions are continually reviewed, reformulated, and changed during the qualitative study. 3 Research questions are also used more frequently in survey projects than hypotheses in experiments in quantitative research to compare variables and their relationships.
Hypotheses are constructed based on the variables identified and as an if-then statement, following the template, ‘If a specific action is taken, then a certain outcome is expected.’ At this stage, some ideas regarding expectations from the research to be conducted must be drawn. 18 Then, the variables to be manipulated (independent) and influenced (dependent) are defined. 4 Thereafter, the hypothesis is stated and refined, and reproducible data tailored to the hypothesis are identified, collected, and analyzed. 4 The hypotheses must be testable and specific, 18 and should describe the variables and their relationships, the specific group being studied, and the predicted research outcome. 18 Hypotheses construction involves a testable proposition to be deduced from theory, and independent and dependent variables to be separated and measured separately. 3 Therefore, good hypotheses must be based on good research questions constructed at the start of a study or trial. 12
In summary, research questions are constructed after establishing the background of the study. Hypotheses are then developed based on the research questions. Thus, it is crucial to have excellent research questions to generate superior hypotheses. In turn, these would determine the research objectives and the design of the study, and ultimately, the outcome of the research. 12 Algorithms for building research questions and hypotheses are shown in Fig. 2 for quantitative research and in Fig. 3 for qualitative research.

EXAMPLES OF RESEARCH QUESTIONS FROM PUBLISHED ARTICLES
- EXAMPLE 1. Descriptive research question (quantitative research)
- - Presents research variables to be assessed (distinct phenotypes and subphenotypes)
- “BACKGROUND: Since COVID-19 was identified, its clinical and biological heterogeneity has been recognized. Identifying COVID-19 phenotypes might help guide basic, clinical, and translational research efforts.
- RESEARCH QUESTION: Does the clinical spectrum of patients with COVID-19 contain distinct phenotypes and subphenotypes? ” 19
- EXAMPLE 2. Relationship research question (quantitative research)
- - Shows interactions between dependent variable (static postural control) and independent variable (peripheral visual field loss)
- “Background: Integration of visual, vestibular, and proprioceptive sensations contributes to postural control. People with peripheral visual field loss have serious postural instability. However, the directional specificity of postural stability and sensory reweighting caused by gradual peripheral visual field loss remain unclear.
- Research question: What are the effects of peripheral visual field loss on static postural control ?” 20
- EXAMPLE 3. Comparative research question (quantitative research)
- - Clarifies the difference among groups with an outcome variable (patients enrolled in COMPERA with moderate PH or severe PH in COPD) and another group without the outcome variable (patients with idiopathic pulmonary arterial hypertension (IPAH))
- “BACKGROUND: Pulmonary hypertension (PH) in COPD is a poorly investigated clinical condition.
- RESEARCH QUESTION: Which factors determine the outcome of PH in COPD?
- STUDY DESIGN AND METHODS: We analyzed the characteristics and outcome of patients enrolled in the Comparative, Prospective Registry of Newly Initiated Therapies for Pulmonary Hypertension (COMPERA) with moderate or severe PH in COPD as defined during the 6th PH World Symposium who received medical therapy for PH and compared them with patients with idiopathic pulmonary arterial hypertension (IPAH) .” 21
- EXAMPLE 4. Exploratory research question (qualitative research)
- - Explores areas that have not been fully investigated (perspectives of families and children who receive care in clinic-based child obesity treatment) to have a deeper understanding of the research problem
- “Problem: Interventions for children with obesity lead to only modest improvements in BMI and long-term outcomes, and data are limited on the perspectives of families of children with obesity in clinic-based treatment. This scoping review seeks to answer the question: What is known about the perspectives of families and children who receive care in clinic-based child obesity treatment? This review aims to explore the scope of perspectives reported by families of children with obesity who have received individualized outpatient clinic-based obesity treatment.” 22
- EXAMPLE 5. Relationship research question (quantitative research)
- - Defines interactions between dependent variable (use of ankle strategies) and independent variable (changes in muscle tone)
- “Background: To maintain an upright standing posture against external disturbances, the human body mainly employs two types of postural control strategies: “ankle strategy” and “hip strategy.” While it has been reported that the magnitude of the disturbance alters the use of postural control strategies, it has not been elucidated how the level of muscle tone, one of the crucial parameters of bodily function, determines the use of each strategy. We have previously confirmed using forward dynamics simulations of human musculoskeletal models that an increased muscle tone promotes the use of ankle strategies. The objective of the present study was to experimentally evaluate a hypothesis: an increased muscle tone promotes the use of ankle strategies. Research question: Do changes in the muscle tone affect the use of ankle strategies ?” 23
EXAMPLES OF HYPOTHESES IN PUBLISHED ARTICLES
- EXAMPLE 1. Working hypothesis (quantitative research)
- - A hypothesis that is initially accepted for further research to produce a feasible theory
- “As fever may have benefit in shortening the duration of viral illness, it is plausible to hypothesize that the antipyretic efficacy of ibuprofen may be hindering the benefits of a fever response when taken during the early stages of COVID-19 illness .” 24
- “In conclusion, it is plausible to hypothesize that the antipyretic efficacy of ibuprofen may be hindering the benefits of a fever response . The difference in perceived safety of these agents in COVID-19 illness could be related to the more potent efficacy to reduce fever with ibuprofen compared to acetaminophen. Compelling data on the benefit of fever warrant further research and review to determine when to treat or withhold ibuprofen for early stage fever for COVID-19 and other related viral illnesses .” 24
- EXAMPLE 2. Exploratory hypothesis (qualitative research)
- - Explores particular areas deeper to clarify subjective experience and develop a formal hypothesis potentially testable in a future quantitative approach
- “We hypothesized that when thinking about a past experience of help-seeking, a self distancing prompt would cause increased help-seeking intentions and more favorable help-seeking outcome expectations .” 25
- “Conclusion
- Although a priori hypotheses were not supported, further research is warranted as results indicate the potential for using self-distancing approaches to increasing help-seeking among some people with depressive symptomatology.” 25
- EXAMPLE 3. Hypothesis-generating research to establish a framework for hypothesis testing (qualitative research)
- “We hypothesize that compassionate care is beneficial for patients (better outcomes), healthcare systems and payers (lower costs), and healthcare providers (lower burnout). ” 26
- Compassionomics is the branch of knowledge and scientific study of the effects of compassionate healthcare. Our main hypotheses are that compassionate healthcare is beneficial for (1) patients, by improving clinical outcomes, (2) healthcare systems and payers, by supporting financial sustainability, and (3) HCPs, by lowering burnout and promoting resilience and well-being. The purpose of this paper is to establish a scientific framework for testing the hypotheses above . If these hypotheses are confirmed through rigorous research, compassionomics will belong in the science of evidence-based medicine, with major implications for all healthcare domains.” 26
- EXAMPLE 4. Statistical hypothesis (quantitative research)
- - An assumption is made about the relationship among several population characteristics ( gender differences in sociodemographic and clinical characteristics of adults with ADHD ). Validity is tested by statistical experiment or analysis ( chi-square test, Students t-test, and logistic regression analysis)
- “Our research investigated gender differences in sociodemographic and clinical characteristics of adults with ADHD in a Japanese clinical sample. Due to unique Japanese cultural ideals and expectations of women's behavior that are in opposition to ADHD symptoms, we hypothesized that women with ADHD experience more difficulties and present more dysfunctions than men . We tested the following hypotheses: first, women with ADHD have more comorbidities than men with ADHD; second, women with ADHD experience more social hardships than men, such as having less full-time employment and being more likely to be divorced.” 27
- “Statistical Analysis
- ( text omitted ) Between-gender comparisons were made using the chi-squared test for categorical variables and Students t-test for continuous variables…( text omitted ). A logistic regression analysis was performed for employment status, marital status, and comorbidity to evaluate the independent effects of gender on these dependent variables.” 27
EXAMPLES OF HYPOTHESIS AS WRITTEN IN PUBLISHED ARTICLES IN RELATION TO OTHER PARTS
- EXAMPLE 1. Background, hypotheses, and aims are provided
- “Pregnant women need skilled care during pregnancy and childbirth, but that skilled care is often delayed in some countries …( text omitted ). The focused antenatal care (FANC) model of WHO recommends that nurses provide information or counseling to all pregnant women …( text omitted ). Job aids are visual support materials that provide the right kind of information using graphics and words in a simple and yet effective manner. When nurses are not highly trained or have many work details to attend to, these job aids can serve as a content reminder for the nurses and can be used for educating their patients (Jennings, Yebadokpo, Affo, & Agbogbe, 2010) ( text omitted ). Importantly, additional evidence is needed to confirm how job aids can further improve the quality of ANC counseling by health workers in maternal care …( text omitted )” 28
- “ This has led us to hypothesize that the quality of ANC counseling would be better if supported by job aids. Consequently, a better quality of ANC counseling is expected to produce higher levels of awareness concerning the danger signs of pregnancy and a more favorable impression of the caring behavior of nurses .” 28
- “This study aimed to examine the differences in the responses of pregnant women to a job aid-supported intervention during ANC visit in terms of 1) their understanding of the danger signs of pregnancy and 2) their impression of the caring behaviors of nurses to pregnant women in rural Tanzania.” 28
- EXAMPLE 2. Background, hypotheses, and aims are provided
- “We conducted a two-arm randomized controlled trial (RCT) to evaluate and compare changes in salivary cortisol and oxytocin levels of first-time pregnant women between experimental and control groups. The women in the experimental group touched and held an infant for 30 min (experimental intervention protocol), whereas those in the control group watched a DVD movie of an infant (control intervention protocol). The primary outcome was salivary cortisol level and the secondary outcome was salivary oxytocin level.” 29
- “ We hypothesize that at 30 min after touching and holding an infant, the salivary cortisol level will significantly decrease and the salivary oxytocin level will increase in the experimental group compared with the control group .” 29
- EXAMPLE 3. Background, aim, and hypothesis are provided
- “In countries where the maternal mortality ratio remains high, antenatal education to increase Birth Preparedness and Complication Readiness (BPCR) is considered one of the top priorities [1]. BPCR includes birth plans during the antenatal period, such as the birthplace, birth attendant, transportation, health facility for complications, expenses, and birth materials, as well as family coordination to achieve such birth plans. In Tanzania, although increasing, only about half of all pregnant women attend an antenatal clinic more than four times [4]. Moreover, the information provided during antenatal care (ANC) is insufficient. In the resource-poor settings, antenatal group education is a potential approach because of the limited time for individual counseling at antenatal clinics.” 30
- “This study aimed to evaluate an antenatal group education program among pregnant women and their families with respect to birth-preparedness and maternal and infant outcomes in rural villages of Tanzania.” 30
- “ The study hypothesis was if Tanzanian pregnant women and their families received a family-oriented antenatal group education, they would (1) have a higher level of BPCR, (2) attend antenatal clinic four or more times, (3) give birth in a health facility, (4) have less complications of women at birth, and (5) have less complications and deaths of infants than those who did not receive the education .” 30
Research questions and hypotheses are crucial components to any type of research, whether quantitative or qualitative. These questions should be developed at the very beginning of the study. Excellent research questions lead to superior hypotheses, which, like a compass, set the direction of research, and can often determine the successful conduct of the study. Many research studies have floundered because the development of research questions and subsequent hypotheses was not given the thought and meticulous attention needed. The development of research questions and hypotheses is an iterative process based on extensive knowledge of the literature and insightful grasp of the knowledge gap. Focused, concise, and specific research questions provide a strong foundation for constructing hypotheses which serve as formal predictions about the research outcomes. Research questions and hypotheses are crucial elements of research that should not be overlooked. They should be carefully thought of and constructed when planning research. This avoids unethical studies and poor outcomes by defining well-founded objectives that determine the design, course, and outcome of the study.
Disclosure: The authors have no potential conflicts of interest to disclose.
Author Contributions:
- Conceptualization: Barroga E, Matanguihan GJ.
- Methodology: Barroga E, Matanguihan GJ.
- Writing - original draft: Barroga E, Matanguihan GJ.
- Writing - review & editing: Barroga E, Matanguihan GJ.
- Search Menu
- Advance articles
- Author Interviews
- Research Curations
- Author Guidelines
- Open Access
- Submission Site
- Why Submit?
- About Journal of Consumer Research
- Editorial Board
- Advertising and Corporate Services
- Self-Archiving Policy
- Dispatch Dates
- Journals on Oxford Academic
- Books on Oxford Academic

Article Contents
Measuring knowledge amount, progress, and research trends, future research agenda, data collection statement, author notes.
- < Previous
How Much Have We Learned about Consumer Research? A Meta-Meta-Analysis

- Article contents
- Figures & tables
- Supplementary Data
Martin Eisend, Gratiana Pol, Dominika Niewiadomska, Joseph Riley, Rick Wedgeworth, How Much Have We Learned about Consumer Research? A Meta-Meta-Analysis, Journal of Consumer Research , Volume 51, Issue 1, June 2024, Pages 180–190, https://doi.org/10.1093/jcr/ucad062
- Permissions Icon Permissions
This meta-meta-analysis study quantifies the development of scientific knowledge in consumer research by summarizing the findings of 222 meta-analyses that together include 2481 meta-analytic effect sizes. The results provide an overview of how much we know and how knowledge has developed in consumer research over time. By explaining 7.8% variance ( r = 0.28) in consumer-relevant dependent variables, the findings show that consumer research, a comparatively young discipline, is relatively effective at knowledge development compared to other disciplines. Furthermore, the accumulation of knowledge is significantly increasing, suggesting that our discipline is still in the growing phase of its life cycle and generating continuously improving explanations of consumer-related phenomena. The development of knowledge varies across consumer-relevant dependent variables, with strong explanations for relationships but significantly weaker ones for memory , affect , and attitudes . Moreover, the knowledge synthesized in meta-analyses is fairly—though not fully—representative of the content of primary research on consumers overall. The findings convey a future research agenda by identifying under-researched areas, advising on the selection of dependent variables, providing indicators for the expected contributions of future studies, suggesting implications for career strategies of consumer researchers, and discussing explanations for the observed knowledge growth effects.
The 50-year anniversary of the Journal of Consumer Research ( JCR ) is a sign of a matured field of inquiry that has accumulated substantial knowledge about consumers, as evidenced by the thousands of published consumer research studies. Several scholars have taken stock of the status of the discipline, painting a big picture of what we have learned about consumers, and how impactful existing research has been. Some scholars agree on a successful history of investigation ( Cohen and Wilkie 2022 ). For instance, using text-mining and citation analysis of all articles published in JCR , Wang et al. (2015) show that social identity research has been flourishing and that consumer culture articles are heavily cited. A bibliometric analysis by Baumgartner (2010) identifies influential research articles and reveals that only few shooting stars exist, while many articles have a long and steady or even accelerating impact, providing little evidence of obsolescence of the field. Other scholars paint a more differentiated picture. Simonson et al. (2001) describe a growing emphasis on substantive phenomena, originality, and theory development over practical applications, while also identifying hot and cold topics and a fragmentation into subareas. MacInnis et al. (2020) highlight the relatively narrow impact of the field and the lack of research significance. These exemplars of big-picture approaches to consumer research all focus on what is done and appreciated by scholars . However, they do not address the crucial questions of what and how much is known .
We do not know how much empirical knowledge—in terms of the variance explained in consumer responses—we have accumulated, how this knowledge differs across consumer variables, and how it compares to knowledge accumulation in other fields. Moreover, as knowledge from primary research gets synthesized and further developed via secondary research studies, it is vital to verify whether such studies are representative of the content of primary research on consumers overall, and where disconnects between primary and secondary research may lie. Answering those questions is important, as they allow researchers to objectively assess the maturity level and knowledge growth trajectory of consumer research, benchmark it against that of related fields, and identify promising future research avenues.
The current study answers the questions of: (1) how much we know about consumers (that is, how well we have explained the variance observed in different consumer responses), (2) how this explained variance varies across (a) time and (b) key research constructs (i.e., dependent variables), (3) how this variance compares to that observed in other research fields, and (4) how well the knowledge synthesized in secondary research is aligned with primary research on consumers. We answer the first three questions by quantitatively measuring knowledge using the meta-analytic effect sizes extracted from all 222 meta-analyses published so far in consumer research. We focus on meta-analyses (and their derived product, meta-meta-analyses), as they represent the “highest level of evidence” in empirical research ( Ioannidis 2017 ), whose benefits include: providing robust conclusions about the size of cause–effect relationships ( Chan and Arvey 2012 ), helping to uncovering explanations for inconsistent findings ( Grewal, Puccinelli, and Monroe 2018 ), and potentially contributing toward alleviating the replication crisis in science ( Ones, Viswesvaran, and Schmidt 2017 ; Sharpe and Poets 2020 ). We answer the fourth question by comparing research trends in meta-analyses (i.e., changes in research topic volume before and after 2014, the year associated with JCR ’s 40th anniversary) with recent research trends observed in primary research ( Wang et al. 2015 ).
In recent years, the number of meta-analyses in marketing and consumer research has increased exponentially ( Grewal et al. 2018 )—a phenomenon consistent with the proliferation of meta-analyses in the behavioral and life sciences ( Ioannidis 2017 ). In view of this expanding body of research, the present work complements the burgeoning practice of evaluating knowledge accumulation in a specific behavioral field via a meta-meta-analytical approach that summarizes all meta-analyses conducted in that field ( Nuijten et al. 2020 ; Siegel et al. 2022 ). Our findings contribute to the consumer research field by quantitatively measuring its knowledge development, comparing it to that of neighboring fields, and investigating the alignment in trends between primary and meta-analytical research topics. The findings differ across dependent variables and research topics, which helps to identify under-researched yet promising topics for future research and funding initiatives. The findings also illustrate what effect sizes are considered normal and should be exceeded in future studies, for such studies to provide substantial research contributions. This knowledge in turn helps researchers, reviewers, and readers better evaluate the merits of future research. Ultimately, as effect sizes are linked to scientific contributions and recognition in the academic community, the findings provide insights for successful career strategies of consumer research scholars.
Knowledge Amount and Progress Measurement
Successful scientific knowledge development rests on the explanatory power of research statements (i.e., hypotheses and theories) that link constructs to one another ( Lehmann 1996 ). Explanatory power is empirically assessed via the effect size , which provides evidence for how strongly two variables are related to or depend on each other—in other words, whether and how well a research question has been answered ( Chan and Arvey 2012 ). Effect sizes indicate the value of scientific explanations and the usefulness of scientific hypotheses and theories: the more variance is explained, the more useful and relevant the underlying theory, and the more valuable the knowledge generated by that theory ( Aguinis et al. 2011 ). Effect sizes also have practical relevance in applied behavioral research, as acting on theories supported by small effects produces results that are likely trivial ( Combs 2010 ). The value of scientific knowledge further depends on the generalization potential of the corresponding effect—that is, being able to identify patterns that are likely to recur in future situations ( Lehmann 1996 ). Because a meta-analysis relies on large samples and repeated tests, averages out sampling error deviations from correct values, and corrects mean values for biases caused by measurement error and other artifacts ( Schmidt 1992 ), the meta-analytic effect size serves as a quantifiable and generalizable measure of the merit of scientific explanations and the value of scientific knowledge ( Aguinis et al. 2011 ). Moreover, summarizing all meta-analytical effect sizes across a particular field—via a so-called meta-meta-analysis ( Ioannidis 2017 ; Schmidt and Oh 2013 )—provides a quantitative, big-picture overview of the amount of knowledge accumulation in that field. A meta-meta-analysis is a straightforward generalization of first-order meta-analytic methods which integrates mean effect sizes across multiple meta-analyses, while modeling the between-meta-analysis variance ( Schmidt and Oh 2013 ).
Changes in observed effect sizes over time can be used to assess how scientific knowledge develops ( Eisend 2015 ), whereby positive changes signal increases in the scope, depth, or precision of a scientific paradigm ( Chan and Arvey 2012 ). Based on a discussion of different science philosophers’ views on the trajectory of scientific progress, Eisend (2015) suggests three knowledge development models: continuous growth , where additional empirical research increases the scope of explanations; discontinuous growth , where strong research contributions occur especially in the beginning of a research program or certain topics exhibit patterns of exhaustion over time; and stasis , where researchers do not build on one another’s work, as no research paradigm is widely accepted and the research environment is selecting problems unsystematically. The three models can be identified by effect size variations over time that follow a linear trend or an exponential growth curve (continuous growth model), a quadratic curve (discontinuous growth), or remain unchanged (stasis).
Measuring Alignment between Primary and Meta-Analytical Research Trends
A meta-analysis is typically conducted when a topic reaches maturity (i.e., research findings around that topic reach a volume and level of complexity or dispersion large enough that evidence synthesis is needed to shed light on the true strength or nature of the observed effects, Paul and Barari 2022 ). As such, the volume of meta-analyses on a topic should closely track the research volume for that topic (albeit with a time delay). We examine the extent to which recent research trends observed in primary studies on consumers line up with research trends observed in meta-analytical research, to identify how representative meta-analysis research is of the consumer research field as a whole, and where re-alignment opportunities lie.
Meta-Analysis Dataset
To investigate the amount and progress of knowledge development in consumer research, we perform a meta-meta-analysis that follows the procedure employed in marketing research ( Eisend 2015 ) and related fields ( Richard, Bond, and Stokes-Zoota 2003 ; Siegel et al. 2022 ; Stanley, Carter, and Doucouliagos 2018 ). To locate all meta-analyses published in consumer research until the end of 2021, we performed the following steps: (1) retrieved all relevant meta-analyses from a prior meta-meta-analysis ( Eisend 2015 ); (2) searched all relevant electronic databases (e.g., ABI/INFORM , EBSCO , INFORMS PubsOnLine ) and Google Scholar , using the keywords “metaanaly*,” “meta-analy*,” “quantitative review,” “synthesis,” and “generalization,” combined with “consumer;” and (3) systematically searched journals that were major outlets of meta-analyses. To be included, a paper had to (1) be a meta-analysis and (2) qualify as a consumer research study. All meta-analytic effect sizes that have as dependent variable a construct that measures a consumer response (i.e., a consumer-related state, evaluation, or behavior) were included. Web appendix A provides full details on the inclusion and exclusion criteria used, including the operationalization of consumer research topic . We chose to focus on dependent variables as the main unit of categorization because dependent variables are considered the central constructs in behavioral research studies ( Larsen et al. 2021 ).
To achieve the broadest generalization, the most highly aggregated meta-analytic effect sizes were selected. If a meta-analysis combined findings from primary studies into several meta-analytic effect sizes rather than a single one, we coded all of those effect sizes. On average, a meta-analysis provides 11.13 effect sizes (median = 6, with 25% of the meta-analyses providing only one effect size). We observed that the number of meta-analytic effect sizes extracted from each meta-analysis is highly correlated with the number of studies included in a meta-analysis, indicating that the number of effect sizes that a meta-analysis contributes is not the effect of randomness or inconsistent dependent variable coding (see web appendix A for further details). We retrieved data from meta-analyses published until 2012 from the dataset reported in Eisend (2015) , which we updated and recoded when necessary. Data from the meta-analyses published after 2012 were coded by a total of 5 coders, who also provided quality checks and oversight. The coding was done using the Cognetto (Hyperthesis) Meta-Extractor ( https://cognetto.ai/meta ), an artificial intelligence (AI)-enabled, interactive data extraction tool for meta-analyses and systematic reviews that allows data coding directly on top of PDF documents, automatically detects and extracts key elements of research papers, organizes the coded data, and links it back to its source location in the text, facilitating fast and accurate data extraction and rapid quality checks.
The correlation coefficient was chosen as the meta-analytic effect size that captures the explained variance in a relationship between two variables. The final dataset includes 2,481 meta-analytic effect sizes extracted from 222 meta-analyses published by the end of 2021 (see web appendix B for the full list). Of the 222 meta-analyses, 93 were included in Eisend (2015) . The meta-analyses include around 14,000 primary studies (62.9 studies per meta-analysis) 1 and more than 100,000 primary effect sizes. Based on the 121 meta-analyses that reported the sample sizes of the included primary studies, we calculated that, on average, a meta-analysis includes data from 58,475 consumers. Assuming roughly the same sample size for the remaining meta-analyses, the full dataset of 222 meta-analyses covers an overall sample size of more than 20 million consumers. Absolute values of the correlation coefficient were coded because we are interested in the size of the effect rather than its direction. 2
Effect Sizes and Dependent Variables
For each effect size, we assigned the dependent variable to a conceptual category. The categories were developed based on a review of the consumer research literature and inspection of the dependent variables in our set of collected meta-analyses. The assignment of each dependent variable to its corresponding category was done via a rule-based computer classification model, which produced a 90.6% agreement rate with a human coder (see table 1 and web appendix C for the categorization details).
META-META-ANALYTIC EFFECT SIZES PER DV CATEGORY AND THE FUNCTIONAL FORMS OF THE RELATIONSHIP BETWEEN EFFECT SIZE AND TIME
Note: All mean r s are significant at p < .01.
Effect Sizes and Time
We assume that the time variable explains effect sizes as a function of consumer research progression over time. To this end, we used a variable that reflects the time when the knowledge included in a meta-analysis was generated. This variable was calculated from the average publication year of the studies included in a meta-analysis, where available. On average, the mean is located after 66% of the time has passed since the publication of the oldest study. If we could not retrieve the full list of studies, the mean value was computed based on the time difference between the publication year of the oldest and the most recently published study of the meta-analysis. 3 To explore the best-fitting model(s) of scientific progress, we estimated different functional forms: a linear function (for continuous, linear progress), a logistic or growth function (for continuous, non-linear progress), and a quadratic function (for discontinuous progress, see Eisend 2015 ). A non-significant effect denotes a static progress model. In cases where more than one model was significant, the additional explained variance of each model was tested against the explained variance of the significant linear model as the base model, to determine the model with the highest explanatory power.
Primary and Meta-Analytical Research Trends
To measure trends in primary research on consumers, we used Wang et al.’s (2015) historical analysis of the content of JCR articles. Published on the occasion of JCR ’s 40th anniversary, 4 the analysis employs a Latent Dirichlet Allocation-based topic modeling procedure to group JCR article abstracts into 16 topics investigated in consumer research. Using these 16 topics, along with Wang et al.’s (2015) list of representative terms for each topic, we trained two coders to manually assign the abstracts of the 222 meta-analyses to each topic. Since each abstract can be represented by a mixture of topics ( Wang et al. 2015 ), coders could categorize an abstract to up to three topics (see web appendix H for an overview of the topics and the categorization criteria used). Inter-rater reliability for each topic (Cohen’s kappa) was sufficiently high (0.81), and differences were resolved through discussions. To determine how well trends in meta-analysis research line up with trends in primary research in consumer behavior, our analysis compares, for each topic, the change in meta-analysis research volume before and after 2014 (where 2014 represents the cutoff point for Wang et al.’s [2015] analysis). We then contrast the observed changes in meta-analysis research volume against the changes identified or predicted by Wang et al. (2015) . Importantly, since the overall number of meta-analyses in consumer research has been exponentially increasing over the years, examining research volume in terms of number of published meta-analyses may not reveal true trends, as publication numbers would be trending up across all topics. Hence, a more informative metric for research volume is the proportion of all meta-analysis publications that is represented by each meta-analysis topic. We therefore examine changes in the proportional representation of each topic.
Overall Effect Sizes
The observed mean effect size (i.e., the meta-meta-analytic effect size, corresponding to the correlation coefficient Pearson’s r ) is 0.28. This effect size indicates 7.8% explained variance (0.28 2 ), leaves 92.2% variance unaccounted for, and counts as a medium-sized effect ( Gignac and Szodorai 2016 ).
Effect Size Variations by Dependent Variable Category
The meta-meta-analytic effect sizes differ based on the dependent variable used, as indicated in table 1 . These differences are substantial, with effect sizes ranging from large to medium to small. The effects for relationship strength (16.5% explained variance) and satisfaction and social behaviors (both 13.2%) stand out as being large in size. They are also significantly stronger ( p < .05) than the effects for the remaining dependent variable categories (except for trust , involvement , choice/decision , willingness-to-pay , and attention ; see web appendix D for all statistical difference tests). Small-sized effects include, among others, effects for attitudes and processing . Among those, the weakest effects are for affect and memor y (4.0% and 4.2%). The differences between the largest and smallest effects are notable, as the largest effect (for relationship strength ) explains more than four times the variance explained by the weakest one (for affect ). Also notable is the finding that effect sizes related to attitudes , one of the most frequently used dependent variables in consumer research (second, in our list of effect frequencies, only to cognitions and behaviors ), are substantially lower ( r = 0.22) compared to effect sizes for conceptually related constructs such as choice or satisfaction . Table 1 reports not only the raw mean but also the median, the mean based on multiple effect sizes from a meta-analysis that were averaged before integration, and a multi-level mean value that accounts for dependencies of multiple effects. The analytical approach is described in more detail in web appendix E , alongside further robustness tests. The comparison of the different mean computation procedures shows that for all effect sizes and for all but two dependent variables the deviation from the raw mean r is less than 0.05. These findings suggest that the results are quite robust, even for variables that are based on sparse data (i.e., less than 10 meta-analyses).
Effect Size Variations over Time
Table 1 additionally provides the results of the curve estimation procedure, with time as the independent variable and effect size as the dependent variable (see detailed results in web appendix F ). The overall relationship between time and all effect sizes is significant and described by a linear and positive trend, suggesting a pattern of continuous knowledge growth. Trend variations, nevertheless, occur across dependent variables. We notice a positive linear trend for attitudes , behavior/intentions , and purchase-related behaviors , a positive growth curve for relationship strength and trust , a negative linear trend for cognitions , and a static (non-significant) trend for all remaining dependent variables. 5 Web appendix G depicts the fitted values of the significant relationships ( p < .1).
Web appendix I shows an overview of the distribution and trends of meta-analyses by research topic and indicates how well those trends align with primary research trends. We find that, for meta-analyses published before 2014, the most frequently researched topics include Persuasion (21%), Advertising (17%), Satisfying Customers (16%), Methodological Issues , Buying Process , and Self-Control and Goals (all at 9%). For meta-analyses published in or after 2014, the most frequently researched topics include Satisfying Customers (29%), Advertising (13%), Persuasion (14%), Social Identity and Influence (12%), and Buying Process (10%). When comparing the changes in meta-analysis research volume distribution before and after 2014 (using χ 2 tests) against the trends predicted by Wang et al. (2015) , we observe a substantial overlap between primary and meta-analytical research trends. For example, consistent with Wang et al. (2015) , there is a significant decline in the share of meta-analysis research on Persuasion and Methodological Issues , a significant increase in the share of Emotional Decision-Making research, and a static development for Contextual Effects. Discrepancies also exist. For Advertising , while primary research volume was on a clearly declining trajectory by 2014, the share of meta-analysis research shows no evidence of decline. For Satisfying Customers , despite speculations that primary research volume may have peaked by 2014, the share of meta-analysis research continued increasing, to the point where it represents the most frequently researched topic after 2014 (29%), with a research volume more than twice as large as the next most frequently researched topic ( Advertising , with 13%). Additionally, while Self-Control and Goals and Social Identity and Influence were expected to deliver healthy streams of consumer research past 2014, the share of meta-analysis research for those topics shows a static development.
The findings show how much is known in consumer research, how this knowledge has progressed over time, and how well its synthesis aligns with the content of primary studies. They provide not only a big picture of the development and current status of a dynamic research field but also a research agenda with implications for knowledge progress.
Distinction from Eisend’s (2015) Meta-Meta-Analysis of Marketing Research
Ninety-three out of the 222 meta-analyses in this article were also included in Eisend’s (2015) meta-meta-analysis of marketing research. When compared to Eisend (2015) , the present investigation offers additional contributions in both methodology and findings. First, our results show an overall effect size of 0.28, which is larger than the 0.24 effect size obtained by Eisend (2015) , suggesting that consumer research is better able to explain its phenomena than the broader area of marketing. Second, while Eisend (2015) finds that marketing research up to 2012 displays knowledge growth but at a decreasing rate, the consumer research knowledge growth trajectory until 2021 is linear and steady, indicating that the field has not yet matured as much as marketing, hence still offering plenty of room for new contributions. The reasons for the observed distinction between consumer and marketing research results are both substantial and methodological. Substantially, consumer research benefits from a constant provision of more varied and innovative research topics that are inspired by a strong interdisciplinary approach, by interactions between different research areas and disciplines, and by fewer challenges brought on by increasing specialization ( Eisend 2015 ; MacInnis and Folkes 2010 ). Compared to the broader area of marketing, consumer research gets published in several journal outlets, which—despite some specializations—still seem to reach the whole research community. As for methodological explanations of knowledge progress, it appears that the advancement of rigorous methods has strengthened the effects obtained in consumer research. This finding is in line with the improvements observed in behavioral research over the recent decades due to the implementation of stronger experimental controls ( Cohen and Wilkie 2022 ). Consumer research hence appears more successful at knowledge development than marketing research overall, which suggests that consumer researchers can capitalize on some of the key characteristics of our field to further accelerate knowledge generation. For example, important knowledge contributions may arise from the exploration of interdisciplinary, boundary-breaking consumer research topics ( MacInnis et al. 2020 ), such as timely investigations at the intersection of consumer and computer science—an area currently being transformed by the adoption of generative AI technologies into mass consumption practices. Finally, the present investigation further extends Eisend’s (2015) approach methodologically, as it breaks findings down per dependent variables, thereby shedding light on individual constructs’ contributions to knowledge development in consumer research and illustrating how the careful, strategic selection of study variables can be key to researchers successfully explaining consumer phenomena of interest.
Contributions
First, by explaining 7.8% variance in the dependent variables, the results offer an objective, standardized measure of how much we have learned in consumer research. While this variance may initially appear small, it allows us to determine how our field’s knowledge development compares to that of other fields, as shown in table 2 . In other behavioral fields—whether across a broad swath of the behavioral sciences (particularly psychology, 3.6%), in specific sub-disciplines such as memory, intelligence, or individual differences research (from 4% to 6.8%), or in applied fields such as organizational psychology (6.8%)—the explained variance is below the level observed in consumer research. The explained variance differences are small, but given the range from 3.6% to 7.8%, a 1% advantage in consumer research is relevant. This represents an encouraging finding, as it suggests that consumer research, a comparatively young discipline, is relatively effective at explaining its phenomena compared to neighboring disciplines. Consumer research also compares favorably to a more remote field such as medical (clinical) research, where effect sizes range from 0.13 (for dichotomous) to 0.15 (for continuous dependent variables).
COMPARISON OF EFFECT SIZES WITH OTHER DISCIPLINES
Second, the findings show that knowledge accumulation in consumer research has been steadily growing and is improving the explanations of phenomena related to consumers, suggesting that the field has still not reached the peak of its knowledge life cycle. This result is notable, because other behavioral and psychological science research indicates either the absence of a time effect ( Schäfer and Schwarz 2019 ) or a downright decline in effect sizes over time in well-established fields such as intelligence research ( Gong and Jiao 2019 ; Pietschnig et al. 2019 ). This insight, combined with the favorable effect size comparison to other disciplines, paints consumer research as a field characterized by dynamism and relatively powerful findings. The effect size increase in consumer research—especially when compared to marketing—can be explained both substantially and methodologically, as discussed in the previous section comparing this investigation to Eisend (2015) .
Third, knowledge development in consumer research varies across dependent variable categories. The field excels at explaining relationship building and maintaining, which is in line with a shift toward relationships in marketing research ( Palmatier et al. 2006 ). The variation in effect sizes across dependent variables suggests that relationship strength is linked closest to behavior and furthest to processing. Less successful explanations refer to variables such as affect , memory , or attitudes . The finding that effect sizes for choice and satisfaction are larger than those for either attitudes or behavior/intentions (in general) suggests that classic hierarchical models (e.g., awareness to attitude to intention [purchase]) may not operate as strongly as previously thought in our field. The observed small effect sizes could also be due to the heterogeneity of measures used for assessing such constructs. For example, affect , cognitions , and behavior/intentions (general) represent combinations of different individual constructs and measures, which could explain their lower effect size estimates compared to more homogenous or standardized constructs like satisfaction , choice , or willingness-to-pay . Of course, the low effect sizes could indicate that those constructs are more difficult to capture by the current measures and manipulations. Finally, knowledge progress also varies across dependent variable categories: relationship variables show an increase in knowledge accumulation, while cognitions even display a decrease. What stands out are purchase and behavioral intentions : although the effects are only medium-sized, their development over time shows an increase, which could be explained by a stronger focus on measuring and explaining managerially relevant variables.
The insights from this meta-meta-analysis provide several substantive, theoretical, and methodological opportunities for future consumer research (points 1–4) and implications for meta-analysis research (point 5).
Mismatch and Its Implications
Assuming that meta-analytic research provides a representative picture of research in a field, 6 the current findings suggest areas of mismatch between research activities and explanatory power in primary research. The constructs that have been investigated the most (i.e., in the largest number of meta-analyses) as dependent variables (e.g., cognitions , attitudes , purchase ) do not provide high explanatory power, while some with high explanatory power are investigated less frequently (e.g., involvement , choice ). As larger effect sizes promise more reliable and replicable effects, the observed mismatch suggests that methodological shifts such as focusing more on incentivized choice rather than attitudes could be fruitful, provided that the theoretical insights are comparable and useful for the field.
Dependent Variable Selection and Career Considerations
Both small and large effect sizes present opportunities for researchers, particularly junior scholars who are starting out their careers. A large effect size means that an effect can more easily be uncovered or replicated. Hence, when the goal is to determine whether an initial research hypothesis should be rejected or not, researchers may want to supplement the dependent variables most frequently examined in consumer research ( attitudes , purchase intentions ) with variables associated with larger effect sizes ( choice , willingness-to-pay , satisfaction ). Alternatively, if a study calls for the use of a dependent variable associated with small effect sizes (e.g., attitudes ), researchers may want to increase the sample size to ensure that the study is adequately powered. We recognize that the former suggestion is not without controversy, in light of the practice of “fishing” for significant effects being discouraged in scientific research. Yet, our results show that not all dependent variables are created equally, as some are significantly better than others at reflecting the impact of various predictors. Hence, we believe that the strategic inclusion of a battery of different dependent variables in a study is warranted, provided that the study authors report all the dependent variables and the effect size-based rationale for their inclusion in the results section.
At the same time, small effect sizes present an opportunity for further theoretical, methodological, or programmatic advances around a given topic. In the case of post-purchase behaviors , for example, the small effect sizes observed likely signal that the topic has not yet reached maturity and is a promising candidate for further research initiatives and funding programs. Small effect sizes suggest the need to develop more precise and nuanced theories of the consumption phenomenon being studied or to explore alternative or complementary methods for studying a phenomenon, for example, by combining different methods or using more sensitive measures. The field could particularly benefit from using consistent constructs and measures to avoid construct fragmentation (i.e., situations where several conceptualizations coexist with no clear shared understanding of the construct among consumer researchers), since such fragmentation hampers knowledge accumulation (as illustrated by the development of the involvement construct; Bergkvist and Eisend 2021 ; Bosco 2022 ).
The findings also provide insights for career considerations of scholars and their strategies in a competitive academic market, as academic career success strongly depends on the contribution one makes to the field. Given that high effect sizes increase a scientific paper’s likelihood to be published in a leading academic journal and its subsequent citation volume ( Eisend and Tarrahi 2014 ) and hence act as a predictor for both research contribution and recognition by the scientific community, the current findings illustrate how the choice of dependent variables can influence a study’s contribution. The findings reveal varying knowledge life cycles and show which variables and thus topics still promise knowledge growth in the future (e.g., relationship-related variables). Junior researchers can use the present findings as a tool for guiding research topics and study design selections for primary research studies.
Minimum Amount of Knowledge Required for Future Research Contributions
The effect sizes observed for different dependent variables can serve as indicators for the amount of knowledge currently produced that is considered “normal” for primary research. They can provide guidance for reviewers and authors regarding the minimum amount of knowledge a future study should provide or exceed, so as to offer a substantial research contribution. For instance, the effect size of 0.32 for attention could serve as an approximation for the expected contribution of new attention-focused consumer studies. Similarly, knowing that the average effect size for satisfaction is about twice as large as for affect could help ensure a fairer evaluation of a study that may report a significant effect for satisfaction, but not for affect.
Need for Further Probing of the Mechanisms Underlying Effect Size Increases
Our findings require further explanations regarding knowledge development in consumer research. While they point toward higher effect sizes over time, the exact reasons for such increases can benefit from in-depth probing. In general, larger meta-analytical effect sizes can be achieved via (1) increasing the precision of a paradigm (using better construct operationalization and measurement), (2) broadening the scope of a paradigm (by extrapolating to other domains), or (3) building consensus in a field (by creating feedback loops between initial and subsequent results) ( Chan and Arvey 2012 ). Hence, larger effect sizes may imply not only larger amounts of knowledge but also “better” knowledge—that is, more confidence in the documented knowledge. For the increases we observed, a plausible explanation involves methodological advances such as stronger manipulations in experiments, better controls of other variables, or the use of dependent variables that are more sensitive to manipulations (in response to journals’ demand for stronger effects that are linked to small p -values). Effects might increase due to publication bias, though our analysis does not support this explanation. 7
Further research can shed light on the exact factors driving the observed effect size increases. Moreover, the finding that effect sizes have been increasing for attitudes , behavioral intentions , and purchase-related behaviors , as well as for trust and relationship strength , but decreasing for cognitions , can also benefit from further examination. It is particularly noteworthy that cognitions—which cover the largest share (23%) of all effect sizes across consumer meta-analyses—are characterized by both low and decreasing effect sizes. Has our field reached maturity when it comes to explaining what drives consumers’ cognitions? Alternatively, as the number and type of cognitions studied in our field keep increasing, the development and adoption of validated measures for such cognitions may not have kept up. If our community has difficulty reaching measurement consensus for the increasingly large number of consumer cognitions being assessed, this could explain why such cognitions show less knowledge accumulation progress compared to other, less heterogeneous constructs ( Bergkvist and Eisend 2021 ). Future research can explore why the pattern for cognitions deviates from that of other major constructs, and which cognitive responses could benefit the most from additional consensus-building measure development efforts.
Opportunities for Future Meta-Analytical Research
Our findings indicate that the trends observed in meta-analysis research are relatively representative of those observed in primary research, suggesting that evidence synthesis in consumer research follows a fairly balanced and rigorous pattern. Nevertheless, an imbalance exists for certain topics, creating opportunities for further meta-analytical research. Notably, meta-analyses on Satisfying Consumers and Advertising appear over-represented compared to primary research on those topics, suggesting saturation on the meta-analytical front, while research on consumers’ Self-control and Goals and Social Identity and Influence appears under-represented, suggesting promising opportunities for additional meta-analytical work. We would also like to point out the relative scarcity of meta-analytical research that measures willingness-to-pay , which was investigated in a single meta-analysis in our dataset, despite its managerial importance.
In summary, the present investigation paints an optimistic picture of the knowledge we have accumulated in consumer research. At the same time, it identifies important research gaps and helps ensure that the next wave of primary and meta-analytic research is well-equipped to provide significant contributions to the consumer research literature.
For meta-analyses published after 2012, the systematic retrieval round took place between April 2021 and September 2021 and was followed by cross-checks for the meta-analyses retrieved from Eisend (2015) , that is, meta-analyses published by 2012. Another round of retrieval for meta-analyses published until December 2021 was performed in March 2022. The data were collected by the first, third, and fourth authors. The data were coded by the second, third, fourth, and fifth authors with the help of two student assistants, from June 2021 to March 2022 and from July to November 2022. The data were analyzed by the first and second authors from February to April 2022 and from September to November 2022. The data are currently stored at ResearchBox.
Martin Eisend ( [email protected] ) is a professor of marketing at the European University Viadrina, Frankfurt (Oder), Germany, and an adjunct professor of marketing at the Copenhagen Business School, Copenhagen, Denmark.
Gratiana Pol ( [email protected] ) is CEO and Co-Founder of Hyperthesis (Cognetto), Sherman Oaks, CA, USA.
Dominika Niewiadomska ( [email protected] ) is a PhD candidate at the European University Viadrina, Frankfurt (Oder), Germany.
Joseph Riley ( [email protected] ) is a PhD candidate at the European University Viadrina, Frankfurt (Oder), Germany.
Rick Wedgeworth ( [email protected] ) is CTO and Co-Founder of Cognetto, Sherman Oaks, CA, USA.
The authors would like to thank everyone who contributed, directly or indirectly, to the development of the software used for annotating the papers included in this meta-analysis, particularly Abishek Borah, whose help was invaluable in getting the software development funded, Roy Nijhof, Luciano Silvi, and Jude Calvillo. Supplementary materials are included in the web appendix accompanying the online version of this article.
We checked for study overlap (i.e., studies that were included in more than one meta-analysis) in meta-analyses for which we could retrieve the study list. We found that 16% of the meta-analyses include only unique, non-overlapping studies and 50% show an overlap of less than 16%. The relationship between the percentage of overlapping studies and the mean effect size of a meta-analysis is not significant ( r = 0.12, p = .11, n = 188). Because our analysis is largely descriptive, the overlap of studies should cause no problem, as the overlap affects only the standard errors and confidence intervals and causes no biases in averages or mean values ( Stanley et al. 2018 ).
Around 10% of the meta-analytic effect sizes were negative and were converted into positive ones (see web appendix E for the exact figures of negative effect sizes). Similar to Eisend (2015) , we found that attenuation-corrected estimates are larger than unattenuated ones (0.31 vs. 0.28, F (1, 479) = 18.11, p < .001), because the correction factor increases unattenuated effect sizes. The average ratio of unattenuated to attenuated effect sizes is 0.89, which was used to correct the estimates from meta-analyses that provide attenuation-corrected estimates (i.e., the estimates were multiplied by 0.89). We found no difference between unweighted and weighted mean values (0.28 vs. 0.27, F (1, 479) = .31, p = .57) and thus did not correct them.
For 24 meta-analyses, neither the study list nor information on the timeframe could be retrieved, and they were therefore excluded from the analysis of temporal developments.
While extrapolating the results from JCR articles to the entirety of the consumer research field is not without limitations, we nevertheless consider JCR , with its “big tent” approach to research, to be representative enough of consumer research developments and trends ( Inman et al. 2018 ) to warrant such extrapolation.
We find a positive relationship between time and the number of studies in a meta-analysis ( r = 0.26, p < .01), suggesting that the overall positive trend results could reflect learning as expressed by the progressively larger number of studies included in consumer research meta-analyses. Thus, we added the number of studies to the models in table 2 and found it to be a significant predictor in several cases. However, the significant effects of time remain unchanged, supporting the robustness of our results.
We explored the assumption that the distribution of dependent variables examined across meta-analyses is representative of those variables’ distribution across primary research studies. To do so, we first separated meta-analyses into two categories: (1) those that focused on a specific dependent variable (either alongside a specified predictor or alongside all predictors examined in relation to that dependent variable) and (2) those that focused on a specific predictor variable and hence captured all available dependent variables examined in the literature in relation to that predictor. We assume that, when taken as a whole, the latter category (which covers 33% of all meta-analyses) provides a representative coverage of the dependent variables examined across primary research. We then compared the latter category of meta-analyses against the full dataset of meta-analyses and observed that the dependent variables have comparable distributions across the two datasets, particularly when it comes to the most and least frequently researched variables. This implies that the distribution of dependent variables examined across all meta-analyses can be considered largely representative of those variables’ distribution across primary research studies. The supporting data for this analysis are provided in web appendix J .
While we find that, as expected, the percentage of unpublished papers in a meta-analysis is negatively related to the magnitude of the effect sizes in a meta-analysis ( r = −0.15, p < .01), this percentage is not related to the average publication year of studies in a meta-analysis ( r = 0.02, p = .51). When controlling the relationship between effect size and time for the percentage of unpublished studies, the relationship becomes weaker compared to the finding in web appendix I but remains significant ( r = 0.05, p = .04). At the same time, we find a highly encouraging change in the application of publication bias tools in consumer research over time, though the use of such tools is not related to effect sizes: We correlated the year variable with several dummy variables indicating whether publication bias tools were used in meta-analyses and found an increase in the general reporting of a publication bias analysis ( r = 0.41, p < .01), the comparison of effect sizes by publication source ( r = 0.16, p = .03), the use of publication source as a moderator in meta-regression ( r = 0.15, p = .03), trim-and-fill analysis ( r = 0.13, p = .08), reporting of funnel plots ( r = 0.29, p < .01), fail safe N ( r = 0.20, p < .01), and the application of other publication bias analysis methods ( r = 0.20, p < .01), but we did not find any relationship between use of publication bias techniques and meta-analytical results.
Aguinis Herman , Dalton Dan R. , Bosco Frank A. , Pierce Charles A. , Dalton Catherine M. ( 2011 ), “ Meta-Analytic Choices and Judgment Calls: Implications for Theory Building and Testing, Obtained Effect Sizes, and Scholarly Impact ,” Journal of Management , 37 ( 1 ), 5 – 38 .
Google Scholar
Baumgartner Hans ( 2010 ), “ Bibliometric Reflections on the History of Consumer Research ,” Journal of Consumer Psychology , 20 ( 3 ), 233 – 8 .
Bergkvist Lars , Eisend Martin ( 2021 ), “ The Dynamic Nature of Marketing Constructs ,” Journal of the Academy of Marketing Science , 49 ( 3 ), 521 – 41 .
Bosco Frank A. ( 2022 ), “ Accumulating Knowledge in the Organizational Sciences ,” Annual Review of Organizational Psychology and Organizational Behavior , 9 ( 1 ), 441 – 64 .
Chan Meow Lan Evelyn , Arvey Richard D. ( 2012 ), “ Meta-Analysis and the Development of Knowledge ,” Perspectives on Psychological Science , 7 ( 1 ), 79 – 92 .
Cohen Joel B. , Wilkie Willam L. ( 2022 ), “Consumer Psychology: Evolving Goals and Research Orientations,” in APA Handbook of Consumer Psychology , ed. Lynn R. Kahle, Tina M. Lowrey, and Joel Huber, Washington, DC : American Psychological Association, 3-45 .
Google Preview
Combs James G. ( 2010 ), “ Big Samples and Small Effects: Let's Not Trade Relevance and Rigor for Power ,” Academy of Management Journal , 53 ( 1 ), 9 – 13 .
Eisend Martin ( 2015 ), “ Have We Progressed Marketing Knowledge? A Meta-Meta-Analysis of Effect Sizes in Marketing Research ,” Journal of Marketing , 79 ( 3 ), 23 – 40 .
Eisend Martin , Tarrahi Farid ( 2014 ), “ Meta-Analysis Selection Bias in Marketing Research ,” International Journal of Research in Marketing , 31 ( 3 ), 317 – 26 .
Gignac Gilles E. , Szodorai Eva T. ( 2016 ), “ Effect Size Guidelines for Individual Differences Researchers ,” Personality and Individual Differences , 102 , 74 – 8 .
Gong Zhun , Jiao Xinian ( 2019 ), “ Are Effect Sizes in Emotional Intelligence Field Declining? A Meta-Meta Analysis ,” Frontiers in Psychology , 10 , 1655 .
Grewal Dhruv , Puccinelli Nancy , Monroe Kent ( 2018 ), “ Meta-Analysis: Integrating Accumulated Knowledge ,” Journal of the Academy of Marketing Science , 46 ( 1 ), 9 – 30 .
Inman Jeffrey J. , Campbell Margaret C. , Kirmani Amna , Price Linda L. ( 2018 ), “ Our Vision for the Journal of Consumer Research: It’s All about the Consumer ,” Journal of Consumer Research , 44 ( 5 ), 955 – 9 .
IntHout Joanna , Ioannidis John P. A. , Borm George F. , Goeman Jelle J. ( 2015 ), “ Small Studies Are More Heterogeneous Than Large Ones: A Meta-Meta-Analysis ,” Journal of Clinical Epidemiology , 68 ( 8 ), 860 – 9 .
Ioannidis John ( 2017 ), “ Next-Generation Systematic Reviews: Prospective Meta-Analysis, Individual-Level Data, Networks and Umbrella Reviews ,” British Journal of Sports Medicine , 51 ( 20 ), 1456 – 8 .
Larsen Kai R. , Ramsay Lauren J. , Godinho Cristina A. , Gershuny Victoria , Hovorka Dirk S. ( 2021 ), “ IC-Behavior: An Interdisciplinary Taxonomy of Behaviors ,” PloS One , 16 ( 9 ), e0252003 .
Lehmann Donald R. ( 1996 ), “Knowledge Generalization and the Convention of Consumer Research: A Study in Inconsistency,” in Advances in Consumer Research , ed. Corfman K. , Lynch J. , Vol. 23 . Provo, UT : Association for Consumer Research, 1–23 .
MacInnis Deborah J. , Folkes Valerie S. ( 2010 ), “ The Disciplinary Status of Consumer Behavior: A Sociology of Science Perspective on Key Controversies ,” Journal of Consumer Research , 36 ( 6 ), 899 – 914 .
MacInnis Deborah J. , Morwitz Vicki G. , Botti Simona , Hoffman Donna L. , Kozinets Robert V. , Lehmann Donald R. , Lynch John G. Jr. , Pechmann Cornelia ( 2020 ), “ Creating Boundary-Breaking, Marketing-Relevant Consumer Research ,” Journal of Marketing , 84 ( 2 ), 1 – 23 .
Nuijten Michèle B. , van Assen Marcel A. L. M. , Augusteijn Hilde E. M. , Crompvoets Elise A. V. , Wicherts Jelte M. ( 2020 ), “ Effect Sizes, Power, and Biases in Intelligence Research: A Meta-Meta-Analysis ,” Journal of Intelligence , 8 ( 4 ), 36 .
Ones Denis S. , Viswesvaran Chockalingam , Schmidt Frank L. ( 2017 ), “ Realizing the Full Potential of Psychometric Meta-Analysis for a Cumulative Science and Practice of Human Resource Management ,” Human Resource Management Review , 27 ( 1 ), 201 – 15 .
Palmatier Robert W. , Dant Rajiv P. , Grewal Dhruv , Evans Kenneth R. ( 2006 ), “ Factors Influencing the Effectiveness of Relationship Marketing: A Meta-Analysis ,” Journal of Marketing , 70 ( 4 ), 136 – 53 .
Paul Justin , Barari Mojtaba ( 2022 ), “ Meta-Analysis and Traditional Systematic Reviews—What, Why, When, Where, and How? ,” Psychology & Marketing , 39 ( 6 ), 1099 – 115 .
Pietschnig Jacob , Siegel Magdalena , Eder Junia Sophia Nur , Gittler Georg ( 2019 ), “ Effect Declines Are Systematic, Strong, and Ubiquitous: A Meta-Meta-Analysis of the Decline Effect in Intelligence Research ,” Frontiers in Psychology , 10 , 2874 .
Richard F. D. , Bond Charles F. , Stokes-Zoota Juli J. ( 2003 ), “ One Hundred Years of Social Psychology Quantitatively Described ,” Review of General Psychology , 7 ( 4 ), 331 – 63 .
Rubio-Aparicio María , Marín-Martínez Fulgenico , Sánchez-Meca Julio , López-López José A. ( 2018 ), “ A Methodological Review of Meta-Analyses of the Effectiveness of Clinical Psychology Treatments ,” Behavior Research Methods , 50 ( 5 ), 2057 – 73 .
Schäfer Thomas , Schwarz Marcus A. ( 2019 ), “ The Meaningfulness of Effect Sizes in Psychological Research: Differences between Sub-Disciplines and the Impact of Potential Biases ,” Frontiers in Psychology , 10 , 813 .
Schmidt Frank L. ( 1992 ), “ What Do Data Really Mean? ” American Psychologist , 47 ( 10 ), 1173 – 81 .
Schmidt Frank L. , Oh In-Sue ( 2013 ), “ Methods for Second Order Meta-Analysis and Illustrative Applications ,” Organizational Behavior and Human Decision Processes , 121 ( 2 ), 204 – 18 .
Sharpe Donald , Poets Sarena ( 2020 ), “ Meta-Analysis as a Response to the Replication Crisis ,” Canadian Psychology / Psychologie Canadienne , 61 ( 4 ), 377 – 87 .
Siegel Magdalena , Eder Junia Sophia Nur , Wicherts Jelte M. , Pietschnig Jakob ( 2022 ), “ Times Are Changing, Bias Isn't: A Meta-Meta-Analysis on Publication Bias Detection Practices, Prevalence Rates, and Predictors ,” The Journal of Applied Psychology , 107 ( 11 ), 2013 – 39 .
Simonson Itamar , Carmon Ziv , Dhar Ravi , Drolet Aimee , Nowlis Stephen ( 2001 ), “ Consumer Research: Search of Identity ,” Annual Review of Psychology , 52 , 249 – 75 .
Stanley T. D. , Carter Evan C. , Doucouliagos Hristos ( 2018 ), “ What Meta-Analyses Reveal about the Replicability of Psychological Research ,” Psychological Bulletin , 144 ( 12 ), 1325 – 46 .
Wang Xin , Bendle Neil T. , Mai Feng , Cotte June ( 2015 ), “ The Journal of Consumer Research at 40: A Historical Analysis ,” Journal of Consumer Research , 42 ( 1 ), 5 – 18 .
Supplementary data
Email alerts, citing articles via.
- Recommend to your Library
Affiliations
- Online ISSN 1537-5277
- Print ISSN 0093-5301
- Copyright © 2024 Journal of Consumer Research Inc.
- About Oxford Academic
- Publish journals with us
- University press partners
- What we publish
- New features
- Open access
- Institutional account management
- Rights and permissions
- Get help with access
- Accessibility
- Advertising
- Media enquiries
- Oxford University Press
- Oxford Languages
- University of Oxford
Oxford University Press is a department of the University of Oxford. It furthers the University's objective of excellence in research, scholarship, and education by publishing worldwide
- Copyright © 2024 Oxford University Press
- Cookie settings
- Cookie policy
- Privacy policy
- Legal notice
This Feature Is Available To Subscribers Only
Sign In or Create an Account
This PDF is available to Subscribers Only
For full access to this pdf, sign in to an existing account, or purchase an annual subscription.
Our lobby is open 9:00-5:00. We also offer virtual appointments.
Our lobby will be closed all day May 31st.
- Undergraduate Students
- Graduate Students
- Recent Graduates & Alumni
- Staff & Faculty
- Managers of On-Campus Student Employees
- Career Fairs
- Online Resume Review
- Drop In Coaching
- Career Coaching Appointments
- Workshops and Events
- Career Courses
- Connect with Employers
- Connect with Alumni & Mentors
- Free Subscriptions for Huskies
- Private Space for Virtual Interviews
- Husky Career Closet
- Professional Headshots
- Find Purpose
- Build Skills
- Get Experience (internships)
- Build Relationships (networking)
- Tell Your Story (profiles, resumes, cover letters, interviews)
- Find Success (jobs, service programs, grad school)
- Arts / Media / Marketing
- Consulting / Business
- Non-profit / Social Justice / Education
- Law / Government / Policy
- Physical & Life Sciences
- Sustainability / Conservation / Energy
- Tech / Data / Gaming
- First Generation Students
- International Students
- LGBTQ+ Students
- Students of Color
- Transfer Students
- Undocumented/DACA Students
- Student Veterans
- Students with Disabilities
- Featured Jobs & Internships
- Handshake Access Details
- Internship Advice
- On-Campus Employment
- Job Search Tips
- For Employers
- Peace Corps
- Diplomat in Residence
- Baldasty Internship Project
- Get Involved
Shirley Ryan AbilityLab
Research associate ii.
- Share This: Share Research Associate II on Facebook Share Research Associate II on LinkedIn Share Research Associate II on X
Job Description Summary
This position will work with the Rehabilitation Research and Training Center (RRTC) within Home and Community-Based services (HCBS). HCBS supports individuals with disability in living in the community. The mission of this NIDLIRR-funded RRTC is to support the improvement of person-centered community living outcomes for people with disabilities who use these services. We are looking for someone to help guide the development of research documents for the studies related to this grant-funded project. We are specifically looking for someone with training in research methodology and manuscript development. For more information about the projects: https://www.sralab.org/research/labs/CROR/projects/home-and-community-based-services.
The Research Associate II conducts research projects/studies in an SRAlab laboratory that requires deep technical expertise in a relevant discipline such as health services & administration, health policy, public health, disabilities studies or other research in other relevant fields. The Research Associate II will typically work as part of a multidisciplinary team that will require the ability to translate one’s discipline to others who do not possess as deep a knowledge base. The Research Associate II should have an explicit understanding of the research methods and measures relevant to laboratory projects, and be able to develop project deliverables independently.
The Research Associate II will consistently demonstrate support of the Shirley Ryan AbilityLab statement of Vision, Mission and Core Values by striving for excellence, contributing to the team efforts and showing respect and compassion for patients and their families, fellow employees, and all others with whom there is contact at or in the interest of the institute.
The Research Associate II will demonstrate Shirley Ryan AbilityLab Core Attributes: Communication, Accountability, Flexibility/Adaptability, Judgment/Problem Solving, Customer Service and Core Values (Hope, Compassion, Discovery, Collaboration, and Commitment to Excellence) while fulfilling job duties.
The Research Associate II will:
- Plans and designs research studies, and leads implementation of key research activities.
- Performs and interprets complex statistical analysis.
- Prepares technical documents describing results of research, both for scientific audiences and funders
- Prepares non-technical documents describing results of research for lay audiences.
- Provides recommendations for and implements quality control and quality improvement of research activities.
- Develops research proposals as appropriate.
- Performs all other duties that may be assigned in the best interest of the Shirley Ryan AbilityLab.
Reporting Relationships
- Reports directly to the Laboratory Director or Project Manager
Knowledge, Skills & Abilities Required
- Work requires a professional level of knowledge acquired by completing a Ph.D. degree in a relevant discipline, or a combination of Master’s degree and significant work experience. Deep knowledge of research methodology is required.
- A high level of analytical ability is necessary in order to design and implement research and to perform complex statistical analysis. Analytic ability may also include mathematical modeling or simulation.
- Interpersonal skills necessary to write detailed explanatory reports and articles and to verbally communicate results of research at meetings and conferences. Proven ability to lead and motivate direct reports.
- Ability to plan the work of, assign work to and follow-up on the work of a research technician, graduate student or laboratory resident.
- Ability to plan and conduct experiments and analyze resulting data while balancing the needs of the laboratory, the institution and the individual.
- Clinical or applied experience working with people with disabilities is ideal.
Working Conditions
- Normal office environment with little or no exposure to dust or extreme temperature.
The above statements are intended to describe the general nature and level of work being performed by people assigned to this classification. They are not intended to be construed as an exhaustive list of all responsibilities, duties and skills required of personnel so classified.
Connect with us:
Contact us: 9a-5p, M-F | 134 Mary Gates Hall | Seattle, WA 98195 | (206) 543-0535 tel | [email protected]
The Division of Student Life acknowledges the Coast Salish people of this land, the land which touches the shared waters of all tribes and bands within the Suquamish, Tulalip, and Muckleshoot Nations. Student Life is committed to developing and maintaining an inclusive climate that honors the diverse array of students, faculty, and staff. We strive to provide pathways for success and to purposefully confront and dismantle existing physical, social, and psychological barriers for minoritized students and communities. We engage in this work while learning and demonstrating cultural humility.

- Font Size A A A

Analytical Accounts of the Exchange Fund
Press releases.
The Hong Kong Monetary Authority (HKMA) released today (14 May) the key analytical accounts of the Exchange Fund at the end of April 2024.
Foreign assets, representing the external assets of the Exchange Fund, decreased during the month by HK$60.9 billion to HK$3,460.2 billion.
The Monetary Base, comprising Certificates of Indebtedness, Government‑issued currency notes and coins in circulation, the balance of the banking system and Exchange Fund Bills and Notes issued, amounted to HK$1,916.2 billion.
Claims on the private sector in Hong Kong amounted to HK$305.1 billion.
Foreign liabilities amounted to HK$22.3 billion.
The analytical accounts of the Exchange Fund are released in accordance with the International Monetary Fund’s Special Data Dissemination Standard (SDDS) and are referred to as the Analytical Accounts of the Central Bank under SDDS ( Annex ).
Hong Kong Monetary Authority 14 May 2024
****************************************************************
At present, four press releases relating to the Exchange Fund’s data are issued by the HKMA each month. Three of these releases are issued to disseminate monetary data in accordance with the International Monetary Fund’s SDDS. The fourth press release, on the Exchange Fund’s Abridged Balance Sheet and Currency Board Account, is made in accordance with the HKMA’s policy of maintaining a high level of transparency. For the month of May 2024, the scheduled dates for issuing the press releases are as follows:
- 14 May 2024 Tentative issuance schedule for Exchange Fund Bills and Notes
- 14 May 2024 Analytical Accounts of the Exchange Fund
- 14 May 2024 Exchange Fund Bills Tender Results
- 14 May 2024 Tender results of the 1-year HONIA-indexed Floating Rate Notes under the Institutional Bond Issuance Programme
- 14 May 2024 Website and Social Media Page Alert – Fraudulent website and social media page related to DBS Bank (Hong Kong) Limited


IMAGES
VIDEO
COMMENTS
Explanatory Research | Definition, Guide, & Examples. Published on December 3, 2021 by Tegan George and Julia Merkus. Revised on November 20, 2023. Explanatory research is a research method that explores why something occurs when limited information is available. It can help you increase your understanding of a given topic, ascertain how or why a particular phenomenon is occurring, and predict ...
Explanatory research is a technique used to gain a deeper understanding of the underlying reasons for, causes of, and relationships behind a particular phenomenon that has yet to be extensively studied. ... Use appropriate analytical methods, such as statistical analysis or thematic coding, to uncover patterns, relationships, and explanations ...
Explanatory research is a type of research that aims to uncover the underlying causes and relationships between different variables. It seeks to explain why a particular phenomenon occurs and how it relates to other factors. This type of research is typically used to test hypotheses or theories and to establish cause-and-effect relationships.
Explanatory Research: Types, Examples, Pros & Cons. Explanatory research is designed to do exactly what it sounds like: explain, and explore. You ask questions, learn about your target market, and develop hypotheses for testing in your study. This article will take you through some of the types of explanatory research and what they are used for.
Explanatory research is a method developed to investigate a phenomenon that has not been studied or explained properly. Its main intention is to provide details about where to find a small amount of information. With this method, the researcher gets a general idea and uses research as a tool to guide them quicker to the issues that we might ...
Types of Research Designs Compared | Guide & Examples. Published on June 20, 2019 by Shona McCombes.Revised on June 22, 2023. When you start planning a research project, developing research questions and creating a research design, you will have to make various decisions about the type of research you want to do.. There are many ways to categorize different types of research.
Results. Scientific thought is represented in both causal and explanatory theories. Explanatory theories are multi-variable constructs used to make sense of complex events and situations; they include basic operating principles of explanation, most importantly: transferring new meaning to complex and confusing phenomena; separating out individual components of an event or situation; unifying ...
For example, it can look into why the value of the Japanese Yen has decreased. This is so that an analytical study can consider "how" and "why" questions. Another example is that someone might conduct analytical research to identify a study's gap. It presents a fresh perspective on your data.
Explanatory research. The primary purpose of explanatory research is to explain why and how phenomena occur and to predict future occurrences. If the focus lies on cause-effect relationships, this study can explain which causes lead to what effects (Yin, 1994). Our primary interest is the casual analysis of how one (set of) variable affects ...
In fact, descriptive research has many useful applications, and you probably rely on findings from descriptive research without even being aware that that is what you are doing. See Table 3.1 for examples. Explanatory research. The third type of research, explanatory research, seeks to answer "why" questions.
Here are the steps to conduct this type of research along with specific explanatory research examples: 1. Develop a research question by identifying the problem or interest
Explanatory research is a research method used to investigate how or why something occurs when only a small amount of information is available pertaining to that topic. It can help you increase your understanding of a given topic. Frequently asked questions: Methodology What is the difference between quantitative and qualitative observations? ...
Research can also be exploratory, descriptive, or explanatory. The classification of research into one of these categories depends on the purpose of the study. Saunders et al. (2009) explain that the purpose of exploratory research is to find out " what is happening ," " seek new insights ," and " assess phenomena in new light ...
In explanatory research, the occurrence relation causally relates one determinant to the occurrence (of an event or a state) taking into account other relevant characteristics (confounders and modifiers). Conflicting results in explanatory research might be (partially) explained by differences in the "theoretical design" or by a mismatch ...
Assessing rigour and quality in qualitative research is challenging because qualitative methods are epistemologically diverse. 20-22 ... comparative qualitative studies in ways that resemble quantitative efforts to identify explanatory ... This should include well-defined procedures including sampling protocols and analytical plans, and ...
In summary, exploratory research is used to gain a deeper understanding of a research problem, while explanatory research is used to explain the relationship between variables or to test hypotheses. Both types of research are important and complement each other in the research process. Exploratory research is usually the first step in a larger ...
Explanatory Research | Definition, Guide & Examples. Published on 7 May 2022 by Tegan George and Julia Merkus. Revised on 20 January 2023. Explanatory research is a research method that explores why something occurs when limited information is available. It can help you increase your understanding of a given topic, ascertain how or why a particular phenomenon is occurring, and predict future ...
Realist epistemology generally underpins the explanatory case study research. In explanatory case study, ... meaningful social phenomena. As a analytical tool, hermeneutics enables a researcher to understand how any specific social phenomenon can be thought of as an expression of human subjectivity (Baronov, 2012, pp. 112-115).
Descriptive research classifies, describes, compares, and measures data. Meanwhile, analytical research focuses on cause and effect. For example, take numbers on the changing trade deficits between the United States and the rest of the world in 2015-2018. This is descriptive research.
Explanatory, analytical and experimental studies. ... In a quasi-experimental study, the research would use an accepted research tool (i.e. a loneliness survey) to measure feelings of loneliness and isolation among a group of residents and then implement the book reading club for some period of time. After the defined period of time has passed ...
Instead of drawing conclusions from a representative statistical sample towards the population, case study research builds on analytical findings from the observed cases (Dubois and Gadde 2002; Eisenhardt and Graebner 2007). Case studies can be descriptive, exploratory, or explanatory (Dubé and Paré 2003).
INTRODUCTION. Scientific research is usually initiated by posing evidenced-based research questions which are then explicitly restated as hypotheses.1,2 The hypotheses provide directions to guide the study, solutions, explanations, and expected results.3,4 Both research questions and hypotheses are essentially formulated based on conventional theories and real-world processes, which allow the ...
In view of this expanding body of research, the present work complements the burgeoning practice of evaluating knowledge accumulation in a specific behavioral field via a meta-meta-analytical approach that summarizes all meta-analyses conducted in that field (Nuijten et al. 2020; Siegel et al. 2022). Our findings contribute to the consumer ...
Exploratory research is a methodology approach that investigates research questions that have not previously been studied in depth. Exploratory research is often qualitative and primary in nature. However, a study with a large sample conducted in an exploratory manner can be quantitative as well. It is also often referred to as interpretive ...
Described as 'the first of its kind in the UK to be dedicated to the study of the environment', the Centre for Multimodal Correlative Microscopy and Spectroscopy (CoreMiS) opened earlier this year. Based at the UK Centre for Ecology & hydrology (UKCEH), the £750,000 lab facility is set up for the study of nanoparticles and nano-scale chemical reactions and brings together Raman Imaging and ...
The Research Associate II conducts research projects/studies in an SRAlab laboratory that requires deep technical expertise in a relevant discipline such as health services & administration, health policy, public health, disabilities studies or other research in other relevant fields. ... A high level of analytical ability is necessary in order ...
The Hong Kong Monetary Authority (HKMA) released today (14 May) the key analytical accounts of the Exchange Fund at the end of April 2024. Foreign assets, representing the external assets of the Exchange Fund, decreased during the month by HK$60.9 billion to HK$3,460.2 billion.