

Difference between Theoretical and Empirical Research

The difference between theoretical and empirical research is fundamental to scientific, scholarly research, as it separates the development of ideas and models from their testing and validation.
These two approaches are used in many different fields of inquiry, including the natural sciences, social sciences, and humanities, and they serve different purposes and employ different methods.
Table of Contents
What is theoretical research.
Theoretical research involves the development of models, frameworks, and theories based on existing knowledge, logic, and intuition.
It aims to explain and predict phenomena, generate new ideas and insights, and provide a foundation for further research.
Theoretical research often takes place at the conceptual level and is typically based on existing knowledge, data, and assumptions.
What is Empirical Research?
In contrast, empirical research involves collecting and analysing data to test theories and models.
Empirical research is often conducted at the observational or experimental level and is based on direct or indirect observation of the world.
Empirical research involves testing theories and models, establishing cause-and-effect relationships, and refining or rejecting existing knowledge.
Theoretical vs Empirical Research
Theoretical research is often seen as the starting point for empirical research, providing the ideas and models that must be tested and validated.
Theoretical research can be qualitative or quantitative and involve mathematical models, simulations, and other computational methods.
Theoretical research is often conducted in isolation, without reference to primary data or observations.
On the other hand, empirical research is often seen as the final stage in the scientific process, as it provides evidence that supports or refutes theoretical models.
Empirical research can be qualitative or quantitative, involving surveys, experiments, observational studies, and other data collection methods.
Empirical research is often conducted in collaboration with others and is based on systematic data collection, analysis, and interpretation.
It is important to note that theoretical and empirical research are not mutually exclusive and can often complement each other.
For example, empirical data can inform the development of theories and models, and theoretical models can guide the design of empirical studies.
The most valuable research combines theoretical and empirical approaches in many fields, allowing for a comprehensive understanding of the studied phenomena.
EMPIRICAL RESEARCH | ||
---|---|---|
Purpose | To develop ideas and models based on existing knowledge, logic, and intuition | To test and validate theories and models using data and observations |
Method | Based on existing knowledge, data, and assumptions | Based on direct or indirect observation of the world |
Focus | Conceptual level, explaining and predicting phenomena | Observational or experimental level, testing and establishing cause-and-effect relationships |
Approach | Qualitative or quantitative, often mathematical or computational | Qualitative or quantitative, often involving surveys, experiments, or observational studies |
Data Collection | Often conducted in isolation, without reference to data or observations | Often conducted in collaboration with others, based on systematic data collection, analysis, and interpretation |
It is important to note that this table is not meant to be exhaustive or prescriptive but rather to provide a general overview of the main difference between theoretical and empirical research.
The boundaries between these two approaches are not always clear, and in many cases, research may involve a combination of theoretical and empirical methods.
What are the Limitations of Theoretical Research?
Assumptions and simplifications may be made that do not accurately reflect the complexity of real-world phenomena, which is one of its limitations. Theoretical research relies heavily on logic and deductive reasoning, which can sometimes be biased or limited by the researcher’s assumptions and perspectives.
Furthermore, theoretical research may not be directly applicable to real-world situations without empirical validation. Applying theoretical ideas to practical situations is difficult if no empirical evidence supports or refutes them.
Furthermore, theoretical research may be limited by the availability of data and the researcher’s ability to access and interpret it, which can further limit the validity and applicability of theories.
What are the Limitations of Empirical Research?
There are many limitations to empirical research, including the limitations of the data available and the quality of the data that can be collected. Data collection can be limited by the resources available to collect the data, accessibility to populations or individuals of interest, or ethical constraints.
The researchers or participants may also introduce biases into empirical research, resulting in inaccurate or unreliable findings.
Lastly, due to confounding variables or other methodological limitations, empirical research may be limited by the inability to establish causal relationships between variables, even when statistical associations are identified.
What Methods Are Used In Theoretical Research?
In theoretical research, deductive reasoning, logical analysis, and conceptual frameworks generate new ideas and hypotheses. To identify gaps and inconsistencies in the present understanding of a phenomenon, theoretical research may involve analyzing existing literature and theories.
To test hypotheses and generate predictions, mathematical or computational models may also be developed.
Researchers may also use thought experiments or simulations to explore the implications of their ideas and hypotheses without collecting empirical data as part of theoretical research.
Theoretical research seeks to develop a conceptual framework for empirically testing and validating phenomena.
What Methods Are Used In Empirical Research?
Methods used in empirical research depend on the research questions, type of data collected, and study design. Surveys, experiments, observations, case studies, and interviews are common methods used in empirical research.
An empirical study tests hypotheses and generates new knowledge about phenomena by systematically collecting and analyzing data.
These methods may utilize standardized instruments or protocols for data collection consistency and reliability. Statistical analysis, content analysis, or qualitative analysis may be used for the data collection type.
As a result of empirical research, the findings can inform theories, models, and practical applications.
Conclusion: Theoretical vs Empirical Research
In conclusion, theoretical and empirical research are two distinct but interrelated approaches to scientific inquiry, and they serve different purposes and employ different methods.
Theoretical research involves the development of ideas and models, while empirical research involves testing and validating these ideas.
Both approaches are essential to research and can be combined to provide a more complete understanding of the world.
- Dictionary.com. “ Empirical vs Theoretical “.
- PennState University Libraries. “ Empirical Research in the Social Sciences and Education “.
- William M. Landes and Richard A. Posner. “ Legal Precedent: A Theoretical and Empirical Analysis “, The Journal of Law and Economics, 1976.
Read more articles

- MAY 16, 2024
What Is Empirical Research? Definition, Types & Samples in 2024

by Imed Bouchrika, Phd
Co-Founder and Chief Data Scientist
How was the world formed? Are there parallel universes? Why does time move forward but never in reverse? These are longstanding questions that have yet to receive definitive answers up to now.
In research, these are called empirical questions, which ask about how the world is, how the world works, etc. Such questions are addressed by a corresponding type of study—called empirical research or the empirical method—which is concerned with actual events and phenomena.
What is an empirical study? Research is empirical if it seeks to find a general story or explanation, one that applies to various cases and across time. The empirical approach functions to create new knowledge about the way the world actually works. This article discusses the empirical research definition, concepts, types, processes, and other important aspects of this method. It also tackles the importance of identifying evidence in research .
I. What is Empirical Research?
A. definitions.
What is empirical evidence? Empirical research is defined as any study whose conclusions are exclusively derived from concrete, verifiable evidence. The term empirical basically means that it is guided by scientific experimentation and/or evidence. Likewise, a study is empirical when it uses real-world evidence in investigating its assertions.
This research type is founded on the view that direct observation of phenomena is a proper way to measure reality and generate truth about the world (Bhattacharya, 2008). And by its name, it is a methodology in research that observes the rules of empiricism and uses quantitative and qualitative methods for gathering evidence.
For instance, a study is being conducted to determine if working from home helps in reducing stress from highly-demanding jobs. An experiment is conducted using two groups of employees, one working at their homes, the other working at the office. Each group was observed. The outcomes derived from this research will provide empirical evidence if working from home does help reduce stress or not. This also applies to entrepreneurs when they use a small business idea generator instead of manual procedures.
It was the ancient Greek medical practitioners who originated the term empirical ( empeirikos which means “experienced") when they began to deviate from the long-observed dogmatic principles to start depending on observed phenomena. Later on, empiricism pertained to a theory of knowledge in philosophy, which follows the belief that knowledge comes from evidence and experience derived particularly using the senses.
What ancient philosophers considered empirical research pertained to the reliance on observable data to design and test theories and reach conclusions. As such, empirical research is used to produce knowledge that is based on experience. At present, the word “empirical" pertains to the gathering of data using evidence that is derived through experience or observation or by using calibrated scientific tools.
Most of today’s outstanding empirical research outputs are published in prestigious journals. These scientific publications are considered high-impact journals because they publish research articles that tend to be the most cited in their fields.
II. Types and Methodologies of Empirical Research
Empirical research is done using either qualitative or quantitative methods.
Qualitative research Qualitative research methods are utilized for gathering non-numerical data. It is used to determine the underlying reasons, views, or meanings from study participants or subjects. Under the qualitative research design, empirical studies had evolved to test the conventional concepts of evidence and truth while still observing the fundamental principles of recognizing the subjects beings studied as empirical (Powner, 2015).
This method can be semi-structured or unstructured. Results from this research type are more descriptive than predictive. It allows the researcher to write a conclusion to support the hypothesis or theory being examined.
Due to realities like time and resources, the sample size of qualitative research is typically small. It is designed to offer in-depth information or more insight regarding the problem. Some of the most popular forms of methods are interviews, experiments, and focus groups.
Quantitative research Quantitative research methods are used for gathering information via numerical data. This type is used to measure behavior, personal views, preferences, and other variables. Quantitative studies are in a more structured format, while the variables used are predetermined.
Data gathered from quantitative studies is analyzed to address the empirical questions. Some of the commonly used quantitative methods are polls, surveys, and longitudinal or cohort studies.
There are situations when using a single research method is not enough to adequately answer the questions being studied. In such cases, a combination of both qualitative and quantitative methods is necessary. Also, papers can also make use of both primary and secondary research methods

III. Qualitative Empirical Research Methods
Some research question examples need to be gathered and analyzed qualitatively or quantitatively, depending on the nature of the study. These not only supply answers to empirical questions but also outline one’s scope of work . Here are the general types of qualitative research methods.
Observational Method
This involves observing and gathering data from study subjects. As a qualitative approach, observation is quite personal and time-intensive. It is often used in ethnographic studies to obtain empirical evidence.
The observational method is a part of the ethnographic research design, e.g., archival research, survey, etc. However, while it is commonly used for qualitative purposes, observation is also utilized for quantitative research, such as when observing measurable variables like weight, age, scale, etc.
One remarkable observational research was conducted by Abbott et al. (2016), a team of physicists from the Advanced Laser Interferometer Gravitational-Wave Observatory who examined the very first direct observation of gravitational waves. According to Google Scholar’s (2019) Metrics ranking, this study is among the most highly cited articles from the world’s most influential journals (Crew, 2019).
This method is exclusively qualitative and is one of the most widely used (Jamshed, 2014). Its popularity is mainly due to its ability to allow researchers to obtain precise, relevant information if the correct questions are asked.
This method is a form of a conversational approach, where in-depth data can be obtained. Interviews are commonly used in the social sciences and humanities, such as for interviewing resource persons.
This method is used to identify extensive information through an in-depth analysis of existing cases. It is typically used to obtain empirical evidence for investigating problems or business studies.
When conducting case studies, the researcher must carefully perform the empirical analysis, ensuring the variables and parameters in the current case are similar to the case being examined. From the findings of a case study, conclusions can be deduced about the topic being investigated.
Case studies are commonly used in studying the experience of organizations, groups of persons, geographic locations, etc.
Textual Analysis
This primarily involves the process of describing, interpreting, and understanding textual content. It typically seeks to connect the text to a broader artistic, cultural, political, or social context (Fairclough, 2003).
A relatively new research method, textual analysis is often used nowadays to elaborate on the trends and patterns of media content, especially social media. Data obtained from this approach are primarily used to determine customer buying habits and preferences for product development, and designing marketing campaigns.
Focus Groups
A focus group is a thoroughly planned discussion guided by a moderator and conducted to derive opinions on a designated topic. Essentially a group interview or collective conversation, this method offers a notably meaningful approach to think through particular issues or concerns (Kamberelis & Dimitriadis, 2011).
This research method is used when a researcher wants to know the answers to “how," “what," and “why" questions. Nowadays, focus groups are among the most widely used methods by consumer product producers for designing and/or improving products that people prefer.
IV. Quantitative Empirical Research Methods
Quantitative methods primarily help researchers to better analyze the gathered evidence. Here are the most common types of quantitative research techniques:
A research hypothesis is commonly tested using an experiment, which involves the creation of a controlled environment where the variables are maneuvered. Aside from determining the cause and effect, this method helps in knowing testing outcomes, such as when altering or removing variables.
Traditionally, experimental, laboratory-based research is used to advance knowledge in the physical and life sciences, including psychology. In recent decades, more and more social scientists are also adopting lab experiments (Falk & Heckman, 2009).
Survey research is designed to generate statistical data about a target audience (Fowler, 2014). Surveys can involve large, medium, or small populations and can either be a one-time event or a continuing process
Governments across the world are among the heavy users of continuing surveys, such as for census of populations or labor force surveys. This is a quantitative method that uses predetermined sets of closed questions that are easy to answer, thus enabling the gathering and analysis of large data sets.
In the past, surveys used to be expensive and time-consuming. But with the advancement in technology, new survey tools like social media and emails have made this research method easier and cheaper.
Causal-Comparative research
This method leverages the strength of comparison. It is primarily utilized to determine the cause and effect relationship among variables (Schenker & Rumrill, 2004).
For instance, a causal-comparative study measured the productivity of employees in an organization that allows remote work setup and compared that to the staff of another organization that does not offer work from home arrangements.
Cross-sectional research
While the observation method considers study subjects at a given point in time, cross-sectional research focuses on the similarity in all variables except the one being studied.
This type does not allow for the determination of cause-effect relationships since subjects are now observed continuously. A cross-sectional study is often followed by longitudinal research to determine the precise causes. It is used mainly by pharmaceutical firms and retailers.
Longitudinal study
A longitudinal method of research is used for understanding the traits or behavior of a subject under observation after repeatedly testing the subject over a certain period of time. Data collected using this method can be qualitative or quantitative in nature.
A commonly-used form of longitudinal research is the cohort study. For instance, in 1951, a cohort study called the British Doctors Study (Doll et al., 2004) was initiated, which compared smokers and non-smokers in the UK. The study continued through 2001. As early as 1956, the study gave undeniable proof of the direct link between smoking and the incidence of lung cancer.
Correlational research
This method is used to determine the relationships and prevalence among variables (Curtis et al., 2016). It commonly employs regression as the statistical treatment for predicting the study’s outcomes, which can only be a negative, neutral, or positive correlation.
A classic example of empirical research with correlational research is when studying if high education helps in obtaining better-paying jobs. If outcomes indicate that higher education does allow individuals to have high-salaried jobs, then it follows that people with less education tend to have lower-paying jobs.

V. Steps for Conducting Empirical Research
Since empirical research is based on observation and capturing experiences, it is important to plan the steps to conduct the experiment and how to analyze it. This will enable the researcher to resolve problems or obstacles, which can occur during the experiment.
Step #1: Establishing the research objective
In this initial step, the researcher must be clear about what he or she precisely wants to do in the study. He or she should likewise frame the problem statement, plans of action, and determine any potential issues with the available resources, schedule, etc. for the research.
Most importantly, the researcher must be able to ascertain whether the study will be more beneficial than the cost it will incur.
Step #2: Reviewing relevant literature and supporting theories
The researcher must determine relevant theories or models to his or her research problem. If there are any such theories or models, they must understand how it can help in supporting the study outcomes.
Relevant literature must also be consulted. The researcher must be able to identify previous studies that examined similar problems or subjects, as well as determine the issues encountered.
Step #3: Framing the hypothesis and measurement
The researcher must frame an initial hypothesis or educated guess that could be the likely outcome. Variables must be established, along with the research context.
Units of measurements should also be defined, including the allowable margin of errors. The researcher must determine if the selected measures will be accepted by other scholars.
Step #4: Defining the research design, methodology, and data collection techniques
Before proceeding with the study, the researcher must establish an appropriate approach for the research. He or she must organize experiments to gather data that will allow him or her to frame the hypothesis.
The researcher should also decide whether he or she will use a nonexperimental or experimental technique to perform the study. Likewise, the type of research design will depend on the type of study being conducted.
Finally, the researcher must determine the parameters that will influence the validity of the research design. Data gathering must be performed by selecting suitable samples based on the research question. After gathering the empirical data, the analysis follows.
Step #5: Conducting data analysis and framing the results
Data analysis is done either quantitatively or qualitatively. Depending on the nature of the study, the researcher must determine which method of data analysis is the appropriate one, or whether a combination of the two is suitable.
The outcomes of this step determine if the hypothesis is supported or rejected. This is why data analysis is considered as one of the most crucial steps in any research undertaking.
Step #6: Making conclusions
A report must be prepared in that it presents the findings and the entire research proceeding. If the researcher intends to disseminate his or her findings to a wider audience, the report will be converted into an article for publication. Aside from including the typical parts from the introduction and literature view, up to the methods, analysis, and conclusions, the researcher should also make recommendations for further research on his or her topic.
To ensure the originality and credibility of the report or research, it is essential to employ a plagiarism checker. By using a reliable plagiarism checker, the researcher can verify the uniqueness of their work and avoid any unintentional instances of plagiarism. This step helps maintain the integrity of the research and ensures that the recommendations for further research are based on the researcher’s own original insights. Incorporating a plagiarism checker into the writing process provides an additional layer of assurance and professionalism, enhancing the impact of the report or article in the academic community. Educators can also check the originality of their students’ research by utilizing a free plagiarism checker for teachers .
VI. Empirical Research Cycle
The empirical research cycle is composed of five phases, with each one considered as important as the next phase (de Groot, 1969). This rigorous and systematic method can consistently capture the process of framing hypotheses on how certain subjects behave or function and then testing them versus empirical data. It is considered to typify the deductive approach to science.
These are the five phases of the empirical research cycle:
1. Observation
During this initial phase, an idea is triggered for presenting a hypothesis. It involves the use of observation to gather empirical data. For example :
Consumers tend to consult first their smartphones before buying something in-store .
2. Induction
Inductive reasoning is then conducted to frame a general conclusion from the data gathered through observation. For example:
As mentioned earlier, most consumers tend to consult first their smartphones before buying something in-store .
A researcher may pose the question, “Does the tendency to use a smartphone indicate that today’s consumers need to be informed before making purchasing decisions?" The researcher can assume that is the case. Nonetheless, since it is still just a supposition, an experiment must be conducted to support or reject this hypothesis.
The researcher decided to conduct an online survey to inquire about the buying habits of a certain sample population of buyers at brick-and-mortar stores. This is to determine whether people always look at their smartphones first before making a purchase.
3. Deduction
This phase enables the researcher to figure out a conclusion out of the experiment. This must be based on rationality and logic in order to arrive at particular, unbiased outcomes. For example:
In the experiment, if a shopper consults first his or her smartphone before buying in-store, then it can be concluded that the shopper needs information to help him or her make informed buying decisions .
This phase involves the researcher going back to the empirical research steps to test the hypothesis. There is now the need to analyze and validate the data using appropriate statistical methods.
If the researcher confirms that in-store shoppers do consult their smartphones for product information before making a purchase, the researcher has found support for the hypothesis. However, it should be noted that this is just support of the hypothesis, not proof of a reality.
5. Evaluation
This phase is often neglected by many but is actually a crucial step to help keep expanding knowledge. During this stage, the researcher presents the gathered data, the supporting contention/s, and conclusions.
The researcher likewise puts forth the limitations of the study and his hypothesis. In addition, the researcher makes recommendations for further studies on the same topic with expanded variables.

VII. Advantages and Disadvantages of Empirical Research
Since the time of the ancient Greeks, empirical research had been providing the world with numerous benefits. The following are a few of them:
- Empirical research is used to validate previous research findings and frameworks.
- It assumes a critical role in enhancing internal validity.
- The degree of control is high, which enables the researcher to manage numerous variables.
- It allows a researcher to comprehend the progressive changes that can occur, and thus enables him to modify an approach when needed.
- Being based on facts and experience makes a research project more authentic and competent.
Disadvantages
Despite the many benefits it brings, empirical research is far from perfect. The following are some of its drawbacks:
- Being evidence-based, data collection is a common problem especially when the research involves different sources and multiple methods.
- It can be time-consuming, especially for longitudinal research.
- Requesting permission to perform certain methods can be difficult, especially when a study involves human subjects.
- Conducting research in multiple locations can be very expensive.
- The propensity of even seasoned researchers to incorrectly interpret the statistical significance. For instance, Amrhein et al. (2019) made an analysis of 791 articles from five journals and found that half incorrectly interpreted that non-significance indicates zero effect.
VIII. Samples of Empirical Research
There are many types of empirical research. And, they can take many formsfrom basic research to action research like community project efforts. Here are some notable empirical research examples:
Professional Research
- Research on Information Technology
- Research on Infectious Diseases
- Research on Occupational Health Psychology
- Research on Infection Control
- Research on Cancer
- Research on Mathematical Science
- Research on Environmental Science
- Research on Genetics
- Research on Climate Change
- Research on Economics
Student Research
- Thesis for B.S. in Computer Science & Engineering
- Thesis for B.S. in Geography
- Thesis for B.S. in Architecture
- Thesis for Master of Science in Electrical Engineering & Computer Science
- Thesis for Master of Science in Artificial Intelligence
- Thesis for Master of Science in Food Science and Nutrition
- Dissertation for Ph.D. in Marketing
- Dissertation for Ph.D. in Social Work
- Dissertation for Ph.D. in Urban Planning
Since ancient times until today, empirical research remains one of the most useful tools in man’s collective endeavor to unlock life’s mysteries. Using meaningful experience and observable evidence, this type of research will continue helping validate myriad hypotheses, test theoretical models, and advance various fields of specialization.
With new forms of deadly diseases and other problems continuing to plague man’s existence, finding effective medical interventions and relevant solutions had never been more important. This is among the reasons why empirical research had assumed a more prominent role in today’s society.
This article was able to discuss the different empirical research methods, the steps for conducting empirical research, the empirical research cycle, and notable examples. All of these contribute to supporting the larger societal cause to help understand how the world really works and make it a better place. Furthermore, being factually accurate is a big part of the criteria of good research , and it serves as the heart of empirical research.
Key Insights
- Definition of Empirical Research: Empirical research is based on verifiable evidence derived from observation and experimentation, aiming to understand how the world works.
- Origins: The concept of empirical research dates back to ancient Greek medical practitioners who relied on observed phenomena rather than dogmatic principles.
- Types and Methods: Empirical research can be qualitative (e.g., interviews, case studies) or quantitative (e.g., surveys, experiments), depending on the nature of the data collected and the research question.
- Empirical Research Cycle: Consists of observation, induction, deduction, testing, and evaluation, forming a systematic approach to generating and testing hypotheses.
- Steps in Conducting Empirical Research: Includes establishing objectives, reviewing literature, framing hypotheses, designing methodology, collecting data, analyzing data, and making conclusions.
- Advantages: Empirical research validates previous findings, enhances internal validity, allows for high control over variables, and is fact-based, making it authentic and competent.
- Disadvantages: Data collection can be challenging and time-consuming, especially in longitudinal studies, and interpreting statistical significance can be problematic.
- Applications: Used across various fields such as IT, infectious diseases, occupational health, environmental science, and economics. It is also prevalent in student research for theses and dissertations.
- What is the primary goal of empirical research? The primary goal of empirical research is to generate knowledge about how the world works by relying on verifiable evidence obtained through observation and experimentation.
- How does empirical research differ from theoretical research? Empirical research is based on observable and measurable evidence, while theoretical research involves abstract ideas and concepts without necessarily relying on real-world data.
- What are the main types of empirical research methods? The main types of empirical research methods are qualitative (e.g., interviews, case studies, focus groups) and quantitative (e.g., surveys, experiments, cross-sectional studies).
- Why is the empirical research cycle important? The empirical research cycle is important because it provides a structured and systematic approach to generating and testing hypotheses, ensuring that the research is thorough and reliable.
- What are the steps involved in conducting empirical research? The steps involved in conducting empirical research include establishing the research objective, reviewing relevant literature, framing hypotheses, defining research design and methodology, collecting data, analyzing data, and making conclusions.
- What are the advantages of empirical research? The advantages of empirical research include validating previous findings, enhancing internal validity, allowing for high control over variables, and being based on facts and experiences, making the research authentic and competent.
- What are some common challenges in conducting empirical research? Common challenges in conducting empirical research include difficulties in data collection, time-consuming processes, obtaining permissions for certain methods, high costs, and potential misinterpretation of statistical significance.
- In which fields is empirical research commonly used? Empirical research is commonly used in fields such as information technology, infectious diseases, occupational health, environmental science, economics, and various academic disciplines for student theses and dissertations.
- Can empirical research use both qualitative and quantitative methods? Yes, empirical research can use both qualitative and quantitative methods, often combining them to provide a comprehensive understanding of the research problem.
- What role does empirical research play in modern society? Empirical research plays a crucial role in modern society by validating hypotheses, testing theoretical models, and advancing knowledge across various fields, ultimately contributing to solving complex problems and improving the quality of life.
- Abbott, B., Abbott, R., Abbott, T., Abernathy, M., & Acernese, F. (2016). Observation of Gravitational Waves from a Binary Black Hole Merger. Physical Review Letters, 116 (6), 061102. https://doi.org/10.1103/PhysRevLett.116.061102
- Akpinar, E. (2014). Consumer Information Sharing: Understanding Psychological Drivers of Social Transmission . (Unpublished Ph.D. dissertation). Erasmus University Rotterdam, Rotterdam, The Netherlands. http://hdl.handle.net/1765/1
- Altmetric (2020). The 2019 Altmetric top 100. Altmetric .
- Amrhein, V., Greenland, S., & McShane, B. (2019). Scientists rise up against statistical significance. Nature, 567 , 305-307. https://doi.org/10.1038/d41586-019-00857-9
- Amrhein, V., Trafimow, D., & Greenland, S. (2019). Inferential statistics as descriptive statistics: There is no replication crisis if we don’t expect replication. The American Statistician, 73 , 262-270. https://doi.org/10.1080/00031305.2018.1543137
- Arute, F., Arya, K., Babbush, R. et al. (2019). Quantum supremacy using a programmable superconducting processor. Nature, 574 , 505510. https://doi.org/10.1038/s41586-019-1666-5
- Bhattacharya, H. (2008). Empirical Research. In L. M. Given (ed.), The SAGE Encyclopedia of Qualitative Research Methods . Thousand Oaks, CA: Sage, 254-255. https://dx.doi.org/10.4135/9781412963909.n133
- Cohn, A., Maréchal, M., Tannenbaum, D., & Zund, C. (2019). Civic honesty around the globe. Science, 365 (6448), 70-73. https://doi.org/10.1126/science.aau8712
- Corbin, J., & Strauss, A. (2015). Basics of Qualitative Research: Techniques and Procedures for Developing Grounded Theory, 4th ed . Thousand Oaks, CA: Sage. ISBN 978-1-4129-9746-1
- Crew, B. (2019, August 2). Google Scholar reveals its most influential papers for 2019. Nature Index .
- Curtis, E., Comiskey, C., & Dempsey, O. (2016). Importance and use of correlational research. Nurse Researcher, 23 (6), 20-25. https://doi.org/10.7748/nr.2016.e1382
- Dashti, H., Jones, S., Wood, A., Lane, J., & van Hees, V., et al. (2019). Genome-wide association study identifies genetic loci for self-reported habitual sleep duration supported by accelerometer-derived estimates. Nature Communications, 10 (1). https://doi.org/10.1038/s41467-019-08917-4
- de Groot, A.D. (1969). Methodology: foundations of inference and research in the behavioral sciences. In Psychological Studies, 6 . The Hague & Paris: Mouton & Co. Google Books
- Doll, R., Peto, R., Boreham, J., & Isabelle Sutherland, I. (2004). Mortality in relation to smoking: 50 years’ observations on male British doctors. BMJ, 328 (7455), 1519-33. https://doi.org/10.1136/bmj.38142.554479.AE
- Fairclough, N. (2003). Analyzing Discourse: Textual Analysis for Social Research . Abingdon-on-Thames: Routledge. Google Books
- Falk, A., & Heckman, J. (2009). Lab experiments are a major source of knowledge in the social sciences. Science, 326 (5952), pp. 535-538. https://doi.org/10.1126/science.1168244
- Fowler, F.J. (2014). Survey Research Methods, 5th ed . Thousand Oaks, CA: Sage. WorldCat
- Gabriel, A., Manalo, M., Feliciano, R., Garcia, N., Dollete, U., & Paler J. (2018). A Candida parapsilosis inactivation-based UV-C process for calamansi (Citrus microcarpa) juice frink. LWT Food Science and Technology, 90 , 157-163. https://doi.org/10.1016/j.lwt.2017.12.020
- Gallus, S., Bosetti, C., Negri, E., Talamini, R., Montella, M., et al. (2003). Does pizza protect against cancer? International Journal of Cancer, 107 (2), pp. 283-284. https://doi.org/10.1002/ijc.11382
- Ganna, A., Verweij, K., Nivard, M., Maier, R., & Wedow, R. (2019). Large-scale GWAS reveals insights into the genetic architecture of same-sex sexual behavior. Science, 365 (6456). https://doi.org/10.1126/science.aat7693
- Gedik, H., Voss, T., & Voss, A. (2013). Money and Transmission of Bacteria. Antimicrobial Resistance and Infection Control, 2 (2). https://doi.org/10.1186/2047-2994-2-22
- Gonzalez-Morales, M. G., Kernan, M. C., Becker, T. E., & Eisenberger, R. (2018). Defeating abusive supervision: Training supervisors to support subordinates. Journal of Occupational Health Psychology, 23 (2), 151-162. https://dx.doi.org/10.1037/ocp0000061
- Google (2020). The 2019 Google Scholar Metrics Ranking . Google Scholar
- Greenberg, D., Warrier, V., Allison, C., & Baron-Cohen, S. (2018). Testing the Empathizing-Systemising theory of sex differences and the Extreme Male Brain theory of autism in half a million people. PNAS, 115 (48), 12152-12157. https://doi.org/10.1073/pnas.1811032115
- Grullon, D. (2019). Disentangling time constant and time-dependent hidden state in time series with variational Bayesian inference . (Unpublished master’s thesis). Massachusetts Institute of Technology, Cambridge, MA. https://hdl.handle.net/1721.1/124572
- He, K., Zhang, X., Ren, S., & Sun, J. (2016). Deep residual learning for image recognition. The IEEE Conference on Computer Vision and Pattern Recognition (CVPR) , 770-778. https://doi.org/10.1109/CVPR.2016.90
- Hviid, A., Hansen, J., Frisch, M., & Melbye, M. (2019). Measles, mumps, rubella vaccination, and autism: A nationwide cohort study. Annals of Internal Medicine, 170 (8), 513-520. https://doi.org/10.7326/M18-2101
- Jamshed, S. (2014). Qualitative research method-interviewing and observation. Journal of Basic and Clinical Pharmacy, 5 (4), 87-88. https://doi.org/10.4103/0976-0105.141942
- Jamshidnejad, A. (2017). Efficient Predictive Model-Based and Fuzzy Control for Green Urban Mobility . (Unpublished Ph.D. dissertation). Delft University of Technology, Delft, Netherlands. DUT
- Kamberelis, G., & Dimitriadis, G. (2011). Focus groups: Contingent articulations of pedagogy, politics, and inquiry. In N. Denzin & Y. Lincoln (Eds.), The SAGE Handbook of Qualitative Research (pp. 545-562). Thousand Oaks, CA: Sage. ISBN 978-1-4129-7417-2
- Knowles-Smith, A. (2017). Refugees and theatre: an exploration of the basis of self-representation . (Unpublished undergraduate thesis). University College London, London, UK. UCL
- Kulp, S.A., & Strauss, B.H. (2019). New elevation data triple estimates of global vulnerability to sea-level rise and coastal flooding. Nature Communications, 10 (4844), 1-12. https://doi.org/10.1038/s41467-019-12808-z
- LeCun, Y., Bengio, Y. & Hinton, G. (2015). Deep learning. Nature, 521 , 436444. https://doi.org/10.1038/nature14539
- Levitt, H. M., Bamberg, M., Creswell, J. W., Frost, D. M., Josselson, R., & Suarez-Orozco, C. (2018). Journal article reporting standards for qualitative primary, qualitative meta-analytic, and mixed methods research in psychology: The APA Publications and Communications Board task force report. American Psychologist, 73 (1), 26-46. https://doi.org/10.1037/amp0000151
- Long, J., Shelhamer, E., & Darrell, T. (2015). Fully convolutional networks for semantic segmentation. 2015 IEEE Conference on Computer Vision and Pattern Recognition (CVPR) , 3431-3440. https://doi.org/10.1109/CVPR.2015.7298965
- Martindell, N. (2014). DCDN: Distributed content delivery for the modern web . (Unpublished undergraduate thesis). University of Washington, Seattle, WA. CSE-UW
- Mora, T. (2019). Transforming Parking Garages Into Affordable Housing . (Unpublished undergraduate thesis). University of Arkansas-Fayetteville, Fayetteville, AK. UARK
- Ng, M., Fleming, T., Robinson, M., Thomson, B., & Graetz, N. (2014). Global, regional, and national prevalence of overweight and obesity in children and adults during 19802013: a systematic analysis for the Global Burden of Disease Study 2013. The Lancet, 384 (9945), 766-781. https://doi.org/10.1016/S0140-6736(14)60460-8
- Ogden, C., Carroll, M., Kit, B., & Flegal, K. (2014). Prevalence of Childhood and Adult Obesity in the United States, 2011-2012. JAMA, 311 (8), 806-14. https://doi.org/10.1001/jama.2014.732
- Powner, L. (2015). Empirical Research and Writing: A Political Science Student’s Practical Guide . Thousand Oaks, CA: Sage, 1-19. https://dx.doi.org/10.4135/9781483395906
- Ripple, W., Wolf, C., Newsome, T., Barnard, P., & Moomaw, W. (2020). World scientists’ warning of a climate emergency. BioScience, 70 (1), 8-12. https://doi.org/10.1093/biosci/biz088
- Schenker, J., & Rumrill, P. (2004). Causal-comparative research designs. Journal of Vocational Rehabilitation, 21 (3), 117-121.
- Shereen, M., Khan, S., Kazmi, A., Bashir, N., & Siddique, R. (2020). COVID-19 infection: Origin, transmission, and characteristics of human coronaviruses. Journal of Advanced Research, 24 , 91-98. https://doi.org/10.1016/j.jare.2020.03.005
- Sipola, C. (2017). Summarizing electricity usage with a neural network . (Unpublished master’s thesis). University of Edinburgh, Edinburgh, Scotland. Project-Archive
- Szegedy, C., Liu, W., Jia, Y., Sermanet, P., Reed, S., Anguelov, D., Erhan, D., Vanhoucke, V., & Rabinovich, A. (2015). Going deeper with convolutions. 2015 IEEE Conference on Computer Vision and Pattern Recognition (CVPR) , 1-9. https://doi.org/10.1109/CVPR.2015.7298594
- Taylor, S. (2017). Effacing and Obscuring Autonomy: the Effects of Structural Violence on the Transition to Adulthood of Street Involved Youth . (Unpublished Ph.D. dissertation). University of Ottawa, Ottawa, Canada. UOttawa
- Vosoughi, S., Roy, D., & Aral, S. (2018). The spread of true and false news online. Science, 359 (6380), 1146-1151. https://doi.org/10.1126/science.aap9559
Related Articles

How to Write a Thesis Statement for a Research Paper in 2024: Steps and Examples

How to Write a Research Paper Abstract in 2024: Guide With Examples

72 Scholarship Statistics: 2024 Data, Facts & Analysis

What Is a University Dissertation: 2024 Structure, Challenges & Writing Tips

Web-Based Research: Tips For Conducting Academic Research

How to Write Research Methodology in 2024: Overview, Tips, and Techniques

EasyChair : Tutorial of how to request an installation for Conference Management System

Levels of Evidence in Research: Examples, Hierachies & Practice in 2024

How to Write a Research Question in 2024: Types, Steps, and Examples

How to Write a Research Proposal in 2024: Structure, Examples & Common Mistakes

Needs Analysis in 2024: Definition, Importance & Implementation
Importing references from google scholar to bibtex.

How to Become a Mental Health Counselor in Maryland in 2024

How to Become a Mental Health Counselor in Georgia in 2024

How to Become a Mental Health Counselor in Connecticut in 2024

How to Become a Mental Health Counselor in Nevada in 2024

How to Become a Mental Health Counselor in Ohio in 2024

How to Become a Mental Health Counselor in New Hampshire in 2024

How to Become a Mental Health Counselor in Kansas in 2024

How to Become a Mental Health Counselor in New Jersey in 2024

How to Become a Mental Health Counselor in West Virginia in 2024
Recently published articles.

Best Online Nursing Degree Programs for Non-Nurses for 2024

Average DNP Salary by State: How Much Do DNPs Make in 2024?

Best Nursing Certifications to Consider for 2024

5 Shortest EdD Online Degree Fast-Track Programs for 2024

How to Become a Private School Teacher in West Virginia: Requirements & Certification in 2024

Quick Medical Certifications that Pay Well in Healthcare in 2024

English Degree Guide: 2024 Costs, Requirements & Job Opportunities

2024 Most Affordable Finance Degree Programs Ranking in Mississippi

What Can You Do With a Clinical Psychology Degree? 2024 Costs & Job Opportunities

2024 Most Affordable Business Degree Programs Ranking in South Dakota

Best Online Associate Degrees: Guide to Online Programs for 2024

Best Online Colleges that Accept FAFSA for 2024

MBA in Healthcare Management Careers: 2024 Guide to Career Paths, Options & Salary

Most Affordable Online Hospitality Management Degree Programs for 2024

Most Affordable Online Master’s in Counseling Degree Programs for 2024

Best Doctorate Degree in Psychology Programs: 2024 Costs & Job Opportunities

Is a Master of Accounting Worth It? 2024 Costs & Job Opportunities

Best Accounting MBA Programs of 2024

2024 Most Valuable Speech Pathology Degree Programs Ranking in the Midwest

2024 Most Valuable Marketing Degree Programs Ranking in Kentucky

Best Online DNP Programs in 2024
Newsletter & conference alerts.
Research.com uses the information to contact you about our relevant content. For more information, check out our privacy policy .

Thank you for subscribing!
Confirmation email sent. Please click the link in the email to confirm your subscription.

Henry Whittemore Library
Research skills hub.
- Click on a section, choose a topic. On computers, page opens on the right. On mobile, scroll past menu to read.
- Who are Our 'Experts'?
- The Information Timeline
- Peer Review
- The Internet vs. Academic Databases
- What kind of Info is in Databases?
- What's in OUR Library's Collection? This link opens in a new window
- Data vs Information
- Popular vs. Scholarly
- Grey Literature
- Primary, Secondary, Tertiary Sources
- What KIND of Info do You Need?
- Find & Narrow Topics
- Keywords from your Topic
- Concept / Mind Mapping
- Advanced Database Features
- Use Boolean Connectors
- Use Phrases
- Use Database Fields
- Use Truncation
- Use Database Limiters
- Use Synonyms
- Use Wildcards
- Use Database Subjects
- Find Better Keywords DURING Your Search
- Google Advanced Search Tricks
- Search Google Scholar
- Reference Mining
- Citation Tracking
- About Call Numbers
- Periodical's Content Not the Same as the Periodical's Website!
- From a scholarly journal - But what IS it??
- Anatomy of a Scientific Research Article
- Quantitative vs Qualitative Research Articles
- Theoretical vs Empirical Research Articles
Theoretical vs Empirical Articles
- Types of Review Articles
- Identify the Research Study Type
- Spotting Bad Science
- Types of Scientific Evidence
- Found it in a newspaper - What is it??
- More About News Evaluation
- Webste Evaluation
- Fear & Loathing on Social Media
- Pseudoscience on Social Media -the Slippery Slope
- Getting Full Text During Library Site Searches
- Find Free Full Text on the Internet
- Got a Citation? Check the Library for Full Text
- Make an Interlibrary Loan (ILL) Request
- Better Organize What You Found
- Organize with Zotero
- Organize with Google Docs
- Organize using personal accounts in databases
- Just store stuff on your laptop?
- Print / Save as PDF Website Info Without the Ads
- Avoid Plagiarism
- Why SO MANY citation systems?
- Why Citations in the Text?
- Citing vs Attribution
- Paraphrase Correctly
- Create a Bibliography
- Create an Annotated Bibliography
- Our Complete Citation Guide This link opens in a new window
Theoretical Research is a logical exploration of a system of beliefs and assumptions, working with abstract principles related to a field of knowledge.
- Essentially...theorizing
Empirical Research is based on real-life direct or indirect observation and measurement of phenomena by a researcher.
- Basically... Collecting data by Observing or Experimenting

- << Previous: Quantitative vs Qualitative Research Articles
- Next: Types of Review Articles >>
- Last Updated: Sep 12, 2024 1:19 PM
- URL: https://library.framingham.edu/research-skills-hub
Library Socials
Contact us:.
[email protected] Phone: (508) 626-4650 [email protected] Phone: (508) 626-4654
Search this site
- Form Builder
- Survey Maker
- AI Form Generator
- AI Survey Tool
- AI Quiz Maker
- Store Builder
- WordPress Plugin

HubSpot CRM

Google Sheets

Google Analytics

Microsoft Excel

- Popular Forms
- Job Application Form Template
- Rental Application Form Template
- Hotel Accommodation Form Template
- Online Registration Form Template
- Employment Application Form Template
- Application Forms
- Booking Forms
- Consent Forms
- Contact Forms
- Donation Forms
- Customer Satisfaction Surveys
- Employee Satisfaction Surveys
- Evaluation Surveys
- Feedback Surveys
- Market Research Surveys
- Personality Quiz Template
- Geography Quiz Template
- Math Quiz Template
- Science Quiz Template
- Vocabulary Quiz Template
Try without registration Quick Start
Read engaging stories, how-to guides, learn about forms.app features.
Inspirational ready-to-use templates for getting started fast and powerful.
Spot-on guides on how to use forms.app and make the most out of it.

See the technical measures we take and learn how we keep your data safe and secure.
- Integrations
- Help Center
- Sign In Sign Up Free
- What is empirical research: Methods, types & examples
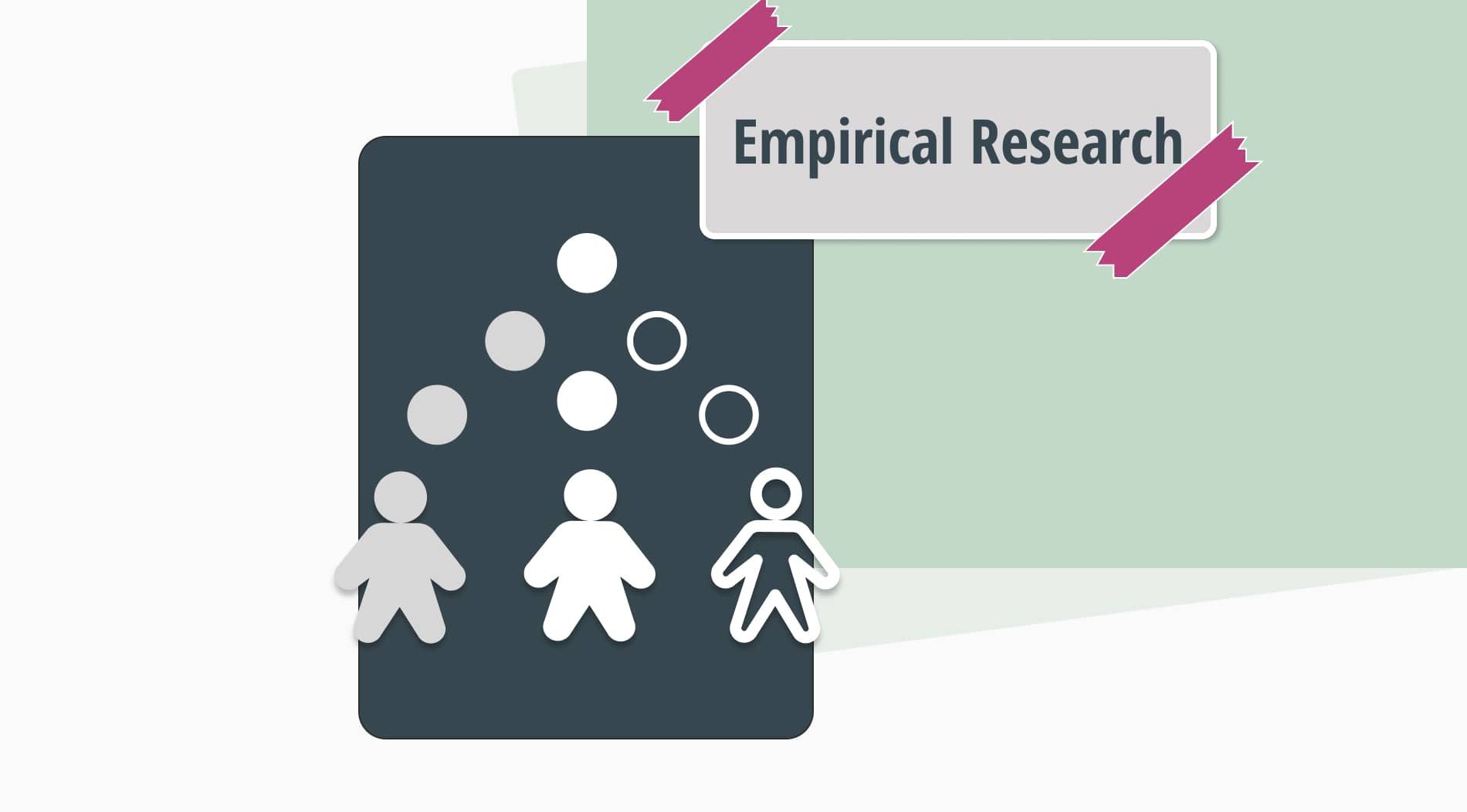
Defne Çobanoğlu
Having opinions on matters based on observation is okay sometimes. Same as having theories on the subject you want to solve. However, some theories need to be tested. Just like Robert Oppenheimer says, “Theory will take you only so far .”
In that case, when you have your research question ready and you want to make sure it is correct, the next step would be experimentation. Because only then you can test your ideas and collect tangible information. Now, let us start with the empirical research definition:
- What is empirical research?
Empirical research is a research type where the aim of the study is based on finding concrete and provable evidence . The researcher using this method to draw conclusions can use both quantitative and qualitative methods. Different than theoretical research, empirical research uses scientific experimentation and investigation.
Using experimentation makes sense when you need to have tangible evidence to act on whatever you are planning to do. As the researcher, you can be a marketer who is planning on creating a new ad for the target audience, or you can be an educator who wants the best for the students. No matter how big or small, data gathered from the real world using this research helps break down the question at hand.
- When to use empirical research?
Empirical research methods are used when the researcher needs to gather data analysis on direct, observable, and measurable data. Research findings are a great way to make grounded ideas. Here are some situations when one may need to do empirical research:
1. When quantitative or qualitative data is needed
There are times when a researcher, marketer, or producer needs to gather data on specific research questions to make an informed decision. And the concrete data gathered in the research process gives a good starting point.
2. When you need to test a hypothesis
When you have a hypothesis on a subject, you can test the hypothesis through observation or experiment. Making a planned study is a great way to collect information and test whether or not your hypothesis is correct.
3. When you want to establish causality
Experimental research is a good way to explore whether or not there is any correlation between two variables. Researchers usually establish causality by changing a variable and observing if the independent variable changes accordingly.
- Types of empirical research
The aim of empirical research is to collect information about a subject from the people by doing experimentation and other data collection methods. However, the methods and data collected are divided into two groups: one collects numerical data, and the other one collects opinion-like data. Let us see the difference between these two types:
Quantitative research
Quantitative research methods are used to collect data in a numerical way. Therefore, the results gathered by these methods will be numbers, statistics, charts, etc. The results can be used to quantify behaviors, opinions, and other variables. Quantitative research methods are surveys, questionnaires, and experimental research.
Qualitiative research
Qualitative research methods are not used to collect numerical answers, instead, they are used to collect the participants’ reasons, opinions, and other meaningful aspects. Qualitative research methods include case studies, observations, interviews, focus groups, and text analysis.
- 5 steps to conduct empirical research
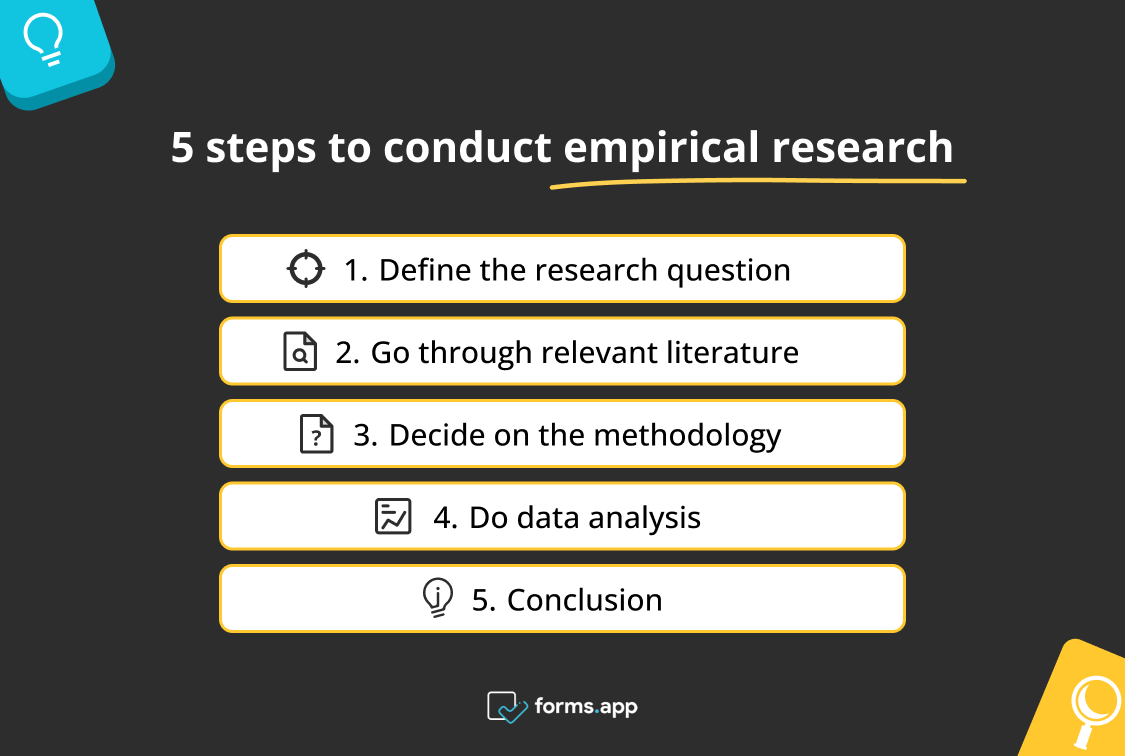
Necessary steps for empirical research
When you want to collect direct and concrete data on a subject, empirical research is a great way to go. And, just like every other project and research, it is best to have a clear structure in mind. This is even more important in studies that may take a long time, such as experiments that take years. Let us look at a clear plan on how to do empirical research:
1. Define the research question
The very first step of every study is to have the question you will explore ready. Because you do not want to change your mind in the middle of the study after investing and spending time on the experimentation.
2. Go through relevant literature
This is the step where you sit down and do a desk research where you gather relevant data and see if other researchers have tried to explore similar research questions. If so, you can see how well they were able to answer the question or what kind of difficulties they faced during the research process.
3. Decide on the methodology
Once you are done going through the relevant literature, you can decide on which method or methods you can use. The appropriate methods are observation, experimentation, surveys, interviews, focus groups, etc.
4. Do data analysis
When you get to this step, it means you have successfully gathered enough data to make a data analysis. Now, all you need to do is look at the data you collected and make an informed analysis.
5. Conclusion
This is the last step, where you are finished with the experimentation and data analysis process. Now, it is time to decide what to do with this information. You can publish a paper and make informed decisions about whatever your goal is.
- Empirical research methodologies
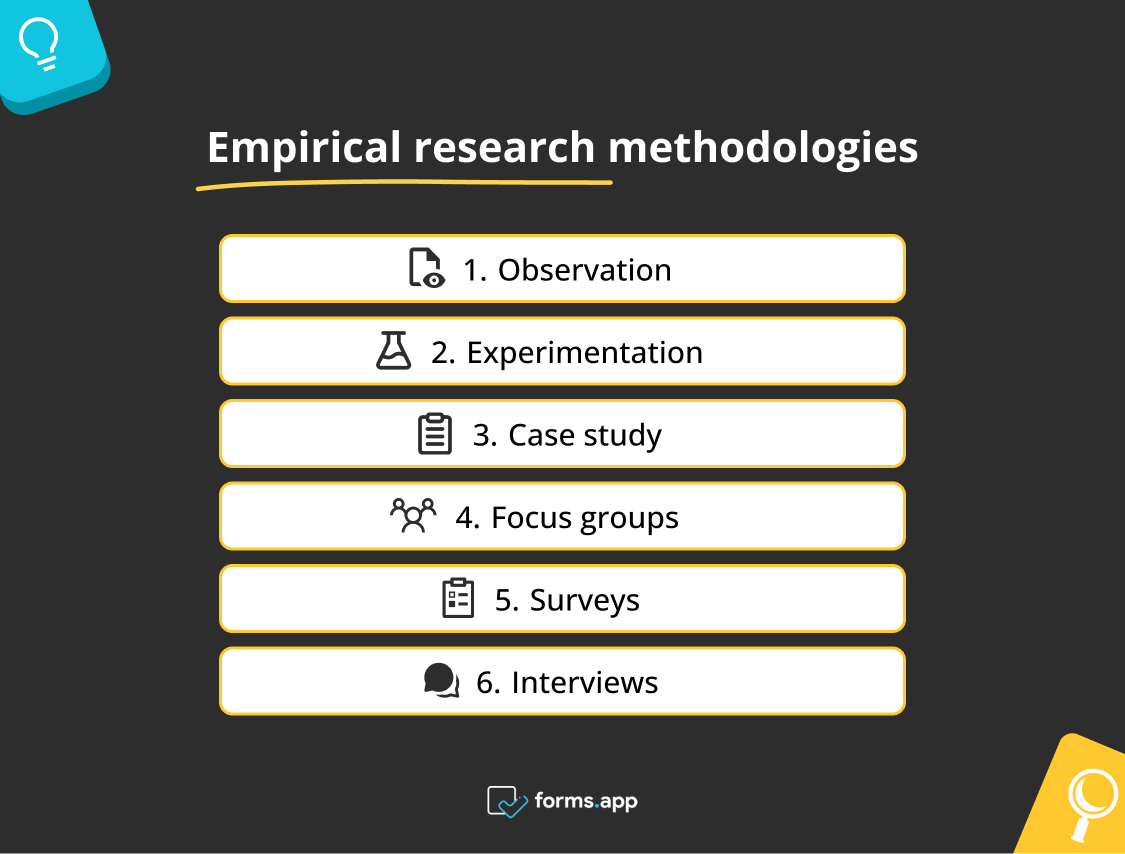
Some essential methodologies to conduct empirical research
The aim of this type of research is to explore brand-new evidence and facts. Therefore, the methods should be primary and gathered in real life, directly from the people. There is more than one method for this goal, and it is up to the researcher to use which one(s). Let us see the methods of empirical research:
- Observation
The method of observation is a great way to collect information on people without the effect of interference. The researcher can choose the appropriate area, time, or situation and observe the people and their interactions with one another. The researcher can be just an outside observer or can be a participant as an observer or a full participant.
- Experimentation
The experimentation process can be done in the real world by intervening in some elements to unify the environment for all participants. This method can also be done in a laboratory environment. The experimentation process is good for being able to change the variables according to the aim of the study.
The case study method is done by making an in-depth analysis of already existing cases. When the parameters and variables are similar to the research question at hand, it is wise to go through what was researched before.
- Focus groups
The case study method is done by using a group of individuals or multiple groups and using their opinions, characteristics, and responses. The scientists gather the data from this group and generalize it to the whole population.
Surveys are an effective way to gather data directly from people. It is a systematic approach to collecting information. If it is done in an online setting as an online survey , it would be even easier to reach out to people and ask their opinions in open-ended or close-ended questions.
Interviews are similar to surveys as you are using questions to collect information and opinions of the people. Unlike a survey, this process is done face-to-face, as a phone call, or as a video call.
- Advantages of empirical research
Empirical research is effective for many reasons, and helps researchers from numerous fields. Here are some advantages of empirical research to have in mind for your next research:
- Empirical research improves the internal validity of the study.
- Empirical evidence gathered from the study is used to authenticate the research question.
- Collecting provable evidence is important for the success of the study.
- The researcher is able to make informed decisions based on the data collected using empirical research.
- Disadvantages of empirical research
After learning about the positive aspects of empirical research, it is time to mention the negative aspects. Because this type may not be suitable for everyone and the researcher should be mindful of the disadvantages of empirical research. Here are the disadvantages of empirical research:
- As it is similar to other research types, a case study where experimentation is included will be time-consuming no matter what. It has more steps and variables than concluding a secondary research.
- There are a lot of variables that need to be controlled and considered. Therefore, it may be a challenging task to be mindful of all the details.
- Doing evidence-based research can be expensive if you need to complete it on a large scale.
- When you are conducting an experiment, you may need some waivers and permissions.
- Frequently asked questions about empirical research
Empirical research is one of the many research types, and there may be some questions in mind about its similarities and differences to other research types.
Is empirical research qualitative or quantitative?
The data collected by empirical research can be qualitative, quantitative, or a mix of both. It is up to the aim of researcher to what kind of data is needed and searched for.
Is empirical research the same as quantitative research?
As quantitative research heavily relies on data collection methods of observation and experimentation, it is, in nature, an empirical study. Some professors may even use the terms interchangeably. However, that does not mean that empirical research is only a quantitative one.
What is the difference between theoretical and empirical research?
Empirical studies are based on data collection to prove theories or answer questions, and it is done by using methods such as observation and experimentation. Therefore, empirical research relies on finding evidence that backs up theories. On the other hand, theoretical research relies on theorizing on empirical research data and trying to make connections and correlations.
What is the difference between conceptual and empirical research?
Conceptual research is about thoughts and ideas and does not involve any kind of experimentation. Empirical research, on the other hand, works with provable data and hard evidence.
What is the difference between empirical vs applied research?
Some scientists may use these two terms interchangeably however, there is a difference between them. Applied research involves applying theories to solve real-life problems. On the other hand, empirical research involves the obtaining and analysis of data to test hypotheses and theories.
- Final words
Empirical research is a good means when the goal of your study is to find concrete data to go with. You may need to do empirical research when you need to test a theory, establish causality, or need qualitative/quantitative data. For example, you are a scientist and want to know if certain colors have an effect on people’s moods, or you are a marketer and want to test your theory on ad places on websites.
In both scenarios, you can collect information by using empirical research methods and make informed decisions afterward. These are just the two of empirical research examples. This research type can be applied to many areas of work life and social sciences. Lastly, for all your research needs, you can visit forms.app to use its many useful features and over 1000 form and survey templates!
Defne is a content writer at forms.app. She is also a translator specializing in literary translation. Defne loves reading, writing, and translating professionally and as a hobby. Her expertise lies in survey research, research methodologies, content writing, and translation.
- Form Features
- Data Collection
Table of Contents
Related posts.
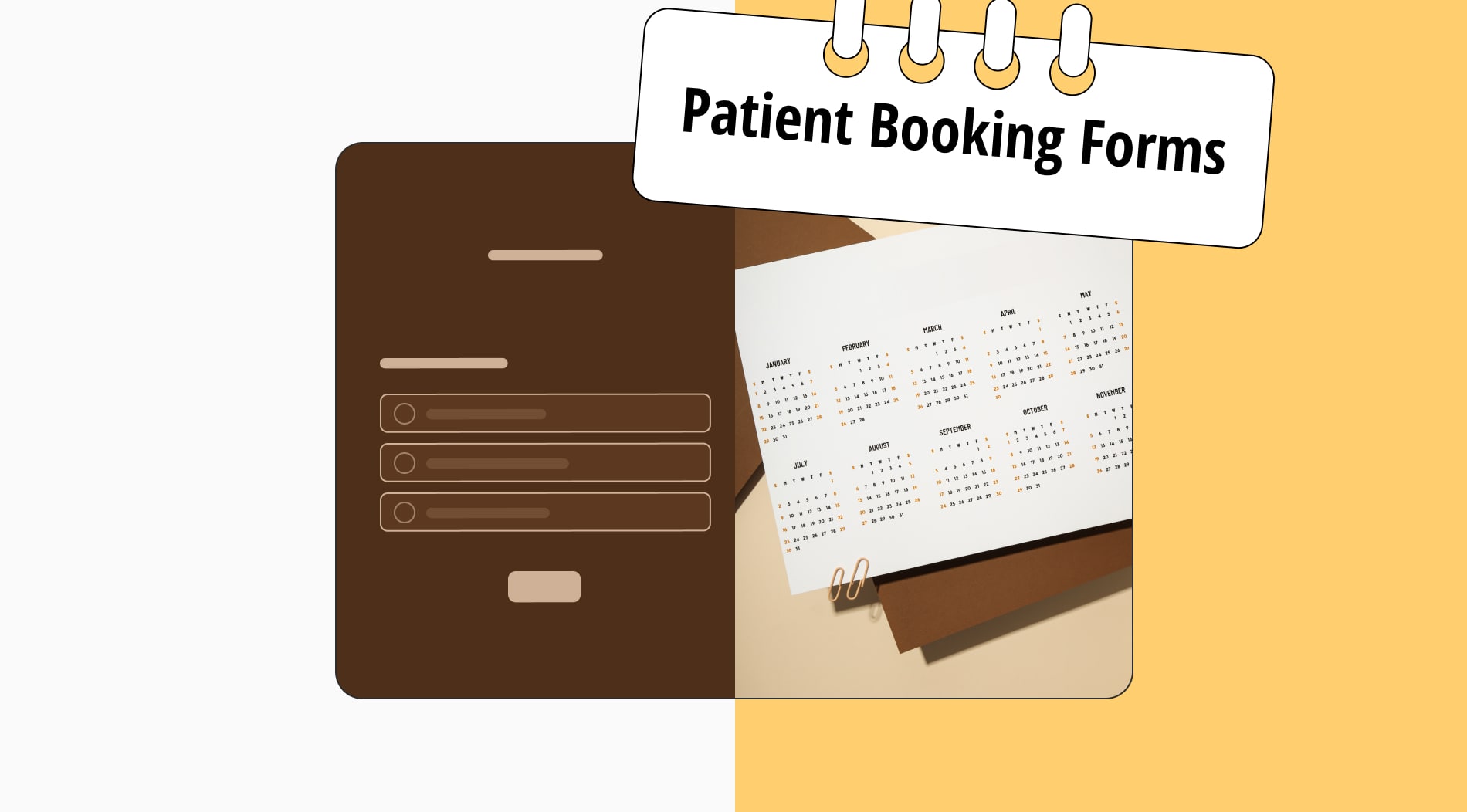
Patient booking forms: Definition, questions and how to create
Yulia Guseva
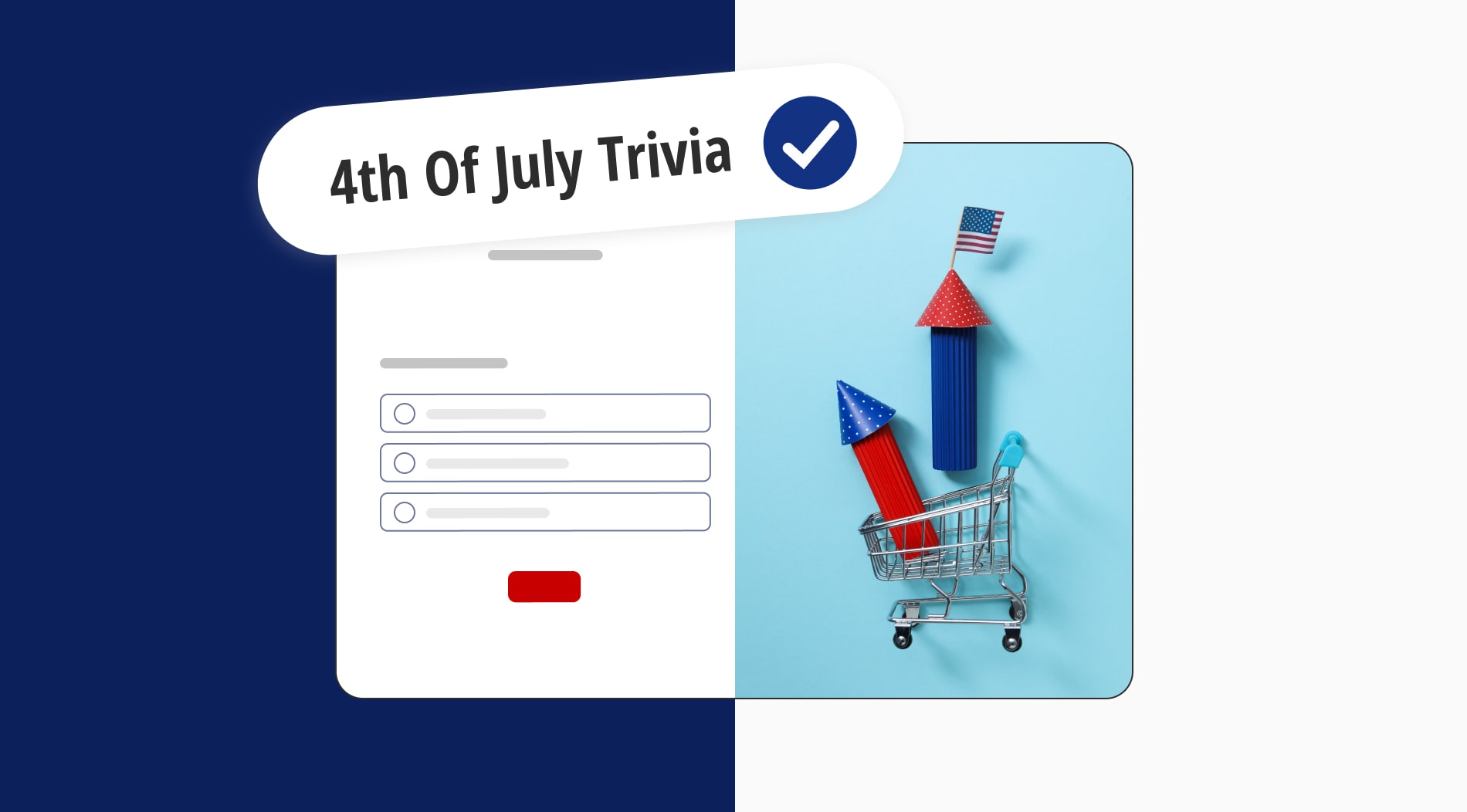
25+ 4th Of July trivia quiz questions & answers
Ayşegül Nacu
.jpg)
What is a lead quiz? (Definition & tips)
Colorado College
Research guides.
Tutt Library Research Guides

- Find Articles
- Search Tips
- Empirical v. Theoretical
- Credibility
- Position Papers
- Curricular Education Databases
- Statistics & Data
- Streaming Films & DVDs
- Print Books
- Professional Development & Careers
- Citation Guide This link opens in a new window
- ED 101 Information
Empirical or Theoretical?
Empirical: Based on data gathered by original experiments or observations.
Theoretical: Analyzes and makes connections between empirical studies to define or advance a theoretical position.
Videos on Finding Empirical Articles
Where Can I Find Empirically-Based Education Articles?

The most direct route is to search PsycInfo, linked above.
This will take you to the Advanced Search, where you can type in your key words at the top. Then scroll down through all the limiting options to the Methodology menu. Select Empirical Study.

In other databases without the Methodology limiter, such as Education Source , try keywords like empirical , study , and research .
How Can I Tell if an Article is Empirical?
Check for these components:
- Peer-reviewed
- Charts, graphs, tables, and/or statistical analyses
- More than 5 pages
- Sections with names like: Abstract, Introduction, Literature Review, Method, Data, Analysis, Results, Discussion, References
Look for visual cues of data collection and analysis:

- << Previous: Search Tips
- Next: Credibility >>
- Last Updated: Mar 27, 2024 10:27 AM
- URL: https://coloradocollege.libguides.com/education
Penn State University Libraries
Empirical research in the social sciences and education.
- What is Empirical Research and How to Read It
- Finding Empirical Research in Library Databases
- Designing Empirical Research
- Ethics, Cultural Responsiveness, and Anti-Racism in Research
- Citing, Writing, and Presenting Your Work
Contact the Librarian at your campus for more help!

Introduction: What is Empirical Research?
Empirical research is based on observed and measured phenomena and derives knowledge from actual experience rather than from theory or belief.
How do you know if a study is empirical? Read the subheadings within the article, book, or report and look for a description of the research "methodology." Ask yourself: Could I recreate this study and test these results?
Key characteristics to look for:
- Specific research questions to be answered
- Definition of the population, behavior, or phenomena being studied
- Description of the process used to study this population or phenomena, including selection criteria, controls, and testing instruments (such as surveys)
Another hint: some scholarly journals use a specific layout, called the "IMRaD" format, to communicate empirical research findings. Such articles typically have 4 components:
- Introduction: sometimes called "literature review" -- what is currently known about the topic -- usually includes a theoretical framework and/or discussion of previous studies
- Methodology: sometimes called "research design" -- how to recreate the study -- usually describes the population, research process, and analytical tools used in the present study
- Results: sometimes called "findings" -- what was learned through the study -- usually appears as statistical data or as substantial quotations from research participants
- Discussion: sometimes called "conclusion" or "implications" -- why the study is important -- usually describes how the research results influence professional practices or future studies
Reading and Evaluating Scholarly Materials
Reading research can be a challenge. However, the tutorials and videos below can help. They explain what scholarly articles look like, how to read them, and how to evaluate them:
- CRAAP Checklist A frequently-used checklist that helps you examine the currency, relevance, authority, accuracy, and purpose of an information source.
- IF I APPLY A newer model of evaluating sources which encourages you to think about your own biases as a reader, as well as concerns about the item you are reading.
- Credo Video: How to Read Scholarly Materials (4 min.)
- Credo Tutorial: How to Read Scholarly Materials
- Credo Tutorial: Evaluating Information
- Credo Video: Evaluating Statistics (4 min.)
- Credo Tutorial: Evaluating for Diverse Points of View
- Next: Finding Empirical Research in Library Databases >>
- Last Updated: Aug 13, 2024 3:16 PM
- URL: https://guides.libraries.psu.edu/emp
What is Empirical Research? Definition, Methods, Examples
Appinio Research · 09.02.2024 · 36min read
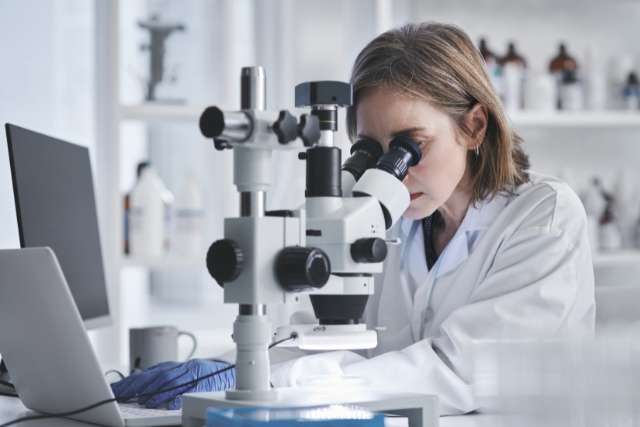
Ever wondered how we gather the facts, unveil hidden truths, and make informed decisions in a world filled with questions? Empirical research holds the key.
In this guide, we'll delve deep into the art and science of empirical research, unraveling its methods, mysteries, and manifold applications. From defining the core principles to mastering data analysis and reporting findings, we're here to equip you with the knowledge and tools to navigate the empirical landscape.
What is Empirical Research?
Empirical research is the cornerstone of scientific inquiry, providing a systematic and structured approach to investigating the world around us. It is the process of gathering and analyzing empirical or observable data to test hypotheses, answer research questions, or gain insights into various phenomena. This form of research relies on evidence derived from direct observation or experimentation, allowing researchers to draw conclusions based on real-world data rather than purely theoretical or speculative reasoning.
Characteristics of Empirical Research
Empirical research is characterized by several key features:
- Observation and Measurement : It involves the systematic observation or measurement of variables, events, or behaviors.
- Data Collection : Researchers collect data through various methods, such as surveys, experiments, observations, or interviews.
- Testable Hypotheses : Empirical research often starts with testable hypotheses that are evaluated using collected data.
- Quantitative or Qualitative Data : Data can be quantitative (numerical) or qualitative (non-numerical), depending on the research design.
- Statistical Analysis : Quantitative data often undergo statistical analysis to determine patterns , relationships, or significance.
- Objectivity and Replicability : Empirical research strives for objectivity, minimizing researcher bias . It should be replicable, allowing other researchers to conduct the same study to verify results.
- Conclusions and Generalizations : Empirical research generates findings based on data and aims to make generalizations about larger populations or phenomena.
Importance of Empirical Research
Empirical research plays a pivotal role in advancing knowledge across various disciplines. Its importance extends to academia, industry, and society as a whole. Here are several reasons why empirical research is essential:
- Evidence-Based Knowledge : Empirical research provides a solid foundation of evidence-based knowledge. It enables us to test hypotheses, confirm or refute theories, and build a robust understanding of the world.
- Scientific Progress : In the scientific community, empirical research fuels progress by expanding the boundaries of existing knowledge. It contributes to the development of theories and the formulation of new research questions.
- Problem Solving : Empirical research is instrumental in addressing real-world problems and challenges. It offers insights and data-driven solutions to complex issues in fields like healthcare, economics, and environmental science.
- Informed Decision-Making : In policymaking, business, and healthcare, empirical research informs decision-makers by providing data-driven insights. It guides strategies, investments, and policies for optimal outcomes.
- Quality Assurance : Empirical research is essential for quality assurance and validation in various industries, including pharmaceuticals, manufacturing, and technology. It ensures that products and processes meet established standards.
- Continuous Improvement : Businesses and organizations use empirical research to evaluate performance, customer satisfaction , and product effectiveness. This data-driven approach fosters continuous improvement and innovation.
- Human Advancement : Empirical research in fields like medicine and psychology contributes to the betterment of human health and well-being. It leads to medical breakthroughs, improved therapies, and enhanced psychological interventions.
- Critical Thinking and Problem Solving : Engaging in empirical research fosters critical thinking skills, problem-solving abilities, and a deep appreciation for evidence-based decision-making.
Empirical research empowers us to explore, understand, and improve the world around us. It forms the bedrock of scientific inquiry and drives progress in countless domains, shaping our understanding of both the natural and social sciences.
How to Conduct Empirical Research?
So, you've decided to dive into the world of empirical research. Let's begin by exploring the crucial steps involved in getting started with your research project.
1. Select a Research Topic
Selecting the right research topic is the cornerstone of a successful empirical study. It's essential to choose a topic that not only piques your interest but also aligns with your research goals and objectives. Here's how to go about it:
- Identify Your Interests : Start by reflecting on your passions and interests. What topics fascinate you the most? Your enthusiasm will be your driving force throughout the research process.
- Brainstorm Ideas : Engage in brainstorming sessions to generate potential research topics. Consider the questions you've always wanted to answer or the issues that intrigue you.
- Relevance and Significance : Assess the relevance and significance of your chosen topic. Does it contribute to existing knowledge? Is it a pressing issue in your field of study or the broader community?
- Feasibility : Evaluate the feasibility of your research topic. Do you have access to the necessary resources, data, and participants (if applicable)?
2. Formulate Research Questions
Once you've narrowed down your research topic, the next step is to formulate clear and precise research questions . These questions will guide your entire research process and shape your study's direction. To create effective research questions:
- Specificity : Ensure that your research questions are specific and focused. Vague or overly broad questions can lead to inconclusive results.
- Relevance : Your research questions should directly relate to your chosen topic. They should address gaps in knowledge or contribute to solving a particular problem.
- Testability : Ensure that your questions are testable through empirical methods. You should be able to gather data and analyze it to answer these questions.
- Avoid Bias : Craft your questions in a way that avoids leading or biased language. Maintain neutrality to uphold the integrity of your research.
3. Review Existing Literature
Before you embark on your empirical research journey, it's essential to immerse yourself in the existing body of literature related to your chosen topic. This step, often referred to as a literature review, serves several purposes:
- Contextualization : Understand the historical context and current state of research in your field. What have previous studies found, and what questions remain unanswered?
- Identifying Gaps : Identify gaps or areas where existing research falls short. These gaps will help you formulate meaningful research questions and hypotheses.
- Theory Development : If your study is theoretical, consider how existing theories apply to your topic. If it's empirical, understand how previous studies have approached data collection and analysis.
- Methodological Insights : Learn from the methodologies employed in previous research. What methods were successful, and what challenges did researchers face?
4. Define Variables
Variables are fundamental components of empirical research. They are the factors or characteristics that can change or be manipulated during your study. Properly defining and categorizing variables is crucial for the clarity and validity of your research. Here's what you need to know:
- Independent Variables : These are the variables that you, as the researcher, manipulate or control. They are the "cause" in cause-and-effect relationships.
- Dependent Variables : Dependent variables are the outcomes or responses that you measure or observe. They are the "effect" influenced by changes in independent variables.
- Operational Definitions : To ensure consistency and clarity, provide operational definitions for your variables. Specify how you will measure or manipulate each variable.
- Control Variables : In some studies, controlling for other variables that may influence your dependent variable is essential. These are known as control variables.
Understanding these foundational aspects of empirical research will set a solid foundation for the rest of your journey. Now that you've grasped the essentials of getting started, let's delve deeper into the intricacies of research design.
Empirical Research Design
Now that you've selected your research topic, formulated research questions, and defined your variables, it's time to delve into the heart of your empirical research journey – research design . This pivotal step determines how you will collect data and what methods you'll employ to answer your research questions. Let's explore the various facets of research design in detail.
Types of Empirical Research
Empirical research can take on several forms, each with its own unique approach and methodologies. Understanding the different types of empirical research will help you choose the most suitable design for your study. Here are some common types:
- Experimental Research : In this type, researchers manipulate one or more independent variables to observe their impact on dependent variables. It's highly controlled and often conducted in a laboratory setting.
- Observational Research : Observational research involves the systematic observation of subjects or phenomena without intervention. Researchers are passive observers, documenting behaviors, events, or patterns.
- Survey Research : Surveys are used to collect data through structured questionnaires or interviews. This method is efficient for gathering information from a large number of participants.
- Case Study Research : Case studies focus on in-depth exploration of one or a few cases. Researchers gather detailed information through various sources such as interviews, documents, and observations.
- Qualitative Research : Qualitative research aims to understand behaviors, experiences, and opinions in depth. It often involves open-ended questions, interviews, and thematic analysis.
- Quantitative Research : Quantitative research collects numerical data and relies on statistical analysis to draw conclusions. It involves structured questionnaires, experiments, and surveys.
Your choice of research type should align with your research questions and objectives. Experimental research, for example, is ideal for testing cause-and-effect relationships, while qualitative research is more suitable for exploring complex phenomena.
Experimental Design
Experimental research is a systematic approach to studying causal relationships. It's characterized by the manipulation of one or more independent variables while controlling for other factors. Here are some key aspects of experimental design:
- Control and Experimental Groups : Participants are randomly assigned to either a control group or an experimental group. The independent variable is manipulated for the experimental group but not for the control group.
- Randomization : Randomization is crucial to eliminate bias in group assignment. It ensures that each participant has an equal chance of being in either group.
- Hypothesis Testing : Experimental research often involves hypothesis testing. Researchers formulate hypotheses about the expected effects of the independent variable and use statistical analysis to test these hypotheses.
Observational Design
Observational research entails careful and systematic observation of subjects or phenomena. It's advantageous when you want to understand natural behaviors or events. Key aspects of observational design include:
- Participant Observation : Researchers immerse themselves in the environment they are studying. They become part of the group being observed, allowing for a deep understanding of behaviors.
- Non-Participant Observation : In non-participant observation, researchers remain separate from the subjects. They observe and document behaviors without direct involvement.
- Data Collection Methods : Observational research can involve various data collection methods, such as field notes, video recordings, photographs, or coding of observed behaviors.
Survey Design
Surveys are a popular choice for collecting data from a large number of participants. Effective survey design is essential to ensure the validity and reliability of your data. Consider the following:
- Questionnaire Design : Create clear and concise questions that are easy for participants to understand. Avoid leading or biased questions.
- Sampling Methods : Decide on the appropriate sampling method for your study, whether it's random, stratified, or convenience sampling.
- Data Collection Tools : Choose the right tools for data collection, whether it's paper surveys, online questionnaires, or face-to-face interviews.
Case Study Design
Case studies are an in-depth exploration of one or a few cases to gain a deep understanding of a particular phenomenon. Key aspects of case study design include:
- Single Case vs. Multiple Case Studies : Decide whether you'll focus on a single case or multiple cases. Single case studies are intensive and allow for detailed examination, while multiple case studies provide comparative insights.
- Data Collection Methods : Gather data through interviews, observations, document analysis, or a combination of these methods.
Qualitative vs. Quantitative Research
In empirical research, you'll often encounter the distinction between qualitative and quantitative research . Here's a closer look at these two approaches:
- Qualitative Research : Qualitative research seeks an in-depth understanding of human behavior, experiences, and perspectives. It involves open-ended questions, interviews, and the analysis of textual or narrative data. Qualitative research is exploratory and often used when the research question is complex and requires a nuanced understanding.
- Quantitative Research : Quantitative research collects numerical data and employs statistical analysis to draw conclusions. It involves structured questionnaires, experiments, and surveys. Quantitative research is ideal for testing hypotheses and establishing cause-and-effect relationships.
Understanding the various research design options is crucial in determining the most appropriate approach for your study. Your choice should align with your research questions, objectives, and the nature of the phenomenon you're investigating.
Data Collection for Empirical Research
Now that you've established your research design, it's time to roll up your sleeves and collect the data that will fuel your empirical research. Effective data collection is essential for obtaining accurate and reliable results.
Sampling Methods
Sampling methods are critical in empirical research, as they determine the subset of individuals or elements from your target population that you will study. Here are some standard sampling methods:
- Random Sampling : Random sampling ensures that every member of the population has an equal chance of being selected. It minimizes bias and is often used in quantitative research.
- Stratified Sampling : Stratified sampling involves dividing the population into subgroups or strata based on specific characteristics (e.g., age, gender, location). Samples are then randomly selected from each stratum, ensuring representation of all subgroups.
- Convenience Sampling : Convenience sampling involves selecting participants who are readily available or easily accessible. While it's convenient, it may introduce bias and limit the generalizability of results.
- Snowball Sampling : Snowball sampling is instrumental when studying hard-to-reach or hidden populations. One participant leads you to another, creating a "snowball" effect. This method is common in qualitative research.
- Purposive Sampling : In purposive sampling, researchers deliberately select participants who meet specific criteria relevant to their research questions. It's often used in qualitative studies to gather in-depth information.
The choice of sampling method depends on the nature of your research, available resources, and the degree of precision required. It's crucial to carefully consider your sampling strategy to ensure that your sample accurately represents your target population.
Data Collection Instruments
Data collection instruments are the tools you use to gather information from your participants or sources. These instruments should be designed to capture the data you need accurately. Here are some popular data collection instruments:
- Questionnaires : Questionnaires consist of structured questions with predefined response options. When designing questionnaires, consider the clarity of questions, the order of questions, and the response format (e.g., Likert scale , multiple-choice).
- Interviews : Interviews involve direct communication between the researcher and participants. They can be structured (with predetermined questions) or unstructured (open-ended). Effective interviews require active listening and probing for deeper insights.
- Observations : Observations entail systematically and objectively recording behaviors, events, or phenomena. Researchers must establish clear criteria for what to observe, how to record observations, and when to observe.
- Surveys : Surveys are a common data collection instrument for quantitative research. They can be administered through various means, including online surveys, paper surveys, and telephone surveys.
- Documents and Archives : In some cases, data may be collected from existing documents, records, or archives. Ensure that the sources are reliable, relevant, and properly documented.
To streamline your process and gather insights with precision and efficiency, consider leveraging innovative tools like Appinio . With Appinio's intuitive platform, you can harness the power of real-time consumer data to inform your research decisions effectively. Whether you're conducting surveys, interviews, or observations, Appinio empowers you to define your target audience, collect data from diverse demographics, and analyze results seamlessly.
By incorporating Appinio into your data collection toolkit, you can unlock a world of possibilities and elevate the impact of your empirical research. Ready to revolutionize your approach to data collection?
Book a Demo
Data Collection Procedures
Data collection procedures outline the step-by-step process for gathering data. These procedures should be meticulously planned and executed to maintain the integrity of your research.
- Training : If you have a research team, ensure that they are trained in data collection methods and protocols. Consistency in data collection is crucial.
- Pilot Testing : Before launching your data collection, conduct a pilot test with a small group to identify any potential problems with your instruments or procedures. Make necessary adjustments based on feedback.
- Data Recording : Establish a systematic method for recording data. This may include timestamps, codes, or identifiers for each data point.
- Data Security : Safeguard the confidentiality and security of collected data. Ensure that only authorized individuals have access to the data.
- Data Storage : Properly organize and store your data in a secure location, whether in physical or digital form. Back up data to prevent loss.
Ethical Considerations
Ethical considerations are paramount in empirical research, as they ensure the well-being and rights of participants are protected.
- Informed Consent : Obtain informed consent from participants, providing clear information about the research purpose, procedures, risks, and their right to withdraw at any time.
- Privacy and Confidentiality : Protect the privacy and confidentiality of participants. Ensure that data is anonymized and sensitive information is kept confidential.
- Beneficence : Ensure that your research benefits participants and society while minimizing harm. Consider the potential risks and benefits of your study.
- Honesty and Integrity : Conduct research with honesty and integrity. Report findings accurately and transparently, even if they are not what you expected.
- Respect for Participants : Treat participants with respect, dignity, and sensitivity to cultural differences. Avoid any form of coercion or manipulation.
- Institutional Review Board (IRB) : If required, seek approval from an IRB or ethics committee before conducting your research, particularly when working with human participants.
Adhering to ethical guidelines is not only essential for the ethical conduct of research but also crucial for the credibility and validity of your study. Ethical research practices build trust between researchers and participants and contribute to the advancement of knowledge with integrity.
With a solid understanding of data collection, including sampling methods, instruments, procedures, and ethical considerations, you are now well-equipped to gather the data needed to answer your research questions.
Empirical Research Data Analysis
Now comes the exciting phase of data analysis, where the raw data you've diligently collected starts to yield insights and answers to your research questions. We will explore the various aspects of data analysis, from preparing your data to drawing meaningful conclusions through statistics and visualization.
Data Preparation
Data preparation is the crucial first step in data analysis. It involves cleaning, organizing, and transforming your raw data into a format that is ready for analysis. Effective data preparation ensures the accuracy and reliability of your results.
- Data Cleaning : Identify and rectify errors, missing values, and inconsistencies in your dataset. This may involve correcting typos, removing outliers, and imputing missing data.
- Data Coding : Assign numerical values or codes to categorical variables to make them suitable for statistical analysis. For example, converting "Yes" and "No" to 1 and 0.
- Data Transformation : Transform variables as needed to meet the assumptions of the statistical tests you plan to use. Common transformations include logarithmic or square root transformations.
- Data Integration : If your data comes from multiple sources, integrate it into a unified dataset, ensuring that variables match and align.
- Data Documentation : Maintain clear documentation of all data preparation steps, as well as the rationale behind each decision. This transparency is essential for replicability.
Effective data preparation lays the foundation for accurate and meaningful analysis. It allows you to trust the results that will follow in the subsequent stages.
Descriptive Statistics
Descriptive statistics help you summarize and make sense of your data by providing a clear overview of its key characteristics. These statistics are essential for understanding the central tendencies, variability, and distribution of your variables. Descriptive statistics include:
- Measures of Central Tendency : These include the mean (average), median (middle value), and mode (most frequent value). They help you understand the typical or central value of your data.
- Measures of Dispersion : Measures like the range, variance, and standard deviation provide insights into the spread or variability of your data points.
- Frequency Distributions : Creating frequency distributions or histograms allows you to visualize the distribution of your data across different values or categories.
Descriptive statistics provide the initial insights needed to understand your data's basic characteristics, which can inform further analysis.
Inferential Statistics
Inferential statistics take your analysis to the next level by allowing you to make inferences or predictions about a larger population based on your sample data. These methods help you test hypotheses and draw meaningful conclusions. Key concepts in inferential statistics include:
- Hypothesis Testing : Hypothesis tests (e.g., t-tests , chi-squared tests ) help you determine whether observed differences or associations in your data are statistically significant or occurred by chance.
- Confidence Intervals : Confidence intervals provide a range within which population parameters (e.g., population mean) are likely to fall based on your sample data.
- Regression Analysis : Regression models (linear, logistic, etc.) help you explore relationships between variables and make predictions.
- Analysis of Variance (ANOVA) : ANOVA tests are used to compare means between multiple groups, allowing you to assess whether differences are statistically significant.
Chi-Square Calculator :
t-Test Calculator :
One-way ANOVA Calculator :
Inferential statistics are powerful tools for drawing conclusions from your data and assessing the generalizability of your findings to the broader population.
Qualitative Data Analysis
Qualitative data analysis is employed when working with non-numerical data, such as text, interviews, or open-ended survey responses. It focuses on understanding the underlying themes, patterns, and meanings within qualitative data. Qualitative analysis techniques include:
- Thematic Analysis : Identifying and analyzing recurring themes or patterns within textual data.
- Content Analysis : Categorizing and coding qualitative data to extract meaningful insights.
- Grounded Theory : Developing theories or frameworks based on emergent themes from the data.
- Narrative Analysis : Examining the structure and content of narratives to uncover meaning.
Qualitative data analysis provides a rich and nuanced understanding of complex phenomena and human experiences.
Data Visualization
Data visualization is the art of representing data graphically to make complex information more understandable and accessible. Effective data visualization can reveal patterns, trends, and outliers in your data. Common types of data visualization include:
- Bar Charts and Histograms : Used to display the distribution of categorical data or discrete data .
- Line Charts : Ideal for showing trends and changes in data over time.
- Scatter Plots : Visualize relationships and correlations between two variables.
- Pie Charts : Display the composition of a whole in terms of its parts.
- Heatmaps : Depict patterns and relationships in multidimensional data through color-coding.
- Box Plots : Provide a summary of the data distribution, including outliers.
- Interactive Dashboards : Create dynamic visualizations that allow users to explore data interactively.
Data visualization not only enhances your understanding of the data but also serves as a powerful communication tool to convey your findings to others.
As you embark on the data analysis phase of your empirical research, remember that the specific methods and techniques you choose will depend on your research questions, data type, and objectives. Effective data analysis transforms raw data into valuable insights, bringing you closer to the answers you seek.
How to Report Empirical Research Results?
At this stage, you get to share your empirical research findings with the world. Effective reporting and presentation of your results are crucial for communicating your research's impact and insights.
1. Write the Research Paper
Writing a research paper is the culmination of your empirical research journey. It's where you synthesize your findings, provide context, and contribute to the body of knowledge in your field.
- Title and Abstract : Craft a clear and concise title that reflects your research's essence. The abstract should provide a brief summary of your research objectives, methods, findings, and implications.
- Introduction : In the introduction, introduce your research topic, state your research questions or hypotheses, and explain the significance of your study. Provide context by discussing relevant literature.
- Methods : Describe your research design, data collection methods, and sampling procedures. Be precise and transparent, allowing readers to understand how you conducted your study.
- Results : Present your findings in a clear and organized manner. Use tables, graphs, and statistical analyses to support your results. Avoid interpreting your findings in this section; focus on the presentation of raw data.
- Discussion : Interpret your findings and discuss their implications. Relate your results to your research questions and the existing literature. Address any limitations of your study and suggest avenues for future research.
- Conclusion : Summarize the key points of your research and its significance. Restate your main findings and their implications.
- References : Cite all sources used in your research following a specific citation style (e.g., APA, MLA, Chicago). Ensure accuracy and consistency in your citations.
- Appendices : Include any supplementary material, such as questionnaires, data coding sheets, or additional analyses, in the appendices.
Writing a research paper is a skill that improves with practice. Ensure clarity, coherence, and conciseness in your writing to make your research accessible to a broader audience.
2. Create Visuals and Tables
Visuals and tables are powerful tools for presenting complex data in an accessible and understandable manner.
- Clarity : Ensure that your visuals and tables are clear and easy to interpret. Use descriptive titles and labels.
- Consistency : Maintain consistency in formatting, such as font size and style, across all visuals and tables.
- Appropriateness : Choose the most suitable visual representation for your data. Bar charts, line graphs, and scatter plots work well for different types of data.
- Simplicity : Avoid clutter and unnecessary details. Focus on conveying the main points.
- Accessibility : Make sure your visuals and tables are accessible to a broad audience, including those with visual impairments.
- Captions : Include informative captions that explain the significance of each visual or table.
Compelling visuals and tables enhance the reader's understanding of your research and can be the key to conveying complex information efficiently.
3. Interpret Findings
Interpreting your findings is where you bridge the gap between data and meaning. It's your opportunity to provide context, discuss implications, and offer insights. When interpreting your findings:
- Relate to Research Questions : Discuss how your findings directly address your research questions or hypotheses.
- Compare with Literature : Analyze how your results align with or deviate from previous research in your field. What insights can you draw from these comparisons?
- Discuss Limitations : Be transparent about the limitations of your study. Address any constraints, biases, or potential sources of error.
- Practical Implications : Explore the real-world implications of your findings. How can they be applied or inform decision-making?
- Future Research Directions : Suggest areas for future research based on the gaps or unanswered questions that emerged from your study.
Interpreting findings goes beyond simply presenting data; it's about weaving a narrative that helps readers grasp the significance of your research in the broader context.
With your research paper written, structured, and enriched with visuals, and your findings expertly interpreted, you are now prepared to communicate your research effectively. Sharing your insights and contributing to the body of knowledge in your field is a significant accomplishment in empirical research.
Examples of Empirical Research
To solidify your understanding of empirical research, let's delve into some real-world examples across different fields. These examples will illustrate how empirical research is applied to gather data, analyze findings, and draw conclusions.
Social Sciences
In the realm of social sciences, consider a sociological study exploring the impact of socioeconomic status on educational attainment. Researchers gather data from a diverse group of individuals, including their family backgrounds, income levels, and academic achievements.
Through statistical analysis, they can identify correlations and trends, revealing whether individuals from lower socioeconomic backgrounds are less likely to attain higher levels of education. This empirical research helps shed light on societal inequalities and informs policymakers on potential interventions to address disparities in educational access.
Environmental Science
Environmental scientists often employ empirical research to assess the effects of environmental changes. For instance, researchers studying the impact of climate change on wildlife might collect data on animal populations, weather patterns, and habitat conditions over an extended period.
By analyzing this empirical data, they can identify correlations between climate fluctuations and changes in wildlife behavior, migration patterns, or population sizes. This empirical research is crucial for understanding the ecological consequences of climate change and informing conservation efforts.
Business and Economics
In the business world, empirical research is essential for making data-driven decisions. Consider a market research study conducted by a business seeking to launch a new product. They collect data through surveys , focus groups , and consumer behavior analysis.
By examining this empirical data, the company can gauge consumer preferences, demand, and potential market size. Empirical research in business helps guide product development, pricing strategies, and marketing campaigns, increasing the likelihood of a successful product launch.
Psychological studies frequently rely on empirical research to understand human behavior and cognition. For instance, a psychologist interested in examining the impact of stress on memory might design an experiment. Participants are exposed to stress-inducing situations, and their memory performance is assessed through various tasks.
By analyzing the data collected, the psychologist can determine whether stress has a significant effect on memory recall. This empirical research contributes to our understanding of the complex interplay between psychological factors and cognitive processes.
These examples highlight the versatility and applicability of empirical research across diverse fields. Whether in medicine, social sciences, environmental science, business, or psychology, empirical research serves as a fundamental tool for gaining insights, testing hypotheses, and driving advancements in knowledge and practice.
Conclusion for Empirical Research
Empirical research is a powerful tool for gaining insights, testing hypotheses, and making informed decisions. By following the steps outlined in this guide, you've learned how to select research topics, collect data, analyze findings, and effectively communicate your research to the world. Remember, empirical research is a journey of discovery, and each step you take brings you closer to a deeper understanding of the world around you. Whether you're a scientist, a student, or someone curious about the process, the principles of empirical research empower you to explore, learn, and contribute to the ever-expanding realm of knowledge.
How to Collect Data for Empirical Research?
Introducing Appinio , the real-time market research platform revolutionizing how companies gather consumer insights for their empirical research endeavors. With Appinio, you can conduct your own market research in minutes, gaining valuable data to fuel your data-driven decisions.
Appinio is more than just a market research platform; it's a catalyst for transforming the way you approach empirical research, making it exciting, intuitive, and seamlessly integrated into your decision-making process.
Here's why Appinio is the go-to solution for empirical research:
- From Questions to Insights in Minutes : With Appinio's streamlined process, you can go from formulating your research questions to obtaining actionable insights in a matter of minutes, saving you time and effort.
- Intuitive Platform for Everyone : No need for a PhD in research; Appinio's platform is designed to be intuitive and user-friendly, ensuring that anyone can navigate and utilize it effectively.
- Rapid Response Times : With an average field time of under 23 minutes for 1,000 respondents, Appinio delivers rapid results, allowing you to gather data swiftly and efficiently.
- Global Reach with Targeted Precision : With access to over 90 countries and the ability to define target groups based on 1200+ characteristics, Appinio empowers you to reach your desired audience with precision and ease.
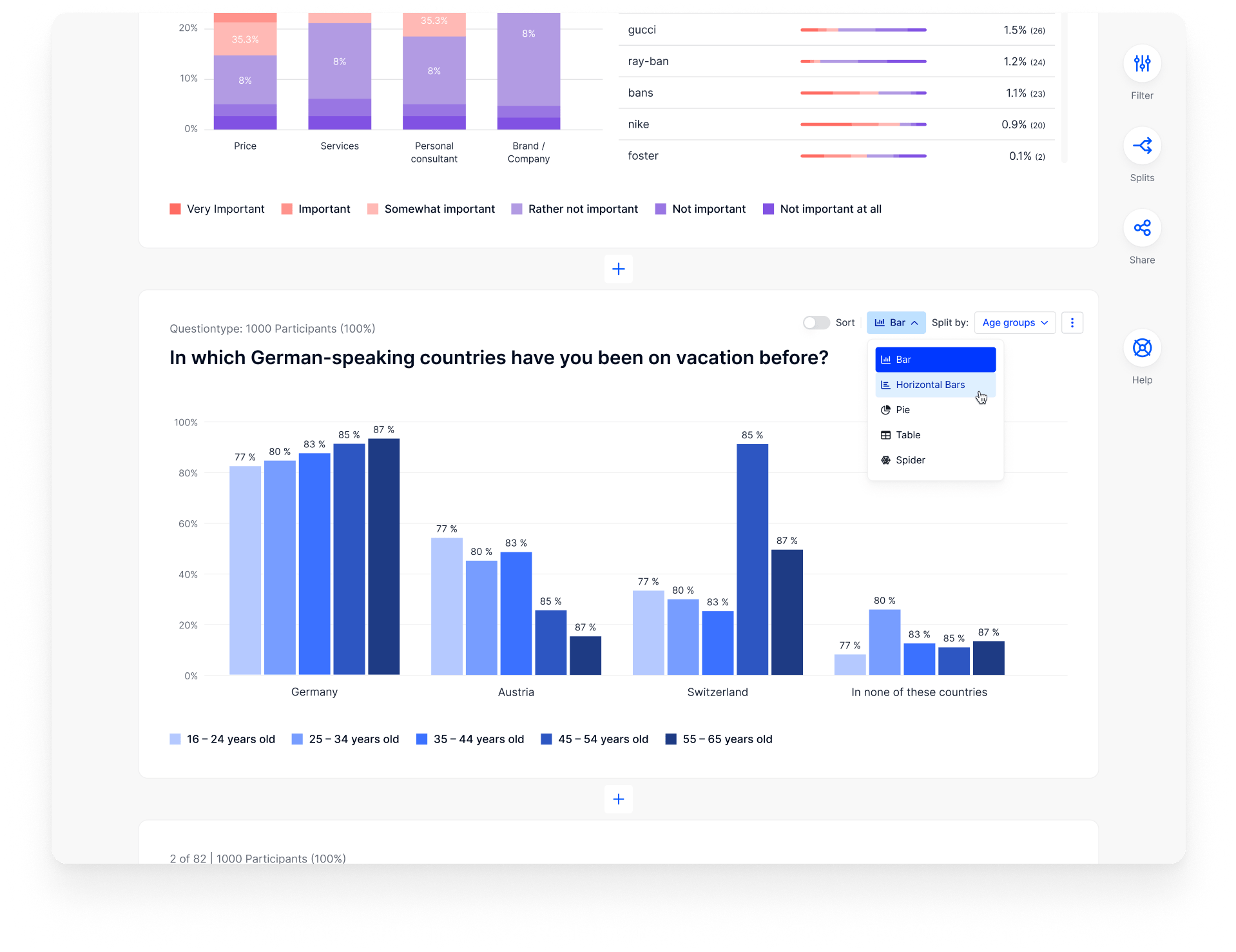
Get free access to the platform!
Join the loop 💌
Be the first to hear about new updates, product news, and data insights. We'll send it all straight to your inbox.
Get the latest market research news straight to your inbox! 💌
Wait, there's more
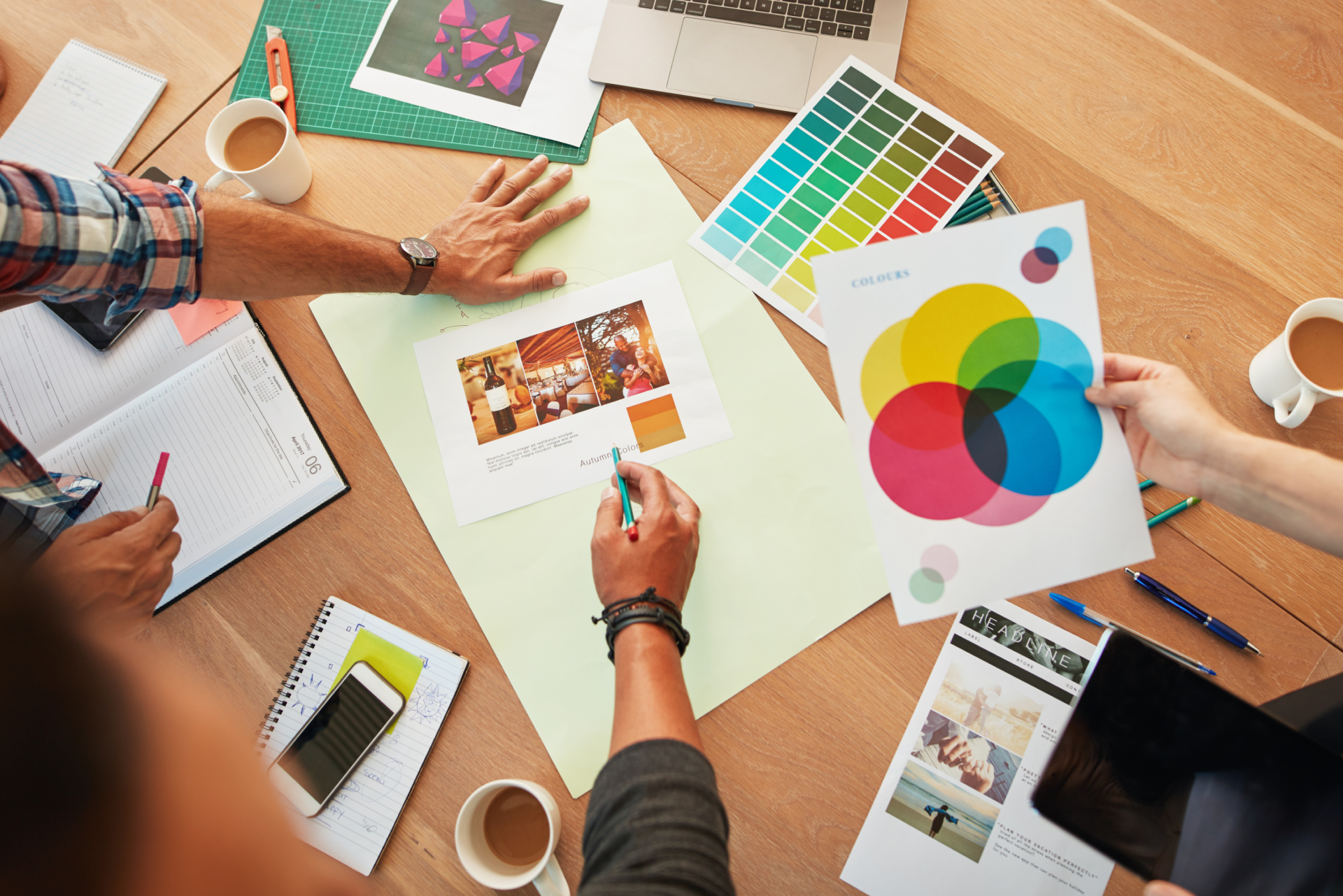
16.09.2024 | 9min read
Creative Checkup – Optimize Advertising Slogans & Creatives for ROI
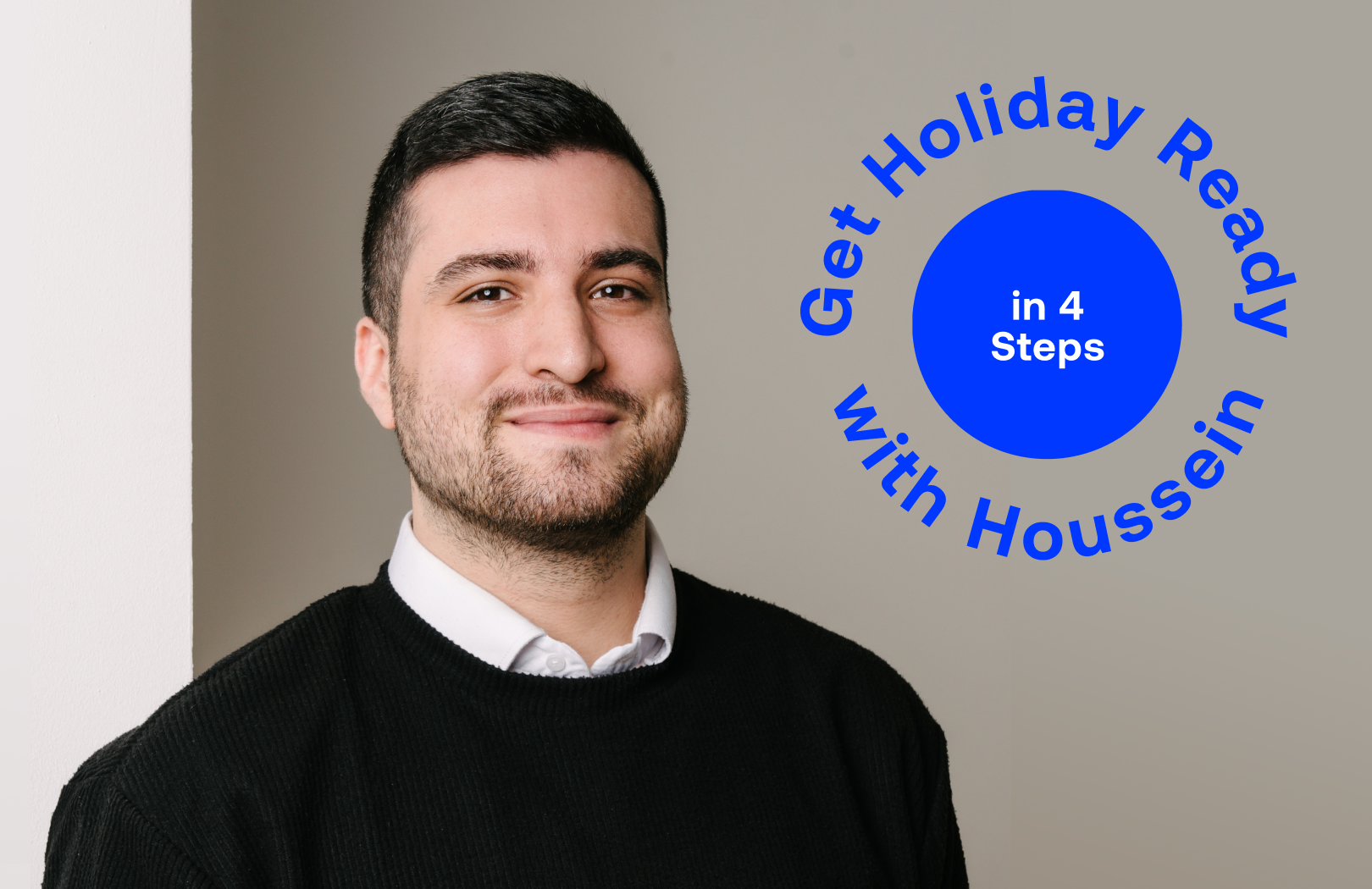
03.09.2024 | 5min read
Get your brand Holiday Ready: 4 Essential Steps to Smash your Q4
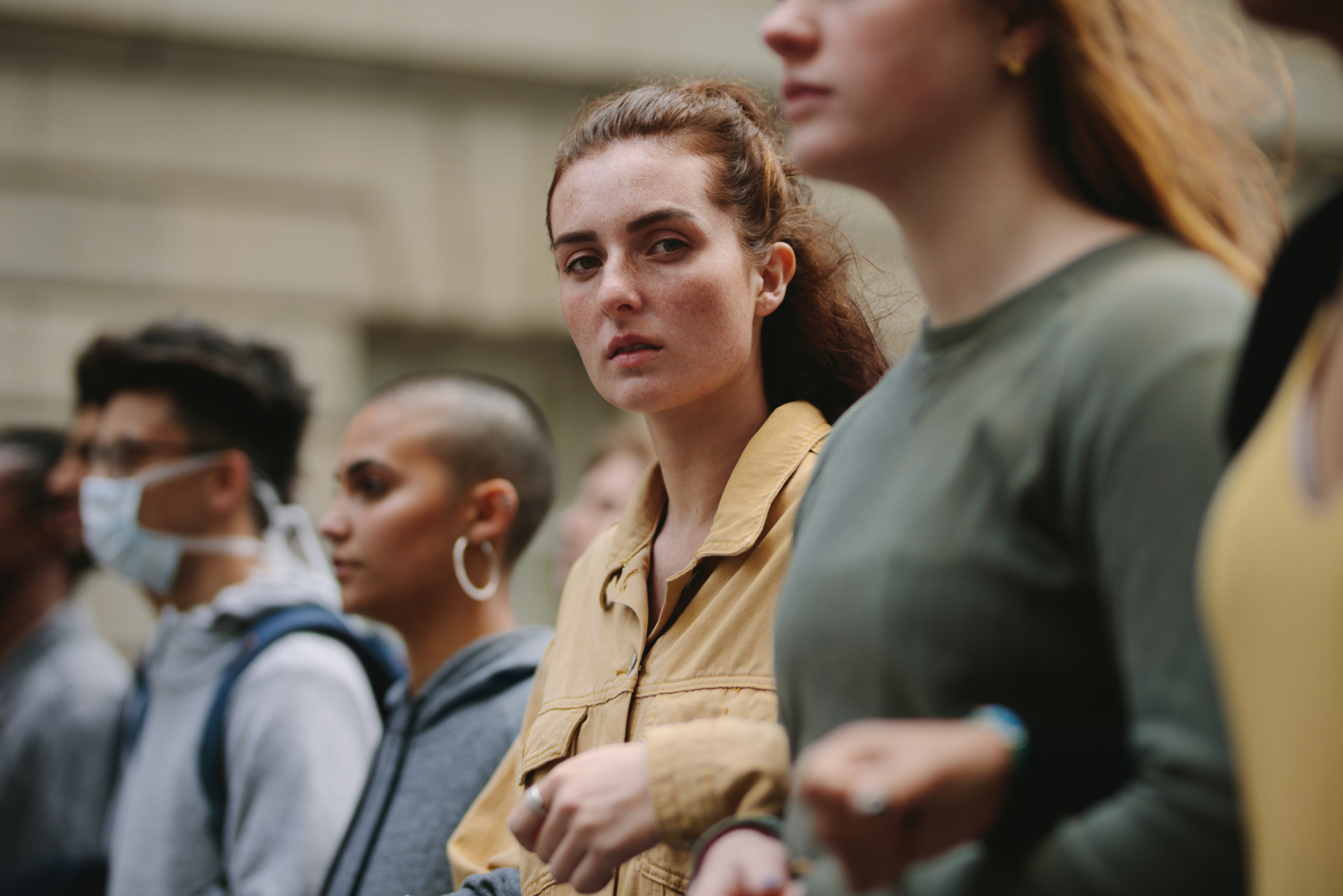
03.09.2024 | 8min read
Beyond Demographics: Psychographics power in target group identification

- Table of Contents
- Random Entry
- Chronological
- Editorial Information
- About the SEP
- Editorial Board
- How to Cite the SEP
- Special Characters
- Advanced Tools
- Support the SEP
- PDFs for SEP Friends
- Make a Donation
- SEPIA for Libraries
- Entry Contents
Bibliography
Academic tools.
- Friends PDF Preview
- Author and Citation Info
- Back to Top
Theory and Observation in Science
Scientists obtain a great deal of the evidence they use by collecting and producing empirical results. Much of the standard philosophical literature on this subject comes from 20 th century logical empiricists, their followers, and critics who embraced their issues while objecting to some of their aims and assumptions. Discussions about empirical evidence have tended to focus on epistemological questions regarding its role in theory testing. This entry follows that precedent, even though empirical evidence also plays important and philosophically interesting roles in other areas including scientific discovery, the development of experimental tools and techniques, and the application of scientific theories to practical problems.
The logical empiricists and their followers devoted much of their attention to the distinction between observables and unobservables, the form and content of observation reports, and the epistemic bearing of observational evidence on theories it is used to evaluate. Philosophical work in this tradition was characterized by the aim of conceptually separating theory and observation, so that observation could serve as the pure basis of theory appraisal. More recently, the focus of the philosophical literature has shifted away from these issues, and their close association to the languages and logics of science, to investigations of how empirical data are generated, analyzed, and used in practice. With this shift, we also see philosophers largely setting aside the aspiration of a pure observational basis for scientific knowledge and instead embracing a view of science in which the theoretical and empirical are usefully intertwined. This entry discusses these topics under the following headings:
1. Introduction
2.1 traditional empiricism, 2.2 the irrelevance of observation per se, 2.3 data and phenomena, 3.1 perception, 3.2 assuming the theory to be tested, 3.3 semantics, 4.1 confirmation, 4.2 saving the phenomena, 4.3 empirical adequacy, 5. conclusion, other internet resources, related entries.
Philosophers of science have traditionally recognized a special role for observations in the epistemology of science. Observations are the conduit through which the ‘tribunal of experience’ delivers its verdicts on scientific hypotheses and theories. The evidential value of an observation has been assumed to depend on how sensitive it is to whatever it is used to study. But this in turn depends on the adequacy of any theoretical claims its sensitivity may depend on. For example, we can challenge the use of a particular thermometer reading to support a prediction of a patient’s temperature by challenging theoretical claims having to do with whether a reading from a thermometer like this one, applied in the same way under similar conditions, should indicate the patient’s temperature well enough to count in favor of or against the prediction. At least some of those theoretical claims will be such that regardless of whether an investigator explicitly endorses, or is even aware of them, her use of the thermometer reading would be undermined by their falsity. All observations and uses of observational evidence are theory laden in this sense (cf. Chang 2005, Azzouni 2004). As the example of the thermometer illustrates, analogues of Norwood Hanson’s claim that seeing is a theory laden undertaking apply just as well to equipment generated observations (Hanson 1958, 19). But if all observations and empirical data are theory laden, how can they provide reality-based, objective epistemic constraints on scientific reasoning?
Recent scholarship has turned this question on its head. Why think that theory ladenness of empirical results would be problematic in the first place? If the theoretical assumptions with which the results are imbued are correct, what is the harm of it? After all, it is in virtue of those assumptions that the fruits of empirical investigation can be ‘put in touch’ with theorizing at all. A number scribbled in a lab notebook can do a scientist little epistemic good unless she can recruit the relevant background assumptions to even recognize it as a reading of the patient’s temperature. But philosophers have embraced an entangled picture of the theoretical and empirical that goes much deeper than this. Lloyd (2012) advocates for what she calls “complex empiricism” in which there is “no pristine separation of model and data” (397). Bogen (2016) points out that “impure empirical evidence” (i.e. evidence that incorporates the judgements of scientists) “often tells us more about the world that it could have if it were pure” (784). Indeed, Longino (2020) has urged that “[t]he naïve fantasy that data have an immediate relation to phenomena of the world, that they are ‘objective’ in some strong, ontological sense of that term, that they are the facts of the world directly speaking to us, should be finally laid to rest” and that “even the primary, original, state of data is not free from researchers’ value- and theory-laden selection and organization” (391).
There is not widespread agreement among philosophers of science about how to characterize the nature of scientific theories. What is a theory? According to the traditional syntactic view, theories are considered to be collections of sentences couched in logical language, which must then be supplemented with correspondence rules in order to be interpreted. Construed in this way, theories include maximally general explanatory and predictive laws (Coulomb’s law of electrical attraction and repulsion, and Maxwellian electromagnetism equations for example), along with lesser generalizations that describe more limited natural and experimental phenomena (e.g., the ideal gas equations describing relations between temperatures and pressures of enclosed gasses, and general descriptions of positional astronomical regularities). In contrast, the semantic view casts theories as the space of states possible according to the theory, or the set of mathematical models permissible according to the theory (see Suppe 1977). However, there are also significantly more ecumenical interpretations of what it means to be a scientific theory, which include elements of diverse kinds. To take just one illustrative example, Borrelli (2012) characterizes the Standard Model of particle physics as a theoretical framework involving what she calls “theoretical cores” that are composed of mathematical structures, verbal stories, and analogies with empirical references mixed together (196). This entry aims to accommodate all of these views about the nature of scientific theories.
In this entry, we trace the contours of traditional philosophical engagement with questions surrounding theory and observation in science that attempted to segregate the theoretical from the observational, and to cleanly delineate between the observable and the unobservable. We also discuss the more recent scholarship that supplants the primacy of observation by human sensory perception with an instrument-inclusive conception of data production and that embraces the intertwining of theoretical and empirical in the production of useful scientific results. Although theory testing dominates much of the standard philosophical literature on observation, much of what this entry says about the role of observation in theory testing applies also to its role in inventing, and modifying theories, and applying them to tasks in engineering, medicine, and other practical enterprises.
2. Observation and data
Reasoning from observations has been important to scientific practice at least since the time of Aristotle, who mentions a number of sources of observational evidence including animal dissection (Aristotle(a), 763a/30–b/15; Aristotle(b), 511b/20–25). Francis Bacon argued long ago that the best way to discover things about nature is to use experiences (his term for observations as well as experimental results) to develop and improve scientific theories (Bacon 1620, 49ff). The role of observational evidence in scientific discovery was an important topic for Whewell (1858) and Mill (1872) among others in the 19th century. But philosophers didn’t talk about observation as extensively, in as much detail, or in the way we have become accustomed to, until the 20 th century when logical empiricists transformed philosophical thinking about it.
One important transformation, characteristic of the linguistic turn in philosophy, was to concentrate on the logic of observation reports rather than on objects or phenomena observed. This focus made sense on the assumption that a scientific theory is a system of sentences or sentence-like structures (propositions, statements, claims, and so on) to be tested by comparison to observational evidence. It was assumed that the comparisons must be understood in terms of inferential relations. If inferential relations hold only between sentence-like structures, it follows that theories must be tested, not against observations or things observed, but against sentences, propositions, etc. used to report observations (Hempel 1935, 50–51; Schlick 1935). Theory testing was treated as a matter of comparing observation sentences describing observations made in natural or laboratory settings to observation sentences that should be true according to the theory to be tested. This was to be accomplished by using laws or lawlike generalizations along with descriptions of initial conditions, correspondence rules, and auxiliary hypotheses to derive observation sentences describing the sensory deliverances of interest. This makes it imperative to ask what observation sentences report.
According to what Hempel called the phenomenalist account , observation reports describe the observer’s subjective perceptual experiences.
… Such experiential data might be conceived of as being sensations, perceptions, and similar phenomena of immediate experience. (Hempel 1952, 674)
This view is motivated by the assumption that the epistemic value of an observation report depends upon its truth or accuracy, and that with regard to perception, the only thing observers can know with certainty to be true or accurate is how things appear to them. This means that we cannot be confident that observation reports are true or accurate if they describe anything beyond the observer’s own perceptual experience. Presumably one’s confidence in a conclusion should not exceed one’s confidence in one’s best reasons to believe it. For the phenomenalist, it follows that reports of subjective experience can provide better reasons to believe claims they support than reports of other kinds of evidence.
However, given the expressive limitations of the language available for reporting subjective experiences, we cannot expect phenomenalistic reports to be precise and unambiguous enough to test theoretical claims whose evaluation requires accurate, fine-grained perceptual discriminations. Worse yet, if experiences are directly available only to those who have them, there is room to doubt whether different people can understand the same observation sentence in the same way. Suppose you had to evaluate a claim on the basis of someone else’s subjective report of how a litmus solution looked to her when she dripped a liquid of unknown acidity into it. How could you decide whether her visual experience was the same as the one you would use her words to report?
Such considerations led Hempel to propose, contrary to the phenomenalists, that observation sentences report ‘directly observable’, ‘intersubjectively ascertainable’ facts about physical objects
… such as the coincidence of the pointer of an instrument with a numbered mark on a dial; a change of color in a test substance or in the skin of a patient; the clicking of an amplifier connected with a Geiger counter; etc. (ibid.)
That the facts expressed in observation reports be intersubjectively ascertainable was critical for the aims of the logical empiricists. They hoped to articulate and explain the authoritativeness widely conceded to the best natural, social, and behavioral scientific theories in contrast to propaganda and pseudoscience. Some pronouncements from astrologers and medical quacks gain wide acceptance, as do those of religious leaders who rest their cases on faith or personal revelation, and leaders who use their political power to secure assent. But such claims do not enjoy the kind of credibility that scientific theories can attain. The logical empiricists tried to account for the genuine credibility of scientific theories by appeal to the objectivity and accessibility of observation reports, and the logic of theory testing. Part of what they meant by calling observational evidence objective was that cultural and ethnic factors have no bearing on what can validly be inferred about the merits of a theory from observation reports. So conceived, objectivity was important to the logical empiricists’ criticism of the Nazi idea that Jews and Aryans have fundamentally different thought processes such that physical theories suitable for Einstein and his kind should not be inflicted on German students. In response to this rationale for ethnic and cultural purging of the German educational system, the logical empiricists argued that because of its objectivity, observational evidence (rather than ethnic and cultural factors) should be used to evaluate scientific theories (Galison 1990). In this way of thinking, observational evidence and its subsequent bearing on scientific theories are objective also in virtue of being free of non-epistemic values.
Ensuing generations of philosophers of science have found the logical empiricist focus on expressing the content of observations in a rarefied and basic observation language too narrow. Search for a suitably universal language as required by the logical empiricist program has come up empty-handed and most philosophers of science have given up its pursuit. Moreover, as we will discuss in the following section, the centrality of observation itself (and pointer readings) to the aims of empiricism in philosophy of science has also come under scrutiny. However, leaving the search for a universal pure observation language behind does not automatically undercut the norm of objectivity as it relates to the social, political, and cultural contexts of scientific research. Pristine logical foundations aside, the objectivity of ‘neutral’ observations in the face of noxious political propaganda was appealing because it could serve as shared ground available for intersubjective appraisal. This appeal remains alive and well today, particularly as pernicious misinformation campaigns are again formidable in public discourse (see O’Connor and Weatherall 2019). If individuals can genuinely appraise the significance of empirical evidence and come to well-justified agreement about how the evidence bears on theorizing, then they can protect their epistemic deliberations from the undue influence of fascists and other nefarious manipulators. However, this aspiration must face subtleties arising from the social epistemology of science and from the nature of empirical results themselves. In practice, the appraisal of scientific results can often require expertise that is not readily accessible to members of the public without the relevant specialized training. Additionally, precisely because empirical results are not pure observation reports, their appraisal across communities of inquirers operating with different background assumptions can require significant epistemic work.
The logical empiricists paid little attention to the distinction between observing and experimenting and its epistemic implications. For some philosophers, to experiment is to isolate, prepare, and manipulate things in hopes of producing epistemically useful evidence. It had been customary to think of observing as noticing and attending to interesting details of things perceived under more or less natural conditions, or by extension, things perceived during the course of an experiment. To look at a berry on a vine and attend to its color and shape would be to observe it. To extract its juice and apply reagents to test for the presence of copper compounds would be to perform an experiment. By now, many philosophers have argued that contrivance and manipulation influence epistemically significant features of observable experimental results to such an extent that epistemologists ignore them at their peril. Robert Boyle (1661), John Herschell (1830), Bruno Latour and Steve Woolgar (1979), Ian Hacking (1983), Harry Collins (1985) Allan Franklin (1986), Peter Galison (1987), Jim Bogen and Jim Woodward (1988), and Hans-Jörg Rheinberger (1997), are some of the philosophers and philosophically-minded scientists, historians, and sociologists of science who gave serious consideration to the distinction between observing and experimenting. The logical empiricists tended to ignore it. Interestingly, the contemporary vantage point that attends to modeling, data processing, and empirical results may suggest a re-unification of observation and intervention under the same epistemological framework. When one no longer thinks of scientific observation as pure or direct, and recognizes the power of good modeling to account for confounds without physically intervening on the target system, the purported epistemic distinction between observation and intervention loses its bite.
Observers use magnifying glasses, microscopes, or telescopes to see things that are too small or far away to be seen, or seen clearly enough, without them. Similarly, amplification devices are used to hear faint sounds. But if to observe something is to perceive it, not every use of instruments to augment the senses qualifies as observational.
Philosophers generally agree that you can observe the moons of Jupiter with a telescope, or a heartbeat with a stethoscope. The van Fraassen of The Scientific Image is a notable exception, for whom to be ‘observable’ meant to be something that, were it present to a creature like us, would be observed. Thus, for van Fraassen, the moons of Jupiter are observable “since astronauts will no doubt be able to see them as well from close up” (1980, 16). In contrast, microscopic entities are not observable on van Fraassen’s account because creatures like us cannot strategically maneuver ourselves to see them, present before us, with our unaided senses.
Many philosophers have criticized van Fraassen’s view as overly restrictive. Nevertheless, philosophers differ in their willingness to draw the line between what counts as observable and what does not along the spectrum of increasingly complicated instrumentation. Many philosophers who don’t mind telescopes and microscopes still find it unnatural to say that high energy physicists ‘observe’ particles or particle interactions when they look at bubble chamber photographs—let alone digital visualizations of energy depositions left in calorimeters that are not themselves inspected. Their intuitions come from the plausible assumption that one can observe only what one can see by looking, hear by listening, feel by touching, and so on. Investigators can neither look at (direct their gazes toward and attend to) nor visually experience charged particles moving through a detector. Instead they can look at and see tracks in the chamber, in bubble chamber photographs, calorimeter data visualizations, etc.
In more contentious examples, some philosophers have moved to speaking of instrument-augmented empirical research as more like tool use than sensing. Hacking (1981) argues that we do not see through a microscope, but rather with it. Daston and Galison (2007) highlight the inherent interactivity of a scanning tunneling microscope, in which scientists image and manipulate atoms by exchanging electrons between the sharp tip of the microscope and the surface to be imaged (397). Others have opted to stretch the meaning of observation to accommodate what we might otherwise be tempted to call instrument-aided detections. For instance, Shapere (1982) argues that while it may initially strike philosophers as counter-intuitive, it makes perfect sense to call the detection of neutrinos from the interior of the sun “direct observation.”
The variety of views on the observable/unobservable distinction hint that empiricists may have been barking up the wrong philosophical tree. Many of the things scientists investigate do not interact with human perceptual systems as required to produce perceptual experiences of them. The methods investigators use to study such things argue against the idea—however plausible it may once have seemed—that scientists do or should rely exclusively on their perceptual systems to obtain the evidence they need. Thus Feyerabend proposed as a thought experiment that if measuring equipment was rigged up to register the magnitude of a quantity of interest, a theory could be tested just as well against its outputs as against records of human perceptions (Feyerabend 1969, 132–137). Feyerabend could have made his point with historical examples instead of thought experiments. A century earlier Helmholtz estimated the speed of excitatory impulses traveling through a motor nerve. To initiate impulses whose speed could be estimated, he implanted an electrode into one end of a nerve fiber and ran a current into it from a coil. The other end was attached to a bit of muscle whose contraction signaled the arrival of the impulse. To find out how long it took the impulse to reach the muscle he had to know when the stimulating current reached the nerve. But
[o]ur senses are not capable of directly perceiving an individual moment of time with such small duration …
and so Helmholtz had to resort to what he called ‘artificial methods of observation’ (Olesko and Holmes 1994, 84). This meant arranging things so that current from the coil could deflect a galvanometer needle. Assuming that the magnitude of the deflection is proportional to the duration of current passing from the coil, Helmholtz could use the deflection to estimate the duration he could not see (ibid). This sense of ‘artificial observation’ is not to be confused e.g., with using magnifying glasses or telescopes to see tiny or distant objects. Such devices enable the observer to scrutinize visible objects. The minuscule duration of the current flow is not a visible object. Helmholtz studied it by cleverly concocting circumstances so that the deflection of the needle would meaningfully convey the information he needed. Hooke (1705, 16–17) argued for and designed instruments to execute the same kind of strategy in the 17 th century.
It is of interest that records of perceptual observation are not always epistemically superior to data collected via experimental equipment. Indeed, it is not unusual for investigators to use non-perceptual evidence to evaluate perceptual data and correct for its errors. For example, Rutherford and Pettersson conducted similar experiments to find out if certain elements disintegrated to emit charged particles under radioactive bombardment. To detect emissions, observers watched a scintillation screen for faint flashes produced by particle strikes. Pettersson’s assistants reported seeing flashes from silicon and certain other elements. Rutherford’s did not. Rutherford’s colleague, James Chadwick, visited Pettersson’s laboratory to evaluate his data. Instead of watching the screen and checking Pettersson’s data against what he saw, Chadwick arranged to have Pettersson’s assistants watch the screen while unbeknownst to them he manipulated the equipment, alternating normal operating conditions with a condition in which particles, if any, could not hit the screen. Pettersson’s data were discredited by the fact that his assistants reported flashes at close to the same rate in both conditions (Stuewer 1985, 284–288).
When the process of producing data is relatively convoluted, it is even easier to see that human sense perception is not the ultimate epistemic engine. Consider functional magnetic resonance images (fMRI) of the brain decorated with colors to indicate magnitudes of electrical activity in different regions during the performance of a cognitive task. To produce these images, brief magnetic pulses are applied to the subject’s brain. The magnetic force coordinates the precessions of protons in hemoglobin and other bodily stuffs to make them emit radio signals strong enough for the equipment to respond to. When the magnetic force is relaxed, the signals from protons in highly oxygenated hemoglobin deteriorate at a detectably different rate than signals from blood that carries less oxygen. Elaborate algorithms are applied to radio signal records to estimate blood oxygen levels at the places from which the signals are calculated to have originated. There is good reason to believe that blood flowing just downstream from spiking neurons carries appreciably more oxygen than blood in the vicinity of resting neurons. Assumptions about the relevant spatial and temporal relations are used to estimate levels of electrical activity in small regions of the brain corresponding to pixels in the finished image. The results of all of these computations are used to assign the appropriate colors to pixels in a computer generated image of the brain. In view of all of this, functional brain imaging differs, e.g., from looking and seeing, photographing, and measuring with a thermometer or a galvanometer in ways that make it uninformative to call it observation. And similarly for many other methods scientists use to produce non-perceptual evidence.
The role of the senses in fMRI data production is limited to such things as monitoring the equipment and keeping an eye on the subject. Their epistemic role is limited to discriminating the colors in the finished image, reading tables of numbers the computer used to assign them, and so on. While it is true that researchers typically use their sense of sight to take in visualizations of processed fMRI data—or numbers on a page or screen for that matter—this is not the primary locus of epistemic action. Researchers learn about brain processes through fMRI data, to the extent that they do, primarily in virtue of the suitability of the causal connection between the target processes and the data records, and of the transformations those data undergo when they are processed into the maps or other results that scientists want to use. The interesting questions are not about observability, i.e. whether neuronal activity, blood oxygen levels, proton precessions, radio signals, and so on, are properly understood as observable by creatures like us. The epistemic significance of the fMRI data depends on their delivering us the right sort of access to the target, but observation is neither necessary nor sufficient for that access.
Following Shapere (1982), one could respond by adopting an extremely permissive view of what counts as an ‘observation’ so as to allow even highly processed data to count as observations. However, it is hard to reconcile the idea that highly processed data like fMRI images record observations with the traditional empiricist notion that calculations involving theoretical assumptions and background beliefs must not be allowed (on pain of loss of objectivity) to intrude into the process of data production. Observation garnered its special epistemic status in the first place because it seemed more direct, more immediate, and therefore less distorted and muddled than (say) detection or inference. The production of fMRI images requires extensive statistical manipulation based on theories about the radio signals, and a variety of factors having to do with their detection along with beliefs about relations between blood oxygen levels and neuronal activity, sources of systematic error, and more. Insofar as the use of the term ‘observation’ connotes this extra baggage of traditional empiricism, it may be better to replace observation-talk with terminology that is more obviously permissive, such as that of ‘empirical data’ and ‘empirical results.’
Deposing observation from its traditional perch in empiricist epistemologies of science need not estrange philosophers from scientific practice. Terms like ‘observation’ and ‘observation reports’ do not occur nearly as much in scientific as in philosophical writings. In their place, working scientists tend to talk about data . Philosophers who adopt this usage are free to think about standard examples of observation as members of a large, diverse, and growing family of data production methods. Instead of trying to decide which methods to classify as observational and which things qualify as observables, philosophers can then concentrate on the epistemic influence of the factors that differentiate members of the family. In particular, they can focus their attention on what questions data produced by a given method can be used to answer, what must be done to use that data fruitfully, and the credibility of the answers they afford (Bogen 2016).
Satisfactorily answering such questions warrants further philosophical work. As Bogen and Woodward (1988) have argued, there is often a long road between obtaining a particular dataset replete with idiosyncrasies born of unspecified causal nuances to any claim about the phenomenon ultimately of interest to the researchers. Empirical data are typically produced in ways that make it impossible to predict them from the generalizations they are used to test, or to derive instances of those generalizations from data and non ad hoc auxiliary hypotheses. Indeed, it is unusual for many members of a set of reasonably precise quantitative data to agree with one another, let alone with a quantitative prediction. That is because precise, publicly accessible data typically cannot be produced except through processes whose results reflect the influence of causal factors that are too numerous, too different in kind, and too irregular in behavior for any single theory to account for them. When Bernard Katz recorded electrical activity in nerve fiber preparations, the numerical values of his data were influenced by factors peculiar to the operation of his galvanometers and other pieces of equipment, variations among the positions of the stimulating and recording electrodes that had to be inserted into the nerve, the physiological effects of their insertion, and changes in the condition of the nerve as it deteriorated during the course of the experiment. There were variations in the investigators’ handling of the equipment. Vibrations shook the equipment in response to a variety of irregularly occurring causes ranging from random error sources to the heavy tread of Katz’s teacher, A.V. Hill, walking up and down the stairs outside of the laboratory. That’s a short list. To make matters worse, many of these factors influenced the data as parts of irregularly occurring, transient, and shifting assemblies of causal influences.
The effects of systematic and random sources of error are typically such that considerable analysis and interpretation are required to take investigators from data sets to conclusions that can be used to evaluate theoretical claims. Interestingly, this applies as much to clear cases of perceptual data as to machine produced records. When 19 th and early 20 th century astronomers looked through telescopes and pushed buttons to record the time at which they saw a star pass a crosshair, the values of their data points depended, not only upon light from that star, but also upon features of perceptual processes, reaction times, and other psychological factors that varied from observer to observer. No astronomical theory has the resources to take such things into account.
Instead of testing theoretical claims by direct comparison to the data initially collected, investigators use data to infer facts about phenomena, i.e., events, regularities, processes, etc. whose instances are uniform and uncomplicated enough to make them susceptible to systematic prediction and explanation (Bogen and Woodward 1988, 317). The fact that lead melts at temperatures at or close to 327.5 C is an example of a phenomenon, as are widespread regularities among electrical quantities involved in the action potential, the motions of astronomical bodies, etc. Theories that cannot be expected to predict or explain such things as individual temperature readings can nevertheless be evaluated on the basis of how useful they are in predicting or explaining phenomena. The same holds for the action potential as opposed to the electrical data from which its features are calculated, and the motions of astronomical bodies in contrast to the data of observational astronomy. It is reasonable to ask a genetic theory how probable it is (given similar upbringings in similar environments) that the offspring of a parent or parents diagnosed with alcohol use disorder will develop one or more symptoms the DSM classifies as indicative of alcohol use disorder. But it would be quite unreasonable to ask the genetic theory to predict or explain one patient’s numerical score on one trial of a particular diagnostic test, or why a diagnostician wrote a particular entry in her report of an interview with an offspring of one of such parents (see Bogen and Woodward, 1988, 319–326).
Leonelli has challenged Bogen and Woodward’s (1988) claim that data are, as she puts it, “unavoidably embedded in one experimental context” (2009, 738). She argues that when data are suitably packaged, they can travel to new epistemic contexts and retain epistemic utility—it is not just claims about the phenomena that can travel, data travel too. Preparing data for safe travel involves work, and by tracing data ‘journeys,’ philosophers can learn about how the careful labor of researchers, data archivists, and database curators can facilitate useful data mobility. While Leonelli’s own work has often focused on data in biology, Leonelli and Tempini (2020) contains many diverse case studies of data journeys from a variety of scientific disciplines that will be of value to philosophers interested in the methodology and epistemology of science in practice.
The fact that theories typically predict and explain features of phenomena rather than idiosyncratic data should not be interpreted as a failing. For many purposes, this is the more useful and illuminating capacity. Suppose you could choose between a theory that predicted or explained the way in which neurotransmitter release relates to neuronal spiking (e.g., the fact that on average, transmitters are released roughly once for every 10 spikes) and a theory which explained or predicted the numbers displayed on the relevant experimental equipment in one, or a few single cases. For most purposes, the former theory would be preferable to the latter at the very least because it applies to so many more cases. And similarly for theories that predict or explain something about the probability of alcohol use disorder conditional on some genetic factor or a theory that predicted or explained the probability of faulty diagnoses of alcohol use disorder conditional on facts about the training that psychiatrists receive. For most purposes, these would be preferable to a theory that predicted specific descriptions in a single particular case history.
However, there are circumstances in which scientists do want to explain data. In empirical research it is often crucial to getting a useful signal that scientists deal with sources of background noise and confounding signals. This is part of the long road from newly collected data to useful empirical results. An important step on the way to eliminating unwanted noise or confounds is to determine their sources. Different sources of noise can have different characteristics that can be derived from and explained by theory. Consider the difference between ‘shot noise’ and ‘thermal noise,’ two ubiquitous sources of noise in precision electronics (Schottky 1918; Nyquist 1928; Horowitz and Hill 2015). ‘Shot noise’ arises in virtue of the discrete nature of a signal. For instance, light collected by a detector does not arrive all at once or in perfectly continuous fashion. Photons rain onto a detector shot by shot on account of being quanta. Imagine building up an image one photon at a time—at first the structure of the image is barely recognizable, but after the arrival of many photons, the image eventually fills in. In fact, the contribution of noise of this type goes as the square root of the signal. By contrast, thermal noise is due to non-zero temperature—thermal fluctuations cause a small current to flow in any circuit. If you cool your instrument (which very many precision experiments in physics do) then you can decrease thermal noise. Cooling the detector is not going to change the quantum nature of photons though. Simply collecting more photons will improve the signal to noise ratio with respect to shot noise. Thus, determining what kind of noise is affecting one’s data, i.e. explaining features of the data themselves that are idiosyncratic to the particular instruments and conditions prevailing during a specific instance of data collection, can be critical to eventually generating a dataset that can be used to answer questions about phenomena of interest. In using data that require statistical analysis, it is particularly clear that “empirical assumptions about the factors influencing the measurement results may be used to motivate the assumption of a particular error distribution”, which can be crucial for justifying the application of methods of analysis (Woodward 2011, 173).
There are also circumstances in which scientists want to provide a substantive, detailed explanation for a particular idiosyncratic datum, and even circumstances in which procuring such explanations is epistemically imperative. Ignoring outliers without good epistemic reasons is just cherry-picking data, one of the canonical ‘questionable research practices.’ Allan Franklin has described Robert Millikan’s convenient exclusion of data he collected from observing the second oil drop in his experiments of April 16, 1912 (1986, 231). When Millikan initially recorded the data for this drop, his notebooks indicate that he was satisfied his apparatus was working properly and that the experiment was running well—he wrote “Publish” next to the data in his lab notebook. However, after he had later calculated the value for the fundamental electric charge that these data yielded, and found it aberrant with respect to the values he calculated using data collected from other good observing sessions, he changed his mind, writing “Won’t work” next to the calculation (ibid., see also Woodward 2010, 794). Millikan not only never published this result, he never published why he failed to publish it. When data are excluded from analysis, there ought to be some explanation justifying their omission over and above lack of agreement with the experimenters’ expectations. Precisely because they are outliers, some data require specific, detailed, idiosyncratic causal explanations. Indeed, it is often in virtue of those very explanations that outliers can be responsibly rejected. Some explanation of data rejected as ‘spurious’ is required. Otherwise, scientists risk biasing their own work.
Thus, while in transforming data as collected into something useful for learning about phenomena, scientists often account for features of the data such as different types of noise contributions, and sometimes even explain the odd outlying data point or artifact, they simply do not explain every individual teensy tiny causal contribution to the exact character of a data set or datum in full detail. This is because scientists can neither discover such causal minutia nor would their invocation be necessary for typical research questions. The fact that it may sometimes be important for scientists to provide detailed explanations of data, and not just claims about phenomena inferred from data, should not be confused with the dubious claim that scientists could ‘in principle’ detail every causal quirk that contributed to some data (Woodward 2010; 2011).
In view of all of this, together with the fact that a great many theoretical claims can only be tested directly against facts about phenomena, it behooves epistemologists to think about how data are used to answer questions about phenomena. Lacking space for a detailed discussion, the most this entry can do is to mention two main kinds of things investigators do in order to draw conclusions from data. The first is causal analysis carried out with or without the use of statistical techniques. The second is non-causal statistical analysis.
First, investigators must distinguish features of the data that are indicative of facts about the phenomenon of interest from those which can safely be ignored, and those which must be corrected for. Sometimes background knowledge makes this easy. Under normal circumstances investigators know that their thermometers are sensitive to temperature, and their pressure gauges, to pressure. An astronomer or a chemist who knows what spectrographic equipment does, and what she has applied it to will know what her data indicate. Sometimes it is less obvious. When Santiago Ramón y Cajal looked through his microscope at a thin slice of stained nerve tissue, he had to figure out which, if any, of the fibers he could see at one focal length connected to or extended from things he could see only at another focal length, or in another slice. Analogous considerations apply to quantitative data. It was easy for Katz to tell when his equipment was responding more to Hill’s footfalls on the stairs than to the electrical quantities it was set up to measure. It can be harder to tell whether an abrupt jump in the amplitude of a high frequency EEG oscillation was due to a feature of the subjects brain activity or an artifact of extraneous electrical activity in the laboratory or operating room where the measurements were made. The answers to questions about which features of numerical and non-numerical data are indicative of a phenomenon of interest typically depend at least in part on what is known about the causes that conspire to produce the data.
Statistical arguments are often used to deal with questions about the influence of epistemically relevant causal factors. For example, when it is known that similar data can be produced by factors that have nothing to do with the phenomenon of interest, Monte Carlo simulations, regression analyses of sample data, and a variety of other statistical techniques sometimes provide investigators with their best chance of deciding how seriously to take a putatively illuminating feature of their data.
But statistical techniques are also required for purposes other than causal analysis. To calculate the magnitude of a quantity like the melting point of lead from a scatter of numerical data, investigators throw out outliers, calculate the mean and the standard deviation, etc., and establish confidence and significance levels. Regression and other techniques are applied to the results to estimate how far from the mean the magnitude of interest can be expected to fall in the population of interest (e.g., the range of temperatures at which pure samples of lead can be expected to melt).
The fact that little can be learned from data without causal, statistical, and related argumentation has interesting consequences for received ideas about how the use of observational evidence distinguishes science from pseudoscience, religion, and other non-scientific cognitive endeavors. First, scientists are not the only ones who use observational evidence to support their claims; astrologers and medical quacks use them too. To find epistemically significant differences, one must carefully consider what sorts of data they use, where it comes from, and how it is employed. The virtues of scientific as opposed to non-scientific theory evaluations depend not only on its reliance on empirical data, but also on how the data are produced, analyzed and interpreted to draw conclusions against which theories can be evaluated. Secondly, it does not take many examples to refute the notion that adherence to a single, universally applicable ‘scientific method’ differentiates the sciences from the non-sciences. Data are produced, and used in far too many different ways to treat informatively as instances of any single method. Thirdly, it is usually, if not always, impossible for investigators to draw conclusions to test theories against observational data without explicit or implicit reliance on theoretical resources.
Bokulich (2020) has helpfully outlined a taxonomy of various ways in which data can be model-laden to increase their epistemic utility. She focuses on seven categories: data conversion, data correction, data interpolation, data scaling, data fusion, data assimilation, and synthetic data. Of these categories, conversion and correction are perhaps the most familiar. Bokulich reminds us that even in the case of reading a temperature from an ordinary mercury thermometer, we are ‘converting’ the data as measured, which in this case is the height of the column of mercury, to a temperature (ibid., 795). In more complicated cases, such as processing the arrival times of acoustic signals in seismic reflection measurements to yield values for subsurface depth, data conversion may involve models (ibid.). In this example, models of the composition and geometry of the subsurface are needed in order to account for differences in the speed of sound in different materials. Data ‘correction’ involves common practices we have already discussed like modeling and mathematically subtracting background noise contributions from one’s dataset (ibid., 796). Bokulich rightly points out that involving models in these ways routinely improves the epistemic uses to which data can be put. Data interpolation, scaling, and ‘fusion’ are also relatively widespread practices that deserve further philosophical analysis. Interpolation involves filling in missing data in a patchy data set, under the guidance of models. Data are scaled when they have been generated in a particular scale (temporal, spatial, energy) and modeling assumptions are recruited to transform them to apply at another scale. Data are ‘fused,’ in Bokulich’s terminology, when data collected in diverse contexts, using diverse methods are combined, or integrated together. For instance, when data from ice cores, tree rings, and the historical logbooks of sea captains are merged into a joint climate dataset. Scientists must take care in combining data of diverse provenance, and model new uncertainties arising from the very amalgamation of datasets (ibid., 800).
Bokulich contrasts ‘synthetic data’ with what she calls ‘real data’ (ibid., 801–802). Synthetic data are virtual, or simulated data, and are not produced by physical interaction with worldly research targets. Bokulich emphasizes the role that simulated data can usefully play in testing and troubleshooting aspects of data processing that are to eventually be deployed on empirical data (ibid., 802). It can be incredibly useful for developing and stress-testing a data processing pipeline to have fake datasets whose characteristics are already known in virtue of having been produced by the researchers, and being available for their inspection at will. When the characteristics of a dataset are known, or indeed can be tailored according to need, the effects of new processing methods can be more readily traced than without. In this way, researchers can familiarize themselves with the effects of a data processing pipeline, and make adjustments to that pipeline in light of what they learn by feeding fake data through it, before attempting to use that pipeline on actual science data. Such investigations can be critical to eventually arguing for the credibility of the final empirical results and their appropriate interpretation and use.
Data assimilation is perhaps a less widely appreciated aspect of model-based data processing among philosophers of science, excepting Parker (2016; 2017). Bokulich characterizes this method as “the optimal integration of data with dynamical model estimates to provide a more accurate ‘assimilation estimate’ of the quantity” (2020, 800). Thus, data assimilation involves balancing the contributions of empirical data and the output of models in an integrated estimate, according to the uncertainties associated with these contributions.
Bokulich argues that the involvement of models in these various aspects of data processing does not necessarily lead to better epistemic outcomes. Done wrong, integrating models and data can introduce artifacts and make the processed data unreliable for the purpose at hand (ibid., 804). Indeed, she notes that “[t]here is much work for methodologically reflective scientists and philosophers of science to do in string out cases in which model-data symbiosis may be problematic or circular” (ibid.)
3. Theory and value ladenness
Empirical results are laden with values and theoretical commitments. Philosophers have raised and appraised several possible kinds of epistemic problems that could be associated with theory and/or value-laden empirical results. They have worried about the extent to which human perception itself is distorted by our commitments. They have worried that drawing upon theoretical resources from the very theory to be appraised (or its competitors) in the generation of empirical results yields vicious circularity (or inconsistency). They have also worried that contingent conceptual and/or linguistic frameworks trap bits of evidence like bees in amber so that they cannot carry on their epistemic lives outside of the contexts of their origination, and that normative values necessarily corrupt the integrity of science. Do the theory and value-ladenness of empirical results render them hopelessly parochial? That is, when scientists leave theoretical commitments behind and adopt new ones, must they also relinquish the fruits of the empirical research imbued with their prior commitments too? In this section, we discuss these worries and responses that philosophers have offered to assuage them.
If you believe that observation by human sense perception is the objective basis of all scientific knowledge, then you ought to be particularly worried about the potential for human perception to be corrupted by theoretical assumptions, wishful thinking, framing effects, and so on. Daston and Galison recount the striking example of Arthur Worthington’s symmetrical milk drops (2007, 11–16). Working in 1875, Worthington investigated the hydrodynamics of falling fluid droplets and their evolution upon impacting a hard surface. At first, he had tried to carefully track the drop dynamics with a strobe light to burn a sequence of images into his own retinas. The images he drew to record what he saw were radially symmetric, with rays of the drop splashes emanating evenly from the center of the impact. However, when Worthington transitioned from using his eyes and capacity to draw from memory to using photography in 1894, he was shocked to find that the kind of splashes he had been observing were irregular splats (ibid., 13). Even curiouser, when Worthington returned to his drawings, he found that he had indeed recorded some unsymmetrical splashes. He had evidently dismissed them as uninformative accidents instead of regarding them as revelatory of the phenomenon he was intent on studying (ibid.) In attempting to document the ideal form of the splashes, a general and regular form, he had subconsciously down-played the irregularity of individual splashes. If theoretical commitments, like Worthington’s initial commitment to the perfect symmetry of the physics he was studying, pervasively and incorrigibly dictated the results of empirical inquiry, then the epistemic aims of science would be seriously undermined.
Perceptual psychologists, Bruner and Postman, found that subjects who were briefly shown anomalous playing cards, e.g., a black four of hearts, reported having seen their normal counterparts e.g., a red four of hearts. It took repeated exposures to get subjects to say the anomalous cards didn’t look right, and eventually, to describe them correctly (Kuhn 1962, 63). Kuhn took such studies to indicate that things don’t look the same to observers with different conceptual resources. (For a more up-to-date discussion of theory and conceptual perceptual loading see Lupyan 2015.) If so, black hearts didn’t look like black hearts until repeated exposures somehow allowed subjects to acquire the concept of a black heart. By analogy, Kuhn supposed, when observers working in conflicting paradigms look at the same thing, their conceptual limitations should keep them from having the same visual experiences (Kuhn 1962, 111, 113–114, 115, 120–1). This would mean, for example, that when Priestley and Lavoisier watched the same experiment, Lavoisier should have seen what accorded with his theory that combustion and respiration are oxidation processes, while Priestley’s visual experiences should have agreed with his theory that burning and respiration are processes of phlogiston release.
The example of Pettersson’s and Rutherford’s scintillation screen evidence (above) attests to the fact that observers working in different laboratories sometimes report seeing different things under similar conditions. It is plausible that their expectations influence their reports. It is plausible that their expectations are shaped by their training and by their supervisors’ and associates’ theory driven behavior. But as happens in other cases as well, all parties to the dispute agreed to reject Pettersson’s data by appealing to results that both laboratories could obtain and interpret in the same way without compromising their theoretical commitments. Indeed, it is possible for scientists to share empirical results, not just across diverse laboratory cultures, but even across serious differences in worldview. Much as they disagreed about the nature of respiration and combustion, Priestley and Lavoisier gave quantitatively similar reports of how long their mice stayed alive and their candles kept burning in closed bell jars. Priestley taught Lavoisier how to obtain what he took to be measurements of the phlogiston content of an unknown gas. A sample of the gas to be tested is run into a graduated tube filled with water and inverted over a water bath. After noting the height of the water remaining in the tube, the observer adds “nitrous air” (we call it nitric oxide) and checks the water level again. Priestley, who thought there was no such thing as oxygen, believed the change in water level indicated how much phlogiston the gas contained. Lavoisier reported observing the same water levels as Priestley even after he abandoned phlogiston theory and became convinced that changes in water level indicated free oxygen content (Conant 1957, 74–109).
A related issue is that of salience. Kuhn claimed that if Galileo and an Aristotelian physicist had watched the same pendulum experiment, they would not have looked at or attended to the same things. The Aristotelian’s paradigm would have required the experimenter to measure
… the weight of the stone, the vertical height to which it had been raised, and the time required for it to achieve rest (Kuhn 1962, 123)
and ignore radius, angular displacement, and time per swing (ibid., 124). These last were salient to Galileo because he treated pendulum swings as constrained circular motions. The Galilean quantities would be of no interest to an Aristotelian who treats the stone as falling under constraint toward the center of the earth (ibid., 123). Thus Galileo and the Aristotelian would not have collected the same data. (Absent records of Aristotelian pendulum experiments we can think of this as a thought experiment.)
Interests change, however. Scientists may eventually come to appreciate the significance of data that had not originally been salient to them in light of new presuppositions. The moral of these examples is that although paradigms or theoretical commitments sometimes have an epistemically significant influence on what observers perceive or what they attend to, it can be relatively easy to nullify or correct for their effects. When presuppositions cause epistemic damage, investigators are often able to eventually make corrections. Thus, paradigms and theoretical commitments actually do influence saliency, but their influence is neither inevitable nor irremediable.
Thomas Kuhn (1962), Norwood Hanson (1958), Paul Feyerabend (1959) and others cast suspicion on the objectivity of observational evidence in another way by arguing that one cannot use empirical evidence to test a theory without committing oneself to that very theory. This would be a problem if it leads to dogmatism but assuming the theory to be tested is often benign and even necessary.
For instance, Laymon (1988) demonstrates the manner in which the very theory that the Michelson-Morley experiments are considered to test is assumed in the experimental design, but that this does not engender deleterious epistemic effects (250). The Michelson-Morley apparatus consists of two interferometer arms at right angles to one another, which are rotated in the course of the experiment so that, on the original construal, the path length traversed by light in the apparatus would vary according to alignment with or against the Earth’s velocity (carrying the apparatus) with respect to the stationary aether. This difference in path length would show up as displacement in the interference fringes of light in the interferometer. Although Michelson’s intention had been to measure the velocity of the Earth with respect to the all-pervading aether, the experiments eventually came to be regarded as furnishing tests of the Fresnel aether theory itself. In particular, the null results of these experiments were taken as evidence against the existence of the aether. Naively, one might suppose that whatever assumptions were made in the calculation of the results of these experiments, it should not be the case that the theory under the gun was assumed nor that its negation was.
Before Michelson’s experiments, the Fresnel aether theory did not predict any sort of length contraction. Although Michelson assumed no contraction in the arms of the interferometer, Laymon argues that he could have assumed contraction, with no practical impact on the results of the experiments. The predicted fringe shift is calculated from the anticipated difference in the distance traveled by light in the two arms is the same, when higher order terms are neglected. Thus, in practice, the experimenters could assume either that the contraction thesis was true or that it was false when determining the length of the arms. Either way, the results of the experiment would be the same. After Michelson’s experiments returned no evidence of the anticipated aether effects, Lorentz-Fitzgerald contraction was postulated precisely to cancel out the expected (but not found) effects and save the aether theory. Morley and Miller then set out specifically to test the contraction thesis, and still assumed no contraction in determining the length of the arms of their interferometer (ibid., 253). Thus Laymon argues that the Michelson-Morley experiments speak against the tempting assumption that “appraisal of a theory is based on phenomena which can be detected and measured without using assumptions drawn from the theory under examination or from competitors to that theory ” (ibid., 246).
Epistemological hand-wringing about the use of the very theory to be tested in the generation of the evidence to be used for testing, seems to spring primarily from a concern about vicious circularity. How can we have a genuine trial, if the theory in question has been presumed innocent from the outset? While it is true that there would be a serious epistemic problem in a case where the use of the theory to be tested conspired to guarantee that the evidence would turn out to be confirmatory, this is not always the case when theories are invoked in their own testing. Woodward (2011) summarizes a tidy case:
For example, in Millikan’s oil drop experiment, the mere fact that theoretical assumptions (e.g., that the charge of the electron is quantized and that all electrons have the same charge) play a role in motivating his measurements or a vocabulary for describing his results does not by itself show that his design and data analysis were of such a character as to guarantee that he would obtain results supporting his theoretical assumptions. His experiment was such that he might well have obtained results showing that the charge of the electron was not quantized or that there was no single stable value for this quantity. (178)
For any given case, determining whether the theoretical assumptions being made are benign or straight-jacketing the results that it will be possible to obtain will require investigating the particular relationships between the assumptions and results in that case. When data production and analysis processes are complicated, this task can get difficult. But the point is that merely noting the involvement of the theory to be tested in the generation of empirical results does not by itself imply that those results cannot be objectively useful for deciding whether the theory to be tested should be accepted or rejected.
Kuhn argued that theoretical commitments exert a strong influence on observation descriptions, and what they are understood to mean (Kuhn 1962, 127ff; Longino 1979, 38–42). If so, proponents of a caloric account of heat won’t describe or understand descriptions of observed results of heat experiments in the same way as investigators who think of heat in terms of mean kinetic energy or radiation. They might all use the same words (e.g., ‘temperature’) to report an observation without understanding them in the same way. This poses a potential problem for communicating effectively across paradigms, and similarly, for attributing the appropriate significance to empirical results generated outside of one’s own linguistic framework.
It is important to bear in mind that observers do not always use declarative sentences to report observational and experimental results. Instead, they often draw, photograph, make audio recordings, etc. or set up their experimental devices to generate graphs, pictorial images, tables of numbers, and other non-sentential records. Obviously investigators’ conceptual resources and theoretical biases can exert epistemically significant influences on what they record (or set their equipment to record), which details they include or emphasize, and which forms of representation they choose (Daston and Galison 2007, 115–190, 309–361). But disagreements about the epistemic import of a graph, picture or other non-sentential bit of data often turn on causal rather than semantical considerations. Anatomists may have to decide whether a dark spot in a micrograph was caused by a staining artifact or by light reflected from an anatomically significant structure. Physicists may wonder whether a blip in a Geiger counter record reflects the causal influence of the radiation they wanted to monitor, or a surge in ambient radiation. Chemists may worry about the purity of samples used to obtain data. Such questions are not, and are not well represented as, semantic questions to which semantic theory loading is relevant. Late 20 th century philosophers may have ignored such cases and exaggerated the influence of semantic theory loading because they thought of theory testing in terms of inferential relations between observation and theoretical sentences.
Nevertheless, some empirical results are reported as declarative sentences. Looking at a patient with red spots and a fever, an investigator might report having seen the spots, or measles symptoms, or a patient with measles. Watching an unknown liquid dripping into a litmus solution an observer might report seeing a change in color, a liquid with a PH of less than 7, or an acid. The appropriateness of a description of a test outcome depends on how the relevant concepts are operationalized. What justifies an observer to report having observed a case of measles according to one operationalization might require her to say no more than that she had observed measles symptoms, or just red spots according to another.
In keeping with Percy Bridgman’s view that
… in general, we mean by a concept nothing more than a set of operations; the concept is synonymous with the corresponding sets of operations (Bridgman 1927, 5)
one might suppose that operationalizations are definitions or meaning rules such that it is analytically true, e.g., that every liquid that turns litmus red in a properly conducted test is acidic. But it is more faithful to actual scientific practice to think of operationalizations as defeasible rules for the application of a concept such that both the rules and their applications are subject to revision on the basis of new empirical or theoretical developments. So understood, to operationalize is to adopt verbal and related practices for the purpose of enabling scientists to do their work. Operationalizations are thus sensitive and subject to change on the basis of findings that influence their usefulness (Feest 2005).
Definitional or not, investigators in different research traditions may be trained to report their observations in conformity with conflicting operationalizations. Thus instead of training observers to describe what they see in a bubble chamber as a whitish streak or a trail, one might train them to say they see a particle track or even a particle. This may reflect what Kuhn meant by suggesting that some observers might be justified or even required to describe themselves as having seen oxygen, transparent and colorless though it is, or atoms, invisible though they are (Kuhn 1962, 127ff). To the contrary, one might object that what one sees should not be confused with what one is trained to say when one sees it, and therefore that talking about seeing a colorless gas or an invisible particle may be nothing more than a picturesque way of talking about what certain operationalizations entitle observers to say. Strictly speaking, the objection concludes, the term ‘observation report’ should be reserved for descriptions that are neutral with respect to conflicting operationalizations.
If observational data are just those utterances that meet Feyerabend’s decidability and agreeability conditions, the import of semantic theory loading depends upon how quickly, and for which sentences reasonably sophisticated language users who stand in different paradigms can non-inferentially reach the same decisions about what to assert or deny. Some would expect enough agreement to secure the objectivity of observational data. Others would not. Still others would try to supply different standards for objectivity.
With regard to sentential observation reports, the significance of semantic theory loading is less ubiquitous than one might expect. The interpretation of verbal reports often depends on ideas about causal structure rather than the meanings of signs. Rather than worrying about the meaning of words used to describe their observations, scientists are more likely to wonder whether the observers made up or withheld information, whether one or more details were artifacts of observation conditions, whether the specimens were atypical, and so on.
Note that the worry about semantic theory loading extends beyond observation reports of the sort that occupied the logical empiricists and their close intellectual descendents. Combining results of diverse methods for making proxy measurements of paleoclimate temperatures in an epistemically responsible way requires careful attention to the variety of operationalizations at play. Even if no ‘observation reports’ are involved, the sticky question about how to usefully merge results obtained in different ways in order to satisfy one’s epistemic aims remains. Happily, the remedy for the worry about semantic loading in this broader sense is likely to be the same—investigating the provenance of those results and comparing the variety of factors that have contributed to their causal production.
Kuhn placed too much emphasis on the discontinuity between evidence generated in different paradigms. Even if we accept a broadly Kuhnian picture, according to which paradigms are heterogeneous collections of experimental practices, theoretical principles, problems selected for investigation, approaches to their solution, etc., connections between components are loose enough to allow investigators who disagree profoundly over one or more theoretical claims to nevertheless agree about how to design, execute, and record the results of their experiments. That is why neuroscientists who disagreed about whether nerve impulses consisted of electrical currents could measure the same electrical quantities, and agree on the linguistic meaning and the accuracy of observation reports including such terms as ‘potential’, ‘resistance’, ‘voltage’ and ‘current’. As we discussed above, the success that scientists have in repurposing results generated by others for different purposes speaks against the confinement of evidence to its native paradigm. Even when scientists working with radically different core theoretical commitments cannot make the same measurements themselves, with enough contextual information about how each conducts research, it can be possible to construct bridges that span the theoretical divides.
One could worry that the intertwining of the theoretical and empirical would open the floodgates to bias in science. Human cognizing, both historical and present day, is replete with disturbing commitments including intolerance and narrow mindedness of many sorts. If such commitments are integral to a theoretical framework, or endemic to the reasoning of a scientist or scientific community, then they threaten to corrupt the epistemic utility of empirical results generated using their resources. The core impetus of the ‘value-free ideal’ is to maintain a safe distance between the appraisal of scientific theories according to the evidence on one hand, and the swarm of moral, political, social, and economic values on the other. While proponents of the value-free ideal might admit that the motivation to pursue a theory or the legal protection of human subjects in permissible experimental methods involve non-epistemic values, they would contend that such values ought not ought not enter into the constitution of empirical results themselves, nor the adjudication or justification of scientific theorizing in light of the evidence (see Intemann 2021, 202).
As a matter of fact, values do enter into science at a variety of stages. Above we saw that ‘theory-ladenness’ could refer to the involvement of theory in perception, in semantics, and in a kind of circularity that some have worried begets unfalsifiability and thereby dogmatism. Like theory-ladenness, values can and sometimes do affect judgments about the salience of certain evidence and the conceptual framing of data. Indeed, on a permissive construal of the nature of theories, values can simply be understood as part of a theoretical framework. Intemann (2021) highlights a striking example from medical research where key conceptual resources include notions like ‘harm,’ ‘risk,’ ‘health benefit,’ and ‘safety.’ She refers to research on the comparative safety of giving birth at home and giving birth at a hospital for low-risk parents in the United States. Studies reporting that home births are less safe typically attend to infant and birthing parent mortality rates—which are low for these subjects whether at home or in hospital—but leave out of consideration rates of c-section and episiotomy, which are both relatively high in hospital settings. Thus, a value-laden decision about whether a possible outcome counts as a harm worth considering can influence the outcome of the study—in this case tipping the balance towards the conclusion that hospital births are more safe (ibid., 206).
Note that the birth safety case differs from the sort of cases at issue in the philosophical debate about risk and thresholds for acceptance and rejection of hypotheses. In accepting an hypothesis, a person makes a judgement that the risk of being mistaken is sufficiently low (Rudner 1953). When the consequences of being wrong are deemed grave, the threshold for acceptance may be correspondingly high. Thus, in evaluating the epistemic status of an hypothesis in light of the evidence, a person may have to make a value-based judgement. However, in the birth safety case, the judgement comes into play at an earlier stage, well before the decision to accept or reject the hypothesis is to be made. The judgement occurs already in deciding what is to count as a ‘harm’ worth considering for the purposes of this research.
The fact that values do sometimes enter into scientific reasoning does not by itself settle the question of whether it would be better if they did not. In order to assess the normative proposal, philosophers of science have attempted to disambiguate the various ways in which values might be thought to enter into science, and the various referents that get crammed under the single heading of ‘values.’ Anderson (2004) articulates eight stages of scientific research where values (‘evaluative presuppositions’) might be employed in epistemically fruitful ways. In paraphrase: 1) orientation in a field, 2) framing a research question, 3) conceptualizing the target, 4) identifying relevant data, 5) data generation, 6) data analysis, 7) deciding when to cease data analysis, and 8) drawing conclusions (Anderson 2004, 11). Similarly, Intemann (2021) lays out five ways “that values play a role in scientific reasoning” with which feminist philosophers of science have engaged in particular:
(1) the framing [of] research problems, (2) observing phenomena and describing data, (3) reasoning about value-laden concepts and assessing risks, (4) adopting particular models, and (5) collecting and interpreting evidence. (208)
Ward (2021) presents a streamlined and general taxonomy of four ways in which values relate to choices: as reasons motivating or justifying choices, as causal effectors of choices, or as goods affected by choices. By investigating the role of values in these particular stages or aspects of research, philosophers of science can offer higher resolution insights than just the observation that values are involved in science at all and untangle crosstalk.
Similarly, fine points can be made about the nature of values involved in these various contexts. Such clarification is likely important for determining whether the contribution of certain values in a given context is deleterious or salutary, and in what sense. Douglas (2013) argues that the ‘value’ of internal consistency of a theory and of the empirical adequacy of a theory with respect to the available evidence are minimal criteria for any viable scientific theory (799–800). She contrasts these with the sort of values that Kuhn called ‘virtues,’ i.e. scope, simplicity, and explanatory power that are properties of theories themselves, and unification, novel prediction and precision, which are properties a theory has in relation to a body of evidence (800–801). These are the sort of values that may be relevant to explaining and justifying choices that scientists make to pursue/abandon or accept/reject particular theories. Moreover, Douglas (2000) argues that what she calls “non-epistemic values” (in particular, ethical value judgements) also enter into decisions at various stages “internal” to scientific reasoning, such as data collection and interpretation (565). Consider a laboratory toxicology study in which animals exposed to dioxins are compared to unexposed controls. Douglas discusses researchers who want to determine the threshold for safe exposure. Admitting false positives can be expected to lead to overregulation of the chemical industry, while false negatives yield underregulation and thus pose greater risk to public health. The decision about where to set the unsafe exposure threshold, that is, set the threshold for a statistically significant difference between experimental and control animal populations, involves balancing the acceptability of these two types of errors. According to Douglas, this balancing act will depend on “whether we are more concerned about protecting public health from dioxin pollution or whether we are more concerned about protecting industries that produce dioxins from increased regulation” (ibid., 568). That scientists do as a matter of fact sometimes make such decisions is clear. They judge, for instance, a specimen slide of a rat liver to be tumorous or not, and whether borderline cases should count as benign or malignant (ibid., 569–572). Moreover, in such cases, it is not clear that the responsibility of making such decisions could be offloaded to non-scientists.
Many philosophers accept that values can contribute to the generation of empirical results without spoiling their epistemic utility. Anderson’s (2004) diagnosis is as follows:
Deep down, what the objectors find worrisome about allowing value judgments to guide scientific inquiry is not that they have evaluative content, but that these judgments might be held dogmatically, so as to preclude the recognition of evidence that might undermine them. We need to ensure that value judgements do not operate to drive inquiry to a predetermined conclusion. This is our fundamental criterion for distinguishing legitimate from illegitimate uses of values in science. (11)
Data production (including experimental design and execution) is heavily influenced by investigators’ background assumptions. Sometimes these include theoretical commitments that lead experimentalists to produce non-illuminating or misleading evidence. In other cases they may lead experimentalists to ignore, or even fail to produce useful evidence. For example, in order to obtain data on orgasms in female stumptail macaques, one researcher wired up females to produce radio records of orgasmic muscle contractions, heart rate increases, etc. But as Elisabeth Lloyd reports, “… the researcher … wired up the heart rate of the male macaques as the signal to start recording the female orgasms. When I pointed out that the vast majority of female stumptail orgasms occurred during sex among the females alone, he replied that yes he knew that, but he was only interested in important orgasms” (Lloyd 1993, 142). Although female stumptail orgasms occurring during sex with males are atypical, the experimental design was driven by the assumption that what makes features of female sexuality worth studying is their contribution to reproduction (ibid., 139). This assumption influenced experimental design in such a way as to preclude learning about the full range of female stumptail orgasms.
Anderson (2004) presents an influential analysis of the role of values in research on divorce. Researchers committed to an interpretive framework rooted in ‘traditional family values’ could conduct research on the assumption that divorce is mostly bad for spouses and any children that they have (ibid., 12). This background assumption, which is rooted in a normative appraisal of a certain model of good family life, could lead social science researchers to restrict the questions with which they survey their research subjects to ones about the negative impacts of divorce on their lives, thereby curtailing the possibility of discovering ways that divorce may have actually made the ex-spouses lives better (ibid., 13). This is an example of the influence that values can have on the nature of the results that research ultimately yields, which is epistemically detrimental. In this case, the values in play biased the research outcomes to preclude recognition of countervailing evidence. Anderson argues that the problematic influence of values comes when research “is rigged in advance” to confirm certain hypotheses—when the influence of values amounts to incorrigible dogmatism (ibid., 19). “Dogmatism” in her sense is unfalsifiability in practice, “their stubbornness in the face of any conceivable evidence”(ibid., 22).
Fortunately, such dogmatism is not ubiquitous and when it occurs it can often be corrected eventually. Above we noted that the mere involvement of the theory to be tested in the generation of an empirical result does not automatically yield vicious circularity—it depends on how the theory is involved. Furthermore, even if the assumptions initially made in the generation of empirical results are incorrect, future scientists will have opportunities to reassess those assumptions in light of new information and techniques. Thus, as long as scientists continue their work there need be no time at which the epistemic value of an empirical result can be established once and for all. This should come as no surprise to anyone who is aware that science is fallible, but it is no grounds for skepticism. It can be perfectly reasonable to trust the evidence available at present even though it is logically possible for epistemic troubles to arise in the future. A similar point can be made regarding values (although cf. Yap 2016).
Moreover, while the inclusion of values in the generation of an empirical result can sometimes be epistemically bad, values properly deployed can also be harmless, or even epistemically helpful. As in the cases of research on female stumptail macaque orgasms and the effects of divorce, certain values can sometimes serve to illuminate the way in which other epistemically problematic assumptions have hindered potential scientific insight. By valuing knowledge about female sexuality beyond its role in reproduction, scientists can recognize the narrowness of an approach that only conceives of female sexuality insofar as it relates to reproduction. By questioning the absolute value of one traditional ideal for flourishing families, researchers can garner evidence that might end up destabilizing the empirical foundation supporting that ideal.
Empirical results are most obviously put to epistemic work in their contexts of origin. Scientists conceive of empirical research, collect and analyze the relevant data, and then bring the results to bear on the theoretical issues that inspired the research in the first place. However, philosophers have also discussed ways in which empirical results are transferred out of their native contexts and applied in diverse and sometimes unexpected ways (see Leonelli and Tempini 2020). Cases of reuse, or repurposing of empirical results in different epistemic contexts raise several interesting issues for philosophers of science. For one, such cases challenge the assumption that theory (and value) ladenness confines the epistemic utility of empirical results to a particular conceptual framework. Ancient Babylonian eclipse records inscribed on cuneiform tablets have been used to generate constraints on contemporary geophysical theorizing about the causes of the lengthening of the day on Earth (Stephenson, Morrison, and Hohenkerk 2016). This is surprising since the ancient observations were originally recorded for the purpose of making astrological prognostications. Nevertheless, with enough background information, the records as inscribed can be translated, the layers of assumptions baked into their presentation peeled back, and the results repurposed using resources of the contemporary epistemic context, the likes of which the Babylonians could have hardly dreamed.
Furthermore, the potential for reuse and repurposing feeds back on the methodological norms of data production and handling. In light of the difficulty of reusing or repurposing data without sufficient background information about the original context, Goodman et al. (2014) note that “data reuse is most possible when: 1) data; 2) metadata (information describing the data); and 3) information about the process of generating those data, such as code, all all provided” (3). Indeed, they advocate for sharing data and code in addition to results customarily published in science. As we have seen, the loading of data with theory is usually necessary to putting that data to any serious epistemic use—theory-loading makes theory appraisal possible. Philosophers have begun to appreciate that this epistemic boon does not necessarily come at the cost of rendering data “tragically local” (Wylie 2020, 285, quoting Latour 1999). But it is important to note the useful travel of data between contexts is significantly aided by foresight, curation, and management for that aim.
In light of the mediated nature of empirical results, Boyd (2018) argues for an “enriched view of evidence,” in which the evidence that serves as the ‘tribunal of experience’ is understood to be “lines of evidence” composed of the products of data collection and all of the products of their transformation on the way to the generation of empirical results that are ultimately compared to theoretical predictions, considered together with metadata associated with their provenance. Such metadata includes information about theoretical assumptions that are made in data collection, processing, and the presentation of empirical results. Boyd argues that by appealing to metadata to ‘rewind’ the processing of assumption-imbued empirical results and then by re-processing them using new resources, the epistemic utility of empirical evidence can survive transitions to new contexts. Thus, the enriched view of evidence supports the idea that it is not despite the intertwining of the theoretical and empirical that scientists accomplish key epistemic aims, but often in virtue of it (ibid., 420). In addition, it makes the epistemic value of metadata encoding the various assumptions that have been made throughout the course of data collection and processing explicit.
The desirability of explicitly furnishing empirical data and results with auxiliary information that allow them to travel can be appreciated in light of the ‘objectivity’ norm, construed as accessibility to interpersonal scrutiny. When data are repurposed in novel contexts, they are not only shared between subjects, but can in some cases be shared across radically different paradigms with incompatible theoretical commitments.
4. The epistemic value of empirical evidence
One of the important applications of empirical evidence is its use in assessing the epistemic status of scientific theories. In this section we briefly discuss philosophical work on the role of empirical evidence in confirmation/falsification of scientific theories, ‘saving the phenomena,’ and in appraising the empirical adequacy of theories. However, further philosophical work ought to explore the variety of ways that empirical results bear on the epistemic status of theories and theorizing in scientific practice beyond these.
It is natural to think that computability, range of application, and other things being equal, true theories are better than false ones, good approximations are better than bad ones, and highly probable theoretical claims are better than less probable ones. One way to decide whether a theory or a theoretical claim is true, close to the truth, or acceptably probable is to derive predictions from it and use empirical data to evaluate them. Hypothetico-Deductive (HD) confirmation theorists proposed that empirical evidence argues for the truth of theories whose deductive consequences it verifies, and against those whose consequences it falsifies (Popper 1959, 32–34). But laws and theoretical generalization seldom if ever entail observational predictions unless they are conjoined with one or more auxiliary hypotheses taken from the theory they belong to. When the prediction turns out to be false, HD has trouble explaining which of the conjuncts is to blame. If a theory entails a true prediction, it will continue to do so in conjunction with arbitrarily selected irrelevant claims. HD has trouble explaining why the prediction does not confirm the irrelevancies along with the theory of interest.
Another approach to confirmation by empirical evidence is Inference to the Best Explanation (IBE). The idea is roughly that an explanation of the evidence that exhibits certain desirable characteristics with respect to a family of candidate explanations is likely to be the true on (Lipton 1991). On this approach, it is in virtue of their successful explanation of the empirical evidence that theoretical claims are supported. Naturally, IBE advocates face the challenges of defending a suitable characterization of what counts as the ‘best’ and of justifying the limited pool of candidate explanations considered (Stanford 2006).
Bayesian approaches to scientific confirmation have garnered significant attention and are now widespread in philosophy of science. Bayesians hold that the evidential bearing of empirical evidence on a theoretical claim is to be understood in terms of likelihood or conditional probability. For example, whether empirical evidence argues for a theoretical claim might be thought to depend upon whether it is more probable (and if so how much more probable) than its denial conditional on a description of the evidence together with background beliefs, including theoretical commitments. But by Bayes’ Theorem, the posterior probability of the claim of interest (that is, its probability given the evidence) is proportional to that claim’s prior probability. How to justify the choice of these prior probability assignments is one of the most notorious points of contention arising for Bayesians. If one makes the assignment of priors a subjective matter decided by epistemic agents, then it is not clear that they can be justified. Once again, one’s use of evidence to evaluate a theory depends in part upon one’s theoretical commitments (Earman 1992, 33–86; Roush 2005, 149–186). If one instead appeals to chains of successive updating using Bayes’ Theorem based on past evidence, one has to invoke assumptions that generally do not obtain in actual scientific reasoning. For instance, to ‘wash out’ the influence of priors a limit theorem is invoked wherein we consider very many updating iterations, but much scientific reasoning of interest does not happen in the limit, and so in practice priors hold unjustified sway (Norton 2021, 33).
Rather than attempting to cast all instances of confirmation based on empirical evidence as belonging to a universal schema, a better approach may be to ‘go local’. Norton’s material theory of induction argues that inductive support arises from background knowledge, that is, from material facts that are domain specific. Norton argues that, for instance, the induction from “Some samples of the element bismuth melt at 271°C” to “all samples of the element bismuth melt at 271°C” is admissible not in virtue of some universal schema that carries us from ‘some’ to ‘all’ but matters of fact (Norton 2003). In this particular case, the fact that licenses the induction is a fact about elements: “their samples are generally uniform in their physical properties” (ibid., 650). This is a fact pertinent to chemical elements, but not to samples of material like wax (ibid.). Thus Norton repeatedly emphasizes that “all induction is local”.
Still, there are those who may be skeptical about the very possibility of confirmation or of successful induction. Insofar as the bearing of evidence on theory is never totally decisive, insofar there is no single trusty universal schema that captures empirical support, perhaps the relationship between empirical evidence and scientific theory is not really about support after all. Giving up on empirical support would not automatically mean abandoning any epistemic value for empirical evidence. Rather than confirm theory, the epistemic role of evidence could be to constrain, for example by furnishing phenomena for theory to systematize or to adequately model.
Theories are said to ‘save’ observable phenomena if they satisfactorily predict, describe, or systematize them. How well a theory performs any of these tasks need not depend upon the truth or accuracy of its basic principles. Thus according to Osiander’s preface to Copernicus’ On the Revolutions , a locus classicus, astronomers “… cannot in any way attain to true causes” of the regularities among observable astronomical events, and must content themselves with saving the phenomena in the sense of using
… whatever suppositions enable … [them] to be computed correctly from the principles of geometry for the future as well as the past … (Osiander 1543, XX)
Theorists are to use those assumptions as calculating tools without committing themselves to their truth. In particular, the assumption that the planets revolve around the sun must be evaluated solely in terms of how useful it is in calculating their observable relative positions to a satisfactory approximation. Pierre Duhem’s Aim and Structure of Physical Theory articulates a related conception. For Duhem a physical theory
… is a system of mathematical propositions, deduced from a small number of principles, which aim to represent as simply and completely, and exactly as possible, a set of experimental laws. (Duhem 1906, 19)
‘Experimental laws’ are general, mathematical descriptions of observable experimental results. Investigators produce them by performing measuring and other experimental operations and assigning symbols to perceptible results according to pre-established operational definitions (Duhem 1906, 19). For Duhem, the main function of a physical theory is to help us store and retrieve information about observables we would not otherwise be able to keep track of. If that is what a theory is supposed to accomplish, its main virtue should be intellectual economy. Theorists are to replace reports of individual observations with experimental laws and devise higher level laws (the fewer, the better) from which experimental laws (the more, the better) can be mathematically derived (Duhem 1906, 21ff).
A theory’s experimental laws can be tested for accuracy and comprehensiveness by comparing them to observational data. Let EL be one or more experimental laws that perform acceptably well on such tests. Higher level laws can then be evaluated on the basis of how well they integrate EL into the rest of the theory. Some data that don’t fit integrated experimental laws won’t be interesting enough to worry about. Other data may need to be accommodated by replacing or modifying one or more experimental laws or adding new ones. If the required additions, modifications or replacements deliver experimental laws that are harder to integrate, the data count against the theory. If the required changes are conducive to improved systematization the data count in favor of it. If the required changes make no difference, the data don’t argue for or against the theory.
On van Fraassen’s (1980) semantic account, a theory is empirically adequate when the empirical structure of at least one model of that theory is isomorphic to what he calls the “appearances” (45). In other words, when the theory “has at least one model that all the actual phenomena fit inside” (12). Thus, for van Fraassen, we continually check the empirical adequacy of our theories by seeing if they have the structural resources to accommodate new observations. We’ll never know that a given theory is totally empirically adequate, since for van Fraassen, empirical adequacy obtains with respect to all that is observable in principle to creatures like us, not all that has already been observed (69).
The primary appeal of dealing in empirical adequacy rather than confirmation is its appropriate epistemic humility. Instead of claiming that confirming evidence justifies belief (or boosted confidence) that a theory is true, one is restricted to saying that the theory continues to be consistent with the evidence as far as we can tell so far. However, if the epistemic utility of empirical results in appraising the status of theories is just to judge their empirical adequacy, then it may be difficult to account for the difference between adequate but unrealistic theories, and those equally adequate theories that ought to be taken seriously as representations. Appealing to extra-empirical virtues like parsimony may be a way out, but one that will not appeal to philosophers skeptical of the connection thereby supposed between such virtues and representational fidelity.
On an earlier way of thinking, observation was to serve as the unmediated foundation of science—direct access to the facts upon which the edifice of scientific knowledge could be built. When conflict arose between factions with different ideological commitments, observations could furnish the material for neutral arbitration and settle the matter objectively, in virtue of being independent of non-empirical commitments. According to this view, scientists working in different paradigms could at least appeal to the same observations, and propagandists could be held accountable to the publicly accessible content of theory and value-free observations. Despite their different theories, Priestley and Lavoisier could find shared ground in the observations. Anti-Semites would be compelled to admit the success of a theory authored by a Jewish physicist, in virtue of the unassailable facts revealed by observation.
This version of empiricism with respect to science does not accord well with the fact that observation per se plays a relatively small role in many actual scientific methodologies, and the fact that even the most ‘raw’ data is often already theoretically imbued. The strict contrast between theory and observation in science is more fruitfully supplanted by inquiry into the relationship between theorizing and empirical results.
Contemporary philosophers of science tend to embrace the theory ladenness of empirical results. Instead of seeing the integration of the theoretical and the empirical as an impediment to furthering scientific knowledge, they see it as necessary. A ‘view from nowhere’ would not bear on our particular theories. That is, it is impossible to put empirical results to use without recruiting some theoretical resources. In order to use an empirical result to constrain or test a theory it has to be processed into a form that can be compared to that theory. To get stellar spectrograms to bear on Newtonian or relativistic cosmology, they need to be processed—into galactic rotation curves, say. The spectrograms by themselves are just artifacts, pieces of paper. Scientists need theoretical resources in order to even identify that such artifacts bear information relevant for their purposes, and certainly to put them to any epistemic use in assessing theories.
This outlook does not render contemporary philosophers of science all constructivists, however. Theory mediates the connection between the target of inquiry and the scientific worldview, it does not sever it. Moreover, vigilance is still required to ensure that the particular ways in which theory is ‘involved’ in the production of empirical results are not epistemically detrimental. Theory can be deployed in experiment design, data processing, and presentation of results in unproductive ways, for instance, in determining whether the results will speak for or against a particular theory regardless of what the world is like. Critical appraisal of the roles of theory is thus important for genuine learning about nature through science. Indeed, it seems that extra-empirical values can sometimes assist such critical appraisal. Instead of viewing observation as the theory-free and for that reason furnishing the content with which to appraise theories, we might attend to the choices and mistakes that can be made in collecting and generating empirical results with the help of theoretical resources, and endeavor to make choices conducive to learning and correct mistakes as we discover them.
Recognizing the involvement of theory and values in the constitution and generation of empirical results does not undermine the special epistemic value of empirical science in contrast to propaganda and pseudoscience. In cases where the influence of cultural, political, and religious values hinder scientific inquiry, it is often the case that they do so by limiting or determining the nature of the empirical results. Yet, by working to make the assumptions that shape results explicit we can examine their suitability for our purposes and attempt to restructure inquiry as necessary. When disagreements arise, scientists can attempt to settle them by appealing to the causal connections between the research target and the empirical data. The tribunal of experience speaks through empirical results, but it only does so through via careful fashioning with theoretical resources.
- Anderson, E., 2004, “Uses of Value Judgments in Science: A General Argument, with Lessons from a Case Study of Feminist Research on Divorce,” Hypatia , 19(1): 1–24.
- Aristotle(a), Generation of Animals in Complete Works of Aristotle (Volume 1), J. Barnes (ed.), Princeton: Princeton University Press, 1995, pp. 774–993
- Aristotle(b), History of Animals in Complete Works of Aristotle (Volume 1), J. Barnes (ed.), Princeton: Princeton University Press, 1995, pp. 1111–1228.
- Azzouni, J., 2004, “Theory, Observation, and Scientific Realism,” British Journal for the Philosophy of Science , 55(3): 371–92.
- Bacon, Francis, 1620, Novum Organum with other parts of the Great Instauration , P. Urbach and J. Gibson (eds. and trans.), La Salle: Open Court, 1994.
- Bogen, J., 2016, “Empiricism and After,”in P. Humphreys (ed.), Oxford Handbook of Philosophy of Science , Oxford: Oxford University Press, pp. 779–795.
- Bogen, J, and Woodward, J., 1988, “Saving the Phenomena,” Philosophical Review , XCVII (3): 303–352.
- Bokulich, A., 2020, “Towards a Taxonomy of the Model-Ladenness of Data,” Philosophy of Science , 87(5): 793–806.
- Borrelli, A., 2012, “The Case of the Composite Higgs: The Model as a ‘Rosetta Stone’ in Contemporary High-Energy Physics,” Studies in History and Philosophy of Science (Part B: Studies in History and Philosophy of Modern Physics), 43(3): 195–214.
- Boyd, N. M., 2018, “Evidence Enriched,” Philosophy of Science , 85(3): 403–21.
- Boyle, R., 1661, The Sceptical Chymist , Montana: Kessinger (reprint of 1661 edition).
- Bridgman, P., 1927, The Logic of Modern Physics , New York: Macmillan.
- Chang, H., 2005, “A Case for Old-fashioned Observability, and a Reconstructive Empiricism,” Philosophy of Science , 72(5): 876–887.
- Collins, H. M., 1985 Changing Order , Chicago: University of Chicago Press.
- Conant, J.B., 1957, (ed.) “The Overthrow of the Phlogiston Theory: The Chemical Revolution of 1775–1789,” in J.B.Conant and L.K. Nash (eds.), Harvard Studies in Experimental Science , Volume I, Cambridge: Harvard University Press, pp. 65–116).
- Daston, L., and P. Galison, 2007, Objectivity , Brooklyn: Zone Books.
- Douglas, H., 2000, “Inductive Risk and Values in Science,” Philosophy of Science , 67(4): 559–79.
- –––, 2013, “The Value of Cognitive Values,” Philosophy of Science , 80(5): 796–806.
- Duhem, P., 1906, The Aim and Structure of Physical Theory , P. Wiener (tr.), Princeton: Princeton University Press, 1991.
- Earman, J., 1992, Bayes or Bust? , Cambridge: MIT Press.
- Feest, U., 2005, “Operationism in psychology: what the debate is about, what the debate should be about,” Journal of the History of the Behavioral Sciences , 41(2): 131–149.
- Feyerabend, P.K., 1969, “Science Without Experience,” in P.K. Feyerabend, Realism, Rationalism, and Scientific Method (Philosophical Papers I), Cambridge: Cambridge University Press, 1985, pp. 132–136.
- Franklin, A., 1986, The Neglect of Experiment , Cambridge: Cambridge University Press.
- Galison, P., 1987, How Experiments End , Chicago: University of Chicago Press.
- –––, 1990, “Aufbau/Bauhaus: logical positivism and architectural modernism,” Critical Inquiry , 16 (4): 709–753.
- Goodman, A., et al., 2014, “Ten Simple Rules for the Care and Feeding of Scientific Data,” PLoS Computational Biology , 10(4): e1003542.
- Hacking, I., 1981, “Do We See Through a Microscope?,” Pacific Philosophical Quarterly , 62(4): 305–322.
- –––, 1983, Representing and Intervening , Cambridge: Cambridge University Press.
- Hanson, N.R., 1958, Patterns of Discovery , Cambridge, Cambridge University Press.
- Hempel, C.G., 1952, “Fundamentals of Concept Formation in Empirical Science,” in Foundations of the Unity of Science , Volume 2, O. Neurath, R. Carnap, C. Morris (eds.), Chicago: University of Chicago Press, 1970, pp. 651–746.
- Herschel, J. F. W., 1830, Preliminary Discourse on the Study of Natural Philosophy , New York: Johnson Reprint Corp., 1966.
- Hooke, R., 1705, “The Method of Improving Natural Philosophy,” in R. Waller (ed.), The Posthumous Works of Robert Hooke , London: Frank Cass and Company, 1971.
- Horowitz, P., and W. Hill, 2015, The Art of Electronics , third edition, New York: Cambridge University Press.
- Intemann, K., 2021, “Feminist Perspectives on Values in Science,” in S. Crasnow and L. Intemann (eds.), The Routledge Handbook of Feminist Philosophy of Science , New York: Routledge, pp. 201–15.
- Kuhn, T.S., The Structure of Scientific Revolutions , 1962, Chicago: University of Chicago Press, reprinted,1996.
- Latour, B., 1999, “Circulating Reference: Sampling the Soil in the Amazon Forest,” in Pandora’s Hope: Essays on the Reality of Science Studies , Cambridge, MA: Harvard University Press, pp. 24–79.
- Latour, B., and Woolgar, S., 1979, Laboratory Life, The Construction of Scientific Facts , Princeton: Princeton University Press, 1986.
- Laymon, R., 1988, “The Michelson-Morley Experiment and the Appraisal of Theories,” in A. Donovan, L. Laudan, and R. Laudan (eds.), Scrutinizing Science: Empirical Studies of Scientific Change , Baltimore: The Johns Hopkins University Press, pp. 245–266.
- Leonelli, S., 2009, “On the Locality of Data and Claims about Phenomena,” Philosophy of Science , 76(5): 737–49.
- Leonelli, S., and N. Tempini (eds.), 2020, Data Journeys in the Sciences , Cham: Springer.
- Lipton, P., 1991, Inference to the Best Explanation , London: Routledge.
- Lloyd, E.A., 1993, “Pre-theoretical Assumptions In Evolutionary Explanations of Female Sexuality,” Philosophical Studies , 69: 139–153.
- –––, 2012, “The Role of ‘Complex’ Empiricism in the Debates about Satellite Data and Climate Models,”, Studies in History and Philosophy of Science (Part A), 43(2): 390–401.
- Longino, H., 1979, “Evidence and Hypothesis: An Analysis of Evidential Relations,” Philosophy of Science , 46(1): 35–56.
- –––, 2020, “Afterward:Data in Transit,” in S. Leonelli and N. Tempini (eds.), Data Journeys in the Sciences , Cham: Springer, pp. 391–400.
- Lupyan, G., 2015, “Cognitive Penetrability of Perception in the Age of Prediction – Predictive Systems are Penetrable Systems,” Review of Philosophical Psychology , 6(4): 547–569. doi:10.1007/s13164-015-0253-4
- Mill, J. S., 1872, System of Logic , London: Longmans, Green, Reader, and Dyer.
- Norton, J., 2003, “A Material Theory of Induction,” Philosophy of Science , 70(4): 647–70.
- –––, 2021, The Material Theory of Induction , http://www.pitt.edu/~jdnorton/papers/material_theory/Material_Induction_March_14_2021.pdf .
- Nyquist, H., 1928, “Thermal Agitation of Electric Charge in Conductors,” Physical Review , 32(1): 110–13.
- O’Connor, C. and J. O. Weatherall, 2019, The Misinformation Age: How False Beliefs Spread , New Haven: Yale University Press.
- Olesko, K.M. and Holmes, F.L., 1994, “Experiment, Quantification and Discovery: Helmholtz’s Early Physiological Researches, 1843–50,” in D. Cahan, (ed.), Hermann Helmholtz and the Foundations of Nineteenth Century Science , Berkeley: UC Press, pp. 50–108.
- Osiander, A., 1543, “To the Reader Concerning the Hypothesis of this Work,” in N. Copernicus On the Revolutions , E. Rosen (tr., ed.), Baltimore: Johns Hopkins University Press, 1978, p. XX.
- Parker, W. S., 2016, “Reanalysis and Observation: What’s the Difference?,” Bulletin of the American Meteorological Society , 97(9): 1565–72.
- –––, 2017, “Computer Simulation, Measurement, and Data Assimilation,” The British Journal for the Philosophy of Science , 68(1): 273–304.
- Popper, K.R.,1959, The Logic of Scientific Discovery , K.R. Popper (tr.), New York: Basic Books.
- Rheinberger, H. J., 1997, Towards a History of Epistemic Things: Synthesizing Proteins in the Test Tube , Stanford: Stanford University Press.
- Roush, S., 2005, Tracking Truth , Cambridge: Cambridge University Press.
- Rudner, R., 1953, “The Scientist Qua Scientist Makes Value Judgments,” Philosophy of Science , 20(1): 1–6.
- Schlick, M., 1935, “Facts and Propositions,” in Philosophy and Analysis , M. Macdonald (ed.), New York: Philosophical Library, 1954, pp. 232–236.
- Schottky, W. H., 1918, “Über spontane Stromschwankungen in verschiedenen Elektrizitätsleitern,” Annalen der Physik , 362(23): 541–67.
- Shapere, D., 1982, “The Concept of Observation in Science and Philosophy,” Philosophy of Science , 49(4): 485–525.
- Stanford, K., 1991, Exceeding Our Grasp: Science, History, and the Problem of Unconceived Alternatives , Oxford: Oxford University Press.
- Stephenson, F. R., L. V. Morrison, and C. Y. Hohenkerk, 2016, “Measurement of the Earth’s Rotation: 720 BC to AD 2015,” Proceedings of the Royal Society A: Mathematical, Physical and Engineering Sciences , 472: 20160404.
- Stuewer, R.H., 1985, “Artificial Disintegration and the Cambridge-Vienna Controversy,” in P. Achinstein and O. Hannaway (eds.), Observation, Experiment, and Hypothesis in Modern Physical Science , Cambridge, MA: MIT Press, pp. 239–307.
- Suppe, F., 1977, in F. Suppe (ed.) The Structure of Scientific Theories , Urbana: University of Illinois Press.
- Van Fraassen, B.C, 1980, The Scientific Image , Oxford: Clarendon Press.
- Ward, Z. B., 2021, “On Value-Laden Science,” Studies in History and Philosophy of Science Part A , 85: 54–62.
- Whewell, W., 1858, Novum Organon Renovatum , Book II, in William Whewell Theory of Scientific Method , R.E. Butts (ed.), Indianapolis: Hackett Publishing Company, 1989, pp. 103–249.
- Woodward, J. F., 2010, “Data, Phenomena, Signal, and Noise,” Philosophy of Science , 77(5): 792–803.
- –––, 2011, “Data and Phenomena: A Restatement and Defense,” Synthese , 182(1): 165–79.
- Wylie, A., 2020, “Radiocarbon Dating in Archaeology: Triangulation and Traceability,” in S. Leonelli and N. Tempini (eds.), Data Journeys in the Sciences , Cham: Springer, pp. 285–301.
- Yap, A., 2016, “Feminist Radical Empiricism, Values, and Evidence,” Hypatia , 31(1): 58–73.
How to cite this entry . Preview the PDF version of this entry at the Friends of the SEP Society . Look up topics and thinkers related to this entry at the Internet Philosophy Ontology Project (InPhO). Enhanced bibliography for this entry at PhilPapers , with links to its database.
- Confirmation , by Franz Huber, in the Internet Encyclopedia of Philosophy .
- Transcript of Katzmiller v. Dover Area School District (on the teaching of intelligent design).
Bacon, Francis | Bayes’ Theorem | constructive empiricism | Duhem, Pierre | empiricism: logical | epistemology: Bayesian | feminist philosophy, topics: perspectives on science | incommensurability: of scientific theories | Locke, John | measurement: in science | models in science | physics: experiment in | science: and pseudo-science | scientific objectivity | scientific research and big data | statistics, philosophy of
Copyright © 2021 by Nora Mills Boyd < nboyd @ siena . edu > James Bogen
- Accessibility
Support SEP
Mirror sites.
View this site from another server:
- Info about mirror sites
The Stanford Encyclopedia of Philosophy is copyright © 2023 by The Metaphysics Research Lab , Department of Philosophy, Stanford University
Library of Congress Catalog Data: ISSN 1095-5054

- Ask a Librarian

Research: Overview & Approaches
- Getting Started with Undergraduate Research
- Planning & Getting Started
- Building Your Knowledge Base
- Locating Sources
- Reading Scholarly Articles
- Creating a Literature Review
- Productivity & Organizing Research
- Scholarly and Professional Relationships
Introduction to Empirical Research
Databases for finding empirical research, guided search, google scholar, examples of empirical research, sources and further reading.
- Interpretive Research
- Action-Based Research
- Creative & Experimental Approaches
Your Librarian

- Introductory Video This video covers what empirical research is, what kinds of questions and methods empirical researchers use, and some tips for finding empirical research articles in your discipline.

- Guided Search: Finding Empirical Research Articles This is a hands-on tutorial that will allow you to use your own search terms to find resources.

- Study on radiation transfer in human skin for cosmetics
- Long-Term Mobile Phone Use and the Risk of Vestibular Schwannoma: A Danish Nationwide Cohort Study
- Emissions Impacts and Benefits of Plug-In Hybrid Electric Vehicles and Vehicle-to-Grid Services
- Review of design considerations and technological challenges for successful development and deployment of plug-in hybrid electric vehicles
- Endocrine disrupters and human health: could oestrogenic chemicals in body care cosmetics adversely affect breast cancer incidence in women?
- << Previous: Scholarly and Professional Relationships
- Next: Interpretive Research >>
- Last Updated: Aug 13, 2024 12:18 PM
- URL: https://guides.lib.purdue.edu/research_approaches

- University of Memphis Libraries
- Research Guides
Empirical Research: Defining, Identifying, & Finding
Defining empirical research, what is empirical research, quantitative or qualitative.
- Introduction
- Database Tools
- Search Terms
- Image Descriptions
Calfee & Chambliss (2005) (UofM login required) describe empirical research as a "systematic approach for answering certain types of questions." Those questions are answered "[t]hrough the collection of evidence under carefully defined and replicable conditions" (p. 43).
The evidence collected during empirical research is often referred to as "data."
Characteristics of Empirical Research
Emerald Publishing's guide to conducting empirical research identifies a number of common elements to empirical research:
- A research question , which will determine research objectives.
- A particular and planned design for the research, which will depend on the question and which will find ways of answering it with appropriate use of resources.
- The gathering of primary data , which is then analysed.
- A particular methodology for collecting and analysing the data, such as an experiment or survey.
- The limitation of the data to a particular group, area or time scale, known as a sample [emphasis added]: for example, a specific number of employees of a particular company type, or all users of a library over a given time scale. The sample should be somehow representative of a wider population.
- The ability to recreate the study and test the results. This is known as reliability .
- The ability to generalize from the findings to a larger sample and to other situations.
If you see these elements in a research article, you can feel confident that you have found empirical research. Emerald's guide goes into more detail on each element.
Empirical research methodologies can be described as quantitative, qualitative, or a mix of both (usually called mixed-methods).
Ruane (2016) (UofM login required) gets at the basic differences in approach between quantitative and qualitative research:
- Quantitative research -- an approach to documenting reality that relies heavily on numbers both for the measurement of variables and for data analysis (p. 33).
- Qualitative research -- an approach to documenting reality that relies on words and images as the primary data source (p. 33).
Both quantitative and qualitative methods are empirical . If you can recognize that a research study is quantitative or qualitative study, then you have also recognized that it is empirical study.
Below are information on the characteristics of quantitative and qualitative research. This video from Scribbr also offers a good overall introduction to the two approaches to research methodology:
Characteristics of Quantitative Research
Researchers test hypotheses, or theories, based in assumptions about causality, i.e. we expect variable X to cause variable Y. Variables have to be controlled as much as possible to ensure validity. The results explain the relationship between the variables. Measures are based in pre-defined instruments.
Examples: experimental or quasi-experimental design, pretest & post-test, survey or questionnaire with closed-ended questions. Studies that identify factors that influence an outcomes, the utility of an intervention, or understanding predictors of outcomes.
Characteristics of Qualitative Research
Researchers explore “meaning individuals or groups ascribe to social or human problems (Creswell & Creswell, 2018, p3).” Questions and procedures emerge rather than being prescribed. Complexity, nuance, and individual meaning are valued. Research is both inductive and deductive. Data sources are multiple and varied, i.e. interviews, observations, documents, photographs, etc. The researcher is a key instrument and must be reflective of their background, culture, and experiences as influential of the research.
Examples: open question interviews and surveys, focus groups, case studies, grounded theory, ethnography, discourse analysis, narrative, phenomenology, participatory action research.
Calfee, R. C. & Chambliss, M. (2005). The design of empirical research. In J. Flood, D. Lapp, J. R. Squire, & J. Jensen (Eds.), Methods of research on teaching the English language arts: The methodology chapters from the handbook of research on teaching the English language arts (pp. 43-78). Routledge. http://ezproxy.memphis.edu/login?url=http://search.ebscohost.com/login.aspx?direct=true&db=nlebk&AN=125955&site=eds-live&scope=site .
Creswell, J. W., & Creswell, J. D. (2018). Research design: Qualitative, quantitative, and mixed methods approaches (5th ed.). Thousand Oaks: Sage.
How to... conduct empirical research . (n.d.). Emerald Publishing. https://www.emeraldgrouppublishing.com/how-to/research-methods/conduct-empirical-research .
Scribbr. (2019). Quantitative vs. qualitative: The differences explained [video]. YouTube. https://www.youtube.com/watch?v=a-XtVF7Bofg .
Ruane, J. M. (2016). Introducing social research methods : Essentials for getting the edge . Wiley-Blackwell. http://ezproxy.memphis.edu/login?url=http://search.ebscohost.com/login.aspx?direct=true&db=nlebk&AN=1107215&site=eds-live&scope=site .
- << Previous: Home
- Next: Identifying Empirical Research >>
- Last Updated: Apr 2, 2024 11:25 AM
- URL: https://libguides.memphis.edu/empirical-research

Theoretical vs Conceptual Framework
By: Derek Jansen (MBA) | Reviewed By: Eunice Rautenbach (DTech) | March 2023
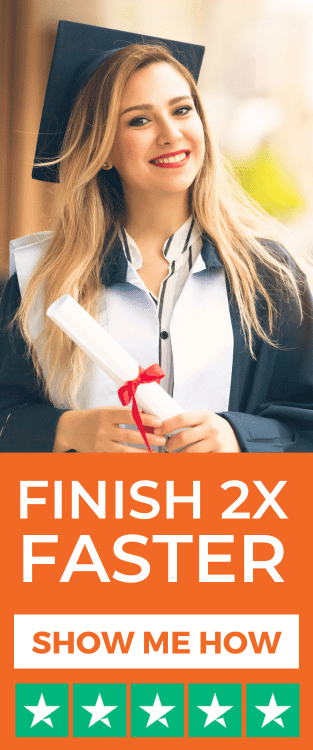
Overview: Theoretical vs Conceptual
What is a theoretical framework, example of a theoretical framework, what is a conceptual framework, example of a conceptual framework.
- Theoretical vs conceptual: which one should I use?
A theoretical framework (also sometimes referred to as a foundation of theory) is essentially a set of concepts, definitions, and propositions that together form a structured, comprehensive view of a specific phenomenon.
In other words, a theoretical framework is a collection of existing theories, models and frameworks that provides a foundation of core knowledge – a “lay of the land”, so to speak, from which you can build a research study. For this reason, it’s usually presented fairly early within the literature review section of a dissertation, thesis or research paper .
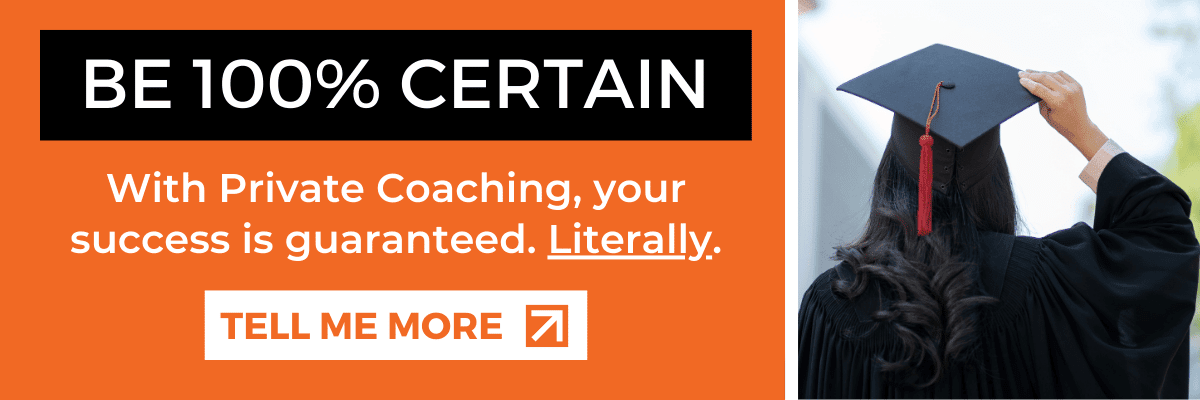
Let’s look at an example to make the theoretical framework a little more tangible.
If your research aims involve understanding what factors contributed toward people trusting investment brokers, you’d need to first lay down some theory so that it’s crystal clear what exactly you mean by this. For example, you would need to define what you mean by “trust”, as there are many potential definitions of this concept. The same would be true for any other constructs or variables of interest.
You’d also need to identify what existing theories have to say in relation to your research aim. In this case, you could discuss some of the key literature in relation to organisational trust. A quick search on Google Scholar using some well-considered keywords generally provides a good starting point.
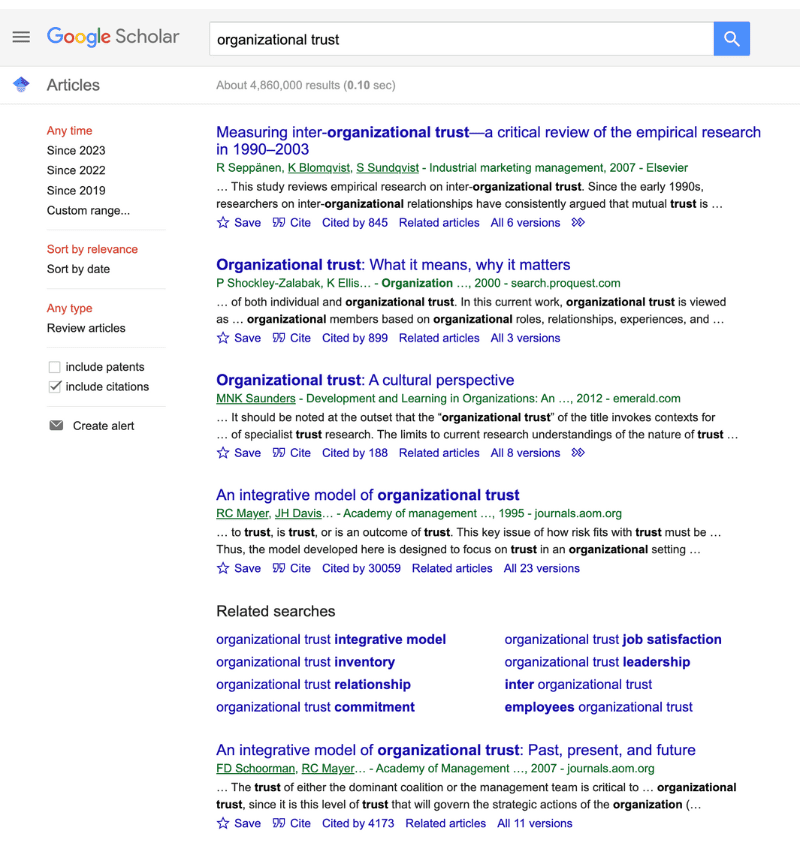
Need a helping hand?
A conceptual framework is typically a visual representation (although it can also be written out) of the expected relationships and connections between various concepts, constructs or variables. In other words, a conceptual framework visualises how the researcher views and organises the various concepts and variables within their study. This is typically based on aspects drawn from the theoretical framework, so there is a relationship between the two.
Quite commonly, conceptual frameworks are used to visualise the potential causal relationships and pathways that the researcher expects to find, based on their understanding of both the theoretical literature and the existing empirical research . Therefore, the conceptual framework is often used to develop research questions and hypotheses .
Let’s look at an example of a conceptual framework to make it a little more tangible. You’ll notice that in this specific conceptual framework, the hypotheses are integrated into the visual, helping to connect the rest of the document to the framework.
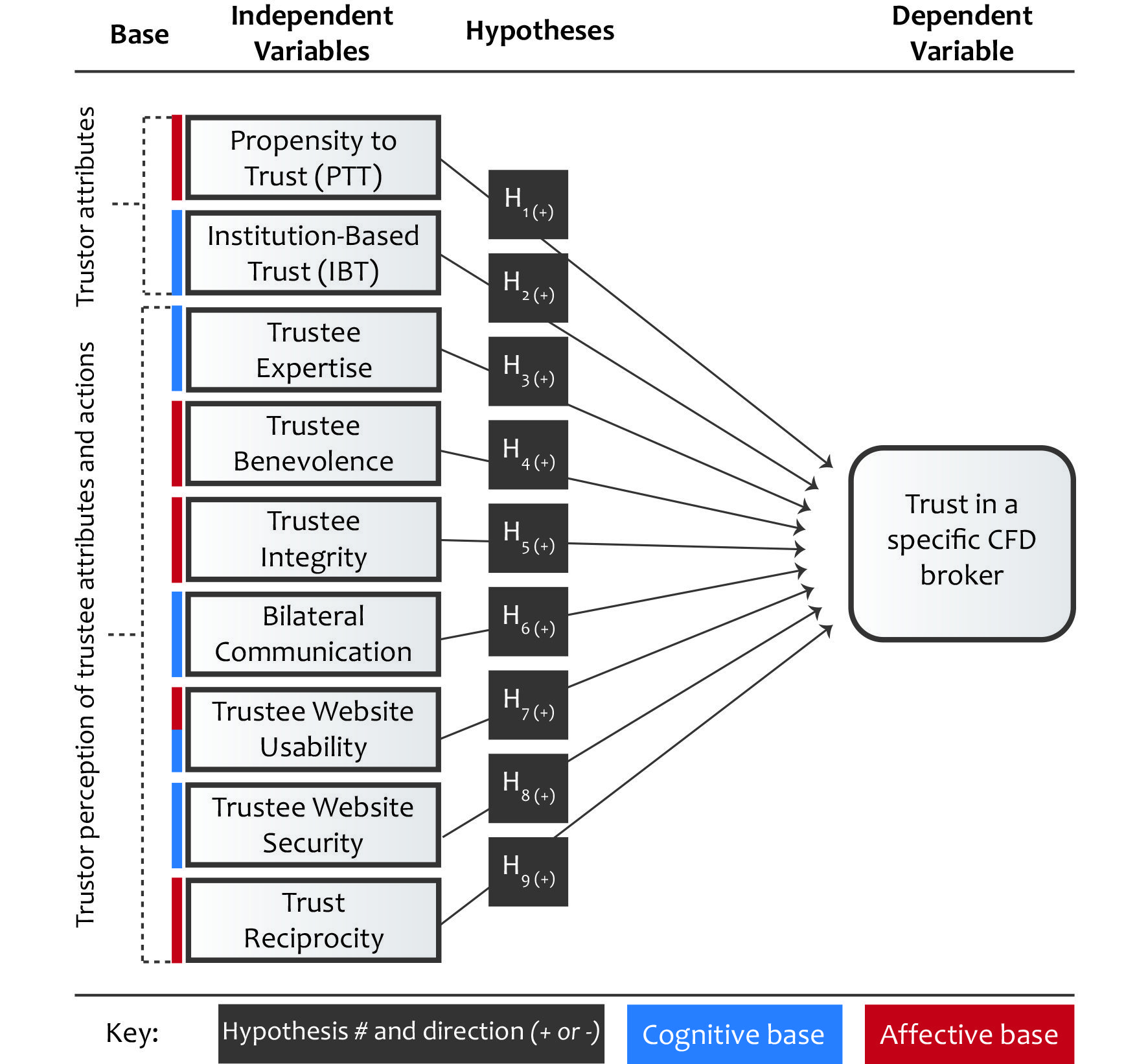
Theoretical framework vs conceptual framework
As you can see, the theoretical framework and the conceptual framework are closely related concepts, but they differ in terms of focus and purpose. The theoretical framework is used to lay down a foundation of theory on which your study will be built, whereas the conceptual framework visualises what you anticipate the relationships between concepts, constructs and variables may be, based on your understanding of the existing literature and the specific context and focus of your research. In other words, they’re different tools for different jobs , but they’re neighbours in the toolbox.
Naturally, the theoretical framework and the conceptual framework are not mutually exclusive . In fact, it’s quite likely that you’ll include both in your dissertation or thesis, especially if your research aims involve investigating relationships between variables. Of course, every research project is different and universities differ in terms of their expectations for dissertations and theses, so it’s always a good idea to have a look at past projects to get a feel for what the norms and expectations are at your specific institution.
Want to learn more about research terminology, methods and techniques? Be sure to check out the rest of the Grad Coach blog . Alternatively, if you’re looking for hands-on help, have a look at our private coaching service , where we hold your hand through the research process, step by step.
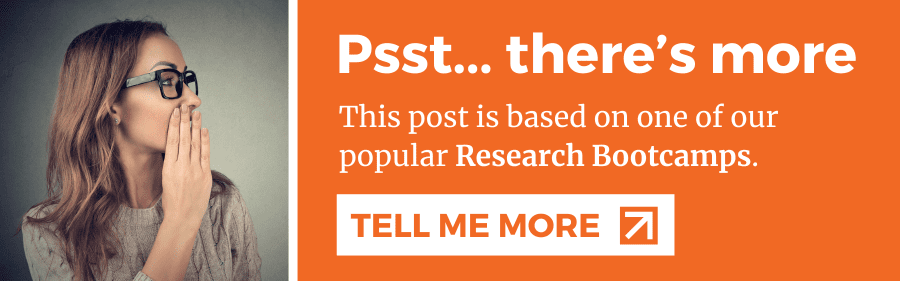
You Might Also Like:
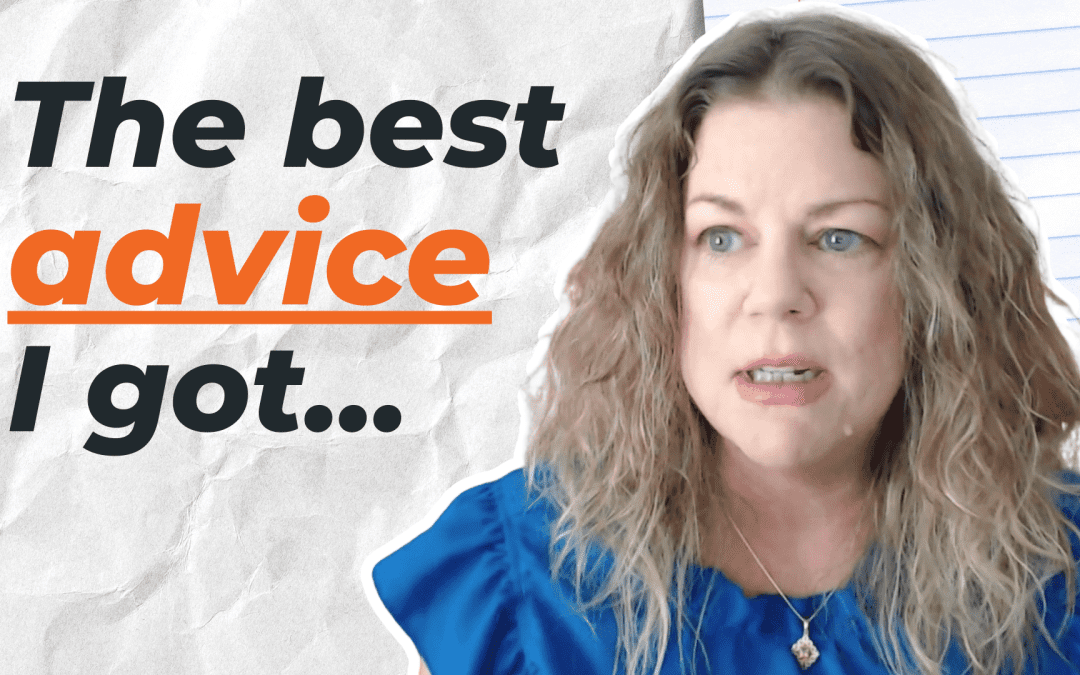
How To Review & Understand Academic Literature Quickly
Learn how to fast-track your literature review by reading with intention and clarity. Dr E and Amy Murdock explain how.
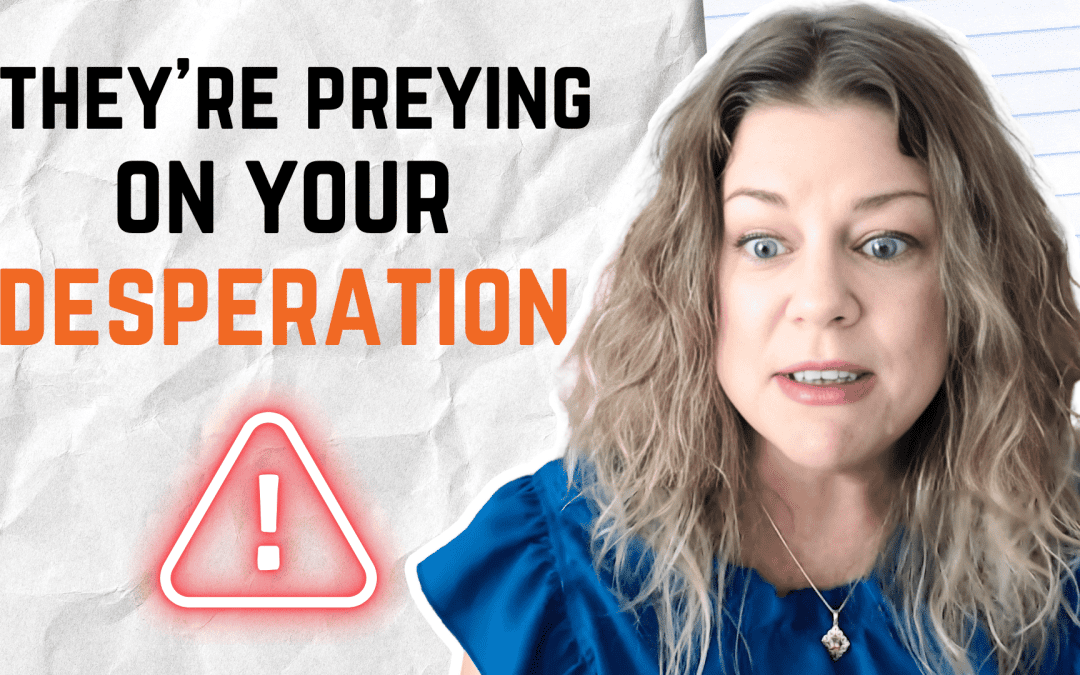
Dissertation Writing Services: Far Worse Than You Think
Thinking about using a dissertation or thesis writing service? You might want to reconsider that move. Here’s what you need to know.
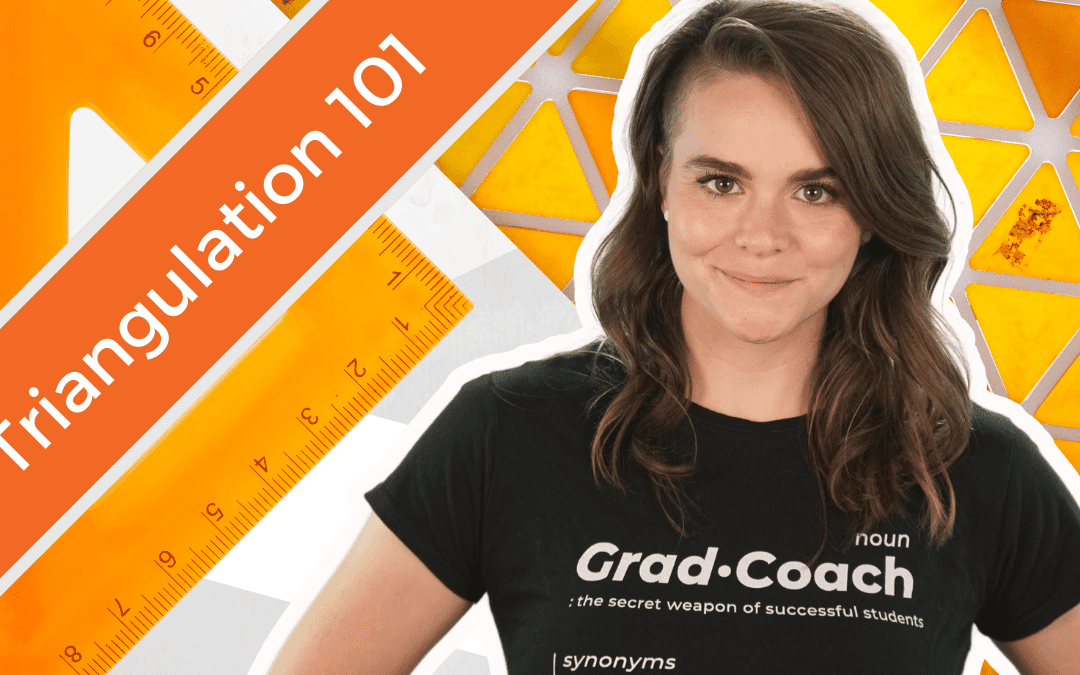
Triangulation: The Ultimate Credibility Enhancer
Triangulation is one of the best ways to enhance the credibility of your research. Learn about the different options here.
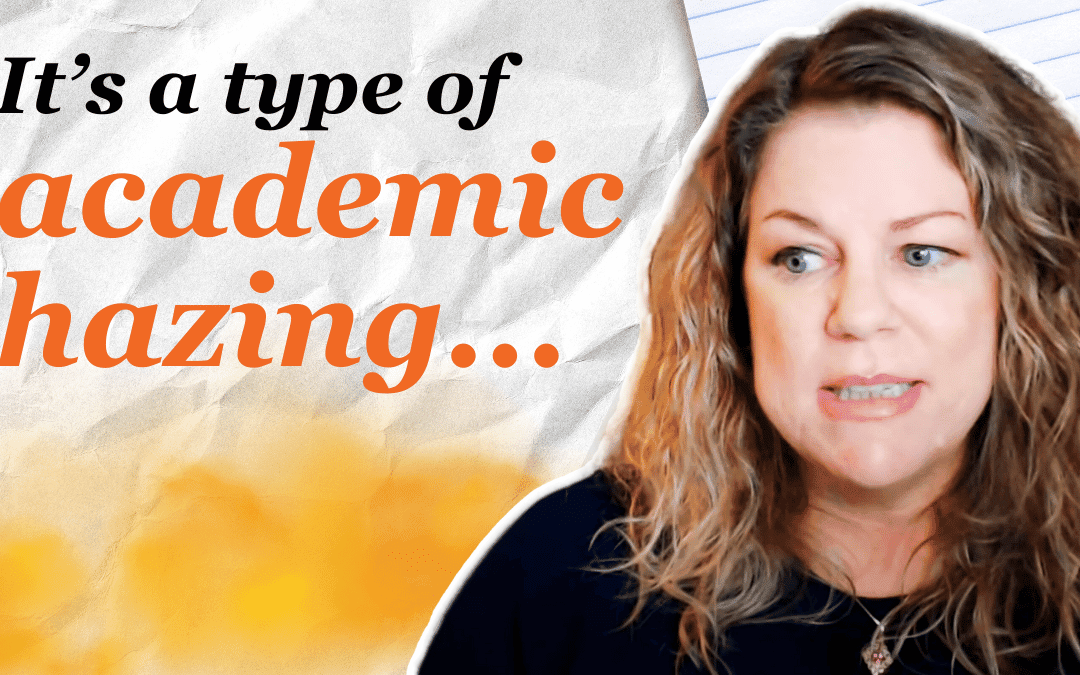
The Harsh Truths Of Academic Research
Dr. Ethar Al-Saraf and Dr. Amy Murdock dive into the darker truths of academic research, so that you’re well prepared for reality.
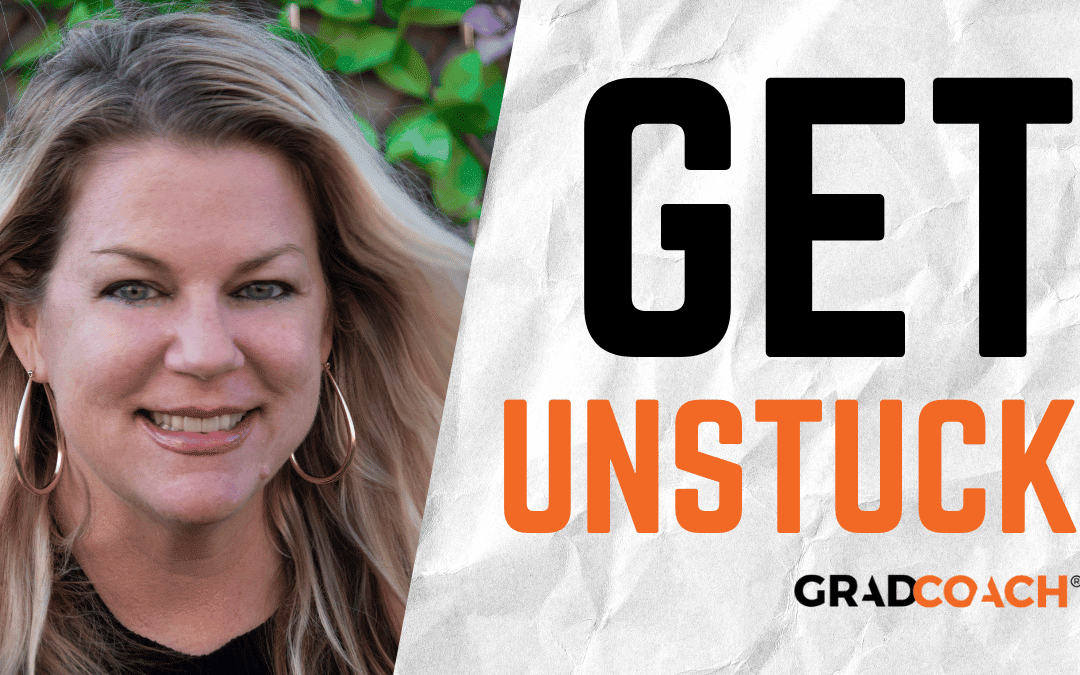
Dissertation Paralysis: How To Get Unstuck
In this episode of the podcast, Dr. Ethar and Dr. Amy Murdock dive into how to get unstuck when you’re facing dissertation paralysis
📄 FREE TEMPLATES
Research Topic Ideation
Proposal Writing
Literature Review
Methodology & Analysis
Academic Writing
Referencing & Citing
Apps, Tools & Tricks
The Grad Coach Podcast
23 Comments
Thank you for giving a valuable lesson
good thanks!
VERY INSIGHTFUL
thanks for given very interested understand about both theoritical and conceptual framework
I am researching teacher beliefs about inclusive education but not using a theoretical framework just conceptual frame using teacher beliefs, inclusive education and inclusive practices as my concepts
good, fantastic
great! thanks for the clarification. I am planning to use both for my implementation evaluation of EmONC service at primary health care facility level. its theoretical foundation rooted from the principles of implementation science.
This is a good one…now have a better understanding of Theoretical and Conceptual frameworks. Highly grateful
Very educating and fantastic,good to be part of you guys,I appreciate your enlightened concern.
Thanks for shedding light on these two t opics. Much clearer in my head now.
Simple and clear!
The differences between the two topics was well explained, thank you very much!
Thank you great insight
Superb. Thank you so much.
Hello Gradcoach! I’m excited with your fantastic educational videos which mainly focused on all over research process. I’m a student, I kindly ask and need your support. So, if it’s possible please send me the PDF format of all topic provided here, I put my email below, thank you!
I am really grateful I found this website. This is very helpful for an MPA student like myself.
I’m clear with these two terminologies now. Useful information. I appreciate it. Thank you
I’m well inform about these two concepts in research. Thanks
I found this really helpful. It is well explained. Thank you.
very clear and useful. information important at start of research!!
Wow, great information, clear and concise review of the differences between theoretical and conceptual frameworks. Thank you! keep up the good work.
thank you so much. Educative and realistic.
Submit a Comment Cancel reply
Your email address will not be published. Required fields are marked *
Save my name, email, and website in this browser for the next time I comment.
Submit Comment
- Print Friendly
- What is Empirical Research Study? [Examples & Method]

The bulk of human decisions relies on evidence, that is, what can be measured or proven as valid. In choosing between plausible alternatives, individuals are more likely to tilt towards the option that is proven to work, and this is the same approach adopted in empirical research.
In empirical research, the researcher arrives at outcomes by testing his or her empirical evidence using qualitative or quantitative methods of observation, as determined by the nature of the research. An empirical research study is set apart from other research approaches by its methodology and features hence; it is important for every researcher to know what constitutes this investigation method.
What is Empirical Research?
Empirical research is a type of research methodology that makes use of verifiable evidence in order to arrive at research outcomes. In other words, this type of research relies solely on evidence obtained through observation or scientific data collection methods.
Empirical research can be carried out using qualitative or quantitative observation methods , depending on the data sample, that is, quantifiable data or non-numerical data . Unlike theoretical research that depends on preconceived notions about the research variables, empirical research carries a scientific investigation to measure the experimental probability of the research variables
Characteristics of Empirical Research
- Research Questions
An empirical research begins with a set of research questions that guide the investigation. In many cases, these research questions constitute the research hypothesis which is tested using qualitative and quantitative methods as dictated by the nature of the research.
In an empirical research study, the research questions are built around the core of the research, that is, the central issue which the research seeks to resolve. They also determine the course of the research by highlighting the specific objectives and aims of the systematic investigation.
- Definition of the Research Variables
The research variables are clearly defined in terms of their population, types, characteristics, and behaviors. In other words, the data sample is clearly delimited and placed within the context of the research.
- Description of the Research Methodology
An empirical research also clearly outlines the methods adopted in the systematic investigation. Here, the research process is described in detail including the selection criteria for the data sample, qualitative or quantitative research methods plus testing instruments.
An empirical research is usually divided into 4 parts which are the introduction, methodology, findings, and discussions. The introduction provides a background of the empirical study while the methodology describes the research design, processes, and tools for the systematic investigation.
The findings refer to the research outcomes and they can be outlined as statistical data or in the form of information obtained through the qualitative observation of research variables. The discussions highlight the significance of the study and its contributions to knowledge.
Uses of Empirical Research
Without any doubt, empirical research is one of the most useful methods of systematic investigation. It can be used for validating multiple research hypotheses in different fields including Law, Medicine, and Anthropology.
- Empirical Research in Law : In Law, empirical research is used to study institutions, rules, procedures, and personnel of the law, with a view to understanding how they operate and what effects they have. It makes use of direct methods rather than secondary sources, and this helps you to arrive at more valid conclusions.
- Empirical Research in Medicine : In medicine, empirical research is used to test and validate multiple hypotheses and increase human knowledge.
- Empirical Research in Anthropology : In anthropology, empirical research is used as an evidence-based systematic method of inquiry into patterns of human behaviors and cultures. This helps to validate and advance human knowledge.
Discover how Extrapolation Powers statistical research: Definition, examples, types, and applications explained.
The Empirical Research Cycle
The empirical research cycle is a 5-phase cycle that outlines the systematic processes for conducting and empirical research. It was developed by Dutch psychologist, A.D. de Groot in the 1940s and it aligns 5 important stages that can be viewed as deductive approaches to empirical research.
In the empirical research methodological cycle, all processes are interconnected and none of the processes is more important than the other. This cycle clearly outlines the different phases involved in generating the research hypotheses and testing these hypotheses systematically using the empirical data.
- Observation: This is the process of gathering empirical data for the research. At this stage, the researcher gathers relevant empirical data using qualitative or quantitative observation methods, and this goes ahead to inform the research hypotheses.
- Induction: At this stage, the researcher makes use of inductive reasoning in order to arrive at a general probable research conclusion based on his or her observation. The researcher generates a general assumption that attempts to explain the empirical data and s/he goes on to observe the empirical data in line with this assumption.
- Deduction: This is the deductive reasoning stage. This is where the researcher generates hypotheses by applying logic and rationality to his or her observation.
- Testing: Here, the researcher puts the hypotheses to test using qualitative or quantitative research methods. In the testing stage, the researcher combines relevant instruments of systematic investigation with empirical methods in order to arrive at objective results that support or negate the research hypotheses.
- Evaluation: The evaluation research is the final stage in an empirical research study. Here, the research outlines the empirical data, the research findings and the supporting arguments plus any challenges encountered during the research process.
This information is useful for further research.
Learn about qualitative data: uncover its types and examples here.
Examples of Empirical Research
- An empirical research study can be carried out to determine if listening to happy music improves the mood of individuals. The researcher may need to conduct an experiment that involves exposing individuals to happy music to see if this improves their moods.
The findings from such an experiment will provide empirical evidence that confirms or refutes the hypotheses.
- An empirical research study can also be carried out to determine the effects of a new drug on specific groups of people. The researcher may expose the research subjects to controlled quantities of the drug and observe research subjects to controlled quantities of the drug and observe the effects over a specific period of time to gather empirical data.
- Another example of empirical research is measuring the levels of noise pollution found in an urban area to determine the average levels of sound exposure experienced by its inhabitants. Here, the researcher may have to administer questionnaires or carry out a survey in order to gather relevant data based on the experiences of the research subjects.
- Empirical research can also be carried out to determine the relationship between seasonal migration and the body mass of flying birds. A researcher may need to observe the birds and carry out necessary observation and experimentation in order to arrive at objective outcomes that answer the research question.
Empirical Research Data Collection Methods
Empirical data can be gathered using qualitative and quantitative data collection methods. Quantitative data collection methods are used for numerical data gathering while qualitative data collection processes are used to gather empirical data that cannot be quantified, that is, non-numerical data.
The following are common methods of gathering data in empirical research
- Survey/ Questionnaire
A survey is a method of data gathering that is typically employed by researchers to gather large sets of data from a specific number of respondents with regards to a research subject. This method of data gathering is often used for quantitative data collection , although it can also be deployed during quantitative research.
A survey contains a set of questions that can range from close-ended to open-ended questions together with other question types that revolve around the research subject. A survey can be administered physically or with the use of online data-gathering platforms like Formplus.
Empirical data can also be collected by carrying out an experiment. An experiment is a controlled simulation in which one or more of the research variables is manipulated using a set of interconnected processes in order to confirm or refute the research hypotheses.
An experiment is a useful method of measuring causality; that is cause and effect between dependent and independent variables in a research environment. It is an integral data gathering method in an empirical research study because it involves testing calculated assumptions in order to arrive at the most valid data and research outcomes.
T he case study method is another common data gathering method in an empirical research study. It involves sifting through and analyzing relevant cases and real-life experiences about the research subject or research variables in order to discover in-depth information that can serve as empirical data.
- Observation
The observational method is a method of qualitative data gathering that requires the researcher to study the behaviors of research variables in their natural environments in order to gather relevant information that can serve as empirical data.
How to collect Empirical Research Data with Questionnaire
With Formplus, you can create a survey or questionnaire for collecting empirical data from your research subjects. Formplus also offers multiple form sharing options so that you can share your empirical research survey to research subjects via a variety of methods.
Here is a step-by-step guide of how to collect empirical data using Formplus:
Sign in to Formplus
In the Formplus builder, you can easily create your empirical research survey by dragging and dropping preferred fields into your form. To access the Formplus builder, you will need to create an account on Formplus.
Once you do this, sign in to your account and click on “Create Form ” to begin.
Unlock the secrets of Quantitative Data: Click here to explore the types and examples.
Edit Form Title
Click on the field provided to input your form title, for example, “Empirical Research Survey”.
Edit Form
- Click on the edit button to edit the form.
- Add Fields: Drag and drop preferred form fields into your form in the Formplus builder inputs column. There are several field input options for survey forms in the Formplus builder.
- Edit fields
- Click on “Save”
- Preview form.
Customize Form
Formplus allows you to add unique features to your empirical research survey form. You can personalize your survey using various customization options. Here, you can add background images, your organization’s logo, and use other styling options. You can also change the display theme of your form.
- Share your Form Link with Respondents
Formplus offers multiple form sharing options which enables you to easily share your empirical research survey form with respondents. You can use the direct social media sharing buttons to share your form link to your organization’s social media pages.
You can send out your survey form as email invitations to your research subjects too. If you wish, you can share your form’s QR code or embed it on your organization’s website for easy access.
Empirical vs Non-Empirical Research
Empirical and non-empirical research are common methods of systematic investigation employed by researchers. Unlike empirical research that tests hypotheses in order to arrive at valid research outcomes, non-empirical research theorizes the logical assumptions of research variables.
Definition: Empirical research is a research approach that makes use of evidence-based data while non-empirical research is a research approach that makes use of theoretical data.
Method: In empirical research, the researcher arrives at valid outcomes by mainly observing research variables, creating a hypothesis and experimenting on research variables to confirm or refute the hypothesis. In non-empirical research, the researcher relies on inductive and deductive reasoning to theorize logical assumptions about the research subjects.
The major difference between the research methodology of empirical and non-empirical research is while the assumptions are tested in empirical research, they are entirely theorized in non-empirical research.
Data Sample: Empirical research makes use of empirical data while non-empirical research does not make use of empirical data. Empirical data refers to information that is gathered through experience or observation.
Unlike empirical research, theoretical or non-empirical research does not rely on data gathered through evidence. Rather, it works with logical assumptions and beliefs about the research subject.
Data Collection Methods : Empirical research makes use of quantitative and qualitative data gathering methods which may include surveys, experiments, and methods of observation. This helps the researcher to gather empirical data, that is, data backed by evidence.
Non-empirical research, on the other hand, does not make use of qualitative or quantitative methods of data collection . Instead, the researcher gathers relevant data through critical studies, systematic review and meta-analysis.
Advantages of Empirical Research
- Empirical research is flexible. In this type of systematic investigation, the researcher can adjust the research methodology including the data sample size, data gathering methods plus the data analysis methods as necessitated by the research process.
- It helps the research to understand how the research outcomes can be influenced by different research environments.
- Empirical research study helps the researcher to develop relevant analytical and observation skills that can be useful in dynamic research contexts.
- This type of research approach allows the researcher to control multiple research variables in order to arrive at the most relevant research outcomes.
- Empirical research is widely considered as one of the most authentic and competent research designs.
- It improves the internal validity of traditional research using a variety of experiments and research observation methods.
Disadvantages of Empirical Research
- An empirical research study is time-consuming because the researcher needs to gather the empirical data from multiple resources which typically takes a lot of time.
- It is not a cost-effective research approach. Usually, this method of research incurs a lot of cost because of the monetary demands of the field research.
- It may be difficult to gather the needed empirical data sample because of the multiple data gathering methods employed in an empirical research study.
- It may be difficult to gain access to some communities and firms during the data gathering process and this can affect the validity of the research.
- The report from an empirical research study is intensive and can be very lengthy in nature.
Conclusion
Empirical research is an important method of systematic investigation because it gives the researcher the opportunity to test the validity of different assumptions, in the form of hypotheses, before arriving at any findings. Hence, it is a more research approach.
There are different quantitative and qualitative methods of data gathering employed during an empirical research study based on the purpose of the research which include surveys, experiments, and various observatory methods. Surveys are one of the most common methods or empirical data collection and they can be administered online or physically.
You can use Formplus to create and administer your online empirical research survey. Formplus allows you to create survey forms that you can share with target respondents in order to obtain valuable feedback about your research context, question or subject.
In the form builder, you can add different fields to your survey form and you can also modify these form fields to suit your research process. Sign up to Formplus to access the form builder and start creating powerful online empirical research survey forms.
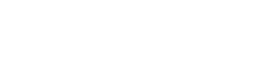
Connect to Formplus, Get Started Now - It's Free!
- advantage of empirical research
- disadvantages of empirical resarch
- empirical research characteristics
- empirical research cycle
- empirical research method
- example of empirical research
- uses of empirical research
- busayo.longe
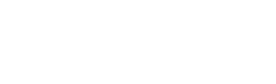
You may also like:
Recall Bias: Definition, Types, Examples & Mitigation
This article will discuss the impact of recall bias in studies and the best ways to avoid them during research.

Research Questions: Definitions, Types + [Examples]
A comprehensive guide on the definition of research questions, types, importance, good and bad research question examples
What is Pure or Basic Research? + [Examples & Method]
Simple guide on pure or basic research, its methods, characteristics, advantages, and examples in science, medicine, education and psychology
Extrapolation in Statistical Research: Definition, Examples, Types, Applications
In this article we’ll look at the different types and characteristics of extrapolation, plus how it contrasts to interpolation.
Formplus - For Seamless Data Collection
Collect data the right way with a versatile data collection tool. try formplus and transform your work productivity today..
Educational resources and simple solutions for your research journey

What is a Theoretical Framework? How to Write It (with Examples)

Theoretical framework 1,2 is the structure that supports and describes a theory. A theory is a set of interrelated concepts and definitions that present a systematic view of phenomena by describing the relationship among the variables for explaining these phenomena. A theory is developed after a long research process and explains the existence of a research problem in a study. A theoretical framework guides the research process like a roadmap for the research study and helps researchers clearly interpret their findings by providing a structure for organizing data and developing conclusions.
A theoretical framework in research is an important part of a manuscript and should be presented in the first section. It shows an understanding of the theories and concepts relevant to the research and helps limit the scope of the research.
Table of Contents
What is a theoretical framework ?
A theoretical framework in research can be defined as a set of concepts, theories, ideas, and assumptions that help you understand a specific phenomenon or problem. It can be considered a blueprint that is borrowed by researchers to develop their own research inquiry. A theoretical framework in research helps researchers design and conduct their research and analyze and interpret their findings. It explains the relationship between variables, identifies gaps in existing knowledge, and guides the development of research questions, hypotheses, and methodologies to address that gap.

Now that you know the answer to ‘ What is a theoretical framework? ’, check the following table that lists the different types of theoretical frameworks in research: 3
Conceptual | Defines key concepts and relationships |
Deductive | Starts with a general hypothesis and then uses data to test it; used in quantitative research |
Inductive | Starts with data and then develops a hypothesis; used in qualitative research |
Empirical | Focuses on the collection and analysis of empirical data; used in scientific research |
Normative | Defines a set of norms that guide behavior; used in ethics and social sciences |
Explanatory | Explains causes of particular behavior; used in psychology and social sciences |
Developing a theoretical framework in research can help in the following situations: 4
- When conducting research on complex phenomena because a theoretical framework helps organize the research questions, hypotheses, and findings
- When the research problem requires a deeper understanding of the underlying concepts
- When conducting research that seeks to address a specific gap in knowledge
- When conducting research that involves the analysis of existing theories
Summarizing existing literature for theoretical frameworks is easy. Get our Research Ideation pack
Importance of a theoretical framework
The purpose of theoretical framework s is to support you in the following ways during the research process: 2
- Provide a structure for the complete research process
- Assist researchers in incorporating formal theories into their study as a guide
- Provide a broad guideline to maintain the research focus
- Guide the selection of research methods, data collection, and data analysis
- Help understand the relationships between different concepts and develop hypotheses and research questions
- Address gaps in existing literature
- Analyze the data collected and draw meaningful conclusions and make the findings more generalizable
Theoretical vs. Conceptual framework
While a theoretical framework covers the theoretical aspect of your study, that is, the various theories that can guide your research, a conceptual framework defines the variables for your study and presents how they relate to each other. The conceptual framework is developed before collecting the data. However, both frameworks help in understanding the research problem and guide the development, collection, and analysis of the research.
The following table lists some differences between conceptual and theoretical frameworks . 5
Based on existing theories that have been tested and validated by others | Based on concepts that are the main variables in the study |
Used to create a foundation of the theory on which your study will be developed | Visualizes the relationships between the concepts and variables based on the existing literature |
Used to test theories, to predict and control the situations within the context of a research inquiry | Helps the development of a theory that would be useful to practitioners |
Provides a general set of ideas within which a study belongs | Refers to specific ideas that researchers utilize in their study |
Offers a focal point for approaching unknown research in a specific field of inquiry | Shows logically how the research inquiry should be undertaken |
Works deductively | Works inductively |
Used in quantitative studies | Used in qualitative studies |
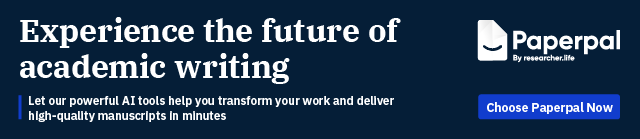
How to write a theoretical framework
The following general steps can help those wondering how to write a theoretical framework: 2
- Identify and define the key concepts clearly and organize them into a suitable structure.
- Use appropriate terminology and define all key terms to ensure consistency.
- Identify the relationships between concepts and provide a logical and coherent structure.
- Develop hypotheses that can be tested through data collection and analysis.
- Keep it concise and focused with clear and specific aims.
Write a theoretical framework 2x faster. Get our Manuscript Writing pack
Examples of a theoretical framework
Here are two examples of a theoretical framework. 6,7
Example 1 .
An insurance company is facing a challenge cross-selling its products. The sales department indicates that most customers have just one policy, although the company offers over 10 unique policies. The company would want its customers to purchase more than one policy since most customers are purchasing policies from other companies.
Objective : To sell more insurance products to existing customers.
Problem : Many customers are purchasing additional policies from other companies.
Research question : How can customer product awareness be improved to increase cross-selling of insurance products?
Sub-questions: What is the relationship between product awareness and sales? Which factors determine product awareness?
Since “product awareness” is the main focus in this study, the theoretical framework should analyze this concept and study previous literature on this subject and propose theories that discuss the relationship between product awareness and its improvement in sales of other products.
Example 2 .
A company is facing a continued decline in its sales and profitability. The main reason for the decline in the profitability is poor services, which have resulted in a high level of dissatisfaction among customers and consequently a decline in customer loyalty. The management is planning to concentrate on clients’ satisfaction and customer loyalty.
Objective: To provide better service to customers and increase customer loyalty and satisfaction.
Problem: Continued decrease in sales and profitability.
Research question: How can customer satisfaction help in increasing sales and profitability?
Sub-questions: What is the relationship between customer loyalty and sales? Which factors influence the level of satisfaction gained by customers?
Since customer satisfaction, loyalty, profitability, and sales are the important topics in this example, the theoretical framework should focus on these concepts.
Benefits of a theoretical framework
There are several benefits of a theoretical framework in research: 2
- Provides a structured approach allowing researchers to organize their thoughts in a coherent way.
- Helps to identify gaps in knowledge highlighting areas where further research is needed.
- Increases research efficiency by providing a clear direction for research and focusing efforts on relevant data.
- Improves the quality of research by providing a rigorous and systematic approach to research, which can increase the likelihood of producing valid and reliable results.
- Provides a basis for comparison by providing a common language and conceptual framework for researchers to compare their findings with other research in the field, facilitating the exchange of ideas and the development of new knowledge.
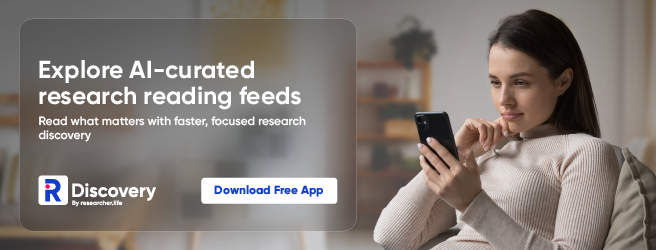
Frequently Asked Questions
Q1. How do I develop a theoretical framework ? 7
A1. The following steps can be used for developing a theoretical framework :
- Identify the research problem and research questions by clearly defining the problem that the research aims to address and identifying the specific questions that the research aims to answer.
- Review the existing literature to identify the key concepts that have been studied previously. These concepts should be clearly defined and organized into a structure.
- Develop propositions that describe the relationships between the concepts. These propositions should be based on the existing literature and should be testable.
- Develop hypotheses that can be tested through data collection and analysis.
- Test the theoretical framework through data collection and analysis to determine whether the framework is valid and reliable.
Q2. How do I know if I have developed a good theoretical framework or not? 8
A2. The following checklist could help you answer this question:
- Is my theoretical framework clearly seen as emerging from my literature review?
- Is it the result of my analysis of the main theories previously studied in my same research field?
- Does it represent or is it relevant to the most current state of theoretical knowledge on my topic?
- Does the theoretical framework in research present a logical, coherent, and analytical structure that will support my data analysis?
- Do the different parts of the theory help analyze the relationships among the variables in my research?
- Does the theoretical framework target how I will answer my research questions or test the hypotheses?
- Have I documented every source I have used in developing this theoretical framework ?
- Is my theoretical framework a model, a table, a figure, or a description?
- Have I explained why this is the appropriate theoretical framework for my data analysis?
Q3. Can I use multiple theoretical frameworks in a single study?
A3. Using multiple theoretical frameworks in a single study is acceptable as long as each theory is clearly defined and related to the study. Each theory should also be discussed individually. This approach may, however, be tedious and effort intensive. Therefore, multiple theoretical frameworks should be used only if absolutely necessary for the study.
Q4. Is it necessary to include a theoretical framework in every research study?
A4. The theoretical framework connects researchers to existing knowledge. So, including a theoretical framework would help researchers get a clear idea about the research process and help structure their study effectively by clearly defining an objective, a research problem, and a research question.
Q5. Can a theoretical framework be developed for qualitative research?
A5. Yes, a theoretical framework can be developed for qualitative research. However, qualitative research methods may or may not involve a theory developed beforehand. In these studies, a theoretical framework can guide the study and help develop a theory during the data analysis phase. This resulting framework uses inductive reasoning. The outcome of this inductive approach can be referred to as an emergent theoretical framework . This method helps researchers develop a theory inductively, which explains a phenomenon without a guiding framework at the outset.

Q6. What is the main difference between a literature review and a theoretical framework ?
A6. A literature review explores already existing studies about a specific topic in order to highlight a gap, which becomes the focus of the current research study. A theoretical framework can be considered the next step in the process, in which the researcher plans a specific conceptual and analytical approach to address the identified gap in the research.
Theoretical frameworks are thus important components of the research process and researchers should therefore devote ample amount of time to develop a solid theoretical framework so that it can effectively guide their research in a suitable direction. We hope this article has provided a good insight into the concept of theoretical frameworks in research and their benefits.
References
- Organizing academic research papers: Theoretical framework. Sacred Heart University library. Accessed August 4, 2023. https://library.sacredheart.edu/c.php?g=29803&p=185919#:~:text=The%20theoretical%20framework%20is%20the,research%20problem%20under%20study%20exists .
- Salomao A. Understanding what is theoretical framework. Mind the Graph website. Accessed August 5, 2023. https://mindthegraph.com/blog/what-is-theoretical-framework/
- Theoretical framework—Types, examples, and writing guide. Research Method website. Accessed August 6, 2023. https://researchmethod.net/theoretical-framework/
- Grant C., Osanloo A. Understanding, selecting, and integrating a theoretical framework in dissertation research: Creating the blueprint for your “house.” Administrative Issues Journal : Connecting Education, Practice, and Research; 4(2):12-26. 2014. Accessed August 7, 2023. https://files.eric.ed.gov/fulltext/EJ1058505.pdf
- Difference between conceptual framework and theoretical framework. MIM Learnovate website. Accessed August 7, 2023. https://mimlearnovate.com/difference-between-conceptual-framework-and-theoretical-framework/
- Example of a theoretical framework—Thesis & dissertation. BacherlorPrint website. Accessed August 6, 2023. https://www.bachelorprint.com/dissertation/example-of-a-theoretical-framework/
- Sample theoretical framework in dissertation and thesis—Overview and example. Students assignment help website. Accessed August 6, 2023. https://www.studentsassignmenthelp.co.uk/blogs/sample-dissertation-theoretical-framework/#Example_of_the_theoretical_framework
- Kivunja C. Distinguishing between theory, theoretical framework, and conceptual framework: A systematic review of lessons from the field. Accessed August 8, 2023. https://files.eric.ed.gov/fulltext/EJ1198682.pdf
Editage All Access is a subscription-based platform that unifies the best AI tools and services designed to speed up, simplify, and streamline every step of a researcher’s journey. The Editage All Access Pack is a one-of-a-kind subscription that unlocks full access to an AI writing assistant, literature recommender, journal finder, scientific illustration tool, and exclusive discounts on professional publication services from Editage.
Based on 22+ years of experience in academia, Editage All Access empowers researchers to put their best research forward and move closer to success. Explore our top AI Tools pack, AI Tools + Publication Services pack, or Build Your Own Plan. Find everything a researcher needs to succeed, all in one place – Get All Access now starting at just $14 a month !
Related Posts

Join Us for Peer Review Week 2024

How Editage All Access is Boosting Productivity for Academics in India
- Skip to main content
- Skip to primary sidebar
- Skip to footer
- QuestionPro

- Solutions Industries Gaming Automotive Sports and events Education Government Travel & Hospitality Financial Services Healthcare Cannabis Technology Use Case AskWhy Communities Audience Contactless surveys Mobile LivePolls Member Experience GDPR Positive People Science 360 Feedback Surveys
- Resources Blog eBooks Survey Templates Case Studies Training Help center

Home Market Research Research Tools and Apps
Theoretical Research: Definition, Methods + Examples
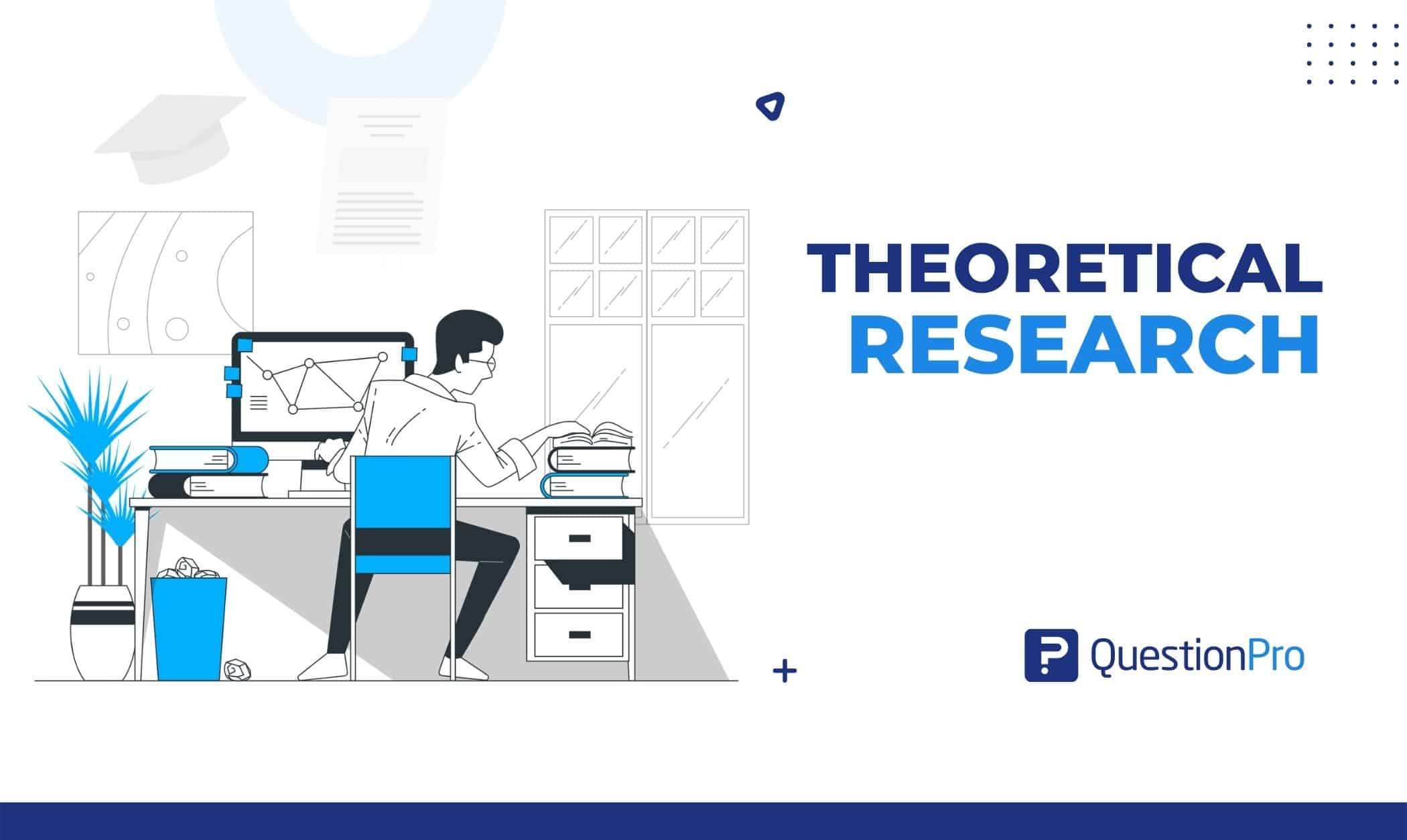
Research is the careful study of a particular research problem or concern using the scientific method. A theory is essential for any research project because it gives it direction and helps prove or disprove something. Theoretical basis helps us figure out how things work and why we do certain things.
Theoretical research lets you examine and discuss a research object using philosophical ideas and abstract theoretical structures.
In theoretical research, you can’t look at the research object directly. With the help of research literature, your research aims to define and sketch out the chosen topic’s conceptual models, explanations, and structures.
LEARN ABOUT: Research Process Steps
This blog will cover theoretical research and why it is essential. In addition to that, we are going to go over some examples.
What is the theoretical research?
Theoretical research is the systematic examination of a set of beliefs and assumptions.
It aims to learn more about a subject and help us understand it better. The information gathered in this way is not used for anything in particular because this kind of research aims to learn more.
All professionals, like biologists, chemists, engineers, architects, philosophers, writers, sociologists, historians, etc., can do theoretical research. No matter what field you work in, theoretical research is the foundation for new ideas.
It tries to answer basic questions about people, which is why this kind of research is used in every field of knowledge.
For example , a researcher starts with the idea that we need to understand the world around us. To do this, he begins with a hypothesis and tests it through experiments that will help him develop new ideas.
What is the theoretical framework?
A theoretical framework is a critical component in research that provides a structured foundation for investigating a specific topic or problem. It encompasses a set of interconnected theories, existing theories, and concepts that guide the entire research process.
The theoretical framework introduces a comprehensive understanding of the subject matter. Also, the theoretical framework strengthens the research’s validity and specifies the key elements that will be explored. Furthermore, it connects different ideas and theories, forming a cohesive structure that underpins the research endeavor.
A complete theoretical framework consists of a network of theories, existing theories, and concepts that collectively shape the direction of a research study.
The theoretical framework is the fundamental principle that will be explored, strengthens the research’s credibility by aligning it with established knowledge, specifies the variables under investigation, and connects different aspects of the research to create a unified approach.
Theoretical frameworks are the intellectual scaffolding upon which the research is constructed. It is the lens through which researchers view their subject, guiding their choice of methodologies, data collection, analysis, and interpretation. By incorporating existing theory, and established concepts, a theoretical framework not only grounds the research but also provides a coherent roadmap for exploring the intricacies of the chosen topic.
Benefits of theoretical research
Theoretical research yields a wealth of benefits across various fields, from social sciences to human resource development and political science. Here’s a breakdown of these benefits while incorporating the requested topics:
Predictive power
Theoretical models are the cornerstone of theoretical research. They grant us predictive power, enabling us to forecast intricate behaviors within complex systems, like societal interactions. In political science, for instance, a theoretical model helps anticipate potential outcomes of policy changes.
Understanding human behavior
Drawing from key social science theories, it assists us in deciphering human behavior and societal dynamics. For instance, in the context of human resource development, theories related to motivation and psychology provide insights into how to effectively manage a diverse workforce.
Optimizing workforce
In the realm of human resource development, insights gleaned from theoretical research, along with the research methods knowledge base, help create targeted training programs. By understanding various learning methodologies and psychological factors, organizations can optimize workforce training for better results.
Building on foundations
It doesn’t exist in isolation; it builds upon existing theories. For instance, within the human resource development handbook, theoretical research expands established concepts, refining their applicability to contemporary organizational challenges.
Ethical policy formulation
Within political science, theoretical research isn’t confined to governance structures. It extends to ethical considerations, aiding policymakers in creating policies that balance the collective good with individual rights, ensuring just and fair governance.
Rigorous investigations
Theoretical research underscores the importance of research methods knowledge base. This knowledge equips researchers in theory-building research methods and other fields to design robust research methodologies, yielding accurate data and credible insights.
Long-term impact
Theoretical research leaves a lasting impact. The theoretical models and insights from key social science theories provide enduring frameworks for subsequent research, contributing to the cumulative growth of knowledge in these fields.
Innovation and practical applications
It doesn’t merely remain theoretical. It inspires innovation and practical applications. By merging insights from diverse theories and fields, practitioners in human resource development devise innovative strategies to foster employee growth and well-being.
Theoretical research method
Researchers follow so many methods when doing research. There are two types of theoretical research methods.
- Scientific methods
- Social science method
Let’s explore them below:
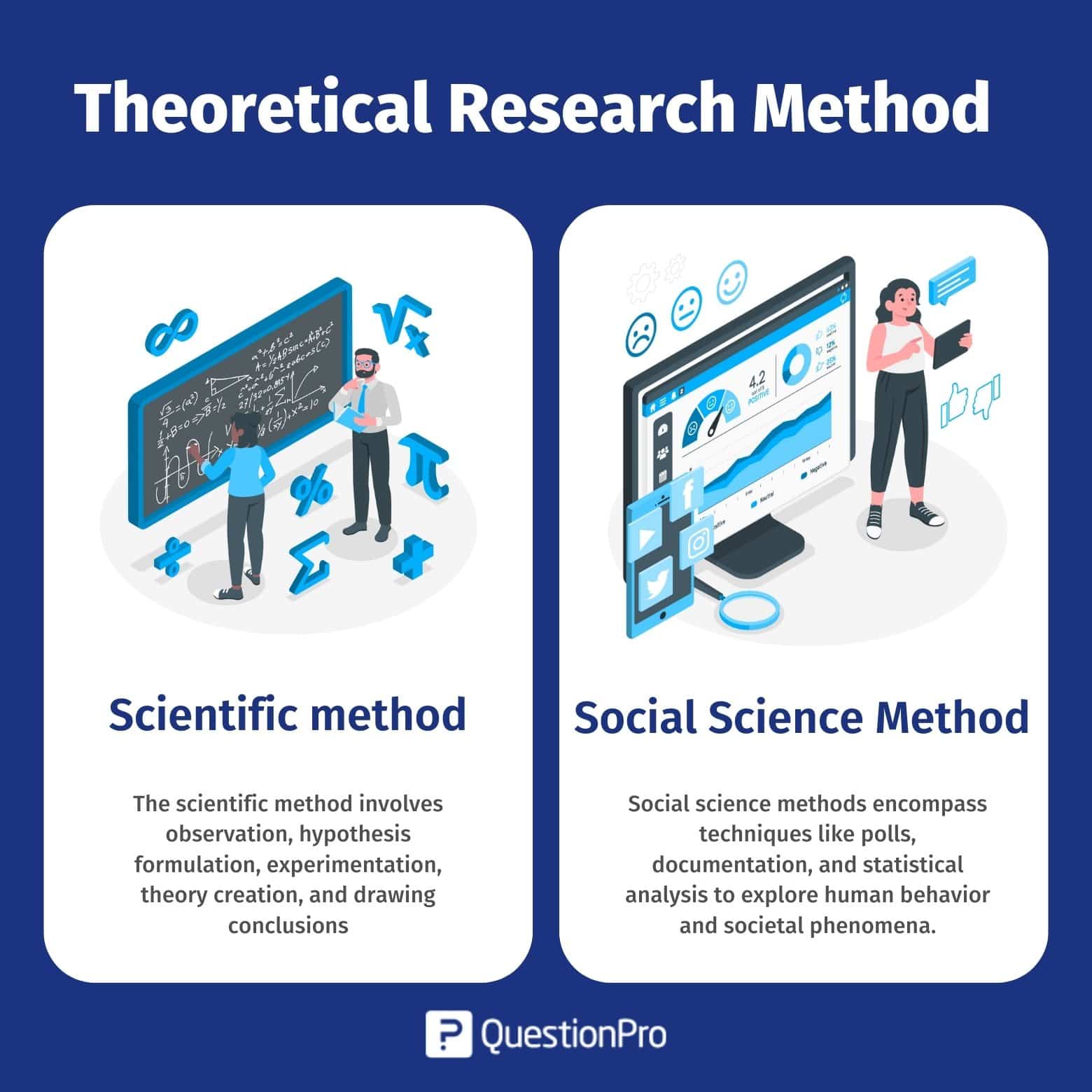
Scientific method
Scientific methods have some important points that you should know. Let’s figure them out below:
- Observation: Any part you want to explain can be found through observation. It helps define the area of research.
- Hypothesis: The hypothesis is the idea put into words, which helps us figure out what we see.
- Experimentation: Hypotheses are tested through experiments to see if they are true. These experiments are different for each research.
- Theory: When we create a theory, we do it because we believe it will explain hypotheses of higher probability.
- Conclusions: Conclusions are the learnings we derive from our investigation.
Social science methods
There are different methods for social science theoretical research. It consists of polls, documentation, and statistical analysis.
- Polls: It is a process whereby the researcher uses a topic-specific questionnaire to gather data. No changes are made to the environment or the phenomenon where the polls are conducted to get the most accurate results. QuestionPro live polls are a great way to get live audiences involved and engaged.
- Documentation: Documentation is a helpful and valuable technique that helps the researcher learn more about the subject. It means visiting libraries or other specialized places, like documentation centers, to look at the existing bibliography. With the documentation, you can find out what came before the investigated topic and what other investigations have found. This step is important because it shows whether or not similar investigations have been done before and what the results were.
- Statistic analysis : Statistics is a branch of math that looks at random events and differences. It follows the rules that are established by probability. It’s used a lot in sociology and language research.
Examples of theoretical research
We talked about theoretical study methods in the previous part. We’ll give you some examples to help you understand it better.
Example 1: Theoretical research into the health benefits of hemp
The plant’s active principles are extracted and evaluated, and by studying their components, it is possible to determine what they contain and whether they can potentially serve as a medication.
Example 2: Linguistics research
Investigate to determine how many people in the Basque Country speak Basque. Surveys can be used to determine the number of native Basque speakers and those who speak Basque as a second language.
Example 3: Philosophical research
Research politics and ethics as they are presented in the writings of Hanna Arendt from a theoretical perspective.
LEARN ABOUT: 12 Best Tools for Researchers
From our above discussion, we learned about theoretical research and its methods and gave some examples. It explains things and leads to more knowledge for the sake of knowledge. This kind of research tries to find out more about a thing or an idea, but the results may take time to be helpful in the real world.
This research is sometimes called basic research. Theoretical research is an important process that gives researchers valuable data with insight.
QuestionPro is a strong platform for managing your data. You can conduct simple surveys to more complex research using QuestionPro survey software.
At QuestionPro, we give researchers tools for collecting data, such as our survey software and a library of insights for any long-term study. Contact our expert team to find out more about it.
LEARN MORE FREE TRIAL
MORE LIKE THIS
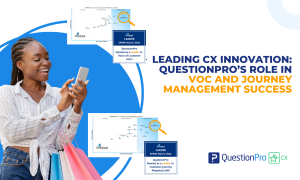
QuestionPro: Leading the Charge in Customer Journey Management and Voice of the Customer Platforms
Sep 17, 2024
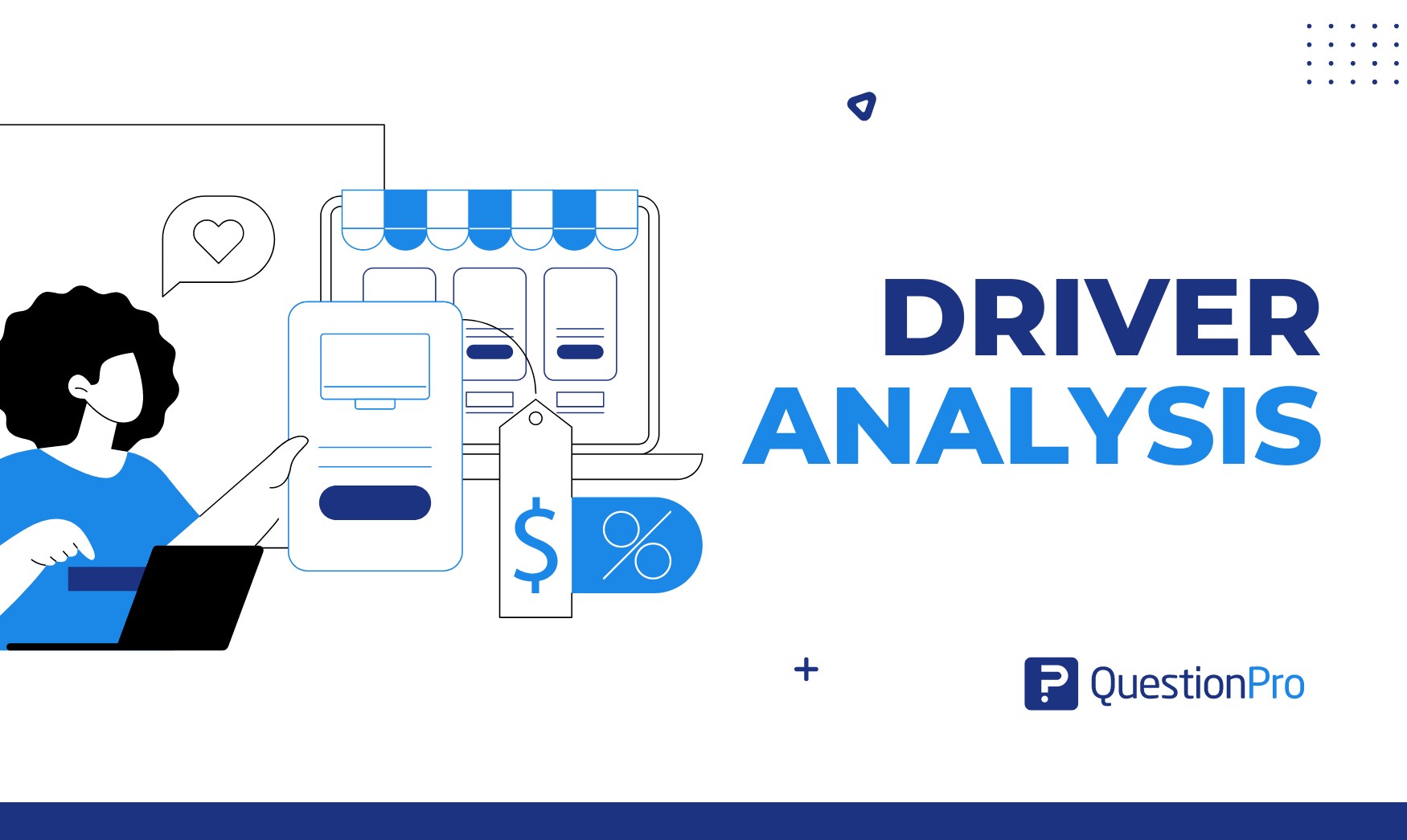
What is Driver Analysis? Importance and Best Practices
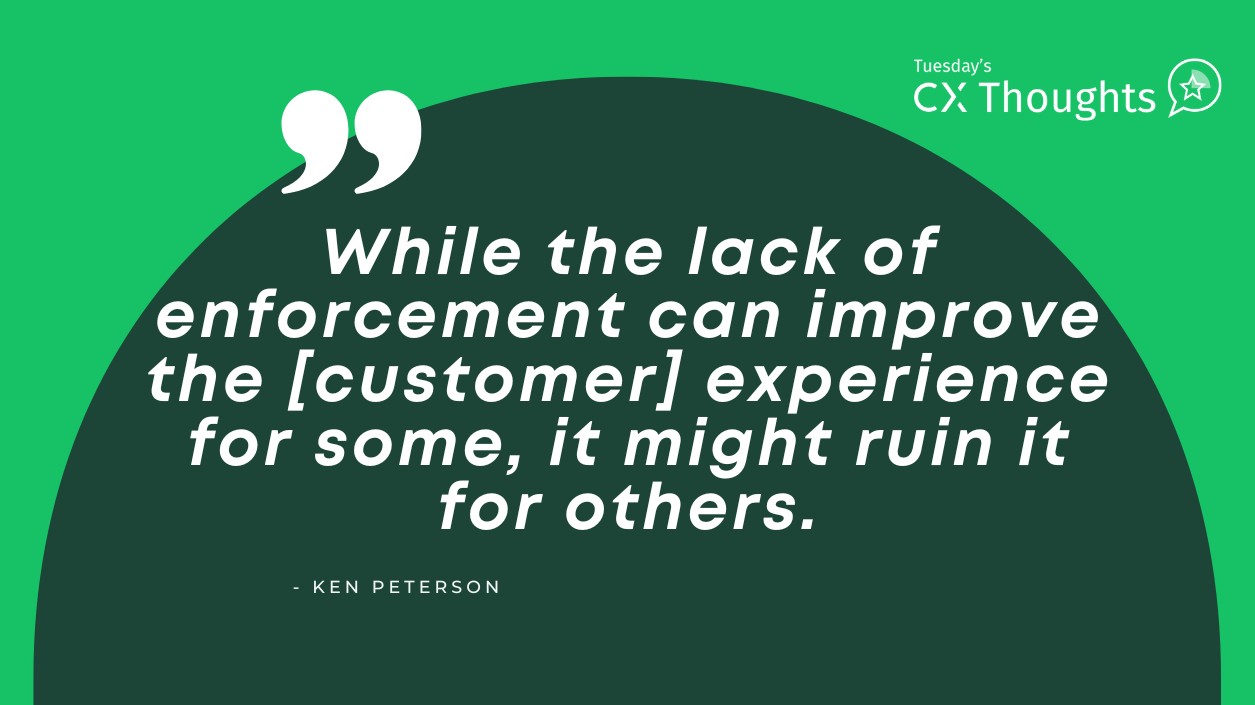
Was The Experience Memorable? (Part II) — Tuesday CX Thoughts
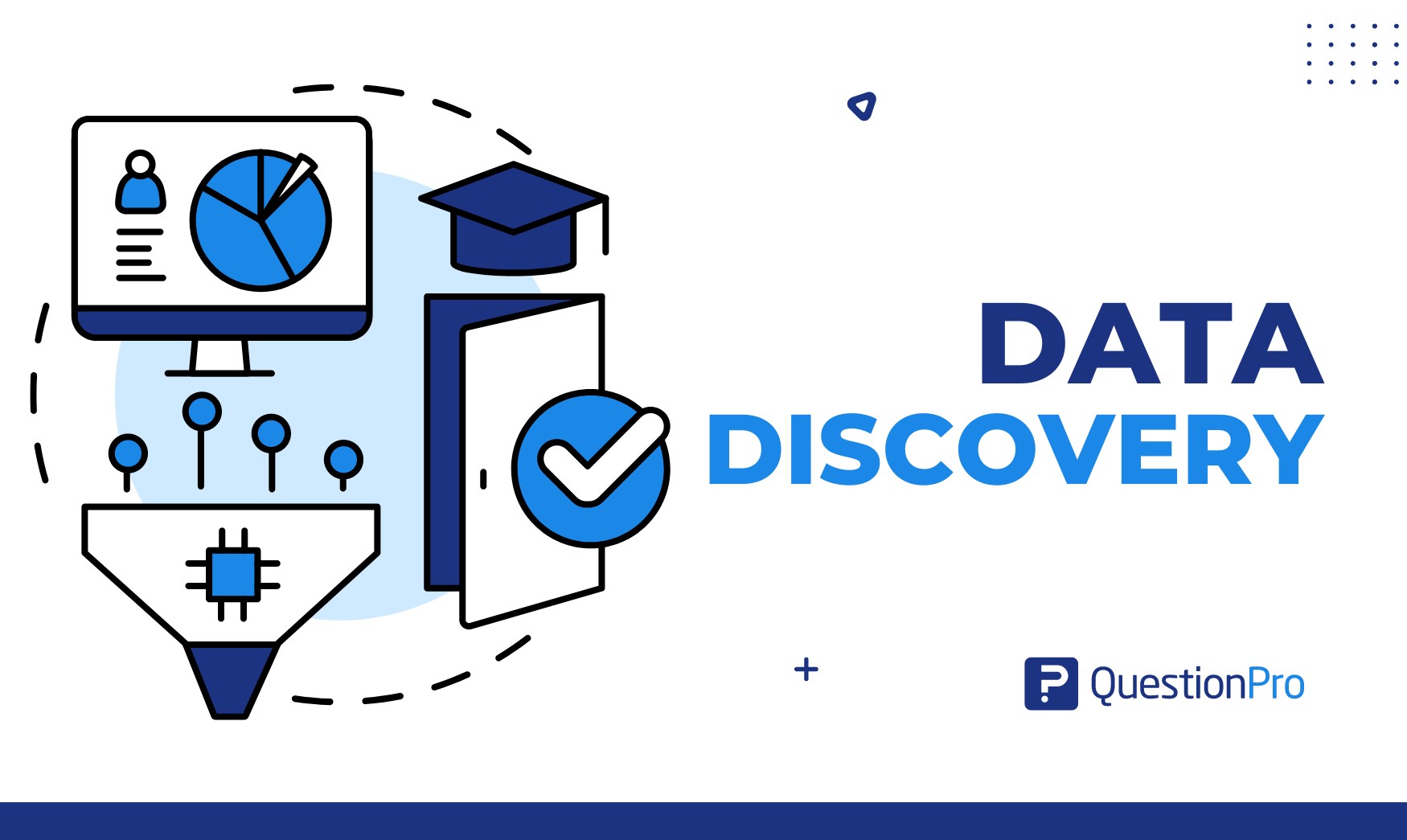
Data Discovery: What it is, Importance, Process + Use Cases
Sep 16, 2024
Other categories
- Academic Research
- Artificial Intelligence
- Assessments
- Brand Awareness
- Case Studies
- Communities
- Consumer Insights
- Customer effort score
- Customer Engagement
- Customer Experience
- Customer Loyalty
- Customer Research
- Customer Satisfaction
- Employee Benefits
- Employee Engagement
- Employee Retention
- Friday Five
- General Data Protection Regulation
- Insights Hub
- Life@QuestionPro
- Market Research
- Mobile diaries
- Mobile Surveys
- New Features
- Online Communities
- Question Types
- Questionnaire
- QuestionPro Products
- Release Notes
- Research Tools and Apps
- Revenue at Risk
- Survey Templates
- Training Tips
- Tuesday CX Thoughts (TCXT)
- Uncategorized
- What’s Coming Up
- Workforce Intelligence

- How to find Psychology Articles
- Using APA Thesaurus
Empirical Articles
- How to Limit to Empirical Articles
- What are they?
- How to Read them?
- Main Sections
Empirical articles are those in which authors report on their own study. The authors will have collected data to answer a research question. Empirical research contains observed and measured examples that inform or answer the research question. The data can be collected in a variety of ways such as interviews, surveys, questionnaires, observations, and various other quantitative and qualitative research methods.
Empirical research is based on observed and measured phenomena and derives knowledge from actual experience rather than from theory or belief.
How do you know if a study is empirical? Read the subheadings within the article, book, or report and look for a description of the research "methodology." Ask yourself: Could I recreate this study and test these results?
Key characteristics to look for:
- Specific research questions to be answered
- Definition of the population, behavior, or phenomena being studied
- Description of the process used to study this population or phenomena, including selection criteria, controls, and testing instruments (such as surveys)
Another hint: some scholarly journals use a specific layout, called the "IMRaD" format, to communicate empirical research findings. Such articles typically have 4 components:
- Introduction : sometimes called "literature review" -- what is currently known about the topic -- usually includes a theoretical framework and/or discussion of previous studies
- Methodology: sometimes called "research design" -- how to recreate the study -- usually describes the population, research process, and analytical tools
- Results : sometimes called "findings" -- what was learned through the study -- usually appears as statistical data or as substantial quotations from research participants
- Discussion : sometimes called "conclusion" or "implications" -- why the study is important -- usually describes how the research results influence professional practices or future studies
General Advice
- Plan to read the article more than once
- Don't read it all the way through in one sitting, read strategically first.
- Identify relevant conclusions and limitations of study
Abstract: Get a sense of the article’s purpose and findings. Use it to assess if the article is useful for your research.
Skim: Review headings to understand the structure and label parts if needed.
Introduction/Literature Review: Identify the main argument, problem, previous work, proposed next steps, and hypothesis.
Methodology: Understand data collection methods, data sources, and variables.
Findings/Results: Examine tables and figures to see if they support the hypothesis without relying on captions.
Discussion/Conclusion: Determine if the findings support the argument/hypothesis and if the authors acknowledge any limitations.
Anatomy of a Research Paper by Richard D. Branson published in Respir Care. 2004 October; 49(10): 1222–1228.
How to Read a Scholarly Chemistry Artricle - Rider tutorial.
How to read and understand a scientific paper - a guide for non-scientists - Violent Metaphors (blog post).
Compare your article to this table to help determine you have located an empirical study/research report.
Look for the following words in the title/abstract: empirical, experiment, research, or study.
|
|
Abstract | A short synopsis of the article’s content |
Introduction | Need and rational of this particular research project with research question, statement, and hypothesis. |
Literature Review (sometimes included in the Introduction) | Supporting their ideas with other scholarly research |
Methods | Describes the methodology including a description of the participants, and a description of the research method, measure, research design, or approach to data analysis. |
Results or Findings | Uses narrative, charts, tables, graphs, or other graphics to describe the findings of the paper |
Discussion/Conclusion/Implications | Provides a discussion, summary, or conclusion, bringing together the research question, statement, |
References | References all the articlesdiscussed and cited in the paper- mostly in the literature or results sections |
- << Previous: Using APA Thesaurus
- Next: How to Limit to Empirical Articles >>
- Last Updated: Sep 16, 2024 2:30 PM
- URL: https://guides.rider.edu/psy

An official website of the United States government
The .gov means it’s official. Federal government websites often end in .gov or .mil. Before sharing sensitive information, make sure you’re on a federal government site.
The site is secure. The https:// ensures that you are connecting to the official website and that any information you provide is encrypted and transmitted securely.
- Publications
- Account settings
The PMC website is updating on October 15, 2024. Learn More or Try it out now .
- Advanced Search
- Journal List
- CBE Life Sci Educ
- v.21(3); Fall 2022
Literature Reviews, Theoretical Frameworks, and Conceptual Frameworks: An Introduction for New Biology Education Researchers
Julie a. luft.
† Department of Mathematics, Social Studies, and Science Education, Mary Frances Early College of Education, University of Georgia, Athens, GA 30602-7124
Sophia Jeong
‡ Department of Teaching & Learning, College of Education & Human Ecology, Ohio State University, Columbus, OH 43210
Robert Idsardi
§ Department of Biology, Eastern Washington University, Cheney, WA 99004
Grant Gardner
∥ Department of Biology, Middle Tennessee State University, Murfreesboro, TN 37132
Associated Data
To frame their work, biology education researchers need to consider the role of literature reviews, theoretical frameworks, and conceptual frameworks as critical elements of the research and writing process. However, these elements can be confusing for scholars new to education research. This Research Methods article is designed to provide an overview of each of these elements and delineate the purpose of each in the educational research process. We describe what biology education researchers should consider as they conduct literature reviews, identify theoretical frameworks, and construct conceptual frameworks. Clarifying these different components of educational research studies can be helpful to new biology education researchers and the biology education research community at large in situating their work in the broader scholarly literature.
INTRODUCTION
Discipline-based education research (DBER) involves the purposeful and situated study of teaching and learning in specific disciplinary areas ( Singer et al. , 2012 ). Studies in DBER are guided by research questions that reflect disciplines’ priorities and worldviews. Researchers can use quantitative data, qualitative data, or both to answer these research questions through a variety of methodological traditions. Across all methodologies, there are different methods associated with planning and conducting educational research studies that include the use of surveys, interviews, observations, artifacts, or instruments. Ensuring the coherence of these elements to the discipline’s perspective also involves situating the work in the broader scholarly literature. The tools for doing this include literature reviews, theoretical frameworks, and conceptual frameworks. However, the purpose and function of each of these elements is often confusing to new education researchers. The goal of this article is to introduce new biology education researchers to these three important elements important in DBER scholarship and the broader educational literature.
The first element we discuss is a review of research (literature reviews), which highlights the need for a specific research question, study problem, or topic of investigation. Literature reviews situate the relevance of the study within a topic and a field. The process may seem familiar to science researchers entering DBER fields, but new researchers may still struggle in conducting the review. Booth et al. (2016b) highlight some of the challenges novice education researchers face when conducting a review of literature. They point out that novice researchers struggle in deciding how to focus the review, determining the scope of articles needed in the review, and knowing how to be critical of the articles in the review. Overcoming these challenges (and others) can help novice researchers construct a sound literature review that can inform the design of the study and help ensure the work makes a contribution to the field.
The second and third highlighted elements are theoretical and conceptual frameworks. These guide biology education research (BER) studies, and may be less familiar to science researchers. These elements are important in shaping the construction of new knowledge. Theoretical frameworks offer a way to explain and interpret the studied phenomenon, while conceptual frameworks clarify assumptions about the studied phenomenon. Despite the importance of these constructs in educational research, biology educational researchers have noted the limited use of theoretical or conceptual frameworks in published work ( DeHaan, 2011 ; Dirks, 2011 ; Lo et al. , 2019 ). In reviewing articles published in CBE—Life Sciences Education ( LSE ) between 2015 and 2019, we found that fewer than 25% of the research articles had a theoretical or conceptual framework (see the Supplemental Information), and at times there was an inconsistent use of theoretical and conceptual frameworks. Clearly, these frameworks are challenging for published biology education researchers, which suggests the importance of providing some initial guidance to new biology education researchers.
Fortunately, educational researchers have increased their explicit use of these frameworks over time, and this is influencing educational research in science, technology, engineering, and mathematics (STEM) fields. For instance, a quick search for theoretical or conceptual frameworks in the abstracts of articles in Educational Research Complete (a common database for educational research) in STEM fields demonstrates a dramatic change over the last 20 years: from only 778 articles published between 2000 and 2010 to 5703 articles published between 2010 and 2020, a more than sevenfold increase. Greater recognition of the importance of these frameworks is contributing to DBER authors being more explicit about such frameworks in their studies.
Collectively, literature reviews, theoretical frameworks, and conceptual frameworks work to guide methodological decisions and the elucidation of important findings. Each offers a different perspective on the problem of study and is an essential element in all forms of educational research. As new researchers seek to learn about these elements, they will find different resources, a variety of perspectives, and many suggestions about the construction and use of these elements. The wide range of available information can overwhelm the new researcher who just wants to learn the distinction between these elements or how to craft them adequately.
Our goal in writing this paper is not to offer specific advice about how to write these sections in scholarly work. Instead, we wanted to introduce these elements to those who are new to BER and who are interested in better distinguishing one from the other. In this paper, we share the purpose of each element in BER scholarship, along with important points on its construction. We also provide references for additional resources that may be beneficial to better understanding each element. Table 1 summarizes the key distinctions among these elements.
Comparison of literature reviews, theoretical frameworks, and conceptual reviews
Literature reviews | Theoretical frameworks | Conceptual frameworks | |
---|---|---|---|
Purpose | To point out the need for the study in BER and connection to the field. | To state the assumptions and orientations of the researcher regarding the topic of study | To describe the researcher’s understanding of the main concepts under investigation |
Aims | A literature review examines current and relevant research associated with the study question. It is comprehensive, critical, and purposeful. | A theoretical framework illuminates the phenomenon of study and the corresponding assumptions adopted by the researcher. Frameworks can take on different orientations. | The conceptual framework is created by the researcher(s), includes the presumed relationships among concepts, and addresses needed areas of study discovered in literature reviews. |
Connection to the manuscript | A literature review should connect to the study question, guide the study methodology, and be central in the discussion by indicating how the analyzed data advances what is known in the field. | A theoretical framework drives the question, guides the types of methods for data collection and analysis, informs the discussion of the findings, and reveals the subjectivities of the researcher. | The conceptual framework is informed by literature reviews, experiences, or experiments. It may include emergent ideas that are not yet grounded in the literature. It should be coherent with the paper’s theoretical framing. |
Additional points | A literature review may reach beyond BER and include other education research fields. | A theoretical framework does not rationalize the need for the study, and a theoretical framework can come from different fields. | A conceptual framework articulates the phenomenon under study through written descriptions and/or visual representations. |
This article is written for the new biology education researcher who is just learning about these different elements or for scientists looking to become more involved in BER. It is a result of our own work as science education and biology education researchers, whether as graduate students and postdoctoral scholars or newly hired and established faculty members. This is the article we wish had been available as we started to learn about these elements or discussed them with new educational researchers in biology.
LITERATURE REVIEWS
Purpose of a literature review.
A literature review is foundational to any research study in education or science. In education, a well-conceptualized and well-executed review provides a summary of the research that has already been done on a specific topic and identifies questions that remain to be answered, thus illustrating the current research project’s potential contribution to the field and the reasoning behind the methodological approach selected for the study ( Maxwell, 2012 ). BER is an evolving disciplinary area that is redefining areas of conceptual emphasis as well as orientations toward teaching and learning (e.g., Labov et al. , 2010 ; American Association for the Advancement of Science, 2011 ; Nehm, 2019 ). As a result, building comprehensive, critical, purposeful, and concise literature reviews can be a challenge for new biology education researchers.
Building Literature Reviews
There are different ways to approach and construct a literature review. Booth et al. (2016a) provide an overview that includes, for example, scoping reviews, which are focused only on notable studies and use a basic method of analysis, and integrative reviews, which are the result of exhaustive literature searches across different genres. Underlying each of these different review processes are attention to the s earch process, a ppraisa l of articles, s ynthesis of the literature, and a nalysis: SALSA ( Booth et al. , 2016a ). This useful acronym can help the researcher focus on the process while building a specific type of review.
However, new educational researchers often have questions about literature reviews that are foundational to SALSA or other approaches. Common questions concern determining which literature pertains to the topic of study or the role of the literature review in the design of the study. This section addresses such questions broadly while providing general guidance for writing a narrative literature review that evaluates the most pertinent studies.
The literature review process should begin before the research is conducted. As Boote and Beile (2005 , p. 3) suggested, researchers should be “scholars before researchers.” They point out that having a good working knowledge of the proposed topic helps illuminate avenues of study. Some subject areas have a deep body of work to read and reflect upon, providing a strong foundation for developing the research question(s). For instance, the teaching and learning of evolution is an area of long-standing interest in the BER community, generating many studies (e.g., Perry et al. , 2008 ; Barnes and Brownell, 2016 ) and reviews of research (e.g., Sickel and Friedrichsen, 2013 ; Ziadie and Andrews, 2018 ). Emerging areas of BER include the affective domain, issues of transfer, and metacognition ( Singer et al. , 2012 ). Many studies in these areas are transdisciplinary and not always specific to biology education (e.g., Rodrigo-Peiris et al. , 2018 ; Kolpikova et al. , 2019 ). These newer areas may require reading outside BER; fortunately, summaries of some of these topics can be found in the Current Insights section of the LSE website.
In focusing on a specific problem within a broader research strand, a new researcher will likely need to examine research outside BER. Depending upon the area of study, the expanded reading list might involve a mix of BER, DBER, and educational research studies. Determining the scope of the reading is not always straightforward. A simple way to focus one’s reading is to create a “summary phrase” or “research nugget,” which is a very brief descriptive statement about the study. It should focus on the essence of the study, for example, “first-year nonmajor students’ understanding of evolution,” “metacognitive prompts to enhance learning during biochemistry,” or “instructors’ inquiry-based instructional practices after professional development programming.” This type of phrase should help a new researcher identify two or more areas to review that pertain to the study. Focusing on recent research in the last 5 years is a good first step. Additional studies can be identified by reading relevant works referenced in those articles. It is also important to read seminal studies that are more than 5 years old. Reading a range of studies should give the researcher the necessary command of the subject in order to suggest a research question.
Given that the research question(s) arise from the literature review, the review should also substantiate the selected methodological approach. The review and research question(s) guide the researcher in determining how to collect and analyze data. Often the methodological approach used in a study is selected to contribute knowledge that expands upon what has been published previously about the topic (see Institute of Education Sciences and National Science Foundation, 2013 ). An emerging topic of study may need an exploratory approach that allows for a description of the phenomenon and development of a potential theory. This could, but not necessarily, require a methodological approach that uses interviews, observations, surveys, or other instruments. An extensively studied topic may call for the additional understanding of specific factors or variables; this type of study would be well suited to a verification or a causal research design. These could entail a methodological approach that uses valid and reliable instruments, observations, or interviews to determine an effect in the studied event. In either of these examples, the researcher(s) may use a qualitative, quantitative, or mixed methods methodological approach.
Even with a good research question, there is still more reading to be done. The complexity and focus of the research question dictates the depth and breadth of the literature to be examined. Questions that connect multiple topics can require broad literature reviews. For instance, a study that explores the impact of a biology faculty learning community on the inquiry instruction of faculty could have the following review areas: learning communities among biology faculty, inquiry instruction among biology faculty, and inquiry instruction among biology faculty as a result of professional learning. Biology education researchers need to consider whether their literature review requires studies from different disciplines within or outside DBER. For the example given, it would be fruitful to look at research focused on learning communities with faculty in STEM fields or in general education fields that result in instructional change. It is important not to be too narrow or too broad when reading. When the conclusions of articles start to sound similar or no new insights are gained, the researcher likely has a good foundation for a literature review. This level of reading should allow the researcher to demonstrate a mastery in understanding the researched topic, explain the suitability of the proposed research approach, and point to the need for the refined research question(s).
The literature review should include the researcher’s evaluation and critique of the selected studies. A researcher may have a large collection of studies, but not all of the studies will follow standards important in the reporting of empirical work in the social sciences. The American Educational Research Association ( Duran et al. , 2006 ), for example, offers a general discussion about standards for such work: an adequate review of research informing the study, the existence of sound and appropriate data collection and analysis methods, and appropriate conclusions that do not overstep or underexplore the analyzed data. The Institute of Education Sciences and National Science Foundation (2013) also offer Common Guidelines for Education Research and Development that can be used to evaluate collected studies.
Because not all journals adhere to such standards, it is important that a researcher review each study to determine the quality of published research, per the guidelines suggested earlier. In some instances, the research may be fatally flawed. Examples of such flaws include data that do not pertain to the question, a lack of discussion about the data collection, poorly constructed instruments, or an inadequate analysis. These types of errors result in studies that are incomplete, error-laden, or inaccurate and should be excluded from the review. Most studies have limitations, and the author(s) often make them explicit. For instance, there may be an instructor effect, recognized bias in the analysis, or issues with the sample population. Limitations are usually addressed by the research team in some way to ensure a sound and acceptable research process. Occasionally, the limitations associated with the study can be significant and not addressed adequately, which leaves a consequential decision in the hands of the researcher. Providing critiques of studies in the literature review process gives the reader confidence that the researcher has carefully examined relevant work in preparation for the study and, ultimately, the manuscript.
A solid literature review clearly anchors the proposed study in the field and connects the research question(s), the methodological approach, and the discussion. Reviewing extant research leads to research questions that will contribute to what is known in the field. By summarizing what is known, the literature review points to what needs to be known, which in turn guides decisions about methodology. Finally, notable findings of the new study are discussed in reference to those described in the literature review.
Within published BER studies, literature reviews can be placed in different locations in an article. When included in the introductory section of the study, the first few paragraphs of the manuscript set the stage, with the literature review following the opening paragraphs. Cooper et al. (2019) illustrate this approach in their study of course-based undergraduate research experiences (CUREs). An introduction discussing the potential of CURES is followed by an analysis of the existing literature relevant to the design of CUREs that allows for novel student discoveries. Within this review, the authors point out contradictory findings among research on novel student discoveries. This clarifies the need for their study, which is described and highlighted through specific research aims.
A literature reviews can also make up a separate section in a paper. For example, the introduction to Todd et al. (2019) illustrates the need for their research topic by highlighting the potential of learning progressions (LPs) and suggesting that LPs may help mitigate learning loss in genetics. At the end of the introduction, the authors state their specific research questions. The review of literature following this opening section comprises two subsections. One focuses on learning loss in general and examines a variety of studies and meta-analyses from the disciplines of medical education, mathematics, and reading. The second section focuses specifically on LPs in genetics and highlights student learning in the midst of LPs. These separate reviews provide insights into the stated research question.
Suggestions and Advice
A well-conceptualized, comprehensive, and critical literature review reveals the understanding of the topic that the researcher brings to the study. Literature reviews should not be so big that there is no clear area of focus; nor should they be so narrow that no real research question arises. The task for a researcher is to craft an efficient literature review that offers a critical analysis of published work, articulates the need for the study, guides the methodological approach to the topic of study, and provides an adequate foundation for the discussion of the findings.
In our own writing of literature reviews, there are often many drafts. An early draft may seem well suited to the study because the need for and approach to the study are well described. However, as the results of the study are analyzed and findings begin to emerge, the existing literature review may be inadequate and need revision. The need for an expanded discussion about the research area can result in the inclusion of new studies that support the explanation of a potential finding. The literature review may also prove to be too broad. Refocusing on a specific area allows for more contemplation of a finding.
It should be noted that there are different types of literature reviews, and many books and articles have been written about the different ways to embark on these types of reviews. Among these different resources, the following may be helpful in considering how to refine the review process for scholarly journals:
- Booth, A., Sutton, A., & Papaioannou, D. (2016a). Systemic approaches to a successful literature review (2nd ed.). Los Angeles, CA: Sage. This book addresses different types of literature reviews and offers important suggestions pertaining to defining the scope of the literature review and assessing extant studies.
- Booth, W. C., Colomb, G. G., Williams, J. M., Bizup, J., & Fitzgerald, W. T. (2016b). The craft of research (4th ed.). Chicago: University of Chicago Press. This book can help the novice consider how to make the case for an area of study. While this book is not specifically about literature reviews, it offers suggestions about making the case for your study.
- Galvan, J. L., & Galvan, M. C. (2017). Writing literature reviews: A guide for students of the social and behavioral sciences (7th ed.). Routledge. This book offers guidance on writing different types of literature reviews. For the novice researcher, there are useful suggestions for creating coherent literature reviews.
THEORETICAL FRAMEWORKS
Purpose of theoretical frameworks.
As new education researchers may be less familiar with theoretical frameworks than with literature reviews, this discussion begins with an analogy. Envision a biologist, chemist, and physicist examining together the dramatic effect of a fog tsunami over the ocean. A biologist gazing at this phenomenon may be concerned with the effect of fog on various species. A chemist may be interested in the chemical composition of the fog as water vapor condenses around bits of salt. A physicist may be focused on the refraction of light to make fog appear to be “sitting” above the ocean. While observing the same “objective event,” the scientists are operating under different theoretical frameworks that provide a particular perspective or “lens” for the interpretation of the phenomenon. Each of these scientists brings specialized knowledge, experiences, and values to this phenomenon, and these influence the interpretation of the phenomenon. The scientists’ theoretical frameworks influence how they design and carry out their studies and interpret their data.
Within an educational study, a theoretical framework helps to explain a phenomenon through a particular lens and challenges and extends existing knowledge within the limitations of that lens. Theoretical frameworks are explicitly stated by an educational researcher in the paper’s framework, theory, or relevant literature section. The framework shapes the types of questions asked, guides the method by which data are collected and analyzed, and informs the discussion of the results of the study. It also reveals the researcher’s subjectivities, for example, values, social experience, and viewpoint ( Allen, 2017 ). It is essential that a novice researcher learn to explicitly state a theoretical framework, because all research questions are being asked from the researcher’s implicit or explicit assumptions of a phenomenon of interest ( Schwandt, 2000 ).
Selecting Theoretical Frameworks
Theoretical frameworks are one of the most contemplated elements in our work in educational research. In this section, we share three important considerations for new scholars selecting a theoretical framework.
The first step in identifying a theoretical framework involves reflecting on the phenomenon within the study and the assumptions aligned with the phenomenon. The phenomenon involves the studied event. There are many possibilities, for example, student learning, instructional approach, or group organization. A researcher holds assumptions about how the phenomenon will be effected, influenced, changed, or portrayed. It is ultimately the researcher’s assumption(s) about the phenomenon that aligns with a theoretical framework. An example can help illustrate how a researcher’s reflection on the phenomenon and acknowledgment of assumptions can result in the identification of a theoretical framework.
In our example, a biology education researcher may be interested in exploring how students’ learning of difficult biological concepts can be supported by the interactions of group members. The phenomenon of interest is the interactions among the peers, and the researcher assumes that more knowledgeable students are important in supporting the learning of the group. As a result, the researcher may draw on Vygotsky’s (1978) sociocultural theory of learning and development that is focused on the phenomenon of student learning in a social setting. This theory posits the critical nature of interactions among students and between students and teachers in the process of building knowledge. A researcher drawing upon this framework holds the assumption that learning is a dynamic social process involving questions and explanations among students in the classroom and that more knowledgeable peers play an important part in the process of building conceptual knowledge.
It is important to state at this point that there are many different theoretical frameworks. Some frameworks focus on learning and knowing, while other theoretical frameworks focus on equity, empowerment, or discourse. Some frameworks are well articulated, and others are still being refined. For a new researcher, it can be challenging to find a theoretical framework. Two of the best ways to look for theoretical frameworks is through published works that highlight different frameworks.
When a theoretical framework is selected, it should clearly connect to all parts of the study. The framework should augment the study by adding a perspective that provides greater insights into the phenomenon. It should clearly align with the studies described in the literature review. For instance, a framework focused on learning would correspond to research that reported different learning outcomes for similar studies. The methods for data collection and analysis should also correspond to the framework. For instance, a study about instructional interventions could use a theoretical framework concerned with learning and could collect data about the effect of the intervention on what is learned. When the data are analyzed, the theoretical framework should provide added meaning to the findings, and the findings should align with the theoretical framework.
A study by Jensen and Lawson (2011) provides an example of how a theoretical framework connects different parts of the study. They compared undergraduate biology students in heterogeneous and homogeneous groups over the course of a semester. Jensen and Lawson (2011) assumed that learning involved collaboration and more knowledgeable peers, which made Vygotsky’s (1978) theory a good fit for their study. They predicted that students in heterogeneous groups would experience greater improvement in their reasoning abilities and science achievements with much of the learning guided by the more knowledgeable peers.
In the enactment of the study, they collected data about the instruction in traditional and inquiry-oriented classes, while the students worked in homogeneous or heterogeneous groups. To determine the effect of working in groups, the authors also measured students’ reasoning abilities and achievement. Each data-collection and analysis decision connected to understanding the influence of collaborative work.
Their findings highlighted aspects of Vygotsky’s (1978) theory of learning. One finding, for instance, posited that inquiry instruction, as a whole, resulted in reasoning and achievement gains. This links to Vygotsky (1978) , because inquiry instruction involves interactions among group members. A more nuanced finding was that group composition had a conditional effect. Heterogeneous groups performed better with more traditional and didactic instruction, regardless of the reasoning ability of the group members. Homogeneous groups worked better during interaction-rich activities for students with low reasoning ability. The authors attributed the variation to the different types of helping behaviors of students. High-performing students provided the answers, while students with low reasoning ability had to work collectively through the material. In terms of Vygotsky (1978) , this finding provided new insights into the learning context in which productive interactions can occur for students.
Another consideration in the selection and use of a theoretical framework pertains to its orientation to the study. This can result in the theoretical framework prioritizing individuals, institutions, and/or policies ( Anfara and Mertz, 2014 ). Frameworks that connect to individuals, for instance, could contribute to understanding their actions, learning, or knowledge. Institutional frameworks, on the other hand, offer insights into how institutions, organizations, or groups can influence individuals or materials. Policy theories provide ways to understand how national or local policies can dictate an emphasis on outcomes or instructional design. These different types of frameworks highlight different aspects in an educational setting, which influences the design of the study and the collection of data. In addition, these different frameworks offer a way to make sense of the data. Aligning the data collection and analysis with the framework ensures that a study is coherent and can contribute to the field.
New understandings emerge when different theoretical frameworks are used. For instance, Ebert-May et al. (2015) prioritized the individual level within conceptual change theory (see Posner et al. , 1982 ). In this theory, an individual’s knowledge changes when it no longer fits the phenomenon. Ebert-May et al. (2015) designed a professional development program challenging biology postdoctoral scholars’ existing conceptions of teaching. The authors reported that the biology postdoctoral scholars’ teaching practices became more student-centered as they were challenged to explain their instructional decision making. According to the theory, the biology postdoctoral scholars’ dissatisfaction in their descriptions of teaching and learning initiated change in their knowledge and instruction. These results reveal how conceptual change theory can explain the learning of participants and guide the design of professional development programming.
The communities of practice (CoP) theoretical framework ( Lave, 1988 ; Wenger, 1998 ) prioritizes the institutional level , suggesting that learning occurs when individuals learn from and contribute to the communities in which they reside. Grounded in the assumption of community learning, the literature on CoP suggests that, as individuals interact regularly with the other members of their group, they learn about the rules, roles, and goals of the community ( Allee, 2000 ). A study conducted by Gehrke and Kezar (2017) used the CoP framework to understand organizational change by examining the involvement of individual faculty engaged in a cross-institutional CoP focused on changing the instructional practice of faculty at each institution. In the CoP, faculty members were involved in enhancing instructional materials within their department, which aligned with an overarching goal of instituting instruction that embraced active learning. Not surprisingly, Gehrke and Kezar (2017) revealed that faculty who perceived the community culture as important in their work cultivated institutional change. Furthermore, they found that institutional change was sustained when key leaders served as mentors and provided support for faculty, and as faculty themselves developed into leaders. This study reveals the complexity of individual roles in a COP in order to support institutional instructional change.
It is important to explicitly state the theoretical framework used in a study, but elucidating a theoretical framework can be challenging for a new educational researcher. The literature review can help to identify an applicable theoretical framework. Focal areas of the review or central terms often connect to assumptions and assertions associated with the framework that pertain to the phenomenon of interest. Another way to identify a theoretical framework is self-reflection by the researcher on personal beliefs and understandings about the nature of knowledge the researcher brings to the study ( Lysaght, 2011 ). In stating one’s beliefs and understandings related to the study (e.g., students construct their knowledge, instructional materials support learning), an orientation becomes evident that will suggest a particular theoretical framework. Theoretical frameworks are not arbitrary , but purposefully selected.
With experience, a researcher may find expanded roles for theoretical frameworks. Researchers may revise an existing framework that has limited explanatory power, or they may decide there is a need to develop a new theoretical framework. These frameworks can emerge from a current study or the need to explain a phenomenon in a new way. Researchers may also find that multiple theoretical frameworks are necessary to frame and explore a problem, as different frameworks can provide different insights into a problem.
Finally, it is important to recognize that choosing “x” theoretical framework does not necessarily mean a researcher chooses “y” methodology and so on, nor is there a clear-cut, linear process in selecting a theoretical framework for one’s study. In part, the nonlinear process of identifying a theoretical framework is what makes understanding and using theoretical frameworks challenging. For the novice scholar, contemplating and understanding theoretical frameworks is essential. Fortunately, there are articles and books that can help:
- Creswell, J. W. (2018). Research design: Qualitative, quantitative, and mixed methods approaches (5th ed.). Los Angeles, CA: Sage. This book provides an overview of theoretical frameworks in general educational research.
- Ding, L. (2019). Theoretical perspectives of quantitative physics education research. Physical Review Physics Education Research , 15 (2), 020101-1–020101-13. This paper illustrates how a DBER field can use theoretical frameworks.
- Nehm, R. (2019). Biology education research: Building integrative frameworks for teaching and learning about living systems. Disciplinary and Interdisciplinary Science Education Research , 1 , ar15. https://doi.org/10.1186/s43031-019-0017-6 . This paper articulates the need for studies in BER to explicitly state theoretical frameworks and provides examples of potential studies.
- Patton, M. Q. (2015). Qualitative research & evaluation methods: Integrating theory and practice . Sage. This book also provides an overview of theoretical frameworks, but for both research and evaluation.
CONCEPTUAL FRAMEWORKS
Purpose of a conceptual framework.
A conceptual framework is a description of the way a researcher understands the factors and/or variables that are involved in the study and their relationships to one another. The purpose of a conceptual framework is to articulate the concepts under study using relevant literature ( Rocco and Plakhotnik, 2009 ) and to clarify the presumed relationships among those concepts ( Rocco and Plakhotnik, 2009 ; Anfara and Mertz, 2014 ). Conceptual frameworks are different from theoretical frameworks in both their breadth and grounding in established findings. Whereas a theoretical framework articulates the lens through which a researcher views the work, the conceptual framework is often more mechanistic and malleable.
Conceptual frameworks are broader, encompassing both established theories (i.e., theoretical frameworks) and the researchers’ own emergent ideas. Emergent ideas, for example, may be rooted in informal and/or unpublished observations from experience. These emergent ideas would not be considered a “theory” if they are not yet tested, supported by systematically collected evidence, and peer reviewed. However, they do still play an important role in the way researchers approach their studies. The conceptual framework allows authors to clearly describe their emergent ideas so that connections among ideas in the study and the significance of the study are apparent to readers.
Constructing Conceptual Frameworks
Including a conceptual framework in a research study is important, but researchers often opt to include either a conceptual or a theoretical framework. Either may be adequate, but both provide greater insight into the research approach. For instance, a research team plans to test a novel component of an existing theory. In their study, they describe the existing theoretical framework that informs their work and then present their own conceptual framework. Within this conceptual framework, specific topics portray emergent ideas that are related to the theory. Describing both frameworks allows readers to better understand the researchers’ assumptions, orientations, and understanding of concepts being investigated. For example, Connolly et al. (2018) included a conceptual framework that described how they applied a theoretical framework of social cognitive career theory (SCCT) to their study on teaching programs for doctoral students. In their conceptual framework, the authors described SCCT, explained how it applied to the investigation, and drew upon results from previous studies to justify the proposed connections between the theory and their emergent ideas.
In some cases, authors may be able to sufficiently describe their conceptualization of the phenomenon under study in an introduction alone, without a separate conceptual framework section. However, incomplete descriptions of how the researchers conceptualize the components of the study may limit the significance of the study by making the research less intelligible to readers. This is especially problematic when studying topics in which researchers use the same terms for different constructs or different terms for similar and overlapping constructs (e.g., inquiry, teacher beliefs, pedagogical content knowledge, or active learning). Authors must describe their conceptualization of a construct if the research is to be understandable and useful.
There are some key areas to consider regarding the inclusion of a conceptual framework in a study. To begin with, it is important to recognize that conceptual frameworks are constructed by the researchers conducting the study ( Rocco and Plakhotnik, 2009 ; Maxwell, 2012 ). This is different from theoretical frameworks that are often taken from established literature. Researchers should bring together ideas from the literature, but they may be influenced by their own experiences as a student and/or instructor, the shared experiences of others, or thought experiments as they construct a description, model, or representation of their understanding of the phenomenon under study. This is an exercise in intellectual organization and clarity that often considers what is learned, known, and experienced. The conceptual framework makes these constructs explicitly visible to readers, who may have different understandings of the phenomenon based on their prior knowledge and experience. There is no single method to go about this intellectual work.
Reeves et al. (2016) is an example of an article that proposed a conceptual framework about graduate teaching assistant professional development evaluation and research. The authors used existing literature to create a novel framework that filled a gap in current research and practice related to the training of graduate teaching assistants. This conceptual framework can guide the systematic collection of data by other researchers because the framework describes the relationships among various factors that influence teaching and learning. The Reeves et al. (2016) conceptual framework may be modified as additional data are collected and analyzed by other researchers. This is not uncommon, as conceptual frameworks can serve as catalysts for concerted research efforts that systematically explore a phenomenon (e.g., Reynolds et al. , 2012 ; Brownell and Kloser, 2015 ).
Sabel et al. (2017) used a conceptual framework in their exploration of how scaffolds, an external factor, interact with internal factors to support student learning. Their conceptual framework integrated principles from two theoretical frameworks, self-regulated learning and metacognition, to illustrate how the research team conceptualized students’ use of scaffolds in their learning ( Figure 1 ). Sabel et al. (2017) created this model using their interpretations of these two frameworks in the context of their teaching.

Conceptual framework from Sabel et al. (2017) .
A conceptual framework should describe the relationship among components of the investigation ( Anfara and Mertz, 2014 ). These relationships should guide the researcher’s methods of approaching the study ( Miles et al. , 2014 ) and inform both the data to be collected and how those data should be analyzed. Explicitly describing the connections among the ideas allows the researcher to justify the importance of the study and the rigor of the research design. Just as importantly, these frameworks help readers understand why certain components of a system were not explored in the study. This is a challenge in education research, which is rooted in complex environments with many variables that are difficult to control.
For example, Sabel et al. (2017) stated: “Scaffolds, such as enhanced answer keys and reflection questions, can help students and instructors bridge the external and internal factors and support learning” (p. 3). They connected the scaffolds in the study to the three dimensions of metacognition and the eventual transformation of existing ideas into new or revised ideas. Their framework provides a rationale for focusing on how students use two different scaffolds, and not on other factors that may influence a student’s success (self-efficacy, use of active learning, exam format, etc.).
In constructing conceptual frameworks, researchers should address needed areas of study and/or contradictions discovered in literature reviews. By attending to these areas, researchers can strengthen their arguments for the importance of a study. For instance, conceptual frameworks can address how the current study will fill gaps in the research, resolve contradictions in existing literature, or suggest a new area of study. While a literature review describes what is known and not known about the phenomenon, the conceptual framework leverages these gaps in describing the current study ( Maxwell, 2012 ). In the example of Sabel et al. (2017) , the authors indicated there was a gap in the literature regarding how scaffolds engage students in metacognition to promote learning in large classes. Their study helps fill that gap by describing how scaffolds can support students in the three dimensions of metacognition: intelligibility, plausibility, and wide applicability. In another example, Lane (2016) integrated research from science identity, the ethic of care, the sense of belonging, and an expertise model of student success to form a conceptual framework that addressed the critiques of other frameworks. In a more recent example, Sbeglia et al. (2021) illustrated how a conceptual framework influences the methodological choices and inferences in studies by educational researchers.
Sometimes researchers draw upon the conceptual frameworks of other researchers. When a researcher’s conceptual framework closely aligns with an existing framework, the discussion may be brief. For example, Ghee et al. (2016) referred to portions of SCCT as their conceptual framework to explain the significance of their work on students’ self-efficacy and career interests. Because the authors’ conceptualization of this phenomenon aligned with a previously described framework, they briefly mentioned the conceptual framework and provided additional citations that provided more detail for the readers.
Within both the BER and the broader DBER communities, conceptual frameworks have been used to describe different constructs. For example, some researchers have used the term “conceptual framework” to describe students’ conceptual understandings of a biological phenomenon. This is distinct from a researcher’s conceptual framework of the educational phenomenon under investigation, which may also need to be explicitly described in the article. Other studies have presented a research logic model or flowchart of the research design as a conceptual framework. These constructions can be quite valuable in helping readers understand the data-collection and analysis process. However, a model depicting the study design does not serve the same role as a conceptual framework. Researchers need to avoid conflating these constructs by differentiating the researchers’ conceptual framework that guides the study from the research design, when applicable.
Explicitly describing conceptual frameworks is essential in depicting the focus of the study. We have found that being explicit in a conceptual framework means using accepted terminology, referencing prior work, and clearly noting connections between terms. This description can also highlight gaps in the literature or suggest potential contributions to the field of study. A well-elucidated conceptual framework can suggest additional studies that may be warranted. This can also spur other researchers to consider how they would approach the examination of a phenomenon and could result in a revised conceptual framework.
It can be challenging to create conceptual frameworks, but they are important. Below are two resources that could be helpful in constructing and presenting conceptual frameworks in educational research:
- Maxwell, J. A. (2012). Qualitative research design: An interactive approach (3rd ed.). Los Angeles, CA: Sage. Chapter 3 in this book describes how to construct conceptual frameworks.
- Ravitch, S. M., & Riggan, M. (2016). Reason & rigor: How conceptual frameworks guide research . Los Angeles, CA: Sage. This book explains how conceptual frameworks guide the research questions, data collection, data analyses, and interpretation of results.
CONCLUDING THOUGHTS
Literature reviews, theoretical frameworks, and conceptual frameworks are all important in DBER and BER. Robust literature reviews reinforce the importance of a study. Theoretical frameworks connect the study to the base of knowledge in educational theory and specify the researcher’s assumptions. Conceptual frameworks allow researchers to explicitly describe their conceptualization of the relationships among the components of the phenomenon under study. Table 1 provides a general overview of these components in order to assist biology education researchers in thinking about these elements.
It is important to emphasize that these different elements are intertwined. When these elements are aligned and complement one another, the study is coherent, and the study findings contribute to knowledge in the field. When literature reviews, theoretical frameworks, and conceptual frameworks are disconnected from one another, the study suffers. The point of the study is lost, suggested findings are unsupported, or important conclusions are invisible to the researcher. In addition, this misalignment may be costly in terms of time and money.
Conducting a literature review, selecting a theoretical framework, and building a conceptual framework are some of the most difficult elements of a research study. It takes time to understand the relevant research, identify a theoretical framework that provides important insights into the study, and formulate a conceptual framework that organizes the finding. In the research process, there is often a constant back and forth among these elements as the study evolves. With an ongoing refinement of the review of literature, clarification of the theoretical framework, and articulation of a conceptual framework, a sound study can emerge that makes a contribution to the field. This is the goal of BER and education research.
Supplementary Material
- Allee, V. (2000). Knowledge networks and communities of learning . OD Practitioner , 32 ( 4 ), 4–13. [ Google Scholar ]
- Allen, M. (2017). The Sage encyclopedia of communication research methods (Vols. 1–4 ). Los Angeles, CA: Sage. 10.4135/9781483381411 [ CrossRef ] [ Google Scholar ]
- American Association for the Advancement of Science. (2011). Vision and change in undergraduate biology education: A call to action . Washington, DC. [ Google Scholar ]
- Anfara, V. A., Mertz, N. T. (2014). Setting the stage . In Anfara, V. A., Mertz, N. T. (eds.), Theoretical frameworks in qualitative research (pp. 1–22). Sage. [ Google Scholar ]
- Barnes, M. E., Brownell, S. E. (2016). Practices and perspectives of college instructors on addressing religious beliefs when teaching evolution . CBE—Life Sciences Education , 15 ( 2 ), ar18. https://doi.org/10.1187/cbe.15-11-0243 [ PMC free article ] [ PubMed ] [ Google Scholar ]
- Boote, D. N., Beile, P. (2005). Scholars before researchers: On the centrality of the dissertation literature review in research preparation . Educational Researcher , 34 ( 6 ), 3–15. 10.3102/0013189x034006003 [ CrossRef ] [ Google Scholar ]
- Booth, A., Sutton, A., Papaioannou, D. (2016a). Systemic approaches to a successful literature review (2nd ed.). Los Angeles, CA: Sage. [ Google Scholar ]
- Booth, W. C., Colomb, G. G., Williams, J. M., Bizup, J., Fitzgerald, W. T. (2016b). The craft of research (4th ed.). Chicago, IL: University of Chicago Press. [ Google Scholar ]
- Brownell, S. E., Kloser, M. J. (2015). Toward a conceptual framework for measuring the effectiveness of course-based undergraduate research experiences in undergraduate biology . Studies in Higher Education , 40 ( 3 ), 525–544. https://doi.org/10.1080/03075079.2015.1004234 [ Google Scholar ]
- Connolly, M. R., Lee, Y. G., Savoy, J. N. (2018). The effects of doctoral teaching development on early-career STEM scholars’ college teaching self-efficacy . CBE—Life Sciences Education , 17 ( 1 ), ar14. https://doi.org/10.1187/cbe.17-02-0039 [ PMC free article ] [ PubMed ] [ Google Scholar ]
- Cooper, K. M., Blattman, J. N., Hendrix, T., Brownell, S. E. (2019). The impact of broadly relevant novel discoveries on student project ownership in a traditional lab course turned CURE . CBE—Life Sciences Education , 18 ( 4 ), ar57. https://doi.org/10.1187/cbe.19-06-0113 [ PMC free article ] [ PubMed ] [ Google Scholar ]
- Creswell, J. W. (2018). Research design: Qualitative, quantitative, and mixed methods approaches (5th ed.). Los Angeles, CA: Sage. [ Google Scholar ]
- DeHaan, R. L. (2011). Education research in the biological sciences: A nine decade review (Paper commissioned by the NAS/NRC Committee on the Status, Contributions, and Future Directions of Discipline Based Education Research) . Washington, DC: National Academies Press. Retrieved May 20, 2022, from www7.nationalacademies.org/bose/DBER_Mee ting2_commissioned_papers_page.html [ Google Scholar ]
- Ding, L. (2019). Theoretical perspectives of quantitative physics education research . Physical Review Physics Education Research , 15 ( 2 ), 020101. [ Google Scholar ]
- Dirks, C. (2011). The current status and future direction of biology education research . Paper presented at: Second Committee Meeting on the Status, Contributions, and Future Directions of Discipline-Based Education Research, 18–19 October (Washington, DC). Retrieved May 20, 2022, from http://sites.nationalacademies.org/DBASSE/BOSE/DBASSE_071087 [ Google Scholar ]
- Duran, R. P., Eisenhart, M. A., Erickson, F. D., Grant, C. A., Green, J. L., Hedges, L. V., Schneider, B. L. (2006). Standards for reporting on empirical social science research in AERA publications: American Educational Research Association . Educational Researcher , 35 ( 6 ), 33–40. [ Google Scholar ]
- Ebert-May, D., Derting, T. L., Henkel, T. P., Middlemis Maher, J., Momsen, J. L., Arnold, B., Passmore, H. A. (2015). Breaking the cycle: Future faculty begin teaching with learner-centered strategies after professional development . CBE—Life Sciences Education , 14 ( 2 ), ar22. https://doi.org/10.1187/cbe.14-12-0222 [ PMC free article ] [ PubMed ] [ Google Scholar ]
- Galvan, J. L., Galvan, M. C. (2017). Writing literature reviews: A guide for students of the social and behavioral sciences (7th ed.). New York, NY: Routledge. https://doi.org/10.4324/9781315229386 [ Google Scholar ]
- Gehrke, S., Kezar, A. (2017). The roles of STEM faculty communities of practice in institutional and departmental reform in higher education . American Educational Research Journal , 54 ( 5 ), 803–833. https://doi.org/10.3102/0002831217706736 [ Google Scholar ]
- Ghee, M., Keels, M., Collins, D., Neal-Spence, C., Baker, E. (2016). Fine-tuning summer research programs to promote underrepresented students’ persistence in the STEM pathway . CBE—Life Sciences Education , 15 ( 3 ), ar28. https://doi.org/10.1187/cbe.16-01-0046 [ PMC free article ] [ PubMed ] [ Google Scholar ]
- Institute of Education Sciences & National Science Foundation. (2013). Common guidelines for education research and development . Retrieved May 20, 2022, from www.nsf.gov/pubs/2013/nsf13126/nsf13126.pdf
- Jensen, J. L., Lawson, A. (2011). Effects of collaborative group composition and inquiry instruction on reasoning gains and achievement in undergraduate biology . CBE—Life Sciences Education , 10 ( 1 ), 64–73. https://doi.org/10.1187/cbe.19-05-0098 [ PMC free article ] [ PubMed ] [ Google Scholar ]
- Kolpikova, E. P., Chen, D. C., Doherty, J. H. (2019). Does the format of preclass reading quizzes matter? An evaluation of traditional and gamified, adaptive preclass reading quizzes . CBE—Life Sciences Education , 18 ( 4 ), ar52. https://doi.org/10.1187/cbe.19-05-0098 [ PMC free article ] [ PubMed ] [ Google Scholar ]
- Labov, J. B., Reid, A. H., Yamamoto, K. R. (2010). Integrated biology and undergraduate science education: A new biology education for the twenty-first century? CBE—Life Sciences Education , 9 ( 1 ), 10–16. https://doi.org/10.1187/cbe.09-12-0092 [ PMC free article ] [ PubMed ] [ Google Scholar ]
- Lane, T. B. (2016). Beyond academic and social integration: Understanding the impact of a STEM enrichment program on the retention and degree attainment of underrepresented students . CBE—Life Sciences Education , 15 ( 3 ), ar39. https://doi.org/10.1187/cbe.16-01-0070 [ PMC free article ] [ PubMed ] [ Google Scholar ]
- Lave, J. (1988). Cognition in practice: Mind, mathematics and culture in everyday life . New York, NY: Cambridge University Press. [ Google Scholar ]
- Lo, S. M., Gardner, G. E., Reid, J., Napoleon-Fanis, V., Carroll, P., Smith, E., Sato, B. K. (2019). Prevailing questions and methodologies in biology education research: A longitudinal analysis of research in CBE — Life Sciences Education and at the Society for the Advancement of Biology Education Research . CBE—Life Sciences Education , 18 ( 1 ), ar9. https://doi.org/10.1187/cbe.18-08-0164 [ PMC free article ] [ PubMed ] [ Google Scholar ]
- Lysaght, Z. (2011). Epistemological and paradigmatic ecumenism in “Pasteur’s quadrant:” Tales from doctoral research . In Official Conference Proceedings of the Third Asian Conference on Education in Osaka, Japan . Retrieved May 20, 2022, from http://iafor.org/ace2011_offprint/ACE2011_offprint_0254.pdf
- Maxwell, J. A. (2012). Qualitative research design: An interactive approach (3rd ed.). Los Angeles, CA: Sage. [ Google Scholar ]
- Miles, M. B., Huberman, A. M., Saldaña, J. (2014). Qualitative data analysis (3rd ed.). Los Angeles, CA: Sage. [ Google Scholar ]
- Nehm, R. (2019). Biology education research: Building integrative frameworks for teaching and learning about living systems . Disciplinary and Interdisciplinary Science Education Research , 1 , ar15. https://doi.org/10.1186/s43031-019-0017-6 [ Google Scholar ]
- Patton, M. Q. (2015). Qualitative research & evaluation methods: Integrating theory and practice . Los Angeles, CA: Sage. [ Google Scholar ]
- Perry, J., Meir, E., Herron, J. C., Maruca, S., Stal, D. (2008). Evaluating two approaches to helping college students understand evolutionary trees through diagramming tasks . CBE—Life Sciences Education , 7 ( 2 ), 193–201. https://doi.org/10.1187/cbe.07-01-0007 [ PMC free article ] [ PubMed ] [ Google Scholar ]
- Posner, G. J., Strike, K. A., Hewson, P. W., Gertzog, W. A. (1982). Accommodation of a scientific conception: Toward a theory of conceptual change . Science Education , 66 ( 2 ), 211–227. [ Google Scholar ]
- Ravitch, S. M., Riggan, M. (2016). Reason & rigor: How conceptual frameworks guide research . Los Angeles, CA: Sage. [ Google Scholar ]
- Reeves, T. D., Marbach-Ad, G., Miller, K. R., Ridgway, J., Gardner, G. E., Schussler, E. E., Wischusen, E. W. (2016). A conceptual framework for graduate teaching assistant professional development evaluation and research . CBE—Life Sciences Education , 15 ( 2 ), es2. https://doi.org/10.1187/cbe.15-10-0225 [ PMC free article ] [ PubMed ] [ Google Scholar ]
- Reynolds, J. A., Thaiss, C., Katkin, W., Thompson, R. J. Jr. (2012). Writing-to-learn in undergraduate science education: A community-based, conceptually driven approach . CBE—Life Sciences Education , 11 ( 1 ), 17–25. https://doi.org/10.1187/cbe.11-08-0064 [ PMC free article ] [ PubMed ] [ Google Scholar ]
- Rocco, T. S., Plakhotnik, M. S. (2009). Literature reviews, conceptual frameworks, and theoretical frameworks: Terms, functions, and distinctions . Human Resource Development Review , 8 ( 1 ), 120–130. https://doi.org/10.1177/1534484309332617 [ Google Scholar ]
- Rodrigo-Peiris, T., Xiang, L., Cassone, V. M. (2018). A low-intensity, hybrid design between a “traditional” and a “course-based” research experience yields positive outcomes for science undergraduate freshmen and shows potential for large-scale application . CBE—Life Sciences Education , 17 ( 4 ), ar53. https://doi.org/10.1187/cbe.17-11-0248 [ PMC free article ] [ PubMed ] [ Google Scholar ]
- Sabel, J. L., Dauer, J. T., Forbes, C. T. (2017). Introductory biology students’ use of enhanced answer keys and reflection questions to engage in metacognition and enhance understanding . CBE—Life Sciences Education , 16 ( 3 ), ar40. https://doi.org/10.1187/cbe.16-10-0298 [ PMC free article ] [ PubMed ] [ Google Scholar ]
- Sbeglia, G. C., Goodridge, J. A., Gordon, L. H., Nehm, R. H. (2021). Are faculty changing? How reform frameworks, sampling intensities, and instrument measures impact inferences about student-centered teaching practices . CBE—Life Sciences Education , 20 ( 3 ), ar39. https://doi.org/10.1187/cbe.20-11-0259 [ PMC free article ] [ PubMed ] [ Google Scholar ]
- Schwandt, T. A. (2000). Three epistemological stances for qualitative inquiry: Interpretivism, hermeneutics, and social constructionism . In Denzin, N. K., Lincoln, Y. S. (Eds.), Handbook of qualitative research (2nd ed., pp. 189–213). Los Angeles, CA: Sage. [ Google Scholar ]
- Sickel, A. J., Friedrichsen, P. (2013). Examining the evolution education literature with a focus on teachers: Major findings, goals for teacher preparation, and directions for future research . Evolution: Education and Outreach , 6 ( 1 ), 23. https://doi.org/10.1186/1936-6434-6-23 [ Google Scholar ]
- Singer, S. R., Nielsen, N. R., Schweingruber, H. A. (2012). Discipline-based education research: Understanding and improving learning in undergraduate science and engineering . Washington, DC: National Academies Press. [ Google Scholar ]
- Todd, A., Romine, W. L., Correa-Menendez, J. (2019). Modeling the transition from a phenotypic to genotypic conceptualization of genetics in a university-level introductory biology context . Research in Science Education , 49 ( 2 ), 569–589. https://doi.org/10.1007/s11165-017-9626-2 [ Google Scholar ]
- Vygotsky, L. S. (1978). Mind in society: The development of higher psychological processes . Cambridge, MA: Harvard University Press. [ Google Scholar ]
- Wenger, E. (1998). Communities of practice: Learning as a social system . Systems Thinker , 9 ( 5 ), 2–3. [ Google Scholar ]
- Ziadie, M. A., Andrews, T. C. (2018). Moving evolution education forward: A systematic analysis of literature to identify gaps in collective knowledge for teaching . CBE—Life Sciences Education , 17 ( 1 ), ar11. https://doi.org/10.1187/cbe.17-08-0190 [ PMC free article ] [ PubMed ] [ Google Scholar ]
A Meta-Analysis of the Relations Between Achievement Goals and Internalizing Problems
- META-ANALYSIS
- Open access
- Published: 16 September 2024
- Volume 36 , article number 109 , ( 2024 )
Cite this article
You have full access to this open access article
- Loredana R. Diaconu-Gherasim ORCID: orcid.org/0000-0003-3598-5375 1 ,
- Andrew J. Elliot ORCID: orcid.org/0000-0002-1664-6426 2 ,
- Alexandra S. Zancu ORCID: orcid.org/0000-0002-1361-6870 1 ,
- Laura E. Brumariu ORCID: orcid.org/0000-0002-3389-4288 3 ,
- Cornelia Măirean ORCID: orcid.org/0000-0001-6895-8627 1 ,
- Cristian Opariuc‑Dan ORCID: orcid.org/0000-0003-4079-0142 4 , 5 &
- Irina Crumpei-Tanasă 1
6 Altmetric
Explore all metrics
This systematic meta-analytic review investigated the relations between achievement goals and internalizing symptoms and disorders, namely, anxiety and depression. The number of samples for each focal relationship ranged from 3 to 36. The results indicated significant effect sizes for the relations between mastery-approach goals and anxiety ( r = − .10) and depression (r = − .18), as well as performance-avoidance goals and anxiety ( r = .25) and depression ( r = .16). A significant effect size was also found for the relation between performance-approach goals and anxiety ( r = .15), and a non-significant effect size was observed for the relation between performance-approach goals and depression ( r = .05). Mastery-avoidance goals were not significantly related to either anxiety ( r = .08) or depression ( r = − .13). Several moderators representing the conceptualization of achievement goals (e.g., theoretical model), sample characteristics (e.g., education level), and methodology- and publication-based characteristics (e.g., year of publication) were significant, and suggested avenues for future research. These findings herein have implications for intervention programs that could focus on reducing the links between achievement goals and internalizing problems.
Similar content being viewed by others
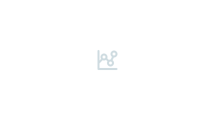
A New Look at Multiple Goal Pursuit: the Promise of a Person-Centered Approach
Goal complexes: integrating achievement goals as standards and self-attributed motives as reasons underlying goal pursuit.
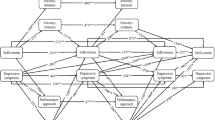
Achievement Goals as Mediators of the Links Between Self-Esteem and Depressive Symptoms From Mid-Adolescence to Early Adulthood
Avoid common mistakes on your manuscript.
The study of achievement goals is central to the achievement motivation literature. Achievement goals are defined as cognitive representations of competence-based end states that individuals strive to approach or avoid (Elliot, 1997 ). Several models of achievement goals (e.g., Dweck, 1986 ; Elliot, 1999 ; Nicholls, 1984 ) have been formulated over the years that vary regarding the focal components or number of achievement goals that individuals adopt in achievement situations. Despite some variation in conceptualization, theorists agree that the definition of competence (i.e., how doing well or poorly is defined) is an important dimension of achievement goals (Dweck & Leggett, 1988 ; Elliot & McGregor, 2001 ; Nicholls, 1984 ). The valence of competence (i.e., the approach-avoidance distinction) is also widely acknowledged as an important dimension of achievement goals (Elliot, 1997 ). Achievement goal frameworks, therefore, center on mastery goals (focusing on the development of competence and task- or self-based standards), performance goals (focusing on the demonstration of competence and other-based standards; Dweck, 1986 ; Nicholls, 1984 ), approach goals (approaching competence), and avoidance goals (avoiding incompetence; Elliot & Harackiewicz, 1996 ). In the present work, we focus on the 2 × 2 model of achievement goals in which the definition of competence is crossed with the valence of competence, resulting in four goals: Mastery-approach (striving to attain task- or self-based competence), performance-approach (striving to attain other-based competence), mastery-avoidance (striving to avoid task- or self-based incompetence), and performance-avoidance (striving to avoid other-based incompetence). This 2 × 2 model is commonly used in theoretical and empirical work in the achievement goal literature, as well as narrative and meta-analytic reviews (Butera et al., 2024 ).
Achievement goals are presumed to guide the way that people engage in achievement situations and how they cognitively, emotionally, and behaviorally respond to these situations (Ames, 1992 ; Dweck & Leggett, 1988 ). A great deal of research has shown that individuals’ achievement goals influence important outcomes such as performance, persistence, intrinsic motivation, and help-seeking behavior (for reviews, see Butera et al., 2024 ; Elliot & Hulleman, 2017 ; Senko, 2016 ). The influence of achievement goals has been extensively documented in a variety of different settings, especially school (e.g., Wirthwein et al., 2013 ), sports (Lochbaum & Gottardy, 2015 ), and work (Payne et al., 2007 ). In the present work we focus on the link between achievement goals and anxiety and depression symptoms and disorders.
Anxiety and depression are the most prevalent mental disorders and symptoms (APA, 2022 ). Theorists use the term “internalizing problems” for the cluster that includes clinical anxiety and depression or a combination of both symptoms or disorders (Yap et al., 2016 ). Anxiety affects a significant number of school-age children, with prevalence rates of an anxiety disorder of 7.1% in middle school and high school children (Ghandour et al., 2019 ), and prevalence rates of symptoms of 32% in college students (Sheldon et al., 2021 ). Depressive disorders have prevalence rates of approximately 3.5% in middle school and high school children (Ghandour et al., 2019 ) and the rate of depressive symptoms in college students reaches 25% (Sheldon et al., 2021 ). The prevalence rates of anxiety in the school-aged population have increased during the past few decades (Bitsko et al., 2018 ; Spoelma et al., 2023 ). Previous research has consistently shown that anxiety (e.g., generalized anxiety, panic disorder, separation anxiety, social anxiety; APA, 2013 ) is related to impairments across domains, such as emotional exhaustion (Koutsimani et al., 2019 ), and relational difficulties (Biswas et al., 2020 ). Similar results are also found for depression (e.g., disruptive mood dysregulation disorder, major depressive disorder; DSM-5; APA, 2013 ; Koutsimani et al., 2019 ; Marx et al., 2023 ). Previous narrative and meta-analytic reviews showed that in the educational context, anxiety and depression are related to a variety of negative outcomes, such as poor school attainment (see Riglin et al., 2014 ), poor school attendance (Finning et al., 2019 ), poor academic competence and greater school dropout (Brumariu et al., 2022 ), and problematic peer functioning (Christina et al., 2021 ), even at non-clinically diagnosed levels. Anxiety and depression show continuity in adulthood and, left untreated, could have severe consequences, even at subclinical levels, across one’s lifespan (e.g., poor quality of life and greater suicide risk; Marx et al., 2023 ).
Several narrative and meta-analytic reviews have tested how achievement goals are related to people’s emotional experiences, conceptualized as achievement emotions or affective states. In their meta-analysis, Payne et al. ( 2007 ) provided evidence of a negative relation between mastery-approach goals and state anxiety, as well as a positive relation between both performance-approach and performance-avoidance goals and state anxiety. Baranik et al.’s ( 2010 ) meta-analysis revealed positive relations of mastery-approach and performance-approach goals with positive affect, as well as positive relations of mastery-avoidance and performance-avoidance goals with negative affect. Huang ( 2011 ) replicated Baranik et al.’s findings, but also found a negative relation between performance-avoidance goals and positive affect, and a positive relation between performance-approach goals and negative affect.
Although these findings reveal that achievement goals are related to state anxiety and overall positive and negative affectivity, they do not address the links between these goals and clinical levels of anxiety and depression, or internalizing problems in general. Importantly, achievement emotions and affective states are conceptually distinct from clinical anxiety and depression, which are not based in a specific situation or event, but instead represent a constellation of symptoms that typically exert a significant impairment on one’s overall functioning (Luttenberger et al., 2018 ). Thus, the question of whether achievement goals are differentially related to internalizing problems has yet to be addressed meta-analytically. A growing number of individual studies have explored how achievement goals are related to internalizing problems (e.g., Madjar et al., 2021 ; Măirean & Diaconu-Gherasim, 2020 ; Sideridis, 2005 ), however, nothing is known about the cumulative nature and strength of these relations. The present meta-analysis advances the literature by synthesizing the existing empirical evidence on the relations between achievement goals and internalizing symptoms and disorders, and by investigating moderators of these links. In our work we focused on clinical anxiety and depression, and the combination of the two (i.e., global internalizing problems), as indicators of internalizing problems (Melton et al., 2016 ).
Basic Components and Models of Achievement Goals
As noted above, several theoretical models have guided research on achievement goals and we will test these different models in the present work. Initially, achievement goals models were dichotomous (Dweck, 1986 ; Nicholls, 1984 ), distinguishing between mastery goals (or task or learning goals) and performance goals (or ego or ability goals). Each goal was conceptualized as focusing on approaching success (Ames, 1992 ). Subsequently, the dichotomous model was extended by including the approach-avoidance distinction. Specifically, Elliot and Harackiewicz ( 1996 ) proposed the trichotomous model which bifurcates performance-based goals into performance-approach (focused on demonstrating other-based competence) and performance-avoidance (focused on avoiding the demonstration of other-based incompetence). In the 2 × 2 model, Elliot ( 1999 ) extended the trichotomous model by applying the approach-avoidance distinction to mastery-based goals; mastery-avoidance goals were conceptualized in terms of avoiding task-based or intrapersonal incompetence (see also Pintrich, 2000 ). Thus, the 2 × 2 model distinguishes among mastery-approach, mastery-avoidance, performance-approach, and performance-avoidance goals. This model implies that the task and self components of mastery-based goals combine together, when at times they may not. Therefore, in the 3 × 2 model (Elliot et al., 2011 ), mastery-based goals were split according to the standards used to evaluate competence, resulting in two approach goals (focused on attaining task-based competence and self-based competence) and two avoidance goals (focused on avoiding task-based incompetence and self-based incompetence). Other-based goals – other-approach and other-avoidance – are the same as the original performance-approach and performance-avoidance goals. The 3 × 2 model has not been tested with regard to internalizing problems, thus we focused on the 2 × 2 model in the present research.
The specific conceptualizations of achievement goals vary across studies in the literature, depending on which component (standpoints or standards) of competence is targeted. The standpoints of competence perspective views competence in terms of developing it vs. demonstrating it, whereas the standards of competence perspective evaluates competence with regard to task/self-based vs. other-based standards (Korn & Elliot, 2016 ). For example, in some studies, mastery-based goals focus on the development of ability, whereas in other studies they focus on task- or self-based standards; likewise for performance-based goals, some studies focus on the demonstration of ability (or appearance goals), whereas other studies focus on other-based standards (or normative goals; see Korn et al., 2019 for an overview).
Furthermore, the terminology and specific content of the goal measures varies somewhat across studies (see Hulleman et al., 2010 for an overview of these types of variation). Specifically, mastery-approach goals are conceptualized and operationalized in terms of fulfillment of one’s potential (e.g., mastery-approach goals, Elliot & McGregor, 2001 ), development of ability/competence (e.g., development-approach goals; Elliot et al., 2011 ), interest and curiosity (e.g., task orientation, Nicholls, 1984 ), or doing better than one has done in the past (e.g., learning goals; Dweck, 1986 ). Mastery-avoidance goals are conceptualized and operationalized in terms of being unable to reach one’s potential (mastery-avoidance goals, Elliot & McGregor, 2001 ), avoiding the development of inability/incompetence (development-avoidance goals, Korn & Elliot, 2016 ), or avoiding task-based incompetence (task-avoidance goals, Elliot et al., 2011 ). Performance-approach goals are conceptualized and operationalized in terms of doing better than others (e.g., performance-approach goals Elliot, 1999 ), appearing competent to others (e.g., demonstration-approach goals, Korn & Elliot, 2016 ), demonstrating ability relative to others (e.g., ego orientation, Nicholls, 1984 ), or confirming one’s ability to an audience (e.g., ability goals, Grant & Dweck, 2003 ). Performance-avoidance goals are conceptualized and operationalized in terms of avoiding doing worse than others (e.g., performance-avoidance goals, Elliot & Church, 1997 ), avoiding the demonstration of incompetence relative to others (e.g., demonstration-avoidance goals, Korn & Elliot, 2016 ), or avoiding negative judgments from others (e.g., avoid orientation, VandeWalle, 1997 ). We attend to this variation in terminology in our meta-analysis.
Theory and Empirical Work on Achievement Goals and Internalizing Problems
Achievement goals are about competence, a basic human need that must be satisfied to sustain mental health and well-being (Elliot et al., 2002 ; Ryan & Deci, 2019 ). Achievement goals represent the cognitive-dynamic lens through which people frame and interpret contexts, events, and outcomes (Dweck, 1986 ; Nicholls, 1984 ). As such, it is sensible to posit that these forms of self-regulation are associated with mental health indicators such as anxiety and depression, and several researchers have proposed links accordingly.
The most influential model of achievement goals and internalizing problems is the goal-orientation model of depression vulnerability (Dykman, 1998 ). Essentially all theorizing in this area is directly or indirectly grounded in this model. The model postulates that achievement goals lead to specific cognitive sets and appraisal patterns that have important implications for people’s mental health. Individuals focused on mastery-based goals are oriented toward growth, learning, and improvement, perceive negative outcomes as opportunities for self-development, and their self-worth is not contingent on performance or social comparison; thus, they experience lower levels of depressive symptoms and are more resilient to failure. Individuals focused on performance-based goals, on the other hand, are orientated toward comparing their success/ability to others and their self-worth is contingent on demonstrating competence relative to others. They tend to evaluate challenging or difficult situations as a reflection of their personal traits (e.g., incompetence, unlikability) and as a test of their ability; thus, they report lower self-worth, are less resilient to failure, and are more vulnerable to depression.
Although the goal orientation model is primarily focused on depression, both Dykman ( 1998 ) and others (e.g., Sideridis, 2007 ) have argued that it is also applicable to anxiety, with comparable patterns expected for depression and anxiety. Furthermore, researchers have extended this analysis to include the approach-avoidance distinction, contending that mastery-approach goals are the most beneficial and performance-avoidance goals are the most detrimental to internalizing problems (Duchesne et al., 2014 ; Van Boekel & Martin, 2014 ; Wang et al., 2021 ). In addition, researchers have noted that anxiety and depression are accompanied by impaired cognitive functioning, lack of access to resources, and poor self-regulation which exert an influence on the type of achievement goals that individuals adopt (e.g., less mastery-approach and more performance-avoidance goals; Duchesne et al., 2014 ; Măirean & Diaconu-Gherasim, 2020 ). In other words, the relation between achievement goals and internalizing problems is posited to be reciprocal.
There is a growing body of research examining the relation between achievement goals and internalizing symptoms, and somewhat inconsistent findings have been reported. Some studies have found that mastery-approach goals are related to a low level of anxiety symptoms (e.g., Ariani, 2017 ; Sideridis, 2005 ; Wei, 2018 ) and depressive symptoms (e.g., Madjar et al., 2021 ; Măirean & Diaconu-Gherasim, 2020 ; Sideridis, 2005 ), while others have not found these relations (e.g., Duchesne et al., 2014 ). Performance-approach and performance-avoidance goals have been linked to higher levels of anxiety (Ariani, 2017 ; Madjar et al., 2021 ) and depressive symptoms (Duchesne et al., 2014 ; Măirean & Diaconu-Gherasim, 2020 ), but null effects have also been reported (Duchesne et al., 2014 ; Madjar et al., 2021 ; Sideridis, 2005 ). Very few studies have investigated the relation between mastery-avoidance goals and internalizing symptoms; mixed findings have been reported (e.g., Liu et al., 2019 ; Wang et al., 2021 ).
Moderators of the Relation Between Achievement Goals and Internalizing Problems
The present meta-analysis also addresses potential moderators of the relation between achievement goals and internalizing problems. Several of these moderator variables (e.g., achievement goal model, achievement goal terminology) have been used in prior achievement goal meta-analyses focused on other outcome measures (Huang, 2011 ; Hulleman et al., 2010 ; Senko & Dawson, 2017 ). We examined four broad moderation categories: Conceptualization of achievement goals, conceptualization of internalizing problems, sample characteristics, and methodology- and publication-based characteristics.
Conceptualization of Achievement Goals
Achievement goal models vary not only in the number of goals but also in the way goals are conceptualized, with some models emphasizing the focus of competence alone (i.e., the dichotomous model) and others placing equal emphasis on the focus and the valence of competence (e.g., the 2 × 2 model; Elliot & Hulleman, 2017 ). Further, the terminology used to refer to the different goal categories varies across studies (e.g., development-approach, learning goals, task orientation for mastery-approach goals; see Hulleman et al., 2010 for an overview of terminology). Thus, in our meta-analysis we tested whether the achievement goal model (dichotomous, trichotomous, 2 × 2) and achievement goal terminology (i.e., the labels used for the different goals) moderated the relation between achievement goals and internalizing problems. Several different scales are used in the literature to assess achievement goals (e.g., the Patterns of Adaptive Learning Scale; PALS; Midgley et al., 1993 ; the Achievement Goal Questionnaire [Revised]; AGQ(-R); Elliot & Church, 1997 ) (see Hulleman et al., 2010 for an overview of scales). The different scales may emphasize different conceptual aspects of the goals (e.g., the PALS measures both normative and appearance aspects, whereas the AGQ-R measures only normative aspects), therefore we tested whether achievement goal scale (PALS, AGQ/AGQ-R, TEOSQ, other scales) moderated the relation between achievement goals and internalizing problems. We also tested whether achievement goal setting (general, specific) served as a moderator. Finally, we also assessed whether the specific type of setting (e.g., academic, sports) moderated the achievement goal-internalizing problem relation.
Conceptualization of Internalizing Problems
Both anxiety and depressive disorders vary in their manifestations and consequences (e.g., avoidance behavior and excessive fear in anxiety disorders; persistent sad mood and change in sleep and appetite in depressive disorders; APA, 2013 ). Thus, we evaluated form of anxiety and depression as a moderator of the relation between achievement goals and anxiety and depression. Furthermore, clinical anxiety and depression involve significant functional impairment (Craske et al., 2017 ), so we also evaluated whether the observed findings were more robust at the diagnostic level.
Sample Characteristics
We evaluated whether the participants’ education level (middle school, high school, college), cultural context where the studies were conducted (Western, Eastern), and participants’ gender and age served as moderators.
Methodology- and Publication-based
We evaluated several methodology- and publication-based characteristics as moderators: Measurement approach (self-report, other report), type of design utilized (cross-sectional, longitudinal), direction of the relation between achievement goals and internalizing problems, year of publication, and publication type (peer reviewed, non-peer reviewed).
The Present Research
The present meta-analysis seeks to synthesize the results of studies that have focused on the associations between achievement goals and internalizing symptoms or disorders, and investigates moderators of these relations. We aimed to evaluate the strength of the relations between the four achievement goals of the 2 × 2 model (i.e., mastery-approach, mastery-avoidance, performance-approach, and performance-avoidance) and internalizing symptoms and disorders (i.e., anxiety, depression, and a combination of both). We predicted, based on the aforementioned theorizing, that high levels of mastery-approach goals would be related to lower levels of internalizing symptoms and disorders, and high levels of performance-approach and performance-avoidance goals would be related to higher levels of internalizing symptoms and disorders. We did not make predictions for mastery-avoidance goals, given that they represent a hybrid of adaptive (mastery) and maladaptive (avoidance) components (Elliot & McGregor, 2001 ). By synthesizing data across studies that varied in several different ways, this meta-analysis promises to yield a more clear and thorough understanding of how achievement goals and internalizing problems are related than any individual study can provide. The relations are important for psychological functioning in general, including affect, cognition, and behavior among children, adolescents, and young adults in educational settings.
We examined four sets of moderators regarding the relations between achievement goals and internalizing problems: Conceptualization of achievement goals, conceptualization of internalizing problems, sample characteristics, and methodology- and publication-based characteristics. We had no a priori hypotheses for these moderators; these analyses are exploratory in nature. Evaluation of these moderators is important in order to understand when achievement goals are associated with anxiety and depression, thus furthering the precision and depth of our knowledge regarding these relations.
Our systematic review was conducted according to the PRISMA 2020 (Preferred Reporting Items for Systematic reviews and Meta-Analyses; Page et al., 2021 ) guidelines. The review and meta-analysis protocol was pre-registered in PROSPERO International Prospective Register of Systematic Reviews (protocol number CRD42022298463).
Literature Search
The initial literature search was conducted in July 2021 in six electronic databases: Web of Science, PsycINFO, PubMed, ERIC, Academic Search Premier (EBSCO), and ProQuest. The final literature search for the work reported herein was conducted in December 2022. The same search strategy was applied in all databases, using the following combination of keywords: ("achievement goal" OR "goal orientation" OR "mastery goal" OR "mastery approach goal" OR "mastery orientation" OR mastery-approach OR "task goal" OR "task orientation" OR "learning goal" OR "development-approach goal" OR "task-approach goal" OR "self-approach goal" OR "mastery avoidance goal" OR mastery-avoid OR "development-avoidance goal" OR "task-avoidance goal" OR "self-avoidance goal" OR "performance goal" OR "performance approach goal" OR "ego goal" OR "ego orientation" OR "ability goal" OR "prove goal orientation" OR "performance-prove orientation" OR "self-enhancing goal orientation" OR "demonstration-approach goal" OR "other-approach goal" OR "performance avoidance goal" OR performance-avoidance OR "avoid goal orientation" OR "self-defeating ego orientation" OR "self-defeating orientation" OR "demonstration-avoidance goal" OR "other-avoidance goal") AND (internalizing OR "internalizing symptoms" OR "internalizing problems" OR "internalizing disorders" OR depression OR depressive OR depressed OR "depressive disorders" OR "depressive problems" OR "depressive symptoms" OR "depression symptoms" OR "mood disorders" OR sadness OR anxiety OR "generalized anxiety" OR "social anxiety" OR "anxiety symptoms" OR "anxiety problems" OR "anxiety disorders" OR anxious OR worry OR worries OR fear OR phobia OR phobic OR panic). The search was restricted to title and abstract fields and to the publication period 1980 (when the achievement goal approach emerged) to December, 2022 (when the final search was conducted). No restrictions were applied for publication type. All types of empirical research reports were eligible, including peer reviewed journal articles, conference papers, book chapters, and dissertation theses. Records and studies published in English, French, Spanish, and German were eligible for inclusion, due to the authors’ language competencies. The reference lists of the studies eligible for inclusion were searched for possible additional relevant studies. Unpublished studies were sought by contacting the authors with two or more published studies eligible for inclusion.
Study Selection
After removing duplicates, all of the records identified during the search stage were screened based on title and abstract by applying the eligibility criteria for inclusion and exclusion described below. Further, all retrieved full-text studies were assessed for eligibility and selected based on the inclusion and exclusion criteria. The same three coders were involved in the screening and selection of studies for inclusion in the systematic review. The coders overlapped with each other on a random sample of 50% of the studies and coded the studies independently. Kappa agreement between coders ranged from 0.76 to 0.91 for the abstract screening and 0.89 to 0.93 for the study selection. Disagreements between coders were resolved by discussion and consensus. Before the formal screening, the selection procedure was piloted on a random sample of studies. The flow diagram of the study selection process (following PRISMA 2020) is depicted in Fig. 1 .
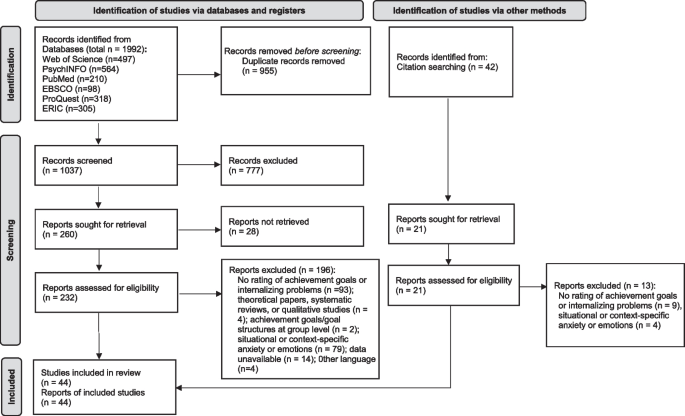
The PRISMA 2020 flow diagram
The original quantitative studies that met the eligibility criteria were selected for inclusion. Inclusion criteria: (1) studies must include ratings of achievement goals (mastery-approach, mastery-avoidance, performance-approach, performance-avoidance, or their variants) and internalizing symptoms and disorders (depression, anxiety, or the combination of the two); if a study included an intervention or quasi-experiment, the achievement goals and internalizing measures had to have been collected prior to the intervention or quasi-experiment; (2) the study was written in English, French, Spanish, or German; (3) statistically relevant information was available for the relations between achievement goals and internalizing symptoms or disorders (e.g., correlation, sample size), allowing for the computation of effect size statistics. For the studies with insufficient reported data, the study’s investigators were contacted and requested to provide additional data (e.g., correlation coefficients).
Exclusion criteria: (1) theoretical papers, systematic reviews or meta-analyses, and qualitative studies; (2) studies that measured achievement goals at the group level (or achievement goal structures), and studies that induced achievement goals situationally; (3) studies measuring situational anxiety or anxiety related to specific settings such as educational or sport settings (e.g., test anxiety, sport anxiety, academic anxiety, learning/classroom anxiety, fear of failure, state anxiety, task anxiety, fear of failure, achievement-related emotions); (4) studies for which no full texts were accessible or sent by the authors upon request; (5) studies in which no statistical values for the relations between achievement goals and internalizing problems were reported or sent by the authors upon request. Reported data had to be independent of other studies included in the meta-analysis.
As depicted in Fig. 1 , the database search resulted in 1992 records. After duplicates were removed ( n = 955), the remaining 1037 unique records were screened based on the abstract, and 777 records were excluded. 260 eligible records were sought for retrieval and the 232 full-text reports that were available were assessed for inclusion, based on the eligibility above criteria. Further, 196 reports were excluded for the following reasons: Did not asses achievement goals or internalizing problems ( n = 93), were not empirical, quantitative studies ( n = 4), examined situational or context-specific anxiety or emotions ( n = 79), assessed achievement goals or goal structures at the group level ( n = 2), statistical data for calculating the effect size was unavailable ( n = 14), and were published in other languages ( n = 4). An additional 21 reports were identified from other sources (i.e., websites, references of included studies) and assessed for eligibility. Overall, 44 reports meeting the eligibility criteria were included in the meta-analysis (see Fig. 1 and Section 7 of the Supplementary Material). The reports include a total of 22,387 participants, in 47 samples of children or adults, between 11 and 60 years old. A summary description of the included studies is presented in Table S1 , Supplementary Material.
Data Extraction and Coding
The following data were extracted and coded from each study: Conceptualization of achievement goals (achievement goal model, achievement goal terminology, achievement goal scale, achievement goal setting, type of informant); conceptualization of internalizing problems (indicators of internalizing problems, type of internalizing problems, form of anxiety, form of depression, type of informant), sample characteristics (education level, cultural context, age, gender); methodological and publication characteristics (type of design, direction of relation, type of publication, year of publication). The data extracted and coded are presented in Table 1 . The correlation coefficients between each achievement goal (mastery-approach, mastery-avoidance, performance-approach, performance-avoidance) and each indicator of internalizing symptoms and disorders (anxiety, depression, their combination) were also extracted. The same three coders from the screening stage extracted and coded the data from the included studies. The coders overlapped on a random sample of 25% of the studies that where coded independently by two coders. Kappa agreement between coders was higher than 94% for all of the categories. Disagreements between the coders were resolved by discussion and consensus coding.
Effect-Size Calculation
Correlation coefficients (i.e., Pearson’s r ) between each type of achievement goal (mastery-approach, mastery-avoidance, performance-approach, and performance-avoidance) and each indicator of internalizing problems (anxiety, depression, their combination) were extracted for effect sizes. When other effect size indicators (e.g., F tests) were reported, we converted them to correlation coefficients.
To ensure the independence of effect sizes, from each study a single effect size derived from a particular sample was included. If a study reported effect size information for different samples (e.g., students), these were considered independent, and the effect sizes from each sample were included. When a study reported more than one effect size from one sample for a particular analysis (e.g., the correlation between performance-avoidance goals and anxiety), several decisions were made to avoid dependency: 1) if a study reported both cross-sectional and longitudinal associations between two indicators, we only included the longitudinal effect size to take advantage of longitudinal research; 2) if a study reported cross-sectional associations from multiple time points between two indicators without reporting longitudinal associations, the coefficients were aggregated into a single effect size; 3) if a study reported the effect sizes for multiple measures of the same indicator (e.g., more than one measure of anxiety) from the same sample and time point, these were aggregated into a single effect size; 4) if a study reported several longitudinal effect sizes between two indicators from multiple time points, these were aggregated into a single effect size.
To test our hypotheses, a random effects meta-analysis model was conducted (Hedges & Olkin, 1985 ). We computed the correlation coefficient for the relation between each type of achievement goal and each indicator of internalizing problems, along with a 95% confidence interval (CI). Egger’s intercept test was used as a publication bias assessment at the global level, testing the funnel plot’s symmetry. Also, a funnel plot analysis was performed for testing publication bias at the moderator level.
Moderator Analysis
Heterogeneity of effect size was computed using Q statistics (Q B; Borenstein, 2009 ) to test whether the relations between the achievement goals and internalizing problems were moderated by the following categorical moderators: 1) conceptualization of achievement goals: Achievement goal model, achievement goal terminology, achievement goal scale, achievement goal setting, and achievement goal informant; 2) conceptualization of internalizing problems: Type of internalizing problems, form of anxiety, form of depression, and internalizing problems informant; 3) sample characteristics: Education level, and cultural context; 4) methodology- and publication-based characteristics of the studies: Type of study design, direction of the relations in longitudinal studies, year of publication, and type of publication. Categorical moderators were evaluated using subgroup analyses. We assessed the significant differences between the categories based on the Q Between test for subgroup differences for a random effect when a moderator had more than two categories. If the moderator had more than two categories, we also conducted a follow-up analysis and compared pairs of categories. For each pair, we assessed the significant differences between categories based on the Q Between test for subgroup differences, as we did in the case of moderators with only two categories. Consistent with previous meta-analytic work (e.g., Brumariu et al., 2022 ), we included a potential moderator only if there were four or more studies available per level. The following moderators were not included in subgroup analyses due to an insufficient number of reports (less than four): Type of informant (all studies used self-reports of achievement goals and internalizing problems), type of internalizing problems (no study assessed disorders), form of depression, and direction of the relation between achievement goals and indicators of internalizing problems. Global internalizing problems (a combination of both depression and anxiety) were examined in a single study, so we were unable to conduct a separate meta-analytic evaluation of the relations between achievement goals and this variable. Not all categories were available for each categorical moderator (e.g., the TEASQ category for the relation between mastery-approach goals and anxiety), thus Tables 2 and 3 present the results of the categorical moderators when each category is at least k = 4. For achievement goal scale the “other scales” category was created, and this category included other validated scales: The Goal Orientation Inventory (Dykman, 1998 ), the Goal Inventory (Roedel et al., 1994 ), the Goal Orientations and Motivational Beliefs scale (Niemivirta, 2002 ), and one author-created scale (Stornes & Bru, 2011 ). The results for follow-up analyses, when a moderator had more than two categories, are presented in the text. Mastery-avoidance goals are not included in any moderator analyses due to an insufficient number of studies. Meta-regression analyses were conducted to evaluate the role of continuous moderators (participant age, percentage of males, and publication year) in the relation between each type of achievement goal and internalizing problems. We present results for these moderators in the text.
The meta-analyses were conducted using R (Version 4.3.2; R Core Team, 2023 ) and the R-packages dmetar (Version 0.1.0; Harrer et al., 2019 ), esc (Version 0.5.1; Lüdecke, 2019 ), MAd (Version 0.8.3; Hoyt, 2014 ), ma ditr (Version 0.8.4; Demin, 2024 ), and meta (Balduzzi et al., 2019 ; Version 6.5.0; Harrer et al., 2019 ).
Associations Between Achievement Goals and Anxiety
As seen in Table 2 , mastery-approach goals were negatively related to anxiety, whereas performance-approach goals were positively related. Performance-avoidance goals were also positively related to anxiety, whereas the relation between mastery-avoidance goals and anxiety was not significant (see Forest plots in Section 2 of Supplementary Material). The significant within-group heterogeneity estimates (Q W values) suggested that there is heterogeneity of the effect sizes.
Moderators of the Relation Between Achievement Goals and Anxiety (see Table 2 )
Mastery-approach goals.
Three of eight categorical variables tested were significant moderators of the negative relation between mastery-approach goals and anxiety. Achievement goal terminology was significant; the negative relation was stronger for development-approach goals than mastery goals/orientation ( Q B = 7.79, p = 0.005), or mastery-approach goals ( Q B = 8.07, p = 0.004), and did not differ from all other terms (all Q B > 0.05). Achievement goal setting was significant; the negative relation was stronger in studies in a general setting than in specific settings. Education level was significant; the negative relation was stronger in studies with college samples than in studies with middle school samples (Q B = 6.89, p = 0.014) or high school samples (Q B = 6.17, p = 0.035). Achievement goal model, achievement goal scale, form of anxiety, type of design and type of publication were not significant. Age ( B = − 0.004), percentage of males ( B = − 0.002), and year of publication ( B = 0.006) were not significant.
Performance-Approach Goals
Three of seven categorical variables tested were significant categorical moderators of the positive relation between performance-approach goals and anxiety. Achievement goal terminology was significant; the positive relation was stronger for demonstration-approach goals than for performance-approach goals. Achievement goal setting was significant; the positive relation was stronger in a general setting than in specific settings. Education level was significant; the positive relation was stronger for college students than for high school (Q B = 6.17, p = 0.013) or middle school (Q B = 6.89, p = 0.009) students. Achievement goal model, achievement goal scale, form of anxiety, and type of publication were not significant moderators. The meta-regression indicated that the percentage of males was a significant moderator; the relation between performance-approach goals and anxiety decreased as the percentage of males increased ( B = − 0.003, p = 0.03). Age ( B = 0.009) and year of publication ( B = − 0.003) were not significant.
Performance-Avoidance Goals
Two of six categorical variables tested were significant categorical moderators of the positive relation between performance-avoidance goals and anxiety. Achievement goal model was significant; the positive relation was stronger in studies using the trichotomous model than in studies using the 2 × 2 model. Achievement goal scale was significant; the positive relation was stronger in studies that used other scales than in studies that used the AGQ/AGQ-R (Q B = 5.94, p = 0.015). Form of anxiety, education level, type of design and type of publication were not significant. The meta-regression for age was significant; the relation between the performance-avoidance goals and anxiety increased as participants’ age increased ( B = 0.008, p = 0.002). Percentage of males ( B = − 0.0003) and year of publication ( B = − 0.005) were not significant.
Associations Between the Achievement Goals and Depression
Mastery-approach goals were negatively related, whereas performance-avoidance goals were positively related to depression (see Table 3 ). Performance-approach goals and mastery-avoidance goals were not significantly related to depression, and mastery-avoidance goals was not significantly related to depression (see Forest plots in Section 3 of Supplementary Material). The significant within-group heterogeneity estimates (Q W values) suggested that there is heterogeneity of the effect sizes.
Moderators of the Relations Between the Achievement Goals and Depression (see Table 3 )
None of categorical variables tested (achievement goal model, achievement goal terminology, achievement goal scale, achievement goal setting, education level, cultural context, type of publication) were significant moderators of the negative relation between mastery-approach goals and depression. The meta-regression for year of publication was significant ( B = − 0.007, p = 0.014); the relation between the mastery-approach goals and depression decreased for more recent publications. Age ( B = − 0.002) and percentage of males ( B = − 0.001) were not significant.
Three of seven categorical variables tested were significant moderators of the relation between performance-approach goals and depression. Achievement goal model was significant; the relation was positive and significant only in studies that used the dichotomous model. Achievement goal scale was significant, but no statistical differences were observed by follow-up analyses (all Q B > 0.05), however, the relation between performance-approach and depression was negative in studies that used the PALS and positive in studies that used all other scales. Cultural context was significant; the relation was positive and significant only in studies conducted in Western countries. Achievement goal terminology, achievement goal setting, education level and type of publication were not significant. The meta-regressions indicated that year of publication was significant ( B = − 0.011, p = 0.042); the relation between the performance-approach goals and depression decreased for more recent publications. Age ( B = 0.01) and percentage of males ( B = − 0.002) were not significant.
None of five categorical variables (achievement goal model, achievement goal scale, education level, type of publication, cultural context) tested were significant moderators of the positive relation between performance-avoidance goals and depression. Meta-regressions indicated that age ( B = 0.0001), percentage of males ( B = − 0.001), and year of publication ( B = 0.003) were not signification moderators.
Publication Bias
Egger’s test for studies evaluating anxiety indicated there was no indication of publication bias for mastery-approach goals, t (32) = − 1.00, p = 0.32, and that the probability of publication bias was significant for performance-approach goals, t (33) = 3.44, p = 0.002, and performance-avoidance goals, t (22) = 2.11, p = 0.04. A trim-and-fill analysis suggested a significant left asymmetry for performance-approach goals, and that an overestimation of the global effect size was plausible (11 powerful studies and one low-powered study should be included to compensate the possible overestimation of the effect; see Funnel plots in Section 4 of Supplementary Material). Similar bias was observed for performance-avoidance goals, suggesting that the effect could be overestimated (2 low-powered studies and 4 medium-powered studies should be included to compensate the possible overestimation of the effect). For depression, there was no indication of publication bias in the studies assessing mastery-approach goals, t (34) = − 1.73, performance-approach goals, t (32) = − 1.21, and performance-avoidance goals, t (22) = − 0.18, all ps > 0.05 (see Funnel plots in Section 5 of Supplementary Material).
In the present meta-analytic work, we evaluated the strength of the relations of the four goals of the 2 × 2 achievement goal model with anxiety and depression. We found significant effect sizes linking mastery-approach goals and performance-avoidance goals to both anxiety and depression, and performance-approach goals to anxiety but not depression; no significant relations were found for mastery-avoidance goals. We also found significant moderation of these relations, indicating variation as a function of conceptualization of achievement goals, sample characteristics, and methodology- and publication-based characteristics.
Direct Relations Between Achievement Goals and Internalizing Problems
Our findings indicate that achievement goals are related to anxiety and depression. The effect sizes are small to medium in magnitude, with the significant relations ranging from r = 0.05 (for performance-avoidance goals and depression) to r = 0.25 (for performance-avoidance goals and anxiety). We found that individuals with higher levels of mastery-approach goals experience lower levels of both anxiety and depression. Individuals with higher levels of performance-approach goals, on the other hand, experience higher levels of anxiety, and exhibit a trend toward higher levels of depression. Those with higher levels of performance-avoidance goals experience higher levels of both anxiety and depression. Mastery-avoidance goals appear to be unrelated to anxiety and depression, although a positive trend is evident for these goals and depression.
These results are in line with and extend the goal-orientation model of depression (Dykman, 1998 ). Individuals who pursue mastery-approach goals are less vulnerable to anxiety and depression, likely because they are focused on growth and self-improvement, and appraise difficult situations/tasks as challenging (Dykman, 1998 ). Individuals who pursue performance-based goals experience higher levels of anxiety and depression, most likely because they are focused on comparing their success/ability to others (Dweck, 1986 ), and interpret difficult situations/tasks as a test of their ability (Dykman, 1998 ). Of note, achievement goals are associated with anxiety as well as depression (Sideridis, 2007 ), and the connection between performance-based goals and internalizing problems is, descriptively, particularly prominent for the avoidance manifestation of such goals (i.e., performance-avoidance). It is also important to note that our findings are mute regarding the causal direction of the relations; it is possible that the relations are bi-direction and feed into one another (Duchesne et al., 2014 ; Măirean & Diaconu-Gherasim, 2020 ).
Although our meta-analysis did not test processes that might explain the observed findings, several cognitive processes seem likely candidates. By encouraging growth and learning, mastery-approach goals might reduce dysfunctional attitudes and negative attributions (e.g., attributing failure to temporary, changeable factors), thus reducing vulnerability to anxiety and depression (Steare et al., 2024 ). Mastery-approach goals might also promote beliefs that ability can be developed through practice (growth mindset) that further encourage adaptive ways of coping with stress and failure that are related to low levels of anxiety and depression (Dykman, 1998 ; Yeager & Dweck, 2023 ). By promoting external standards based on social comparison, performance-based goals might lead to more negative attributions (e.g., attributing difficulty to internal, stable factors) and interpreting failure as a threat to one’s self-worth, facilitating stress and rumination, and elevated levels of anxiety and depression (Steare et al., 2024 ). Performance-based goals might also promote beliefs that ability cannot be developed through practice (fixed mindset), which is related to increased risk of anxiety and depression (Yeager & Dweck, 2023 ). Finally, to the degree that anxiety and depression themselves reduce mastery-approach and promote performance-based goals pursuit, they may do so by impairing cognitive functioning, coping, and self-regulation processes (Duchesne et al., 2014 ).
Mastery-avoidance goals are not significantly related to anxiety and depression which at first glance may suggest that these goals are not relevant to this type of psychopathology. However, these goals are focused on not losing one’s skills and abilities, and thus may be particularly prevalent among and applicable to older people (Elliot & McGregor, 2001 ). The vast majority of studies included in this meta-analysis were conducted on samples of middle school, high school, and college students, and the observed relations may be stronger in samples where age is more evenly distributed. It is also important to take care in interpreting the mastery-avoidance goal results given the small number of existing studies on anxiety (k = 4) and depression (k = 4). Although the results did not reach significance for mastery-avoidance goals and depression, the effect size was similar to those for mastery-approach and performance-avoidance goals and depression; thus, more research is needed to more clearly determine the precise nature of the mastery-avoidance goal-depression relation.
Considering the findings for the four achievement goals together, the pattern of results between achievement goals and internalizing problems is similar to the pattern found for other achievement-relevant processes and outcomes in the literature. Meta-analyses on variables such as achievement, intrinsic motivation, help seeking, etc. have consistently revealed that mastery-approach goals have the most adaptive pattern of relations, performance-avoidance goals have the least adaptive pattern, and the pattern for performance-approach and mastery-avoidance goals lies in between (e.g., Baranik et al., 2010 ; Hulleman et al., 2010 ; Wirthwein et al., 2013 ; see also Butera et al., 2024 for a narrative review). Perhaps most relevant to the current work, prior meta-analytic work has indicated that mastery-approach goals are negatively, and performance-approach, performance-avoidance, and mastery-avoidance goals are positively, related to negative achievement emotions (Huang, 2011 ). Our findings are consistent with this pattern for all but mastery-avoidance goals (which produce null results herein); this is interesting as it suggests that the mastery-avoidance goal pursuit may be less pernicious, with regard to emotional experience, than the two performance-based goals. Critically, our findings extend the prior work on negative affect by linking achievement goals to clinical anxiety and depression marked by persistent and intense emotional experience. Thus, the present work expands the achievement goal nomological network to include broad outcomes beyond the achievement domain and relevant to overall psychological functioning and mental health.
From a theoretical standpoint, our work may be seen as contributing to an understanding of the links between approach and avoidance motivation on one hand and anxiety and depression on the other. Gray’s Reinforcement Sensitivity Theory (Fowles, 1994 ; Gray, 1982 ) focuses on two basic, biologically-based motivational systems, the behavioral inhibition system (BIS; avoidance motivation) and the behavioral activation system (BAS; approach motivation). In this theory, the BIS is sensitive to stimuli representing nonreward, punishment, and novelty and involves moving away from (avoiding) or inhibiting undesirable affective states; high BIS sensitivity is positively associated with both anxiety and depression. The BAS is sensitive to stimuli representing reward and escape from punishment and involves moving toward (approaching) or maintaining desirable affective states; high BAS sensitivity is negatively association with depression, but not anxiety (see Katz et al., 2020 for further elaboration on these constructs and findings). Our findings at the goal level indicate that it is one type of avoidance motivation that is positively associated with anxiety, namely performance-avoidance goals, and that it is one type of approach motivation that is negatively associated with depression, namely mastery-approach goals. In addition, our findings indicate that one type of approach goals, performance-approach, is positively associated with anxiety. Thus, our findings show that the motivation-internalizing symptoms relations become more nuanced and specific as people regulate their basic energization tendencies with more concrete directional aims (Elliot & Thrash, 2002 ; see also Dickson & MacLeod, 2004 for related work on personal goals and internalizing symptoms).
Moderators of the Relations Between Achievement Goals and Internalizing Problems
Several of the tested moderator variable candidates were significant. Below we highlight what we perceive to be the most informative moderator variable findings.
Achievement goal model was a robust moderator across types of goals and internalizing problems. Specifically, the findings were significantly stronger for the dichotomous model than for the trichotomous and 2 × 2 models for the positive relation between performance-approach goals and depression (and they were descriptively stronger for the negative relation between mastery-approach goals and anxiety, and the positive relation performance-approach goals and anxiety). Further, the findings were significantly stronger for the trichotomous model than for the 2 × 2 model for the positive relations between performance-avoidance goals and both anxiety and depression (and they were descriptively stronger for the negative relations between mastery-approach goals and both anxiety and depression, and the positive relation between performance-approach goals and anxiety). Operationally, the achievement goals in the trichotomous model contain content other than goal standards per se, including a preference for challenge (mastery-approach), a desire to impress important others (performance-approach), and worries and fears (performance-avoidance; see Elliot & Church, 1997 ; Middleton & Midgley, 1997 ; Skaalvik, 1997 ; Vandewalle, 1997 ). These added components essentially create goal complexes that encompass both the goal standard and the reasons for pursuing that standard, and these reasons likely add to the predictive power of the standard (Senko & Tropiano, 2016 ; Sommet & Elliot, 2017 ). In essence (that is, heuristically, not technically), one can compare the trichotomous model effect sizes to those for the 2 × 2 model to get a rough estimate of the predictive utility gained by adding reason-based content to the goal standard content (for more on achievement goal complexes, see Sommet et al., 2021 ; Liem & Senko, 2022 ).
Another robust moderator variable was achievement goal terminology. We found that the goals using “development” and “demonstration” terminology showed the strongest relations when there were sufficient sample sizes for this moderator to be tested. Specifically, the negative relation between development-approach goals and anxiety (and, descriptively, depression), as well as the positive relation between demonstration-approach goals and anxiety were stronger than the relations observed for other achievement goal terms. It is interesting to note that the development and demonstration labels aren’t just distinct terms, but they also represent somewhat distinct content. That is, mastery-based goals include both task-based and self-based competence standards, whereas development-based goals focus on self-based standards only (Elliot et al., 2011 ; Korn & Elliot, 2016 ). The stronger associations of development- and demonstration-based goals are mirrored in the significant moderation for achievement goal scale. Studies using the PALS (Midgley et al., 1993 ) and the AGQ/AGQ-R (Elliot & Church, 1997 ) showed weaker associations than studies using other scales (e.g., Dykman, 1998 ). For example, the PALS measured both the normative and appearance aspect of performance-approach goals, the AGQ/AGQ-R measured only normative goals, whereas other scales measured performance goals as demonstration/appearance goals. Our results thus suggest a stronger negative impact of the development or demonstration/appearance component on anxiety and depression. As such, our results suggest that it is appetitive temporal striving – trying to increase one’s competence (development-approach) – that is most strongly linked to lower anxiety and depression. Likewise, performance-approach goals include other-based standards, whereas demonstration-based goals focus more on showing one’s ability to others. As such, our results suggest that a focus on appearance and demonstration may create a propensity for external motivators and a potential reliance on others for validation, which is likely to perpetuate anxious/depressed thoughts (see Hulleman et al., 2010 ; Senko & Dawson, 2017 for related work).
A third robust moderator variable was achievement goal setting. We found that the goals focusing on competence in general relative to those focusing on specific domains showed the strongest relations when there were sufficient sample sizes for this moderator to be tested. That is, domain-general mastery-approach goals had a stronger negative relation with both anxiety and depression than domain-specific mastery-approach goals. Likewise, domain-general performance-approach goals had a stronger positive relation with anxiety than domain-specific performance-approach goals, and domain-general mastery-approach goals had a stronger negative relation with anxiety (and, descriptively, depression) than domain-specific mastery-approach goals. This pattern of findings likely reflects the correspondence principle, which states that the relationship between two variables will be strongest when they are matched in level of generality-specificity (Ajzen & Fishbein, 1977 ). The anxiety and depression variables that were the focus of the present research represent broad, domain-general indicators of mental health, so it is sensible that they would be more strongly related to broad, domain-general indicators of achievement goals than narrow, domain-specific indicators. Achievement goal researchers (and researchers across domains and disciplines) would do well to attend to this often-overlooked principle in their work (for an empirical demonstration of the importance of correspondence in work on other achievement motivation constructs, see Chan et al., 2023 ).
A final point that we would like to highlight concerns the robustness of the performance-avoidance goal findings across moderators. The analyses did show that the performance-avoidance goals links to both anxiety and depression were moderated by a number of difference variables. However, this moderation almost exclusively revealed differences in the relative strength of significant findings, rather than revealing a significant finding under one condition but not another. For example, for each tested moderator of the positive relation between performance-avoidance goals and anxiety, the relation was significant and positive, only varying in magnitude; in fact, all but two of the eleven observed effect sizes for this relation dropped below the r = 0.30 mark. This robustness across moderators was unique to performance-avoidance goals, testifying to how this type of self-regulation represents a particularly pervasive and pernicious mental health vulnerability (Elliot & Hulleman, 2017 ).
It is important to also note that some other moderators – achievement goal scale, participants’ education level, cultural context, and type of design – were also relevant, even if only for a small number of relations. For example, with regard to cultural context, we found evidence supporting a universalist perspective and evidence supporting cultural differences (see Zusho & Clayton, 2011 ). Findings indicated that mastery-approach goals were positively and performance-avoidance goals negatively related to depression across cultures (a universalist finding), and findings also indicated that performance-approach goals were positively related to depression for Western Cultures but unrelated for Eastern cultures (a cultural difference). There were not enough existing studies to test for mastery-avoidance goal differences. Several things are noteworthy here. First, prior work showing cultural differences regarding achievement goals has tended to find differences for performance-avoidance goals. These goals fit the stronger collectivistic emphasis on avoiding negative outcomes in Eastern, relative to Western cultures, which accounts for why performance-avoidance goals are sometimes not detrimental and can even be beneficial in such contexts (Elliot et al., 2001 ). Importantly, this has been found for performance-based outcomes but not experience-based outcomes such as intrinsic motivation (see Hulleman et al., 2010 ). It may be that performance-avoidance goals afford performance benefits in Eastern contexts, but that the stress of regulating according to a negative normative possibility still exacts a toll on experience and well-being (Roskes et al., 2014 ). Second, our finding that performance-approach goals are detrimental for depression in Western but not Eastern cultures may also be a function of individualistic and collectivistic emphases. In Western cultures, individualistic values emphasize personal achievement and success relative to others, perhaps amplifying the impact of performance-approach goals on mental health. In Eastern cultures, on the other hand, collectivistic values emphasize in-group (e.g. family) achievement and success, and thus performance-approach goals may have fewer implications for how the self is construed (King et al., 2017 ), mitigating the impact of these goals on depression. Third, the fact that there were not enough existing studies to test for cultural differences for the mastery-avoidance goal to depression link nor for cultural differences for any achievement goal and anxiety highlights the clear need for more research in this important area.
In sum, the moderator variable analyses yielded several informative findings that provide a more precise and rich empirical picture than that gleaned from the omnibus relations alone. Nevertheless, two cautions are in order. First, some of the moderator tests should be interpreted with caution given modest numbers of available studies (e.g., mastery-approach goal, task orientation goal, and learning goal orientation variants of mastery-approach goals); furthermore, in some instances there were not enough samples to test for moderation (e.g., ego goal, ability goal, and prove goal variants of performance-approach goals). Thus, some effect sizes may be unstable (note that we highlighted the particularly robust findings above), some differences in associations between moderator levels are practically negligible albeit significantly different (e.g., stronger correlation between performance-avoidance goals and depression measured with other scales than with the AGQ/AGQ-R), and some important moderator information may be missing altogether (e.g., performance-avoidance terminology, form of depression). Second, there is conceptual overlap in some of the moderators tested, especially regarding achievement goal conceptualization (e.g., achievement goal model – dichotomous, trichotomous, 2 × 2 – clearly has some overlap with achievement goal terminology – mastery goal/orientation, mastery-approach goal, etc.). As such, the number of significant moderators may be somewhat misleading, as some of the significant findings may emerge from nonindependent tests. Regardless, it is clear from the present work that there is considerable complexity and nuance underlying the direct relations between achievement goals and internalizing problems documented herein, performance-avoidance goals being the exceptional case.
Limitations, Future Directions, and Implications
Limitations of the present work should be noted; these limitations point to additional avenues for future research. First, we evaluated each achievement goal separately. However, achievement goals are not mutually exclusive and people commonly simultaneously adopt multiple goals (Barron & Harackiewicz, 2001 ; Pintrich, 2000 ). Future studies would do well to evaluate how multiple goals (e.g., high mastery-approach and performance-approach goals) are related to anxiety and depression. Second, all studies in our meta-analytic work used self-reported scales of achievement goals, anxiety, and depression; as such, the results might be affected by common method variance. Future studies would do well to use other informants (e.g., teachers’ reports of students’ achievement goals, clinical interviews to assess depression and anxiety) in order to more definitively document the focal relations. Third, there were not sufficient longitudinal data incorporating multiple measurements of both achievement goals and internalizing problems for us to test direction of causality. Future research would do well to attend to this important issue, particularly given the differing theoretical emphases on this matter. Fourth, although our research documented direct relations between achievement goals and internalizing problems, it did not document the mechanisms responsible for these relations. Future research is needed to test purported mediation of the observed links, such as cognitive appraisals (Dykman, 1998 ), perceived stress (Wang et al., 2021 ), and rumination (Van Boekel & Martin, 2014 ). Finally, the present work revealed minimal or no existing research on the following: Mastery-avoidance goals, different types of anxiety (e.g., generalized anxiety, agoraphobia) and depression (e.g., disruptive mood dysregulation disorder), and older adults. These issues are in clear need of future research attention.
The findings from the present research join the growing corpus of findings indicating that mastery-approach goals are beneficial for psychological functioning, whereas performance-avoidance goals are detrimental. The results reveal a new and promising perspective for prevention efforts, with achievement goals as sensible entry points to prevent poor mental health. Achievement goals are modifiable through interventions targeting school environment by, for example, emphasizing students’ personal growth and learning (evoking mastery-approach goals) (see Elliot & Hulleman, 2017 for a review). School-based interventions are needed to address the structural aspects of the educational system by emphasizing the development of abilities, understanding of material, and promoting mistakes as opportunities for growth (Liu et al., 2024 ; Steare et al., 2024 ). Accordingly, the take-home message from this research is similar to that of other achievement goal research: Teachers, coaches, employers, and parents would do well to structure their instructions, incentives, and feedback to those under their charge in ways that facilitate and support the pursuit of mastery-approach goals, and discourage and disrupt the pursuit of performance-avoidance goals (Bardach et al., 2020 ; Korn et al., 2019 ; Senko, 2016 ). The data are not yet clear enough for emphatic statements about performance-approach or mastery-avoidance goals. Of course, these recommendations must be made while acknowledging the relatively small number of studies conducted and the relatively modest overall effect sizes.
Concluding Thoughts
A clear and unequivocal conclusion that may be drawn from the present meta-analytic work is that achievement goals and internalizing problems are systematically related to each other. This conclusion is of conceptual and applied importance. Conceptually, it means that competence pursuits are relevant to mental health in general, they are not just relevant to competence-specific (e.g., achievement, intrinsic motivation) or domain-specific (e.g., school, work) outcomes, or affective states (e.g., achievement-relevant emotions). Competence is a basic human need, so it makes sense that competence-based pursuits would be linked to broad health and well-being indices (Elliot et al., 2002 ; Ryan & Deci, 2019 ). Further, researchers might consider achievement goals as an explanation for why depression and anxiety are related to individuals’ achievement and adjustment in various settings, including educational, work, and sport settings. In terms of application, it means that greater attention needs to be allocated – in the classroom, the workplace, and the ballfield – to the achievement goal-mental health nexus. Attending to the whole person, not just the individual’s short-term achievements, will likely pay dividends for both long-term accomplishment and overall flourishing and functioning. Accordingly, we believe there is strong reason to sound the call for increased research attention to the relation between achievement goal pursuit and internalizing problems.
Data Availability
The data is available on OSF on the following link: https://osf.io/beu6h/?view_only=b463289949aa413a936f8349ab2a702a
Ajzen, I., & Fishbein, M. (1977). Attitude–behavior relations: A theoretical analysis and review of empirical research. Psychological Bulletin, 84 , 888–918. https://doi.org/10.1037/0033-2909.84.5.888
Article Google Scholar
American Psychiatric Association. (2013). Diagnostic and statistical manual of mental disorders (5th ed.). https://doi.org/10.1176/appi.books.9780890425596
American Psychiatric Association. (2022). Diagnostic and statistical manual of mental disorders (5th ed., text rev.). https://doi.org/10.1176/appi.books.9780890425787
Ames, C. (1992). Classrooms: Goals, structures, and student motivation. Journal of Educational Psychology, 84 , 261–271. https://doi.org/10.1037/0022-0663.84.3.261
Ariani, D. W. (2017). Self-determined motivation, achievement goals and anxiety of economic and business students in Indonesia. Educational Research and Reviews, 12 , 1154–1166. https://doi.org/10.5897/ERR2017.3381
Balduzzi, S., Rücker, G., & Schwarzer, G. (2019). How to perform a meta-analysis with R:43A practical tutorial. Evidence-Based Mental Health, 22 , 153–160. https://doi.org/10.1136/ebmental-2019-300117
Baranik, L. E., Stanley, L. J., Bynum, B. H., & Lance, C. E. (2010). Examining the construct validity of mastery-avoidance achievement goals: A meta-analysis. Human Performance, 23 , 265–282. https://doi.org/10.1080/08959285.2010.488463
Bardach, L., Oczlon, S., Pietschnig, J., & Lüftenegger, M. (2020). Has achievement goal theory been right? A meta-analysis of the relation between goal structures and personal achievement goals. Journal of Educational Psychology, 112 , 1197–1220. https://doi.org/10.1037/edu0000419
Barron, K. E., & Harackiewicz, J. M. (2001). Achievement goals and optimal motivation: Testing multiple goal models. Journal of Educational Psychology, 80 , 706–722. https://doi.org/10.1037/0022-3514.80.5.706
Biswas, T., Scott, J. G., Munir, K., Renzaho, A. M., Rawal, L. B., Baxter, J., & Mamun, A. A. (2020). Global variation in the prevalence of suicidal ideation, anxiety and their correlates among adolescents: A population based study of 82 countries. E-Clinical Medicine, 24 , 100395. https://doi.org/10.1016/j.eclinm.2020.100395
Bitsko, R. H., Holbrook, J. R., Ghandour, R. M., Blumberg, S. J., Visser, S. N., Perou, R., & Walkup, J. T. (2018). Epidemiology and impact of health care provider–diagnosed anxiety and depression among US children. Journal of Developmental and Behavioral Pediatrics, 39 , 395–403. https://doi.org/10.1097/DBP.0000000000000571
Borenstein,. (2009). Effect sizes for continuous data. In H. Cooper, L. Hedges, & J. Valentine (Eds.), The handbook of research synthesis and meta-analysis (pp. 279–293). Sage.
Google Scholar
Brumariu, L., Waslin, S., Gastelle, M., Kochendorfer, L., & Kerns, K. (2022). Anxiety, academic achievement, and academic self-concept: Meta-analytic syntheses of their relations across developmental periods. Development and Psychopathology, 35 , 1597–1613. https://doi.org/10.1017/S0954579422000323
Butera, F., Dompnier, B., & Darnon, C. (2024). Achievement goals: A social influence cycle. Annual Review of Psychology, 75 , 527–554. https://doi.org/10.1146/annurev-psych-013123-102139
Chan, H. S., Chiu, C. Y., Lee, S. L., Tong, Y. Y., & Leung. (2023). Improving the predictor-criterion consistency of mindset measures: Application of the correspondence principle. Journal of Pacific Rim Psychology, 17 , 1–11. https://doi.org/10.1177/18344909231166964
Christina, S., Magson, N. R., Kakar, V., & Rapee, R. M. (2021). The bidirectional relationships between peer victimization and internalizing problems in school-aged children: An updated systematic review and meta-analysis. Clinical Psychology Review, 85 , 101979. https://doi.org/10.1016/j.cpr.2021.101979
Craske, M. G., Stein, M. B., Eley, T. C., Milad, M. R., Holmes, A., Rapee, R. M., & Wittchen, H. U. (2017). Anxiety Disorders. Nature Reviews Disease Primers, 3 , 17024. https://doi.org/10.1038/nrdp.2017.24
Demin, G. (2024). Maditr: Fast data aggregation, modification, and filtering with pipes and ’data.table’. https://CRAN.R-project.org/package=maditr
Dickson, J. M., & MacLeod, A. K. (2004). Approach and avoidance goals and plans: Their relationship to anxiety and depression. Cognitive Therapy and Research, 28 , 415–432. https://doi.org/10.1023/B:COTR.0000031809.20488.ee
Duchesne, S., Ratelle, C. F., & Feng, B. (2014). Developmental trajectories of achievement goal orientations during the middle school transition: The contribution of emotional and behavioral dispositions. The Journal of Early Adolescence, 34 , 486–517. https://doi.org/10.1177/0272431613495447
Dweck, C. S. (1986). Motivational processes affecting learning. American Psychologist, 41 , 1040–1048. https://doi.org/10.1037/0003-066X.41.10.1040
Dweck, C. S., & Leggett, E. L. (1988). A social-cognitive approach to motivation and personality. Psychological Review, 95 , 256–273. https://doi.org/10.1037/0033-295X.95.2.256
Dykman, B. M. (1998). Integrating cognitive and motivational factors in depression: Initial tests of a goal-orientation approach. Journal of Personality and Social Psychology, 74 , 139–158. https://doi.org/10.1037/0022-3514.74.1.139
Elliot, A. (1997). Integrating the ‘classic’ and ‘contemporary’ approaches to achievement motivation: A hierarchical model of approach and avoidance achievement motivation. In M. Maehr & P. Pintrich (Eds.), Advances in motivation and achievement (Vol. 10, pp. 143–179). JAI Press.
Elliot, A. J., Chirkov, V. I., Kim, Y., & Sheldon, K. M. (2001). A cross-cultural analysis of avoidance (relative to approach) personal goals. Psychological Science, 12 , 505–510. https://doi.org/10.1111/1467-9280.00393
Elliot, A. J., & Hulleman, C. S. (2017). Achievement goals. In A. Elliot, C. Dweck, & D. Yeager (Eds.), Handbook of competence and motivation. Theory and application (pp. 43–60). Guilford Press.
Elliot, A. J. (1999). Approach and avoidance motivation and achievement goals. Educational Psychologist, 34 , 169–189. https://doi.org/10.1207/s15326985ep3403_3
Elliot, A. J., & Church, M. A. (1997). A hierarchical model of approach and avoidance achievement motivation. Journal of Personality and Social Psychology, 72 , 218–232. https://doi.org/10.1037/0022-3514.72.1.218
Elliot, A. J., & Harackiewicz, J. M. (1996). Approach and avoidance achievement goals and intrinsic motivation: A mediational analysis. Journal of Personality and Social Psychology, 70 , 461–475. https://doi.org/10.1037/0022-0663.100.3.613
Elliot, A. J., & McGregor, H. A. (2001). A 2×2 achievement goal framework. Journal of Personality and Social Psychology, 80 , 501–519. https://doi.org/10.1037/0022-3514.80.3.501
Elliot, A. J., McGregor, H. A., & Thrash, T. M. (2002). The need for competence. In E. Deci & R. Ryan (Eds.), Handbook of self-determination research (pp. 361–387). University of Rochester Press.
Elliot, A. J., Murayama, K., & Pekrun, R. (2011). A 3 × 2 achievement goal model. Journal of Educational Psychology, 103 (3), 632–648. https://doi.org/10.1037/a0023952
Elliot, A. J., & Thrash, T. M. (2002). Approach–avoidance motivation in personality: Approach and avoidance temperaments and goals. Journal of Personality and Social Psychology, 82 , 804–818. https://doi.org/10.1037/0022-3514.82.5.804
Finning, K., Ukoumunne, O. C., Ford, T., Danielsson-Waters, E., Shaw, L., De Jager, I. R., ..., & Moore, D. A. (2019). The association between child and adolescent depression and poor attendance at school: A systematic review and meta-analysis. Journal of Affective Disorders , 245 , 928–938. https://doi.org/10.1016/j.jad.2018.11.055
Fowles, D. C. (1994). A motivational theory of psychopathology. In W. Spaulding (Ed.), Integrative views of motivation, cognition, and emotion (pp. 181–238). University of Nebraska Press.
Ghandour, R. M., Sherman, L. J., Vladutiu, C. J., Ali, M. M., Lynch, S. E., Bitsko, R. H., & Blumberg, S. J. (2019). Prevalence and treatment of depression, anxiety, and conduct problems in US children. The Journal of Pediatrics, 206 , 256–267. https://doi.org/10.1016/j.jpeds.2018.09.021
Grant, H., & Dweck, C. S. (2003). Clarifying achievement goals and their impact. Journal of Personality and Social Psychology, 85 , 541–553. https://doi.org/10.1037/0022-3514.85.3.541
Gray, J. A. (1982). The neuropsychology of anxiety: An enquiry into the functions of the septo-hippocampal system . Oxford University Press.
Harrer, M., Cuijpers, P., Furukawa, T., & Ebert, D. D. (2019). Dmetar: Companion r package for the guide ’doing meta-analysis in r’. http://dmetar.protectlab.org/
Hedges, L. V., & Olkin, I. (1985). Statistical methods for meta-analysis . Academic Press. https://doi.org/10.1016/C2009-0-03396-0
Book Google Scholar
Hoyt, ACDR&WT. (2014). MAd: Meta-analysis with mean differences. https://CRAN.R-project.org/package=MAd
Huang, C. (2011). Achievement goals and achievement emotions: A meta-analysis. Educational Psychology Review, 23 , 359–388. https://doi.org/10.1007/s10648-011-9155-x
Hulleman, C. S., Schrager, S. M., Bodmann, S. M., & Harackiewicz, J. M. (2010). A meta-analytic review of achievement goal measures: Different labels for the same constructs or different constructs with similar labels? Psychological Bulletin, 136 , 422–449. https://doi.org/10.1037/a0018947
Katz, B. A., Matanky, K., Aviram, G., & Yovel, I. (2020). Reinforcement sensitivity, depression and anxiety: A meta-analysis and meta-analytic structural equation model. Clinical Psychology Review, 77 , 101842. https://doi.org/10.1016/j.cpr.2020.101842
King, R. B., McInerney, D. M., & Nasser, R. (2017). Different goals for different folks: A cross-cultural study of achievement goals across nine cultures. Social Psychology of Education, 20 , 619–642. https://doi.org/10.1007/s11218-017-9381-2
Korn, R. M., & Elliot, A. J. (2016). The 2 x 2 standpoints model of achievement goals. Frontiers in Psychology, 7 , 1–12. https://doi.org/10.3389/fpsyg.2016.00742
Korn, R. M., Elliot, A. J., & Daumiller, M. (2019). Back to the roots: The 2 × 2 standpoints and standards achievement goal model. Learning and Individual Differences, 72 , 92–102. https://doi.org/10.1016/j.lindif.2019.04.009
Koutsimani, P., Montgomery, A., & Georganta, K. (2019). The relationship between burnout, depression, and anxiety: A systematic review and meta-analysis. Frontiers in Psychology, 10 , 284. https://doi.org/10.3389/fpsyg.2019.00284
Liem, G. A. D., & Senko, C. (2022). Goal complexes: A new approach to studying the coordination, consequences, and social contexts of pursuing multiple goals. Educational Psychology Review, 34 , 2167–2195. https://doi.org/10.1007/s10648-022-09701-5
Liu, X., Gao, X., & Ping, S. (2019). Post-1990s college students academic sustainability: the role of negative emotions, achievement goals, and self-efficacy on academic performance. Sustainability, 11 (3), 775. https://doi.org/10.3390/su11030775
Liu, X., Zhang, Y., Cao, X., et al. (2024). Does anxiety consistently affect the achievement goals of college students? A four-wave longitudinal investigation from China. Current Psychology, 43 , 10495–10508. https://doi.org/10.1007/s12144-023-05184-x
Lochbaum, M., & Gottardy, J. (2015). A meta-analytic review of the approach-avoidance achievement goals and performance relationships in the sport psychology literature. Journal of Sport and Health Science, 4 , 164–173. https://doi.org/10.1016/j.jshs.2013.12.004
Lüdecke, D. (2019). Esc: Effect size computation for meta analysis (version 0.5.1). https://doi.org/10.5281/zenodo.1249218
Luttenberger, S., Wimmer, S., & Paechter, M. (2018). Spotlight on math anxiety. Psychology Research and Behavior Management , 311–322. https://doi.org/10.2147/PRBM.S141421
Madjar, N., Ratelle, C. F., & Duchesne, S. (2021). A longitudinal analysis of the relationships between students’ internalized symptoms and achievement goals. Motivation Science, 7 , 207–218. https://doi.org/10.1037/mot0000195
Măirean, C., & Diaconu-Gherasim, L. R. (2020). Depressive symptoms and achievement goals: Parental rejection as a moderator. The Journal of Early Adolescence, 40 , 1369–1396. https://doi.org/10.1177/0272431619858417
Marx, W., Penninx, B. W., Solmi, M., Furukawa, T. A., Firth, J., Carvalho, A. F., & Berk, M. (2023). Major depressive disorder. Nature Reviews Disease Primers, 9 , 44. https://doi.org/10.1038/s41572-023-00454-1
Melton, T. H., Croarkin, P. E., Strawn, J. R., & McClintock, S. M. (2016). Comorbid anxiety and depressive symptoms in children and adolescents: A systematic review and analysis. Journal of Pychiatric Practice, 22 , 84–98. https://doi.org/10.1097/PRA.0000000000000132
Middleton, M. J., & Midgley, C. (1997). Avoiding the demonstration of lack of ability: An underexplored aspect of goal theory. Journal of Educational Psychology, 89 , 710–718. https://doi.org/10.1037/0022-0663.89.4.710
Midgley, C., Maehr, M. L., & Urdan, T. (1993). Manual for the Patterns of Adaptive Learning Survey (PALS) . University of Michigan.
Nicholls, J. G. (1984). Achievement Motivation: Conceptions of ability, subjective experience, task choice, and performance. Psychological Review, 91 , 328–346. https://doi.org/10.1037/0033-295X.91.3.328
Niemivirta, M. (2002). Motivation and performance in context: The influence of goal orientations and instructional setting on situational appraisals and task performance. Psychologia, 45 , 250–270.
Page, M. J., McKenzie, J. E., Bossuyt, P. M., Boutron, I., Hoffmann, T. C., Mulrow, C. D., ..., & Moher, D. (2021). The PRISMA 2020 statement: An updated guideline for reporting systematic reviews. International Journal of Surgery , 88 , 105906. https://doi.org/10.1016/j.ijsu.2021.105906
Payne, S. C., Youngcourt, S. S., & Beaubien, J. M. (2007). A meta-analytic examination of the goal orientation nomological net. Journal of Applied Psychology, 92 , 128–150. https://doi.org/10.1037/0021-9010.92.1.128
Pintrich, P. R. (2000). An achievement goal theory perspective on issues in motivation terminology, theory, and research. Contemporary Educational Psychology, 25 , 92–104. https://doi.org/10.1006/ceps.1999.1017
R Core Team. (2023). R: A language and environment for statistical computing. R Foundation for Statistical Computing. https://www.R-project.org/
Riglin, L., Petrides, K. V., Frederickson, N., & Rice, F. (2014). The relationship between emotional problems and subsequent school attainment: A meta-analysis. Journal of Adolescence, 37 , 335–346. https://doi.org/10.1016/j.adolescence.2014.02.010
Roedel, T. D., Schraw, G., & Plake, B. S. (1994). Validation of a measure of learning and performance goal orientations. Educational and Psychological Measurement, 54 , 1013–1021.
Roskes, M., Elliot, A. J., & De Dreu, C. K. W. (2014). Why is avoidance motivation problematic, and what can be done about it? Current Directions in Psychological Science, 23 , 133–138. https://doi.org/10.1177/0963721414524224
Ryan, R. M., & Deci, E. L. (2019). Brick by brick: The origins, development, and future of self-determination theory. In A. Elliot (Ed.), Advances in motivation science (Vol. 6, pp. 111–156). Elsevier.
Senko, C. (2016). Achievement goal theory: A story of early promises, eventual discords, and future possibilities. In K. Wentzel & D. Miele (Eds), Handbook of motivation at school (2nd ed., pp. 75–95). Routledge.
Senko, C., & Dawson, B. (2017). Performance-approach goal effects depend on how they are defined: Meta-analytic evidence from multiple educational outcomes. Journal of Educational Psychology, 109 , 574–598. https://doi.org/10.1037/edu0000160
Senko, C., & Tropiano, K. L. (2016). Comparing three models of achievement goals: Goal orientations, goal standards, and goal complexes. Journal of Educational Psychology, 108 , 1178–1192. https://doi.org/10.1037/edu0000114
Sheldon, E., Simmonds-Buckley, M., Bone, C., Mascarenhas, T., Chan, N., Wincott, M., ..., & Barkham, M. (2021). Prevalence and risk factors for mental health problems in university undergraduate students: A systematic review with meta-analysis. Journal of Affective Disorders , 287 , 282–292. https://doi.org/10.1016/j.jad.2021.03.054
Sideridis, G. D. (2005). Goal orientation, academic achievement, and depression: Evidence in favor of a revised goal theory framework. Journal of Educational Psychology, 97 , 366–375. https://doi.org/10.1037/0022-0663.97.3.366
Sideridis, G. D. (2007). Why are students with LD depressed? A goal orientation model of depression vulnerability. Journal of Learning Disabilities, 40 (6), 526–539. https://doi.org/10.1177/00222194070400060401
Skaalvik, E. M. (1997). Self-enhancing and self-defeating ego orientation: Relations with task and avoidance orientation, achievement, self-perceptions, and anxiety. Journal of Educational Psychology, 89 , 71–81. https://doi.org/10.1037/P022-0663.89.1.71
Sommet, N., Elliot, A. J., & Sheldon, K. M. (2021). The “what” and “why” of achievement motivation: Conceptualization, operationalization, and consequences of self-determination derived achievement goal complexes. In R. Robbins & O. John (Eds.), Handbook of personality psychology: Theory and research, 4 th Ed (pp, 104–121). Guilford Press.
Sommet, N., & Elliot, A. J. (2017). Achievement goals, reasons for goal pursuit, and achievement goal complexes as predictors of beneficial outcomes: Is the influence of goals reducible to reasons? Journal of Educational Psychology, 109 , 1141–1162. https://doi.org/10.1037/edu0000199
Spoelma, M. J., Sicouri, G. L., Francis, D. A., Songco, A. D., Daniel, E. K., & Hudson, J. L. (2023). Estimated prevalence of depressive disorders in children from 2004 to 2019: A systematic review and meta-analysis. JAMA Pediatrics., 177 , 1017–1027. https://doi.org/10.1001/jamapediatrics.2023.3221
Steare, T., Lewis, G., Lange, K., & Lewis, G. (2024). The association between academic achievement goals and adolescent depressive symptoms: A prospective cohort study in Australia. Lancet Child Adolescent Health, 8 , 413–421. https://doi.org/10.1016/S2352-4642(24)00051-8
Stornes, T., & Bru, E. (2011). Perceived motivational climates and self-reported emotional and behavioural problems among Norwegian secondary school students. School Psychology International, 32 , 425–438. https://doi.org/10.1177/0143034310397280
Van Boekel, M., & Martin, J. M. (2014). Examining the relation between academic rumination and achievement goal orientation. Individual Differences Research, 12 (4-A), 153–169.
Vandewalle, D. (1997). Development and validation of a work domain goal orientation instrument. Educational and Psychological Measurement, 57 , 995–1015. https://doi.org/10.1177/0013164497057006009
Wang, Y., Liu, L., Ding, N., Li, H., & Wen, D. (2021). The mediating role of stress perception in pathways linking achievement goal orientation and depression in Chinese medical students. Frontiers in Psychology, 12 , 614787. https://doi.org/10.3389/fpsyg.2021.614787
Wei, J. (2018). Academic contingent self-worth of adolescents in mainland China: Distinguishing between success and failure as a basis of self-worth. The Chinese College of Hong Kong. ProQuest Dissertations Publishing, 10805400.
Wirthwein, L., Sparfeldt, J. R., Pinquart, M., Wegerer, J., & Steinmayr, R. (2013). Achievement goals and academic achievement: A closer look at moderating factors. Educational Research Review, 10 , 66–89. https://doi.org/10.1016/j.edurev.2013.07.001
Yap, M. B., Morgan, A. J., Cairns, K., Jorm, A. F., Hetrick, S. E., & Merry, S. (2016). Parents in prevention: A meta-analysis of randomized controlled trials of parenting interventions to prevent internalizing problems in children from birth to age 18. Clinical Psychology Review, 50 , 138–158. https://doi.org/10.1016/j.cpr.2016.10.003
Yeager, D. S., & Dweck, C. S. (2023). Mindsets and adolescent mental health. Nature Mental Health, 1 , 79–81. https://doi.org/10.1038/s44220-022-00009-5
Zusho, A., & Clayton, K. (2011). Culturalizing achievement goal theory and research. Educational Psychologist, 46 , 239–260. https://doi.org/10.1080/00461520.2011.614526
Download references
Acknowledgements
The study was supported by a grant of the Romanian Ministry of Education and Research, CNCS-UEFISCDI, project number PN-III-P4-ID-PCE-2020-2963, within PNCDI III.
Author information
Authors and affiliations.
Department of Psychology and Educational Sciences, Alexandru Ioan Cuza University, 3 Toma Cozma, 700554, Iasi, Romania
Loredana R. Diaconu-Gherasim, Alexandra S. Zancu, Cornelia Măirean & Irina Crumpei-Tanasă
Department of Psychology, University of Rochester, Rochester, NY, USA
Andrew J. Elliot
Gordon F. Derner School of Psychology, Adelphi University, Garden City, NY, USA
Laura E. Brumariu
Department of Psychology and Educational Sciences, University of Bucharest, Bucharest, Romania
Cristian Opariuc‑Dan
Ovidius University, Constanta, Romania
You can also search for this author in PubMed Google Scholar
Corresponding author
Correspondence to Loredana R. Diaconu-Gherasim .
Ethics declarations
Competing interests.
The authors declare no competing interests.
Additional information
Publisher's note.
Springer Nature remains neutral with regard to jurisdictional claims in published maps and institutional affiliations.
Supplementary Information
Below is the link to the electronic supplementary material.
Supplementary file1 (DOCX 295 KB)
Rights and permissions.
Open Access This article is licensed under a Creative Commons Attribution-NonCommercial-NoDerivatives 4.0 International License, which permits any non-commercial use, sharing, distribution and reproduction in any medium or format, as long as you give appropriate credit to the original author(s) and the source, provide a link to the Creative Commons licence, and indicate if you modified the licensed material. You do not have permission under this licence to share adapted material derived from this article or parts of it. The images or other third party material in this article are included in the article’s Creative Commons licence, unless indicated otherwise in a credit line to the material. If material is not included in the article’s Creative Commons licence and your intended use is not permitted by statutory regulation or exceeds the permitted use, you will need to obtain permission directly from the copyright holder. To view a copy of this licence, visit http://creativecommons.org/licenses/by-nc-nd/4.0/ .
Reprints and permissions
About this article
Diaconu-Gherasim, L.R., Elliot, A.J., Zancu, A.S. et al. A Meta-Analysis of the Relations Between Achievement Goals and Internalizing Problems. Educ Psychol Rev 36 , 109 (2024). https://doi.org/10.1007/s10648-024-09943-5
Download citation
Accepted : 23 August 2024
Published : 16 September 2024
DOI : https://doi.org/10.1007/s10648-024-09943-5
Share this article
Anyone you share the following link with will be able to read this content:
Sorry, a shareable link is not currently available for this article.
Provided by the Springer Nature SharedIt content-sharing initiative
- Achievement goals
- Internalizing problems
- Find a journal
- Publish with us
- Track your research
Empirical Referent Concept: Bridging Theory and Reality
How it works
Concepts are like the building blocks that help us ask questions, do research, and understand stuff. Among these, there’s something called an “empirical referent.” It’s super important because it links abstract ideas with the real world. This essay talks about what empirical referents are, why they’re important, and how they help make our knowledge solid and trustworthy.
- 1 Getting What Empirical Referents Are
- 2 How Empirical Referents Help in Research
- 3 Challenges and Things to Think About
- 4 Impact on Theory and Practice
Getting What Empirical Referents Are
Empirical referents are things we can see or measure that help us understand abstract concepts. They provide the proof we need to test theories, check models, and improve ideas.
According to logical positivism, a concept only makes sense if we can verify it with evidence or it’s logically true. So, empirical referents are like the glue that sticks theoretical ideas to real-world science.
Take “intelligence” in psychology, for example. Intelligence is a big, complex idea. But by using things like IQ scores, cognitive tests, and problem-solving tasks, psychologists can measure and study it. This turns the abstract idea of intelligence into something we can actually analyze.
How Empirical Referents Help in Research
Empirical referents are super important in research. They give us a way to test theories and check if they’re right. They help researchers go from just thinking about ideas to actually testing them in the real world.
For example, in social sciences, the idea of “social capital” is measured by things like the size of social networks, how often people interact, and their involvement in community groups. These measurements help researchers study social capital and see how it affects things like economic growth, health, and happiness. Without these measurements, social capital would just be a vague idea that’s hard to study.
Empirical referents also make it easier to repeat studies. By providing clear, measurable indicators, they ensure that studies can be repeated in different places and with different people. This makes research findings more reliable. In medical research, for instance, using standard diagnostic criteria and biomarkers helps ensure that studies on diseases, treatments, and patient outcomes can be consistently repeated and verified.
Challenges and Things to Think About
While empirical referents are really useful, they’re not always easy to work with. One big challenge is making sure the referents really capture what the abstract concept is all about. If the referents aren’t good enough, the research can be flawed.
For instance, in education research, “student engagement” can be measured by attendance, participation in class, and self-reported interest. But if we only look at attendance, we might miss other important parts of engagement, like how interested students are or how much they’re thinking about the material. So, researchers need to pick their referents carefully to make sure they’re getting the whole picture.
Another challenge is that abstract concepts can change over time. As we learn more, the empirical referents might need to change too. This means researchers have to keep updating their referents to make sure they’re still relevant. In technology adoption studies, for example, new tech means we have to keep updating how we measure user acceptance and usage patterns.
Impact on Theory and Practice
Using empirical referents well can have a big impact on both theories and practical applications. For theories, good empirical referents help develop strong, testable ideas that can stand up to real-world testing. They help us investigate and improve theoretical concepts, building a solid body of knowledge.
In practice, empirical referents make research findings more useful and relevant. By grounding abstract ideas in real-world evidence, they help turn research into practical insights and actions. In public health, for example, empirical referents like vaccination rates, disease incidence, and health behaviors provide crucial data that helps shape policies, programs, and resource allocation.
Empirical referents also promote collaboration between different fields. By providing common ground, they help researchers from various disciplines work together on shared concepts and measures. In environmental studies, for instance, the idea of “sustainability” can be measured by carbon footprint, resource use, and biodiversity. This allows ecologists, economists, and social scientists to work together on solving big environmental problems.
In the end, empirical referents are key to connecting theory with the real world. They provide the proof needed to test, validate, and refine ideas, advancing both theory and practice. While there are challenges in identifying and using them, careful selection and ongoing updates are crucial. Effective use of empirical referents builds a strong, credible knowledge base, driving scientific progress and informing real-world decisions.

Cite this page
Empirical Referent Concept: Bridging Theory and Reality. (2024, Sep 17). Retrieved from https://papersowl.com/examples/empirical-referent-concept-bridging-theory-and-reality/
"Empirical Referent Concept: Bridging Theory and Reality." PapersOwl.com , 17 Sep 2024, https://papersowl.com/examples/empirical-referent-concept-bridging-theory-and-reality/
PapersOwl.com. (2024). Empirical Referent Concept: Bridging Theory and Reality . [Online]. Available at: https://papersowl.com/examples/empirical-referent-concept-bridging-theory-and-reality/ [Accessed: 18 Sep. 2024]
"Empirical Referent Concept: Bridging Theory and Reality." PapersOwl.com, Sep 17, 2024. Accessed September 18, 2024. https://papersowl.com/examples/empirical-referent-concept-bridging-theory-and-reality/
"Empirical Referent Concept: Bridging Theory and Reality," PapersOwl.com , 17-Sep-2024. [Online]. Available: https://papersowl.com/examples/empirical-referent-concept-bridging-theory-and-reality/. [Accessed: 18-Sep-2024]
PapersOwl.com. (2024). Empirical Referent Concept: Bridging Theory and Reality . [Online]. Available at: https://papersowl.com/examples/empirical-referent-concept-bridging-theory-and-reality/ [Accessed: 18-Sep-2024]
Don't let plagiarism ruin your grade
Hire a writer to get a unique paper crafted to your needs.
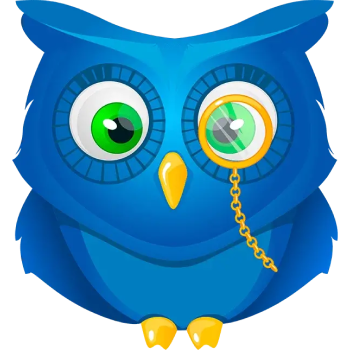
Our writers will help you fix any mistakes and get an A+!
Please check your inbox.
You can order an original essay written according to your instructions.
Trusted by over 1 million students worldwide
1. Tell Us Your Requirements
2. Pick your perfect writer
3. Get Your Paper and Pay
Hi! I'm Amy, your personal assistant!
Don't know where to start? Give me your paper requirements and I connect you to an academic expert.
short deadlines
100% Plagiarism-Free
Certified writers

IMAGES
VIDEO
COMMENTS
For example, empirical data can inform the development of theories and models, and theoretical models can guide the design of empirical studies. The most valuable research combines theoretical and empirical approaches in many fields, allowing for a comprehensive understanding of the studied phenomena.
Empirical research is defined as any study whose conclusions are exclusively derived from concrete, verifiable evidence. The term empirical basically means that it is guided by scientific experimentation and/or evidence. Likewise, a study is empirical when it uses real-world evidence in investigating its assertions.
Empirical research is defined as any research where conclusions of the study is strictly drawn from concretely empirical evidence, and therefore "verifiable" evidence. This empirical evidence can be gathered using quantitative market research and qualitative market research methods. For example: A research is being conducted to find out if ...
Theoretical Research is a logical exploration of a system of beliefs and assumptions, working with abstract principles related to a field of knowledge.. Essentially...theorizing; vs. Empirical Research is based on real-life direct or indirect observation and measurement of phenomena by a researcher.. Basically... Collecting data by Observing or Experimenting
Empirical research methods are used when the researcher needs to gather data analysis on direct, observable, and measurable data. Research findings are a great way to make grounded ideas. Here are some situations when one may need to do empirical research: 1. When quantitative or qualitative data is needed.
The most direct route is to search PsycInfo, linked above. This will take you to the Advanced Search, where you can type in your key words at the top. Then scroll down through all the limiting options to the Methodology menu. Select Empirical Study. In other databases without the Methodology limiter, such as Education Source, try keywords like ...
Another hint: some scholarly journals use a specific layout, called the "IMRaD" format, to communicate empirical research findings. Such articles typically have 4 components: Introduction: sometimes called "literature review" -- what is currently known about the topic -- usually includes a theoretical framework and/or discussion of previous studies
Empirical research is the cornerstone of scientific inquiry, providing a systematic and structured approach to investigating the world around us. It is the process of gathering and analyzing empirical or observable data to test hypotheses, answer research questions, or gain insights into various phenomena.
Scientists conceive of empirical research, collect and analyze the relevant data, and then bring the results to bear on the theoretical issues that inspired the research in the first place. However, philosophers have also discussed ways in which empirical results are transferred out of their native contexts and applied in diverse and sometimes ...
Furthermore, variables may be independent, dependent, mediating, or moderating, as discussed in Chapter 2. The distinction between constructs (conceptualised at the theoretical level) and variables (measured at the empirical level) is shown in Figure 4.1. Figure 4.1 Distinction between theoretical and empirical concepts
Examples of Empirical Research. Study on radiation transfer in human skin for cosmetics. ... Strategies for Empirical Research in Writing is a particularly accessible approach to both qualitative and quantitative empirical research methods, helping novices appreciate the value of empirical research in writing while easing their fears about the ...
Definition and explanation. Empirical evidence is the evidence that we directly observe and get from our senses. This might be contrasted to philosophical or theoretical reasoning, which can be done without any direct observation of 'real life'. Empirical evidence is related to the philosophical distinction between a priori and a posteriori ...
Empirical research methodologies can be described as quantitative, qualitative, or a mix of both (usually called mixed-methods). Ruane (2016) (UofM login required) gets at the basic differences in approach between quantitative and qualitative research: Quantitative research -- an approach to documenting reality that relies heavily on numbers both for the measurement of variables and for data ...
In empirical research, knowledge is developed from factual experience as opposed to theoretical assumption and usually involved the use of data sources like datasets or fieldwork, but can also be based on observations within a laboratory setting. Testing hypothesis or answering definite questions is a primary feature of empirical research.
A theoretical framework (also sometimes referred to as a foundation of theory) is essentially a set of concepts, definitions, and propositions that together form a structured, comprehensive view of a specific phenomenon.. In other words, a theoretical framework is a collection of existing theories, models and frameworks that provides a foundation of core knowledge - a "lay of the land ...
Empirical research is a type of research methodology that makes use of verifiable evidence in order to arrive at research outcomes. In other words, this type of research relies solely on evidence obtained through observation or scientific data collection methods. Empirical research can be carried out using qualitative or quantitative ...
A theoretical framework guides the research process like a roadmap for the study, so you need to get this right. Theoretical framework 1,2 is the structure that supports and describes a theory. A theory is a set of interrelated concepts and definitions that present a systematic view of phenomena by describing the relationship among the variables for explaining these phenomena.
It follows the rules that are established by probability. It's used a lot in sociology and language research. Examples of theoretical research. We talked about theoretical study methods in the previous part. We'll give you some examples to help you understand it better. Example 1: Theoretical research into the health benefits of hemp
A scientist gathering data for her research. Empirical research is research using empirical evidence.It is also a way of gaining knowledge by means of direct and indirect observation or experience. Empiricism values some research more than other kinds. Empirical evidence (the record of one's direct observations or experiences) can be analyzed quantitatively or qualitatively.
Page ID. Jenkins-Smith et al. University of Oklahoma via University of Oklahoma Libraries. This book is concerned with the connection between theoretical claims and empirical data. It is about using statistical modeling; in particular, the tool of regression analysis, which is used to develop and refine theories.
scientific theory. belief. empirical evidence, information gathered directly or indirectly through observation or experimentation that may be used to confirm or disconfirm a scientific theory or to help justify, or establish as reasonable, a person's belief in a given proposition. A belief may be said to be justified if there is sufficient ...
The authors will have collected data to answer a research question. Empirical research contains observed and measured examples that inform or answer the research question. The data can be collected in a variety of ways such as interviews, surveys, questionnaires, observations, and various other quantitative and qualitative research methods ...
Theoretical frameworks are defined, according to Anfara and Mertz, as "any empirical or quasi-empirical theory of social/ and/or psychological processes, at a variety of levels (e.g., grand, mid-range, explanatory), that can be applied to the understanding of the phenomena" (p. 15).
A study by Jensen and Lawson (2011) provides an example of how a theoretical framework connects different parts of the study. They compared undergraduate biology students in heterogeneous and homogeneous groups over the course of a semester. ... Standards for reporting on empirical social science research in AERA publications: American ...
Recipient financial need is a crucial factor in donation decisions. This study proposes a novel model for determining financial donations, incorporating consumption levels of both donor and recipient within a societal context. Solving our model's utility maximization problem reveals how consumption, donation, and savings are interlinked. Empirical evidence reinforces these findings, aligning ...
This systematic meta-analytic review investigated the relations between achievement goals and internalizing symptoms and disorders, namely, anxiety and depression. The number of samples for each focal relationship ranged from 3 to 36. The results indicated significant effect sizes for the relations between mastery-approach goals and anxiety (r = − .10) and depression (r = − .18), as well ...
In a sample of cognitively unimpaired old people (N = 1,189), three out of four subscales reached sufficient internal consistency, and the factorial structure of the 28-item questionnaire was confirmed using an exploratory factor analysis. In a sample of 245 people with Parkinson's disease, the four-factor structure could not be proven.
Comparative school-to-work research has long emphasised the role of institutions in shaping youth labour market integration. This paper provides an overview of this research stream, consisting of four main sections. The first section introduces a variety of labour market outcomes of young graduates within Europe and identifies country clusters with higher and lower outcomes; this empirical ...
For theories, good empirical referents help develop strong, testable ideas that can stand up to real-world testing. They help us investigate and improve theoretical concepts, building a solid body of knowledge. In practice, empirical referents make research findings more useful and relevant.
What kind of impact does the government's housing support expenditure have on residents' consumption? This is a topic that deserves in-depth study and is of practical significance. This study constructs provincial equilibrium panel data based on China's guaranteed housing construction and financial expenditures on housing support data from 1999-2009 and 2000-2021. It applies the ...