
An official website of the United States government
The .gov means it’s official. Federal government websites often end in .gov or .mil. Before sharing sensitive information, make sure you’re on a federal government site.
The site is secure. The https:// ensures that you are connecting to the official website and that any information you provide is encrypted and transmitted securely.
- Publications
- Account settings
Preview improvements coming to the PMC website in October 2024. Learn More or Try it out now .
- Advanced Search
- Journal List
- Taylor & Francis Open Select


Tobacco smoking: Health impact, prevalence, correlates and interventions
Robert west.
a Department of Behavioural Science and Health , University College London , London, UK
Background and objectives : Despite reductions in prevalence in recent years, tobacco smoking remains one of the main preventable causes of ill-health and premature death worldwide. This paper reviews the extent and nature of harms caused by smoking, the benefits of stopping, patterns of smoking, psychological, pharmacological and social factors that contribute to uptake and maintenance of smoking, the effectiveness of population and individual level interventions aimed at combatting tobacco smoking, and the effectiveness of methods used to reduce the harm caused by continued use of tobacco or nicotine in some form.
Results and conclusions : Smoking behaviour is maintained primarily by the positive and negative reinforcing properties of nicotine delivered rapidly in a way that is affordable and palatable, with the negative health consequences mostly being sufficiently uncertain and distant in time not to create sufficient immediate concern to deter the behaviour. Raising immediate concerns about smoking by tax increases, social marketing and brief advice from health professionals can increase the rate at which smokers try to stop. Providing behavioural and pharmacological support can improve the rate at which those quit attempts succeed. Implementing national programmes containing these components are effective in reducing tobacco smoking prevalence and reducing smoking-related death and disease.
Introduction
The continued popularity of tobacco smoking appears to defy rational explanation. Smokers mostly acknowledge the harm they are doing to themselves and many report that they do not enjoy it – yet they continue to smoke (Fidler & West, 2011 ; Ussher, Brown, Rajamanoharan, & West, 2014 ). The reason is that nicotine from cigarettes generates strong urges to smoke that undermine and overwhelm concerns about the negative consequences of smoking, and the resolve not to smoke in those trying to stop (West & Shiffman, 2016 ). Progress is being made in many countries in reducing smoking prevalence but it remains one of the main causes of ill health and premature death worldwide (Gowing et al., 2015 ).
This paper provides a broad overview of smoking in terms of: the health effects, benefits of stopping, prevalence and patterns of use, psychological, pharmacological and social factors leading to uptake and maintenance of the behaviour, effectiveness of population level and individual level interventions to combat it, and methods used to reduce the harm despite continued use of tobacco or nicotine.
Definitions of smoking and smoking cessation
Tobacco smoking consists of drawing into the mouth, and usually the lungs, smoke from burning tobacco (West & Shiffman, 2016 ). The type of product smoked is most commonly cigarettes, but can also include cigarillos, cigars, pipes or water pipes. ‘Smokeless’ tobacco is also popular in some parts of the world. This typically involves using tobacco preparations for chewing, sniffing into the nose or placing as a wad in the mouth between the cheeks and gums (Critchley & Unal, 2003 ). Smokeless tobacco use has features that are similar to smoking and can carry significant health risks (Critchley & Unal, 2003 ); however, this article focuses on smoked tobacco only as this has been the subject of by far the largest volume of research and is the most harmful form of tobacco use.
Stopping smoking usually involves an intention not to smoke any more cigarettes from a given point in time (a ‘quit attempt’), followed by self-conscious resistance of urges to smoke resulting in a period of abstinence. If someone making a quit attempt smokes one or more cigarettes on an occasion but then resumes abstinence, this is usually termed a ‘lapse’. If this person resumes smoking on a regular basis s/he is said to have ‘relapsed’. ‘Short-term abstinence’ is commonly defined in terms of achieving up to 4 weeks of abstinence. ‘Long-term abstinence’ often refers to abstinence for at least 6 months but more typically involves abstinence for at least 12 months. There is no agreed criterion for deciding when someone has ‘stopped smoking’ so it is essential when using the term to be clear about how long the abstinence period has been.
Health impact of smoking and the benefits of stopping
Tobacco smoking increases the risk of contracting a wide range of diseases, many of which are fatal. Stopping smoking at any age is beneficial compared with continuing to smoke. For some diseases, the risk can be reversed while for others the risk is approximately frozen at the point when smoking stopped.
Health impact of smoking
Table Table1 1 lists the main causes of death from smoking. Tobacco smoking is estimated to lead to the premature death of approximately 6 million people worldwide and 96,000 in the UK each year (Action on Smoking and Health, 2016b ; World Health Organization, 2013 ). A ‘premature death from smoking’ is defined as a death from a smoking-related disease in an individual who would otherwise have died later from another cause. On average, these premature deaths involve 10 years of life years lost (US Department of Health and Human Services, 2004 ). Many of these deaths occur in people who have stopped smoking but whose health has already been harmed by smoking. It also happens to be the case that smokers who do not stop smoking lose an average of 10 years of life expectancy compared with never-smokers and they start to suffer diseases of old age around 10 years earlier than non-smokers (Jha & Peto, 2014 ).
Most smoking-related deaths arise from cancers (mainly lung cancer), respiratory disease (mainly chronic obstructive pulmonary disease – COPD), and cardiovascular disease (mainly coronary heart disease) (Action on Smoking and Health, 2016b ). Smoking is an important risk factor for stroke, blindness, deafness, back pain, osteoporosis, and peripheral vascular disease (leading to amputation) (US Department of Health and Human Services, 2004 ). After the age of 40, smokers on average have higher levels of pain and disability than non-smokers (US Department of Health and Human Services, 2004 ).
Smoking in both women and men reduces fertility (Action on Smoking and Health, 2013 ). Smoking in pregnancy causes underdevelopment of the foetus and increases the risk of miscarriage, neonatal death, respiratory disease in the offspring, and is probably a cause of mental health problems in the offspring (Action on Smoking and Health, 2013 ).
People used to think that smoking was protective against Alzheimer’s disease but we now know that the opposite is the case: it is a major risk factor for both Alzheimer’s and vascular dementia (Ferri et al., 2011 ; US Department of Health and Human Services, 2004 ).
There is a positive association between average daily cigarette consumption and risk of smoking-related disease, but in the case of cardiovascular disease the association is non-linear, so that low levels of cigarette consumption carry a higher risk than would be expected from a simple linear relationship (US Department of Health and Human Services, 2004 ).
Tobacco smoke contains biologically significant concentrations of known carcinogens as well as many other toxic chemicals. Some of these, including a number of tobacco-specific nitrosamines (particularly NNK and NNN) are constituents of tobacco, largely as a result of the way it is processed, while others such as benzopyrine result from combustion of tobacco (Action on Smoking and Health, 2014b ). These chemicals form part of the particulate matter in smoke. Tobacco smoke also contains the gas, carbon monoxide (CO). CO is a potent toxin, displacing oxygen from haemoglobin molecules. However, acutely the amount of CO in tobacco smoke is too small to lead to hypoxia and the body produces increased numbers of red blood cells to compensate.
The nicotine in tobacco smoke may cause a small part of the increase in cardiovascular disease but none or almost none of the increase in risk of respiratory disease or cancer (Benowitz, 1997 , 1998 ). It is the other components of cigarette smoke that do almost all the damage. It has been proposed on the basis of studies with other species that nicotine damages the adolescent brain but there is no evidence for clinically significant deficits in cognition or emotion in adults who smoked during adolescence and then stopped (US Department of Health and Human Services, 2004 ).
Exposure to second-hand smoke carries a significant risk for both children and adults. Thus, non-smokers who are exposed to a smoky environment have an increased risk of cancer, heart disease and respiratory disease (Action on Smoking and Health, 2014a ).
Benefits of stopping smoking
Table Table1 1 lists the main benefits of stopping smoking. Smokers who stop before their mid-30s have approximately the same life expectancy as never smokers (Doll, Peto, Boreham, & Sutherland, 2004 ; Pirie, Peto, Reeves, Green, & Beral, 2013 ). After the age of 35 years or so, stopping smoking recovers 2–3 months of healthy life expectancy for every year of smoking avoided, or 4–6 h for every day (Jha & Peto, 2014 ).
Stopping smoking has different effects on different smoking-related diseases. Excess risk of heart attack caused by smoking reduces by 50% within 12 months of stopping smoking. Stopping smoking returns the rate of decline in lung function to the normal age-related decline, but does not reverse this; it reduces the frequency of ‘exacerbations’ (acute attacks of breathing difficulty resulting in death or hospitalisation) in COPD patients (US Surgeon General, 1990 ). Stopping smoking ‘freezes’ the risk of smoking-related cancers at the level experienced when stopping occurs but does not decrease it in absolute terms (US Surgeon General, 1990 ).
Smokers who stop show reduced levels of stress and mood disorder than those who continue (Royal College of Physicians and Royal College of Psychiatrists, 2013 ). They also report higher levels of happiness and life satisfaction than those who continue (Shahab & West, 2009 , 2012 ). This suggests that smoking may harm mental health, though other explanations cannot be ruled out on the current evidence.
Prevalence and patterns of smoking
Smoking prevalence.
There are estimated to be approximately 1 billion tobacco smokers worldwide (Eriksen, Mackay, & Ross, 2013 ), amounting to approximately 30% of men and 7% of women (Gowing et al., 2015 ).
Cigarette smoking prevalence in Great Britain was estimated to be 16.9% in 2015, the most recent year for which figures are available at the time of writing: slightly lower in women than men (Office of National Satistics, 2016 ). Smoking in Great Britain has declined by 0.7 percentage points per year since 2001 (from 26.9% of adults in 2001). In Australia, daily cigarette smoking has declined by 0.6 percentage points per year over a similar time period (from 22.4% of adults aged 18 + years in 2001 to 14.5% in 2015) (Australian Bureau of Statistics, 2015 ). However, international comparisons are confused by different countries using a different definition of what counts as being a smoker, and different methods for assessing prevalence. Australia only counts daily smokers in their headline figures. The situation in the US is even more misleading. The headline prevalence figure for the US is below 16%, but this does not include occasional smokers and people who smoke cigarillos which are essentially cigarettes in all but name and which have become increasingly popular in recent years. So the figure for prevalence that is most comparable to the figure for Great Britain is 20% (Jamal, 2016 ).
With the above caveats in mind, the figures in Table Table2 2 for smoking prevalence in world regions in men and women provide very broad estimates (Gowing et al., 2015 ). Most noteworthy is that smoking prevalence in men is more than four times that in women globally but that the difference is much less in most parts of Europe, and that Eastern Europe as a whole has the highest smoking prevalence of any region in the world.
Note: Current smoking of any tobacco product, adults aged 15 years and older, age-standardised rate, by gender. ‘Tobacco smoking’ includes cigarettes, cigars, pipes or any other smoked tobacco products. ‘Current smoking’ includes both daily and non-daily or occasional smoking. From Gowing et al. ( 2015 ).
Smoking patterns
The most common age of first trying a cigarette in countries that have been studied is 10–15 years (Action on Smoking and Health, 2015b ; Talip, Murang, Kifli, & Naing, 2016 ); take up of regular smoking usually continues up to early 20s (Dierker et al., 2008 ).
Average daily cigarette consumption among smokers in the US and UK has declined steadily since the 1970s. In the UK, it is currently 11 cigarettes per day, and non-daily smoking is very rare (Action on Smoking and Health, 2016c ; Jarvis, Giovino, O’Connor, Kozlowski, & Bernert, 2014 ). Smokers take in an average of 1–1.5 mg of nicotine per cigarette (US Department of Health Human Services, 2014 ). The US figures on patterns of smoking are distorted by not counting ‘cigarillos’ and other smoked tobacco products which are used very much like cigarettes, whose prevalence has increased in recent years (Jamal et al., 2015 ). The reduction in daily cigarette consumption has not been accompanied by a reduction in daily nicotine intake (Jarvis et al., 2014 ). This could be due to the use of other smoked tobacco products (in the case of the US) or smokers smoking their cigarettes more intensively (taking more, deeper or longer puffs).
Smokers in England spend an average of £23 per week on cigarettes and this figure is slowly rising (West & Brown, 2015 ). In the UK, hand-rolled cigarettes have become increasingly popular with 34% of smokers currently reporting use of these products (Action on Smoking and Health, 2016c ). Men and people in more deprived socio-economic groups are more likely to smoke hand-rolled cigarettes (Action on Smoking and Health, 2016c ).
In most countries, there are strong negative associations between smoking prevalence and educational level, affluence and mental health; and positive associations with alcohol use disorder and substance use disorder (Action on Smoking and Health, 2016a , 2016c ; Royal College of Physicians and Royal College of Psychiatrists, 2013 ; Talati, Keyes, & Hasin, 2016 ). In the UK, average daily cigarette consumption is higher for men than women, and higher in smokers in more deprived socio-economic groups and those with mental health problems (Action on Smoking and Health, 2016c ).
Psychological, pharmacological and social factors involved in smoking and smoking cessation
The natural history of smoking can be modelled as states and factors that influence the transition between these. Figure Figure1 1 shows transitions that have been researched – the variables identified in the diagram are listed descriptively without attempting to explain how they may be connected.

Factors associated with transitions in the natural history of smoking (parentheses indicate negative associations).
Smoking initiation
Important factors predicting initiation in western societies are: having friends who smoke, having parents who smoke, low social grade, tendency to mental health problems and impulsivity (Action on Smoking and Health, 2015b ). Transition to daily smoking follows a highly variable pattern sometimes being very rapid and sometimes taking several years (Schepis & Rao, 2005 ). Important factors predicting transition to regular smoking are: having friends who smoke, weak academic orientation, low parental support, pro-smoking attitudes, drinking alcohol and low socio-economic status (Action on Smoking and Health, 2015b ).
Smoking initiation has a ‘heritability’ (the proportion of variance in a characteristic that is attributable to genetic rather than environmental variance) of approximately 30–50% in western societies (Vink, Willemsen, & Boomsma, 2005 ). This means that differences in genetic make-up account for almost half of the difference in likelihood of starting smoking between individuals. This does not mean that environmental factors do not also play a crucial role as is evident from the very large decline in smoking initiation since the 1970s in many western countries.
The heritability of cigarette addiction (as distinct from smoking) is approximately 70–80% in western societies (Vink et al., 2005 ). Cigarette addiction here refers to the extent to which someone experiences a strong need to smoke. It is usually indexed by a combination of number of cigarettes per day and time from waking to smoking the first cigarette of the day (Kozlowski, Porter, Orleans, Pope, & Heatherton, 1994 ). It can also be indexed by the self-reported strength of urges to smoke (Fidler, Shahab, & West, 2011 ). Heritability of cigarette addiction, as indexed by failure of attempts to stop, is higher than the heritability for smoking and for initiation of smoking. This suggests that differences in genetic inheritance play a larger role in being able to stop smoking than in starting to smoke.
Cigarette addiction
Cigarette addiction stems from the fact that smoking provides highly controllable doses of the drug, nicotine, rapidly to the brain in a form that is accessible, affordable and palatable (West, 2009 ; West & Shiffman, 2016 ). Nicotine provided more slowly, for example by the nicotine transdermal patch, is much less addictive. It is possible that one or more mono-amine oxidase inhibitors in cigarette smoke add to, or synergise, the addictive properties of nicotine (Hogg, 2016 ).
The psychopharmacology of cigarette addiction is complex and far from fully understood. The following paragraphs summarise the current narrative.
Nicotine resembles the naturally occurring neurotransmitter, acetylcholine, sufficiently to attach itself to a subset of neuronal receptors for this neurotransmitter in the brain. These are called ‘nicotinic acetylcholine receptors’. When it does this with receptors in the ventral tegmental area in the midbrain, it causes an increased rate of firing of the nerves projecting forward from that area to another part of the brain called the nucleus accumbens. This causes release of another neurotransmitter called dopamine in the nucleus accumbens.
Dopamine release and uptake by neurones in the nucleus accumbens is believed to be central to all addictive behaviours. It acts as a neural ‘teaching signal’ which causes the brain to form an association between the current situation as perceived and the impulse to engage in whatever action immediately preceded this release. In the case of smoking, this creates an urge to smoke in situations in which smoking frequently occurs. These are often referred to as ‘cue-driven smoking urges’ or ‘situational cravings’ (West, 2009 ; West & Shiffman, 2016 ). This explains why even non-daily smokers often find it difficult to stop smoking altogether.
Repeated ingestion of nicotine from cigarettes causes changes to the functioning of the ventral tegmental area and nucleus accumbens such that when brain concentrations of nicotine are lower than usual, there is an abnormally low level of neural activity in these regions. This leads to feelings of need for behaviours that have in the past restored normal functioning, typically smoking. This feeling of need can be thought of as a kind of ‘nicotine hunger’, also called ‘background craving’ (West, 2009 ; West & Shiffman, 2016 ). This is probably why time between waking and first cigarette of the day is a useful predictor of difficulty stopping smoking (Vangeli, Stapleton, Smit, Borland, & West, 2011 ). So ‘cue-driven smoking urges’ and ‘nicotine hunger’ are important factors contributing to smoking behaviour and thought to be the primary mechanisms underpinning cigarette addiction (West, 2009 ; West & Shiffman, 2016 ).
When smokers abstain from cigarettes, within a few hours many of them start to experience nicotine withdrawal symptoms. Withdrawal symptoms from a drug are temporary symptoms that arise when the drug dose is reduced or use is terminated. They arise from neural adaptation to the presence of the drug in the central nervous system. For smoking, the most common early onset symptoms are: irritability, restlessness and difficult concentrating. Depression and anxiety have also been observed in some smokers. These symptoms typically last 1 to 4 weeks (West, 2009 ; West & Shiffman, 2016 ).
After a day or two of stopping smoking, many smokers experience other symptoms: increased appetite, constipation, mouth ulcers, cough, and weight gain. Increased appetite tends to last for at least 3 months; weight gain (averaging around 6 kg) tends to be permanent; other symptoms tend to last a few weeks. The increased appetite, weight gain and constipation arise from termination of nicotine intake but the others are probably related to other effects of stopping smoking (West, 2009 ; West & Shiffman, 2016 ).
Any of the above effects of abstinence may in individual cases promote resumption of smoking following a quit attempt but statistically the association is inconsistent and weak; the main factors driving relapse appear to be cue-driven smoking urges and nicotine hunger (Fidler & West, 2011 ; West, 2009 ; West & Shiffman, 2016 ).
Many smokers report that smoking helps them cope with stress and increases their ability to concentrate. However, this appears to be because when they go for a period without smoking they experience nicotine withdrawal symptoms that are relieved by smoking. Long-term smokers who stop report lower levels of stress than when they were smoking and no reduction in ability to concentrate (West, 2009 ; West & Shiffman, 2016 ).
It is commonly thought that smokers with mental health problems are using cigarettes to ‘self-medicate’ or treat their psychological symptoms. However, the evidence indicates that neither nicotine nor smoking improves psychological symptoms, and people with serious mental health disorders who stop smoking do not experience a worsening of mental health. In fact some studies have found an improvement (Royal College of Physicians and Royal College of Psychiatrists, 2013 ).
Smoking cessation
For most smokers, cessation requires a determined attempt to stop and then sufficient resolve in the following weeks and months to overcome what are often powerful urges to smoke. Factors that predict quit attempts differ from those that predict the success of those attempts (Vangeli et al., 2011 ). Approximately 5% of unaided quit attempts succeed for at least 6 months (Hughes, Keely, & Naud, 2004 ). Relapse after this point is estimated to be around 50% over subsequent years (Stapleton & West, 2012 ).
The most common self-reported reasons for smoking are stress relief and enjoyment, with around half of smokers reporting these smoking motives. Weight control, aiding concentration and socialising are also quite commonly cited (Fidler & West, 2009 ). Smoking for supposed stress relief, improved concentration, weight control or other functions has not been found to be related to attempts to stop or success of attempts to stop (Fidler & West, 2009 ). Smokers who report enjoying smoking are less likely to try to stop but not less likely to succeed if they do try (Fidler & West, 2011 ). In addition, having a positive smoker identity (liking being a smoker) predicts not trying to quit, over and above enjoyment of smoking (Fidler & West, 2009 ).
No clear association has been found between the number of times smokers have tried to stop in the past and their chances of success the next time they try (Vangeli et al., 2011 ). However, having tried to stop in the past few months is predictive of failure of the next quit attempt (Zhou et al., 2009 ). Belief in the harm caused by smoking is predictive of smokers making quit attempts but not the success of those attempts (Vangeli et al., 2011 ).
Some clinical studies have found that women were less likely to succeed in quit attempts than men but large population studies have found no difference in success rates between the genders (Vangeli et al., 2011 ) so it may be the case that women who seek help with stopping have greater difficulty than men who seek help with stopping.
Number of cigarettes smoked per day, time between waking and the first cigarette of the day and rated strength of urges to smoke prior to a quit attempt have been found to predict success of quit attempts (Vangeli et al., 2011 ).
Quit attempts that involve gradual reduction are less likely to succeed than those that involve quitting abruptly, even after controlling statistically for measures of cigarette addiction, confidence in quitting, other methods used to quit (e.g. nicotine replacement therapy) and sociodemographic factors (Lindson-Hawley et al., 2016 ).
Interventions to combat smoking
There is extensive evidence on interventions that can reduce smoking prevalence, either by reducing initiation or promoting cessation. Table Table3 3 lists those that have the strongest evidence.
Population-level interventions
Increasing the financial cost of smoking through tax increases and control of illicit supply on average reduces overall consumption with a typical price elasticity globally of 0.4 (meaning that for every 10% increase in the real cost there is a 4% decrease in the number of cigarettes purchased). Most of the effect is in getting smokers to reduce their daily cigarette consumption so the effect on smoking prevalence has been found to be an average of a 1–2 percentage point prevalence reduction for every 10% increase in the real cost (Levy, Huang, Havumaki, & Meza, 2016 ). It has been claimed that increasing taxes on tobacco increases the amount of smuggling of cheap tobacco, but the evidence does not support this (Action on Smoking and Health, 2015a ; Joossens & Raw, 2003 ).
Social marketing campaigns (e.g. TV advertising) can prevent smoking uptake, increase the rate at which smokers try to quit and improve the chances of success. This can lead to a reduction in smoking prevalence. Their effectiveness varies considerably with intensity, type of campaign and context (Bala, Strzeszynski, Topor-Madry, & Cahill, 2013 ; Hoffman & Tan, 2015 ).
Legislating to ban smoking in all indoor public areas may have a one-off effect on reducing smoking prevalence but findings are inconsistent across different countries (Bala et al., 2013 ). For example, in countries such as France it was not possible to detect an effect while in England, there did appear to be a decline in prevalence following the ban.
Although it is hard to show conclusively, circumstantial evidence suggests that banning tobacco advertising and putting large graphic health warnings on cigarette packets may have reduced smoking prevalence in some countries (Hoffman & Tan, 2015 ; Noar et al., 2016 ).
Individual-level interventions to promote smoking cessation
Brief advice.
Brief advice to stop smoking from a physician and offer of support to all smokers, regardless of motivation to quit, has been found in randomised trials to increase rate of quitting by an average of 2 percentage points of all those receiving it, whether or not they were initially interested in quitting (Stead et al., 2013 ). The offer of support appears to be more effective in getting smokers to try to quit than just advising smokers to stop (Aveyard, Begh, Parsons, & West, 2012 ).
Pharmacotherapy
Using a form of nicotine replacement therapy (NRT: transdermal patch, chewing gum, nasal spray, mouth spray, lozenge, inhalator, dissolvable strip) for at least 6 weeks from the start of a quit attempt increases the chances of long-term success of that quit attempt by about 3–7 percentage points if the user is under the care of a health professional or provided as part of a structured support programme (Stead et al., 2012 ). Some studies have found that NRT when bought from a shop and used without any additional structured support does not improve the chances of success at stopping (Kotz, Brown, & West, 2014a , 2014b ). A small proportion of people who use NRT to stop smoking continue to use it for months or even years after stopping smoking, but NRT appears to carry minimal risk to long-term users (Royal College of Physicians, 2016 ; Stead et al., 2012 ).
Data are sparse but at present, using an electronic cigarette in a quit attempt appears to increase the chances of success at stopping on average by an amount broadly similar to that from NRT; the variety of products available and the greater similarity to smoking appear to make them more attractive to many smokers as a means of stopping than NRT (McNeill et al., 2015 ; Royal College of Physicians, 2016 ). Electronic cigarettes deliver nicotine to users by heating a liquid containing nicotine, propylene glycol or glycerol and usually flavourings to create a vapour that is inhaled. They appear to carry minimal acute risk to users. If they are used long-term, their risk is almost certainly much less than that of smoking (based on concentrations of chemicals in the vapour) (McNeill et al., 2015 ; Royal College of Physicians, 2016 ).
‘Dual-form NRT’ (combining a transdermal NRT patch and one of the other forms) increases the chances of success at stopping more than ‘single-form NRT’ (just using one of the products) (Stead et al., 2012 ). Starting to use a nicotine transdermal patch several weeks before the target quit date may improve the chances of success at quitting compared with starting on the quit date (Stead et al., 2012 ).
Taking the prescription anti-depressant, bupropion (brand name Zyban), improves the chances of success of quit attempts by a similar amount to single-form NRT (Hughes, Stead, Hartmann-Boyce, Cahill, & Lancaster, 2014 ). Bupropion often leads to sleep disturbance and carries a very small risk of seizure. Bupropion probably works by reducing urges to smoke rather than any effect on depressed mood, but how it does this is not known. It is contra-indicated in pregnant smokers and people with an elevated seizure risk or history of eating disorder (Hughes et al, 2014 ). Taking the tricyclic anti-depressant, nortriptyline also improves the chances of success of quit attempts, probably by about the same amount as bupropion and NRT (Hughes et al., 2014 ). Its mechanism of action is not known. Nortriptyline often leads to dry mouth and sleep disorder and can be fatal in overdose (Hughes et al., 2014 ).
Taking the nicotinic-acetylcholine receptor partial agonist, varenicline (brand name Chantix in the US and Champix elsewhere), improves the chances of success by about 50% more than bupropion or single-form NRT (Cahill, Lindson-Hawley, Thomas, Fanshawe, & Lancaster, 2016 ). This is true for smokers with or without a psychiatric disorder (Anthenelli et al., 2016 ). Varenicline appears to work both by reducing urges to smoke and the rewarding effect of nicotine should a lapse occur (West, Baker, Cappelleri, & Bushmakin, 2008 ). Varenicline often leads to sleep disturbance and nausea. Serious neuropsychiatric and cardiovascular adverse reactions have been reported, but in comparative studies these have not been found to be more common than placebo or NRT (Anthenelli et al., 2016 ; Cahill et al., 2016 ; Sterling, Windle, Filion, Touma, & Eisenberg, 2016 ).
Taking the nicotinic-acetylcholine receptor partial agonist, cytisine, appears to improve the chances of success at least as much as single-form NRT and probably more (Cahill et al., 2016 ). Cytisine often causes nausea. No serious adverse reactions have been reported to date (Cahill et al., 2016 ). Where it is licensed for sale, cytisine is less than 1/10th the cost of other smoking cessation medications (Cahill et al., 2016 ).
Behavioural support
There is good evidence that behavioural interventions of many kinds, delivered though several modalities can help smokers to stop. Thus, behavioural support (encouragement, advice and discussion) from a trained stop-smoking specialist, provided at least weekly until at least 4 weeks following the target quit date can increase the chances of long-term success of a quit attempt by about 3–7 percentage points, whether it is given by phone or face-to-face (Lancaster & Stead, 2005 ). Group behavioural support (specialist-led groups of smokers stopping together and engaging in a structured discussion about their experiences), involving at least weekly sessions lasting until at least 4 weeks after the target quit date can increase the chances of success of a quit attempt by a similar amount or possibly more than individual support (Stead & Lancaster, 2005 ). Scheduled, multi-session telephone support can improve rates of success at stopping smoking by a broadly similar amount (Stead, Hartmann-Boyce, Perera, & Lancaster, 2013 ) but some large studies have failed to detect an effect so contextual factors and/or the precise type of support could be crucial to success. The effects of behavioural support and medication/NRT on success at stopping smoking appear to combine roughly additively (Stead, Koilpillai, & Lancaster, 2015 ). Smoking cessation support appears to be effective in primary care, secondary care and worksite settings (Cahill & Lancaster, 2014 ; West et al., 2015 ). Financial incentives, in the form of vouchers, have been found to increase smoking cessation rates for as long as they are in place (Cahill, Hartmann-Boyce, & Perera, 2015 ; Higgins & Solomon, 2016 ). Printed self-help materials can improve the chances of success at stopping long term by around 1–2 percentage points (Hartmann-Boyce, Lancaster, & Stead, 2014 ).
There is still relatively limited evidence on the effectiveness of digital support interventions for smoking cessation. Thus, while there is evidence that tailored, interactive websites can improve the chances of success at stopping smoking compared with no support, brief written materials or static information websites, many of those tested have not been found to be effective and it is not clear what differentiates those that are effective from those that are not (Graham et al., 2016 ). Text messaging programmes have been found to increase the chances of success of quit attempts by about 2–7 percentage points (Whittaker, McRobbie, Bullen, Rodgers, & Gu, 2016 ). There is currently insufficient evidence to know whether smartphone applications can improve success rates of quit attempts, although preliminary data suggest that they might (Whittaker et al., 2016 ). Evidence on alternative and complementary therapies is not sufficient to make confident statements about their effectiveness as aids to smoking cessation (Barnes et al., 2010 ; White, Rampes, Liu, Stead, & Campbell, 2014 ).
Overall, the highest smoking cessation rates appear to be achieved using specialist face-to-face behavioural support together with either varenicline or dual form NRT. With this support, continuous abstinence rates up to 52 weeks, verified by expired-air carbon monoxide tests, of more than 40% have been achieved (Kralikova et al., 2013 ). More commonly, 52-week continuous abstinence rates with this treatment are between 15 and 25% (West et al., 2015 ).
Smoking cessation support for pregnant smokers
In pregnant smokers, there is some evidence that NRT can help promote smoking cessation but evidence for an effect sustained to end of pregnancy is not conclusive (Sterling et al., 2016 ). There is also evidence that written self-help materials and face-to-face behavioural support can aid smoking cessation (Jones, Lewis, Parrott, Wormall, & Coleman, 2016 ), and financial incentives have also been found to improve quitting rates among pregnant smokers (Tappin et al., 2015 ). Almost half of women who stop smoking during pregnancy as a result of a clinical intervention relapse to smoking within 6 months of the birth (Jones et al., 2016 ).
Effectiveness of programmes to reduce smoking uptake
School-based programmes that involve both social competence training and peer-led social influence have been found to reduce smoking uptake (Georgie, Sean, Deborah, Matthew, & Rona, 2016 ) but educational programmes have not (Thomas, McLellan, & Perera, 2013 ). Mass media campaigns and increasing the financial cost of smoking reduce smoking uptake (Brinn, Carson, Esterman, Chang, & Smith, 2012 ; van Hasselt et al., 2015 ).
Reducing the harm from tobacco and nicotine use
Smokers who report that they are reducing their cigarette consumption smoke only 1–2 fewer cigarettes per day on average than when they say they are not (Beard et al., 2013 ). Clinical trials have found that use of NRT while smoking can substantially reduce cigarette consumption compared with placebo (Royal College of Physicians, 2016 ) but national surveys show very little reduction in cigarette consumption when smokers take up use of NRT in real-world settings (Beard et al., 2013 ). The benefit from using NRT while continuing to smoke appears to be in promoting subsequent smoking cessation. Using NRT (or varenicline) to reduce cigarette smoking with no immediate plans to quit leads to increased rates of quitting subsequently (Wu, Sun, He, & Zeng, 2015 ).
‘Snus’, a form of tobacco that is placed between the gums and the cheek and which is prepared in a way that is very low in carcinogens, gives high doses of nicotine but without evidence of an increase in risk of major tobacco-related cancers and either no, or a small, increase in risk of heart disease. It does appear to increase risk of periodontal disease, however. Snus is very popular in Sweden. Sweden has very low rates of smoking and tobacco-related disease indicating that a form of nicotine intake other than smoking can become popular and suggesting that this can contribute to a substantial reduction in tobacco-related harm (Royal College of Physicians, 2016 ).
The introduction of complete bans on smoking in indoor public areas can also be considered as a harm reduction measure. In this case, the main issue is harm to non-tobacco users. The evidence shows that such bans have been rapidly followed in the UK and several other jurisdictions by a reduction in heart attacks in non-smokers (Action on Smoking and Health, 2014a ).
Conclusions
Tobacco smoking causes death and disability on a huge scale and only about half of smokers report enjoying it. Despite this, approximately 1 billion adults engage in this behaviour worldwide and only around 5% of unaided quit attempts succeed for 6 months or more. The main reason appears to be that cigarettes deliver nicotine rapidly to the brain in a form that is convenient, and palatable. Nicotine acts on the brain to create urges to smoke in situations where smoking would normally occur and when brain nicotine levels become depleted. Concern about the harm from, and financial cost of, smoking are mostly not sufficient to counter this.
Governments can reduce smoking prevalence by raising the cost of smoking through taxation, mounting sustained social marketing campaigns, ensuring that health professionals routinely advise smokers to stop and offer support for quitting, and make available pharmacological and behavioural support for stopping.
Statement of competing interests
RW has, within the past 3 years, undertaken research and consultancy for companies that develop and manufacture smoking cessation medications (Pfizer, GSK, and J&J). He is an unpaid advisor to the UK’s National Centre for Smoking cessation and Training. His salary is funded by Cancer Research UK.
Disclosure statement
No potential conflict of interest was reported by the author.
This work was supported by Cancer Research UK [grant number C1417/A22962].
- Action on Smoking and Health (2013). Smoking and reproduction . London: ASH; Retrieved from http://www.ash.org.uk/files/documents/ASH_112.pdf [ Google Scholar ]
- Action on Smoking and Health (2014a). Secondhand smoke . London: ASH; Retrieved from http://www.ash.org.uk/files/documents/ASH_113.pdf [ Google Scholar ]
- Action on Smoking and Health (2014b). What’s in a cigarette? London: ASH; Available from http://www.ash.org.uk/files/documents/ASH_117.pdf [ Google Scholar ]
- Action on Smoking and Health (2015a). Illicit trade in tobacco . London: ASH; Available from http://www.ash.org.uk/files/documents/ASH_122.pdf [ Google Scholar ]
- Action on Smoking and Health (2015b). Young people and smoking . London: ASH; Retrieve from http://www.ash.org.uk/files/documents/ASH_108.pdf [ Google Scholar ]
- Action on Smoking and Health (2016a). Smoking and mental health . London: ASH; Retrieved from http://www.ash.org.uk/files/documents/ASH_120.pdf [ Google Scholar ]
- Action on Smoking and Health (2016b). Smoking statistics: Illness and death . London: ASH; Retrieved from http://www.ash.org.uk/files/documents/ASH_107.pdf [ Google Scholar ]
- Action on Smoking and Health (2016c). Smoking statistics: Who smokes and how much? London: ASH; Retrieved from http://www.ash.org.uk/files/documents/ASH_106.pdf [ Google Scholar ]
- Anthenelli R. M., Benowitz N. L., West R., St Aubin L., McRae T., Lawrence D., & Evins A. E. (2016). Neuropsychiatric safety and efficacy of varenicline, bupropion, and nicotine patch in smokers with and without psychiatric disorders (EAGLES): A double-blind, randomised, placebo-controlled clinical trial . The Lancet , , 2507–2520. doi: 10.1016/s0140-6736(16)30272-0 [ PubMed ] [ CrossRef ] [ Google Scholar ]
- Australian Bureau of Statistics (2015). National health survey . Canberra: Author. [ Google Scholar ]
- Aveyard P., Begh R., Parsons A., & West R. (2012). Brief opportunistic smoking cessation interventions: a systematic review and meta-analysis to compare advice to quit and offer of assistance . Addiction , , 1066–1073. doi: 10.1111/j.1360-0443.2011.03770.x [ PubMed ] [ CrossRef ] [ Google Scholar ]
- Bala M. M., Strzeszynski L., Topor-Madry R., & Cahill K (2013). Mass media interventions for smoking cessation in adults . Cochrane Database of Systematic Reviews , , Cd004704. doi: 10.1002/14651858.CD004704.pub3 [ PubMed ] [ CrossRef ] [ Google Scholar ]
- Barnes J., Dong C. Y., McRobbie H., Walker N., Mehta M., & Stead L. F. (2010). Hypnotherapy for smoking cessation . Cochrane Database of Systematic Reviews , , Cd001008. doi: 10.1002/14651858.CD001008.pub2 [ PubMed ] [ CrossRef ] [ Google Scholar ]
- Beard E., McNeill A., Aveyard P., Fidler J., Michie S., & West R. (2013). Association between use of nicotine replacement therapy for harm reduction and smoking cessation: A prospective study of English smokers . Tobacco Control , , 118–122. 10.1136/tobaccocontrol-2011-050007 [ PubMed ] [ CrossRef ] [ Google Scholar ]
- Benowitz N. L. (1997). The role of nicotine in smoking-related cardiovascular disease . Preventive Medicine , , 412–417. 10.1006/pmed.1997.0175 [ PubMed ] [ CrossRef ] [ Google Scholar ]
- Benowitz N. L. (1998). Nicotine safety and toxicity . Oxford: Oxford University Press. [ Google Scholar ]
- Brinn M. P., Carson K. V., Esterman A. J., Chang A. B., & Smith B. J. (2012). Cochrane review: Mass media interventions for preventing smoking in young people . Evidence-based Child Health: A Cochrane Review Journal , , 86–144. 10.1002/ebch.v7.1 [ PubMed ] [ CrossRef ] [ Google Scholar ]
- Cahill K., Hartmann-Boyce J., & Perera R (2015). Incentives for smoking cessation . Cochrane Database of Systematic Reviews , , Cd004307. doi: 10.1002/14651858.CD004307.pub5 [ PubMed ] [ CrossRef ] [ Google Scholar ]
- Cahill K., & Lancaster T (2014). Workplace interventions for smoking cessation . Cochrane Database of Systematic Reviews , , Cd003440. doi: 10.1002/14651858.CD003440.pub4 [ PubMed ] [ CrossRef ] [ Google Scholar ]
- Cahill K., Lindson-Hawley N., Thomas K. H., Fanshawe T. R., & Lancaster T (2016). Nicotine receptor partial agonists for smoking cessation . Cochrane Database of Systematic Reviews , , Cd006103. doi: 10.1002/14651858.CD006103.pub7 [ PMC free article ] [ PubMed ] [ CrossRef ] [ Google Scholar ]
- Critchley J., & Unal B. (2003). Health effects associated with smokeless tobacco: A systematic review . Thorax , , 435–443. 10.1136/thorax.58.5.435 [ PMC free article ] [ PubMed ] [ CrossRef ] [ Google Scholar ]
- Dierker L., He J., Kalaydjian A., Swendsen J., Degenhardt L., Glantz M., & Merikangas K. (2008). The importance of timing of transitions for risk of regular smoking and nicotine dependence . Annals of Behavioral Medicine , , 87–92. doi: 10.1007/s12160-008-9051-x [ PMC free article ] [ PubMed ] [ CrossRef ] [ Google Scholar ]
- Doll R., Peto R., Boreham J., & Sutherland I. (2004). Mortality in relation to smoking: 50 years’ observations on male British doctors . British Medical Journal , , 1519. doi: 10.1136/bmj.38142.554479.AE [ PMC free article ] [ PubMed ] [ CrossRef ] [ Google Scholar ]
- Eriksen M., Mackay J., & Ross H (2013). The tobacco atlas . New York, NY: American Cancer Society. [ Google Scholar ]
- Ferri C. P., West R., Moriyama T. S., Acosta D., Guerra M., Huang Y., … Prince M. J. (2011). Tobacco use and dementia: Evidence from the 1066 dementia population-based surveys in Latin America, China and India . International Journal of Geriatric Psychiatry , , 1177–1185. doi: 10.1002/gps.2661 [ PubMed ] [ CrossRef ] [ Google Scholar ]
- Fidler J., Shahab L., & West R. (2011). Strength of urges to smoke as a measure of severity of cigarette dependence: Comparison with the fagerström test for nicotine dependence and its components . Addiction , , 631–638. 10.1111/add.2011.106.issue-3 [ PubMed ] [ CrossRef ] [ Google Scholar ]
- Fidler J., & West R. (2009). Self-perceived smoking motives and their correlates in a general population sample . Nicotine & Tobacco Research , , 1182–1188. doi: 10.1093/ntr/ntp120 [ PubMed ] [ CrossRef ] [ Google Scholar ]
- Fidler J., & West R. (2011). Enjoyment of smoking and urges to smoke as predictors of attempts and success of attempts to stop smoking: A longitudinal study . Drug and Alcohol Dependence , , 30–34. doi: 10.1016/j.drugalcdep.2010.10.009 [ PubMed ] [ CrossRef ] [ Google Scholar ]
- Georgie J. M., Sean H., Deborah M. C., Matthew H., & Rona C. (2016). Peer-led interventions to prevent tobacco, alcohol and/or drug use among young people aged 11–21 years: a systematic review and meta-analysis . Addiction , , 391–407. doi: 10.1111/add.13224 [ PMC free article ] [ PubMed ] [ CrossRef ] [ Google Scholar ]
- Gowing L. R., Ali R. L., Allsop S., Marsden J., Turf E. E., West R., & Witton J. (2015). Global statistics on addictive behaviours: 2014 status report . Addiction , , 904–919. doi: 10.1111/add.12899 [ PubMed ] [ CrossRef ] [ Google Scholar ]
- Graham A. L., Carpenter K. M., Cha S., Cole S., Jacobs M. A., Raskob M., & Cole-Lewis H. (2016). Systematic review and meta-analysis of internet interventions for smoking cessation among adults . Substance Abuse and Rehabilitation , , 55–69. doi: 10.2147/sar.s101660 [ PMC free article ] [ PubMed ] [ CrossRef ] [ Google Scholar ]
- Hartmann-Boyce J., Lancaster T., & Stead L. F (2014). Print-based self-help interventions for smoking cessation . Cochrane Database of Systematic Reviews , , Cd001118. doi: 10.1002/14651858.CD001118.pub3 [ PubMed ] [ CrossRef ] [ Google Scholar ]
- Higgins S. T., & Solomon L. J. (2016). Some recent developments on financial incentives for smoking cessation among pregnant and newly postpartum women . Current Addiction Reports , , 9–18. doi: 10.1007/s40429-016-0092-0 [ PMC free article ] [ PubMed ] [ CrossRef ] [ Google Scholar ]
- Hoffman S. J., & Tan C. (2015). Overview of systematic reviews on the health-related effects of government tobacco control policies . BMC Public Health , , 744. doi: 10.1186/s12889-015-2041-6 [ PMC free article ] [ PubMed ] [ CrossRef ] [ Google Scholar ]
- Hogg R. C. (2016). Contribution of monoamine oxidase inhibition to tobacco dependence: A review of the evidence . Nicotine & Tobacco Research , , 509–523. doi: 10.1093/ntr/ntv245 [ PubMed ] [ CrossRef ] [ Google Scholar ]
- Hughes J. R., Keely J., & Naud S. (2004). Shape of the relapse curve and long-term abstinence among untreated smokers . Addiction , , 29–38. 10.1111/add.2004.99.issue-1 [ PubMed ] [ CrossRef ] [ Google Scholar ]
- Hughes J. R., Stead L. F., Hartmann-Boyce J., Cahill K., & Lancaster T (2014). Antidepressants for smoking cessation . Cochrane Database of Systematic Reviews , , Cd000031. doi: 10.1002/14651858.CD000031.pub4 [ PMC free article ] [ PubMed ] [ CrossRef ] [ Google Scholar ]
- Jamal A. (2016). Current cigarette smoking among adults – United States, 2005–2015 . Morbidity and Mortality Weekly Report , , 1205–1211 [ PubMed ] [ Google Scholar ]
- Jamal A., Homa D., O’Connor E., Babb S., Caraballo R., Singh T., & King B. (2015). Current cigarette smoking among adults – United States, 2005–2014 . Morbidity and Mortality Weekly Report , , 1233–1240. 10.15585/mmwr.mm6444a2 [ PubMed ] [ CrossRef ] [ Google Scholar ]
- Jarvis M. J., Giovino G. A., O’Connor R. J., Kozlowski L. T., & Bernert J. T. (2014). Variation in nicotine intake among U.S. cigarette smokers during the past 25 years: Evidence from NHANES surveys . Nicotine & Tobacco Research , , 1620–1628. doi: 10.1093/ntr/ntu120 [ PubMed ] [ CrossRef ] [ Google Scholar ]
- Jha P., & Peto R. (2014). Global effects of smoking, of quitting, and of taxing tobacco . New England Journal of Medicine , , 60–68. doi: 10.1056/NEJMra1308383 [ PubMed ] [ CrossRef ] [ Google Scholar ]
- Jones M., Lewis S., Parrott S., Wormall S., & Coleman T. (2016). Re-starting smoking in the postpartum period after receiving a smoking cessation intervention: A systematic review . Addiction , , 981–990. doi: 10.1111/add.13309 [ PMC free article ] [ PubMed ] [ CrossRef ] [ Google Scholar ]
- Joossens L., & Raw M. (2003). Turning off the tap: The real solution to cigarette smuggling . International Journal of Tuberculosis and Lung Disease , , 214–222. [ PubMed ] [ Google Scholar ]
- Kotz D., Brown J., & West R. (2014a). Prospective cohort study of the effectiveness of smoking cessation treatments used in the “real world” . Mayo Clinic Proceedings , , 1360–1367. doi: 10.1016/j.mayocp.2014.07.004 [ PMC free article ] [ PubMed ] [ CrossRef ] [ Google Scholar ]
- Kotz D., Brown J., & West R. (2014b). ‘Real-world’ effectiveness of smoking cessation treatments: A population study . Addiction , , 491–499. doi: 10.1111/add.12429 [ PubMed ] [ CrossRef ] [ Google Scholar ]
- Kozlowski L. T., Porter C. Q., Orleans C. T., Pope M. A., & Heatherton T. (1994). Predicting smoking cessation with self-reported measures of nicotine dependence: FTQ, FTND, and HSI . Drug and Alcohol Dependence , , 211–216. 10.1016/0376-8716(94)90158-9 [ PubMed ] [ CrossRef ] [ Google Scholar ]
- Kralikova E., Kmetova A., Stepankova L., Zvolska K., Davis R., & West R. (2013). Fifty-two-week continuous abstinence rates of smokers being treated with varenicline versus nicotine replacement therapy . Addiction , , 1497–1502. doi: 10.1111/add.12219 [ PubMed ] [ CrossRef ] [ Google Scholar ]
- Lancaster T., & Stead L. F (2005). Individual behavioural counselling for smoking cessation . Cochrane Database of Systematic Reviews , , Cd001292. doi: 10.1002/14651858.CD001292.pub2 [ PubMed ] [ CrossRef ] [ Google Scholar ]
- Levy D. T., Huang A. T., Havumaki J. S., & Meza R. (2016). The role of public policies in reducing smoking prevalence: Results from the Michigan SimSmoke tobacco policy simulation model . Cancer Causes and Control , , 615–625. doi: 10.1007/s10552-016-0735-4 [ PMC free article ] [ PubMed ] [ CrossRef ] [ Google Scholar ]
- Lindson-Hawley N., Banting M., West R., Michie S., Shinkins B., & Aveyard P. (2016). Gradual versus abrupt smoking cessation: A randomized, controlled noninferiority trial . Annals of Internal Medicine , , 585–592. doi: 10.7326/m14-2805 [ PubMed ] [ CrossRef ] [ Google Scholar ]
- McNeill A., Brose L., Calder R., Hitchman S., Hajek P., & McRobbie H. (2015). E-cigarettes: An evidence update. A report commissioned by Public Health England . London: Public Health England. [ Google Scholar ]
- Noar S. M., Francis D. B., Bridges C., Sontag J. M., Ribisl K. M., & Brewer N. T. (2016). The impact of strengthening cigarette pack warnings: Systematic review of longitudinal observational studies . Social Science & Medicine , , 118–129. doi: 10.1016/j.socscimed.2016.06.011 [ PMC free article ] [ PubMed ] [ CrossRef ] [ Google Scholar ]
- Office of National Satistics (2016). Smoking prevalence in Great Britain . London: ONS; Retrieved from https://www.ons.gov.uk/peoplepopulationandcommunity/healthandsocialcare/drugusealcoholandsmoking/datasets/adultsmokinghabitsingreatbritain [ Google Scholar ]
- Royal College of Physicians and Royal College of Psychiatrists (2013). Smoking and mental health . London: RCP. [ Google Scholar ]
- Pirie K., Peto R., Reeves G. K., Green J., & Beral V. (2013). The 21st century hazards of smoking and benefits of stopping: A prospective study of one million women in the UK . Lancet , , 133–141. doi: 10.1016/s0140-6736(12)61720-6 [ PMC free article ] [ PubMed ] [ CrossRef ] [ Google Scholar ]
- Royal College of Physicians. (2016). Nicotine without smoke: Tobacco harm reduction . London: RCP. [ Google Scholar ]
- Schepis T. S., & Rao U. (2005). Epidemiology and etiology of adolescent smoking . Current Opinion in Pediatrics , , 607–612. 10.1097/01.mop.0000176442.49743.31 [ PubMed ] [ CrossRef ] [ Google Scholar ]
- Shahab L., & West R. (2009). Do ex-smokers report feeling happier following cessation? Evidence from a cross-sectional survey . Nicotine & Tobacco Research , , 553–557. doi: 10.1093/ntr/ntp031 [ PubMed ] [ CrossRef ] [ Google Scholar ]
- Shahab L., & West R. (2012). Differences in happiness between smokers, ex-smokers and never smokers: cross-sectional findings from a national household survey . Drug and Alcohol Dependence , , 38–44. doi: 10.1016/j.drugalcdep.2011.08.011 [ PubMed ] [ CrossRef ] [ Google Scholar ]
- Stapleton J. A., & West R. (2012). A direct method and ICER tables for the estimation of the cost-effectiveness of smoking cessation interventions in general populations: application to a new cytisine trial and other examples . Nicotine & Tobacco Research , , 463–471. doi: 10.1093/ntr/ntr236 [ PubMed ] [ CrossRef ] [ Google Scholar ]
- Stead L. F., Buitrago D., Preciado N., Sanchez G., Hartmann-Boyce J., & Lancaster T (2013). Physician advice for smoking cessation . Cochrane Database of Systematic Reviews , , Cd000165. doi: 10.1002/14651858.CD000165.pub4 [ PMC free article ] [ PubMed ] [ CrossRef ] [ Google Scholar ]
- Stead L. F., Hartmann-Boyce J., Perera R., & Lancaster T (2013). Telephone counselling for smoking cessation . Cochrane Database of Systematic Reviews , , Cd002850. doi: 10.1002/14651858.CD002850.pub3 [ PubMed ] [ CrossRef ] [ Google Scholar ]
- Stead L. F., Koilpillai P., & Lancaster T (2015). Additional behavioural support as an adjunct to pharmacotherapy for smoking cessation . Cochrane Database of Systematic Reviews , , Cd009670. doi: 10.1002/14651858.CD009670.pub3 [ PubMed ] [ CrossRef ] [ Google Scholar ]
- Stead L. F., & Lancaster T (2005). Group behaviour therapy programmes for smoking cessation . Cochrane Database of Systematic Reviews , , Cd001007. doi: 10.1002/14651858.CD001007.pub2 [ PubMed ] [ CrossRef ] [ Google Scholar ]
- Stead L. F., Perera R., Bullen C., Mant D., Hartmann-Boyce J., Cahill K., & Lancaster T. (2012). Nicotine replacement therapy for smoking cessation . Cochrane Database of Systematic Reviews , , Cd000146. doi: 10.1002/14651858.CD000146.pub4 [ PubMed ] [ CrossRef ] [ Google Scholar ]
- Sterling L. H., Windle S. B., Filion K. B., Touma L., & Eisenberg M. J. (2016). Varenicline and adverse cardiovascular events: A systematic review and meta-analysis of randomized controlled trials . Journal of the American Heart Association , , doi: 10.1161/jaha.115.002849 [ PMC free article ] [ PubMed ] [ CrossRef ] [ Google Scholar ]
- Talati A., Keyes K., & Hasin D. (2016). Changing relationships between smoking and psychiatric disorders across twentieth century birth cohorts: Clinical and research implications . Molecular Psychiatry , , 464–471. doi: 10.1038/mp.2015.224 [ PMC free article ] [ PubMed ] [ CrossRef ] [ Google Scholar ]
- Talip T., Murang Z., Kifli N., & Naing L. (2016). Systematic review of smoking initiation among Asian Adolescents, 2005–2015: Utilizing the frameworks of triadic influence and planned behavior . Asian Pacific Journal of Cancer Prevention , , 3341–3355. [ PubMed ] [ Google Scholar ]
- Tappin D., Bauld L., Purves D., Boyd K., Sinclair L., MacAskill S., & Coleman T. (2015). Financial incentives for smoking cessation in pregnancy: Randomised controlled trial . British Medical Journal , , h134. doi: 10.1136/bmj.h134 [ PubMed ] [ CrossRef ] [ Google Scholar ]
- Thomas R. E., McLellan J., & Perera R. (2013). School-based programmes for preventing smoking . Evidence-based Child Health: A Cochrane Review Journal , , 1616–2040. 10.1002/ebch.v8.5 [ CrossRef ] [ Google Scholar ]
- US Department of Health and Human Services (2004). The health consequences of smoking: a report of the surgeon general (p. 62). Atlanta, GA: US Department of Health and Human Services, Centers for Disease Control and Prevention, National Center for Chronic Disease Prevention and Health Promotion, Office on Smoking and Health. [ Google Scholar ]
- US Department of Health Human Services (2014). The health consequences of smoking – 50 years of progress: A report of the surgeon general (p. 17). Atlanta, GA: US Department of Health and Human Services, Centers for Disease Control and Prevention, National Center for Chronic Disease Prevention and Health Promotion, Office on Smoking and Health. [ Google Scholar ]
- US Surgeon General. (1990). The health benefits of smoking cessation . Washington, DC: Department of Health and Human Services. [ Google Scholar ]
- Ussher M., Brown J., Rajamanoharan A., & West R. (2014). How do prompts for attempts to quit smoking relate to method of quitting and quit success? Annals of Behavioral Medicine , , 358–368. doi: 10.1007/s12160-013-9545-z [ PubMed ] [ CrossRef ] [ Google Scholar ]
- van Hasselt M., Kruger J., Han B., Caraballo R. S., Penne M. A., Loomis B., & Gfroerer J. C. (2015). The relation between tobacco taxes and youth and young adult smoking: What happened following the 2009 US federal tax increase on cigarettes? Addictive Behaviors , , 104–109. 10.1016/j.addbeh.2015.01.023 [ PMC free article ] [ PubMed ] [ CrossRef ] [ Google Scholar ]
- Vangeli E., Stapleton J., Smit E. S., Borland R., & West R. (2011). Predictors of attempts to stop smoking and their success in adult general population samples: A systematic review . Addiction , , 2110–2121. doi: 10.1111/j.1360-0443.2011.03565.x [ PubMed ] [ CrossRef ] [ Google Scholar ]
- Vink J. M., Willemsen G., & Boomsma D. I. (2005). Heritability of smoking initiation and nicotine dependence . Behavior Genetics , , 397–406. doi: 10.1007/s10519-004-1327-8 [ PubMed ] [ CrossRef ] [ Google Scholar ]
- West R. (2009). The multiple facets of cigarette addiction and what they mean for encouraging and helping smokers to stop . Chronic Obstructive Pulmonary Disease , , 277–283. 10.1080/15412550903049181 [ PubMed ] [ CrossRef ] [ Google Scholar ]
- West R., Baker C. L., Cappelleri J. C., & Bushmakin A. G. (2008). Effect of varenicline and bupropion SR on craving, nicotine withdrawal symptoms, and rewarding effects of smoking during a quit attempt . Psychopharmacology (Berl) , , 371–377. doi: 10.1007/s00213-007-1041-3 [ PubMed ] [ CrossRef ] [ Google Scholar ]
- West R., & Brown J. (2015). Smoking in England 2007–2014 . Retrieved from www.smokinginengland.info/latest-statistics/ [ Google Scholar ]
- West R., Raw M., McNeill A., Stead L., Aveyard P., Bitton J., & Borland R. (2015). Health-care interventions to promote and assist tobacco cessation: A review of efficacy, effectiveness and affordability for use in national guideline development . Addiction , , 1388–1403. doi: 10.1111/add.12998 [ PMC free article ] [ PubMed ] [ CrossRef ] [ Google Scholar ]
- West R., & Shiffman S (2016). Smoking cessation (3rd ed.). Abingdon: Health Press. [ Google Scholar ]
- White A. R., Rampes H., Liu J. P., Stead L.F., & Campbell J (2014). Acupuncture and related interventions for smoking cessation . Cochrane Database of Systematic Reviews , , Cd000009. doi: 10.1002/14651858.CD000009.pub4 [ PMC free article ] [ PubMed ] [ CrossRef ] [ Google Scholar ]
- Whittaker R., McRobbie H., Bullen C., Rodgers A., & Gu Y. (2016). Mobile phone-based interventions for smoking cessation . Cochrane Database of Systematic Reviews , , Cd006611. doi: 10.1002/14651858.CD006611.pub4 [ PMC free article ] [ PubMed ] [ CrossRef ] [ Google Scholar ]
- World Health Organization (2013). WHO report on the global tobacco epidemic, 2013: enforcing bans on tobacco advertising, promotion and sponsorship . Geneva: WHO. [ Google Scholar ]
- Wu L., Sun S., He Y., & Zeng J. (2015). Effect of smoking reduction therapy on smoking cessation for smokers without an intention to quit: An updated systematic review and meta-analysis of randomized controlled . International Journal of Environmental Research and Public Health , , 10235–10253. doi: 10.3390/ijerph120910235 [ PMC free article ] [ PubMed ] [ CrossRef ] [ Google Scholar ]
- Zhou X., Nonnemaker J., Sherrill B., Gilsenan A. W., Coste F., & West R. (2009). Attempts to quit smoking and relapse: Factors associated with success or failure from the ATTEMPT cohort study . Addictive Behaviors , , 365–373. doi: 10.1016/j.addbeh.2008.11.013 [ PubMed ] [ CrossRef ] [ Google Scholar ]
Thank you for visiting nature.com. You are using a browser version with limited support for CSS. To obtain the best experience, we recommend you use a more up to date browser (or turn off compatibility mode in Internet Explorer). In the meantime, to ensure continued support, we are displaying the site without styles and JavaScript.
- View all journals
- Explore content
- About the journal
- Publish with us
- Sign up for alerts
- NEWS AND VIEWS
- 14 February 2024
Smoking’s lasting effect on the immune system
- Yang Luo ORCID: http://orcid.org/0000-0001-7385-6166 0 &
- Simon Stent 1
Yang Luo is at the Kennedy Institute of Rheumatology, University of Oxford, Oxford OX3 7FY, UK.
You can also search for this author in PubMed Google Scholar
Simon Stent is a research scientist based in Oxford, UK.
When our bodies encounter pathogens such as bacteria and viruses, immune cells release molecules called cytokines to coordinate the body’s defence mechanisms. These cytokines send signals to other immune cells to mount an appropriate response against the invading pathogens. The secretion of cytokines can vary among individuals and is influenced by both environmental and inherited factors. Writing in Nature , Saint-André et al . 1 report their examination of data from the Milieu Intérieur project 2 , a research initiative designed to study the variability in the immune system among 1,000 healthy individuals.
Access options
Access Nature and 54 other Nature Portfolio journals
Get Nature+, our best-value online-access subscription
24,99 € / 30 days
cancel any time
Subscribe to this journal
Receive 51 print issues and online access
185,98 € per year
only 3,65 € per issue
Rent or buy this article
Prices vary by article type
Prices may be subject to local taxes which are calculated during checkout
Nature 626 , 724-725 (2024)
doi: https://doi.org/10.1038/d41586-024-00232-3
Saint-André, V. et al. Nature 626 , 827–835 (2024).
Article Google Scholar
Thomas, S. et al. Clin. Immunol. 157 , 277–293 (2015).
Article PubMed Google Scholar
Burgos, M. et al. Ther. Adv. Med. Oncol . 14 , 17588359211072621 (2022).
Prüss-Ustün, A. et al. BMJ 364 , l265 (2019).
Download references
Reprints and permissions
Competing Interests
The authors declare no competing interests.
Related Articles
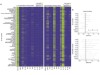
Gut clues to weight gain after quitting smoking
See all News & Views
- Medical research
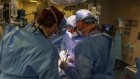
Pig-organ transplants: what three human recipients have taught scientists
News 17 MAY 24
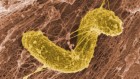
Gut microbes linked to fatty diet drive tumour growth
News 16 MAY 24
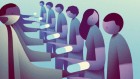
Neglecting sex and gender in research is a public-health risk
Comment 15 MAY 24
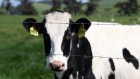
Could bird flu in cows lead to a human outbreak? Slow response worries scientists
Imprinting of serum neutralizing antibodies by Wuhan-1 mRNA vaccines
Article 15 MAY 24
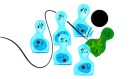
How to kill the ‘zombie’ cells that make you age
News Feature 15 MAY 24
Postdoc in CRISPR Meta-Analytics and AI for Therapeutic Target Discovery and Priotisation (OT Grant)
APPLICATION CLOSING DATE: 14/06/2024 Human Technopole (HT) is a new interdisciplinary life science research institute created and supported by the...
Human Technopole
Research Associate - Metabolism
Houston, Texas (US)
Baylor College of Medicine (BCM)
Postdoc Fellowships
Train with world-renowned cancer researchers at NIH? Consider joining the Center for Cancer Research (CCR) at the National Cancer Institute
Bethesda, Maryland
NIH National Cancer Institute (NCI)
Faculty Recruitment, Westlake University School of Medicine
Faculty positions are open at four distinct ranks: Assistant Professor, Associate Professor, Full Professor, and Chair Professor.
Hangzhou, Zhejiang, China
Westlake University
PhD/master's Candidate
PhD/master's Candidate Graduate School of Frontier Science Initiative, Kanazawa University is seeking candidates for PhD and master's students i...
Kanazawa University
Sign up for the Nature Briefing newsletter — what matters in science, free to your inbox daily.
Quick links
- Explore articles by subject
- Guide to authors
- Editorial policies
- Open access
- Published: 09 December 2021
Socio-environmental and psychosocial predictors of smoking susceptibility among adolescents with contrasting socio-cultural characteristics: a comparative analysis
- Christopher Tate 1 ,
- Rajnish Kumar 2 ,
- Jennifer M. Murray 1 ,
- Sharon Sanchez-Franco 3 ,
- Shannon C. Montgomery 4 ,
- Felipe Montes 5 ,
- Laura Dunne 6 ,
- Olga L. Sarmiento 3 ,
- Frank Kee 1 na1 &
- Ruth F. Hunter 1 na1
BMC Public Health volume 21 , Article number: 2240 ( 2021 ) Cite this article
4551 Accesses
7 Citations
7 Altmetric
Metrics details
Despite a steady decline in adolescent smoking globally, it remains a prevalent risk factor for non-communicable disease. Previous research points to differences in socio-environmental and psychosocial risk factors for smoking and how they vary across different settings with disparate social and cultural characteristics. As a result, smoking rates have remained disproportionately higher in some settings while decreasing in others. This study explored the socio-environmental and psychosocial risk factors for smoking susceptibility in a high-income and upper-middle income setting.
Cross-sectional data were obtained from 1,573 male and female adolescents aged 11-15 years who completed self-administered questionnaires in schools in Northern Ireland and Bogotá, Colombia. Using logistic regression analysis, we examined how socio-environmental and psychosocial predictors of smoking susceptibility compared across the two countries.
In Northern Ireland, reduced odds of smoking susceptibility were significantly associated with less family smoking (OR: 0.64, 95% CI: 0.41-1.00); having access to information about smoking in school (OR: 0.75, 95% CI: 0.59-0.96); negative attitudes towards smoking (OR: 0.35, 95% CI: 0.23-0.51); higher levels of openness (OR: 0.59, 95% CI: 0.50-0.69); and higher levels of self-reported wellbeing (OR: 0.57, 95% CI: 0.44-0.74). Increased odds of smoking susceptibility were associated with reporting less smoking of a mother (OR: 1.37, 95% CI: 1.06-1.76); higher levels of extraversion (OR: 1.40, 95% CI: 1.04-1.90); and receiving pocket money (OR: 1.20, 95% CI: 1.06-1.37). In Bogotá, reduced odds of smoking susceptibility were significantly associated with reporting less smoking among friends (OR: 0.86, 95% CI: 0.76-0.98); higher levels of self-efficacy (OR: 0.58, 95% CI: 0.40-0.83); greater perceived behavioural control to quit smoking (OR: 0.71, 95% CI: 0.56-0.90); and lower levels of truancy (OR: 0.69, 95% CI: 0.52-0.92). In Bogotá, no factors were associated with increased odds of smoking susceptibility in the final model.
Conclusions
The findings illustrate that there were differences in predictors of adolescent smoking susceptibility across the two settings. By using a comparative approach we demonstrate that smoking interventions and policies must be sensitive to the cultural and normative context within which they are implemented.
Peer Review reports
Smoking is an important modifiable risk factor for non-communicable diseases (NCD) in both high-income countries as well as low- and middle-income countries (LMICs), not least because of its role as a precursor and accessory to other risk behaviours among younger populations [ 1 , 2 , 3 ]. In Northern Ireland, a high-income constituent country of the United Kingdom, it is estimated that current cigarette consumption amongst adolescents aged 11-16 years is 4% [ 4 ]. By comparison, in Colombia, an upper-middle income country, data suggests 8.1% of adolescents aged 12-18 years smoke, and in the capital city, Bogotá, estimates place this figure at 13.1% [ 5 ].
Smoking susceptibility (the absence of a firm commitment not to smoke) is inherently linked to experimentation which predisposes adolescents to subsequent cigarette smoking [ 6 , 7 ]. The importance of susceptibility to smoking within the developmental context of adolescence is significant given the role of behavioural intentions in predicting future behaviour [ 8 ]. Studies have harnessed smoking susceptibility both as a predictor of future smoking [ 9 , 10 ] as well as an outcome of various social and behavioural risk factors [ 11 , 12 ]. As such, there is value in understanding the factors that predict smoking susceptibility due to the implications for the subsequent formation of smoking habits that can extend into adulthood.
Various studies point to the role of social norms (both descriptive and injunctive) in adolescent smoking. Descriptive norms are the perception of what behaviours are performed by others [ 13 ]. Injunctive norms correspond to the perceived pressures to conform to a behaviour to avoid social sanctions [ 14 ]. The perceived prevalence and perceived acceptability of smoking among peers and family members have been shown to predict adolescent smoking [ 15 , 16 , 17 , 18 ].
Studies have also investigated the proximal social factors in family and peer contexts [ 19 , 20 ], as well as distal (upstream) influences emerging from cultural spheres of influence such as exposure to smoking-related media content [ 21 ]. For example, it has been shown that adolescents who report higher levels of exposure to smoking in movies are at a greater risk of commencing smoking [ 22 , 23 ]. However, the socio-environmental factors conducive to smoking are not universal across all contexts, therefore, it cannot be assumed that smoking intentions are regulated entirely by external factors.
Self-efficacy, the belief an individual holds about their ability to exercise control over their own actions and associated outcomes [ 24 ], is well established as a determinant of adolescent smoking [ 25 , 26 , 27 ]. For example, refusal self-efficacy has been shown to predict smoking among adolescent boys in a study of Chinese youth [ 28 ]. Further, another study [ 29 ] found that self-efficacy mediated the association between smoking behaviour and social influence.
Cognitive constructs, such as perceived behavioural control (PBC) and attitude towards smoking, have also been shown to be associated with adolescent’s intentions to smoke [ 30 ]. Furthermore, adolescents knowledge of the potential side effects and perceived benefits of smoking can alter their intentions. For example, adolescent smokers report that they perceive themselves as being less likely to become addicted [ 31 ], less likely to suffer negative health-related side effects [ 32 ], and perceive greater social benefits of smoking [ 33 ].
Personality factors were examined in an earlier study [ 34 ] that used the five personality dimensions (or “Big Five”) [ 35 ]. It was reported that students who scored higher on the extraversion dimension and lower on the emotional stability dimension were consistently more likely to smoke. This finding was reiterated in another study [ 36 ] that found adolescents who exhibited more extraverted behavioural traits were at higher risk of smoking, whereas greater emotional stability was protective.
Research indicates that other psychosocial factors such as emotional well-being [ 37 ] and life-satisfaction [ 38 ] are also protective factors against adolescent smoking. Conversely, depressive symptoms [ 39 ], low self-esteem [ 40 ], emotional or behavioural problems [ 41 ], low life satisfaction [ 42 ], and high levels of anxiety [ 43 ] are reported to be predictive of adolescent smoking initiation.
This study adds a cross-cultural perspective to the wealth of existing evidence that already highlights the importance of both socio-environmental and individual-level factors that contribute to smoking among adolescents. Importantly, by providing a direct comparison between settings characterised by distinct socio-cultural and normative characteristics, cross-cultural research offers insight into potential ways of optimising intervention policies and preventative strategies to accommodate the variability of risk factors for smoking across settings. Using data obtained from adolescents in Northern Ireland and Bogotá, Colombia, the objective of this study was to compare and contrast socio-environmental and individual-level factors associated with smoking susceptibility in a high-income setting and upper-middle income setting.
Study sample
Study participants were a cross-sectional sample from the first wave of data collection of the Mechanisms of Networks and Norms Influence on Smoking in Schools (MECHANISMS) study. The MECHANISMS study was a school-based study designed to further understanding of social norms based mechanisms of action related to smoking in high- and middle-income settings. Baseline data collection took place in Northern Ireland and Bogotá, Colombia before students participated in school-based smoking prevention interventions.
Cross-sectional data were collected from 1,573 students aged 11-15 years in a post-primary educational setting in schools in Northern Ireland, UK ( n = 7) and Bogotá ( n = 8). In Northern Ireland, the sample of schools served urban and rural catchments, and maximum variation sampling was used to ensure there was an adequate balance of schools with high and low proportions of pupils eligible for free school meals. Eight public schools in Bogotá were identified using a comparable maximum variation sampling approach. Sampling of schools in Bogotá was performed in three steps: first, 40 private and public schools were identified based on health risks by the Education and Health Departments of Bogotá; second, 13 schools were shortlisted for inclusion in the study if they were situated in an urban area, were mixed-gender, and had an enrolment of 90-150 students in year 7; third, six schools accepted the invitation to participate in the study and were subsequently selected.
Participants (50% female) completed a baseline self-report survey measuring a range of variables pertaining to socio-environmental risk factors for smoking, smoking-related cognitions, and psychosocial traits. The self-administered questionnaire was based conceptually on key variables from the Theory of Planned Behaviour [ 44 ], namely attitudes, subjective norms, and PBC. Theory of Planned Behaviour constructs were supplemented with additional measures identified in the literature as having a significant bearing on adolescent smoking intentions. A description of the scales used is included in the Appendix . The questionnaire items are shown in Table 1 in the Appendix .
Ethical considerations
All pupils were required to complete consent forms indicating whether they agree or decline to participate. A parental opt-out procedure was used whereby parents/guardians who did not wish their child to take part were asked to return completed opt-out forms. Pupils who consented to participate were asked to complete a baseline assessment. Ethical approval was obtained prior to the first wave of data collection. Ethical approval for this study was granted by the Queen's University Belfast, School of Medicine, Dentistry and Biomedical Sciences Ethics Committee in September 2018, and Research Committee of the Universidad de Los Andes, Bogotá in July 2018 (see the study protocol [ 45 ] for full details of the study design).
Smoking susceptibility
Susceptibility to smoking was defined as the absence of a firm commitment not to smoke [ 6 ]. Participants were classified as susceptible or not based on three items measuring intentions to smoke:
Do you think you will try a cigarette soon?
If one of your best friends were to offer you cigarette, would you smoke it?
If you don’t currently smoke, do you intend to take up smoking in the next 6 months?
The student was coded as not susceptible if they answered ‘No’ (from three choices), ‘Definitely not’ (from five choices), and ‘Definitely not’ (from six choices) respectively to these questions. The student was coded as susceptible with any other set of responses.
Socio-demographic factors
Socio-demographic data collected in the baseline survey included gender, age, socioeconomic level based on country-specific measures, ethnicity and family structure. Student and school deprivation ranks were obtained for Northern Ireland from Northern Ireland and Statistics Research Agency data [ 46 ]. Student and school socioeconomic level indexes for Bogotá were obtained from data published by the Colombian Institute for the Evaluation of Education [ 47 ].
Socio-environmental factors
Injunctive norms were assessed with seven subscales and descriptive norms were assessed with eight subscales [ 48 ]. Exposure to advertising in the media was assessed with eight items [ 49 ]. Exposure to tobacco advertising in shops was measured using four items [ 50 ]. School smoking information was assessed with a single item asking, “Do you think your school has given you enough information on smoking?”.
Smoking-related cognitions
Self-efficacy was assessed using three subscales: (i) emotional; (ii) friends; and (iii) opportunity (Cronbach’s α: 0.981) [ 51 , 52 ]. PBC was assessed with two items that assessed PBC to quit and PBC to avoid smoking [ 30 ]. Perceived risks and benefits of tobacco-use were assessed using two separate scales: perceived risks (13 items; α = 0.864); and perceived benefits (five items; α = 0.774) [ 31 ]. Attitudes towards smoking were assessed a 12-item scale [ 53 ] (α = 0.787). Knowledge of health effects of smoking was assessed with the 6-item scale [ 48 ].
Psychosocial characteristics and personality traits
Need to belong was measured using 10 items (α = 0.813) [ 54 , 55 ]. Fear of negative evaluation was assessed with 12 items (α = 0.894) [ 55 , 56 , 57 ]. The Prosocial Behaviour score was derived from 5 items (α = 0.733) [ 55 , 58 ]. We assessed personality traits [ 35 ] by using the Big Five Personality Trait Short Questionnaire (BFPTSQ). Each dimension was measured using a 10-item subscale: openness (α = 0.798); extraversion (α = 0.776); agreeableness (α = 0.700); conscientiousness (α = 0.700); and emotional stability (α = 0.745). In Northern Ireland, we used the questionnaire validated for English-speaking adolescents [ 59 ]. In Bogotá, we used the questionnaire validated for Spanish-speaking adults [ 60 ]. Self-perceived wellbeing was measured using five items (α = 0.821) [ 61 ]. Truancy, and access to and disposal of pocket money were assessed using questions adapted from an earlier study [ 50 ].
Statistical analyses
The analysis used univariate and multivariate logistic regression modelling adjusted for clustering at country and school level to test the probability of a participant being either susceptible or not susceptible to smoking based on the variables outlined above. Three independent regressions were performed on: the whole sample ( n = 1,573); the Northern Ireland sub-sample ( n = 701); and the Bogotá sub-sample ( n = 872). To account for differences in scales used to measure the independent variables a new scale was calculated using z-scores. Interaction analysis was used to determine if there was a statistically significant different in predictors of susceptibility according to country.
A Hosmer-Lemeshow goodness-of-fit test and receiver operating characteristic analysis were used to evaluate the predictive accuracy of the final model. The conceptual framework that guided the analysis is presented in Fig. 1 . All statistical analyses were conducted using Stata 16.1 (StataCorp, 2019, Stata Statistical Software: Release 16. College Station, TX: StataCorp LLC.).
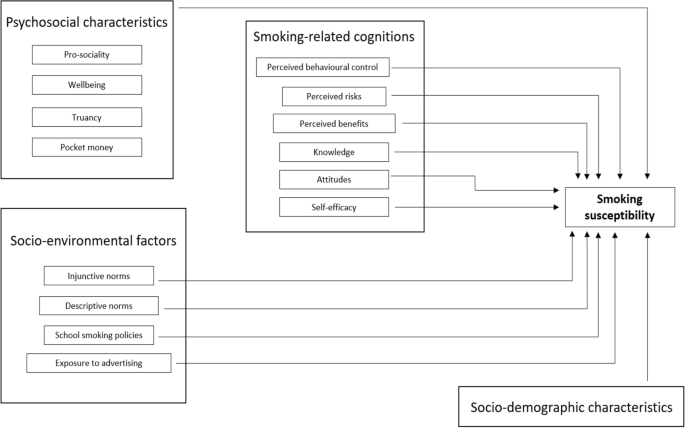
Conceptual framework of socio-environmental and psychosocial factors contributing to smoking susceptibility
A Pearson’s product-moment correlation matrix was used to assess if there was any potential associations among the predictor variables. The strength of the association between independent variables was assessed using Cohen’s [ 62 ] guidelines for interpreting the magnitude of correlation coefficients. As an additional tool to check for multicollinearity, variation inflation factors (VIF) (VIF ≥ 10 suggests that variables are measuring similar constructs) and tolerance scores were analysed post-hoc [ 63 ].
Table 1 shows the socio-demographic characteristics of the student sample. Both samples demonstrate similar socioeconomic characteristics, with the majority of students being categorised in low – middle socioeconomic ranking scales. A smaller proportion of the students in the Bogotá sample live with both parents (55%) when compared to the Northern Ireland sample (80%).
The smoking characteristics of the sample are shown in Table 2 . A smaller proportion of students in the Bogotá cohort reported having never smoked (80%) compared to Northern Ireland (85%). A larger proportion of students in Bogotá (43%) were classified as susceptible compared to the Northern Ireland cohort (31%).
The unadjusted odds ratios (OR), odds ratios adjusted for sociodemographic characteristics, and multivariate-adjusted odds ratios are reported in Tables 2, 3, and 4 respectively in the Appendix . Corresponding 95% confidence intervals (CI) and p -values are also reported.
Factors associated with smoking susceptibility in both countries
In the unadjusted model (Table 2 in the Appendix ), all socio-environmental factors demonstrated statistically significant associations with smoking susceptibility (p < 0.05). Self-efficacy, PBC to avoid smoking, and perceived risks of smoking were significantly negatively associated with the odds of being susceptible in the unadjusted model. Conversely, perceived benefits was positively associated with the odds of being susceptible. Students who held more negative attitudes towards smoking and had greater knowledge of the health effects of smoking were less likely to be susceptible. A higher score on each of the Big Five personality dimensions significantly predicted a reduced likelihood of being susceptible.
Students from Bogotá were statistically more likely to be susceptible to smoking, as were those who were older.
After adjusting for sociodemographic factors (Table 3 in the Appendix ), the odds ratios for smoking susceptibility remained lower for adolescents who reported fewer injunctive norms favourable to smoking and fewer descriptive smoking norms. The odds of being susceptible remained higher for students reporting more frequent exposure to smoking in media content and smoking advertising in shops. Higher levels on the fear of negative evaluation scale significantly increased the odds of being susceptible, after adjusting for sociodemographic factors. Only PBC to quit smoking, need to belong, and receiving pocket money did not significantly predict the odds of being susceptible in this model.
The results of the multivariate-adjusted analysis (Table 4 in the Appendix ) differed from those of the univariate analysis in a number of ways. After adjusting for all variables, descriptive norms pertaining to sister(s) smoking (OR: 1.12, 95% CI: 1.06 - 1.18) significantly predicted the odds of being susceptible, as did injunctive norms for important people (OR: 0.86, 95% CI: 0.76 - 0.97), father (OR: 1.15, 95% CI: 1.00 - 1.33), sister(s) (OR: 0.94, 95% CI: 0.91 - 0.98), and friends (OR: 0.79, 95% CI: 0.76 - 0.82). Additionally, cigarette advertising in shops remained a significant socio-environmental predictor of smoking susceptibility (OR: 1.07, 95% CI: 1.03 - 1.11). Greater self-efficacy (OR: 0.59, 95% CI: 0.53 - 0.65), perceiving more risks associated with smoking (OR: 0.86, 95% CI: 0.85 - 0.86), and more negative attitudes towards smoking (OR: 0.62, 95% CI: 0.47 - 0.80) significantly reduced the odds of being susceptible in the fully adjusted model.
Among the psychosocial factors, scoring higher on the need to belong scale positively predicted the odds of smoking susceptibility (OR: 1.09, 95% CI: 1.01 - 1.16). In contrast, a higher score on the prosociality scale (OR: 0.95, 95% CI: 0.95 - 0.96) and conscientiousness scale (OR: 0.92, 95% CI: 0.90 - 0.94) significantly reduced the odds of being susceptible as well as lower rates of truancy (OR: 0.72, 95% CI: 0.67 - 0.78). Students who reported that they were restricted with regards to how they spent pocket money were also less likely to be susceptible (OR: 0.92, 95% CI: 0.89 - 0.94).
Age (OR: 1.04, 95% CI: 1.03 - 1.04) and country (OR: 1.50, 95% CI: 1.04 - 2.15) were the only sociodemographic factors that significantly predicted the odds of being susceptible in the fully adjusted model.
Factors associated with smoking susceptibility across countries
In the univariate model (Table 2 in the Appendix ), examining the results from the Northern Ireland and Bogotá cohorts separately showed minimal deviation from the results obtained with the whole sample. All socio-environmental factors significantly predicted the odds of being susceptible in Northern Ireland. In Bogotá, injunctive norms from the family context (excluding mother) were not significant, nor was access to information about smoking in school. The demographic factors age, socioeconomic status and school socioeconomic status were significant in Bogotá, while no sociodemographic factors were significant in Northern Ireland.
After adjusting for socio-demographic factors (Table 3 in the Appendix ), all socio-environmental factors significantly predicted smoking susceptibility in Northern Ireland, with the exception of father injunctive norms. In Bogotá, two types of injunctive norm (father and brother), sister(s) descriptive norms, and school smoking information were non-significant.
In the fully adjusted model (Table 4 in the Appendix ), descriptive norms from two sources (mother (OR: 1.37, 95% CI: 1.06 - 1.76) and family (OR: 0.64, 95% CI: 0.41 - 1.00)) and school smoking information (OR: 0.75, 95% CI: 0.59 - 0.96) significantly predicted the odds of being susceptible in Northern Ireland. By comparison, friend descriptive norms (OR: 0.86, 95% CI: 0.76 - 0.98) was the only significant socio-environmental variable in Bogotá. Interaction analysis confirmed that school smoking information differed significantly across the two settings (OR: 0.75, p = 0.024 in Northern Ireland compared to OR: 1.09, p = 0.313 in Bogotá).
There was some variation in smoking-related cognitions as predictors of smoking susceptibility across the two countries. In Northern Ireland, the univariate analysis showed self-efficacy, perceived risks of smoking, perceived benefits of smoking, PBC to avoid smoking, and attitudes towards smoking significantly predicted susceptibility. In Bogotá, self-efficacy, perceived risks of smoking, PBC to avoid smoking, attitudes towards smoking, and knowledge of the health effects significantly predicted susceptibility.
Adjusting for sociodemographic factors produced no significant change in the estimates for smoking-related cognitions in either country.
In the fully adjusted model, attitude (OR: 0.35, 95% CI: 0.23 - 0.51) maintained a significant association with smoking susceptibility in Northern Ireland. In Bogotá, self-efficacy (OR: 0.58, 95% CI: 0.40 - 0.83) and PBC to quit smoking (OR: 0.71, 95% CI: 0.56 - 0.90) significantly predicted susceptibility. In this model, attitude toward smoking was the only smoking-related cognition that differed significantly between the two countries (OR: 0.35, p = 0.000 in Northern Ireland compared to OR: 0.68, p = 0.100 in Bogotá).
Of the Big Five personality dimensions, only extraversion was statistically non-significant in Northern Ireland in the univariate model, while higher scores on the remaining Big Five subscales were associated with lower odds of being susceptible in both countries. Students who reported higher levels of wellbeing in Northern Ireland and Bogotá were less likely to be susceptible. Similarly, students who reported lower levels of truancy had lower odds of being susceptible to smoking in both Northern Ireland and Bogotá in the univariate model.
After adjusting for sociodemographic factors, fear of negative evaluation was no longer a significant predictor in Northern Ireland. Adjusting for sociodemographic factors produced no change in the variables that predicted smoking susceptibility in Bogotá.
In the multivariate-adjusted model, openness (OR: 0.59, 95% CI: 0.50 - 0.69), extraversion (OR: 1.40, 95% CI: 1.04 - 1.90), wellbeing (OR: 0.57, 95% CI: 0.44 - 0.74), and receiving pocket money (OR: 1.20, 96% CI: 1.06 - 1.37) demonstrated a significant association with smoking susceptibility in Northern Ireland, while truancy (OR: 0.69, 95% CI: 0.52 - 0.92) was the only psychosocial variable that significantly predicted susceptibility in Bogotá. OR estimates for agreeableness, wellbeing and receiving pocket money differed significantly across countries in the final model.
As shown in the Pearson’s product-moment correlation matrix for both countries (Table 5 in the Appendix ), a high proportion of the independent variables were correlated, however the strength of the association was small for most. Self-efficacy was positively correlated with both injunctive and descriptive norms (p < 0.05), however, the strength of the association was small for most subscales (r < .3). The VIF and tolerance scores for the independent variables included in the final analysis for both countries indicated that no variables exhibited signs of meaningful collinearity in our analysis (Table 6 in the Appendix ).
Previous research has shown that a disproportionate number of those aged 15 years and over who smoke (approximately 80%) live in LMICs [ 64 ]. This is concerning given the role of initiation during early adolescence as a risk factor for subsequent smoking [ 65 ]. This study investigated differences between the socio-environmental and individual-level risk factors for smoking susceptibility in a high-income country (Northern Ireland) and upper-middle income country (Bogotá, Colombia). Findings from logistic regression analyses illustrated differences between the two settings regarding descriptive norms, smoking-related cognitions, and psychosocial traits. In Northern Ireland, adolescents who reported that fewer family members smoked were less likely to be susceptible to smoking. In Bogotá, reporting that fewer friends smoked reduced the odds of being susceptible. Reduced odds of being susceptible to smoking were significantly associated with negative attitudes towards smoking in Northern Ireland, while higher levels of self-efficacy and PBC to quit were associated with reduced odds in Bogotá. When psychosocial traits were examined, higher levels of openness and self-reported wellbeing significantly reduced the odds of being susceptible in Northern Ireland. Conversely, higher levels of extraversion increased the odds of being susceptible in Northern Ireland. In Bogotá, students who did not skip school were less likely to be classified as susceptible to future smoking.
Descriptive and injunctive norms are reported in the literature as risk factors for smoking among adolescents [ 66 , 67 , 68 ]. We did find evidence of a significant association between injunctive norms and smoking susceptibility in both countries after adjusting for sociodemographic factors, however, this relationship was not significant in our final model. In the composite sample, injunctive norms favourable to smoking from important people, father, sister(s), and friends were significant in the final model.
We did find support for the role of descriptive norms in predicting smoking susceptibility in the final model. Students in Bogotá who reported less smoking among friends were less likely to be susceptible. In addition, fewer descriptive smoking norms among family reduced the odds of being susceptible in Northern Ireland. Interestingly, reporting less smoking by a mother increased the odds of being susceptible in Northern Ireland in the final model.
In agreement with other studies pointing to a link between pro-smoking messages in media content and an elevated risk of susceptibility to smoking [ 69 , 70 ], we found a significant correlation between exposure to smoking-related media content and smoking susceptibility in both countries after adjusting for sociodemographic factors. However, this association was no longer significant in the final model. This is in contrast to another study of LMIC settings which found that adolescents who were exposed to smoking in electronic media were more likely to be smokers [ 71 ]. Similar to the results of other studies [ 72 , 73 ], we found exposure to cigarette advertising in shops was a significant predictor of susceptibility in both countries after adjusting for sociodemographic factors. In the final model, this finding was limited to the composite sample.
Consistent with previous research [ 74 ] that showed refusal self-efficacy was protective against smoking initiation, we found a statistically significant association between refusal self-efficacy and smoking susceptibility. Additionally, our results concur with the findings of an earlier study [ 31 ] that found students who perceived greater risks associated with smoking were at less risk for future initiation. However, when the data were disaggregated by country in the final model, the association was no longer significant. In line with previous findings [ 33 ], we found a statistically significant link between perceived benefits of smoking and susceptibility after adjusting for sociodemographic factors in Northern Ireland. Further, our results confirmed a significant correlation between knowledge of the harmful effects of smoking and susceptibility in Bogotá. However, in the final model, knowledge was not a significant predictor of susceptibility in either country. This echoes the findings from an earlier study [ 75 ], but contrasts with others [ 16 ] who found that lack of knowledge about the harms of smoking predicted ever-smoking.
Of the Big Five Personality factors, only two (openness and extraversion) significantly predicted the odds of being susceptible in Northern Ireland in the final model. In Northern Ireland, our results show that students who were more receptive to new ideas or experiences and expressed a greater tendency to be curious were less likely to be susceptible. This finding coincides with an earlier study [ 76 ] that reported lower levels of openness were associated with intentions to smoke. The current analysis also showed that more extraverted students were at greater risk of being susceptible to smoking, adding to the results of two earlier studies [ 34 , 36 ].
Self-reported wellbeing was a protective factor against smoking susceptibility in both countries after adjusting for sociodemographic factors, and in Northern Ireland in the final model. While studies have shown that a direct inverse relationship exists between life-satisfaction and smoking behaviour [ 38 , 77 ], our final model did not demonstrate this in Bogotá. We did, however, find a significant positive association between truancy and susceptibility in Bogotá, reinforcing the findings from previous studies [ 78 , 79 ].
The results reaffirm that refusal self-efficacy and adolescents’ attitudes towards smoking are important targets for prevention interventions in both LMICs and high-income countries. Interventions directed at younger populations should focus on mitigating pro-smoking social influences such as exposure to tobacco advertising by providing appropriate education about the negative side-effects of smoking and equipping adolescents with the necessary skills to refuse cigarettes. Moreover, the results highlight the differences in risk factors for smoking across the two countries, further emphasising the need for smoking prevention policies to be sensitive to the normative and cultural context within which they are implemented.
Strengths and limitations
There were several limitations of this study. Firstly, responses from the survey may be subject to social desirability bias which is not uncommon for self-report surveys [ 80 ]. As a result, students may have underreported smoking behaviors [ 81 ]. However, studies have shown self-reports of smoking behaviour are reliable [ 82 ]. Secondly, estimates of the smoking of friends and family members would potentially be subject to individual biases or “pluralistic ignorance” [ 83 ]. Thirdly, students who did not participate were potentially more likely to be smokers who did not want to report their behaviour [ 84 ].
The findings from this study may not generalise to other populations due to cultural and social factors unique to the two settings. However, the study used robust maximum variation sampling to ensure there was sufficient heterogeneity between schools in both countries serving urban and rural areas. To ensure the validity of student’s responses participants were assured their responses would not be shared with other students or teachers. Students were also assigned a unique identification number to anonymise their responses.
In the fully adjusted model for both countries, the Hosmer-Lemeshow goodness of fit test yielded a p-value of .1752 indicating that the model fit the data well. This was also true for the Bogotá ( p = 0.8623) model but not the Northern Ireland model ( p = 0.0112). Receiver operating characteristic (ROC) analysis was performed to evaluate the discriminative accuracy of our final models which included data from both countries. This is demonstrated by the ROC curve which was plotted to visually illustrate the concordance between model estimates of susceptibility and observed susceptibility to smoking (referred to as the C-statistic; Figures 1, 2, and 3 in the Appendix ). The C-statistics were .838, .903, and .828 for the dual-country model, Northern Ireland model, and Bogotá model respectively, indicating that the models achieved acceptable levels of discrimination [ 85 ].
In sum, the results of the present study suggest there are differences in socio-environmental and psychosocial correlates of smoking susceptibility in the high-income setting of Northern Ireland and upper-middle income setting of Bogotá. For example, reporting fewer descriptive smoking norms among friends was protective against smoking susceptibility in Bogotá, but not Northern Ireland. Students who reported that their school provided information about smoking were less at risk in Northern Ireland, but not in Bogotá. Greater self-efficacy was significantly associated with a lower risk of smoking in Bogotá, highlighting the importance of self-efficacy as a mitigating factor against socio-environmental influences, such as being offered a cigarette by a friend. Exploration of how group identities that prescribe behavioural norms in each country may provide potential insights into the mechanisms underlying the formation of these behaviours within the intragroup context and the impact this has on an individual’s self-efficacy [ 86 ].
We affirm that the cultural, normative and social factors unique to each setting provided a good basis for comparison of risk factors across the socioecological spectrum.
Availability of data and materials
The datasets used and/or analysed during the current study are available from the corresponding author on reasonable request.
Abbreviations
Low- and middle-income country
Non-communicable disease
Chen X, Unger JB, Palmer P, Weiner MD, Johnson CA, Wong MM, et al. Prior cigarette smoking initiation predicting current alcohol use: evidence for a gateway drug effect among California adolescents from eleven ethnic groups. Addict Behav. 2002;27(5):799–817.
Article PubMed Google Scholar
Siqueira LM, Brook JS. Tobacco use as a predictor of illicit drug use and drug-related problems in Colombian youth. J Adolesc Heal. 2003;32(1):50–7.
Article Google Scholar
Oppong Asante K, Kugbey N. Alcohol use by school-going adolescents in Ghana: Prevalence and correlates. Ment Heal Prev. 2019;13:75–81.
Foster C, Scarlett M, Stewart B. Young persons’ behaviour and attitude survey 2019 - substance use [Internet]. 2020 [cited 2020 Nov 3]. Available from: https://www.health-ni.gov.uk/publications/young-persons-behaviour-attitudes-survey-2019-substance-use
Google Scholar
Ministry of Justice and Law, Ministry of National Education M of H and SP. National Study of psychoactive substance use in school population Colombia [Internet]. 2016 [cited 2020 Oct 8]. Available from: https://www.unodc.org/documents/colombia/2018/Junio/CO03142016_estudio_consumo_escolares_2016.pdf .
Pierce JP, Choi WS, Gilpin EA, Farkas AJ, Merritt RK. Validation of susceptibility as a predictor of which adolescents take up smoking in the United States. Heal Psychol. 1996;15(5):355–61.
Article CAS Google Scholar
Jackson C. Cognitive susceptibility to smoking and initiation of smoking during childhood: a longitudinal study. Prev Med. 1998;27(1):129–34.
Article CAS PubMed Google Scholar
Ajzen I. Predicting and changing behavior: a reasoned action approach. In: Ajzen I, Albarracin D, Hornik R, editors. Prediction and change of health behavior: applying the reasoned action approach. New Jersey: Erlbaum; 2007. p. 3–21.
Chapter Google Scholar
Dube SR, Arrazola RA, Lee J, Engstrom M, Malarcher A. Pro-tobacco influences and susceptibility to smoking cigarettes among middle and high school students--United States, 2011. J Adolesc Heal. 2013;52(5 Suppl):S45–51.
Lechner WV, Murphy CM, Colby SM, Janssen T, Rogers ML, Jackson KM. Cognitive risk factors of electronic and combustible cigarette use in adolescents. Addict Behav. 2018;82:182–8.
Article PubMed PubMed Central Google Scholar
Owotomo O, Maslowsky J. Adolescent smoking susceptibility in the current tobacco context: 2014-2016. Am J Health Behav. 2018;42(3):102–13.
Kamke K, Sabado-Liwag M, Rodriquez EJ, Pérez-Stable EJ, El-Toukhy S. Adolescent smoking susceptibility: gender-stratified racial and ethnic differences, 1999-2018. Am J Prev Med. 2020;58(5):666–74.
Cialdini RB, Kallgren CA, Reno RR. A focus theory of normative conduct: a theoretical refinement and reevaluation of the role of norms in human behavior. In: Zanna MP, editor. Advances in experimental social psychology: Academic Press; 1991. p. 201–34.
Cialdini RB, Reno RR, Kallgren CA. A focus theory of normative conduct: recycling the concept of norms to reduce littering in public places. J Pers Soc Psychol. 1990;58(6):1015–26.
Chen J, Ho SY, Wang MP, Lam TH. Parental smoking, rejection of parental smoking, and smoking susceptibility and behaviors in Hong Kong adolescents. Addict Behav. 2018;82:19–22.
Ho SY, Chen J, Leung LT, Mok HY, Wang L, Wang MP, et al. Adolescent smoking in Hong Kong: prevalence, psychosocial correlates, and prevention. J Adolesc Heal. 2019;64(6 Suppl):S19–27.
Vitória P, Pereira SE, Muinos G, De Vries H, Lima ML. Parents modelling, peer influence and peer selection impact on adolescent smoking behavior: a longitudinal study in two age cohorts. Addict Behav. 2020;100:106131.
Zaleski AC, Aloise-Young PA. Using peer injunctive norms to predict early adolescent cigarette smoking intentions. J Appl Soc Psychol. 2013;43(Suppl 1):E124–31.
Mercken L, Candel M, Willems P, de Vries H. Disentangling social selection and social influence effects on adolescent smoking: the importance of reciprocity in friendships. Addiction. 2007;102(9):1483–92.
Wang Y, Tian L, Huebner ES. Parental control and Chinese adolescent smoking and drinking: The mediating role of refusal self-efficacy and the moderating role of sensation seeking. Child Youth Serv Rev. 2019;102:63–72.
McCool JP, Cameron LD, Petrie KJ. The influence of smoking imagery on the smoking intentions of young people: testing a media interpretation model. J Adolesc Heal. 2005;36(6):475–85.
Dalton MA, Sargent JD, Beach ML, Titus-Ernstoff L, Gibson JJ, Ahrens MB, et al. Effect of viewing smoking in movies on adolescent smoking initiation: a cohort study. Lancet. 2003;362(9380):281–5.
Sargent JD, Beach ML, Adachi-Mejia AM, Gibson JJ, Titus-Ernstoff LT, Carusi CP, et al. Exposure to movie smoking: its relation to smoking initiation among US adolescents. Pediatrics. 2005;116(5):1183–91.
Bandura A. Social cognitive theory of self-regulation. Organ Behav Hum Decis Process. 1991;50(2):248–87.
Engels RCME, Hale WW 3rd, Noom M, De Vries H. Self-efficacy and emotional adjustment as precursors of smoking in early adolescence. Subst Use Misuse. 2005;40(12):1883–93.
Hiemstra M, Otten R, de Leeuw RNH, van Schayck OCP, Engels RCME. The changing role of self-efficacy in adolescent smoking initiation. J Adolesc Heal. 2011;48(6):597–603.
Veselska Z, Madarasova Geckova A, Reijneveld SA, Van Dijk JP. Self-efficacy, affectivity and smoking behavior in adolescence. Eur Addict Res. 2011;17(4):172–7.
Ma H, Unger JB, Chou C-P, Sun P, Palmer PH, Zhou Y, et al. Risk factors for adolescent smoking in urban and rural China: findings from the China seven cities study. Addict Behav. 2008;33(8):1081–5.
Vitória PD, Salgueiro MF, Silva SA, de Vries H. Social influence, intention to smoke, and adolescent smoking behaviour longitudinal relations. Br J Health Psychol. 2011;16(4):779–98.
Smith BN, Bean MK, Mitchell KS, Speizer IS, Fries EA. Psychosocial factors associated with non-smoking adolescents’ intentions to smoke. Health Educ Res. 2007;22(2):238–47.
Halpern-Felsher BL, Biehl M, Kropp RY, Rubinstein ML. Perceived risks and benefits of smoking: differences among adolescents with different smoking experiences and intentions. Prev Med. 2004;39(3):559–67.
Aryal UR, Petzold M, Krettek A. Perceived risks and benefits of cigarette smoking among Nepalese adolescents: a population-based cross-sectional study. BMC Public Health. 2013;13(1):187.
Song AV, Morrell HER, Cornell JL, Ramos ME, Biehl M, Kropp RY, et al. Perceptions of smoking-related risks and benefits as predictors of adolescent smoking initiation. Am J Public Health. 2009;99(3):487–92.
Harakeh Z, Scholte RHJ, de Vries H, Engels RCME. Association between personality and adolescent smoking. Addict Behav. 2006;31(2):232–45.
Goldberg LR. An alternative “description of personality”: the big-five factor structure. J Pers Soc Psychol. 1990;59(6):1216–29.
de Leeuw RNH, Scholte RHJ, Sargent JD, Vermulst AA, Engels RCME. Do interactions between personality and social-environmental factors explain smoking development in adolescence? J Fam Psychol. 2010;24(1):68–77.
Scal P, Ireland M, Borowsky IW. Smoking among American adolescents: a risk and protective factor analysis. J Community Health. 2003;28(2):79–97.
Piko BF, Luszczynska A, Gibbons FX, Tekozel M. A culture-based study of personal and social influences of adolescent smoking. Eur J Public Health. 2005;15(4):393–8.
Patton GC, Carlin JB, Coffey C, Wolfe R, Hibbert M, Bowes G. Depression, anxiety, and smoking initiation: a prospective study over 3 years. Am J Public Health. 1998;88(10):1518–22.
Article CAS PubMed PubMed Central Google Scholar
Wilkinson D, Abraham C. Constructing an integrated model of the antecedents of adolescent smoking. Br J Health Psychol. 2004;9(Pt 3):315–33.
Giannakopoulos G, Tzavara C, Dimitrakaki C, Kolaitis G, Rotsika V, Tountas Y. Emotional, behavioural problems and cigarette smoking in adolescence: findings of a Greek cross-sectional study. BMC Public Health. 2010;10:57.
Topolski TD, Patrick DL, Edwards TC, Huebner CE, Connell FA, Mount KK. Quality of life and health-risk behaviors among adolescents. J Adolesc Heal. 2001;29(6):426–35.
Weiss JW, Palmer PH, Chou C-P, Mouttapa M, Johnson CA. Association between psychological factors and adolescent smoking in seven cities in China. Int J Behav Med. 2008;15(2):149–56.
Ajzen I. The theory of planned behavior. Organ Behav Hum Decis Process. 1991;50(2):179–211.
Hunter RF, Montes F, Murray JM, Sanchez-Franco SC, Montgomery SC, Jaramillo J, et al. MECHANISMS Study: using game theory to assess the effects of social norms and social networks on adolescent smoking in schools—study protocol. Front Public Heal. 2020;8.
Northern Ireland Statistics and Research Agency. Northern Ireland multiple deprivation measure 2017 (NIMDM2017) [Internet]. 2017 [cited 8 Oct 2020]. Available from: https://www.nisra.gov.uk/statistics/deprivation/northern-ireland-multiple-deprivation-measure-2017-nimdm2017 .
Colombian Institute for the Evaluation of Education. Informe nacional de resultados del examen saber 11 - 2014-2 - 2016-2 [Internet]. 2017 [cited 8 Oct 2020]. Available from: http://www.icfes.gov.co/documents/20143/193784/Informe-nacional-de-resultados-del-examen-saber-11-2014-2-2016-2.pdf .
Cremers H-P, Mercken L, Oenema A, de Vries H. A web-based computer-tailored smoking prevention programme for primary school children: intervention design and study protocol. BMC Public Health. 2012;12:277.
Stigler MH, Perry CL, Arora M, Reddy KS. Why are urban Indian 6th graders using more tobacco than 8th graders? Findings from Project MYTRI. Tob Control. 2006;15(Suppl 1):i54–60.
PubMed Google Scholar
Dunne L, Thurston A, Gildea A, Kee F, Lazenbatt A. Protocol: a randomised controlled trial evaluation of cancer focus NI’s ‘Dead Cool’ smoking prevention programme in post-primary schools. Int J Educ Res. 2016;75:24–30.
Lawrance L. Validation of a self-efficacy scale to predict adolescent smoking. Health Educ Res. 1989;4(3):351–60.
Condiotte MM, Lichtenstein E. Self-efficacy and relapse in smoking cessation programs. J Consult Clin Psychol. 1981;49(5):648–58.
Ganley BJ, Rosario DI. The smoking attitudes, knowledge, intent, and behaviors of adolescents and young adults: implications for nursing practice. J Nurs Educ Pract. 2013;3(1).
Leary MR, Kelly KM, Cottrell CA, Schreindorfer LS. Construct validity of the need to belong scale: mapping the nomological network. J Pers Assess. 2013;95(6):610–24.
Bevelander KE, Smit CR, van Woudenberg TJ, Buijs L, Burk WJ, Buijzen M. Youth’s social network structures and peer influences: study protocol MyMovez project - Phase I. BMC Public Health. 2018;18(1):504.
Collins KA, Westra HA, Dozois DJA, Stewart SH. The validity of the brief version of the fear of negative evaluation scale. J Anxiety Disord. 2005;19(3):345–59.
Leary MR. A brief version of the fear of negative evaluation scale. Personal Soc Psychol Bull. 1983;9(3):371–5.
Goodman R, Meltzer H, Bailey V. The strengths and difficulties questionnaire: a pilot study on the validity of the self-report version. Int Rev Psychiatry. 2003;15(1–2):173–7.
Morizot J. Construct validity of adolescents’ self-reported big five personality traits: importance of conceptual breadth and initial validation of a short measure. Assessment. 2014;21(5):580–606.
Ortet G, Martínez T, Mezquita L, Morizot J, Ibáñez MI. Big five personality trait short questionnaire: preliminary validation with spanish adults. Span J Psychol. 2017;20:E7.
Rees G, Bradshaw J, Goswami H, Keung A. Understanding children’s well-being: a national survey of young people’s well-being. London: Children’s Society; 2010.
Cohen J. Statistical power analysis for the behavioral sciences. 2nd ed. Hillsdale: Erlbaum; 1988.
Chatterjee S, Price B. Regression diagnostic. New York: John Wiley; 1991.
Action on Smoking and Health. ASH fact sheet: tobacco and the developing world [Internet]. 2019 [cited 8 Oct 2020]. Available from: https://ash.org.uk/information-and-resources/fact-sheets/economics-regulation/tobacco-and-the-developing-world/ .
Reidpath DD, Davey TM, Kadirvelu A, Soyiri IN, Allotey P. Does one cigarette make an adolescent smoker, and is it influenced by age and age of smoking initiation? Evidence of association from the U.S. Youth Risk Behavior Surveillance System (2011). Prev Med. 2014;59:37–41.
Su X, Li L, Griffiths SM, Gao Y, Lau JTF, Mo PKH. Smoking behaviors and intentions among adolescents in rural China: the application of the theory of planned behavior and the role of social influence. Addict Behav. 2015;48:44–51.
Xi B, Liang Y, Liu Y, Yan Y, Zhao M, Ma C, et al. Tobacco use and second-hand smoke exposure in young adolescents aged 12-15 years: data from 68 low-income and middle-income countries. Lancet Glob Heal. 2016;4(11):e795–805.
Ra JS, Cho YH. Psychosocial factors associated with smoking intention in Korean male middle school students. J Sch Nurs. 2017;33(5):355–63.
Villanti A, Boulay M, Juon H-S. Peer, parent and media influences on adolescent smoking by developmental stage. Addict Behav. 2011;36(1–2):133–6.
Morgenstern M, Sargent JD, Engels RCME, Florek E, Hanewinkel R. Smoking in European adolescents: relation between media influences, family affluence, and migration background. Addict Behav. 2013;38(10):2589–95.
Mishu MP, Siddiqui F, Shukla R, Kanaan M, Dogar O, Siddiqi K. Predictors of cigarette smoking, smokeless tobacco consumption, and use of both forms in adolescents in South Asia: a secondary analysis of the global youth tobacco surveys. Nicotine Tob Res. 2021;23(6):956–65.
Weiss JW, Cen S, Schuster DV, Unger JB, Johnson CA, Mouttapa M, et al. Longitudinal effects of pro-tobacco and anti-tobacco messages on adolescent smoking susceptibility. Nicotine Tob Res. 2006;8(3):455–65.
Cruz TB, McConnell R, Low BW, Unger JB, Pentz MA, Urman R, et al. Tobacco marketing and subsequent use of cigarettes, e-cigarettes, and hookah in adolescents. Nicotine Tob Res. 2019;21(7):926–32.
Chang F-C, Lee C-M, Lai H-R, Chiang J-T, Lee P-H, Chen W-J. Social influences and self-efficacy as predictors of youth smoking initiation and cessation: a 3-year longitudinal study of vocational high school students in Taiwan. Addiction. 2006;101(11):1645–55.
Mohammadi S, Ghajari H, Valizade R, Ghaderi N, Yousefi F, Taymoori P, et al. Predictors of smoking among the secondary high school boy students based on the health belief model. Int J Prev Med. 2017;8:24.
Conner M, Grogan S, Fry G, Gough B, Higgins AR. Direct, mediated and moderated impacts of personality variables on smoking initiation in adolescents. Psychol Health. 2009;24(9):1085–104.
Jung S, Choi E. Life satisfaction and delinquent behaviors among Korean adolescents. Pers Individ Dif. 2017;104:104–10.
Tomori M, Zalar B, Kores Plesnicar B, Ziherl S, Stergar E. Smoking in relation to psychosocial risk factors in adolescents. Eur Child Adolesc Psychiatry. 2001;10(2):143–50.
Lee LK, Paul CYC, Kam CW, Jagmohni K. Smoking among secondary school students in Negeri Sembilan, Malaysia. Asia-Pacific J Public Heal. 2005;17(2):130–6.
Dolcini MM, Adler NE, Ginsberg D. Factors influencing agreement between self-reports and biological measures of smoking among adolescents. J Res Adolesc. 1996;6(4):515–42.
Kandel DB, Schaffran C, Griesler PC, Hu M-C, Davies M, Benowitz N. Salivary cotinine concentration versus self-reported cigarette smoking: three patterns of inconsistency in adolescence. Nicotine Tob Res. 2006;8(4):525–37.
Yeager DS, Krosnick JA. The validity of self-reported nicotine product use in the 2001-2008 National Health and Nutrition Examination Survey. Med Care. 2010;48(12):1128–32.
Miller DT, McFarland C. When social comparison goes awry: The case of pluralistic ignorance. In: Social comparison: contemporary theory and research. Hillsdale: Lawrence Erlbaum Associates, Inc; 1991. p. 287–313.
McCormick LK, Crawford M, Anderson RH, Gittelsohn J, Kingsley B, Upson D. Recruiting adolescents into qualitative tobacco research studies: experiences and lessons learned. J Sch Health. 1999;69(3):95–9.
Swets JA. Measuring the accuracy of diagnostic systems. Science. 1988;240(4857):1285–93.
Spears R. Social influence and group identity. Annu Rev Psychol. 2021;72:367–90.
Download references
Acknowledgements
The authors wish to thank the teachers and pupils in participating schools in each country. We also wish to acknowledge the support from our partners Cancer Focus Northern Ireland and Evidence to Impact.
This study was funded by a grant from the Medical Research Council Population and Systems Medicine Board (reference number MR/R011176/1). CT was funded by a Ph.D. studentship from the Department for the Economy, Northern Ireland.
Author information
Frank Kee and Ruth F. Hunter are joint last authors.
Authors and Affiliations
Centre for Public Health, Institute of Clinical Sciences Block B, Queen’s University Belfast, Royal Victoria Hospital, Grosvenor Road, Belfast, BT12 6BA, UK
Christopher Tate, Jennifer M. Murray, Frank Kee & Ruth F. Hunter
Queen’s Management School, Queen’s University Belfast, Riddel Hall, 185 Stranmillis Road, Belfast, BT9 5EE, UK
Rajnish Kumar
Department of Public Health, School of Medicine, Universidad de Los Andes, Carrera 1 No 18 A – 10, Bloque Q Piso 8, Bogotá, Postal Code: 57, Colombia
Sharon Sanchez-Franco & Olga L. Sarmiento
Department of Family and Child Sciences, Florida State University, Sandels Building, 675 W Call St, Tallahassee, FL, 32304, USA
Shannon C. Montgomery
Department of Industrial Engineering, Social and Health Complexity Center, Universidad de Los Andes, Bogotá, Colombia
Felipe Montes
Centre for Evidence and Social Innovation, School of Social Sciences, Education and Social Work, Queen’s University Belfast, 2-8 Fitzwilliam Street, Belfast, BT9 6AW, UK
Laura Dunne
You can also search for this author in PubMed Google Scholar
Contributions
CT is a Ph.D. student who proposed and initiated the current piece of research with RH, RK, and FK. CT led the statistical analysis and drafted the manuscript. OLS, FK and RH are Principal Investigators on the MECHANISMS study from which the data were obtained and helped draft the manuscript. All authors reviewed and approved the final manuscript.
Corresponding author
Correspondence to Christopher Tate .
Ethics declarations
Ethics approval and consent to participate.
The studies involving human participants were reviewed and approved. Ethical approval has been granted from the Queen's University Belfast, School of Medicine, Dentistry and Biomedical Sciences ethics committee (reference number 18.43; v3 Sept 2018), and Research committee of the Universidad de Los Andes, Bogotá (937 - July 30, 2018). Written informed consent to participate in this study was provided by the participants' legal guardian/next of kin.
The methods were carried out in accordance with the relevant guidelines and regulations set out in the Declaration of Helsinki.
Consent for publication
Not applicable.
Competing interests
The authors declare that they have no competing interests.
Additional information
Publisher’s note.
Springer Nature remains neutral with regard to jurisdictional claims in published maps and institutional affiliations.
Supplementary Information
Additional file 1., rights and permissions.
Open Access This article is licensed under a Creative Commons Attribution 4.0 International License, which permits use, sharing, adaptation, distribution and reproduction in any medium or format, as long as you give appropriate credit to the original author(s) and the source, provide a link to the Creative Commons licence, and indicate if changes were made. The images or other third party material in this article are included in the article's Creative Commons licence, unless indicated otherwise in a credit line to the material. If material is not included in the article's Creative Commons licence and your intended use is not permitted by statutory regulation or exceeds the permitted use, you will need to obtain permission directly from the copyright holder. To view a copy of this licence, visit http://creativecommons.org/licenses/by/4.0/ . The Creative Commons Public Domain Dedication waiver ( http://creativecommons.org/publicdomain/zero/1.0/ ) applies to the data made available in this article, unless otherwise stated in a credit line to the data.
Reprints and permissions
About this article
Cite this article.
Tate, C., Kumar, R., Murray, J.M. et al. Socio-environmental and psychosocial predictors of smoking susceptibility among adolescents with contrasting socio-cultural characteristics: a comparative analysis. BMC Public Health 21 , 2240 (2021). https://doi.org/10.1186/s12889-021-12351-x
Download citation
Received : 14 April 2021
Accepted : 18 November 2021
Published : 09 December 2021
DOI : https://doi.org/10.1186/s12889-021-12351-x
Share this article
Anyone you share the following link with will be able to read this content:
Sorry, a shareable link is not currently available for this article.
Provided by the Springer Nature SharedIt content-sharing initiative
- Social norms
- Risk factors
- Psychosocial
BMC Public Health
ISSN: 1471-2458
- Submission enquiries: [email protected]
- General enquiries: [email protected]

Log in using your username and password
- Search More Search for this keyword Advanced search
- Latest content
- For authors
- Browse by collection
- BMJ Journals More You are viewing from: Google Indexer
You are here
- Volume 12, Issue 6
- Systematic review of changed smoking behaviour, smoking cessation and psychological states of smokers according to cigarette type during the COVID-19 pandemic
- Article Text
- Article info
- Citation Tools
- Rapid Responses
- Article metrics

- http://orcid.org/0000-0002-2567-2845 Hae-ryoung Chun 1 ,
- Eunsil Cheon 1 ,
- http://orcid.org/0000-0002-5094-6107 Ji-eun Hwang 2
- 1 Graduate School of Public Health , Seoul National University , Gwanak-gu , Seoul , Republic of Korea
- 2 College of health science , Dankook University , Chungnam , Republic of Korea
- Correspondence to Dr Ji-eun Hwang; loshjeve{at}gmail.com
Objectives Although the global COVID-19 pandemic has increased interest in research involving high-risk smokers, studies examining changed smoking behaviours, cessation intentions and associated psychological states among smokers are still scarce. This study aimed to systematically review the literature related to this subject.
Design A systematic review of published articles on cigarettes and COVID-19 -related topics
Data sources Our search was conducted in January 2021. We used the keywords COVID-19, cigarettes, electronic cigarettes (e-cigarettes) and psychological factors in PubMed and ScienceDirect and found papers published between January and December 2020.
Data selection We included articles in full text, written in English, and that surveyed adults. The topics included smoking behaviour, smoking cessation, psychological state of smokers and COVID-19-related topics.
Data extraction and synthesis Papers of low quality, based on quality assessment, were excluded. Thirteen papers were related to smoking behaviour, nine papers were related to smoking cessation and four papers were related to psychological states of smokers.
Results Owing to the COVID-19 lockdown, cigarette users were habituated to purchasing large quantities of cigarettes in advance. Additionally, cigarette-only users increased their attempts and willingness to quit smoking, compared with e-cigarette-only users.
Conclusions Owing to the COVID-19 outbreak, the intention to quit smoking was different among smokers, according to cigarette type (cigarette-only users, e-cigarette-only users and dual users). With the ongoing COVID-19 pandemic, policies and campaigns to increase smoking cessation intentions and attempts to quit smoking among smokers at high risk of COVID-19 should be implemented. Additionally, e-cigarette-only users with poor health-seeking behaviour require interventions to increase the intention to quit smoking.
- public health
- mental health
Data availability statement
All data relevant to the study are included in the article or uploaded as supplementary information.
This is an open access article distributed in accordance with the Creative Commons Attribution 4.0 Unported (CC BY 4.0) license, which permits others to copy, redistribute, remix, transform and build upon this work for any purpose, provided the original work is properly cited, a link to the licence is given, and indication of whether changes were made. See: https://creativecommons.org/licenses/by/4.0/ .
https://doi.org/10.1136/bmjopen-2021-055179
Statistics from Altmetric.com
Request permissions.
If you wish to reuse any or all of this article please use the link below which will take you to the Copyright Clearance Center’s RightsLink service. You will be able to get a quick price and instant permission to reuse the content in many different ways.
Strengths and limitations of this study
This study is the first to systematically review the literature regarding changes in smoking behaviours, cessation intentions and psychological states of smokers, based on the study period, country and age of participants during the COVID-19 pandemic.
According to the Risk of Bias Assessment Tool for Non-randomised Studies, three researchers cross-checked and evaluated the articles’ quality and excluded papers with a high risk of bias.
Using the backward snowballing method, screening for article selection was strictly performed by checking for any additional papers.
Since the articles collected for this systematic review were only cross-sectional designs, it was difficult to determine causality; therefore, only associations were presented.
Introduction
On 30 January 2020, the WHO declared COVID-19, an infectious disease of the respiratory tract characterised by SARS, 1 a public health emergency of international concern. 2 In 2020, a total of 350 594 336 cases of COVID-19 were reported, including 7 893 839 deaths. 3 4 Due to the pandemic, different standards and steps to control the spread of the disease have been adopted worldwide, but almost all countries have implemented lockdown and social distancing measures to prevent COVID-19 infection.
Smoking is a high-risk factor for COVID-19 infection, and many systematic reviews and meta-analyses report the association between COVID-19 and smoking. 5 6 Since tobacco is associated with chronic lung disease, physicians could expect more cigarette users to develop a severe form of COVID-19, 5 given the effect of tobacco on respiratory disease and immune function. 7 Smoking is associated with negative progression and side effects of COVID-19. 6 Smoking, including current or previous smoking history, significantly increases the severity and risk of death from COVID-19. 8 Similar to cigarette use, e-cigarette use can cause oxidative stress and inflammatory response in the lungs, making e-cigarette users more susceptible to bacterial or viral infections. 9 Although no literature indicates that cigarettes are the direct cause of COVID-19 infection, smokers have a higher risk of severe COVID-19 symptoms than non-smokers. 10 Previous studies have suggested several public health messages to continue focusing on smoking cessation efforts; however, smoking rates have not decreased. 11
Few investigations have focused on whether smoking behavior 12 and cessation efforts 13 have changed during the COVID-19 pandemic. Previous studies have shown that smoking has decreased 12 and quitting attempts have increased 13 during the pandemic. Since lockdowns and various social distancing measures were implemented in the early stages of the COVID-19 pandemic, they have the potential to affect smoking behaviour and cessation intention. In some countries, it is expected that smoking behaviour will change given the difficulty in freely purchasing tobacco products as non-essential items; individuals are locked down under the pandemic and are required to stay at home for prolonged periods. 14 Changes in daily life due to social distancing and lockdowns are expected to help reduce smoking consumption. 15 Therefore, it is necessary to examine the factors influencing smoking behaviour and cessation intention in the early stages of the pandemic, which have been investigated in the literature.
There are insufficient studies on smokers’ negative psychological states during the COVID-19 pandemic. Further research is needed because the relationship between negative psychological states and health behaviour is unclear. 16 People experience higher stress levels than usual because of social isolation, employment insecurity, finances uncertainty, responsibilities and concerns about illness from the virus. 17 Therefore, many cigarette users smoke to relieve stress and negative emotions, 18 but it is often counterproductive. 19 Previous research has shown that negative psychological states, such as stress, anxiety and depression, can influence tobacco-use behaviour. 20
There are insufficient studies to compare the smoking behaviour, cessation intention and psychological state of cigarette and e-cigarette users during the COVID-19 pandemic. 14 The daily use of e-cigarettes among e-cigarette users slightly increased during the COVID-19 lockdown 21 ; therefore, it is necessary to investigate whether smoking behaviour changes according to the cigarette type. Cigarette users are aware that they engage in harmful health behaviours. However, it is unclear whether e-cigarettes help cigarette users quit smoking. 22 E-cigarette users believe that e-cigarettes are less harmful to their health as they contain relatively fewer harmful ingredients than cigarettes. 23 Therefore, they believe that smoking e-cigarettes is an effort to quit smoking. 23
In addition, after the COVID-19 pandemic, smoking behaviour may change by age depending on the cigarette type. The use of e-cigarettes increases among persons in their early 20s compared with old persons. 24 In addition, it is known that older persons are more vulnerable to COVID-19 infection and can develop severe infection symptoms. 25 Therefore, there may be an increase in the older persons’ intention to quit smoking cigarettes. However, young people who use e-cigarettes may not be less willing to quit smoking.
Many studies have investigated whether smoking is a high-risk factor for COVID-19. 6 8 However, relatively few studies have been published on whether smoking behaviour, cessation intention and psychological state have changed during the COVID-19 pandemic. In the future, it is expected that this study will establish a scientific basis and policy response to smoking behaviour, cessation intention and psychological state based on cigarette type, both during and post COVID-19. Therefore, we conducted a systematic review based on the hypothesis that there would be changes in smoking behaviour, cessation intention and psychological states of adult smokers, depending on the tobacco product used during the COVID-19 pandemic.
Literature search strategy
We systematically reviewed the data according to the Preferred Reporting Items for Systematic Reviews and Meta-Analyses, shown in the Supplementary file. We searched articles using MeSH terms ‘COVID-19, depression, stress, psychological, anxiety, tobacco, tobacco products, tobacco use and electronic nicotine delivery system’ in January 2021. Only papers published between January and December 2020 were included in the study. All search terms are listed in online supplemental table 1 .
Supplemental material
A literature search was conducted on PubMed using the following MeSH terms: ‘(depression OR stress OR anxiety OR psychology) AND ((tobacco OR tobacco products OR tobacco use OR electronic nicotine delivery system) AND COVID-19))’ in the title or the abstract in 2020. A literature search was conducted on ScienceDirect using the following search terms: ‘COVID-19 AND (e-cigarette OR “electronic cigarette” OR “electronic nicotine delivery” OR “vaping” OR “heat not burn” OR “heated tobacco product”)’, as well as COVID-19 AND (tobaccos OR cigarette OR kretek OR bidis OR “pipe tobacco” OR cigarillos).
Inclusion and exclusion criteria
Figure 1 shows a flowchart of the database searches, followed by the exclusion/inclusion strategy. The inclusion criteria were cases that did not meet the exclusion criteria. In the first phase ( figure 1 ), certain types of articles were excluded, including comments, letters, editorials, viewpoints, correspondence and articles without full text. In addition, duplicate works and those not written in English and studies that did not include humans or adults were excluded. In the next phase ( figure 1 ), studies unrelated to tobacco or COVID-19 were excluded. In addition, articles were excluded if the topics were not related to changes in tobacco use and mental health caused by COVID-19. Finally, articles with a high risk of bias were excluded using the Risk of Bias Assessment Tool for Non-randomised Studies (RoBANS) ( figures 2 and 3 ). 26
- Download figure
- Open in new tab
- Download powerpoint
Flowchart diagram. The flowchart shows the article selection process, the criteria for exclusion of articles and the number of articles excluded.
Assessment of the risk of bias in identified studies using the RoBANS questionnaire. Author judgement for each RoBANS domain for the included articles. Green indicates a low risk of bias, yellow indicates unclear risk and red indicates a high risk of bias. The six domains are ‘comparability of groups, participant selection, confounding variables, measurement of exposure, blinding of the outcome, incomplete outcome data, selective outcome reporting’. RoBANS, Risk of Bias Assessment Tool for Non-randomised Studies.
Assessment summary of the risk of bias in identified studies using the RoBANS questionnaire. Author judgement for each RoBANS domain for the included articles. Green circles indicate low risk of bias, yellow circles indicate unclear risk and red circles indicate high risk of bias. The study numbers indicate the order of the articles presented in table 1 . RoBANS, Risk of Bias Assessment Tool for Non-randomised Studies.
Data extraction
Two independent reviewers (H-rC and J-eH) screened the literature and assessed each paper by reading the titles, abstracts and full texts. Three authors extracted data from studies that fulfilled our inclusion criteria independently (H-rC, J-eH and EC). The primary data points included were the following: study details (author, journal, publication date, country, study design, study period and funding), the total number of participants, types of cigarettes, changes in smoking behaviour, cessation intention and psychological state.
Two authors (H-rC and EC) independently assessed the quality of included studies using the RoBANS. 26 If the opinions of the two authors were different, the quality evaluation was completed through further discussion with another researcher (J-eH). The RoBANS contains six domains: selection of participants, confounding variables, measurement of exposure, blinding of outcome assessments, incomplete outcome data and selective outcome reporting ( figures 2 and 3 ). The risk of bias was divided into three stages (high, unclear or low risk). When the risk of bias in a study was high, it was excluded.
Using the backward snowballing method, 27 28 we checked the references of the articles that met the inclusion criteria. We checked the titles, journals, abstracts and full texts of the references of the articles. We excluded articles that did not meet the inclusion criteria. In addition, articles not related to the subject of this study were excluded. As a result, no additional papers were added.
Literature search and literature selection results
A total of 1659 papers were found in PubMed and ScienceDirect, and 905 non-original papers were excluded. Eleven papers without full text and four papers not written in English were excluded. In addition, 413 papers were selected, excluding 102 papers that were not conducted in humans, 114 papers that were not conducted in adults and 110 duplicate papers after the first screening phase. Papers unrelated to tobacco (n=200) and COVID-19 (n=21) were excluded. In addition, a total of 18 papers were included, excluding 166 papers that were not related to tobacco behaviour and psychological states that changed during the COVID-19 pandemic as well as eight papers targeting COVID-19 patients or medical personnel in the first screening phase.
Assessment result of the risk of bias
Thirteen papers were selected after excluding five papers with a high risk of bias, as determined through quality evaluation of the 18 papers using the RoBANS ( figures 2 and 3 ).
Characteristics of included papers by three subjects
All 13 selected papers were published in 2020; three were conducted in the USA, three in the UK, two in Italy, two in Turkey and one in China, India and Australia. All were cross-sectional design studies that used surveys. Comments, letters, editorials, viewpoints and correspondence were not included. In the included papers, the number of study participants varied from 93 to 52 002, and all participants were adults. We also presented the average age of the participants in the papers or the ratio by age group. Eight papers targeted cigarette-only users, and five reviewed e-cigarette and cigarette users together ( table 1 ). Table 1 shows that smokers’ smoking behaviour, cessation intentions and psychological states changed due to the COVID-19 pandemic, presented in all 13 papers. Seven papers studied changes in smoking behaviour, eight papers examined changes in smoking cessation and five papers examined changes in smokers’ psychological state after the COVID-19 outbreak ( table 1 ).
- View inline
Integrating results of the 13 selected papers
Changes in frequency of smoking occurred in the early stages of the COVID-19 pandemic, regardless of cigarette type. However, smoking cessation intentions and attempts were higher among cigarette-only users than e-cigarette-only users. When the average age of participants was presented, and only papers surveyed according to the cigarette type were examined, the smoking behaviour of cigarette-only users was found to increase in the paper with an average age of 22. In a study targeting participants on average in their 50s or older, cigarette-only users showed a higher intention to quit smoking than e-cigarette-only users. Regardless of the average age of participants, in most studies, the amount of e-cigarette-only users’ usage did not decrease, and the intention to quit smoking did not increase. In addition, it was found that all smokers, regardless of the tobacco product used, had increased stress and anxiety during the COVID-19 pandemic, and the more they did not intend to quit, the more stressed they were. We present detailed information on smoking behaviour, smoking cessation and psychological state changes according to cigarette type during the COVID-19 pandemic in the following six subheadings.
Changes in smoking behaviour in e-cigarette users (or dual users) during the COVID-19 outbreak
According to Soule et al , 29 the higher the reliance on e-cigarettes, the higher the incidence of cigarette use. There have also been increasing efforts to reduce e-cigarette use among dual users. However, mastery of e-cigarette-specific behaviours during the additional time spent at home improved e-cigarette skills and increased e-cigarette use. 29 According to the article by Caponnetto et al , 14 daily cigarette consumption by dual users of cigarettes and e-cigarettes and cigarette users decreased slightly. Due to the COVID-19 outbreak, purchasing products, including tobacco products, has changed; individuals buy large numbers of products at once.
Changes in smoking behaviour in cigarette-only users during the COVID-19 outbreak
According to the results of articles by Cancello et al 11 and Ren et al , 30 only cigarette consumption increased by 38% and 30%, respectively. Compared with before the COVID-19 pandemic, Chopra et al showed a significant decrease in smoking consumption (0.02 (0.03), p<0.05). 12 However, in the study by Ozcelik and Yilmaz Kara, 31 67.5% of participants reported no change in smoking behaviour, which cannot be interpreted as a significant change in smoking behaviour during the COVID-19 pandemic.
Changes in intentions and attempts to quit smoking among e-cigarette users (or dual users) during the COVID-19 outbreak
Tattan-Birch et al 13 found that a few cigarette-only users and e-cigarette-only users attempted to quit smoking due to the COVID-19 outbreak. Approximately, 1 in 10 current e-cigarette-only users reported an attempt to quit vaping because of COVID-19. Soule et al reported that users with high reliance on e-cigarettes exerted less effort to reduce product usage than users with low reliance. 29 According to a study by Caponnetto et al , while most cigarette-only users have considered quitting smoking, most e-cigarette-only users have not considered stopping e-cigarette use. 14 According to a study by Chertok et al , 36.7% of all smokers attempted to quit smoking during the pandemic regardless of cigarette type; people who quit smoking due to COVID-19 perceived smoking as a high-risk factor COVID-19 infection. 32
Changes in intentions and attempts to quit smoking among cigarette users during the COVID-19 outbreak
According to Jackson et al , 33 COVID-19 shutdowns have increased attempts to quit smoking. According to the results of the article by Kayhan Tetik et al , 34 which compared the success rates of smoking cessation before and during the pandemic, the COVID-19 outbreak effectively promoted smoking cessation. Only 12.8% of people who quit smoking during the COVID-19 outbreak did not restart smoking. According to Ozcelik and Yilmaz Kara, 31 people with coronaphobia exhibited a significantly higher decrease or cessation in smoking than those with no change in smoking behaviour or increased smoking consumption. A study of cigarette-only users found that the number of attempts to quit smoking had increased in the USA. 32 35 In the UK, all studies conducted on cigarette-only users found that attempts to quit smoking had increased. 13 36 In the case of cigarette-only users, there are more successful quitting smoking cases than e-cigarette-only users. 13
The association between smoking and psychological changes in e-cigarette users (or dual users) during the COVID-19 outbreak
According to Soule et al , the greater the dependence on e-cigarettes, the higher the concern about COVID-19 infection than those with low dependence. 29 In the article by Kowitt et al , the higher the awareness regarding the risk of contracting COVID-19, regardless of cigarette type, the greater the willingness to quit (B=0.38, p<0.001) and the attempt to quit smoking (OR: 1.31, 95% CI 1.04 to 1.64). 35 In addition, as social distancing (B=0.11, p=0.01) was enforced more strongly, the willingness to quit smoking increased. 35
The association between smoking and psychological changes in cigarette users during the COVID-19 outbreak
Stanton et al found that people with increased tobacco consumption had a higher risk of depression, anxiety and stress. 16 Jackson et al reported that compared with non-smokers, current and former cigarette-only users had increased stress from COVID-19. 37 Ozcelik et al indicated that among people with coronaphobia, those who reduced or stopped smoking felt more anxious than those who increased their smoking frequency. 31
We examined the changes in smoking behaviour, cessation intentions and psychological states caused by the COVID-19 outbreak in smokers (cigarette-only users, e-cigarette-only users and dual users). Regardless of the cigarette type, the amount of smoking either increased or decreased in some cases. However, compared with e-cigarette-only users, most cigarette-only users’ intentions and attempts to cease smoking increased. In addition, regardless of the type of cigarette, smokers showed a negative psychological state due to COVID-19. It was found that attempts and intentions to cease smoking increased as dependence on cigarettes decreased. Additionally, social distancing strengthened and awareness about COVID-19 increased.
The amount of smoking done by e-cigarette-only users increased with increased dependency. For dual users, those who use cigarettes and e-cigarettes, efforts to reduce product usage increased; however, there was a tendency to buy cigarettes in bulk, increase e-cigarette usage skills and increase their use. This is thought to result from a tendency to recognise that using e-cigarettes is relatively less harmful to health than smoking cigarettes. 23 According to previous studies, many e-cigarette users recognise that e-cigarettes are relatively less harmful to health, their own and others, 23 because they contain far fewer harmful components 38 than cigarettes. However, it is not yet clear how e-cigarettes can help cigarette users quit smoking. 22 For this reason, e-cigarette users should consider the risks of using e-cigarettes as much as cigarettes.
Cigarette-only users’ efforts to reduce product usage and quit smoking during the pandemic increased, especially when the average age group was high. In other words, the higher the age group, the higher the risk of COVID-19 infection and symptom severity. Therefore, it was found that the use of cigarettes decreased. 25 Efforts to reduce product usage by e-cigarette users, regardless of age, decreased. In particular, the higher the dependence of e-cigarette users, the higher were the worries related to COVID-19; however, efforts to reduce the amount of smoking were lower among users with low e-cigarette dependence. This could be because e-cigarette users think that using e-cigarettes is an attempt to quit smoking. 23 Therefore, e-cigarette users’ intentions to quit smoking have not increased, even during the pandemic. A cohort analysis conducted in the USA found that e-cigarette users did not quit smoking, compared with cigarette-only users, and more than half of e-cigarette users still used e-cigarettes a year later. 39 In another study, it was unclear whether e-cigarette users were more likely to quit smoking. 40 Since many studies are still being conducted on the cessation effect of e-cigarettes, it should be recognised that e-cigarette use could be harmful to health.
Regardless of the cigarette type, the higher the awareness of the risks associated with contracting COVID-19, the higher the level of social distancing since the start of COVID-19 and intent to quit smoking. In the case of cigarette-only users, coronaphobia increased smoking cessation intentions. Among them, those who reduced smoking felt more anxious than those who increased their amount of smoking. Smokers were more likely to quit after understanding the health risks of smoking due to the ‘vulnerability hypothesis’, wherein smokers who care about their health would be inclined to quit smoking when they know it makes them vulnerable to certain diseases. 41 42 This presents the same context as the results of this systematic review.
In contrast, previous studies have shown that smokers have higher levels of depression, anxiety, stress and increased smoking amounts. According to a study by Klemperer et al , 43 28.3% of patients reduced their tobacco use due to fear of infectious diseases, while 30.3% showed increased tobacco use and anxiety levels in both groups. However, in the literature surveyed in this study, it was found that the higher the level of anxiety, the higher the attempt to quit smoking. Therefore, further research exploring the relationship between mental health and smoking behaviours during the COVID-19 pandemic using standardised tools to measure mental health conditions, such as anxiety, is needed.
Increased awareness of COVID-19 risk has led to increased smoking cessation attempts, which can be considered a desirable outcome for physical health in the long run. However, for mental health, including anxiety, depression and increased stress during the pandemic, governments’ response to address the increasing trend of smoking should be to conduct campaigns for risk awareness to protect their citizens’ mental health. Research on the association between cigarette smoking and COVID-19 increases; the WHO also suggests that smokers may be considered at high risk of contracting COVID-19. However, because of the lack of research on the relationship between the use of liquid e-cigarettes and COVID-19, the willingness or attempts to quit e-cigarette use has not increased compared with cigarette-only users or dual users. Therefore, this study was able to review only cross-sectional studies. As smokers have a high risk of contracting certain diseases, both during and after the COVID-19 pandemic, further studies on the risk of e-cigarettes and their impact on smoking cessation, especially regarding COVID-19 infection, should be conducted.
This study is the first to systematically review the literature regarding changes in smoking behaviours, cessation intentions and psychological states of smokers, based on the study period, country and age of participants during the COVID-19 pandemic. Since this study aimed to investigate people’s smoking behaviour, smoking cessation and psychological state in the early stages of the COVID-19 pandemic in 2020, all studies used questionnaire tools. There were no articles that had conducted qualitative or interventional studies on this topic. Therefore, only cross-sectional studies were included. This study only systematically reviewed articles published between January and December 2020. According to the results of this study, as social distancing and lockdown were strengthened in the early stages of the pandemic, smoking behaviour, smoking cessation intention and psychological changes appeared. However, in 2021, as vaccinations began to occur worldwide, social distancing decreased, as did people’s willingness to participate in social distancing and stay-at-home. 44 Accordingly, smoking behaviour, smoking cessation and psychological status in 2021 may differ from that in 2020. When reviewing articles published in 2021, it is necessary to examine the changes in smoking behaviour, smoking cessation and psychological state of smokers while considering vaccine variables.
However, it is meaningful that this study looked at smoking usage behaviour during the COVID-19 pandemic based on cigarette type. This study could serve as primary data to establish effective strategies that focus on specific tobacco-product users by suggesting different smoking behaviours, smoking cessation intentions and psychological changes based on cigarette type.
In this study, we examined changes in smoking behaviours, smoking cessation intentions and psychological states of smokers during COVID-19 according to cigarette type. We conducted a systematic review of 13 papers to suggest future research and policy directions that the government may implement for COVID-19 and smokers. During the COVID-19 pandemic, cigarette-only users’ consumption did not change significantly, but dual users and e-cigarette-only users with a high dependence on e-cigarettes showed increased consumption. Cigarette users’ quit intentions, attempts and cessation success rates increased, and even those with coronaphobia showed increased intent to quit smoking. On the other hand, users of e-cigarettes demonstrated fewer attempts to quit. As the COVID-19 pandemic continues, policies and campaigns should be implemented to increase the intent and attempt to stop smoking. Research and interventions on COVID-19, smoking and e-cigarette use should also be conducted in the medium to long term.
Ethics statements
Patient consent for publication.
Not applicable.
Ethics approval
- Le Faou A-L , et al
- Organization WH
- Our World in Data
- Vardavas C ,
- Umnuaypornlert A ,
- Kanchanasurakit S ,
- Lucero-Prisno DEI , et al
- Sladewski K , et al
- Lungarella G ,
- Cancello R ,
- Soranna D ,
- Zambra G , et al
- Singh V , et al
- Tattan-Birch H ,
- Jackson S , et al
- Caponnetto P ,
- Inguscio L ,
- Saitta C , et al
- Stanton R ,
- Khalesi S , et al
- Fancourt D ,
- ↵ Collision of the COVID-19 and addiction epidemics. American College of physicians . Ann Intern Med 2020 . doi:10.7326/M20-1212
- García-Álvarez L ,
- Fuente-Tomás LDla ,
- Adriaens K ,
- Van Gucht D ,
- Etter J-F ,
- Schroeder R ,
- Jensen JW , et al
- Garibaldi BT , et al
- Lee YJ , et al
- Greenhalgh T ,
- Snipes W , et al
- Li Z , et al
- Ozcelik N ,
- Yilmaz Kara B
- Chertok IRA
- Jackson SE ,
- Garnett C ,
- Kayhan Tetik B ,
- Tekinemre G ,
- Kowitt SD ,
- Cornacchione Ross J ,
- Jarman KL , et al
- Shahab L , et al
- Goniewicz ML ,
- Gawron M , et al
- Pierce JP ,
- Benmarhnia T ,
- Chen R , et al
- Pasquereau A ,
- Guignard R ,
- Andler R , et al
- Borrelli B ,
- Dunsiger S , et al
- Weinstein ND
- Klemperer EM ,
- Peasley-Miklus C , et al
- Andersson O ,
- Campos-Mercade P ,
- Meier AN , et al
- Tekinemre IG ,
Supplementary materials
Supplementary data.
This web only file has been produced by the BMJ Publishing Group from an electronic file supplied by the author(s) and has not been edited for content.
- Data supplement 1
Contributors H-rC designed the study, analysed and interpreted the data, and wrote the manuscript. J-eH and EC contributed to the study design and interpretation of the results. H-rC and J-eH took full responsibility for the study and access to all data and controlled the decision to publish.
Funding This study was supported by the National Research Foundation of Korea (NRF) grant, funded by the Ministry of Science and ICT (MSIT) (Number 2020R1C1C1012562).
Competing interests None declared.
Patient and public involvement Patients and/or the public were not involved in the design, or conduct, or reporting, or dissemination plans of this research.
Provenance and peer review Not commissioned; externally peer reviewed.
Supplemental material This content has been supplied by the author(s). It has not been vetted by BMJ Publishing Group Limited (BMJ) and may not have been peer-reviewed. Any opinions or recommendations discussed are solely those of the author(s) and are not endorsed by BMJ. BMJ disclaims all liability and responsibility arising from any reliance placed on the content. Where the content includes any translated material, BMJ does not warrant the accuracy and reliability of the translations (including but not limited to local regulations, clinical guidelines, terminology, drug names and drug dosages), and is not responsible for any error and/or omissions arising from translation and adaptation or otherwise.
Read the full text or download the PDF:
Click through the PLOS taxonomy to find articles in your field.
For more information about PLOS Subject Areas, click here .
Loading metrics
Open Access
Peer-reviewed
Research Article
Associations between smoking to relieve stress, motivation to stop and quit attempts across the social spectrum: A population survey in England
Roles Conceptualization, Data curation, Formal analysis, Investigation, Methodology, Project administration, Writing – original draft, Writing – review & editing
* E-mail: [email protected]
Affiliation Research Department of Behavioural Science and Health, University College London, London, United Kingdom

Roles Conceptualization, Writing – review & editing
Roles Conceptualization, Funding acquisition, Writing – review & editing
- Olga Perski,
- Maria Theodoraki,
- Sharon Cox,
- Loren Kock,
- Lion Shahab,
- Jamie Brown
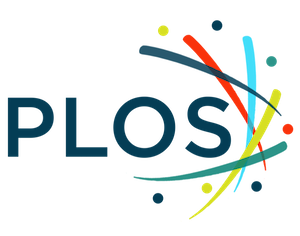
- Published: May 17, 2022
- https://doi.org/10.1371/journal.pone.0268447
- Peer Review
- Reader Comments
Smoking prevalence in several high-income countries is steadily declining but remains persistently high in ‘lower’ socioeconomic position (SEP) groups, contributing to inequities in morbidity and mortality. Smoking to relieve stress is a commonly endorsed motive for continued smoking; however, it remains unclear whether smoking to relieve stress has a negative impact on motivation to stop and future quit attempts and if so, whether associations are moderated by SEP. This was an observational study with cross-sectional and prospective survey data from the nationally representative Smoking Toolkit Study in England. A total of 1,135 adult smokers were surveyed at baseline, with 153 (13.5%) respondents followed up at 12 months. Respondents provided information on demographic, social and smoking characteristics. A series of multivariable logistic regression analyses was conducted. Bayes Factors (BFs) were calculated to explore non-significant associations. Smoking to relieve stress was commonly endorsed by respondents from both ‘lower’ (43.2% [95% CI = 39.4%, 47.0%]) and ‘higher’ (40.5% [95% CI = 35.9%, 45.1%]) SEP groups ( p = 0.39). Smoking to relieve stress was associated with high motivation to stop at baseline (OR adj = 1.48, 95% CI = 1.03–2.12, p = 0.035) but not significantly with the odds of making a quit attempt at a 12-month follow-up, although the magnitude and direction of the effect was similar to that observed for high motivation to stop (OR adj = 1.49, 95% CI = 0.69–3.20, p = 0.3). Data were insensitive to detect moderation effects of SEP (BF = 0.90 and BF = 1.65, respectively). Smoking to relieve stress is a commonly endorsed motive and is associated with high motivation to stop but not significantly with the odds of making a quit attempt in the next 12 months, although the magnitude and direction of the effect was similar for both outcomes. There was no clear evidence of moderation by SEP, although data were insensitive to distinguish the alternative from the null hypothesis.
Citation: Perski O, Theodoraki M, Cox S, Kock L, Shahab L, Brown J (2022) Associations between smoking to relieve stress, motivation to stop and quit attempts across the social spectrum: A population survey in England. PLoS ONE 17(5): e0268447. https://doi.org/10.1371/journal.pone.0268447
Editor: Jesse T. Kaye, University of Wisconsin-Madison, UNITED STATES
Received: June 4, 2021; Accepted: April 29, 2022; Published: May 17, 2022
Copyright: © 2022 Perski et al. This is an open access article distributed under the terms of the Creative Commons Attribution License , which permits unrestricted use, distribution, and reproduction in any medium, provided the original author and source are credited.
Data Availability: The dataset underpinning the analyses is available via Figshare: https://figshare.com/articles/dataset/Associations_between_smoking_to_relieve_stress_motivation_to_stop_and_future_quit_attempts_across_the_social_spectrum_A_population_survey_in_England/14731029 .
Funding: Cancer Research UK is the main contributor (C1417/A22962), but the UK Department of Health, Pfizer, GlaxoSmithKline and Johnson and Johnson have also all contributed funding to the data collection for the Smoking Toolkit Study. The Society for the Study of Addiction and the National Institute for Health Research’s School for Public Health Research have contributed funding to the data collection for the Alcohol Toolkit Study. JB, LS, OP, SC and LK are members of SPECTRUM, a UK Prevention Research Partnership Consortium (MR/S037519/1). UKPRP is an initiative funded by the UK Research and Innovation Councils, the Department of Health and Social Care (England) and the UK devolved administrations, and leading health research charities. The funders had no final role in the study design; in the collection, analysis and interpretation of data; in the writing of the report; or in the decision to submit the paper for publication. All researchers listed as authors are independent from the funders and all final decisions about the research were taken by the investigators and were unrestricted.
Competing interests: OP, MT, SC and LK have no conflicts of interest to declare. LS has received a research grant and honoraria for a talk and travel expenses from manufacturers of smoking cessation medications (Pfizer and Johnson & Johnson). JB has received unrestricted research funding from Pfizer to study smoking cessation. This does not alter our adherence to PLOS ONE’s policies on sharing data and materials.
Introduction
Tobacco smoking remains one of the leading causes of premature illness and death, with global smoking-related deaths approximating 8 million people per year in 2019 [ 1 ]. Smoking prevalence in a number of high-income countries, including England, has been steadily declining for the past decade but remains persistently high in ‘lower’ socioeconomic position (SEP) groups [ 2 ]. In England, ‘lower’ SEP is associated with increased morbidity and mortality, of which smoking is a significant contributor [ 3 ]. To help reduce observed health inequities between ‘higher’ and ‘lower’ SEP groups [ 4 ], supporting people to stop smoking–particularly those from priority SEP groups–is urgently needed. The development of effective policies and interventions requires the identification of key barriers to smoking cessation, such as beliefs or motives that hinder smoking cessation attempts.
Motivational factors, including desire or intention to stop, have been identified as key predictors of smoking cessation attempts [ 5 ]. Although longitudinal studies show that smoking cessation (as compared with continued smoking) is associated with reduced stress, anxiety and depression–with equal improvements to mental wellbeing observed in adults with and without a mental health diagnosis [ 6 ]–approximately 40% of smokers in England indicate that they smoke to cope with stress or anxiety [ 7 ]. However, it remains unclear whether smoking to relieve stress undermines motivation to stop and subsequent quit attempts. According to the PRIME (Plans, Responses, Impulses, Motives, Evaluations) Theory of Motivation [ 8 ], plans to stop smoking influence behaviour only if they generate sufficiently strong impulses at relevant moments in time. However, competing motives (including the belief that smoking helps to relieve stress) may act to undermine both plans and subsequent quit attempts by generating strong, opposing impulses. It is therefore plausible that the belief that smoking relieves stress may lead to reduced motivation to stop or it may undermine intentions to stop by generating strong, competing impulses which contribute to continued smoking. Previous research has examined whether the belief that one is addicted to smoking (‘perceived addiction’) undermines motivation to stop and future quit attempts. However, contrary to predictions, perceived addiction to smoking was recently found to be positively associated with motivation to stop [ 9 ] and future quit attempts [ 10 ].
SEP refers to social and economic factors that influence the position that individuals inhabit within the structures of society, and which in turn influence their health [ 11 ]. These factors typically include (but are not limited to) income, educational attainment, home ownership/tenure, occupation and employment status. The term ‘lower’ SEP is used in this study to reflect the relatively average lower income and status of those in routine and manual occupational trades and those who are unemployed. Smokers from lower SEP groups face multiple environmental and social stressors, including financial and occupational hardship and instability, as well as poor physical and mental health [ 11 ]. They also face smoking-specific stressors such as a lack of social support and higher nicotine dependence (compared with smokers from more advantaged groups) [ 12 , 13 ], which means that negative affect from withdrawal is likely experienced as more intense and stressful, also highlighting the role of negative affect control through continued smoking [ 14 ].
Several qualitative studies have highlighted the role of smoking to cope with stress in particular subgroups of smokers, including adults experiencing homelessness [ 15 ], smokers living with psychiatric conditions [ 16 ], heavy smokers [ 17 ] and older smokers [ 18 ]. However, it remains unclear whether smoking to relieve stress is more commonly endorsed in smokers from lower (compared with higher) SEP groups, but it is plausible given the frequent occurrence of high stress levels in lower SEP groups, coupled with the widely held belief that smoking relieves stress. In addition, the belief that smoking is beneficial for stress is likely weighed against perceived benefits of quitting, such as improved health and financial savings (which could help alleviate financial hardship) [ 19 ]. It is therefore important to examine not only whether smoking to relieve stress has a negative impact on motivation to stop and subsequent attempts to quit, but also whether these associations are moderated by SEP. Recent research indicates that although motivation to stop appears similar by housing tenure–a reliable indicator of SEP and predictor of smoking status [ 20 ]–and prevalence of past-year quit attempts are higher in adults living in homes that belong to a housing association or are rented from local authority (compared with other housing), people from lower SEP groups are less likely to succeed in quitting [ 21 ].
We therefore aimed to examine, in the nationally representative Smoking Toolkit Study, the associations of smoking to relieve stress with motivation to stop (measured at baseline) and quit attempts at a 12-month follow-up in England, and whether these associations are moderated by SEP. Specifically, we aimed to address the following research questions:
- Do smokers who indicate that they smoke to relieve stress have reduced odds of high (vs. low) motivation to stop smoking, without and with adjustment for age, sex, SEP, children in the household, cigarettes smoked per day and number of quit attempts in the past year?
- Do smokers who indicate that they smoke to relieve stress have reduced odds of making a quit attempt at a 12-month follow-up, without and with adjustment for age, sex, SEP, children in the household, cigarettes smoked per day and number of quit attempts in the past year?
- Does SEP moderate any associations between smoking to relieve stress and a) high motivation to stop and b) quit attempts at a 12-month follow-up, without and with adjustment for age, sex, SEP, children in the household, cigarettes per day and number of quit attempts in the past year?
Study design and setting
This was an observational study involving cross-sectional and prospective survey data. The STROBE guidelines were used in the design and reporting of this study [ 22 ].
The study is part of the ongoing Smoking Toolkit Study (STS), which involves monthly, face-to-face, computer-assisted household surveys of adults aged 16+ in England [ 23 , 24 ]. The sample is a hybrid of a random probability and quota sample, which results in a sample that is representative of the adult population of smokers in England. Interviews are held with one household member in selected geographic output areas until quotas are fulfilled. The quotas are based on factors influencing the probability of being at home (i.e. working status, age and gender). This hybrid form of random probability and quota sampling is considered superior to conventional quota sampling. Here, the choice of households to approach is limited by the random allocation of small output areas and rather than being sent to specific households in advance, interviewers can choose which households within these small geographic areas are most likely to fulfil their quotas. Therefore, unlike random probability sampling, it is not appropriate to record the response rate in the STS. Data included in the present study were collected across four waves (139–142) from respondents surveyed between April and July 2018 (when smoking to relieve stress was included in the STS). Attempts to recontact consenting past-year smokers via telephone are made 12 months later. Follow-up respondents are not remunerated. Up to eight attempts are made to follow up each consenting respondent, with calls made at different times of day across weekdays and weekends.
Initial analyses were conducted as part of a student project. An extended study protocol and analysis plan were pre-registered on the Open Science Framework prior to conducting the analyses ( https://osf.io/efbn2 ).
Inclusion criteria
Respondents were included in the analyses if they were aged 16+ years and smoked cigarettes at the time of the baseline survey. Respondents who indicated that they did not smoke cigarettes but did smoke tobacco of some kind were not included (~0.4%) as they do not complete equivalent measures of dependence (e.g. cigarettes per day). Respondents with missing data on any of the variables of interest were excluded from the analyses.
Ethics statement
Ethical approval was granted by UCL’s Research Ethics Committee (0498/001). Respondents provided verbal informed consent prior to each interview.
Outcome variables.
The first outcome variable was motivation to stop, measured at baseline with the validated Motivation To Stop Scale [ 25 , 26 ]. Respondents are asked: “Which of the following best describes you?” Response options include: 1) I don’t want to stop smoking; 2) I think I should stop smoking but don’t really want to; 3) I want to stop smoking but haven’t thought about when; 4) I REALLY want to stop smoking, but I don’t know when I will; 5) I want to stop smoking and hope to soon; 6) I REALLY want to stop smoking and intend to in the next 3 months; 7) I REALLY want to stop smoking and intend to in the next month. To aid interpretation, responses were dichotomised into low (response options 1–5) and high (response options 6–7) motivation to stop, as previously done in studies using this variable [ 27 , 28 ].
The second outcome variable was quit attempts at a 12-month follow-up, measured with a single item: “How many serious attempts to stop smoking have you made in the last 12 months? By serious attempt I mean you decided that you would try to make sure you never smoked again.” Responses were coded 0 for respondents who reported no quit attempt and 1 for those who reported 1+ quit attempts.
Explanatory variable.
The explanatory variable was smoking to relieve stress, measured at baseline with a single item asking respondents to select from a list the motives that they believe are important in keeping them smoking. Those endorsing the response option “It helps me cope with stress or anxiety” were coded as 1, the rest as 0.
Covariates.
Covariates measured at baseline included: age (16–24 years, 25–34 years, 35–44 years, 45–54 years, 55–64 years, 65+ years); sex (male, female); SEP, operationalised as occupational social grade and measured with the British National Readership Survey’s Social Grade Classification Tool [ 29 ], which comprises the categories AB (higher and intermediate managerial, administrative and professional), C1 (supervisory, clerical and junior managerial, administrative and professional), C2 (skilled manual workers), D (semi-skilled and unskilled manual workers) and E (state pensioners, casual and lowest-grade workers, unemployed with state benefits), with responses grouped into ‘lower’ (C2DE) and ‘higher’ (ABC1) social grade; children in the household (no vs. yes); cigarettes per day (converted to daily consumption for non-daily smokers who reported the number of cigarettes smoked per week); and number of quit attempts in the past year (0, 1, 2, 3, 4+).
We also captured housing tenure as an alternative indicator of SEP [ 20 ]. Respondents whose homes belonged to a housing association or were rented from local authority were categorised as ‘lower’ SEP. All other responses (i.e. houses which were ‘bought on a mortgage’, ‘owned outright by household’, ‘rented from private landlord’, ‘other’) were categorised as ‘higher’ SEP.
Data analysis
Analyses were conducted in rstudio v.1.2.5033..
Data were weighted with the rim (marginal) technique [ 30 ] to match the analytic sample to the proportions of the English population profile on the dimensions of age, social grade, region, housing tenure, ethnicity and working status within sex. We report descriptive statistics for the baseline and follow-up samples. Any differences between the baseline and follow-up samples were assessed with Chi-square or t -tests, as appropriate.
To address RQ1 and RQ2, unweighted logistic regression analyses were conducted, without and with adjustment for all covariates. To address RQ3, the analyses were repeated, including the two-way interaction between smoking to relieve stress and SEP. As a planned sensitivity analysis, the analyses were repeated with housing tenure used as the indicator of SEP [ 20 ].
Bayes Factors.
In the event of non-significant interactions, we had planned to calculate Bayes Factors (using an online calculator; www.bayesfactor.info ) to examine whether the non-significant associations could best be characterised as evidence of no association, evidence of an association, or whether the data were insensitive to detect an association. As there was limited evidence to inform what effect sizes to expect for the potential interactions with SEP, we set the expected effect sizes to OR = 0.7, informed by previous research examining the association between enjoyment of smoking and future quit attempts [ 31 ]. The alternative hypothesis was conservatively represented by a half-normal distribution. Bayes Factors (BFs) can be interpreted as follows: BFs >3 lend support to the alternative hypothesis (over the null), BFs <1/3 lend support to the null, and BFs in-between reflect data being insensitive to distinguish the two.
A total of 1,203 cigarette smokers were surveyed between April and July 2018, of whom 1,135 (94.3%; unweighted) had complete data on all variables of interest. A total of 153 (13.5%; unweighted) respondents provided data at the 12-month follow-up (see Table 1 ). Compared with the baseline sample, those responding to the 12-month follow-up survey were older ( p < 0.001), more likely to be from higher SEP groups ( p < 0.001) and less likely to have children in the household ( p = 0.02). At baseline, a total of 43.2% (95% CI = 39.4%, 47.0%) of respondents from lower SEP groups indicated that they smoke to relieve stress, compared with 40.5% (95% CI = 35.9%, 45.1%) of respondents from higher SEP groups ( p = 0.39).
- PPT PowerPoint slide
- PNG larger image
- TIFF original image
https://doi.org/10.1371/journal.pone.0268447.t001
In unadjusted and adjusted analyses without the interaction, smoking to relieve stress was associated with 48% greater odds of high motivation to stop (OR adj = 1.48, 95% CI = 1.03–2.12, p = 0.035; see Table 2 ). The association between smoking to relieve stress and quit attempts at the 12-month follow-up was not significant (OR adj = 1.49, 95% CI = 0.69–3.20, p = 0.3). The adjusted associations between the exposure and outcome variables were unchanged in the sensitivity analyses with housing tenure (see S1 Table ). In an unplanned sensitivity analysis with cigarettes per day coded as a categorical variable, the associations were similar (see S2 Table ).
https://doi.org/10.1371/journal.pone.0268447.t002
In unadjusted and adjusted analyses, the two-way interactive effects of smoking to relieve stress and SEP on high motivation to stop (OR adj = 1.66, 95% CI = 0.14–24.3, p = 0.7) and quit attempts at the 12-month follow-up (OR adj = 0.31, 95% CI = 0.1–1.4, p = 0.12) were not significant (see S3 and S4 Tables for the ORs stratified by SEP). Results remained non-significant in the planned sensitivity analyses with housing tenure (OR adj = 0.34, 95% CI = 0.03–4.31, p = 0.4 and OR adj = 0.72, 95% CI = 0.14–3.8, p = 0.7, respectively).
Bayes Factors (BFs)
The calculation of BFs indicated that the data on the two-way interactive effects of smoking to relieve stress and SEP on high motivation to stop and quit attempts at the 12-month follow-up were insensitive to detect an association (BF = 0.90 and BF = 1.65, respectively). As an unplanned sensitivity analysis, we also calculated a BF for the non-significant association between smoking to relieve stress and quit attempts at the 12-month follow-up using the same prior as the above analyses, which indicated that data were insensitive to detect an association (BF = 0.46).
Principal findings
This study aimed to examine whether smoking to relieve stress is associated with high motivation to stop at baseline and quit attempts at a 12-month follow-up, and whether any associations are moderated by SEP. Contrary to expectations, we found that smoking to relieve stress was associated with high motivation to stop smoking, but not significantly with the odds of making a quit attempt at the 12-month follow-up. Although non-significant, the direction and magnitude of the association of smoking to relieve stress with future quit attempts were similar to those observed for the association with high motivation to stop. We did not observe significant moderation by SEP; however, the Bayes Factors indicated that data were insensitive to detect an association (as opposed to lending support to the null hypothesis of no association). For this to be known, other studies with larger samples need to be conducted to detect any potential moderation effects by SEP.
The finding that smoking to relieve stress is associated with high (as opposed to low) motivation to stop is consistent with previous research indicating that the belief that one is addicted to smoking is positively associated with motivation to stop [ 9 ]. There are several plausible explanations for this finding. First, people who smoke to relieve stress may evaluate their smoking experience as biological or instrumental (as opposed to pleasurable), which may be perceived as unpleasant or annoying, hence leading to greater motivation to stop. Second, those who smoke to relieve stress may find smoking enjoyable but at the same time, find it a source of dissonance to use smoking as a ‘crutch’ to cope with stress, hence leading to greater motivation to stop. Third, it is also possible that there is a common factor (e.g., depression) that gives rise both to the belief that smoking helps to relieve stress and high motivation to stop. Previous research has found that smokers with more severe (compared with less severe) levels of depression report lower quitting self-efficacy (which may be influenced by positive outcome expectations, such as the belief that smoking helps to cope with stress) but higher motivation to stop [ 32 ]. Fourth, as both smoking to relieve stress and motivation to stop were measured at the same time, there is potential for reverse causation, whereby those highly motivated to stop are also more likely to identify motives for smoking that some people may judge negatively (e.g. they may judge it unpleasant to feel that smoking is needed to relieve stress).
The finding that smokers from both higher and lower SEP groups endorse the belief that smoking helps to relieve stress may indicate that smokers are not only more/less likely to endorse this belief depending on their level of actual stressors (which may, on average, be higher in smokers from lower SEP groups). Instead, this may be related to people’s perceived ability to cope with stressors. Smokers across the social spectrum may perceive themselves to be better/worse equipped to cope with stress (irrespective of whether they actually are), thus influencing their beliefs about the role of smoking (e.g. positive outcome expectancies).
Strengths and limitations
This study was strengthened by examining associations in a nationally representative sample of smokers in England. To our knowledge, this was the first study to examine the associations of smoking to relieve stress, motivation to stop and future quit attempts in addition to differences in smoking to relieve stress by SEP. However, our study also had several limitations. First, the sample size for the prospective association of smoking to relieve stress and future quit attempts was small, which led to imprecision. However, this was pre-determined by the period for which the funding was available (i.e., the question about smoking motives–including smoking to relieve stress–was included only in a few STS waves), and we attempted to mitigate this by calculating Bayes Factors to elucidate whether the data provided evidence of no association or whether they were insensitive to detect an association. Second, the follow-up sample differed from the baseline sample on important characteristics, including SEP. This means that, had our findings pertaining to the follow-up sample been statistically significant, they may not be generalisable to the general population of smokers. In addition, if those who were more likely to be followed up (i.e. older smokers, men, those from higher SEP groups) were also more or less likely to endorse the belief that they smoke to relieve stress, this may have distorted any association with the outcome. Third, although the Smoking Toolkit Study is a nationally representative survey on key sociodemographic and smoking characteristics, it may not be representative with regards to psychological characteristics such as motivation to stop or the motive that smoking helps to relieve stress and it also may not reach smokers who are the most disadvantaged. Similar to the previous point about the follow-up sample, this may mean our findings are not generalisable to the general population of smokers. Fourth, the survey during this study did not collect data on perceived stress levels; this would be a useful variable to consider in future research. Fifth, smokers may not have recalled (and reported) all quit attempts made in the 12 months preceding the follow-up survey [ 33 , 34 ]. Sixth, the analyses pertaining to motivation to stop were cross-sectional and therefore the direction of the association is unclear.
Implications for research, policy and practice
Future research to unpick the potential explanations for the positive association between smoking to relieve stress and high motivation to stop would benefit from deploying qualitative methods and/or Ecological Momentary Assessments in smokers’ daily lives, allowing a closer examination of the temporal dynamics of competing (or synergistic) motives, evaluations and impulses [ 8 , 35 ]. Further understanding of the relationship between smoking to relieve stress and future quit attempts is also required in studies with larger sample sizes. As many quit attempts are, on average, needed before quitting smoking successfully [ 36 ], a positive relationship between smoking to relieve stress and making a quit attempt in the future would likely have a positive impact on public health.
The finding that smoking to relieve stress is a commonly endorsed motive in smokers from both lower and higher SEP groups indicates that public health interventions may benefit from tackling such misperceptions about smoking and stress relief, with evidence from a recent systematic review and meta-analysis of longitudinal studies indicating that smoking cessation (compared with continued smoking) is associated with reduced stress, anxiety and depression [ 6 ]. Although the finding that smoking to relieve stress is associated with high motivation to stop (and possibly the likelihood of making a quit attempt in the future) may be perceived as a positive finding that does not warrant intervention, it may be more ethically defensible to highlight to smokers the large body of work indicating that smoking cessation leads to positive mental health outcomes and use this as a motive for stopping, thus correcting common misperceptions. In addition, if smoking is normatively perceived as a stress reducer, then it may encourage people to take up smoking. This merits further investigation.
At the same time (although not measured in the present study), research shows that many mental health professionals believe that smoking helps to alleviate stress and therefore refrain from offering appropriate smoking cessation support to their clients [ 37 ]. This suggests that the tackling of common misperceptions also needs to occur among practitioners. Public health campaigns have often focused on the well-documented physical health aspects of smoking, with less attention paid to the mental health benefits of quitting, including reduced stress, anxiety and depression [ 6 ] but also increased levels of happiness [ 38 , 39 ]. With increasing evidence for how to best counter misperceptions without inadvertently reinforcing them in the areas of vaccine hesitancy and climate change (e.g. normative feedback, framing messages in terms of gain) [ 40 – 42 ], interventions targeting healthcare professionals may benefit from drawing on this growing literature.
Finally, prior research shows that although smokers from lower SEP groups try to quit at a similar rate to smokers from higher SEP groups, they are less likely to succeed [ 43 ]. It is plausible that people from lower SEP groups endorse a confluence of motivational, cognitive and social factors for continued smoking (e.g. smoking to relieve stress and boredom, lower quitting self-efficacy, social aspects of smoking), which may interact to make it more difficult to quit [ 44 ]. Future research should examine the potential clustering of such factors and whether certain patterns are more pronounced in lower (compared with higher) SEP groups, as this is needed to help reduce smoking-related health inequalities.
Smoking to relieve stress is a commonly endorsed motive and is associated with high motivation to stop but not significantly with the odds of making a future quit attempt, although the magnitude and direction of the effect was similar for both outcomes. There was no clear evidence of moderation by SEP, although data were insensitive to distinguish the alternative from the null hypothesis.
Supporting information
S1 table. unadjusted and adjusted odds ratios for the associations of smoking to relieve stress with i) high motivation to stop and ii) quit attempts in the next 12 months in the planned sensitivity analysis with housing tenure..
https://doi.org/10.1371/journal.pone.0268447.s001
S2 Table. Unadjusted and adjusted odds ratios for the associations of smoking to relieve stress with i) high motivation to stop and ii) quit attempts in the next 12 months in the unplanned sensitivity analysis with cigarettes per day coded as a categorical variable.
https://doi.org/10.1371/journal.pone.0268447.s002
S3 Table. Unadjusted and adjusted odds ratios for the associations of smoking to relieve stress with i) high motivation to stop and ii) quit attempts in the next 12 months in the higher SEP group.
https://doi.org/10.1371/journal.pone.0268447.s003
S4 Table. Unadjusted and adjusted odds ratios for the associations of smoking to relieve stress with i) high motivation to stop and ii) quit attempts in the next 12 months in the lower SEP group.
https://doi.org/10.1371/journal.pone.0268447.s004
Acknowledgments
We gratefully acknowledge the funding listed.
- View Article
- Google Scholar
- PubMed/NCBI
- 8. West R, Brown J. Theory of Addiction. 2nd ed. Wiley-Blackwell; 2013.
- 29. National Readership Survey. Social Grade. Available: http://www.nrs.co.uk/nrs-print/lifestyle-and-classification-data/social-grade/
Log in using your username and password
- Search More Search for this keyword Advanced search
- Latest content
- Current issue
- Hosted content
- BMJ Journals More You are viewing from: Google Indexer
You are here
- Online First
- Association of time spent on social media with youth cigarette smoking and e-cigarette use in the UK: a national longitudinal study
- Article Text
- Article info
- Citation Tools
- Rapid Responses
- Article metrics

- http://orcid.org/0000-0003-3235-0454 Nicholas S Hopkinson 1 ,
- http://orcid.org/0000-0002-6187-0638 Charlotte Vrinten 2 ,
- http://orcid.org/0000-0002-4385-2153 Jennie C Parnham 2 ,
- Márta K Radó 3 ,
- http://orcid.org/0000-0002-2101-2559 Filippos Filippidis 2 ,
- Eszter P Vamos 2 ,
- http://orcid.org/0000-0003-1318-8439 Anthony A Laverty 2
- 1 National Heart and Lung Institute , Imperial College London , London , UK
- 2 Department of Primary Care and Public Health , Imperial College London School of Public Health , London , UK
- 3 Department of Medical Epidemiology and Biostatistics, Karolinska Institutet , Stockholm , Sweden
- Correspondence to Dr Anthony A Laverty, Department of Primary Care and Public Health, Imperial College London School of Public Health, London, W6 8RP, UK; a.laverty{at}imperial.ac.uk
Background Social media may influence children and young people’s health behaviour, including cigarette and e-cigarette use.
Methods We analysed data from participants aged 10–25 years in the UK Household Longitudinal Study 2015–2021. The amount of social media use reported on a normal weekday was related to current cigarette smoking and e-cigarette use. Generalised estimating equation (GEE) logistic regression models investigated associations of social media use with cigarette smoking and e-cigarette use. Models controlled for possible confounders including age, sex, country of UK, ethnicity, household income and use of cigarette/e-cigarettes by others within the home.
Results Among 10 808 participants with 27 962 observations, current cigarette smoking was reported by 8.6% of participants for at least one time point, and current e-cigarette use by 2.5% of participants. In adjusted GEE models, more frequent use of social media was associated with greater odds of current cigarette smoking. This was particularly apparent at higher levels of use (eg, adjusted odds ratio (AOR) 3.60, 95% CI 2.61 to 4.96 for ≥7 hours/day vs none). Associations were similar for e-cigarettes (AOR 2.73, 95% CI 1.40 to 5.29 for ≥7 hours/day social media use vs none). There was evidence of dose–response in associations between time spent on social media and both cigarette and e-cigarette use (both p<0.001). Analyses stratified by sex and household income found similar associations for cigarettes; however, for e-cigarettes associations were concentrated among males and those from higher household income groups.
Conclusions Social media use is associated with increased risk of cigarette smoking and e-cigarette use. There is a need for greater research on this issue as well as potential policy responses.
- Tobacco control
Data availability statement
Data are available in a public, open access repository. Data available from UK Data Service https://ukdataservice.ac.uk .
This is an open access article distributed in accordance with the Creative Commons Attribution 4.0 Unported (CC BY 4.0) license, which permits others to copy, redistribute, remix, transform and build upon this work for any purpose, provided the original work is properly cited, a link to the licence is given, and indication of whether changes were made. See: https://creativecommons.org/licenses/by/4.0/ .
https://doi.org/10.1136/thorax-2023-220569
Statistics from Altmetric.com
Request permissions.
If you wish to reuse any or all of this article please use the link below which will take you to the Copyright Clearance Center’s RightsLink service. You will be able to get a quick price and instant permission to reuse the content in many different ways.
WHAT IS ALREADY KNOWN ON THIS TOPIC
There is substantial use of social media among children and young people, which has had debated impacts on health outcomes. There are studies examining social media use and associations with cigarette and e-cigarette use in the US but only two such studies in the UK. One study was cross-sectional, while one previous cohort study of data from 2014 to 2018 found that social media use at age 14 years was associated with a greater likelihood of cigarette smoking at age 17 years. This study did not, however, assess the use of e-cigarettes.
WHAT THIS STUDY ADDS
This study examined daily use of social media among 10–25-year-olds from 2015 to 2021. It found that time spent on social media is associated, in a dose-dependent manner, with likelihood both of cigarette smoking and vaping. Those using social media for ≥7 hours/day were more than two and a half times more likely to use both cigarettes and e-cigarettes than those not using social media.
HOW THIS STUDY MIGHT AFFECT RESEARCH, PRACTICE OR POLICY
This study highlights that more frequent social media use is associated with increased likelihood of using both cigarettes and e-cigarettes in the UK. This reinforces concerns that social media is a vector of direct and indirect marketing and promotion of these products and that policies to curtail this may be warranted.
Introduction
Understanding the mechanisms that drive uptake and use of cigarettes and e-cigarettes is key to developing strategies to prevent harm. The use of social media has been identified as a novel potential vector, with substantial increases in time spent in this activity by young people. 1–5 Social media use increases with age, and girls are more likely to spend longer periods of time on social media than boys. 6 Social media may be driving cigarette smoking and e-cigarette use through both direct, targeted advertisements and the use of paid influencers by the tobacco industry. 7 To date, most evidence on the impact of social media on cigarette and e-cigarette use has focused on America. 8–10 This has found associations with uptake, regular use and reduced perceptions of harm and has included assessment of engagement with different platforms. 11–13 The only two previous UK studies include a cohort study which found that social media use at age 14 years was associated with greater likelihood of cigarette use at age 17 years. 14 A second cross-national study from 42 countries including the UK concluded that there was a link between social media use and substance use but did not examine cigarette use separately from other substances. 15
Previous research has identified links between social media use and both cigarette and e-cigarette use. For example, analyses of Instagram have identified networks of influencers promoting e-cigarettes, often without disclosing financial relationships; while Juul has recently settled a lawsuit over marketing of e-cigarettes to teens, including on social media. 16 17 Comparative analyses in the UK have found good compliance with advertising standards for e-cigarettes on traditional media, but high levels of breaches on social media. 18 Analyses of 11 of the most popular social media platforms have highlighted high levels of tobacco promotion, with few platforms having policies to deal with novel forms of promotion such as sponsored or influencer content. 19 A systematic review of exposure to tobacco promotion and use identified 29 studies (none from the UK) and concluded that there is a need for greater regulation. 20 Any proposal to regulate social media needs to be justified and based on evidence. To contribute to this, we examined the longitudinal relationship of social media use with cigarette smoking and e-cigarette use among children and young people in the UK.
Data come from participants of the UK Household Longitudinal Study (UKHLS), also known as Understanding Society. 21 This is a longitudinal household panel study with annual surveys starting in 2009. The original sample consisted of a clustered and stratified probability sample of approximately 28 000 households in the UK. Data are collected via face-to-face interviews carried out by a trained interviewer in the respondent’s home and via online, self-completion questionnaires. Adults over the age of 16 years or above are asked to complete an individual questionnaire, including a self-completion questionnaire. Household members aged 10–15 years are asked to fill in a shorter self-completion questionnaire, with permission from their parent or carer.
We have focused on children and young adults aged 10–25 years using data from 2015/2016 to 2020/2021 (wave 7 to wave 12). Questions on e-cigarette use were added to UKHLS in 2015/2016. Participation in the panel is voluntary, with a gift voucher sent to encourage completion of questionnaires and a further gift voucher sent when these are completed. All participants provided consent to be interviewed. The University of Essex Ethics Committee approved all data collection. 22
Outcomes and exposure
We used three separate binary outcomes: current cigarette smoking, current e-cigarette use and current dual use of both products. Participants were classified as current cigarette smokers if they responded “I usually smoke between one and six cigarettes a week” or “I usually smoke more than six cigarettes a week”. All other responses were coded as non-users. The same question was used for all waves of data and for all ages.
Current e-cigarette use was first assessed in 2015/2016 with the question “Do you ever use electronic cigarettes (e-cigarettes)?” with response options “Yes” and “No”. From wave 8 (2016/2017) onwards participants were classified as current (weekly) e-cigarette users if they responded “I use e-cigarettes at least once a week”. All other responses were coded as non-users. Dual use was classified as participants currently using both products, with those using only one or no products classed as non-dual users.
The main exposure variable was social media use. Participants were first asked “Do you belong to any social networking websites?” (Yes/No), and if “Yes”, they were also asked how many hours they spend chatting or interacting with friends through a social website on a normal weekday, with the following response options: “None”, “<1 hour/day”, “1–3 hours”, “4–6 hours” and “≥7 hours”. We combined those reporting “None” along with those who were not a member of a social media website into a reference category of “Not a member or no use”. 6
We considered a range of potentially relevant sociodemographics: age, sex, country in UK, self-defined ethnic group (collapsed into White vs non-White due to low numbers in the non-White category), an indicator of living in an urban or rural areas (derived from Office for National Statistics Rural and Urban Classification of Output Areas) and equivalised household net income (based on the Organisation for Economic Co-operation and Development (OECD) equivalence scale, which was used to adjust household income by household composition 23 ).
Statistical analyses
We compared differences in sociodemographics between categories of social media use using ANOVA. We used binary generalised estimating equation (GEE) regression models (family: binomial; link: logit; correlation matrix: exchangeable) to assess relationships between social media use and product use, using separate models for each outcome: cigarette smoking, e-cigarette use and dual use. GEE models assess changes over time and account for the correlation caused by observations being from the same individuals. 24 We also present tests for trend based on frequency of social media use. Analyses were adjusted for time (categorical) as well as the sociodemographic variables listed above. Models of cigarette smoking were additionally adjusted for parental tobacco use, models of e-cigarette use were adjusted for parental e-cigarette use, and models of dual use were adjusted for both. Analyses used survey weights designed by the UKHLS survey team to account for clustered and stratified probability sampling and non-response bias. 25
We tested for interactions of social media use with age (split into above and below 18 years of age), sex and household income (in three groups). This was due to possible differences between those above and below the legal age of sale, greater social media use among women, and potentially differential effects by socioeconomic groups. All interactions were p<0.001 and so we present stratified analyses. Due to the small numbers, we did not test interactions for dual use.
Sensitivity analyses
We performed a range of sensitivity analyses to test the robustness of our findings. As it is possible that those not using social media at all are atypical, we repeated our analyses excluding these participants. Our main analyses used household income as a marker of socioeconomic status. We also performed our analyses using Index of Multiple Deprivation (IMD) (in five groups) as an alternative marker of socioeconomic status. We performed analyses categorising current e-cigarette use as participants who reported using e-cigarettes at least monthly. We also performed analyses controlling for a measure of mental health (the 12-item General Health Questionnaire (GHQ-12)) to consider whether this is a possible pathway, whereby social media impacts mental health, which is then linked to cigarette and e-cigarette use.
Finally, we used fixed effects analyses to directly test if changes in social media use corresponded to uptake of cigarette smoking and e-cigarette use. These adjusted for the time-varying variables parental cigarette/e-cigarette use and household income. These models were on a smaller subset of individuals who were not product users when entering the study and who were found to change their social media use over time.
Outcomes and covariates across categories of social media use are shown in table 1 . Overall, 8.6% of the sample reported current cigarette smoking at one or more data point, 2.5% reported current e-cigarette use, and 1.1% of participants were dual users at one or more data point. Social media use frequency broken down by covariates is shown in online supplemental appendix table 1 .
Supplemental material
- View inline
Description of sample observations by social media use 2015–2021
Cigarette smoking, e-cigarette use and dual use were all more common among participants reporting greater social media use (all p<0.001) ( figure 1 ). Some 2.0% of participants who used social media “None or not a member” reported being a current cigarette smoker compared with 15.7% among those using social media for ≥7 hours/day. Current e-cigarette use ranged from 0.8% among those not using social media to 2.5% among those using it for ≥7 hours/day.
- Download figure
- Open in new tab
- Download powerpoint
Cigarette smoking, e-cigarette use and dual use by social media use.
Differences between categories of social media use were apparent for all variables studied (all p<0.001). Males were less likely to be in higher social media use groups than females (57.5% of the “None or not a member” social media group compared with 39.9% of the “≥7 hours/day” group). Social media use was more frequent at older ages (mean age of “None or not a member” social media group 12.0 years vs 17.7 years for the “≥7 hours/day” group). Parental cigarette smoking was more common among those using social media more frequently (17.0% for the “None or not a member” social media group vs 25.2% for the “≥7 hours/day” group) as was parental e-cigarette use (7.3% and 10.5%, respectively).
Table 2 shows results of our GEE models of social media use and cigarette smoking. Cigarette smoking was more common among those using social media more frequently (p for trend <0.001). Those using social media for “<1 hour/day” were more likely to be current cigarette smokers than those using social media “None or not a member” (adjusted odds ratio (AOR) 1.92, 95% CI 1.43 to 2.58) ( table 2 ). Those using social media for “≥7 hours/day” were substantially more likely to be current cigarette smokers than those using social media “None or not a member” (AOR 3.60, 95% CI 2.61 to 4.96).
Associations of social media use with current cigarette use from generalised estimating equation model
Table 3 shows results for e-cigarette use. E-cigarette use was more common among those using social media more frequently (p for trend <0.001). E-cigarette use was more common among those using social media “1–3 hours per day” compared with those using it “None or not a member” (AOR 1.92, 95% CI 1.07 to 3.46). E-cigarette use was considerably more likely among participants using social media “≥7 hours/day” than those using social media “None or not a member” (AOR 2.73, CI 1.40 to 5.29).
Associations of social media use and current e-cigarette use from generalised estimating equation model
Table 4 shows results for dual cigarette and e-cigarette use. Models have wide confidence intervals reflecting low levels of dual use. Those using social media more frequently were more likely to be dual users (p for trend <0.001). Those using social media “1–3 hours per day” were more likely to be dual users compared with those using it “None or not a member” (AOR 3.28, 95% CI 1.24 to 8.70). Dual use was more likely among participants using social media “≥7 hours/day” than among those using social media “None or not a member” (AOR 4.96, 95% CI 1.71 to 14.34).
Associations of social media use with current e-cigarette and cigarette dual use from generalised estimating equation model
Interactions of social media and sex were statistically significant for both cigarettes and e-cigarettes (both p<0.001). In stratified models ( table 5 ) AORs were similar between the sexes for current cigarette smoking. For e-cigarettes, associations between social media use and e-cigarette use were statistically significant for males but not for females (AOR 4.10, 95% CI 1.90 to 8.87 for males for “≥7 hours/day” vs “None or not a member” social media use).
Associations of social media use with current e-cigarette and cigarette use from gender stratified generalised estimating equation models
Interactions with household income categories were statistically significant (p<0.001 for both cigarettes and e-cigarettes) ( table 6 ). In stratified analyses of cigarette smoking, point estimates for the richest income group were higher than for the lowest income group, although these overlapped (eg, AOR 5.22 for “≥7 hours/day”, 95% CI 2.82 to 9.67 for the richest income group vs AOR 4.17, 95% CI 2.27 to 7.65 for the lowest income group). For e-cigarette use, associations were statistically significant for the highest income groups (eg, AOR 7.85, 95% CI 1.72 to 35.82 for “≥7 hours/day” vs no social media use) but were not statistically significant for the lowest income group.
Associations of social media use with current e-cigarette and cigarette use from household income stratified generalised estimating equation models
Analyses stratified by age found similar results to main analyses for cigarettes ( online supplemental appendix table 2 ). Models for e-cigarette use were only statistically significant among those <18 years old.
GEE analyses excluding those not using any social media were similar to main analyses ( online supplemental appendix table 3 ). Analyses using IMD as a marker of socioeconomic status rather than household income also gave similar results ( online supplemental appendix table 4 ). Analyses classifying current e-cigarette use as participants using them at least monthly also gave similar results although with larger point estimates ( online supplemental appendix table 5 ). Analyses controlling for GHQ-12 as a measure of mental health were similar for cigarettes but did not find statistically significant associations between social media and e-cigarette or dual use. This may indicate that social media use impacts mental health, which in turn impacts likelihood of using cigarettes or e-cigarettes, although this result should be treated with caution ( online supplemental appendix table 6 ).
Fixed effect analyses gave similar results to main analyses for uptake of cigarette smoking ( online supplemental appendix table 7 ). It should be noted that sample size was much reduced for this model (n=864). These analyses found some evidence that changes in social media use are linked to uptake of cigarette smoking in a dose–response manner (p for trend=0.053). For example, changing to using social media for ≥7 hours/day was associated with more than double the odds of taking up cigarette smoking (AOR 2.33, 95% CI 1.28 to 4.24).
Associations between changes in social media use and uptake of e-cigarettes did not reveal associations between changes in social media use and uptake of e-cigarettes. These analysis models had even lower sample sizes (n=564). For example, AORs of e-cigarette uptake ranged from 0.71 (95% CI 0.34 to 1.48) for participants using social media “<1 hour/day” to AOR 0.84 (95% CI 0.38 to 1.85) for those using social media “≥7 hours/day”. The test for trend was not statistically significant (p=0.584).
The main finding of the present study is that in children and young adults more frequent social media use was associated with a higher likelihood of both current use of cigarettes and e-cigarettes. This association was independent of other factors associated with increasing smoking and vaping including age, gender, socioeconomic status and parental smoking and vaping. These findings were robust to sensitivity analyses, while in stratified analyses there were more consistent associations for e-cigarette use among those under the legal age of sale, males and those with higher household incomes.
While we were unable to assess use of specific social media platforms or what content was being accessed, we propose a number of possible, non-exclusive explanations for this relationship. First, and most straightforwardly, there is evidence that the corporations behind cigarette smoking and vaping make use of social media to advertise and promote their products. 8–10 16 This includes direct advertising which is algorithmically targeted and the use of paid social media influencers who present smoking and vaping as a fashionable and desirable activity. Greater time spent on social media is likely to increase exposure to these forms of influence. While cigarettes and e-cigarettes are likely promoted differently, we found association with use of both products, highlighting the need for greater understanding of such corporate behaviours. Second, social media use has been shown to have features in common with reward-seeking addictive behaviour. 26 High social media use may increase susceptibility to other addictive behaviours like smoking. Alternatively, both behaviours may be driven by a common susceptibility. Third, as a space that is largely unsupervised by parents/caregivers, social media use may encourage behaviours that are transgressive, including cigarette smoking and vaping. There is evidence that peer smoking is a strong influence on child uptake of smoking 27 and social media is one of the ways in which peer smoking and vaping will be experienced, both by seeing others’ behaviour and by sharing “influencer content” that promotes these behaviours.
Stratified analyses revealed more consistent associations for cigarettes, while for e-cigarettes statistically significant associations were only found for those under the legal age of sale, among males, and those from richer households. Analyses of cigarette smoking did not identify changes over time, which fits with other evidence that smoking prevalence has been reasonably consistent over this time frame. 28 Analyses of e-cigarette use found reduced odds of these outcomes after 2015/2016, likely caused by changes in e-cigarette use ascertainment, although our main findings were robust to reclassification to examine monthly use. Our main analyses focused on weekly use of e-cigarettes; as any health impacts are probably related to amounts of vapour inhaled, this measure of regular use is more important for health than e-cigarette experimentation.
Strengths and limitations
This study uses a nationally representative cohort to examine social media use and use of cigarettes and e-cigarettes over time. UKHLS households are sampled based on geographical areas, population densities and ethnic composition, with survey weight adjusting for differential non-response across groups. 29 We conducted a range of sensitivity analyses, although other potential factors such as education may also be important. All data are based on self-report, and specifically we do not have information about which social media platforms were being used or how individuals were using them, for example, the extent to which they are interacting socially with individuals they know or consuming content from influencers, personalities or media corporations, etc. Precise pathways remain to be fully elucidated: our sensitivity analyses point to a possible role for mental health, although it should be noted that a formal mediation analysis was outside the scope of this article. As cigarette smoking is linked to poorer mental health, these relationships could well be bidirectional. 30 This, as well as potential targeted advertising, are among pathways that should be investigated in both quantitative and qualitative research.
Policy implications
Although we do not have data on the specific platforms used or content used, there is compelling evidence that vape companies are using social media to market their products. 2–5 The content that social media users are exposed to is to a substantial extent algorithmically controlled, both through targeted advertising and by the promotion of material that maximises engagement in order to increase revenue to the platform. This can be controlled. For example, far right imagery which is otherwise widely available is largely inaccessible in Germany, as a consequence of German law which social media platforms are bound to enforce. The companies that own social media platforms have substantial power to modify exposure to material that promotes smoking and vaping if they choose to or are compelled to. Voluntary codes seem unlikely to achieve this, and the introduction and enforcement on bans on material that promote this should be considered. In general, we think that algorithms should not be promoting products to individuals that they cannot legally buy. Legislation and enforcement around this and other corporate determinants of health concerns should be considered a core part of online safety and child protection.
This longitudinal analysis of children and young people in the UK found that more frequent social media use is associated with an increased risk of cigarette and e-cigarette use.
Ethics statements
Patient consent for publication.
Not applicable.
Ethics approval
- Royal Society for Public Health
- LaVallee H ,
- Rams A , et al
- O’Brien EK ,
- Hoffman L ,
- Navarro MA , et al
- Buckton C ,
- Patterson C , et al
- McNamee P ,
- Mendolia S ,
- ↵ Tobacco Tactics . Social media , Available : https://tobaccotactics.org/wiki/social-media/ [Accessed 3 Jun 2023 ].
- Soto D , et al
- Vannucci A ,
- Simpson EG ,
- Gagnon S , et al
- Stanton CA ,
- Pericot-Valverde I , et al
- Galimov A ,
- Kennedy CJ , et al
- Vrinten C ,
- Parnham JC ,
- Filippidis FT , et al
- Boniel-Nissim M ,
- van den Eijnden RJJM ,
- Furstova J , et al
- Valente T ,
- Barker J , et al
- Angus K , et al
- Laestadius L ,
- Vassey J , et al
- Donaldson SI ,
- Dormanesh A ,
- Perez C , et al
- Institute for Social and Economic Research
- ↵ Understanding Society: The UK Household Longitudinal Study . Consent information , Available : https://www.understandingsociety.ac.uk/documentation/mainstage/consents [Accessed 3 Jun 2023 ].
- Liang K-Y ,
- Lindström B ,
- Bellander M ,
- Schultner DT , et al
- Laverty AA ,
- Filippidis FT ,
- Taylor-Robinson D , et al
- Office for National Statistics
- Benzeval M ,
- Bollinger CR ,
- Burton J , et al
- McNeill A ,
- Girling A , et al
Supplementary materials
Supplementary data.
This web only file has been produced by the BMJ Publishing Group from an electronic file supplied by the author(s) and has not been edited for content.
- Data supplement 1
X @COPDdoc, @cvrinten, @anthonylav
Contributors AAL conceived the project. JCP, CV and AAL cleaned data and performed the analyses with guidance from FF and MKR. AAL and NSH wrote the first draft and all authors contributed to this process. AAL is guarantor
Funding This study was supported by Cancer Research UK (CRUK PPRCTAGPJT\100005).
Competing interests NSH is Chair of Action on Smoking and Health and Medical Director of Asthma and Lung UK. AAL is a Trustee of Action on Smoking and Health.
Provenance and peer review Not commissioned; externally peer reviewed.
Supplemental material This content has been supplied by the author(s). It has not been vetted by BMJ Publishing Group Limited (BMJ) and may not have been peer-reviewed. Any opinions or recommendations discussed are solely those of the author(s) and are not endorsed by BMJ. BMJ disclaims all liability and responsibility arising from any reliance placed on the content. Where the content includes any translated material, BMJ does not warrant the accuracy and reliability of the translations (including but not limited to local regulations, clinical guidelines, terminology, drug names and drug dosages), and is not responsible for any error and/or omissions arising from translation and adaptation or otherwise.
Linked Articles
- Editorial Strengthening the evidence base to support stronger regulation of social media based advertising of e-cigarette products to youth Kim L Lavoie Thorax 2024; - Published Online First: 16 May 2024. doi: 10.1136/thorax-2023-221169
Read the full text or download the PDF:

IMAGES
VIDEO
COMMENTS
Health impact of smoking. Table Table1 1 lists the main causes of death from smoking. Tobacco smoking is estimated to lead to the premature death of approximately 6 million people worldwide and 96,000 in the UK each year (Action on Smoking and Health, 2016b; World Health Organization, 2013).A 'premature death from smoking' is defined as a death from a smoking-related disease in an ...
age of 35 years or so, stopping smoking recovers 2-3 months of healthy life expectancy for every year of smoking avoided, or 4-6 h for every day (Jha & Peto, 2014). Stopping smoking has different effects on different smoking-related diseases. Excess risk of heart attack caused by smoking reduces by 50% within 12 months of stopping smoking.
We identified three outcomes with a 4-star association with smoking: COPD (72% increase in risk based on the BPRF, 0.54 ROS), lower respiratory tract infection (54%, 0.43) and pancreatic cancer ...
Decades after its ill effects on human health were first documented, tobacco smoking remains one of the major global drivers of premature death and disability. In 2017, smoking was responsible for ...
3.3 Trends in prevalence of tobacco smoking 3.3.1 at global level 3.3.2 numbers of smokers 3.4 Levels of tobacco use, smoking and cigarette use among adults in 2018 3.5 Smokeless tobacco use among people aged 15 years and older 3.6 Smokeless tobacco use among adolescents aged 13-15 years
Smoking's lasting effect on the immune system. It emerges from a study of human cells that smoking can influence certain immune responses to the same extent as can age or genetics. Smoking can ...
Despite a steady decline in adolescent smoking globally, it remains a prevalent risk factor for non-communicable disease. Previous research points to differences in socio-environmental and psychosocial risk factors for smoking and how they vary across different settings with disparate social and cultural characteristics. As a result, smoking rates have remained disproportionately higher in ...
Objectives Although the global COVID-19 pandemic has increased interest in research involving high-risk smokers, studies examining changed smoking behaviours, cessation intentions and associated psychological states among smokers are still scarce. This study aimed to systematically review the literature related to this subject. Design A systematic review of published articles on cigarettes and ...
A review was conducted on 12 May 2020 on smoking and COVID-19, using MEDLINE, EMBASE, Cochrane Library, and WHO Global Database Quantitative primary research on adults or secondary analyses of such studies were included. Individual studies . included in meta-analyses that were not otherwise identified in the search were sought.
tobacco smoking remains one of the main preventable causes of ill-health and. premature death worldwide. This paper reviews the extent and nature of harms. caused by smoking, the bene fits of ...
Present study aims to investigate the role of several personal and social factors on smoking behavior among young adults. Methods: This is a cross-sectional survey study with 466 university ...
Study design and setting. This was an observational study involving cross-sectional and prospective survey data. The STROBE guidelines were used in the design and reporting of this study [].The study is part of the ongoing Smoking Toolkit Study (STS), which involves monthly, face-to-face, computer-assisted household surveys of adults aged 16+ in England [23, 24].
Smoking and COVID-19 . Scientific brief 30 June 2020 . This is an update to the Scientific Brief Smoking and COVIDentitled '-19,' originally published on 26 May 2020. its Since publication, a study entitled ' Cardiovascular Disease, Drug Therapy, and Mortality in Covid-19' by Mehra et al. has been retracted
estimated that smoking increases the risk of. coronary heart disease about 2-4 times, stroke 2-4 times, lung cancer 25 times in. men, and 25.7 times in women. Be sides, smoking can lead to an ...
The overview highlights the current lack of scientific research into the environmental impact of tobacco, including the health and economic consequences that result from the cultivation, production, distribution, and waste of what is a highly addictive and unnecessary product. The costs
Smoking is the leading cause of death (Center for Disease Control and Prevention, 2002), and the detri-mental effects of smoking on health, such as bronchitis, asthma, and cancer are well known (Arday et al., 1995). In addition, economic loss linked to smoking-related health issues among adults accounted for 8% of person-al health care ...
The prevalence of smoking in our study reached 55.4%, which was higher than the rate reported in Lebanon in 2018 (42.1%) 19 and higher than the ones reported in some studies done in Taiwan and Germany, on individuals aged more than 40 years old and more than 10 years old respectively 20,21 and lower than the rate expressed in a Chinese study ...
Many studies have been done on the health risks of smoking and driving. In a survey of 'the effects of smoking on society: a case study', Gupta and Kumar (2018) observe that smoking is very ...
Smoking alone causes 90% of lung cancer, 80%. of chronic bronchitis, and 25% of other lung-related. diseases. None of the organs in the body are safe from. the harmful effects of tobacco. Smoking ...
Background Social media may influence children and young people's health behaviour, including cigarette and e-cigarette use. Methods We analysed data from participants aged 10-25 years in the UK Household Longitudinal Study 2015-2021. The amount of social media use reported on a normal weekday was related to current cigarette smoking and e-cigarette use. Generalised estimating equation ...
57-63; doi 10.35198/01-2020-001-0009. Smoking Behaviour of Univ ersity. Students: a Descriptiv e Study. BACKGROUND: The theoretical background of the. study shows the importance of the issue of ...