User Preferences
Content preview.
Arcu felis bibendum ut tristique et egestas quis:
- Ut enim ad minim veniam, quis nostrud exercitation ullamco laboris
- Duis aute irure dolor in reprehenderit in voluptate
- Excepteur sint occaecat cupidatat non proident

Keyboard Shortcuts
2.4 - simple random sampling and other sampling methods, sampling methods section .
Sampling Methods can be classified into one of two categories:
- Probability Sampling : Sample has a known probability of being selected
- Non-probability Sampling : Sample does not have known probability of being selected as an inconvenience or voluntary response surveys
Probability Sampling Section
In probability sampling, it is possible to both determine which sampling units belong to which sample and the probability that each sample will be selected. The following sampling methods are examples of probability sampling :
- Simple Random Sampling (SRS)
- Stratified Sampling
- Cluster Sampling
- Systematic Sampling
- Multistage Sampling (in which some of the methods above are combined in stages)
Of the five methods listed above, students have the most trouble distinguishing between stratified sampling and cluster sampling .
Stratified Sampling is possible when it makes sense to partition the population into groups based on a factor that may influence the variable that is being measured. These groups are then called strata. An individual group is called a stratum. With stratified sampling one should:
- partition the population into groups (strata)
- obtain a simple random sample from each group (stratum)
- collect data on each sampling unit that was randomly sampled from each group (stratum)
Stratified sampling works best when a heterogeneous population is split into fairly homogeneous groups. Under these conditions, stratification generally produces more precise estimates of the population percents than estimates that would be found from a simple random sample. Table 2.2 shows some examples of ways to obtain a stratified sample.
Example 1 | Example 2 | Example 3 | |
---|---|---|---|
Population | All people in the U.S. | All PSU intercollegiate athletes | All elementary students in the local school district |
Groups (Strata) | 4 Time Zones in the U.S. (Eastern, Central, Mountain, Pacific) | 26 PSU intercollegiate teams | 11 different elementary schools in the local school district |
Obtain a Simple Random Sample | 500 people from each of the 4 time zones | 5 athletes from each of the 26 PSU teams | 20 students from each of the 11 elementary schools |
Sample | 4 × 500 = 2000 selected people | 26 × 5 = 130 selected athletes | 11 × 20 = 220 selected students |
Cluster Sampling is very different from Stratified Sampling. With cluster sampling , one should
- divide the population into groups (clusters).
- obtain a simple random sample of so many clusters from all possible clusters.
- obtain data on every sampling unit in each of the randomly selected clusters.
It is important to note that, unlike with the strata in stratified sampling, the clusters should be microcosms, rather than subsections, of the population. Each cluster should be heterogeneous. Additionally, the statistical analysis used with cluster sampling is not only different but also more complicated than that used with stratified sampling.
Example 1 | Example 2 | Example 3 | |
---|---|---|---|
Population | All people in the U.S. | All PSU intercollegiate athletes | All elementary students in a local school district |
Groups (Clusters) | 4 Time Zones in the U.S. (Eastern, Central, Mountain, Pacific.) | 26 PSU intercollegiate teams | 11 different elementary schools in the local school district |
Obtain a Simple Random Sample | 2 time zones from the 4 possible time zones | 8 teams from the 26 possible teams | 4 elementary schools from the l1 possible elementary schools |
Sample | every person in the 2 selected time zones | every athlete on the 8 selected teams | every student in the 4 selected elementary schools |
Each of the three examples that are found in Tables 2.2 and 2.3 was used to illustrate how both stratified and cluster sampling could be accomplished. However, there are obviously times when one sampling method is preferred over the other. The following explanations add some clarification about when to use which method.
- With Example 1 : Stratified sampling would be preferred over cluster sampling, particularly if the questions of interest are affected by time zone. For example, the percentage of people watching a live sporting event on television might be highly affected by the time zone they are in. Cluster sampling really works best when there are a reasonable number of clusters relative to the entire population. In this case, selecting 2 clusters from 4 possible clusters really does not provide many advantages over simple random sampling.
- With Example 2 : Either stratified sampling or cluster sampling could be used. It would depend on what questions are being asked. For instance, consider the question "Do you agree or disagree that you receive adequate attention from the team of doctors at the Sports Medicine Clinic when injured?" The answer to this question would probably not be team dependent, so cluster sampling would be fine. In contrast, if the question of interest is "Do you agree or disagree that weather affects your performance during an athletic event?" The answer to this question would probably be influenced by whether or not the sport is played outside or inside. Consequently, stratified sampling would be preferred.
- With Example 3 : Cluster sampling would probably be better than stratified sampling if each individual elementary school appropriately represents the entire population as in a school district where students from throughout the district can attend any school. Stratified sampling could be used if the elementary schools had very different locations and served only their local neighborhood (i.e., one elementary school is located in a rural setting while another elementary school is located in an urban setting.) Again, the questions of interest would affect which sampling method should be used.
The most common method of carrying out a poll today is using Random Digit Dialing in which a machine random dials phone numbers. Some polls go even farther and have a machine conduct the interview itself rather than just dialing the number! Such " robocall polls " can be very biased because they have extremely low response rates (most people don't like speaking to a machine) and because federal law prevents such calls to cell phones. Since the people who have landline phone service tend to be older than people who have cell phone service only, another potential source of bias is introduced. National polling organizations that use random digit dialing in conducting interviewer based polls are very careful to match the number of landline versus cell phones to the population they are trying to survey.
Non-probability Sampling Section
The following sampling methods that are listed in your text are types of non-probability sampling that should be avoided :
- volunteer samples
- haphazard (convenience) samples
Since such non-probability sampling methods are based on human choice rather than random selection, a statistical theory cannot explain how they might behave and potential sources of bias are rampant. In your textbook, the two types of non-probability samples listed above are called "sampling disasters."
Read the article: " How Polls are Conducted " by the Gallup organization available in Canvas.
The article provides great insight into how major polls are conducted. When you are finished reading this article you may want to go to the Gallup Poll Website and see the results from recent Gallup polls. Another excellent source of public opinion polls on a wide variety of topics using solid sampling methodology is the Pew Research Center Website . When you read one of the summary reports on the Pew site, there is a link (in the upper right corner) to the complete report giving more detailed results and a full description of their methodology as well as a link to the actual questionnaire used in the survey so you can judge whether there might be bias in the wording of their survey.
It is important to be mindful of margin or error as discussed in this article. We all need to remember that public opinion on a given topic cannot be appropriately measured with one question that is only asked on one poll. Such results only provide a snapshot at that moment under certain conditions. The concept of repeating procedures over different conditions and times leads to more valuable and durable results. Within this section of the Gallup article, there is also an error: "in 95 out of those 100 polls, his rating would be between 46% and 54%." This should instead say that in an expected 95 out of those 100 polls, the true population percent would be within the confidence interval calculated. In 5 of those surveys, the confidence interval would not contain the population percent.
Random Assignment in Psychology: Definition & Examples
Julia Simkus
Editor at Simply Psychology
BA (Hons) Psychology, Princeton University
Julia Simkus is a graduate of Princeton University with a Bachelor of Arts in Psychology. She is currently studying for a Master's Degree in Counseling for Mental Health and Wellness in September 2023. Julia's research has been published in peer reviewed journals.
Learn about our Editorial Process
Saul McLeod, PhD
Editor-in-Chief for Simply Psychology
BSc (Hons) Psychology, MRes, PhD, University of Manchester
Saul McLeod, PhD., is a qualified psychology teacher with over 18 years of experience in further and higher education. He has been published in peer-reviewed journals, including the Journal of Clinical Psychology.
Olivia Guy-Evans, MSc
Associate Editor for Simply Psychology
BSc (Hons) Psychology, MSc Psychology of Education
Olivia Guy-Evans is a writer and associate editor for Simply Psychology. She has previously worked in healthcare and educational sectors.
In psychology, random assignment refers to the practice of allocating participants to different experimental groups in a study in a completely unbiased way, ensuring each participant has an equal chance of being assigned to any group.
In experimental research, random assignment, or random placement, organizes participants from your sample into different groups using randomization.
Random assignment uses chance procedures to ensure that each participant has an equal opportunity of being assigned to either a control or experimental group.
The control group does not receive the treatment in question, whereas the experimental group does receive the treatment.
When using random assignment, neither the researcher nor the participant can choose the group to which the participant is assigned. This ensures that any differences between and within the groups are not systematic at the onset of the study.
In a study to test the success of a weight-loss program, investigators randomly assigned a pool of participants to one of two groups.
Group A participants participated in the weight-loss program for 10 weeks and took a class where they learned about the benefits of healthy eating and exercise.
Group B participants read a 200-page book that explains the benefits of weight loss. The investigator randomly assigned participants to one of the two groups.
The researchers found that those who participated in the program and took the class were more likely to lose weight than those in the other group that received only the book.
Importance
Random assignment ensures that each group in the experiment is identical before applying the independent variable.
In experiments , researchers will manipulate an independent variable to assess its effect on a dependent variable, while controlling for other variables. Random assignment increases the likelihood that the treatment groups are the same at the onset of a study.
Thus, any changes that result from the independent variable can be assumed to be a result of the treatment of interest. This is particularly important for eliminating sources of bias and strengthening the internal validity of an experiment.
Random assignment is the best method for inferring a causal relationship between a treatment and an outcome.
Random Selection vs. Random Assignment
Random selection (also called probability sampling or random sampling) is a way of randomly selecting members of a population to be included in your study.
On the other hand, random assignment is a way of sorting the sample participants into control and treatment groups.
Random selection ensures that everyone in the population has an equal chance of being selected for the study. Once the pool of participants has been chosen, experimenters use random assignment to assign participants into groups.
Random assignment is only used in between-subjects experimental designs, while random selection can be used in a variety of study designs.
Random Assignment vs Random Sampling
Random sampling refers to selecting participants from a population so that each individual has an equal chance of being chosen. This method enhances the representativeness of the sample.
Random assignment, on the other hand, is used in experimental designs once participants are selected. It involves allocating these participants to different experimental groups or conditions randomly.
This helps ensure that any differences in results across groups are due to manipulating the independent variable, not preexisting differences among participants.
When to Use Random Assignment
Random assignment is used in experiments with a between-groups or independent measures design.
In these research designs, researchers will manipulate an independent variable to assess its effect on a dependent variable, while controlling for other variables.
There is usually a control group and one or more experimental groups. Random assignment helps ensure that the groups are comparable at the onset of the study.
How to Use Random Assignment
There are a variety of ways to assign participants into study groups randomly. Here are a handful of popular methods:
- Random Number Generator : Give each member of the sample a unique number; use a computer program to randomly generate a number from the list for each group.
- Lottery : Give each member of the sample a unique number. Place all numbers in a hat or bucket and draw numbers at random for each group.
- Flipping a Coin : Flip a coin for each participant to decide if they will be in the control group or experimental group (this method can only be used when you have just two groups)
- Roll a Die : For each number on the list, roll a dice to decide which of the groups they will be in. For example, assume that rolling 1, 2, or 3 places them in a control group and rolling 3, 4, 5 lands them in an experimental group.
When is Random Assignment not used?
- When it is not ethically permissible: Randomization is only ethical if the researcher has no evidence that one treatment is superior to the other or that one treatment might have harmful side effects.
- When answering non-causal questions : If the researcher is just interested in predicting the probability of an event, the causal relationship between the variables is not important and observational designs would be more suitable than random assignment.
- When studying the effect of variables that cannot be manipulated: Some risk factors cannot be manipulated and so it would not make any sense to study them in a randomized trial. For example, we cannot randomly assign participants into categories based on age, gender, or genetic factors.
Drawbacks of Random Assignment
While randomization assures an unbiased assignment of participants to groups, it does not guarantee the equality of these groups. There could still be extraneous variables that differ between groups or group differences that arise from chance. Additionally, there is still an element of luck with random assignments.
Thus, researchers can not produce perfectly equal groups for each specific study. Differences between the treatment group and control group might still exist, and the results of a randomized trial may sometimes be wrong, but this is absolutely okay.
Scientific evidence is a long and continuous process, and the groups will tend to be equal in the long run when data is aggregated in a meta-analysis.
Additionally, external validity (i.e., the extent to which the researcher can use the results of the study to generalize to the larger population) is compromised with random assignment.
Random assignment is challenging to implement outside of controlled laboratory conditions and might not represent what would happen in the real world at the population level.
Random assignment can also be more costly than simple observational studies, where an investigator is just observing events without intervening with the population.
Randomization also can be time-consuming and challenging, especially when participants refuse to receive the assigned treatment or do not adhere to recommendations.
What is the difference between random sampling and random assignment?
Random sampling refers to randomly selecting a sample of participants from a population. Random assignment refers to randomly assigning participants to treatment groups from the selected sample.
Does random assignment increase internal validity?
Yes, random assignment ensures that there are no systematic differences between the participants in each group, enhancing the study’s internal validity .
Does random assignment reduce sampling error?
Yes, with random assignment, participants have an equal chance of being assigned to either a control group or an experimental group, resulting in a sample that is, in theory, representative of the population.
Random assignment does not completely eliminate sampling error because a sample only approximates the population from which it is drawn. However, random sampling is a way to minimize sampling errors.
When is random assignment not possible?
Random assignment is not possible when the experimenters cannot control the treatment or independent variable.
For example, if you want to compare how men and women perform on a test, you cannot randomly assign subjects to these groups.
Participants are not randomly assigned to different groups in this study, but instead assigned based on their characteristics.
Does random assignment eliminate confounding variables?
Yes, random assignment eliminates the influence of any confounding variables on the treatment because it distributes them at random among the study groups. Randomization invalidates any relationship between a confounding variable and the treatment.
Why is random assignment of participants to treatment conditions in an experiment used?
Random assignment is used to ensure that all groups are comparable at the start of a study. This allows researchers to conclude that the outcomes of the study can be attributed to the intervention at hand and to rule out alternative explanations for study results.
Further Reading
- Bogomolnaia, A., & Moulin, H. (2001). A new solution to the random assignment problem . Journal of Economic theory , 100 (2), 295-328.
- Krause, M. S., & Howard, K. I. (2003). What random assignment does and does not do . Journal of Clinical Psychology , 59 (7), 751-766.

Have a language expert improve your writing
Run a free plagiarism check in 10 minutes, generate accurate citations for free.
- Knowledge Base
Methodology
- What Is Probability Sampling? | Types & Examples
What Is Probability Sampling? | Types & Examples
Published on July 5, 2022 by Kassiani Nikolopoulou . Revised on June 22, 2023.
Probability sampling is a sampling method that involves randomly selecting a sample, or a part of the population that you want to research. It is also sometimes called random sampling.
To qualify as being random, each research unit (e.g., person, business, or organization in your population) must have an equal chance of being selected. This is usually done through a random selection process, like a drawing.
Table of contents
Types of probability sampling, examples of probability sampling methods, probability vs. non-probability sampling, advantages and disadvantages of probability sampling, other interesting articles, frequently asked questions about probability sampling.
There are four commonly used types of probability sampling designs:
Simple random sampling
- Stratified sampling
Systematic sampling
- Cluster sampling
Simple random sampling gathers a random selection from the entire population, where each unit has an equal chance of selection. This is the most common way to select a random sample.
To compile a list of the units in your research population, consider using a random number generator. There are several free ones available online, such as random.org , calculator.net , and randomnumbergenerator.org .
Writing down the names of all 4,000 inhabitants by hand to randomly draw 100 of them would be impractical and time-consuming, as well as questionable for ethical reasons. Instead, you decide to use a random number generator to draw a simple random sample.
Stratified sampling collects a random selection of a sample from within certain strata, or subgroups within the population. Each subgroup is separated from the others on the basis of a common characteristic, such as gender, race, or religion. This way, you can ensure that all subgroups of a given population are adequately represented within your sample population.
For example, if you are dividing a student population by college majors, Engineering, Linguistics, and Physical Education students are three different strata within that population.
To split your population into different subgroups, first choose which characteristic you would like to divide them by. Then you can select your sample from each subgroup. You can do this in one of two ways:
- By selecting an equal number of units from each subgroup
- By selecting units from each subgroup equal to their proportion in the total population
If you take a simple random sample, children from urban areas will have a far greater chance of being selected, so the best way of getting a representative sample is to take a stratified sample.
First, you divide the population into your strata: one for children from urban areas and one for children from rural areas. Then, you take a simple random sample from each subgroup. You can use one of two options:
- Select 100 urban and 100 rural, i.e., an equal number of units
- Select 80 urban and 20 rural, which gives you a representative sample of 100 people
Systematic sampling draws a random sample from the target population by selecting units at regular intervals starting from a random point. This method is useful in situations where records of your target population already exist, such as records of an agency’s clients, enrollment lists of university students, or a company’s employment records. Any of these can be used as a sampling frame.
To start your systematic sample, you first need to divide your sampling frame into a number of segments, called intervals. You calculate these by dividing your population size by the desired sample size.
Then, from the first interval, you select one unit using simple random sampling. The selection of the next units from other intervals depends upon the position of the unit selected in the first interval.
Let’s refer back to our example about the political views of the municipality of 4,000 inhabitants. You can also draw a sample of 100 people using systematic sampling. To do so, follow these steps:
- Determine your interval: 4,000 / 100 = 40. This means that you must select 1 inhabitant from every 40 in the record.
- Using simple random sampling (e.g., a random number generator), you select 1 inhabitant.
- Let’s say you select the 11th person on the list. In every subsequent interval, you need to select the 11th person in that interval, until you have a sample of 100 people.
Cluster sampling is the process of dividing the target population into groups, called clusters. A randomly selected subsection of these groups then forms your sample. Cluster sampling is an efficient approach when you want to study large, geographically dispersed populations. It usually involves existing groups that are similar to each other in some way (e.g., classes in a school).
There are two types of cluster sampling:
- Single (or one-stage) cluster sampling, when you divide the entire population into clusters
- Multistage cluster sampling, when you divide the cluster further into more clusters, in order to narrow down the sample size
Clusters are pre-existing groups, so each high school is a cluster, and you assign a number to each one of them. Then, you use simple random sampling to further select clusters. How many clusters you select will depend on the sample size that you need.
Multi-stage sampling is a more complex form of cluster sampling, in which smaller groups are successively selected from larger populations to form the sample population used in your study.
First, you take a simple random sample of departments. Then, again using simple random sampling, you select a number of units. Based on the size of the population (i.e., how many employees work at the company) and your desired sample size, you establish that you need to include 3 units in your sample.
In stratified sampling , you divide your population in groups (strata) that share a common characteristic and then select some members from every group for your sample. In cluster sampling , you use pre-existing groups to divide your population into clusters and then include all members from randomly selected clusters for your sample.
Prevent plagiarism. Run a free check.
There are a few methods you can use to draw a random sample. Here are a few examples:
- The fishbowl draw
- A random number generator
- The random number function
Fishbowl draw
You are investigating the use of a popular portable e‐reader device among library and information science students and its effects on individual reading practices. You write the names of 25 students on pieces of paper, put them in a jar, and then, without looking, randomly select three students to be interviewed for your research.
All students have equal chances of being selected and no other consideration (such as personal preference) can influence this selection. This method is suitable when your total population is small, so writing the names or numbers of each unit on a piece of paper is feasible.
Random number generator
Suppose you are researching what people think about road safety in a specific residential area. You make a list of all the suburbs and assign a number to each one of them. Then, using an online random number generator, you select four numbers, corresponding to four suburbs, and focus on them.
This works best when you already have a list with the total population and you can easily assign every individual a number.
RAND function in Microsoft Excel
If your data are in a spreadsheet, you can also use the random number function (RAND) in Microsoft Excel to select a random sample.
Suppose you have a list of 4,000 people and you need a sample of 300. By typing in the formula =RAND() and then pressing enter, you can have Excel assign a random number to each name on the list. For this to work, make sure there are no blank rows.
This video explains how to use the RAND function.
Depending on the goals of your research study, there are two sampling methods you can use:
- Probability sampling : Sampling method that ensures that each unit in the study population has an equal chance of being selected
- Non-probability sampling : Sampling method that uses a non-random sample from the population you want to research, based on specific criteria, such as convenience
Probability sampling
In quantitative research , it is important that your sample is representative of your target population. This allows you to make strong statistical inferences based on the collected data. Having a sufficiently large random probability sample is the best guarantee that the sample will be representative and the results are generalizable and free from research biases like selection bias and sampling bias .
Non-probability sampling
Non-probability sampling designs are used in both quantitative and qualitative research when the number of units in the population is either unknown or impossible to individually identify. It is also used when you want to apply the results only to a certain subsection or organization rather than the general public. Non-probability sampling is at higher risk than probability sampling for research biases like sampling bias .
You are unlikely to be able to compile a list of every practicing organizational psychologist in the country, but you could compile a list with all the experts in your area and select a few to interview.
It’s important to be aware of the advantages and disadvantages of probability sampling, as it will help you decide if this is the right sampling method for your research design.
Advantages of probability sampling
There are two main advantages to probability sampling.
- Samples selected with this method are representative of the population at large. Due to this, inferences drawn from such samples can be generalized to the total population you are studying.
- As some statistical tests, such as multiple linear regression , t test , or ANOVA , can only be applied to a sample size large enough to approximate the true distribution of the population, using probability sampling allows you to establish correlation or cause-and-effect relationship between your variables.
Disadvantages of probability sampling
Choosing probability sampling as your sampling method comes with some challenges, too. These include the following:
- It may be difficult to access a list of the entire population, due to ethical or privacy concerns, or a full list may not exist. It can be expensive and time-consuming to compile this yourself.
- Although probability sampling reduces the risk of sampling bias , it can still occur. When your selected sample is not inclusive enough, representation of the full population is skewed .
Receive feedback on language, structure, and formatting
Professional editors proofread and edit your paper by focusing on:
- Academic style
- Vague sentences
- Style consistency
See an example
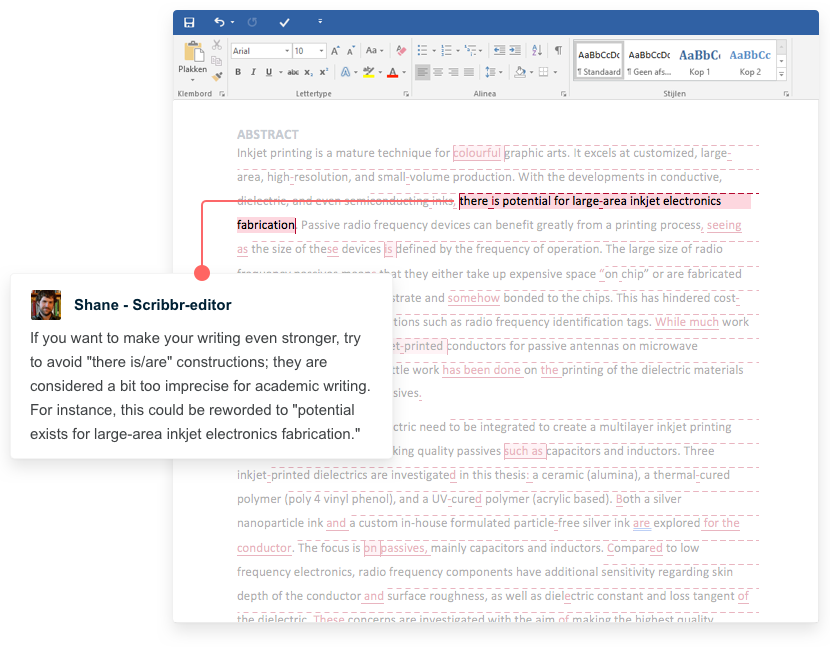
If you want to know more about statistics , methodology , or research bias , make sure to check out some of our other articles with explanations and examples.
- Student’s t -distribution
- Normal distribution
- Null and Alternative Hypotheses
- Chi square tests
- Confidence interval
- Quartiles & Quantiles
- Data cleansing
- Reproducibility vs Replicability
- Peer review
- Prospective cohort study
Research bias
- Implicit bias
- Cognitive bias
- Placebo effect
- Hawthorne effect
- Hindsight bias
- Affect heuristic
- Social desirability bias
When your population is large in size, geographically dispersed, or difficult to contact, it’s necessary to use a sampling method .
This allows you to gather information from a smaller part of the population (i.e., the sample) and make accurate statements by using statistical analysis. A few sampling methods include simple random sampling , convenience sampling , and snowball sampling .
Stratified and cluster sampling may look similar, but bear in mind that groups created in cluster sampling are heterogeneous , so the individual characteristics in the cluster vary. In contrast, groups created in stratified sampling are homogeneous , as units share characteristics.
Relatedly, in cluster sampling you randomly select entire groups and include all units of each group in your sample. However, in stratified sampling, you select some units of all groups and include them in your sample. In this way, both methods can ensure that your sample is representative of the target population .
A sampling frame is a list of every member in the entire population . It is important that the sampling frame is as complete as possible, so that your sample accurately reflects your population.
Cite this Scribbr article
If you want to cite this source, you can copy and paste the citation or click the “Cite this Scribbr article” button to automatically add the citation to our free Citation Generator.
Nikolopoulou, K. (2023, June 22). What Is Probability Sampling? | Types & Examples. Scribbr. Retrieved September 3, 2024, from https://www.scribbr.com/methodology/probability-sampling/
Is this article helpful?
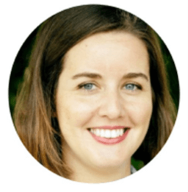
Kassiani Nikolopoulou
Other students also liked, population vs. sample | definitions, differences & examples, sampling methods | types, techniques & examples, what is a research design | types, guide & examples, get unlimited documents corrected.
✔ Free APA citation check included ✔ Unlimited document corrections ✔ Specialized in correcting academic texts
- Skip to secondary menu
- Skip to main content
- Skip to primary sidebar
Statistics By Jim
Making statistics intuitive
Random Assignment in Experiments
By Jim Frost 4 Comments
Random assignment uses chance to assign subjects to the control and treatment groups in an experiment. This process helps ensure that the groups are equivalent at the beginning of the study, which makes it safer to assume the treatments caused any differences between groups that the experimenters observe at the end of the study.

Huh? That might be a big surprise! At this point, you might be wondering about all of those studies that use statistics to assess the effects of different treatments. There’s a critical separation between significance and causality:
- Statistical procedures determine whether an effect is significant.
- Experimental designs determine how confidently you can assume that a treatment causes the effect.
In this post, learn how using random assignment in experiments can help you identify causal relationships.
Correlation, Causation, and Confounding Variables
Random assignment helps you separate causation from correlation and rule out confounding variables. As a critical component of the scientific method , experiments typically set up contrasts between a control group and one or more treatment groups. The idea is to determine whether the effect, which is the difference between a treatment group and the control group, is statistically significant. If the effect is significant, group assignment correlates with different outcomes.
However, as you have no doubt heard, correlation does not necessarily imply causation. In other words, the experimental groups can have different mean outcomes, but the treatment might not be causing those differences even though the differences are statistically significant.
The difficulty in definitively stating that a treatment caused the difference is due to potential confounding variables or confounders. Confounders are alternative explanations for differences between the experimental groups. Confounding variables correlate with both the experimental groups and the outcome variable. In this situation, confounding variables can be the actual cause for the outcome differences rather than the treatments themselves. As you’ll see, if an experiment does not account for confounding variables, they can bias the results and make them untrustworthy.
Related posts : Understanding Correlation in Statistics , Causation versus Correlation , and Hill’s Criteria for Causation .
Example of Confounding in an Experiment

- Control group: Does not consume vitamin supplements
- Treatment group: Regularly consumes vitamin supplements.
Imagine we measure a specific health outcome. After the experiment is complete, we perform a 2-sample t-test to determine whether the mean outcomes for these two groups are different. Assume the test results indicate that the mean health outcome in the treatment group is significantly better than the control group.
Why can’t we assume that the vitamins improved the health outcomes? After all, only the treatment group took the vitamins.
Related post : Confounding Variables in Regression Analysis
Alternative Explanations for Differences in Outcomes
The answer to that question depends on how we assigned the subjects to the experimental groups. If we let the subjects decide which group to join based on their existing vitamin habits, it opens the door to confounding variables. It’s reasonable to assume that people who take vitamins regularly also tend to have other healthy habits. These habits are confounders because they correlate with both vitamin consumption (experimental group) and the health outcome measure.
Random assignment prevents this self sorting of participants and reduces the likelihood that the groups start with systematic differences.
In fact, studies have found that supplement users are more physically active, have healthier diets, have lower blood pressure, and so on compared to those who don’t take supplements. If subjects who already take vitamins regularly join the treatment group voluntarily, they bring these healthy habits disproportionately to the treatment group. Consequently, these habits will be much more prevalent in the treatment group than the control group.
The healthy habits are the confounding variables—the potential alternative explanations for the difference in our study’s health outcome. It’s entirely possible that these systematic differences between groups at the start of the study might cause the difference in the health outcome at the end of the study—and not the vitamin consumption itself!
If our experiment doesn’t account for these confounding variables, we can’t trust the results. While we obtained statistically significant results with the 2-sample t-test for health outcomes, we don’t know for sure whether the vitamins, the systematic difference in habits, or some combination of the two caused the improvements.
Learn why many randomized clinical experiments use a placebo to control for the Placebo Effect .

Experiments Must Account for Confounding Variables
Your experimental design must account for confounding variables to avoid their problems. Scientific studies commonly use the following methods to handle confounders:
- Use control variables to keep them constant throughout an experiment.
- Statistically control for them in an observational study.
- Use random assignment to reduce the likelihood that systematic differences exist between experimental groups when the study begins.
Let’s take a look at how random assignment works in an experimental design.
Random Assignment Can Reduce the Impact of Confounding Variables
Note that random assignment is different than random sampling. Random sampling is a process for obtaining a sample that accurately represents a population .

Random assignment uses a chance process to assign subjects to experimental groups. Using random assignment requires that the experimenters can control the group assignment for all study subjects. For our study, we must be able to assign our participants to either the control group or the supplement group. Clearly, if we don’t have the ability to assign subjects to the groups, we can’t use random assignment!
Additionally, the process must have an equal probability of assigning a subject to any of the groups. For example, in our vitamin supplement study, we can use a coin toss to assign each subject to either the control group or supplement group. For more complex experimental designs, we can use a random number generator or even draw names out of a hat.
Random Assignment Distributes Confounders Equally
The random assignment process distributes confounding properties amongst your experimental groups equally. In other words, randomness helps eliminate systematic differences between groups. For our study, flipping the coin tends to equalize the distribution of subjects with healthier habits between the control and treatment group. Consequently, these two groups should start roughly equal for all confounding variables, including healthy habits!
Random assignment is a simple, elegant solution to a complex problem. For any given study area, there can be a long list of confounding variables that you could worry about. However, using random assignment, you don’t need to know what they are, how to detect them, or even measure them. Instead, use random assignment to equalize them across your experimental groups so they’re not a problem.
Because random assignment helps ensure that the groups are comparable when the experiment begins, you can be more confident that the treatments caused the post-study differences. Random assignment helps increase the internal validity of your study.
Comparing the Vitamin Study With and Without Random Assignment
Let’s compare two scenarios involving our hypothetical vitamin study. We’ll assume that the study obtains statistically significant results in both cases.
Scenario 1: We don’t use random assignment and, unbeknownst to us, subjects with healthier habits disproportionately end up in the supplement treatment group. The experimental groups differ by both healthy habits and vitamin consumption. Consequently, we can’t determine whether it was the habits or vitamins that improved the outcomes.
Scenario 2: We use random assignment and, consequently, the treatment and control groups start with roughly equal levels of healthy habits. The intentional introduction of vitamin supplements in the treatment group is the primary difference between the groups. Consequently, we can more confidently assert that the supplements caused an improvement in health outcomes.
For both scenarios, the statistical results could be identical. However, the methodology behind the second scenario makes a stronger case for a causal relationship between vitamin supplement consumption and health outcomes.
How important is it to use the correct methodology? Well, if the relationship between vitamins and health outcomes is not causal, then consuming vitamins won’t cause your health outcomes to improve regardless of what the study indicates. Instead, it’s probably all the other healthy habits!
Learn more about Randomized Controlled Trials (RCTs) that are the gold standard for identifying causal relationships because they use random assignment.
Drawbacks of Random Assignment
Random assignment helps reduce the chances of systematic differences between the groups at the start of an experiment and, thereby, mitigates the threats of confounding variables and alternative explanations. However, the process does not always equalize all of the confounding variables. Its random nature tends to eliminate systematic differences, but it doesn’t always succeed.
Sometimes random assignment is impossible because the experimenters cannot control the treatment or independent variable. For example, if you want to determine how individuals with and without depression perform on a test, you cannot randomly assign subjects to these groups. The same difficulty occurs when you’re studying differences between genders.
In other cases, there might be ethical issues. For example, in a randomized experiment, the researchers would want to withhold treatment for the control group. However, if the treatments are vaccinations, it might be unethical to withhold the vaccinations.
Other times, random assignment might be possible, but it is very challenging. For example, with vitamin consumption, it’s generally thought that if vitamin supplements cause health improvements, it’s only after very long-term use. It’s hard to enforce random assignment with a strict regimen for usage in one group and non-usage in the other group over the long-run. Or imagine a study about smoking. The researchers would find it difficult to assign subjects to the smoking and non-smoking groups randomly!
Fortunately, if you can’t use random assignment to help reduce the problem of confounding variables, there are different methods available. The other primary approach is to perform an observational study and incorporate the confounders into the statistical model itself. For more information, read my post Observational Studies Explained .
Read About Real Experiments that Used Random Assignment
I’ve written several blog posts about studies that have used random assignment to make causal inferences. Read studies about the following:
- Flu Vaccinations
- COVID-19 Vaccinations
Sullivan L. Random assignment versus random selection . SAGE Glossary of the Social and Behavioral Sciences, SAGE Publications, Inc.; 2009.
Share this:

Reader Interactions
November 13, 2019 at 4:59 am
Hi Jim, I have a question of randomly assigning participants to one of two conditions when it is an ongoing study and you are not sure of how many participants there will be. I am using this random assignment tool for factorial experiments. http://methodologymedia.psu.edu/most/rannumgenerator It asks you for the total number of participants but at this point, I am not sure how many there will be. Thanks for any advice you can give me, Floyd
May 28, 2019 at 11:34 am
Jim, can you comment on the validity of using the following approach when we can’t use random assignments. I’m in education, we have an ACT prep course that we offer. We can’t force students to take it and we can’t keep them from taking it either. But we want to know if it’s working. Let’s say that by senior year all students who are going to take the ACT have taken it. Let’s also say that I’m only including students who have taking it twice (so I can show growth between first and second time taking it). What I’ve done to address confounders is to go back to say 8th or 9th grade (prior to anyone taking the ACT or the ACT prep course) and run an analysis showing the two groups are not significantly different to start with. Is this valid? If the ACT prep students were higher achievers in 8th or 9th grade, I could not assume my prep course is effecting greater growth, but if they were not significantly different in 8th or 9th grade, I can assume the significant difference in ACT growth (from first to second testing) is due to the prep course. Yes or no?
May 26, 2019 at 5:37 pm
Nice post! I think the key to understanding scientific research is to understand randomization. And most people don’t get it.
May 27, 2019 at 9:48 pm
Thank you, Anoop!
I think randomness in an experiment is a funny thing. The issue of confounding factors is a serious problem. You might not even know what they are! But, use random assignment and, voila, the problem usually goes away! If you can’t use random assignment, suddenly you have a whole host of issues to worry about, which I’ll be writing about in more detail in my upcoming post about observational experiments!
Comments and Questions Cancel reply
4 Types of Random Sampling Techniques Explained
Collect unbiased data utilizing these four types of random sampling techniques: systematic, stratified, cluster, and simple random sampling.

Random sampling means choosing a subset of a larger population where each sample has an equal probability of being chosen. Random samples are used in statistical and scientific research to reduce sampling bias and get sample data that is generally representative of a population, which help form unbiased conclusions.
4 Types of Random Sampling Techniques
- Simple random sampling.
- Stratified random sampling.
- Cluster random sampling.
- Systematic random sampling.
More From Terence Shin 10 Advanced SQL Concepts You Should Know for Data Science Interviews
What Is Random Sampling?
Random sampling simply describes a state wherein every element in a population has an equal chance of being chosen for the sample. Sounds simple, right? Well, it’s a lot easier said than done because you must consider a lot of logistics in order to minimize bias.
Why Is Random Sampling Important?
If you’re a data scientist and want to develop models, or a researcher who wants to analyze a population, you need data. And if you need data, someone needs to collect that data. And if someone is collecting data, they need to make sure that it isn’t biased or it will be extremely costly in the long run.
Therefore, if you want to collect unbiased data and create more accurate data models , then you need to know about random sampling and how it works.
More on Data Science Importance Sampling Explained
Types of Random Sampling
There are four main types of random sampling techniques: simple random sampling, stratified random sampling, cluster random sampling and systematic random sampling. Each is used for different sampling situations.
1. Simple Random Sampling
Simple random sampling requires the use of randomly generated numbers to choose a sample. More specifically, it initially requires a sampling frame, which is a list or database of all members of a population. You can then randomly generate a number for each element, using Excel for example, and take the first n number of samples that you require.
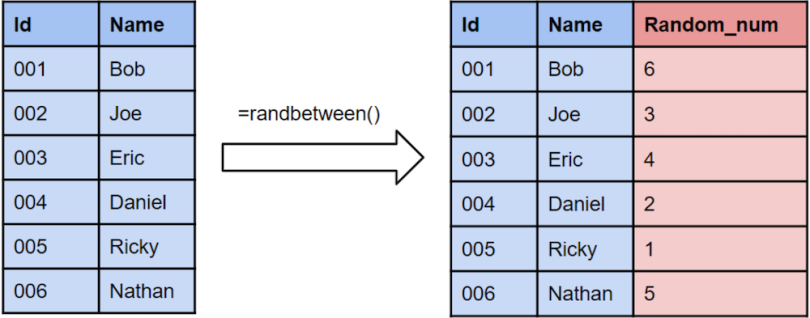
To give an example, imagine the table on the right was your sampling frame. Using software like Excel, you can then generate random numbers for each element in the sampling frame. If you need a sample size of three, then you would take the samples with the random numbers from one to three.
2. Stratified Random Sampling
Stratified random sampling involves dividing a population into groups with similar attributes and randomly sampling each group.
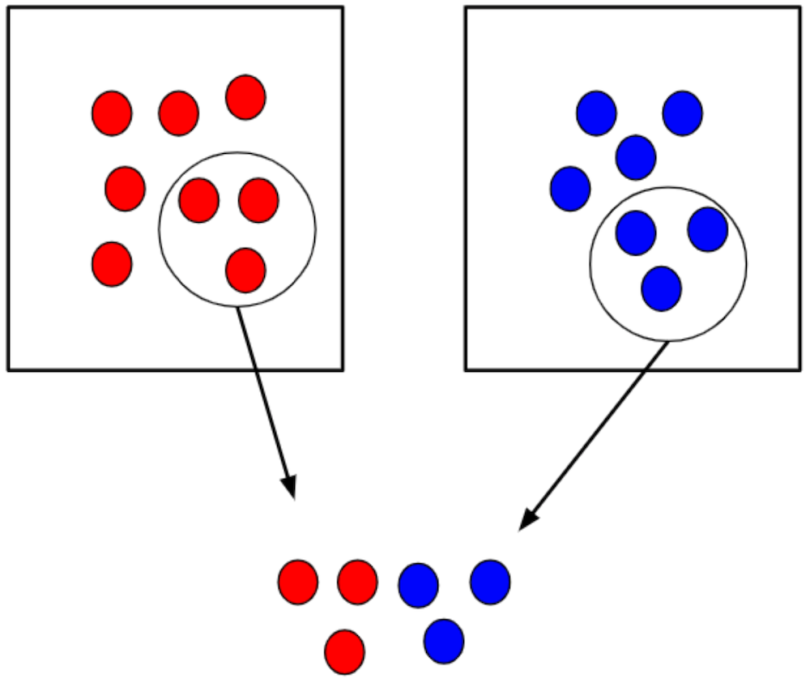
This method ensures that different segments in a population are equally represented. To give an example, imagine a survey is conducted at a school to determine overall satisfaction. Here, stratified random sampling can equally represent the opinions of students in each department.
3. Cluster Random Sampling
Cluster sampling starts by dividing a population into groups or clusters. What makes this different from stratified sampling is that each cluster must be representative of the larger population. Then, you randomly select entire clusters to sample.
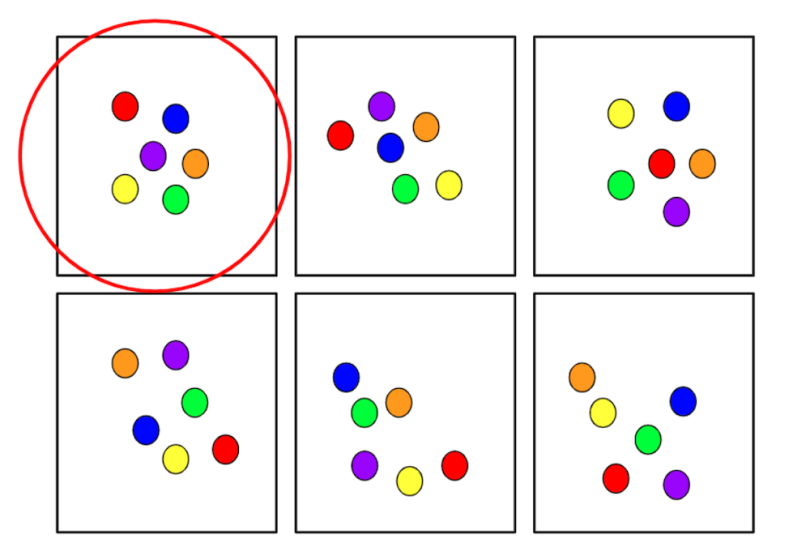
For example, if a school had five different eighth grade classes, cluster random sampling means any one class would serve as a sample.
4. Systematic Random Sampling
Systematic random sampling is a common technique in which you sample every k th element. For example, if you were conducting surveys at a mall, you might survey every 100th person that walks in.
If you have a sampling frame, then you would divide the size of the frame, N , by the desired sample size, n , to get the index number, k . You would then choose every k th element in the frame to create your sample.
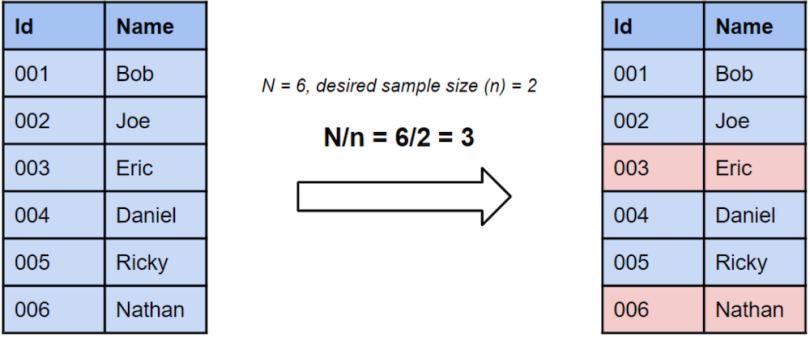
Using the same charts from the first example, if we wanted a sample size of two this time, then we would take every third row in the sampling frame.
Frequently Asked Questions
What is random sampling.
Random sampling involves collecting a subset of samples from a population in a way where each sample has an equal chance of being chosen. Random samples are used to ensure a sample adequately represents the larger population and to minimize sampling bias in research results.
What are the 4 types of simple random sampling?
The 4 main types of random sampling are:
- Simple random sampling
- Stratified random sampling
- Cluster random sampling
- Systematic random sampling
Which is an example of a random sample?
An example of a random sample would be randomly choosing the names of 10 people from a hat containing the names from a group of 100 people.
Recent Big Data Articles
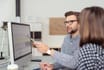
Random Sampling vs. Random Assignment
Random sampling and random assignment are fundamental concepts in the realm of research methods and statistics. However, many students struggle to differentiate between these two concepts, and very often use these terms interchangeably. Here we will explain the distinction between random sampling and random assignment.
Random sampling refers to the method you use to select individuals from the population to participate in your study. In other words, random sampling means that you are randomly selecting individuals from the population to participate in your study. This type of sampling is typically done to help ensure the representativeness of the sample (i.e., external validity). It is worth noting that a sample is only truly random if all individuals in the population have an equal probability of being selected to participate in the study. In practice, very few research studies use “true” random sampling because it is usually not feasible to ensure that all individuals in the population have an equal chance of being selected. For this reason, it is especially important to avoid using the term “random sample” if your study uses a nonprobability sampling method (such as convenience sampling).
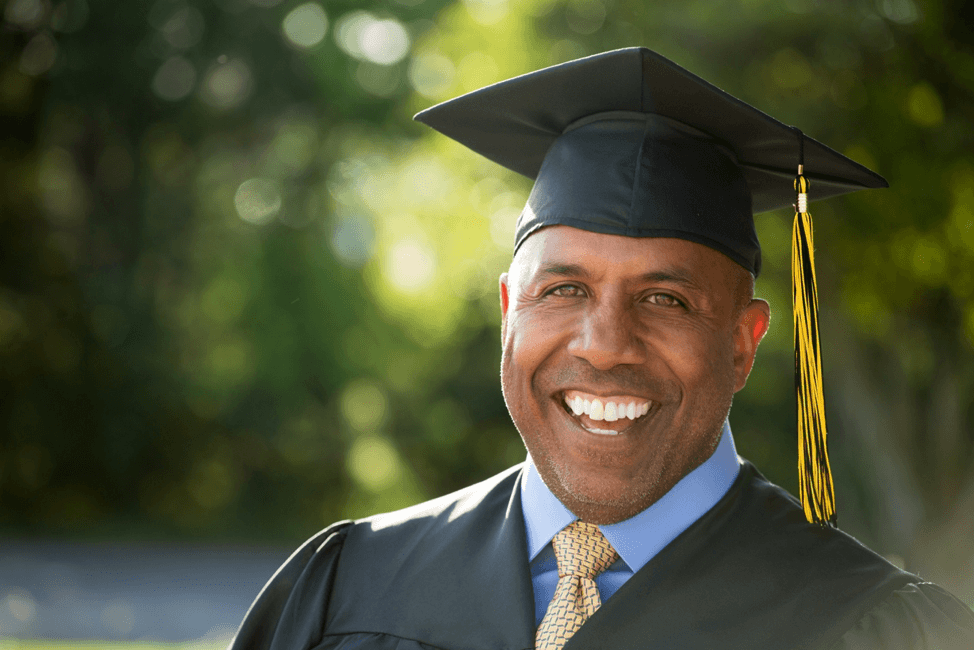
Discover How We Assist to Edit Your Dissertation Chapters
Aligning theoretical framework, gathering articles, synthesizing gaps, articulating a clear methodology and data plan, and writing about the theoretical and practical implications of your research are part of our comprehensive dissertation editing services.
- Bring dissertation editing expertise to chapters 1-5 in timely manner.
- Track all changes, then work with you to bring about scholarly writing.
- Ongoing support to address committee feedback, reducing revisions.
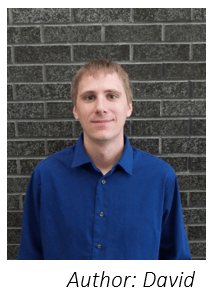
Random assignment refers to the method you use to place participants into groups in an experimental study. For example, say you are conducting a study comparing the blood pressure of patients after taking aspirin or a placebo. You have two groups of patients to compare: patients who will take aspirin (the experimental group) and patients who will take the placebo (the control group). Ideally, you would want to randomly assign the participants to be in the experimental group or the control group, meaning that each participant has an equal probability of being placed in the experimental or control group. This helps ensure that there are no systematic differences between the groups before the treatment (e.g., the aspirin or placebo) is given to the participants. Random assignment is a fundamental part of a “true” experiment because it helps ensure that any differences found between the groups are attributable to the treatment, rather than a confounding variable.
So, to summarize, random sampling refers to how you select individuals from the population to participate in your study. Random assignment refers to how you place those participants into groups (such as experimental vs. control). Knowing this distinction will help you clearly and accurately describe the methods you use to collect your data and conduct your study.
- Search Search Please fill out this field.
What Is Simple Random Sampling?
- Simple Random Sample
- Disadvantages
- Random Sampling FAQs
The Bottom Line
Simple random sampling definition, advantages and disadvantage.
:max_bytes(150000):strip_icc():format(webp)/picture-53894-1440689455-5bfc2a8846e0fb00260af532.jpg)
Simple random sampling is a technique in which a researcher selects a random subset of people from a larger group or population. In simple random sampling, each member of the group has an equal chance of getting selected. The method is commonly used in statistics to obtain a sample that is representative of the larger population.
Statistics is a branch of applied mathematics that helps us learn about large datasets by studying smaller events or objects. Put simply, you can make inferences about a large population by examining a smaller sample. Statistical analysis is commonly used to identify trends in many different areas, including business and finance. Individuals can use findings from statistical research to make better decisions about their money, businesses, and investments.
The simple random sampling method allows researchers to statistically measure a subset of individuals selected from a larger group or population to approximate a response from the entire group. This research method has both benefits and drawbacks. We highlight these pros and cons in this article, along with an overview of simple random sampling.
Key Takeaways
- A simple random sample is one of the methods researchers use to choose a sample from a larger population.
- This method works if there is an equal chance that any of the subjects in a population will be chosen.
- Researchers choose simple random sampling to make generalizations about a population.
- Major advantages include its simplicity and lack of bias.
- Among the disadvantages are difficulty gaining access to a list of a larger population, time, costs, and that bias can still occur under certain circumstances.
Simple Random Sample: An Overview
As noted above, simple random sampling involves choosing a smaller subset of a larger population. This is done randomly. But the catch here is that there is an equal chance that any of the samples in the subset will be chosen. Researchers tend to choose this method of sampling when they want to make generalizations about the larger population.
Simple random sampling can be conducted by using:
- The lottery method. This method involves assigning a number to each member of the dataset then choosing a prescribed set of numbers from those members at random.
- Technology. Using software programs like Excel makes it easier to conduct random sampling. Researchers just have to make sure that all the formulas and inputs are correctly laid out.
For simple random sampling to work, researchers must know the total population size. They must also be able to remove all hints of bias as simple random sampling is meant to be a completely unbiased approach to garner responses from a large group.
Keep in mind that there is room for error with random sampling. This is noted by adding a plus or minus variance to the results. In order to avoid any errors, researchers must study the entire population, which for all intents and purposes, isn't always possible.
To ensure bias does not occur, researchers must acquire responses from an adequate number of respondents, which may not be possible due to time or budget constraints.
Advantages of a Simple Random Sample
Simple random sampling may be simple to perform (as the name suggests) but it isn't used that often. But that doesn't mean it shouldn't be used. As long as it is done properly, there are certain distinct advantages to this sampling method.
Lack of Bias
The use of simple random sampling removes all hints of bias —or at least it should. Because individuals who make up the subset of the larger group are chosen at random, each individual in the large population set has the same probability of being selected. In most cases, this creates a balanced subset that carries the greatest potential for representing the larger group as a whole.
Here's a simple way to show how a researcher can remove bias when conducting simple random sampling. Let's say there are 100 bingo balls in a bowl, from which the researcher must choose 10. In order to remove any bias, the individual must close their eyes or look away when choosing the balls.
As its name implies, producing a simple random sample is much less complicated than other methods . There are no special skills involved in using this method, which can result in a fairly reliable outcome. This is in contrast to other sampling methods like stratified random sampling . This method involves dividing larger groups into smaller subgroups that are called strata. Members are divided up into these groups based on any attributes they share. As mentioned, individuals in the subset are selected randomly and there are no additional steps.
Less Knowledge Required
We've already established that simple random sampling is a very simple sampling method to execute. But there's also another, similar benefit: It requires little to no special knowledge. This means that the individual conducting the research doesn't need to have any information or knowledge about the larger population in order to effectively do their job.
Be sure that the sample subset from the larger group is inclusive enough. A sample that doesn't adequately reflect the population as a whole will result in a skewed result.
Disadvantages of a Simple Random Sample
Although there are distinct advantages to using a simple random sample, it does come with inherent drawbacks. These disadvantages include the time needed to gather the full list of a specific population, the capital necessary to retrieve and contact that list, and the bias that could occur when the sample set is not large enough to adequately represent the full population. We go into more detail below.
Difficulty Accessing Lists of the Full Population
An accurate statistical measure of a large population can only be obtained in simple random sampling when a full list of the entire population to be studied is available. Think of a list of students at a university or a group of employees at a specific company.
The problem lies in the accessibility of these lists. As such, getting access to the whole list can present challenges. Some universities or colleges may not want to provide a complete list of students or faculty for research. Similarly, specific companies may not be willing or able to hand over information about employee groups due to privacy policies.
Time Consuming
When a full list of a larger population is not available, individuals attempting to conduct simple random sampling must gather information from other sources. If publicly available, smaller subset lists can be used to recreate a full list of a larger population, but this strategy takes time to complete.
Organizations that keep data on students, employees, and individual consumers often impose lengthy retrieval processes that can stall a researcher's ability to obtain the most accurate information on the entire population set.
In addition to the time it takes to gather information from various sources, the process may cost a company or individual a substantial amount of capital. Retrieving a full list of a population or smaller subset lists from a third-party data provider may require payment each time data is provided.
If the sample is not large enough to represent the views of the entire population during the first round of simple random sampling, purchasing additional lists or databases to avoid a sampling error can be prohibitive.
Sample Selection Bias
Although simple random sampling is intended to be an unbiased approach to surveying, sample selection bias can occur. When a sample set of the larger population is not inclusive enough, representation of the full population is skewed and requires additional sampling techniques.
Data Quality Is Reliant on Researcher Qualify
The success of any sampling method relies on the researcher's willingness to thoroughly do their job. Someone who isn't willing to follow the rules or deviates from the task at hand won't help get a reliable result. For instance, there may be issues if a researcher doesn't ask the appropriate questions or asks the wrong ones. This could create implicit bias, ending up in a skewed study.
The term simple random sampling refers to a smaller section of a larger population. There is an equal chance that each member of this section will be chosen. For this reason, a simple random sampling is meant to be unbiased in its representation of the larger group. There is normally room for error with this method, which is indicated by a plus or minus variant. This is known as a sampling error.
How Is Simple Random Sampling Conducted?
Simple random sampling involves the study of a larger population by taking a smaller subset. This subgroup is chosen at random and studied to get the desired result. In order for this sampling method to work, the researcher must know the size of the larger population. The selection of the subset must be unbiased.
What Are the 4 Types of Random Sampling?
There are four types of random sampling. Simple random sampling involves an unbiased study of a smaller subset of a larger population. Stratified random sampling uses smaller groups derived from a larger population that is based on shared characteristics and attributes. Systematic sampling is a method that involves specific members of a larger dataset. These samples are selected based on a random starting point using a fixed, periodic interval. The final type of random sampling is cluster sampling, which takes members of a dataset and places them into clusters based on shared characteristics. Researchers then randomly select clusters to study.
When Is It Best to Use Simple Random Sampling?
It's always a good idea to use simple random sampling when you have smaller data sets to study. This allows you to produce better results that are more representative of the overall population. Keep in mind that this method requires each member of the larger population is identified and selected individually, which can often be challenging and time consuming.
Studying large populations can be very difficult. Getting information from each individual member can be costly and time-consuming. That's why researchers turn to random sampling to help reach the conclusions they need to make key decisions, whether that means helping provide the services that residents need, making better business decisions, or executing changes in an investor's portfolio.
Simple random sampling is relatively easy to conduct as long as you remove any and all hints of bias. Doing so means you must have information about each member of the larger population at your disposal before you conduct your research. This can be relatively simple and require very little knowledge. But keep in mind that the process can be costly and it may be hard trying to get access to information about all of the members of the population.
Pressbooks. " Significant Statistics: 1.5 Sampling Techniques and Ethics ."
:max_bytes(150000):strip_icc():format(webp)/thinkstockphotos-186299182-5bfc351cc9e77c00519c7317.jpg)
- Terms of Service
- Editorial Policy
- Privacy Policy

Students also viewed


IMAGES
VIDEO
COMMENTS
Simple Random Sample Assignment A researcher wants to generate an SRS to study the blood pressures of a population of 100 athletes on four high school athletic teams.
Study with Quizlet and memorize flashcards containing terms like What is a frame?, Define simple random sampling., What does it mean when sampling is done without replacement? and more.
In simple random sampling, researchers randomly choose subjects from a population with equal probability to create representative samples.
Simple random sampling is a technique in which each member of a population has an equal chance of being chosen through an unbiased selection method. Each subject in the sample is given a number, and then the sample is chosen randomly.
Simple Random Samples and Statistics We formulate the notion of a (simple) random sample, which is basic to much of classical statistics. Once formulated, we may apply probability theory to exhibit several basic ideas of statistical analysis. We begin with the notion of a population distribution.
A simple random sample is a subset of a statistical population in which each member of the subset has an equal probability of being chosen.
Learn how to conduct simple random sampling, a method that selects a random subset of a population for data analysis, with steps and examples.
Assignment for Stats Learn with flashcards, games, and more — for free.
With simple random assignment, every member of the sample has a known or equal chance of being placed in a control group or an experimental group. Studies that use simple random assignment are also called completely randomized designs. Random assignment is a key part of experimental design.
The following sampling methods are examples of probability sampling: Simple Random Sampling (SRS) Stratified Sampling. Cluster Sampling. Systematic Sampling. Multistage Sampling (in which some of the methods above are combined in stages) Of the five methods listed above, students have the most trouble distinguishing between stratified sampling ...
Study with Quizlet and memorize flashcards containing terms like Random sampling, 4 basic sampling techniques, Simple random sampling and more.
Random selection (also called probability sampling or random sampling) is a way of randomly selecting members of a population to be included in your study. On the other hand, random assignment is a way of sorting the sample participants into control and treatment groups. Random selection ensures that everyone in the population has an equal ...
Probability sampling is a sampling method that involves randomly selecting a sample, or a part of the population that you want to research. It is also sometimes called random sampling. To qualify as being random, each research unit (e.g., person, business, or organization in your population) must have an equal chance of being selected.
Random Assignment Can Reduce the Impact of Confounding Variables Note that random assignment is different than random sampling. Random sampling is a process for obtaining a sample that accurately represents a population. Random assignment uses a chance process to assign subjects to experimental groups.
Collect unbiased data utilizing these four types of random sampling techniques: systematic, stratified, cluster, and simple random sampling.
Random sampling and random assignment are fundamental concepts in the realm of research methods and statistics. However, many students struggle to differentiate between these two concepts, and very often use these terms interchangeably. Here we will explain the distinction between random sampling and random assignment.
Study with Quizlet and memorize flashcards containing terms like replication, What is the goal of sampling?, target population and more.
Random sampling allows us to obtain a sample representative of the population. Therefore, results of the study can be generalized to the population. Random assignment allows us to make sure that the only difference between the various treatment groups is what we are studying. For example, in the serif/sans serif example, random assignment helps ...
A simple explanation of the difference between random selection and random assignment along with several examples.
Study with Quizlet and memorize flashcards containing terms like Courtney would like to estimate the net worth of all people in the US. The distribution of net worth of people in the US is strongly skewed to the right. Courtney selects an SRS of size n = 15 from this population and calculates the sample mean. What is the shape of the distribution of the sample mean for all possible simple ...
Researchers use the simple random sample methodology to choose a subset of individuals from a larger population. While easier to implement than other methods, it can be costly and time-consuming.
Study with Quizlet and memorize flashcards containing terms like 1. All members of a population have an equal chance of being selected as subjects in a research project. This is called: 1. Randomization 2. Random assignment 3. Random sample 4. Random quota, 2. You decide to select a sample of 100 subjects of whom 10% will be African American, 10% Hispanic, and 80% white. Identify the ...
There are four major types of probability sample designs: simple random sampling, stratified sampling, systematic sampling, and cluster sampling (see Figure 5.1). Simple random sampling is the most recognized probability sam-pling procedure. Stratified sampling offers significant improvement to simple random sampling.