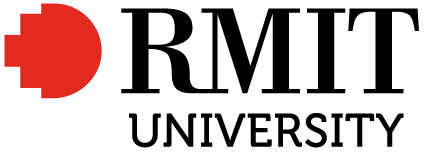
Want to create or adapt books like this? Learn more about how Pressbooks supports open publishing practices.

What is a claim?
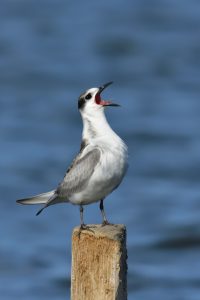
A claim is a statement that presents an idea or series of ideas as arguments. Arguments therefore consist of claims, or another way to put it is, to say that claims are the building blocks of a good argument.
In research writing, claims will be the backbone that form a thesis or a hypothesis (here the term ‘hypothesis’ refers to the argument that is evidenced within the scope of the work).
According to Heady (2013) “Claims are the points you want to prove, interpretations you want to offer, and assertions you want to make” (p. 74). Importantly, in academia claims are statements that can be supported by evidence.
‘Traditional classroom teaching is boring’
For example, claiming that traditional classroom teaching is boring is not a good claim because it lacks definition (what does ‘traditional classroom teaching’ actually mean? and how do we measure ‘boring’)? It may also be a ‘sweeping statement’ (meaning it’s far too general in scope). However, claiming that “traditional teaching methods, like didactic instruction, do not provide sufficient interaction with students and lead to poor learning outcomes” is a good argumentative claim, because it can be investigated and measured.
Characteristics of a good claim
In order to make effective claims it is important to understand the difference between statements and sentences. While a statement is also a sentence (in that it is a grammatical unit with subject, verb, object clause), not all sentences are statements (in other words, not all sentences consist of a stance or a position).
The following provides examples of the difference between sentences and statements. The statements present a stance or position about the topic under discussion. This is important to understand as all claims must consist of a stance towards the topic.
Function of claims
The function of claims in academic writing is to provoke, analyse, or interpret rather than merely describe or present facts. They can do this by affirming, acknowledging, confirming, or refuting the proposition being made. In this way, claims do the job of building an overall argument or thesis in a piece of work (i.e. each claim progresses the key argument). It is for this reason that claims will appear in topic sentences, thesis statements, introductory and concluding sentences/paragraphs.
Check your understanding
Research and Writing Skills for Academic and Graduate Researchers Copyright © 2022 by RMIT University is licensed under a Creative Commons Attribution-NonCommercial 4.0 International License , except where otherwise noted.
Share This Book
Accessibility Links
- Skip to content
- Skip to search IOPscience
- Skip to Journals list
- Accessibility help
- Accessibility Help
Click here to close this panel.
Purpose-led Publishing is a coalition of three not-for-profit publishers in the field of physical sciences: AIP Publishing, the American Physical Society and IOP Publishing.
Together, as publishers that will always put purpose above profit, we have defined a set of industry standards that underpin high-quality, ethical scholarly communications.
We are proudly declaring that science is our only shareholder.
Claims in Construction Projects: A Comprehensive Literature Review
H H Shaikh 1 , N Y Zainun 1 and S H Khahro 2
Published under licence by IOP Publishing Ltd IOP Conference Series: Earth and Environmental Science , Volume 498 , The 5th International Conference on Civil and Environmental Engineering for Sustainability 19-20 December 2019, Johor, Malaysia Citation H H Shaikh et al 2020 IOP Conf. Ser.: Earth Environ. Sci. 498 012095 DOI 10.1088/1755-1315/498/1/012095
Article metrics
2673 Total downloads
Share this article
Author e-mails.
Author affiliations
1 Jamilus Research Center, Faculty of Civil Engineering & Built Environment, Universiti Tun Hussein Onn Malaysia, 86400 Parit Raja, Batu Pahat, Johor
2 Department of Engineering Management, Prince Sultan University, Riyadh, KSA
Buy this article in print
Construction industry plays main part in the financial development of a country and occupies an essential position in the state's growth policies. Construction industry generates thousands of jobs into different sectors to accommodate people according to its capabilities. On the contrary, it faces huge number of claims, as a result it gives birth to various problems including additional cost and time to complete the project. Therefore, this paper aims to identify the common types and causes of claims generally occur in construction industry and specifically in large scale projects. A detailed literature review has been carried out for this research and covers past research papers of the last two decades. The results showed delay in claims and extra work claim submitted by the parties, they are the main types of claims followed by contract ambiguity claims, extension of time claim and suspension of work claims. Delay of payment is the most important cause of claim followed by incomplete design, variation order, change order by owner, lack of communication and in last poor project management. It is concluded that proper contract documentation should be done and early error identification in contract documents, drawings and specifications should be highlighted. Finally, it is recommended to adopt proper claim management policy and procedures to address the queries by all parties. This paper will be used to design future guidelines in managing claims in large scale construction projects to improve the performance of construction industry.
Export citation and abstract BibTeX RIS
Content from this work may be used under the terms of the Creative Commons Attribution 3.0 licence . Any further distribution of this work must maintain attribution to the author(s) and the title of the work, journal citation and DOI.
We use cookies to give you the best experience possible. By continuing we’ll assume you’re on board with our cookie policy
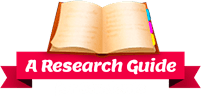
- A Research Guide
- Writing Guide
- Assignment Writing
How to Write a Claim
- What is a claim in a thesis?
Types of claim used in the thesis
How to craft a great claim statement.
- How to write your claim in a right way
What is a claim in a thesis?
Cause and effect, the claim of solutions or policies, factual or definitive.
Read also: Find research paper writers hire services to take care of your papers.
Claim of value
Choose and explore the topic of your interest, set a question and answer it with your thesis.
Read also: A reliable thesis statement maker will help you create a perfect thesis.
Define a goal of your paper
Take a stand for a single issue, take a different approach to the topic, write your claim in a right way, choose what you can prove, write your claim in a correct way, use a definitive yet firm tone.
Useful information: Dissertation writers for hire will help you complete your dissertation.
Place your claim in a proper place
By clicking "Log In", you agree to our terms of service and privacy policy . We'll occasionally send you account related and promo emails.
Sign Up for your FREE account
A semi-parametric claims reserving model with monotone splines
- Original Research
- Open access
- Published: 06 May 2024
Cite this article
You have full access to this open access article
- Le Chang 1 ,
- Guangyuan Gao 2 &
- Yanlin Shi ORCID: orcid.org/0000-0001-8049-5290 3
100 Accesses
Explore all metrics
Stochastic reserving models used in the insurance industry are usually based on an assumed distribution of claim amounts. Despite their popularity, such models may unavoidably be affected by the misspecification issue given that it is likely that the underlying distribution will be different from that assumed. In this paper, we incorporate monotone splines to ensure the expected monotonically increasing pattern of cumulative development factors (CDFs) to develop a new semi-parametric reserving model that does not require a density assumption. To allow the maximum utilization of available information, we also propose an enhanced sampling approach that greatly increases the size of unbiased CDFs, particularly in later development periods. Based on the enhanced samples, a bootstrap technique is employed in the estimation of monotone splines, from which incurred-but-not-reported (IBNR) reserves and prediction errors can be obtained. Associated technical features, such as the consistency of estimator, are discussed and demonstrated. Our simulation studies suggest that the new model improves the accuracy of IBNR reserving, compared with a range of classic competing models. A real data analysis produces many consistent findings, thus supporting the usefulness of the monotone spline model in actuarial and insurance practice.
Similar content being viewed by others
A review of bayesian asymptotics in general insurance applications.
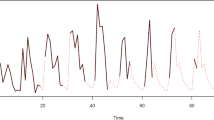
Holt–Winters method for run-off triangles in claims reserving
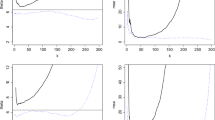
Conditional tail moment and reinsurance premium estimation under random right censoring
Avoid common mistakes on your manuscript.
1 Introduction
The outstanding claims reserve is usually the largest liability faced by an insurer. This provides information for both investors and regulators, and it is associated with ratemaking and solvency monitoring. Therefore, accurate estimation of an outstanding claims reserve, also known as the incurred-but-not-reported (IBNR) reserving, is critical in actuarial practice. This is particularly important for property and casualty insurers because their claims can develop over periods of more than one decade. It is worth noting that outstanding claims of an insurer include both IBNR and reported-but-not-settled (RBNS) claims. In this paper, we focus on cumulative claim amounts, rather than on counts, with only one run-off triangle. Consequently, IBNR and RBNS claims cannot be distinguished from each other, and the term (generalized) IBNR claims is used to indicate all the outstanding claim amounts.
In existing studies, relevant IBNR reserving models can be categorized into three areas. First, univariate multiplicative reserving models, which include the classic chain ladder (CL) method and its stochastic extensions, such as the overdispersed Poisson model (Renshaw & Verrall, 1998 ). Second, multivariate reserving models, which work with more than one run-off triangle (Wüthrich, 2003 ; Miranda et al., 2012 ). Third, micro-level reserving models, which, rather than working with run-off triangles as do the other two categories, investigate the development of individual claims (Antonio & Plat, 2014 ; Gabrielli & Wüthrich, 2018 ).
This paper contributes to developing univariate multiplicative reserving models, which constitute the most studied area of reserving modeling in the literature. As implied by the name, a common feature of such models is that the mean of cumulative paid claims until the next development period is assumed to be a multiplication of the development factor (DF) and the most recent cumulative claim. Over the past several decades, various parametric models have been developed as stochastic extensions of the classic CL method (e.g., Wright, 1990 ; Mack & Venter, 2000 ; England & Verrall, 2002 ; Wüthrich & Merz, 2008 ; Kuang et al., 2015 ; Kuang & Nielsen, 2020 ; Gao et al., 2021 ). Many of these extensions are based on a generalized linear model (GLM) framework with a Poisson family distribution. To allow more complicated features, such as tail factor extrapolation, parametric curves (Clark, 2003 ) or non-parametric splines (Gao & Meng, 2018 ) are fitted to the development pattern of the outstanding claims.
Despite the popularity in existing research, there are several inherent issues. First, all the parametric and Bayesian models depend on the reliableness of assumed distributions and/or associated parameters. Thus, if a model is misspecified, the estimated IBNR reserves and/or its prediction error might be unreliable. Second, most Bayesian models employ a complicated modeling structure. This can cause problems because the computational cost could be considerably high due to the employed MCMC algorithm. Further, the complicated parametric frameworks may reduce their applicability in actuarial practice. As Gisler ( 2019 ) argues, “A methodology whose mechanism is understood and which is sufficiently accurate is mostly preferred to a highly sophisticated methodology whose mechanism is difficult to understand and to see through.”
To resolve this, we adopt assumptions and technical findings in the seminal works of Mack ( 1993 , 1999 ) and explore a semi-parametric reserving model. Compared with a distribution-based parametric model, our model does not require an assumed density for the underlying variables, and it is usually more robust against the misspecification issue in the assumed distribution. In addition, this less complicated model is easy to follow and understand, and it has a much lower computational cost than Bayesian models.
Based on three fundamental assumptions, Mack ( 1993 , 1999 ) derives closed-form formula for both the prediction errors of IBNR reserves obtained by the classic CL method. Unlike modeling the (incremental) claims as in GLM, Mack’s model estimates the cumulative development factor (CDF) and age-to-age DF. Subsequent influential research has focused on revisiting the proposed prediction errors. For example, Diers et al. ( 2016 ) estimate the prediction error using bootstrap techniques. Röhr ( 2016 ) rewrites the prediction error of ultimate run-off risks as a function of the individual future CL DFs. Gisler ( 2019 ) provides simplified uncertainty estimators and compares them with those of Mack’s model and the model of Merz and Wuthrich ( 2015 ). Lindholm et al. ( 2020 ) use Akaike’s final prediction error in the uncertainty estimator, which is claimed to nest Mack’s model as a special case.
Unlike existing research, this study presents a framework that directly estimates forward-factors in the diagonal direction of a run-off triangle. This enables one to make the best use of the available information about CDFs/DFs and of the reliable “prior” belief using monotone splines. Specifically, in actuarial practice, negative incremental claims in a subsequent development period may inevitably arise as a result of salvage recoveries; payments from third parties; total or partial cancellation of outstanding claims due to initial overestimation of the loss or a jury decision in favor of the insurer; rejection by the insurer; or simply errors (De Alba, 2006 ). Despite the possibility of such occurrences, these negative values are believed to be rare/random events, and the mean development would usually be positive. That is, the CDFs are expected to monotonically increase with the development periods. Therefore, this belief strongly motivates the application of monotone splines on the modeling of CDFs.
Monotone splines belong to the popular spline functions. The application of spline functions has become an influential method in statistical regression analysis, particularly since the seminal work of Hastie and Tibshirani ( 1986 ). To ensure the monotone shapes, we employ the I-spline function (also known as an integrated B-spline) basis. As introduced by De Boor ( 1978 ), the B-spline basis is one of the most popular spline functions and is commonly associated with a special parametrization of cubic splines. Given the number of knots, the I-spline basis is fully determined for a regressor, and the estimation can be easily performed in a usual linear regression fashion, and this does not require an assumed density. To ensure the (increasing) monotonicity, we need to impose only the inequality constraints of the coefficients, which are expected to be non-negative. The estimation can be efficiently completed via sophisticated algorithms, as discussed in Gill et al. ( 2019 ).
However, applying the monotone splines to the original sample of CDFs is not optimal. As is illustrated in Fig. 1 and will be discussed in Sect. 3.2 , the original sample has fewer observations in the later development periods. This “unbalanced” structure will make observations at the tails unnecessarily influential in introducing large uncertainty. To resolve this issue, based on the fundamental assumptions of Mack ( 1993 , 1999 ), we first demonstrate that DFs across all accident periods are unbiased and uncorrelated. Consequently, a compound multiplication of DFs sampled from associated development periods will naturally produce a large unbiased sample of CDFs at later development periods. We name this new and (much) larger sample the “enhanced sample”. In particular, following Mack ( 1993 ), the sampling procedure may use probabilities that depend on the cumulative claim amounts. Under certain conditions, we prove that the sample mean of such CDFs in the enhanced sample will coincide with the classic CL estimates. Working with the enhanced sample, a bootstrap technique is employed to obtain the mean and standard errors of estimated DFs on the diagonal of a run-off triangle. The final IBNR reserve and prediction error can then be produced following the principles of Mack ( 1993 , 1999 ).
To demonstrate the effectiveness of our proposed model, we conduct systematically designed simulation studies. Apart from the monotone spline model, we consider four popular and classic competitors: GLM (based on CL); Mack’s model; bootstrap CL (England & Verrall, 2002 ); and Clark’s loss DF model (Clark, 2003 ). Using the simulation machine proposed in Gabrielli and Wüthrich ( 2018 ), we demonstrate that our model consistently outperforms all competitors. Specifically, our model results in the smallest estimate of prediction error, averaged over all simulated replicates. More importantly, this prediction error comes close to the root mean square prediction error (RMSPE) by contrasting point estimates and true values. Thus, the produced small prediction error is reliable and does not come at the cost of overfitting. To demonstrate its practical usefulness, the classic dataset of Taylor and Ashe ( 1983 ) is further investigated using all five models. Our findings are consistent with those obtained from the simulation study.
The contributions of this paper to the existing reserving methodology are threefold. First, we propose a framework that incorporates reliable beliefs and allows maximum utilization of available information. Footnote 1 The adopted monotone spline can easily deal with negative incremental values and does not require adjustment in the estimation iteration, which is often needed for parametric approaches with log transformation (e.g., GLM). Footnote 2 More importantly, the spline method ensures the monotone increasing shape of CDFs and thus reduces the influence of unavoidable random errors and improves credibility. In addition, the enhanced sample consists of CDFs naturally composed of the actual DFs. This greatly increases the sample space of CDFs than the original data, and thus may effectively reduce the uncertainty. Second, we present the technical properties of the enhanced sample and estimators of monotone splines. Without imposing additional assumptions, the unbiasedness of CDFs in the enhanced sample validates its applicability to being modeled compared with the original scarce CDFs. This means that the employed bootstrap technique can provide consistent estimators of both IBNR reserves and the prediction errors. Third, our model exhibits desirable technical features that are superior to those of existing models. Compared with parametric approaches, our model retains the property of a distribution-free framework to be robust against the misspecification issue. Compared with Bayesian models, the computation based on the monotone spline can be efficiently completed at a much lower cost. In addition, the monotone spline can be easily and reliably interpolated/extrapolated. This can be used to estimate claim developments at intermediate steps (e.g., for a development quarter whereas yearly data are modeled) and tail factors. Such features significantly complement Mack’s model and related extensions. Note that a continuous CL model developed by Miranda et al. ( 2013 ) also enables DFs to be estimated at continuous time points. As will be detailed in Sect. 3 , our model complements such in-sample density forecasting models by ensuring the monotone increasing shape of estimated CDFs and ease of calculation of prediction errors.
The remainder of this paper is structured as follows. Section 2 introduces existing claims reserving models. Section 3 proposes the monotone spline model and discusses the technical properties. Section 4 presents the simulations studies. Section 5 performs a real data analysis. Section 6 concludes the paper.
2 Existing claims reserving methods
Most existing IBNR claims reserving models focus on the aggregated paid claims organized in a so-called run-off triangle . In this triangle, claims are grouped into cohorts by their accident years (AY). Each cohort is then subsequently settled by development years (DY), which are usually organized in calendar years. However, months and quarters are also popular units of temporal periods. A claims cohort with the earliest AY has the longest development history, while that with the latest AY has only one DY of the last AY. Such a run-off triangle with an incremental claim in each DY is presented below, where \(Y_{i,j}\) is the claims paid in AY i and settled in DY j , with \(i,j\in \{1,2,...,I\}\) . Note that the numbers of AY and DY can be different, in which case all the methods examined in this paper still apply. In this section, we briefly introduce the classic CL method and its parametric GLM specification, as well as a distribution-free model developed in Mack ( 1993 ).
2.1 Classic CL method and GLM
The classic CL method calculates DFs to estimate the IBNR claims of each AY. The CL method employs the cumulative claims to compute DFs and CDFs. Let \(C_{i,j}\) denote the cumulative claims corresponding to AY i and DY j , which gives \(C_{i,j}=\sum _{l=1}^{j}Y_{i,l}\) . In addition, the claims on the diagonal with the suffix \(c=i+j-1\) represent the claims paid in the calendar year c . For example, \(C_{1,2}\) defines the paid cumulative claims in the second DY, that is, one year after the second AY from which the claim arises. Hence, it is clear that the claims in the upper triangle with \(i+j-1\le I\) , as presented in the Table 1, are observed historical claims. The claims in the lower triangle with \(i+j-1> I\) are IBNR claims to be predicted.
Without considering a risk margin, the total of the incremental IBNR claims in the lower triangle is then the overall reserve that an insurer must hold. Specifically, the outstanding incremental IBNR reserve for each AY \(i=2, 3, \ldots , I\) is defined as:
The sum of all outstanding incremental IBNR reserve is therefore:
To estimate R , one of the most commonly used models is the CL method, which estimates DFs derived from historical data for the calculation. Using the same notations as in Mack ( 1993 ) and Mack ( 1999 ), the DF corresponding to DY k is estimated here:
Future cumulative claims can be predicted by multiplying these DFs with the latest observed cumulative claims in each AY, such that:
Then, \(R_i\) defined in ( 1 ) can be estimated by \({\widehat{C}}_{i,I}-C_{i,I-i+1}=C_{i,I-i+1}(\prod ^{I-1}_{k=I-i+1}{\hat{f}}_k-1)\) for \(i=1,2,...,I\) .
It can be shown that the above estimators of the classic CL model are unbiased, under the assumptions adopted in Mack ( 1993 ). However, apart from the estimated IBNR reserve, the associated uncertainty or prediction error is usually required to determine the risk margin for prudence (England & Verrall, 2002 ). Unfortunately, the deterministic CL method as presented in ( 4 ) cannot be used for this purpose without additional assumptions.
To derive the uncertainty measure for CL, there are two popular approaches in the literature. The first (detailed in Sect. 2.2 ) is discussed in the seminal works of Mack ( 1993 , 1999 ), which employ the same distribution-free assumption as the classic CL. The second is embedded within a GLM framework, which requires an assumption for the density of claims. For the GLM approach, since stochastic errors are allowed, the prediction error can then be obtained. To enable a GLM estimation of IBNR reserves, we consider a multiplicative model for the incremental claims as follows:
where \({c_i}\) is the expected claims amounts for AY i , and \(b_j\) is the proportion of claims paid in DY j for a given accident year. Note that the mean of \(Y_{i,j}\) is structured in a multiplicative fashion, such that \(E[Y_{i,j}]= {c_i}b_j\) . Consequently, applying a logarithmic transformation would enable ( 5 ) to be estimated in an additive fashion that can be accommodated via a GLM. The associated log-linear specification of such a GLM is then as follows:
where \(\mu \) is the intercept, \(\alpha _i\) is a parameter for each AY i and \(\beta _j\) is the associated coefficient for each DY j . As a convention, we impose the corner constraint, such that \(\alpha _1=\beta _1=0\) to achieve the aim of identification. Footnote 3 The predictor vector, or \({\varvec{x}}_{i,j}\in 1\times \{0,1\}^{2I-2}\) , includes the constant 1 (the intercept) and the indicators of each AY and DY starting from 2. The parameter vector \(\varvec{\theta }\) then consists of \((\mu , \alpha _2, \ldots , \alpha _I, \beta _2, \ldots , \beta _I)\) .
With an estimated parameter vector \(\widehat{\varvec{\theta }}\) , the outstanding IBNR claims can be predicted as \(\exp {({\varvec{x}}^\top _{i,j}\widehat{\varvec{\theta }})}\) . The GLM specification ( 6 ) can therefore be viewed as a stochastic CL. One of the most desirable features of the above GLM framework is that the predicted claims exactly coincide with those obtained from ( 4 ), or the deterministic counterpart (see Renshaw & Verrall, 1998 ; England & Verrall, 2002 , for proofs).
However, due to the employed Poisson distribution, the original GLM specified in ( 6 ) assumes that the variance of the response variable is equal to its mean. In actuarial literature, it is widely acknowledged that this assumption is too strong, and the variance of the claim is usually (much) greater than the mean. Within the same parametric framework, To allow for a more flexible mean-variance relationship, overdispersed Poisson models are examined. An additional parameter known as the dispersion is employed to estimate more accurately the prediction error in the stochastic claims reserving. More details regarding those parametric extensions can be found in Renshaw and Verrall ( 1998 ), England and Verrall ( 2002 ), Wüthrich and Merz ( 2008 ) and Gao et al. ( 2021 ).
2.2 Mack’s distribution-free model
Unlike the density-based GLM extensions, the classic CL model does not assume a distribution for the claims. To derive an associated uncertainty measure of the reserves estimate, in his seminal works, Mack ( 1993 , 1999 ) makes two major contributions by demonstrating the following: 1) the classic CL estimators of the DFs are standard weighted least squares estimators; and 2) an estimator of the conditional mean squared error (MSE) of predicted reserves.
Consistent with the classic CL model, no distribution assumption is required, and the following three fundamental assumptions are needed Footnote 4 :
where \(F_{i,k}=C_{i,k+1}/C_{i,k}\) is the AY-specific DF in AY i and DY k , \(i=1,...,I\) and \(k=1,...,I-1\) .
Mack’s model focuses on the estimation of DFs directly, rather than on modeling claims as in a GLM framework. The first contribution of Mack ( 1993 , 1999 ) means that \(f_k\) as estimated by ( 3 ) for the CL method is performed in a standard weighted-average fashion. Using Assumption (II), it is further demonstrated that the estimator of ( 3 ) is the minimum variance unbiased linear estimator of \(f_k\) . Unlike in a deterministic approach such as the classic CL, by proposing Assumptions (I)–(III), the second contribution details how a prediction error can be estimated following usual statistical principles. Specifically, using Assumption (III), it is first shown which \({\hat{f}}_k\) obtained via ( 3 ) is unbiased and uncorrelated (Mack, 1993 ). Based on this, together with Assumptions (I)–(III), the following estimators are developed (Mack, 1999 ):
For the last four equations, we have \(i,k=1,...,I-1\) . The (estimated) uncertainty measured by \({\widehat{Var}}({\hat{R}}_i)\) is also known as the (estimated) MSE of predicted outstanding claims in AY i to indicate the prediction error of the model, accounting for both process and parameter risks. The total prediction error is therefore measured by \({\widehat{Var}}({\hat{R}})\) .
Note that for the Mack’s estimators to be valid, we need to test for the so-called calendar year effect (for AYs) and the correlation between DFs (Mack, 1994 ). These effects/correlations are not claimed to be underlying assumptions of the classic CL method.
3 A semi-parametric model using monotone splines
Past research has developed many extensions of Mack’s model (e.g., Merz & Wuthrich, 2015 ; Röhr, 2016 ; Gisler, 2019 ; Lindholm et al., 2020 ). Most of these studies focus on developing new MSE formulas based on different statistical principles. In this section, using Assumptions (I)–(III) and findings of ( 8 ), we aim to utilize more information from the historical data and reliable “prior” beliefs. For example, although incremental claims could be negative for reasons such as salvage recoveries, cumulative claims would increase under “normal” circumstances. Thus, while no distribution is assumed, CDFs might be fitted using monotone splines for this belief.
3.1 Monotone splines
The application of spline functions has become an influential and popular tool in statistical regression analysis, stemming from the seminal work of Hastie and Tibshirani ( 1986 ). Simply speaking, the spline function employs piecewise polynomials, which are continuously differentiable at the knots, to model a sequence of data. In addition, splines can be spanned using different basis functions. Introduced by De Boor ( 1978 ) and other scholars, B-spline basis is one of the most popular basis functions, and a common practice is associated with a special parametrization of cubic splines (i.e., piecewise polynomials up to cubic order).
To restrict fitted splines to being monotone increasing, we employ the integrated B-splines, also known as the I-splines. For a quadratic I-spline with three consecutive knots \(t_j\) , \(t_{j+1}\) and \(t_{j+2}\) , with \(t_j<t_{j+1}<{t_{j+2}}\) , the I-spline basis for a given value x is described below Footnote 5 :
To fit I-splines of a regressor X to a response variable Y , a linear regression can be fitted by replacing original values of X by its corresponding I-spline basis, given all predetermined knots.
As defined in an integrated form, it can be shown that the I-spline basis is non-negative (e.g., the above quadratic case). In addition, with the increasing value of inputs, each corresponding basis of a partition will be monotone increasing, with lower limit 0 and upper limit 1. For example (this is the same I-spline to be used in Section 4), for DY 1-11 with 4 knots (evenly located), the cubic I-spline basis is displayed in Table 2 . Consequently, to ensure fitted piecewise polynomials are monotone increasing (decreasing) with the regressor values, it is sufficient to impose non-negative (non-positive) constraints on each coefficient in the linear regression. In the usual case of a squared loss, the estimation is equivalent to a quadratic optimization issue with inequality constraints. This can be solved via efficient iteration-based algorithms (Gill et al., 2019 ).
3.2 Modeling claim DFs using monotone splines: preliminary analyses
We now explore how monotone splines can be employed to obtain claim DFs. To facilitate the exploration, we employ an illustrative example for the remainder of this section. The historical cumulative claims and associated AY-specific CDFs (denoted by \(A_{i,k}\) = \(C_{i,k+1}/C_{i,1}=\prod _{j=1}^{k}F_{i,j}\) for AY i and DY k ) are presented in Table 3 . The incremental claims and AY-specific DFs (denoted by \(F_{i,k}\) for AY i and DY k ) are presented in Table 4 . To perform the simulation, we implement the individual claims history simulation machine proposed by Gabrielli and Wüthrich ( 2018 ) to create 1,000 run-off rectangles with 12 accident years and 12 development years. Specifically, a synthetic insurance portfolio of features, including the claims code, AY, age of injured, and injured body part, is first generated. Please see Appendix B of Gabrielli and Wüthrich ( 2018 ) for the detailed procedure of generating this synthetic portfolio. Then, based on this synthetic portfolio of claims features, individual claims cash flows are simulated 1,000 times using neural networks. The design and calibration of these neural networks can be found in Sections 2 and 3 of Gabrielli and Wüthrich ( 2018 ), respectively. Finally, those individual claims cash flows are aggregated into 1,000 run-off rectangles with 12 AYs and 12 DYs, and each triangle is an aggregation of 50,000 individual claims.
Following common practices, such as the GLM, we now fit monotone splines to the “unbalanced” raw data of CDFs. Specifically, we have \(I-k\) observations for DY \(k=1,...,I-1\) . Note that unlike with the GLM (accounting for both AY and DY), the spline function would be applied to DYs only. This is due to the inference that \(F_{i,k}\) and \(A_{i,k}\) should be independent across AYs, which is demonstrated below:
Under Assumptions (I) and (III) described in ( 7 ), AY-specific \(F_{i,k}\) ’s ( \(F_{i,k}=C_{i,k+1}/C_{i,k}\) ) are unbiased estimators of \(f_k\) , and are uncorrelated for \(i=1,...,I\) and \(k=1,...,I-1\) .
See Appendix B.1 . \(\square \)
Under Assumptions (I) and (III) described in ( 7 ), AY-specific CDFs, or \(A_{i,k}\) ’s ( \(A_{i,k}=C_{i,k+1}/C_{i,1}\) ), are unbiased estimators of \(a_k\) , and are uncorrelated across AYs, regardless of k being the same or not.
This stems straightforwardly using Lemma 1 and from the fact that \(A_{i,k}=\prod _{j=1}^{k}F_{i,j}\) . \(\square \)
The last issue to consider when fitting monotone splines is the selection of knots. As briefly explained in Remark 4 , m knots will create \(m+1\) partitions in one regressor. In a usual linear regression case, the monotone spline model to fit \(A_{i,j}\) is specified below:
where \(c_j\) is the intercept of DY j , \({\varvec{I}}^\top _m(j)\) is the \(1 \times (m+1)\) I-spline basis of DY j with m knots, \(\varvec{\beta }\) is the corresponding \((m+1) \times 1\) coefficient vector, and \(\varepsilon _{i,j}\) is the error term. To ensure the monotonicity of fitted CDFs, as stated in Remark 4 , we require that \(\varvec{\beta }\) is non-negative. Footnote 6 The estimation is performed via quadratic optimization with no other constraints. The range of m is from 2 to I . To avoid the overfitting (i.e., choosing the largest m ), the selection of knots number can follow a cross-validation principle. Specifically, m is selected in the following steps, where all knots are evenly located:
For a given value of m , fit all \(\{A_{i,j}\}\) excluding \(j=2\) in ( 9 );
Use the fitted model in 1, backcast the CDF in DY 2, or \(\widehat{a^s_{2}}\) ;
Calculate the mean squared error in DY 2, or \(e^2_2=\sum _{i=1}^{I-2}(A_{i,2}-\widehat{a^s_{2}})^2\) ;
Repeat steps 1–3, until all \(e^2_i\) for \(i=2,...,I-2\) are produced Footnote 7 ; and
Select m such that the root of mean squared error (RMSPE) \(\sqrt{\sum _{i=2}^{I-2}e^2_i}\) is minimized.
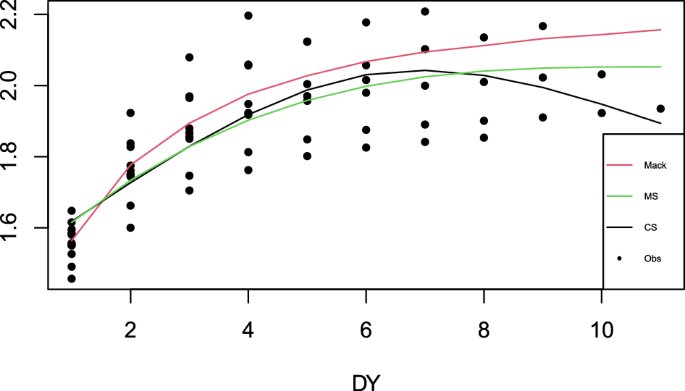
Illustrative example: fitted CDFs. Solid back dots are the CDFs of the actual data. Red curve is the estimated CDFs of Mack’s method. Black curve is the estimated CDFs of cubic splines without monotone constraint. Green curve is the estimated CDFs of monotone splines. (Color figure online)
To explore the appropriateness of this “naive” modeling approach, we fit monotone splines Footnote 8 to the raw dataset of AY-specific CDFs. According to the steps described above, six knots are selected in the monotone spline basis. All raw data points, together with fitted monotone splines, are plotted in Fig. 1 . For comparison, we also present the fitted CDFs of Mack’s model (identical to those of a classic CL or a GLM approach) and fitted values using cubic splines without monotone constraint. First, we compare the two curves resulting from splines. The cubic splines lead to undesirable fitted CDFs, which are decreasing from DY 8. This is due to the relatively small AY-specific CDFs of the first two AYs. For example, \(A_{1,11}\) is only 1.935, which is even smaller than the \(A_{8,4}\) of 2.197. After imposing the monotone constraint, the resulting curve is more desirable and closer to that of Mack’s model. However, because of the unbalanced feature, the small AY-specific CDFs at larger DYs still significantly affect the fitted curve, which causes distinct differences from Mack’s model. Footnote 9
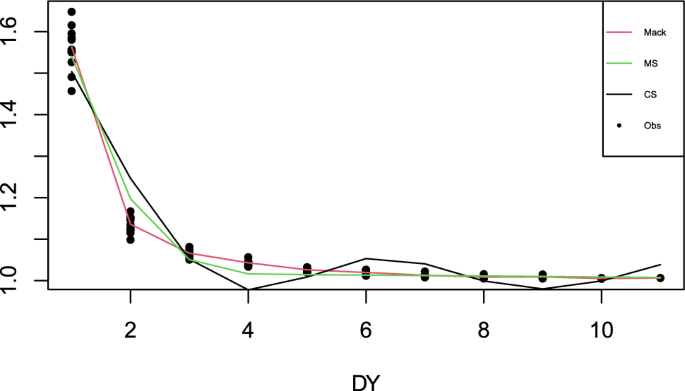
Illustrative example: fitted DFs. Solid back dots are the DFs of the actual data. Red curve is the estimated DFs of the Mack method. Black curve is the estimated DFs of natural splines. Green curve is the estimated DFs of monotone splines. Note that both Figs. 1 and 2 concern the data presented in Table 4 . (Color figure online)
Many similar observations are made if AY-specific DFs ( \(F_{i,k}\) ) are examined instead of the results that are plotted in Fig. 2 . In this case, although the prior belief of the monotone trend is not as strong as it is for the CDFs, we employ monotone decreasing splines for illustration. Consequently, although supported by Theorem 1 , fitting monotone splines to raw CDFs or DFs may lead to drastically different results from the classic CL approach. This may indicate that the associated uncertainty would be (much) larger, therefore leading to greater prediction error than the CL and/or Mack models.

3.3 Modeling claim DFs using enhanced samples
We now explore the possibility of enhancing the credibility using limited historical observations. As can be inferred from Lemma 1 , AY-specific \(F_{i,k}\) would be uncorrelated and have an identical mean. Footnote 10 Therefore, the following result can be obtained.
A total of \(I-k\) distinct samples can be randomly drawn from the set \(\{F_{i,k}:i=1,\ldots ,I-k+1\}\) for each \(k=1,...,I-1\) . Denote \(F^*_{k_n}\) as the n th distinct sample drawn for the set of DY k , and \(A^*_{k_n}\) as the corresponding n th distinct CDF sample, with \(k=1,...,I-1\) . Footnote 11 \(A^*_{k_n}\) is then a CDF consisting of the product of corresponding DFs not necessarily of the same AY. Under Assumptions (I) and (III) described in ( 7 ), such \(A^*_{k_n}\) ’s are all unbiased estimators of the true CDFs \(a_k=\prod _{j=1}^{k}f_j\) .
This follows straightforwardly using Lemma 1 . \(\square \)
Theorem 2 implies that using the samples randomly drawn from the raw dataset can substantially increase the size of distinctive CDFs. To see this, at DY k , instead of observing \(I-k+1\) distinct \(A_{i,k}\) , we now have \(\prod _{j=1}^{k}(I-j+1)\) distinct and unbiased \(A^*_{k_n}\) that can be used. This drastically increased sample size, together with the reliable belief of monotone increasing trend, can improve estimation accuracy for IBNR reserves. Moreover, unlike working with the raw \(A_{i,k}\) dataset, modeling such \(A^*_{k_n}\) will result in the desirable identical mean IBNR reserves as in the classic CL model. This is discussed below.
Note that the enhancement to increase available and reliable (unbiased) sample size is only possible for CDFs. This is a natural result because CDFs are products of associated DFs. Apart from the stronger belief that CDFs would usually be monotone increasing with DYs, this is another and more important reason to work with CDFs rather than incremental DFs (as per most existing studies) in this study.
With the following additional assumptions, we discuss important features of the estimators based on the enhanced samples.
Essentially, Assumption (IV) indicates that \(F^*_{k_n}\) is sampled from \(F_{i,k}\) corresponding to AY i and DY k with a probability of \(C_{i,k}/\sum _{i=1}C_{i,k}\) , for \(i=1,...,I-k\) . Assumption (V) means that for DY k , the enhanced sample \(\{A^*_{k_n}\}\) consists of all distinct DFs over DYs 1 to k , or \(\{F_{i,j}\}\) with \(i=1,...,I-j\) and \(j=1,...,k\) . In other words, \(\{A^*_{k_n}\}\) is the CDF set of all distinct samples.
Under Assumptions (I) and (III)–(V) described in ( 7 ) and ( 10 ), the mean in the empirical distribution of \(\{A^*_{k_n}\}\) with sample size \(N_k=(I-1)!/(I-k-1)!\) , denoted by \(\overline{A_k^*}\) , is identical to \(\prod _{j=1}^{k}{\widehat{f}}_j\) , where \({\widehat{f}}_j\) is defined as in ( 3 ).
See Appendix B.2 . \(\square \)
With a sufficiently large size, rather than modeling the raw AY-specific CDFs directly as in Sect. 3.2 , Lemma 2 supports that the enhanced sample of \(\{A^*_{k_n}\}\) may be modeled to improve the estimation. This is because its mean in the empirical distribution \(\overline{A^*_k}\) coincides with the classic CL estimate of CDFs, which is clearly not true for the raw AY-specific CDFs. Therefore, we will apply monotone splines to this enhanced sample for the remainder of this study.
As seen in Mack ( 1993 ), eventually, incremental DFs are needed to perform the IBNR reserving and produce the prediction error. Using the enhanced sample of CDFs with monotone splines, the estimated DFs can be then obtained. Specifically, suppose that the enhanced sample \(\{A^*_{k_n}\}\) as described in Assumption (V) is collected, monotone splines defined in ( 9 ) is then employed to model those \(A^*_{k_n}\) ’s as in a usual linear regression, or
where \(\widehat{A^*_{k}}=c_k+{\varvec{I}}^\top _m(k)\varvec{\beta }\) is the fitted CDF in DY k , and \(\varepsilon _{n,k}\) is the corresponding residual. Our estimated DF in DY k is then \(\widehat{f^*_1}=\widehat{A^*_1}\) for \(k=1\) and \(\widehat{f^*_k}=\widehat{A^*_k}/\widehat{A^*_{k-1}}\) for \(k>1\) . Features of estimators \(\widehat{f^*_k}\) are discussed below, which requires a final assumption of the residual. Footnote 12
Under Assumptions (I) and (III)–(VI) described in ( 7 ), ( 10 ) and ( 12 ), the monotone splines estimators \(\widehat{f^*_k}\) are unbiased estimators of \(f_k\) ’s for all \(k=1,...,I-1\) .
See Appendix B.3 . \(\square \)
It is worth noting that the distinct number of \(\{A^*_{k_n}\}\) increases quickly with k . For instance, if the DY is limited to 10 years, the distinct number for the last CDF is up to 9!=362,880. Given that insurance claims can develop over a long period, it might not be computationally practical to consider all distinct samples in each AY.
Alternative to using all distinct samples in \(\{A^*_{k_n}\}\) , we sample a fixed number of N observations in each DY. Under Assumptions (I), (III) and (IV), when \(N \rightarrow \infty \) , the corresponding estimator of DF in DY k is expected to converge to \(\widehat{f^*_k}=\widehat{A^*_k}/\widehat{A^*_{k-1}}\) , the estimator when all distinct \(A^*_{k_n}\) ’s are used. Further, when \(I \rightarrow \infty \) , Footnote 13 \(\overline{A^*_k}\) , the mean of the empirical distribution of \(\{A^*_{k_n}\}\) , is expected to converge in probability to \(a_k\) , the population CDF in DY k .
With Assumption (VI) and as proved in Appendix B.3 , we have that \(\widehat{A^*_k}=\overline{A^*_k}-\overline{\varepsilon _k}\) and \(E(\overline{\varepsilon _k})=0\) . Thus, we would expect the monotone spline estimator of DF in DY k with N samples will converge in probability to \(f_k=a_k/a_{k-1}\) , when both N and I go to infinity. In other words, our estimator is asymptotically consistent, when \(N,I \rightarrow \infty \) . Although a rigorous proof is beyond the scope of this paper, we provide simulation results in Appendix C . Also see Sect. 4.2 for discussions related to estimated claims amounts.
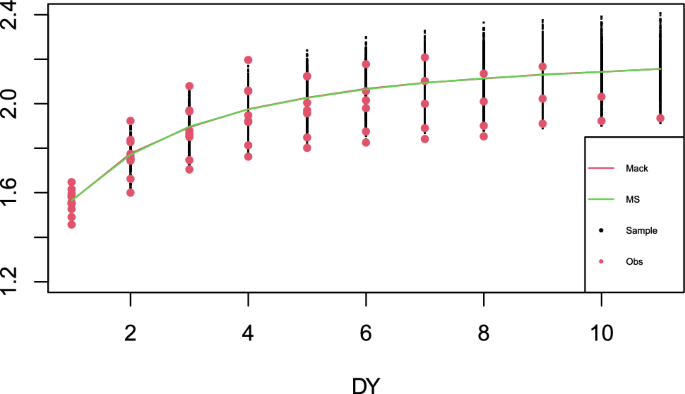
Illustrative example: fitted CDFs with an enhanced sample. Solid back dots are the sampled CDFs. Solid red dots are the CDFs of the actual data. Red curve is the estimated CDFs of the Mack method. Green curve is the estimated CDFs of monotone splines. Note that although visually identically, estimates of Mack and monotone splines differ slightly for each DY. (Color figure online)
We now illustrate the effectiveness of the enhanced sample using the same example discussed in Sect. 3.2 . Simply speaking, we sample a total of 1000 AY-specific DFs for each DY, the product of which then composes the sampled CDFs. The specific sampling procedure follows that described in Sect. 3.4 when the bootstrap replicate is introduced. Our monotone spline method is then fitted to this sample, with six knots as explained in Sect. 3.2 , and the estimated CDFs are plotted in Fig. 3 . For comparison, the estimated CDFs of Mack’s model (identical to the classic CL estimates) are also presented. Clearly, the enhanced sample effectively complements the scarcity of raw CDFs and “balances” the distribution, which have “dragged down” the estimated curve of monotone splines. The fitted curve using this enhanced sample is highly identical to the Mack model, which preliminarily supports its reliability. Thus, the corresponding IBNR reserves would be obtained following ( 4 ), with \({\widehat{f}}_k\) replaced by \(\widehat{f^*_k}\) , or the fitted DFs of monotone splines.
3.4 Estimation of prediction errors via bootstrap replicates and the final model
Finally, we need to estimate prediction errors of the IBNR reserve. As discussed in Mack ( 1993 ), estimators of \(\sigma _{k}^2\) and \(Var(\widehat{f^*_{k}})\) are needed, as presented in ( 8 ). We now discuss the estimator of \(Var(\widehat{f^*_{k}})\) , or the uncertainty in the estimated DFs. Note that as \(\widehat{f^*_{k}}\) is obtained using the monotone spline model rather than the dataset itself, Mack’s estimator in ( 8 ), or \({\hat{\sigma }}_k^2/\sum _{j=1}^{I-k}C_{j,k}\) , is not directly applicable. In our case, given that \(\widehat{f^*_k}=\widehat{A^*_k}/\widehat{A^*_{k-1}}\) , the covariance matrix of the fitted CDFs ( \(\widehat{A^*_{k}}\) ) is then needed to estimate the variance of \(\widehat{f^*_k}\) . This covariance matrix is based on the enhanced sample using monotone splines. However, unlike a usual linear regression, the algorithm to solve the constrained quadratic optimization (Gill et al., 2019 ) cannot produce a standard error for prediction. Therefore, an alternative estimation method is needed.
The bootstrap technique, as employed in related literature such as England and Verrall ( 2002 ), is an effective approach for obtaining the covariance matrix of \(\widehat{A^*_{k}}\) . Nevertheless, bootstrap replicates can also be naturally employed to produce the enhanced sample. Simply speaking, with a predetermined number of observations for each DY, we can first resample the corresponding DFs. Those realizations are then multiplied together to produce the bootstrap replicates of CDFs. However, to resample DF from \(\{F_{i,j}\}\) , it is important to note that Assumption (II) suggests that DFs of the same DY are heteroskedastic across different AYs. Since bootstrap replicates need to be drawn from an identically distributed dataset, standardization is required before resampling. Specifically, suppose that there are N observations to be sampled for each of the I DYs, the resampling steps are described below.
For DY 1, we sample one realization \(r^b_{1,1}\) with replacement from the standardized “residuals” \(\{r_{i,1}\}\) for \(i=1,...,I-1\) , where \(r_{i,1}=\sqrt{C_{i,1}}(F_{i,1}-{\hat{f}}_1)\) . Footnote 14
A realization of DF is then created by \(F^b_{1,1}=r^b_{1,1}/\sqrt{C^b_{1,1}}+{\hat{f}}_1\) , where \(C^b_{1,1}\) is sampled with replacement from \(\{C_{i,1}\}\) for \(i=1,...,I-1\) . The probability of selecting \(C_{i,1}\) is equal to \(C_{i,1}/\sum _{i}C_{i,1}\) .
Repeat steps 1–2 for all the \(I-1\) DYs.
In DY k , the first sampled CDF is then \(A^b_{1,k}=\prod _{j}^kF^b_{1,j}\) .
Repeat steps 1–4 N times, a bootstrap sample then consists of \(\{A^b_{i,k}\}\) with \(i=1,...,N\) and \(k=1,...,I-1\) .
Repeat the above steps B times and fit monotone splines for each bootstrap sample; we then obtain B realizations of \(\widehat{A^*_{k}}\) for each \(k=1,...,I-1\) , with each fitted from ( 11 ) to model a bootstrap replicate \(\{A^b_{i,k}\}\) . Their \((I-1) \times (I-1)\) covariance matrix \(\widehat{\varvec{\Sigma }_A}\) can then be estimated as the corresponding sample covariance using the B realizations. Footnote 15 Given that all AY-specific \(\{r_{i,j}\}\) will have finite variances, or \(\sigma _k<\infty \) shall hold as in Assumption (II) of ( 7 ), the estimated \(\widehat{\varvec{\Sigma }_A}\) using those bootstrap samples constructed above will produce consistent estimators of the underlying covariances of \(\{\widehat{A^*_{k}}\) }. (Bickel & Freedman, 1981 ). Finally, the variance of DF estimator can be straightforwardly obtained following the usual delta method. Footnote 16 To improve the performance, although DFs estimated in a single unadjusted enhanced sample could be sufficiently accurate, we use the sample mean of the B realizations produced in the bootstrap replicates. That is, this sample mean is employed as our final estimator, denoted by \(\widehat{f^s_k}\) , to obtain the IBNR reserve for the proposed monotone spline reserving method.
Note that unlike with existing bootstrap approaches (e.g., England & Verrall, 2002 ; Peremans et al., 2017 ), an underlying distribution of claims is not needed in the resampling procedure. Therefore, the associated process error (i.e., adopting an incorrect distribution) is avoided. That is, our approach is likely to be robust against the potential misspecification issue of the density of claims.
The last estimator that remains unknown is analogous to \({\hat{\sigma }}_k\) , as in ( 8 ) for Mack’s model. Note that Mack’s estimator described therein follows from Assumption (II) in ( 7 ) and the prediction of \({\hat{C}}_{i,k}\) described in ( 4 ). As discussed above, since our final estimator \(\widehat{f^s_{k}}\) using the bootstrap samples is expected to be unbiased (or at least consistent), we estimate \({\sigma }_k\) as:
For \(\widehat{\sigma ^s_{I-1}}\) , the variety at the last DY, Mack ( 1993 ) adopts a simple extrapolation method displayed in ( 8 ). Given that those varieties usually exponentially decrease with DYs, we fit monotone decreasing splines on the obtained \(\widehat{\sigma ^s_{k}}\) for \(k=1,..,I-2\) , and \(\widehat{\sigma ^s_{I-1}}\) is produced as the predicted value from the fitted splines. Following the reasons discussed above, it is straightforward to demonstrate that those \(\{\widehat{\sigma ^s_{k}}\}\) estimated using monotone splines and bootstrap replicates are consistent estimators of \(\{\sigma _k\}\) .
The proposed reserving method using the monotone splines is summarized below.
Select m knots of monotone splines using the original dataset \(\{A_{i,j}\}\) , as discussed in Sect. 3.2 ;
Produce B bootstrap replicates with N observations in individual DYs of each sample, following the procedure described in Sect. 3.4 ;
Within each bootstrap replicate, fit monotone splines with m knots and obtain \(\{\widehat{A^*_{k}}\}\) and \(\{\widehat{f^*_{k}}\}\) ;
The final estimator of DFs is \(\{\widehat{f^s_{k}}\}\) , the sample mean of the B \(\{\widehat{f^*_{k}}\}\) for each \(k=1,...,I-1\) , and calculate the sample covariance of \(\{\widehat{A^*_{k}}\}\) , or \(\widehat{\varvec{\Sigma }_A}\) ;
Obtain \(\widehat{\sigma ^s_k}\) as in ( 13 ) and \({\widehat{Var}}(\widehat{f^s_k})\) using the delta method Footnote 17 and \(\widehat{\varvec{\Sigma }_A}\) , i.e., \({\widehat{Var}}(\widehat{f^s_k})= \hat{{\varvec{g}}}_f' \widehat{\varvec{\Sigma }_A} \hat{{\varvec{g}}}_f\) , where \(k=2,...,I-1\) , \(\hat{{\varvec{g}}}_f\) is a function of estimated DF calculated from estimated CDF in vector; Footnote 18 and
The final IBNR reserves and prediction errors are derived from the following equations.
Compared with existing models, such as the popular GLM and Mack models, there are some advantages of our approach for IBNR reserving. First, without imposing additional assumptions, we have made the best use of available information by working with enhanced samples. All additional samples of CDFs naturally consist of the AY-specific DFs, which are based on reliable actual data. Second, the monotone splines adopt the common and appropriate belief such that CDFs would usually monotonically increase with DYs. Similar to Mack’s model, our framework remains distribution free, but it has utilized reliable “prior” beliefs. Compared with the GLM, our model can incorporate the negative incremental claims without changing the iterations of estimation. In addition, the distribution-free feature avoids the issue of assuming and fitting the most appropriate dispersion as is required with the GLM (e.g., Wüthrich, 2003 ; Boucher & Davidov, 2011 ; Gao et al., 2021 ), and is robust against the inappropriate choice of distribution. Those two advantages enable our monotone spline model to produce more accurate IBNR reserves. This is discussed in Sect. 4 with evidence from a simulation. Third, considering the efficiency of the employed optimization algorithm, the computational cost of our model is not high. This is preferable to the reserving models based on a Bayesian approach (e.g., Gao & Meng, 2018 ), which require the complex Markov Chain Monte Carlo algorithm. Fourth, the fitted monotone spline can be easily interpolated and extrapolated. This is particularly useful for computing tail factors, as well as for calculating intermediate DFs (e.g., DF of a development quarter). The selection of knots effectively avoids the overfitting issue, which leads to credible extrapolation/interpolation results. Fifth, the modeling framework is flexible and extensible. For example, a general additive model (GAM) may be employed instead of linear regression. In addition, rather than assuming the usual squared loss, robust loss function could be used to prepare reserving models that are more robust to outliers.
It is worth noting that a continuous CL model developed by Miranda et al. ( 2013 ) also enables DFs to be estimated at continuous time points. The employed method is also known as in-sample density forecasting (Lee et al., 2015 , 2017 , 2018 ), which usually adopts density smoothers such as kernels (Mammen et al., 2015 ). Compared with this approach, there are some critical differences:
When applied to non-life insurance reserving, the continuous CL method focuses on working with the DY and AY effects separately, similar to the GLM framework described in ( 6 ); and
The prediction error, or an associated uncertainty measure, is not explicitly discussed therein.
In our proposed model, we focus on the CDFs, rather than on the DY and AY effects. This may be understood as working directly with forward-factors in the diagonal direction of a run-off triangle. As explained, the benefit is the ability to adopt the prior belief of non-decreasing patterns of such CDFs, which cannot be guaranteed in the continuous CL model. In addition, based on ( 8 ), the estimator of prediction error is comprehensively addressed in our model. The measure of prediction error is critical to the precision of ratemaking and the efficiency of solvency management for a property and casualty insurer.
4 Simulation evidence
In this section, we first present the fitted results of monotone splines of the illustrative example used in Sect. 3 . We also discuss the accuracy of prediction errors and the relation of estimation variety with the sample sizes of bootstrap replicates. Further, with a more comprehensive collection of simulated data, we compare the prediction accuracy of the IBNR reserves of our model with several popular existing models.
4.1 Illustrative example: fitted results using the monotone spline model
We now follow the steps described in Sect. 3.4 to fit the monotone splines to the example introduced in Table 3 . Note that the efficiency of linear regressions depends on the independence of error terms. To verify this for the monotone spline model using bootstrap samples, we first present the fitted autocorrelation functions (ACFs) of DY-dependent errors. Those errors are analogous to \(\varepsilon \) defined in ( 9 ). Recall that for each of B bootstrap sample, we have N observations in each DY. For the b th bootstrap sample, denote the sample mean over those N errors in DY k by \(\overline{\varepsilon ^*_{b,k}}\) . We then calculate the b th realization of ACFs (up to lags of \(I-2\) ) of bootstrap replicates using the sample ACFs of the \(I-1\) \(\{\overline{\varepsilon ^*_{b,k}}\}\) . Thus, the point estimate and 95% confidence interval of the ACFs of error terms are estimated by the mean, and 2.5th and 97.5h percentiles, respectively, out of the B estimates. Let \(N=1,000\) and \(B=1,000\) ; the results corresponding to our illustrative example are presented in Fig. 4 . Clearly, since 0 is covered by 95% confidence intervals at all lags, the independence of error terms across DYs is preliminarily supported.
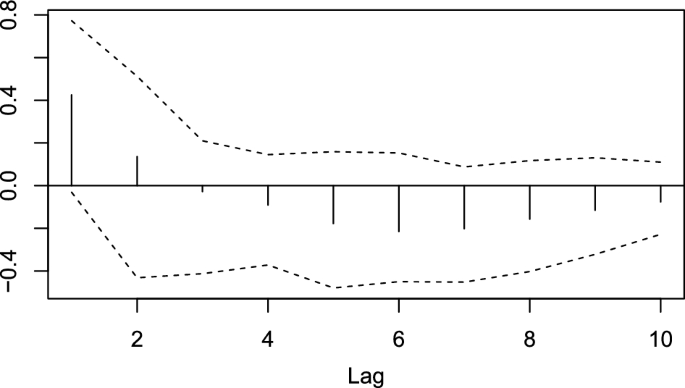
Illustrative example: fitted ACFs of bootstrap residuals. Solid bar is the mean ACFs of bootstrap residuals. Dashed curves are the 95% bootstrap confidence intervals of the ACFs of bootstrap residuals
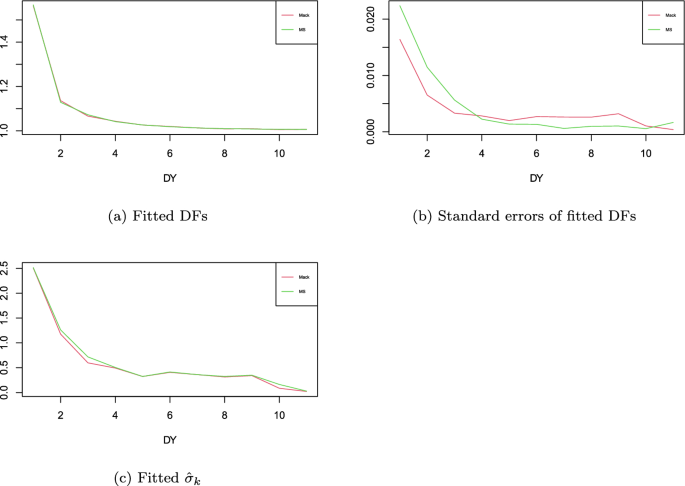
Illustrative example: Mack v. monotone estimates. Red curve is the estimates of Mack’s method. Green curve is the estimates of the monotone splines. (Color figure online)
We now present a comprehensive discussion of the estimates obtained from our monotone spline model. Our final estimates of DFs ( \(\widehat{f^s_{k}}\) ), their associated standard errors ( \(SE(\widehat{f^s_{k}})\) ), and \(\widehat{\sigma ^s_k}\) are plotted in Fig. 5 a–c, respectively. The Mack’s estimates are also presented for comparison. As expected and preliminarily demonstrated in Fig. 3 , the point estimates of the two models are close to each other at all DYs. This also explains the similarity of estimated \(\sigma _k\) , given that the only source of difference (other than for the last DY) is the estimate of \(f_k\) . Finally, there are some noticeable differences on the estimated standard errors of DFs. Those of monotone splines almost monotonically decline, which is consistent the expected exponential decay with DY (Mack, 1993 ). For the Mack method, we observe some “bounce-up” over DYs 6–9. As outlined in Remark 8 , despite some similarities, our monotone spline model may result in more accurate IBNR reserving.
Nevertheless, it is important to understand the influence of N and B in our model. We consider three choices of N , a similar size of the raw dataset (10), an intermediate size (100), and a large size (1000). Small (100) and large (1000) numbers of bootstrap replicates B are further compared, which results in a total of six unique combinations of N and B . For each combination, we repeat the entire estimation process 1000 times. To demonstrate the variety affected by N and B , we report the average and standard deviation of the total IBNR reserve ( \({\hat{R}}\) ) and its prediction error ( \({\widehat{Var}}({\hat{R}})\) ) in Table 5 . Clearly, compared with N , the number of bootstrap replicates is more influential. With \(B=1000\) , the standard deviation of prediction error among all simulations is as small as 1.0 for \(N=10\) . Further increasing N to 100 and 1000 will not significantly reduce this variety. For the total IBNR reserve, increasing N will more obviously improve the stability in estimation. Specifically, when \(B=1000\) , standard deviations are 4.8, 1.6, and 0.6 for \(N=\) 100, 100, and 1000, respectively. Nevertheless, the average of estimates is almost identical across all combinations. The average estimate of R is approximately 7370, whereas the associated average prediction error is approximately 350.
Next, we compare prediction accuracy across popular competing models via simulation studies. Apart from the proposed monotone spline method, our ensemble includes four classic models: the GLM, Mack’s model, CL based on bootstrap replicates (England & Verrall, 2002 ), and Clark’s loss DF method (Clark, 2003 ). Footnote 19 The illustrative triangle is then fitted by all the six models, and results are presented in Table 6 . Considering our model and Mack’s model, the results are much consistent with those observed in Fig. 5 . For instance, the estimated reserves in each AY are relatively close to each other. The predicted error of monotone splines is larger in AY 2–3 and 10–11 and smaller in the rest, compared to the error of Mack’s model. Despite the similarity, we find that our model produces the largest total estimated reserve (more conservative), and the total prediction error 351 is the smallest (most accurate). Specifically, the total prediction error of our model is around 20%, 10%, 20% and 5% smaller than those of CL, Mack’s, bootstrap CL and Clark’s models, respectively. This motivates us to perform a more comprehensive study, preferably with true values, to evaluate the monotone splines and other competing models.
4.2 Comparisons of the prediction accuracy
This section comprehensively compares the performance of models concerned in Sect. 4.1 via simulations. We firstly continue to examine the illustrative example as a preliminary analysis under an ideal scenario (true model is CL). Next, a more sophisticated analysis is carried out with simulations replicating real-life claims (true model is not one of the competitors).
We now preliminarily demonstrate the preferred performance of our monotone spline method using the same illustrative example examined. The accuracy comparison is based on 1000 simulations. Specifically, simulations are produced via the following steps:
Fit the triangle presented in Table 3 via GLM, find the fitted Pearson residuals, and calculate their sample mean and standard deviations in each DY \(k=1,...,I-1\) , denoted by \(x_k\) and \(s_k\) , respectively;
With the fitted model, collect both in- and out-of-sample incremental claims (i.e., \({\hat{Y}}_{i,k}\) for \(i=1,...,I\) and \(k=1,...,I\) );
For each DY k , simulate I realization (or \(e_{i,k}\) ) from \(N(x_k,s_k^2)\) , or a normal distribution with the same sample mean and standard error of the fitted Pearson residuals in DY k from step 1;
Simulated realizations \(e_{i,k}\) are then added to fitted \({\hat{Y}}_{i,k}\) , which then forms a complete simulated triangle, with both training (upper-triangle) and test (lower-triangle) datasets; and
Repeat steps 3–4 until 1000 triangles are produced.
In short, the simulation assumes that GLM is a true model, and Pearson residuals are normally distributed. The five competing models are then fitted to the simulated training data, with N and B both set to 1000 for the monotone splines for its lowest variety, as presented in Table 5 . This is repeated for all 1000 simulations, and we compute the RMSPE of total IBNR reserve as \(\sqrt{\sum _{i=1}^{1000}(\widehat{R^i}-R^i)^2/1000}\) , where \(\widehat{R^i}\) is the point estimate for the i th simulation of one model, and \(R^i\) is the true value. In addition, the average total prediction error across all 1000 simulations is produced for each model.
In Table 7 , we report the RMSPE and average prediction error. These statistics enable comparison of the prediction accuracy of the total IBNR reserve across the five models. First, all RMSPEs are close to each other. This is as expected given that the principle of all models is to produce equivalent CL point estimates. Despite this, by utilizing more information, our monotone spline model results in slightly more accurate point estimates of the total IBNR reserves. More distinctive differences are observed for the prediction errors. The GLM and bootstrap CL model lead to the largest prediction errors, which are approximately 20% larger than their corresponding RMSPEs. In contrast, the average prediction error of our model is the smallest among all competitors and is also very close to the RMSPE. This result strongly supports that the small prediction error does not occur as a cost of overfitting, but rather as evidence of improved prediction accuracy. Nevertheless, it is worth noting that the prediction error of 323 of our model is somewhat different from 350, as presented in Table 5 . This can be explained by the fact that GLM (with Gaussian Pearson errors) is not the actual data-generation process used to produce this illustrative example.
We now more comprehensively compare the prediction accuracy. In an ideal case, if the true test data were known, the prediction accuracy of competing reserving models can be explicitly measured as above. As outlined in Sect. 3.2 we use the simulation machine proposed in Gabrielli and Wüthrich ( 2018 ), and historical claims are simulated repeatedly until 1000 run-off rectangles (including the true test data or the lower triangle) are obtained. As was conducted above, we will focus on the prediction of total IBNR claims.
In Table 8 , out of the 1000 simulated datasets, both the mean and 95th percentile of the true total data of estimated total IBNR claims are reported. Despite the similarity of the estimates, there are some noticeable differences. Of all the models, Clark’s model results in the least accurate estimates. The mean of R is below 7600, whereas the 95th percentile is approximately 8450. The estimates of the GLM/Mack’s model and bootstrap CL are largely identical, with a mean of approximately 7700 and \(Q_{95}\) of 8680. In comparison, our monotone model produces the most accurate mean and \(Q_{95}\) , both of which are closest to the true values among those estimates. Hence, as measured by RMSPE, our model is the preferred approach for prediction accuracy. The same conclusion can be drawn when employing the alternative metric of the mean absolute prediction error (MAPE), which considers the absolute deviation from the true value.
Contrasting Tables 7 and 8 , one may argue that all models underperform when GLM is not the true model, and a more complicated data generation process (DGP) is employed instead. Despite the expected unbiasedness, we do observe an 5% average underestimation of the total reserves. As stated in Remark 6 , the asymptotics of monotone splines will require \(I \rightarrow \infty \) , and the same argument may hold for other models as well. In Appendix C , we present results using DGPs set to GLM and to the simulation machine of Gabrielli and Wüthrich ( 2018 ) with more available AYs. Two conclusions can be made in those cases. First, when DGP is different from the true model, more available data are needed to achieve a desirable level of convergence. Second, desirable asymptotics are observed for all models (i.e., prediction accuracy is improved with more AYs). This supports the common regulated actuarial practice, such that risk margins (i.e., additional capital to the estimated total reserve) need to be held to combat against the uncertainty in DGP and scarcity in historical data. Nevertheless, it is also worth pointing that in all cases, our model produces the best prediction accuracy among all competitors.
Thus, using simulation studies, we demonstrate that the proposed monotone spline model can improve the prediction accuracy of IBNR reserving. Despite the similarity, utilizing more information and the prior belief of a monotone trend, the point estimate is relatively more accurate in our model than it is in the existing models that we used for comparison. Additionally, we demonstrate that the smaller estimated prediction error of our proposed model is much close to the error produced using the true values. This supports that the proposed monotone spline model can provide more accurate point estimates of IBNR reserves with reliable prediction errors.
5 Real data study
In this section, we explore the famous dataset of Taylor and Ashe ( 1983 ) to compare the performance of the five competing models. This dataset has been frequently examined in seminal research (e.g., Mack, 1993 ) and recent literature (e.g., Gao et al., 2021 ). The run-off triangle of cumulative claims and AY-specific CDFs are presented in Table 9 . The incremental claims and AY-specific DFs are presented in Table 10 . As stated in Remark 3 , we must test for the AY effect and correlation between DFs. Using the stats proposed in Mack ( 1994 ), at the 5% significance level, we do not reject the null hypotheses, such that those assumptions hold for the examined dataset. This validates the subsequent analyses using the five competing models.
The proposed monotone spline method is first fitted to the dataset. Let \(N=1000\) and \(B=1000\) ; we follow the steps described in Sect. 4.1 to obtain the point estimate and 95% confidence intervals of ACFs of DY-dependent error terms. It can be seen in Fig. 6 that the independence of those errors cannot be rejected.
Consistent with our discussion in Sect. 4.1 , the related estimates of DFs produced by Mack’s model and the monotone splines are compared. Specifically, we plot \({\widehat{f}}_{k}\) and \(\widehat{f^s_{k}}\) , \(SE({\widehat{f}}_{k})\) and \(SE(\widehat{f^s_{k}})\) , and \({\widehat{\sigma }}_k\) and \(\widehat{\sigma ^s_k}\) in Fig. 7 a–c, respectively. Most of our observations for the illustrative example made from Fig. 5 consistently hold for this real dataset. It is worth noting that DFs fitted by monotone splines in DYs 3 and 8 are relatively lower than they are in the Mack model. This is enlarged after being multiplied by the corresponding cumulative claims, which may cause the notable difference in the estimated standard errors in DYs 3 and 8.
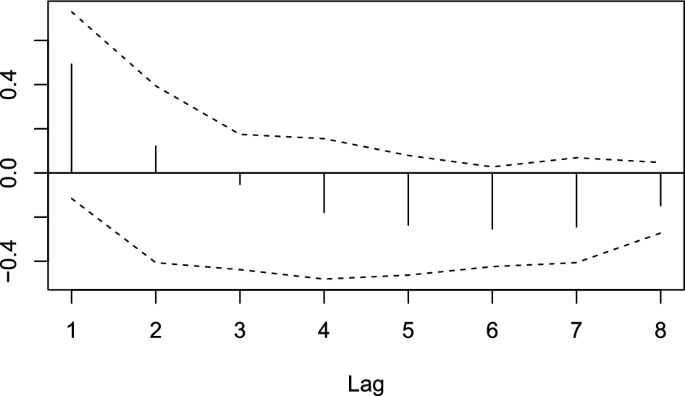
Real data illustration: fitted ACFs with bootstrap residuals. Solid bar is the mean ACFs of bootstrap residuals. Dashed curves are the 95% bootstrap confidence intervals of the ACFs of bootstrap residuals
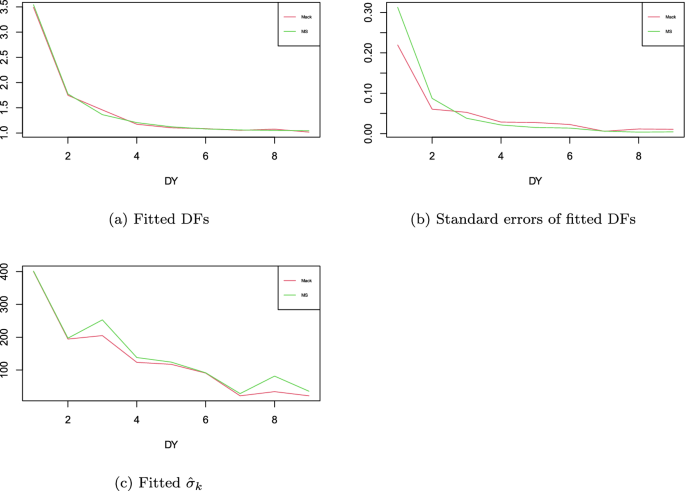
Read data illustration: Mack v. monotone estimates. Red curve is the estimates of Mack’s method. Green curve is the estimates of the monotone splines. (Color figure online)
We now compare the IBNR reserves estimated by the five competing models. The AY-specific cumulative IBNR reserves in the DY 10, the total IBNR reserve, and their associated prediction errors are reported in Table 11 . For the AY-specific reserves, the GLM and Mack’s model result in identical point estimates. Those produced by the bootstrap CL model are uniformly greater, although the differences are rather small. Apart from in several cases, Clark’s model and our monotone splines lead to larger AY-specific reserves. The largest relative difference exists for AY 2. While the estimates of the GLM/Mack’s model and bootstrap CL are all below 100 (measured in thousands), the estimate of Clark’s model is approximately 210, and that of our monotone spline model is almost 250. This can be explained by the difference of estimated DF of DY 10. For example, \({\widehat{f}}_{10}\) is 1.017 using Mack’s model, and \(\widehat{f^s_{10}}\) is 1.046 using the monotone spline method. Footnote 20 Given that the Mack’s/GLM estimate of the last DF is based on one observation only, the estimate produced using our approach might be more reliable because the enhanced samples utilize much more information. Aggregating all those AY-specific results, Clark’s model produces the largest estimate of the total IBNR reserve (19682.38), followed by the monotone spline model (19317.75), with GLM and Mack’s model ranked last (18680.86).
As summarized in Sect. 4 , the monotone spline approach uses more available information and is thus expected to produce a lower prediction error. The relative magnitudes of AY-specific errors are mixed across models. Roughly speaking, our model and Mack’s method have similar results and both lead to the smallest AY-specific prediction errors for all AYs excluding 6–8, for which GLM and bootstrap CL result in the smallest errors. The similarity of prediction errors of monotone spline and Mack’s models are consistent with our observations in Fig. 7 . However, Clark’s model tends to cause the largest prediction errors in almost all AYs. For the coefficient of variation (CV), or the ratio of prediction error over IBNR reserve, the values of our monotone spline model are consistently small and below 0.40, suggesting a low level of dispersion. Aggregating all AY-specific values, our monotone spline model has both the smallest prediction error measured by dollar unit and as relative to the point estimate (i.e., measured by CV). Specifically, the monotone spline prediction error of total IBNR reserve is 2343.88, which is approximately 5%, 20%, 25%, and 30% smaller than the prediction errors of Mack’s model, GLM, bootstrap CL, and Clark’s method, respectively. The associated CV of our model is 0.12, close to 0.13 of Mack’s model and relatively lower than 0.16 \(-\) 0.17 of GLM, bootstrap CL, and Clark’s model. As evidenced in Table 7 , and discussed in Sect. 4.2 , such a smaller error would not be the result of overfitting. Consequently, this desirable observation supports the potential effectiveness of our proposed monotone spline model in actuarial practice. In particular, we propose that monotone splines may be a widely useful tool to improve the accuracy of IBNR reserving.
6 Concluding remarks
Following the fundamental assumptions and findings of Mack ( 1993 , 1999 ), we propose a semi-parametric IBNR reserving model using monotone spline and enhanced bootstrap replicates. As with Mack’s model, we do not assume an underlying distribution, nor is any associated dispersion parameter imposed. This distribution-free approach is expected to be more robust against model misspecification of a parametric approach, including GLM and its extensions. Three key conclusions can be drawn from our research.
First, the proposed monotone spline method has desirable technical properties. By working with the enhanced sample of CDFs, we demonstrate that the fitted CDFs and DFs of our method are unbiased and/or asymptotically consistent. Under certain conditions, the estimates may coincide with those of the classic CL method. Further, the bootstrap technique provides consistent estimators of the corresponding prediction errors.
Second, without imposing additional assumptions, the proposed method makes the best use of available information and could improve the accuracy of IBNR reserving. Specifically, the enhanced sample considers much more comprehensive combinations of DFs to produce sampled CDFs, all of which are proven to be unbiased. The reliable “prior” belief, such that all CDFs would usually be monotone increasing, is further adopted. Thus, our model is expected to reduce the prediction uncertainty of the Mack model.
Third, via our simulation studies and real data analysis, the effectiveness of our model is systematically demonstrated. Compared with the four popular classic models examined in our study-GLM, Mack’s model, bootstrap CL (England & Verrall, 2002 ), and Clark’s model Clark ( 2003 )-the prediction error of our model is the smallest averaged over all simulated replicates. This reduced uncertainty is highly reliable and not a result of overfitting given that its value is close to the RMSPE produced using point estimates and true values. Using the popular dataset of Taylor and Ashe ( 1983 ), the improved prediction accuracy of our proposed model is consistently demonstrated. Thus, our model could be a competitive tool to help improve IBNR reserving accuracy in actuarial practice.
There are some directions that are worth exploring for future research. First, the monotone spline method can be integrated into a GAM framework (Wood, 2017 ). In a general case, Pya and Wood ( 2015 ) explore a shape constrained additive model, which may incorporate monotone splines. Compared with a linear regression method, GAM enables smoothness and may further reduce the overfitting issue. Early attempts to investigate GAM for IBNR reserving can be found in Verrall ( 1996 ) and England and Verrall ( 2002 ). Second, instead of the usual squared loss, a more robust loss function (e.g., Hampel et al., 2011 ) could be employed in the estimation. The importance of robust techniques for IBNR reserving is discussed in Verdonck and Debruyne ( 2011 ) and Peremans et al. ( 2017 ), which reduces the estimation sensitivity to outliers, such as the recent 2021 North American winter storm. Integrating a robust method into the monotone spline framework would therefore improve this proposed model’s feasibility in more complicated conditions. Third, future analysis may be conducted to compare the effectiveness of monotone splines in estimating the tail factors. In addition, as presented in Fig. 7 c, without further restrictions, \(\widehat{\sigma ^s_k}\) appears to be not strictly monotone declining. Employing the monotone decreasing spline in the estimation may help remove this irregularity. Nevertheless, a more rigorous discussion on the asymptotics of monotone splines estimators as noted in Remark 6 is worth exploration.
Note that this paper concerns the aggregated claim data, such that the individual claims are not considered there. Admittedly, if individual data are available, more information would be utilized to improve the reserving accuracy.
Since log transformation requires positive inputs, negative incremental values will need to be adjusted in the estimation iteration implemented by GLM. The corresponding estimation is known as the quasi maximum likelihood approach (Nelder & Lee, 1992 ) Also, it is known that GLM may incorporate individual negativity, but the estimation will fail in the case of a negative mean development. Fortunately, as can be inferred from Sect. 3 , our proposed model can accommodate both single and mean negativity.
Note that since \(E[Y_{i,j}]= {c_i}b_j\) , \(c_i\) and \(b_j\) have a confounding impact on the constant \(E[Y_{i,j}]\) , and cannot be identified without constraints.
Note that Mack ( 1999 ) has extended Assumption (II) to allow more flexible relationships, which does not affect the new model proposed in this paper and therefore is not discussed further.
The choice of quadratic order is for the purpose of illustration. The algebraic structure of an I-spline basis with an arbitrary order can be found in Ramsay ( 1988 ).
Note that despite this imposed non-negative constraint on coefficients of regressors, for the usual least square loss, it is well known that the quadratic optimization will still lead to errors with 0 mean. This validates the relevant assumption in Theorem 3 (i.e., \(E(\varepsilon _{i,k})=0\) ).
Note that CDFs of the first and last DY are excluded in the cross-validation process, such that the monotone splines are not extrapolated for prediction. The results including all CDFs are highly robust.
All monotone splines analyzed in this study are up to the cubic order, which is commonly used in practice as a balance of accurate data fitting and non-overfitting.
The same conclusion will hold even if we consider weights (i.e., \(C_{i,k}/\sum _{i}C_{i,k}\) for \(A_{i,k}\) ) in the loss function to fit monotone splines, which also applies to the case if \(F_{i,k}\) s are to be fitted.
Note that those AY-specific DFs are not identically distributed given that the associated variances are dependent on \(C_{i,k}\) as per Assumption (II). However, if an unadjusted enhanced sample (introduced in this section) is to be worked with, it will not affect the unbiasedness/consistency of the estimators. To derive the standard errors of the estimator, adjustments are made in the bootstrap procedure to ensure the resampled realizations are identically distributed. This is detailed in Sect. 3.4 .
In this case, the subscript \(k_n\) denotes the n th distinct sample drawn for DY k . This notation is to distinguish from the subscript ( i , k ), indicating the observation of AY i and DY k . It is easily seen that for the CDF, the total number of distinct samples in DY k is the number of k -combinations with \(I-1\) elements, or \((I-1)!/[k!(I-k)!]\) .
Intuitively, a sufficient condition for the independence in Assumption (VI) is that \(\varepsilon _{n,k}\) is independent of \(\widehat{A^*_{k-1}}\) , \(\widehat{A^*_{k}}\) and \(\widehat{A^*_{k+1}}\) . In other words, residuals in DY k are independent of fitted values in DYs from \(k-1\) to \(k+1\) .
Although we assume that the total numbers of AY and DY are identical, the asymptotic statement is only related to the number of AYs. This is more closely linked to actuarial practice, since a tail factor is used after a few DYs, whereas the number of AY can go large with a sufficiently long claim history.
From Assumptions (I)–(III), it can be verified that such residuals are independently and identically distributed with a (conditional) mean of 0 and a (conditional) variance of \(\sigma _k^2\) . Essentially, we are assuming CL is the true model to perform the residual-based bootstrap.
Note that the degree of freedom needs to be adjusted to account for the difference between sizes of a bootstrap sample and the raw dataset. The rationale is that we have a total of \((I-1)!\) actual observations, rather than \(N\times (I-1)\) as in an enhanced (bootstrap) sample.
Note that as outlined in Remark 6 , the asymptotic framework in this case requires that both N and I go to infinity. When \(N \rightarrow \infty \) only, the delta method’s estimator will converge to the estimator as if all distinct CDFs are modeled. Furthered with \(I \rightarrow \infty \) the delta method’s estimator will converge to the true variances of DFs.
See footnote 16 for the asymptotic framework and expected features of the delta method.
Note that the DF and CDF at DY 1 are identical, so will be their variances. For \(k \ge 2\) , essentially the delta method implies that
where \(\widehat{A^s_{k}}\) is the bootstrap sample mean of CDF estimates, and \((\widehat{\varvec{\Sigma }_A})_{k-1,k}\) is the \(k-1\) to k th row and \(k-1\) to k th column elements (a \(2 \times 2\) matrix) of \(\widehat{\varvec{\Sigma }_A}\) .
Given that we do not consider tail factors, the largest DY is set to 12 in Clark’s model.
Our simulation study also suggests that there is more likely for DF estimators from different models to differ at later DYs. The results are available upon request.
Antonio, K., & Plat, R. (2014). Micro-level stochastic loss reserving for general insurance. Scandinavian Actuarial Journal, 7 , 649–669.
Article Google Scholar
Bickel, P. J., & Freedman, D. A. (1981). Some asymptotic theory for the bootstrap. The Annals of Statistics, 9 (6), 1196–1217.
Boucher, J. P., & Davidov, D. (2011). On the importance of dispersion modeling for claims reserving: An application with the tweedie distribution. Variance, 5 (2), 158–172.
Google Scholar
Clark, D. R. (2003). Ldf curve-fitting and stochastic reserving: A maximum likelihood approach. CAS Forum Citeseer, 3 , 41–92.
De Alba, E. (2006). Claims reserving when there are negative values in the runoff triangle: Bayesian analysis using the three-parameter log-normal distribution. North American Actuarial Journal, 10 (3), 45–59.
De Boor, C. (1978). A practical guide to splines . New York: Springer.
Book Google Scholar
Diers, D., Linde, M., & Hahn, L. (2016). Quantification of multi-year non-life insurance risk in chain ladder reserving models. Insurance: Mathematics and Economics, 67 , 187–199.
England, P. D., & Verrall, R. J. (2002). Stochastic claims reserving in general insurance. British Actuarial Journal, 8 (3), 443–518.
Gabrielli, A., & Wüthrich, M. (2018). An individual claims history simulation machine. Risks, 6 (2), 29.
Gao, G., & Meng, S. (2018). Stochastic claims reserving via a bayesian spline model with random loss ratio effects. ASTIN Bulletin: The Journal of the IAA, 48 (1), 55–88.
Gao, G., Meng, S., & Shi, Y. (2021). Dispersion modelling of outstanding claims with double Poisson regression models. Insurance: Mathematics and Economics, 101 , 572–586.
Gill, P. E., Murray, W., & Wright, M. H. (2019). Practical optimization . SIAM.
Gisler, A. (2019). The reserve uncertainties in the chain ladder model of mack revisited. ASTIN Bulletin: The Journal of the IAA, 49 (3), 787–821.
Hampel, F. R., Ronchetti, E. M., Rousseeuw, P. J., & Stahel, W. A. (2011). Robust statistics: The approach based on influence functions, (Vol. 196). Wiley.
Hastie, T., & Tibshirani, R. (1986). Generalized additive models. Statistical Science, 1 (3), 297–310.
Kuang, D., & Nielsen, B. (2020). Generalized log-normal chain-ladder. Scandinavian Actuarial Journal, 2020 (6), 553–576.
Kuang, D., Nielsen, B., & Nielsen, J. P. (2015). The geometric chain-ladder. Scandinavian Actuarial Journal, 2015 (3), 278–300.
Lee, Y., Mammen, E., Nielsen, J., & Park, B. (2018). In-sample forecasting: A brief review and new algorithms. ALEA-Latin American Journal of Probability and Mathematical Statistics, 15 , 875–895.
Lee, Y. K., Mammen, E., Nielsen, J. P., & Park, B. U. (2015). Asymptotics for in-sample density forecasting. The Annals of Statistics, 43 (2), 620–651.
Lee, Y. K., Mammen, E., Nielsen, J. P., & Park, B. U. (2017). Operational time and in-sample density forecasting. The Annals of Statistics, 45 (3), 1312–1341.
Lindholm, M., Lindskog, F., & Wahl, F. (2020). Estimation of conditional mean squared error of prediction for claims reserving. Annals of Actuarial Science, 14 (1), 93–128.
Mack, T. (1993). Distribution-free calculation of the standard error of chain ladder reserve estimates. ASTIN Bulletin: The Journal of the IAA, 23 (2), 213–225.
Mack, T. (1994). Measuring the variability of chain ladder reserve estimates. Casualty Actuarial Society Forum, 1 , 101–83.
Mack, T. (1999). The standard error of chain ladder reserve estimates: Recursive calculation and inclusion of a tail factor. ASTIN Bulletin: The Journal of the IAA, 29 (2), 361–366.
Mack, T., & Venter, G. (2000). A comparison of stochastic models that reproduce chain ladder reserve estimates. Insurance: Mathematics and economics, 26 (1), 101–107.
Mammen, E., Miranda, M. D. M., & Nielsen, J. P. (2015). In-sample forecasting applied to reserving and mesothelioma mortality. Insurance: Mathematics and Economics, 61 , 76–86.
Merz, M., & Wuthrich, M. V. (2015). Claims run-off uncertainty: The full picture. Swiss Finance Institute Research Paper (14-69)
Miranda, M. D. M., Nielsen, J. P., & Verrall, R. (2012). Double chain ladder. ASTIN Bulletin: The Journal of the IAA, 42 (1), 59–76.
Miranda, M. D. M., Nielsen, J. P., Sperlich, S., & Verrall, R. (2013). Continuous chain ladder: Reformulating and generalizing a classical insurance problem. Expert Systems with Applications, 40 (14), 5588–5603.
Nelder, J., & Lee, Y. (1992). Likelihood, quasi-likelihood and pseudolikelihood: some comparisons. Journal of the Royal Statistical Society: Series B (Methodological), 54 (1), 273–284.
Peremans, K., Segaert, P., Van Aelst, S., & Verdonck, T. (2017). Robust bootstrap procedures for the chain-ladder method. Scandinavian Actuarial Journal, 10 , 870–897.
Pya, N., & Wood, S. N. (2015). Shape constrained additive models. Statistics and Computing, 25 (3), 543–559.
Ramsay, J. O. (1988). Monotone regression splines in action. Statistical Science, 1 , 425–441.
Renshaw, A. E., & Verrall, R. J. (1998). A stochastic model underlying the chain-ladder technique. British Actuarial Journal, 4 (4), 903–923.
Röhr, A. (2016). Chain ladder and error propagation. ASTIN Bulletin: The Journal of the IAA, 46 (2), 293–330.
Taylor, G. C., & Ashe, F. R. (1983). Second moments of estimates of outstanding claims. Journal of Econometrics, 23 (1), 37–61.
Verdonck, T., & Debruyne, M. (2011). The influence of individual claims on the chain-ladder estimates: Analysis and diagnostic tool. Insurance: Mathematics and Economics, 48 (1), 85–98.
Verrall, R. (1996). Claims reserving and generalised additive models. Insurance: Mathematics and Economics, 19 (1), 31–43.
Wood, S. N. (2017). Generalized additive models: An introduction with R . CRC Press.
Wright, T. S. (1990). A stochastic method for claims reserving in general insurance. Journal of the Institute of Actuaries, 117 (3), 677–731.
Wüthrich, M. V. (2003). Claims reserving using Tweedie’s compound Poisson model. ASTIN Bulletin: The Journal of the IAA, 33 (2), 331–346.
Wüthrich, M. V., & Merz, M. (2008). Stochastic claims reserving methods in insurance (Vol. 435). Wiley.
Download references
Acknowledgements
The authors would like to thank the Australian National University, Renmin University, and Macquarie University for the research support. This work is supported by the MOE Project of Key Research Institute of Humanities and Social Sciences [22JJD910003]. We particularly thank the Lead Guest Editor (Rita Laura D’Ecclesia) and two anonymous referees for providing valuable and insightful comments on earlier drafts. The usual disclaimer applies.
Open Access funding enabled and organized by CAUL and its Member Institutions.
Author information
Authors and affiliations.
Research School of Finance, Actuarial Studies and Statistics, Australian National University, Canberra, ACT, 2601, Australia
Center for Applied Statistics and School of Statistics, Renmin University of China, Beijing, People’s Republic of China
Guangyuan Gao
Department of Actuarial Studies and Business Analytics, Macquarie University, Sydney, NSW, 2109, Australia
You can also search for this author in PubMed Google Scholar
Corresponding author
Correspondence to Yanlin Shi .
Ethics declarations
Conflict of interest.
We have no conflict of interest to disclose.
Additional information
Publisher's note.
Springer Nature remains neutral with regard to jurisdictional claims in published maps and institutional affiliations.
Le Chang and Guangyuan Gao are co-first authors.
List of notations used in monotone splines estimators
Proof of lemmas and theorems, 1.1 proof of lemma 1.
The argued uncorrelatedness in different DYs can be straightforwardly demonstrated using the proof of Theorem 2 in Mack ( 1993 ) for the uncorrelation of \(F_{i,k}\) and \(F_{j,s}\) , where \(k \ne s\) , and i and j can be equal or not.
In addition, from Assumption (I), \(E(E(F_{i,k}|C_{i,1},...,C_{i,k}))=f_k\) proves the unbiasedness. Then for \(i \ne j\) , we have that
The second equality stems from the fact that \(F_{i,k}=C_{i,k+1}/{C_{i,k}}\) , and the third equality is due to Assumption (III), which completes the proof of uncorrelation for DFs of the same DY but different AY.
1.2 Proof of Lemma 2
The proof follows a usual mathematical induction.
When \(k=1\) , since \( \{F^*_{k_n}\}=\{F_{i,1}\}\) for \(i=1,...,I-1\) , it is clear that:
where \(C_{n,1}/\sum _{j}C_{n,1}\) is the associate probability of \(F_{n,1}\) in calculating the empirical sample mean \(\overline{A^*_1}\) , and \({\widehat{f}}_1\) is defined as in ( 3 ).
Denote \({\widehat{a}}_s=\prod _{i=1}^{s}{\widehat{f}}_s\) , we now assume that when \(k=s\) , \(\overline{A^*_s}={\widehat{a}}_s\) .
When \(k=s+1\) , it follows that \(\{A^*_{k_n}\}\) consists of all possible products of \(\{A^*_{s_n}\}\) and \(\{F^*_{s_n}\}\) . Thus, denote \(A^*_{s_n}\) as the n th element in \(\{A^*_{s_n}\}\) with the corresponding probability of \(w_{n,s}\) to be sampled to form a CDF in DY \(s+1\) ; we have that:
which completes the proof.
1.3 Proof of Theorem 3
When \(k=1\) , we have that:
Consider all samples with their associated probabilities/weights, we have that:
where \(\overline{\varepsilon _{1}}\) is the empirical (weighted sample) mean of residuals. Use Lemma 2 , the assumption \(E(\varepsilon _{i,1})=0\) and the fact \(\widehat{f^*_1}=\widehat{A^*_1}\) , it is clear that:
The equation and associated notations in ( B.1 ) can be extended to all DYs. For DY \(k>1\) , use Lemma 2 , we have that:
From Assumption (VI), it can be inferred that \(\overline{\varepsilon _{k-1}}\) is independent of \({\widehat{f}}_k\) and \(\overline{\varepsilon _{k}}\) is independent of \(\widehat{A^*_{k-1}}\) . For a usual linear regression, the error term is orthogonal with regressors. Thus, we further have that \(\overline{\varepsilon _{k-1}}\) is independent of \(\widehat{A^*_{k-1}}\) . It is then clear that:
Hence, we have that
Additional simulation results
1.1 df estimators.
This section presents simulation evidence to preliminarily support the asymptotics of monotone spline estimators of DF. We firstly consider the case with increasing N and fixed I . The scenario with increased I and fixed N is then concerned. In both cases, the simulation machine of Gabrielli and Wüthrich ( 2018 ) is employed to produce a total of 1000 triangles, with true \(f_k\) known. The procedure of simulation is outlined in Sect. 3.2 .
Table 12 presents the results with \(I=8\) (8 DYs and 8 AYs) and N allowed to increase. When \(I=8\) , the total number of distinct CDFs is 7!=5040, a large but manageable number for computation. The aim of this table is to explore the performance of monotone splines estimators of DF with N observations in each DY. Specifically, recall in Remark 6 , it is expected that such estimators would converge to counterparts using the full enhanced sample (i.e., \((I-1)!/(I-k-1)!\) observations in DY k ). Thus, for each of the 1000 simulated triangle, we firstly fit the monotone splines with the full enhanced sample. A subsample model, with N observations in each DY as explained in Sect. 3.3 , is then fitted. The absolute differences of two sets of DY estimates are recorded, and their sample mean is reported in Table 12 for each DY. Similarly, we also compare bootstrap standard error estimators of DF estimators using the enhanced sample and subsample, with 1000 replicates in all cases. As expected, both absolute differences between DF estimates and those between the corresponding standard error estimates reduce uniformly in each DY, with N increased from 10 to 50 and 100. This preliminarily supports the asymptotic convergence using the subsample approach and the full enhanced sample.
Table 13 uses the same simulation setting in the paper, with the baseline case with 12 AYs and 12 DYs. Similarly, we use the simulation machine of Gabrielli and Wüthrich ( 2018 ) to simulate 1000 triangles, with true DF in each DY, or \(f_k\) known. Thus, the absolute bias of the monotone spline estimators, or \(\widehat{f^s_{k}}\) , can be produced. To consider the asymptotic convergence, we fix the DY number as 12, and increase the AY number I from 12 to 24 and 60. This is to simulate the case that more historical data are available, whereas it is believed all claims will fully develop in 12 years. In addition, we consider the asymptotic convergence of \(\sqrt{{\widehat{Var}}(\widehat{f_{k}^s})}\) , the estimated standard error of \(\widehat{f^s_{k}}\) , and use the sample standard deviation of \(\widehat{f^s_{k}}\) as a benchmark. In all cases, N is set to 1000 with 1000 bootstrap replicates. Two promising conclusions can be drawn. First, the absolute bias in each DY uniformly reduces with the increased I from 12 to 24 and 60. Second, both SD and SE decline with I , with decreased differences between SD and SE. Those results preliminarily support the asymptotic consistency of \(\widehat{f^s_{k}}\) and \(\sqrt{{\widehat{Var}}(\widehat{f_{k}^s})}\) of the monotone splines proposed in this paper.
1.2 Reserve estimators
First, we work with the same 1000 triangles simulated using the method proposed by Gabrielli and Wüthrich ( 2018 ). Note that we only keep the training triangle rather than the complete dataset in each case. Next, we follow the same procedure as outlined in Sect. 4.2 . Complete datasets are then produced with GLM being the true DGP. Both the numbers of AYs and DYs are set to 12. The results are listed in Table 14 . Second, we employ the simulation machine of Gabrielli and Wüthrich ( 2018 ) to generate complete datasets. With the same 12 DYs, the number of AY is increased to 24 and 60, and we present results in Panels A and B of Table 15 , respectively. In summary, when the true DGP is GLM, Table 14 suggests that all models produce results that are much comparable to those in Table 7 , and are much more accurate than those in Table 8 . When the number of DY increases to 24 and 60, Table 15 suggests that more available data leading to uniformly improved results for all models. In particular, when the number of AY is 60, the results of simulation machine DGP are comparable to those of GLM DGP with 12 AYs, as presented in Table 14 .
Computational codes
Illustrative computational codes used in this paper can be found in https://osf.io/xmnt2/ .
Rights and permissions
Open Access This article is licensed under a Creative Commons Attribution 4.0 International License, which permits use, sharing, adaptation, distribution and reproduction in any medium or format, as long as you give appropriate credit to the original author(s) and the source, provide a link to the Creative Commons licence, and indicate if changes were made. The images or other third party material in this article are included in the article's Creative Commons licence, unless indicated otherwise in a credit line to the material. If material is not included in the article's Creative Commons licence and your intended use is not permitted by statutory regulation or exceeds the permitted use, you will need to obtain permission directly from the copyright holder. To view a copy of this licence, visit http://creativecommons.org/licenses/by/4.0/ .
Reprints and permissions
About this article
Chang, L., Gao, G. & Shi, Y. A semi-parametric claims reserving model with monotone splines. Ann Oper Res (2024). https://doi.org/10.1007/s10479-024-06007-3
Download citation
Received : 21 October 2022
Accepted : 18 January 2024
Published : 06 May 2024
DOI : https://doi.org/10.1007/s10479-024-06007-3
Share this article
Anyone you share the following link with will be able to read this content:
Sorry, a shareable link is not currently available for this article.
Provided by the Springer Nature SharedIt content-sharing initiative
- Claims reserving
- Chain-ladder technique
- Monotone splines
- Find a journal
- Publish with us
- Track your research
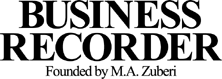
Mid-term pipeline projects
New projects identified, Korean EXIM Bank team told RECORDER REPORT ISLAMABAD: Minister for Economic Affairs Ahad Khan Cheema has informed the programme mission of the EXIM Bank Korea that as part of planning for mid-term pipeline projects, the ministry has identified new projects in priority sectors such as Information and Communication Technology (ICT), climate change, construction, and transport. On Thursday, the minister chaired the kickoff meeting of the Economic Development Cooperation Fund (EDCF) Country Programme Mission, led by Bonhyun Koo, Director of Asia Team, from the Export-Import Bank of Korea (EXIM Bank) on Thursday. The meeting included members of the EDCF Project Management Consultant (PMC), secretary EAD and senior officials from the Ministry of Economic Affairs, and other key stakeholders. The minister welcomed the delegation and expressed gratitude for the continued support provided by the EXIM Bank and the Government of the Republic of Korea. The minister noted that seven ongoing projects, totaling US$ 522 million, are currently being implemented across key sectors, including road infrastructure, information technology, and health. He also commended EXIM Bank for signing the Framework Arrangement (2022-26) and expressed the hope for full utilisation of the US$ 1,000 million committed under this agreement. Furthermore, it was noted that 10 pipeline projects have already been shared with the EDCF and their prompt implementation is anticipated. Cheema further apprised the mission that as part of planning for Mid-term Pipeline Projects, the ministry has identified new projects in priority sectors such as ICT, climate change, construction, and transport. In his concluding remarks, Cheema reiterated Government of Pakistan’s commitment to strengthening cooperation with the EDCF and expressed confidence that with improved efficiency in project preparation and implementation, both countries can maximise their collaborative potential. The meeting concluded with the minister thanking all participants for their valuable contributions.
More from the page
Qatari team lays focus on energy, mining and aviation: fo, scientists win world food prize for work on global seed vault, modi skips election in iiojk as critics dispute integration claims, centcom chief meets coas, ke’s fca of nine months, sbp’s reserves up $1.114bn to $9.120bn.
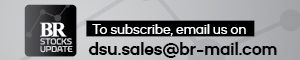

Thousands Believe Covid Vaccines Harmed Them. Is Anyone Listening?
All vaccines have at least occasional side effects. But people who say they were injured by Covid vaccines believe their cases have been ignored.
Shaun Barcavage, 54, a nurse practitioner in New York City, said that ever since his first Covid shot, standing up has sent his heart racing. Credit... Hannah Yoon for The New York Times
Supported by
- Share full article

By Apoorva Mandavilli
Apoorva Mandavilli spent more than a year talking to dozens of experts in vaccine science, policymakers and people who said they had experienced serious side effects after receiving a Covid-19 vaccine.
- Published May 3, 2024 Updated May 4, 2024
Within minutes of getting the Johnson & Johnson Covid-19 vaccine, Michelle Zimmerman felt pain racing from her left arm up to her ear and down to her fingertips. Within days, she was unbearably sensitive to light and struggled to remember simple facts.
She was 37, with a Ph.D. in neuroscience, and until then could ride her bicycle 20 miles, teach a dance class and give a lecture on artificial intelligence, all in the same day. Now, more than three years later, she lives with her parents. Eventually diagnosed with brain damage, she cannot work, drive or even stand for long periods of time.
“When I let myself think about the devastation of what this has done to my life, and how much I’ve lost, sometimes it feels even too hard to comprehend,” said Dr. Zimmerman, who believes her injury is due to a contaminated vaccine batch .
The Covid vaccines, a triumph of science and public health, are estimated to have prevented millions of hospitalizations and deaths . Yet even the best vaccines produce rare but serious side effects . And the Covid vaccines have been given to more than 270 million people in the United States, in nearly 677 million doses .
Dr. Zimmerman’s account is among the more harrowing, but thousands of Americans believe they suffered serious side effects following Covid vaccination. As of April, just over 13,000 vaccine-injury compensation claims have been filed with the federal government — but to little avail. Only 19 percent have been reviewed. Only 47 of those were deemed eligible for compensation, and only 12 have been paid out, at an average of about $3,600 .
Some scientists fear that patients with real injuries are being denied help and believe that more needs to be done to clarify the possible risks.
“At least long Covid has been somewhat recognized,” said Akiko Iwasaki, an immunologist and vaccine expert at Yale University. But people who say they have post-vaccination injuries are “just completely ignored and dismissed and gaslighted,” she added.

In interviews and email exchanges conducted over several months, federal health officials insisted that serious side effects were extremely rare and that their surveillance efforts were more than sufficient to detect patterns of adverse events.
“Hundreds of millions of people in the United States have safely received Covid vaccines under the most intense safety monitoring in U.S. history,” Jeff Nesbit, a spokesman for the Department of Health and Human Services, said in an emailed statement.
But in a recent interview, Dr. Janet Woodcock, a longtime leader of the Food and Drug Administration, who retired in February, said she believed that some recipients had experienced uncommon but “serious” and “life-changing” reactions beyond those described by federal agencies.
“I feel bad for those people,” said Dr. Woodcock, who became the F.D.A.’s acting commissioner in January 2021 as the vaccines were rolling out. “I believe their suffering should be acknowledged, that they have real problems, and they should be taken seriously.”
“I’m disappointed in myself,” she added. “I did a lot of things I feel very good about, but this is one of the few things I feel I just didn’t bring it home.”
Federal officials and independent scientists face a number of challenges in identifying potential vaccine side effects.
The nation’s fragmented health care system complicates detection of very rare side effects, a process that depends on an analysis of huge amounts of data. That’s a difficult task when a patient may be tested for Covid at Walgreens, get vaccinated at CVS, go to a local clinic for minor ailments and seek care at a hospital for serious conditions. Each place may rely on different health record systems.
There is no central repository of vaccine recipients, nor of medical records, and no easy to way to pool these data. Reports to the largest federal database of so-called adverse events can be made by anyone, about anything. It’s not even clear what officials should be looking for.
“I mean, you’re not going to find ‘brain fog’ in the medical record or claims data, and so then you’re not going to find” a signal that it may be linked to vaccination, Dr. Woodcock said. If such a side effect is not acknowledged by federal officials, “it’s because it doesn’t have a good research definition,” she added. “It isn’t, like, malevolence on their part.”
The government’s understaffed compensation fund has paid so little because it officially recognizes few side effects for Covid vaccines. And vaccine supporters, including federal officials, worry that even a whisper of possible side effects feeds into misinformation spread by a vitriolic anti-vaccine movement.
‘I’m Not Real’
Patients who believe they experienced serious side effects say they have received little support or acknowledgment.
Shaun Barcavage, 54, a nurse practitioner in New York City who has worked on clinical trials for H.I.V. and Covid, said that ever since his first Covid shot, merely standing up sent his heart racing — a symptom suggestive of postural orthostatic tachycardia syndrome , a neurological disorder that some studies have linked to both Covid and, much less often, vaccination .
He also experienced stinging pain in his eyes, mouth and genitals, which has abated, and tinnitus, which has not.
“I can’t get the government to help me,” Mr. Barcavage said of his fruitless pleas to federal agencies and elected representatives. “I am told I’m not real. I’m told I’m rare. I’m told I’m coincidence.”
Renee France, 49, a physical therapist in Seattle, developed Bell’s palsy — a form of facial paralysis, usually temporary — and a dramatic rash that neatly bisected her face. Bell’s palsy is a known side effect of other vaccines, and it has been linked to Covid vaccination in some studies.
But Dr. France said doctors were dismissive of any connection to the Covid vaccines. The rash, a bout of shingles, debilitated her for three weeks, so Dr. France reported it to federal databases twice.
“I thought for sure someone would reach out, but no one ever did,” she said.
Similar sentiments were echoed in interviews, conducted over more than a year, with 30 people who said they had been harmed by Covid shots. They described a variety of symptoms following vaccination, some neurological, some autoimmune, some cardiovascular.
All said they had been turned away by physicians, told their symptoms were psychosomatic, or labeled anti-vaccine by family and friends — despite the fact that they supported vaccines.
Even leading experts in vaccine science have run up against disbelief and ambivalence.
Dr. Gregory Poland, 68, editor in chief of the journal Vaccine, said that a loud whooshing sound in his ears had accompanied every moment since his first shot, but that his entreaties to colleagues at the Centers for Disease Control and Prevention to explore the phenomenon, tinnitus, had led nowhere.
He received polite responses to his many emails, but “I just don’t get any sense of movement,” he said.
“If they have done studies, those studies should be published,” Dr. Poland added. In despair that he might “never hear silence again,” he has sought solace in meditation and his religious faith.
Dr. Buddy Creech, 50, who led several Covid vaccine trials at Vanderbilt University, said his tinnitus and racing heart lasted about a week after each shot. “It’s very similar to what I experienced during acute Covid, back in March of 2020,” Dr. Creech said.
Research may ultimately find that most reported side effects are unrelated to the vaccine, he acknowledged. Many can be caused by Covid itself.
“Regardless, when our patients experience a side effect that may or may not be related to the vaccine, we owe it to them to investigate that as completely as we can,” Dr. Creech said.
Federal health officials say they do not believe that the Covid vaccines caused the illnesses described by patients like Mr. Barcavage, Dr. Zimmerman and Dr. France. The vaccines may cause transient reactions, such as swelling, fatigue and fever, according to the C.D.C., but the agency has documented only four serious but rare side effects .
Two are associated with the Johnson & Johnson vaccine, which is no longer available in the United States: Guillain-Barré syndrome , a known side effect of other vaccines , including the flu shot; and a blood-clotting disorder.
The C.D.C. also links mRNA vaccines made by Pfizer-BioNTech and Moderna to heart inflammation, or myocarditis, especially in boys and young men. And the agency warns of anaphylaxis, or severe allergic reaction, which can occur after any vaccination.
Listening for Signals
Agency scientists are monitoring large databases containing medical information on millions of Americans for patterns that might suggest a hitherto unknown side effect of vaccination, said Dr. Demetre Daskalakis, director of the C.D.C.’s National Center for Immunization and Respiratory Diseases.
“We toe the line by reporting the signals that we think are real signals and reporting them as soon as we identify them as signals,” he said. The agency’s systems for monitoring vaccine safety are “pretty close” to ideal, he said.

Those national surveillance efforts include the Vaccine Adverse Event Reporting System (VAERS). It is the largest database, but also the least reliable: Reports of side effects can be submitted by anyone and are not vetted, so they may be subject to bias or manipulation.
The system contains roughly one million reports regarding Covid vaccination, the vast majority for mild events, according to the C.D.C.
Federal researchers also comb through databases that combine electronic health records and insurance claims on tens of millions of Americans. The scientists monitor the data for 23 conditions that may occur following Covid vaccination. Officials remain alert to others that may pop up, Dr. Daskalakis said.
But there are gaps, some experts noted. The Covid shots administered at mass vaccination sites were not recorded in insurance claims databases, for example, and medical records in the United States are not centralized.
“It’s harder to see signals when you have so many people, and things are happening in different parts of the country, and they’re not all collected in the same system,” said Rebecca Chandler, a vaccine safety expert at the Coalition for Epidemic Preparedness Innovations.
An expert panel convened by the National Academies concluded in April that for the vast majority of side effects, there was not enough data to accept or reject a link.
Asked at a recent congressional hearing whether the nation’s vaccine-safety surveillance was sufficient, Dr. Peter Marks, director of the F.D.A.’s Center for Biologics Evaluation and Research, said, “I do believe we could do better.”
In some countries with centralized health care systems, officials have actively sought out reports of serious side effects of Covid vaccines and reached conclusions that U.S. health authorities have not.
In Hong Kong, the government analyzed centralized medical records of patients after vaccination and paid people to come forward with problems. The strategy identified “a lot of mild cases that other countries would not otherwise pick up,” said Ian Wong, a researcher at the University of Hong Kong who led the nation’s vaccine safety efforts.
That included the finding that in rare instances — about seven per million doses — the Pfizer-BioNTech vaccine triggered a bout of shingles serious enough to require hospitalization.
The European Medicines Agency has linked the Pfizer and Moderna vaccines to facial paralysis, tingling sensations and numbness. The E.M.A. also counts tinnitus as a side effect of the Johnson & Johnson vaccine, although the American health agencies do not. There are more than 17,000 reports of tinnitus following Covid vaccination in VAERS.
Are the two linked? It’s not clear. As many as one in four adults has some form of tinnitus. Stress, anxiety, grief and aging can lead to the condition, as can infections like Covid itself and the flu.
There is no test or scan for tinnitus, and scientists cannot easily study it because the inner ear is tiny, delicate and encased in bone, said Dr. Konstantina Stankovic, an otolaryngologist at Stanford University.
Still, an analysis of health records from nearly 2.6 million people in the United States found that about 0.04 percent , or about 1,000, were diagnosed with tinnitus within three weeks of their first mRNA shot. In March, researchers in Australia published a study linking tinnitus and vertigo to the vaccines .
The F.D.A. is monitoring reports of tinnitus, but “at this time, the available evidence does not suggest a causal association with the Covid-19 vaccines,” the agency said in a statement.
Despite surveillance efforts, U.S. officials were not the first to identify a significant Covid vaccine side effect: myocarditis in young people receiving mRNA vaccines. It was Israeli authorities who first raised the alarm in April 2021. Officials in the United States said at the time that they had not seen a link.
On May 22, 2021, news broke that the C.D.C. was investigating a “relatively few” cases of myocarditis. By June 23, the number of myocarditis reports in VAERS had risen to more than 1,200 — a hint that it is important to tell doctors and patients what to look for.
Later analyses showed that the risk for myocarditis and pericarditis, a related condition, is highest after a second dose of an mRNA Covid vaccine in adolescent males aged 12 to 17 years.
In many people, vaccine-related myocarditis is transient. But some patients continue to experience pain, breathlessness and depression, and some show persistent changes on heart scans . The C.D.C. has said there were no confirmed deaths related to myocarditis, but in fact there have been several accounts of deaths reported post-vaccination .
Pervasive Misinformation
The rise of the anti-vaccine movement has made it difficult for scientists, in and out of government, to candidly address potential side effects, some experts said. Much of the narrative on the purported dangers of Covid vaccines is patently false, or at least exaggerated, cooked up by savvy anti-vaccine campaigns.
Questions about Covid vaccine safety are core to Robert F. Kennedy Jr.’s presidential campaign. Citing debunked theories about altered DNA, Florida’s surgeon general has called for a halt to Covid vaccination in the state.
“The sheer nature of misinformation, the scale of misinformation, is staggering, and anything will be twisted to make it seem like it’s not just a devastating side effect but proof of a massive cover-up,” said Dr. Joshua Sharfstein, a vice dean at Johns Hopkins University.
Among the hundreds of millions of Americans who were immunized for Covid, some number would have had heart attacks or strokes anyway. Some women would have miscarried. How to distinguish those caused by the vaccine from those that are coincidences? The only way to resolve the question is intense research .
But the National Institutes of Health is conducting virtually no studies on Covid vaccine safety, several experts noted. William Murphy, a cancer researcher who worked at the N.I.H. for 12 years, has been prodding federal health officials to initiate these studies since 2021.
The officials each responded with “that very tired mantra: ‘But the virus is worse,’” Dr. Murphy recalled. “Yes, the virus is worse, but that doesn’t obviate doing research to make sure that there may be other options.”
A deeper understanding of possible side effects, and who is at risk for them, could have implications for the design of future vaccines, or may indicate that for some young and healthy people, the benefit of Covid shots may no longer outweigh the risks — as some European countries have determined.
Thorough research might also speed assistance to thousands of Americans who say they were injured.
The federal government has long run the National Vaccine Injury Compensation Program , designed to compensate people who suffer injuries after vaccination. Established more than three decades ago, the program sets no limit on the amounts awarded to people found to have been harmed.
But Covid vaccines are not covered by that fund because Congress has not made them subject to the excise tax that pays for it. Some lawmakers have introduced bills to make the change.
Instead, claims regarding Covid vaccines go to the Countermeasures Injury Compensation Program . Intended for public health emergencies, this program has narrow criteria to pay out and sets a limit of $50,000, with stringent standards of proof.
It requires applicants to prove within a year of the injury that it was “the direct result” of getting the Covid vaccine, based on “compelling, reliable, valid, medical, and scientific evidence.”
The program had only four staff members at the beginning of the pandemic, and now has 35 people evaluating claims. Still, it has reviewed only a fraction of the 13,000 claims filed, and has paid out only a dozen.
Dr. Ilka Warshawsky, a 58-year-old pathologist, said she lost all hearing in her right ear after a Covid booster shot. But hearing loss is not a recognized side effect of Covid vaccination.
The compensation program for Covid vaccines sets a high bar for proof, she said, yet offers little information on how to meet it: “These adverse events can be debilitating and life-altering, and so it’s very upsetting that they’re not acknowledged or addressed.”
Dr. Zimmerman, the neuroscientist, submitted her application in October 2021 and provided dozens of supporting medical documents. She received a claim number only in January 2023.
In adjudicating her claim for workers’ compensation, Washington State officials accepted that Covid vaccination caused her injury, but she has yet to get a decision from the federal program.
One of her therapists recently told her she might never be able to live independently again.
“That felt like a devastating blow,” Dr. Zimmerman said. “But I’m trying not to lose hope there will someday be a treatment and a way to cover it.”
Apoorva Mandavilli is a reporter focused on science and global health. She was a part of the team that won the 2021 Pulitzer Prize for Public Service for coverage of the pandemic. More about Apoorva Mandavilli
Advertisement

IMAGES
VIDEO
COMMENTS
A claim is a statement that presents an idea or series of ideas as arguments. Arguments therefore consist of claims, or another way to put it is, to say that claims are the building blocks of a good argument. In research writing, claims will be the backbone that form a thesis or a hypothesis (here the term 'hypothesis' refers to the ...
Abstract. For research evidence to inform decision making, an appraisal needs to be made of whether the claims are justified and whether they are useful to the decisions being made. This chapter provides a high level framework of core issues relevant to appraising the "fitness for purpose" of evidence claims.
A claim defines your paper‟s goals, direction, scope, and exigence and is supported by evidence, quotations, argumentation, expert opinion, statistics, and telling details. ... —A claim can be substantiated with research, evidence, testimony, and academic reasoning. —A claim is something more than statement and support: an arguable claim ...
As compared with physicians who had one previous paid claim, physicians who had two paid claims had almost twice the risk of having another one (hazard ratio, 1.97; 95% confidence interval [CI], 1 ...
Although we write in terms of empirical papers, these strategies are also relevant for review papers and talks. ... We believe that this chapter—focusing on the appropriate way to make research-based claims—will be of particular benefit to fledgling psychologists. We admire Neal Miller's aphorism: "Be bold in what you try, cautious in ...
The paper used the data of policyholders from Delhi/NCR, India, who have availed the cashless claims in the last three years. In the process, a total of 790 questionnaires were sought to be.
It does this by accurately describing the practice, precisely defining what it claims to do, and determining readiness, capacity, and resources involved in further research or evaluation. 8 The second method is the REAL, which is a streamlined, efficient systematic review process conducted to determine the quantity, quality, and strength of ...
A "claim" (also known as a "thesis statement" or "argument") is the central idea of your paragraph or essay and should appear in the first sentence. Effective Claims are: In most cases, writing ability could be your ticket in—or it could be your ticket out. U.S. NATIONAL COMMISSION. ON WRITING'S SURVEY OF 120 MAJOR CORPORATIONS ...
Faster Claim Settlements: The time it takes for health insurance claims to be settled is one of the main difficulties for both policyholders and insurers. This might be due to lengthy manual processes or bogus claims. ... The authors identified 245 published research papers from 2017 to the present date from IEEE, ACM, Inderscience, Elsevier ...
Descriptive Statistics for Claim Frequency & Severity data From the table above, a summary of the results of the descriptive data analysis of the claim data is shown. USD 5058.00 is the minimum ...
Claims data contain information on insurance payment as well as clinical diagnoses and procedure codes to ascertain medical conditions and treatments, making them valuable sources for economic evaluation research. This paper offers an introductory overview of the use of claims data for oncology-related cost-of-illness, cost comparison, and cost ...
Research Methodology The methodology of research is purely doctrinal in nature having analytical and descriptive approach. The author has studied the scope of arbitration in insurance cases studying the related legislations, regulations and case laws along with the UNCITRAL Model Law. ... Whether a claim made falls within the arbitration clause ...
However, if progress is to be made in depression research, evidence must be provided upon which claims are made (84). Therefore, while data-sharing policies should be implemented and encouraged ...
Therefore, this paper aims to identify the common types and causes of claims generally occur in construction industry and specifically in large scale projects. A detailed literature review has been carried out for this research and covers past research papers of the last two decades. The results showed delay in claims and extra work claim ...
1.2. Statement of the problem. The main purpose of this paper is to come up with a model to be used to find out if a certain insurance claim made is a fraud or not. The model will be designed after testing multiple algorithms to come up with the best model that can detect if a claim is fraudulent or not.
The placement of claim in writing of an essay or research paper may also depend on how lengthy it is or how long your paper is. But it is good to place where your readers get it without reading to much. So placing it at the end of the first paragraph is more expected. Remember to keep your claim statement short, ideally of about two lines.
A claim is the main argument of an essay. It is the most important part of an academic paper. A claim defines the paper's goals, direction, and scope. It is supported by evidence. A claim must be argumentative. A good claim makes a focused argument (Because of the growing obesity epidemic, elementary schools
This video provides an introduction to and brief explanation for writing a claim with evidence and reasoning. The CER framework is a key concept used across ...
Just what is a claim in writing? It's not all that far off from a claim you might make out loud. Learn more about when you're making a claim right here.
Stochastic reserving models used in the insurance industry are usually based on an assumed distribution of claim amounts. Despite their popularity, such models may unavoidably be affected by the misspecification issue given that it is likely that the underlying distribution will be different from that assumed. In this paper, we incorporate monotone splines to ensure the expected monotonically ...
A study on factors influencing. claims in general insurance. business in India. T. Joji Rao and Krishan K. Pandey. College of Management and Economic Studies, University of Petroleum & Energy ...
Table of contents. Step 1: Introduce your topic. Step 2: Describe the background. Step 3: Establish your research problem. Step 4: Specify your objective (s) Step 5: Map out your paper. Research paper introduction examples. Frequently asked questions about the research paper introduction.
1. A good thesis sentence will make a claim. This doesn't mean that you have to reduce an idea to an "either/or" proposition and then take a stand. Rather, you need to develop an interesting perspective that you can support and defend. This perspective must be more than an observation.
New projects identified, Korean EXIM Bank team told RECORDER REPORT ISLAMABAD: Minister for Economic Affairs Ahad Khan Cheema has informed the programme mission of the EXIM Bank Korea that as part of planning for mid-term pipeline projects, the ministry has identified new projects in priority sectors such as Information and Communication Technology (ICT), climate change, construction, and ...
Tabled Paper Final report - Workers compensation claims management.pdf: Document Type: Statutory Report: Under the Act: Government Sector Audit Act 1983
The past research papers have been studied to find the m ost comm on causes and types of claim s. These research papers have b een collected from the s ources including Science Direct, Elsevier, and
Instead, claims regarding Covid vaccines go to the Countermeasures Injury Compensation Program. Intended for public health emergencies, this program has narrow criteria to pay out and sets a limit ...