Understanding and Implementing Research Data Management
- Living reference work entry
- First Online: 01 May 2018
- Cite this living reference work entry
- Sebastian Netscher 4 &
- Alexia Katsanidou 4
Part of the book series: Springer Reference Sozialwissenschaften ((SRS))
487 Accesses
3 Altmetric
Research Data Management is an integral part of good scientific practice. Its relevance increases as funders, journals, and other scientific outlets place it as a requirement in the context of sharing data and concepts on data harmonization. Consequently, it has become imperative that researchers know what research data management is, how it supports their research project and fosters satisfying funders’ requirements on sharing. This chapter introduces research data management, describes activities of data handling, and highlights legal issues. Based on this introduction, we discuss different objectives of research data management, such as ensuring replicability or sharing of research data generated in a research project as well as sharing concepts on data harmonizing and merging in secondary analysis.
This is a preview of subscription content, log in via an institution to check access.

Access this chapter
Institutional subscriptions
Similar content being viewed by others
Current Trends in Research Data Management
Research Data Stewardship for Healthcare Professionals
A computer science method that automatically links available data over the internet.
Also most research institutes have automated back-up processes, nowadays, researcher should be aware of how these procedures work and how to access back-ups if needed.
It is important to note that licenses can be irrevocable as well as not suitable for personal data, requiring confidentiality.
The Consortium of European Social Science Data Archives (CESSDA) is a pan-European Research Infrastructure (ERIC) that brings together social science data archives across Europe to provides large scale, integrated, and sustainable data services to the social sciences.
At least, researchers should not avoid data sharing by restrictive formulations in the consent form, such as ‘data will only be used within the research project’ (Doorn 2010 ).
In some cases, data anonymization might not be appropriate due to a loss of information. In such cases, researchers might share their data weakly or even non-anonymized, controlling data access and re-use by a restrictive license or even by sharing data via a so called secure data center.
BDSG. 1990. Bundesdatenschutzgesetz. https://www.gesetze-im-internet.de/bdsg_1990/ . Accessed 22 Feb 2018.
Beagrie, Charles. 2011. User guide for keeping research data safe. Assessing Costs/Benefits of Research Data Management, Preservation and Re-Use. Version 2.0. https://beagrie.com/KeepingResearchDataSafe_UserGuide_v2.pdf . Accessed 22 Feb 2018.
CESSDA. ERIC 2017. Consortium or European Social Science Data Archive. https://www.cessda.eu/ .
Corti, Louise, Veerle van den Eynden, Libby Bishop, and Matthew Woollard. 2014. Managing and sharing research data. A guide to good practice . London: Sage publications.
Google Scholar
DataCite Metadata Working Group. 2016. DataCite Metadata schema documentation for the publication and citation of research data. Version 4.0. DataCite e.V. https://doi.org/10.5438/0012 .
DDI Allicane. 2017. Data documentation initiative. http://www.ddialliance.org/ . Accessed 22 Feb 2018.
DFG-Deutsche Forschungsgemeinschaft. 2013. Sicherung guter wissenschaftlicher Praxis. Denkschrift, Empfehlungen der Kommission „Selbstkontrolle in der Wissenschaft“ . Weinheim: WILEY-VCH Verlag. http://www.dfg.de/foerderung/grundlagen_rahmenbedingungen/gwp/ .
Book Google Scholar
Doorn, Peter K. 2010. Preparing data for sharing. Guide to social science data archiving . DANS Data Guide 8. Amsterdam: Pallas Publications/Amsterdam University Press.
Elliot, Mark, Elaine Mackey, Kieron O’Hara, and Caroline Tudor. 2016. The anonymization decision-making framework . Manchester: University of Manchester.
Force11. 2017. The FAIR data principles. https://www.force11.org/group/fairgroup/fairprinciples . Accessed 22 Feb 2018.
GDPR. 2016. Regulation (EU) 2016/679 of the European Parliament and of the Council on the Protection of Natural Persons with Regard to the Processing of Personal Data and on the Free Movement of Such Data (General Data Protection Regulation). http://eur-lex.europa.eu/legal-content/DE/ALL/?uri=CELEX%3A32016R0679 . Accessed 22 Feb 2018.
GESIS. 2017. Replikationsserver. http://www.gesis.org/replikationsserver/home/ .
Horizon 2020 Programme. 2016. Guidelines on FAIR Data Management in Horizon 2020. Version 3.0. http://ec.europa.eu/research/participants/data/ref/h2020/grants_manual/hi/oa_pilot/h2020-hi-oa-data-mgt_en.pdf . Accessed 22 Feb 2018.
Huschka, Denis, Claudia Oellers, Notburga Ott, and Gert G. Wagner. 2011. Datenmanagement und Data Sharing- Erfahrungen in den Sozial- und Wirtschaftswissenschaften. In Handbuch Forschungsdatenmanagement , eds. S. Büttner, H.-C. Hobohm, and L. Müller, 35–48. Bad Honnef: Bock+Herchen Verlag.
ISCED. 2011. International standard classification of education. http://uis.unesco.org/en/documents . Accessed 22 Feb 2018.
Jensen, Uwe, Alexia Katsanidou, and Wolfgang Zenk-Möltgen. 2011. Metadaten und Standards. In Handbuch Forschungsdatenmanagement , eds. S. Büttner, H.-C. Hobohm, and L. Müller, 83–100. Bad Honnef: Bock+Herchen Verlag.
Kimpton, Michele, and Carol Minton Morris. 2014. Managing and archiving research data: Local repositories and cloud-based practices. In Research data management. Practical strategies for information professionals , ed. J. M. Ray, 223–238. West Lafayette: Purdue University Press.
Recker, Astrid, Stefan Müller, Jessica Trixa, and Natascha Schumann. 2015. Paving the way for data-centric, open science: An example from the social sciences. Journal of Librarianship and Scholarly Communication 3(2). https://doi.org/10.7710/2162-3309.1227 .
Wallace, David A. 2001. Archiving metadata forum: Report from the recordkeeping metadata working meeting, June 2000. Archival Science 1: 253–269.
Article Google Scholar
Winters, Kristi, and Sebastian Netscher. 2016. Proposed standards for variable harmonization documentation and referencing: A case study using QuickCharmStats 1.1. PLoS ONE 11(2). https://doi.org/10.1371/journal.pone.0147795 .
Download references
Author information
Authors and affiliations.
Abteilung Datenarchiv für Sozialwissenschaften, GESIS - Leibniz Institut für Sozialwissenschaften, Köln, Deutschland
Sebastian Netscher & Alexia Katsanidou
You can also search for this author in PubMed Google Scholar
Corresponding author
Correspondence to Alexia Katsanidou .
Editor information
Editors and affiliations.
Methodenzentrum Sozialwissenschaft, Goethe-Universität Frankfurt Methodenzentrum Sozialwissenschaft, Frankfurt, Hessen, Germany
Claudius Wagemann
Institute for Political Sciences, University of Duisburg-Essen Institute for Political Sciences, Duisburg, Germany
Achim Goerres
Institut für Politikwissenschaft, Goethe-Universität Frankfurt am Main Institut für Politikwissenschaft, Frankfurt, Hessen, Germany
Markus Siewert
Rights and permissions
Reprints and permissions
Copyright information
© 2018 Springer Fachmedien Wiesbaden GmbH, ein Teil von Springer Nature
About this entry
Cite this entry.
Netscher, S., Katsanidou, A. (2018). Understanding and Implementing Research Data Management. In: Wagemann, C., Goerres, A., Siewert, M. (eds) Handbuch Methoden der Politikwissenschaft. Springer Reference Sozialwissenschaften. Springer VS, Wiesbaden. https://doi.org/10.1007/978-3-658-16937-4_4-1
Download citation
DOI : https://doi.org/10.1007/978-3-658-16937-4_4-1
Received : 22 February 2018
Accepted : 22 February 2018
Published : 01 May 2018
Publisher Name : Springer VS, Wiesbaden
Print ISBN : 978-3-658-16937-4
Online ISBN : 978-3-658-16937-4
eBook Packages : Springer Referenz Sozialwissenschaften und Recht
- Publish with us
Policies and ethics
- Find a journal
- Track your research
Using the Library
- Teaching & publication support
- Ask a Librarian
Research Data Management
- Research Data Management at UVA
- About Research Data Management
- Data Privacy and Human Subjects
- File Management Best Practices
- Metadata and Documentation
- Data Storage, Backup and Security
- Data Citations
- Licensing Data & Code
- Data Rights and Policies
- Open Science and Open Data
- 2022 OSTP Public Access Policy Memo
Your Research Data Management Team
Senior research data management librarian.

What is Research Data Management?
Defining Research Data Management
Research Data Management (RDM) refers to a set of principles, processes, and best practices for handling research data both during and after the completion of a research project. The intention of this guide is to help researchers better understand and implement these principles and practices, including:
- How to write a data management plan
- How to best organize and manage files
- How to write quality documentation and create effective metadata
- How to keep data safe and secure
- How to preserve and share data at the end of a project
- How to conduct more open and reproducible research
Following research data management recommendations helps make the researcher an effective steward of their research data, ensuring it is accessible, comprehensible, and fit for use and reuse.
How is the concept of research data defined? One commonly cited definition, used in federal regulations , is "the recorded factual material commonly accepted in the scientific community as necessary to validate research findings." We understand that data is a concept that extends outside the scientific fields, however, so we might choose to say that research data consists of materials or other sources that form the basis for your conclusions and serve to justify scholarly claims and/or findings.
Why do RDM?
Why should you work to improve your data management? One reason is compliance: the norms and expectations for research are shifting and requirements that data be stored and shared for later reuse are increasingly common and will eventually apply to most (if not all) federally funded research. Additionally, data management plans are now a required component of many grant applications.
However, it is our view that good research data management simply makes for better research. Data is now commonly viewed as a first-class research output alongside the journal article, and well-managed data both facilitates and reflects well-conducted studies. Some specific benefits of research data management can be found below .
- Managing Your Data: Project Checklist (UC Davis) A checklist from UC Davis Library providing a useful outline of research data management activities throughout a project.
- Research Data Management Workbook An open resource by librarian Kristin Briney containing a collection of exercises intended to help researchers improve their research data management.
- Managing Data (QDR) A series of pages covering data management from the Qualitative Data Repository.
The Research Data Lifecycle
Researchers will be familiar with the "research lifecycle" - the broadly accepted outline of steps that constitute the research process, from devising the research questions and securing funding to disseminating the findings via publication. The research data lifecycle is closely related to the research lifecycle. Different models include or emphasize different steps, but they typically cover similar ground, including:
- Project planning
- Data collection and/or generation
- Data processing and analysis
- Data storage and security
- Preparing data for preservation
- Data sharing
The various activities of effective research data management play their part in different stages of the model; for instance, writing a data management plan and preparing a dataset for deposit into a data repository occur at different points in the research process. Other practices, such as writing and updating documentation and consistently following an organization scheme, extend across multiple stages.

( UVA Library Research Data Lifecycle model courtesy of Sherry Lake)
We introduce the research data lifecycle to highlight that research data management is a set of activities that span the entire research project, from inception to completion (and potentially beyond). We recommend taking a look at several research data lifecycle models, and while you are going through this guide, considering where each practice/activity is situated in the data lifecycle. We hope that this additional context helps research data management seem like a coherent process of caring for and attending to your data throughout a project rather than merely additional tasks to complete.
- Princeton Library Research Data Lifecycle Guide A page from Princeton Library's research data management guide that offers an alternative data lifecycle model, as well as motivations for RDM.
- University of Illinois Self-Guided Data Management Workshop This self-guided workshop, available in both Prezi and text/html formats, is a useful RDM resource organized around the research data lifecycle.
- DCC Curation Lifecycle Model A version of the data lifecycle model provided by the Digital Curation Centre with a curation-focused viewpoint.
- USGS Science Data Lifecycle Model A data lifecycle model by the United States Geological Survey. This model distinguishes between sequential steps and "cross-cutting" elements that span the entire lifecycle.
Benefits of Good Data Management
Some of the many benefits of practicing good research data management include:
- Compliance : As noted above, at least some aspects of research data management such as data sharing are increasingly required by funders and peer-reviewed journals. Other practices may be recommended or required by your institution.
- Credit : Collecting/generating and managing data is a part of the research process that is gaining more recognition as an undervalued part of scholarship. Shared data is now itself cited in the literature, along with increasing the visibility of the associated journal articles.
- Protection from loss : Data can be easily lost due to a multitude of factors, including natural disaster, hardware failure, software failure, obsolescence, human error, or simply neglect. Good practices around storage and backups can safeguard against losing critical files.
- Efficiency : Good data management practices around files, organization, and documentation result in greater efficiency for workflows and other research processes. Following good data management recommendations may also save time in the long run by making it easier to locate and understand data and files from earlier in the project.
- Transparency and reproducibility : Well-documented and planned research is both more transparent and more reproducible, qualities we should strive for as scholarly ideals that uphold research integrity. Reproducible research also helps eliminate wasteful duplicate data collection.
- Improved research and data quality : Effective research data management may reduce the chance of errors, mistakes, or other blemishes on your research caused by inattentive habits or practices around handling files, data, and code.
- Easier collaboration : Careful planning and consistent organization agreed on by all investigators helps smooth out the occasionally rocky experience of conducting collaborative and interdisciplinary studies.
- Enables preservation, sharing, and reuse : Preparing a dataset for preservation, sharing and reuse takes considerable effort, including data cleaning, file transformations, reorganization, writing documentation, and generating metadata. Following data management best practices throughout a study simplifies this process and makes your life easier when it comes time to deposit, helping to ensure your data is actually fit for long-term archival and has the potential for fruitful reuse down the line.
- << Previous: Research Data Management at UVA
- Next: Elements of a Data Management & Sharing Plan >>
- Last Updated: Oct 17, 2024 12:00 PM
- URL: https://guides.lib.virginia.edu/RDM
- 434-924-3021
- [email protected]
- UVA Shannon Library P.O. Box 400113 160 McCormick Road Charlottesville, VA 22904
About the Library
- Staff Directory
- Fellowships
- Library Use Policies
- Off-Grounds Access
- ITS Computing Accounts
- Accessibility Services
- Emergency Information
- UVA Privacy Policy
- Tracking Opt-out
Other Sites
- Cavalier Advantage
- Library Staff Site
Thank you for visiting nature.com. You are using a browser version with limited support for CSS. To obtain the best experience, we recommend you use a more up to date browser (or turn off compatibility mode in Internet Explorer). In the meantime, to ensure continued support, we are displaying the site without styles and JavaScript.
- View all journals
- Explore content
- About the journal
- Publish with us
- Sign up for alerts
- CAREER FEATURE
- 13 March 2018
Data management made simple
- Quirin Schiermeier
You can also search for this author in PubMed Google Scholar
When Marjorie Etique learnt that she had to create a data-management plan for her next research project, she was not sure exactly what to do.
Access options
Access Nature and 54 other Nature Portfolio journals
Get Nature+, our best-value online-access subscription
24,99 € / 30 days
cancel any time
Subscribe to this journal
Receive 51 print issues and online access
185,98 € per year
only 3,65 € per issue
Rent or buy this article
Prices vary by article type
Prices may be subject to local taxes which are calculated during checkout
Nature 555 , 403-405 (2018)
doi: https://doi.org/10.1038/d41586-018-03071-1
See Editorial: Everyone needs a data-management plan
Related Articles
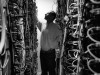
The FAIR Guiding Principles for scientific data management and stewardship
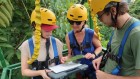
How to run a successful internship programme
Career Feature 23 OCT 24
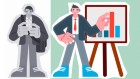
How job-seeking scientists should walk the line between high-calibre and humble
Career Feature 21 OCT 24
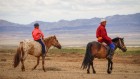
How I created a film festival to explore climate communication
Career Column 18 OCT 24
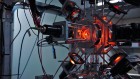
Consider the ethical impacts of quantum technologies in defence — before it’s too late
Comment 22 OCT 24
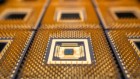
Fixing AI’s energy crisis
Outlook 17 OCT 24
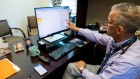
Can AI shake-up translational research?
Spotlight 16 OCT 24

Post-doctoral researcher (m/f/d) in (Marine) Microbial Natural Product Chemistry
GEOMAR Helmholtz Centre for Ocean Research Kiel, Wischhofstraße 1-3, 24148 Kiel, Germany
Helmholtz Centre for Ocean Research Kiel (GEOMAR)
Qiushi Chair Professor
Distinguished scholars with notable achievements and extensive international influence.
Hangzhou, Zhejiang, China
Zhejiang University
ZJU 100 Young Professor
Promising young scholars who can independently establish and develop a research direction.
Faculty Positions at the Center for Machine Learning Research (CMLR), Peking University
CMLR's goal is to advance machine learning-related research across a wide range of disciplines.
Beijing, China
Center for Machine Learning Research (CMLR), Peking University
Postdoctoral Research Fellows at Suzhou Institute of Systems Medicine (ISM)
ISM, based on this program, is implementing the reserve talent strategy with postdoctoral researchers.
Suzhou, Jiangsu, China
Suzhou Institute of Systems Medicine (ISM)
Sign up for the Nature Briefing newsletter — what matters in science, free to your inbox daily.
Quick links
- Explore articles by subject
- Guide to authors
- Editorial policies

Research Data Management
Research Data Management (RDM) is a term used to describe the management of research data throughout the entirety of a research project. This webpage offers tools, resources, and guidance to help researchers manage their data effectively and prepare it for sharing, and reuse.
Data Management Planning
Create a data management and storage plan.
- Data Management Plans are formalized documents outlining how research data will be collected, analyzed, stored and shared throughout a project.
- Generating a Data Management Plan at the start of a project or when entering a new lab can save time, funding, and effort in the long run, as it ensures research data is organized, findable, and shareable. Many funding agencies now require submission of a data management and/or sharing plan with grant applications.
- Harvard specific guidance provided in the DMPTool, a template for creating DMSPs offered through Harvard Library
Review data policies and procedures
- Research Data Ownership Policy
- Harvard Research Data Security Policy (HRDSP)
- Research Safety Application (Sensitive Research)
- Data Use Agreements (DUA): A binding contract governing access to nonpublic data, often required by external parties.
- Retention and Maintenance of Research Records and Data Frequently Asked Questions (“FAQs”) : General institutional policy stipulates that ‘essential research records’ need to be retained, for a period of no fewer than seven (7) years after the end of a research project or activity.
- Harvard University General Records Schedule
- Harvard NIH DMSP Budgeting and Application Instructions – Tip Sheet (09/21/2023)
- NIH Genomic Data Sharing
- NSF Data Management Plan Requirements and Data Sharing Policy
- GDPR Research Guidance
Develop standardized data organizational procedures
- Create a framework for naming files that describes what the files contain and how they relate to one other.
- File naming conventions can help with data organization and the identification of records, especially when working in a collaborative environment.
- Example: ExperimentName_InstrumentName_CaptureTime_ImageID.tif
- Folder structures should correspond to how the records are generated and how they function, while complementing proposed or existing workflows.
Assign roles and responsibilities within the lab, identifying data stewards
- PI Responsibilities on the Cluster: https://docs.rc.fas.harvard.edu/kb/pi-responsibilities-at-fas-rc/
- A lab Data Manager can also help to promote and support data management best practices, organize directory structures, establish file naming conventions, and identify data for retention, long term storage, or deletion.
Data Storage and Security
Review storage options.
- Identify where to store research data based on its behavior, performance, and means of access. A master copy of raw data should be retained, with further changes to subsequent versions well documented.
- FASSE Cluster : A secure cluster environment providing Harvard researchers with access to a secure enclave for analysis of sensitive datasets (level 3).
- Home Directories : Every user with a FASRC account when receiving cluster access will be granted a 100 GB home directory. Designed as a personal storage space for analysis, scripts, or documentation.
- Tier 0 : High performance storage with no backups, designed as a location for data analysis, as it has high read/write speeds.
- Tier 1 : Lab storage, ideal for general file sharing. The primary storage location for the lab, as it maintains backups. Best utilized for irrecoverable data like raw datasets.
- Tier 2 : Less active storage location for lab data. Not designed for high throughput jobs as it has lower read/write speeds.
- Data moved to Cold Storage must be in the proper format. File sizes should range from 100MB to 1 TB, and will be allocated in 20TB increments. If the data is not within these size ranges, the files will need to be tarred.
- Google Drive storage request form : This form can be used to request a new personal or shared Google Drive, or request additional storage space for an existing Google Drive. The default Google Shared Drive storage limit is 5 GB. Eligible users may request additional storage using the form. The requests will need to be approved by FAS, as they are currently responsible for the costs.
Connect to your new storage folder from a desktop computer
Request a new storage allocation or increase an existing storage allocation in coldfront.
- Coldfront is a resource allocation management system FASRC adapted to manage allocations on the FASRC cluster. The platform enables the viewing and management of lab groups (Projects) and storage or cluster allocations (Allocations).
View information about storage folders associated with a group/lab
- Utilize the Starfish Zones tool to view key information about your group or labs storage folders
- The Starfish Zone User Interface is a self-service visual tool that enables users to view group storage amounts and locations. Users can navigate folder structures to access detailed information about files and storage. Labs and groups are strongly recommended to utilize this tool to assist with their data organization and cleanup efforts.
Storage Service Center Overview and Pricing
- Service Center and Billing FAQ
Review and comply with data safety and security requirements
- Harvard University Information Security
- Cluster security levels
- Harvard Data Safety Website
- Data Safety System
Data Migrations
Transferring data between research platforms can be challenging. However, we’ve provided a list of possible tools that can assist with data transfers; the selection of the tool will depend on the size of dataset, the data security level, and who will need access to the dataset.
Data transfer tools:
- Transferring data on the cluster
- Using Globus to transfer data
- Rsync : A fast and versatile file-copying tool. Migrates only modified files from source to destination.
- Filezilla : An open-source client that is available cross platform (i.e. Mac, Windows, Linux)
- Rclone : A convenient and performant command-line tool for transferring files and synchronizing directories directly between FAS RC filesystems and Google Drive
Data Sharing
Researchers should make every effort to ensure research data is available to others for reuse when completing a project or study. It has become increasingly common, and often a requirement of a publisher or funder, that data be shared following the completion of a project.
There are many resources and tools available that researchers can use to share datasets, including government-sponsored repositories, disciplinary repositories, third-party repositories, and Harvard Dataverse , a free data repository open to all researchers at Harvard. A key aspect of data sharing means also making public the code and other materials that support the research. Data repositories are a centralized place to hold, share, and organize data in a logical manner.
Review data repository options and select one for each project and publication
- Global registry of research data repositories (re3data.org)
- Harvard Dataverse
- FASRC suggestions for sharing data
Additional resources
- SEAS Research Data Management : Support and consultation on Data Management Plans
- Longwood Research Data Management (RDM): Information and resources on NIH Data Management Plans
- DMPTool : Web-based platform to assist with the creation and sharing of Data Management Plans. DMPTool provides step-by-step guidance for drafting DMPs, including NIH-specific templates and samples to address specific requirements.
- Harvard Library Research Data Management Program : Connects members of the Harvard community to services and resources that span the research data lifecycle, to help ensure that Harvard’s multi-disciplinary research data is findable, accessible, interoperable, and reusable ( FAIR )
For additional support, please contact Sarah Marchese, Research Data Manager for FASRC: [email protected]
Research Data Management
What is research data management.
- Data Management Plans (DMP)
- Data Curation
- "Readme" Style Metadata
- Data Citation
- Tabular Data Guidelines
- Data Storage and Backup
- Finding a Data Repository
- Funder and Publisher Data Mandates
- Data Management Glossary
Research data management (RDM) is the care and maintenance of data produced during a research cycle. It includes how you:
- create and organize data
- process data
- analyze data
- preserve and archive data
- give access to data
Managing your research data well is one best ways to ensure standardization, reproducibility, and the ability to disseminate your research to others with minimal efforts and time.
Data Sharing and Management Snafu
This video (4:40 minutes) portrays a data management cautionary tale of what should not happen when a researcher makes a data sharing request. Topics included are: storage, documentation, and file formats.
- << Previous: Home
- Next: Data Management Plans (DMP) >>
- Last Updated: Aug 19, 2024 1:54 PM
- URL: https://researchguides.njit.edu/data-management

IMAGES
VIDEO
COMMENTS
Research Data Management is the process of providing the appropriate labeling, storage, and access for data at all stages of a research project.
This week introduces multiple types of research data in an array of contexts as well as important data management concepts including metadata and the research data lifecycle.
What is research data management? RDM is a set of practices designed to keep data accessible and secure. Organizations practicing effective RDM are those that are preserving all the key research output resulting from their research efforts, while archiving or disposing of content in line with their individual fields' requirements and guidelines.
Based on this introduction, we discuss different objectives of research data management, such as ensuring replicability or sharing of research data generated in a research project as well as sharing concepts on data harmonizing and merging in secondary analysis.
Research data management (RDM), a term that encompassess activities related to the storage, organization, documentation, and dissemination of data*1, is central to efforts aimed at maximizing the value of scientific investment (e.g. Holdren 2013) and addressing
Research Data Management (RDM) refers to a set of principles, processes, and best practices for handling research data both during and after the completion of a research project. The intention of this guide is to help researchers better understand and implement these principles and practices, including:
A data-management plan explains how researchers will handle their data during and after a project, and encompasses creating, sharing and preserving research data of any type, including...
By implementing foundational practices of data management, researchers set themselves up for success by formalizing processes and reducing common errors in data handling, which can free up more time for research. This paper provides an introduction to best practices for managing all types of data. Keywords.
Research Data Management (RDM) is a term used to describe the management of research data throughout the entirety of a research project. This webpage offers tools, resources, and guidance to help researchers manage their data effectively and prepare it for sharing, and reuse.
Research data management (RDM) is the care and maintenance of data produced during a research cycle. It includes how you: create and organize data. process data. analyze data. preserve and archive data. give access to data. reuse data.