
- Weill Cornell Medicine


Institute of Artificial Intelligence for Digital Health
Our mission is to advance individualized health and healthcare with data-driven insights derived from applying artificial intelligence technologies. The institute will establish a community of experts across multiple disciplines in computational science and clinical medicine, facilitate collaborations, promote multi-disciplinary research, and engage in entrepreneurship and technology commercialization.
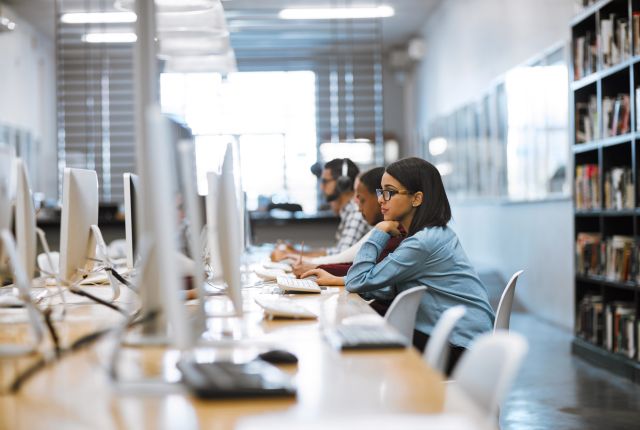
Institute of AI for Digital Health
402 E 67th St New York, NY 10065
.jpg)
Sowing the Future of Primary Care with the AI/ML Bootcamp at 2023 NAPCRG Annual Meeting Read More
.png)
Unleashing the power of AI in Primary Care Learn More

Toward Equitable Innovation in Health and Medicine with National Academies Press Read More

Stanford Medicine wins HIMSS Davies Award of Excellence Read More
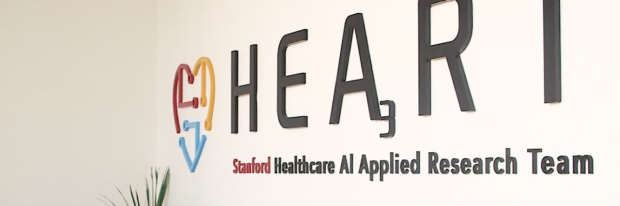
Code to Bedside: November 2023 Newsletter Read More

Our work covers a broad range of aims centered on the development and integration of artificial intelligence (AI) technologies that solve important, practical problems for patients, providers and health systems.
We work with clinical, operational, and technical teams to advance the development of clinically relevant models, leveraging quality improvement, implementation science, design thinking, and traditional research methods.
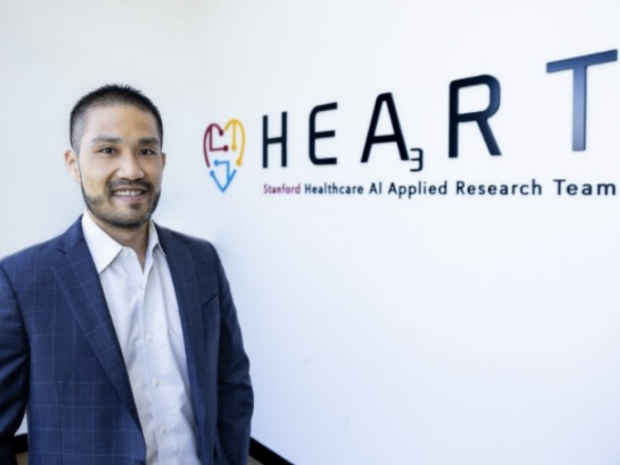
Director's Message
Read a message from HEA 3 RT's Executive Director Dr. Steven Lin and learn about the mission and vision of our program

Work With Us
Learn about ways to engage with HEA 3 RT, whether you're a prospective partner from industry, academia, or the non-profit world

Explore our projects studying the implementation of AI technologies in healthcare to support patients, providers, and health systems
Recent News & Publications
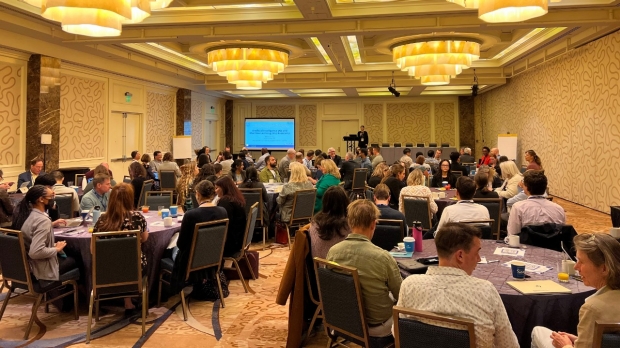
Sowing the Future of Primary Care with the AI/ML Bootcamp at NAPCRG
North American Primary Care Research Group (NAPCRG) 51st Annual Meeting, October 2023.
At this year's NAPCRG meeting, HEA3RT, supported by the generous sponsorship of the Gordon and Betty Moore Foundation and the American Board of Family Medicine Foundation, led a momentous event — a sold-out AI/ML Bootcamp that drew a diverse crowd of primary care researchers, clinicians, and healthcare professionals. Each attendee arrived with a shared objective: to delve into the vast potential that AI holds for primary care. For more insights, check out the E-participant packet from the workshop here .

HEA3RT featured in Stanford Medicine Annual Report
Stanford Medicine Annual Report, October 2023.
In the 2023 Stanford Medicine Annual Report, Dr. Steven Lin, HEA3RT's Executive Director, discusses the organization's threefold mission: accelerating the application of artificial intelligence and machine learning into the primary care space, supporting rigorous scientific AI implementation research, and addressing issues of diversity, equity, and inclusion in AI development. HEA3RT's goal is to serve as a bridge between data scientists and the front lines of health care. Read the full report here .
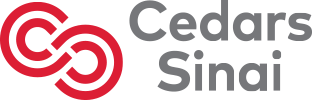
- Departments
- Anesthesiology
- Biomedical Sciences
- Cardiac Surgery
- Computational Biomedicine
- Neurosurgery
- Obstetrics & Gynecology
- Orthopaedics
- Pathology & Laboratory Medicine
- Physical Medicine & Rehabilitation
- Psychiatry & Behavioral Neurosciences
- Radiation Oncology
- Board of Governors Regenerative Medicine Institute
- F. Widjaja Inflammatory Bowel Disease Institute
- Samuel Oschin Comprehensive Cancer Institute
- Smidt Heart Institute
- Advanced Clinical Biosystems Research Institute
- Biomedical Imaging Research Institute
- Diabetes & Obesity Research Institute
- Geri & Richard Brawerman Nursing Institute
- Human Microbiome Research Institute
- Kao Autoimmunity Institute
- Maxine Dunitz Neurosurgical Institute
- Women's Guild Lung Institute
- Research Topics
- Laboratories
- Research Cores
- Clinical Trials
- Office of Research Administration
- Technology & Innovations
- Clinical & Translational Research Center
- News & Breakthroughs
- Graduate Medical Education
- Graduate School of Biomedical Sciences
- Continuing Medical Education
- Professional Training Programs
- Women's Guild Simulation Center
- Center for the Arts and Humanities in Medicine
- Medical Library
- Campus Life
- Office of the Dean
- Academic Calendar
- Back to PhD Program in Health Artificial Intelligence
Training & Curriculum
- Faculty & Administration
The curriculum of the PhD in Health AI emphasizes an active learning approach that will be used to teach six required courses, including AI, ethical AI, machine learning, natural language processing, clinical applications of AI and biomedical informatics. Students will gain healthcare experience through clinical rotations, clinical collaborations and access to clinical data from the electronic health record.
Cedars-Sinai 's PhD in Health AI is pending WSCUC accreditation.
Program Overview
This program will provide doctoral students with the knowledge and practical experience to develop, evaluate and apply cutting-edge AI algorithms and methods for improving patient care. We utilize a hands-on, active learning approach to teaching that reinforces AI concepts through short projects completed during class. Students will be exposed to hospital rotations to better understand how AI might be used in healthcare. Students will work with clinical collaborators and will have access to data from the electronic health record. Graduates of the program will be positioned to improve healthcare and patient outcomes through the rigorous development and deployment of AI algorithms and software.
- Computational Biomedicine: This course will provide a broad introduction to the field of computational biomedicine including the analysis, interpretation, and use of biomedical and clinical data for improving patient care.
- Artificial Intelligence: This course will cover foundational concepts in artificial intelligence, including history, logic, semantics, knowledge engineering, rule-based learning, probability, search and machine learning.
- Ethical Artificial Intelligence: This course will introduce and discuss the ethical issues associated with the application of artificial intelligence methods to clinical data and the deployment of artificial intelligence in the clinic for patient care.
- Machine Learning: This course will cover key concepts and methods in machine learning, including feature selection, feature engineering, model selection, prediction, evaluation and interpretation.
- Natural Language Processing: This course will introduce algorithms, methods, and software for the processing of text and language.
- Clinical Artificial Intelligence: This course will introduce and discuss the challenges and opportunities of using artificial intelligence for patient care with practical examples and use cases.
- Electives: Two electives will be selected by the student to provide a specialization or focus. Examples include Biostatistics, Computational Biology, and Image Analysis.
Clinical Rotations
All students are required to fulfill a minimum of 20 hours of clinical rotations across one or more specialties. During these rotations, students will shadow doctors during patient encounters and observe interactions, utilizing electronic health records and decision-support tools.
Research Rotations
All students will complete three rotations during the first year in candidate dissertation research labs. This process will culminate in identifying a willing research mentor to supervise a dissertation research project.
Dissertation Research
Students are expected to conduct a dissertation research project that generates new knowledge at the intersection of AI and healthcare. The project will facilitate collaboration between AI experts and clinicians, culminating in several peer-reviewed publications.
Have Questions or Need Help?
If you have questions or wish to learn more about the PhD program in Health AI, call us or send a message.
- Who’s Teaching What
- Subject Updates
- MEng program
- Opportunities
- Minor in Computer Science
- Resources for Current Students
- Program objectives and accreditation
- Graduate program requirements
- Admission process
- Degree programs
- Graduate research
- EECS Graduate Funding
- Resources for current students
- Student profiles
- Instructors
- DEI data and documents
- Recruitment and outreach
- Community and resources
- Get involved / self-education
- Rising Stars in EECS
- Graduate Application Assistance Program (GAAP)
- MIT Summer Research Program (MSRP)
- Sloan-MIT University Center for Exemplary Mentoring (UCEM)
- Electrical Engineering
- Computer Science
- Artificial Intelligence + Decision-making
- AI and Society
- AI for Healthcare and Life Sciences
- Artificial Intelligence and Machine Learning
- Biological and Medical Devices and Systems
- Communications Systems
- Computational Biology
- Computational Fabrication and Manufacturing
- Computer Architecture
- Educational Technology
- Electronic, Magnetic, Optical and Quantum Materials and Devices
- Graphics and Vision
- Human-Computer Interaction
- Information Science and Systems
- Integrated Circuits and Systems
- Nanoscale Materials, Devices, and Systems
- Natural Language and Speech Processing
- Optics + Photonics
- Optimization and Game Theory
- Programming Languages and Software Engineering
- Quantum Computing, Communication, and Sensing
- Security and Cryptography
- Signal Processing
- Systems and Networking
- Systems Theory, Control, and Autonomy
- Theory of Computation
- Departmental History
- Departmental Organization
- Visiting Committee
- Explore all research areas
Our goal is to develop AI technologies that will change the landscape of healthcare and the life sciences. This includes the whole span from the discovery of biological mechanisms to early disease diagnostics, drug discovery, care personalization and management. Building on MIT’s pioneering history in artificial intelligence and life sciences, we are working on algorithms suitable for modeling biological and clinical data across a range of modalities including imaging, text and genomics.
While achieving this goal, we strive to make new discoveries in machine learning, biology, chemistry and clinical sciences, and translate our discoveries into technologies that can improve people’s lives. While the Jameel Clinic focuses primarily on AI and Health, other research labs and centers affiliated with EECS have groups engaged in AI for healthcare and life sciences, including IMES , CSAIL , LIDS , and the Eric and Wendy Schmidt Center at the Broad Institute .
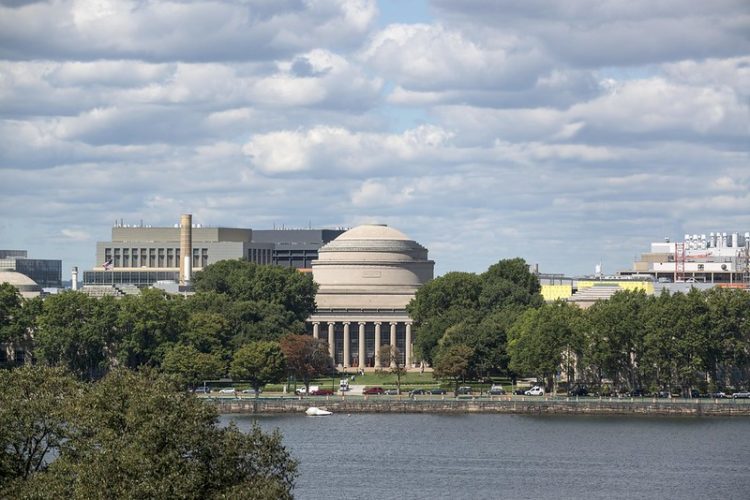
Latest news in AI for healthcare and life sciences
To build a better ai helper, start by modeling the irrational behavior of humans.
A new technique can be used to predict the actions of human or AI agents who behave suboptimally while working toward unknown goals.
Priya Donti named AI2050 Early Career Fellow
Assistant Professor Priya Donti has been named an AI2050 Early Career Fellow by Schmidt Sciences, a philanthropic initiative from Eric and Wendy Schmidt aimed at helping to solve hard problems in AI.
Using generative AI to improve software testing
MIT spinout DataCebo helps companies bolster their datasets by creating synthetic data that mimic the real thing.
Student Spotlight: Victory Yinka-Banjo
Victory Yinka-Banjo is a junior majoring in 6-7: Computer Science and Molecular Biology.
Department of EECS Announces 2024 Promotions
The Department of Electrical Engineering and Computer Science (EECS) is proud to announce multiple promotions.
Upcoming events
Doctoral thesis: from systemic to regional: personal health and medical monitoring systems that adapt to individual variance, doctoral thesis: learning to model atoms across scales, doctoral thesis: design of high-speed, high-specific-power motor drives for megawatt aircraft applications, doctoral thesis: a class of high-efficiency air-core power transformers with flux-guiding resonators, career inspiration series: rich miner.
Machine Learning - CMU
Phd program in machine learning.
Carnegie Mellon University's doctoral program in Machine Learning is designed to train students to become tomorrow's leaders through a combination of interdisciplinary coursework, hands-on applications, and cutting-edge research. Graduates of the Ph.D. program in Machine Learning will be uniquely positioned to pioneer new developments in the field, and to be leaders in both industry and academia.
Understanding the most effective ways of using the vast amounts of data that are now being stored is a significant challenge to society, and therefore to science and technology, as it seeks to obtain a return on the huge investment that is being made in computerization and data collection. Advances in the development of automated techniques for data analysis and decision making requires interdisciplinary work in areas such as machine learning algorithms and foundations, statistics, complexity theory, optimization, data mining, etc.
The Ph.D. Program in Machine Learning is for students who are interested in research in Machine Learning. For questions and concerns, please contact us .
The PhD program is a full-time in-person committment and is not offered on-line or part-time.
PhD Requirements
Requirements for the phd in machine learning.
- Completion of required courses , (6 Core Courses + 1 Elective)
- Mastery of proficiencies in Teaching and Presentation skills.
- Successful defense of a Ph.D. thesis.
Teaching Ph.D. students are required to serve as Teaching Assistants for two semesters in Machine Learning courses (10-xxx), beginning in their second year. This fulfills their Teaching Skills requirement.
Conference Presentation Skills During their second or third year, Ph.D. students must give a talk at least 30 minutes long, and invite members of the Speaking Skills committee to attend and evaluate it.
Research It is expected that all Ph.D. students engage in active research from their first semester. Moreover, advisor selection occurs in the first month of entering the Ph.D. program, with the option to change at a later time. Roughly half of a student's time should be allocated to research and lab work, and half to courses until these are completed.
Master of Science in Machine Learning Research - along the way to your PhD Degree.
Other Requirements In addition, students must follow all university policies and procedures .
Rules for the MLD PhD Thesis Committee (applicable to all ML PhDs): The committee should be assembled by the student and their advisor, and approved by the PhD Program Director(s). It must include:
- At least one MLD Core Faculty member
- At least one additional MLD Core or Affiliated Faculty member
- At least one External Member, usually meaning external to CMU
- A total of at least four members, including the advisor who is the committee chair
Financial Support
Application Information
For applicants applying in Fall 2023 for a start date of August 2024 in the Machine Learning PhD program, GRE Scores are REQUIRED. The committee uses GRE scores to gauge quantitative skills, and to a lesser extent, also verbal skills.
Proof of English Language Proficiency If you will be studying on an F-1 or J-1 visa, and English is not a native language for you (native language…meaning spoken at home and from birth), we are required to formally evaluate your English proficiency. We require applicants who will be studying on an F-1 or J-1 visa, and for whom English is not a native language, to demonstrate English proficiency via one of these standardized tests: TOEFL (preferred), IELTS, or Duolingo. We discourage the use of the "TOEFL ITP Plus for China," since speaking is not scored. We do not issue waivers for non-native speakers of English. In particular, we do not issue waivers based on previous study at a U.S. high school, college, or university. We also do not issue waivers based on previous study at an English-language high school, college, or university outside of the United States. No amount of educational experience in English, regardless of which country it occurred in, will result in a test waiver.
Submit valid, recent scores: If as described above you are required to submit proof of English proficiency, your TOEFL, IELTS or Duolingo test scores will be considered valid as follows: If you have not received a bachelor’s degree in the U.S., you will need to submit an English proficiency score no older than two years. (scores from exams taken before Sept. 1, 2021, will not be accepted.) If you are currently working on or have received a bachelor's and/or a master's degree in the U.S., you may submit an expired test score up to five years old. (scores from exams taken before Sept. 1, 2018, will not be accepted.)
Graduate Online Application
- Early Application Deadline – November 29, 2023 (3:00 p.m. EST)
- Final Application Deadline - December 13, 2023 (3:00 p.m. EST)
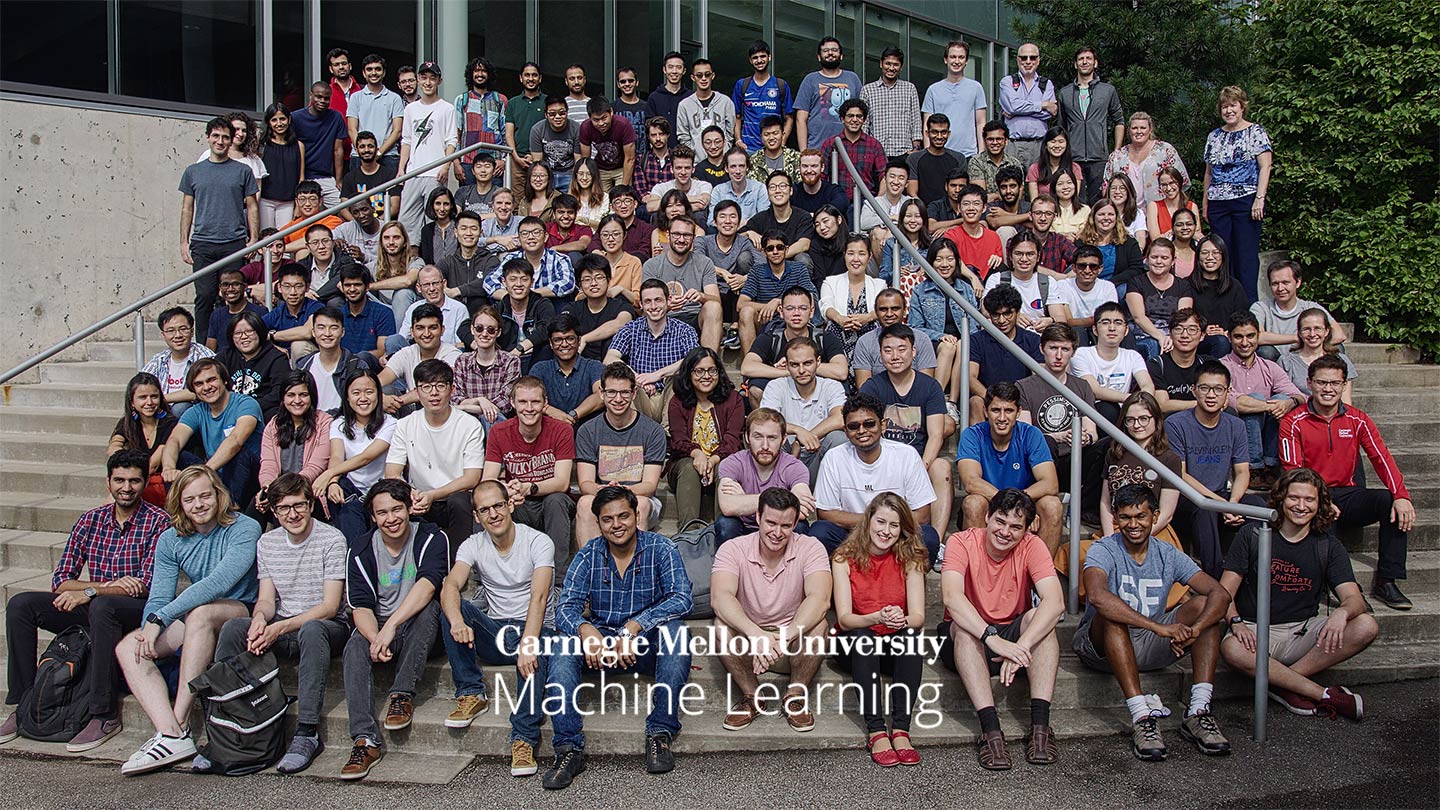
AI for Health Care: Concepts and Applications
Related programs, implementing health care ai into clinical practice, innovation with ai in health care, related articles, how health care leaders can build effective, bias free ai, to meet future needs, health care leaders must look at the data (science), the implementation gap: using artificial intelligence to help patients in clinical practices.
Interested in this course? Subscribe for all the latest updates.
This program is not currently available for registration. Click subscribe to be notified when information becomes available.
All information is subject to change.
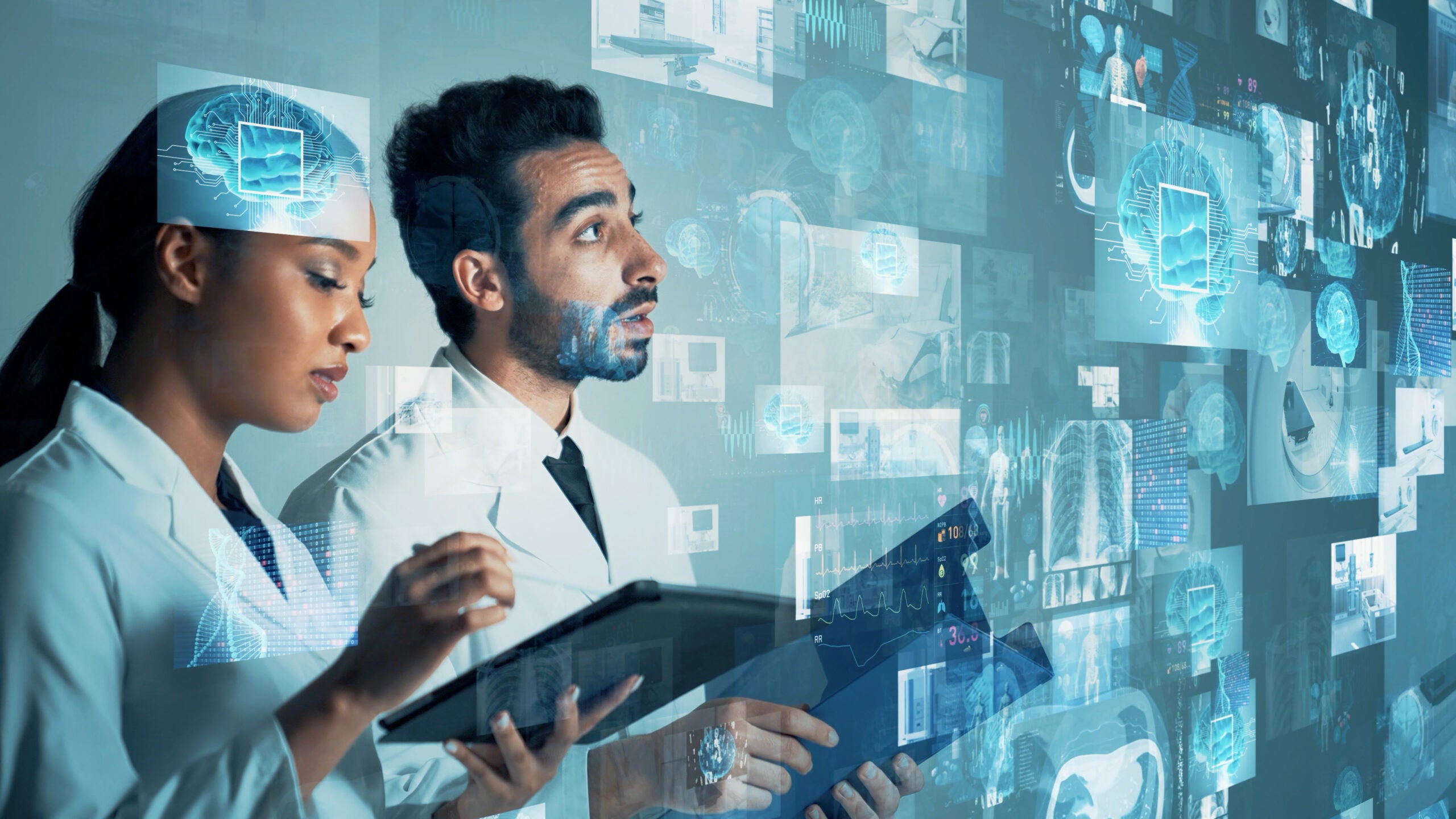
- Objectives & Highlights
Credits and Logistics
Who should participate.
Large Language Models (LLMs) and Generative Artificial Intelligence (AI) have captured the public imagination and have potential to drive significant change in healthcare. This course, AI for Health Care: Concepts and Applications , aims to unveil the core principles of AI, the capabilities of Large Language Models and Generative AI, and their profound implications for health care.
Under the tutelage of distinguished Harvard faculty, AI for Health Care: Concepts and Applications offers a conduit to transition from traditional health care paradigms to a more data-driven and AI-augmented approach. Adopting a “zero-to-AI” strategy, this course is crafted to equip health care professionals with foundational concepts, fine-tuned for health care applications. The curriculum navigates real-world health care dynamics, exploring AI’s potential to transform the doctor-patient relationship, and establishing a foundation for ethical AI deployment within healthcare.
Take the Next Step in Your Career Evolution with the Business Applications for AI in Health Care Certificate of Specialization
AI for Health Care: Concepts and Applications contributes to the Business Applications for AI in Health Care Certificate of Specialization , designed for all health care stakeholders, from the doctor’s office to the business suite. To obtain your Certificate , choose two of three online programs created and taught by Harvard Faculty and pioneers of AI in health care to prepare you for the future of an ever-changing health care industry.
For more information, visit our Business Applications for AI in Health Care Certificate of Specialization page .
Immerse yourself in a stimulating learning environment encompassing group discussions, active learning strategies, case studies, and master classes that probe into the genesis of AI, tackle implementation challenges, evaluate viable business models for AI in health care, and forecast the field’s evolution over the next five years. The program further cultivates a conducive networking atmosphere, promoting enduring collaboration among participants, which will act as a robust resource post-program.
Tackling AI Challenges in Health Care:
- Diagnosis AI’s prowess in harnessing large multimodal reservoirs of health data accelerates accurate diagnoses, thereby reducing misdiagnosis rates and easing clinician workload.
- Precision Medicine With AI as an ally, precision medicine transitions from generic treatment models to a more patient-centric approach, managing extensive data sets to formulate personalized treatment plans, thus enhancing patient care and resource allocation.
- Prediction Models Leveraging prediction models, clinicians can perform comparative analyses aiding in precise prognostics, which are instrumental in creating patient-specific care plans, mitigating risks, and optimizing resource utilization.To fully harness the potential of these formidable technologies and avert the potential harms, it’s pivotal for practitioners to be well-versed and proficient in the work that needs to be done before and after algorithm development. A proactive approach towards mitigating issues like algorithmic bias is crucial to ensure AI acts as a benefactor to the communities it serves. This course accentuates these dimensions, offering a public health lens to AI, and empowers students with the insight to catalyze meaningful transformations in patient care and organizational efficiency.
Objectives & Highlights
Learning objectives .
- Reinforce the first principles of AI in health care from the previous course or other related courses.
- Discuss how large language models can and have been applied in health care
- Detail and implement prompt engineering and tuning and optimizing large language models
- Explain how AI can be used to solve clinical problems
- Describe the process of successfully implementing AI projects in large health care organizations
- Identify future challenges and opportunities in generative AI
Introduction to AI: Definitions and Terminology
- Introduction to AI
- How AI is transforming society and daily life
- How healthcare needs to benefit from AI too
Foundational Concepts and Current State of the Art
- Key Concepts in AI
- What is possible today using AI
- What will be possible in the near future
- Further Foundation Concepts in AI
- How AI works in practice
Masterclass: Emily Melton, AI in Clinical Medicine
- How AI can revolutionize clinical medicine
Digital Health and AI
- How AI is supercharging digital health
- How to build robust AI-enabled digital health systems
AI in the Life Sciences
- How biopharma are being impacted by AI
- How to build, lead, and develop data science teams
Masterclass: Tich Changamire, How to Create Artificial Intelligence
- The key ingredients and high level recipes required to create artificial intelligence
- Common pitfalls and considerations
When AI Goes Wrong: Algorithmic Bias
- Types of Bias
- How algorithms can perpetuate or exacerbate existing biases in datasets and society
- The need to build bias-mitigating or bias-free systems
Collaborative and Open Data Science
- The difficulty of generalizing AI models
- The importance of collaboration and open datasets to build robust AI
Masterclass: Javier Tordable, AI and Strategy
- AI from the perspective of the C-suite
- How AI enabled businesses have the edge
Business Models for Healthcare AI
- How the innovations in AI and subtleties of healthcare combine to produce unique business models for healthcare AI
- Practical exercises to think through some of the novel business models for healthcare AI
Masterclass: Lisa Maki, AI Ethics
- Ethical ramifications of AI specific to healthcare
- Difficulties and uncertainties
AI and Global Health Systems
- How health systems across the world differ in culture, funding, scope and delivery
- How these differences lead to different opportunities for AI
- The importance and difficulties of regulating AI in healthcare
- How the traditional regulatory models don’t work, and novel work being done in this area
Continuing Education Credit
All participants will receive a Certificate of Participation upon completion of the program.
This online program is designed for senior managers and executives who are responsible for developing and implementing AI strategy in their organizations and are looking to understand AI, its current state of the art, and future.
Participants will come from a range of organizational functions including health care delivery, health care technology, primary care systems, payers, and governments. Some titles represented in the program will include:
- Chief Executive Officer
- Chief Information Officer
- Chief Innovation Officer
- Chief Medical Informatics Officer
- Chief Medical Officer
- Clinician
- Data Scientist
- Innovation Specialist
- Finance Professional
- Product Manager
- Project Manager
- Venture Capital Investor

Centre for Doctoral Training in AI-enabled Healthcare
Partnerships
- How to apply and funding
- News & Success Stories
- Publications

UKRI UCL Centre for Doctoral Training in AI-enabled Healthcare Systems
The UKRI Centre for Doctoral Training (CDT) in AI-enabled Healthcare Systems combines UCL’s excellence both in AI and computational science, and in biomedical research. We offer a unique programme consisting of a 1 year MRes followed by a 3 year PhD embedded within an NHS setting. Applications now open.

How to apply
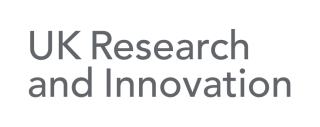
UCL is home to cutting-edge research not only in artificial intelligence in healthcare, but also the whole spectrum of AI innovation from education, law and social science to engineering, computer science and biology. By joining our CDT, you will have the opportunity to work with inspirational world-leading academics to transform healthcare systems using AI for patient benefit. Professor Geraint Rees, CDT Director and UCL Pro-Vice-Provost (AI)
Keep up to date with all matters AI related. On the form please select: PhD as the level of study and Health Informatics as the subject of interest.
Register your interest
Antonia Coote, CDT Manager
Follow @cdt_ai_health
UKRI Centre for Doctoral Training in AI for Healthcare

Duke AI Health
Duke ethical and equitable data science.
Developing, evaluating and implementing artificial intelligence for health at Duke and beyond

QUALITY | DATA | EVALUATION | EDUCATION | RESEARCH
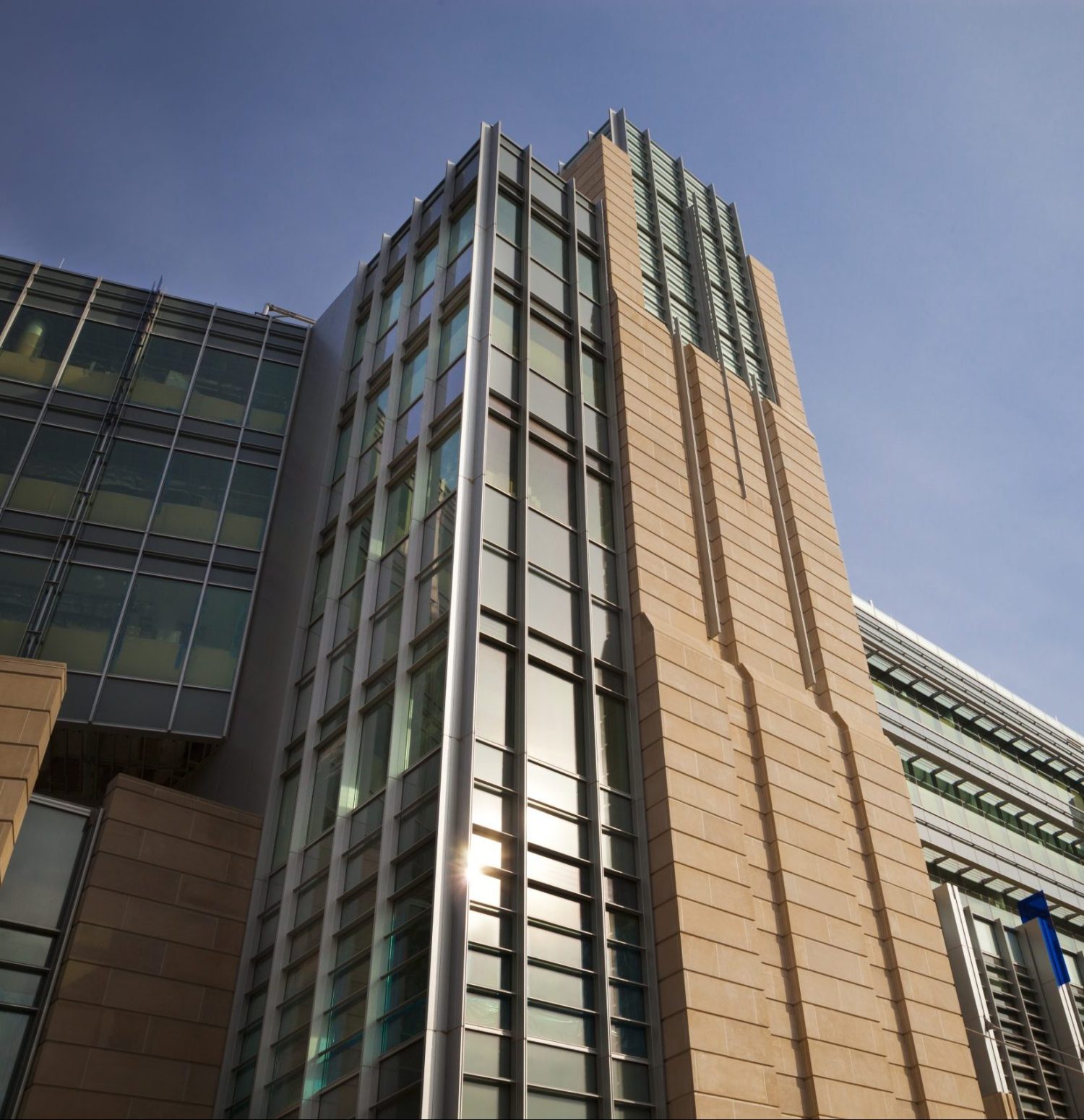
Instilling Ubiquitous Data Science Excellence Across Duke
Duke AI Health connects, strengthens, amplifies, and grows multiple streams of theoretical and applied research on artificial intelligence and machine learning at the University in order to answer the most urgent and difficult challenges in medicine and population health.
Designed as a multidisciplinary, campus-spanning initiative, AI Health harnesses expertise and insights across multiple schools, centers, and institutes at Duke to bring to bear the power of machine learning and related quantitative fields on medicine, healthcare delivery, and the health of individuals and communities.
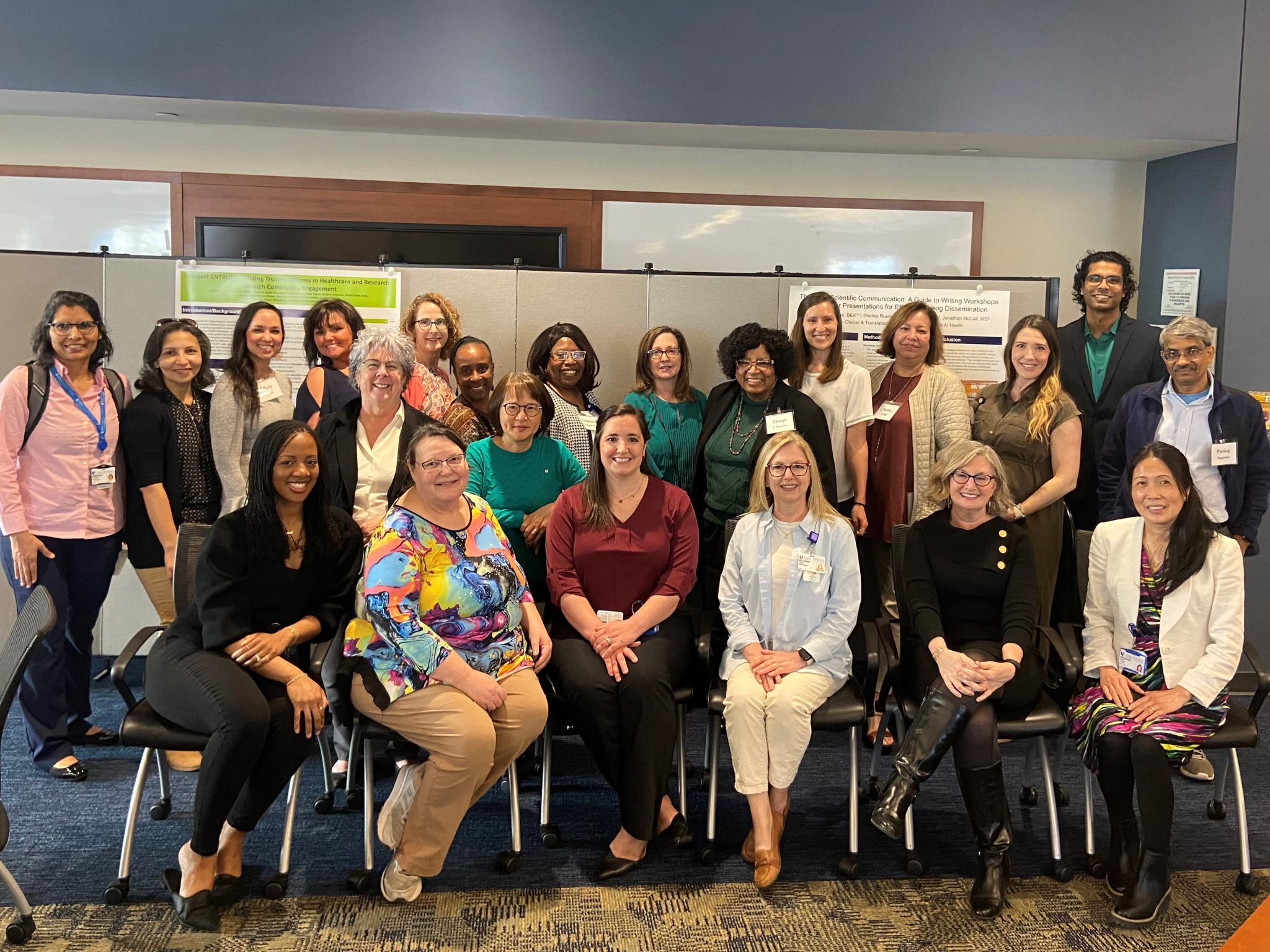

Scientific Writing Workshop Series Empowers Staff

Registration now open for the March 13-14 Duke Symposium on Fostering AI/ML Research for Health Equity and Learning Transformation (FAIR HEALTH™)
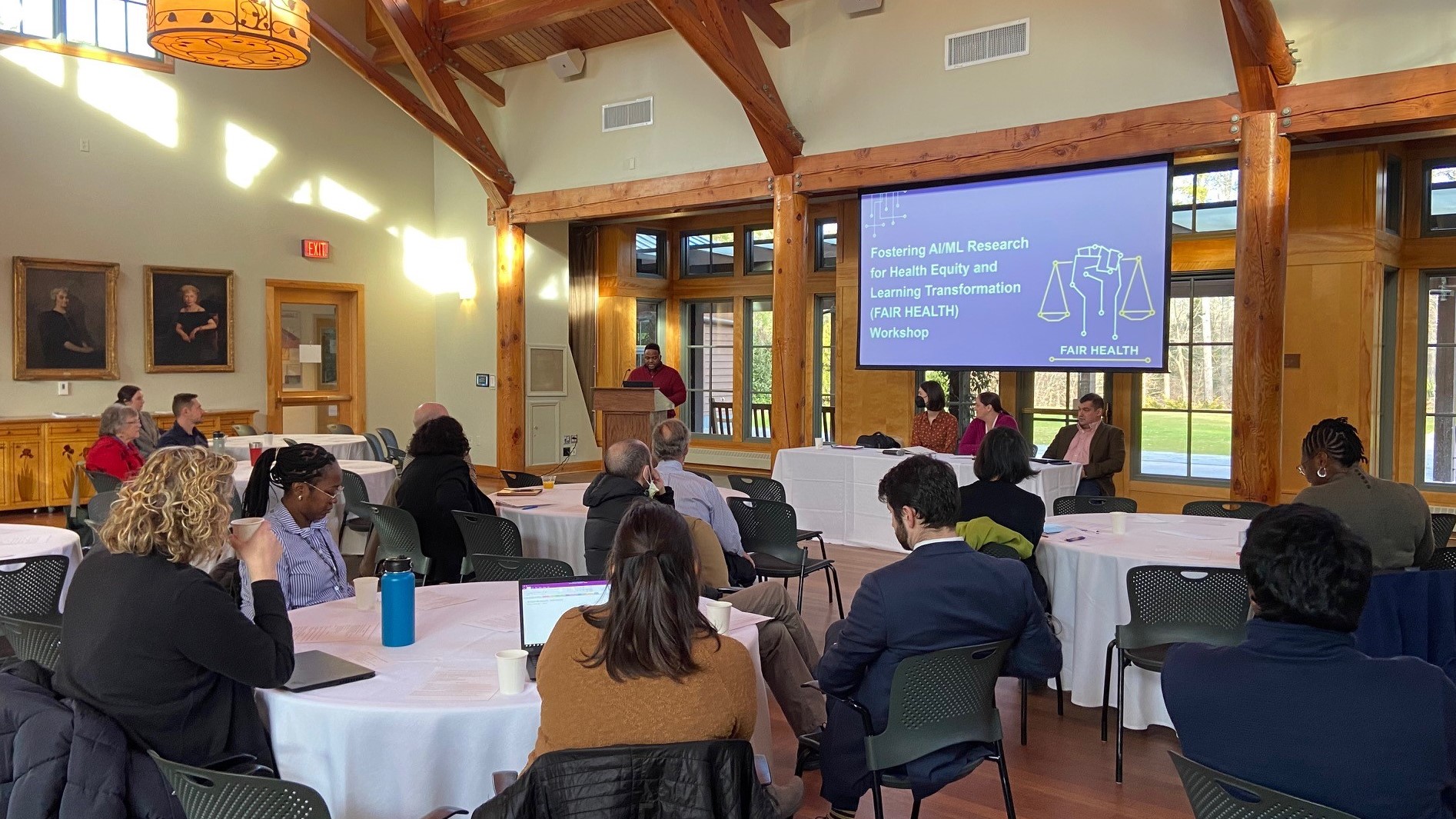
Advancing Healthcare Equity through AI/ML Innovation: Duke hosts FAIR HEALTH Workshop on Algorithmic Bias in Healthcare
Ai health leadership.

Michael Pencina

Shelley Rusincovitch
Managing Director

Ben Goldstein
Director of Data Science

Richard Shannon
Senior Advisory Board
Skip to Content
Healthcare research & technology advancements
Our team of clinicians, researchers, and engineers are all working together to create new AI and discover opportunities to increase the availability and accuracy of healthcare technologies globally, to realize long-term health technology potential.
Meet Med-PaLM 2, our large language model designed for the medical domain
Developing AI that can answer medical questions accurately has been a challenge for several decades. With Med-PaLM 2 , a version of PaLM 2 fine-tuned for the medical domain, we showed state-of-the-art performance in answering medical licensing exam questions. With thorough human evaluation, we’re exploring how Med-PaLM 2 can help healthcare organizations by drafting responses, summarizing documents, and providing insights. Learn more .
Expanding the power of AI in medicine
We are building and testing AI models with the goal of helping alleviate the global shortages of physicians, as well as the low access to modern imaging and diagnostic tools in certain parts of the world. With improved tech, we hope to increase accessibility and help more patients receive timely and accurate diagnoses and care.
How DeepVariant is improving the accuracy of genomic analysis
Sequencing genomes enables us to identify variants in a person’s DNA that indicate genetic disorders such as an elevated risk for breast cancer. DeepVariant is an open-source variant caller that uses a deep neural network to call genetic variants from next-generation DNA sequencing data.
Healthcare research led by scientists, enhanced by Google
Google Health is providing secure technology to partners that helps doctors, nurses, and other healthcare professionals conduct research and help improve our understanding of health. If you are a researcher interested in working with Google Health to conduct health research, enter your details to be notified when Google Health is available for research partnerships.
Using AI to give doctors a 48-hour head start on life-threatening illness
In this research in Nature , we demonstrated how artificial intelligence could accurately predict acute kidney injuries (AKI) in patients up to 48 hours earlier than it is currently diagnosed. Notoriously difficult to spot, AKI affects up to one in five hospitalized patients in the US and UK, and deterioration can happen quickly. Read the article
Deep Learning
Protecting patients, deep learning for electronic health records.
In a paper published in npj Digital Medicine , we used deep learning models to make a broad set of predictions relevant to hospitalized patients using de-identified electronic health records, and showed how that model could be used to render an accurate prediction 24 hours after a patient was admitted to the hospital. Read the article
Protecting patients from medication errors
Research shows that 2% of hospitalized patients experience serious preventable medication-related incidents that can be life-threatening, cause permanent harm, or result in death. Published in Clinical Pharmacology and Therapeutics , our best-performing AI model was able to anticipate physician’s actual prescribing decisions 75% of the time, based on de-identified electronic health records and the doctor’s prescribing records. This is an early step towards testing the hypothesis that machine learning can support clinicians in ways that prevent mistakes and help to keep patients safe. Read the article
Discover the latest
Learn more about our most recent developments from Google’s health-related research and initiatives.
Detecting Signs of Disease from External Images of the Eye
Detecting abnormal chest x-rays using deep learning, improving genomic discovery with machine learning, how ai is advancing science and medicine.
Google researchers have been exploring ways technologies could help advance the fields of medicine and science, working with scientists, doctors, and others in the field. In this video, we share a few research projects that have big potential.
We are continuously publishing new research in health
- Crimson Careers
- For Employers
- Harvard College
- Harvard Kenneth C. Griffin Graduate School of Arts & Sciences
- Harvard Extension School
- Premed / Pre-Health
- Families & Supporters
- Faculty & Staff
- Prospective Students
- First Generation / Low Income
- International Students
- Students of Color
- Students with Disabilities
- Undocumented Students
- Explore Interests & Make Career Decisions
- Create a Resume/CV or Cover Letter
- Expand Your Network
- Engage with Employers
- Search for a Job
- Find an Internship
- January Experiences (College)
- Find & Apply for Summer Opportunities Funding
- Prepare for an Interview
- Negotiate an Offer
- Apply to Graduate or Professional School
- Access Resources
- AI for Professional Development and Exploration
- Arts & Entertainment
- Business & Entrepreneurship
- Climate, Sustainability, Environment, Energy
- Government, Int’l Relations, Education, Law, Nonprofits
- Life Sciences & Health
- Technology & Engineering
- Still Exploring
- Talk to an Advisor
UnitedHealth Group
Optum ai phd intern – remote.
- Share This: Share Optum AI PhD Intern – Remote on Facebook Share Optum AI PhD Intern – Remote on LinkedIn Share Optum AI PhD Intern – Remote on X
Perform research which advances the state of the art in AI and ML Implement algorithms and evaluate their performance on healthcare data Communicate results across an interdisciplinary team and explore use cases which improve real world healthcare outcomes Document research internally and externally, including publications in machine learning and other scientific conferences and journals
PhD student in Health Data Science
Job information, offer description.
The department of Medical Informatics is looking for a PhD student to work on cutting-edge health AI and data science topics. This research will be performed in close collaboration with the Observational Health Data Sciences and Informatics (OHDSI) initiative, which is a global, multi-stakeholder, interdisciplinary collaborative to bring out the value of health data through large-scale analytics ( www.ohdsi.org ) which develop frameworks to generate reliable real-world evidence.
As a PhD student, you will be responsible for the research on using federated data networks to improve best practices around the development and validation of prediction models. You will lead and contribute to projects conducting methodological research within the field of machine learning in healthcare. Research will be performed in clinical settings, feature engineering methods, deep-learning, and other advanced machine-learning methods to support personalized medicine. The research will focus on using large-scale federated data networks made possible by the standardization of health data to the OMOP Common Data Model. Impact assessments of these new approaches on patient care are part of the research agenda.
Requirements
- We encourage applications from candidates with a background in Natural Sciences, Informatics, Economics, Sociology and Data Science.
- Interest in data mining, deep-learning, machine learning, regression analysis and programming is required.
- We invite candidates who are working in the regulatory domain or are interested in applying health data science in their clinical setting, to propose research topics. These candidates preferably have a medical background.
- The candidates must be highly motivated, willing to learn and demonstrate initiative in assigned tasks.
- Good written and verbal communication skills are desired for this international project.
Additional Information
- You will receive a temporary position for 4 years.The gross monthly salary is € 3.017 ,- in the 1st year and increases to € 3.824,- in the 4th year (scale OIO).
- Excellent fringe benefits, such as a 13th month that is already paid out in November and a individual travel expense package.
- Pension insurance with ABP. We take care of approximately 70% of the monthly contribution.
- Special benefits, such as a incompany physiotherapist and bicycle repairer. And there is also a gym where you can work on your fitness after work.
For more information about this position, please contact Ross D. Williams, project lead, via +31 (0)10 704 30 50 or e-mail [email protected] . See also www.healthdatascience.nl .
If you are excited by the thought of this position and would like to apply, please do so by using the application form on our website.
Work Location(s)
Where to apply.
Mobile Menu Overlay
The White House 1600 Pennsylvania Ave NW Washington, DC 20500
Biden- Harris Administration Announces Key AI Actions 180 Days Following President Biden’s Landmark Executive Order
Six months ago, President Biden issued a landmark Executive Order to ensure that America leads the way in seizing the promise and managing the risks of artificial intelligence (AI). Since then, agencies all across government have taken vital steps to manage AI’s safety and security risks, protect Americans’ privacy, advance equity and civil rights, stand up for consumers and workers, promote innovation and competition, advance American leadership around the world, and more. Today, federal agencies reported that they completed all of the 180-day actions in the E.O. on schedule, following their recent successes completing each 90-day, 120-day, and 150-day action on time. Agencies also progressed on other work tasked by the E.O. over longer timeframes. Actions that agencies reported today as complete include the following: Managing Risks to Safety and Security: Over 180 days, the Executive Order directed agencies to address a broad range of AI’s safety and security risks, including risks related to dangerous biological materials, critical infrastructure, and software vulnerabilities. To mitigate these and other threats to safety, agencies have:
- Established a framework for nucleic acid synthesis screening to help prevent the misuse of AI for engineering dangerous biological materials. This work complements in-depth study by the Department of Homeland Security (DHS), Department of Energy (DOE) and Office of Science and Technology Policy on AI’s potential to be misused for this purpose, as well as a DHS report that recommended mitigations for the misuse of AI to exacerbate chemical and biological threats. In parallel, the Department of Commerce has worked to engage the private sector to develop technical guidance to facilitate implementation. Starting 180 days after the framework is announced, agencies will require that grantees obtain synthetic nucleic acids from vendors that screen.
- Released for public comment draft documents on managing generative AI risks, securely developing generative AI systems and dual-use foundation models, expanding international standards development in AI, and reducing the risks posed by AI-generated content. When finalized, these documents by the National Institute of Standards and Technology (NIST) will provide additional guidance that builds on NIST’s AI Risk Management Framework, which offered individuals, organizations, and society a framework to manage AI risks and has been widely adopted both in the U.S. and globally.
- Developed the first AI safety and security guidelines for critical infrastructure owners and operators. These guidelines are informed by the completed work of nine agencies to assess AI risks across all sixteen critical infrastructure sectors.
- Launched the AI Safety and Security Board to advise the Secretary of Homeland Security, the critical infrastructure community, other private sector stakeholders, and the broader public on the safe and secure development and deployment of AI technology in our nation’s critical infrastructure. The Board’s 22 inaugural members include representatives from a range of sectors, including software and hardware company executives, critical infrastructure operators, public officials, the civil rights community, and academia.
- Piloted new AI tools for identifying vulnerabilities in vital government software systems. The Department of Defense (DoD) made progress on a pilot for AI that can find and address vulnerabilities in software used for national security and military purposes. Complementary to DoD’s efforts, DHS piloted different tools to identify and close vulnerabilities in other critical government software systems that Americans rely on every hour of every day.
Standing up for Workers, Consumers, and Civil Rights The Executive Order directed bold steps to mitigate other risks from AI—including risks to workers, to consumers, and to Americans’ civil rights—and ensure that AI’s development and deployment benefits all Americans. Today, agencies reported that they have:
- Developed bedrock principles and practices for employers and developers to build and deploy AI safely and in ways that empower workers. Agencies all across government are now starting work to establish these practices as requirements, where appropriate and authorized by law, for employers that receive federal funding.
- Released guidance to assist federal contractors and employers comply with worker protection laws as they deploy AI in the workplace. The Department of Labor (DOL) developed a guide for federal contractors and subcontractors to answer questions and share promising practices to clarify federal contractors’ legal obligations, promote equal employment opportunity, and mitigate the potentially harmful impacts of AI in employment decisions. DOL also provided guidance regarding the application of the Fair Labor Standards Act and other federal labor standards as employers increasingly use of AI and other automated technologies in the workplace.
- Released resources for job seekers, workers, and tech vendors and creators on how AI use could violate employment discrimination laws. The Equal Employment Opportunity Commission’s resources clarify that existing laws apply the use of AI and other new technologies in employment just as they apply to other employment practices.
- Issued guidance on AI’s nondiscriminatory use in the housing sector. In two guidance documents, the Department of Housing and Urban Development affirmed that existing prohibitions against discrimination apply to AI’s use for tenant screening and advertisement of housing opportunities, and it explained how deployers of AI tools can comply with these obligations.
- Published guidance and principles that set guardrails for the responsible and equitable use of AI in administering public benefits programs. The Department of Agriculture’s guidance explains how State, local, Tribal, and territorial governments should manage risks for uses of AI and automated systems in benefits programs such as SNAP. The Department of Health and Human Services (HHS) released a plan with guidelines on similar topics for benefits programs it oversees. Both agencies’ documents prescribe actions that align with the Office of Management and Budget’s policies, published last month, for federal agencies to manage risks in their own use of AI and harness AI’s benefits.
- Announced a final rule clarifying that nondiscrimination requirements in health programs and activities continue to apply to the use of AI, clinical algorithms, predictive analytics, and other tools. Specifically, the rule applies the nondiscrimination principles under Section 1557 of the Affordable Care Act to the use of patient care decision support tools in clinical care, and it requires those covered by the rule to take steps to identify and mitigate discrimination when they use AI and other forms of decision support tools for care.
- Developed a strategy for ensuring the safety and effectiveness of AI deployed in the health care sector. The strategy outlines rigorous frameworks for AI testing and evaluation, and it outlines future actions for HHS to promote responsible AI development and deployment.
Harnessing AI for Good President Biden’s Executive Order also directed work to seize AI’s enormous promise, including by advancing AI’s use for scientific research, deepening collaboration with the private sector, and piloting uses of AI. Over the past 180 days, agencies have done the following:
- Announced DOE funding opportunities to support the application of AI for science , including energy-efficient AI algorithms and hardware.
- Prepared convenings for the next several months with utilities, clean energy developers, data center owners and operators, and regulators in localities experiencing large load growth. Today, DOE announced new actions to assess the potential energy opportunities and challenges of AI, accelerate deployment of clean energy, and advance AI innovation to manage the growing energy demand of AI.
- Launched pilots, partnerships, and new AI tools to address energy challenges and advance clean energy. For example, DOE is piloting AI tools to streamline permitting processes and improving siting for clean energy infrastructure, and it has developed other powerful AI tools with applications at the intersection of energy, science, and security. Today, DOE also published a report outlining opportunities AI brings to advance the clean energy economy and modernize the electric grid.
- Initiated a sustained effort to analyze the potential risks that deployment of AI may pose to the grid. DOE has started the process of convening energy stakeholders and technical experts over the coming months to collaboratively assess potential risks to the grid, as well as ways in which AI could potentially strengthen grid resilience and our ability to respond to threats—building off a new public assessment .
- Authored a report on AI’s role in advancing scientific research to help tackle major societal challenges, written by the President’s Council of Advisors on Science and Technology.
Bringing AI Talent into Government The AI and Tech Talent Task Force has made substantial progress on hiring through the AI Talent Surge. Since President Biden signed the E.O., federal agencies have hired over 150 AI and AI-enabling professionals and, along with the tech talent programs, are on track to hire hundreds by Summer 2024. Individuals hired thus far are already working on critical AI missions, such as informing efforts to use AI for permitting, advising on AI investments across the federal government, and writing policy for the use of AI in government.
- The General Services Administration has onboarded a new cohort of Presidential Innovation Fellows (PIF) and also announced their first-ever PIF AI cohort starting this summer.
- DHS has launched the DHS AI Corps , which will hire 50 AI professionals to build safe, responsible, and trustworthy AI to improve service delivery and homeland security.
- The Office of Personnel Management has issued guidance on skills-based hiring to increase access to federal AI roles for individuals with non-traditional academic backgrounds.
- For more on the AI Talent Surge’s progress, read its report to the President . To explore opportunities, visit https://ai.gov/apply The table below summarizes many of the activities that federal agencies have completed in response to the Executive Order.
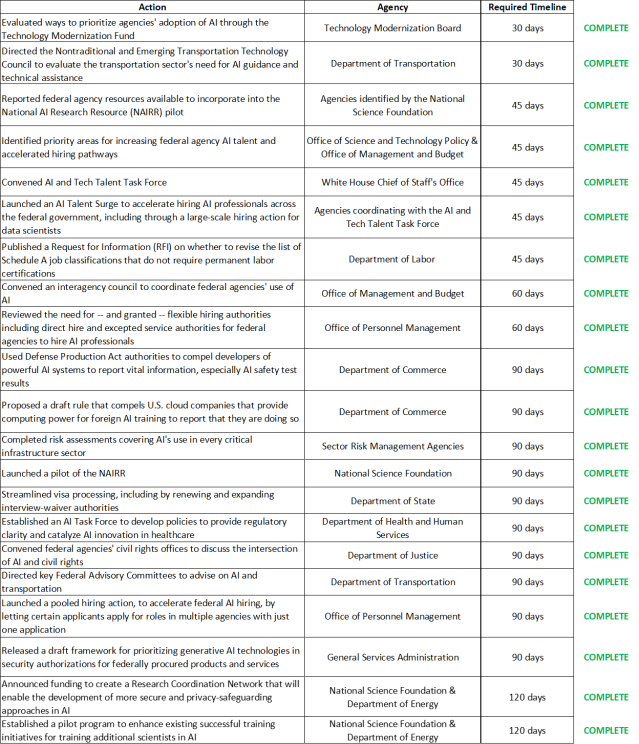
Stay Connected
We'll be in touch with the latest information on how President Biden and his administration are working for the American people, as well as ways you can get involved and help our country build back better.
Opt in to send and receive text messages from President Biden.
- Penn Engineering Online Degrees
- Penn Engineering Online Dual Dual Degree
- Online Graduate Certificates
- Take a Course
- On-Demand Learning
- MSE-AI Online
- MSE-AI Academics
- Request Info
Next-Level Opportunities With AI
Help shape the future of a revolutionary technology. Our online Master of Science in Engineering (MSE) in Artificial Intelligence (AI) degree program gives you the technical foundation you need to lead the development and implementation of AI systems — along with the broad perspective to deploy new tools in an ethical and responsible way.
Taught by some of the world’s leading experts in artificial intelligence, the program combines Ivy League excellence with the convenience of an entirely online curriculum. No matter where in the world you live, you’ll learn at your own pace and on your schedule.
The MSE-AI program explores the mathematical, computational and algorithmic foundation for AI and develops strong competencies in machine learning, statistical modeling and optimization. Students will be equipped with the latest knowledge on the data center infrastructures that are powering the AI revolution, namely distributed systems, networking and GPU programming. At the same time, gain understanding of the ethical implications of AI and prepare to mitigate its risks.
“AI is one of the most important areas in technology today. It’s generating an incredible amount of enthusiasm and a massive amount of investment. With this program, our goal is to educate a new generation of engineers who have the skills to analyze trends as they emerge — not only from the technological perspective, but also from the societal and ethical perspective. ”
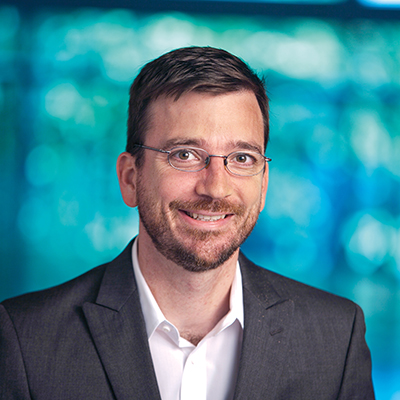
Chris Callison-Burch
- Professor, Computer and Information Science Department
- Program Director, MSE-AI Online
“We’ve specifically designed our curriculum to provide both cutting-edge technical skills and an understanding of the impact this technology will have on people’s lives. Students will not only learn about the algorithms and models underpinning AI applications, they will also be equipped with the latest knowledge on the data center infrastructures that are powering the AI revolution, namely distributed systems, networking and GPU programming”
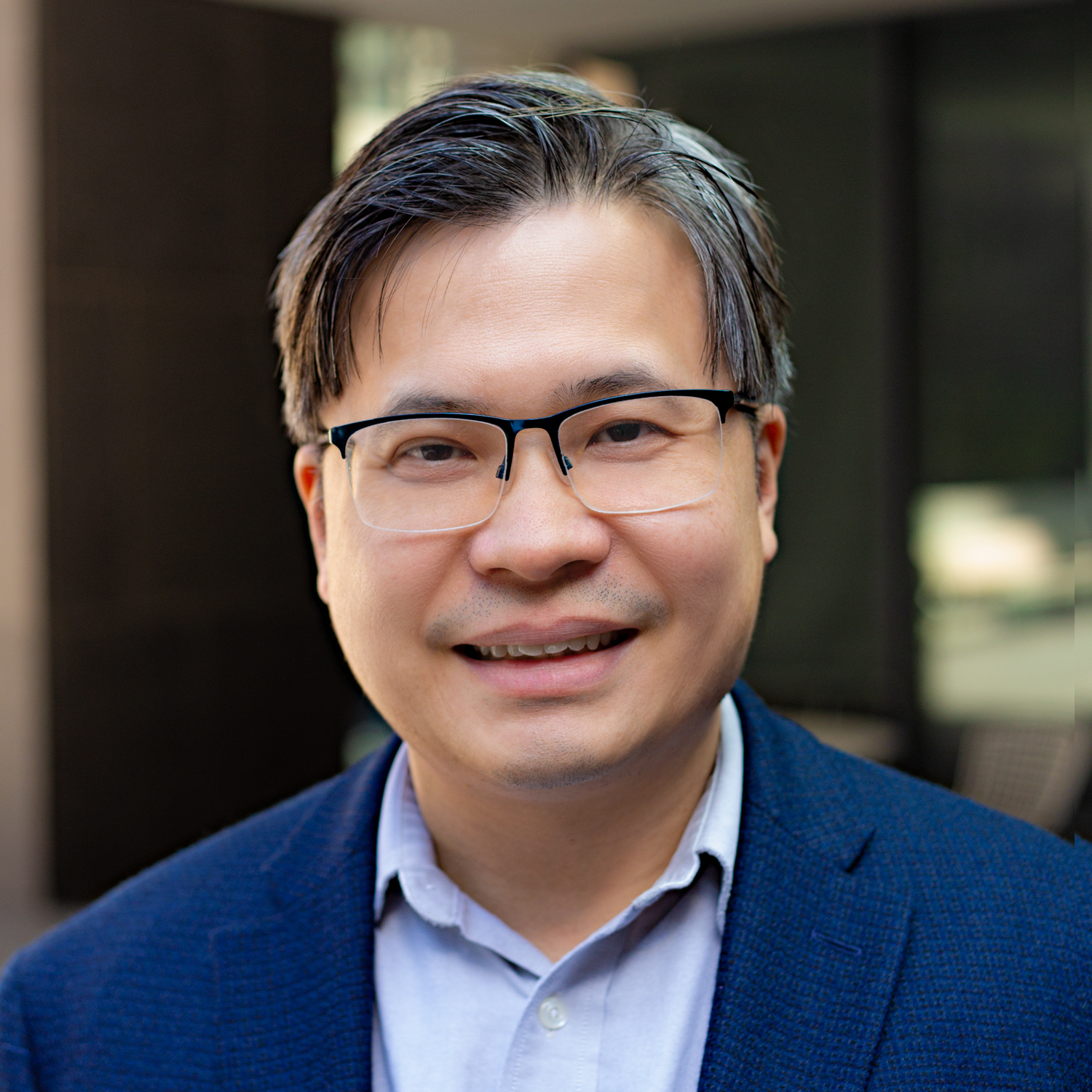
Boon Thau Loo
- RCA Professor, Computer and Information Science Department
- Associate Dean for Graduate Programs
- Director, Distributed Systems Laboratory
MSE-AI Online Academic Journey
Mse-ai online course catalog.
Explore our extensive course catalog, providing a comprehensive overview of available courses, including detailed descriptions and prerequisites.
MSE-AI Online Sample Degree Plan
Students benefit from the support of a dedicated Student Success Advising Team, available to assist them in crafting tailored degree pathways and navigating various options for degree completion.
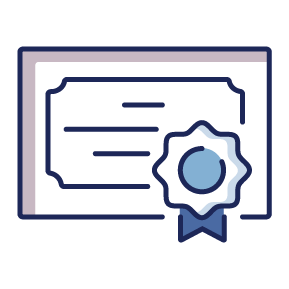
Expanding Your Academic Journey
Mse-ai online + online graduate certificate.
Gain expertise in crucial computer science areas and expand your career horizons with our certificates in Data Science and Software Systems.
Eligibility – Graduates of MSE-AI Online.
Academic Plan – If you graduated in the last five (5) years, you may transfer in two courses for your Certificate. Certificates consist of four courses from the certificate plan.
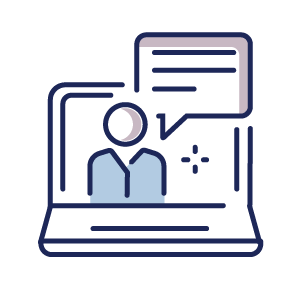
MSE-AI Online + Take a Course
Discover newly launched elective courses that delve into the latest advancements in technology. Embrace a shift in your learning focus to explore emerging areas, expanding your horizons to unveil a myriad of career possibilities.
Eligibility – Graduates of MSE-AI Online
Academic Plan – Join Penn Engineering Online for a single semester to boost your knowledge and discover new areas.
Try Before You Apply
Not sure if MSE-AI Online is for you? Try an open-enrollment specialization to find out if this interests you.
Artificial Intelligence and Machine Learning Essentials with Python Specialization
- The Graduate School >
- Graduate News >
AI smartphones may help monitor chronic wounds from home
research news

Approximately 8.2 million Americans suffer from chronic wounds that stem from a multitude of conditions.
By LAURIE KAISER
Published May 1, 2024

Individuals increasingly can check on their health conditions at home. Devices that measure blood pressure, glucose and blood oxygen levels, for example, enable patients’ providers to make decisions for their care.
In the near future, patients may also be able to monitor their chronic wounds from the comfort of their homes through mobile artificial intelligence (AI) technology being developed at UB.
Wenyao Xu, professor and associate chair of the Department of Computer Science and Engineering, is leading a team that recently was awarded $3 million from the National Institutes of Health for the four-year research project, “Mobile Health Technologies for Assessing Blood Perfusion in Chronic Wounds.”
Approximately 8.2 million Americans suffer from chronic wounds that stem from a multitude of conditions, including diabetes and major surgery. This technology — accessible through a smartphone — will help streamline treatment, Xu says.
“Patients with these conditions frequently visit their health care providers or specialty wound care clinics and, in and some cases, need to be hospitalized,” Xu explains. “This can be a financial burden and a time burden to both the patient and the health care system.”
Empowering patients, regardless of income, location
While the new technology will benefit all patients, it will particularly help individuals who have barriers to transportation or who live in rural areas where wound care services are limited or unavailable, he says.
“The approach proposed in this project is innovative,” he says, “because it will explore and validate new mobile computing and data-driven techniques for wound care and blood perfusion assessment based on evidence-based truth.”
The goal is to empower patients by giving them free, usable technology they can download that will provide exact measurements on how the wound is healing — and keep them out of the hospital.
“Chronic wounds often result from poor blood flow to the affected area, which can lead to a lack of oxygen and nutrients necessary for the healing process,” Xu says. “The long-term objective of this project is to improve wound care and impact wound treatment services by developing low-cost technologies that aid with chronic wound treatment and care.”
Other UB researchers on the project are Jun Xia, professor in the Department of Biomedical Engineering; Linda M. Harris, professor of surgery and chief of UB’s Vascular Surgery Division; Saptarshi Chakraborty, assistant professor in the Department of Biostatistics; Praveen Arany, associate professor in the Department of Oral Biology; and Heamchand Subryan, director of interaction design at the Center for Inclusive Design and Environmental Access (IDEA Center). They are also working with a research team at NEC Laboratories America, based in Princeton, N.J.
“We are engaging with an industry partner early on to pave the way for mass production of this technology,” Xu notes.
Combining AI, mobile optics
The research began about five years ago, stemming from a conversation Xu had with Xia on how to advance AI and mobile optics together — mobile AI — to improve health care, Xu explains.
“We then connected with Dr. Harris, a professor of surgery at UB who has a specialty in vascular surgery,” Xu says. “This is an underexplored area for advanced mobile AI technology.”
Currently, they are collaborating with vascular clinics in the UBMD Physicians’ Group, where the team recruits patients in the technology evaluation and clinical study.
“The AI technology analyzes the mobile images and predicts if the wound is on the right track for healing,” Xu says. “If it’s not, the patients can make an appointment to see the doctor.”
First stage: fine-tuning technology
The work with the clinics is the first in a two-stage process and will focus on fine-tuning the prototype and determining what needs to be improved over the next two years. It will involve more than 200 participants in Western New York.
“We will build upon this work to enhance a commodity smartphone with a low-cost multispectral imaging sensor. An app will be available to download on users’ phones to obtain perfusion-related measures,” Xu says. “We are developing machine learning algorithms that extract the biomarkers of healing and rigorously assess the tools for usability.”
In early 2026, the team will implement the second stage: a participatory design, feedback and usability study that will include patients and human-computer interaction experts to assess and improve the technology’s usability.
The hope is that eventually anyone who is suffering from a chronic wound can access this technology, Xu says.
“This AI technology has the potential to advance the current standard of wound care for recovery after discharge from clinical therapies,” he says. “These contributions are significant because they can extend the health self-management of our society through proactive health care and real-time intervention and reduce subjective bias and financial burden for patients, their caregivers and society.”

IMAGES
VIDEO
COMMENTS
The Artificial Intelligence in Medicine (AIM) PhD track, newly developed by the Department of Biomedical Informatics (DBMI) at Harvard Medical School, will enable future academic, clinical, industry, and government leaders to rapidly transform patient care, improve health equity and outcomes, and accelerate precision medicine by creating new AI technologies that reason across massive-scale ...
If you have questions or wish to learn more about the PhD program in Health AI, call us or send a message. 310‐423‐3391. SEND A MESSAGE. The PhD program in Health Artificial Intelligence at Cedars-Sinai prepares students with rigorous training in AI algorithms and methods to improve patient care.
AIET will also work in close conjunction with BMEII, led by Founding Director Zahi A. Fayad, PhD.BMEII will serve as a catalyst for creating novel medical inventions in the fields of imaging, nanomedicine, artificial intelligence, and computer vision technologies, such as virtual reality, augmented reality, and extended reality.
Artificial intelligence (AI) has transformed industries around the world, and has the potential to radically alter the field of healthcare. Imagine being able to analyze data on patient visits to the clinic, medications prescribed, lab tests, and procedures performed, as well as data outside the health system -- such as social media, purchases made using credit cards, census records, Internet ...
Artificial Intelligence (AI) has the potential to transform health and healthcare systems globally, yet few individuals have the required skills and training. To address this challenge, our Centre For Doctoral Training (CDT) in AI-Enabled Healthcare Systems will create a unique interdisciplinary environment to train the brightest and best healthcare artificial intelligence
Artificial Intelligence in Medicine. Scroll. Artificial Intelligence in Medicine Program. An academic program designed to accelerate AI solutions into clinic practice. Our latest Updates and News. Featured. publication. 2024. LLMs for social determinants of health.
PhD Program Toggle PhD Program menu options. Program Faculty; ... Institute of Artificial Intelligence for Digital Health. Our mission is to advance individualized health and healthcare with data-driven insights derived from applying artificial intelligence technologies. The institute will establish a community of experts across multiple ...
Code to Bedside: November 2023 Newsletter. Our work covers a broad range of aims centered on the development and integration of artificial intelligence (AI) technologies that solve important, practical problems for patients, providers and health systems. We work with clinical, operational, and technical teams to advance the development of ...
Artificial intelligence (AI) and a variety of other powerful technologies are paving the way for a new era of biomedical research, offering unparalleled opportunities to improve human health. The Artificial Intelligence and Emerging Technologies in Medicine multidisciplinary training area of the PhD in Biomedical Sciences program offers ...
Training & Curriculum. The curriculum of the PhD in Health AI emphasizes an active learning approach that will be used to teach six required courses, including AI, ethical AI, machine learning, natural language processing, clinical applications of AI and biomedical informatics. Students will gain healthcare experience through clinical rotations ...
Our goal is to develop AI technologies that will change the landscape of healthcare and the life sciences. This includes the whole span from the discovery of biological mechanisms to early disease diagnostics, drug discovery, care personalization and management. Building on MIT's pioneering history in artificial intelligence and life sciences ...
These days, engineers, computer scientists, and healthcare professionals are excited about the potential of artificial intelligence to improve patient outcomes. Nigam Shah, MBBS, PhD, Professor of Medicine, (Biomedical Informatics) at Stanford and Associate CIO for Data Science at Stanford Healthcare, shares this excitement but brings a unique ...
The Machine Learning (ML) Ph.D. program is a fully-funded doctoral program in machine learning (ML), designed to train students to become tomorrow's leaders through a combination of interdisciplinary coursework, and cutting-edge research. Graduates of the Ph.D. program in machine learning are uniquely positioned to pioneer new developments in the field, and to be leaders in both industry and ...
Under the tutelage of distinguished Harvard faculty, AI for Health Care: Concepts and Applications offers a conduit to transition from traditional health care paradigms to a more data-driven and AI-augmented approach. Adopting a "zero-to-AI" strategy, this course is crafted to equip health care professionals with foundational concepts, fine ...
T he University has announced 50 fully-funded PhD researcher places to unlock the potential of Artificial Intelligence (AI) in medical diagnosis and care.. A new centre for doctoral training (CDT) will be created, with a focus on the early detection, diagnosis, treatment and care of cancers. It follows newly created government funding from UK Research and Innovation, to aid in training the ...
The UKRI Centre for Doctoral Training (CDT) in AI-enabled Healthcare Systems combines UCL's excellence both in AI and computational science, and in biomedical research. We offer a unique programme consisting of a 1 year MRes followed by a 3 year PhD embedded within an NHS setting. Applications now open.
UKRI Centre for Doctoral Training in AI for Healthcare. Imperial College London.
The institute focuses on research and education in data science, machine learning, and artificial intelligence across diverse fields, including neuroscience, precision medicine, climate resilience, sustainability, public-sector innovation, and social sciences. About Us. Collaborate to develop data science and AI, and accelerate breakthroughs ...
Designed as a multidisciplinary, campus-spanning initiative, AI Health harnesses expertise and insights across multiple schools, centers, and institutes at Duke to bring to bear the power of machine learning and related quantitative fields on medicine, healthcare delivery, and the health of individuals and communities. ...
Research shows that 2% of hospitalized patients experience serious preventable medication-related incidents that can be life-threatening, cause permanent harm, or result in death. Published in Clinical Pharmacology and Therapeutics, our best-performing AI model was able to anticipate physician's actual prescribing decisions 75% of the time ...
Offered by Stanford University. Artificial intelligence (AI) has transformed industries around the world, and has the potential to radically alter the field of healthcare. Imagine being able to analyze data on patient visits to the clinic, medications prescribed, lab tests, and procedures performed, as well as data outside the health system -- such as social media, purchases made using credit ...
Artificial intelligence (AI) will likely have an impact on virtually every sector of the economy, but one of its greatest benefits will come from deploying it in healthcare. While some researchers have anticipated these benefits for years, there is an emerging body of scientific evidence that these capabilities may soon be ready to move from ...
Optum, part of the UnitedHealth Group family of companies, is a leading health services and innovation company dedicated to helping make the health system work better for everyone. With more than 94,000 people collaborating worldwide, Optum combines people, technology, data and action to improve the delivery, quality and efficiency of health care.
University of Sheffield Department of Computer Science. Artificial intelligence (AI) has permeated various aspects of modern life, significantly influencing the healthcare sector. Fuelled by this momentum, remote patient monitoring facilitated by mobile sensing technology is on the way to changing how patients are monitored and treated. Read ...
The precision of radiologists improved from 65.9 to 81.9% and recall improved from 17.5 to 71.75 when assistance with AI was provided. AI showed 92% accuracy in classifying "normal" CXR into COVID or non-COVID. Analysis of attention maps revealed attention on the cardiac shadow in these "normal" radiographs.
Perform research which advances the state of the art in AI and ML Implement algorithms and evaluate their performance on healthcare data Communicate results across an interdisciplinary team and explore use cases which improve real world healthcare outcomes Document research internally and externally, including publications in machine learning and other scientific conferences and journals
The department of Medical Informatics is looking for a PhD student to work on cutting-edge health AI and data science topics. This research will be performed in close collaboration with the Observational Health Data Sciences and Informatics (OHDSI) initiative, which is a global, multi-stakeholder, interdisciplinary collaborative to bring out the value of health data through large-scale ...
Developed a strategy for ensuring the safety and effectiveness of AI deployed in the health care sector. The strategy outlines rigorous frameworks for AI testing and evaluation, and it outlines ...
MSE-AI Online + Online Graduate Certificate. Gain expertise in crucial computer science areas and expand your career horizons with our certificates in Data Science and Software Systems. Eligibility - Graduates of MSE-AI Online. Academic Plan - If you graduated in the last five (5) years, you may transfer in two courses for your Certificate ...
The research began about five years ago, stemming from a conversation Xu had with Xia on how to advance AI and mobile optics together — mobile AI — to improve health care, Xu explains. "We then connected with Dr. Harris, a professor of surgery at UB who has a specialty in vascular surgery," Xu says.