Click through the PLOS taxonomy to find articles in your field.
For more information about PLOS Subject Areas, click here .
Loading metrics
Open Access
Peer-reviewed
Research Article

Recent quantitative research on determinants of health in high income countries: A scoping review
Roles Conceptualization, Data curation, Formal analysis, Investigation, Methodology, Project administration, Software, Visualization, Writing – original draft, Writing – review & editing
* E-mail: [email protected]
Affiliation Centre for Health Economics Research and Modelling Infectious Diseases, Vaccine and Infectious Disease Institute, University of Antwerp, Antwerp, Belgium

Roles Conceptualization, Data curation, Funding acquisition, Project administration, Resources, Supervision, Validation, Visualization, Writing – review & editing
- Vladimira Varbanova,
- Philippe Beutels
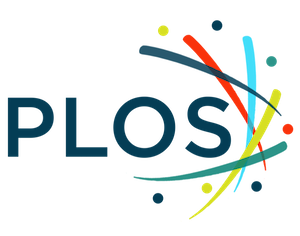
- Published: September 17, 2020
- https://doi.org/10.1371/journal.pone.0239031
- Peer Review
- Reader Comments
Identifying determinants of health and understanding their role in health production constitutes an important research theme. We aimed to document the state of recent multi-country research on this theme in the literature.
We followed the PRISMA-ScR guidelines to systematically identify, triage and review literature (January 2013—July 2019). We searched for studies that performed cross-national statistical analyses aiming to evaluate the impact of one or more aggregate level determinants on one or more general population health outcomes in high-income countries. To assess in which combinations and to what extent individual (or thematically linked) determinants had been studied together, we performed multidimensional scaling and cluster analysis.
Sixty studies were selected, out of an original yield of 3686. Life-expectancy and overall mortality were the most widely used population health indicators, while determinants came from the areas of healthcare, culture, politics, socio-economics, environment, labor, fertility, demographics, life-style, and psychology. The family of regression models was the predominant statistical approach. Results from our multidimensional scaling showed that a relatively tight core of determinants have received much attention, as main covariates of interest or controls, whereas the majority of other determinants were studied in very limited contexts. We consider findings from these studies regarding the importance of any given health determinant inconclusive at present. Across a multitude of model specifications, different country samples, and varying time periods, effects fluctuated between statistically significant and not significant, and between beneficial and detrimental to health.
Conclusions
We conclude that efforts to understand the underlying mechanisms of population health are far from settled, and the present state of research on the topic leaves much to be desired. It is essential that future research considers multiple factors simultaneously and takes advantage of more sophisticated methodology with regards to quantifying health as well as analyzing determinants’ influence.
Citation: Varbanova V, Beutels P (2020) Recent quantitative research on determinants of health in high income countries: A scoping review. PLoS ONE 15(9): e0239031. https://doi.org/10.1371/journal.pone.0239031
Editor: Amir Radfar, University of Central Florida, UNITED STATES
Received: November 14, 2019; Accepted: August 28, 2020; Published: September 17, 2020
Copyright: © 2020 Varbanova, Beutels. This is an open access article distributed under the terms of the Creative Commons Attribution License , which permits unrestricted use, distribution, and reproduction in any medium, provided the original author and source are credited.
Data Availability: All relevant data are within the manuscript and its Supporting Information files.
Funding: This study (and VV) is funded by the Research Foundation Flanders ( https://www.fwo.be/en/ ), FWO project number G0D5917N, award obtained by PB. The funder had no role in study design, data collection and analysis, decision to publish, or preparation of the manuscript.
Competing interests: The authors have declared that no competing interests exist.
Introduction
Identifying the key drivers of population health is a core subject in public health and health economics research. Between-country comparative research on the topic is challenging. In order to be relevant for policy, it requires disentangling different interrelated drivers of “good health”, each having different degrees of importance in different contexts.
“Good health”–physical and psychological, subjective and objective–can be defined and measured using a variety of approaches, depending on which aspect of health is the focus. A major distinction can be made between health measurements at the individual level or some aggregate level, such as a neighborhood, a region or a country. In view of this, a great diversity of specific research topics exists on the drivers of what constitutes individual or aggregate “good health”, including those focusing on health inequalities, the gender gap in longevity, and regional mortality and longevity differences.
The current scoping review focuses on determinants of population health. Stated as such, this topic is quite broad. Indeed, we are interested in the very general question of what methods have been used to make the most of increasingly available region or country-specific databases to understand the drivers of population health through inter-country comparisons. Existing reviews indicate that researchers thus far tend to adopt a narrower focus. Usually, attention is given to only one health outcome at a time, with further geographical and/or population [ 1 , 2 ] restrictions. In some cases, the impact of one or more interventions is at the core of the review [ 3 – 7 ], while in others it is the relationship between health and just one particular predictor, e.g., income inequality, access to healthcare, government mechanisms [ 8 – 13 ]. Some relatively recent reviews on the subject of social determinants of health [ 4 – 6 , 14 – 17 ] have considered a number of indicators potentially influencing health as opposed to a single one. One review defines “social determinants” as “the social, economic, and political conditions that influence the health of individuals and populations” [ 17 ] while another refers even more broadly to “the factors apart from medical care” [ 15 ].
In the present work, we aimed to be more inclusive, setting no limitations on the nature of possible health correlates, as well as making use of a multitude of commonly accepted measures of general population health. The goal of this scoping review was to document the state of the art in the recent published literature on determinants of population health, with a particular focus on the types of determinants selected and the methodology used. In doing so, we also report the main characteristics of the results these studies found. The materials collected in this review are intended to inform our (and potentially other researchers’) future analyses on this topic. Since the production of health is subject to the law of diminishing marginal returns, we focused our review on those studies that included countries where a high standard of wealth has been achieved for some time, i.e., high-income countries belonging to the Organisation for Economic Co-operation and Development (OECD) or Europe. Adding similar reviews for other country income groups is of limited interest to the research we plan to do in this area.
In view of its focus on data and methods, rather than results, a formal protocol was not registered prior to undertaking this review, but the procedure followed the guidelines of the PRISMA statement for scoping reviews [ 18 ].
We focused on multi-country studies investigating the potential associations between any aggregate level (region/city/country) determinant and general measures of population health (e.g., life expectancy, mortality rate).
Within the query itself, we listed well-established population health indicators as well as the six world regions, as defined by the World Health Organization (WHO). We searched only in the publications’ titles in order to keep the number of hits manageable, and the ratio of broadly relevant abstracts over all abstracts in the order of magnitude of 10% (based on a series of time-focused trial runs). The search strategy was developed iteratively between the two authors and is presented in S1 Appendix . The search was performed by VV in PubMed and Web of Science on the 16 th of July, 2019, without any language restrictions, and with a start date set to the 1 st of January, 2013, as we were interested in the latest developments in this area of research.
Eligibility criteria
Records obtained via the search methods described above were screened independently by the two authors. Consistency between inclusion/exclusion decisions was approximately 90% and the 43 instances where uncertainty existed were judged through discussion. Articles were included subject to meeting the following requirements: (a) the paper was a full published report of an original empirical study investigating the impact of at least one aggregate level (city/region/country) factor on at least one health indicator (or self-reported health) of the general population (the only admissible “sub-populations” were those based on gender and/or age); (b) the study employed statistical techniques (calculating correlations, at the very least) and was not purely descriptive or theoretical in nature; (c) the analysis involved at least two countries or at least two regions or cities (or another aggregate level) in at least two different countries; (d) the health outcome was not differentiated according to some socio-economic factor and thus studied in terms of inequality (with the exception of gender and age differentiations); (e) mortality, in case it was one of the health indicators under investigation, was strictly “total” or “all-cause” (no cause-specific or determinant-attributable mortality).
Data extraction
The following pieces of information were extracted in an Excel table from the full text of each eligible study (primarily by VV, consulting with PB in case of doubt): health outcome(s), determinants, statistical methodology, level of analysis, results, type of data, data sources, time period, countries. The evidence is synthesized according to these extracted data (often directly reflected in the section headings), using a narrative form accompanied by a “summary-of-findings” table and a graph.
Search and selection
The initial yield contained 4583 records, reduced to 3686 after removal of duplicates ( Fig 1 ). Based on title and abstract screening, 3271 records were excluded because they focused on specific medical condition(s) or specific populations (based on morbidity or some other factor), dealt with intervention effectiveness, with theoretical or non-health related issues, or with animals or plants. Of the remaining 415 papers, roughly half were disqualified upon full-text consideration, mostly due to using an outcome not of interest to us (e.g., health inequality), measuring and analyzing determinants and outcomes exclusively at the individual level, performing analyses one country at a time, employing indices that are a mixture of both health indicators and health determinants, or not utilizing potential health determinants at all. After this second stage of the screening process, 202 papers were deemed eligible for inclusion. This group was further dichotomized according to level of economic development of the countries or regions under study, using membership of the OECD or Europe as a reference “cut-off” point. Sixty papers were judged to include high-income countries, and the remaining 142 included either low- or middle-income countries or a mix of both these levels of development. The rest of this report outlines findings in relation to high-income countries only, reflecting our own primary research interests. Nonetheless, we chose to report our search yield for the other income groups for two reasons. First, to gauge the relative interest in applied published research for these different income levels; and second, to enable other researchers with a focus on determinants of health in other countries to use the extraction we made here.
- PPT PowerPoint slide
- PNG larger image
- TIFF original image
https://doi.org/10.1371/journal.pone.0239031.g001
Health outcomes
The most frequent population health indicator, life expectancy (LE), was present in 24 of the 60 studies. Apart from “life expectancy at birth” (representing the average life-span a newborn is expected to have if current mortality rates remain constant), also called “period LE” by some [ 19 , 20 ], we encountered as well LE at 40 years of age [ 21 ], at 60 [ 22 ], and at 65 [ 21 , 23 , 24 ]. In two papers, the age-specificity of life expectancy (be it at birth or another age) was not stated [ 25 , 26 ].
Some studies considered male and female LE separately [ 21 , 24 , 25 , 27 – 33 ]. This consideration was also often observed with the second most commonly used health index [ 28 – 30 , 34 – 38 ]–termed “total”, or “overall”, or “all-cause”, mortality rate (MR)–included in 22 of the 60 studies. In addition to gender, this index was also sometimes broken down according to age group [ 30 , 39 , 40 ], as well as gender-age group [ 38 ].
While the majority of studies under review here focused on a single health indicator, 23 out of the 60 studies made use of multiple outcomes, although these outcomes were always considered one at a time, and sometimes not all of them fell within the scope of our review. An easily discernable group of indices that typically went together [ 25 , 37 , 41 ] was that of neonatal (deaths occurring within 28 days postpartum), perinatal (fetal or early neonatal / first-7-days deaths), and post-neonatal (deaths between the 29 th day and completion of one year of life) mortality. More often than not, these indices were also accompanied by “stand-alone” indicators, such as infant mortality (deaths within the first year of life; our third most common index found in 16 of the 60 studies), maternal mortality (deaths during pregnancy or within 42 days of termination of pregnancy), and child mortality rates. Child mortality has conventionally been defined as mortality within the first 5 years of life, thus often also called “under-5 mortality”. Nonetheless, Pritchard & Wallace used the term “child mortality” to denote deaths of children younger than 14 years [ 42 ].
As previously stated, inclusion criteria did allow for self-reported health status to be used as a general measure of population health. Within our final selection of studies, seven utilized some form of subjective health as an outcome variable [ 25 , 43 – 48 ]. Additionally, the Health Human Development Index [ 49 ], healthy life expectancy [ 50 ], old-age survival [ 51 ], potential years of life lost [ 52 ], and disability-adjusted life expectancy [ 25 ] were also used.
We note that while in most cases the indicators mentioned above (and/or the covariates considered, see below) were taken in their absolute or logarithmic form, as a—typically annual—number, sometimes they were used in the form of differences, change rates, averages over a given time period, or even z-scores of rankings [ 19 , 22 , 40 , 42 , 44 , 53 – 57 ].
Regions, countries, and populations
Despite our decision to confine this review to high-income countries, some variation in the countries and regions studied was still present. Selection seemed to be most often conditioned on the European Union, or the European continent more generally, and the Organisation of Economic Co-operation and Development (OECD), though, typically, not all member nations–based on the instances where these were also explicitly listed—were included in a given study. Some of the stated reasons for omitting certain nations included data unavailability [ 30 , 45 , 54 ] or inconsistency [ 20 , 58 ], Gross Domestic Product (GDP) too low [ 40 ], differences in economic development and political stability with the rest of the sampled countries [ 59 ], and national population too small [ 24 , 40 ]. On the other hand, the rationales for selecting a group of countries included having similar above-average infant mortality [ 60 ], similar healthcare systems [ 23 ], and being randomly drawn from a social spending category [ 61 ]. Some researchers were interested explicitly in a specific geographical region, such as Eastern Europe [ 50 ], Central and Eastern Europe [ 48 , 60 ], the Visegrad (V4) group [ 62 ], or the Asia/Pacific area [ 32 ]. In certain instances, national regions or cities, rather than countries, constituted the units of investigation instead [ 31 , 51 , 56 , 62 – 66 ]. In two particular cases, a mix of countries and cities was used [ 35 , 57 ]. In another two [ 28 , 29 ], due to the long time periods under study, some of the included countries no longer exist. Finally, besides “European” and “OECD”, the terms “developed”, “Western”, and “industrialized” were also used to describe the group of selected nations [ 30 , 42 , 52 , 53 , 67 ].
As stated above, it was the health status of the general population that we were interested in, and during screening we made a concerted effort to exclude research using data based on a more narrowly defined group of individuals. All studies included in this review adhere to this general rule, albeit with two caveats. First, as cities (even neighborhoods) were the unit of analysis in three of the studies that made the selection [ 56 , 64 , 65 ], the populations under investigation there can be more accurately described as general urban , instead of just general. Second, oftentimes health indicators were stratified based on gender and/or age, therefore we also admitted one study that, due to its specific research question, focused on men and women of early retirement age [ 35 ] and another that considered adult males only [ 68 ].
Data types and sources
A great diversity of sources was utilized for data collection purposes. The accessible reference databases of the OECD ( https://www.oecd.org/ ), WHO ( https://www.who.int/ ), World Bank ( https://www.worldbank.org/ ), United Nations ( https://www.un.org/en/ ), and Eurostat ( https://ec.europa.eu/eurostat ) were among the top choices. The other international databases included Human Mortality [ 30 , 39 , 50 ], Transparency International [ 40 , 48 , 50 ], Quality of Government [ 28 , 69 ], World Income Inequality [ 30 ], International Labor Organization [ 41 ], International Monetary Fund [ 70 ]. A number of national databases were referred to as well, for example the US Bureau of Statistics [ 42 , 53 ], Korean Statistical Information Services [ 67 ], Statistics Canada [ 67 ], Australian Bureau of Statistics [ 67 ], and Health New Zealand Tobacco control and Health New Zealand Food and Nutrition [ 19 ]. Well-known surveys, such as the World Values Survey [ 25 , 55 ], the European Social Survey [ 25 , 39 , 44 ], the Eurobarometer [ 46 , 56 ], the European Value Survey [ 25 ], and the European Statistics of Income and Living Condition Survey [ 43 , 47 , 70 ] were used as data sources, too. Finally, in some cases [ 25 , 28 , 29 , 35 , 36 , 41 , 69 ], built-for-purpose datasets from previous studies were re-used.
In most of the studies, the level of the data (and analysis) was national. The exceptions were six papers that dealt with Nomenclature of Territorial Units of Statistics (NUTS2) regions [ 31 , 62 , 63 , 66 ], otherwise defined areas [ 51 ] or cities [ 56 ], and seven others that were multilevel designs and utilized both country- and region-level data [ 57 ], individual- and city- or country-level [ 35 ], individual- and country-level [ 44 , 45 , 48 ], individual- and neighborhood-level [ 64 ], and city-region- (NUTS3) and country-level data [ 65 ]. Parallel to that, the data type was predominantly longitudinal, with only a few studies using purely cross-sectional data [ 25 , 33 , 43 , 45 – 48 , 50 , 62 , 67 , 68 , 71 , 72 ], albeit in four of those [ 43 , 48 , 68 , 72 ] two separate points in time were taken (thus resulting in a kind of “double cross-section”), while in another the averages across survey waves were used [ 56 ].
In studies using longitudinal data, the length of the covered time periods varied greatly. Although this was almost always less than 40 years, in one study it covered the entire 20 th century [ 29 ]. Longitudinal data, typically in the form of annual records, was sometimes transformed before usage. For example, some researchers considered data points at 5- [ 34 , 36 , 49 ] or 10-year [ 27 , 29 , 35 ] intervals instead of the traditional 1, or took averages over 3-year periods [ 42 , 53 , 73 ]. In one study concerned with the effect of the Great Recession all data were in a “recession minus expansion change in trends”-form [ 57 ]. Furthermore, there were a few instances where two different time periods were compared to each other [ 42 , 53 ] or when data was divided into 2 to 4 (possibly overlapping) periods which were then analyzed separately [ 24 , 26 , 28 , 29 , 31 , 65 ]. Lastly, owing to data availability issues, discrepancies between the time points or periods of data on the different variables were occasionally observed [ 22 , 35 , 42 , 53 – 55 , 63 ].
Health determinants
Together with other essential details, Table 1 lists the health correlates considered in the selected studies. Several general categories for these correlates can be discerned, including health care, political stability, socio-economics, demographics, psychology, environment, fertility, life-style, culture, labor. All of these, directly or implicitly, have been recognized as holding importance for population health by existing theoretical models of (social) determinants of health [ 74 – 77 ].
https://doi.org/10.1371/journal.pone.0239031.t001
It is worth noting that in a few studies there was just a single aggregate-level covariate investigated in relation to a health outcome of interest to us. In one instance, this was life satisfaction [ 44 ], in another–welfare system typology [ 45 ], but also gender inequality [ 33 ], austerity level [ 70 , 78 ], and deprivation [ 51 ]. Most often though, attention went exclusively to GDP [ 27 , 29 , 46 , 57 , 65 , 71 ]. It was often the case that research had a more particular focus. Among others, minimum wages [ 79 ], hospital payment schemes [ 23 ], cigarette prices [ 63 ], social expenditure [ 20 ], residents’ dissatisfaction [ 56 ], income inequality [ 30 , 69 ], and work leave [ 41 , 58 ] took center stage. Whenever variables outside of these specific areas were also included, they were usually identified as confounders or controls, moderators or mediators.
We visualized the combinations in which the different determinants have been studied in Fig 2 , which was obtained via multidimensional scaling and a subsequent cluster analysis (details outlined in S2 Appendix ). It depicts the spatial positioning of each determinant relative to all others, based on the number of times the effects of each pair of determinants have been studied simultaneously. When interpreting Fig 2 , one should keep in mind that determinants marked with an asterisk represent, in fact, collectives of variables.
Groups of determinants are marked by asterisks (see S1 Table in S1 Appendix ). Diminishing color intensity reflects a decrease in the total number of “connections” for a given determinant. Noteworthy pairwise “connections” are emphasized via lines (solid-dashed-dotted indicates decreasing frequency). Grey contour lines encircle groups of variables that were identified via cluster analysis. Abbreviations: age = population age distribution, associations = membership in associations, AT-index = atherogenic-thrombogenic index, BR = birth rate, CAPB = Cyclically Adjusted Primary Balance, civilian-labor = civilian labor force, C-section = Cesarean delivery rate, credit-info = depth of credit information, dissatisf = residents’ dissatisfaction, distrib.orient = distributional orientation, EDU = education, eHealth = eHealth index at GP-level, exch.rate = exchange rate, fat = fat consumption, GDP = gross domestic product, GFCF = Gross Fixed Capital Formation/Creation, GH-gas = greenhouse gas, GII = gender inequality index, gov = governance index, gov.revenue = government revenues, HC-coverage = healthcare coverage, HE = health(care) expenditure, HHconsump = household consumption, hosp.beds = hospital beds, hosp.payment = hospital payment scheme, hosp.stay = length of hospital stay, IDI = ICT development index, inc.ineq = income inequality, industry-labor = industrial labor force, infant-sex = infant sex ratio, labor-product = labor production, LBW = low birth weight, leave = work leave, life-satisf = life satisfaction, M-age = maternal age, marginal-tax = marginal tax rate, MDs = physicians, mult.preg = multiple pregnancy, NHS = Nation Health System, NO = nitrous oxide emissions, PM10 = particulate matter (PM10) emissions, pop = population size, pop.density = population density, pre-term = pre-term birth rate, prison = prison population, researchE = research&development expenditure, school.ref = compulsory schooling reform, smoke-free = smoke-free places, SO = sulfur oxide emissions, soc.E = social expenditure, soc.workers = social workers, sugar = sugar consumption, terror = terrorism, union = union density, UR = unemployment rate, urban = urbanization, veg-fr = vegetable-and-fruit consumption, welfare = welfare regime, Wwater = wastewater treatment.
https://doi.org/10.1371/journal.pone.0239031.g002
Distances between determinants in Fig 2 are indicative of determinants’ “connectedness” with each other. While the statistical procedure called for higher dimensionality of the model, for demonstration purposes we show here a two-dimensional solution. This simplification unfortunately comes with a caveat. To use the factor smoking as an example, it would appear it stands at a much greater distance from GDP than it does from alcohol. In reality however, smoking was considered together with alcohol consumption [ 21 , 25 , 26 , 52 , 68 ] in just as many studies as it was with GDP [ 21 , 25 , 26 , 52 , 59 ], five. To aid with respect to this apparent shortcoming, we have emphasized the strongest pairwise links. Solid lines connect GDP with health expenditure (HE), unemployment rate (UR), and education (EDU), indicating that the effect of GDP on health, taking into account the effects of the other three determinants as well, was evaluated in between 12 to 16 studies of the 60 included in this review. Tracing the dashed lines, we can also tell that GDP appeared jointly with income inequality, and HE together with either EDU or UR, in anywhere between 8 to 10 of our selected studies. Finally, some weaker but still worth-mentioning “connections” between variables are displayed as well via the dotted lines.
The fact that all notable pairwise “connections” are concentrated within a relatively small region of the plot may be interpreted as low overall “connectedness” among the health indicators studied. GDP is the most widely investigated determinant in relation to general population health. Its total number of “connections” is disproportionately high (159) compared to its runner-up–HE (with 113 “connections”), and then subsequently EDU (with 90) and UR (with 86). In fact, all of these determinants could be thought of as outliers, given that none of the remaining factors have a total count of pairings above 52. This decrease in individual determinants’ overall “connectedness” can be tracked on the graph via the change of color intensity as we move outwards from the symbolic center of GDP and its closest “co-determinants”, to finally reach the other extreme of the ten indicators (welfare regime, household consumption, compulsory school reform, life satisfaction, government revenues, literacy, research expenditure, multiple pregnancy, Cyclically Adjusted Primary Balance, and residents’ dissatisfaction; in white) the effects on health of which were only studied in isolation.
Lastly, we point to the few small but stable clusters of covariates encircled by the grey bubbles on Fig 2 . These groups of determinants were identified as “close” by both statistical procedures used for the production of the graph (see details in S2 Appendix ).
Statistical methodology
There was great variation in the level of statistical detail reported. Some authors provided too vague a description of their analytical approach, necessitating some inference in this section.
The issue of missing data is a challenging reality in this field of research, but few of the studies under review (12/60) explain how they dealt with it. Among the ones that do, three general approaches to handling missingness can be identified, listed in increasing level of sophistication: case-wise deletion, i.e., removal of countries from the sample [ 20 , 45 , 48 , 58 , 59 ], (linear) interpolation [ 28 , 30 , 34 , 58 , 59 , 63 ], and multiple imputation [ 26 , 41 , 52 ].
Correlations, Pearson, Spearman, or unspecified, were the only technique applied with respect to the health outcomes of interest in eight analyses [ 33 , 42 – 44 , 46 , 53 , 57 , 61 ]. Among the more advanced statistical methods, the family of regression models proved to be, by and large, predominant. Before examining this closer, we note the techniques that were, in a way, “unique” within this selection of studies: meta-analyses were performed (random and fixed effects, respectively) on the reduced form and 2-sample two stage least squares (2SLS) estimations done within countries [ 39 ]; difference-in-difference (DiD) analysis was applied in one case [ 23 ]; dynamic time-series methods, among which co-integration, impulse-response function (IRF), and panel vector autoregressive (VAR) modeling, were utilized in one study [ 80 ]; longitudinal generalized estimating equation (GEE) models were developed on two occasions [ 70 , 78 ]; hierarchical Bayesian spatial models [ 51 ] and special autoregressive regression [ 62 ] were also implemented.
Purely cross-sectional data analyses were performed in eight studies [ 25 , 45 , 47 , 50 , 55 , 56 , 67 , 71 ]. These consisted of linear regression (assumed ordinary least squares (OLS)), generalized least squares (GLS) regression, and multilevel analyses. However, six other studies that used longitudinal data in fact had a cross-sectional design, through which they applied regression at multiple time-points separately [ 27 , 29 , 36 , 48 , 68 , 72 ].
Apart from these “multi-point cross-sectional studies”, some other simplistic approaches to longitudinal data analysis were found, involving calculating and regressing 3-year averages of both the response and the predictor variables [ 54 ], taking the average of a few data-points (i.e., survey waves) [ 56 ] or using difference scores over 10-year [ 19 , 29 ] or unspecified time intervals [ 40 , 55 ].
Moving further in the direction of more sensible longitudinal data usage, we turn to the methods widely known among (health) economists as “panel data analysis” or “panel regression”. Most often seen were models with fixed effects for country/region and sometimes also time-point (occasionally including a country-specific trend as well), with robust standard errors for the parameter estimates to take into account correlations among clustered observations [ 20 , 21 , 24 , 28 , 30 , 32 , 34 , 37 , 38 , 41 , 52 , 59 , 60 , 63 , 66 , 69 , 73 , 79 , 81 , 82 ]. The Hausman test [ 83 ] was sometimes mentioned as the tool used to decide between fixed and random effects [ 26 , 49 , 63 , 66 , 73 , 82 ]. A few studies considered the latter more appropriate for their particular analyses, with some further specifying that (feasible) GLS estimation was employed [ 26 , 34 , 49 , 58 , 60 , 73 ]. Apart from these two types of models, the first differences method was encountered once as well [ 31 ]. Across all, the error terms were sometimes assumed to come from a first-order autoregressive process (AR(1)), i.e., they were allowed to be serially correlated [ 20 , 30 , 38 , 58 – 60 , 73 ], and lags of (typically) predictor variables were included in the model specification, too [ 20 , 21 , 37 , 38 , 48 , 69 , 81 ]. Lastly, a somewhat different approach to longitudinal data analysis was undertaken in four studies [ 22 , 35 , 48 , 65 ] in which multilevel–linear or Poisson–models were developed.
Regardless of the exact techniques used, most studies included in this review presented multiple model applications within their main analysis. None attempted to formally compare models in order to identify the “best”, even if goodness-of-fit statistics were occasionally reported. As indicated above, many studies investigated women’s and men’s health separately [ 19 , 21 , 22 , 27 – 29 , 31 , 33 , 35 , 36 , 38 , 39 , 45 , 50 , 51 , 64 , 65 , 69 , 82 ], and covariates were often tested one at a time, including other covariates only incrementally [ 20 , 25 , 28 , 36 , 40 , 50 , 55 , 67 , 73 ]. Furthermore, there were a few instances where analyses within countries were performed as well [ 32 , 39 , 51 ] or where the full time period of interest was divided into a few sub-periods [ 24 , 26 , 28 , 31 ]. There were also cases where different statistical techniques were applied in parallel [ 29 , 55 , 60 , 66 , 69 , 73 , 82 ], sometimes as a form of sensitivity analysis [ 24 , 26 , 30 , 58 , 73 ]. However, the most common approach to sensitivity analysis was to re-run models with somewhat different samples [ 39 , 50 , 59 , 67 , 69 , 80 , 82 ]. Other strategies included different categorization of variables or adding (more/other) controls [ 21 , 23 , 25 , 28 , 37 , 50 , 63 , 69 ], using an alternative main covariate measure [ 59 , 82 ], including lags for predictors or outcomes [ 28 , 30 , 58 , 63 , 65 , 79 ], using weights [ 24 , 67 ] or alternative data sources [ 37 , 69 ], or using non-imputed data [ 41 ].
As the methods and not the findings are the main focus of the current review, and because generic checklists cannot discern the underlying quality in this application field (see also below), we opted to pool all reported findings together, regardless of individual study characteristics or particular outcome(s) used, and speak generally of positive and negative effects on health. For this summary we have adopted the 0.05-significance level and only considered results from multivariate analyses. Strictly birth-related factors are omitted since these potentially only relate to the group of infant mortality indicators and not to any of the other general population health measures.
Starting with the determinants most often studied, higher GDP levels [ 21 , 26 , 27 , 29 , 30 , 32 , 43 , 48 , 52 , 58 , 60 , 66 , 67 , 73 , 79 , 81 , 82 ], higher health [ 21 , 37 , 47 , 49 , 52 , 58 , 59 , 68 , 72 , 82 ] and social [ 20 , 21 , 26 , 38 , 79 ] expenditures, higher education [ 26 , 39 , 52 , 62 , 72 , 73 ], lower unemployment [ 60 , 61 , 66 ], and lower income inequality [ 30 , 42 , 53 , 55 , 73 ] were found to be significantly associated with better population health on a number of occasions. In addition to that, there was also some evidence that democracy [ 36 ] and freedom [ 50 ], higher work compensation [ 43 , 79 ], distributional orientation [ 54 ], cigarette prices [ 63 ], gross national income [ 22 , 72 ], labor productivity [ 26 ], exchange rates [ 32 ], marginal tax rates [ 79 ], vaccination rates [ 52 ], total fertility [ 59 , 66 ], fruit and vegetable [ 68 ], fat [ 52 ] and sugar consumption [ 52 ], as well as bigger depth of credit information [ 22 ] and percentage of civilian labor force [ 79 ], longer work leaves [ 41 , 58 ], more physicians [ 37 , 52 , 72 ], nurses [ 72 ], and hospital beds [ 79 , 82 ], and also membership in associations, perceived corruption and societal trust [ 48 ] were beneficial to health. Higher nitrous oxide (NO) levels [ 52 ], longer average hospital stay [ 48 ], deprivation [ 51 ], dissatisfaction with healthcare and the social environment [ 56 ], corruption [ 40 , 50 ], smoking [ 19 , 26 , 52 , 68 ], alcohol consumption [ 26 , 52 , 68 ] and illegal drug use [ 68 ], poverty [ 64 ], higher percentage of industrial workers [ 26 ], Gross Fixed Capital creation [ 66 ] and older population [ 38 , 66 , 79 ], gender inequality [ 22 ], and fertility [ 26 , 66 ] were detrimental.
It is important to point out that the above-mentioned effects could not be considered stable either across or within studies. Very often, statistical significance of a given covariate fluctuated between the different model specifications tried out within the same study [ 20 , 49 , 59 , 66 , 68 , 69 , 73 , 80 , 82 ], testifying to the importance of control variables and multivariate research (i.e., analyzing multiple independent variables simultaneously) in general. Furthermore, conflicting results were observed even with regards to the “core” determinants given special attention, so to speak, throughout this text. Thus, some studies reported negative effects of health expenditure [ 32 , 82 ], social expenditure [ 58 ], GDP [ 49 , 66 ], and education [ 82 ], and positive effects of income inequality [ 82 ] and unemployment [ 24 , 31 , 32 , 52 , 66 , 68 ]. Interestingly, one study [ 34 ] differentiated between temporary and long-term effects of GDP and unemployment, alluding to possibly much greater complexity of the association with health. It is also worth noting that some gender differences were found, with determinants being more influential for males than for females, or only having statistically significant effects for male health [ 19 , 21 , 28 , 34 , 36 , 37 , 39 , 64 , 65 , 69 ].
The purpose of this scoping review was to examine recent quantitative work on the topic of multi-country analyses of determinants of population health in high-income countries.
Measuring population health via relatively simple mortality-based indicators still seems to be the state of the art. What is more, these indicators are routinely considered one at a time, instead of, for example, employing existing statistical procedures to devise a more general, composite, index of population health, or using some of the established indices, such as disability-adjusted life expectancy (DALE) or quality-adjusted life expectancy (QALE). Although strong arguments for their wider use were already voiced decades ago [ 84 ], such summary measures surface only rarely in this research field.
On a related note, the greater data availability and accessibility that we enjoy today does not automatically equate to data quality. Nonetheless, this is routinely assumed in aggregate level studies. We almost never encountered a discussion on the topic. The non-mundane issue of data missingness, too, goes largely underappreciated. With all recent methodological advancements in this area [ 85 – 88 ], there is no excuse for ignorance; and still, too few of the reviewed studies tackled the matter in any adequate fashion.
Much optimism can be gained considering the abundance of different determinants that have attracted researchers’ attention in relation to population health. We took on a visual approach with regards to these determinants and presented a graph that links spatial distances between determinants with frequencies of being studies together. To facilitate interpretation, we grouped some variables, which resulted in some loss of finer detail. Nevertheless, the graph is helpful in exemplifying how many effects continue to be studied in a very limited context, if any. Since in reality no factor acts in isolation, this oversimplification practice threatens to render the whole exercise meaningless from the outset. The importance of multivariate analysis cannot be stressed enough. While there is no “best method” to be recommended and appropriate techniques vary according to the specifics of the research question and the characteristics of the data at hand [ 89 – 93 ], in the future, in addition to abandoning simplistic univariate approaches, we hope to see a shift from the currently dominating fixed effects to the more flexible random/mixed effects models [ 94 ], as well as wider application of more sophisticated methods, such as principle component regression, partial least squares, covariance structure models (e.g., structural equations), canonical correlations, time-series, and generalized estimating equations.
Finally, there are some limitations of the current scoping review. We searched the two main databases for published research in medical and non-medical sciences (PubMed and Web of Science) since 2013, thus potentially excluding publications and reports that are not indexed in these databases, as well as older indexed publications. These choices were guided by our interest in the most recent (i.e., the current state-of-the-art) and arguably the highest-quality research (i.e., peer-reviewed articles, primarily in indexed non-predatory journals). Furthermore, despite holding a critical stance with regards to some aspects of how determinants-of-health research is currently conducted, we opted out of formally assessing the quality of the individual studies included. The reason for that is two-fold. On the one hand, we are unaware of the existence of a formal and standard tool for quality assessment of ecological designs. And on the other, we consider trying to score the quality of these diverse studies (in terms of regional setting, specific topic, outcome indices, and methodology) undesirable and misleading, particularly since we would sometimes have been rating the quality of only a (small) part of the original studies—the part that was relevant to our review’s goal.
Our aim was to investigate the current state of research on the very broad and general topic of population health, specifically, the way it has been examined in a multi-country context. We learned that data treatment and analytical approach were, in the majority of these recent studies, ill-equipped or insufficiently transparent to provide clarity regarding the underlying mechanisms of population health in high-income countries. Whether due to methodological shortcomings or the inherent complexity of the topic, research so far fails to provide any definitive answers. It is our sincere belief that with the application of more advanced analytical techniques this continuous quest could come to fruition sooner.
Supporting information
S1 checklist. preferred reporting items for systematic reviews and meta-analyses extension for scoping reviews (prisma-scr) checklist..
https://doi.org/10.1371/journal.pone.0239031.s001
S1 Appendix.
https://doi.org/10.1371/journal.pone.0239031.s002
S2 Appendix.
https://doi.org/10.1371/journal.pone.0239031.s003
- View Article
- Google Scholar
- PubMed/NCBI
- 75. Dahlgren G, Whitehead M. Policies and Strategies to Promote Equity in Health. Stockholm, Sweden: Institute for Future Studies; 1991.
- 76. Brunner E, Marmot M. Social Organization, Stress, and Health. In: Marmot M, Wilkinson RG, editors. Social Determinants of Health. Oxford, England: Oxford University Press; 1999.
- 77. Najman JM. A General Model of the Social Origins of Health and Well-being. In: Eckersley R, Dixon J, Douglas B, editors. The Social Origins of Health and Well-being. Cambridge, England: Cambridge University Press; 2001.
- 85. Carpenter JR, Kenward MG. Multiple Imputation and its Application. New York: John Wiley & Sons; 2013.
- 86. Molenberghs G, Fitzmaurice G, Kenward MG, Verbeke G, Tsiatis AA. Handbook of Missing Data Methodology. Boca Raton: Chapman & Hall/CRC; 2014.
- 87. van Buuren S. Flexible Imputation of Missing Data. 2nd ed. Boca Raton: Chapman & Hall/CRC; 2018.
- 88. Enders CK. Applied Missing Data Analysis. New York: Guilford; 2010.
- 89. Shayle R. Searle GC, Charles E. McCulloch. Variance Components: John Wiley & Sons, Inc.; 1992.
- 90. Agresti A. Foundations of Linear and Generalized Linear Models. Hoboken, New Jersey: John Wiley & Sons Inc.; 2015.
- 91. Leyland A. H. (Editor) HGE. Multilevel Modelling of Health Statistics: John Wiley & Sons Inc; 2001.
- 92. Garrett Fitzmaurice MD, Geert Verbeke, Geert Molenberghs. Longitudinal Data Analysis. New York: Chapman and Hall/CRC; 2008.
- 93. Wolfgang Karl Härdle LS. Applied Multivariate Statistical Analysis. Berlin, Heidelberg: Springer; 2015.
Your browser is not supported
Sorry but it looks as if your browser is out of date. To get the best experience using our site we recommend that you upgrade or switch browsers.
Find a solution
- Skip to main content
- Skip to navigation
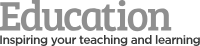
- Back to parent navigation item
- Primary teacher
- Secondary/FE teacher
- Early career or student teacher
- Higher education
- Curriculum support
- Literacy in science teaching
- Periodic table
- Interactive periodic table
- Climate change and sustainability
- Resources shop
- Collections
- Remote teaching support
- Starters for ten
- Screen experiments
- Assessment for learning
- Microscale chemistry
- Faces of chemistry
- Classic chemistry experiments
- Nuffield practical collection
- Anecdotes for chemistry teachers
- On this day in chemistry
- Global experiments
- PhET interactive simulations
- Chemistry vignettes
- Context and problem based learning
- Journal of the month
- Chemistry and art
- Art analysis
- Pigments and colours
- Ancient art: today's technology
- Psychology and art theory
- Art and archaeology
- Artists as chemists
- The physics of restoration and conservation
- Ancient Egyptian art
- Ancient Greek art
- Ancient Roman art
- Classic chemistry demonstrations
- In search of solutions
- In search of more solutions
- Creative problem-solving in chemistry
- Solar spark
- Chemistry for non-specialists
- Health and safety in higher education
- Analytical chemistry introductions
- Exhibition chemistry
- Introductory maths for higher education
- Commercial skills for chemists
- Kitchen chemistry
- Journals how to guides
- Chemistry in health
- Chemistry in sport
- Chemistry in your cupboard
- Chocolate chemistry
- Adnoddau addysgu cemeg Cymraeg
- The chemistry of fireworks
- Festive chemistry
- Education in Chemistry
- Teach Chemistry
- On-demand online
- Live online
- Selected PD articles
- PD for primary teachers
- PD for secondary teachers
- What we offer
- Chartered Science Teacher (CSciTeach)
- Teacher mentoring
- UK Chemistry Olympiad
- Who can enter?
- How does it work?
- Resources and past papers
- Top of the Bench
- Schools' Analyst
- Regional support
- Education coordinators
- RSC Yusuf Hamied Inspirational Science Programme
- RSC Education News
- Supporting teacher training
- Interest groups
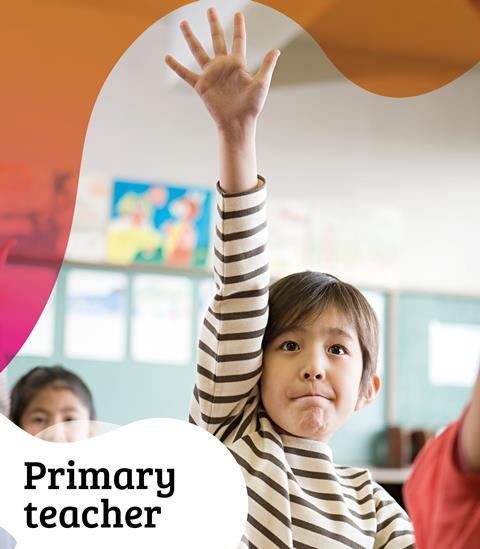
- More navigation items
All Quantitative research articles
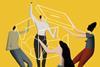
Harness self-regulation to nurture independent study skills
2020-10-29T10:15:00Z
Follow these tips to engage students with learning processes
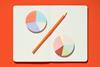
Why declining science scores are no reason to panic
2020-02-05T10:31:00Z
PISA provides an interesting background to teaching, but is it only for policymakers?
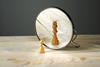
Dunning-Kruger: the gap between prediction and performance
2018-03-19T14:15:00Z
Improve expectations to improve learning
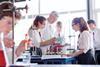
Encouraging inquiry-based approaches
2016-09-28T00:00:00Z
Manage the load for students
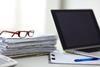
Transforming education research
2016-09-14T00:00:00Z
New project to investigate the opportunities and challenges for teachers and researchers
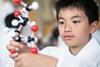
The value of modelling molecules
2016-08-10T00:00:00Z
Challenge of visual-spatial representations
![articles using quantitative research Education research shutterstock 139305425 300tb[1]](https://d1ymz67w5raq8g.cloudfront.net/Pictures/100x67/5/6/4/113564_educationresearch_shutterstock_139305425_300tb1.jpg)
Why don't teachers use education research in teaching?
2016-08-09T07:57:00Z
Paul MacLellan digs into the problem with research from Durham, a secondary school teacher and a journal editor
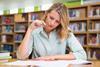
What influences future science study?
2016-07-27T00:00:00Z
Study beyond GCSE linked to confidence and perceptions
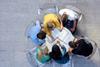
It’s good to talk
2016-06-08T00:00:00Z
Facilitating peer group learning
![articles using quantitative research Micer shutterstock 348717923 300tb[1]](https://d1ymz67w5raq8g.cloudfront.net/Pictures/100x67/5/9/9/113599_micer_shutterstock_348717923_300tb1.jpg)
The community of chemistry education research
2016-03-03T15:11:00Z
Michael Seery talks about being part of the chemistry education research community in the UK and Ireland
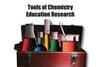
Tools of chemistry education research
2015-11-09T00:00:00Z
Methods and strategies
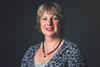
Understanding education
2015-11-06T00:00:00Z
Raising awareness of teaching and learning opportunities all around us
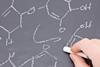
Organic confusion
Rote memorising v deep understanding
![articles using quantitative research Img 0013 300tb[1]](https://d1ymz67w5raq8g.cloudfront.net/Pictures/100x67/6/3/3/113633_img_0013_300tb1.jpg)
Variety in Chemistry Education 2015
2015-08-24T16:14:00Z
Michael Seery reports from the conference for chemistry teaching and learning in higher education
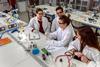
The case against inquiry-based learning
2015-05-26T10:44:00Z
Michael Seery takes a critical look at inquiry-based learning
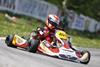
Rationalising reasoning
2015-05-11T00:00:00Z
Is contextualisation the best solution?
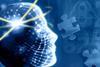
Analysing analogies
Teacher CPD could support analogical thinking
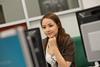
Flipped chemistry revisited
2015-03-05T00:00:00Z
Successful organic chemistry teaching
![articles using quantitative research Sl india 300tb[1]](https://d1ymz67w5raq8g.cloudfront.net/Pictures/100x67/6/8/1/113681_slindia_300tb1.jpg)
International Conference on Education in Chemistry, 2014
2015-01-20T13:20:00Z
Simon Lancaster reports on his visit to ICEC-2014 in Mumbai
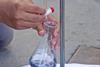
Moles and titrations
2015-01-06T00:00:00Z
Dorothy Warren describes some of the difficulties with teaching this topic and shows how you can help your students to master aspects of quantitative chemistry
- Previous Page
- Contributors
- Email alerts
Site powered by Webvision Cloud

An official website of the United States government
The .gov means it’s official. Federal government websites often end in .gov or .mil. Before sharing sensitive information, make sure you’re on a federal government site.
The site is secure. The https:// ensures that you are connecting to the official website and that any information you provide is encrypted and transmitted securely.
- Publications
- Account settings
- My Bibliography
- Collections
- Citation manager
Save citation to file
Email citation, add to collections.
- Create a new collection
- Add to an existing collection
Add to My Bibliography
Your saved search, create a file for external citation management software, your rss feed.
- Search in PubMed
- Search in NLM Catalog
- Add to Search
Quantitative research
Affiliation.
- 1 Faculty of Health and Social Care, University of Hull, Hull, England.
- PMID: 25828021
- DOI: 10.7748/ns.29.31.44.e8681
This article describes the basic tenets of quantitative research. The concepts of dependent and independent variables are addressed and the concept of measurement and its associated issues, such as error, reliability and validity, are explored. Experiments and surveys – the principal research designs in quantitative research – are described and key features explained. The importance of the double-blind randomised controlled trial is emphasised, alongside the importance of longitudinal surveys, as opposed to cross-sectional surveys. Essential features of data storage are covered, with an emphasis on safe, anonymous storage. Finally, the article explores the analysis of quantitative data, considering what may be analysed and the main uses of statistics in analysis.
Keywords: Experiments; measurement; nursing research; quantitative research; reliability; surveys; validity.
PubMed Disclaimer
Similar articles
- Experimental designs. Behi R, Nolan M. Behi R, et al. Br J Nurs. 1996 Jun 27-Jul 10;5(12):754-6. doi: 10.12968/bjon.1996.5.12.754. Br J Nurs. 1996. PMID: 8718332
- Rigour in quantitative research. Claydon LS. Claydon LS. Nurs Stand. 2015 Jul 22;29(47):43-8. doi: 10.7748/ns.29.47.43.e8820. Nurs Stand. 2015. PMID: 26198528 Review.
- Searching the biomedical literature: research study designs and critical appraisal. Callas PW. Callas PW. Clin Lab Sci. 2008 Winter;21(1):42-8. Clin Lab Sci. 2008. PMID: 18335861
- Understanding and critiquing quantitative research papers. Lee P. Lee P. Nurs Times. 2006 Jul 11-17;102(28):28-30. Nurs Times. 2006. PMID: 16869219
- Methodological challenges in meditation research. Caspi O, Burleson KO. Caspi O, et al. Adv Mind Body Med. 2007 Winter;22(3-4):36-43. Adv Mind Body Med. 2007. PMID: 20664132 Review.
- Impact of floral and geographical origins on honey quality parameters in Saudi Arabian regions. Alaerjani WMA, Mohammed MEA. Alaerjani WMA, et al. Sci Rep. 2024 Apr 15;14(1):8720. doi: 10.1038/s41598-024-59359-y. Sci Rep. 2024. PMID: 38622258 Free PMC article.
- The Influence of Emotional Intelligence on Quality of Life in Patients Undergoing Chronic Hemodialysis Focused on Age and Gender. Masià-Plana A, Sitjar-Suñer M, Mantas-Jiménez S, Suñer-Soler R. Masià-Plana A, et al. Behav Sci (Basel). 2024 Mar 8;14(3):220. doi: 10.3390/bs14030220. Behav Sci (Basel). 2024. PMID: 38540523 Free PMC article.
- Technology Integration in Higher Education During COVID-19: An Assessment of Online Teaching Competencies Through Technological Pedagogical Content Knowledge Model. Akram H, Yingxiu Y, Al-Adwan AS, Alkhalifah A. Akram H, et al. Front Psychol. 2021 Aug 26;12:736522. doi: 10.3389/fpsyg.2021.736522. eCollection 2021. Front Psychol. 2021. PMID: 34512488 Free PMC article.
- Search in MeSH
- Citation Manager
NCBI Literature Resources
MeSH PMC Bookshelf Disclaimer
The PubMed wordmark and PubMed logo are registered trademarks of the U.S. Department of Health and Human Services (HHS). Unauthorized use of these marks is strictly prohibited.
Have a language expert improve your writing
Run a free plagiarism check in 10 minutes, generate accurate citations for free.
- Knowledge Base
Methodology
- What Is Quantitative Research? | Definition, Uses & Methods
What Is Quantitative Research? | Definition, Uses & Methods
Published on June 12, 2020 by Pritha Bhandari . Revised on June 22, 2023.
Quantitative research is the process of collecting and analyzing numerical data. It can be used to find patterns and averages, make predictions, test causal relationships, and generalize results to wider populations.
Quantitative research is the opposite of qualitative research , which involves collecting and analyzing non-numerical data (e.g., text, video, or audio).
Quantitative research is widely used in the natural and social sciences: biology, chemistry, psychology, economics, sociology, marketing, etc.
- What is the demographic makeup of Singapore in 2020?
- How has the average temperature changed globally over the last century?
- Does environmental pollution affect the prevalence of honey bees?
- Does working from home increase productivity for people with long commutes?
Table of contents
Quantitative research methods, quantitative data analysis, advantages of quantitative research, disadvantages of quantitative research, other interesting articles, frequently asked questions about quantitative research.
You can use quantitative research methods for descriptive, correlational or experimental research.
- In descriptive research , you simply seek an overall summary of your study variables.
- In correlational research , you investigate relationships between your study variables.
- In experimental research , you systematically examine whether there is a cause-and-effect relationship between variables.
Correlational and experimental research can both be used to formally test hypotheses , or predictions, using statistics. The results may be generalized to broader populations based on the sampling method used.
To collect quantitative data, you will often need to use operational definitions that translate abstract concepts (e.g., mood) into observable and quantifiable measures (e.g., self-ratings of feelings and energy levels).
Research method | How to use | Example |
---|---|---|
Control or manipulate an to measure its effect on a dependent variable. | To test whether an intervention can reduce procrastination in college students, you give equal-sized groups either a procrastination intervention or a comparable task. You compare self-ratings of procrastination behaviors between the groups after the intervention. | |
Ask questions of a group of people in-person, over-the-phone or online. | You distribute with rating scales to first-year international college students to investigate their experiences of culture shock. | |
(Systematic) observation | Identify a behavior or occurrence of interest and monitor it in its natural setting. | To study college classroom participation, you sit in on classes to observe them, counting and recording the prevalence of active and passive behaviors by students from different backgrounds. |
Secondary research | Collect data that has been gathered for other purposes e.g., national surveys or historical records. | To assess whether attitudes towards climate change have changed since the 1980s, you collect relevant questionnaire data from widely available . |
Note that quantitative research is at risk for certain research biases , including information bias , omitted variable bias , sampling bias , or selection bias . Be sure that you’re aware of potential biases as you collect and analyze your data to prevent them from impacting your work too much.
Receive feedback on language, structure, and formatting
Professional editors proofread and edit your paper by focusing on:
- Academic style
- Vague sentences
- Style consistency
See an example
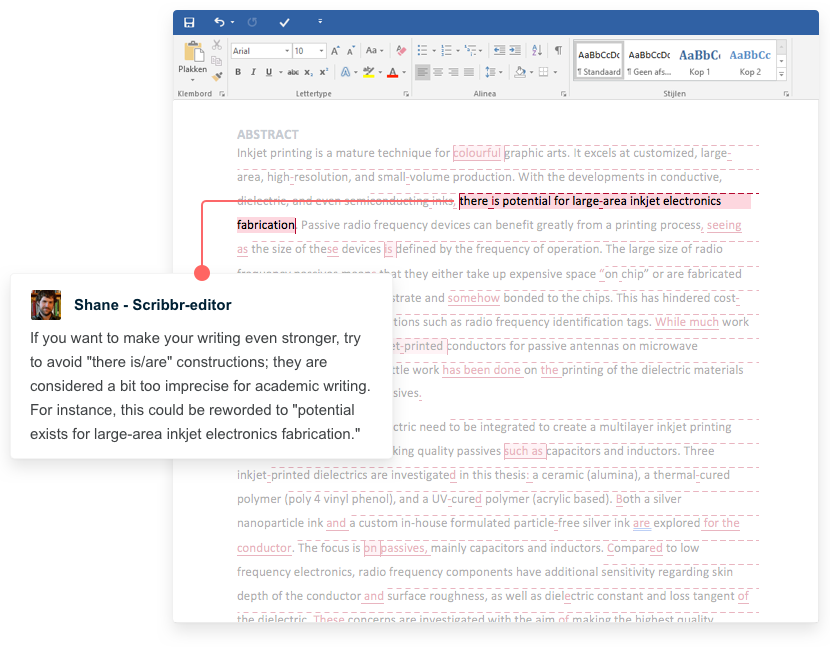
Once data is collected, you may need to process it before it can be analyzed. For example, survey and test data may need to be transformed from words to numbers. Then, you can use statistical analysis to answer your research questions .
Descriptive statistics will give you a summary of your data and include measures of averages and variability. You can also use graphs, scatter plots and frequency tables to visualize your data and check for any trends or outliers.
Using inferential statistics , you can make predictions or generalizations based on your data. You can test your hypothesis or use your sample data to estimate the population parameter .
First, you use descriptive statistics to get a summary of the data. You find the mean (average) and the mode (most frequent rating) of procrastination of the two groups, and plot the data to see if there are any outliers.
You can also assess the reliability and validity of your data collection methods to indicate how consistently and accurately your methods actually measured what you wanted them to.
Quantitative research is often used to standardize data collection and generalize findings . Strengths of this approach include:
- Replication
Repeating the study is possible because of standardized data collection protocols and tangible definitions of abstract concepts.
- Direct comparisons of results
The study can be reproduced in other cultural settings, times or with different groups of participants. Results can be compared statistically.
- Large samples
Data from large samples can be processed and analyzed using reliable and consistent procedures through quantitative data analysis.
- Hypothesis testing
Using formalized and established hypothesis testing procedures means that you have to carefully consider and report your research variables, predictions, data collection and testing methods before coming to a conclusion.
Despite the benefits of quantitative research, it is sometimes inadequate in explaining complex research topics. Its limitations include:
- Superficiality
Using precise and restrictive operational definitions may inadequately represent complex concepts. For example, the concept of mood may be represented with just a number in quantitative research, but explained with elaboration in qualitative research.
- Narrow focus
Predetermined variables and measurement procedures can mean that you ignore other relevant observations.
- Structural bias
Despite standardized procedures, structural biases can still affect quantitative research. Missing data , imprecise measurements or inappropriate sampling methods are biases that can lead to the wrong conclusions.
- Lack of context
Quantitative research often uses unnatural settings like laboratories or fails to consider historical and cultural contexts that may affect data collection and results.
Here's why students love Scribbr's proofreading services
Discover proofreading & editing
If you want to know more about statistics , methodology , or research bias , make sure to check out some of our other articles with explanations and examples.
- Chi square goodness of fit test
- Degrees of freedom
- Null hypothesis
- Discourse analysis
- Control groups
- Mixed methods research
- Non-probability sampling
- Inclusion and exclusion criteria
Research bias
- Rosenthal effect
- Implicit bias
- Cognitive bias
- Selection bias
- Negativity bias
- Status quo bias
Quantitative research deals with numbers and statistics, while qualitative research deals with words and meanings.
Quantitative methods allow you to systematically measure variables and test hypotheses . Qualitative methods allow you to explore concepts and experiences in more detail.
In mixed methods research , you use both qualitative and quantitative data collection and analysis methods to answer your research question .
Data collection is the systematic process by which observations or measurements are gathered in research. It is used in many different contexts by academics, governments, businesses, and other organizations.
Operationalization means turning abstract conceptual ideas into measurable observations.
For example, the concept of social anxiety isn’t directly observable, but it can be operationally defined in terms of self-rating scores, behavioral avoidance of crowded places, or physical anxiety symptoms in social situations.
Before collecting data , it’s important to consider how you will operationalize the variables that you want to measure.
Reliability and validity are both about how well a method measures something:
- Reliability refers to the consistency of a measure (whether the results can be reproduced under the same conditions).
- Validity refers to the accuracy of a measure (whether the results really do represent what they are supposed to measure).
If you are doing experimental research, you also have to consider the internal and external validity of your experiment.
Hypothesis testing is a formal procedure for investigating our ideas about the world using statistics. It is used by scientists to test specific predictions, called hypotheses , by calculating how likely it is that a pattern or relationship between variables could have arisen by chance.
Cite this Scribbr article
If you want to cite this source, you can copy and paste the citation or click the “Cite this Scribbr article” button to automatically add the citation to our free Citation Generator.
Bhandari, P. (2023, June 22). What Is Quantitative Research? | Definition, Uses & Methods. Scribbr. Retrieved August 29, 2024, from https://www.scribbr.com/methodology/quantitative-research/
Is this article helpful?
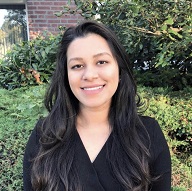
Pritha Bhandari
Other students also liked, descriptive statistics | definitions, types, examples, inferential statistics | an easy introduction & examples, "i thought ai proofreading was useless but..".
I've been using Scribbr for years now and I know it's a service that won't disappoint. It does a good job spotting mistakes”
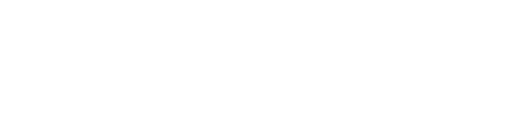
Archer Library
Nursing resources: finding quantitative research articles.
- Online Medical Reference E-Books
- Key word search or subject search?
- Finding Qualitative Research Articles
- Database Tutorials
- Finding Quantitative Research Articles
- Research Tools
- Copyright Resources This link opens in a new window
- Transcultural
- Public Health
- Evidence Based Nursing
What is Quantitative Research?
"Quantitative research is a systematic process used to gather and statistically analyze information that has been measured by an instrument. Instruments are used to convert information into numbers. It studies only quantifiable concepts (concepts that can be measured and turned into numbers)." It examines phenomenon through the numerical representation of observations and statistical analysis.
Langford, R. ( 2000). Navigating the Maze of Nursing Research . Elsevier.
Tips for Finding Quantitative Articles with a Keyword Search
If you want to limit your search to quantitative studies, first try "quantitative" as a keyword, then try using one of the following terms/phrases in your search (example: lactation AND statistics):
Correlational design*
Effect size
Empirical research
Experiment*
Quasi-experiment*
Reliability
- << Previous: Database Tutorials
- Next: Internet Resources >>
- Last Updated: Apr 16, 2024 2:41 PM
- URL: https://libguides.ashland.edu/nursing
Archer Library • Ashland University © Copyright 2023. An Equal Opportunity/Equal Access Institution.
- Privacy Policy

Home » Quantitative Research – Methods, Types and Analysis
Quantitative Research – Methods, Types and Analysis
Table of Contents

Quantitative Research
Quantitative research is a type of research that collects and analyzes numerical data to test hypotheses and answer research questions . This research typically involves a large sample size and uses statistical analysis to make inferences about a population based on the data collected. It often involves the use of surveys, experiments, or other structured data collection methods to gather quantitative data.
Quantitative Research Methods

Quantitative Research Methods are as follows:
Descriptive Research Design
Descriptive research design is used to describe the characteristics of a population or phenomenon being studied. This research method is used to answer the questions of what, where, when, and how. Descriptive research designs use a variety of methods such as observation, case studies, and surveys to collect data. The data is then analyzed using statistical tools to identify patterns and relationships.
Correlational Research Design
Correlational research design is used to investigate the relationship between two or more variables. Researchers use correlational research to determine whether a relationship exists between variables and to what extent they are related. This research method involves collecting data from a sample and analyzing it using statistical tools such as correlation coefficients.
Quasi-experimental Research Design
Quasi-experimental research design is used to investigate cause-and-effect relationships between variables. This research method is similar to experimental research design, but it lacks full control over the independent variable. Researchers use quasi-experimental research designs when it is not feasible or ethical to manipulate the independent variable.
Experimental Research Design
Experimental research design is used to investigate cause-and-effect relationships between variables. This research method involves manipulating the independent variable and observing the effects on the dependent variable. Researchers use experimental research designs to test hypotheses and establish cause-and-effect relationships.
Survey Research
Survey research involves collecting data from a sample of individuals using a standardized questionnaire. This research method is used to gather information on attitudes, beliefs, and behaviors of individuals. Researchers use survey research to collect data quickly and efficiently from a large sample size. Survey research can be conducted through various methods such as online, phone, mail, or in-person interviews.
Quantitative Research Analysis Methods
Here are some commonly used quantitative research analysis methods:
Statistical Analysis
Statistical analysis is the most common quantitative research analysis method. It involves using statistical tools and techniques to analyze the numerical data collected during the research process. Statistical analysis can be used to identify patterns, trends, and relationships between variables, and to test hypotheses and theories.
Regression Analysis
Regression analysis is a statistical technique used to analyze the relationship between one dependent variable and one or more independent variables. Researchers use regression analysis to identify and quantify the impact of independent variables on the dependent variable.
Factor Analysis
Factor analysis is a statistical technique used to identify underlying factors that explain the correlations among a set of variables. Researchers use factor analysis to reduce a large number of variables to a smaller set of factors that capture the most important information.
Structural Equation Modeling
Structural equation modeling is a statistical technique used to test complex relationships between variables. It involves specifying a model that includes both observed and unobserved variables, and then using statistical methods to test the fit of the model to the data.
Time Series Analysis
Time series analysis is a statistical technique used to analyze data that is collected over time. It involves identifying patterns and trends in the data, as well as any seasonal or cyclical variations.
Multilevel Modeling
Multilevel modeling is a statistical technique used to analyze data that is nested within multiple levels. For example, researchers might use multilevel modeling to analyze data that is collected from individuals who are nested within groups, such as students nested within schools.
Applications of Quantitative Research
Quantitative research has many applications across a wide range of fields. Here are some common examples:
- Market Research : Quantitative research is used extensively in market research to understand consumer behavior, preferences, and trends. Researchers use surveys, experiments, and other quantitative methods to collect data that can inform marketing strategies, product development, and pricing decisions.
- Health Research: Quantitative research is used in health research to study the effectiveness of medical treatments, identify risk factors for diseases, and track health outcomes over time. Researchers use statistical methods to analyze data from clinical trials, surveys, and other sources to inform medical practice and policy.
- Social Science Research: Quantitative research is used in social science research to study human behavior, attitudes, and social structures. Researchers use surveys, experiments, and other quantitative methods to collect data that can inform social policies, educational programs, and community interventions.
- Education Research: Quantitative research is used in education research to study the effectiveness of teaching methods, assess student learning outcomes, and identify factors that influence student success. Researchers use experimental and quasi-experimental designs, as well as surveys and other quantitative methods, to collect and analyze data.
- Environmental Research: Quantitative research is used in environmental research to study the impact of human activities on the environment, assess the effectiveness of conservation strategies, and identify ways to reduce environmental risks. Researchers use statistical methods to analyze data from field studies, experiments, and other sources.
Characteristics of Quantitative Research
Here are some key characteristics of quantitative research:
- Numerical data : Quantitative research involves collecting numerical data through standardized methods such as surveys, experiments, and observational studies. This data is analyzed using statistical methods to identify patterns and relationships.
- Large sample size: Quantitative research often involves collecting data from a large sample of individuals or groups in order to increase the reliability and generalizability of the findings.
- Objective approach: Quantitative research aims to be objective and impartial in its approach, focusing on the collection and analysis of data rather than personal beliefs, opinions, or experiences.
- Control over variables: Quantitative research often involves manipulating variables to test hypotheses and establish cause-and-effect relationships. Researchers aim to control for extraneous variables that may impact the results.
- Replicable : Quantitative research aims to be replicable, meaning that other researchers should be able to conduct similar studies and obtain similar results using the same methods.
- Statistical analysis: Quantitative research involves using statistical tools and techniques to analyze the numerical data collected during the research process. Statistical analysis allows researchers to identify patterns, trends, and relationships between variables, and to test hypotheses and theories.
- Generalizability: Quantitative research aims to produce findings that can be generalized to larger populations beyond the specific sample studied. This is achieved through the use of random sampling methods and statistical inference.
Examples of Quantitative Research
Here are some examples of quantitative research in different fields:
- Market Research: A company conducts a survey of 1000 consumers to determine their brand awareness and preferences. The data is analyzed using statistical methods to identify trends and patterns that can inform marketing strategies.
- Health Research : A researcher conducts a randomized controlled trial to test the effectiveness of a new drug for treating a particular medical condition. The study involves collecting data from a large sample of patients and analyzing the results using statistical methods.
- Social Science Research : A sociologist conducts a survey of 500 people to study attitudes toward immigration in a particular country. The data is analyzed using statistical methods to identify factors that influence these attitudes.
- Education Research: A researcher conducts an experiment to compare the effectiveness of two different teaching methods for improving student learning outcomes. The study involves randomly assigning students to different groups and collecting data on their performance on standardized tests.
- Environmental Research : A team of researchers conduct a study to investigate the impact of climate change on the distribution and abundance of a particular species of plant or animal. The study involves collecting data on environmental factors and population sizes over time and analyzing the results using statistical methods.
- Psychology : A researcher conducts a survey of 500 college students to investigate the relationship between social media use and mental health. The data is analyzed using statistical methods to identify correlations and potential causal relationships.
- Political Science: A team of researchers conducts a study to investigate voter behavior during an election. They use survey methods to collect data on voting patterns, demographics, and political attitudes, and analyze the results using statistical methods.
How to Conduct Quantitative Research
Here is a general overview of how to conduct quantitative research:
- Develop a research question: The first step in conducting quantitative research is to develop a clear and specific research question. This question should be based on a gap in existing knowledge, and should be answerable using quantitative methods.
- Develop a research design: Once you have a research question, you will need to develop a research design. This involves deciding on the appropriate methods to collect data, such as surveys, experiments, or observational studies. You will also need to determine the appropriate sample size, data collection instruments, and data analysis techniques.
- Collect data: The next step is to collect data. This may involve administering surveys or questionnaires, conducting experiments, or gathering data from existing sources. It is important to use standardized methods to ensure that the data is reliable and valid.
- Analyze data : Once the data has been collected, it is time to analyze it. This involves using statistical methods to identify patterns, trends, and relationships between variables. Common statistical techniques include correlation analysis, regression analysis, and hypothesis testing.
- Interpret results: After analyzing the data, you will need to interpret the results. This involves identifying the key findings, determining their significance, and drawing conclusions based on the data.
- Communicate findings: Finally, you will need to communicate your findings. This may involve writing a research report, presenting at a conference, or publishing in a peer-reviewed journal. It is important to clearly communicate the research question, methods, results, and conclusions to ensure that others can understand and replicate your research.
When to use Quantitative Research
Here are some situations when quantitative research can be appropriate:
- To test a hypothesis: Quantitative research is often used to test a hypothesis or a theory. It involves collecting numerical data and using statistical analysis to determine if the data supports or refutes the hypothesis.
- To generalize findings: If you want to generalize the findings of your study to a larger population, quantitative research can be useful. This is because it allows you to collect numerical data from a representative sample of the population and use statistical analysis to make inferences about the population as a whole.
- To measure relationships between variables: If you want to measure the relationship between two or more variables, such as the relationship between age and income, or between education level and job satisfaction, quantitative research can be useful. It allows you to collect numerical data on both variables and use statistical analysis to determine the strength and direction of the relationship.
- To identify patterns or trends: Quantitative research can be useful for identifying patterns or trends in data. For example, you can use quantitative research to identify trends in consumer behavior or to identify patterns in stock market data.
- To quantify attitudes or opinions : If you want to measure attitudes or opinions on a particular topic, quantitative research can be useful. It allows you to collect numerical data using surveys or questionnaires and analyze the data using statistical methods to determine the prevalence of certain attitudes or opinions.

Purpose of Quantitative Research
The purpose of quantitative research is to systematically investigate and measure the relationships between variables or phenomena using numerical data and statistical analysis. The main objectives of quantitative research include:
- Description : To provide a detailed and accurate description of a particular phenomenon or population.
- Explanation : To explain the reasons for the occurrence of a particular phenomenon, such as identifying the factors that influence a behavior or attitude.
- Prediction : To predict future trends or behaviors based on past patterns and relationships between variables.
- Control : To identify the best strategies for controlling or influencing a particular outcome or behavior.
Quantitative research is used in many different fields, including social sciences, business, engineering, and health sciences. It can be used to investigate a wide range of phenomena, from human behavior and attitudes to physical and biological processes. The purpose of quantitative research is to provide reliable and valid data that can be used to inform decision-making and improve understanding of the world around us.
Advantages of Quantitative Research
There are several advantages of quantitative research, including:
- Objectivity : Quantitative research is based on objective data and statistical analysis, which reduces the potential for bias or subjectivity in the research process.
- Reproducibility : Because quantitative research involves standardized methods and measurements, it is more likely to be reproducible and reliable.
- Generalizability : Quantitative research allows for generalizations to be made about a population based on a representative sample, which can inform decision-making and policy development.
- Precision : Quantitative research allows for precise measurement and analysis of data, which can provide a more accurate understanding of phenomena and relationships between variables.
- Efficiency : Quantitative research can be conducted relatively quickly and efficiently, especially when compared to qualitative research, which may involve lengthy data collection and analysis.
- Large sample sizes : Quantitative research can accommodate large sample sizes, which can increase the representativeness and generalizability of the results.
Limitations of Quantitative Research
There are several limitations of quantitative research, including:
- Limited understanding of context: Quantitative research typically focuses on numerical data and statistical analysis, which may not provide a comprehensive understanding of the context or underlying factors that influence a phenomenon.
- Simplification of complex phenomena: Quantitative research often involves simplifying complex phenomena into measurable variables, which may not capture the full complexity of the phenomenon being studied.
- Potential for researcher bias: Although quantitative research aims to be objective, there is still the potential for researcher bias in areas such as sampling, data collection, and data analysis.
- Limited ability to explore new ideas: Quantitative research is often based on pre-determined research questions and hypotheses, which may limit the ability to explore new ideas or unexpected findings.
- Limited ability to capture subjective experiences : Quantitative research is typically focused on objective data and may not capture the subjective experiences of individuals or groups being studied.
- Ethical concerns : Quantitative research may raise ethical concerns, such as invasion of privacy or the potential for harm to participants.
About the author
Muhammad Hassan
Researcher, Academic Writer, Web developer
You may also like

Qualitative Research – Methods, Analysis Types...

Survey Research – Types, Methods, Examples

Descriptive Research Design – Types, Methods and...

One-to-One Interview – Methods and Guide

Mixed Methods Research – Types & Analysis

Research Methods – Types, Examples and Guide

- Schools & departments

Advanced Quantitative Research in Education
The Advanced Quantitative Research in Education Thematic Hub builds interdisciplinary connections and creates grounds for cross-fertilisation among researchers using advanced quantitative methods in policy-relevant topics.
This is a space where academics with quantitative methods expertise and an interest in education issues come together to discuss their research; find support in addressing data, methodological and conceptual challenges; and develop collaborative and inter-disciplinary work on topics relevant to policy and practice. The Hub is open to researchers using mixed methods and from different substantive research areas.
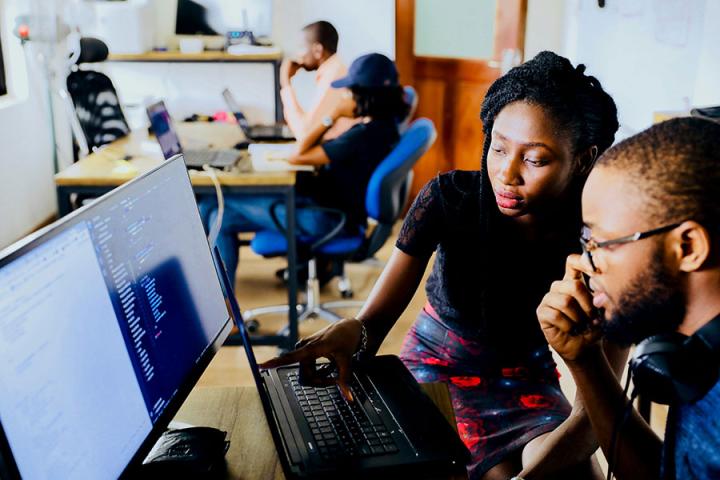
Our members' research interests span many areas, including:
- social inequalities in education and the labour market
- social mobility
- school-to-work transitions
- comparative education and international development
- teacher education
- higher education
- research on children and youth
Our methodological expertise includes:
the analysis of data from complex survey designs and linked administrative sources
- longitudinal data analysis
- multilevel modelling
- quasi-experimental approaches such as inverse probability weighting
- propensity score matching
- regression discontinuity
- latent variable modelling techniques
- log-linear modelling
Postgraduate research
See what research students in our Hub are working on, and find information about applying for a PGR programme at Moray House School of Education and Sport.
Research students in our Hub
- Find out about our current students' research on our 'Members' page
Applying to study with us
Find information about our programmes, finding a supervisor, writing a research proposal and applying for PGR study at Moray House School of Education and Sport.
- Learn more about applying for postgraduate research
AQRiE members
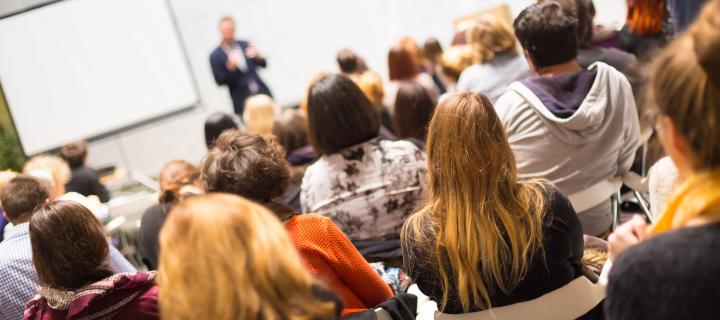
AQRiE events

Centres, Groups and Networks
- Centre for Statistics
The Centre for Statistics unites data-driven researchers from across the University of Edinburgh and Associated Institutions, building the capacity needed to address key scientific and societal challenges.
Comparative Education and International Development (CEID) Research Group
CEID serves as a hub for critical reflection on and evaluation of education's direct and indirect potential by exploring its complex, interdependent relationship with social and political contexts.
- Data for Children Collaborative with UNICEF
This partnership between UNICEF, The Scottish Government and the University of Edinburgh’s Data Driven Innovation Programme seeks to improve outcomes for children locally, nationally and globally. We draw on the strengths of all partners to bring insight and solve problems using data and data science techniques.
Edinburgh Centre for Data, Culture & Society (CDCS)
The Edinburgh Centre for Data, Culture & Society (CDCS) provides a locus for data-driven and digitally engaged research across the disciplines and forms the foundation of an open and inclusive community in which such research can grow and thrive.
- Edinburgh Centre for Data, Culture and Society (CDCS)
Edinburgh Q-STEP Centre
Our mission at Edinburgh Q-Step Centre is to equip students with the quantitative skills needed in tomorrow’s social scientists.
- Research-led Teacher Education Network (RTEN)
RTEN is an interdisciplinary network for dialogue and collaboration in research on teachers, teaching and teacher education.
- Research Training Centre
At the Research Training Centre, we apply expertise in a diverse set of social science methods through teaching and research to contribute new insights to the key challenges in society.
- Understanding Inequalities
Understanding Inequalities (UI) is an innovative and ambitious programme of interdisciplinary research funded by the Economic and Social Research Council (ESRC) which provides robust evidence to inform and develop new policy solutions to tackle multiple forms of inequality.
Get in touch
Follow us on X @AQRiE_Hub
- Open access
- Published: 28 August 2024
Perspectives of midwives on the use of Kaligutim (local oxytocin) for induction of labour among pregnant women in the government hospitals in Tamale
- Ahmad Sukerazu Alhassan 1 ,
- Shivera Dakurah 2 &
- Joseph Lasong 1
BMC Pregnancy and Childbirth volume 24 , Article number: 561 ( 2024 ) Cite this article
Metrics details
The use of herbal medicine and/or its products is common throughout the world. In Tamale Metropolis, pregnant women frequently use local oxytocin to induce labour, as shown by the fact that 90% of midwives reported managing patients who used kaligutim (local oxytocin) to speed up labour. Early career midwives are also aware of this and have personally observed it being used by their clients. The purpose of the study was to assess midwives’ opinions on pregnant women’s use of the well-known kaligutim (local oxytocin) for labour induction in the Tamale Metropolis.
A facility-based, quantitative, cross-sectional research design was used for the study. A total of 214 working midwives from Tamale’s three main public hospitals participated. Data for the study were gathered through a standardized questionnaire. For the analysis and presentation of the data, descriptive and analytical statistics, such as basic frequencies, percentages, Fisher’s exact test, chi square test and multivariate analysis, were employed.
According to the findings of this study, the safety, dosages, and contraindications of kaligutim during pregnancy and labour are unknown. The cessation of contractions was reported by 44 (22.4%) of the respondents whose clients used local oxytocin. The study also revealed that women in Tamale metropolis use “walgu”, a spiritual form of oxytocin, to induce and augment labour. Respondents who responded, “yes” to baby admission to the new-born care unit were 25% more likely to use kaligutim (local oxytocin) than were those who responded, “no” to baby admission to the new-born care unit (AOR = 0.25 95% CI (0.01, 0.53), P = 0.021).
Conclusions
It can be concluded that using kaligutim to start labour has negative effects on both the mother and the foetus. Additional research is required to evaluate the efficacy, effectiveness, biochemical makeup, and safety of these herbal medicines, particularly during pregnancy and delivery, as well as the spiritual significance of kaligutim (Walgu) and its forms.
Peer Review reports
Introduction
Herbal medicines, traditional treatments, and traditional practitioners are the main source of health care for many millions of people, and sometimes the only source of care [ 1 ]. Herbal medicines include herbs, herbal materials, herbal preparations and finished herbal products, that contain as active ingredients parts of plants, or other plant materials, or combinations [ 1 , 2 ]. Women in both developed and developing countries use herbal medicine before pregnancy and during pregnancy and delivery, which has several consequences [ 3 ]. The use of herbal medicine has a long history, tracing its roots back to ancient and biblical days when there was no Orthodox medicine. Currently, both developed and developing countries use herbal medicine due to the presence of many traditional medicine practitioners [ 4 ].
Many cultures worldwide use herbal medicine to induce or accelerate labour, and the incidence of labour induction to shorten the duration of labour is on the rise. Most herbal medicine users are pregnant women who have no formal education, who have a low level of income and who mostly stay far from health facilities [ 5 ]. The majority of pregnant women use herbal medicine through the oral route and have confidence in its efficacy, safety and effectiveness [ 6 ]. Herbal medicine is used by women for maternal health-related issues, such as to induce abortion and labour, to correct infertility, for the treatment of pregnancy-related issues, for breast milk secretion and for general wellbeing during pregnancy [ 5 ].
Women who use herbal medicine during pregnancy and/or labour usually have a high risk of postpartum complications [ 7 ]. The use of herbal uterotonics can lead to hyperstimulation of the uterus, foetal asphyxia and several other adverse effects of labour [ 8 ]. Moreover, traditional medicine used by pregnant women is associated with several complications, including a ruptured uterus, a fresh still birth, a macerated still birth, a caesarean section and even death [ 9 ]. These herbal medicines have both uterotonic and nonuterotonic effects on labour and delivery and are mostly used to induce or augment labour in prolonged labour or postdate or to relax or widen the pelvis for delivery [ 8 ].
Maternal and neonatal deaths are still major challenges for most developing countries, with obstetric complications, especially postpartum haemorrhage (P.P.H.) being the major cause of maternal mortality [ 10 ]. The delivery of healthcare services is still poor quality in developing nations [ 11 ]. Maternal and foetal mortality and morbidity have remained high due to inadequate health services and inadequate emergency obstetric treatment. Childbirth is accompanied by numerous customs that are subject to ethnological research and are often rooted in traditional medicine or religion. Cultural influences and sociodemographic characteristics play an important role in a woman’s decision to seek maternal and child health services.
The induction of labour is the process of artificially starting labour by stimulating the uterus with oxytocin or manually through the rupture of amniotic membranes. This process is usually not risk free, and most women find it to be uncomfortable [ 12 ]. The induction of labour is an obstetric procedure recommended when the benefits to the baby and mother outweigh the benefits of continuing the pregnancy. The procedure usually involves complications and failures and must be performed under close monitoring, proper selection of clients and good preparation [ 13 ].
Labour induction also changes the normal physiological processes that accompany childbirth and increases the risk of adverse pregnancy outcomes such as postpartum haemorrhage, neonatal mortality, foetal distress, uterine rapture and premature birth [ 14 ]. Oxytocin is a natural hormone produced by the hypothalamus and is responsible for the activation of sensory nerves during labour and breastfeeding [ 15 ]. Clinically, commercially manufactured synthetic oxytocin is administered to commence or increase uterine activity to reduce the duration of labour [ 16 ].
The induction of labour is not free from risk and must be performed with caution because the procedure involves hyperstimulation of the uterus and foetal distress. Herbal medicine used by pregnant women has long-term effects on both mothers and babies [ 17 ]. Many pregnant women in the Tamale Metropolis use prepackaged herbal medicine before and during pregnancy [ 18 ]. Health-related factors such as cost, distance, access and unavailability of medications influence the utilization of herbal medicine by pregnant women [ 17 ].
All women should be given a prophylactic dose of oxytocin as soon as they give birth. If they start to haemorrhage, they should also be given a treatment dose of oxytocin, which is greater than the prophylactic dose [ 19 ]. There is also a traditional manufactured form of oxytocin (kaligutim) that pregnant women use to start labour. Kaligutim is the local name for the mixture of some special plant parts or a combination of plants prepared and given to pregnant women to start or accelerate the process of labour in the northern part of Ghana [ 17 ].
Ideally, women should take medical drugs during pregnancy (folic acid and fersolate) to help prevent birth defects and congenital malformations such as neural tube defects of the foetus and spinal bifida during pregnancy [ 20 ]. However, in recent decades, women worldwide have used herbal medications during pregnancy and labour, with some taking both herbal medicine and orthodox medicine at the same time [ 21 ]. However, little is known about the use and safety of these medicines, especially during pregnancy, and their dosages, indications and contraindications are not known [ 22 ].
There are studies on herbal medicine use by women during pregnancy and labour, but there is currently no literature on the use of Kaligutim (local oxytocin) for labour induction among pregnant women in Ghana, but similar studies have been conducted in Uganda, Malawi, Tanzania, and Nigeria. Despite the efforts of the government and other nongovernmental organizations to ensure maximum coverage of skilled delivery to help reduce maternal and neonatal mortalities, women still use locally prepared oxytocin to induce labour. Although herbal medicine is commonly used by pregnant women, healthcare providers, especially midwives, are often unprepared to communicate effectively with patients or make proper decisions concerning complementary and alternative medicine use, especially during pregnancy and labour [ 23 ].
It is well known that herbs have played a vital role since the precolonial era during pregnancy, delivery and postpartum care in many parts of the country, but there are still few data on the use of herbs among pregnant women in Ghana [ 24 ]. Towards the end of pregnancy, many women are tired and eager to welcome their babies into the world. Moreover, as the expected date of delivery approaches, these women are given local oxytocin by their mothers’ in-laws, grandmothers, mothers, or TBAs or even by the women themselves to start labour at home before going to the health facility [ 25 ].
Medicinal plants that are used to hasten or speed up labour are mostly taken towards the end of pregnancy or the beginning of labour [ 26 ]. Even after delivery, these herbs may be found in small amounts in the mother’s breast, and some may cross the placental barrier and have harmful effects on the baby. The use of herbal medication by pregnant women is inevitable given that up to 80% of people who live in developing nations rely on traditional medicine for their healthcare needs [ 18 ].
The situation in Ghana, especially Northern Ghana, is not different, as pregnant women continue to use herbs despite the availability of health facilities [ 24 ]. The use of herbal medicine (kaligutim) among the Ghanaian population is alarming. Pregnant women in Tamale use herbal products at a rate of 42.5% prior to pregnancy and 52.7% during pregnancy [ 27 ]. Residents of Tamales who seek healthcare services in hospitals or herbal clinics are therefore at a greater risk of experiencing adverse consequences from drug-herb interactions [ 28 ].
Herbal product manufacturers should clearly state that pregnancy is a contraindication, and vendors should use caution when selling these items to pregnant women [ 27 ]. The use of Kaligutim (local oxytocin) by pregnant women is a maternal and child health problem. Herbal medicine used by pregnant women has long-term effects on both mothers and babies [ 17 ]. Unfortunately, maternal, and neonatal deaths may occur, and hence, there is a need to examine midwives’ perspectives on local oxytocin use during labour, its effects on the progress and outcome of labour, and the relationship between kaligutim use and birth outcomes among pregnant women in the three major government hospitals in Tamale Metropolis.
Theoretical foundation
This study adopted and adapted Andersen’s (1968) behavioural model of healthcare service utilization (use and nonuse of health services [ 29 ]. Andersen’s healthcare utilization model is a conceptual model aimed at demonstrating the factors that lead to the use/nonuse of health services [ 29 ]. This study was guided by Andersen’s behavioural model of health service use as a theoretical framework to identify the effects of Kaligutim on the progress and outcome of labour and to establish the relationship between the use of Kaligutim and nonuse of kaligutim and birth outcomes. The behavioural model is a multilevel model that incorporates both individual and contextual determinants of health service use.
Conceptual framework
Many people rely on products made from medicinal plants to maintain their health or treat illness, and current general development trends in developing and developed countries suggest that the consumption of medicinal plants is unlikely to decline in the short to medium term because of the benefits to consumers, producers, and society as a whole [ 29 ]. Therefore, there is a need to increase our understanding of what motivates the consumption of medicinal plants, despite the barriers to the establishment of solid evidence on the safety and efficacy of herbal medicines and related products [ 29 ].
This unified conceptual framework offers a step towards establishing a comprehensive approach to understanding the experiences midwives encounter when their clients use herbal medicine to induce their labour. The exposure variable in this study refers to kaligutim (local oxytocin) used by pregnant women in the three major government hospitals to induce labour through several routes, including oral, rectal, and vaginal routes, among others. When oxytocin is used by pregnant women, it can produce several results that can be immediate or late.
The results elicited on labour are termed the outcome variables, which can be immediate outcomes (the progress of labour) or outcomes after delivery (the outcome of labour). The progression of labour includes three stages: progressive dilatation of the cervix from 1 cm to 10 cm, delivery of the baby and expulsion of the placenta. Several factors can be used to determine the progress of labour (obstructed labour, prolonged labour, nature of uterine contractions, precipitated labour, foetal distress, and poor progress of labour).
The outcome of labour on the hand refers to what happens during the delivery of the baby, how the baby was delivered, foetal conditions and maternal conditions. The following factors were used for the purpose of this study to determine the outcome of labour (mode of delivery, postpartum haemorrhage, ruptured uterus, cervical tear, birth asphyxia, uterine atony, maternal mortality, and neonatal mortality). This study focused on the immediate effects of Kaligutim (on labour progress) and the effects of Kaligutim after delivery (on labour outcomes) and the relationship between the use of Kaligutim and birth outcomes.
The study was carried out in Tamale, which is the capital city of the northern region of Ghana. According to the 2021 World Urbanization Review, Tamales has an estimated population of 671,812 people. Tamale still has a blend of typical rural and urban communities, although it has attained the status of a metropolitan area. There are three major government hospitals in Tamale: Tamale Central Hospital, Tamale West Hospital and Tamale Teaching Hospital. The Tamale Teaching Hospital is the only tertiary facility in the northern region and serves as the main reference centre for the five regions of the north.
Study population
The main study population was midwives working in Tamale Metropolis. The sampling frame was all midwives practicing in the three major hospitals in Tamale Metropolis who were willing to participate in the study.
Study design
A facility-based cross-sectional research design was used for this study. A cross-sectional study is a type of observational study design carried out at one point in time or over a short period of time to estimate the prevalence of the outcome of interest for a given population for the purpose of public health planning [ 30 ]. This study adopted a quantitative research approach to obtain information.
Sampling technique
A purposive sampling technique was used for this study. Purposive sampling is a nonprobability sampling method in which participants are selected for inclusion in the sample based on their characteristics, knowledge, or experiences. This is because of the midwives’ knowledge, experiences, and objective of the study.
Sample size calculation
Total number of midwives = 458
Yamane formula (1967) was used with a confidence interval of 95% and a margin of error of 5%.
N = population size (458).
n = the sample size (?)
e = margin of error (5%).
n \(\:=\frac{N}{1\:+N\left(e\right)2}\)
n= \(\:\frac{458}{1\:+458\left(0.05\right)2}\)
n = 214.01 = 214.
Sample size = 214 midwives.
Inclusion criteria
All midwives practicing in the three major government hospitals in Tamale Metropolis who were willing to participate in the study were included.
All midwives in the three-government hospital with experience with kaligutim use during labour were also included in the study.
Exclusion criteria
All midwives who were not practising at the three major government hospitals in Tamale Metropolis were excluded from the study.
Midwives who were practicing at the three major government hospitals in Tamale and who were not willing to participate in the study were also excluded from the study.
All midwives who did not have experience with kaligutim use for the induction of labour were excluded from the study.
Data collection instrument
The data collection tool that was used for the study was a standardized questionnaire. The questionnaire was constructed by reviewing various documents, including existing questionnaires that have been used in previous research. Close-ended questions with few open-ended questions were used as the question format. It was designed in line with the objectives of the study to help obtain the necessary information needed for the study. The questionnaire was pretested with midwives before the actual data collection took place.
Data management and analysis
Data collected from the field were coded, cleaned, and entered into the Statistical Package for Social Services (SPSS) version 21.0. Descriptive and analytical statistics, including simple frequencies and percentages, were used for the analysis and presentation of the data. The relationships between predictor and outcome variables were assessed by means of bivariate (chi-square test) analysis to determine potential predictors of kaligutim (local oxytocin) at p values less than 0.05. Adjusted odds ratios were reported, and p values less than 0.05 were deemed to indicate statistical significance at the 95% confidence level after multivariate analysis.
Ethical consideration
The following ethical principles guided this study: respect for persons, beneficence, and justice for all. These principles are based on the human rights that must be protected during any research project, including the right to self-determination, privacy, anonymity, confidentiality, fair treatment and protection from discomfort and harm. First, an introductory letter was obtained from the University for Development Studies authorities. This letter was then presented to the authorities of the three major government hospitals in Tamale, namely, Tamale West Hospital (T.W.H.), Tamale Central Hospital (T.C.H.) and Tamale Teaching Hospital (TTH.), to seek permission to undertake the study. Ethical clearance was also obtained from the Kwame Nkrumah University of Science and Technology (KNUST) (CHRPE/AP/332/22).
Permission was once sought through a consent form to which participants were asked to consent if they were willing to participate in the study. The participants were assured of the confidentiality of all the information they were going to provide. They were also encouraged to participate in the study as much as they could but were also made aware that the study was voluntary and that they could withdraw at any point in time during the process if needed. There was no compensation for the study participants.
The study revealed that 45% of the respondents were between the ages of 20 and 30. Most of the respondents were in their twenties or thirties. Those who were in the first half of their work life constituted 73% of the respondents, while 17% were in the second half of their working life. The majority of the respondents were diploma midwives, representing 48% of the respondents; post basic midwives, constituting 32%; and degree and master’s holders, representing 19% and 1%, respectively. Staff midwives composed the largest group of respondents, while Principal Midwifery officers composed the group with the lowest participation in the study. The lowest rank in midwifery practice in the study was staff midwives, and the highest was principal midwifery officers. This is presented in Table 1 .
The experience of using local oxytocin to induce labour
Approximately 90% of the respondents have prior knowledge or heard that some of their clients take local oxytocin at home to start labour, and only 10% of respondents have no prior knowledge of that. Approximately 63.4% of the respondents encountered local oxytocin cases more than three times every week. This is presented in Fig. 1 .
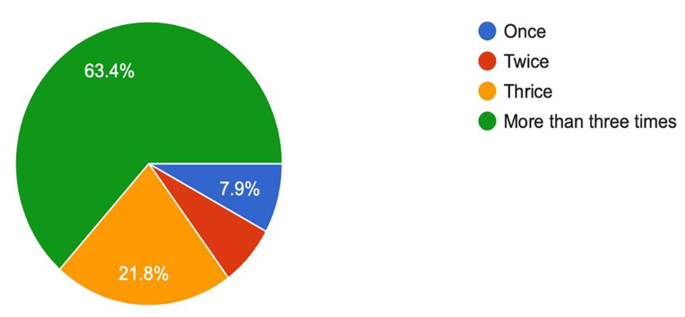
Average number of local oxytocin cases per week
Approximately 72.9% of the respondents said that their clients had ever induced labour during the previous C/S, and 59.6% of the respondents said that they met clients who also induced their labour during twin pregnancy. Another 64.5% of the respondents said that they also met clients with large babies who also induced labour using local oxytocin, while 86.2% of the respondents said that they also met clients who induced labour with local oxytocin even when they had grand multiparity. Another 11.3% of the respondents said that they met clients who used local oxytocin to induce labour during transverse lies, and 15.3% of the respondents said that they had experienced when clients with mal presentations used local oxytocin to induce labour. This is presented in Fig. 2 .
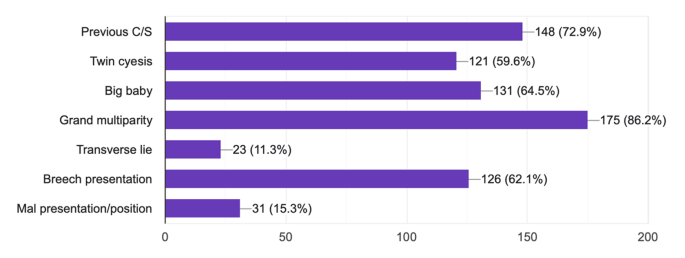
Induction of labour by clients through local oxytocin under certain conditions
The study additionally asked midwives to report on how pregnant women who had taken local oxytocin to induce labour coped during their care. Midwives were expected to respond whether the women they cared for experienced good, difficult, bad, painful, life-threatening, terrible, or normal labour. As shown in Fig. 3 , generally, the experience that pregnant women experience when they use local oxytocin to induce labour is not good. A total of 93.5% of the respondents said that the women who used local oxytocin had very bad experiences.
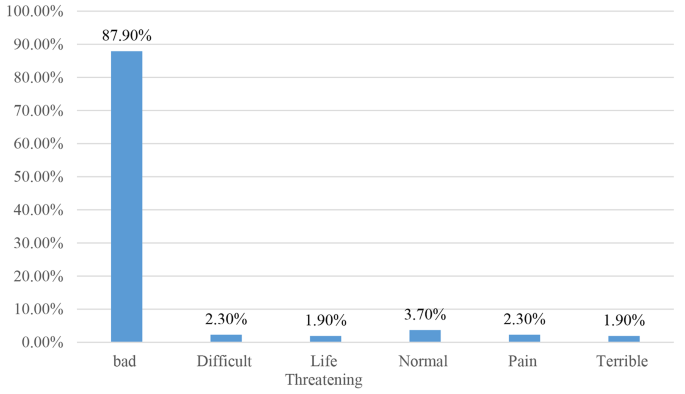
Experience of using local oxytocin to induce labour
The study further revealed that 15.2% of the respondents had experienced situations where some pregnant women died because of the use of local oxytocin.
Effects of local oxytocin on the progress of labour
The effects of local oxytocin (Kaligutim) on the progress of labour were diverse. The study revealed that the effects of Kaligutim on the progress of labour are negative, as it causes prolonged labour for some, obstructed labour for others, precipitated labour, and poor progress of labour for others. With obstructed labour being the leading effect of kaligutim on the progress of labour, most of the respondents chose caesarean section as the preferred delivery for most clients who used kaligutim at birth. The use of local oxytocin also has some effect on the amniotic fluid of pregnant women, as 99% of the midwives who responded to the study said that there were some levels of stain of the amniotic fluid, and only 1% said it was clear. It is evident from the study that for most pregnant women who use local oxytocin, there is hyperstimulation of the uterus, as most of the midwives confirmed this for the study. Most pregnant women who use kaligutim suffer excessive contractions, which could have an effect on both mothers and babies. Again, more than half (53.75) of the respondents also said that their foetal heart rate was above 160 bpm. The majority (77.65) of the respondents said that there was no cessation of the contractions for those who took the local oxytocin. The results are presented in Table 2 .
Impact of local oxytocin on the outcome of labour
To understand how local oxytocin impacts labour, the study went further to ask participants what the mode of delivery was for those who used Kaligutim. According to the data, caesarean section is the mode of delivery for most women (56.5%) who use local oxytocin, and most are unable to achieve spontaneous delivery. This has contributed to the increasing number of caesarean sections recorded daily. Most of the babies had an Apgar score of 4/10 to 6/10. Many babies born to mothers who used herbal oxytocin were born with moderate birth asphyxia (69.6%) and severe birth asphyxia (24%). The study also reported that 20.8% of midwives reported that hysterectomy was carried out on their clients who had used herbal preparations to induce or hasten labour. This is alarming because many women have their uterus removed as a result of herbal oxytocin (kaligutm) usage. Most clients who used Kaligutim experienced postpartum haemorrhage after delivery. It was also evident that some pregnant women (34.5%) had uterine atony, although it cannot be said that Kaligutim was the cause of uterine atony. Several pregnant women (65.3 years old) who used Kaligutim also developed a ruptured uterus. See Table 3 .
Relationship between kaligutim (local oxytocin) use and birth outcome
Table 4 shows the associations between kaligutim (local oxytocin) use and birth outcomes among the respondents. Fisher’s exact test and the chi-square test showed that several birth outcome variables were significantly associated with kaligutim (local oxytocin). Do women who go through the normal process of labour and those who use kaligutim to induce their labour have the same birth outcome? (P value = 0.021), what was the foetal wellbeing? (P value = 0.041), When do most neonates whose mothers have taken Kaligutim die? (P value = 0.038), was baby admitted at the Newborn Care Unit? (P value = 0.001), were significantly associated with kaligutim. Additionally, having recorded a maternal death because of the use of Kaligutim (p value = 0.002) was also significantly associated with kaligutim, as presented in Table 4 .
Multivariate analysis of birth outcome predictors of Kaligutim (local oxytocin) among pregnant women in three major government hospitals in Tamale metropolis
In Table 5 , three birth outcome variables strongly depicted kaligutim use among the respondents: foetal wellbeing, admission to the new-born care unit, and death of most neonates because of the use of Kaligutim by their mothers. Respondents who responded, “yes” to baby admission to the Newborn Care Unit were 25% more likely to use kaligutim (local oxytocin) than were those who responded “no” to baby admission to the Newborn Care Unit [(AOR = 0.25 95% CI (0.01, 0.53), P = 0.021)].
Discussions
Although the respondents cut across with regard to the number of years of experience, most of the respondents were early career midwives. The fact that these early career midwives are familiar with and have experienced the use of local oxytocin by their clients shows that it is widely used by pregnant women in the Tamale metropolis. Approximately 90% of respondents were aware of the usage of kaligutim (local oxytocin) for inducing labour at home before going to the hospital for delivery. However, a study conducted in the Ashanti region of Ghana revealed that midwives and other healthcare professionals lack proper knowledge about herbal medicine usage among pregnant women, even though this information is urgently needed so that appropriate action may be taken to address the issue [ 31 ]. The study findings also demonstrated that pregnant women frequently utilize local oxytocin and that many of them are unaware of the potential negative effects that these herbs may have on them in certain circumstances. Figure 2 shows that the use of local oxytocin was not limited to only one condition. These findings further show that the use of local oxytocin by pregnant women is widespread and that pregnant women do not know the effect that local oxytocin can have on them when they have certain conditions. Additionally, pregnant women are ignorant of the fact that local oxytocin can be contraindicated under certain conditions and must be avoided. Hence, it may put the life of the pregnant mother and her baby in danger.
Although herbal medicines are natural, not all herbs are safe to use while pregnant. Thus, expectant mothers should consult their midwives for guidance before taking herbal remedies. The experience that pregnant women have when they use local oxytocin to induce labour is not a positive one. A total of 188 respondents, or 93.5% of the respondents, stated that the women who used local oxytocin had a very unpleasant experience. This is supported by additional research results showing that between 50 and 80% of pregnant women use traditional plant remedies, which could have adverse perinatal effects [ 32 ]. The statistics indicate that local oxytocin is frequently used by pregnant women in the Tamale Metropolis. Most of the midwives reported seeing these cases virtually daily. This finding supports a study conducted in Ghana’s Ashanti region (Kumasi), which revealed that knowledge of herbal medicine is widely shared and that there is evidence of an increase in the usage of herbs [ 33 ].
The study revealed that local oxytocin (Kaligutim) has a diverse range of effects on the progress of labour, including precipitating labour, prolonging labour, obstructing labour, and slowing labour. The partograph is a great tool for keeping track of labour progress and serving as a warning system for abnormalities in normal labour, which helps to prevent obstructed labour and improves maternal and foetal outcomes [ 34 ]. This is supported by the study’s findings, which indicate that using a partograph to monitor labour progress and identify any deviations is essential [ 34 ].
According to this study, most midwives, who make up 65.2% of the respondents, also claimed that pregnant women who use local oxytocin (Kaligutim) have excessive contractions, while only 71 of them, or 34.8% of the respondents, claimed that they do not notice excessive contractions in their clients. This is supported by research performed in Zambia, which revealed that these herbal medicines also elicit greater than normal uterine contractions [ 26 ].
Most pregnant women who use kaligutim experience excessive contractions, which may have an impact on both the mother and the unborn child. Similarly, other authors have also claimed that using herbal remedies during labour causes stronger and more frequent uterine contractions, which do not necessarily result in cervical dilatation [ 35 ]. This was confirmed in the study’s findings, which also noted that herbal oxytocin not only produces excessive uterine contractions but also may cause contractions to cease, as 44 (22.4%) of the respondents reported that those who took local oxytocin had a halt in contractions. Intravenous fluids such as normal saline and Ringer’s lactate are used to flush out the local oxytocin in the system and CS in the case of an emergency. Nifedipine is also given in certain circumstances to prevent contractions.
According to the study, 121 midwives, or 59.6% of the respondents, stated that caesarean sections were the preferred method of delivery for women who used kaligutim to induce labour. Both [ 36 ] in South Africa and [ 34 ] in Western Uganda reported these findings. Moderate birth asphyxia (69.6%) and severe birth asphyxia (23%) are common in newborns whose mothers utilize herbal oxytocin. According to the survey, 20.8% of midwives said they had performed hysterectomy procedures on clients who had utilized herbal induction or hastening methods to induce labour.
One of the main causes of maternal deaths worldwide, including in Ghana, is postpartum haemorrhage [ 10 ]. 91% of midwives said that when their patients use herbal oxytocin during labour, more of them suffer from postpartum haemorrhage. This is corroborated by research by Frank (2018), who found a connection between postpartum haemorrhage and the use of herbal medications during labour [ 37 ]. In contrast, other studies [ 38 ] have shown that using herbal medication during childbirth is linked to a lower risk of postpartum haemorrhage. Individuals who experienced postpartum haemorrhage were managed with uterine massage, intravenous fluids, Cytotec, repairs to tears, expulsion of retained products, blood transfusions, cervical repairs, and catheter use.
This report supports the findings of a study conducted in the Ugandan village of Kiganda, where the researcher [ 37 ] reported that the use of herbal medicines has been linked to labour induction, which can cause significant birth canal tearing, postpartum haemorrhage, uterine atony, a raptured uterus, and, if untreated, maternal mortality. Medical experts who are aware of the dangers of herbal remedies and who are obliged to advise patients against using them do so themselves. The majority of women who use herbal preparations during pregnancy have a high school education or higher, according to evidence showing that more than 57.5% of pregnant women who use herbs have a high school diploma or higher, which is consistent with findings from Saudi Arabia by [ 39 ] that show that formal education cannot even prevent women from taking herbs during pregnancy and labour.
Kaligutim also causes excessive uterine contractions, foetal discomfort, excessive uterine stimulation, uterine atony, PPH, birth hypoxia, and premature bearing down, claims this study. This is supported by the results of a study carried out in Europe, where researchers [ 40 ] found that the majority of herbal drugs taken by pregnant women have undesirable side effects. An Iranian study, however, revealed that utilizing herbal treatments during labour can lessen discomfort, speed up the process, and enhance both the quality of a woman’s delivery experience and her odds of having a healthy baby [ 41 ].
According to the study’s findings, three birth outcome variables strongly affected kaligutim (local oxytocin) use among the respondents: foetal wellbeing, admission to the newborn care unit, and death of most neonates as a result of the use of Kaligutim by their mothers. Respondents who responded, “yes to baby” and were admitted to the new-born care unit were 25% more likely to use kaligutim (local oxytocin) than were those who responded, “no to baby” and were admitted to the new-born care unit (AOR = 0.25 95% CI (0.01, 0.53), P = 0.021). This is probably one of the effects of taking local oxytocin. These infants were hospitalized for a variety of reasons, including asphyxia, respiratory distress, and low Apgar scores.
Additionally, the study results indicated that respondents who responded that a still birth outcome affected foetal wellbeing were 1.9 times more likely to use kaligutim (local oxytocin) than those who responded no to having live births were (AOR = 1.9 95% CI (0.01, 1.21), P = 0.047)]. This finding is consistent with findings from a sub-Saharan African study that showed that herbal medications used to speed up and induce labour have uterotonic effects and increase the risk of neonatal asphyxia attributable to uterine hyperstimulation [ 42 ]. This could be ascribed to the fact that the respondents wanted fast and easy delivery, which subsequently caused this effect.
Another interesting finding was that respondents who responded that having a birth asphyxia outcome to foetal wellbeing were 0.16 times more likely to use kaligutim (local oxytocin) than were those who responded no to having live births (AOR = 0.16, 95% CI (0.08, 3.08), P = 0.047). This result is similar to that of [ 42 ], who conducted their study in sub-Saharan Africa. This could be a result of the effects of kaligutim on foetal well-being, which results in birth asphyxia.
Furthermore, newborns whose mothers used kaligutim during labour and who died within the first hour of birth were 3.4 times more likely to use kaligutim (local oxytocin) than those whose mothers used kaligutim during labour [AOR = 3.4 95% CI (0.74, 1.5), P = 0.045]. In support of the findings from this study, a study on the consumption of herbal drugs among pregnant women in rural Malawi revealed that consumption was linked to pregnancy-related issues and that users had a greater risk of neonatal mortality/morbidity within the first hour of life than nonusers [ 14 ]. This could be attributed to the dangers this herb poses to the foetus during delivery.
Newborns whose mothers used kaligutim during labor and who died within the first week of life were 2.23 times more likely to use kaligutim (local oxytocin) than those whose mothers used intrauterine kaligutim [(AOR = 2.23 95% CI (0.00, 0.02), P = 0.045)]. This is supported by findings from a Malawian study that revealed that the use of labour-inducing plants during pregnancy has negative effects on obstetric and labour outcomes, such as uterine rapture, which can cause neonatal mortality and morbidity [ 35 ]. This could be attributed to the fact that PPH, uterine rapture, cervical tear, DIC, and hypoxia were the main causes of death.
Every life matter, which is why mothers’ lives and that of their newborn babies must be safeguarded at all costs. A sufficient level of knowledge is always vital since it exacerbates doubt. Therefore, it is crucial that people are informed of their rights, their health, and the services they can utilize to maintain and improve health to have a healthy increasing population. Although herbal medicine could be effective in treating certain ailments associated with pregnancy and delivery and is easily accessible to pregnant women, especially in rural communities, the possibility of overdose, drug-herb interactions, contraindications, and the unhygienic conditions under which they are prepared may influence both maternal and neonatal conditions.
The results showed that the use of kaligutim by pregnant women in Tamale Metropolis is on the rise. This means that much needs to be done to do away with the use of kaligutim, and this must start with midwives. Pregnancies and births can be improved with a healthy and qualified midwifery care model in improving and protecting women’s and newborn health in Tamale.
It can be concluded that the use of this herbal medicine (Kaligutim) poses a greater long-term health challenge for mothers and their babies. Midwives and other healthcare workers in the Tamale Metropolis must therefore intensify their public health campaigns against the use of Kaligutim for labour induction.
Recommendations
The findings of the study have important implications for maternal and child health. The nonuse of kaligutim (local oxytocin) for the induction of labour is the best option for pregnant women. Pregnant women should visit the hospital for all their health needs during the entire pregnancy. This will help prevent adverse pregnancy and labour outcomes as well as maternal and neonatal mortalities and morbidities.
Future researchers should perform further studies on the spiritual aspects of kaligutim (Walgu) and its types. Like synthetic oxytocin, an Islamic form of oxytocin is prepared by Mallams and causes uterine contractions and dilates the cervix.
However, studies should also be conducted on the efficiency, effectiveness and biochemical composition of these herbal preparations and their safety, especially during pregnancy and delivery. Samples of these herbal preparations should be taken for laboratory investigations.
Data availability
All data generated or analysed during this study are included in this article and its supplementary information files are available from the corresponding author on reasonable request.
World Health Organization. WHO traditional medicine strategy: 2014–2023. World Health Organization;2013. Accessed 20 Nov 2023.
Fokunang CN, Ndikum V, Tabi OY, Jiofack RB, Ngameni B, Guedje NM et al. Traditional medicine: past, present and future research and development prospects and integration in the National Health System of Cameroon. Afr J Trad Complement Alt Med. 2011;8(3).
John LJ, Shantakumari N. Herbal medicines use during pregnancy: a review from the Middle East. Oman Med J. 2015;30(4):229.
Article CAS PubMed PubMed Central Google Scholar
Mekuria AB, Erku DA, Gebresillassie BM, Birru EM, Tizazu B, Ahmedin A. Prevalence and associated factors of herbal medicine use among pregnant women on antenatal care follow-up at University of Gondar referral and teaching hospital, Ethiopia: a cross-sectional study. BMC Complement Alt Med. 2017;17:1–7.
Google Scholar
Shewamene Z, Dune T, Smith CA. The use of traditional medicine in maternity care among African women in Africa and the diaspora: a systematic review. BMC Complement Alt Med. 2017;17:1–6.
Nyeko R, Tumwesigye NM, Halage AA. Prevalence and factors associated with use of herbal medicines during pregnancy among women attending postnatal clinics in Gulu district, Northern Uganda. BMC Preg Childbirth. 2016;1–12.
Fukunaga R, Morof D, Blanton C, Ruiz A, Maro G, Serbanescu F. Factors associated with local herb use during pregnancy and labor among women in Kigoma region, Tanzania, 2014–2016. BMC Preg Childbirth. 2020;20:1–1.
Article Google Scholar
Tripathi V, Stanton C, Anderson FW. Traditional preparations used as uterotonics in Sub-saharan Africa and their pharmacologic effects. Int J Gynecol Obstet. 2013;120(1):16–22.
Maliwichi-Nyirenda CP, Maliwichi LL. Medicinal plants used to induce labour and traditional techniques used in determination of onset of labour in pregnant women in Malawi: a case study of Mulanje district. J Med Plants Res. 2010;4(24):2609.
Amanuel T, Dache A, Dona A. Postpartum Hemorrhage and its Associated factors among women who gave birth at Yirgalem General Hospital, Sidama Regional State, Ethiopia. Health Res Serv Manag Epidemiol. 2021;8:1–7.
James PB, Wardle J, Steel A, Adams J. Traditional, complementary and alternative medicine use in Sub-saharan Africa: a systematic review. BMJ Glob Health. 2018;3(5).
Coates D, Homer C, Wilson A, Deady L, Mason E, Foureur M, et al. Induction of labour indications and timing: a systematic analysis of clinical guidelines. Women Birth. 2020;33(3):219–30.
Article PubMed Google Scholar
Lawani OL, Onyebuchi AK, Iyoke CA, Okafo CN, Ajah LO. Obstetric outcome and significance of labour induction in a health resource poor setting. Obstet Gynecol Int. 2014;2014(1):419621.
PubMed PubMed Central Google Scholar
Zamawe C, King C, Jennings HM, Fottrell E. Associations between the use of herbal medicines and adverse pregnancy outcomes in rural Malawi: a secondary analysis of randomised controlled trial data. BMC Complement Alt Med. 2018;18:1–8.
Roopasree B, Joseph J, Mukkadan JK. Oxytocin-functions: an overview. MOJ Anat Physiol. 2019;6:128–33.
Espada-Trespalacios X, Ojeda F, Perez-Botella M, Milà Villarroel R, Bach Martinez M, Figuls Soler H, et al. Oxytocin administration in low-risk women, a retrospective analysis of birth and neonatal outcomes. Int J Environ Res Public Health. 2021;18(8):4375.
Article PubMed PubMed Central Google Scholar
Ayelyini B, Yidana A, Ziblim SD. The Use of Indigenous Medicine among women during pregnancy and labour in rural Ghana. Cent Afr J Public Health. 2019;5(3):120–8. https://doi.org/10.11648/j.cajph.20190503.14 .
Kwame Ameade EP, Zakaria AP, Abubakar L, Sandow R. Herbal medicine usage before and during pregnancy – a study in Northern Ghana. Int J Complement Alt Med. 2018;11(4).
Jhpiego. Business Case: Investing in production of high-quality oxytocin for low-resource settings–Final report December 2014. 2014. http://www.conceptfoundation.org/wp-content/uploads/2015/06/BusinessCase_Oxytocin_web.pdf . Accesed 25 Jan 2024.
Barišić T, Pecirep A, Milićević R, Vasilj A, Tirić D. What do pregnant women know about harmful effects of medication and herbal remedies use during pregnancy? Psychiatr Danub. 2017;29(1):804–11.
PubMed Google Scholar
Ameade EPK, Ibrahim M, Ibrahim HS, Habib RH, Gbedema SY. Concurrent Use of Herbal and Orthodox Medicines among Residents of Tamale, Northern Ghana, Who Patronize Hospitals and Herbal Clinics. Evidence-based Complement Alt Med. 2018;2018(2002).
Illamola SM, Amaeze OU, Krepkova LV, Birnbaum AK, Karanam A, Job KM, et al. Use of herbal medicine by pregnant women: what physicians need to know. Front Pharmacol. 2020;10:1483.
Bahall M, Legall G. Knowledge, attitudes, and practices among health care providers regarding complementary and alternative medicine in Trinidad and Tobago. BMC Complement Alt Med. 2017;17:1–9.
Peprah P, Agyemang-duah W, Arthur-holmes F, Budu HI, Abalo EM, Okwei R et al. ‘ we are nothing without herbs ’ : a story of herbal remedies use during pregnancy in rural Ghana. BMC Complement Altern Med.2019;1–12.
Kamatenesi-Mugisha M, Oryem-Origa H. Medicinal plants used to induce labour during childbirth in western Uganda. J Ethnopharmacol. 2007;109(1):1–9.
Ngoma CM. Use of Herbal Medicines to induce labour by pregnant women: a systematic review of literature. JOJ Nurs Heal Care. 2017;2(3):7–12.
Ameade EK, Zakaria AP, Abubakar L, Sandow R. Herbal medicine usage before and during pregnancy—a study in Northern Ghana. Int J Complement Alt Med. 2018;11(4):235–42.
Ameade EP, Ibrahim M, Ibrahim HS, Habib RH, Gbedema SY. Concurrent use of herbal and orthodox medicines among residents of Tamale, Northern Ghana, who patronize hospitals and herbal clinics. Evidence-Based Complement Alt Med. 2018;2018(1):1289125.
Andersen RM. Revisiting the behavioral Model and Access to Medical Care: does it Matter? J Health Soc Behav. 1995;1–10. https://doi.org/10.2307/2137284 .
Smith-Hall C, Larsen HO, Pouliot M. People, plants and health: a conceptual framework for assessing changes in medicinal plant consumption. J Ethnobiolo Ethnomed. 2012;8:1–1.
Levin KA. Study design III: cross-sectional studies. Evid-Based Dent. 2006;7(1):24–5.
Beste J, Asanti D, Nsabimana D, Anastos K, Mutimura E, Merkatz I, et al. Use of Traditional Botanical Medicines during pregnancy in Rural Rwanda. J Glob Health Perspect. 2015;2015:1–10.
Adusi-Poku Y, Vanotoo L, Detoh E, Oduro J, Nsiah R, Natogmah A. Type of herbal medicines utilized by pregnant women attending ante-natal clinic in Offinso North district: are orthodox prescribers aware? Ghana Med J. 2016;49(4):227.
Agyei-Baffour P, Kudolo A, Quansah DY, Boateng D. Integrating herbal medicine into mainstream healthcare in Ghana: clients’ acceptability, perceptions and disclosure of use. BMC Complement Alt Med. 2017;17(1):1–9.
Mukasa PK, Kabakyenga J, Senkungu JK, Ngonzi J, Kyalimpa M, Roosmalen VJ. Uterine rupture in a teaching hospital in Mbarara, western Uganda, unmatched case-control study. Reprod Health. 2013;10(1):1–6.
Lampiao F, Maliwichi-Nyirenda C, Mponda J, Tembo L, Clements C. A preliminary investigation of the effects of labour inducing plant, cissampelos mucronata, on the outcomesof pregnancy using rat models. Malawi Med J. 2018;30(3):159–61.
Kekana LS, Sebitloane MH. Ingestion of herbal medication during pregnancy and adverse perinatal outcomes. S Afr J Obstet Gynaecol. 2020;26(2):1–5.
Buyondo BF. Use of Herbal Medicines in Preparation for Labour and its determinants among pregnant women at Kiganda Health Centre Iv-Mubende District. Angew Chemie Int Edu. 2018;6(11):951–2.
Koh LM, Percival B, Pauley T, Pathak S. Complementary therapy and alternative medicine: effects on induction of labour and pregnancy outcome in low risk post-dates women. Heliyon. 2019;5(11). https://doi.org/10.1016/j.heliyon.2019.e02787 .
Al-Ghamdi S, Aldossari K, Al-Zahrani J, Al-Shaalan F, Al-Sharif S, Al-Khurayji H, et al. Prevalence, knowledge and attitudes toward herbal medication use by Saudi women in the central region during pregnancy, during labor and after delivery. BMC Complement Alt Med. 2017;17(1):1–9.
Gruber CW, O’Brien M. Uterotonic plants and their bioactive constituents. Planta Med. 2011;77(03):207–20.
Article CAS PubMed Google Scholar
Zahra A. Lavender aromatherapy massages in reducing labor pain and duration of labor: a randomized controlled trial. Afr J Pharm Pharmacol. 2013;7(8):456–430.
Download references
Acknowledgements
We thank the Management and Healthcare Staff of the Tamale West Hospital (T.W.H), Tamale Central Hospital (T.C.H) and Tamale Teaching Hospital (TTH) for their support throughout the data collection process. We acknowledge the contributions of all the midwives who shared their knowledge and experiences with us, your efforts are well appreciated.
No funding was available for the study.
Author information
Authors and affiliations.
Department of Population and Reproductive Health, School of Public Health, University for Development Studies, P. O. Box 1883, Tamale, Northern Region, Ghana
Ahmad Sukerazu Alhassan & Joseph Lasong
Nandom Nursing and Midwifery Training College, Upper West Region, Nandom, Ghana
Shivera Dakurah
You can also search for this author in PubMed Google Scholar
Contributions
ASA and SD conceptualised and drafted the research proposal. ASA, SD, and JL performed the statistical analysis, assisted with interpretation of the results, and co-drafted the manuscript. All authors contributed to the discussion of the paper, read, and approved the final manuscript.
Corresponding author
Correspondence to Ahmad Sukerazu Alhassan .
Ethics declarations
Ethics approval and consent to participate.
An introductory letter from the University for Development Studies was presented to the three government hospitals, Tamale West Hospital (T.W.H), Tamale Central Hospital (T.C.H) and Tamale Teaching Hospital (TTH) to seek for permission to undertake the study. Ethical clearance was also obtained from the Kwame Nkrumah University of Science and Technology (KNUST) with reference number (CHRPE/AP/332/22). Permission was also sought through a consent form of which participants were asked to consent to if they were willing to participate in the study. They were assured of confidentiality of every information they were going to provide. All other methods were performed in accordance with relevant guidelines and regulations on subject selection and participation.
Consent for publication
Not applicable.
Competing interests
The authors declare no competing interests.
Additional information
Publisher’s note.
Springer Nature remains neutral with regard to jurisdictional claims in published maps and institutional affiliations.
Rights and permissions
Open Access This article is licensed under a Creative Commons Attribution-NonCommercial-NoDerivatives 4.0 International License, which permits any non-commercial use, sharing, distribution and reproduction in any medium or format, as long as you give appropriate credit to the original author(s) and the source, provide a link to the Creative Commons licence, and indicate if you modified the licensed material. You do not have permission under this licence to share adapted material derived from this article or parts of it. The images or other third party material in this article are included in the article’s Creative Commons licence, unless indicated otherwise in a credit line to the material. If material is not included in the article’s Creative Commons licence and your intended use is not permitted by statutory regulation or exceeds the permitted use, you will need to obtain permission directly from the copyright holder. To view a copy of this licence, visit http://creativecommons.org/licenses/by-nc-nd/4.0/ .
Reprints and permissions
About this article
Cite this article.
Alhassan, A.S., Dakurah, S. & Lasong, J. Perspectives of midwives on the use of Kaligutim (local oxytocin) for induction of labour among pregnant women in the government hospitals in Tamale. BMC Pregnancy Childbirth 24 , 561 (2024). https://doi.org/10.1186/s12884-024-06745-z
Download citation
Received : 15 April 2024
Accepted : 08 August 2024
Published : 28 August 2024
DOI : https://doi.org/10.1186/s12884-024-06745-z
Share this article
Anyone you share the following link with will be able to read this content:
Sorry, a shareable link is not currently available for this article.
Provided by the Springer Nature SharedIt content-sharing initiative
- Local oxytocin
- Herbal medicine
- Traditional medicine
BMC Pregnancy and Childbirth
ISSN: 1471-2393
- Submission enquiries: [email protected]
- General enquiries: [email protected]

An official website of the United States government
The .gov means it’s official. Federal government websites often end in .gov or .mil. Before sharing sensitive information, make sure you’re on a federal government site.
The site is secure. The https:// ensures that you are connecting to the official website and that any information you provide is encrypted and transmitted securely.
- Publications
- Account settings
Preview improvements coming to the PMC website in October 2024. Learn More or Try it out now .
- Advanced Search
- Journal List
- HHS Author Manuscripts

Quantitative Approaches for the Evaluation of Implementation Research Studies
Justin d. smith.
1 Center for Prevention Implementation Methodology (Ce-PIM) for Drug Abuse and HIV, Department of Psychiatry and Behavioral Sciences, Northwestern University Feinberg School of Medicine, 750 N Lake Shore Dr., Chicago, Illinois, USA.
Mohamed Hasan
2 Center for Healthcare Studies, Institute of Public Health and Medicine, Northwestern University Feinberg School of Medicine, 633 N St. Claire St., Chicago, Illinois, USA.
Authors’ contributions
Implementation research necessitates a shift from clinical trial methods in both the conduct of the study and in the way that it is evaluated given the focus on the impact of implementation strategies. That is, the methods or techniques to support the adoption and delivery of a clinical or preventive intervention, program, or policy. As strategies target one or more levels within the service delivery system, evaluating their impact needs to follow suit. This article discusses the methods and practices involved in quantitative evaluations of implementation research studies. We focus on evaluation methods that characterize and quantify the overall impacts of an implementation strategy on various outcomes. This article discusses available measurement methods for common quantitative implementation outcomes involved in such an evaluation—adoption, fidelity, implementation cost, reach, and sustainment—and the sources of such data for these metrics using established taxonomies and frameworks. Last, we present an example of a quantitative evaluation from an ongoing randomized rollout implementation trial of the Collaborative Care Model for depression management in a large primary healthcare system.
1. Background
As part of this special issue on implementation science, this article discusses quantitative methods for evaluating implementation research studies and presents an example of an ongoing implementation trial for illustrative purposes. We focus on what is called “summative evaluation,” which characterizes and quantifies the impacts of an implementation strategy on various outcomes ( Gaglio & Glasgow, 2017 ). This type of evaluation involves aggregation methods conducted at the end of a study to assess the success of an implementation strategy on the adoption, delivery, and sustainment of an evidence-based practice (EBP), and the cost associated with implementation ( Bauer, Damschroder, Hagedorn, Smith, & Kilbourne, 2015 ). These results help decision makers understand the overall worth of an implementation strategy and whether to upscale, modify, or discontinue ( Bauer et al., 2015 ). This topic complements others in this issue on formative evaluation (Elwy et al.) and qualitative methods (Hamilton et al.), which are also used in implementation research evaluation.
Implementation research, as defined by the United States National Institutes of Health (NIH), is “the scientific study of the use of strategies [italics added] to adopt and integrate evidence-based health interventions into clinical and community settings in order to improve patient outcomes and benefit population health. Implementation research seeks to understand the behavior of healthcare professionals and support staff, healthcare organizations, healthcare consumers and family members, and policymakers in context as key influences on the adoption, implementation and sustainability of evidence-based interventions and guidelines” ( Department of Health and Human Services, 2019 ). Implementation strategies are methods or techniques used to enhance the adoption, implementation, and sustainability of a clinical program or practice ( Powell et al., 2015 ).
To grasp the evaluation methods used in implementation research, one must appreciate the nature of this research and how the study designs, aims, and measures differ in fundamental ways from those methods with which readers will be most familiar—that is, evaluations of clinical efficacy or effectiveness trials. First, whereas clinical intervention research focuses on how a given clinical intervention—meaning a pill, program, practice, principle, product, policy, or procedure ( Brown et al., 2017 )—affects a health outcome at the patient level, implementation research focuses on how systems can take that intervention to scale in order to improve health outcomes of the broader community ( Colditz & Emmons, 2017 ). Thus, when implementation strategies are the focus, the outcomes evaluated are at the system level. Figure 1 illustrates the emphasis (foreground box) of effectiveness versus implementation research and the corresponding outcomes that would be included in the evaluation. This difference can be illustrated by “hybrid trials” in which effectiveness and implementation are evaluated simultaneously but with different outcomes for each aim ( Curran, Bauer, Mittman, Pyne, & Stetler, 2012 ; also see Landes et al., this issue).

Emphasis and Outcomes Evaluated in Clinical Effectiveness versus Implementation Research
Note. Adapted from a slide developed by C. Hendricks Brown.
2. Design Considerations for Evaluating Implementation Research Studies
The stark contrast between the emphasis in implementation versus effectiveness trials occurs largely because implementation strategies most often, but not always, target one or more levels within the system that supports the adoption and implementation of the intervention, such as the provider, clinic, school, health department, or even state or national levels ( Powell et al., 2015 ). Implementation strategies are discussed in this issue by Kirchner and colleagues. With the focus on levels within which patients who receive the clinical or preventive intervention are embedded, research designs in implementation research follow suit. The choice of a study design to evaluate an implementation strategy influences the confidence in the association drawn between a strategy and an observed effect ( Grimshaw, Campbell, Eccles, & Steen, 2000 ). Strong designs and methodologically-robust studies support the validity of the evaluations and provide evidence likely to be used by policy makers. Study designs are generally classified into observational (descriptive) and experimental/quasi-experimental.
Brown et al. (2017) described three broad types of designs for implementation research. ( 1 ) Within-site designs involve evaluation of the effects of implementation strategies within a single service system unit (e.g., clinic, hospital). Common within-site designs include post, pre-post, and interrupted time series. While these designs are simple and can be useful for understanding the impact in a local context ( Cheung & Duan, 2014 ), they contribute limited generalizable knowledge due to the biases inherent small-sample studies with no direct comparison condition. Brown et al. describe two broad design types can be used to create generalizable knowledge as they inherently involve multiple units for aggregation and comparison using the evaluation methods described in this article. ( 2 ) Between-site designs involve comparison of outcomes between two or more service system units or clusters/groups of units. While they commonly involve the testing of a novel implementation strategy compared to routine practice (i.e., implementation as usual), they can also be head-to-head tests of two or more novel implementation strategies for the same intervention, which we refer to as a comparative implementation trial (e.g., Smith et al., 2019 ). ( 3 ) Within- and between-site designs add a time-based crossover for each unit in which they begin in one condition—usually routine practice—and then move to a second condition involving the introduction of the implementation strategy. We refer to this category as rollout trials, which includes the stepped-wedge and dynamic wait-list design ( Brown et al., 2017 ; Landsverk et al., 2017 ; Wyman, Henry, Knoblauch, & Brown, 2015 ). Designs for implementation research are discussed in this issue by Miller and colleagues.
3. Quantitative Methods for Evaluating Implementation Outcomes
While summative evaluation is distinguishable from formative evaluation (see Elwy et al. this issue ), proper understanding of the implementation strategy requires using both methods, perhaps at different stages of implementation research ( The Health Foundation, 2015 ). Formative evaluation is a rigorous assessment process designed to identify potential and actual influences on the effectiveness of implementation efforts ( Stetler et al., 2006 ). Earlier stages of implementation research might rely solely on formative evaluation and the use of qualitative and mixed methods approaches. In contrast, later stage implementation research involves powered tests of the effect of one or more implementation strategies and are thus likely to use a between-site or a within- and between-site research design with at least one quantitative outcome. Quantitative methods are especially important to explore the extent and variation of change (within and across units) induced by the implementation strategies.
Proctor and colleagues (2011) provide a taxonomy of available implementation outcomes, which include acceptability, adoption, appropriateness, feasibility, fidelity, implementation cost, penetration/reach, and sustainability/sustainment. Table 1 in this article presents a modified version of Table 1 from Proctor et al. (2011) , focusing only on the quantitative measurement characteristics of these outcomes. Table 1 also includes the additional metrics of speed and quantity, which will be discussed in more detail in the case example. As noted in Table 1 , and by Proctor et al. (2011) , certain outcomes are more applicable at earlier versus later stages of implementation research. A recent review of implementation research in the field of HIV indicated that earlier stage implementation research was more likely to focus on acceptability and feasibility, whereas later stage testing of implementation strategies focused less on these and more on adoption, cost, penetration/reach, fidelity, and sustainability ( Smith et al., 2019 ). These sources of quantitative information are at multiple levels in the service delivery system, such as the intervention delivery agent, leadership, and key stakeholders in and outside of a particular delivery system ( Brown et al., 2013 ).
Quantitative Measurement Characteristics of Common Implementation Outcomes
Implementation outcome | Level of analysis | Other terms in the literature | Salience by implementation stage | Quantitative measurement method | Example from the published literature |
---|---|---|---|---|---|
Acceptability | Individual provider Individual consumer | Satisfaction with various aspects of the innovation (e.g. content, complexity, comfort, delivery, and credibility) | Early for adoption Ongoing for penetration Late for sustainability | Survey Administrative data Refused/blank | Auslander, W., McGinnis, H., Tlapek, S., Smith, P., Foster, A., Edmond, T., & Dunn, J. (2017). Adaptation and implementation of a trauma-focused cognitive behavioral intervention for girls in child welfare. 87(3), 206–215. doi : |
Adoption | Individual provider Organization or setting | Uptake; utilization; initial implementation; intention to try | Early to mid | Administrative data Observation Survey | Knudsen, H. K., & Roman, P. M. (2014). Dissemination, adoption, and implementation of acamprosate for treating alcohol use disorders. Journal of studies on alcohol and drugs, 75(3), 467–475. doi : |
Appropriateness | Individual provider Individual consumer Organization or setting | Perceived fit; relevance; compatibility; suitability; usefulness; practicability | Early (prior to adoption) | Survey | Proctor, E., Ramsey, A. T., Brown, M. T., Malone, S., Hooley, C., & McKay, V. (2019). Training in Implementation Practice Leadership (TRIPLE): evaluation of a novel practice change strategy in behavioral health organizations. Implementation science: IS, 14(1), 66. doi: |
Feasibility | Individual providers Organization or setting | Actual fit or utility; suitability for everyday use; practicability | Early (during adoption) | Survey Administrative data | Lyon, A. R., Bruns, E. J., Ludwig, K., Stoep, A. V., Pullmann, M. D., Dorsey, S.,… McCauley, E. (2015). The Brief Intervention for School Clinicians (BRISC): A mixed-methods evaluation of feasibility, acceptability, and contextual appropriateness. School mental health, 7(4), 273–286. doi: |
Fidelity | Individual provider Program | Delivered as intended; adherence; integrity; quality of program delivery | Early to mid | Observation Checklists Self-report | Smith, J. D., Dishion, T. J., Shaw, D. S., & Wilson, M. N. (2013). Indirect effects of fidelity to the family check-up on changes in parenting and early childhood problem behaviors. Journal of consulting and clinical psychology, 81(6), 962–974. doi : |
Implementation cost | Provider or providing Institution Payor Individual consumer | Marginal cost; cost-effectiveness; cost-benefit | Early for adoption and feasibility Mid for penetration Late for sustainability | Administrative data | Jordan N, Graham AK, Berkel C, Smith JD (2019). Budget impact analysis of preparing to implement the Family Check-Up 4 Health in primary care to reduce pediatric obesity. 20(5), 655–664. doi: |
Penetration/Reach | Organization or setting | Level of institutionalization? Spread? Service access? | Mid to late | Case audit Checklists | Emily M. Woltmann, M. S. W., Rob Whitley, P. D., Gregory J. McHugo, P. D., Mary Brunette, M. D., William C. Torrey, M. D., Laura Coots, M. S.,… Robert E. Drake, M. D., Ph.D.,. (2008). The Role of Staff Turnover in the Implementation of Evidence-Based Practices in Mental Health Care. 59(7), 732737. doi : |
Sustainability | Administrators Organization or setting | Maintenance; continuation; durability; incorporation; integration; sustained use; institutionalization; routinization; | Late | Case audit Checklists Survey | Scudder, A. T., Taber-Thomas, S. M., Schaffner, K., Pemberton, J. R., Hunter, L., & Herschell, A. D. (2017). A mixed-methods study of system-level sustainability of evidence-based practices in 12 large-scale implementation initiatives. 15(1), 102. doi: |
Quantity | Organization or setting | Proportion; quantity | Early through late | Administrative data Observation | Brown, C. H., Chamberlain, P., Saldana, L., Padgett, C., Wang, W., & Cruden, G. (2014). Evaluation of two implementation strategies in 51 child county public service systems in two states: results of a cluster randomized head-to-head implementation trial. 134. doi: |
Speed | Organization or setting | Duration (speed) | Early through late | Administrative data Observation | Saldana, L., Chamberlain, P., Wang, W., & Hendricks Brown, C. (2012). Predicting program start-up using the stages of implementation measure. 39(6), 419–425. doi: |
Note. This table is modeled after Table 1 in the Proctor et al. (2011) article.
Methods for quantitative data collection include structured surveys; use of administrative records, including payor and health expenditure records; extraction from the electronic health record (EHR); and direct observation. Structured surveys are commonly used to assess attitudes and perceptions of providers and patients concerning such factors as the ability to sustain the intervention and a host of potential facilitators and barriers to implementation (e.g., Bertrand, Holtgrave, & Gregowski, 2009 ; Luke, Calhoun, Robichaux, Elliott, & Moreland-Russell, 2014 ). Administrative databases and the EHR are used to assess aspects of intervention delivery that result from the implementation strategies ( Bauer et al., 2015 ). Although the EHR supports automatic and cumulative data acquisition, its utility for measuring implementation outcomes is limited depending on the type of implementation strategy and the intervention. For example, it is well suited for gathering data on EHR-based implementation strategies, such as clinical decision supports and symptom screening, but less useful for behaviors that would not otherwise be documented in the EHR (e.g., effects of a learning collaborative on adoption of a cognitive behavioral therapy protocol). Last, observational assessment of implementation is fairly common but resource intensive, which limits its use outside of funded research. This is particularly germane to assessing fidelity of implementation, which is commonly observational in funded research but is rarely done when the intervention is adopted under real-world circumstances ( Schoenwald et al., 2011 ). The costs associated with observational fidelity measurement has led to promising efforts to automate this process with machine learning methods (e.g., Imel et al., 2019 ).
Quantitative evaluation of implementation research studies most commonly involves assessment of multiple outcome metrics to garner a comprehensive appraisal of the effects of the implementation strategy. This is due in large part to the interrelatedness and interdependence of these metrics. A shortcoming of the Proctor et al. (2011) taxonomy is that it does not specify relations between outcomes, rather they are simply listed. The RE-AIM evaluation framework ( Gaglio, Shoup, & Glasgow, 2013 ; Glasgow, Vogt, & Boles, 1999 ) is commonly used and includes consideration of the interrelatedness between both the implementtion outcomes and the clinical effectiveness of the intervention being implemented. Thus, it is particularly well-suited for effectiveness-implementation hybrid trials ( Curran et al., 2012 ; also see Landes et al., this issue). RE-AIM stands for Reach, Effectiveness (of the clinical or preventive intervention), Adoption, Implementation, and Maintenance. Each metric is important for determining the overall public health impact of the implementation, but they are somewhat interdependent. As such, RE-AIM dimensions can be presented in some combination, such as the “public health impact” metric (reach rate multiplied by the effect size of the intervention) ( Glasgow, Klesges, Dzewaltowski, Estabrooks, & Vogt, 2006 ). RE-AIM is one in a class of evaluation frameworks. For a review, see Tabak, Khoong, Chambers, and Brownson (2012) .
4. Resources for Quantitative Evaluation in Implementation Research
There are a number of useful resources for the quantitative measures used to evaluate implementation research studies. First is the Instrument Review Project affiliated with the Society for Implementation Research Collaboration ( Lewis, Stanick, et al., 2015 ). The results of this systematic review of measures indicated significant variability in the coverage of measures across implementation outcomes and salient determinants of implementation (commonly referred to as barriers and facilitators). The authors reviewed each identified measure for the psychometric properties of internal consistency, structural validity, predictive validity, having norms, responsiveness, and usability (pragmatism). Few measures were deemed high-quality and psychometrically sound due in large part to not using gold-standard measure development methods. This review is ongoing and a website ( https://societyforimplementationresearchcollaboration.org/sirc-instrument-project/ ) is continuously updated to reflect completed work, as well as emerging measures in the field, and is available to members of the society. A number of articles and book chapters provide critical discussions of the state of measurement in implementation research, noting the need for validation of instruments, use across studies, and pragmatism ( Emmons, Weiner, Fernandez, & Tu, 2012 ; Lewis, Fischer, et al., 2015 ; Lewis, Proctor, & Brownson, 2017 ; Martinez, Lewis, & Weiner, 2014 ; Rabin et al., 2016 ).
The RE-AIM website also includes various means of operationalizing the components of this evaluation framework ( http://www.re-aim.org/resources-and-tools/measures-and-checklists/ ) and recent reviews of the use of RE-AIM are also helpful when planning a quantitative evaluation ( Gaglio et al., 2013 ; Glasgow et al., 2019 ). Additionally, the Grid-Enabled Measures Database (GEM), hosted by the National Cancer Institute, has an ever-growing list of implementation-related measures (130 as of July, 2019) with a general rating by users ( https://www.gem-measures.org/public/wsmeasures.aspx?cat=8&aid=1&wid=11 ). Last, Rabin et al. (2016) provide an environmental scan of resources for measures in implementation and dissemination science.
5. Pragmatism: Reducing Measurement Burden
An emphasis in the field has been on finding ways to reduce the measurement burden on implementers, and to a lesser extent on implementation researchers to reduce costs and increase the pace of dissemination ( Glasgow et al., 2019 ; Glasgow & Riley, 2013 ). Powell et al. (2017) established criteria for pragmatic measures that resulted in four distinct categories: (1) acceptable, (2) compatible, (3) easy, and (4) useful; next steps are to develop consensus regarding the most important criteria and developing quantifiable rating criteria for assessing implementation measures on their pragmatism. Advancements have occurred using technology for the evaluation of implementation ( Brown et al., 2015 ). For example, automated and unobtrusive implementation measures can greatly reduce stakeholder burden and increase response rates. As an example, our group ( Wang et al., 2016 ) conducted a proof-of-concept demonstrating the use text analysis to automatically classify the completion of implementation activities using communication logs between implementer and implementing agency. As mentioned earlier in this article, researchers have begun to automate the assessment of implementation fidelity to such evidence-based interventions as motivational interviewing (e.g., Imel et al., 2019 ; Xiao, Imel, Georgiou, Atkins, & Narayanan, 2015 ), and this work is expanding to other intervention protocols to aid in implementation quality ( Smith et al., 2018 ).
6. Example of a Quantitative Evaluation of an Implementation Research Study
We now present the quantitative evaluation plan for an ongoing hybrid type II effectiveness-implementation trial (see Landes et al., this issue ) examining the effectiveness and implementation of the Collaborative Care Model (CCM; Unützer et al., 2002 ) for the management of depression in adult primary care clinics of Northwestern Medicine (Principal Investigator: Smith). CCM is a structure for population-based management of depression involving the primary care provider, a behavioral care manager, and a consulting psychiatrist. A meta-analysis of 79 randomized trials (n=24,308), concluded that CCM is more effective than standard care for short- and long-term treatment of depression ( Archer et al., 2012 ). CCM has also been shown to provide good economic value ( Jacob et al., 2012 ).
Our study involves 11 primary care practices in a rollout implementation design (see Figure 2 ). Randomization in roll-out designs occurs by start time of the implementation strategy, and ensures confidence in the results of the evaluation because known and unknown biases are equally distributed in the case and control groups ( Grimshaw et al., 2000 ). Rollout trials are both powerful and practical as many organizations feel it is unethical to withhold effective interventions, and roll-out designs reduce the logistic and resource demands of delivering the strategy to all units simultaneously. The co-primary aims of the study concern the effectiveness of CCM and its implementation, respectively: 1) Test the effectiveness of CCM to improve depression symptomatology and access to psychiatric services within the primary care environment; and 2) Evaluate the impact of our strategy package on the progressive improvement in speed and quantity of CCM implementation over successive clinics. We will use training and educational implementation strategies, provided to primary care providers, support staff (e.g., nurses, medical assistants), and to practice and system leadership, as well as monitoring and feedback to the practices. Figure 3 summarizes the quantitative evaluation being conducted in this trial using the RE-AIM framework.

Design and Timeline of Randomized Rollout Implementation Trial of CCM
Note. CCM = Collaborative Care Model. Clinics will have a staggered start every 3–4 months randomized using a matching scheme. Pre-implementation assessment period is 4 months. Evaluation of CCM implementation will be a minimum of 24 months at each clinic.

Summative Evaluation Metrics of CCM Implementation Using the RE-AIM Framework
Note. CCM = Collaborative Care Model. EHR = electronic health record.
7.1. EHR and other administrative data sources
As this is a Type 2effectiveness-implementation hybrid trial, Aim 1 encompasses both reach —an implementation outcome—of depression management by CCM within primary care—and the effectiveness of CCM at improving patient and service outcomes. Within RE-AIM, the Public Health Impact metric is effectiveness (effect size) multiplied by reach rate. EHR and administrative data are being used to evaluate the primary implementation outcomes of reach (i.e., the proportion of patients in the practice who are eligible for CCM and who are referred). The reach rates achieved after implementation of CCM can be compared to rates of mental health contact for patients with depression prior to implementation as well as to that achieved by other CCM implementation evaluations in the literature.
The primary effectiveness outcome of CCM is the reduction of patients’ depression symptom severity. De-identified longitudinal patient outcome data from the EHR—principally depression diagnosis and scores on the PHQ-9 ( Kroenke, Spitzer, & Williams, 2001 )—will be analyzed to evaluate the impact of CCM. Other indicators of the effectiveness of CCM will be evaluated as well but are not discussed here as they are likely to be familiar to most readers with knowledge of clinical trials. Service outcomes, from the Institute of Medicine’s Standards of Care ( Institute of Medicine Committee on Crossing the Quality Chasm, 2006 ), centered on providing care that is effective (providing services based on scientific knowledge to all who could benefit and refraining from providing services to those not likely to benefit), timely (reducing waits and sometimes harmful delays for both those who receive and those who give care), and equitable (providing care that does not vary in quality because of personal characteristics such as gender, ethnicity, geographic location, and socioeconomic status). We also sought to provide care that is safe, patient-centered, and efficient.
EHR data will also be used to determine adoption of CCM (i.e., the number of providers with eligible patients who refer to CCM). This can be accomplished by tracking patient screening results and intakes completed by the CCM behavioral care manager within the primary care clinician’s encounter record.
7.2. Speed and quantity of implementation
Achievement of Aim 2 requires an evaluation approach and an appropriate trial design to obtain results that can contribute to generalizable knowledge. A rigorous rollout implementation trial design, with matched-pair randomization to when the practice would change from usual care to CCM was devised. Figure 2 provides a schematic of the design with the timing of the crossover from standard practice to CCM implementation. The first thing one will notice about the design is that the sequential nature of the rollout in which implementation at earlier sites precedes the onset of implementation in later sites. This suggests the potential to learn from successes and challenges to improve implementation efficiency (speed) over time. We will use the Universal SIC® ( Saldana, Schaper, Campbell, & Chapman, 2015 ), a date-based, observational measure, to capture the speed of implementation of various activities needed to successfully implement CCM, such as “establishing a workflow”, “preparing for training”, and “behavioral care manager hired.” This measure is completed by practice staff and members of the implementation team based on their direct knowledge of precisely when the activity was completed. Using the completion date of each activity, we will analyze the time elapsed in each practice to complete each stage (Duration Score). Then, we will calculate the percentage of stages completed (Proportion Score). These scores can then be used in statistical analyses to understand the factors that contributed to timely stage completion, the number of stages that are important for successful program implementation by relating the SIC to other implementation outcomes, such as reach rate; and simply whether there was a degree of improvement in implementation efficiency and scale as the rollout took place. That is, were more stages completed more quickly by later sites compared to earlier ones in the rollout schedule. This analysis comprises the implementation domain of RE-AIM. It will be used in combination with other metrics from the EHR to determine the fidelity of implementation, which is consistent with RE-AIM.
7.3. Surveys
To understand the process and the determinants of implementation—the factors that impede or promote adoption and delivery with fidelity—a battery of surveys was administered at multiple time-points to key staff members in each practice. One challenge with large-scale implementation research is the need for measures to be both psychometrically sound as well as pragmatic. With this in mind, we adapted a set of questions for the current trial that were developed and validated in prior studies. This low-burden assessment is comprised of items from four validated implementation surveys concerning factors at the inner setting of the organization: the Implementation Leadership Scale ( Aarons, Ehrhart, & Farahnak, 2014 ), the Evidence-Based Practice Attitude Scale ( Aarons, 2004 ), the Clinical Effectiveness and Evidence-Based Practice Questionnaire ( Upton & Upton, 2006 ), and the Organizational Change Recipient’s Belief Scale ( Armenakis, Bernerth, Pitts, & Walker, 2007 ). In a prior study, we used confirmatory factor analysis to evaluate the four scales after shortening for pragmatism and tailoring the wording of the items (when appropriate) to the context under investigation in the study (Smith et al., under review). Further, different versions of the survey were created for administration to the various professional roles in the organization. Results showed that the scales were largely replicated after shortening and tailoring; internal consistencies were acceptable; and the factor structures were statistically invariant across professional role groups. The same process was undertaken for this study with versions of the battery developed for providers, practice leadership, support staff, and the behavioral care managers. The survey was administered immediately after initial training in the model and then again at 4, 12, and 24 months. Items were added after the baseline survey regarding the process of implementation thus far and the most prominent barriers and facilitators to implementation of CCM in the practice. Survey-based evaluation of maintenance in RE-AIM, also called sustainability, will occur via the Clinical Sustainability Assessment Tool ( Luke, Malone, Prewitt, Hackett, & Lin, 2018 ) to key decision makers at multiple levels in the healthcare system.
7.4. Cost of implementation
The costs incurred when adopting and delivering a new clinical intervention are a top reason attributed to lack of adoption of behavioral interventions ( Glasgow & Emmons, 2007 ). While cost-effectiveness and cost-benefit analyses demonstrate the long-term economic benefits associated with the effects of these interventions, they rarely consider the costs to the implementer associated with these endeavors as a unique component ( Ritzwoller, Sukhanova, Gaglio, & Glasgow, 2009 ). As such, decision makers value different kinds of economic evaluations, such as budget impact analysis, which assesses the expected short-term changes in expenditures for a health care organization or system in adopting a new intervention ( Jordan, Graham, Berkel, & Smith, 2019 ), and cost-effectiveness analysis from the perspective of the implementing system and not simply the individual recipient of the evidence-based intervention being implemented ( Raghavan, 2017 ). Eisman and colleagues ( this issue ) discuss economic evaluations in implementation research.
In our study, our economic approach focuses on the cost to Northwestern Medicine to deliver CCM and will incorporate reimbursement from payors to ensure that the costs to the system are recouped in such a way that it can be sustained over time under current models of compensated care. The cost-effectiveness of CCM has been established ( Jacob et al., 2012 ), but we will also quantify the cost of achieving salient health outcomes for the patients involved, such as cost to achieve remission as well as projected costs that would increase remission rates.
7. Conclusions
The field of implementation research has developed methods for conducting quantitative evaluation to summarize the overall, aggregate impact of implementation strategies on salient outcomes. Methods are still emerging to aid researchers in the specification and planning of evaluations for implementation studies (e.g., Smith, 2018 ). However, as noted in the case example, evaluations focused only on the aggregate results of a study should not be done in the absence of ongoing formative evaluations, such as in-protocol audit and feedback and other methods (see Elwy et al., this issue ),and mixed and/or qualitative methods (see Hamilton et al., this issue ). Both of which are critical for interpreting the results of evaluations that aggregate the results of a large trial and gaging the generalizability of the findings. In large part, the intent of quantitative evaluations of large trials in implementation research aligns with its clinical-level counterparts, but with the emphasis on the factors in the service delivery system associated with adoption and delivery of the clinical intervention rather than on the direct recipients of that intervention (see Figure 1 ). The case example shows how both can be accomplished in an effectiveness-implementation hybrid design (see Landes et al., this issue ). This article shows current thinking on quantitative outcome evaluation in the context of implementation research. Given the quickly-evolving nature of the field of implementation research, it is imperative for interested readers to consult the most up-to-date resources for guidance on quantitative evaluation.
- Quantitative evaluation can be conducted in the context of implementation research to determine impact of various strategies on salient outcomes.
- The defining characteristics of implementation research studies are discussed.
- Quantitative evaluation frameworks and measures for key implementation research outcomes are presented.
- Application is illustrated using a case example of implementing collaborative care for depression in primary care practices in a large healthcare system.
Acknowledgements
The authors wish to thank Hendricks Brown who provided input on the development of this article and to the members of the Collaborative Behavioral Health Program research team at Northwestern: Lisa J. Rosenthal, Jeffrey Rado, Grace Garcia, Jacob Atlas, Michael Malcolm, Emily Fu, Inger Burnett-Zeigler, C. Hendricks Brown, and John Csernansky. We also wish to thank the Woman’s Board of Northwestern Memorial Hospital, who generously provided a grant to support and evaluate the implementation and effectiveness of this model of care as it was introduced to the Northwestern Medicine system, and our clinical, operations, and quality partners in Northwestern Medicine’s Central Region.
This study was supported by a grant from the Woman’s Board of Northwestern Memorial Hospital and grant P30DA027828 from the National Institute on Drug Abuse, awarded to C. Hendricks Brown. The opinions expressed herein are the views of the authors and do not necessarily reflect the official policy or position of the Woman’s Board, Northwestern Medicine, the National Institute on Drug Abuse, or any other part of the US Department of Health and Human Services.
List of Abbreviations
CCM | collaborative care model |
HER | electronic health record |
Competing interests
None declared.
Declarations
Ethics approval and consent to participate
Not applicable. This study did not involve human subjects.
Availability of data and material
Not applicable.
Publisher's Disclaimer: This is a PDF file of an unedited manuscript that has been accepted for publication. As a service to our customers we are providing this early version of the manuscript. The manuscript will undergo copyediting, typesetting, and review of the resulting proof before it is published in its final citable form. Please note that during the production process errors may be discovered which could affect the content, and all legal disclaimers that apply to the journal pertain.
- Aarons GA (2004). Mental health provider attitudes toward adoption of evidence-based practice: the Evidence-Based Practice Attitude Scale (EBPAS) . Ment Health Serv Res , 6 ( 2 ), 61–74. [ PMC free article ] [ PubMed ] [ Google Scholar ]
- Aarons GA, Ehrhart MG, & Farahnak LR (2014). The implementation leadership scale (ILS): Development of a brief measure of unit level implementation leadership . Implementation Science , 9 . doi: 10.1186/1748-5908-9-45 [ PMC free article ] [ PubMed ] [ CrossRef ] [ Google Scholar ]
- Archer J, Bower P, Gilbody S, Lovell K, Richards D, Gask L,… Coventry P (2012). Collaborative care for depression and anxiety problems . Cochrane Database of Systematic Reviews(10) doi: 10.1002/14651858.CD006525.pub2 [ PubMed ] [ CrossRef ] [ Google Scholar ]
- Armenakis AA, Bernerth JB, Pitts JP, & Walker HJ (2007). Organizational Change Recipients Beliefs Scale: Development of an Assessmetn Instrument . The Journal of Applied Behavioral Science , 42 , 481–505. doi:DOI: 10.1177/0021886307303654 [ CrossRef ] [ Google Scholar ]
- Bauer MS, Damschroder L, Hagedorn H, Smith J, & Kilbourne AM (2015). An introduction to implementation science for the non-specialist . BMC Psychology , 3 ( 1 ), 32. doi: 10.1186/s40359-015-0089-9 [ PMC free article ] [ PubMed ] [ CrossRef ] [ Google Scholar ]
- Bertrand JT, Holtgrave DR, & Gregowski A (2009). Evaluating HIV/AIDS programs in the US and developing countries In Mayer KH & Pizer HF (Eds.), HIV Prevention (pp. 571–590). San Diego: Academic Press. [ Google Scholar ]
- Brown CH, Curran G, Palinkas LA, Aarons GA, Wells KB, Jones L,… Cruden G (2017). An overview of research and evaluation designs for dissemination and implementation . Annual Review of Public Health , 38 ( 1 ), null. doi:doi: 10.1146/annurev-publhealth-031816-044215 [ PMC free article ] [ PubMed ] [ CrossRef ] [ Google Scholar ]
- Brown CH, Mohr DC, Gallo CG, Mader C, Palinkas L, Wingood G,… Poduska J (2013). A computational future for preventing HIV in minority communities: how advanced technology can improve implementation of effective programs . J Acquir Immune Defic Syndr , 63 . doi: 10.1097/QAI.0b013e31829372bd [ PMC free article ] [ PubMed ] [ CrossRef ] [ Google Scholar ]
- Brown CH, PoVey C, Hjorth A, Gallo CG, Wilensky U, & Villamar J (2015). Computational and technical approaches to improve the implementation of prevention programs . Implementation Science , 10 ( Suppl 1 ), A28. doi: 10.1186/1748-5908-10-S1-A28 [ CrossRef ] [ Google Scholar ]
- Cheung K, & Duan N (2014). Design of implementation studies for quality improvement programs: An effectiveness-cost-effectiveness framework . American Journal of Public Health , 104 ( 1 ), e23–e30. doi: 10.2105/ajph.2013.301579 [ PMC free article ] [ PubMed ] [ CrossRef ] [ Google Scholar ]
- Colditz GA, & Emmons KM (2017). The promise and challenges of dissemination and implementation research In Brownson RC, Colditz GA, & Proctor EK (Eds.), Dissemination and implementation research in health: Translating science to practice . New York, NY: Oxford University Press. [ Google Scholar ]
- Curran GM, Bauer M, Mittman B, Pyne JM, & Stetler C (2012). Effectiveness-implementation hybrid designs: Combining elements of clinical effectiveness and implementation research to enhance public health impact . Medical Care , 50 ( 3 ), 217–226. doi: 10.1097/MLR.0b013e3182408812 [ PMC free article ] [ PubMed ] [ CrossRef ] [ Google Scholar ]
- Department of Health and Human Services. (2019). PAR-19–274: Dissemination and Implementation Research in Health (R01 Clinical Trial Optional) . Retrieved from https://grants.nih.gov/grants/guide/pa-files/PAR-19-274.html
- Emmons KM, Weiner B, Fernandez ME, & Tu S (2012). Systems antecedents for dissemination and implementation: a review and analysis of measures . Health Educ Behav , 39 . doi: 10.1177/1090198111409748 [ PMC free article ] [ PubMed ] [ CrossRef ] [ Google Scholar ]
- Gaglio B, & Glasgow RE (2017). Evaluation approaches for dissemination and implementation research In Brownson R, Colditz G, & Proctor E (Eds.), Dissemination and Implementation Research in Health: Translating Science into Practice (2nd ed., pp. 317–334). New York: Oxford University Press. [ Google Scholar ]
- Gaglio B, Shoup JA, & Glasgow RE (2013). The RE-AIM Framework: A systematic review of use over time . American Journal of Public Health , 103 ( 6 ), e38–e46. doi: 10.2105/ajph.2013.301299 [ PMC free article ] [ PubMed ] [ CrossRef ] [ Google Scholar ]
- Glasgow RE, & Emmons KM (2007). How can we increase translation of research into practice? Types of evidence needed . Annual Review of Public Health , 28 , 413–433. [ PubMed ] [ Google Scholar ]
- Glasgow RE, Harden SM, Gaglio B, Rabin B, Smith ML, Porter GC,… Estabrooks PA (2019). RE-AIM Planning and Evaluation Framework: Adapting to New Science and Practice With a 20-Year Review . Frontiers in Public Health , 7 ( 64 ). doi: 10.3389/fpubh.2019.00064 [ PMC free article ] [ PubMed ] [ CrossRef ] [ Google Scholar ]
- Glasgow RE, Klesges LM, Dzewaltowski DA, Estabrooks PA, & Vogt TM (2006). Evaluating the impact of health promotion programs: using the RE-AIM framework to form summary measures for decision making involving complex issues . Health Education Research , 21 ( 5 ), 688–694. doi: 10.1093/her/cyl081 [ PubMed ] [ CrossRef ] [ Google Scholar ]
- Glasgow RE, & Riley WT (2013). Pragmatic measures: what they are and why we need them . Am J Prev Med , 45 . doi: 10.1016/j.amepre.2013.03.010 [ PubMed ] [ CrossRef ] [ Google Scholar ]
- Glasgow RE, Vogt TM, & Boles SM (1999). Evaluating the public health impact of health promotion interventions: The RE-AIM framework . American Journal of Public Health , 89 ( 9 ), 1322–1327. doi: 10.2105/AJPH.89.9.1322 [ PMC free article ] [ PubMed ] [ CrossRef ] [ Google Scholar ]
- Grimshaw J, Campbell M, Eccles M, & Steen N (2000). Experimental and quasi-experimental designs for evaluating guideline implementation strategies . Family practice , 17 Suppl 1 , S11–16. doi: 10.1093/fampra/17.suppl_1.s11 [ PubMed ] [ CrossRef ] [ Google Scholar ]
- Imel ZE, Pace BT, Soma CS, Tanana M, Hirsch T, Gibson J,… Atkins, D. C. (2019). Design feasibility of an automated, machine-learning based feedback system for motivational interviewing . Psychotherapy , 56 ( 2 ), 318–328. doi: 10.1037/pst0000221 [ PMC free article ] [ PubMed ] [ CrossRef ] [ Google Scholar ]
- Institute of Medicine Committee on Crossing the Quality Chasm. (2006). Adaption to mental health and addictive disorder: Improving the quality of health care for mental and substanceuse conditions . Retrieved from Washington, D.C.: [ Google Scholar ]
- Jacob V, Chattopadhyay SK, Sipe TA, Thota AB, Byard GJ, & Chapman DP (2012). Economics of collaborative care for management of depressive disorders: A community guide systematic review . American Journal of Preventive Medicine , 42 ( 5 ), 539–549. doi: 10.1016/j.amepre.2012.01.011 [ PubMed ] [ CrossRef ] [ Google Scholar ]
- Jordan N, Graham AK, Berkel C, & Smith JD (2019). Budget impact analysis of preparing to implement the Family Check-Up 4 Health in primary care to reduce pediatric obesity . Prevention Science , 20 ( 5 ), 655–664. doi: 10.1007/s11121-018-0970-x [ PMC free article ] [ PubMed ] [ CrossRef ] [ Google Scholar ]
- Kroenke K, Spitzer R, & Williams JW (2001). The PHQ-9 . Journal of General Internal Medicine , 16 ( 9 ), 606–613. doi: 10.1046/j.1525-1497.2001.016009606.x [ PMC free article ] [ PubMed ] [ CrossRef ] [ Google Scholar ]
- Landsverk J, Brown CH, Smith JD, Chamberlain P, Palinkas LA, Ogihara M,… Horwitz SM (2017). Design and analysis in dissemination and implementation research In Brownson RC, Colditz GA, & Proctor EK (Eds.), Dissemination and implementation research in health: Translating research to practice (2nd ed., pp. 201–227). New York: Oxford University Press. [ Google Scholar ]
- Lewis CC, Fischer S, Weiner BJ, Stanick C, Kim M, & Martinez RG (2015). Outcomes for implementation science: an enhanced systematic review of instruments using evidence-based rating criteria . Implementation Science , 10 ( 1 ), 155. doi: 10.1186/s13012-015-0342-x [ PMC free article ] [ PubMed ] [ CrossRef ] [ Google Scholar ]
- Lewis CC, Proctor EK, & Brownson RC (2017). Measurement issues in dissemination and implementation research In Brownson RC, Colditz GA, & Proctor EK (Eds.), Dissemination and implementation research in health: Translating research to practice (2nd ed., pp. 229–244). New York: Oxford University Press. [ Google Scholar ]
- Lewis CC, Stanick CF, Martinez RG, Weiner BJ, Kim M, Barwick M, & Comtois KA (2015). The Society for Implementation Research Collaboration Instrument Review Project: A methodology to promote rigorous evaluation . Implementation Science , 10 ( 1 ), 2. doi: 10.1186/s13012-014-0193-x [ PMC free article ] [ PubMed ] [ CrossRef ] [ Google Scholar ]
- Luke DA, Calhoun A, Robichaux CB, Elliott MB, & Moreland-Russell S (2014). The Program Sustainability Assessment Tool: A new instrument for public health programs . Preventing Chronic Disease , 11 , E12. doi: 10.5888/pcd11.130184 [ PMC free article ] [ PubMed ] [ CrossRef ] [ Google Scholar ]
- Luke DA, Malone S, Prewitt K, Hackett R, & Lin J (2018). The Clinical Sustainability Assessment Tool (CSAT): Assessing sustainability in clinical medicine settings . Paper presented at the Conference on the Science of Dissemination and Implementation in Health, Washington, DC. [ Google Scholar ]
- Martinez RG, Lewis CC, & Weiner BJ (2014). Instrumentation issues in implementation science . Implement Sci , 9 . doi: 10.1186/s13012-014-0118-8 [ PMC free article ] [ PubMed ] [ CrossRef ] [ Google Scholar ]
- Powell BJ, Stanick CF, Halko HM, Dorsey CN, Weiner BJ, Barwick MA,… Lewis CC (2017). Toward criteria for pragmatic measurement in implementation research and practice: a stakeholder-driven approach using concept mapping . Implementation Science , 12 ( 1 ), 118. doi: 10.1186/s13012-017-0649-x [ PMC free article ] [ PubMed ] [ CrossRef ] [ Google Scholar ]
- Powell BJ, Waltz TJ, Chinman MJ, Damschroder LJ, Smith JL, Matthieu MM,… Kirchner JE (2015). A refined compilation of implementation strategies: results from the Expert Recommendations for Implementing Change (ERIC) project . Implement Sci , 10 . doi: 10.1186/s13012-015-0209-1 [ PMC free article ] [ PubMed ] [ CrossRef ] [ Google Scholar ]
- Proctor E, Silmere H, Raghavan R, Hovmand P, Aarons G, Bunger A,… Hensley M (2011). Outcomes for implementation research: conceptual distinctions, measurement challenges, and research agenda . Adm Policy Ment Health Ment Health Serv Res , 38 . doi: 10.1007/s10488-010-0319-7 [ PMC free article ] [ PubMed ] [ CrossRef ] [ Google Scholar ]
- Rabin BA, Lewis CC, Norton WE, Neta G, Chambers D, Tobin JN,… Glasgow RE (2016). Measurement resources for dissemination and implementation research in health . Implementation Science , 11 ( 1 ), 42. doi: 10.1186/s13012-016-0401-y [ PMC free article ] [ PubMed ] [ CrossRef ] [ Google Scholar ]
- Raghavan R (2017). The role of economic evaluation in dissemination and implementation research In Brownson RC, Colditz GA, & Proctor EK (Eds.), Dissemination and implementation research in health: Translating science to practice (2nd ed.). New York: Oxford University Press. [ Google Scholar ]
- Ritzwoller DP, Sukhanova A, Gaglio B, & Glasgow RE (2009). Costing behavioral interventions: a practical guide to enhance translation . Annals of Behavioral Medicine , 37 ( 2 ), 218–227. [ PubMed ] [ Google Scholar ]
- Saldana L, Schaper H, Campbell M, & Chapman J (2015). Standardized Measurement of Implementation: The Universal SIC . Implementation Science , 10 ( 1 ), A73. doi: 10.1186/1748-5908-10-s1-a73 [ CrossRef ] [ Google Scholar ]
- Schoenwald S, Garland A, Chapman J, Frazier S, Sheidow A, & Southam-Gerow M (2011). Toward the effective and efficient measurement of implementation fidelity . Admin Policy Mental Health Mental Health Serv Res , 38 . doi: 10.1007/s10488-010-0321-0 [ PMC free article ] [ PubMed ] [ CrossRef ] [ Google Scholar ]
- Smith JD (2018). An implementation research logic model: A step toward improving scientific rigor, transparency, reproducibility, and specification . Implementation Science , 14 ( Supp 1 ), S39. [ Google Scholar ]
- Smith JD, Berkel C, Jordan N, Atkins DC, Narayanan SS, Gallo C,… Bruening MM (2018). An individually tailored family-centered intervention for pediatric obesity in primary care: Study protocol of a randomized type II hybrid implementation-effectiveness trial (Raising Healthy Children study) . Implementation Science , 13 ( 11 ), 1–15. doi: 10.1186/s13012-017-0697-2 [ PMC free article ] [ PubMed ] [ CrossRef ] [ Google Scholar ]
- Smith JD, Li DH, Hirschhorn LR, Gallo C, McNulty M, Phillips GI,… Benbow ND (2019). Landscape of HIV implementation research funded by the National Institutes of Health: A mapping review of project abstracts (submitted for publication) . [ PMC free article ] [ PubMed ] [ Google Scholar ]
- Smith JD, Rafferty MR, Heinemann AW, Meachum MK, Villamar JA, Lieber RL, & Brown CH (under review). Evaluation of the factor structure of implementation research measures adapted for a novel context and multiple professional roles . [ PMC free article ] [ PubMed ] [ Google Scholar ]
- Stetler CB, Legro MW, Wallace CM, Bowman C, Guihan M, Hagedorn H,… Smith JL (2006). The role of formative evaluation in implementation research and the QUERI experience . Journal of General Internal Medicine , 21 ( 2 ), S1. doi : 10.1007/s11606-006-0267-9 [ PMC free article ] [ PubMed ] [ CrossRef ] [ Google Scholar ]
- Tabak RG, Khoong EC, Chambers DA, & Brownson RC (2012). Bridging research and practice: Models for dissemination and implementation research . American Journal of Preventive Medicine , 43 ( 3 ), 337–350. [ PMC free article ] [ PubMed ] [ Google Scholar ]
- The Health Foundation. (2015). Evaluation: What to consider. Commonly asked questions about how to approach evaluation of quality improvement in health care . Retrieved from London, England: https://www.health.org.uk/sites/default/files/EvaluationWhatToConsider.pdf [ Google Scholar ]
- Unützer J, Katon W, Callahan CM, Williams J, John W, Hunkeler E, Harpole L,… Investigators, f. t. I. (2002). Collaborative care management of late-life depression in the primary care setting: A randomized controlled trial . JAMA , 288 ( 22 ), 2836–2845. doi: 10.1001/jama.288.22.2836 [ PubMed ] [ CrossRef ] [ Google Scholar ]
- Upton D, & Upton P (2006). Development of an evidence-based practice questionnaire for nurses . Journal of Advanced Nursing , 53 ( 4 ), 454–458. [ PubMed ] [ Google Scholar ]
- Wang D, Ogihara M, Gallo C, Villamar JA, Smith JD, Vermeer W,… Brown CH (2016). Automatic classification of communication logs into implementation stages via text analysis . Implementation Science , 11 ( 1 ), 1–14. doi: 10.1186/s13012-016-0483-6 [ PMC free article ] [ PubMed ] [ CrossRef ] [ Google Scholar ]
- Wyman PA, Henry D, Knoblauch S, & Brown CH (2015). Designs for testing group-based interventions with limited numbers of social units: The dynamic wait-listed and regression point displacement designs . Prevention Science , 16 ( 7 ), 956–966. doi : 10.1007/s11121-014-0535-6 [ PMC free article ] [ PubMed ] [ CrossRef ] [ Google Scholar ]
- Xiao B, Imel ZE, Georgiou PG, Atkins DC, & Narayanan SS (2015). “Rate My Therapist”: Automated detection of empathy in drug and alcohol counseling via speech and language processing . PLoS ONE , 10 ( 12 ), e0143055. doi: 10.1371/journal.pone.0143055 [ PMC free article ] [ PubMed ] [ CrossRef ] [ Google Scholar ]

IMAGES
VIDEO
COMMENTS
Background Identifying determinants of health and understanding their role in health production constitutes an important research theme. We aimed to document the state of recent multi-country research on this theme in the literature. Methods We followed the PRISMA-ScR guidelines to systematically identify, triage and review literature (January 2013—July 2019). We searched for studies that ...
INTRODUCTION. Scientific research is usually initiated by posing evidenced-based research questions which are then explicitly restated as hypotheses.1,2 The hypotheses provide directions to guide the study, solutions, explanations, and expected results.3,4 Both research questions and hypotheses are essentially formulated based on conventional theories and real-world processes, which allow the ...
Quantitative research usually aims to provide precise, unbiased estimates of parameters of interest for the entire population which requires a large, randomly selected sample. Brett et al 4 reported a positive impact of PPI on recruitment in studies, but the representativeness of the sample is as important in quantitative research as sample ...
Quantitative Research for the Qualitative Researcher. 2014. SAGE Knowledge. Book chapter . Issues in Validity and Reliability. Show details Hide details. Daniel J. Boudah. Conducting Educational Research: Guide to Completing a Major Project. 2011. SAGE Knowledge. Entry . Quantitative Research.
Funding: The author(s) disclosed receipt of the following financial support for the research, authorship, and/or publication of this article: This study was funded by the Agency for Healthcare Research and Quality (AHRQ) under contract HHSA-290-2013-00002-C and through AHRQ intramural research funds. The views expressed herein are those of the ...
Quantitative research explains phenomena by collecting numerical unchanging d etailed data t hat. are analyzed using mathematically based methods, in particular statistics that pose questions of ...
Abstract. In an era of data-driven decision-making, a comprehensive understanding of quantitative research is indispensable. Current guides often provide fragmented insights, failing to offer a holistic view, while more comprehensive sources remain lengthy and less accessible, hindered by physical and proprietary barriers.
The study was quantitative in design with data collected using a semi-structured mixed questionnaire with an option for patients to add their own comments to the questions. ... The results of the interviews were analysed by the research group to identify crucial experiences of having a chronic disease. The research group then constructed the ...
Psychology-based journals are not new to issues dedicated to quantitative methods. Many special issues and key invited articles have highlighted important advancements in methodology, each helping to promote methodological rigor.For example, the journal Health Psychology Review recently published an issue (2017, Volume 11, Issue 3) on statistical tools that can benefit the subdiscipline of ...
Moles and titrations. 5 January 2015. Dorothy Warren describes some of the difficulties with teaching this topic and shows how you can help your students to master aspects of quantitative chemistry. Previous. 1. 2. Next. All Quantitative research articles in RSC Education.
quantitative research are: Describing a problem statement by presenting the need for an explanation of a variable's relationship. Offering literature, a significant function by answering research ...
Quantitative research as defined in this study uses empirical assessment based on numerical measurements while qualitative methods involve the interpretation of text or other materials without relying on numerical measurement (Zikmund, Babin, Carr, & Griffin, 2013). Within the quantitative methods, survey was the most used data collection process.
Quantitative and qualitative methods are the engine behind evidence-based outcomes. For decades, one of the popular phenomena that troubled young researchers is that which appropriate research ...
Quantitative research in the social sciences is undergoing a change. After years of scholarship on the oppressive history of quantitative methods, quantitative scholars are grappling with the ways that our preferred methodology reinforces social injustices (Zuberi, 2001).Among others, the emerging fields of CritQuant (critical quantitative studies) and QuantCrit (quantitative critical race ...
We conclude that quantitative research may benefit from a bottom-up generalization strategy as it is employed in most qualitative research programs. Inductive reasoning forces us to think about the boundary conditions of our theories and provides a framework for generalization beyond statistical testing. In this perspective, failed replications ...
This article describes the basic tenets of quantitative research. The concepts of dependent and independent variables are addressed and the concept of measurement and its associated issues, such as error, reliability and validity, are explored. Experiments and surveys - the principal research designs in quantitative research - are described ...
Revised on June 22, 2023. Quantitative research is the process of collecting and analyzing numerical data. It can be used to find patterns and averages, make predictions, test causal relationships, and generalize results to wider populations. Quantitative research is the opposite of qualitative research, which involves collecting and analyzing ...
Multidimensional analysis of the linguistic phenomena improves the analytic potential. This article focuses on the application of quantitative methods in schoolscape research, including a discussion of its advantages and disadvantages. This article seeks to rehabilitate the quantitative by re-theorizing the landscape in linguistic landscape (LL ...
Tips for Finding Quantitative Articles with a Keyword Search. If you want to limit your search to quantitative studies, first try "quantitative" as a keyword, then try using one of the following terms/phrases in your search (example: lactation AND statistics): Correlational design*. Effect size. Empirical research. Experiment*.
Here are some key characteristics of quantitative research: Numerical data: Quantitative research involves collecting numerical data through standardized methods such as surveys, experiments, and observational studies. This data is analyzed using statistical methods to identify patterns and relationships.
This is a space where academics with quantitative methods expertise and an interest in education issues come together to discuss their research; find support in addressing data, methodological and conceptual challenges; and develop collaborative and inter-disciplinary work on topics relevant to policy and practice.
SPSC Quantitative Stock Analysis August 31, 2024 — 04:00 pm EDT. ... Additional Research Links. Top NASDAQ 100 Stocks. Top Technology Stocks. Top Large-Cap Growth Stocks. High Momentum Stocks.
SEARCH FOR RELEVANT ARTICLES. To identify articles relevant to this topic, we adhered to the search strategy recommended by Gasparyan et al.7 We searched through PubMed, Scopus, Directory of Open Access Journals, and Google Scholar databases using the following keywords: quantitative research, qualitative research, mixed-method research, deductive reasoning, inductive reasoning, study design ...
Introduction. Recognition has grown that while quantitative methods remain vital, they are usually insufficient to address complex health systems related research questions. 1 Quantitative methods rely on an ability to anticipate what must be measured in advance. Introducing change into a complex health system gives rise to emergent reactions, which cannot be fully predicted in advance.
The use of herbal medicine and/or its products is common throughout the world. In Tamale Metropolis, pregnant women frequently use local oxytocin to induce labour, as shown by the fact that 90% of midwives reported managing patients who used kaligutim (local oxytocin) to speed up labour. Early career midwives are also aware of this and have personally observed it being used by their clients.
3. Quantitative Methods for Evaluating Implementation Outcomes. While summative evaluation is distinguishable from formative evaluation (see Elwy et al. this issue), proper understanding of the implementation strategy requires using both methods, perhaps at different stages of implementation research (The Health Foundation, 2015).Formative evaluation is a rigorous assessment process designed ...