
Move fast, think slow: How financial services can strike a balance with GenAI
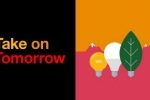
Take on Tomorrow @ the World Economic Forum in Davos: Energy demand
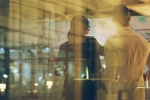
PwC’s Global Investor Survey 2023
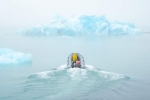
Climate risk, resilience and adaptation

Business transformation
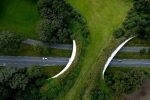
Sustainability assurance
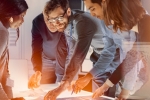
The Leadership Agenda
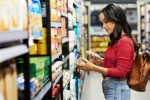
PwC’s Voice of the Consumer Survey 2024
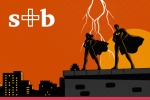
The s+b digital issue: Corporate “power changers”

The New Equation

PwC’s Global Annual Review

Committing to Net Zero

The Solvers Challenge
Loading Results
No Match Found

Case studies
Demonstrating our purpose in our work.
PwC's purpose is to "build trust in society and solve important problems." Our Partners and staff demonstrate a commitment to this purpose every day in the work we do with our Health Services clients. We do so because of a deep and passionate recognition of the importance of health to society - because health matters. The following case studies are an example of just a few engagements that show our strong desire to make a difference.
Working with clients to make an impact
We work side by side with health organizations and community residents, to improve health and wellness. The stories featured here illustrate the importance of working together to solve the complex health challenges we face. When we collaborate, our strengths are amplified. See how we are working together to solve complex health challenges.
{{filterContent.facetedTitle}}
{{item.publishDate}}
{{item.title}}
{{item.text}}

Ron Chopoorian
Global Health Industries Leader, Partner, PwC United States
© 2017 - 2024 PwC. All rights reserved. PwC refers to the PwC network and/or one or more of its member firms, each of which is a separate legal entity. Please see www.pwc.com/structure for further details.
- Legal notices
- Cookie policy
- Legal disclaimer
- Terms and conditions
- Open access
- Published: 15 May 2024
Learning together for better health using an evidence-based Learning Health System framework: a case study in stroke
- Helena Teede 1 , 2 na1 ,
- Dominique A. Cadilhac 3 , 4 na1 ,
- Tara Purvis 3 ,
- Monique F. Kilkenny 3 , 4 ,
- Bruce C.V. Campbell 4 , 5 , 6 ,
- Coralie English 7 ,
- Alison Johnson 2 ,
- Emily Callander 1 ,
- Rohan S. Grimley 8 , 9 ,
- Christopher Levi 10 ,
- Sandy Middleton 11 , 12 ,
- Kelvin Hill 13 &
- Joanne Enticott ORCID: orcid.org/0000-0002-4480-5690 1
BMC Medicine volume 22 , Article number: 198 ( 2024 ) Cite this article
2 Altmetric
Metrics details
In the context of expanding digital health tools, the health system is ready for Learning Health System (LHS) models. These models, with proper governance and stakeholder engagement, enable the integration of digital infrastructure to provide feedback to all relevant parties including clinicians and consumers on performance against best practice standards, as well as fostering innovation and aligning healthcare with patient needs. The LHS literature primarily includes opinion or consensus-based frameworks and lacks validation or evidence of benefit. Our aim was to outline a rigorously codesigned, evidence-based LHS framework and present a national case study of an LHS-aligned national stroke program that has delivered clinical benefit.
Current core components of a LHS involve capturing evidence from communities and stakeholders (quadrant 1), integrating evidence from research findings (quadrant 2), leveraging evidence from data and practice (quadrant 3), and generating evidence from implementation (quadrant 4) for iterative system-level improvement. The Australian Stroke program was selected as the case study as it provides an exemplar of how an iterative LHS works in practice at a national level encompassing and integrating evidence from all four LHS quadrants. Using this case study, we demonstrate how to apply evidence-based processes to healthcare improvement and embed real-world research for optimising healthcare improvement. We emphasize the transition from research as an endpoint, to research as an enabler and a solution for impact in healthcare improvement.
Conclusions
The Australian Stroke program has nationally improved stroke care since 2007, showcasing the value of integrated LHS-aligned approaches for tangible impact on outcomes. This LHS case study is a practical example for other health conditions and settings to follow suit.
Peer Review reports
Internationally, health systems are facing a crisis, driven by an ageing population, increasing complexity, multi-morbidity, rapidly advancing health technology and rising costs that threaten sustainability and mandate transformation and improvement [ 1 , 2 ]. Although research has generated solutions to healthcare challenges, and the advent of big data and digital health holds great promise, entrenched siloes and poor integration of knowledge generation, knowledge implementation and healthcare delivery between stakeholders, curtails momentum towards, and consistent attainment of, evidence-and value-based care [ 3 ]. This is compounded by the short supply of research and innovation leadership within the healthcare sector, and poorly integrated and often inaccessible health data systems, which have crippled the potential to deliver on digital-driven innovation [ 4 ]. Current approaches to healthcare improvement are also often isolated with limited sustainability, scale-up and impact [ 5 ].
Evidence suggests that integration and partnership across academic and healthcare delivery stakeholders are key to progress, including those with lived experience and their families (referred to here as consumers and community), diverse disciplines (both research and clinical), policy makers and funders. Utilization of evidence from research and evidence from practice including data from routine care, supported by implementation research, are key to sustainably embedding improvement and optimising health care and outcomes. A strategy to achieve this integration is through the Learning Health System (LHS) (Fig. 1 ) [ 2 , 6 , 7 , 8 ]. Although there are numerous publications on LHS approaches [ 9 , 10 , 11 , 12 ], many focus on research perspectives and data, most do not demonstrate tangible healthcare improvement or better health outcomes. [ 6 ]
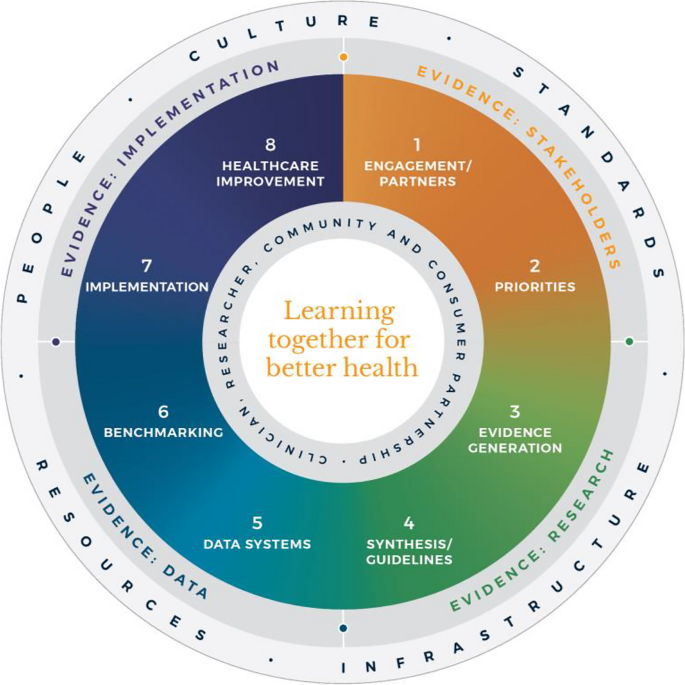
Monash Learning Health System: The Learn Together for Better Health Framework developed by Monash Partners and Monash University (from Enticott et al. 2021 [ 7 ]). Four evidence quadrants: Q1 (orange) is evidence from stakeholders; Q2 (green) is evidence from research; Q3 (light blue) is evidence from data; and, Q4 (dark blue) is evidence from implementation and healthcare improvement
In developed nations, it has been estimated that 60% of care provided aligns with the evidence base, 30% is low value and 10% is potentially harmful [ 13 ]. In some areas, clinical advances have been rapid and research and evidence have paved the way for dramatic improvement in outcomes, mandating rapid implementation of evidence into healthcare (e.g. polio and COVID-19 vaccines). However, healthcare improvement is challenging and slow [ 5 ]. Health systems are highly complex in their design, networks and interacting components, and change is difficult to enact, sustain and scale up. [ 3 ] New effective strategies are needed to meet community needs and deliver evidence-based and value-based care, which reorients care from serving the provider, services and system, towards serving community needs, based on evidence and quality. It goes beyond cost to encompass patient and provider experience, quality care and outcomes, efficiency and sustainability [ 2 , 6 ].
The costs of stroke care are expected to rise rapidly in the next decades, unless improvements in stroke care to reduce the disabling effects of strokes can be successfully developed and implemented [ 14 ]. Here, we briefly describe the Monash LHS framework (Fig. 1 ) [ 2 , 6 , 7 ] and outline an exemplar case in order to demonstrate how to apply evidence-based processes to healthcare improvement and embed real-world research for optimising healthcare. The Australian LHS exemplar in stroke care has driven nationwide improvement in stroke care since 2007.
An evidence-based Learning Health System framework
In Australia, members of this author group (HT, AJ, JE) have rigorously co-developed an evidence-based LHS framework, known simply as the Monash LHS [ 7 ]. The Monash LHS was designed to support sustainable, iterative and continuous robust benefit of improved clinical outcomes. It was created with national engagement in order to be applicable to Australian settings. Through this rigorous approach, core LHS principles and components have been established (Fig. 1 ). Evidence shows that people/workforce, culture, standards, governance and resources were all key to an effective LHS [ 2 , 6 ]. Culture is vital including trust, transparency, partnership and co-design. Key processes include legally compliant data sharing, linkage and governance, resources, and infrastructure [ 4 ]. The Monash LHS integrates disparate and often siloed stakeholders, infrastructure and expertise to ‘Learn Together for Better Health’ [ 7 ] (Fig. 1 ). This integrates (i) evidence from community and stakeholders including priority areas and outcomes; (ii) evidence from research and guidelines; (iii) evidence from practice (from data) with advanced analytics and benchmarking; and (iv) evidence from implementation science and health economics. Importantly, it starts with the problem and priorities of key stakeholders including the community, health professionals and services and creates an iterative learning system to address these. The following case study was chosen as it is an exemplar of how a Monash LHS-aligned national stroke program has delivered clinical benefit.
Australian Stroke Learning Health System
Internationally, the application of LHS approaches in stroke has resulted in improved stroke care and outcomes [ 12 ]. For example, in Canada a sustained decrease in 30-day in-hospital mortality has been found commensurate with an increase in resources to establish the multifactorial stroke system intervention for stroke treatment and prevention [ 15 ]. Arguably, with rapid advances in evidence and in the context of an ageing population with high cost and care burden and substantive impacts on quality of life, stroke is an area with a need for rapid research translation into evidence-based and value-based healthcare improvement. However, a recent systematic review found that the existing literature had few comprehensive examples of LHS adoption [ 12 ]. Although healthcare improvement systems and approaches were described, less is known about patient-clinician and stakeholder engagement, governance and culture, or embedding of data informatics into everyday practice to inform and drive improvement [ 12 ]. For example, in a recent review of quality improvement collaborations, it was found that although clinical processes in stroke care are improved, their short-term nature means there is uncertainty about sustainability and impacts on patient outcomes [ 16 ]. Table 1 provides the main features of the Australian Stroke LHS based on the four core domains and eight elements of the Learning Together for Better Health Framework described in Fig. 1 . The features are further expanded on in the following sections.
Evidence from stakeholders (LHS quadrant 1, Fig. 1 )
Engagement, partners and priorities.
Within the stroke field, there have been various support mechanisms to facilitate an LHS approach including partnership and broad stakeholder engagement that includes clinical networks and policy makers from different jurisdictions. Since 2008, the Australian Stroke Coalition has been co-led by the Stroke Foundation, a charitable consumer advocacy organisation, and Stroke Society of Australasia a professional society with membership covering academics and multidisciplinary clinician networks, that are collectively working to improve stroke care ( https://australianstrokecoalition.org.au/ ). Surveys, focus groups and workshops have been used for identifying priorities from stakeholders. Recent agreed priorities have been to improve stroke care and strengthen the voice for stroke care at a national ( https://strokefoundation.org.au/ ) and international level ( https://www.world-stroke.org/news-and-blog/news/world-stroke-organization-tackle-gaps-in-access-to-quality-stroke-care ), as well as reduce duplication amongst stakeholders. This activity is built on a foundation and culture of research and innovation embedded within the stroke ‘community of practice’. Consumers, as people with lived experience of stroke are important members of the Australian Stroke Coalition, as well as representatives from different clinical colleges. Consumers also provide critical input to a range of LHS activities via the Stroke Foundation Consumer Council, Stroke Living Guidelines committees, and the Australian Stroke Clinical Registry (AuSCR) Steering Committee (described below).
Evidence from research (LHS quadrant 2, Fig. 1 )
Advancement of the evidence for stroke interventions and synthesis into clinical guidelines.
To implement best practice, it is crucial to distil the large volume of scientific and trial literature into actionable recommendations for clinicians to use in practice [ 24 ]. The first Australian clinical guidelines for acute stroke were produced in 2003 following the increasing evidence emerging for prevention interventions (e.g. carotid endarterectomy, blood pressure lowering), acute medical treatments (intravenous thrombolysis, aspirin within 48 h of ischemic stroke), and optimised hospital management (care in dedicated stroke units by a specialised and coordinated multidisciplinary team) [ 25 ]. Importantly, a number of the innovations were developed, researched and proven effective by key opinion leaders embedded in the Australian stroke care community. In 2005, the clinical guidelines for Stroke Rehabilitation and Recovery [ 26 ] were produced, with subsequent merged guidelines periodically updated. However, the traditional process of periodic guideline updates is challenging for end users when new research can render recommendations redundant and this lack of currency erodes stakeholder trust [ 27 ]. In response to this challenge the Stroke Foundation and Cochrane Australia entered a pioneering project to produce the first electronic ‘living’ guidelines globally [ 20 ]. Major shifts in the evidence for reperfusion therapies (e.g. extended time-window intravenous thrombolysis and endovascular clot retrieval), among other advances, were able to be converted into new recommendations, approved by the Australian National Health and Medical Research Council within a few months of publication. Feedback on this process confirmed the increased use and trust in the guidelines by clinicians. The process informed other living guidelines programs, including the successful COVID-19 clinical guidelines [ 28 ].
However, best practice clinical guideline recommendations are necessary but insufficient for healthcare improvement and nesting these within an LHS with stakeholder partnership, enables implementation via a range of proven methods, including audit and feedback strategies [ 29 ].
Evidence from data and practice (LHS quadrant 3, Fig. 1 )
Data systems and benchmarking : revealing the disparities in care between health services. A national system for standardized stroke data collection was established as the National Stroke Audit program in 2007 by the Stroke Foundation [ 30 ] following various state-level programs (e.g. New South Wales Audit) [ 31 ] to identify evidence-practice gaps and prioritise improvement efforts to increase access to stroke units and other acute treatments [ 32 ]. The Audit program alternates each year between acute (commencing in 2007) and rehabilitation in-patient services (commencing in 2008). The Audit program provides a ‘deep dive’ on the majority of recommendations in the clinical guidelines whereby participating hospitals provide audits of up to 40 consecutive patient medical records and respond to a survey about organizational resources to manage stroke. In 2009, the AuSCR was established to provide information on patients managed in acute hospitals based on a small subset of quality processes of care linked to benchmarked reports of performance (Fig. 2 ) [ 33 ]. In this way, the continuous collection of high-priority processes of stroke care could be regularly collected and reviewed to guide improvement to care [ 34 ]. Plus clinical quality registry programs within Australia have shown a meaningful return on investment attributed to enhanced survival, improvements in quality of life and avoided costs of treatment or hospital stay [ 35 ].
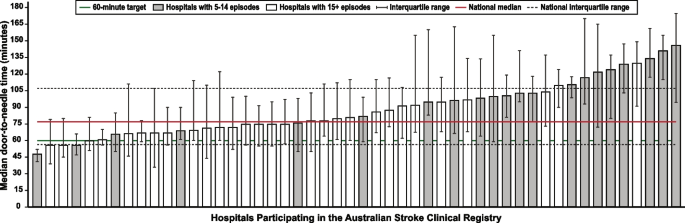
Example performance report from the Australian Stroke Clinical Registry: average door-to-needle time in providing intravenous thrombolysis by different hospitals in 2021 [ 36 ]. Each bar in the figure represents a single hospital
The Australian Stroke Coalition endorsed the creation of an integrated technological solution for collecting data through a single portal for multiple programs in 2013. In 2015, the Stroke Foundation, AuSCR consortium, and other relevant groups cooperated to design an integrated data management platform (the Australian Stroke Data Tool) to reduce duplication of effort for hospital staff in the collection of overlapping variables in the same patients [ 19 ]. Importantly, a national data dictionary then provided the common data definitions to facilitate standardized data capture. Another important feature of AuSCR is the collection of patient-reported outcome surveys between 90 and 180 days after stroke, and annual linkage with national death records to ascertain survival status [ 33 ]. To support a LHS approach, hospitals that participate in AuSCR have access to a range of real-time performance reports. In efforts to minimize the burden of data collection in the AuSCR, interoperability approaches to import data directly from hospital or state-level managed stroke databases have been established (Fig. 3 ); however, the application has been variable and 41% of hospitals still manually enter all their data.
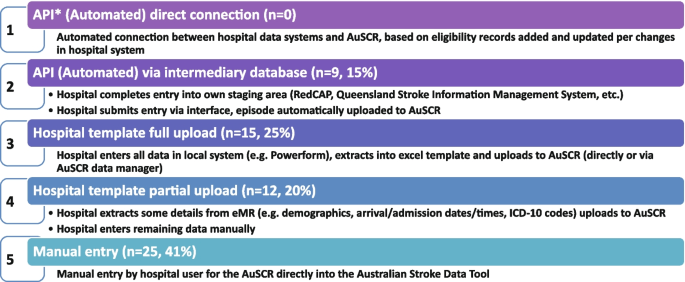
Current status of automated data importing solutions in the Australian Stroke Clinical Registry, 2022, with ‘ n ’ representing the number of hospitals. AuSCR, Australian Stroke Clinical Registry; AuSDaT, Australian Stroke Data Tool; API, Application Programming Interface; ICD, International Classification of Diseases; RedCAP, Research Electronic Data Capture; eMR, electronic medical records
For acute stroke care, the Australian Commission on Quality and Safety in Health Care facilitated the co-design (clinicians, academics, consumers) and publication of the national Acute Stroke Clinical Care Standard in 2015 [ 17 ], and subsequent review [ 18 ]. The indicator set for the Acute Stroke Standard then informed the expansion of the minimum dataset for AuSCR so that hospitals could routinely track their performance. The national Audit program enabled hospitals not involved in the AuSCR to assess their performance every two years against the Acute Stroke Standard. Complementing these efforts, the Stroke Foundation, working with the sector, developed the Acute and Rehabilitation Stroke Services Frameworks to outline the principles, essential elements, models of care and staffing recommendations for stroke services ( https://informme.org.au/guidelines/national-stroke-services-frameworks ). The Frameworks are intended to guide where stroke services should be developed, and monitor their uptake with the organizational survey component of the Audit program.
Evidence from implementation and healthcare improvement (LHS quadrant 4, Fig. 1 )
Research to better utilize and augment data from registries through linkage [ 37 , 38 , 39 , 40 ] and to ensure presentation of hospital or service level data are understood by clinicians has ensured advancement in the field for the Australian Stroke LHS [ 41 ]. Importantly, greater insights into whole patient journeys, before and after a stroke, can now enable exploration of value-based care. The LHS and stroke data platform have enabled focused and time-limited projects to create a better understanding of the quality of care in acute or rehabilitation settings [ 22 , 42 , 43 ]. Within stroke, all the elements of an LHS culminate into the ready availability of benchmarked performance data and support for implementation of strategies to address gaps in care.
Implementation research to grow the evidence base for effective improvement interventions has also been a key pillar in the Australian context. These include multi-component implementation interventions to achieve behaviour change for particular aspects of stroke care, [ 22 , 23 , 44 , 45 ] and real-world approaches to augmenting access to hyperacute interventions in stroke through the use of technology and telehealth [ 46 , 47 , 48 , 49 ]. The evidence from these studies feeds into the living guidelines program and the data collection systems, such as the Audit program or AuSCR, which are then amended to ensure data aligns to recommended care. For example, the use of ‘hyperacute aspirin within the first 48 h of ischemic stroke’ was modified to be ‘hyperacute antiplatelet…’ to incorporate new evidence that other medications or combinations are appropriate to use. Additionally, new datasets have been developed to align with evidence such as the Fever, Sugar, and Swallow variables [ 42 ]. Evidence on improvements in access to best practice care from the acute Audit program [ 50 ] and AuSCR is emerging [ 36 ]. For example, between 2007 and 2017, the odds of receiving intravenous thrombolysis after ischemic stroke increased by 16% 9OR 1.06 95% CI 1.13–1.18) and being managed in a stroke unit by 18% (OR 1.18 95% CI 1.17–1.20). Over this period, the median length of hospital stay for all patients decreased from 6.3 days in 2007 to 5.0 days in 2017 [ 51 ]. When considering the number of additional patients who would receive treatment in 2017 in comparison to 2007 it was estimated that without this additional treatment, over 17,000 healthy years of life would be lost in 2017 (17,786 disability-adjusted life years) [ 51 ]. There is evidence on the cost-effectiveness of different system-focussed strategies to augment treatment access for acute ischemic stroke (e.g. Victorian Stroke Telemedicine program [ 52 ] and Melbourne Mobile Stroke Unit ambulance [ 53 ]). Reciprocally, evidence from the national Rehabilitation Audit, where the LHS approach has been less complete or embedded, has shown fewer areas of healthcare improvement over time [ 51 , 54 ].
Within the field of stroke in Australia, there is indirect evidence that the collective efforts that align to establishing the components of a LHS have had an impact. Overall, the age-standardised rate of stroke events has reduced by 27% between 2001 and 2020, from 169 to 124 events per 100,000 population. Substantial declines in mortality rates have been reported since 1980. Commensurate with national clinical guidelines being updated in 2007 and the first National Stroke Audit being undertaken in 2007, the mortality rates for men (37.4 deaths per 100,000) and women (36.1 deaths per 100,0000 has declined to 23.8 and 23.9 per 100,000, respectively in 2021 [ 55 ].
Underpinning the LHS with the integration of the four quadrants of evidence from stakeholders, research and guidelines, practice and implementation, and core LHS principles have been addressed. Leadership and governance have been important, and programs have been established to augment workforce training and capacity building in best practice professional development. Medical practitioners are able to undertake courses and mentoring through the Australasian Stroke Academy ( http://www.strokeacademy.com.au/ ) while nurses (and other health professionals) can access teaching modules in stroke care from the Acute Stroke Nurses Education Network ( https://asnen.org/ ). The Association of Neurovascular Clinicians offers distance-accessible education and certification to develop stroke expertise for interdisciplinary professionals, including advanced stroke co-ordinator certification ( www.anvc.org ). Consumer initiative interventions are also used in the design of the AuSCR Public Summary Annual reports (available at https://auscr.com.au/about/annual-reports/ ) and consumer-related resources related to the Living Guidelines ( https://enableme.org.au/resources ).
The important success factors and lessons from stroke as a national exemplar LHS in Australia include leadership, culture, workforce and resources integrated with (1) established and broad partnerships across the academic-clinical sector divide and stakeholder engagement; (2) the living guidelines program; (3) national data infrastructure, including a national data dictionary that provides the common data framework to support standardized data capture; (4) various implementation strategies including benchmarking and feedback as well as engagement strategies targeting different levels of the health system; and (5) implementation and improvement research to advance stroke systems of care and reduce unwarranted variation in practice (Fig. 1 ). Priority opportunities now include the advancement of interoperability with electronic medical records as an area all clinical quality registry’s programs needs to be addressed, as well as providing more dynamic and interactive data dashboards tailored to the need of clinicians and health service executives.
There is a clear mandate to optimise healthcare improvement with big data offering major opportunities for change. However, we have lacked the approaches to capture evidence from the community and stakeholders, to integrate evidence from research, to capture and leverage data or evidence from practice and to generate and build on evidence from implementation using iterative system-level improvement. The LHS provides this opportunity and is shown to deliver impact. Here, we have outlined the process applied to generate an evidence-based LHS and provide a leading exemplar in stroke care. This highlights the value of moving from single-focus isolated approaches/initiatives to healthcare improvement and the benefit of integration to deliver demonstrable outcomes for our funders and key stakeholders — our community. This work provides insight into strategies that can both apply evidence-based processes to healthcare improvement as well as implementing evidence-based practices into care, moving beyond research as an endpoint, to research as an enabler, underpinning delivery of better healthcare.
Availability of data and materials
Not applicable
Abbreviations
Australian Stroke Clinical Registry
Confidence interval
- Learning Health System
World Health Organization. Delivering quality health services . OECD Publishing; 2018.
Enticott J, Braaf S, Johnson A, Jones A, Teede HJ. Leaders’ perspectives on learning health systems: A qualitative study. BMC Health Serv Res. 2020;20:1087.
Article PubMed PubMed Central Google Scholar
Melder A, Robinson T, McLoughlin I, Iedema R, Teede H. An overview of healthcare improvement: Unpacking the complexity for clinicians and managers in a learning health system. Intern Med J. 2020;50:1174–84.
Article PubMed Google Scholar
Alberto IRI, Alberto NRI, Ghosh AK, Jain B, Jayakumar S, Martinez-Martin N, et al. The impact of commercial health datasets on medical research and health-care algorithms. Lancet Digit Health. 2023;5:e288–94.
Article CAS PubMed PubMed Central Google Scholar
Dixon-Woods M. How to improve healthcare improvement—an essay by Mary Dixon-Woods. BMJ. 2019;367: l5514.
Enticott J, Johnson A, Teede H. Learning health systems using data to drive healthcare improvement and impact: A systematic review. BMC Health Serv Res. 2021;21:200.
Enticott JC, Melder A, Johnson A, Jones A, Shaw T, Keech W, et al. A learning health system framework to operationalize health data to improve quality care: An Australian perspective. Front Med (Lausanne). 2021;8:730021.
Dammery G, Ellis LA, Churruca K, Mahadeva J, Lopez F, Carrigan A, et al. The journey to a learning health system in primary care: A qualitative case study utilising an embedded research approach. BMC Prim Care. 2023;24:22.
Foley T, Horwitz L, Zahran R. The learning healthcare project: Realising the potential of learning health systems. 2021. Available from https://learninghealthcareproject.org/wp-content/uploads/2021/05/LHS2021report.pdf . Accessed Jan 2024.
Institute of Medicine. Best care at lower cost: The path to continuously learning health care in America. Washington: The National Academies Press; 2013.
Google Scholar
Zurynski Y, Smith CL, Vedovi A, Ellis LA, Knaggs G, Meulenbroeks I, et al. Mapping the learning health system: A scoping review of current evidence - a white paper. 2020:63
Cadilhac DA, Bravata DM, Bettger J, Mikulik R, Norrving B, Uvere E, et al. Stroke learning health systems: A topical narrative review with case examples. Stroke. 2023;54:1148–59.
Braithwaite J, Glasziou P, Westbrook J. The three numbers you need to know about healthcare: The 60–30-10 challenge. BMC Med. 2020;18:1–8.
Article Google Scholar
King D, Wittenberg R, Patel A, Quayyum Z, Berdunov V, Knapp M. The future incidence, prevalence and costs of stroke in the UK. Age Ageing. 2020;49:277–82.
Ganesh A, Lindsay P, Fang J, Kapral MK, Cote R, Joiner I, et al. Integrated systems of stroke care and reduction in 30-day mortality: A retrospective analysis. Neurology. 2016;86:898–904.
Lowther HJ, Harrison J, Hill JE, Gaskins NJ, Lazo KC, Clegg AJ, et al. The effectiveness of quality improvement collaboratives in improving stroke care and the facilitators and barriers to their implementation: A systematic review. Implement Sci. 2021;16:16.
Australian Commission on Safety and Quality in Health Care. Acute stroke clinical care standard. 2015. Available from https://www.safetyandquality.gov.au/our-work/clinical-care-standards/acute-stroke-clinical-care-standard . Accessed Jan 2024.
Australian Commission on Safety and Quality in Health Care. Acute stroke clinical care standard. Sydney: ACSQHC; 2019. Available from https://www.safetyandquality.gov.au/publications-and-resources/resource-library/acute-stroke-clinical-care-standard-evidence-sources . Accessed Jan 2024.
Ryan O, Ghuliani J, Grabsch B, Hill K, G CC, Breen S, et al. Development, implementation, and evaluation of the Australian Stroke Data Tool (AuSDaT): Comprehensive data capturing for multiple uses. Health Inf Manag. 2022:18333583221117184.
English C, Bayley M, Hill K, Langhorne P, Molag M, Ranta A, et al. Bringing stroke clinical guidelines to life. Int J Stroke. 2019;14:337–9.
English C, Hill K, Cadilhac DA, Hackett ML, Lannin NA, Middleton S, et al. Living clinical guidelines for stroke: Updates, challenges and opportunities. Med J Aust. 2022;216:510–4.
Cadilhac DA, Grimley R, Kilkenny MF, Andrew NE, Lannin NA, Hill K, et al. Multicenter, prospective, controlled, before-and-after, quality improvement study (Stroke123) of acute stroke care. Stroke. 2019;50:1525–30.
Cadilhac DA, Marion V, Andrew NE, Breen SJ, Grabsch B, Purvis T, et al. A stepped-wedge cluster-randomized trial to improve adherence to evidence-based practices for acute stroke management. Jt Comm J Qual Patient Saf. 2022.
Elliott J, Lawrence R, Minx JC, Oladapo OT, Ravaud P, Jeppesen BT, et al. Decision makers need constantly updated evidence synthesis. Nature. 2021;600:383–5.
Article CAS PubMed Google Scholar
National Stroke Foundation. National guidelines for acute stroke management. Melbourne: National Stroke Foundation; 2003.
National Stroke Foundation. Clinical guidelines for stroke rehabilitation and recovery. Melbourne: National Stroke Foundation; 2005.
Phan TG, Thrift A, Cadilhac D, Srikanth V. A plea for the use of systematic review methodology when writing guidelines and timely publication of guidelines. Intern Med J . 2012;42:1369–1371; author reply 1371–1362
Tendal B, Vogel JP, McDonald S, Norris S, Cumpston M, White H, et al. Weekly updates of national living evidence-based guidelines: Methods for the Australian living guidelines for care of people with COVID-19. J Clin Epidemiol. 2021;131:11–21.
Grimshaw JM, Eccles MP, Lavis JN, Hill SJ, Squires JE. Knowledge translation of research findings. Implement Sci. 2012;7:50.
Harris D, Cadilhac D, Hankey GJ, Hillier S, Kilkenny M, Lalor E. National stroke audit: The Australian experience. Clin Audit. 2010;2:25–31.
Cadilhac DA, Purvis T, Kilkenny MF, Longworth M, Mohr K, Pollack M, et al. Evaluation of rural stroke services: Does implementation of coordinators and pathways improve care in rural hospitals? Stroke. 2013;44:2848–53.
Cadilhac DA, Moss KM, Price CJ, Lannin NA, Lim JY, Anderson CS. Pathways to enhancing the quality of stroke care through national data monitoring systems for hospitals. Med J Aust. 2013;199:650–1.
Cadilhac DA, Lannin NA, Anderson CS, Levi CR, Faux S, Price C, et al. Protocol and pilot data for establishing the Australian Stroke Clinical Registry. Int J Stroke. 2010;5:217–26.
Ivers N, Jamtvedt G, Flottorp S, Young J, Odgaard-Jensen J, French S, et al. Audit and feedback: Effects on professional practice and healthcare outcomes. Cochrane Database Syst Rev . 2012
Australian Commission on Safety and Quality in Health Care. Economic evaluation of clinical quality registries. Final report. . 2016:79
Cadilhac DA, Dalli LL, Morrison J, Lester M, Paice K, Moss K, et al. The Australian Stroke Clinical Registry annual report 2021. Melbourne; 2022. Available from https://auscr.com.au/about/annual-reports/ . Accessed 6 May 2024.
Kilkenny MF, Kim J, Andrew NE, Sundararajan V, Thrift AG, Katzenellenbogen JM, et al. Maximising data value and avoiding data waste: A validation study in stroke research. Med J Aust. 2019;210:27–31.
Eliakundu AL, Smith K, Kilkenny MF, Kim J, Bagot KL, Andrew E, et al. Linking data from the Australian Stroke Clinical Registry with ambulance and emergency administrative data in Victoria. Inquiry. 2022;59:469580221102200.
PubMed Google Scholar
Andrew NE, Kim J, Cadilhac DA, Sundararajan V, Thrift AG, Churilov L, et al. Protocol for evaluation of enhanced models of primary care in the management of stroke and other chronic disease (PRECISE): A data linkage healthcare evaluation study. Int J Popul Data Sci. 2019;4:1097.
CAS PubMed PubMed Central Google Scholar
Mosalski S, Shiner CT, Lannin NA, Cadilhac DA, Faux SG, Kim J, et al. Increased relative functional gain and improved stroke outcomes: A linked registry study of the impact of rehabilitation. J Stroke Cerebrovasc Dis. 2021;30: 106015.
Ryan OF, Hancock SL, Marion V, Kelly P, Kilkenny MF, Clissold B, et al. Feedback of aggregate patient-reported outcomes (PROs) data to clinicians and hospital end users: Findings from an Australian codesign workshop process. BMJ Open. 2022;12:e055999.
Grimley RS, Rosbergen IC, Gustafsson L, Horton E, Green T, Cadigan G, et al. Dose and setting of rehabilitation received after stroke in Queensland, Australia: A prospective cohort study. Clin Rehabil. 2020;34:812–23.
Purvis T, Middleton S, Craig LE, Kilkenny MF, Dale S, Hill K, et al. Inclusion of a care bundle for fever, hyperglycaemia and swallow management in a national audit for acute stroke: Evidence of upscale and spread. Implement Sci. 2019;14:87.
Middleton S, McElduff P, Ward J, Grimshaw JM, Dale S, D’Este C, et al. Implementation of evidence-based treatment protocols to manage fever, hyperglycaemia, and swallowing dysfunction in acute stroke (QASC): A cluster randomised controlled trial. Lancet. 2011;378:1699–706.
Middleton S, Dale S, Cheung NW, Cadilhac DA, Grimshaw JM, Levi C, et al. Nurse-initiated acute stroke care in emergency departments. Stroke. 2019:STROKEAHA118020701.
Hood RJ, Maltby S, Keynes A, Kluge MG, Nalivaiko E, Ryan A, et al. Development and pilot implementation of TACTICS VR: A virtual reality-based stroke management workflow training application and training framework. Front Neurol. 2021;12:665808.
Bladin CF, Kim J, Bagot KL, Vu M, Moloczij N, Denisenko S, et al. Improving acute stroke care in regional hospitals: Clinical evaluation of the Victorian Stroke Telemedicine program. Med J Aust. 2020;212:371–7.
Bladin CF, Bagot KL, Vu M, Kim J, Bernard S, Smith K, et al. Real-world, feasibility study to investigate the use of a multidisciplinary app (Pulsara) to improve prehospital communication and timelines for acute stroke/STEMI care. BMJ Open. 2022;12:e052332.
Zhao H, Coote S, Easton D, Langenberg F, Stephenson M, Smith K, et al. Melbourne mobile stroke unit and reperfusion therapy: Greater clinical impact of thrombectomy than thrombolysis. Stroke. 2020;51:922–30.
Purvis T, Cadilhac DA, Hill K, Reyneke M, Olaiya MT, Dalli LL, et al. Twenty years of monitoring acute stroke care in Australia from the national stroke audit program (1999–2019): Achievements and areas of future focus. J Health Serv Res Policy. 2023.
Cadilhac DA, Purvis T, Reyneke M, Dalli LL, Kim J, Kilkenny MF. Evaluation of the national stroke audit program: 20-year report. Melbourne; 2019.
Kim J, Tan E, Gao L, Moodie M, Dewey HM, Bagot KL, et al. Cost-effectiveness of the Victorian Stroke Telemedicine program. Aust Health Rev. 2022;46:294–301.
Kim J, Easton D, Zhao H, Coote S, Sookram G, Smith K, et al. Economic evaluation of the Melbourne mobile stroke unit. Int J Stroke. 2021;16:466–75.
Stroke Foundation. National stroke audit – rehabilitation services report 2020. Melbourne; 2020.
Australian Institute of Health and Welfare. Heart, stroke and vascular disease: Australian facts. 2023. Webpage https://www.aihw.gov.au/reports/heart-stroke-vascular-diseases/hsvd-facts/contents/about (accessed Jan 2024).
Download references
Acknowledgements
The following authors hold National Health and Medical Research Council Research Fellowships: HT (#2009326), DAC (#1154273), SM (#1196352), MFK Future Leader Research Fellowship (National Heart Foundation #105737). The Funders of this work did not have any direct role in the design of the study, its execution, analyses, interpretation of the data, or decision to submit results for publication.
Author information
Helena Teede and Dominique A. Cadilhac contributed equally.
Authors and Affiliations
Monash Centre for Health Research and Implementation, 43-51 Kanooka Grove, Clayton, VIC, Australia
Helena Teede, Emily Callander & Joanne Enticott
Monash Partners Academic Health Science Centre, 43-51 Kanooka Grove, Clayton, VIC, Australia
Helena Teede & Alison Johnson
Stroke and Ageing Research, Department of Medicine, School of Clinical Sciences at Monash Health, Monash University, Level 2 Monash University Research, Victorian Heart Hospital, 631 Blackburn Rd, Clayton, VIC, Australia
Dominique A. Cadilhac, Tara Purvis & Monique F. Kilkenny
Stroke Theme, The Florey Institute of Neuroscience and Mental Health, University of Melbourne, Heidelberg, VIC, Australia
Dominique A. Cadilhac, Monique F. Kilkenny & Bruce C.V. Campbell
Department of Neurology, Melbourne Brain Centre, Royal Melbourne Hospital, Parkville, VIC, Australia
Bruce C.V. Campbell
Department of Medicine, Faculty of Medicine, Dentistry and Health Sciences, University of Melbourne, Victoria, Australia
School of Health Sciences, Heart and Stroke Program, University of Newcastle, Hunter Medical Research Institute, University Drive, Callaghan, NSW, Australia
Coralie English
School of Medicine and Dentistry, Griffith University, Birtinya, QLD, Australia
Rohan S. Grimley
Clinical Excellence Division, Queensland Health, Brisbane, Australia
John Hunter Hospital, Hunter New England Local Health District and University of Newcastle, Sydney, NSW, Australia
Christopher Levi
School of Nursing, Midwifery and Paramedicine, Australian Catholic University, Sydney, NSW, Australia
Sandy Middleton
Nursing Research Institute, St Vincent’s Health Network Sydney and and Australian Catholic University, Sydney, NSW, Australia
Stroke Foundation, Level 7, 461 Bourke St, Melbourne, VIC, Australia
Kelvin Hill
You can also search for this author in PubMed Google Scholar
Contributions
HT: conception, design and initial draft, developed the theoretical formalism for learning health system framework, approved the submitted version. DAC: conception, design and initial draft, provided essential literature and case study examples, approved the submitted version. TP: revised the manuscript critically for important intellectual content, approved the submitted version. MFK: revised the manuscript critically for important intellectual content, provided essential literature and case study examples, approved the submitted version. BC: revised the manuscript critically for important intellectual content, provided essential literature and case study examples, approved the submitted version. CE: revised the manuscript critically for important intellectual content, provided essential literature and case study examples, approved the submitted version. AJ: conception, design and initial draft, developed the theoretical formalism for learning health system framework, approved the submitted version. EC: revised the manuscript critically for important intellectual content, approved the submitted version. RSG: revised the manuscript critically for important intellectual content, provided essential literature and case study examples, approved the submitted version. CL: revised the manuscript critically for important intellectual content, provided essential literature and case study examples, approved the submitted version. SM: revised the manuscript critically for important intellectual content, provided essential literature and case study examples, approved the submitted version. KH: revised the manuscript critically for important intellectual content, provided essential literature and case study examples, approved the submitted version. JE: conception, design and initial draft, developed the theoretical formalism for learning health system framework, approved the submitted version. All authors read and approved the final manuscript.
Authors’ Twitter handles
@HelenaTeede
@DominiqueCad
@Coralie_English
@EmilyCallander
@EnticottJo
Corresponding authors
Correspondence to Helena Teede or Dominique A. Cadilhac .
Ethics declarations
Ethics approval and consent to participate, consent for publication, competing interests, additional information, publisher's note.
Springer Nature remains neutral with regard to jurisdictional claims in published maps and institutional affiliations.
Rights and permissions
Open Access This article is licensed under a Creative Commons Attribution 4.0 International License, which permits use, sharing, adaptation, distribution and reproduction in any medium or format, as long as you give appropriate credit to the original author(s) and the source, provide a link to the Creative Commons licence, and indicate if changes were made. The images or other third party material in this article are included in the article's Creative Commons licence, unless indicated otherwise in a credit line to the material. If material is not included in the article's Creative Commons licence and your intended use is not permitted by statutory regulation or exceeds the permitted use, you will need to obtain permission directly from the copyright holder. To view a copy of this licence, visit http://creativecommons.org/licenses/by/4.0/ . The Creative Commons Public Domain Dedication waiver ( http://creativecommons.org/publicdomain/zero/1.0/ ) applies to the data made available in this article, unless otherwise stated in a credit line to the data.
Reprints and permissions
About this article
Cite this article.
Teede, H., Cadilhac, D.A., Purvis, T. et al. Learning together for better health using an evidence-based Learning Health System framework: a case study in stroke. BMC Med 22 , 198 (2024). https://doi.org/10.1186/s12916-024-03416-w
Download citation
Received : 23 July 2023
Accepted : 30 April 2024
Published : 15 May 2024
DOI : https://doi.org/10.1186/s12916-024-03416-w
Share this article
Anyone you share the following link with will be able to read this content:
Sorry, a shareable link is not currently available for this article.
Provided by the Springer Nature SharedIt content-sharing initiative
- Evidence-based medicine
- Person-centred care
- Models of care
- Healthcare improvement
BMC Medicine
ISSN: 1741-7015
- Submission enquiries: [email protected]
- General enquiries: [email protected]
Insights on Healthcare
Read McKinsey's latest insights on the changes that matter most for navigating the future of healthcare.
Provider Insights | Payer Insights | Healthcare Services & Technology Insights | Business Building in Healthcare | Consumer Health Insights | Behavioral Health Insights | Public Health Insights
Featured Insight

Value creation through business model innovation in US healthcare
Featured article.

The future of Medicare Advantage
Most recent articles.

Consumers rule: Driving healthcare growth with a consumer-led strategy

Ensuring the financial sustainability of academic medical centers

How price transparency could affect US healthcare markets

How to bridge the experience gap by supporting nurses of all tenures
Featured collections.

The Future of Nursing: McKinsey’s Frontline Insights

McKinsey on Healthcare: Innovate to thrive

The Future of Healthcare

McKinsey on Healthcare Podcast
Our conversations.

Speaking to hearts and minds in healthcare

Beyond the numbers: Creating a more diverse future for health

Meeting the member where they are: What is the future of health equity?

Aging reframed: Seeing aging as an opportunity in healthcare
Provider insights.
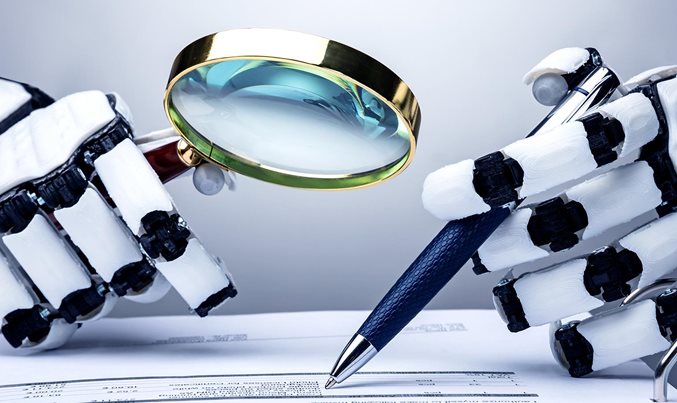
Payment integrity in the age of AI and value-based care

2024 health systems outlook: A host of challenges ahead

Understanding and prioritizing nurses’ mental health and well-being
Payer insights.

2024 payers outlook: Opportunities abound
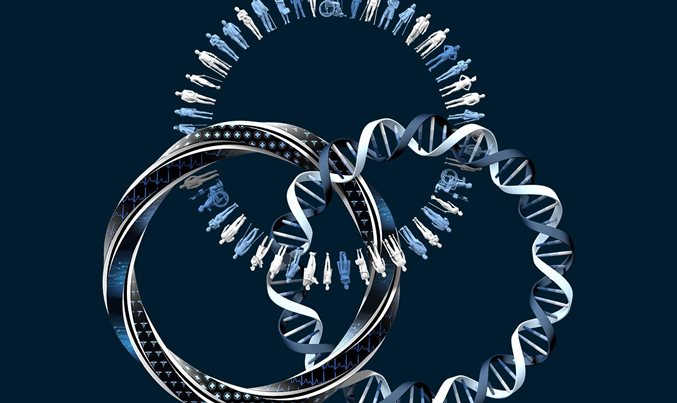
Leadership rundown: How US healthcare leaders are scaling innovation and transformation
Healthcare services & technology insights.

The potential benefits of AI for healthcare in Canada
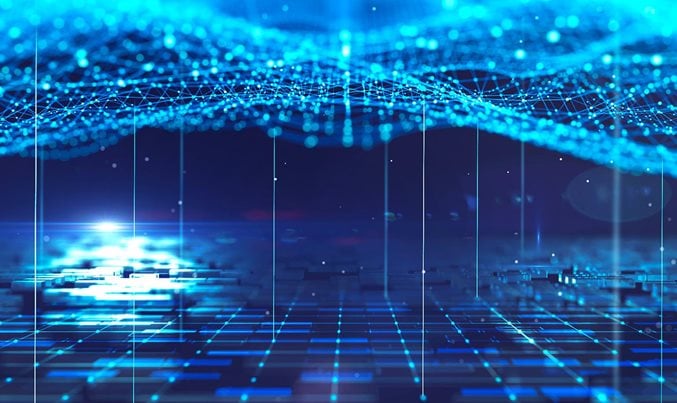
2024 healthcare services outlook: Challenges and opportunities

Untapped opportunities for health system pharmacies

Setting the revenue cycle up for success in automation and AI
Want to learn more about how we help clients in healthcare practice, business building in healthcare.

Women in the healthcare industry: An update
Consumer health insights.

Healthcare in Latin America: What are consumers looking for?

Consumers’ perceptions of their health-related social needs

Meeting changing consumer needs: The US retail pharmacy of the future
Behavioral health insights.

Working nine to thrive

Reframing employee health: Moving beyond burnout to holistic health

Gen Z mental health: The impact of tech and social media
Public health insights.

Public health’s inflection point with generative AI
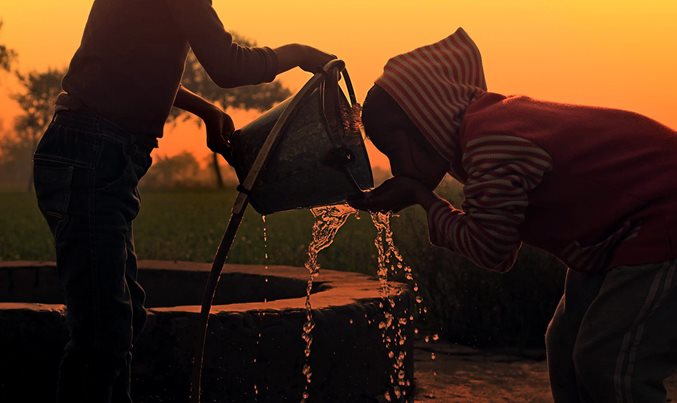
Future-proofing health systems for climate risks and pandemics

The end of the COVID-19 public health emergency: What’s next?

Virtual health for all: Closing the digital divide to expand access
More insights.

Early adoption of generative AI in commercial life sciences

Redesigning for speed: Addressing life cycle compression in biopharma

The business of healthcare: ‘I’m always optimistic about what’s possible’

‘We need to invest in health workers between major pandemics—not just when we have a pandemic’

Healthcare’s next chapter: What’s ahead for the US healthcare industry

What early-stage investing reveals about biotech innovation

Digital diagnostics: A path forward for IVD

Aging with purpose: Why meaningful engagement with society matters

RELATED PRACTICE
- Life Sciences
- Public & Social Sector
Connect with our Healthcare Practice
- Research article
- Open access
- Published: 14 November 2019
Organisational change in hospitals: a qualitative case-study of staff perspectives
- Chiara Pomare ORCID: orcid.org/0000-0002-9118-7207 1 ,
- Kate Churruca 1 ,
- Janet C. Long 1 ,
- Louise A. Ellis 1 &
- Jeffrey Braithwaite 1
BMC Health Services Research volume 19 , Article number: 840 ( 2019 ) Cite this article
42k Accesses
21 Citations
8 Altmetric
Metrics details
Organisational change in health systems is common. Success is often tied to the actors involved, including their awareness of the change, personal engagement and ownership of it. In many health systems, one of the most common changes we are witnessing is the redevelopment of long-standing hospitals. However, we know little about how hospital staff understand and experience such potentially far-reaching organisational change. The purpose of this study is to explore the understanding and experiences of hospital staff in the early stages of organisational change, using a hospital redevelopment in Sydney, Australia as a case study.
Semi-structured interviews were conducted with 46 clinical and non-clinical staff working at a large metropolitan hospital. Hospital staff were moving into a new building, not moving, or had moved into a different building two years prior. Questions asked staff about their level of awareness of the upcoming redevelopment and their experiences in the early stage of this change. Qualitative data were analysed using thematic analysis.
Some staff expressed apprehension and held negative expectations regarding the organisational change. Concerns included inadequate staffing and potential for collaboration breakdown due to new layout of workspaces. These fears were compounded by current experiences of feeling uninformed about the change, as well as feelings of being fatigued and under-staffed in the constantly changing hospital environment. Nevertheless, balancing this, many staff reported positive expectations regarding the benefits to patients of the change and the potential for staff to adapt in the face of this change.
Conclusions
The results of this study suggest that it is important to understand prospectively how actors involved make sense of organisational change, in order to potentially assuage concerns and alleviate negative expectations. Throughout the processes of organisational change, such as a hospital redevelopment, staff need to be engaged, adequately informed, trained, and to feel supported by management. The use of champions of varying professions and lead departments, may be useful to address concerns, adequately inform, and promote a sense of engagement among staff.
Peer Review reports
Change is a common experience in complex health care systems. Staff, patients and visitors come and go [ 1 ]; leadership, models of care, workforce and governing structures are reshaped in response to policy and legislative change [ 2 ], and new technologies and equipment are introduced or retired [ 3 ]. In addition to these common changes experienced throughout health care, the acute sector in many countries is constantly undergoing major changes to the physical hospital infrastructure [ 4 , 5 ]. In New South Wales, Australia, several reports have described the increase in hospital redevelopment projects as a ‘hospital building boom’ [ 4 , 6 ], with approximately 100 major health capital projects (i.e., projects over AUD$10 million) currently in train [ 7 ]. In addition to meeting the needs of a growing and ageing population [ 8 ], the re-design and refurbishment of older hospital infrastructure is supported by a range of arguments and anecdotal evidence highlighting the positive relationship between the hospital physical environment and patient [ 9 ] and staff outcomes [ 10 ]. While there are many reasons why hospital redevelopments are taking place, we know little about how hospital staff prospectively perceive change, and their experiences, expectations, and concerns. Hospital staff encapsulates any employee working in the hospital context. This includes clinical and non-clinical staff who provide care, support, cleaning, catering, managerial and administrative duties to patients and the broader community.
One reason as to why little research has explored the perspectives of hospital staff during a redevelopment may be because hospital redevelopment is often considered a physical, rather than organisational change. Organisational change means that not only the physical environment is altered, but also the behavioural operations, structural relationships and roles, and the hospital organisational culture may transform. For example, changing the physical health care environment can affect job satisfaction, stress, intention to leave [ 11 ], and the way staff work together [ 12 ].
Redeveloping a hospital can be both an exciting and challenging time for staff. In a recent notable example of opening a new hospital building in Australia, staff attitudes shifted from appreciation and excitement in the early stages of change to frustration and angst as the development progressed [ 13 ]. Similar experiences have been reported elsewhere, such as in a study describing the consequences for staff of hospital change in South Africa [ 14 ]. However, these examples explored staff attitudes towards change retrospectively and considered the change as a physical redevelopment, rather than organisational change. Such retrospective reports may be limited in validity [ 15 ] as prospective experiences and understanding of change reported by staff may be conflated with the final outcome of the change. The hospital redevelopment literature has also prospectively assessed health impacts of proposed redevelopment plans as a means to predetermine the impact of a large change on the population [ 16 ]; while prospective, this research again considers redevelopment as a physical modification, rather than an organisational change. Thus, while the literature has reported retrospective accounts of staff experiences in large hospital change and prospective assessment of the impact of the change, there is little research examining the understanding and experiences of staff in the early stages of redevelopmental change in hospitals through a lens of organisational change.
Seminal research in the organisational change literature highlights that the role of frontline workers (in this case hospital staff) is crucial to implementation of any process or change [ 17 , 18 ]. Specifically, that the support of actors (understanding, owning, and engaging) can determine the success of a change [ 19 ]. This is consistent with complexity science accounts which suggest that any improvement and transformation of health systems is dependent upon the actors involved, and the extent and quality of their interactions, their emergent behaviours, and localised responses [ 1 , 20 ]. In health care, change can be resisted when it is imposed on actors (in this case, hospital staff), but may be better accepted when people are involved and adopt a sense of ownership of the changes that will affect them [ 21 ]. This may include being involved in the design process. For this reason, it is important to examine the understanding and experiences of actors involved in a change (i.e., hospital staff in a redevelopment), in order to understand and potentially address their concerns, alleviating negative expectations prior to the change.
This study is part of a larger project exploring how hospital redevelopment influences the organisation, staff and patients involved [ 22 ]. The present study aimed to explore the understanding and experiences of staff prior to moving into a new building as one stage in a multidimensional organisational change project. The research questions were: How do staff make sense of this organisational change? How well informed do they feel? What are their expectations and concerns? What are the implications for hospitals undergoing organisational change, particularly redevelopment?
The study protocol has been published elsewhere [ 22 ]. The Consolidated criteria for reporting qualitative research (COREQ) guidelines were used to ensure comprehensive reporting of the qualitative study results (Additional file 1 ) [ 23 ] .
Study setting and participants
This study was conducted at a large metropolitan, publicly-funded hospital in Sydney, Australia. The facility is undergoing a multimillion-dollar development project to meet the growing needs of the community. This hospital has undergone a number of other changes over the last two decades, including incremental increases in size. Since its opening in the mid 1990s (with approximately 150 beds), several buildings have been added over the years. The hospital now has multiple buildings and over 500 beds.
During the time of this study, the hospital was in the second stage of the multi-stage redevelopment. This stage included: the opening of a new acute services building, the relocation of several wards to this new building (e.g., Intensive care unit (ICU) and Maternity), increases in resources (e.g., equipment, staffing), and the adoption of new ways of working (e.g., activity-based workspaces for support staff). Essentially, the redevelopment involves the opening of a new state-of-the-art building which will include moving services (and staff) from the old to the new building, with some wards staying in the old building. For the wards moving into the new building, this change does not initially involve more patients in existing services, but is intended to increase the number of staff because there will be more physical space to cover and new models of care introduced (e.g., ICU changing to single-bed rooms, more staff needed to individually attend to patients). The current redevelopment includes space for future expansion to account for the growing population. In addition to the redevelopment of the physical infrastructure, the way staff work together is also planned to change. Hospital leadership is aiming to foster a cultural shift towards greater cohesion and unity; highlighting that the hospital redevelopment can be conceptualised as an organisational change of considerable importance and magnitude.
Participants were hospital staff (clinical and non-clinical) working at the hospital under investigation. Staff working on four wards were targeted for interviews, with the intention to capture diverse experiences of the redevelopment and the broader organisational change; two of these wards would be moving into the new building (ICU and Maternity), one ward was not moving (Surgical), and one ward had moved into a new building two years prior (Respiratory). Interviews were also conducted with staff who held responsibilities across wards (e.g., General Services Department: cleaners, porters). The hospital staff were purposively recruited by department heads and snowballed from participants. Fifty staff members were approached (until data saturation was met) with four refusing to participate because they did not have the time.
Semi-structured interviews
Semi-structured interviews were conducted in private settings at the participants’ place of work (e.g., ward interview rooms, private offices). In the event a participant was unable to meet the researcher in person, interviews were conducted over the phone. A semi-structured interview guide was created in collaboration with key stakeholders from the hospital under investigation and following a literature review. The guide (Additional file 2 ) included questions aimed at exploring participants’: (1) understanding of the hospital’s culture and current ways of working; (2) understanding of the redevelopment and other hospital changes; and (3) concerns or expectations about the organisational change. The interviews were audio-taped and transcribed verbatim by the first author who is trained and experienced in conducting semi-structured interviews. No field notes were made during the interview nor were transcripts returned to participants for comment or correction due to the time poor characteristics of the study participants (hospital staff). Participants were informed that the research was part of the first author’s doctoral studies.
Interview data were analysed via thematic analysis [ 24 ] using NVivo [ 25 ]. This approach followed Braun and Clarke’s (2006) six phases of thematic analysis: familiarise, generate initial codes, develop themes, review potential themes, define and name themes, produce the report. Data were initially read multiple times by the first author, then descriptively and iteratively coded according to semantic features. The analysis included the use of inductive coding to identify patterns driven by the data, together with deductive coding, keeping the research questions in mind. Through examination of codes and coded data, themes were developed. The broader research team (KC, LAE, JCL) were included throughout each stage of the analysis process, with frequent discussions concerning the categorization of codes and themes. This process of having one researcher responsible for the analysis while other researchers then checked and clarified emerging themes throughout contributes to the trustworthiness of the findings [ 26 ].
In presenting the results, extracts have been edited minimally to enhance readability, without altering meaning or inference. Where extracts are presented, staff are coded according to their department (G: General – works across several wards; ICU: Intensive care unit; MAT: Maternity ward; RES: Respiratory ward; SUR: Surgical ward) and profession (AD: Administrative staff; CHGTEAM: Change management team staff; DR: Medical staff; GS: General services staff; MW: Midwifery staff; N: Nursing staff; OTH: Other profession).
Forty-six staff members participated in the semi-structured interviews. Interviews were typically conducted face-to-face ( n = 41; 89.1%), with five interviews conducted over the phone. No differences were discerned in content between these different mediums. Hospital staff taking part in interviews included those from: nursing and midwifery, medical, general services, administrative, and change management (Table 1 ). Change management staff are external to the hospital staff, and do not report to hospital executives. Interviews ranged from seven to 33 min in length ( M = 17 min). Participating staff had worked at the hospital for on average 10.5 years (range 5 months and 30 years).
Five themes were identified related to hospital staff’s understanding and experiences (i.e., expectations and concerns) of the change: staffing; benefits to patients; collaboration; fatigue; and adaptability. These expectations and concerns are schematically presented in Fig. 1 , with shades of red indicating negative expectations and concerns associated with the theme, and green representing positive expectations. Intensity of the colour demonstrated the frequency of positivity or negativity associated with that theme (i.e., deeper shades of red indicate frequency of negative discussion of this theme by different hospital staff). This figure also highlights the complexity and interrelatedness of these themes (e.g., the concern of inadequate staffing for the new building was linked with concerns about patient care, which could possibly impede the way the team work together, leading to staff feeling overworked and worn out; these expectations were all mitigated by the staff member’s understanding and awareness of the change). Explanations and examples are presented below.
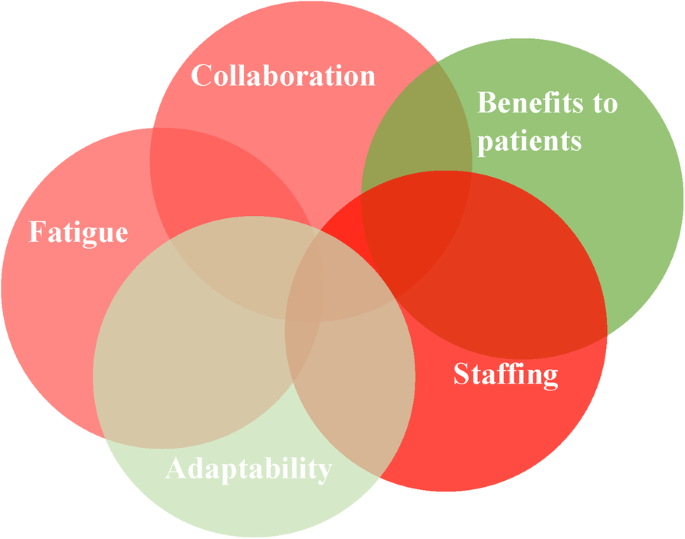
Thematic visualisation of staff understanding and expectations of the change
Hospital staff consistently held staffing to be a major concern in this redevelopment. To them, the opening of the new building, and with it the increase in physical size and addition of new services, meant that an increase in staff was crucial to successfully implement the change: “ My biggest uncertainty at the moment is the fact that I’m really concerned about whether I’m actually going to get enough staff ” (GS1). Many participants suggested that this issue would determine the success of the new hospital building. This was particularly important for staff moving into the new building with a bigger work space: “ We just need more staff. Yeah I think that’s the main issue - if we fix that then I believe everything should be smooth ” (ICUN4). For the most part, staff were unaware about how many new staff they would have in the new building. This uncertainty involved two related issues: (1) will we get the budget for new staff that we need? And if so, (2) where will we find all these new staff to employ?
On the first point, staff reported concerns that they would not have enough staff to cover the increased physical space and new ways of working within the new building. This lingering uncertainty was the result of external factors, specifically unresolved budget issues: “ But I suppose some of the issues stem from the fact that you never know how many beds we are able to open based on the funding from the government, and that is what is still up in the air ” (ICUDR1).
Regarding the second point, staff noted that even if budgetary issues were resolved, and there was enough money to hire new staff to fill the new building, a challenge would be finding the staff to recruit: “ I don’t know where these new staff are going to come from” (GN3). Some participants suggested that they already encountered difficulties with employing enough appropriately qualified staff and reported concerns that this issue would be compounded when they moved into the new building: “ Excitement will be way gone. It’s more to deal with that stress and the workload of other staff ” (ICUN4). Participants working on wards that were not moving into the new building also reported concerns about staffing. They noted that, despite not being directly involved in previous stages of the redevelopment, they had still been affected by these changes, because their colleagues were taken from their ward without consultation and moved into a new area. Hence, even staff not moving in the next stage of the redevelopment had concerns that their staffing levels would be affected: “ We have been told that we are not moving in there. And hopefully they don’t take our staff there ” (SURN5).
Benefits to patients
Many hospital staff expressed a positive expectation of the move related to benefits for patients. This was consistent across wards, departments and professions. Staff expected patients to experience benefits including reductions in infection rates and improved satisfaction, due to staying in a well-controlled and physically appealing environment with natural light: “ Any new place will give some joy or some happiness to people… The major change will be that because there are individual rooms, the infection rate will be lower and that I’m very pleased with” (ICUDR1).
Despite these participants reporting the improved physical environment was expected to positively affect patients, they also raised concerns that being in the new building might negatively affect patient safety because the increased physical space could introduce more room for error with the greater workload: “ Brings with it the fear, of how will we treat so many patients with nursing when you have one to one and the rooms are closed. That is a constant worry ” (ICUDR1). Participants indicated that this issue would be compounded if staffing levels were not increased.
Collaboration
Staff expressed multiple negative expectations or concerns about how their ways of working together would be affected by moving into the new building. Staff understood the change as more than just a physical expansion, but as an organisational change that would affect their ways of working. This understanding led to concern regarding how to work together in the new building. Specifically, staff moving into the new building were worried about the new layout of ICU, where nurses would be working alone in rooms with single patients. This would disrupt their ability to easily ask for support currently done by asking the nurse at an adjacent bed, or signalling to someone visible across the room: “ Single rooms are great for patients and everything but I think it becomes a bit more isolated for staffing ” (ICUOTH1). These concerns were also recognised among staff working in the change management team, who may not be directly affected by the change, but acknowledged that this is a major consequence of the move into the new building: “ All the beds, they were able to see each other all the time whereas now it’s a different work environment. They’re a bit more isolated… So that’s what we find is the challenge” (CHGTEAM2). Further, staff were concerned about working in open plan spaces that limit opportunity for private discussions, for example with other staff about workplace conflict or personal matters: “ I’m very concerned about insufficient space for private stuff ” (ICUAD1).
Staff reported negative expectations of collaboration breakdown not only within wards, but across the hospital. The organisational change will include far-flung staff and expanded infrastructure, which may decrease opportunity to collaborate directly. For several participants, the growing size of the hospital was seen as a fracturing of the positive, cohesive culture of what was once a smaller hospital—“ It used to be that the general manager would walk through and know everybody by name, the cleaner, maintenance crew, everybody knew everybody’s name ” (GN1)—into more disconnected, subunits: “ Now we’re very separate ” (ICUOTH1).
During interviews, many participants reported feeling over-worked and under-resourced. While some described being fatigued and unhappy at work, the redevelopment was, nevertheless, clearly a positive: “ We’re not happy because we’re under so much pressure and stress. But, you know, we are looking forward to the new build, it’ll be a beautiful building” (GN3). For others, there were concerns that their feelings of being over-worked would not subside with the opening of the new hospital building and that there was a lack of time to even consider the change. This was expressed by staff moving in to the new building, as well as those not moving:
Who has got the time to go and look at those decorative things ! (SURN5).
I can’t see how it will make a big difference to me… I don’t pay a lot of attention to the looks (MATDR1).
It doesn’t really matter… I could be providing it [patient care] in a tent or a building . (MATMW2).
Further, hospital staff expressed frustration in having to endure poor resourcing, which tempered their excitement for the new building: “We’ve all put up with whatever since whenever and I’m done, I’m so done” (ICUAD1). Some participants reported negative expectations related to the increase in physical space in the new building, as adding to the work load of clinical staff and requiring they travel further to get supplies and attend to patients: “They are worried about, hang on I’m going to have to do so many more laps” (ICUAD1). Similarly, an issue expressed on behalf of staff in the General Services Department was whether they will be able to adequately clean and cater for physically larger areas: “ I’m sitting here and looking at [a previous building that was opened] and seeing how filthy it is ” (CHGTEAM3). Concerns about being over-worked in the face of the redevelopment were further emphasised by some interviewees who discussed a problem with turnover: “ We’ve actually had a few people, I have had three people, which is unusual for us, who have looked for other jobs and are probably resigning. You know which is sort of the opposite of what we’d expect at this time, we’d expect they’d be excited for the new building ” (ICUN5). However, most staff in more junior positions had not seen the new building and thus were unaware of the layout and the degree to which it may impact their work: “ Because I have not seen the actual structure of the area, and I don’t know what they based it on and how they figured out a way to be friendly for both staff and patients at the same time ” (ICUN3). The unawareness and lack of understanding accentuated concerns and negative expectations among staff as they expected the worst.
Also contributing to reports of experiencing fatigue, staff described numerous other large changes taking place at the hospital over the years, in addition to the redevelopment: “ Basically for seven years we’ve been undergoing changes since I’ve been here. It is utterly exhausting having this many changes all the time ” (GS1). This highlights that while this study captures prospective insights to the change, change is constant in health care. While the move into the new building has not yet occurred, the move is part of a broader organisational change grander than the physical expansion of infrastructure. While this was a major concern for many staff, some of the senior medical staff dismissed this as being an issue, suggesting constant change is part of health care and should not lead to staff feeling worn out: “ I think once you get to my level you get good at kind of jumping through hoops… As you get more experienced, you just go with the flow a bit more” (SURDR2).
Adaptability
An additional theme involved staff’s positive expectation that they would be able to adapt to the changes brought about by the move into the new building. Reflecting on past experiences of organisational and infrastructure changes at the hospital, staff expressed that it could take time to adapt and see the benefits of the change: “ At the beginning, of course, everybody was scared of the changes and stuff like that, but eventually we got used to it. ” (SURN3). However, some staff reported that they saw adapting to the new building as a concern, potentially because of a lack of knowledge pertaining to what the new building entails: “ I just don’t know. I’m worried because I don’t know what we’re walking in to ” (ICUN2). In general, staff expressed an understanding of the change as one of physical growth (hospital redevelopment) and changes in ways of working (organisational change): “Getting bigger. So, basically taking all of our acute services and putting it in a brand spanking new building where they’re significantly expanding” (GN1); “ The biggest change is changing the way they work. Changing the way they deliver care .” (CHGTEAM2). When asked why the change was happening, hospital staff were consistent in attributing the need for redevelopment to population growth: “ To develop more resources to accommodate for the growing number of patients ” (SURDR3).
Feeling uninformed and uncertain about the change was expressed by staff of different professions and different levels throughout the hospital. In fact, even wards that were not moving to the new building were unsure if this was the case: “ There’s been no communication from anyone really. I hear from different people yes we are moving and then somebody says no we’re not. We’re staying here in the old building. So, I’m not sure exactly who’s going” (SURN1).
Our findings suggest that in the early stages of hospital redevelopment, staff experience both positive and negative expectations that are dependent upon the level of personal understanding, awareness of the change to come, and how well-resourced they already feel. Interviews with hospital staff highlighted a general understanding of the change as involving physical expansion of the hospital. However, participants also reported feeling inadequately informed about what is to come and described a range of sometimes differing expectations about the organisational effects of this change (e.g., on collaboration, for patients). This supports the conceptualisation of hospital redevelopment as not only a physical change, but an organisational one too.
The present study is the first to empirically explore the experiences and understanding of staff in the early stages of a hospital redevelopment, and conceptualised this as an organisational change. This conceptualisation is an important contribution to the organisational change literature because we show that change, even when based on the best evidence-based design, can be disappointing and bring about negative experiences for staff. The concerns and negative expectations of the change expressed by staff in the present study echo past research that retrospectively explored the experiences of staff during a hospital change, in Australia [ 13 ], and elsewhere [e.g., 14]. In the present study, staffing was a major concern reported by hospital staff. This is consistent with other reports of hospital redevelopment in the Australian context. For example, in a report into the opening of a new children’s hospital, staff were frustrated about the progression of the change and that a lack of staffing impacted on service planning. Staffing was also emphasised as an issue in another Australian hospital redevelopment project, where the building opened with insufficient staffing and resources [ 27 ]. Additionally, hospital staff in the present study indicated that they felt fatigued, so much so that excitement for the opening of the new building was diminishing. Reports of low staff morale in hospital redevelopment projects has also been documented in other Australian and international studies [ 13 , 14 ]. Further, participants in this study reported a lack of awareness of the redevelopment, something that appears to be common with a report of hospital revitalisation in the United States reporting a similar finding [ 28 ].
One source of many of the issues expressed by staff was uncertainty, a common and often inevitable experience in health care [ 29 ], for example, systems uncertainty about staffing levels and uncertainty about whether collaboration and support would break down as the hospital expands. While some types of uncertainty cannot be eradicated, it is important to manage uncertainty in times where information is available. One way to do this is to make sure front-line actors have a platform to seek information and ask questions during organisational change; having access to information is a predictor of success for organisational change in healthcare [ 30 ]. This may help alleviate stress associated with change and make the transition period less uncertain for staff, particularly in early stages where uncertainty may be greater. While it is not always possible for all the concerns and expectations of staff to be individually acknowledged and addressed by those coordinating the change (e.g., change management team or hospital executives), an alternative is through the use of ‘champions’ or ‘opinion leaders’. Opinion leaders are actors with a brokerage role; they carry information across social boundaries, such as between groups of professionals or different hospital wards [ 31 ]. Otherwise referred to as a ‘champion’, by virtue of their trustworthiness and connectedness, these actors are able to lead the opinions of others and are integral in the adoption and diffusion of new phenomena. Successful champions are enthusiastic and motivated about the change they are promoting [ 32 ]. In this case, a successful champion in a hospital undergoing organisational change is a staff member who can inform others and influence acceptance, and provide a positive frame for the change.
Implications
While findings may be localised to the hospital we researched, it is important to note that the hospital redevelopment under investigation is similar to other hospital redevelopments in metropolitan cities in Australia [ 7 ] and worldwide [ 5 ]. Specifically, the redevelopment is an expansion of infrastructure to meet the growing needs of the community which the hospital serves. The perceptions and experiences maintained by hospital staff will differ dependent on the state of the new facilities; these findings broadly generalise to any hospital redevelopment where a newer, larger building is opened. The implications of this study provide broad suggestions for other hospitals undergoing this type of hospital redevelopment.
Firstly, hospital redevelopment should be considered as more than physical change, but as an organisational change, in order to recognise the ripple effects of changing the infrastructure and how this may influence social and behavioural processes. From this study’s findings of the expectations and present experiences of organisational change, we recommend four strategies to aid in the early stages of hospital redevelopment: engage actors; plan and train; learn from the past; and increase managerial engagement (see Table 2 ). These recommendations correspond with suggestions from a past review examining transforming systems in health care [ 33 ]. Effort must be taken to ensure staff are informed of the change and rectify any confusions about who, what, when, and how the change is taking place. This is consistent with organisational change theory that maintains that large scale change requires significant effort and planning to ensure its success [ 19 ]. Therefore, an implication of this study lies in the importance of exploring the understanding and expectations of staff preceding a large organisational change in order to aid in the acceptance of, rather than resistance to, the change [ 21 ]. Further, this study also highlights the importance of studying the experiences of actors not directly involved in the organisational change but who are a part of the broader system (i.e., wards not moving implied they will be affected).
Strengths and limitations
A strength of this study lies in the number of participants and variability in the professions that contribute to the transferability of the study findings. Further, checking and clarifying themes with other researchers throughout the coding process increases the trustworthiness of the findings [ 26 ]. As to limitations, interviews were on average 17 min long, with the shortest interview lasting seven minutes. While this may be perceived as a short duration for collecting interview data it was appropriate for participants who were incredibly time poor (e.g., nurses on shift who could only get a 10 min break to talk to the researcher). It is important that the opinions of these busy staff are captured to reflect the true nature of a sample of varied hospital staff. Further, the findings may not be generalisable to other instances of organisational change and may be specific to the four wards and hospital examined in this study. Wards were purposively chosen rather than randomised. While findings may be specific to the hospital under investigation, the research has been designed to optimise research credibility in this qualitative analysis. Further, considerable context was provided to help readers infer relevance to different settings. This in-depth analysis of how staff understand and interpret organisational change in hospitals provides the opportunity to uncover theoretical insights into the processes of change in the health care system and the perspectives of staff during times of organisational change.
This study explored the prospective understanding and experiences of staff in organisational change in hospitals, using an Australian hospital redevelopment as a case exemplar. Findings indicated that staff were concerned about staffing levels, fatigue, and the potential for a breakdown of current collaborative working. These concerns are similar to past reports of redevelopment in hospitals. This paper presents recommendations for the early stages of organisational change in hospitals. For present and future hospital organisational change projects, it is important that staff concerns are addressed and that staff are informed adequately about the ongoing changes in order to improve their engagement and ownership of the change.
Availability of data and materials
The datasets analysed during the current study are not publicly available due to individual privacy, but are available from the corresponding author on reasonable request.
Abbreviations
Administrative staff
Change management team staff
Medical staff
General – works across several wards
General services staff
Intensive care unit
Maternity ward
Midwifery staff
Nursing staff
Other profession
Respiratory ward
Surgical ward
Braithwaite J, Churruca K, Ellis LA, Long JC, Clay-Williams R, Damen N, et al. Complexity science in healthcare-aspirations, approaches, applications and accomplishments: a white paper. Sydney, Australia: Macquarie University; 2017.
Google Scholar
Braithwaite J, Wears RL, Hollnagel E. Resilient health care: turning patient safety on its head. Int J Qual Health Care. 2015;27(5):418–20.
Article Google Scholar
Malkin RA. Design of health care technologies for the developing world. Annu Rev Biomed Eng. 2007;9:567–87.
Article CAS Google Scholar
Ritchie E. NSW budget 2017: ‘hospital building boom’ at heart of $23bn deal. The Australian. 2017.
Carpenter D, Hoppszallern S. Hospital building report. The boom goes on. Hospitals Health Networks. 2006;80(3):48–50 2-4, 2.
PubMed Google Scholar
Aubusson K. Berejiklian government pledges $750 million for Sydney's RPA Hospital 2019 [5 Mar]. Available from: https://www.smh.com.au/national/nsw/berejiklian-government-pledges-750-million-for-sydney-s-rpa-hospital-20190304-p511n4.html .
NSW Government. Health Infrastructure 2018 [Available from: https://www.hinfra.health.nsw.gov.au/our-projects/project-search .
Australian Institute of Health and Welfare. Australia's health 2016. Canberra: AIHW; 2016.
Schweitzer M, Gilpin L, Frampton S. Healing spaces: elements of environmental design that make an impact on health. J Altern Complement Med. 2004;10:71–83.
Rechel B, Buchan J, McKee M. The impact of health facilities on healthcare workers’ well-being and performance. Int J Nurs Stud. 2009;46(7):1025–34.
Berry LL, Parish JT. The impact of facility improvements on hospital nurses. HERD. 2008;1(2):5–13.
Gharaveis A, Hamilton DK, Pati D. The impact of environmental design on teamwork and communication in healthcare facilities: a systematic literature review. HERD. 2018;11(1):119–37.
Children's Health Queensland Hospital and Health Service. Lady Cilento Children's Hospital clinical review. 2015.
Lourens G, Ballard H. The consequences of hospital revitalisation on staff safety and wellness. Occup Health Southern Africa. 2016;22(6):13–8.
Schwarz N, Sudman S. Autobiographical memory and the validity of retrospective reports. New York: Springer-Verlag; 2012.
Dannenberg AL, Bhatia R, Cole BL, Heaton SK, Feldman JD, Rutt CD. Use of health impact assessment in the US: 27 case studies, 1999–2007. Am J Prev Med. 2008;34(3):241–56.
Austin MJ, Ciaassen J. Impact of organizational change on organizational culture: implications for introducing evidence-based practice. J Evid Based Soc Work. 2008;5(1–2):321–59.
Fitzgerald L, McDermott A. Challenging perspectives on organizational change in health care: Taylor & Francis; 2017.
Book Google Scholar
Todnem BR. Organisational change management: a critical review. J Chang Manag. 2005;5(4):369–80.
Plsek PE, Greenhalgh T. Complexity science: the challenge of complexity in health care. Br Med J. 2001;323(7313):625.
Braithwaite J. Changing how we think about healthcare improvement. Br Med J. 2018;361:k2014.
Pomare C, Churruca K, Long JC, Ellis LA, Gardiner B, Braithwaite J. Exploring the ripple effects of an Australian hospital redevelopment: a protocol for a longitudinal, mixed-methods study. BMJ Open. 2019;9(7):e027186.
Tong A, Sainsbury P, Craig J. Consolidated criteria for reporting qualitative research (COREQ): a 32-item checklist for interviews and focus groups. Int J Qual Health Care. 2007;19(6):349–57.
Braun V, Clarke V. Using thematic analysis in psychology. Qual Res Psychol. 2006;3(2):77–101.
Castleberry A. NVivo 10 [software program]. Version 10. QSR International; 2012. American journal of pharmaceutical education. 2014;78(1).
Elo S, Kääriäinen M, Kanste O, Pölkki T, Utriainen K, Kyngäs H. Qualitative content analysis: a focus on trustworthiness. SAGE Open. 2014;4(1):2158244014522633.
Braithwaite J. How to fix a sick hospital: attend to its stressed health carers the Sydney morning herald; 2018.
Baker JG. The perspective of the staff regarding facility revitalization at Walter reed Army medical center. Army Medical Material Agency Fort Detrick MD; 2004.
Pomare C, Churruca K, Ellis LA, Long JC, Braithwaite J. A revised model of uncertainty in complex healthcare settings: a scoping review. J Eval Clin Pract. 2019;25(2):176–82.
Kash BA, Spaulding A, Johnson CE, Gamm L. Success factors for strategic change initiatives: a qualitative study of healthcare administrators' perspectives. J Healthc Manag. 2014;59(1):65–81.
Long JC, Cunningham FC, Braithwaite J. Bridges, brokers and boundary spanners in collaborative networks: a systematic review. BMC Health Serv Res. 2013;13(1):158.
Damschroder L, Banaszak-Holl J, Kowalski CP, Forman J, Saint S, Krein S. The role of the “champion” in infection prevention: results from a multisite qualitative study. BMJ Qual Saf. 2009;18(6):434–40.
Best A, Greenhalgh T, Lewis S, Saul JE, Carroll S, Bitz J. Large-system transformation in health care: a realist review. Milbank Q. 2012;90(3):421–56.
Download references
Acknowledgements
We thank the hospital executives, ward directors and nursing unit managers for their support in recruitment of interview participants. The authors also thank and acknowledge the interview participants.
CP was funded by the Australian Government Research Training Program (RTP) PhD Scholarship. JB is supported by multiple grants, including the National Health and Medical Research Council (NHMRC) Partnership Grant for Health Systems Sustainability (ID: 9100002). The funders had no role in the design, analysis and drafting of the manuscript.
Author information
Authors and affiliations.
Centre for Healthcare Resilience and Implementation Science, Australian Institute of Health Innovation, Macquarie University, 75 Talavera Rd, Macquarie Park, Australia
Chiara Pomare, Kate Churruca, Janet C. Long, Louise A. Ellis & Jeffrey Braithwaite
You can also search for this author in PubMed Google Scholar
Contributions
CP and JB conceptualised the project. CP collected and analysed the data, and drafted the manuscript. KC, LAE and JCL assisted in the coding and interpretation of data. All authors read and approved the final manuscript.
Corresponding author
Correspondence to Chiara Pomare .
Ethics declarations
Ethics approval and consent to participate.
The study was approved by the relevant Ethics Committee in Sydney, New South Wales, Australia (no: 18/233). Due to ethical requirements, the committee cannot be named because it may lead to the identification of the study site. Informed consent was obtained from all study participants.
Consent for publication
Not applicable.
Competing interests
The authors declare that they have no competing interests.
Additional information
Publisher’s note.
Springer Nature remains neutral with regard to jurisdictional claims in published maps and institutional affiliations.
Supplementary information
Additional file 1..
Consolidated criteria for reporting qualitative studies (COREQ): 32-item checklist.
Additional file 2.
Semi-structured interview guide.
Rights and permissions
Open Access This article is distributed under the terms of the Creative Commons Attribution 4.0 International License ( http://creativecommons.org/licenses/by/4.0/ ), which permits unrestricted use, distribution, and reproduction in any medium, provided you give appropriate credit to the original author(s) and the source, provide a link to the Creative Commons license, and indicate if changes were made. The Creative Commons Public Domain Dedication waiver ( http://creativecommons.org/publicdomain/zero/1.0/ ) applies to the data made available in this article, unless otherwise stated.
Reprints and permissions
About this article
Cite this article.
Pomare, C., Churruca, K., Long, J.C. et al. Organisational change in hospitals: a qualitative case-study of staff perspectives. BMC Health Serv Res 19 , 840 (2019). https://doi.org/10.1186/s12913-019-4704-y
Download citation
Received : 23 May 2019
Accepted : 31 October 2019
Published : 14 November 2019
DOI : https://doi.org/10.1186/s12913-019-4704-y
Share this article
Anyone you share the following link with will be able to read this content:
Sorry, a shareable link is not currently available for this article.
Provided by the Springer Nature SharedIt content-sharing initiative
- Organisational change
- Health systems change
- Hospital redevelopment
- Hospital expansion
- Staff expectations
BMC Health Services Research
ISSN: 1472-6963
- General enquiries: [email protected]

- SUGGESTED TOPICS
- The Magazine
- Newsletters
- Managing Yourself
- Managing Teams
- Work-life Balance
- The Big Idea
- Data & Visuals
- Reading Lists
- Case Selections
- HBR Learning
- Topic Feeds
- Account Settings
- Email Preferences
Healthcare sector

Equitable Health Care Requires Inclusive Language
- Nkem Chukwumerije
- July 19, 2022
Biogen Unchained
- David Bovet
- Joseph Martha
- From the May–June 2000 Issue

Why Less Choice is More in Health Insurance Exchanges
- Sanjay B Saxena
- Nate Holobinko
- October 09, 2013

Redesigning Care for High-Cost, High-Risk Patients
- Zubin J. Eapen
- Sachin H. Jain
- February 07, 2017
Match Your Sales Force Structure to Your Business Life Cycle
- Andris A. Zoltners
- Prabhakant Sinha
- Sally E. Lorimer
- From the July–August 2006 Issue

The Harvard Contest That's Trying to Improve Health Care Delivery
- Richard G. Hamermesh
- Robert S. Huckman
- Barbara McNeil
- Joseph P Newhouse
- Cara Sterling
- Joseph Newhouse
- October 02, 2015
All Strategy Is Local
- Bruce C. Greenwald
- September 01, 2005
Who Goes, Who Stays? (HBR Case Study)
- David A. Light
- January 01, 2001

How Tech Companies Can Help Fix U.S. Health Care
- Hemant Taneja
- April 28, 2020
Personalized Technology Will Upend the Doctor-Patient Relationship
- Sundar Subramanian
- Carl Dumont
- Christoph Dankert
- Audris Wong
- June 19, 2015
Innovate at Your Own Risk: Deborah Wince-Smith on Competitiveness
- Gardiner Morse
- From the May 2005 Issue

How We Transformed Emergency Care at Our Hospital
- Richard Zane, MD
- December 17, 2015

Preparing Hospitals for the Next Pandemic
- Regina E. Herzlinger
- Regina Herzlinger
- Barak Richman
- June 10, 2021
Leading Change from the Top Line: The HBR Interview
- Fred Hassan
- Thomas A. Stewart
- David Champion

Where Both the ACA and AHCA Fall Short, and What the Health Insurance Market Really Needs
- David Blumenthal
- Sara Collins
- March 21, 2017

What It Takes to Lead a Disease Research Foundation
- Kathy Giusti
- August 18, 2020

A New Generation of Drug Therapies Requires New Business Strategies
- Jeff Holder
- Adam Siebert
- February 27, 2024
Conquering a Culture of Indecision (HBR Classic)
- January 01, 2006

Can AI Address Health Care’s Red-Tape Problem?
- Minoo Javanmardian
- Aditya Lingampally
- November 05, 2018

How New Health Care Platforms Will Improve Patient Care
- Ashwini M. Zenooz
- October 11, 2019

From operational data maintenance to strategic data architecture: Master data management at Chr. Hansen
- Attila Marton
- Lasse Friis Nielsen
- Nina Baekbo Andersen
- December 21, 2023
Cialis Lifecycle Management: Lilly's BPH Dilemma
- Natalie Kindred
- September 26, 2012
Flexibility at Genentech: Developing Versatile Domain Experts and Deploying Flexible Resources at One U.S. Medical Affairs Unit
- Homa Bahrami
- Stuart Evans
- October 01, 2016
Hope Noah: Is All-Inclusive Pricing an Effective Strategy for the Medical Tourism Industry?
- Dongsheng Zhou
- Liyang Ruan
- June 29, 2019
Uncertainty in Surgical Supplies-A Cardinal Error (D): Uncertainty in Everything as COVID-19 Arrives
- G. Paul Matherne
- February 17, 2022
Merck & Company: Product KL-798
- Sherwood C. Frey
- Samuel E Bodily
- July 09, 2002
Pfizer's Centers for Therapeutic Innovation (CTI)
- Gary P. Pisano
- James Weber
- Kaitlyn Szydlowski
- September 02, 2014
Procurement at Betapharm Corp. (C)
- Taylor Randall
- March 15, 2005
The unlikely inventor and the reluctant manufacturer - Coloplast's start-up story
- Louise Skyggebjerg
Moodcafe: From India Conception to Raising Funds
- Chitra Singla
- Shridhar Sethuram
- Sanjay Kumar Jena
- October 24, 2021
Merck: Managing Vioxx (F)
- Robert Simons
- April 20, 2009
U.S. Health Care Reform
- Katherine Casey
- Christy Johnson
- May 04, 2020
Pfizer: Letter from the Chairman (A)
- July 07, 2009
Kurt Landgraf and Du Pont Merck Pharmaceutical Co. (A)
- Mary Gentile
- Sarah B. Gant
- June 01, 1994
James Burke: A Career in American Business, Video
- Richard S. Tedlow
- February 15, 1990
Impax Laboratories: Executing Accretive Acquisitions (A)
- Benjamin C. Esty
- Daniel Fisher
- October 31, 2019
Marketing Antidepressants: Prozac and Paxil
- Youngme Moon
- Kerry Herman
- May 14, 2002
ImmuLogic Pharmaceutical Corp. (B4): Phillip Gross
- Josh Lerner
- April 28, 1993
Imprimis (B)
- Ramon Casadesus-Masanell
- Karen Elterman
- April 03, 2017
Graham's Pharmacy: Traditional Care, Modern Solutions
- Nicole Berube
- R. Frank Delanghe
- October 05, 2016

Professionalization of Ujwal Bharati, Teaching Note
- K. Ramachandran
- June 03, 2010
From operational data maintenance to strategic data architecture: Master data management at Chr. Hansen, Teaching Note
Pandemic_analysis on italy cyclicality: into the abyss and back: covid-19 and the economy, instructor spreadsheet.
- Gabriele Martegiani
- Nicolas Vincent
- Pierre Yared
- January 25, 2021
Popular Topics
Partner center.
- Browse All Articles
- Newsletter Sign-Up
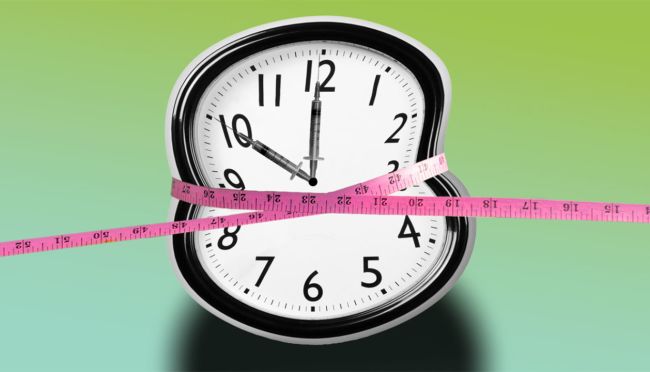
- 09 Feb 2024
Slim Chance: Drugs Will Reshape the Weight Loss Industry, But Habit Change Might Be Elusive
Medications such as Ozempic, Wegovy, and Mounjaro have upended a $76 billion industry that has long touted lifestyle shifts as a means to weight loss. Regina Herzlinger says these drugs might bring fast change, especially for busy professionals, but many questions remain unanswered.
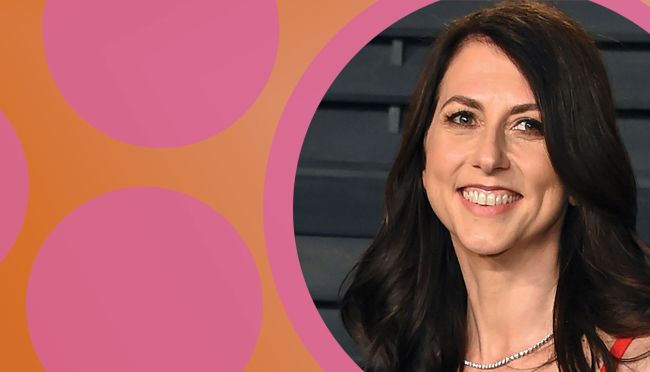
- 19 Dec 2023
- Research & Ideas
$15 Billion in Five Years: What Data Tells Us About MacKenzie Scott’s Philanthropy
Scott's hands-off approach and unparalleled pace—helping almost 2,000 organizations and counting—has upended the status quo in philanthropy. While her donations might seem scattershot, an analysis of five years of data by Matthew Lee, Brian Trelstad, and Ethan Tran highlights clear trends and an emerging strategy.
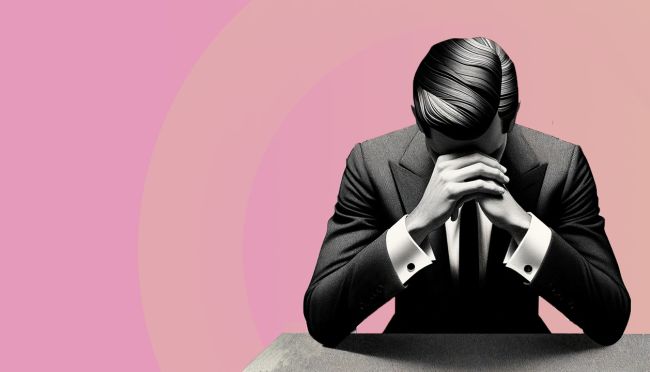
- 09 Nov 2023
What Will It Take to Confront the Invisible Mental Health Crisis in Business?
The pressure to do more, to be more, is fueling its own silent epidemic. Lauren Cohen discusses the common misperceptions that get in the way of supporting employees' well-being, drawing on case studies about people who have been deeply affected by mental illness.
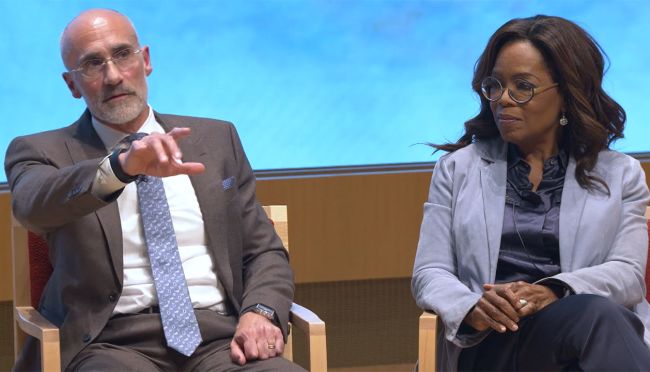
- 03 Oct 2023
- Research Event
Build the Life You Want: Arthur Brooks and Oprah Winfrey Share Happiness Tips
"Happiness is not a destination. It's a direction." In this video, Arthur C. Brooks and Oprah Winfrey reflect on mistakes, emotions, and contentment, sharing lessons from their new book.
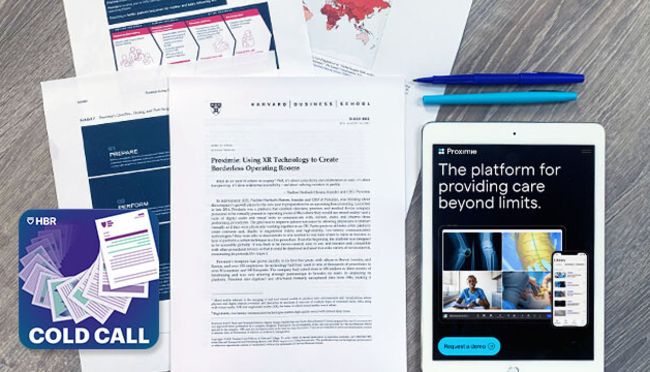
- 12 Sep 2023
- Cold Call Podcast
Can Remote Surgeries Digitally Transform Operating Rooms?
Launched in 2016, Proximie was a platform that enabled clinicians, proctors, and medical device company personnel to be virtually present in operating rooms, where they would use mixed reality and digital audio and visual tools to communicate with, mentor, assist, and observe those performing medical procedures. The goal was to improve patient outcomes. The company had grown quickly, and its technology had been used in tens of thousands of procedures in more than 50 countries and 500 hospitals. It had raised close to $50 million in equity financing and was now entering strategic partnerships to broaden its reach. Nadine Hachach-Haram, founder and CEO of Proximie, aspired for Proximie to become a platform that powered every operating room in the world, but she had to carefully consider the company’s partnership and data strategies in order to scale. What approach would position the company best for the next stage of growth? Harvard Business School associate professor Ariel Stern discusses creating value in health care through a digital transformation of operating rooms in her case, “Proximie: Using XR Technology to Create Borderless Operating Rooms.”
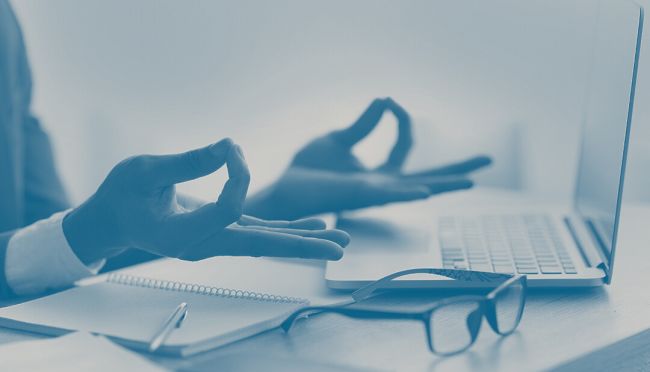
- 28 Aug 2023
How Workplace Wellness Programs Can Give Employees the Energy Boost They Need
At a time when many workers are struggling with mental health issues, workplace wellness programs need to go beyond providing gym discounts and start offering employees tailored solutions that improve their physical and emotional well-being, says Hise Gibson.
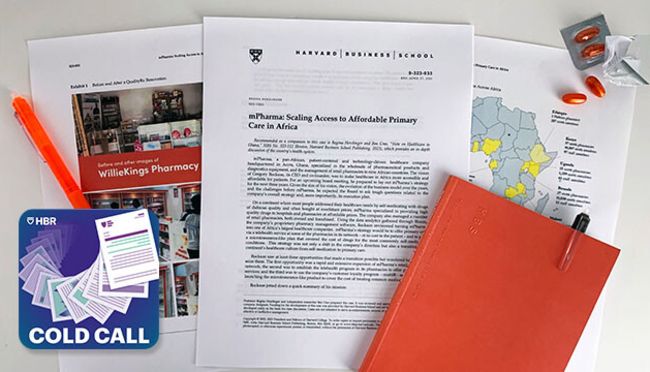
- 01 Aug 2023
Can Business Transform Primary Health Care Across Africa?
mPharma, headquartered in Ghana, is trying to create the largest pan-African health care company. Their mission is to provide primary care and a reliable and fairly priced supply of drugs in the nine African countries where they operate. Co-founder and CEO Gregory Rockson needs to decide which component of strategy to prioritize in the next three years. His options include launching a telemedicine program, expanding his pharmacies across the continent, and creating a new payment program to cover the cost of common medications. Rockson cares deeply about health equity, but his venture capital-financed company also must be profitable. Which option should he focus on expanding? Harvard Business School Professor Regina Herzlinger and case protagonist Gregory Rockson discuss the important role business plays in improving health care in the case, “mPharma: Scaling Access to Affordable Primary Care in Africa.”
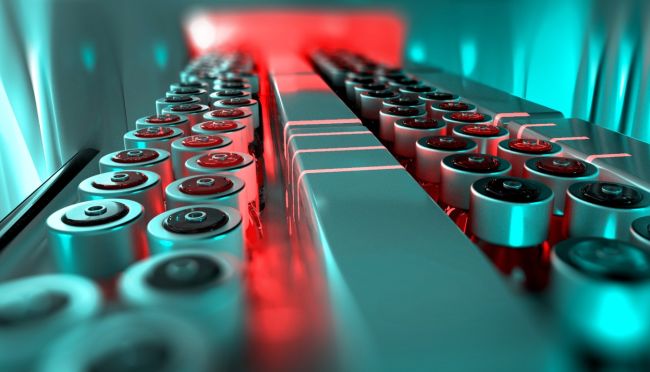
- 25 Jul 2023
Could a Business Model Help Big Pharma Save Lives and Profit?
Gilead Sciences used a novel approach to help Egypt address a public health crisis while sustaining profits from a key product. V. Kasturi Rangan and participants at a recent seminar hosted by the Institute for the Study of Business in Global Society discussed what it would take to apply the model more widely.
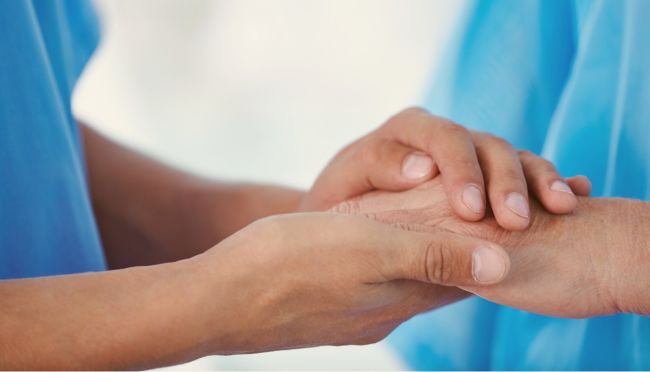
- 23 Jun 2023
This Company Lets Employees Take Charge—Even with Life and Death Decisions
Dutch home health care organization Buurtzorg avoids middle management positions and instead empowers its nurses to care for patients as they see fit. Tatiana Sandino and Ethan Bernstein explore how removing organizational layers and allowing employees to make decisions can boost performance.
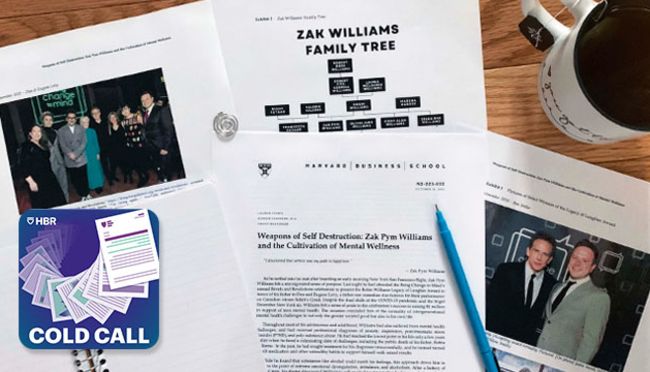
- 09 May 2023
Can Robin Williams’ Son Help Other Families Heal Addiction and Depression?
Zak Pym Williams, son of comedian and actor Robin Williams, had seen how mental health challenges, such as addiction and depression, had affected past generations of his family. Williams was diagnosed with generalized anxiety disorder, depression, and post-traumatic stress disorder (PTSD) as a young adult and he wanted to break the cycle for his children. Although his children were still quite young, he began considering proactive strategies that could help his family’s mental health, and he wanted to share that knowledge with other families. But how can Williams help people actually take advantage of those mental health strategies and services? Professor Lauren Cohen discusses his case, “Weapons of Self Destruction: Zak Pym Williams and the Cultivation of Mental Wellness.”
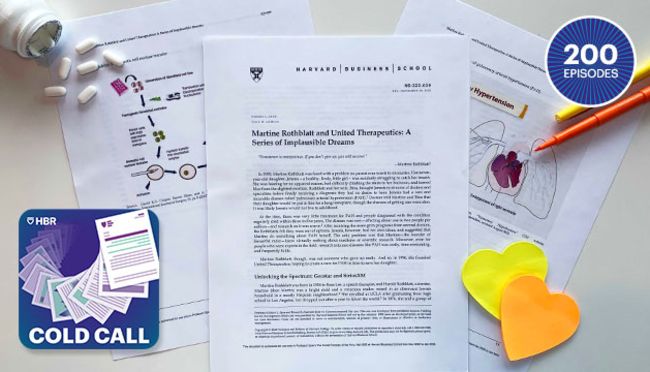
- 26 Apr 2023
How Martine Rothblatt Started a Company to Save Her Daughter
When serial entrepreneur Martine Rothblatt (founder of Sirius XM) received her seven-year-old daughter’s diagnosis of Pulmonary Arterial Hypertension (PAH), she created United Therapeutics and developed a drug to save her life. When her daughter later needed a lung transplant, Rothblatt decided to take what she saw as the logical next step: manufacturing organs for transplantation. Rothblatt’s entrepreneurial career exemplifies a larger debate around the role of the firm in creating solutions for society’s problems. If companies are uniquely good at innovating, what voice should society have in governing the new technologies that firms create? Harvard Business School professor Debora Spar debates these questions in the case “Martine Rothblatt and United Therapeutics: A Series of Implausible Dreams.” As part of a new first-year MBA course at Harvard Business School, this case examines the central question: what is the social purpose of the firm?
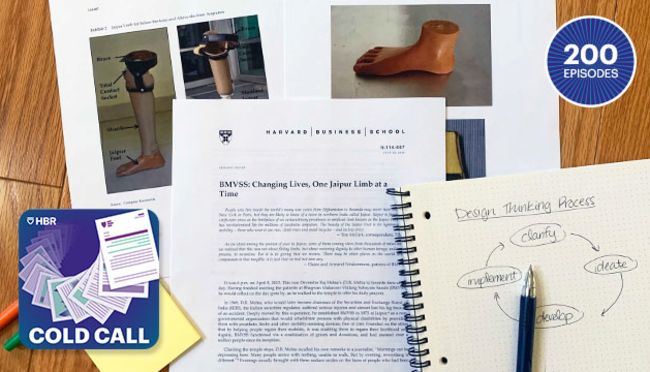
- 25 Apr 2023
Using Design Thinking to Invent a Low-Cost Prosthesis for Land Mine Victims
Bhagwan Mahaveer Viklang Sahayata Samiti (BMVSS) is an Indian nonprofit famous for creating low-cost prosthetics, like the Jaipur Foot and the Stanford-Jaipur Knee. Known for its patient-centric culture and its focus on innovation, BMVSS has assisted more than one million people, including many land mine survivors. How can founder D.R. Mehta devise a strategy that will ensure the financial sustainability of BMVSS while sustaining its human impact well into the future? Harvard Business School Dean Srikant Datar discusses the importance of design thinking in ensuring a culture of innovation in his case, “BMVSS: Changing Lives, One Jaipur Limb at a Time.”
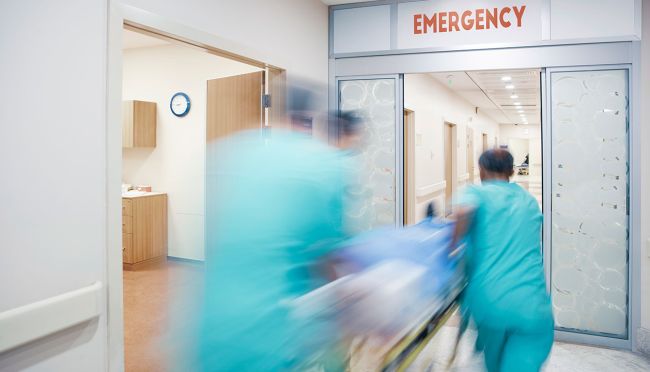
- 31 Mar 2023
Can a ‘Basic Bundle’ of Health Insurance Cure Coverage Gaps and Spur Innovation?
One in 10 people in America lack health insurance, resulting in $40 billion of care that goes unpaid each year. Amitabh Chandra and colleagues say ensuring basic coverage for all residents, as other wealthy nations do, could address the most acute needs and unlock efficiency.
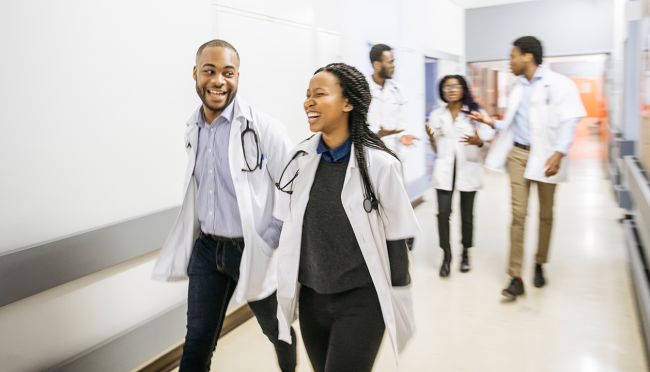
- 13 Mar 2023
The Power of Personal Connections: How Shared Experiences Boost Performance
Doctors who train together go on to provide better patient care later in their careers. What could teams in other industries learn? Research by Maximilian Pany and J. Michael McWilliams.
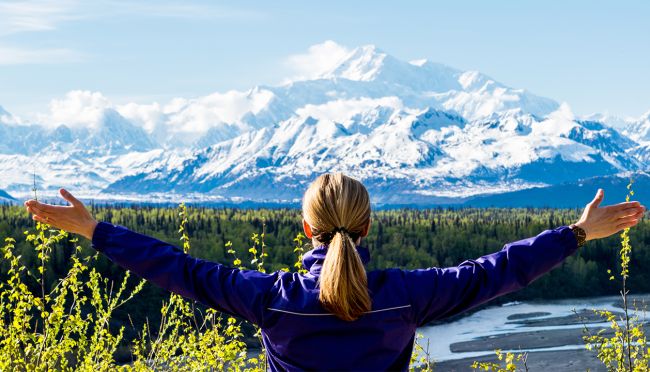
- 14 Feb 2023
When a Vacation Isn’t Enough, a Sabbatical Can Recharge Your Life—and Your Career
Burning out and ready to quit? Consider an extended break instead. Drawing from research inspired by his own 900-mile journey, DJ DiDonna offers practical advice to help people chart a new path through a sabbatical.
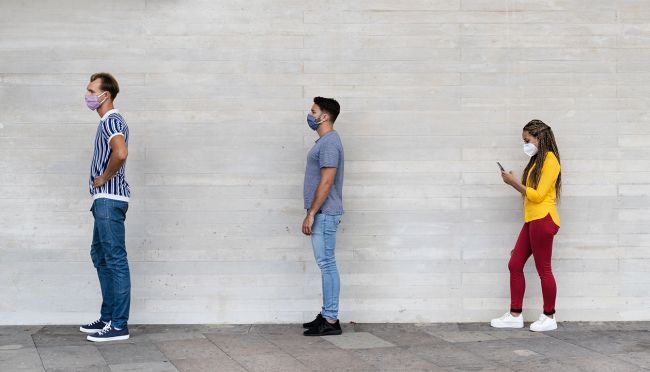
- 10 Feb 2023
COVID-19 Lessons: Social Media Can Nudge More People to Get Vaccinated
Social networks have been criticized for spreading COVID-19 misinformation, but the platforms have also helped public health agencies spread the word on vaccines, says research by Michael Luca and colleagues. What does this mean for the next pandemic?
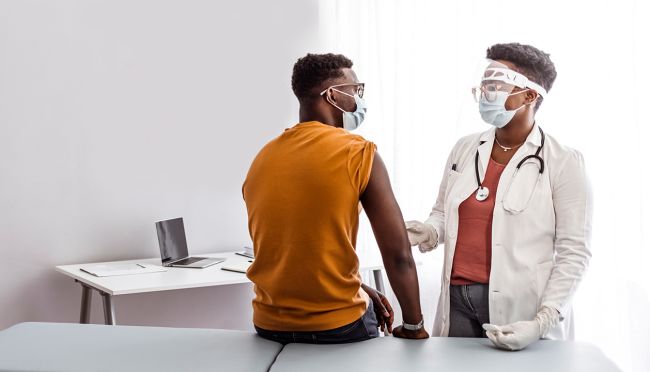
- 12 Dec 2022
Buy-In from Black Patients Suffers When Drug Trials Don’t Include Them
Diversifying clinical trials could build trust in new treatments among Black people and their physicians. Research by Joshua Schwartzstein, Marcella Alsan, and colleagues probes the ripple effects of underrepresentation in testing, and offers a call to action for drugmakers.
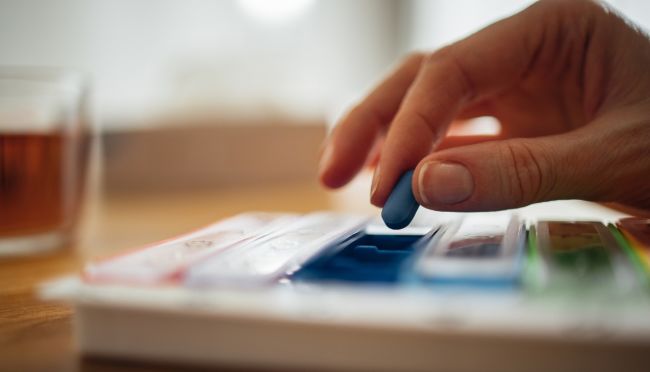
- 06 Sep 2022

Curbing an Unlikely Culprit of Rising Drug Prices: Pharmaceutical Donations
Policymakers of every leaning have vowed to rein in prescription drug costs, with little success. But research by Leemore Dafny shows how closing a loophole on drugmaker donations could eliminate one driver of rising expenses.
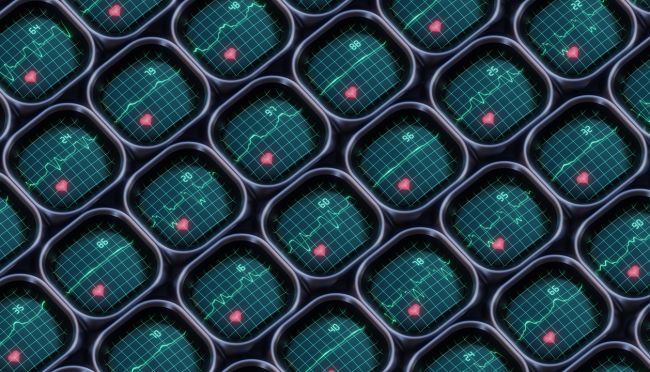
- 22 Aug 2022
Can Amazon Remake Health Care?
Amazon has disrupted everything from grocery shopping to cloud computing, but can it transform health care with its One Medical acquisition? Amitabh Chandra discusses company's track record in health care and the challenges it might face.
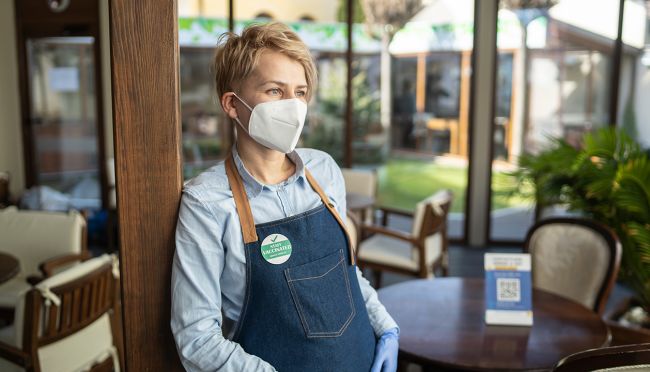
- 24 Mar 2022
Why Cutting Jobless Aid Isn't the Answer to Worker Shortages
Many policymakers thought that halting COVID-related unemployment insurance would be a "silver bullet" to addressing worker shortages. In reality, cutting aid undermined consumer spending, says research by Raymond Kluender. Open for comment; 0 Comments.
- Search Menu
- Advance articles
- Editor's Choice
- Supplements
- French Abstracts
- Portuguese Abstracts
- Spanish Abstracts
- Author Guidelines
- Submission Site
- Open Access
- About International Journal for Quality in Health Care
- About the International Society for Quality in Health Care
- Editorial Board
- Advertising and Corporate Services
- Journals Career Network
- Self-Archiving Policy
- Dispatch Dates
- Contact ISQua
- Journals on Oxford Academic
- Books on Oxford Academic

Article Contents
Introduction, acknowledgements, data availability.
- < Previous
Case study: international healthcare service quality, building a model for cultivating cultural sensitivity
- Article contents
- Figures & tables
- Supplementary Data
Ya-Ting Yang, Yi-Hsin Elsa Hsu, Kung-Pei Tang, Christine Wang, Stephen Timmon, Wen-Ta Chiu, Saileela Annavajjula, Jan-Show Chu, Case study: international healthcare service quality, building a model for cultivating cultural sensitivity, International Journal for Quality in Health Care , Volume 32, Issue 9, November 2020, Pages 639–642, https://doi.org/10.1093/intqhc/mzaa097
- Permissions Icon Permissions
In the context of medical tourism, cultural differences and language barriers are unneglectable factors, which compromise the shared decision-making between doctor and patients.
This study constructs a cultural sensitivity cultivation (CSC) model that could be used to train medical professionals in the sector of medical tourism.
Since 2016, there have been explorations in new strategies to offer better services. A critical step added is to include clients’ perspectives in the re-examining process as a way to cultivate cultural sensitivity among the service providers. This practice expands to the sector of medical tourism. In our case study, we are able to conclude a new model that could yield quality international healthcare services.
The steps of our CSC model include (i) ‘Promote Awareness’ for shifting mindset, (ii) ‘Share Scenarios’ for developing empathy and compassion, (iii) ‘Review Process’ for collecting detail feedback, (iv) ‘Identify Gaps’ for targeting areas for improvement and (v) ‘Improve Systems,’ for changing standard operation procedures (SOPs) based on the strategies through Assmann’s theory with a cultural–anthropological approach.
After Kuang Tien General Hospital (KTGH) implemented the new model for 1 year, the number of international patients has increased by 64%. More research could be done in the future to cover all the important aspects of providing international medical services and could apply the CSC model to different healthcare settings.
To optimize the shared decision-making between the doctor and medical traveler patients, healthcare providers should not only overcome language and cultural barriers but also should avoid unnecessary gestures in terms of status respect. Inviting patients to be co-investigator for quality improvement is a viable solution.
In the context of medical tourism, cultural differences and language barriers are unneglectable factors, which compromise the shared decision-making between doctor and patients. This situation has raised the demand for cultural competency training for medical providers. In recent years, Taiwan is gradually being recognized as a destination of choice for high-quality and affordable healthcare [ 1 ].
Therefore, there is an immediate need to rectify the problem. This study aims to construct a cultural sensitivity cultivation (CSC) model to train Taiwanese medical professionals to enhance shared decision-making and healthcare service for medical tourists.
The study was conducted at Kuang Tien General Hospital (KTGH), a regional hospital founded more than a decade ago in Taiwan. In order to develop the CSC model using a systematic approach, a combination of literature studies, case report analyses, in-depth interviews and statistical comparisons was applied. In addition to the literature studies, we undertook an exploratory qualitative study to investigate the concept of international healthcare service quality focusing on cultural sensitivity cultivating. Adopting multiple qualitative and quantitative approaches provides a sound foundation for generating empirical conceptual frameworks of complex concepts. There are three experienced researchers reviewed applied this concept of respect when reviewing five extensive case reports provided by the KTGH. The reports include detailed information such as patients’ previsit, visit, and postvisit information (follow-up and testimonials). Also, the Chief Strategy Officer and members of the hospital participated in in-person interviews. The results of literature reviews have indicated a lack of standardization in medical tourism regulation. This has led to difficulties relating to ethical issues such as informed consent and health equity control [ 2 ]. On the other hand, the healthcare providers’ commitment to improving their cultural sensitivities is the essential component to ensure high quality of medical tourism services [ 3 ]. However, there are very few studies that address the basic theories needed in order to establish the ideal relationship between international medical seekers and healthcare providers. Hence, a cultural–anthropological approach by Assmann [ 4 ] is adopted to develop this CSC model. This is because Assmann’s theory can transcend the economic colonialist globalization framework. Assmann’s theory addresses five forms of respect: (i) Status respect ‘respect due to the position and office,’ (ii) Respect for achievement ‘respect that distinguishes individual according to their ability and achievements,’ (iii) Social respect ‘raising emotional temperature of society surplus personal appearance, abilities or possessions, which is focusing of recognition and admiration,’ (iv) Cultural respect ‘affirmative action on a global level of intercultural communication and digital self-expression within a larger political framework of decolonization’ (v) Civil respect ‘multicultural and multiethnic society stimulate new forms of social perception, interaction and empathy.’
Findings and Results
From the interviews and analysis, we discovered several fundamental strategies that KTGH employs in improving their staff’s ability to care for international patients by based on the strategies through Assmann’s theory with a cultural–anthropological approach between medical professionals and international patients such as they include practicing ‘social respect’ and ‘civil respect’ through the improvement of verbal and nonverbal communication skills; embodying the ‘cultural respect’ through sharing experience among different ethnic groups and hosting international holiday promotional events to promote mutual understandings among global citizens and also applied the Assmann’s respect theory with the international patients’ visit journey from promote awareness, share scenarios, review process, identify gaps and improve system to connected with CSC model. Since 2016, KTGH has started the efforts of exploring new strategies to offer better services, and they have especially paid attention to cultivating staffs’ intercultural competencies. During each service delivery to an international patient, staff records details on patient–doctor interactions. They use the recordings to create case studies or scenarios to develop hospital-wide training to cultivate empathy for all. To decrease the conventional ‘status respect’ between the doctor-patient relationship, the research team invited ‘the clients,’ the international healthcare seekers, to work as the ‘co-investigators.’ The goal is to include patients’ perspectives when examining behaviors occurred during the healthcare-seeking process.
After implementing these new strategies for 1 year, KTGH received testimonials from international patients on the high quality of their healthcare services. In addition, the number of international patients increased by 64% in the following year. Witnessing KTGH process and success in cultivating cultural awareness, we have used its practice to form the CSC model in our study, as shown in Figure 1 .

The stage of ‘Promoting Awareness’ emphasizes the importance of educating and introducing staff to cultural differences and characteristics. The ‘Share Scenarios’ stage promotes empathy and compassion through sharing through the previous experiences and lessons learned between one and another. The ‘Review Process’ stage consists of collecting service feedback from teams and discussing step-by-step experiences with patients. The ‘Identify Gaps’ stage is to target areas for improvement in each step: pre-, during- and postvisit experiences with patients. Lastly, under ‘Improve Systems,’ the model emphasizes the point in shaping patients’ experiences systemically through discussions with involved departments to enhance standard operation procedures (SOPs).
The proposed CSC model provides a conceptual framework and a systematic application process in the area where little research was conducted previously. According to the research analyzing publications in the IJQHC over the past 3 years, one of the leading keywords is ‘quality improvement’ [ 5 ]. There are various approaches to quality improvement, with different levels, from quality indicators to systematic monitoring [ 6 ]. The CSC model indicates that besides the apparent reason of language abilities that contribute to quality service, other important elements such as creating cultural awareness and using previous experiences to develop training materials are also crucial to improve service quality and experience for international patients. Although the CSC model is developed based on experiences in a single healthcare facility that offers international healthcare, we have made valuable observations that could lead to quality healthcare service in this specific facility. Attention to data can itself significantly improve data quality [ 7 ]; we have observed in this case that focusing on the internal process of delivering healthcare services could also improve the quality of the service itself. Based on the CSC model, we encourage more research that could be done in the future to cover all aspects of providing international medical services and consider applying the CSC model in different healthcare settings. Further implementation and investigation of the effects of the CSC model are suggested.
The authors thank CSF and staff members of KTGH who assisted in providing the data for this study.
We have interviewed subjects, and all data are available upon request to reviewers and editors. No new data were generated or analyzed.
Stephano RM . Health & Wellness Destination Guide . Taiwan : Global Health Insurance Publications , 2014 . http://taiwan.medicaltourism.com/online-view/document.pdf
Google Scholar
Google Preview
Foley BM , Haglin JM , Tanzer JR et al. Patient care without borders: a systematic review of medical and surgical tourism . J Travel Med 2019 ; 26 :6.
Rokni L , Park S-H , Avci T . Improving medical tourism services through human behaviour and cultural competence . Iran J Public Health 2019 ; 48 : 1988 – 96 .
Assmann A . Civilizing societies: recognition and respect in a global world . New Lit Hist 2013 ; 44 : 69 – 91 .
Iqbal U , Yu CY , Li J . What are the leading keywords of IJQHC in last 3 years? Int J Qual Health Care 2015 ; 27 : 163 – 4 .
Geboers H , Mokkink H , Montfort P et al. . Continuous quality improvement in small general medical practices: the attitudes of general practitioners and other practice staff . Int J Qual Health Care 2001 ; 13 : 391 – 7 .
Mate KS , Bennett B , Mphatswe W et al. Challenges for routine health system data management in a large public programme to prevent mother-to-child HIV transmission in South Africa . PLos One 2009 ; 4 :e5483.
Author notes
# Equal Contribution.
- health personnel
- central serous chorioretinopathy
- cultural differences
- quality improvement
- language barriers
- medical tourism
- shared decision making
- standard operating procedure
- cultural sensitivity
Email alerts
Citing articles via.
- Recommend to your Library
Affiliations
- Online ISSN 1464-3677
- Print ISSN 1353-4505
- Copyright © 2024 International Society for Quality in Health Care and Oxford University Press
- About Oxford Academic
- Publish journals with us
- University press partners
- What we publish
- New features
- Open access
- Institutional account management
- Rights and permissions
- Get help with access
- Accessibility
- Advertising
- Media enquiries
- Oxford University Press
- Oxford Languages
- University of Oxford
Oxford University Press is a department of the University of Oxford. It furthers the University's objective of excellence in research, scholarship, and education by publishing worldwide
- Copyright © 2024 Oxford University Press
- Cookie settings
- Cookie policy
- Privacy policy
- Legal notice
This Feature Is Available To Subscribers Only
Sign In or Create an Account
This PDF is available to Subscribers Only
For full access to this pdf, sign in to an existing account, or purchase an annual subscription.

“Case Studies in Healthcare: Success Stories and Lessons Learned”

Table of Contents
The healthcare industry is an ever-evolving field with innovations and improvements happening daily. As healthcare providers strive to deliver the best care possible, case studies have become a valuable resource for learning and growth. In this article, we will explore various case studies in healthcare, highlighting both success stories and the lessons learned along the way. By analyzing what works and why, we can gain insight into the practices that lead to triumphs in healthcare and potentially replicate these successes in our own organizations.
Case Studies in Healthcare: A Closer Look at Triumphs and Takeaways
Healthcare case studies provide a unique opportunity to dissect real-world scenarios, understand the decisions made, and measure the outcomes of those choices. One notable success story is the implementation of telemedicine in rural areas. By leveraging technology, healthcare providers have successfully expanded access to care for patients who would otherwise have to travel long distances for treatment. Lessons learned include the importance of investing in reliable technology and training staff to effectively use telemedicine platforms.
Another critical case study involves the management of electronic health records (EHRs). When a large hospital system transitioned to a new EHR system, they faced significant resistance from physicians who were accustomed to the old way of doing things. However, by involving physicians in the planning and implementation process, the hospital successfully integrated the new system, leading to improved efficiency and patient care. This case study highlights the value of stakeholder engagement and effective change management.
In the fight against infectious diseases, case studies have shown the significance of swift and coordinated responses. An example of this is the containment of Ebola in West Africa. Through international collaboration and the rapid deployment of healthcare resources, the spread of the virus was effectively limited. This case study underscores the importance of preparedness, communication, and teamwork in tackling healthcare crises.
Success Stories in Healthcare: Analyzing What Works and Why
Understanding why certain strategies succeed is crucial for replicating positive results in the healthcare industry. For instance, one hospital’s initiative to reduce patient readmissions focused on comprehensive discharge planning and follow-up care. By ensuring patients had clear instructions and support after leaving the hospital, readmission rates dropped significantly. This case study emphasizes the role of thorough patient education and post-discharge care in improving outcomes.
In the realm of preventive care, a primary care clinic introduced a program to increase vaccination rates among its patient population. By actively reaching out to patients due for immunizations and offering flexible scheduling options, the clinic saw a dramatic increase in vaccination rates. The takeaway from this case study is the impact of proactive patient engagement and removing barriers to care.
Lastly, a healthcare organization’s embrace of continuous quality improvement (CQI) led to enhanced patient safety and satisfaction. By fostering a culture of open communication and ongoing learning, the organization identified areas for improvement and systematically implemented changes. This case study demonstrates the power of a commitment to CQI as a driver for excellence in healthcare.
The healthcare industry is rich with case studies that provide valuable insights and lessons learned. By analyzing and understanding these success stories, healthcare providers can apply similar strategies to achieve positive outcomes in their own organizations. Whether it’s through technology, stakeholder engagement, or quality improvement initiatives, these case studies offer a blueprint for triumph and provide a roadmap for future success in the ever-changing landscape of healthcare.
Why are case studies valuable in the healthcare industry, and how do they provide insights into successful decision-making and problem-solving within healthcare organizations?
Case studies are valuable as they offer real-world examples of challenges and solutions in healthcare. They provide insights into successful decision-making, problem-solving, and strategies that can be applied by healthcare professionals and organizations facing similar scenarios.
How does the article select and present case studies, and what criteria are considered to ensure the relevance and applicability of the showcased success stories to a diverse audience?
The article discusses the criteria for selecting case studies, such as their impact on healthcare outcomes, innovation, or overcoming significant challenges. It highlights the diversity of cases to ensure relevance to a broad audience, considering different healthcare settings, specialties, and contexts.
Can you provide examples of healthcare case studies featured in the article, and how do these stories illustrate successful decision-making or lessons learned that can benefit readers in the healthcare field?
Certainly! Examples may include cases where innovative technologies improved patient outcomes, or instances where strategic decisions enhanced operational efficiency. The article presents these stories to illustrate valuable lessons learned and best practices that readers can apply in their own healthcare settings.
In what ways do case studies contribute to professional development and learning opportunities for healthcare professionals, and how can organizations leverage these stories for continuous improvement and staff training?
The article explores how case studies offer learning opportunities, allowing healthcare professionals to gain insights from others’ experiences. Organizations can leverage these stories for staff training, fostering a culture of continuous improvement and encouraging employees to apply lessons learned to their daily practices.
For healthcare leaders seeking to implement successful strategies within their organizations, what recommendations and actionable insights does the article provide based on the analysis of the showcased case studies?
The article offers recommendations based on the case studies, such as the importance of collaboration, data-driven decision-making, and embracing innovation. It provides actionable insights that healthcare leaders can use to inform their decision-making processes and drive positive outcomes within their organizations.
Leave a comment Cancel reply
Save my name, email, and website in this browser for the next time I comment.
I agree that my submitted data is being collected and stored . *
+1 (800) 782 1768
Design Thinking in Health Care
SYSTEMATIC REVIEW — Volume 15 — September 27, 2018
Myra Altman, PhD 1 ,2,3 ; Terry T.K. Huang, PhD, MPH, MBA 4 ; Jessica Y. Breland, PhD 2 ( View author affiliations )
Suggested citation for this article: Altman M, Huang TT, Breland JY. Design Thinking in Health Care. Prev Chronic Dis 2018;15:180128. DOI: http://dx.doi.org/10.5888/pcd15.180128 external icon .
PEER REVIEWED
Introduction
Acknowledgments, author information.
Applying Design Thinking to health care could enhance innovation, efficiency, and effectiveness by increasing focus on patient and provider needs. The objective of this review is to determine how Design Thinking has been used in health care and whether it is effective.
We searched online databases (PubMed, Medline, Web of Science, CINAHL, and PyscINFO) for articles published through March 31, 2017, using the terms “health,” “health care,” or “healthcare”; and “Design Thinking,” “design science,” “design approach,” “user centered design,” or “human centered design.” Studies were included if they were written in English, were published in a peer-reviewed journal, provided outcome data on a health-related intervention, and used Design Thinking in intervention development, implementation, or both. Data were collected on target users, health conditions, intervention, Design Thinking approach, study design or sample, and health outcomes. Studies were categorized as being successful (all outcomes improved), having mixed success (at least one outcome improved), or being not successful (no outcomes improved).
Twenty-four studies using Design Thinking were included across 19 physical health conditions, 2 mental health conditions, and 3 systems processes. Twelve were successful, 11 reported mixed success, and one was not successful. All 4 studies comparing Design Thinking interventions to traditional interventions showed greater satisfaction, usability, and effectiveness.
Design Thinking is being used in varied health care settings and conditions, although application varies. Design Thinking may result in usable, acceptable, and effective interventions, although there are methodological and quality limitations. More research is needed, including studies to isolate critical components of Design Thinking and compare Design Thinking–based interventions with traditionally developed interventions.
Health care systems require continuous innovation to meet the needs of patients and providers (1,2). However, these stakeholders are not always considered when new interventions or system processes are designed, which results in products that remain unused because they do not account for human context, need, or fallibility (3,4). This approach also likely contributes to the decades-long gaps between intervention development and implementation (5). Design Thinking offers a way to close that gap by helping investigators incorporate user needs and feedback throughout the development process.
Design Thinking is an approach that prioritizes developing empathy for users, working in collaborative multidisciplinary teams, and using “action-oriented rapid prototyping” of solutions (2,6). It is an iterative process, with innovation emerging only after cycling through several rounds of ideation, prototyping, and testing, which distinguishes it from the traditional linear and often top-down approach to health intervention design (Figure 1) (1,2,4). Design Thinking has been used across sectors to solve complex problems, including the redesign of an elementary school curriculum to enhance student engagement (7), and in domains such as aviation (8) that, like health care, have high levels of risk. Design Thinking is similar to both “user-centered design” and “human-centered design,” which are both referred to as “Design Thinking” in this article.
Figure 1. Design Thinking process, stages of design thinking and examples of exercises used and questions asked in each stage, systematic review on Design Thinking in health care, search results through March 31, 2017. [A text description of this figure is available.]
There is much enthusiasm for the use of Design Thinking in health care, from intervention development to large-scale organizational and systems changes (9). However, health care settings present different challenges than do other domains, so it is important to consider these challenges in assessing whether Design Thinking provides added benefit over traditional approaches. With this in mind, the purpose of this review was to answer the questions, “How has Design Thinking been used to design interventions in health care settings, and have these interventions been effective?”
Data sources
Studies published through March 31, 2017, were identified through searches of online databases (PubMed, Medline, Web of Science, CINAHL, and PyscINFO) using the following search terms: “health,” “health care,” or “healthcare”; and “Design Thinking,” “design science,” “design approach,” “user centered design,” or “human centered design.” Additional articles were included if they were referenced as original research articles in existing articles. To provide an overview of the range of uses of the Design Thinking approach, we did not limit our review to specific populations or conditions and included articles addressing multiple health promotion and disease prevention topics. Given the search terms, the likely target populations for inclusion were patients and health care professionals and the settings in which they work or seek care.
Study selection
We reviewed selected articles using PRISMA guidelines (10,11) and entered citations into a reference manager, which removed duplicates. To be eligible for inclusion, studies had to be written in English, be published in a peer-reviewed journal, provide outcome data on a health-related intervention, and use Design Thinking in intervention development, implementation or both.
There are multiple definitions of Design Thinking, so we focused on the key principles common to most descriptions of the approach; thus, the list of Design Thinking approaches is not exhaustive. Studies were considered to use Design Thinking if they 1) described user/needs assessment, 2) involved iterative prototyping/testing of the intervention with user feedback, and 3) tested the intervention with target users (2,4). The user/needs assessment could include contextual observation of users in the setting in which they would interact with the innovation, interviews, narrative accounts, and documentation from users, gathering extreme user/outlier stories or a review of existing literature and work (2,6). Prototyping included activities such as creating a series of low-fidelity and high-fidelity prototypes of the potential innovation and refining it multiple times through iterative cycles of feedback from end users, stakeholders, and experts. Testing the intervention with target users included implementing and testing the innovation while continuing to refine it on the basis of user feedback and data (1,2,4). Design Thinking is also similar to other techniques, such as plan-do-study-act cycles and formative evaluations. We considered the emphasis on empathizing with the user and the use of low-fidelity prototyping to be key distinguishing features of Design Thinking, so only articles that explicitly indicate their use of these approaches were included. Initial screening was completed for all selected abstracts, and a second round of screening was completed on eligible full-text articles.
Data abstraction
Data were collected on target users, health conditions, objective of the intervention, details on the Design Thinking process, study design and sample, and reported health outcomes. If information was not reported in the article, we contacted the study authors. Studies were also evaluated to determine whether the intervention improved all targeted outcomes (successful), at least one targeted outcome (mixed success), or no targeted outcomes (not successful). Data quality was assessed using the National Institutes of Health’s (NIH’s) National Heart, Lung, and Blood Institute Study Quality Assessment Tools (12).
Study extraction
Figure 2 presents study flow based on the PRISMA study guidelines (10,11). After the initial search, the authors separately screened all abstracts based on the eligibility criteria. One author reviewed all full-text articles (N = 297), and a second author reviewed roughly 15% as a reliability check. Agreement on inclusion/exclusion was more than 80%. Any abstracts or articles for which there was disagreement or uncertainty were reviewed by 2 authors and discussed until consensus was reached. A total of 26 papers representing 24 interventions were included in the analysis. Two authors reviewed all included studies.
Figure 2. PRISMA 2009 flow diagram, systematic review on Design Thinking in health care, search results through March 31, 2017. [A text description of this figure is available.]
Study characteristics
A summary of all included studies is provided in ( Table 1 ). Eleven studies were successful (13–25), 12 reported mixed success (26–37), and one reported no success (38) (Table 1 and Table 2 ). Sample sizes of included studies ranged from 12 to 291, but most studies were small; 14 studies had fewer than 40 participants. Eleven (45.8%) used a control group (15,17,18,24,26–29,31,33,35,38,42), and 4 (16.6%) compared a design-thinking intervention to an intervention designed using traditional methods (17,18,24,26,35). Two of the studies included were “good” quality, 13 were “fair” quality, and 9 were “poor” quality. All studies used Design Thinking methodology in intervention development, and 3 also used it for implementation (16,20,25,43)
The 24 included interventions targeted a range of conditions, including 19 related to physical health (17 unique conditions), 2 related to mental health, and 3 related to systems processes. Approximately two-thirds of the interventions were mobile telephone–based or tablet-based.
Summary of findings by target user
Patient-facing interventions (n = 11). Five interventions were successful: 4 with a pre/post design (13,19,22,23,44) and 1 pilot randomized control trial (RCT) (15). Five reported mixed success, including one pre/post design (31), one pilot RCT (29), one RCT (28), one cohort study (47), and one unblinded, randomized crossover design (33). One, a pilot RCT, was not successful (46).
Provider-facing interventions (n = 9). Six were successful, including 3 studies using a pre/post design (16,20,25), one field experiment (14), one using a quasi-experimental crossover design (24), and one cross-sectional study (21). Three had mixed success, including 2 studies with an experimental crossover design (17,18,26) and one with primarily a pre/post design, one portion of which was a randomized crossover design (35).
Patient-facing and provider-facing interventions (n = 2). Both reported mixed success and were pre/post designs (30,34,45).
Caregiver-facing or family-facing interventions (n = 2). Both reported mixed success, one in a pilot RCT (27,39) and one using a pre/post design (32).
Summary of Randomized-Controlled Trials (K = 5). Of the RCTs and pilot RCTs reviewed, one demonstrated success on all outcomes (15,40), 3 showed mixed success (27–29,39,41), and one reported no enduring significant results (38,46).
Summary of studies directly testing Design Thinking methodology
Four studies directly compared interventions created with Design Thinking to interventions created with traditional methods. In one study with a within-sample experimental crossover design (26), a Design Thinking–based graphical information display to improve nurses’ ability to detect changes in patients’ physiological states in an intensive care unit (ICU) was compared with a conventional display in commercial, electronic ICU charting systems. The Design Thinking intervention resulted in improved detection of changes in patient states and greater ease of use, usefulness, satisfaction, and support of understanding, but no differences in workload for nurses (26). Another study using an experimental crossover design compared 2 computer interfaces designed to display drug interaction alerts, one developed using Design Thinking and one using traditional software (17,18). Whereas the design of the traditional software was not described, the traditional display included only basic text information. In this study, users (ICU nurses) were more efficient and effective, and reported higher satisfaction with the Design Thinking interface. Another study using a quasi-experimental crossover design used Design Thinking to develop an application to guide clinicians in detecting and scoring the severity of graft versus host disease (GvHD) (24). When compared with paper-based NIH guidelines, users of the application (app) signficantly improved diagnostic and scoring accuracy. A final study compared a Design Thinking–based app that provided nurses with information about antibiotic use with regular information sources (which were not described) (35). In the randomized portion of this study, nurses using the app found information on antibiotic use more quickly; however, the app did not enhance their ability to improve antibiotic-related behaviors. (Only 7 participants were included in the randomized portion of the study.) Whereas the development of the control intervention was not fully described in these papers, based on the limited descriptions given, it is likely that it did not include key elements of Design Thinking such as user feedback and prototyping.
The 24 interventions summarized in this review provide an overview of the breadth of Design Thinking’s applicability in health care and demonstrate that it is feasible and applicable to multiple health care domains. It has been applied across a range of diverse patient populations and conditions, including chronic obstructive pulmonary disease (28,34), diabetes (34,47), caregiver stress (27), and posttraumatic stress disorder (22). It also has been applied to systems process changes, such as nursing handoffs (16) and drug–drug interaction alerts (17,18). Results also demonstrate that, although it is often applied to electronic interventions, Design Thinking is feasible for use in other modalities (eg, on paper, in person).
Initial results of the interventions included in this review are promising; all but one demonstrated positive effects on at least one identified outcome, and half showed positive effects on all measured outcomes. In addition, in the studies that directly compared the Design Thinking intervention with a traditional intervention, the Design Thinking intervention generally demonstrated improved outcomes and higher usability and satisfaction.
However, none of these studies were RCTs with large sample sizes. Design Thinking interventions have been tested primarily in pre/post designs or pilot RCTs with small samples. Furthermore, most studies included were poor or fair quality, with only 2 being considered good quality. Importantly, the criteria used to assess quality were based on traditional research approaches, and many of the features of poor-quality studies were included by design; some had small sample sizes to generate insights and to test assumptions rapidly, and some were pilot studies. This feature of Design Thinking also may account for the limited use of large RCTs; however, this poses a challenge when evaluating the effectiveness of the approach. More work in this area using more rigorous methods and larger samples is critical to fully understanding the benefits of Design Thinking. Although many studies that used Design Thinking were excluded from our review because they did not include sufficient outcome data (n = 131), full-scale trials of many of these interventions are under way, results of which will provide more evidence about the effectiveness of this approach in health care. In addition, no studies measured Design Thinking directly to explain how or what components of Design Thinking lead to improved usability and effectiveness, limiting the field’s ability to disseminate the most effective components and refine the Design Thinking approach for health care.
Design Thinking methods varied among the studies reviewed. For example, only 6 studies conducted contextual observations of users during the needs assessment phase, no studies reported a brainstorming stage, 10 studies did not use low-fidelity prototypes, and some reported a small number of iterations (eg, one mixed-success trial had 4 intervention iterations, but only 2 iterations were evaluated with target users [27]). Using more thorough and structured Design Thinking methodology may have resulted in more consistent and enhanced outcomes. At the same time, Design Thinking is meant to be flexibly applied. Future work should balance that flexibility with the potential benefits of a more systematic approach.
Our results suggest that one area where Design Thinking could be especially useful is in designing interventions for underserved populations whose needs may be overlooked by other approaches. For example, the study of a mobile health tool for detecting and managing cardiovascular disease in rural India required significant feedback from the end users — minimally trained health workers — to ensure that the intervention was suited to their level of technological familiarity as well as the inconsistent technical infrastructure (eg, creating a one-touch navigation system) (21). Using Design Thinking allowed the multidisciplinary team to question assumptions and biases and develop an intervention that was successful, acceptable, and feasible to the actual users, an outcome that may not have been possible using traditional methods (21). Another study evaluated the impact of an education tool to enhance long-acting contraceptive use in a clinic serving mostly African American patients who were included early in the usability testing process to ensure the tool met their needs. Several changes were made as a result, such as including more peer testimonials, which likely increased the tool’s impact and relevance (29). In this way, Design Thinking could also pair well with other approaches that prioritize the inclusion of users in service of reducing health disparities, such as community-based participatory research (48).
Tensions when using Design Thinking in health care
In their text and through our analysis, the studies included in this review show several challenges to consider when applying Design Thinking to health care. First, there is the possibility of tension between what users want and what providers and researchers believe to be beneficial based on research and expertise (49). Whereas in industry, where an innovation designer may prioritize customers’ preferences to maximize profits, in health care a balance must be struck between creating interventions that are effective and sufficiently palatable and feasible so that they will be used by providers and patients.
Second, tension may exist between the needs assessment, a fundamental step of Design Thinking, and existing literature and evidence base for some conditions. That is, given the evidence, intervention developers may not be willing or see it necessary to conduct their own needs assessment using observation or interview strategies or to brainstorm creative solutions. Indeed, 7 of the studies included in this review reported literature reviews, and possibly expert consultation, as their only needs assessment steps, and none reported brainstorming. One way to overcome this tension is to view evidence as a set of design constraints in which needs assessment, brainstorming, ideation, and prototyping should occur.
A third possible tension relates to balancing the Design Thinking approach of understanding the narrative of outliers with traditional health research methods that prioritize statistics on large samples to produce generalizable results. Conclusions drawn from small user samples should be tested in broader populations to ensure their applicability. Mixed-methods approaches that use both strategies may reduce this tension. For example, a research team that uses a qualitative Design Thinking approach early in the research process (eg, user observations, focus groups, and usability tests with small groups of target users) may be able to generate insights into the key needs of the target population. This approach may also find ways to address these needs, and subsequent quantitative testing of the developed interventions in broader samples will allow the group to evaluate whether their assumptions generalize to the broader population, and the intervention will be more effective as a result.
Fourth, there is inherent tension between a central philosophy of the prototyping process in Design Thinking — to rapidly move through low-fidelity then high-fidelity iterations to fail early and often to more quickly reach a better design (50) — and the risk of serious negative outcomes due to health care failures (eg, death). Many of the studies did not use low-fidelity prototyping or multiple rapid iterations, perhaps because of this tension. However, although there may be some reluctance to experiment with low-fidelity prototypes in health care where morbidity and mortality are at stake, there are low-stakes approaches to low-fidelity prototyping that may minimize risk and improve the pace of innovation (eg, storyboards to illustrate a new clinic process).
Intervention development and implementation: case example
Considering the role of Design Thinking is important, not only in efficacious intervention development but also in effective implementation into practice (5). Only 3 of the included interventions addressed implementation, but this limited implementation provides insights. For example, in designing a new process for facilitating nurse handoffs between shifts, Lin and colleagues conducted an extensive 6-month intervention development design process that was user-focused and empathic and had rapid iteration in pilot sites (43). However, despite this strong preliminary work, the intervention was not readily accepted when implemented in other clinics. As a participant stated:
After the concepts had been co-developed and field tested with our pilot units . . . we assumed the units were “bought in” to the idea of the change. . . . Surprisingly, our approach to the training resulted in criticism and created skepticism [at other clinics]. . . . They attributed this to “not made here” sentiments from those units not involved in the original design.
To overcome this tension, the team involved additional stakeholders to develop a more user-centered process for the implementation of their Design Thinking innovation, after which they successfully implemented the innovation across 125 nursing units in 14 hospitals over 2 years (16). This study highlights the importance of understanding the context of the setting and users, both when developing and implementing an intervention using a Design Thinking approach. It should also be noted that this process required significant time and energy from stakeholders. One stakeholder commented, “Don’t get me wrong. What we did was fantastic. But it took a lot out of us” (43). This study highlights the importance of staying true to the user-centered nature of Design Thinking throughout the process — from development to implementation — to maximize implementation success. It also highlights the challenges in using this approach. Teams using Design Thinking should be prepared for a more intensive process than traditional, less iterative and user-centered methods.
Limitations
Given the varied outcomes included in the review and the inconsistent reporting of qualitative outcomes it was difficult to make comparisons across studies. The range of study types and limited number of large scale RCTs testing intervention effects also made it difficult to draw definitive conclusions about effectiveness. At the same time, given that there was only one study with a null result, there was likely publication bias, which may have led to overestimation of the effectiveness of Design Thinking. It is also possible that investigators used methods but did not report them (eg, prototyping). In addition, we did not assess the use of Design Thinking in other health care areas where it may be beneficial, such as the design of physical spaces. Finally, Design Thinking–based health care innovations that were developed and implemented outside research contexts may exist and are thus not reported in the literature.
Conclusions
Design Thinking is being used in varied health care settings and health conditions, and more studies are forthcoming. This review suggests that Design Thinking may result in more usable, acceptable, and effective interventions compared with traditional expert-driven methods. However, there is inconsistent use of the methodology and significant limitations inherent in the studies, which limits our ability to draw conclusions about this approach. Future studies may benefit from focusing on comparing interventions developed using Design Thinking methods with traditionally developed interventions, including those with RCT designs, and identifying the most useful components of Design Thinking methods.
Overall, Design Thinking is a promising approach to intervention development, implementation, and dissemination that may increase the acceptability and effectiveness of health care interventions by actively engaging patients and providers in the design process and rapidly iterating innovation prototypes to maximize success.
The research was supported by the Department of Veterans Affairs (VA), Veterans Health Administration, Health Services Research and Development Service (HSR&D). Dr Breland is a VA HSR&D Career Development awardee at the VA Palo Alto (15-257). The views expressed in this article are those of the authors and do not necessarily represent the views of the Department of Veterans Affairs. There is no copyrighted material used in this article, and no copyrighted surveys, instruments, or tools were used in this article.
The authors thank Ms Elon Hailu for her help editing the manuscript. At the time of the research, Dr Altman was affiliated with the Department of Psychology, Washington University in St. Louis, St. Louis, Missouri, and the VA Palo Alto Health Care System, Menlo Park, California.
Corresponding Author: Myra Altman, PhD, Postdoctoral Design Fellow, Clinical Excellence Research Center, Stanford University, 75 Alta Rd, Stanford, CA 94305. Telephone: 603-306-6231. Email: [email protected] .
Author Affiliations: 1 Washington University in St. Louis, St. Louis, Missouri. 2 VA Palo Alto Health Care System, Menlo Park, California. 3 Stanford University, Stanford, California. 4 Center for Systems and Community Design, Graduate School of Public Health and Health Policy, City University of New York, New York, New York.
- MacFadyen JS. Design thinking. Holist Nurs Pract 2014;28(1):3–5. CrossRef external icon PubMed external icon
- Roberts JP, Fisher TR, Trowbridge MJ, Bent C. A design thinking framework for healthcare management and innovation. Healthc (Amst) 2016;4(1):11–4. CrossRef external icon PubMed external icon
- Searl MM, Borgi L, Chemali Z. It is time to talk about people: a human-centered healthcare system. Health Res Policy Syst 2010;8(1):35. CrossRef external icon PubMed external icon
- Lyon AR, Koerner K. User-centered design for psychosocial intervention development and implementation. Clin Psychol (New York) 2016;23(2):180–200. CrossRef external icon PubMed external icon
- Munro CL, Savel RH. Narrowing the 17-year research to practice gap. Am J Crit Care 2016;25(3):194–6. CrossRef external icon PubMed external icon
- Ferreira FK, Song EH, Gomes H, Garcia EB, Ferreira LM. New mindset in scientific method in the health field: Design Thinking. Clinics (Sao Paulo) 2015;70(12):770–2. CrossRef external icon PubMed external icon
- Kelley D, Kelley T. Creative confidence: unleashing the creative potential within us all. Danvers (MA): Crown Publications; 2013.
- Hall A, Mayer T, Wuggetzer I, Childs PRN. Future aircraft cabins and design thinking: optimisation vs. win-win scenarios. Propulsion and Power Research 2013;2(2):85–95. CrossRef external icon
- Bevan H, Robert G, Bate P, Maher L, Wells J. Using a design approach to assist large-scale organizational change: “10 High Impact Changes” to improve the National Health Service in England. J Appl Behav Sci 2007;43(1):135–52. CrossRef external icon
- Stewart LA, Clarke M, Rovers M, Riley RD, Simmonds M, Stewart G, et al. ; PRISMA-IPD Development Group. Preferred reporting items for a systematic review and meta-analysis of individual participant data: The prisma-ipd statement. JAMA 2015;313(16):1657–65. CrossRef external icon PubMed external icon
- Moher D, Liberati A, Tetzlaff J, Altman DG; PRISMA Group. Preferred reporting items for systematic reviews and meta-analyses: the PRISMA statement. PLoS Med 2009;6(7):e1000097. CrossRef external icon PubMed external icon
- National Institutes of Health, National Heart Lung, and Blood Institute. Study quality assessment tools. https://www.nhlbi.nih.gov/health-topics/study-quality-assessment-tools. Accessed May 4, 2018.
- Adirim T, Chafranskaia A, Nyhof-Young J. Investigating the impact of socioeconomic status on the effectiveness of a pamphlet on achieving and maintaining bone health in breast cancer survivors: a patient education resource development primer. J Cancer Educ 2012;27(1):54–8. CrossRef external icon PubMed external icon
- Kuipers DA, Wartena BO, Dijkstra BH, Terlouw G, van T Veer JT, van Dijk HW, et al. iLift: A health behavior change support system for lifting and transfer techniques to prevent lower-back injuries in healthcare. Int J Med Inform 2016;96:11–23. CrossRef external icon PubMed external icon
- DeVito Dabbs A, Dew MA, Myers B, Begey A, Hawkins R, Ren D, et al. Evaluation of a hand-held, computer-based intervention to promote early self-care behaviors after lung transplant. Clin Transplant 2009;23(4):537–45. CrossRef external icon PubMed external icon
- Lin M, Heisler S, Fahey L, McGinnis J, Whiffen TL. Nurse knowledge exchange plus: human-centered implementation for spread and sustainability. Jt Comm J Qual Patient Saf 2015;41(7):303–12. CrossRef external icon PubMed external icon
- Luna D, Otero C, Risk M, Stanziola E, González Bernaldo de Quirós F. impact of participatory Design for Drug-Drug Interaction Alerts. A comparison study between two interfaces. Stud Health Technol Inform 2016;228:68–72. PubMed external icon
- Luna DR, Rizzato Lede DA, Otero CM, Risk MR, González Bernaldo de Quirós F. User-centered design improves the usability of drug-drug interaction alerts: Experimental comparison of interfaces. J Biomed Inform 2017;66:204–13. CrossRef external icon PubMed external icon
- McGaffey A, Hughes K, Fidler SK, D’Amico FJ, Stalter MN. Can Elvis Pretzley and the Fitwits improve knowledge of obesity, nutrition, exercise, and portions in fifth graders? Int J Obes 2010;34(7):1134–42. CrossRef external icon PubMed external icon
- Pottenger BC, Davis RO, Miller J, Allen L, Sawyer M, Pronovost PJ. Comprehensive Unit-based Safety Program (CUSP) to Improve Patient Experience: how a hospital enhanced care transitions and discharge processes. quality management in health care 2016;25(4):197-202.
- Raghu A, Praveen D, Peiris D, Tarassenko L, Clifford G. Engineering a mobile health tool for resource-poor settings to assess and manage cardiovascular disease risk: SMARThealth study. BMC Med Inform Decis Mak 2015;15(1):36. CrossRef external icon PubMed external icon
- Rizzo A, Difede J, Rothbaum BO, et al. Development and early evaluation of the Virtual Iraq/Afghanistan exposure therapy system for combat-related PTSD. In: Barchas JD, Difede J, editors. Psychiatric and Neurologic Aspects of War. Vol. 1208. Hoboken (NJ): John Wiley and Sons; 2010. p. 114–25.
- Sánchez-Morillo D, Crespo M, León A, Crespo Foix LF. A novel multimodal tool for telemonitoring patients with COPD. Inform Health Soc Care 2015;40(1):1–22. CrossRef external icon PubMed external icon
- Schoemans H, Goris K, Durm RV, Vanhoof J, Wolff D, Greinix H, et al. Development, preliminary usability and accuracy testing of the EBMT ‘eGVHD App’ to support GvHD assessment according to NIH criteria-a proof of concept. Bone Marrow Transplant 2016;51(8):1062–5. CrossRef external icon PubMed external icon
- Trail-Mahan T, Heisler S, Katica M. Quality improvement project to improve patient satisfaction with pain management using human-centered design. J Nurs Care Qual 2016;31(2):105–12, quiz 113–4. CrossRef external icon PubMed external icon
- Anders S, Albert R, Miller A, Weinger MB, Doig AK, Behrens M, et al. Evaluation of an integrated graphical display to promote acute change detection in ICU patients. Int J Med Inform 2012;81(12):842–51. CrossRef external icon PubMed external icon
- Cristancho-Lacroix V, Moulin F, Wrobel J, Batrancourt B, Plichart M, De Rotrou J, et al. A web-based program for informal caregivers of persons with Alzheimer’s disease: an iterative user-centered design. JMIR Res Protoc 2014;3(3):e46–46. CrossRef external icon PubMed external icon
- Farmer A, Williams V, Velardo C, Shah SA, Yu LM, Rutter H, et al. Self-management support using a digital health system compared with usual care for chronic obstructive pulmonary disease: randomized controlled trial. J Med Internet Res 2017;19(5):e144. CrossRef external icon PubMed external icon
- Gilliam ML, Martins SL, Bartlett E, Mistretta SQ, Holl JL. Development and testing of an iOS waiting room “app” for contraceptive counseling in a Title X family planning clinic. Am J Obstet Gynecol 2014;211(5):481.e1–8. CrossRef external icon PubMed external icon
- Hartzler AL, Izard JP, Dalkin BL, Mikles SP, Gore JL. Design and feasibility of integrating personalized PRO dashboards into prostate cancer care. J Am Med Inform Assoc 2016;23(1):38–47. CrossRef external icon PubMed external icon
- Kamal N, Fels S, Fergusson M. Online social networks for health behaviour change: designing to increase socialization. Comput Human Behav 2014;41:444–53. CrossRef external icon
- Koehly LM, Morris BA, Skapinsky K, Goergen A, Ludden A. Evaluation of the Families SHARE workbook: an educational tool outlining disease risk and healthy guidelines to reduce risk of heart disease, diabetes, breast cancer and colorectal cancer. BMC Public Health 2015;15(1):1120. CrossRef external icon PubMed external icon
- van Besouw RM, Oliver BR, Grasmeder ML, Hodkinson S, Solheim H. Evaluation of an interactive music awareness program for cochlear implant recipients. Music Percept 2016;33(4):493–508. CrossRef external icon
- Verwey R, van der Weegen S, Spreeuwenberg M, Tange H, van der Weijden T, de Witte L. Technology combined with a counseling protocol to stimulate physical activity of chronically ill patients in primary care. Stud Health Technol Inform 2014;201:264–70. PubMed external icon
- Wentzel J, van Drie-Pierik R, Nijdam L, Geesing J, Sanderman R, van Gemert-Pijnen JE. Antibiotic information application offers nurses quick support. Am J Infect Control 2016;44(6):677–84. CrossRef external icon PubMed external icon
- Yu CH, Parsons JA, Mamdani M, Lebovic G, Hall S, Newton D, et al. A web-based intervention to support self-management of patients with type 2 diabetes mellitus: effect on self-efficacy, self-care and diabetes distress. BMC Med Inform Decis Mak 2014;14(1):117. CrossRef external icon PubMed external icon
- Wachter SB, Agutter J, Syroid N, Drews F, Weinger MB, Westenskow D. The employment of an iterative design process to develop a pulmonary graphical display. J Am Med Inform Assoc 2003;10(4):363–72. CrossRef external icon PubMed external icon
- Welch JL, Astroth KS, Perkins SM, Johnson CS, Connelly K, Siek KA, et al. Using a mobile application to self-monitor diet and fluid intake among adults receiving hemodialysis. Res Nurs Health 2013;36(3):284–98. CrossRef external icon PubMed external icon
- Cristancho-Lacroix V, Wrobel J, Cantegreil-Kallen I, Dub T, Rouquette A, Rigaud A-S. A web-based psychoeducational program for informal caregivers of patients with Alzheimer’s disease: a pilot randomized controlled trial. J Med Internet Res 2015;17(5):e117. CrossRef external icon PubMed external icon
- DeVito Dabbs A, Myers B, McCurry K. User-centered design and the development of interactive health communication applications. Comput Nurs 2009;27(3):175.
- Velardo C, Shah SA, Gibson O, Clifford G, Heneghan C, Rutter H, et al. ; EDGE COPD Team. Digital health system for personalised COPD long-term management. BMC Med Inform Decis Mak 2017;17(1):19. CrossRef external icon PubMed external icon
- Kamal N, Fels S, Blackstock M, Ho K. VivoSpace: towards health behavior change using social gaming. Paper presented at: Entertainment Computing — ICEC 2011. Berlin, Germany, and Heidelberg, Germany; 2011.
- Lin MC, Hughes BL, Katica MK, Dining-Zuber C, Plsek PE. Service design and change of systems: human-centered approaches to implementing and spreading service design. Int J Des 2011;5(2):73–86.
- McLay RN, Graap K, Spira J, Perlman K, Johnston S, Rothbaum BO, et al. Development and testing of virtual reality exposure therapy for post-traumatic stress disorder in active duty service members who served in Iraq and Afghanistan. Mil Med 2012;177(6):635–42. CrossRef external icon PubMed external icon
- van der Weegen S, Verwey R, Spreeuwenberg M, Tange H, van der Weijden T, de Witte L. The development of a mobile monitoring and feedback tool to stimulate physical activity of people with a chronic disease in primary care: a user-centered design. JMIR Mhealth Uhealth 2013;1(2):e8. CrossRef external icon PubMed external icon
- Welch JL, Siek KA, Connelly KH, Astroth KS, McManus MS, Scott L, et al. Merging health literacy with computer technology: self-managing diet and fluid intake among adult hemodialysis patients. Patient Educ Couns 2010;79(2):192–8. CrossRef external icon PubMed external icon
- Yu CH, Parsons JA, Hall S, Newton D, Jovicic A, Lottridge D, et al. User-centered design of a web-based self-management site for individuals with type 2 diabetes – providing a sense of control and community. BMC Med Inform Decis Mak 2014;14(1):60. CrossRef external icon PubMed external icon
- Henderson VA, Barr KL, An LC, Guajardo C, Newhouse W, Mase R, et al. Community-based participatory research and user-centered design in a diabetes medication information and decision tool. Prog Community Health Partnersh 2013;7(2):171–84. CrossRef external icon PubMed external icon
- Witteman HO, Dansokho SC, Colquhoun H, Coulter A, Dugas M, Fagerlin A, et al. User-centered design and the development of patient decision aids: protocol for a systematic review. Syst Rev 2015;4(1):11. CrossRef external icon PubMed external icon
- Thoring K. M RM, #252, ller. Understanding the creative mechanisms of design thinking: an evolutionary approach. Procedings of the Second Conference on Creativity and Innovation in Design. Eindhoven, Netherlands; 2011.
Abbreviation: DNR, did not report; NA, not applicable; RCT, randomized control trial; a If 2 studies are cited, the earlier article is the intervention development methodology and the later article is the evaluation study.
Abbreviations: App, application; CI, confidence interval; COPD, chronic obstructive pulmonary disease; GvHD, graft vs host disease; HBA1c, hemoglobin A1c; HCAHPS, Hospital Consumer Assessment of Healthcare Providers and Systems; HRQOL, health-related quality of life; IGID, integrated graphical information display; IUD, intrauterine device; NKE, nurse knowledge exchange plus; PTSD, posttraumatic stress disorder; SD, standard deviation a All results significant at P < .05.
The opinions expressed by authors contributing to this journal do not necessarily reflect the opinions of the U.S. Department of Health and Human Services, the Public Health Service, the Centers for Disease Control and Prevention, or the authors’ affiliated institutions.
Exit Notification / Disclaimer Policy
- The Centers for Disease Control and Prevention (CDC) cannot attest to the accuracy of a non-federal website.
- Linking to a non-federal website does not constitute an endorsement by CDC or any of its employees of the sponsors or the information and products presented on the website.
- You will be subject to the destination website's privacy policy when you follow the link.
- CDC is not responsible for Section 508 compliance (accessibility) on other federal or private website.
Log in using your username and password
- Search More Search for this keyword Advanced search
- Latest content
- Current issue
- BMJ Journals More You are viewing from: Google Indexer
You are here
- Volume 7, Issue 2
- Design thinking as an approach for innovation in healthcare: systematic review and research avenues
- Article Text
- Article info
- Citation Tools
- Rapid Responses
- Article metrics

- http://orcid.org/0000-0002-2759-8036 Mariana Oliveira ,
- http://orcid.org/0000-0001-8361-0637 Eduardo Zancul ,
- http://orcid.org/0000-0003-4937-0339 André Leme Fleury
- School of Engineering, Industrial Engineering Department , Universidade de São Paulo (USP) , Sao Paulo , Brazil
- Correspondence to Dr Eduardo Zancul, School of Engineering, Industrial Engineering Department, Universidade de São Paulo (USP), Sao Paulo, Brazil; ezancul{at}usp.br
Design thinking has been increasingly adopted as an approach to support innovation in healthcare. Recent publications report design thinking application to various innovation projects, across medical specialties, including paediatrics, psychiatry, radiology, gastroenterology, oncology, orthopaedics and surgery, as well as to innovation in hospital operations and healthcare management. Current literature in the area typically focuses on single case descriptions. With the recent increase in the number of cases, there is an opportunity to assess multiple cases to identify patterns and avenues for further research. This study provides a systematic review of published design thinking projects in healthcare. The aim of the study is to provide an overview of how design thinking has been applied in the healthcare sector. Data collection was based on Institute of Scientific Information (ISI) Web of Science, PubMed and Scopus databases. The systematic review followed Preferred Reporting Items for Systematic Reviews and Meta-Analyses guidelines. A total of 32 original pieces of research was selected for analysis, being classified and assessed. The paper presents current status of research and practice from various perspectives, including the design thinking progression phase—inspiration, ideation, implementation—and the prevalence of design thinking tools. Avenues for further research include the need to increase focus on the inspiration phase, the opportunity for platforms for leveraging the integration of individuals in innovation projects, and the opportunity to enhance the role of lead users in healthcare innovation.
- diagnostics
Data availability statement
All data relevant to the study are included in the article or uploaded as supplemental information. Data reuse is permitted.
https://doi.org/10.1136/bmjinnov-2020-000428
Statistics from Altmetric.com
Request permissions.
If you wish to reuse any or all of this article please use the link below which will take you to the Copyright Clearance Center’s RightsLink service. You will be able to get a quick price and instant permission to reuse the content in many different ways.
Summary box
What is already known.
Design thinking has been adopted in healthcare innovation projects in several domains, with reports of positive outcomes.
What are the new findings?
The research details the design thinking processes and tools applied in healthcare based on multiple case reports.
Design thinking provides a frame for addressing the development of healthcare innovation by balancing contextual factors (eg, users, stakeholders, resources) and clinical evidence.
Design thinking is an ally for democratising access to healthcare through innovative solutions in low-resource settings.
Opportunities for further research include: (a) increased focus on the inspiration stage, (b) creation of platforms for leveraging the integration of individuals in health innovation projects, (c) e-health focused user research and (d) lead user involvement.
Introduction
Healthcare is increasingly applying design knowledge and competence to deal with challenges, 1 as design provides a frame for understanding and developing a subject or business and its related policies, products, resources and services. 2 As a matter of fact, innovation is required to address the changing environments (eg, ageing of the population) and guarantee the financial sustainability of health services; 3 this may be achieved by improving health outcomes at a good value, reducing cost for care or tracking health outcomes. 4 In this scenario, design thinking emerges as an approach for incorporating innovation in medical practice in public and private sectors. 5 Clinical outcomes of healthcare interventions that claim to have employed design thinking have proven to be positive. 6 Design thinking application may potentially benefit the design of new health devices, products and processes, and the implementation of evidence-based practices. 7
Brown 8 popularised the term design thinking and promoted a significant increase in its published research literature. Despite the increase in research, there is still a lack of standardisation regarding the definition and understanding of what is design thinking. 9–11 In convergence with trends in the literature, we define design thinking as a human-centred approach for solving complex problems employing attributes such as creativity, user involvement, multidisciplinary teamwork, iteration, prototyping and user centredness. 9–11 Many toolkits 12 13 and practical guides 14 presenting design thinking processes have been published; despite of using different terms to refer to the design thinking phases, they follow the same overall logic for problem-solving. 9–11 15 16 Practically, design thinking may be portrayed in three iterative phases: inspiration, ideation and implementation.
Inspiration is the first phase and it is based on need-finding: understanding the core issue of the problem by empathising with the user and discovering their explicit and non-explicit needs. Users and stakeholders identification is critical for innovation success; 17 18 in healthcare, this task has an increased complexity due to the various paying systems structures. 4 Ethnographic research techniques, such as observation and interviewing, are recommended at the inspiration phase. 16 After the need is defined, data analysis and solution conceptualisation start at the second phase, ideation; many strategies may be used to foster concept generation and free-of-judgement creativity at this second phase. 10 Studies acknowledge the positive effects of a visually stimulating environment on problem-solving; 19 low-fidelity prototyping is used as a source of ideas and a tool for concept validation; 15 sensemaking tools, like mind-mapping, are used to support brainstorming. 16 The aims of the third and final phase, implementation, are to refine and build the concept validated during the second phase and draw a marketing strategy for the final product. Prototyping is again required at this phase, but with higher fidelity as testing will also be required. 16
Previous works have analysed the impacts of solutions developed using a design thinking approach on health outcomes both in broad 1 and deep 6 accounts. However, rigorous evaluations on how design thinking is operationalised in the health sector from a process perspective remain an opportunity for further integrating design knowledge into health research. 1 This article aims to appraise the final results of solutions developed using design thinking in healthcare and the course of actions and tools that took place throughout development. As the enactment of the design thinking approach is context-dependent, 10 20 the format of a systematic literature search and review are aligned with the aim of this research; 21 22 an exhaustive search allows for an aggregate appreciation of the literature, and capturing several configurations in which design thinking is adopted.
We contribute to the literature by consolidating previous reports on how design thinking has been applied in the healthcare sector and drawing conclusions from these reports. This article is also directed to practitioners as it presents tools used when applying design thinking. We will analyse articles reporting solutions ranging from the early stages of their development to solutions that are available to the market. By reviewing articles that report developing solutions, we aim to capture perspectives on every phase in the development process and avoid publication bias. We will review and tabulate aspects of each study, such as the nature of the innovation intervention, which design thinking tools were employed, team multidisciplinarity and stakeholder involvement. Finally, we will discuss the contents of the studies analysed and possible avenues for research. We aim to provide an overview of the best practices on design thinking in healthcare.
Data collection began with a search in Institute of Scientific Information (ISI) Web of Science, PubMed and Scopus databases without start date constraint (ie, from their inception) until October 2019; the earliest publication record found dated from February 2003. The three databases were chosen to provide a comprehensive search on journals focused on the disciplines of interest of this paper (eg, design, business, engineering, health sciences). The search strings used were ‘“design think*” or “user-cent* design” or “user cent* design” or “human-cent* design” or “human cent* design”’ + ‘innovation’ + ‘“health*” or “medical”’ included on title, abstract or keywords. In spite of subtle differences among the terms user-centred design, human-centred design and design thinking, 1 there is a conceptual overlap between these terms. In accordance with previous works, we will use them as synonyms. 1 6
The systematic review followed Preferred Reporting Items for Systematic Reviews and Meta-Analyses guidelines (see online supplemental file exhibit A1 ). 23 Only primary peer-reviewed studies were eligible for the study. Search was restricted to papers published in English. A total of 224 articles and reviews were identified in database search, of which 150 came to be non-duplicate documents. Scopus yielded 89 unique results to our search, the Web of Science (WoS) database yielded 32 non-duplicate results when compared with Scopus results, and the PubMed database yielded 29 non-duplicate results when compared with Scopus and WoS results.
Supplemental material
An initial selection process was conducted aiming to filter documents that were not aligned with the research scope through title and abstract analysis, followed by a full-text review of the selected articles. Our research targets articles describing experiences, perceptions and assessment on the development of innovative health-related solutions, specifically on medical devices, products and processes following a design thinking approach. In this review, medical devices refer to hardware solutions, medical products refer to innovative treatments or service offerings solutions (eg, mobile health (m-health) solutions), and processes refer to untangible routines, whether these routines are visible to the patients or not. 24 25 Articles unrelated were discarded. Most articles discarded in title and abstract review regarded pharmaceutical solutions and health aids to be used by the patients without an interface to a health professional. In full-text review, the articles discarded included theoretical reports without an associated solution development, literature reviews, event descriptions, and articles that were not focused on the solution development (eg, design theory, design teaching, testing routines).
After title and abstract review, 65 articles were selected for full-text review. This sample was submitted to bibliometric analysis to identify the main references in their cocitation network, which resulted in the addition of eight references. Finally, following a full-text review, 32 references were selected for analysis. Selection process is made available ( online supplemental file exhibit A2 ).
Literature review results
The final 32 studies were reviewed and summarised ( online supplemental file exhibits A3 and A4 ). As design thinking has no unique coded language, 9 some of the objects of interest in this review were coded for analysis and comparison purposes ( online supplemental file exhibit A5 presents our codes and their correspondance with each of the papers in our sample). A few codes (eg, prototyping) are present in more than one design thinking phase; when evaluating the papers, we took into consideration reports given by the authors to assess the maturity of the activities and whether these activities would fall into one phase or another (eg, cardboard prototypes were considered an ideation phase activity, while functional prototypes were considered implementation phase activities).
Solution status was classified according to what is reported in their studies; due to design thinking’s iterative nature, it is possible that one intervention has performed an ‘implementation’ phase activity, but its status is still at the ideation stage. At the time of publishing, five of the solutions were at the inspiration stage of design thinking and had finalised their need assessments, 26–29 or had study protocols established. 30 Eighteen of the 32 solutions were at the ideation stage, having either a visual prototype, 31 a design concept 32–35 or a functional prototype 36–48 finalised. Regarding the implementation stage, out of eight solutions, one had a final product developed but not implemented, 3 six were fully implemented, 49–54 and one had been implemented and failed. 55 One solution was discontinued due to resource limitations. 56
Regarding medical specialty, of the 32 studies, 10 discussed initiatives to manage chronic disease, 3 32 35 37 38 40 41 46 50 55 4 brought solutions for hospital management, 26 34 47 49 4 on paediatrics, 43 44 51 53 3 on psychiatry, 30 31 48 2 on radiology, 27 39 2 on geriatrics, 29 43 and single articles pulverised in multiple areas, such as addiction, 36 family health, 28 gastroenterology, 52 general practice, 42 oncology, 54 orthopaedics 33 and surgery. 45
A noteworthy theme across our sample is the creation and use of cloud-based multipurpose digital platforms. 35 38 41 43 46 This type of intervention aims to provide an actionable use of information by patients, health professionals and providers while optimising resource allocation (eg, one of the papers presents two solutions for medication management targetting two different populations using a shared architecture for personal health record systems). 43
Four of the papers in our sample provide solutions that aim to address more than one target condition; 28 31 50 51 these works elicited from both user and desk research that these conditions were intertwined and could benefit from being treated as a whole rather than as separate parts. For example, one of the solutions developed a clinical decision support for addressing tuberculosis prevention and treatment considering the high prevalence of HIV infection among the local population. 50
Another recurring theme is the systematisation of stakeholder involvement across various specialties and target conditions, such as orthopaedics, 33 surgical rounds 26 and pharmacy management. 34 One of the papers even reported an increase in its engagement metrics after the refinement of the intervention based on stakeholder feedback. 48
The vast majority of the papers in our sample report interventions in the form of software tools. Only six of the papers report the development of medical devices; we assume this happens due to resource constraints and a longer time to market of medical devices when compared with other types of interventions (eg, one of the papers reported a 48-month project duration). 39 Isolated papers report the creation of events (eg, creation of a seasonal community market to generate income aiming to address social determinants of health inequities), 53 timetables (eg, collaborative creation of a timetable balancing employees’ preferences and nursing home needs), 49 toolkits and decision support systems. The following sections present the main elucidations resulting from the systematic review.
Tools employed
Each phase of the design thinking approach and their objectives is presented in figure 1 ; for each phase, we listed the five most reported tools in our sample and their prevalence rate.
- Download figure
- Open in new tab
- Download powerpoint
Three phases of the design thinking approach, objectives for each phase and main tools employed.
As for the tools employed in the inspiration stage by the authors in our sample, they emphasise the bystander roles of the researchers or individuals when first starting a new project applying design thinking. At this stage, the designer—or any professional acting as a designer—must put aside his/her convictions about the problem addressed. Only then he/she is ready to effectively absorb relevant information regarding the context in which the solution is going to be developed. It is fundamental to consider this context as broadly as possible (considering time and resource limitations) to visualise the actors impacted, possible side problems that could interfere, previous documentation to improve the understanding of the situation, and any other relevant information.
Interview is the most employed tool in the inspiration stage. We assume this happens because an introductory interview is easy to perform, easy to gain access to, may have multiple formats (eg, by telephone, 33 semistructured, 27 28 30 34 39 40 unstructured 26 ) and are greatly clarifying. Observations 26 29 53 and reviews of various sorts (eg, clinical practice review, 28 32 54 literature review 30 51 ) are also clarifying and, after the initial contact is made, require little effort from the user involved in the research. Focus groups 31 36 56 and user empathy tools (eg, clinical immersion, 54 experience maps 31 ) could bring substantial information to the project but have the downside of requiring significant time and effort from both the research team and possible users or stakeholders of the intervention. Tools that do not rely solely on spoken accounts of the users or stakeholders, such as observations, do have the advantage of allowing the research team to uncover opportunities for innovation that the users or stakeholders do not perceive as valuable or achievable; we refer to these opportunities as the user’s unspoken needs.
The ideation phase gathers data collected at the immersion phase and makes sense of it by creating inputs and specifications for the solution. In other words, the users’ spoken and unspoken needs will be translated into the solution’s technical requirements. However, this ‘translation’ and data analysis is not always obvious. 34 39 50 To initialise the design of a solution, conceptualisation 40 43 45 and correlated tools such as brainstorming 27 33 49 are strongly recommended to keep the ideas as broad and fluid as possible. Other user empathy tools (eg, personas, 29 36 45 experience maps 33 47 ) may be used to support this stage. After this initial wave of ideas, the most promising ones are selected for prototyping, 36 37 40 48 which is used as a tool for concept visualisation. Design thinking postulates that prototyping helps the design team to perceive the strengths and weaknesses of their solution early in the design process and even get feedback 3 34 37 40 42 43 from the users. Anchoring the conceptualisation activities in low-fidelity prototypes promotes a quick escalation in the attributes of the concept and smart allocation of resources in ideas that are worth pursuing.
The implementation phase, which aims to refine the ideated concept into a viable solution, was the least reported among our sample, as a significant portion of the articles did not report reaching this phase. Some of those who had reached it focused their reports on assessing the intervention and not describing their development process, 51–53 and a couple of articles reported that they would not disclose these issues due to commercial confidentiality. 27 39 Among the references that did report tools employed in the implementation stage, testing was the most mentioned tool (eg, user testing, 37 42 44 requirements testing 34 45 ), followed by prototyping, 31 34 36 38 40 45 47 53 interviews, 33 36 42 50 54 55 solution evaluation, 36 44 46 50 and solution feedback. 3 34 38 44 It caught our attention that commercial analysis was reported by only three articles in our sample. 33 53 54 If the solution is meant to be commercially viable, this aspect must be addressed in a diligent manner.
Disciplines and stakeholders involved
Although combining different competences and backgrounds is a best practice for design thinking, 8 more than half of the articles in our sample did not report multidisciplinarity in their design thinking teams. This is problematic as diverse teams are more likely to promote relevant innovative solutions. 10 Among the literature that mentioned disciplines and areas involved in their teams, the most cited were health-related disciplines, 3 27 30 32 37 38 49 50 54 design, 30 33 38 49 53 54 Information Technology (IT), 38 50 55 56 Research and Development (R&D) 32 33 37 50 and engineering. 27 32 54
Besides congregating multiple areas of knowledge, it is necessary to gather different perspectives. Managing stakeholders in the healthcare sector is not trivial as healthcare users vary in their roles as device operators, patients or decision-makers. 29 Understanding who the stakeholders are and their roles is a key factor for achieving relevant results and requires an understanding of the business model around the product. 29 33 A solution development focused on technical issues and neglecting stakeholders' perspectives is susceptible to barriers in implementation. 39 55 Stakeholder participation assessment tools 57 and frameworks for listening to the voices of the customer, business and technology 33 are strategies to promote effective stakeholder involvement.
Developing medical devices and products must follow regulatory requirements. In the USA, the Food and Drug Administration (FDA) is the main body of regulation for medical devices. 58
Even though regulatory issues are inherently critical to the implementation of medical devices and products, only 12% of the articles in our sample mention the FDA or another regulator, 27 33 54 56 with only one of them stating the class their devices were fitted in. 33 Our attempt to stratify the findings in our sample according to regulation status or classification was not successful, as we found that a number of our references did not address regulatory issues. This might indicate a lack of maturity of research—or even awareness—in this topic. Design thinking brings the possibility of everyone being a part of the design process on the table, but one individual must own the process and be accountable for design feasibility and regulatory issues. Additionally, two articles did not go into detail on their developments claiming commercial confidentiality. 27 39
Discussion and avenues for research
Drawing attention to the inspiration stage.
Regarding the reportings on the tools employed in the inspiration phase, it was noted that solutions that were in more advanced stages of development—ranging from having a functional prototype to being fully implemented and commercialised—often failed to report the tools employed in the inspiration stage (19% of the sample) or lacked detail about this stage. We believe that this bias is due to the fact that researchers often prioritise describing the intervention developed to the detriment of reporting the development process.
We perceive this ‘setting aside’ of the initial development stage as counterproductive for the replication of design thinking: the engagement and understanding of the final user which is acquired from the inspiration stage are essential for developing appropriate solutions, at the risk of developing solutions that relieve the symptoms of a problem without addressing its root causes. 59 In fact, it is more crucial for the direction of the intervention that users and stakeholders are involved in the early stages when compared with the late stages of the innovation process. 18 60 If the body of literature on design thinking does not consider the relevance of this stage, there is a tendency that individuals learning from this body of literature will have the same perception. This may incur professionals involved in projects employing the design thinking approach neglecting information collected in the inspiration stage, and realising that their solutions do not fulfil user needs. 39 55 Although exhibits from the literature present a systematisation of how to incorporate the results of the inspiration phase and user-centred research throughout the development process, 27 29 due to the variety of stakeholders, users and types of problems in healthcare, further studies seeking to formalise the incorporation of inspiration phase data throughout development would be beneficial to the theory and practice of health research involving design.
Research groups, networks and common platforms for healthcare innovation
One thing that caught our attention was the establishment of research groups and software platforms for improving synergy in the development of healthcare solutions. UK-based Multidisciplinary Assessment of Technology Centre for Healthcare—a publicly funded research group with close collaboration with medical device industries—presents substantial results on research regarding the role of the user in medical device development. 61 Project HealthDesign was a sponsored multiyear, multisite project that gathered design teams across the USA to develop e-health applications using a common back-end platform. 35 41 43 Tidepool is an open-access platform designed to host and integrate applications related to diabetes management, counting with open-source development to augment and sustain the platform. 38
How to make these fruitful connections happen? Norman et al 62 propose the Complex Network Electronic Knowledge Translation Research (CoNEKTR) model for integrating individuals from distinct backgrounds by their common interest in promoting innovation in healthcare; we could not find evidence of CoNEKTR’s applicability and performance outcomes. A proven effective model for leveraging the integration of individuals around healthcare innovation will certainly be a major contribution to this research field.
The future of e-health
Approximately 56% of the articles in our sample reported a healthcare solution using e-health, with the major amount of those discussing m-health. Regarding technology usage, a part of the papers in our sample reported the development of auxiliary technologies for telemedicine, 52 56 and data-gathering technologies, such as personal health records, 29 35 41 43 55 patient self-monitoring 3 40 46 and patient motivation trackers. 32 48
Developing functional and usable e-health applications is not trivial, as there is a need to create an in-depth understanding of the user’s needs, desires, limitations, preferences, attitudes and behaviours through a user model that will serve as a common point for the different individuals involved in the development process. 29 However, capturing these psychological and psychosocial nuances is not possible with the ‘traditional’ application of user-centred methods like user profiles and personas, as they tend to rely on demographic data and shallow caricatures of user groups. 29 Not employing the rigour, time and collective sense of the importance of user research may doom user research to become an unactionable or overlooked work. 39 55
In-depth user research is necessary to address users’ underlying cognitive and behavioural patterns, user subgroups and characteristics unique to different conditions (eg, knowledge about the disease, support network, comorbidities); capturing the amount of data necessary to build actionable user profiles and personas is resource consuming, but its benefits outweigh its costs. 29 Design thinking may provide a framework for aligning healthcare system needs, user needs and software requirements towards healthcare innovation. 34 There are numerous conceptual layers from which the development of successful e-health solutions can be studied: system integration, wearables, user heuristics and interface design are just a few of them.
User involvement
von Hippel 63 introduced the concept of lead users as composed of two main characteristics: the first is that lead users face needs that will be general in the market-place prior to the bulk of that market-place; the second is that they could benefit by obtaining a solution to their needs and thus are highly motivated to seek one. These users play an active role in the development process, beyond the passive role implied by expert-driven user-centred practices, such as interviews, personas and journey mapping. There is evidence of the potential benefits of involving lead users in the co-creation and development of solutions in healthcare. 18 Involving these users could potentially increase development rates and expertise in pioneer technologies and boost commercial performance. Consequently, it could increase manufacturers’ profits by reducing time to market and development costs. 18 Even though there are generic suggestions in the literature of how to retain these lead users, 64 further research on identifying and contacting lead users in the healthcare sector may benefit future development projects.
Another discussion regarding user involvement in the healthcare industry is motivated by understanding who is the user of interest. While there are more obvious contexts where we can identify the main user (eg, a mobile app for patient self-monitoring 3 29 30 46 ), in other cases, such as a medical imaging device, 27 39 it is not clear if the main user is the patient or the healthcare professional and it is not trivial to counterbalance their needs. On top of this, there is a third stakeholder—the payer—which could be either a provider or a healthcare organisation. Further discussion on whether and how design thinking is a suitable approach to manage these user layers would be a contribution to the literature.
Design thinking is a flexible approach for innovation which is being used to develop healthcare solutions. Considering healthcare, our research shows evidence that design thinking is an approach to innovation in clinical and managerial settings, across a wide range of medical specialties. Our research findings endorse that design thinking provides a frame for addressing the development of innovation in healthcare by balancing contextual factors (eg, users, stakeholders, resources) and clinical evidence. Additionally, our sample shows that design thinking is an ally for democratising access to healthcare through innovative solutions in low-resource settings. Design thinking provides an arsenal of tools for problem-solving across the phases of inspiration, ideation and implementation.
With this review, we aimed to present a selection of practical applications of design thinking in healthcare, highlighting the most common practices among them. We present this selection of practice and tools as a guide, rather than as a toolset. The selection of 32 papers shows that design thinking is not a one-size-fits-all approach and that it may be adapted to different circumstances. To further advance this field, future research should follow more rigorous procedures for reporting health research involving design; this could be achieved by following structured guidelines. 65 Additionally, future research on emerging technologies in service of health should address user-centred design, providing replicable procedures on how to identify and address user needs. Finally, once a more consistent body of literature is consolidated, with standardised report procedures, a research agenda for quantitatively assessing the relationship between design choices and clinical outcomes may provide more assertive recommendations for the incorporation of design knowledge into health innovation.
Strengths and limitations
Despite our efforts to establish clear selection criteria, sample selection and subsequent codification were subjected to the authors’ bias. The lack of standards in reporting health research involving design, and the variability of studies in our sample both in their objects of study and development stages refrained this review from assessing criteria such as design success rate, design success critical paths, optimal team composition for design success and types of intervention (eg, devices, products, processes) for which design thinking may be more suitable. This may be interpreted as a clash between design and health sciences underlying research traditions and epistemologies. To address this issue and enable further analysis in future literature reviews, we recommend future works that report interventions on the intersection of design and health to consider following of systematic guidelines. 65
- Bazzano AN ,
- Hicks E , et al
- Valentine L ,
- Bruce F , et al
- Cummings E ,
- Duff J , et al
- Peiffer V ,
- Yock PG , et al
- Roberts JP ,
- Fisher TR ,
- Trowbridge MJ , et al
- Huang TTK ,
- Parisi KE ,
- Munson SA , et al
- Fleury AL ,
- Stabile H ,
- Carvalho MM
- Micheli P ,
- Wilner SJS ,
- Bhatti SH , et al
- Johansson-Sköldberg U ,
- Woodilla J ,
- Çetinkaya M
- Seidel VP ,
- Vaquero Martín M ,
- Reinhardt R ,
- Goldschmidt G ,
- Carlgren L ,
- Liberati A ,
- Tetzlaff J , et al
- Schultz C ,
- Zippel-Schultz B ,
- Labitzke G ,
- Svoboda S ,
- Sammann A ,
- Patel D , et al
- Martin JL ,
- Morgan SP , et al
- Cunningham PM ,
- Cunningham M ,
- Van Greunen D
- LeRouge C ,
- Sneha S , et al
- Irmansyah I ,
- Lovell K , et al
- Mulvale G ,
- Miatello A , et al
- van der Weegen S ,
- Spreeuwenberg M , et al
- de Ana FJ ,
- Umstead KA ,
- Phillips GJ , et al
- Carroll N ,
- Richardson I
- Brennan PF ,
- Downs S , et al
- Vilardaga R ,
- Zeng E , et al
- Ramadas A ,
- Oldenburg B , et al
- Neinstein A ,
- Look H , et al
- Predmore Z , et al
- Coley KC , et al
- Johnson KB ,
- Siek KA , et al
- Stephens K , et al
- Lalloo C , et al
- Schlosser D ,
- Campellone T ,
- Kim D , et al
- Catalani C ,
- Owiti P , et al
- Källander K ,
- Strachan D ,
- Soremekun S , et al
- Rossos PG ,
- Purdy B , et al
- Vechakul J ,
- Shrimali BP ,
- Langell A ,
- Pickett T ,
- Mangum C , et al
- Greenhalgh T ,
- Stramer K , et al
- Maniatopoulos G ,
- Martin M , et al
- Hendricks S ,
- Douglas TS , et al
- Barnett J ,
- Kuljis J , et al
- Noktehdan M ,
- Shahbazpour M ,
- Zare MR , et al
- Grocott P ,
- Norman CD ,
- Charnaw-Burger J ,
- Yip AL , et al
- von Hippel E
- Martin J , et al
Supplementary materials
Supplementary data.
This web only file has been produced by the BMJ Publishing Group from an electronic file supplied by the author(s) and has not been edited for content.
- Data supplement 1
Contributors ALF and MO planned the study. MO conducted the data gathering and literature review analysis. ALF and EZ guided the research method and revised the manuscript.
Funding This study was financed by the Coordenação de Aperfeiçoamento de Pessoal de Nível Superior (CAPES) – Finance Code 001 and by the Ocean R&D Programme.
Competing interests None declared.
Provenance and peer review Not commissioned; externally peer reviewed.
Supplemental material This content has been supplied by the author(s). It has not been vetted by BMJ Publishing Group Limited (BMJ) and may not have been peer-reviewed. Any opinions or recommendations discussed are solely those of the author(s) and are not endorsed by BMJ. BMJ disclaims all liability and responsibility arising from any reliance placed on the content. Where the content includes any translated material, BMJ does not warrant the accuracy and reliability of the translations (including but not limited to local regulations, clinical guidelines, terminology, drug names and drug dosages), and is not responsible for any error and/or omissions arising from translation and adaptation or otherwise.
Read the full text or download the PDF:
Supply Chain Management in Healthcare: A Literature Review
- Conference paper
- First Online: 25 October 2019
- Cite this conference paper
- Tuğçe Beldek 3 ,
- Aziz Kemal Konyalıoğlu 3 &
- Hatice Camgöz Akdağ 3
Part of the book series: Lecture Notes in Mechanical Engineering ((LNME))
Included in the following conference series:
- International Conference on Production Research
- Proceedings of the International Symposium for Production Research
1742 Accesses
1 Citations
Supply chain management becomes more important in the developing world in order to provide a material flow with an optimum cost and time generally. Many sectors focus on supply chain management optimization and qualifications to construct a better network of suppliers for their end consumers. Especially in healthcare sector, not only for pharmaceutical products but also for hospital materials, supply chain management gets importance for service quality and patient satisfaction. Thus, there are many studies about supply chain management in healthcare to emphasize its importance. In this study, it is aimed to put forward a literature review of supply chain management in healthcare in order to give a perspective by expressing the important cases and researches which are parallel to the latest studies. Furthermore, this literature review will assess a perspective in order to understand how to manage a complex supply chain in healthcare sector by investigating studies in the literature.
This is a preview of subscription content, log in via an institution to check access.
Access this chapter
- Available as PDF
- Read on any device
- Instant download
- Own it forever
- Available as EPUB and PDF
- Compact, lightweight edition
- Dispatched in 3 to 5 business days
- Free shipping worldwide - see info
- Durable hardcover edition
Tax calculation will be finalised at checkout
Purchases are for personal use only
Institutional subscriptions
Similar content being viewed by others
Electronic Supply Chain: A Bibliographic and Descriptive Literature Review
Hospital Supply Chain Management and Quality of Services Within Hospitals: A Preliminary Review
Big Data Analytics for Supply Chain Transformation: A Systematic Literature Review Using SCOR Framework
Londe, La, Bernard, J., Masters, James M.: Emerging logistics strategies: blueprints for the next century. Int. J. Phys. Distrib. Logistics Manag. 24 (7), 35–47 (1994)
Article Google Scholar
Baltacioglu, T., Ada, E., Kaplan, M.D., Yurt And, O., Cem Kaplan, Y.: A new framework for service supply chains. Serv. Ind. J. 27 (2), 105–124 (2007)
Giunipero, L.C., Brand, R.R.: Purchasing’s role in supply chain management. Int. J. Logistics Manag. 7 (1), 29–38 (1996)
Hervani, A.A., Helms, M.M., Sarkis, J.: Performance measurement for green supply chain management. Benchmarking Int. J. 12 (4), 330–353 (2005)
Lambert, D.M., Cooper, M.C.: Issues in supply chain management. Ind. Mark. Manage. 29 (1), 65–83 (2000)
De Vries, J., Huijsman, R.: Supply chain management in health services: an overview. Supply Chain Manag. Int. J. 16 (3), 159–165 (2011)
Kumar, A., Ozdamar, L., Ning Zhang, C.: Supply chain redesign in the healthcare industry of Singapore. Supply Chain Manage. Int. J. 13 (2), 95–103 (2008)
Meijboom, B., Schmidt-Bakx, S., Westert, G.: Supply chain management practices for improving patient-oriented care. Supply Chain Manage. Int. J. 16 (3), 166–175 (2011)
Kumar, S., Swanson, E., Tran, T.: RFID in the healthcare supply chain: usage and application. Int. J. Health Care Qual. Assur. 22 (1), 67–81 (2009)
Attaran, M.: Critical success factors and challenges of implementing RFID in supply chain management. J. Supply Chain Oper. Manage. 10 (1), 144–167 (2012)
Google Scholar
Uthayakumar, R., Priyan, S.: Pharmaceutical supply chain and inventory management strategies: Optimization for a pharmaceutical company and a hospital. Oper. Res. Health Care 2 (3), 52–64 (2013)
Settanni, E., Harrington, T.S., Srai, J.S.: Pharmaceutical supply chain models: a synthesis from a system view of operations research. Oper. Res. Perspect. 4 , 74–95 (2017)
Aronsson, H., Abrahamsson, M., Spens, K.: Developing lean and agile health care supply chains. Supply Chain Manage. Int. J. 16 (3), 176–183 (2011)
Shah, R., Goldstein, S.M., Unger, B.T., Henry, T.D.: Explaining anomalous high performance in a health care supply chain. Decis. Sci. 39 (4), 759–789 (2008)
Sinha, K.K., Kohnke, E.J.: Health care supply chain design: toward linking the development and delivery of care globally. Decis. Sci. 40 (2), 197–212 (2009)
Cook, J., DeBree, K., Feroleto, A.: From raw materials to customers: supply chain management in the service industry. SAM Adv. Manage. J. (2001)
Bhakoo, V., Chan, C.: Collaborative implementation of e-business processes within the health-care supply chain: the Monash Pharmacy Project. Supply Chain Manage. Int. J. 16 (3), 184–193 (2011)
Chandra, C., Kachhal, S.K.: Managing health care supply chain: trends, issues, and solutions from a logistics perspective. In: Proceedings of the Sixteenth Annual Society of Health Systems Management Engineering Forum, pp. 20–21, February 2004
Kim, S.M.: An empirical investigation of the impact of electronic commerce on supply chain management: a study in the healthcare industry (2004)
McKone-Sweet, K.E., Hamilton, P., Willis, S.B.: The ailing healthcare supply chain: a prescription for change. J. Supply Chain Manage. 41 (1), 4–17 (2005)
Kim, D.: An integrated supply chain management system: a case study in healthcare sector. In: International Conference on Electronic Commerce and Web Technologies, pp. 218–227. Springer, Heidelberg, August 2005
Langabeer, J.: The evolving role of supply chain management technology in healthcare. J. Healthcare Inf. Manage JHIM 19 (2), 27–33 (2005)
Zheng, J., Bakker, E., Knight, L., Gilhespy, H., Harland, C., Walker, H.: A strategic case for e-adoption in healthcare supply chains. Int. J. Inf. Manage. 26 (4), 290–301 (2006)
Kitsiou, S., Matopoulos, A., Manthou, V., Vlachopoulou, M.: Evaluation of integration technology approaches in the healthcare supply chain. Int. J. Value Chain Manage. 1 (4), 325–343 (2007)
Sousa, R.T., Liu, S., Papageorgiou, L.G., Shah, N.: Global supply chain planning for pharmaceuticals. Chem. Eng. Res. Des. 89 (11), 2396–2409 (2011)
Rahimnia, F., Moghadasian, M.: Supply chain leagility in professional services: how to apply decoupling point concept in healthcare delivery system. Supply Chain Manage. Int. J. 15 (1), 80–91 (2010)
Walker, H., Di Sisto, L., McBain, D.: Drivers and barriers to environmental supply chain management practices: Lessons from the public and private sectors. J. Purchasing Supply Manage. 14 (1), 69–85 (2008)
Bhakoo, V., Choi, T.: The iron cage exposed: Institutional pressures and heterogeneity across the healthcare supply chain. J. Oper. Manage. 31 (6), 432–449 (2013)
Kwon, I.W.G., Kim, S.H., Martin, D.G.: Healthcare supply chain management; strategic areas for quality and financial improvement. Technol. Forecast. Soc. Chang. 113 , 422–428 (2016)
Kogan, K., Leu, J., Chernonog, T.: Healthcare supply chain operations: Why are doctors reluctant to consolidate? Oper. Res. Health Care 3 (3), 101–115 (2014)
Ishii, L., Demski, R., Lee, K.K., Mustafa, Z., Frank, S., Wolisnky, J.P., Cohenh, D., Khanna, J., Ammerman, J., Khanuja, H.S., Unger, A.S., Gould, L., Wachter, P.A., Stearns, L., Werthman, R., Pronovost, P.: Improving healthcare value through clinical community and supply chain collaboration. Healthcare 5 (1–2), 1–5 (2017)
Imran, M., Kang, C., Ramzan, M.B.: Medicine supply chain model for an integrated healthcare system with uncertain product complaints. J. Manuf. Syst. 46 , 13–28 (2018)
Syahrir, I., Vanany, I.: Healthcare and disaster supply chain: literature review and future research. Procedia Manuf. 4 , 2–9 (2015)
Zepeda, E.D., Nyaga, G.N., Young, G.J.: Supply chain risk management and hospital inventory: effects of system affiliation. J. Oper. Manage. 44 , 30–47 (2016)
Rossetti, C.L., Handfield, R., Dooley, K.J.: Forces, trends, and decisions in pharmaceutical supply chain management. Int. J. Phys. Distrib. Logistics Manage. 41 (6), 601–622 (2011)
Moons, K., Waeyenbergh, G., Pintelon, L.: Measuring the logistics performance of internal hospital supply chains–a literature study. Omega 82 , 205–217 (2019)
Dobrzykowski, D., Deilami, V.S., Hong, P., Kim, S.C.: A structured analysis of operations and supply chain management research in healthcare (1982–2011). Int. J. Prod. Econ. 147 , 514–530 (2014)
Narayana, S.A., Pati, R.K., Vrat, P.: Managerial research on the pharmaceutical supply chain–a critical review and some insights for future directions. J. Purchasing Supply Manage. 20 (1), 18–40 (2014)
Chen, D.Q., Preston, D.S., Xia, W.: Enhancing hospital supply chain performance: a relational view and empirical test. J. Oper. Manage. 31 (6), 391–408 (2013)
Bishara, R.H.: Cold chain management–an essential component of the global pharmaceutical supply chain. Am. Pharm. Rev. 9 (1), 105–109 (2006)
Bagchi, P., Lejeune, M.A., Alam, A.: How supply competency affects FDI decisions: some insights. Int. J. Prod. Econ. 147 , 239–251 (2014)
Kelle, P., Woosley, J., Schneider, H.: Pharmaceutical supply chain specifics and inventory solutions for a hospital case. Oper. Res. Health Care 1 (2–3), 54–63 (2012)
Stecca, G., Baffo, I., Kaihara, T.: Design and operation of strategic inventory control system for drug delivery in healthcare industry. IFAC-PapersOnLine 49 (12), 904–909 (2016)
Download references
Author information
Authors and affiliations.
Management Engineering Department, Istanbul Technical University, Istanbul, Turkey
Tuğçe Beldek, Aziz Kemal Konyalıoğlu & Hatice Camgöz Akdağ
You can also search for this author in PubMed Google Scholar
Corresponding author
Correspondence to Hatice Camgöz Akdağ .
Editor information
Editors and affiliations.
IFT, Technische Universität Wien, Vienna, Wien, Austria
Numan M. Durakbasa
Faculty of Engineering, Istanbul Aydın University, Istanbul, Turkey
M. Güneş Gençyılmaz
Rights and permissions
Reprints and permissions
Copyright information
© 2020 Springer Nature Switzerland AG
About this paper
Cite this paper.
Beldek, T., Konyalıoğlu, A.K., Akdağ, H.C. (2020). Supply Chain Management in Healthcare: A Literature Review. In: Durakbasa, N., Gençyılmaz, M. (eds) Proceedings of the International Symposium for Production Research 2019. ISPR ISPR 2019 2019. Lecture Notes in Mechanical Engineering. Springer, Cham. https://doi.org/10.1007/978-3-030-31343-2_50
Download citation
DOI : https://doi.org/10.1007/978-3-030-31343-2_50
Published : 25 October 2019
Publisher Name : Springer, Cham
Print ISBN : 978-3-030-31342-5
Online ISBN : 978-3-030-31343-2
eBook Packages : Engineering Engineering (R0)
Share this paper
Anyone you share the following link with will be able to read this content:
Sorry, a shareable link is not currently available for this article.
Provided by the Springer Nature SharedIt content-sharing initiative
- Publish with us
Policies and ethics
- Find a journal
- Track your research
- Survey paper
- Open access
- Published: 19 June 2019
Big data in healthcare: management, analysis and future prospects
- Sabyasachi Dash 1 na1 ,
- Sushil Kumar Shakyawar 2 , 3 na1 ,
- Mohit Sharma 4 , 5 &
- Sandeep Kaushik 6
Journal of Big Data volume 6 , Article number: 54 ( 2019 ) Cite this article
454k Accesses
713 Citations
103 Altmetric
Metrics details
‘Big data’ is massive amounts of information that can work wonders. It has become a topic of special interest for the past two decades because of a great potential that is hidden in it. Various public and private sector industries generate, store, and analyze big data with an aim to improve the services they provide. In the healthcare industry, various sources for big data include hospital records, medical records of patients, results of medical examinations, and devices that are a part of internet of things. Biomedical research also generates a significant portion of big data relevant to public healthcare. This data requires proper management and analysis in order to derive meaningful information. Otherwise, seeking solution by analyzing big data quickly becomes comparable to finding a needle in the haystack. There are various challenges associated with each step of handling big data which can only be surpassed by using high-end computing solutions for big data analysis. That is why, to provide relevant solutions for improving public health, healthcare providers are required to be fully equipped with appropriate infrastructure to systematically generate and analyze big data. An efficient management, analysis, and interpretation of big data can change the game by opening new avenues for modern healthcare. That is exactly why various industries, including the healthcare industry, are taking vigorous steps to convert this potential into better services and financial advantages. With a strong integration of biomedical and healthcare data, modern healthcare organizations can possibly revolutionize the medical therapies and personalized medicine.
Introduction
Information has been the key to a better organization and new developments. The more information we have, the more optimally we can organize ourselves to deliver the best outcomes. That is why data collection is an important part for every organization. We can also use this data for the prediction of current trends of certain parameters and future events. As we are becoming more and more aware of this, we have started producing and collecting more data about almost everything by introducing technological developments in this direction. Today, we are facing a situation wherein we are flooded with tons of data from every aspect of our life such as social activities, science, work, health, etc. In a way, we can compare the present situation to a data deluge. The technological advances have helped us in generating more and more data, even to a level where it has become unmanageable with currently available technologies. This has led to the creation of the term ‘big data’ to describe data that is large and unmanageable. In order to meet our present and future social needs, we need to develop new strategies to organize this data and derive meaningful information. One such special social need is healthcare. Like every other industry, healthcare organizations are producing data at a tremendous rate that presents many advantages and challenges at the same time. In this review, we discuss about the basics of big data including its management, analysis and future prospects especially in healthcare sector.
The data overload
Every day, people working with various organizations around the world are generating a massive amount of data. The term “digital universe” quantitatively defines such massive amounts of data created, replicated, and consumed in a single year. International Data Corporation (IDC) estimated the approximate size of the digital universe in 2005 to be 130 exabytes (EB). The digital universe in 2017 expanded to about 16,000 EB or 16 zettabytes (ZB). IDC predicted that the digital universe would expand to 40,000 EB by the year 2020. To imagine this size, we would have to assign about 5200 gigabytes (GB) of data to all individuals. This exemplifies the phenomenal speed at which the digital universe is expanding. The internet giants, like Google and Facebook, have been collecting and storing massive amounts of data. For instance, depending on our preferences, Google may store a variety of information including user location, advertisement preferences, list of applications used, internet browsing history, contacts, bookmarks, emails, and other necessary information associated with the user. Similarly, Facebook stores and analyzes more than about 30 petabytes (PB) of user-generated data. Such large amounts of data constitute ‘ big data ’. Over the past decade, big data has been successfully used by the IT industry to generate critical information that can generate significant revenue.
These observations have become so conspicuous that has eventually led to the birth of a new field of science termed ‘ Data Science ’. Data science deals with various aspects including data management and analysis, to extract deeper insights for improving the functionality or services of a system (for example, healthcare and transport system). Additionally, with the availability of some of the most creative and meaningful ways to visualize big data post-analysis, it has become easier to understand the functioning of any complex system. As a large section of society is becoming aware of, and involved in generating big data, it has become necessary to define what big data is. Therefore, in this review, we attempt to provide details on the impact of big data in the transformation of global healthcare sector and its impact on our daily lives.
Defining big data
As the name suggests, ‘big data’ represents large amounts of data that is unmanageable using traditional software or internet-based platforms. It surpasses the traditionally used amount of storage, processing and analytical power. Even though a number of definitions for big data exist, the most popular and well-accepted definition was given by Douglas Laney. Laney observed that (big) data was growing in three different dimensions namely, volume, velocity and variety (known as the 3 Vs) [ 1 ]. The ‘big’ part of big data is indicative of its large volume. In addition to volume, the big data description also includes velocity and variety. Velocity indicates the speed or rate of data collection and making it accessible for further analysis; while, variety remarks on the different types of organized and unorganized data that any firm or system can collect, such as transaction-level data, video, audio, text or log files. These three Vs have become the standard definition of big data. Although, other people have added several other Vs to this definition [ 2 ], the most accepted 4th V remains ‘veracity’.
The term “ big data ” has become extremely popular across the globe in recent years. Almost every sector of research, whether it relates to industry or academics, is generating and analyzing big data for various purposes. The most challenging task regarding this huge heap of data that can be organized and unorganized, is its management. Given the fact that big data is unmanageable using the traditional software, we need technically advanced applications and software that can utilize fast and cost-efficient high-end computational power for such tasks. Implementation of artificial intelligence (AI) algorithms and novel fusion algorithms would be necessary to make sense from this large amount of data. Indeed, it would be a great feat to achieve automated decision-making by the implementation of machine learning (ML) methods like neural networks and other AI techniques. However, in absence of appropriate software and hardware support, big data can be quite hazy. We need to develop better techniques to handle this ‘endless sea’ of data and smart web applications for efficient analysis to gain workable insights. With proper storage and analytical tools in hand, the information and insights derived from big data can make the critical social infrastructure components and services (like healthcare, safety or transportation) more aware, interactive and efficient [ 3 ]. In addition, visualization of big data in a user-friendly manner will be a critical factor for societal development.
Healthcare as a big-data repository
Healthcare is a multi-dimensional system established with the sole aim for the prevention, diagnosis, and treatment of health-related issues or impairments in human beings. The major components of a healthcare system are the health professionals (physicians or nurses), health facilities (clinics, hospitals for delivering medicines and other diagnosis or treatment technologies), and a financing institution supporting the former two. The health professionals belong to various health sectors like dentistry, medicine, midwifery, nursing, psychology, physiotherapy, and many others. Healthcare is required at several levels depending on the urgency of situation. Professionals serve it as the first point of consultation (for primary care), acute care requiring skilled professionals (secondary care), advanced medical investigation and treatment (tertiary care) and highly uncommon diagnostic or surgical procedures (quaternary care). At all these levels, the health professionals are responsible for different kinds of information such as patient’s medical history (diagnosis and prescriptions related data), medical and clinical data (like data from imaging and laboratory examinations), and other private or personal medical data. Previously, the common practice to store such medical records for a patient was in the form of either handwritten notes or typed reports [ 4 ]. Even the results from a medical examination were stored in a paper file system. In fact, this practice is really old, with the oldest case reports existing on a papyrus text from Egypt that dates back to 1600 BC [ 5 ]. In Stanley Reiser’s words, the clinical case records freeze the episode of illness as a story in which patient, family and the doctor are a part of the plot” [ 6 ].
With the advent of computer systems and its potential, the digitization of all clinical exams and medical records in the healthcare systems has become a standard and widely adopted practice nowadays. In 2003, a division of the National Academies of Sciences, Engineering, and Medicine known as Institute of Medicine chose the term “ electronic health records ” to represent records maintained for improving the health care sector towards the benefit of patients and clinicians. Electronic health records (EHR) as defined by Murphy, Hanken and Waters are computerized medical records for patients any information relating to the past, present or future physical/mental health or condition of an individual which resides in electronic system(s) used to capture, transmit, receive, store, retrieve, link and manipulate multimedia data for the primary purpose of providing healthcare and health-related services” [ 7 ].
Electronic health records
It is important to note that the National Institutes of Health (NIH) recently announced the “All of Us” initiative ( https://allofus.nih.gov/ ) that aims to collect one million or more patients’ data such as EHR, including medical imaging, socio-behavioral, and environmental data over the next few years. EHRs have introduced many advantages for handling modern healthcare related data. Below, we describe some of the characteristic advantages of using EHRs. The first advantage of EHRs is that healthcare professionals have an improved access to the entire medical history of a patient. The information includes medical diagnoses, prescriptions, data related to known allergies, demographics, clinical narratives, and the results obtained from various laboratory tests. The recognition and treatment of medical conditions thus is time efficient due to a reduction in the lag time of previous test results. With time we have observed a significant decrease in the redundant and additional examinations, lost orders and ambiguities caused by illegible handwriting, and an improved care coordination between multiple healthcare providers. Overcoming such logistical errors has led to reduction in the number of drug allergies by reducing errors in medication dose and frequency. Healthcare professionals have also found access over web based and electronic platforms to improve their medical practices significantly using automatic reminders and prompts regarding vaccinations, abnormal laboratory results, cancer screening, and other periodic checkups. There would be a greater continuity of care and timely interventions by facilitating communication among multiple healthcare providers and patients. They can be associated to electronic authorization and immediate insurance approvals due to less paperwork. EHRs enable faster data retrieval and facilitate reporting of key healthcare quality indicators to the organizations, and also improve public health surveillance by immediate reporting of disease outbreaks. EHRs also provide relevant data regarding the quality of care for the beneficiaries of employee health insurance programs and can help control the increasing costs of health insurance benefits. Finally, EHRs can reduce or absolutely eliminate delays and confusion in the billing and claims management area. The EHRs and internet together help provide access to millions of health-related medical information critical for patient life.
Digitization of healthcare and big data
Similar to EHR, an electronic medical record (EMR) stores the standard medical and clinical data gathered from the patients. EHRs, EMRs, personal health record (PHR), medical practice management software (MPM), and many other healthcare data components collectively have the potential to improve the quality, service efficiency, and costs of healthcare along with the reduction of medical errors. The big data in healthcare includes the healthcare payer-provider data (such as EMRs, pharmacy prescription, and insurance records) along with the genomics-driven experiments (such as genotyping, gene expression data) and other data acquired from the smart web of internet of things (IoT) (Fig. 1 ). The adoption of EHRs was slow at the beginning of the 21st century however it has grown substantially after 2009 [ 7 , 8 ]. The management and usage of such healthcare data has been increasingly dependent on information technology. The development and usage of wellness monitoring devices and related software that can generate alerts and share the health related data of a patient with the respective health care providers has gained momentum, especially in establishing a real-time biomedical and health monitoring system. These devices are generating a huge amount of data that can be analyzed to provide real-time clinical or medical care [ 9 ]. The use of big data from healthcare shows promise for improving health outcomes and controlling costs.
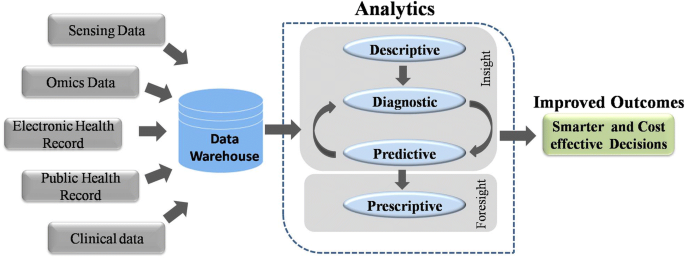
Workflow of Big data Analytics. Data warehouses store massive amounts of data generated from various sources. This data is processed using analytic pipelines to obtain smarter and affordable healthcare options
Big data in biomedical research
A biological system, such as a human cell, exhibits molecular and physical events of complex interplay. In order to understand interdependencies of various components and events of such a complex system, a biomedical or biological experiment usually gathers data on a smaller and/or simpler component. Consequently, it requires multiple simplified experiments to generate a wide map of a given biological phenomenon of interest. This indicates that more the data we have, the better we understand the biological processes. With this idea, modern techniques have evolved at a great pace. For instance, one can imagine the amount of data generated since the integration of efficient technologies like next-generation sequencing (NGS) and Genome wide association studies (GWAS) to decode human genetics. NGS-based data provides information at depths that were previously inaccessible and takes the experimental scenario to a completely new dimension. It has increased the resolution at which we observe or record biological events associated with specific diseases in a real time manner. The idea that large amounts of data can provide us a good amount of information that often remains unidentified or hidden in smaller experimental methods has ushered-in the ‘- omics ’ era. The ‘ omics ’ discipline has witnessed significant progress as instead of studying a single ‘ gene ’ scientists can now study the whole ‘ genome ’ of an organism in ‘ genomics ’ studies within a given amount of time. Similarly, instead of studying the expression or ‘ transcription ’ of single gene, we can now study the expression of all the genes or the entire ‘ transcriptome ’ of an organism under ‘ transcriptomics ’ studies. Each of these individual experiments generate a large amount of data with more depth of information than ever before. Yet, this depth and resolution might be insufficient to provide all the details required to explain a particular mechanism or event. Therefore, one usually finds oneself analyzing a large amount of data obtained from multiple experiments to gain novel insights. This fact is supported by a continuous rise in the number of publications regarding big data in healthcare (Fig. 2 ). Analysis of such big data from medical and healthcare systems can be of immense help in providing novel strategies for healthcare. The latest technological developments in data generation, collection and analysis, have raised expectations towards a revolution in the field of personalized medicine in near future.
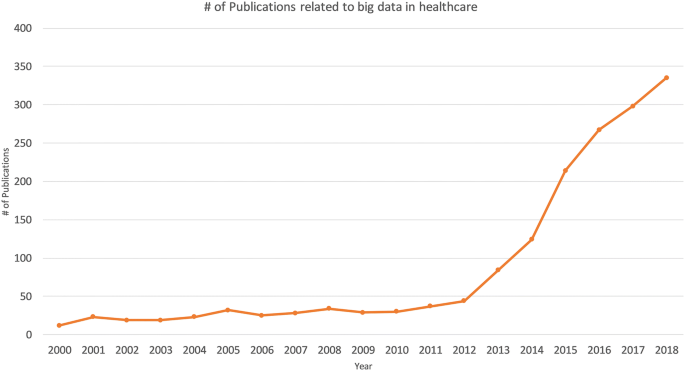
Publications associated with big data in healthcare. The numbers of publications in PubMed are plotted by year
Big data from omics studies
NGS has greatly simplified the sequencing and decreased the costs for generating whole genome sequence data. The cost of complete genome sequencing has fallen from millions to a couple of thousand dollars [ 10 ]. NGS technology has resulted in an increased volume of biomedical data that comes from genomic and transcriptomic studies. According to an estimate, the number of human genomes sequenced by 2025 could be between 100 million to 2 billion [ 11 ]. Combining the genomic and transcriptomic data with proteomic and metabolomic data can greatly enhance our knowledge about the individual profile of a patient—an approach often ascribed as “individual, personalized or precision health care”. Systematic and integrative analysis of omics data in conjugation with healthcare analytics can help design better treatment strategies towards precision and personalized medicine (Fig. 3 ). The genomics-driven experiments e.g., genotyping, gene expression, and NGS-based studies are the major source of big data in biomedical healthcare along with EMRs, pharmacy prescription information, and insurance records. Healthcare requires a strong integration of such biomedical data from various sources to provide better treatments and patient care. These prospects are so exciting that even though genomic data from patients would have many variables to be accounted, yet commercial organizations are already using human genome data to help the providers in making personalized medical decisions. This might turn out to be a game-changer in future medicine and health.
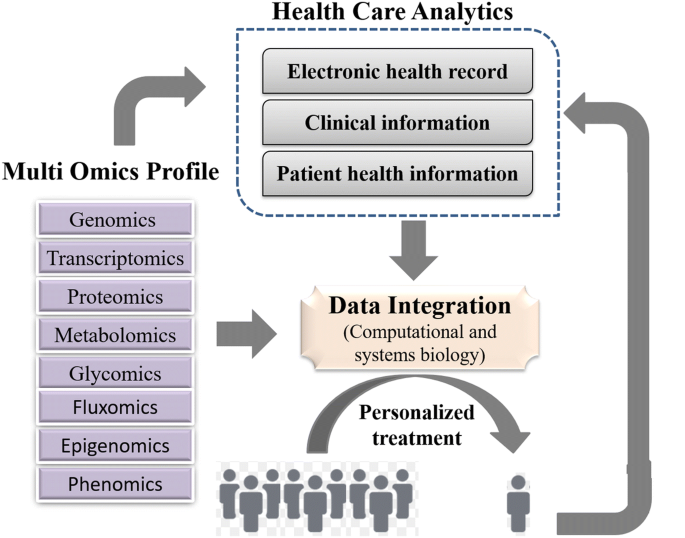
A framework for integrating omics data and health care analytics to promote personalized treatment
Internet of Things (IOT)
Healthcare industry has not been quick enough to adapt to the big data movement compared to other industries. Therefore, big data usage in the healthcare sector is still in its infancy. For example, healthcare and biomedical big data have not yet converged to enhance healthcare data with molecular pathology. Such convergence can help unravel various mechanisms of action or other aspects of predictive biology. Therefore, to assess an individual’s health status, biomolecular and clinical datasets need to be married. One such source of clinical data in healthcare is ‘internet of things’ (IoT).
In fact, IoT is another big player implemented in a number of other industries including healthcare. Until recently, the objects of common use such as cars, watches, refrigerators and health-monitoring devices, did not usually produce or handle data and lacked internet connectivity. However, furnishing such objects with computer chips and sensors that enable data collection and transmission over internet has opened new avenues. The device technologies such as Radio Frequency IDentification (RFID) tags and readers, and Near Field Communication (NFC) devices, that can not only gather information but interact physically, are being increasingly used as the information and communication systems [ 3 ]. This enables objects with RFID or NFC to communicate and function as a web of smart things. The analysis of data collected from these chips or sensors may reveal critical information that might be beneficial in improving lifestyle, establishing measures for energy conservation, improving transportation, and healthcare. In fact, IoT has become a rising movement in the field of healthcare. IoT devices create a continuous stream of data while monitoring the health of people (or patients) which makes these devices a major contributor to big data in healthcare. Such resources can interconnect various devices to provide a reliable, effective and smart healthcare service to the elderly and patients with a chronic illness [ 12 ].
Advantages of IoT in healthcare
Using the web of IoT devices, a doctor can measure and monitor various parameters from his/her clients in their respective locations for example, home or office. Therefore, through early intervention and treatment, a patient might not need hospitalization or even visit the doctor resulting in significant cost reduction in healthcare expenses. Some examples of IoT devices used in healthcare include fitness or health-tracking wearable devices, biosensors, clinical devices for monitoring vital signs, and others types of devices or clinical instruments. Such IoT devices generate a large amount of health related data. If we can integrate this data with other existing healthcare data like EMRs or PHRs, we can predict a patients’ health status and its progression from subclinical to pathological state [ 9 ]. In fact, big data generated from IoT has been quiet advantageous in several areas in offering better investigation and predictions. On a larger scale, the data from such devices can help in personnel health monitoring, modelling the spread of a disease and finding ways to contain a particular disease outbreak.
The analysis of data from IoT would require an updated operating software because of its specific nature along with advanced hardware and software applications. We would need to manage data inflow from IoT instruments in real-time and analyze it by the minute. Associates in the healthcare system are trying to trim down the cost and ameliorate the quality of care by applying advanced analytics to both internally and externally generated data.
Mobile computing and mobile health (mHealth)
In today’s digital world, every individual seems to be obsessed to track their fitness and health statistics using the in-built pedometer of their portable and wearable devices such as, smartphones, smartwatches, fitness dashboards or tablets. With an increasingly mobile society in almost all aspects of life, the healthcare infrastructure needs remodeling to accommodate mobile devices [ 13 ]. The practice of medicine and public health using mobile devices, known as mHealth or mobile health, pervades different degrees of health care especially for chronic diseases, such as diabetes and cancer [ 14 ]. Healthcare organizations are increasingly using mobile health and wellness services for implementing novel and innovative ways to provide care and coordinate health as well as wellness. Mobile platforms can improve healthcare by accelerating interactive communication between patients and healthcare providers. In fact, Apple and Google have developed devoted platforms like Apple’s ResearchKit and Google Fit for developing research applications for fitness and health statistics [ 15 ]. These applications support seamless interaction with various consumer devices and embedded sensors for data integration. These apps help the doctors to have direct access to your overall health data. Both the user and their doctors get to know the real-time status of your body. These apps and smart devices also help by improving our wellness planning and encouraging healthy lifestyles. The users or patients can become advocates for their own health.
Nature of the big data in healthcare
EHRs can enable advanced analytics and help clinical decision-making by providing enormous data. However, a large proportion of this data is currently unstructured in nature. An unstructured data is the information that does not adhere to a pre-defined model or organizational framework. The reason for this choice may simply be that we can record it in a myriad of formats. Another reason for opting unstructured format is that often the structured input options (drop-down menus, radio buttons, and check boxes) can fall short for capturing data of complex nature. For example, we cannot record the non-standard data regarding a patient’s clinical suspicions, socioeconomic data, patient preferences, key lifestyle factors, and other related information in any other way but an unstructured format. It is difficult to group such varied, yet critical, sources of information into an intuitive or unified data format for further analysis using algorithms to understand and leverage the patients care. Nonetheless, the healthcare industry is required to utilize the full potential of these rich streams of information to enhance the patient experience. In the healthcare sector, it could materialize in terms of better management, care and low-cost treatments. We are miles away from realizing the benefits of big data in a meaningful way and harnessing the insights that come from it. In order to achieve these goals, we need to manage and analyze the big data in a systematic manner.
Management and analysis of big data
Big data is the huge amounts of a variety of data generated at a rapid rate. The data gathered from various sources is mostly required for optimizing consumer services rather than consumer consumption. This is also true for big data from the biomedical research and healthcare. The major challenge with big data is how to handle this large volume of information. To make it available for scientific community, the data is required to be stored in a file format that is easily accessible and readable for an efficient analysis. In the context of healthcare data, another major challenge is the implementation of high-end computing tools, protocols and high-end hardware in the clinical setting. Experts from diverse backgrounds including biology, information technology, statistics, and mathematics are required to work together to achieve this goal. The data collected using the sensors can be made available on a storage cloud with pre-installed software tools developed by analytic tool developers. These tools would have data mining and ML functions developed by AI experts to convert the information stored as data into knowledge. Upon implementation, it would enhance the efficiency of acquiring, storing, analyzing, and visualization of big data from healthcare. The main task is to annotate, integrate, and present this complex data in an appropriate manner for a better understanding. In absence of such relevant information, the (healthcare) data remains quite cloudy and may not lead the biomedical researchers any further. Finally, visualization tools developed by computer graphics designers can efficiently display this newly gained knowledge.
Heterogeneity of data is another challenge in big data analysis. The huge size and highly heterogeneous nature of big data in healthcare renders it relatively less informative using the conventional technologies. The most common platforms for operating the software framework that assists big data analysis are high power computing clusters accessed via grid computing infrastructures. Cloud computing is such a system that has virtualized storage technologies and provides reliable services. It offers high reliability, scalability and autonomy along with ubiquitous access, dynamic resource discovery and composability. Such platforms can act as a receiver of data from the ubiquitous sensors, as a computer to analyze and interpret the data, as well as providing the user with easy to understand web-based visualization. In IoT, the big data processing and analytics can be performed closer to data source using the services of mobile edge computing cloudlets and fog computing. Advanced algorithms are required to implement ML and AI approaches for big data analysis on computing clusters. A programming language suitable for working on big data (e.g. Python, R or other languages) could be used to write such algorithms or software. Therefore, a good knowledge of biology and IT is required to handle the big data from biomedical research. Such a combination of both the trades usually fits for bioinformaticians. The most common among various platforms used for working with big data include Hadoop and Apache Spark. We briefly introduce these platforms below.
Loading large amounts of (big) data into the memory of even the most powerful of computing clusters is not an efficient way to work with big data. Therefore, the best logical approach for analyzing huge volumes of complex big data is to distribute and process it in parallel on multiple nodes. However, the size of data is usually so large that thousands of computing machines are required to distribute and finish processing in a reasonable amount of time. When working with hundreds or thousands of nodes, one has to handle issues like how to parallelize the computation, distribute the data, and handle failures. One of most popular open-source distributed application for this purpose is Hadoop [ 16 ]. Hadoop implements MapReduce algorithm for processing and generating large datasets. MapReduce uses map and reduce primitives to map each logical record’ in the input into a set of intermediate key/value pairs, and reduce operation combines all the values that shared the same key [ 17 ]. It efficiently parallelizes the computation, handles failures, and schedules inter-machine communication across large-scale clusters of machines. Hadoop Distributed File System (HDFS) is the file system component that provides a scalable, efficient, and replica based storage of data at various nodes that form a part of a cluster [ 16 ]. Hadoop has other tools that enhance the storage and processing components therefore many large companies like Yahoo, Facebook, and others have rapidly adopted it. Hadoop has enabled researchers to use data sets otherwise impossible to handle. Many large projects, like the determination of a correlation between the air quality data and asthma admissions, drug development using genomic and proteomic data, and other such aspects of healthcare are implementing Hadoop. Therefore, with the implementation of Hadoop system, the healthcare analytics will not be held back.
Apache Spark
Apache Spark is another open source alternative to Hadoop. It is a unified engine for distributed data processing that includes higher-level libraries for supporting SQL queries ( Spark SQL ), streaming data ( Spark Streaming ), machine learning ( MLlib ) and graph processing ( GraphX ) [ 18 ]. These libraries help in increasing developer productivity because the programming interface requires lesser coding efforts and can be seamlessly combined to create more types of complex computations. By implementing Resilient distributed Datasets (RDDs), in-memory processing of data is supported that can make Spark about 100× faster than Hadoop in multi-pass analytics (on smaller datasets) [ 19 , 20 ]. This is more true when the data size is smaller than the available memory [ 21 ]. This indicates that processing of really big data with Apache Spark would require a large amount of memory. Since, the cost of memory is higher than the hard drive, MapReduce is expected to be more cost effective for large datasets compared to Apache Spark. Similarly, Apache Storm was developed to provide a real-time framework for data stream processing. This platform supports most of the programming languages. Additionally, it offers good horizontal scalability and built-in-fault-tolerance capability for big data analysis.
Machine learning for information extraction, data analysis and predictions
In healthcare, patient data contains recorded signals for instance, electrocardiogram (ECG), images, and videos. Healthcare providers have barely managed to convert such healthcare data into EHRs. Efforts are underway to digitize patient-histories from pre-EHR era notes and supplement the standardization process by turning static images into machine-readable text. For example, optical character recognition (OCR) software is one such approach that can recognize handwriting as well as computer fonts and push digitization. Such unstructured and structured healthcare datasets have untapped wealth of information that can be harnessed using advanced AI programs to draw critical actionable insights in the context of patient care. In fact, AI has emerged as the method of choice for big data applications in medicine. This smart system has quickly found its niche in decision making process for the diagnosis of diseases. Healthcare professionals analyze such data for targeted abnormalities using appropriate ML approaches. ML can filter out structured information from such raw data.
Extracting information from EHR datasets
Emerging ML or AI based strategies are helping to refine healthcare industry’s information processing capabilities. For example, natural language processing (NLP) is a rapidly developing area of machine learning that can identify key syntactic structures in free text, help in speech recognition and extract the meaning behind a narrative. NLP tools can help generate new documents, like a clinical visit summary, or to dictate clinical notes. The unique content and complexity of clinical documentation can be challenging for many NLP developers. Nonetheless, we should be able to extract relevant information from healthcare data using such approaches as NLP.
AI has also been used to provide predictive capabilities to healthcare big data. For example, ML algorithms can convert the diagnostic system of medical images into automated decision-making. Though it is apparent that healthcare professionals may not be replaced by machines in the near future, yet AI can definitely assist physicians to make better clinical decisions or even replace human judgment in certain functional areas of healthcare.
Image analytics
Some of the most widely used imaging techniques in healthcare include computed tomography (CT), magnetic resonance imaging (MRI), X-ray, molecular imaging, ultrasound, photo-acoustic imaging, functional MRI (fMRI), positron emission tomography (PET), electroencephalography (EEG), and mammograms. These techniques capture high definition medical images (patient data) of large sizes. Healthcare professionals like radiologists, doctors and others do an excellent job in analyzing medical data in the form of these files for targeted abnormalities. However, it is also important to acknowledge the lack of specialized professionals for many diseases. In order to compensate for this dearth of professionals, efficient systems like Picture Archiving and Communication System (PACS) have been developed for storing and convenient access to medical image and reports data [ 22 ]. PACSs are popular for delivering images to local workstations, accomplished by protocols such as digital image communication in medicine (DICOM). However, data exchange with a PACS relies on using structured data to retrieve medical images. This by nature misses out on the unstructured information contained in some of the biomedical images. Moreover, it is possible to miss an additional information about a patient’s health status that is present in these images or similar data. A professional focused on diagnosing an unrelated condition might not observe it, especially when the condition is still emerging. To help in such situations, image analytics is making an impact on healthcare by actively extracting disease biomarkers from biomedical images. This approach uses ML and pattern recognition techniques to draw insights from massive volumes of clinical image data to transform the diagnosis, treatment and monitoring of patients. It focuses on enhancing the diagnostic capability of medical imaging for clinical decision-making.
A number of software tools have been developed based on functionalities such as generic, registration, segmentation, visualization, reconstruction, simulation and diffusion to perform medical image analysis in order to dig out the hidden information. For example, Visualization Toolkit is a freely available software which allows powerful processing and analysis of 3D images from medical tests [ 23 ], while SPM can process and analyze 5 different types of brain images (e.g. MRI, fMRI, PET, CT-Scan and EEG) [ 24 ]. Other software like GIMIAS, Elastix, and MITK support all types of images. Various other widely used tools and their features in this domain are listed in Table 1 . Such bioinformatics-based big data analysis may extract greater insights and value from imaging data to boost and support precision medicine projects, clinical decision support tools, and other modes of healthcare. For example, we can also use it to monitor new targeted-treatments for cancer.
Big data from omics
The big data from “omics” studies is a new kind of challenge for the bioinformaticians. Robust algorithms are required to analyze such complex data from biological systems. The ultimate goal is to convert this huge data into an informative knowledge base. The application of bioinformatics approaches to transform the biomedical and genomics data into predictive and preventive health is known as translational bioinformatics. It is at the forefront of data-driven healthcare. Various kinds of quantitative data in healthcare, for example from laboratory measurements, medication data and genomic profiles, can be combined and used to identify new meta-data that can help precision therapies [ 25 ]. This is why emerging new technologies are required to help in analyzing this digital wealth. In fact, highly ambitious multimillion-dollar projects like “ Big Data Research and Development Initiative ” have been launched that aim to enhance the quality of big data tools and techniques for a better organization, efficient access and smart analysis of big data. There are many advantages anticipated from the processing of ‘ omics’ data from large-scale Human Genome Project and other population sequencing projects. In the population sequencing projects like 1000 genomes, the researchers will have access to a marvelous amount of raw data. Similarly, Human Genome Project based Encyclopedia of DNA Elements (ENCODE) project aimed to determine all functional elements in the human genome using bioinformatics approaches. Here, we list some of the widely used bioinformatics-based tools for big data analytics on omics data.
SparkSeq is an efficient and cloud-ready platform based on Apache Spark framework and Hadoop library that is used for analyses of genomic data for interactive genomic data analysis with nucleotide precision
SAMQA identifies errors and ensures the quality of large-scale genomic data. This tool was originally built for the National Institutes of Health Cancer Genome Atlas project to identify and report errors including sequence alignment/map [SAM] format error and empty reads.
ART can simulate profiles of read errors and read lengths for data obtained using high throughput sequencing platforms including SOLiD and Illumina platforms.
DistMap is another toolkit used for distributed short-read mapping based on Hadoop cluster that aims to cover a wider range of sequencing applications. For instance, one of its applications namely the BWA mapper can perform 500 million read pairs in about 6 h, approximately 13 times faster than a conventional single-node mapper.
SeqWare is a query engine based on Apache HBase database system that enables access for large-scale whole-genome datasets by integrating genome browsers and tools.
CloudBurst is a parallel computing model utilized in genome mapping experiments to improve the scalability of reading large sequencing data.
Hydra uses the Hadoop-distributed computing framework for processing large peptide and spectra databases for proteomics datasets. This specific tool is capable of performing 27 billion peptide scorings in less than 60 min on a Hadoop cluster.
BlueSNP is an R package based on Hadoop platform used for genome-wide association studies (GWAS) analysis, primarily aiming on the statistical readouts to obtain significant associations between genotype–phenotype datasets. The efficiency of this tool is estimated to analyze 1000 phenotypes on 10 6 SNPs in 10 4 individuals in a duration of half-an-hour.
Myrna the cloud-based pipeline, provides information on the expression level differences of genes, including read alignments, data normalization, and statistical modeling.
The past few years have witnessed a tremendous increase in disease specific datasets from omics platforms. For example, the ArrayExpress Archive of Functional Genomics data repository contains information from approximately 30,000 experiments and more than one million functional assays. The growing amount of data demands for better and efficient bioinformatics driven packages to analyze and interpret the information obtained. This has also led to the birth of specific tools to analyze such massive amounts of data. Below, we mention some of the most popular commercial platforms for big data analytics.
Commercial platforms for healthcare data analytics
In order to tackle big data challenges and perform smoother analytics, various companies have implemented AI to analyze published results, textual data, and image data to obtain meaningful outcomes. IBM Corporation is one of the biggest and experienced players in this sector to provide healthcare analytics services commercially. IBM’s Watson Health is an AI platform to share and analyze health data among hospitals, providers and researchers. Similarly, Flatiron Health provides technology-oriented services in healthcare analytics specially focused in cancer research. Other big companies such as Oracle Corporation and Google Inc. are also focusing to develop cloud-based storage and distributed computing power platforms. Interestingly, in the recent few years, several companies and start-ups have also emerged to provide health care-based analytics and solutions. Some of the vendors in healthcare sector are provided in Table 2 . Below we discuss a few of these commercial solutions.
Ayasdi is one such big vendor which focuses on ML based methodologies to primarily provide machine intelligence platform along with an application framework with tried & tested enterprise scalability. It provides various applications for healthcare analytics, for example, to understand and manage clinical variation, and to transform clinical care costs. It is also capable of analyzing and managing how hospitals are organized, conversation between doctors, risk-oriented decisions by doctors for treatment, and the care they deliver to patients. It also provides an application for the assessment and management of population health, a proactive strategy that goes beyond traditional risk analysis methodologies. It uses ML intelligence for predicting future risk trajectories, identifying risk drivers, and providing solutions for best outcomes. A strategic illustration of the company’s methodology for analytics is provided in Fig. 4 .
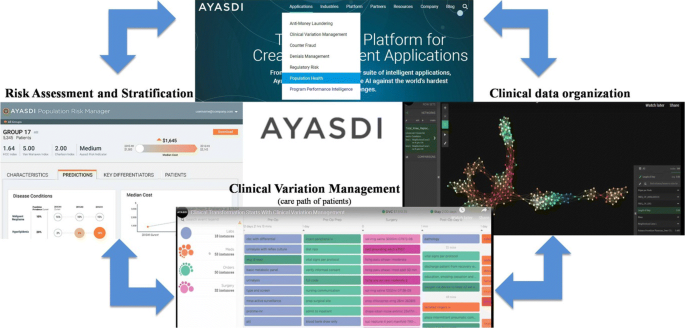
Illustration of application of “Intelligent Application Suite” provided by AYASDI for various analyses such as clinical variation, population health, and risk management in healthcare sector
Linguamatics
It is an NLP based algorithm that relies on an interactive text mining algorithm (I2E). I2E can extract and analyze a wide array of information. Results obtained using this technique are tenfold faster than other tools and does not require expert knowledge for data interpretation. This approach can provide information on genetic relationships and facts from unstructured data. Classical, ML requires well-curated data as input to generate clean and filtered results. However, NLP when integrated in EHR or clinical records per se facilitates the extraction of clean and structured information that often remains hidden in unstructured input data (Fig. 5 ).
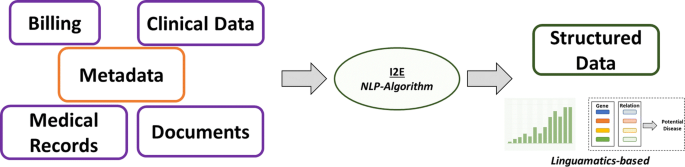
Schematic representation for the working principle of NLP-based AI system used in massive data retention and analysis in Linguamatics
This is one of the unique ideas of the tech-giant IBM that targets big data analytics in almost every professional sector. This platform utilizes ML and AI based algorithms extensively to extract the maximum information from minimal input. IBM Watson enforces the regimen of integrating a wide array of healthcare domains to provide meaningful and structured data (Fig. 6 ). In an attempt to uncover novel drug targets specifically in cancer disease model, IBM Watson and Pfizer have formed a productive collaboration to accelerate the discovery of novel immune-oncology combinations. Combining Watson’s deep learning modules integrated with AI technologies allows the researchers to interpret complex genomic data sets. IBM Watson has been used to predict specific types of cancer based on the gene expression profiles obtained from various large data sets providing signs of multiple druggable targets. IBM Watson is also used in drug discovery programs by integrating curated literature and forming network maps to provide a detailed overview of the molecular landscape in a specific disease model.
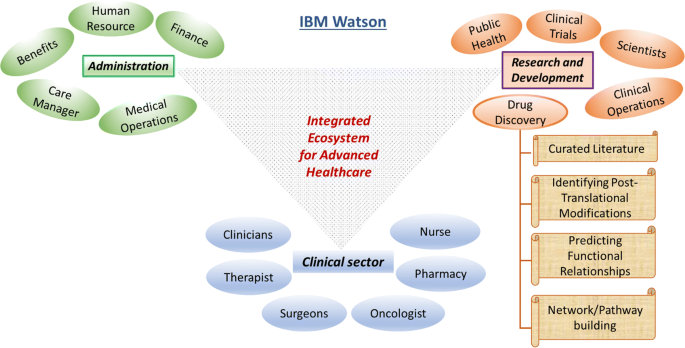
IBM Watson in healthcare data analytics. Schematic representation of the various functional modules in IBM Watson’s big-data healthcare package. For instance, the drug discovery domain involves network of highly coordinated data acquisition and analysis within the spectrum of curating database to building meaningful pathways towards elucidating novel druggable targets
In order to analyze the diversified medical data, healthcare domain, describes analytics in four categories: descriptive, diagnostic, predictive, and prescriptive analytics. Descriptive analytics refers for describing the current medical situations and commenting on that whereas diagnostic analysis explains reasons and factors behind occurrence of certain events, for example, choosing treatment option for a patient based on clustering and decision trees. Predictive analytics focuses on predictive ability of the future outcomes by determining trends and probabilities. These methods are mainly built up of machine leaning techniques and are helpful in the context of understanding complications that a patient can develop. Prescriptive analytics is to perform analysis to propose an action towards optimal decision making. For example, decision of avoiding a given treatment to the patient based on observed side effects and predicted complications. In order to improve performance of the current medical systems integration of big data into healthcare analytics can be a major factor; however, sophisticated strategies need to be developed. An architecture of best practices of different analytics in healthcare domain is required for integrating big data technologies to improve the outcomes. However, there are many challenges associated with the implementation of such strategies.
Challenges associated with healthcare big data
Methods for big data management and analysis are being continuously developed especially for real-time data streaming, capture, aggregation, analytics (using ML and predictive), and visualization solutions that can help integrate a better utilization of EMRs with the healthcare. For example, the EHR adoption rate of federally tested and certified EHR programs in the healthcare sector in the U.S.A. is nearly complete [ 7 ]. However, the availability of hundreds of EHR products certified by the government, each with different clinical terminologies, technical specifications, and functional capabilities has led to difficulties in the interoperability and sharing of data. Nonetheless, we can safely say that the healthcare industry has entered into a ‘post-EMR’ deployment phase. Now, the main objective is to gain actionable insights from these vast amounts of data collected as EMRs. Here, we discuss some of these challenges in brief.
Storing large volume of data is one of the primary challenges, but many organizations are comfortable with data storage on their own premises. It has several advantages like control over security, access, and up-time. However, an on-site server network can be expensive to scale and difficult to maintain. It appears that with decreasing costs and increasing reliability, the cloud-based storage using IT infrastructure is a better option which most of the healthcare organizations have opted for. Organizations must choose cloud-partners that understand the importance of healthcare-specific compliance and security issues. Additionally, cloud storage offers lower up-front costs, nimble disaster recovery, and easier expansion. Organizations can also have a hybrid approach to their data storage programs, which may be the most flexible and workable approach for providers with varying data access and storage needs.
The data needs to cleansed or scrubbed to ensure the accuracy, correctness, consistency, relevancy, and purity after acquisition. This cleaning process can be manual or automatized using logic rules to ensure high levels of accuracy and integrity. More sophisticated and precise tools use machine-learning techniques to reduce time and expenses and to stop foul data from derailing big data projects.
Unified format
Patients produce a huge volume of data that is not easy to capture with traditional EHR format, as it is knotty and not easily manageable. It is too difficult to handle big data especially when it comes without a perfect data organization to the healthcare providers. A need to codify all the clinically relevant information surfaced for the purpose of claims, billing purposes, and clinical analytics. Therefore, medical coding systems like Current Procedural Terminology (CPT) and International Classification of Diseases (ICD) code sets were developed to represent the core clinical concepts. However, these code sets have their own limitations.
Some studies have observed that the reporting of patient data into EMRs or EHRs is not entirely accurate yet [ 26 , 27 , 28 , 29 ], probably because of poor EHR utility, complex workflows, and a broken understanding of why big data is all-important to capture well. All these factors can contribute to the quality issues for big data all along its lifecycle. The EHRs intend to improve the quality and communication of data in clinical workflows though reports indicate discrepancies in these contexts. The documentation quality might improve by using self-report questionnaires from patients for their symptoms.
Image pre-processing
Studies have observed various physical factors that can lead to altered data quality and misinterpretations from existing medical records [ 30 ]. Medical images often suffer technical barriers that involve multiple types of noise and artifacts. Improper handling of medical images can also cause tampering of images for instance might lead to delineation of anatomical structures such as veins which is non-correlative with real case scenario. Reduction of noise, clearing artifacts, adjusting contrast of acquired images and image quality adjustment post mishandling are some of the measures that can be implemented to benefit the purpose.
There have been many security breaches, hackings, phishing attacks, and ransomware episodes that data security is a priority for healthcare organizations. After noticing an array of vulnerabilities, a list of technical safeguards was developed for the protected health information (PHI). These rules, termed as HIPAA Security Rules, help guide organizations with storing, transmission, authentication protocols, and controls over access, integrity, and auditing. Common security measures like using up-to-date anti-virus software, firewalls, encrypting sensitive data, and multi-factor authentication can save a lot of trouble.
To have a successful data governance plan, it would be mandatory to have complete, accurate, and up-to-date metadata regarding all the stored data. The metadata would be composed of information like time of creation, purpose and person responsible for the data, previous usage (by who, why, how, and when) for researchers and data analysts. This would allow analysts to replicate previous queries and help later scientific studies and accurate benchmarking. This increases the usefulness of data and prevents creation of “data dumpsters” of low or no use.
Metadata would make it easier for organizations to query their data and get some answers. However, in absence of proper interoperability between datasets the query tools may not access an entire repository of data. Also, different components of a dataset should be well interconnected or linked and easily accessible otherwise a complete portrait of an individual patient’s health may not be generated. Medical coding systems like ICD-10, SNOMED-CT, or LOINC must be implemented to reduce free-form concepts into a shared ontology. If the accuracy, completeness, and standardization of the data are not in question, then Structured Query Language (SQL) can be used to query large datasets and relational databases.
Visualization
A clean and engaging visualization of data with charts, heat maps, and histograms to illustrate contrasting figures and correct labeling of information to reduce potential confusion, can make it much easier for us to absorb information and use it appropriately. Other examples include bar charts, pie charts, and scatterplots with their own specific ways to convey the data.
Data sharing
Patients may or may not receive their care at multiple locations. In the former case, sharing data with other healthcare organizations would be essential. During such sharing, if the data is not interoperable then data movement between disparate organizations could be severely curtailed. This could be due to technical and organizational barriers. This may leave clinicians without key information for making decisions regarding follow-ups and treatment strategies for patients. Solutions like Fast Healthcare Interoperability Resource (FHIR) and public APIs, CommonWell (a not-for-profit trade association) and Carequality (a consensus-built, common interoperability framework) are making data interoperability and sharing easy and secure. The biggest roadblock for data sharing is the treatment of data as a commodity that can provide a competitive advantage. Therefore, sometimes both providers and vendors intentionally interfere with the flow of information to block the information flow between different EHR systems [ 31 ].
The healthcare providers will need to overcome every challenge on this list and more to develop a big data exchange ecosystem that provides trustworthy, timely, and meaningful information by connecting all members of the care continuum. Time, commitment, funding, and communication would be required before these challenges are overcome.
Big data analytics for cutting costs
To develop a healthcare system based on big data that can exchange big data and provides us with trustworthy, timely, and meaningful information, we need to overcome every challenge mentioned above. Overcoming these challenges would require investment in terms of time, funding, and commitment. However, like other technological advances, the success of these ambitious steps would apparently ease the present burdens on healthcare especially in terms of costs. It is believed that the implementation of big data analytics by healthcare organizations might lead to a saving of over 25% in annual costs in the coming years. Better diagnosis and disease predictions by big data analytics can enable cost reduction by decreasing the hospital readmission rate. The healthcare firms do not understand the variables responsible for readmissions well enough. It would be easier for healthcare organizations to improve their protocols for dealing with patients and prevent readmission by determining these relationships well. Big data analytics can also help in optimizing staffing, forecasting operating room demands, streamlining patient care, and improving the pharmaceutical supply chain. All of these factors will lead to an ultimate reduction in the healthcare costs by the organizations.
Quantum mechanics and big data analysis
Big data sets can be staggering in size. Therefore, its analysis remains daunting even with the most powerful modern computers. For most of the analysis, the bottleneck lies in the computer’s ability to access its memory and not in the processor [ 32 , 33 ]. The capacity, bandwidth or latency requirements of memory hierarchy outweigh the computational requirements so much that supercomputers are increasingly used for big data analysis [ 34 , 35 ]. An additional solution is the application of quantum approach for big data analysis.
Quantum computing and its advantages
The common digital computing uses binary digits to code for the data whereas quantum computation uses quantum bits or qubits [ 36 ]. A qubit is a quantum version of the classical binary bits that can represent a zero, a one, or any linear combination of states (called superpositions ) of those two qubit states [ 37 ]. Therefore, qubits allow computer bits to operate in three states compared to two states in the classical computation. This allows quantum computers to work thousands of times faster than regular computers. For example, a conventional analysis of a dataset with n points would require 2 n processing units whereas it would require just n quantum bits using a quantum computer. Quantum computers use quantum mechanical phenomena like superposition and quantum entanglement to perform computations [ 38 , 39 ].
Quantum algorithms can speed-up the big data analysis exponentially [ 40 ]. Some complex problems, believed to be unsolvable using conventional computing, can be solved by quantum approaches. For example, the current encryption techniques such as RSA, public-key (PK) and Data Encryption Standard (DES) which are thought to be impassable now would be irrelevant in future because quantum computers will quickly get through them [ 41 ]. Quantum approaches can dramatically reduce the information required for big data analysis. For example, quantum theory can maximize the distinguishability between a multilayer network using a minimum number of layers [ 42 ]. In addition, quantum approaches require a relatively small dataset to obtain a maximally sensitive data analysis compared to the conventional (machine-learning) techniques. Therefore, quantum approaches can drastically reduce the amount of computational power required to analyze big data. Even though, quantum computing is still in its infancy and presents many open challenges, it is being implemented for healthcare data.
Applications in big data analysis
Quantum computing is picking up and seems to be a potential solution for big data analysis. For example, identification of rare events, such as the production of Higgs bosons at the Large Hadron Collider (LHC) can now be performed using quantum approaches [ 43 ]. At LHC, huge amounts of collision data (1PB/s) is generated that needs to be filtered and analyzed. One such approach, the quantum annealing for ML (QAML) that implements a combination of ML and quantum computing with a programmable quantum annealer, helps reduce human intervention and increase the accuracy of assessing particle-collision data. In another example, the quantum support vector machine was implemented for both training and classification stages to classify new data [ 44 ]. Such quantum approaches could find applications in many areas of science [ 43 ]. Indeed, recurrent quantum neural network (RQNN) was implemented to increase signal separability in electroencephalogram (EEG) signals [ 45 ]. Similarly, quantum annealing was applied to intensity modulated radiotherapy (IMRT) beamlet intensity optimization [ 46 ]. Similarly, there exist more applications of quantum approaches regarding healthcare e.g. quantum sensors and quantum microscopes [ 47 ].
Conclusions and future prospects
Nowadays, various biomedical and healthcare tools such as genomics, mobile biometric sensors, and smartphone apps generate a big amount of data. Therefore, it is mandatory for us to know about and assess that can be achieved using this data. For example, the analysis of such data can provide further insights in terms of procedural, technical, medical and other types of improvements in healthcare. After a review of these healthcare procedures, it appears that the full potential of patient-specific medical specialty or personalized medicine is under way. The collective big data analysis of EHRs, EMRs and other medical data is continuously helping build a better prognostic framework. The companies providing service for healthcare analytics and clinical transformation are indeed contributing towards better and effective outcome. Common goals of these companies include reducing cost of analytics, developing effective Clinical Decision Support (CDS) systems, providing platforms for better treatment strategies, and identifying and preventing fraud associated with big data. Though, almost all of them face challenges on federal issues like how private data is handled, shared and kept safe. The combined pool of data from healthcare organizations and biomedical researchers have resulted in a better outlook, determination, and treatment of various diseases. This has also helped in building a better and healthier personalized healthcare framework. Modern healthcare fraternity has realized the potential of big data and therefore, have implemented big data analytics in healthcare and clinical practices. Supercomputers to quantum computers are helping in extracting meaningful information from big data in dramatically reduced time periods. With high hopes of extracting new and actionable knowledge that can improve the present status of healthcare services, researchers are plunging into biomedical big data despite the infrastructure challenges. Clinical trials, analysis of pharmacy and insurance claims together, discovery of biomarkers is a part of a novel and creative way to analyze healthcare big data.
Big data analytics leverage the gap within structured and unstructured data sources. The shift to an integrated data environment is a well-known hurdle to overcome. Interesting enough, the principle of big data heavily relies on the idea of the more the information, the more insights one can gain from this information and can make predictions for future events. It is rightfully projected by various reliable consulting firms and health care companies that the big data healthcare market is poised to grow at an exponential rate. However, in a short span we have witnessed a spectrum of analytics currently in use that have shown significant impacts on the decision making and performance of healthcare industry. The exponential growth of medical data from various domains has forced computational experts to design innovative strategies to analyze and interpret such enormous amount of data within a given timeframe. The integration of computational systems for signal processing from both research and practicing medical professionals has witnessed growth. Thus, developing a detailed model of a human body by combining physiological data and “-omics” techniques can be the next big target. This unique idea can enhance our knowledge of disease conditions and possibly help in the development of novel diagnostic tools. The continuous rise in available genomic data including inherent hidden errors from experiment and analytical practices need further attention. However, there are opportunities in each step of this extensive process to introduce systemic improvements within the healthcare research.
High volume of medical data collected across heterogeneous platforms has put a challenge to data scientists for careful integration and implementation. It is therefore suggested that revolution in healthcare is further needed to group together bioinformatics, health informatics and analytics to promote personalized and more effective treatments. Furthermore, new strategies and technologies should be developed to understand the nature (structured, semi-structured, unstructured), complexity (dimensions and attributes) and volume of the data to derive meaningful information. The greatest asset of big data lies in its limitless possibilities. The birth and integration of big data within the past few years has brought substantial advancements in the health care sector ranging from medical data management to drug discovery programs for complex human diseases including cancer and neurodegenerative disorders. To quote a simple example supporting the stated idea, since the late 2000′s the healthcare market has witnessed advancements in the EHR system in the context of data collection, management and usability. We believe that big data will add-on and bolster the existing pipeline of healthcare advances instead of replacing skilled manpower, subject knowledge experts and intellectuals, a notion argued by many. One can clearly see the transitions of health care market from a wider volume base to personalized or individual specific domain. Therefore, it is essential for technologists and professionals to understand this evolving situation. In the coming year it can be projected that big data analytics will march towards a predictive system. This would mean prediction of futuristic outcomes in an individual’s health state based on current or existing data (such as EHR-based and Omics-based). Similarly, it can also be presumed that structured information obtained from a certain geography might lead to generation of population health information. Taken together, big data will facilitate healthcare by introducing prediction of epidemics (in relation to population health), providing early warnings of disease conditions, and helping in the discovery of novel biomarkers and intelligent therapeutic intervention strategies for an improved quality of life.
Availability of data and materials
Not applicable.
Laney D. 3D data management: controlling data volume, velocity, and variety, Application delivery strategies. Stamford: META Group Inc; 2001.
Google Scholar
Mauro AD, Greco M, Grimaldi M. A formal definition of big data based on its essential features. Libr Rev. 2016;65(3):122–35.
Article Google Scholar
Gubbi J, et al. Internet of Things (IoT): a vision, architectural elements, and future directions. Future Gener Comput Syst. 2013;29(7):1645–60.
Doyle-Lindrud S. The evolution of the electronic health record. Clin J Oncol Nurs. 2015;19(2):153–4.
Gillum RF. From papyrus to the electronic tablet: a brief history of the clinical medical record with lessons for the digital Age. Am J Med. 2013;126(10):853–7.
Reiser SJ. The clinical record in medicine part 1: learning from cases*. Ann Intern Med. 1991;114(10):902–7.
Reisman M. EHRs: the challenge of making electronic data usable and interoperable. Pharm Ther. 2017;42(9):572–5.
Murphy G, Hanken MA, Waters K. Electronic health records: changing the vision. Philadelphia: Saunders W B Co; 1999. p. 627.
Shameer K, et al. Translational bioinformatics in the era of real-time biomedical, health care and wellness data streams. Brief Bioinform. 2017;18(1):105–24.
Service, R.F. The race for the $1000 genome. Science. 2006;311(5767):1544–6.
Stephens ZD, et al. Big data: astronomical or genomical? PLoS Biol. 2015;13(7):e1002195.
Yin Y, et al. The internet of things in healthcare: an overview. J Ind Inf Integr. 2016;1:3–13.
Moore SK. Unhooking medicine [wireless networking]. IEEE Spectr 2001; 38(1): 107–8, 110.
MathSciNet Google Scholar
Nasi G, Cucciniello M, Guerrazzi C. The role of mobile technologies in health care processes: the case of cancer supportive care. J Med Internet Res. 2015;17(2):e26.
Apple, ResearchKit/ResearchKit: ResearchKit 1.5.3. 2017.
Shvachko K, et al. The hadoop distributed file system. In: Proceedings of the 2010 IEEE 26th symposium on mass storage systems and technologies (MSST). New York: IEEE Computer Society; 2010. p. 1–10.
Dean J, Ghemawat S. MapReduce: simplified data processing on large clusters. Commun ACM. 2008;51(1):107–13.
Zaharia M, et al. Apache Spark: a unified engine for big data processing. Commun ACM. 2016;59(11):56–65.
Gopalani S, Arora R. Comparing Apache Spark and Map Reduce with performance analysis using K-means; 2015.
Ahmed H, et al. Performance comparison of spark clusters configured conventionally and a cloud servicE. Procedia Comput Sci. 2016;82:99–106.
Saouabi M, Ezzati A. A comparative between hadoop mapreduce and apache Spark on HDFS. In: Proceedings of the 1st international conference on internet of things and machine learning. Liverpool: ACM; 2017. p. 1–4.
Strickland NH. PACS (picture archiving and communication systems): filmless radiology. Arch Dis Child. 2000;83(1):82–6.
Article MathSciNet Google Scholar
Schroeder W, Martin K, Lorensen B. The visualization toolkit. 4th ed. Clifton Park: Kitware; 2006.
Friston K, et al. Statistical parametric mapping. London: Academic Press; 2007. p. vii.
Li L, et al. Identification of type 2 diabetes subgroups through topological analysis of patient similarity. Sci Transl Med. 2015;7(311):311ra174.
Valikodath NG, et al. Agreement of ocular symptom reporting between patient-reported outcomes and medical records. JAMA Ophthalmol. 2017;135(3):225–31.
Fromme EK, et al. How accurate is clinician reporting of chemotherapy adverse effects? A comparison with patient-reported symptoms from the Quality-of-Life Questionnaire C30. J Clin Oncol. 2004;22(17):3485–90.
Beckles GL, et al. Agreement between self-reports and medical records was only fair in a cross-sectional study of performance of annual eye examinations among adults with diabetes in managed care. Med Care. 2007;45(9):876–83.
Echaiz JF, et al. Low correlation between self-report and medical record documentation of urinary tract infection symptoms. Am J Infect Control. 2015;43(9):983–6.
Belle A, et al. Big data analytics in healthcare. Biomed Res Int. 2015;2015:370194.
Adler-Milstein J, Pfeifer E. Information blocking: is it occurring and what policy strategies can address it? Milbank Q. 2017;95(1):117–35.
Or-Bach, Z. A 1,000x improvement in computer systems by bridging the processor-memory gap. In: 2017 IEEE SOI-3D-subthreshold microelectronics technology unified conference (S3S). 2017.
Mahapatra NR, Venkatrao B. The processor-memory bottleneck: problems and solutions. XRDS. 1999;5(3es):2.
Voronin AA, Panchenko VY, Zheltikov AM. Supercomputations and big-data analysis in strong-field ultrafast optical physics: filamentation of high-peak-power ultrashort laser pulses. Laser Phys Lett. 2016;13(6):065403.
Dollas, A. Big data processing with FPGA supercomputers: opportunities and challenges. In: 2014 IEEE computer society annual symposium on VLSI; 2014.
Saffman M. Quantum computing with atomic qubits and Rydberg interactions: progress and challenges. J Phys B: At Mol Opt Phys. 2016;49(20):202001.
Nielsen MA, Chuang IL. Quantum computation and quantum information. 10th anniversary ed. Cambridge: Cambridge University Press; 2011. p. 708.
Raychev N. Quantum computing models for algebraic applications. Int J Scientific Eng Res. 2015;6(8):1281–8.
Harrow A. Why now is the right time to study quantum computing. XRDS. 2012;18(3):32–7.
Lloyd S, Garnerone S, Zanardi P. Quantum algorithms for topological and geometric analysis of data. Nat Commun. 2016;7:10138.
Buchanan W, Woodward A. Will quantum computers be the end of public key encryption? J Cyber Secur Technol. 2017;1(1):1–22.
De Domenico M, et al. Structural reducibility of multilayer networks. Nat Commun. 2015;6:6864.
Mott A, et al. Solving a Higgs optimization problem with quantum annealing for machine learning. Nature. 2017;550:375.
Rebentrost P, Mohseni M, Lloyd S. Quantum support vector machine for big data classification. Phys Rev Lett. 2014;113(13):130503.
Gandhi V, et al. Quantum neural network-based EEG filtering for a brain-computer interface. IEEE Trans Neural Netw Learn Syst. 2014;25(2):278–88.
Nazareth DP, Spaans JD. First application of quantum annealing to IMRT beamlet intensity optimization. Phys Med Biol. 2015;60(10):4137–48.
Reardon S. Quantum microscope offers MRI for molecules. Nature. 2017;543(7644):162.
Download references
Acknowledgements
Author information.
Sabyasachi Dash and Sushil Kumar Shakyawar contributed equally to this work
Authors and Affiliations
Department of Pathology and Laboratory Medicine, Weill Cornell Medicine, New York, 10065, NY, USA
Sabyasachi Dash
Center of Biological Engineering, University of Minho, Campus de Gualtar, 4710-057, Braga, Portugal
Sushil Kumar Shakyawar
SilicoLife Lda, Rua do Canastreiro 15, 4715-387, Braga, Portugal
Postgraduate School for Molecular Medicine, Warszawskiego Uniwersytetu Medycznego, Warsaw, Poland
Mohit Sharma
Małopolska Centre for Biotechnology, Jagiellonian University, Kraków, Poland
3B’s Research Group, Headquarters of the European Institute of Excellence on Tissue Engineering and Regenerative Medicine, AvePark - Parque de Ciência e Tecnologia, Zona Industrial da Gandra, Barco, 4805-017, Guimarães, Portugal
Sandeep Kaushik
You can also search for this author in PubMed Google Scholar
Contributions
MS wrote the manuscript. SD and SKS further added significant discussion that highly improved the quality of manuscript. SK designed the content sequence, guided SD, SS and MS in writing and revising the manuscript and checked the manuscript. All authors read and approved the final manuscript.
Corresponding author
Correspondence to Sandeep Kaushik .
Ethics declarations
Ethics approval and consent to participate, consent for publication, competing interests.
The authors declare that they have no competing interests.
Additional information
Publisher's note.
Springer Nature remains neutral with regard to jurisdictional claims in published maps and institutional affiliations.
Rights and permissions
Open Access This article is distributed under the terms of the Creative Commons Attribution 4.0 International License ( http://creativecommons.org/licenses/by/4.0/ ), which permits unrestricted use, distribution, and reproduction in any medium, provided you give appropriate credit to the original author(s) and the source, provide a link to the Creative Commons license, and indicate if changes were made.
Reprints and permissions
About this article
Cite this article.
Dash, S., Shakyawar, S.K., Sharma, M. et al. Big data in healthcare: management, analysis and future prospects. J Big Data 6 , 54 (2019). https://doi.org/10.1186/s40537-019-0217-0
Download citation
Received : 17 January 2019
Accepted : 06 June 2019
Published : 19 June 2019
DOI : https://doi.org/10.1186/s40537-019-0217-0
Share this article
Anyone you share the following link with will be able to read this content:
Sorry, a shareable link is not currently available for this article.
Provided by the Springer Nature SharedIt content-sharing initiative
- Biomedical research
- Big data analytics
- Internet of things
- Personalized medicine
- Quantum computing
Top 18 AI Use Cases in Healthcare Industry in 2024

Cem is the principal analyst at AIMultiple since 2017. AIMultiple informs hundreds of thousands of businesses (as per Similarweb) including 60% of Fortune 500 every month.
Cem's work has been cited by leading global publications including Business Insider, Forbes, Washington Post, global firms like Deloitte, HPE, NGOs like World Economic Forum and supranational organizations like European Commission. You can see more reputable companies and media that referenced AIMultiple.
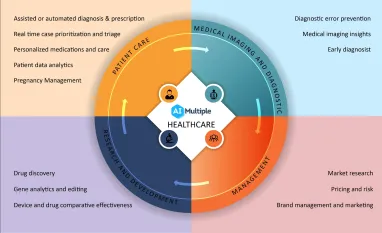
As the interest in AI in the healthcare industry continues to grow , there are numerous current AI applications in healthcare, and more use cases will emerge in the future.
We have identified the top 18 artificial intelligence use cases and vendors in the healthcare industry and structured them around typical processes that are used in the healthcare industry.
The purpose of this article is to shed light on healthcare-related AI application cases. We, therefore, anticipate that professionals will stay informed of new developments and take into account current technologies/applications when making strategic decisions.
Patient Care
- 1- Assisted or automated diagnosis & prescription: Chatbots can help patients self-diagnose or assist doctors in diagnosis.
- 2- Prescription auditing: AI audit systems can help minimize prescription errors.
- 3- Pregnancy Management: Monitor mother and fetus to reduce mother’s worries and enable early diagnosis
- Jvion : The Cognitive Clinical Success Machine precisely and comprehensively foresees risk delivering the recommended actions that improve outcomes.
- Wellframe : Wellframe flips the script by delivering interactive care programs directly to patients on a mobile device. Its portfolio of clinical modules, developed based on evidence-based care, enables the Care Team to provide a personalized experience for any patient.
- Enlitic : Patient triaging solutions scan the incoming cases for multiple clinical findings, determine their priority, and route them to the most appropriate doctor in the network.
- GNS Healthcare : The company uses machine learning to match the patients with the treatments that prove the most effective for them.
- Oncora Medicals: The software structure analyzes and learns from the data that health systems have to enable them to provide personalized treatment.
- Zakipoint Health : The company displays all the relevant healthcare data at a member level on a dashboard to transparently display the risk and cost, provide tailored programs, and improve on patient engagement
- We have a great article covering top 20 healthcare analytics vendors if you want to learn more. Also, you can check our sortable list of healthcare analytics companies on our website.
- 7-Surgical robots: Robot-assisted surgeries combine AI and collaborative robots. These robots are well-suited for procedures that require the same repetitive movements as they can work without fatigue. AI can identify patterns within surgical procedures to improve best practices and to improve surgical robots’ control accuracy to sub-millimeter precision.
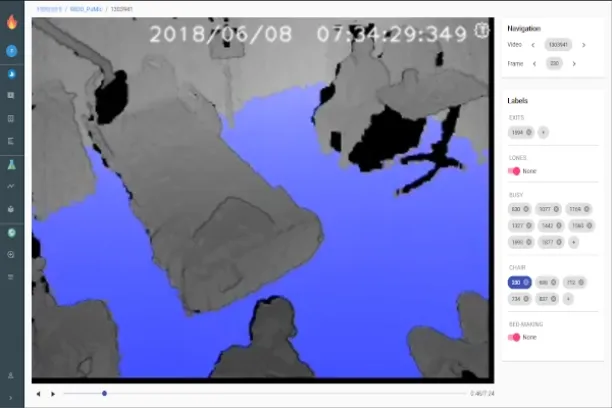
Medical Imaging and Diagnostic
- Ezra: Ezra leverages AI while analyzing full-body MRI scans to support clinicians in the early detection of cancer.
- SkinVision: SkinVision enables you to diagnose skin cancer early by taking photos of your skin with your phone and getting it to a doctor at the right time.
- AI-powered medical imaging is also widely used in diagnosing COVID-19 cases and identifying patients who require ventilator support. For example, a Chinese company, Huiying Medical , has developed an AI-powered medical imaging solution with a 96% accuracy rate.
Research & Development
- NuMedii : Biopharma company, NuMedii, has built the AIDD (Artificial Intelligence for Drug Discovery) technology that harnesses Big Data and AI to rapidly discover connections between drugs and diseases at a systematic level.
- 11- Gene analysis and editing: Understand genes and their components. Predict the impact of gene edits.
- 4Quant: The company utilizes the latest Big Data and Deep Learning technology to extract meaningful, actionable information from images and videos for experiment design to help pick and choose which components make the most sense for the needs.
Healthcare Management
- Healint: The company’s product, Migraine Buddy, has recorded terabytes of data that helps patients, doctors and researchers better understand the real-world causes and effects of neurological disorders.
- 14- Pricing and risk: Determine the optimal price for treatment and other services according to competition and other market conditions.
- MD Analytics: MD analytics is a global provider of health and pharmaceutical marketing research solutions.
- 16- Operations: Process automation technologies such as intelligent automation and RPA help hospitals automate routine front-office and back-office operations such as reporting.
- 17- Customer service chatbots: Customer service chatbots allow patients to ask questions regarding bill payment, appointments, or medication refills.
- 18- Fraud detection: Patients may make false claims. Leveraging AI-pow ered fraud detection tools can help hospital managers identify fraudsters.
There are too many possible AI use cases in healthcare to be listed here, and they can be identified by the practitioners. A machine learning-based solution can be built in areas where significant training data is available and the problem statement can be formulated clearly. In these areas, AI can benefit healthcare providers by:
- Enabling data-driven decision making
- Time & cost saving
We help companies identify partners for building such custom machine learning / AI solutions :
Further reading
- 43 Healthtech AI vendors by area of focus & geography
- Top 4 Challenges of AI in Healthcare & How to Overcome Them
- Digitizing Healthcare: Customer-centric Health Services
If you have any questions, feel free to contact us:
- MobileHealthNews
This article was originally written by former AIMultiple industry analyst Atakan Kantarci and reviewed by Cem Dilmegani

Throughout his career, Cem served as a tech consultant, tech buyer and tech entrepreneur. He advised enterprises on their technology decisions at McKinsey & Company and Altman Solon for more than a decade. He also published a McKinsey report on digitalization.
He led technology strategy and procurement of a telco while reporting to the CEO. He has also led commercial growth of deep tech company Hypatos that reached a 7 digit annual recurring revenue and a 9 digit valuation from 0 within 2 years. Cem's work in Hypatos was covered by leading technology publications like TechCrunch and Business Insider.
Cem regularly speaks at international technology conferences. He graduated from Bogazici University as a computer engineer and holds an MBA from Columbia Business School.
AIMultiple.com Traffic Analytics, Ranking & Audience , Similarweb. Why Microsoft, IBM, and Google Are Ramping up Efforts on AI Ethics , Business Insider. Microsoft invests $1 billion in OpenAI to pursue artificial intelligence that’s smarter than we are , Washington Post. Data management barriers to AI success , Deloitte. Empowering AI Leadership: AI C-Suite Toolkit , World Economic Forum. Science, Research and Innovation Performance of the EU , European Commission. Public-sector digitization: The trillion-dollar challenge , McKinsey & Company. Hypatos gets $11.8M for a deep learning approach to document processing , TechCrunch. We got an exclusive look at the pitch deck AI startup Hypatos used to raise $11 million , Business Insider.
To stay up-to-date on B2B tech & accelerate your enterprise:
Next to Read
Top 6 radiology ai use cases in 2024, top 4 ai use cases in the pharmaceutical sector in 2024, healthcare intelligent automation: use cases & examples [2024].
Your email address will not be published. All fields are required.
Related research
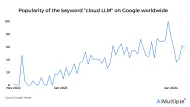
In-Depth Guide to Cloud Large Language Models (LLMs) in 2024
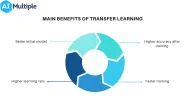
Transfer Learning in 2024: What It Is & How It Works
Artificial intelligence is being used in healthcare for everything from answering patient questions to assisting with surgeries and developing new pharmaceuticals.
According to Statista , the artificial intelligence (AI) healthcare market, which is valued at $11 billion in 2021, is projected to be worth $187 billion in 2030. That massive increase means we will likely continue to see considerable changes in how medical providers, hospitals, pharmaceutical and biotechnology companies, and others in the healthcare industry operate.
Better machine learning (ML) algorithms, more access to data, cheaper hardware, and the availability of 5G have contributed to the increasing application of AI in the healthcare industry, accelerating the pace of change. AI and ML technologies can sift through enormous volumes of health data—from health records and clinical studies to genetic information—and analyze it much faster than humans.
Healthcare organizations are using AI to improve the efficiency of all kinds of processes, from back-office tasks to patient care. The following are some examples of how AI might be used to benefit staff and patients:
- Administrative workflow: Healthcare workers spend a lot of time doing paperwork and other administrative tasks. AI and automation can help perform many of those mundane tasks, freeing up employee time for other activities and giving them more face-to-face time with patients. For example, generative AI can help clinicians with note-taking and content summarization that can help keep medical records as thoroughly as possible. AI might also help with accurate coding and sharing of information between departments and billing.
- Virtual nursing assistants: One study found that 64% of patients are comfortable with the use of AI for around-the-clock access to answers that support nurses provide. AI virtual nurse assistants—which are AI-powered chatbots, apps, or other interfaces—can be used to help answer questions about medications, forward reports to doctors or surgeons and help patients schedule a visit with a physician. These sorts of routine tasks can help take work off the hands of clinical staff, who can then spend more time directly on patient care, where human judgment and interaction matter most.
- Dosage error reduction: AI can be used to help identify errors in how a patient self-administers medication. One example comes from a study in Nature Medicine , which found that up to 70% of patients don’t take insulin as prescribed. An AI-powered tool that sits in the patient’s background (much like a wifi router) might be used to flag errors in how the patient administers an insulin pen or inhaler.
- Less invasive surgeries: AI-enabled robots might be used to work around sensitive organs and tissues to help reduce blood loss, infection risk and post-surgery pain.
- Fraud prevention: Fraud in the healthcare industry is enormous, at $380 billion/year, and raises the cost of consumers’ medical premiums and out-of-pocket expenses. Implementing AI can help recognize unusual or suspicious patterns in insurance claims, such as billing for costly services or procedures that are not performed, unbundling (which is billing for the individual steps of a procedure as though they were separate procedures), and performing unnecessary tests to take advantage of insurance payments.
A recent study found that 83% of patients report poor communication as the worst part of their experience, demonstrating a strong need for clearer communication between patients and providers. AI technologies like natural language processing (NLP), predictive analytics, and speech recognition might help healthcare providers have more effective communication with patients. AI might, for instance, deliver more specific information about a patient’s treatment options, allowing the healthcare provider to have more meaningful conversations with the patient for shared decision-making.
According to Harvard’s School of Public Health , although it’s early days for this use, using AI to make diagnoses may reduce treatment costs by up to 50% and improve health outcomes by 40%.
One use case example is out of the University of Hawaii , where a research team found that deploying deep learning AI technology can improve breast cancer risk prediction. More research is needed, but the lead researcher pointed out that an AI algorithm can be trained on a much larger set of images than a radiologist—as many as a million or more radiology images. Also, that algorithm can be replicated at no cost except for hardware.
An MIT group developed an ML algorithm to determine when a human expert is needed. In some instances, such as identifying cardiomegaly in chest X-rays, they found that a hybrid human-AI model produced the best results.
Another published study found that AI recognized skin cancer better than experienced doctors. US, German and French researchers used deep learning on more than 100,000 images to identify skin cancer. Comparing the results of AI to those of 58 international dermatologists, they found AI did better.
As health and fitness monitors become more popular and more people use apps that track and analyze details about their health. They can share these real-time data sets with their doctors to monitor health issues and provide alerts in case of problems.
AI solutions—such as big data applications, machine learning algorithms and deep learning algorithms—might also be used to help humans analyze large data sets to help clinical and other decision-making. AI might also be used to help detect and track infectious diseases, such as COVID-19, tuberculosis, and malaria.
One benefit the use of AI brings to health systems is making gathering and sharing information easier. AI can help providers keep track of patient data more efficiently.
One example is diabetes. According to the Centers for Disease Control and Prevention , 10% of the US population has diabetes. Patients can now use wearable and other monitoring devices that provide feedback about their glucose levels to themselves and their medical team. AI can help providers gather that information, store, and analyze it, and provide data-driven insights from vast numbers of people. Using this information can help healthcare professionals determine how to better treat and manage diseases.
Organizations are also starting to use AI to help improve drug safety. The company SELTA SQUARE, for example, is innovating the pharmacovigilance (PV) process , a legally mandated discipline for detecting and reporting adverse effects from drugs, then assessing, understanding, and preventing those effects. PV demands significant effort and diligence from pharma producers because it’s performed from the clinical trials phase all the way through the drug’s lifetime availability. Selta Square uses a combination of AI and automation to make the PV process faster and more accurate, which helps make medicines safer for people worldwide.
Sometimes, AI might reduce the need to test potential drug compounds physically, which is an enormous cost-savings. High-fidelity molecular simulations can run on computers without incurring the high costs of traditional discovery methods.
AI also has the potential to help humans predict toxicity, bioactivity, and other characteristics of molecules or create previously unknown drug molecules from scratch.
As AI becomes more important in healthcare delivery and more AI medical applications are developed, ethical, and regulatory governance must be established. Issues that raise concern include the possibility of bias, lack of transparency, privacy concerns regarding data used for training AI models, and safety and liability issues.
“AI governance is necessary, especially for clinical applications of the technology,” said Laura Craft, VP Analyst at Gartner . “However, because new AI techniques are largely new territory for most [health delivery organizations], there is a lack of common rules, processes, and guidelines for eager entrepreneurs to follow as they design their pilots.”
The World Health Organization (WHO) spent 18 months deliberating with leading experts in ethics, digital technology, law, and human rights and various Ministries of Health members to produce a report that is called Ethics & Governance of Artificial Intelligence for Health . This report identifies ethical challenges to using AI in healthcare, identifies risks, and outlines six consensus principles to ensure AI works for the public’s benefit:
- Protecting autonomy
- Promoting human safety and well-being
- Ensuring transparency
- Fostering accountability
- Ensuring equity
- Promoting tools that are responsive and sustainable
The WHO report also provides recommendations that ensure governing AI for healthcare both maximizes the technology’s promise and holds healthcare workers accountable and responsive to the communities and people they work with.
AI provides opportunities to help reduce human error, assist medical professionals and staff, and provide patient services 24/7. As AI tools continue to develop, there is potential to use AI even more in reading medical images, X-rays and scans, diagnosing medical problems and creating treatment plans.
AI applications continue to help streamline various tasks, from answering phones to analyzing population health trends (and likely, applications yet to be considered). For instance, future AI tools may automate or augment more of the work of clinicians and staff members. That will free up humans to spend more time on more effective and compassionate face-to-face professional care.
When patients need help, they don’t want to (or can’t) wait on hold. Healthcare facilities’ resources are finite, so help isn’t always available instantaneously or 24/7—and even slight delays can create frustration and feelings of isolation or cause certain conditions to worsen.
IBM® watsonx Assistant™ AI healthcare chatbots can help providers do two things: keep their time focused where it needs to be and empower patients who call in to get quick answers to simple questions.
IBM watsonx Assistant is built on deep learning, machine learning and natural language processing (NLP) models to understand questions, search for the best answers and complete transactions by using conversational AI.
Get email updates about AI advancements, strategies, how-tos, expert perspective and more.
See IBM watsonx Assistant in action and request a demo
Get our newsletters and topic updates that deliver the latest thought leadership and insights on emerging trends.
- Open access
- Published: 17 May 2024
Ensuring universal access to quality care for persons with presumed tuberculosis reaching the private sector: lessons from Kerala
- P. S. Rakesh 1 , 2 &
- Mohd Shannawaz 1
International Journal for Equity in Health volume 23 , Article number: 101 ( 2024 ) Cite this article
1 Altmetric
Metrics details
More than half of the people with Tuberculosis (TB) symptoms in India seek care from the private sector. People with TB getting treatment from private sector in India are considered to be at a higher risk for receiving suboptimal quality of care in terms of incorrect diagnosis and treatment, lack of treatment adherence support with a high loss to follow-up rate that could eventually increase their risk of drug resistance. The current study aims at documenting the approach and efforts taken by the Kerala state to partner with the private health care delivery providers for ensuring quality TB care to the people with presumed TB reaching them.
A case study approach was adopted with review of all available literature followed by five Key Informant Interviews to understand the case through a primary descriptive exploration. Grounded theory approach was used to generating the single theory of the case itself that explains it.
Kerala state has taken a variety of interventions to ensure universal access to TB care for citizens reaching the private sector with documented improvement in the quality of TB care. Key learnings from these initiatives were (i) patients need to be at the centre of partnerships, (ii) good governance is essential for ensuring Universal Health Coverage in a mixed health system, (iii) data intelligence is required to guide partnerships, (iv) identification of the correct ‘problems’ is crucial for effective design of partnerships and (v) a platform for meaningful dialogue of key stakeholders is needed.
Kerala experience demonstrated that if governments take a proactive role in engaging the private sector, in an informed and evidence-based way, they can leverage the advantages of the private sector while protecting the public health interest.
The Standards for TB Care in India (STCI), which is a locally customized version of the International Standards of Tuberculosis Care , mentions 26 standards of care that every citizen of India should receive irrespective of the sector of treatment [ 1 ]. STCI deliberate on standard tools and strategies for early and complete detection of TB, standards of treatment in terms of drugs and regimens for best patient outcome, standards of public health actions and patient support such as adherence monitoring, clinical follow up, contact investigations and TB Preventive Therapy to the family. STCI lays down what is expected for quality TB care from the Indian healthcare system.
More than half of the people with TB symptoms in India seek care from the private sector [ 2 , 3 ]. Gaps in the TB care cascade such as people with TB not having access to correct and complete diagnosis, people diagnosed with TB not being started on correct treatment; and people started on treatment not completing treatment, were observed more among people with TB who were diagnosed in the private sector compared to the public sector [ 4 , 5 , 6 , 7 ]. There are also concerns about the suboptimal quality of care including incorrect diagnosis and non-standardized treatment regimens, lack of systems for treatment adherence support resulting in high loss to follow-up rate increasing the risk of drug resistance among the people who seek care from the private sector in India [ 4 , 5 , 6 , 7 ]. Many services assured in the public sector, such as free diagnostics including rapid molecular tests and drug susceptibility testing, free quality assured drugs, treatment adherence support and monitoring, contact investigation and TB preventive therapy, linkages to social welfare schemes rarely reach the patients who are treated in the private sector. Not having access to full range of quality TB care when and where the people need them, could be a hindrance to achieve Universal health coverage (UHC).
Realising the urgent need to engage private sector, the National Strategic Plan (NSP) for TB Elimination in India (2017–2025) enlisted various strategies to ensure that patients reaching the private sector receive care as per the STCI [ 8 ]. National TB Elimination Program (NTEP) has taken a variety of approaches including trainings, regulations, provisions of free services such as drugs and diagnostics, incentives and partnership schemes to engage with private sector [ 9 ]. Government of India has issued directives making TB notification mandatory as a first step to ensure STCI to all people affected with TB [ 10 ]. Provisions have been developed in Ni-kshay, the real time case-based web-based management information system of NTEP, where private providers can directly log in to the system using their user credentials and notify TB and report outcomes [ 11 ]. NTEP provides 500 INR (IUSD = 85 INR) as an incentive to the private provider to notify each TB patient and another 500 INR to report the treatment outcome [ 12 ]. Anti TB medicines have been included in schedule H1 which can only be sold on prescription of a Registered Medical Practitioner and details of the prescriber, the patient and the drug sold needs to be maintained by the chemists [ 13 ]. NTEP has also provisions for supplying free quality assured anti- TB drugs and free molecular tests and drug susceptibility tests to the patients reaching the private sector. Directly and through various agencies, NTEP intends to provide support for contact investigations and treatment adherence to all patients reaching private sector. NTEP guidance document on partnership (2019) details out about the purchase of various services from private sector [ 14 ]. Patient Provider Support Agencies (PPSA), predominantly Non-Governmental Agencies, are contracted in with the task of engaging with private sector for notifying cases to NTEP and coordinating with NTEP for free drugs and follow-up.
Despite all these initiatives in India, TB notifications and ensuring public health actions for people with TB have not been really converted into routine practice in private sector. NSP intended to achieve a target of two million TB notification from private sector in 2021; there is a gap of 1.3 million TB cases who are ‘missing’ in the surveillance system [ 2 , 9 ]. Studies, annual reports and evaluations revealed that Kerala state has successful ensured quality TB care to the people reaching the private sector [ 9 , 15 , 16 ]. The current study aims at documenting the approach and efforts taken by the Kerala TB Elimination program to partner with the ‘for profit’ formal private health care delivery providers for ensuring quality TB care with special emphasis on potential lessons to be learnt from the state. This is expected to help policy makers and programme managers in other parts of India and in other countries who face challenges in partnering with private sector for ensuring universal access to quality TB care.
Local setting
Kerala has experienced a 7.5% annual decline in the TB incidence since 2015 and has been certified for the same by Government of India under its sub-national TB free certification process [ 17 , 18 ]. The state TB program has been appreciated by the Joint Monitoring Mission (JMM) 2019, led by the World Health Organization and global developmental partners, for providing patient-centric TB care through systematic interventions [ 15 ]. Various efforts by the state for providing support to the people and family with TB were documented [ 19 ]. The private health care sector in Kerala accounts for more than 70% of all facilities and 60% of all inpatient beds. A significant proportion of people from low socio economic status also avail health care from private hospitals in the state for various reason such as long waiting time in public facilities, perceived low quality of care in public sector and proximity of health facilities [ 20 ]. National TB Prevalence survey (NTPS) revealed that among those with TB symptoms and sought health care in Kerala, 38% consulted the private sector first [ 2 ].
A case study approach was used to understand the case through a primary descriptive exploration. Review of all available literature followed by five Key Informant Interviews (KIIs) were conducted.
Desk review
Desk review of all available documents and studies were attempted first. All documents such as policy documents, operational plans, guidelines, annual reports and evaluation studies available in public domain in official websites of health department were referred to look for (1) policy and plan for engagement of private sector for TB care and (2) outcomes and data related to TB patients reaching the private sector. A high level of objectivity and sensitivity was maintained during the process; the authenticity of the documents were assessed, and the goals and biases were explored before examining the content. Literature search was done in MEDLINE, Embase and Web of Science using the key words (TB OR Tuberculosis) AND (Private hospital OR Private facility OR Private Partnership OR Private Practise OR Private Sector OR Public Private Partnership) AND Kerala. The search was carried out on 21st December 2022, and all studies including descriptive and analytical studies were included irrespective of the publication type. Studies published since 2000–2022 were included as our interest was in the recent situation, and earlier publications were difficult to access. Reference lists of papers identified in the searches, were scanned. Information was obtained about studies from state TB cell from where permissions for using TB-related data are sanctioned.
We used the operational definition provided by World Health Organisation which defined private health sector as ‘the individuals and organizations that are neither owned nor directly controlled by governments and are involved in provision of health services’ [ 21 ]. Reading abstracts, studies related to private sector and TB done in Kerala were included. We focussed on ‘for-profit’ healthcare providers, because they are more numerous and difficult to engage. We included only health service providers rather than manufacturers or distributors of medical equipment, technologies, consumables or drugs. All identified articles were screened for full-text review. Articles not focussing on ‘for-profit’ health sector and ‘quality of TB care’ were excluded.
Key informant interviews
Based on the literature review, additional five KIIs were conducted to fill in gaps in the information, to explore additional documents to be reviewed and to validate the observations from the literature. Key informants were persons with long experience in the field of TB control, who had broad knowledge of the TB scenario in the state, were aware of the TB programme activities over the years and who were involved in policy formulation and implementation related to TB and private sector. They were selected as they were the persons with the longest experience, each having more than 15 years of experience, in the field and had a broad overview of the TB and private sector engagement in the state. They were (i) a senior bureaucrat, involved in policy making (ii) a state-level programme manager responsible for TB programme activity implementation, (iii) a technical consultant for TB elimination from a global partner agency, responsible for guiding the state in TB policy formulation, implementation and supervision (iv) a senior leader of Indian Medical Association who was associated with TB projects implemented through IMA and (v) director of a 600 bedded private hospital. Participants were approached by phone, the purpose of the interviews were communicated and the prerequisites during the interview (stable internet connection, calm environment and with video switched on) were communicated. Two interviews were conducted in English and the rest in Malayalam, by PS (male, medical doctor with post-graduation in public health with 10 years of research experience), who was experienced in conducting qualitative studies and was fluent in the local language. All KIIs were conducted online using Zoom (Zoom Video Communications, San Jose, CA, USA) with video. One researcher recorded the proceedings, identifying key themes and monitored the verbal and non-verbal interactions. All participants who were contacted could participate. Major themes discussed were initiatives taken by the Government to engage private sector, major outcomes of such strategies and reasons for changing strategies. Each interview lasted for approximately 45 min (range: 25–75). No repeat interviews were attempted. KIIs were later transcribed verbatim and translated into English. Transcripts were returned to participants for comments.
Grounded theory approach was used to develop theory. We did not attempt to generate strong theories rather focussed on generating the single theory of the case itself that explains it. This was a deliberate attempt in order not to draw away our attention from features for understanding the case.
The transcripts were then manually coded by PS, emerging themes were identified. Citations with similar coding were grouped according to the predetermined themes. Themes were derived from the data. Recurrent themes were marked as important. All the flagged statements were put together and synthesised. The team went through the transcripts and notes and reached a consensus. Data triangulation was done comparing information from literature and qualitative interviews. Inductive thematic saturation was reached and no new codes emerged after the fourth interview. The study was approved by the Institutional Ethics Committee of Amity University, Noida, India.
PRISMA flow chart for the literature review were presented as Fig. 1 .
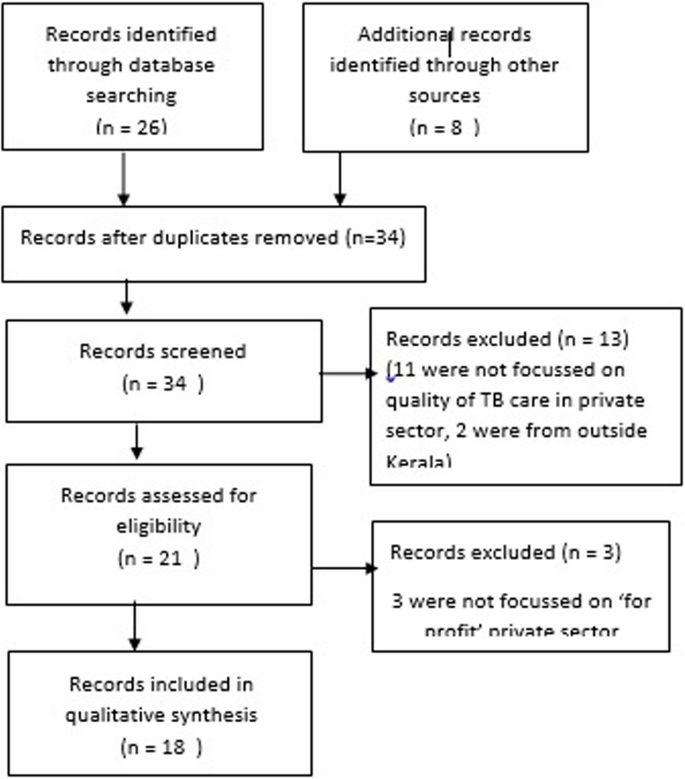
PRISMA flowchart indicating the results of literature search
The results of desk review and the KIIs are presented below.
Efforts for private sector engagement for TB care in Kerala
Many public-private mix strategies for TB care started in Kerala during the initial years of implementation of the National TB Elimination Program (NTEP), then called Revised National TB Control Program (RNTCP). Milestones of various initiatives by the state TB program to engage private sector for TB care ares described in Fig. 2 . Details of major initiatives by NTEP directly or in partnership with various stakeholders are compiled in Table 1 .
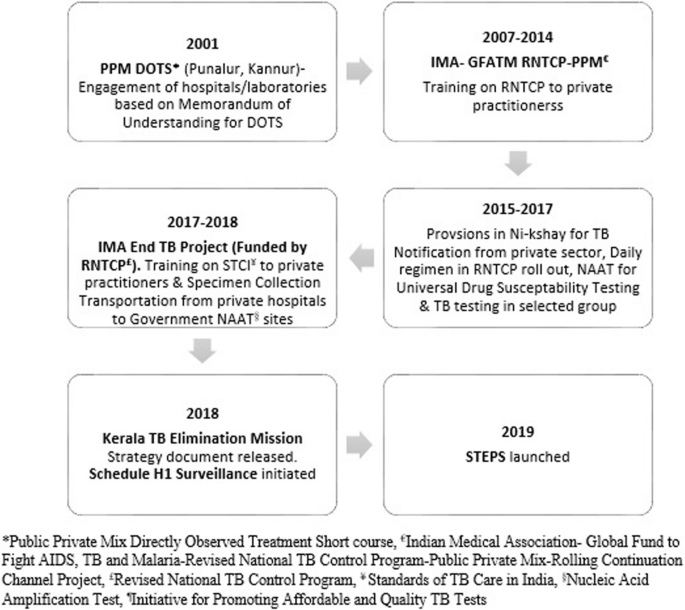
Milestone of Private Partnership for TB Care in Kerala
In 2016, even after the NTEP implemented a daily anti-TB regimen—the absence of which was cited as the major reason by the private sector for not participating—there was not much improvement in the private sector participation in terms of contributions towards TB notification or partnering with NTEP on signed partnership schemes. Operations researches conducted through the NTEP mechanisms, revealed many challenges for sub-optimal private sector participation [ 32 , 33 ]. Efforts were also put in place to understand the real problems of patients reaching the private sector [ 34 ]. A study that followed up a cohort of TB patients treated in private facilities in Kerala reported a loss to follow-up of 20–30% [ 34 ]. Limited ability to monitor and promote treatment adherence remained a major challenge in the private sector. Although NTEP documents the treatment outcome of every patient diagnosed/enrolled for treatment, such documentation is rare in the private sector. Lack of a network of field staff in the private sector limits the ability to monitor and support adherence to standards of care. STEPS (System for TB Elimination in Private Sector) evolved as a solution for ensuring standards of TB care in a patient-centric way for all patients accessing the private sector, addressing the concerns of the public and private sector [ 29 , 30 ]. A pilot STEPS centre in a private tertiary care centre in Kerala demonstrated that establishing STEPS centre within the hospital ensured 100% TB notification with a 4-fold increase in the number of patients notified over 6 months [ 35 ].Two interventions which emerged as prominent and replicable ones in our review are described below.
STEPS model
STEPS was envisioned as an equal partnership between the public and private sector for benefit of the society with improving standards of TB care as the outcome. Concept and evolution of STEPS are documented in detail elsewhere [ 30 ]. STEPS has three components: (i) a private hospitals TB consortium at district and state level consisting of hospital managements for policy support and review (ii) a coalition of medical professional associations at state and district levels for advocating with doctors and (iii) a STEPS centre in each private hospital. STEPS centre within a private hospital is a single window for diagnostic and treatment services, notification, patient linkage with social welfare, contact investigation, TB Preventive Therapy and treatment adherence support. A central person (STEPS lead) nominated by the hospital management, work together with contact persons (STEPS links) for each in-house department in a hub-and-spoke model. The STEPS lead and links are typically staff nurses. STEPS Links from various in-house clinical departments transfer the patients and related information to the STEPS Lead. Patients visit STEPS centre where education, counselling, support and linkages for molecular diagnostics, anti TB treatment initiation, contact investigations, TB Preventive Therapy, social welfare schemes and air borne infection control counselling are provided. STEPS Lead follows up the patient periodically over telephone and provides treatment adherence support, monitors adverse drug reactions, reminds clinical follow up and schedules reviews. Patient visits the concerned in house departments for clinical follow up. Through STEPS, private hospitals proactively supported patients to make decisions and participate in their own care. It also fosters customer loyalty. STEPS Lead enters information in NI-KSHAY. NTEP through its field staff and also through the five city officers of project JEET, a PPSA worked in the state for 2 years (2018–2019), provided customised and need based support to the patients reaching private hospitals, through the STEPS centres, including free diagnostics such as molecular tests and drug susceptibility testing, transportation of specimen from the hospitals for testing, free drugs, support for contact investigation, TB Preventive Therapy, Direct Benefit Transfer, linkages to social welfare services and retrieval if there is a lost to follow up. STEPS was implemented in all 14 districts of Kerala state, India since January 2019. Of the 446 hospitals mapped which manages more than 80% of the TB patients in the state, 318 established STEPS centres during 2019 and the remaining in 2020 [ 17 , 30 ].
Outcomes of STEPS
JMM (2019 November, India) visited Kerala and recommended supporting the establishment of STEPS Centres in all private health care facilities [ 15 ]. A formal evaluation of STEPS was conducted by a multi-disciplinary team in 2021 by (i) visiting 30 randomly selected STEPS centres for assessing infrastructure and process using a checklist, (ii) validating the patient data with Ni-kshay by telephonic interview of 57 TB patients (iii) analysing the quality of patient care indicators (iv) conducting in-depth interviews with 33 beneficiaries and stakeholders to understand their satisfaction and perceived benefits and (v) a cost analysis from the perspective of NTEP, private hospital and patients [ 31 ] .
Evaluation concluded that STEPS is a low cost, ‘patient centric’ model where the patient can approach any provider according to his/her choice and get uniform high quality TB care. Evaluation also revealed that STEPS was acceptable to all stakeholders and patient satisfaction was good. There was significant improvement in the quality of TB care indicators for patients diagnosed in private hospitals over the years in terms of notification, proportion with a microbiological confirmation of diagnosis, known HIV status, beneficiary receiving baseline rifampicin testing, Direct Benefit Transfer and the documented treatment success rate (Fig. 3 ).
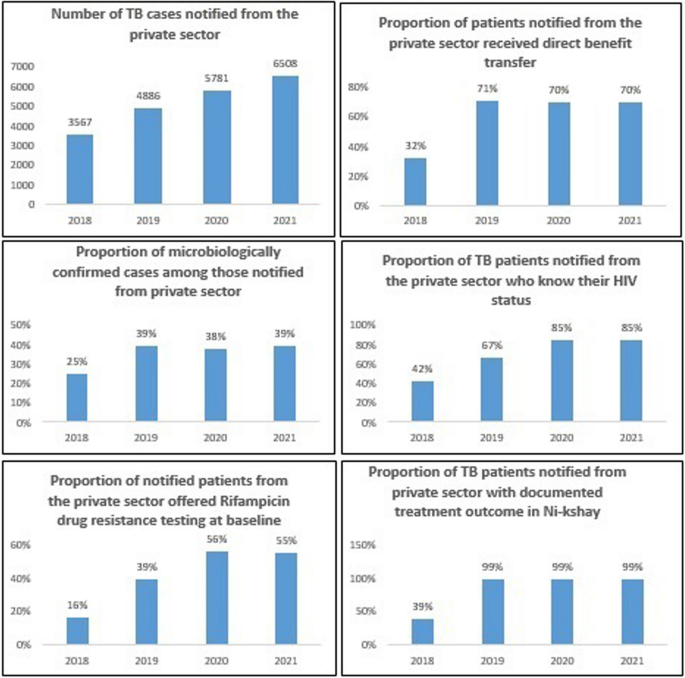
Trend in the indicator for ‘quality of care’ among the patients with TB who availed care from private sector in Kerala (2018–2021)
Number of specimens from private sector tested using molecular testing machines at public laboratories increased from 7606 in 2018 to 14,940 in 2021 [ 9 ]. STEPS led to a shift from using private anti-TB drugs to NTEP-supplied drugs and there was a drastic drop in the sale of anti-TB drugs in the state from 1.6 million rifampicin units in 2018 to 0.5 million rifampicin units in 2019 [ 16 , 17 , 31 ]. STEPS had also demonstrated good resilience in ensuring TB services during COVID-19 pandemic period [ 36 ].
Schedule H1 surveillance
Since 2016, the Government of Kerala has enforced Schedule H1 implementation for anti-TB drugs as a joint venture by the drugs control department and state TB Elimination program, with monitoring from the top administrative level. From 2018 onwards, the state program managers of NTEP have modified it into a Schedule H1 surveillance system [ 28 ]. The process of schedule H1 surveillance and its benefits were documented through in-depth interviews of the drugs control department enforcement officers, chemist shop owners, private sector doctors, leaders of professional medical associations, and program managers and key staff of the TB Elimination Program in Kerala [ 28 ]. The TB Elimination Program of Kerala used the information from the Schedule H1 drug register to identify the missing TB cases from the surveillance system, identify providers who prescribed the anti TB drugs and extended support to them for ensuring STCI to their patients, and provide feedback to providers regarding prescription practices. The estimated number of un-notified TB cases per 100,000 people based on the total sales of rifampicin-containing products in Kerala showed an annual decline of 22% over the last few years, closing the gap in the surveillance system [ 17 ]. The major initiatives taken by the state for ensuring quality care to the patients reaching the private sector is represented schematically in Fig. 4 .
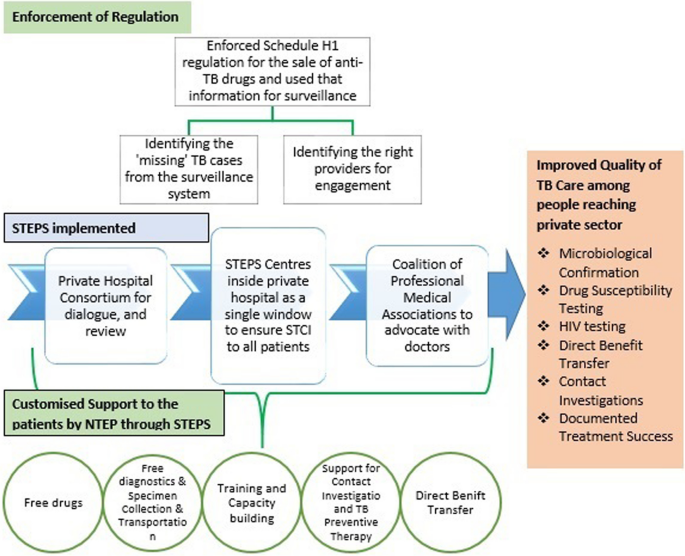
Schematic representation of major initiatives by Kerala to improve the quality of TB care among people reaching the private sector
Learnings from Kerala for engaging private sector for TB care
Vision of partnership: Most of the partnership models implemented in the country were mostly business-centred like incentive-based or service-purchase models that were similar to a client-vendor relationship rather than an equal partnership between the public and private sectors. Several models implemented in India that have successfully increased private case notifications were difficult to expand due to lesser emphasis on creating lasting partnerships and huge short-term financial implications [ 37 , 38 ]. As the intermediary agency who interacts with the private sector withdraws, the model collapses. Kerala state has literally redefined the ‘private sector engagement’ as a partnership for shared health outcomes. The vision of partnership that the state implemented through STEPS is in line with the recent vision shared by World Health Organization which described partnership as a means to “bring together a set of actors for the common goal of improving the health of populations based on mutually agreed roles and principles based on the principles of relative equality between the partners, mutual benefits to the stakeholders, autonomy, accountability and mutual commitment to agreed objectives” [ 39 ]. The state has proved that partnership with private sector is possible in the form of a relationship and not mere as contracts or purchase.
Strategic policy direction: Having a clear policy and strategy will help to avoid confusions among the stakeholders and will enable them to execute better. It should clearly define the goals and objectives of private sector engagement, clarify roles of all stakeholders, describe the institutional arrangements for engagement, outline the feasible strategies and arrangements to monitor performance. Health system decision makers need to know “where they are going” to be able to make efficient use of finite resources. The state has set a clear strategic policy direction with regard to engagement of private sector and trained all program managers regarding the same [ 29 ]
Strong governance mechanism: In Kerala, the state government focused on the governance of the whole health system – both private and public – to ensure access to quality TB care. The government efforts were successful in aligning the private sector with a common goal and making them commit to work to support the agenda. Both the sectors collectively delivered on the realization to ensure access and quality of TB care. Institutional mechanisms for periodic interaction between the public health system and the private sector were established. There was a clear understanding and delineation of the roles based on each stakeholder’s skills and expertise and there was mechanism to ensure accountability of both sectors. There was also a strong regulatory environment with regard to implementation of schedule H1 for anti-TB drugs, at the same time the information through the process has been used for generating data intelligence and ‘nudging’ positive behaviors among the private sector. The governance mechanisms ensured that the actors have the powers to do their jobs and to ensure that others do theirs. The government proactively promoted initiatives like IPAQT where TB tests are made available at affordable prices in the private sector [ 40 ]. Through ‘Coalition of Professional Medical Associations’ the government tried to bring in a social regulation too promoting STCI to every citizen.
Understanding the ‘problem’: Clear understanding of the ‘problem’ that is to be solved through partnership is critical in any successful partnership. The partnership needs to be tailor made to address the identified problems. Similarly understanding the behaviour of stakeholders and involving them from the planning phase are also important before developing any strategy. In Kerala, efforts were put in formally through operations research to identify the problem clearly and document the perceptions and concerns of different stakeholders [ 32 , 33 ]. STEPS evolved as a solution to address those concerns. Major challenges for ‘partnerships’ identified through various operations research done in the state and how that have been addressed through the STEPS is summarised in Table 2 . Kerala experience also reiterates that it is better to avoid over-reliance on pre-designed solutions when it comes to designing partnerships.
Generate data intelligence: There need to be systematic efforts for collection, compilation and analysis of data to guide the priorities for action. In Kerala, overall data regarding the sales of anti-TB drugs helped in (1) estimating the load of patients being treated in private sector and (2) monitor the trends. Granular information from schedule H1 data helped to (1) identify the potential providers for engagement and (2) identify the cases missed from the official surveillance system [ 28 ]. Experiences from the state iterate that pharmacy based surveillance of anti-TB drug sales has immense potential to improve the overall quality of TB care.
Enable stakeholders, foster relations and align structures: In Kerala, organizational structures were aligned towards the policy objectives that empowered the actors. ‘Private hospital consortium’ and ‘coalition of professional medical associations’ at state and district level enabled actors to work openly, sustainably, and effectively together, with trust. The consortium became the face of the private sector which facilitated dialogues between partners, catalyzed the implementation of policies and ensured that both the sectors are held accountable for their actions for the population. There were also district and sub-district wise WhatsApp groups with STEPS leads of all hospitals and the NTEP staff which facilitated smooth and easy communication. All such efforts to foster relations allowed stakeholders to move beyond simply understanding one another, to being able to work together.
Openness to change : Important strategic change that contributed to rapid scale-up and acceptance of STEPS were the attempts to gain the trust of hospital management, involvement of nurses for documentation and counselling, efforts for quality control through a coalition of professional medical associations, lack of formal memoranda of understanding, and lack of major financial transactions between partners [ 30 ]. Such flexibility and openness to change is crucial while governing mixed health systems.
Patient needs to be at the centre of every partnership: All public private partnership need to be ‘patient centric’ and their specific health needs and desired health outcomes should be the driving force. STEPS is a patient centric partnership where patient is the central figure in the continuum of care [ 30 , 31 ]. STEPS tells us that partnerships need to be designed after understanding the needs, preferences and circumstances of patients.
Equipping the public sector with the necessary skills and understanding to effectively engage with private sector: The state has undertaken a behavior change strategy for the NTEP staff to have a uniform outlook with regard to private sector engagement. Capacity of program managers and peripheral staff were built to deal with private sector in a more efficient way [ 31 ].
Incorporating UHC principles into a business model is possible: STEPS is a model based upon a self-initiated business promotion focusing on the concepts of ‘quality of care’ and ‘customer loyalty’ blended with the social responsibility of the private sector. STEPS centers used ‘after-sales support’ by following up every client, which could also be viewed as a business strategy which typically leads to higher customer satisfaction, brand loyalty, and even word-of-mouth marketing. Cost estimates revealed that implementing STEPS will be of benefit to private hospitals in terms of business returns [ 31 ].
The current study documents various approaches and efforts taken by the Kerala TB Elimination program to partner with the‘for profit’ formal private health care delivery providers for ensuring quality TB care. The current study attempts to synthesise the evidences generated from various studies conducted over different time periods and narrates a story which explains the changes in strategies, outcomes and the reasons for the same. Though the results are not meant to be generalized to other settings, it provides lot of insights to strengthen the private sector engagement in the country.
Though, Kerala state was proactive in engaging hospital and laboratories for DOTS (Directly Observed Treatment Short course) through formal memorandum of understanding through various partnership schemes and various efforts for capacity building, a significant chunk of private hospitals could not be engaged until 2018 resulting in sub-optimal quality of care to a significant proportion of people reaching the private sector in terms of not being offered treatment adherence support, baseline susceptibility testing for Rifampicin, contact investigations and linkages to social welfare schemes. After thorough understanding of the problem and the characteristics of the private sector and in consultation with the stakeholders, the state initiated STEPS which is an equal partnership model where both sectors have been made accountable to ensure uniform standards of TB care to the citizens reaching the private sector. Through enforcing schedule H1 regulations for the sales of anti-TB drugs, the state generated data and established an intelligence system for prioritising actions for partnerships and monitoring the outcomes. The implementation resulted in improvement in the quality of TB care among the people with TB reaching the private sector too.
Through the initiatives, Kerala has literality reframed ‘private sector engagement’ as ‘a partnership where both the sectors come together for the benefit of the society’. STEPS ensured that public resources are not diverted too much; and whatever is diverted through the STEPS is for the patient. In a country like India where most of the patients seek care from the private sector and the program managers are thriving to ensure quality of care in public sector with the limited resources, ‘purchasing’ of services and ‘incentivising’ of the private sector may not be the best way to create sustainable partnerships. Instead, the commitments to offer quality TB services need to be considered as the prime partnership option. Private sector will be more than willing to improve the quality of care to their clients as it improves patient satisfaction and provides good marketing advantage in the competitive healthcare. High out of pocket health expenditure will be the only concern then, which could be addressed to the needy through public provision of services through the private partner, promoting schemes such as IPAQT where TB tests are made available at affordable prices in the private sector or hospitals getting reimbursed for drugs and diagnostics through national health insurance schemes.
Kerala demonstrated that if Governments take a proactive role in engaging the private sector, in an informed and evidence-based way, they can leverage the advantages of the private sector while protecting the public health interest. The case study reiterate that a good governance is essential for ensuring care in a mixed health system. The pursuit of UHC requires Governments to take ownership of healthcare, irrespective of where a person seeks care. WHO Advisory Group on the Governance of the Private Sector for Universal Health Coverage also emphasise a fundamental shift in the Governance behaviours to do business in a new way [ 39 ].
Data intelligence is crucial to successful governance. Good governance require data to identify whom to engage, monitor the progress and measure the impact. The state has used data coming out from the drug sales for the surveillance purpose. The state demonstrated that pharmacy based surveillance of anti-TB drug sales has immense potential to help the TB programs in improving the quality of care in private sector.
Success of STEPS also emphasises that successful private sector engagement initiatives need to have a platform for meaningful dialogue. Strengthening platforms, or structures for dialogue, and communication between sectors is important in building trust, and co-development of policies. Medical College Task Force is an example of a structured mechanism where NTEP interacts with all medical colleges [ 41 ]. It has state, zonal and national level structures. Similarly, a ‘Private Health Sector Task Force/consortium’ created at district, state, zonal and national level need to be established at the country level.
Kerala state has taken proactive role to partner with private sector after ensuring the quality of care in the public system. The model need to be customised and contextually adapted following the principles and approach and shall not be replicated as such, especially in settings where health systems are weak. It has also to be emphasised that the current experiences with the STEPS model in Kerala are with private hospitals and not with individual practitioners. It is also recommended that Kerala needs to systematically capture the patient’s cost and document the same for more meaningful insights.
Kerala state has taken a variety of interventions through STEPS to ensure universal access to TB care for citizens reaching the private sector with documented improvement in the quality of TB care. Kerala demonstrated that if Governments take a proactive role in engaging the private sector, in an informed and evidence-based way, they can leverage the advantages of the private sector while protecting the public health interest. The case study reiterates that a good governance is essential for ensuring care in a mixed health system and such successful governance requires good data intelligence. Kerala reminds us that identifying the correct ‘problems’ are critical for effective design of partnerships and over reliance on pre designed solutions may not work.
Availability of data and materials
Data are available upon reasonable request. Data collected for the study, including individual de-identified participant data and a data dictionary defining each field in the set, study protocol, informed consent forms will be made available to others for a period of three years from the date of publication of the article, with a signed data access agreement and on due approval from the institutional ethics committee of Amity University, India, on submission of request to the principal investigator explaining the purpose for which the data will be used along with other relevant supporting documents.
World Health Organization (WHO) country Office for India. Standards for TB Care in India. WHO; 2014. [Accessed March 1, 2023]. Available from: https://apps.who.int/iris/bitstream/handle/10665/208894/stci_book.pdf
Indian Council of Medical Research. National TB prevalence survey 2019–2021. ICMR;2022[Accessed March 1 2023]. Available from: https://tbcindia.gov.in/showfile.php?lid=3659
Arinaminpathy N, Batra D, Khaparde S. The number of privately treated tuberculosis cases in India: an estimation from drug sales data. Lancet Infect Dis. 2016;16(11):1255–60.
Article PubMed PubMed Central Google Scholar
Stallworthy G, Dias HM, Pai M. Quality of tuberculosis care in the private health sector. J Clin Tuberc Other Mycobact Dis. 2020;20:100171.
Kwan A, Daniels B, Saria V. Variations in the quality of tuberculosis care in urban India: a cross-sectional, standardized patient study in two cities. PLoS Med. 2018;15(9):e1002653.
Rupani MP, Shah CJ, Dave JD, Trivedi AV, Mehta KG. ‘We are not aware of notification of tuberculosis’: A mixed-methods study among private practitioners from western India. Int J Health Plann Manag. 2021;36(4):1052–68.
Article Google Scholar
Satyanarayana S, Subbaraman R, Shete P, et al. Quality of tuberculosis care in India: a systematic review. Int J Tuberc Lung Dis. 2015;19(7):751–63.
Article CAS PubMed Google Scholar
Government of India. Ministry of health and family welfare (MOHFW). Central TB Division. National Strategic Plan for tuberculosis elimination 2017–2025. MOHFW; 2017. [Accessed March 1, 2023]. Available from: https://tbcindia.gov.in/WriteReadData/NSP%20Draft%2020.02.2017%201.pdf
Ministry of Health and Family Welfare. India TB Report 2022.CTD. [Accessed January 10,2023]. Available from: https://tbcindia.gov.in/WriteReadData/IndiaTBReport2022/TBAnnaulReport2022.pdf
Government of India. Ministry of Health and Family Welfare. Gazette of India notification G.S.R. 588(E). 2013. Drugs and cosmetics (fourth amendment) rules, 2013 [Accessed January 1, 2023].Available from: https://thehealthmaster.com/wp-content/uploads/2020/10/GSR-No.-588E-Dt-30-08-2013-Schedule-H1-Drugs-DC-fourth-Amendment-Rules-2013.pdf
Government of India. Ministry of Health and Family Welfare. National Tuberculosis Elimination Programme. NIKSHAY. [Accessed March 1, 2023]. Available from: https://www.nikshay.in/
Government of India. Ministry of Health and Family Welfare. Direct Benet Transfer Manual for National Tuberculosis Elimination Programme. [Accessed March 1, 2023]. Available from: https://tbcindia.gov.in/WriteReadData/l892s/23294204DBTManualForNTEP.pdf
Government of India. Ministry of health and family welfare. Gazette of India notification GSR 588(E). 2013. [Accessed March 1, 2023] https://thehealthmaster.com/wp-content/uploads/2020/10/GSR-No.-588E-Dt-30-08-2013-Schedule-H1-Drugs-DC-fourth-Amendment-Rules-2013.pdf
Government of India. Ministry of health & family welfare. Guidance document on partnerships 2019. [Accessed March 1, 2023] Available from: https://tbcindia.gov.in/WriteReadData/l892s/9531588006Guidance%20Doucument%20on%20Partnerships%20RNTCP%202019.pdf
Government of India, Ministry of Health & Family Welfare (MOHFW), Central TB Division. Report of the joint monitoring Mission. Revised National Tuberculosis Control Programme November 2019. MOHFW; 2019. [Accessed March 5, 2023]. Available from: https://tbcindia.gov.in/showfile.php?lid=3536
Government of Kerala Department of health and family welfare. Making the SDG a reality. Government of Kerala; 2021. [Accessed March 1, 2023]. https://www.keralahealthconference.in/e-Book.pdf
Government of Kerala. Kerala Health Conference-Making the SDG A reality—moving towards TB elimination—4 march 2021. [Video Recording]. Thiruvananthapuram: GOK. 2021. [Accessed March 4, 2023]. Available from: https://www.youtube.com/watch?v=C4Yr_FTMdgQ&t=3452s
Jeyashree K, Thangaraj J, Rade K, Modi B, Selvaraju S, Velusamy S, et al. Subnational TB claims verification group. Estimation of tuberculosis incidence at subnational level using three methods to monitor progress towards ending TB in India, 2015-2020. BMJ Open. 2022;12(7):e060197. https://doi.org/10.1136/bmjopen-2021-060197 .
Article PubMed Google Scholar
George LS, Rakesh PS, Sunilkumar M, Vijayakumar K, Kunoor A, Kumar VA. TB patient support systems in Kerala: A qualitative analysis. Indian J Tuberc. 2021;68(1):9–15. https://doi.org/10.1016/j.ijtb.2020.11.005 . Epub 2020 Nov 7
Manju R Nair, Ravi Prasad Varma. Availability, distribution and utilisation of health care services in Kerala. Thiruvananthapuram. 2021. [Accessed March 7, 2023] Available from: https://spb.kerala.gov.in/sites/default/files/inline-files/AvailDistribUtilisationHSKerala.pdf
World Health Organisation. The private health sector: an operational definition. Geneva. 2020. [Accessed on March 7, 2023]. Available from: https://www.who.int/docs/default-source/health-system-governance/private-health-sector-an-operational-definition.pdf
Kumar MK, Dewan PK, Nair PK, et al. Improved tuberculosis case detection through public-private partnership and laboratory-based surveillance, Kannur District, Kerala, India, 2001–2002. Int J Tuberc Lung Dis. 2005;9(8):870–6.
CAS PubMed Google Scholar
World Health Organization (WHO). Public–Private Mix for DOTS Global Progress. Report of the Second Meeting of the PPM Subgroup for DOTS Expansion . WHO; 2004. [Accessed April 17, 2023].Available from: https://apps.who.int/iris/handle/10665/68619
Ferroussier O, Kumar MK, Dewan PK, Nair PK, Sahu S, Wares DF, et al. Cost and cost-effectiveness of a public-private mix project in Kannur District, Kerala, India, 2001-2002. Int J Tuberc Lung Dis. 2007 Jul;11(7):755–61.
Greaves F, Ouyang H, Pefole M, MacCarthy S, Cash RA. Compliance with DOTS diagnosis and treatment recommendations by private practitioners in Kerala, India. Int J Tuberc Lung Dis. 2007;11(1):110–2.
Chugh S. IMA GFATM RNTCP PPM project: milestones in sight one year after. J Indian Med Assoc. 2008;106(7):454–8.
PubMed Google Scholar
Rakesh PS, Balakrishnan S, Jayasankar S, Asokan RV. TB management by private practitioners – is it bad everywhere? Indian J Tuberc. 2016;63(4):251–4. https://doi.org/10.1016/j.ijtb.2016.09.009 .
Ps R, Balakrishnan S, Ramachandran R, Nandhan S, Samuel NI, Pp P, et al. Using a pharmacy-based surveillance system to improve standards for TB Care in Kerala. India Glob Health Sci Pract. 2021;9(4):846–54. https://doi.org/10.9745/GHSP-D-21-00346 .
Government of Kerala. Kerala TB Elimination Mission. August 2017. [Accessed on March 2, 2023] Available online: https://dhs.kerala.gov.in/wp-content/uploads/2021/01/KeralaTB-Elimination-Mission_Strategy-activity-plan-and-budget..pdf .
Balakrishnan S, Rakesh PS, Sunilkumar M, Sankar B, Ramachandran R, Ameer KA, et al. STEPS: A solution for ensuring standards of TB Care for Patients Reaching Private Hospitals in India. Glob Health Sci Pract. 2021;9(2):286–95. https://doi.org/10.9745/GHSP-D-20-00449 .
Rakesh PS, Balakrishnan S, Sunilkumar M, Alexander KG, Vijayan S, Roddawar V, et al. STEPS - a patient centric and low-cost solution to ensure standards of TB care to patients reaching private sector in India. BMC Health Serv Res. 2022;2(1):2. https://doi.org/10.1186/s12913-021-07342-y .
Rakesh PS, Nair S, Philip S, Varma RP. Barriers for involvement of private doctors in RNTCP – qualitative study from Kerala, India. J Family Med Prim Care. 2019;8(1):160–5. https://doi.org/10.4103/jfmpc.jfmpc_208_18 .
Philip S, Isaakidis P, Sagili KD, Meharunnisa A, Mrithyunjayan S, Kumar AM. "They know, they agree, but they don't do"--the paradox of tuberculosis case notification by private practitioners in Alappuzha district, Kerala, India. PLoS One. 2015;10(4):e0123286. https://doi.org/10.1371/journal.pone.0123286 .
Article CAS PubMed PubMed Central Google Scholar
Kunoor A, James PT, Raj M, Rajesh CT, Nair P, Rakesh PS. Outcomes of patients treated with individualised anti-tuberculosis regimens in a tertiary care center in India. Public Health Action. 2017;7(4):308. https://doi.org/10.5588/pha.17.0062 .
Nair P, James PT, Kunoor A, Rakesh PS. How we ensured 100% TB notification: experiences from a private tertiary care hospital in India. Public Health Action. 2017;7(2):179–80. https://doi.org/10.5588/pha.16.0128 .
Khobragade RN, Kelkar RU, Sunilkumar M, Cency B, Murthy N, Surendran D, et al. Health system resilience: ensuring TB services during COVID-19 pandemic in Kerala. India Indian J Tuberc. 2022;69(4):427–31. https://doi.org/10.1016/j.ijtb.2021.10.004 . Epub 2021 Oct 13y
Government of India, Ministry of Health & family welfare, National Tuberculosis Elimination Programme (NTEP). Universal access to TB care (UATBC): concurrent assessment report. NTEP; 2016. [Accessed March 9, 2021]. Available from: https://tbcindia.gov.in/index1.php?sublinkid=4711&level=3&lid=3265&lang=1.
Shibu V, Daksha S, Rishabh C, Sunil K, Devesh G, Lal S, et al. Tapping private health sector for public health program? Findings of a novel intervention to tackle TB in Mumbai. India Indian J Tuberc. 2020;67(2):189–201.
World Health Organisation. Engaging the private health service delivery sector through governance in mixed health systems: strategy report of the WHO advisory group on the governance of the private sector for universal health coverage. Geneva: WHO; 2020. [Accessed on Feb 3,2023]. Available from: https://www.who.int/activities/strengthening-private-sector-engagement-for-uhc
Initiative for Promoting Affordable and Quality TB Tests (IPAQT). [Online] Available at: https://healthmarketinnovations.org/program/initiative-promoting-affordable-and-quality-tb-tests-ipaqt
Central TB Division. Training modules (5-9) for programme managers & medical officers. New Delhi July 2020. [Accessed on March 3, 2023] Available from: https://tbcindia.gov.in/WriteReadData/NTEPTrainingModules5to9.pdf
Download references
Acknowledgements
This research received no external funding.
Author information
Authors and affiliations.
Amity Institute of Public Health & Hospital Administration, Amity University, Noida, India
P. S. Rakesh & Mohd Shannawaz
The Union South East Asia Office, New Delhi, India
P. S. Rakesh
You can also search for this author in PubMed Google Scholar
Contributions
PSR & MS have substantial participation in the conception, design, collection of data, analysis and interpretation of the data. PSR wrote the manuscript, MS reviewed and edited it. Both approved the final version of the paper.
Corresponding author
Correspondence to P. S. Rakesh .
Ethics declarations
Ethics approval and consent to participate.
This study involves human participants. The study was approved by institutional review board and ethics committee of Amity University, Noida, India. Participants gave written informed consent to participate in the study before taking part.
Consent for publication
Not Applicable as no personal information regarding participants are revealed.
Competing interests
PS Rakesh was closely associated with the state TB Elimination program, Kerala in multiple roles such as WHO consultant & State Technical consultant for IMA-GFATM-RNTCP-PPM Project and was part of all major recent initiatives for private sector engagement. He also contributed to most of the studies and evaluations regarding private sector engagement for TB care in the state, which were mentioned in the current study. This could be a potential personal conflict of interest as his views and ideologies can influence this research.
Additional information
Publisher’s note.
Springer Nature remains neutral with regard to jurisdictional claims in published maps and institutional affiliations.
Supplementary Information
Supplementary material 1..
Abstract in Malayalam.
Rights and permissions
Open Access This article is licensed under a Creative Commons Attribution 4.0 International License, which permits use, sharing, adaptation, distribution and reproduction in any medium or format, as long as you give appropriate credit to the original author(s) and the source, provide a link to the Creative Commons licence, and indicate if changes were made. The images or other third party material in this article are included in the article's Creative Commons licence, unless indicated otherwise in a credit line to the material. If material is not included in the article's Creative Commons licence and your intended use is not permitted by statutory regulation or exceeds the permitted use, you will need to obtain permission directly from the copyright holder. To view a copy of this licence, visit http://creativecommons.org/licenses/by/4.0/ . The Creative Commons Public Domain Dedication waiver ( http://creativecommons.org/publicdomain/zero/1.0/ ) applies to the data made available in this article, unless otherwise stated in a credit line to the data.
Reprints and permissions
About this article
Cite this article.
Rakesh, P.S., Shannawaz, M. Ensuring universal access to quality care for persons with presumed tuberculosis reaching the private sector: lessons from Kerala. Int J Equity Health 23 , 101 (2024). https://doi.org/10.1186/s12939-024-02151-1
Download citation
Received : 11 March 2023
Accepted : 15 March 2024
Published : 17 May 2024
DOI : https://doi.org/10.1186/s12939-024-02151-1
Share this article
Anyone you share the following link with will be able to read this content:
Sorry, a shareable link is not currently available for this article.
Provided by the Springer Nature SharedIt content-sharing initiative
- Public private partnership
- Private sector engagement
- Schedule H1
- Standards of TB care
- Universal access to TB care
International Journal for Equity in Health
ISSN: 1475-9276
- General enquiries: [email protected]
Wegovy users keep weight off for 4 years, new analysis finds
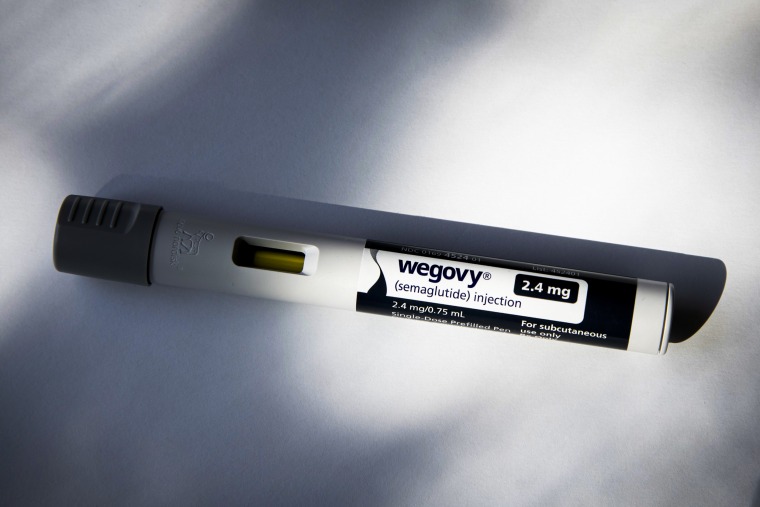
Patients taking Novo Nordisk’s Wegovy obesity treatment maintained an average of 10% weight loss after four years, potentially boosting the drugmaker’s case to insurers and governments to cover the cost of the effective but expensive drug.
The Danish drugmaker presented the new long-term data on Tuesday at the European Congress on Obesity in Venice, Italy, in a new analysis from a large study for which substantial results had been published last year.
“This is the longest study we’ve conducted so far of semaglutide for weight loss,” Martin Holst Lange, Novo’s head of development, said in an interview, referring to the active ingredient in Wegovy and the company’s diabetes drug Ozempic.
“We see that once the majority of the weight loss is accrued, you don’t go back and start to increase in weight if you stay on the drug,” he added.
The data could go some way to convince insurers and governments to reimburse Wegovy, which ranges from $200 to almost $2,000 a month in the 10 countries it has been launched in so far.
Wegovy was the first to market from a newer generation of medicines known as GLP-1 agonists, originally developed for diabetes, that provide a new way to address record obesity rates. Eli Lilly launched its rival drug Zepbound in the United States in December. Neither company has been able to produce enough to meet unprecedented demand .
Dr. Simon Cork, Senior Lecturer in Physiology from Anglia Ruskin University, said Britain’s public health service’s decision to limit coverage of the medicine to two years was “because of questionable long-term effectiveness”.
The new data showing benefits continuing to four years may go some way to negating that argument, he said.
How Wegovy benefits the heart
The 17,604-patient trial tested Wegovy not for weight loss but for its heart protective benefits for overweight and obese patients who had pre-existing heart disease but not diabetes. Participants were not required to track diet and exercise because it was not an obesity study.
Around 17% of trial participants stopped using Wegovy due to side effects, the most common of which was nausea, Novo said in another analysis in the trial published by the drugmaker on Tuesday.
Patients in the trial, called Select, lost an average of nearly 10% of their total body weight after 65 weeks on Wegovy. That percentage weight-loss was roughly sustained year-on-year until the end of about four years, where weight loss stood at 10.2%, the company said.
A third new analysis on Select published by Novo on Tuesday showed that the heart protective benefits of Wegovy to patients in the trial occurred regardless of their weight before starting on the drug and regardless of how much weight they lose on it.
“We now also understand that while we know that body weight loss is important, it’s not the only thing driving the cardiovascular benefit of semaglutide treatment”, Lange told Reuters in the interview.
The Select study, released in August, showed that Wegovy reduced the risk of a major cardiovascular event such as a stroke by 20% in overweight or obese people with a history of heart disease.
Novo says researchers are still working to understand the mechanisms of the cardiovascular protection that semaglutide provides.
Wegovy and Zepbound are being tested to assess their benefits in a variety of other medical uses such as lowering heart attack risk and for sleep apnea and kidney disease.
The weight loss in the heart trial was less than the average of 15% weight loss in earlier Wegovy obesity studies before the drug was launched in the United States in June 2021.

An official website of the United States government
The .gov means it’s official. Federal government websites often end in .gov or .mil. Before sharing sensitive information, make sure you’re on a federal government site.
The site is secure. The https:// ensures that you are connecting to the official website and that any information you provide is encrypted and transmitted securely.
- Publications
- Account settings
Preview improvements coming to the PMC website in October 2024. Learn More or Try it out now .
- Advanced Search
- Journal List
- Elsevier - PMC COVID-19 Collection

Lean six sigma in the healthcare sector: A systematic literature review
Rajeev rathi.
a School of Mechanical Engineering, Lovely Professional University, Phagwara 144001, India
Ammar Vakharia
b School of Electrical and Electronics Engineering, Lovely Professional University, Phagwara 144001, India
Mohd Shadab
Healthcare is a very important sector as our lives depend on it. During the novel corona virus pandemic, it was evident that our healthcare organizations still lack in terms of efficiency and productivity. Especially in the developing nations, the problems were much bigger. Lean Six Sigma (LSS) is a methodology which when implemented in an organization, helps to increase the process capability and the efficiency, by reducing the defects and wastes. The present study systematically reviews the research studies conducted on LSS in the healthcare sector. It was found that comparatively less studies are focused on improving the medical processes, most of the studies targeted the management processes. Moreover, lesser number of studies were being conducted for developing nations, but now it seems that the focus of research scholars has shifted towards the developing nations also. But it was observed that the studies in these nations were majorly empirical in nature, very few studies were conceptual or exploratory. There is a need for guiding healthcare professionals on creating a continuous improvement environment, which sustains the improvements achieved after LSS implementation.
1. Introduction
There is little room for error when it comes to healthcare. Simple mistakes can impact hundreds of people and can lead to fatalities. According to the journal of healthcare finance, medical errors cost the US over nineteen point five billion dollars in 2008. The Institute of Medicine in 1998 estimated that 98 thousand deaths could have been prevented that year due to medical errors. While in 2008 that figure had ballooned to 200 thousand deaths a year, that’s five hundred and forty-eight deaths a day or 1 death almost every two minutes in the US alone [1] . Lean Six Sigma can go in a long way in reducing these devastating preventable deaths. A healthcare practitioner who understands how to use the tools and methodology to systematically resolve problems and improve the quality of care is well-positioned to become a highly valued asset to their organization [2] . They not only prevent deaths but also improve the quality of patient care and reduce operating costs. There are plenty of opportunities for improvement in healthcare. Lean Six Sigma combines the strategies of Lean and Six sigma. Lean is famous for its ability to handle waste and Six sigma is known for process improvement [3] . It is well known that six sigma stands for standard deviation. Therefore, to improve the efficiency and quality of the process combining these philosophies will eliminate waste and reduce variability [4] . First lean methodology is used to eliminates the waste then through six sigma tools we can improve process variation so these two methods go hand in hand in today’s time [5] . Combination of lean and six sigma also gives good results in improving the process flow [6] . Principles of six sigma and lean have a lot of similarities, and that’s why lean and six sigma has been practiced together. Both of them, at the end of the day deliver the same kind of value to the customer and to the businesses [7] . We know that lean and six sigma both sort of originated out of manufacturing, a lot of it from manufacturing of automotive. But today it’s used in the public sector, in customer service, in healthcare and can be used everywhere. Lean thinking goes back a long way. Henry Ford kind of established it early on the first mass production system by combining standard parts conveyors and workflow. Later on, Kiichiro Toyota implemented new concepts, like they came up with things like value stream and Kanban, which become to known as Toyota production system in the 90 s [8] . It became more extensively recognized as a solution that works. Lean in manufacturing was introduced by James Womack who works at the lean institute. When taking a lean approach, the general focus is on the qualitative tools. This is one of the reasons that most lean six sigma activity begin with lean, as qualitative tools are little more intuitive. The concept of lean and six sigma is a little easier to understand then to implement [9] .
Day to day rising in cost of healthcare and decrease in reimbursement rates what can hospitals do to ensure operational efficiencies, quality patient safety, and employee engagement while still meeting the bottom line. Lean and Six Sigma have a combine relationship with each other in improving the quality of services by reducing costs and wastes and gives a good result in healthcare improvement [10] . Lean six sigma is not just a methodology or not just having tools that are needed to make an improvement but it is having mentality and psychology to make change happen. As healthcare is people intensive and process driven industry so this is the perfect environment for lean and six sigma. Worldwide, LSS is being implemented in various service as well as manufacturing organisations. Still, healthcare sector is not much familiar with the sustainable benefits of LSS strategy, especially in developing nation like India, Pakistan, Sri Lanka etc. This study has presented a systematic literature review of LSS in developing nations healthcare organisations and the opportunities was explored. With this study, the healthcare professionals will be able to focus on grey areas to effectively improve their organization performance.
2. Literature review
Exploring the literature for a topic or a field is a very essential process. As soon as a person starts with reviewing the literature, a picture forms slowly with each step ahead. It represents all the major contributions for developing the field. Knowledge of various techniques, scientific methodologies and new technologies is gained as a result of the literature review [11] . Further, it navigates towards the direction where more research work and study are required, in order to achieve advancements in the sector. There are three steps in the research methodology for the current study. In the first step, the SLR is conducted. It includes three sub steps of accessing the articles from various sources, then excluding the articles according to the exclusion criteria of SLR and then finally categorizing them according to various parameters. In the second step, the articles are analysed and some of the trends are observed, like which department of a healthcare organization is given an utmost importance in research perspective. Lastly, the future implications are noted, which can act as a useful guide for healthcare professionals to look for in the upcoming time.
2.1. Systematic literature review
Systematic Literature Review (SLR) on the other hand, is different from the traditional literature review method. It is an exhaustive process, where the literature is explored in a systematic manner, as represented in Fig. 1 . It was first introduced by Tranfield, Denyer, & Smart in their research paper published in the year 2003 [12] . SLR is carried out in 3 phases. The first phase deals with planning of the review, while in the second phase the review is conducted after defining few inclusion criteria. Finally, the articles are reviewed in the third phase [13] .To search for the articles, there is a criterion which is mainly focused on the topic related to lean six sigma methodology implication, LSS framework in healthcare and healthcare sector itself to include/exclude the articles. Moreover, the language of the articles is also considered for the inclusion/extrusion of the articles. In spite of this formal search and collection strategy, was needed to ensure the thoroughness of the literature review which is mainly focused on the topic (healthcare sector). As a result, articles that simply did not discuss the issue of lean six sigma in the healthcare sector were excluded. So, for the current study, hundred and forty articles were analysed but some of them were extruded due to the following barriers:
- • Language of the article (means articles must be only in English).
- • Article must have the concept of lean six sigma.
- • Article must be on healthcare sector.

Systematic Literature Review.
The research articles can be identified according to three main characteristics, namely place, research category and time, as shown in Fig. 2 . Place depicts the country where the research study is focused on. A country can be a developed nation, or a developing nation. While the research category means the type of article or the method used in the article. Research category can be categorized further into 4 types, namely conceptual, descriptive, empirical and exploratory. A conceptual research represents theories and ideas, which are developed using observations. A scholar may introduce a new concept or upgrade an existing methodology, but no experimentation work is generally required here [14] . Whereas a descriptive research study is an inclusive research work which represents all the major contributions, changes or things happening in relation to the field under scope of the project [15] . In order to collect data, surveys are conducted which makes it's obvious that the whole study is navigated solely by the data received and the research scholars have no control over it. Empirical research study is more of an experimental study, which uses observational methods to collect data and then experimentation is done to verify that observations [16] . While an exploratory study tries to address a new topic or a problem whose solution is not yet obtained or is at preliminary stage of development. Exploratory study helps in designing methods which may lead towards a solution or help better understand the field [17] . The time indicates the year in which the study was conducted or published.

Characteristics of research papers.
2.2. Systematic literature review of LSS in healthcare
Healthcare is categorized as a service sector, where service in the form of medical aid is provided. It is very important to rigorously upgrade the healthcare system, in terms of efficiency and productivity. As the healthcare is not just merely a business, but a system which has an impact on all lives. After the introduction of Six Sigma (SS) methodology in 1986 by Bill Smith, and Lean methodology in 1988 by John Krafcik, it took nearly a decade for these individual methodologies to be introduced in the healthcare sector [18] . Very few research studies were conducted for the Lean and SS in healthcare during the early days. In the year 2001, Lean and Six Sigma integration was in the trend. Yet, the use of LSS methodology in healthcare was not seen before the year 2005 [19] . The integration of Lean with Six Sigma results in a method which can reduce wastes and improve productivity, as well as reduce errors and improve patient satisfaction level [20] .A total of 80 articles are considered for the present study. Table 1 lists 47 articles which addresses the developed nations, and the articles which were not written specifically for any nation. While Table 2 contains 33 articles whose main focus was on developing nations, and the healthcare organizations in that countries.
LSS in Healthcare: Developed Nations and Global.
2. LSS in Healthcare: Developing Nations.
Empirical studies were conducted while focusing on a particular hospital or a particular department in a hospital, like Usha Manjunath et. al. 2007 [64] implemented LSS methodology in an Indian hospital, while Giovanni Improta et al 2019 [59] implemented LSS to reduce the length of stay of patients in an Italian hospital, as listed in the table 1 . Research studies focusing on reviewing the literature plays a crucial part in the development of a field, because it shows the complete picture which tells about the overall advancements and the areas where still more study is required. Similarly, for the healthcare sector studies have been conducted to review the literature available. For an example, Peimbert-García et al 2019 [ 55 ] conducted a review and directed the research scholars towards the areas in healthcare which required more focus. Whereas, the scholars have also focused on topics which makes the implementation of LSS in healthcare much easier. Like, V. Vaishnavi et al 2020 [ 90 ] identified 16 readiness factors for LSS implementation in healthcare. These factors should be fulfilled by a healthcare organization before starting with the implementation phase of LSS [96] .Certain crucial factors which affect the implementation of LSS are -:
- • Management willingness and effective leadership
- • Resource capabilities
- • Employee training and trust
- • Financial capabilities
- • Availability of expertise and knowledge
3. Results and discussions
LSS implementation in the healthcare began not earlier than 2005. A lot of research has already been done on LSS in healthcare all over the world, but when it comes to developing nations like India and Brazil, comparatively less research has been conducted [97] .The time constraint trend in catagorisation of the articles, is crucial to indicates how the trends is changing over the year and how many researches are conducted in a year to overcome the problem. Moreover, the time also indicates the change of area of focus of the researchers with changing time. Until the year 2013, research scholars focused majorly on improving the healthcare sector of developed nations, as evident from Fig. 3 .However, commencing from 2014 the focus shifted to developing nations, as they are the ones which require more efficient systems.41 percent of the studies targeted the organizations in developing nations, and further 30 percent of articles from the developing nations category addressed the Indian healthcare organizations, as shown in Fig. 4 .It is important to note this trend because a study related to a particular place demonstrates the importance and need of the study for a unique problem and its solution related to that place. Because as the places differ problems also varies. So that it could be defined as per the requirement related to that particular place.

Year wise distribution of the research articles.

Categorization of the articles according to place.
Fig. 5 helps to distribute the articles according to the type of process in the healthcare organization they target, namely medical processes, management processes and general processes. Further, the articles are also divided according to the place they have focused on. It is evident from the Fig. 5 that comparatively less articles have addressed the medical processes. Most of the research studies conducted in the developing nations were empirical, as depicted in Fig. 6 . Whereas, there is a gap when it comes to exploratory studies and conceptual studies. This trend is significant because, if in a particular country there are less exploratory studies carried out, than the problems unique to that particular country won’t be decomposed. So, there must be an exploratory study to demonstrate the simple and feasible solution. The researchers need to devise new concepts and address the problems which are still not solved yet, while targeting not just one organization but the whole nation. For an example, Sreeranga Bhatt et al 2014 [73] implemented the LSS methodology using DMAIC in a hospital, similarly some other researchers have successfully implemented LSS in one or in some cases up to five hospitals. But this successful research work method cannot be applied to all the hospitals, as all have their own unique problems.

Characteristics of articles according to process.

Research category in developing nations.
3.1. Implications and future opportunities
After analysing the articles included for the current study, some areas or topics were found which lacked in terms of research. Moreover, these can be taken as future opportunities for the research scholar and the healthcare professionals to work on. Healthcare practitioners may be able to identify fields that need more study and, as a result, better strengthen their organizations. The following are the topics which will set direction for future research:
- 1. Medical process: More study is needed to apply the LSS approach in order to minimize defects and wastes associated with surgical procedures such as therapies and operating rooms. The majority of studies were found to be either for managerial processes or were conducted aiming the whole organizational structure.
- 2. Continuous improvement culture: After the implementation of the LSS approach, healthcare managers must be motivated in developing a continuous improvement philosophy in order to sustain the gains made and identify potential prospects. As per the research done by the authors, no studies were found to address this topic.
- 3. Sustainability: It is important to understand environmental factors as well, as the healthcare industry generates bio hazardous wastes that harm the ecosystem. LSS can be integrated with green technologies to have a sustainable improvement framework.
- 4. Supply chain: As evident during the pandemic, the supply chain of the healthcare sector was not quite effective and ready for these kinds of uncertain situations. But the bad times always gives a lesson, and hence it is the best time to reflect on the problems and build an LSS framework which can help even during pandemic.
- 5. More research work is required in developing nations. Moreover, most of the studies were conducted in India, very less aimed at other developing nations like Sri Lanka, Pakistan, Bhutan, and Bangladesh.
- 6. Most of the studies in the developing nations were empirical in nature, more conceptual and exploratory are required. Because these types of studies have the potential to address the unique problems for each individual country. As each country has their own policies and rules, which could produce a challenge for a LSS model which was successful in other nation.
There is a need for improving the performance of healthcare organizations. These organizations faced a lot of difficulty during the corona virus pandemic. Therefore, to avoid such situations in future it has become very critical for the healthcare managers to implement the process improvement methodologies like Lean Six Sigma. Moreover, the managers and their staff are motivated to implement LSS to reduce the defects and wastes related to medical processes like during the surgeries and in operation theatres. After the initiation of LSS methodology, the healthcare managers need to create a continuous improvement culture in their organization, so as to maintain the improvements done and find new opportunities. Lastly, the healthcare professionals and managers need to consider the environmental aspects too, as healthcare sector has bio hazardous wastes which has damaging effects on environment. Lean Six Sigma can be combined with green methodology in order to deal with this [98] . The integrated green lean six sigma could become an effective booster to enhance the quality, effectiveness and efficiency of a particular organisation. Therefore, the management of the healthcare organizations are motivated to implement this method to develop their organization in a sustainable manner [99] . Yet, it is evident that still the journey for LSS implementation in the healthcare sector is a long way ahead, especially for the developing nations.
4. Conclusion
Healthcare is a very crucial and complex sector, it involves several departments, and a failure in one department may have a negative impact on patient's care. It was also evident during the pandemic due to the Covid-19, that we need an operational excellence in healthcare operations. Lean Six Sigma is a methodology which can reduce wastes and variation in an organization with the help of lean and six sigma tool set. Lean Six Sigma is a well-established methodology, mostly used in the manufacturing sector [100] . However, in the past decade it is being extensively used for the non-manufacturing industry also, like the service sector. There has been a shift in number of studies being conducted according to place. The researchers have acknowledged the gap, when it comes to developing nations, and the number of studies conducted has increased for these nations [101] . Present study shows that till, in healthcare sector, LSS is mainly limited in management of operations, and more research is needed in eliminating waste and defects in surgical and operation theatre activities. Furthermore, guidance should be provided to the healthcare professionals on how to create a continuous improvement culture, and then sustain the environment and the improved processes. Researchers are working tirelessly in order to make the healthcare organizations perfect and efficient, so that everyone's life can be improved. Indeed, it is the healthcare sector on which we are dependant for life support.
5. Limitations
An exhaustive systematic literature review was conducted for the present study. The major limitation of this study is that during the exhaustive literature review a number of articles were filtered out which are in language other than English, not related to the healthcare sector, not using the LSS methodology, and not yet published. As a result, a very clear scenario of LSS in the healthcare sector might not be presented. Secondly, the study has not included a LSS framework which can guide the healthcare professionals to implement the LSS methodology in their organizations, but this can be a part in a consecutive paper.
CRediT authorship contribution statement
Rajeev Rathi: Supervision, Conceptualization, Resources, Writing - review & editing. Ammar Vakharia: Conceptualization, Formal analysis, Investigation, Visualization, Validation, Writing - original draft. Mohd. Shadab: Data curation, Formal analysis, Investigation, Visualization, Validation, Writing - original draft.
Declaration of Competing Interest
The authors declare that they have no known competing financial interests or personal relationships that could have appeared to influence the work reported in this paper.

IMAGES
VIDEO
COMMENTS
A case study approach [ 47, 48] was adopted here to understand the deployment of a whole system change in the acute hospital along the four dimensions of STS outlined above. A case study is an approach that is used to generate an in-depth, multi-faceted understanding of a complex issue in its real-life context [ 49 ].
Health System Innovation in Lesotho: Design and early operations of the Maseru Public Private Integrated Partnership. This PwC case study details the design and launch of an innovative partnership to dramatically improve delivery of healthcare in Lesotho in southern Africa. 07 Jun 2012.
Using this case study, we demonstrate how to apply evidence-based processes to healthcare improvement and embed real-world research for optimising healthcare improvement. ... This is compounded by the short supply of research and innovation leadership within the healthcare sector, ... D.A., Purvis, T. et al. Learning together for better health ...
Learn how McKinsey helps private and public healthcare leaders make healthcare better, more affordable, and more accessible for millions of people around the world. ... December 19, 2023 - The healthcare services sector has seen rapid growth over recent years. Despite the challenges organizations may face in 2024,...
Change is a common experience in complex health care systems. Staff, patients and visitors come and go []; leadership, models of care, workforce and governing structures are reshaped in response to policy and legislative change [], and new technologies and equipment are introduced or retired [].In addition to these common changes experienced throughout health care, the acute sector in many ...
Healthcare sector. Follow this topic. Following ... 5% of patients drive half of the health care spending in the U.S. Save; Share; February 07, 2017 ... Management Case Study. David Barrett; Hong ...
The healthcare industry is growing at a very fast pace with global healthcare spending projected to touch USD 8.7 trillion in 2020. 1 The healthcare sector comprises the industries that specialize in products and services that provide health and medical care to patients. The sector can be broadly classified under four main subsectors displayed in Figure 5-1.
One in 10 people in America lack health insurance, resulting in $40 billion of care that goes unpaid each year. Amitabh Chandra and colleagues say ensuring basic coverage for all residents, as other wealthy nations do, could address the most acute needs and unlock efficiency. 13 Mar 2023. Research & Ideas.
combination of policies and services, of the health sector and health sector-led initiatives, and of involving local people, the social economy and wider society. The cases are structured using the following headings: Description of the intervention; How the case study
The healthcare sector represents one of the largest and most exciting areas for digital transformation. The World Bank has estimated that global spending on health will increase from US$9.21 trillion in 2014 to $24.24 trillion in 2040 (Global Burden of Disease Health Financing Collaborator Network 2017, with an uncertainty interval from $20.47 trillion to $29.72 trillion).
PFM in the health sector is complex because of the unpredictable nature of healthcare needs, its life and death consequences and the ever-shifting policies aiming to improve health outcomes. ... This research used a case study design with ethnographic methods. 28 This approach facilitates immersion into the local context and a deep ...
Since 2004, the agency has developed more than 400 Impact Case Studies that illustrate AHRQ's contributions to healthcare improvement. Available online and searchable via an interactive map , the Impact Case Studies help to tell the story of how AHRQ-funded research findings, data and tools have made an impact on the lives of millions of ...
Ya-Ting Yang, Yi-Hsin Elsa Hsu, Kung-Pei Tang, Christine Wang, Stephen Timmon, Wen-Ta Chiu, Saileela Annavajjula, Jan-Show Chu, Case study: international healthcare service quality, building a model for cultivating cultural sensitivity, International Journal for Quality in Health Care, Volume 32, Issue 9, November 2020, Pages 639-642, https ...
This case study demonstrates the power of a commitment to CQI as a driver for excellence in healthcare. The healthcare industry is rich with case studies that provide valuable insights and lessons learned. By analyzing and understanding these success stories, healthcare providers can apply similar strategies to achieve positive outcomes in ...
Design Thinking is being used in varied health care settings and conditions, although application varies. Design Thinking may result in usable, acceptable, and effective interventions, although there are methodological and quality limitations. More research is needed, including studies to isolate critical components of Design Thinking and ...
1. Introduction. Artificial intelligence (AI) is defined as the intelligence of machines, as opposed to the intelligence of humans or other living species [1], [2].AI can also be defined as the study of "intelligent agents"—that is, any agent or device that can perceive and understand its surroundings and accordingly take appropriate action to maximize its chances of achieving its ...
From this brief discussion, the importance of Industry 4.0 in the healthcare sector is obvious. Thus, this review highlights state-of-the-art digitalisation of medicine and healthcare and alludes to the sharp transition this sector is facing while moving towards Industry 5.0. ... Case study in Barnett, Marcellus, Fayetteville, and Haynesville ...
The aim of the study is to provide an overview of how design thinking has been applied in the healthcare sector. Data collection was based on Institute of Scientific Information (ISI) Web of Science, PubMed and Scopus databases. ... Adoption, non-adoption, and abandonment of a personal electronic health record: case study of HealthSpace. BMJ ...
Kim, D.: An integrated supply chain management system: a case study in healthcare sector. In: International Conference on Electronic Commerce and Web Technologies, pp. 218-227. Springer, Heidelberg, August 2005. Google Scholar Langabeer, J.: The evolving role of supply chain management technology in healthcare. J.
It then turns to a case study of the use of AI for healthcare purposes in India, discussing key applications, challenges and risks in this context. ... Simply adding AI applications to a fragmented system will not create sustainable change (ibid.). The healthcare sector has also traditionally been resistant to the permeation of information and ...
Abstract and Figures. A case studies brief by Cenacle Research presenting a variety of Healthcare Analytics solutions crafted to the needs of: + Individuals (Patients) Personalized Healthcare ...
'Big data' is massive amounts of information that can work wonders. It has become a topic of special interest for the past two decades because of a great potential that is hidden in it. Various public and private sector industries generate, store, and analyze big data with an aim to improve the services they provide. In the healthcare industry, various sources for big data include hospital ...
A new AHA Trustee Services resource, Building a Governing Board Strategy on Diversity and Health Equity, includes a series of case studies interviewing nine executives and board leaders, highlighting their hospital or health system's strong commitment to diversity and health equity and underlining their integration into the fabric of their organization's culture and governance strategy.
17- Customer service chatbots: Customer service chatbots allow patients to ask questions regarding bill payment, appointments, or medication refills. 18- Fraud detection: Patients may make false claims. Leveraging AI-pow ered fraud detection tools can help hospital managers identify fraudsters.
A recent study found that 83% of patients report poor communication as the worst part of their experience, demonstrating a strong need for clearer communication between patients and providers. AI technologies like natural language processing (NLP), predictive analytics, and speech recognition might help healthcare providers have more effective communication with patients.
Disparities in care delivery, driven by factors such structural racism and social determinants of health (SDOH), are pervasive and can have a substantial impact on patient care. Many health care providers are tackling the difficult task of reducing disparities and providing more equitable care. In a February 2024 survey of NEJM Catalyst ...
The six countries included in the study are Bangladesh, Kenya, Nigeria, Pakistan, Senegal, and Tunisia. The case studies were conducted by the Local Production and Assistance (LPA) Unit, within the Innovation and Emerging Technologies (IET) Department, part of the Access to Medicines and Health Products Division, at WHO. The LPA Unit's mission ...
The private health care sector in Kerala accounts for more than 70% of all facilities and 60% of all inpatient beds. ... they can leverage the advantages of the private sector while protecting the public health interest. The case study reiterates that a good governance is essential for ensuring care in a mixed health system and such successful ...
The Select study, released in August, showed that Wegovy reduced the risk of a major cardiovascular event such as a stroke by 20% in overweight or obese people with a history of heart disease.
Investigated how Lean Six Sigma methodologies would help the health-care sector. (2017) Empirical: India : 18: An ISM method for identifying factors that can influence lean adoption in healthcare sector. (2017) Conceptual: India : 19: A case study conducted to determine if healthcare organizations are ready to adopt lean. (2018) Descriptive ...