

The Future of AI Research: 20 Thesis Ideas for Undergraduate Students in Machine Learning and Deep Learning for 2023!
A comprehensive guide for crafting an original and innovative thesis in the field of ai..
By Aarafat Islam on 2023-01-11
“The beauty of machine learning is that it can be applied to any problem you want to solve, as long as you can provide the computer with enough examples.” — Andrew Ng
This article provides a list of 20 potential thesis ideas for an undergraduate program in machine learning and deep learning in 2023. Each thesis idea includes an introduction , which presents a brief overview of the topic and the research objectives . The ideas provided are related to different areas of machine learning and deep learning, such as computer vision, natural language processing, robotics, finance, drug discovery, and more. The article also includes explanations, examples, and conclusions for each thesis idea, which can help guide the research and provide a clear understanding of the potential contributions and outcomes of the proposed research. The article also emphasized the importance of originality and the need for proper citation in order to avoid plagiarism.
1. Investigating the use of Generative Adversarial Networks (GANs) in medical imaging: A deep learning approach to improve the accuracy of medical diagnoses.
Introduction: Medical imaging is an important tool in the diagnosis and treatment of various medical conditions. However, accurately interpreting medical images can be challenging, especially for less experienced doctors. This thesis aims to explore the use of GANs in medical imaging, in order to improve the accuracy of medical diagnoses.
2. Exploring the use of deep learning in natural language generation (NLG): An analysis of the current state-of-the-art and future potential.
Introduction: Natural language generation is an important field in natural language processing (NLP) that deals with creating human-like text automatically. Deep learning has shown promising results in NLP tasks such as machine translation, sentiment analysis, and question-answering. This thesis aims to explore the use of deep learning in NLG and analyze the current state-of-the-art models, as well as potential future developments.
3. Development and evaluation of deep reinforcement learning (RL) for robotic navigation and control.
Introduction: Robotic navigation and control are challenging tasks, which require a high degree of intelligence and adaptability. Deep RL has shown promising results in various robotics tasks, such as robotic arm control, autonomous navigation, and manipulation. This thesis aims to develop and evaluate a deep RL-based approach for robotic navigation and control and evaluate its performance in various environments and tasks.
4. Investigating the use of deep learning for drug discovery and development.
Introduction: Drug discovery and development is a time-consuming and expensive process, which often involves high failure rates. Deep learning has been used to improve various tasks in bioinformatics and biotechnology, such as protein structure prediction and gene expression analysis. This thesis aims to investigate the use of deep learning for drug discovery and development and examine its potential to improve the efficiency and accuracy of the drug development process.
5. Comparison of deep learning and traditional machine learning methods for anomaly detection in time series data.
Introduction: Anomaly detection in time series data is a challenging task, which is important in various fields such as finance, healthcare, and manufacturing. Deep learning methods have been used to improve anomaly detection in time series data, while traditional machine learning methods have been widely used as well. This thesis aims to compare deep learning and traditional machine learning methods for anomaly detection in time series data and examine their respective strengths and weaknesses.
Photo by Joanna Kosinska on Unsplash
6. Use of deep transfer learning in speech recognition and synthesis.
Introduction: Speech recognition and synthesis are areas of natural language processing that focus on converting spoken language to text and vice versa. Transfer learning has been widely used in deep learning-based speech recognition and synthesis systems to improve their performance by reusing the features learned from other tasks. This thesis aims to investigate the use of transfer learning in speech recognition and synthesis and how it improves the performance of the system in comparison to traditional methods.
7. The use of deep learning for financial prediction.
Introduction: Financial prediction is a challenging task that requires a high degree of intelligence and adaptability, especially in the field of stock market prediction. Deep learning has shown promising results in various financial prediction tasks, such as stock price prediction and credit risk analysis. This thesis aims to investigate the use of deep learning for financial prediction and examine its potential to improve the accuracy of financial forecasting.
8. Investigating the use of deep learning for computer vision in agriculture.
Introduction: Computer vision has the potential to revolutionize the field of agriculture by improving crop monitoring, precision farming, and yield prediction. Deep learning has been used to improve various computer vision tasks, such as object detection, semantic segmentation, and image classification. This thesis aims to investigate the use of deep learning for computer vision in agriculture and examine its potential to improve the efficiency and accuracy of crop monitoring and precision farming.
9. Development and evaluation of deep learning models for generative design in engineering and architecture.
Introduction: Generative design is a powerful tool in engineering and architecture that can help optimize designs and reduce human error. Deep learning has been used to improve various generative design tasks, such as design optimization and form generation. This thesis aims to develop and evaluate deep learning models for generative design in engineering and architecture and examine their potential to improve the efficiency and accuracy of the design process.
10. Investigating the use of deep learning for natural language understanding.
Introduction: Natural language understanding is a complex task of natural language processing that involves extracting meaning from text. Deep learning has been used to improve various NLP tasks, such as machine translation, sentiment analysis, and question-answering. This thesis aims to investigate the use of deep learning for natural language understanding and examine its potential to improve the efficiency and accuracy of natural language understanding systems.
Photo by UX Indonesia on Unsplash
11. Comparing deep learning and traditional machine learning methods for image compression.
Introduction: Image compression is an important task in image processing and computer vision. It enables faster data transmission and storage of image files. Deep learning methods have been used to improve image compression, while traditional machine learning methods have been widely used as well. This thesis aims to compare deep learning and traditional machine learning methods for image compression and examine their respective strengths and weaknesses.
12. Using deep learning for sentiment analysis in social media.
Introduction: Sentiment analysis in social media is an important task that can help businesses and organizations understand their customers’ opinions and feedback. Deep learning has been used to improve sentiment analysis in social media, by training models on large datasets of social media text. This thesis aims to use deep learning for sentiment analysis in social media, and evaluate its performance against traditional machine learning methods.
13. Investigating the use of deep learning for image generation.
Introduction: Image generation is a task in computer vision that involves creating new images from scratch or modifying existing images. Deep learning has been used to improve various image generation tasks, such as super-resolution, style transfer, and face generation. This thesis aims to investigate the use of deep learning for image generation and examine its potential to improve the quality and diversity of generated images.
14. Development and evaluation of deep learning models for anomaly detection in cybersecurity.
Introduction: Anomaly detection in cybersecurity is an important task that can help detect and prevent cyber-attacks. Deep learning has been used to improve various anomaly detection tasks, such as intrusion detection and malware detection. This thesis aims to develop and evaluate deep learning models for anomaly detection in cybersecurity and examine their potential to improve the efficiency and accuracy of cybersecurity systems.
15. Investigating the use of deep learning for natural language summarization.
Introduction: Natural language summarization is an important task in natural language processing that involves creating a condensed version of a text that preserves its main meaning. Deep learning has been used to improve various natural language summarization tasks, such as document summarization and headline generation. This thesis aims to investigate the use of deep learning for natural language summarization and examine its potential to improve the efficiency and accuracy of natural language summarization systems.
Photo by Windows on Unsplash
16. Development and evaluation of deep learning models for facial expression recognition.
Introduction: Facial expression recognition is an important task in computer vision and has many practical applications, such as human-computer interaction, emotion recognition, and psychological studies. Deep learning has been used to improve facial expression recognition, by training models on large datasets of images. This thesis aims to develop and evaluate deep learning models for facial expression recognition and examine their performance against traditional machine learning methods.
17. Investigating the use of deep learning for generative models in music and audio.
Introduction: Music and audio synthesis is an important task in audio processing, which has many practical applications, such as music generation and speech synthesis. Deep learning has been used to improve generative models for music and audio, by training models on large datasets of audio data. This thesis aims to investigate the use of deep learning for generative models in music and audio and examine its potential to improve the quality and diversity of generated audio.
18. Study the comparison of deep learning models with traditional algorithms for anomaly detection in network traffic.
Introduction: Anomaly detection in network traffic is an important task that can help detect and prevent cyber-attacks. Deep learning models have been used for this task, and traditional methods such as clustering and rule-based systems are widely used as well. This thesis aims to compare deep learning models with traditional algorithms for anomaly detection in network traffic and analyze the trade-offs between the models in terms of accuracy and scalability.
19. Investigating the use of deep learning for improving recommender systems.
Introduction: Recommender systems are widely used in many applications such as online shopping, music streaming, and movie streaming. Deep learning has been used to improve the performance of recommender systems, by training models on large datasets of user-item interactions. This thesis aims to investigate the use of deep learning for improving recommender systems and compare its performance with traditional content-based and collaborative filtering approaches.
20. Development and evaluation of deep learning models for multi-modal data analysis.
Introduction: Multi-modal data analysis is the task of analyzing and understanding data from multiple sources such as text, images, and audio. Deep learning has been used to improve multi-modal data analysis, by training models on large datasets of multi-modal data. This thesis aims to develop and evaluate deep learning models for multi-modal data analysis and analyze their potential to improve performance in comparison to single-modal models.
I hope that this article has provided you with a useful guide for your thesis research in machine learning and deep learning. Remember to conduct a thorough literature review and to include proper citations in your work, as well as to be original in your research to avoid plagiarism. I wish you all the best of luck with your thesis and your research endeavors!
Continue Learning
Is ai-generated content grounds for plagiarism, beginner’s guide to openai’s gpt-3.5-turbo model.
From GPT-3 to GPT-3.5-Turbo: Understanding the Latest Upgrades in OpenAI’s Language Model API.
The Depth I: Stereo Calibration and Rectification
Znote ai: the perfect sandbox for prototyping and deploying code, future trends in conversational ai: what's next.
The future of Conversational AI: from NLU advancements to ethical considerations. Discover emerging trends shaping software development.
Llama 2: A New LLMs Family Has Arrived
Use Llama 2 LLMs with Hugging Face and Transformers

Research Topics & Ideas
Artifical Intelligence (AI) and Machine Learning (ML)
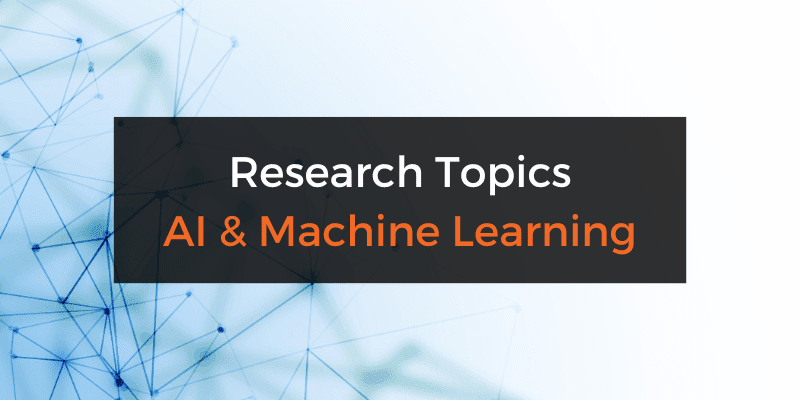
If you’re just starting out exploring AI-related research topics for your dissertation, thesis or research project, you’ve come to the right place. In this post, we’ll help kickstart your research topic ideation process by providing a hearty list of research topics and ideas , including examples from past studies.
PS – This is just the start…
We know it’s exciting to run through a list of research topics, but please keep in mind that this list is just a starting point . To develop a suitable research topic, you’ll need to identify a clear and convincing research gap , and a viable plan to fill that gap.
If this sounds foreign to you, check out our free research topic webinar that explores how to find and refine a high-quality research topic, from scratch. Alternatively, if you’d like hands-on help, consider our 1-on-1 coaching service .
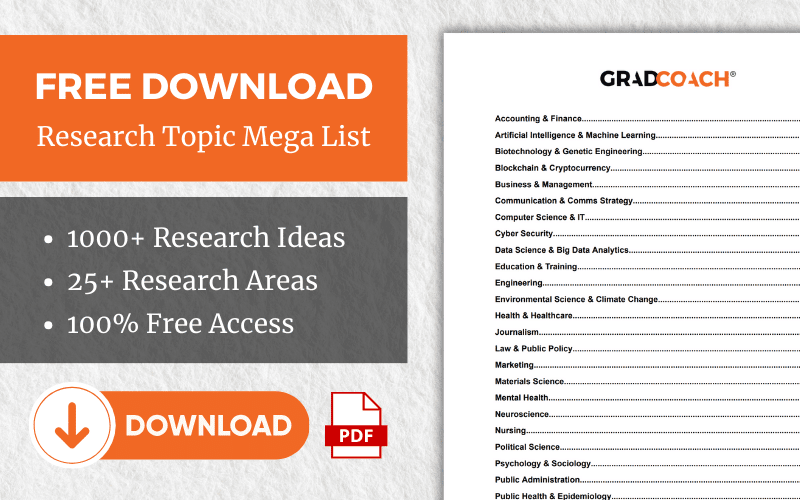
AI-Related Research Topics & Ideas
Below you’ll find a list of AI and machine learning-related research topics ideas. These are intentionally broad and generic , so keep in mind that you will need to refine them a little. Nevertheless, they should inspire some ideas for your project.
- Developing AI algorithms for early detection of chronic diseases using patient data.
- The use of deep learning in enhancing the accuracy of weather prediction models.
- Machine learning techniques for real-time language translation in social media platforms.
- AI-driven approaches to improve cybersecurity in financial transactions.
- The role of AI in optimizing supply chain logistics for e-commerce.
- Investigating the impact of machine learning in personalized education systems.
- The use of AI in predictive maintenance for industrial machinery.
- Developing ethical frameworks for AI decision-making in healthcare.
- The application of ML algorithms in autonomous vehicle navigation systems.
- AI in agricultural technology: Optimizing crop yield predictions.
- Machine learning techniques for enhancing image recognition in security systems.
- AI-powered chatbots: Improving customer service efficiency in retail.
- The impact of AI on enhancing energy efficiency in smart buildings.
- Deep learning in drug discovery and pharmaceutical research.
- The use of AI in detecting and combating online misinformation.
- Machine learning models for real-time traffic prediction and management.
- AI applications in facial recognition: Privacy and ethical considerations.
- The effectiveness of ML in financial market prediction and analysis.
- Developing AI tools for real-time monitoring of environmental pollution.
- Machine learning for automated content moderation on social platforms.
- The role of AI in enhancing the accuracy of medical diagnostics.
- AI in space exploration: Automated data analysis and interpretation.
- Machine learning techniques in identifying genetic markers for diseases.
- AI-driven personal finance management tools.
- The use of AI in developing adaptive learning technologies for disabled students.
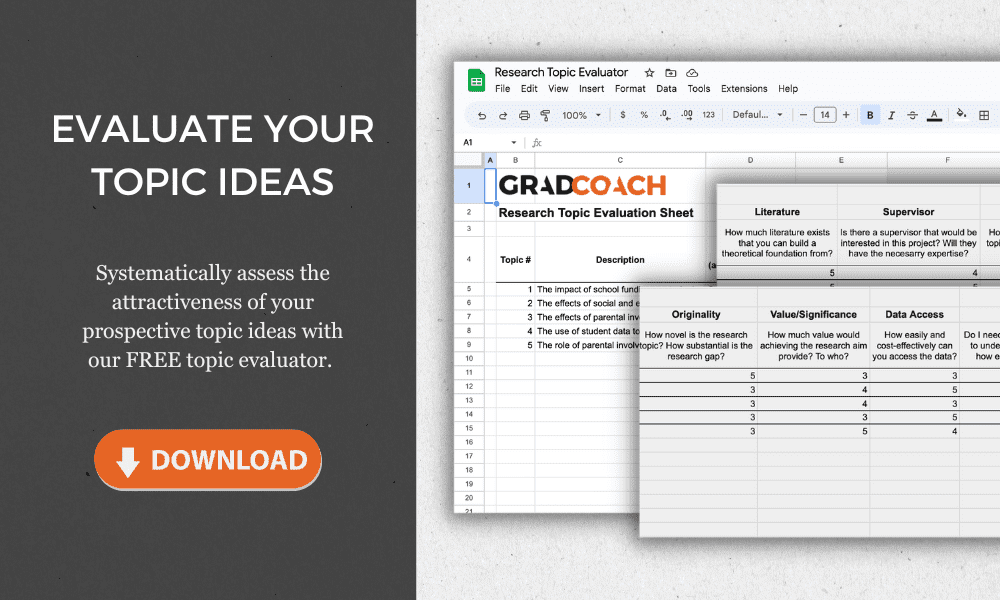
AI & ML Research Topic Ideas (Continued)
- Machine learning in cybersecurity threat detection and response.
- AI applications in virtual reality and augmented reality experiences.
- Developing ethical AI systems for recruitment and hiring processes.
- Machine learning for sentiment analysis in customer feedback.
- AI in sports analytics for performance enhancement and injury prevention.
- The role of AI in improving urban planning and smart city initiatives.
- Machine learning models for predicting consumer behaviour trends.
- AI and ML in artistic creation: Music, visual arts, and literature.
- The use of AI in automated drone navigation for delivery services.
- Developing AI algorithms for effective waste management and recycling.
- Machine learning in seismology for earthquake prediction.
- AI-powered tools for enhancing online privacy and data protection.
- The application of ML in enhancing speech recognition technologies.
- Investigating the role of AI in mental health assessment and therapy.
- Machine learning for optimization of renewable energy systems.
- AI in fashion: Predicting trends and personalizing customer experiences.
- The impact of AI on legal research and case analysis.
- Developing AI systems for real-time language interpretation for the deaf and hard of hearing.
- Machine learning in genomic data analysis for personalized medicine.
- AI-driven algorithms for credit scoring in microfinance.
- The use of AI in enhancing public safety and emergency response systems.
- Machine learning for improving water quality monitoring and management.
- AI applications in wildlife conservation and habitat monitoring.
- The role of AI in streamlining manufacturing processes.
- Investigating the use of AI in enhancing the accessibility of digital content for visually impaired users.
Recent AI & ML-Related Studies
While the ideas we’ve presented above are a decent starting point for finding a research topic in AI, they are fairly generic and non-specific. So, it helps to look at actual studies in the AI and machine learning space to see how this all comes together in practice.
Below, we’ve included a selection of AI-related studies to help refine your thinking. These are actual studies, so they can provide some useful insight as to what a research topic looks like in practice.
- An overview of artificial intelligence in diabetic retinopathy and other ocular diseases (Sheng et al., 2022)
- HOW DOES ARTIFICIAL INTELLIGENCE HELP ASTRONOMY? A REVIEW (Patel, 2022)
- Editorial: Artificial Intelligence in Bioinformatics and Drug Repurposing: Methods and Applications (Zheng et al., 2022)
- Review of Artificial Intelligence and Machine Learning Technologies: Classification, Restrictions, Opportunities, and Challenges (Mukhamediev et al., 2022)
- Will digitization, big data, and artificial intelligence – and deep learning–based algorithm govern the practice of medicine? (Goh, 2022)
- Flower Classifier Web App Using Ml & Flask Web Framework (Singh et al., 2022)
- Object-based Classification of Natural Scenes Using Machine Learning Methods (Jasim & Younis, 2023)
- Automated Training Data Construction using Measurements for High-Level Learning-Based FPGA Power Modeling (Richa et al., 2022)
- Artificial Intelligence (AI) and Internet of Medical Things (IoMT) Assisted Biomedical Systems for Intelligent Healthcare (Manickam et al., 2022)
- Critical Review of Air Quality Prediction using Machine Learning Techniques (Sharma et al., 2022)
- Artificial Intelligence: New Frontiers in Real–Time Inverse Scattering and Electromagnetic Imaging (Salucci et al., 2022)
- Machine learning alternative to systems biology should not solely depend on data (Yeo & Selvarajoo, 2022)
- Measurement-While-Drilling Based Estimation of Dynamic Penetrometer Values Using Decision Trees and Random Forests (García et al., 2022).
- Artificial Intelligence in the Diagnosis of Oral Diseases: Applications and Pitfalls (Patil et al., 2022).
- Automated Machine Learning on High Dimensional Big Data for Prediction Tasks (Jayanthi & Devi, 2022)
- Breakdown of Machine Learning Algorithms (Meena & Sehrawat, 2022)
- Technology-Enabled, Evidence-Driven, and Patient-Centered: The Way Forward for Regulating Software as a Medical Device (Carolan et al., 2021)
- Machine Learning in Tourism (Rugge, 2022)
- Towards a training data model for artificial intelligence in earth observation (Yue et al., 2022)
- Classification of Music Generality using ANN, CNN and RNN-LSTM (Tripathy & Patel, 2022)
As you can see, these research topics are a lot more focused than the generic topic ideas we presented earlier. So, in order for you to develop a high-quality research topic, you’ll need to get specific and laser-focused on a specific context with specific variables of interest. In the video below, we explore some other important things you’ll need to consider when crafting your research topic.
Get 1-On-1 Help
If you’re still unsure about how to find a quality research topic, check out our Research Topic Kickstarter service, which is the perfect starting point for developing a unique, well-justified research topic.
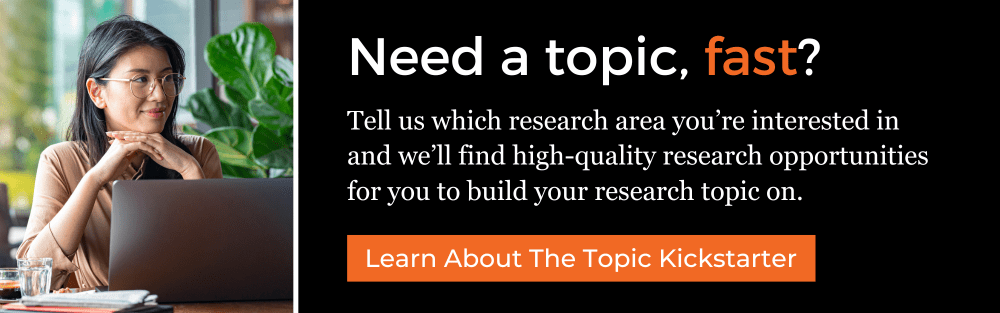
can one come up with their own tppic and get a search
can one come up with their own title and get a search
Submit a Comment Cancel reply
Your email address will not be published. Required fields are marked *
Save my name, email, and website in this browser for the next time I comment.
- Print Friendly
Available Master's thesis topics in machine learning
Main content.
Here we list topics that are available. You may also be interested in our list of completed Master's theses .
Learning and inference with large Bayesian networks
Most learning and inference tasks with Bayesian networks are NP-hard. Therefore, one often resorts to using different heuristics that do not give any quality guarantees.
Task: Evaluate quality of large-scale learning or inference algorithms empirically.
Advisor: Pekka Parviainen
Sum-product networks
Traditionally, probabilistic graphical models use a graph structure to represent dependencies and independencies between random variables. Sum-product networks are a relatively new type of a graphical model where the graphical structure models computations and not the relationships between variables. The benefit of this representation is that inference (computing conditional probabilities) can be done in linear time with respect to the size of the network.
Potential thesis topics in this area: a) Compare inference speed with sum-product networks and Bayesian networks. Characterize situations when one model is better than the other. b) Learning the sum-product networks is done using heuristic algorithms. What is the effect of approximation in practice?
Bayesian Bayesian networks
The naming of Bayesian networks is somewhat misleading because there is nothing Bayesian in them per se; A Bayesian network is just a representation of a joint probability distribution. One can, of course, use a Bayesian network while doing Bayesian inference. One can also learn Bayesian networks in a Bayesian way. That is, instead of finding an optimal network one computes the posterior distribution over networks.
Task: Develop algorithms for Bayesian learning of Bayesian networks (e.g., MCMC, variational inference, EM)
Large-scale (probabilistic) matrix factorization
The idea behind matrix factorization is to represent a large data matrix as a product of two or more smaller matrices.They are often used in, for example, dimensionality reduction and recommendation systems. Probabilistic matrix factorization methods can be used to quantify uncertainty in recommendations. However, large-scale (probabilistic) matrix factorization is computationally challenging.
Potential thesis topics in this area: a) Develop scalable methods for large-scale matrix factorization (non-probabilistic or probabilistic), b) Develop probabilistic methods for implicit feedback (e.g., recommmendation engine when there are no rankings but only knowledge whether a customer has bought an item)
Bayesian deep learning
Standard deep neural networks do not quantify uncertainty in predictions. On the other hand, Bayesian methods provide a principled way to handle uncertainty. Combining these approaches leads to Bayesian neural networks. The challenge is that Bayesian neural networks can be cumbersome to use and difficult to learn.
The task is to analyze Bayesian neural networks and different inference algorithms in some simple setting.
Deep learning for combinatorial problems
Deep learning is usually applied in regression or classification problems. However, there has been some recent work on using deep learning to develop heuristics for combinatorial optimization problems; see, e.g., [1] and [2].
Task: Choose a combinatorial problem (or several related problems) and develop deep learning methods to solve them.
References: [1] Vinyals, Fortunato and Jaitly: Pointer networks. NIPS 2015. [2] Dai, Khalil, Zhang, Dilkina and Song: Learning Combinatorial Optimization Algorithms over Graphs. NIPS 2017.
Advisors: Pekka Parviainen, Ahmad Hemmati
Estimating the number of modes of an unknown function
Mode seeking considers estimating the number of local maxima of a function f. Sometimes one can find modes by, e.g., looking for points where the derivative of the function is zero. However, often the function is unknown and we have only access to some (possibly noisy) values of the function.
In topological data analysis, we can analyze topological structures using persistent homologies. For 1-dimensional signals, this can translate into looking at the birth/death persistence diagram, i.e. the birth and death of connected topological components as we expand the space around each point where we have observed our function. These observations turn out to be closely related to the modes (local maxima) of the function. A recent paper [1] proposed an efficient method for mode seeking.
In this project, the task is to extend the ideas from [1] to get a probabilistic estimate on the number of modes. To this end, one has to use probabilistic methods such as Gaussian processes.
[1] U. Bauer, A. Munk, H. Sieling, and M. Wardetzky. Persistence barcodes versus Kolmogorov signatures: Detecting modes of one-dimensional signals. Foundations of computational mathematics17:1 - 33, 2017.
Advisors: Pekka Parviainen , Nello Blaser
Causal Abstraction Learning
We naturally make sense of the world around us by working out causal relationships between objects and by representing in our minds these objects with different degrees of approximation and detail. Both processes are essential to our understanding of reality, and likely to be fundamental for developing artificial intelligence. The first process may be expressed using the formalism of structural causal models, while the second can be grounded in the theory of causal abstraction [1]. This project will consider the problem of learning an abstraction between two given structural causal models. The primary goal will be the development of efficient algorithms able to learn a meaningful abstraction between the given causal models. [1] Rubenstein, Paul K., et al. "Causal consistency of structural equation models." arXiv preprint arXiv:1707.00819 (2017).
Advisor: Fabio Massimo Zennaro
Causal Bandits
"Multi-armed bandit" is an informal name for slot machines, and the formal name of a large class of problems where an agent has to choose an action among a range of possibilities without knowing the ensuing rewards. Multi-armed bandit problems are one of the most essential reinforcement learning problems where an agent is directly faced with an exploitation-exploration trade-off. This project will consider a class of multi-armed bandits where an agent, upon taking an action, interacts with a causal system [1]. The primary goal will be the development of learning strategies that takes advantage of the underlying causal system in order to learn optimal policies in a shortest amount of time. [1] Lattimore, Finnian, Tor Lattimore, and Mark D. Reid. "Causal bandits: Learning good interventions via causal inference." Advances in neural information processing systems 29 (2016).
Causal Modelling for Battery Manufacturing
Lithium-ion batteries are poised to be one of the most important sources of energy in the near future. Yet, the process of manufacturing these batteries is very hard to model and control. Optimizing the different phases of production to maximize the lifetime of the batteries is a non-trivial challenge since physical models are limited in scope and collecting experimental data is extremely expensive and time-consuming [1]. This project will consider the problem of aggregating and analyzing data regarding a few stages in the process of battery manufacturing. The primary goal will be the development of algorithms for transporting and integrating data collected in different contexts, as well as the use of explainable algorithms to interpret them. [1] Niri, Mona Faraji, et al. "Quantifying key factors for optimised manufacturing of Li-ion battery anode and cathode via artificial intelligence." Energy and AI 7 (2022): 100129.
Advisor: Fabio Massimo Zennaro , Mona Faraji Niri
Reinforcement Learning for Computer Security
The field of computer security presents a wide variety of challenging problems for artificial intelligence and autonomous agents. Guaranteeing the security of a system against attacks and penetrations by malicious hackers has always been a central concern of this field, and machine learning could now offer a substantial contribution. Security capture-the-flag simulations are particularly well-suited as a testbed for the application and development of reinforcement learning algorithms [1]. This project will consider the use of reinforcement learning for the preventive purpose of testing systems and discovering vulnerabilities before they can be exploited. The primary goal will be the modelling of capture-the-flag challenges of interest and the development of reinforcement learning algorithms that can solve them. [1] Erdodi, Laszlo, and Fabio Massimo Zennaro. "The Agent Web Model--Modelling web hacking for reinforcement learning." arXiv preprint arXiv:2009.11274 (2020).
Advisor: Fabio Massimo Zennaro , Laszlo Tibor Erdodi
Approaches to AI Safety
The world and the Internet are more and more populated by artificial autonomous agents carrying out tasks on our behalf. Many of these agents are provided with an objective and they learn their behaviour trying to achieve their objective as better as they can. However, this approach can not guarantee that an agent, while learning its behaviour, will not undertake actions that may have unforeseen and undesirable effects. Research in AI safety tries to design autonomous agent that will behave in a predictable and safe way [1]. This project will consider specific problems and novel solution in the domain of AI safety and reinforcement learning. The primary goal will be the development of innovative algorithms and their implementation withing established frameworks. [1] Amodei, Dario, et al. "Concrete problems in AI safety." arXiv preprint arXiv:1606.06565 (2016).
Reinforcement Learning for Super-modelling
Super-modelling [1] is a technique designed for combining together complex dynamical models: pre-trained models are aggregated with messages and information being exchanged in order synchronize the behavior of the different modles and produce more accurate and reliable predictions. Super-models are used, for instance, in weather or climate science, where pre-existing models are ensembled together and their states dynamically aggregated to generate more realistic simulations.
This project will consider how reinforcement learning algorithms may be used to solve the coordination problem among the individual models forming a super-model. The primary goal will be the formulation of the super-modelling problem within the reinforcement learning framework and the study of custom RL algorithms to improve the overall performance of super-models.
[1] Schevenhoven, Francine, et al. "Supermodeling: improving predictions with an ensemble of interacting models." Bulletin of the American Meteorological Society 104.9 (2023): E1670-E1686.
Advisor: Fabio Massimo Zennaro , Francine Janneke Schevenhoven
Multilevel Causal Discovery
Modelling causal relationships between variables of interest is a crucial step in understanding and controlling a system. A common approach is to represent such relations using graphs with directed arrows discriminating causes from effects.
While causal graphs are often built relying on expert knowledge, a more interesting challenge is to learn them from data. In particular, we want to consider the case where data might have been collected at multiple levels, for instance, with sensor with different resolutions. In this project we want to explore how these heterogeneous data can help the process of inferring causal structures.
[1] Anand, Tara V., et al. "Effect identification in cluster causal diagrams." Proceedings of the 37th AAAI Conference on Artificial Intelligence. Vol. 82. 2023.
Advisor: Fabio Massimo Zennaro , Pekka Parviainen
Manifolds of Causal Models
Modelling causal relationships is fundamental in order to understand real-world systems. A common formalism is offered by structural causal models (SCMs) which represent these relationships graphical. However, SCMs are complex mathematical objects entailing collections of different probability distributions In this project we want to explore a differential geometric perspective on structural causal models [1]. We will model an SCM and the probability distributions it generates in terms of manifold, and we will study how this modelling encodes causal properties of interest and how relevant quantities may be computed in this framework. [1] Dominguez-Olmedo, Ricardo, et al. "On data manifolds entailed by structural causal models." International Conference on Machine Learning. PMLR, 2023.
Advisor: Fabio Massimo Zennaro , Nello Blaser
Abstraction for Epistemic Logic
Weighted Kripke models constitute a powerful formalism to express the evolving knowledge of an agent; it allows to express known facts and beliefs, and to recursively model the knowledge of an agent about another agent. Moreover, such relations of knowledge can be given a graphical expression using suitable diagrams on which to perform reasoning. Unfortunately, such graphs can quickly become very large and inefficient to process.
This project consider the reduction of epistemic logic graph using ideas from causal abstraction [1]. The primary goal will be the development of ML models that can learn to output small epistemic logic graph still satisfying logical and consistency constraints.
[1] Zennaro, Fabio Massimo, et al. "Jointly learning consistent causal abstractions over multiple interventional distributions." Conference on Causal Learning and Reasoning. PMLR, 2023
Advisor: Fabio Massimo Zennaro , Rustam Galimullin
Optimal Transport for Public Transportation
Modelling public transportation across cities is critical in order to improve viability, provide reliable services and increase reliance on greener form of mass transport. Yet cities and transportation networks are complex systems and modelling often has to rely on incomplete and uncertain data.
This project will start from considering a concrete challenge in modelling commuter flows across the city of Bergen. In particular, it will consider the application of the mathematical framework of optimal transport [1] to recover statistical patterns in the usage of the main transportation lines across different periods.
[1] Peyré, Gabriel, and Marco Cuturi. "Computational optimal transport: With applications to data science." Foundations and Trends in Machine Learning 11.5-6 (2019): 355-607.
Finalistc Models
The behavior of an agent may be explained both in causal terms (what has caused a certain behavior) or in finalistic terms (what aim justifies a certain behaviour). While causal reasoning is well explained by different mathematical formalism (e.g., structural causal models), finalistic reasoning is still object of research.
In this project we want to explore how a recently-proposed framework for finalistic reasoning [1] may be used to model intentions and counterfactuals in a causal bandit setting, or how it could be used to enhance inverse reinforcement learning.
[1] Compagno, Dario. "Final models: A finalistic interpretation of statistical correlation." arXiv preprint arXiv:2310.02272 (2023).
Automatic hyperparameter selection for isomap
Isomap is a non-linear dimensionality reduction method with two free hyperparameters (number of nearest neighbors and neighborhood radius). Different hyperparameters result in dramatically different embeddings. Previous methods for selecting hyperparameters focused on choosing one optimal hyperparameter. In this project, you will explore the use of persistent homology to find parameter ranges that result in stable embeddings. The project has theoretic and computational aspects.
Advisor: Nello Blaser
Topological Ancombs quartet
This topic is based on the classical Ancombs quartet and families of point sets with identical 1D persistence ( https://arxiv.org/abs/2202.00577 ). The goal is to generate more interesting datasets using the simulated annealing methods presented in ( http://library.usc.edu.ph/ACM/CHI%202017/1proc/p1290.pdf ). This project is mostly computational.
Persistent homology vectorization with cycle location
There are many methods of vectorizing persistence diagrams, such as persistence landscapes, persistence images, PersLay and statistical summaries. Recently we have designed algorithms to in some cases efficiently detect the location of persistence cycles. In this project, you will vectorize not just the persistence diagram, but additional information such as the location of these cycles. This project is mostly computational with some theoretic aspects.
Divisive covers
Divisive covers are a divisive technique for generating filtered simplicial complexes. They original used a naive way of dividing data into a cover. In this project, you will explore different methods of dividing space, based on principle component analysis, support vector machines and k-means clustering. In addition, you will explore methods of using divisive covers for classification. This project will be mostly computational.
Learning Acquisition Functions for Cost-aware Bayesian Optimization
This is a follow-up project of an earlier Master thesis that developed a novel method for learning Acquisition Functions in Bayesian Optimization through the use of Reinforcement Learning. The goal of this project is to further generalize this method (more general input, learned cost-functions) and apply it to hyperparameter optimization for neural networks.
Advisors: Nello Blaser , Audun Ljone Henriksen
Stable updates
This is a follow-up project of an earlier Master thesis that introduced and studied empirical stability in the context of tree-based models. The goal of this project is to develop stable update methods for deep learning models. You will design sevaral stable methods and empirically compare them (in terms of loss and stability) with a baseline and with one another.
Advisors: Morten Blørstad , Nello Blaser
Multimodality in Bayesian neural network ensembles
One method to assess uncertainty in neural network predictions is to use dropout or noise generators at prediction time and run every prediction many times. This leads to a distribution of predictions. Informatively summarizing such probability distributions is a non-trivial task and the commonly used means and standard deviations result in the loss of crucial information, especially in the case of multimodal distributions with distinct likely outcomes. In this project, you will analyze such multimodal distributions with mixture models and develop ways to exploit such multimodality to improve training. This project can have theoretical, computational and applied aspects.
Wet area segmentation for rivers
NORCE LFI is working on digitizing wetted areas in rivers. You will apply different machine learning techniques for distinguishing water bodies (rivers) from land based on drone aerial (RGB) pictures. This is important for water management and assessing effects of hydropower on river ecosystems (residual flow, stranding of fish and spawning areas). We have a database of approximately 100 rivers (aerial pictures created from totally ca. 120.000 single pictures with Structure from Motion, single pictures available as well) and several of these rivers are flown at 2-4 different discharges, taken in different seasons and with different weather patterns. For ca. 50 % of the pictures the wetted area is digitized for training (GIS shapefile), most (>90 % of single pictures) cover water surface and land. Possible challenges include shading, reflectance from the water surface, different water/ground colours and wet surfaces on land. This is an applied topic, where you will try many different machine learning techniques to find the best solution for the mapping tasks by NORCE LFI.
Advisor: Nello Blaser , Sebastian Franz Stranzl
Learning a hierarchical metric
Often, labels have defined relationships to each other, for instance in a hierarchical taxonomy. E.g. ImageNet labels are derived from the WordNet graph, and biological species are taxonomically related, and can have similarities depending on life stage, sex, or other properties.
ArcFace is an alternative loss function that aims for an embedding that is more generally useful than softmax. It is commonly used in metric learning/few shot learning cases.
Here, we will develop a metric learning method that learns from data with hierarchical labels. Using multiple ArcFace heads, we will simultaneously learn to place representations to optimize the leaf label as well as intermediate labels on the path from leaf to root of the label tree. Using taxonomically classified plankton image data, we will measure performance as a function of ArcFace parameters (sharpness/temperature and margins -- class-wise or level-wise), and compare the results to existing methods.
Advisor: Ketil Malde ( [email protected] )
Self-supervised object detection in video
One challenge with learning object detection is that in many scenes that stretch off into the distance, annotating small, far-off, or blurred objects is difficult. It is therefore desirable to learn from incompletely annotated scenes, and one-shot object detectors may suffer from incompletely annotated training data.
To address this, we will use a region-propsal algorithm (e.g. SelectiveSearch) to extract potential crops from each frame. Classification will be based on two approaches: a) training based on annotated fish vs random similarly-sized crops without annotations, and b) using a self-supervised method to build a representation for crops, and building a classifier for the extracted regions. The method will be evaluated against one-shot detectors and other training regimes.
If successful, the method will be applied to fish detection and tracking in videos from baited and unbaited underwater traps, and used to estimate abundance of various fish species.
See also: Benettino (2016): https://link.springer.com/chapter/10.1007/978-3-319-48881-3_56
Representation learning for object detection
While traditional classifiers work well with data that is labeled with disjoint classes and reasonably balanced class abundances, reality is often less clean. An alternative is to learn a vectors space embedding that reflects semantic relationships between objects, and deriving classes from this representation. This is especially useful for few-shot classification (ie. very few examples in the training data).
The task here is to extend a modern object detector (e.g. Yolo v8) to output an embedding of the identified object. Instead of a softmax classifier, we can learn the embedding either in a supervised manner (using annotations on frames) by attaching an ArcFace or other supervised metric learning head. Alternatively, the representation can be learned from tracked detections over time using e.g. a contrastive loss function to keep the representation for an object (approximately) constant over time. The performance of the resulting object detector will be measured on underwater videos, targeting species detection and/or indiviual recognition (re-ID).
Time-domain object detection
Object detectors for video are normally trained on still frames, but it is evident (from human experience) that using time domain information is more effective. I.e., it can be hard to identify far-off or occluded objects in still images, but movement in time often reveals them.
Here we will extend a state of the art object detector (e.g. yolo v8) with time domain data. Instead of using a single frame as input, the model will be modified to take a set of frames surrounding the annotated frame as input. Performance will be compared to using single-frame detection.
Large-scale visualization of acoustic data
The Institute of Marine Research has decades of acoustic data collected in various surveys. These data are in the process of being converted to data formats that can be processed and analyzed more easily using packages like Xarray and Dask.
The objective is to make these data more accessible to regular users by providing a visual front end. The user should be able to quickly zoom in and out, perform selection, export subsets, apply various filters and classifiers, and overlay annotations and other relevant auxiliary data.
Learning acoustic target classification from simulation
Broadband echosounders emit a complex signal that spans a large frequency band. Different targets will reflect, absorb, and generate resonance at different amplitudes and frequencies, and it is therefore possible to classify targets at much higher resolution and accuracy than before. Due to the complexity of the received signals, deriving effective profiles that can be used to identify targets is difficult.
Here we will use simulated frequency spectra from geometric objects with various shapes, orientation, and other properties. We will train ML models to estimate (recover) the geometric and material properties of objects based on these spectra. The resulting model will be applied to read broadband data, and compared to traditional classification methods.
Online learning in real-time systems
Build a model for the drilling process by using the Virtual simulator OpenLab ( https://openlab.app/ ) for real-time data generation and online learning techniques. The student will also do a short survey of existing online learning techniques and learn how to cope with errors and delays in the data.
Advisor: Rodica Mihai
Building a finite state automaton for the drilling process by using queries and counterexamples
Datasets will be generated by using the Virtual simulator OpenLab ( https://openlab.app/ ). The student will study the datasets and decide upon a good setting to extract a finite state automaton for the drilling process. The student will also do a short survey of existing techniques for extracting finite state automata from process data. We present a novel algorithm that uses exact learning and abstraction to extract a deterministic finite automaton describing the state dynamics of a given trained RNN. We do this using Angluin's L*algorithm as a learner and the trained RNN as an oracle. Our technique efficiently extracts accurate automata from trained RNNs, even when the state vectors are large and require fine differentiation.arxiv.org
Scaling Laws for Language Models in Generative AI
Large Language Models (LLM) power today's most prominent language technologies in Generative AI like ChatGPT, which, in turn, are changing the way that people access information and solve tasks of many kinds.
A recent interest on scaling laws for LLMs has shown trends on understanding how well they perform in terms of factors like the how much training data is used, how powerful the models are, or how much computational cost is allocated. (See, for example, Kaplan et al. - "Scaling Laws for Neural Language Models”, 2020.)
In this project, the task will consider to study scaling laws for different language models and with respect with one or multiple modeling factors.
Advisor: Dario Garigliotti
Applications of causal inference methods to omics data
Many hard problems in machine learning are directly linked to causality [1]. The graphical causal inference framework developed by Judea Pearl can be traced back to pioneering work by Sewall Wright on path analysis in genetics and has inspired research in artificial intelligence (AI) [1].
The Michoel group has developed the open-source tool Findr [2] which provides efficient implementations of mediation and instrumental variable methods for applications to large sets of omics data (genomics, transcriptomics, etc.). Findr works well on a recent data set for yeast [3].
We encourage students to explore promising connections between the fiels of causal inference and machine learning. Feel free to contact us to discuss projects related to causal inference. Possible topics include: a) improving methods based on structural causal models, b) evaluating causal inference methods on data for model organisms, c) comparing methods based on causal models and neural network approaches.
References:
1. Schölkopf B, Causality for Machine Learning, arXiv (2019): https://arxiv.org/abs/1911.10500
2. Wang L and Michoel T. Efficient and accurate causal inference with hidden confounders from genome-transcriptome variation data. PLoS Computational Biology 13:e1005703 (2017). https://journals.plos.org/ploscompbiol/article?id=10.1371/journal.pcbi.1005703
3. Ludl A and and Michoel T. Comparison between instrumental variable and mediation-based methods for reconstructing causal gene networks in yeast. arXiv:2010.07417 https://arxiv.org/abs/2010.07417
Advisors: Adriaan Ludl , Tom Michoel
Space-Time Linkage of Fish Distribution to Environmental Conditions
Conditions in the marine environment, such as, temperature and currents, influence the spatial distribution and migration patterns of marine species. Hence, understanding the link between environmental factors and fish behavior is crucial in predicting, e.g., how fish populations may respond to climate change. Deriving this link is challenging because it requires analysis of two types of datasets (i) large environmental (currents, temperature) datasets that vary in space and time, and (ii) sparse and sporadic spatial observations of fish populations.
Project goal
The primary goal of the project is to develop a methodology that helps predict how spatial distribution of two fish stocks (capelin and mackerel) change in response to variability in the physical marine environment (ocean currents and temperature). The information can also be used to optimize data collection by minimizing time spent in spatial sampling of the populations.
The project will focus on the use of machine learning and/or causal inference algorithms. As a first step, we use synthetic (fish and environmental) data from analytic models that couple the two data sources. Because the ‘truth’ is known, we can judge the efficiency and error margins of the methodologies. We then apply the methodologies to real world (empirical) observations.
Advisors: Tom Michoel , Sam Subbey .
Towards precision medicine for cancer patient stratification
On average, a drug or a treatment is effective in only about half of patients who take it. This means patients need to try several until they find one that is effective at the cost of side effects associated with every treatment. The ultimate goal of precision medicine is to provide a treatment best suited for every individual. Sequencing technologies have now made genomics data available in abundance to be used towards this goal.
In this project we will specifically focus on cancer. Most cancer patients get a particular treatment based on the cancer type and the stage, though different individuals will react differently to a treatment. It is now well established that genetic mutations cause cancer growth and spreading and importantly, these mutations are different in individual patients. The aim of this project is use genomic data allow to better stratification of cancer patients, to predict the treatment most likely to work. Specifically, the project will use machine learning approach to integrate genomic data and build a classifier for stratification of cancer patients.
Advisor: Anagha Joshi
Unraveling gene regulation from single cell data
Multi-cellularity is achieved by precise control of gene expression during development and differentiation and aberrations of this process leads to disease. A key regulatory process in gene regulation is at the transcriptional level where epigenetic and transcriptional regulators control the spatial and temporal expression of the target genes in response to environmental, developmental, and physiological cues obtained from a signalling cascade. The rapid advances in sequencing technology has now made it feasible to study this process by understanding the genomewide patterns of diverse epigenetic and transcription factors as well as at a single cell level.
Single cell RNA sequencing is highly important, particularly in cancer as it allows exploration of heterogenous tumor sample, obstructing therapeutic targeting which leads to poor survival. Despite huge clinical relevance and potential, analysis of single cell RNA-seq data is challenging. In this project, we will develop strategies to infer gene regulatory networks using network inference approaches (both supervised and un-supervised). It will be primarily tested on the single cell datasets in the context of cancer.
Developing a Stress Granule Classifier
To carry out the multitude of functions 'expected' from a human cell, the cell employs a strategy of division of labour, whereby sub-cellular organelles carry out distinct functions. Thus we traditionally understand organelles as distinct units defined both functionally and physically with a distinct shape and size range. More recently a new class of organelles have been discovered that are assembled and dissolved on demand and are composed of liquid droplets or 'granules'. Granules show many properties characteristic of liquids, such as flow and wetting, but they can also assume many shapes and indeed also fluctuate in shape. One such liquid organelle is a stress granule (SG).
Stress granules are pro-survival organelles that assemble in response to cellular stress and important in cancer and neurodegenerative diseases like Alzheimer's. They are liquid or gel-like and can assume varying sizes and shapes depending on their cellular composition.
In a given experiment we are able to image the entire cell over a time series of 1000 frames; from which we extract a rough estimation of the size and shape of each granule. Our current method is susceptible to noise and a granule may be falsely rejected if the boundary is drawn poorly in a small majority of frames. Ideally, we would also like to identify potentially interesting features, such as voids, in the accepted granules.
We are interested in applying a machine learning approach to develop a descriptor for a 'classic' granule and furthermore classify them into different functional groups based on disease status of the cell. This method would be applied across thousands of granules imaged from control and disease cells. We are a multi-disciplinary group consisting of biologists, computational scientists and physicists.
Advisors: Sushma Grellscheid , Carl Jones
Machine Learning based Hyperheuristic algorithm
Develop a Machine Learning based Hyper-heuristic algorithm to solve a pickup and delivery problem. A hyper-heuristic is a heuristics that choose heuristics automatically. Hyper-heuristic seeks to automate the process of selecting, combining, generating or adapting several simpler heuristics to efficiently solve computational search problems [Handbook of Metaheuristics]. There might be multiple heuristics for solving a problem. Heuristics have their own strength and weakness. In this project, we want to use machine-learning techniques to learn the strength and weakness of each heuristic while we are using them in an iterative search for finding high quality solutions and then use them intelligently for the rest of the search. Once a new information is gathered during the search the hyper-heuristic algorithm automatically adjusts the heuristics.
Advisor: Ahmad Hemmati
Machine learning for solving satisfiability problems and applications in cryptanalysis
Advisor: Igor Semaev
Hybrid modeling approaches for well drilling with Sintef
Several topics are available.
"Flow models" are first-principles models simulating the flow, temperature and pressure in a well being drilled. Our project is exploring "hybrid approaches" where these models are combined with machine learning models that either learn from time series data from flow model runs or from real-world measurements during drilling. The goal is to better detect drilling problems such as hole cleaning, make more accurate predictions and correctly learn from and interpret real-word data.
The "surrogate model" refers to a ML model which learns to mimic the flow model by learning from the model inputs and outputs. Use cases for surrogate models include model predictions where speed is favoured over accuracy and exploration of parameter space.
Surrogate models with active Learning
While it is possible to produce a nearly unlimited amount of training data by running the flow model, the surrogate model may still perform poorly if it lacks training data in the part of the parameter space it operates in or if it "forgets" areas of the parameter space by being fed too much data from a narrow range of parameters.
The goal of this thesis is to build a surrogate model (with any architecture) for some restricted parameter range and implement an active learning approach where the ML requests more model runs from the flow model in the parts of the parameter space where it is needed the most. The end result should be a surrogate model that is quick and performs acceptably well over the whole defined parameter range.
Surrogate models trained via adversarial learning
How best to train surrogate models from runs of the flow model is an open question. This master thesis would use the adversarial learning approach to build a surrogate model which to its "adversary" becomes indistinguishable from the output of an actual flow model run.
GPU-based Surrogate models for parameter search
While CPU speed largely stalled 20 years ago in terms of working frequency on single cores, multi-core CPUs and especially GPUs took off and delivered increases in computational power by parallelizing computations.
Modern machine learning such as deep learning takes advantage this boom in computing power by running on GPUs.
The SINTEF flow models in contrast, are software programs that runs on a CPU and does not happen to utilize multi-core CPU functionality. The model runs advance time-step by time-step and each time step relies on the results from the previous time step. The flow models are therefore fundamentally sequential and not well suited to massive parallelization.
It is however of interest to run different model runs in parallel, to explore parameter spaces. The use cases for this includes model calibration, problem detection and hypothesis generation and testing.
The task of this thesis is to implement an ML-based surrogate model in such a way that many surrogate model outputs can be produced at the same time using a single GPU. This will likely entail some trade off with model size and maybe some coding tricks.
Uncertainty estimates of hybrid predictions (Lots of room for creativity, might need to steer it more, needs good background literature)
When using predictions from a ML model trained on time series data, it is useful to know if it's accurate or should be trusted. The student is challenged to develop hybrid approaches that incorporates estimates of uncertainty. Components could include reporting variance from ML ensembles trained on a diversity of time series data, implementation of conformal predictions, analysis of training data parameter ranges vs current input, etc. The output should be a "traffic light signal" roughly indicating the accuracy of the predictions.
Transfer learning approaches
We're assuming an ML model is to be used for time series prediction
It is possible to train an ML on a wide range of scenarios in the flow models, but we expect that to perform well, the model also needs to see model runs representative of the type of well and drilling operation it will be used in. In this thesis the student implements a transfer learning approach, where the model is trained on general model runs and fine-tuned on a most representative data set.
(Bonus1: implementing one-shot learning, Bonus2: Using real-world data in the fine-tuning stage)
ML capable of reframing situations
When a human oversees an operation like well drilling, she has a mental model of the situation and new data such as pressure readings from the well is interpreted in light of this model. This is referred to as "framing" and is the normal mode of work. However, when a problem occurs, it becomes harder to reconcile the data with the mental model. The human then goes into "reframing", building a new mental model that includes the ongoing problem. This can be seen as a process of hypothesis generation and testing.
A computer model however, lacks re-framing. A flow model will keep making predictions under the assumption of no problems and a separate alarm system will use the deviation between the model predictions and reality to raise an alarm. This is in a sense how all alarm systems work, but it means that the human must discard the computer model as a tool at the same time as she's handling a crisis.
The student is given access to a flow model and a surrogate model which can learn from model runs both with and without hole cleaning and is challenged to develop a hybrid approach where the ML+flow model continuously performs hypothesis generation and testing and is able to "switch" into predictions of a hole cleaning problem and different remediations of this.
Advisor: Philippe Nivlet at Sintef together with advisor from UiB
Explainable AI at Equinor
In the project Machine Teaching for XAI (see https://xai.w.uib.no ) a master thesis in collaboration between UiB and Equinor.
Advisor: One of Pekka Parviainen/Jan Arne Telle/Emmanuel Arrighi + Bjarte Johansen from Equinor.
Explainable AI at Eviny
In the project Machine Teaching for XAI (see https://xai.w.uib.no ) a master thesis in collaboration between UiB and Eviny.
Advisor: One of Pekka Parviainen/Jan Arne Telle/Emmanuel Arrighi + Kristian Flikka from Eviny.
If you want to suggest your own topic, please contact Pekka Parviainen , Fabio Massimo Zennaro or Nello Blaser .
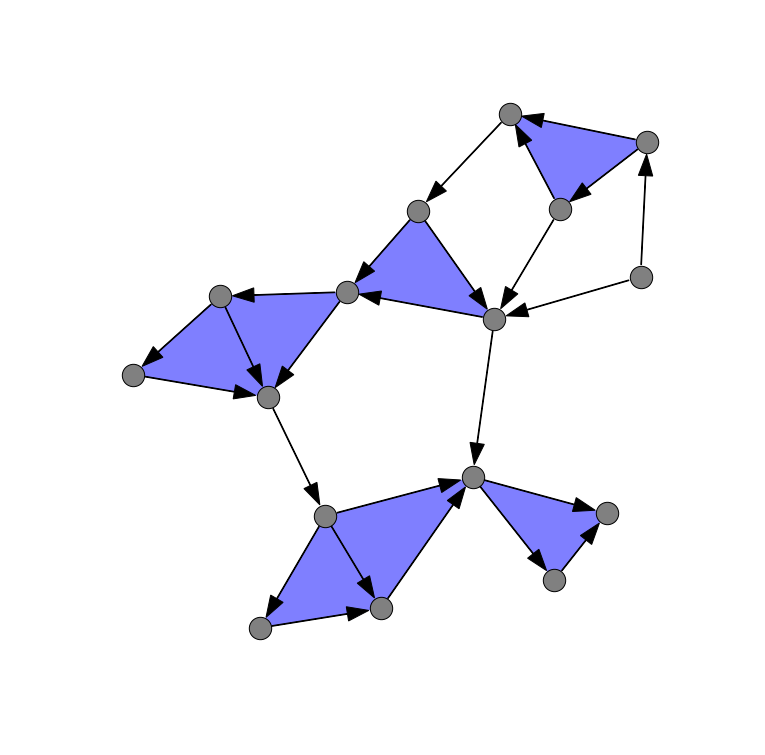
- Latest News
.png)
- Cryptocurrencies
- White Papers
Top 10 Research and Thesis Topics for ML Projects in 2022
This article features the top 10 research and thesis topics for ml projects for students to try in 2022.
In this tech-driven world, selecting research and thesis topics in machine learning projects is the first choice of masters and Doctorate scholars. Selecting and working on a thesis topic in machine learning is not an easy task as machine learning uses statistical algorithms to make computers work in a certain way without being explicitly programmed. Achieving mastery over machine learning (ML) is becoming increasingly crucial for all the students in this field. Both artificial intelligence and machine learning complement each other. So, if you are a beginner, the best thing you can do is work on some ML projects. This article features the top 10 research and thesis topics for ML projects for students to try in 2022.
Text Mining and Text Classification
Text mining (also referred to as text analytics) is an artificial intelligence (AI) technology that uses natural language processing (NLP) to transform the free (unstructured) text in documents and databases into normalized, structured data suitable for analysis or to drive machine learning (ML) algorithms. Text classification tools categorize text by understanding its overall meaning, without predefined categories being explicitly present within the text. This is one of the best research and thesis topics for ML projects.
Image-Based Applications
An image-based test consists of a sequence of operations on UI elements in your tested application: clicks (for desktop and web applications), touches (for mobile applications), drag and drop operations, checkpoints, and so on. In image applications, one must first get familiar with masks, convolution, edge, and corner detection to be able to extract useful information from images and further use them for applications like image segmentation, keypoints extraction, and more.
Machine Vision
Using machine learning -based/mathematical techniques to enable machines to do specific tasks. For example, watermarking, face identification from datasets of images with rotation and different camera angles, criminals identification from surveillance cameras (video and series of images), handwriting and personal signature classification, object detection/recognition.
Clustering or cluster analysis is a machine learning technique, which groups the unlabeled dataset. It can be defined as "A way of grouping the data points into different clusters, consisting of similar data points. For example Graph clustering, data clustering, density-based clustering, and more. Clustering is one of the best research and thesis topics for ML projects.
Optimization
A) Population-based optimization inspired from a natural mechanism: Black-box optimization, multi/many-objective optimization, evolutionary methods (Genetic Algorithm, Genetic Programming, Memetic Programming), Metaheuristics (e.g., PSO, ABC, SA)
B) Exact/Mathematical Models: Convex optimization, Bi-Convex, and Semi-Convex optimization, Gradient Descent, Block Coordinate Descent, Manifold Optimization, and Algebraic Models
Voice Classification
Voice classification or sound classification can be referred to as the process of analyzing audio recordings. Voice and Speech Recognition, Signal Processing, Message Embedding, Message Extraction from Voice Encoded, and more are the best research and thesis topics for ML projects.
Sentiment Analysis
Sentiment analysis is one of the best Machine Learning projects well-known to uncover emotions in the text. By analyzing movie reviews, customer feedback, support tickets, companies may discover many interesting things. So learning how to build sentiment analysis models is quite a practical skill. There is no need to collect the data yourself. To train and test your model, use the biggest open-source database for sentiment analysis created by IMDb.
Recommendation Framework Project
This a rich dataset assortment containing a different scope of datasets accumulated from famous sites like Goodreads book audits, Amazon item surveys, online media, and so forth You will probably fabricate a recommendation engine (like the ones utilized by Amazon and Netflix) that can create customized recommendations for items, films, music, and so on, because of client inclinations, needs, and online conduct.
Mall Customers' Project
As the name suggests, the mall customers' dataset includes the records of people who visited the mall, such as gender, age, customer ID, annual income, spending score, etc. You will build a model that will use this data to segment the customers into different groups based on their behavior patterns. Such customer segmentation is a highly useful marketing tactic used by brands and marketers to boost sales and revenue while also increasing customer satisfaction.
Object Detection with Deep Learning
Object Detection with Deep Learning is one of the interesting machine learning projects to create. When it comes to image classification, Deep Neural Networks (DNNs) should be your go-to choice. While DNNs are already used in many real-world image classification applications, it is one of the best ML projects that aims to crank it up a notch. In this Machine Learning project, you will solve the problem of object detection by leveraging DNNs.
Disclaimer: Analytics Insight does not provide financial advice or guidance. Also note that the cryptocurrencies mentioned/listed on the website could potentially be scams, i.e. designed to induce you to invest financial resources that may be lost forever and not be recoverable once investments are made. You are responsible for conducting your own research (DYOR) before making any investments. Read more here.
Related Stories

Machine Learning - CMU

PhD Dissertations
[all are .pdf files].
The Neurodynamic Basis of Real World Face Perception Arish Alreja, 2024
Towards More Powerful Graph Representation Learning Lingxiao Zhao, 2024
Robust Machine Learning: Detection, Evaluation and Adaptation Under Distribution Shift Saurabh Garg, 2024
UNDERSTANDING, FORMALLY CHARACTERIZING, AND ROBUSTLY HANDLING REAL-WORLD DISTRIBUTION SHIFT Elan Rosenfeld, 2024
Representing Time: Towards Pragmatic Multivariate Time Series Modeling Cristian Ignacio Challu, 2024
Foundations of Multisensory Artificial Intelligence Paul Pu Liang, 2024
Advancing Model-Based Reinforcement Learning with Applications in Nuclear Fusion Ian Char, 2024
Learning Models that Match Jacob Tyo, 2024
Improving Human Integration across the Machine Learning Pipeline Charvi Rastogi, 2024
Reliable and Practical Machine Learning for Dynamic Healthcare Settings Helen Zhou, 2023
Automatic customization of large-scale spiking network models to neuronal population activity (unavailable) Shenghao Wu, 2023
Estimation of BVk functions from scattered data (unavailable) Addison J. Hu, 2023
Rethinking object categorization in computer vision (unavailable) Jayanth Koushik, 2023
Advances in Statistical Gene Networks Jinjin Tian, 2023 Post-hoc calibration without distributional assumptions Chirag Gupta, 2023
The Role of Noise, Proxies, and Dynamics in Algorithmic Fairness Nil-Jana Akpinar, 2023
Collaborative learning by leveraging siloed data Sebastian Caldas, 2023
Modeling Epidemiological Time Series Aaron Rumack, 2023
Human-Centered Machine Learning: A Statistical and Algorithmic Perspective Leqi Liu, 2023
Uncertainty Quantification under Distribution Shifts Aleksandr Podkopaev, 2023
Probabilistic Reinforcement Learning: Using Data to Define Desired Outcomes, and Inferring How to Get There Benjamin Eysenbach, 2023
Comparing Forecasters and Abstaining Classifiers Yo Joong Choe, 2023
Using Task Driven Methods to Uncover Representations of Human Vision and Semantics Aria Yuan Wang, 2023
Data-driven Decisions - An Anomaly Detection Perspective Shubhranshu Shekhar, 2023
Applied Mathematics of the Future Kin G. Olivares, 2023
METHODS AND APPLICATIONS OF EXPLAINABLE MACHINE LEARNING Joon Sik Kim, 2023
NEURAL REASONING FOR QUESTION ANSWERING Haitian Sun, 2023
Principled Machine Learning for Societally Consequential Decision Making Amanda Coston, 2023
Long term brain dynamics extend cognitive neuroscience to timescales relevant for health and physiology Maxwell B. Wang, 2023
Long term brain dynamics extend cognitive neuroscience to timescales relevant for health and physiology Darby M. Losey, 2023
Calibrated Conditional Density Models and Predictive Inference via Local Diagnostics David Zhao, 2023
Towards an Application-based Pipeline for Explainability Gregory Plumb, 2022
Objective Criteria for Explainable Machine Learning Chih-Kuan Yeh, 2022
Making Scientific Peer Review Scientific Ivan Stelmakh, 2022
Facets of regularization in high-dimensional learning: Cross-validation, risk monotonization, and model complexity Pratik Patil, 2022
Active Robot Perception using Programmable Light Curtains Siddharth Ancha, 2022
Strategies for Black-Box and Multi-Objective Optimization Biswajit Paria, 2022
Unifying State and Policy-Level Explanations for Reinforcement Learning Nicholay Topin, 2022
Sensor Fusion Frameworks for Nowcasting Maria Jahja, 2022
Equilibrium Approaches to Modern Deep Learning Shaojie Bai, 2022
Towards General Natural Language Understanding with Probabilistic Worldbuilding Abulhair Saparov, 2022
Applications of Point Process Modeling to Spiking Neurons (Unavailable) Yu Chen, 2021
Neural variability: structure, sources, control, and data augmentation Akash Umakantha, 2021
Structure and time course of neural population activity during learning Jay Hennig, 2021
Cross-view Learning with Limited Supervision Yao-Hung Hubert Tsai, 2021
Meta Reinforcement Learning through Memory Emilio Parisotto, 2021
Learning Embodied Agents with Scalably-Supervised Reinforcement Learning Lisa Lee, 2021
Learning to Predict and Make Decisions under Distribution Shift Yifan Wu, 2021
Statistical Game Theory Arun Sai Suggala, 2021
Towards Knowledge-capable AI: Agents that See, Speak, Act and Know Kenneth Marino, 2021
Learning and Reasoning with Fast Semidefinite Programming and Mixing Methods Po-Wei Wang, 2021
Bridging Language in Machines with Language in the Brain Mariya Toneva, 2021
Curriculum Learning Otilia Stretcu, 2021
Principles of Learning in Multitask Settings: A Probabilistic Perspective Maruan Al-Shedivat, 2021
Towards Robust and Resilient Machine Learning Adarsh Prasad, 2021
Towards Training AI Agents with All Types of Experiences: A Unified ML Formalism Zhiting Hu, 2021
Building Intelligent Autonomous Navigation Agents Devendra Chaplot, 2021
Learning to See by Moving: Self-supervising 3D Scene Representations for Perception, Control, and Visual Reasoning Hsiao-Yu Fish Tung, 2021
Statistical Astrophysics: From Extrasolar Planets to the Large-scale Structure of the Universe Collin Politsch, 2020
Causal Inference with Complex Data Structures and Non-Standard Effects Kwhangho Kim, 2020
Networks, Point Processes, and Networks of Point Processes Neil Spencer, 2020
Dissecting neural variability using population recordings, network models, and neurofeedback (Unavailable) Ryan Williamson, 2020
Predicting Health and Safety: Essays in Machine Learning for Decision Support in the Public Sector Dylan Fitzpatrick, 2020
Towards a Unified Framework for Learning and Reasoning Han Zhao, 2020
Learning DAGs with Continuous Optimization Xun Zheng, 2020
Machine Learning and Multiagent Preferences Ritesh Noothigattu, 2020
Learning and Decision Making from Diverse Forms of Information Yichong Xu, 2020
Towards Data-Efficient Machine Learning Qizhe Xie, 2020
Change modeling for understanding our world and the counterfactual one(s) William Herlands, 2020
Machine Learning in High-Stakes Settings: Risks and Opportunities Maria De-Arteaga, 2020
Data Decomposition for Constrained Visual Learning Calvin Murdock, 2020
Structured Sparse Regression Methods for Learning from High-Dimensional Genomic Data Micol Marchetti-Bowick, 2020
Towards Efficient Automated Machine Learning Liam Li, 2020
LEARNING COLLECTIONS OF FUNCTIONS Emmanouil Antonios Platanios, 2020
Provable, structured, and efficient methods for robustness of deep networks to adversarial examples Eric Wong , 2020
Reconstructing and Mining Signals: Algorithms and Applications Hyun Ah Song, 2020
Probabilistic Single Cell Lineage Tracing Chieh Lin, 2020
Graphical network modeling of phase coupling in brain activity (unavailable) Josue Orellana, 2019
Strategic Exploration in Reinforcement Learning - New Algorithms and Learning Guarantees Christoph Dann, 2019 Learning Generative Models using Transformations Chun-Liang Li, 2019
Estimating Probability Distributions and their Properties Shashank Singh, 2019
Post-Inference Methods for Scalable Probabilistic Modeling and Sequential Decision Making Willie Neiswanger, 2019
Accelerating Text-as-Data Research in Computational Social Science Dallas Card, 2019
Multi-view Relationships for Analytics and Inference Eric Lei, 2019
Information flow in networks based on nonstationary multivariate neural recordings Natalie Klein, 2019
Competitive Analysis for Machine Learning & Data Science Michael Spece, 2019
The When, Where and Why of Human Memory Retrieval Qiong Zhang, 2019
Towards Effective and Efficient Learning at Scale Adams Wei Yu, 2019
Towards Literate Artificial Intelligence Mrinmaya Sachan, 2019
Learning Gene Networks Underlying Clinical Phenotypes Under SNP Perturbations From Genome-Wide Data Calvin McCarter, 2019
Unified Models for Dynamical Systems Carlton Downey, 2019
Anytime Prediction and Learning for the Balance between Computation and Accuracy Hanzhang Hu, 2019
Statistical and Computational Properties of Some "User-Friendly" Methods for High-Dimensional Estimation Alnur Ali, 2019
Nonparametric Methods with Total Variation Type Regularization Veeranjaneyulu Sadhanala, 2019
New Advances in Sparse Learning, Deep Networks, and Adversarial Learning: Theory and Applications Hongyang Zhang, 2019
Gradient Descent for Non-convex Problems in Modern Machine Learning Simon Shaolei Du, 2019
Selective Data Acquisition in Learning and Decision Making Problems Yining Wang, 2019
Anomaly Detection in Graphs and Time Series: Algorithms and Applications Bryan Hooi, 2019
Neural dynamics and interactions in the human ventral visual pathway Yuanning Li, 2018
Tuning Hyperparameters without Grad Students: Scaling up Bandit Optimisation Kirthevasan Kandasamy, 2018
Teaching Machines to Classify from Natural Language Interactions Shashank Srivastava, 2018
Statistical Inference for Geometric Data Jisu Kim, 2018
Representation Learning @ Scale Manzil Zaheer, 2018
Diversity-promoting and Large-scale Machine Learning for Healthcare Pengtao Xie, 2018
Distribution and Histogram (DIsH) Learning Junier Oliva, 2018
Stress Detection for Keystroke Dynamics Shing-Hon Lau, 2018
Sublinear-Time Learning and Inference for High-Dimensional Models Enxu Yan, 2018
Neural population activity in the visual cortex: Statistical methods and application Benjamin Cowley, 2018
Efficient Methods for Prediction and Control in Partially Observable Environments Ahmed Hefny, 2018
Learning with Staleness Wei Dai, 2018
Statistical Approach for Functionally Validating Transcription Factor Bindings Using Population SNP and Gene Expression Data Jing Xiang, 2017
New Paradigms and Optimality Guarantees in Statistical Learning and Estimation Yu-Xiang Wang, 2017
Dynamic Question Ordering: Obtaining Useful Information While Reducing User Burden Kirstin Early, 2017
New Optimization Methods for Modern Machine Learning Sashank J. Reddi, 2017
Active Search with Complex Actions and Rewards Yifei Ma, 2017
Why Machine Learning Works George D. Montañez , 2017
Source-Space Analyses in MEG/EEG and Applications to Explore Spatio-temporal Neural Dynamics in Human Vision Ying Yang , 2017
Computational Tools for Identification and Analysis of Neuronal Population Activity Pengcheng Zhou, 2016
Expressive Collaborative Music Performance via Machine Learning Gus (Guangyu) Xia, 2016
Supervision Beyond Manual Annotations for Learning Visual Representations Carl Doersch, 2016
Exploring Weakly Labeled Data Across the Noise-Bias Spectrum Robert W. H. Fisher, 2016
Optimizing Optimization: Scalable Convex Programming with Proximal Operators Matt Wytock, 2016
Combining Neural Population Recordings: Theory and Application William Bishop, 2015
Discovering Compact and Informative Structures through Data Partitioning Madalina Fiterau-Brostean, 2015
Machine Learning in Space and Time Seth R. Flaxman, 2015
The Time and Location of Natural Reading Processes in the Brain Leila Wehbe, 2015
Shape-Constrained Estimation in High Dimensions Min Xu, 2015
Spectral Probabilistic Modeling and Applications to Natural Language Processing Ankur Parikh, 2015 Computational and Statistical Advances in Testing and Learning Aaditya Kumar Ramdas, 2015
Corpora and Cognition: The Semantic Composition of Adjectives and Nouns in the Human Brain Alona Fyshe, 2015
Learning Statistical Features of Scene Images Wooyoung Lee, 2014
Towards Scalable Analysis of Images and Videos Bin Zhao, 2014
Statistical Text Analysis for Social Science Brendan T. O'Connor, 2014
Modeling Large Social Networks in Context Qirong Ho, 2014
Semi-Cooperative Learning in Smart Grid Agents Prashant P. Reddy, 2013
On Learning from Collective Data Liang Xiong, 2013
Exploiting Non-sequence Data in Dynamic Model Learning Tzu-Kuo Huang, 2013
Mathematical Theories of Interaction with Oracles Liu Yang, 2013
Short-Sighted Probabilistic Planning Felipe W. Trevizan, 2013
Statistical Models and Algorithms for Studying Hand and Finger Kinematics and their Neural Mechanisms Lucia Castellanos, 2013
Approximation Algorithms and New Models for Clustering and Learning Pranjal Awasthi, 2013
Uncovering Structure in High-Dimensions: Networks and Multi-task Learning Problems Mladen Kolar, 2013
Learning with Sparsity: Structures, Optimization and Applications Xi Chen, 2013
GraphLab: A Distributed Abstraction for Large Scale Machine Learning Yucheng Low, 2013
Graph Structured Normal Means Inference James Sharpnack, 2013 (Joint Statistics & ML PhD)
Probabilistic Models for Collecting, Analyzing, and Modeling Expression Data Hai-Son Phuoc Le, 2013
Learning Large-Scale Conditional Random Fields Joseph K. Bradley, 2013
New Statistical Applications for Differential Privacy Rob Hall, 2013 (Joint Statistics & ML PhD)
Parallel and Distributed Systems for Probabilistic Reasoning Joseph Gonzalez, 2012
Spectral Approaches to Learning Predictive Representations Byron Boots, 2012
Attribute Learning using Joint Human and Machine Computation Edith L. M. Law, 2012
Statistical Methods for Studying Genetic Variation in Populations Suyash Shringarpure, 2012
Data Mining Meets HCI: Making Sense of Large Graphs Duen Horng (Polo) Chau, 2012
Learning with Limited Supervision by Input and Output Coding Yi Zhang, 2012
Target Sequence Clustering Benjamin Shih, 2011
Nonparametric Learning in High Dimensions Han Liu, 2010 (Joint Statistics & ML PhD)
Structural Analysis of Large Networks: Observations and Applications Mary McGlohon, 2010
Modeling Purposeful Adaptive Behavior with the Principle of Maximum Causal Entropy Brian D. Ziebart, 2010
Tractable Algorithms for Proximity Search on Large Graphs Purnamrita Sarkar, 2010
Rare Category Analysis Jingrui He, 2010
Coupled Semi-Supervised Learning Andrew Carlson, 2010
Fast Algorithms for Querying and Mining Large Graphs Hanghang Tong, 2009
Efficient Matrix Models for Relational Learning Ajit Paul Singh, 2009
Exploiting Domain and Task Regularities for Robust Named Entity Recognition Andrew O. Arnold, 2009
Theoretical Foundations of Active Learning Steve Hanneke, 2009
Generalized Learning Factors Analysis: Improving Cognitive Models with Machine Learning Hao Cen, 2009
Detecting Patterns of Anomalies Kaustav Das, 2009
Dynamics of Large Networks Jurij Leskovec, 2008
Computational Methods for Analyzing and Modeling Gene Regulation Dynamics Jason Ernst, 2008
Stacked Graphical Learning Zhenzhen Kou, 2007
Actively Learning Specific Function Properties with Applications to Statistical Inference Brent Bryan, 2007
Approximate Inference, Structure Learning and Feature Estimation in Markov Random Fields Pradeep Ravikumar, 2007
Scalable Graphical Models for Social Networks Anna Goldenberg, 2007
Measure Concentration of Strongly Mixing Processes with Applications Leonid Kontorovich, 2007
Tools for Graph Mining Deepayan Chakrabarti, 2005
Automatic Discovery of Latent Variable Models Ricardo Silva, 2005
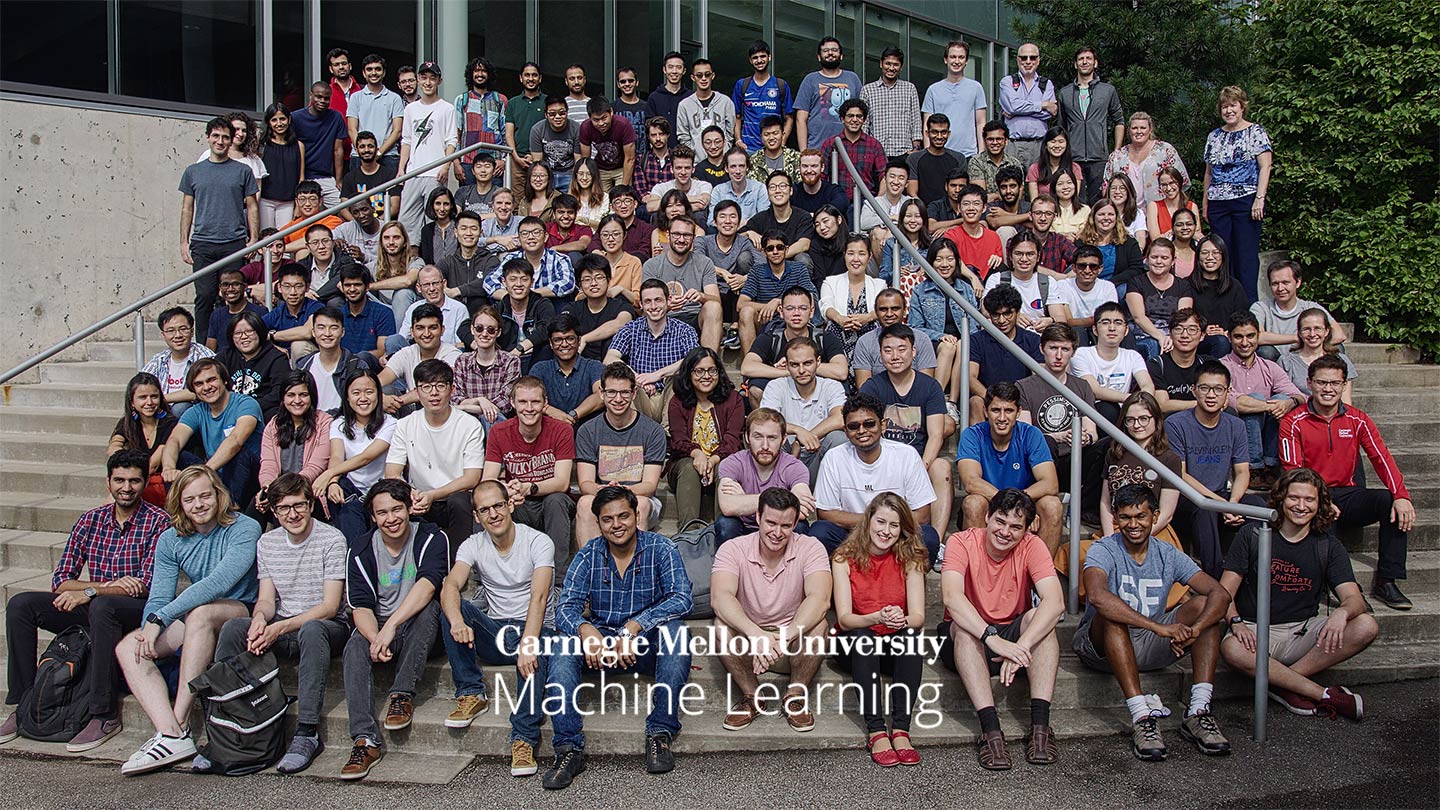

Exploring 250+ Machine Learning Research Topics

In recent years, machine learning has become super popular and grown very quickly. This happened because technology got better, and there’s a lot more data available. Because of this, we’ve seen lots of new and amazing things happen in different areas. Machine learning research is what makes all these cool things possible. In this blog, we’ll talk about machine learning research topics, why they’re important, how you can pick one, what areas are popular to study, what’s new and exciting, the tough problems, and where you can find help if you want to be a researcher.
Whether you’re delving into popular areas or tackling tough problems, our ‘ ‘ service is here to support your research journey.” |
Why Does Machine Learning Research Matter?
Table of Contents
Machine learning research is at the heart of the AI revolution. It underpins the development of intelligent systems capable of making predictions, automating tasks, and improving decision-making across industries. The importance of this research can be summarized as follows:
Advancements in Technology
The growth of machine learning research has led to the development of powerful algorithms, tools, and frameworks. Numerous industries, including healthcare, banking, autonomous cars, and natural language processing, have found use for these technology.
As researchers continue to push the boundaries of what’s possible, we can expect even more transformative technologies to emerge.
Real-world Applications
Machine learning research has brought about tangible changes in our daily lives. Voice assistants like Siri and Alexa, recommendation systems on streaming platforms, and personalized healthcare diagnostics are just a few examples of how this research impacts our world.
By working on new research topics, scientists can further refine these applications and create new ones.
Economic and Industrial Impacts
The economic implications of machine learning research are substantial. Companies that harness the power of machine learning gain a competitive edge in the market.
This creates a demand for skilled machine learning researchers, driving job opportunities and contributing to economic growth.
How to Choose the Machine Learning Research Topics?
Selecting the right machine learning research topics is crucial for your success as a machine learning researcher. Here’s a guide to help you make an informed decision:
- Understanding Your Interests
Start by considering your personal interests. Machine learning is a broad field with applications in virtually every sector. By choosing a topic that aligns with your passions, you’ll stay motivated and engaged throughout your research journey.
- Reviewing Current Trends
Stay updated on the latest trends in machine learning. Attend conferences, read research papers, and engage with the community to identify emerging research topics. Current trends often lead to exciting breakthroughs.
- Identifying Gaps in Existing Research
Sometimes, the most promising research topics involve addressing gaps in existing knowledge. These gaps may become evident through your own experiences, discussions with peers, or in the course of your studies.
- Collaborating with Experts
Collaboration is key in research. Working with experts in the field can help you refine your research topic and gain valuable insights. Seek mentors and collaborators who can guide you.
250+ Machine Learning Research Topics: Category-wise
Supervised learning.
- Explainable AI for Decision Support
- Few-shot Learning Methods
- Time Series Forecasting with Deep Learning
- Handling Imbalanced Datasets in Classification
- Regression Techniques for Non-linear Data
- Transfer Learning in Supervised Settings
- Multi-label Classification Strategies
- Semi-Supervised Learning Approaches
- Novel Feature Selection Methods
- Anomaly Detection in Supervised Scenarios
- Federated Learning for Distributed Supervised Models
- Ensemble Learning for Improved Accuracy
- Automated Hyperparameter Tuning
- Ethical Implications in Supervised Models
- Interpretability of Deep Neural Networks.
Unsupervised Learning
- Unsupervised Clustering of High-dimensional Data
- Semi-Supervised Clustering Approaches
- Density Estimation in Unsupervised Learning
- Anomaly Detection in Unsupervised Settings
- Transfer Learning for Unsupervised Tasks
- Representation Learning in Unsupervised Learning
- Outlier Detection Techniques
- Generative Models for Data Synthesis
- Manifold Learning in High-dimensional Spaces
- Unsupervised Feature Selection
- Privacy-Preserving Unsupervised Learning
- Community Detection in Complex Networks
- Clustering Interpretability and Visualization
- Unsupervised Learning for Image Segmentation
- Autoencoders for Dimensionality Reduction.
Reinforcement Learning
- Deep Reinforcement Learning in Real-world Applications
- Safe Reinforcement Learning for Autonomous Systems
- Transfer Learning in Reinforcement Learning
- Imitation Learning and Apprenticeship Learning
- Multi-agent Reinforcement Learning
- Explainable Reinforcement Learning Policies
- Hierarchical Reinforcement Learning
- Model-based Reinforcement Learning
- Curriculum Learning in Reinforcement Learning
- Reinforcement Learning in Robotics
- Exploration vs. Exploitation Strategies
- Reward Function Design and Ethical Considerations
- Reinforcement Learning in Healthcare
- Continuous Action Spaces in RL
- Reinforcement Learning for Resource Management.
Natural Language Processing (NLP)
- Multilingual and Cross-lingual NLP
- Contextualized Word Embeddings
- Bias Detection and Mitigation in NLP
- Named Entity Recognition for Low-resource Languages
- Sentiment Analysis in Social Media Text
- Dialogue Systems for Improved Customer Service
- Text Summarization for News Articles
- Low-resource Machine Translation
- Explainable NLP Models
- Coreference Resolution in NLP
- Question Answering in Specific Domains
- Detecting Fake News and Misinformation
- NLP for Healthcare: Clinical Document Understanding
- Emotion Analysis in Text
- Text Generation with Controlled Attributes.
Computer Vision
- Video Action Recognition and Event Detection
- Object Detection in Challenging Conditions (e.g., low light)
- Explainable Computer Vision Models
- Image Captioning for Accessibility
- Large-scale Image Retrieval
- Domain Adaptation in Computer Vision
- Fine-grained Image Classification
- Facial Expression Recognition
- Visual Question Answering
- Self-supervised Learning for Visual Representations
- Weakly Supervised Object Localization
- Human Pose Estimation in 3D
- Scene Understanding in Autonomous Vehicles
- Image Super-resolution
- Gaze Estimation for Human-Computer Interaction.
Deep Learning
- Neural Architecture Search for Efficient Models
- Self-attention Mechanisms and Transformers
- Interpretability in Deep Learning Models
- Robustness of Deep Neural Networks
- Generative Adversarial Networks (GANs) for Data Augmentation
- Neural Style Transfer in Art and Design
- Adversarial Attacks and Defenses
- Neural Networks for Audio and Speech Processing
- Explainable AI for Healthcare Diagnosis
- Automated Machine Learning (AutoML)
- Reinforcement Learning with Deep Neural Networks
- Model Compression and Quantization
- Lifelong Learning with Deep Learning Models
- Multimodal Learning with Vision and Language
- Federated Learning for Privacy-preserving Deep Learning.
Explainable AI
- Visualizing Model Decision Boundaries
- Saliency Maps and Feature Attribution
- Rule-based Explanations for Black-box Models
- Contrastive Explanations for Model Interpretability
- Counterfactual Explanations and What-if Analysis
- Human-centered AI for Explainable Healthcare
- Ethics and Fairness in Explainable AI
- Explanation Generation for Natural Language Processing
- Explainable AI in Financial Risk Assessment
- User-friendly Interfaces for Model Interpretability
- Scalability and Efficiency in Explainable Models
- Hybrid Models for Combined Accuracy and Explainability
- Post-hoc vs. Intrinsic Explanations
- Evaluation Metrics for Explanation Quality
- Explainable AI for Autonomous Vehicles.
Transfer Learning
- Zero-shot Learning and Few-shot Learning
- Cross-domain Transfer Learning
- Domain Adaptation for Improved Generalization
- Multilingual Transfer Learning in NLP
- Pretraining and Fine-tuning Techniques
- Lifelong Learning and Continual Learning
- Domain-specific Transfer Learning Applications
- Model Distillation for Knowledge Transfer
- Contrastive Learning for Transfer Learning
- Self-training and Pseudo-labeling
- Dynamic Adaption of Pretrained Models
- Privacy-Preserving Transfer Learning
- Unsupervised Domain Adaptation
- Negative Transfer Avoidance in Transfer Learning.
Federated Learning
- Secure Aggregation in Federated Learning
- Communication-efficient Federated Learning
- Privacy-preserving Techniques in Federated Learning
- Federated Transfer Learning
- Heterogeneous Federated Learning
- Real-world Applications of Federated Learning
- Federated Learning for Edge Devices
- Federated Learning for Healthcare Data
- Differential Privacy in Federated Learning
- Byzantine-robust Federated Learning
- Federated Learning with Non-IID Data
- Model Selection in Federated Learning
- Scalable Federated Learning for Large Datasets
- Client Selection and Sampling Strategies
- Global Model Update Synchronization in Federated Learning.
Quantum Machine Learning
- Quantum Neural Networks and Quantum Circuit Learning
- Quantum-enhanced Optimization for Machine Learning
- Quantum Data Compression and Quantum Principal Component Analysis
- Quantum Kernels and Quantum Feature Maps
- Quantum Variational Autoencoders
- Quantum Transfer Learning
- Quantum-inspired Classical Algorithms for ML
- Hybrid Quantum-Classical Models
- Quantum Machine Learning on Near-term Quantum Devices
- Quantum-inspired Reinforcement Learning
- Quantum Computing for Quantum Chemistry and Drug Discovery
- Quantum Machine Learning for Finance
- Quantum Data Structures and Quantum Databases
- Quantum-enhanced Cryptography in Machine Learning
- Quantum Generative Models and Quantum GANs.
Ethical AI and Bias Mitigation
- Fairness-aware Machine Learning Algorithms
- Bias Detection and Mitigation in Real-world Data
- Explainable AI for Ethical Decision Support
- Algorithmic Accountability and Transparency
- Privacy-preserving AI and Data Governance
- Ethical Considerations in AI for Healthcare
- Fairness in Recommender Systems
- Bias and Fairness in NLP Models
- Auditing AI Systems for Bias
- Societal Implications of AI in Criminal Justice
- Ethical AI Education and Training
- Bias Mitigation in Autonomous Vehicles
- Fair AI in Financial and Hiring Decisions
- Case Studies in Ethical AI Failures
- Legal and Policy Frameworks for Ethical AI.
Meta-Learning and AutoML
- Neural Architecture Search (NAS) for Efficient Models
- Transfer Learning in NAS
- Reinforcement Learning for NAS
- Multi-objective NAS
- Automated Data Augmentation
- Neural Architecture Optimization for Edge Devices
- Bayesian Optimization for AutoML
- Model Compression and Quantization in AutoML
- AutoML for Federated Learning
- AutoML in Healthcare Diagnostics
- Explainable AutoML
- Cost-sensitive Learning in AutoML
- AutoML for Small Data
- Human-in-the-Loop AutoML.
AI for Healthcare and Medicine
- Disease Prediction and Early Diagnosis
- Medical Image Analysis with Deep Learning
- Drug Discovery and Molecular Modeling
- Electronic Health Record Analysis
- Predictive Analytics in Healthcare
- Personalized Treatment Planning
- Healthcare Fraud Detection
- Telemedicine and Remote Patient Monitoring
- AI in Radiology and Pathology
- AI in Drug Repurposing
- AI for Medical Robotics and Surgery
- Genomic Data Analysis
- AI-powered Mental Health Assessment
- Explainable AI in Healthcare Decision Support
- AI in Epidemiology and Outbreak Prediction.
AI in Finance and Investment
- Algorithmic Trading and High-frequency Trading
- Credit Scoring and Risk Assessment
- Fraud Detection and Anti-money Laundering
- Portfolio Optimization with AI
- Financial Market Prediction
- Sentiment Analysis in Financial News
- Explainable AI in Financial Decision-making
- Algorithmic Pricing and Dynamic Pricing Strategies
- AI in Cryptocurrency and Blockchain
- Customer Behavior Analysis in Banking
- Explainable AI in Credit Decisioning
- AI in Regulatory Compliance
- Ethical AI in Financial Services
- AI for Real Estate Investment
- Automated Financial Reporting.

AI in Climate Change and Sustainability
- Climate Modeling and Prediction
- Renewable Energy Forecasting
- Smart Grid Optimization
- Energy Consumption Forecasting
- Carbon Emission Reduction with AI
- Ecosystem Monitoring and Preservation
- Precision Agriculture with AI
- AI for Wildlife Conservation
- Natural Disaster Prediction and Management
- Water Resource Management with AI
- Sustainable Transportation and Urban Planning
- Climate Change Mitigation Strategies with AI
- Environmental Impact Assessment with Machine Learning
- Eco-friendly Supply Chain Optimization
- Ethical AI in Climate-related Decision Support.
Data Privacy and Security
- Differential Privacy Mechanisms
- Federated Learning for Privacy-preserving AI
- Secure Multi-Party Computation
- Privacy-enhancing Technologies in Machine Learning
- Homomorphic Encryption for Machine Learning
- Ethical Considerations in Data Privacy
- Privacy-preserving AI in Healthcare
- AI for Secure Authentication and Access Control
- Blockchain and AI for Data Security
- Explainable Privacy in Machine Learning
- Privacy-preserving AI in Government and Public Services
- Privacy-compliant AI for IoT and Edge Devices
- Secure AI Models Sharing and Deployment
- Privacy-preserving AI in Financial Transactions
- AI in the Legal Frameworks of Data Privacy.
Global Collaboration in Research
- International Research Partnerships and Collaboration Models
- Multilingual and Cross-cultural AI Research
- Addressing Global Healthcare Challenges with AI
- Ethical Considerations in International AI Collaborations
- Interdisciplinary AI Research in Global Challenges
- AI Ethics and Human Rights in Global Research
- Data Sharing and Data Access in Global AI Research
- Cross-border Research Regulations and Compliance
- AI Innovation Hubs and International Research Centers
- AI Education and Training for Global Communities
- Humanitarian AI and AI for Sustainable Development Goals
- AI for Cultural Preservation and Heritage Protection
- Collaboration in AI-related Global Crises
- AI in Cross-cultural Communication and Understanding
- Global AI for Environmental Sustainability and Conservation.
Emerging Trends and Hot Topics in Machine Learning Research
The landscape of machine learning research topics is constantly evolving. Here are some of the emerging trends and hot topics that are shaping the field:
As AI systems become more prevalent, addressing ethical concerns and mitigating bias in algorithms are critical research areas.
Interpretable and Explainable Models
Understanding why machine learning models make specific decisions is crucial for their adoption in sensitive areas, such as healthcare and finance.
Meta-learning algorithms are designed to enable machines to learn how to learn, while AutoML aims to automate the machine learning process itself.
Machine learning is revolutionizing the healthcare sector, from diagnostic tools to drug discovery and patient care.
Algorithmic trading, risk assessment, and fraud detection are just a few applications of AI in finance, creating a wealth of research opportunities.
Machine learning research is crucial in analyzing and mitigating the impacts of climate change and promoting sustainable practices.
Challenges and Future Directions
While machine learning research has made tremendous strides, it also faces several challenges:
- Data Privacy and Security: As machine learning models require vast amounts of data, protecting individual privacy and data security are paramount concerns.
- Scalability and Efficiency: Developing efficient algorithms that can handle increasingly large datasets and complex computations remains a challenge.
- Ensuring Fairness and Transparency: Addressing bias in machine learning models and making their decisions transparent is essential for equitable AI systems.
- Quantum Computing and Machine Learning: The integration of quantum computing and machine learning has the potential to revolutionize the field, but it also presents unique challenges.
- Global Collaboration in Research: Machine learning research benefits from collaboration on a global scale. Ensuring that researchers from diverse backgrounds work together is vital for progress.
Resources for Machine Learning Researchers
If you’re looking to embark on a journey in machine learning research topics, there are various resources at your disposal:
- Journals and Conferences
Journals such as the “Journal of Machine Learning Research” and conferences like NeurIPS and ICML provide a platform for publishing and discussing research findings.
- Online Communities and Forums
Platforms like Stack Overflow, GitHub, and dedicated forums for machine learning provide spaces for collaboration and problem-solving.
- Datasets and Tools
Open-source datasets and tools like TensorFlow and PyTorch simplify the research process by providing access to data and pre-built models.
- Research Grants and Funding Opportunities
Many organizations and government agencies offer research grants and funding for machine learning projects. Seek out these opportunities to support your research.
Machine learning research is like a superhero in the world of technology. To be a part of this exciting journey, it’s important to choose the right machine learning research topics and keep up with the latest trends.
Machine learning research makes our lives better. It powers things like smart assistants and life-saving medical tools. It’s like the force driving the future of technology and society.
But, there are challenges too. We need to work together and be ethical in our research. Everyone should benefit from this technology. The future of machine learning research is incredibly bright. If you want to be a part of it, get ready for an exciting adventure. You can help create new solutions and make a big impact on the world.
Related Posts

Tips on How To Tackle A Machine Learning Project As A Beginner
Here in this blog, CodeAvail experts will explain to you tips on how to tackle a machine learning project as a beginner step by step…

Artificial Intelligence and Machine Learning Basics for Beginners
Here in this blog, CodeAvail experts will explain to you Artificial Intelligence and Machine Learning basics for beginners in detail step by step. What is…
Thank you for visiting nature.com. You are using a browser version with limited support for CSS. To obtain the best experience, we recommend you use a more up to date browser (or turn off compatibility mode in Internet Explorer). In the meantime, to ensure continued support, we are displaying the site without styles and JavaScript.
- View all journals
Machine learning articles from across Nature Portfolio
Machine learning is the ability of a machine to improve its performance based on previous results. Machine learning methods enable computers to learn without being explicitly programmed and have multiple applications, for example, in the improvement of data mining algorithms.
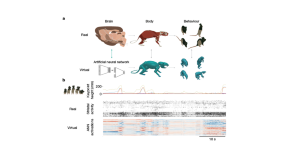
A virtual rat tests theories of motor control
How the brain controls complex movements has been a mystery. Advances in artificial intelligence now make it possible to simulate this process in virtual animals. Comparing activations in artificial control networks with brain activity in real animals enables long-standing theories of motor control at the level of neural circuits to be probed.
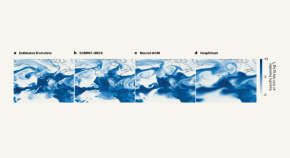
Weather and climate predicted accurately — without using a supercomputer
A cutting-edge global model of the atmosphere combines machine learning with a numerical model based on the laws of physics. This ‘hybrid’ system accurately predicts the weather — and even shows promise for climate simulations.
- Oliver Watt-Meyer
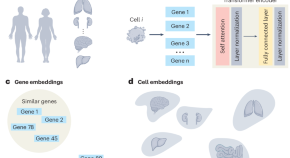
Toward learning a foundational representation of cells and genes
Inspired by the success of large-scale machine learning models in natural language, several groups are adapting these models for cellular data using massive single-cell datasets.
- Mohammad Lotfollahi
Latest Research and Reviews

Focal liver lesion diagnosis with deep learning and multistage CT imaging
The distinction of liver lesions is critical for the accurate diagnosis and treatment of liver cancer. Here, the authors develop LiLNet, a deep learning-based system to identify focal liver lesions as well as benign and malignant liver tumours from CT images with high accuracy across multiple patient cohorts.
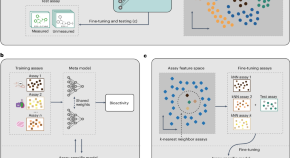
A bioactivity foundation model using pairwise meta-learning
Traditional machine learning methods for drug development struggle with bioactivity prediction due to the limited number of compounds in each assay and assay incompatibilities. Feng et al. developed ActFound, a bioactivity foundation model trained by pairwise learning and meta-learning, that improves the accuracy and generalization of bioactivity prediction.
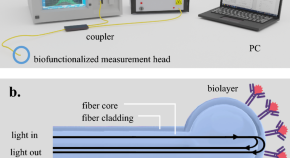
C-reactive protein (CRP) evaluation in human urine using optical sensor supported by machine learning
- Kacper Cierpiak
- Paweł Wityk
- Małgorzata Szczerska
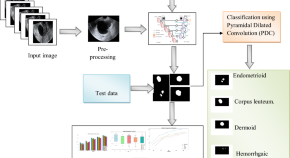
Segmentation of ovarian cyst in ultrasound images using AdaResU-net with optimization algorithm and deep learning model
- Mohemmed Sha
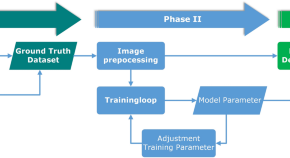
Development and performance evaluation of fully automated deep learning-based models for myocardial segmentation on T1 mapping MRI data
- Mathias Manzke
- Simon Iseke
- Felix G. Meinel
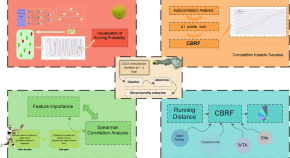
Momentum prediction models of tennis match based on CatBoost regression and random forest algorithms
- Xingchen Lv
News and Comment
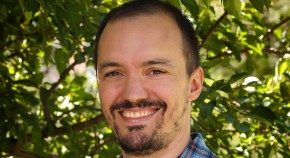
Chatbots in science: What can ChatGPT do for you?
Milton Pividori spent a year and a half studying how best to use ChatGPT in research. Here, he highlights three key lessons.
- Milton Pividori
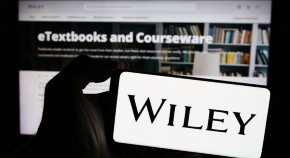
Has your paper been used to train an AI model? Almost certainly
Artificial-intelligence developers are buying access to valuable data sets that contain research papers — raising uncomfortable questions about copyright.
- Elizabeth Gibney
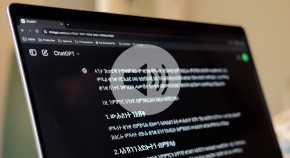
ChatGPT has a language problem — but science can fix it
The Large Language Models that power chatbots are known to struggle in languages outside of English — this podcast explores how this challenge can be overcome.
- Nick Petrić Howe
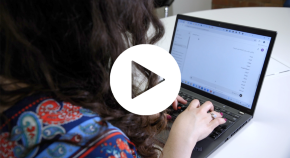
Why ChatGPT can't handle some languages
In a test of the chatbot's language abilities it fails at certain languages.
Quick links
- Explore articles by subject
- Guide to authors
- Editorial policies


- [email protected]
- +91-97 91 62 64 69
Thesis Topics for Machine Learning
Machine learning is one of the recently growing fields of research for classification, clustering, and prediction of input data . The techniques of ensembles and hybridization have contributed to the improvement of machine learning models . As a result, the speed of computation, operation, accuracy, and robustness of the machine learning models are enhanced. Through this article, you can get an overview of novel machine learning models, their design, performance, merits, and uses explained via a new taxonomic approach . Also, you can get all essential details regarding any thesis topics for machine learning from this page.
At present, there are many new ensembles and hybridized machine learning models being introduced and developed . Here the essentials of thesis writing are presented to you by our world-class certified writers and developers. What are the essential elements of a thesis statement?
- First of all you have to understand that thesis statement writing is the most crucial process which involves a lot of time and thinking
- Enough research data and evidence have to be gathered before writing a thesis statement
- The main Idea or the objective has to be presented clearly with supporting evidence
- Also remember that the thesis statement should be in accordance with the argument where adjustments are allowed
Usually, research scholars interact with our writers and experts for all aspects of thesis writing in machine learning. So we insist that you contact us much before you start your thesis so that you can have a clear-cut vision and well-strategized approach towards writing the best thesis.

Let us now have an idea about various headings to be included in any thesis topics for machine learning.
- Introduction – overview of the thesis
- Related / Existing works – presents of existing research
- Problems definition/statements – identify and highlight the problems
- Research methodology – convey the proposed concepts
- Results and Discussion – discuss the results of the proposed works with previous works
- Conclusion and future work – present the results of the proposed work
The introduction is the very first part of your thesis. It is the way by which you tend to create the first impression in the minds of the readers. What are the parts of the introduction in the thesis?
- The issue under examination is the core of an overview
- Main Idea and assertion has to be mentioned clearly
- Thesis statement and argument forms the fundamental aspect here
- Address the audience to prove to them that they are at the right place
- Scope of your paper should be mentioned satisfactorily
- The Planning based approach that you used to conduct research
In general, the choice of words, tone, approach, and language decide the quality of a thesis likewise the introduction. Our technical team and expert writers have gained enough experience writing thesis topics in machine learning. The amount of field knowledge and expertise that we gathered is quite large which will be of great use to you. Let us now talk about the next most important topic of a thesis called the issue
What are the guidelines for thesis writing?
Under the heading of the issue, the following aspects of research are to be included
- The background history about your result issue or concern solving which is stated as your objective
- The impact of the issue in this field
- Important characteristic features that affect the issue
- Potential research solutions that are undertaken for research
With the massive amount of reliable and authentic research materials that we provide, you can surely get all the necessary information to include in the issues part of your thesis. Also, our engineers and technical team are here to solve any kind of technical queries that you may get. Let us now talk about the literature review
LITERATURE REVIEW
- With important references and constructs from standard textbooks journals and relevant publications you need to make the following descriptions
- Relevant theory
- Issue explanation
- Potential solution
- Theoretical constructs
- Explanation on major theories
- Empirical literature from journal articles are considered for the following aspects
- Explanation on latest empirical studies
- Summary of the methodology adopted
- Important findings of the study
- Constraints associated with your findings
- The pathway of your research study has to be organized in line with the literature review to make keynotes on the following
- The referred definitions and concepts
- Unique aspects of the issues under examination
- Suitable method of your research
If you are searching for the best and most reliable online research guide for all kinds of thesis topics in machine learning then you are here at the right place. You can get professional and customized research support aligned with your institutional format from our experts. Let us now look into the method section in detail below
The following are the different aspects that you need to incorporate in the methods section of your thesis
- The research questions and issues under your examination
- Description of proposed works like data collection
- Rationale justification for the method of your choice
In addition to these aspects, you need to provide a clear description of all the research methods that you adopt in your study. For this purpose here are our research experts who will provide you with details on novel and innovative approaches useful for your research . You can also get concise and precise quantitative research data from us. Let us now look into this section of results
RESULTS AND DISCUSSION
On the page of results and discussion you need to incorporate the following aspects
- Description of major findings
- Visualization tools like charts, graphs, and tables to present the findings
- Relevant previous studies and results
- Creative and new results that you obtained
- Scopes to expand the previous studies with your findings
- Constraints of your study
The support of technical experts can help you do the best research work in machine learning . The interested researcher plus reliable and experienced research support makes the best PhD work possible. With our guidance, you get access to the best combo needed to carry out your research. Let’s now discuss the conclusions part
Conclusion and recommendation
In the part of conclusion, you need to include the following aspects
- Recap of issues being discussed
- Methods used and major findings
- Comparison between the original objective and accomplished results
- Scope for future expansion of your research
For each and every aspect of your machine learning PhD thesis , you can get complete support from our experts. In this respect let us now look to the topmost machine learning thesis topics below
Top 5 Thesis Topics for machine learning
- Machine learning is of great importance to physicians in the following perspectives
- Chatbots for speech recognition
- Pattern recognition for disease detection
- Treatment recommendation
- Detecting cancerous cells
- Body fluid analysis
- Identification of phenotypes in case of rare diseases
- Classifying data into groups for fault detection is possible using machine learning
- The following are some real-time examples for predictive analysis
- Fraudulent and legitimate transaction
- Improvement of prediction mechanism for detecting faults
- From the basics of developing products to predicting the stock market and real estate prices, predictive analytics is of greater importance
- Using a trading algorithm that makes use of a proper strategy for financing huge volumes of security is called statistical arbitrage
- Real-time examples of statistical arbitrage
- Analysis of huge data sets
- Algorithm-based trading for market microstructural analysis
- Real-time arbitrage possibilities
- Machine learning is used to enhance the strategy for statistical arbitrage as a result of which advanced results can be obtained
- In order to help the predictive analytics mechanisms to obtain increased accuracy feature extraction using machine learning plays a significant role
- Dataset annotations can be performed with greater significance using machine learning extraction methods where structured data can be extracted from unstructured information
- Real-time examples of machine learning-based feature extraction include the following
- Vocal cord disorder prediction
- Mechanism for prevention diagnosis and treatment of many disorders
- Detecting and solving many physiological problems in a Swift manner
- Extraction of critical information becomes easy with machine learning even when large volumes of data are being processed
- Machine learning methodologies can be used for translating speech into texts
- Recorded speech and real-time voice can be converted into text using machine learning systems designed for this purpose
- Speech can also be classified based on intensity, time, and frequency
- Voice search, appliance control, and voice dialing are the main real-time examples of speech recognition
In order to get confidential research guidance from world-class experts on all these thesis topics for machine learning, you can feel free to contact us. With more than 15 years of customer satisfaction, we are providing in-depth Research and advanced project support for all thesis topics for machine learning . Our thesis writing support also includes the following aspects
- Multiple revisions
- Complete grammatical check
- Formatting and editing
- Benchmark reference and citations from topmost journals
- Work privacy
- Internal review
We ensure all these criteria are conferred to you by world-class certified engineers, developers, and writers. So you can avail of our services with elevated confidence. We are here to support you fully. Let us now see some important machine learning methods in the following
Machine learning methods
Machine learning techniques are most often used in cases of making automatic decisions for any kind of input that they are trained and implemented for. Therefore machine learning approaches are expected to support the following aspects in decision making.
- Maximum accuracy of recommendations
- In-depth understanding and analysis before deciding to increase the trustworthiness
The decision-making approach using machine learning methods provides for higher accuracy in prediction and advanced comprehensible models respectively in implicit and explicit learning. For all your doubts and queries regarding the above-mentioned machine learning and decision-making approaches , you may feel free to contact us at any time of your convenience. Our technical team is highly experienced and skilled in resolving any kind of queries . Let us now see the important machine learning algorithms
Machine learning algorithms
Machine learning algorithms are very much diverse that they can be oriented into various objectives and goals for which machine learning methods are frequently adopted
- One rule, zero rule, and cubist
- RIPPER or Repeated Incremental Pruning to Produce Error Reduction
- Random forest, boosting, and AdaBoost
- Gradient Boosted Regression Trees and the Stacked Generalization
- Gradient Boosting Machines and Bootstrapped Aggregation
- Convolutional Neural Networks and Stacked Autoencoders
- Deep Boltzmann Machine and Deep Belief Networks
- Projection Pursuit and Sammon Mapping
- Principal Component Analysis and Partial Least Square Discriminant Analysis
- Quadratic Discriminant Analysis and Flexible Discriminant Analysis
- Partial Least Squares Regression and Multidimensional Scaling
- Principal Component Regression and Mixture Discriminant Analysis
- Regularized Discriminant Analysis and Linear Discriminant Analysis
- K means and K medians
- Expectation Maximization and Hierarchical Clustering
- Ridge Regression and Elastic Net
- Least Angle Regression and the LASSO or Least Absolute Shrinkage and Selection Operator
- Hopfield Network and perception
- Black Propagation and Radian Basis Function Network
- Naive Bayes and Bayesian Network
- Averaged One Dependents Estimators and Gaussian Naive Bayes
- Bayesian Belief Networks and Multinomial Naive Bayes
- Logistic, stepwise, and linear regression
- Locally Estimated Scatterplot Smoothing and Ordinary Least Squares Regression
- Multivariate Adaptive Regression Splines
- MS, C 4.5, C 5.0, and Decision stump
- Conditional Decision Trees and Iterative Dichotomiser 3
- Chi-squared Automatic Interaction Detection
- Classification and regression tree
- K Nearest Neighbour and Self Organising Map
- Locally Weighted Learning and Learning Vector Quantization
You can get a complete technical explanation and tips associated with the usage of these algorithms from our website. The selection of your thesis topic for machine learning becomes easier than before when you look into the various aspects of these algorithms and get to choose the best one based on your interests and needs. For this purpose, you can connect with us. We are here to assist you by giving proper expert consultation support for topic selection and allocating a highly qualified team of engineers to carry out your project successfully. Let us now talk about linear regression in detail
What is the process of linear regression?
The following are the three important stages in the process of linear regression analysis
- Data correlation and directionality analysis
- Model estimation based on linear fitting
- Estimation of validity and assessing the merits of the model
It is important that certain characteristic features are inherent in a model for the proper working of an algorithm. Feature engineering is the process by which essential features from raw data are obtained for the better functioning of an algorithm. With the most appropriate features extracted the algorithms become simple. Thus as a result accuracy of results is obtained even in the case of nonideal algorithms. What are the objectives of feature engineering?
- Preparation of input data for Better compatibility with the chosen machine learning algorithm
- Enhancement of the efficiency and working of machine learning models
With these goals, feature engineering becomes one of the important aspects of a machine learning research project. Talk to engineers for more details on the methods and algorithms used in extracting the necessary features. What are the techniques used in feature engineering?
- Imputation and binning
- Log transform and feature split
- Outliers handling and grouping functions
- One hot encoding and scaling
- Data extraction
Usually, we provide practical explanations in easy to understand words to our customers so that all their doubts are cleared even before they start their research. For this purpose, we make use of the real-time implemented models and our successful projects . Check out our website for all our machine learning project details. Let us now talk about hybrid machine learning models.
HYBRID MACHINE LEARNING MODELS
- When the machine learning methods are integrated with other methods such as optimization approaches, soft computing, and so on drastic improvement can be observed in the machine learning model.
- The ensemble methods are the culmination of grouping methods like boosting and bagging in case of multiple machine learning classifiers.
Our experts claim that the success of machine learning is dependent on ensemble and hybrid methods advancements. In this regard let us have a look into some of the hybrid methods below
- NBTree and functional tree
- Hybrid fuzzy with decision tree
- Logistic model tree and hybrid hoeffding tree
Most importantly these hybrid models and ensemble-based approaches in machine learning are on a rising scale and our technical team always stays updated about such novelties. So we are highly capable of providing you with the best support in all thesis topics for machine learning. Let us now look into the metrics used in analyzing the performance of machine learning models
Performance analysis of machine learning
Confusion metrics are prominently used for analyzing the machine learning models. The following are the fundamental terms associated with machine learning confusion metrics
- Contradiction of actual and predicted classes
- Correct prediction of negative values consisting of ‘no’ results for both actual and predicted classes
- Correct prediction of positive values consisting of ‘yes’ results for both actual and prediction classes
Using these fundamental parameters the essential values for calculation of efficiency and performance of the machine learning models are obtained as follows.
- Procession is considered as the ratio between the number of accurate positives predicted and the total number of positives claimed
- Recall is the ratio of all The true positive rate (in actual class being yes)
- F1 Score is the average between recall and precision hence taking into account all the false positives and false negatives
- Uneven distribution of classes require F1 Score to be evaluated than the accuracy, about which we will discuss below
- Accuracy can be considered in cases of similar false positives and false negatives.
- For different cost values of false positives and false negatives, it is recommended that you choose to recall and precision for performance evaluation
- Accuracy is the ratio between correct predictions and the total observations
- Also accuracy is considered as one of the most important and intuitive measures for analyzing the machine learning system performance
It becomes significant to note here that at thesis topics for machine learning ; our experts have produced excellent results in all these performance metrics. Contact our experts’ team for more details on the approaches that are considered to produce such the best outcomes . We work 24/7 to assist you.

Opening Hours
- Mon-Sat 09.00 am – 6.30 pm
- Lunch Time 12.30 pm – 01.30 pm
- Break Time 04.00 pm – 04.30 pm
- 18 years service excellence
- 40+ country reach
- 36+ university mou
- 194+ college mou
- 6000+ happy customers
- 100+ employees
- 240+ writers
- 60+ developers
- 45+ researchers
- 540+ Journal tieup
Payment Options

Our Clients

Social Links

- Terms of Use

Opening Time
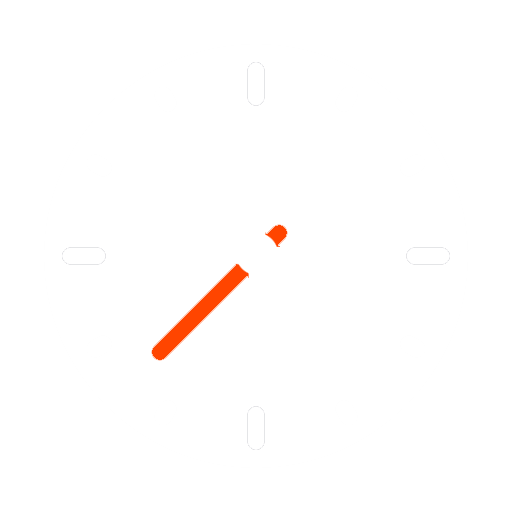
Closing Time
- We follow Indian time zone

Thesis Topic Proposals 2022-2023
Thesis information form.
Below you can see the thesis topics for 2022-2023. We offer 3 different thesis formats: - Format 1 : Regular thesis (fully supervised by KU Leuven) - Format 2 : Thesis in cooperation with a company (supervised by KU Leuven and the company) - Format 3 : Thesis with a company project within a company (supervised by the company)
NOTE: Additional late proposals are still added in October. Please regularly check this page for updates.
X = Thesis topic is taken
[NEW] = added less than a week ago
Company projects (format 3) for ECS or BDA
- X Accurate predictive sales forecasting model in the Belgian hearing aid market
- X AI-based identification of confident data driven virtual channels
- Applying multimodal transformer architectures (DONUT/LayoutLM) for document understanding in real life applications
- X Arbitrage of electricity prices between the Day-ahead market and the real time imbalance market
- [NEW] Continual Learning with a mix of labeled and unlabeled data
- X Data augmentation methods for audio ML
- Determination of crop characteristics using radar images and deep learning approaches
- Digital Twin process optimization
- Dynamic measurement of the ankle pose on a mobile device
- Edge computing on board of drone platform for real-time monitoring
- Embedded neural network based modality detection for medical displays
- X Generating Synthetic Intra Oral Scan Data
- ML based anomaly detection on timeseries data (generated in the additive manufacturing context) for root cause analysis
- Multiple company projects proposed by Dataroots
- One-shot Indirect Time-of-Flight Depth Sensing by Phase Demosaicking
- Quantum Computing for Earth Observation
- X Real time electricity price forecasting in the Belgian electricity market
- Robust image calibration for AI object detection system in the waste industry
- Selection of a state of the art object detection techniques and full end to end implementation into an intelligent document processing product
- Self learning strategies in healthcare to generate a representable feature space
- X Spot-NeRF: Extending Neural Radiance Fields with ToF Sensor Data
- Supply chain optimization and inventory projections
- X Titanium 3D printing: computer vision based anomaly detection and classification as quality inspection for critical components
- Towards automatic self-learning, self-MLOps & sustainable computer vision systems
- Towards generalization of runtime prediction for High Performance Computing tasks on cloud
- Valuation of With-profit Insurance Liabilities using Machine Learning techniques
- X Vision Transformers for Depth Completion on Mobile Devices
- Visual anomaly detection for industrial object detection system
Theses proposed by the Department of Computer Science
- Bypassing NIDS with adversarial examples
- Realistic Poisoning Attacks against Active Learning approaches for Cybersecurity Systems
- X Analyzing the important features for embedding models via data augmentation
- Automation of EU Tenders Electronic Daily TED transformation to Knowledge Graphs
- Better Similarity metrics for Time Series Clustering
- Discovering manipulation of experimental results in machine learning
- X Exploiting the structure of the problem in Interactive Constraint Acquisition
- X Generating residential electricity consumption scenarios with conditional generative neural networks
- How to improve data efficiency of deep reinforcement learning in ATARI games?
- Recovering serialized objects from binary CArchive files
- X Tunify: predicting business types based on website photos
- X Updating cluster assignments in COBRAS to improve query efficiency
- Using the right tools: Learning Hyperparameters for Knowledge Compilation
- What do you really know? Disentangling expertise in scientific collaborations
- An integrated end-to-end solution for aspect-based sentiment analysis
- Case study in linguistically informed interpretability
- Case study in NLP for truly low-resource languages
- Casual Explanation for multi-agent cooperation
- Enhancing the evaluation of flooding natural hazards and their societal impact using machine learning
- Meta Learning optimized Dynamic Memory Network for Task Free Continual Learning
- Multitask federated learning for entities and relations extraction from clinical records
- NVIDIA cuOpt – GPU accelerated AI route optimization
- Radius formulations for a selection of p-center problem variants
Theses proposed by the Department of Electrical Engineering
- Anonymous trading using MPC
- Comparative Tool/Platform for Cryptographic Designs
- X Electric vehicle charging data analytics and flexibility modeling
- Care about patients with dysarthria Adapting Spoken Language Understanding model with limited dysarthric speech data
- Data quality assessment methods for speech ML applications
- X Exploiting spatial relations for object detection with transformers
- X Few-shot Segmentation of Craniofacial Complexes
- Generating CT from MRI images using cycle GANs
- X Making deep learning models suitable for edge devices
- X Multi-output regression for prediction of tree species ratios in mixed forest stands in Flanders
- Multimodal Geometric Deep Learning to Link Face and Brain Shape
- X Statistical methods in homomorphic encryption
- What do self-supervised trained models learn about speech?
- What is wrong with Word Error Rate Exploration of semantically-aware metrics to evaluate speech transcriptions?
- A General Post-processing Framework for Change Point Detection Approaches based on Time-invariant Representations
- X Advancing gravitational wave simulations with machine learning
- X Automated mapping of medical terminologies to terms in a known vocabulary
- Classification of dynamic daily life activities through hipworn accelerometry
- X Data shuffling strategies in time series forecasting
- Deciphering lyrics by combining object detection and natural language processing
- X Deep learning on electrocardiographic data to improve non-invasive risk stratification for sudden cardiac death
- Development of an algorithm to detect and quantify stair climbing performance using accelerometry
- Effects of Different Data Partitioning Scenarios on Training Accuracy using Federated Learning
- Efficient Multi-view Kernel Spectral Clustering for Large-scale Data
- X Federated synthetic data generation for privacy preserving lung disease classification
- FedLearn Privacy-preserving: Federated Machine Learning for Drug Discovery
- X Filling the gaps in time-series: Generative models applied to air-quality data imputation
- X Functional Output Regression Beyond Square Loss: A Kernel Approach
- Gaussian graphical models and dynamical modeling of gene expression for Gene Regulatory Network inference
- GNN-based Change Point Detection method for Multi-channel Time Series
- Hierarchical Multi-view Kernel Spectral Clustering
- X Human-like decision-making in dense highway traffic using hybrid reinforcement learning policies
- X Model Performance in Prediction of Hospitalized Patients using Federated Learning versus Pooled and local dataset
- Multi-View Restricted Kernel Machines for Time-Series Forecasting
- Multimodal automatic image annotation for decision support systems
- X Multimodal motor seizure detection based on wearable sensors
- X Nonconvex and constrained optimization problems as neural network layers for arbitrarily-structured predictions
- Regularization Schemes for the Joint One-Class SVM and their Relationship to Density Level Sets Estimation
- Representation learning for high-dimensional cancer data
- X Representation learning in histopathological images via Deep Learning based models
- X Robust and Sample-Efficient Offline Reinforcement Learning
- X Segmentation of kidney tissue images using stainings as labels
- Semi-supervised Multiway Clustering in the Restricted Kernel Machines Framework
- X Skeleton-based deep learning approach for eating event detection from video signals
- Towards end-to-end modeling of cell-free DNA sequencing reads with read-level kernel functions
- X Transfer Learning Models for Predicting Clinical Outcomes Across Diverse Types of Cancer with the use of EHRs
- Transforming event detection: Building a better signal event detector
- X Utilization of convolutional kernels in deep restricted kernel machines for image tasks
WaveCORE, Networked Systems
- Sustainable AI
Theses proposed by the Computational Neuroscience Research Group
- X Averting a crisis: a predictive model for dementia using magnetoencephalography and AI
- Comparison of deep learning-based EEG motor imagery decoders with real-life BCI datasets
- Continuous sequence-to-sequence classification for imagined movement using a transformer
- X Linear Discriminant Analysis in the combined space, time and frequency domain for Brain-Computer Interfaces
- Predicting BCI illiteracy, is there an indication of BCI illiteracy in resting state EEG data?
- Steady-State Motion Visual Evoked Potentials (SSMVEP) using spatiotemporal beamforming
- X The effect of the analytic beamforming transformation on deep learning classification towards a plug and play brain computer interface
Theses proposed by the Centre for Computational Linguistics -- Faculty of Arts
- An Exploration of Generative Restricted Kernel Machines for the Induction of Latent Semantic Representations from Text
- X An Exploration of Neural Transformer Models for the Prediction of Cognitive Affect
- X An Investigation of Neural Network Architectures for Sign-Gloss to Text Translation
- X Automated rating of Exams Dutch as a Foreign Language
- Automatical (verification of) dating of Dutch historical documents
- Enriching Open Dutch Wordnet through Knowledge Graph Completion
- X How Well Do Neural Language Models Correlate with Human Measures on Narrative Understanding Tasks?
- Linguistic enrichment of historical Dutch using deep learning
- Multilingual Textual Similarity for the Investigation of Science News Circulation
- X PostASR Language Identification
Theses proposed by the Deparment of Mechanical Engineering
Students are welcome to contact prof. Herman Bruyninckx with a suggestion of their own, as long as it focusses on the integration of explicit formal "knowledge" with the sensor-based control of robots.
- Forward Model of Spray-based Material Deposition
- X Instrumentation of a soft robot hand with wireless microsensors
- X Make it fly! Achieving stable hovering of a hummingbird-like nano drone using reinforcement learning
- Seeing through Dust by Fusing Data from Long- and Short-wavelength Sensors
Theses proposed by Philosophy
Contemporary issues in Deontic Logic
Additionally, students are welcome to contact prof. Stef Frijters with a suggestion of their own, as long as it focusses on the use of formal logic within the domain of AI or on one of the following topics
- Epistemic logic
- Dynamic logic
- Bitstring semantics
- Temporal logic
- Formal semantics
Students are also welcome to contact prof. L ode Lauwaert with a suggestion of their own, as long as it focusses on one of the following topics
- Machine consciousness
- AI and the responsibility gap
- Singularity and AI
- Fairness in AI
- Agency of AI systems
- Value alignment
- Machine ethics
- Ethical algorithms
- Value by design
OTHER THESIS TOPICS
Natural sciences.
Topics proposed by
Dept. of Biomedical Sciences - Cardiovascular Imaging and Dynamics
- Interaction-aware network for interactive medical image segmentations using deep learning and B-spline explicit active surfaces
Cell Stress & Immunity laboratory
- Microbiome machine learning colorectal cancer classifier
Dept. of Earth and Environmental Sciences - Forest, Nature & Landscape
- X Deepfaking ecosystem response to climate extremes
- Integration of spatio-temporal context in remote sensing image classification
- Regional Climate Model Emulator based on Deep Learning over the Antarctic Peninsula
- X Chicken call detector using machine learning techniques
- X Chicken health monitoring by sneeze detection in noisy environments using deep learning
- X Estimating body temperature of a group of chickens based on surface temperature from thermal images
- Few-Shot Bird calls detection and classification as a pre-processing step for animal welfare monitoring through vocalization
- Improve the animals monitoring system in different environment by semantic transfer and GAN
Dept. of Mathematics - Plasma-astrophysics
- X Autoencoding of solar images and generation of artificial active regions
- Discovering physical laws from computer simulations of plasmas using machine learning
- Unsupervised ML Classification of velocity distributions in space
Nerf - KU Leuven, Imec, VIB
- X Uncovering neuronal population dynamics in the superior colliculus using latent variable models
VIB-KU Leuven Center for Brain&Disease research
- X Deciphering the DNA regulatory code of human brain cell types
Social Sciences
the laboratory of Experimental Psychology
- X A data-driven approach for completing 2D shape occlusions
- AI-assisted drawing style conversion for comics a deep learning approach
- X AI-based DeepGaze neural network to extract visual features and to predict gaze fixations for aesthetics judgments
Faculty of Economics and Business - LIRIS
- X Graph Neural Networks for Process Model Forecasting
KULAK - Public health and Primary care
- Bridging the gap between extreme multi-label classification and recommender systems
- How can I build a predictive model using little labeled data? A study of active learning applied to multi-target regression
- How can we process text in Dutch? A deep learning application for multi-target regression in education
- Rating elicitation using active learning to improve collaborative filtering performance
Faculty of Engineering - Tutorial services
- X Conversational Agent for Academic Advising
- X Explainable Learning Analytics opening the black box in the prediction of student success
- X Improved understanding of gait compensations to explore new pathways of gait rehabilitation in growing children with Duchenne muscular dystrophy
- X Learning Analytics how important and supportive is reflection for academic achievement
- Detection of Adult Spinal Deformation using various AI techniques on EMG signals
- X Development of algorithms which predict blood glucose values of type 1 diabetes patients
- X Swarm Intelligence via the Internet of Things
Propose your own thesis topic or company project
Students with other interests than the topics proposed by the programme can propose their own topic.
- Thesis topic proposal (Format 1 and 2) : The student can take contact with a lecturer of whom they expect that the topic lies in the expertise of this lecturer, or they can contact [email protected] to get help on finding a potential promotor for the topic. Typically, if a lecturer can be found who is interested in guiding the thesis topic, this will still require some discussion to polish the proposal, in order for it to reach a number of criteria (achievable goals, sufficient research questions, manageable, within the interests and expertise of the lecturer, sufficient AI dimension). If you found a promotor, you can fill out the following template and send it to [email protected] In case your proposal is in cooperation with a company, you can use this template and send it to [email protected]
- Company project proposal (Format 3) : The student can take contact with a company of their interest. Together with the company, you can fill in the project template and send it to [email protected]. If the proposed project is positively evaluated, an academic promotor will be sought by the program director. Please find the "requirements for the company" here . ! It is not allowed for working students to do a company project nor a thesis within their own company.
Bachelor and Master Thesis Opportunities
Finding a supervisor.
Our group supervises as many students as possible, but we often do not have the capacity to supervise all interested students.
Preliminaries
Prerequisites for thesis candidates.
Applicants must have significant experience in machine learning, e.g., as acquired through courses offered by our group (passed with a grade of “good” or better) or an equivalent qualification. This often includes a deep conceptual understanding of machine learning and significant programming experience. The necessary skills vary depending on the thesis topic, e.g., purely theoretical theses require more mathematical than programming skills. Candidates must possess the necessary abilities before applying. Cooperation with an external party (like a company or research institute) is usually only possible when the collaborating party does not significantly reduce scientific autonomy (e.g., regarding data access, topic selection, and thesis trajectory).
Application form
Interested students can contact us with the thesis application form as a PDF, a curriculum vitae or resume, and a cover letter. Please provide evidence of relevant skills. Degree theses are often connected to ongoing research in our group. However, it is also possible for students to suggest their own topics/ideas.
Application channels
1. contacting researchers directly.
Candidates can apply to potential supervisors directly using the standardized form above. In your email, please detail your reasons for reaching out to the particular individual.
How to find suitable researchers:
- Filter researchers by interests using this platform .
- Browse our team overview and research profile .
- Contact the researchers from the section “Thesis supervision offerings” below.
Students may send a follow-up email if a researcher does not respond, as messages can sometimes be overlooked amidst numerous emails. Please wait at least two weeks before writing such a follow-up mail.
2. Applying via a mailing list
In addition to targeted applications via channel (1), candidates can send their application to thesis(∂)ml.tu-berlin.de using the standardized form above. This distribution list reaches most researchers in our group. Responses will be provided only if your application aligns well with the needs of at least one researcher in our group.
3. Contacting the Thesis Coordination Team
If previous application attempts via the outlined channels are unsuccessful, and you meet the necessary thesis candidate prerequisites, you are encouraged to contact the Thesis Domain Coordinators. Please include a brief summary of your prior application attempts in your communication.
Thesis Domain Coordinators:
- Quantum Chemistry / ML for Physical Sciences: Stefan Chmiela
- Explainable AI: Grégoire Montavon
- Probabilistic ML: Shinichi Nakajima
- Biomedical Sensing and Neurotechnology: Alexander von Lühmann
- Kernel Methods: Andreas Ziehe
- Computational Neuroscience: Mina Jamshidi Idaji
- Digital Pathology: Julius Hense
- Multimodal Learning: Jannik Wolff
- Anomaly Detection: Alexander Bauer
- NLP and Digital Humanities: Oliver Eberle
- Graph Neural Networks: Thomas Schnake
For topics not covered by the above domains, or for general inquiries and feedback regarding the application process, please direct your correspondence to thesis.coordination(∂)ml.tu-berlin.de. If students do not receive a reply from the Thesis Domain Coordinators or the mailing list, they may send a follow-up email after a waiting period of at least two weeks.
Thesis supervision offerings
Please be aware that we often keep the titles of available theses confidential, as they frequently pertain to our current, yet-to-be-published research. Group members can access our internal database .
High-level overview of inquiring researchers
Supervisor and email address | High-level topic(s) | Bachelor / Master |
---|---|---|
Alexander von Lühmann (vonluehmann(∂)tu-berlin.de) | Biomedical Sensing | Master |
Shinichi Nakajima (nakajima(∂)tu-berlin.de) | Probabilistic ML | Master |
Sidney Bender (s.bender(∂)tu-berlin.de) | Generative Modelling for XAI | Master |
Thomas Schnake (t.schnake(∂)tu-berlin.de) | XAI | Master |
Mihail Bogojeski (m.bogojeski(∂)tu-berlin.de) | Quantum Chemistry | Master |
Stefan Gugler (stefan.gugler(∂)tu-berlin.de) | Quantum Chemistry | Master |
Pengzhou (Abel) Wu (pengzhou.wu(∂)tu-berlin.de | Probabilistic ML | Master |
Publicly advertised thesis topics
MS/BS | Topic | Supervisor + email address | Date of entry | Additional information |
---|---|---|---|---|
- | - | - | - | - |
Next steps after having found a supervisor
General information (link)
After having found a supervisor, students prepare a thesis proposal that includes
- the research question and its context/motivation,
- related work,
- preliminary methodological and/or experimental results,
- and formalities such as the number of ECTS credits and the writing time specified in the student’s examination regulations.
The supervisor can help the student with writing the proposal. Students may register their thesis with the examination office once Prof. Müller or an authorized senior researcher approves the proposal. We encourage students not to underestimate the time required for writing the proposal. Furthermore, consider that we may require some time to review the proposal. Therefore, it is helpful to apply to our group early.
- Python Course
- Python Basics
- Interview Questions
- Python Quiz
- Popular Packages
- Python Projects
- Practice Python
- AI With Python
- Learn Python3
- Python Automation
- Python Web Dev
- DSA with Python
- Python OOPs
- Dictionaries
8 Best Topics for Research and Thesis in Artificial Intelligence
Imagine a future in which intelligence is not restricted to humans!!! A future where machines can think as well as humans and work with them to create an even more exciting universe. While this future is still far away, Artificial Intelligence has still made a lot of advancement in these times. There is a lot of research being conducted in almost all fields of AI like Quantum Computing, Healthcare, Autonomous Vehicles, Internet of Things , Robotics , etc. So much so that there is an increase of 90% in the number of annually published research papers on Artificial Intelligence since 1996.
Keeping this in mind, if you want to research and write a thesis based on Artificial Intelligence, there are many sub-topics that you can focus on. Some of these topics along with a brief introduction are provided in this article. We have also mentioned some published research papers related to each of these topics so that you can better understand the research process.
Table of Content
1. Machine Learning
2. deep learning, 3. reinforcement learning, 4. robotics, 5. natural language processing (nlp), 6. computer vision, 7. recommender systems, 8. internet of things.
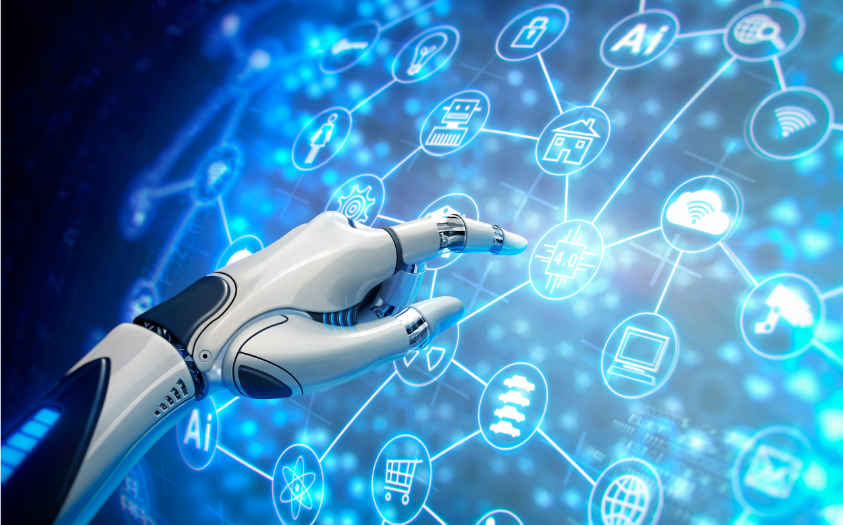
So without further ado, let’s see the different Topics for Research and Thesis in Artificial Intelligence!
Machine Learning involves the use of Artificial Intelligence to enable machines to learn a task from experience without programming them specifically about that task. (In short, Machines learn automatically without human hand holding!!!) This process starts with feeding them good quality data and then training the machines by building various machine learning models using the data and different algorithms. The choice of algorithms depends on what type of data do we have and what kind of task we are trying to automate.
However, generally speaking, Machine Learning Algorithms are generally divided into 3 types: Supervised Machine Learning Algorithms , Unsupervised Machine Learning Algorithms , and Reinforcement Machine Learning Algorithms . If you are interested in gaining practical experience and understanding these algorithms in-depth, check out the Data Science Live Course by us.
Deep Learning is a subset of Machine Learning that learns by imitating the inner working of the human brain in order to process data and implement decisions based on that data. Basically, Deep Learning uses artificial neural networks to implement machine learning. These neural networks are connected in a web-like structure like the networks in the human brain (Basically a simplified version of our brain!).
This web-like structure of artificial neural networks means that they are able to process data in a nonlinear approach which is a significant advantage over traditional algorithms that can only process data in a linear approach. An example of a deep neural network is RankBrain which is one of the factors in the Google Search algorithm.
Reinforcement Learning is a part of Artificial Intelligence in which the machine learns something in a way that is similar to how humans learn. As an example, assume that the machine is a student. Here the hypothetical student learns from its own mistakes over time (like we had to!!). So the Reinforcement Machine Learning Algorithms learn optimal actions through trial and error.
This means that the algorithm decides the next action by learning behaviors that are based on its current state and that will maximize the reward in the future. And like humans, this works for machines as well! For example, Google’s AlphaGo computer program was able to beat the world champion in the game of Go (that’s a human!) in 2017 using Reinforcement Learning.
Robotics is a field that deals with creating humanoid machines that can behave like humans and perform some actions like human beings. Now, robots can act like humans in certain situations but can they think like humans as well? This is where artificial intelligence comes in! AI allows robots to act intelligently in certain situations. These robots may be able to solve problems in a limited sphere or even learn in controlled environments.
An example of this is Kismet , which is a social interaction robot developed at M.I.T’s Artificial Intelligence Lab. It recognizes the human body language and also our voice and interacts with humans accordingly. Another example is Robonaut , which was developed by NASA to work alongside the astronauts in space.
It’s obvious that humans can converse with each other using speech but now machines can too! This is known as Natural Language Processing where machines analyze and understand language and speech as it is spoken (Now if you talk to a machine it may just talk back!). There are many subparts of NLP that deal with language such as speech recognition, natural language generation, natural language translation , etc. NLP is currently extremely popular for customer support applications, particularly the chatbot . These chatbots use ML and NLP to interact with the users in textual form and solve their queries. So you get the human touch in your customer support interactions without ever directly interacting with a human.
Some Research Papers published in the field of Natural Language Processing are provided here. You can study them to get more ideas about research and thesis on this topic.
The internet is full of images! This is the selfie age, where taking an image and sharing it has never been easier. In fact, millions of images are uploaded and viewed every day on the internet. To make the most use of this huge amount of images online, it’s important that computers can see and understand images. And while humans can do this easily without a thought, it’s not so easy for computers! This is where Computer Vision comes in.
Computer Vision uses Artificial Intelligence to extract information from images. This information can be object detection in the image, identification of image content to group various images together, etc. An application of computer vision is navigation for autonomous vehicles by analyzing images of surroundings such as AutoNav used in the Spirit and Opportunity rovers which landed on Mars.
When you are using Netflix, do you get a recommendation of movies and series based on your past choices or genres you like? This is done by Recommender Systems that provide you some guidance on what to choose next among the vast choices available online. A Recommender System can be based on Content-based Recommendation or even Collaborative Filtering.
Content-Based Recommendation is done by analyzing the content of all the items. For example, you can be recommended books you might like based on Natural Language Processing done on the books. On the other hand, Collaborative Filtering is done by analyzing your past reading behavior and then recommending books based on that.
Artificial Intelligence deals with the creation of systems that can learn to emulate human tasks using their prior experience and without any manual intervention. Internet of Things , on the other hand, is a network of various devices that are connected over the internet and they can collect and exchange data with each other.
Now, all these IoT devices generate a lot of data that needs to be collected and mined for actionable results. This is where Artificial Intelligence comes into the picture. Internet of Things is used to collect and handle the huge amount of data that is required by the Artificial Intelligence algorithms. In turn, these algorithms convert the data into useful actionable results that can be implemented by the IoT devices.
Please Login to comment...
Similar reads.
- AI-ML-DS Blogs
Improve your Coding Skills with Practice
What kind of Experience do you want to share?
M.Tech/Ph.D Thesis Help in Chandigarh | Thesis Guidance in Chandigarh
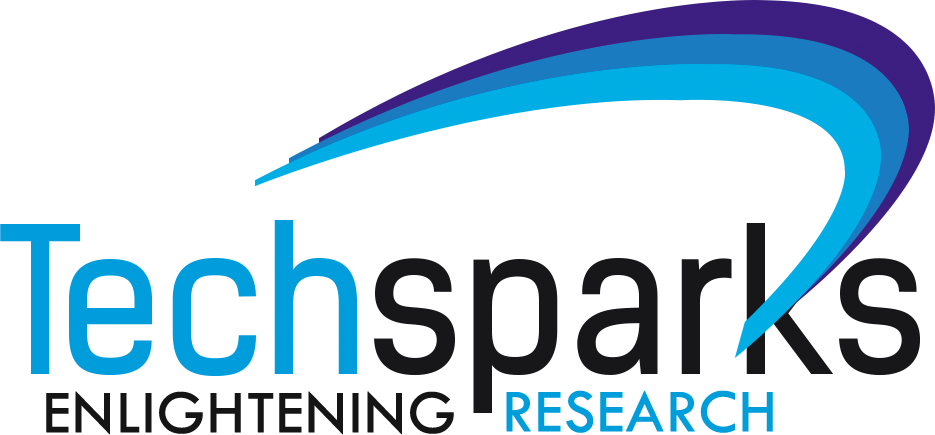

+91-9465330425

Latest thesis topics in Machine Learning for research scholars:
Choosing a research and thesis topics in Machine Learning is the first choice of masters and Doctorate scholars now a days. Though, choosing and working on a thesis topic in machine learning is not an easy task as Machine learning uses certain statistical algorithms to make computers work in a certain way without being explicitly programmed. The algorithms receive an input value and predict an output for this by the use of certain statistical methods. The main aim of machine learning is to create intelligent machines which can think and work like human beings. Achieving the above mentioned goals is surely not very easy because of which students who choose research topic in machine learning face difficult challenges and require professional thesis help in their thesis work.
Below is the list of the latest thesis topics in Machine learning for research scholars:
- The classification technique for the face spoof detection in artificial neural networks using concepts of machine learning .
- The iris detection and reorganization system using classification and glcm algorithm in machine learning.
- Using machine learning algorithms in the detection of pattern system using algorithm of textual feature analysis and classification
- The plant disease detection using glcm and KNN classification in neural networks merged with the concepts of machine learning
- Using the algorithms of machine learning to propose technique for the prediction analysis in data mining
- The sentiment analysis technique using SVM classifier in data mining using machine learning approach
- The heart disease prediction using technique of classification in machine learning using the concepts of data mining.
So let’s start with machine learning.
First of all…
What exactly is machine learning?
Find the link at the end to download the latest topics for thesis and research in Machine Learning
What is Machine Learning?
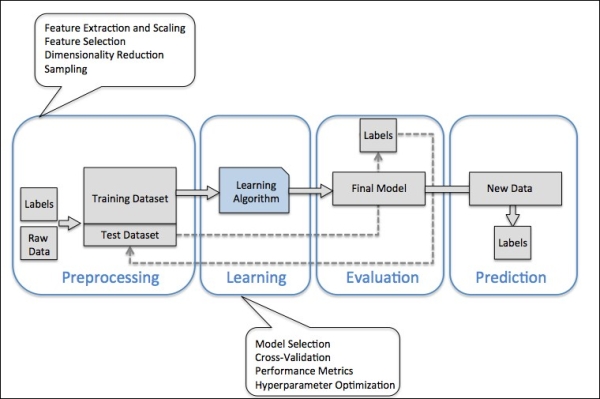
Machine Learning is a branch of artificial intelligence that gives systems the ability to learn automatically and improve themselves from the experience without being explicitly programmed or without the intervention of human. Its main aim is to make computers learn automatically from the experience.
Requirements of creating good machine learning systems
So what is required for creating such machine learning systems? Following are the things required in creating such machine learning systems:
Data – Input data is required for predicting the output.
Algorithms – Machine Learning is dependent on certain statistical algorithms to determine data patterns.
Automation – It is the ability to make systems operate automatically.
Iteration – The complete process is iterative i.e. repetition of process.
Scalability – The capacity of the machine can be increased or decreased in size and scale.
Modeling – The models are created according to the demand by the process of modeling.
Methods of Machine Learning
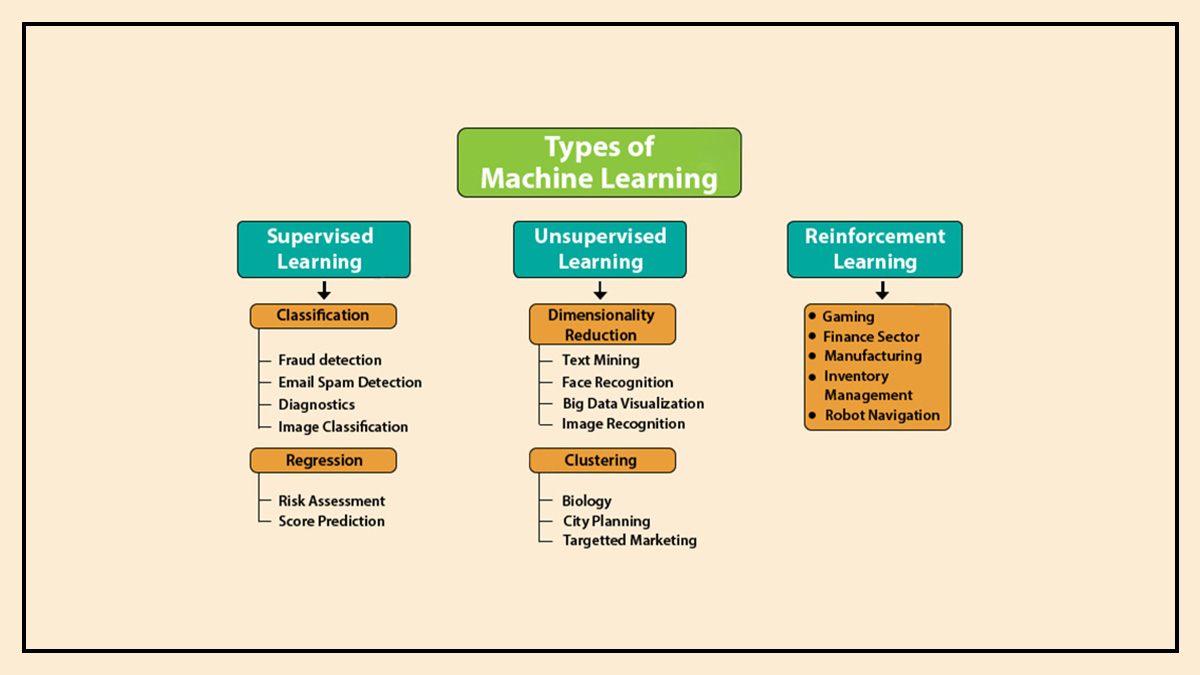
Machine Learning methods are classified into certain categories These are:
- Supervised Learning
- Unsupervised Learning
Reinforcement Learning
Supervised Learning – In this method, input and output is provided to the computer along with feedback during the training. The accuracy of predictions by the computer during training is also analyzed. The main goal of this training is to make computers learn how to map input to the output.
Unsupervised Learning – In this case, no such training is provided leaving computers to find the output on its own. Unsupervised learning is mostly applied on transactional data. It is used in more complex tasks. It uses another approach of iteration known as deep learning to arrive at some conclusions.
Reinforcement Learning – This type of learning uses three components namely – agent, environment, action. An agent is the one that perceives its surroundings, an environment is the one with which an agent interacts and acts in that environment. The main goal in reinforcement learning is to find the best possible policy.
How does machine learning work?
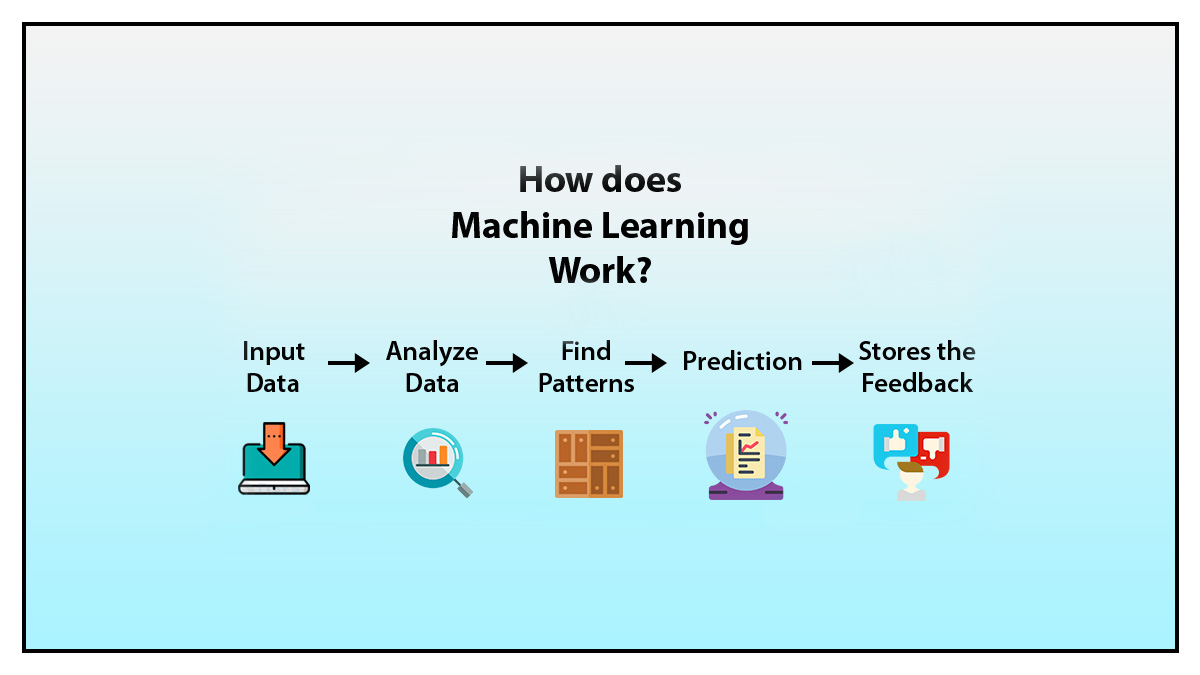
Machine learning makes use of processes similar to that of data mining. Machine learning algorithms are described in terms of target function(f) that maps input variable (x) to an output variable (y). This can be represented as:
There is also an error e which is the independent of the input variable x. Thus the more generalized form of the equation is:
In machine the mapping from x to y is done for predictions. This method is known as predictive modeling to make most accurate predictions. There are various assumptions for this function.
Benefits of Machine Learning
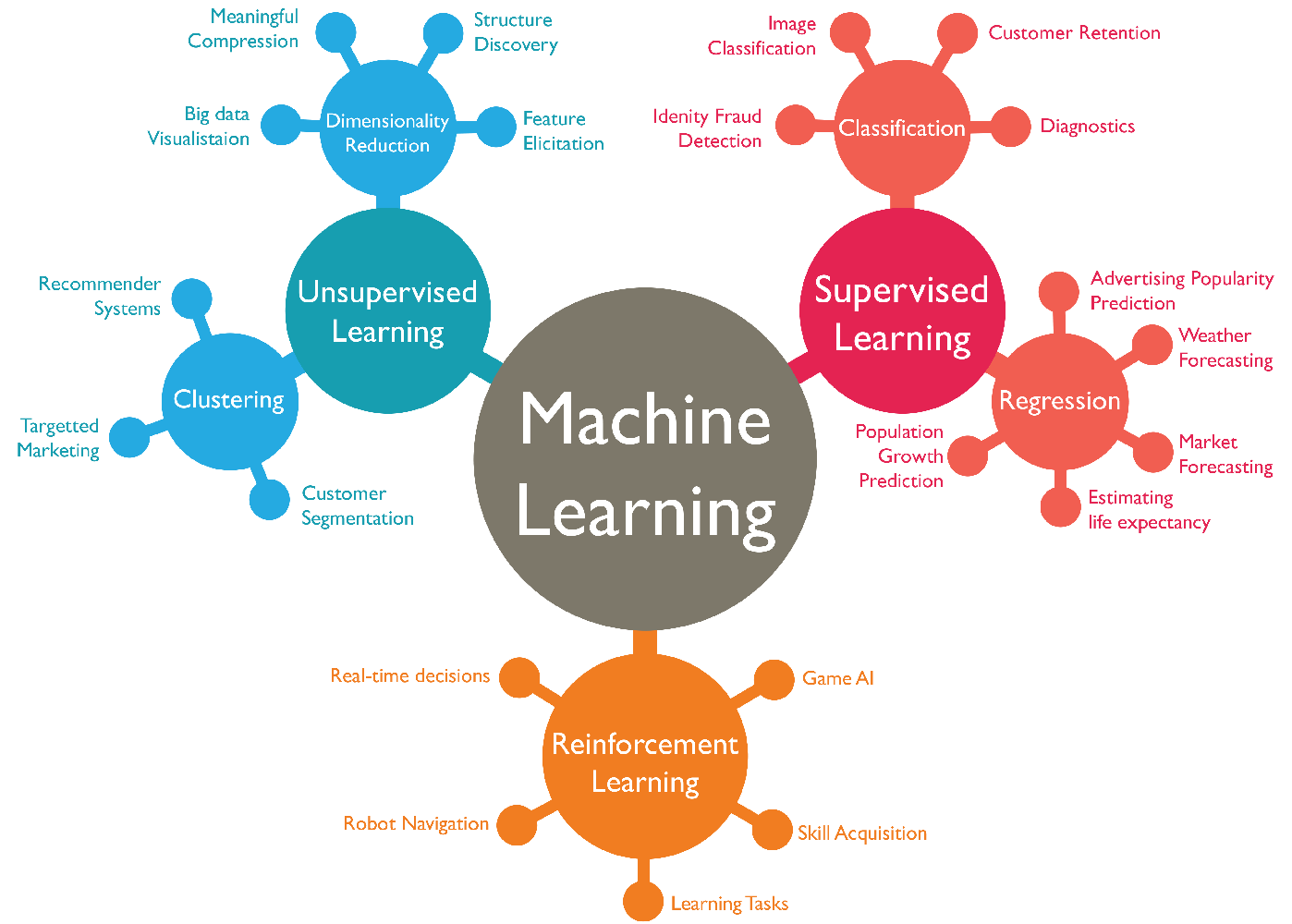
Everything is dependent on machine learning. Find out what are the benefits of machine learning.
Decision making is faster – Machine learning provides the best possible outcomes by prioritizing the routine decision-making processes.
Adaptability – Machine Learning provides the ability to adapt to new changing environment rapidly. The environment changes rapidly due to the fact that data is being constantly updated.
Innovation – Machine learning uses advanced algorithms that improve the overall decision-making capacity. This helps in developing innovative business services and models.
Insight – Machine learning helps in understanding unique data patterns and based on which specific actions can be taken.
Business growth – With machine learning overall business process and workflow will be faster and hence this would contribute to the overall business growth and acceleration.
Outcome will be good – With machine learning the quality of the outcome will be improved with lesser chances of error.
Branches of Machine Learning
- Computational Learning Theory
- Adversarial Machine Learning
- Quantum Machine Learning
- Robot Learning
- Meta-Learning
Computational Learning Theory – Computational learning theory is a subfield of machine learning for studying and analyzing the algorithms of machine learning. It is more or less similar to supervised learning.
Adversarial Machine Learning – Adversarial machine learning deals with the interaction of machine learning and computer security. The main aim of this technique is to look for safer methods in machine learning to prevent any form of spam and malware. It works on the following three principles:
Finding vulnerabilities in machine learning algorithms.
Devising strategies to check these potential vulnerabilities.
Implementing these preventive measures to improve the security of the algorithms.
Quantum Machine Learning – This area of machine learning deals with quantum physics. In this algorithm, the classical data set is translated into quantum computer for quantum information processing. It uses Grover’s search algorithm to solve unstructured search problems.
Predictive Analysis – Predictive Analysis uses statistical techniques from data modeling, machine learning and data mining to analyze current and historical data to predict the future. It extracts information from the given data. Customer relationship management(CRM) is the common application of predictive analysis.
Robot Learning – This area deals with the interaction of machine learning and robotics. It employs certain techniques to make robots to adapt to the surrounding environment through learning algorithms.
Grammar Induction – It is a process in machine learning to learn formal grammar from a given set of observations to identify characteristics of the observed model. Grammar induction can be done through genetic algorithms and greedy algorithms.
Meta-Learning – In this process learning algorithms are applied on meta-data and mainly deals with automatic learning algorithms.
Best Machine Learning Tools
Here is a list of artificial intelligence and machine learning tools for developers:
ai-one – It is a very good tool that provides software development kit for developers to implement artificial intelligence in an application.
Protege – It is a free and open-source framework and editor to build intelligent systems with the concept of ontology. It enables developers to create, upload and share applications.
IBM Watson – It is an open-API question answering system that answers questions asked in natural language. It has a collection of tools which can be used by developers and in business.
DiffBlue – It is another tool in artificial intelligence whose main objective is to locate bugs, errors and fix weaknesses in the code. All such things are done through automation.
TensorFlow – It is an open-source software library for machine learning. TensorFlow provides a library of numerical computations along with documentation, tutorials and other resources for support.
Amazon Web Services – Amazon has launched toolkits for developers along with applications which range from image interpretation to facial recognition.
OpenNN – It is an open-source, high-performance library for advanced analytics and is written in C++ programming language. It implements neural networks. It has a lot of tutorials and documentation along with an advanced tool known as Neural Designer.
Apache Spark – It is a framework for large-scale processing of data. It also provides a programming tool for deep learning on various machines.
Caffe – It is a framework for deep learning and is used in various industrial applications in the area of speech, vision and expression.
Veles – It is another deep learning platform written in C++ language and make use of python language for interaction between the nodes.
Machine Learning Applications
Following are some of the applications of machine learning:
Cognitive Services
Medical Services
Language Processing
Business Management
Image Recognition
Face Detection
Video Games
Computer Vision
Pattern Recognition
Machine Learning in Bioinformatics
Bioinformatics term is a combination of two terms bio, informatics. Bio means related to biology and informatics means information. Thus bioinformatics is a field that deals with processing and understanding of biological data using computational and statistical approach. Machine Learning has a number of applications in the area of bioinformatics. Machine Learning find its application in the following subfields of bioinformatics:
Genomics – Genomics is the study of DNA of organisms. Machine Learning systems can help in finding the location of protein-encoding genes in a DNA structure. Gene prediction is performed by using two types of searches named as extrinsic and intrinsic. Machine Learning is used in problems related to DNA alignment.
Proteomics – Proteomics is the study of proteins and amino acids. Proteomics is applied to problems related to proteins like protein side-chain prediction, protein modeling, and protein map prediction.
Microarrays – Microarrays are used to collect data about large biological materials. Machine learning can help in the data analysis, pattern prediction and genetic induction. It can also help in finding different types of cancer in genes.
System Biology – It deals with the interaction of biological components in the system. These components can be DNA, RNA, proteins and metabolites. Machine Learning help in modeling these interactions.
Text mining – Machine learning help in extraction of knowledge through natural language processing techniques.
Deep Learning
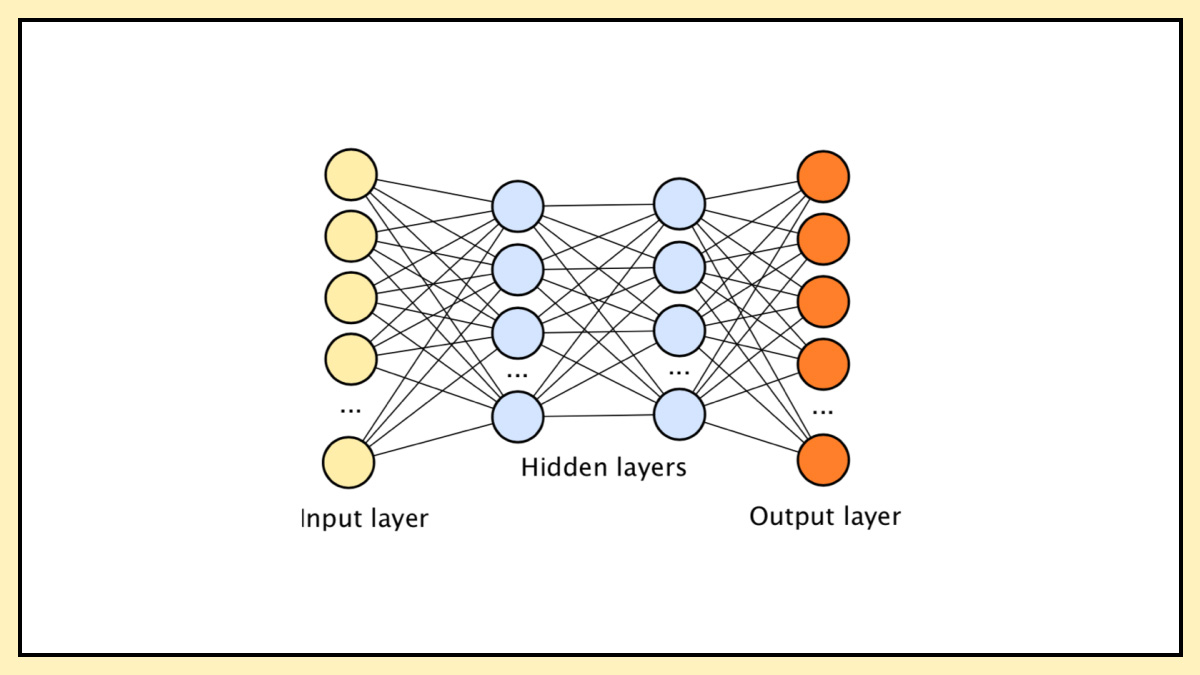
Deep Learning is a part of the broader field machine learning and is based on data representation learning. It is based on the interpretation of artificial neural network. Deep Learning algorithm uses many layers of processing. Each layer uses the output of previous layer as an input to itself. The algorithm used can be supervised algorithm or unsupervised algorithm. Deep Learning is mainly developed to handle complex mappings of input and output. It is another hot topic for M.Tech thesis and project along with machine learning.
Deep Neural Network
Deep Neural Network is a type of Artificial Neural Network with multiple layers which are hidden between the input layer and the output layer. This concept is known as feature hierarchy and it tends to increase the complexity and abstraction of data. This gives network the ability to handle very large, high-dimensional data sets having millions of parameters. The procedure of deep neural networks is as follows:
Consider some examples from a sample dataset.
Calculate error for this network.
Improve weight of the network to reduce the error.
Repeat the procedure.
Applications of Deep Learning
Here are some of the applications of Deep Learning:
Automatic Speech Recognition
Natural Language Processing
Customer Relationship Management
Bioinformatics
Mobile Advertising
Advantages of Deep Learning
Deep Learning helps in solving certain complex problems with high speed which were earlier left unsolved. Deep Learning is very useful in real world applications. Following are some of the main advantages of deep learning:
Eliminates unnecessary costs – Deep Learning helps to eliminate unnecessary costs by detecting defects and errors in the system.
Identifies defects which otherwise are difficult to detect – Deep Learning helps in identifying defects which left untraceable in the system.
Can inspect irregular shapes and patterns – Deep Learning can inspect irregular shapes and patterns which is difficult for machine learning to detect.
From this introduction, you must have known that why this topic is called as hot for your M.Tech thesis and projects. This was just the basic introduction to machine learning and deep learning. There is more to explore in these fields. You will get to know more once you start doing research on this topic for your M.Tech thesis. You can get thesis assistance and guidance on this topic from experts specialized in this field.
Research and Thesis Topics in Machine Learning
Here is the list of current research and thesis topics in Machine Learning :
Machine Learning Algorithms
Supervised Machine Learning
Unsupervised Machine Learning
Neural Networks
Predictive Learning
Bayesian Network
Data Mining
For starting with Machine Learning, you need to know some algorithms. Machine Learning algorithms are classified into three categories which provide the base for machine learning. These categories of algorithms are supervised learning, unsupervised learning, and reinforcement learning. The choice of algorithms depends upon the type of tasks you want to be done along with the type, quality, and nature of data present. The role of input data is crucial in machine learning algorithms.
Computer Vision is a field that deals with making systems that can read and interpret images. In simple terms, computer vision is a method of transmitting human intelligence and vision in machines. In computer vision, data is collected from images which are imparted to systems. The system will take action according to the information it interprets from what it sees.
It is a good topic for machine learning masters thesis. It is a type of machine learning algorithm in which makes predictions based on known data-sets. Input and output is provided to the system along with feedback. Supervised Learning is further classified into classification and regression problems. In the classification problem, the output is a category while in regression problem the output is a real value.
It is another category of machine learning algorithm in which input is known but the output is not known. Prior training is not provided to the system as in case of supervised learning. The main purpose of unsupervised learning is to model the underlying structure of data. Clustering and Association are the two types of unsupervised learning problems. k-means and Apriori algorithm are the examples of unsupervised learning algorithms.
Deep Learning is a hot topic in Machine Learning. It is already explained above. It is a part of the family of machine learning and deals with the functioning of the artificial neural network. Neural Networks are used to study the functioning of the human brain. It is one of the growing and exciting field. Deep learning has made it possible for the practical implementation of various machine learning applications.
Neural Networks are the systems to study the biological neural networks. It is an important application of machine learning and a good topic for masters thesis and research. The main purpose of Artificial Neural Network is to study how the human brain works. It finds its application in computer vision, speech recognition, machine translation etc. Artificial Neural Network is a collection of nodes which represent neurons.
Reinforcement Learning is a category of machine learning algorithms. Reinforcement Learning deals with software agents to study how these agents take actions in an environment in order to maximize their performance. Reinforcement Learning is different from supervised learning in the sense that correct input and output parameters are not provided.
Predictive Learning is another good topic for thesis in machine learning. In this technique, a model is built by an agent of its environment in which it performs actions. There is another field known as predictive analytics which is used to make predictions about future events which are unknown. For this, techniques like data mining, statistics, modeling, machine learning, and artificial intelligence are used.
It is a network that represents probabilistic relationships via Directed Acyclic Graph(DAG). There are algorithms in Bayesian Network for inference and learning. In the network, a probability function is there for each node which takes an input to give probability to the value associated with the node. Bayesian Network finds its application in bioinformatics, image processing, and computational biology.
Data Mining is the process of finding patterns from large data-sets to extract valuable information to make better decisions. It is a hot area of research. This technology use method from machine learning, statistics, and database systems for processing. There exist data mining techniques like clustering, association, decision trees, classification for the data mining process.
Click on the following link to download the latest thesis and research topics in Machine Learning
Latest Thesis and Research Topics on Machine Learning(pdf)
For more details Contact Us. You can call us on this number +91-9465330425 or drop an email at [email protected] for any type of dissertation help in India. You can also fill the query form on the website.
You can also visit our website Techsparks and follow us on Pinterest , Facebook , Twitter, YouTube and Linkedin for latest thesis blog.
Leave a Reply Cancel reply
Your email address will not be published. Required fields are marked *
Save my name, email, and website in this browser for the next time I comment.
Quick Enquiry
Get a quote, share your details to get free.
Academia.edu no longer supports Internet Explorer.
To browse Academia.edu and the wider internet faster and more securely, please take a few seconds to upgrade your browser .
Enter the email address you signed up with and we'll email you a reset link.
- We're Hiring!
- Help Center
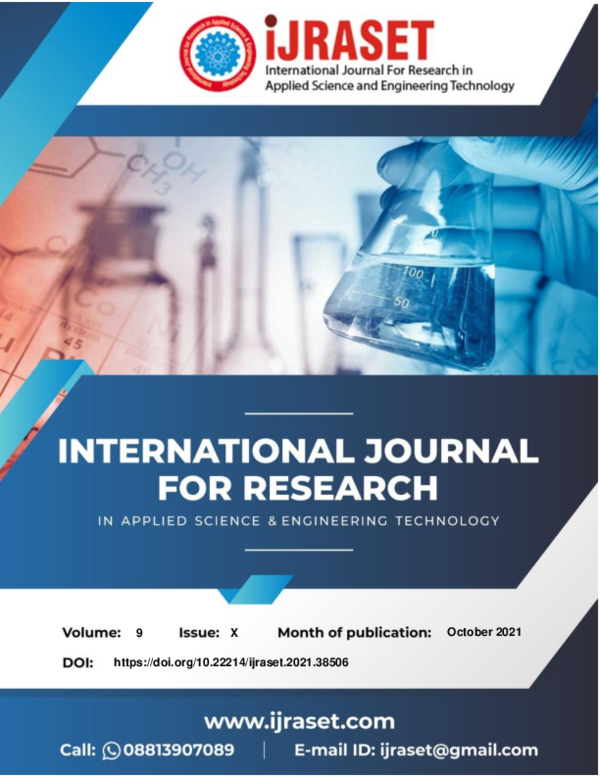
Thesis on Machine Learning Methods and Its Applications

2021, IJRASET
In the 1950s, the concept of machine learning was discovered and developed as a subfield of artificial intelligence. However, there were no significant developments or research on it until this decade. Typically, this field of study has developed and expanded since the 1990s. It is a field that will continue to develop in the future due to the difficulty of analysing and processing data as the number of records and documents increases. Due to the increasing data, machine learning focuses on finding the best model for the new data that takes into account all the previous data. Therefore, machine learning research will continue in correlation with this increasing data. This research focuses on the history of machine learning, the methods of machine learning, its applications, and the research that has been conducted on this topic. Our study aims to give researchers a deeper understanding of machine learning, an area of research that is becoming much more popular today, and its applications.
Related Papers
Manisha More
Machine learning is the fastest growing areas of computer science. It has the ability to lets the computer to create the program. It is a subset of Artificial Intelligence (AI), and consists of the more advanced techniques and models that enable computers to figure things out from the data and deliver. It is a field of learning and broadly divided into supervised learning, unsupervised learning, and reinforcement learning. There are many fields where the Machine learning algorithms are used. The objective of the paper is to represent the ML objectives, explore the various ML techniques and algorithms with its applications in the various fields from published papers, workshop materials & material collected from books and material available online on the World Wide Web.
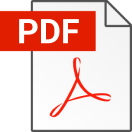
Pattern Recognition Letters
Ramon Lopez De Mantaras
pankaj verma
The field of machine learning is introduced at a conceptual level. The main goal of machine learning is how computers automatically learn without any human invention or assistance so that they can adjust their action accordingly. We are discussing mainly three types of algorithms in machine learning and also discussed ML's features and applications in detail. Supervised ML, In this typeof algorithm, the machine applies what it has learned in its past to new data, in which they use labeled examples, so that they predict future events. Unsupervised ML studies how systems can infer a function, so that they can describe a hidden structure from unlabeled data. Reinforcement ML, is a type of learning method, which interacts with its environment, produces action, as well as discovers errors and rewards.
Journal of Advances in Mathematical & Computational Science. Vol 10, No.3. Pp 1 – 14.
Jerry Sarumi
Machine learning and associated algorithms occupies a pride of place in the execution of automation in the field of computing and its application to addressing contemporary and human-centred problems such as predictions, evaluations, deductions, analytics and analysis. This paper presents types of data and machine learning algorithms in a broader sense. We briefly discuss and explain different machine learning algorithms and real-world application areas based on machine learning. We highlight several research issues and potential future directions
IJESRT Journal
Machine learning [1], a branch of artificial intelligence, that gives computers the ability to learn without being explicitly programmed, means it gives system the ability to learn from data. There are two types of learning techniques: supervised learning and unsupervised learning [2]. This paper summarizes the recent trends of machine learning research.
International Journal for Research in Applied Science & Engineering Technology (IJRASET)
Dr. Manish Kumar Singh
Machine learning has become one of the most envisaged areas of research and development field in modern times. But the area of research related to machine learning is not new. The term machine learning was coined by Arthur Samuel in 1952 and since then lots of developments have been made in this field. The data scientists and the machine learning enthusiasts have developed myriad algorithms from time to time to let the benefit of machine learning reach to each and every field of human endeavors. This paper is an effort to put light on some of the most prominent algorithms that have been used in machine learning field on frequent basis since the time of its inception. Further, we will analyze their area of applications.
International Journal of Advanced Technology and Engineering Exploration
Akash Badone
International Journal of Engineering Applied Sciences and Technology
vishal bari
Today, huge amounts of data are available everywhere. Therefore, analyzing this data is very important to derive useful information from it and develop an algorithm based on this analysis. This can be achieved through data mining and machine learning. Machine learning is an essential part of artificial intelligence used to design algorithms based on data trends and past relationships between data. Machine learning is used in a variety of areas such as bioinformatics, intrusion detection, information retrieval, games, marketing, malware detection, and image decoding. This paper shows the work of various authors in the field of machine learning in various application areas.
Ioannis Vlahavas
IJRASET Publication
This paper describes essential points of machine learning and its application. It seamlessly turns around and teach about the pros and cons of the ML. As well as it covers the real-life application where the machine learning is being used. Different types of machine learning and its algorithms. This paper is giving the detail knowledge about the different algorithms used in machine learning with their applications. There is brief explanation about the Weather Prediction application using the machine learning and also the comparison between various machine learning algorithms used by various researchers for weather prediction.
Loading Preview
Sorry, preview is currently unavailable. You can download the paper by clicking the button above.
RELATED PAPERS
Sumeet Agarwal
JMSS, A2Z Journals
Journal of Management and Service Science (JMSS), A 2 Z Journals
Applied Sciences
Grzegorz Dudek
Pooja Ambatkar
Journal of Physics: Conference Series
Jafar Alzubi
IRJET Journal
Kostantinos Demertzis
International Journal of Computer Applications
IJERA Journal
International journal of engineering research and technology
Dr Nitin Rajvanshi
International Journal of Engineering Research and Advanced Technology
rama prasad
International Journal of Scientific Research in Computer Science, Engineering and Information Technology
International Journal of Scientific Research in Computer Science, Engineering and Information Technology IJSRCSEIT
Zachary Barillaro
International Journal of Innovative Technology and Exploring Engineering
atul kathole
Iqbal Muhammad
Artificial Intelligence
mplab.ucsd.edu
Paul Ruvolo
Foundation of Computer Applications
Editor IJATCA , nikhil katoch
International Journal of Scientific Research in Science, Engineering and Technology
International Journal of Scientific Research in Science, Engineering and Technology IJSRSET
RELATED TOPICS
- We're Hiring!
- Help Center
- Find new research papers in:
- Health Sciences
- Earth Sciences
- Cognitive Science
- Mathematics
- Computer Science
- Academia ©2024

Member-only story
Office Hours
How to write a great data science thesis, some tips and tricks to help make you write your thesis as smoothly as possible.

Max van der Werf
Towards Data Science
There are probably more than a thousand manuals on how to write a great thesis (some of my favorites can be found here , here and here ). They will stress the importance of structure, substance and style. They will urge you to write down your methodology and results first, then progress to the literature review, introduction and conclusions and to write the summary or abstract last. To write clearly and directly with the reader’s expectations always in mind. All of these tips are very valuable, but which tips apply to writing academically in the domain of data science?
Data science students originate from all kinds of fields and are not necessarily experienced writers of technical papers. Thus, which specific tips should one read before they proceed to write a great data science thesis? Having finished my data science master’s two weeks ago, I feel like somewhat of an expert in this field. Most of me and my peers’ struggles are still quite fresh in my mind, and I would like to convey the most practical solutions here.
Choosing a Research Topic

Written by Max van der Werf
Aspiring data scientist interested in sports, psychology, economics and everything in between.
Text to speech
- Bibliography
- More Referencing guides Blog Automated transliteration Relevant bibliographies by topics
- Automated transliteration
- Relevant bibliographies by topics
- Referencing guides
TechRepublic
Account information.
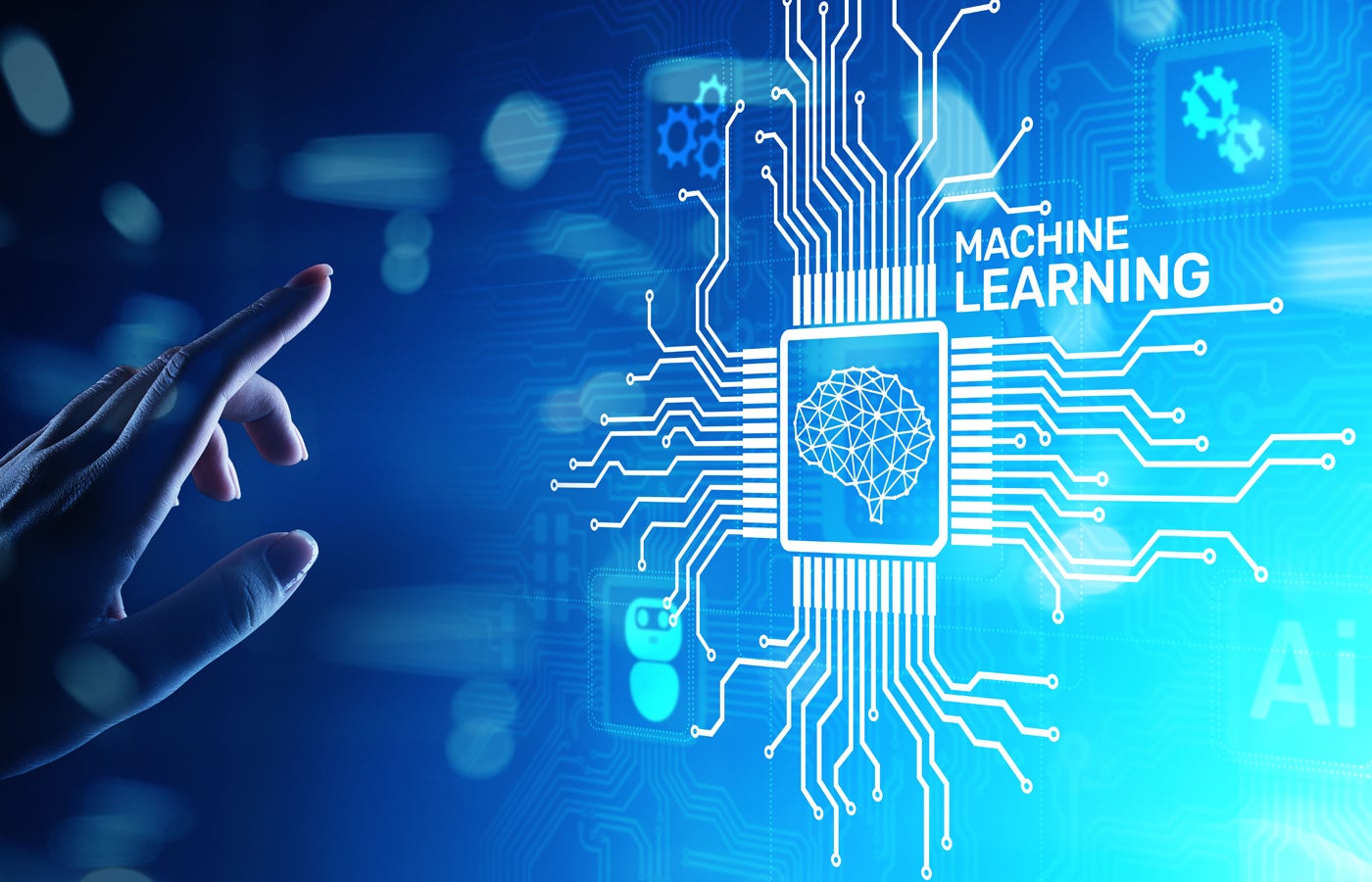
Share with Your Friends
The 5 Best Machine Learning Courses for 2024
Your email has been sent
Machine learning may sound relatively old-fashioned in the age of AI, but it remains a valuable and oft-used skill. Machine learning is the use of algorithms in computer systems to “learn” from data, allowing those systems to take on autonomous tasks. Manufacturing, engineering, programming, data science and more can include machine learning.
The field is distinct from AI in its approach, methods and underlying structure, and it often makes headlines in physics and other science applications. To discover more about machine learning, you can take online courses from a variety of businesses or institutions.
- Best for complete beginners: Introduction to Machine Learning (Google)
- Best for data scientists: Data Science - Machine Learning (Harvard on edX)
- Best for a traditional university education: Cornell University’s Machine Learning Certificate Program (Cornell)
- Best for building neural network applications: Stanford Machine Learning Specialization (Coursera)
- Best for aspiring data scientists: IBM Introduction to Machine Learning Specialization (Coursera)
Best Machine Learning Courses: Comparison table
Course | ||||
---|---|---|---|---|
Introduction to Machine Learning (Google): Best for complete beginners
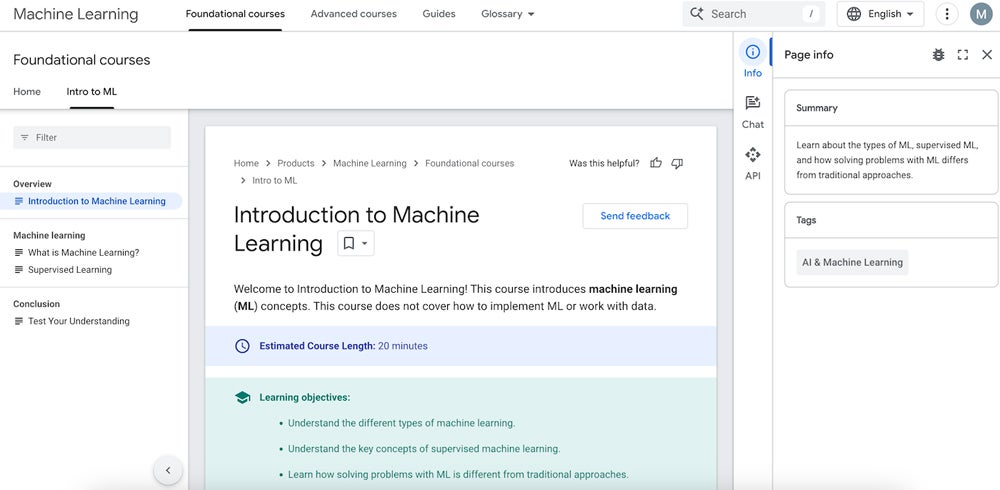
For beginners, Google’s Introduction to Machine Learning is a clear-cut, low-commitment option. This course is the first entry in a longer sequence of Google “foundational courses” on machine learning. That makes it easy to explore as much or as little of the topic as you want.
This course is free.
This course can be completed in 20 minutes.
Pros | Cons |
---|---|
Prerequisites
There are no prerequisites for this course.
Data Science - Machine Learning (Harvard on edX): Best for data scientists
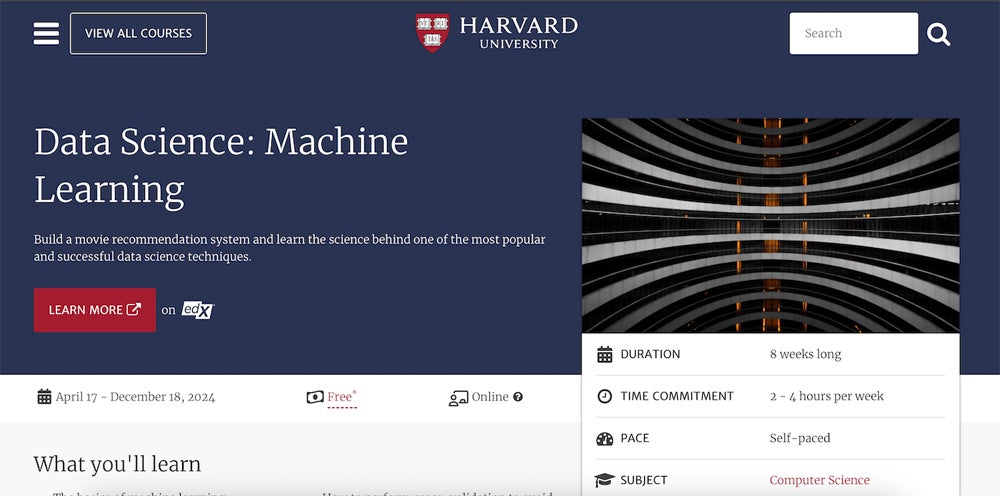
Harvard University has some of the brightest minds in education behind its online courses — contributing to our selection of “Data Science: Machine Learning.” This course is a section in Harvard’s larger online data science course. It’s appropriate for people with some professional experience in data science, placing machine learning in the context of existing, practical work. This course results in a project the learner can use or show to current or prospective employers — namely, a movie recommendation system showing mastery of predictive algorithms.
“Data Science: Machine Learning” can be “audited” for free. Paying $149 adds a certification of completion and unlimited access to the course materials.
This course is self-paced. It has enough content for about eight weeks of work if done at 2 to 4 hours per week.
It is recommended to take the previous courses in Professional Certificate Program in Data Science before taking this course.
Cornell University’s Machine Learning Certificate Program (Cornell): Best for a traditional university education
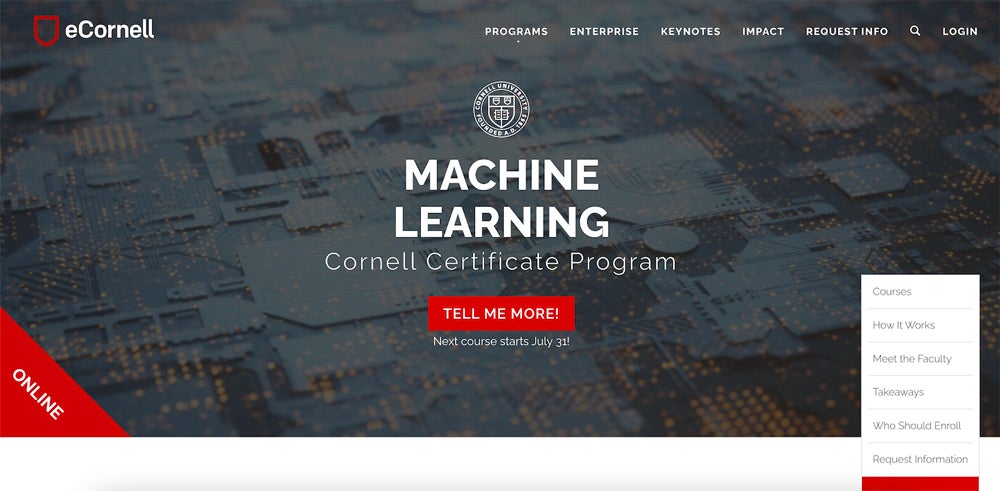
While this certification includes self-paced elements, it also offers live discussions with peers and educators. Participants will get feedback on their work. The course includes projects suitable for a resume or other real-world demonstrations. It covers the math involved in machine learning — including linear algebra and probability distributions — and computing aspects, including kernel machines and neural networks.
This certification costs $3,750.
This course can be completed in 3.5 months at 6-9 hours of study per week.
Cornell University recommends that learners taking this course have a background in “math, including familiarity with Python, probability theory, statistics, multivariate calculus and linear algebra.” Completing some projects requires using the NumPy library and Jupyter Notebooks.
Stanford Machine Learning Specialization (Coursera): Best for building neural network applications
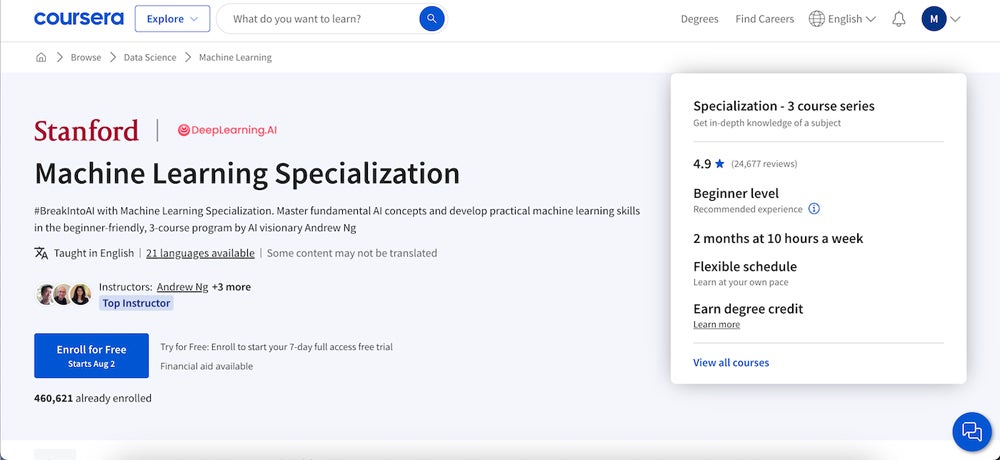
Andrew Ng is often referred to as one of the best instructors of artificial intelligence. An adjunct professor at Stanford University and co-founder of Coursera, he has built a brand on conveying complex information in a useful, actionable way for people who want to progress in their tech careers. The Machine Learning Specialization contains three separate courses and covers neural networks, deep reinforcement learning and more.
This course is accessible through a Coursera Plus subscription at $59 per month.
Coursera estimates this self-paced course will take 2 months at 10 hours per week.
Coursera recommends that learners taking this course have a background in “Basic coding (for loops, functions, if/else statements) and high school-level math (arithmetic, algebra).”
IBM Introduction to Machine Learning Specialization (Coursera): Best for aspiring data scientists
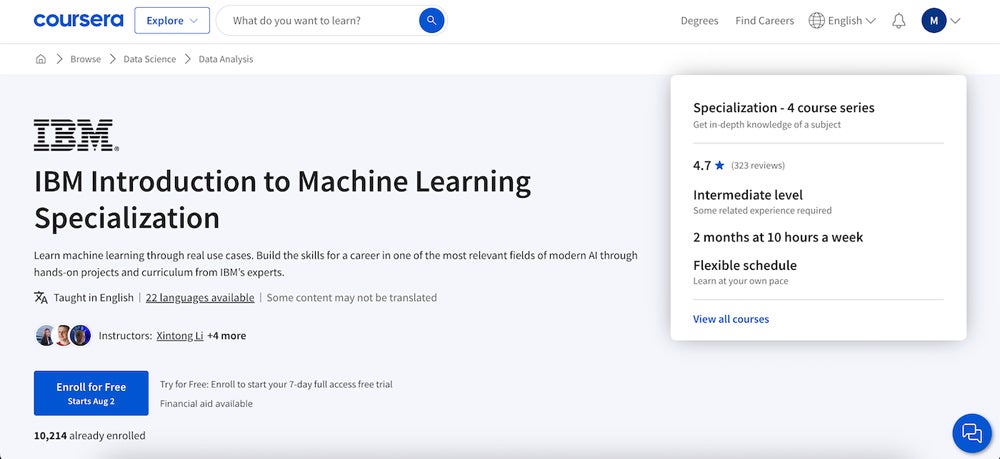
IBM instructors teach this machine learning course, which comprises four smaller courses:
- Exploratory Data Analysis for Machine Learning.
- Supervised Machine Learning: Regression.
- Supervised Machine Learning: Classification.
- Unsupervised Machine Learning.
This specialization includes hands-on exercises in SQL, regression, classification and other tools and techniques useful in ML. By the end of the course, you will be able to design ML systems to glean insights from data sets that lack a target or labeled variable. Upon completing the specialization, learners will earn a career certificate from IBM.
This specialization is accessible through a Coursera Plus subscription at $59 per month.
This specialization takes two months at 10 hours per week to complete.
Learners pursuing this specialization should have some experience in coding, particularly in Python, as well as be comfortable with calculus, linear algebra, probability and statistics.
Methodology
In choosing these courses, we looked at universities and online learning platforms well-known in the tech world. We sought to provide a mix of beginner, intermediate and advanced courses and certifications.
Subscribe to the Developer Insider Newsletter
From the hottest programming languages to commentary on the Linux OS, get the developer and open source news and tips you need to know. Delivered Tuesdays and Thursdays
- 5 Best Online Course Platforms for 2024
- The 10 Best AI Courses That Are Worth Taking in 2024
- The 10 Best Python Courses That are Worth Taking in 2024
- Programming languages and developer career resources
Create a TechRepublic Account
Get the web's best business technology news, tutorials, reviews, trends, and analysis—in your inbox. Let's start with the basics.
* - indicates required fields
Sign in to TechRepublic
Lost your password? Request a new password
Reset Password
Please enter your email adress. You will receive an email message with instructions on how to reset your password.
Check your email for a password reset link. If you didn't receive an email don't forgot to check your spam folder, otherwise contact support .
Welcome. Tell us a little bit about you.
This will help us provide you with customized content.
Want to receive more TechRepublic news?
You're all set.
Thanks for signing up! Keep an eye out for a confirmation email from our team. To ensure any newsletters you subscribed to hit your inbox, make sure to add [email protected] to your contacts list.
Information
- Author Services
Initiatives
You are accessing a machine-readable page. In order to be human-readable, please install an RSS reader.
All articles published by MDPI are made immediately available worldwide under an open access license. No special permission is required to reuse all or part of the article published by MDPI, including figures and tables. For articles published under an open access Creative Common CC BY license, any part of the article may be reused without permission provided that the original article is clearly cited. For more information, please refer to https://www.mdpi.com/openaccess .
Feature papers represent the most advanced research with significant potential for high impact in the field. A Feature Paper should be a substantial original Article that involves several techniques or approaches, provides an outlook for future research directions and describes possible research applications.
Feature papers are submitted upon individual invitation or recommendation by the scientific editors and must receive positive feedback from the reviewers.
Editor’s Choice articles are based on recommendations by the scientific editors of MDPI journals from around the world. Editors select a small number of articles recently published in the journal that they believe will be particularly interesting to readers, or important in the respective research area. The aim is to provide a snapshot of some of the most exciting work published in the various research areas of the journal.
Original Submission Date Received: .
- Active Journals
- Find a Journal
- Proceedings Series
- For Authors
- For Reviewers
- For Editors
- For Librarians
- For Publishers
- For Societies
- For Conference Organizers
- Open Access Policy
- Institutional Open Access Program
- Special Issues Guidelines
- Editorial Process
- Research and Publication Ethics
- Article Processing Charges
- Testimonials
- Preprints.org
- SciProfiles
- Encyclopedia
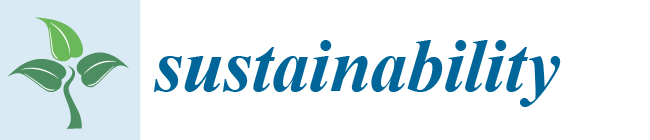
Article Menu
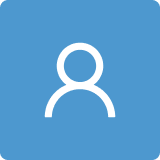
- Subscribe SciFeed
- Recommended Articles
- Google Scholar
- on Google Scholar
- Table of Contents
Find support for a specific problem in the support section of our website.
Please let us know what you think of our products and services.
Visit our dedicated information section to learn more about MDPI.
JSmol Viewer
Tracking the dynamics and uncertainties of soil organic carbon in agricultural soils based on a novel robust meta-model framework using multisource data.
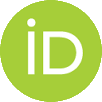
1. Introduction
- Process-based simulation models, e.g., such as MIcrobial-MIneral Carbon Stabilization model (MIMICS, [ 21 ]), DeNitrification-DeComposition model (DNDC, [ 22 ]), and Environmental Policy Integrated Climate model (EPIC [ 23 , 24 , 25 , 26 , 27 , 28 , 29 ]), which represent key dynamic processes affecting soil nutrients, land emissions, and productivity (yields);
- Statistical and machine learning models, which estimate functional relationships between historical observations of climate, soil characteristics, nutrient composition, and land productivity [ 30 , 31 , 32 , 33 , 34 ].
2. Modeling SOC Dynamics: Process-Based vs. Statistical Models
2.1. soc analysis and modeling, 2.2. modeling soc dynamics: processed-based vs. statistical models, 2.2.1. process-based epic model, 2.2.2. statistical and machine learning models, 2.2.3. statistical and machine learning models, 3. estimating soc level dependencies on land practices and climate changes, 3.1. experimental design, 3.3. machine learning quantile regression meta-model, 3.3.1. linear regression model, 3.3.2. quantile regression (qr) model, 4. selected results, results discussion, 5. conclusions, author contributions, institutional review board statement, informed consent statement, data availability statement, conflicts of interest.
- Alexander, P.; Paustian, K.; Smith, P.; Moran, D. The economics of soil C sequestration and agricultural emissions abatement. Soil 2015 , 1 , 331–339. [ Google Scholar ] [ CrossRef ]
- Batjes, N.H. Mitigation of atmospheric CO 2 concentrations by increased carbon sequestration in the soil. Biol. Fertil. Soils 1988 , 27 , 230–235. [ Google Scholar ] [ CrossRef ]
- Batjes, N.H.; Ribeiro, E.; Van Oostrum, A. Standardized soil profile data to support global mapping and modelling (WoSIS snapshot 2019). Earth Syst. Sci. Data Discuss. 2019 , 12 , 299–320. [ Google Scholar ] [ CrossRef ]
- Amelung, W.; Bossio, D.; de Vries, W.; Kögel-Knabner, I.; Lehmann, J.; Amundson, R.; Bol, R.; Collins, C.; Lal, R.; Leifeld, J.; et al. Towards a global-scale soil climate mitigation strategiy. Nat. Commun. 2020 , 11 , 5427. [ Google Scholar ] [ CrossRef ]
- Cowie, A. Guidelines for Land Degradation Neutrality. A report prepared for the Scientific and Technical Advisory Panel of the Global Environment Facility, 2020. Available online: https://catalogue.unccd.int/1474_LDN_Technical_Report_web_version.pdf (accessed on 10 May 2024).
- Panagos, P.; Montanarella, L. Soil thematic strategy: An important contribution to policy support, research, data development and raising the awareness. Curr. Opin. Environ. Sci. Health 2018 , 5 , 38–41. [ Google Scholar ] [ CrossRef ]
- Panagos, P.; Montanarella, L.; Barbero, M.; Schneegans, A.; Aguglia, L. Soil priorities in the European Union. Geoderma Reg. 2022 , 29 , e00510. [ Google Scholar ] [ CrossRef ]
- Álvaro-Fuentes, J.; Easter, M.; Paustian, K. Climate change effects on organic carbon storage in agricultural soils of northeastern Spain. Agric. Ecosyst. Environ. 2012 , 155 , 87–94. [ Google Scholar ] [ CrossRef ]
- Schimel, D.S.; Braswell, B.H.; Holland, E.A.; McKeon, R.; Ojima, D.S.; Painter, T.H.; Parton, W.J.; Townsend, A.R. Climatic, edaphic, and biotic controls over storage and turnover of carbon in soils. Glob. Biogeochem. Cycles 1994 , 8 , 279–293. [ Google Scholar ] [ CrossRef ]
- Wu, Z.T.; Dijkstra, P.; Koch, G.W.; Peñuelas, J.; Hungate, B.A. Responses of terrestrial ecosystems to temperature and precipitation change: A meta-analysis of experimental manipulation. Glob. Chang. Biol. 2011 , 17 , 927–942. [ Google Scholar ] [ CrossRef ]
- Poll, C.; Marhan, S.; Back, F.; Niklaus, P.A.; Kandeler, E. Field-scale manipulation of soil temperature and precipitation change soil CO 2 flux in a temperate agricultural ecosystem. Agric. Ecosyst. Environ. 2013 , 165 , 88–97. [ Google Scholar ] [ CrossRef ]
- USDA Natural Resources Conservation Service. Carbon to Nitrogen Ratios in Cropping Systems. 2011. Available online: https://www.nrcs.usda.gov/conservation-basics/natural-resource-concerns/soil/soil-science (accessed on 1 February 2024).
- Carbon to Nitrogen Ratio (C:N). Soil Health Nexus. Available online: https://soilhealthnexus.org/resources/soil-properties/soil-chemical-properties/carbon-to-nitrogen-ratio-cn/ (accessed on 8 July 2024).
- Rocci, K.S.; Lavallee, J.M.; Stewart, C.E.; Cotrufo, M.F. Soil organic carbon response to global environmental change depends on its distribution between mineral-associated and particulate organic matter: A meta-analysis. Sci. Total Environ. 2021 , 793 , 148569. [ Google Scholar ] [ CrossRef ] [ PubMed ]
- Tang, B.; Rocci, K.S.; Lehmann, A.; Rillig, M.C. Nitrogen increases soil organic carbon accrual and alters its functionality. Glob. Chang. Biol. 2023 , 29 , 1971–1983. [ Google Scholar ] [ CrossRef ] [ PubMed ]
- Manzoni, S.; Taylor, P.; Richter, A.; Porporato, A.; Ågren, G.I. Environmental and stoichiometric controls on microbial carbon-use efficiency in soils. New Phytol. 2012 , 196 , 7991. [ Google Scholar ] [ CrossRef ]
- Nakhavali, M.A.; Lauerwald, R.; Regnier, P.; Friedlingstein, P. Predicting future trends of terrestrial dissolved organic carbon transport to global river systems. Earth’s Future 2024 , 12 , e2023EF004137. [ Google Scholar ] [ CrossRef ]
- Zhu, K.; Ran, H.; Wang, F.; Ye, X.; Niu, L.; Schulin, R.; Wang, G. Conservation tillage facilitated soil carbon sequestration through diversified carbon conservation. Agric. Ecosyst. Environ. 2022 , 337 , 108080. [ Google Scholar ] [ CrossRef ]
- Aditi, K.; Abbhishek, K.; Chander, G.; Singh, A.; Thomas Falk, T.; Mequanint, M.B.; Cuba, P.; Anupama, G.; Mandapati, R.; Nagaraji, S. Assessing residue and tillage management options for carbon sequestration in future climate change scenarios. Curr. Res. Environ. Sustain. 2023 , 5 , 100210. [ Google Scholar ] [ CrossRef ]
- Havlík, P.; Schneider, U.A.; Schmid, E.; Boettcher, H.; Fritz, S.; Skalský, R.; Aoki, K.; de Cara, S.; Kindermann, G.; Kraxner, F.; et al. Global land-use implications of first and second generation biofuel targets. Energy Policy 2011 , 39 , 5690–5702. [ Google Scholar ] [ CrossRef ]
- Kyker-Snowman, E.; Wieder, W.R.; Frey, S.D.; Grandy, A.S. Stoichiometrically coupled carbon and nitrogen cycling in the MIcrobial-MIneral Carbon Stabilization model version 1.0 (MIMICS-CN v1.0). Geosci. Model Dev. 2020 , 13 , 4413–4434. [ Google Scholar ] [ CrossRef ]
- Li, C.; Frolking, S.; Frolking, T.A. A model of N 2 O evolution from soil driven by rainfall events: 1. Model structure and sensitivity. J. Geophys. Res. 1992 , 97 , 9759–9776. [ Google Scholar ] [ CrossRef ]
- Balkovič, J.; Madaras, M.; Skalsky, R.; Folberth, C.; Smatanova, M.; Schmid, E.; van der Velde, M.; Kraxner, F.; Obersteiner, M. Verifiable soil organic carbon modelling to facilitate regional reporting of cropland carbon change: A test case in the Czech Republic. J. Environ. Manag. 2020 , 274 , 111206. [ Google Scholar ] [ CrossRef ]
- Balkovič, J.; van der Velde, M.; Schmid, E.; Skalský, R.; Khabarov, N.; Obersteiner, M.; Stürmer, B.; Xiong, W. Pan-European crop modelling with EPIC: Implementation, up-scaling and regional crop yield validation. Agric. Syst. 2013 , 120 , 61–75. [ Google Scholar ] [ CrossRef ]
- Jones, C.A.; Dyke, P.T.; Williams, J.R.; Kiniry, J.R.; Benson, V.W.; Griggs, R.H. EPIC: An operational model for evaluation of agricultural sustainability. Agric. Syst. 1991 , 37 , 341–350. [ Google Scholar ] [ CrossRef ]
- Jones, R.J.A.; Hiederer, R.; Rusco, E.; Loveland, P.J.; Montanarella, L. Estimating organic carbon in the soils of Europe for policy support. Eur. J. Soil. Sci. 2015 , 56 , 655–671. [ Google Scholar ] [ CrossRef ]
- Jones, J.W.; Antle, J.M.; Basso, B.; Boote, K.J.; Conant, R.T.; Foster, I.; Godfray, H.C.J.; Herrero, M.; Howitt, R.E.; Janssen, S.; et al. Toward a new generation of agricultural system data, models, and knowledge products: State of agricultural systems science. Agric. Syst. 2017 , 155 , 269–288. [ Google Scholar ] [ CrossRef ] [ PubMed ]
- Williams, J.R.; Jones, C.A.; Dyke, P.T. A modelling approach to determining the relationship between erosion and soil productivity. Trans. ASAE 1984 , 27 , 129–144. [ Google Scholar ] [ CrossRef ]
- Williams, J.R. The erosion productivity impact calculator (EPIC) model: A case history. Phil. Trans. Roy. Soc. 1990 , 329 , 421–428. [ Google Scholar ]
- Drummond, S.T.; Sudduth, K.A.; Joshi, A.; Birrell, S.J.; Kitchen, N.R. Statistical and neural methods for site-specific yield prediction. Trans. ASAE 2003 , 46 , 5. [ Google Scholar ] [ CrossRef ]
- Van Klompenburg, T.; Kassahun, A.; Catal, C. Crop yield prediction usingmachine learning: A systematic literature review. Comput. Electron. Agric. 2020 , 177 , 105709. [ Google Scholar ] [ CrossRef ]
- Lobell, D.B.; Burke, M.B. On the use of statistical models to predict crop yield responses to climate change. Agric. Forest Meteorol. 2010 , 150 , 1443–1452. [ Google Scholar ] [ CrossRef ]
- Micale, F.; Genovese, G. Methodology of the MARS Crop Yield Forecasting System ; Statistical data collectoin, processing and analysis; EUR.; No. 21291 EN/4; EC: Luxenburg, 2004; Volume 4, ISBN 9789289481830. [ Google Scholar ]
- Zayani, H.; Fouad, Y.; Michot, D.; Kassouk, Z.; Baghdadi, N.; Vaudour, E.; Lili-Chabaane, Z.; Walter, C. Using Machine-Learning Algorithms to Predict Soil Organic Carbon Content from Combined Remote Sensing Imagery and Laboratory Vis-NIR Spectral Datasets. Remote Sens. 2023 , 15 , 4264. [ Google Scholar ] [ CrossRef ]
- Rosenzweig, C.; Jones, J.W.; Hatfield, J. The Agricultural Model Intercomparison and Improvement Project (AgMIP): Protocols and pilot studies. Agric. For. Meteorol. 2013 , 170 , 166–182. [ Google Scholar ] [ CrossRef ]
- Schlenker, W.; Roberts, M.J. Nonlinear effects of weather on corn yields. Rev. Agr. Econ. 2006 , 28 , 391–398. [ Google Scholar ] [ CrossRef ]
- Izaurralde, R.C.; Williams, J.R.; McGill, W.B.; Rosenberg, N.J.; Jakas, M.C.Q. Simulating soil C dynamics with EPIC: Model description and testing against long-term data. Ecol. Modell. 2006 , 192 , 362–384. [ Google Scholar ] [ CrossRef ]
- Causarano, H.J.; Doraiswamy, P.C.; McCarty, G.W.; Hatfield, J.L.; Milak, S.; Stern, A.J. EPIC Modeling of Soil Organic Carbon Sequestration in Croplands of Iowa ; USDA-ARS/UNL Faculty: Washington, DC, USA, 2008; p. 1363. Available online: https://digitalcommons.unl.edu/usdaarsfacpub/1363 (accessed on 24 April 2024).
- Ermolieva, T.; Ermoliev, Y.; Havlik, P.; Lessa-Dersi-Augustynczik, A.; Kahil, T.; Balkovic, J.; Skalsky, R.; Folberth, C.; Knopov, P.S.; Wang, G. Connections between robust statistical estimation, robust decision making withtwo-stage stochastic optimization, and robust machine learning problems. Cybern. Syst. Anal. 2023 , 59 , 33–47. [ Google Scholar ] [ CrossRef ]
- Ermolieva, T.; Havlik, P.; Derci Augustynczik, A.L.; Boere, E.; Frank, S.; Kahil, T.; Wang, G.; Balkovič, J.; Skalský, R.; Folberth, C.; et al. A Novel Robust Meta-Model Framework for Predicting Crop Yield Probability Distributions Using Multisource Data. Cybern. Syst. Anal. 2023 , 59 , 844–858. [ Google Scholar ] [ CrossRef ]
- Liu, T.; Wang, L.; Feng, X.; Zhang, J.; Ma, T.; Wang, X.; Liu, Z. Comparing soil carbon loss through respiration and leaching under extreme precipitation events in arid and semiarid grasslands. Biogeosciences 2018 , 15 , 1627–1641. [ Google Scholar ] [ CrossRef ]
- Ermolieva, T.; Havlík, P.; Ermoliev, Y.; Mosnier, A.; Obersteiner, M.; Leclere, D.; Khabarov, N.; Valin, H.; Reuter, W. Integrated management of land use systems under systemic risks and security targets: A Stochastic Global Biosphere Management Model. J. Agric. Econ. 2016 , 67 , 584–601. [ Google Scholar ] [ CrossRef ]
- Ermolieva, T.; Havlik, P.; Frank, S.; Kahil, T.; Balkovic, J.; Skalsky, R.; Ermoliev, Y.; Knopov, P.S.; Borodina, O.M.; Gorbachuk, V.M. A Risk-Informed Decision-Making Framework for Climate Change Adaptation through Robust Land Use and Irrigation Planning. Sustainability 2022 , 14 , 1430. [ Google Scholar ] [ CrossRef ]
- FAO. Global Soil Partnership: RECSOIL, Recarbonization of Global Agricultural Soils ; FAO: Rome, Italy, 2023; Available online: https://www.fao.org/global-soil-partnership/areas-of-work/recsoil/what-is-soc/en/ (accessed on 20 December 2023).
- Liptzin, D.; Norris, C.E.; Cappellazzi, C.B.; Bean, G.M.; Cope, M.; Greub, K.L.H.; Rieke, E.L.; Tracy, R.W.; Aberle, E.; Ashworth, A.; et al. An evaluation of carbon indicators of soil health in long-term agricultural experiments. Soil Biol. Biochem. 2022 , 172 , 108708. [ Google Scholar ] [ CrossRef ]
- European Commission. A Soil Deal for Europe ; European Commission: Brussels, Belgium, 2021; Available online: https://research-and-innovation.ec.europa.eu/document/download/1517488e-767a-4f47-94a0-bd22197d18fa_en?filename=soil_mission_implementation_plan_final.pdf (accessed on 20 December 2023).
- Oldfield, E.E.; Bradford, M.A.; Wood, S.A. Global meta-analysis of the relationship between soil organic matter and crop yields. SOIL 2019 , 5 , 15–32. [ Google Scholar ] [ CrossRef ]
- Bruni, E.; Guenet, B.; Clivot, H.; Kaetterer, T.; Martin, M.; Virto, I.; Chenu, C. Defining quantitative targets for topsoil organic carbon stock increase in European croplands: Case studies with exogenous organic matter inputs. Front. Environ. Sci. 2020 , 10 , 824724. [ Google Scholar ] [ CrossRef ]
- Goidts, E.; van Wesemael, B. Regional Assessment of Soil Organic Carbon Changes under Agriculture in Southern Belgium (1955–2005). Geoderma 2007 , 141 , 341–354. [ Google Scholar ] [ CrossRef ]
- Meersmans, J.; Van Wesemael, B.; Goidts, E.; Van Molle, M.; De Baets, S.; De Ridder, F. Spatial Analysis of Soil Organic Carbon Evolution in Belgian Croplands and Grasslands, 1960-2006. Spat. Anal. Soil Org. Carbon Evol. 2011 , 17 , 466–479. [ Google Scholar ] [ CrossRef ]
- Smith, P.; Smith, J.U.; Powlson, D.S.; McGill, W.B.; Arah, J.R.M.; Chertov, O.G.; Coleman, K.; Franko, U.; Frolking, S.; Jenkinson, D.S.; et al. A comparison of the performance of nine soil organic matter models using datasets from seven long-term experiments. Geoderma 1997 , 81 , 153–225. [ Google Scholar ] [ CrossRef ]
- Guo, L.; Falloon, P.; Coleman, K.; Zhou, B.; Li, Y.; Lin, E.; Zhang, F. Application of the RothC model to the results of long-term experiments on typical upland soils in northern China. Soil Use Manag. 2007 , 23 , 63–70. [ Google Scholar ] [ CrossRef ]
- Gilhespy, S.L.; Anthony, S.; Cardenas, L.; Chadwick, D.; del Prado, A.; Li, C.; Misselbrook, T.; Rees, R.M.; Salas, W.; Sanz-Cobena, A.; et al. First 20 years of DNDC (DeNitrification DeComposition): Model evolution. Ecol. Model. 2014 , 292 , 51–62. [ Google Scholar ] [ CrossRef ]
- Li, C. Biogeochemical concepts and methodologies: Development of the DNDC model. Quat. Sci. 2001 , 2 , 89–99. [ Google Scholar ]
- Li, C.; Frolking, S.; Harriss, R. Modeling carbon biogeochemistry in agricultural soils. Glob. Biogeochem. Cycles 1994 , 8 , 237–254. [ Google Scholar ] [ CrossRef ]
- Parton, W.J.; Schimel, D.S.; Cole, C.V.; Ojima, D.S. Analysis of factors controlling soil organic matter levels in Great Plains grasslands. Soil Sci. Soc. Am. J. 1987 , 51 , 1173–1179. [ Google Scholar ] [ CrossRef ]
- Parton, W.J.; Scurlock, J.M.O.; Ojima, D.S.; Gilmanov, T.G.; Scholes, R.J.; Schimel, D.S.; Kirchner, T.; Menaut, J.-C.; Seastedt, T.; Garcia Moya, E.; et al. Observations and modelling of biomass and soil organic matter dynamics for the grassland biome worldwide. Glob. Biogeochem. Cycles 1993 , 7 , 785–809. [ Google Scholar ] [ CrossRef ]
- Müller, C.; Elliott, J.; Chryssanthacopoulos, J.; Arneth, A.; Balkovic, J.; Ciais, P.; Deryng, D.; Folberth, C.; Glotter, M.; Hoek, S.; et al. Global Gridded Crop Model evaluation: Benchmarking, skills, deficiencies and implications. Geosci. Model Dev. Discuss. (GMDD) 2016 , 1–39. [ Google Scholar ] [ CrossRef ]
- Lembaid, I.; Moussadek, R.; Mrabet, R.; Bouhaouss, A. Soil organic carbon changes under alternative climatic scenarios and soil properties using DNDC model as a semi-arid Mediterranean environment. Climate 2022 , 10 , 23. [ Google Scholar ] [ CrossRef ]
- Kahil, M.T.; Dinar, A.; Albiac, J. Modeling water scarcity and droughts for policy adaptation to climate change in arid and semiarid regions. J. Hydrol. 2015 , 522 , 95–109. [ Google Scholar ] [ CrossRef ]
- Kahil, M.T.; Connor, J.D.; Albiac, J. Efficient water management policies for irrigation adaptation to climate change in Southern Europe. Ecol. Econ. 2015 , 120 , 226–233. [ Google Scholar ] [ CrossRef ]
- Schnecker, J.; Baldaszti, L.; Gündler, P.; Pleitner, M.; Sandén, T.; Simon, E.; Spiegel, F.; Spiegel, H.; Malo, C.U.; Zechmeister-Boltenstern, S.; et al. Seasonal dynamics of soil microbial growth, respiration, biomass, and carbon use efficiency in temperate soils. Geoderma 2023 , 440 , 116693. [ Google Scholar ] [ CrossRef ]
- Zheng, Q.; Hu, Y.; Zhang, S.; Noll, L.; Böckle, T.; Dietrich, M.; Herbold, C.W.; Eichorst, S.A.; Woebken, D.; Richter, A.; et al. Soil multifunctionality is affected by the soil environment and by microbial community composition and diversity. Soil Biol. Biochem. 2019 , 136 , 07521. [ Google Scholar ] [ CrossRef ] [ PubMed ]
- Pietikäinen, J.; Pettersson, M.; Bååth, E. Comparison of temperature effects on soil respiration and bacterial and fungal growth rates. FEMS Microbiol. Ecol. 2005 , 52 , 49–58. [ Google Scholar ] [ CrossRef ] [ PubMed ]
- Burke, I.C.; Yonker, C.M.; Parton, W.J.; Cole, C.V.; Schimel, D.S.; Flach, K. Texture, Climate, and Cultivation Effects on Soil Organic Matter Content in U.S. Grassland Soils. Soil Sci. Soc. Am. J. 1989 , 53 , 800. [ Google Scholar ] [ CrossRef ]
- Haddad, A.N. Evaluating the Relationship between Soil Texture and Soil Organic Carbon across California Grasslands. Soil Clay Content Soil Carbon 2017. Available online: https://nature.berkeley.edu/classes/es196/projects/2017final/HaddadA_2017.pdf (accessed on 4 January 2024).
- Bengough, A.G.; Bransby, M.F.; Hans, J.; McKenna SJRoberts, T.J.; Valentine, T.A. Root responses to soil physical conditions; growth dynamics from field to cell. J. Exp. Bot. 2006 , 57 , 437–447. [ Google Scholar ] [ CrossRef ]
- Soldatova, E.; Krasilnikov, S.; Kuzyakov, Y. Soil organic matter turnover: Global implications from δ13C and δ15N signatures. Sci. Total Environ. 2023 , 912 , 169423. [ Google Scholar ] [ CrossRef ]
- Wang, C.; Kuzyakov, Y. Soil organic matter priming: The pH effects. Glob. Chang. Biol. 2023 , 30 , e17349. [ Google Scholar ] [ CrossRef ] [ PubMed ]
- Mahal, N.K.; Osterholz, W.R.; Miguez, F.E.; Poffenbarger, H.J.; Sawyer, J.E.; Olk, D.C.; Archontoulis, S.V.; Castellano, M.J. Nitrogen Fertilizer Suppresses Mineralization of Soil Organic Matter in Maize Agroecosystems. Front. Ecol. Evol. 2019 , 7 , 59. [ Google Scholar ] [ CrossRef ]
- Smit, M.J.; van Leeuwen, E.S.; Florax, R.J.G.M.; de Groot, H.L.F. Rural development funding and agricultural labour productivity: A spatial analysis of the European Union at the NUTS2 level. Ecol. Indic. 2015 , 59 , 6–18. [ Google Scholar ] [ CrossRef ]
- Scholtz, R.; Tarasovičová, Z.; Balkovič, J.; Schmid, E.; Fuchs, M.; Moltchanova, E.; Kindermann, G.; Scholtz, P. GEOBENE Global Database for Bio-Physical Modeling. GEOBENE Project 2008. Available online: https://geo-bene.project-archive.iiasa.ac.at/files/Deliverables/Geo-BeneGlbDb10(DataDescription).pdf (accessed on 1 June 2023).
- Werner, M. Shuttle Radar Topography Mission (SRTM), Mission overview. J. Telecom. 2001 , 55 , 75–79. [ Google Scholar ] [ CrossRef ]
- Wösten, J.H.M.; Lilly, A.; Nemes, A.; Le Bas, C. Development and use of a database of hydraulic properties of Europen soils. Geoderma 1999 , 90 , 169–185. [ Google Scholar ] [ CrossRef ]
- Jones, R.J.A.; Hiederer, R.; Rusco, E.; Loveland, P.J.; Montanarella, L. The Map of Organic Carbon in Topsoils in Europe, Version 1.2, September 2003: Explanation of Special Publication Ispra 2004 No.72 (S.P.I.04.72) ; European Soil Bureau Research Report 2004, No.17, EUR 21209 EN, 26pp. and 1 map in ISO B1 format; Office for Official Publications of the European Communities: Luxembourg, 2003. [ Google Scholar ]
- IFA; IFD; IPI; PPI; FAO. Fertiliser Use by Crop ; FAO: Rome, Italy, 2002. [ Google Scholar ]
- Wriedt, G.; van der Velde, M.; Aloe, A.; Bouraoui, F. A European irrigation map for spatially distributed agricultural modelling. Agric. Water Manag. 2009 , 96 , 771–789. [ Google Scholar ] [ CrossRef ]
- Rodríguez-Lado, L.; Martínez-Cortizas, A. Modelling and mapping organic carbon content of topsoils in an Atlantic area of southwestern Europe (Galicia, NW-Spain). Geoderma 2015 , 245–246 , 65–73. [ Google Scholar ] [ CrossRef ]
- Evans, C.D.; Monteith, D.T.; Cooper, D.M. Long-term increases in surface water dissolved organic carbon: Observations, possible causes and environmental impacts. Environ. Pollut. 2005 , 137 , 55–71. [ Google Scholar ] [ CrossRef ]
- Chen, Z.; Wei, X.; Ni, X.; Wu, F.; Liao, S. Changing presipitation effect on forest soil carbon dynamics is driven by different attributes between dry and wet areas. Geoderma 2023 , 429 , 116279. [ Google Scholar ] [ CrossRef ]
- Poeplau, C.; Bolinder, M.A.; Eriksson, J.O.; Lundblad, M.; Kätterer, T. Increasing organic carbon stocks in Swedish agricultural soils due to unexpected socio-economic drivers. Geophys. Res. Abstr. 2015 , 17 , EGU2015-9264. [ Google Scholar ]
Click here to enlarge figure
The statements, opinions and data contained in all publications are solely those of the individual author(s) and contributor(s) and not of MDPI and/or the editor(s). MDPI and/or the editor(s) disclaim responsibility for any injury to people or property resulting from any ideas, methods, instructions or products referred to in the content. |
Share and Cite
Ermolieva, T.; Havlik, P.; Lessa-Derci-Augustynczik, A.; Frank, S.; Balkovic, J.; Skalsky, R.; Deppermann, A.; Nakhavali, M.; Komendantova, N.; Kahil, T.; et al. Tracking the Dynamics and Uncertainties of Soil Organic Carbon in Agricultural Soils Based on a Novel Robust Meta-Model Framework Using Multisource Data. Sustainability 2024 , 16 , 6849. https://doi.org/10.3390/su16166849
Ermolieva T, Havlik P, Lessa-Derci-Augustynczik A, Frank S, Balkovic J, Skalsky R, Deppermann A, Nakhavali M, Komendantova N, Kahil T, et al. Tracking the Dynamics and Uncertainties of Soil Organic Carbon in Agricultural Soils Based on a Novel Robust Meta-Model Framework Using Multisource Data. Sustainability . 2024; 16(16):6849. https://doi.org/10.3390/su16166849
Ermolieva, Tatiana, Petr Havlik, Andrey Lessa-Derci-Augustynczik, Stefan Frank, Juraj Balkovic, Rastislav Skalsky, Andre Deppermann, Mahdi (Andrè) Nakhavali, Nadejda Komendantova, Taher Kahil, and et al. 2024. "Tracking the Dynamics and Uncertainties of Soil Organic Carbon in Agricultural Soils Based on a Novel Robust Meta-Model Framework Using Multisource Data" Sustainability 16, no. 16: 6849. https://doi.org/10.3390/su16166849
Article Metrics
Article access statistics, further information, mdpi initiatives, follow mdpi.
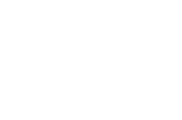
Subscribe to receive issue release notifications and newsletters from MDPI journals

IMAGES
COMMENTS
This article provides a list of 20 potential thesis ideas for an undergraduate program in machine learning and deep learning in 2023. Each thesis idea includes an introduction, which presents a brief overview of the topic and the research objectives. The ideas provided are related to different areas of machine learning and deep learning, such ...
Get 1-On-1 Help. If you're still unsure about how to find a quality research topic, check out our Research Topic Kickstarter service, which is the perfect starting point for developing a unique, well-justified research topic. A comprehensive list of research topics ideas in the AI and machine learning area. Includes access to a free webinar ...
Potential thesis topics in this area: a) Compare inference speed with sum-product networks and Bayesian networks. Characterize situations when one model is better than the other. ... and machine learning could now offer a substantial contribution. Security capture-the-flag simulations are particularly well-suited as a testbed for the ...
In this tech-driven world, selecting research and thesis topics in machine learning projects is the first choice of masters and Doctorate scholars. Selecting and working on a thesis topic in machine learning is not an easy task as machine learning uses statistical algorithms to make computers work in a certain way without being explicitly ...
PhD Dissertations [All are .pdf files] Probabilistic Reinforcement Learning: Using Data to Define Desired Outcomes, and Inferring How to Get There Benjamin Eysenbach, 2023. Data-driven Decisions - An Anomaly Detection Perspective Shubhranshu Shekhar, 2023. METHODS AND APPLICATIONS OF EXPLAINABLE MACHINE LEARNING Joon Sik Kim, 2023. Applied Mathematics of the Future Kin G. Olivares, 2023
Based on this background, the aim of this thesis is to select and implement a machine learning process that produces an algorithm, which is able to detect whether documents have been translated by humans or computerized systems. This algorithm builds the basic structure for an approach to evaluate these documents. 1.2 Related Work
This dissertation explores three topics related to random forests: tree aggregation, variable importance, and robustness. 10. Climate Data Computing: Optimal Interpolation, Averaging, Visualization and Delivery. This dissertation solves two important problems in the modern analysis of big climate data.
This machine learning dissertation comprises four chapters. The first is an introduction to the topics of the dissertation and the remaining chapters contain the main results. Chapter 2 gives new results for consistency of maximum likelihood estimators with a focus on multivariate mixed models.
Machine learning (ML) systems are remarkably successful on a variety of benchmarks across sev- ... This thesis focuses on an extreme version of this brittleness, adversarial examples, where ... Progress on this widely-studied topic has been limited by the following critical roadblocks which we address in this thesis. Challenge 1: Worst-case ...
that a machine can be made to simulate it." [3] In the AI field, there are several terms. Artificial intelligence is the largest collection, machine learning is a subset of artificial intelligence, and deep learning is a subset of machine learning, as shown in Exhibit 2.3 [4]. This thesis mainly
The importance of this research can be summarized as follows: Advancements in Technology. The growth of machine learning research has led to the development of powerful algorithms, tools, and frameworks. Numerous industries, including healthcare, banking, autonomous cars, and natural language processing, have found use for these technology.
Advancements in machine learning techniques have encouraged scholars to focus on convolutional neural network (CNN) based solutions for object detection and pose estimation tasks. Most … Year: 2020 Contributor: Derman, Can Eren (creator) Bahar, Iris (thesis advisor) Taubin, Gabriel (reader) Brown University. School of Engineering (sponsor ...
Machine learning is the ability of a machine to improve its performance based on previous results. Machine learning methods enable computers to learn without being explicitly programmed and have ...
Ph.D. candidates are highly motivated to choose research topics that establish new and creative paths toward discovery in their field of study. In this article, I present 10 compelling machine learning dissertations that I found interesting in terms of my own areas of pursuit. I hope you'll find several of them that match your own interests.
Let us now have an idea about various headings to be included in any thesis topics for machine learning. Introduction - overview of the thesis; Related / Existing works - presents of existing research; Problems definition/statements - identify and highlight the problems; Research methodology - convey the proposed concepts; Results and Discussion - discuss the results of the proposed ...
Below you can see the thesis topics for 2022-2023. We offer 3 different thesis formats: - Format 1 : Regular thesis (fully supervised by KU Leuven) - Format 2 : Thesis in cooperation with a company (supervised by KU Leuven and the company) - Format 3 : Thesis with a company project within a company (supervised by the company) NOTE: Additional ...
This often includes a deep conceptual understanding of machine learning and significant programming experience. The necessary skills vary depending on the thesis topic, e.g., purely theoretical theses require more mathematical than programming skills. Candidates must possess the necessary abilities before applying.
So without further ado, let's see the different Topics for Research and Thesis in Artificial Intelligence! 1. Machine Learning. Machine Learning involves the use of Artificial Intelligence to enable machines to learn a task from experience without programming them specifically about that task. (In short, Machines learn automatically without ...
Supervised Machine Learning. It is a good topic for machine learning masters thesis. It is a type of machine learning algorithm in which makes predictions based on known data-sets. Input and output is provided to the system along with feedback. Supervised Learning is further classified into classification and regression problems.
Machine learning is the fastest growing areas of computer science. It has the ability to lets the computer to create the program. It is a subset of Artificial Intelligence (AI), and consists of the more advanced techniques and models that enable computers to figure things out from the data and deliver.
Or, you might have to come up with a research topic all by yourself. At an external organization. Writing your thesis at a company simplifies the task of choosing a research topic as you are likely to be put to work on a particular business or research problem. However, some other particular issues apply here that you should be aware of.
This thesis proposes a mode of inquiry that considers the inter- active qualities of what machine learning does, as opposed the tech- nical specifications of what machine learning is. A shift in focus from the technicality of ML to the artifacts it creates allows the interaction designer to situate its existing skill set, affording it to engage ...
For example, perhaps take a walk through a park, take pictures of all of the plants of one species, and see if you can use machine learning that can figure out things like degree of branching, age, pest prevalence, etc., from images of the plant. Undergrad ML TA. I suggest you find a researcher at your university, preferably in biology ...
With the rapid development of artificial intelligence, machine learning is gradually becoming popular for predictions in all walks of life. In meteorology, it is gradually competing with traditional climate predictions dominated by physical models. This survey aims to consolidate the current understanding of Machine Learning (ML) applications in weather and climate prediction—a field of ...
Topics include machine learning, deep generative models, neural networks, and natural language processing and understanding. View Courses & Programs. AI for Business Professionals. These courses and programs are tailored for leaders, founders, team managers, and product professionals looking to create and implement AI strategies. This portfolio ...
A new study introduced a machine learning-powered clustering model that incorporates both basic features and target properties, successfully grouping over 1,000 inorganic materials.
The new AWS Certified Machine Learning Engineer - Associate, a role-based, technical certification, validates skills in developing, deploying, and operating ML systems on AWS. "The exam covers topics across the full ML lifecycle - data preparation, model training, workflow orchestration, monitoring, and more," explained Taylor.
Machine learning approach helps researchers design better gene-delivery vehicles for gene therapy The method could accelerate the development of more effective adeno-associated viruses (AAVs)
Data Science - Machine Learning (Harvard on edX): Best for data scientists. Harvard has a strong stable of courses hosted on edX. Image: Harvard University
Monitoring and estimating spatially resolved changes in soil organic carbon (SOC) stocks are necessary for supporting national and international policies aimed at assisting land degradation neutrality and climate change mitigation, improving soil fertility and food production, maintaining water quality, and enhancing renewable energy and ecosystem services. In this work, we report on the ...