- Internet of Things
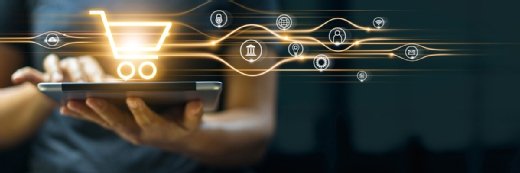
Getty Images/iStockphoto

The future of the food industry: Food tech explained
Food tech shows how technology can improve the way the world grows, produces, distributes and supplies food by using technology such as ai and automation..
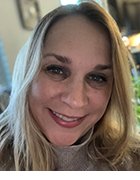
- Amanda Hetler, Senior Editor
As businesses turn to technology to fight inflation and improve efficiency, food tech is reshaping the food industry using the latest technology to manage production, distribution and consumption.
The food industry is worth more than $1 trillion in the U.S. gross domestic product, according to the U.S. Department of Agriculture. With an industry this large, there are several challenges, including food sustainability. Food tech is leading the way in transforming the global food sector.
What is food tech?
Food tech is any technology that improves food production, distribution and supply, and it affects the way people sell, produce and distribute food.
Even though this term may sound new, technology and food have been connected since the Industrial Revolution in the late 1700s and early 1800s . This period led to the emergence of industrialized agriculture and set the standards for farming. During this time, industry leaders and inventors worked together to help increase food production and quality. Major developments included the use of artificial fertilizer, creation of pesticides, development of electric power, and the start of horse-powered and then steam-powered machines.
But in the last few years, food tech has become its own sector with the rise of big data, AI and the internet of things ( IoT ). Food tech helps the food industry to be more sustainable by using IoT in all stages.
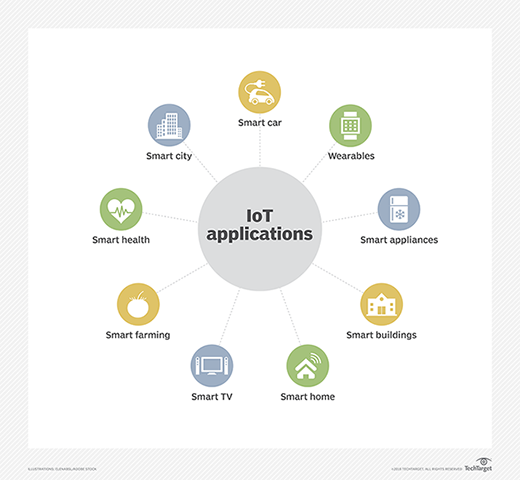
Challenges of food sustainability
Food systems are responsible for nearly one-third of the world's greenhouse gas admissions, according to a study by Nature Food. These emissions continue to rise due to land-use change, waste management, raising livestock, production and packaging.
The world also wastes about one-third of its food, according to a report by the Food and Agriculture Organization of the United Nations. Even with all this food waste, the World Health Organization estimates that nearly 828 million people don't have enough food.
Farming, the food industry and people's diets affect the environment with the energy consumed for food production and waste generation. Farming affects the land and strips it of its nutrients. Along with water shortages, the world faces a reduction in arable land to produce food.
Food tech is looking to address some of these issues. Startups are taking the newest technology and applying it to various points of the food cycle to create jobs, reduce hunger, and promote responsible production and consumption.
Impact of food tech
The global food tech market was worth $220.32 billion in 2019, according to Emergen Research , and is estimated to grow to $342.52 billion by 2027.
Food tech is increasing food production to help reduce the rate of hunger and feed the world. Agriculture is becoming more automated by using digital and advanced technology to produce food and raw materials with smart farming . Some uses of technology in food production include the following:
- Genetically modified organisms. GMOs are inserted in a plant's genes to help it become disease resistant and grow in areas not favorable for production. GMOs are used in large crops such as rice, wheat and corn.
- Drones. Drones can provide satellite imagery to monitor crop growth and deal with problem areas.
- Meat industry technology. AI is effective in poultry production where it helps detect health issues with birds by the sounds they make. AI robots can work at poultry farms to collect eggs or assist with butchering.
- Crop monitoring. Along with the use of drones, AI can detect pests and diseases in crops. Digital apps -- such as AgroPestAlert, Farm Scout Pro and IPM Toolkit -- can help detect pest infestation and changing soil conditions to prevent large losses.
- 3D food printer. Food printers can create food -- such as pizza, snacks and candy -- at a faster pace. AI helps design the layers and structure of the food by placing one ingredient at a time. This could eliminate waste, as leftover ingredients can be reused.
Various sectors in food tech
In addition to lab-grown meats and vertical farms, food tech is a broad ecosystem of technologies that can be divided into subcategories for each food cycle.
Startups work to increase the quality of crops with technologies such as sensors, drones and software that replace manual labor. AI and machine learning are used to understand how plants and fungi grow and how they can grow effectively. Other parts of ag tech include fertilizer management, automated machinery , soil sensors and water solutions.
Ag tech can help farmers practice regenerative agriculture, which goes beyond preserving the environment and aims to actively improve it through agricultural practices. With enhanced data and automation provided by ag tech, regenerative agriculture can mitigate climate change, restore biodiversity and improve the work environment for farmers.
Food science
Startups are researching new ways to develop products that are both environmentally friendly and can address health concerns. Plant-based meat substitutes -- such as Beyond -- are an example of a recent product in this category. Scientists work with high-moisture extrusion and shear cell technology to find meal replacements -- such as using vegetable proteins in place of meat -- for individuals with health issues, and to find ways to remove common allergies such as lactose intolerance.
Food service
Food-related businesses -- including restaurants, cafeterias, hotels and cafes -- are looking at automation to help them run more efficiently. Robotics is being researched for use by restaurants of the future to help prepare and serve food, such as at the 2022 Beijing Olympics .
Restaurants are also using IoT technology to manage supply orders and track ingredients from initial order to arrival. Using sensors, restaurant owners can track the temperature of storeroom shelves and delivery trucks. They can watch the entire journey to ensure all safety standards are followed.
Smart appliances help make cooking easier with the use of meat temperature sensors and set-and-forget technology so staff can maximize their time while they wait for items to cook. Kitchen automation systems can also help chefs manage time and orders by tracking what needs to be cooked or how long something has been in a hot bin.
Businesses face the challenge of transporting food due to supply chain disruptions. With the growing demand for direct delivery to consumers, including restaurant and grocery delivery and meal kits, technology is needed to track and ensure food is packaged and delivered safely.
Consumer services
Consumers are looking to technology apps to improve diets, find restaurants, search for recipes, and track allergy or specialized diet information. For example, there are apps to find restaurants that meet certain dietary requirements so consumers can avoid allergens and follow their diets.
There are also startups working to educate people on their food choices and the benefits of proper nutrition to manage chronic health conditions and other personal fitness goals.
Food safety
Technology can help restaurants, grocers and other food suppliers manage the shelf life of food. This includes using technology to trace ingredients and check for recalls.
Some businesses are turning to blockchain technology to manage their supply distribution. For example, a grocery store can track a package of chicken to ensure it came from an antibiotic-free supplier.
Surplus and waste management
Technology is working to help reduce waste and improve sustainability in the food industry. Technology -- such as LeanPath -- combines software, smart scales and cameras to monitor and calculate food waste in kitchens. Staff uses the scale to classify and weigh all food thrown out, and the software identifies patterns to help minimize waste.
Manufacturers are turning to more sustainable packaging made of biopolymers that can also help extend the shelf life of a product. Drones and smart sensors can oversee display shelves and take inventory in real time to track product movement and shelf life.
Related Resources
- Managed Workplace Package With Smart Spaces For Manufacturing –Spectrum Enterprise
- World’s Leading Planet-Protecting Technology Secured by Trend Micro Security ... –Trend Micro
- 5 Questions to ASK When Selecting An Advanced Analytics Solution For Process ... –Seeq
- A Proactive Approach To Field Service 5 Priorities For Implementing Strong ... –ServiceNow
Dig Deeper on Internet of Things
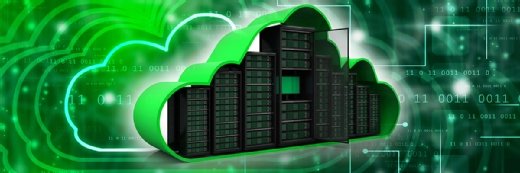
French datacentre operator to prototype using site's waste heat to generate renewable energy
Government hands over £12.5m to farming tech innovators
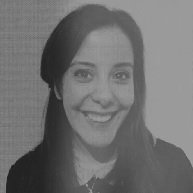
How the US Farm Bill Contributes to Adverse Human Health Outcomes
A URL (Uniform Resource Locator) is a unique identifier used to locate a resource on the internet.
File Transfer Protocol (FTP) is a network protocol for transmitting files between computers over TCP/IP connections.
A virtual private network (VPN) is a service that creates a safe, encrypted online connection.
Cloud computing requires a security approach that is different than traditional protections. Where does cloud detection and ...
An endpoint protection platform (EPP) is a security technology that safeguards endpoint devices.
Endpoint security is the protection of endpoint devices against cybersecurity threats.
Project planning is a project management discipline that addresses how to complete a project in a certain time frame, usually ...
Executive leadership is the ability of those who manage or direct employees in an organization to influence and guide these ...
Transfer learning is a machine learning (ML) technique where an already developed ML model is reused in another task.
Employee self-service (ESS) is a widely used human resources technology that enables employees to perform many job-related ...
Diversity, equity and inclusion is a term used to describe policies and programs that promote the representation and ...
Payroll software automates the process of paying salaried, hourly and contingent employees.
Customer segmentation is the practice of dividing a customer base into groups of individuals that have similar characteristics ...
Customer experience (CX) is the sum total of customers' perceptions and feelings resulting from interactions with a brand's ...
A buyer persona is a composite representation of a specific type of customer in a market segment.
More From Forbes
How technology is transforming the food industry.
- Share to Facebook
- Share to Twitter
- Share to Linkedin
When it comes to food, tech isn’t always the first thing that comes to mind. However, technology over the years has changed how we produce and find our food through applications, robotics, data and processing techniques.
According to a recent report from ING, technology helps food manufacturers produce more efficiently for a growing world population. There are 7.5 billion people in the world right now and that means a higher demand for food each year. By using tech to improve processing and packaging, it can improve the shelf life and safety of food.
Robotics and Machines
The use of machines in the food industry also ensures quality and affordability. By using machines, it drives down the costs of keeping the food fresh and increases productivity. According to the report, “The rise of robotics in the food industry is a tangible example of food tech. The number of robots in the European food industry is well over 30,000, while the number of robots per 10,000 employees rose from 62 in 2013 to 84 in 2017. Although Germany is the largest market, robot density is relatively highest in Sweden, Denmark, the Netherlands and Italy.”
Robotic machines can help to eliminate safety issues for the more dangerous jobs in the food industry. In 2016, a tech company rolled out a program for butchery. By using robots to cut the more difficult of the meats, they can save many work injuries. This is just one of the many ways technology can improve the industry.
3D Printing
In the past few years, 3D printing has really taken off across many industries and the food industry is one of them. There have been several applications of 3D printing food from NASA printing a pizza to creating soft foods for those who cannot chew hard food to consume. It opens the door for innovation being able to create many things that we were unable to before while also being able to help with food sustainability. There are many ways 3D printing is shaking up the industry.
Precision agriculture is a major player when it comes to how technology can make a difference. It is the use of GPS tracking systems and satellite imagery to monitor crop yields, soil levels, and weather patterns to increase efficiency on the farm. Not only can they see all that is happening across the fields, but they can also use analysis from the findings to test the soil and the health of the crops. A major way they are doing this is through the use of drones. These drones can locate and identify diseased or damaged crops and tend to them immediately. The use of these robots does not eliminate the need for food workers but helps them be more efficient with their work. With strict product requirements at large volumes and demand for lower pricing, the robotic elements help create a faster environment to produce more goods than regular labor.
Packaging and Waste
One of the biggest concerns for consumers right now is having healthy and sustainable goods. Consumers pay attention to labels and harmful ingredients, especially with social media, there is not much that companies can get away with anymore. Many companies use technology to help them “go green.” By using robotics and digitizing, companies in the food industry are able to find alternatives to plastics and other harmful packaging to the environment.
There are many different ways we are using technology in packaging now from edible packaging, micro packaging and even bacteria fighting packaging .
Consumers are also looking for where companies are sourcing their products and how they are handling their waste. Currently, 40% of America’s food is thrown away each year. With the help of technology, there are strides being taken to reduce that number and utilize the extra food.
One app, Copia uses its extensive food waste reduction dashboard to connect businesses with surplus food to local shelters, after-school programs, and other nonprofit organizations. Copia’s analytic software manages and tracks their surplus to save money and reduce their overall food waste.
With all these advancements in technology, there are so many different ways that it can really change how we produce food. Evolving technology could be the key to eliminating world hunger and solving our waste problem- we will just have to wait and see.
- Editorial Standards
- Reprints & Permissions

How Technology Impacts the Food Industry
- Ossian Muscad
- April 21, 2022
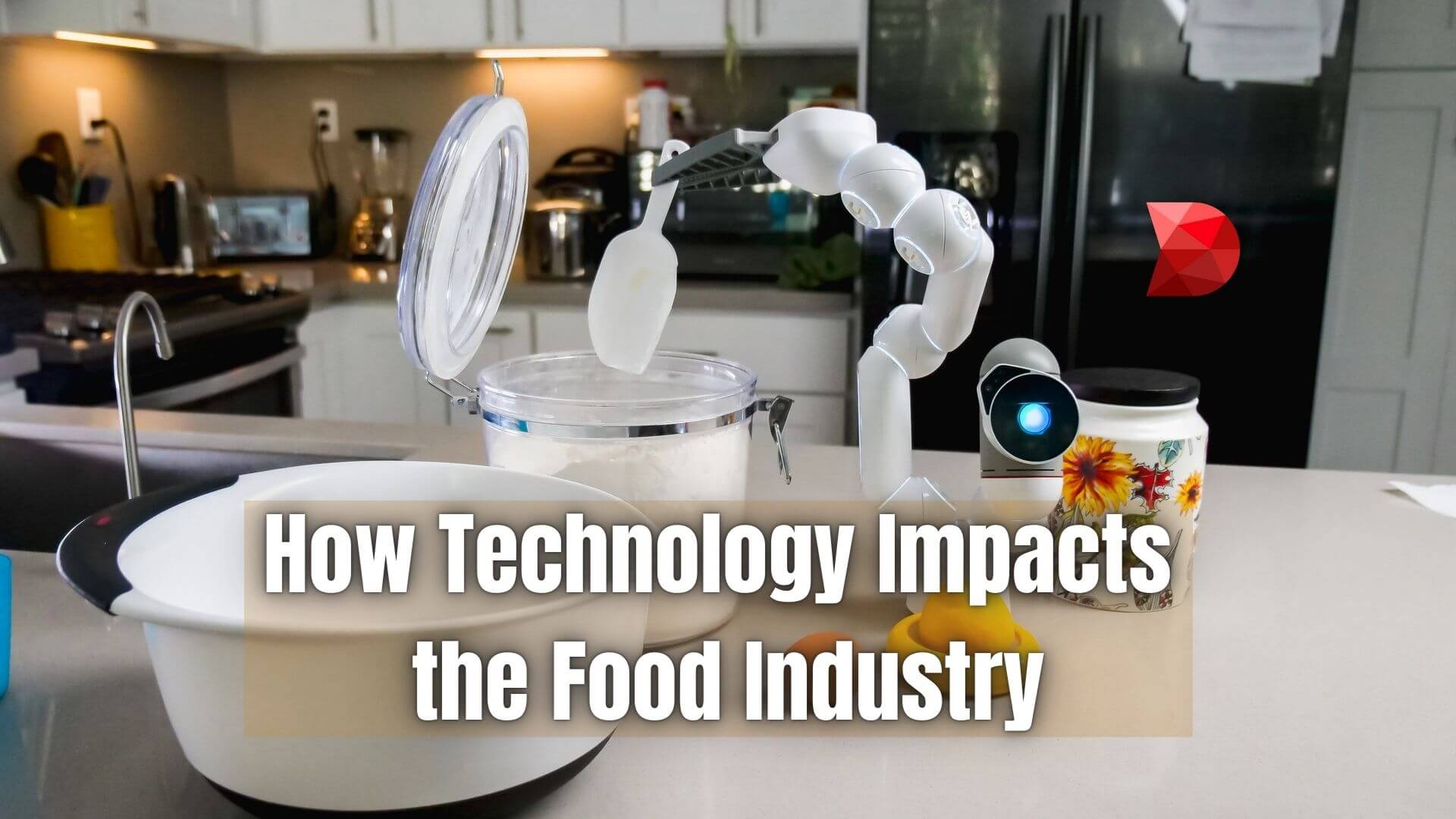
Last Updated on January 7, 2024 by Ossian Muscad
Technology has been increasingly prominent in the food industry in recent years. Though it might not be the first thing that comes to mind when you think of food, companies are always looking for new ways to improve and optimize their production.
Sustainability, health, and freshness are becoming more important to consumers, so businesses have to adjust to meet these demands. But just how impactful is technology in the food industry, and what changes can we expect to see in the future? Let’s find out!
Technology and the Food Industry
Technology and the food industry have certainly developed a symbiotic relationship. As the industry evolves, technology adapts and provides solutions for new challenges. In return, the food industry pushes technology to innovate and evolve.
Strengthening Production and Supply Chain
For instance, technology has greatly enhanced food production and distribution. Advanced machinery and automation technologies have increased efficiency, reducing the time it takes to produce food and minimizing waste. Moreover, sophisticated supply chain management systems, fueled by the Internet of Things (IoT) and predictive analytics, ensure that food products reach consumers on time, maintaining freshness and reducing spoilage.
Innovations in Food Safety and Quality
Technology also plays a critical role in ensuring food safety and improving food quality. For example, food safety technology such as blockchain is being used to track food from farm to table, enhancing transparency and traceability in the food supply chain. Additionally, innovations like modified atmosphere packaging (MAP) have extended the shelf life of food, preserving its quality and freshness for a longer period of time.
Enhancing Consumer Experience
Lastly, technology has revolutionized the way consumers interact with the food industry. Technology has made it easier for consumers to access, prepare, and enjoy food, from online food ordering and delivery platforms to smart kitchen appliances that help prepare meals. This has not only improved the consumer experience but also created new opportunities for businesses in the food industry.
How Technology Affects the Food Industry
The presence of technology in the food industry has grown exponentially in recent years. And as technology advances, so too does the food industry’s ability to meet the ever-changing demands of consumers.
Some of the most notable impacts of technology on the food industry include:
- Increased efficiency and productivity in food production: technology has allowed for a more streamlined and efficient production process, from planting and harvesting to manufacturing and packaging.
- Change in food consumption habits: technology has also changed people’s food consumption habits. For example, the rise of online grocery shopping has made it easier and more convenient for people to buy food.
- Greater focus on sustainability and health: with technology, businesses can track and monitor their environmental impact and make changes to reduce their carbon footprint. In addition, technology has also allowed for a greater focus on food safety and quality control.
- Improved food safety and quality control: technology has made it easier to track food safety and quality and identify and recall contaminated products.
- More environmentally friendly practices: technology has allowed businesses to adopt more environmentally friendly practices, such as using solar power or investing in energy-efficient equipment.
- Increased transparency and traceability: technology has made it easier for businesses to track their ingredients and ensure sourcing from sustainable and ethical suppliers.
- Enhanced customer experience: technology has allowed businesses to create a personalized and interactive experience, from online ordering and delivery to in-store technology such as self-checkout and digital menus.
As we can see, technology has a positive impact on the industry. And with continued technological advancements, we can only expect these positive impacts to continue.
Technologies that Impact the Food Industry
The only constant in technology is change, and the food industry is no exception. As new technologies emerge, businesses constantly adapt to stay ahead of the curve. The following technological advancements are currently making an impact in the food industry and will continue to shape the industry’s future:
Robots are crucial in automating many processes in the food industry, from harvesting and sorting to packaging and shipping. They enhance efficiency, reduce human error, and can work non-stop, resulting in increased productivity. Utilizing robots also allows for reallocating human labor to more intricate tasks.
Drones are used in agriculture for precision farming, helping farmers monitor crop health and irrigation systems, and even assisting in planting and harvesting. They reduce the time and effort required for these tasks and provide detailed aerial data for better farm management. When incorporated into the food supply chain, drones can also help with inventory management and delivery of products to remote or hard-to-reach areas.
Eco-friendly Packaging and Waste Reduction
Innovative technologies have led to the development of biodegradable and eco-friendly packaging materials. In addition, technologies like waste-to-energy conversion and smart waste management systems help reduce waste and promote sustainability in the food industry. As a result, businesses can reduce their environmental impact and meet the changing demands of environmentally conscious consumers.
Blockchain Methods
Blockchain technology is enhancing transparency and traceability in the food supply chain. It allows real-time food tracking from farm to table, ensuring food safety and preventing fraud. At the same time, blockchain technologies promote ethical sourcing and sustainability in the industry.
Smart Sensors
Smart sensors are used for quality control in the food industry. They monitor temperature, humidity, and other critical factors in real time during food storage and transportation, ensuring food safety and reducing spoilage.
3D Printing
3D printing is used in the food industry to create customized food items, particularly confectionery and catering. It allows chefs and food manufacturers to shape food in ways that were not previously possible. With 3D printing, there is also less waste and more efficient utilization of ingredients.
AI-based Solutions
Artificial Intelligence (AI) is used in the food industry for predictive analysis, demand forecasting, and personalizing customer experiences. AI algorithms can analyze large amounts of data and provide insights that help businesses make informed decisions. The power of AI also enables businesses to optimize processes and minimize costs, ultimately increasing productivity and efficiency.
Hackathons, or problem-solving events, are utilized in the food industry to spur innovation and solve industry challenges. They bring programmers, designers, and industry specialists together to create innovative solutions in a limited timeframe. This innovative approach creates a space for generating out-of-the-box ideas, promoting industry-wide progress.
Internet of Things (IoT)
IoT devices are being used to monitor and control processes in the food supply chain. They collect real-time data that aids decision-making, improves efficiency, and ensures food quality. The influence and integration of IoT devices in the food industry are expected to grow further in the coming years as technological advancements continue.
Data Utilization
Data analytics is helping food companies understand customer behavior, optimize operations, and predict trends. It provides valuable insights that drive decision-making and strategy development in the food industry. By utilizing data, businesses can improve the customer experience, increase efficiency, and stay competitive. At the same time, data utilization also promotes sustainable practices by identifying areas for improvement and reducing waste.
Online Marketing
Online marketing tools and platforms are used to reach consumers directly, promote products, and build brand loyalty. They allow food businesses to engage with customers, receive feedback, and personalize their marketing efforts. Through social media, email marketing, and other online strategies, companies can build a solid online presence and connect with customers in new ways.
Benefits of Using Technology in the Food Industry
The integration of technology into the food industry is not only transforming operations but also providing a multitude of benefits. It is revolutionizing how businesses operate – from the farm to the table – by making processes more efficient, safer, and customer-centric. Here are some of the prominent benefits of using technology in the food industry:
Accurate Predictions for Supply and Demand
With the help of AI and machine learning algorithms, food businesses can predict supply and demand trends with greater accuracy. This predictive analysis reduces waste, optimizes inventory, and effectively helps businesses cater to consumer needs.
Reduced Risk of Perishable Goods
Smart sensors and IoT devices allow for real-time monitoring of food items during storage and transportation, significantly reducing the risk associated with perishable goods. These technologies help maintain optimal conditions, ensuring the freshness and quality of food items, thereby minimizing spoilage and waste.
Flexibility in Restaurants
Technology provides restaurants with unprecedented flexibility in their operations. For instance, digital menus allow for swift changes in offerings based on availability, seasonality, or customer preferences. Similarly, online ordering systems provide the flexibility to efficiently manage takeout and delivery orders.
Better Customer Experience
Technology enhances the customer experience in many ways. Online platforms facilitate easy ordering and delivery services, while AI-driven data analytics enable personalized marketing and recommendations. Furthermore, self-ordering kiosks and mobile payment options improve the speed and convenience of service.
Safer and More Sanitized Food Production Environment
Technologies like automation and robotics ensure a safer and more sanitized food production environment. Robots, being non-biological, drastically reduce the risk of food contamination. Additionally, automated cleaning systems provide a thoroughly sanitized production area, contributing to overall food safety.
Predictions for the Future
As we navigate the 21st century, technology’s role in the food and beverage industry is expected to become even more prominent. The integration of advanced technologies is set to revolutionize every aspect of the industry, from production and processing to service and consumption. Here are several predictions that showcase the future trajectory of technology in the food and beverage industry:
Digital Dining Experience
The continual rise in food delivery services and the integration of technology into the dining experience is rapidly transforming the food industry. This shift makes delivery and takeaway services a crucial part of food businesses. Technologies such as online ordering systems, mobile apps, and third-party delivery platforms enable customers to enjoy meals in the comfort of their homes. This not only expands the customer reach of restaurants but also enhances the customer experience by providing convenience and choice.
Keeping an Eye on Virtual Food Trends
Technology also facilitates the monitoring of virtual food trends. Social media platforms, influencer marketing, and online food blogs play a significant role in shaping and spreading food trends. By keeping an eye on these virtual trends, businesses can stay ahead of the curve, adapt their offerings to current customer preferences, and effectively engage with their audience. Additionally, AI-driven algorithms can analyze online data to anticipate upcoming trends, giving businesses a competitive edge.
Streamlining New Staff Onboarding Process
Technology is also expected to streamline the staff onboarding process in the food industry. Digital training programs, e-learning modules, and virtual reality techniques can provide comprehensive and efficient training for new staff. These tools allow consistent training that can be easily updated to incorporate new procedures or information. Moreover, digital onboarding reduces the reliance on in-person training, thus saving time and resources.
Rise in Eco-friendly Packaging
The rise in eco-friendly packaging is a significant technology trend in the food and beverage industry. As environmental awareness grows, businesses are exploring innovative, sustainable packaging solutions. Technologies are being developed to produce biodegradable, recyclable, and edible packaging, reducing the industry’s environmental impact. This move towards eco-friendly packaging meets the growing demand for sustainable practices and paves the way for a more sustainable future in the food industry.
How the Food Industry Can Adapt to these Changes
As the impact of technology in the food industry continues to evolve, businesses must adapt to keep pace with these changes. They must embrace new customer expectations, improve efficiency through technology, and transition to eco-friendly practices. These changes boost operational effectiveness, enhance customer satisfaction, and contribute to a sustainable environment.
Adapt to New Customer Expectations
In the digital age, customer expectations are constantly evolving. Customers now demand seamless online ordering, quick delivery services, and personalized experiences. Food businesses must leverage technology to meet these expectations. AI-driven data analytics can help understand customer preferences and digital platforms can facilitate easy ordering and delivery services. Additionally, interactive digital menus and mobile payment options can enhance the dining experience, meeting the growing customer demand for convenience and personalization.
Improve Efficiency with Technology
Technology can significantly enhance operational efficiency in the food industry. Automation in food production and processing can speed up operations and reduce manual labor. Similarly, AI and machine learning can streamline inventory management by accurately predicting supply and demand trends. This not only optimizes resources but also reduces waste. Moreover, digital platforms can efficiently manage online orders, improving service speed and customer satisfaction.
Transition to Eco-friendly Practices
Transitioning to eco-friendly practices is crucial in today’s environment-conscious society. The food industry can leverage technology to make this transition. For instance, businesses can implement energy-efficient machines and appliances to reduce their carbon footprint. Additionally, innovative technologies are being developed to create eco-friendly, biodegradable, and recyclable packaging options. By embracing these practices, food businesses can contribute to environmental sustainability and appeal to the growing customer demand for environmentally friendly businesses.
Frequently Asked Questions (FAQs)
Q1: how does technology contribute to food safety.
Technology plays a vital role in enhancing food safety. Advances such as food tracking and traceability technology help ensure the quality of food by enabling the tracking of food products throughout the entire supply chain. Furthermore, automated cleaning systems and robotics help maintain high standards of hygiene, reducing the risk of food contamination.
Q2: How does technology impact food waste?
Technology can significantly reduce food waste through innovations like smart packaging and intelligent inventory management systems. These can detect when a product is about to spoil, allowing for timely consumption or disposal. AI can also predict demand trends, ensuring optimal stocking and reducing wastage due to overstocking.
Q3: How is the dining experience changing with modern technology?
With the advent of technology, the dining experience is becoming increasingly digitized. Customers now have the convenience of online ordering, mobile payments, and interactive digital menus. In addition, AI can provide personalized recommendations based on a customer’s past preferences, enhancing the overall dining experience.
Q4: What role does technology play in creating eco-friendly packaging in the food industry?
Technology is central to the development of eco-friendly packaging in the food industry. Researchers use technology to create sustainable packaging solutions such as biodegradable or recyclable materials. There are also innovations in edible packaging that reduce waste and environmental impact.
Q5: How can technology help in staff training in the food industry?
Digital training programs, e-learning modules, and virtual reality techniques are transforming staff training in the food industry. These tools provide:
- Comprehensive, easily updated training.
- Reducing reliance on in-person sessions.
- Saving time.
- Improving the consistency of the training.
Q6: How does technology aid in tracking food trends?
Technology, specifically AI-driven algorithms, can analyze data from social media platforms, influencer marketing, and online food blogs to identify and predict food trends. This can give businesses a competitive edge, allowing them to adapt their offerings to current preferences and engage more effectively with their audience.
Streamline Your Food Business Operations with DATAMYTE
DATAMYTE is a quality management platform with low-code capabilities. Our Digital Clipboard , in particular, is a low-code workflow automation software that features a workflow, checklist, and smart form builder. This tool lets you streamline multiple processes to create customized workflows, forms, and checklists for your food business operations. By automating your processes, you can improve efficiency, reduce errors, and foster a culture of continuous improvement.
DATAMYTE also lets you conduct layered process audits, a high-frequency evaluation of critical process steps, focusing on areas with the highest failure risk or non-compliance. Conducting LPA with DATAMYTE lets you effectively identify and correct potential defects before they become major quality issues.
With DATAMYTE, you have an all-in-one solution for your food business operations that can help you stay competitive, adapt to changing customer expectations, and contribute to a sustainable future. Book a demo now to learn more.
Technology holds transformative potential for the contemporary food industry, positively impacting every facet – from enhancing customer experience to optimizing operational efficiency, ensuring food safety, reducing waste, facilitating eco-friendly practices, staff training, and keeping pace with ever-evolving food trends.
The use of AI, machine learning, and digital platforms proves instrumental in delivering personalized services, streamlining inventory management, reducing carbon footprint, and predicting demand trends. As we continue to navigate this technologically driven era, integrating these innovations into your food business operations is more than a luxury — it’s a necessity.
While change may seem daunting, embracing these technologies can strategically position your business for success, giving you a competitive edge and aiding in creating a sustainable future. Explore the multitude of ways technology can revolutionize your food industry operations and take that crucial step towards optimization and innovation today.
Related Articles:
- CCTV Maintenance: 10 Tips to Keep Your Security Cameras Running
- 11 Tips for Accurate CNA Charting or Documentation
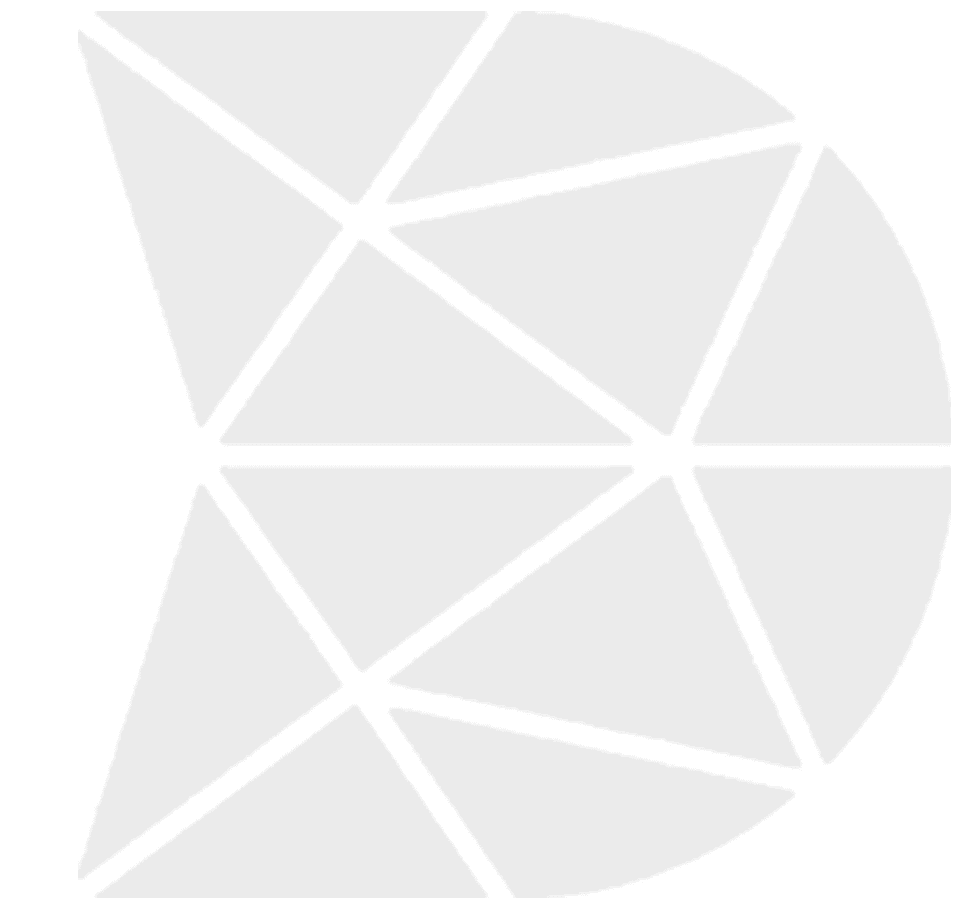
- Implementation
- Case-Studies
- White Papers
- Knowledge Base
Experts in the Connected Factory
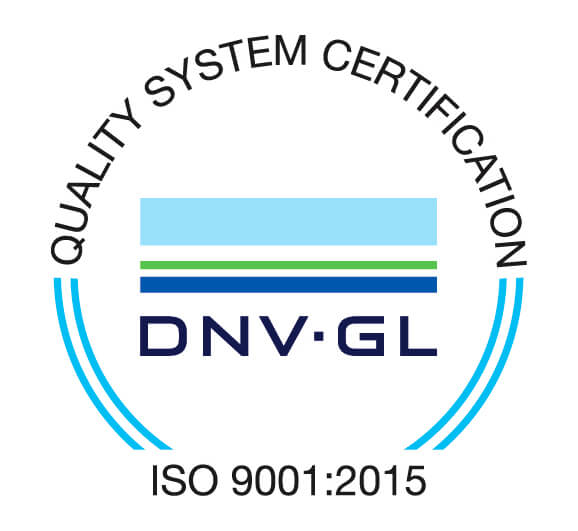
- 1-800-455-4359
- (763) 553-0455 ext. 1
- [email protected]

An official website of the United States government
The .gov means it’s official. Federal government websites often end in .gov or .mil. Before sharing sensitive information, make sure you’re on a federal government site.
The site is secure. The https:// ensures that you are connecting to the official website and that any information you provide is encrypted and transmitted securely.
- Publications
- Account settings
Preview improvements coming to the PMC website in October 2024. Learn More or Try it out now .
- Advanced Search
- Journal List
- Springer Nature - PMC COVID-19 Collection

Application of Artificial Intelligence in Food Industry—a Guideline
Nidhi rajesh mavani.
1 Department of Chemical and Process Engineering, Faculty of Engineering & Built Environment, Universiti Kebangsaan Malaysia, UKM, Selangor 43600 Bangi, Malaysia
Jarinah Mohd Ali
Suhaili othman.
2 Department of Biological and Agricultural Engineering, Faculty of Engineering, Universiti Putra Malaysia, UPM Serdang, 43400 Selangor, Malaysia
M. A. Hussain
3 Department of Chemical Engineering, Faculty of Engineering, University of Malaya, 50603 Kuala Lumpur, Malaysia
Haslaniza Hashim
4 Department of Food Sciences, Faculty of Science & Technology, Universiti Kebangsaan Malaysia, UKM, Selangor 43600 Bangi, Malaysia
Norliza Abd Rahman
Artificial intelligence (AI) has embodied the recent technology in the food industry over the past few decades due to the rising of food demands in line with the increasing of the world population. The capability of the said intelligent systems in various tasks such as food quality determination, control tools, classification of food, and prediction purposes has intensified their demand in the food industry. Therefore, this paper reviews those diverse applications in comparing their advantages, limitations, and formulations as a guideline for selecting the most appropriate methods in enhancing future AI- and food industry–related developments. Furthermore, the integration of this system with other devices such as electronic nose, electronic tongue, computer vision system, and near infrared spectroscopy (NIR) is also emphasized, all of which will benefit both the industry players and consumers .
Introduction
Artificial intelligence (AI) is defined as a field in computer science that imitates human thinking processes, learning ability, and storage of knowledge [ 1 , 2 ]. AI can be categorized into two types which are strong AI and weak AI. The weak AI principle is to construct the machine to act as an intelligent unit where it mimics the human judgments, while the strong AI principle states that the machine can actually represent the human mind [ 3 ]. However, strong AI does not exist yet and the study on this AI is still in progress. The gaming industry, weather forecasting, heavy industry, process industry, food industry, medical industry, data mining, stem cells, and knowledge representation are among the areas that have been utilizing AI methods [ 4 – 11 ]. AI has a variety of algorithms to choose from such as reinforcement learning, expert system, fuzzy logic (FL), swarm intelligence, Turing test, cognitive science, artificial neural network (ANN), and logic programming [ 3 ]. The alluring performance of AI has made it the most favorable tool to apply in industries including decision making and process estimation aiming at overall cost reduction, quality enhancement, and profitability improvement [ 7 , 12 ].
As the population in the world is rising, food demand is predicted to rise from 59 to 98% by 2050 [ 13 ]. Thus, to cater for this food demand, AI has been applied such as in management of the supply chain, food sorting, production development, food quality improvement, and proper industrial hygiene [ 14 – 16 ]. Sharma stated that the food processing and handling industries are expected to grow about CAGR of 5% at least until 2021 [ 15 ]. ANN has been used as a tool in aiding real complex problem solving in the food industry according to Funes and coworkers [ 17 ], while based on Correa et al., the classification and prediction of parameters are simpler when using ANN, which leads to higher usage demand of ANN over the past years [ 18 ]. Besides, FL and ANN have also acted as controllers in ensuring food safety, quality control, yield increment, and production cost reduction [ 19 , 20 ]. AI technologies have also known to be beneficial in food drying technology and as process control for the drying process [ 21 – 23 ].
Previous studies have shown many usages of AI in food industries focusing on individual target and aims. A study has been conducted on the various ANN applications in food process modeling where it has only highlighted the food process modeling using ANN [ 24 ]. Apart from that, the implementation of AI such as ANN, FL, and expert system in food industries have been reviewed but specifically focusing on the drying of fresh fruits [ 23 ]. A review has been conducted on how food safety has been one of the main concerns in the food industry which leads to the development of smart packaging systems to fulfill the requirements of the food supply chain. Intelligent packaging monitors the condition of foods to give details on the quality of the food during storage and transportation [ 25 ]. Another study reviewed on intelligent packaging as a tool to minimize food waste where about 45 recent advances in the field of optical systems for freshness monitoring have been reported. Meat, fish products, fruits, and vegetables were covered in the study as they are the most representative fields of application [ 25 ]. Few different studies have been conducted on intelligent packaging, and these studies proved that the usage of intelligent packaging systems plays an important role in the food factory in the context of the food chain as they are able to monitor the freshness of food products and crops [ 23 , 26 – 30 ].
There are also several other studies that have been conducted on the application of AI and sensors in food; however, the coverage is rather limited. Therefore, a comprehensive review that assembles all AI applications in the food industry as well as its combinations with appropriate sensor will be a great advantage, all of which are unavailable as to the knowledge of the author. Such review will assist in gathering the advantages, limitations, and methodologies as a one-stop guideline and reference for food industry players, practitioners, and academicians. To be exact, different types of AI and their recent application in food industries will be highlighted which comprises several AI techniques including expert system, fuzzy logic, ANN, and machine learning. In addition, the integration of AI with electronic nose (E-nose), electronic tongue (E-tongue), near infrared spectroscopy (NIRS), and computer vision system (CVS) is also provided. This paper is organized as follows. The introduction of AI is explained in the first section followed by the application of different types of AI in the food industry. Following that, the fusion of the AI with the external sensors in the food industry is presented. In the latter part, a critical review is conducted where discussion on the main application of the AI algorithms in the food industry is carried out. A flowchart is presented to assist the researchers on establishing the most appropriate AI model based on their specific case study. Then, the trends on the application of AI in the food industry are illustrated after that section. Finally, a brief conclusion is discussed in this paper.
AI in Food Industry
The application of AI in the food industry has been growing for years due to various reasons such as food sorting, classification and prediction of the parameters, quality control, and food safety. Expert system, fuzzy logic, ANN, adaptive neuro-fuzzy inference system (ANFIS), and machine learning are among the popular techniques that have been utilized in the food industries. Prior to AI implementation, studies related to food have been going on over the years to educate the public about food knowledge as well as to improve the final outcomes related to food properties and the production of foods [ 31 – 36 ]. A lot of benefits can be obtained by using the AI method, and its implementation in the food industry has been going on since decades ago and has been increasing till today [ 37 – 39 , 31 , 32 ]. Nevertheless, this paper will focus on the application of AI in food industries from the year 2015 onwards since tremendous increase and innovation are seen in the implementation recently. It is worth noting that several methods such as partial least square, gastrointestinal unified theoretical framework, in silico models, empirical models, sparse regression, successive projections algorithms, and competitive adaptive reweighted sampling which have been used for prediction and enhancement of the food industries are not discussed here; instead it is narrowed down to the wide application of AI in the food industry.
Knowledge-based Expert System in Food Industry
The knowledge-based system is a computer program that utilizes knowledge from different sources, information, and data to solve complicated problems. It can be classified into three categories which are expert systems, knowledge-based artificial intelligence, and knowledge-based engineering. The breakdown of the knowledge-based system is presented in Fig. 1 . The knowledge-based expert system which is widely used in the industries is a decisive and collective computer system that is able to imitate the decision-making ability of human expert [ 40 ]. It is a type of knowledge-based system that is known as among the first successful AI models. This system depends on experts for solving the complicated issues in a particular domain. It has two sub-systems, which are knowledge base and inference engine. The facts about the world are stored in the knowledge base, and the inference engine represents the rules and conditions regarding the world which are usually expressed in terms of the IF–THEN rules [ 41 ]. Normally, it is able to resolve complicated issues by the aid of a human expert. This system is based on the knowledge from the experts. The main components of the expert system (ES) are human expert, knowledge engineer, knowledge base, inference engine, user interface, and the user. The flow of the expert system is shown in Fig. 2 .

Knowledge-based system

Expert system
The food industry has been utilizing ES for various objectives as this system is proven to be useful especially in the decision-making process. The knowledge-based expert system has been applied in white winemaking during the fermentation process for the supervision, intelligent control, and data recovery [ 42 ]. Apart from that, a web-based application was developed by implementing the ES to calculate the nutritional value of the food for the users, and the development of ES was able to help the SMIs in obtaining the details required for the qualification in obtaining the food production certificates [ 43 ]. Food safety is very important in the food industry,thus, the application of ES that is linked closely to food safety has been used extensively ranging from process design, safety management, quality of food, and risk assessment [ 44 ]. Furthermore, a prototype information technology tool and guidelines with corrective actions that considered ES in the model were developed for the food industry where few essential factors such as food safety, nutrition, quality, and cost were studied [ 45 ]. In addition, a digital learning tool, namely, MESTRAL, was developed to assist people in food processing by using models developed from research in food science and technology and simulators. This tool is based on the knowledge engineering and reflected real applications which can be mapped with the system scale and knowledge frameworks [ 46 ]. A comprehensive review was conducted by Leo Kumar on the application of the knowledge-based expert system in manufacturing planning. The paper has also discussed the utilization of ES in decision making in three wide areas which are the process planning activities, diverse applications, and manufacturing planning [ 41 ]. Moreover, Table Table1 1 gathers some of the recent application of ES in the food industry ranging from the raw material to the final production as well as the food safety.
Application of expert system in food industry
Application | Objective | Category in the industry | Important outcomes | References |
---|---|---|---|---|
Banana | To identify the banana disease and methods to overcome them | Raw material/agriculture | (i) The system was able to diagnose the diseases in the plants based on the stems, leaves, and roots which assists in preventing from the diseases happening | Budiyanto et al. [ ] |
Barley | To classify the barley grains | Quality control | (i) The classification accuracy by using the ES method was above 72% which was considered satisfactory | Szturo & Szczypinski [ ] |
Coffee | To determine the appropriate process on the dry mill | Production/quality control | (i) ES was able to select the correct production process for a specific coffee with an accuracy of 93% | Hernández-Vera et al. [ ] |
Coffee beans | To identify the quality grading of the coffee beans | Sensory evaluation | (i) The developed fuzzy ES, namely, AI Cupper, was able to grade the coffee with an accuracy of 95% | Livio & Hodhod [ ] |
Corn | To detect the corn pests and diseases | Agriculture/raw material | (i) The system was able to detect the pests and disease with an accuracy of 76.6% and also provide ways to control them (ii) The developed system was able to provide facility explanation regarding the diagnosis results | Sumaryanti et al. [ ] |
Food additives | To identify the halal safety rating for food additives | Food dafety | (i) The developed Halal Food Additive using ES provides the consumers with safety rating key according to their past food consumption record experience (ii) It provides consumers in finding nutritional foods and at the same time fulfilling the Halalan-Toyyiban criteria | Zakaria et al. [ ] |
Food products | To monitor and forecast the product quality in the production process | Production/food quality | (i) An intelligent ES was developed which is able to monitor the product quality indicators and make changes to the existing methods; recommendations for the products and the defects for the final product can be identified by this system | Blagoveshchenskiy et al. [ ] |
Fresh food | To optimize the distribution networks of the fresh foods | Sustainability | (i) The proposed expert system, Food Distribution Planner, was able to develop the most effective distribution method which reduces the emission of carbon dioxide by 9.6%, increase of 2.7% in operating cost, and no waste produced during the delivery time due to the preservation method utilized during the shipment | Bortolini et al. [ ] |
Livestock production (milk, meat) | To analyze the outcome of various variables on the performance of the livestock production | Production/raw material | (i) ES can be utilized as a decision support system for livestock producers for identifying the best practices for the livestock which maximizes the production of the meat and milk (ii) The greatest impact on the production is the type of grazing being fed to the cattle as the diet affects the health of the cow who produces the milk and the meat | Vásquez et al. [ ] |
Red wine and rum | To forecast the key aroma compounds for foods without using the human olfactory system | Sensory evaluation/quality control | (i) The developed rapid method system, Sensomics Based Expert System, resulted in a good agreement in the key odorants for the food aroma distillate | Nicolotti et al. [ ] |
Rice crops | To aid the farmers in making decisions for the rice crops | Agriculture/ production | (i) The farmers are assisted by the ES in selecting the seeds and tackling the pests and diseases for the rice crops which eventually will improve the production of the rice crops | Kharisma et al. [ ] |
Soybean | To identify the diseases on soybeans | Agriculture | (i) The study successfully designed an ES to identify soybean diseases by comparing the accuracy using the frame-based representation and rule-based representation method (ii) Frame-based representation ES has shown a higher accuracy compared to the rule-based ES | Rajendra et al. [ ] |
White winemaking | To develop a knowledge-based ES for the alcoholic fermentation process of the white winemaking | Processing/sustainability | (i) A cost-efficiency advanced control system through the knowledge-based ES was developed for the alcoholic fermentation process which was used for the supervision, control, and data recovery software of the bioreactor (ii) It was proven to be applied in winemaking at the industrial scale and can be adjusted for few areas in the food manufacturing sectors | Sipos [ ] |
Wine | To measure the environmental impact of viticulture at wine estate | Sustainability | (i) ES integrated with the geographic information system software was able to measure the environmental impact of viticulture in a comprehensive way (ii) The model is said to be an environmental support system in supporting policy and decision-making in the management | Lamastra et al. [ ] |
Fuzzy Logic Technique in the Food Industry
Fuzzy logic (FL) was first introduced by Zadeh in 1965 based on the impeccable capability of human intellect in decision making and unraveling the imprecise, uncertain, and ambiguous data while solving problems [ 47 , 48 ]. The fuzzy set theory is recognized in such a manner that an element belongs to a fuzzy set with a certain degree of membership which has a real number in the interval [0, 1] [ 49 ]. FL models consist of several steps which are fuzzification, inference system, and defuzzification process [ 50 , 51 ]. Fuzzification is a process where the crisp value is converted into a degree of membership and yields the fuzzy input sets. The corresponding degree in the membership functions is normally between 0 and 1. [ 52 ]. There are a variety of membership functions to choose from, whereby the commonly used ones are triangular, Z-shaped, S-shaped, trapezoidal, and Gaussian-shaped [ 52 ]. The inference system is where the fuzzy input is being translated to get output by using the fuzzy rules. The fuzzy rules are known as IF–THEN rules where it is written such IF premise, THEN consequent whereby the IF comprises input parameters and THEN is the output parameters [ 53 ]. The inference system consists of the style which is either the Mamdani or Takagi–Sugeno Kang (TSK). Defuzzification is the ultimate phase in the fuzzy logic model where the crisp values are obtained [ 54 ]. There are different methods of defuzzification which are center of gravity, mean of maximum, smallest of maximum, largest of maximum, center of maximum, and centroid of area [ 55 ].
FL has been long utilized in the industry due to its simplicity and ability to solve problems in a fast and accurate manner. FL has been employed in the food industry in food modeling, control, and classification and in addressing food-related problems by managing human reasoning in linguistic terms [ 56 ]. The food manufacturing system has improved by the implementation of the fuzzy logic where about 7% of electricity losses has been reduced compared to the conventional regulation method [ 57 ]. Sensory evaluation of the food is also one of the most common parts where FL plays an important role. Furthermore, a quicker solution to problems can be performed by using a system involving fuzzy rules [ 58 ]. Table Table2 2 shows previous applications of FL in the food industry and their attributes. From a previous study, FL has been proven to successfully maintain the quality of the foods, and it acts as a prediction tool and control system for food production processes.
Application of fuzzy logic in the food industry
Application | Objective | Fuzzy inference system | Membership function | Important outcomes | References |
---|---|---|---|---|---|
Aromatic foods | To rank the sensory attributes of aromatic foods packed in films made from corn starch | Mamdani | Triangular | (i) Overall ranking for tea and tastemaker and the important quality attributes of the food materials in general, and the samples were able to be done using FL (ii) Aroma and taste of tea leaf and tastemaker in general were assessed as “Highly important” sensory attributes | Chowdhury & Das [ ] |
Beetroot candy | To rank the candy with various content ratios | Mamdani | Triangular | (i) The developed model was able to optimize and perform the ranking of the candy involving different ingredient ratios | Fatma et al. [ ] |
Canned food | To control sterilization temperature by using fuzzy logic and making online corrections in autoclave operation | Mamdani | Triangular | (i) The sterilizing temperature with an accuracy of ± 0.5 ℃ can be maintained by a fuzzy controller (ii) Batch processing can be completed using the proposed system with less time, steam consumption, and risk of over-sterilization | Chung et al. [ ] |
Coffee | To determine the suitable process on a dry mill according to customer requirements using an expert system based on fuzzy logic | Mamdani | Triangular | (i) The developed system will be useful for the correct decision process between two different types of coffee (ii) Validation was carried out by comparing the process values by the model with the real process data, and the coefficient of determination obtained was 93% | Hernández-Vera et al. [ ] |
Coffee beans | To introduce a control system for the roasting machine | Mamdani | Triangular | (i) The consistent roasting level of the beans is able to be produced by the proposed model | Harsawardana et al. [ ] |
Cupcakes | To rank the cupcakes according to their quality attributes | Mamdani | Triangular | (i) The system was able to determine the best condition for their cupcakes with respect to their sensory attributes (ii) The ranking of the quality attributes was able to be performed by the system | Singh et al. [ ] |
Dough | To implement the FL to act as a controller system in bread making | Mamdani | Triangular, trapezoidal | (i) The settling time and the response of the FL controller showed a better performance than the proportional-integral-derivative (PID) controller (ii) The FL controller system was established successfully for the proofing process in bread making | Yousefi-Darani et al. [ ] |
Fava beans | To predict the physical parameters of the beans with various moisture contents | Mamdani | Triangular | (i) The model was able to predict thirteen parameters of the beans with the moisture content ranging from 9.3 to 31.3% in the input (ii) Comparison was done between the FL results and the experimental value where a high correlation value which is 0.999 and mean standard deviation ranging 1.23–12.56% were obtained as an effective system and can be used to develop a model for controlling and managing various stages during processing | Farzaneh et al. ( , |
Flixweed seed | To rank different ways of extraction in preparing the seeds | Mamdani | Triangular | (i) Ranking based on the properties, extraction output, and duration was able to determine the best method for the preparation of the seeds | Shahidi et al. [ ] |
Fresh mango juice (litchi juice) | To study the effect of high pressure processes (HPP) on sensory attributes of fresh mango juice and litchi juice | Mamdani | Triangular | (i) The model was able to determine that the HPP effect depended on the type of products and domain of pressure–temperature (ii) HPP is proven to be a promising method for the preservation of fruit products | Kaushik et al. [ ] |
Hydrogel colloidosomes | To estimate the release of caffeine from hydrogel colloidosome | Mamdani | Type S, type Z | (i) The proposed diffusional-fuzzy model able to describe the caffeine release from hydrogel colloidosomes (ii) The model has a higher precision, better handling of uncertainty property and better generalization capability | Amiryousefi et al. [ ] |
Onions | To predict the drying kinetics of the onions | Mamdani | Triangular | (i) The model was able to predict the moisture ratio at varying conditions with high performance where the value of R obtained was 0.9999 and the low root mean square error (RMSE) was 0.004157 | Jafari et al. [ ] |
Pineapple Rasgulla | To rank the pineapple Rasgulla with respect to the parameters | TSK | Triangular | (i) Sensory evaluation for different concentrations of pineapple Rasgulla and ranking of the samples was performed successfully by using the FL model | Sarkar et al. [ ] |
Pizza production industry | To develop a system in order to improve the production system | Mamdani | Triangular | (i) The developed FL control system is able to identify the amounts of workers and ovens needed in pizza production which improves the customer’s satisfactory level by reducing the waiting time as well as reducing the wastage | Blasi [ ] |
Salt | To estimate the production of salt by variables that affect it | TSK | Triangular | (i) By using the Sugeno zero-order model, the time for production of salt could be estimated with a minor error value of 0.0917 | Yulianto et al. [ ] |
Sardine | To assess fish quality by biogenic amines using a fuzzy logic model | Mamdani | Triangular | (i) The model was able to determine the quality of the fish at the initial stages of storage (ii) The Pearson correlation r value obtained was greater than 0.95 at different temperatures | Zare & Ghazali [ ] |
Treated raw apple juice | To carry out a sensory evaluation of raw apple juice treated with raw betel leaf essential oil | Mamdani | Triangular | (i) The FL approach by applying similarity analysis gave an insight into variation into customers’ acceptability on treated apple juice | Basak [ ] |
Wheat dough | To develop an effective sheeting of wheat dough | Mamdani | Trapezoidal | (i) Able to decide the best program with the least number of rolling steps based on the quality of the dough which improves the sheeting process of the dough | Mahadevappa et al. [ ] |
White mulberry | To forecast the moisture ratio of the mulberry during the drying process | Mamdani | Triangular | (i) The model could predict the moisture ratio of mulberry under varying conditions with a high accuracy where the value of equals to 0.9996 and RMSE value of 0.01095 | Jahedi Rad et al. [ ] |
ANN Technique in the Food Industry
ANN is another AI element, which is also commonly applied in the food industry. ANN is designed to mimic the human brain and be able to gain knowledge through learning and the inter-neuro connections which are known as synaptic weights [ 59 , 60 ]. Gandhi and coworkers have stated that the configuration of ANN is designed in such a way that it will accommodate certain application such as data classification or pattern recognition [ 61 ]. According to Gonzalez-Fernandez, ANN is applicable to a different kind of problems and situations, adaptable, and flexible. In addition, Gonzalez et al. (2019) have also stated that ANN is suitable to model most non-linear systems and is adaptable to new situations even though adjustments are needed. Moreover, the most outstanding features of ANN is its non-linear regression [ 62 ]. There are several types of ANN including feedforward neural network, radial basis function neural network, Kohonen self-organizing neural network, recurrent neural network, convolutional neural network, and modular neural network [ 63 ]. Multilayer perceptron (MLP), radial basis function networks (RBFNN), and Kohonen self-organizing algorithms are the most effective types of NN when it comes to solving real problems [ 61 ]. The most common network that is used for prediction and pattern recognition is the multilayer perceptron [ 18 , 64 , 65 ]. Besides that, ANN learning could be classified into supervised and unsupervised depending on the learning techniques [ 17 ]. In general, the structure of ANN consisted of an input layer, hidden layer, and output layer, either single or many layers [ 66 – 68 ]. The architecture comprises activation functions, namely, the feed-forward or feedback [ 69 ]. The backpropagation learning algorithm is normally used as it is able to minimize the prediction error by feeding it back as an input until the minimum acceptable error is obtained [ 18 ]. An additional input known as bias is added to neurons which allows a portrayal of phenomena having thresholds [ 70 , 71 ]. In ANN, the dataset is normally associated with a learning algorithm which trained the network and could be categorized into three groups specifically supervised, unsupervised, and reinforcement learning [ 72 ]. Then, the data will undergo training and testing for analyzing the outputs. The general structure for the ANN is shown in Fig. 3 . The output data can be calculated by using the equation shown based on Fig. 4 .

ANN structure in general

General calculation in ANN
Previous studies have highlighted the utilization of ANN in numerous tasks within the food industry. This includes the assessment and classification of the samples, complex calculation such as heat and mass transfer, and analysis of the existing data for control purposes as well as for prediction purposes which are listed in Table Table3. 3 . All applications have shown satisfactory performances based on the R 2 values, showing that ANN can provide results in an accurate and reliable manner.
Application of ANN in the food industry
Application | Objectives | Types of ANN | Outcomes | References |
---|---|---|---|---|
Cocoa powder | To predict the effect of process parameters on the properties of cocoa mixtures | MLP | (i) The model could predict the effect of different parameters changes on the physical and chemical properties of the cocoa mixtures with a high accuracy where the value of derived was 0.934 with low error of 0.053 | Benković et al. [ ] |
Dried vegetables | To identify the quality of dried carrots and classification of such dried products | MLP | (i) Suitable to be used for assessment and classification of dry carrots (ii) Enable the selection of important representative characteristics for the quality assessment (iii) Comparison between algorithms was done, and the backpropagation is the most successful algorithm compared to other algorithms | Koszela et al. [ ] |
Eggplant | To describe the mass transfer kinetics of eggplants in osmotic dehydration (OD) | MLP | (i) High volume of complicated problems is able to be modeled and analyzed by using ANN, and it is the most suitable software for calculation problems (ii) The developed model achieved the highest value of 0.9825 | Bahmani et al. [ ] |
Extra virgin olive oils | To evaluate the influence of light exposure conditions and packaging material on the stability of physicochemical characteristics of extra virgin olive oils | MLP | (i) ANN showed a high classification performance with an accuracy of greater than 90% for the test data and greater than 85% for the training set (ii) Showed the robustness of the model which indicates the suitability for solving clustering, pattern recognition, classification, and adulteration issues regarding extra virgin olive oils | S. F. Silva et al. ( , |
Garlic | To forecast the sensory quality of garlic | MLP | (i) A model with the best prediction of the sensory quality of the garlic and garlic products was developed with an value of 0.9866 | Liu et al. [ ] |
Honey | To predict the stability of the Indian honey crystallization of different component ratios | MLP | (i) The ANN model was able to forecast the stability of the honey consisting of different compositions with high accuracy where the value obtained was 0.9994 | Naik et al. [ ] |
Mango | To estimate the weight of the mango | MLP | (i) Estimation of the weight of the mangoes was able to be done by applying thirteen different parameters in the model | Dang et al. [ ] |
Mushrooms | To develop an ANN model that can predict the moisture content of the mushrooms during the drying process | MLP | (i) The ANN model was able to predict the moisture content of the mushrooms during the drying process with an value of 0.9817 | Omari et al. [ ] |
Mushrooms | To predict the temperature variety of mushroom growing hall based on the parameters affecting the room temperature | MLP, RBF | (i) The prediction by using the RBF method has a higher accuracy compared to that of MLP where the value of correlation achieved was 0.996 and 0.9612, respectively | Ardabili et al. [ ] |
Onions | To estimate the drying behavior of onion | MLP | (i) The develop model was able to forecast the drying kinetics of onions at different temperatures and times with a high performance where the value achieved a value of 0.99956 | Jafari et al. [ ] |
Potato cubes | To carry out analysis in a fluidized bed dryer for the drying of potato cubes under different conditions | MLP | (i) The developed model was able to carry out the analysis of energy and exergy losses in the dryer for the drying of the potato cubes (ii) All the analysis done by using the ANN model obtained a high value of which is greater than 0.98 and the average value obtained was 0.99 | Azadbakht et al. [ ] |
Potato peels | To model a system that can predict and optimize the extracted conditions by using the response surface methodology and ANN | MLP | (i) This study with the aid of the ANN model actually helped to determine that the potato peels which are often thrown away as a waste are actually beneficial (ii) The value achieved by the ANN model for the analysis of three different extracted values were greater than 0.93 | Anastácio et al. [ ] |
Quince fruit | To determine the moisture ratio of the fruit during the drying process and test the performance of the developed model | MLP | (i) By implementing different ANN structures, the moisture content ratio during the drying process for the quince fruits was modeled successfully (ii) The developed model was able to predict the moisture ratio with high correlation value where the obtained was greater than 99% | Chasiotis et al. [ ] |
Rice crop | To predict rice production yield and investigate the factors affecting the rice crop yield | MLP | (i) A good alternative to predict the rice production yield compared to traditional linear regression methods (ii) The accuracy obtained by the developed algorithm was 97.54%, with the sensitivity of 96.33% and specificity of 98.12% | Gandhi et al. [ ] |
Sausage | To forecast the benzo[a]pyrene (BaP) content of smoked sausage | MLP | (i) The model was able to predict the BaP content in smoked sausages and create a control system for the smoking to reduce the BaP contamination in smoked sausages (ii) The model has a high accuracy where the overall prediction was greater than 0.90 | Chen et al. [ ] |
Vegetable oils | To classify vegetable oils: canola, sunflower, corn, and soybean using a very few mathematical manipulation and ANN | MLP | (i) Able to simplify the vegetable oil classification with high accuracy (ii) A fast-network training and uses very few mathematical manipulations in the spectra data | Silva et al. [ ], Da et al. [ ] |
Machine Learning Techniques
Machine learning (ML) is known to be the subset of AI [ 73 , 74 ]. It is a computer algorithm that advances automatically with experiences. ML can be classified into three broad categories which are supervised learning, unsupervised learning, and reinforcement learning [ 11 , 75 ]. Supervised learning aims to predict the desired target or output by applying the given set of inputs [ 76 ]. On the other hand, unsupervised learning does not have any outputs to be predicted and this method is utilized to classify the given data and determine the naturally occurring patterns [ 77 ]. Reinforcement learning is when there is an interaction between the program and the environment in reaching certain goals [ 78 ]. Among the known models in machine learning are ANN, decision trees (DT), support vector machines (SVM), regression analysis, Bayesian networks, genetic algorithm, kernel machines, and federated learning [ 76 , 79 ]. ML has been commonly used for handling complex tasks and huge amount of data as well as variety of variables where no pre-formula or existing formula is available for the problem. Other than that, ML models have the additional ability to learn from examples instead of being programmed with rules [ 80 ].
Among the ML methods that are used in the food industry include ordinary least square regression (OLS-R), stepwise linear regression (SL-R), principal component regression (PC-R), partial least square regression (PLS-R), support vector regression (SVM-R), boosted logistic regression (BLR), random forest regression (RF-R), and k-nearest neighbors’ regression (kNN-R) [ 81 ]. Studies showed that the usage of ML has helped in reducing the sensory evaluation cost, in decision making, and in enhancing business strategies so as to cater users’ need [ 82 ]. Long short-term memory (LSTM) which is an artificial recurrent neural network has been employed in the food industry as pH detection in the cheese fermentation process [ 83 ]. On the other hand, GA has been utilized for finding the optimum parameters in food whereas NN has been occupied to predict the final fouling rate in food processing [ 84 ]. ML has shown to be advantageous in predicting the food insecurity in the UK [ 85 ]. Apart from that, ML has also proven to have predicted the trend of sales in the food industry [ 86 ] In addition to that, ML was also able to predict the food waste generated and give an insight to the production system [ 87 ]. Major applications of ML in the food industry and its positive highlights are briefly emphasized in Table Table4 4 .
Application of machine learning in food industries
Application | ML methods | Important outcomes | References |
---|---|---|---|
Apple | Linear discriminant analysis, adaptive boosting | (i) The ML was able to classify the apples accurately with a rate of 100% using the collected acoustic emission signals | Li et al. [ ] |
Artichoke | MLP, RF, BLR | (i) The characteristics of ion patterns were done by the ML models to be set up for each enzyme with a high prediction rate of 95% above | Sabater et al. [ ] |
Beer | ANN | (i) The ANN model was able to classify the chemical components in the beer with a high overall accuracy of 95% | Claudia Gonzalez et al. [ ] |
Biscuits | Convolutional neural network | (i) The developed model was able to classify and evaluate the quality of different types of biscuits with an accuracy up to 99% | De Sousa Silva et al. [ ] |
Cheese | LSTM | (i) The combination of mechanistic modeling with LSTM was able to describe the changes in lactic acid, lactose, and biomass with high accuracy where the value of obtained is greater than 0.99 (ii) The prediction of pH for the cheese fermentation is able to be done by using the developed model | Li et al. [ ] |
(Mosambi peel) | SVM-ANN, SVM-Gaussian process regression (SVM-GPR) | (i) The SVM model was able to predict and classify all the results for the taste of lime powder that has been treated by the salt with an accuracy of 1.0 (ii) Optimization by using the ML tool allows to maintain the taste and retain the polyphenol content in the lime | Younis et al. [ ] |
Fruits ( fruits) | RF, SVM, ANN | (i) The stability of the extracts in the fruits in terms of aqueous and powder systems was able to be done by the ML methods with overall coefficient in the range of 0.9128 and 0.9912 for the best models chosen | Astray et al. [ ] |
Lamb meat | SVM | (i) The classification accuracy of the lamb meat fat increased from 89.70 to 93.89% by using the SVM method | Alaiz-Rodriguez & Parnell [ ] |
Mangoes | Naive Bayes, SVM | (i) The system is able to detect the maturity of the mangoes based on their quality attributes | Pise & Upadhye [ ] |
Meat | OLS-R, SL-R, PC-R, PLS-R, SVM-R, RF-R, and kNN-R | (i) Different kinds of microorganisms causing the beef spoilage could be detected by using the regression ML that obtained the data from five different analytical methods (ii) All the methods were able to predict in all cases accurately with the rank order of RF-R, PLS-R, kNN-R, PC-R, and SVM-R | Estelles-Lopez et al. [ ] |
Milk | SVM | (i) Presence and the level of antibiotics concentration in the cow milk was determined by using the SVM classifiers with high accuracy rate of greater than 83% and greater sensitivity compared to the typical metrics | Gutiérrez et al. [ ] |
Salmon | TreeBagger | (i) The established model was able to classify the normal and freezer burnt categories with high accuracy where the correct classification rate yielded 0.914 for validation and 0.978 in cross validation | Xu & Sun [ ] |
Wine | SVM, RF, MLP | (i) The comparison among the three algorithms were done in the evaluation of wine quality and the best result was obtained by the RF method with an average accuracy of 81.96% meanwhile others delivered a low accuracy late. This indicates the RF algorithm can be used to evaluate the quality of the wine | Shaw et al. [ ] |
Adaptive Neuro Fuzzy Inference System (ANFIS) Techniques
ANFIS is a type of AI where FL and ANN are combined in such a way that it integrates the human-like reasoning style of the FL system with the computational and learning capabilities of ANN [ 56 ]. In ANFIS, the learning procedure is transferred from the neural network into the FL system where a set of fuzzy rules with suitable membership functions from the data obtained is developed [ 88 ]. Mamat et al. [ 89 ] stated that uncertainty data could be processed and gain higher accuracy when ANFIS is applied [ 89 ]. Besides, ANFIS is also known as a fast and robust method in solving problems [ 90 ]. Not only that, Sharma et al. [ 91 ] also claimed that ANFIS has a higher performance compared to other models such as ANN and multiple regression models in their study [ 91 ]. ANFIS is a fuzzy reasoning system and combination of the parameters trained by ANN-based algorithms. The fuzzy inference system that is normally used is Takagi Sugeno Kang in the ANFIS model with the feedforward neural network consisting of the learning algorithms [ 92 ]. The structure of ANFIS is made up of five layers which are fuzzy layer, product layer, normalized layer, defuzzification layer, and total output layer [ 93 , 31 , 32 ]. The backpropagation algorithm has been normally applied in the model in order to avoid over-fitting from occurring [ 92 ]. A high correlation value ( R 2 ) indicates that the developed model has high accuracy and is suitable for industrial applications. The general structure of the ANFIS model is illustrated in Fig. 5 .

General structure of ANFIS
The first layer in ANFIS has nodes that are adjustable, and it is called as the premise parameters [ 56 ]. The second layer in ANFIS has fixed nodes, and the output is the product of all incoming signals. Every output node represents the firing strength of the rule. The third layer consists of fixed node labeled as N. The outputs of the third layer are called normalized firing strengths. Every node in the fourth layer is an adaptive node with a node function, and the parameters in this layer are called as the subsequent parameters [ 56 ]. The final layer in the ANFIS layer has a fixed single node which calculates the overall output as the summation of all the incoming signals. The calculation involved in each layer is shown below. The output of the ith model in layer 1 is denoted as 0 1 , i.
Layer 1: O 1 , i = μ Ai x , f o r i = 1 , 2 atau O 1 , i = μ B i - 2 y , f o r i = 3 , 4 .
Layer 2: O 2 , i = w i = μ Ai x μ Bi y , f o r i = 1 , 2 .
Layer 3: O 3 , i = w ¯ = w i w 1 + w 2 , i = 1 , 2 .
Layer 4: O 4 , i = w ¯ f i = w ¯ i ( p i x + q i y + r i ); w i is the normalized firing strength from layer 3 and.
{ p i , q i , r i } is the parameter set of this node.
Layer 5: O 5 , 1 = ∑ i w ¯ i f i = ∑ i w i f i ∑ i w i .
The ANFIS model is attractive enough that it could solve problems related to the food industry, which are complicated, practical, and barely solved by other methods and has been widely used in the food industry for prediction and classification purposes. ANFIS has been applied in various food processing involving recent technology which comprised five main categories which are food property prediction, drying of food, thermal process modeling, microbial growth, and quality control of food as well as food rheology [ 56 ]. The utilization of ANFIS in the food industry has been commenced years ago, and Table Table5 5 describes those applications.
Application of ANFIS in the food industry
Applications | Outcomes | References |
---|---|---|
Fish oil | (i) A model was developed to estimate the oxidation parameters using three different algorithms which are, ANFIS, multilinear perceptron, and multiple linear regression, and it was found that ANFIS model had the best accuracy in predicting the parameters | Asnaashari et al. [ ] |
Ice cream | (i) The sensory attributes of ice cream were investigated by using the ANFIS model to predict the acceptability of taste with respect to the input parameters and the model achieved a minimum error of 5.11% and high correlation value of 0.93 | Bahram-Parvar et al. [ ] |
Indian sweets ( ) | (i) The prediction of the heat transfer coefficient during the frying of using the ANFIS model yielded a high value of 0.9984, and this prediction is important for designing the process equipment as well as saving energy in commercial production | Neethu et al. [ ] |
Orange | (i) The developed ANFIS model was able to predict the orange taste and has higher performance when compared to multiple regression model | Mokarram et al. [ ] |
Quince fruits | (i) The ANFIS model was able to predict the moisture ratio, energy utilization, energy utilization ratio, exergy loss, and exergy efficiency of quince fruit during the drying process with high accuracy of with value of 0.9997, 0.9989, 0.9988,0.9986, and 0.9978, respectively (ii) The ANFIS model was compared with the ANN model, and the results obtained showed that the ANFIS model has higher accuracy compared to the ANN model where the value of was higher with a lower error value in the ANFIS model | Abbaspour-Gilandeh et al. [ ] |
Rapeseed oil | (i) The developed ANFIS could predict the different outputs of rapeseed oil process by oil extraction and cooking at industrial scale, and the model achieved a high correlation coefficient which is around 0.99 | Farzaneh et al. [ , ] |
(i) Prediction of the inactivation of by ultrasound was able to be done by the developed ANFIS model with a good accuracy where the correlation coefficient obtained was 0.974 (ii) This study was known to be important in the food industry as the bacteria can cause food poisoning if proper detection is not being done | Soleimanzadeh et al. [ ] | |
Taro | (i) The optimization of extraction conditions of antioxidants from the taro flour can be done by using the developed ANFIS model coupled with response surface methodology (ii) The prediction values obtained from the developed model were validated by comparing with the experimental values, and the results were almost consistent with prediction values from the developed model | Kumar & Sharma [ ] |
Vegetables (cantaloupe, garlic, potatoes) | (i) The developed model by using the ANFIS system was able to predict the effective moisture diffusivity, specific energy consumption, moisture ratio, and drying rate of the vegetables with a high regression coefficient of 0.9990, 0.9917, 0.9974, and 0.9901, respectively, with minimum error value (ii) Comparison between the ANFIS model and ANN model was carried out, and the results showed that the ANFIS model possess a higher efficiency than that of ANN model | Kaveh et al. [ ] |
Virgin olive oil | (i) The ANFIS model was able to predict the quality of virgin olive oil samples with high accuracy where the coefficient determination obtained was greater than 0.998 (ii) It was also able to visualize the effects of temperature, time, total polyphenol, fatty acid profile, and tocopherol on the oxidative stability of virgin olive oil | Arabameri et al. [ ] |
Yam | (i) The prediction of the yam moisture ratio during the drying process showed a high value with 0.98226 by using the developed ANFIS model | Ojediran et al. [ ] |
Integrating AI with External Sensors for Real-time Detection in Food Industry
FL or ANN is often integrated with several sensors for real-time detection such as electronic nose (E-nose), electronic tongue (E-tongue), machine learning (ML), computer vision system (CVS), and near infrared spectroscopy (NIRS) for real-time detection and to obtain higher accuracy results in a shorter time. These detectors have also combined their elements together for enhancing their accuracy and targeted results. The integration of these sensors with the artificial intelligence methods has been shown quite a number in food industries over the past few years.
Electronic nose also known as E-nose is an instrument created to sense odors or flavors in analogy to the human nose. It consists of an array of electronic chemical sensors where it is able to recognize both simple and complex odors [ 94 ]. E-nose has been used in gas sensing where the analysis of each component or mixture of gases/vapors is required. Besides, it plays an important role in the food industry for controlling the quality of the products. Due to its ability to detect complex odors, it has been employed as an environment protection tool and detection of explosives materials [ 95 ]. An array of non-specific gas sensors is known to be the main hardware component of E-nose where the sensors will interact with a variety of chemicals with differing strengths. It then stimulates the sensors in the array where characteristic response is extracted known as a fingerprint [ 94 ]. The main software component of E-nose is its feature extraction and pattern recognition algorithms where the response is processed, important details are elicited and then chosen. Thus, the software component of the E-nose is greatly important to stimulate its performance. In general, E-nose is divided into three main parts, namely, sample delivery system, a detection system, and a computing system. ANN, FL, and pattern recognitions are the examples of the methodology employed in E-nose [ 96 ]. The general system of E-nose is shown in Fig. 6 .

E-nose system
E-nose has been widely used to aid in both quality control and assurance in the food industries. Wines, grains, cooking oils, eggs, dairy products, meat and dairy products, meat, fish products, fresh-cut and processed vegetables, tea, coffee, and juices have successfully applied e-nose for sampling classification, detection, and quality control. E-nose has successfully classified samples with different molecular compounds [ 97 ]. Besides, Sanaeifar et al. have reviewed and confirmed that e-nose was able to detect defects and contamination in foodstuffs [ 98 ]. Classification and differentiation of different fruits have also determined by using e-nose [ 99 ]. A review has been conducted on the application of the E-nose for monitoring the authenticity of food [ 100 ]. Adding to this, Mohamed et al. have carried out a comprehensive review on the classification of food freshness by using e-nose integrated with the FL and ANN method [ 101 ]. Recent application of e-nose with computing methods involving AI in food industries is shown in Table Table6 6 .
Application of E-nose with AI in food industries
Application | Objectives | AI technique | Outcomes/impacts | References |
---|---|---|---|---|
Beef | To classify the beef samples | Adaptive FL system (AFLS); ANFIS | (i) The accuracy of the AFLS model was very good which was 94.28% for overall correct classification which shows that it is able to tackle complex, non-linear problems like meat spoilage (ii) By using the ANFIS method, the results were satisfactory but were obtained with high computational cost (iii) E-nose with appropriate machine learning tools proved that it is useful to monitor the spoilage of the meat during aerobic stage at various temperatures | Kodogiannis & Alshejari [ ] |
Cocoa | To figure out and classify the fermentation time of the cocoa beans | DT, boosted tree RF, ANN, KNN, naïve Baiyes (NB) | (i) The ANN and Boosted tree algorithm manage to obtain an acceptable classification rate while the fermentation time was not able to be determined by the KNN and NB algorithm | Tan et al. [ ] |
Coffee beans | To forecast the level of acidity in fresh roasted beans | ANN | (i) The model was able to predict the acidity level values with an accuracy around 95% based on human sourness level scores | Thazin et al. [ ] |
Chicken meat | To classify the fresh and freeze-thawed chicken meat | Fuzzy K-nearest neighbors algorithm (FK-NN) | (i) The FK-NN algorithm showed a high performance, and it can be used in e-nose to identify and classify the fresh and frozen-thawed chicken meat | Mirzaee-Ghaleh et al. [ ] |
Cow ghee | To detect the adulteration of the margarine in cow ghee | ANN | (i) The ANN model was able to analyze the data obtained from the e-nose with high accuracy | Ayari et al. [ ] |
Edible oil | To detect the adulteration in oxidized and non-oxidized edible oil | ANN | (i) The developed ANN model with e-nose was able to detect the adulteration in the edible oil with high accuracy (ii) The classification of the system was compared with other methods and it was given that ANN had the highest classification rate with 97.3% | Karami et al. [ ] |
Fish | To identify and classify the fish spoilage | ANN, PCA | (i) The developed model using the PCA and ANN was able to classify the fish according to their spoilage group with an accuracy of 96.87% | Vajdi et al. [ ] |
Fruits | To apply Kernel extreme learning machines in E-nose for recognition and perception of fruit odors | Kernel extreme machine learning | (i) The proposed system performed significantly good in odor recognition (ii) It achieved higher testing accuracy and smallest value of training time and testing time compared to other systems that were used for the comparison for recognizing the fruit odors | Uçar & Özalp [ ] |
Fruits | To apply artificial bee colony (ABC) algorithm in ANN to classify data from electronic nose and evaluate its performance | ANN & artificial bee colony | (i) ABC-ANN is more successful in odor classification of e-nose data and has higher performance compared to the backpropagation algorithm that was used (ii) The system was able to classify four different aroma which are the aroma of the lemon, cherry, strawberry, and melon | Adak & Yumusak [ ] |
Fruit juice | To figure out the amount of the food additives in the fruit juice | RF, SVM, ELM, PLSR | (i) The additives which are benzoic acid and chitosan in the juice could be predicted with accurately by using the ELM and RF methods with value of 0.92 and 0.91, respectively (ii) ELM and RF method has a higher accuracy in predicting the additives when compared to ELM and PLSR where the value obtained by ELM and PLSR are 0.72 and 0.51, respectively | Qiu & Wang [ ] |
Honey | To determine the best classifier in forecasting the physicochemical properties of Iranian ziziphus honey samples | ANN, SVM | (i) Both the ANN and SVM model combined with the e-nose showed a good prediction rate in determining the physicochemical properties of the honey sample (ii) The developed model using ANN method shower a higher accuracy in the prediction compared to that of with SVM method | Faal et al. [ ] |
Lemon | To characterize and predict the quality of various lemon slices | ELM, RF, SVM | (i) Prediction using the ELM method obtained the highest accuracy of 0.959 followed by RF and SVM with 0.935 and 0.922 respectively which indicates that ELM integrated with the E-nose is the best classification model | Guo et al. [ ] |
Pear | To enhance the food quality by optimize the drying process of balsam pear | FL | (i) The drying time was able to be shortened to retain the aroma and enhance the product quality (ii) Designation of industrialized control method was established to simplify the control and has good drying effects | Li et al. [ ] |
Pork meat | To differentiate between the fresh and frozen-thawed meat | BPANN | (i) Combined ANN with E-nose was able to distinguish three types of meat which are loin, neck and ham (ii) The model was able to differentiate the fresh meat from spoiled meat and frozen meat with overall sensitivity of 85.1% and 97.5% specificity | Górska-Horczyczak et al. [ ] |
Rice grains | To detect the infestation in stored rice grains | FL ARTMAP PCA, MLR | (i) E-nose with the application of FL ARTMAP is useful when the data are exhaustive and deductions about analysis are needed to be done (ii) The model was able to classify the grains of infested, non-infested, required treatment and others (iii) The hybrid system is beneficial in the food and grain industry where early detection of infestation in grain can be done to minimize the post-harvest losses | Srivastava et al. [ ] |
Rice grains | To classify the infested rice grains and recognized them | ANN | (i) BPANN with e-nose system gave the highest value which is 0.98 compared to other methods that were used with the e-nose for the classification process and it has the highest accuracy compared to the rest (ii) The system was able to predict the infested rice grains for different days | Srivastava et al. [ ] |
Shelled peanuts | To assess the storage quality of shelled peanuts | FL | (i) The FL analysis able to screen and rank the e-nose sensors and the discard time for the shelled peanuts was able to be determined (ii) The storage time prediction of shelled peanuts using sensors closely matched with the conventional methods (iii) It can be an eco-friendly alternative as it is rapid and not destructive | Raigar et al. [ ] |
Salmon | To classify the level of freshness of the salmon samples | PCA, CNN, SVM | (i) The developed system was able to detect, cluster, and classify the salmon according to their freshness level, and the overall accuracy obtained by the CNN-SVM model was greater than 90% | Feng et al. [ ] |
Saffron | To detect the adulteration of saffron samples | ANN | (i) The classification of original and adulterated saffron was successfully done by using e-nose with the pattern recognition method with 100 and 86.87% accuracy, respectively | Heidarbeigi et al. [ ] |
Saffron | To identify different aromas of Iranian saffron | ES, PLS, ANN | (i) E-nose coupled with ANN successfully obtained 100% for the classification of saffron samples | Kiani et al. [ ] |
Spinach | To detect the postharvest freshness of spinach | ANN | (i) Combination of E-nose with the BPNN method proves to be effective for a fast and non-destructive way to detect the spinach freshness with an accuracy of 93.75% for the classification accuracy | Huang et al. [ , ] |
Strawberry juice | To classify and carry out analysis by using E-nose with neural networks and other learning methods as well to evaluate the performance of the system | ANN, ML | (i) E-nose could differentiate each treatment type and be able to predict the quality parameters (ii) ELM network was able to classify the strawberry juice and is much faster compared to other modelling networks that were used (iii) ELM also showed a better performance compared to other modelling techniques that were being used | Qiu et al. [ ] |
Sunflower oil (SO) | To determine the frying disposal point of SO | FL | (i) E-nose combined with FL was able to assess the frying disposal time of fried SO blend (ii) The combination of e-nose with FL has potential for other fried product platforms | Upadhyay et al. [ ] |
Wheat grain | To determine the granary weevil infestation in stored wheat grains | FL | (i) The most responsive sensors and specific VOCs generated by insect-infected wheat grains were able to be screened out by the e-noses sensor associated with the fuzzy logic analysis (ii) E-nose was proven to be a potential method for accurate and rapid in monitoring the infestation in stored wheat grain. It is also a reliable method for industries to determine the quality of the product throughout the storage period | Mishra et al. [ ] |
Electronic tongue (E-tongue) is an instrument that is able to determine and analyze taste. Several low-selective sensors are available in E-tongue which is also known as “a multisensory system,” and advanced mathematical technique is being used to process the signal based on pattern recognition (PARC) and multivariate data analysis [ 102 ]. For example, different types of chemical substances in the liquid phase samples can be segregated using E-tongue. About seven sensors of electronic instruments are equipped in E-tongue, which enabled it to identify the organic and inorganic compounds. A unique fingerprint is formed from the combination of all sensors that has a spectrum of reactions that differ from one another. The statistical software of E-tongue enables the recognition and the perception of the taste. E-tongue comprises three elements specifically the sample-dispensing chamber or automatic sample dispenser, an array of sensors of different selectivity, and image recognition system for data processing (Ekezie, 2015). Samples in liquid forms could be analyzed directly without any preparation while the samples in solid forms have to undergo preliminary dissolution before measurement is carried out. The process of E-tongue system is shown in Fig. 7 below. The ability to sense any taste like a human olfactory system makes it one of the important devices in the food industry, especially for quality control and assurance of food and beverages [ 103 ]. In addition, E-tongue has been used to identify the aging of flavor in beverages [ 104 ], identify the umami taste in the mushrooms [ 105 ], and assess the bitterness of drinks or dissolved compounds [ 102 ]. Jiang et al. performed a summarized review on the application of e-nose in the sensory and safety index detection of foods [ 106 ]. Moreover, the demand of E-tongue in the food industry market has risen due to the awareness on delivering safe and higher-quality products. The details of recent applications of E-tongue in the food industry are shown in Table Table7 7 .

E-tongue system
Application of E-tongue with AI in food industries
Application | Objectives | AI technique | Outcomes/impacts | References |
---|---|---|---|---|
Ham | To monitor the salt processing of hams salted differently with different formulations | Simplified fuzzy ARTMAP neural network | (i) The data obtained from the e-tongue was able to be analyzed and classified using ANN (ii) The data was classified using two processing variables which are the processing time and salt formulation (iii) Optimum parameters value for SFAM neural networks were drawn out to be used in the microcontroller device | Gil-Sánchez et al. [ ] |
Honey | To differentiate different types of honey according to their antioxidant level | Fuzzy ARTMAP neural network (FAM) | (i) The proposed E-tongue system was able to differentiate different types of honey as well as their total antioxidant capacity level (ii) The ANN fuzzy art map type analysis had a high classification success rate of 100% which indicates that it is a good measurement system | Marisol et al. [ ] |
Liquor | To classify different types of Chinese liquor flavor using e-tongue with fuzzy evaluation and prediction by SVM | SVM & fuzzy evaluation | (i) E-nose with the SVM system was able to classify four different flavors of liquor with an accuracy of 100% (ii) The developed system is able to discriminate the samples accurately and the output evaluation language in line with the human perception | Jingjing et al. [ ] |
Milk | To detect the adulteration of raw milk | SVM | (i) The developed model was able to determine the adulteration in the samples with a high accuracy values which are all greater than 87% for different types of adulterants in the milk | Tohidi et al. [ ] |
Peanut meal | To assess the taste attributes of peanut and compare the predictive abilities of the methods used | ANN, partial least square (PLS) | (i) Good stability and repeatability with respect to the measured signals were exhibited by the sensors in the E-tongue (ii) Different concentrations with the same taste (five types of taste) were able to be discriminated by the E-tongue (iii) RBFNN has a better prediction ability with lower error and higher correlation coefficients than those of the PLS method | Wang et al. [ , ] |
Pineapple | To classify the pineapples according to their sweetness level and determine the best algorithm | SVM, KNN, ANN, RF | (i) Different machine learning algorithms were employed in determining the sweetness of the pineapple, and the best algorithm obtained was the KNN method where it achieved an accuracy of 0.820 (ii) The developed model will be beneficial in industry when the selection of pineapples in large quantities is required | Hasan et al. [ ] |
Rice | To discriminate and predict the solid foods as well as to provide an assessment tool for food industries | ANN | (i) RBFNN was able to distinguish different types of rice with 95% accuracy in classification (ii) Voltametric E-tongue is useful for the qualitative analysis for rice | Wang et al. [ , ] |
Rice | To develop similarity analysis combined with artificial neural networks (SA-ANN) in e-tongue for the prediction of rice sensory quality | ANN | (i) SA-ANN in E-tongue was able to predict the rice sensory quality and carry out systematic analysis (ii) Comparison was carried out between PCA-ANN and SA-ANN, and it was found that SA-ANN has better precision and accuracy compared to PCA-ANN (iii) SA-ANN is a less-labor intensive, quicker method and has potential for rapid and big scale prediction of rice sensory property | Lu et al. [ ] |
Sugarcane | To characterize and apply voltametric e-tongue for the analysis of glucose from the sugarcane | ANN | (i) Multilayer ANN with wavelet information was able to process complex responses from the E-tongue (ii) The proposed model is suitable to be used for hydrolyzed samples from sugarcane bases | De Sá et al. [ ] |
Tangerine peel | To classify tangerine peel of different ages | BPNN, ELM | (i) The model was able to classify the tangerine peel samples of different ages (ii) Comparison was done for few linear models and non-linear models, and it was obtained that non-linear models exhibited better performance than linear models (iii) ELM was the best for the classification of the samples with high accuracy followed by BPNN | Shi et al. [ , ] |
Teas | To distinguish different types of teas | ANN, SVM | (i) Different types of teas were able to be distinguished by using the developed system and the compositions of the tea also could be identified | Huang et al. [ , ] |
Tilapia fillets | To predict the changes in freshness of tilapia fillets at different temperatures using the combined techniques | ANN-PCA | (i) E-tongue is able to distinguish the extracts of tilapia fillets stored at different days and different temperatures (ii) The model set up is able to predict the freshness of tilapia fillets stored at different temperatures ranging from 0 to 10 °C | Shi et al. [ , ] |
The computer vision system (CVS) is a branch of AI that combines the image processing and pattern recognition techniques. It is a non-destructive method that allows the examination and extraction of image’s features to facilitate and design the classification pattern [ 107 ]. It is also recognized as a useful tool in extracting the external feature measurement such as the size, shape, color, and defects. In general, it comprised a digital camera, a lighting system, and a software to process the images and carry out the analysis [ 108 ]. The system can be divided into two types which are 2D and 3D versions. Its usage is not restricted to various applications in food industries such as evaluating the stages of ripeness in apples [ 107 ], predicting the color attributes of the pork loin [ 109 ], detecting the roasting degree of the coffee [ 110 ], evaluating the quality of table grapes [ 111 ], and detecting the defects in the pork [ 112 ]. The combination of CVS with soft computing techniques has been said as a valuable and important tool in the food industry. This is because the combination of these systems offers good advantages such as an accurate prediction in a fast manner can be achieved. Table Table8 8 shows the combination of CVS and soft computing that has been used in the food industry. Figure 8 shows the working principle of CVS. An example on the utilization of CVS for the quality control is shown in Fig. 9 [ 113 ].
Application of CVS with AI in food industries
Application | Objectives | AI technique | Important outcomes | References |
---|---|---|---|---|
Apple | To sort the defective and normal apples | CNN, SVM | (i) The developed CNN with the CVS model was able to classify the apples with a high accuracy rate of 96.5%, and it was proven to be more effective than the conventional image processing method which was combined with the SVM classifier where the accuracy rate was 87.1% | Fan et al. [ ] |
Apple slices | To study the drying effects on the changing color of the apple slices | ANN | (i) CVS was able to track the color changes during the drying process, and the combination with the ANN was able to estimate the quality of the apple during the drying process ii) The developed model achieved values of greater than 0.92 for all the analyses | Nadian et al. [ ] |
Banana | To classify the banana according to its ripeness | ANN, SVM, KNN, DT | (i) The ANN-based model system has a higher classification rate compared to the other algorithms with the highest overall recognition rate of 97.75% | Mazen & Nashat [ ] |
Barley flour | To predict the barley flour based on the improvised method | SVM, KNN, DT, RF | (i) The developed model of CVS with different learning algorithms was improvised by using the spatial pyramid partition ensemble method for the classification of the barley flour where the accuracy achieved was 75% (KNN), 95% (SVM & RF), and 100% (DT) | Lopes et al. [ ] |
Beer | To forecast the beer acceptability based on different sensory parameters | ANN, ML | (i) Seventeen ML algorithms were used to find the best model with a good performance was carried out, and the results showed that Bayesian regularization had the best accuracy where the value obtained was 0.92 (ii) The combination of RoboBEER, CVS, and ANN algorithms allowed to determine the beer making based on its acceptability of customers and its quality | Gonzalez Viejo et al. [ ] |
Bell pepper | To describe the ripeness level of bell pepper automatically | ANN, FL | (i) An artificial vision system was able to be developed by using the CVS and ANN/FL in predicting the maturity of the bell pepper (ii) The model using RBF-ANN has a higher classification accuracy compared to FL where the maximum accuracy obtained by both models are 100% and 88%, respectively | Villaseñor-Aguilar et al. [ ] |
Cape gooseberry | To classify the ripeness of cape gooseberry | ANN, SVM, DT, KNN | (i) All the models were able to classify the ripeness of the cape gooseberry with a high accuracy where the accuracy obtained by all the models was greater than 86% using different color spaces, which indicates that it is a good classifier system | Castro et al. [ ] |
Coffee beans | To develop a system that can classify the coffee beans | ANN | (i) Green coffee beans were able to be analyzed and classified by using the developed system (ii) ANN were used in this system as a color space transformation model where the transformed value was later used for the classification purposes | De Oliveira et al. [ ] |
Dry beans | To classify different types of seed from the production | ANN, KNN, DT, SVM | (i) The classification of the beans was able to be done by all the ML algorithms with SVM which achieved the highest overall classification rates of 93.13% followed by the DT, ANN, and KNN where the classification rates were 92.52%, 91.73%, and 87.92%, respectively | Koklu & Ozkan [ ] |
Eggs | To predict the volume of eggs | ANN | (i) The volume of the eggs was predicted by a developed system with a good linear coefficient of 0.9738 with the actual volume and relative absolute error of 2.2078% which indicates that the developed system is an efficient model | Siswantoro et al. [ ] |
Figs | To classify fig fruits based on its visual features | Decision tree-FL | (i) Comparison was carried out between three different types of decision tree for the data obtained from CVS, and it was shown the REP decision tree had the highest value of and lower RMSE values and hence was selected to be implemented in the fuzzy system (ii) The developed system was able to classify the fig fruits into five qualitative grades with a high accuracy | Khodaei & Behroozi-khazaei [ ] |
Fish | To identify the freshness of the fish | ANN, PCA | (i) The developed system was able to classify the freshness of fish with a success rate of 94.17% in the training set and 90.00% in the prediction set | Huang et al. [ ] |
Gluten-free cake | To develop a system for quality control of celiac-friendly products | FL | (i) The developed system was able to study the texture of the cake when different amounts of materials were added to it, and the optimal ingredients value suitable for the gluten-free cake were able to be determined | Rezagholi & Hesarinejad [ ] |
Lime | To predict the weight of Indian lime fruits | ANFIS | (i) Different clustering methods were fused with the ANFIS model to improve the accuracy in the classification system, and it was found that the fuzzy C-means clustering (FCM) was the best in predicting the weight of the sweet lime (ii) The developed system was able to predict the weight of the Indian sweet lime fruits accurately | Phate et al. [ ] |
Mango | To estimate the fruit mass of the mango | ANN | (i) The data obtained from the CVS was used as the input parameters in the developed ANN model, and the model was able to estimate the fruit mass successfully (ii) The developed system has the highest success rate of 97% and the efficiency coefficient of 0.99 by applying two input parameters or three input parameters | Utai et al. [ ] |
Mushrooms | To determine the appearance quality of mushrooms | ANN, FL | (i) The accuracy obtained by the image processing system was 95.6% (ii) The artificial neural network was able to determine the weight of the mushrooms, and the fuzzy logic used the data from the CVS and was able to determine the quality of the mushroom | Nadim et al. [ ] |
Passion fruits | To classify the passion fruits based on their ripeness level | Multi-class SVM (MCSVM) | The developed system was able to classify the ripeness level passion fruits with an accuracy of 93.3% within 0.94128 s | Sidehabi et al. [ ] |
Pork loin | To assess the quality of the pork loin according to the industry demand | SVM | (i) The model was able to predict the quality of the pork loin based on their color and quality attributes based on the industries’ demand | Sun et al. [ ] |
Potatoes | To develop a grading system for potatoes | FL | (i) Combination of CVS and fuzzy logic allows faster grading of the potatoes as well as reduces the cost required for the manual grading | Bhagat & Markande [ ] |
Rice | To carry out the qualitative grading of milled rice | FL | (i) The study was able to conclude that the developed hybrid system can be used in the processing industry for automatic grading of milled rice (ii) Comparison was done between the developed system and experts’ judgment, and around 89.80% overall confidence was obtained (iii) The fuzzy system has obtained 89.83% total sensitivity and 97.45% specificity for the quality grading of milled rice | Zareiforoush et al. [ ] |
Rice | To control the performance of rice whitening machines | FL | (i) The developed automatic control system had an average of 31.3% higher performance speed than that of a normal human operator, and there was an improvement in the quality of the output based on the decision made by the system (ii) The setup flexibility of the system allows alteration to be done according to the preference of each rice mill operator | Zareiforoush et al. [ ] |
Tea | To classify the Iranian green and black tea | DT-FL | (i) REP decision tree was shown to be more convenient compared to the J48 tree for developing a fuzzy classifier system (ii) The research successfully showed that DT-based fuzzy systems can be applied for automated intelligent classification of Iranian green tea and black tea | Bakhshipour et al. [ ] |
Tomato | To detect the maturity of the fresh tomato | ANN | (i) The maturity of the tomato was able to be detected by using the developed system with an accuracy of 99.31% and 1.2% of standard deviation | Wan et al. [ ] |
Vegetable seeds | To classify the vegetable seeds | FL | (i) The system can classify the two different types of seeds that look similar which are cauliflower seed and Chinese cabbage seed | Garcia et al. [ ] |

Working Principle of CVS

CVS-based quality control process
Near infrared spectroscopy (NIRS) is another technique in the food industry as there is no usage of chemicals and results can be obtained accurately as well as precisely within minutes or even continuously [ 114 ]. In addition, it is known to be non-destructive, cost effective, quick, and straightforward which makes it a good alternative for the traditional techniques which are expensive and labor intensive and consumes a lot of time [ 115 ]. The chemical-free method by NIRS makes it suitable to be used as a sustainable alternative since it will not endanger the environment or the human health. It has a wide range of quantitative and qualitative analysis of gases, materials, slurries, powders, and solid materials. Furthermore, samples are not required to be grounded when light passes through it and certain features or characteristics that are unique to the class of the sample are revealed by the spectra of the light. Complex physical and chemical information on the vibrational of molecular bonds such as C–H, N–H, and O–H groups and N–O, C–N, C–O, and C–C groups in organic materials can be provided by the spectra which can be recorded in reflection, interactance, or in transmission modes [ 114 ].
The basic working principle for NIRS is shown in Fig. 10 . Recently, NIRS has become an interest in food industries to inspect food quality, controlling the objective of the study and evaluating the safety of the food [ 114 , 116 – 119 ]. Several researchers have applied the NIRS in food to obtain its properties for multiple reasons including determining the fatty acid profile of the milk as well as fat groups in goat milk [ 120 ]. Apart from that, it is able to aid in the prediction of salted meat composition at different temperatures [ 121 ] and in the prediction of sodium contents in processed meat products [ 122 ]. The detection and grading of the wooden breast syndrome in chicken fillet in the process line was also able to be performed by using the NIRS technique [ 123 ]. Not only that, it is proven to be efficient in determining the maturity of the avocado based on their oil content [ 124 ], predicting the acrylamide content in French-fried potato and in the potato flour model system [ 125 ], and determining the composition of fatty acid in lamb [ 126 ]. There has been a review conducted on the application of the ANN combined with the near-infrared spectroscopy for the detection and authenticity of the food [ 127 ]. The ability of the NIRS system in detecting the physical and chemical properties coupled with soft computing techniques such as ANN, FL, and ML allows the classification and prediction of the samples to be performed rapidly and accurately. Table Table9 9 shows the application of NIRS coupled with AI techniques in the food industry.

Basic working principle of CVS
Application of NIRS with AI in food industries
Application | Objectives | AI technique | Important outcomes | References |
---|---|---|---|---|
Cheese | To determine the characteristics of controlled-processing cheese | PCA-ANN | (i) Sensory attributes of cheese have been determined with sufficient evidence on well-defined population which was known to be cost effective and useful method for quality control | Curto et al. [ ] |
Chicken meat | To classify chicken meat with respect to their quality grades | Decision tree (DTree) | (i) The assessment of the poultry meat was able to be done by using the spectral analysis and the classification using the DTree models (REPTree) had a better performance compared to the support vector machine and decision table model that were carried out for comparison purposes | Barbon et al. [ ] |
Chocolate | To develop a model for the quality assessment of chocolate | ANN | (i) NIRS was used to obtain the fingerprint of the chocolate using the five taste senses and fused with ANN for the prediction of chocolate quality based on chemical, physical, and sensory properties and accurate prediction was achieved by the developed model | Gunaratne et al. [ ] |
Civet coffee | To detect any alteration in the civet coffee | ANN, SVM, KNN | (i) The combination of trained ANN and NIRS method was able to discriminate the civet coffee from non-civet coffee successfully with an accuracy in the range of 95–100% (ii) The performance of classification using ANN was compared with other learning algorithms such as decision trees, discriminant analysis, SVM, KNN, and ensemble classifiers, and it was proven that ANN has better accuracy compared to the others | Arboleda [ ] |
Gelatin | To detect the adulteration in edible gelatin | ANN, SVM | (i) NIRS fused with both methods which were done separately was able to detect the adulteration in edible gelatin (ii) SVM model showed as the best recognition methods among the other models that were used with an accuracy of 100% meanwhile the recognition rate obtained by the ANN model was 97.44% | Zhang et al. [ ] |
Eggs | To determine the freshness of the egg | RBFNN, PCA | (i) The system was able to classify the egg freshness, and the model is appropriate to be implemented for the rough screening of the eggs | Aboonajmi et al. [ ] |
Food powders | To classify different types of food powders | SVM | (i) The system was able to classify the food powders varying of whole wheat flour, organic wheat flour, tapioca starch, corn starch, and rice flour with a very high accuracy rate of 100% | Mohamed et al. [ ] |
Keemun black tea | To develop a model for the discrimination of different grades of keemun black tea in China | ANN, least square SVM (LSSVM), Random Forest | (i) Three different methods which are ANN and LSSVM were used to develop the model and all the methods were able to distinguish the different grades of keemun tea (ii) It was identified that the LSSVM method has a higher performance and predictivity compared to the rest | Ren et al. [ ] |
Meat | To create a system for the detection of meat spoilage | AFLS | (i) The AFLS model was able to classify the meat into three classes which are fresh, semi-fresh, and spoiled by using the data provided by the FTIR spectrometer (ii) The model achieved a high percentage with a value of 95.94% of correct classification overall which indicates that it can be an effective tool for the detection of meat spoilage | Alshejari & Kodogiannis [ ] |
Olive oil | To detect the adulteration in olive oils | SVM | (i) The fusion of NIRS and Raman spectral data could identify the adulterated olive oils effectively and the SVM model was able to predict the dopant contents in olive oil accurately | Xu et al. [ ] |
Pears | To determine the soluble solid contents in pear | ELM | (i) The proposed successive projection algorithm and extreme learning machine (SPA-ELM) was able to predict the contents better than the conventional PCA-ELM method | Lu et al. [ ] |
Rice | To classify the rice according to the compositions and processing parameters | RF, PCA, LDA, PLS | (i) All the ML techniques were able to identify and classify the rice based on its composition (amylose-based, glycemic index) and the hydrothermal treatment severity with a good performance | Rizwana & Hazarika [ ] |
Wheat flour | To predict the wheat flour quality using the NIRS | Multitarget coupled with SVM, RF | (i) The use of multitarget over partial least squares coupled with machine learning algorithm offers more advantage for the parameter prediction from NIRS (ii) The prediction using random forest overcomes the performance of SVM | Barbon Junior et al. [ ] |
White asparagus | To predict the origin of the asparagus and distinguish the German from imported products | SVM | (i) The linear SVM could predict the country of origin of white asparagus with an accuracy of 89% and also was able to distinguish the German and non-German products | Richter et al. [ ] |
Summary on the Application of AI in the Food Industry
From the review so far, it can be shown that AI has been used for various reasons in food industries such as for detection, safety, prediction, control tool, quality analysis, and classification purposes. Ranking of sensory attributes in the foods can be done easily by using the FL model. Not only that, fuzzy logic can be used for classification, control, and non-linear food modeling in the food industry. ES is widely used in the food industry for decision-making process. On the other hand, ANN model is applied widely in the food industry for prediction, classification, and control task as well as for food processing and technology. The supervised ANN method has the ability to learn from examples which allows for the prediction process to be done accurately. Meanwhile, the unsupervised method of ANN is found to be more common for the classification task. Another method that has been utilized for the prediction and classification of the food samples is by using the machine learning (ML) method. ML can be used in solving complicated tasks which involves a huge amount of data and variables but does not have pre-existing equations or formula. This method is known to be useful when the rules are too complex and constantly changing or when the data keep changing and require adaptation. Furthermore, the adaptive neuro fuzzy inference system (ANFIS) is another hybrid AI method that can be used to solve sophisticated and practical problems in the food industry. However, decent data are required for the model to learn in order to perform well. In addition to that, this model is useful for solving analytical mathematical models in the food industry such as studies involving mass and heat transfer coefficients. ANFIS is recommended to be used when complex systems where time-varying processes or complex functional relationships and multivariable are involved. Apart from that, it can be used in descriptive sensory evaluation.
These AI algorithms can be combined with other sensors such as the electronic nose, electronic tongue, computer vision system, and near infrared spectroscopy to glean the data from the samples. Both the E-nose and E-tongue have shown to enhance the quality characteristics in comparison to the traditional detection approach [ 128 ]. E-nose can be used to sense the odors or gases while the E-tongue can be applied for the identification of the organic and inorganic compounds. Studies involving the examination and drawing out the features of the samples like shape, color, defects, and size can be carried out by using the CVS sensors. NIRS can be utilized to determine the properties or contents in the samples. The data obtained from these sensors is then merged with the AI algorithms and utilizing their computing strengths to accomplish the desired studies.
Advantages and Disadvantages of AI
AI has been used widely in the industry as it offers a lot of advantages compared to the traditional method. All the algorithms are known to be accurate and reliable, but careful selection should be made by considering the advantages and limitations of the algorithms. The different algorithms have their own strengths and weakness, hence choosing them for a particular application in the food industry needs to be looked on a case-to-case basis. The guideline to choose the most appropriate method is given in the next section. The benefits and constraints that each of the algorithm exhibits are explained briefly in Table Table10 10 .
Advantages and limitations of AI algorithms
AI algorithm | Advantages | Limitations |
---|---|---|
ES | (i) Reliable and understandable (ii) Highly responsive (iii) High performance (iv) Error rate is lower than human errors (v) Better use of production capacities | (i) The construction and designing of the ES are expensive and rare as it requires expert engineers (ii) Vocabulary utilized by the experts is limited and often is difficult to be understood |
FL | (i) Imprecise, incomplete, and uncertain information can be solved (ii) Simpler and direct results can be obtained (iii) Accountable, noise tolerant, and robust to disturbances (iv) Faster interpretation than ANN and support vector machine method (v) The knowledge base can be extended easily with the extension of the rules (vi) Saves costs and time (vii) Can improve the quality and safety of the products | (i) Generalization is not possible as it can only deduce the given rules (ii) Sometimes requires the knowledge of an expert in creating the rules |
ANN | (i) Able to model complex functions or problems accurately and easily (ii) Accurate, robust to disturbances, and noise tolerant (iii) Has the ability to learn from the patterns or examples (iv) Has the generalization ability (v) Affordable, noise tolerant, easy, and flexible method (vi) Solving non-linear problems are more appropriate by using this method (vii) Useful as prediction, classification, and control tool | (i) The performance of the model is hard to be explained compared to the others as it appears as a black box model (ii) Requires more time compared to the FL as the suitable number of layers should be determined (iii) Need sufficient and reliable data |
ANFIS | (i) Able to merge details from various resources (ii) Noise tolerant, accurate, and effective method in solving complex problems (iii) It has a higher performance compared to ANN and FL methods (iv) Possesses the benefits from both ANN and FL method (v) Classification and prediction tasks can be done more conveniently (vi) Able to save time and cost overall in general compared to manual methods | (i) The data available should be reliable to avoid any confusion or misinformation during the training process as it will affect the final results |
Guidelines on Choosing the Appropriate AI Method
Selecting the appropriate algorithm is important when developing the AI model as it can aid the user to attain an accurate, rapid, and cost-saving results. Therefore, a guideline given in Fig. 3 is an important asset prior to achieving best performances in a case study. The primary step in the selection process is that users should define and finalize the objective of using AI in their research or implementation. Prediction, classification, quality control, detection of adulterants, and estimation are among the common objectives of AI applications in the food industries. Next, decision should be made whether sensors such as E-tongue, E-nose, CVS, and NIRS are required to collect the sampling data or not for collecting the data from the samples. Normally, integration with those sensors is conducted to obtain the parameters and characteristics of the samples to be included in the AI algorithms for sample testing purposes. Upon deciding the necessity of the sensors, users should compare and choose the fitting algorithm with respect to their study. Among the most common AI algorithms that have been employed include the FL, ANN, ANFIS, and ML methods. ANFIS has shown to have a higher accuracy, but the complexity of the model makes it less favorable compared to the other algorithms. It is advisable for the users to determine the complexity of the research in selecting the most appropriate algorithm for their studies. Once the selection of the algorithm has been confirmed, the data available are integrated with the AI algorithms. Finally, the testing and validation based on R 2 and MSE are done to analyze the performance of the established model. The AI model has been created successfully once the validation is accepted; otherwise, users should return to the previous step and reselect the algorithm. Figure Figure11 shows 11 shows the guideline in choosing and development of the AI model in food industry application.

Flowchart for developing AI model
Trends on the Application of AI in the Food Industry in the Future
The overall trend on the application of AI in the food industry is shown in Fig. Fig.12. 12 . From the studies within the past few years, the usage of the AI methods has been observed to increase from 2015 to 2020 and is predicted to rise for the next 10 years based on the current trends. Among the rising factors for the application of AI in the food industry is the introduction of Industrial Revolution 4.0 (IR 4.0). The merging of technologies or intelligent systems into conventional industry is what is known as IR 4.0 and can also be called smart factory [ 129 , 130 ]. AI which is categorized under the IR 4.0 technologies focuses on the development of intelligent machines that functions like the humans [ 131 ]. IR 4.0 makes a great impact in the product recalls due to the inspections or complains in the food industries. The implementation of the AI integrated in the sensors able to detect the errors during the manufacturing process and rectify the problems efficiently. Apart from that, IR 4.0 also plays a big role in the human behavior as consumers in the twenty-first century often discover information regarding the foods in the internet. The rising concerns on the food quality allow more usage of AI as they are able to enhance the quality of the food and aids during the production process. The highest amount of application of AI in the food industry was seen in the year 2020 as more researchers are carrying out studies using the AI method, and it is believed to continue rising for the upcoming years due to increasing in food demand and the concern on the safety of the foods which are being produced.

Application of AI in the food industry
The comparison between the AI integration with and without sensors for real-time monitoring in the food industry is displayed in Fig. 13 . Integration with external sensors has a higher percentage compared to those without the integration of the sensors in the food industries. The purpose of external sensors was to obtain the data from the samples which are then employed into the AI algorithms to carry out various tasks such as classification, prediction, quality control, and others that have been stated earlier. However, the data collection for the year 2017 showed that the percentage for the AI without the external sensors is greater than that with integration with the sensors. This is due to the high amount of research which was conducted without using the external sensors which are listed in this paper. Based on the evaluation carried out during this study, it was found that a high amount of research was done on the integration of CVS sensors with the AI methods. It is explainable as CVS sensors are able to provide important parameters such as the shape, size, colors, and defects which are essential for the quality control in the food industry. However, the integration of the system is mainly dependent on the objectives of the researcher and the industrial players and the availability of the data.

Comparison between integration of AI for real-time monitoring in the food industry
In short, as the AI world is heading towards 2.0 [ 132 ], it can be predicted that the rise in the usage of AI in the food industry is definite and inevitable because of the advantages that they can offer such as saving in terms of time, money, and energy as well as the accuracy in predicting the main factors which are affecting the food industries. Apart from that, in the recent pandemic situation due to the Covid-19 virus, it is predicted that more companies will opt for the usage of AI in their industries to cut down the costs and boost the performance of their company. There have been reports by some of the SMEs that their earnings have dropped and some SMEs have claimed that they could only survive for about 1 to 3 months. The high demand of food and the tight standard operating procedure in the companies during the pandemic situation will encourage the industry players to find an alternative to their problems and AI will be one of them to ensure a smooth operation.
Conclusion and Future Outlook
In conclusion, AI has been playing a major role in the food industry for various intents such as for modeling, prediction, control tool, food drying, sensory evaluation, quality control, and solving complex problems in the food processing. Apart from that, AI is able to enhance the business strategies due to its ability in conducting the sales prediction and allowing the yield increment. AI is recognized widely due to its simplicity, accuracy, and cost-saving method in the food industry. The applications of AI, its advantages, and limitations as well as the integration of the algorithms with different sensors such as E-nose and E-tongue in the food industry are critically summarized. Moreover, a guideline has been proposed as a step-by-step procedure in developing the appropriate algorithm prior to using the AI model in the food industry–related field, all of which will aid and encourage researchers and industrial players to venture into the current technology that has been proven to provide better outcome.
The authors were supported by the Universiti Kebangsaan Malaysia under grant GUP-2019–012.
Declarations
The authors declare no competing interests.
Publisher's Note
Springer Nature remains neutral with regard to jurisdictional claims in published maps and institutional affiliations.
Information
- Author Services
Initiatives
You are accessing a machine-readable page. In order to be human-readable, please install an RSS reader.
All articles published by MDPI are made immediately available worldwide under an open access license. No special permission is required to reuse all or part of the article published by MDPI, including figures and tables. For articles published under an open access Creative Common CC BY license, any part of the article may be reused without permission provided that the original article is clearly cited. For more information, please refer to https://www.mdpi.com/openaccess .
Feature papers represent the most advanced research with significant potential for high impact in the field. A Feature Paper should be a substantial original Article that involves several techniques or approaches, provides an outlook for future research directions and describes possible research applications.
Feature papers are submitted upon individual invitation or recommendation by the scientific editors and must receive positive feedback from the reviewers.
Editor’s Choice articles are based on recommendations by the scientific editors of MDPI journals from around the world. Editors select a small number of articles recently published in the journal that they believe will be particularly interesting to readers, or important in the respective research area. The aim is to provide a snapshot of some of the most exciting work published in the various research areas of the journal.
Original Submission Date Received: .
- Active Journals
- Find a Journal
- Proceedings Series
- For Authors
- For Reviewers
- For Editors
- For Librarians
- For Publishers
- For Societies
- For Conference Organizers
- Open Access Policy
- Institutional Open Access Program
- Special Issues Guidelines
- Editorial Process
- Research and Publication Ethics
- Article Processing Charges
- Testimonials
- Preprints.org
- SciProfiles
- Encyclopedia
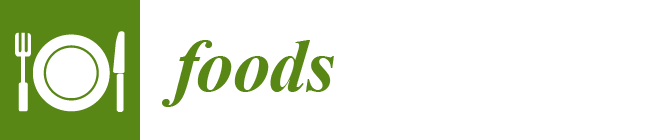
Article Menu
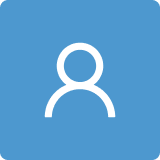
- Subscribe SciFeed
- Recommended Articles
- Author Biographies
- PubMed/Medline
- Google Scholar
- on Google Scholar
- Table of Contents
Find support for a specific problem in the support section of our website.
Please let us know what you think of our products and services.
Visit our dedicated information section to learn more about MDPI.
JSmol Viewer
The application of artificial intelligence and big data in the food industry.
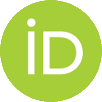
1. Introduction
1.1. the early situation in the food industry, 1.2. the current state of the food industry, 1.3. the importance of food safety, 1.4. digital transformation in the food industry, 2. big data in the food industry, 2.1. applications of big data in the food industry, 2.1.1. application of personalized marketing and recommendation system, 2.1.2. consumer behavior analysis and forecasting, 2.1.3. the utilization of big data analytics in supply chain management, 2.1.4. application of forecasting models and machine learning algorithms in demand forecasting, 2.2. the bottleneck of big data applications for the food industry, 2.3. blockchain technology, 3. artificial intelligence in the food sector, 3.1. knowledge-based expert systems in the food industry, the future and challenges of expert systems, 3.2. fuzzy logic systems, 3.3. adaptive neuro-fuzzy inference system (anfis) technology, 3.4. near-infrared spectroscopy technology combined with artificial intelligence, 3.5. application of computer vision systems in the food industry, 3.6. artificial intelligence combined with smart sensors for real-time inspection in the food industry, 4. future trends and challenges for artificial intelligence applications in the food field, 4.1. future development direction and outlook, 4.1.1. application prospects of emerging technologies, 4.1.2. possible directions for innovation and improvement, 4.1.3. exploration of feasibility and sustainability issues, 4.1.4. future challenges ahead, 5. conclusions, author contributions, institutional review board statement, informed consent statement, data availability statement, acknowledgments, conflicts of interest.
- Akoka, J.; Comyn-Wattiau, I.; Laoufi, N. Research on Big Data—A systematic mapping study. Comput. Stand. Interfaces 2017 , 54 , 105–115. [ Google Scholar ] [ CrossRef ]
- Schroeder, R. Big data business models: Challenges and opportunities. Cogent Soc. Sci. 2016 , 2 , 1166924. [ Google Scholar ] [ CrossRef ]
- Misra, N.; Dixit, Y.; Al-Mallahi, A.; Bhullar, M.S.; Upadhyay, R.; Martynenko, A. IoT, big data, and artificial intelligence in agriculture and food industry. IEEE Int. Things J. 2020 , 9 , 6305–6324. [ Google Scholar ] [ CrossRef ]
- Sahu, M.; Gupta, R.; Ambasta, R.K.; Kumar, P. Artificial intelligence and machine learning in precision medicine: A paradigm shift in big data analysis. Prog. Mol. Biol. Transl. Sci. 2022 , 190 , 57–100. [ Google Scholar ] [ CrossRef ] [ PubMed ]
- Ramakrishna, R.R.; Abd Hamid, Z.; Zaki, W.M.D.W.; Huddin, A.B.; Mathialagan, R. Stem cell imaging through convolutional neural networks: Current issues and future directions in artificial intelligence technology. PeerJ 2020 , 8 , e10346. [ Google Scholar ] [ CrossRef ] [ PubMed ]
- Serazetdinova, L.; Garratt, J.; Baylis, A.; Stergiadis, S.; Collison, M.; Davis, S. How should we turn data into decisions in AgriFood? J. Sci. Food Agric. 2019 , 99 , 3213–3219. [ Google Scholar ] [ CrossRef ] [ PubMed ]
- Ben Ayed, R.; Hanana, M. Artificial Intelligence to Improve the Food and Agriculture Sector. J. Food Qual. 2021 , 2021 , 7. [ Google Scholar ] [ CrossRef ]
- Corney, D. Food bytes: Intelligent systems in the food industry. Br. Food J. 2002 , 104 , 787–805. [ Google Scholar ] [ CrossRef ]
- Qian, C.; Murphy, S.; Orsi, R.; Wiedmann, M. How can AI help improve food safety? Annu. Rev. Food Sci. Technol. 2023 , 14 , 517–538. [ Google Scholar ] [ CrossRef ]
- Camarena, S. Artificial intelligence in the design of the transitions to sustainable food systems. J. Clean. Prod. 2020 , 271 , 122574. [ Google Scholar ] [ CrossRef ]
- Mavani, N.R.; Ali, J.M.; Othman, S.; Hussain, M.; Hashim, H.; Rahman, N.A. Application of artificial intelligence in food industry—A guideline. Food Eng. Rev. 2022 , 14 , 134–175. [ Google Scholar ] [ CrossRef ]
- Brookes, B.C. Theory of the Bradford law. J. Doc. 1977 , 33 , 180–209. [ Google Scholar ] [ CrossRef ]
- Kumar, M.V.; Iyengar, N.C.S. A framework for Blockchain technology in rice supply chain management. Adv. Sci. Technol. Lett. 2017 , 146 , 125–130. [ Google Scholar ] [ CrossRef ]
- Zhong, R.; Xu, X.; Wang, L. Food supply chain management: Systems, implementations, and future research. Ind. Manag. Data Syst. 2017 , 117 , 2085–2114. [ Google Scholar ] [ CrossRef ]
- Jagtap, S.; Bader, F.; Garcia-Garcia, G.; Trollman, H.; Fadiji, T.; Salonitis, K. Food logistics 4.0: Opportunities and challenges. Logistics 2020 , 5 , 2. [ Google Scholar ] [ CrossRef ]
- van Asselt, E.D.; Van der Fels-Klerx, H.; Marvin, H.; Van Bokhorst-van de Veen, H.; Groot, M.N. Overview of food safety hazards in the European dairy supply chain. Compr. Rev. Food Sci. Food Saf. 2017 , 16 , 59–75. [ Google Scholar ] [ CrossRef ]
- Aung, M.M.; Chang, Y.S. Traceability in a food supply chain: Safety and quality perspectives. Food Control 2014 , 39 , 172–184. [ Google Scholar ] [ CrossRef ]
- Fung, F.; Wang, H.S.; Menon, S. Food safety in the 21st century. Biomed. J. 2018 , 41 , 88–95. [ Google Scholar ] [ CrossRef ]
- Janjarasskul, T.; Suppakul, P. Active and intelligent packaging: The indication of quality and safety. Crit. Rev. Food Sci. Nutr. 2018 , 58 , 808–831. [ Google Scholar ] [ CrossRef ]
- Chen, K.; Tan, H.; Gao, J.; Lu, Y. Big data based design of food safety cloud platform. Appl. Mech. Mater. 2014 , 536 , 583–587. [ Google Scholar ] [ CrossRef ]
- Hassoun, A.; Jagtap, S.; Garcia-Garcia, G.; Trollman, H.; Pateiro, M.; Lorenzo, J.M.; Trif, M.; Rusu, A.V.; Aadil, R.M.; Simat, V.; et al. Food quality 4.0: From traditional approaches to digitalized automated analysis. J. Food Eng. 2023 , 337 , 16. [ Google Scholar ] [ CrossRef ]
- Aguilar, E.; Bolaños, M.; Radeva, P. Exploring food detection using CNNs. In Proceedings of the Computer Aided Systems Theory–EUROCAST 2017: 16th International Conference, Las Palmas de Gran Canaria, Spain, 19–24 February 2017; Revised Selected Papers, Part II 16. Springer: Berlin/Heidelberg, Germany, 2018; pp. 339–347. [ Google Scholar ]
- Ding, H.; Wilson, D.I.; Yu, W.; Young, B.R. An investigation of the relative impact of process and shape factor variables on milk powder quality. Food Bioprod. Process. 2021 , 126 , 62–72. [ Google Scholar ] [ CrossRef ]
- Ding, H.; Wilson, D.I.; Yu, W.; Young, B.R. Assessing and Quantifying the Surface Texture of Milk Powder Using Image Processing. Foods 2022 , 11 , 1519. [ Google Scholar ] [ CrossRef ] [ PubMed ]
- Ding, H.; Wilson, D.I.; Yu, W.; Young, B.R.; Cui, X. Application of Three-Dimensional Digital Photogrammetry to Quantify the Surface Roughness of Milk Powder. Foods 2023 , 12 , 967. [ Google Scholar ] [ CrossRef ] [ PubMed ]
- Zhong, J.; Sun, L.; Zuo, E.; Chen, C.; Chen, C.; Jiang, H.; Li, H.; Lv, X. An ensemble of AHP-EW and AE-RNN for food safety risk early warning. PLoS ONE 2023 , 18 , e0284144. [ Google Scholar ] [ CrossRef ] [ PubMed ]
- Do, H.-D.; Kim, D.-E.; Lam, M.B.; Chung, W.-Y. Self-powered food assessment system using LSTM network and 915 MHz RF energy harvesting. IEEE Access 2021 , 9 , 97444–97456. [ Google Scholar ] [ CrossRef ]
- Dayıoğlu, M.A.; Turker, U. Digital transformation for sustainable future-agriculture 4.0: A review. J. Agric. Sci. 2021 , 27 , 373–399. [ Google Scholar ] [ CrossRef ]
- Chen, J.; Chen, Y.; Du, X.; Li, C.; Lu, J.; Zhao, S.; Zhou, X. Big data challenge: A data management perspective. Front. Comput. Sci. 2013 , 7 , 157–164. [ Google Scholar ] [ CrossRef ]
- Li, Z.-H.; Wang, G.-R.; Zhou, A.-Y. Research progress and trends of big data from a database perspective. Comput. Eng. Sci./Jisuanji Gongcheng Yu Kexue 2013 , 35 , 19–30. [ Google Scholar ] [ CrossRef ]
- Mohamed, A.; Najafabadi, M.K.; Wah, Y.B.; Zaman, E.A.K.; Maskat, R. The state of the art and taxonomy of big data analytics: View from new big data framework. Artif. Intell. Rev. 2020 , 53 , 989–1037. [ Google Scholar ] [ CrossRef ]
- Géczy, P. Big data characteristics. Macrotheme Rev. 2014 , 3 , 94–104. [ Google Scholar ] [ CrossRef ]
- Sravanthi, K.; Reddy, T.S. Applications of big data in various fields. Int. J. Comput. Sci. Inf. Technol. 2015 , 6 , 4629–4632. [ Google Scholar ] [ CrossRef ]
- Du, X.; Lu, W.; Zhang, F. History, Present, and Future of Big Data Management Systems. J. Softw. 2019 , 30 , 127–141. [ Google Scholar ] [ CrossRef ]
- Torre-Bastida, A.I.; Del Ser, J.; Lana, I.; Ilardia, M.; Bilbao, M.N.; Campos-Cordobes, S. Big Data for transportation and mobility: Recent advances, trends and challenges. Iet Intell. Transp. Syst. 2018 , 12 , 742–755. [ Google Scholar ] [ CrossRef ]
- Chen, J.; Ding, Z.; Gao, X. Survey of Big Data Hot Techniques. J. Beijing Univ. Technol. 2017 , 43 , 358–367. [ Google Scholar ] [ CrossRef ]
- Guo, P.; Wang, K.; Luo, A.; Xue, M. Computational Intelligence for Big Data Analysis: Current Status and Future Prospect. J. Softw. 2015 , 26 , 3010–3025. [ Google Scholar ] [ CrossRef ]
- Zuheros, C.; Martínez-Cámara, E.; Herrera-Viedma, E.; Herrera, F. Sentiment analysis based multi-person multi-criteria decision making methodology using natural language processing and deep learning for smarter decision aid. Case study of restaurant choice using TripAdvisor reviews. Inf. Fusion 2021 , 68 , 22–36. [ Google Scholar ] [ CrossRef ]
- Delanoy, N.; Kasztelnik, K. Business Open Big Data Analytics to Support Innovative Leadership Decision in Canada. Bus. Ethics Leadersh. 2020 , 4 , 56–74. [ Google Scholar ] [ CrossRef ]
- Zhou, Q.; Zhang, H.; Wang, S. Artificial intelligence, big data, and blockchain in food safety. Int. J. Food Eng. 2021 , 18 , 1–14. [ Google Scholar ] [ CrossRef ]
- Li, W.; Gu, C.; Chen, J.; Ma, C.; Zhang, X.; Chen, B.; Wan, S. DLS-GAN: Generative Adversarial Nets for Defect Location Sensitive Data Augmentation. IEEE Trans. Autom. Sci. Eng. 2023 , 33 , 1–17. [ Google Scholar ] [ CrossRef ]
- Ding, H.; Li, B.; Boiarkina, I.; Wilson, D.I.; Yu, W.; Young, B.R. Effects of morphology on the bulk density of instant whole milk powder. Foods 2020 , 9 , 1024. [ Google Scholar ] [ CrossRef ] [ PubMed ]
- Ding, H.; Yu, W.; Boiarkina, I.; Depree, N.; Young, B.R. Effects of morphology on the dispersibility of instant whole milk powder. J. Food Eng. 2020 , 276 , 109841. [ Google Scholar ] [ CrossRef ]
- Schakel, S.F.; Buzzard, I.M.; Gebhardt, S.E. Procedures for estimating nutrient values for food composition databases. J. Food Compos. Anal. 1997 , 10 , 102–114. [ Google Scholar ] [ CrossRef ]
- Marvin, H.J.; Janssen, E.M.; Bouzembrak, Y.; Hendriksen, P.J.; Staats, M. Big data in food safety: An overview. Crit. Rev. Food Sci. Nutr. 2017 , 57 , 2286–2295. [ Google Scholar ] [ CrossRef ] [ PubMed ]
- Kazama, M.; Sugimoto, M.; Hosokawa, C.; Matsushima, K.; Varshney, L.R.; Ishikawa, Y. A neural network system for transformation of regional cuisine style. Front. ICT 2018 , 5 , 14. [ Google Scholar ] [ CrossRef ]
- Kalra, J.; Batra, D.; Diwan, N.; Bagler, G. Nutritional profile estimation in cooking recipes. In Proceedings of the 2020 IEEE 36th International Conference on Data Engineering Workshops (ICDEW), Dallas, TX, USA, 14–20 April 2020; IEEE: Piscataway, NJ, USA, 2020; pp. 82–87. [ Google Scholar ]
- Whitehouse, S.; Yordanova, K.; Paiement, A.; Mirmehdi, M. Recognition of unscripted kitchen activities and eating behaviour for health monitoring. In Proceedings of the 2nd IET International Conference on Technologies for Active and Assisted Living (TechAAL 2016), London, UK, 24–25 October 2016; IET: London, UK, 2016; pp. 1–6. [ Google Scholar ]
- Lee, M.; Kwon, W.; Back, K.-J. Artificial intelligence for hospitality big data analytics: Developing a prediction model of restaurant review helpfulness for customer decision-making. Int. J. Contemp. Hosp. Manag. 2021 , 33 , 2117–2136. [ Google Scholar ] [ CrossRef ]
- Maru, A.; Berne, D.; De Beer, J.; Ballantyne, P.; Pesce, V.; Kalyesubula, S.; Fourie, N.; Addison, C.; Collett, A.; Chaves, J. Digital and data-driven agriculture: Harnessing the power of data for smallholders. F1000Research 2018 , 7 , 525. [ Google Scholar ] [ CrossRef ]
- Kamble, S.S.; Gunasekaran, A.; Gawankar, S.A. Achieving sustainable performance in a data-driven agriculture supply chain: A review for research and applications. Int. J. Prod. Econ. 2020 , 219 , 179–194. [ Google Scholar ] [ CrossRef ]
- Liao, S.-H.; Yang, C.-A. Big data analytics of social network marketing and personalized recommendations. Soc. Netw. Anal. Min. 2021 , 11 , 1–19. [ Google Scholar ] [ CrossRef ]
- Mariani, M.M.; Wamba, S.F. Exploring how consumer goods companies innovate in the digital age: The role of big data analytics companies. J. Bus. Res. 2020 , 121 , 338–352. [ Google Scholar ] [ CrossRef ]
- Verma, J.P.; Patel, B.; Patel, A. Big data analysis: Recommendation system with Hadoop framework. In Proceedings of the 2015 IEEE International Conference on Computational Intelligence & Communication Technology, Ghaziabad, India, 13–14 February 2015; IEEE: Piscataway, NJ, USA, 2015; pp. 92–97. [ Google Scholar ]
- Le, T.M.; Liaw, S.-Y. Effects of pros and cons of applying big data analytics to consumers’ responses in an e-commerce context. Sustainability 2017 , 9 , 798. [ Google Scholar ] [ CrossRef ]
- Ji, G.; Hu, L.; Tan, K.H. A study on decision-making of food supply chain based on big data. J. Syst. Sci. Syst. Eng. 2017 , 26 , 183–198. [ Google Scholar ] [ CrossRef ]
- Rabl, T.; Sadoghi, M.; Jacobsen, H.-A.; Gómez-Villamor, S.; Muntés-Mulero, V.; Mankowskii, S. Solving big data challenges for enterprise application performance management. arXiv 2012 , arXiv:1208.4167. [ Google Scholar ] [ CrossRef ]
- Joshi, A.; Khosravy, M.; Gupta, N. Machine learning for predictive analysis. Proc. ICTIS 2020 , 13 , 225. [ Google Scholar ] [ CrossRef ]
- Cheng, Y.; Chen, K.; Sun, H.; Zhang, Y.; Tao, F. Data and knowledge mining with big data towards smart production. J. Ind. Inf. Integr. 2018 , 9 , 1–13. [ Google Scholar ] [ CrossRef ]
- Hofmann, E.; Rutschmann, E. Big data analytics and demand forecasting in supply chains: A conceptual analysis. Int. J. Logist. Manag. 2018 , 29 , 739–766. [ Google Scholar ] [ CrossRef ]
- Djekic, I.; Sanjuán, N.; Clemente, G.; Jambrak, A.R.; Tonda, A. Review on environmental models in the food chain-Current status and future perspectives. J. Clean. Prod. 2018 , 176 , 1012–1025. [ Google Scholar ] [ CrossRef ]
- Bronson, K.; Knezevic, I. Big Data in food and agriculture. Big Data Soc. 2016 , 3 , 2053951716648174. [ Google Scholar ] [ CrossRef ]
- Ducange, P.; Pecori, R.; Mezzina, P. A glimpse on big data analytics in the framework of marketing strategies. Soft Comput. 2018 , 22 , 325–342. [ Google Scholar ] [ CrossRef ]
- Mehmood, A.; Natgunanathan, I.; Xiang, Y.; Hua, G.; Guo, S. Protection of big data privacy. IEEE Access 2016 , 4 , 1821–1834. [ Google Scholar ] [ CrossRef ]
- Deepa, N.; Pham, Q.-V.; Nguyen, D.C. A survey on blockchain for big data: Approaches, opportunities, and future directions. Future Gener. Comput. Syst. 2022 , 131 , 209–226. [ Google Scholar ] [ CrossRef ]
- Khan, S.N.; Loukil, F.; Ghedira-Guegan, C.; Benkhelifa, E.; Bani-Hani, A. Blockchain smart contracts: Applications, challenges, and future trends. Peer–Peer Netw. Appl. 2021 , 14 , 2901–2925. [ Google Scholar ] [ CrossRef ] [ PubMed ]
- Puthal, D.; Malik, N.; Mohanty, S.P.; Kougianos, E.; Yang, C. The blockchain as a decentralized security framework [future directions]. IEEE Consum. Electron. Mag. 2018 , 7 , 18–21. [ Google Scholar ] [ CrossRef ]
- De Filippi, P. The interplay between decentralization and privacy: The case of blockchain technologies. J. Peer Prod. Issue 2016 , 14 , 508–527. [ Google Scholar ]
- Macrinici, D.; Cartofeanu, C.; Gao, S. Smart contract applications within blockchain technology: A systematic mapping study. Telemat. Inform. 2018 , 35 , 2337–2354. [ Google Scholar ] [ CrossRef ]
- Ahmed, S.; ten Broek, N. Blockchain could boost food security. Nature 2017 , 550 , 43. [ Google Scholar ] [ CrossRef ]
- Feng, H.; Wang, X.; Duan, Y.Q.; Zhang, J.; Zhang, X.S. Applying blockchain technology to improve agri-food traceability: A review of development methods, benefits and challenges. J. Clean. Prod. 2020 , 260 , 15. [ Google Scholar ] [ CrossRef ]
- Jaison, F.; Ramaiah, N.S. A survey on traceability in food safety system using blockchain. J. Discret. Math. Sci. Cryptogr. 2022 , 25 , 793–799. [ Google Scholar ] [ CrossRef ]
- Kim, H.M.; Laskowski, M. Toward an ontology-driven blockchain design for supply-chain provenance. Intell. Syst. Account. Financ. Manag. 2018 , 25 , 18–27. [ Google Scholar ] [ CrossRef ]
- Demestichas, K.; Peppes, N.; Alexakis, T.; Adamopoulou, E. Blockchain in Agriculture Traceability Systems: A Review. Appl. Sci. 2020 , 10 , 4113. [ Google Scholar ] [ CrossRef ]
- Lin, Q.; Wang, H.; Pei, X.; Wang, J. Food safety traceability system based on blockchain and EPCIS. IEEE Access 2019 , 7 , 20698–20707. [ Google Scholar ] [ CrossRef ]
- Mohan, T. Improve Food Supply Chain Traceability Using Blockchain. Master’s Thesis, The Pennsylvania State University, State College, PA, USA, 2018. [ Google Scholar ]
- Mao, D.H.; Hao, Z.H.; Wang, F.; Li, H.S. Innovative Blockchain-Based Approach for Sustainable and Credible Environment in Food Trade: A Case Study in Shandong Province, China. Sustainability 2018 , 10 , 3149. [ Google Scholar ] [ CrossRef ]
- Smith, B.G. Developing sustainable food supply chains. Philos. Trans. R. Soc. B-Biol. Sci. 2008 , 363 , 849–861. [ Google Scholar ] [ CrossRef ] [ PubMed ]
- Ariemma, L.; De Carlo, N.; Pennino, D.; Pizzonia, M.; Vitaletti, A.; Zecchini, M. Blockchain for the supply chain of the Italian craft beer sector: Tracking and discount coupons. Int. J. Parallel Emergent Distrib. Syst. 2023 , 16 , 1–34. [ Google Scholar ] [ CrossRef ]
- Cao, S.; Powell, W.; Foth, M.; Natanelov, V.; Miller, T.; Dulleck, U. Strengthening consumer trust in beef supply chain traceability with a blockchain-based human-machine reconcile mechanism. Comput. Electron. Agric. 2021 , 180 , 105886. [ Google Scholar ] [ CrossRef ]
- Lucena, P.; Binotto, A.P.; Momo, F.d.S.; Kim, H. A case study for grain quality assurance tracking based on a Blockchain business network. arXiv 2018 , arXiv:1803.07877. [ Google Scholar ] [ CrossRef ]
- Kshetri, N. Blockchain and the economics of food safety. It Prof. 2019 , 21 , 63–66. [ Google Scholar ] [ CrossRef ]
- Bai, C.; Sarkis, J. A supply chain transparency and sustainability technology appraisal model for blockchain technology. Int. J. Prod. Res. 2020 , 58 , 2142–2162. [ Google Scholar ] [ CrossRef ]
- Zheng, Z.; Xie, S.; Dai, H.-N.; Chen, X.; Wang, H. Blockchain challenges and opportunities: A survey. Int. J. Web Grid Serv. 2018 , 14 , 352–375. [ Google Scholar ] [ CrossRef ]
- Marvin, H.J.; Bouzembrak, Y.; Van der Fels-Klerx, H. Digitalisation and Artificial Intelligence for sustainable food systems. Trends Food Sci. Technol. 2022 , 120 , 344–348. [ Google Scholar ] [ CrossRef ]
- Li, W.; Chen, J.; Cao, J.; Ma, C.; Wang, J.; Cui, X.; Chen, P. EID-GAN: Generative adversarial nets for extremely imbalanced data augmentation. IEEE Transac. Ind. Inform. 2022 , 19 , 3208–3218. [ Google Scholar ] [ CrossRef ]
- Kaula, R.; Lander, L.C. A Module-Based Conceptual-Framework For Large-Scale Expert-Systems. Ind. Manag. Data Syst. 1995 , 95 , 15–23. [ Google Scholar ] [ CrossRef ]
- Yang, X.; Zhu, D.-Q.; Sang, Q.-B. Research and prospect of expert system. Jisuanji Yingyong Yanjiu/Appl. Res. Comput. 2007 , 24 , 4–9. [ Google Scholar ] [ CrossRef ]
- Valavanis, K.P.; Kokkinaki, A.I.; Tzafestas, S.G. Knowledge-Based (Expert) Systems In Engineering Applications—A Survey. J. Intell. Robot. Syst. 1994 , 10 , 113–145. [ Google Scholar ] [ CrossRef ]
- Myers, W. Introduction to expert systems. IEEE Intell. Syst. 1986 , 1 , 100–109. [ Google Scholar ] [ CrossRef ]
- Filter, M.; Appel, B.; Buschulte, A. Expert systems for food safety. Curr. Opin. Food Sci. 2015 , 6 , 61–65. [ Google Scholar ] [ CrossRef ]
- Vasquez, R.P.; Aguilar-Lasserre, A.A.; Lopez-Segura, M.V.; Rivero, L.C.; Rodriguez-Duran, A.A.; Rojas-Luna, M.A. Expert system based on a fuzzy logic model for the analysis of the sustainable livestock production dynamic system. Comput. Electron. Agric. 2019 , 161 , 104–120. [ Google Scholar ] [ CrossRef ]
- Blagoveshchenskiy, I.; Blagoveshchenskiy, V.; Besfamilnaya, E.; Sumerin, V. Development of databases of intelligent expert systems for automatic control of product quality indicators. In Journal of Physics: Conference Series ; IOP Publishing: Bristol, UK, 2020; p. 012019. [ Google Scholar ]
- Hernández-Vera, B.; Aguilar Lasserre, A.A.; Gastón Cedillo-Campos, M.; Herrera-Franco, L.E.; Ochoa-Robles, J. Expert system based on fuzzy logic to define the production process in the coffee industry. J. Food Process Eng. 2017 , 40 , e12389. [ Google Scholar ] [ CrossRef ]
- Saibene, A.; Assale, M.; Giltri, M. Expert systems: Definitions, advantages and issues in medical field applications. Expert Syst. Appl. 2021 , 177 , 114900. [ Google Scholar ] [ CrossRef ]
- Wagner, W.P. Trends in expert system development: A longitudinal content analysis of over thirty years of expert system case studies. Expert Syst. Appl. 2017 , 76 , 85–96. [ Google Scholar ] [ CrossRef ]
- Castillo, O.; Amador-Angulo, L.; Castro, J.R.; Garcia-Valdez, M. A comparative study of type-1 fuzzy logic systems, interval type-2 fuzzy logic systems and generalized type-2 fuzzy logic systems in control problems. Inf. Sci. 2016 , 354 , 257–274. [ Google Scholar ] [ CrossRef ]
- Eerikainen, T.; Linko, P.; Linko, S.; Siimes, T.; Zhu, Y.-H. Fuzzy-Logic and Neural-Network Applications In Food-Science And Technology. Trends Food Sci. Technol. 1993 , 4 , 237–242. [ Google Scholar ] [ CrossRef ]
- Li, H. Output-back fuzzy logic systems and equivalence with feedback neural networks. Chin. Sci. Bull. 2000 , 45 , 592–596. [ Google Scholar ] [ CrossRef ]
- Farzaneh, V.; Ghodsvali, A.; Bakhshabadi, H.; Ganje, M.; Dolatabadi, Z.; Carvalho, I.S. Modelling of the Selected Physical Properties of the Fava Bean with Various Moisture Contents UsingFuzzy Logic Design. J. Food Process Eng. 2017 , 40 , 9. [ Google Scholar ] [ CrossRef ]
- Zadeh, L.A. The calculus of fuzzy if-then rules. In Proceedings of the Fuzzy Engineering toward Human Friendly Systems—Proceedings of the International Fuzzy Engineering Symposium, Leipzig, Germany, 20–22 September 2023; pp. 11–12. [ Google Scholar ]
- Mahadevappa, J.; Gross, F.; Delgado, A. Fuzzy logic based process control strategy for effective sheeting of wheat dough in small and medium-sized enterprises. J. Food Eng. 2017 , 199 , 93–99. [ Google Scholar ] [ CrossRef ]
- Tanima, C.; Madhusweta, D. Sensory evaluation of aromatic foods packed in developed starch based films using fuzzy logic. Int. J. Food Stud. 2015 , 4 , 29–48. [ Google Scholar ] [ CrossRef ]
- Farzaneh, V.; Bakhshabadi, H.; Gharekhani, M.; Ganje, M.; Farzaneh, F.; Rashidzadeh, S.; Carvalho, I.S. Application of an adaptive neuro_fuzzy inference system (ANFIS) in the modeling of rapeseeds’ oil extraction. J. Food Process Eng. 2017 , 40 , 8. [ Google Scholar ] [ CrossRef ]
- Samodro, B.; Mahesworo, B.; Suparyanto, T.; Atmaja, D.B.S.; Pardamean, B. Maintaining the quality and aroma of coffee with fuzzy logic coffee roasting machine. In IOP Conference Series: Earth and Environmental Science ; IOP Publishing: Bristol, UK, 2020; p. 012148. [ Google Scholar ]
- Yulianto, T.; Komariyah, S.; Ulfaniyah, N. Application of fuzzy inference system by Sugeno method on estimating of salt production. In AIP Conference Proceedings ; AIP Publishing: Melville, NY, USA, 2017. [ Google Scholar ]
- Basak, S. The use of fuzzy logic to determine the concentration of betel leaf essential oil and its potency as a juice preservative. Food Chem. 2018 , 240 , 1113–1120. [ Google Scholar ] [ CrossRef ]
- Vivek, K.; Subbarao, K.; Routray, W.; Kamini, N.; Dash, K.K. Application of fuzzy logic in sensory evaluation of food products: A comprehensive study. Food Bioprocess Technol. 2020 , 13 , 1–29. [ Google Scholar ] [ CrossRef ]
- Pelsmaeker, S.; Gellynck, X.; Delbaere, C.; Declercq, N.; Dewettinck, K. Consumer-driven product development and improvement combined with sensory analysis: A case-study for European filled chocolates. Food Qual. Prefer. 2015 , 41 , 20–29. [ Google Scholar ] [ CrossRef ]
- Shahbazi, Z.; Byun, Y.-C. A procedure for tracing supply chains for perishable food based on blockchain, machine learning and fuzzy logic. Electronics 2020 , 10 , 41. [ Google Scholar ] [ CrossRef ]
- Azeem, M.F.; Hanmandlu, M.; Ahmad, N. Generalization of adaptive neuro-fuzzy inference systems. IEEE Trans. Neural Netw. 2000 , 11 , 1332–1346. [ Google Scholar ] [ CrossRef ] [ PubMed ]
- Arabameri, M.; Nazari, R.R.; Abdolshahi, A.; Abdollahzadeh, M.; Mirzamohammadi, S.; Shariatifar, N.; Barba, F.J.; Mousavi Khaneghah, A. Oxidative stability of virgin olive oil: Evaluation and prediction with an adaptive neuro-fuzzy inference system (ANFIS). J. Sci. Food Agric. 2019 , 99 , 5358–5367. [ Google Scholar ] [ CrossRef ] [ PubMed ]
- Abbaspour-Gilandeh, Y.; Jahanbakhshi, A.; Kaveh, M. Prediction kinetic, energy and exergy of quince under hot air dryer using ANNs and ANFIS. Food Sci. Nutr. 2020 , 8 , 594–611. [ Google Scholar ] [ CrossRef ] [ PubMed ]
- Kaveh, M.; Sharabiani, V.R.; Chayjan, R.A.; Taghinezhad, E.; Abbaspour-Gilandeh, Y.; Golpour, I. ANFIS and ANNs model for prediction of moisture diffusivity and specific energy consumption potato, garlic and cantaloupe drying under convective hot air dryer. Inf. Process. Agric. 2018 , 5 , 372–387. [ Google Scholar ] [ CrossRef ]
- Mokarram, M.; Amin, H.; Khosravi, M.R. Using adaptive neuro-fuzzy inference system and multiple linear regression to estimate orange taste. Food Sci. Nutr. 2019 , 7 , 3176–3184. [ Google Scholar ] [ CrossRef ] [ PubMed ]
- Kumar, V.; Sharma, H.K. Process optimization for extraction of bioactive compounds from taro (Colocasia esculenta), using RSM and ANFIS modeling. J. Food Meas. Charact. 2017 , 11 , 704–718. [ Google Scholar ] [ CrossRef ]
- Ojediran, J.O.; Okonkwo, C.E.; Adeyi, A.J.; Adeyi, O.; Olaniran, A.F.; George, N.E.; Olayanju, A.T. Drying characteristics of yam slices ( Dioscorea rotundata ) in a convective hot air dryer: Application of ANFIS in the prediction of drying kinetics. Heliyon 2020 , 6 , 3. [ Google Scholar ] [ CrossRef ]
- Weeranantanaphan, J.; Downey, G.; Allen, P.; Sun, D.-W. A review of near infrared spectroscopy in muscle food analysis: 2005–2010. J. Near Infrared Spectrosc. 2011 , 19 , 61–124. [ Google Scholar ] [ CrossRef ]
- Rivera, N.V.; Gomez-Sanchis, J.; Chanona-Perez, J. Early detection of mechanical damage in mango using NIR hyperspectral images and machine learning. Biosyst. Eng. 2014 , 122 , 91–98. [ Google Scholar ] [ CrossRef ]
- Curto, B.; Moreno, V.; Garcia-Esteban, J.A.; Blanco, F.J.; Gonzalez, I.; Vivar, A.; Revilla, I. Accurate Prediction of Sensory Attributes of Cheese Using Near-Infrared Spectroscopy Based on Artificial Neural Network. Sensors 2020 , 20 , 3566. [ Google Scholar ] [ CrossRef ] [ PubMed ]
- Gunaratne, T.M.; Gonzalez Viejo, C.; Gunaratne, N.M.; Torrico, D.D.; Dunshea, F.R.; Fuentes, S. Chocolate quality assessment based on chemical fingerprinting using near infra-red and machine learning modeling. Foods 2019 , 8 , 426. [ Google Scholar ] [ CrossRef ] [ PubMed ]
- Qiao, J.; Ngadi, M.O.; Wang, N.; Gariepy, C.; Prasher, S.O. Pork quality and marbling level assessment using a hyperspectral imaging system. J. Food Eng. 2007 , 83 , 10–16. [ Google Scholar ] [ CrossRef ]
- Alshejari, A.; Kodogiannis, V.S. An intelligent decision support system for the detection of meat spoilage using multispectral images. Neural Comput. Appl. 2017 , 28 , 3903–3920. [ Google Scholar ] [ CrossRef ]
- Kakani, V.; Nguyen, V.H.; Kumar, B.P.; Kim, H.; Pasupuleti, V.R. A critical review on computer vision and artificial intelligence in food industry. J. Agric. Food Res. 2020 , 2 , 12. [ Google Scholar ] [ CrossRef ]
- Lopes, J.F.; Ludwig, L.; Barbin, D.F.; Grossmann, M.V.E.; Barbon, S. Computer Vision Classification of Barley Flour Based on Spatial Pyramid Partition Ensemble. Sensors 2019 , 19 , 2953. [ Google Scholar ] [ CrossRef ] [ PubMed ]
- Phate, V.R.; Malmathanraj, R.; Palanisamy, P. Clustered ANFIS weighing models for sweet lime ( Citrus limetta ) using computer vision system. J. Food Process Eng. 2019 , 42 , 16. [ Google Scholar ] [ CrossRef ]
- Nadim, M.; Ahmadifar, H.; Mashkinmojeh, M. Application of image processing techniques for quality control of mushroom. Casp. J. Health Res. 2019 , 4 , 72–75. [ Google Scholar ] [ CrossRef ]
- Villaseñor-Aguilar, M.-J.; Bravo-Sánchez, M.-G.; Padilla-Medina, J.-A.; Vázquez-Vera, J.L.; Guevara-González, R.-G.; García-Rodríguez, F.-J.; Barranco-Gutiérrez, A.-I. A maturity estimation of bell pepper ( Capsicum annuum L.) by artificial vision system for quality control. Appl. Sci. 2020 , 10 , 5097. [ Google Scholar ] [ CrossRef ]
- Siswantoro, J.; Hilman, M.; Widiasri, M. Computer vision system for egg volume prediction using backpropagation neural network. In IOP Conference Series: Materials Science and Engineering ; IOP Publishing: Bristol, UK, 2017; p. 012002. [ Google Scholar ]
- Bakhshipour, A.; Zareiforoush, H.; Bagheri, I. Application of decision trees and fuzzy inference system for quality classification and modeling of black and green tea based on visual features. J. Food Meas. Charact. 2020 , 14 , 1402–1416. [ Google Scholar ] [ CrossRef ]
- Mazen, F.M.; Nashat, A.A. Ripeness classification of bananas using an artificial neural network. Arab. J. Sci. Eng. 2019 , 44 , 6901–6910. [ Google Scholar ] [ CrossRef ]
- Wan, P.; Toudeshki, A.; Tan, H.; Ehsani, R. A methodology for fresh tomato maturity detection using computer vision. Comput. Electron. Agric. 2018 , 146 , 43–50. [ Google Scholar ] [ CrossRef ]
- Bhagat, N.B.; Markande, S. Automatic grading of potatoes with fuzzy logic. In Proceedings of the 2016 Online International Conference on Green Engineering and Technologies (IC-GET), Coimbatore, India, 19 November 2016; IEEE: Piscataway, NJ, USA, 2016; pp. 1–4. [ Google Scholar ]
- Garcia, J.A.A.; Arboleda, E.R.; Galas, E.M. Identification of visually similar vegetable seeds using image processing and fuzzy logic. Int. J. Sci. Technol. Res. 2020 , 9 , 4925–4928. [ Google Scholar ] [ CrossRef ]
- Koklu, M.; Ozkan, I.A. Multiclass classification of dry beans using computer vision and machine learning techniques. Comput. Electron. Agric. 2020 , 174 , 105507. [ Google Scholar ] [ CrossRef ]
- Zareiforoush, H.; Minaei, S.; Alizadeh, M.R.; Banakar, A. A hybrid intelligent approach based on computer vision and fuzzy logic for quality measurement of milled rice. Measurement 2015 , 66 , 26–34. [ Google Scholar ] [ CrossRef ]
- Yu, Z.; Jung, D.; Park, S.; Hu, Y. Smart traceability for food safety. Crit. Rev. Food Sci. Nutr. 2022 , 62 , 905–916. [ Google Scholar ] [ CrossRef ]
- Di Rosa, A.R.; Leone, F.; Cheli, F.; Chiofalo, V. Fusion of electronic nose, electronic tongue and computer vision for animal source food authentication and quality assessment—A review. J. Food Eng. 2017 , 210 , 62–75. [ Google Scholar ] [ CrossRef ]
- Jafarzadeh, S.; Nooshkam, M.; Zargar, M.; Garavand, F.; Ghosh, S.; Hadidi, M.; Forough, M. Green synthesis of nanomaterials for smart biopolymer packaging: Challenges and outlooks. J. Nanostruct. Chem. 2015 , 24 , 1–24. [ Google Scholar ] [ CrossRef ]
- McVey, C.; Elliott, C.T.; Cannavan, A.; Kelly, S.D.; Petchkongkaew, A.; Haughey, S.A. Portable spectroscopy for high throughput food authenticity screening: Advancements in technology and integration into digital traceability systems. Trends Food Sci. Technol. 2021 , 118 , 777–790. [ Google Scholar ] [ CrossRef ]
- Ndisya, J.; Gitau, A.; Mbuge, D.; Arefi, A.; Badulescu, L.; Pawelzik, E.; Hensel, O.; Sturm, B. Vis-NIR Hyperspectral Imaging for Online Quality Evaluation during Food Processing: A Case Study of Hot Air Drying of Purple-Speckled Cocoyam ( Colocasia esculenta (L.) Schott). Processes 2021 , 9 , 1804. [ Google Scholar ] [ CrossRef ]
- Abedi-Firoozjah, R.; Yousefi, S.; Heydari, M.; Seyedfatehi, F.; Jafarzadeh, S.; Mohammadi, R.; Rouhi, M.; Garavand, F. Application of Red Cabbage Anthocyanins as pH-Sensitive Pigments in Smart Food Packaging and Sensors. Polymers 2022 , 14 , 1629. [ Google Scholar ] [ CrossRef ] [ PubMed ]
- Sanaeifar, A.; ZakiDizaji, H.; Jafari, A.; de la Guardia, M. Early detection of contamination and defect in foodstuffs by electronic nose: A review. TrAC Trends Anal. Chem. 2017 , 97 , 257–271. [ Google Scholar ] [ CrossRef ]
- Feng, H.; Zhang, M.; Liu, P.; Liu, Y.; Zhang, X. Evaluation of IoT-enabled monitoring and electronic nose spoilage detection for salmon freshness during cold storage. Foods 2020 , 9 , 1579. [ Google Scholar ] [ CrossRef ] [ PubMed ]
- Wadehra, A.; Patil, P.S. Application of electronic tongues in food processing. Anal. Methods 2016 , 8 , 474–480. [ Google Scholar ] [ CrossRef ]
- Defelice, S.L. The Nutraceutial Revolution—Its Impact On Food-Industry R-And-D. Trends Food Sci. Technol. 1995 , 6 , 59–61. [ Google Scholar ] [ CrossRef ]
- Cheein, F.A.A.; Carelli, R. Agricultural Robotics Unmanned Robotic Service Units in Agricultural Tasks. IEEE Ind. Electron. Mag. 2013 , 7 , 48–58. [ Google Scholar ] [ CrossRef ]
- Maddikunta, P.K.R.; Hakak, S.; Alazab, M.; Bhattacharya, S.; Gadekallu, T.R.; Khan, W.Z.; Pham, Q.V. Unmanned Aerial Vehicles in Smart Agriculture: Applications, Requirements, and Challenges. IEEE Sens. J. 2021 , 21 , 17608–17619. [ Google Scholar ] [ CrossRef ]
- Quan, H.Y.; Zhang, T.; Xu, H.; Luo, S.; Nie, J.; Zhu, X.Q. Photo-curing 3D printing technique and its challenges. Bioact. Mater. 2020 , 5 , 110–115. [ Google Scholar ] [ CrossRef ]
- Tao, F.; Xiao, B.; Qi, Q.L.; Cheng, J.F.; Ji, P. Digital twin modeling. J. Manuf. Syst. 2022 , 64 , 372–389. [ Google Scholar ] [ CrossRef ]
- Zhuang, Y.; Wang, W.; Yun, W. Status and development of robotic control technologies based on networks. Robot 2002 , 24 , 276–282. [ Google Scholar ] [ CrossRef ]
- Griffith, C.J.; Livesey, K.M.; Clayton, D. The assessment of food safety culture. Br. Food J. 2010 , 112 , 439–456. [ Google Scholar ] [ CrossRef ]
- Mauer, W.A.; Kaneene, J.B.; DeArman, V.T.; Roberts, C.A.; Miller, R.; Pong, L.; Dickey, T.E. Ethnic-food safety concerns: An online survey of food safety professionals. J. Environ. Health 2006 , 68 , 32–38. [ Google Scholar ] [ CrossRef ] [ PubMed ]
- Milic, D.C.; Dujmenovic, I.; Peko, M. An Approach to the Application of the Internet of Things in Logistics. Teh. Glas.-Technol. J. 2023 , 17 , 134–140. [ Google Scholar ] [ CrossRef ]
- Tudora, E.; Tirziu, E.; Gheorghe-Moisii, M. Internet of Things in energy: Technologies, applications, challenges and future trends. Rom. J. Inf. Technol. Autom. Control.-Rev. Romana Inform. Autom. 2021 , 31 , 45–58. [ Google Scholar ] [ CrossRef ]
- Ismail, A.A.; Hassan, R. Technical Competencies in Digital Technology towards Industrial Revolution 4.0. J. Technol. Educ. Train. 2019 , 11 , 56–62. [ Google Scholar ] [ CrossRef ]
- Kim, S.S.; Kim, S. Impact and prospect of the fourth industrial revolution in food safety: Mini-review. Food Sci. Biotechnol. 2022 , 31 , 399–406. [ Google Scholar ] [ CrossRef ] [ PubMed ]
- Niszczota, P.; Rybicka, I.; Kamini, N. The credibility of dietary advice formulated by ChatGPT: Robo-diets for people with food allergies. Nutrition 2023 , 112 , 112076. [ Google Scholar ] [ CrossRef ]
- Paul, J.; Ueno, A.; Dennis, C. ChatGPT and consumers: Benefits, Pitfalls and Future Research Agenda. Int. J. Consum. Stud. 2022 , 13 , 1213–1225. [ Google Scholar ] [ CrossRef ]
- Cheng, L.; Liu, F.; Yao, D. Enterprise data breach: Causes, challenges, prevention, and future directions. Wiley Interdiscip. Rev. Data Min. Knowl. Discov. 2017 , 7 , e1211. [ Google Scholar ] [ CrossRef ]
- Anagnostopoulos, I.; Zeadally, S.; Exposito, E. Handling big data: Research challenges and future directions. J. Supercomput. 2016 , 72 , 1494–1516. [ Google Scholar ] [ CrossRef ]
- Massaro, A.; Calicchio, A.; Maritati, V.; Galiano, A.; Birardi, V.; Pellicani, L.; Gutierrez Millan, M.; Dalla Tezza, B.; Bianchi, M.; Vertua, G. A case study of innovation of an information communication system and upgrade of the knowledge base in industry by ESB, artificial intelligence, and big data system integration. Int. J. Artif. Intell. Appl. (IJAIA) 2018 , 9 , 27–43. [ Google Scholar ] [ CrossRef ]
- Frank, B. Artificial intelligence-enabled environmental sustainability of products: Marketing benefits and their variation by consumer, location, and product types. J. Clean. Prod. 2021 , 285 , 125242. [ Google Scholar ] [ CrossRef ]
Click here to enlarge figure
Products | Objectives | Projects/Companies Involved |
---|---|---|
Beer | Tracking the entire production process of beer to reveal its relevant ingredients. (Downstream is the first company to apply blockchain technology to beer.) | Downstream Brewing Company [ ] |
Beef | Implement blockchain technology to detect its supply chain process and prevent food fraud. | BeefLedger Corporation [ ] |
Grain | Identify the entire supply chain. | Agri-Digital [ ] |
Mango | Guarantee the traceability of the mango production chain. | IBM, Wal-Mart, Nestle, etc. [ ] |
High fructose corn syrup | Supervision and management. | The Coca-Cola Company |
Chicken | Ensure its traceability. | Gogochicken, OriginTrail Inc. [ ] |
Food waste | Monitoring and management, waste forecasting. | Plastic Bank, Agora Technology Labs |
Rice | Supervision and to ensure the quality of rice during transportation. | “Agri-Food Blockchain” Project [ ] |
Milk | Traceability to prevent food fraud in the dairy production process. | “Agri-Food Blockchain” Project |
Authors | Research Subjects | Expected Goals | Experimental Results |
---|---|---|---|
Arabameri et al. [ ] | Olive Oil | Prediction of the quality of olive oil samples and determination of the influence of other factors | Highly accurate prediction of olive oil quality and successful prediction of the effects of time, temperature, and phenolics on its stability |
Kaveh et al. [ ] | Potatoes, garlic, and cantaloupe | Predicted moisture diffusion rate and energy consumption ratio | Successful use of the ANFIS model for accurate prediction of its water content |
Mokarram et al. [ ] | Orange | Predicting orange flavor | Successful use of the ANFIS model for accurate prediction of orange flavor |
Abbaspour-Gilandeh et al. [ ] | Quince | Prediction of kinetic energy and energy of quince under hot air drying | Accurate prediction of kinetic energy of quince using the ANFIS model and multiple linear regression |
Kumar et al. [ ] | Taro | Optimization of the extraction process of taro | Successful optimization of extraction process of taro bioactive compounds using response surface methodology and ANFIS |
Ojediran JO et al. [ ] | Yam | Predicting the drying characteristics of yam | Accurate prediction of drying characteristics of yam slices in convective hot air desiccant using ANFIS |
Authors | Research Subjects | Objectives | Experimental Results |
---|---|---|---|
Lopes et al. [ ] | Barley flour | Forecast for barley flour | Classification using spatial pyramid segmentation method, the final prediction with SVM is 95% |
Siswantoro et al. [ ] | Eggs | Predicting egg volume | Successfully predicted egg volume with ANN model with a 97.38% success rate |
Villager-Aguilar et al. [ ] | Sweet pepper | Predicting the ripening status of bell peppers | Successfully developed an artificial vision system using CVS and ANN/FL to predict the ripeness of bell peppers with a maximum accuracy of 88% for FL and 100% for ANN |
Bakhshipour et al. [ ] | Iranian black tea and green tea | Classification of black and green teas in Iran | Successful classification of both with REP decision trees |
Mazen et al. [ ] | Banana | Predicting the ripening of bananas | Successfully used SVM and ANN algorithms to accurately predict the ripening level of bananas with an accuracy of 98% |
Wan et al. [ ] | Tomato | Predicting the ripeness of fresh tomatoes | Accurate detection of tomato ripeness with ANN algorithm with 99% accuracy |
Markande et al. [ ] | Potatoes | Grade classification of potatoes | A combination of CVS technology and fuzzy logic system successfully classifies potatoes and reduces costs |
Garcia et al. [ ] | Vegetable seeds | Sorting vegetable seeds | Successful classification of spinach seeds and cabbage seeds with ANN technology |
Ozkan et al. [ ] | Dry beans | Classification of different types of dry bean seeds | Successful classification of dry bean seeds with SVM, DT, ANN, and KNN algorithms |
Zareiforoush et al. [ ] | Rice | Grading the quality of rice | Successfully developed a system to grade rice quality with 97% accuracy |
Feature | Conventional Laboratory Instruments | Electronic Nose | Electronic Tongue | Computer Vision | Sensory Analysis |
---|---|---|---|---|---|
Fast detection | √ | √ | √ | √ | × |
Low-cost analysis | √ | √ | √ | √ | × |
Chemical free analysis | × | × | × | × | × |
Objectivity | √ | √ | √ | √ | × |
Non-destructive measurement | √ | √ | × | √ | √ |
Sample pre-treatment | × | × | √ | × | × |
simple | √ | √ | √ | √ | × |
Single operator | √ | √ | √ | √ | × |
Permanent data storage | √ | √ | √ | √ | √ |
The statements, opinions and data contained in all publications are solely those of the individual author(s) and contributor(s) and not of MDPI and/or the editor(s). MDPI and/or the editor(s) disclaim responsibility for any injury to people or property resulting from any ideas, methods, instructions or products referred to in the content. |
Share and Cite
Ding, H.; Tian, J.; Yu, W.; Wilson, D.I.; Young, B.R.; Cui, X.; Xin, X.; Wang, Z.; Li, W. The Application of Artificial Intelligence and Big Data in the Food Industry. Foods 2023 , 12 , 4511. https://doi.org/10.3390/foods12244511
Ding H, Tian J, Yu W, Wilson DI, Young BR, Cui X, Xin X, Wang Z, Li W. The Application of Artificial Intelligence and Big Data in the Food Industry. Foods . 2023; 12(24):4511. https://doi.org/10.3390/foods12244511
Ding, Haohan, Jiawei Tian, Wei Yu, David I. Wilson, Brent R. Young, Xiaohui Cui, Xing Xin, Zhenyu Wang, and Wei Li. 2023. "The Application of Artificial Intelligence and Big Data in the Food Industry" Foods 12, no. 24: 4511. https://doi.org/10.3390/foods12244511
Article Metrics
Article access statistics, further information, mdpi initiatives, follow mdpi.
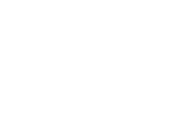
Subscribe to receive issue release notifications and newsletters from MDPI journals
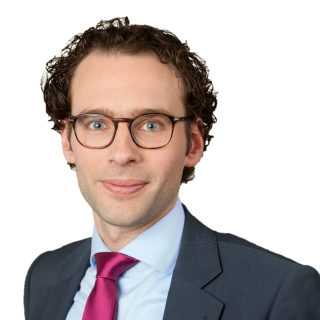
Senior Sector Economist, Commodities, Food & Agri
Food tech: Technology in the food industry
Technology is increasingly contributing to food’s journey from the farm to the fork. In this report, we look at the driving forces behind ‘food tech’ and the most promising food tech applications, robotics, data technology and novel processing techniques
Download pdf
Executive summary
- More and better food through food tech
Technology helps food manufacturers to produce more efficiently for a growing world population. Improving shelf life and food safety revolves around technology, and greater use of machines and software ensures affordability and consistent quality. The importance of technology for manufacturers continues to grow.
- Demanding customers, consumers and society
Food industry customers, like retailers, set stricter product requirements and require larger volumes at low prices. Higher efficiency and labour productivity are essential to remaining competitive. At the same time, consumers and society are demanding in terms of health and sustainability. Robotisation, digitalisation and novel processing methods enable companies to respond to this demand.
- Food industry embraces robotics
The rise of robotics in the food industry is a tangible example of food tech. The number of robots in the European food industry is well over 30,000, while the number of robots per 10,000 employees rose from 62 in 2013 to 84 in 2017. Although Germany is the largest market, robot density is relatively highest in Sweden, Denmark, the Netherlands and Italy.
- Impact of technology on the labour market
Robotisation and digitalisation increase the complexity of production processes and this impacts the labour market. Employees’ duties change and the required level of education increases. Applying food tech, therefore, requires both investments in capital goods and attention to training current staff and recruiting new staff.
The Role of Science, Technology and Innovation in Transforming Food Systems Globally
- Open Access
- First Online: 02 January 2023
Cite this chapter
You have full access to this open access chapter
- Robin Fears 5 &
- Claudia Canales 5
21k Accesses
1 Citations
6 Altmetric
Although much progress has been made in past decades, the prospects for food and nutrition security are now deteriorating and the converging crises of climate change and Covid-19 present major risks for nutrition and health, and challenges to the development of sustainable food systems. In 2018, the InterAcademy Partnership published a report on the scientific opportunities and challenges for food and nutrition security and agriculture based on four regional reports by academy networks in Africa, Asia, the Americas and Europe. The present chapter draws on new evidence from the regions reaffirming the continuing rapid pace of science, technology and innovation and the need to act urgently worldwide to capitalise on the new opportunities to transform food systems. We cover issues around sustainable, healthy food systems in terms of the whole food value chain, including consumption and waste, the interconnections between agriculture and natural resources, and the objectives for developing a more balanced food production strategy (for land and sea) to deliver nutritional, social and environmental benefits. Our focus is on science, and we discuss a range of transdisciplinary research opportunities that can underpin the UN FSS Action Tracks, inform the introduction of game-changers, and provide core resources to stimulate innovation, inform practice and guide policy decisions. Academies of science, with their strengths of scientific excellence, inclusiveness, diversity and the capacity to link the national, regional and global levels, are continuing to support the scientific community’s a key role in catalysing action. Our recommendations concentrate on priorities around building the science base – including the recognition of the importance of fundamental research – to generate diverse yet equitable solutions for providing sustainable, healthy diets that are culturally sensitive and attend to the needs of vulnerable populations. We also urge better use of the transdisciplinary science base to advise policymaking, and suggest that this would be greatly advanced by constituting an international advisory Panel for Food and Nutrition Security, with particular emphasis on sustainable food systems.
You have full access to this open access chapter, Download chapter PDF
Similar content being viewed by others
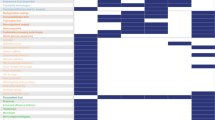
Innovation can accelerate the transition towards a sustainable food system
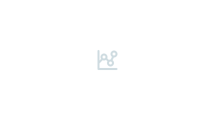
Contested agri-food futures: Introduction to the Special Issue
The Food Industry as a Partner for Public Health?
1 introduction: the transformation of food systems.
The world is not on track to meet the Sustainable Development Goal (SDG) targets linked to hunger and food and nutrition security. According to FAO data (FAO 2020 ), the number of hungry people has increased by 10% in the past 5 years and 3 billion people cannot afford a healthy diet. Some countries in Asia and Africa have made significant progress in increasing food and nutrition security alongside reducing poverty in the past decade, but others have not (EIU 2020 ). The risks continue to be compounded by the impacts of population growth, urbanisation, climate and other environmental changes, market instability and economic inequality. Furthermore, the Covid-19 pandemic has exacerbated problems and imposed disproportionate effects on the economically vulnerable, including marginalised groups in urban areas and smallholder farmers in rural areas (FAO 2020 ; EIU 2020 ). However, while there are unprecedented challenges, there are also unprecedented opportunities to capitalise on science, technology and innovation for the purpose of transforming food systems.
In 2018, the InterAcademy Partnership (IAP), the global network of more than 140 academies of science, engineering and medicine, published a global report on food and nutrition security and agriculture, drawing on information from four regional reports prepared by academy networks in Africa (NASAC), Asia (AASSA), the Americas (IANAS) and Europe (EASAC) and emphasising the value of taking a transdisciplinary approach. In the present chapter, we present an update on some of the issues from that global report linked to the assessments made in the chapters in this volume prepared by the regional academy networks for the UNFSS.
The work of the academies has adopted an integrative food systems approach that considers all points along the value chain, encompassing food processing, transport, retail, consumption, and recycling, as well as agricultural production. Moreover, in the transformation of food systems towards economic, social and environmental sustainability, setting agricultural priorities must take account of climate change and pressures on other critical natural resources, particularly water soil and energy, and the continuing need to avoid further loss in ecosystem biodiversity. Interest worldwide in the sustainability of food systems is accelerating (e.g., Global Panel 2020 ; IFPRI 2020 ; Food Systems Dashboard 2020 ; von Braun et al. 2021 ).
In this chapter, which covers the opportunities and challenges for food systems in tackling malnutrition in all its forms (undernutrition, micronutrient deficiencies, overweight and obesity), we frame the contribution that science can make to the local-global connectivity of food systems: (i) to strengthen and safeguard international public goods, i.e., those goods and services that have to be provided at a scale beyond that of individual countries or that can be better achieved collectively; (ii) to understand and tackle environmental and institutional risks in an increasingly uncertain world; and (iii) to help to address the SDGs by resolving complexities within evidence-based policies and programmes and their potential conflicts.
2 Regional Heterogeneity
Inevitably, in a summary of the global position, it is difficult to capture the diversity within and between regions relating to the challenges for food systems. The regional chapters are indicative of the territorial dimension in analysing obstacles to food and nutrition security, emphasising specific contexts for marginalised peoples and smallholder farmers, e.g., for the Hindu Kush Himalayan region (AASSA 2021 ). In Africa, although remarkable progress has been made over the last two decades in reducing extreme hunger, there are increasing pressures on food systems that require radical action (discussed in detail in NASAC 2021 ). Most African Union member states are not on track to achieve the Comprehensive Africa Agricultural Development Plan goals (African Union 2020 ). In the comprehensive publication on country-level data in the Americas that accompanied the regional report on food and nutrition security and agriculture (IANAS 2017 , regional update IANAS 2021 ), there was detailed discussion of diversities within the region and of variation in the social determinants of food and nutrition security, e.g., related to gender. Other regional assessments find moderate-severe food insecurity (SDG Indicator 2.1.2) across the FAO Europe-Central Asia region, varying from 6.7% in the EU to 19% in the Caucasus. Obesity throughout this region is higher than the world average, Footnote 1 a challenge that has been examined by EASAC ( 2021 ).
3 Agriculture-Environment Nexus
IAP defines the desired outcome for food systems as access for all to a healthy and affordable diet that is environmentally sustainably produced and culturally acceptable. The IAP report from 2018 cautioned that an emphasis on increasing total factor productivity (TFP, the efficiency in the use of labour, land, capital and other inputs) is not warranted if such a focus leads to reductions in environmental protection. Since then, there has been continuing interest in using research to leverage TFP for sustainable and resilient farming (e.g., Coomes et al. 2019 ). In particular, the paradox of productivity has been highlighted (Benton and Bailey 2019 ), whereby agricultural productivity may generate food system inefficiency. That is, productivity, when leading to the increased availability of cheaper calories, may help to promote obesity, although nutritional content matters as much as calories. Current global competition policies incentivise producers who can produce the most food for the least amount of money, typically with accompanying environmental damage, including biodiversity loss (Chatham House 2021 ). The strategic focus of research and development, as well as production systems, should shift from staple crops, with the current emphasis on production of a narrow range of calorie-intensive staples, to a balanced strategy for crops that are of more value in terms of nutritional, social and environmental benefits, including fruit, vegetables, seeds, nuts and legumes (as food and feed, NASAC 2021 ).
Reform of food systems requires decision-makers to recognise the interdependence of supply-side and demand-side (including dietary change and waste reduction) actions. There must be further consideration given to strengthening coherence between global agreements, e.g., on responsible investment, and national action (Chatham House 2021 ). And, the continuing food system sustainability challenge of balancing production objectives for agricultural exports with satisfying domestic food and nutrition requirements is an issue for some countries (e.g., IANAS 2021 ).
Current intensive agricultural production depends heavily on fertilisers, pesticides, energy, land and water, with negative consequences for environmental sustainability. Changing environmental conditions and competition for key resources such as land and water provoke violence and conflict, exacerbating the vicious circle of hunger and poverty (NASAC 2021 ). Discussion in the NASAC ( 2021 ) Policy Brief exemplifies some of the particular issues for managing water demand, including conservation and the recycling of waste water, and notes the opportunities for science, technology and innovation in new irrigation schemes. Research and innovation play a crucial role in the transformation to sustainable food systems that produce more efficiently by environmentally friendly means. The options for the convergence of technological and societal innovation (including outputs from biotechnology, AI, digitalisation, and from social and cognitive sciences), exemplified later in this chapter, help to underpin the objectives for sustainable food systems.
Agro-ecology encompasses various approaches to using nature-based solutions for regenerative agriculture innovation (HLPE 2019 ) and systems research is still needed to help strengthen the evidence base for agro-ecological (nature-based) approaches. For example, agroforestry in sub-Saharan Africa has the potential to help tackle health concerns associated with a lack of food and nutrition security (non-communicable diseases) and with human migration, but requires additional research to characterise any increased risk from infectious disease alongside the beneficial outcomes (Rosenstock et al. 2019 ).
Developing diverse and resilient production systems worldwide is important in preparing for the likelihood of cumulative threats from extreme weather events through spillover across multiple food sectors on land and sea (Cottrell et al. 2019 ). In this context, it is relevant to note the interest in the potential of oceans for sustainable economies in addressing food security, biodiversity and climate change. One of the UK Presidency’s core themes for UN FCCC COP26 is “Nature,” with objectives for sustainable land use, sustainable and resilient agriculture, and increasing ambition and awareness of the ocean’s potential. This potential is also of great importance for the UN FSS Action Track on nature-positive production. By contrast with difficulties in expanding land-based agriculture, the potential for the sustainable production of fish and other seafood is increasingly recognised (Lubchenco et al. 2020 ; Costello et al. 2020 ) and brings new possibilities for local livelihoods. Fish supplies provide 19% of the animal protein in African diets (Chan et al. 2019 ; NASAC 2021 ). However, currently, one-third of the world’s marine fish stocks are overfished (FAO 2020 ). Realising the potential of the oceans requires technological innovation and policy reform for fishery management and governance, to restore wild fish stocks, eliminate illegal and unregulated fishing, and ensure sustainable mariculture so as to minimise environmental impacts. Oceans can contribute to climate change mitigation as well as to improved food systems, but it is important to be aware of inadvertent consequences of policy action, e.g., adoption of industrial-scale aquaculture can be associated with rapid growth in GHGs (in China, Yuan et al. 2019 ). Genetic improvement of fish species may help to reduce the environmental footprint of aquaculture (for example, in Africa, where aquaculture has been expanding at a faster rate than in some other places, NASAC 2021 ). This exemplifies a general point about seeking co-ordinated policy across sectors to avoid unintended effects and negative trade-offs. Another example is provided by poorly-designed land use policies to increase bioenergy production, which drive increases in land rent with negative implications for food and nutrition security (Fujimori et al. 2019 ).
4 Delivering Healthy Diets, Sustainably Produced, Under Climate Change
An accumulating evidence base demonstrates that climate change exacerbates food insecurity in all regions by reducing crop yield and nutritional content and by posing additional food safety risks from toxins and microbial contamination (e.g., IPCC 2019 ; Park et al. 2019 ; Ray et al. 2019 ; Watts et al. 2021 ). The effects are most pronounced in those groups who are already vulnerable, e.g., children, because of reduced nutrient intake (Park et al. 2019 ) or a decline in dietary diversity (Niles et al. 2021 ). A systematic review of the literature identified climate change and violent conflict as the most consistent predictors of child malnutrition (Brown et al. 2020 ). By increasing the volatility of risks in the global food system, climate change may also reduce the incentive to invest (IAP 2018 ), and rising heat- and humidity-induced declines in labour productivity reduce the income of subsistence farmers (Andrews et al. 2018 ).
Although better international integration of food trade can be a key component of climate change adaptation at the global scale, it requires sensitive implementation to benefit all regions (Janssens et al. 2020 ): in hunger-affected export-oriented regions, partial trade integration may exacerbate food and nutrition insecurity by increasing exports at the expense of domestic food availability. When assessing trade implications, it is also important to appreciate that climate change presents a risk to global port operations, with the greatest risk being projected for ports located in the Pacific Islands, the Caribbean Sea, the Indian Ocean, the Arabian Peninsula and the African Mediterranean (Izaguirre et al. 2021 ).
There are twin, overarching challenges for food systems: how can they adapt to climate change and, at the same time, reduce their own contribution to it, including in regard to GHG emissions? These intertwined challenges are discussed in all of the regional assessments. Multiple scientific opportunities have been identified to adapt by developing climate-resilient agriculture, e.g., from the application of biosciences to breed improved crop varieties resistant to biotic and abiotic stresses, as well as for the social sciences to understand and influence the behaviour of farmers, manufacturers and consumers in responding to climate change (see, for example, EASAC 2021 ). Combining evidence-based measures will also be essential to mitigate GHG emissions from the sector (currently contributing approximately 30% of global GHGs, Watts et al. 2021 ), including improving agronomic practices, reducing waste, and shifting to diets with a lower carbon footprint. For example, a background paper prepared in 2020 for the Subsidiary Body for Scientific and Technological Advice (SBSTA) of UN FCCC COP Footnote 2 explored agronomic case studies (in South America, Asia, Africa and Europe) for managing nitrogen pollution (including the powerful GHG nitrous oxide) and improving manure management so as to decrease GHGs and benefit the environment. Capitalising on such research requires better connections between science and the broader community, along with relevant policy processes. There is particular need to dismantle obstacles to the transferability of practices and the scaling up of local research results to guide decision-making at the national and regional levels.
One major mitigation opportunity discussed by IAP ( 2018 ) and in all of the regional assessments relates to the potential to adjust dietary consumption patterns so as to reduce GHGs and, at the same time, gain significant potential health benefits (see Neufeld et al. 2021 for discussion of the definition of a healthy diet). For example, there is evidence that reducing red meat consumption, where it is excessive, can improve population health (Willett et al. 2019 ; systematic review of the literature in Jarmul et al. 2020 ). Red meat supplies only 1% of calories worldwide, while accounting for 25% of all land use emissions (Hong et al. 2021 ), though meat is an important source of protein, minerals and vitamins. The policies for reaching such consumption adjustments require more research to actually identify solutions. The proportion of excess deaths attributable to excess red meat consumption is highest in Europe, the Eastern Mediterranean, the Americas and the Western Pacific (Watts et al. 2021 ). However, some populations consume sustainable diets that are meat-based, e.g., the Inuit Indigenous People in the Canadian Arctic: proposals for dietary change must be carefully designed, evidence-based and culturally sensitive in being adapted to circumstances and protecting nutrient supplies for the most vulnerable groups. It should also be acknowledged that the efficiency of livestock production varies according to farming system, such that conclusions, e.g., about the sustainability of pastoral cattle production, may be different from those for feed-lot cattle production (Adeosogen et al. 2019 ; AASSA 2021 ), and that livestock may be the only agricultural activity possible in dryland regions that do not support the cultivation of crops.
Although Africa accounts for the smallest regional share of total anthropogenic GHG emissions, about half of this is linked to agriculture, and the continent is experiencing the fastest increase of all regions (Tongwane and Moeletsi 2018 ; Latin America and South East Asia are also demonstrating rapid growth, Hong et al. 2021 ). As part of the whole systems approach, formulation of mitigation solutions must decouple increases in livestock productivity (and cereal productivity, Loon et al. 2019 ) from increases in GHGs. Progress is being made (e.g., in China, Cui et al. 2018 ; AASSA 2021 ), and decoupling can be informed by better use of the research evidence available, e.g., for improving herd management and animal health, breeding new varieties (with better feed conversion and energy utilisation efficiencies), improving forage provision (e.g., NASAC 2021 ) and strengthening targeted social protection mechanisms, alongside more generic recommendations for dietary change (EASAC 2021 ).
There are unprecedented scientific opportunities coming within range, but there are also multiple obstacles to mainstreaming climate change solutions into food system development planning. Evaluation of obstacles in India (Singh et al. 2017 ) highlights the limited access to finance, difficulties in accessing research and education, and delays in accessing weather information. Systematic review of the literature on smallholder production systems in South Asia (Aryal et al. 2020 ) notes weaknesses in the institutional infrastructure for implementing and disseminating available solutions: the application of science requires institutional change. At the global scale, there is a need for enhanced access to climate information and services around climate-resilient food security actions (WMO 2019 ), e.g., to aid decisions on the most suitable crops and planting times.
5 Responding to Covid-19
Climate change and Covid-19 are converging crises for health in many respects (Anon 2021 ), including food and nutrition security. Observations early in the pandemic Footnote 3 indicated that the production of staple food crops during critical periods (planting and harvesting) was vulnerable to interruptions in labour supply; food processing, transport and retail were also affected early on, particularly the relatively perishable, nutritionally-important fresh fruit and vegetables (Ali et al. 2020 ). Subsequent comprehensive assessment of consequences for global food security (Swinner and McDermott 2020 ) has evaluated how adverse effects on local practice and routines are transmitted to longer-term impacts on poverty and food systems worldwide in increasingly interconnected trade and markets. In some cases, supply disruption has been aggravated by national decisions to restrict the export of food. Footnote 4 The combined effects of Covid-19 in regard to economic recession and food system disruption are particularly detrimental to the poor (Ali et al. 2020 ; Swinner and McDermott 2020 , which includes case studies in Ethiopia, China, Egypt and Myanmar; NASAC 2021 ). However, in some regions, food systems proved relatively resilient (IANAS 2021 ), and there are also examples of good practice in new safety net programmes, including school feeding programmes that should be more widely shared and implemented. Tackling the consequences for child malnutrition has been identified as a particular priority for action (Fore et al. 2020 ), as has attention to gender bias, whereby women are suffering more adverse effects as a consequence of Covid-19-changed household and community dynamics (Swinner and McDermott 2020 ).
As emphasised by EASAC ( 2021 ), the pandemic has exposed the vulnerability of over-reliance on just-in-time and lean delivery systems, globalised food production and distribution based on complex value chains. Therefore, opportunities for increasing the localisation of production systems should be re-examined. However, there is often a mismatch in the timescale needed to adapt to Covid-19 between the imperative for early action to protect vulnerable groups and the relatively slow policy responses (Savary et al. 2020 ). Capitalising on the scientific opportunities may help to minimise this mismatch, e.g., improving food safety and reducing post-harvest losses (IAP 2018 ), implementing evidence-based social protection measures and using Information and Communication Technologies for e-commerce, food supply resilience, early warning systems, and health delivery. Post-Covid-19 initiatives on novel foods, and urban and peri-urban farming systems, can also strengthen food supply chains and create new livelihoods for expanding urban populations, although it is also important to understand and manage inadvertent consequences for rural employment and the environment (Ali et al. 2020 ).
6 Using Science, Technology and Innovation to Promote and Evaluate Action
Continuing with business as usual will not meet the objectives for transformative change. To reaffirm a core message from IAP ( 2018 ): there is urgent need to use currently available evidence to strengthen policies and programmes, and to invest in initiatives to gain new knowledge. Examples of what is possible are discussed extensively elsewhere (e.g., Fanzo et al. 2020 ; Lillford and Hermansson 2020 ). Footnote 5 It is not the purpose here to provide a detailed assessment of transdisciplinary research priorities, but in Table 1 , we map some onto the UN FSS Action Tracks to emphasise new opportunities that are coming within range and the need for science to achieve its potential. Examples are illustrative, not comprehensive; more detail on these and other research priorities are provided in IAP ( 2018 ), the regional chapters and in Sects. 1 , 2 , 3 , and 4 of this chapter. There are also, of course, many interactions between research streams and objectives that cannot be captured in Table 1 .
Several general recommendations can be made:
There is a need to increase the commitment to invest in fundamental science, and then connect that to applications and align it all with development priorities. There is also an important priority to develop improved methodologies for understanding the levers of change, including the attributes of “game-changers.” That is, how to attribute outcomes and impact to investments chosen and scientific or other actions undertaken.
There are new opportunities to improve collaboration and coordination worldwide, as well as build partnerships among the public and private sectors, NGOs and other stakeholders to co-design and conduct research. Transdisciplinary approaches should be encouraged. There is increasing entrepreneurial activity worldwide, e.g., in the Latin America region, a wide range of start-up company activities includes novel foods, novel production systems, and novel approaches to the optimisation of water and other natural resources (IANAS 2021 ). There are also considerable opportunities in Africa for action on agriculture to stimulate economic growth, reducing poverty while also increasing food and nutrition security (Baumuller et al. 2021 ; NASAC 2021 ).
Training and mentoring the next generation of researchers worldwide is essential: academies of science have a key role in encouraging younger scientists.
Obstacles, especially in low- and middle-income countries, in the use and production of data and in the scaling up of applications must be addressed. For example, although big data/mobile-based communications bring significant benefits (e.g., IANAS 2021 ; NASAC 2021 ) and there have been advances in using mobile technology to deliver climate services for agriculture in Africa (Dayamba et al. 2018 ), more should be done to increase access for small-scale farmers (Mehrabi et al. 2021 ). A digital inclusion agenda is needed for governments and the private sector to increase access to data-driven agriculture.
In addition to generating excellent science, it is vital to reduce the delay in translating research outputs into innovation, public policy and practice (IAP 2018 ). Time lags may arise from negative attitudes associated with perceived risks, from excessive regulatory requirements in some countries or from an absence of regulation in others. This leads to fragmentation in the capture of benefits. For example, there is current heterogeneity in considering whether new plant-breeding techniques – such as those based on genome editing – should be included within older legislation governing genetically modified organisms. Scientific advances are occurring worldwide, e.g., collaborative work in Colombia, Germany, France, the Philippines and the USA to develop rice that is resistant to bacterial blight (Oliva et al. 2019 ; IANAS 2021 ). The controversy created by a situation in which regulatory frameworks are disconnected from robust science is discussed by EASAC ( 2021 ). Figure 1 demonstrates the resulting incoherence that acts to deter science, innovation and competitiveness, creates non-tariff barriers to trade and undermines collective action to enhance food and nutrition security. This may have particular adverse consequences for those already suffering malnutrition; for example, the acceptance of gene-based technologies has been mixed in Africa, even though there may be considerable scientific opportunities for using biotechnology in crop breeding programmes to increase resistance to biotic and abiotic stress and to improve nutrient content and nitrogen use efficiency (NASAC 2021 ).
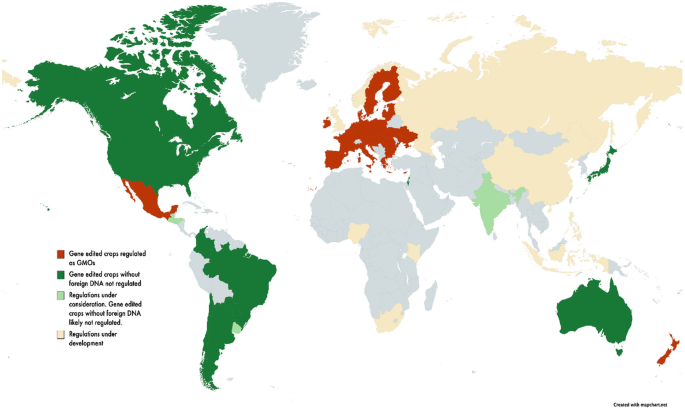
Variation in the regulation of genome editing for plant breeding
7 Strengthening the Contribution of Research to Policymaking
Alongside action to accelerate investment in agriculture and food systems research (von Braun et al. 2020 ), there must be transdisciplinary integration of priorities at the science-policy interface across all relevant sectors (Fears et al. 2019 ), including agriculture, the environment, health and social care, rural and urban development, and fiscal policy. There must also be linkage of policy at the local, regional and global levels (Fears et al. 2020 ), while taking account of local values and circumstances and recognising the challenges for coordination. One recent example from Asia (Islam and Kieu 2020 ) of developing critical mass in regional policy for climate change and food security discusses criteria for successive steps in policy planning, implementation, cooperation and legal obligation, and observes that the latter two steps often present fundamental barriers to moving from the priorities in a national development agenda to regional coherence. In the African region, the recent Joint Ministerial Declaration and Action Agenda (AU 2020 ) calls upon governments to build greater productive capacity in agriculture and strengthen resilience throughout Africa’s agri-food systems.
Scaling efforts for critical mass requires individual countries to recognise that their policy decisions may have an impact on other countries and regions. For example, some countries export their lack of environmental sustainability by increasing food imports from elsewhere (IAP 2018 ).
Academies and others within the scientific community (STCMG 2020 ) have a key role in overcoming obstacles to effective policy by working together across disciplines to show the value of an inclusive approach, e.g., to the SDGs. Moreover, systematic review of the literature indicates that public support for a policy can be increased by communicating evidence of its effectiveness (Reynolds et al. 2020 ; Fears et al. 2020 ). Therefore, the work of academies in using the evidence base to inform policy development and implementation can help to provide the bridge between policymakers and the public.
What are the implications for the UN FSS? UN FSS discussions have highlighted the place of “game-changers” in driving transformative action, and the scientific community has much to contribute in exploring the potential of game-changers to underpin transformation at the science-policy interface (see AASSA 2021 ). For example, a recent commentary on Action Track 1 Footnote 6 identified some key precepts that can be illustrated by academies’ work at the regional and global levels (Table 2 ).
We suggest that there is an additional game-changer, applicable to all Action Tracks: the development of a new international science advisory Panel on Food and Nutrition Security (IAP 2018 ), with a broad remit for food systems, focused on shaping policy choices and strengthening governance mechanisms. A new Panel, recognising the new opportunities and challenges for food system governance, could help to streamline research efficiency in its linkage to policy action and increase the legitimacy of that science advice by using robust assessment procedures (Global Panel 2020 ). The impetus created by the UN FSS requires the coordination and management of food systems by more sectors of government and stakeholders than had been the case for food security, creating an unprecedented opportunity to develop a framework for greater transparency, accountability and the sharing of knowledge. By consolidating the present myriad, fragmented, array of panels and advisory committees, the proposed international advisory Panel could draw on the large scientific community already working on these topics – including academies – and should be asked to address the most pressing issues for transformative change in the face of the mounting global challenges. Food and nutrition security, particularly for high-risk groups, must be a top priority on every country’s national agenda, yet many countries do not have a national security strategy in place (EIU 2020 ). Furthermore, as already noted, advisory capacities, governance policies, and institutions are sometimes weak at the regional level (AASSA 2021 ; NASAC 2021 ). Thus, in addition to building the critical mass for evaluating complex issues at the global scale, an international advisory Panel could help to drive momentum for a national food system strategy in all countries and engender regional-level initiatives in policy development and implementation.
IAP recommends that the UN FSS now consider options for constituting a new international advisory Panel, so as to make best use of the rapid advances in science, technology and innovation, and to motivate evidence-based policymaking at all levels. IAP and its regional academy networks are eager to be involved.

8 Conclusions
Achieving food and nutrition security worldwide by transforming food systems remains a major challenge, compounded by recent pressures from climate change and the Covid-19 pandemic. Actions to promote food systems are relevant to multiple SDGs. It is essential to identify opportunities for synergies and trade-offs while avoiding inadvertent negative consequences, and to engage everybody, in order to enable change. This requires advances in complex food system modelling.
Food systems are diverse and heterogeneous. Continuing research is needed to inform diverse yet equitable solutions for sustainable, healthy diets that are culturally sensitive, focusing on vulnerable groups. That calls for stronger connections between local and international research entities. The opportunities for complex and innovative remote sensing and web-based data should also be explored for this purpose.
Greater transdisciplinarity is needed in research to progress from the current scientific agenda, which is still too often focused on individual components of food systems or on agriculture separate from its environmental context. Social science research must be better integrated with other disciplines, e.g., to understand and inform consumer, farmer and manufacturer behaviours and to guide policies to deliver objectives for social justice. The development of improved methodologies for understanding the attribution of impact is also a critical research priority.
Science is a public good, yet the conduct and use of basic and other research is often fragmented. There is still much to be done to build critical mass worldwide, to share skills and a research infrastructure, and to collaborate in agreeing upon and addressing research priorities and avoiding unnecessary duplication. There is a continued convening role for academies of science to facilitate the exploration of opportunities and tackle the obstacles to research collaboration between disciplines and between the public and private research communities.
There are also opportunities to improve science-policy interfaces and integrate policy development at the local, regional and global levels. One game-changer would be to constitute an international advisory Panel on Food and Nutrition Security with new emphasis on food systems to make better use of the best science to inform, motivate and implement evidence-based policymaking at all levels.
FAO ( 2020 ) “Sustainable food systems and healthy diets in Europe and Central Asia.” ERC/20/2, on www.fao.org/3/nc226en/nc2262n.pdf . This report discusses multiple issues around diversified and sustainable food systems, improving supply chains and reducing food loss and waste.
SBSTA 52nd Session 2020. “Improved nutrient use and manure management towards sustainable and resilient agricultural systems”. FCCC/SB/2020/1.
CGIAR’s response to COVID-19. www.cgiar.org/news-events/all-news/our-response-to-covid-19
International Monetary Fund “Policy responses to COVID-19”. https://www.imf.org/en/Topics/imf-and-covid-19/Policy-Responses-to-COVID-19
See also repositories of recent literature, e.g., Sustainable solutions to end hunger ( https://www.nature.com/collections/dhiggjeagd ); Sustainable nutrition ( https://www.nature.com/collections/fibbgbiebc ); and Socio-technical innovation bundles for agri-food transformation ( https://www.nature.com/documents/Bundles_agrifood_transformation.pdf ).
Haddad, L. 2021 “Food systems “game changers”: reflections so far”, on https://un-food-systems.medium.com/food-systems-game-changers-reflections-so-far-d4c8200c5663
AASSA (2021) Regional brief for UN FSSS
Google Scholar
Adesogan AT, Havelaar AH, McKune SL, Eilitta M, Dahl GE (2019) Animal source foods: sustainability problem or malnutrition and sustainability solution? Perspective matters. Glob Food Secur 25:100325. https://doi.org/10.1016/j.gfs.2019.100325
Article Google Scholar
Ali Z, Green R, Zougmore RB et al (2020) Long-term impact of West African food system responses to COVID-19. Nat Food 1:768–770
Article CAS Google Scholar
Andrews O, Le Quere C, Kjellstrom T, Lemke B, Haines A (2018) Implications for workability and survivability in populations exposed to extreme heat under climate change: a modelling study. Lancet Planet Health 2:e540–e547
Anon. (2021) Climate and COVID-19 converging crises. Lancet 397:71
Aryal JP, Sapkota TB, Khurana R, Khatri-Chhetri A, Rahut DB, Jat ML (2020) Climate change and agriculture in South Asia: adaptation options in smallholder production systems. Environ Dev Sustain 22:504505075
AU (African Union) (2020) Joint virtual meeting of the African Ministers responsible for agriculture, trade and finance on the impact of COVID-19 on food and nutrition security in Africa, 27 July 2020. Joint Ministerial Declaration and Action Agenda. AU, Addis Ababa
Basso B, Antle J (2020) Digital agriculture to design sustainable agricultural systems. Nat Sustain 3:254–256
Baumüller K, Admassie A, Hendriks S, Tadesse G, von Braun J (eds) (2021) From potentials to reality: transforming Africa’s food production – investment and policy priorities for sufficient, nutritious and sustainable food supplies. Peter Lang Publ. (Forthcoming, an earlier draft is available at https://www.zef.de/fileadmin/downloads/ZEF_Akademiya2063.pdf )
Benton TG, Bailey R (2019) The paradox of productivity: agricultural productivity promotes food system inefficiency. Glob Sustain 2:e6
Brown ME, Backer D, Billing T et al (2020) Empirical studies of factors associated with child malnutrition: highlighting the evidence about climate and conflict shocks. Food Secur. https://doi.org/10.1007/s12571-020-01041-y
Chan C, Tran N, Pethiyagoda S, Crissman C, Sulser T, Phillips M (2019) Prospects and challenges of fish for food security in Africa. Glob Food Sec 20:17–25
Chatham House (2021) Food system impacts on biodiversity. ISBN: 978 1 78413 433 4
Coomes OT, Barham BL, MacDonald GK, Ramankutty N, Chavas J-P (2019) Leveraging total factor productivity growth for sustainable and resilient farming. Nat Sustain 2:22–28
Costello C, Cao L, Gelcich S et al (2020) The future of food from the sea. Nature 588:95–100
Cottrell RS, Nash KL, Halpern BS et al (2019) Food production shocks across land and sea. Nat Sustain 2:130–137
Cuevas RP, de Guia A, Demont M (2017) Developing a framework of gastronomic systems research to unravel drivers of food choice. Int J Gastron Food Sci 9:86–99
Cui Z, Zhang H, Chen X et al (2018) Pursuing sustainable productivity with millions of smallholder farmers. Nature 555:363–366
Dayamba DS, Ky-Dembele C, Bayala J et al (2018) Assessment of the use of Participatory Integrated Climate Services for Agriculture (PICSA) approach by farmers to manage climate risk in Mali and Senegal. Clim Serv 12:27–35
EASAC (2021) Regional brief for UN FSSS
Economist Intelligence Unit (2020) 2020 Global food security index. https://foodsecurityindex.eiu.com/index
Eker S, Reese G, Obersteiner M (2019) Modelling the drivers of a widespread shift to sustainable diets. Nat Sustain 2:725–735
Fanzo J, Covic N, Dobermann A et al (2020) A research vision for food systems in the 2020s: defying the status quo. Glob Food Sec 26:100397
FAO (2019) Tracking progress on food and agriculture-related SDG indicators. FAO
FAO (2020) State of food security and nutrition in the world. FAO
Fears R, ter Meulen V, von Braun J (2019) Global food and nutrition security needs more and new science. Sci Adv 5:eaba2946
Fears R, Canales Holzeis C, ter Meulen V (2020) Designing inter-regional engagement to inform cohesive policy making. Palgrave Commun 6:107
Food systems Dashboard (2020) A food systems framework. https://foodsystemsdashboard.org/about-food-system
Fore HH, Dongyu O, Beasley DM, Ghebreyesus TA (2020) Child malnutrition and COVID-19: the time to act is now. Lancet 396:517–518
Fujimori S, Hasegawa T, Krey V et al (2019) A multi-model assessment of food security implications of climate change mitigation. Nat Sustain 2:386–396
Global Panel on Agriculture and Food systems for Nutrition (2020) Future food systems: for people, our planet, and prosperity. Foresight 2.0
High Level Panel of Experts on Food Security and Nutrition (2019) Agroecological and other innovative approaches for sustainable agriculture and food systems that enhance food security and nutrition. Committee on World Food Security
Hodson De Jaramillo E, Henry G, Trigo E (2019) La Bioeconomía. Nuevo marco para el crecimiento sostenible en América Latina/Bioeconomy. New Framework for Sustainable Growth in Latin America. Editorial Pontificia Universidad Javeriana, Bogotá. ISBN 978-958-781-378-4. Available at https://repository.javeriana.edu.co/handle/10554/43705
Hong C, Burney JA, Pongratz J et al (2021) Global and regional drivers of land-use emissions in 1961-2017. Nature 589:554–561
IANAS (2017) Challenges and opportunities for food and nutrition security in the Americas. The view of the academies of sciences
IANAS (2021). Regional brief for UN FSS
IAP (2018) Opportunities for future research and innovation on food and nutrition security and agriculture. The InterAcademy Partnership’s global perspective
IFPRI (2020) Building inclusive food systems. Global Food Policy Report
IPCC (2019) Climate change and land. In: An IPCC special report on climate change, desertification, land degradation, sustainable land management, food security, and greenhouse gas fluxes in terrestrial ecosystems
Islam MS, Kieu E (2020) Tackling regional climate change impacts and food security issues: a critical analysis across ASEAN, PIF, and SAARC. Sustainability 12:883
Izaguirre C, Losada IJ, Camus P, Vigh JL, Stenek V (2021) Climate change risk to global port operations. Nat Clim Chang 11:14–20
Jain M, Singh B, Rao P et al (2019) The impact of agricultural interventions can be doubled by using satellite data. Nat Sustain 2:931–934
Janssens C, Havlik P, Krisztin T et al (2020) Global hunger and climate change adaptation through international trade. Nat Clim Chang 10:829–835
Jarmul S, Dangour AD, Green R et al (2020) Climate change mitigation through dietary change: a systematic review of empirical and modelling studies on the environmental footprints and health effects of “sustainable diets”. Environ Res Lett 15:123014
Kongerslev TT, Bertram HC, Bonjour J-P et al (2017) Whole dairy matrix or single nutrients in an assessment of health effects: current evidence and knowledge gaps. Am J Clin Nutr 105:1033–1045
Laar A, Barnes A, Aryeetey R et al (2020) Implementation of healthy food environment policies to prevent nutrition-related non-communicable diseases in Ghana: national experts’ assessment of government action. Food Policy 93:101907
Lillford P, Hermansson A-M (2020) Global missions and the critical needs of food science and technology. Trends Food Sci Technol. https://doi.org/10.1016/j.tifs.2020.04.009
Lubchenco J, Haugan P, Pangestu ME (2020) Five priorities for a sustainable ocean economy. Nature 588:30–32
Mabhaudhi T, Vimbayi V, Chimonyo G et al (2019) Prospects of orphan crops in climate change. Planta 250:695–708
Mehrabi Z, McDowell MJ, Ricciardi V et al (2021) The global divide in data-driven farming. Nat Sustain 4:154–160
NASAC (2021) Regional brief for UN FSS
Neufeld LM, Hendriks S, Hugas M (2021) Healthy diet: a definition for the United Nations Food Systems Summit 2021. Scientific Group Report. https://sc-fss2021.org
Niles MT, Emery BF, Wiltshire S et al (2021) Climate impacts associated with reduced diet diversity in children across nineteen countries. Environ Res Lett 16:015010
Nuffield Council on Bioethics (2019) Meat alternatives. Bioethics Briefing Note
Oliva R, Ji C, Atienza-Grande G et al (2019) Broad-spectrum resistance to bacterial blight in rice using genome editing. Nat Biotechnol 37:1344–1350
Park CS, Vogel E, Larson LM et al (2019) The global effect of extreme weather events on nutrient supply: a superposed epoch analysis. Lancet Planet Health 3:e429–e438
Pironen S, Etherington TR, Borrell JS et al (2019) Potential adaptive strategies for 29 sub-Saharan crops under future climate change. Nat Clim Chang 9:758–763
Ray DK, West PC, Clark M et al (2019) Climate change has likely already affected global food production. PLoS One. https://doi.org/10.1371/journal.pone.0217148
Reynolds JP, Stautz K, Pilling M et al (2020) Communicating the effectiveness and ineffectiveness of government policies and their impact on public support: a systematic review with meta-analysis. R Soc Open Sci 7. https://doi.org/10.1098/rsos.190522
Rosenstock TS, Dawson IK, Aynekulu E et al (2019) A planetary health perspective on agroforestry in sub-Saharan Africa. One Earth 1:330–344
Savary S, Akter S, Almekinders C et al (2020) Mapping disruption and resilience mechanisms in food systems. Food Secur 12:695–717
Scientific and Technological Community Major Group (2020) Position paper on the theme of the 2020 High-Level Political Forum. https://council.science/wp-content/uploads/2020/06/Position-Paper-STC-29_June.pdf
Singh NP, Arathy A, Pavithra S et al (2017) Mainstreaming climate change adaptation into development planning, Policy Paper 32. ICAR – National Institute of Agricultural Economics and Policy Research, New Delhi
Singh BK, Trivedi P, Egidi E et al (2020) Crop microbiome and sustainable agriculture. Nat Rev Microbiol 18:601–602
Smith J, Andersson G, Gourlay R et al (2016) Balancing competing policy demands: the case of sustainable public sector food procurement. J Clean Prod 112:249–256
Swinnen J, McDermott J (eds) (2020) COVID-19 and global food security. IFPRI
Thorrez L, Vandenburg H (2019) Challenges in the quest for “clean meat”. Nat Biotechnol 37:215–216
Tongwane MI, Moeletsi ME (2018) A review of greenhouse gas emissions from the agriculture sector in Africa. Agric Syst 166:124–134
van Loon MP, Hijbeek R, ten Berge HFM et al (2019) Impacts of intensifying or expanding cereal cropping in sub-Saharan Africa on greenhouse gas emissions and food security. Glob Chang Biol. https://doi.org/10.1111/gcb.14783
von Braun J, Chichaibelu BB, Torero CM, Laborde D, Smaller C (2020) Ending hunger by 2030 – policy actions and costs. ZEF Policy Brief
von Braun J, Afsano K, Fresco L, Hassan M, Torero M (2021) Food systems – definition, concept and application for the UN Food Systems Summit. Scientific Group Report. https://sc-fss2021.org
Watts N, Amann M, Arnell N et al (2021) The 2020 report of The Lancet Countdown on health and climate change: responding to converging crises. Lancet 397:129–170
Willett W, Rockstrom J, Loken B et al (2019) Food in the Anthropocene: the EAT-Lancet Commission on healthy diets from sustainable food systems. Lancet 393:447–492
WMO (2019) 2019 State of climate services. Report WMO-No. 1242
Yuan J, Xiang J, Liu D et al (2019) Rapid growth in greenhouse gas emissions from the adoption of industrial-scale aquaculture. Nat Clim Chang 9:318–322
Download references
Acknowledgements
This IAP Brief was drafted by Robin Fears and Claudia Canales in discussion with Volker ter Meulen. We thank Sheryl Hendriks (NASAC), Elizabeth Hodson (IANAS) and Paul Moughan (AASSA) for their helpful advice.
Author information
Authors and affiliations.
European Academies’ Science Advisory Council’s (EASAC) Biosciences Programme, Halle (Saale), Germany
Robin Fears & Claudia Canales
You can also search for this author in PubMed Google Scholar
Corresponding author
Correspondence to Claudia Canales .
Editor information
Editors and affiliations.
Center for Development Research, University of Bonn, Bonn, Nordrhein-Westfalen, Germany
Joachim von Braun
James P Grant School of Public Health, BRAC University, Dhaka, Bangladesh
Kaosar Afsana
Wageningen University and Research, Wageningen, Gelderland, The Netherlands
Louise O. Fresco
The World Academy of Sciences for the Advancement of Science in Developing Countries (TWAS), Trieste, Italy
Mohamed Hag Ali Hassan
Rights and permissions
Open Access This chapter is licensed under the terms of the Creative Commons Attribution 4.0 International License ( http://creativecommons.org/licenses/by/4.0/ ), which permits use, sharing, adaptation, distribution and reproduction in any medium or format, as long as you give appropriate credit to the original author(s) and the source, provide a link to the Creative Commons license and indicate if changes were made.
The images or other third party material in this chapter are included in the chapter's Creative Commons license, unless indicated otherwise in a credit line to the material. If material is not included in the chapter's Creative Commons license and your intended use is not permitted by statutory regulation or exceeds the permitted use, you will need to obtain permission directly from the copyright holder.
Reprints and permissions
Copyright information
© 2023 The Author(s)
About this chapter
Fears, R., Canales, C. (2023). The Role of Science, Technology and Innovation in Transforming Food Systems Globally. In: von Braun, J., Afsana, K., Fresco, L.O., Hassan, M.H.A. (eds) Science and Innovations for Food Systems Transformation. Springer, Cham. https://doi.org/10.1007/978-3-031-15703-5_44
Download citation
DOI : https://doi.org/10.1007/978-3-031-15703-5_44
Published : 02 January 2023
Publisher Name : Springer, Cham
Print ISBN : 978-3-031-15702-8
Online ISBN : 978-3-031-15703-5
eBook Packages : Biomedical and Life Sciences Biomedical and Life Sciences (R0)
Share this chapter
Anyone you share the following link with will be able to read this content:
Sorry, a shareable link is not currently available for this article.
Provided by the Springer Nature SharedIt content-sharing initiative
- Publish with us
Policies and ethics
- Find a journal
- Track your research
Thank you for visiting nature.com. You are using a browser version with limited support for CSS. To obtain the best experience, we recommend you use a more up to date browser (or turn off compatibility mode in Internet Explorer). In the meantime, to ensure continued support, we are displaying the site without styles and JavaScript.
- View all journals
- Explore content
- About the journal
- Publish with us
- Sign up for alerts
- Review Article
- Open access
- Published: 09 October 2018
Review of the sustainability of food systems and transition using the Internet of Food
- Nicholas M. Holden 1 ,
- Eoin P. White 2 ,
- Matthew. C. Lange ORCID: orcid.org/0000-0002-6148-7962 3 &
- Thomas L. Oldfield 1
npj Science of Food volume 2 , Article number: 18 ( 2018 ) Cite this article
34k Accesses
53 Citations
44 Altmetric
Metrics details
- Agriculture
Environmental impact
Many current food systems are unsustainable because they cause significant resource depletion and unacceptable environmental impacts. This problem is so severe, it can be argued that the food eaten today is equivalent to a fossil resource. The transition to sustainable food systems will require many changes but of particular importance will be the harnessing of internet technology, in the form of an ‘Internet of Food’, which offers the chance to use global resources more efficiently, to stimulate rural livelihoods, to develop systems for resilience and to facilitate responsible governance by means of computation, communication, education and trade without limits of knowledge and access. A brief analysis of the evidence of resource depletion and environmental impact associated with food production and an outline of the limitations of tools like life cycle assessment, which are used to quantify the impact of food products, indicates that the ability to combine data across the whole system from farm to human will be required in order to design sustainable food systems. Developing an Internet of Food, as a precompetitive platform on which business models can be built, much like the internet as we currently know it, will require agreed vocabularies and ontologies to be able to reason and compute across the vast amounts of data that are becoming available. The ability to compute over large amounts of data will change the way the food system is analysed and understood and will permit a transition to sustainable food systems.
Similar content being viewed by others
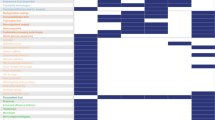
Innovation can accelerate the transition towards a sustainable food system
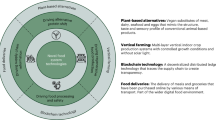
A systematic scoping review of the sustainability of vertical farming, plant-based alternatives, food delivery services and blockchain in food systems
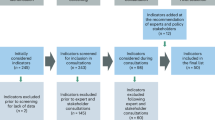
The state of food systems worldwide in the countdown to 2030
Introduction.
The food we eat today is unsustainable for two reasons: the food system causes unacceptable environmental impacts and it is depleting non-renewable resources. Our food can be regarded as ‘fossil food’ because its production relies on fossil fuel, non-renewable mineral resources, depletion of groundwater reserves and excessive soil loss. The idea of sustainable food systems is at the heart of global efforts to manage and regulate human food supply. 1 The sustainable development goals focus on a number of critical global issues, but Goal 2 (‘end hunger, achieve food security and improved nutrition and promote sustainable agriculture’), Goal 12 (‘ensure sustainable consumption and production patterns’) and Goal 13 (‘take urgent action to combat climate change and its impacts’) are intimately related to the need to transition global food systems from fossil to sustainable. To understand how to meet the challenge presented by these goals, it is necessary to consider what is meant by ‘sustainable’ in the context of a food system. In 1989, the Food and Agriculture Organisation (FAO) council defined sustainable development as ‘the management and conservation of the natural resource base, and the orientation of technological and institutional change in such a manner as to ensure the attainment and continued satisfaction of human needs for present and future generations. Such sustainable development (in the agriculture, forestry and fisheries sectors) conserves land, water, plant and animal genetic resources, is environmentally non-degrading, technically appropriate, economically viable and socially acceptable’. 2 The important ideas in this definition are working within the planetary boundary (‘the natural resource base’), having a future-proof system (‘continued satisfaction’, ‘present and future generations’), limiting impacts to those manageable by the buffering capacity of the planet (‘environmentally non-degrading’), considering the financial needs of business stakeholders (‘economically viable’) and compatible with local needs and customs (‘socially acceptable’).
Five principles have been identified to support a common vision for sustainable agriculture and food. 3 These are: (1) resource efficiency; (2) action to conserve, protect and enhance natural resources; (3) rural livelihood protection and social well-being; (4) enhanced resilience of people, communities and ecosystems; and (5) responsible governance. The aim of this paper is to outline the case for why food systems are not sustainable and to define the case for using technology, specifically internet technologies (hardware and software combined to make the ‘Internet of Food’) to enable the transition of the food system from fossil to sustainable. Increasing population, increasing consumption, a billion malnourished people and agriculture that is concurrently degrading land, water, biodiversity and climate on a global scale 4 combine to indicate that the fossil food systems we currently rely on are not fit-for-purpose. It is suggested that halting agricultural expansion, closing yield gaps, increasing efficiency, changing diets and reducing waste could lead to a doubling of food production with reduced environmental impacts of agriculture. 4 To achieve these changes, it is going to be necessary to harness internet technology, in the form of an ‘Internet of Food’, which offers the chance to use global resources more efficiently, to stimulate rural livelihoods, to develop systems for resilience and to facilitate responsible governance by means of computation, communication, education and trade without limits of knowledge and access.
The concept of ‘Internet of Food’ first appeared in peer-reviewed literature in 2011 (based on a search of scopus.com using ‘Internet of Food’ as the search term). It was described by the idea of food items having an ‘IP identify’, which raised the question of how this might influence our eating habits. 5 Their focus was very much on how the technology could influence food choices and predicted that by 2020 it would be possible to monitor and control food objects remotely through the Internet. It is this technological control of the food system that has real potential to help societal stakeholders (consumers, retailers, processors, producers, shareholders, landowners, indigenous peoples and so on) to engage in the transition of our food system from being fossil to sustainable. The ubiquitous physical tagging and sensing of mass and energy flow in the food system linked to a formal semantic web will allow computation over the whole system to answer questions such as: What was the resource depletion of this product? What is the social impact of eating this product? What food safety procedures have been employed for this product? What and where has wealth been created by the value chain of this product? When these questions can be answered for specific instances of food product types and predicted for new products, then it will be possible to determine whether a specific food system is sustainable or not. The stakeholders demanding answers to these questions are likely to be governance and policy makers and consumers. When these questions can be answered, it will be possible to plan how to manage the evolution of the fundamental life support system (food) from fossil to sustainable in order to support a growing global population.
Current food systems
To understand the need for a systematic transformation of the food system, it is necessary to detail exactly why it is unsustainable. An overwhelming case can be made for the environmental dimension of the system, but there are also social and economic issues as well. This paper will focus the environmental case (resource depletion and adverse environmental impact that relate to the ‘continued satisfaction’ and ‘environmentally non-degrading’ criteria for sustainable food systems), but similar cases can be made for important social and economic issues as well.
Resource depletion
The resource depletion case can be made with respect to energy, nutrients, water, soil and land. Each will be summarised in turn. To date, the agri-food system has converted non-renewable fossil fuel energy into food by enabling mechanisation, amplified fertiliser production, improved food processing and safe global transportation. 6 According to FAO, 7 the agri-food sector accounts for around 30% of the world’s total energy consumption, with Europe alone accounting for 17% of gross energy consumption in 2013. 8 Agriculture, including crop cultivation and animal rearing, is the most energy-intense phase of the food system, accounting for nearly one third of the total energy consumed in the food production chain. 9 To date, renewable energy has had limited penetration of the agri-food sector with fossil fuels accounting for almost 79% of the energy consumed by the food sector. 8 From an energy perspective, the food system can be regarded as unsustainable (cannot meet the ‘continued satisfaction’ requirement) due to its reliance on fossil energy sources.
By the end of the 20th Century, it was estimated that US-produced ammonia represented 32% of fertiliser nitrogen (N) demand, which was produced by extracting N from the atmosphere as ammonia by a process using hydrogen from natural gas (fossil fuel). 9 The vast majority of N fertilisers consumed today are still created using fossil fuels and cannot be regarded as sustainable until such times as new technological approaches emerge, which are currently in their infancy. 10 , 11 A review of mineral fertiliser reserves concluded that potash reserves (the source of most potassium (K) fertilisers) are of great concern and that it is time to start evaluating other sources of K for agriculture 12 but others concluded that ‘modern agriculture is currently relying on a non-renewable resource and future phosphate rock is likely to yield lower quality P at a higher price’. 13 If significant physical and institutional changes are not made to the way we currently use and source P, agricultural yields will be severely compromised in the future. Estimates for when world peak P will be reached range from 2027 14 to 2033. 13 Variations in estimations of when peak P will occur are due the constant changing of reserve levels. 12 The ‘power imbalance’ where just three countries controlling >85% of the known global phosphorus reserves, 15 a concentration of power far greater than that of crude oil, is also of concern, and it has been concluded that the combined impact increasing demand, dwindling reserves and geopolitical constraints could result in reduced production and supply of chemical P fertilisers and increased global P price. 16 It is clear that over time horizons of around 50 years the agri-food system is going to face a major nutrient crisis unless reliance of fossil mineral resources is significantly reduced and ultimately eliminated. From a nutrient management point-of-view, the food system can be regarded as unsustainable (cannot meet the ‘continued satisfaction’ requirement) due to its reliance on fossil mineral resources.
Modern food production is reliant on irrigation to a great extent, which according to the UN water programme, accounts for 70% of freshwater withdrawals worldwide. 17 Excessive removal of groundwater for irrigation is leading to rapid depletion of aquifers in key food-producing regions, such as North-Western India, the North China Plain, Central USA and California. 18 Aquifers replenish so slowly that they are effectively a non-renewable resource. The depletion of these large freshwater stocks threatens food production and security locally and globally via international food trade. Non-sustainable groundwater abstraction contributed to 20% of global gross irrigation water demand in the year 2000 with this demand having tripled over the period 1960–2000. 19 For many countries, irrigation is sustained by non-renewable groundwater, and it has been highlighted that ‘a vast majority of the world’s population lives in countries sourcing nearly all their staple crop imports from partners who deplete groundwater to produce these crops’. 18 Countries who both produce and import food irrigated from rapidly depleting aquifers are particularly at risk, such as USA, Mexico, Iran and China. It has been estimated that India, soon to be the most populous country in the world, will be unable to meet water requirements within 300 years and emerging pressures may reduce this time horizon considerably. 20 Given the interaction of water supply with energy, this situation may become even worse. For example, in California, 20% of electricity production is used for moving and pumping water for agriculture, 21 and as water becomes more difficult to access, the energy demand will increase. From a water management point-of-view, the food system can be regarded as unsustainable (cannot meet the ‘continued satisfaction’ requirement) due to its reliance on non-renewable water resources.
Over 20 years ago, it was estimated that around one third of the world’s agricultural land had been lost to erosion and the rate of loss was about 10 Mha/year 22 Calculations suggest that soil erosion rates under ploughed cultivation are one to two orders of magnitude greater than soil production rates. 23 This rate of soil loss is not compatible with the ‘continued satisfaction’ requirement for a sustainable food system. It is also linked with other environmental impacts, such as loss of carbon, gaseous emissions, non-point source pollution and sedimentation of waterways, 24 therefore it is not compatible with the ‘environmentally non-degrading’ criteria as well. Given projections for expansion of dryland areas to around 50% of total land surface, with 78% of dryland expansion in areas supporting 50% of population growth in the coming decades, 25 the control of soil erosion and its related impacts is going to be a major requirement for sustainable food systems. From a soil management perspective, the food system can be regarded as unsustainable (cannot meet the ‘continued satisfaction’ requirement).
Having considered the energy, nutrient, water, soil and land requirements for food production, it must be concluded that the food system is unsustainable and needs to change because the natural resource base, future satisfaction and environmentally non-degrading requirements cannot be met. It is reasonable to describe food as ‘fossil food’ because of the reliance of non-renewable (and rapidly depleting) resources to supply much of the world’s population. A complete transformation of the food system is required, one that can perhaps be best driven by harnessing appropriate technology to monitor, control and regulate the different types of food system by unleashing the potential benefits of being able to compute over the vast amounts of data that can be obtained from the activities along the food value chain.
Modern industrial agriculture was made possible through land clearing and habitat disruption. Some recognised consequences of this were fragmentation and loss of biodiversity, significant greenhouse gas (GHG) emissions from land clearing and adverse impact on marine and freshwater ecosystems. 26 An estimate suggests that the global food system, from fertiliser manufacture to food storage and packaging, is responsible for up to one third of all human-caused GHG emissions. 27 Using data from 2005, 2007 and 2008, agricultural production is also estimated to be responsible for a significant share of GHG emissions from the food system, releasing ~12,000 Mt CO 2 e/year representing about 86% of all food-related anthropogenic GHG emissions, followed by fertiliser manufacture at ~575 Mt CO 2 e/year and refrigeration at ~490 Mt CO 2 e/year. 28 The impacts of such emissions are already being felt 29 including negative feedbacks on crop yield and health. Reducing this impact will be critical to transitioning from unsustainable fossil food to a sustainable future-proof food system. 28 , 30
The eutrophication of surface waters has become an endemic global problem. 31 From the 1950s to the 1990s, agriculture was associated with a 6.87-fold increase in nitrogen fertilisation, a 3.48-fold increase in phosphorus fertilisation, a 1.68-fold increase in the amount of irrigated cropland and a 1.1-fold increase in land in cultivation. 26 Agricultural production has been identified as a major underlying and persistent cause of eutrophication in many catchments around the world 32 , 33 Nutrient loadings from agriculture are a major driver of water quality deterioration, but it is unclear what level of on-farm control is necessary to achieve water quality improvements. 31 Smart agriculture and precision farming will drive improvement by increasing resource use efficiency and by harnessing technology to determine current conditions, future weather conditions and the correct intervention. 34 , 35
Similar cases can be made for acidification, 36 biodiversity, 37 ecosystem toxicity 38 and other environmental impacts. 39 Taking just the limited number of examples presented above, it is clear that the ‘environmentally non-degrading’ requirement for a sustainable food system is not being met by current food supply systems and a radical change is needed. From an environmental perspective (resource depletion and adverse impact), it can be concluded that food systems are not sustainable (in general), and if we work from a strong sustainability perspective of working within planetary boundaries, 40 they cannot become sustainable until this adverse situation is rectified.
Life cycle thinking methods and the need for an Internet of Food
Life cycle thinking is increasingly being used to assess food system sustainability. 41 It is an approach used to assess products, processes or services in terms of their place in the world, the full life cycle that is required for them to serve human society and environmental, social and economic consequences of that service. The method has been recognised as the leading approach for including sustainability in decision-making in the United States of America, 42 Europe 43 and elsewhere in the world. The quantitative tool used to implement life cycle thinking is life cycle assessment (LCA), which is formalised by international standard (ISO 14040/14044) 44 and has been widely used to assess food production systems. 45 LCA is one of the most important methodologies used to assess the impact (pollution and resource depletion) of the food system by using mass and energy flow accounting to model the system and agreed scientific models to calculate resource depletion and specific types of environmental impacts.
It has been suggested that LCA can lead to practitioners focus on the ‘eco-efficiency’ of inherently unsustainable products, and this can lead to increased consumption, because of the LCA paradigm of reducing negatives rather than increasing positives 46 The cradle-to-cradle (C2C) concept tends to focus more on linking resource consumption and waste creation with sustainability status rather than minimisation of specific impacts. One conclusion is that the best attributes of both approaches should be harnessed. 46 All such methods (e.g. LCA, C2C) depend on being able to collect sufficient data to characterise a system of interest or the use of publicly funded or commercial databases when site-specific data are not available. It was noted that ‘the practicality of adopting LCA to support decision-making can be limited by the generic nature of the assessment and the resource-intensive nature of data collection and life cycle inventory modelling’, 47 which is the key limitation for developing tools to facilitate the transition from fossil to sustainable food. The need to share data between stakeholders in increasingly important for the creation of useful information about the food system.
A number of issues associated with using LCA to better understand and manage food systems have been noted, 41 including (i) the variability of food production, supply chain and consumption globally; (ii) uncertainty related to the specification of data 48 and the system; 49 (iii) identifying the boundary between technosphere and ecosphere because agriculture relies on exploiting the ecosphere; 50 (iv) correctly identifying the real function 51 of the food system in order to select the most useful functional unit; (v) the multi-functionality of the system; (vi) capturing or modelling inventory data (which requires cooperation between stakeholders for food system applications); (vii) the geo-temporal specificity of background data from LCA databases; (viii) capturing the role of different stakeholders (e.g. consumers, government, industry); (ix) the role of diet choices and (x) handling ‘waste’. These issues are seen in the lack of comparability of LCA studies of the same type of product. 52 Furthermore, the scope of LCA as a global tool to quantify environmental impacts over the whole life cycle creates limitations. 53 LCA by its nature, focusses on the global scale and on steady-state, linear homogenous modelling, making it ‘very difficult to include varying spatial and temporal characteristics and nonlinear characteristics of large numbers of processes that occur all over the world’. 53 There are inherent limitations of inventory because of loss of spatial, temporal, dose–response and threshold information, which reduced the accuracy of impact assessments. 54 The ‘Internet of Food’ would transform our understanding of the food system and how they are modelled using LCA, provided data sharing is possible. Of the issues affecting food LCA, 41 most could be directly addressed by the ability to collect data and compute across the whole food chain: variability, uncertainty, multi-functionality, inventory data, databases, stakeholder influence, diet and waste, and the other two, boundary and function, could probably be better understood based on discernible activity. The examples of data mining of U.S. Environmental Protection Agency (EPA) data sets, 47 potential for avoiding excessive simplification 55 and use of big data in industrial ecology 56 indicate that this is the way forward.
Internet of Food: an enabling technology for the transition from fossil to sustainable
The deployment of sensor networks in the food system have historically been stage-specific and typically designed for monitoring and decision-making at a specific site and time, despite the potential for system integration having been recognised more than a decade ago. 57 Many sensors have been developed that could be used for the food chain, for example, for soil monitoring, for precision agriculture purposes, 58 for post-harvest storage monitoring, 59 for process control, 60 for retail logistics monitoring 61 and in some cases for domestic use. 62 A key requirement to create an ‘Internet of Food’ will be to make the data from these sensors interoperable and to be able to compute across the data set they create. A notable limitation is lack of integration caused by the current mix of open and closed data, communications, hardware standards and a lack of willingness to share data between stakeholders. It has been noted that an ‘…ontology-driven architecture for developing hybrid systems [that] consists of various entities including software components, hardware components (sensors, actuators and controllers), datastores (knowledge base, raw data, metadata), biological elements (plants[or animals]) and environmental context…’ 63 would permit the development of precision agriculture applications, and by logical extension this is required to utilise information across the whole food system (i.e. the Internet of Food). The proposal here is that the ‘hybrid-system’ needs to be extended to cover the whole food system, thus permitting production, process, logistics, retail, purchasing, consumption, nutrition and health outcomes to be integrated through information and computation. Where it is not possible to integrate data of the whole system that delivers a product, it will be very difficult to use Internet of Food for best advantage because its strength is determined by the data available.
A critical requirement will be the development of related ontologies. An ontology is the formal naming of concepts (e.g. types, properties, inter-relationships) within a domain and it is used to describe or infer properties of that domain. In order to be able to draw upon a range of data sources (sensors) and databases (knowledge silos), it is necessary to label data with unique identifiers that permit computers to reason with or compute over those data sets. This is where the real value of Internet of Things, and more specifically Internet of Food lies. To achieve the paradigm shift from fossil food to sustainable food systems, such a shift is needed, facilitated by the ability to reason with such data. As noted, 63 an ontology-driven architecture is needed to enable the ‘Internet of Food’. Ecologists have recognised the importance of big data in ecological research 64 in order to address major scientific and societal issues, and to answer the major question facing food (how to achieve a sustainable food system?), an agreed vocabulary and language structure (ontology) is needed. To take simple examples, the word ‘buttermilk’ originally referred to liquid left after churning butter is now also used to describe a fermented or cultured milk drink, so until the language describing these two concepts is standardised it is not possible to compute from diverse data sets within the domain of dairy processing, never mind across domains, where words such as slurry, matrix and texture all mean very different things depending on context. A noted rapid growth of Internet of Things requires standardisation to lower the entry barriers for the new services, to improve interoperability of systems and to allow better services performance. 65 They noted that this is particularly important for security, communication and identification where interoperability, and particularly semantic interoperability, will be critical. It has been recognised that a proliferation of ad hoc coded data systems will be an impediment to developing data-centric systems that can transform farming, 66 so sharing of data, agreement of standards and stakeholder cooperation will be required to achieve food systems transformation.
Food ontologies can be used with the specific aim of identifying gaps and for purposes beyond the initial, relatively simple applications, such as recognising foods, 67 with a contextual focus on diet, food selection, health and wellbeing being possible, 68 which is a critical component of a sustainable food system, and just as important are the social, economic and environmental impacts and benefits. There are untold opportunities to develop specific services targeted in these areas as well as the potential for integration, with tools such as life cycle sustainability assessment to evaluate the true sustainability of specific food products, meal combinations, whole diets and food systems. These ideas have been evaluated in the context of mining U.S. EPA data for assessing chemical manufacturing, 47 which identified that automating data access was a challenge because the data are incompatible with semantic queries. Data need to be described using ontologies to relate those data that need to be linked and to introduce LCA concepts to the descriptions. A framework for integrating ‘big data’ with LCA has been suggested 69 and it was also noted that development of semantic web standards for ecological data have greatly enhanced interoperability in that domain. 70 The same is required for the food system. It has been suggested that when food (and water) domain descriptors have been developed, this will enable ‘IT support [for] improved production, distribution and sales of foodstuffs [and water]’, 71 but the development of the domain models for the food chain is perhaps not a task for commerce or industry, rather for public, international research.
The opportunities that will be created by the Internet of Food are immense. One important shift will be from a descriptive, inferential approach to analysing food systems to a ‘big data’ approach. 68 ‘Big data’ can be characterised in terms of volume (data sets too large for conventional database management), velocity (acquiring, understanding and interpreting data in real time) and variety (the vast array of sources and types of data beyond the conventional rows and columns of numbers describing transactions). 72 Examples have already emerged where ‘big data’ has been used to provide data useful for LCA including agricultural resource survey 73 and resource use and emissions associated with U.S. electric power generation. 74 It is worth pointing out that much of the data relied on for LCA studies is drawn from commonly used databases (e.g. EcoInvent, ELCD, NREL) and are reliant on ‘small data’ and limited observations, which has resulted in reported error (multiple orders of magnitude), 47 while ‘big data’ offers a means to answering questions about environmental impact or food safety that simply cannot be contemplated in the context of controlled experiments. 71
Authors have considered ‘big data’ and ‘internet of things’ in the context of specific parts of the food chain. For example, ‘big data’ in ‘smart farming’ (i.e. the production stage of the food system) is now being used to make predictive insights about farm operations, to support operational decisions, to redesign business processes and to change business models. 75 To leverage this value at the farm level required extension along the food chain beyond the farm, but two scenarios are emerging: closed proprietary systems and open collaborative systems, 74 such as Food Industry Intelligence Network, 76 Food Innovation Network 77 and European Institute of Innovation & Technology (EIT) Food. 78 Priority should be given to development of data and applications infrastructure and at the same time to organisational issues concerning governance and business models for data sharing. 74 In the context of circular economy (i.e. the end of life, non-consumed part of the food system), it was found that, despite the concept of circular economy being discussed for decades, it has not become an adopted business model. 79 An analysis of literature from 2006 to 2015 found only 70 publications at the intersection of circular economy and ‘big data’/‘internet of things’, but nearly half (34) had been published in 2015. 75 It was suggested that technology encompassed by ‘big data’ and ‘internet of things’ is what is needed to enable such change, 75 which is the same argument being put forward here for the Internet of Food in the context of sustainable food systems. Two implications of relevance for the Internet of Food are: there is a gap between scientific research and corporate initiatives, which needs to be closed, and the search of literature was limited by the keywords available, and more specifically the lack of structured taxonomy to describe the circular economy. It is reasonable to conclude that if these ideas are relevant to one small component of the Internet of Food, then they are probably relevant to the concept as a whole.
These two recent reviews highlight the importance of developing the Internet of Food as a precompetitive platform on which business models can be built, much like the internet as we currently know it, and to achieve this we need to define agreed vocabularies and ontologies to be able to reason and compute across the vast amounts of data that are and will be available in the future. The ability to compute over large amounts of data will change the way the food system is analysed and understood. Biological scientists have noted how important data curation is, because as curated data become available the way science is conducted changes. 80 A key requirement of data curation is the connection of data from different sources in a human- and machine-comprehensible way. Another key change is the processing of multiple sources of complex data (‘big data’) using inference programs. While this might lead to new ways of conducting experimental (hypothesis driven) research, it is also unlocking the door to data-driven research, i.e. extracting new knowledge and understanding from data without experimentation or preconceived ideas, and providing new management approaches based on information and better decision-making capabilities. 71
The Internet of Food offers substantial opportunities for understanding the limits and constraints to sustainable food systems and thus supporting decisions about the transition from fossil to sustainable food. It is essential that all stakeholders engage with the development of Internet of Food to ensure harmonious development of a technology that can be used for both pre-competitive applications and commercial exploitation, if it is to be fully developed over the coming 5–10 years. In addition to the technical issues highlighted here, there are considerations of data ownership, privacy, ethical use of data, market control and other application domains (e.g. food safety, traceability, personal nutrition, security, fraud and policy) that need to be developed with stakeholder contributions alongside the technical advances considered here.
Conclusions
In order to transition to a sustainable food system, we need specific technology infrastructure to allow high-quality data to be collected about the food system that will permit the best possible decision-making. Key requirements are: standard vocabularies and ontologies to allow integration of data sets across the internet; proliferation of low cost sensing to allow orders of magnitude change in the supply of empirical observation data into LCA models; and new analytical methods to collate, curate, analyse and utilise data across the whole food production system. We need an Internet of Food to monitor conditions and analyse data to derive knowledge that can be combined with the means to implement control of the system to enable a step change in how we think about food systems. This technology will give us the chance to transition from fossil food to sustainable food systems.
United Nations. Transforming our world: the 2030 Agenda for Sustainable Development, Resolution adopted by the General Assembly on 25 September 2015, available at https://www.un.org/ga/search/view_doc.asp?symbol=A/RES/70/1&Lang=E , Accessed 16 Oct 2017.
The State of Food and Agriculture 1998 (Food and Agriculture Organization of the United Nations, Rome, 1998).
Building a Common Vision for Sustainable Food and Agriculture: Principles and Approaches (Food and Agriculture Organization of the United Nations, Rome, 2014).
Foley, J. A. et al. Solutions for a cultivated planet. Nature 478 , 337–342 (2011).
Article CAS Google Scholar
Kouma, J. P. & Lui, L. Internet of Food. In 2011 IEEE International Conferences on Internet of Things, and Cyber, Physical and Social Computing . https://doi.org/10.1109/iThings/CPSCom.2011.120 , 2011.
Kucukvar, M. & Samadi, H. Linking national food production to global supply chain impacts for the energy-climate challenge: the cases of the EU-27 and Turkey. J. Clean. Prod. 108 , 395–408 (2015).
Article Google Scholar
FAO. Energy Smart Food for People and Climate - Issue Paper (Food and Agriculture Organization of the United Nations, Rome, 2011).
Google Scholar
Monforti-Ferrario, F. et al. Energy Use in the EU Food Sector: State of Play and Opportunities for Improvement (Publications Office, Luxembourg, 2015).
Jasinski, S. M., Kramer, D. A., Ober, J. A. & Searls, J. P. Fertilizers—sustaining global food supplies. United States Geological Survey Fact Sheet FS-155-99 (1999).
Zou, Z., Ye, J., Sayama, K. & Arakawa, H. Direct splitting of water under visible light irradiation with an oxide semiconductor photocatalyst. Nature 414 , 625–627 (2001).
Kudo, A. & Miseki, Y. Heterogeneous photocatalyst materials for water splitting. Chem. Soc. Rev. 38.1 , 253–278 (2009).
Manning, D. A. C. How will minerals feed the world in 2050? Proc. Geologists Assoc. 126 , 14–17 (2015).
Cordell, D., Drangert, J.-O. & White., S. The story of phosphorus: global food security and food for thought. Glob. Environ. Change 19 , 292–305 (2009).
Mohr, S. & Evans, G. Projections of future phosphorus production. Philica - The Instant, Open access Journal of Everything (2013).
Elser, J. & Bennett, E. Phosphorus cycle: a broken biogeochemical cycle. Nature 478 , 29–31 (2011).
Chowdhury, R. B., Moore, G. A., Weatherley, A. J. & Arora, M. Key sustainability challenges for the global phosphorus resource, their implications for global food security, and options for mitigation. J. Clean. Prod. 140 , 945–963 (2017).
Water and Jobs - The United Nations World Water Development Report 2016 (United Nations Educational, Scientific and Cultural Organization, Paris, 2016).
Dalin, C., Wada, Y., Kastner, T. & Puma., M. J. Groundwater depletion embedded in international food trade. Nature 543 , 700–704 (2017).
Wada, Y., Van Beek, L. P. H. & Bierkens, F. P. M. Nonsustainable groundwater sustaining irrigation: a global assessment. Water Resourc. Res. https://doi.org/10.1029/2011WR010562 (2012).
Goswami, P. & Nishad, S. Virtual water trade and time scales for loss of water sustainability: a comparative regional analysis. Sci. Rep. 5 , 1 (2015).
Schwabe, K., Knapp, K. & Luviano, I. In Competition for Water Resources - Experiences and Management Approaches in the US and Europe (eds Ziolkowska, J. & Peterson, J.) Ch. 2.1.2 (Elsevier, Cambridge, United States, 2017).
Pimentel, D. et al. Environmental and economic costs of soil erosion and conservation benefits. Science 267.5201 , 1117–1123 (1995).
Montgomery, D. R. Soil erosion and agricultural sustainability. Proc. Natl. Acad. Sci. 104 , 13268–13272 (2007).
Lal, R. Soil degradation by erosion. Land Degrad. Dev. 12 , 519–539 (2001).
Huang, J., Yu, H., Guan, X., Wang, G. & Guo, R. Accelerated dryland expansion under climate change. Nat. Clim. Change 6 , 166–171 (2015).
Tilman, D. Global environmental impacts of agricultural expansion: the need for sustainable and efficient practices. Proc. Natl. Acad. Sci. 96.11 , 5995–6000 (1999).
Gilbert, N. One-third of our greenhouse gas emissions come from agriculture. Nature (31 Oct 2012).
Vermeulen, S. J., Campbell, B. M. & Ingram., J. S. Climate change and food systems. Annu. Rev. Environ. Resour. 37 , 195–222 (2012).
Intergovernmental Panel on Climate Change [Core Writing Team, Pachauri, R. K. & Meyer, L]. (2014) CLIMATE CHANGE 2014 Synthesis Report Summary for Policymakers . https://www.ipcc.ch/pdf/assessment-report/ar5/syr/AR5_SYR_FINAL_SPM.pdf , Accessed 01 Oct 2018.
Thornton, P. K. Recalibrating food production in the developing world: global warming will change more than just the climate. Handle Proxy 2012. Web. 15 June 2017.
Withers, P., Neal, C., Jarvie, H. & Doody, D. Agriculture and eutrophication: where do we go from here? Sustainability 6 , 5853–5875 (2014).
Carpenter, S. R. Eutrophication of aquatic ecosystems: bistability and soil phosphorus. Proc. Natl. Acad. Sci. 102 , 10002–10005 (2005).
Moss, B. Water pollution by agriculture. Philos. Trans. R. Soc. Lond. B Biol. Sci. 363 , 659–666 (2008).
Ruiz-Garcia, L., Lunadei, L., Barreiro, P. & Robla, I. A review of wireless sensor technologies and applications in agriculture and food industry: state of the art and current trends. Sensors 9 , 4728–4750 (2009).
Rawat, P., Singh, K. D., Chaouchi, H. & Bonnin, J. M. Wireless sensor networks: a survey on recent developments and potential synergies. J. Supercomput. 68 , 1–48 (2013).
Bouwman, A. F., Van Vuuren, D. P., Derwent, R. G. & Posch, M. A global analysis of acidification and eutrophication of terrestrial ecosystems. Water Air Soil Pollut. 141 , 349–382 (2002).
Green, R. E. Farming and the fate of wild nature. Science 307 , 550–555 (2005).
Nagajyoti, P. C., Lee, K. D. & Sreekanth, T. V. M. Heavy metals, occurrence and toxicity for plants: a review. Environ. Chem. Lett. 8.3 , 199–216 (2010).
Foley, J. A. Global consequences of land use. Science 309 , 570–574 (2005).
Rockström, J. et al. Planetary boundaries: exploring the safe operating space for humanity. Ecol. Soc. 14 , 32 (2009).
Notarnicola, B., Sala, S., Anton, A., Mclaren, S. J., Saouter, E. & Sonesson, U. The role of life cycle assessment in supporting sustainable agri-food systems: a review of the challenges. J. Clean. Prod. 140 , 399–409 (2017).
National Research Council. Sustainability Concepts in Decision-Making: Tools and Approaches for the US Environmental Protection Agency (The National Academies Press, Washington, DC, 2014). https://doi.org/10.17226/18949 .
Wolf M.-A., Pant, R., Chomkhamsri, K., Sala, S. & Pennington, D. The International Reference Life Cycle Data System (ILCD) Handbook (European Commission, Joint Research Centre, Ispra, Italy, 2012).
ISO. Environmental Management: Life Cycle Assessment- Illustrative Examples on How to Apply ISO14044 to Impact Assessment Situations (International Organization for Standardization, Geneva, 2012).
Roy, P. et al. A review of life cycle assessment (LCA) on some food products. J. Food Eng. 90 , 1–10 (2009).
Bjørn, A. & Michael, Z. H. Absolute versus relative environmental sustainability. J. Ind. Ecol. 17 , 321–332 (2012).
Cashman, S. A. et al. Mining available data from the united states environmental protection agency to support rapid life cycle inventory modeling of chemical manufacturing. Environ. Sci. Technol. 50 , 9013–9025 (2016).
Groen, E. A. & Heijungs, R. Ignoring correlation in uncertainty and sensitivity analysis in life cycle assessment: what is the risk? Environ. Impact Assess. Rev. 62 , 98–109 (2017).
Li, T., Zhang, H., Liu, Z., Ke, Q. & Alting, L. A system boundary identification method for life cycle assessment. Int. J. Life Cycle Assess. 19 , 646–660 (2013).
Tillman, A.-M., Ekvall, T., Baumann, H. & Rydberg, T. Choice of system boundaries in life cycle assessment. J. Clean. Prod. 2 , 21–29 (1994).
Cooper, J. S. Specifying functional units and reference flows for comparable alternatives. Int. J. Life Cycle Assess. 8 , 337–349 (2003).
Yan, M.-J., Humphreys, J. & Holden, N. M. An evaluation of life cycle assessment of european milk production. J. Environ. Manag. 92 , 372–379 (2011).
Udo de Haes, H. A., Heijungs, R., Suh, S. & Huppes, G. Three strategies to overcome the limitations of life-cycle assessment. J. Ind. Ecol. 8.3 , 19–32 (2004).
Owens, J. W. Life-cycle assessments: constraints on moving from inventory to impact assessment. J. Ind. Ecol. 1 , 37–50 (1997).
Hellweg, S., Mila, L. & Canals, I. Emerging approaches, challenges and opportunities in life cycle assessment. Science 344 , 1109–1113 (2014).
Xu, M., Cai, H. & Liang, S. Big data and industrial ecology. J. Ind. Ecol. 19 , 205–210 (2015).
Wang, N., Zhang, N. & Wang, M. Wireless sensors in agriculture and food industry—recent development and future perspective. Comput. Electron. Agric. 50 , 1–14 (2006).
Corwin, D. L. & Lesch, S. M. Application of soil electrical conductivity to precision agriculture. Agron. J. 95 , 455 (2003).
Pérez-Marín, D., Paz, P., Guerrero, J.-E., Garrido-Varo, A. & Sánchez., M.-T. Miniature handheld NIR sensor for the on-site non-destructive assessment of post-harvest quality and refrigerated storage behavior in plums. J. Food Eng. 99 , 294–302 (2010).
Woodcock, T., Fagan, C. C., O’Donnell, C. P. & Downey, G. Application of near and mid-infrared spectroscopy to determine cheese quality and authenticity. Food Bioprocess Technol. 1 , 117–129 (2007).
Abad, E. et al. RFID smart tag for traceability and cold chain monitoring of foods: demonstration in an intercontinental fresh fish logistic chain. J. Food Eng. 93 , 394–399 (2009).
Chi, P.-Y. (Peggy)., Chen, J.-H., Chu, H.-H. & Lo, J.-L. Enabling calorie-aware cooking in a smart kitchen. In Persuasive Technology . PERSUASIVE 2008 (eds Oinas-Kukkonen, H., Hasle, P., Harjumaa, M., Segerståhl, K. & Øhrstrøm, P.) 116–127 (Springer, Berlin, Hiedelberg, 2008).
Goumopoulos, C., Achilles, D. K. & Cassells, A. An ontology-driven system architecture for precision agriculture applications. Int. J. Metadata Semant. Ontol. 4 , 72 (2009).
Hampton, S. et al. Big data and the future of ecology. Front. Ecol. Environ. 11 , 156–162 (2013).
Xu, L. D., He, W. & Li, S. Internet of Things in industries: a survey. IEEE Trans. Ind. Inform. 10 , 2233–2243 (2014).
Iftikhar, N., Pedersen, T. B. Flexible exchange of farming device data. Comput. Electron. Agric. 75 , 52–63 (2011).
Boulos, M., Yassine, A., Shirmohammadi, S., Namahoot, C. & Brückner, M. Towards an “Internet of Food”: food ontologies for the Internet of Things. Future Internet 7 , 372–392 (2015).
Kouma, J.-P. & Liu, L. Internet of Food. In International Conference on Internet of Things and 4th International Conference on Cyber , Physical and Social Computing (eds. Xia, F., Chen, Z., Pan, G., Yang, L. T. & Ma, J.) (IEEE Computer Society, Los Alamitos, California, Washington, Tokyo, 2011). https://ieeexplore.ieee.org/stamp/stamp.jsp?tp=&arnumber=6142168 , Accessed 01 Oct 2018.
Cooper, J., Noon, M., Jones, C., Kahn, E. & Arbuckle, P. Big data in life cycle assessment. J. Ind. Ecol. 17 , 796–799 (2013).
Hampton, S. E. et al. Big data and the future of ecology. Front. Ecol. Environ. 11 , 156–162 (2013).
Zander, J. & Mosterman, P. J. Computation for Humanity: Information Technology to Advance Society (CRC, Taylor & Francis Group, Boca Raton, 2014).
Sonka, S. Big data and the Ag sector: more than lots of numbers. Int. Food Agribusiness Manage. Rev. 17 , 2014.
ARMS Farm Financial and Crop Production Practices. USDA ERS - ARMS Farm Financial and Crop Production Practices . Web. 07 June 2017.
Emissions & Generation Resource Integrated Database (eGRID). EPA. Environmental Protection Agency . 01 June 2017. Web. 07 June 2017.
Wolfert, S., Ge, L., Verdouw, C. & Bogaardt, M.-J. Big data in smart farming – a review. Agric. Syst. 153 , 69–80 (2017).
Food Industry Intelligence Network, available at http://www.campdenbri.co.uk/news/fiin.php , Accessed 25th May 2018
Food Innovation Network, available at http://foodinnovationnetwork.co.uk , Accessed 29th March 2018.
European Institute of Innovation & Technology Food, available at http://www.eitfood.eu , Accessed 29th March 2018.
Nobre, G. C. & Tavares, E. Scientific literature analysis on big data and internet of things applications on circular economy: a bibliometric study. Scientometrics 111 , 463–492 (2017).
Howe, D. et al. Big data: the future of biocuration. Nature 455 , 47–50 (2008).
Download references
Acknowledgements
The authors would like to acknowledge the support and funding from the UCD Institute of Food and Health, UC Davis, Food for Health Institute and the IC3-Foods Conference.
Author information
Authors and affiliations.
UCD School of Biosystems and Food Engineering, University College Dublin, Belfield, Dublin, 4, Ireland
Nicholas M. Holden & Thomas L. Oldfield
Orbas CTR Ltd, Dublin, Ireland
Eoin P. White
Department of Food Science and Technology, University of California, Davis, CA, 95616, USA
Matthew. C. Lange
You can also search for this author in PubMed Google Scholar
Contributions
The ideas and initial draft for this paper were drawn together by N.M.H. and consolidated at the inaugural IC3-Foods Conference in UC Davis, November 2016, following extensive discussion with M.C.L. T.L.O. and E.P.W. contributed to background research and additional draft text. M.C.L. refined the technology discussion.
Corresponding author
Correspondence to Nicholas M. Holden .
Ethics declarations
Competing interests.
The authors declare no competing interests.
Additional information
Publisher's note: Springer Nature remains neutral with regard to jurisdictional claims in published maps and institutional affiliations.
Rights and permissions
Open Access This article is licensed under a Creative Commons Attribution 4.0 International License, which permits use, sharing, adaptation, distribution and reproduction in any medium or format, as long as you give appropriate credit to the original author(s) and the source, provide a link to the Creative Commons license, and indicate if changes were made. The images or other third party material in this article are included in the article’s Creative Commons license, unless indicated otherwise in a credit line to the material. If material is not included in the article’s Creative Commons license and your intended use is not permitted by statutory regulation or exceeds the permitted use, you will need to obtain permission directly from the copyright holder. To view a copy of this license, visit http://creativecommons.org/licenses/by/4.0/ .
Reprints and permissions
About this article
Cite this article.
Holden, N.M., White, E.P., Lange, M.C. et al. Review of the sustainability of food systems and transition using the Internet of Food. npj Sci Food 2 , 18 (2018). https://doi.org/10.1038/s41538-018-0027-3
Download citation
Received : 31 January 2018
Revised : 10 September 2018
Accepted : 12 September 2018
Published : 09 October 2018
DOI : https://doi.org/10.1038/s41538-018-0027-3
Share this article
Anyone you share the following link with will be able to read this content:
Sorry, a shareable link is not currently available for this article.
Provided by the Springer Nature SharedIt content-sharing initiative
This article is cited by
Estimating the carbon footprint of mexican food consumption based on a high-resolution environmentally extended input-output model.
Environmental Science and Pollution Research (2024)
Nutritional-environmental trade-offs in potato storage and processing for a sustainable healthy diet
- Aubin Payne
- Ebenezer M. Kwofie
- Jamie I. Baum
npj Science of Food (2023)
Mapping the consumer foodshed of the Kampala city region shows the importance of urban agriculture
- Lisa-Marie Hemerijckx
- Gloria Nsangi Nakyagaba
- Anton Van Rompaey
npj Urban Sustainability (2023)
The nutritional profile, mineral content and heavy metal uptake of yellow mealworm reared with supplementation of agricultural sidestreams
- Isabelle Noyens
- Floris Schoeters
- Sabine Van Miert
Scientific Reports (2023)
End-to-end Precision Agriculture UAV-Based Functionalities Tailored to Field Characteristics
- Emmanuel K. Raptis
- Marios Krestenitis
- Elias B. Kosmatopoulos
Journal of Intelligent & Robotic Systems (2023)
Quick links
- Explore articles by subject
- Guide to authors
- Editorial policies
Sign up for the Nature Briefing: Anthropocene newsletter — what matters in anthropocene research, free to your inbox weekly.

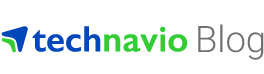
Discover Market Opportunities
- Food and Beverage
Is Food Technology the Future of the Food Industry?
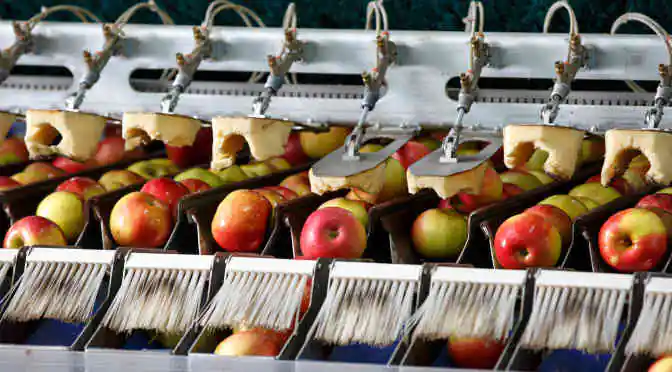
T he global food and food processing industry is on the threshold of a major alteration. Food technologies like bio-innovation, robotics, gene editing, and AI have redesigned the way in which we produce food and manage demand. As the population has increased at a rapid pace, the quantity of agricultural yield has gained a lot of attention. Therefore, industry experts are promoting food technology as the savior of the food industry’s future .
Why food technology? Are we running out of food?
As far-fetched as it sounds, just as with water and agricultural soil, we might run out of food as well. By 2030, per the UN, the global population is expected to number around 8.5 billion. Consequently, there is a need for focused action to increase food yield.
With a third of arable land being degraded and the food sector alone accounting for 70% of global water usage, the threat of running out of food seems to be quite real. And, this could happen sooner than predicted.
The only way to face the glaring threat of food deficit is by deploying sustainable modes of food production . Through food fabrication, nerd farming, and waste recycling, food technology can help all the major stakeholders in the industry to meet the growing demand for food. As a result, food technology is afforded its fair share of funding and R&D.
3D-printed food : An innovative technology with immense potential
By integrating technology and digital gastronomy techniques, 3D food printers manufacture customized food products. Herein, the potential benefits of 3D food printing — like conversion of algae and beet leaves into proteins — are what make it a promising food technology.
3D-printed food also helps save time and effort and is the key to sustainable food creation . Unlike traditional food manufacturing systems, food printers minimize waste with the use of hydrocolloid cartridges that form gels when combined with water.
Already, restaurants like Food Ink and Melissa are innovating their cuisine with the help of 3D food printers. Even hospitals have turned to 3D-printed food to cater to the special needs of their patients. For instance, the Isala Hospital in the Netherlands has switched to 3D-printed meals for their aged care patients. This 3D-printed food has better nutritional content and is customized for patients who have difficulty chewing food.
The takeaway: Food technology is the future, provided…
Food technology has surely changed the way in which food is produced and consumed. Even though the future does not totally belong to technology-driven food production, these techniques do have a critical role to play. Consequently, in the long term, it will be a mix of government policies and business ethics which will have a major influence on the food industry.
According to our expert analysts, the governments of the world should:
- Provide smaller farmers with capital to invest in technology
- Create an environment which fosters collaboration between farmers, universities, and tech companies
- Encourage tech innovation which will help small farmers in developing economies to bridge the ‘last mile’ problem
- Embrace technologies like big data and satellites to increase the adoption of smart farming techniques .
Source the latest insights into the global food & beverages industry. Download relevant reports HERE .
Related news.
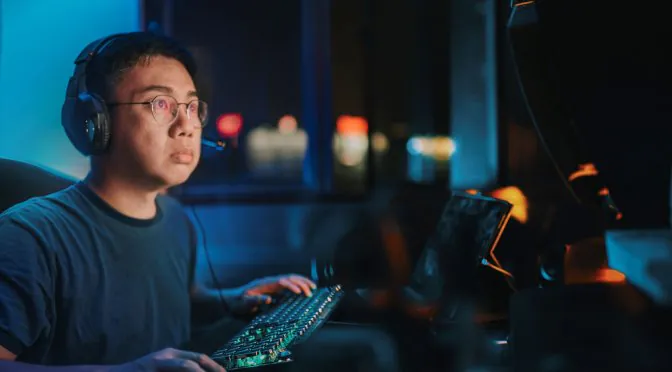
Top 14 Vendors in the Global E-Commerce Market
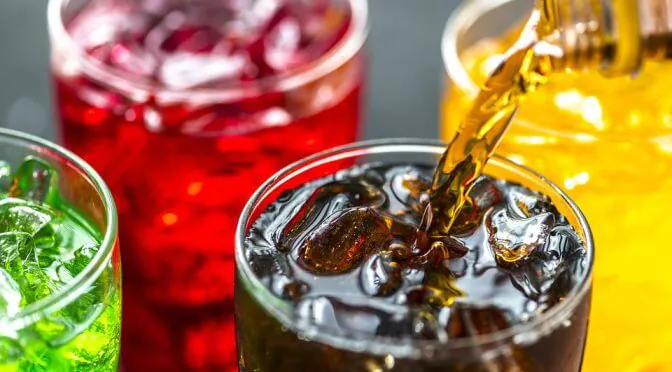
Top 10 Soft Drink Companies in the World
Manage settings.
Technology and Communications in the Global Food Industry Essay
- To find inspiration for your paper and overcome writer’s block
- As a source of information (ensure proper referencing)
- As a template for you assignment
Literature Review
Summary and critique.
Nowadays, supply chains attract more attention of researchers all over the world as they remain an important part of the business in many fields. Speaking about the industries whose primary aim lies in food supply and distribution, it is important to mention that their role in the life of the human society cannot be overstated. As for the fourteenth chapter of the textbook which has been chosen for the previous assignment, it contains a lot of important information on technical aspects of supply chain management and various technologies facilitating the work of specialists related to the sphere of activity.
Considering that the topic of technology in different industries is extremely significant for the future specialist, it is necessary to pay more attention to the most recent studies devoted to the role of technology and communications in the global food industry. As is clear from the review, traceability systems, RFID, intelligent packaging technologies, and MOLP approach are seen as major enablers in the food supply industry.
In reference to the most recent studies that can shed light on the role of technology and communications as enablers, it is important to mention the work by Aung and Chang (2014) which is devoted to the principles of work of food traceability systems, acting as enablers in the food industry. According to the authors, it is a common truth that various specialists related to the food industry are likely to implement a more customer-oriented approach in order to improve the quality of service and customer satisfaction.
At the same time, considering that modern business is a changing world which tends to swallow up the companies whose employees make crucial mistakes, GTS systems described in the article are extremely important as they enable specialists to spend less time in order to identify possible sources of complaints and prevent distribution and production of goods that do not correspond to basic quality standards. According to the discussed research, traceability is regarded as a tool used in numerous industries that allows checking the quality of a product on a regular basis. Apart from that, the properties of traceability systems tend to vary depending on the type of production.
Thus, working with meat, specialists use the combination of RFID and material handling systems to ensure high quality of distributed production. Just like the authors of the previous article, Piramuthu, Farahani, and Grunow (2013) agree that improvements related to food traceability are supposed to be more effective than labelling. Thus, the former helps to ensure that all goods are authentic and that they do not pose a threat to customers’ health whereas the latter has weaknesses related to this problem.
At the same time, the researchers insist that RFID technologies need to be improved due to the fact that larger production volumes always entail the increasing number of cases of food loss; apart from that, in certain food supply networks, the opportunities for the employees to perform instant information exchange are limited due to different reasons. Therefore, with a lack of improved RFID technology, it becomes more difficult for employees to identify contamination sources as soon as possible and prevent further deterioration of alimentary products. Similarly, the effectiveness of food traceability systems within the context of the global food industry is also confirmed by Kim, Zhang, and Yoon (2016) who claim that the experience of East Asian countries such as South Korea and China indicates that the use of FTS helps to mitigate risks related to product quality.
The general effects of the implementation of RFID that confirm its effectiveness are described by Ha, Song, Chung, Lee, and Park (2014) who have conducted their research on the recent changes within the logistics industry. According to them, the use of RFID helps to create a more collaborative environment for employees and, therefore, acts as an enabler in the food supply industry. Apart from that, as it follows from the conclusions made by Mohammed and Wang (2017), RFID definitely helps to improve performance of companies in food supply industry even though many researchers still find the effectiveness of the technology to be a controversial topic.
Technology helping to improve traceability is also studied in the article by Costa et al. (2013) that provides a substantial review of methods enhancing food control. According to the researchers, traceability is mandatory for the food industry as it acts as a factor ensuring client safety. In particular, the authors focus on the role of RFID in the food industry, claiming that the latter is able to bring numerous benefits to business owners and customers.
As is clear from the analysis conducted by the researchers, RFID technology is becoming increasingly popular when it comes to the food supply, but the opportunities for its implementation are still limited due to economic and technical factors which act as the barriers to progress in different countries. Despite the fact that the authors agree that RFID technologies enable companies to improve the quality of products, they acknowledge that their implementation can entail negative consequences. RFID tools may be too expensive for smaller businesses and their implementation involves the use of additional equipment which may be costly or difficult to find.
According to Bibi, Guillaume, Gontard, and Sorli (2017), RFID technology can be regarded as a tool which is more preferred than the barcode because it can be used to fulfil a wide range of tasks, including the control of external factors (such as humidity or temperature) which may have an impact on product quality. At the same time, the researchers suppose that one of the important objectives for modern developers is the necessity to provide the opportunity for better monitoring with the help of sensors capable of working in combination with RFID tags. To the authors, it seems that the development of improved RFID technology involving the use of sensors acts as a key to the problem of food waste.
Traceability issues related to the food industry are also studied in the work by Dabbene, Gay, and Tortia (2014) which is focused on technologies promoting safety and quality in the food industry. As is clear from the research conducted by the authors, traceability systems present a critical tool for specialists in the field as they significantly increase the effectiveness of food supply chains. In their article, the authors review the approaches to optimise traceability systems to be used in the food industry so that they would act as real enablers. The approaches can be divided into different groups based on the type of goods involved.
For instance, when it comes to perishable foods, the most of the researchers whose works are reviewed suppose that the use of mixed-integer linear programming would be the most appropriate as it helps to optimise traceability systems based on the information concerning expenses. At the same time, the authors state that reducing the costs of product recall remains an important problem in the field. Unlike the authors of the previous article, Soysal, Bloemhof-Ruwaard, and Van der Vorst (2014) focus on the development and implementation of MOLP model which is expected to minimise the costs of logistic operations, make transportation safer for the environment, and, therefore, improve the reputation of a company using this model.
The effectiveness of such an approach is also confirmed by Kannan, Khodaverdi, Olfat, Jafarian, and Diabat (2013) who report the positive results of its implementation in the automobile industry. Despite the different requirements to product quality that are used in various industries, MOLP model has already demonstrated its effectiveness in the case with perishable alimentary products such as meat.
Continuing on the future of technology in food supply, Kumar, Heustis, and Graham (2015) highlight that a little part of technical solutions is thoroughly studied in the United States due to the lack of resources. Within the frame of their research, the authors were focusing on global standards related to a product recall. As is clear from their position, in order to improve product quality and traceability, specialists related to the field should pay more attention to instant communication between employees on different levels.
The packaging is another important problem in the food supply industry. In their work, Vanderroost, Ragaert, Devlieghere, and De Meulenaer (2014) study basic functions of packaging in the food industry and the ways to improve it to ensure better protection. According to them, intelligent packaging is becoming increasingly popular as it can be seen as an enabler in the industry. The use of intelligent packaging can be regarded as a factor enabling business owners to improve HACCP systems used in their companies.
Among other things, the latter is supposed to act as important tools which are used to detect products of inappropriate quality which may be dangerous for customers, reduce the number of health risks for employees and consumers, and single out working processes which may influence the products quality. Understanding that it would be extremely important to create an integrated system that would control FSCs, product flows, and data stream simultaneously, the researchers acknowledge that modern developers are still unable to perform this task. Understanding the great role of sensors in the industry, the authors suppose that their implementation on the global scale is still impossible due to low flexibility of production, high production costs, and relatively low resistance to external factors such as temperature changes in the industry.
Therefore, the question remains open. The interest in intelligent packaging in the field can be illustrated by the fact that numerous researchers have studied the topic. Thus, Dobrucka and Cierpiszewski (2014) have conducted their research to study technologies of food packaging. According to the researchers, the use of technology in the food supply industry is closely interconnected with client preferences that tend to change with unfailing regularity.
As is clear from the analysis conducted by the authors, the most important trends related to packaging technology in the food industry are connected with the development of eco-friendly packaging which is expected to have a reduced impact on the environment and help to improve social image of food suppliers and manufacturers. In their work, the authors describe a number of technical solutions aimed at improving the performance of companies in the industry.
Among them, it is possible to single out the so-called “electronic nose” which propels smell recognition to the next level and helps companies to reduce the number of items of inappropriate quality. As is clear from the article by Fang, Zhao, Warner, and Johnson (2017), the use of intelligent packaging in meat industry helps to improve control of FSCs, minimise quality deterioration of meat products, and prevent the distribution of counterfeited foods. The use of new packaging technologies acts as a factor which improves both the quality of goods and the reputation of a company as proper tools significantly reduce the number of dissatisfied clients and distributors.
At the same time, the development of effective packaging technology is complicated by legislative problems related to safe materials and unstable customer preferences. As is stated by Ghaani, Cozzolino, Castelli, and Farris (2016) in their article, intelligent packaging technologies that possess the largest potential to change the global industry for the better include the use of sensory elements, electronic data carriers such as barcodes, and other devices aimed at improving product safety and customer satisfaction.
Such as authors of many articles that have been mentioned, these researchers believe that the use of intelligent packaging technology provides managers and customers with numerous benefits but there is an important weakness that needs to be paid more attention to during further research in the field: earning capacity for its users should be improved and implementation of these systems should not have an obvious impact on prices of the products as it can entail massive customer outflow.
Evaluating these findings reported by the researchers, it is necessary to notice that there is no significant difference in views when it comes to the effectiveness of the technologies mentioned. In fact, all authors whose works were reviewed tend to highlight the role of RFID, intelligent packaging, traceability systems, and MOLP in global food supply industry as numerous experiments show that all these technologies act as enablers, improve customer satisfaction, and reduce food waste.
Personally, I agree with the points covered by the authors as technologies studied by them are actively used despite the opinions of hard observers, who tend to exaggerate the risks of their implementation. At the same time, the opinions related to questions that need further research tend to be different. For instance, the majority of authors state that cost-effectiveness of RFID systems needs to be improved and additional sensors are to be implemented to reduce food waste on the global scale, and I agree with these points as prevention of food loss is the primary task related to the industry. Speaking of intelligent packaging, different authors single out such problems as the necessity to comply with environmental standards which may be different and the need to reduce costs of implementation of the technology.
To conclude, the fourteenth chapter of the textbook is devoted to the role of technology in supply chains, and the review demonstrates that the primary enablers in global food supply industry include intelligent packaging, the use of RFID technologies, food traceability systems, and MOLP model. In fact, there is a wide range of problems existing in the field that are already solved, but, based on the weaknesses of technologies reported by the authors, it is possible to single out suggestions for further research.
In general, the latter include studying measures, allowing to reduce the costs of these technologies’ implementation so that they could be effectively used all over the world. More than that, future researchers in the field need to pay focused attention to possible improvements to RFID technologies, helping to synchronise them with sensors to prevent possible mistakes.
Aung, M. M., & Chang, Y. S. (2014). Traceability in a food supply chain: Safety and quality perspectives. Food Control , 39 , 172-184.
Bibi, F., Guillaume, C., Gontard, N., & Sorli, B. (2017). A review: RFID technology having sensing aptitudes for food industry and their contribution to tracking and monitoring of food products. Trends in Food Science & Technology . Web.
Costa, C., Antonucci, F., Pallottino, F., Aguzzi, J., Sarriá, D., & Menesatti, P. (2013). A review on agri-food supply chain traceability by means of RFID technology. Food and Bioprocess Technology , 6 (2), 353-366.
Dabbene, F., Gay, P., & Tortia, C. (2014). Traceability issues in food supply chain management: A review. Biosystems Engineering , 120 , 65-80.
Dobrucka, R., & Cierpiszewski, R. (2014). Active and intelligent packaging food – Research and development – A review. Polish Journal of Food and Nutrition Sciences , 64 (1), 7-15.
Fang, Z., Zhao, Y., Warner, R. D., & Johnson, S. K. (2017). Active and intelligent packaging in meat industry. Trends in Food Science & Technology , 61 (1), 60-71.
Ghaani, M., Cozzolino, C. A., Castelli, G., & Farris, S. (2016). An overview of the intelligent packaging technologies in the food sector. Trends in Food Science & Technology , 51 (1), 1-11.
Ha, O. K., Song, Y. S., Chung, K. Y., Lee, K. D., & Park, D. (2014). Relation model describing the effects of introducing RFID in the supply chain: Evidence from the food and beverage industry in South Korea. Personal and Ubiquitous Computing , 18 (3), 553-561.
Kannan, D., Khodaverdi, R., Olfat, L., Jafarian, A., & Diabat, A. (2013). Integrated fuzzy multi criteria decision making method and multi-objective programming approach for supplier selection and order allocation in a green supply chain. Journal of Cleaner Production , 47 , 355-367.
Kim, R. B., Zhang, Q., & Yoon, D. H. (2016). Traceability system as perceived-uncertainty mitigator for sustainable global food trade. Quality Innovation Prosperity , 20 (1), 18-39.
Kumar, S., Heustis, D., & Graham, J. M. (2015). The future of traceability within the US food industry supply chain: A business case. International Journal of Productivity and Performance Management , 64 (1), 129-146.
Mohammed, A., & Wang, Q. (2017). Multi-criteria optimization for a cost-effective design of an RFID-based meat supply chain. British Food Journal , 119 (3), 676-689.
Piramuthu, S., Farahani, P., & Grunow, M. (2013). RFID-generated traceability for contaminated product recall in perishable food supply networks. European Journal of Operational Research , 225 (2), 253-262.
Soysal, M., Bloemhof-Ruwaard, J. M., & Van der Vorst, J. G. A. J. (2014). Modelling food logistics networks with emission considerations: The case of an international beef supply chain. International Journal of Production Economics , 152 , 57-70.
Vanderroost, M., Ragaert, P., Devlieghere, F., & De Meulenaer, B. (2014). Intelligent food packaging: The next generation. Trends in Food Science & Technology , 39 (1), 47-62.
- The UAE IT Market Project
- Australian Wine Production and Advantage Theories
- Adopting RFID Technology in Supermarkets
- Ways in Which NFTs Can Benefit Companies
- NFR+ Framework as a Systematic Approach
- Reducing the Cost of the Wedding Industry
- Kuwaiti Food Industry and Its Development
- Saudi Electricity Sector and Its Unsustainability
- Competition in the U.S. Cosmetics Industry
- Solar Energy Industry in the UAE
- Chicago (A-D)
- Chicago (N-B)
IvyPanda. (2020, November 13). Technology and Communications in the Global Food Industry. https://ivypanda.com/essays/technology-and-communications-in-the-global-food-industry/
"Technology and Communications in the Global Food Industry." IvyPanda , 13 Nov. 2020, ivypanda.com/essays/technology-and-communications-in-the-global-food-industry/.
IvyPanda . (2020) 'Technology and Communications in the Global Food Industry'. 13 November.
IvyPanda . 2020. "Technology and Communications in the Global Food Industry." November 13, 2020. https://ivypanda.com/essays/technology-and-communications-in-the-global-food-industry/.
1. IvyPanda . "Technology and Communications in the Global Food Industry." November 13, 2020. https://ivypanda.com/essays/technology-and-communications-in-the-global-food-industry/.
Bibliography
IvyPanda . "Technology and Communications in the Global Food Industry." November 13, 2020. https://ivypanda.com/essays/technology-and-communications-in-the-global-food-industry/.
- Discovery Platform
- Innovation Scouting
- Startup Scouting
- Technology Scouting
- Tech Supplier Scouting
- Venture Clienting
- Startup Program
- Trend Intelligence
- Business Intelligence
- All Industries
- Industry 4.0
- Manufacturing
- Case Studies
- Research & Development
- Corporate Strategy
- Corporate Innovation
- Open Innovation
- New Business Development
- Product Development
- Agriculture
- Construction
- Sustainability
- All Startups
- Circularity
- All Innovation
- Business Trends
- Emerging Tech
- Innovation Intelligence
- New Companies
- Scouting Trends
- Startup Programs
- Supplier Scouting
- Tech Scouting
- Top AI Tools
- Trend Tracking
- All Reports [PDF]
- Circular Economy
- Engineering
- Oil & Gas
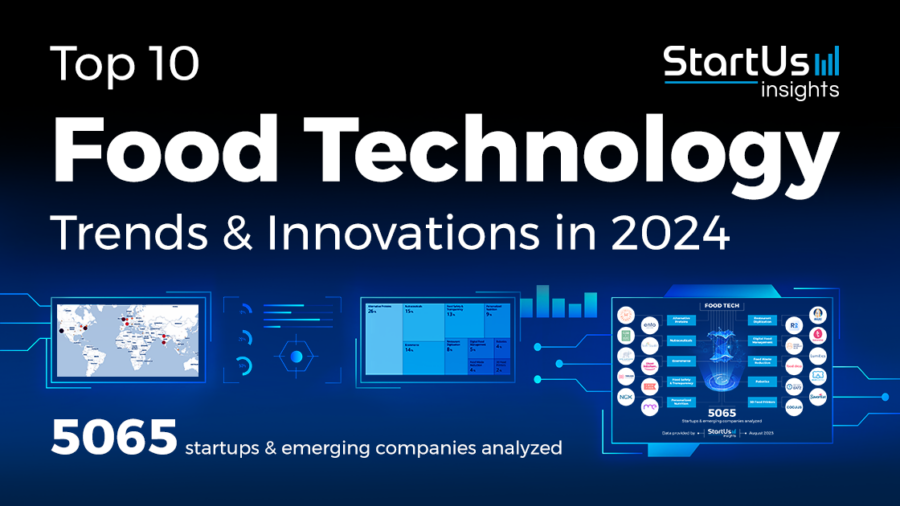
Share this:
- Click to share on Facebook (Opens in new window)
- Click to share on Twitter (Opens in new window)
- Click to share on LinkedIn (Opens in new window)
Discover 10 Emerging Food Industry Trends in 2025
How are the latest innovations in food technology tackling food shortage and ensuring climate-conscious eating habits? Explore our data-driven industry research on the current food trends based on our analysis of 5000+ companies. These trends include alternative proteins, 3D food printing, personalized nutrition, food robotics & more.
The food industry trends indicate a shift towards sustainable and personalized food choices, including alternative protein sources, local foods, nutraceuticals, and personalized nutrition. Concerns over environmental impacts drive FoodTech startups and brands to integrate waste reduction practices and zero-waste workflows. The COVID-19 pandemic also accelerated the adoption of food industry 4.0 technologies to improve food quality and efficiency throughout the food value chain. Further, food producers digitize production floors with robotics, eCommerce, and digital food management while restaurants employ robots for hospitality and cooking. These advances address the continuing impact of the COVID-19 pandemic as well as foster efficient, transparent, and sustainable operations within the food industry.
This article was last updated in August 2024.
What are the latest trends in the food industry?
- Alternative Proteins
- Nutraceuticals
- Food Safety & Transparency
- Personalized Nutrition
- Restaurant Digitization
- Digital Food Management
- Food Waste Reduction
- 3D Food Printers
Innovation Map outlines the Top 10 Food Industry Trends & 20 Promising Startups
For this in-depth research on the Top Food Technology Trends & Startups, we analyzed a sample of 5065 global startups & scaleups. This data-driven research provides innovation intelligence that helps you improve strategic decision-making by giving you an overview of emerging technologies in the food industry. In the Food Tech Innovation Map, you get a comprehensive overview of the innovation trends & startups that impact your company.
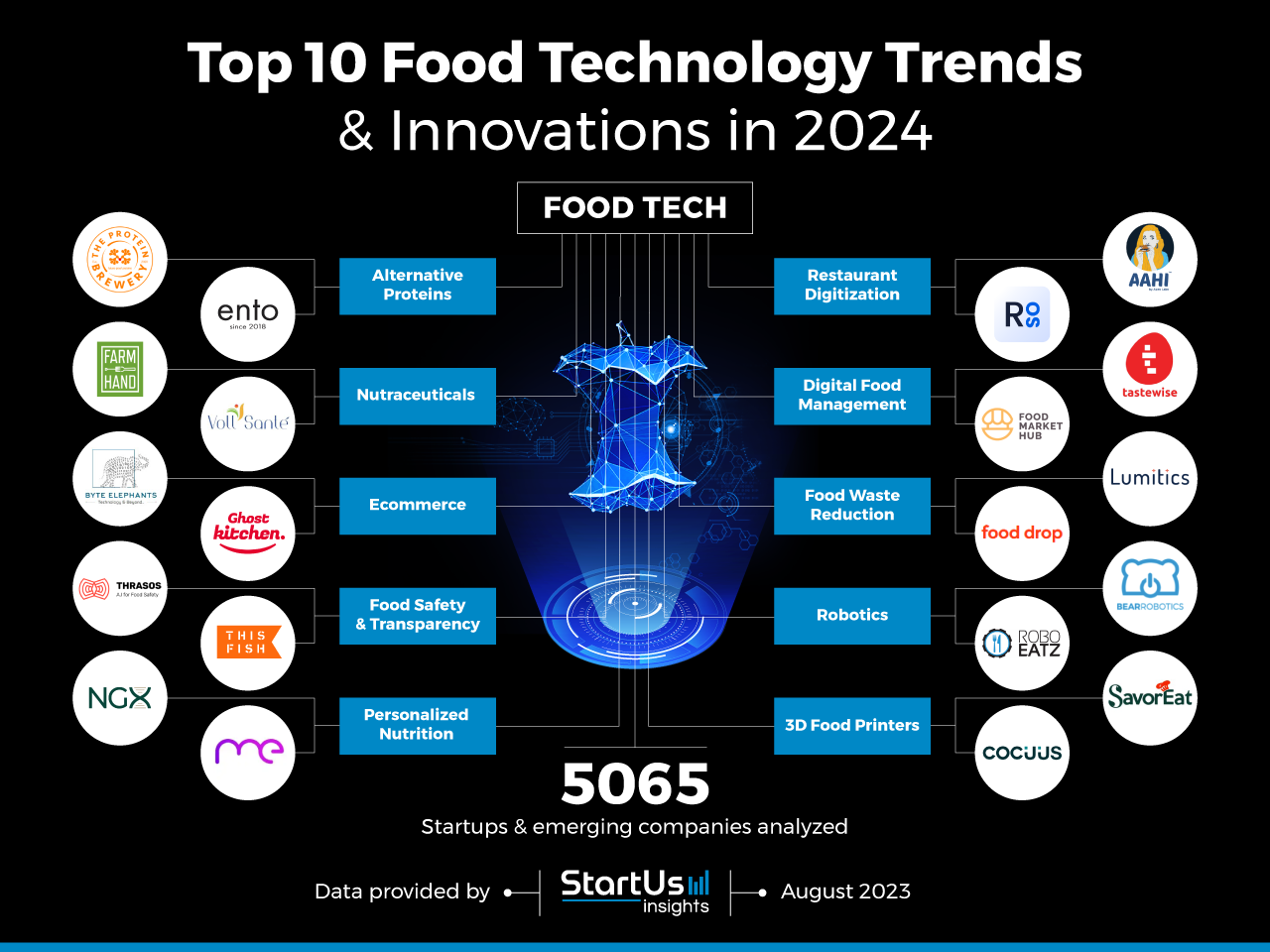
Want to explore all food industry innovations & trends?
Request Sample Database
These insights are derived by working with our Big Data & Artificial Intelligence-powered StartUs Insights Discovery Platform , covering 4.7M+ startups & scaleups globally. As the world’s largest resource for data on emerging companies, the SaaS platform enables you to identify relevant technologies and industry trends quickly & exhaustively.
Tree Map reveals the Impact of the Top 10 Food Technology Trends in 2025
The Tree Map below illustrates the Top Food Trends that will impact companies in 2025. Consumers shifting to alternative protein sources, including lab-grown food, is the most significant trend in the food industry. Further, the increased awareness of nutrition and health concerns during the pandemic drives the demand for nutraceuticals and personalized nutrition. Food e-commerce is another big trend that is fueled by the COVID-19 situation. Also, there is a substantial rise in food safety concerns directly impacting food transparency across the food value chain.
With digitization being an integral part of the food and beverage industry, companies are adopting restaurant digitization, digital food management, and food robotics to improve operations. Food brands are also focussing on food waste reduction and are adopting zero-waste practices. 3D food printers are also a part of the trends in food science and technology, enabling many other major trends such as meat alternatives and personalized nutrition.
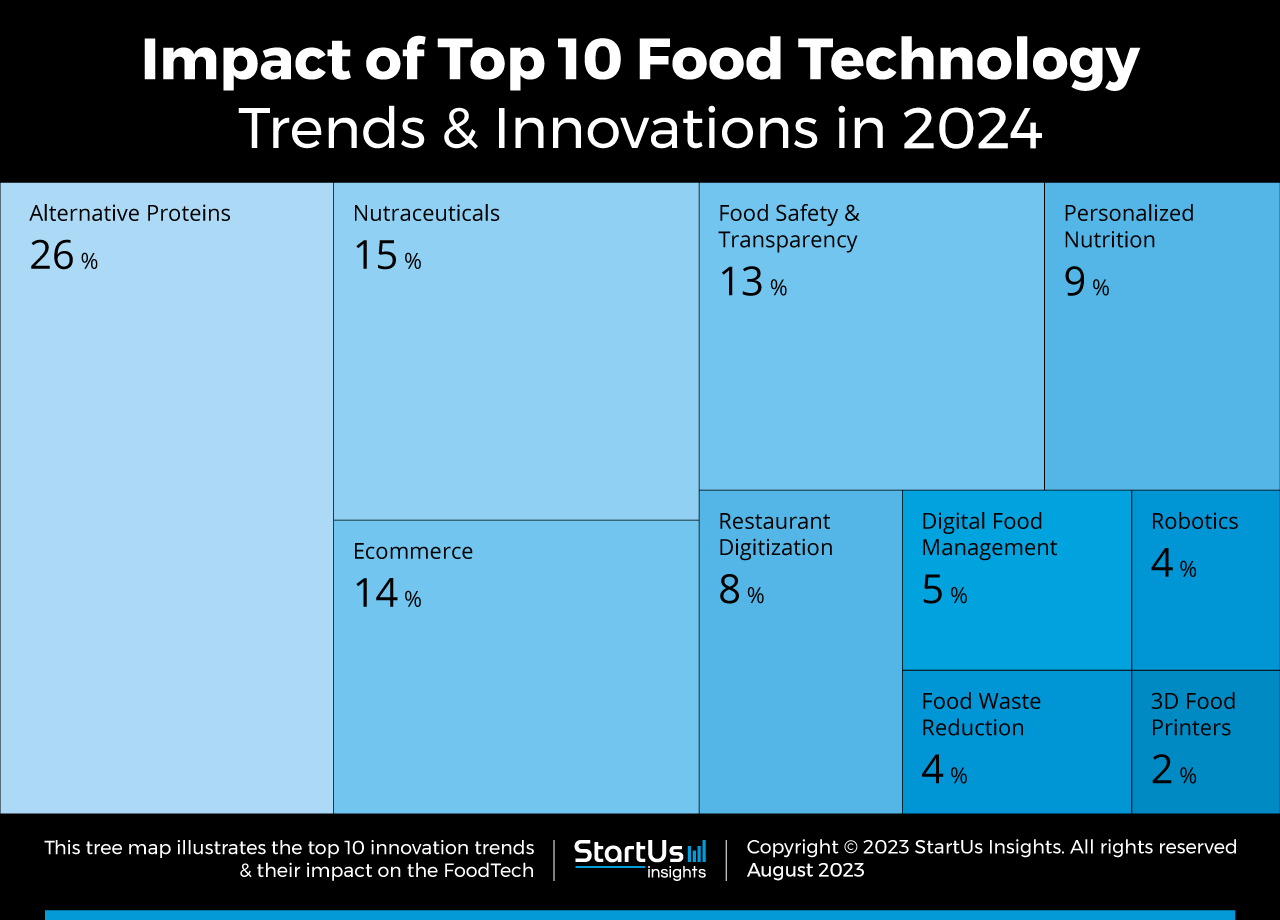
Click to download
Global Startup Heat Map covers 5065 Food Technology Startups & Scaleups
The Global Startup Heat Map below highlights the global distribution of the 5065 exemplary startups & scaleups that we analyzed for this research. Created through the StartUs Insights Discovery Platform , the Heat Map reveals that the US is home to most of these companies while we also observe increased activity in Europe, particularly in the UK and France.
Below, you get to meet 20 out of these 5065 promising startups & scaleups as well as the solutions they develop. These 20 startups were hand-picked based on criteria such as founding year, location, funding raised, and more. Depending on your specific needs, your top picks might look entirely different.
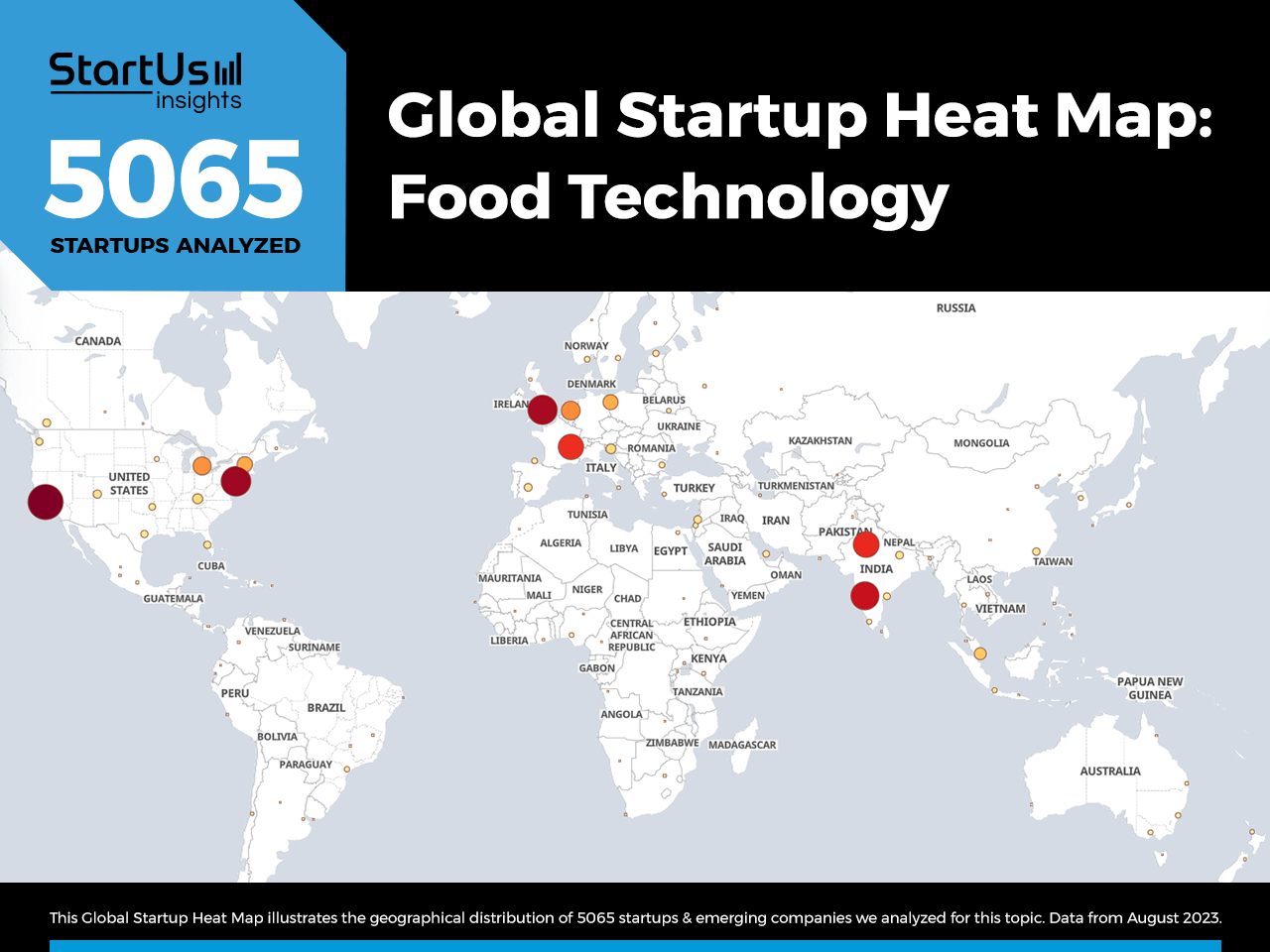
Top 10 Trends in the Food Industry (2025)
1. alternative proteins.
Consumers are increasingly shifting towards alternative protein sources , driven by health and environmental considerations. This makes it a prominent trend in food technology. These alternatives include cultured meat, lab-grown food, plant-based nutrition, edible insects, and mycoprotein. They have high nutritional richness and resource efficiency from production to consumption, a marked contrast to traditional livestock-based protein.
Further, alternative protein sources offer cost advantages due to their minimal dietary requirements and health monitoring. The ongoing advances in 3D printing, fermentation, and molecular biology also empower startups to develop sustainable solutions for protein production. This, in turn, allows food companies to address ethical concerns and reduce the carbon footprint associated with conventional meat production.
The Protein Brewery offers Animal-Free Protein
The Protein Brewery, a Dutch startup, develops FERMOTEIN , a proprietary animal-free lab-grown food. The startup brews FERMOTEIN using non-allergenic crops and fungi, infusing them with essential amino acids and fiber. This protein alternative has a 10% fat content and high water-binding capabilities, resulting in a meat-like flavor profile.
FERMOTEIN stands out as a natural ingredient, comprising solely brewed fibrous protein. The startup’s solution enables sustainable food developers to mitigate additional protein processing and expedite food product development.
ento manufactures Insect-based Protein
Malaysian startup ento farms crickets in a controlled environment to develop nutritious cricket-based food products. The startup’s insect-based alternative protein offers more proteins per gram than beef and contains all nine essential amino acids.
Also, ento’s cricket farming solution requires less land, water, and food than traditional livestock production, reducing greenhouse gas emissions. This enables food producers to save production costs.
2. Nutraceuticals
There is an increasing concern about the impact of food habits on health and a growing need for essential nutrients for a healthy lifestyle. With the COVID-19 pandemic, consumers are focusing more on eating healthy, making nutraceuticals a top trend in the food industry. This is a critical element in driving the demand for nutraceuticals.
Nutriceuticals include nutritional supplements, functional foods, medicinal foods, and gut microbiome-enhancing foods like prebiotics, probiotics, and postbiotics. Scientific research on nutraceuticals underscores their potential health benefits. Importantly, their use in addressing oxidative stress-related disorders such as allergies, diabetes, and immune-related conditions.
Farmhand Organics provides Fermented Foods
Based in the US, FoodTech startup Farmhand Organics develops hand-crafted, naturally probiotic, plant-based, fermented foods using organic ingredients. The startup’s organic krauts and kimchi facilitate healthy digestion, as well as maintain sleep and immunity balance.
Further, Farmhand Organics’ fermented vegetables are nutrient-rich and flavorful. The startup sources its ingredients directly from family farms and other local suppliers, reducing the carbon footprint due to transport.
Voll Sante makes Functional Food
Indian startup Voll Sante provides functional foods and beverages as well as nutraceuticals. The startup’s products utilize natural ingredients to prevent and manage malnutrition. Its nutraceutical products assist consumers in preventing chronic diseases.
The startup’s nutraceuticals also reduce healthcare expenditures and turn the focus of healthcare from reactive to preventive. They are available as protein and vitamin supplements, immunity boosters, and breakfast cereals.
3. eCommerce
eCommerce has long been a focal point in the food and beverage industry, but the COVID-19 pandemic has accelerated innovations within food supply chains. Food brands are harnessing digital platforms to provide on-demand online delivery services and connect with customers through direct-to-customer (D2C) distribution models. Additionally, heightened safety concerns during the pandemic are fueling the growth of ghost kitchens or cloud kitchens, exclusively offering food takeout and delivery services.
In tandem with D2C strategies, brands are prioritizing omnichannel distribution to enhance the customer experience and boost sales. This integrated approach ensures that customers access products both online and in brick-and-mortar stores, catering to diverse shopping preferences. Further, eCommerce empowers food manufacturers to establish stronger connections with their customers while ensuring product availability. This direct engagement allows manufacturers to gather valuable feedback and data, enabling them to tailor their offerings to consumer preferences.
Byte Elephant builds a Food E-Commerce Platform
Byte Elephant is an Indian startup that develops BETs , an e-commerce platform for food companies. It allows multi-brand, multi-location businesses to manage all orders in a centralized ecosystem. The platform features a quick customer onboarding workflow and is customizable based on specific brand requirements.
The startup’s platform thus enables food businesses to mitigate upfront IT development costs and offer online service quickly. It also enables them to scale operations quickly. This allows food businesses to address customer demands and stay ahead of the competition.
Ghost Kitchen offers Kitchen Spaces
South Korean startup Ghost Kitchen provides individual kitchen spaces to quickly start delivery businesses. At present, the startup owns 143 kitchens with adequate hygiene management and workflow from material storage to cooking, packaging, and delivery.
Also, Ghost Kitchen enables data-driven planning services through shared data collection from the entire individual kitchens. In effect, the startup minimizes expenses for contracts and interiors by directly offering standalone kitchens for restaurants and other food delivery entrepreneurs.
4. Food Safety & Transparency
Consumers prioritize the quality and safety of food products, leading to heightened food safety concerns. To address these concerns, consumers now have access to smart labels and standalone food grading devices, empowering them to make informed decisions when selecting food items. Additionally, advancements in blockchain technology and real-time food monitoring via Internet of Things (IoT) devices allow food brands to offer comprehensive end-to-end traceability.
Further, startups are contributing to food safety and transparency by creating cost-effective and scalable monitoring solutions. These innovations foster trust between food producers and consumers, increasing brand credibility and sales. Startups also play a crucial role in driving continuous improvement in food safety practices and ensuring that consumers have access to reliable information about the products they consume.
Thrasos simplifies Cleaning Protocol Scanning
Thrasos is a French startup that offers an AI-based solution to scan cleaning protocols in food production lines. The startup’s food safety management system utilizes a deep learning network to analyze numerous food safety parameters to validate the compliance of hygiene procedures.
This approach enables food businesses to leverage food safety management as a service as well as monitor and assess the efficacy of cleaning operations. As a result, food companies are able to ensure food-safe production lines and improve customer safety.
ThisFish develops Seafood Traceability Software
ThisFish is a Canadian startup that develops Tally , a software solution for seafood traceability. The startup digitizes data collection in real time on the food manufacturing floor using electronic scales, barcode printers, and scanners. Also, Tally allows seafood manufacturers to upload catch certificates and compliance documents.
The startup’s approach strengthens traceability while eliminating data errors and building trust among customers. Furthermore, ThisFish assists seafood producers in streamlining their operations for overall cost reduction, from fleet management and port unloading to production and quality control.
5. Personalized Nutrition
Growing consumer awareness about nutrition is fueling the demand for personalized nutrition solutions . These solutions encompass nutrigenomics-based diets and also cater to individual preferences such as sugar-free, gluten-free, vegan diets, and clean-label food products. Technological advancements like 3D printing and the integration of robotics into food production lines enable food manufacturers to offer personalized nutrition on a large scale.
At-home blood and urine testing kits empower consumers to identify dietary choices that align with their genetic profiles. Tracking devices further allow users to monitor their diets and health conditions, streamlining their nutritional choices. This level of customization enhances customer convenience and drives sales. Furthermore, ongoing research and development efforts are continuously expanding the range of personalized nutrition offerings. This ensures that consumers have access to dietary options to suit their needs.
NGX provides Genetically Personalized Meal-Shakes
NGX is a British startup that develops genetically personalized meal shakes. The startup offers at-home DNA nutrition tests and utilizes the test results to personalize nutrition intake for each individual. NGX’s products are plant-based breakfast and snack shakes that contain 30 essential nutrients including valine, isoleucine, leucine, lysine, and more.
Also, the startup’s products have minimal calories, fat, and carbohydrate value as well as zero added sugars. NGX’s meal shakes enable customers to reach their fitness goals quicker and lead healthier lives.
Mefood Omics develops an Online Precision Nutrition Platform
Mefood Omics is a French startup that builds an online precision nutrition platform for nutrition professionals. It combines nutrigenetics, artificial intelligence, and machine learning to design personalized dietary plans and monitor patients. Patients use the platform to create appointments and track billing as well as support diet customization and dynamic patient tracking.
The company also provides a nutrigenetic test and syncs the results with patient profiles. This way, the platform integrates nutrigenetic information as the last layer of personalization in generating the diet plan. This allows nutrition professionals and patients to leverage data-driven eating habits tailored to patient-specific diet goals.
Find out how 10 emerging technologies shape your industry!
6. Restaurant Digitization
Digitization in restaurants enhances the customer experience and also streamlines operational management, leading to improved efficiency. It empowers restaurant brands to collect valuable data at every stage, facilitating data-driven decision-making across operations. The upheaval caused by the COVID-19 pandemic has accelerated the adoption of digital management systems throughout the food and beverage supply chain. To minimize direct human-to-human contact, restaurants are integrating digital menus, self-service kiosks, and cashless payment methods.
Additionally, emerging technologies like chatbots and voice bots are gaining prominence for assisting customers with food orders and addressing restaurant-related inquiries. Leveraging customer preference and behavior data, AI-enabled tools provide personalized food recommendations and even contribute to new recipe development. This transformation underscores the pivotal role of digital solutions in the evolving restaurant landscape.
AAHI develops a Customer Engagement Platform
AAHI is a Czech startup that provides a customer engagement platform for restaurants. The startup’s contactless ordering platform uses digital menus and QR codes, supports multiple languages, and is tailorable to brand specifications. This offers a unique interactive experience for customers along with minimizing direct contact between personnel and customers.
Also, the platform optimizes customer-restaurant interactions, thereby increasing order frequency. AAHI’s Ecological Marketplace further connects restaurants with customers interested in sustainability and promotes special offers. This allows businesses to turn unsold and less popular items into revenue, reducing food wastage.
RestaurantOS optimizes Restaurant Operations
RestaurantOS is a Canadian startup that creates a restaurant management platform. Its AI assistant automates back-office tasks like order creation, reservation management, menu pricing, and more. The platform also offers guest analytics and supports inventory management and customer loyalty programs.
Through its solution, RestaurantOS enables restaurant chains to optimize operations, save time, and cut costs. Its pay-as-you-go business model further alleviates upfront and per-order platform fees for food businesses.
7. Digital Food Management
The integration of big data analytics and AI, coupled with real-time monitoring, facilitates comprehensive digital food management from farm to fork. Innovative startups are developing food management solutions that optimize manufacturing processes and streamline supply chain operations. Further, the digitization of restaurants empowers businesses to analyze customer behavior and forecast demand based on consumer preferences.
Collectively, these digital solutions empower food producers to gain a deeper understanding of market requirements and anticipate potential disruptions. This minimizes losses and improves surplus food inventory management. For instance, the utilization of quantum computing allows startups to swiftly analyze critical disruptions, including pandemics, and simulate market fluctuations accurately. Additionally, customer and market intelligence enables brands to streamline marketing strategies and drive increased sales.
Tastewise develops a Food Intelligence AI Engine
Tastewise is an Israeli startup that provides customer intelligence. The startup’s food intelligence AI engine collects customer data from social media interactions and digital restaurant platforms. It then cleans and classifies the data using historical consumer behavior and restaurant activities.
Through this process, the platform identifies critical insights into customer preferences and overall trends. It visualizes these insights as dashboards and reports, enabling restaurant owners to formulate strategies for product innovation, content marketing, and customer retention.
Food Market Hub provides a Procurement and Inventory System
Food Market Hub , a Malaysian startup, provides a cloud-based procurement and inventory system for the food and beverage industry. It streamlines the supply chain by offering real-time inventory tracking and automated purchase order generation. It also emails the purchase orders to the suppliers.
Using its companion smartphone app, restaurant managers centralize all procurement activities in one accessible platform. Moreover, Food Market Hub enhances inventory control by leveraging data analysis from various sources, like weather reports and historical data, to make more accurate purchase forecasts.
8. Food Waste Reduction
Addressing food wastage is paramount in the global effort to combat food insecurity and reduce the environmental footprint associated with the food industry. Food entrepreneurs and major corporations are prioritizing food waste reduction as a means to achieve sustainability and cut operational costs. The implementation of advanced food monitoring solutions empowers food producers, restaurants, and smart cities to proactively mitigate food waste.
Further, there is a notable shift towards adopting zero-waste approaches in food manufacturing. This emphasizes the upcycling and reuse of food waste to create value and resonate with environmentally-conscious consumers. A prime example of this shift is the emergence of 3D food printing solutions that utilize food waste to produce edible food products. Such means effectively curb food waste at restaurants and other food outlets while promoting sustainability.
Lumitics develops a Smart Food Waste Tracker
Lumitics is a Singaporean startup that tracks food waste. The startup’s smart food waste tracker, Insight , uses proprietary AI-based image recognition technology. It offers chefs insights into food waste, fostering feedback loops that enhance food quality based on customer preferences.
This dynamic approach empowers restaurants and food establishments to optimize production and tailor menus to meet customer demands effectively. Lumitics’ Insight reduces food waste and costs in hotels, airplanes, cruise ships, and more.
Food Drop connects Surplus Food to Local Charities
British startup Food Drop connects stores with unsold or surplus food to local charities, schools, and community groups. The startup’s online platform allows stores and charities to sign up, which then matches the stores and local charities.
Food Drop’s automated platform ensures a supply of charities to collect the surplus from stores at the end of the day. Also, if a charity is unavailable for pick up, the platform notifies stores in real time. The startup’s solution provides monthly reports on donated food and carbon savings.
9. Robotics
In the food and beverage industry, robotics plays a pivotal role across the entire value chain. It improves efficiency, consistency, and scalability throughout food production processes. Hospitality robots find their place in hotels and restaurants, elevating customer convenience and safety. Robotic chefs and food processing robots further solidify food robotics as a prominent trend in food technology.
Autonomous drones and vehicles are emerging as efficient alternatives to manual delivery services, increasing cost savings. These drones and other food-handling robots facilitate rapid and cost-effective food tagging and monitoring in warehouses and grocery stores. In effect, the robotics bloom in the food industry is expediting food production revenue with enhanced speed and precise food quality control.
Bear Robotics manufactures Autonomous Food Service Robots
US-based startup Bear Robotics develops Servi , an autonomous food-serving robot. It complements waitstaff by delivering dishes and beverages in tight spaces using advanced camera and laser sensor navigation, allowing it to navigate without encountering blind spots.
By prioritizing safety, Servi promptly stops or adjusts its course when encountering obstacles. Bear Robotics’ solutions offer a transformative approach to hospitality, enhancing operational efficiency and service quality in the industry.
ROBOEATZ provides Autonomous Robotic Kitchens
ROBOEATZ is a Canadian startup developing AI-based autonomous robotic kitchens for restaurants, grocery stores, and office spaces. The startup’s ARK 03 robot uses proprietary software for autonomous operation of kitchen tasks, including hot and cold food storage, preparation, plating, cleaning, and sanitizing.
Additionally, ARK 03 features automatic ordering and inventory control that keeps a tab on ingredient supply and automatically requests refills from wholesalers. ROBOEATZ’s solution improves the efficiency of food production while requiring minimal human intervention and adhering to effective food safety practices.
10. 3D Food Printers
3D food printers open up possibilities for personalized diets and the creation of alternative protein-based meals, offering precision and consistency in nutrition. While material extrusion remains the most widely used method in food printing, startups are exploring innovative approaches such as laser and inkjet food printing, as well as bioprinting. These novel methods enhance the quality and accuracy of 3D-printed food products.
With an emphasis on meeting specific product requirements and ensuring consistent quality, ongoing research in 3D food printing is geared toward large-scale food production. As a result, 3D food printing simplifies production processes and reduces costs. Further, it empowers food brands to deliver personalized food products at scale without the need for additional tooling and operational expenses.
SavorEat prints Plant-based Meat
SavorEat is an Israeli startup that develops plant-based meat. The startup combines chef robots, proprietary 3D printing, and non-GMO ingredients to print meat. At present, the startup produces meatless burgers and plans to expand to steaks, kebabs, and alternative seafood.
SavorEat’s meat alternatives replicate the authentic taste, texture, and experience of real meat. They are also customizable to suit specific preferences, dietary needs, and lifestyles. This 3D printing technology ensures rapid, precise, and consistent results, making it ideal for food trucks, restaurants, and hotels.
COCUUS manufactures Bioinks for 3D Food Printers
Spanish startup COCUUS produces plant- or cell-based animal protein analogs using 2D/3D laser printing, bioprinting, and robotics. The startup offers LEVEL-UP inkjet 3D printers and LASERGLOW laser 2D/3D printers for food producers. The LASERGLOW is accurate to design specifications and supports 3D shapes as well as hyper-personalization.
On the other hand, LEVEL-UP is suitable for product customization with surface filling at scale. The startup’s 3D printers allow restaurants and food brands to personalize their products without tooling changes and help in improving the brand reach, boosting sales.
Discover all Food Tech Trends & Startups
The top 10 food technology trends drive sustainable food sources and food waste reduction, with Industry 4.0 technologies enhancing efficiency and scalability. 3D food printing emerges as a promising technology, complementing other key trends and prompting continued investment in 3D food printers and bio-inks. Furthermore, advances in AI and big data analytics play a crucial role in optimizing food industry operations.
In synergy, the food and beverage sector is making strides towards sustainability while elevating overall operational efficiency. The Food Industry Trends & Startups outlined in this report only scratch the surface of trends that we identified during our data-driven innovation & startup scouting process. Identifying new opportunities & emerging technologies to implement into your business goes a long way in gaining a competitive advantage.

Your Name Business Email Company
Get our free newsletter on technology and startups.
Protected by reCAPTCHA and the Google Privacy Policy and Terms of Service apply.
Discover our Free FoodTech Report 21 pages
Get free updates on Global Startups, Technologies & Trends!
Join 25k subscribers.
Business Email
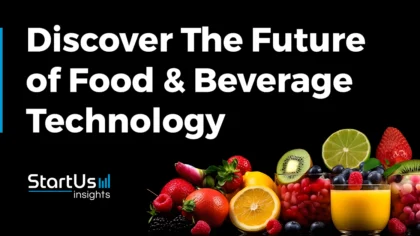
FoodTech 21 pages report
Beverage technology 21 pages report, agritech 22 pages report, biotech 22 pages report.
Leverage our unparalleled data advantage to quickly and easily find hidden gems among 4.7M+ startups, scaleups. Access the world's most comprehensive innovation intelligence and stay ahead with AI-powered precision.
Get in touch
Your Name Business Email Company How can we support you? (optional)
Explore 30+ Emerging Tech Startups for Free
Get ahead with our interactive Innovation Map, featuring 30+ of the most promising tech startups. Download now to discover top tech companies and accelerate your innovation agenda.

Protected by reCAPTCHA and the Google Privacy Policy and Terms of Service apply.
IEEE Account
- Change Username/Password
- Update Address
Purchase Details
- Payment Options
- Order History
- View Purchased Documents
Profile Information
- Communications Preferences
- Profession and Education
- Technical Interests
- US & Canada: +1 800 678 4333
- Worldwide: +1 732 981 0060
- Contact & Support
- About IEEE Xplore
- Accessibility
- Terms of Use
- Nondiscrimination Policy
- Privacy & Opting Out of Cookies
A not-for-profit organization, IEEE is the world's largest technical professional organization dedicated to advancing technology for the benefit of humanity. © Copyright 2024 IEEE - All rights reserved. Use of this web site signifies your agreement to the terms and conditions.
We’re sorry, this feature is currently unavailable. We’re working to restore it. Please try again later.
- Our network
The Sydney Morning Herald
- Life & relationships
Kamala’s secret sauce: Working in fast food sets you up for success
Shona hendley, save articles for later.
Add articles to your saved list and come back to them any time.
McPresident Kamala Harris? While I’m personally lovin’ the sound of that – despite Harris holding a part-time position at McDonald’s during college – I’m sure she’d prefer the title without the prefix.
If Harris gets the votes this November, she will become the first US president who has worked behind the McDonald’s counter, but she is far from the only successful person to do so. Pink, Pharrell Williams, Shania Twain and Jay Leno have also worn a fast-food uniform before making it big.
Amazon founder Jeff Bezos is another, often publicly reflecting on his first job flipping burgers. “You learn a lot as a teenager working at McDonald’s. It’s different from what you learn in school. Don’t underestimate the value of that,” he said in an interview with Wired .
This value, which I like to call the “secret sauce” of working in fast food, has served me well too.
Harris could become the first US president who has worked at McDonald’s. Credit: Bloomberg via Getty Images
As someone who donned my red, Colonel Sanders embroidered polo shirt, black slacks and matching cap to work behind the register of my local KFC for over five years through high school and into university, there’s no doubt that the skills I learnt, many mistakes I made and encounters I had – like those with “extra salt Sally”, a slightly aggressive regular who always demanded blood pressure rising levels of salt – set me up for my future working life.
As the fast food industry’s name suggests, it’s fast-paced and can be intense, especially for teenagers and younger people who, according to the Australian Bureau of Statistics, make up a significant portion of fast-food employees.
Yes, this can be challenging – impatient customers lining up all the way to the door is never a walk in the park – but it is also formative and greatly beneficial. I may not have Harris’ level of professional responsibility, but like her, I do (usually) remain calm under pressure. If you’re looking for someone who can salt chips while listening to a grown adult melt down over how long they’ve waited for a piece of chicken, you know, just the multitasker to call.
In addition to the fast-food industry’s inherent nature, HR and people strategist Sophie Firmanger says that many foundational skills, like teamwork and time management, lay the groundwork for success in virtually any career.
“Time management is a universal skill, essential for balancing responsibilities in your professional life and teamwork is the backbone of any successful organisation, and problem-solving is critical in leadership roles,” says Firmanger.
Skills like problem-solving and resilience can also be used outside of work, says clinical psychologist and author Dr Rebecca Ray.
“The ability to think on your feet and make quick decisions becomes a real asset when tackling everyday challenges, whether at home or in your personal life and facing demanding customers or hectic shifts builds emotional resilience, which helps in managing stress in other parts of life.”
And that is something I can vouch for. Many of the lessons I learnt working in fast food have proven surprisingly beneficial.
Every job has its shortcomings
No matter how good it sounds, the reality is, whether you’re president, pop star or a parent, there will be downsides to your job.
In my gig, this often involved cleaning the male toilet. This, along with the regular burns inflicted at the corn cob station, taught me very early on in my working life that there will always be tasks that you won’t enjoy doing and, in some cases, may literally scar you for life.
It also taught me that, unfortunately, you must still do them. (Or, in the case of cleaning the toilet, teach your kids how to do it.)
Working in the fast-food industry leaves you with an unexpected set of skills. Credit: iStock
People can be very difficult
The first rule of any customer service job is that the customer is always right. However, as anyone who has ever worked in customer service knows, this isn’t always the case. One former customer was adamant that our cook altered the Colonel’s Secret Herbs and Spices recipe just to annoy her after she complained about a batch of the chicken the week before.
Learning how to manage difficult people professionally and navigating conflict is one of the greatest skills that that job has ever taught me. Because no matter where you work, you will have to deal with tricky, sometimes unpleasant situations (and people) and need to resolve them without completely losing it.
You will work with all sorts of people
While I predominantly worked alongside other students, the team also included members from various age brackets and different cultural, religious, and socioeconomic backgrounds.
Away from the neon bucket of the restaurant, this group never would have mixed. Starting my career, understanding that teamwork is only possible if you embrace the differences of everyone in the team has made me a much more understanding person to work with.
Unexpected skills can become useful
And then there are the skills you never imagined you would use again after you’ve hung up your branded visor.
As I was learning the “first in, first out” system of food storage or how to make a Twister wrap that won’t fall apart, I assumed these skills would be useless outside the fast-food industry.
But fast-forward 20 years, and as I pack school lunches and pack my groceries away (old vegies at the top, new underneath) I realise these skills couldn’t be more valuable. You never know what skill you’re going to need when, only that you come away from these jobs knowing a lot more than when you started.
While it appears Harris has taken many similar learnings with her throughout her career – from McDonald’s to politics – I wonder whether the same could be said of Donald Trump.
As Harris’s running mate, Tim Walz, said: “Can you simply picture Donald Trump working at a McDonald’s, trying to make a McFlurry or something? He couldn’t run that damn McFlurry machine if it cost him anything.”
Now that’s fast food for thought.
Make the most of your health, relationships, fitness and nutrition with our Live Well newsletter . Get it in your inbox every Monday .
- Kamala Harris
- For subscribers
Most Viewed in Lifestyle

IMAGES
COMMENTS
Learn more about food tech and how technology can improve food sustainability, speed up production and enhance efficiency in the food service industry.
When it comes to food, tech isn't always the first thing that comes to mind. However, technology over the years has changed how we produce and find our food through applications, robotics, data ...
Uncover the transformative role of technology in the food industry. Learn how advancements are reshaping production and consumption.
Artificial intelligence (AI) has embodied the recent technology in the food industry over the past few decades due to the rising of food demands in line with the increasing of the world population. The capability of the said intelligent systems in various tasks such as food quality determination, control tools, classification of food, and prediction purposes has intensified their demand in the ...
Artificial intelligence (AI) has embodied the recent technology in the food industry over the past few decades due to the rising of food demands in line with the increasing of the world population. The capability of the said intelligent systems in various tasks such as food quality determination, control tools, classification of food, and ...
Food sustainability: Here's how the food industry can use digital technology to address the complex challenges of producing enough to feed an ever-growing global population.
Over the past few decades, the food industry has undergone revolutionary changes due to the impacts of globalization, technological advancements, and ever-evolving consumer demands. Artificial intelligence (AI) and big data have become pivotal in strengthening food safety, production, and marketing. With the continuous evolution of AI technology and big data analytics, the food industry is ...
Executive summary. Technology helps food manufacturers to produce more efficiently for a growing world population. Improving shelf life and food safety revolves around technology, and greater use of machines and software ensures affordability and consistent quality. The importance of technology for manufacturers continues to grow.
The present chapter draws on new evidence from the regions reaffirming the continuing rapid pace of science, technology and innovation and the need to act urgently worldwide to capitalise on the new opportunities to transform food systems.
The transition to sustainable food systems will require many changes but of particular importance will be the harnessing of internet technology, in the form of an 'Internet of Food', which ...
The takeaway: Food technology is the future, provided…. Food technology has surely changed the way in which food is produced and consumed. Even though the future does not totally belong to technology-driven food production, these techniques do have a critical role to play. Consequently, in the long term, it will be a mix of government ...
To synthesize the main research topics and highlight possible lines for future research, this work aims to develop a bibliometric analysis of technological innovation in the field of the food ...
Highlights • The adoption of RAS in the food industry is reviewed from the supply chain perspective. • 54 papers were collected from a nine-step systematic review process. • The adoption of RAS was analysed based on five major themes of food supply chain. • The role of RAS, the data availability, cybersecurity, skill capability, and financial costs are main issues in the adoption ...
"Food processing 4.0" concept denotes processing food in the current digital era by harnessing fourth industrial revolution (called Industry 4.0) technologies to improve quality and safety of processed food products, reduce production costs and time, save energy and resources, as well as diminish food loss and waste. Industry 4.0 technologies have been gaining great attention in recent ...
Conclusion. Technology has had a significant impact on the food industry, from farm to table. It has allowed us to produce new types of foods and distribute them across the globe, while also ...
Given the increasing worldwide trade in food and heterogeneous food preferences, market orientation has become a driving force in the food industry. Together with advances in the novelty of foods produced, innovative technologies of food production are being developed to address consumer demand and acceptance.
This paper pays attention to the most recent studies devoted to the role of technology and communications in the global food industry.
Discover 10 Emerging Food Industry Trends in 2025 How are the latest innovations in food technology tackling food shortage and ensuring climate-conscious eating habits? Explore our data-driven industry research on the current food trends based on our analysis of 5000+ companies.
ICT applications for the food industry Chapter 35 619. Cloud computing's role is indispensable in several technologies and is also associated with Industry 4.0, such as big data. analysis, IoT ...
The objective of this article is to determine the good and bad impacts will human globally face when utilizing the modern technology on food. The investigation first focuses on history and ...
Efficient and reliable management of the business operations within the food supply chain is crucial to ensure the quality, safety, traceability, and security of food products. Streamlining different food supply chain management operations by utilizing cutting-edge technologies such as Cloud computing and the Internet of Things (IoT) has gained enough attention in recent years. Most of the ...
The Institute of Food Technologists (IFT) is a community of professionals and students passionate about the essential role science and innovation play in ensuring that our global food system is safe, nutritious, and sustainable. - Feeding the Minds that Feed the World.
The food providers will provide information to customers based on their needs and preferences, while customers will benchmark the available providers and receive both marketing and factual word-of-mouth on the aspects of services, food, aesthetic and service quality.
Essay On Food Technology. Food science and technology is a branch of science that deals with the study of processing of food. It is not limited to manufacturing but also includes methods of food preservation, food packaging, food safety, nutrition and many more subject areas related to food. The United States is one of the best countries to ...
In addition to the fast-food industry's inherent nature, HR and people strategist Sophie Firmanger says that many foundational skills, like teamwork and time management, lay the groundwork for ...