Thank you for visiting nature.com. You are using a browser version with limited support for CSS. To obtain the best experience, we recommend you use a more up to date browser (or turn off compatibility mode in Internet Explorer). In the meantime, to ensure continued support, we are displaying the site without styles and JavaScript.
- View all journals
- Explore content
- About the journal
- Publish with us
- Sign up for alerts
- NATURE INDEX
- 09 December 2020

Six researchers who are shaping the future of artificial intelligence
- Gemma Conroy ,
- Hepeng Jia ,
- Benjamin Plackett &
You can also search for this author in PubMed Google Scholar
Andy Tay is a science writer in Singapore.
As artificial intelligence (AI) becomes ubiquitous in fields such as medicine, education and security, there are significant ethical and technical challenges to overcome.
CYNTHIA BREAZEAL: Personal touch
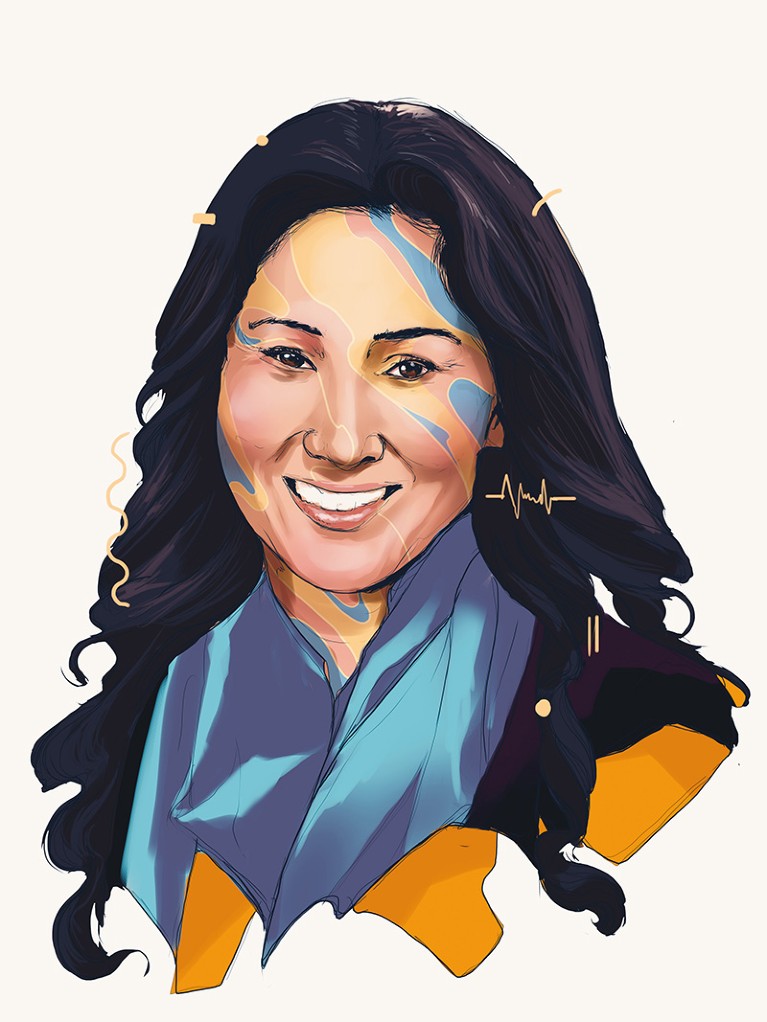
Credit: Taj Francis
While the credits to Star Wars drew to a close in a 1970s cinema, 10-year-old Cynthia Breazeal remained fixated on C-3PO, the anxious robot. “Typically, when you saw robots in science fiction, they were mindless, but in Star Wars they had rich personalities and could form friendships,” says Breazeal, associate director of the Massachusetts Institute of Technology (MIT) Media Lab in Cambridge, Massachusetts. “I assumed these robots would never exist in my lifetime.”
A pioneer of social robotics and human–robot interaction, Breazeal has made a career of conceptualizing and building robots with personality. As a master’s student at MIT’s Humanoid Robotics Group, she created her first robot, an insectile machine named Hannibal that was designed for autonomous planetary exploration and funded by NASA.
Some of the best-known robots Breazeal developed as a young researcher include Kismet, one of the first robots that could demonstrate social and emotional interactions with humans; Cog, a humanoid robot that could track faces and grasp objects; and Leonardo, described by the Institute of Electrical and Electronics Engineers in New Jersey as “one of the most sophisticated social robots ever built”.
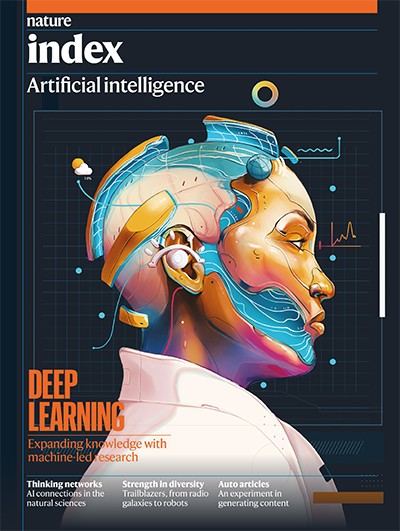
Nature Index 2020 Artificial intelligence
In 2014, Breazeal founded Jibo, a Boston-based company that launched her first consumer product, a household robot companion, also called Jibo. The company raised more than US$70 million and sold more than 6,000 units. In May 2020, NTT Disruption, a subsidiary of London-based telecommunications company, NTT, bought the Jibo technology, and plans to explore the robot’s applications in health care and education.
Breazeal returned to academia full time this year as director of the MIT Personal Robots Group. She is investigating whether robots such as Jibo can help to improve students’ mental health and wellbeing by providing companionship. In a preprint published in July, which has yet to be peer-reviewed, Breazeal’s team reports that daily interactions with Jibo significantly improved the mood of university students ( S. Jeong et al . Preprint at https://arxiv.org/abs/2009.03829; 2020 ). “It’s about finding ways to use robots to help support people,” she says.
In April 2020, Breazeal launched AI Education, a free online resource that teaches children how to design and use AI responsibly. “Our hope is to turn the hundreds of students we’ve started with into tens of thousands in a couple of years,” says Breazeal. — by Benjamin Plackett
CHEN HAO: Big picture
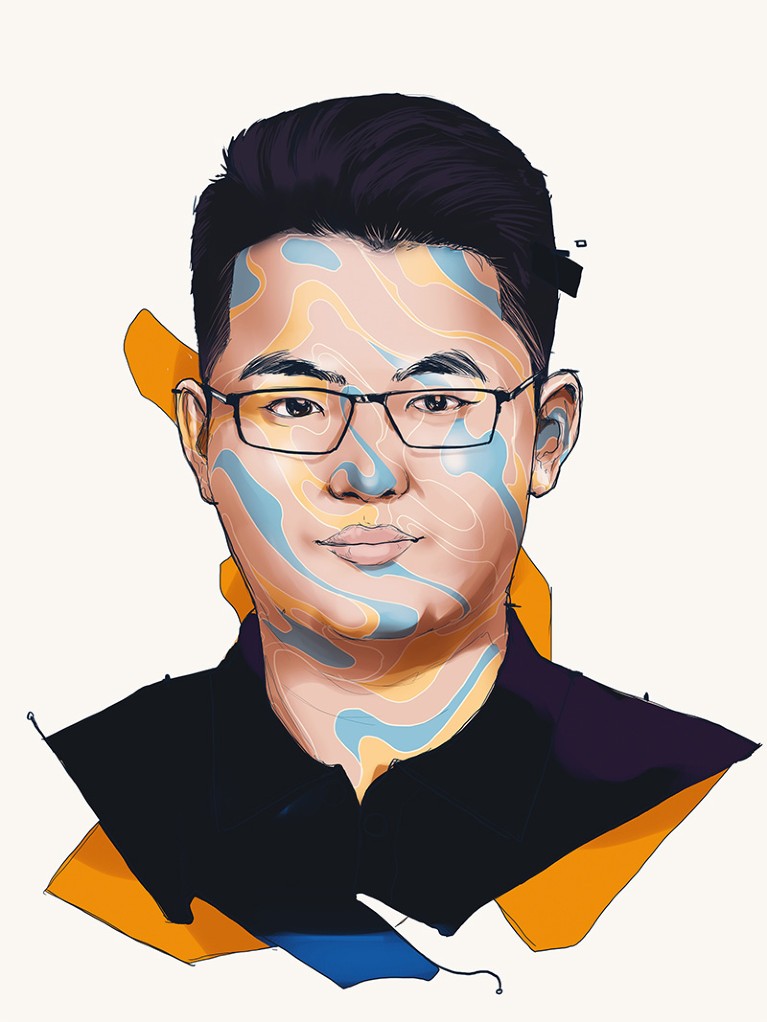
Analysing medical images is an intensive and technical task, and there is a shortage of pathologists and radiologists to meet demands. In a 2018 survey by the UK’s Royal College of Pathologists, just 3% of the National Health Service histopathology departments (which study diseases in tissues) said they had enough staff. A June 2020 report published by the Association of American Medical Colleges found that the United States’ shortage of physician specialists could climb to nearly 42,000 by 2033.
AI systems that can automate part of the process of medical imaging analysis could be the key to easing the burden on specialists. They can reduce tasks that usually take hours or days to seconds, says Chen Hao, founder of Imsight, an AI medical imaging start-up based in Shenzhen, China.
Launched in 2017, Imsight’s products include Lung-Sight, which can automatically detect and locate signs of disease in CT scans, and Breast-Sight, which identifies and measures the metastatic area in a tissue sample. “The analysis allows doctors to make a quick decision based on all of the information available,” says Chen.
Since the outbreak of COVID-19, two of Shenzhen’s largest hospitals have been using Imsight’s imaging technology to analyse subtle changes in patients’ lungs caused by treatment, which enables doctors to identify cases with severe side effects.
In 2019, Chen received the Young Scientist Impact Award from the Medical Image Computing and Computer-Assisted Intervention Society, a non-profit organization in Rochester, Minnesota. The award recognized a paper he led that proposed using a neural network to process fetal ultrasound images ( H. Chen et al. in Medical Image Computing and Computer-Assisted Intervention — MICCAI 2015 (eds N. Navab et al. ) 507–514; Springer, 2015 ). The technique, which has since been adopted in clinical practice in China, reduces the workload of the sonographer.
Despite the rapid advancement of AI’s role in health care, Chen rejects the idea that doctors can be easily replaced. “AI will not replace doctors,” he says. “But doctors who are better able to utilize AI will replace doctors who cannot.” — by Hepeng Jia
ANNA SCAIFE: Star sifting
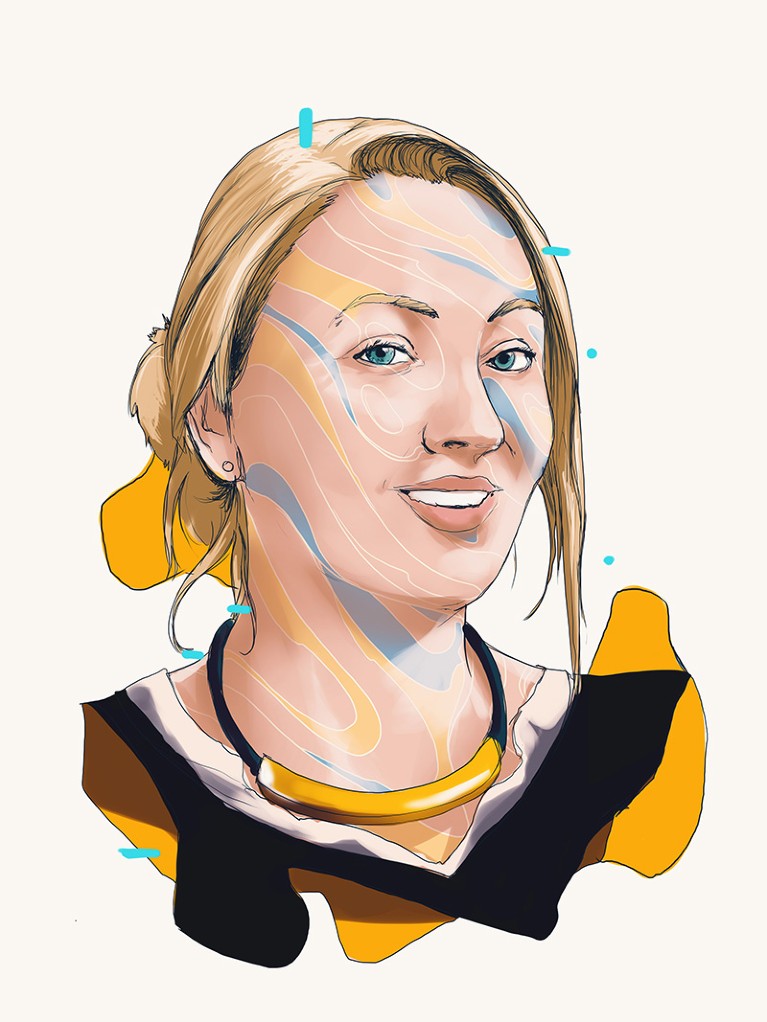
When construction of the Square Kilometre Array (SKA) is complete , it will be the world’s largest radio telescope. With roughly 200 radio dishes in South Africa and 130,000 antennas in Australia expected to be installed by the 2030s, it will produce an enormous amount of raw data, more than current systems can efficiently transmit and process.
Anna Scaife, professor of radio astronomy at the University of Manchester, UK, is building an AI system to automate radio astronomy data processing. Her aim is to reduce manual identification, classification and cataloguing of signals from astronomical objects such as radio galaxies, active galaxies that emit more light at radio wavelengths than at visible wavelengths.
In 2019, Scaife was the recipient of the Jackson-Gwilt Medal, one of the highest honours bestowed by the UK Royal Astronomical Society (RAS). The RAS recognized a study led by Scaife, which outlined data calibration models for Europe’s Low Frequency Array (LOFAR) telescope, the largest radio telescope operating at the lowest frequencies that can be observed from Earth ( A. M. M. Scaife and G. H. Heald Mon. Not. R. Astron. Soc. 423 , L30–L34; 2012 ). The techniques in Scaife’s paper underpin most low-frequency radio observations today.
“It’s a very peculiar feeling to win an RAS medal,” says Scaife. “It’s a mixture of excitement and disbelief, especially because you don’t even know that you were being considered, so you don’t have any opportunity to prepare yourself. Suddenly, your name is on a list that commemorates more than 100 years of astronomy history, and you’ve just got to deal with that.”
Scaife is the academic co-director of Policy@Manchester, the University of Manchester’s policy engagement institute, where she helps researchers to better communicate their findings to policymakers. She also runs a data science training network that involves South African and UK partner universities, with the aim to build a team of researchers to work with the SKA once it comes online. “I hope that the training programmes I have developed can equip young people with skills for the data science sector,” says Scaife. — by Andy Tay
TIMNIT GEBRU: Algorithmic bias
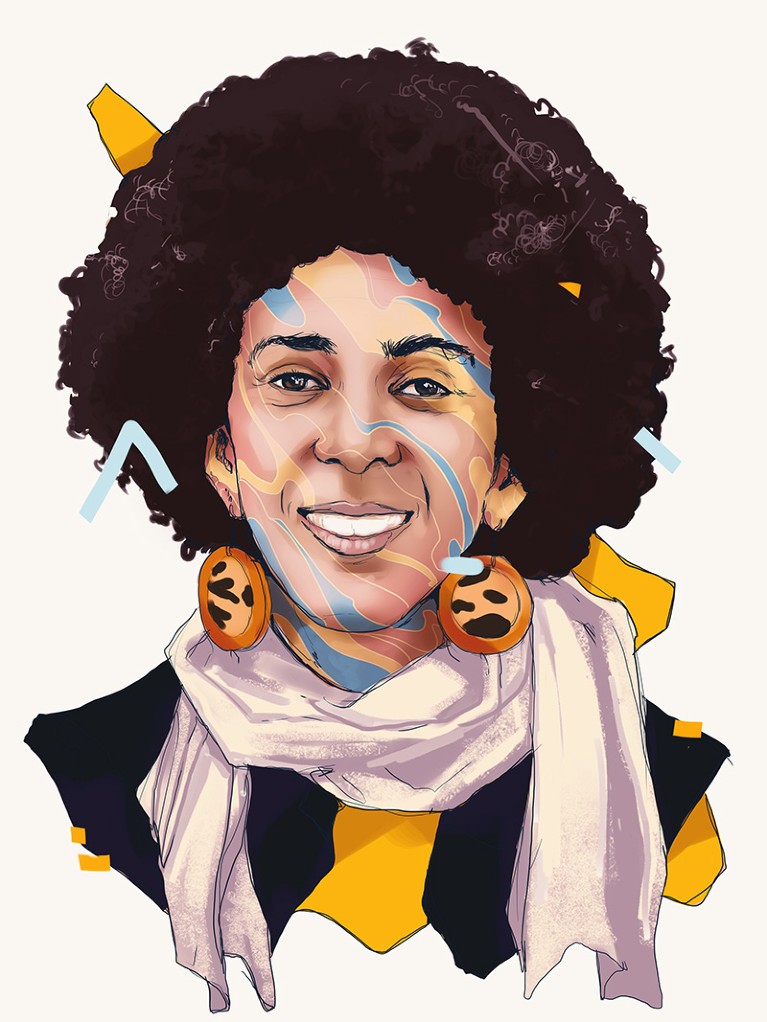
Computer vision is one of the most rapidly developing areas of AI. Algorithms trained to read and interpret images are the foundation of technologies such as self-driving cars, surveillance and augmented reality.
Timnit Gebru, a computer scientist and former co-lead of the Ethical AI Team at Google in Mountain View, California, recognizes the promise of such advances, but is concerned about how they could affect underrepresented communities, particularly people of colour . “My research is about trying to minimize and mitigate the negative impacts of AI,” she says.
In a 2018 study , Gebru and Joy Buolamwini, a computer scientist at the MIT Media Lab, concluded that three commonly used facial analysis algorithms drew overwhelmingly on data obtained from light-skinned people ( J. Buolamwini and T. Gebru. Proc. Mach. Learn. Res. 81 , 77–91; 2018 ). Error rates for dark-skinned females were found to be as high as 34.7% , due to a lack of data, whereas the maximum error rate for light-skinned males was 0.8%. This could result in people with darker skin getting inaccurate medical diagnoses, says Gebru. “If you’re using this technology to detect melanoma from skin photos, for example, then a lot of dark-skinned people could be misdiagnosed.”
Facial recognition used for government surveillance, such as during the Hong Kong protests in 2019, is also highly problematic , says Gebru, because the technology is more likely to misidentify a person with darker skin. “I’m working to have face surveillance banned,” she says. “Even if dark-skinned people were accurately identified, it’s the most marginalized groups that are most subject to surveillance.”
In 2017, as a PhD student at Stanford University in California under the supervision of Li Fei-Fei , Gebru co-founded the non-profit, Black in AI, with Rediet Abebe, a computer scientist at Cornell University in Ithaca, New York. The organization seeks to increase the presence of Black people in AI research by providing mentorship for researchers as they apply to graduate programmes, navigate graduate school, and enter and progress through the postgraduate job market. The organization is also advocating for structural changes within institutions to address bias in hiring and promotion decisions. Its annual workshop calls for papers with at least one Black researcher as the main author or co-author. — by Benjamin Plackett
YUTAKA MATSUO: Internet miner
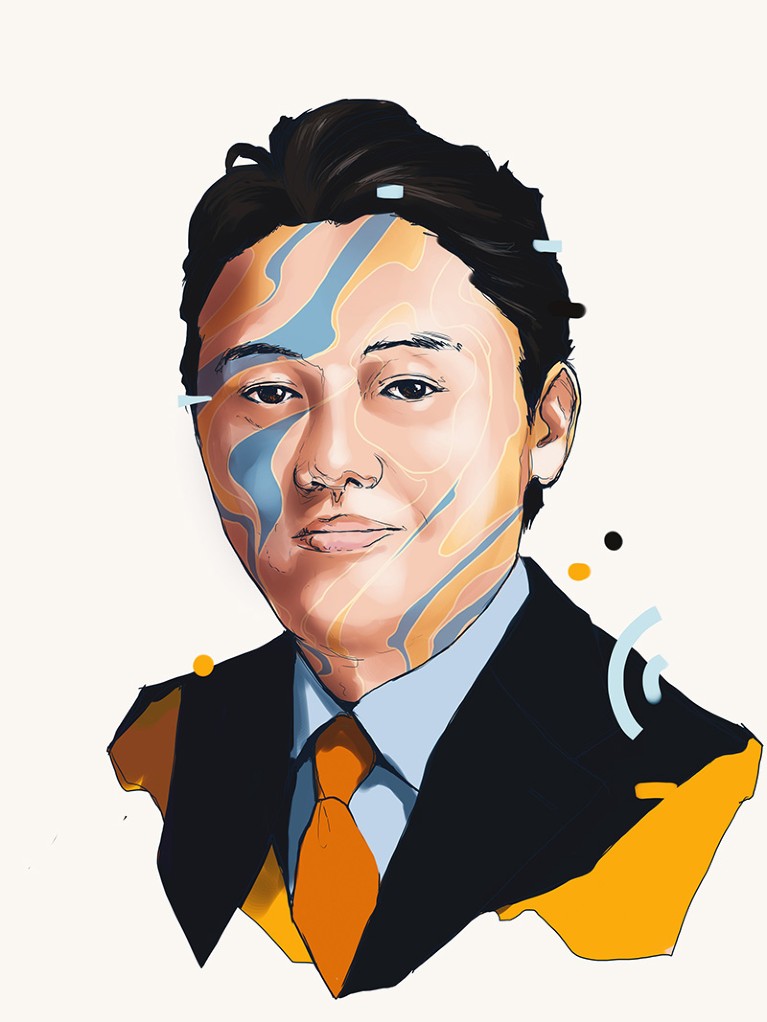
In 2010, Yutaka Matsuo created an algorithm that could detect the first signs of earthquakes by monitoring Twitter for mentions of tremors. His system not only detected 96% of the earthquakes that were registered by the Japan Meteorological Agency (JMA), it also sent e-mail alerts to registered users much faster than announcements could be broadcast by the JMA.
He applied a similar web-mining technique to the stock market. “We were able to classify news articles about companies as either positive or negative,” says Matsuo. “We combined that data to accurately predict profit growth and performance.”
Matsuo’s ability to extract valuable information from what people are saying online has contributed to his reputation as one of Japan’s leading AI researchers. He is a professor at the University of Tokyo’s Department of Technology Management and president of the Japan Deep Learning Association, a non-profit organization that fosters AI researchers and engineers by offering training and certification exams. In 2019, he was the first AI specialist added to the board of Japanese technology giant Softbank.
Over the past decade, Matsuo and his team have been supporting young entrepreneurs in launching internationally successful AI start-ups. “We want to create an ecosystem like Silicon Valley, which Japan just doesn’t have,” he says.
Among the start-ups supported by Matsuo is Neural Pocket, launched in 2018 by Roi Shigematsu, a University of Tokyo graduate. The company analyses photos and videos to provide insights into consumer behaviour.
Matsuo is also an adviser for ReadyFor, one of Japan’s earliest crowd-funding platforms. The company was launched in 2011 by Haruka Mera, who first collaborated with Matsuo as an undergraduate student at Keio University in Tokyo. The platform is raising funds for people affected by the COVID-19 pandemic, and reports that its total transaction value for donations rose by 4,400% between March and April 2020.
Matsuo encourages young researchers who are interested in launching AI start-ups to seek partnerships with industry. “Japanese society is quite conservative,” he says. “If you’re older, you’re more likely to get a large budget from public funds, but I’m 45, and that’s still considered too young.” — by Benjamin Plackett
DACHENG TAO: Machine visionary
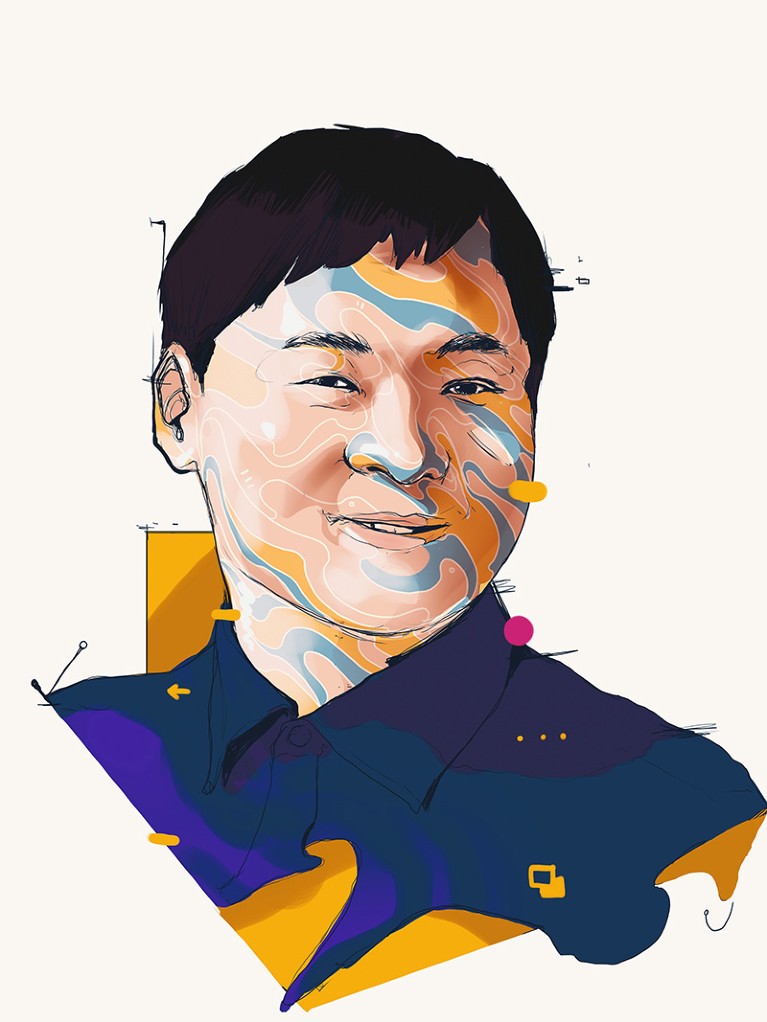
By 2030, an estimated one in ten cars globally will be self-driving. The key to getting these autonomous vehicles on the road is designing computer-vision systems that can identify obstacles to avoid accidents at least as effectively as a human driver .
Neural networks, sets of AI algorithms inspired by neurological processes that fire in the human cerebral cortex, form the ‘brains’ of self-driving cars. Dacheng Tao, a computer scientist at the University of Sydney, Australia, designs neural networks for computer-vision tasks. He is also building models and algorithms that can process videos captured by moving cameras, such as those in self-driving cars.
“Neural networks are very useful for modelling the world,” says Tao, director of the UBTECH Sydney Artificial Intelligence Centre, a partnership between the University of Sydney and global robotics company UBTECH.
In 2017, Tao was awarded an Australian Laureate Fellowship for a five-year project that uses deep-learning techniques to improve moving-camera computer vision in autonomous machines and vehicles. A subset of machine learning, deep learning uses neural networks to build systems that can ‘learn’ through their own data processing.
Since launching in 2018, Tao’s project has resulted in more than 40 journal publications and conference papers. He is among the most prolific researchers in AI research output from 2015 to 2019, as tracked by the Dimensions database, and is one of Australia’s most highly cited computer scientists. Since 2015, Tao’s papers have amassed more than 42,500 citations, as indexed by Google Scholar. In November 2020, he won the Eureka Prize for Excellence in Data Science, awarded by the Australian Museum.
In 2019, Tao and his team trained a neural network to construct 3D environments using a motion-blurred image, such as would be captured by a moving car. Details, including the motion, blurring effect and depth at which it was taken, helped the researchers to recover what they describe as “the 3D world hidden under the blurs”. The findings could help self-driving cars to better process their surroundings. — by Gemma Conroy
Nature 588 , S114-S117 (2020)
doi: https://doi.org/10.1038/d41586-020-03411-0
This article is part of Nature Index 2020 Artificial intelligence , an editorially independent supplement. Advertisers have no influence over the content.
Related Articles
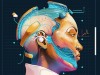
Partner content: Advancing precision medicine using AI and big data
Partner content: Using AI to accelerate drug discovery
Partner content: Using AI to make healthcare more human
Partner content: Strengthening links in the discovery chain
Partner content: LMU Munich harnesses AI to drive discovery
Partner content: Breaking AI out of the computer science bubble
Partner content: Discovering a theory to visualize the world
Partner content: Supporting the technology game-changers
Partner content: Data-driven AI illuminates the future
Partner content: The humans at the heart of AI
Partner content: New reach for computer imaging
Partner content: Building natural trust in artificial intelligence
Partner content: Raising a global centre for deep learning
Partner content: Japan’s new centre of gravity for clinical data science
Partner content: AI researchers wanted in Germany
- Computer science
- Institutions
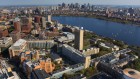
AlphaFold3 — why did Nature publish it without its code?
Editorial 22 MAY 24
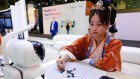
AI now beats humans at basic tasks — new benchmarks are needed, says major report
News 15 APR 24
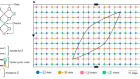
High-threshold and low-overhead fault-tolerant quantum memory
Article 27 MAR 24
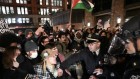
Protests over Israel-Hamas war have torn US universities apart: what’s next?
News Explainer 22 MAY 24
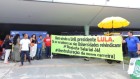
Dozens of Brazilian universities hit by strikes over academic wages
News 08 MAY 24
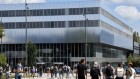
France’s research mega-campus faces leadership crisis
News 03 MAY 24
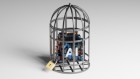
Guidelines for academics aim to lessen ethical pitfalls in generative-AI use
Nature Index 22 MAY 24
Internet use and teen mental health: it’s about more than just screen time
Correspondence 21 MAY 24
Social-media influence on teen mental health goes beyond just cause and effect
Recruitment of Global Talent at the Institute of Zoology, Chinese Academy of Sciences (IOZ, CAS)
The Institute of Zoology (IOZ), Chinese Academy of Sciences (CAS), is seeking global talents around the world.
Beijing, China
Institute of Zoology, Chinese Academy of Sciences (IOZ, CAS)
Full Professorship (W3) in “Organic Environmental Geochemistry (f/m/d)
The Institute of Earth Sciences within the Faculty of Chemistry and Earth Sciences at Heidelberg University invites applications for a FULL PROFE...
Heidelberg, Brandenburg (DE)
Universität Heidelberg
Postdoc: deep learning for super-resolution microscopy
The Ries lab is looking for a PostDoc with background in machine learning.
Vienna, Austria
University of Vienna
Postdoc: development of a novel MINFLUX microscope
The Ries lab is developing super-resolution microscopy methods for structural cell biology. In this project we will develop a fast, simple, and robust
Postdoctoral scholarship in Structural biology of neurodegeneration
A 2-year fellowship in multidisciplinary project combining molecular, structural and cell biology approaches to understand neurodegenerative disease
Umeå, Sweden
Umeå University
Sign up for the Nature Briefing newsletter — what matters in science, free to your inbox daily.
Quick links
- Explore articles by subject
- Guide to authors
- Editorial policies
share this!
May 16, 2024
This article has been reviewed according to Science X's editorial process and policies . Editors have highlighted the following attributes while ensuring the content's credibility:
fact-checked
peer-reviewed publication
trusted source
AI reveals critical gaps in global antimicrobial resistance research
by Newcastle University

Artificial intelligence (AI) has helped identify knowledge, methodological and communication gaps in global antimicrobial resistance (AMR) research.
In a new study carried out by the Chinese Academy of Sciences and Newcastle University under the co-leadership of Professor Yong-Guan Zhu and Professor David W. Graham, respectively, experts compiled a comprehensive database of 254,738 articles spanning two decades, shedding light on patterns of AMR research worldwide.
They found that the terminology and methods used in AMR research significantly differ across the medical, veterinary, food safety , plant agriculture, and environmental sectors. The semantic and methodological differences result in limited valuation work between sectors and limited cross-sectoral communication, resulting in inconsistent messages to decision-makers.
Through sophisticated AI-based analysis, the team developed global maps showcasing regional, methodological, and sectoral AMR research activities. The findings confirm a stark lack of interdisciplinary collaboration, particularly in low-income countries, where the burden of increasing AMR is most acute.
Published in the journal Environment International , the findings explain why solutions to AMR based on One Health are not developing as needed. The results could play a critical role in providing guidance on how and where to better integrate AMR surveillance across sectors and regions worldwide.
Professor David W. Graham, Emeritus Professor of Engineering at Newcastle University, said, "The findings highlight the urgent need for greater coordination in research methods across sectors and regions. For instance, the medical and veterinary communities need information about living AMR infectious pathogens to prioritize decisions, whereas environmental researchers often focus on genetic targets. Our work shows that culturing microbiology and isolate sequencing, and metagenomics must be performed in tandem in all future work, and more context data must be collected to relate results from different sectors.
"Our paper's findings support key messages from UN Environment Program and World Health Organization that emphasize the best way to mitigate AMR is through prevention and integrated surveillance, which is key to prioritizing solutions."
This is being addressed by the United Nations Quadripartite Technical Group on Integrated Surveillance on Antimicrobial Use and Resistance, of which both Prof Zhu and Graham are members.
Graham continued, "This work was only possible due its novel use of artificial intelligence and natural language processing to intelligently search an extensive and living database, an archive we make openly available for public use and contributions. This paper represents the first in a series of joint manuscripts leveraging AI to guide future AMR and other research agenda."
Professor Yong-Guan Zhu, Professor of Environmental Sciences, Chinese Academy of Sciences, added, "The framework of One Health is of critical importance in safeguarding human and ecosystem health, but it needs roadmaps to implement; this study timely identifies [a] path forward. The study also demonstrates that multidisciplinary and international collaboration is essential in solving global challenges, and we should embrace emerging technologies, such as AI."
Both scientists recommend future research and increased investment in capacity development, especially in low-income countries , to address the pressing AMR challenges in these regions.
Journal information: Environment International
Provided by Newcastle University
Explore further
Feedback to editors

Study identifies high-performance alternative to conventional ferroelectrics
6 hours ago

Study reveals key role of plant-bacteria communication for assembly of a healthy plant microbiome
7 hours ago

Crows can deliberately plan how many calls to make, study shows

Novel liquid crystals produced by stacking antiaromatic units could lead to advances in organic semiconductors

Stress bragging may make you seem less competent, less likable at work
8 hours ago

Parents of the year: Scavenging raptors demonstrate high level of collaboration in raising chicks

Birdsong and human voice built from same genetic blueprint

Team develops an intelligent nanodevice based on a component of cinnamon essential oil as an antimicrobial agent

New 'atlas' provides unprecedented insights on how genes function in early embryo development

Researchers reveal dynamic structure of FLVCR proteins and their function in nutrient transport
9 hours ago
Relevant PhysicsForums posts
Dna-maternity test - could you see other relationship than mother.
May 19, 2024
And Now, here comes COVID-19 version BA.2, BA.4, BA.5,...
May 17, 2024
Is it usual for vaccine injection site to hurt again during infection?
A brief biography of dr virgina apgar, creator of the baby apgar test.
May 12, 2024
Who chooses official designations for individual dolphins, such as FB15, F153, F286?
May 9, 2024
The Cass Report (UK)
May 1, 2024
More from Biology and Medical
Related Stories

Urgent environmental action needed to limit the spread of superbugs, says new report
Feb 9, 2023

Quantifying antibiotic resistance genes in environmental samples
Aug 14, 2023

How to slow the spread of deadly 'superbugs'
Oct 24, 2023

Researchers say nature recovery must be integrated across all sectors to bend the curve of biodiversity loss
May 2, 2024

Experts call for global genetic warning system to combat the next pandemic and antimicrobial resistance
Apr 25, 2024

Holes in 'One Health Network' coverage leave vulnerable communities in Global South
Mar 14, 2023
Recommended for you

Finding the beat of collective animal motion: Scientists show reciprocity is key to driving coordinated movements
10 hours ago

Researchers identify the pathogen causing sea urchin mass mortalities in the Red Sea
12 hours ago

Genetic mutation responsible for new coat pattern in cats in Finland identified
13 hours ago

Scientists discover primary wound signal that promotes plant regeneration
Let us know if there is a problem with our content.
Use this form if you have come across a typo, inaccuracy or would like to send an edit request for the content on this page. For general inquiries, please use our contact form . For general feedback, use the public comments section below (please adhere to guidelines ).
Please select the most appropriate category to facilitate processing of your request
Thank you for taking time to provide your feedback to the editors.
Your feedback is important to us. However, we do not guarantee individual replies due to the high volume of messages.
E-mail the story
Your email address is used only to let the recipient know who sent the email. Neither your address nor the recipient's address will be used for any other purpose. The information you enter will appear in your e-mail message and is not retained by Phys.org in any form.
Newsletter sign up
Get weekly and/or daily updates delivered to your inbox. You can unsubscribe at any time and we'll never share your details to third parties.
More information Privacy policy
Donate and enjoy an ad-free experience
We keep our content available to everyone. Consider supporting Science X's mission by getting a premium account.
E-mail newsletter
A comprehensive analysis of the role of artificial intelligence in aligning tertiary institutions academic programs to the emerging digital enterprise
- Published: 10 May 2024
Cite this article
- Duncan Nyale ORCID: orcid.org/0000-0003-0059-7655 1 ,
- Simon Karume 1 &
- Andrew Kipkebut 2
52 Accesses
1 Altmetric
Explore all metrics
The study explores the use of Artificial Intelligence (AI) frameworks in transforming academic programs into adaptive, industry-relevant programs. The paper explores the development, validation, and effectiveness of artificial intelligence (AI) frameworks in aligning academic programs with the digital enterprise while highlighting the importance of these frameworks in enhancing graduates' digital skills and employability. Through a comprehensive analysis of existing literature, the paper highlights the significance of AI frameworks in bridging the gap between academia and industry requirements. It also presents case studies and empirical evidence to demonstrate the effectiveness of these frameworks in enhancing graduates' digital competencies. The findings emphasize the need for educational institutions to adopt AI frameworks to equip graduates with the necessary skills for success in the digital age. The research highlights the need for educational institutions to adapt to the rapidly changing digital landscape. The study shows significant improvements in graduates' digital literacy, problem-solving abilities, and adaptability to technological advancements. The real-world implications of these AI-driven educational interventions highlight the transformative potential of integrating AI technologies in education.
This is a preview of subscription content, log in via an institution to check access.
Access this article
Price includes VAT (Russian Federation)
Instant access to the full article PDF.
Rent this article via DeepDyve
Institutional subscriptions
Similar content being viewed by others
The Use of Artificial Intelligence in Higher Education – Systematic Review
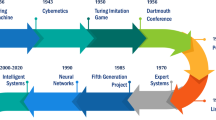
An artificial intelligence educational strategy for the digital transformation
Artificial Intelligence in the Context of Digital Learning Environments (DLEs): Towards Adaptive Learning
Data availability.
The data and materials used in this paper are available upon request. The comprehensive list of included studies, along with relevant data extracted from these studies, is available from the corresponding author upon request.
Akojie, P., & Haynes, S. (2023). A Framework to Enhance Graduate Employability. International Journal of Doctoral Studies, 18 , 055–075. https://doi.org/10.28945/5090
Alhothali, A., Albsisi, M., Assalahi, H., & Aldosemani, T. (2022). Predicting student outcomes in online courses Using Machine Learning techniques: A review. Sustainability, 14 (10), 6199. https://doi.org/10.3390/su14106199
Article Google Scholar
Anderson, M., & Perrin, A. (2021). Mobile Technology and Home Broadband 2021. Pew Research Center. https://www.pewresearch.org/internet/2021/06/03/mobile-technology-and-home-broadband-2021/ . Accessed 5/7/23.
Bagai, R., & Mane, V. (2023). Designing an AI-Powered Mentorship Platform for Professional Development: Opportunities and Challenges. International Journal of Computer Trends and Technology, 71 (4), 108–114. https://doi.org/10.14445/22312803/ijctt-v71i4p114
Barboutidis, G., & Stiakakis, E. (2023). Identifying the Factors to Enhance Digital Competence of Students at Vocational Training Institutes. Tech Know Learn, 2 (28), 613–650. https://doi.org/10.1007/s10758-023-09641-1
Carlisle, S., Ivanov, S. H., & Dijkmans, C. (2021). The Digital Skills Divide: Evidence from the European Tourism Industry. Journal of Tourism F, 2 (9), 240–266. https://doi.org/10.1108/jtf-07-2020-0114
Cevikbas, M., & Kaiser, G. (2022). Promoting Personalized Learning in Flipped Classrooms: A Systematic Review Study. Sustainability, 14 (18), 11393. https://doi.org/10.3390/su141811393
Chaudhry, M. N., & Kazim, E. (2021). Artificial Intelligence in Education (Aied): A High-level Academic and Industry Note 2021. AI Ethics, 1 (2), 157–165. https://doi.org/10.1007/s43681-021-00074-z
Columbus, L. (n.d.). IBM predicts demand for data scientists will soar 28% by 2020. Forbes . https://www.forbes.com/sites/louiscolumbus/2017/05/13/ibm-predicts-demand-for-data-scientists-will-soar-28-by-2020/ . Accessed 7/7/23.
Crompton, H. (2023). Artificial Intelligence in Higher Education: The State of the Field. International Journal of Educational Technology in Higher Education, 1 (20). https://doi.org/10.1186/s41239-023-00392-8
Darling-Hammond, L., Flook, L., Cook-Harvey, C. M., Barron, B., & Osher, D. (2019). Implications for Educational Practice of the Science of Learning and Development. Applied Developmental Science, 2 (24), 97–140. https://doi.org/10.1080/10888691.2018.1537791
Ezell S. (2021). Assessing the State of Digital Skills in the U.S. Economy. Itif.org. https://itif.org/publications/2021/11/29/assessing-state-digital-skills-us-economy/ . Accessed 7/7/23.
Feijao, C., Flanagan, I., Van Stolk, C., & Gunashekar, S. (2021). Current trends and future directions The global digital skills gap. https://www.rand.org/content/dam/rand/pubs/research_reports/RRA1500/RRA1533-1/RAND_RRA1533-1.pdf . Accessed 6/7/23.
Guan, X., Feng, X., & Islam, A. Y. M. (2023). The Dilemma and Countermeasures of Educational Data Ethics in The Age of Intelligence. Humanities and Social Sciences Communications, 1 (10). https://doi.org/10.1057/s41599-023-01633-x
Halder, S., Saha, S. (2023). Artificial Intelligence in Education. The Routledge Handbook of Education Technology , 390–399. https://doi.org/10.4324/9781003293545-33
Hecker, I., & Loprest, P. (2019). Foundational Digital Skills for Career Progress. Urban Institute. https://www.urban.org/sites/default/files/publication/100843/foundational_digital_skills_for_career_progress_2.pdf . Accessed 6/7/23.
Hooda, M., Rana, C., Dahiya, O., Rizwan, A., & Hossain, M. S. (2022). Artificial Intelligence for Assessment and Feedback to Enhance Student Success in Higher Education. Mathematical Problems in Engineering, 2022 , 1–19. https://doi.org/10.1155/2022/5215722
Hootsuite. (2022). Digital 2022 - Social Media Marketing & Management Dashboard. Hootsuite. https://www.hootsuite.com/resources/digital-trends . Accessed 6/7/23.
ILO. (2021). Digital Skills Assessment Guidebook . ITU Publications. https://academy.itu.int/sites/default/files/media2/file/D-PHCB-CAP_BLD.04-2020-PDF-E_02%20June%202020.pdf . Accessed 10/4/23.
Khan, I., Ahmad, A. R., Jabeur, N., & Mahdi, M. N. (2021a). An Artificial Intelligence Approach to Monitor Student Performance and Devise Preventive Measures. Smart Learning Environments, 1 (8). https://doi.org/10.1186/s40561-021-00161-y
Khan, N., Khan, S., Tan, B. C., & Loon, C. H. (2021b). Driving Digital Competency Model Towards Ir 4.0 In Malaysia. Journal of Physics: Conference Series, 1 (1793), 012049. https://doi.org/10.1088/1742-6596/1793/1/012049
Khosravi, H., Shum, S. B., Chen, G., Gašević, D., Kay, J., Knight, S. R., & Tsai, Y. S. (2022). Explainable Artificial Intelligence in Education. Computers and Education: Artificial Intelligence, 3 , 100074. https://doi.org/10.1016/j.caeai.2022.100074
Kim, J., Lee, H., & Cho, Y. M. (2022). Learning Design to Support Student-AI Collaboration: Perspectives of Leading Teachers for AI in Education. Education and Information Technologies, 5 (27), 6069–6104. https://doi.org/10.1007/s10639-021-10831-6
Kousa, P., & Niemi, H. (2023). Artificial Intelligence Ethics from the Perspective of Educational Technology Companies and Schools. In H. Niemi, R. D. Pea, & Y. Lu (Eds.), AI in Learning: Designing the Future. Cham: Springer. https://doi.org/10.1007/978-3-031-09687-7_17
Chapter Google Scholar
Llego, M. A. (2023). The AI-Driven Holistic Development Framework for Education: A Comprehensive Approach to Integrating AI in Learning Environments. TeacherPH . https://www.teacherph.com/ai-driven-holistic-development-framework-education/ . Accessed 11/7/23.
Natarajan, P., & Szeto, A. (2023). A new paradigm for partnership between industry and academia in the age of AI. (2023, March 23). Amazon Science. https://www.amazon.science/news-and-features/a-new-paradigm-for-partnership-between-industry-and-academia-in-the-age-of-ai . Accessed 11/7/23.
Ng, D. T. K., Leung, J. K. L., Su, J., Ng, R. C. W., & Chu, S. K. W. (2023). Teachers’ Ai Digital Competencies and Twenty-first Century Skills in The Post-Pandemic World. Educational Technology Research and Development, 1 (71), 137–161. https://doi.org/10.1007/s11423-023-10203-6
Nguyen, A., Ngo, H. N., Hong, Y., Dang, B., & Nguyen, B. T. (2022). Ethical Principles for Artificial Intelligence in Education. Education and Information Technologies, 4 (28), 4221–4241. https://doi.org/10.1007/s10639-022-11316-w
OECD. (2021). Artificial Intelligence, Machine Learning and Big Data in Finance Opportunities, Challenges and Implications for Policy Makers . OECD Publishing. https://www.oecd.org/finance/financial-markets/Artificial-intelligence-machine-learning-big-data-in-finance.pdf . Accessed 8/7/23.
Ojajuni, O. P., Ayeni, F., Akodu, O., Ekanoye, F., Adewole, S., Ayo, T., & Mbarika, V. (2021). Predicting Student Academic Performance Using Machine Learning. Computational Science and Its Applications – ICCSA 2021 , 481–491. https://doi.org/10.1007/978-3-030-87013-3_36
Pantanowitz, L., Bui, M. M., Chauhan, C., ElGabry, E. A., Hassell, L. A., Li, Z., & Becich, M. J. (2022). Rules of engagement: promoting academic-industry partnership in the era of digital pathology and artificial Intelligence. Academic Pathology, 1 (9), 100026. https://doi.org/10.1016/j.acpath.2022.100026
Raji, N. A. S., Busson-Crowe, D. A., & Dommett, E. J. (2023). University-wide Digital Skills Training: A Case Study Evaluation. Education Sciences, 4 (13), 333. https://doi.org/10.3390/educsci13040333
Ramesh, D., & Sanampudi, S. K. (2021). An automated essay scoring systems: A systematic literature review. Artificial Intelligence Review, 55 , 1–33. https://doi.org/10.1007/s10462-021-10068-2
Sarker, I. H. (2021). Machine Learning: Algorithms, Real-world Applications and Research Directions. SN Computer Science, 3 (2). https://doi.org/10.1007/s42979-021-00592-x
Seo, K., Tang, J., Roll, I., Fels, S., & Yoon, D. (2021). The Impact of Artificial Intelligence On Learner–instructor Interaction in Online Learning. International Journal of Educational Technology in Higher Education, 1 (18). https://doi.org/10.1186/s41239-021-00292-9
Suarta, I. M., & Suwintana, I. K. (2021). The New Framework of Employability Skills for Digital Business. Journal of Physics: Conference Series, 1 (1833), 012034. https://doi.org/10.1088/1742-6596/1833/1/012034
Swiecki, Z., Khosravi, H., Chen, G., Martinez-Maldanado, R., Lodge, J. M., Milligan, S., & Gašević, D. (2022). Assessment in the Age of Artificial Intelligence. Computers and Education: Artificial Intelligence, 3 , 100075. https://doi.org/10.1016/j.caeai.2022.100075
Tapalova, O., Zhiyenbayeva, N., & Gura, D. (2022). Artificial Intelligence in Education: AIEd for Personalised Learning Pathways. Electronic Journal of e-Learning, 20 , 639–653. https://doi.org/10.34190/ejel.20.5.2597
Thongprasit, J., & Wannapiroon, P. (2022). Framework of Artificial Intelligence Learning Platform for Education. IES, 1 (15), 76. https://doi.org/10.5539/ies.v15n1p76
Zhang, Z., Xia, Y., & Abula, K. (2023). How Digital Skills Affect Rural Labor Employment Choices: Evidence from Rural China. Sustainability, 7 (15), 6050. https://doi.org/10.3390/su15076050
Zhou, X., Tong, Y., Lan, X., Zheng, K., Zhan, Z. (2021). AI Education in Massive Open Online Courses: A Content Analysis. 3rd International Conference on Computer Science and Technologies in Education (CSTE). https://doi.org/10.1109/cste53634.2021.00023
Download references
Acknowledgements
Not applicable.
The authors declare that this research paper did not receive any funding from external organizations. The study was conducted independently and without financial support from any source. The authors have no financial interests or affiliations that could have influenced the design, execution, analysis, or reporting of the research.
Author information
Authors and affiliations.
School of Computing and Mathematics, The Co-operative University of Kenya, Nairobi, Kenya
Duncan Nyale & Simon Karume
Department of Computer Science & Information Technology, Kabarak University, Nakuru, Kenya
Andrew Kipkebut
You can also search for this author in PubMed Google Scholar
Corresponding author
Correspondence to Duncan Nyale .

Ethics declarations
Competing interests.
Authors have no competing interests to declare.
Additional information
Publisher's note.
Springer Nature remains neutral with regard to jurisdictional claims in published maps and institutional affiliations.
Rights and permissions
Springer Nature or its licensor (e.g. a society or other partner) holds exclusive rights to this article under a publishing agreement with the author(s) or other rightsholder(s); author self-archiving of the accepted manuscript version of this article is solely governed by the terms of such publishing agreement and applicable law.
Reprints and permissions
About this article
Nyale, D., Karume, S. & Kipkebut, A. A comprehensive analysis of the role of artificial intelligence in aligning tertiary institutions academic programs to the emerging digital enterprise. Educ Inf Technol (2024). https://doi.org/10.1007/s10639-024-12743-7
Download citation
Received : 02 February 2024
Accepted : 24 April 2024
Published : 10 May 2024
DOI : https://doi.org/10.1007/s10639-024-12743-7
Share this article
Anyone you share the following link with will be able to read this content:
Sorry, a shareable link is not currently available for this article.
Provided by the Springer Nature SharedIt content-sharing initiative
- Digital skills
- Digital competencies
- Employability
- Machine learning
- Find a journal
- Publish with us
- Track your research
Our websites may use cookies to personalize and enhance your experience. By continuing without changing your cookie settings, you agree to this collection. For more information, please see our University Websites Privacy Notice .
UConn Today
- School and College News
- Arts & Culture
- Community Impact
- Entrepreneurship
- Health & Well-Being
- Research & Discovery
- UConn Health
- University Life
- UConn Voices
- University News
May 22, 2024 | Christine Buckley - College of Liberal Arts and Sciences
Geoscientist Among First Projects Approved by National Artificial Intelligence Research Resource (NAIRR) Pilot
Lijing Wang, who joins UConn in August, will develop AI models for mountain water flow that aid in climate change predictions
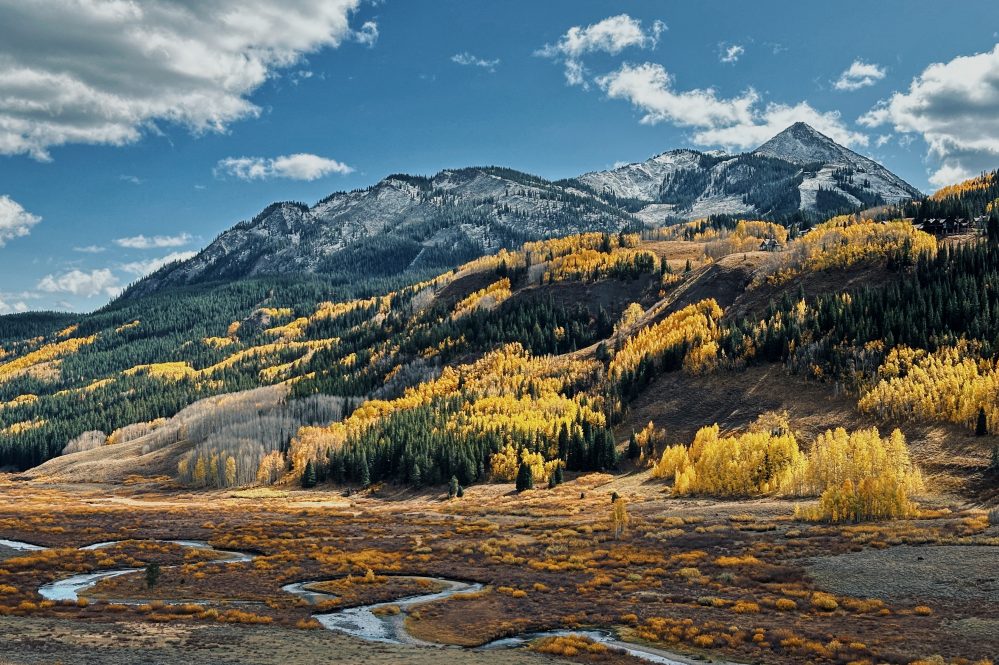
The East River Watershed during an October 2023 research trip (Photo courtesy of Lijing Wang)
Lijing Wang, assistant professor of Earth Sciences in the College of Liberal Arts and Sciences, is among the first scientists in the U.S. to earn support from the National Artificial Intelligence Research Resource (NAIRR) Pilot, a nationwide infrastructure that connects U.S. researchers to the computational data, software, models, and training they need to conduct paradigm-shfting AI research.
The U.S. National Science Foundation (NSF) and the Department of Energy (DOE) announced last week the first 35 projects awarded computational time through the project, marking what it calls a significant milestone in fostering responsible AI research and democratizing access to AI tools across the country.
The NAIRR Pilot will support fundamental, translational and use-inspired AI-related research with emphasis on societal challenges. Initial priority topics include safe, secure and trustworthy AI; human health; and environment and infrastructure.
Wang, who will join UConn as assistant professor of Earth Sciences in the fall, received 10,500 node hours at the DOE Argonne National Laboratory AI Testbed. A node hour is the cumulative amount of time that computing resources equivalent to one individual node, or a single computer within a larger network or cluster, have been active or utilized for computation.
Her project studies water flow in mountainous areas where the lack of data about snow melt and water movement makes predictions about the area’s future water flow difficult to compute or inaccurate.
“Mountainous watersheds provide significant water resources,” says Wang. “Conducting intensive monitoring is key to understanding water availability, but it’s not feasible in every catchment. Together with monitoring, an AI tool could help us evaluate these water variations more efficiently in the face of climate change.”
The work will simulate water movement across multiple mountain slopes under different conditions, and the results will form a dataset for an AI model to predict snow melt, water flow, and groundwater levels. Her results will lead to more rapid water forecasting, which will improve water management and climate change studies.
Of the 35 projects, 27 will be supported through the NSF-funded advanced computing systems, and eight projects will utilize DOE-supported systems.
Recent Articles
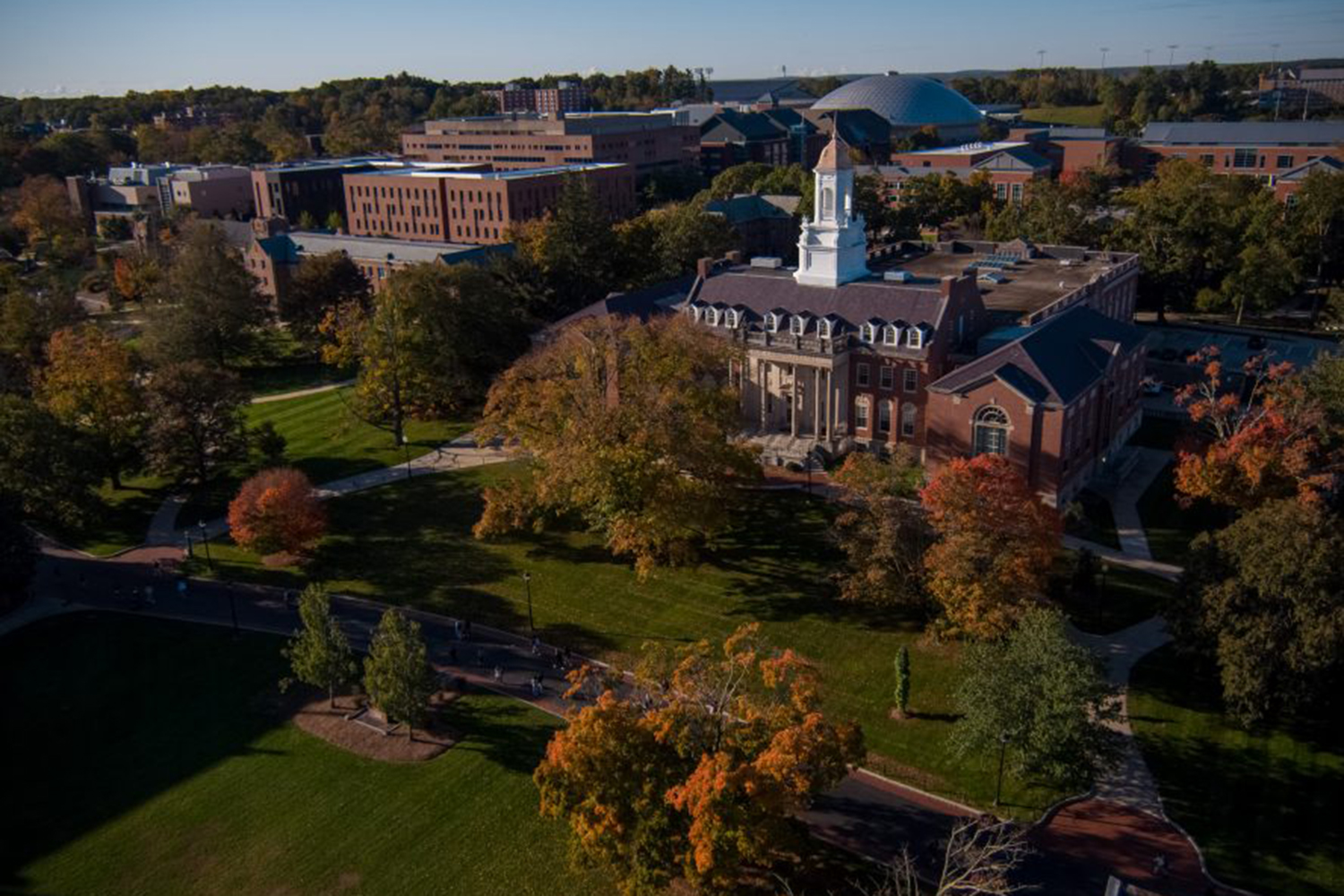
May 23, 2024
Announcing the Provost’s 2024 Alumni Faculty Excellence Awards
Read the article
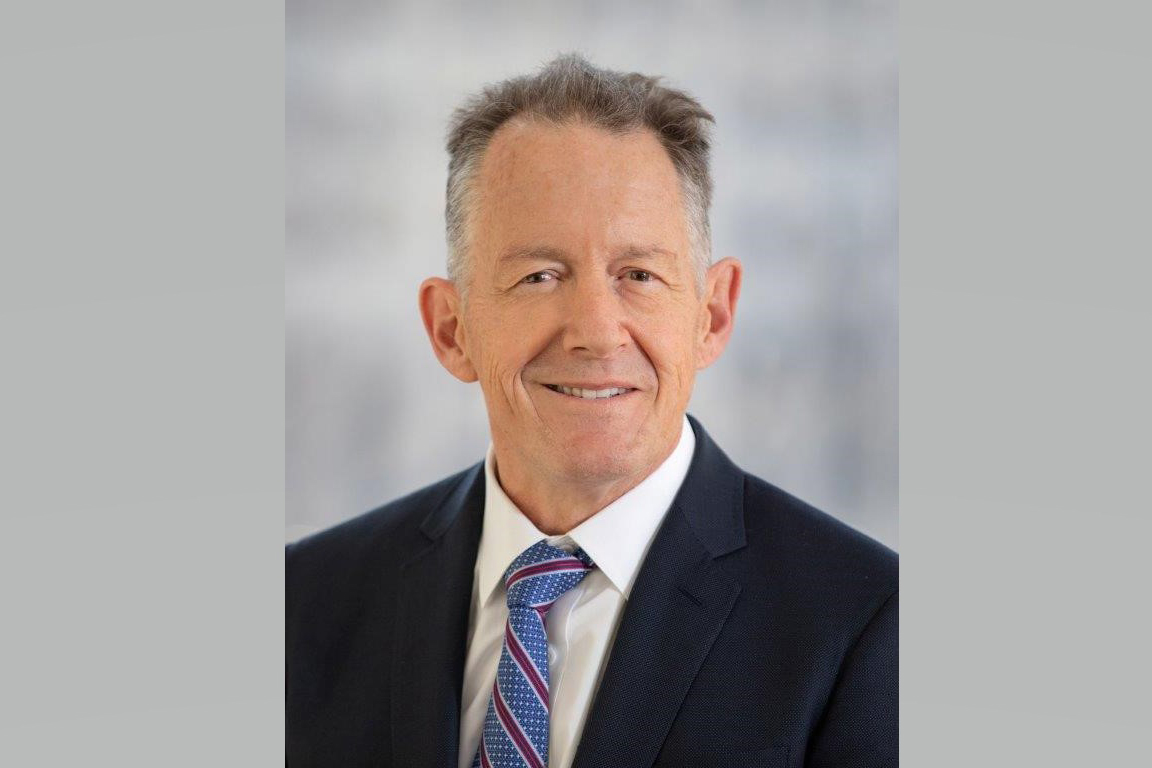
UConn Law Grad Starts Teaching Fellowship
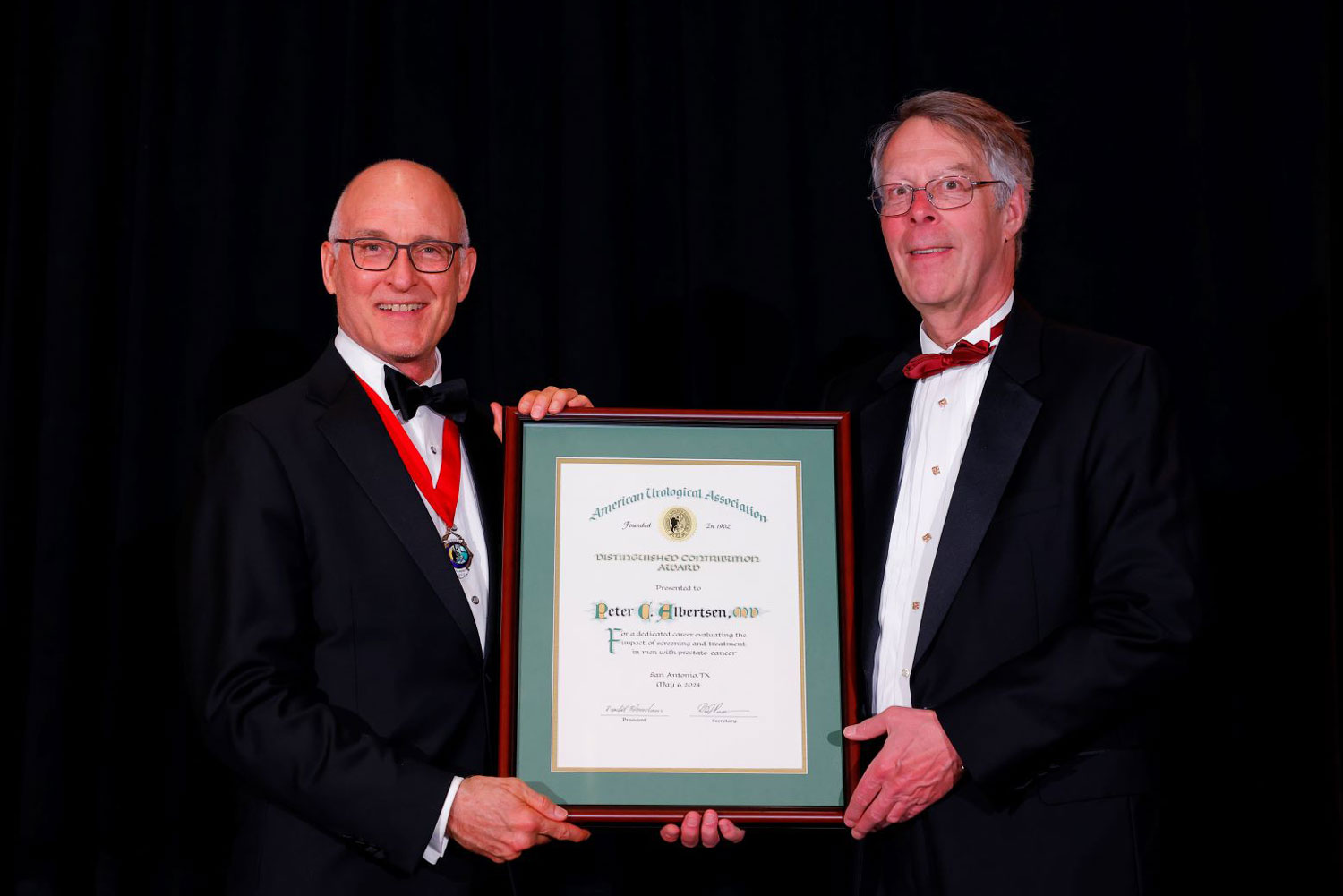
American Urology Association Honors Dr. Peter Albertsen
Subscribe to our newsletter
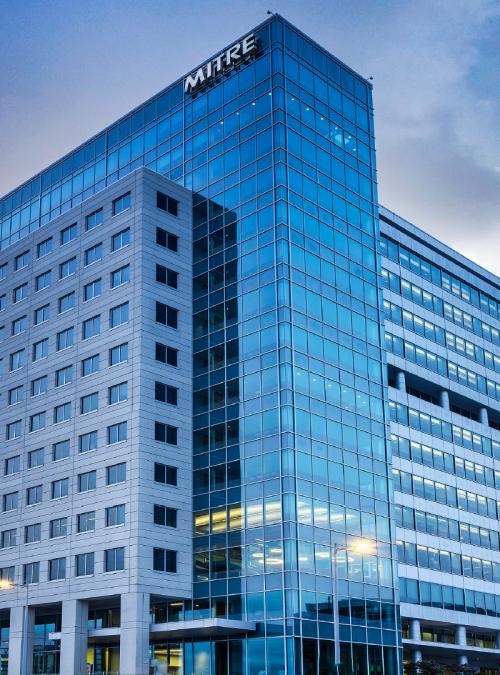
- Enhancing Acquisition Outcomes through Leveraging of Artificial Intelligence
The extraordinary speed of the advancement and impact of artificial intelligence (AI), combined with the surge in private- and public-sector demand, has caused confusion among U.S. regulators and senior leadership on how to approach possible integration of AI within government systems. A small amount of guidance has emerged since 2018, with 2023 being an inflection point—“the year of implementation.” In that year, both the European Union and the United States issued legislation calling for action by both the public and private sectors as AI’s pivotal role in the great power competition becomes more widely recognized.
Download Resources
Despite the “sudden” arrival of AI in the mainstream, many early pioneers within the government ecosystem have already worked directly or experimented indirectly with AI. An understanding of the current state—disjointed though it might be—is necessary to comprehend the important successes and failures as the collective federal government seeks to offer varying degrees of top-down guidance. This analysis of the current state will put AI’s impact on acquisition into perspective, highlighting the existing gaps and known areas of opportunity and risk and recognizing that for every known area there are likely to be a plethora of unknowns.
The implementation of new technology immediately alerts acquisition professionals that demand for new products and services will surge. However, implementing AI in a responsible and safe way is the dimension that will make developing, acquiring, maintaining, implementing, using, incorporating, etc. AI systems far different from approaches to previous adoption of any other technology by the federal acquisition community. It is in the acquisition community’s best interest to become involved and have a voice in formulating new regulations that govern how to meet the goals of AI implementation. The acquisition community must ensure that its regulations are compatible with existing processes and address ways in which AI can improve notoriously inefficient acquisition processes—without displacing human insight and expertise. In other words, acquisition professionals should not think only about how to acquire AI but also about how to integrate it within the federal acquisition workflow. They must work toward this future state now, to adopt AI at the speed of relevance and maintain America’s strategic advantage.
The recommendations in this paper, therefore, focus on how the government can reach this future state. It puts forth and advocates actionable insights based on independent, successful instances of AI use within government as well as industry suggestions based on accelerated implementation of AI in the private sector.
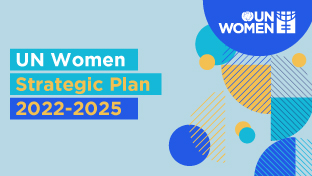
Artificial Intelligence and gender equality
- Share to Facebook
- Share to Twitter
- Share to LinkedIn
- Share to E-mail
The world has a gender equality problem, and Artificial Intelligence (AI) mirrors the gender bias in our society.
Although globally more women are accessing the internet every year , in low-income countries, only 20 per cent are connected . The gender digital divide creates a data gap that is reflected in the gender bias in AI.
Who creates AI and what biases are built into AI data (or not), can perpetuate, widen, or reduce gender equality gaps.
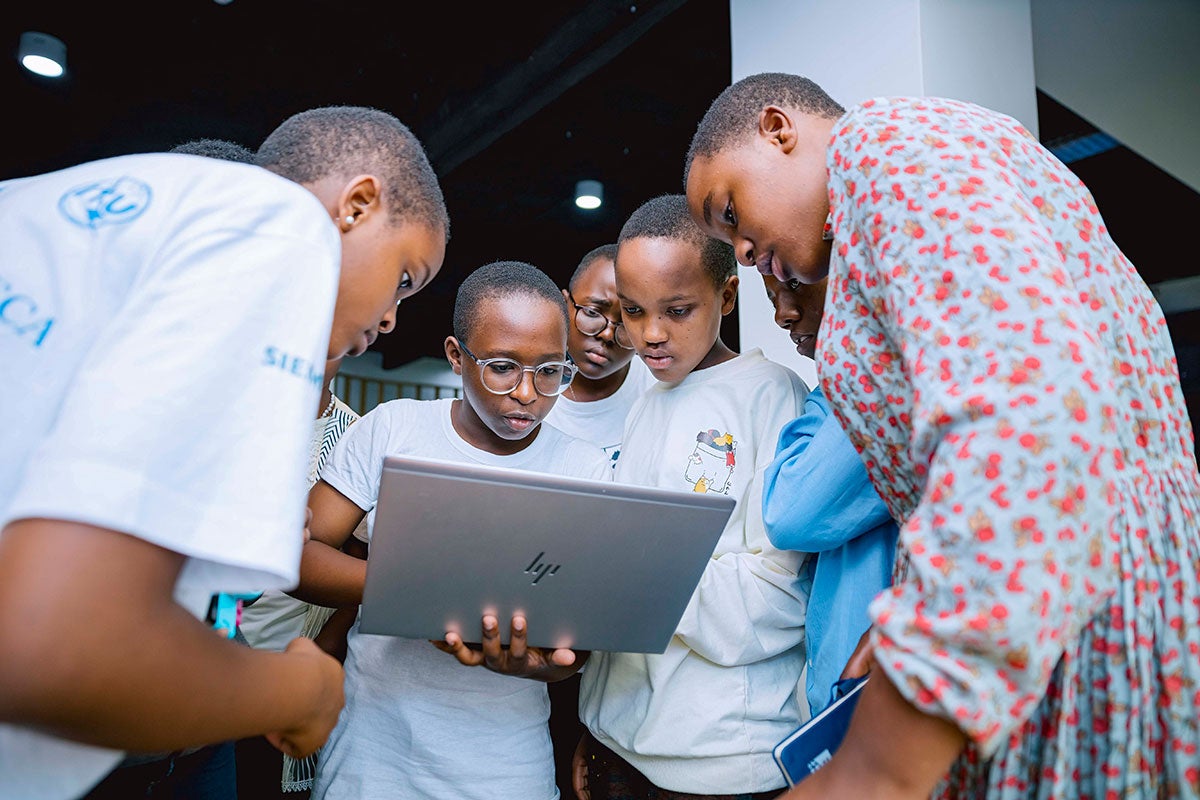
What is AI gender bias?
A study by the Berkeley Haas Center for Equity, Gender and Leadership analysed 133 AI systems across different industries and found that about 44 per cent of them showed gender bias , and 25 per cent exhibited both gender and racial bias.
Beyza Doğuç, an artist from Ankara, Turkey, encountered gender bias in Generative AI when she was researching for a novel and prompted it to write a story about a doctor and a nurse. Generative AI creates new content (text, images, video, etc.) inspired by similar content and data that it was trained on, often in response to questions or prompts by a user.
The AI made the doctor male and the nurse female. Doğuç continued to give it more prompts, and the AI always chose gender stereotypical roles for the characters and associated certain qualities and skills with male or female characters. When she asked the AI about the gender bias it exhibited, the AI explained it was because of the data it had been trained on and specifically, “word embedding” – which means the way certain words are encoded in machine learning to reflect their meaning and association with other words – it’s how machines learn and work with human language. If the AI is trained on data that associates women and men with different and specific skills or interests, it will generate content reflecting that bias.
“Artificial intelligence mirrors the biases that are present in our society and that manifest in AI training data,” said Doğuç, in a recent interview with UN Women.
Who develops AI, and what kind of data it is trained on, has gender implications for AI-powered solutions.
Sola Mahfouz, a quantum computing researcher at Tufts University, is excited about AI, but also concerned. “Is it equitable? How much does it mirror our society’s patriarchal structures and inherent biases from its predominantly male creators,” she reflected.
Mahfouz was born in Afghanistan, where she was forced to leave school when the Taliban came to her home and threatened her family. She eventually escaped Afghanistan and immigrated to the U.S. in 2016 to attend college.
As companies are scrambling for more data to feed AI systems, researchers from Epoch claim that tech companies could run out of high-quality data used by AI by 2026 .
Natacha Sangwa is a student from Rwanda who participated in the first coding camp organized under the African Girls Can Code Initiative last year. “I have noticed that [AI] is mostly developed by men and trained on datasets that are primarily based on men,” said Sangwa, who saw first-hand how that impacts women’s experience with the technology. “When women use some AI-powered systems to diagnose illnesses, they often receive inaccurate answers, because the AI is not aware of symptoms that may present differently in women.”
If current trends continue, AI-powered technology and services will continue lacking diverse gender and racial perspectives, and that gap will result in lower quality of services, biased decisions about jobs, credit, health care and more.
How to avoid gender bias in AI?
Removing gender bias in AI starts with prioritizing gender equality as a goal, as AI systems are conceptualized and built. This includes assessing data for misrepresentation, providing data that is representative of diverse gender and racial experiences, and reshaping the teams developing AI to make them more diverse and inclusive.
According to the Global Gender Gap Report of 2023, there are only 30 per cent women currently working in AI .
“When technology is developed with just one perspective, it’s like looking at the world half-blind,” concurred Mahfouz. She is currently working on a project to create an AI-powered platform that would connect Afghan women with each other.
“More women researchers are needed in the field. The unique lived experiences of women can profoundly shape the theoretical foundations of technology. It can also open new applications of the technology,” she added.
“To prevent gender bias in AI, we must first address gender bias in our society,” said Doğuç from Turkey.
There is a critical need for drawing upon diverse fields of expertise when developing AI, including gender expertise, so that machine learning systems can serve us better and support the drive for a more equal and sustainable world.
In a rapidly advancing AI industry, the lack of gender perspectives, data, and decision-making can perpetuate profound inequality for years to come.
The AI field needs more women, and that requires enabling and increasing girls’ and women’s access to and leadership in STEM and ICT education and careers.
The World Economic Forum reported in 2023 that women accounted for just 29 per cent of all science, technology, engineering and math (STEM) workers. Although more women are graduating and entering STEM jobs today than ever before, they are concentrated in entry level jobs and less likely to hold leadership positions.
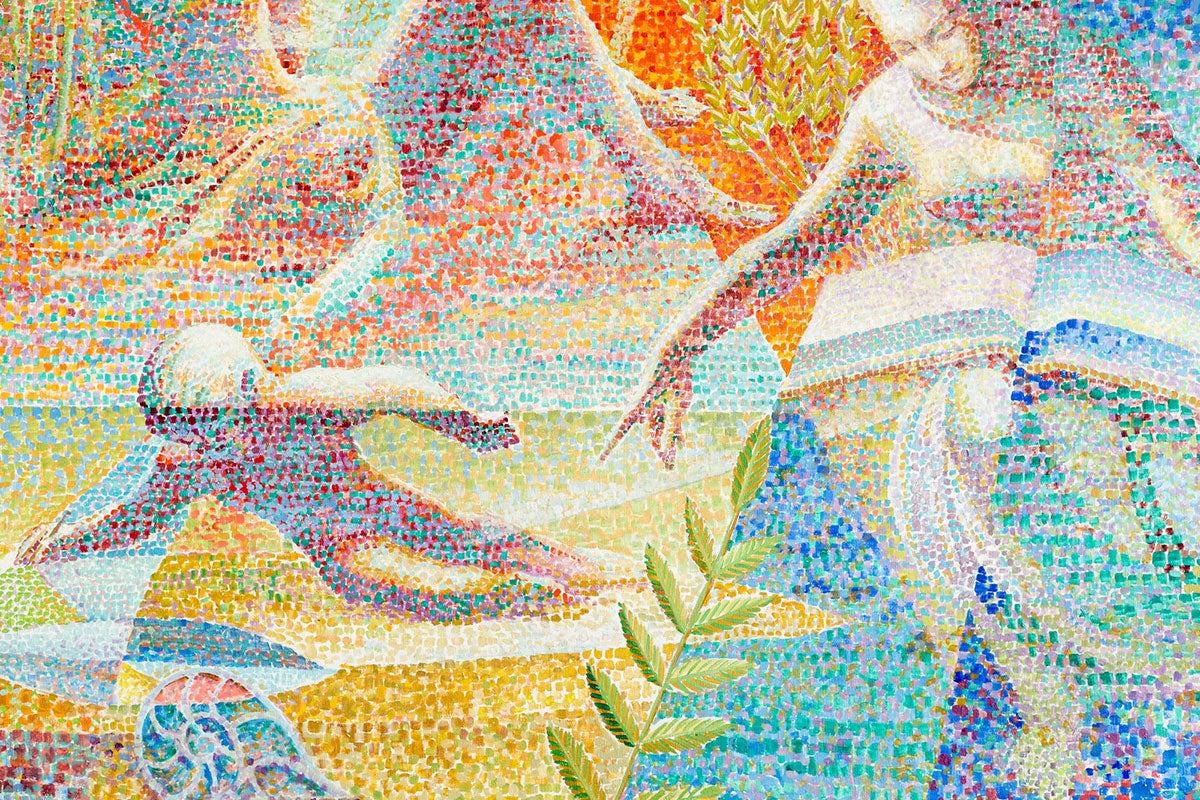
How can AI governance help accelerate progress towards gender equality?
International cooperation on digital technology has focused on technical and infrastructural issues and the digital economy, often at the expense of how technological developments were affecting society and generating disruption across all its layers – especially for the most vulnerable and historically excluded. There is a global governance deficit in addressing the challenges and risks of AI and harnessing its potential to leave no one behind.
“Right now, there is no mechanism to constrain developers from releasing AI systems before they are ready and safe. There’s a need for a global multistakeholder governance model that prevents and redresses when AI systems exhibit gender or racial bias, reinforce harmful stereotypes, or does not meet privacy and security standards,” said Helene Molinier, UN Women’s Advisor on Digital Gender Equality Cooperation in a recent interview with Devex.
In the current AI architecture, benefits and risks are not equitably distributed, with power concentrated in the hands of a few corporations, States and individuals, who control talent, data and computer resources. There is also no mechanism to look at broader considerations, like new forms of social vulnerability generated by AI, the disruption of industries and labour markets, the propensity for emerging technology to be used as a tool of oppression, the sustainability of the AI supply chain, or the impact of AI on future generations.
In 2024, the negotiation of the Global Digital Compact (GDC) offers a unique opportunity to build political momentum and place gender perspectives on digital technology at the core of a new digital governance framework. Without it, we face the risk of overlaying AI onto existing gender gaps, causing gender-based discrimination and harm to be left unchanged – and even amplified and perpetuated by AI systems.
UN Women position paper on the GDC provide concrete recommendations to harness the speed, scale, and scope of digital transformation for the empowerment of women and girls in all their diversity, and to trigger transformations that set countries on paths to an equitable digital future for all.
- Science and technology for development
- Innovation and technology
Related content
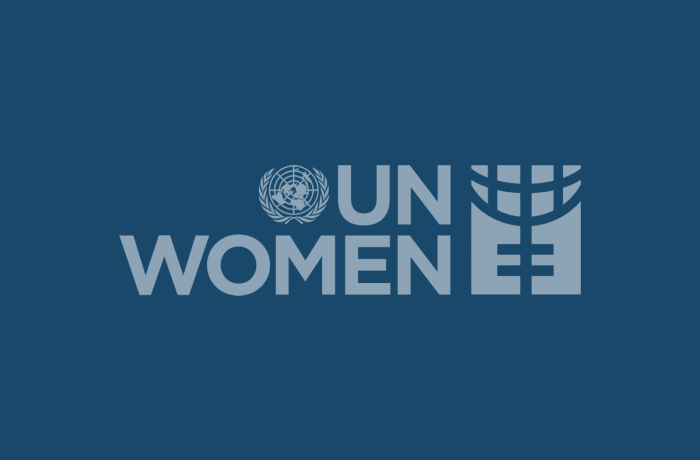
UN Women statement for the International Girls in ICT Day 2024
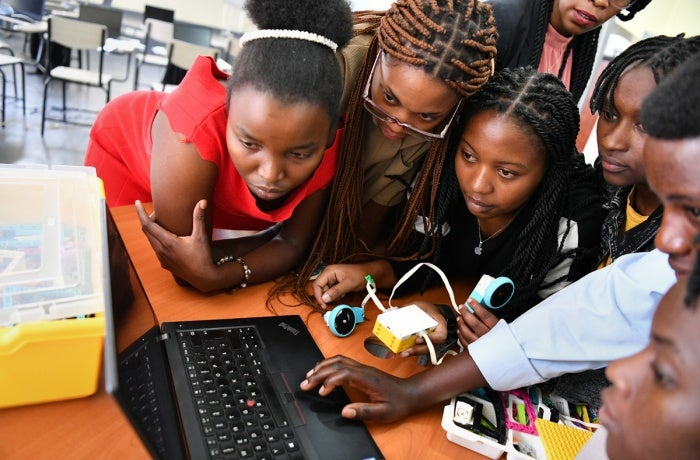
UN Women statement for the International Day for Women and Girls in Science
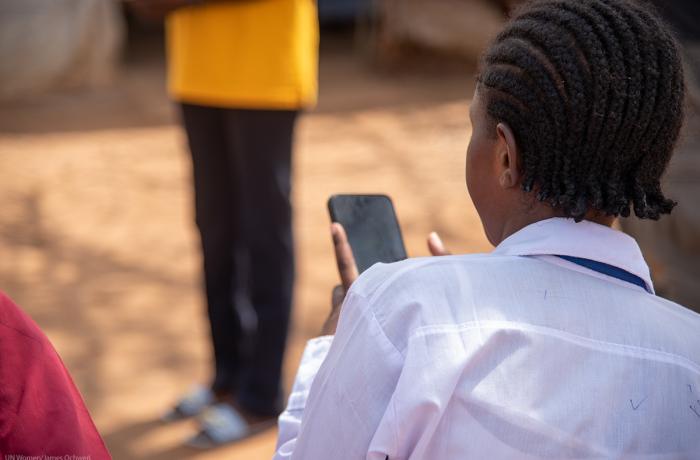
Creating safe digital spaces free of trolls, doxing, and hate speech

An official website of the United States government
The .gov means it’s official. Federal government websites often end in .gov or .mil. Before sharing sensitive information, make sure you’re on a federal government site.
The site is secure. The https:// ensures that you are connecting to the official website and that any information you provide is encrypted and transmitted securely.
- Publications
- Account settings
Preview improvements coming to the PMC website in October 2024. Learn More or Try it out now .
- Advanced Search
- Journal List
- BMC Health Serv Res

Challenges to implementing artificial intelligence in healthcare: a qualitative interview study with healthcare leaders in Sweden
Lena petersson.
1 School of Health and Welfare, Halmstad University, Box 823, 301 18 Halmstad, Sweden
Ingrid Larsson
Jens m. nygren.
2 Department of Health, Medicine and Caring Sciences, Division of Public Health, Faculty of Health Sciences, Linköping University, Linköping, Sweden
Margit Neher
3 Department of Rehabilitation, School of Health Sciences, Jönköping University, Jönköping, Sweden
Julie E. Reed
Daniel tyskbo, petra svedberg, associated data.
Empirical material generated and/or analyzed during the current study are not publicly available, but are available from the corresponding author on reasonable request.
Artificial intelligence (AI) for healthcare presents potential solutions to some of the challenges faced by health systems around the world. However, it is well established in implementation and innovation research that novel technologies are often resisted by healthcare leaders, which contributes to their slow and variable uptake. Although research on various stakeholders’ perspectives on AI implementation has been undertaken, very few studies have investigated leaders’ perspectives on the issue of AI implementation in healthcare. It is essential to understand the perspectives of healthcare leaders, because they have a key role in the implementation process of new technologies in healthcare. The aim of this study was to explore challenges perceived by leaders in a regional Swedish healthcare setting concerning the implementation of AI in healthcare.
The study takes an explorative qualitative approach. Individual, semi-structured interviews were conducted from October 2020 to May 2021 with 26 healthcare leaders. The analysis was performed using qualitative content analysis, with an inductive approach.
The analysis yielded three categories, representing three types of challenge perceived to be linked with the implementation of AI in healthcare: 1) Conditions external to the healthcare system; 2) Capacity for strategic change management; 3) Transformation of healthcare professions and healthcare practice.
Conclusions
In conclusion, healthcare leaders highlighted several implementation challenges in relation to AI within and beyond the healthcare system in general and their organisations in particular. The challenges comprised conditions external to the healthcare system, internal capacity for strategic change management, along with transformation of healthcare professions and healthcare practice. The results point to the need to develop implementation strategies across healthcare organisations to address challenges to AI-specific capacity building. Laws and policies are needed to regulate the design and execution of effective AI implementation strategies. There is a need to invest time and resources in implementation processes, with collaboration across healthcare, county councils, and industry partnerships.
The use of artificial intelligence (AI) in healthcare can potentially enable solutions to some of the challenges faced by healthcare systems around the world [ 1 – 3 ]. AI generally refers to a computerized system (hardware or software) that is equipped with the capacity to perform tasks or reasoning processes that we usually associate with the intelligence level of a human being [ 4 ]. AI is thus not one single type of technology but rather many different types within various application areas, e.g., diagnosis and treatment, patient engagement and adherence, and administrative activities [ 5 , 6 ]. However, when implementing AI technology in practice, certain problems and challenges may require an optimization of the method in combination with the specific setting. We may therefore define AI as complex sociotechnical interventions as their success in a clinical healthcare setting depends on more than the technical performance [ 7 ]. Research suggests that AI technology may be able to improve the treatment of many health conditions, provide information to support decision-making, minimize medical errors and optimize care processes, make healthcare more accessible, provide better patient experiences and care outcomes as well as reduce the per capita costs of healthcare [ 8 – 10 ]. Even if the expectations for AI in healthcare are great [ 2 ], the potential of its use in healthcare is far from having been realized [ 5 , 11 , 12 ].
Most of the research on AI in healthcare focuses heavily on the development, validation, and evaluation of advanced analytical techniques, and the most significant clinical specialties for this are oncology, neurology, and cardiology [ 2 , 3 , 11 , 13 , 14 ]. There is, however, a current research gap between the development of robust algorithms and the implementation of AI systems in healthcare practice. The conclusion in newly published reviews addressing regulation, privacy and legal aspects [ 15 , 16 ], ethics [ 16 – 18 ], clinical and patient outcomes [ 19 – 21 ] and economic impact [ 22 ], is that further research is needed in a real-world clinical setting although the clinical implementation of AI technology is still at an early stage. There are no studies describing implementation frameworks or models that could inform us concerning the role of barriers and facilitators in the implementation process and relevant implementation strategies of AI technology [ 23 ]. This illustrates a significant knowledge gap on how to implement AI in healthcare practice and how to understand the variation of acceptance of this technology among healthcare leaders, healthcare professionals, and patients [ 14 ]. It is well established in implementation and innovation research that novel technologies, such as AI, are often resisted by healthcare leaders, which contributes to their slow and variable uptake [ 13 , 24 – 26 ]. New technologies often fail to be implemented and embedded in practice because healthcare leaders do not consider how they fit with or impact existing healthcare work practices and processes [ 27 ]. Although, understanding how AI technologies should be implemented in healthcare practice is unexplored.
Based on literature from other scientific fields, we know that the leaders’interest and commitment is widely recognized as an important factor for successful implementation of new innovations and interventions [ 28 , 29 ]. The implementation of AI in healthcare is thus supposed to require leaders who understand the state of various AI systems. The leaders have to drive and support the introduction of AI systems, the integration into existing or altered work routines and processes, and how AI systems can be deployed to improve efficiency, safety, and access to healthcare services [ 30 , 31 ]. There is convincing evidence from outside the healthcare field of the importance of leadership for organizational culture and performance [ 32 ], the implementation of planned organizational change [ 33 ], and the implementation and stimulation of organizational innovation [ 34 ]. The relevance of leadership to implementing new practices in healthcare is reflected in many of the theories, frameworks, and models used in implementation research that analyses barriers to and facilitators of its implementation [ 35 ]. For example, Promoting Action on Research Implementation in Health Services [ 36 ], Consolidated Framework for Implementation Research (CFIR) [ 37 ], Active Implementation Frameworks [ 38 ], and Tailored Implementation for Chronic Diseases [ 39 ] all refer to leadership as a determinant of successful implementation. Although these implementation models are available and frequently used in healthcare research, they are highly abstract and not tailored to the implementation of AI systems in healthcare practices. We thus do not know if these models are applicable to AI as a socio-technical system or if other determinants are important for the implementation process. Likewise, based on a new literature study, we found no AI-specific implementation theories, frameworks, or models that could provide guidance for how leaders could facilitate the implementation and realize the potential of AI in healthcare [ 23 ]. We thus need to understand what the unique challenges are when implementing AI in healthcare practices.
Research on various types of stakeholder perspectives on AI implementation in healthcare has been undertaken, including studies involving professionals [ 40 – 43 ], patients [ 44 ], and industry partners [ 42 ]. However, very few studies have investigated the perspectives of healthcare leaders. This is a major shortcoming, given that healthcare leaders are expected to have a key role in the implementation and use of AI for the development of healthcare. Petitgand et al.’s study [ 45 ] serves as a notable exception. They interviewed healthcare managers, providers, and organizational developers to identify barriers to integrating an AI decision-support system to enhance diagnostic procedures in emergency care. However, the study did not focus on the leaders’ perspectives, and the study was limited to one particular type of AI solution in one specific care department. Our present study extends beyond any specific technology and encompasses the whole socio-technical system around AI technology. The present study thus aimed to explore challenges perceived by leaders in a regional Swedish healthcare setting regarding implementation of AI systems in healthcare.
This study took an explorative qualitative approach to understanding healthcare leaders’ perceptions in contexts in which AI will be developed and implemented. The knowledge generated from this study will inform the development of strategies to support an AI implementation and help avoid potential barriers. The analysis was based on qualitative content analysis, with an inductive approach [ 46 ]. Qualitative content analysis is widely used in healthcare research [ 46 ] to find similarities and differences in the data, in order to understand human experiences [ 47 ]. To ensure trustworthiness, the study is reported in accordance with the Consolidated Criteria for Reporting Qualitative Research 32‐item checklist [ 48 ].
The study was conducted in a county council (also known as “region”) in the south of Sweden. The Swedish healthcare system is publicly financed based on local taxation; residents are insured by the state and there is a vision that healthcare should be equally accessible across the population. Healthcare responsibility is decentralized to 21 county councils, whose responsibilities include healthcare provision and promotion of good health for citizens.
The county council under investigation has since 2016 invested financial, personnel and service resources to enable agile analysis (based on machine learning models) of clinical and administrative data of patients in healthcare [ 49 , 50 ]. The ambition is to gain more value from the data, utilizing insights drawn from machine learning on healthcare data to make facts-based decisions on how healthcare is managed, organized, and structured in routines and processes. The focus is thus on overall issues around management, staffing, planning and standardization for optimization of resource use, workflows, patient trajectories and quality improvement at system level. This includes several layers within the socio-technical ecosystem around the technology, dealing with: a) generating, cleaning, and labeling data, b) developing models, verifying, assuring, and auditing AI tools and algorithms, c) incorporating AI outputs into clinical decisions and resource allocation, and d) the shaping of new organizational structures, roles, and practices. Given that AI thus extends beyond any specific technology and encompasses the whole socio-technical system around the technology, in the context of this article, it is hereafter referred to generically as ‘AI systems’. We deliberately sought to understand the broad perspectives on healthcare leaders in a region that has a high level of support for AI developments and our study thus focuses on the potential of a wide range of AI systems that could emerge from the regional investments, rather than a specific AI application or AI algorithms.
Participants
Given the focus on understanding healthcare leaders’ perceptions, we purposively recruited leaders who were in a position to potentially influence the implementation and use of AI systems in relation to the setting described above. To achieve potential variability, these leaders belonged to three groups: politicians at the highest county council level, managers at various levels, such as the hospital director, manager for primary care, manager for knowledge and evidence, head of research and development center, and quality developers and strategists with responsibilities for strategy-based work at county council level or development work in various divisions in the county council healthcare organization.
The ambition was to include leaders who had a range of experiences, interests and with different mandates and responsibilities in relation to funding, running, and sustaining the implementation of AI systems in practice. A sample of 28 healthcare leaders was invited through snowball recruitment; two declined and 26 agreed to participate (Table (Table1). 1 ). This sample comprised five individuals originally identified on the basis of their knowledge and insights. They were interviewed and they then identified and suggested other leaders to interview.
Participants’ characteristics ( n = 26)
Data collection
Individual semi-structured interviews were conducted between October 2020 and May 2021 via phone or video communication by one of the authors (LP or DT). We start from a broad perspective on AI focusing on healthcare leaders’ perceptions bottom-up and not on the views of AI experts or healthcare professionals who work with specific AI algortihms in clinical practice. The interviews were based on an interview guide, structured around: 1) the roles and previous experiences of the informants regarding the application of AI systems in practice, 2) the opportunities and problems that need to be considered to support implementation of AI systems, 3) beliefs and attitudes towards the possibilities of using AI systems to support healthcare improvements, and 4) the obstacles, opportunities and facilitating factors that need to be considered to enable AI systems to fit into existing processes, methods and systems. The interview guide was thus based on important factors previously identified in terms of implementing technology in healthcare [ 51 , 52 ]. Interviews lasted between 30 and 120 min, with a total length of 23 h and 49 min and were audio-recorded.
Data analysis
An inductive qualitative content analysis [ 46 ] was used to analyze the data. First, the interviews were transcribed verbatim and read several times by the first (LP) and second (IL) authors, to gain familiarity. Then, the first (LP) and second (IL) authors conducted the initial analyses of the interviews, by identifying and extracting meaning units and/or phrases with information relevant to the object of the study. The meaning units were then abstracted into codes, subcategories, and categories. The analytical process was discussed continuously between authors (LP, IL, JMN, PN, MN, PS). Finally, all authors, who are from different disciplines, reviewed and discussed the analysis to increase the trustworthiness and rigour of the analysis. To further strengthen the trustworthiness, the leaders’ quotations used in this paper were translated from Swedish to English by a native English-speaking professional proofreader and were edited only slightly to improve readability.
Three categories consisting of nine sub-categories emerged from the analysis of the interviews with the healthcare leaders (Fig. 1 ). Conditions external to the healthcare system concern various exogenous conditions and circumstances beyond the direct control of the healthcare system that the leaders believed could affect AI implementation. Capacity for strategic change management reflects endogenous influences and internal requirements related to the healthcare system that the leaders suggested could pose challenges to AI implementation. Transformation of healthcare professions and healthcare practice concerns challenges to AI implementation observed by the leaders, in terms of how AI might change professional roles and relations and its impact on existing work practices and routines.

Categories and subcategories
Conditions external to the healthcare system
Addressing liability issues and legal information sharing.
The healthcare leaders described the management of existing laws and policies for the implementation of AI systems in healthcare as a challenge and an issue that was essential to address. According to them, the existing laws and policies have not kept pace with technological developments and the organization of healthcare in today’s society and need to be revised to ensure liability.
The accountability held among individuals, organizations, and AI systems regarding decisions based on support from an AI algorithm was perceived as a risk and an element that needs to be addressed. However, accountability is not addressed in existing laws, which were perceived by the leaders to present problematic uncertainties in terms of responsibilities. They raised concerns about where responsibilities lie in relation to decisions made by AI algorithms, such as when an AI algorithm run in one part of the system identifies actions that should be taken in another part of the system. For example, if a patient is given AI-based advice from a county council-operated patient portal for triaging suggesting self-care, and the advice instead should have been to visit the emergency department, who has the responsibility, is it the AI system itself, the developers of the system or the county council. Additionally, concerns were raised about accountability, if it turns out that the advice was not accurate.
The issue of accountability is a very difficult one. If I agree with what doctor John (AI systems) recommended, where does the burden of proof lie? I may have looked at this advice and thought that it worked quite well. I chose to follow this advice, but can I blame Doctor John? The legislation is a risk that we have to deal with. Leader 7.
Concerns were raised as to how errors would be handled when AI systems contributed to decision making, highlighting the need for clear laws and policies. The leaders emphasized that, if healthcare professionals made erroneous decisions based on AI systems, they could be reported to the Patients Advisory Committee or have their medical license revoked. This impending threat could lead to a stressful situation for healthcare professionals. The leaders expressed major concerns about whether AI systems would be support systems for healthcare professionals’ decisions or systems that could take automated and independent decisions. They believed based on the latter interpretation that there would be a need for changes in the laws before they could be implemented in practice. Nevertheless, some leaders anticipated a development where some aspects of care could be provided without any human involvement.
If the legislation is changed so that the management information can be automated, that is to say that they start acting themselves, but they’re not allowed to do that yet. It could, however, be so that you open an app in a few years’ time, then you furnish the app with the information that it needs about your health status. Then the app can write a prescription for medication for you, because it has all the information that is needed. That is not allowed at present, because the judicial authority still need an individual to blame when something goes wrong. But even that aspect will be gradually developed. Leader 2.
According to the leaders, legislation and policies also constituted obstacles to the foundation in the implementation of AI systems in healthcare: collecting, using, merging, and analyzing patient information. The limited opportunities to legally access and share information about patients within and between organizations were described as a crucial obstacle in implementing and using AI systems. Another issue was the legal problems when a care provider wanted to merge information about patients from different providers, such as the county council and a municipality. For this to take place, it was perceived that a considerable change of the laws regulating the possibilities of sharing information across different care providers would be required. Additionally, there are challenges in the definition of personal data in laws regulating personal integrity and in the risk of individuals being identified when the data is used for computerized advanced analytics. The law states that it is not legal to share personal data, but the boundaries of what is constituted by personal data in today’s society are changing, due to the increasing amounts of data and opportunities for complex and intelligent analysis.
You are not allowed to share any personal information. No, we understand that but what is personal information and when is personal information no longer personal information? Because legally speaking it is definitely not just the case of removing the personal identity number and the name, as a computer can still identify who you are at an individual level. When can it not do that? Leader 2.
Thus, according to the healthcare leaders, laws and regulations presented challenges for an organization that want to implement AI systems in healthcare practice, as laws and regulations have different purposes and oppose each other, e.g., the Health and Medical Services Act, the Patient Act and the Secrecy Act. Leaders described how outdated laws and regulations are handled in healthcare practice, by stretching current regulations and attempts to contribute to changing laws . They aimed to not give up on visions and ideas, but to try to find gaps in existing laws and to use rather than break the laws. When possible, another way to approach this was to try to influence decision-makers on the national political level to change the laws. The leaders reported that civil servants and politicians in the county council do this lobbying work in different contexts, such as the parliament or the Swedish Association of Local Authorities and Regions (SALAR).
We discuss this regularly with our members of parliament with the aim of influencing the legislative work towards an enabling of the flow of information over boundaries. It’s all a bit old-fashioned. Leader 16.
Complying with standards and quality requirements
The healthcare leaders believed it could be challenging to follow standardized care processes when AI systems are implemented in healthcare. Standardized care processes are an essential feature that has contributed to development and improved quality in Swedish healthcare. However, some leaders expressed that the implementation of AI systems could be problematic because of uncertainties regarding when an AI algorithm is valid enough to be a part of a standardized care process. They were uncertain about which guarantees would be required for a product or service before it would be considered “good enough” and safe to use in routine care. An important legal aspect for AI implementation is the updated EU regulation for medical devices (MDR) that came into force in May 2021. According to one of the leaders, this regulation could be problematic for small innovative companies, as they are not used to these demands and will not always have the resources needed to live up to the requirements. Therefore, the leaders perceived that the county council should support AI companies to navigate these demands, if they are to succeed in bringing their products or services to implementation in standardized care processes.
We have to probably help the narrow, supersmart and valuable ideas to be realized, so that there won’t be a cemetery of ideas with things that could have been good for our patients, if only the companies had been given the conditions and support to live up to the demands that the healthcare services have and must have in terms of quality and security. Leader 2.
Integrating AI-relevant learning in higher education for healthcare staff
The healthcare leaders described that changes needed to be made in professional training, so that new healthcare professionals would be prepared to use digital technology in their practical work. Some leaders were worried that basic level education for healthcare professionals, such as physicians, nurses, and assistant nurses has too little focus on digital technology in general, and AI systems in particular. They stated that it is crucial that these educational programs are restructured and adapted to prepare students for the ongoing digitalization of the healthcare sector. Otherwise, recently graduated healthcare professionals will not be ready to take part in utilizing and implementing new AI systems in practice.
I am fundamentally quite concerned that our education, mainly when it comes to the healthcare services. Both for doctors and nurses and also assistant nurses for that matter. That it isn’t sufficiently proactive and prepare those who educate themselves for what will come in the future. // I can feel a certain concern for the fact that our educations do not actually sufficiently prepare our future co-workers for what everybody is talking now about that will take place in the healthcare services. Leader 15.
Capacity for strategic change management
Developing a systematic approach to ai implementation.
The healthcare leaders described that there is a need for a systematic approach and shared plans and strategies at the county council level, in order to meet the challenge of implementing AI systems in practice. They recognized that it will not be successful if the change is built on individual interests, instead of organizational perspectives. According to the leaders, the county council has focused on building the technical infrastructure that enables the use of AI algorithms. The county council have tried to establish a way of working with multi-professional teams around each application area for AI-based analysis. However, the leaders expressed that it is necessary to look beyond the technology development and plan for the implementation at a much earlier stage in the development process. They believed that their organization generally underestimated the challenges of implementation in practice. Therefore, the leaders believed that it was essential that the politicians and the highest leadership in the county council both support and prioritize the change process. This requires an infrastructure for strategic change management together with clear leadership that has the mandate and the power to prioritize and support both development of AI systems and implementation in practice. This is critical for strategic change to be successful.
If the County Council management does not believe in this, then nothing will come of it either, the County Council management have to indicate in some way that this is a prioritized issue. It is this we are going to work with, then it’s not sufficient for a single executive director who pursues this and who thinks it’s interesting. It has to start at the top and then filter right through, but then the politicians have to also believe in this and think that it’s important. Leader 4.
Additionally, the healthcare leaders experienced that there was increasing interest among unit managers within the organization in using data for AI-based analysis and that there might be a need to make more prioritizations of requests for data analysis in the future. The leaders expressed that it would not be enough to simply have a shared core facility supporting this. Instead, management at all levels should also be involved and active in prioritization, based on their needs. They also perceived that the implementation of AI systems will demand skilled and structured change management that can prioritize and that is open to new types of leadership and decision-making processes. Support for innovative work will be needed, but also caution so that change does not proceed too quickly and is sufficiently anchored among the staff. The implementation of AI systems in healthcare was anticipated to challenge old routines and replace them with new ones, and that, as a result, would meet resistance from the staff. Therefore, a prepared plan at the county council level was perceived to be required for the purpose of “anchoring” with managers at the unit level, so that the overall strategy would be aligned with the needs and views of those who would have to implement it and supported by the knowledge needed to lead the implementation work.
It’s in the process of establishing legitimacy that we have often erred, where we’ve made mistakes and mistakes and mistakes all the time, I’ve said. That we’re not at the right level to make the decisions and that we don’t follow up and see that they understand what it’s about and take it in. It’s from the lowest manager to the middle manager to executive directors to politicians, the decisions have to have been gained legitimacy otherwise we’ll not get the impetus. Leader 21.
The leaders believed that it was essential to consider how to evaluate different parts of the implementation process. They expressed that method development is required within the county council, because, at the moment, there is a lack of knowledge and guidelines on how to evidence-base the use of AI systems in practice. There will be a need for a support organization spanning different levels within the county council, to guide and supervise units in the systematic evaluation of AI implementations. There will also be a need for quantitative evaluation of the clinical and organizational effects and qualitative assessment that focuses on how healthcare professionals and patients experience the implementation. Additionally, validation and evaluation of AI algorithms will be needed, both before they can be used in routine care, and afterwards, to provide evidence of quality improvements and optimizations of resources.
I believe that one needs to get an approval in some way, perhaps not from the Swedish Medical Products Agency, but the AI Agency or something similar. I don’t know. The Swedish National Board of Health and Welfare or some agency needs to go in and check that it is a sufficiently good foundation that they have based this algorithm on. So that it can be approved for clinical use. Leader 10.
Furthermore, the leaders described a challenge around how the implementation of AI systems in practice could be sustainable and last over time. They expressed that the county council should develop strategies in the organization so that they are readied for sustainability and long-term implementation. At the same time, this is an area with fast development and high uncertainty about the future, and thus what AI systems and services will look like in five or ten years, and how healthcare professionals and patients will use them. This is a challenge and requires that both leaders and staff are prepared to adjust and change their ways of working during the implementation process, including continuous improvements and uptake, updating and evolution of technologies and work practices.
The rate of change where digitalization, technology, new technology and AI is concerned is so high and the rate of implementation is low, so this will entail that as soon as we are about to implement something then there is something else in the market that is better. So I think it’s important to dare to implement something that is a little further on in the future. Leader 13.
Ascertaining resources for AI implementation
The leaders emphasized the importance of training for implementation of AI systems in healthcare. The county council should provide customized training at the workplace and extra knowledge support for certain professions. This could result in difficult decisions regarding what and whom to prioritize. The leaders discussed whether there was a need to provide all staff with basic training on AI systems or if it would be enough to train some of them, such as quality developers, and provide targeted training for some healthcare professionals who are close to the implementation of the AI system at a care unit. Furthermore, the leaders described that the training had to be connected to implementing the AI system at a specific care unit, which could present a challenge for the planning and realization. They emphasized that it could be a waste of resources to educate the staff beforehand. They need to be educated in close connection to the implementation of a specific AI system in their workplace, which thus demands organizational resources and planning.
I think that we often make the mistake of educating first, and then you have to use it. But you have been educated, so now you should know this? Yes, but it is not until we use something that the questions arise. Leader 13.
There could also be a need for patient education and patient guidance, if they are to use AI systems for self-care or remote monitoring. Thus, it is vital to give all citizens the same opportunities to access and utilize new technical solutions in healthcare.
We treat all our patients equally now, everyone will receive the same invitation, and everyone will need to ring about their appointment, although 99% could really book and do this themselves. Then we should focus on that, and thus return the impetus and the power to the patient and the population for them to take care of this themselves to a greater extent. But then of course information is needed and that in turn needs intuitive systems. That is not something we are known for. Leader 14.
Many of the healthcare leaders found financial resources and time, especially the prioritization of time, to be critical to the implementation process of AI system. There is already time pressure in many care units, and it can be challenging to set aside time and other resources for the implementation.
Involving staff throughout the implementation process of AI systems
The healthcare leaders stated that anchoring and involving staff and citizens is crucial to the successfully implementation of AI systems. The management has to be responsible for the implementation process but also ensure that the staff are aware of and interested in the implementation, based on their needs. Involvement of the staff together with representatives from patient groups was considered key to successful implementation and to limit risks of perceiving the AI system as unnecessary and erroneously used. At the same time, the leaders described that it would be important for unit managers to “stand up” for the change that is required, if their staff questioned the implementation.
I think for example that if you’re going to make a successful implementation then you have to perhaps involve the co-workers. You can’t involve all of them, but a representative sample of co-workers and patients and the population who are part of it. // We mess it up time after time, and something comes that we have to implement with short notice. So we try to force it on the organization, so we forget that we need to get the support of the co-workers. Leader 4.
The propensity for change differs both among individuals and within the organization. According to the leaders, that could pose a challenge, since the support and needs differ between individuals. The motivational aspect could also vary between different actors, and some leaders claim that it is crucial to arouse curiosity among healthcare professionals. If the leaders are not motivated and do not believe that the change benefits them, implementation will not be successful. To increase healthcare professionals’ motivation and engagement, the value that will be created for the clinicians has to be made obvious, along with whether the AI system will support them in their daily work.
It has to be beneficial for the clinics otherwise it’s meaningless so to speak. A big risk with AI is that you work and work with data and then algorithms emerge that are sort of obvious. Everyone can do this. It’s why it’s important to have clinical staff in the small agile teams, that there really is a clinical benefit, this actually improves it. Leader 10.
Developing new strategies for internal and external collaboration
The healthcare leaders believed that there was a need for new forms of collaboration and communication within the county council, at both organizational and professional levels. Professionals need to interact with professions other than their own, thus enabling new teamwork and new knowledge. The challenge is for different groups to talk to each other, since they do not always have the same professional language. However, it was perceived that, when these kinds of team collaborations are successful, there will be benefits, such as automation of care processes that are currently handled by humans.
To be successful in getting a person with expert knowledge in computer science to talk to a person with expert knowledge in integrity legislation, to a one who has expert knowledge in the clinical care of a patient. Even if all of them go to work with exactly the same objective, that one person or a few people can live a bit longer or feel a bit better, then it’s difficult to talk with each other because they use essentially different languages. They don’t know much about what knowledge the other has, so just getting that altogether. Leader 2.
Leaders’ views the implementation of AI systems would require the involvement and collaboration of several departments in the county council across organizational boundaries, and with external actors. A perceived challenge was that half of the primary care units are owned by private care providers, where the county council has limited jurisdiction, which challenges the dissemination of common ways of working. Additionally, the organization in the county council and its boundaries might have to be reviewed to enable different professions to work together and interact on an everyday basis.
The complexity in terms of for example apps is very, very, very much greater, we see that now. Besides there being this app, so perhaps the procurement department must be involved, the systems administration must definitely be involved, the knowledge department must be involved and the digitalization department, there are so many and the finance department of course and the communication department, the system is thus so complex. Leader 9.
There was also consensus among the healthcare leaders that the county council should collaborate with companies in AI systems implementation and should not handle such processes on their own. An eco-system of actors working in AI systems implementation is required, who have shared goals for the joint work. The leaders expressed that companies must be supported and invited to collaborate within the county council’s organization at an early stage. In that way, pitfalls regarding legal or technical aspects can be discovered early in product development. Similar relations and dialogues are also needed with patients to succeed with implementation that is not primarily based on technical possibilities, but patients’ needs. Transparency is essential to patients’ awareness of AI systems’ functions and for the reliability in outcomes.
This is born out of a management philosophy, which is based on the principle of not being able to command everything oneself, one has to be humble, perceptive about not being able to do it. One needs to invite others to be there and help with the solution. Leader 16.
Transformation of healthcare professions and healthcare practices
Managing new roles in care processes.
The healthcare leaders described a need for new professions and professional roles in healthcare for AI systems implementation. All professional groups in today’s healthcare sector were expected to be affected by these changes, particularly the work unit managers responsible for daily work processes and the physicians accountable for the medical decisions. The leaders argued that the changes could challenge traditions, hierarchies, conventional professional roles and division of labour. There might be changes regarding the responsibilities for specific work tasks, changes in professional roles, a need for new professions that do not exist in today’s labour market and the AI systems might replace some work tasks and even professions. A change towards more combined positions at both the county council and a company or a university might also be a result of the development and implementation of AI systems. However, the leaders perceived that, for some healthcare professionals, these ideas are unthinkable, and it may take several years before these changes in roles and care processes become a reality in the healthcare sector.
I think I will be seeing other professions in the healthcare services who have perhaps not received a healthcare education. It will be a culture shock, I think. It also concerns that you may perhaps not need to be medically trained, for sitting there and checking those yellow flags or whatever they are, or it could perhaps be another type of professional group. I think that it would actually be good. We have to start economizing with the competencies we now have and it’s difficult enough to manage. Leader 15.
The acceptance of the AI systems may vary within and between professional groups, ages, and areas of specialized care. The leaders feared that the implementation of AI systems would change physicians’ knowledge base and that there would be a loss of knowledge that could be problematic in the long run. The leaders argued that younger, more recently graduated physicians would never be able to accumulate the experience-based knowledge to the extent that their older colleagues have done, as they will rely more on AI systems to support their decisions. Thus, on one hand, professional roles and self-images might be threatened when output from the AI systems is argued to be more valid than the recommendation by an experienced physician. However, on the other hand, physicians who do not “work with their hands” can utilize such output as decision support to complement their experience-based knowledge. Thus, it is important that healthcare professionals have trust in recommendations from the AI systems in clinical practice. If some healthcare professionals do not trust the AI systems and their output, there is a risk that they will not use them in clinical practice and continue to work in the way they are used to, resulting in two parallel systems. This might be problematic, both for the work environment and the healthcare professionals’ wellbeing. The leaders emphasized that this would represent a challenge for the implementation of AI systems in healthcare.
We can’t add anything more today without taking something else away, I’d say it was impossible. // The level of burden is so high today so it’s difficult to see, it’s not sufficient to say that this will be of use to us in two years’ time. Leader 20.
Implementing AI systems can change existing care processes and change the role of the patient. The leaders described that, in primary care, AI systems have the best potential to change existing work processes and make care more efficient, for example through an automatic AI-based triage for patients. The AI system could take the anamnesis, instead of the healthcare professionals, and do this when patients still are at home, so the healthcare professionals will not meet the patient unless the AI system has decided that it is necessary. The AI system can also autonomously discover something in a patient’s health status and suggest that the patient contact healthcare staff for follow-up. This use of AI systems could open up opportunities for more proactive and personalized care.
The leaders also described that the implementation of AI systems in practice could facilitate an altered patient role. The development that is taking place in the healthcare sector with, for instance, patient-reported data, enables and, in some cases, requires an active and committed patient that takes part in his or her care process. The leaders mentioned that there might be a need for patient support. Otherwise, there might be a risk that only patients with high digital literacy would be able to participate with valid data. The leaders described that AI systems could facilitate this development, by recommending self-care advice to patients or empowering them to make decisions. Still, there were concerns that not all patients would benefit from AI systems, due to variations in patients’ capabilities and literacy.
We also deal with people who are ill, we must also have respect for that. Everyone will not be able to use these tools. Leader 7.
Building trust for AI systems acceptance in clinical practice
A challenge and prerequisite for implementing AI systems in healthcare is that the technology meets expectations on quality to support the healthcare professionals in their practical work, such as having a solid evidence base, being thoroughly validated and meeting requirements for equality. It is important to have confidence in the validity of the data, the algorithms and their output. A key challenge pointed out was the need to have a sufficiently large population base, the “right” type of data and the right populations to build valid AI systems. For common conditions, where rich data exists to base AI algorithms, leaders believed the reliability would be high. For unusual conditions, there were concerns that there would be lower accuracy. Questions were also raised about how AI systems take aspects around equity and equality into account, such as gender and ethnicity. The leaders expressed concern that, due to these obstacles, in relation to certain unusual or complex conditions AI systems might not be suitable.
Then there is a challenge with the new technology, whether it’s Ok to apply it. Because it’s people who are affected, people’s health and lives that are affected by the new technology. How can we guarantee that it delivers what it says it will deliver? It must be safe and reviewed, validated and evidence-based in order for us to be able to use it. If a bug is built in then the consequences can be enormous. Leader 2.
Lack of confidence in the reliability of AI systems was also described and will place higher demands and requirements on their accuracy than on similar assessments made by humans. Thus, acceptance depends on confidence in AI systems as highly sensitive and that they can diagnose conditions at earlier stages than skilled healthcare professionals. The leaders perceived that the “black box” needs to be understood in order to be reliable, i.e. what the AI algorithms calculations are based on. Thus, reliance on the outputs from AI algorithms depends on reliance on the algorithm itself and the data used for its calculation.
There are a number of inherent problems with AI. It’s a little black box. AI looks at all the data. AI is not often easy to explain, “oh, you’ve got a risk, that it passed the cut-off value for that person or patient”, no because it weighs up perhaps a hundred different dimensions in a mathematical model. AI models are often called a black box and there have been many attempts at opening that box. The clinics are a bit skeptical then when they are not able to, they just get a risk score, I would say. Leader 10.
Big data sets are important for quality, but the leaders stated that too much information about a patient also could be problematic. There is a risk that information about a patient is available to healthcare professionals who should not have that information. The leaders believed that this could already be a problem today, but that it would be an increased risk in the future. This challenge needs to be handled as the amount of patient information increases, and as more healthcare professionals get access to such information when it’s being used in AI systems, regardless of the reason for the patient’s contact with the healthcare unit. Another challenge and prerequisite for implementing AI systems in healthcare is that the technology is user-friendly and create value for both healthcare professionals and patients. The leaders expected AI systems to be user-friendly, self-instructing, and easy to use, without requiring too much prior knowledge or training. In addition to being easy to use, the AI systems must also be time-saving and never time-consuming or dependent on the addition of yet more digital operative systems to work with. Using AI systems should, in some cases, be equated with having a second opinion from a colleague, when it comes to simplicity and time consumption.
An easy way to receive this support is needed. One needs to ask a number of questions in order to receive the correct information. But it mustn’t be too complicated, and it mustn’t take time, then nothing will come of it. Leader 4.
The leaders expected that AI systems would place the patients in focus and thereby contribute to more person-centred care. These expectations are based on a large amount of data on which AI algorithms are built, which leaders perceive will make it possible to individualize assessments and treatment options. AI systems would enable more person-centred and value-creating care for patients. AI systems could potentially contribute to making healthcare efficient without compromising quality. It was seen as an opportunity to meet future increasing needs for care among the citizens, combined with a reduced number of healthcare professionals. Smart and efficient AI systems used in investigations, assessments, and treatments can streamline care and allow more patients to receive care. Making healthcare efficient was also about the idea that AI systems should contribute to improved communication within and between caregivers for both public and private care. Using AI systems to follow up the given care and to evaluate the quality of care with other caregivers was highlighted, along with the risk that the increased efficiency provided by AI systems could result in a loss of essential values for healthcare and in impaired care.
I think that automatization via AI would be a safe way and it would be perfect for the primary care services. It would have entailed that we have more hands, that we can meet the patients who need to be met and that we can meet more often and for longer periods and perhaps do more house calls and just be there where we are needed a little more and help these a bit more easily. Leader 13.
The perspectives of the challenges described by leaders in the present study are an important contribution to improving knowledge regarding the determinants influencing the implementation of AI systems in healthcare. Our results showed that healthcare leaders perceived challenges to AI implementation concerning the handling of conditions external to the healthcare system, the building of internal capacity for strategic change management and the transformation of professional roles and practices. While implementation science has advanced the knowledge concerning determinants for successful implementation of digital technology in healthcare [ 53 ], our study is one of the few that have investigated leaders’ perceptions of the implementation of AI systems in healthcare. Our findings demonstrate that the leaders concerns do not lie so much with the specific technological nuances of AI, but with the more general factors relating to how such AI systems can be channeled into routine service organization, regulation and practice delivery. These findings demonstrate the breadth of concerns that leaders perceive are important for the successful application of AI systems and therefore suggest areas for further advancements in research and practice. However, the findings also demonstrate a potential risk that, even in a county council where there is a high level of investment and strategic support for AI systems, there is a lack of technical expertise and awareness of AI specific challenges that might be encountered. This could cause challenges to the collaboration between the developers of AI systems and healthcare leaders if there is a cognitive dissonance about the nature and scope of the problem they are seeking to address, and the practical and technical details of both AI systems and healthcare operational issues [ 7 ]. This suggests the need for people who are conversant in languages of both stakeholder groups maybe necessary to facilitate communication and collaboration across professional boundaries [ 54 ]. Importantly, these findings demonstrate that addressing the technological challenges of AI alone is unlikely to be sufficient to support their adoption into healthcare services, and AI developers are likely to need to collaborate with those with expertise in healthcare implementation and improvement scientists in order to address the wider systems issues that this study has identified.
The healthcare leaders perceived challenges resulting from external conditions and circumstances, such as ambiguities in existing laws and sharing data between organizations. The external conditions highlighted in our study resonate with the outer setting in the implementation framework CFIR [ 37 ], which is described in terms of governmental and other bodies that exercise control, with the help of policies and incentives that influence readiness to implement innovations in practice. These challenges described in our study resulted in uncertainties concerning responsibilities in relation to the development and implementation of AI systems and what one was allowed to do, giving rise to legal and ethical considerations. The external conditions and circumstances were recognized by the leaders as having considerable impact on the possibility of implementing AI systems in practice although they recognized that these were beyond their direct influence. This suggests that, when it comes to the implementation of AI systems, the influence of individual leaders is largely restricted and bounded. Healthcare leaders in our study perceived that policy and regulation cannot keep up with the national interest in implementing AI systems in healthcare. Here, concerted and unified national authority initiatives are required according to the leaders. Despite the fact that the introduction of AI systems in healthcare appears to be inevitable, the consideration of existing regulatory and ethical mechanisms appears to be slow [ 16 , 18 ]. Additionally, another challenge attributable to the setting was the lack of to increase the competence and expertise among professionals in AI systems, which could be a potential barrier to the implementation of AI in practice. The leaders reflected on the need for future higher education programs to provide healthcare professionals with better knowledge of AI systems and its use in practice. Although digital literacy is described as important for healthcare professionals [ 55 , 56 ], higher education faces many challenges in meeting emerging requirements and demands of society and healthcare.
The healthcare leaders addressed the fact that the healthcare system’s internal capacity for strategic change management is a hugh challenge, but at the same time of great importance for successful and sustainable implementation of AI systems in the county council. The leaders highlighted the need to create an infrastructure and joint venture, with common structures and processes for the promotion of the capability to work with implementation strategies of AI systems at a regional level. This was needed to obtain a lasting improvement throughout the organization and to meet organizational goals, objectives, and missions. Thus, this highlights that the implementation of change within an organization is a complex process that does not solely depend on individual healthcare professionals’ change responses [ 57 ]. We need to focus on factors such as organisational capacity, climate, culture and leadership, which are common factors within the “inner context” in CFIR [ 37 ]. The capacity to put the innovations into practice consists of activities related to maintaining a functioning organization and delivery system [ 58 ]. Implementation research has most often focused on implementation of various individual, evidence-based practices, typically (digitally) health interventions [ 59 ]. However, AI implementation represents a more substantial and more disruptive form of change than typically involved in implementing new practices in healthcare [ 60 ]. Although there are likely many similarities between AI systems and other new digital technologies implemented in healthcare, there may also be important differences. For example, our results and other AI research has acknowledged that the lack of transparency (i.e. the “black box” problem) might yield resistance to some AI systems [ 61 ]. This problem is probably less apparent when implementing various evidence-based practices based on empirical research conducted according to well-established principles to be trustworthy [ 62 ]. Ethical and trust issues were also highlighted in our study as playing a more prominent role in AI implementation, perhaps more prominently than in “traditional” implementation of evidence-based practices. There might thus be AI-specific characteristics that are not really part of existing frameworks and models currently used in implementation science.
Transformation of healthcare professions and healthcare practice
The healthcare leaders perceived that the use of AI in practice could transform professional roles and practices and this could be an implementation challenge. They reflected on how the implementation of AI systems would potentially impact provider-patient relationships and how the shifts in professional roles and responsibilities in the service system could potentially lead to changes in clinical processes of care. The leaders’ concerns related to the compatibility of new ways of working with existing practice, which is an important innovation characteristic highlighted in the Diffusion of Innovation theory [ 63 ]. According to the theory, compatibility with existing values and past experiences facilitates implementation. The leaders in our study also argued that it was important to see the value of AI systems for both professionals and service-users. Unless the benefits of using AI systems are observable healthcare professionals will be reluctant to drive the implementation forward. The importance of observability for adoption of innovations is also addressed in the Diffusion of Innovation theory [ 63 ], being the degree to which the results of an innovation are visible to the users. The leaders in our study conveyed the importance for healthcare professionals of having trust and confidence in the use of AI systems. They discussed uncertainties regarding accountability and liability in situations where AI systems impacts directly or indirectly on human healthcare, and how ambiguity and uncertainty about AI systems could lead to healthcare workers having a lack of trust in the technology. Trust in relation to AI systems is well reflected on as a challenge in research in healthcare [ 30 , 41 , 64 – 66 ]. The leaders also perceived that the expectations of patient-centeredness and usability (efficacy and usefulness) for service users could be a potential challenge in connection with AI implementation. Their concerns are echoed in a review by Buchanan et al. [ 67 ], in which it was observed that the use of AI systems could serve to weaken the person-centred relationships between healthcare professionals and patients.
In summary, the expectations for AI in healthcare are high in society and the technological impetus is strong. A lack of “translation” of the technology is in some ways part of the initial difficulties of implementing AI, because implementation strategies still need to be developed that might facilitate testing and clinical use of AI to demonstrate its value in regular healthcare practice. Our results relate well to the implementation science literature, identifying implementation challenges attributable to both external and internal conditions and circumstances [ 37 , 68 , 69 ] and the characteristics of the innovation [ 37 , 63 ]. However, the leaders in our study also pointed out the importance of establishing an infrastructure and common strategies for change management on the system level in healthcare. Thus, introducing AI systems and the required changes in healthcare practice should not only be dependent on early adopters at the particular units. This resonates with the Theory of Organizational Readiness for Change [ 70 ], which emphasizes the importance of an organization being both willing and able to implement an innovation [ 71 ]. The theory posits that, although organizational willingness is one of the factors that may facilitate the introduction of an innovation into practice, both the organization’s general capacities and its innovation-specific capacities for adoption and sustained use of an innovation are key to all phases in the implementation process [ 71 ].
Methodological considerations
In qualitative research, the concepts credibility, dependability, and transferability are used to describe different aspects of trustworthiness [ 72 ]. Credibility was strengthened by the purposeful sample of participants with various experiences and a crucial role in any implementation process. It is considered of great relevance to investigate the challenges that leaders in the county council expressed concerning the implementation of various AI systems in healthcare, albeit the preparation for implementing AI systems is a current issue in many Swedish county councils. Furthermore, the research team members’ familiarity with the methodology, together with their complementary knowledge and backgrounds enabled a more nuanced and profound, in-depth analysis of the empirical material and was another strength of the study.
Dependability was strengthened by using an interview guide to ensure that the same opening questions were put to all participants and that they were encouraged to talk openly. Because this study took place during the COVID-19 pandemic, the interviews were performed either at a distance, using the Microsoft Teams application, or face-to-face, the variation might be a limitation. However, according to Archibald et al. [ 73 ], distance interviewing with videoconferencing services, such as Microsoft Teams, could be beneficial and even preferred. Based on the knowledge gap regarding implementation of AI systems in healthcare, the authors chose to use an inductive qualitative approach to the exploration of healthcare leaders’ perceptions of implementation challenges. It might be that the implementation of AI systems largely aligns with the implementation of other digital technologies or techniques in healthcare. A strength of our study is that it focuses on perceptions on AI systems in general regardless of the type of AI algorithm or the context or area of application. However, one potential limitation of this approach is the possibility that more specific AI systems and or areas of applications may become associated with somewhat different challenges. Further studies specifying such boundaries will provide more specific answers but will probably also require the investigation be conducted in connection with the actual implementation of a specific AI systems and based on participants' experiences of having participated in the implementation process. With this in mind, we encourage future research to take this into account when deciding upon study designs.
Transferability was strengthened by a rich presentation of the results along with appropriate quotations. However, a limitation could be that all healthcare leaders work in the same county council, so transferability to other county councils must be considered with caution. In addition, an important contextual factor that might have an impact on whether, and how, the findings observed in this study will occur in other settings as well, concerns the nature of, and approach to, AI implementation. AI could be considered a rather broad concept, and while we adopted a broad and general approach to AI systems in order to understand healthcare leader’s perceptions, we would, perhaps, expect that more specific AI systems and or areas of applications become associated with different challenges. Taken together, these are aspects that may affect the possibilities for our results to be portable or transferred to other contexts. We thus suggest that the perceptions of healthcare leaders in other empirical contexts and the involvement of both more specific and broader AI systems are utilized in the study designs of future research.
In conclusion, the healthcare leaders highlighted several implementation challenges in relation to AI within the healthcare system and beyond the healthcare organization. The challenges comprised conditions external to the healthcare system, internal capacity for strategic change management, and transformation of healthcare professions and healthcare practice. Based on our findings, there is a need to see the implementation of AI system in healthcare as a changing learning process at all organizational levels, necessitating a healthcare system that applies more nuanced systems thinking. It is crucial to involve and collaborate with stakeholders and users inside the regional healthcare system itself and other actors outside the organization in order to succeed in developing and applying system thinking on implementation of AI. Given that the preparation for implementing AI systems is a current and shared issue in many (Swedish) county councils and other countries, and that our study is limited to one specific county council context, we encourage future studies in other contexts, in order to corroborate the findings.
Acknowledgements
The authors would like to thank the participants who contributed to this study with their experiences.
All authors belong to the Healthcare Improvement Research Group at Halmstad University, https://hh.se/english/research/our-research/research-at-the-school-of-health-and-welfare/healthcare-improvement.html
Authors’ contributions
LP, JMN, JR, DT and PS together identified the research question and designed the study. Applications for funding and coproduction agreements were put in place by PS and JMN. Data collection (the interviews) was carried out by LP and DT. Data analysis was performed by LP, IL, JMN, PN, MN and PS and then discussed with all authors. The manuscript was drafted by LP, IL, JMN, PN, MN and PS. JR and DT provided critical revision of the paper in terms of important intellectual content. All authors have read and approved the final submitted version.
Open access funding provided by Halmstad University. The funders for this study are the Swedish Government Innovation Agency Vinnova (grant 2019–04526) and the Knowledge Foundation (grant 20200208 01H). The funders were not involved in any aspect of study design, collection, analysis, interpretation of data, or in the writing or publication process.
Availability of data and materials
Declarations.
The study conforms to the principles outlined in the Declaration of Helsinki (74) and was approved by the Swedish Ethical Review Authority (no. 2020–06246). The study fulfilled the requirements of Swedish research: information, consent, confidentiality, and safety of the participants and is guided by the ethical principles of: autonomy, beneficence, non-maleficence, and justice (75). The participants were first informed about the study by e-post and, at the same time, were asked if they wanted to participate in the study. If they agreed to participate, they were verbally informed at the beginning of the interview about the purpose and the structure of the study and that they could withdraw their consent to participate at any time. Participation was voluntary and the respondents were informed about the ethical considerations of confidentiality. Informed consent was obtained from all participants prior to the interview.
Not applicable.
The authors declare that they have no potential conflicts of interest with respect to the research, authorship, and publication of this article.
Publisher’s Note
Springer Nature remains neutral with regard to jurisdictional claims in published maps and institutional affiliations.
- Facts & Figures
- Accreditations
- Maps and Directions
- Faculty Distinctions, Awards and Honors
- Engineering Honors
- Computer Engineering
- Global Programs
- Student Organizations
- Peer Teachers
- Fast Track: Optimizing the transition from Undergraduate to Graduate Studies
- Admissions and Aid
- Entry to a Major Process
- Scholarships and Financial Aid
- Research Areas
- Undergraduate Research
- Seminars and Distinguished Lectures
- Industry Capstone Program
- Industrial Affiliates Program
Online Artificial Intelligence and Machine Learning Certificate
Gain a competitive edge with our graduate-level Artificial Intelligence and Machine Learning Certificate. This program equips both novices and seasoned professionals with the essential skills to harness the power of modern Artificial Intelligence and Machine Learning in their domain. Upon completion, participants will master statistical analysis and machine learning techniques, enabling them to dissect complex data sets. Armed with the ability to synthesize and evaluate AI models, graduates will confidently tackle real-world challenges, leveraging cutting-edge tools to derive actionable insights and drive innovation in their respective fields.
I'm ready to apply! Request more information

Certificate Overview
The Artificial Intelligence and Machine Learning certificate is a 12-credit program that equips novices and seasoned professionals with the essential skills to harness the power of modern Artificial Intelligence and Machine Learning in their respective fields of operation.
Technical Qualifications
To be successful in this program, prospective students must demonstrate an understanding of core concepts in computer science or equivalent covered in the categories below:
- Program Design and Concepts : programming proficiency through problem-solving with a high-level programming language, emphasizing computational thinking, data types, object-oriented design, dynamic memory management, and error handling for robust program development.
- Data Structures : implementing essential abstract data types and algorithms covering stacks, queues, sorting, searching, graphs, and hashing; examining performance trade-offs, analyzing runtime and memory usage.
- Algorithms : computer algorithms for numeric and non-numeric problems; design paradigms; analysis of time and space requirements of algorithms; correctness of algorithms.
- Discrete Structures for Computing : foundations from discrete mathematics for algorithm analysis, focusing on correctness and performance; introducing models like finite state machines and Turing machines.
- Mathematical Foundations : Calculus, Probability, and Linear Algebra.
Students must take four out of five possible courses to complete this certificate. See course information below.
Information
To qualify for this certificate, you must complete 12 semester credit hours (SCH) of coursework from the following list of courses. All courses must be completed with a grade of C or above. Each course is linked to its course description within the catalog.
Courses (12 credits):
Select four of the following:*
- CSCE 625 - Artificial Intelligence
- CSCE 633 - Machine Learning
- CSCE 635 - AI Robotics
- CSCE 636 - Deep Learning
- CSCE 642 - Deep Reinforcement Learning
* Additional courses are available with the consultation of an academic advisor.
For more information, please see the course catalog .
Why choose Engineering Online
Advance your career with our Engineering Online program! Backed by the university's esteemed reputation and national recognition in engineering education, you'll engage directly with industry leaders and a rigorous curriculum. Beyond graduation, tap into the extensive Aggie Alumni Network, offering invaluable connections to propel your career forward.
Engineering Online Benefits
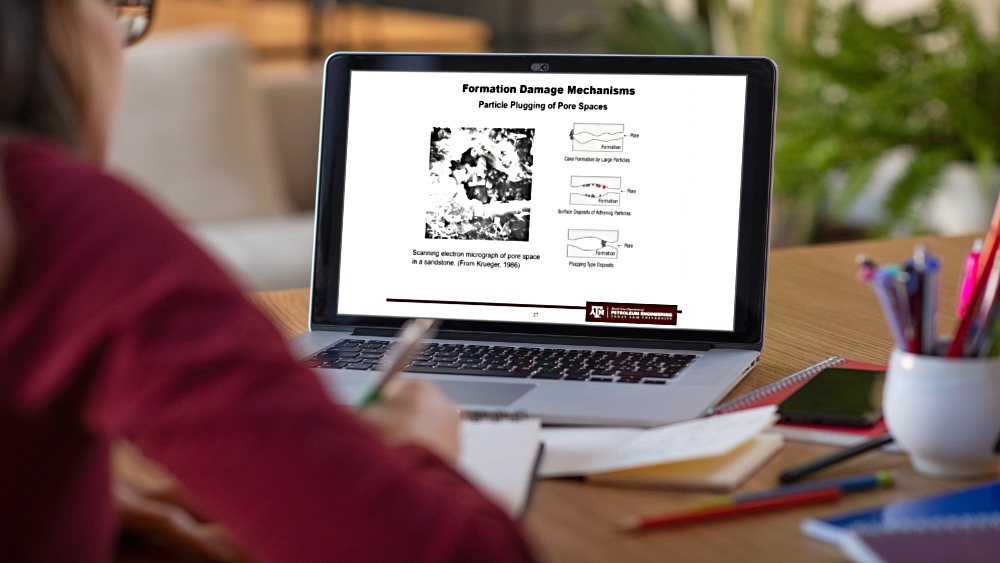
Certificate Highlights
Related academics.
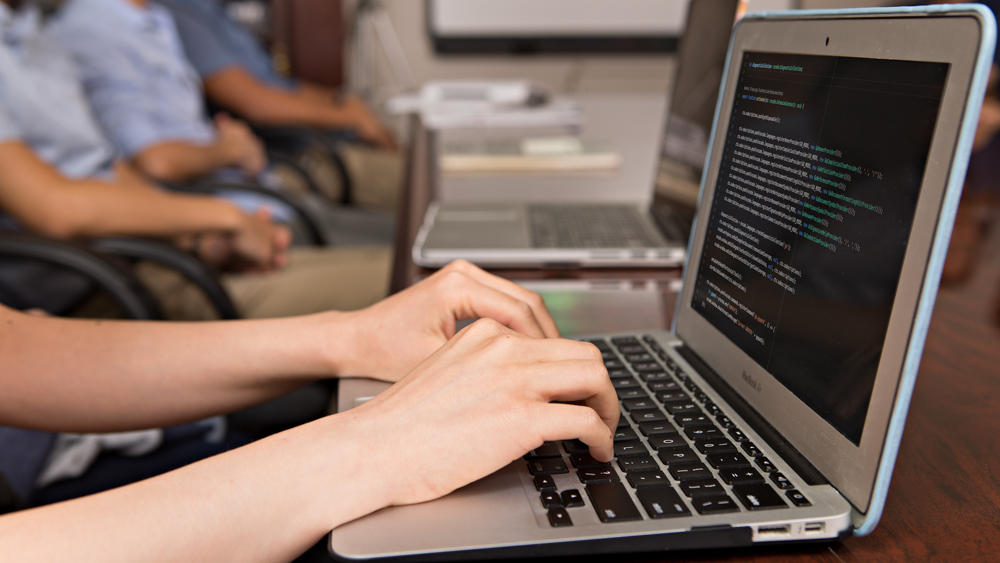
Online Master of Computer Science
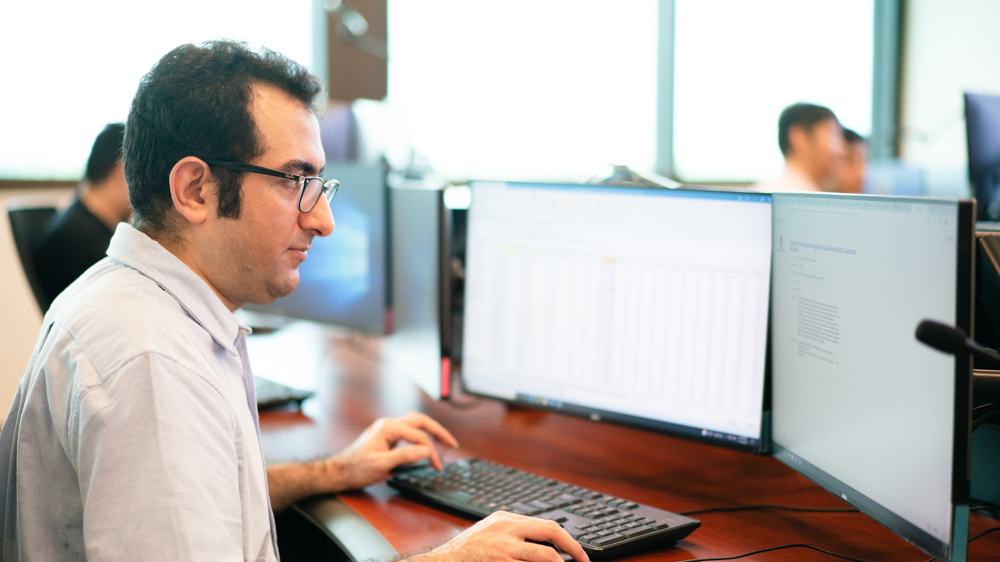
Online Master of Engineering in Computer Engineering
Frequently asked questions.
Discover answers to frequently asked questions tailored to assist you in making informed decisions regarding your education with Engineering Online.
Graduate Admissions
Use EngineeringCAS to apply for the distance education version of the certificate. Follow the provided instructions, as they may differ from certificate to certificate.
Graduate Tuition Calculator
To calculate cost, select the semester you’ll start, choose “Engineering” from the drop-down menu, and slide “Hours” to how many you’ll take each semester. Your total cost is Tuition and Required Fees + Engineering Program Fee (Remote).
Questions? Email [email protected] !

IMAGES
VIDEO
COMMENTS
The paper calls for further multi-disciplinary research initiatives that explore new ways to close the analytical gap between technical and social approaches to AI. Humanities and Social Sciences ...
Projects that new ways to connect technological and social find analyses will be better equipped to understand and in uence how fl AI changes society. This commentary paper has focused on drawing ...
1. Introduction. Artificial Intelligence in Education (AIEd) concerns mainly about the development of "computers which perform cognitive tasks, usually associated with human minds, particularly learning and problem-solving (p. 10)" (Baker and Smith (2019).AIEd has become a field of scientific research for more than 30 years (Luckin, Holmes, Griffiths, & Forcier, 2016).
By doing this, the article aims to identify research gaps that can guide future investigations. A total of 85 peer-reviewed articles from 2020 to 2023 were used in the analysis. ... The third examined theme is artificial intelligence conceptualisation. Articles [[6], [17], [41], [49], [50]] in this domain seek to provide a substructure and a ...
Gemma Conroy, Hepeng Jia, Benjamin Plackett &. Andy Tay. As artificial intelligence (AI) becomes ubiquitous in fields such as medicine, education and security, there are significant ethical and ...
challenges of AI research relate to the gap between technological and social. analyses, and it proposes steps ahead for how to practically achieve prosperous. collaborations for future AI research ...
Artificial Intelligence (AI), which typically refers to the artificial creation of human-like intelligence that can learn, perceive and process information, is rapidly becoming a powerful tool for solving image recognition, document classification ( Vapkin, 1995; LeCun et al., 2015) as well as for the advancement of interdisciplinary problems.
Literature review. Artificial Intelligence (AI) has dramatically altered the landscape of academic research, acting as a catalyst for both methodological innovation and broader shifts in scholarly paradigms (Pal, Citation 2023).Its transformative power is evident across multiple disciplines, enabling researchers to engage with complex datasets and questions at a scale previously unimaginable ...
Artificial intelligence (AI) is beginning to transform traditional research practices in many areas. In this context, literature reviews stand out because they operate on large and rapidly growing volumes of documents, that is, partially structured (meta)data, and pervade almost every type of paper published in information systems research or related social science disciplines.
For instance, AI might identify a gap in research related to the long-term impact of new insulin analogues on different age groups. ... Artificial Intelligence (AI) represents and essential productivity tool which substantially revolutionises academic writing and research across six domains, as identified in this review. ...
In this comprehensive study, insights from 1389 scholars across the US, UK, Germany, and Switzerland shed light on the multifaceted perceptions of artificial intelligence (AI). AI's burgeoning integration into everyday life promises enhanced efficiency and innovation. The Trustworthy AI principles by the European Commission, emphasising data safeguarding, security, and judicious governance ...
Bridging the Gap Between Artificial Intelligence Research and Clinical Practice in Cardiovascular Science: What the Clinician Needs to Know. Emily Shipley, 1,,2 Martha Joddrell, 1,,2 Gregory YH Lip, 1,,2 and Yalin Zheng 1,,3 Author ... Artificial intelligence (AI) and its applications in cardiovascular science have rapidly grown in recent years ...
There has been a gap between research ethics and AI research, inconsistent standards regarding AI regulation and guidelines, and a lack of knowledge and training in these new technologies has been widely noticed. ... Machine learning and artificial intelligence research for patient benefit: 20 critical questions on transparency, replicability ...
the growing use of artificial intelligence, machine learning, and empirical modeling in engineering and scientific applications, there is no standard process for ... Foundational Research Gaps and Future Directions for Digital Twins (2023). The study was sponsored by the Department of Defense, the Department
There is growing consensus and appreciation for the importance of trust in the development of Artificial Intelligence (AI) technologies; however, there is a reliance on principles-based frameworks. Recent research has highlighted the principles/practice gap, where principles alone are not actionable, and may not be wholly effective in ...
The particularly important issue is further corroborated by other studies that suggest that there are certain gaps in artificial intelligence applications used in outcomes research across therapeutic areas and further considerations will be needed before artificial intelligence usage can be incorporated into health technology assessment ...
The notion of "responsibility gap" with artificial intelligence (AI) was originally introduced in the philosophical debate to indicate the concern that "learning automata" may make more difficult or impossible to attribute moral culpability to persons for untoward events. Building on literature in moral and legal philosophy, and ethics of technology, the paper proposes a broader and ...
DOI: 10.1016/j.envint.2024.108680. Artificial intelligence (AI) has helped identify knowledge, methodological and communication gaps in global antimicrobial resistance (AMR) research. In a new ...
An expanding body of information systems research is adopting a design perspective on artificial intelligence (AI), wherein researchers prescribe solutions to problems using AI approaches rather than describing or explaining AI-related phenomena being studied. In this editorial, we address some of the challenges faced in publishing design ...
The study explores the use of Artificial Intelligence (AI) frameworks in transforming academic programs into adaptive, industry-relevant programs. The paper explores the development, validation, and effectiveness of artificial intelligence (AI) frameworks in aligning academic programs with the digital enterprise while highlighting the importance of these frameworks in enhancing graduates ...
Lijing Wang, assistant professor of Earth Sciences in the College of Liberal Arts and Sciences, is among the first scientists in the U.S. to earn support from the National Artificial Intelligence Research Resource (NAIRR) Pilot, a nationwide infrastructure that connects U.S. researchers to the computational data, software, models, and training they need to conduct paradigm-shfting AI research.
Artificial Intelligence (AI) is increasingly adopted by organizations to innovate, and this is ever more reflected in scholarly work. To illustrate, assess and map research at the intersection of AI and innovation, we performed a Systematic Literature Review (SLR) of published work indexed in the Clarivate Web of Science (WOS) and Elsevier Scopus databases (the final sample includes 1448 ...
Artificial Intelligence A small amount of guidance has emerged since 2018, with 2023 being an inflection point—"the year of implementation." In that year, both the European Union and the United States issued legislation calling for action by both the public and private sectors as AI's pivotal role in the great power competition becomes ...
Artificial Intelligence (AI) has transformed many industries, but because AI models are inherently opaque, it can be difficult to understand how they make decisions. Explainable AI (XAI) aims to reduce this comprehension gap between AI models and humans by offering comprehensible justifications for actions made by AI.
The world has a gender equality problem, and Artificial Intelligence (AI) mirrors the gender bias in our society. Although globally more women are accessing the internet every year, in low-income countries, only 20 per cent are connected. The gender digital divide creates a data gap that is reflected in the gender bias in AI. Who creates AI and what biases are built into AI data (or not), can ...
Artificial intelligence (AI) for healthcare presents potential solutions to some of the challenges faced by health systems around the world. ... There is, however, a current research gap between the development of robust algorithms and the implementation of AI systems in healthcare practice. The conclusion in newly published reviews addressing ...
Online Artificial Intelligence and Machine Learning Certificate. Gain a competitive edge with our graduate-level Artificial Intelligence and Machine Learning Certificate. This program equips both novices and seasoned professionals with the essential skills to harness the power of modern Artificial Intelligence and Machine Learning in their domain.
The rapid development of Artificial Intelligence (AI) has facilitated the digital generation and cross-media dissemination of artworks, which has led to an explosion of artistic information and increased aesthetic opportunities. However, this also presents a dilemma for the sustainable development of generative AI artworks in media communication.
Working together, NASA and IBM Research have developed a new artificial intelligence model to support a variety of weather and climate applications. The new model - known as the Prithvi-weather-climate foundational model - uses artificial intelligence (AI) in ways that could vastly improve the resolution we'll be able to get, opening the ...