
An official website of the United States government
The .gov means it’s official. Federal government websites often end in .gov or .mil. Before sharing sensitive information, make sure you’re on a federal government site.
The site is secure. The https:// ensures that you are connecting to the official website and that any information you provide is encrypted and transmitted securely.
- Publications
- Account settings
Preview improvements coming to the PMC website in October 2024. Learn More or Try it out now .
- Advanced Search
- Journal List
- J Korean Med Sci
- v.37(16); 2022 Apr 25


A Practical Guide to Writing Quantitative and Qualitative Research Questions and Hypotheses in Scholarly Articles
Edward barroga.
1 Department of General Education, Graduate School of Nursing Science, St. Luke’s International University, Tokyo, Japan.
Glafera Janet Matanguihan
2 Department of Biological Sciences, Messiah University, Mechanicsburg, PA, USA.
The development of research questions and the subsequent hypotheses are prerequisites to defining the main research purpose and specific objectives of a study. Consequently, these objectives determine the study design and research outcome. The development of research questions is a process based on knowledge of current trends, cutting-edge studies, and technological advances in the research field. Excellent research questions are focused and require a comprehensive literature search and in-depth understanding of the problem being investigated. Initially, research questions may be written as descriptive questions which could be developed into inferential questions. These questions must be specific and concise to provide a clear foundation for developing hypotheses. Hypotheses are more formal predictions about the research outcomes. These specify the possible results that may or may not be expected regarding the relationship between groups. Thus, research questions and hypotheses clarify the main purpose and specific objectives of the study, which in turn dictate the design of the study, its direction, and outcome. Studies developed from good research questions and hypotheses will have trustworthy outcomes with wide-ranging social and health implications.
INTRODUCTION
Scientific research is usually initiated by posing evidenced-based research questions which are then explicitly restated as hypotheses. 1 , 2 The hypotheses provide directions to guide the study, solutions, explanations, and expected results. 3 , 4 Both research questions and hypotheses are essentially formulated based on conventional theories and real-world processes, which allow the inception of novel studies and the ethical testing of ideas. 5 , 6
It is crucial to have knowledge of both quantitative and qualitative research 2 as both types of research involve writing research questions and hypotheses. 7 However, these crucial elements of research are sometimes overlooked; if not overlooked, then framed without the forethought and meticulous attention it needs. Planning and careful consideration are needed when developing quantitative or qualitative research, particularly when conceptualizing research questions and hypotheses. 4
There is a continuing need to support researchers in the creation of innovative research questions and hypotheses, as well as for journal articles that carefully review these elements. 1 When research questions and hypotheses are not carefully thought of, unethical studies and poor outcomes usually ensue. Carefully formulated research questions and hypotheses define well-founded objectives, which in turn determine the appropriate design, course, and outcome of the study. This article then aims to discuss in detail the various aspects of crafting research questions and hypotheses, with the goal of guiding researchers as they develop their own. Examples from the authors and peer-reviewed scientific articles in the healthcare field are provided to illustrate key points.
DEFINITIONS AND RELATIONSHIP OF RESEARCH QUESTIONS AND HYPOTHESES
A research question is what a study aims to answer after data analysis and interpretation. The answer is written in length in the discussion section of the paper. Thus, the research question gives a preview of the different parts and variables of the study meant to address the problem posed in the research question. 1 An excellent research question clarifies the research writing while facilitating understanding of the research topic, objective, scope, and limitations of the study. 5
On the other hand, a research hypothesis is an educated statement of an expected outcome. This statement is based on background research and current knowledge. 8 , 9 The research hypothesis makes a specific prediction about a new phenomenon 10 or a formal statement on the expected relationship between an independent variable and a dependent variable. 3 , 11 It provides a tentative answer to the research question to be tested or explored. 4
Hypotheses employ reasoning to predict a theory-based outcome. 10 These can also be developed from theories by focusing on components of theories that have not yet been observed. 10 The validity of hypotheses is often based on the testability of the prediction made in a reproducible experiment. 8
Conversely, hypotheses can also be rephrased as research questions. Several hypotheses based on existing theories and knowledge may be needed to answer a research question. Developing ethical research questions and hypotheses creates a research design that has logical relationships among variables. These relationships serve as a solid foundation for the conduct of the study. 4 , 11 Haphazardly constructed research questions can result in poorly formulated hypotheses and improper study designs, leading to unreliable results. Thus, the formulations of relevant research questions and verifiable hypotheses are crucial when beginning research. 12
CHARACTERISTICS OF GOOD RESEARCH QUESTIONS AND HYPOTHESES
Excellent research questions are specific and focused. These integrate collective data and observations to confirm or refute the subsequent hypotheses. Well-constructed hypotheses are based on previous reports and verify the research context. These are realistic, in-depth, sufficiently complex, and reproducible. More importantly, these hypotheses can be addressed and tested. 13
There are several characteristics of well-developed hypotheses. Good hypotheses are 1) empirically testable 7 , 10 , 11 , 13 ; 2) backed by preliminary evidence 9 ; 3) testable by ethical research 7 , 9 ; 4) based on original ideas 9 ; 5) have evidenced-based logical reasoning 10 ; and 6) can be predicted. 11 Good hypotheses can infer ethical and positive implications, indicating the presence of a relationship or effect relevant to the research theme. 7 , 11 These are initially developed from a general theory and branch into specific hypotheses by deductive reasoning. In the absence of a theory to base the hypotheses, inductive reasoning based on specific observations or findings form more general hypotheses. 10
TYPES OF RESEARCH QUESTIONS AND HYPOTHESES
Research questions and hypotheses are developed according to the type of research, which can be broadly classified into quantitative and qualitative research. We provide a summary of the types of research questions and hypotheses under quantitative and qualitative research categories in Table 1 .
Research questions in quantitative research
In quantitative research, research questions inquire about the relationships among variables being investigated and are usually framed at the start of the study. These are precise and typically linked to the subject population, dependent and independent variables, and research design. 1 Research questions may also attempt to describe the behavior of a population in relation to one or more variables, or describe the characteristics of variables to be measured ( descriptive research questions ). 1 , 5 , 14 These questions may also aim to discover differences between groups within the context of an outcome variable ( comparative research questions ), 1 , 5 , 14 or elucidate trends and interactions among variables ( relationship research questions ). 1 , 5 We provide examples of descriptive, comparative, and relationship research questions in quantitative research in Table 2 .
Hypotheses in quantitative research
In quantitative research, hypotheses predict the expected relationships among variables. 15 Relationships among variables that can be predicted include 1) between a single dependent variable and a single independent variable ( simple hypothesis ) or 2) between two or more independent and dependent variables ( complex hypothesis ). 4 , 11 Hypotheses may also specify the expected direction to be followed and imply an intellectual commitment to a particular outcome ( directional hypothesis ) 4 . On the other hand, hypotheses may not predict the exact direction and are used in the absence of a theory, or when findings contradict previous studies ( non-directional hypothesis ). 4 In addition, hypotheses can 1) define interdependency between variables ( associative hypothesis ), 4 2) propose an effect on the dependent variable from manipulation of the independent variable ( causal hypothesis ), 4 3) state a negative relationship between two variables ( null hypothesis ), 4 , 11 , 15 4) replace the working hypothesis if rejected ( alternative hypothesis ), 15 explain the relationship of phenomena to possibly generate a theory ( working hypothesis ), 11 5) involve quantifiable variables that can be tested statistically ( statistical hypothesis ), 11 6) or express a relationship whose interlinks can be verified logically ( logical hypothesis ). 11 We provide examples of simple, complex, directional, non-directional, associative, causal, null, alternative, working, statistical, and logical hypotheses in quantitative research, as well as the definition of quantitative hypothesis-testing research in Table 3 .
Research questions in qualitative research
Unlike research questions in quantitative research, research questions in qualitative research are usually continuously reviewed and reformulated. The central question and associated subquestions are stated more than the hypotheses. 15 The central question broadly explores a complex set of factors surrounding the central phenomenon, aiming to present the varied perspectives of participants. 15
There are varied goals for which qualitative research questions are developed. These questions can function in several ways, such as to 1) identify and describe existing conditions ( contextual research question s); 2) describe a phenomenon ( descriptive research questions ); 3) assess the effectiveness of existing methods, protocols, theories, or procedures ( evaluation research questions ); 4) examine a phenomenon or analyze the reasons or relationships between subjects or phenomena ( explanatory research questions ); or 5) focus on unknown aspects of a particular topic ( exploratory research questions ). 5 In addition, some qualitative research questions provide new ideas for the development of theories and actions ( generative research questions ) or advance specific ideologies of a position ( ideological research questions ). 1 Other qualitative research questions may build on a body of existing literature and become working guidelines ( ethnographic research questions ). Research questions may also be broadly stated without specific reference to the existing literature or a typology of questions ( phenomenological research questions ), may be directed towards generating a theory of some process ( grounded theory questions ), or may address a description of the case and the emerging themes ( qualitative case study questions ). 15 We provide examples of contextual, descriptive, evaluation, explanatory, exploratory, generative, ideological, ethnographic, phenomenological, grounded theory, and qualitative case study research questions in qualitative research in Table 4 , and the definition of qualitative hypothesis-generating research in Table 5 .
Qualitative studies usually pose at least one central research question and several subquestions starting with How or What . These research questions use exploratory verbs such as explore or describe . These also focus on one central phenomenon of interest, and may mention the participants and research site. 15
Hypotheses in qualitative research
Hypotheses in qualitative research are stated in the form of a clear statement concerning the problem to be investigated. Unlike in quantitative research where hypotheses are usually developed to be tested, qualitative research can lead to both hypothesis-testing and hypothesis-generating outcomes. 2 When studies require both quantitative and qualitative research questions, this suggests an integrative process between both research methods wherein a single mixed-methods research question can be developed. 1
FRAMEWORKS FOR DEVELOPING RESEARCH QUESTIONS AND HYPOTHESES
Research questions followed by hypotheses should be developed before the start of the study. 1 , 12 , 14 It is crucial to develop feasible research questions on a topic that is interesting to both the researcher and the scientific community. This can be achieved by a meticulous review of previous and current studies to establish a novel topic. Specific areas are subsequently focused on to generate ethical research questions. The relevance of the research questions is evaluated in terms of clarity of the resulting data, specificity of the methodology, objectivity of the outcome, depth of the research, and impact of the study. 1 , 5 These aspects constitute the FINER criteria (i.e., Feasible, Interesting, Novel, Ethical, and Relevant). 1 Clarity and effectiveness are achieved if research questions meet the FINER criteria. In addition to the FINER criteria, Ratan et al. described focus, complexity, novelty, feasibility, and measurability for evaluating the effectiveness of research questions. 14
The PICOT and PEO frameworks are also used when developing research questions. 1 The following elements are addressed in these frameworks, PICOT: P-population/patients/problem, I-intervention or indicator being studied, C-comparison group, O-outcome of interest, and T-timeframe of the study; PEO: P-population being studied, E-exposure to preexisting conditions, and O-outcome of interest. 1 Research questions are also considered good if these meet the “FINERMAPS” framework: Feasible, Interesting, Novel, Ethical, Relevant, Manageable, Appropriate, Potential value/publishable, and Systematic. 14
As we indicated earlier, research questions and hypotheses that are not carefully formulated result in unethical studies or poor outcomes. To illustrate this, we provide some examples of ambiguous research question and hypotheses that result in unclear and weak research objectives in quantitative research ( Table 6 ) 16 and qualitative research ( Table 7 ) 17 , and how to transform these ambiguous research question(s) and hypothesis(es) into clear and good statements.
a These statements were composed for comparison and illustrative purposes only.
b These statements are direct quotes from Higashihara and Horiuchi. 16
a This statement is a direct quote from Shimoda et al. 17
The other statements were composed for comparison and illustrative purposes only.
CONSTRUCTING RESEARCH QUESTIONS AND HYPOTHESES
To construct effective research questions and hypotheses, it is very important to 1) clarify the background and 2) identify the research problem at the outset of the research, within a specific timeframe. 9 Then, 3) review or conduct preliminary research to collect all available knowledge about the possible research questions by studying theories and previous studies. 18 Afterwards, 4) construct research questions to investigate the research problem. Identify variables to be accessed from the research questions 4 and make operational definitions of constructs from the research problem and questions. Thereafter, 5) construct specific deductive or inductive predictions in the form of hypotheses. 4 Finally, 6) state the study aims . This general flow for constructing effective research questions and hypotheses prior to conducting research is shown in Fig. 1 .

Research questions are used more frequently in qualitative research than objectives or hypotheses. 3 These questions seek to discover, understand, explore or describe experiences by asking “What” or “How.” The questions are open-ended to elicit a description rather than to relate variables or compare groups. The questions are continually reviewed, reformulated, and changed during the qualitative study. 3 Research questions are also used more frequently in survey projects than hypotheses in experiments in quantitative research to compare variables and their relationships.
Hypotheses are constructed based on the variables identified and as an if-then statement, following the template, ‘If a specific action is taken, then a certain outcome is expected.’ At this stage, some ideas regarding expectations from the research to be conducted must be drawn. 18 Then, the variables to be manipulated (independent) and influenced (dependent) are defined. 4 Thereafter, the hypothesis is stated and refined, and reproducible data tailored to the hypothesis are identified, collected, and analyzed. 4 The hypotheses must be testable and specific, 18 and should describe the variables and their relationships, the specific group being studied, and the predicted research outcome. 18 Hypotheses construction involves a testable proposition to be deduced from theory, and independent and dependent variables to be separated and measured separately. 3 Therefore, good hypotheses must be based on good research questions constructed at the start of a study or trial. 12
In summary, research questions are constructed after establishing the background of the study. Hypotheses are then developed based on the research questions. Thus, it is crucial to have excellent research questions to generate superior hypotheses. In turn, these would determine the research objectives and the design of the study, and ultimately, the outcome of the research. 12 Algorithms for building research questions and hypotheses are shown in Fig. 2 for quantitative research and in Fig. 3 for qualitative research.

EXAMPLES OF RESEARCH QUESTIONS FROM PUBLISHED ARTICLES
- EXAMPLE 1. Descriptive research question (quantitative research)
- - Presents research variables to be assessed (distinct phenotypes and subphenotypes)
- “BACKGROUND: Since COVID-19 was identified, its clinical and biological heterogeneity has been recognized. Identifying COVID-19 phenotypes might help guide basic, clinical, and translational research efforts.
- RESEARCH QUESTION: Does the clinical spectrum of patients with COVID-19 contain distinct phenotypes and subphenotypes? ” 19
- EXAMPLE 2. Relationship research question (quantitative research)
- - Shows interactions between dependent variable (static postural control) and independent variable (peripheral visual field loss)
- “Background: Integration of visual, vestibular, and proprioceptive sensations contributes to postural control. People with peripheral visual field loss have serious postural instability. However, the directional specificity of postural stability and sensory reweighting caused by gradual peripheral visual field loss remain unclear.
- Research question: What are the effects of peripheral visual field loss on static postural control ?” 20
- EXAMPLE 3. Comparative research question (quantitative research)
- - Clarifies the difference among groups with an outcome variable (patients enrolled in COMPERA with moderate PH or severe PH in COPD) and another group without the outcome variable (patients with idiopathic pulmonary arterial hypertension (IPAH))
- “BACKGROUND: Pulmonary hypertension (PH) in COPD is a poorly investigated clinical condition.
- RESEARCH QUESTION: Which factors determine the outcome of PH in COPD?
- STUDY DESIGN AND METHODS: We analyzed the characteristics and outcome of patients enrolled in the Comparative, Prospective Registry of Newly Initiated Therapies for Pulmonary Hypertension (COMPERA) with moderate or severe PH in COPD as defined during the 6th PH World Symposium who received medical therapy for PH and compared them with patients with idiopathic pulmonary arterial hypertension (IPAH) .” 21
- EXAMPLE 4. Exploratory research question (qualitative research)
- - Explores areas that have not been fully investigated (perspectives of families and children who receive care in clinic-based child obesity treatment) to have a deeper understanding of the research problem
- “Problem: Interventions for children with obesity lead to only modest improvements in BMI and long-term outcomes, and data are limited on the perspectives of families of children with obesity in clinic-based treatment. This scoping review seeks to answer the question: What is known about the perspectives of families and children who receive care in clinic-based child obesity treatment? This review aims to explore the scope of perspectives reported by families of children with obesity who have received individualized outpatient clinic-based obesity treatment.” 22
- EXAMPLE 5. Relationship research question (quantitative research)
- - Defines interactions between dependent variable (use of ankle strategies) and independent variable (changes in muscle tone)
- “Background: To maintain an upright standing posture against external disturbances, the human body mainly employs two types of postural control strategies: “ankle strategy” and “hip strategy.” While it has been reported that the magnitude of the disturbance alters the use of postural control strategies, it has not been elucidated how the level of muscle tone, one of the crucial parameters of bodily function, determines the use of each strategy. We have previously confirmed using forward dynamics simulations of human musculoskeletal models that an increased muscle tone promotes the use of ankle strategies. The objective of the present study was to experimentally evaluate a hypothesis: an increased muscle tone promotes the use of ankle strategies. Research question: Do changes in the muscle tone affect the use of ankle strategies ?” 23
EXAMPLES OF HYPOTHESES IN PUBLISHED ARTICLES
- EXAMPLE 1. Working hypothesis (quantitative research)
- - A hypothesis that is initially accepted for further research to produce a feasible theory
- “As fever may have benefit in shortening the duration of viral illness, it is plausible to hypothesize that the antipyretic efficacy of ibuprofen may be hindering the benefits of a fever response when taken during the early stages of COVID-19 illness .” 24
- “In conclusion, it is plausible to hypothesize that the antipyretic efficacy of ibuprofen may be hindering the benefits of a fever response . The difference in perceived safety of these agents in COVID-19 illness could be related to the more potent efficacy to reduce fever with ibuprofen compared to acetaminophen. Compelling data on the benefit of fever warrant further research and review to determine when to treat or withhold ibuprofen for early stage fever for COVID-19 and other related viral illnesses .” 24
- EXAMPLE 2. Exploratory hypothesis (qualitative research)
- - Explores particular areas deeper to clarify subjective experience and develop a formal hypothesis potentially testable in a future quantitative approach
- “We hypothesized that when thinking about a past experience of help-seeking, a self distancing prompt would cause increased help-seeking intentions and more favorable help-seeking outcome expectations .” 25
- “Conclusion
- Although a priori hypotheses were not supported, further research is warranted as results indicate the potential for using self-distancing approaches to increasing help-seeking among some people with depressive symptomatology.” 25
- EXAMPLE 3. Hypothesis-generating research to establish a framework for hypothesis testing (qualitative research)
- “We hypothesize that compassionate care is beneficial for patients (better outcomes), healthcare systems and payers (lower costs), and healthcare providers (lower burnout). ” 26
- Compassionomics is the branch of knowledge and scientific study of the effects of compassionate healthcare. Our main hypotheses are that compassionate healthcare is beneficial for (1) patients, by improving clinical outcomes, (2) healthcare systems and payers, by supporting financial sustainability, and (3) HCPs, by lowering burnout and promoting resilience and well-being. The purpose of this paper is to establish a scientific framework for testing the hypotheses above . If these hypotheses are confirmed through rigorous research, compassionomics will belong in the science of evidence-based medicine, with major implications for all healthcare domains.” 26
- EXAMPLE 4. Statistical hypothesis (quantitative research)
- - An assumption is made about the relationship among several population characteristics ( gender differences in sociodemographic and clinical characteristics of adults with ADHD ). Validity is tested by statistical experiment or analysis ( chi-square test, Students t-test, and logistic regression analysis)
- “Our research investigated gender differences in sociodemographic and clinical characteristics of adults with ADHD in a Japanese clinical sample. Due to unique Japanese cultural ideals and expectations of women's behavior that are in opposition to ADHD symptoms, we hypothesized that women with ADHD experience more difficulties and present more dysfunctions than men . We tested the following hypotheses: first, women with ADHD have more comorbidities than men with ADHD; second, women with ADHD experience more social hardships than men, such as having less full-time employment and being more likely to be divorced.” 27
- “Statistical Analysis
- ( text omitted ) Between-gender comparisons were made using the chi-squared test for categorical variables and Students t-test for continuous variables…( text omitted ). A logistic regression analysis was performed for employment status, marital status, and comorbidity to evaluate the independent effects of gender on these dependent variables.” 27
EXAMPLES OF HYPOTHESIS AS WRITTEN IN PUBLISHED ARTICLES IN RELATION TO OTHER PARTS
- EXAMPLE 1. Background, hypotheses, and aims are provided
- “Pregnant women need skilled care during pregnancy and childbirth, but that skilled care is often delayed in some countries …( text omitted ). The focused antenatal care (FANC) model of WHO recommends that nurses provide information or counseling to all pregnant women …( text omitted ). Job aids are visual support materials that provide the right kind of information using graphics and words in a simple and yet effective manner. When nurses are not highly trained or have many work details to attend to, these job aids can serve as a content reminder for the nurses and can be used for educating their patients (Jennings, Yebadokpo, Affo, & Agbogbe, 2010) ( text omitted ). Importantly, additional evidence is needed to confirm how job aids can further improve the quality of ANC counseling by health workers in maternal care …( text omitted )” 28
- “ This has led us to hypothesize that the quality of ANC counseling would be better if supported by job aids. Consequently, a better quality of ANC counseling is expected to produce higher levels of awareness concerning the danger signs of pregnancy and a more favorable impression of the caring behavior of nurses .” 28
- “This study aimed to examine the differences in the responses of pregnant women to a job aid-supported intervention during ANC visit in terms of 1) their understanding of the danger signs of pregnancy and 2) their impression of the caring behaviors of nurses to pregnant women in rural Tanzania.” 28
- EXAMPLE 2. Background, hypotheses, and aims are provided
- “We conducted a two-arm randomized controlled trial (RCT) to evaluate and compare changes in salivary cortisol and oxytocin levels of first-time pregnant women between experimental and control groups. The women in the experimental group touched and held an infant for 30 min (experimental intervention protocol), whereas those in the control group watched a DVD movie of an infant (control intervention protocol). The primary outcome was salivary cortisol level and the secondary outcome was salivary oxytocin level.” 29
- “ We hypothesize that at 30 min after touching and holding an infant, the salivary cortisol level will significantly decrease and the salivary oxytocin level will increase in the experimental group compared with the control group .” 29
- EXAMPLE 3. Background, aim, and hypothesis are provided
- “In countries where the maternal mortality ratio remains high, antenatal education to increase Birth Preparedness and Complication Readiness (BPCR) is considered one of the top priorities [1]. BPCR includes birth plans during the antenatal period, such as the birthplace, birth attendant, transportation, health facility for complications, expenses, and birth materials, as well as family coordination to achieve such birth plans. In Tanzania, although increasing, only about half of all pregnant women attend an antenatal clinic more than four times [4]. Moreover, the information provided during antenatal care (ANC) is insufficient. In the resource-poor settings, antenatal group education is a potential approach because of the limited time for individual counseling at antenatal clinics.” 30
- “This study aimed to evaluate an antenatal group education program among pregnant women and their families with respect to birth-preparedness and maternal and infant outcomes in rural villages of Tanzania.” 30
- “ The study hypothesis was if Tanzanian pregnant women and their families received a family-oriented antenatal group education, they would (1) have a higher level of BPCR, (2) attend antenatal clinic four or more times, (3) give birth in a health facility, (4) have less complications of women at birth, and (5) have less complications and deaths of infants than those who did not receive the education .” 30
Research questions and hypotheses are crucial components to any type of research, whether quantitative or qualitative. These questions should be developed at the very beginning of the study. Excellent research questions lead to superior hypotheses, which, like a compass, set the direction of research, and can often determine the successful conduct of the study. Many research studies have floundered because the development of research questions and subsequent hypotheses was not given the thought and meticulous attention needed. The development of research questions and hypotheses is an iterative process based on extensive knowledge of the literature and insightful grasp of the knowledge gap. Focused, concise, and specific research questions provide a strong foundation for constructing hypotheses which serve as formal predictions about the research outcomes. Research questions and hypotheses are crucial elements of research that should not be overlooked. They should be carefully thought of and constructed when planning research. This avoids unethical studies and poor outcomes by defining well-founded objectives that determine the design, course, and outcome of the study.
Disclosure: The authors have no potential conflicts of interest to disclose.
Author Contributions:
- Conceptualization: Barroga E, Matanguihan GJ.
- Methodology: Barroga E, Matanguihan GJ.
- Writing - original draft: Barroga E, Matanguihan GJ.
- Writing - review & editing: Barroga E, Matanguihan GJ.
Want to create or adapt books like this? Learn more about how Pressbooks supports open publishing practices.
Module 3 Chapter 4: Overview of Quantitative Study Variables
The first thing we need to understand is the nature of variables and how variables are used in a study’s design to answer the study questions. In this chapter you will learn:
- different types of variables in quantitative studies,
- issues surrounding the unit of analysis question.
Understanding Quantitative Variables
The root of the word variable is related to the word “vary,” which should help us understand what variables might be. Variables are elements, entities, or factors that can change (vary); for example, the outdoor temperature, the cost of gasoline per gallon, a person’s weight, and the mood of persons in your extended family are all variables. In other words, they can have different values under different conditions or for different people.
We use variables to describe features or factors of interest. Examples might include the number of members in different households, the distance to healthful food sources in different neighborhoods, the ratio of social work faculty to students in a BSW or MSW program, the proportion of persons from different racial/ethnic groups incarcerated, the cost of transportation to receive services from a social work program, or the rate of infant mortality in different counties. In social work intervention research, variables might include characteristics of the intervention (intensity, frequency, duration) and outcomes associated with the intervention.
Demographic Variables . Social workers are often interested in what we call demographic variables . Demographic variables are used to describe characteristics of a population, group, or sample of the population. Examples of frequently applied demographic variables are
- national origin,
- religious affiliation,
- sexual orientation,
- marital/relationship status,
- employment status,
- political affiliation,
- geographical location,
- education level, and
At a more macro level, the demographics of a community or organization often includes its size; organizations are often measured in terms of their overall budget.
Independent and Dependent Variables . A way that investigators think about study variables has important implications for a study design. Investigators make decisions about having them serve as either independent variables or as dependent variables . This distinction is not something inherent to a variable, it is based on how the investigator chooses to define each variable. Independent variables are the ones you might think of as the manipulated “input” variables, while the dependent variables are the ones where the impact or “output” of that input variation would be observed.
Intentional manipulation of the “input” (independent) variable is not always involved. Consider the example of a study conducted in Sweden examining the relationship between having been the victim of child maltreatment and later absenteeism from high school: no one intentionally manipulated whether the children would be victims of child maltreatment (Hagborg, Berglund, & Fahlke, 2017). The investigators hypothesized that naturally occurring differences in the input variable (child maltreatment history) would be associated with systematic variation in a specific outcome variable (school absenteeism). In this case, the independent variable was a history of being the victim of child maltreatment, and the dependent variable was the school absenteeism outcome. In other words, the independent variable is hypothesized by the investigator to cause variation or change in the dependent variable. This is what it might look like in a diagram where “ x ” is the independent variable and “ y ” is the dependent variable (note: you saw this designation earlier, in Chapter 3, when we discussed cause and effect logic):
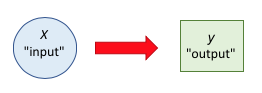
For another example, consider research indicating that being the victim of child maltreatment is associated with a higher risk of substance use during adolescence (Yoon, Kobulsky, Yoon, & Kim, 2017). The independent variable in this model would be having a history of child maltreatment. The dependent variable would be risk of substance use during adolescence. This example is even more elaborate because it specifies the pathway by which the independent variable (child maltreatment) might impose its effects on the dependent variable (adolescent substance use). The authors of the study demonstrated that post-traumatic stress (PTS) was a link between childhood abuse (physical and sexual) and substance use during adolescence.
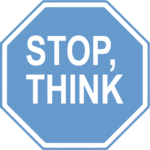
Take a moment to complete the following activity.
Types of Quantitative Variables
There are other meaningful ways to think about variables of interest, as well. Let’s consider different features of variables used in quantitative research studies. Here we explore quantitative variables as being categorical, ordinal, or interval in nature. These features have implications for both measurement and data analysis.
Categorical Variables. Some variables can take on values that vary, but not in a meaningful numerical way. Instead, they might be defined in terms of the categories which are possible. Logically, these are called categorical variables . Statistical software and textbooks sometimes refer to variables with categories as nominal variables . Nominal can be thought of in terms of the Latin root “nom” which means “name,” and should not be confused with number. Nominal means the same thing as categorical in describing variables. In other words, categorical or nominal variables are identified by the names or labels of the represented categories. For example, the color of the last car you rode in would be a categorical variable: blue, black, silver, white, red, green, yellow, or other are categories of the variable we might call car color.
What is important with categorical variables is that these categories have no relevant numeric sequence or order. There is no numeric difference between the different car colors, or difference between “yes” or “no” as the categories in answering if you rode in a blue car. There is no implied order or hierarchy to the categories “Hispanic or Latino” and “Not Hispanic or Latino” in an ethnicity variable; nor is there any relevant order to categories of variables like gender, the state or geographical region where a person resides, or whether a person’s residence is owned or rented.
If a researcher decided to use numbers as symbols related to categories in such a variable, the numbers are arbitrary—each number is essentially just a different, shorter name for each category. For example, the variable gender could be coded in the following ways, and it would make no difference, as long as the code was consistently applied.
Race and ethnicity. One of the most commonly explored categorical variables in social work and social science research is the demographic referring to a person’s racial and/or ethnic background. Many studies utilize the categories specified in past U.S. Census Bureau reports. Here is what the U.S. Census Bureau has to say about the two distinct demographic variables, race and ethnicity ( https://www.census.gov/mso/www/training/pdf/race-ethnicity-onepager.pdf ):
What is race? The Census Bureau defines race as a person’s self-identification with one or more social groups. An individual can report as White, Black or African American, Asian, American Indian and Alaska Native, Native Hawaiian and Other Pacific Islander, or some other race. Survey respondents may report multiple races. What is ethnicity? Ethnicity determines whether a person is of Hispanic origin or not. For this reason, ethnicity is broken out into two categories, Hispanic or Latino and Not Hispanic or Latino. Hispanics may report as any race.
In other words, the Census Bureau defines two categories for the variable called ethnicity (Hispanic or Latino and Not Hispanic or Latino), and seven categories for the variable called race. While these variables and categories are often applied in social science and social work research, they are not without criticism.
Based on these categories, here is what is estimated to be true of the U.S. population in 2016:
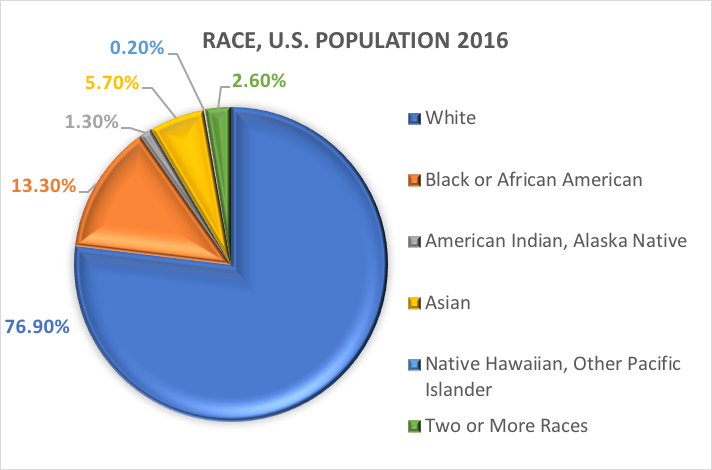
Dichotomous variables. There exists a special category of categorical variable with implications for certain statistical analyses. Categorical variables comprised of exactly two options, no more and no fewer are called dichotomous variables . One example was the U.S. Census Bureau dichotomy of Hispanic/Latino and Non-Hispanic/Non-Latino ethnicity. For another example, investigators might wish to compare people who complete treatment with those who drop out before completing treatment. With the two categories, completed or not completed, this treatment completion variable is not only categorical, it is dichotomous. Variables where individuals respond “yes” or “no” are also dichotomous in nature.
The past tradition of treating gender as either male or female is another example of a dichotomous variable. However, very strong arguments exist for no longer treating gender in this dichotomous manner: a greater variety of gender identities are demonstrably relevant in social work for persons whose identity does not align with the dichotomous (also called binary) categories of man/woman or male/female. These include categories such as agender, androgynous, bigender, cisgender, gender expansive, gender fluid, gender questioning, queer, transgender, and others.
Ordinal Variables. Unlike these categorical variables, sometimes a variable’s categories do have a logical numerical sequence or order. Ordinal, by definition, refers to a position in a series. Variables with numerically relevant categories are called ordinal variables. For example, there is an implied order of categories from least-to-most with the variable called educational attainment. The U.S. Census data categories for this ordinal variable are:
- 1st-4thgrade
- 5th-6thgrade
- 7th-8thgrade
- high school graduate
- some college, no degree
- associate’s degree, occupational
- associate’s degree academic
- bachelor’s degree
- master’s degree
- professional degree
- doctoral degree
In looking at the 2016 Census Bureau estimate data for this variable, we can see that females outnumbered males in the category of having attained a bachelor’s degree: of the 47,718,000 persons in this category, 22,485,000 were male and 25,234,000 were female. While this gendered pattern held for those receiving master’s degrees, the pattern was reversed for receiving doctoral degrees: more males than females obtained this highest level of education. It is also interesting to note that females outnumbered males at the low end of the spectrum: 441,000 females reported no education compared to 374,000 males.
Here is another example of using ordinal variables in social work research: when individuals seek treatment for a problem with alcohol misuse, social workers may wish to know if this is their first, second, third, or whatever numbered serious attempt to change their drinking behavior. Participants enrolled in a study comparing treatment approaches for alcohol use disorders reported that the intervention study was anywhere from their first to eleventh significant change attempt (Begun, Berger, Salm-Ward, 2011). This change attempt variable has implications for how social workers might interpret data evaluating an intervention that was not the first try for everyone involved.
Rating scales. Consider a different but commonly used type of ordinal variable: rating scales. Social, behavioral, and social work investigators often ask study participants to apply a rating scale to describe their knowledge, attitudes, beliefs, opinions, skills, or behavior. Because the categories on such a scale are sequenced (most to least or least to most), we call these ordinal variables.
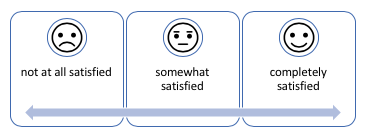
Examples include having participants rate:
- how much they agree or disagree with certain statements (not at all to extremely much);
- how often they engage in certain behaviors (never to always);
- how often they engage in certain behaviors (hourly, daily, weekly, monthly, annually, or less often);
- the quality of someone’s performance (poor to excellent);
- how satisfied they were with their treatment (very dissatisfied to very satisfied)
- their level of confidence (very low to very high).
Interval Variables. Still other variables take on values that vary in a meaningful numerical fashion. From our list of demographic variables, age is a common example. The numeric value assigned to an individual person indicates the number of years since a person was born (in the case of infants, the numeric value may indicate days, weeks, or months since birth). Here the possible values for the variable are ordered, like the ordinal variables, but a big difference is introduced: the nature of the intervals between possible values. With interval variables the “distance” between adjacent possible values are equal. Some statistical software packages and textbooks use the term scale variable : this is exactly the same thing as what we call an interval variable.
For example, in the graph below, the 1 ounce difference between this person consuming 1 ounce or 2 ounces of alcohol (Monday, Tuesday) is exactly the same as the 1 ounce difference between consuming 4 ounces or 5 ounces (Friday, Saturday). If we were to diagram the possible points on the scale, they would all be equidistant; the interval between any two points is measured in standard units (ounces, in this example).
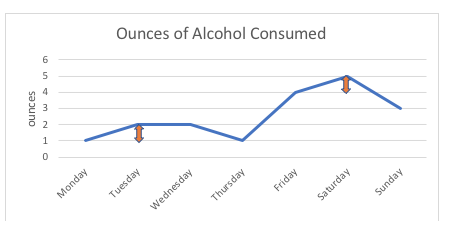
With ordinal variables, such as a rating scales, no one can say for certain that the “distance” between the response options of “never” and “sometimes” is the same as the “distance” between “sometimes” and “often,” even if we used numbers to sequence these response options. Thus, the rating scale remains ordinal, not interval.
What might become a tad confusing is that certain statistical software programs, like SPSS, refer to an interval variable as a “scale” variable. Many variables used in social work research are both ordered and have equal distances between points. Consider for example, the variable of birth order. This variable is interval because:
- the possible values are ordered (e.g., the third-born child came after the first- and second-born and before the fourth-born), and
- the “distances” or intervals are measured in equivalent one-person units.
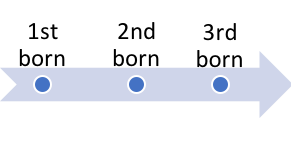
Continuous variables. There exists a special type of numeric interval variable that we call continuous variables. A variable like age might be treated as a continuous variable. Age is ordinal in nature, since higher numbers mean something in relation to smaller numbers. Age also meets our criteria for being an interval variable if we measure it in years (or months or weeks or days) because it is ordinal and there is the same “distance” between being 15 and 30 years old as there is between being 40 and 55 years old (15 calendar years). What makes this a continuous variable is that there are also possible, meaningful “fraction” points between any two intervals. For example, a person can be 20½ (20.5) or 20¼ (20.25) or 20¾ (20.75) years old; we are not limited to just the whole numbers for age. By contrast, when we looked at birth order, we cannot have a meaningful fraction of a person between two positions on the scale.
The Special Case of Income . One of the most abused variables in social science and social work research is the variable related to income. Consider an example about household income (regardless of how many people are in the household). This variable could be categorical (nominal), ordinal, or interval (scale) depending on how it is handled.
Categorical Example: Depending on the nature of the research questions, an investigator might simply choose to use the dichotomous categories of “sufficiently resourced” and “insufficiently resourced” for classifying households, based on some standard calculation method. These might be called “poor” and “not poor” if a poverty line threshold is used to categorize households. These distinct income variable categories are not meaningfully sequenced in a numerical fashion, so it is a categorical variable.
Ordinal Example: Categories for classifying households might be ordered from low to high. For example, these categories for annual income are common in market research:
- Less than $25,000.
- $25,000 to $34,999.
- $35,000 to $49,999.
- $50,000 to $74,999.
- $75,000 to $99,999.
- $100,000 to $149,999.
- $150,000 to $199,999.
- $200,000 or more.
Notice that the categories are not equally sized—the “distance” between pairs of categories are not always the same. They start out in about $10,000 increments, move to $25,000 increments, and end up in about $50,000 increments.
Interval Example . If an investigator asked study participants to report an actual dollar amount for household income, we would see an interval variable. The possible values are ordered and the interval between any possible adjacent units is $1 (as long as dollar fractions or cents are not used). Thus, an income of $10,452 is the same distance on a continuum from $9,452 and $11,452—$1,000 either way.
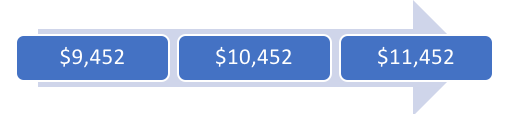
The Special Case of Age . Like income, “age” can mean different things in different studies. Age is usually an indicator of “time since birth.” We can calculate a person’s age by subtracting a date of birth variable from the date of measurement (today’s date minus date of birth). For adults, ages are typically measured in years where adjacent possible values are distanced in 1-year units: 18, 19, 20, 21, 22, and so forth. Thus, the age variable could be a continuous type of interval variable.
However, an investigator might wish to collapse age data into ordered categories or age groups. These still would be ordinal, but might no longer be interval if the increments between possible values are not equivalent units. For example, if we are more interested in age representing specific human development periods, the age intervals might not be equal in span between age criteria. Possibly they might be:
- Infancy (birth to 18 months)
- Toddlerhood (18 months to 2 ½ years)
- Preschool (2 ½ to 5 years)
- School age (6 to 11 years)
- Adolescence (12 to 17 years)
- Emerging Adulthood (18 to 25 years)
- Adulthood (26 to 45 years)
- Middle Adulthood (46 to 60 years)
- Young-Old Adulthood (60 to 74 years)
- Middle-Old Adulthood (75 to 84 years)
- Old-Old Adulthood (85 or more years)
Age might even be treated as a strictly categorical (non-ordinal) variable. For example, if the variable of interest is whether someone is of legal drinking age (21 years or older), or not. We have two categories—meets or does not meet legal drinking age criteria in the United States—and either one could be coded with a “1” and the other as either a “0” or “2” with no difference in meaning.
What is the “right” answer as to how to measure age (or income)? The answer is “it depends.” What it depends on is the nature of the research question: which conceptualization of age (or income) is most relevant for the study being designed.
Alphanumeric Variables. Finally, there are data which do not fit into any of these classifications. Sometimes the information we know is in the form of an address or telephone number, a first or last name, zipcode, or other phrases. These kinds of information are sometimes called alphanumeric variables . Consider the variable “address” for example: a person’s address might be made up of numeric characters (the house number) and letter characters (spelling out the street, city, and state names), such as 1600 Pennsylvania Ave. NW, Washington, DC, 20500.
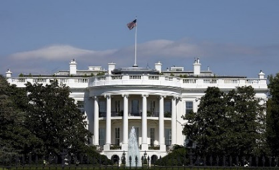
Actually, we have several variables present in this address example:
- the street address: 1600 Pennsylvania Ave.
- the city (and “state”): Washington, DC
- the zipcode: 20500.
This type of information does not represent specific quantitative categories or values with systematic meaning in the data. These are also sometimes called “string” variables in certain software packages because they are made up of a string of symbols. To be useful for an investigator, such a variable would have to be converted or recoded into meaningful values.
A Note about Unit of Analysis
An important thing to keep in mind in thinking about variables is that data may be collected at many different levels of observation. The elements studied might be individual cells, organ systems, or persons. Or, the level of observation might be pairs of individuals, such as couples, brothers and sisters, or parent-child dyads. In this case, the investigator may collect information about the pair from each individual, but is looking at each pair’s data. Thus, we would say that the unit of analysis is the pair or dyad, not each individual person. The unit of analysis could be a larger group, too: for example, data could be collected from each of the students in entire classrooms where the unit of analysis is classrooms in a school or school system. Or, the unit of analysis might be at the level of neighborhoods, programs, organizations, counties, states, or even nations. For example, many of the variables used as indicators of food security at the level of communities, such as affordability and accessibility, are based on data collected from individual households (Kaiser, 2017). The unit of analysis in studies using these indicators would be the communities being compared. This distinction has important measurement and data analysis implications.
A Reminder about Variables versus Variable Levels
A study might be described in terms of the number of variable categories, or levels, that are being compared. For example, you might see a study described as a 2 X 2 design—pronounced as a two by two design. This means that there are 2 possible categories for the first variable and 2 possible categories for the other variable—they are both dichotomous variables. A study comparing 2 categories of the variable “alcohol use disorder” (categories for meets criteria, yes or no) with 2 categories of the variable “illicit substance use disorder” (categories for meets criteria, yes or no) would have 4 possible outcomes (mathematically, 2 x 2=4) and might be diagrammed like this (data based on proportions from the 2016 NSDUH survey, presented in SAMHSA, 2017):
Reading the 4 cells in this 2 X 2 table tells us that in this (hypothetical) survey of 540 individuals, 500 did not meet criteria for either an alcohol or illicit substance use disorder (No, No); 26 met criteria for an alcohol use disorder only (Yes, No); 10 met criteria for an illicit substance use disorder only (No, Yes), and 4 met criteria for both an alcohol and illicit substance use disorder (Yes, Yes). In addition, with a little math applied, we can see that a total of 30 had an alcohol use disorder (26 + 4) and 14 had an illicit substance use disorder (10 + 4). And, we can see that 40 had some sort of substance use disorder (26 + 10 + 4).
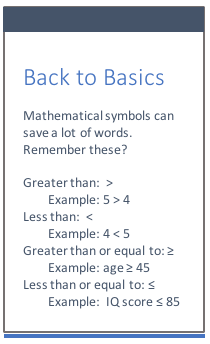
Thus, when you see a study design description that looks like two numbers being multiplied, that is essentially telling you how many categories or levels of each variable there are and leads you to understand how many cells or possible outcomes exist. A 3 X 3 design has 9 cells, a 3 X 4 design has 12 cells, and so forth. This issue becomes important once again when we discuss sample size in Chapter 6.
Interactive Excel Workbook Activities
Complete the following Workbook Activity:
- SWK 3401.3-4.1 Beginning Data Entry
Chapter Summary
In summary, investigators design many of their quantitative studies to test hypotheses about the relationships between variables. Understanding the nature of the variables involved helps in understanding and evaluating the research conducted. Understanding the distinctions between different types of variables, as well as between variables and categories, has important implications for study design, measurement, and samples. Among other topics, the next chapter explores the intersection between the nature of variables studied in quantitative research and how investigators set about measuring those variables.
Social Work 3401 Coursebook Copyright © by Dr. Audrey Begun is licensed under a Creative Commons Attribution-NonCommercial-NoDerivatives 4.0 International License , except where otherwise noted.
Share This Book
Variables in Quantitative Research: A Beginner's Guide (COUN)
Quantitative variables.
Because quantitative methodology requires measurement, the concepts being investigated need to be defined in a way that can be measured. Organizational change, reading comprehension, emergency response, or depression are concepts, but they cannot be measured as such. Frequency of organizational change, reading comprehension scores, emergency response time, or types of depression can be measured. They are variables (concepts that can vary).
- Independent variables (IV).
- Dependent variables (DV).
- Sample variables.
- Extraneous variables.
Independent Variables (IV)
Independent variables (IV) are those that are suspected of being the cause in a causal relationship. If you are asking a cause and effect question, your IV will be the variable (or variables) that you suspect causes the effect.
There are two main sorts of IV—active independent variables and attribute independent variables:
- Active IV are interventions or conditions that are being applied to the participants. A special tutorial for the third graders, a new therapy for clients, or a new training program being tested on employees would be active IVs.
- Attribute IV are intrinsic characteristics of the participants that are suspected of causing a result. For example, if you are examining whether gender which is intrinsic to the participants results in higher or lower scores on some skill, gender is an attribute IV.
- Both types of IV can have what are called levels. For example:
- In the example above, the active IV special tutorial , receiving the tutorial is one level, and tutorial withheld (control) is a second level.
- In the same example, being a third grader would be an attribute IV. It could be defined as only one level—being in third grade or you might wish to define it with more than one level, such as first half of third grade and second half of third grade. Indeed, that attribute IV could take many more, for example, if you wished to look at each month of third grade.
Independent variables are frequently called different things depending on the nature of the research question. In predictive questions, where a variable is thought to predict another but it is not yet appropriate to ask whether it causes the other, the IV is usually called a predictor or criterion variable rather than an independent variable.
Dependent Variables (DV)
- Dependent variables are variables that depend on or are influenced by the independent variables.
- They are outcomes or results of the influence of the independent variable.
- Dependent variables answer the question, "What do I observe happening when I apply the intervention?"
- The dependent variable receives the intervention.
In questions where full clausation is not assumed, such as a predictive question or a question about differences between groups but no manipulation of an IV, the dependent variables are usually called outcome variable s, and the independent variables are usually called the predictor or criterion variables.
Sample Variables
In some studies, some characteristic of the participants must be measured for some reason, but that characteristic is not the IV or the DV. In this case, these are called sample variables. For example, suppose you are investigating whether amount of sleep affects level of concentration in depressed people. In order to obtain a sample of depressed people, a standard test of depression will be given. So the presence or absence of depression will be a sample variable. That score is not used as an IV or a DV, but simply to get the appropriate people into the sample.
When there is no measure of a characteristic of the participants, the characteristic is called a sample characteristic . When the characteristic must be measured, it is called a sample variable .
Extraneous Variables
Extraneous variables are not of interest to the study, but may influence the dependent variable. For this reason, most quantitative studies attempt to control extraneous variables. The literature should inform you what extraneous variables to account for. For example, in the study of third graders' reading scores, variables such as noise levels in the testing room, the size or lighting or temperature of the room, and whether the children had had a good breakfast might be extraneous variables.
There is a special class of extraneous variables called confounding variables. These are variables that can cause the effect we are looking for if they are not controlled for, resulting in a false finding that the IV is effective when it is not. In a study of changes in skill levels in a group of caseworkers after a training program, if the follow-up measure is taken relatively late after the training, the simple effect of practicing the skills might explain improved scores, and the training might be mistakenly thought to be successful when it was not.
There are many details about variables not covered in this handout. Please consult any text on research methods for a more comprehensive review.
Doc. reference: phd_t2_coun_u02s2_h02_quantvar.html
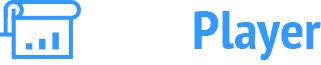
- My presentations
Auth with social network:
Download presentation
We think you have liked this presentation. If you wish to download it, please recommend it to your friends in any social system. Share buttons are a little bit lower. Thank you!
Presentation is loading. Please wait.
Introduction to the Quantitative Research Process
Published by Job Thompson Modified over 9 years ago
Similar presentations
Presentation on theme: "Introduction to the Quantitative Research Process"— Presentation transcript:
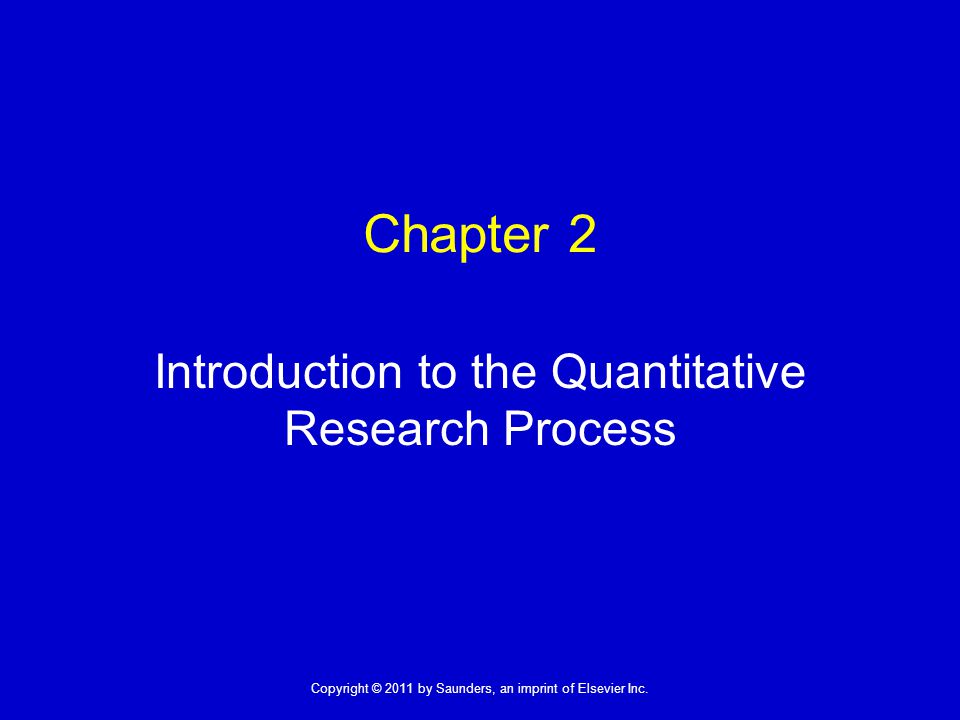
Critical Reading Strategies: Overview of Research Process
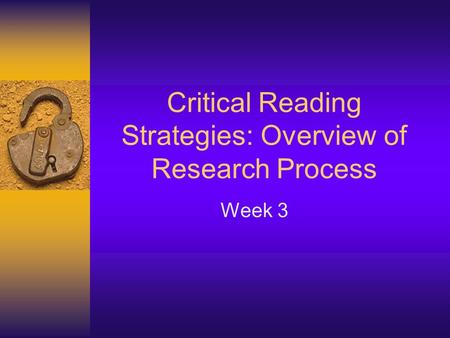
Andrea M. Landis, PhD, RN UW LEAH
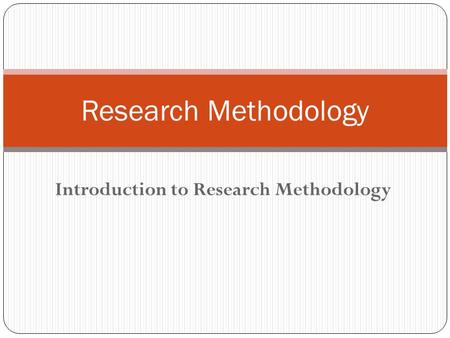
Introduction to Research Methodology
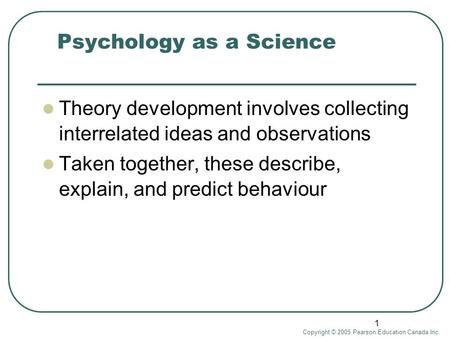
Copyright © 2005 Pearson Education Canada Inc. 1 Psychology as a Science Theory development involves collecting interrelated ideas and observations Taken.
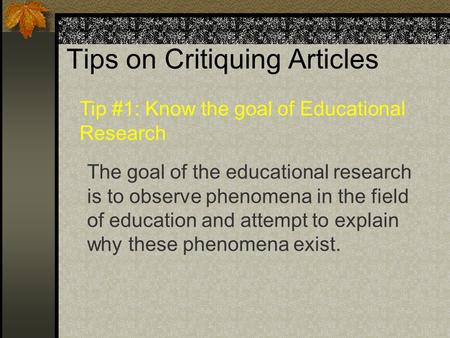
Tips on Critiquing Articles The goal of the educational research is to observe phenomena in the field of education and attempt to explain why these phenomena.
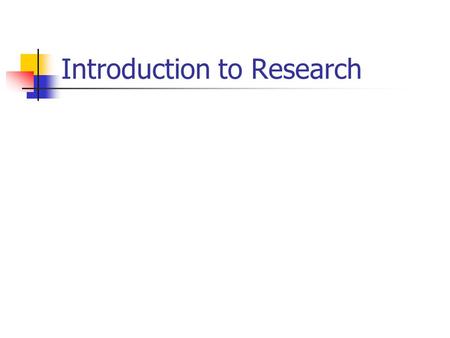
Introduction to Research
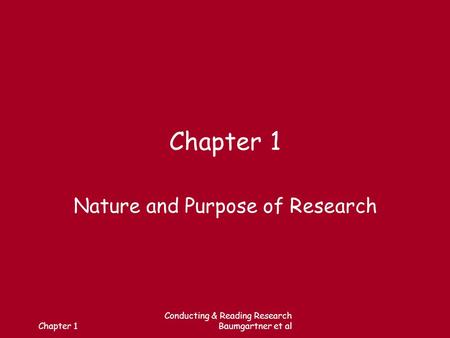
Chapter 1 Conducting & Reading Research Baumgartner et al Chapter 1 Nature and Purpose of Research.
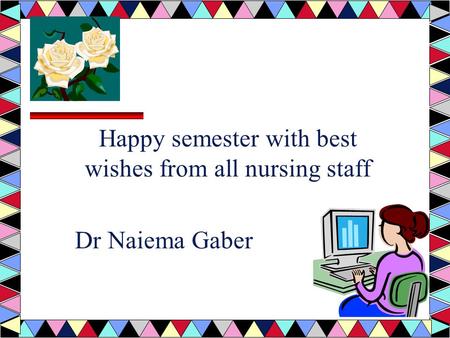
Happy semester with best wishes from all nursing staff Dr Naiema Gaber
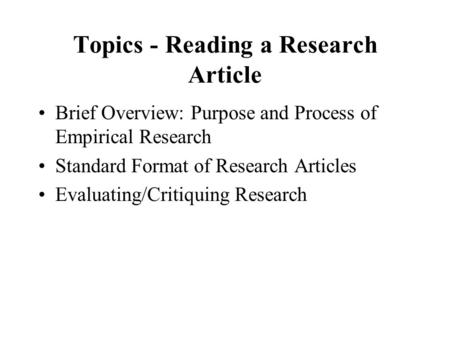
Topics - Reading a Research Article Brief Overview: Purpose and Process of Empirical Research Standard Format of Research Articles Evaluating/Critiquing.
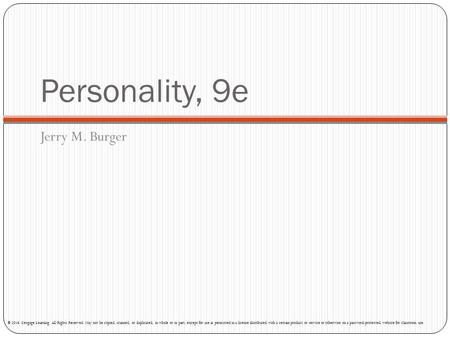
Personality, 9e Jerry M. Burger
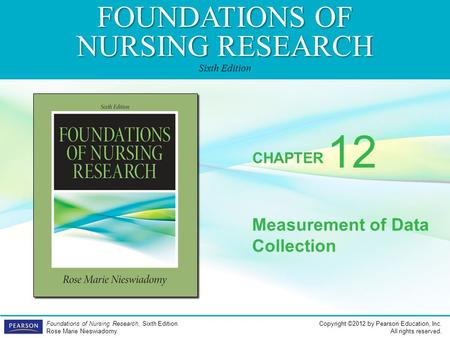
FOUNDATIONS OF NURSING RESEARCH Sixth Edition CHAPTER Copyright ©2012 by Pearson Education, Inc. All rights reserved. Foundations of Nursing Research,
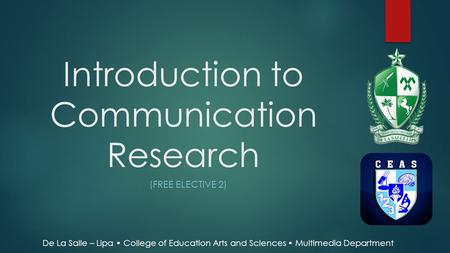
Introduction to Communication Research
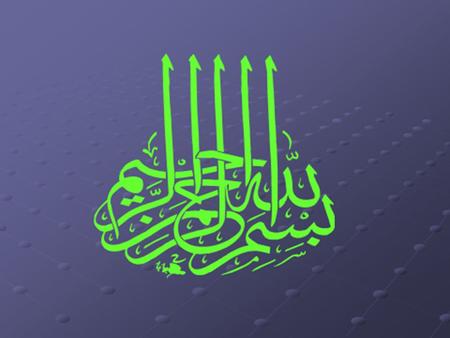
Research problem, Purpose, question
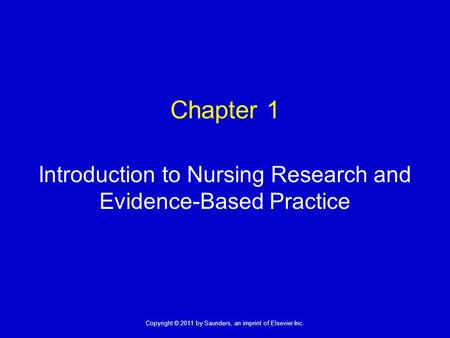
Introduction to Nursing Research and Evidence-Based Practice
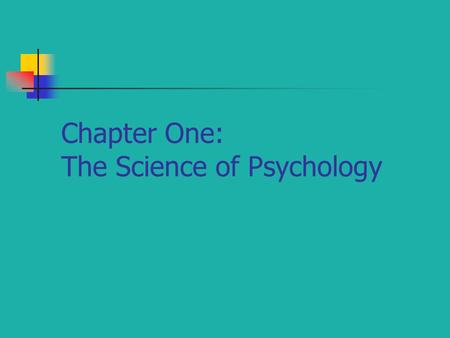
Chapter One: The Science of Psychology
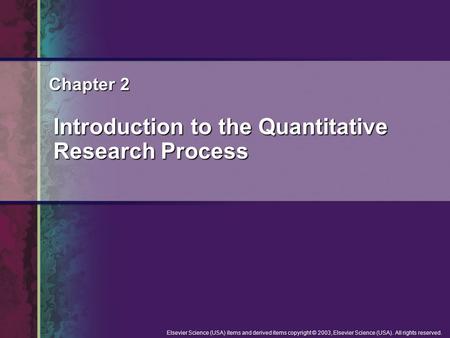
Elsevier Science (USA) items and derived items copyright © 2003, Elsevier Science (USA). All rights reserved. Chapter 2 Introduction to the Quantitative.
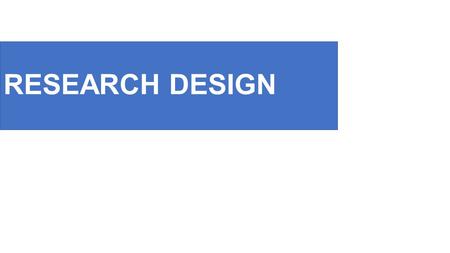
RESEARCH DESIGN.
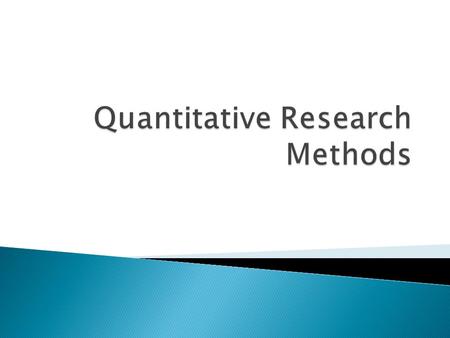
Fig Theory construction. A good theory will generate a host of testable hypotheses. In a typical study, only one or a few of these hypotheses can.
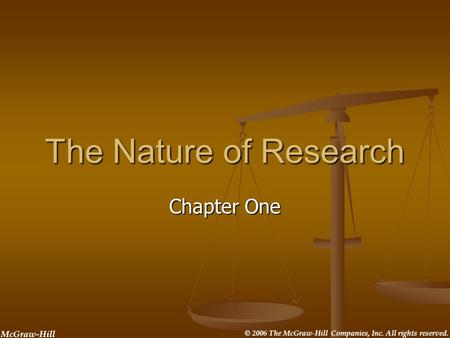
McGraw-Hill © 2006 The McGraw-Hill Companies, Inc. All rights reserved. The Nature of Research Chapter One.
About project
© 2024 SlidePlayer.com Inc. All rights reserved.
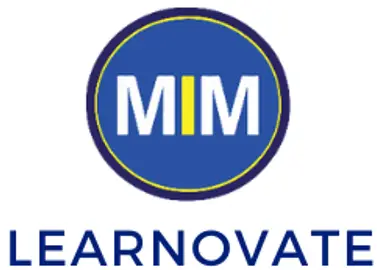
10 Types of Variables in Research | Examples | PPT
In any research study, there are variables. Variables are any characteristics that can be measured or observed. There are many great uses for these variables, but it is important to know what they are!
Understanding the difference between different types of variables is key to more research.
In this article, you will learn the basics of variables and will give you a clear picture of the types of variables that exist in the social sciences and statistics and how they can be used.
Find out what the types of variables are, how they are measured, and how to choose one for your research project.
- Table of Contents
What is a Variable?
In statistics and research, variables are items that you can measure, modify, and control. Every study looks at a variable, which can be a person, location, thing, or idea. The value of a variable can change between groups or over time.
In research, variables are the factors that are manipulated to measure their effects on an outcome variable.
- A variable is any characteristic of an individual, group, organization or social phenomenon that changes.
- A variable is something which varies and can have more than one value.
- A Variable is attribute or characteristic of an entity.
Example of Variable
1- Suppose lets take organization as an example. Organization is an entity. Name, Size, Type, Learning, Innovation are the attributes of an organization. So all these attributes are the variables.
2- Other example is that Employee is also an entity. Name, Age, Gender, Experience, Stress level, satisfaction, Performance are the attributes of an employee. So all these attributes are the variables.
3- For example, if a person’s skin color is the variable in an experiment, its value can range from brown to pale to white, from individual to individual.
- Operationalization of Variables Examples | Benefits, Drawbacks | Table
- Measurement of Scale | Examples | PPT
- Types of Validity in Research | Examples | PPT
- Types of Reliability in Research | Example | PPT
- Quasi-Experimental Design Example, Types | PPT
- Mixed Method Research: What It Is & Why You Should Use It
10 Types of Variables
Researchers classify variables into several categories, the most popular of which are,
- Independent variable
- Dependent variable
- Moderating variable
- Mediating variable
- Control variable
- Qualitative variable
- Quantitative variable
- Extraneous variable
- Composite variable
- Confounding variable
Independent Variable
The variable that is manipulated to measure its effects on an outcome variable. Independent variables are the variable that is being manipulated by the researcher.
- The independent variable is the factor that the researcher purposely change or control in order to see what effect it has.
- The variable which causes affect on dependent variable.
- It is also called predictor or explanatory variable.
Example of Independent Variable
The amount of sugar, added to each cup of orange juice.
Dependent Variable
The dependent variable is a variable that represents the experiment’s outcome. The variable that is measured in order to determine the effect of an independent variable. The dependent variable is the variable being measured.
- It is the variable which the researcher is studying and is being measured.
- It is affected by the change in Independent variable.
- It is also called criterion or outcome variable.
Example of Dependent Variable
Any measurement, of human health, and growth.
Mediating Variable
A mediating variable is a theoretical variable used by a researcher to explain a reason or relationship between other study factors, typically dependent and independent variables. Mediating variables are those that exist between the independent and dependent variable and could potentially affect the relationship between them.
- It is the variable that is between independent variable and dependent variable.
- It explains the relationship between Independent Variable and Dependent Variable.
- It is also called intervening variable.
Example of Mediating Variable
For example, if income is the independent variable and longevity is the dependent variable, the researcher could postulate that access to quality healthcare is the mediating variable that connects income and longevity.
Moderating Variable
A moderating variable, also known as a moderator variable, modifies the link between dependent and independent factors by strengthening or diminishing the effect of the mediating variable.
Moderating variables are those that moderate or change the relationship between the independent and dependent variable.
- It is the variable that effects the relationship between independent variable and dependent variable.
- This variable either weaken or strengthen the relationship of IV and DV.
While social media use can predict isolation, this association may be higher in teens than in older persons. Age is a moderator here.
Control Variables
Control variables are those that remain constant throughout the experiment. Variables that are held constant in order to isolate the effect of a given independent variable.
- A control variable strongly influences values
- It is held constant to test the relative impact of independent variables.
- It is also called constant variable
Example of Control Variables
The temperature, and light, in the room the plants are kept in, and the volume of water, given to each plant.
Developing Conceptual Framework with variables
Now I am going to give example to explain further. Suppose, Organizational learning has an impact on innovation. Organizational learning and innovation are variables.
Organization learning is IV that is independent variable and innovation is DV dependent Variable.
Organizational learning enhances that impact of innovation in the firm. So there is positive relation between two variables. Note that DV is always on the right side whereas IV is on the left side
Lets take creativity as a mediator because organizational learning brings creativity which will help employees to be more innovative. So creativity is explaining the relationship between organizational learning and innovation and creativity helps to achieve innovation.
Lets add moderator between organizational learning and innovation. Take leadership as a moderator as it will strengthen the relationship of IV and DV.
Leadership influences employees to produce creative ideas, products, and services hence bringing innovation in the firm.
These three variables organizational learning, leadership , creativity effects innovation but there could be other variables that affects innovation like firm size and firm age.
The larger firms need to be more innovative as compared to the smaller firms. So, these variables, by default have impact on firm’s innovation. So, we should control these variables in order to see whether IV, mediator and moderator have an effect on the dependent variable.
Quantitative Variable
A quantitative variable is any data set that contains numbers or amounts. There are two kinds of quantitative variables:
- Discrete variables
- Continuous variables
Example of Quantitative Variable
Quantity of things like weigh, Height, distance
Discrete Variable
Any numerical variables, you can realistically count. Discrete variables are those that can only take on a limited number of values. In research, discrete variables are often used to represent categorical data, such as gender or race. Discrete variables are often represented by integers.
Example of Discrete Variable
1- As an example, consider the money in your pocket or the funds in your savings account.

Continuous Variable
A continuous variable is a variable that can take on any value within a certain range. In research, a continuous variable is often used to measure things like opinion or behavior.
- Continuous variables are important because they allow researchers to get more detailed information about a population.
- Continuous variables are also useful for measuring change over time.
Example of Continuous Variable
1- If researchers want to know how people feel about a new product, they can use a continuous variable to measure how much people like the product on a scale of 1 to 10.
2- If researchers want to know whether people’s opinions about a product are changing, they can use a continuous variable to measure how people’s opinions change from month to month.
Qualitative Variables
Qualitative variables, often known as categorical variables, are non-numerical values or categories. You can realistically count any numerical variables.
Example of Qualitative Variables
Eye color, skin color or hair color.
There are three kinds of qualitative variables.
- Binary variable
- Nominal variable
- Ordinal variable
Binary Variable
A binary variable is a variable that can take on only two values, usually 0 and 1. In research, binary variables are often used to represent the presence or absence of something,
Binary variables can be either categorical or numerical. Categorical binary variables are also called dichotomous variables. A numerical binary variable is one where the two values represent different levels of a quantitative variable, such as small and large.
Binary variables are often used in logistic regression, which is a type of statistical analysis that is used to predict the probability of an event occurring.
Example of Binary Variable
1- To know whether a person has a disease (0 = no, 1 = yes).
2- Male or female, black or white.
Nominal Variable
A nominal variable is a type of variable that is used to label or categorize data. Nominal variables are often used in research to create groups or categories for analysis. There is no rank or order between these groups. e.g. Species names and brand names.
Nominal variables are sometimes called categorical variables. Nominal variables are usually coded with numbers, but the numbers do not have any mathematical meaning. In other words, the order of the numbers does not matter.
1- If you were coding gender with the numbers 1 and 2, it would not matter if you coded 1 as male and 2 as female, or vice versa.
2- A researcher may use a nominal variable to group participants by gender, age, or race.
Ordinal Variable
In research, an ordinal variable is a variable that is used to rank items. They are the groups that are arranged in a particular order. Ordinal variables are often used in surveys.
Example of Ordinal Variable
1- A survey might ask respondents to rate their satisfaction with a product on a scale of 1 to 5, with 1 being very dissatisfied and 5 being very satisfied. In this case, the responses would be an ordinal variable.
2. If you were researching the most popular books among teenagers, you would use an ordinal variable to rank the books from most popular to least popular.
Extraneous Variable
Extraneous variables are factors that affect the dependent variable but were not originally considered by the researcher while designing the experiment. These unexpected variables can alter the outcomes of a study or how a researcher perceives the results.
An extraneous variable is a variable that is not related to the main independent or dependent variables in a study. This can be a problem because it can skew the results of the study, making it difficult to interpret. There are ways to control for extraneous variables, however, by using experimental design and/or statistical methods.
Example of Extraneous Variable
A study could be conducted to determine if private tutoring or online courses are more helpful at improving students’ Spanish test scores. Parental support, prior understanding of a foreign language, or socioeconomic background are examples of extraneous elements that may unintentionally influence the outcome.
Latent Variable
A latent variable is a variable that is not directly observed but is instead inferred from other variables that are observed.
- used in statistical models to explain the relationships between observed variables.
- useful for understanding the relationships between different factors in a complex system.
- help to explain why some people are more successful than others.
Example of Latent Variable
1- In a study of the relationship between IQ and success, latent variables could be used to represent factors such as motivation and opportunity.
2- in a study of the relationship between job satisfaction and job performance, latent variables could be used to represent factors such as motivation and ability.
Confounding Variable
A variable in your experiment that conceals the true influence of another variable. This can occur when another variable is strongly related to a variable of interest but is not controlled in your experiment.
A confounding variable is an extraneous variable in a statistical model that correlates with both the dependent and independent variables . This confounds the interpretation of the results because it is not possible to tell which variables are causing the relationship. The most common way to control for confounding variables is to include them in the model as covariates.
Confounding variables are a problem in observational studies because they can lead to biased estimates of the exposure-outcome association. It is important to be aware of potential confounding variables when interpreting results from observational studies.
Example of Confounding Variable
1- Suppose there is an association between smoking and lung cancer. If age is a confounder, then this means that older people are more likely to get lung cancer, but they are also more likely to smoke. Therefore, the true association between smoking and lung cancer may be underestimated if age is not taken into account.
2- Pot size and soil type, for example, may affect plant longevity as much as or more than salt doses. These potential confounders would be controlled in an experiment by holding them constant.
Composite Variable
Composite variables are those that are created by combining two or more other variables. They can be useful because they can provide a more accurate representation of what you’re trying to measure.
Example of Composite Variable
A composite variable could be created by combining the variables “height” and “weight” to create the variable “BMI.”
The variable “BMI” is a better measure of body fat than either “height” or “weight” alone.
Other articles
Please read through some of our other articles with examples and explanations if you’d like to learn more about research methodology.
- PLS-SEM model
- Principal Components Analysis
- Multivariate Analysis
- Friedman Test
- Chi-Square Test (Χ²)
- Effect Size
Methodology
- Research Methods
- Quantitative Research
- Qualitative Research
- Case Study Research
- Survey Research
- Conclusive Research
- Descriptive Research
- Cross-Sectional Research
- Theoretical Framework
- Conceptual Framework
- Triangulation
- Grounded Theory
- Quasi-Experimental Design
- Mixed Method
- Correlational Research
- Randomized Controlled Trial
- Stratified Sampling
- Ethnography
- Ghost Authorship
- Secondary Data Collection
- Primary Data Collection
- Ex-Post-Facto
- Dissertation Topic
- Thesis Statement
- Research Proposal
- Research Questions
- Research Problem
- Research Gap
- Types of Research Gaps
- Operationalization of Variables
- Literature Review
- Research Hypothesis
- Questionnaire
- Reliability
- Measurement of Scale
- Sampling Techniques
- Acknowledgements
Related Posts
Survey sampling: what it is, types & tips, cluster sampling | method and examples, who is a good peer reviewer, peer review | types of peer review, ethics in research: safeguarding integrity and credibility, advantages and disadvantages of snowball sampling, exploring qualitative researcher skills: what they are and how to develop them, difference between quota sampling and stratified sampling, how effective laboratory design impacts health, safety, and productivity, why is laboratory safety important in research.
Comments are closed.
Academia.edu no longer supports Internet Explorer.
To browse Academia.edu and the wider internet faster and more securely, please take a few seconds to upgrade your browser .
Enter the email address you signed up with and we'll email you a reset link.
- We're Hiring!
- Help Center
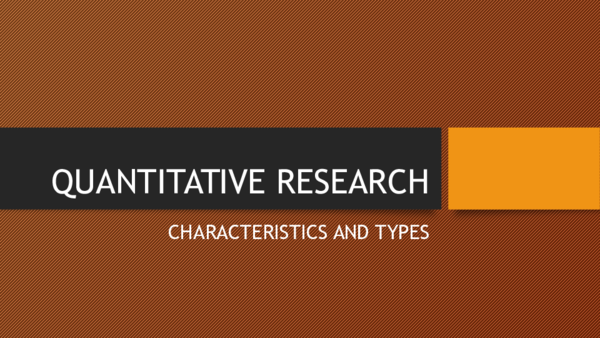
QUANTITATIVE RESEARCH METHODS AND DESIGN

AN OVERVIEW OF THE QUANTITIATIVE RESEARCH METHODS. EXPERIMENTAL RESEARCH. SINGE SUBJECT RESEARCH. CORRELATIONAL RESEARCH. CAUSAL COMPARATIVE RESEARCH. DESCRIPTIVE RESEARCH. RESEARCH TOOL AND OBJECTIVES
Related Papers
Regina Oliver , Joseph Wehby
Disruptive behavior in schools has been a source of concern for school systems for several years. Indeed, the single most common request for assistance from teachers is related to behavior and classroom management (Rose & Gallup, 2005). Classrooms with frequent disruptive behaviors have less academic engaged time, and the students in disruptive classrooms tend to have lower grades and do poorer on standardized tests (Shinn, Ramsey, Walker, Stieber, & O’Neill, 1987). Furthermore, attempts to control disruptive behaviors cost considerable teacher time at the expense of academic instruction. Effective classroom management focuses on preventive rather than reactive procedures and establishes a positive classroom environment in which the teacher focuses on students who behave appropriately (Lewis & Sugai, 1999). Rules and routines are powerful preventative components to classroom organization and management plans because they establish the behavioral context of the classroom by specifying what is expected, what will be reinforced, and what will be retaught if inappropriate behavior occurs (Colvin, Kame’enui, & Sugai, 1993). This prevents problem behavior by giving students specific, appropriate behaviors to engage in. Monitoring student behavior allows the teacher to acknowledge students who are engaging in appropriate behavior and prevent misbehavior from escalating (Colvin et al., 1993). Research on classroom management has typically focused on the identification of individual practices that have some level of evidence to support their adoption within classrooms. These practices are then combined under the assumption that, if individual practices are effective, combining these practices into a package will be equally, if not more, effective. Textbooks are written and policies and guidelines are disseminated to school personnel based on these assumptions. Without research that examines classroom management as an efficient package of effective practices, a significant gap in our current knowledge base still exists. Understanding the components that make up the most effective and efficient classroom management system as well as identifying the effects teachers and administrators can expect from implementing effective classroom management strategies represent some of these gaps. A meta-analysis of classroom management which identifies more and less effective approaches to universal, whole-class, classroom management as a set of practices is needed to provide the field with clear research-based standards. This review examines the effects of teachers’ universal classroom management practices in reducing disruptive, aggressive, and inappropriate behaviors. The specific research questions addressed are: Do teacher’s universal classroom management practices reduce problem behavior in classrooms with students in kindergarten through 12th grade? What components make up the most effective and efficient classroom management programs? Do differences in effectiveness exist between grade levels? Do differences in classroom management components exist between grade levels? Does treatment fidelity affect the outcomes observed? These questions were addressed through a systematic review of the classroom management literature and a meta-analysis of the effects of classroom management on disruptive or aggressive student behavior. Twelve studies of universal classroom management programs were included in the review. The classroom-level mean effect size for the 12 programs was positive and statistically significant (d=.80 with an ICC=.05; d=.71 with an ICC=.10; p<.05). Note that cluster adjustments were required due to differences in reporting measures between classroom level outcomes and individual student level outcomes. The resulting effect sizes index classroom-level differences and cannot be compared to the typical student-level effect sizes commonly reported in the literature. Due to a lack of power to detect heterogeneity and lack of information reported in the studies reviewed, only the first research question could be addressed. Teacher’s classroom management practices have a significant, positive effect on decreasing problem behavior in the classroom. Students in the treatment classrooms in all 12 studies located for the review showed less disruptive, inappropriate, and aggressive behavior in the classroom compared to untreated students in the control classrooms. The overall mean classroom effect size of either .71 or .80 indicates a positive effect that significantly impacts the classroom environment. To put our classroom-level mean effect sizes into a comparable format with the more typical effect sizes, we back-transformed our mean effect sizes using the original adjustment formulas (Hedges, 2007). Thus, the classroom-level mean effect sizes of .80 and .71 are roughly comparable to student level effect sizes of .18 and .22 for ICC=.05 and ICC=.10, respectively. Teachers who use effective classroom management can expect to experience improvements in student behavior and improvements that establish the context for effective instructional practices to occur.
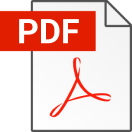
Michele Terry Thompson , Gordon S. Gibb , Darlene Anderson
Research suggests a compelling correlation between teacher behavior and effective learning environments. Focusing on the evidence-based teaching skill of offering behavior-specific praise (BSP), the researchers worked with three elementary-level general educators in a tiered model of training generally known as response to intervention (RtI). Although RtI commonly provides targeted instructional support to students, this study used the RtI framework to provide professional development instruction to teachers. The researchers also tracked the behavior of three students identified by the teachers as having behavioral difficulties, who became the focus of each teacher’s BSP. Results showed increases in rates of BSP following the Tier 2 and Tier 3 interventions (video self-monitoring and peer coaching), but not following the Tier 1 intervention (school-wide in-service training). Averagesfor all three students’ on-task behavior increased with increased teacher BSP.
Journal of Education for Students Placed at Risk (JESPAR)
Raymond Burke
Michelle Parker-Tomlin
Abigail Camus
Paul Caldarella , Krystine Jolstead , Leslie Williams
Challenging behavior in preschool is a serious concern for teachers. Positive behavior interventions and supports (PBIS) has been shown to be effective in reducing such behaviors. Class-Wide Function-Related Intervention Teams (CW-FIT) is a specific multi-tiered intervention for implementing effective classroom management strategies using PBIS practices. CW-FIT has been shown to be effective in elementary classrooms but has not yet been evaluated with younger age groups. CW-FIT Tier 1 is a group contingency utilizing social skills training, teacher praise, and positive reinforcement to improve student behavior. The present study examined the effects of CW-FIT Tier 1 implementation on student group on-task behavior and on teacher praise and reprimand rates in four preschool classrooms. A single-subject delayed multiple-baseline design with embedded reversals was used to evaluate impact. Results indicated the intervention increased student group on-task behavior and teacher praise to reprimand ratios. Both teachers and children found CW-FIT Tier 1 to be socially valid. Limitations and implications of this study for researchers and practitioners are discussed.
amira lamira
Paul Caldarella , Leslie Williams
Comprehensive evidence-based interventions are needed to help early childhood educators manage challenging student behaviors. One such intervention, class-wide function-related intervention teams (CW-FIT), is a multi-tiered behavioral intervention program based on positive behavior support principles, including four main elements: (a) teaching socially appropriate communication skills, (b) using differential reinforcement with an interdependent group contingency, (c) extinguishing or eliminating potential reinforcement for problem behavior, and (d) implementing individual interventions using self-management, help cards, and/or functional assessment. This study implemented CW-FIT with five kindergarten through second grade teachers. Results demonstrated efficacy of the program as early childhood teachers increased their praise rates and decreased their reprimand rates, and students responded with increased on-task behavior and fewer classroom disruptions. Study implications and limitations are addressed.
IOSR Journal of Nursing and Health Science
Hassanat R A M A D A N Abdel-Aziz
Background: Poor sleep is reported in 50% of older adults, calling for an important need for identifying safe and effective alternatives to improve their sleep quality. Aim of the study: The present study aimed to evaluate the effect of cognitive behavioral (CB) intervention on improving sleep quality in older adults. Design: A quasi-experimental pretest-and-posttest design was utilized to conduct this study. Setting: The study was conducted in the geriatric social club in Zagazig City. Sample: A purposive sample of 75 elderly subjects who fulfilled the study inclusion criteria. Tools: Three tools were used in the present study; the first tool was a structured interview questionnaire consisted of two parts, the second tool was The Sleep Hygiene Awareness and Practice Scale, and the third tool was The Pittsburgh Sleep Quality Index (PSQI). Results: The results revealed post-intervention statistically significant improvements in PSQI components scores, PSQI global score, sleep hygiene knowledge, caffeine knowledge, sleep hygiene practice, and other sleep parameters as sleep latency and sleep efficiency. Conclusion: CB intervention is effective in improving elderly's sleep quality, and can be considered a safe and effective non-pharmacological approach to improve elderly's sleep quality. Recommendations: The developed CB intervention should be implemented in the study setting on a long term basis to test its sustainability, and in similar settings to confirm its effectiveness and for further improvements.
Journal of Early and Intensive Behavioral Intervention,
Tracy L Morris
Sorry, this document isn't available for viewing at this time.
In the meantime, you can download the document by clicking the 'download' button above., related papers.
Rikki Wheatley
Administration and Policy in Mental Health and Mental Health Services Research
Keith Herman
Children and Youth Services Review
Carisa Wilsie
Jacqueline Rodriguez
Cut Eka Para Samya
Jonathan Green , Catherine Kay
Drug and Alcohol Dependence
Jesse Dallery , Steven Meredith
Journal of Child Psychology and Psychiatry
Sabine Landau
Teacher Education and Special Education: The Journal of the Teacher Education Division of the Council for Exceptional Children
Madeleine Gregg
Journal of Behavioral Education
Paul Caldarella , Robyn Orr
Child & Family Behavior Therapy
Molly Murphy
Journal of Early and Intensive Behavior Intervention
Stephen Larmar
A Context for Science with a Commitment to Behavior …
John Blackledge
Herbert Quay
Psychology in the Schools
Thomas Kehle
Regina Oliver
Joe Cautilli
American Journal on Intellectual and Developmental Disabilities
Mark Durand
Carla Matias
JBI Reports 2(4) :115-83.
Emily Haesler
Unpublished PhD Thesis - King's College London
Journal of Graduate Medical Education
Sumant Ranji
Child Care in Practice
Mickey Keenan , Karola Dillenburger
Tonya Davis
Journal of School Psychology
David Daley
Dr. Marissa N Mroz-Cline
Child psychiatry and human development
Ata Ghaderi
Nicole Zarrett , Carl Sorensen
Pelin Kovanci
Adrian Treves
Journal of Emotional and Behavioral Disorders
Monica Carr
Children's Health Care
Shauna Lynne
What Works Clearinghouse
Douglas Cullinan
Journal of Applied Behavior Analysis
Gregory Hanley , Einar Ingvarsson
Jaja Delano
Scott Ardoin
RELATED TOPICS
- We're Hiring!
- Help Center
- Find new research papers in:
- Health Sciences
- Earth Sciences
- Cognitive Science
- Mathematics
- Computer Science
- Academia ©2024
Presentation of Quantitative Research Findings
- First Online: 30 August 2023
Cite this chapter
- Jan Koetsenruijter 3 &
- Michel Wensing 3
470 Accesses
Valid and clear presentation of research findings is an important aspect of health services research. This chapter presents recommendations and examples for the presentation of quantitative findings, focusing on tables and graphs. The recommendations in this field are largely experience-based. Tables and graphs should be tailored to the needs of the target audience, which partly reflects conventional formats. In many cases, simple formats of tables and graphs with precise information are recommended. Misleading presentation formats must be avoided, and uncertainty of findings should be clearly conveyed in the presentation. Research showed that the latter does not reduce trust in the presented data.
This is a preview of subscription content, log in via an institution to check access.
Access this chapter
- Available as EPUB and PDF
- Read on any device
- Instant download
- Own it forever
- Durable hardcover edition
- Dispatched in 3 to 5 business days
- Free shipping worldwide - see info
Tax calculation will be finalised at checkout
Purchases are for personal use only
Institutional subscriptions
Similar content being viewed by others
Quantitative Methods in Global Health Research
Quantitative Research
Recommended readings.
Designing tables: (Boers, 2018b) (from an article series in BMJ Heart).
Google Scholar
Practical guidelines for designing graphs: http://www.perceptualedge.com (Stephen Few).
Aronson, J. K., Barends, E., Boruch, R., et al. (2019). Key concepts for making informed choices. Nature , 572(7769), 303–306.
Boers, M. (2018a). Designing effective graphs to get your message across. Annals of the Rheumatic Diseases , 77(6), 833–839.
Boers, M. (2018b). Graphics and statistics for cardiology: designing effective tables for presentation and publication. Heart , 104(3), 192–200.
Bramwell, R., West, H., & Salmon, P. (2006). Health professionals’ and service users’ interpretation of screening test results: experimental study. British Medical Journal , 333 (7562), 284.
Cukier, K. (2010). Data, Data Everywhere: A Special Report on Managing Information . The Economist, 394, 3–5.
Duke, S. P., Bancken, F., Crowe, B., et al. (2015). Seeing is believing: Good graphic design principles for medical research. Statistics in Medicine , 34 (22), 3040–3059.
Few, S. (2005). Effectively Communicating Numbers: Selecting the Best Means and Manner of Display [White Paper]. Retrieved December 8, 2021, from http://www.perceptualedge.com/articles/Whitepapers/Communicating_Numbers.pdf
Fischhoff, B., & Davis, A. L. (2014). Communicating scientific uncertainty. Proceedings of the National Academy of Sciences , 111 (supplement_4), 13664–13671.
Gustafson, A., & Rice, R. E. (2020). A review of the effects of uncertainty in public science communication. Public Understanding of Science , 29 (6), 614–633.
Han, P. K. J., Klein, W. M. P., Lehman, T., et al. (2011). Communication of uncertainty regarding individualized cancer risk estimates: Effects and influential factors. Medical Decision Making , 31 (2), 354–366.
Johnston, B. C., Alonso-Coello, P., Friedrich, J. O., et al. (2016). Do clinicians understand the size of treatment effects? A randomized survey across 8 countries. Canadian Medical Association Journal , 188(1), 25–32.
Kelleher, C., & Wagener, T. (2011). Ten guidelines for effective data visualization in scientific publications. Environmental Modelling and Software , 26(6), 822–827.
Khasnabish, S., Burns, Z., Couch, M., et al. (2020). Best practices for data visualization: Creating and evaluating a report for an evidence-based fall prevention program. Journal of the American Medical Informatics Association , 27 (2), 308–314.
Lavis, J., Davies, H. T. O., Oxman, A., et al. (2005). Towards systematic reviews that inform health care management and policy-making. Journal of Health Services Research and Policy . 35–48.
Lopez, K. D., Wilkie, D. J., Yao, Y., et al. (2016). Nurses’ numeracy and graphical literacy: Informing studies of clinical decision support interfaces. Journal of Nursing Care Quality , 31 (2), 124–130.
Norton, E. C., Dowd, B. E., & Maciejewski, M. L. (2018). Odds ratios-current best practice and use. JAMA – Journal of the American Medical Association , 320 (1), 84–85.
Oudhoff, J. P., & Timmermans, D. R. M. (2015). The effect of different graphical and numerical likelihood formats on perception of likelihood and choice. Medical Decision Making , 35(4), 487–500.
Rougier, N. P., Droettboom, M., & Bourne, P. E. (2014). Ten simple rules for better figures. PLoS Computational Biology , 10 (9), 1–7.
Schmidt, C. O., & Kohlmann, T. (2008). Risk quantification in epidemiologic studies. International Journal of Public Health , 53 (2), 118–119.
Springer (2022). Writing a Journal Manuscript: Figures and tables. Retrieved April 10, 2022, from https://www.springer.com/gp/authors-editors/authorandreviewertutorials/writing-a-journal-manuscript/figures-and-tables/10285530
Trevena, L. J., Zikmund-Fisher, B. J., Edwards, A., et al. (2013). Presenting quantitative information about decision outcomes: A risk communication primer for patient decision aid developers. BMC Medical Informatics and Decision Making , 13 (SUPPL. 2), 1–15.
Tufte, E. R. (1983). The visual display of quantitative information. https://www.edwardtufte.com/tufte/books_vdqi
Wensing, M., Szecsenyi, J., Stock, C., et al. (2017). Evaluation of a program to strengthen general practice care for patients with chronic disease in Germany. BMC Health Services Research , 17, 62.
Wronski, P., Wensing, M., Ghosh, S., et al. (2021). Use of a quantitative data report in a hypothetical decision scenario for health policymaking: a computer-assisted laboratory study. BMC Medical Informatics and Decision Making , 21 (1), 32.
Download references
Author information
Authors and affiliations.
Department of General Practice and Health Services Research, Heidelberg University Hospital, Heidelberg, Germany
Jan Koetsenruijter & Michel Wensing
You can also search for this author in PubMed Google Scholar
Corresponding author
Correspondence to Jan Koetsenruijter .
Editor information
Editors and affiliations.
Michel Wensing
Charlotte Ullrich
Rights and permissions
Reprints and permissions
Copyright information
© 2023 The Author(s), under exclusive license to Springer Nature Switzerland AG
About this chapter
Koetsenruijter, J., Wensing, M. (2023). Presentation of Quantitative Research Findings. In: Wensing, M., Ullrich, C. (eds) Foundations of Health Services Research. Springer, Cham. https://doi.org/10.1007/978-3-031-29998-8_5
Download citation
DOI : https://doi.org/10.1007/978-3-031-29998-8_5
Published : 30 August 2023
Publisher Name : Springer, Cham
Print ISBN : 978-3-031-29997-1
Online ISBN : 978-3-031-29998-8
eBook Packages : Medicine Medicine (R0)
Share this chapter
Anyone you share the following link with will be able to read this content:
Sorry, a shareable link is not currently available for this article.
Provided by the Springer Nature SharedIt content-sharing initiative
- Publish with us
Policies and ethics
- Find a journal
- Track your research
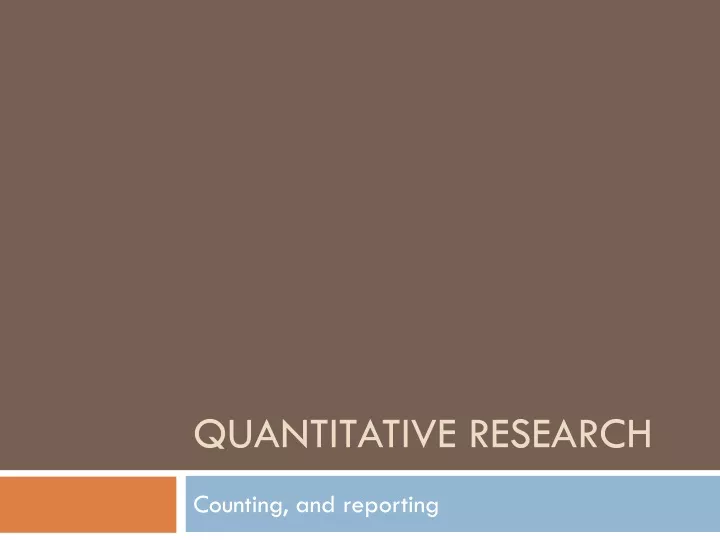
Quantitative Research
Jan 01, 2020
520 likes | 764 Views
Quantitative Research. Counting, and reporting. Quantitative Research. Numbers-based – Quantitative research refers to the manipulation of numbers to make claims, provide evidence, describe phenomena, determine relationships, or determine causation.
Share Presentation
- research question
- quantitative research
- descriptive statistics
- good research question
- experienced rpg mmorpg players
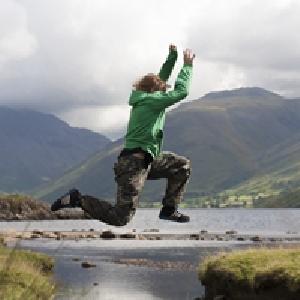
Presentation Transcript
Quantitative Research Counting, and reporting
Quantitative Research • Numbers-based – Quantitative research refers to the manipulation of numbers to make claims, provide evidence, describe phenomena, determine relationships, or determine causation. • Deductive – usually tests a hypothesis based on previous research. Numbers are important to determine when a hypothesis has been confirmed or not. You are looking FOR something. • Generalizable – through statistical or mathematical modeling, can make predictions about future events.
Quantitative Research Ideas and Controversy
Goals • The intention of the “empirical/quantitative” part of the research tradition course goal is to consider the rhetorical and research benefits (and drawbacks) of using numbers as evidence. • It is less about advanced quantitative reasoning and more about teaching an appreciation of what it means to produce, analyze, and report numbers in support of an argument or to answer a research question.
Quantitative research • Descriptive Research – describe a phenomenon using numbers • Quantitative research is good at this because it shows aggregates (combined instances) in an easy to see way. • Inferential research – make a prediction or comparison about what you found using numbers • Quantitative research is good at this because numbers are precise, they can be used for comparison better than qualitative research (cold v. cool OR 45 v 55)
Experimental Research • Experimental – testing whether a “thing” (independent variable) applied to a subject/group has an effect (dependant variable) • Two equal groups, one control, one experimental • Pre-test, post-test • Quasi-experimental – testing whether a “thing” applied to a subject/group has an effect but without being able to • Actively apply the “thing” • Control for other variables
Top 4 things • Numbers are a semiotic system. As such, they are arbitrary. They aren’t inherently more “true” than any other sign. • Numbers are precise, which makes them really good for specificity and measuring. • Numbers are mutually exclusive, so they are good for comparisons. • Numbers can be manipulated using formula to discover causes, make predictions, and generalize from samples to populations.
Quantitative Controversy • Some scholars believe that human experience, attitudes and beliefs cannot be quantified. • A person’s “feelings” can change from day to day with little conscious thought of the fact • Some scholars believe that quantitative research is trusted more than it should be • Bridges still collapse, spaceships still get lost, new cars still break down, pharmaceutical companies still produce harmful drugs, computers still crash • Some scholars believe that quantitative research is reductive • Statistically speaking, your SAT has already predicted what your final college GPA is going to be. Will you only ever be 1400 or 3.6 smart? • Some scholars believe that quantitative research misses important nuances • In a ChangeWave survey, the iPhone had the highest customer satisfaction with 79% of the sample “very satisfied.”
Fieldwork methods • Observations • count the number of people who hold the door open for another at Driscoll or the library • count the number of people looking at their phones while with other people in the dining halls • count the number of people who return shopping carts to the stalls. • Surveys • hand out paper or online surveys related to a research question. • Experiments • use online personality test to find a Meyers-Briggs type and then ask participants to complete a word search, keeping track of how long they take and what words they find first, second, third… • blind, soda taste test or pizza test after giving them different descriptions about what they are about to taste.
Designing studies
Research Plan • As we follow this scientific method, recognize that it really is just a research plan, but in a more focused manner. You would still benefit much from working out the following BEFORE you conduct your study • Research Question • Method • Plan • Timeline
Define the Question • Defining the question, often called your research question, determines the scope of what you are able to research. A good research question should be (FINER): • Feasible – is it a realistic question to ask? • Interesting – will we learn something from it? • Novel – have very few people done it? • Ethical – does it respect the participants? • Relevant – will we be able to do something with the findings? Hulley S, Cummings S. (Eds ) Designing Clinical Research. Willimas & Wilkins: Baltimore, 1988
Defining the Question • To create a Quantitative Research Question • Define your participants • Define your issue • Define the variables of that issue • Ask a question of the participants, issue, and variable • Do DU students have part-time jobs that they enjoy? • Are college major and writing anxiety in undergraduatewriters correlated?
Gather Information and Resources • Text-Based Research is useful in helping you define your expectation (hypothesis). You want to find what has come before in the topic or related topics. You will rarely find your exact study (if you do, then your research question isn’t novel). You are looking for elements, pieces of your topic that have come before. • Previous studies have determined that experienced female MMORPG players have a primary motivation to play for social reasons (Yee, 2007). Other studies have shown no gender differences when looking at more than a single primary motivation (Tychsen, Hitchens, & Brolund, 2008). In these studies, the intention was to look at motivation in experienced RPG/MMORPG players—in my current study, I intend to look at motivation to play MMORPG World of Warcraft by non-experienced gamers.
Gather Information and Resources • Your experiences as well as those experiences of your friends can also be useful in helping you gather information and resources.
Form Hypothesis • What is your best guess as to the outcome of your Research Question • The hypothesis is based on your Research Question, but it is not phrased as a question – it is phrased as your best guess as to the outcome of that question • DU students do not enjoy their part-time work • College major and writing anxiety in undergraduate writers is not related. • You might create sub-hypotheses to account for other variables that you might consider relevant (e.g. gender, class-standing). You tag these on to the end of your initial hypothesis. • Seniors tend to enjoy their part-time work more than first-year students enjoy their work. • Female undergraduates experience more anxiety than males.
Design Experiment • Determine what best would address the research question you are asking. In quantitative studies, a survey works the best because you can control responses. • Determine triangulation questions or observations for two reasons: • You don’t want it to be obvious what you are asking • Other variables may be affecting the outcome. • Refer to Chapter 8 in Situating Research (or the handout Conducting Surveys) when coming up with your Survey Questions • It’s a good idea to playtest your survey with one or more people so that they can give you feedback about what questions might be confusing.
Design Experiment • Likert-type scale (1-5) will allow you to “quantify” human beliefs, attitudes, and experiences. Likert-type scales are used often in social science, descriptive studies. • Usually ask positive questions, and then follow with whether the person agrees or disagrees. • Usually scaled so that higher numbers are positive/agreement, lower numbers negative/disagreement “A good writing class should consist of lectures on grammar” 1-strongly disagree, 2-disagree, 3-agree, 4-strongly agree “How satisfied are you with University of Denver’s dorms” 1-very unsatisfied, 2-unsatisfied, 3-satisfied, 4-very satisfied
Design Experiment • Planning your survey, your hypothesis, your plan is vital BEFORE you conduct your survey because you only get one shot at the survey.
The Final Four • Collect Data (Perform Experiment, conduct survey, conduct observation) – remember to be professional, take notes (you never know what might effect your results), and ethical. • Analyze Data – Keep track of your data, put it in a spreadsheet, and work the numbers. We will be talking a bit about statistics here, but really, all you will be expected to do is descriptive analysis • Interpret Data and Draw Conclusions • Publish Results (see IMRAD PowerPoint)
Very Short Guide to Stats for Quantitative Research Papers Basics of aggregate and statistical data
Inferential v. Descriptive • Descriptive statistics “describe” the data of a sample or population. They are usually aggregate data • Average (Mean) GPA • Standard Deviation of SAT score • Inferential statistics “infer” (i.e. conclude) relationships between a sample AND a population, or “infer” past, present or future results of a sample/population based on its data. • Regression/correlation analysis of GPA and SAT (relationship between SAT and GPA, and SAT can be used to predict GPA)
N = number of participants • In inferential statistics, you would refer to the number of participants in your survey as N. If it is a sample or part of a whole, it is n (lowercase), and if it is a total population, it is N (uppercase). • Population: N = 4,432 • Sample: n = 100 • In descriptive studies and descriptive statistics, it is common to refer to participants as N, subgroups of those participants as n • Of the total students surveyed (N = 100), only 10% (n = 10) were male.
Measures of central tendency • Central Tendency measures common “middles” • Mean is the arithmetic average of items or values • Mode is the most occurring item or value • Median is the item or value of which 50% are greater and 50% are less. • Sometimes GPA or time can be used as a measure, but another measure is one of attitudes and beliefs using a Likert-type scale. • Standard Deviation is a measure of the spread of items or values in a series. Understanding the variation can help you see how close a particular item or value is to other numbers. • Distribution (Histogram) is a visual representation of the number of a particular result in an array of numbers. In this series (number of hours I played WoW over break):8, 0, 0, 3, 2, 10, 0 • Mean = 3.29, Mode = 0, Median = 2, SD = 4.11 In this series (number of hours I worked this week):8, 8, 8, 8, 6, 6, 5 • Mean = 7, Mode = 8, Median = 8, SD = 1.29
Correlations and comparisons Student’s t-test The “basic” comparison test between a sample and a population. Designed at Guinness beer in 1908. T Tests are reported like, t(degrees of freedom) = t statistic P = significance level. The t-statistic is less important than the significance level. Pearson Correlation The “basic” correlation test between two samples. Designed by publisher Pearson to compare test scores. Pearson correlations are reported like, r = correlation score between 1.00 (positive correlation) and -1.00 (negative correlation).
Using Excel to do your stats • Mean { =average(range) } • You can compute mode { =mode(range) } or median {=median(range) }, but they might not be as useful in this project. • Standard Deviation { =stdev(range) } • You can also count the number of instances of a value including instances of text: { =countif(range,”value”) } • The following example would count every instance of “male” in the range: =countif(A2:A7,”male”) • Pearson’s correlation { =correl(range1,range2) } • Student’s t-test { =ttest(range1,range2,tails,type) } • You can create frequency distribution histograms by using Tools -> Data Analysis, then Historgram. Histograms count the number of instances of a result in a given array. You can also find these commands by using Insert -> Function. There are also far more complex inferential statistics available in Excel • You can do a complete Descriptive Stats Summary by selecting Tools > Data Analysis (If you don’t see a Data Analysis, then (Excel 2003) Tools > Add-ins > Analysis ToolPak; (Excel 2007) Excel Options > Add-ins > Manage Add-ins > Analysis ToolPak
Writing Stats in APA • Standard Deviation = SD • Mean = M • Descriptive statistics are often written in parentheses after an item that the statistic refers to, and symbols and numbers should be separated by a space • In a survey of DU students, participants (N = 100) responded that money was more important (M = 4.2, SD = .9) than experience (M = 3.5, SD = .76) in selecting a summer job. • In a survey of computer game addicts, females (n = 15) were more likely to be depressed during withdrawal (M = 5.2, SD = .45) than males were (n = 78, M = 3.2, SD = .98) • Chapter 8 in Situating Research has more about this.
Charts and Graphs It’s important in doing graphs that you compute an aggregate (sum, average, SD, something) before graphing information. You cannot just Select All of data and make a graph out of it. • Pie graphs – good for showing distributions of a total population (you will have to compute aggregates first) • Line graphs – good for showing time-based, linear progression • Column/Bar graphs – good for showing distribution of individual responses (you will have to create aggregates first) • Y-Axis (vertical) for variables, X-Axis (horizontal) for participants.
- More by User
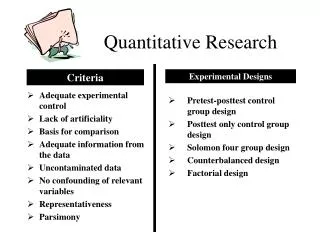
Adequate experimental control Lack of artificiality Basis for comparison Adequate information from the data Uncontaminated data No confounding of relevant variables Representativeness Parsimony. Pretest-posttest control group design Posttest only control group design
622 views • 14 slides
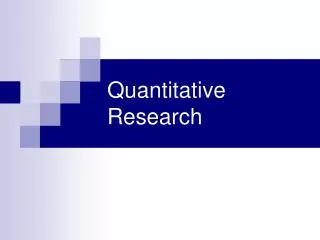
Quantitative Research. March 8. Quiz Assignments Experimental Research Quantitative Research Emotional Intelligence Survey Validity, Reliability, Error Characteristics of Surveys. Laboratory Experiments Artificial – low realism Few extraneous variables High control Low cost
1.33k views • 71 slides
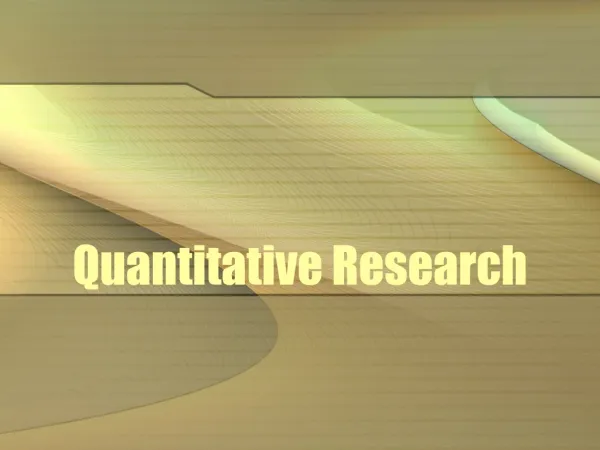
Quantitative Research. Overview. Observational. Definition - Directly observing naturally occurring behavior unobtrusively, typically in the field, but can also take place in laboratory settings Pros
688 views • 16 slides
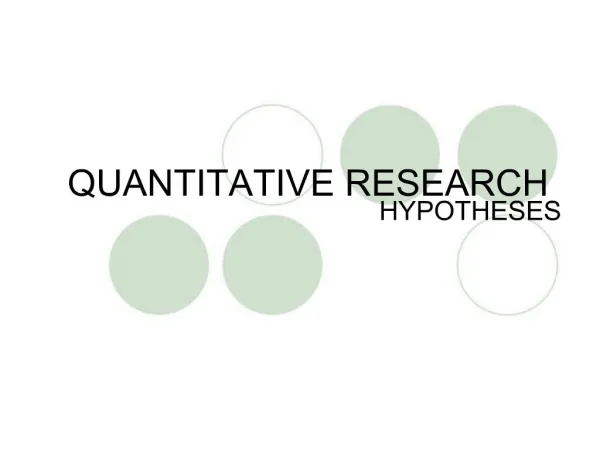
QUANTITATIVE RESEARCH
HYPOTHESES[see pp. 176-179]. I. Defining hypotheses A. Statements posing a relationship between 2 or more variables.B. Usually emerge out of substantial literature review. C. Types of relationships.1. Covariance (correlative)a. Vary together but not causal. b. Can be positive or negati
605 views • 14 slides
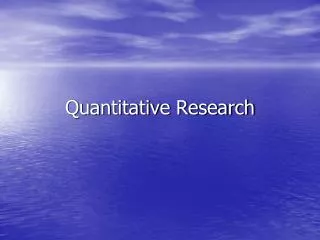
Quantitative Research. Descriptive Research. Also called “Survey Research” Describes the way things exist Data collected Test hypothesis Or to classify opinions on issues/topics. Descriptive Research. Most common research Surveys conducted
657 views • 44 slides
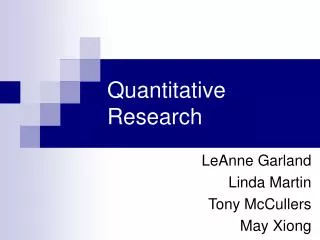
Quantitative Research. LeAnne Garland Linda Martin Tony McCullers May Xiong. Image from: http://www.ryerson.ca/~mjoppe/ResearchProcess/QuantitativeResearch.htm . Definition.
875 views • 14 slides
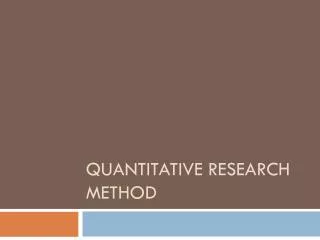
Quantitative Research Method
Quantitative Research Method . 4.2 Quantitative Research Methods. Uses deductive method of knowledge acquisition Intends to falsifies an existing theory Tries to generate generalizable knowledge Accepts objectivity of knowledge Uses a standard measurement instruments
427 views • 28 slides
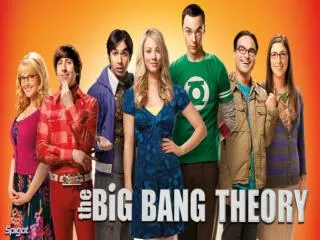
Quantitative Audience Research
Quantitative Audience Research . My chosen show is The Big Bang Theory Season 1-Average audience of 8.31 Million Season 2-Average audience of 10 Million Season 3-Average audience of 14.14 Million Season 4-Average audience of 13.14 Million Season 5-Average audience of 15.82 Million
161 views • 8 slides
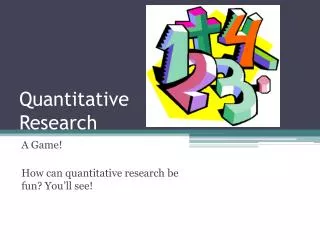
Quantitative Research. A Game! How can quantitative research be fun? You’ll see!. Goals for the Game. Choose a button. Each button includes: Points Question When you answer the question correctly, you get the points! You answer incorrectly, you get the points taken away.
223 views • 16 slides
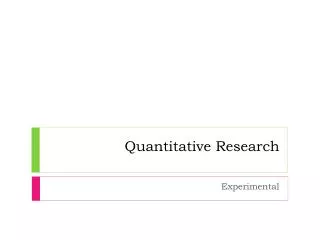
Quantitative Research. Experimental. Experimental Research. Cause and effect relationships are established by manipulating the INDEPENDENT variable(s) and observing the effect on the DEPENDENT variable.
263 views • 18 slides
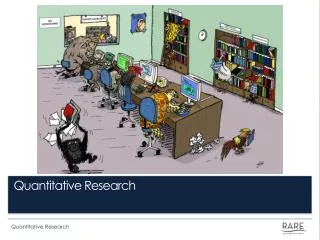
Quantitative Research. In this lesson, we will deepen our skills in quantitative research methods to: Explain how survey tools can be used to evaluate your campaign’s impact. Describe the value of sample size for your quantitative research and measurement of impact.
731 views • 32 slides
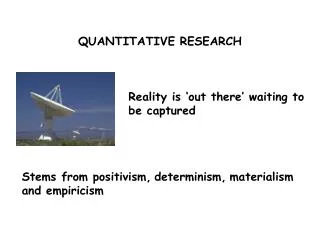
Reality is ‘out there’ waiting to be captured. QUANTITATIVE RESEARCH. Stems from positivism, determinism, materialism and empiricism. POSITIVISM. The universe consists of real, independent phenomena that we can come to know through direct observation.
542 views • 27 slides
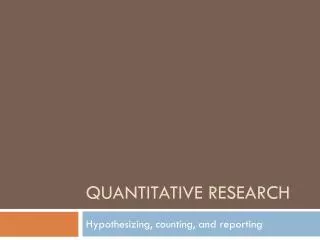
Quantitative Research. Hypothesizing, counting, and reporting. Quantitative Research. Numbers-based – Quantitative research refers to the manipulation of numbers to make claims, provide evidence, describe phenomena, determine relationships, or determine causation.
464 views • 16 slides
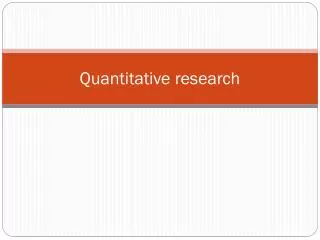
Quantitative research
Quantitative research. DEFINITION. Quantitative research is a formal, objective, systematic process in which numerical data are used to obtain information about the world. This research method is used: to describe variables; to examine relationships among variables;
536 views • 41 slides
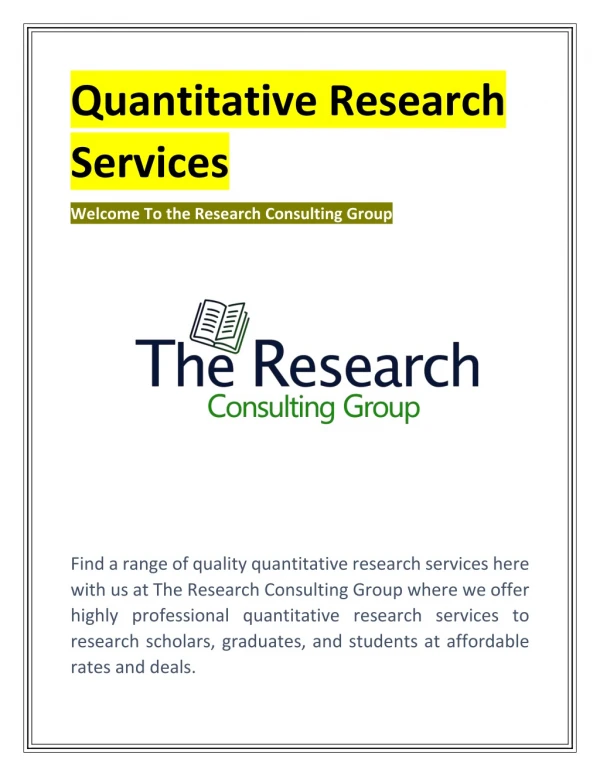
Quantitative Research Services
Looking for Quantitative Research Services? If yes, we are for you! We are The Research Consulting Group and we offer a range of highly professional quantitative research services that you can trust and rely on. We are a qualified team dedicated to providing you with the best services. Visit us now Click at https://www.theresearchconsultinggroup.com
74 views • 4 slides
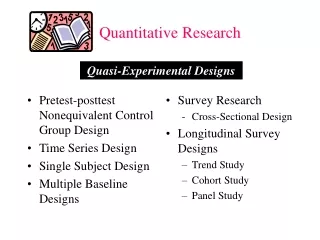
Pretest-posttest Nonequivalent Control Group Design Time Series Design Single Subject Design Multiple Baseline Designs. Survey Research Cross-Sectional Design Longitudinal Survey Designs Trend Study Cohort Study Panel Study. Quantitative Research. Quasi-Experimental Designs.
280 views • 8 slides
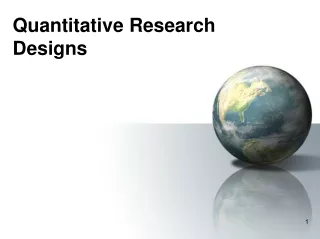
Quantitative Research Designs
Quantitative Research Designs. Questions for Thought. What is the difference between experimental, quasi-experimental, and nonexperimental ?. Research Design. Research Design It is the researcher’s overall plan for Answering the research question Testing the research hypotheses.
565 views • 48 slides
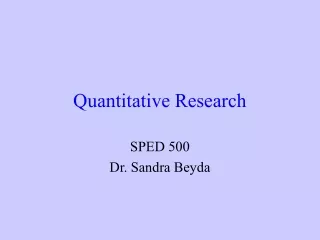
Quantitative Research. SPED 500 Dr. Sandra Beyda. Designs that maximize objectivity by using numbers, statistics, structure, and experimenter control. Modes Experimental mode Non-experimental mode. Quantitative Research. Structure of a Data Based Study. Abstract Introduction Method
303 views • 25 slides
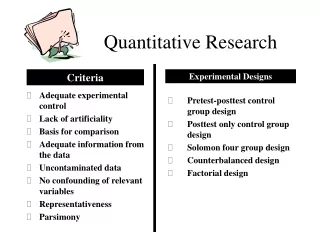
690 views • 14 slides
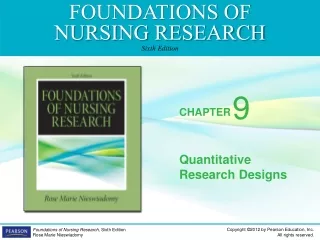
9. Quantitative Research Designs. Learning Objectives. Identify Criteria For Exploratory, Descriptive, And Explanatory Studies Define Experimental Research Differentiate Between Internal And External Validity In Experimental Designs Identify Six Threats To Internal Validity
871 views • 80 slides
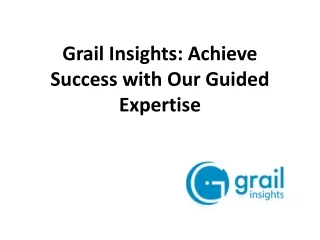
Quantitative Market Research |Quantitative Market Research | Qualitative Market Research
Grain Insights understands that to be a leader in the industry, you continuously need to be monitoring your marketing efforts to improve your approach. With its Qualitative Market Research, it is easy to understand consumer behavior, changing preference patterns and events that impact markets.
103 views • 5 slides

COMMENTS
Solution 1. Independent variable: Level of schooling, four categories - primary, upper primary, secondary and junior college. Dependent variable: Score on a classroom observation inventory, which measures teacher - student interaction 2. Independent variable: Gender of the teacher - male, female.
Jan 11, 2016 • Download as PPTX, PDF •. 164 likes • 178,782 views. Tooba Kanwal. This presentation is about Quantitative Research, its types and important aspects including advantages and disadvantages, characteristics and definitions. Education. 1 of 32. Download now. Quantitative research - Download as a PDF or view online for free.
In these cases you may call the preceding variable (i.e., the rainfall) the predictor variable and the following variable (i.e. the mud) the outcome variable. Other common types of variables Once you have defined your independent and dependent variables and determined whether they are categorical or quantitative, you will be able to choose the ...
Types of Variables. Aug 26, 2015 • Download as PPT, PDF •. 244 likes • 243,024 views. Ali Mustafa. Statistics Methodology's Presentation. Data & Analytics. Download now. Types of Variables - Download as a PDF or view online for free.
In quantitative research, hypotheses predict the expected relationships among variables.15 Relationships among variables that can be predicted include 1) between a single dependent variable and a single independent variable (simple hypothesis) or 2) between two or more independent and dependent variables (complex hypothesis).4,11 Hypotheses may ...
Among other topics, the next chapter explores the intersection between the nature of variables studied in quantitative research and how investigators set about measuring those variables. Take a moment to complete the following activity. Previous/next navigation. Previous: Module 3 Chapter 3: Overview of Quantitative Traditions ...
Frequency of organizational change, reading comprehension scores, emergency response time, or types of depression can be measured. They are variables (concepts that can vary). Quantitative research involves many kinds of variables. There are four main types discussed in further detail below: Independent variables (IV). Dependent variables (DV).
University of Texas at El Paso ... á #«
Part 1: quantitative designs. The purpose of this study is to compare and contrast two quantitative scholarly articles, identify and analyze the designs in each one, summarize the rationale of the design for each, briefly state the results of each article, and also critique both of them.
Presentation Transcript. Quantitative Research Methodology Session 2 Variables, Population, and Sampling. Variable A characteristic that varies • Independent Variable "the factor that is measured, manipulated, or selected by the experimenter to determine its relationship with an observed phenomenon" (Tuckman, 1999, p.93) Variable (2) 2.
Constructing a Histogram. Step 1 - Choose the Classes. Step 2 - Count. Step 3 - Draw the Histogram. Identifying Identifiers • Identifier variables are categorical variables with exactly one individual in each category. • Examples: Social Security Number, ISBN, FedEx Tracking Number • Don't be tempted to analyze identifier variables.
Download ppt "Introduction to the Quantitative Research Process". Quantitative Research Formal, objective, rigorous, systematic process for generating information Describes new situations, events, or concepts Examines relationships among variables Determines the effectiveness of treatments.
Presentation Transcript. Quantitative Research Experimental. Experimental Research • Cause and effect relationships are established by manipulating the INDEPENDENT variable (s) and observing the effect on the DEPENDENTvariable. • Research design must control for the possible effects of extraneous variables that could mask, enhance, or in ...
Simply defined as, variable is a concept that varies. It can be in Quantity, Intensity, Amount and Types. It takes two or more values. Variable is a measurable characteristics that varies. It may change from group to group, person to person or within person over time. In research science, variable refer to factor or condition that can change ...
Organization is an entity. Name, Size, Type, Learning, Innovation are the attributes of an organization. So all these attributes are the variables. 2- Other example is that Employee is also an entity. Name, Age, Gender, Experience, Stress level, satisfaction, Performance are the attributes of an employee.
Aim of the study: The present study aimed to evaluate the effect of cognitive behavioral (CB) intervention on improving sleep quality in older adults. Design: A quasi-experimental pretest-and-posttest design was utilized to conduct this study. Setting: The study was conducted in the geriatric social club in Zagazig City.
Steps in Quantitative Research 7. Formulate the Hypothesis or Research Question • A hypothesis predicts the relationship between two or more variables. • The hypothesis contains the population and the variables. • Independent Variable is the "cause" or the variable that is thought to influence the dependent variable.
Introduction to Quantitative Research Methods. Introduction to Quantitative Research Methods. MEd Support Session 25 March 2013 Dr Surette van Staden. Research Methodology. Quantitative Research Inferring evidence for a theory through measurement of variables that produce numeric outcomes. Qualitative research. 2.08k views • 39 slides
The use of graphs in research presentation and the communication of results makes it possible to synthesise large amounts of data and enables users to comprehend the information more easily than if it were presented in mere words or numbers (Cukier 2010).Tufte refers to well-designed graphics as instruments for reasoning about quantitative information and considers them the most powerful way ...
When collecting and analyzing data, quantitative research deals with numbers and statistics, while qualitative research deals with words and meanings. Both are important for gaining different kinds of knowledge. Quantitative research. Quantitative research is expressed in numbers and graphs. It is used to test or confirm theories and assumptions.
Quantitative Data Analysis. Nov 21, 2013 • Download as PPTX, PDF •. 203 likes • 406,367 views. Asma Muhamad. A simple summary for introduction to quantitative data analysis. It is made for research methodology sub-topic. Education Technology. 1 of 30. Download now.
PR2 Q1W2L2 - PPT - RESEARCH VARIABLES - Free download as Powerpoint Presentation (.ppt / .pptx), PDF File (.pdf), Text File (.txt) or view presentation slides online. Variables are measurable characteristics that can change or vary. There are two main types of variables: discrete and continuous. Discrete variables assume a finite set of values that can be counted, like the number of errors in ...
Presentation Transcript. Quantitative Research Counting, and reporting. Quantitative Research • Numbers-based - Quantitative research refers to the manipulation of numbers to make claims, provide evidence, describe phenomena, determine relationships, or determine causation. • Deductive - usually tests a hypothesis based on previous ...