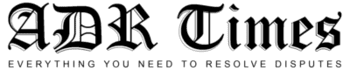

Common Problem-Solving Models & How to Use Them
Problem – solving models are step-by-step processes that provide a framework for addressing challenges. Problems arise in every facet of life. From work. to home. to friends and family, problems and conflicts can make life difficult and interfere with our physical and mental well-being. Understanding how to approach problems when they arise and implementing problem-solving techniques can make the journey through a problem less onerous on ourselves and those around us.
By building a structured problem-solving process, you can begin to build muscle memory by repeatedly practicing the same approach, and eventually, you may even begin to find yourself solving complex problems . Building a problem-solving model for each of the situations where you may encounter a problem can give you a path forward, even when the most difficult of problems arise.
This article will explore the concept of problem-solving models and dive into examples of such models and how to use them. It will also outline the benefits of implementing a problem-solving model in each area of life and why these problem-solving methods can have a large impact on your overall well-being. The goal of this article is to help you identify effective problem-solving strategies and develop critical thinking to generate solutions for any problem that comes your way.
Problem-Solving Model Defined
The first step in creating a problem-solving plan is to understand what we mean when we say problem-solving models. A problem-solving model is a step-by-step process that helps a team identify and effectively solve problems that they may encounter. This problem-solving approach gives the team the muscle memory and guide to address a conflict and resolve disputes quickly and effectively.
There are common problem-solving models that many teams have implemented, but there is also the freedom to shape a method to fit the needs of a specific situation. These models often rely on various problem-solving techniques to identify the root cause of the issue and find the best solution. This article will explore some common problem-solving models as well as general problem-solving techniques to help a team engage with and solve problems effectively.
Benefits of Implementing Problem-Solving Models
Before we discuss the exact models for problem-solving, it can be helpful to discuss why problem-solving models are beneficial in the first place. There are a variety of benefits to having a plan in place when a problem arises, but a few important benefits are listed below.
Guide Posts
When a team encounters a problem and has a guide for how to approach and solve the problem, it can be a relief to know that they have a process to fall back on when the issue cannot be resolved quickly from the beginning. A problem-solving strategy will serve as a guide for the parties to know which steps to take next and how to identify the appropriate solution.
It can also clarify when the issue needs to stay within the team, and when the issue needs to be escalated to someone in a position with more authority. It can also help the entire team solve complex problems without creating an issue out of the way the team solves the problem. It gives the team a blueprint to work from and encourages them to find a good solution.
Creative Solutions That Last
When the team or family has a way to fall back on to solve a problem, it takes some of the pressure off of coming up with the process and allows the parties to focus on identifying the relevant information and coming up with various potential solutions to the issue. By using a problem-solving method, the parties can come up with different solutions and find common ground with the best solution. This can be stifled if the team is too focused on figuring out how to solve the problem.
Additionally, the solutions that the parties come up with through problem-solving tools will often address the root cause of the issue and stop the team from having to revisit the same problem over and over again. This can lead to overall productivity and well-being and help the team continue to output quality work. By encouraging collaboration and creativity, a problem-solving technique will often keep solving problems between the parties moving forward and possibly even address them before they show up.
Common Models to Use in the Problem-Solving Process
Several models can be applied to a complex problem and create possible solutions. These range from common and straightforward to creative and in-depth to identify the most effective ways to solve a problem. This section will discuss and break down the problem-solving models that are most frequently used.
Standard Problem-Solving Process
When you search for a problem-solving technique, chances are you will find the standard model for saving problems. This model identifies and uses several important steps that will often be used in other models as well, so it can be helpful to begin the model-building process with an understanding of this model as a base. Other models often draw from this process and adapt one or more of the steps to help create additional options. Each of these steps works to accomplish a specific goal in furtherance of a solution.
Define the Problem
The first step in addressing a problem is to create a clear definition of the issue at hand. This will often require the team to communicate openly and honestly to place parameters around the issue. As the team defines the problem, it will be clear what needs to be solved and what pieces of the conflict are ancillary to the major issue. It helps to find the root causes of the issue and begin a process to address that rather than the symptoms of the problem. The team can also create a problem statement, which outlines the parameters of the problem and what needs to be fixed.
In addition to open and honest communication, other techniques can help to identify the root cause and define the problem. This includes a thorough review of the processes and steps that are currently used in the task and whether any of those steps are directly or indirectly causing the problem.
This includes reviewing how tasks are done, how communication is shared, and the current partners and team members that work together to identify if any of those are part of the issue. It is also the time to identify if some of the easy fixes or new tools would solve the problem and what the impact would be.
It is also important to gain a wide understanding of the problem from all of the people involved. Many people will have opinions on what is going on, but it is also important to understand the facts over the opinions that are affecting the problem. This can also help you identify if the problem is arising from a boundary or standard that is not being met or honored. By gathering data and understanding the source of the problem, the process of solving it can begin.
Generate Solutions
The next step in the basic process is to generate possible solutions to the problem. At this step, it is less important to evaluate how each of the options will play out and how they may change the process and more important to identify solutions that could address the issue. This includes solutions that support the goals of the team and the task, and the team can also identify short and long-term solutions.
The team should work to brainstorm as many viable solutions as possible to give them the best options to consider moving forward. They cannot pick the first solution that is proposed and consider it a successful problem-solving process.
Evaluate and Select
After a few good options have been identified, the next step is to evaluate the options and pick the most viable option that also supports the goals of the team or organization. This includes looking at each of the possible solutions and determining how they would either encourage or hinder the goals and standards of the team. These should evaluated without bias toward the solution proposed or the person putting forward the solution. Additionally, the team should consider both actual outcomes that have happened in the past and predicted instances that may occur if the solution is chosen.
Each solution should be evaluated by considering if the solution would solve the current problem without causing additional issues, the willingness of the team to buy in and implement the solution, and the actual ability of the team to implement the solution.
Participation and honesty from all team members will make the process go more smoothly and ensure that the best option for everyone involved is selected. Once the team picks the option they would like to use for the specific problem, they should clearly define what the solution is and how it should be implemented. There should also be a strategy for how to evaluate the effectiveness of the solution.
Implement the Solution and Follow Up
Once a solution is chosen, a team will often assume that the work of solving problems is complete. However, the final step in the basic model is an important step to determine if the matter is resolved or if additional options are needed. After the solution has been implemented by the team, the members of the team must provide feedback and identify any potential obstacles that may have been missed in the decision-making process.
This encourages long-term solutions for the problem and helps the team to continue to move forward with their work. It also gives the team a sense of ownership and an example of how to evaluate an idea in the future.
If the solution is not working the way that it should, the team will often need to adapt the option, or they may get to the point where they scrap the option and attempt another. Solving a problem is not always a linear process, and encouraging reform and change within the process will help the team find the answer to the issues that they face.
GROW Method
Another method that is similar to the standard method is the G.R.O.W. method. This method has very similar steps to the standard method, but the catchiness of the acronym helps a team approach the problem from the same angle each time and work through the method quickly.
The first step in the method is to identify a goal, which is what the “g” stands for in “grow.” To establish a goal, the team will need to look at the issues that they are facing and identify what they would like to accomplish and solve through the problem-solving process. The team will likely participate in conversations that identify the issues that they are facing and what they need to resolve.
The next step is to establish the current reality that the group is facing. This helps them to determine where they currently are and what needs to be done to move them forward. This can help the group establish a baseline for where they started and what they would like to change.
The next step is to find any obstacles that may be blocking the group from achieving their goal. This is where the main crux of the issues that the group is facing will come out. This is also helpful in giving the group a chance to find ways around these obstacles and toward a solution.
Way Forward
After identifying the obstacles and potential ways to avoid them, the group will then need to pick the best way to move forward and approach their goal together. Here, they will need to create steps to move forward with that goal.
Divide and Conquer
Another common problem-solving method is the divide-and-conquer method. Here, instead of the entire team working through each step of the process as a large group, they split up the issue into smaller problems that can be solved and have individual members or small groups work through the smaller problems. Once each group is satisfied with the solution to the problem, they present it to the larger group to consider along with the other options.
This process can be helpful if there is a large team attempting to solve a large and complex problem. It is also beneficial because it can be used in teams with smaller, specialized teams within it because it allows each smaller group to focus on what they know best.
However, it does encourage the parties to shy away from collaboration on the overall issue, and the different solutions that each proposes may not be possible when combined and implemented.
For this reason, it is best to use this solution when approaching complex problems with large teams and the ability to combine several problem-solving methods into one.
Six Thinking Hats
The Six Thinking Hats theory is a concept designed for a team with a lot of differing conflict styles and problem-solving techniques. This method was developed to help sort through the various techniques that people may use and help a team find a solution that works for everyone involved. It helps to organize thinking and lead the conversation to the best possible solution.
Within this system, there are six different “hats” that identify with the various aspects of the decision-making process: the overall process, idea generation, intuition and emotions, values, information gathering, and caution or critical thinking. The group agrees to participate in the process by agreeing on which of the hats the group is wearing at a given moment. This helps set parameters and expectations around what the group is attempting to achieve at any moment.
This system is particularly good in a group with different conflict styles or where people have a hard time collecting and organizing their thoughts. It can be incredibly beneficial for complex problems with many moving parts. It can also help groups identify how each of the smaller sections relates to the big picture and help create new ideas to answer the overall problem.
However, it can derail if the group focuses too heavily or for too long on one of the “hats.” The group should ensure that they have a facilitator to guide them through the process and ensure that each idea and section is considered adequately.
Trial and Error
The trial and error process takes over the evaluation and selection process and instead chooses to try out each of the alternatives to determine what the best option would be. It allows the team to gather data on each of the options and how they apply practically. It also provides the ability for the team to have an example of each possible answer to help a decision-maker determine what the best option is.
Problem-solving methods that focus on trial and error can be helpful when a team has a simple problem or a lot of time to test potential solutions, gather data, and determine an answer to the issue.
It can also be helpful when the team has a sense of the best guess for a solution but wants to test it out to determine if the data supports that option, or if they have several viable options and would like to identify the best one. However, it can be incredibly time-consuming to test each of the options and evaluate how they went. Time can often be saved by evaluating each option and selecting the best to test.
Other Problem-Solving Skills
In addition to the methods outlined above, other problem-solving skills can be used regardless of the model that is used. These techniques can round out the problem-solving process and help address either specific steps in the overall method or alter the step in some way to help it fit a specific situation.
Ask Good Questions
One of the best ways to work through any of the problem-solving models is to ask good questions. This will help the group find the issue at the heart of the problem and address that issue rather than the symptoms. The best questions will also help the group find viable solutions and pick the solution that the group can use to move forward. The more creative the questions , the more likely that they will produce innovative solutions.
Take a Step Back
Occasionally, paying attention to a problem too much can give the group tunnel vision and harm the overall processes that the group is using. Other times, the focus can lead to escalations in conflict. When this happens, it can be helpful to set aside the problem and give the group time to calm down. Once they have a chance to reconsider the options and how they apply, they can approach the issue with a new sense of purpose and determination. This can lead to additional creative solutions that may help the group find a new way forward.
Final Thoughts
Problem-solving can be a daunting part of life. However, with a good problem-solving method and the right techniques, problems can be addressed well and quickly. Applying some of these options outlined in this article can give you a head start in solving your next problem and any others that arise.
To learn more about problem-solving models, problem-solving activities, and more, contact ADR Times !
Must-read Articles:

- Recent Posts
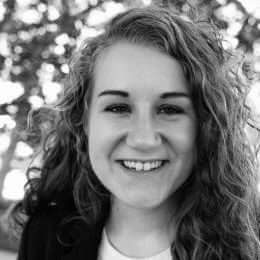
- Personal Injury Settlement Amounts Examples - August 12, 2024
- Writing an Effective Problem Statement - August 5, 2024
- 14 Home Insurance Claim Adjuster Secret Tactics - July 29, 2024
Inspiration & Information for Self-Improvement
Problem Solving Models (List & Applications)
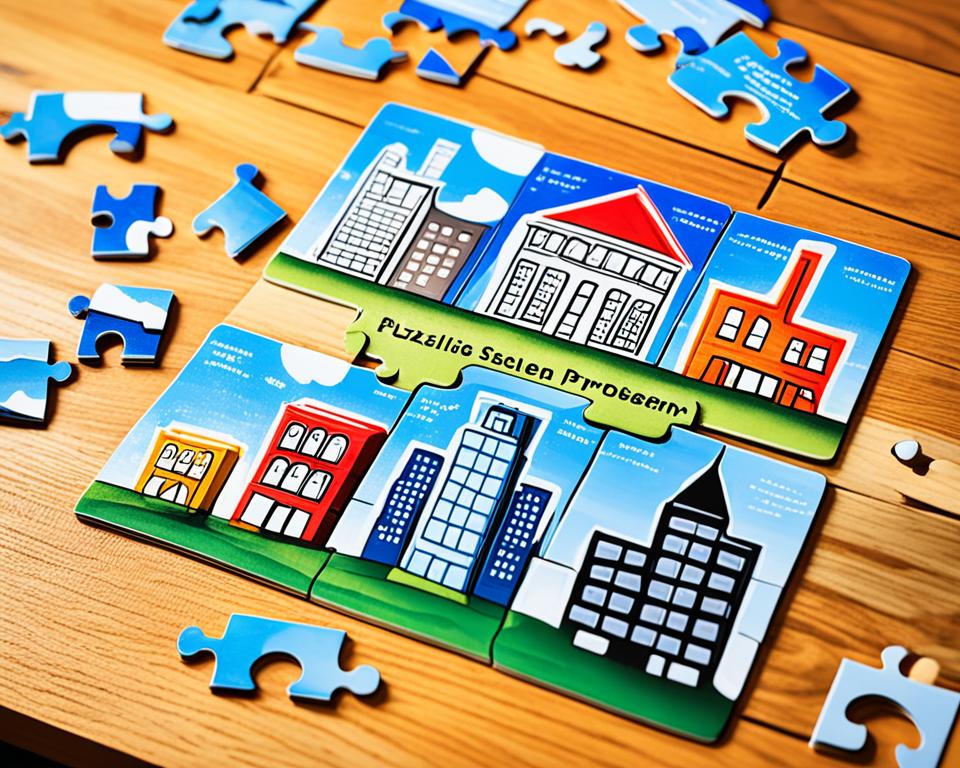
In today’s fast-paced business environment, effective problem-solving techniques are essential for teams and organizations to tackle challenges and resolve critical issues. Whether it’s identifying the root cause of a recurring problem or finding innovative solutions to complex issues, having a structured approach can greatly enhance problem-solving outcomes. This article explores different problem-solving models, techniques, and strategies that can empower individuals and teams to navigate through obstacles and find optimal solutions.
Key Takeaways:
- Problem-solving techniques are crucial for teams and organizations to overcome challenges and achieve desired outcomes.
- Clear problem definition and analysis play a vital role in the problem-solving process .
- Using problem-solving tools can help generate effective solutions and test their viability.
- Well-designed problem-solving workshops provide a structured approach and foster open discussions.
- Tips such as defining the problem, trying different approaches, and involving the right people enhance the problem-solving process .
Table of Contents
Importance of Problem Identification and Analysis
Before finding solutions , accurately identifying and defining the problem is crucial. Allowing all team members to contribute their views in an open and safe manner is essential for effective problem identification and analysis. Creating a problem statement that clearly describes the problem and sets the goal for the problem-solving process is also vital.
Problem Solving Tools for Generating Solutions
When it comes to problem-solving, having the right tools and methods can make all the difference. This section introduces a range of problem-solving tools that can help teams generate effective solutions. By leveraging these tools, teams can navigate through complex challenges and find viable options to address the problem at hand.
The problem-solving process is often iterative, requiring teams to constantly test and refine their solutions. This iterative approach allows teams to learn from their mistakes, make necessary adjustments, and roadtest viable solutions for optimal outcomes.
One effective tool for generating solutions is the use of consensus tools and methods. Consensus tools encourage teams to tap into the collective intelligence of the group, promoting collaboration, and aligning everyone towards a common goal. By involving all team members in the decision-making process, consensus tools can facilitate effective problem-solving and help teams reach agreements that everyone can support.
To illustrate the value of problem-solving tools , the following table provides a comparison of different consensus tools along with their applications.
Consensus Tool | Application |
---|---|
Dot Voting | Quickly prioritize ideas or solutions based on team consensus. |
Deliometer | Evaluate the desirability, feasibility, and viability of solutions. |
Five Whys | Dive deeper into root causes of problems to develop comprehensive solutions. |
Multi-Voting | Narrow down a list of options by allowing each team member to vote on their preferred choices. |
By leveraging problem-solving tools and embracing an iterative process , teams can enhance their problem-solving capabilities and find innovative solutions to even the most complex challenges.
Designing Effective Problem Solving Workshops
When it comes to solving complex problems, well-designed workshops can be instrumental in fostering collaborative brainstorming and effective problem-solving sessions. This section explores the key aspects of designing problem-solving workshops, including agenda design , workshop planning , facilitation skills , and creating a psychologically safe space for open and constructive discussions.
Agenda Design
An effective agenda sets the foundation for a successful problem-solving workshop. It provides a clear framework and structure to guide participants through the process, ensuring that all stages of problem exploration and solution implementation are covered. Additionally, a well-designed agenda allows for flexibility and adaptation to the specific needs of the participants and the problem at hand.
Consider including the following elements in your agenda:
- Introduction and icebreaker activities to create a positive and inclusive atmosphere
- Problem definition and analysis to ensure all participants have a common understanding of the problem
- Idea generation and exploration to encourage creativity and diverse perspectives
- Evaluation of potential solutions through critical thinking and analysis
- Action planning to define next steps and assign responsibilities
- Closing and reflection to gather feedback and insights from participants
Workshop Planning
Effective workshop planning involves careful consideration of logistical and practical aspects to ensure the smooth execution of the session. Some key factors to consider include:
- Choosing an appropriate venue that accommodates the number of participants and fosters collaboration
- Providing necessary materials, such as flip charts, sticky notes, and markers, to facilitate idea sharing and visual representation
- Ensuring availability of technical equipment, if needed, for presentations or visual aids
- Scheduling the workshop at a time when participants are most likely to be engaged and focused
- Setting realistic timeframes for each agenda item to maintain momentum and avoid unnecessary delays
Facilitation Skills
Effective facilitation is key to guiding participants through the problem-solving process and maximizing engagement and collaboration. A skilled facilitator should:
- Establish clear communication channels and encourage equal participation from all participants
- Create a safe and nonjudgmental environment that promotes open sharing of ideas and perspectives
- Listen actively to participants’ input, ensuring everyone feels heard and respected
- Facilitate consensus-building and encourage collaboration among participants
- Manage time effectively to keep the workshop on track and ensure all agenda items are addressed
Remember that the facilitator plays a crucial role in maintaining the energy and momentum throughout the workshop, ensuring that each participant feels valued and contributing to the problem-solving session .
Psychologically Safe Space
Creating a psychologically safe space is essential for participants to feel comfortable expressing their thoughts and perspectives openly. This can be achieved by:
- Establishing ground rules that encourage respectful and constructive communication
- Creating an inclusive environment that values diverse perspectives and experiences
- Emphasizing that all ideas are valuable and should be considered without judgment
- Encouraging active listening and building upon each other’s ideas
- Acknowledging and appreciating the contributions of all participants
An environment that promotes psychological safety enables participants to engage in meaningful discussions, challenge assumptions, and generate innovative solutions.
Benefits of Well-Designed Problem Solving Workshops | |
---|---|
Enhanced collaboration and teamwork | Create a conducive environment for collaboration, allowing participants to harness the collective intelligence of the group. |
Improved problem definition and analysis | Facilitate a structured approach to problem exploration, ensuring a clear understanding of the problem and its root causes. |
Increased creativity and | Encourage diverse thinking and the generation of innovative solutions through interactive workshops and activities. |
Effective decision-making | Provide a platform for evaluating potential solutions, enabling data-driven decision-making and critical thinking. |
Greater ownership and commitment | Engage participants in collaborative problem-solving, resulting in increased commitment to implementing solutions. |
Problem Solving Tips for Success
When faced with a problem, it’s essential to approach it with a clear and strategic mindset. Here are some problem-solving tips that can help you achieve successful outcomes:
- Clearly define the problem: Before diving into solutions, take the time to clearly define the problem at hand. Break it down into manageable parts and ensure everyone involved has a solid understanding of the issue.
- Avoid jumping to conclusions: It’s easy to make assumptions and jump to conclusions when faced with a problem. Instead, take a step back, gather all the relevant information, and analyze it objectively before drawing conclusions. Avoid letting biases cloud your judgment.
- Try different approaches: Don’t get stuck in a single approach. Be open to exploring different angles and perspectives. Consider brainstorming sessions or using problem-solving techniques like the Six Thinking Hats to encourage creative thinking and generate diverse solutions.
- Don’t take it personally: Problem-solving is a collaborative process that involves different viewpoints. It’s important not to take criticism or differing opinions personally. Embrace constructive feedback and use it to refine your ideas and strategies.
- Get the right people in the room: Assemble a team of individuals with diverse skills and expertise that are relevant to the problem at hand. Having the right people involved ensures a broader range of perspectives and a higher chance of finding effective solutions.
- Document everything: Keep a record of the entire problem-solving process, including discussions, decisions, and possible solutions. This documentation not only provides valuable insights but also helps track progress and enables effective communication with stakeholders.
- Bring a facilitator: Having a neutral facilitator can greatly enhance the problem-solving process. A facilitator ensures that the discussions remain focused, encourages participation from all team members, and helps maintain a productive atmosphere.
- Develop problem-solving skills: Continuously work on developing your problem-solving skills. Seek out opportunities to learn new techniques, attend workshops, or engage in professional development programs. Problem-solving is a skill that can be honed and improved over time.
- Design a great agenda: A well-planned and structured agenda sets the tone for an effective problem-solving session . It provides a roadmap and ensures that the discussion stays on track. Include time for brainstorming, idea evaluation , and decision-making to maximize productivity.
By following these problem-solving tips , you can increase your chances of finding innovative and effective solutions to complex problems.
Problem Solving Models and Strategies: Polya’s Four-Step Model
In this section, we will explore Polya’s four-step problem-solving model, a versatile approach that can be applied to various types of problems. This model provides a systematic framework for understanding, analyzing, and solving problems effectively.
Step 1: Understand the Problem
The first step in Polya’s model is to gain a clear understanding of the problem at hand. This involves thoroughly analyzing the problem statement , identifying any constraints or limitations, and determining what needs to be achieved.
Step 2: Devise a Plan
Once the problem is understood, the next step is to devise a plan or strategy to solve it. This may involve breaking down the problem into smaller, more manageable parts, identifying relevant concepts or theories, and exploring possible approaches or techniques.
Step 3: Carry Out the Plan
With a well-defined plan in place, it’s time to execute it. This step involves taking action according to the devised plan, implementing the chosen strategy, and applying problem-solving techniques to address the problem effectively.
Step 4: Look Back
After carrying out the plan, it is crucial to reflect on the solution and evaluate its effectiveness. This step involves reviewing the outcomes, assessing the strengths and weaknesses of the approach, and identifying any lessons learned for future problem-solving endeavors.
Polya’s four-step model provides a structured and iterative approach to problem solving, allowing individuals and teams to navigate complex challenges with clarity and confidence. By promoting a systematic analysis of problems and emphasizing reflection, this model enhances problem-solving skills and facilitates continuous improvement.
Let’s take a closer look at how Polya’s model can be applied to real-life scenarios and academic contexts:
Real-Life Problem | Academic Problem |
---|---|
Alexander wants to improve sales for his small business. | Sara is struggling with a complex math problem. |
1. : Analyze market trends, customer preferences, and potential obstacles. | 1. : Break down the problem, identify relevant mathematical concepts, and analyze given data. |
2. : Develop a marketing strategy, introduce new products, and enhance customer experience. | 2. : Apply appropriate formulas, develop a step-by-step approach, and consider alternative methods. |
3. : Implement the marketing strategy, measure sales performance, and gather feedback. | 3. : Solve the problem systematically, double-check calculations, and revise as necessary. |
4. : Evaluate the impact of the implemented strategy, identify areas for improvement, and adjust accordingly. | 4. : Verify the solution, reflect on problem-solving techniques used, and seek additional practice if needed. |
The application of Polya’s four-step model in these scenarios demonstrates its effectiveness in guiding problem-solving processes and facilitating successful outcomes, whether in business or educational settings.
Problem Solving Models and Strategies: IDEAL Model
In problem-solving, having a structured approach can greatly enhance effectiveness. The IDEAL model , developed by Bransford and Stein, provides a comprehensive framework for understanding and solving problems in both educational and professional settings.
Identify the Problem
The first step of the IDEAL model is to identify the problem at hand. It involves recognizing and clearly defining the core issue that needs to be addressed. By honing in on the specific problem, you can direct your efforts towards finding an appropriate solution.
Define an Outcome
Once the problem is identified, the next step is to define the desired outcome or goal. This requires envisioning the ideal state or solution that you aim to achieve. Clearly defining the outcome helps guide the problem-solving process and aligns the efforts of the team.
Explore Possible Strategies
With the problem and desired outcome in mind, the next step is to explore possible strategies or approaches to solve the problem. This involves brainstorming and considering different perspectives and ideas. By exploring a range of strategies, you increase the likelihood of finding innovative and effective solutions.
Anticipate Outcomes and Act
After evaluating the different strategies, it is essential to anticipate the potential outcomes of each option. This step involves assessing the pros, cons, risks, and benefits associated with each strategy. Once the analysis is complete, it is time to make a decision and take action.
Look and Learn
The final step of the IDEAL model is to reflect on the problem-solving process and learn from it. This step includes evaluating the effectiveness of the chosen strategy, identifying areas for improvement, and capturing lessons learned for future problem-solving endeavors. By actively engaging in this reflection, you enhance your problem-solving skills over time.
The IDEAL model offers a structured and systematic approach to problem-solving. By following these steps – identifying the problem, defining an outcome, exploring possible strategies, anticipating outcomes and acting, and reflecting on the process – individuals and teams can enhance their problem-solving capabilities and achieve better outcomes.
Advantages of the IDEAL Model | Disadvantages of the IDEAL Model |
---|---|
1. Offers a structured approach to problem-solving | 1. May require more time and resources |
2. Helps identify specific problem areas | 2. May not be suitable for all types of problems |
3. Guides decision-making processes | 3. Requires effective communication and collaboration |
4. Encourages critical thinking and analysis | 4. May limit creativity and flexibility |
Problem Solving Examples: Polya’s Four-Step Model
In this section, we will explore some examples of problem solving using Polya’s four-step model . These examples will demonstrate how the model can be applied to solve everyday problems, as well as mathematical and academic problems. By understanding the problem, devising a plan, executing the plan, and reflecting on the solution, the problem-solving process becomes more structured and effective.
Example 1: Everyday Problem
Let’s consider a common everyday problem: organizing a family gathering. The problem is to find a suitable date, venue, and activities that accommodate everyone’s preferences and availability. Using Polya’s four-step model, we can break down the problem-solving process:
- Understanding the problem: Analyze the requirements, preferences, and constraints of all family members.
- Devising a plan: Create a checklist of potential dates, venues, and activities. Consider the logistics, costs, and feasibility of each option.
- Executing the plan: Discuss the options with family members and gather their input. Negotiate and find common ground to finalize the details.
- Reflecting on the solution: Evaluate the success of the gathering based on the feedback and experience of the participants. Identify areas for improvement in future events.
Using Polya’s model helps ensure that all aspects of the problem are considered and addressed, leading to a more inclusive and successful family gathering.
Example 2: Mathematical Problem
Let’s now apply Polya’s four-step model to a mathematical problem. Consider the following example:
“Find the value of x in the equation 3x + 7 = 22.”
By following Polya’s four-step model, we can approach this problem systematically:
- Understanding the problem: Recognize that the equation represents a linear relationship and that the goal is to find the value of x that satisfies the equation.
- Devising a plan: Isolate the variable x on one side of the equation by performing the necessary arithmetic operations.
- Executing the plan: Apply the plan by subtracting 7 from both sides of the equation and then dividing by 3 to isolate x.
- Reflecting on the solution: Verify the calculated value of x by substituting it back into the original equation. Check if it satisfies the equation and provides a logical solution.
Through the application of Polya’s four-step model, we can solve mathematical problems with clarity and confidence.
Polya’s four-step model provides a systematic approach to problem-solving. The examples discussed in this section demonstrate the practical application of the model in solving everyday and mathematical problems. By understanding the problem, devising a plan, executing the plan, and reflecting on the solution, individuals and teams can enhance their problem-solving process and achieve effective outcomes.
Problem Solving Examples: IDEAL Model
This section provides practical examples of problem solving using the IDEAL model. Through these examples, you will gain a better understanding of how the IDEAL model can be applied in various contexts to analyze and solve problems effectively.
Example 1: Improving Customer Satisfaction
A customer service team in a retail company noticed a decline in customer satisfaction ratings. Following the IDEAL model, they identified the problem: low customer satisfaction. They defined the outcome they wanted to achieve: increase customer satisfaction by 15% within three months. Next, the team explored possible strategies such as enhancing training programs, implementing a customer feedback system, and improving communication channels. They anticipated the outcomes of each strategy, considering factors like resource allocation, customer response, and employee engagement. After careful evaluation, they decided to implement a comprehensive customer feedback system. The team acted upon their decision and rolled out the new system, collecting customer feedback and analyzing the data regularly. They closely monitored customer satisfaction metrics, made refinements based on feedback, and ensured timely resolution of customer issues. Throughout the process, the team reflected on the effectiveness of their strategies and made necessary adjustments. As a result, customer satisfaction improved by 20% within the target timeline, exceeding their initial goal.
Example 2: Optimizing Project Management
A project management team in a software development company faced challenges with meeting project deadlines and maintaining quality standards. Applying the IDEAL model, they took a systematic approach to address these issues. They identified the problem: missed project deadlines and compromised quality. Defining the outcome, they aimed to improve on-time project completion and deliver high-quality products. The team explored various strategies, including enhancing project planning and tracking tools, implementing agile project management methodologies, and fostering cross-functional collaboration. They anticipated the outcomes of each strategy, considering factors like stakeholder satisfaction, resource utilization, and project productivity. After careful evaluation, they decided to adopt an agile project management approach. The team took action and implemented agile practices, such as daily stand-up meetings, sprint planning, and continuous feedback loops. These practices enabled them to adapt quickly to changing project requirements and effectively manage resources. Throughout the project, the team continuously looked back, reflecting on the project’s progress and identifying areas for improvement. The iterative nature of the IDEAL model allowed them to make necessary adjustments, resulting in improved project performance and increased customer satisfaction.
Example 3: Enhancing Employee Engagement
A human resources team in a multinational corporation aimed to enhance employee engagement and improve overall organizational culture. They utilized the IDEAL model to guide their problem-solving process. They first identified the problem: low employee engagement and poor organizational culture. Defining the outcome, they aimed to increase employee engagement by 10% and foster a positive work environment. The team explored strategies such as implementing employee recognition programs, promoting work-life balance, and providing leadership development opportunities. They anticipated the outcomes of each strategy, considering factors like employee satisfaction, retention rates, and productivity. After careful evaluation, they prioritized the implementation of employee recognition programs. The team took proactive steps and launched a company-wide recognition program, acknowledging and appreciating employee contributions. They encouraged open communication and collaboration, creating a supportive and inclusive work environment. Throughout the implementation process, the team regularly evaluated the program’s effectiveness and gathered feedback from employees. They made adjustments based on the feedback received and ensured continuous improvement. As a result of their efforts, employee engagement increased by 12%, and the overall organizational culture improved, leading to enhanced productivity and employee satisfaction.
Example 4: Streamlining Supply Chain Operations
A supply chain management team in a manufacturing company faced inefficiencies in their operations, leading to delays in product delivery and increased costs. They applied the IDEAL model to overcome these challenges. They identified the problem: supply chain inefficiencies causing delays and increased costs. Defining the outcome, they aimed to streamline supply chain operations, reduce delivery lead times by 20%, and minimize costs. The team explored strategies such as implementing advanced inventory management systems, optimizing transportation routes, and enhancing supplier relationships. They anticipated the outcomes of each strategy, considering factors like cost savings, lead time reductions, and customer satisfaction. After careful evaluation, they decided to optimize transportation routes to reduce delivery lead times. The team took action and collaborated with logistics partners to streamline transportation routes, eliminate bottlenecks, and enhance delivery efficiency. They monitored performance indicators, such as on-time delivery rates and transportation costs, to measure the effectiveness of the changes. Throughout the process, the team reflected on the impact of their actions and continuously looked for opportunities to further optimize the supply chain operations. As a result, they successfully reduced delivery lead times by 25% and achieved significant cost savings.
In each of these examples, the IDEAL problem-solving model played a crucial role in guiding teams to effectively define problems, explore strategies, anticipate outcomes, take action, and reflect on the process. By utilizing the IDEAL model, teams can enhance their problem-solving capabilities and achieve desirable outcomes in diverse scenarios.
Art of Problem Solving: Slowing Down and Asking Questions
In the art of problem solving , one key element that often gets overlooked is the importance of slowing down and adopting a problem-solving mindset . In our fast-paced world, we tend to rush through challenges, seeking quick solutions without taking the time to fully understand the problem at hand.
Slowing down allows us to step back, analyze the situation, and gain a deeper understanding of the problem. By taking a moment to pause and reflect, we can identify any assumptions or biases that may be clouding our judgment. It allows us to approach the problem-solving process with a clear and open mind, ready to explore new possibilities.
One effective way to slow down and gain a fresh perspective is to ask great questions. Questions have the power to stimulate creativity, challenge preconceived notions, and generate multiple perspectives. They help us to delve deeper into the problem, uncovering hidden complexities and uncovering new insights.
“The important thing is not to stop questioning. Curiosity has its own reason for existing.” – Albert Einstein
Benefits of Slowing Down and Asking Questions
When we slow down and ask questions, we open up a world of possibilities. Here are some key benefits of adopting this approach:
- Stimulates creativity: Asking questions encourages out-of-the-box thinking, sparking new ideas and innovative solutions.
- Challenges assumptions: By questioning our assumptions, we can uncover biases and limitations that may be hindering our problem-solving process.
- Explores different perspectives: Asking questions helps us consider diverse viewpoints, leading to a more comprehensive understanding of the problem.
- Overcomes stress and limitations: Slowing down and being curious reduces stress and allows for a more mindful problem-solving process, enabling us to navigate challenges more effectively.
By adopting a problem-solving mindset that prioritizes slowing down and asking questions , we can unlock our full problem-solving potential and approach challenges with clarity and confidence.
Benefits of Slowing Down and Asking Questions |
---|
Stimulates creativity |
Challenges assumptions |
Explores different perspectives |
Overcomes stress and limitations |
Problem Solving Techniques: Brainstorming and Six Thinking Hats
In problem-solving, it’s crucial to have effective techniques that stimulate creativity, generate ideas, and evaluate potential solutions. Two widely used problem-solving techniques are brainstorming and the six thinking hats method.
Brainstorming is a collaborative idea generation process that encourages participants to think freely and generate as many ideas as possible. The goal is to create a nonjudgmental environment where all ideas are welcomed and explored. By suspending judgment and fostering an open mindset, brainstorming enables teams to uncover innovative solutions and overcome obstacles.
The six thinking hats method, developed by Edward de Bono, provides a structured approach to problem solving. This technique divides thinking into six distinct perspectives, each associated with a specific color hat. The different hats represent different modes of thinking and allow individuals to look at a problem from multiple angles. The six hats are:
- White Hat : Focusing on the available information and facts
- Red Hat : Exploring emotions, intuitions, and feelings
- Black Hat : Identifying potential risks, obstacles, and critical judgment
- Yellow Hat : Identifying benefits, opportunities, and positive aspects
- Green Hat : Stimulating creativity and generating alternative ideas
- Blue Hat : Managing the thinking process and facilitating discussions
The six thinking hats method allows teams to wear different hats at different moments, encouraging diverse perspectives and avoiding bias. This technique enhances idea generation, problem analysis , and decision-making by ensuring that all relevant aspects are considered.
Brainstorming | |
By combining brainstorming and the six thinking hats method, teams can benefit from both free-flowing idea generation and structured analysis. This dynamic approach can lead to innovative solutions that address complex problems effectively.
Next, we’ll explore the concept of “How Might We?” ( HMW ) questions, which further enhance idea generation and problem-solving.
In conclusion, problem-solving models, strategies, and techniques play a crucial role in addressing challenges and achieving desired outcomes in various fields. By utilizing effective problem-solving methods, teams and organizations can navigate complex issues, identify innovative solutions, and drive positive change.
Developing problem-solving skills is essential for individuals and teams to approach problems with clarity and confidence. Through clear problem identification , thorough analysis, and the application of problem-solving tools, teams can work collaboratively to generate viable solutions and make informed decisions.
Creating a supportive environment that encourages open communication, diverse perspectives, and an iterative approach to problem-solving is key to success. By fostering a culture that values curiosity, creativity, and continuous learning, organizations can empower their employees to tackle challenges head-on and drive meaningful progress.
In summary, problem-solving is not just a necessary skill, but also an art. By leveraging problem-solving models, techniques, and strategies, individuals and teams can confidently navigate complex issues, overcome obstacles, and achieve optimal outcomes. With a clear problem-solving process, a supportive environment, and a commitment to continuous improvement, problem-solving becomes a powerful tool for success in any context.
What is the importance of problem identification and analysis?
Problem identification and analysis are crucial in the problem-solving process as they help in accurately defining the problem, setting a clear goal, and understanding the underlying factors contributing to the problem.
What are some problem-solving tools for generating solutions?
There are various problem-solving tools available, including brainstorming, consensus tools, and iterative processes. These tools aid in generating solutions by encouraging creativity, tapping into collective intelligence, and refining ideas through testing and feedback.
How can I design effective problem-solving workshops?
To design effective problem-solving workshops, focus on agenda design , facilitation skills , and creating a psychologically safe space. It is essential to plan the workshop, provide structure, encourage open discussions, and guide the group towards solution implementation.
What are some problem-solving tips for success?
Some problem-solving tips for success include clearly defining the problem, avoiding hasty conclusions, trying different approaches, maintaining a constructive and collaborative mindset, getting the right people involved, documenting the process, and considering the role of a facilitator.
What is Polya’s four-step problem-solving model?
Polya’s four-step problem-solving model involves understanding the problem, devising a plan, executing the plan, and reflecting on the solution. It is a versatile model applicable to both academic and real-life problems.
What is the IDEAL problem-solving model?
The IDEAL problem-solving model includes identifying the problem, defining an outcome, exploring strategies, anticipating outcomes, and reflecting on the process. It is a comprehensive model for understanding and solving problems in various settings.
Can you provide examples of problem solving using Polya’s four-step model?
Sure! Examples of problem solving using Polya’s four-step model include solving everyday problems and academic/mathematical problems. These examples demonstrate the importance of understanding the problem, devising a plan, executing the plan, and reflecting on the solution.
Can you provide examples of problem solving using the IDEAL model?
Of course! Examples of problem solving using the IDEAL model cover various contexts and demonstrate the importance of identifying the problem, defining an outcome, exploring strategies, anticipating outcomes, and reflecting on the process for effective problem solving.
How does slowing down and asking questions contribute to problem solving?
Slowing down and adopting a problem-solving mindset allows for asking great questions, stimulating creativity, challenging assumptions, and generating multiple perspectives. This approach helps overcome stress and limitations, leading to better problem-solving outcomes.
What are some problem-solving techniques I can use?
Some problem-solving techniques include brainstorming and the six thinking hats method. Brainstorming fosters creativity, idea generation, and evaluation, while the six thinking hats method encourages considering different perspectives and exploring possibilities.
What are the key takeaways from this article on problem-solving?
The key takeaways include understanding the importance of problem-solving models, strategies, and techniques for tackling challenges, developing problem-solving skills, creating a supportive environment, and using a structured approach for successful outcomes.
Related Posts
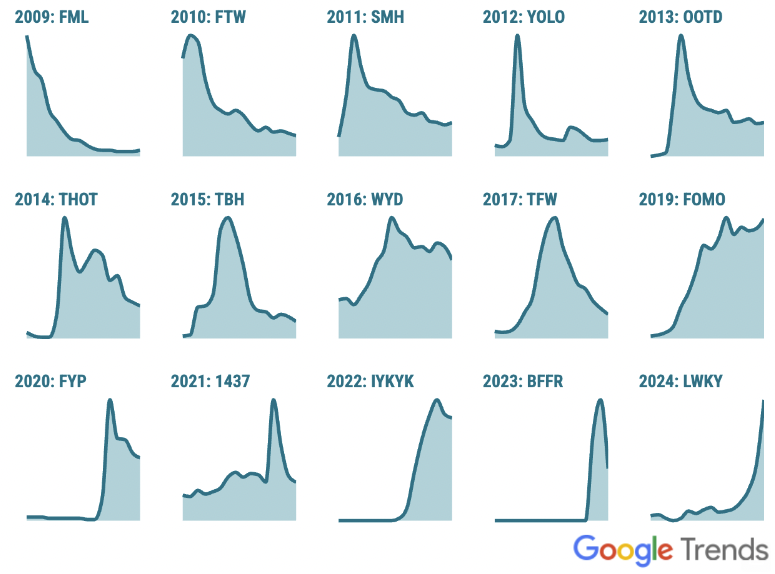
65+ Common Text Abbreviations
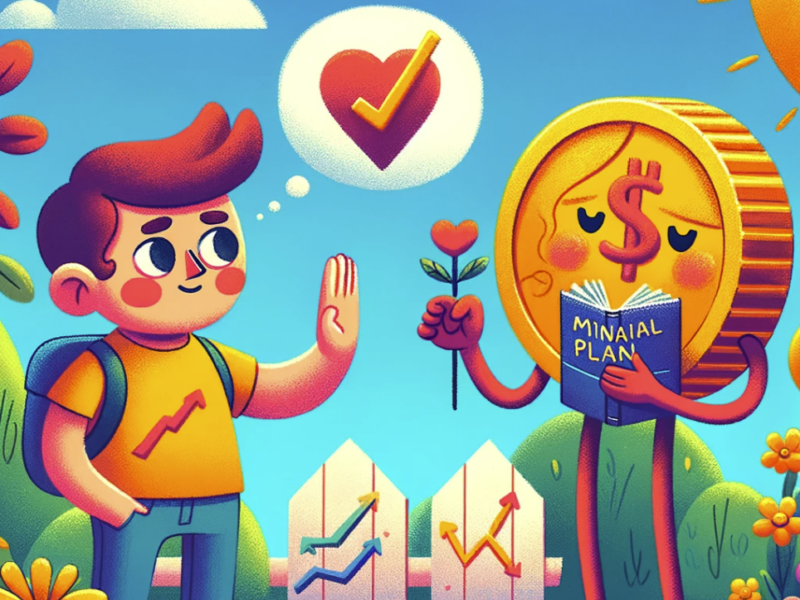
47+ Examples to Turn Down Financial Advice from Friends & Family (Polite)
40 problem-solving techniques and processes
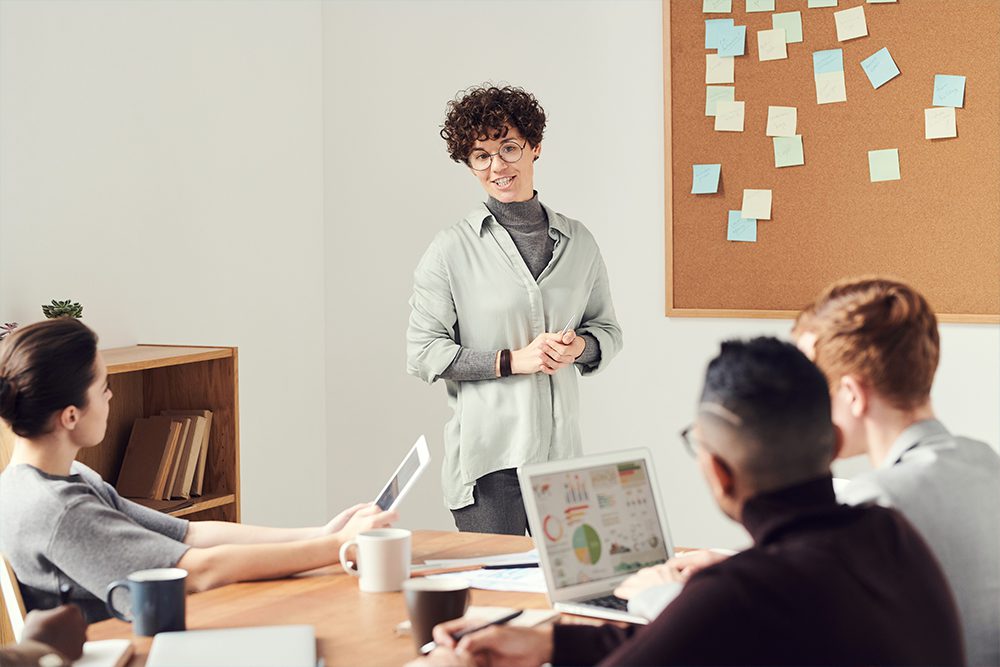
All teams and organizations encounter challenges. Approaching those challenges without a structured problem solving process can end up making things worse.
Proven problem solving techniques such as those outlined below can guide your group through a process of identifying problems and challenges , ideating on possible solutions , and then evaluating and implementing the most suitable .
In this post, you'll find problem-solving tools you can use to develop effective solutions. You'll also find some tips for facilitating the problem solving process and solving complex problems.
Design your next session with SessionLab
Join the 150,000+ facilitators using SessionLab.
Recommended Articles
A step-by-step guide to planning a workshop, 54 great online tools for workshops and meetings, how to create an unforgettable training session in 8 simple steps.
- 18 Free Facilitation Resources We Think You’ll Love
What is problem solving?
Problem solving is a process of finding and implementing a solution to a challenge or obstacle. In most contexts, this means going through a problem solving process that begins with identifying the issue, exploring its root causes, ideating and refining possible solutions before implementing and measuring the impact of that solution.
For simple or small problems, it can be tempting to skip straight to implementing what you believe is the right solution. The danger with this approach is that without exploring the true causes of the issue, it might just occur again or your chosen solution may cause other issues.
Particularly in the world of work, good problem solving means using data to back up each step of the process, bringing in new perspectives and effectively measuring the impact of your solution.
Effective problem solving can help ensure that your team or organization is well positioned to overcome challenges, be resilient to change and create innovation. In my experience, problem solving is a combination of skillset, mindset and process, and it’s especially vital for leaders to cultivate this skill.
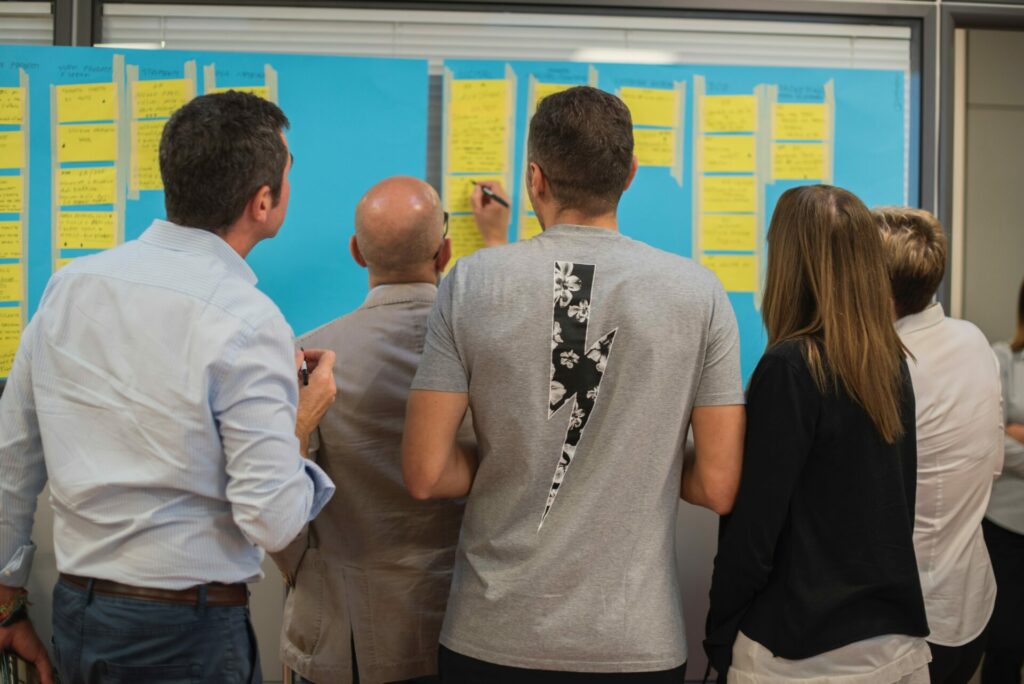
What is the seven step problem solving process?
A problem solving process is a step-by-step framework from going from discovering a problem all the way through to implementing a solution.
With practice, this framework can become intuitive, and innovative companies tend to have a consistent and ongoing ability to discover and tackle challenges when they come up.
You might see everything from a four step problem solving process through to seven steps. While all these processes cover roughly the same ground, I’ve found a seven step problem solving process is helpful for making all key steps legible.
We’ll outline that process here and then follow with techniques you can use to explore and work on that step of the problem solving process with a group.
The seven-step problem solving process is:
1. Problem identification
The first stage of any problem solving process is to identify the problem(s) you need to solve. This often looks like using group discussions and activities to help a group surface and effectively articulate the challenges they’re facing and wish to resolve.
Be sure to align with your team on the exact definition and nature of the problem you’re solving. An effective process is one where everyone is pulling in the same direction – ensure clarity and alignment now to help avoid misunderstandings later.
2. Problem analysis and refinement
The process of problem analysis means ensuring that the problem you are seeking to solve is the right problem . Choosing the right problem to solve means you are on the right path to creating the right solution.
At this stage, you may look deeper at the problem you identified to try and discover the root cause at the level of people or process. You may also spend some time sourcing data, consulting relevant parties and creating and refining a problem statement.
Problem refinement means adjusting scope or focus of the problem you will be aiming to solve based on what comes up during your analysis. As you analyze data sources, you might discover that the root cause means you need to adjust your problem statement. Alternatively, you might find that your original problem statement is too big to be meaningful approached within your current project.
Remember that the goal of any problem refinement is to help set the stage for effective solution development and deployment. Set the right focus and get buy-in from your team here and you’ll be well positioned to move forward with confidence.
3. Solution generation
Once your group has nailed down the particulars of the problem you wish to solve, you want to encourage a free flow of ideas connecting to solving that problem. This can take the form of problem solving games that encourage creative thinking or techniquess designed to produce working prototypes of possible solutions.
The key to ensuring the success of this stage of the problem solving process is to encourage quick, creative thinking and create an open space where all ideas are considered. The best solutions can often come from unlikely places and by using problem solving techniques that celebrate invention, you might come up with solution gold.
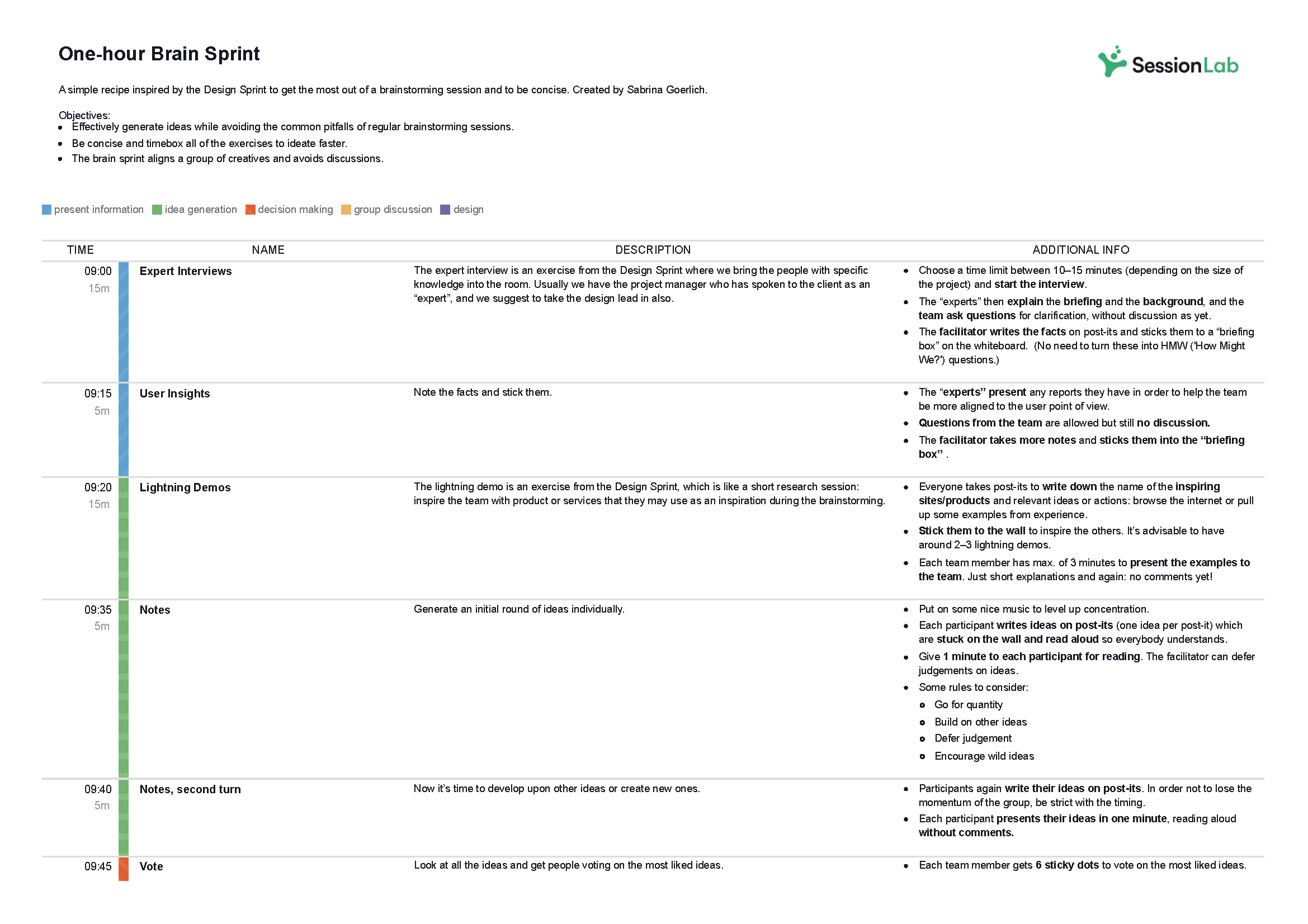
4. Solution development
No solution is perfect right out of the gate. It’s important to discuss and develop the solutions your group has come up with over the course of following the previous problem solving steps in order to arrive at the best possible solution. Problem solving games used in this stage involve lots of critical thinking, measuring potential effort and impact, and looking at possible solutions analytically.
During this stage, you will often ask your team to iterate and improve upon your front-running solutions and develop them further. Remember that problem solving strategies always benefit from a multitude of voices and opinions, and not to let ego get involved when it comes to choosing which solutions to develop and take further.
Finding the best solution is the goal of all problem solving workshops and here is the place to ensure that your solution is well thought out, sufficiently robust and fit for purpose.
5. Decision making and planning
Nearly there! Once you’ve got a set of possible, you’ll need to make a decision on which to implement. This can be a consensus-based group decision or it might be for a leader or major stakeholder to decide. You’ll find a set of effective decision making methods below.
Once your group has reached consensus and selected a solution, there are some additional actions that also need to be decided upon. You’ll want to work on allocating ownership of the project, figure out who will do what, how the success of the solution will be measured and decide the next course of action.
Set clear accountabilities, actions, timeframes, and follow-ups for your chosen solution. Make these decisions and set clear next-steps in the problem solving workshop so that everyone is aligned and you can move forward effectively as a group.
Ensuring that you plan for the roll-out of a solution is one of the most important problem solving steps. Without adequate planning or oversight, it can prove impossible to measure success or iterate further if the problem was not solved.
6. Solution implementation
This is what we were waiting for! All problem solving processes have the end goal of implementing an effective and impactful solution that your group has confidence in.
Project management and communication skills are key here – your solution may need to adjust when out in the wild or you might discover new challenges along the way. For some solutions, you might also implement a test with a small group and monitor results before rolling it out to an entire company.
You should have a clear owner for your solution who will oversee the plans you made together and help ensure they’re put into place. This person will often coordinate the implementation team and set-up processes to measure the efficacy of your solution too.
7. Solution evaluation
So you and your team developed a great solution to a problem and have a gut feeling it’s been solved. Work done, right? Wrong. All problem solving strategies benefit from evaluation, consideration, and feedback.
You might find that the solution does not work for everyone, might create new problems, or is potentially so successful that you will want to roll it out to larger teams or as part of other initiatives.
None of that is possible without taking the time to evaluate the success of the solution you developed in your problem solving model and adjust if necessary.
Remember that the problem solving process is often iterative and it can be common to not solve complex issues on the first try. Even when this is the case, you and your team will have generated learning that will be important for future problem solving workshops or in other parts of the organization.
It’s also worth underlining how important record keeping is throughout the problem solving process. If a solution didn’t work, you need to have the data and records to see why that was the case. If you go back to the drawing board, notes from the previous workshop can help save time.
What does an effective problem solving process look like?
Every effective problem solving process begins with an agenda . In our experience, a well-structured problem solving workshop is one of the best methods for successfully guiding a group from exploring a problem to implementing a solution.
The format of a workshop ensures that you can get buy-in from your group, encourage free-thinking and solution exploration before making a decision on what to implement following the session.
This Design Sprint 2.0 template is an effective problem solving process from top agency AJ&Smart. It’s a great format for the entire problem solving process, with four-days of workshops designed to surface issues, explore solutions and even test a solution.
Check it for an example of how you might structure and run a problem solving process and feel free to copy and adjust it your needs!
For a shorter process you can run in a single afternoon, this remote problem solving agenda will guide you effectively in just a couple of hours.
Whatever the length of your workshop, by using SessionLab, it’s easy to go from an idea to a complete agenda . Start by dragging and dropping your core problem solving activities into place . Add timings, breaks and necessary materials before sharing your agenda with your colleagues.
The resulting agenda will be your guide to an effective and productive problem solving session that will also help you stay organized on the day!
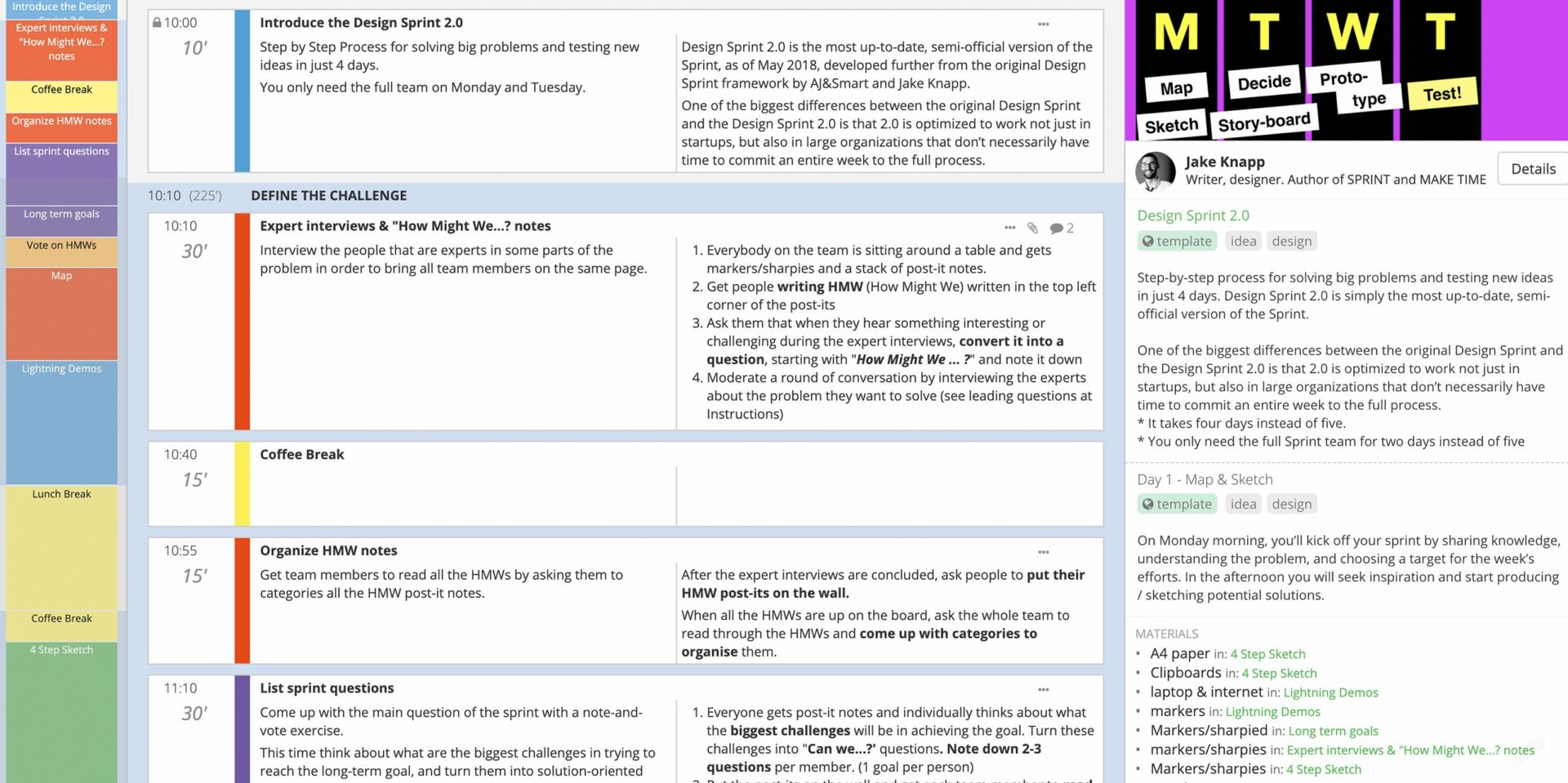
Complete problem-solving methods
In this section, we’ll look at in-depth problem-solving methods that provide a complete end-to-end process for developing effective solutions. These will help guide your team from the discovery and definition of a problem through to delivering the right solution.
If you’re looking for an all-encompassing method or problem-solving model, these processes are a great place to start. They’ll ask your team to challenge preconceived ideas and adopt a mindset for solving problems more effectively.
Six Thinking Hats
Individual approaches to solving a problem can be very different based on what team or role an individual holds. It can be easy for existing biases or perspectives to find their way into the mix, or for internal politics to direct a conversation.
Six Thinking Hats is a classic method for identifying the problems that need to be solved and enables your team to consider them from different angles, whether that is by focusing on facts and data, creative solutions, or by considering why a particular solution might not work.
Like all problem-solving frameworks, Six Thinking Hats is effective at helping teams remove roadblocks from a conversation or discussion and come to terms with all the aspects necessary to solve complex problems.
The Six Thinking Hats #creative thinking #meeting facilitation #problem solving #issue resolution #idea generation #conflict resolution The Six Thinking Hats are used by individuals and groups to separate out conflicting styles of thinking. They enable and encourage a group of people to think constructively together in exploring and implementing change, rather than using argument to fight over who is right and who is wrong.
Lightning Decision Jam
Featured courtesy of Jonathan Courtney of AJ&Smart Berlin, Lightning Decision Jam is one of those strategies that should be in every facilitation toolbox. Exploring problems and finding solutions is often creative in nature, though as with any creative process, there is the potential to lose focus and get lost.
Unstructured discussions might get you there in the end, but it’s much more effective to use a method that creates a clear process and team focus.
In Lightning Decision Jam, participants are invited to begin by writing challenges, concerns, or mistakes on post-its without discussing them before then being invited by the moderator to present them to the group.
From there, the team vote on which problems to solve and are guided through steps that will allow them to reframe those problems, create solutions and then decide what to execute on.
By deciding the problems that need to be solved as a team before moving on, this group process is great for ensuring the whole team is aligned and can take ownership over the next stages.
Lightning Decision Jam (LDJ) #action #decision making #problem solving #issue analysis #innovation #design #remote-friendly It doesn’t matter where you work and what your job role is, if you work with other people together as a team, you will always encounter the same challenges: Unclear goals and miscommunication that cause busy work and overtime Unstructured meetings that leave attendants tired, confused and without clear outcomes. Frustration builds up because internal challenges to productivity are not addressed Sudden changes in priorities lead to a loss of focus and momentum Muddled compromise takes the place of clear decision- making, leaving everybody to come up with their own interpretation. In short, a lack of structure leads to a waste of time and effort, projects that drag on for too long and frustrated, burnt out teams. AJ&Smart has worked with some of the most innovative, productive companies in the world. What sets their teams apart from others is not better tools, bigger talent or more beautiful offices. The secret sauce to becoming a more productive, more creative and happier team is simple: Replace all open discussion or brainstorming with a structured process that leads to more ideas, clearer decisions and better outcomes. When a good process provides guardrails and a clear path to follow, it becomes easier to come up with ideas, make decisions and solve problems. This is why AJ&Smart created Lightning Decision Jam (LDJ). It’s a simple and short, but powerful group exercise that can be run either in-person, in the same room, or remotely with distributed teams.
Problem Definition Process
While problems can be complex, the problem-solving methods you use to identify and solve those problems can often be simple in design.
By taking the time to truly identify and define a problem before asking the group to reframe the challenge as an opportunity, this method is a great way to enable change.
Begin by identifying a focus question and exploring the ways in which it manifests before splitting into five teams who will each consider the problem using a different method: escape, reversal, exaggeration, distortion or wishful. Teams develop a problem objective and create ideas in line with their method before then feeding them back to the group.
This method is great for enabling in-depth discussions while also creating space for finding creative solutions too!
Problem Definition #problem solving #idea generation #creativity #online #remote-friendly A problem solving technique to define a problem, challenge or opportunity and to generate ideas.
The 5 Whys
Sometimes, a group needs to go further with their strategies and analyze the root cause at the heart of organizational issues. An RCA or root cause analysis is the process of identifying what is at the heart of business problems or recurring challenges.
The 5 Whys is a simple and effective method of helping a group go find the root cause of any problem or challenge and conduct analysis that will deliver results.
By beginning with the creation of a problem statement and going through five stages to refine it, The 5 Whys provides everything you need to truly discover the cause of an issue.
The 5 Whys #hyperisland #innovation This simple and powerful method is useful for getting to the core of a problem or challenge. As the title suggests, the group defines a problems, then asks the question “why” five times, often using the resulting explanation as a starting point for creative problem solving.
World Cafe is a simple but powerful facilitation technique to help bigger groups to focus their energy and attention on solving complex problems.
World Cafe enables this approach by creating a relaxed atmosphere where participants are able to self-organize and explore topics relevant and important to them which are themed around a central problem-solving purpose. Create the right atmosphere by modeling your space after a cafe and after guiding the group through the method, let them take the lead!
Making problem-solving a part of your organization’s culture in the long term can be a difficult undertaking. More approachable formats like World Cafe can be especially effective in bringing people unfamiliar with workshops into the fold.
World Cafe #hyperisland #innovation #issue analysis World Café is a simple yet powerful method, originated by Juanita Brown, for enabling meaningful conversations driven completely by participants and the topics that are relevant and important to them. Facilitators create a cafe-style space and provide simple guidelines. Participants then self-organize and explore a set of relevant topics or questions for conversation.
Discovery & Action Dialogue (DAD)
One of the best approaches is to create a safe space for a group to share and discover practices and behaviors that can help them find their own solutions.
With DAD, you can help a group choose which problems they wish to solve and which approaches they will take to do so. It’s great at helping remove resistance to change and can help get buy-in at every level too!
This process of enabling frontline ownership is great in ensuring follow-through and is one of the methods you will want in your toolbox as a facilitator.
Discovery & Action Dialogue (DAD) #idea generation #liberating structures #action #issue analysis #remote-friendly DADs make it easy for a group or community to discover practices and behaviors that enable some individuals (without access to special resources and facing the same constraints) to find better solutions than their peers to common problems. These are called positive deviant (PD) behaviors and practices. DADs make it possible for people in the group, unit, or community to discover by themselves these PD practices. DADs also create favorable conditions for stimulating participants’ creativity in spaces where they can feel safe to invent new and more effective practices. Resistance to change evaporates as participants are unleashed to choose freely which practices they will adopt or try and which problems they will tackle. DADs make it possible to achieve frontline ownership of solutions.
Design Sprint 2.0
Want to see how a team can solve big problems and move forward with prototyping and testing solutions in a few days? The Design Sprint 2.0 template from Jake Knapp, author of Sprint, is a complete agenda for a with proven results.
Developing the right agenda can involve difficult but necessary planning. Ensuring all the correct steps are followed can also be stressful or time-consuming depending on your level of experience.
Use this complete 4-day workshop template if you are finding there is no obvious solution to your challenge and want to focus your team around a specific problem that might require a shortcut to launching a minimum viable product or waiting for the organization-wide implementation of a solution.
Open space technology
Open space technology- developed by Harrison Owen – creates a space where large groups are invited to take ownership of their problem solving and lead individual sessions. Open space technology is a great format when you have a great deal of expertise and insight in the room and want to allow for different takes and approaches on a particular theme or problem you need to be solved.
Start by bringing your participants together to align around a central theme and focus their efforts. Explain the ground rules to help guide the problem-solving process and then invite members to identify any issue connecting to the central theme that they are interested in and are prepared to take responsibility for.
Once participants have decided on their approach to the core theme, they write their issue on a piece of paper, announce it to the group, pick a session time and place, and post the paper on the wall. As the wall fills up with sessions, the group is then invited to join the sessions that interest them the most and which they can contribute to, then you’re ready to begin!
Everyone joins the problem-solving group they’ve signed up to, record the discussion and if appropriate, findings can then be shared with the rest of the group afterward.
Open Space Technology #action plan #idea generation #problem solving #issue analysis #large group #online #remote-friendly Open Space is a methodology for large groups to create their agenda discerning important topics for discussion, suitable for conferences, community gatherings and whole system facilitation
Techniques to identify and analyze problems
Using a problem-solving method to help a team identify and analyze a problem can be a quick and effective addition to any workshop or meeting.
While further actions are always necessary, you can generate momentum and alignment easily, and these activities are a great place to get started.
We’ve put together this list of techniques to help you and your team with problem identification, analysis, and discussion that sets the foundation for developing effective solutions.
Let’s take a look!
Fishbone Analysis
Organizational or team challenges are rarely simple, and it’s important to remember that one problem can be an indication of something that goes deeper and may require further consideration to be solved.
Fishbone Analysis helps groups to dig deeper and understand the origins of a problem. It’s a great example of a root cause analysis method that is simple for everyone on a team to get their head around.
Participants in this activity are asked to annotate a diagram of a fish, first adding the problem or issue to be worked on at the head of a fish before then brainstorming the root causes of the problem and adding them as bones on the fish.
Using abstractions such as a diagram of a fish can really help a team break out of their regular thinking and develop a creative approach.
Fishbone Analysis #problem solving ##root cause analysis #decision making #online facilitation A process to help identify and understand the origins of problems, issues or observations.
Problem Tree
Encouraging visual thinking can be an essential part of many strategies. By simply reframing and clarifying problems, a group can move towards developing a problem solving model that works for them.
In Problem Tree, groups are asked to first brainstorm a list of problems – these can be design problems, team problems or larger business problems – and then organize them into a hierarchy. The hierarchy could be from most important to least important or abstract to practical, though the key thing with problem solving games that involve this aspect is that your group has some way of managing and sorting all the issues that are raised.
Once you have a list of problems that need to be solved and have organized them accordingly, you’re then well-positioned for the next problem solving steps.
Problem tree #define intentions #create #design #issue analysis A problem tree is a tool to clarify the hierarchy of problems addressed by the team within a design project; it represents high level problems or related sublevel problems.
SWOT Analysis
Chances are you’ve heard of the SWOT Analysis before. This problem-solving method focuses on identifying strengths, weaknesses, opportunities, and threats is a tried and tested method for both individuals and teams.
Start by creating a desired end state or outcome and bare this in mind – any process solving model is made more effective by knowing what you are moving towards. Create a quadrant made up of the four categories of a SWOT analysis and ask participants to generate ideas based on each of those quadrants.
Once you have those ideas assembled in their quadrants, cluster them together based on their affinity with other ideas. These clusters are then used to facilitate group conversations and move things forward.
SWOT analysis #gamestorming #problem solving #action #meeting facilitation The SWOT Analysis is a long-standing technique of looking at what we have, with respect to the desired end state, as well as what we could improve on. It gives us an opportunity to gauge approaching opportunities and dangers, and assess the seriousness of the conditions that affect our future. When we understand those conditions, we can influence what comes next.
Agreement-Certainty Matrix
Not every problem-solving approach is right for every challenge, and deciding on the right method for the challenge at hand is a key part of being an effective team.
The Agreement Certainty matrix helps teams align on the nature of the challenges facing them. By sorting problems from simple to chaotic, your team can understand what methods are suitable for each problem and what they can do to ensure effective results.
If you are already using Liberating Structures techniques as part of your problem-solving strategy, the Agreement-Certainty Matrix can be an invaluable addition to your process. We’ve found it particularly if you are having issues with recurring problems in your organization and want to go deeper in understanding the root cause.
Agreement-Certainty Matrix #issue analysis #liberating structures #problem solving You can help individuals or groups avoid the frequent mistake of trying to solve a problem with methods that are not adapted to the nature of their challenge. The combination of two questions makes it possible to easily sort challenges into four categories: simple, complicated, complex , and chaotic . A problem is simple when it can be solved reliably with practices that are easy to duplicate. It is complicated when experts are required to devise a sophisticated solution that will yield the desired results predictably. A problem is complex when there are several valid ways to proceed but outcomes are not predictable in detail. Chaotic is when the context is too turbulent to identify a path forward. A loose analogy may be used to describe these differences: simple is like following a recipe, complicated like sending a rocket to the moon, complex like raising a child, and chaotic is like the game “Pin the Tail on the Donkey.” The Liberating Structures Matching Matrix in Chapter 5 can be used as the first step to clarify the nature of a challenge and avoid the mismatches between problems and solutions that are frequently at the root of chronic, recurring problems.
Organizing and charting a team’s progress can be important in ensuring its success. SQUID (Sequential Question and Insight Diagram) is a great model that allows a team to effectively switch between giving questions and answers and develop the skills they need to stay on track throughout the process.
Begin with two different colored sticky notes – one for questions and one for answers – and with your central topic (the head of the squid) on the board. Ask the group to first come up with a series of questions connected to their best guess of how to approach the topic. Ask the group to come up with answers to those questions, fix them to the board and connect them with a line. After some discussion, go back to question mode by responding to the generated answers or other points on the board.
It’s rewarding to see a diagram grow throughout the exercise, and a completed SQUID can provide a visual resource for future effort and as an example for other teams.
SQUID #gamestorming #project planning #issue analysis #problem solving When exploring an information space, it’s important for a group to know where they are at any given time. By using SQUID, a group charts out the territory as they go and can navigate accordingly. SQUID stands for Sequential Question and Insight Diagram.
To continue with our nautical theme, Speed Boat is a short and sweet activity that can help a team quickly identify what employees, clients or service users might have a problem with and analyze what might be standing in the way of achieving a solution.
Methods that allow for a group to make observations, have insights and obtain those eureka moments quickly are invaluable when trying to solve complex problems.
In Speed Boat, the approach is to first consider what anchors and challenges might be holding an organization (or boat) back. Bonus points if you are able to identify any sharks in the water and develop ideas that can also deal with competitors!
Speed Boat #gamestorming #problem solving #action Speedboat is a short and sweet way to identify what your employees or clients don’t like about your product/service or what’s standing in the way of a desired goal.
The Journalistic Six
Some of the most effective ways of solving problems is by encouraging teams to be more inclusive and diverse in their thinking.
Based on the six key questions journalism students are taught to answer in articles and news stories, The Journalistic Six helps create teams to see the whole picture. By using who, what, when, where, why, and how to facilitate the conversation and encourage creative thinking, your team can make sure that the problem identification and problem analysis stages of the are covered exhaustively and thoughtfully. Reporter’s notebook and dictaphone optional.
The Journalistic Six – Who What When Where Why How #idea generation #issue analysis #problem solving #online #creative thinking #remote-friendly A questioning method for generating, explaining, investigating ideas.
Individual and group perspectives are incredibly important, but what happens if people are set in their minds and need a change of perspective in order to approach a problem more effectively?
Flip It is a method we love because it is both simple to understand and run, and allows groups to understand how their perspectives and biases are formed.
Participants in Flip It are first invited to consider concerns, issues, or problems from a perspective of fear and write them on a flip chart. Then, the group is asked to consider those same issues from a perspective of hope and flip their understanding.
No problem and solution is free from existing bias and by changing perspectives with Flip It, you can then develop a problem solving model quickly and effectively.
Flip It! #gamestorming #problem solving #action Often, a change in a problem or situation comes simply from a change in our perspectives. Flip It! is a quick game designed to show players that perspectives are made, not born.
LEGO Challenge
Now for an activity that is a little out of the (toy) box. LEGO Serious Play is a facilitation methodology that can be used to improve creative thinking and problem-solving skills.
The LEGO Challenge includes giving each member of the team an assignment that is hidden from the rest of the group while they create a structure without speaking.
What the LEGO challenge brings to the table is a fun working example of working with stakeholders who might not be on the same page to solve problems. Also, it’s LEGO! Who doesn’t love LEGO!
LEGO Challenge #hyperisland #team A team-building activity in which groups must work together to build a structure out of LEGO, but each individual has a secret “assignment” which makes the collaborative process more challenging. It emphasizes group communication, leadership dynamics, conflict, cooperation, patience and problem solving strategy.
What, So What, Now What?
If not carefully managed, the problem identification and problem analysis stages of the problem-solving process can actually create more problems and misunderstandings.
The What, So What, Now What? problem-solving activity is designed to help collect insights and move forward while also eliminating the possibility of disagreement when it comes to identifying, clarifying, and analyzing organizational or work problems.
Facilitation is all about bringing groups together so that might work on a shared goal and the best problem-solving strategies ensure that teams are aligned in purpose, if not initially in opinion or insight.
Throughout the three steps of this game, you give everyone on a team to reflect on a problem by asking what happened, why it is important, and what actions should then be taken.
This can be a great activity for bringing our individual perceptions about a problem or challenge and contextualizing it in a larger group setting. This is one of the most important problem-solving skills you can bring to your organization.
W³ – What, So What, Now What? #issue analysis #innovation #liberating structures You can help groups reflect on a shared experience in a way that builds understanding and spurs coordinated action while avoiding unproductive conflict. It is possible for every voice to be heard while simultaneously sifting for insights and shaping new direction. Progressing in stages makes this practical—from collecting facts about What Happened to making sense of these facts with So What and finally to what actions logically follow with Now What . The shared progression eliminates most of the misunderstandings that otherwise fuel disagreements about what to do. Voila!
Journalists
Problem analysis can be one of the most important and decisive stages of all problem-solving tools. Sometimes, a team can become bogged down in the details and are unable to move forward.
Journalists is an activity that can avoid a group from getting stuck in the problem identification or problem analysis stages of the process.
In Journalists, the group is invited to draft the front page of a fictional newspaper and figure out what stories deserve to be on the cover and what headlines those stories will have. By reframing how your problems and challenges are approached, you can help a team move productively through the process and be better prepared for the steps to follow.
Journalists #vision #big picture #issue analysis #remote-friendly This is an exercise to use when the group gets stuck in details and struggles to see the big picture. Also good for defining a vision.
Problem-solving techniques for brainstorming solutions
Now you have the context and background of the problem you are trying to solving, now comes the time to start ideating and thinking about how you’ll solve the issue.
Here, you’ll want to encourage creative, free thinking and speed. Get as many ideas out as possible and explore different perspectives so you have the raw material for the next step.
Looking at a problem from a new angle can be one of the most effective ways of creating an effective solution. TRIZ is a problem-solving tool that asks the group to consider what they must not do in order to solve a challenge.
By reversing the discussion, new topics and taboo subjects often emerge, allowing the group to think more deeply and create ideas that confront the status quo in a safe and meaningful way. If you’re working on a problem that you’ve tried to solve before, TRIZ is a great problem-solving method to help your team get unblocked.
Making Space with TRIZ #issue analysis #liberating structures #issue resolution You can clear space for innovation by helping a group let go of what it knows (but rarely admits) limits its success and by inviting creative destruction. TRIZ makes it possible to challenge sacred cows safely and encourages heretical thinking. The question “What must we stop doing to make progress on our deepest purpose?” induces seriously fun yet very courageous conversations. Since laughter often erupts, issues that are otherwise taboo get a chance to be aired and confronted. With creative destruction come opportunities for renewal as local action and innovation rush in to fill the vacuum. Whoosh!
Mindspin
Brainstorming is part of the bread and butter of the problem-solving process and all problem-solving strategies benefit from getting ideas out and challenging a team to generate solutions quickly.
With Mindspin, participants are encouraged not only to generate ideas but to do so under time constraints and by slamming down cards and passing them on. By doing multiple rounds, your team can begin with a free generation of possible solutions before moving on to developing those solutions and encouraging further ideation.
This is one of our favorite problem-solving activities and can be great for keeping the energy up throughout the workshop. Remember the importance of helping people become engaged in the process – energizing problem-solving techniques like Mindspin can help ensure your team stays engaged and happy, even when the problems they’re coming together to solve are complex.
MindSpin #teampedia #idea generation #problem solving #action A fast and loud method to enhance brainstorming within a team. Since this activity has more than round ideas that are repetitive can be ruled out leaving more creative and innovative answers to the challenge.
The Creativity Dice
One of the most useful problem solving skills you can teach your team is of approaching challenges with creativity, flexibility, and openness. Games like The Creativity Dice allow teams to overcome the potential hurdle of too much linear thinking and approach the process with a sense of fun and speed.
In The Creativity Dice, participants are organized around a topic and roll a dice to determine what they will work on for a period of 3 minutes at a time. They might roll a 3 and work on investigating factual information on the chosen topic. They might roll a 1 and work on identifying the specific goals, standards, or criteria for the session.
Encouraging rapid work and iteration while asking participants to be flexible are great skills to cultivate. Having a stage for idea incubation in this game is also important. Moments of pause can help ensure the ideas that are put forward are the most suitable.
The Creativity Dice #creativity #problem solving #thiagi #issue analysis Too much linear thinking is hazardous to creative problem solving. To be creative, you should approach the problem (or the opportunity) from different points of view. You should leave a thought hanging in mid-air and move to another. This skipping around prevents premature closure and lets your brain incubate one line of thought while you consciously pursue another.
Idea and Concept Development
Brainstorming without structure can quickly become chaotic or frustrating. In a problem-solving context, having an ideation framework to follow can help ensure your team is both creative and disciplined.
In this method, you’ll find an idea generation process that encourages your group to brainstorm effectively before developing their ideas and begin clustering them together. By using concepts such as Yes and…, more is more and postponing judgement, you can create the ideal conditions for brainstorming with ease.
Idea & Concept Development #hyperisland #innovation #idea generation Ideation and Concept Development is a process for groups to work creatively and collaboratively to generate creative ideas. It’s a general approach that can be adapted and customized to suit many different scenarios. It includes basic principles for idea generation and several steps for groups to work with. It also includes steps for idea selection and development.
Problem-solving techniques for developing and refining solutions
The success of any problem-solving process can be measured by the solutions it produces. After you’ve defined the issue, explored existing ideas, and ideated, it’s time to develop and refine your ideas in order to bring them closer to a solution that actually solves the problem.
Use these problem-solving techniques when you want to help your team think through their ideas and refine them as part of your problem solving process.
Improved Solutions
After a team has successfully identified a problem and come up with a few solutions, it can be tempting to call the work of the problem-solving process complete. That said, the first solution is not necessarily the best, and by including a further review and reflection activity into your problem-solving model, you can ensure your group reaches the best possible result.
One of a number of problem-solving games from Thiagi Group, Improved Solutions helps you go the extra mile and develop suggested solutions with close consideration and peer review. By supporting the discussion of several problems at once and by shifting team roles throughout, this problem-solving technique is a dynamic way of finding the best solution.
Improved Solutions #creativity #thiagi #problem solving #action #team You can improve any solution by objectively reviewing its strengths and weaknesses and making suitable adjustments. In this creativity framegame, you improve the solutions to several problems. To maintain objective detachment, you deal with a different problem during each of six rounds and assume different roles (problem owner, consultant, basher, booster, enhancer, and evaluator) during each round. At the conclusion of the activity, each player ends up with two solutions to her problem.
Four Step Sketch
Creative thinking and visual ideation does not need to be confined to the opening stages of your problem-solving strategies. Exercises that include sketching and prototyping on paper can be effective at the solution finding and development stage of the process, and can be great for keeping a team engaged.
By going from simple notes to a crazy 8s round that involves rapidly sketching 8 variations on their ideas before then producing a final solution sketch, the group is able to iterate quickly and visually. Problem-solving techniques like Four-Step Sketch are great if you have a group of different thinkers and want to change things up from a more textual or discussion-based approach.
Four-Step Sketch #design sprint #innovation #idea generation #remote-friendly The four-step sketch is an exercise that helps people to create well-formed concepts through a structured process that includes: Review key information Start design work on paper, Consider multiple variations , Create a detailed solution . This exercise is preceded by a set of other activities allowing the group to clarify the challenge they want to solve. See how the Four Step Sketch exercise fits into a Design Sprint
Ensuring that everyone in a group is able to contribute to a discussion is vital during any problem solving process. Not only does this ensure all bases are covered, but its then easier to get buy-in and accountability when people have been able to contribute to the process.
1-2-4-All is a tried and tested facilitation technique where participants are asked to first brainstorm on a topic on their own. Next, they discuss and share ideas in a pair before moving into a small group. Those groups are then asked to present the best idea from their discussion to the rest of the team.
This method can be used in many different contexts effectively, though I find it particularly shines in the idea development stage of the process. Giving each participant time to concretize their ideas and develop them in progressively larger groups can create a great space for both innovation and psychological safety.
1-2-4-All #idea generation #liberating structures #issue analysis With this facilitation technique you can immediately include everyone regardless of how large the group is. You can generate better ideas and more of them faster than ever before. You can tap the know-how and imagination that is distributed widely in places not known in advance. Open, generative conversation unfolds. Ideas and solutions are sifted in rapid fashion. Most importantly, participants own the ideas, so follow-up and implementation is simplified. No buy-in strategies needed! Simple and elegant!
15% Solutions
Some problems are simpler than others and with the right problem-solving activities, you can empower people to take immediate actions that can help create organizational change.
Part of the liberating structures toolkit, 15% solutions is a problem-solving technique that focuses on finding and implementing solutions quickly. A process of iterating and making small changes quickly can help generate momentum and an appetite for solving complex problems.
Problem-solving strategies can live and die on whether people are onboard. Getting some quick wins is a great way of getting people behind the process.
It can be extremely empowering for a team to realize that problem-solving techniques can be deployed quickly and easily and delineate between things they can positively impact and those things they cannot change.
15% Solutions #action #liberating structures #remote-friendly You can reveal the actions, however small, that everyone can do immediately. At a minimum, these will create momentum, and that may make a BIG difference. 15% Solutions show that there is no reason to wait around, feel powerless, or fearful. They help people pick it up a level. They get individuals and the group to focus on what is within their discretion instead of what they cannot change. With a very simple question, you can flip the conversation to what can be done and find solutions to big problems that are often distributed widely in places not known in advance. Shifting a few grains of sand may trigger a landslide and change the whole landscape.
Problem-solving techniques for making decisions and planning
After your group is happy with the possible solutions you’ve developed, now comes the time to choose which to implement. There’s more than one way to make a decision and the best option is often dependant on the needs and set-up of your group.
Sometimes, it’s the case that you’ll want to vote as a group on what is likely to be the most impactful solution. Other times, it might be down to a decision maker or major stakeholder to make the final decision. Whatever your process, here’s some techniques you can use to help you make a decision during your problem solving process.
How-Now-Wow Matrix
The problem-solving process is often creative, as complex problems usually require a change of thinking and creative response in order to find the best solutions. While it’s common for the first stages to encourage creative thinking, groups can often gravitate to familiar solutions when it comes to the end of the process.
When selecting solutions, you don’t want to lose your creative energy! The How-Now-Wow Matrix from Gamestorming is a great problem-solving activity that enables a group to stay creative and think out of the box when it comes to selecting the right solution for a given problem.
Problem-solving techniques that encourage creative thinking and the ideation and selection of new solutions can be the most effective in organisational change. Give the How-Now-Wow Matrix a go, and not just for how pleasant it is to say out loud.
How-Now-Wow Matrix #gamestorming #idea generation #remote-friendly When people want to develop new ideas, they most often think out of the box in the brainstorming or divergent phase. However, when it comes to convergence, people often end up picking ideas that are most familiar to them. This is called a ‘creative paradox’ or a ‘creadox’. The How-Now-Wow matrix is an idea selection tool that breaks the creadox by forcing people to weigh each idea on 2 parameters.
Impact and Effort Matrix
All problem-solving techniques hope to not only find solutions to a given problem or challenge but to find the best solution. When it comes to finding a solution, groups are invited to put on their decision-making hats and really think about how a proposed idea would work in practice.
The Impact and Effort Matrix is one of the problem-solving techniques that fall into this camp, empowering participants to first generate ideas and then categorize them into a 2×2 matrix based on impact and effort.
Activities that invite critical thinking while remaining simple are invaluable. Use the Impact and Effort Matrix to move from ideation and towards evaluating potential solutions before then committing to them.
Impact and Effort Matrix #gamestorming #decision making #action #remote-friendly In this decision-making exercise, possible actions are mapped based on two factors: effort required to implement and potential impact. Categorizing ideas along these lines is a useful technique in decision making, as it obliges contributors to balance and evaluate suggested actions before committing to them.
If you’ve followed each of the problem-solving steps with your group successfully, you should move towards the end of your process with heaps of possible solutions developed with a specific problem in mind. But how do you help a group go from ideation to putting a solution into action?
Dotmocracy – or Dot Voting -is a tried and tested method of helping a team in the problem-solving process make decisions and put actions in place with a degree of oversight and consensus.
One of the problem-solving techniques that should be in every facilitator’s toolbox, Dot Voting is fast and effective and can help identify the most popular and best solutions and help bring a group to a decision effectively.
Dotmocracy #action #decision making #group prioritization #hyperisland #remote-friendly Dotmocracy is a simple method for group prioritization or decision-making. It is not an activity on its own, but a method to use in processes where prioritization or decision-making is the aim. The method supports a group to quickly see which options are most popular or relevant. The options or ideas are written on post-its and stuck up on a wall for the whole group to see. Each person votes for the options they think are the strongest, and that information is used to inform a decision.
Straddling the gap between decision making and planning, MoSCoW is a simple and effective method that allows a group team to easily prioritize a set of possible options.
Use this method in a problem solving process by collecting and summarizing all your possible solutions and then categorize them into 4 sections: “Must have”, “Should have”, “Could have”, or “Would like but won‘t get”.
This method is particularly useful when its less about choosing one possible solution and more about prioritorizing which to do first and which may not fit in the scope of your project. In my experience, complex challenges often require multiple small fixes, and this method can be a great way to move from a pile of things you’d all like to do to a structured plan.
MoSCoW #define intentions #create #design #action #remote-friendly MoSCoW is a method that allows the team to prioritize the different features that they will work on. Features are then categorized into “Must have”, “Should have”, “Could have”, or “Would like but won‘t get”. To be used at the beginning of a timeslot (for example during Sprint planning) and when planning is needed.
When it comes to managing the rollout of a solution, clarity and accountability are key factors in ensuring the success of the project. The RAACI chart is a simple but effective model for setting roles and responsibilities as part of a planning session.
Start by listing each person involved in the project and put them into the following groups in order to make it clear who is responsible for what during the rollout of your solution.
- Responsibility (Which person and/or team will be taking action?)
- Authority (At what “point” must the responsible person check in before going further?)
- Accountability (Who must the responsible person check in with?)
- Consultation (Who must be consulted by the responsible person before decisions are made?)
- Information (Who must be informed of decisions, once made?)
Ensure this information is easily accessible and use it to inform who does what and who is looped into discussions and kept up to date.
RAACI #roles and responsibility #teamwork #project management Clarifying roles and responsibilities, levels of autonomy/latitude in decision making, and levels of engagement among diverse stakeholders.
Problem-solving warm-up activities
All facilitators know that warm-ups and icebreakers are useful for any workshop or group process. Problem-solving workshops are no different.
Use these problem-solving techniques to warm up a group and prepare them for the rest of the process. Activating your group by tapping into some of the top problem-solving skills can be one of the best ways to see great outcomes from your session.
Check-in / Check-out
Solid processes are planned from beginning to end, and the best facilitators know that setting the tone and establishing a safe, open environment can be integral to a successful problem-solving process. Check-in / Check-out is a great way to begin and/or bookend a problem-solving workshop. Checking in to a session emphasizes that everyone will be seen, heard, and expected to contribute.
If you are running a series of meetings, setting a consistent pattern of checking in and checking out can really help your team get into a groove. We recommend this opening-closing activity for small to medium-sized groups though it can work with large groups if they’re disciplined!
Check-in / Check-out #team #opening #closing #hyperisland #remote-friendly Either checking-in or checking-out is a simple way for a team to open or close a process, symbolically and in a collaborative way. Checking-in/out invites each member in a group to be present, seen and heard, and to express a reflection or a feeling. Checking-in emphasizes presence, focus and group commitment; checking-out emphasizes reflection and symbolic closure.
Doodling Together
Thinking creatively and not being afraid to make suggestions are important problem-solving skills for any group or team, and warming up by encouraging these behaviors is a great way to start.
Doodling Together is one of our favorite creative ice breaker games – it’s quick, effective, and fun and can make all following problem-solving steps easier by encouraging a group to collaborate visually. By passing cards and adding additional items as they go, the workshop group gets into a groove of co-creation and idea development that is crucial to finding solutions to problems.
Doodling Together #collaboration #creativity #teamwork #fun #team #visual methods #energiser #icebreaker #remote-friendly Create wild, weird and often funny postcards together & establish a group’s creative confidence.
Show and Tell
You might remember some version of Show and Tell from being a kid in school and it’s a great problem-solving activity to kick off a session.
Asking participants to prepare a little something before a workshop by bringing an object for show and tell can help them warm up before the session has even begun! Games that include a physical object can also help encourage early engagement before moving onto more big-picture thinking.
By asking your participants to tell stories about why they chose to bring a particular item to the group, you can help teams see things from new perspectives and see both differences and similarities in the way they approach a topic. Great groundwork for approaching a problem-solving process as a team!
Show and Tell #gamestorming #action #opening #meeting facilitation Show and Tell taps into the power of metaphors to reveal players’ underlying assumptions and associations around a topic The aim of the game is to get a deeper understanding of stakeholders’ perspectives on anything—a new project, an organizational restructuring, a shift in the company’s vision or team dynamic.
Constellations
Who doesn’t love stars? Constellations is a great warm-up activity for any workshop as it gets people up off their feet, energized, and ready to engage in new ways with established topics. It’s also great for showing existing beliefs, biases, and patterns that can come into play as part of your session.
Using warm-up games that help build trust and connection while also allowing for non-verbal responses can be great for easing people into the problem-solving process and encouraging engagement from everyone in the group. Constellations is great in large spaces that allow for movement and is definitely a practical exercise to allow the group to see patterns that are otherwise invisible.
Constellations #trust #connection #opening #coaching #patterns #system Individuals express their response to a statement or idea by standing closer or further from a central object. Used with teams to reveal system, hidden patterns, perspectives.
Draw a Tree
Problem-solving games that help raise group awareness through a central, unifying metaphor can be effective ways to warm-up a group in any problem-solving model.
Draw a Tree is a simple warm-up activity you can use in any group and which can provide a quick jolt of energy. Start by asking your participants to draw a tree in just 45 seconds – they can choose whether it will be abstract or realistic.
Once the timer is up, ask the group how many people included the roots of the tree and use this as a means to discuss how we can ignore important parts of any system simply because they are not visible.
All problem-solving strategies are made more effective by thinking of problems critically and by exposing things that may not normally come to light. Warm-up games like Draw a Tree are great in that they quickly demonstrate some key problem-solving skills in an accessible and effective way.
Draw a Tree #thiagi #opening #perspectives #remote-friendly With this game you can raise awarness about being more mindful, and aware of the environment we live in.
Closing activities for a problem-solving process
Each step of the problem-solving workshop benefits from an intelligent deployment of activities, games, and techniques. Bringing your session to an effective close helps ensure that solutions are followed through on and that you also celebrate what has been achieved.
Here are some problem-solving activities you can use to effectively close a workshop or meeting and ensure the great work you’ve done can continue afterward.
One Breath Feedback
Maintaining attention and focus during the closing stages of a problem-solving workshop can be tricky and so being concise when giving feedback can be important. It’s easy to incur “death by feedback” should some team members go on for too long sharing their perspectives in a quick feedback round.
One Breath Feedback is a great closing activity for workshops. You give everyone an opportunity to provide feedback on what they’ve done but only in the space of a single breath. This keeps feedback short and to the point and means that everyone is encouraged to provide the most important piece of feedback to them.
One breath feedback #closing #feedback #action This is a feedback round in just one breath that excels in maintaining attention: each participants is able to speak during just one breath … for most people that’s around 20 to 25 seconds … unless of course you’ve been a deep sea diver in which case you’ll be able to do it for longer.
Who What When Matrix
Matrices feature as part of many effective problem-solving strategies and with good reason. They are easily recognizable, simple to use, and generate results.
The Who What When Matrix is a great tool to use when closing your problem-solving session by attributing a who, what and when to the actions and solutions you have decided upon. The resulting matrix is a simple, easy-to-follow way of ensuring your team can move forward.
Great solutions can’t be enacted without action and ownership. Your problem-solving process should include a stage for allocating tasks to individuals or teams and creating a realistic timeframe for those solutions to be implemented or checked out. Use this method to keep the solution implementation process clear and simple for all involved.
Who/What/When Matrix #gamestorming #action #project planning With Who/What/When matrix, you can connect people with clear actions they have defined and have committed to.
Response cards
Group discussion can comprise the bulk of most problem-solving activities and by the end of the process, you might find that your team is talked out!
Providing a means for your team to give feedback with short written notes can ensure everyone is head and can contribute without the need to stand up and talk. Depending on the needs of the group, giving an alternative can help ensure everyone can contribute to your problem-solving model in the way that makes the most sense for them.
Response Cards is a great way to close a workshop if you are looking for a gentle warm-down and want to get some swift discussion around some of the feedback that is raised.
Response Cards #debriefing #closing #structured sharing #questions and answers #thiagi #action It can be hard to involve everyone during a closing of a session. Some might stay in the background or get unheard because of louder participants. However, with the use of Response Cards, everyone will be involved in providing feedback or clarify questions at the end of a session.
Tips for effective problem solving
Problem-solving activities are only one part of the puzzle. While a great method can help unlock your team’s ability to solve problems, without a thoughtful approach and strong facilitation the solutions may not be fit for purpose.
Let’s take a look at some problem-solving tips you can apply to any process to help it be a success!
Clearly define the problem
Jumping straight to solutions can be tempting, though without first clearly articulating a problem, the solution might not be the right one. Many of the problem-solving activities below include sections where the problem is explored and clearly defined before moving on.
This is a vital part of the problem-solving process and taking the time to fully define an issue can save time and effort later. A clear definition helps identify irrelevant information and it also ensures that your team sets off on the right track.
Don’t jump to conclusions
It’s easy for groups to exhibit cognitive bias or have preconceived ideas about both problems and potential solutions. Be sure to back up any problem statements or potential solutions with facts, research, and adequate forethought.
The best techniques ask participants to be methodical and challenge preconceived notions. Make sure you give the group enough time and space to collect relevant information and consider the problem in a new way. By approaching the process with a clear, rational mindset, you’ll often find that better solutions are more forthcoming.
Try different approaches
Problems come in all shapes and sizes and so too should the methods you use to solve them. If you find that one approach isn’t yielding results and your team isn’t finding different solutions, try mixing it up. You’ll be surprised at how using a new creative activity can unblock your team and generate great solutions.
Don’t take it personally
Depending on the nature of your team or organizational problems, it’s easy for conversations to get heated. While it’s good for participants to be engaged in the discussions, ensure that emotions don’t run too high and that blame isn’t thrown around while finding solutions.
You’re all in it together, and even if your team or area is seeing problems, that isn’t necessarily a disparagement of you personally. Using facilitation skills to manage group dynamics is one effective method of helping conversations be more constructive.
Get the right people in the room
Your problem-solving method is often only as effective as the group using it. Getting the right people on the job and managing the number of people present is important too!
If the group is too small, you may not get enough different perspectives to effectively solve a problem. If the group is too large, you can go round and round during the ideation stages.
Creating the right group makeup is also important in ensuring you have the necessary expertise and skillset to both identify and follow up on potential solutions. Carefully consider who to include at each stage to help ensure your problem-solving method is followed and positioned for success.
Create psychologically safe spaces for discussion
Identifying a problem accurately also requires that all members of a group are able to contribute their views in an open and safe manner.
It can be tough for people to stand up and contribute if the problems or challenges are emotive or personal in nature. Try and create a psychologically safe space for these kinds of discussions and where possible, create regular opportunities for challenges to be brought up organically.
Document everything
The best solutions can take refinement, iteration, and reflection to come out. Get into a habit of documenting your process in order to keep all the learnings from the session and to allow ideas to mature and develop. Many of the methods below involve the creation of documents or shared resources. Be sure to keep and share these so everyone can benefit from the work done!
Bring a facilitator
Facilitation is all about making group processes easier. With a subject as potentially emotive and important as problem-solving, having an impartial third party in the form of a facilitator can make all the difference in finding great solutions and keeping the process moving. Consider bringing a facilitator to your problem-solving session to get better results and generate meaningful solutions!
Develop your problem-solving skills
It takes time and practice to be an effective problem solver. While some roles or participants might more naturally gravitate towards problem-solving, it can take development and planning to help everyone create better solutions.
You might develop a training program, run a problem-solving workshop or simply ask your team to practice using the techniques below. Check out our post on problem-solving skills to see how you and your group can develop the right mental process and be more resilient to issues too!
Design a great agenda
Workshops are a great format for solving problems. With the right approach, you can focus a group and help them find the solutions to their own problems. But designing a process can be time-consuming and finding the right activities can be difficult.
Check out our workshop planning guide to level-up your agenda design and start running more effective workshops. Need inspiration? Check out templates designed by expert facilitators to help you kickstart your process!
Save time and effort creating an effective problem solving process
A structured problem solving process is a surefire way of solving tough problems, discovering creative solutions and driving organizational change. But how can you design for successful outcomes?
With SessionLab, it’s easy to design engaging workshops that deliver results. Drag, drop and reorder blocks to build your agenda. When you make changes or update your agenda, your session timing adjusts automatically , saving you time on manual adjustments.
Collaborating with stakeholders or clients? Share your agenda with a single click and collaborate in real-time. No more sending documents back and forth over email.
Explore how to use SessionLab to design effective problem solving workshops or watch this five minute video to see the planner in action!
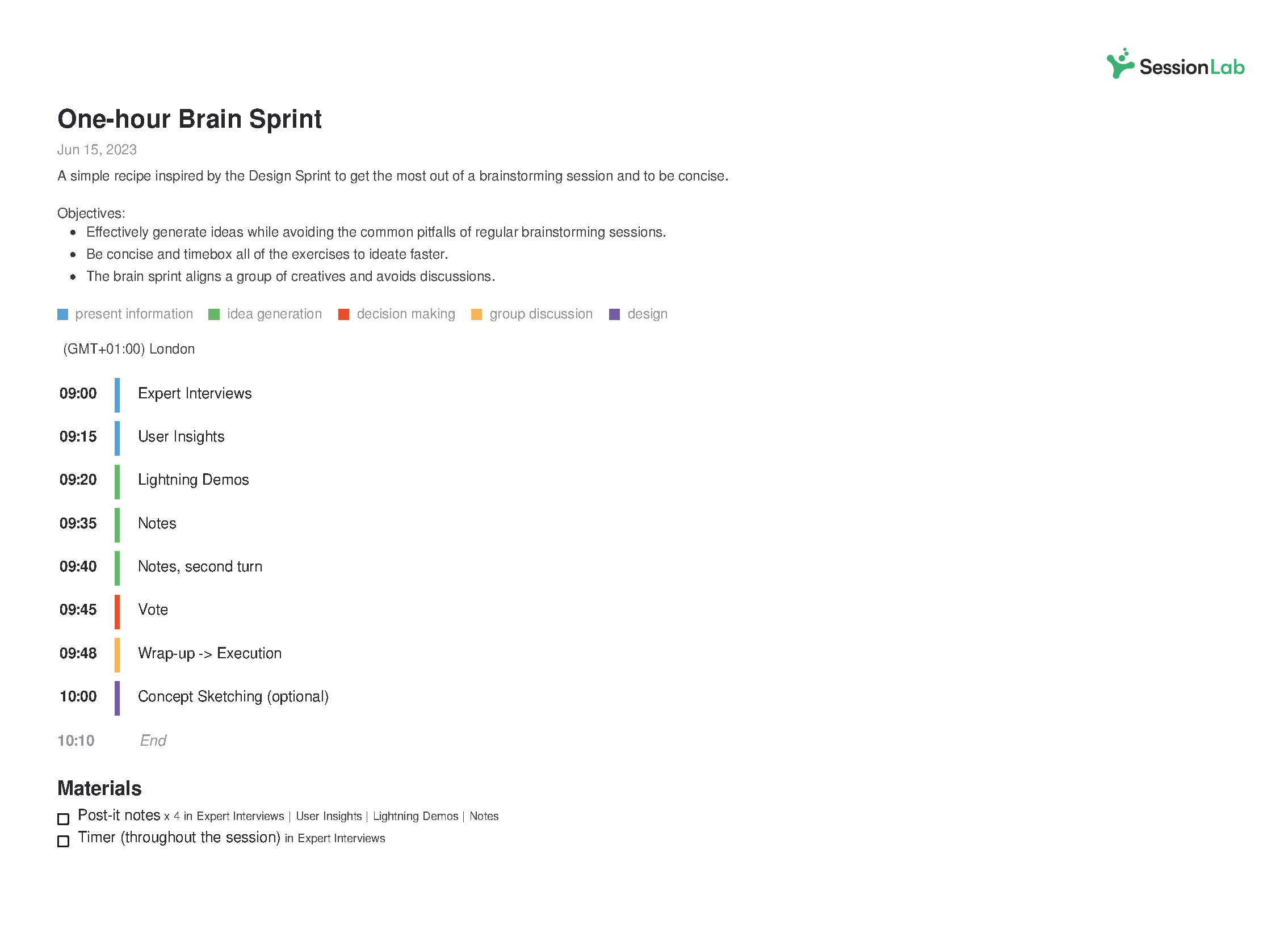
Over to you
The problem-solving process can often be as complicated and multifaceted as the problems they are set-up to solve. With the right problem-solving techniques and a mix of exercises designed to guide discussion and generate purposeful ideas, we hope we’ve given you the tools to find the best solutions as simply and easily as possible.
Is there a problem-solving technique that you are missing here? Do you have a favorite activity or method you use when facilitating? Let us know in the comments below, we’d love to hear from you!
James Smart is Head of Content at SessionLab. He’s also a creative facilitator who has run workshops and designed courses for establishments like the National Centre for Writing, UK. He especially enjoys working with young people and empowering others in their creative practice.
thank you very much for these excellent techniques
Certainly wonderful article, very detailed. Shared!
Your list of techniques for problem solving can be helpfully extended by adding TRIZ to the list of techniques. TRIZ has 40 problem solving techniques derived from methods inventros and patent holders used to get new patents. About 10-12 are general approaches. many organization sponsor classes in TRIZ that are used to solve business problems or general organiztational problems. You can take a look at TRIZ and dwonload a free internet booklet to see if you feel it shound be included per your selection process.
Leave a Comment Cancel reply
Your email address will not be published. Required fields are marked *
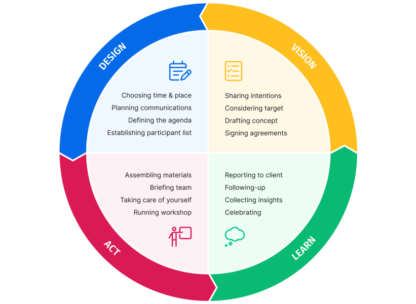
Going from a mere idea to a workshop that delivers results for your clients can feel like a daunting task. In this piece, we will shine a light on all the work behind the scenes and help you learn how to plan a workshop from start to finish. On a good day, facilitation can feel like effortless magic, but that is mostly the result of backstage work, foresight, and a lot of careful planning. Read on to learn a step-by-step approach to breaking the process of planning a workshop into small, manageable chunks. The flow starts with the first meeting with a client to define the purposes of a workshop.…
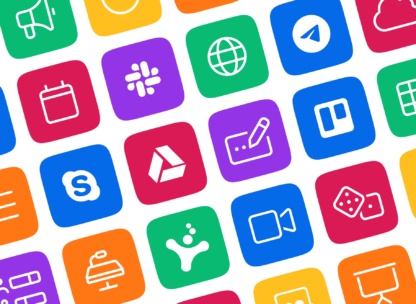
Effective online tools are a necessity for smooth and engaging virtual workshops and meetings. But how do you choose the right ones? Do you sometimes feel that the good old pen and paper or MS Office toolkit and email leaves you struggling to stay on top of managing and delivering your workshop? Fortunately, there are plenty of great workshop tools to make your life easier when you need to facilitate a meeting and lead workshops. In this post, we’ll share our favorite online tools you can use to make your life easier and run better workshops and meetings. In fact, there are plenty of free online workshop tools and meeting…
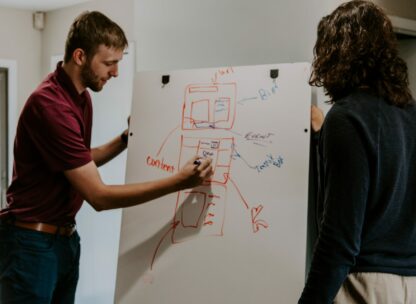
How does learning work? A clever 9-year-old once told me: “I know I am learning something new when I am surprised.” The science of adult learning tells us that, in order to learn new skills (which, unsurprisingly, is harder for adults to do than kids) grown-ups need to first get into a specific headspace. In a business, this approach is often employed in a training session where employees learn new skills or work on professional development. But how do you ensure your training is effective? In this guide, we'll explore how to create an effective training session plan and run engaging training sessions. As team leader, project manager, or consultant,…
Design your next workshop with SessionLab
Join the 150,000 facilitators using SessionLab
Sign up for free
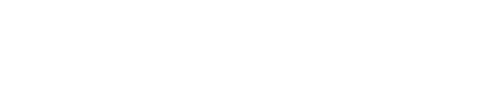
Or search by topic
Number and algebra.
- Place value and the number system
- Fractions, decimals, percentages, ratio and proportion
- Calculations and numerical methods
- Algebraic expressions, equations and formulae
- Coordinates, functions and graphs
- Patterns, sequences and structure
- Properties of numbers
Geometry and measure
- 3D geometry, shape and space
- Transformations and constructions
- Vectors and matrices
- Measuring and calculating with units
- Pythagoras and trigonometry
- Angles, polygons, and geometrical proof
Probability and statistics
- Handling, processing and representing data
- Probability (spec_group)
Working mathematically
- Thinking mathematically
- Mathematical mindsets

Advanced mathematics
- Decision mathematics and combinatorics
- Advanced probability and statistics
For younger learners
- Early years foundation stage
Problem Solving, Using and Applying and Functional Mathematics
Problem solving.
The problem-solving process can be described as a journey from meeting a problem for the first time to finding a solution, communicating it and evaluating the route. There are many models of the problem-solving process but they all have a similar structure. One model is given below. Although implying a linear process from comprehension through to evaluation, the model is more of a flow backward and forward, revisiting and revising on the problem-solving journey.
Comprehension
Representation.
- Can they represent the situation mathematically?
- What is it that they are trying to find?
- What do they think the answer might be (conjecturing and hypothesising)?
- What might they need to find out before they can get started?
Planning, analysis and synthesis
Having understood what the problem is about and established what needs finding, this stage is about planning a pathway to the solution. It is within this process that you might encourage pupils to think about whether they have seen something similar before and what strategies they adopted then. This will help them to identify appropriate methods and tools. Particular knowledge and skills gaps that need addressing may become evident at this stage.
Execution and communication
During the execution phase, pupils might identify further related problems they wish to investigate. They will need to consider how they will keep track of what they have done and how they will communicate their findings. This will lead on to interpreting results and drawing conclusions.
Pupils can learn as much from reflecting on and evaluating what they have done as they can from the process of solving the problem itself. During this phase pupils should be expected to reflect on the effectiveness of their approach as well as other people's approaches, justify their conclusions and assess their own learning. Evaluation may also lead to thinking about other questions that could now be investigated.
Using and Applying Mathematics
Aspects of using and applying reflect skills that can be developed through problem solving. For example:
In planning and executing a problem, problem solvers may need to:
- select and use appropriate and efficient techniques and strategies to solve problems
- identify what further information may be required in order to pursue a particular line of enquiry and give reasons for following or rejecting particular approaches
- break down a complex calculation problem into simpler steps before attempting a solution and justify their choice of methods
- make mental estimates of the answers to calculations
- present answers to sensible levels of accuracy; understand how errors are compounded in certain calculations.
During problem solving, solvers need to communicate their mathematics for example by:
- discussing their work and explaining their reasoning using a range of mathematical language and notation
- using a variety of strategies and diagrams for establishing algebraic or graphical representations of a problem and its solution
- moving from one form of representation to another to get different perspectives on the problem
- presenting and interpreting solutions in the context of the original problem
- using notation and symbols correctly and consistently within a given problem
- examining critically, improve, then justifying their choice of mathematical presentation
- presenting a concise, reasoned argument.
Problem solvers need to reason mathematically including through:
- exploring, identifying, and using pattern and symmetry in algebraic contexts, investigating whether a particular case may be generalised further and understanding the importance of a counter-example; identifying exceptional cases
- understanding the difference between a practical demonstration and a proof
- showing step-by-step deduction in solving a problem; deriving proofs using short chains of deductive reasoning
- recognising the significance of stating constraints and assumptions when deducing results
- recognising the limitations of any assumptions that are made and the effect that varying the assumptions may have on the solution to a problem.
Functional Mathematics
Functional maths requires learners to be able to use mathematics in ways that make them effective and involved as citizens, able to operate confidently in life and to work in a wide range of contexts. The key processes of Functional Skills reflect closely the problem solving model but within three phases:
- Making sense of situations and representing them
- Processing and using the mathematics
- Interpreting and communicating the results of the analysis
Your browser is not supported
Sorry but it looks as if your browser is out of date. To get the best experience using our site we recommend that you upgrade or switch browsers.
Find a solution
- Skip to main content
- Skip to navigation
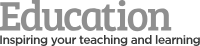
- Back to parent navigation item
- Collections
- Sustainability in chemistry
- Simple rules
- Teacher well-being hub
- Women in chemistry
- Global science
- Escape room activities
- Decolonising chemistry teaching
- Teaching science skills
- Get the print issue
- RSC Education
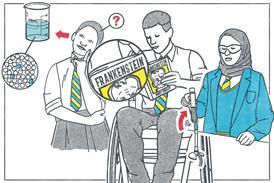
- More navigation items
A model approach to solving problems

- No comments
Use models in your teaching to help students learn how to find solutions
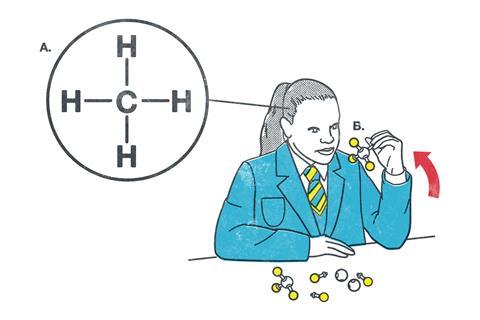
Source: © Tobatron
Models help students solve problems in the science classroom, a skill they will take into the wider world
In science, as in everyday life, there are many problems to solve. As science teachers, we use models all the time in our lessons, not just because they are explicitly mentioned in exam board specifications, but also because they help make abstract concepts more concrete. How do we help our students use models to problem solve in their science education?
Problem solving in everyday life
As a starting point, we can link models and problem solving to everyday life. In class, a student can successfully neutralise an acid using an alkali. We can gain student buy-in by applying this to situations outside the classroom, for example the need to brush our teeth.
Introduce routines around problem solving to motivate students
Taking it further, we can use their classroom experience of models when thinking about future careers. We can emphasise that problem solving is a fundamental skill in all jobs. We can embed the idea that problem solving isn’t simply something being learned in the classroom. Rather, it is a skill that will help them be successful in their later lives. To help them understand this concept, ask students to name a job and then explain how problem solving would be successfully used in that job.
Introduce routines around problem solving to motivate students into believing they have a strategy to attempt a task. Teach your students to use a textbook if they’re stuck. Teach them how to use the contents and index pages. This reinforces a skill that encourages independence and will benefit them if they run out of mobile data.
Promote engagement with students needing more encouragement by using a simple rhyme and choral response: ‘What do we do if we’re stuck?’ – ‘We look in a textbook’. If students find a task too hard and can’t solve the problem, motivation will fall and possibly lead to behavioural issues. While this approach needs a big input of teacher direction to begin with, over time the skill will embed.
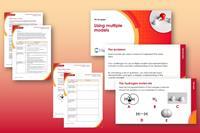
Download this
Evaluate multiple models activity, for age range 14–16
Enhance your learners' skills interpreting and evaluating models with this set of examples showing a hydrogen molecule.
Download the teacher notes as MS Word or pdf , slides as PowerPoint or pdf and student worksheet as MS Word or pdf.
Multiple-models activity, for age range 14–16 years
Enhance your learners’ skills interpreting and evaluating models with this set of examples showing a hydrogen molecule, including classroom slides and a worksheet.
Download the activity from the Education in Chemistry website: rsc.li/3x7j2KW
Using models
While models are scattered throughout the curriculum, there are some best bets of where they routinely apply. When discussing JJ Thomson’s plum pudding model, students could choose a different model that represents the same idea, for example, a chocolate chip cookie. After a chromatography practical, students can be encouraged to identify an unknown substance based on different Rf values.
Acronyms are a useful model that students can learn to apply to similar questions. For example, to aid students in successfully drawing covalent bonding , introduce them to GROSO:
- g roup number
- r equired shared pairs
- o verlap circles
- s hared pairs drawn in
- o ther outer electrons
Acronyms are a useful model that students can learn to apply to similar questions. For example, to aid students in successfully drawing covalent bonding, introduce them to GROSO: g roup number; r equired shared pairs; o verlap circles; s hared pairs drawn in; o ther outer electrons.
Initially, this would need lots of practice and quick checks using mini whiteboards. Once the routine is embedded though, students can take this model and apply it to other covalent questions.
Worked examples also support students by providing a scaffold of how to answer a question. By referencing a completed question, students can attempt a question by following the steps.
More recommended resources
- Use Johnstone’s triangle to help learners of all ages and stages model their thinking.
- Our online Problem solving tutor will help your 16–18 learners to structure and develop their problem solving skills in quantitative chemistry.
- Find out how to use post-16 models to inform 14–16 understanding .
- Show your students how models can help to predict reactions and influence the taste and texture of chocolate and biscuits, by sharing a profile of food scientist, Robert .
- Use Johnstone’s triangle to help learners of all ages and stages model their thinking: rsc.li/3mLA282
- Our online Problem solving tutor will help your 16–18 learners to structure and develop their problem solving skills in quantitative chemistry: rsc.li/3DJRJtG
- Find out how to use post-16 models to inform 14–16 understanding: rsc.li/3HYxHy6
- Show your students how models can help to predict reactions and influence the taste and texture of chocolate and biscuits, by sharing a profile of food scientist, Robert: rsc.li/3RCMMZl
Focusing on problem solving is also a brilliant opportunity to work with other departments. If multiple departments are using the same routine, students are more likely to commit this to long-term memory, thus reducing cognitive load.
The Ofsted research review into science contains a section on coherence between the mathematics and science departments describing how to share similar language around tackling equations. If students can problem solve in maths, we can show them how to carry that skill across by using the same method in science. Many maths departments teach the balance method to solve equations. You can apply this to many chemistry calculations, such as titration.
The Ofsted research review into science contains a section on coherence between the mathematics and science departments describing how to share similar language around tackling equations (rsc.li/3Yx4yjf). If students can problem solve in maths, we can show them how to carry that skill across by using the same method in science. Many maths departments teach the balance method to solve equations. You can apply this to many chemistry calculations, such as titration.
By using these strategies and questions with our students, we can teach them the importance of problem solving. These are skills, once learned, that students can carry with them for future success.

This article is part of our Teaching science skills series, bringing together strategies and classroom activities to help your learners develop essential scientific skills, from literacy to risk assessment and more.
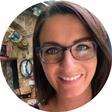
More Holly Walsh
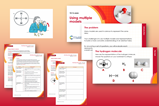
Using multiple models in science | 14–16 years
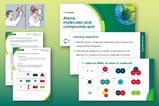
Atoms, molecules and compounds quiz | 11–14 years
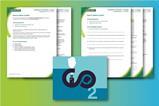
How is carbon cycled? | 11–14 years
- Problem solving
Related articles
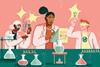
Your guide to the UK Chemistry Olympiad
2024-06-05T07:00:00Z By Nina Notman
Discover how your school can easily participate in the leading annual chemistry competition for secondary school learners
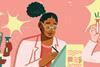
How to run a successful Chemistry Olympiad club
2024-06-05T07:00:00Z By Lynne Maxwell
Discover effective approaches and essential resources to prepare learners for this competition (and others), recommended by two heads of science
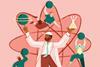
Why you and your students should get involved in the Olympiad
Challenge your learners and ignite a passion for chemistry
No comments yet
Only registered users can comment on this article..
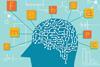
3 ways to help students master metacognition
2024-07-30T05:21:00Z By Louise Glynn
Improve learners’ thinking about their thinking to help them successfully answer multistep questions
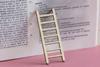
Four super replicable steps to embed new vocabulary
2024-07-17T07:00:00Z By Omer Pazar
Effective tips for teaching tier 2 and 3 terms that’ll improve learning outcomes
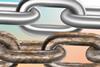
4 ways to teach redox in terms of electrons
2024-07-03T05:06:00Z By Kristy Turner
Use these teacher-tested approaches to help learners gain a deeper understanding of redox reactions
- Contributors
- Print issue
- Email alerts
Site powered by Webvision Cloud
Teach Starter, part of Tes Teach Starter, part of Tes
Search everything in all resources
What Is a Bar Model? How to Use This Math Problem-Solving Method in Your Classroom
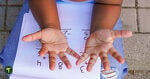
Written by B
Teaching mathematical problem-solving to your students is a crucial element of mathematical understanding. They must be able to decipher word problems and recognize the correct operation to use in order to solve the problem correctly (rather than freaking out as soon as they see a mathematical problem in words!!!). But what is the best strategy for embedding this knowledge into your students? Enter the Bar Model Method , also known in education as a tape diagram, strip diagram, or tape model.
The model has gained traction in classrooms around the world, so you may be thinking it’s time to introduce it in your classroom. But what is the bar model all about, and what are the advantages of using this method with elementary students? The math teachers on the Teach Starter team are here with a quick primer to help you decide if this is the right path for your math class!
Explore the latest math teaching resources from Teach Starter!
What Is a Bar Model?
The Bar Model is a mathematical diagram that is used to represent and solve problems involving quantities and their relationships to one another.
It was developed in Singapore in the 1980s when data showed Singapore’s elementary school students were lagging behind their peers in math. An analysis of testing data at the time showed less than half of Singapore’s students in grades 2-4 could solve word problems that were presented without keywords such as “altogether” or “left.” Something had to be done, and that something was the introduction of the bar model, which has been widely credited with rocketing the kids of Singapore to the top of math scores for kids all around the globe.
At its core, the bar model is an explicit teaching and learning strategy for problem-solving. The actual bar model consists of a set of bars or rectangles that represent the quantities in the problem, and the operations are represented by the lengths and arrangements of the bars. Among its strengths is the fact that it can be applied to all operations, including multiplication and division. They’re also useful when it comes to teaching students more advanced math concepts, such as ratios and proportionality.
The Bar Model combines the concrete (drawings) and the abstract (algorithms or equations) to help the student solve the problem.
How to Use a Bar Model in Math
Whether you’re calling it a bar model, a strip diagram, or a tape diagram, the concept is the same – you have rectangular bars (or strips) that are laid out horizontally to represent quantities and the relationships between them.
- The bars themselves — horizontal rectangles— represent the problem.
- The length of the bar(s) represents the quantity.
- The locations of the bars show the relationship between the quantities.
Visualizing this relationship helps students decide which operation to use to solve the problem. The student then labels the known quantities with numbers and labels the unknown quantities with question marks.
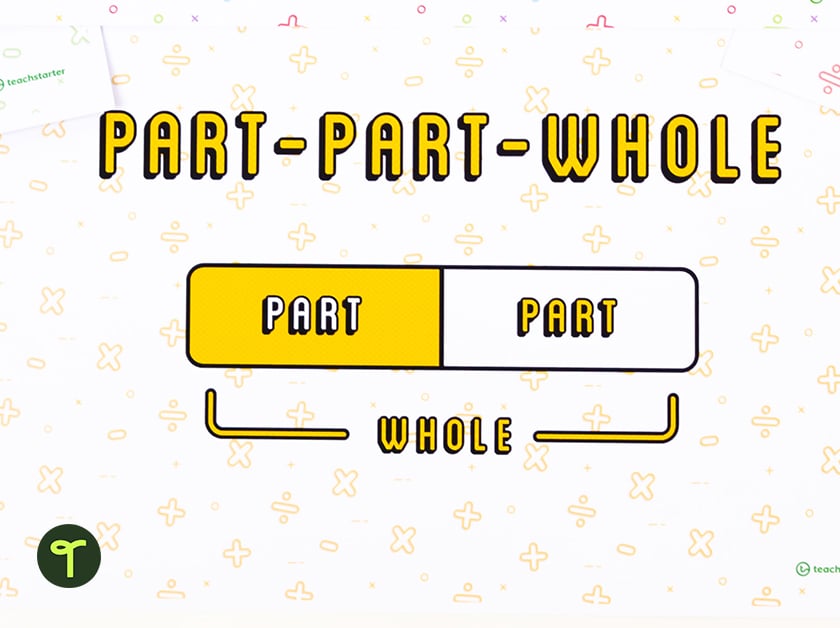
The three basic structures are:
- Part-Part-Whole
- Equal Parts
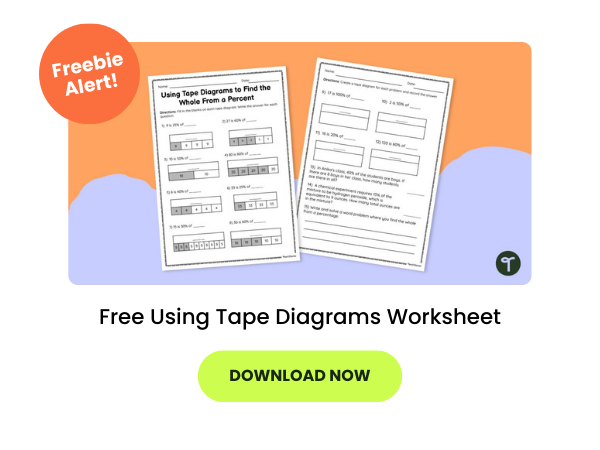
Many teachers (particularly in the elementary grades) will recognize elements of the Bar Model as being similar to the Part-Part-Whole method we’ve been teaching forever.
The Bar Model could be looked at as an extension of this concept. It can be used by students right through elementary school (and beyond) not only to solve addition and subtraction problems but to tackle multiplication and division word-based problems as well.
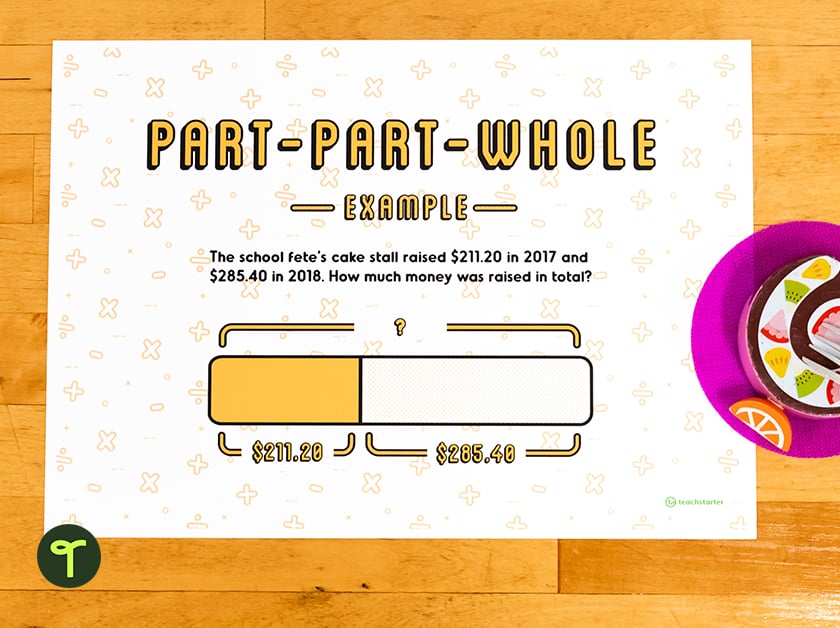
Teaching with the Bar Model
The Bar Model can easily be incorporated into your elementary math instruction, from simple addition to more complex multiplication and division and so on. Here are just a few ideas from our teacher team:
- Use part–whole bar models to show word problems with a missing number element to teach addition or subtraction.
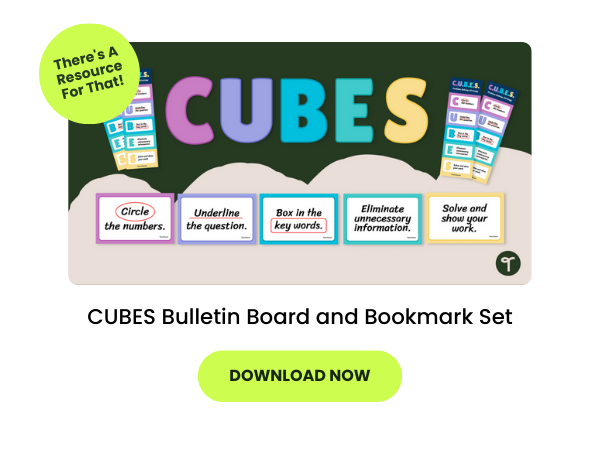
- Use the bar model method to help students see how a bar must be cut into equal parts when multiplying and dividing.
- Help students visualize fractions with the bar model — Use the rectangles to help students see how the fractions relate to whole numbers by showing the relationship between the numerator and denominator.
Explore our complete collection of curriculum-aligned resources for teaching about operations !
Banner image via Shutterstock/Komuso Colorsandia
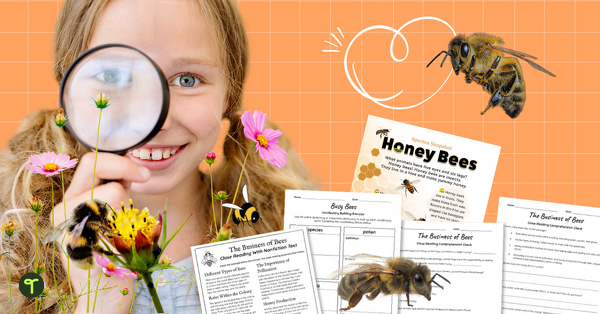
30 Buzzing Facts About Bees to Excite Kids About Nature
Everyone benefits from the busyness of bees which is why these bee facts will help inspire your students to appreciate and protect them!
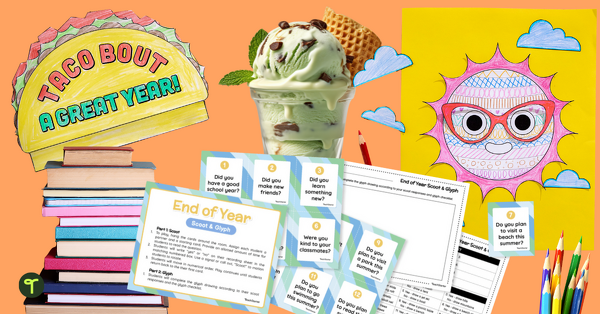
21 Epic Last Day of School Activities to Kick Off Summer Break
Looking for easy last day of school ideas for elementary or middle school? These quick and fun end of year classroom activities will help you finish off your year just right!
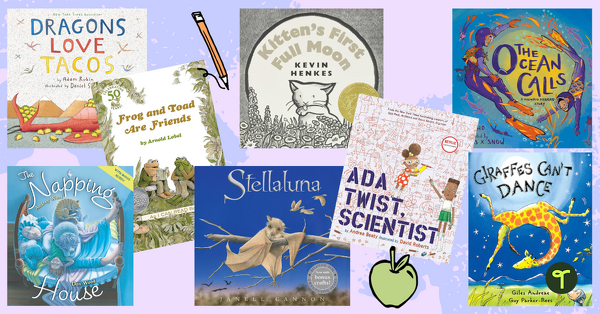
70 1st Grade Books to Add to Your Classroom Reading Corner This Year
Wondering which 1st grade books you should add to your classroom library? Look no further! We have a list of 70 that are teacher (and student) approved!
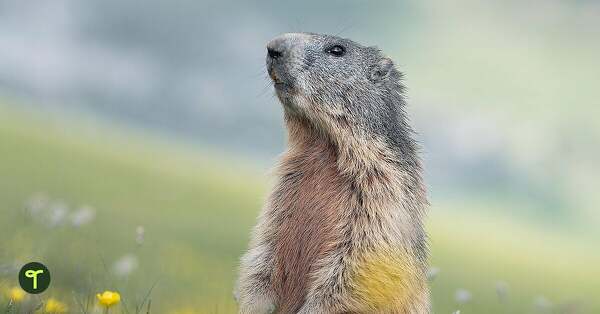
22 Fun Groundhog Facts to Share With Your Class on Groundhog Day
Need fun groundhog facts to share with your students this Groundhog Day? Find out what groundhogs eat, where they live and why we let them predict the weather!
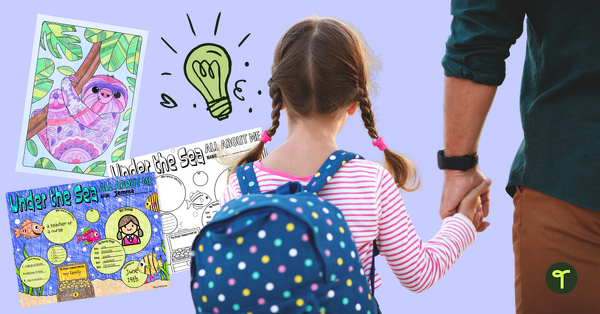
How to Teach 1st Grade — The Ultimate Guide to What to Do, What to Buy and What to Teach
Looking for tips on teaching first grade? Our comprehensive 1st grade teacher guide will answer all your questions from what to buy to how to prepare for the first day of school and what to do throughout the school year.
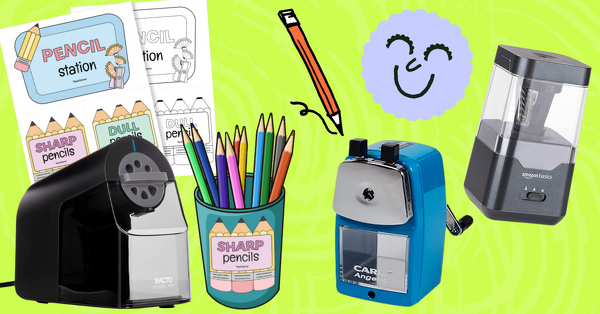
10 Best Pencil Sharpeners for the Classroom — Recommended by Teachers
Need new pencil sharpeners in your classroom? We've rounded up 10 best pencil sharpeners recommended by teachers to get you started.
Get more inspiration delivered to your inbox!
Sign up for a free membership and receive tips, news and resources directly to your email!
- Our Mission
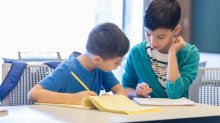
Using Mathematical Modeling to Get Real With Students
Unlike canned word problems, mathematical modeling plunges students into the messy complexities of real-world problem solving.
How do you bring math to life for kids? Illustrating the boundless possibilities of mathematics can be difficult if students are only asked to examine hypothetical situations like divvying up a dessert equally or determining how many apples are left after sharing with friends, writes third- and fourth- grade teacher Matthew Kandel for Mathematics Teacher: Learning and Teaching PK-12 .
In the early years of instruction, it’s not uncommon for students to think they’re learning math for the sole purpose of being able to solve word problems or help fictional characters troubleshoot issues in their imaginary lives, Kandel says. “A word problem is a one-dimensional world,” he writes. “Everything is distilled down to the quantities of interest. To solve a word problem, students can pick out the numbers and decide on an operation.”
But through the use of mathematical modeling, students are plucked out of the hypothetical realm and plunged into the complexities of reality—presented with opportunities to help solve real-world problems with many variables by generating questions, making assumptions, learning and applying new skills, and ultimately arriving at an answer.
In Kandel’s classroom, this work begins with breaking students into small groups, providing them with an unsharpened pencil and a simple, guiding question: “How many times can a pencil be sharpened before it is too small to use?”
Setting the Stage for Inquiry
The process of tackling the pencil question is not unlike the scientific method. After defining a question to investigate, students begin to wonder and hypothesize—what information do we need to know?—in order to identify a course of action. This step is unique to mathematical modeling: Whereas a word problem is formulaic, leading students down a pre-existing path toward a solution, a modeling task is “free-range,” empowering students to use their individual perspectives to guide them as they progress through their investigation, Kandel says.
Modeling problems also have a number of variables, and students themselves have the agency to determine what to ignore and what to focus their attention on.
After inter-group discussions, students in Kandel’s classroom came to the conclusion that they’d need answers to a host of other questions to proceed with answering their initial inquiry:
- How much does the pencil sharpener remove?
- What is the length of a brand new, unsharpened pencil?
- Does the pencil sharpener remove the same amount of pencil each time it is used?
Introducing New Skills in Context
Once students have determined the first mathematical question they’d like to tackle (does the pencil sharpener remove the same amount of pencil each time it is used?), they are met with a roadblock. How were they to measure the pencil if the length did not fall conveniently on an inch or half inch? Kandel took the opportunity to introduce a new target skill which the class could begin using immediately: measuring to the nearest quarter inch.
“One group of students was not satisfied with the precision of measuring to the nearest quarter inch and asked to learn how to measure to the nearest eighth of an inch,” Kandel explains. “The attention and motivation exhibited by students is unrivaled by the traditional class in which the skill comes first, the problem second.”
Students reached a consensus and settled on taking six measurements total: the initial length of the new, unsharpened pencil as well as the lengths of the pencil after each of five sharpenings. To ensure all students can practice their newly acquired skill, Kandel tells the class that “all group members must share responsibility, taking turns measuring and checking the measurements of others.”
Next, each group created a simple chart to record their measurements, then plotted their data as a line graph—though exploring other data visualization techniques or engaging students in alternative followup activities would work as well.
“We paused for a quick lesson on the number line and the introduction of a new term—mixed numbers,” Kandel explains. “Armed with this new information, students had no trouble marking their y-axis in half- or quarter-inch increments.”
Sparking Mathematical Discussions
Mathematical modeling presents a multitude of opportunities for class-wide or small-group discussions, some which evolve into debates in which students state their hypotheses, then subsequently continue working to confirm or refute them.
Kandel’s students, for example, had a wide range of opinions when it came to answering the question of how small of a pencil would be deemed unusable. Eventually, the class agreed that once a pencil reached 1 ¼ inch, it could no longer be sharpened—though some students said they would be able to still write with it.
“This discussion helped us better understand what it means to make an assumption and how our assumptions affected our mathematical outcomes,” Kandel writes. Students then indicated the minimum size with a horizontal line across their respective graphs.
Many students independently recognized the final step of extending their line while looking at their graphs. With each of the six points representing their measurements, the points descended downward toward the newly added horizontal “line of inoperability.”
With mathematical modeling, Kandel says, there are no right answers, only models that are “more or less closely aligned with real-world observations.” Each group of students may come to a different conclusion, which can lead to a larger class discussion about accuracy. To prove their group had the most accurate conclusion, students needed to compare and contrast their methods as well as defend their final result.
Developing Your Own Mathematical Models
The pencil problem is a great starting point for introducing mathematical modeling and free-range problem solving to your students, but you can customize based on what you have available and the particular needs of each group of students.
Depending on the type of pencil sharpener you have, for example, students can determine what constitutes a “fair test” and set the terms of their own inquiry.
Additionally, Kandel suggests putting scaffolds in place to allow students who are struggling with certain elements to participate: Simplified rulers can be provided for students who need accommodations; charts can be provided for students who struggle with data collection; graphs with prelabeled x- and y-axes can be prepared in advance.
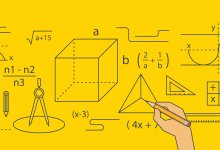
.css-1sk4066:hover{background:#d1ecfa;} 7 Real-World Math Strategies
Students can also explore completely different free-range problem solving and real world applications for math . At North Agincourt Jr. Public School in Scarborough, Canada, kids in grades 1-6 learn to conduct water audits. By adding, subtracting, finding averages, and measuring liquids—like the flow rate of all the water foundations, toilets, and urinals—students measure the amount of water used in their school or home in a single day.
Or you can ask older students to bring in common household items—anything from a measuring cup to a recipe card—and identify three ways the item relates to math. At Woodrow Petty Elementary School in Taft, Texas, fifth-grade students display their chosen objects on the class’s “real-world math wall.” Even acting out restaurant scenarios can provide students with an opportunity to reinforce critical mathematical skills like addition and subtraction, while bolstering an understanding of decimals and percentages. At Suzhou Singapore International School in China, third- to fifth- graders role play with menus, ordering fictional meals and learning how to split the check when the bill arrives.
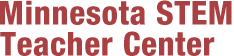
Modeling Word Problems
Using models is a critical step in helping students transition from concrete manipulative work with word problems to the abstract step of generating an equation to solve contextual problems. By learning to use simple models to represent key mathematical relationships in a word problem, students can more easily make sense of word problems, recognize both the number relationships in a given problem and connections among types of problems, and successfully solve problems with the assurance that their solutions are reasonable.
Why is modeling word problems important?
Mr. Alexander and teachers from his grade level team were talking during their Professional Learning Community (PLC) meeting about how students struggle with word problems. Everyone felt only a few of their students seem to be able to quickly generate the correct equation to solve the problem. Many students just seem to look for some numbers and do something with them, hoping they solve the problem.
Mr. Alexander had recently learned about using modeling for word problems in a workshop he had attended. He began to share the model diagrams with his teammates and they were excited to see how students might respond to this approach. They even practiced several model diagrams among themselves as no one had ever learned to use models with word problems. Since part of their PLC work freed them up to observe lessons in each others' rooms, they decided they would watch Mr. Alexander introduce modeling to his students.
So, two days later they gathered in Mr. Alexander's room for the math lesson. Mr. Alexander presented the following problem:
Lily and her brother, Scotty, were collecting cans for the recycling drive. One weekend they collected 59 cans and the next weekend they collected 85 cans. How many cans were collected in all?
Mr. Alexander went over the problem and drew a rectangular bar divided into two parts on the board, explaining that each part of the rectangle was for the cans collected on one of the weekends and the bracket indicated how many cans were collected in all. Reviewing the problem, Mr. Alexander asked students what was not known, and where the given numbers would go and why. This resulted in the following bar model:
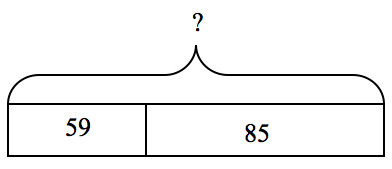
The class then discussed what equations made sense given the relationship of the numbers in the bar model. This time many students wrote the equation, 59 + 85 = ?, and solved the problem. In their discussion after the lesson, Mr. Alexander's teammates mentioned that they noticed a much higher degree of interest and confidence in problem solving when Mr. Alexander introduced the bar model. Everyone noticed that many more students were successful in solving problems once modeling was introduced and encouraged. As the class continued to do more word problems, the diagrams appeared to be a helpful step in scaffolding success with word problems.
Forsten, 2010, p.1 | Students often have regarded each word problem as a new experience, and fail to connect a given problem to past problems of a similar type. Students need to sort out the important information in a word problem, and identify the relationships among the numbers involved in the situation. A model can help students organize their thinking about a given problem, and identify an equation that would be helpful in solving the problem. Models are a kind of graphic organizer for the numbers in a word problem, and may connect to students' work with graphic organizers in other subjects. |
Leinwand, 2009, p.19 | Modeling can begin with young learners with basic addition, subtraction, multiplication, and division problems. Modeling can be extended to ratio, rate, percent, multi-step, and other complex problems in the upper grades. Utilizing modeling on a routine basis in early grades can lay an important foundation for later work, including the transition to algebra, by stressing patterns, generalizations, and how numbers relate to each other. |
Knowledge can be represented both linguistically and nonlinguistically. An increase in nonlinguistic representations allows students to better recall knowledge and has a strong impact on student achievement (Marzano, et. al., 2001, Section 5). In classic education research, Bruner (1961) identified three modes of learning: enactive (manipulating concrete objects), iconic (pictures or diagrams), and symbolic (formal equation). The iconic stage, using pictures and diagrams, is an important bridge to abstracting mathematical ideas using the symbols of an equation. Research has also validated that students need to see an idea in multiple representations in order to identify and represent the common core (Dienes, undated). For word problems involving the operation of addition, students need to experience several types of problems to generalize that when two parts are joined they result in a total or a quantity that represents the whole. Whether the items are bears, balloons, or cookies no longer matters as the students see the core idea of two subsets becoming one set. Dienes discovered that this abstraction is only an idea; therefore it is hard to represent. Diagrams can capture the similarity students notice in addition/joining problems where both addends are known and the total or whole is the unknown. Diagrams will also be useful for missing addend situations. Like Bruner, Dienes saw diagrams as an important bridge to abstracting and formalizing mathematical ideas.
Along with Bruner and Dienes, Skemp (1993) identified the critical middle step in moving from a real-life situation to the abstractness of an equation. While students need to experience many real-life situations they will get bogged down with the "noise" of the problem such as names, locations, kinds of objects, and other details. It is the teacher's role to help students sort through the noise to capture what matters most for solving the problem. A diagram can help students capture the numerical information in a problem, and as importantly, the relationship between the numbers, e.g. Do we know both the parts, or just one of the parts and the whole? Are the parts similar in size, or is one larger than the other? Once students are comfortable with one kind of diagram, they can think about how to relate it to a new situation. A student who has become proficient with using a part-part-whole bar model diagram when the total or whole is unknown, (as in the collecting cans problem in Mr. Alexander's class), cannot only use the model in other part-part-whole situations, but can use it in new situations, for example, a missing addend situation. Given several missing addend situations, students may eventually generalize that these will be subtractive situations, solvable by either a subtraction or adding on equation.
The work of Bruner, Dienes and Skemp informed the development of computation diagrams in some elementary mathematics curriculum materials in the United States. Interestingly, it also informed the development of curriculum in Singapore, as they developed the "Thinking Schools, Thinking Nation" era of reforming their educational model and instructional strategies (Singapore Ministry of Education, 1997). The bar model is a critical part of "Singapore Math." It is used and extended across multiple grades to capture the relationships within mathematical problems. Singapore has typically scored near the top of the world on international assessments, a possible indicator of the strong impact of including the visual diagram step to represent and solve mathematical problems.
What is modeling word problems?
Models at any level can vary from simple to complex, realistic to representational. Young students often solve beginning word problems, acting them out, and modeling them with the real objects of the problem situation, e.g. teddy bears or toy cars. Over time they expand to using representational drawings, initially drawing pictures that realistically portray the items in a problem, and progressing to multi-purpose representations such as circles or tally marks. After many concrete experiences with real-life word problems involving joining and separating, or multiplying and dividing objects, teachers can transition students to inverted-V and bar model drawings which are multi-purpose graphic organizers tied to particular types of word problems.
Modeling Basic Number Relationships
Simple diagrams, sometimes known as fact triangles, math mountains, situation diagrams, or representational diagrams have appeared sporadically in some curriculum materials. But students' problem solving and relational thinking abilities would benefit by making more routine use of these diagrams and models.
Young children can begin to see number relationships that exist within a fact family through the use of a model from which they derive equations. An inverted-V is one simple model that helps students see the addition/subtraction relationships in a fact family, and can be used with word problems requiring simple joining and separating. The inverted-V model can be adapted for multiplication and division fact families. For addition, students might think about the relationships among the numbers in the inverted-V in formal terms, addend and sum , or in simpler terms, part and total , as indicated in the diagrams below.
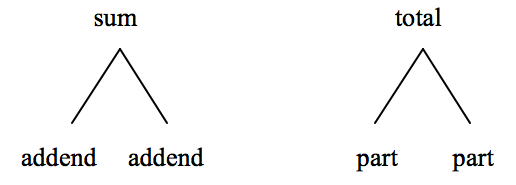
A specific example for a given sum of 10 would be the following, depending on which element of the problem is unknown.
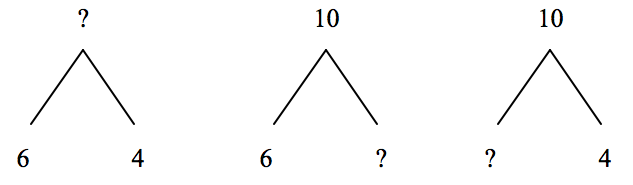
6 + 4 = ? 6 + ? = 10 ? + 4 = 1
4 + 6 = ? 10 - 6 = ? 10 - 4 = ?
While often used with fact families, and the learning of basic facts, inverted-V diagrams can also work well with solving word problems. Students need to think about what they know and don't know in a word problem - are both the parts known, or just one of them? By placing the known quantities correctly into the inverted-V diagram, students are more likely to determine a useful equation for solving the problem, and see the result as reasonable for the situation. For example, consider the following problem:
Zachary had 10 train cars. Zachary gave 3 train cars to his brother. How many train cars does Zachary have now?
Students should determine they know how many Zachary started with ( total or whole ), and how many he gave away ( part of the total ). So, they need to find out how many are left ( other part of the total ). The following inverted-V diagram represents the relationships among the numbers of this problem:

3 + ? = 10 or 10 - 3 = ?, so Zachary had 7 train cars left.
As students move on to multiplication and division, the inverted-V model can still be utilized in either the repeated addition or multiplicative mode. Division situations do not require a new model; division is approached as the inverse of multiplication or a situation when one of the factors is unknown.

Again, the inverted-V diagram can be useful in solving multiplication and division word problems. For example, consider the following problem:
Phong planted 18 tomato plants in 3 rows. If each row had the same number of plants, how many plants were in each row?
Students can see that they know the product and the number OF rows. The number IN A row is unknown. Either diagram below may help solve this problem, convincing students that 6 in a row is a reasonable answer.
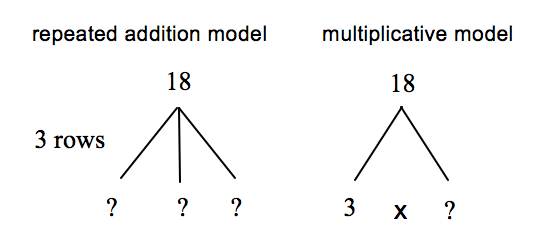
While the inverted-V diagram can be extended to multi-digit numbers, it has typically been used with problems involving basic fact families. Increasing the use of the inverted-V model diagram should heighten the relationship among numbers in a fact family making it a useful, quick visual for solving simple word problems with the added benefit of using and increasing the retention of basic facts.
Models and Problem Types for Computation
As children move to multi-digit work, teachers can transition students to bar model drawings, quick sketches that help students see the relationships among the important numbers in a word problem and identify what is known and unknown in a situation.
Carpenter, et.al, 1999, p. 7 | Bar models work well with recognition of problem types. There are four basic types for addition and subtraction word problems: 1) join (addition), 2) separate (subtraction), 3) part-part-whole, and 4) comparison (Carpenter, Fennema, Franke, Levi, & Empson, 1999, Chapter 2). Within each of the first three types, either the sum (whole or total), or one of the addends (parts) can be the unknown. For a comparison problem, either the larger quantity, smaller quantity, or the difference can be unknown.
By introducing students to bar models a teacher has an important visual to facilitate student thinking about the mathematical relationships among the numbers of a given word problem. |
With bar models the relationships among numbers in all these types of problems becomes more transparent, and helps bridge student thinking from work with manipulatives and drawing pictures to the symbolic stage of writing an equation for a situation. With routine use of diagrams and well-facilitated discussions by teachers, student will begin to make sense of the parts of a word problem and how the parts relate to each other.
Part-Part-Whole Problems. Part-Part-Whole problems are useful with word problems that are about sets of things, e.g. collections. They are typically more static situations involving two or more subsets of a whole set. Consider the problem,
Cole has 11 red blocks and 16 blue blocks. How many blocks does Cole have in all?
Students may construct a simple rectangle with two parts to indicate the two sets of blocks that are known (parts/addends). It is not important to have the parts of the rectangle precisely proportional to the numbers in the problem, but some attention to their relative size can aid in solving the problem. The unknown in this problem is how many there are altogether (whole/total/sum), indicated by a bracket (or an inverted-V) above the bar, indicating the total of the 2 sets of blocks. The first bar model below reflects the information in the problem about Cole's blocks.
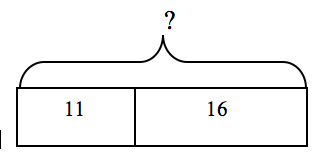
11 + 16 = ? so Cole has 27 blocks in all.
A similar model would work for a problem where the whole amount is known, but one of the parts (a missing addend) is the unknown. For example:
Cole had 238 blocks. 100 of them were yellow. If all Cole's blocks are either blue or yellow, how many were blue?
The following bar model would be useful in solving this problem.
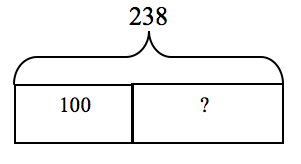
100 + ? = 238 or 238 - 100 = ? so Cole has 138 blue blocks.
The answer has to be a bit more than 100 because 100 + 100 is 200 but the total here is 238 so the blue blocks have to be a bit more than 100.
The part-part-whole bar model can easily be expanded to large numbers, and other number types such as fractions and decimals. Consider the problem:
Leticia read 7 ½ books for the read-a-thon.She wants to read 12 books in all. How many more books does she have to read?
The first diagram below reflects this problem. Any word problem that can be thought of as parts and wholes is responsive to bar modeling diagrams. If a problem has multiple addends, students just draw enough parts in the bar to reflect the number of addends or parts, and indicate whether one of the parts, or the whole/sum, is the unknown, as shown in the second figure below.

12 - 7 ½ = ? or 7 ½ + ? = 12 so Leticia needs to read 4 ½ more books.
Join (Addition) and Separate (Subtraction) Problems.
Leinwand, 2009, p. 23 | Some addition and subtraction problems have a stated action - something is added to or separated from a beginning quantity. While often considered a different problem type from the more static part-part-whole problems, join and separate problems can also use a rectangular bar model to represent the quantities involved. Students need to think about whether something is being joined (added) to an amount, or if something is being separated (subtracted). In addition the bracket indicates the total that will result when the additive action is completed. In whole number subtraction, a starting quantity is indicated by the bracket. It is decreased by an amount that is separated or taken away, resulting in a number that indicates what is remaining. |
Consider this joining problem:
Maria had $20. She got $11 more dollars for babysitting. How much money does she have now?
Students can identify that the starting amount of $20 is one of the parts, $11 is another part (the additive amount), and the unknown is the sum/whole amount, or how much money she has now. The first diagram below helps represent this problem.
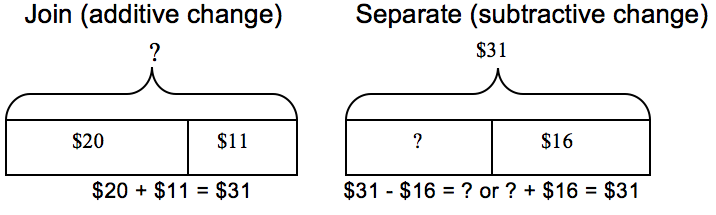
Consider the related subtractive situation:
Maria had $31. She spent some of her money on a new CD. Maria now has $16 left.
The second diagram above represents this situation. Students could use the model to help them identify that the total or sum is now $31, one of the parts (the subtractive change) is unknown, so the other part is the $16 she has left.
Comparison Problems. Comparison problems have typically been seen as difficult for children. This may partially be due to an emphasis on subtraction as developed in word problems that involve "take away" situations rather than finding the "difference" between two numbers. Interestingly, studies in countries that frequently use bar models have determined that students do not find comparison problems to be much more difficult than part-part-whole problems (Yeap, 2010, pp. 88-89).
A double bar model can help make comparison problems less mysterious. Basically, comparison problems involve two quantities (either one quantity is greater than the other one, or they are equal), and a difference between the quantities. Two bars, one representing each quantity, can be drawn with the difference being represented by the dotted area added onto the lesser amount. For example, given the problem:
Tameka rode on 26 county fair rides. Her friend, Jackson, rode on 19 rides. How many more rides did Tameka ride on than Jackson?
Students might generate the comparison bars diagram shown below, where the greater quantity, 26, is the longer bar. The dotted section indicates the difference between Jackson's and Tameka's quantities, or how much more Tameka had than Jackson, or how many more rides Jackson would have had to have ridden to have the same number of rides as Tameka.
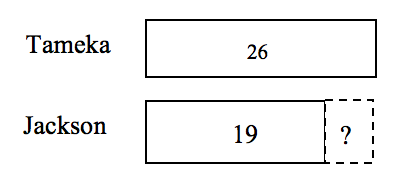
26 - 19 = ? or 19 + ? = 26; the difference is 7 so Tameka rode 7 more rides.
Comparison problems express several differently worded relationships. If Tameka rode 7 more rides than Jackson, Jackson rode 7 fewer rides than Tameka. Variations of the double bar model diagram can make differently worded relationships more visual for students. It is often helpful for students to recognize that at some point both quantities have the same amount, as shown in the model below by the dotted line draw up from the end of the rectangle representing the lesser quantity. But one of the quantities has more than that, as indicated by the area to the right of the dotted line in the longer bar. The difference between the quantities can be determined by subtracting 19 from 26, or adding up from 19 to 26 and getting 7, meaning 26 is 7 more than 19 or 19 is 7 less than 26.
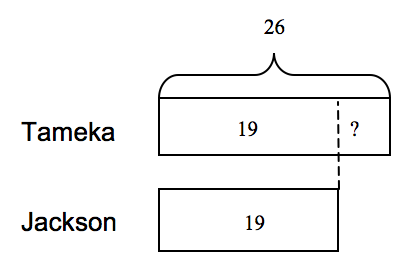
Comparison word problems are especially problematic for English Learners as the question can be asked several ways. Modifying the comparison bars may make the questions more transparent. Some variations in asking questions about the two quantities of rides that Tameka and Jackson rode might be:
- How many more rides did Tameka ride than Jackson?
- How many fewer rides did Jackson go on than Tameka?
- How many more rides would Jackson have had to ride to have ridden the same number of rides as Tameka?
- How many fewer rides would Tameka have had to ride to have ridden the same number of rides as Jackson?
Comparisons may also be multiplicative. Consider the problem:
Juan has 36 CDs in his collection. This is 3 times the amount of CDs that his brother, Marcos, has. How many CDs does Marcos have?
In this situation, students would construct a bar model, shown below on the left, with 3 parts. Students could divide the 36 into 3 equal groups to show the amount that is to be taken 3 times to create 3 times as many CDs for Juan.
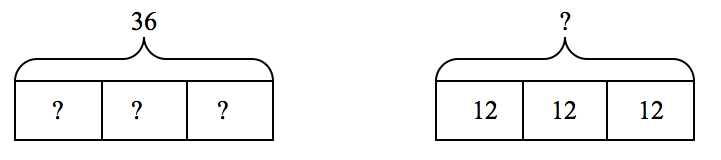
36 ¸ 3 = ? or 3 x ? = 36 12 + 12 + 12 = ? (or 3 x 12 = ?)
so Marcos has 12 CDs. so Juan has 36 CDs.
A similar model can be used if the greater quantity is unknown, but the lesser quantity, and the multiplicative relationship are both known. If the problem was:
Juan has some CDs. He has 3 times as many CDs as Marcos who has 12 CDs. How many CDs does Juan have?
As seen in the diagram above on the right, students could put 12 in a box to show the number of CDs Marcos has; then duplicate that 3 times to sow that Juan has 3 times as many CDs. Then the total number that Juan has would be the sum of those 3 parts.
Multiplication and Division Problems. The same model used for multiplicative comparisons will also work for basic multiplication word problems, beginning with single digit multipliers. Consider the problem:
Alana had 6 packages of gum. Each package holds 12 pieces of gum. How many pieces of gum does Alana have in all?
The following bar model uses a repeated addition view of multiplication to visualize the problem.
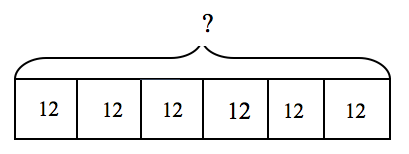
12 + 12 + 12 + 12 + 12 + 12 = 72 (or 6 x 12 = 72)
so Alana has 72 pieces of gum.
As students move into multi-digit multipliers, they can use a model that incorporates an ellipsis to streamline the bar model. For example:
Sam runs 32 km a day during April to get ready for a race. If Sam runs every day of the month, how many total kilometers did he run in April?
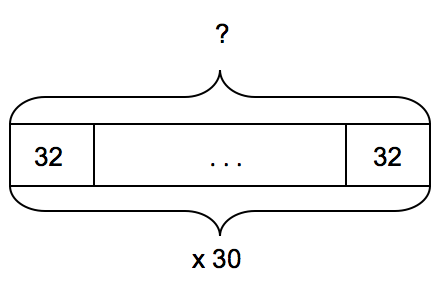
30 x 32 km = 30 x 30 km + 30 x 2 km = 960 km
Sam ran 960 km during the 30 days of April.
Since division is the inverse of multiplication, division word problems will utilize the multiplicative bar model where the product (dividend) is known, but one of the factors (divisor or quotient) is the unknown.
Problems Involving Rates, Fractions, Percent & Multiple Steps. As students progress through the upper grades, they can apply new concepts and multi-step word problems to bar model drawings. Skemp (1993) identified the usefulness of relational thinking as critical to mathematical development. A student should be able to extend their thinking based on models they used earlier, by relating and adapting what they know to new situations.
Consider this rate and distance problem:
Phong traveled 261 miles to see her grandmother. She averaged 58 mph. How long did it take her to get to her grandmother's house?
The following model builds off of the part-part-whole model using a repeated addition format for multiplication and division. It assumes that students have experience with using the model for division problems whose quotients are not just whole numbers. As they build up to (or divide) the total of 261 miles, they calculate that five 58's will represent 5 hours of travel, and the remaining 29 miles would be represented by a half box, so the solution is it would take Phong 5½ hours of driving time to get to her grandmother's house.
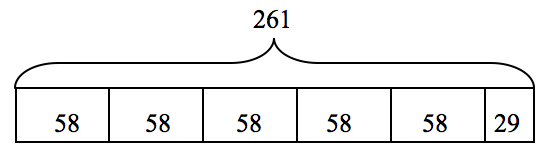
Even a more complex rate problem can be captured with a combination of similar models. Consider this problem:
Sue and her friend Anne took a trip together. Sue drove the first 2/5 of the trip and Anne drove 210 miles for the last 3/5 of the trip. Sue averaged 60 mph and Anne averaged 70 mph. How long did the trip take them?
There are several ways students might combine or modify a basic bar model. One solution might be the following, where the first unknown is how many miles Sue drove. A bar divided into fifths represents how to calculate the miles Sue drove. Since we know that the 210 miles Anne drove is 3/5 of the total trip, each one of Anne's boxes, each representing 1/5 of the trip, is 70 miles. Therefore, Sue drove two 70 mile parts, or 140 miles, to equal 2/5 of the total trip.
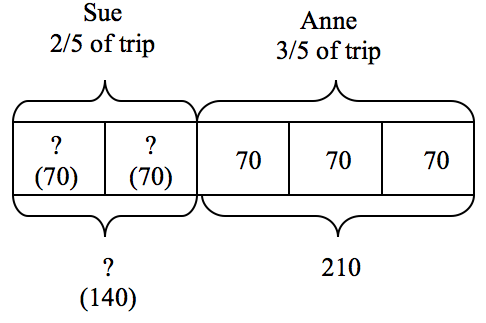
The diagram now needs to be extended to show how to calculate the number of hours. Anne's 210 mile segment, divided by her 70 mph rate will take 3 hours, as recorded on the following extension of the diagram. Sue's distance of 140 miles now needs to be divided into 60 mph segments to determine her driving time of 2 1/3 hours. So, the total trip of 350 miles would take 5 1/3 hours of driving time, considering the two driving rates.
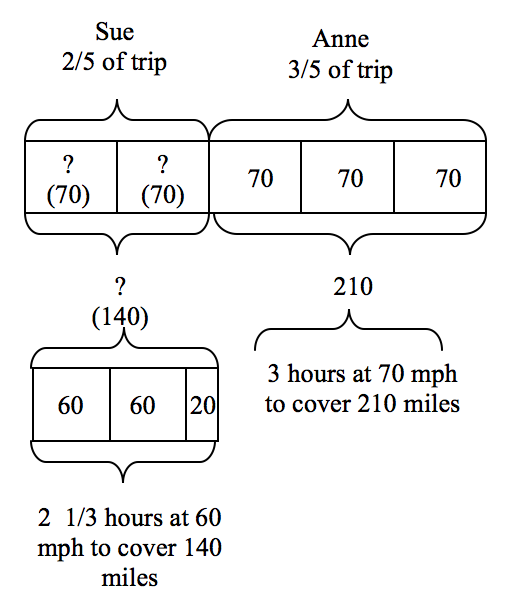
Certainly, a foundation of using simple bar model drawings needs to be well developed in early grades for students to extend diagrams with understanding in later grades. The Sue and Anne rate-time-distance problem would not be the place to begin using bar models! But, by building on work in earlier grades with models, this extended model makes the mathematics of this complex problem more transparent, and helps students think through the steps.
Consider a simpler multi-step problem:
Roberto purchased 5 sports drinks at $1.25 each. Roberto gave the cashier $20. How much change did he get back?
Again, there may be student variations when they begin to extend the use of diagrams in multi-step or more complex problems. Some students might use two diagrams at once, as show below on the left. Others may indicate computation within one diagram, as shown in the diagram on the right.
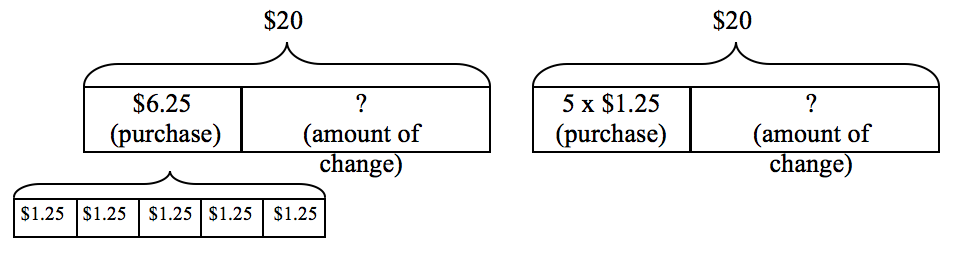
With routine experience with bar modeling, students can extend the use of the models to problems involving relationships that can be expressed with variables. Consider this simple problem that could be represented algebraically:
Callan and Avrielle collected a total of 190 bugs for a science project. Callan collected 10 more bugs than Avrielle. How many bugs did Callan collect?
Let n equal the number of bugs Avrielle collected, and n + 10 equal the number of bugs Callan collected. The following model might be created by students:
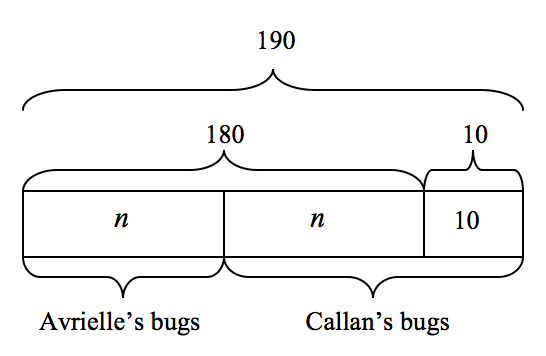
Since n + n = 180 (or 2 n = 180), n = 90. Therefore, Callan collected 90 + 10 or 100 bugs and Avrielle collected 90 bugs for a total of 190 bugs collected together.
Yaep, 2010. p.162 | Understanding the structure of a word problem involves knowing how the mathematical information in a given word problem is related, and how to extract the components needed for solving the problem. Bar model drawings can help students become more proficient at identifying the variables involved in a problem as well as the relationship(s) between them. This ability to focus on the relationships among the numbers in a given problem, and to recognize the mathematical structure as a particular type of problem, is part of relational thinking - a critical skill for success in algebra. Building inverted-V and bar models into pre-algebraic work in grades K-7 can make students more powerfully ready for the formal study of algebra. |
Planning and Instruction
How do I intentionally plan for and use modeling?
If modeling is not a way you learned to identify the important information and numerical relationships in word problems, you may want to review some of the resources on problem types (see Carpenter's book in References and Resources section below), or bar modeling (see books by Forsten, Walker, or Yeap in the References and Resources section below). You may also want to practice the different types of models. Decide which are most accessible for your students, and start with introducing one model at a time, helping students determine what is unknown in the problem, and where that unknown and the other numerical information should be placed in the bar model. A question mark, box, or a variable can be used for the unknown. As students become comfortable with that model, introduce, and compare and contrast a second model with the known model.
You might introduce bar model drawings, or inverted-V diagrams, when there is a unit in your curriculum that contains several word problems. If word problems are sporadic in your curriculum, you might introduce a "Word-Problem-of-the-Day" format where students solve a problem, or cluster of related problems, each day.
To emphasize model drawings, you might have students take a set of problems, and classify them as to which model would help them solve the problem, or do a matching activity between word problems and model drawings. Ask students to explain why a particular equation matches a model and would be useful in finding the solution. Another activity is to present a bar model with some numerical information and an unknown. Then ask students to write a word problem that could logically be solved using that model. Ask students to explain why the word problem created matches a diagram well. As students use models for solving word problems, they may generate different equations to solve a problem even though their models are the same. Plan for class discussions where students may discuss why there can be different equations from the same bar model.
Several studies have shown that students who can visualize a word problem through modeling increase their problem solving ability and accuracy. This has been particularly documented in Singapore and other high performing countries where bar modeling is used extensively across grades. Students are more likely to solve problems correctly when they incorporate bar model drawings. On difficult problems, students who have been able to easily generate equations with simple problems often find that bar model drawings are especially helpful in increasing accuracy as problems increase in difficulty or involve new concepts (Yeap, 2010, pp. 87-89).
TALK: Reflection and Discussion
- Are there particular types of word problems that your students solve more easily than others? What characterizes these problems?
- Identify some basic facts with which your students struggle. How could you incorporate those facts into word problems, and how might the use of the inverted-V model help?
- How do bar model drawings help extract and represent the mathematical components and numerical relationships of a word problem?
- With which type of word problems would you begin to show your students the use of bar model drawings?
DO: Action Plans
- Select several story problems from your curriculum, MCA sample test items, or the Forsten, Walker, or Yeap resources on bar model drawing. Practice creating a bar model for several problems. Compare your models with others in your grade level, team, or PLC group. Practice until you feel comfortable with various model drawings.
- Investigate the types of multiplication and division problems, and how bar models can be used with different types such as measurement and partitive division, arrays, equal groups, rates. The Carpenter resource may be helpful.
- Select some problems from your curriculum that are of a similar type. Which bar model would be helpful in solving this type of problem? Practice using the model yourself with several problems of this type. How will you introduce the model to your students?
- Identify some basic facts with which your students struggle. Craft some rich word problems utilizing these fact families. Introduce the inverted-V diagrams with the word problems to make sense of the information in the word problem, and discuss strategies for solving the problems.
- Initiate a "Word-Problem-of-the-Day". Students might want to keep problem solving notebooks. Begin with problems of a particular type, and show students how to use a bar model to represent the information in a problem. Cluster several problems of a given type during the week. What improvements do you see in student selection of appropriate equations, accuracy of solutions, and ability to estimate or justify their answers as they increase the use of bar models to solve the word problems? A quick way to disseminate the "Word-Problem-of-the-Day" is to duplicate the problem on each label on a sheet of address labels. Students can just peel off the daily problem, add it to their problem solving notebook or a sheet of paper and solve away.
- When your district is doing a curriculum materials review, advocate to include a criteria that requires the use of visual models in helping students make sense of mathematical problems.
- Watch some of the videos of students using models on the Powerful Practices CD (see Carpenter and Romberg in References and Resources Section).
References and Resources
Bruner, J. S. (1961). The act of discovery. Harvard Educational Review, 31, pp. 21-32, in Yeap, Ban Har. (2010). Bar modeling: A problem solving tool. Singapore: Marshall Cavendish Education.
Carpenter, T. P., Fennema, E., Franke, M. L., Levi, L. & Empson, S. B. (1999). Children's mathematics: Cognitively guided instruction. Portsmouth, NH: Heinemann. (Book and CD)
Carpenter, T. P. & Romberg. T. A. (2004). Modeling, generalization, and justification in mathematics cases, in Powerful practices in mathematics & science. Madison, WI: National Center for Improving Student Learning and Achievement in Mathematics and Science. www.wcer.wisc.edu/ncisla (Booklet and CD)
Dienes, Z. (undated). Zoltan Dienes' six-state theory of learning mathematics. Retrieved from http://www.zoltandienes.com
Forsten, C. (2009). Step-by-step model drawing: Solving math problems the Singapore way. Peterborough, NH: SDE: Crystal Spring Books. http:// www.crystalspringsbooks.com
Hoven, J. & Garelick, B. (2007). Singapore math: Simple or complex? Educational Leadership, 65 (3), 28-31.
Leinwand, S. (2009). Accessible mathematics: 10 instructional shifts that raise student achievement. Portsmouth, NH: Heinemann.
Marzano, R. J., Norford, J. S., Paynter, D. E., Pickering, D. J., & Gaddy, B. B. (2001). A handbook for classroom instruction that works. Alexandria, VA: Association for Supervision and Curriculum Development.
Singapore Ministry of Education. (1997). Retrieved http://moe.gov.sg
Skemp, R. R. (January, 1993). "Theoretical foundations of problem solving: A position paper." University of Warwick. Retrieved from http://www.grahamtall.co.uk/skemp/sail/theops.html
Walker, L. (2010). Model drawing for challenging word problems: Finding solutions the Singapore way. Peterborough, NH: SDE: Crystal Spring Books. http:// www.crystalspringsbooks.com
Yeap, B. H. (2010). Bar modeling: A problem solving tool. Singapore: Marshall Cavendish Education. http:// www.singaporemath.com
Thank you for visiting nature.com. You are using a browser version with limited support for CSS. To obtain the best experience, we recommend you use a more up to date browser (or turn off compatibility mode in Internet Explorer). In the meantime, to ensure continued support, we are displaying the site without styles and JavaScript.
- View all journals
- Explore content
- About the journal
- Publish with us
- Sign up for alerts
- Open access
- Published: 17 August 2024
Three layered sparse dictionary learning algorithm for enhancing the subject wise segregation of brain networks
- Muhammad Usman Khalid 1 ,
- Malik Muhammad Nauman 2 ,
- Sheeraz Akram 1 &
- Kamran Ali 2
Scientific Reports volume 14 , Article number: 19070 ( 2024 ) Cite this article
Metrics details
- Computational biology and bioinformatics
- Computational neuroscience
- Image processing
- Mathematics and computing
- Statistical methods
Independent component analysis (ICA) and dictionary learning (DL) are the most successful blind source separation (BSS) methods for functional magnetic resonance imaging (fMRI) data analysis. However, ICA to higher and DL to lower extent may suffer from performance degradation by the presence of anomalous observations in the recovered time courses (TCs) and high overlaps among spatial maps (SMs). This paper addressed both problems using a novel three-layered sparse DL (TLSDL) algorithm that incorporated prior information in the dictionary update process and recovered full-rank outlier-free TCs from highly corrupted measurements. The associated sequential DL model involved factorizing each subject’s data into a multi-subject (MS) dictionary and MS sparse code while imposing a low-rank and a sparse matrix decomposition restriction on the dictionary matrix. It is derived by solving three layers of feature extraction and component estimation. The first and second layers captured brain regions with low and moderate spatial overlaps, respectively. The third layer that segregated regions with significant spatial overlaps solved a sequence of vector decomposition problems using the proximal alternating linearized minimization (PALM) method and solved a decomposition restriction using the alternating directions method (ALM). It learned outlier-free dynamics that integrate spatiotemporal diversities across brains and external information. It differs from existing DL methods owing to its unique optimization model, which incorporates prior knowledge, subject-wise/multi-subject representation matrices, and outlier handling. The TLSDL algorithm was compared with existing dictionary learning algorithms using experimental and synthetic fMRI datasets to verify its performance. Overall, the mean correlation value was found to be \(26\%\) higher for the TLSDL than for the state-of-the-art subject-wise sequential DL (swsDL) technique.
Similar content being viewed by others
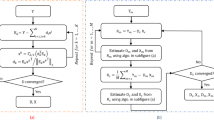
A novel subject-wise dictionary learning approach using multi-subject fMRI spatial and temporal components
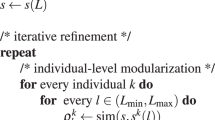
Iterative consensus spectral clustering improves detection of subject and group level brain functional modules
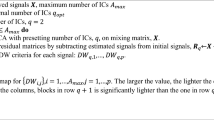
CW_ICA: an efficient dimensionality determination method for independent component analysis
Introduction.
During cognition or rest, fMRI measurements capture brain activity dynamics that are renowned for being noisy, which makes drawing statistical inferences a significant challenge 1 . Despite eliminating the noise sources due to machine, head motion, and physiology, the anatomical localization of neuronal responses triggered by the same stimulus might differ across brains 2 . This is further exacerbated by the experimental response’s divergence from the expected response due to variations in hemodynamics response (HR) across brains 3 . This makes hypothesis-driven univariate methodology such as the general linear model (GLM) in statistical parametric mapping (SPM) toolbox 4 an inferior approach. Mainly, the regressors for the design matrix of GLM constructed by convolving the HR function (HRF) with stimulus are incapable of adapting to variations across brains and their regions.
In contrast, a multivariate data-driven approach, for instance, blind source separation based matrix factorization, that recovers an approximation of the original signals, is fully adaptable without requiring prior knowledge about the mixing process of the training data. Diversity measures such as probabilistic distribution 5 , statistical attributes 6 , geometrical structures 7 , and sparse representation 8 have yielded several successful outcomes for fMRI analysis by unmasking hidden brain structures 9 , 10 , 11 . In particular, multivariate data matrix decomposition approaches have proven very beneficial for resting state functional connectivity studies and task-based activation identification 12 , 13 , 14 , 15 , 16 , 17 , 18 . Despite accounting for the idiosyncrasy, these constraint signal separation problems lack the estimation power for subject-wise analysis due to limited training data and the mathematical model’s inadequacy to take into consideration functional–anatomical brain differences and complexities, which other brains in group studies may offer 19 .
While group ICA 20 , multi-subject DL 21 , compressed online DL 22 , hybrid concatenation scheme for DL 23 , shared and subject-specific DL 24 , sparse group bases for DL 25 , and low-rank Tucker 2 model (LRT-2) 26 offer excellent analysis for group studies, some of them are irrelevant for resting state data or unable to yield subject-wise unique brain activity. Their model design endeavours to either (i) extract shared/individual dynamics where resting state data is complex to model due to the absence of shared temporal dynamics or (ii) aggregate dynamics to retrieve common variations where subject-wise analysis is exasperating to achieve because the model itself is ineffective.
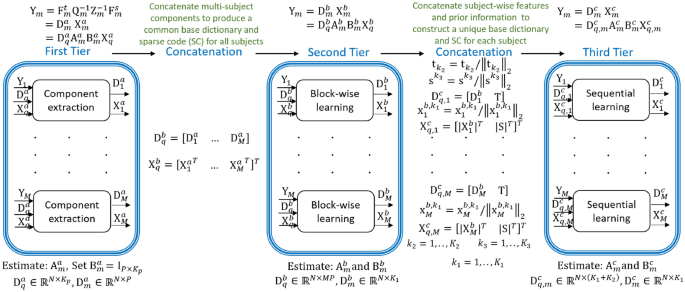
A flowchart describing the proposed multi-tier approach. The details of the mathematical notations that appeared in this figure are given in the method section.
Motivated by the shared neural response modeling 2 , 27 , the aforementioned problems were addressed and resolved in the recently proposed swsDL algorithm and its block variant swbDL 19 , which consisted of extracting the spatiotemporal components across brains using computationally efficient sparse spatiotemporal BSS (ssBSS) 28 method and integrating them through the multi-subject base dictionary, base sparse code, and sparse representation matrices (RMs). Combining common variations and separating unique ones from multiple subjects via sparse RMs provide statistical strength to subject-wise dictionary learning. However, due to minor segregation offered by ssBSS spatial components and the presence of background noise (outliers) in TCs, this approach may result in poor subject-wise discrimination potential for both swsDL and swbDL. One procedure to resolve these problems is to provide these algorithms with (i) atleast moderately segregated spatial activations, (ii) spatial and temporal prior information, and (iii) an outlier elimination scheme with automated suppression capability that down-weighs all those data points that are distant from the bulk of the data.
To address the inherent limitations of the BSS techniques compared to model-driven GLM, some data-driven approaches have allowed incorporating prior information 29 , 30 , 31 . Previous research has provided much evidence and discussion supporting the validity of the sparse assumption for the separation of fMRI signals 32 , 33 , 34 , 35 , and the benefits gained by including the prior information in the learning process in the form of modeled hemodynamic response functions (MHRs) and intrinsic brain networks (IBNs) 36 , 37 , 38 .
In this paper, instead of fixing the model-driven dictionary atoms to extract highly overlapped spatial maps as in the case of supervised dictionary learning 36 , a different approach is adopted where at the first two levels, multi-subject components from the ssBSS method are fed into the block-wise dictionary learning algorithm to extract subject-wise dictionary and sparse codes with moderate spatial overlaps, which are then concatenated with the normalized MHRs and IBNs, respectively. At the third level, sequential learning is performed using these concatenated dynamics. Moreover, the imposition of dictionary atom decomposition at the third level assures decomposition stability when facing variations (anomaly) from the Gaussian noise model. Here, we define the anomaly in the fMRI time series as time points showing increased noise levels, artifacts, and outliers. Anomalies may occur due to magnetic field instabilities, head movement, physiological influences, and various preprocessing steps involved in managing fMRI data.
It is noteworthy that only this three-level learning strategy can accommodate the proposed model for sequential learning given by equation ( 8 ) to perform (i) the decomposition of subject-wise data into multi-subject TCs and SMs, (ii) the incorporation of prior TCs and SMs information, and (iii) the elimination of outliers in the TCs. Analytically, it is observed that the three-level strategy enhances the separation of brain regions with high spatial overlaps. Overall, there is an increase in the likelihood of extracting source signals more closely resembling the ground truth. The three-level strategy is explained with the help of a block diagram in Fig. 1 .
Our proposed multi-factor matrix factorization approach is somewhat similar to the adaptive distribution modeling-based collaborative clustering approach (ADCoC) in regards to mathematical formulation; for instance, it decomposes the feature matrix into a pair of semi-orthogonal dictionaries and their corresponding coefficient matrix. However, there is a significant difference as our approach aims to decompose each subject’s whole-brain fMRI data into multi-subject dictionary/sparse and their corresponding representation matrices, whereas the ADCoC focuses on clustering subjects and features through non-negative matrix factorization 39 .
Methods and materials
Scalar values are indicated by lowercase or uppercase italic letters, vectors are designated by lowercase letters, and matrices are represented by uppercase letters. Vectors with subscripts and superscripts denote the particular column and row of the matrix, accordingly. Subscript m attached to a matrix/vector indicates the m th subject whose values are in this range \(m=\{1,\hdots ,M\}\) . Superscripts a , b , and c attached to a matrix/vector signify the first, second, and third level dictionary/sparse code/RMs, respectively. Subscript q attached to a matrix/vector defines a base dictionary/sparse code. Matrices \(\text {S} \in \mathbb {R}^{N\times K_3}\) and \(\text {T} \in \mathbb {R}^{N\times K_2}\) contain the IBNs and MHRs, respectively.
Why three level learning
There is no redundancy for decomposing each subject’s data using a three-level approach, as we are constantly adding features to both second and third-tier learning. In the second layer, the gain is derived from the multi-subject dynamics learned in the previous level. In the third tier, the gain is derived from preexisting neuroscience knowledge.
Why not add multi-subject features and prior information at the second level and replace three-level learning with two? This could have resulted in an inferior approach because spatial features obtained from ssBSS are not mature enough in terms of spatial localization to be on equal footing with the prior information. Dynamics that have been atleast moderately segregated would gain more by adding the prior information.
Using ssBSS at the first tier can also not be avoided due to the requirement of constructing a multi-subject base dictionary/sparse code while keeping the numerical cost minimal.
Another valid question is why not use sequential learning on both the second and third levels. The main reason to avoid this is the computing burden of sequential learning.
Why robust principle component analysis
Utilizing robust function through \(l_1\) data fidelity term 40 , a capped \(l_1\) norm loss function 41 , \(\alpha \) -divergence based data fidelity term 42 results in a computationally intensive weighted least squares (WLS) operation. Therefore, an alternative approach based on robust principal component analysis is considered and achieved using principal component pursuit (PCP).
Conventional robust learning using WLS may not work well when there is a base matrix involved because instead of the dictionary getting updated, only the representation matrix gets updated while the base dictionary keeps retaining the outliers.
Using PCP, all samples in the dictionary atoms away from the bulk of the data are automatically clipped based on a threshold value during each iteration of the sequential learning.
Three tier learning
Specifically, (i) at the first layer, the ssBSS method, for each subject, solved using alternating minimization optimization, produced preliminary spatiotemporal components that had minor spatial overlaps, (ii) at the second layer, solved using alternating minimization optimization, the swbDL algorithm exploited multi-subject bases assembled by the previous layer to produce intermediary spatial bases that were moderately but not entirely separated, and iii) at the third layer, the sequential learning algorithm solved using coordinate descent optimization first constructed the base dictionary and sparse code by concatenating subject-wise temporal and spatial components from the previous layer with MHRs and IBNs, respectively, then utilized this base dictionary to produce segregated spatial maps and outlier free temporal dynamics via alternating directions method for each subject.
First tier learning
The ssBSS method 28 was chosen at the first tier for its computational efficiency in constructing the multi-subject base dictionary for second-level learning. The first tier decomposition of fMRI data matrix \(\text {Y}_m \in \mathbb {R}^{N\times V}\) from m th subject into temporal source matrix \(\text {D}^a_m \in \mathbb {R}^{N\times P}\) and spatial source matrix \(\text {X}_m^a \in \mathbb {R}^{P\times V}\) was accomplished by considering the following dictionary learning optimization model with double sparsity 28 , 43
The smoothness of the blood-oxygen-level-dependent (BOLD) signal is explained by \(\text {D}_m^a=\text {D}_q^a\text {A}^a_m\) , which stores discrete cosine transform (DCT) bases in \(\text {D}_q^a \in \mathbb {R}^{N\times K_p}\) . The p -th column of the sparse representation matrix \(\text {A}^a_m\) , \(P<K_p<N\) , is given by \(\text {a}^a_{m,p}\) . The \(l_0\) norm, denoted as \(\left\Vert .\right\Vert _0\) , induced sparsity by quantifying the number of non-zero elements. The \(l_1\) norm on \(\text {X}^a_m\) is specified as \(\left\Vert \text {X}^a_m\right\Vert _1\) , and defined as \(\sum _{p=1}^{P} \sum _{j=1}^{V}|x^{a,p}_{m,j}|\) . Its associated sparsity hyperparameter \(\lambda _1\) controlled the values of the coefficients. The numerical burden associated with equation ( 1 ) became a significant concern when it had to be availed for second-level learning. To circumvent this issue, the blind source separation theory was used to decompose equation ( 1 ) into two distinct spatial and temporal source separation problems, enabling a computationally efficient solution as
where the matrices \(\text {F}_{m}^t \in \mathbb {R}^{N\times K}\) and \(\text {F}_{m}^s \in \mathbb {R}^{K\times V}\) obtained using principal component analysis (PCA) represented the temporal and spatial features in the reduced dimension, respectively. The mixing matrices \(\text {Q}_m \in \mathbb {R}^{P\times K}\) and \(\text {Z}_m \in \mathbb {R}^{K\times P}\) were unknowns. The sparsity regularization parameter \(\lambda _1\) was estimated using brute force with reference to correlation strength. However, cross-validation could have also been availed to fine-tune this parameter 44 . Equation ( 2 ) was solved until convergence using an alternating minimization approach for the unknown model variables via Neumann’s alternating projection lemma 45 .
Second tier learning
At this tier, the block design dictionary learning technique extracted subject-wise TCs and SMs using multi-subject dictionary/sparse codes as described in novel subject-wise learning method 19 . In the present situation, using the first tier components, the second tier base dictionary and sparse code were constructed as \(\text {D}_q^b = [\text {D}_1^a, \text {D}_2^a, \hdots , \text {D}_M^a] \in \mathbb {R}^{N\times MP}\) , and \(\text {X}_q^b = [\text {X}_1^{a^\top }, \text {X}_2^{a^\top }, \hdots , \text {X}_M^{a^\top }]^\top \in \mathbb {R}^{MP\times V}\) and their respective non-sparse representation matrices \(\text {A}_m^b \in \mathbb {R}^{MP\times K_1}\) and \(\text {B}_m^b \in \mathbb {R}^{K_1\times MP}\) were estimated using the following minimization
where tuning parameter \(\alpha \) is used to penalize the temporal correlation structure between the current \(\text {D}_m^b=\text {D}_q^b\text {A}_m^b\) and the lagged dictionary \(\text {D}_0\) , and \(\lambda _2\) is the sparsity tuning parameter. A combination of soft thresholding and least squares was used to estimate \(\text {B}_m^b\) as
Using a relaxation variable \(\text {U}\) , Eq. ( 3 ) can be reformulated as
The alternating directions method of multipliers (ADMM) technique was used to tackle this problem. It offered a closed-form solution for both \(\text {D}^b_m\) and \(\text {U}\) , required only one tuning parameter, and converged for all possible values 46 . The augmented Lagrangian for Eq. ( 5 ) lead us to the following closed-form solution for all unknown variables
where \(\beta _1\) is the tuning parameter and \(\mathscr {W}_1\) is the Lagrangian multiplier. Due to a dictionary-wide normalization restriction, every iteration involved normalizing every column in \(\text {D}^b_m\) and \(\text {U}\) . After initializing \(\text {U}\) and \(\mathscr {W}_1\) to zero, the above expressions were computed till convergence. Overall, Eq. ( 2 ) was solved using Eqs. ( 4 ) and ( 6 ) until convergence in an alternating minimization manner 19 .
Third tier learning
At third tier, the base dictionary and the base sparse code were constructed by the concatenation of subject-wise dictionary/sparse code from the previous tier and MHRs/IBNs as \(\text {D}^c_{q,m} = [\text {D}^b_m, \text {T}] \in \mathbb {R}^{N\times (K_1+K_2)}\) , and \(\text {X}^c_{q,m} = [\text {X}_m^{b^\top },\text {S}^\top ]^\top \in \mathbb {R}^{(K_1+K_3)\times V}\) . Their respective representation matrices were denoted as \(\text {A}_m^c \in \mathbb {R}^{(K_1+K_2)\times K_1}\) and \(\text {B}_m^c \in \mathbb {R}^{K_1\times (K_1+K_3)}\) . From Fig. 1 , the m th subject fMRI dataset can be decomposed as
Although \(\text {B}_m^a\) did not exist for our model, it could if we consider the point spread functions 47 along with the pre-defined network templates 48 . If there existed inverse matrices \(\text {Q}_m^{-1}\) and \(\text {Z}_m^{-1}\) that could factorize PCA features to spatial and temporal components (source matrices) such that \(K>P\) , then the above equation can be further decomposed to
From the above equation, one can draw an analogy between PCA+ICA, PCA+Canonical correlation analysis and dictionary learning. We decomposed each subject’s data into principal components, prior information, and representation (mixing) matrices using the proposed approach. Equations ( 2 ), ( 4 ), and ( 6 ) can be used to learn some of these mixing matrices, and the remaining can be learned by considering the proposed model for sequential learning. It decomposed each subject’s data into a dictionary (a superposition of a low-rank and a sparse matrix \(\text {H}_m+\Psi _m\) ) and a sparse code. This is presented as
This model may lead to redundant decomposition because we are not interested in the respective spatial maps of the sparse matrix \(\Psi _m\) , which only contains background noise. Moreover, it has been observed that the least absolute shrinkage and selection operator (LASSO) method may not be entirely efficient 49 , and its outcomes for model selection may lack consistency 50 . To resolve this, an analogous matrix version of the adaptive LASSO penalty 50 was introduced in the above equation where a data-driven regularization parameter \(\lambda ^{k_1}_{3,j}\) was allocated to each entry of \(\text {x}^{c,k_1}_{m,j}\) . This would allow the application of a more significant amount of shrinkage to entries near zero and a lesser amount to entries with substantial values, resulting in improved outcomes. In terms of sparse representation matrices, dictionary decomposition constraint in the form of \(\text {D}_m^c = \text {H}_m+\Psi _m\) , and adaptive sparse penalty for a fairer assignment of penalty to each entry in \(\text {X}_m^c\) , the above model can be reformulated as
where \(\text {D}^c_m=\text {D}^c_{q,m}\text {A}_{m}^c\) and \(\text {X}^c_m=\text {B}_{m}^c\text {X}^c_{q,m}\) . This objective function decomposed each subject’s data into third tier dictionary, sparse code, and their associated RMs, while imposing sparsity constraint on the sparse code, sparsity constraint on the RMs, and a low-rank and a sparse matrix decomposition constraint on \(\text {D}^c_m\) . This approach allowed us to remove outliers from the recovered TCs and enhance the segregation of highly overlapped spatial maps. However, Eq. ( 8 ) is not convex with respect to unknown matrices \(\text {A}_{m}^c\) , \(\text {B}_{m}^c\) , \(\text {H}_{m}\) , and \(\Psi _{m}\) but convex with respect to each of them when the others are fixed. Also, solving this problem is intractable because the unknowns \(\text {H}_m\) and \(\Psi _m\) do not appear in the cost function. Therefore, we split this objective function into two separate optimization problems by exploiting their relationship through \(\text {D}^c_m=\text {D}^c_{q,m}\text {A}_{m}^c\) given as
The PALM algorithm 51 is a classical optimization method used in sparse BSS, which iteratively updates both the dictionary and the sparse code using proximal gradient steps. It can be deployed to solve Eq. ( 9 ). It was preferred in our case due to the non-convexity (the overall optimization problem is nonconvex) and non-smoothness (contains absolute value function) of Eq. ( 9 ). Its fast convergence was guaranteed because it was shown that the sequence generated by PALM has a finite length property due to Kurdyka-Lojasiewicz (KL) inequality 51 . Whereas, the ALM algorithm, which in fewer iterations achieved higher accuracy than accelerated proximal gradient 52 can be used to solve the convex PCP problem for robust PCA given by Eq. ( 10 ) by repeatedly updating the low rank (full rank in our case, the rank can be ensured by carefully selecting the threshold value), sparse matrix and the Lagrange multiplier matrix. Specifically, ignoring the constraints on the mixing matrices, Eq. ( 9 ) according to PALM can be solved as
where \(\mathscr {S}_{\tau }(\text {x})\) denotes the shrinkage operator given as \({\textrm sgn}(\text {x})\circ (|\text {x}|-\tau )_+\) , and \(\Pi _{\left\Vert .\right\Vert _2\le 1}\) is the proximal operator of the oblique constraint given as a normalization constraint on each dictionary atom. However, for a sequential atom-wise update above equations can be re-written as
where the data-driven tuning parameters \(\lambda ^{k_1}_{3,j}\) are obtained as \(\lambda _3^{\dagger }=\lambda _3\text {1}/\Big (\text {d}_{m,k_1}^{c^\top }(\text {Y}_m-\text {D}_{m}^c\text {X}_m^{c}+\text {d}_{m,k_1}^c\text {x}_m^{c,k_1})\Big )\) . Here \(\text {1}\) is the vector of ones. On the other hand Eq. ( 10 ), which is the principal component pursuit comprising the minimization of \(l_1\) and nuclear norm 52 can be solved by considering the augmented Lagrangian as
which boils down to the following closed-form solution
where \(\mathscr {D}_{\beta }(\mathscr {M})=\text {U}\mathscr {S}_{\beta }(\Sigma )\text {V}^\top \) is a singular value thresholding operator, while \(\mathscr {M} = \text {U}\Sigma \text {V}^\top \) is the SVD of \(\mathscr {M}\) , and \(\mathscr {S}_{\tau }(x)\) denotes the shrinkage operator given as \(\textrm{sgn}(x)\circ (|x|-\tau )_+\) . This strategy would allow to decompose \(\text {D}^c_m\) during each iteration of the algorithm into low-rank and sparse components to remove gross corruptions from each of its recovered time courses.
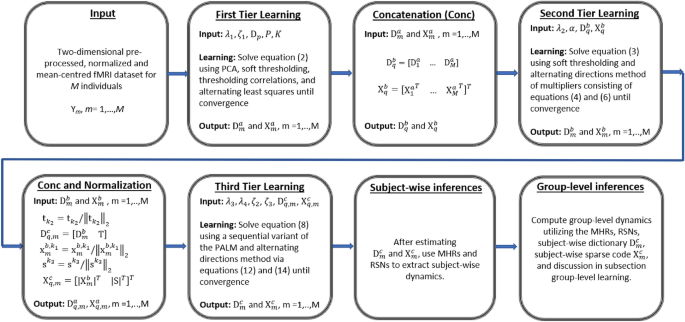
A block diagram of the multi-tier dictionary where first and second-tier learning extracted preliminary and intermediary components at low computational cost, enabling the third-tier learning to reconstruct the ground truth with high precision.
Group level learning
Since the proposed model (Eq. 8 ) was applied to many subjects, group-level analysis was also performed in addition to the subject-level data decomposition. This was accomplished by using neuroscience knowledge, which consisted of convolving task stimuli and the canonical HRF to construct modelled HRFs (MHRs) for task-related analysis, and acquiring resting-state network templates (RSNs) for resting-state analysis 53 . Firstly, the task-related group components were assembled by concatenating dictionary atoms/sparse codes using MHRs as \(\text {D}^c_r = [\text {d}^c_{1,j_1(r)}, \text {d}^c_{2,j_2(r)},\hdots ,\text {d}^c_{M,j_M(r)}]\) and \(\text {X}^c_r = \Big [\text {x}_1^{c,j_1(r)^\top }, \text {x}_2^{c,j_2(r)^\top },\hdots ,\text {x}_M^{c,j_M(r)^\top }\Big ]^\top \) . Secondly, the concatenated dictionary atoms and sparse codes were subjected to rank-1 decomposition, which was expressed as \( \frac{1}{M}[\text {D}^c_{r}\text {X}^c_r]=\omega _r\delta _r\gamma _r^\top \) , \(\text {d}^c_{g,r} = \omega _r\) , and \(\text {x}_{g}^{c,r} = \delta _r\gamma _r^\top \) .
Here \(r =\{1,\hdots ,R\}\) , the number of MHRs is denoted by R , the indices of the most associated atom in the m -th dictionary with the r -th MHR are indicated by \(j_m(r)\) , \(m=\{1,\hdots ,M\}\) , the group-level dictionary is \(\text {D}^c_g\) , the group-level sparse code is \(\text {X}^c_g\) , and the number of subjects is M . Alternatively, for the resting-state components, only sparse code is built as \(\Pi _r=\Big [\text {x}_1^{c,j_1(r)^\top }, \text {x}_2^{c,j_1(r)^\top }, \hdots , \text {x}_M^{c,j_M(r)^\top }\Big ]^\top \) and \(\text {x}_g^{c,r}=\frac{1}{M}\sum _{m=1}^{M} \pi _r^m\) .
Summing it all up, the TLSDL algorithm to solve the three-layered dictionary learning framework is presented in Fig. 2 . The third tier of the TLSDL algorithm for estimating robust dictionary and sparse code sequentially is described in Table 1 . In order to keep both recovered dynamics and prior information on equal footing, the MHRs, TCs, IBNs, and SMs were normalized, and the absolute values of IBNs and SMs were considered.
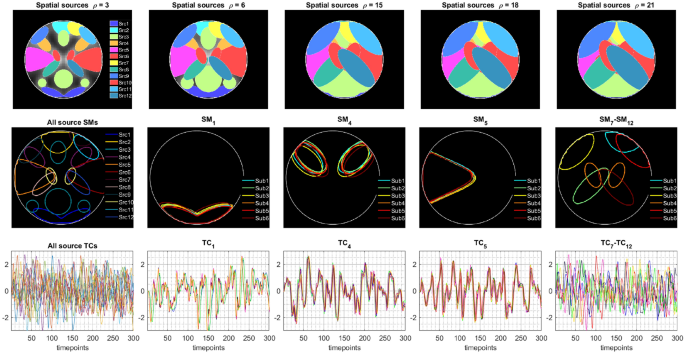
In the top row, spatial sources with moderate to significant overlaps are created by varying the size of twelve distinct activation blobs using seven different values of the spread parameter \(\rho \) , of which only five are shown, the first subfigure of the second row displays the SMs of the first subject and all unique sources from other subjects, while the other subfigures illustrate the position, shape, and variability of common spatial sources among subjects, as well as distinct spatial sources, and the first subfigure of the bottom row displays the respective TCs of the first subject and all unique sources from other subjects, while the other subfigures demonstrate the temporal variability of common sources, each with its own distinct temporal pattern, and unique temporal sources in the last subfigure.
Experiments
This section evaluated and validated the proposed algorithm by comparing it to the existing state-of-the-art source retrieval data-driven algorithms. This was accomplished by means of fMRI based data analysis, for which, two different fMRI datasets were considered, (i) simulated fMRI dataset for six subjects created using Simtb toolbox 54 , and (ii) block design experimental fMRI dataset for eight participants acquired from quarter 3 release of the Human Connectome Project (HCP) 55 , 56 . Notably, the proposed algorithm is applicable to task-related and resting state fMRI data, but only task-related dataset results have been provided in the paper to avoid increasing its length. The results of the resting state dataset are submitted as part of the Supplementary Material .
The sparse group independent component analysis (sgICA) 20 , 57 , compressed online dictionary learning (CODL) 22 , adaptive consistent sequential dictionary (ACSD) 58 , sparse spatiotemporal blind source separation (ssBSS) method 28 , subject-wise sequential dictionary learning (swsDL) 19 , and subject-wise block dictionary learning (swbDL) 19 are incorporated along with the proposed three-layered sparse dictionary learning (TLSDL) in the comparison study. The effectiveness of each algorithm was assessed based on how successfully it retrieved the spatial and temporal ground truth using the synthetic and experimental fMRI datasets. All algorithms were implemented in Matlab.
Synthetic dataset generation
In line with earlier fMRI research investigations 19 , 28 , a dataset of six individuals was generated in MATLAB using the Simtb toolbox to replicate the experimental fMRI data. The synthetic fMRI dataset was produced for six individuals, using twelve distinct temporal sources, each comprising 300 timepoints with a repetition time (TR) of 1 second and twelve different spatial sources, each containing a grid of 50 by 50 voxels. The synthetic brain was mapped using the source IDs \(\{3, 6, 8, 10, 22, 23, 26, 30, 4, 12, 5, 29\}\) to produce spatial components. For each individual, a total of seven spatiotemporal sources were used to generate the fMRI data from the source signals. As elaborated in 25 , the first six spatiotemporal sources were universally seen in all six subjects, although with some degree of intersubject variability. Conversely, the last six sources were distinct, with each subject being assigned one.
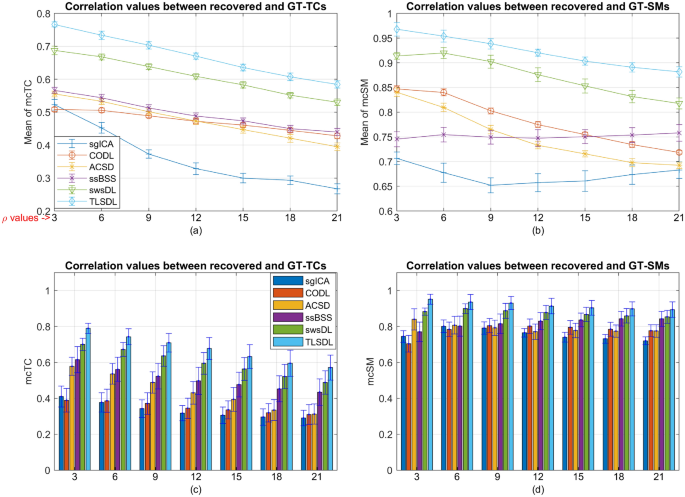
Correlation values shown with respect to GT-SMs for ( a,b ) subject-wise analysis computed over 7 source components, 6 subjects, 30 realizations, and 3 temporal noise variance cases, and ( c,d ) group-level analysis computed over 7 components, 30 realizations and 3 temporal noise variance case. In both cases, the deviation from the mean values has been plotted as error bars. \(\rho \) values along the x-axis indicate the extent of spatial overlap.
The HRF parameters, including delay, response dispersion, and undershoot, were modified with respect to shared temporal sources to introduce variability across subjects. Similarly, the variability across subjects for the common spatial maps was created by adjusting the parameters of the Gaussian distribution, namely the mean ( \(\mu \) ) and the deviation from the mean ( \(\sigma \) ). These parameters allowed for manipulation of the activations’ direction, position, and spread. This was achieved by applying a random translation with a mean of 0 and a standard deviation of 1 in both the x and y axes, a random rotation with a mean of 0 and a standard deviation of 0.9, and a random scaling with a mean of \(\rho \) and a standard deviation of 0.05, as seen in the middle row of Fig. 3 . The spread parameter, denoted by \(\rho \) , determines the structural range of the activations and generates seven unique instances of spatial overlaps. The values of \(\rho \) are \(\{3,6,9,12,15,18,21\}\) , representing the Gaussian distribution’s mean. The top row of Fig. 3 shows the spatial sources range, demonstrating a degree of interdependence from moderate to large.
The first subject contributes to the six common and one distinct sources, while the next five subjects each provide one distinct spatiotemporal sources. These sources were concatenated to create the ground truth time courses (TCs) and spatial maps (SMs) for group-level analysis, as seen in the left most column and bottom two rows of Fig. 3 serving as the primary case for the ground truth. The following subfigures illustrate the temporal and spatial sources used to generate each of the six datasets, represented by the equation \(\text {Y}_m=\sum _{i=1}^{7}(\text {tc}_i+\psi _i)(\text {sm}^i+\phi ^i)\) . The noise-generating matrices, \(\Psi \in \mathbb {R}^{300\times 7}\) and \(\Phi \in \mathbb {R}^{7\times 2500}\) , were created using Laplacian distributions with parameters \(\mathscr {L}(0,\,n_t)\) and \(\mathscr {L}(0,\,0.01)\) , respectively. All datasets were generated using Laplacian noise and some outliers were manually added at time points 60, 120, 180, 240. Subsequently, the datasets \(\text {Y}_{m=1}^M\) (with \(M=6\) ) were created and used by all source retrieval methods, contingent upon the values of \(\rho \) , \(n_t\) , and trial number.
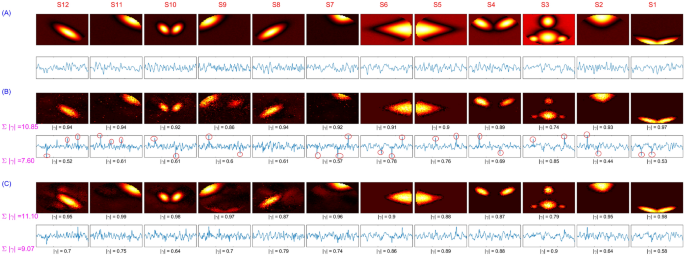
( A ) Spatial and temporal sources that were artificially generated, ( B ) spatial and temporal sources retrieved using swsDL, and ( C ) spatial and temporal sources restored using TLSDL. The absolute temporal and spatial correlation ( \(\gamma \) ) are shown for each source, with the total sum of correlation values on the left side. The red circles on the temporal sources derived from swsDL indicate the outliers that were effectively suppressed by TLSDL but were not reduced in intensity by swsDL.
Synthetic dataset dictionary learning
The same parameter values were used across all algorithms to provide an impartial comparison wherever feasible. In the case of sgICA and CODL, the desired number of components to be obtained was determined as 12 since they were applied to the grouped/concatenated data where 18 components were retained using the subject-wise PCA. However, in the case of other algorithms, it was acknowledged that the exact number of underlying sources for experimental fMRI data is unknown. Consequently, more components were allowed to be trained for the simulated dataset instead of learning an equal number of components as the number of generating sources. The components were configured to a value of 12 for ACSD, ssBSS, swsDL, and TLSDL, and these values were applied on a per-subject basis. These algorithms, including CODL, were executed for a total of 30 iterations. The optimal initialization for each method was determined by evaluating the initialization scenario utilizing the data, random number generator, and DCT bases separately. The ssBSS method used random numbers generated from the standard normal distribution, whereas the ACSD, swsDL, and TLSDL algorithms employed DCT bases, and CODL utilized initialization from the concatenated data.
We conducted several trials with various tuning parameter configurations. We selected the ones that produced the most favourable outcomes in terms of the similarity between the ground truth and the estimated sources. In the case of sgICA, twelve components were preserved after the second PCA reduction, although the first PCA yielded 18 components for each participant. The best values for sparsity and smoothing in the case of sgICA were found to be 3 and 50000, respectively. The tuning parameters for ssBSS were set as \(\zeta _1=60\) , \(\lambda _1=3\) , \(K_p=150\) , \(K=14\) , and \(P=7\) . The best sparsity parameter for ACSD was found to be 12. The best tuning parameters for swbDL were found to be \(\lambda _2=8\) , \(\alpha =100\) and \(K_1=12\) . The optimal tuning parameters for swsDL were determined to be \(\zeta _2=\zeta _3=24\) , \(\lambda _2=16\) , and \(K=12\) . The optimal parameters for TLSDL were found to be \(\zeta _2=\zeta _3=8\) , \(\lambda _3=8\) , \(\lambda _4=0.1\) , \(\alpha =150\) , and \(K_1=12\) .
Synthetic dataset results
The process of generating the synthetic dataset and learning from it using the participating algorithms was done several times for various instances of noise and spatial overlap to showcase the resilience and reliability of the proposed algorithm. In order to accomplish this, the experiment was repeated under the following conditions: (i) three different variance values (the square of the standard deviation) of the temporal noise, denoted as \(n_t=\{0.6, 0.9, 1.2\}\) , (ii) seven values of \(\rho \) ranging from 3 to 21, which were gradually increased to enlarge the activation overlaps as depicted in the top row of Fig. 3 , and (iii) a total of 30 trials.
Furthermore, the source recovery was conducted for both subject-level and group-level analysis. The underlying source TCs/SMs were acquired by selecting the indices with the greatest correlation values after correlating each algorithm’s trained dictionary atoms/sparse code rows with the ground truth TCs/SMs. The correlation values were calculated based on the ground truth SMs (GT-SMs) and were stored as cTC/sSM. For each of the participating algorithms and seven instances of spatial overlap, we calculated and saved mcTC/mcSM, which is the average of the cTC/cSM values over all seven spatiotemporal sources, three realizations of temporal noise, and thirty trials of the experiment. For both temporal and spatial sources, the mean of mcTC/mcSM values over 6 subjects is plotted in Fig. 4 a, b in the case of subject-wise analysis, and just the mcTC/mcSM values in the case of group-level analysis, because there was no need for the calculation of mean of correlation values over the subjects. Figure 5 shows the results of component-wise analysis related to the experiment that was repeated for \(\rho =8\) and \(n_t=0.6\) using swsDL and TLSDL to highlight the strength of the proposed algorithm. Figure 6 displays the pace at which the suggested algorithms converge and the evolution of average correlation values as a function of the number of algorithm iterations. It also shows the values recovered by the sparse matrix \(\Psi _m\) during the tenth iteration for the first subject.
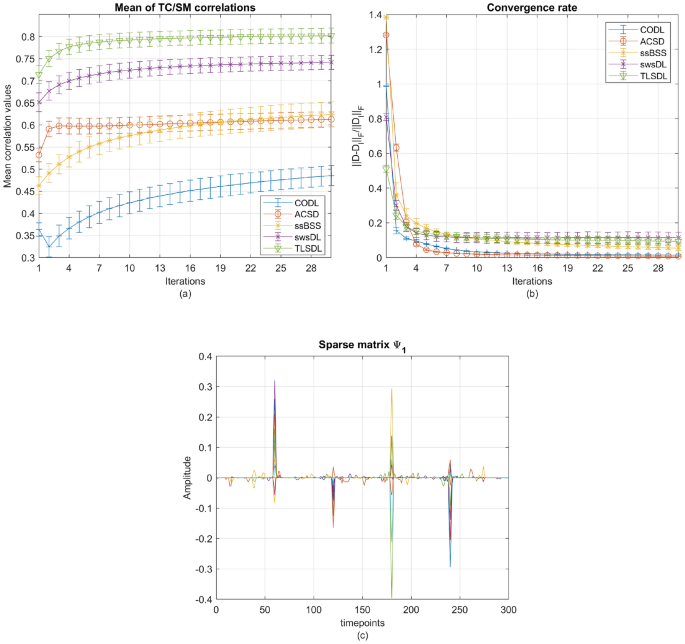
Based on the number of times the algorithm is run, the average of the ( a ) correlation values for all spatiotemporal sources and ( b ) convergence rate. The deviation from the mean values has also been plotted as error bars. Temporal background noise recovered by the sparse matrix during the tenth iteration of the algorithm is shown in subfigure ( c ).
Based on the results shown in Fig. 4 , it can be inferred that the proposed TLSDL repeatedly achieved better results than all other algorithms in various source recovery situations comprising spatial/temporal features, subject-wise/group-level analysis, and instances involving different spatial overlaps. By also taking Table 2 into account, it was revealed that TLSDL had superior recovery performance for all spatiotemporal sources without exception. Despite the rise in noise intensity and spatial overlaps, its performance remained superior to all other competing algorithms. Its competitor swsDL came in second place for the subject-wise analysis. It retained its place as the runner-up for both subject-wise and group-level analysis. Surprisingly, ssBSS, in the case of spatial source recovery, outperformed ACSD, CODL, and sgICA when spatial overlaps started to increase. Furthermore, it is worth noting that the proposed algorithm consistently exhibited a considerably higher level of performance for both subject-wise and group-level analysis without exception.
Figure 4 further revealed that the TLSDL algorithm consistently outperformed all other algorithms when it came to source recovery circumstances for both spatial/temporal feature instances and spatial overlap cases. It had the highest recovery rate, despite the fact that there was a significant degree of spatial dependency among sources and a high amount of temporal noise. It is important to note that despite the fact that its retrieval capability reduced as the number of spatial overlaps increased, it continued to retain its greater recovery performance. In contrast, the swsDL method came out on top as a runner-up, despite the fact that it had relatively lower deviations from the mean correlation value for both the spatial and temporal recovery scenarios than TLSDL. The ssBSS method, much like the swsDL algorithm, displayed reduced standard deviations; nonetheless, the findings of its mean correlation were not very spectacular. Overall, as spatial overlaps kept increasing, the TLSDL algorithm performance stayed relatively better.
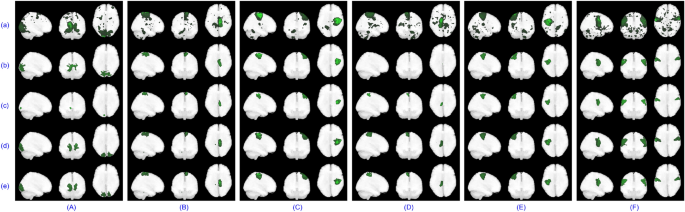
The visual cue ( A ), left toe ( B ), left finger ( C ), right toe ( D ), right finger ( E ), and tongue ( F ) movement tasks of the block design dataset were analyzed using five different methods: ( a ) CODL, ( b ) ACSD, ( c ) swbDL, ( d ) swsDL, and ( e ) TLSDL. The extracted common activation maps were thresholded at a significance level of \(p<0.001\) using random field correction. The correlation values that correspond to these spatial maps are provided in Table 3 .
Based on the information provided in Fig. 5 C, it can be inferred that TLSDL effectively eliminated all outliers and, as a result, achieved a better overall temporal correlation strength compared to swsDL. In addition to this, one can also observe that SMs recovered by TLSDL had better correlation strength because of its higher capability of segregating spatial maps.
Figure 6 c shows the background noise eliminated from dictionary atoms during the tenth iteration of the algorithm. It clearly clipped the outliers from the TCs. It can be deduced from the data shown in Fig. 6 b that both the TLSDL algorithm and the swsDL approach converged at an equal pace. This tendency was also seen in Fig. 6 a, where it was observed that the correlation strength for both TLSDL and swsDL practically stopped rising after the tenth iteration. On the other hand, when the number of iterations increased, both ACSD and ssBSS continued to demonstrate progress in source recovery, although the ssBSS technique converged slowly.
A source-wise group-level analysis was performed using 30 trials, 3 temporal noise instances, and 7 spatial dependence situations on 6 algorithms, and the findings are shown in Table 2 . In general, it is clear from this data that TLSDL emerged as the victor in the source recovery of each spatiotemporal component, with swsDL coming in as the runner-up.
Block design dataset
The motor task’s 3T MRI raw block design dataset was acquired from the HCP’s quarter 3 release. The experimental specifics may be found in the reference 19 , 55 , 56 . The dataset, which depicts the brain’s motor cortex, was obtained during a 204-second experiment. The experiment provided the participants with instructions to react to visual stimuli by tapping either their right or left fingers, pinching their right or left toes, or moving their tongues. Participants performed a specific motor task lasting 12 seconds after a three-second visual cue. A total of ten movement tasks were taken into account, including two tongue motions, movements of the left and right fingers, and movements of the left and right toes. There were a total of 13 blocks, three of which were fixation blocks lasting for 15 seconds each. Six simulated MHRs were generated using the standard hemodynamic response function and task stimuli associated with five distinct forms of movement: tongue (T), left toe (LT), right toe (RT), left finger (LF), right finger (RF), and visual type cue (VC). The purpose was to get accurate ground truth time courses (TCs). After normalisation, these six MHRs were also stored in the matrix \(\text {T}\) to be used by the propose method.
The fMRI scan for each individual was acquired using a Siemens 3 Tesla (3T) scanner. The following were the acquisition parameters: The data acquisition parameters for the MRI scan are as follows: there were 72 consecutive slices with isotropic voxels measuring \(2 \ \textrm{mm}\) each. The echo time (TE) is \(33.1 \ \textrm{ms}\) , the repetition time (TR) is \(0.72 \ \textrm{s}\) , and the field of view (FOV) is \(208 \times 180 \ \textrm{mm}\) . The flip angle (FA) is 52 \(^\circ \) , and the matrix size is 104 by 90. The slice thickness is 2 mm, and the echo spacing is \(0.58 \ \textrm{ms}\) . A total of 284 EPI volumes were collected, and the first five scans were discarded. The dataset used in our analysis comprised eight people, ages spanning from 22 to 35.
Block design dataset preprocessing
The block design dataset was preprocessed using the SPM-12 toolbox 4 , as mentioned in the paper 19 . The dataset’s preparation processes, which include masking, realignment, normalizing, and spatial smoothing, are extensively described in 25 , 59 . To mitigate motion artifacts, functional images were aligned with the first image. Subsequently, all images were subjected to spatial smoothing using a Gaussian kernel with a full-width at half-maximum (FWHM) of \(6\times 6 \times 6\) \(\textrm{mm}^3\) . This was done after spatial normalization to a Tailarach template and resampling to \(2 \times 2 \times 2\) \(\textrm{mm}^3\) voxels. Next, an endeavour was made to exclude data beyond the scalp during the masking phase. The dataset with four dimensions was then transformed and saved as a two-dimensional matrix called \(\text {Y}\) for each subject to be regarded as a complete brain dataset.
After completing this phase, a dataset \(\text {Y}\) was generated with a size of \(279 \times 236115\) for the m -th subject, where m ranges from 1 to 8. Subsequently, temporal filtering was implemented on the \(\text {Y}\) matrix derived from all individuals. This included the implementation of a low-pass filter using FWHM to eliminate high-frequency physiological noise and a high-pass filter utilizing DCT to eliminate low-frequency trends. An FWHM cutoff of 1 second and a DCT filter cutoff of 1/150 Hz were used. Following the previously explained methodology, the variable \(\text {Y}\) was normalized to have a mean of zero and a variance of one.
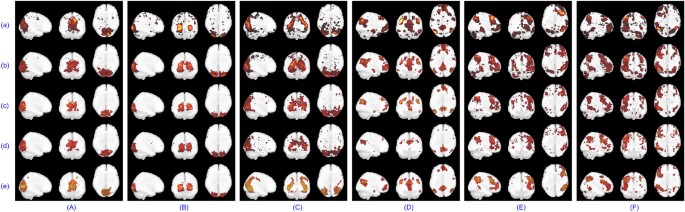
The group-level activation maps using five different methods: ( a ) CODL, ( b ) ACSD, ( c ) swbDL, ( d ) swsDL, and ( e ) TLSDL that were threshold using a random field correction with a significance level of \(p<0.001\) . The activation maps were derived for six different templates: ( A ) medial visual, ( B ) occipital pole visual, ( C ) lateral visual, ( D ) default mode network, ( E ) frontoparietal right, and ( F ) frontoparietal left.The correlation values corresponding to these spatial maps are reported in Table 3 .
Block design dataset dictionary learning
We experimented with a diverse array of tuning parameter combinations. The ones that yielded the most accurate results in terms of the degree of similarity between the recovered sources and the ground truth were considered. The dictionary initialization process used concatenated data, random numbers, and DCT bases for CODL, ssBSS, and ACSD/swbDL/swsDL/TLSDL, respectively. These initializations were agreed upon after testing each for the competing algorithms. The dictionary learning algorithms were executed for 15 iterations, except for the CODL and ssBSS algorithms, which were executed for 30 iterations due to their slow convergence.
During the process of dimensionality reduction for sgICA, a total of 100 components were preserved after using PCA for the first time. Subsequently, when PCA was used for the second time, only 60 components were maintained. The exact number of components was also retrieved using sgICA. The sparsity parameter for sgICA was set to 5, while the smoothing value was set at 50000. For the ssBSS algorithm, a total of 60 components were selected from the PCA, and 40 of them were retained, and its remaining parameters were assigned the values of \(\lambda _1=16\) , \(\zeta _1=50\) , \(K_p=60\) , \(K=60\) , \(P=40\) . The CODL training process included training 70 dictionary atoms, with a sparsity parameter set to 6 and a batch size set to the data dimension. The ACSD algorithm was used to train 40 dictionary atoms for each subject, with a sparsity parameter set to 60. Both swbDL and swsDL were trained using a total of 40 atoms. The tuning parameters for swbDL were set as \(\lambda _2=12\) and \(\alpha =3000\) , while for swsDL, the tuning parameters were set as \(\zeta _2=\zeta _3=48\) and \(\lambda _2=25\) . The optimal parameters for TLSDL were found to be \(\lambda _4=0.15\) , \(\alpha =150\) , \(\lambda _3=12\) , \(\zeta _2=\zeta _3=8\) , and \(K_1=40\) .
Block design dataset results
In this section, the unavailability of task-related activation maps prompted us to use temporal analysis with six created MHRs as our chosen method of investigation. Similarly, we used Smith’s templates because resting state networks (RSNs) do not include temporal profiles. These MHRs and RSNs also became the entries of matrices \(\text {T}\) and \(\text {S}\) , respectively. Both subject-wise and group-level techniques served as the basis for the study; however, for the sake of this article, we will only be discussing group-level analysis. When doing the subject-wise analysis, the TCs and SMs for each subject obtained from ACSD, swbDL, swsDL, and TLSDL were considered. Meanwhile, group-level TCs and SMs were trained for CODL and sgICA, and the individual TCs/SMs were obtained through back reconstruction. On the other hand, the group-level TCs and SMs produced by each competing algorithm were used as a reference for the group-level evaluation. The common TCs and SMs for ACSD, swbDL, swsDL, and TLSDL were extracted for the purpose of group-level analysis. The discussions in the subsection on group-level TLSDL were used to extract these TCs and SMs. Ultimately, these common TCs were correlated with MHRs and averaged SMs were correlated with RSNs. We retained the atoms and sparse codes with the most significant correlation values. One may consult the ideas mentioned in 25 to get further information on this extraction.
Only a limited number of activation maps and temporal dynamics have been shown to avoid prolonging the study, even though there were quite a few of them for the block design dataset. Figure 7 shows a series of two-dimensional images blended to illustrate three-dimensional volume for various group-level movement activities. Similarly, the results of the resting-state study, in this instance, only represent a subset of the total. For example, refer to Fig. 8 , which presents the SMs for the first four and last two resting state network templates. According to the data shown in Table 3 , the proposed TLSDL algorithm surpassed all other algorithms in terms of overall performance. It generated atoms and sparse codes with the greatest correlation with the ground truth, while the swsDL algorithm came in second place. The TLSDL algorithm successfully recovered all task-related and resting-state networks with highest specificity.
The spatial maps shown in Fig. 7 make it abundantly evident that, in comparison to the activations discovered by other approaches, the activations discovered by swbDL, swsDL, and TLSDL are more exclusively confined to the motor area. According to Table 3 , it is possible to assert that, when considering correlation values, TLSDL yielded superior results compared to the other approaches. This was also supported graphically by Fig. 8 , demonstrating that the resting state networks recovered by TLSDL were more specific and consistent in terms of sensitivity than those generated by other methods.
The correlation strength accumulation and convergence rate of the CODL, ACSD, swbDL, swsDL, and TLSDL algorithms are shown in Fig. 9 , which is presented in terms of algorithm iterations. From this vantage point, it is clear that the TLSDL algorithm gradually gained an advantage over its contenders. The outliers recovered by the TLSDL in the sparse matrix for the first subject during the fifteenth iteration are also shown in this figure.
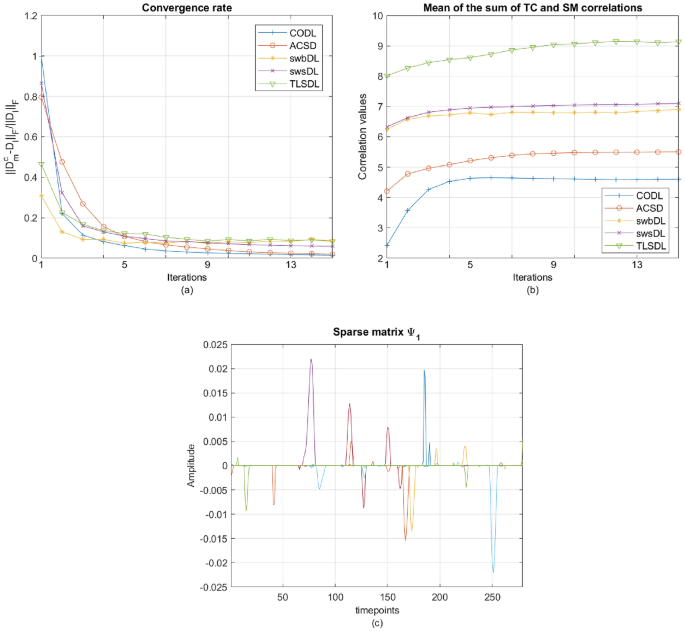
The mean ( a ) correlation values, and ( b ) convergence rate for subject-wise dictionary learning calculated as a function of algorithm iterations. Temporal background noise recovered by the sparse matrix during the fifteenth iteration of the algorithm is shown in subfigure ( c ).
A novel multi-tier semi-blind (hypothesis and data-driven) approach that ameliorates subject-wise dictionary learning has been presented in this paper. Using sparse assumption, three types of learning approaches that are (i) reduced dimension, (ii) block-wise, and (iii) sequential, realized in a multi-tier framework, allowed the extraction of spatial and temporal components that were more comparable to the ground truth and produced better group-level and subject-wise atoms/sparse codes. This is probably because components with significant spatial overlaps have higher chances of segregation when the prior information and outliers removal scheme, in addition to sparse assumption, are incorporated into the model. The primary advantage of this proposed strategy is that, in contrast to swsDL, it recovers very localized fMRI networks and produces noise-free dictionary atoms. It achieves this at the cost of slightly more computational complexity. Like swsDL, it can be used for both resting-state connectivity analysis and task-related activation detection of fMRI datasets. However, this paper has not considered resting-state experimental data to avoid increasing the paper length.
Moreover, the proposed algorithm deploys a unique technique for subject-wise BSS by using the underlying spatiotemporal dynamics from several subjects and prior information in a multi-tier manner while avoiding redundancy at every level. This approach allows for the inclusion of comparable components from the reduced-dimension space in order to exploit the spatiotemporal heterogeneity offered by other subjects, resulting in increased statistical power of the recovered components, which are adequately separated from each other. When combined with prior information, these components result in even better segregation in the presence of high spatial dependence. When integrated with outlier removal scheme, the recovered TCs show promising resemblance with the ground truth. The proposed model draws an analogy between PCA+ICA and dictionary learning (PCA+RMs), emphasising the training of spatiotemporal representation matrices at three layers using PCA based components. The efficacy of the suggested approach has been shown using both synthetic and experimental fMRI datasets, and its performance is found to be robust and consistent across datasets. Empirically, it is shown that the TLSDL algorithm achieved convergence for all cases, and can be considered a plausible alternative to swsDL.
Data and code availability
The experimental fMRI datasets used in this study are open-access and shared on the human connectome website. The Matlab code implemented for this study will be available from the corresponding author upon reasonable request.
Friston, K. J. Modalities, modes, and models in functional neuroimaging. Science 326 , 399–403. https://doi.org/10.1126/science.1174521 (2009).
Article ADS PubMed Google Scholar
Huang, J. et al. Learning shared neural manifolds from multi-subject FMRI data. In EEE International Workshop on Machine Learning for Signal Processing (MLSP) . 01–06 (2022).
Aguirre, G. K., Zarahn, E. & D’esposito, M. The variability of human, BOLD hemodynamic responses. Neuroimage 8 , 360–369. https://doi.org/10.1006/nimg.1998.0369 (1998).
Article PubMed Google Scholar
Penny, W., Friston, K., Ashburner, J., Kiebel, S. & Nichols, T. Statistical Parametric Mapping: The Analysis of Functional Brain Images (Academic Press, 2007).
Moussaoui, S., Brie, D., Caspary, O. & Mohammad-Djafari, A. A Bayesian method for positive source separation. In IEEE International Conference on Acoustics, Speech, and Signal Processing . vol. 5. V-485 (2004).
Hyvärinen, A. & Oja, E. Independent component analysis: Algorithms and applications. Neural Netw. 13 , 411–430. https://doi.org/10.1016/S0893-6080(00)00026-5 (2000).
Zhuang, X., Yang, Z. & Cordes, D. A technical review of canonical correlation analysis for neuroscience applications. Human Brain Mapp. 41 , 3807–3833. https://doi.org/10.1002/hbm.25090 (2020).
Article Google Scholar
Aharon, M., Elad, M. & Bruckstein, A. K-SVD: An algorithm for designing overcomplete dictionaries for sparse representation. IEEE Trans. Signal Process. 54 , 4311–4322. https://doi.org/10.1109/TSP.2006.881199 (2006).
Article ADS Google Scholar
Khalid, M. U. & Seghouane, A.-K. Constrained maximum likelihood based efficient dictionary learning for fMRI analysis. In IEEE International Symposium on Biomedical Imaging (ISBI) . 45–48 (2014).
Khalid, M. U. & Seghouane, A.-K. Unsupervised detrending technique using sparse dictionary learning for fMRI preprocessing and analysis. In 2015 IEEE International Conference on Acoustics, Speech and Signal Processing (ICASSP) . 917–921 (2015).
Khalid, M. U. & Seghouane, A.-K. Multi-subject fMRI connectivity analysis using sparse dictionary learning and multiset canonical correlation analysis. In IEEE International Symposium on Biomedical Imaging (ISBI) . 683–686 (2015).
McKeown, M. J. et al. Spatially independent activity patterns in functional MRI data during the stroop color-naming task. Proc. Natl. Acad. Sci. USA 95 , 803–810. https://doi.org/10.1073/pnas.95.3.803 (1998).
Article ADS PubMed PubMed Central Google Scholar
Friman, O., Borga, M., Lundberg, P. & Knutsson, H. Exploratory fMRI analysis by autocorrelation maximization. Neuroimage 16 , 454–464. https://doi.org/10.1006/nimg.2002.1067 (2002).
Khalid, M. U., Shah, A. & Seghouane, A.-K. Adaptive 2DCCA based approach for improving spatial specificity of activation detection in functional MRI. In International Conference on Digital Image Computing Techniques and Applications (DICTA) . 1–6 (2012).
Khalid, M. U. & Seghouane, A.-K. Improving functional connectivity detection in fMRI by combining sparse dictionary learning and canonical correlation analysis. In IEEE International Symposium on Biomedical Imaging . 286–289 (2013).
Shah, A., Khalid, M. U. & Seghouane, A.-K. Comparing causality measures of fMRI data using PCA, CCA and vector autoregressive modelling. In International Conference of the IEEE Engineering in Medicine and Biology Society . 6184–6187 (2012).
Khalid, M. U., Shah, A. & Seghouane, A.-K. Sparse dictionary learning for fMRI analysis using autocorrelation maximization. In Annual International Conference of the IEEE Engineering in Medicine and Biology Society (EMBC) . 4286–4289 (2015).
Lin, W., Wu, H., Liu, Y., Lv, D. & Yang, L. A CCA and ICA-based mixture model for identifying major depression disorder. IEEE Trans. Med. Imaging 36 , 745–756. https://doi.org/10.1109/TMI.2016.2631001 (2017).
Khalid, M. U. & Nauman, M. M. A novel subject-wise dictionary learning approach using multi-subject fMRI spatial and temporal components. Sci. Rep. 13 , 20201. https://doi.org/10.1038/s41598-023-47420-1 (2023).
Calhoun, V. D., Adali, T., Pearlson, G. D. & Pekar, J. J. A method for making group inferences from functional MRI data using independent component analysis. Hum. Brain Mapp. 14 , 140–151. https://doi.org/10.1002/hbm.1048 (2001).
Article PubMed PubMed Central Google Scholar
Varoquaux, G., Gramfort, A., Pedregosa, F., Michel, V. & Thirion, B. Multi-subject dictionary learning to segment an atlas of brain spontaneous activity. Inf. Process Med. Imaging 22 , 562–573. https://doi.org/10.1007/978-3-642-22092-0_46 (2011).
Mensch, A., Varoquaux, G. & Thirion, B. Compressed online dictionary learning for fast resting-state fMRI decomposition. In 2016 IEEE 13th International Symposium on Biomedical Imaging (ISBI) . 1282–1285 (2016).
Iqbal, A. & Seghouane, A.-K. A dictionary learning algorithm for multi-subject fMRI analysis based on a hybrid concatenation scheme. Digital Signal Process. 83 , 249–260. https://doi.org/10.1016/j.dsp.2018.09.007 (2018).
Iqbal, A., Seghouane, A.-K. & Adali, T. Shared and subject-specific dictionary learning (ShSSDL) algorithm for multisubject fMRI data analysis. IEEE Trans. Biomed. Eng. 65 , 2519–2528. https://doi.org/10.1109/TBME.2018.2806958 (2018).
Khalid, M. U. Sparse group bases for multisubject fMRI data. IEEE Access 10 , 83379–83397. https://doi.org/10.1109/ACCESS.2022.3194651 (2022).
Han, Y. et al. Low-rank Tucker-2 model for multi-subject fMRI data decomposition with spatial sparsity constraint. IEEE Trans. Med. Imag. 41 , 667–679. https://doi.org/10.1109/TMI.2021.3122226 (2022).
Chen, P.-H. C. et al. A reduced-dimension fMRI shared response model. In Advances in Neural Information Processing Systems . Vol. 28 (Curran Associates, Inc., 2015).
Khalid, M. U., Khawaja, B. A. & Nauman, M. M. Efficient blind source separation method for fMRI using autoencoder and spatiotemporal sparsity constraints. IEEE Access 11 , 50364–50381. https://doi.org/10.1109/ACCESS.2023.3277543 (2023).
Hu, D. et al. Unified SPM-ICA for fMRI analysis. Neuroimage 25 , 746–755. https://doi.org/10.1016/j.neuroimage.2004.12.031 (2005).
Calhoun, V. D., Adali, T., Stevens, M. C., Kiehl, K. A. & Pekar, J. J. Semi-blind ICA of fMRI: A method for utilizing hypothesis-derived time courses in a spatial ICA analysis. Neuroimage 25 , 527–538. https://doi.org/10.1016/j.neuroimage.2004.12.012 (2005).
Wang, Z., Xia, M., Jin, Z., Yao, L. & Long, Z. Temporally and spatially constrained ICA of fMRI data analysis. PLoS One 9 , e94211. https://doi.org/10.1371/journal.pone.0094211 (2014).
Daubechies, I. et al. Independent component analysis for brain fMRI does not select for independence. Proc. Natl. Acad. Sci. USA 106 , 10415–10422. https://doi.org/10.1073/pnas.0903525106 (2009).
Lee, K., Tak, S. & Ye, J.C. A data-driven sparse GLM for fMRI analysis using sparse dictionary learning with MDL criterion. IEEE Trans. Med. Imaging 30 , 1076–1089 https://doi.org/10.1109/TMI.2010.2097275 (2011).
Seghouane, A.-K. & Khalid, M. U. Hierarchical sparse brain network estimation. In 2012 IEEE International Workshop on Machine Learning for Signal Processing . 1–6 (2012).
Seghouane, A.-K. & Khalid, M. U. Learning dictionaries from correlated data: Application to fMRI data analysis. In IEEE International Conference on Image Processing (ICIP) . 2340–2344 (2016).
Zhao, S. et al. Supervised dictionary learning for inferring concurrent brain networks. IEEE Trans. Med. Imaging 34 , 2036–2045. https://doi.org/10.1109/TMI.2015.2418734 (2015).
Long, Z., Liu, L., Gao, Z., Chen, M. & Yao, L. A semi-blind online dictionary learning approach for fMRI data. J. Neurosci. Methods 323 , 1–12. https://doi.org/10.1016/j.jneumeth.2019.03.014 (2019).
Morante, M., Kopsinis, Y., Theodoridis, S. & Protopapas, A. Information assisted dictionary learning for fMRI data analysis. IEEE Access 8 , 90052–90068. https://doi.org/10.1109/ACCESS.2020.2994276 (2020).
Liu, H. et al. ADCoC: Adaptive distribution modeling based collaborative clustering for disentangling disease heterogeneity from neuroimaging data. IEEE Trans. Emerg. Top. Comput. Intell. 7 , 308–318. https://doi.org/10.1109/TETCI.2021.3136587 (2023).
Lu, C., Shi, J. & Jia, J. Online robust dictionary learning. In 2013 IEEE Conference on Computer Vision and Pattern Recognition . 415–422 https://doi.org/10.1109/CVPR.2013.60 (2013).
Jiang, W., Nie, F. & Huang, H. Robust dictionary learning with capped l1-norm. In Proceedings of the 24th International Conference on Artificial Intelligence , IJCAI’15. 3590–3596 (AAAI Press, 2015).
Iqbal, A. & Seghouane, A.-K. Robust dictionary learning using \(\alpha \) -divergence. In ICASSP 2019—2019 IEEE International Conference on Acoustics, Speech and Signal Processing (ICASSP) . 2972–2976 https://doi.org/10.1109/ICASSP.2019.8683210 (2019).
Seghouane, A.-K. & Iqbal, A. Basis expansion approaches for regularized sequential dictionary learning algorithms with enforced sparsity for fMRI data analysis. IEEE Trans. Med. Imaging 36 , 1796–1807. https://doi.org/10.1109/TMI.2017.2699225 (2017).
Stone, M. An asymptotic equivalence of choice of model by cross-validation and Akaike’s criterion. J. R. Stat. Soc. Ser. B (Methodol.) 39 , 44–47 (1977).
von Neumann, J. Functional Operators Volume 2: The Geometry of Orthogonal Spaces. (Princeton University Press, 1951).
Lin, Z., Chen, M. & Ma, Y. The augmented Lagrange multiplier method for exact recovery of corrupted low-rank matrices. J. Struct. Biol. 181 , 116–127 https://doi.org/10.1016/j.jsb.2012.10.010 (2013).
Progress in Optics (Elsevier, 2008).
Vanasse, T. J. et al. BrainMap VBM: An environment for structural meta-analysis. Hum. Brain Mapp. 39 , 3308–3325. https://doi.org/10.1002/hbm.24078 (2018).
Fan, J. & Li, R. Variable selection via nonconcave penalized likelihood and its oracle properties. J. Am. Stat. Assoc. 96 (1348–1360), 3085904 (2001).
MathSciNet Google Scholar
Zou, H. The adaptive Lasso and its oracle properties. J. Am. Stat. Assoc. 101 , 1418–1429. https://doi.org/10.1198/016214506000000735 (2006).
Article MathSciNet Google Scholar
Bolte, J., Sabach, S. & Teboulle, M. Proximal alternating linearized minimization for nonconvex and nonsmooth problems. Math. Prog. 146 , 459–494. https://doi.org/10.1007/s10107-013-0701-9 (2014).
Candes, E. J., Li, X., Ma, Y. & Wright, J. Robust Principal Component Analysis? https://doi.org/10.48550/arXiv.0912.3599 (2009).
Smith, S. M. et al. Correspondence of the brain’s functional architecture during activation and rest. Proc. Natl. Acad. Sci. 106 , 13040–13045. https://doi.org/10.1073/pnas.0905267106 (2009).
Erhardt, E., Allen, E., Wei, Y., Eichele, T. & Calhoun, V. SimTB, a simulation toolbox for fMRI data under a model of spatiotemporal separability. NeuroImage 59 , 4160–7. https://doi.org/10.1016/j.neuroimage.2011.11.088 (2011).
Van Essen, D. C. et al. The human connectome project: A data acquisition perspective. Neuroimage 62 , 2222–2231 (2012).
Barch, D. M. et al. Function in the human connectome: Task-fMRI and individual differences in behavior. Neuroimage 80 , 169–189. https://doi.org/10.1016/j.neuroimage.2013.05.033 (2013).
Boukouvalas, Z., Levin-Schwartz, Y., Calhoun, V. D. & Adalı, T. Sparsity and independence: Balancing two objectives in optimization for source separation with application to fMRI analysis. J. Franklin Inst. 355 , 1873–1887. https://doi.org/10.1016/j.jfranklin.2017.07.003 (2018).
Seghouane, A.-K. & Iqbal, A. Consistent adaptive sequential dictionary learning. Signal Process. 153 , 300–310. https://doi.org/10.1016/j.sigpro.2018.07.018 (2018).
Khalid, M. U. Dictionary Learning Algorithms for Functional Magnetic Resonance Imaging . Ph.D. thesis, Australian National University (2015).
Download references
Acknowledgements
The work presented in the article is financially supported by Universiti Brunei Darussalam, Brunei Darussalam, through its University Research Grant scheme (grant number: UBD/RSCH/1.3/FICBF(b)/2022/019) The block design fMRI data was provided [in part] by the Human Connectome Project, WU-Minn Consortium (Principal Investigators: David Van Essen and Kamil Ugurbil; 1U54MH091657) funded by the 16 NIH Institutes and Centers that support the NIH Blueprint for Neuroscience Research; and by the McDonnell Center for Systems Neuroscience at Washington University.
Author information
Authors and affiliations.
College of Computer and Information Sciences, Imam Mohammad Ibn Saud Islamic University, 11564, Riyadh, Saudi Arabia
Muhammad Usman Khalid & Sheeraz Akram
Faculty of Integrated Technologies, Universiti Brunei Darussalam, Bandar Seri Begawan, BE1410, Brunei
Malik Muhammad Nauman & Kamran Ali
You can also search for this author in PubMed Google Scholar
Contributions
Idea, Conception, Methods, Implementation: Muhammad Usman Khalid Experiments: Sheeraz Akram Supervision, Management: Malik Muhammad Nauman Funding, Management: Kamran Ali All authors reviewed the manuscript.
Corresponding author
Correspondence to Kamran Ali .
Ethics declarations
Competing interests.
The authors declare no competing interests.
Additional information
Publisher's note.
Springer Nature remains neutral with regard to jurisdictional claims in published maps and institutional affiliations.
Supplementary Information
Supplementary information., rights and permissions.
Open Access This article is licensed under a Creative Commons Attribution-NonCommercial-NoDerivatives 4.0 International License, which permits any non-commercial use, sharing, distribution and reproduction in any medium or format, as long as you give appropriate credit to the original author(s) and the source, provide a link to the Creative Commons licence, and indicate if you modified the licensed material. You do not have permission under this licence to share adapted material derived from this article or parts of it. The images or other third party material in this article are included in the article’s Creative Commons licence, unless indicated otherwise in a credit line to the material. If material is not included in the article’s Creative Commons licence and your intended use is not permitted by statutory regulation or exceeds the permitted use, you will need to obtain permission directly from the copyright holder. To view a copy of this licence, visit http://creativecommons.org/licenses/by-nc-nd/4.0/ .
Reprints and permissions
About this article
Cite this article.
Khalid, M.U., Nauman, M.M., Akram, S. et al. Three layered sparse dictionary learning algorithm for enhancing the subject wise segregation of brain networks. Sci Rep 14 , 19070 (2024). https://doi.org/10.1038/s41598-024-69647-2
Download citation
Received : 14 March 2024
Accepted : 07 August 2024
Published : 17 August 2024
DOI : https://doi.org/10.1038/s41598-024-69647-2
Share this article
Anyone you share the following link with will be able to read this content:
Sorry, a shareable link is not currently available for this article.
Provided by the Springer Nature SharedIt content-sharing initiative
By submitting a comment you agree to abide by our Terms and Community Guidelines . If you find something abusive or that does not comply with our terms or guidelines please flag it as inappropriate.
Quick links
- Explore articles by subject
- Guide to authors
- Editorial policies
Sign up for the Nature Briefing: AI and Robotics newsletter — what matters in AI and robotics research, free to your inbox weekly.

Using the Systematic Problem-Solving Method If you have a difficult decision to make, try this well-tested method | ||||
The Systematic Problem-Solving (SPS) Method:
Make better decisions tom g. stevens phd.
Solving problems is important in every area of human thinking. Learning general problem-solving skills can therefore help you improve your ability to cope with every area of your life. All disciplines of philosophy, business, science, and humanities have developed their own approach to solving problems. Remarkably, the problem-solving models developed by each of these areas are strikingly similar. I describe a simple problem-solving process that you can use to solve almost all problems.
Stages of the problem-solving process. The famous psychologist, Dr. Carl Rogers, was one of the first to help us understand how important self-exploration and problem-solving are for overcoming all types of personal, psychological, and daily-living problems. (1);
Consciously going through each of these four stages when solving any complex problem can be very useful. Following are the five stages of the problem-solving method.
STAGE 1: EXPLORATION OF THE PROBLEM
STAGE 2: EXPLORING ALTERNATIVE SOLUTIONS (Routes to Happiness);
STAGE 3: CHOOSING THE BEST ALTERNATIVE
STAGE 4: PLANNING AND ACTION
STAGE 5: EXPERIMENTING AND GATHERING FEEDBACK
During this stage, we gather all of the information we can about both external aspects of the problem and internal aspects. Good information gathering is not an easy process. Scientists spend their whole lives trying to learn about some very small piece of the world. The type of information-gathering process we use will depend upon the type of problem we are trying to solve. For information about the world the following are powerful skills to use.
- Library reference skills
- Observational skills
- Informational interviewing skills
- Critical thinking skills
- Scientific method skills
- Data analysis and statistical skills
Learning how to become an expert at identifying problems and finding causes is essential to become an expert in any field. The above skills are useful in solving many types of problems--even intra-personal ones. However, the focus of this book is how to be happy; and the key to happiness almost always involves not just external causes but internal ones as well.
It is usually much easier for most of us to observe an external event than an internal one. We have our external sensory organs to see and hear external events, but not internal ones. How do we observe that which we cannot see? We can learn to be better observers of our emotions, self-talk, and images.
The self-exploration process described above provides enough information to make you an expert at self-exploration. That is one of the most essential parts of developing your own inner therapist.
STAGE 2: EXPLORING ALTERNATIVE SOLUTIONS OR ROUTES TO HAPPINESS
Gather all the best information you can about possible solutions. Use brainstorming techniques, observe and consult with people who have overcome similar problems, read relevant material, consult experts, and recall your own relevant past experience. Look at both internal and external solutions.
Once you learn so many different routes to happiness, then you will be truly free to choose to be happy in almost any situation you face in life. The actual choice is made in stage 3 of the /problem-solving process. The appendix contains a very useful decision-making model for helping you make complex choices such as choosing a career or relationship. The following is a simple approach to making a decision between alternatives. (See Carkhuff Decision-Making Model, below, for a method for making complex decisions--for career or life planning.);
(1); List all the alternatives you are considering
(2); List all of the values or criteria that will be affected by the decision
(3); Evaluate each alternative by each criteria or value
(4); Choose the alternative which you predict will satisfy the criteria the best and lead to your greatest overall happiness
STAGE 4: PLANNING AND ACTION (Experimenting);
Many decisions are made, but never implemented. See that you follow-up with good planning. Once you have made your choice, you can use some of the planning methods suggested in the O-PATSM method from chapter 11 to make sure that you follow through with your decision.
This is the stage of acting on your decision. Many people fear making mistakes and failure as if these were some terrible sins that they should never commit. That view of life of life makes every decision and action seem very serious and they often become very timid people who lack creativity and are plagued by guilt and fear of failure. Instead we can view every action as an experiment. If one of our overall goals in life is learning and growth, then we can never fail to learn. All people who have accomplished great happiness for themselves and contributed to others have shared the courage to act on their beliefs.
STAGE 5: GATHERING FEEDBACK
Many people hate to be evaluated and dread finding out the results of what they have done out of fear that the feedback will be negative. These fears can be serious impediments to the growth that can only happen through getting open, accurate feedback.
However, once learning and growth are important goals, then getting feedback becomes highly desirable. How else can we learn? Even negative outcomes can provide valuable information. Of course, almost everyone would rather have outcomes that maximize happiness; but when we don't, we can at least try to maximize our learning. Learning can help maximize happiness in the future.
We can also make the mistakes of dwelling on past mistakes that goes beyond constructive learning and reasonable reparations to victims and of punishing ourselves unnecessarily. Normally, there is no value to punishment--once a lesson has been learned. (2); Keep clear at all times that this problem-solving process is only a tool to serve the overall life goals of increased health, growth, and happiness.
CARKHUFF DECISION-MAKING MODEL: This particular decision-making model is based upon one by Dr. Robert Carkhuff and follows the general guidelines of a considerable amount of research on how people can make more effective decisions. It can also be used for any other type of decision--from buying a new car to choosing a mate.
EXAMPLE OF USING THE DECISION-MAKING MODEL
The decision-making model will be illustrated in a way which you can use aa an analogy for making your own career decision. In this example, Henry is trying to decide whether to major in psychology or in computer science. Thus he has narrowed his alternatives to the following two:
1); majoring in psychology with a career goal of either going into high school counseling or teaching or 2); majoring in computer science with a possible goal of working as a computer programmer.
These are represented along the top axis of the following matrix.
|
|
|
|
|
|
|
|
|
|
|
|
|
|
|
|
|
|
|
|
|
|
|
|
|
|
|
|
|
|
|
|
|
|
|
|
|
|
|
|
|
|
|
|
|
|
|
|
|
|
|
|
|
|
|
|
|
|
|
|
|
|
|
|
|
|
|
|
|
|
|
|
|
|
|
|
|
|
|
|
7 | ||||
|
|
|
|
** is the WINNER-it has the most points of the two alternatives
STEPS TO USING THE DECISION-MAKING MODEL--(use above example);
STEP 1-- LIST YOUR CAREER ALTERNATIVES. This is your refined list of alternatives of which majors or occupations you are trying to decide between. Remember, that you can list as many as you want. You can list unusual combinations of simpler alternatives. For Henry those narrowed alternatives were psychology and computer science.
STEP 2--CAREER SELECTION CRITERIA. Review your Career Selection Criteria list and write all the important career selection criteria in the far left column. Note that repeating the same idea or leaving out an important idea can affect the decision outcome.
STEP 3-- CRITERIA WEIGHTS. Evaluate the relative importance to you of each of your Career Selection Criteria on a scale of 1 to 10 (10 being the most important);. Write your answer in the column next to the selection criteria.
STEP 4--ALTERNATIVE EVALUATION SCALE. Each alternative is to be evaluated from the point of view of each selection criterion. You need to think about what this means for each selection criterion. For example Henry determined that for the selection criteria of income , a "minimally acceptable" income would be $25,000 starting with prospects of making up to $50,000 eventually. An outstanding salary would be starting at about $40, 000 with prospects of making up to $100,000.
+5 = Maximum evaluation--outstanding (example: income begin $40,000 go to $100,000 +4, +3, +2, +1 = intermediate values
0 = Minimally acceptable value. (example: income = begin $20,000 go to $45,000);
-1, -2, -3, -4 = intermediate values
-5 = Minimum evaluation--worst possible (example: income < $10,000
STEP 5--EVALUATE EACH ALTERNATIVE BY EACH SELECTION CRITERION. Use the evaluation scale from step 3 to evaluate each alternative from the point of view of each Career Selection Criterion. Give it rating from -5 to +5. In the example above, both alternatives were evaluated on the criterion of "income": Henry gave the psychology income an evaluation of "+2" and computer science income an evaluation of " +4."
STEP 6--MULTIPLY THE CRITERIA WEIGHTS TIMES THE EVALUATIONS. In the example above for the selection criterion of "income," Henry multiplied the criterion weight of "9" times the evaluation of " +2" for "PSYCH" to get a result of "18." That is its SCORE OR POINTS for psychology on the criterion of income. Put it inside of the parentheses. This score of 18 is an overall prediction much Henry's income in psychology will contribute to his overall happiness. Since he had a score of 36 in computer science, he his predicting that he will be much happier with his income in that field.
STEP 7--FIND THE OVERALL SUM OF THE SCORES FOR EACH ALTERNATIVE. Add together the numbers inside the parentheses for each alternative. In the example above, the overall sum for the "PSYCH" alternative is "405."
STEP 8--COMPARE THE ALTERNATIVES WITH EACH OTHER AND WITH THE "IDEAL." The "ideal" is the maximum possible number of points. Once you have determined all the totals and compared them to each other, try to figure out why one alternative came out ahead of another--where it got its points. Play with the points until you think the points match your true feelings and values.
* The alternative with the most points is the one you are predicting will make you the happiest person.
1. 1 Some might argue that Freud was the first. He clearly did describe many helpful techniques. I think that some of his free association techniques are still very useful for helping to find underlying beliefs, images, or cognitive systems which are related to the problem. However, Rogers was the one that more clearly described the stages of self-exploration and problem-solving and the conditions of unconditional positive regard, empathetic understanding, and genuineness on the part of the therapist which seem to be important to the therapeutic process or to any person attempting to feel better.
Robert Carkhuff (one of Roger's pupils); has developed a structured training system for helping people learn these skills. Robert Cash, a personal friend, has further elaborated these skills in his own courses and introduced me to this process. There is a good deal of research supporting the effectiveness of these techniques.
2. 2 This statement does not address the use of punishment as a deterrent to prevent some persons from profiting from their dysfunctional behaviors. For example if behaviors such as murder, robbery, or selling drugs are not given sufficient punishment, some people will engage in these behaviors. A person whose ultimate concern is money and pleasure may deal drugs to make money with little regard to how it affects others. Increasing the cost for a person with those beliefs can reduce the chances they will sell drugs.
Self-Help and other resources on this website (and site map)
Copyright 2024 Tom G. Stevens PhD
- Creating Environments Conducive to Social Interaction
- Thinking Ethically: A Framework for Moral Decision Making
- Developing a Positive Climate with Trust and Respect
- Developing Self-Esteem, Confidence, Resiliency, and Mindset
- Developing Ability to Consider Different Perspectives
- Developing Tools and Techniques Useful in Social Problem-Solving
- Leadership Problem-Solving Model
- A Problem-Solving Model for Improving Student Achievement
Six-Step Problem-Solving Model
- Hurson’s Productive Thinking Model: Solving Problems Creatively
- The Power of Storytelling and Play
- Creative Documentation & Assessment
- Materials for Use in Creating “Third Party” Solution Scenarios
- Resources for Connecting Schools to Communities
- Resources for Enabling Students
weblink: http://www.yale.edu/bestpractices/resources/docs/problemsolvingmodel.pdf
This six-step model is designed for the workplace, but is easily adaptable to other settings such as schools and families. It emphasizes the cyclical , continuous nature of the problem-solving process . The model describes in detail the following steps:
Step One: Define the Problem
Step Two: Determine the Root Cause(s) of the Problem
Step Three: Develop Alternative Solutions
Step Four: Select a Solution
Step Five: Implement the Solution
Step Six: Evaluate the Outcome

Critical & Creative Thinking - OER & More Resources: IDEAL problem solving
- Self evaluation
- Creating goals
- Creating personal mission statement
- Creative Thinking
- Problem Solving
- IDEAL problem solving
- CRITICAL THINKING
- Critical Thinking Tips
- Logic Terms
- Logic Traps
- Free OER Textbooks
- More Thinking: OER
- Ethics - OER Textbooks
- Evidence-Based Critical Thinking
- BELIEFS & BIAS
- Limits of Perception
- Reality & Assumptions
- Stereotypes & Race
- MAKING YOUR CASE
- Argument (OER)
- Inductive Arguments
- Information Literacy: Be Savvy about your Sources
- Persuasive Speaking (OER)
- Philosophy & Thinking
- WiPhi Philosophy Project
- Browse All Guides
VM: I had to inter-library loan this item to read the original content. This is highly cited throughout literature, so I wanted to have a good grasp on what it covered. Here are my notes and commentary:
- Full text From TNtech.edu: "Ideal Problem Solver, 2 ed." (c) 1984, 1993 more... less... Thanks to Center for Assessment & Improvement of Learning - Reports & Publications"
- Full text from ERIC: The IDEAL Workplace: Strategies for Improving Learning, Problem Solving, and Creativity
- Show your support: The Ideal Problem Solver: A Guide to Improving Thinking, Learning, and Creativity Second Edition
The reason you should learn the IDEAL method is so you don't need to avoid problems. The more know about and practice problem solving, the easier it gets. It is learnable skill. It also prompts you to look for problems and solutions instead of just doing things the same old way.
Improvement of problem solving skills.
Model for analyzing the processes that underlie effective problem solving.
IDEAL Model for improving problem solving (Verbatim copy of Fig 2.1; p.12)
I = Identifying the problem.
D = Define and represent the problem.
E = Explore possible strategies.
A = Act on the strategies.
L = Look back and evaluate the effects of your activities.
ELABORATION:
I = Identifying that there is a problem that, once described as a problem, may be solved or improved.
D = Define and represent the problem. Draw it instead of trying to imagine it.
E = Explore possible strategies & alternative approaches or viewpoints.
General strategies: Break problem down into small simple problems. Working a problem backwards. Build scale model Try simulation experiment, with smaller or simpler sets.
A = Act on the strategies. Try, then reflect or recall. Actively try learning strategy.
L = Look back and evaluate the effects of your activities. Look at results of learning strategy used: Does it work to allow full recall?
"Many students make the mistake of assuming that they have "learned" adequately if the information seems to make sense as they read it in a textbook or hear it in a lecture." (p. 23" Must use or practice, recall, or paraphrase - in order to evaluate effectiveness of learning.
Math: Do example problems before looking at solution to practice concepts. Look at solution to see where you went wrong (or not).
Don't let the test be the first time you evaluate your understanding of material
Problem identification and definition.
Proof of concept - act/look/evaluate.
To find an answer to a problem, you can dig deeper, or dig somewhere else.
Question assumptions about limits The old - think outside the box- strategy.
When memorizing, know what you need to remember Definitions? Concepts? Graphs? Dates? each teacher has different priorities...ask them what to focus on
Ways to solve problem of learning new information.
Techniques for improving memory.
Short term meomory
Long term memory
Remembering people's names
Studying for an essay test.
Using cues to retrieve information. For example, you can remember IDEAL first and that will help you reconstruct the idea of how to solve problems.
Some strategies for remembering information:
Make a story full of memorable images.
Funny obnoxious "vivid images" or "mental pictures" are more memorabl e. (Ex: random words in a list, passwords, people's names. Banana vomit haunts me.)
Rehearse over and over - over learn. (Ex: Memorizing a phone number 867-5309 )
Rehearse words in groups - chunking. (Ex: Memorizing a part in a play, poems, pledges, short stories.)
Organize words into conceptual categories - Look for unifying relationships. (Recall, order not important. Ex: Shopping list, points in an essay.)
Look for similarities and coincidences in the words themselves. (Ex: How many words have e's, or 2 syllables, or have pun-ishing homonyms)
The feet that use the manual transmission car pedals are, from left to right: C ( L eft-foot) utch , the B( R ight-foot) ake , and the A ccelerato ( R ight-foot)
Does order mimic alphabetical order? The manual transmission car pedals are, from left to right, the C lutch, the B rake, and the A ccelerator )
Use Acronyms I dentify D efine E xplore A ct L ook
Acronym- easily remembered word: FACE

Acrostic- easily remembered phrase: E very G ood B oy D eserves F udge
- Modified image source: Commons.wikimedia.org
Don't waste time studying what you already know
Image - Name Strategy:
What is unique about the person? What is unique about their name?
Find a relationship between the two.
Other Pairing Strategies:
method of loci: arranging words to be remembered in association with familiar location or path .
Peg-word method: arranging words to be remembered in association with number order or alphabet letter order .
Strategies to comprehend new information.
more difficult than
Strategies to memorize new information.
Learning with understanding - comprehending new information.
Knowledge of CORE CONCEPTS in a field SIMPLIFIES problem solving.
Ways to approach a problem of learning information that seems to be arbitrary:
Over-learn: rehearse the facts until they are mastered. 2+2=4
Find relationships between images or words that are memorable: story telling, silmilarieties, vivid images, pegging, etc.
When a concept seems unclear, learn more about it.
Memory- can be of seemingly arbitrary words or numbers: ROTE (Ex. Facts and relationships) appearance
Comprehension - is understanding significance or relationships or function
Novices often forced to memorize information until they learn enough (related concepts and context) to understand it.
The mere memorization of information rarely provides useful conceptual tools that enable one to solve new problems later on. (p. 61,69)
Taking notes will not necessarily lead to effective recall prompts. How do you know when you understand material? Self-test by trying to explain material to another person.That will expose gaps in understanding.
Recall answers or solve problems out of order to be sure you know which concepts to apply and why.
Look at mistakes made as soon as possible, and learn where you went wrong.
Uses of information require more or less precision in understanding, depending on context. (A pilot must know more about an airplane than a passenger.)
Evaluation basics: evaluate factual claims look for flaws in logic question assumptions that form the basis of the argument
Correlation does not necessarily prove cause and effect.
Importance of being able to criticize ideas and generate alternatives.
Strategies for effective criticism.
Strategies for formulating creative solutions.
Finding/understanding implicit assumptions that hamper brainstorming.
Strategies for making implicit assumptions explicit.
"The uncreative mind can spot wrong answers, but it takes a creative mnd to spot wrong questions ." Emphasis added. - Anthony Jay, (p.93)
Making implicit assumptions explicit: look for inconsistencies question assumptions make predictions analyze worst case get feedback & criticism from others
Increase generation of novel ideas: break down problem into smaller parts analyze properties on a simpler level use analogies use brainstorming give it a rest, sleep on it don't be in a hurry, let ideas incubate: talk to others, read, keep the problem in the back of your mind try to communicate your ideas as clearly as possible, preferably in writing. attempting to write or teach an idea can function as a discovery technique
Strategies for Effective Communication
What we are trying to accomplish (goal)
Evaluating communication fro effectiveness:
Identify and Define: Have you given audience basis to understand different points of view about a topic? Different problem definitions can lead to different solutions. Did you Explore pros and cons of different strategies? Did you take Action and then Look at consequences? Did you organize your content into main points that are easy to identify and remeber?
Did you use analogies and background information to put facts into context?
Did you make sure your facts were accurate and did you avoid making assumptions?Always check for logical fallacies and inconsistencies. Did you include information that is novel and useful, instead of just regurgitating what everyone already knows?
After you communicate, get feedback and evaluate your strategies. Look for effects, and learn from your mistakes. (p. 117)
Identify and Define what (problem) you want to communicate, with respect to your audience and your goals. Explore strategies for communicating your ideas.Act - based on your strategies. Look at effects.
Summaries of Useful Attitudes and Strategies: Anybody can use the IDEAL system to improve their problem solving skills.
Related Resources:
- Teaching The IDEAL Problem-Solving Method To Diverse Learners Written by: Amy Sippl
- << Previous: Problem Solving
- Next: CRITICAL THINKING >>
- Last Updated: Aug 13, 2024 8:34 AM
- URL: https://library.fvtc.edu/Thinking
About Us • Contact Us • FVTC Terms of Service • Sitemap FVTC Mission, Vision, Values & Purposes • FVTC Privacy Statement • FVTC Library Services Accessibility Statement DISCLAIMER: Any commercial mentions on our website are for instructional purposes only. Our guides are not a substitute for professional legal or medical advice. Fox Valley Technical College • Library Services • 1825 N. Bluemound Drive • Room G113 Appleton, WI 54912-2277 • United States • (920) 735-5653 © 2024 • Fox Valley Technical College • All Rights Reserved.
The https://library.fvtc.edu/ pages are hosted by SpringShare. Springshare Privacy Policy.
Grab your spot at the free arXiv Accessibility Forum
Help | Advanced Search
Computer Science > Artificial Intelligence
Title: nl2or: solve complex operations research problems using natural language inputs.
Abstract: Operations research (OR) uses mathematical models to enhance decision-making, but developing these models requires expert knowledge and can be time-consuming. Automated mathematical programming (AMP) has emerged to simplify this process, but existing systems have limitations. This paper introduces a novel methodology that uses recent advances in Large Language Model (LLM) to create and edit OR solutions from non-expert user queries expressed using Natural Language. This reduces the need for domain expertise and the time to formulate a problem. The paper presents an end-to-end pipeline, named NL2OR, that generates solutions to OR problems from natural language input, and shares experimental results on several important OR problems.
Subjects: | Artificial Intelligence (cs.AI); Human-Computer Interaction (cs.HC) |
Cite as: | [cs.AI] |
(or [cs.AI] for this version) | |
Focus to learn more arXiv-issued DOI via DataCite (pending registration) |
Submission history
Access paper:.
- HTML (experimental)
- Other Formats

References & Citations
- Google Scholar
- Semantic Scholar
BibTeX formatted citation

Bibliographic and Citation Tools
Code, data and media associated with this article, recommenders and search tools.
- Institution
arXivLabs: experimental projects with community collaborators
arXivLabs is a framework that allows collaborators to develop and share new arXiv features directly on our website.
Both individuals and organizations that work with arXivLabs have embraced and accepted our values of openness, community, excellence, and user data privacy. arXiv is committed to these values and only works with partners that adhere to them.
Have an idea for a project that will add value for arXiv's community? Learn more about arXivLabs .
Information
- Author Services
Initiatives
You are accessing a machine-readable page. In order to be human-readable, please install an RSS reader.
All articles published by MDPI are made immediately available worldwide under an open access license. No special permission is required to reuse all or part of the article published by MDPI, including figures and tables. For articles published under an open access Creative Common CC BY license, any part of the article may be reused without permission provided that the original article is clearly cited. For more information, please refer to https://www.mdpi.com/openaccess .
Feature papers represent the most advanced research with significant potential for high impact in the field. A Feature Paper should be a substantial original Article that involves several techniques or approaches, provides an outlook for future research directions and describes possible research applications.
Feature papers are submitted upon individual invitation or recommendation by the scientific editors and must receive positive feedback from the reviewers.
Editor’s Choice articles are based on recommendations by the scientific editors of MDPI journals from around the world. Editors select a small number of articles recently published in the journal that they believe will be particularly interesting to readers, or important in the respective research area. The aim is to provide a snapshot of some of the most exciting work published in the various research areas of the journal.
Original Submission Date Received: .
- Active Journals
- Find a Journal
- Proceedings Series
- For Authors
- For Reviewers
- For Editors
- For Librarians
- For Publishers
- For Societies
- For Conference Organizers
- Open Access Policy
- Institutional Open Access Program
- Special Issues Guidelines
- Editorial Process
- Research and Publication Ethics
- Article Processing Charges
- Testimonials
- Preprints.org
- SciProfiles
- Encyclopedia
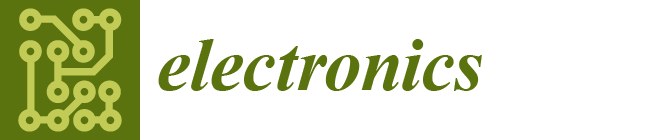
Article Menu

- Subscribe SciFeed
- Recommended Articles
- Google Scholar
- on Google Scholar
- Table of Contents
Find support for a specific problem in the support section of our website.
Please let us know what you think of our products and services.
Visit our dedicated information section to learn more about MDPI.
JSmol Viewer
Solving the vehicle routing problem with stochastic travel cost using deep reinforcement learning.
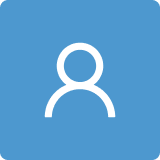
1. Introduction
2. preliminary, 2.1. vrp-stc, 2.2. reinforcement learning framework, 3.1. formulation of drl.
- State: Status s t = ( R t , C t ) is a part of the solution, for instance, G ( Q , q , R ) created at time step t . Here, R t (for t ≠ 0) is the group of customers receiving services, which includes all of the chosen customer locations up to step t , C t is the set of candidate nodes at step t , Q is the maximum capacity of each vehicle, q is the demand of customers.
- Action: Action a t indicates that at step t , the candidate node π t is chosen from the candidate node set C t and added to the service customer set R t .
- Transition: With the action a t , a modern fractional solution is obtained as the following state, i.e., s t+ 1 = ( R t+ 1 , C t+ 1 ). Within the updated state, R t+ 1 includes π t in addition to the nodes chosen so far, whereas C t+ 1 consists of the candidate nodes from C t with π t expelled.
- Reward: To minimize the total cost, we define the value of the objective function at step t as Obj t = min E( cost ), and the reward at step t as r t = Obj t−1 − Obj t .
- Policy: The strategy P θ is parameterized using θ within the GAT-AM model. At each step t , a candidate node is automatically chosen as the service customer node until all service customer nodes are chosen, resulting in the final solution π = { π 1 , π 2 , …, π n ,} generated by the policy.
3.3. Encoder
3.4. decoder, 3.5. algorithm.
REINFORCE with Rollout Baseline |
number of epochs E, steps per epoch T, batch size B, significance α Initialize θ, θ ← θ epoch = 1, …, E step = 1, …, T Test (P , P ) < α θ ← θ |
3.6. Stochastic Travel Costs
4. experiments, 4.1. experimental settings, 4.2. baseline methods and evaluation metrics, 4.3. comparison analysis, 4.4. model convergence performance, 4.5. visualization, 5. conclusions.
- Algorithmic Refinement and Generalization: The model currently exhibits certain limitations. Enhancing the algorithm’s generalization capacity to accommodate a broader spectrum of environments and various problem scenarios represents a pivotal area for future investigation.
- Real-time Dynamic Planning: With the growing demand for practical applications, implementing real-time dynamic planning within the model to accommodate the evolving logistics demands and traffic conditions is a pressing challenge awaiting resolution.
- Multi-objective Optimization: The present model primarily focuses on minimizing the total travel cost. In the future, exploration could be extended to achieve a balance among multiple objectives, such as service level assurance and minimizing environmental impacts.
Author Contributions
Data availability statement, acknowledgments, conflicts of interest.
- Khalil, E.; Dai, H.; Zhang, Y.; Dilkina, B.; Song, L. Learning combinatorial optimization algorithms over graphs. Adv. Neural Inf. Process. Syst. 2017 , 30 , 6348–6358. [ Google Scholar ]
- Bengio, Y.; Lodi, A.; Prouvost, A. Machine learning for combinatorial optimization: A methodological tour d’horizon. Eur. J. Oper. Res. 2021 , 290 , 405–421. [ Google Scholar ] [ CrossRef ]
- Glorot, X.; Bengio, Y. Understanding the difficulty of training deep feedforward neural networks. In Proceedings of the Thirteenth International Conference on Artificial Intelligence and Statistics, Sardinia, Italy, 13–15 May 2010; pp. 249–256. [ Google Scholar ]
- Sutton, R.S.; Barto, A.G. Reinforcement Learning: An Introduction. IEEE Trans. Neural Netw. 1998 , 9 , 1054. [ Google Scholar ] [ CrossRef ]
- Silver, D.; Schrittwieser, J.; Simonyan, K.; Antonoglou, I.; Huang, A. Mastering the game of Go without human knowledge. Nature 2017 , 550 , 354–359. [ Google Scholar ] [ CrossRef ] [ PubMed ]
- Mnih, V.; Kavukcuoglu, K.; Silver, D.; Rusu, A.A.; Veness, J.; Bellemare, M.G.; Graves, A.; Riedmiller, M.; Fidjeland, A.K.; Ostrovski, G. Human-level control through deep reinforcement learning. Nature 2015 , 518 , 529–533. [ Google Scholar ] [ CrossRef ]
- Karimi-Mamaghan, M.; Mohammadi, M.; Meyer, P.; Karimi-Mamaghan, A.M.; Talbi, E.-G. Machine learning at the service of meta-heuristics for solving combinatorial optimization problems: A state-of-the-art. Eur. J. Oper. Res. 2022 , 296 , 393–422. [ Google Scholar ] [ CrossRef ]
- Watkins, C.J.; Dayan, P. Q-learning. Mach. Learn. 1992 , 8 , 279–292. [ Google Scholar ] [ CrossRef ]
- Williams, R.J. Simple statistical gradient-following algorithms for connectionist reinforcement learning. Mach. Learn. 1992 , 8 , 229–256. [ Google Scholar ] [ CrossRef ]
- Konda, V.; Tsitsiklis, J. Actor-critic algorithms. Adv. Neural Inf. Process. Syst. 1999 , 12 , 1008–1014. [ Google Scholar ]
- Silver, D.; Lever, G.; Heess, N.; Degris, T.; Wierstra, D.; Riedmiller, M. Deterministic policy gradient algorithms. In Proceedings of the International Conference on Machine Learning, Beijing, China, 22–24 June 2014; pp. 387–395. [ Google Scholar ]
- Schulman, J.; Levine, S.; Abbeel, P.; Jordan, M.; Moritz, P. Trust region policy optimization. In Proceedings of the International Conference on Machine Learning, Lille, France, 7–9 July 2015; pp. 1889–1897. [ Google Scholar ]
- Lillicrap, T.P.; Hunt, J.J.; Pritzel, A.; Heess, N.; Erez, T.; Tassa, Y.; Silver, D.; Wierstra, D. Continuous control with deep reinforcement learning. arXiv 2015 , arXiv:1509.02971. [ Google Scholar ]
- Schulman, J.; Wolski, F.; Dhariwal, P.; Radford, A.; Klimov, O. Proximal policy optimization algorithms. arXiv 2017 , arXiv:1707.06347. [ Google Scholar ]
- Babaeizadeh, M.; Frosio, I.; Tyree, S.; Clemons, J.; Kautz, J. Reinforcement learning through asynchronous advantage actor-critic on a gpu. arXiv 2016 , arXiv:1611.06256. [ Google Scholar ]
- Levine, S.; Finn, C.; Darrell, T.; Abbeel, P. End-to-end training of deep visuomotor policies. J. Mach. Learn. Res. 2016 , 17 , 1334–1373. [ Google Scholar ]
- Deng, Y.; Bao, F.; Kong, Y.; Ren, Z.; Dai, Q. Deep direct reinforcement learning for financial signal representation and trading. IEEE Trans. Neural Netw. Learn. Syst. 2016 , 28 , 653–664. [ Google Scholar ] [ CrossRef ]
- Zheng, G.; Zhang, F.; Zheng, Z.; Xiang, Y.; Yuan, N.J.; Xie, X.; Li, Z. DRN: A deep reinforcement learning framework for news recommendation. In Proceedings of the 2018 World Wide Web Conference, Lyon, France, 23–27 April 2018; pp. 167–176. [ Google Scholar ]
- Silver, D.; Huang, A.; Maddison, C.J.; Guez, A.; Sifre, L.; Van Den Driessche, G.; Schrittwieser, J.; Antonoglou, I.; Panneershelvam, V.; Lanctot, M. Mastering the game of Go with deep neural networks and tree search. Nature 2016 , 529 , 484–489. [ Google Scholar ] [ CrossRef ] [ PubMed ]
- Silver, D.; Hubert, T.; Schrittwieser, J.; Antonoglou, I.; Lai, M.; Guez, A.; Lanctot, M.; Sifre, L.; Kumaran, D.; Graepel, T. A general reinforcement learning algorithm that masters chess, shogi, and Go through self-play. Science 2018 , 362 , 1140–1144. [ Google Scholar ] [ CrossRef ]
- Schrittwieser, J.; Antonoglou, I.; Hubert, T.; Simonyan, K.; Sifre, L.; Schmitt, S.; Guez, A.; Lockhart, E.; Hassabis, D.; Graepel, T. Mastering atari, go, chess and shogi by planning with a learned model. Nature 2020 , 588 , 604–609. [ Google Scholar ] [ CrossRef ]
- Vinyals, O.; Fortunato, M.; Jaitly, N. Pointer networks. Adv. Neural Inf. Process. Syst. 2015 , 28 . [ Google Scholar ] [ CrossRef ]
- Lu, H.; Zhang, X.; Yang, S. A learning-based iterative method for solving vehicle routing problems. In Proceedings of International Conference on Learning Representations. Available online: https://openreview.net/forum?id=BJe1334YDH (accessed on 13 August 2024).
- Manchanda, S.; Mittal, A.; Dhawan, A.; Medya, S.; Ranu, S.; Singh, A. Learning heuristics over large graphs via deep reinforcement learning. arXiv 2019 , arXiv:1903.03332. [ Google Scholar ]
- Mazyavkina, N.; Sviridov, S.; Ivanov, S.; Burnaev, E. Reinforcement learning for combinatorial optimization: A survey. Comput. Oper. Res. 2021 , 134 , 105400. [ Google Scholar ] [ CrossRef ]
- Cappart, Q.; Chételat, D.; Khalil, E.B.; Lodi, A.; Morris, C.; Veličković, P. Combinatorial optimization and reasoning with graph neural networks. J. Mach. Learn. Res. 2023 , 24 , 1–61. [ Google Scholar ]
- Kool, W.; Van Hoof, H.; Welling, M. Attention, learn to solve routing problems! arXiv 2018 , arXiv:1803.08475. [ Google Scholar ]
- Nowak, A.; Villar, S.; Bandeira, A.S.; Bruna, J. A note on learning algorithms for quadratic assignment with graph neural networks. Stat 2017 , 1050 , 22. [ Google Scholar ]
- Li, Z.; Chen, Q.; Koltun, V. Combinatorial optimization with graph convolutional networks and guided tree search. Adv. Neural Inf. Process. Syst. 2018 , 31 . Available online: https://proceedings.neurips.cc/paper_files/paper/2018/file/8d3bba7425e7c98c50f52ca1b52d3735-Paper.pdf (accessed on 13 August 2024).
- Drori, I.; Kharkar, A.; Sickinger, W.R.; Kates, B.; Ma, Q.; Ge, S.; Dolev, E.; Dietrich, B.; Williamson, D.P.; Udell, M. Learning to solve combinatorial optimization problems on real-world graphs in linear time. In Proceedings of the 2020 19th IEEE International Conference on Machine Learning and Applications (ICMLA), Miami, FL, USA, 14–17 December 2020; pp. 19–24. [ Google Scholar ]
- Lodi, A.; Mossina, L.; Rachelson, E. Learning to handle parameter perturbations in combinatorial optimization: An application to facility location. EURO J. Transp. Logist. 2020 , 9 , 100023. [ Google Scholar ] [ CrossRef ]
- Xidias, E.; Zacharia, P.; Nearchou, A. Intelligent fleet management of autonomous vehicles for city logistics. Appl. Intell. 2022 , 52 , 18030–18048. [ Google Scholar ] [ CrossRef ]
- Luo, H.; Dridi, M.; Grunder, O. A branch-price-and-cut algorithm for a time-dependent green vehicle routing problem with the consideration of traffic congestion. Comput. Ind. Eng. 2023 , 177 , 109093. [ Google Scholar ] [ CrossRef ]
- Bai, R.; Chen, X.; Chen, Z.-L.; Cui, T.; Gong, S.; He, W.; Jiang, X.; Jin, H.; Jin, J.; Kendall, G. Analytics and machine learning in vehicle routing research. Int. J. Prod. Res. 2023 , 61 , 4–30. [ Google Scholar ] [ CrossRef ]
- Puterman, M.L. Markov decision processes. Handb. Oper. Res. Manag. Sci. 1990 , 2 , 331–434. [ Google Scholar ]
- Veličković, P.; Cucurull, G.; Casanova, A.; Romero, A.; Lio, P.; Bengio, Y. Graph attention networks. arXiv 2017 , arXiv:1710.10903. [ Google Scholar ]
- He, K.; Zhang, X.; Ren, S.; Sun, J. Deep residual learning for image recognition. In Proceedings of the IEEE Conference on Computer Vision and Pattern Recognition, Las Vegas, NV, USA, 27–30 June 2016; pp. 770–778. [ Google Scholar ]
- Vaswani, A.; Shazeer, N.; Parmar, N.; Uszkoreit, J.; Jones, L.; Gomez, A.N.; Kaiser, Ł.; Polosukhin, I. Attention is all you need. arXiv 2017 , arXiv:1706.03762. [ Google Scholar ]
- Rennie, S.J.; Marcheret, E.; Mroueh, Y.; Ross, J.; Goel, V. Self-critical sequence training for image captioning. In Proceedings of the IEEE Conference on Computer Vision and Pattern Recognition, Honolulu, HI, USA, 21–26 July 2017; pp. 7008–7024. [ Google Scholar ]
- Liu, Z.; Li, X.; Khojandi, A. The flying sidekick traveling salesman problem with stochastic travel time: A reinforcement learning approach. Transp. Res. Part E Logist. Transp. Rev. 2022 , 164 , 102816. [ Google Scholar ] [ CrossRef ]
- Kingma, D.P.; Ba, J. Adam: A method for stochastic optimization. arXiv 2014 , arXiv:1412.6980. [ Google Scholar ]
- Helsgaun, K. An extension of the Lin-Kernighan-Helsgaun TSP solver for constrained traveling salesman and vehicle routing problems. Rosk. Rosk. Univ. 2017 , 12 , 966–980. [ Google Scholar ]
- Bello, I.; Pham, H.; Le, Q.V.; Norouzi, M.; Bengio, S. Neural combinatorial optimization with reinforcement learning. arXiv 2016 , arXiv:1611.09940. [ Google Scholar ]
Click here to enlarge figure
VRP20 | VRP50 | VRP100 | |||||||
---|---|---|---|---|---|---|---|---|---|
Method | Obj | Gap | Time | Obj | Gap | Time | Obj | Gap | Time |
LKH3 | 6.14 | 0.00% | (7 h) | 10.38 | 0.00% | (7 h) | 15.65 | 0.00% | (13 h) |
OR Tools | 6.42 | 4.84% | (-) | 11.22 | 8.12% | (-) | 17.14 | 9.34% | (-) |
AM (greedy) | 6.40 | 4.57% | (1 s) | 10.98 | 5.78% | (3 s) | 16.80 | 7.34% | (8 s) |
AM (sampling) | 6.25 | 2.12% | (6 m) | 10.62 | 2.31% | (28 m) | 16.23 | 3.72% | (2 h) |
GAT-AM (greedy) | 6.35 | 3.76% | (1 s) | 10.88 | 4.82% | (2 s) | 16.13 | 2.89% | (5 s) |
GAT-AM (sampling) | 6.18 | 0.98% | (5 m) | 10.51 | 1.25% | (11 m) | 15.89 | 1.53% | (23 m) |
VRP-STC20 | VRP-STC50 | VRP-STC100 | ||||
---|---|---|---|---|---|---|
Method | Obj | Time | Obj | Time | Obj | Time |
AM (greedy) | 9.54 | (2 s) | 16.36 | (8 s) | 25.08 | (23 s) |
AM (sampling) | 9.31 | (13 m) | 15.98 | (1 h) | 24.68 | (4.5 h) |
GAT-AM (greedy) | 9.34 | (2 s) | 16.01 | (6 s) | 24.67 | (13 s) |
GAT-AM (sampling) | 9.18 | (7 m) | 15.68 | (16 m) | 24.36 | (46 m) |
The statements, opinions and data contained in all publications are solely those of the individual author(s) and contributor(s) and not of MDPI and/or the editor(s). MDPI and/or the editor(s) disclaim responsibility for any injury to people or property resulting from any ideas, methods, instructions or products referred to in the content. |
Share and Cite
Cai, H.; Xu, P.; Tang, X.; Lin, G. Solving the Vehicle Routing Problem with Stochastic Travel Cost Using Deep Reinforcement Learning. Electronics 2024 , 13 , 3242. https://doi.org/10.3390/electronics13163242
Cai H, Xu P, Tang X, Lin G. Solving the Vehicle Routing Problem with Stochastic Travel Cost Using Deep Reinforcement Learning. Electronics . 2024; 13(16):3242. https://doi.org/10.3390/electronics13163242
Cai, Hao, Peng Xu, Xifeng Tang, and Gan Lin. 2024. "Solving the Vehicle Routing Problem with Stochastic Travel Cost Using Deep Reinforcement Learning" Electronics 13, no. 16: 3242. https://doi.org/10.3390/electronics13163242
Article Metrics
Article access statistics, further information, mdpi initiatives, follow mdpi.
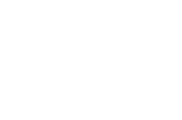
Subscribe to receive issue release notifications and newsletters from MDPI journals
Leveraging two-level deep learning classifiers for 2D shape recognition to automatically solve geometry math word problems
- Original Article
- Published: 14 August 2024
- Volume 27 , article number 102 , ( 2024 )
Cite this article
- Archana Boob 1 &
- Mansi Radke 1
13 Accesses
Explore all metrics
In mathematics, closed-domain systems for Question Answering (QA) have shown a distinct advantage over open-domain systems, primarily due to their focused use of supporting knowledge bases. This advantage is particularly salient in the era of online and hybrid tutoring, where automatic QA systems have become vital in addressing complex mathematical problems. This paper focuses on the challenge of geometric shape recognition in math word problems (MWPs) accompanied by figures that aid in the solution process. Existing systems rely on manually inputted shape information, which is less efficient. In this work, a novel customized two-layer deep learning model ‘2DGeoShapeNet’ for 2D geometric shape recognition has been developed. At the first level, it recognizes images in broad categories such as circles, quadrilaterals, or triangles. At the second level, the subtypes of quadrilaterals and triangles are detected. The proposed 2D shape detection model is trained and tested on a newly created integrated dataset, ‘GeoCQT’ (Circle, Quadrilateral, and Triangle), consisting of 6K+ images. The proposed deep learning technique achieved 93.98% accuracy on the ‘GeoCQT’ dataset. The performance of the proposed techniques is also evaluated on other geometry math word problem solver datasets such as GeoS, Geometry3K, GeoQA, PGDP5K, and PGPS9K. The proposed technique is compared with the already-published work that employed traditional image processing techniques for 2D shape detection. Findings highlight the superiority of two-level deep learning classifiers in detecting geometric shapes, marking a significant advancement in automated geometry problem-solving.
This is a preview of subscription content, log in via an institution to check access.
Access this article
Subscribe and save.
- Get 10 units per month
- Download Article/Chapter or eBook
- 1 Unit = 1 Article or 1 Chapter
- Cancel anytime
Price includes VAT (Russian Federation)
Instant access to the full article PDF.
Rent this article via DeepDyve
Institutional subscriptions
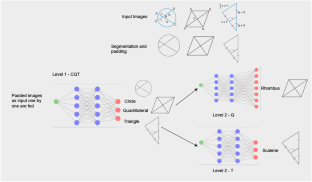
Similar content being viewed by others
RingNet: Geometric Deep Representation Learning for 3D Multi-domain Protein Shape Retrieval
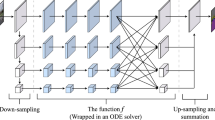
A transformer-based neural ODE for dense prediction
Deep Learning Architecture for Off-Line Recognition of Handwritten Math Symbols
Explore related subjects.
- Artificial Intelligence
Data availability
Data will be made available on request.
https://www.kaggle.com/code/stpeteishii/geometric-shapes-classify-densenet201/data .
https://www.kaggle.com/datasets/smeschke/four-shapes .
https://www.kaggle.com/datasets/dineshpiyasamara/geometric-shapes-dataset .
https://docs.scipy.org/doc/scipy/
Manjunath TN, Yogish D, Mahalakshmi S, Yogish HK (2023) Smart question answering system using vectorization approach and statistical scoring method. Mater Today Proc 80:3719–3725. https://doi.org/10.1016/j.matpr.2021.07.369
Article Google Scholar
Zhang D, Xu N, Dai BT, Shen H (2020) The gap of semantic parsing: a survey on automatic math word problem solvers. IEEE Trans Pattern Anal Mach Intell 42(9):2287–2305. https://doi.org/10.1109/TPAMI.2019.2914054
Chaudhuri D, Kushwaha NK, Sharif I, Gohri V (2012) Unique measure for geometrical shape object detection-based on area matching. Def Sci J 62(1):58–66. https://doi.org/10.14429/dsj.62.942
Rege S, Memane R, Phatak M, Agarwal P (2013) 2D geometric shape and color recognition using digital image processing. Int J Adv Res Electr Electron Instrum Eng 2(6):2479–2487
Google Scholar
Al-Eqabie HJ (2014) Recognition of primary geometric shapes using image segmentation and blobs. Eur Acad Res 2(5):6067–6075
Lestriandoko NH, Sadikin R (2016) Circle detection based on Hough transform and Mexican Hat Filter. In: IEEE international conference on computer, control, informatics and its applications, Tangerang, Indonesia, pp. 153–157. https://doi.org/10.1109/IC3INA.2016.7863041
Seo M, Hajisherzi H, Farhadui A, Etzioni O, Malcoln C (2015) Solving geometry problems: Combining text and diagram interpretation. In: Proceedings of conference on empirical methods in NLP, ACL, Lisbon, Portugal. https://doi.org/10.18653/v1/D15-1171
Sachan M, Dubey K, Xing E (2017) From textbooks to knowledge: a case study in harvesting axiomatic knowledge from textbooks to solve geometry problems. In: Palmer M, Hwa R, Riedel S (eds) Proceedings of the 2017 conference on empirical methods in natural language processing, pp. 773–784. Association for Computational Linguistics, Copenhagen, Denmark. https://doi.org/10.18653/v1/D17-1081 . https://aclanthology.org/D17-1081
Lu P, Gong R, Jiang S, Qiu L, Huang S, Liang X, Zhu S-C (2021) Inter-GPS: interpretable geometry problem solving with formal language and symbolic reasoning. In: The joint conference of the 59th annual meeting of the association for computational linguistics and the 11th international joint conference on natural language processing (ACL-IJCNLP 2021), pp. 6774–6786. https://doi.org/10.48550/arXiv.2105.04165
Chen J, Tang J, Qin J, Liang X, Liu L, Xing EnP, Lin L (2021) GeoQA: a geometric question answering benchmark towards multimodal numerical reasoning. Findings of the association for computational linguistics: ACL-IJCNLP 2021, pp. 513–523. arXiv:2105.14517v3 , https://doi.org/10.48550/arXiv.2105.14517
Boob A, Radke MA (2023) 2D shape detection for solving geometry word problems. IETE J Res. https://doi.org/10.1080/03772063.2023.2274914
Lin X, Shimotsuji S, Minoh M, Sakai T (1985) Efficient diagram understanding with characteristic pattern detection. Comput Vis Gr Image Process 30(1):84–106. https://doi.org/10.1016/0734-189X(85)90020-9
Lagunovsky D, Ablameyko S (1999) Straight-line-based primitive extraction in grey-scale object recognition. Pattern Recognit Lett 20:1005–1014. https://doi.org/10.1016/S0167-8655(99)00067-7
Pratt WK, Jr J E A (2007) Digital image processing, 4th Edn. J Electron Imaging 16(2):029901. https://doi.org/10.1117/1.2744044
Marr H (1980) Theory of edge detection. Proceedings of the royal society of London. Series B, Containing papers of a biological character. Royal Society (Great Britain), 187–217. https://doi.org/10.1098/rspb.1980.0020
Bharadwaj S, Mittal A (2012) A survey on various edge detector technique. Proc Technol 4:220–226. https://doi.org/10.1016/j.protcy.2012.05.033
Canny J (1986) Member, IEEE: a computational approach to edge detection. IEEE Trans Pattern Anal Mach Intell 8(6):679–698. https://doi.org/10.1109/TPAMI.1986.4767851
Moon H, Chellappa R, Rosenfeld A (2002) Optimal edge-based shape detection. IEEE Trans Image Process 11(11):1209–1226. https://doi.org/10.1109/TIP.2002.800896
Article MathSciNet Google Scholar
Chen A, Dong G, Liu N (2013) An algorithm for detecting lines based on primitive connection. In: 2013 IEEE third international conference on instrumentation, measurement, computer, communication and control, Shenyang, China, 479–482. IEEE. https://doi.org/10.1109/IMCCC.2013.109
Kitti T, Jaruwan T, Chaiyapon T (2012) An object recognition and identification system using the Harris corner detection method. Int J Mach Learn Comput 2(4):462–465. https://doi.org/10.7763/IJMLC.2012.V2.168
Lazin MF, ul Masood MR (2019) A fast interactive geometric shape recognition method. In: IEEE international conference on computer and information technology (ICCIT), Dhaka, Bangladesh, 2479–2487. https://doi.org/10.1109/ICCIT48885.2019.9038246
Fu Z, Han Y (2012) A circle detection algorithm based on mathematical morphology and chain code. In: International conference on computing, measurement, control and sensor network, Taiyuan, China, 253–256. https://doi.org/10.1109/CMCSN.2012.61
Seethalakshmi D, Sumathi PM (2014) Geometric shapes recognition using morphological operations in digital image processing. Int J Sci Res Comput Sci Appl Manag Stud 3(4)
Chhaya SV, Khera S, Kumar P (2015) Basic geometric shape and primary colour detection using image processing on MATLAB. IJRET Int J Res Eng Technol 4(5):679–698. https://doi.org/10.15623/IJRET.2015.0405094
Toshev A, Taskar B, Daniilidis K (2012) Shape-based object detection via boundary structure segmentation. Int J Comput Vis 99:123–146. https://doi.org/10.1007/s11263-012-0521-z
Manzanera A, Nguyen TP, Xu X (2016) Line and circle detection using dense one to one Hough transforms on grey scale images. EURASIP J Image Video Process 2016:1–18. https://doi.org/10.1186/s13640-016-0149-y
Jung CR, Schramm R (2004) Rectangle detection based on a windowed Hough transform. In: XVII Brazilian symposium on computer graphics and image processing (SIBGRAPI’04), Curitiba, Brazil. https://doi.org/10.1109/SIBGRA.2004.1352951
He JP, Ma Y (2009) Triangle detection based on windowed Hough transform. In: Proceedings of the 2009 international conference on wavelet analysis and pattern recognition, Baoding, 95–100. https://doi.org/10.1109/ICWAPR.2009.5207484
Papari G, Petkov N (2011) Edge and line oriented contour detection: state of the art. Image Vis Comput 29(2–3):79–103. https://doi.org/10.1016/j.imavis.2010.08.009
Mihu IZ, Gellert A, Caprita HV (2004) Improved methods of geometric shape recognition using Fuzzy and neural techniques. In: Periodica politechnica, transactions on automatic control and computer science 49(63)
Abdulateef S, Salman M (2021) A comprehensive review of image segmentation techniques. Iraqi J Electr Electron Eng 17:166–175. https://doi.org/10.37917/ijeee.17.2.18
Chutisant K (2014) Hybrid model of human hand motion for cybernetics application. In: 2014 IEEE international conference on systems, man, and cybernetics (SMC), pp 2367–2372. https://doi.org/10.1109/SMC.2014.6974280
Postnikov VV, Krohina DA, Prun, VE (2015) Road shape recognition based on scene self-similarity. In: Seventh international conference on machine vision (ICMV 2014), vol 9445, p 944502. SPIE
Md Sallah SS, Hussin FA, Yusoff MZ (2010) Shape-based road sign detection and recognition for embedded application using MATLAB. In: 2010 international conference on intelligent and advanced systems, pp 1–5. https://doi.org/10.1109/ICIAS.2010.5716193
Lahmyed R, Ansari ME, Kerkaou Z (2022) Automatic road sign detection and recognition based on neural network. Soft Comput 26(4):1743–1764
A-masiri P, Kerdvibulvech C (2023) Anime face recognition to create awareness. Int J Inf Technol 15:3507–3512. https://doi.org/10.1007/s41870-023-01391-8
Chen Z, Luo Y, Wang Z, Baktashmotlagh M, Huang Z (2023) Revisiting domain-adaptive 3D object detection by reliable, diverse and class-balanced pseudo-labeling. In: Proceedings of the IEEE/CVF international conference on computer vision, pp 3714–3726
Qi S, Ning X, Yang G, Zhang L, Long P, Cai W, Li W (2021) Review of multi-view 3D object recognition methods based on deep learning. Displays 69:102053. https://doi.org/10.1016/j.displa.2021.102053
Cao J, Yu L, Ling BW-K, Yao Z, Dai Q (2024) Mhsan: multi-view hierarchical self-attention network for 3D shape recognition. Pattern Recognit 150:110315. https://doi.org/10.1016/j.patcog.2024.110315
El Korchi A, Ghanou Y (2020) 2D geometric shapes dataset for machine learning and pattern recognition. Data Brief 32:106090. https://doi.org/10.1016/j.dib.2020.106090
Hao Y, Zhang M, Yin F, Huang L-L (2022) PGDP5K: a diagram parsing dataset for plane geometry problems. In: 2022 26th international conference on pattern recognition (ICPR), pp 1763–1769. IEEE. https://doi.org/10.1109/ICPR56361.2022.9956397
Zhang M-L, Yin F, Liu C-L (2023) A multi-modal neural geometric solver with textual clauses parsed from diagram. arXiv:2302.11097
Niu Z, Li H (2019) Research and analysis of threshold segmentation algorithms in image processing. J Phys Conf Ser 1237:022122. https://doi.org/10.1088/1742-6596/1237/2/022122
Sankur B, Sezgin M (2001) Image thresholding techniques: a survey over categories. Pattern Recogn 34:1573–1583
Szegedy C, Vanhoucke V, Ioffe S, Shlens J, Wojna Z (2016) Rethinking the inception architecture for computer vision. In: 2016 IEEE conference on computer vision and pattern recognition (CVPR), pp 2818–2826. https://doi.org/10.1109/CVPR.2016.308
Howard AG, Zhu M, Chen B, Kalenichenko D, Wang W, Weyand T, Andreetto M, Adam H (2017) MobileNets: efficient convolutional neural networks for mobile vision applications. https://doi.org/10.48550/arXiv.1704.04861 , arXiv:1704.04861
Chollet F (2017) Xception: deep learning with depthwise separable convolutions. In: Proceedings of the IEEE conference on computer vision and pattern recognition, pp 1251–1258. https://doi.org/10.1109/CVPR.2017.195
Huang G, Liu Z, Van Der Maaten L, Weinberger KQ (2017) Densely connected convolutional networks. In: Proceedings of the IEEE conference on computer vision and pattern recognition, pp 4700–4708. https://doi.org/10.1109/CVPR.2017.243
Download references
Not Applicable.
Author information
Authors and affiliations.
Computer Science and Engineering, Visvesvaraya National Institute of Technology, South Ambazari Road, Nagpur, Maharashtra, 440010, India
Archana Boob & Mansi Radke
You can also search for this author in PubMed Google Scholar
Contributions
A.B. wrote the main manuscript text and prepared figures. M.R. reviewed the manuscript and refined it further.
Corresponding author
Correspondence to Archana Boob .
Ethics declarations
Conflict of interest.
The authors declare that they have no Conflict of interest.
Additional information
Publisher's note.
Springer Nature remains neutral with regard to jurisdictional claims in published maps and institutional affiliations.
Rights and permissions
Springer Nature or its licensor (e.g. a society or other partner) holds exclusive rights to this article under a publishing agreement with the author(s) or other rightsholder(s); author self-archiving of the accepted manuscript version of this article is solely governed by the terms of such publishing agreement and applicable law.
Reprints and permissions
About this article
Boob, A., Radke, M. Leveraging two-level deep learning classifiers for 2D shape recognition to automatically solve geometry math word problems. Pattern Anal Applic 27 , 102 (2024). https://doi.org/10.1007/s10044-024-01321-9
Download citation
Received : 06 February 2024
Accepted : 01 August 2024
Published : 14 August 2024
DOI : https://doi.org/10.1007/s10044-024-01321-9
Share this article
Anyone you share the following link with will be able to read this content:
Sorry, a shareable link is not currently available for this article.
Provided by the Springer Nature SharedIt content-sharing initiative
- 2D geometric shape
- Classification
- Deep Learning
- Question Answering (QA)
- Math Word Problems (MWPs)
- Convolutional Neural Network (CNN)
- Find a journal
- Publish with us
- Track your research

Hey! You are using an outdated browser. Please upgrade your browser to improve your experience.
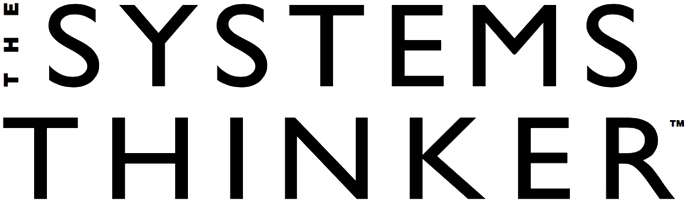
The Common Flaw of All Problem-Solving Models
W e suspect that every reader of this newsletter has been steeped in articles and classes on a myriad of problem-solving techniques. We have all been exposed to techniques such as:
- GROW Model (Goal, Reality, Obstacles/Options, Way Forward)
- Decision Analysis
- Divide & Conquer
- Brainstorming
- Etc, etc, etc… ad nauseum
Unfortunately, abundant data doesn’t necessarily help leaders develop insight or see the interrelatedness of the information.
For the benefit of those who played hooky or who don’t like to read, the underlying message of problem-solving workshops and best practice articles is, ironically, that:
1, Most models do not deliver on the promise of actually solving organizational problems. 2. And the reason for this is usually not due to “operator error.”
… But I Love Models!
Although problem-solving models can be elegant in theory, they are often less helpful in practice. The linear, equation-like approach to problem solving offers hard-edge clarity and precision that is very comforting. However, with complex problems (those involving humans), models typically result in little or no lasting change to the problem.
On the plus side, the problem-solving processes have a strong focus on data gathering. Information on all the parts and pieces of a problem, and occasionally on all of the internal/external forces that impact the problem, is usually a key part of any problem-solving process.
Unfortunately, abundant data doesn’t necessarily help leaders develop insight or see the interrelatedness of the information. In fact, sometimes it results in information overload and actually inhibits problem solving. But as the gambler says, “The game may be crooked but it’s the only game in town.”
At the most basic level, problem solving in an organization is about:
- Making quality decisions
- Executing effectively on those decisions
- Oh yeah, and solutions should reflect an understanding of all of the variables.
No sweat, right? Unfortunately, what seems so simple in theory is anything but in practice.
The Monkey Wrench of Problem Solving
A key condition that makes this seemingly simple and logical process of organizational problem solving so difficult is complexity.
Pardon the geeky definition, but “Complexity refers to interesting patterns and structures that arise from the dynamic interaction of independent elements”—and people are a major element!
In enterprises of any significant size, complexity is alive and well. In fact, complex organizational problems can be so dynamic and adaptive, they can feel alive. They can also feel like they are teetering on the edge of chaos and apt to spin out of control at any moment!
But We Are the Best and Brightest
There is no doubt about the amazing talent that rises to leadership positions in competitive organizations. We have the honor of working with many of the best and brightest daily.
The problem is that even the best leaders have little time for the reflection and analysis that good problem solving requires. And many have only been exposed to linear problem-solving techniques. Good stuff, but applying linear problem-solving tools to complex, dynamic problems will have you drooling on your BlackBerry® and stuttering in no time.
There is no easy fix for complex, dynamic problems. All the problems with easy fixes get taken care of lower down on the organizational chain. Only the most persistent, vexing, and seemingly irresolvable challenges land on your desk—that’s why you get the big bucks.
But don’t despair.
Six Steps to Resolving Complex Dynamic Problems
People can solve complex and dynamic business problems. So, how do they do it? Our favorite approach is using the tools and methodology of systems thinking. Oh no, another model? But I thought you said…
Taking a systemic approach to problem solving offers a practical and pragmatic way to begin to:
- Understand the value of exploring the contributing factors of a problem
- Assess potential intended and unintended consequences
- Redefine the problem based on a closer look at all of the contributing factors
- Select solutions that can deliver the greatest degree of change
Go through the six steps and judge for yourself whether this might provide a breakthrough in your problem solving approach. One suggestion as you contemplate the value of these steps: remember, systems thinking is a team sport. This approach blossoms from multiple perspectives.
Step One: Select a Problem Determine if the problem is complex, dynamic, and interconnected, or straight-forward and linear.* You can use the following five criteria with your team to determine if a situation fits the definition of a complex, systemic issue:
1. It has a pattern. There is a clear history of this problem happening over time, sometimes to the point of acceptance.
2. It has no easy answer and seems to impact a number of other issues and/or departments. No
_________ * A problem can be very complicated, with a fundamentally high level of intricacies—such as determining the tensile strength of the steel required to build a tank—and not have the kind of dynamic complexity that makes it a systemic issue. The techniques of lean manufacturing are superb for resolving linear problems, even if the linear problems are highly complicated!
one wants to take on the problem because it seems too daunting.
3. People are involved. The success of a process depends on human input or judgment.
4. Fixes have been tried but the problem persists. You may have tried to fix the problem or implemented workarounds, but the problem remains and as a result of the “fix,” new problems may have been created.
5. It is multi-dimensional. The issue or problem may involve several functions or possibly customers or suppliers.
Step Two: Identify Contribution Factors
Every problem has some things that you and your team believe are reinforcing, driving, or contributing to the issue in some fashion. The “Systemic Curiosity Worksheet” offers a simple format to use to record the team’s input.
SYSTEMIC CURIOSITY WORKSHEET
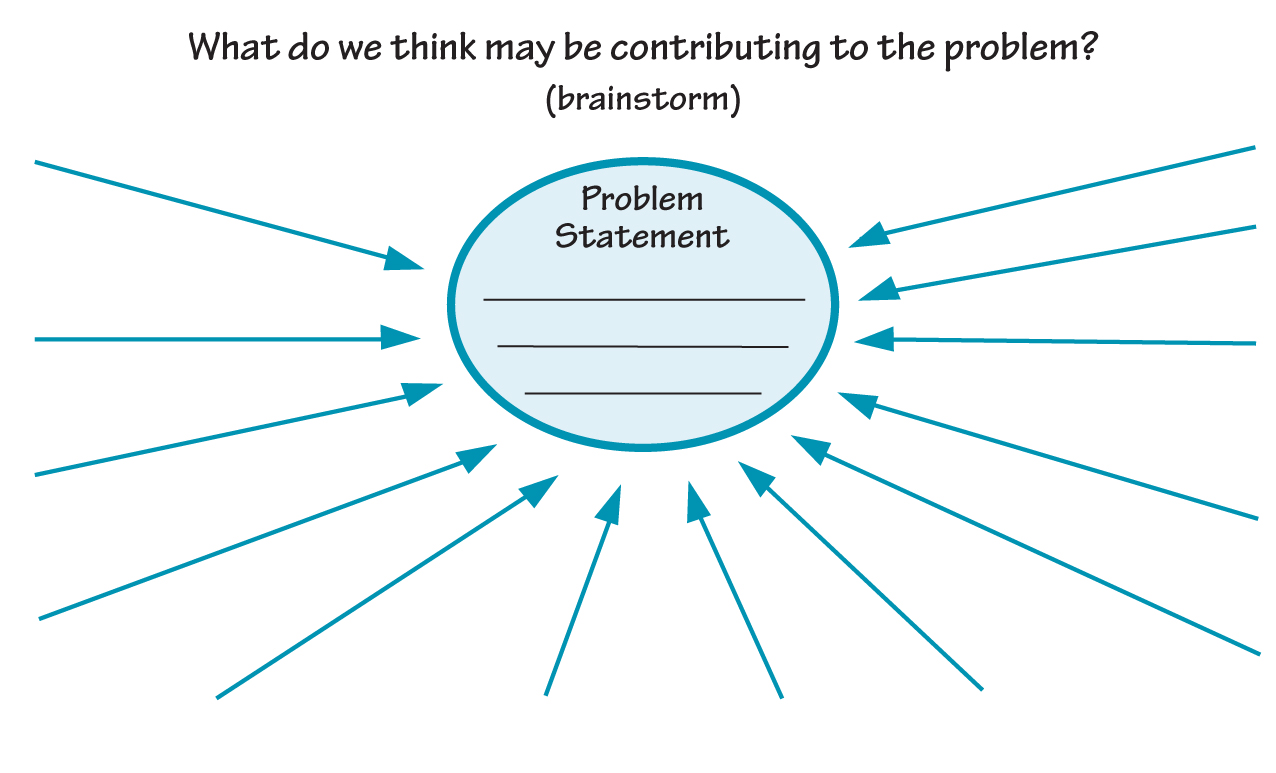
The “Systemic Curiosity Worksheet” offers a simple format to use to record the team’s beliefs on the things that are reinforcing, driving, or contributing to the problem.
Step Three: Review the Problem
Based on the identification of the factors contributing to the problem, review the problem statement and determine if it needs to be restated. The perspectives shared in the discussion about contributing factors frequently lead to a fuller and more complete understanding of the dimensions of the issues to be addressed.
Step Four: Establish Solutions
From a review and discussion of the contributing factors, and the potentially restated problem statement, work with the team to identify at least three ways to solve (influence) the problem.
Step Five: Frame Implications
Determine what the consequences could be of implementing each of the chosen solutions. Describe both intended and unintended consequences, outlining what could happen that the solution is designed to produce, as well as what could happen that is unplanned.
The “Implications Wheel” worksheet is a handy visual to use.
IMPLICATIONS WHEEL
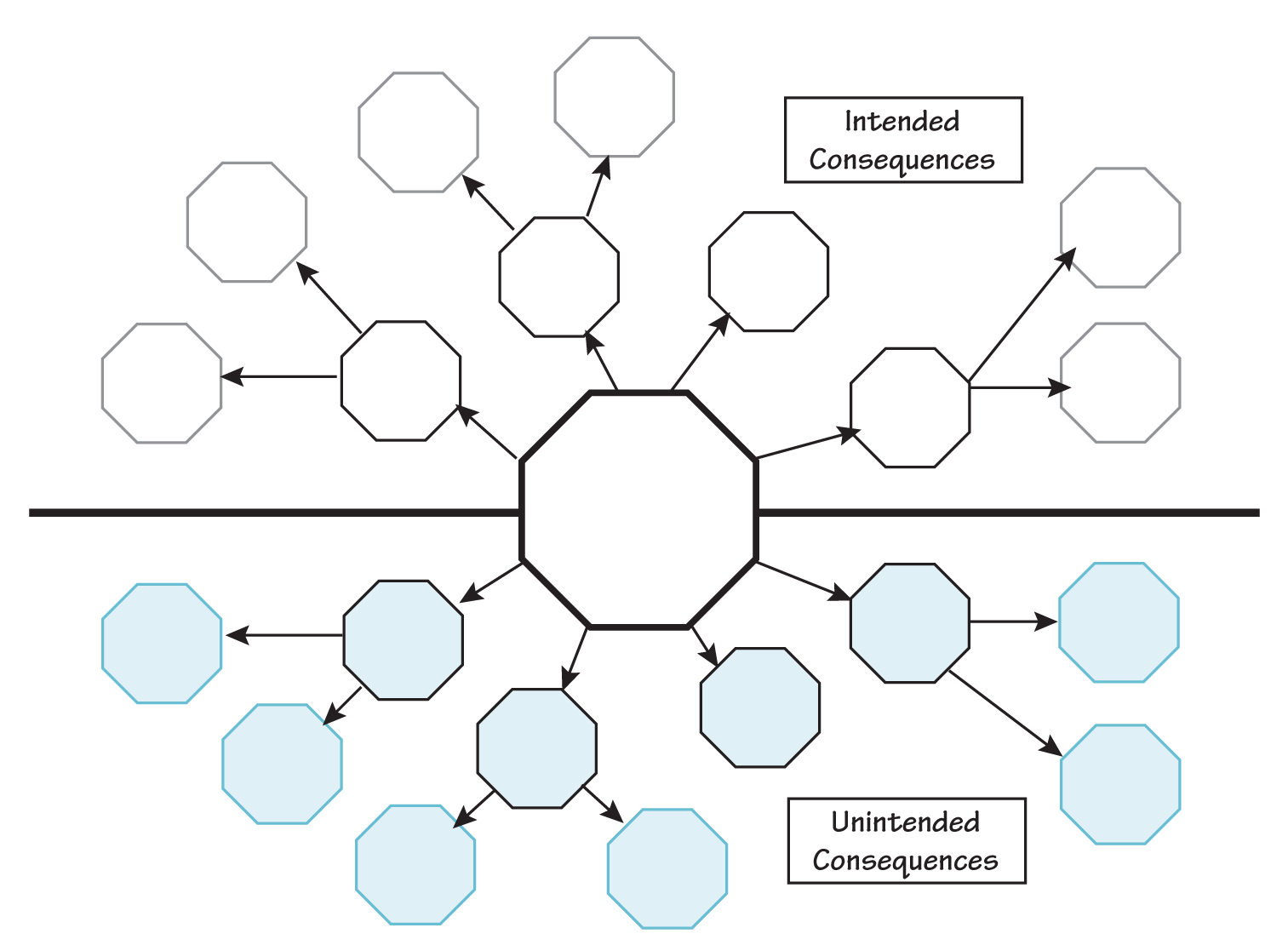
Use the “Implications Wheel” to determine what the intended and unintended consequences could be of implementing each of the chosen solutions.
Step Six: Select Solutions
With a more thorough understanding of the system that is producing the current problem, as well as having identified and tested a number of potential “right answers,” you and your team are now able to select the solutions that can deliver the highest degree of change.
Bonus Outcome
In addition to resolving complex-dynamic problems, this six-step process has the added benefit of:
Encouraging team interaction
► which generates shared team understanding
► which helps develop significant insights around the chosen issue that were not previously apparent.
Paul R. Plotczyk is president of Work Systems Affiliates International, Inc., a leadership and organization development consulting firm. For more than 25 years, he has helped a wide range of clients address tough challenges. Paul has taught and lectured at universities and professional conferences in the United States and Europe. He has co-authored a number of publications, including Best Practices in Executing Projects, Best Practices in Teams, Best Practices in Tools, and Best Practices in Process Improvement.
Related Articles
Learning and leading through the badlands.
We hear a lot about complexity in the business world today — specifically, that increasing complexity is making…
All Methods Are Wrong. Some Methods Are Useful.
Interest in systems concepts is reviving and broadening. However, the sheer size of the field poses a dilemma…
A Pioneer on the Next Frontier: An Interview with Jay Forrester
DIANE CORY: This first question is from a manager at Xerox: “How can I help overcome the common perception…
Integrating Systems Thinking and Design Thinking
As readers of this newsletter are aware, systems thinking is evolving as an alternative to the old paradigms.
Sign up to stay in the loop
Receive updates of new articles and save your favorites..
- First Name *
- Last Name *
- Password * Enter Password Confirm Password

IMAGES
COMMENTS
Common Models to Use in the Problem-Solving Process. Several models can be applied to a complex problem and create possible solutions. These range from common and straightforward to creative and in-depth to identify the most effective ways to solve a problem. This section will discuss and break down the problem-solving models that are most ...
Most problem-solving models rely on data to inform decisions, which helps to maintain objectivity and fairness throughout the process. By using problem-solving methods to hear the opinions of everyone, you can eliminate bias when solving a problem. In addition, implementing problem-solving models can lead to more effective, thoughtful solutions.
Problem Solving Models and Strategies: Polya's Four-Step Model. In this section, we will explore Polya's four-step problem-solving model, a versatile approach that can be applied to various types of problems. This model provides a systematic framework for understanding, analyzing, and solving problems effectively.
Problem-solving games that help raise group awareness through a central, unifying metaphor can be effective ways to warm-up a group in any problem-solving model. Draw a Tree is a simple warm-up activity you can use in any group and which can provide a quick jolt of energy.
Problem Solving. The problem-solving process can be described as a journey from meeting a problem for the first time to finding a solution, communicating it and evaluating the route. There are many models of the problem-solving process but they all have a similar structure. One model is given below.
When we do problem definition well in classic problem solving, we are demonstrating the kind of empathy, at the very beginning of our problem, that design thinking asks us to approach. When we ideate—and that's very similar to the disaggregation, prioritization, and work-planning steps—we do precisely the same thing, and often we use ...
Our online Problem solving tutor will help your 16-18 learners to structure and develop their problem solving skills in quantitative chemistry. Find out how to use post-16 models to inform 14-16 understanding. Show your students how models can help to predict reactions and influence the taste and texture of chocolate and biscuits, by ...
The FOCUS Model is a simple quality-improvement tool commonly used in the health care industry. You can use it to improve any process, but it is particularly useful for processes that span different departments. The five steps in FOCUS are as follows: F ind the problem. O rganize a team. C larify the problem.
The Bar Model is a mathematical diagram that is used to represent and solve problems involving quantities and their relationships to one another. It was developed in Singapore in the 1980s when data showed Singapore's elementary school students were lagging behind their peers in math. An analysis of testing data at the time showed less than ...
To solve a word problem, students can pick out the numbers and decide on an operation.". But through the use of mathematical modeling, students are plucked out of the hypothetical realm and plunged into the complexities of reality—presented with opportunities to help solve real-world problems with many variables by generating questions ...
Along with his colleague Mary Boone, he published the framework in the November 2007 issue of the Harvard Business Review. The Cynefin framework (Figure 1 below) is a problem-solving tool that helps you put situations into five "domains" defined by cause-and-effect relationships. This helps you assess your situation more accurately and respond ...
The Six Step Problem Solving Model isn't just a method; it's a mindset. A mindset that ensures problems are tackled systematically and collaboratively, driving teams towards effective ...
The Productive Thinking Model was developed by author and creativity theorist, Tim Hurson, and was published in his 2007 book, " Think Better ." [1] The model presents a structured framework for solving problems creatively. You can use it on your own or in a group. The model consists of six steps, as follows:
The 8D problem solving model establishes a permanent corrective action based on statistical analysis of the problem and focuses on the origin of the problem by determining its root causes. Although it originally comprised eight stages, or disciplines, the eight disciplines system was later augmented by an initial planning stage. ...
Using models is a critical step in helping students transition from concrete manipulative work with word problems to the abstract step of generating an equation to solve contextual problems. By learning to use simple models to represent key mathematical relationships in a word problem, students can more easily make sense of word problems ...
This paper addressed both problems using a novel three-layered sparse DL (TLSDL) algorithm that incorporated prior information in the dictionary update process and recovered full-rank outlier-free ...
The McKinsey guide to problem solving. Become a better problem solver with insights and advice from leaders around the world on topics including developing a problem-solving mindset, solving problems in uncertain times, problem solving with AI, and much more.
Remarkably, the problem-solving models developed by each of these areas are strikingly similar. I describe a simple problem-solving process that you can use to solve almost all problems. Stages of the problem-solving process. The famous psychologist, Dr. Carl Rogers, was one of the first to help us understand how important self-exploration and ...
This six-step model is designed for the workplace, but is easily adaptable to other settings such as schools and families. It emphasizes the cyclical, continuous nature of the problem-solving process. The model describes in detail the following steps: Step One: Define the Problem. Step Two: Determine the Root Cause(s) of the Problem
Improvement of problem solving skills. Model for analyzing the processes that underlie effective problem solving. IDEAL Model for improving problem solving (Verbatim copy of Fig 2.1; p.12) ... Recall answers or solve problems out of order to be sure you know which concepts to apply and why. Look at mistakes made as soon as possible, and learn ...
Operations research (OR) uses mathematical models to enhance decision-making, but developing these models requires expert knowledge and can be time-consuming. Automated mathematical programming (AMP) has emerged to simplify this process, but existing systems have limitations. This paper introduces a novel methodology that uses recent advances in Large Language Model (LLM) to create and edit OR ...
have probably already engaged in solving problems, you have probably used many different approaches in order to achieve a solution. Issues and operational problems in a committee can be solved more easily and with better results by using a problem solving model, i.e. a structured, systematic approach to solving problems and making improvements.
In insight problem-solving, the cognitive processes that help you solve a problem happen outside your conscious awareness. 4. Working backward. Working backward is a problem-solving approach often ...
The Vehicle Routing Problem (VRP) is a classic combinatorial optimization problem commonly encountered in the fields of transportation and logistics. This paper focuses on a variant of the VRP, namely the Vehicle Routing Problem with Stochastic Travel Cost (VRP-STC). In VRP-STC, the introduction of stochastic travel costs increases the complexity of the problem, rendering traditional ...
The '2DGeoShapeNet' model was developed using TensorFlow and Keras libraries, leveraging advanced CNN architectures for feature extraction and classification. ... The implementation of the '2DGeoShapeNet' model addresses a significant gap in the field of automated geometry problem-solving by enhancing the performance of geometric shape ...
The model follows a very simple seven-step process: [1] 1. Assemble a Team. Gather together people who are familiar with the specifics of the problem, and with the process that you're trying to fix. Include someone to act as a facilitator, who can keep the team focused on identifying effective counter-measures. 2.
Problem-solving is the capacity to identify and describe a problem and generate solutions to fix it. Problem-solving involves other executive functioning behaviors as well, including attentional control, planning, and task initiation. Individuals might use time management, emotional control, or organization skills to solve problems as well.
Step One: Select a Problem Determine if the problem is complex, dynamic, and interconnected, or straight-forward and linear.*. You can use the following five criteria with your team to determine if a situation fits the definition of a complex, systemic issue: 1. It has a pattern.
Lessons from Will Hunting and McGayverIn the world of data science, data cleaning is often seen as one of the most time-consuming and least glamorous tasks. Yet, it's also one of the most critical. Without clean data, even the most sophisticated algorithms and models can produce misleading results. This is where the janitor package in R comes into play, serving as the unsung hero that ...