- Previous Article
- Next Article

Settlements and slides: a large landslide case study from the Central Cordillera of the Philippines
* Correspondence: [email protected]
- View This Citation
- Add to Citation Manager
- Permissions
- Search Site
Gareth James Hearn , Jonathan Roy Hart; Settlements and slides: a large landslide case study from the Central Cordillera of the Philippines. Quarterly Journal of Engineering Geology and Hydrogeology 2019;; 53 (1): 62–73. doi: https://doi.org/10.1144/qjegh2019-050
Download citation file:
- Ris (Zotero)
The Central Cordillera of Luzon in the Philippines is home to some of the most complex geology, active plate margin tectonism, heaviest rainfall and steepest terrain in the world. It also hosts a thriving agricultural community that has developed its agrarian, municipal and transport infrastructure in a landscape of marginal stability and extreme geomorphological sensitivity. The village of Pilando occupies a saddle on a prominent ridge where it is crossed by the Halsema Highway. As a result of landslide displacements, both the highway and the village have been subsiding for several decades and many dwellings have had to be abandoned. From topographical survey and visual observation, average rates of settlement vary between c. 0.1 and 0.4 m a −1 . The apparent depth and areal extent of ground movement are such that attempts to stabilize the area would be both impracticable and uneconomic. Realignment and relocation are options that might be considered but many adjacent slopes are unstable or have failed in the past and those that have not are highly sensitive to earthworks and drainage disturbance. Unfortunately, there are many areas of the Central Cordillera that are affected by similar slope instability problems. The interpretation of satellite imagery available on Google Earth, supported by geomorphological field observation, can assist in the delineation and assessment of these notably unstable areas for land use planning and slope management purposes.
Shibboleth Sign In
OpenAthens Sign In
Institutional Sign In
Email alerts
- active margins
- case studies
- catastrophes
- Central Cordillera
- climate effects
- earthquake prediction
- earthquakes
- erosion features
- foundations
- geologic hazards
- Luzon earthquake 1990
- mass movements
- mobile belts
- natural hazards
- Philippine Islands
- radar methods
- slope stability
- Abra River Fault
- Digdig Fault
- Halsema Highway
- N12°31'00" - N18°34'60", E119°45'00" - E124°13'60"

Citing articles via
Affiliations.
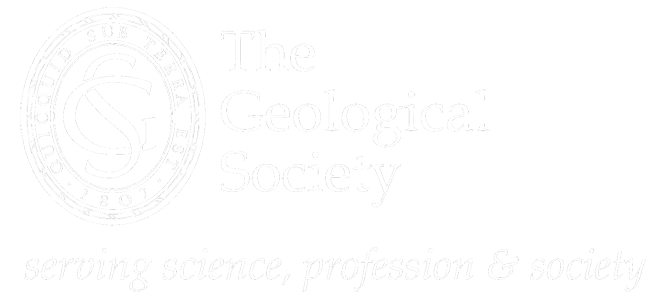
- Current Issue
- Early Publication
- Online ISSN 2041-4803
- ISSN 1470-9236
- Copyright © 2024 Geological Society of London
- How to Subscribe
- Privacy Policy
- For Librarians
- For Industry
- For Society Members
- For Authors
- Terms & Conditions
- 1750 Tysons Boulevard, Suite 1500
- McLean, VA 22102
- Telephone: 1-800-341-1851
- Copyright © 2024 GeoScienceWorld
This Feature Is Available To Subscribers Only
Sign In or Create an Account
Effects of Digital Elevation Models on Spatial Characterisation of Landslides in the Kalka-Shimla Region of the Indian Himalayas
- Research Article
- Published: 05 July 2024
Cite this article
- Ankur Sharma ORCID: orcid.org/0000-0002-0271-984X 1 &
- Har Amrit Singh Sandhu 1
Landslides are complex geohazards responsible for damage to life, the natural environment, and essential infrastructures like buildings, roads, and transmission lines in mountainous regions. The modeling of topographic input parameters for landslide-related investigations is often based on Digital Elevation Models (DEMs), which serve as a crucial geospatial data source. The present study attempts to analyze the effects of DEMs, obtained from different sources and varying in spatial resolution, on terrain feature estimation and spatial characterization of landslide-affected areas in the Indian Himalayas. Carto-DEM version 3R1 and ALOS PALSAR DEM are used to generate two geodatabases of DEM-derived landslide causative factors, each including digital maps of Elevation, Slope, Aspect, Curvature, Terrain Ruggedness Index, and Distance to Drainage. The generated geodatabases are utilized for conducting a spatial frequency distribution analysis to characterize the selected area into spatial bins with similar topographic characteristics. A comparative study of this analysis reveals that both the DEMs exhibited comparable topographic characteristics on a general level. However, considerable variations are observed when both the geodatabases are scrutinized closely. The results of this study highlight that the quality of the DEM used may affect its usability in a specific investigation and hope to add to the scientific discourse on the effects of DEM on landslide-related studies.
This is a preview of subscription content, log in via an institution to check access.
Access this article
Subscribe and save.
- Get 10 units per month
- Download Article/Chapter or Ebook
- 1 Unit = 1 Article or 1 Chapter
- Cancel anytime
Price includes VAT (Russian Federation)
Instant access to the full article PDF.
Rent this article via DeepDyve
Institutional subscriptions
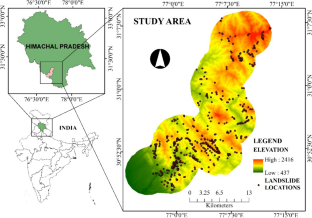
Amatya, P., Kirschbaum, D., & Stanley, T. (2019). Use of very high-resolution optical data for landslide mapping and susceptibility analysis along the Karnali highway, Nepal. Remote Sensing . https://doi.org/10.3390/rs11192284
Article Google Scholar
Asmare, D. (2023). Application and validation of AHP and FR methods for landslide susceptibility mapping around choke mountain, Northwestern, Ethiopia. Scientific African . https://doi.org/10.1016/j.sciaf.2022.e01470
Ayalew, L., Yamagishi, H., & Ugawa, N. (2004). Landslide susceptibility mapping using GIS-based weighted linear combination, the case in Tsugawa area of Agano River, Niigata Prefecture, Japan. Landslides, 1 (1), 73–81. https://doi.org/10.1007/s10346-003-0006-9
Batar, A. K., & Watanabe, T. (2021). Landslide susceptibility mapping and assessment using geospatial platforms and weights of evidence (WoE) method in the Indian Himalayan region: Recent developments, gaps, and future directions. ISPRS International Journal of Geo-Information, . https://doi.org/10.3390/ijgi10030114
Batar, A. K., Watanabe, T., & Kumar, A.(2017). Assessment of land-use/land-cover change and forest fragmentation in the Garhwal Himalayan region of India. Environments—MDPI, 4 (2), 1–16. https://doi.org/10.3390/environments4020034
Bureau of Indian Standards New Delhi. (2002). Criteria for earthquake resistant design of structures—general provisions and buildings part-1. Bureau of Indian Standards, New Delhi, Part, 1 , 1–39.
Google Scholar
Chawla, A., Chawla, S., Pasupuleti, S., Rao, A. C. S., Sarkar, K., & Dwivedi, R. Landslide susceptibility mapping in Darjeeling Himalayas, India. Advances in Civil Engineering . https://doi.org/10.1155/2018/6416492
Chawla, A., Pasupuleti, S., Chawla, S., Rao, A. C. S., Sarkar, K., & Dwivedi, R. (2019). Landslide susceptibility zonation mapping: A Case study from Darjeeling District, Eastern Himalayas, India. Journal of the Indian Society of Remote Sensing, 47 (3), 497–511. https://doi.org/10.1007/s12524-018-0916-6
Chawla, S., Chawla, A., & Pasupuleti, S.(2017). A feasible approach for landslide susceptibility map using GIS 101–110. https://doi.org/10.1061/9780784480717.010
Chang, Z., Du, Z., Zhang, F., Huang, F., Chen, J., Li, W., & Guo, Z. (2020). Landslide susceptibility prediction based on remote sensing images and GIS: Comparisons of supervised and unsupervised machine learning models. Remote Sensing . https://doi.org/10.3390/rs12030502
Chow, T. E., & Hodgson, M. E. (2009). Effects of lidar post-spacing and DEM resolution to mean slope estimation. International Journal of Geographical Information Science, 23 (10), 1277–1295. https://doi.org/10.1080/13658810802344127
Conforti, M., Pascale, S., Robustelli, G., & Sdao, F. (2014). Evaluation of prediction capability of the artificial neural networks for mapping landslide susceptibility in the Turbolo River catchment (northern Calabria, Italy). CATENA, 113 , 236–250. https://doi.org/10.1016/j.catena.2013.08.006
Cruden, D. M. (1991). A simple definition of a landslide. Bulletin of the International Association of Engineering Geology-Bulletin De L’association Internationale De Géologie De L’ingénieur, 43 (1), 27–29. https://doi.org/10.1007/BF02590167
Dahal, R. K., Hasegawa, S., Masuda, T., & Yamanaka, M. (2006). Roadside Slope Failures in Nepal during Torrential Rainfall and their Mitigation Road construction practice in Nepal. In Proceedings of the Interpaevent international symposium on Niijigata 2006, disaster mitigation of debris flow, slope failures and landslides (pp. 503–514).
Dai, F. C., Lee, C. F., & Ngai, Y. Y. (2002). Landslide risk assessment and management: An overview. Engineering Geology, 64 (1), 65–87. https://doi.org/10.1016/S0013-7952(01)00093-X
Deng, Y., Wilson, J. P., & Bauer, B. O. (2007). DEM resolution dependencies of terrain attributes across a landscape. International Journal of Geographical Information Science, 21 (2), 187–213. https://doi.org/10.1080/13658810600894364
El Jazouli, A., Barakat, A., & Khellouk, R. (2019). GIS-multicriteria evaluation using AHP for landslide susceptibility mapping in Oum Er Rbia high basin (Morocco). Geoenvironmental Disasters . https://doi.org/10.1186/s40677-019-0119-7
Fell, R., Corominas, J., Bonnard, C., Cascini, L., Leroi, E., & Savage, W. Z. (2008). Guidelines for landslide susceptibility, hazard and risk zoning for land use planning. Engineering Geology, 102 (3–4), 85–98. https://doi.org/10.1016/j.enggeo.2008.03.022
Geertsema, M., & Pojar, J. J. (2007). Influence of landslides on biophysical diversity—A perspective from British Columbia. Geomorphology, 89 (1–2 SPEC. ISS), 55–69. https://doi.org/10.1016/j.geomorph.2006.07.019
Glade, T. (2002). Landslide occurrence as a response to land use change: A review of evidence from New Zealand. CATENA, 51 , 297–314.
Gorsevski, P. V., Gessler, P. E., Foltz, R. B., & Elliot, W. J. (2006). Spatial prediction of landslide hazard using logistic regression and ROC analysis. Transactions in GIS, 10 (3), 395–415. https://doi.org/10.1111/j.1467-9671.2006.01004.x
Hao, L., A., R., Van Westen, C., K. S., S., Ranjan Martha, T., Jaiswal, P., & G. McAdoo, B. (2020). ‘Constructing a complete landslide inventory dataset for the 2018 monsoon disaster in Kerala, India, for land use change analysis. Earth System Science Data, 12 (4), 2899–2918. https://doi.org/10.5194/essd-12-2899-2020
Huang, F., Yin, K., Huang, J., Gui, L., & Wang, P. (2017). Landslide susceptibility mapping based on self-organizing-map network and extreme learning machine. Engineering Geology, 223 , 11–22. https://doi.org/10.1016/j.enggeo.2017.04.013
Huang, F., Cao, Z., Guo, J., Jiang, S. H., Li, S., & Guo, Z. (2020). Comparisons of heuristic, general statistical and machine learning models for landslide susceptibility prediction and mapping. CATENA . https://doi.org/10.1016/j.catena.2020.104580
Jones, S., Kasthurba, A. K., Bhagyanathan, A., & Binoy, B. V. (2021). Landslide susceptibility investigation for Idukki district of Kerala using regression analysis and machine learning. Arabian Journal of Geosciences . https://doi.org/10.1007/s12517-021-07156-6
Kamiński, M. (2020). The impact of quality of digital elevation models on the result of landslide susceptibility modeling using the method of weights of evidence. Geosciences (switzerland), 10 , 1–21. https://doi.org/10.3390/geosciences10120488
Kanungo, D. P., Arora, M. K., Sarkar, S., & Gupta, R. P. (2006). A comparative study of conventional, ANN black box, fuzzy and combined neural and fuzzy weighting procedures for landslide susceptibility zonation in Darjeeling Himalayas. Engineering Geology, 85 (3–4), 347–366. https://doi.org/10.1016/j.enggeo.2006.03.004
Keefer, D. K. (1984). Landslides caused by earthquakes. Geological Society of America Bulletin, 95 (4), 406–421. http://pubs.er.usgs.gov/publication/70014049
Kim, J. C., Lee, S., Jung, H. S., & Lee, S. (2018). Landslide susceptibility mapping using random forest and boosted tree models in Pyeong-Chang, Korea. Geocarto International, 33 (9), 1000–1015. https://doi.org/10.1080/10106049.2017.1323964
Lin, G. F., Chang, M. J., Huang, Y. C., & Ho, J. Y. (2017). Assessment of susceptibility to rainfall-induced landslides using improved self-organizing linear output map, support vector machine, and logistic regression. Engineering Geology, 224 , 62–74. https://doi.org/10.1016/j.enggeo.2017.05.009
Mahalingam, R., & Olsen, M. J. (2016). Evaluation of the influence of source and spatial resolution of DEMs on derivative products used in landslide mapping. Geomatics, Natural Hazards and Risk, 7 (6), 1835–1855. https://doi.org/10.1080/19475705.2015.1115431
Meusburger, K., & Alewell, C. (2008). Impacts of anthropogenic and environmental factors on the occurrence of shallow landslides in an alpine catchment (Urseren Valley, Switzerland). Natural Hazards and Earth System Science, 8 (3), 509–520. https://doi.org/10.5194/nhess-8-509-2008
Naidu, S., Sajinkumar, K. S., Oommen, T., Anuja, V. J., Samuel, R. A., & Muraleedharan, C. (2018). Early warning system for shallow landslides using rainfall threshold and slope stability analysis. Geoscience Frontiers, 9 (6), 1871–1882. https://doi.org/10.1016/j.gsf.2017.10.008
Naseer, S., Haq, T. U., Khan, A., Tanoli, J. I., Khan, N. G., Qaiser, F. ur R., & Shah, S. T. H. (2021). GIS-based spatial landslide distribution analysis of district Neelum, AJ&K, Pakistan. Natural Hazards, 106 (1), 965–989. https://doi.org/10.1007/s11069-021-04502-5
National Disaster Management Authority (2019) A publication of the National Disaster Management Authority, Government of India .
Niu, R., Wu, X., Yao, D., Peng, L., Ai, L., & Peng, J. (2014). Susceptibility assessment of landslides triggered by the Lushan earthquake, April 20, 2013, China. IEEE Journal of Selected Topics in Applied Earth Observations and Remote Sensing, 7 (9), 3979–3992. https://doi.org/10.1109/JSTARS.2014.2308553
Pandit, K., Singh, M., Sharma, S., Sandhu, H. A. S., & Sahoo, J. P. (2021). Back-analysis of a debris slope through numerical methods and field observations of slope displacements. Indian Geotechnical Journal, 51 (4), 811–828. https://doi.org/10.1007/s40098-021-00553-4
Park, S., & Kim, J. (2019). Landslide susceptibility mapping based on random forest and boosted regression tree models, and a comparison of their performance. Applied Sciences (switzerland) . https://doi.org/10.3390/app9050942
Peethambaran, B., Anbalagan, R., Shihabudheen, K. V., & Goswami, A. (2019). Robustness evaluation of fuzzy expert system and extreme learning machine for geographic information system-based landslide susceptibility zonation: A case study from Indian Himalaya. Environmental Earth Sciences . https://doi.org/10.1007/s12665-019-8225-0
Pham, B. T., Tien Bui, D., Prakash, I., & Dholakia, M. B. (2016). Rotation forest fuzzy rule-based classifier ensemble for spatial prediction of landslides using GIS. Natural Hazards, 83 (1), 97–127. https://doi.org/10.1007/s11069-016-2304-2
Pourghasemi, H. R., Gayen, A., Park, S., Lee, C. W., & Lee, S. (2018). “Assessment of landslide-prone areas and their zonation using logistic regression, LogitBoost, and naïvebayes machine-learning algorithms. Sustainability (switzerland) . https://doi.org/10.3390/su10103697
Pradhan, B. (2013). A comparative study on the predictive ability of the decision tree, support vector machine and neuro-fuzzy models in landslide susceptibility mapping using GIS. Computers and Geosciences, 51 , 350–365. https://doi.org/10.1016/j.cageo.2012.08.023
Pradhan, B., & Lee, S. (2010). Landslide susceptibility assessment and factor effect analysis: Backpropagation artificial neural networks and their comparison with frequency ratio and bivariate logistic regression modelling. Environmental Modelling and Software, 25 (6), 747–759. https://doi.org/10.1016/j.envsoft.2009.10.016
Promper, C., Puissant, A., Malet, J. P., & Glade, T. (2014). Analysis of land cover changes in the past and the future as contribution to landslide risk scenarios. Applied Geography, 53 , 11–19. https://doi.org/10.1016/j.apgeog.2014.05.020
Rabby, Y. W., Ishtiaque, A., & Rahman, M. S. (2020). Evaluating the effects of digital elevation models in landslide susceptibility mapping in rangamati district, Bangladesh. Remote Sensing . https://doi.org/10.3390/RS12172718
Raghuvanshi, T. K., Ibrahim, J., & Ayalew, D. Slope stability susceptibility evaluation parameter (SSEP) rating scheme—An approach for landslide hazard zonation. Journal of African Earth Sciences, 99 (PA2), 595–612. https://doi.org/10.1016/j.jafrearsci.2014.05.004
Rawat, M. S., Uniyal, D. P., Dobhal, R., Joshi, V., Rawat, B. S., Bartwal, A., Singh, D., & Aswal, A. (2015). Study of landslide hazard zonation in Mandakini Valley, Rudraprayag district, Uttarakhand using remote sensing and GIS. Current Science, 109 (1).
Riley, S. J., DeGloria, S. D., & Elliot, R. (1999). A terrain ruggedness index that qauntifies topographic heterogeneity. Intermountain Journal of Sciences, 5 (1–4), 23–27.
Roy, J., & Saha, S. (2019). Landslide susceptibility mapping using knowledge driven statistical models in Darjeeling District, West Bengal, India. Geoenvironmental Disasters . https://doi.org/10.1186/s40677-019-0126-8
Saha, A., Pal, S. C., Chowdhuri, I., Chakrabortty, R., & Roy, P. (2022). Understanding the scale effects of topographical variables on landslide susceptibility mapping in Sikkim Himalaya using deep learning approaches. Geocarto International, 37 (27), 17826–17852. https://doi.org/10.1080/10106049.2022.2136255
Saha, A. K., Gupta, R. P., & Arora, M. K. (2002). GIS-based Landslide Hazard Zonation in the Bhagirathi (Ganga) Valley, Himalayas. International Journal of Remote Sensing, 23 (2), 357–369. https://doi.org/10.1080/01431160010014260
Sarkar, S., & Kanungo, D. P. (2004). An integrated approach for landslide susceptibility mapping using remote sensing and GIS. Photogrammetric Engineering and Remote Sensing, 70 (5), 617–625. https://doi.org/10.14358/PERS.70.5.617
Sarkar, S., Kanungo, D. P., & Mehrotra, G. S. (1995). Landslide Hazard Zonation: A case study in Garhwal Himalaya, India. Mountain Research and Development, 15 (4), 301–309.
Sarkar, S., Roy, A. K., & Martha, T. R. (2013). Landslide susceptibility assessment using Information Value Method in parts of the Darjeeling Himalayas. Journal of the Geological Society of India, 82 (4), 351–362. https://doi.org/10.1007/s12594-013-0162-z
Sarma, C. P., Dey, A., & Krishna, A. M. (2020). Influence of digital elevation models on the simulation of rainfall-induced landslides in the hillslopes of Guwahati, India. Engineering Geology . https://doi.org/10.1016/j.enggeo.2020.105523
Schuster, R. L., & Fleming, R. W. (1986). Economic losses and fatalities due to landslides. Bulletin of the Association of Engineering Geologists . https://doi.org/10.2113/gseegeosci.xxiii.1.11
Schuster, R. L., & Highland, L. M. (2007). The third hans cloos lecture. Urban landslides: Socioeconomic impacts and overview of mitigative strategies. Bulletin of Engineering Geology and the Environment, 66 (1), 1–27. https://doi.org/10.1007/s10064-006-0080-z
Shirzadi, A., Soliamani, K., Habibnejhad, M., Kavian, A., Chapi, K., Shahabi, H., Chen, W., Khosravi, K., Pham, B. T., Pradhan, B., Ahmad, A., Bin Ahmad, B., & Bui, D. T. (2018). Novel GIS based machine learning algorithms for shallow landslide susceptibility mapping. Sensors (switzerland), 18 (11), 234. https://doi.org/10.3390/s18113777
Soria, D., Garibaldi, J. M., Ambrogi, F., Biganzoli, E. M., & Ellis, I. O. (2011). A ‘non-parametric’ version of the naive Bayes classifier. Knowledge-Based Systems, 24 (6), 775–784. https://doi.org/10.1016/j.knosys.2011.02.014
Tan, Q., Wang, P., Hu, J., Zhou, P., Bai, M., & Hu, J. (2020). The application of multi-sensor target tracking and fusion technology to the comprehensive early warning information extraction of landslide multi-point monitoring data. Measurement: Journal of the International Measurement Confederation, 166 , 108044. https://doi.org/10.1016/j.measurement.2020.108044
Tien Bui, D., Nguyen, Q. P., Hoang, N. D., & Klempe, H. (2017). A novel fuzzy K-nearest neighbor inference model with differential evolution for spatial prediction of rainfall-induced shallow landslides in a tropical hilly area using GIS. Landslides, 14 (1), 1–17. https://doi.org/10.1007/s10346-016-0708-4
Tropeano, D., & Turconi, L. (2002). Using historical documents for landslide, debris flow and stream flood prevention. Applications in Northern Italy. Natural Hazards, 31 (October 2004), 663–679.
van Beek, L. P. H., & van Asch, T. W. J. (2004). Regional assessment of the effects of land-use change on landslide hazard by means of physically based modelling. Natural Hazards, 31 (1), 289–304. https://doi.org/10.1023/B:NHAZ.0000020267.39691.39
Xu, C., Xu, X., Shyu, J. B. H., Zheng, W., & Min, W. (2014). Landslides triggered by the 22 July 2013 Minxian-Zhangxian, China, Mw 5.9 earthquake: Inventory compiling and spatial distribution analysis. Journal of Asian Earth Sciences, 92 (July 2013), 125–142. https://doi.org/10.1016/j.jseaes.2014.06.014
Zare, M., Pourghasemi, H. R., Vafakhah, M., & Pradhan, B. (2013). Landslide susceptibility mapping at Vaz Watershed (Iran) using an artificial neural network model: A comparison between multilayer perceptron (MLP) and radial basic function (RBF) algorithms. Arabian Journal of Geosciences, 6 (8), 2873–2888. https://doi.org/10.1007/s12517-012-0610-x
Zhao, X., & Chen, W. (2020). Optimization of computational intelligence models for landslide susceptibility evaluation. Remote Sensing . https://doi.org/10.3390/rs12142180
Download references
Acknowledgements
The authors would like to sincerely thank the anonymous reviewers and members of the editorial team for their comments and contributions.
The authors declare that no funds, grants, or other support were received during the preparation of this manuscript.
Author information
Authors and affiliations.
Department of Civil Engineering, Punjab Engineering College, Sector 12, Chandigarh, 160012, India
Ankur Sharma & Har Amrit Singh Sandhu
You can also search for this author in PubMed Google Scholar
Contributions
AS Conceptualisation, Methodology, Software, Validation, Formal analysis, Investigation, Data curation, Writing—Original Draft. HASS Supervision, Writing—Reviewing and editing.
Corresponding author
Correspondence to Ankur Sharma .
Ethics declarations
Conflict of interest.
The authors declared that they have no conflict of interest.
Data Availability
The data that support the findings of this study are available from the corresponding author, Ankur Sharma, upon reasonable request.
Additional information
Publisher's note.
Springer Nature remains neutral with regard to jurisdictional claims in published maps and institutional affiliations.
About this article
Sharma, A., Sandhu, H.A.S. Effects of Digital Elevation Models on Spatial Characterisation of Landslides in the Kalka-Shimla Region of the Indian Himalayas. J Indian Soc Remote Sens (2024). https://doi.org/10.1007/s12524-024-01938-7
Download citation
Received : 07 June 2022
Accepted : 21 June 2024
Published : 05 July 2024
DOI : https://doi.org/10.1007/s12524-024-01938-7
Share this article
Anyone you share the following link with will be able to read this content:
Sorry, a shareable link is not currently available for this article.
Provided by the Springer Nature SharedIt content-sharing initiative
- Landslide inventory
- Topographical characterization
- Spatial frequency distribution analysis
- Digital elevation model (DEM)
- Landslide zonation mapping
- Geographical information system (GIS)
- Find a journal
- Publish with us
- Track your research

- Unit 4: Anatomy of a tragic slide: Oso Landslide case study
Sarah Hall (College of the Atlantic) Becca Walker (Mt. San Antonio College)
This activity was selected for the On the Cutting Edge Reviewed Teaching Collection
This activity has received positive reviews in a peer review process involving five review categories. The five categories included in the process are
For more information about the peer review process itself, please see https://serc.carleton.edu/teachearth/activity_review.html .
Landslides can have profound societal consequences, such as did the slide that occurred near Oso, Washington in 2014. Forty-three people were killed and entire rural neighborhood was destroyed. In this unit, students consider the larger-scale tectonic and climatic setting for the landslide and subsequently use lidar and SRTM (Shuttle Radar Topography Mission) hillshade images, topographic maps, and InSAR (interferometric synthetic aperture radar) to determine relationships between landscape characteristics and different types of mass-wasting events. They conclude by considering the societal costs of such a disaster and ways that communities in similar situations may mitigate their risk.
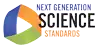
Expand for more detail and links to related resources
Activity Classification and Connections to Related Resources Collapse
Grade level.
View Standards Details »
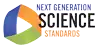
Science and Engineering Practices
Obtaining, Evaluating, and Communicating Information: Compare, integrate and evaluate sources of information presented in different media or formats (e.g., visually, quantitatively) as well as in words in order to address a scientific question or solve a problem. HS-P8.2:
Constructing Explanations and Designing Solutions: Apply scientific ideas, principles, and/or evidence to provide an explanation of phenomena and solve design problems, taking into account possible unanticipated effects. HS-P6.3:
Analyzing and Interpreting Data: Analyze data using tools, technologies, and/or models (e.g., computational, mathematical) in order to make valid and reliable scientific claims or determine an optimal design solution. HS-P4.1:
Cross Cutting Concepts
Stability and Change: Change and rates of change can be quantified and modeled over very short or very long periods of time. Some system changes are irreversible. HS-C7.2:
Patterns: Empirical evidence is needed to identify patterns. HS-C1.5:
Cause and effect: Cause and effect relationships can be suggested and predicted for complex natural and human designed systems by examining what is known about smaller scale mechanisms within the system. HS-C2.2:
Disciplinary Core Ideas
The Roles of Water in Earth's Surface Processes: The abundance of liquid water on Earth’s surface and its unique combination of physical and chemical properties are central to the planet’s dynamics. These properties include water’s exceptional capacity to absorb, store, and release large amounts of energy, transmit sunlight, expand upon freezing, dissolve and transport materials, and lower the viscosities and melting points of rocks. HS-ESS2.C1:
Plate Tectonics and Large-Scale System Interactions: Plate tectonics is the unifying theory that explains the past and current movements of the rocks at Earth’s surface and provides a framework for understanding its geologic history. Plate movements are responsible for most continental and ocean-floor features and for the distribution of most rocks and minerals within Earth’s crust. HS-ESS2.B2:
Performance Expectations
Earth and Human Activity: Construct an explanation based on evidence for how the availability of natural resources, occurrence of natural hazards, and changes in climate have influenced human activity. HS-ESS3-1:
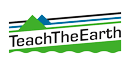
Learning Goals
Unit 4 learning outcomes × div[id^='image-'] {position:static}div[id^='image-'] div.hover{position:static} a view from a washington army national guard helicopter shows trees that were at the very top of the hill when the mudslide occurred now lie across the slide's width like a woven belt. provenance: photo by sgt. matthew sissel joint forces headquarters, washington national guard https://www.dvidshub.net/image/1209685/oso-mudslide reuse: this item is in the public domain and maybe reused freely without restriction..
- Students will identify several environmental characteristics of a natural landscape that increase an area's mass-wasting susceptibility.
- Students will create a map with appropriate symbology illustrating the morphological characteristics of a mass-wasting event.
- Students will use geodetic data to compare the appearance, existence, and morphology of surface features before and after a mass-wasting event.
Unit 4 Teaching Objectives
- Cognitive: Provide opportunities for students to use climate and geodetic data to characterize the evolution of landscape features after a mass-wasting event has occurred.
- Behavioral: Facilitate student ability to qualitatively assess and map the changes in geomorphic features before and after a mass-wasting event.
- Affective: Facilitate student ability to analyze the societal impact of mass-wasting events.
Context for Use
The content in Unit 4 is appropriate for introductory geology, hazards, and other geoscience courses; sophomore-level courses in which geodesy and/or geomorphology/surface processes are being introduced; or non-geoscience courses where infrastructure planning, Earth hazards, and/or the nature and methods of science are being investigated. Unit 4 activities can be adapted to serve small or large-enrollment classes and can be executed in lecture and lab settings as an in-class activity in which students work in small groups, a lab exercise, or as part of a ~2 week investigation of the use of geodesy to understand surface-process hazards and decision making using the entire Surface Process Hazards module.
This unit precedes Unit 5: Mitigating future disasters—developing a mass-wasting hazard map in which students create a hazard map and report on an area susceptible to mass wasting. If the entire two-week module will not be used, we recommend pairing Unit 4 with Unit 5 to give students the opportunity to use geodetic data to identify landscape features associated with a slow-slip mass-wasting event and classify low, medium, and high-risk areas based on landscape characteristics. However, Unit 4 does build on skills from Unit 2: Reading the landscape and Unit 3: Understanding landslide factors so you may need to have to give some background practice or information on these topics.
Description and Teaching Materials
Part 1: preparatory activity.
- In each of the five study sites that students have looked at in Units 2 and 3, there is a significant mass wastage somewhere in the map area. In order to prepare for Unit 4, have students prepare five-minute presentations on one those mass-wastage events (except the Northern Washington one, as the rest of Unit 4 is about the Oso Landslide in that region). If you have a large class, you may want to have the students work in groups or randomly select one person/team from each of the four sites to present. This process helps students understand more about the societal cost of mass wasting and the different types of ones that can occur.
- Unit 4 preparation student exercise (Microsoft Word 2007 (.docx) 304kB Jun20 17)
- USGS: History of Landslides at the Base of Bare Mountain, Tully Valley, Onondaga County, New York Report
- USGS: Landslide Susceptibility in the Tully Valley Area, Finger Lakes Region, New York
- USGS: Report of the Thistle Slide
- Utah Geological Survey: Geosights: Thistle Landslide Revisited
- USGS: Rockslide-debris Avalanche of May18, 1980, Mt St Helens Volcano
- USGS: Mt St Helens 1980 Cataclysmic Eruption
- AK Dept of Fish & Game: [link https://pubs.er.usgs.gov/publication/70168586 Part 2: Setting the stage 'A submarine landslide source for the devastating 1964 Chenega tsunami, southern Alaska']
- Earthquake, Landslide and Tsunami Hazards in the Port Valdez area, Alaska
In order to place the Oso Landslide in a larger geologic context, students will review the environmental and built characteristics of the northwest Washington locality using the maps from Units 2 and 3.
A) Plate tectonic setting Seismic and volcanic hazards exist along all of eastern Washington state related to the subduction of the Juan de Fuca Plate beneath the North American Plate. This is a good opportunity to relate the plate tectonic setting to the landscape features (faults, linear ridges/streams, mountains) and present hazards. Use the same map-set for Northern Washington as you did in Unit 3. Northern Washington Maps Unit 3-4 (Acrobat (PDF) 41.3MB Oct22 20)
We suggest one of the following already-existing plate tectonics activities:
- GPS Data and Earthquake Hazards in Cascadia - Students learn to read GPS timeseries to calculate velocities and then analyze what the changing velocity across Cascadia means for earthquake hazard.
- Analyzing Plate Motion Using EarthScope GPS Data - Student use a spreadsheet of GPS data from the Plate Boundary Observatory (PBO) to measure the motion of GPS stations in the Pacific Northwest. They generate and map annual velocity vectors of GPS stations.
B) Climatic setting Washington state has an excellent example of high-precipitation region and a rain shadow. High seasonal precipitation focused on the western slopes of the Cascades and arid conditions prevailing over eastern Washington. In this activity students looks at climate data from near Oso, Washington, as well as a precipitation map of Washington to learn more about the general climate of the area as well as in the time leading up to the slide itself.
Washington precipitation map (Acrobat (PDF) 1.2MB Feb25 16) Unit 4: Oso Washington climate assessment student exercise (Microsoft Word 2007 (.docx) 426kB Jun20 17) Unit 4: Oso Washington climate assessment student exercise PDF (Acrobat (PDF) 361kB Aug28 17) Unit 4: Oso Washington climate assessment student exercise answer key -- private instructor-only file Hide Unit 4: Oso Washington climate assessment student exercise answer key This file is only accessible to verified educators. If you are a teacher or faculty member and would like access to this file please enter your email address to be verified as belonging to an educator. Email Adress Submit Unit 4: Oso Washington climate assessment instructor calculations -- private instructor-only file Hide Unit 4: Oso Washington climate assessment instructor calculations This file is only accessible to verified educators. If you are a teacher or faculty member and would like access to this file please enter your email address to be verified as belonging to an educator. Email Adress Submit
Part 3. Mass-wasting anatomy—Oso, WA, Case Study
Along with the assignment below and the map set they have already used, students should be given a lidar hillshade image onto which they will identify, map, and label morphological features of the mass-wasting event. They will answer qualitative questions and will color code their maps to show regions of high/moderate/low risk for damage due to slip. Students will then be shown spatial data of the region at various timesteps (including SRTM [Shuttle Radar Topography Mission], lidar, and InSAR maps of the locality) and answer questions related to the geology and societal impacts of the event.
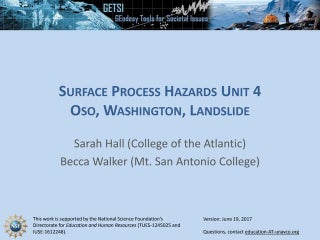
- Northern Washington Maps Unit 3-4 (Acrobat (PDF) 41.3MB Oct22 20)
- Unit 4 Oso Landslide Exercise (Microsoft Word 2007 (.docx) 34MB Oct21 20) Unit 4 Oso landslide exercise PDF (Acrobat (PDF) 16.4MB Oct21 20)
This file is only accessible to verified educators. If you are a teacher or faculty member and would like access to this file please enter your email address to be verified as belonging to an educator. Email Adress Submit
Teaching Notes and Tips
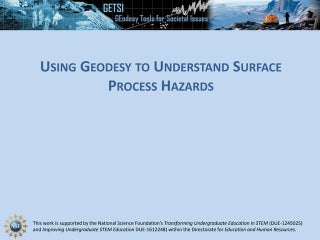
- This unit seems to go forward in a pretty straightforward way. Although it takes a little extra time, we really do recommend the brief student presentations in Part 1. If students have never done PowerPoint presentations (or other presentation software), you may need to spend a little time explaining what to do or give guidance on what each slide should contain.
Formative Assessment
Most likely, formative assessment will be gathered through discussion with students and observation of students as they work through the exercise. By monitoring classroom activity, the instructor can gauge how students are progressing toward the learning outcomes.
Summative Assessment
The primary summative assessment for the module is the Oso Landslide Exercise. In addition, in order to assess whether students have achieved a sufficient understanding of the societal side of the unit, one of the following questions could be used on a test.
Level 2: Besides the mass of material that buried the town, the Oso mass-wasting event left a mark on the geographic and societal landscape both locally and regionally. Students will be asked to answer the following questions:
- Name and describe two examples of a local indirect effect of the Oso mass-wasting event—near to the town of Oso.
- Name and describe two examples of a regional indirect effect of the Oso mass-wasting event—downstream of the event?
Level 3: Students will research what has been done to plan for the future in the aftermath of the Oso event. They will prepare a one-page narrative describing how the community and/or state is working to prevent or preparing for the future in this landslide-prone region.
References and Resources
- Jin-Woo Kim, Zhong Lu, Feifei Qu & Xie Hu (2015) Pre-2014 mudslides at Oso revealed by InSAR and multi-source DEM analysis , Geomatics, Natural Hazards and Risk, 6:3, 184-194, DOI: 10.1080/1947705.2015.1016556
- Haugerud, R. A. (2014) Preliminary Interpretation of Pre-2014 Landslide Deposits in the Vicinity of Oso, Washington , USGS Open-File Report 2014–1065
- USGS Follow-up March 2015: One Year Later—The Oso Landslide in Washington .
- Wikipedia Oso Mudslide
- Introduction to Geodesy and Surface Process Hazards Presentation (PowerPoint 2007 (.pptx) 14.7MB Jul11 17)
- https://www.oregongeology.org/Landslide/Landslidehome.htm
- http://www.seattle.gov/dpd/aboutus/whoweare/emergencymanagement/
- http://www.portlandoregon.gov/pbem/53935
- http://landslides.usgs.gov/
- http://landslides.usgs.gov/learn/prepare.php
- http://pubs.usgs.gov/circ/1325/
« Previous Page Next Page »
- Module Overview
- Unit 1: Slip-sliding away: case study landslides in Italy and Peru
- Unit 2: Reading the landscape
- Unit 3: Understanding landslide factors
- Unit 5: Mitigating future disasters: developing a mass-wasting hazard map
- Student Materials
- Instructor Stories
- Join the Community
Already used some of these materials in a course? Let us know and join the discussion »
Considering using these materials with your students? Get advice for using GETSI modules in your courses » Get pointers and learn about how it's working for your peers in their classrooms »
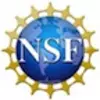
- About this Project
- Accessibility
We encourage the reuse and dissemination of the material on this site for noncommercial purposes as long as attribution to the original material on the GETSI site is retained.
Citing and Terms of Use
Material on this page is offered under a Creative Commons license unless otherwise noted below.
Show terms of use for text on this page »
Show terms of use for media on this page »
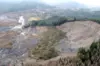
- Initial Publication Date: October 2, 2017 | Reviewed: December 10, 2020
- Short URL: https://serc.carleton.edu/189147 What's This?
ENCYCLOPEDIC ENTRY
A landslide is the movement of rock, earth, or debris down a sloped section of land.
Earth Science, Geology, Geography, Human Geography, Physical Geography
Loading ...

A landslide is the movement of rock , earth , or debris down a sloped section of land. Landslides are caused by rain , earthquakes , volcanoes , or other factors that make the slope unstable . Geologists , scientists who study the physical formations of Earth, sometimes describe landslides as one type of mass wasting . A mass wasting is any downward movement in which Earth's surface is worn away. Other types of mass wasting include rockfalls and the flow of shore deposits called alluvium . Near populated areas, landslides present major hazards to people and property. Landslides cause an estimated 25 to 50 deaths and $3.5 billion in damage each year in the United States.
What Causes Landslides?
Landslides have three major causes: geology , morphology , and human activity.
Geology refers to characteristics of the material itself. The earth or rock might be weak or fractured , or different layers may have different strength and stiffness.
Morphology refers to the structure of the land. For example, slopes that lose their vegetation to fire or drought are more vulnerable to landslides. Vegetation holds soil in place, and without the root systems of trees , bushes , and other plants , the land is more likely to slide away.
A classic morphological cause of landslides is erosion , or weakening of earth due to water. In April 1983, the U.S. town of Thistle, Utah, experienced a devastating landslide brought on by heavy rains and rapidly melting snow . A mass of earth eventually totaling 305 meters (1,000 feet) wide, 61 meters (200 feet) thick, and 1.6 kilometers (one mile) long slid across the nearby Spanish Fork River, damming it and severing railroad and highway lines. The landslide was the costliest in U.S. history, causing over $400 million in damage and destroying Thistle, which remains an evacuated ghost town today.
Human activity, such as agriculture and construction , can increase the risk of a landslide. Irrigation , deforestation , excavation , and water leakage are some of the common activities that can help destabilize, or weaken, a slope.
Types of Landslides
There are many ways to describe a landslide. The nature of a landslide's movement and the type of material involved are two of the most common.
Landslide Movement
There are several ways of describing how a landslide moves. These include falls , topples , translational slides , lateral spreads , and flows. In falls and topples, heavy blocks of material fall after separating from a very steep slope or cliff. Boulders tumbling down a slope would be a fall or topple. In translational slides, surface material is separated from the more stable underlying layer of a slope. An earthquake may shake the loosen top layer of soil from the harder earth beneath in this type of landslide. A lateral spread or flow is the movement of material sideways, or laterally. This happens when a powerful force, such as an earthquake, makes the ground move quickly, like a liquid.
Landslide Material
A landslide can involve rock, soil, vegetation, water, or some combination of all these. A landslide caused by a volcano can also contain hot volcanic ash and lava from the eruption . A landslide high in the mountains may have snow and snowmelt . Volcanic landslides, also called lahars , are among the most devastating type of landslides. The largest landslide in recorded history took place after the 1980 eruption of Mount St. Helens in the U.S. state of Washington. The resulting flow of ash, rock, soil, vegetation and water, with a volume of about 2.9 cubic kilometers (0.7 cubic miles), covered an area of 62 square kilometers (24 square miles).
Other Factors
Another factor that might be important for describing landslides is the speed of the movement. Some landslides move at many meters per second, while others creep along at an centimeter or two a year. The amount of water, ice , or air in the earth should also be considered. Some landslides include toxic gases from deep in Earth expelled by volcanoes. Some landslides, called mudslides , contain a high amount of water and move very quickly. Complex landslides consist of a combination of different material or movement types.
Martian Landslide In December 2008, scientists announced that they had found evidence of the largest landslide ever. Because of a giant asteroid impact billions of years ago, the smooth northern hemisphere of Mars is sharply separated from the irregular southern highlands. Arabia Terra, a previously unexplained plateau between the two regions, is thought to have been formed by an enormous landslide immediately after the impact. The land mass that slid north to form Arabia Terra was the size of the entire United States!
Media Credits
The audio, illustrations, photos, and videos are credited beneath the media asset, except for promotional images, which generally link to another page that contains the media credit. The Rights Holder for media is the person or group credited.
Illustrators
Educator reviewer, last updated.
April 26, 2024
User Permissions
For information on user permissions, please read our Terms of Service. If you have questions about how to cite anything on our website in your project or classroom presentation, please contact your teacher. They will best know the preferred format. When you reach out to them, you will need the page title, URL, and the date you accessed the resource.
If a media asset is downloadable, a download button appears in the corner of the media viewer. If no button appears, you cannot download or save the media.
Text on this page is printable and can be used according to our Terms of Service .
Interactives
Any interactives on this page can only be played while you are visiting our website. You cannot download interactives.
Related Resources
ORIGINAL RESEARCH article
Seismic landslide susceptibility evaluation model based on historical data and its application to areas with similar environmental settings.
- 1 Sichuan Earthquake Administration, Chengdu, Sichuan, China
- 2 Institute of Geology, China Earthquake Administration, Beijing, China
- 3 Institute for Disaster Management and Reconstruction, Sichuan University, Chengdu, Sichuan, China
- 4 Remote Sensing Center of Yunnan Province, Yunnan, China
Seismic landslide susceptibility evaluation models are usually built on the basis of historical sample data; however, the evaluation results are often unsatisfactory when the environmental settings differ between the historical sample data region and application region. Therefore, similarity between the environmental settings is important for the application of such models. In this paper, a seismic landslide susceptibility evaluation model was first built using data from the 2008 Ms 8.0 Wenchuan earthquake-induced landslide, and the model was then used to evaluate the 2022 Ms 6.8 Luding earthquake area. In addition, the grade of susceptibility is typically represented by the landslide density, which is insufficient for capturing the details of landslides, such as their sizes, frequencies, and spatial distribution patterns. The authors therefore use a large and concentrated landslide as the susceptibility grade for the Luding earthquake area. The test results demonstrate that these two areas have similar background environments. The area under the curve (AUC) value of the receiver operating characteristics (ROC) curve of the evaluation accuracy for the model applied to the Luding earthquake area is 0.889, which indicates relatively high accuracy. Besides, the results also demonstrate that the evaluations are consistent with the disaster situation of the Moxi Platform, Wandong Village, as well as the Dagangshan Hydropower Station area. Therefore, it is reliable to apply the susceptibility evaluation model based on the Wenchuan earthquake data to the Luding earthquake area. These results show that better evaluations can be obtained based on environmental similarity tests between the areas used for historical data modeling and areas to which the models are applied.
1 Introduction
Earthquake-induced landslides are the result of multiple factors, such as ground motions, geology, and geomorphology ( Xu et al., 2009 , 2013 ; Havenith, 2022 ). Seismic landslide susceptibility evaluations are helpful for reducing the losses caused by landslides and are also the focus of the present research on prevention and mitigation of earthquake-induced disasters ( Micu et al., 2023 ; Yuan et al., 2013 ; Li et al., 2013 ; Dai et al., 2023 ). Current susceptibility evaluation models are usually based on historical sample data from around the world, but background similarity tests between the historical sample regions and current application regions are not performed often before implementing these models ( Ullah et al., 2022 ; Ozdemir et al., 2013 ; Chen et al., 2020 ; Quesada et al., 2019 , Quesada RA, 2021a ; Quesada RA, 2021b ; Quesada RA, et al., 2021c ; Arroyo Solórzano et al., 2022 ; Porras et al., 2021 ). Therefore, we attempted to conduct background environment similarity tests before model application to ensure that the susceptibility evaluation model used is reasonable; this study involved assessment of the evaluation model developed for the Wenchuan earthquake region for application to the Luding earthquake region that has a similar background environment. In addition, susceptibility evaluation models currently use landslide density as the index representing susceptibility grade ( Nowicki et al., 2014 ; Wang et al., 2017 ; Fan et al., 2018a ; Sun et al., 2018 ; Sun et al., 2020 ; Liu et al., 2021a ). Landslide characteristics such as scale, frequency, and spatial distribution pattern are often quite different in different regions; thus, the landslide density index cannot comprehensively reflect the landslide characteristics ( Liu et al., 2021b ). The spatial distribution pattern of a landslide is hence used as the evaluation index in this work to reflect more detailed characteristics that could improve the evaluation results. Furthermore, the evaluation model implemented in this work uses only the significant influencing factors and not all factors that improve the accuracy of the evaluation results.
2 Study area
The southeastern edge of the Tibetan Plateau is a region with many active tectonics, large terrain differences, and frequent earthquakes. Since the 2008 Wenchuan Ms 8.0 earthquake, several earthquakes have occurred in the region, such as the Ms 7.0 Lushan earthquake in 2013, Ms 6.5 Ludian earthquake in 2014, Ms 7.0 Jiuzhaigou earthquake in 2017, and Ms 6.8 Luding earthquake in 2022 ( Huang et al., 2008 ; Zhang et al., 2008 ; Fan et al., 2018b ; Liu et al., 2023 ). As the earthquake with the highest magnitude in southwest China in recent years, the 2008 Wenchuan Ms 8.0 earthquake induced the largest number of landslides, causing serious disasters. In the early period following this earthquake, researchers counted nearly 8,000 landslides in the region; five months later, more than 10,000 landslides had occurred ( Huang et al., 2009 ). In the 6-year period following the earthquake, a total of 197,481 landslides were reported, whose data included both the locations and size of the landslides ( Xu et al., 2014c ) that provide details for studying the landslide distribution pattern.
The 2008 Wenchuan earthquake originated in the Longmen Mountain thrust tectonic belt on the eastern edge of the Tibetan Plateau and induced two reverse faults ( Xu et al., 2008 ), namely, the Beichuan-Yingxiu and Guanxian-Jiangyou faults. This earthquake area has high terrain in the northwest and low terrain in the southeast, including alluvial plains (elevation of approximately 500 m), alpine landforms (elevation of approximately 2,000–5,000 m), and plateau landforms (elevation of approximately 4,000–5,000 m). The relative altitude difference in this area is more than 4,000 m, and the slope ranges between 20° and 50° ( Qiao et al., 2009 ). The earthquake-induced landslides were distributed in an elliptical zone of area approximately 110,000 km 2 along the Beichuan-Yingxiu surface rupture, and the high-density landslides were concentrated in an area southwest of this surface rupture. The number, scale, and degree of concentration of these landslides in the hanging wall of the fault were significantly larger than those in the footwall, indicating the hanging wall effect.
3 Landslide clustering analysis
The characteristics of landslide distribution are usually described as “concentrated in a certain zone” or “concentrated along the fault,” which do not reflect the “clustering degree,” “clustering scale,” and “clustering location” of the landslides. In this paper, the clustering characteristics of landslides were quantified via clustering analysis, and the susceptibility evaluation model of the large-scale concentrated landslide was constructed. Large-scale concentrated landslides are quantified by local spatial autocorrelation analysis, which is also called as landslide clustering analysis. This method can obtain the clustering locations of large and small landslides; additionally, it can obtain the locations where large landslides are surrounded by small landslides as well as locations where small landslides are surrounded by large landslides. This analysis method involves calculating the local Moran’s I value (Eq. 1 ), z-score, p -value, and clustering type of each landslide [Copyright(C) 1995–2013 Esri].
where X i is the area value of landslide i; X ¯ is the average area value of the landslide in the study zone; 𝟂 i,j is the spatial weight between landslides i and j, where i and j are not the same landslide; n is the total number of landslides in the area.
The score of the statistics is calculated as Eq. 2
The Z Ii score is statistically calculated as Eqs 3 – 5
In the study area, the results of landslide clustering analysis showed that 1) the zone extending along the Beichuan-Yingxiu surface rupture, from the epicenter to the southwest, was predominantly characterized by small concentrated landslides. 2) The zone extending from the epicenter to the north was characterized by large landslides surrounded by smaller ones. 3) The zone along the Minjiang River was predominantly characterized by large concentrated landslides. 4) The zone extending along the Beichuan-Yingxiu surface rupture, from the epicenter to the northeast, showed a transition from “small scattered pattern” to “small concentrated pattern” until the Xiaoyudong surface rupture that interrupts the Beichuan-Yingxiu surface rupture. 5) The Beichuan-Yingxiu surface rupture reemerged on the northeast side of the Xiaoyudong surface rupture and continued to extend further northeast while a parallel Guanxian-Jiangyou surface rupture became apparent simultaneously; the pattern of landslides observed on the hanging walls of these two parallel surface ruptures indicated a concentration of large landslides, with the smaller landslides being surrounded by the larger landslides. 6) The location where the Guanxian-Jiangyou surface rupture terminated was the point at which the largest single landslide of the Wenchuan earthquake, known as the Daguangbao landslide, occurred ( Tan et al., 2013 ) ( Figure 1 ).
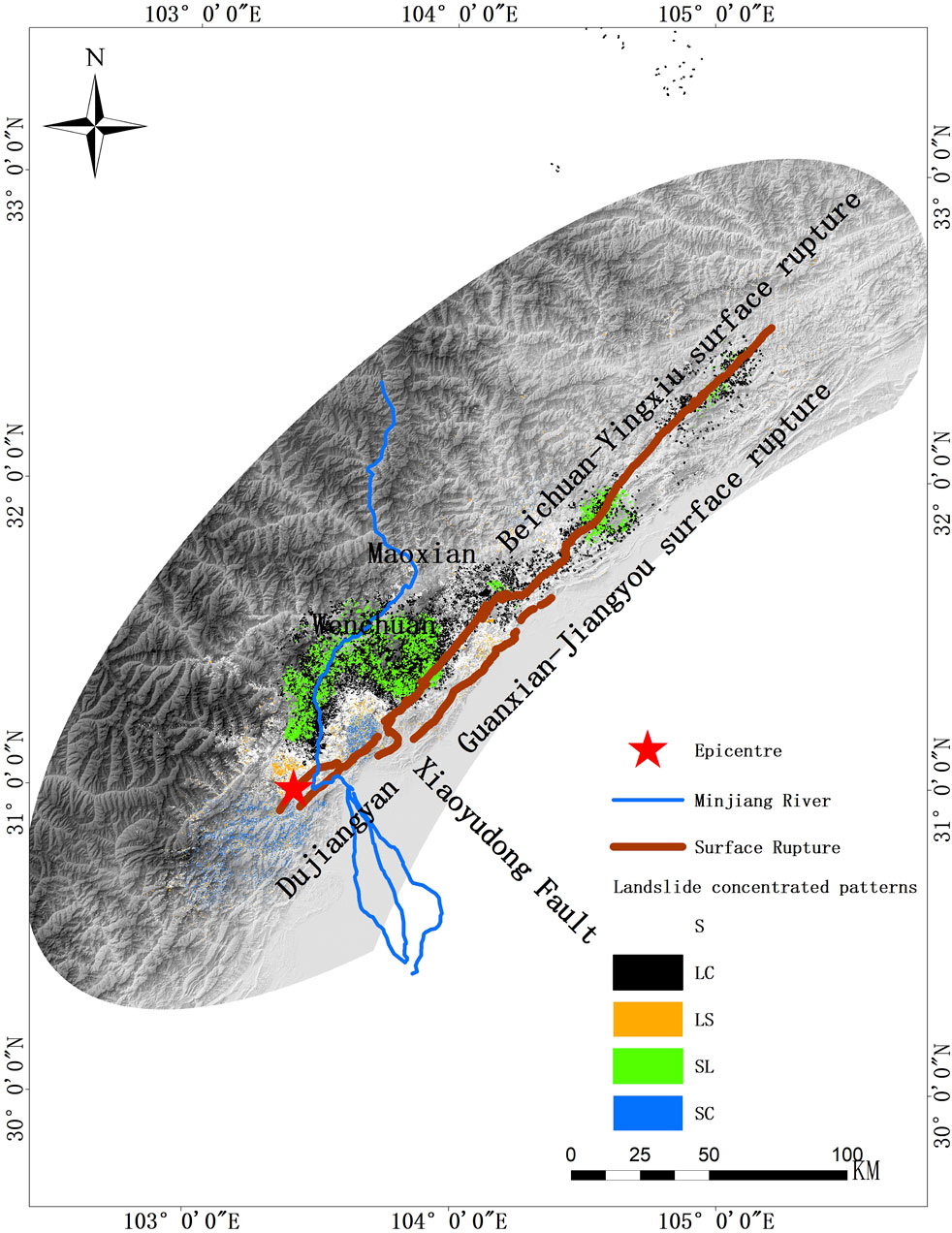
Figure 1 . Spatial distribution of landslides in the Wenchuan earthquake area. Note: S for scattered landslides; LC for large concentrated landslides; LS for large landslide surrounded by small landslides; SL for small landslide surrounded by large landslides; SC for small concentrated landslides.
4 Landslide susceptibility evaluation model
Along the Beichuan-Yingxiu surface rupture, the density of landslides on the hanging wall was higher than that on the footwall; there were approximately 150,000 landslides on the hanging wall along the Beichuan-Yingxiu surface rupture that constituted about 80% of the total number of landslides recorded. The study area encompassed a 20 km range on both sides of the surface rupture. The study area accounted for approximately 100,000 landslides, constituting 52% of the total number of events reported. The study area also exhibited various landslide patterns, including large concentrated pattern, small concentrated pattern, large landslides surrounded by small landslides, and large single landslides. These diverse patterns provide valuable foundational data for the present study ( Figure 2 ).
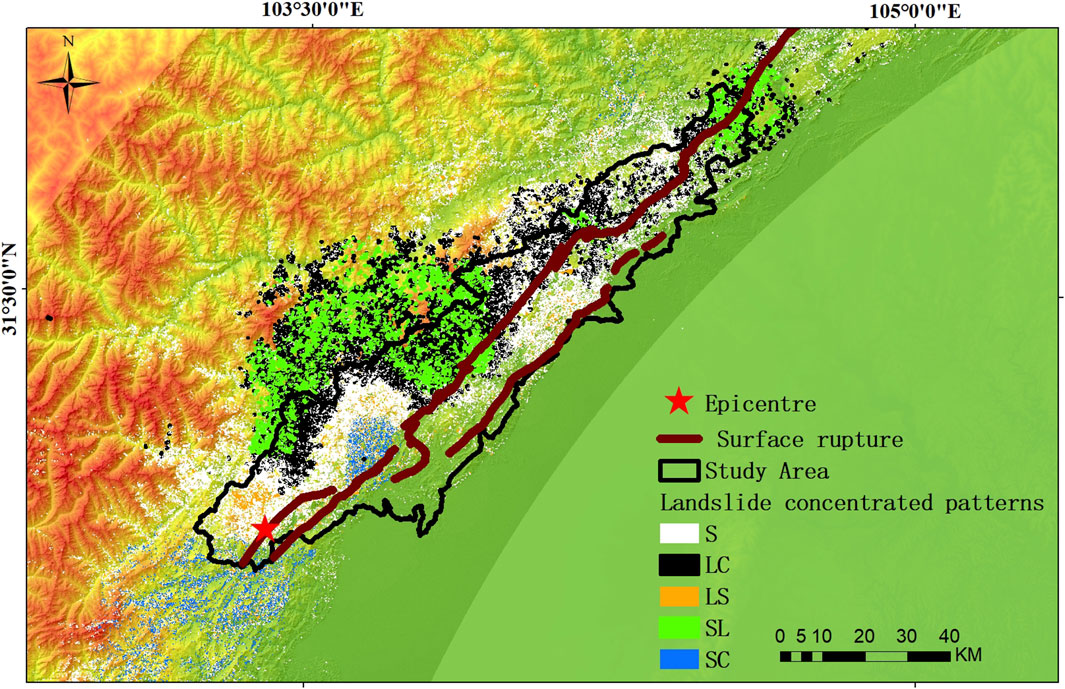
Figure 2 . Different landslide patterns in the Wenchuan earthquake study area. Note: S for scattered landslides; LC for large concentrated landslides; LS for large landslide surrounded by small landslides; SL for small landslide surrounded by large landslides; SC for small concentrated landslides.

4.1 Collinearity diagnosis
Based on the landslide clustering analysis, five landslide patterns were obtained as follows: 1) large concentrated landslides; 2) large landslide surrounded by small landslides; 3) small landslide surrounded by large landslides; 4) small concentrated landslides; 5) scattered landslides. Among these five patterns, the large concentrated landslides were considered to be the most hazardous, so more attention was focused on this particular pattern. In this paper, these five patterns were divided into two evaluation indexes to construct the susceptibility evaluation model. Patterns 1) and 2) were categorized into the large concentrated landslide (LCL) evaluation index, while the remaining three patterns were classified into the non-large concentrated landslide (NLCL) evaluation index. The values of the indexes were considered to be dependent variables, where 1 represented the LCL and 0 represented the NLCL evaluation indexes.
The evaluation model incorporated 10 influencing factors, including slope aspect, slope angle, elevation, peak ground acceleration (PGA), intensity, surface rupture distance, fault-locked segment, duration of strong motions, lithology, and river distance. Before constructing the model, the collinearity of the influencing factors was tested to identify and exclude those factors that exhibited multicollinearity; collinearity diagnosis by regression analysis was employed to assess the presence of multicollinearity among the influencing factors. Factors exhibiting high levels of multicollinearity were excluded from the model to ensure the independence and reliability of the remaining factors. In the diagnosis results, if the tolerance value of a factor was less than 0.1 or the variance inflation factor (VIF) exceeded 10, it was considered to be multicollinear. In such cases, the corresponding factor was excluded from the model to mitigate the effects of multicollinearity and ensure the reliability of the remaining factors. From the results presented in Table 1 , all 10 influencing factors were found to have tolerance values greater than 0.1 and VIF values less than 10, indicating the absence of multicollinearity. Thus, all 10 factors were utilized to construct the model without the need for exclusion.
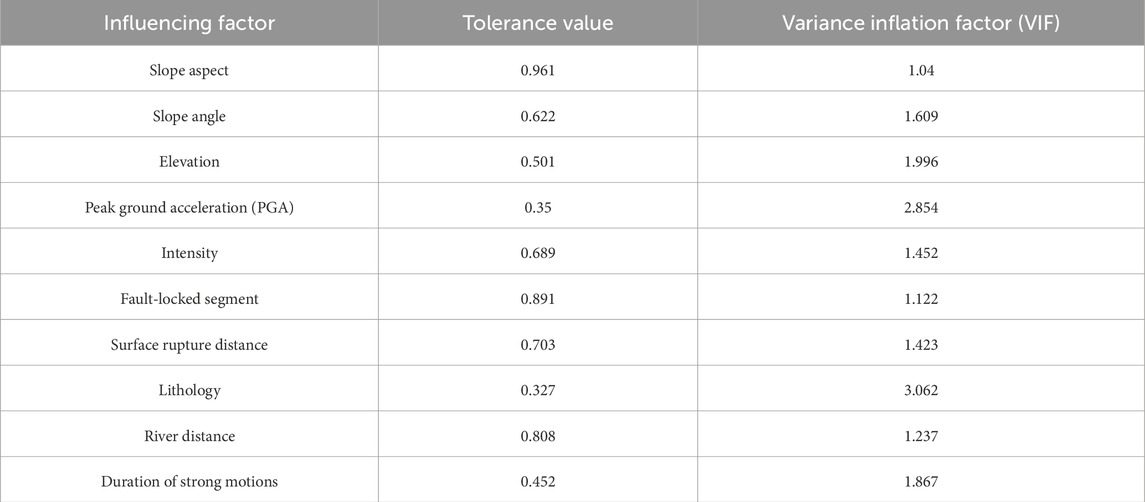
Table 1 . Collinearity diagnosis results of the influencing factors.
4.2 Significant independent variables selection
We used watershed as the evaluation unit for this selection. There were a total of 231 watershed regions within the designated zone. Based on the landslide clustering analysis, 83 watershed regions exhibited the characteristics of a large landslide concentrated distribution, and the remaining 148 watershed areas were characterized as having a non-large landslide concentrated distribution ( Figure 3 ). These two characteristics corresponded to the two evaluation indexes LCL and NLCL described above, which help in understanding the spatial distributions and patterns of landslides in the study area. Among the watershed areas, 70% were designated as training samples, and the remaining 30% were used as verification samples. In the evaluation model, the landslide concentrated evaluation index of each watershed area was considered to be the dependent variable, while the 10 influencing factors were treated as the independent variables. Logistic regression analysis was then used to identify the significant independent variables in the evaluation model; this statistical technique is particularly suitable when the dependent variable is binary or dichotomous, as is the case with the landslide concentrated evaluation indexes in this work. Logistic regression helps to assess the relationships between the independent variables (influencing factors) and probability of a watershed area being classified into one of the two categories. By analyzing the coefficients and significance levels of the independent variables, we determined the variables that had significant impacts on the predictions of the dependent variables. The non-significant independent variables were excluded from the model by evaluating the statistical significance of each independent variable and removing those that did not contribute significantly to the model’s predictive power. By eliminating the non-significant variables, the model is more focused and robust, including only those variables that have meaningful impacts on the dependent variables. In the model construction process, six significant independent variables were finally selected to construct the model: slope aspect, elevation, intensity, fault-locked segment, duration of strong motions, and river distance ( Table 2 ). The regression coefficients associated with these factors were used as the weights in the construction of the model.
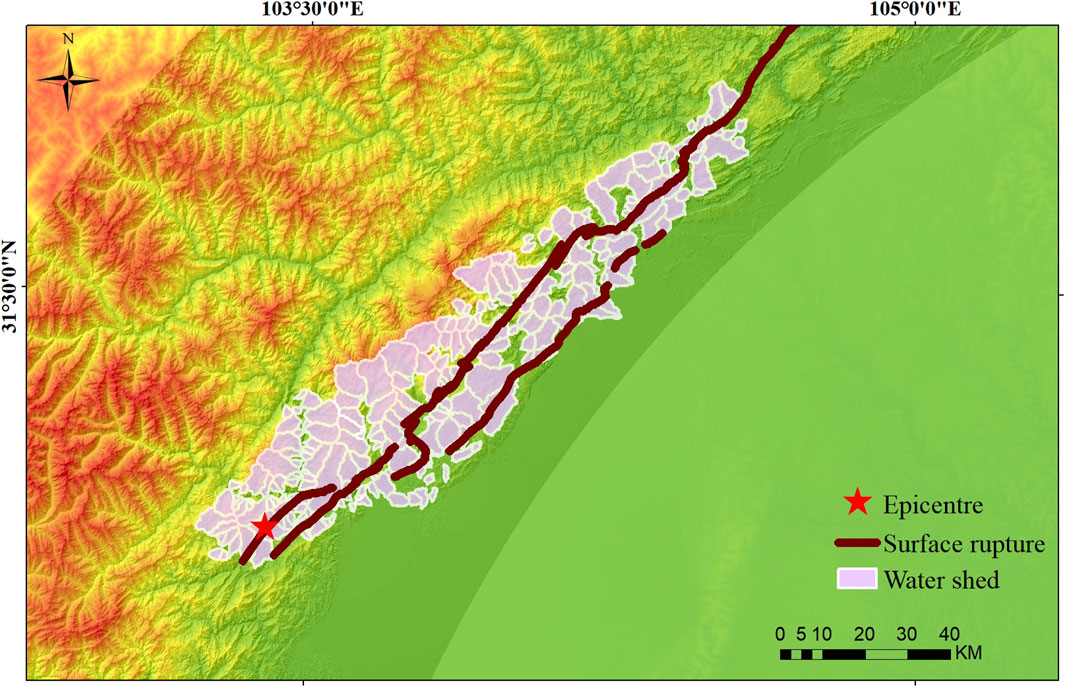
Figure 3 . Distribution of watershed regions in the Wenchuan earthquake study area.
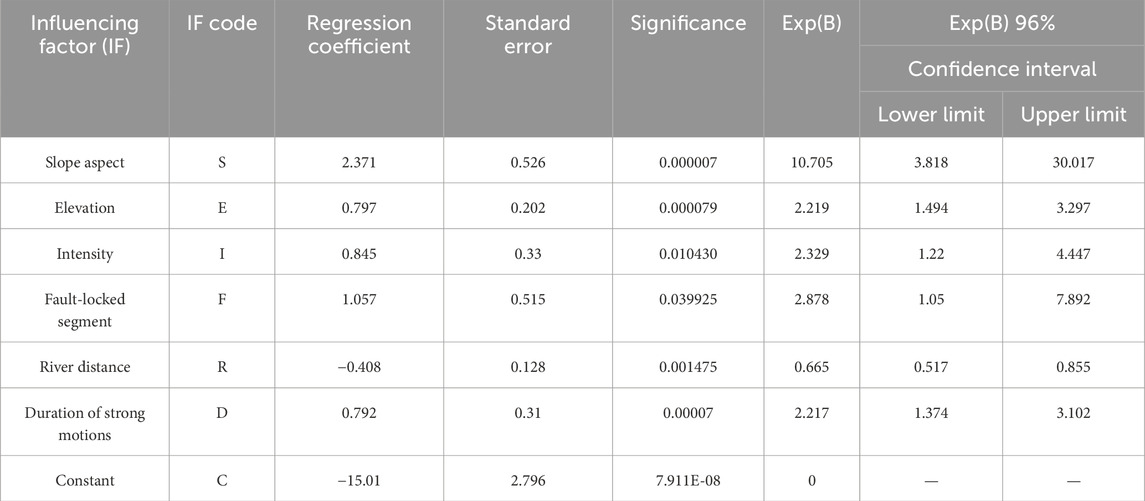
Table 2 . Significant independent variable analysis results.
Here, P WC is the evaluation probability of the LCL, and its value is between 0 and 1; a higher value of P WC indicates a greater possibility of LCL occurrence in the watershed area. Z WC is the weighted sum of the influencing factors. These influencing factors were indicated by letters as follows: S for aspect, E for elevation, I for intensity, F for fault-locking section, R for river distance, and D for strong movement duration.
5 Model application
5.1 background environment similarity test.
The Luding area is situated at the boundary of the Sichuan-Yunnan rhombic block, which is considered as a potential seismic area. In this study, the Luding earthquake region was used as the application region for the susceptibility evaluation model for earthquake prevention evaluation. The landslides induced by the 2022 Ms 6.8 Luding earthquake were used as the verification samples for the model. Before applying the model, a background environment similarity test was conducted between the Wenchuan and Luding earthquake regions to assess the similarities or comparability of the environmental conditions and factors. The comparisons were made between the factors influencing the Wenchuan earthquake study area and Luding earthquake application area.
5.1.1 Seismic factor similarity test
The Wenchuan earthquake area is situated at the eastern edge of the Bayankala block, which is known for its high seismic activity and considered as one of the most active blocks within the Tibetan Plateau. The Luding earthquake area is located at the eastern edge of the Sichuan-Yunnan block, which is also recognized as one of the most active blocks within the Tibetan Plateau and characterized by significant tectonic activity.
The epicenter of the 2022 Luding earthquake was located in the Hailuogou Glacier Forest Park at a depth of 16 km. A surface rupture along the Moxi fault is observed south of the epicenter, with a length of 15–25 km, exhibiting a nearly straight dip and northwest strike, consistent with the movement and strike of the Xianshuihe fault ( Xu et al., 2022 ; Li et al., 2023 ). The Xianshuihe fault is indeed located at the boundary between the Bayankala and Sichuan-Yunnan blocks, spanning a total length of approximately 400 km; this fault begins in Ganzi and extends through Luhuo County, Daofu County, Kangding County, Moxi Town, before ending south of Xinmin Town ( Deng et al., 2003 ; Zhang et al., 2003 ). The Xianshuihe fault and the southwest part of the Longmenshan fault interact at Moxi Town in Luding County. The seismic faults associated with the Wenchuan earthquake are the Guanxian-Jiangyou and Beichuan-Yingxiu faults. Both these faults are characterized as thrust faults with right-lateral strike-slip components. The Beichuan-Yingxiu fault dips to the northwest at an angle greater than 70°.
The Luding earthquake fault is a left-lateral strike-slip fault. Generally, there are differences between thrust and strike-slip earthquakes in terms of the distribution and attenuation of ground motions. In strike-slip earthquakes, the ground motions on the active plate are typically stronger than those on the passive plate. Ground motions from strike-slip earthquakes are rapidly attenuated with distance, particularly beyond zones that are approximately 5 km away from the fault. As a result, landslides induced by strike-slip earthquakes tend to be concentrated within a narrow region. The occurrence of landslides decreases as the distance from the fault increases. In a thrust fault, the ground motions are generally larger at the hanging wall than at the footwall; this is because the hanging wall experiences greater compression and uplift during an earthquake. Additionally, the rate of attenuation of ground motions for a thrust fault is typically lower than that for a strike-slip fault. This means that the intensity of ground shaking from a thrust earthquake decreases more slowly with distance from the fault. As a result, landslides induced by thrust faults can occur over a wider region compared to those induced by strike-slip faults ( Figures 4 , 5 ).
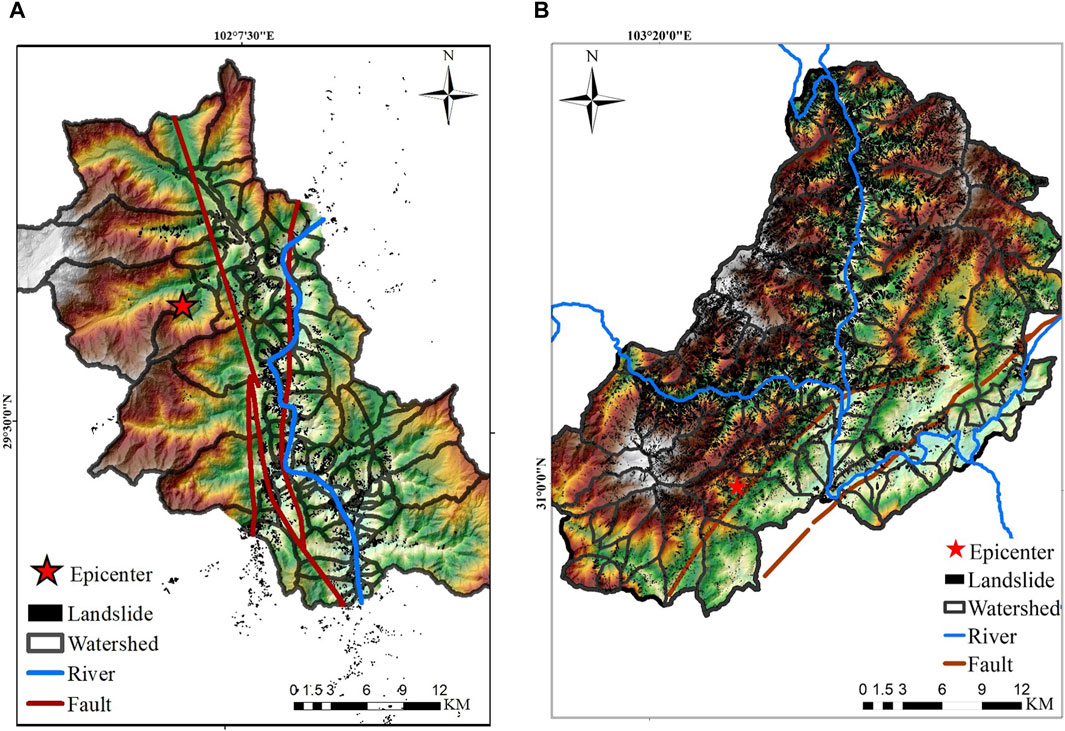
Figure 4 . Surface ruptures and landslides in the (A) Luding and (B) Wenchuan earthquake typical zones.
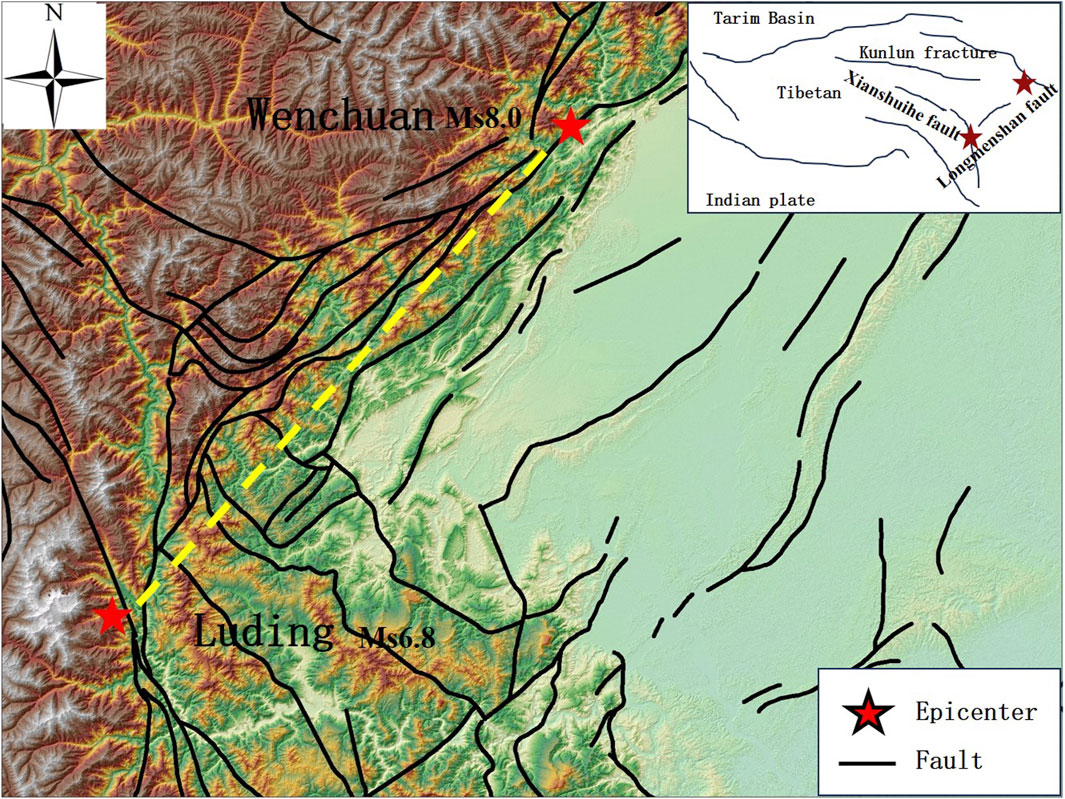
Figure 5 . Epicenters of the Wenchuan and Luding earthquakes.
Despite the differences in magnitude, intensity, ground motion characteristics, landslide numbers, and scale between the Wenchuan and Luding earthquakes, both zones were near surface ruptures and are considered as areas with slow ground motion attenuation. In these zones, the ground motions do not attenuate rapidly with distance from the epicenter or fault rupture, meaning that the intensity of ground shaking remains relatively high at areas near the surface rupture. These zones are characterized by relatively strong ground motions compared to other areas and are therefore considered as typical zones in this paper. The spatial distribution pattern of landslides is similar for these two earthquake typical zones. It is observed that landslides tend to align along the faults and exhibit a high density of occurrence in both cases. In addition, the dip angles of both surface ruptures are large ( Table 3 ). The steep dip angle of the surface rupture in conjunction with the characteristics of the lithology plays a significant role in increasing the probability of landslide occurrences in both earthquake areas. The high dip angles generate significant stresses, which can lead to the development of joints and cracks in brittle rocks. The dominant lithology is metamorphic volcanic rock in the Wenchuan earthquake area, while that in the Luding earthquake area is granite. Both these lithologies are characterized as hard rocks with well-developed structural planes that serve as potential sliding surfaces. Based on an analysis of the seismic influencing factors, including the attenuation rate of ground motions and dip angle of the surface rupture, it is concluded that the typical zones in both earthquake areas have similar influences on landslides.
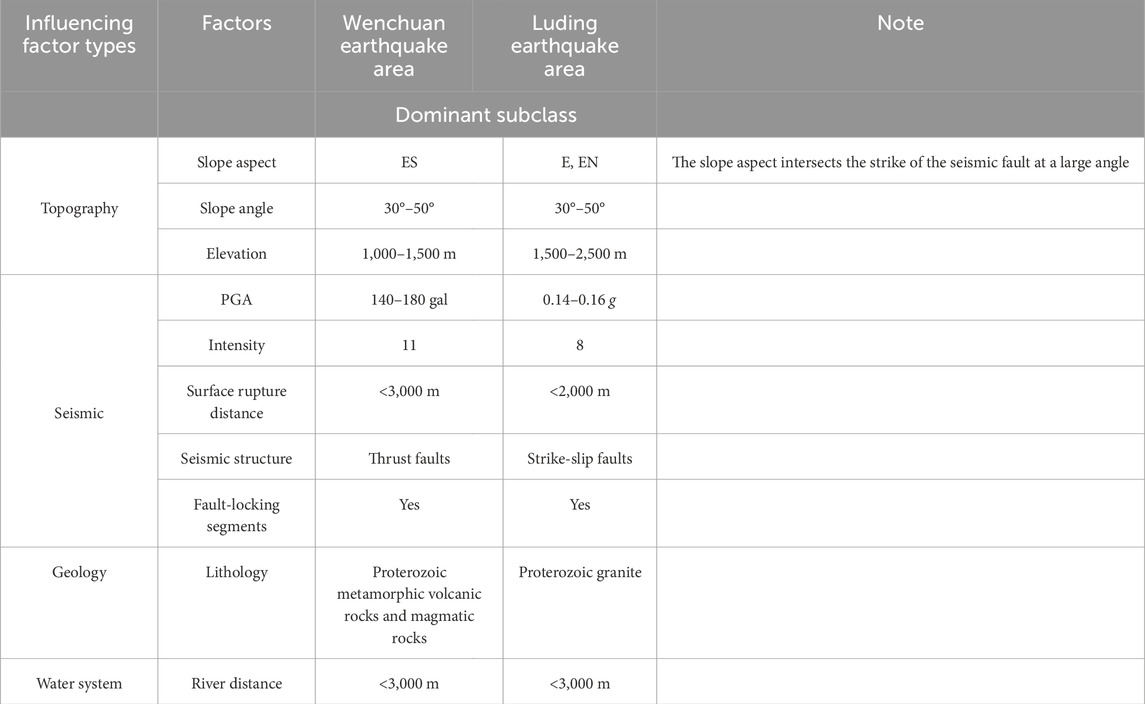
Table 3 . Comparison of background factors influencing the Wenchuan and Luding earthquake typical zones.
5.1.2 Topographic and geological factor similarity test
The Luding earthquake occurred in a high mountain area with a very large altitude difference of approximately 6,570 m and the highest altitude being 7,556 m, indicating steep slopes and varied topography within the region. This area is traversed by the Dadu River, which is the largest tributary of the Minjiang River. The dominant lithology in this zone includes proterozoic granite, permian quartzite, and quaternary gravel and sand. When the dominant slope aspects intersect the strike of the seismic fault at a large angle, they result in strong destructive effects on slopes during earthquakes. Further, the combination of mountainous valley landforms, well-developed rivers, hard lithology, and cracked rocks increases the possibility of occurrence of large landslides in both the Wenchuan and Luding earthquake typical zones ( Table 3 ).
5.2 Evaluation model application
5.2.1 model construction.
For the purpose of this paper, the zones along both sides of Dadu River in the Luding earthquake area are selected as the application zones, and these are within 10 km from the fault, covering an area of approximately 884.5562 km 2 . The Luding earthquake area zone includes approximately 78 watershed regions of similar sizes. The model based on the Wenchuan earthquake (Eqs. (6 , 7) ) was used to construct the evaluation model of the Luding application zone (Eqs. (8 , 9) ). The evaluation model is based on 10 influencing factors, namely, slope aspect, slope angle, elevation, PGA, intensity, surface rupture distance, fault-locked segment, duration of strong motions, lithology, and river distance ( Figure 6 ). The landslide susceptibility of the Luding earthquake zone is classified on the basis of the susceptibility evaluation grade ( Figure 7 ). The receiver operating characteristics (ROC) curve test was performed for the landslide samples, and the results show that the area under the curve (AUC) value was 0.889; this indicates that the model evaluation result is high ( Figure 8 ).
where P LD is the evaluation probability of LCLs in the Luding earthquake zone having a value between 0 and 1; the larger the value of this probability, the greater is the possibility of LCL occurrence. Z LD is the weighted sum of the influencing factors, where S indicates slope aspect, E is the elevation, I is the intensity, F is the fault-locked segment, R is the river distance, and D is the duration of strong motions.
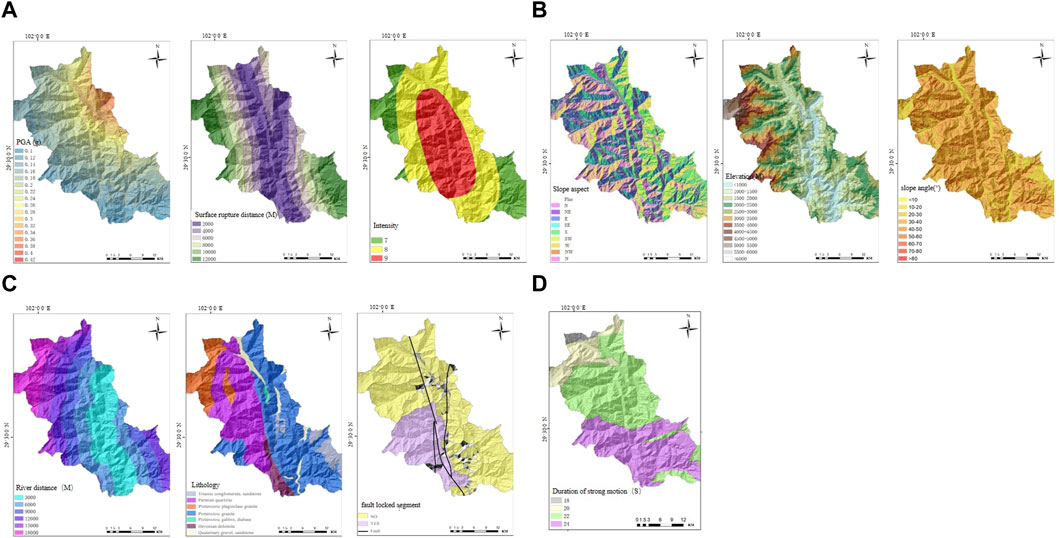
Figure 6 . Ten types of factors influencing the Luding earthquake zone: (A) peak ground acceleration (PGA), surface rupture distance, and intensity; (B) slope aspect, elevation, and slope angle; (C) river distance, lithology, and fault-locked segment; (D) duration of strong motions.
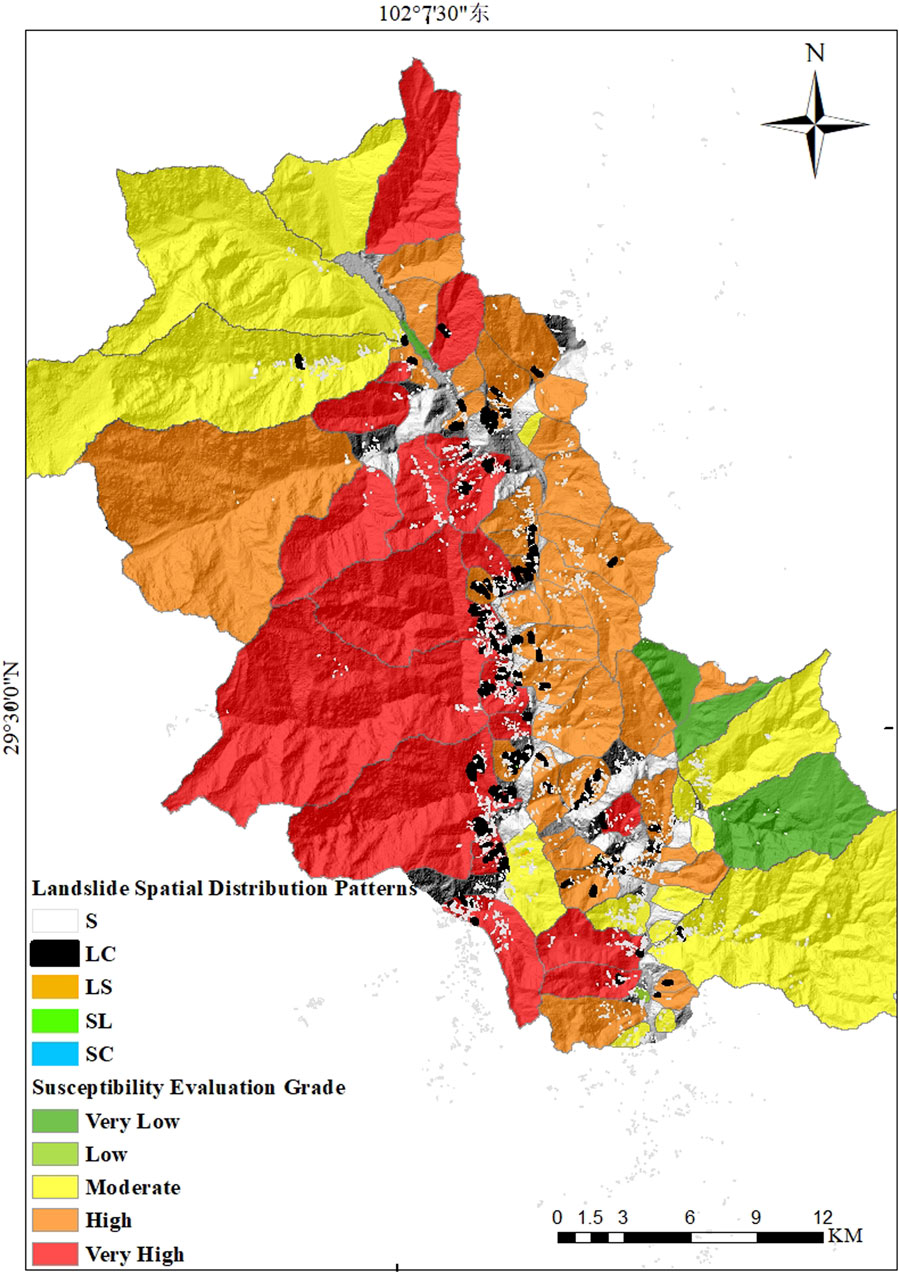
Figure 7 . Evaluation results of large concentrated landslides in the Luding earthquake zone.
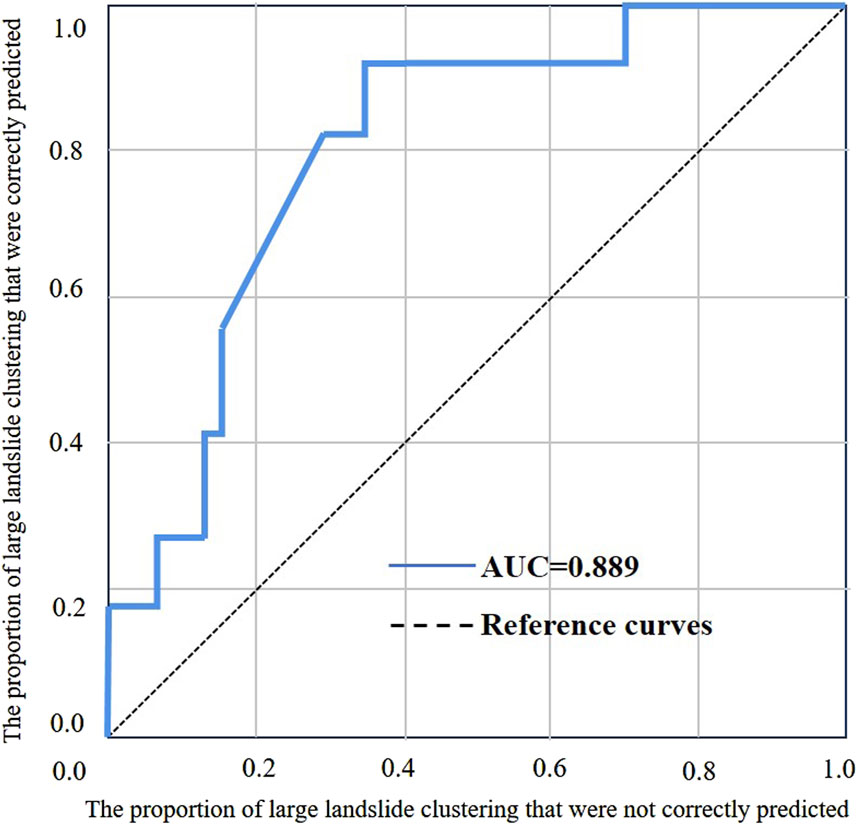
Figure 8 . Receiver operating characteristics (ROC) curve of the large concentrated landslides in the Luding earthquake zone.
5.2.2 On-site investigation
Upon occurrence of the Luding earthquake, our research team promptly arrived at the affected site to carry out investigations. The specific locations investigated were Moxi Town and Detuo Town in Luding County as well as Wanggangping Town in Shimian County. Most of the large-scale landslides in the Luding earthquake area were distributed along the river and roads, resulting in road burial, collapse, and roadbed damage. In this paper, we compared the on-site investigation results and model evaluation results of LCLs in Moxi Platform, Wandong Village of Detuo Town, and Wanggangping Town ( Figure 9 ). The details of these evaluations are as follows:
1) The landslide disaster in Wandong Village was more serious than that at the other two sites. The Wandong River channel was blocked by the large earthquake-induced avalanche, which created a dammed lake and endangered the safety of downstream areas. Many houses were buried near the Shenyun Hot Spring Villa of Wandong Village. Here, the alluvium from the upper stream accumulated to a height of nearly 10 m; the area is approximately 1 km from the dammed lake, and the road to the village was buried by the landslides and debris flows, resulting in diversion of the original river path ( Figure 10 ). We compared the on-site investigation results with those of the landslide susceptibility evaluations in Wandong Village and found that both of these were well-matched with “very high” grade ( Figures 9 , 10 ).
2) The scale and number of landslides in Wanggangping Town was smaller than that at the other two sites. There were some small landslides at the exit of Taoba Tunnel and south side of Dagangshan hydropower station. Moreover, scattered landslides were also noted on both sides of the road from Taoba Tunnel to Aiguo Village. The on-site investigations were consistent with the landslide susceptibility evaluation results, with the evaluation grade being “high” ( Figures 9 , 11 ).
3) Although Moxi Platform was the closest site to the epicenter, the scale of landslides in this area was not as large as that in Wandong Village. The landslides were concentrated on both sides of the river. The on-site findings were consistent with the landslide susceptibility evaluation results, with the evaluation grade being “high” ( Figures 9 , 12 ).
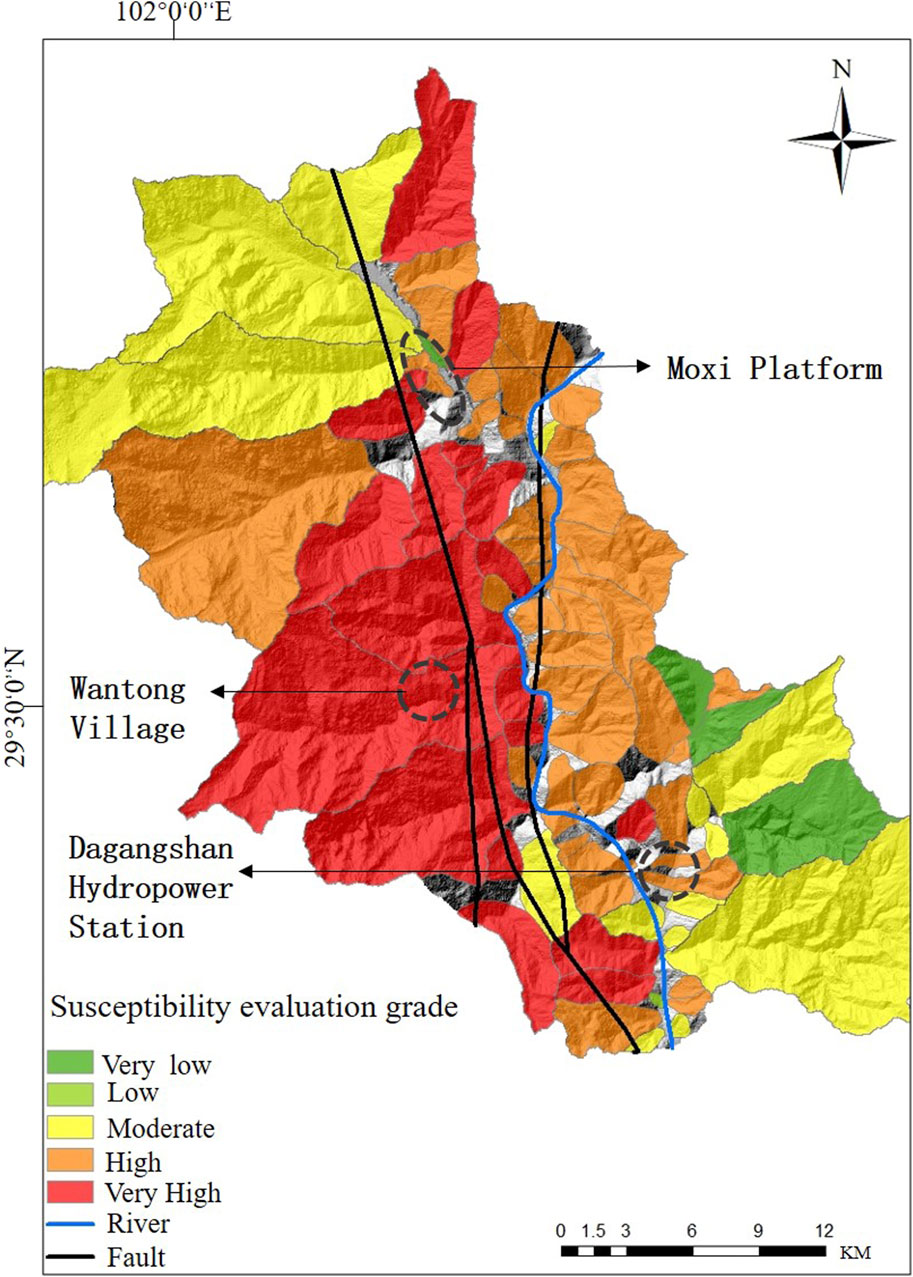
Figure 9 . Locations of Moxi platform, Wantong village of Detuo town, and Dagangshan hydropower station.
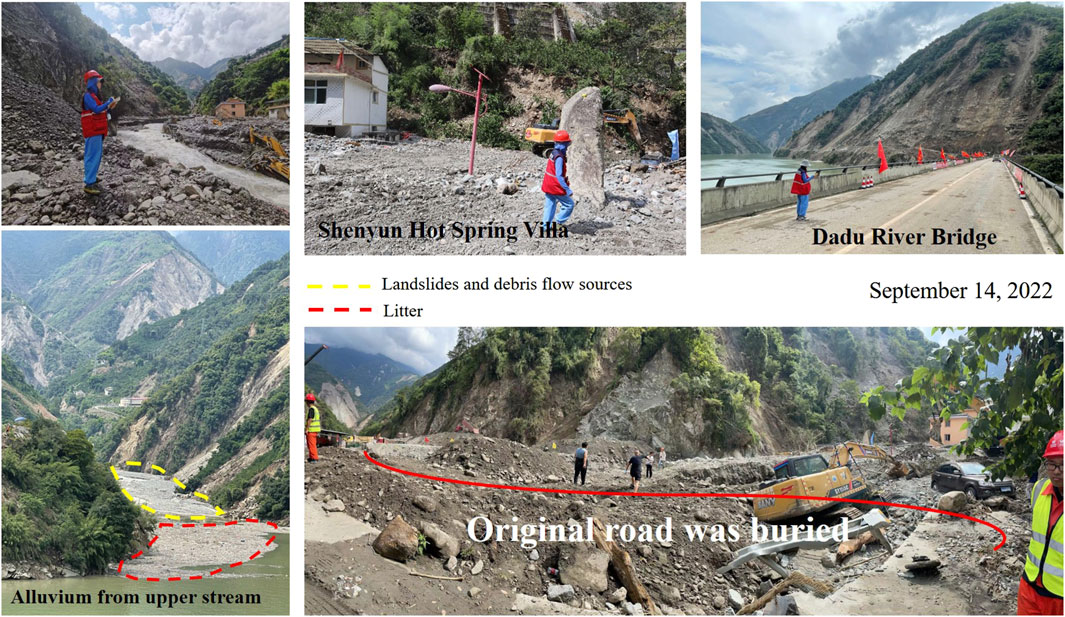
Figure 10 . On-site investigations in Wandong village.
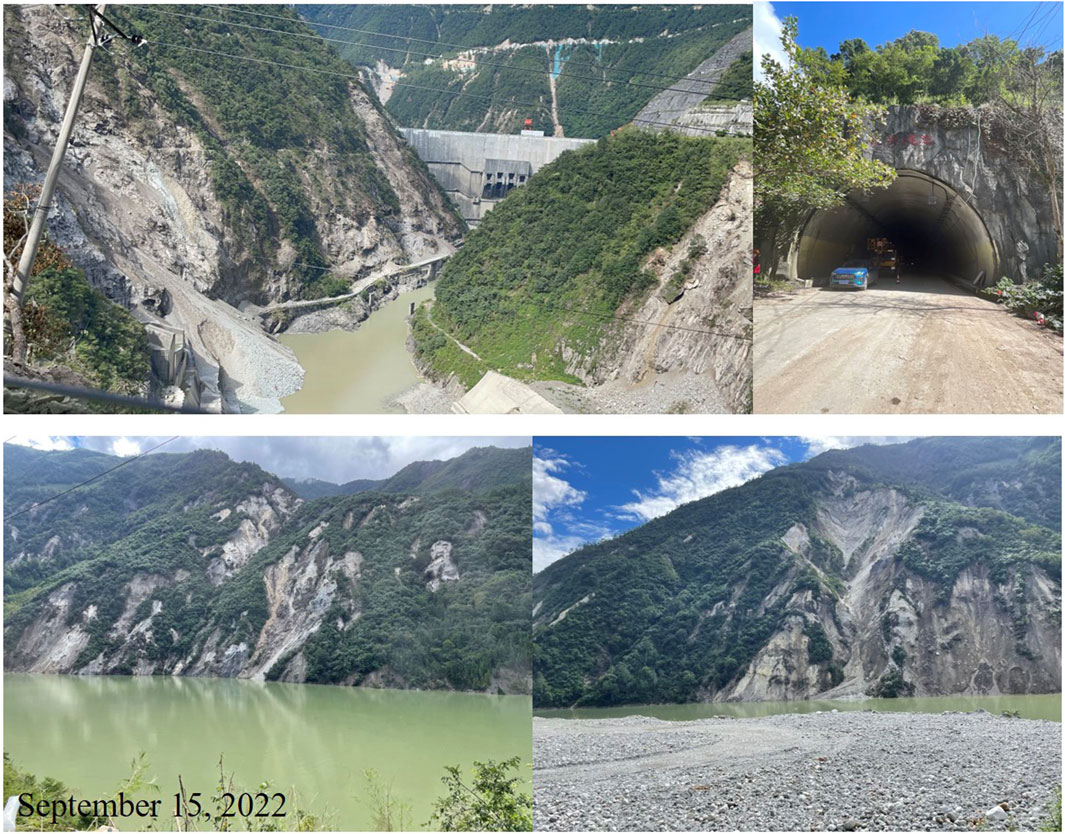
Figure 11 . On-site investigations in Wanggangping town.
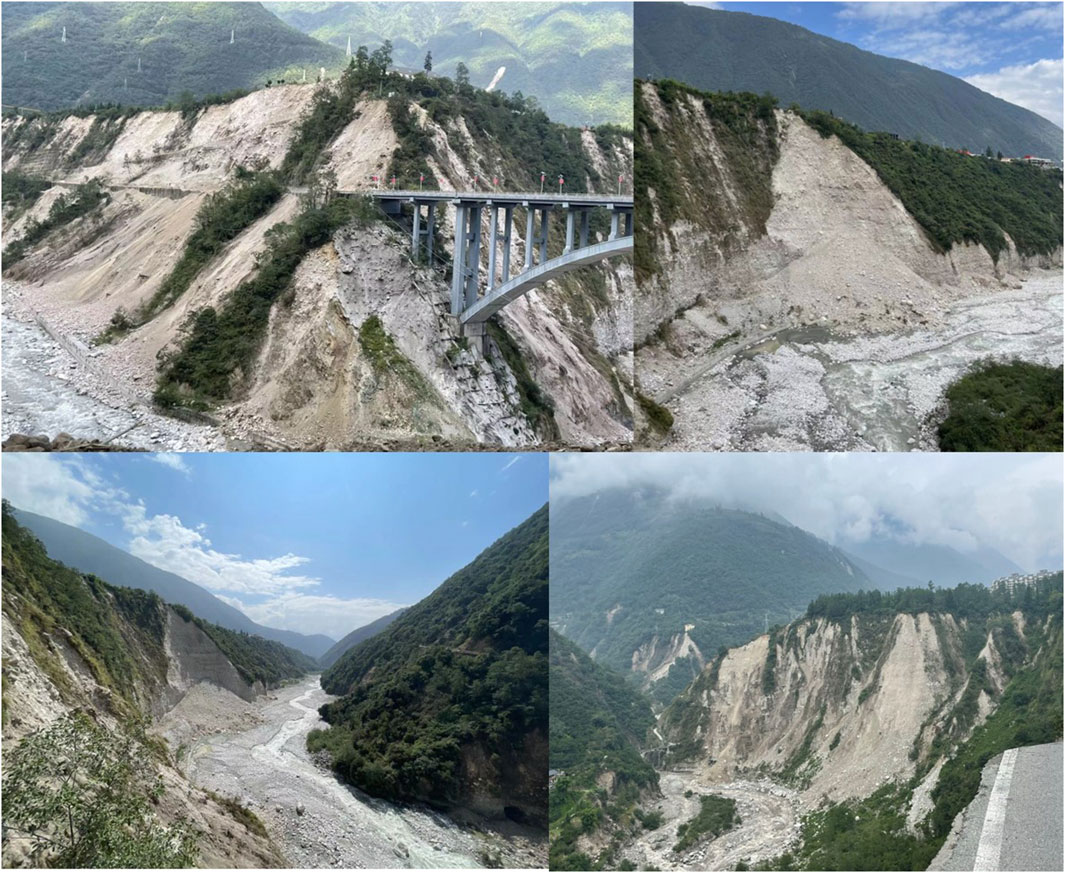
Figure 12 . On-site investigations in the Moxi platform.
By comparing the on-site and evaluation results for the three investigation sites, two sites were found to match well, and one site was almost completely matched. It is thus concluded that the model used for the Luding earthquake area is applicable. The technical procedures used to develop the susceptibility evaluation model based on the Wenchuan earthquake area can thus be used in other potential earthquake areas with similar background environments.
6 Discussion
Nowadays, most of the seismic landslide susceptibility evaluation models are not implemented based on similarities in the background environments. The extant models were built using historical data from all over the world and are being applied to different regions; however, these regions may have different background environments, meaning that the factors influencing the models may not be suitable for all regions. Only regions with similar background environments have higher probabilities of success when applying such models. Most of the available studies have not addressed this issue and have not conducted tests for similarities in the background environments. Our research focuses on this issue and such similarity tests. Conducting a background environment similarity test imposes specific requirements on both the historical sample region and current application region; both regions should have similar geologies, topographies, and landform characteristics. Additionally, it is preferable for both regions to be in close proximity.
In recent years, researchers have made great efforts to build and preserve historical samples in their original forms. Their findings have provided valuable data for this research ( Martinez et al., 2021 ; Gnyawali et al., 2016 ; Xu et al., 2014a ; Xu C, et al., 2014b ; Xu C, et al., 2014c ; Ferrario et al., 2019 ; Li et al., 2014 ). However, finding suitable samples still remains a challenge. The two regions studied herein are one of the few pairs of samples that meet the required criteria. Hence, this research was conducted with the hope that the findings could provide ideas for future research endeavors. As more landslide samples are restored, this type of research can be expanded further in the future.
7 Conclusion
In this paper, the susceptibility evaluation model based on the Wenchuan earthquake area was applied to the Luding earthquake area that has a similar background environment. The evaluation model results demonstrate good accuracy. Additionally, we utilized LCLs as the evaluation index instead of landslide density. This choice allowed us to highlight the spatial distribution characteristics of the significant landslides, which is crucial for post-earthquake relief efforts. Therefore, the evaluation results presented in this paper can provide more valuable information. The main conclusions of this work are as follows:
1) Before using historical sample data to construct susceptibility evaluation models for earthquake-induced landslides, it is important to conduct background environment similarity tests between the historical and application areas. Accurate results can then be obtained if the model is applied to an area with a similar background environment.
2) Different earthquakes can trigger different landslide distribution characteristics. Landslide density often reflects only the total number of landslides, and the distribution degree of the landslide scale reflects the spatial distribution characteristics of the landslides. It is found that the evaluation index should be based on the pattern of LCLs, rather than relying solely on the landslide density. This approach allows obtaining more useful evaluation results.
3) The susceptibility evaluation model used in this work considers only the significant influencing factors rather than all factors to enhance model efficiency. Thus, six significant independent variables, namely, slope aspect, elevation, intensity, fault-locked segment, duration of strong motions, and river distance, were used to construct the model.
Data availability statement
The original contributions presented in the study are included in the article/supplementary material; further inquiries can be directed to the corresponding authors.
Author contributions
XL: conceptualization, formal analysis, investigation, methodology, visualization, writing–original draft, and writing–review and editing. XY: writing–review and editing and project administration. RY: funding acquisition and writing–review and editing. RX: methodology and writing–review and editing. CL: writing–review and editing.
The authors declare that financial support was received for the research, authorship, and/or publication of this article. This research was financially supported by the National Non-profit Fundamental Research Grant of China, Institute of Geology, China Earthquake Administration (grant no. IGCEA2202), Youth Key Projects of Earthquake Emergency and Information (grant no. CEAEDEM202316), and Science and Technology Department of Sichuan Province (grant no. 2022JDRC0002).
Conflict of interest
The authors declare that the research was conducted in the absence of any commercial or financial relationships that could be construed as a potential conflict of interest.
Publisher’s note
All claims expressed in this article are solely those of the authors and do not necessarily represent those of their affiliated organizations, or those of the publisher, the editors, and the reviewers. Any product that may be evaluated in this article, or claim that may be made by its manufacturer, is not guaranteed or endorsed by the publisher.
Arroyo Solórzano, M., Quesada, R. A., and Barrantes Castillo, G. (2022). Seismic and geomorphic assessment for coseismic landslides zonation in tropical volcanic contexts. Nat. Hazards 114 (3), 2811–2837. doi:10.1007/s11069-022-05492-8
CrossRef Full Text | Google Scholar
Chen, W., Chen, Y., Tsangaratos, P., Ilia, I., and Wang, X. (2020). Combining evolutionary algorithms and machine learning models in landslide susceptibility assessments. Remote Sens. 12, 3854. doi:10.3390/rs12233854
Dai, L., Fan, X., Wang, X., Fang, C., Zou, C., Tang, X., et al. (2023). Coseismic landslides triggered by the 2022 Luding Ms6.8 earthquake, China. Landslides 20, 1277–1292. doi:10.1007/s10346-023-02061-3
Deng, Q. D., Zhang, P., Ran, Y., Yang, X., Min, W., and Chu, Q. (2003). Basic characteristics of active tectonics of China. Sci. China Ser. D-Earth Sci. 46, 356–372. doi:10.1360/03yd9032
Fan, X. M., Domènech, G., Scaringi, G., Huang, R., Xu, Q., Tristram, C., et al. (2018a). Spatio-temporal evolution of mass wasting after the 2008 Mw 7.9 Wenchuan earthquake revealed by a detailed multi-temporal inventory. Landslides 15, 2325–2341. doi:10.1007/s10346-018-1054-5
Fan, X. M., Scaringi, G., Xu, Q., Zhan, W., Dai, L., Li, Y., et al. (2018b). Coseismic landslides triggered by the 8th August 2017 Ms 7.0 Jiuzhaigou earthquake (Sichuan, China): factors controlling their spatial distribution and implications for the seismogenic blind fault identification. Landslides 15, 967–983. doi:10.1007/s10346-018-0960-x
Ferrario, M. F. (2019). Landslides triggered by multiple earthquakes: insights from the 2018 Lombok (Indonesia) events. Nat. Hazards 98, 575–592. doi:10.1007/s11069-019-03718-w
Gnyawali, K. R., and Adhikari, B. R. (2016). Spatial relations of earthquake induced landslides triggered by 2015 Gorkha earthquake Mw 7.8. Eur. Geosci. Union General Assem. Conf. Abstr. 18, 18429.
Google Scholar
Havenith, H. B. (2022). “Recent earthquake-triggered landslide events in central asia, evidence of seismic landslides in the lesser caucasus and the carpathians,” in Coseismic landslides. Springer natural Hazards. Editors I. Towhata, G. Wang, Q. Xu, and C. Massey (Singapore: Springer ).
Huang, R. Q., and Li, W. L. (2009). Analysis of the geo-hazards triggered by the 12 may 2008 wenchuan earthquake, China. Bull. Eng. Geol. Environ. 68 (3), 363–371. doi:10.1007/s10064-009-0207-0
Huang, R. Q. R., and Qiang, Xu (2008). Typical catastrophic landslides in China. J. Eng. Geol. 16 (5), 1.
Li, C. Y., Sun, K., Jun, Ma, Junjie, Li, Liang, M., and Fang, L. (2023). The 2022 M6.8 LuDing Earthquake: a complicated event by faulting of the MoXi segment of the XianShuiHe fault zone. Seismol. Geol. 44 (6), 1648–1666.
Li, G., West, A. J., Densmore, A. L., Jin, Z., Parker, R. N., and Hilton, R. G. (2014). Seismic mountain building: landslides associated with the 2008 Wenchuan earthquake in the context of a generalized model for earthquake volume balance. Geochem. Geophys. Geosystems 15, 833–844. doi:10.1002/2013gc005067
Li, W. L., Huang, R., Xu, Q., and Tang, C. (2013). Rapid prediction of co-seismic landslides triggered by Lushan earthquake, Sichuan, China . Journal of Chengdu University of Technology Science and Technology Edition , 264–274. 040.003.
Liu, X. M., Su, P., Li, Y., Xia, Z., Ma, S., Xu, R., et al. (2023). Spatial distribution of landslide shape induced by Luding Ms6.8 earthquake, Sichuan, China: case study of the Moxi Town. Landslides 20, 1667–1678. doi:10.1007/s10346-023-02070-2
Liu, X. M., Su, P., Li, Y., Zhang, J., and Yang, T. (2021a). Susceptibility assessment of small, shallow and clustered landslide. Earth Sci. Inf. 14, 2347–2356. doi:10.1007/s12145-021-00687-2
Liu, X. M., Su, P., Yong, Li, Rui, Xu, Jun, Z., Yang, T., et al. (2021b). Spatial patterns and scaling distributions of earthquake-induced landslides—a case study of landslides in watersheds along dujiangyan–wenchuan highway. Front. Earth Sci. 9 (10), 3389. doi:10.3389/feart.2021.659152
Martinez, S. N., Allstadt, K. E., Slaughter, S. L., Schmitt, R., Collins, E., Schaefer, L. N., et al. (2021). Landslides triggered by the august 14, 2021, magnitude 7.2 nippes, Haiti, earthquake: U.S. Geological survey open-file Report.17 , 1112.
Micu, M., Micu, D., and Havenith, H. B. (2023). Earthquake-induced landslide hazard assessment in the vrancea seismic region (eastern carpathians, Romania): constraints and perspectives. Geomorphology 427, 108635. doi:10.1016/j.geomorph.2023.108635
Nowicki, M. A., Wald, D. J., Hamburger, M. W., Hearne, M., and Thompson, E. M. (2014). Development of a globally applicable model for near real-time prediction of seismically induced landslides. Eng. Geol. 173, 54–65. doi:10.1016/j.enggeo.2014.02.002
Ozdemir, A., and Altural, T. (2013). A comparative study of frequency ratio, weights of evidence and logistic regression methods for landslide susceptibility mapping: sultan mountains, SW Turkey. J. Asian Earth Sci. 64, 180–197. doi:10.1016/j.jseaes.2012.12.014
Porras, J., Massin, F., Arroyo Solórzano, M., Arroyo, I., Linkimer, L., Böse, M., et al. (2021). Preliminary results of an earthquake early warning system in Costa Rica. Front. Earth Sci. 9, 700843. doi:10.3389/feart.2021.700843
Qiao, B. C., Li, Y., Dong, S., Yan, L., Chen, H., Ma, B. L., et al. (2009). The surface rupture of the M_s8.0 wenchuan earthquake on the northern segment of central fault. China Earthq. Eng. J. 31 (4), 333–348. doi:10.1042/BSR20080061
Quesada, R. A. (2021a). Review of the geomorphological effects of the 1991 Limón earthquake. Rev. Geol. América Cent. 65, 370–395.
Quesada, R. A. (2021b). Landslides and floods zonation using geomorphological analyses in a dynamic basin of Costa Rica. Rev. cartográfica 102, 125–138. doi:10.35424/rcarto.i102.901
Quesada, R. A., Castro-Chacón, J. P., and Boraschi, S. F. (2021c). Geomorphology, land use, and environmental impacts in a densely populated urban catchment of Costa Rica. J. S. Am. Earth Sci. 112, 103560. doi:10.1016/j.jsames.2021.103560
Quesada, R. A., Fallas López, B., Hernández, E. K., Stoffel, M., and Ballesteros-Cánovas, J. A. (2019). Relationships between earthquakes, hurricanes, and landslides in Costa Rica. Landslides 16, 1539–1550. doi:10.1007/s10346-019-01209-4
Sun, X. H., Chen, J., Bao, Y., Han, X., Zhan, J., and Peng, W. (2018). Landslide susceptibility mapping using logistic regression analysis along the jinsha river and its tributaries close to derong and deqin county, southwestern China. ISPRS Int. J. Geo-Information 7 (11), 438. doi:10.3390/ijgi7110438
Sun, X. H., Chen, J., Han, X., Bao, Y., Zhou, X., and Peng, W. (2020). Landslide susceptibility mapping along the upper Jinsha River, south-western China: a comparison of hydrological and curvature watershed methods for slope unit classification. Bull. Eng. Geol. Environ. 79, 4657–4670. doi:10.1007/s10064-020-01849-0
Tan, X. B., Yuan, R., Xu, X., Chen, guihua, and Chang, chunpai (2013). Co-seismic rupture and displacement in the XiaoYuDong area produced by the 2008 wenchuan earthquake, China, and its mechanism. Seismol. Geol . 0253-496702-0247-14. (In China).
Ullah, I., Aslam, B., Shah, S. H. I. A., Tariq, A., Qin, S., Majeed, M., et al. (2022). An integrated approach of machine learning, remote sensing, and GIS data for the landslide susceptibility mapping. Remote Sens. GIS Data Landslide Susceptibility Mapp. Land 11 (8), 1265. doi:10.3390/land11081265
Wang, F., Xu, P., Wang, C., Wang, N., and Jiang, N. (2017). Application of a GIS-based slope unit method for landslide susceptibility mapping along the longzi river, southeastern Tibetan plateau, China. ISPRS Int. J. Geo-Information. 6, 172. doi:10.3390/ijgi6060172
Xu, C., Dai, F., suning, Xu, xiwei, Xu, He, H., Wu, X., et al. (2013). Application of logistic regression model on the Wenchuan earthquake triggered landslide hazard mapping and its validation. Hydrogeology Eng. Geol. 3, 7.
Xu, C., Dai, F., Yao, X., Chen, J., Tu, X., Sun, Yu, et al. (2009). GIS-Based landslide susceptibility assessment using analytical hierarchy process in WenChuan earthquake region. Chin. J. Rock Mech. Eng. 28 (S2).
Xu, C., Xu, X., Shen, L., Dou, S., Wu, S., Tian, Y., et al. (2014b). Inventory of landslides triggered by the 2014 Ms 6.5 Ludian earthquake and its implications on several earthquake parameters. Seismol. Geol. 36 (4), 1186–1203.
Xu, C., Xu, X., Shyu, J. B. H., Zheng, W., and Min, W. (2014c). Landslides triggered by the 22 July 2013 Minxian–Zhangxian, China, Mw 5.9 earthquake: inventory compiling and spatial distribution analysis. J. Asian Earth Sci. 92, 125–142. doi:10.1016/j.jseaes.2014.06.014
Xu, C., Xu, X., Yao, X., and Dai, F. (2014a). Three (nearly) complete inventories of landslides triggered by the May 12, 2008 Wenchuan Mw 7.9 earthquake of China and their spatial distribution statistical analysis. Landslides 11, 441–461. doi:10.1007/s10346-013-0404-6
Xu, T. R., Dai, D., Yang, Z., Xi, N., Deng, W., Zhang, Y. J., et al. (2022). Preliminary study results of the 6.8 magnitude earthquake in luding, sichuan province on september 5, 2022. Earthq. Res. China 38 (3), 412–424. (In China).
Xu, X. W., Wen, X., Ye, J. Q., Ma, B. Q., Cheng, J., Zhou, R. Q., et al. (2008). Wenchuan Ms8.0 earthquake surface rupture zone and its seismic structure. Seismol. Geol. 03. (In China).
Yuan, R. M., Deng, Q. H., Cunningham, D., Xu, C., Xu, X. W., and Chang, C. P. (2013). Density distribution of landslides triggered by the 2008 wenchuan earthquake and their relationships to peak ground acceleration. Bull. Seismol. Soc. Am. 103 (4), 2344–2355. doi:10.1785/0120110233
Zhang, P. Z., Deng, Q., Zhang, G., jin, Ma, Weijun, G., Wei, M., et al. (2003). Strong seismic activity and active plate in China. Sci. China (Series D) 33, 9.
Zhang, Y., Feng, W., Xu, L., Zhou, C., and Chen, Y. (2008). The spatiotemporal rupture process of the 2008 Wenchuan earthquake. Sci. China Press 38 (10), 9.
Keywords: seismic landslide, Wenchuan earthquake, Luding earthquake, susceptibility evaluation, influencing factor
Citation: Liu X, Yang X, Yuan R, Xu R and Liu C (2024) Seismic landslide susceptibility evaluation model based on historical data and its application to areas with similar environmental settings. Front. Earth Sci. 12:1419851. doi: 10.3389/feart.2024.1419851
Received: 19 April 2024; Accepted: 20 May 2024; Published: 03 July 2024.
Reviewed by:
Copyright © 2024 Liu, Yang, Yuan, Xu and Liu. This is an open-access article distributed under the terms of the Creative Commons Attribution License (CC BY). The use, distribution or reproduction in other forums is permitted, provided the original author(s) and the copyright owner(s) are credited and that the original publication in this journal is cited, in accordance with accepted academic practice. No use, distribution or reproduction is permitted which does not comply with these terms.
*Correspondence: Xianhe Yang, [email protected] ; Renmao Yuan, [email protected]
Disclaimer: All claims expressed in this article are solely those of the authors and do not necessarily represent those of their affiliated organizations, or those of the publisher, the editors and the reviewers. Any product that may be evaluated in this article or claim that may be made by its manufacturer is not guaranteed or endorsed by the publisher.
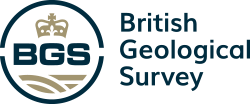
Quick links
- Climate change
- Multiple hazards
- Decarbonisation
Holbeck Hall, Scarborough
Landslide case study
The Holbeck Hall landslide, south of Scarborough in North Yorkshire, attracted considerable interest when it destroyed the four-star Holbeck Hall Hotel between 3 and 5 June 1993. A rotational landslide involving about 1 million tonnes of glacial till cut back the 60 m-high cliff by 70 m. It flowed across the beach to form a semicircular promontory 200 m wide projecting 135 m outward from the foot of the cliff.
The landslide is National Landslide Database ID 10741/1.
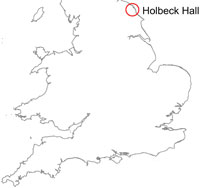
Holbeck Hall location map. BGS © UKRI.
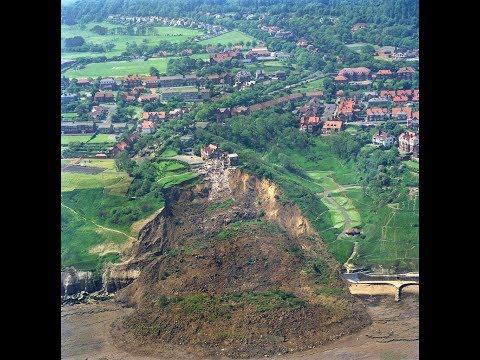
Holbeck Hall landslide aerial survey. Previously unseen aerial footage of the survey carried out by BGS of the landslide that affected the Holbeck Hall hotel in June 1993. BGS © UKRI.
Landslide details
The first signs of movement on the cliff were seen six weeks before the main failure, when cracks developed in the tarmac surface of footpaths running across the cliffs. These were filled to stop ingress of water to the cliff, but when the cracks reopened shortly before the main failure, the council closed the cliff paths below the hotel. At this time, a small part of the hotel garden was also observed to have suffered a minor movement.
There was originally 70 m of garden between the hotel and the cliff edge. At 6 am on 4 June, a guest saw that 55 m of the garden had disappeared. The hotel was evacuated and the landslide continued to develop, culminating in the collapse of the east wing of the hotel by the evening of 5 June.
The likely cause of the landslide was a combination of:
- rainfall of 140 mm in the two months before the slide took place
- issues related to the drainage of the slope
- pore water pressure build up in the slope
The landslide is a rotational landslide degrading to a mud/debris flow which covered the rocks on the beach (platform).
The cliff consists of glacial till (sandy, silty clay) resting on a low cliff of the Middle Jurassic Scalby Formation . The Scalby Formation comprises the Scalby Mudstone and the Moor Grit (sandstone).
Forster, A. 1993. Scarborough Landslip. Geoscientist , Vol. 3(5), 2–3.
Forster, A, and Culshaw, M. 2004. Implications of climate change for hazardous ground conditions in the UK , GeologyToday , Vol. 20(2), 61–66.
Lee, E M. 1999. Coastal planning and management: the impact of the 1993 Holbeck Hall landslide, Scarborough. East Midlands Geographer , Vol. 21(2) and Vol. 22(1), 78–91.
Unseen video footage — the Holbeck Hall landslide 25 years on by Catherine Pennington. Looking back to the Holbeck Hall landslide with unseen video footage taken at the time from an aeroplane – drones hadn’t been invented yet…
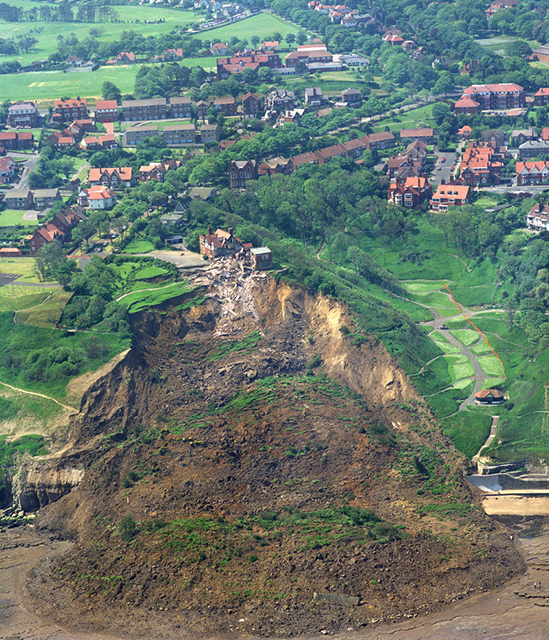
Holbeck Hall landslide from the air. BGS © UKRI.
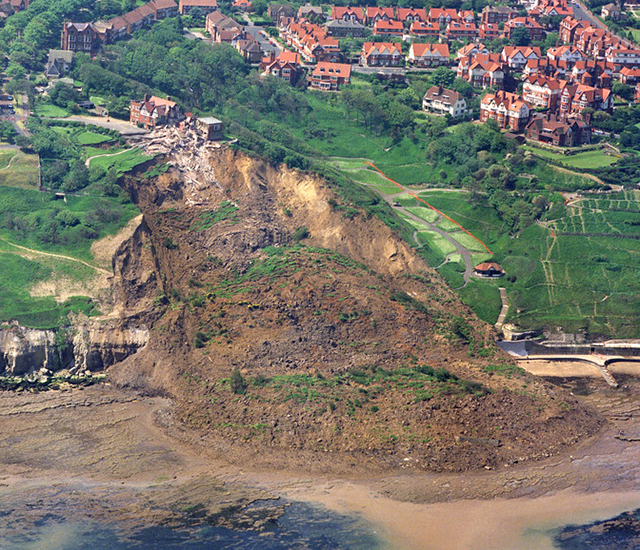
Top of the landslide showing damage to the Holbeck Hall Hotel. BGS © UKRI.
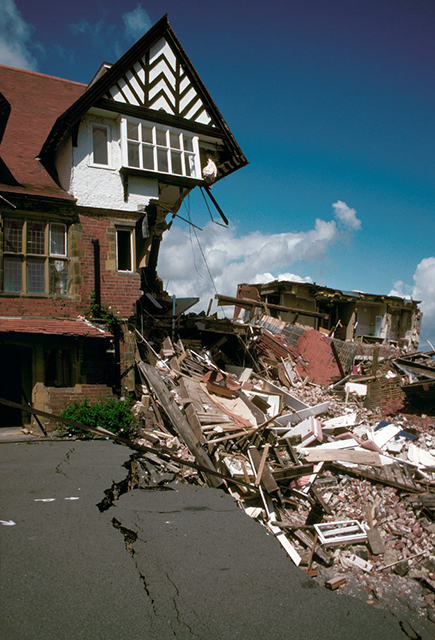
Damage to the hotel. BGS © UKRI.
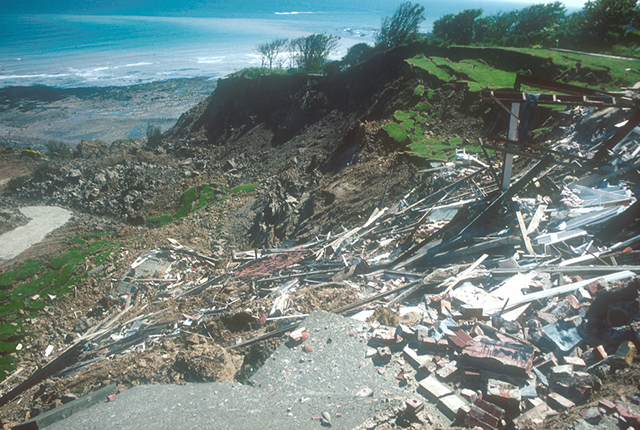
Looking down the landslide. BGS © UKRI.
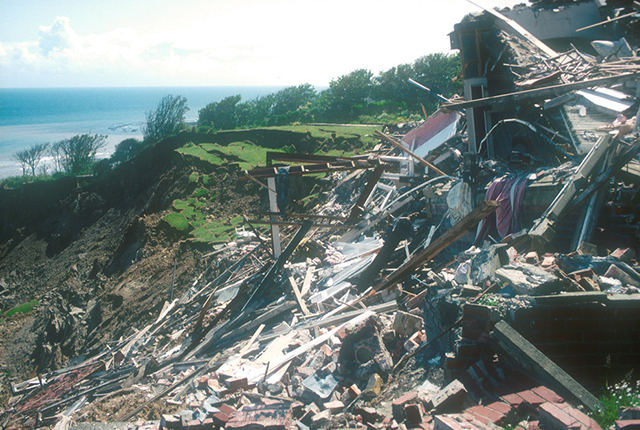
Debris from the hotel. BGS © UKRI.
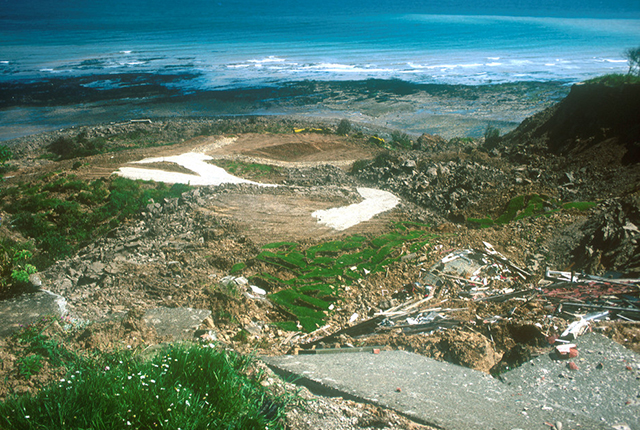
View of inside. BGS © UKRI.
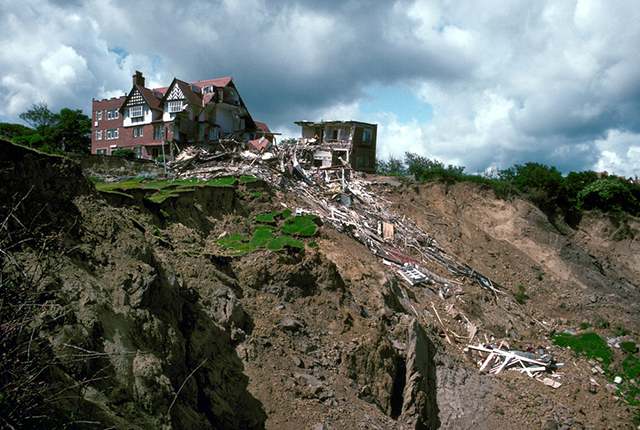
View of the Holbeck Hall Hotel and the top of the landslide. BGS © UKRI.
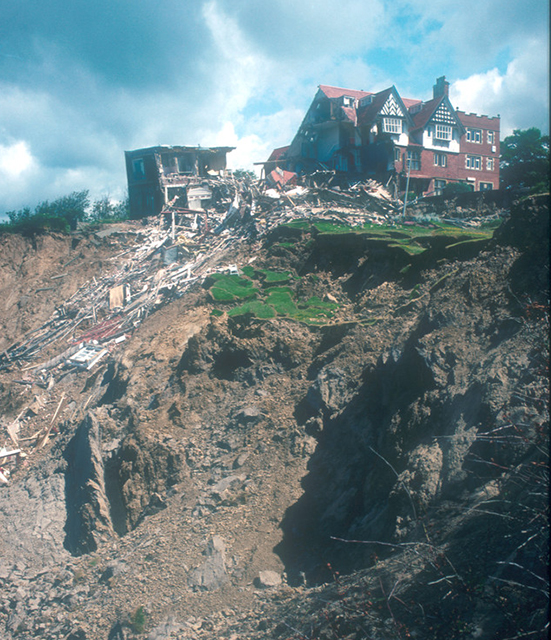
Holbeck Hall Hotel landslide. BGS © UKRI.
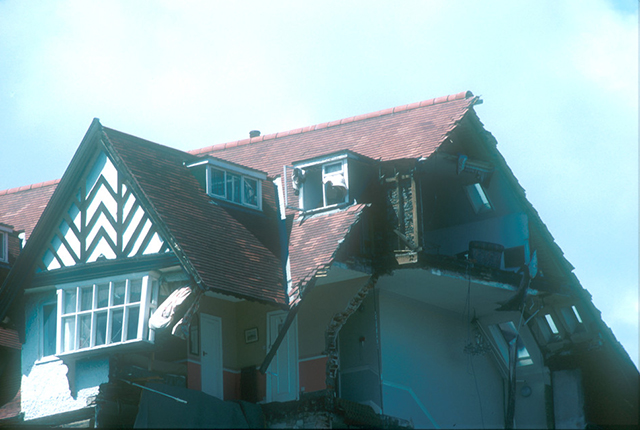
Damage to the roof. BGS © UKRI.
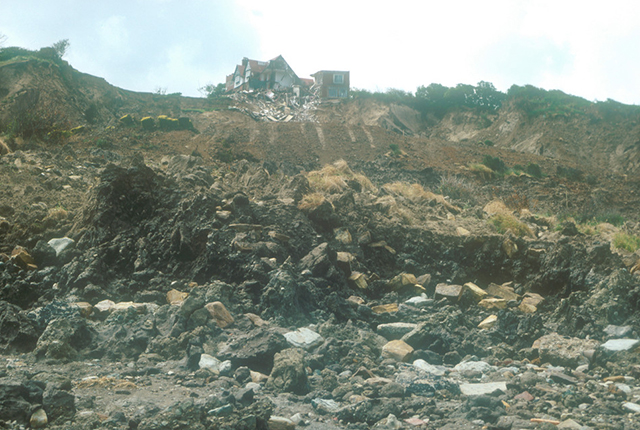
hotel from the bottom of the landslide looking up towards the hotel. BGS © UKRI.
Contact the Landslide Response Team
You may also be interested in.
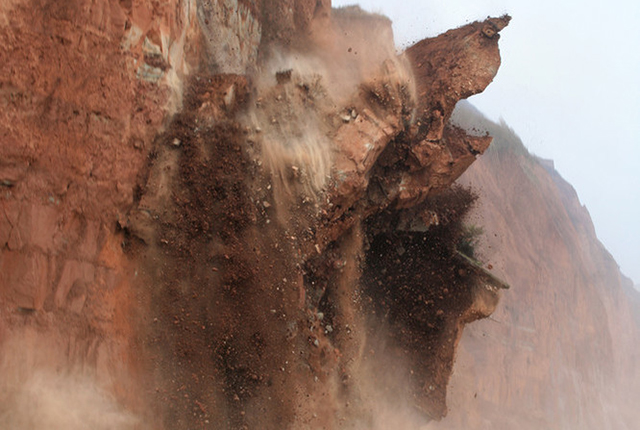
Landslide case studies
The landslides team at the BGS has studied numerous landslides. This work informs our geological maps, memoirs and sheet explanations and provides data for our National Landslide Database, which underpins much of our research.
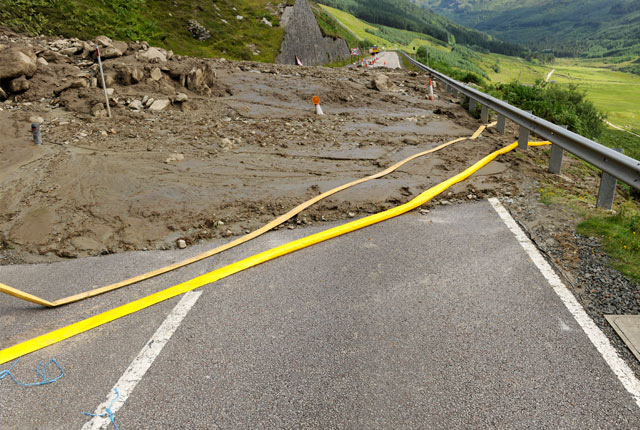
Understanding landslides
What is a landslide? Why do landslides happen? How to classify a landslide. Landslides in the UK and around the world.
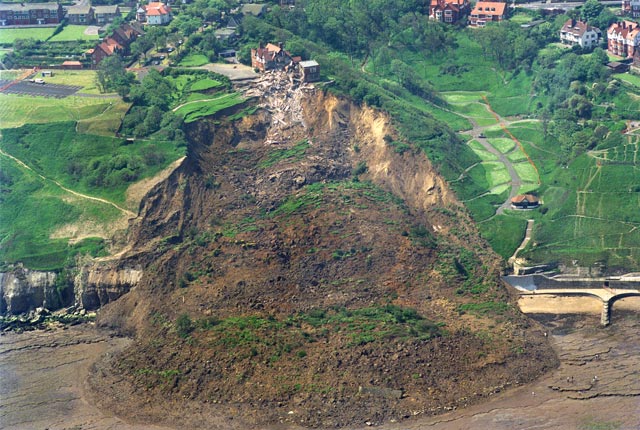
How to classify a landslide
Landslides are classified by their type of movement. The four main types of movement are falls, topples, slides and flows.
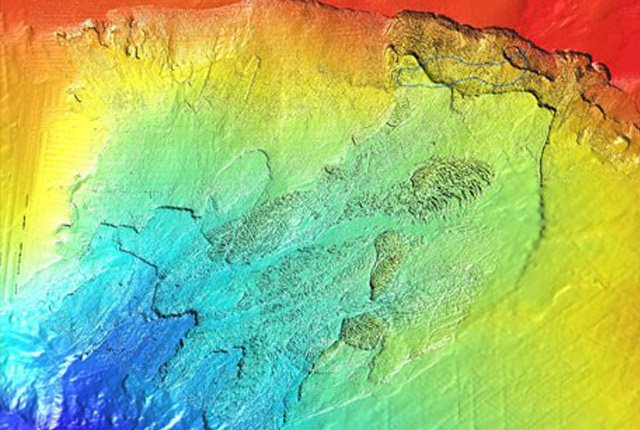
Landslides in the UK and around the world
Landslides in the UK, around the world and under the sea.
Was this page helpful?
How can we make this section better?*
- How can we make this section better? *
Please select a reason*
- Please select a reason * Select reason Didn't answer my question Too confusing Links are broken/Doesn't work
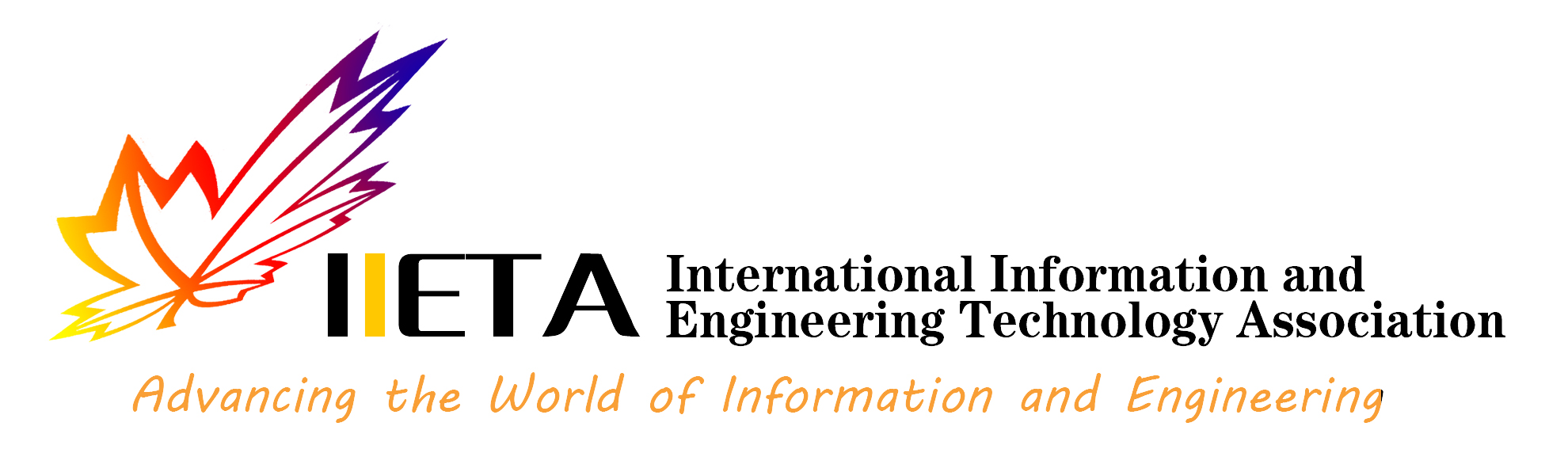
Search form
Post-landslide liquefaction analysis: a case study in the kupang regency area, indonesia.
Copyright: ©2024 The authors. This article is published by IIETA and is licensed under the CC BY 4.0 license ( http://creativecommons.org/licenses/by/4.0/ ).
OPEN ACCESS
Landslide disasters in Indonesia often cause significant damage to public facilities, agricultural lands, and result in human casualties. This study was conducted to deeply understand the dynamics and causes of landslides and to develop effective disaster mitigation strategies in areas vulnerable to soil movement. The focus of the research is on the rotational landslide incident that occurred on the Trans Timor National Road KM 73, Noelmina Village, Takari District, Kupang Regency, East Nusa Tenggara Province, on February 17, 2023. Through a methodological approach that includes geomorphological analysis, evaluation of hydrological conditions, and land use study, this research identifies the main factors causing the landslide, including the changeable nature of Bobonaro clay soil, increased soil saturation due to hydrological conditions, and increased water load in the basin area due to land use. The findings emphasize the importance of efficient hydrological management, careful land use planning, and strengthening the capacity of local communities in landslide disaster mitigation. Recommendations for disaster mitigation include the development of infrastructure that is resistant to soil movement, vegetation planting for slope stabilization, and community education on sustainable land use practices. This research provides a significant contribution to disaster mitigation efforts in Indonesia by highlighting an approach based on a comprehensive understanding of the causes of landslides and effective adaptation strategies.
investigation, landslide, disaster, mitigation, geomorphology, hydrology, land use
Landslides represent a critical and recurrent natural disaster globally, posing significant challenges to sustainable development, especially in regions like Indonesia, where the confluence of geographical, climatic, and anthropogenic factors exacerbates their frequency and impact. Indonesia, a country characterized by its archipelagic geography and tropical climate, experiences a high incidence of landslides, affecting both rural and urban communities across its diverse landscapes. These disasters not only cause immediate loss of life and destruction of infrastructure but also have long-term repercussions on agricultural productivity and socio-economic stability. The escalating pattern of landslide occurrences is closely linked to human activities such as deforestation, land-use changes, and improper agricultural practices, alongside natural triggers like heavy rainfall and seismic events [1-7].
The geological processes underpinning landslides involve the movement of soil or rock masses on slopes under the influence of gravity, which may be precipitated by natural vibrations or earthquakes. This dynamic earth surface process, part of the broader category of mass wasting, challenges our understanding of the natural landscape's stability and evolution. The study of these processes, from the initial detachment of material from a slope to its eventual deposition, reveals critical insights into the natural balance of forces and materials shaping the earth's surface. Landslides occur when this balance is disturbed, leading to shear failure along a plane within the soil or rock mass. This failure is often a complex interplay between the material's inherent shear strength and the external stresses applied to it, including those from natural or human-induced changes in the environment [8-15].
This research targets a comprehensive exploration of the causative factors behind landslides in Noelmina Village, Takari District, Kupang Regency, aiming to dissect the intricate web of geomorphological, soil, hydrological, and land use characteristics contributing to slope instability. The investigation focuses on the Bobonaro clay formations, known for their susceptibility to sliding, evaluating how variations in slope geometry, soil properties, rainfall patterns, and anthropogenic interventions such as land clearing since 2016 influence landslide risk. By integrating geotechnical analysis with environmental and socio-economic evaluations, this study aspires to delineate the multifactorial dimensions of landslide hazards and devise effective mitigation strategies to safeguard vulnerable communities.
Amidst a backdrop of increasing landslide incidents attributed to both natural and human factors, this research posits a hypothesis that underscores the critical interplay between Indonesia's unique environmental conditions and the expanding footprint of human activities. It hypothesizes that a strategic blend of land management practices and community-based educational initiatives can substantially mitigate the adverse effects of landslides. Through a methodological approach that employs predictive modeling to assess landslide vulnerability, incorporating both environmental and human variables, this study aims to advance our understanding of landslide dynamics and inform more resilient spatial planning and disaster management practices. The novelty of this research lies in its holistic approach to examining landslide phenomena, offering a novel perspective on integrating quantitative and qualitative methodologies to enhance the predictive accuracy of landslide vulnerability assessments and the effectiveness of mitigation measures in Indonesia's wet tropical regions.
The interaction between Indonesia's unique natural environmental conditions and the expansion of human activities, especially deforestation, land-use changes, and improper agricultural practices, increases the risk and frequency of landslides. This study hypothesizes that the implementation of strategic land management practices and community-based educational initiatives can significantly reduce the adverse effects of landslides. Utilizing predictive modeling that incorporates environmental and human variables, this study aims to deepen the understanding of landslide dynamics and provide a foundation for more resilient spatial planning and disaster management practices.
The assessment of vulnerability levels in landslide-prone areas involves a multidimensional approach, integrating geological, hydrological, and land use data along with human activities and socioeconomic factors. This methodology employs a combination of field surveys, remote sensing, and GIS technologies to systematically categorize areas based on their susceptibility to landslides. Such an integrated approach ensures a comprehensive understanding of both natural predispositions and anthropogenic impacts on landslide vulnerability.
It is essential to clarify the interaction between natural conditions, such as soil composition and slope gradient, and human factors, including changes in land use and deforestation, in determining vulnerability levels. These elements collectively contribute to the landscape's susceptibility to landslides, where natural conditions prepare the stage for potential geological movements, and human activities can mitigate or exacerbate this vulnerability.
Illustrating the practical application of this framework, case studies highlight its utility. For instance, areas with high vulnerability might include regions with steep slopes and poor vegetation cover, worsened by unsustainable agricultural practices. Conversely, areas with low vulnerability could feature gentle slopes with substantial forest cover and well-managed watershed areas, demonstrating effective land use planning and conservation efforts.
Recognizing the influence of socioeconomic factors adds another layer of depth to this assessment. Populations with limited resources are often more vulnerable due to inadequate access to early warning systems, less robust infrastructure, and a lack of alternatives to high-risk land use practices. This socioeconomic dimension emphasizes the importance of an integrated approach that combines geological assessment with community engagement and resilience building.
Variation in vulnerability across different areas could significantly enhance the understanding and communication of these assessments. If data allows, these visual aids can facilitate the identification of priority areas for intervention, supporting targeted and effective landslide risk reduction strategies.
This hypothesis reflects the research assumption linking the causative factors of landslides to potential interventions that can mitigate the risks and impacts. It is based on previous observations about the impact of human activities and natural conditions on the occurrence of landslides, as well as the importance of a multidisciplinary approach in reducing the risks of natural disasters.
In summary, this extended background section endeavors to encapsulate a broader spectrum of considerations relevant to understanding and managing landslide risks in Indonesia, setting the stage for a detailed examination of the specific case of Noelmina Village. Through a critical review of existing literature and the introduction of new research questions and methodologies, this study seeks to contribute valuable insights to the ongoing discourse on natural disaster management and resilience building in vulnerable regions.
Landslides represent intricate phenomena, shaped by a combination of geological, morphological, hydrological aspects, environmental conditions, and human interventions [16]. A myriad of elements including soil properties, geological context, climatic conditions, atmospheric dynamics, and human contributions play a role in determining landslide vulnerability [17]. Understanding the spatial distribution of landslides and their influencing factors is pivotal for assessing landslide susceptibility [18]. The process of mapping landslide susceptibility necessitates amalgamating diverse factors such as the angle and composition of slopes, seismic activities, drainage configurations, and vegetation cover [19]. The employment of logistic regression alongside fuzzy analytical hierarchy processes for assigning weights to these factors in susceptibility maps has shown effectiveness [20]. Predicting landslide movements requires considering the intricate interplay among various elements, with models like GA-LSSVM being suggested for forecasting landslide progression [21]. Studies have delved into how lithology, slope gradients, orientations, peak ground accelerations, and proximity to faults influence the occurrence of co-seismic landslides [14]. Furthermore, the utility of bivariate statistical methods in correlating landslide inventories with parameters influencing landslides for susceptibility analysis has been documented [22].
The nomenclature surrounding landslides has sparked discussion among scholars. slope movement as a more precise term to describe the downward or outward movement of slope materials under gravity's pull [23]. Myers et al. [24] introduced "mass movement" as a term that more accurately captures the essence of slope material movements compared to "landslide".
Terranova et al. [25] developed a hydrological model based on genetic algorithms to predict landslide activations, showcasing its utility in forecasting and providing early warnings for civil protection. Similarly, Santangelo et al. [26] demonstrated the efficiency of remote landslide mapping techniques using laser rangefinder binoculars and GPS, proving that geographical data gathered remotely is comparable to that collected through on-ground GPS surveys.
Cui et al. [27] focused on assessing ecological risks in landscapes using remote sensing imagery and DEM data across different timelines, showing remote sensing's potential in tracking landscape alterations over time. These studies underline the critical role of advanced technologies and methodologies in comprehending, predicting landslide events, and evaluating ecological risks due to landscape transformations.
Recent investigations have shed light on various critical aspects such as the analysis of slope stability, the impact of land use alterations, rainfall effects, and community-based mitigation strategies [27-31]. Grasping the geometric facets of landslides, including rock formations, properties of slope materials, and external influences on slope stability, is vital for understanding landslides and devising effective prevention measures [32, 33]. This geometric insight is indispensable not only for researchers but also for practitioners and policymakers in formulating strategies for landslide prevention and management [34, 35].
Hungr et al. [36] stressed the necessity of adopting a multidisciplinary approach to devise holistic solutions for mitigating landslide risks and crafting prevention mechanisms Li et al. [14], Bucknam et al. [37], and Glade et al. [38] further emphasized the multidisciplinary strategy by discussing the application of empirical models to refine rainfall thresholds that trigger landslides.
The pivotal role of rainfall as a primary catalyst for landslides, especially in tropical regions, has been explored by Chang et al. [30], Nugroho and Kristanto [39] with the latter analyzing the link between typhoon-induced landslides and critical rainfall conditions. Highlighted precipitation as a likely trigger for most landslides, underscoring the significance of rainfall in landslide initiation [31].
Shabbir et al. [40] investigated the impact of environmental changes on landslides, revealing seasonal patterns in fatal landslides and the influence of environmental factors on their occurrence (the study examined the role of citrus groves in exacerbating rainfall-induced landslide hazards, highlighting vegetation's impact on landslide events) [41].
Recent research emphasizes the necessity of comprehending landslide mechanisms and triggers, particularly highlighting rainfall's role as a primary catalyst. The adoption of a multidisciplinary approach and a deep understanding of landslides' geometric properties are essential for developing effective preventive measures and comprehensive solutions to reduce landslide disaster risks. Consequently, the body of literature on landslides suggests that embracing a holistic approach and a thorough grasp of geotechnical and environmental factors are crucial for managing the challenges and consequences of landslides.
Landslide-prone areas are characterized by specific conditions such as high average rainfall levels, steep slope angles, and the presence of waterways and springs near rivers. These areas are often fertile and extensively used for cultivation and residential purposes. However, the lack of awareness and understanding among the community regarding the vulnerability of these areas to landslides leads to inadequate preparedness for disaster mitigation, resulting in greater impacts when landslides occur [42].
In addition to the general characteristics, specific areas prone to landslides include slopes at river bends, slope bend areas, and areas traversed by fault structures. Slopes at river bends are susceptible to erosion by river flow, leading to instability. Slope bend areas, which transition from steep to gentle slopes, accumulate water from steeper parts, increasing pore water pressure and weakening soil bonds, thus triggering landslides. Areas traversed by fault structures, characterized by steep slopes and fractured rocks with numerous springs, are prone to slope instability due to disrupted rock stability caused by water seepage and vibrations [42].
The classification of soil/rock mass movements includes translational landslides, rotational landslides, block movements, rockfalls, soil creep, and debris flows. Translational and rotational landslides are the most common types in Indonesia, with debris flows causing the highest human casualties. Landslides occur when the driving force on the slope exceeds the resisting force, influenced by factors such as slope angle, water, load, and rock/soil density. The understanding of these factors is crucial for landslide susceptibility assessment and mitigation.
The references provide various methodologies and models for landslide susceptibility mapping, including frequency ratio, fuzzy logic, logistic regression, weights of evidence, and artificial neural network models. These models integrate causative factors such as elevation, slope, lithology, distance to river, precipitation, and land cover to assess landslide susceptibility. These approaches have been applied in different geographical locations, including the Himalayas, Indonesia, Ethiopia, and Portugal, demonstrating their versatility and effectiveness in assessing landslide susceptibility. These methodologies and models play a crucial role in identifying potential landslide-prone areas, providing valuable information for disaster preparedness and mitigation efforts. By considering various causative factors and employing advanced techniques such as remote sensing, GIS, and statistical analysis, researchers and authorities can effectively assess and manage landslide susceptibility, ultimately reducing the impact of landslides on communities and infrastructure.
The process of assessing landslide vulnerability adopts a comprehensive multidisciplinary approach, beginning with the identification and mapping of areas potentially susceptible to landslides through remote sensing techniques and spatial analysis. The evaluation of the geotechnical conditions of slopes, including soil composition, moisture, and structural stability, is the initial step to determine the physical vulnerability of these areas. Human factors, such as land use intensity, agricultural practices, and infrastructure development, are also analyzed to understand how human interventions could increase landslide risks. This approach is strengthened with case studies providing insights into the relationship between natural conditions and human activities causing landslides.
A mass movement of land in the form of a rotational landslide occurred on the Trans Timor National Road at KM 73, Noelmina Village, Takari District, Kupang Regency, East Nusa Tenggara Province. Geographically, this location is at coordinates 9°58'52.05" South Latitude and 124°05'20.92" East Longitude. Topographically, Noelmina Village is situated at an elevation ranging from 132 m to 323 meters and is an area vulnerable to ground movement.
The interaction between vulnerable natural conditions and human pressures, such as deforestation and infrastructure development without considering geological conditions, illustrates how these factors collectively increase vulnerability to landslides. Understanding this relationship is crucial for clear articulation in vulnerability assessment. Providing case studies from areas classified at various vulnerability levels illustrates the practical application of this framework, highlighting the importance of identifying and managing landslide risks in real-world contexts.
Socio-economic factors, such as population density and the adaptive capacity of local communities, are also significant in assessing vulnerability. An approach that considers socio-economic factors enables the development of inclusive and sustainable mitigation solutions. The use of visualization, such as vulnerability maps, facilitates rapid identification of high-risk areas and supports strategic planning for landslide disaster mitigation.
This integrated approach, combining the analysis of natural conditions, human influences, and socio-economic factors, along with visualization tools, offers an effective method in assessing and managing landslide risks. Through the integration of data and multidisciplinary analysis, the assessment of landslide vulnerability becomes more accurate, informative, and relevant for reducing the impact of disasters on communities and infrastructure at risk. This approach emphasizes the importance of a comprehensive understanding of landslide dynamics and targeted mitigation strategies to reduce vulnerability and enhance resilience against landslides in Noelmina Village and similar areas. The location of the Takari Landslide is shown in Figure 1.
A field visit was carried out on February 21 2023 to the landslide location in Takari by the Disaster Mitigation Team formed by the Chancellor of Nusa Cendana University. The team consists of 8 experts with multidisciplinary knowledge to investigate landslides and is accompanied by assistant staff to carry out investigations at the Takari landslide location. From Kupang City to Noelmina Village, Takari District, Kupang Regency can be reached in ±1.5 hours by road with a distance of ±85 km.
In the Research Methodology segment A description of the assessment procedures or protocols implemented by the team is presented. This process begins with a comprehensive evaluation of areas potentially susceptible to landslides, incorporating the use of remote sensing techniques as well as direct field inspections. The applied protocol includes the identification of geological characteristics, soil composition, and signs of previous landslides. Data collection activities encompass technical measurements and interviews with the local community to gain an understanding of historical events and transformations in land use patterns.
Regarding safety protocols during field surveys, the team adheres to strict safety standards to ensure the security of members and the conservation of the natural environment. The use of safety equipment such as helmets, safety boots, and reflective vests is mandated. Furthermore, the team coordinates with local authorities for activity notification and to obtain the necessary permissions or guidance. Ethical aspects include respecting private property and securing consent from local residents for interviews. The team also strives to ensure that their activities do not disrupt local ecosystems or communities.
The application of these methodologies and protocols is crucial in conducting research that is not only thorough and responsible but also ensures accurate results while respecting local conditions and communities. The integration of technical, social, and ethical aspects in the research methodology underscores the complexity of evaluating vulnerability to landslides and highlights the need for a multidisciplinary approach.
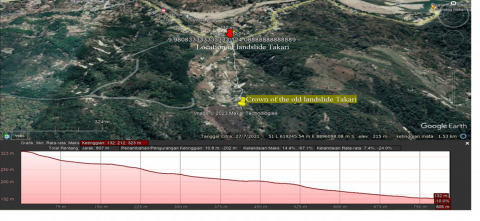
Source: Google Earth, 2023
Figure 1. Location of the Takari landslide 17 February 2023
4 .1 Field observation identification
Upon conducting field investigations, it was determined that the landslide phenomenon experienced in Noelmina Village, situated within the Takari District of Kupang Regency, adhered to the rotational/slide type, specifically delineated as a curvilinear slide, as depicted in Figure 2. This event was precipitated by the translocation of soil masses comprising silt and clay dimensions, which proceeded to glide across the slip plane associated with Bobonaro clay, characterized as Expansive Clay. The arrival of the rainy season exacerbates this condition, as the notably low permeability of Bobonaro clay impedes water filtration, resulting in the overlying soil strata reaching a state of saturation. This saturation induces a reduction in friction, thereby rendering the area prone to slipperiness and instigating landslide occurrences, culminating in the downward movement of the upper soil mass. Contributory factors to landslides within Noelmina Village include the lithological characteristics, geological structures and soil taxonomy, the gradient of the terrain, alongside hydrological influences, notably the incidence of elevated rainfall.
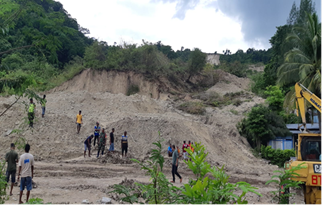
(Source: Investigation Team Documentation 21 February 2023)
Figure 2. Rotational landslide or curved slide on the Takari landslide

(Source: Documentation by Mr. Beni Polin, February 16 2023)
Figure 3. Springs that formed at the foot of the slope before the Takari landslide occurred
In the days leading up to the landslide event in Takari, as recounted by a local observer, Mr. Beni Polin, the area experienced rainfall, and notably, a spring emerged at the slope's base adjacent to the roadway, as detailed in Figure 3. This phenomenon suggests that the soil strata reached a saturation point, with its pores fully inundated, causing rainwater infiltration to manifest as spring runoff. The underlying stratum, composed of Bobonaro Clay - a layer impervious to water-further impedes the downward movement of rainwater. Consequently, the emergence of springs can be attributed to the saturation of soil and its minimal permeability. Such conditions serve as preliminary signs of potential soil mass movements. This is due to an increased load on the soil from infiltrating rainwater, coupled with a reduction in soil shear strength from water-induced softening, facilitating the disintegration of inter-particle bonds within the soil and its slip plane. These processes lead to the formation of increasingly slippery layers of Bobonaro silt and clay, thereby instigating landslides.
4 .2 Causes of land movement/landslides
Land movement represents a geological phenomenon arising from the interplay among various factors, including morphology, geology, geological structures, hydrogeology, and land use. Triggers for such movements encompass both natural and anthropogenic processes capable of altering the stability of slopes from a state of susceptibility to actual displacement [1]). Drone-based aerial photogrammetry has revealed that the volume of material displaced during the Takari landslide amounted to 175,000 m³, with an additional 16,000 m³ affecting the road and its vicinity. Field investigations have identified multiple factors contributing to soil displacement in Noelmina Village, Takari District.
The geomorphological context of Noelmina Village, located within the Takari District, is predominantly mountainous, characterized by slopes ranging between 30º-35º and elevations spanning 130-325 meters above sea level. This region's topography features steep mountains and undulating terrains, rendering it susceptible to soil movement. The Ground Movement Forecast Map for February 2023 in the Province of East Nusa Tenggara (Geological Agency, Center for Volcanology and Geological Hazard Mitigation, 2023) classifies the Takari District as being within a medium to high potential zone for ground movement (Figure 4). The medium ground movement potential indicates that such events may occur following above-normal rainfall, particularly near river valleys, escarpments, and roadside cliffs if slope stability is compromised. Conversely, the high movement potential denotes areas where soil displacement is likely under similar rainfall conditions, with the added possibility of reactivating previous soil movements.
4 .3 Condition of the soil/rock that makes up the slope
Based on the information provided from the Geological Map Sheet of Kupang-Atambua, Timor, as documented [43] accessible via the Geological Agency's website, particularly the Center for Volcanology and Geological Disaster Mitigation (2023), Figure 5, reveals the disaster site to be constituted of lithologies from the Bobonaro Complex (Tmb), Batuputih Formation (Tmpb), and the Noele Formation (QTn). The Bobonaro Complex is delineated by its composition of boulder-sized fragments within a Foraminifera-bearing clay matrix. Conversely, the Batuputih Formation is characterized by its components of calcilutite, tuff, minor marl, and arenite limestone, which is intricately associated with the Noele Formation, noted for its interbedded sandstone, conglomerate, and tuff. The geological structure at the ground movement site demonstrates considerable complexity, predominantly defined by normal faults with a west-east orientation, intersected by left-lateral faults that exhibit a north-south direction, as evidenced by the findings of [44-46].
Upon the collection and laboratory analysis of soil samples from the landslide site, including both debris and native soil from the base of the landslide, the study has elucidated the physical and mechanical properties of the undisturbed soil samples retrieved from the base, pinpointing the stratum responsible for the initiation of the landslide, as well as the disturbed soil samples, representing the landslide's material aftermath. Referenced in Figure 6 and detailed in Table 1, the findings contribute a crucial insight into the landslide dynamics.
The undisturbed samples, embodying the intact geological conditions at the landslide's genesis, present a distinctive set of properties when juxtaposed against the disturbed samples, which have undergone alteration through the landslide process. The differentiation in properties between these sample types not only delineates the landslide's impact on soil stability but also emphasizes the inherent vulnerability of the site's geological makeup.
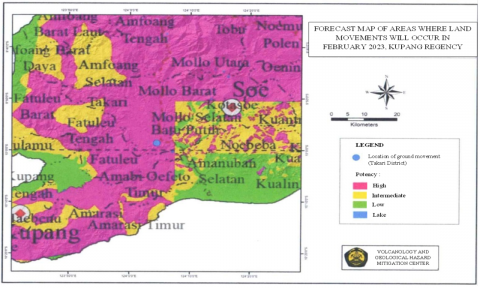
(Source: Geological Agency, Center for Volcanology and Geological Disaster Mitigation, 2023)
Figure 4. Forecast map of areas of land movement occurrence in February 2023 in Kupang Regency, Prov. NTT

Figure 5. Forecast map of areas where land movement will occur in February 2023 in Kupang Regency, Prov. NTT
Table 1. Soil physical and mechanical properties
|
|
| ||
|
|
| ||
1 | Water content (%) | 30.26 | 46.06 | 46.63 |
2 | Specific Gravity | 2.67 | 2.61 | 2.62 |
3 | Liquid Limit (%) | 73.64 | 53.53 | 54.25 |
Plastic Limit (%) | 15.86 | 15.85 | 15.29 | |
Shrinkage Limit (%) | 7.25 | 24.64 | 32.35 | |
4 | Granule Analysis (Passing sieve no.200) (%) | 90.27 | 51.50 | 58.53 |
5 | Plasticity Index (%) | 57.77 | 37.68 | 38.96 |
6 | Optimum Water Content (%) | - | 38.55 | 43.32 |
7 | Maximum Dry Volume Weight (g/cm³) | - | 0.96 | 0.90 |
8 | Internal Friction Angle | 10.95 | - | - |
9 | Cohesion, c (kg/cm ) | 0 | - | - |
10 | Unconfined compressive strength, qu (kg/cm ) | 0.418 | - | - |
11 | Soil permeability, K (cm/sec) | 2.6×10 | 1.74×10 | 4.77×10 |
Source: Analysis results, 2023

(a) Original soil sampling (UDS)
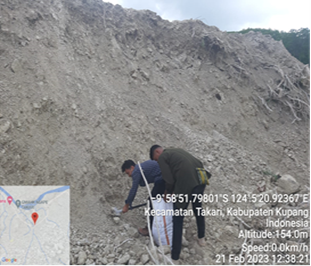
(b) Point landslide debris sampling 1
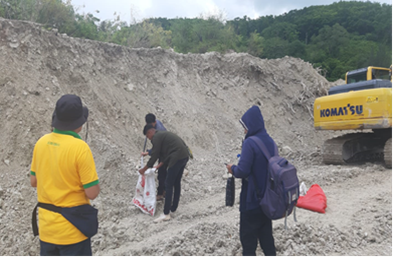
(c) Point sampling of landslide debris 2
Figure 6. Soil sampling for laboratory
The analysis aligns with existing literature, suggesting that variations in soil mechanical and physical properties significantly influence landslide susceptibility. Specifically, the study's findings resonate highlighted the critical role of soil composition and structure in landslide initiation, particularly in areas characterized by complex geological formations similar to those described by the Bobonaro Complex, Batuputih Formation, and the Noele Formation. The critical erosive shear stress is calculated as 2.8 Pa, and it has been highlighted that the breaching process of a landslide dam is influenced by the variations in soil erodibility along depth, especially for the breach initiation time [33] found that the emergence of weak regions induced by spatial variations of soil type and initial water content resulted in early triggering of landslides with smaller volumes of released mass relative to a homogeneous slope. Timing and patterns of landslide initiations were closely related to their initial dry densities, and the initiation processes were accompanied by a variation of dry density and void ratio. Additionally, the mechanical state of gravel soil in the mobilization of rainfall-induced landslides was found to be influenced by the migration of fine particles and the rearrangement of coarse-fine particles, contributing to the reorganization of the microscopic structure. In conclusion, the literature supports the assertion that variations in soil mechanical and physical properties significantly influence landslide susceptibility, particularly in areas characterized by complex geological formations. The studies provide valuable insights into the critical role of soil composition, structure, and erodibility in landslide initiation and the subsequent impact on vegetation colonization.
Furthermore, the intensive geological structure, marked by the presence of normal and left-lateral faults as identified in the region [44, 46], compounds the area's susceptibility to landslides. This study's results, therefore, not only validate the significant influence of soil properties on landslide mechanisms but also underscore the need for comprehensive geological and soil assessments in landslide-prone areas.
Based on laboratory analyses of undisturbed soil samples (UDS) collected from the base of the Takari landslide and adjacent disturbed areas, the debris material predominantly consisted of fine-grained soil (silt and clay). The sieve analysis revealed that the percentage of material passing through sieve No. 200 ranged from 51.50% to 90.27%, with the native soil (UDS) from the primary slip zone displaying a significantly high proportion of fine-grained soil at 90.27%. This characteristic resulted in an exceptionally low permeability rate for the soil, quantified at 2.6×10 -7 cm/second, thereby hindering water infiltration. Consequently, overlying layers with higher permeability (landslide debris) became saturated upon water ingress, leading to a reduction in intergranular cohesion. This phenomenon was corroborated by shear strength tests on the UDS, indicating a cohesion value of 0 kg/cm 2 and a consequent diminution in soil shear strength. This degradation in shear strength, precipitated by increased pore water pressure due to rainwater infiltration, directly contributed to landslide occurrences (T. Disturbed 1 and T. Disturbed 2). Classification of these soil samples, based on their Liquid Limit (LL) and Plasticity Index (PI) values within the Unified Soil Classification System (USCS), places them in the CH category, denoting non-organic clays with high plasticity (Figure 7).
The shear plane or sliding surface in the Bobonaro clay soil (expansive clay, UDS) manifests due to its high plasticity index value of 57.77%, significantly exceeding the 35% threshold. The inherent properties of Bobonaro clay soil, characterized by pronounced swelling and shrinkage with variations in moisture content, exacerbate this condition. During arid periods, desiccation and subsequent cracking occur as moisture evaporates from soil pores, whereas the wet season sees these cracks facilitating water infiltration, leading to soil expansion. Such hydrological dynamics at the slope's base weaken inter-particle cohesion, precipitating landslides as a result of diminished soil shear strength, rendering the soil exceedingly malleable.
In the landslide strata, composed of debris material, notably low soil density values were recorded, with disturbed soil samples 1 and 2 yielding densities of 0.96 g/cm 3 and 0.90 g/cm 3 , respectively. This suggests an augmentation in soil porosity, thereby enhancing water permeability. Empirical evidence of this dynamic is found in the moisture content of landslide-affected disturbed soil 1, which was 46.06%, surpassing the optimal moisture content of 38.55%, and in disturbed soil 2, where the moisture content at the time of the landslide was 46.63%, exceeding the optimal moisture content of 43.32%. Such conditions, where moisture content exceeds optimal levels, lead to reduced soil density or a diminished capacity of the soil to bear loads, contributing to landslide incidents [47-51].
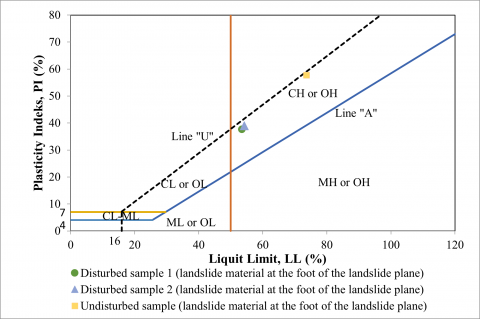
Figure 7. Soil classification based on the USCS system
Prolonged rainfall in the region further escalates soil moisture content. The groundwater table responds sensitively to rainwater infiltration, with elevated groundwater levels increasing pore water pressure and weakening the bonds between soil particles, thereby facilitating the downward or sliding movement of the soil mass.
4 .4 Hydrological conditions
4.4.1 Location of the Takari Sub-watershed
The Takari Sub-watershed, situated within the Noelmina Watershed as depicted in Figure 8, is subject to hydrological monitoring facilitated by data from BMKG Lasiana in Kupang City and BWS NT II. Within the Takari District, two proximal rainfall observation stations, namely the Hueknutu and Benu Rain Posts, are instrumental in gathering precipitation data. It is noteworthy that the Benu Rain Post corresponds to the location identified as the Kumlol Rain Post in the context of water allocation plan development for the Noelmina Watershed.
4.4.2 Ground station rain data
Based on Figure 8, the upstream rain post closest to the landslide location is the Hueknutu Rain Post. Rain data taken from BMKG information (No: B/KL.01.00/021/KKPN/II/2023) is obtained as in Table 2.
The search did not yield any results for the term "Ground Station Rain Data" within the provided document. Based on the image you've shared, I can provide an interpretation and synthesis of the rainfall data in the context of a research study, which can be articulated in academic language as follows:
The hydrological assessment of the Takari Sub-watershed, as delineated in Figure 8, involves meticulous analysis of precipitation data sourced from the closest upstream rain gauge, the Hueknutu Rain Post. As per the data presented in Table 2, a discernible pattern of daily rainfall during the month of February can be observed, with measurements ranging from minimal to significant levels of precipitation. Notably, the data indicates a fluctuating trend, with the highest recorded daily rainfall of 22 mm, suggesting variable weather patterns during this period. It is imperative to note the occurrence of rain on certain days where the quantity was non-quantifiable, denoted by the symbol '(-)', underscoring the limitations of measurement during certain events. The gathered data is crucial for establishing a correlation between rainfall intensity and landslide activity in the region, providing a foundation for further investigation into the hydrological triggers of slope instability.
As can be seen from Figure 9, there is unmeasured rain data and this makes it difficult for subsequent hydrological analysis. The initial moisture value (antecedent moisture conditions) can determine whether the moisture condition is in dry conditions (AMC I), normal conditions (AMC II) and wet conditions (AMC III). This can be seen in Table 3.
Table 2. Daily rain data at the Hueknutu rain post
|
|
| 13.3 |
| 22 |
| 5.7 |
| 12.8 |
| - |
| 1.3 |
| 1.3 |
Description: (-) there is rain but it is immeasurable
Table 3. AMC value for each class
|
| |
|
| |
I | < 13 mm | < 36 mm |
II | 13 mm – 28 mm | 36 mm – 53 mm |
III | >28 mm | > 53 mm |
Source: Triatmodjo, 2009
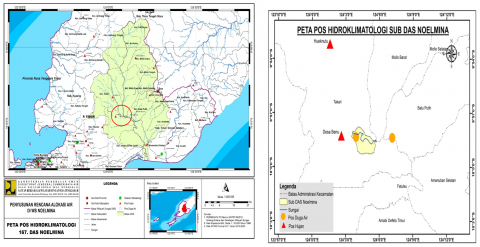
Figure 8. Location of landslides in the Takari sub-watershed in the Noelmina watershed
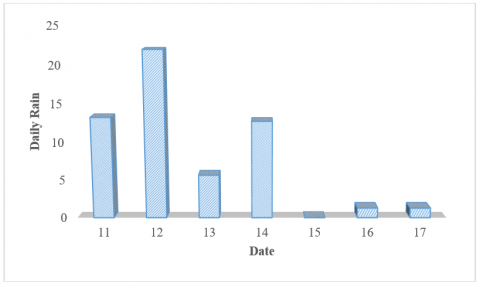
Figure 9. Location of landslides in the Takari sub-watershed in the Noelmina watershed
Figure 10 shows that soil moisture conditions tend to be wetter, but this cannot be concluded with certainty because there is data that was not measured on February 15 2023. To get more accurate data from conditions in the field, GPM satellite data was downloaded for 1 last week before the landslide occurred.
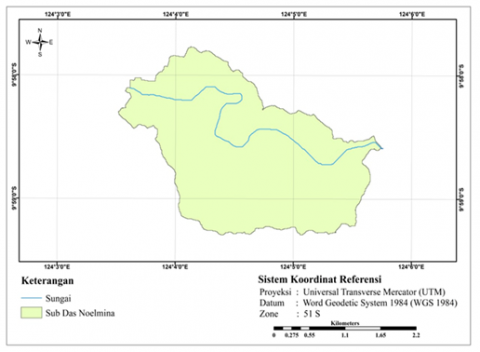
Figure 10. Grid for downloading satellite rainfall data in the Takari sub-catchment
For GPM rain post data in the Takari Sub-watershed area, it is multiplied by a correction factor for watersheds on Timor Island (Bam Engineering Center) as in Table 4.
Table 4. Correction factors for watersheds on Timor Island
|
|
5 | 0.50 |
10 | 0.90 |
30 | 1.00 |
50 | 1.05 |
80 | 1.10 |
110 | 1.20 |
> 110 | 1.25 |
Based on Figure 11, it can be seen that 6 days before the landslide, the amount of rain reached 74,008 mm and after correction it became 68,142 mm. This amount of rain is higher than 53 mm. Continuous rain in the Takari sub-watershed causes soil moisture to become higher and the soil to become more saturated with water. This also results in the soil's ability to absorb water also decreasing. Continuous water pressure on this layer of soil will also influence the strength of the soil to become unstable.
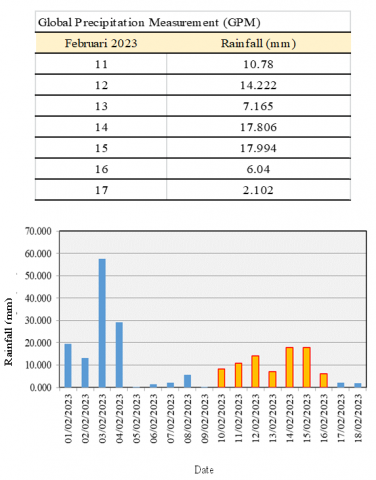
Figure 11. Satellite rainfall data in the Takari sub-catchment
The data presented in Table 5 details the calibration of daily rainfall measurements obtained from the Global Precipitation Measurement (GPM) satellite data for a seven-day period in February 2023. The original satellite-derived rainfall values (in mm) are systematically adjusted by applying a correction factor, resulting in the corrected rainfall values. This table exemplifies the necessity for correction in satellite rainfall data, with the highest recorded uncorrected daily rainfall being 17.806 mm on the 14th, which after calibration is reduced to 16.721 mm, and the lowest recorded at 2.102 mm on the 17th, adjusted to 1.809 mm. These corrections are crucial for achieving higher accuracy in rainfall data, which has significant implications for hydrological studies and water resource management.
Table 5. Corrected rainfall based on GPM satellite data
|
|
|
|
11 | 10.78 | 0.904 | 9.744 |
12 | 14.222 | 0.921 | 13.1 |
13 | 7.165 | 0.886 | 6.347 |
14 | 17.806 | 0.939 | 16.721 |
15 | 17.994 | 0.940 | 16.914 |
16 | 6.04 | 0.880 | 5.317 |
17 | 2.102 | 0.861 | 1.809 |
The Takari landslide incident underscores the profound impact of hilltop land clearing activities initiated since 2016 on the hydrological and geotechnical stability of the region. Notably, an ancient landslide scar at the summit forms a depression, functioning as a catchment area for rainwater and surface runoff, exacerbating the condition of the already porous basin. This particular landslide zone features dual channels extending towards the slope's base, serving as conduits for the infiltration of water from steeper inclines, thereby imposing additional strain and undermining the structural integrity of the slope's foothold.
Land clearing activities in hilly or sloped areas significantly impact the hydrological stability of the region. These initiatives, often started for development or agricultural activities, alter the landscape and soil characteristics, affecting the water cycle. Before land clearing, native vegetation plays a crucial role in absorbing rainwater and reducing surface runoff, facilitating infiltration into the soil and enhancing its capacity to retain water.
The removal of vegetation cover decreases the soil's function as an absorber and regulator of rainwater flow, leading to increased surface runoff and reduced water infiltration into the soil. The absence of vegetation increases erosion, carrying soil particles and sediment into water systems, reducing water quality and disturbing aquatic habitats, and heightens the risk of flooding due to decreased soil capacity to absorb rainwater.
Furthermore, the reduction of vegetation and root structures diminishes the mechanical strength of the soil, increasing susceptibility to landslides, particularly in areas with significant slope inclinations. Soil that becomes more porous and loses structure is more prone to water saturation, triggering soil movement or landslides, especially during or after heavy rainfall.
Land clearing impacts water availability for ecosystems and human activities by reducing water infiltration into the soil and groundwater availability. Therefore, a comprehensive environmental evaluation before land clearing and sustainable land and water management strategies, such as reforestation and terracing construction, are crucial to mitigate negative impacts and support hydrological stability.
The analysis of water accumulation dynamics in the basin area and soil mechanics indicates that without vegetation, infiltration decreases, and the potential for water accumulation increases. The loss of root structure leads to reduced soil porosity, decreasing its ability to absorb water and increasing erosion risk. Maintaining soil stability is critical to reducing flood and landslide risks, necessitating the restoration of vegetation and soil structure.
An integrated understanding of hydrology and soil mechanics is essential to address the impacts of land clearing on the hydrological and geotechnical stability of the basin area. This approach ensures the strategic design of management interventions to support environmental sustainability.
The cessation of vegetation cover due to land clearing endeavors drastically diminishes the landscape's capacity for rainwater absorption and soil structure maintenance. Vegetation is instrumental in mitigating surface runoff and water accumulation, thus safeguarding against the escalation of hydrostatic pressure within the subsoil layers, which could precipitate elevated water saturation levels [52]. During precipitation events, the augmented flow of water towards the basin amplifies the hydrostatic pressure exerted on the underlying soil strata, fostering conditions conducive to heightened water saturation.
This interplay between reduced vegetative cover and increased water saturation magnifies the susceptibility of slopes to landslides, particularly in regions with underlying vulnerabilities such as pre-existing landslide scars or depressions that serve as water collection basins. The phenomenon observed in the Takari landslide incident exemplifies the critical linkage between deforestation or land clearing practices and the destabilization of hilltop areas, underscoring the necessity for integrated land and water management strategies that prioritize the preservation of vegetation cover as a means to bolster hydrological stability and prevent soil erosion [53].
In summary, the Takari landslide incident offers a poignant illustration of how anthropogenic alterations to the natural landscape, specifically through the removal of vegetative cover on hilltops, can precipitate significant disruptions to the hydrological equilibrium of a region. These disruptions, characterized by increased surface runoff, water accumulation, and heightened hydrostatic pressure, culminate in the degradation of slope stability, thereby elevating the risk of landslides. This case study reinforces the imperative for adopting sustainable land management practices that recognize and preserve the critical role of vegetation in maintaining hydrological and geotechnical stability [54].
The dynamics of water accumulation in the basin area due to land clearing can have critical implications. The absence of vegetation leads to faster surface flow towards the basin, increasing saturation and resulting in greater hydrostatic pressure on the soil layers. The low permeability of the Bobonaro clay further complicates the issue by inhibiting water infiltration, thereby increasing the risk of saturation above the Bobonaro clay layer [55].
The presence of vegetation significantly influences the flow dynamics and hydrological processes. Vegetation affects the turbulence characteristics, shear stress, and sediment transport in channel flows [56-58]. The interaction between irregularly distributed submerged rigid vegetation and flow within a straight pool was found to be influenced by sweep and ejection events, indicating the momentum transfer between flow, bedform, and vegetated elements [59]. Additionally, the flow-retarding effects of vegetation and geotextiles on soil detachment during concentrated flow were observed, suggesting the need to treat flow shear force dissipation on soil surface roughness and roughness elements as separate processes [60].
Furthermore, the impact of vegetation complexity on subsurface flow paths has been studied, indicating that soil development, rainfall intensity, and vegetation complexity play crucial roles in shaping subsurface flow paths [61]. The presence of vegetation also affects sediment transport capacity based on slope gradients and flow discharge, as observed in laboratory experiments conducted on different underlying surfaces [15].
In addition to the physical effects, the presence of vegetation also influences the hydrogeological conditions in a given area. For instance, the investigation of hydrogeological conditions in the Manikin Dam Diversion Tunnel, Timor Island, Indonesia, revealed the presence of scaly clay and carbonate sandstone units in the study area.
In conclusion, the presence or absence of vegetation significantly impacts the dynamics of water accumulation, flow characteristics, and sediment transport in basin areas. Understanding these effects is crucial for effective land management and water resource conservation.
Based on the results of field and laboratory investigations, it can be concluded as the landslide incident in Noelmina Village, Takari District, Kupang Regency, characterized as a rotational slide within the Bobonaro clay soil domain, epitomizes the intricate interplay of geomorphological, soil, hydrological, and anthropogenic influences on land stability. This study delineates the village's predisposition to landslides, situated in mountainous terrain with slopes between 30º-35º and altitudes ranging from 130 to 325 meters above sea level. The critical threshold for slope angles instigating landslides, identified at 18° for steep slopes with horizontal planes, underscores the geomorphological vulnerability. The composition of the slopes, incorporating the Bobonaro Complex, Batuputih Formation, and Noele Formation, reveals that the landslide areas predominantly occur within the Bobonaro clay layers. These layers exhibit significant swelling and shrinkage behavior in response to moisture variability, coupled with minimal permeability. Consequently, rainwater infiltration leads to saturation, diminishing the soil's shear strength through the weakening of intergranular cohesion and elevation of pore water pressure, precipitating soil displacement.
Hydrological contributions to the landslide phenomena are highlighted by rainfall data exceeding 53 mm from local rain posts, indicating a shift from unsaturated to saturated soil conditions due to preceding light to moderate rainfall events. This transition facilitates gravitational soil movement, exacerbated by the additional load from water-induced softening. Furthermore, anthropogenic activities, specifically land clearing activities since 2016, have significantly altered the landscape. The presence of a depression at the hill's summit, a remnant of a prior landslide, becomes a receptacle for rainwater, thereby increasing saturation and the mass of the soil in the basin area. This alteration in the physical state of the soil at the slope's base acts as a catalyst for subsequent landslides. This investigation into the landslide at Noelmina Village not only elucidates the multifaceted causes behind such geophysical events but also accentuates the critical need for comprehensive land management and disaster preparedness strategies. These strategies should incorporate geomorphological and hydrological assessments alongside sustainable land use practices to mitigate future landslide risks effectively.
The research introduces valuable suggestions from reviewers aimed at enhancing the scope and depth of the paper's content, particularly concerning landslide mitigation and management. These suggestions encompass various pertinent aspects that can enrich readers' comprehension of the subject matter. In this study, our objective is to provide significant insights into understanding and confronting the challenges associated with landslide mitigation. We intend to achieve this by implementing the following recommendations, Firstly, we will focus on evaluating the effectiveness of proposed mitigation strategies. This will involve conducting a comparative analysis, drawing from relevant case studies or empirical data, to elucidate the success of these strategies in mitigating landslide risks and impacts. By doing so, we aim to offer a comprehensive understanding of the suitability of these strategies in different contexts. Secondly, we will assess the potential negative consequences of implementing mitigation recommendations. This analysis will include identifying possible compromises or side effects, accompanied by suitable mitigation strategies. Through this approach, readers will gain a balanced perspective on the implications of implementing these recommendations. Additionally, we will address the challenges associated with implementing these recommendations. This entails discussing technical, financial, and social barriers, along with proposed solutions to overcome them. By directly tackling these challenges, readers will develop a clearer understanding of the implementation process for mitigation recommendations. Finally, we will advocate for the development of advanced monitoring and early warning systems. These systems will be designed to detect early indicators of potential landslides, facilitating timely preventive actions to mitigate losses. Furthermore, we will explore the latest technology and innovative approaches to enhance the effectiveness of these mitigation efforts.
These findings have broader implications for disaster risk management and sustainable development, especially in the context of climate change, which increases the frequency and intensity of extreme weather phenomena. Knowledge of the factors affecting slope stability and landslide dynamics can guide more effective spatial planning and natural resource management, reducing community vulnerability to landslides. Additionally, improved understanding of the interactions between hydrological, geomorphological, and anthropogenic factors in triggering landslides offers crucial insights for the development of monitoring technologies and early warning systems, which can strengthen community adaptation and mitigation capacities against natural disaster risks. Lessons from this study also underscore the importance of a multidisciplinary approach in natural disaster research, integrating knowledge from various scientific fields to develop comprehensive solutions that enhance environmental and community resilience and sustainability.
This research, titled "Post-Landslide Liquefaction Analysis: A Case Study in the Kupang Regency Area, Indonesia," would not have been possible without the support and contributions from various parties. Therefore, we would like to express our deepest gratitude and appreciation to everyone who has assisted in this research process.
First and foremost, we would like to thank our Research Board who has provided guidance, advice, and constructive criticism throughout this study. Their mentorship not only shaped the framework of this research but also inspired us to pursue excellence in our work.
We are also grateful to the local government and the community in the Kupang Regency for granting permissions, access, and unwavering support while we conducted our field research. Without their hospitality and openness, the collection of data and field observations could not have been successfully executed.
[1] Attewell, P.B., Farmer, I.W. (2012). Principles of Engineering Geology. Springer Science & Business Media. [2] Nikolaidou, S., Klöti, T., Tappert, S., Drilling, M. (2016). Urban gardening and green space governance: Towards new collaborative planning practices. Urban Planning, 1(1): 5-19. https://doi.org/10.17645/up.v1i1.520 [3] Ji, R., Wang, K., Zhou, M., Zhang, Y., Bai, Y., Wu, X., Yan, H., Zhao, Z.Q., Ye, H. (2023). Green space compactness and configuration to reduce carbon emissions from energy use in buildings. Remote Sensing, 15(6): 1502. https://doi.org/10.3390/rs15061502 [4] Popescu, M. E. (2002). Landslide causal factors and landslide remediatial options. In 3rd International Conference on Landslides, Slope Stability and Safety of Infra-Structures, pp. 61-81. CI-Premier PTE LTD Singapore. [5] Rakhman, A.N., Maulana, F.W. (2021). Edukasi pendekatan selidik sifat fisik batuan lapuk untuk Penanganan Bencana Longsor dalam Masa Pandemi COVID-19 di RT 02 Pedukuhan Cengkehan, Wukirsari, Kapanewon Imogiri, Kabupaten Bantul. Jurnal Pengabdian Masyarakat As-Salam, 1(2): 99-108. https://doi.org/10.37249/jpma.v1i2.319 [6] Thapa, P.S., Chaudhary, S., Dasgupta, P. (2022). Contribution of integrated watershed management (IWM) to disaster risk reduction and community development: Lessons from Nepal. International Journal of Disaster Risk Reduction, 76(4): 103029. https://doi.org/10.1016/j.ijdrr.2022.103029 [7] Arfani, M. (2022). Kolaborasi pentahelix dalam upaya pengurangan risiko bencana pada destinasi wisata di desa kalanganyar sidoarjo. Jurnal Syntax Transformation, 3(1): 104-120. https://doi.org/10.46799/jst.v3i1.497 [8] Esposito, M., Palma, L., Belli, A., Sabbatini, L., Pierleoni, P. (2022). Recent advances in Internet of Things solutions for early warning systems: A review. Sensors, 22(6): 2124. https://doi.org/10.3390/s22062124 [9] Siad, L. (2001). Stability analysis of jointed rock slopes reinforced by passive, fully grouted bolts. Computers and Geotechnics, 28(5): 325-347. https://doi.org/10.1016/S0266-352X(01)00004-0 [10] Dai, F.C., Lee, C.F. (2002). Landslide characteristics and slope instability modeling using GIS, Lantau Island, Hong Kong. Geomorphology, 42(3-4): 213-228. https://doi.org/10.1016/S0169-555X(01)00087-3 [11] Qin, Z., Agarwal, V., Gee, D., Marsh, S., Grebby, S., Chen, Y., Meng, N. (2021). Study of ground movement in a mining area with geological faults using FDM analysis and a stacking InSAR method. Frontiers in Environmental Science, 9: 787053. https://doi.org/10.3389/fenvs.2021.787053 [12] Ma, K., Tang, C.A., Li, L.C., Ranjith, P.G., Cai, M., Xu, N.W. (2013). 3D modeling of stratified and irregularly jointed rock slope and its progressive failure. Arabian Journal of Geosciences, 6: 2147-2163. https://doi.org/10.1007/s12517-012-0578-6 [13] Tang, C.A., Tang, S. (2020). Applications of rock failure process analysis (RFPA) to rock engineering. Modelling Rock Fracturing Processes, Springer, Cham, 421-459. https://doi.org/10.1007/978-3-030-35525-8_17 [14] Li, J., Wang, W.D., Li, Y., Han, Z., Chen, G.Q. (2021). Spatiotemporal landslide susceptibility mapping incorporating the effects of heavy rainfall: A case study of the heavy rainfall in August 2021 in Kitakyushu, Fukuoka, Japan. Water, 13(22): 3312. https://doi.org/10.3390/w13223312 [15] Li, J., Li, B., He, K., Gao, Y., Wan, J.W., Wu, W.L., Zhang, H. (2022). Failure mechanism analysis of mining-induced landslide based on geophysical investigation and numerical modelling using distinct element method. Remote Sensing, 14(23): 6071. https://doi.org/10.3390/rs14236071 [16] Hungr, O., Evans, S.G., Bovis, M.J., Hutchinson, J.N. (2001). A review of the classification of landslides of the flow type. Environmental & Engineering Geoscience, 7(3): 221-238. https://doi.org/10.2113/gseegeosci.7.3.221 [17] Alcántara-Ayala, I., Parteli, E.J.R., Pradhan, B., Cuomo, S., Vieira, B.C. (2023). Physics and modelling of landslides. Frontiers in Physics, 11: 83. https://doi.org/10.3389/fphy.2023.1146166 [18] Erener, A., Düzgün, H.S.B. (2010). Improvement of statistical landslide susceptibility mapping by using spatial and global regression methods in the case of More and Romsdal (Norway). Landslides, 7: 55-68. https://doi.org/10.1007/s10346-009-0188-x [19] Rahman, G., Rahman, A., Ullah, S., Miandad, M., Collins, A.E. (2019). Spatial analysis of landslide susceptibility using failure rate approach in the Hindu Kush region, Pakistan. Journal of Earth System Science, 128: 59. https://doi.org/10.1007/s12040-019-1066-0 [20] Shu, H.P., Guo, Z.Z., Qi, S., Song, D.Q., Pourghasemi, H.R., Ma, J.C. (2021). Integrating landslide typology with weighted frequency ratio model for landslide susceptibility mapping: A case study from Lanzhou city of northwestern China. Remote Sensing, 13(18): 3623. https://doi.org/10.3390/rs13183623 [21] Wen, T., Tang, H.M., Wang, Y.K., Lin, C.Y., Xiong, C.R. (2017). Landslide displacement prediction using the GA-LSSVM model and time series analysis: A case study of three gorges reservoir, China. Natural Hazards and Earth System Science, 17(12): 2181-2198. https://doi.org/10.5194/nhess-17-2181-2017 [22] Shen, L., Xu, C., Liu, L. (2015). Interaction among controlling factors for landslides triggered by the 2008 Wenchuan, China mw 7.9 earthquake. Frontiers of Earth Science, 10: 264-273. https://doi.org/10.1007/s11707-015-0517-4 [23] Brown, S., Marley, A.A.J., Donkin, C., Heathcote, A. (2008). An integrated model of choices and response times in absolute identification. Psychological Review, 115(2): 396-425. https://doi.org/10.1037/0033-295X.115.2.396 [24] Myers, P.G., Kulan, N., Ribergaard, M.H. (2007). Irminger water variability in the west Greenland current. Geophysical Research Letters, 34(17). https://doi.org/10.1029/2007GL030419 [25] Terranova, O.G., Gariano, S.L., Iaquinta, P., Iovine, G.G.R. (2015). GASAKe: Forecasting landslide activations by a genetic-algorithms-based hydrological model. Geoscientific Model Development, 8(7): 1955-1978. https://doi.org/10.5194/gmd-8-1955-2015 [26] Santangelo, M., Cardinali, M., Rossi, M., Mondini, A.C., Guzzetti, F. (2010). Remote landslide mapping using a laser rangefinder binocular and GPS. Natural Hazards and Earth System Science, 10(12): 2539-2546. https://doi.org/10.5194/nhess-10-2539-2010 [27] Cui, L., Zhao, Y.H., Liu, J.C., Han, L., Ao, Y., Yin, S. (2018). Landscape ecological risk assessment in Qinling mountain. Geological Journal, 53(S1): 342-351. https://doi.org/10.1002/gj.3115 [28] Rossi, M., Witt, A., Guzzetti, F., Malamud, B., Peruccacci, S. (2010). Analysis of historical landslide time series in the Emilia-Romagna region, northern Italy. Earth Surface Processes and Landforms, 35(10): 1123-1137. https://doi.org/10.1002/esp.1858 [29] Prastica, R., Apriatresnayanto, R., Marthanty, D. (2019). Structural and green infrastructure mitigation alternatives prevent Ciliwung river from water-related landslide. International Journal on Advanced Science Engineering and Information Technology, 9(6): 1825-1832. https://doi.org/10.18517/ijaseit.9.6.8413 [30] Chang, K.T., Chiang, S.H., Lu, F. (2007). Analysing the relationship between typhoon‐triggered landslides and critical rainfall conditions. Earth Surface Processes and Landforms, 33(8): 1261-1271. https://doi.org/10.1002/esp.1611 [31] LaHusen, S., Duvall, A., Booth, A., Grant, A., Mishkin, B., Montgomery, D. (2020). Rainfall triggers more deep-seated landslides than Cascadia earthquakes in the Oregon coast range, USA. Science Advances, 6(38). https://doi.org/10.1126/sciadv.aba6790 [32] Crozier, M.J. (1999). Prediction of rainfall-triggered landslides: A test of the antecedent water status model. Earth Surface Processes and Landforms, 24(9): 825-833. https://doi.org/10.1002/(SICI)1096-9837(199908)24:9<825::AID-ESP14>3.0.CO;2-M [33] Chen, Y.C., Chang, K.T., Chiu, Y.J., Lau, S.M., Lee, H.Y. (2012). Quantifying rainfall controls on catchment‐scale landslide erosion in Taiwan. Earth Surface Processes and Landforms, 38(4): 372-382. https://doi.org/10.1002/esp.3284 [34] Hong, Y., Adler, R., Huffman, G. (2006). Evaluation of the potential of NASA multi‐satellite precipitation analysis in global landslide hazard assessment. Geophysical Research Letters, 33(22). https://doi.org/10.1029/2006GL028010 [35] Gordo, C., Zêzere, J.L., Marques, R. (2019). Landslide susceptibility assessment at the basin scale for rainfall- and earthquake-triggered shallow slides. Geosciences, 9(6): 268. https://doi.org/10.3390/geosciences9060268 [36] Hungr, O., Evans, S.G., Bovis, M.J., Hutchinson, J.N. (2001). A review of the classification of landslides of the flow type. Environmental and Engineering Geoscience, 7(3): 221-238. https://doi.org/10.2113/gseegeosci.7.3.221 [37] Bucknam, R.C., Coe, J.A., Chavarria, M.M., Godt, J.W., Tarr, A.C., Bradley, L.A., Rafferty, S.A., Hancock, D., Dart, R.L., Johnson, M.L. (2001). Landslides triggered by hurricane mitch in Guatemala - inventory and discussion. Report. https://doi.org/10.3133/ofr01443 [38] Glade, T., Crozier, M., Smith, P. (2000). Applying probability determination to refine landslide-triggering rainfall thresholds using an empirical “antecedent daily rainfall model”. Pure and Applied Geophysics, 157: 1059-1079. https://doi.org/10.1007/s000240050017 [39] Nugroho, N.E., Kristanto, W.A.D. (2020). Kajian tingkat risiko tanah longsor desa hargomulyo, kecamatan kokap, kabupaten kulonprogo. Jurnal Ilmiah Lingkungan Kebumian, 1(2): 9-25. https://doi.org/10.31315/jilk.v1i2.3281.g2541 [40] Shabbir, W., Omer, T., Pilz, J. (2023). The impact of environmental change on landslides, fatal landslides, and their triggers in Pakistan (2003-2019). Environmental Science and Pollution Research, 30: 33819-33832. https://doi.org/10.1007/s11356-022-24291-z [41] Lusiana, N., Shinohara, Y. (2022). The role of citrus groves in rainfall-triggered landslide hazards in Uwajima, Japan. Water, 14(13): 2113. https://doi.org/10.3390/w14132113 [42] Samia, J., Temme, A., Bregt, A., Wallinga, J., Guzzetti, F., Ardizzone, F., Rossi, M. (2017). Do landslides follow landslides? Insights in path dependency from a multi-temporal landslide inventory. Landslides, 14: 547-558. https://doi.org/10.1007/s10346-016-0739-x [43] Suwitodirjo, T., Tjokrosapoetro, S. (1996). Geological map of the Kupang–Atambua sheet. Timor, Center for Geological Research and Development. [44] Tintakorn, N., Pananont, P., Mahattanachai, T., Charusiri, P. (2012). Analysis of geologic structures in the southern Mergui Basin, Andaman Sea. Bulletin of Earth Sciences of Thailand, 5(1): 68-88. [45] Ford, M., Hemelsdaël, R., Mancini, M., Palyvos, N. (2016). Rift migration and lateral propagation: Evolution of normal faults and sediment-routing systems of the western Corinth rift (Greece). Geological Society, London, Special Publications, 439: 131-168. https://doi.org/10.1144/SP439.15 [46] Handayanto, E., Muddarisna, N., Fiqri, A. (2017). Soil Fertility Management. Brawijaya University Press. [47] Hardiyatmo, H.C. (2006). Handling Landslides and Erosion. Gadjah Mada University Press. [48] McKenna, J.P., Santi, P.M., Amblard, X., Negri, J. (2012). Effects of soil-engineering properties on the failure mode of shallow landslides. Landslides, 9: 215-228. https://doi.org/10.1007/s10346-011-0295-3 [49] Sorbino, G., Nicotera, M.V. (2013). Unsaturated soil mechanics in rainfall-induced flow landslides. Engineering Geology, 165: 105-132. https://doi.org/10.1016/j.enggeo.2012.10.008 [50] Bogaard, T.A., Greco, R. (2016). Landslide hydrology: From hydrology to pore pressure. Wiley Interdisciplinary Reviews: Water, 3(3): 439-459. https://doi.org/10.1002/wat2.1126 [51] Pei, P., Zhao, Y.L., Ni, P.P., Mei, G.X. (2020). A protective measure for expansive soil slopes based on moisture content control. Engineering Geology, 269: 105527. https://doi.org/10.1016/j.enggeo.2020.105527 [52] Dietrich, W.E., Perron, J.T. (2006). The search for a topographic signature of life. Nature, 439(7075): 411-418. https://doi.org/10.1038/nature04452. [53] Montgomery, D.R., Brandon, M.T. (2002). Topographic controls on erosion rates in tectonically active mountain ranges. Earth and Planetary Science Letters, 201(3-4): 481-489. https://doi.org/10.1016/S0012-821X(02)00725-2 [54] Schuster, R.L., Highland, L.M. (2001). Socioeconomic and environmental impacts of landslides in the western hemisphere (No. 2001-276). Open-File Report 2001-276. https://doi.org/10.3133/ofr01276 [55] Fatima, I.M.D.D., Putra, D., Pranantya, P.A., Sidharno, W. (2024). Investigation of hydrogeology condition in manikin dam diversion tunnel, Timor Island, Indonesia: A case study. E3S Web of Conferences, 479: 02004. https://doi.org/10.1051/e3sconf/202447902004 [56] Loheide, S.P., Gorelick, S.M. (2007). Riparian hydroecology: A coupled model of the observed interactions between groundwater flow and meadow vegetation patterning. Water Resources Research, 43(7). https://doi.org/10.1029/2006WR005233 [57] Penna, N., Coscarella, F., D’Ippolito, A., Gaudio, R. (2020). Bed roughness effects on the turbulence characteristics of flows through emergent rigid vegetation. Water, 12(9): 2401. https://doi.org/10.3390/w12092401 [58] Kothyari, U.C., Hashimoto, H., Hayashi, K. (2009). Effect of tall vegetation on sediment transport by channel flows. Journal of Hydraulic Research, 47(6): 700-710. https://doi.org/10.3826/jhr.2009.3317 [59] Nosrati, K., Afzalimehr, H., Sui, J.Y. (2022). Interaction of irregular distribution of submerged rigid vegetation and flow within a straight pool. Water, 14(13): 2036. https://doi.org/10.3390/w14132036 [60] Knapen, A., Smets, T., Poesen, J. (2009). Flow‐retarding effects of vegetation and geotextiles on soil detachment during concentrated flow. Hydrological Processes, 23(17): 2427-2437. https://doi.org/10.1002/hyp.7360 [61] Hartmann, A., Weiler, M., Greinwald, K., Blume, T. (2021). The impact of soil development, rainfall intensity and vegetation complexity on subsurface flow paths along a glacial chronosequence of 10 millennia. Hydrology and Earth System Sciences Discussions, 1-35. https://doi.org/10.5194/hess-2021-242
Phone: + 1 825 436 9306
Email: [email protected]
Subscription
Language support
Please sign up to receive notifications on new issues and newsletters from IIETA
Select Journal/Journals:
Copyright © 2024 IIETA. All Rights Reserved.
IMAGES
VIDEO
COMMENTS
1. Introduction. Landslide is a common natural disaster often in hilly terrain, which causes huge loss of natural resources and human life (Ering & Babu, Citation 2016).It is mainly attributed by natural factors, such as earthquakes and rainfall occurred on these regions (Collins & Znidarcic, Citation 2004; Rahardjo, Li, Toll, & Leong, Citation 2001).A sudden downward movement of the ...
A landslide is defined as movement of rock or soil mass, or both caused by the effects of gravitational force (Highland and Bobrowsky 2008).It is a common and quite complex natural phenomenon in mountainous area causes by geological, hydrological, anthropological, environmental factors (Ahmed et al. 2014; Marana 2017).In other words, it is a mass displacement of surface of the earth's slope ...
Landslide case studies. The landslides team at the BGS has studied numerous landslides. This work informs our geological maps, memoirs and sheet explanations and provides data for our National Landslide Database , which underpins much of our research. If you've seen a landslide or want to contact us about landslides, you can email us at ...
As a result of landslide displacements, both the highway and the village have been subsiding for several decades and many dwellings have had to be abandoned. From topographical survey and visual observation, average rates of settlement vary between c. 0.1 and 0.4 m a −1 .
Landslide case study. Share this article Facebook Twitter Pinterest WhatsApp Email Copy Link. On 21 October 1966, the worst mining-related disaster in British history took place in Aberfan, a small village in South Wales. This harrowing and tragic event started when the nearby colliery ran out of tipping space in the valley floor in 1916.
The landslides in study area were detected based on remote sensing images; (4) ... remote sensing imagery has found broad application in physical geography and environmental research, particularly in landslide detection and geohazard management. ... A case study along the g318 linzhi section. Sensors, 23 (2023), p. 6608, 10.3390/s23146608.
IB Geography - Hazards & Disasters Case Study Summary Sheet for Ponzano Landslide, Italy (HIC) Where did it happen? Ponzano is a small village found in the Abruzzo region of Southern Italy. It has a population of 200. Geo Fact Box - Italy Need To Know Indicator Values (2017 estimated) GDP per capita PPP $32000
Landslides are complex geohazards responsible for damage to life, the natural environment, and essential infrastructures like buildings, roads, and transmission lines in mountainous regions. The modeling of topographic input parameters for landslide-related investigations is often based on Digital Elevation Models (DEMs), which serve as a crucial geospatial data source. The present study ...
Part 1: Preparatory activity. In each of the five study sites that students have looked at in Units 2 and 3, there is a significant mass wastage somewhere in the map area. In order to prepare for Unit 4, have students prepare five-minute presentations on one those mass-wastage events (except the Northern Washington one, as the rest of Unit 4 is ...
Key Landslide Triggers_ A Case Study of Upper Alaknanda Valley, Uttarakhand Himalaya, India[#973414]-1888079.pdf ... Journal of Geography and Natural Disasters 8 (2), 1-10. Khanduri, S., 2021 ...
A landslide is the movement of rock, earth, or debris down a sloped section of land. Landslides are caused by rain, earthquakes, volcanoes, or other factors that make the slope unstable. Geologists, scientists who study the physical formations of Earth, sometimes describe landslides as one type of mass wasting.A mass wasting is any downward movement in which Earth's surface is worn away.
School of Geography, Faculty of Social Sciences, University of Costa Rica, Costa Rica ... as is the case with the landslide concentrated evaluation indexes in this work. ... Xu, Jun, Z., Yang, T., et al. (2021b). Spatial patterns and scaling distributions of earthquake-induced landslides—a case study of landslides in watersheds along ...
At least 180 people in Zimbabwe known to have been killed by landslides triggered by Idai. Nasa satellite images depict the extensive landslide activity associated with Cyclone Idai. The landslides were partly caused by deforestation. People were still being rescued a week and a half after the storm.
IB Geography - Hazards & Disasters Case Study Summary Sheet for Freetown Landslide, Sierra Leone (LIC) Where did it happen? Freetown is the capital and largest city of Sierra Leone. It is a major port city on the Atlantic Ocean and is located in the Western Area of the country. Freetown is Sierra Leone's major urban, economic, financial ...
A landslide inventory of 1101 landslides over a 570 km2 area is used to study the geomorphological characteristics and land cover more prone to landsliding as well as the rainfall and soil ...
The resulting LNRF model generates a landslide susceptibility map, classifying over 35% of the study region's land area as having a high probability of landslides.
Landslide case study. Share this article Facebook Twitter Pinterest WhatsApp Email Copy Link. The Holbeck Hall landslide, south of Scarborough in North Yorkshire, attracted considerable interest when it destroyed the four-star Holbeck Hall Hotel between 3 and 5 June 1993. A rotational landslide involving about 1 million tonnes of glacial till ...
Indonesia, a country characterized by its archipelagic geography and tropical climate, experiences a high incidence of landslides, affecting both rural and urban communities across its diverse landscapes. ... This research, titled "Post-Landslide Liquefaction Analysis: A Case Study in the Kupang Regency Area, Indonesia," would not have been ...
Landslide Risk and Mitigation Strategy: A Case Study of Kullu Valley, India Anand Malik1 1. Dr Anand Malik, Associate Professor, Geography, SSN College (University of Delhi). Abstract: Landslides are defined as the movement of rocks, debris, and landfills under the influence of gravity. Slide is one of the most common types of accidents on ...
Sixty-seven people were killed and 20 injured when an Occupied 12-storey private apartment building was demolished under the impact of an extremely rapid flowslide (Hong Kong Government 1972b). The landslip, illustrated in Figure 2, was initiated on the hillside above by the collapse of a steep cutting in a works site for a private building.
DOI: 10.1080/10106049.2024.2361714 Corpus ID: 270874819; Comparison of informative modelling and machine learning methods in landslide vulnerability evaluation - a case study of Wenchuan County, China
The L'Aquila Earthquake - Background. On 6 April 2009, a magnitude 6.3 earthquake struck L'Aquila in central Italy, killing 309 people. The main shock happened in the early morning hours at 3.32 am when most people were sleeping, extensively damaging the 13th-century city of L'Aquila, located only about 60 miles (100 km) northeast of Rome.
The place of Malin village that lies between Latitude 73o40' N to 73o45' N and Longitude 19o10' E to 19o5' E. This area surrounded by mountain terrain has an average altitude of 850 meters from mean sea level. The average temperature is 200 to 280 C and average annual precipitation is 1133.73 mm (Monsoon season).
Death toll= 23 people and injured 163 people (hospitalised) Debris flows were up to 15 feet in height of mud, tree branches and boulders and moved at over 20 mph into the lower areas of Montecito. 20,000 people lost power. A 30-mile section of Route 101 from Santa Barbara to Ventura was shut as it filled with 2 feet of mud and debris.
A map to show the location of Nepal in Asia. At 11.26 am on Saturday, 25th of April 2015, a magnitude 7.9 earthquake struck Nepal. The focus was only eight kilometres deep, and the epicentre was just 60 kilometres northwest of Kathmandu, the capital city of Nepal. At the time of the earthquake, Kathmandu had 800,000+ inhabitants.
20million m3. How did the landslide occur? The slopes were covered in snow at the top, however global warming caused this snow to melt, and lubricating the slope, there were also two significant earthquakes and few days before. The geothermal steam softened the base and the rock wall gave way.
Created on: 08-05-15 18:46. Landslide Case Studies Word Document 218 Kb. Geography. Case studies Natural hazards. AS. Edexcel. Download. Save to favourites.